Numbers, Facts and Trends Shaping Your World
Read our research on:
Full Topic List
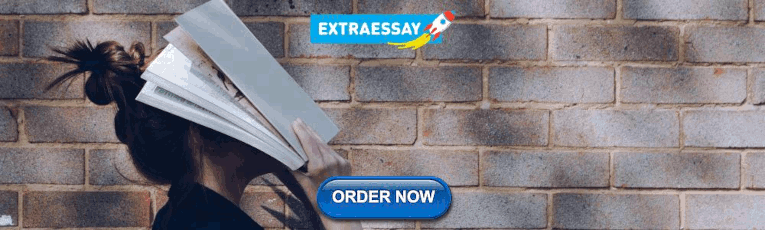
Regions & Countries
- Publications
- Our Methods
- Short Reads
- Tools & Resources
Read Our Research On:
Obesity and poverty don’t always go together
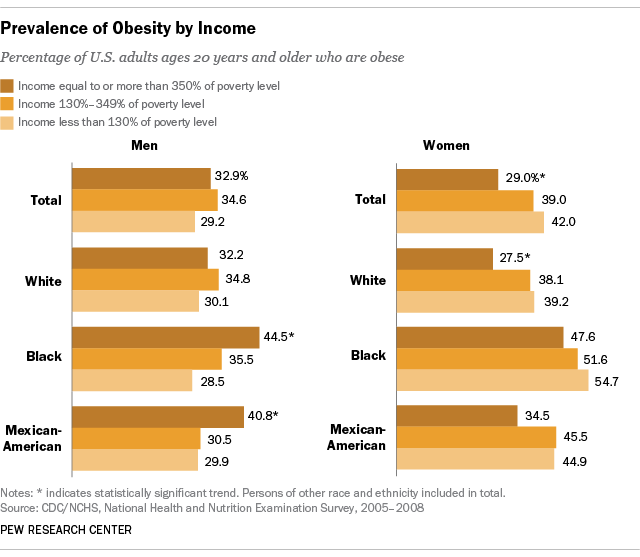
Americans overwhelmingly see obesity as a very serious public health problem, one with consequences not just for individuals but society as a whole, a new Pew Research Center report finds. But just who is obese? Research on obesity and socioeconomic status from the Centers for Disease Control and Prevention throws a few twists into the common wisdom that, in the U.S. at least, obesity is primarily a disease of the poor .
Public health researchers define obesity, and overweight more generally, in terms of body mass index (BMI). A person’s BMI is his or her weight (in kilograms) divided by the square of his or her height (in meters), rounded to one decimal place. A BMI of 25 or more is considered overweight; 30 or more is considered obese.
As we first reported back in 2006 , Americans appear to have a sliding scale (so to speak) when it comes to weight, consistently underestimating their own relative to others’. For instance, only 31% of people surveyed by Pew Research earlier this month said they were overweight; most of those said they were “somewhat” or “only a little” overweight, while 63% termed their weight “just about right.” But according to the CDC, 68% of Americans are overweight, and more than a third are obese. The CDC data come from the National Health and Nutrition Examination Survey , an ongoing program of studies that combines interviews with standardized physical exams, including height and weight measurements.
Obesity varies considerably depending on gender, race, ethnicity and socioeconomic factors. In 2010, CDC researchers (using data from 2005-08) found that among black and Mexican-American men, obesity increased with income: 44.5% and 40.8% of those men are obese, respectively, at the highest income level, compared with 28.5% and 29.9% at the lowest level. Beyond that, though, the researchers found little correlation between obesity prevalence among men and either income or education.
Among women, obesity was indeed most prevalent at lower income levels: 42% of women living in households with income below 130% of the poverty level were obese, compared with 29% of women in households at or above 350% of poverty. But the correlation was significant only for white women, though the trend was similar for all racial and ethnic groups studied. ( Poverty thresholds are set every year by the Census Bureau , and vary by family size and composition. In 2008, for instance, the poverty threshold for a single person under 65 was $11,201; for a two-adult, two-child household the threshold was $21,834.)
The most recent government report , covering 2011-12, found that obesity was significantly more common among black women (56.8%) than black men (37.1%). There was far less obesity among Asian Americans than other racial/ethnic groups: 10.8%, compared with 32.6% for whites, 42.5% for Hispanics and 47.8% for blacks. However, body shape and normal build varies by gender, race and ethnicity, and members of different demographic groups can have more or less body fat, even at the same BMI. For instance, as the researchers noted, “at a given BMI, Asian adults may have more body fat than white adults.”
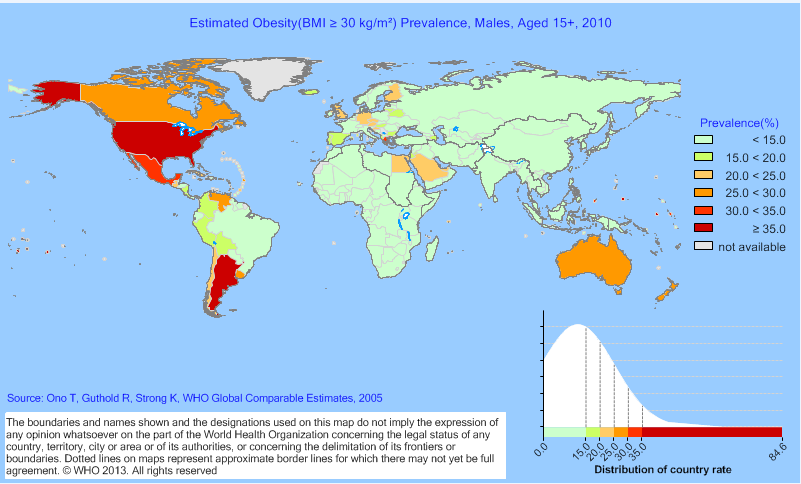
After rising sharply in the 1980s and 1990s, obesity rates in the U.S. appear to have mostly leveled off in recent years. More than a third (34.9%) of U.S. adults, or 78.6 million, were obese in 2011-12, according to government data — not significantly different from the 35.7% obesity rate found in 2009-10 or the 34.2% rate found in 2007-08 .
Globally, obesity is overwhelmingly a problem of developed rather than developing nations, and in few places more than in the United States. Using 2010 data, the World Health Organization estimated that 44.2% of U.S. males and 48.3% of U.S. females aged 15 and above were obese.
- Health Policy
- Medicine & Health
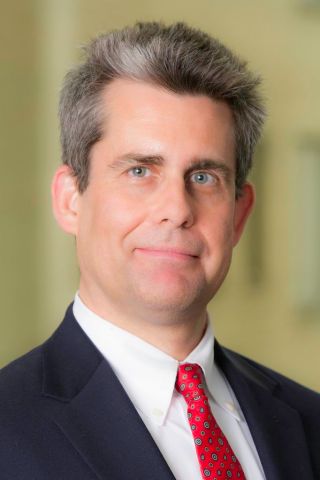
Drew DeSilver is a senior writer at Pew Research Center .
9 facts about Americans and marijuana
Most americans favor legalizing marijuana for medical, recreational use, how americans view the coronavirus, covid-19 vaccines amid declining levels of concern, americans’ top policy priority for 2024: strengthening the economy, 5 facts about black americans and health care , most popular.
1615 L St. NW, Suite 800 Washington, DC 20036 USA (+1) 202-419-4300 | Main (+1) 202-857-8562 | Fax (+1) 202-419-4372 | Media Inquiries
Research Topics
- Email Newsletters
ABOUT PEW RESEARCH CENTER Pew Research Center is a nonpartisan fact tank that informs the public about the issues, attitudes and trends shaping the world. It conducts public opinion polling, demographic research, media content analysis and other empirical social science research. Pew Research Center does not take policy positions. It is a subsidiary of The Pew Charitable Trusts .
Copyright 2024 Pew Research Center
- Search Menu
- Sign in through your institution
- Advance articles
- Featured articles
- Virtual Issues
- Prize-Winning Articles
- Browse content in A - General Economics and Teaching
- Browse content in A1 - General Economics
- A11 - Role of Economics; Role of Economists; Market for Economists
- A12 - Relation of Economics to Other Disciplines
- Browse content in B - History of Economic Thought, Methodology, and Heterodox Approaches
- Browse content in B4 - Economic Methodology
- B41 - Economic Methodology
- Browse content in C - Mathematical and Quantitative Methods
- Browse content in C1 - Econometric and Statistical Methods and Methodology: General
- C10 - General
- C11 - Bayesian Analysis: General
- C12 - Hypothesis Testing: General
- C13 - Estimation: General
- C14 - Semiparametric and Nonparametric Methods: General
- C18 - Methodological Issues: General
- Browse content in C2 - Single Equation Models; Single Variables
- C22 - Time-Series Models; Dynamic Quantile Regressions; Dynamic Treatment Effect Models; Diffusion Processes
- C23 - Panel Data Models; Spatio-temporal Models
- C26 - Instrumental Variables (IV) Estimation
- Browse content in C3 - Multiple or Simultaneous Equation Models; Multiple Variables
- C31 - Cross-Sectional Models; Spatial Models; Treatment Effect Models; Quantile Regressions; Social Interaction Models
- C32 - Time-Series Models; Dynamic Quantile Regressions; Dynamic Treatment Effect Models; Diffusion Processes; State Space Models
- C33 - Panel Data Models; Spatio-temporal Models
- C36 - Instrumental Variables (IV) Estimation
- Browse content in C4 - Econometric and Statistical Methods: Special Topics
- C40 - General
- C44 - Operations Research; Statistical Decision Theory
- C45 - Neural Networks and Related Topics
- C49 - Other
- Browse content in C5 - Econometric Modeling
- C52 - Model Evaluation, Validation, and Selection
- C53 - Forecasting and Prediction Methods; Simulation Methods
- C55 - Large Data Sets: Modeling and Analysis
- Browse content in C6 - Mathematical Methods; Programming Models; Mathematical and Simulation Modeling
- C61 - Optimization Techniques; Programming Models; Dynamic Analysis
- C62 - Existence and Stability Conditions of Equilibrium
- C63 - Computational Techniques; Simulation Modeling
- Browse content in C7 - Game Theory and Bargaining Theory
- C70 - General
- C71 - Cooperative Games
- C72 - Noncooperative Games
- C73 - Stochastic and Dynamic Games; Evolutionary Games; Repeated Games
- C78 - Bargaining Theory; Matching Theory
- Browse content in C8 - Data Collection and Data Estimation Methodology; Computer Programs
- C81 - Methodology for Collecting, Estimating, and Organizing Microeconomic Data; Data Access
- Browse content in C9 - Design of Experiments
- C90 - General
- C91 - Laboratory, Individual Behavior
- C92 - Laboratory, Group Behavior
- C93 - Field Experiments
- C99 - Other
- Browse content in D - Microeconomics
- Browse content in D0 - General
- D00 - General
- D01 - Microeconomic Behavior: Underlying Principles
- D02 - Institutions: Design, Formation, Operations, and Impact
- D03 - Behavioral Microeconomics: Underlying Principles
- Browse content in D1 - Household Behavior and Family Economics
- D11 - Consumer Economics: Theory
- D12 - Consumer Economics: Empirical Analysis
- D13 - Household Production and Intrahousehold Allocation
- D14 - Household Saving; Personal Finance
- D15 - Intertemporal Household Choice: Life Cycle Models and Saving
- D18 - Consumer Protection
- Browse content in D2 - Production and Organizations
- D21 - Firm Behavior: Theory
- D22 - Firm Behavior: Empirical Analysis
- D23 - Organizational Behavior; Transaction Costs; Property Rights
- D24 - Production; Cost; Capital; Capital, Total Factor, and Multifactor Productivity; Capacity
- Browse content in D3 - Distribution
- D30 - General
- D31 - Personal Income, Wealth, and Their Distributions
- D33 - Factor Income Distribution
- Browse content in D4 - Market Structure, Pricing, and Design
- D40 - General
- D42 - Monopoly
- D43 - Oligopoly and Other Forms of Market Imperfection
- D44 - Auctions
- D47 - Market Design
- Browse content in D5 - General Equilibrium and Disequilibrium
- D50 - General
- D52 - Incomplete Markets
- Browse content in D6 - Welfare Economics
- D60 - General
- D61 - Allocative Efficiency; Cost-Benefit Analysis
- D62 - Externalities
- D63 - Equity, Justice, Inequality, and Other Normative Criteria and Measurement
- D64 - Altruism; Philanthropy
- Browse content in D7 - Analysis of Collective Decision-Making
- D70 - General
- D71 - Social Choice; Clubs; Committees; Associations
- D72 - Political Processes: Rent-seeking, Lobbying, Elections, Legislatures, and Voting Behavior
- D73 - Bureaucracy; Administrative Processes in Public Organizations; Corruption
- D74 - Conflict; Conflict Resolution; Alliances; Revolutions
- D78 - Positive Analysis of Policy Formulation and Implementation
- Browse content in D8 - Information, Knowledge, and Uncertainty
- D80 - General
- D81 - Criteria for Decision-Making under Risk and Uncertainty
- D82 - Asymmetric and Private Information; Mechanism Design
- D83 - Search; Learning; Information and Knowledge; Communication; Belief; Unawareness
- D84 - Expectations; Speculations
- D85 - Network Formation and Analysis: Theory
- D86 - Economics of Contract: Theory
- D87 - Neuroeconomics
- D89 - Other
- Browse content in D9 - Micro-Based Behavioral Economics
- D90 - General
- D91 - Role and Effects of Psychological, Emotional, Social, and Cognitive Factors on Decision Making
- D92 - Intertemporal Firm Choice, Investment, Capacity, and Financing
- Browse content in E - Macroeconomics and Monetary Economics
- Browse content in E0 - General
- E01 - Measurement and Data on National Income and Product Accounts and Wealth; Environmental Accounts
- E02 - Institutions and the Macroeconomy
- E1 - General Aggregative Models
- Browse content in E2 - Consumption, Saving, Production, Investment, Labor Markets, and Informal Economy
- E20 - General
- E21 - Consumption; Saving; Wealth
- E22 - Investment; Capital; Intangible Capital; Capacity
- E23 - Production
- E24 - Employment; Unemployment; Wages; Intergenerational Income Distribution; Aggregate Human Capital; Aggregate Labor Productivity
- E25 - Aggregate Factor Income Distribution
- E26 - Informal Economy; Underground Economy
- E27 - Forecasting and Simulation: Models and Applications
- Browse content in E3 - Prices, Business Fluctuations, and Cycles
- E30 - General
- E31 - Price Level; Inflation; Deflation
- E32 - Business Fluctuations; Cycles
- E37 - Forecasting and Simulation: Models and Applications
- Browse content in E4 - Money and Interest Rates
- E43 - Interest Rates: Determination, Term Structure, and Effects
- E44 - Financial Markets and the Macroeconomy
- Browse content in E5 - Monetary Policy, Central Banking, and the Supply of Money and Credit
- E51 - Money Supply; Credit; Money Multipliers
- E52 - Monetary Policy
- E58 - Central Banks and Their Policies
- Browse content in E6 - Macroeconomic Policy, Macroeconomic Aspects of Public Finance, and General Outlook
- E61 - Policy Objectives; Policy Designs and Consistency; Policy Coordination
- E62 - Fiscal Policy
- E63 - Comparative or Joint Analysis of Fiscal and Monetary Policy; Stabilization; Treasury Policy
- E65 - Studies of Particular Policy Episodes
- Browse content in F - International Economics
- Browse content in F0 - General
- F01 - Global Outlook
- F02 - International Economic Order and Integration
- Browse content in F1 - Trade
- F10 - General
- F11 - Neoclassical Models of Trade
- F12 - Models of Trade with Imperfect Competition and Scale Economies; Fragmentation
- F13 - Trade Policy; International Trade Organizations
- F14 - Empirical Studies of Trade
- F15 - Economic Integration
- F16 - Trade and Labor Market Interactions
- F17 - Trade Forecasting and Simulation
- Browse content in F2 - International Factor Movements and International Business
- F20 - General
- F21 - International Investment; Long-Term Capital Movements
- F22 - International Migration
- F23 - Multinational Firms; International Business
- Browse content in F3 - International Finance
- F31 - Foreign Exchange
- F32 - Current Account Adjustment; Short-Term Capital Movements
- F33 - International Monetary Arrangements and Institutions
- F34 - International Lending and Debt Problems
- F35 - Foreign Aid
- Browse content in F4 - Macroeconomic Aspects of International Trade and Finance
- F41 - Open Economy Macroeconomics
- F42 - International Policy Coordination and Transmission
- F43 - Economic Growth of Open Economies
- F45 - Macroeconomic Issues of Monetary Unions
- Browse content in F5 - International Relations, National Security, and International Political Economy
- F50 - General
- F51 - International Conflicts; Negotiations; Sanctions
- F52 - National Security; Economic Nationalism
- F55 - International Institutional Arrangements
- Browse content in F6 - Economic Impacts of Globalization
- F60 - General
- F61 - Microeconomic Impacts
- F66 - Labor
- F68 - Policy
- Browse content in G - Financial Economics
- Browse content in G0 - General
- G01 - Financial Crises
- Browse content in G1 - General Financial Markets
- G10 - General
- G11 - Portfolio Choice; Investment Decisions
- G12 - Asset Pricing; Trading volume; Bond Interest Rates
- G15 - International Financial Markets
- Browse content in G2 - Financial Institutions and Services
- G20 - General
- G21 - Banks; Depository Institutions; Micro Finance Institutions; Mortgages
- G24 - Investment Banking; Venture Capital; Brokerage; Ratings and Ratings Agencies
- G28 - Government Policy and Regulation
- Browse content in G3 - Corporate Finance and Governance
- G30 - General
- G31 - Capital Budgeting; Fixed Investment and Inventory Studies; Capacity
- G32 - Financing Policy; Financial Risk and Risk Management; Capital and Ownership Structure; Value of Firms; Goodwill
- G33 - Bankruptcy; Liquidation
- G34 - Mergers; Acquisitions; Restructuring; Corporate Governance
- Browse content in G4 - Behavioral Finance
- G41 - Role and Effects of Psychological, Emotional, Social, and Cognitive Factors on Decision Making in Financial Markets
- Browse content in G5 - Household Finance
- G51 - Household Saving, Borrowing, Debt, and Wealth
- G53 - Financial Literacy
- Browse content in H - Public Economics
- Browse content in H0 - General
- H00 - General
- Browse content in H1 - Structure and Scope of Government
- H11 - Structure, Scope, and Performance of Government
- H12 - Crisis Management
- Browse content in H2 - Taxation, Subsidies, and Revenue
- H20 - General
- H21 - Efficiency; Optimal Taxation
- H22 - Incidence
- H23 - Externalities; Redistributive Effects; Environmental Taxes and Subsidies
- H24 - Personal Income and Other Nonbusiness Taxes and Subsidies; includes inheritance and gift taxes
- H25 - Business Taxes and Subsidies
- H26 - Tax Evasion and Avoidance
- Browse content in H3 - Fiscal Policies and Behavior of Economic Agents
- H30 - General
- H31 - Household
- Browse content in H4 - Publicly Provided Goods
- H41 - Public Goods
- H42 - Publicly Provided Private Goods
- H44 - Publicly Provided Goods: Mixed Markets
- Browse content in H5 - National Government Expenditures and Related Policies
- H50 - General
- H51 - Government Expenditures and Health
- H52 - Government Expenditures and Education
- H53 - Government Expenditures and Welfare Programs
- H54 - Infrastructures; Other Public Investment and Capital Stock
- H55 - Social Security and Public Pensions
- H56 - National Security and War
- H57 - Procurement
- Browse content in H6 - National Budget, Deficit, and Debt
- H62 - Deficit; Surplus
- H63 - Debt; Debt Management; Sovereign Debt
- H68 - Forecasts of Budgets, Deficits, and Debt
- Browse content in H7 - State and Local Government; Intergovernmental Relations
- H71 - State and Local Taxation, Subsidies, and Revenue
- H72 - State and Local Budget and Expenditures
- H75 - State and Local Government: Health; Education; Welfare; Public Pensions
- H76 - State and Local Government: Other Expenditure Categories
- H77 - Intergovernmental Relations; Federalism; Secession
- Browse content in H8 - Miscellaneous Issues
- H87 - International Fiscal Issues; International Public Goods
- Browse content in I - Health, Education, and Welfare
- Browse content in I1 - Health
- I10 - General
- I11 - Analysis of Health Care Markets
- I12 - Health Behavior
- I14 - Health and Inequality
- I15 - Health and Economic Development
- I18 - Government Policy; Regulation; Public Health
- I19 - Other
- Browse content in I2 - Education and Research Institutions
- I20 - General
- I21 - Analysis of Education
- I22 - Educational Finance; Financial Aid
- I23 - Higher Education; Research Institutions
- I24 - Education and Inequality
- I25 - Education and Economic Development
- I26 - Returns to Education
- I28 - Government Policy
- Browse content in I3 - Welfare, Well-Being, and Poverty
- I30 - General
- I31 - General Welfare
- I32 - Measurement and Analysis of Poverty
- I38 - Government Policy; Provision and Effects of Welfare Programs
- Browse content in J - Labor and Demographic Economics
- Browse content in J0 - General
- J00 - General
- J01 - Labor Economics: General
- J08 - Labor Economics Policies
- Browse content in J1 - Demographic Economics
- J10 - General
- J11 - Demographic Trends, Macroeconomic Effects, and Forecasts
- J12 - Marriage; Marital Dissolution; Family Structure; Domestic Abuse
- J13 - Fertility; Family Planning; Child Care; Children; Youth
- J14 - Economics of the Elderly; Economics of the Handicapped; Non-Labor Market Discrimination
- J15 - Economics of Minorities, Races, Indigenous Peoples, and Immigrants; Non-labor Discrimination
- J16 - Economics of Gender; Non-labor Discrimination
- J17 - Value of Life; Forgone Income
- J18 - Public Policy
- Browse content in J2 - Demand and Supply of Labor
- J20 - General
- J21 - Labor Force and Employment, Size, and Structure
- J22 - Time Allocation and Labor Supply
- J23 - Labor Demand
- J24 - Human Capital; Skills; Occupational Choice; Labor Productivity
- J26 - Retirement; Retirement Policies
- J28 - Safety; Job Satisfaction; Related Public Policy
- Browse content in J3 - Wages, Compensation, and Labor Costs
- J31 - Wage Level and Structure; Wage Differentials
- J32 - Nonwage Labor Costs and Benefits; Retirement Plans; Private Pensions
- J33 - Compensation Packages; Payment Methods
- Browse content in J4 - Particular Labor Markets
- J42 - Monopsony; Segmented Labor Markets
- J43 - Agricultural Labor Markets
- J45 - Public Sector Labor Markets
- J46 - Informal Labor Markets
- J47 - Coercive Labor Markets
- Browse content in J5 - Labor-Management Relations, Trade Unions, and Collective Bargaining
- J50 - General
- J51 - Trade Unions: Objectives, Structure, and Effects
- J53 - Labor-Management Relations; Industrial Jurisprudence
- Browse content in J6 - Mobility, Unemployment, Vacancies, and Immigrant Workers
- J61 - Geographic Labor Mobility; Immigrant Workers
- J62 - Job, Occupational, and Intergenerational Mobility
- J63 - Turnover; Vacancies; Layoffs
- J64 - Unemployment: Models, Duration, Incidence, and Job Search
- J65 - Unemployment Insurance; Severance Pay; Plant Closings
- J68 - Public Policy
- Browse content in J7 - Labor Discrimination
- J71 - Discrimination
- Browse content in K - Law and Economics
- Browse content in K1 - Basic Areas of Law
- K10 - General
- K12 - Contract Law
- K14 - Criminal Law
- Browse content in K3 - Other Substantive Areas of Law
- K36 - Family and Personal Law
- Browse content in K4 - Legal Procedure, the Legal System, and Illegal Behavior
- K40 - General
- K41 - Litigation Process
- K42 - Illegal Behavior and the Enforcement of Law
- Browse content in L - Industrial Organization
- Browse content in L0 - General
- L00 - General
- Browse content in L1 - Market Structure, Firm Strategy, and Market Performance
- L11 - Production, Pricing, and Market Structure; Size Distribution of Firms
- L12 - Monopoly; Monopolization Strategies
- L13 - Oligopoly and Other Imperfect Markets
- L14 - Transactional Relationships; Contracts and Reputation; Networks
- L15 - Information and Product Quality; Standardization and Compatibility
- L16 - Industrial Organization and Macroeconomics: Industrial Structure and Structural Change; Industrial Price Indices
- Browse content in L2 - Firm Objectives, Organization, and Behavior
- L22 - Firm Organization and Market Structure
- L25 - Firm Performance: Size, Diversification, and Scope
- L26 - Entrepreneurship
- Browse content in L3 - Nonprofit Organizations and Public Enterprise
- L31 - Nonprofit Institutions; NGOs; Social Entrepreneurship
- L32 - Public Enterprises; Public-Private Enterprises
- L33 - Comparison of Public and Private Enterprises and Nonprofit Institutions; Privatization; Contracting Out
- Browse content in L4 - Antitrust Issues and Policies
- L41 - Monopolization; Horizontal Anticompetitive Practices
- L42 - Vertical Restraints; Resale Price Maintenance; Quantity Discounts
- L44 - Antitrust Policy and Public Enterprises, Nonprofit Institutions, and Professional Organizations
- Browse content in L5 - Regulation and Industrial Policy
- L51 - Economics of Regulation
- L52 - Industrial Policy; Sectoral Planning Methods
- Browse content in L6 - Industry Studies: Manufacturing
- L60 - General
- L66 - Food; Beverages; Cosmetics; Tobacco; Wine and Spirits
- L67 - Other Consumer Nondurables: Clothing, Textiles, Shoes, and Leather Goods; Household Goods; Sports Equipment
- Browse content in L8 - Industry Studies: Services
- L81 - Retail and Wholesale Trade; e-Commerce
- L82 - Entertainment; Media
- L83 - Sports; Gambling; Recreation; Tourism
- L86 - Information and Internet Services; Computer Software
- Browse content in L9 - Industry Studies: Transportation and Utilities
- L91 - Transportation: General
- L94 - Electric Utilities
- L96 - Telecommunications
- L98 - Government Policy
- Browse content in M - Business Administration and Business Economics; Marketing; Accounting; Personnel Economics
- Browse content in M1 - Business Administration
- M10 - General
- M12 - Personnel Management; Executives; Executive Compensation
- M13 - New Firms; Startups
- Browse content in M2 - Business Economics
- M21 - Business Economics
- Browse content in M3 - Marketing and Advertising
- M30 - General
- M31 - Marketing
- Browse content in M5 - Personnel Economics
- M50 - General
- M51 - Firm Employment Decisions; Promotions
- M52 - Compensation and Compensation Methods and Their Effects
- M55 - Labor Contracting Devices
- Browse content in N - Economic History
- Browse content in N1 - Macroeconomics and Monetary Economics; Industrial Structure; Growth; Fluctuations
- N10 - General, International, or Comparative
- N12 - U.S.; Canada: 1913-
- N13 - Europe: Pre-1913
- N14 - Europe: 1913-
- N15 - Asia including Middle East
- Browse content in N2 - Financial Markets and Institutions
- N20 - General, International, or Comparative
- N23 - Europe: Pre-1913
- N26 - Latin America; Caribbean
- Browse content in N3 - Labor and Consumers, Demography, Education, Health, Welfare, Income, Wealth, Religion, and Philanthropy
- N30 - General, International, or Comparative
- N32 - U.S.; Canada: 1913-
- N33 - Europe: Pre-1913
- N34 - Europe: 1913-
- N35 - Asia including Middle East
- Browse content in N4 - Government, War, Law, International Relations, and Regulation
- N40 - General, International, or Comparative
- N41 - U.S.; Canada: Pre-1913
- N42 - U.S.; Canada: 1913-
- N43 - Europe: Pre-1913
- N44 - Europe: 1913-
- N45 - Asia including Middle East
- Browse content in N5 - Agriculture, Natural Resources, Environment, and Extractive Industries
- N50 - General, International, or Comparative
- N51 - U.S.; Canada: Pre-1913
- N53 - Europe: Pre-1913
- N55 - Asia including Middle East
- N57 - Africa; Oceania
- Browse content in N6 - Manufacturing and Construction
- N63 - Europe: Pre-1913
- Browse content in N7 - Transport, Trade, Energy, Technology, and Other Services
- N70 - General, International, or Comparative
- N71 - U.S.; Canada: Pre-1913
- N72 - U.S.; Canada: 1913-
- N73 - Europe: Pre-1913
- N75 - Asia including Middle East
- Browse content in N9 - Regional and Urban History
- N90 - General, International, or Comparative
- N92 - U.S.; Canada: 1913-
- N94 - Europe: 1913-
- N95 - Asia including Middle East
- Browse content in O - Economic Development, Innovation, Technological Change, and Growth
- Browse content in O1 - Economic Development
- O10 - General
- O11 - Macroeconomic Analyses of Economic Development
- O12 - Microeconomic Analyses of Economic Development
- O13 - Agriculture; Natural Resources; Energy; Environment; Other Primary Products
- O14 - Industrialization; Manufacturing and Service Industries; Choice of Technology
- O15 - Human Resources; Human Development; Income Distribution; Migration
- O16 - Financial Markets; Saving and Capital Investment; Corporate Finance and Governance
- O17 - Formal and Informal Sectors; Shadow Economy; Institutional Arrangements
- O18 - Urban, Rural, Regional, and Transportation Analysis; Housing; Infrastructure
- O19 - International Linkages to Development; Role of International Organizations
- Browse content in O2 - Development Planning and Policy
- O22 - Project Analysis
- O24 - Trade Policy; Factor Movement Policy; Foreign Exchange Policy
- O25 - Industrial Policy
- Browse content in O3 - Innovation; Research and Development; Technological Change; Intellectual Property Rights
- O30 - General
- O31 - Innovation and Invention: Processes and Incentives
- O32 - Management of Technological Innovation and R&D
- O33 - Technological Change: Choices and Consequences; Diffusion Processes
- O34 - Intellectual Property and Intellectual Capital
- O38 - Government Policy
- O39 - Other
- Browse content in O4 - Economic Growth and Aggregate Productivity
- O40 - General
- O41 - One, Two, and Multisector Growth Models
- O43 - Institutions and Growth
- O44 - Environment and Growth
- O47 - Empirical Studies of Economic Growth; Aggregate Productivity; Cross-Country Output Convergence
- Browse content in O5 - Economywide Country Studies
- O50 - General
- O52 - Europe
- O53 - Asia including Middle East
- O55 - Africa
- Browse content in P - Economic Systems
- Browse content in P0 - General
- P00 - General
- Browse content in P1 - Capitalist Systems
- P14 - Property Rights
- P16 - Political Economy
- Browse content in P2 - Socialist Systems and Transitional Economies
- P26 - Political Economy; Property Rights
- Browse content in P3 - Socialist Institutions and Their Transitions
- P39 - Other
- Browse content in P4 - Other Economic Systems
- P48 - Political Economy; Legal Institutions; Property Rights; Natural Resources; Energy; Environment; Regional Studies
- Browse content in P5 - Comparative Economic Systems
- P50 - General
- P51 - Comparative Analysis of Economic Systems
- Browse content in Q - Agricultural and Natural Resource Economics; Environmental and Ecological Economics
- Browse content in Q1 - Agriculture
- Q12 - Micro Analysis of Farm Firms, Farm Households, and Farm Input Markets
- Q14 - Agricultural Finance
- Q15 - Land Ownership and Tenure; Land Reform; Land Use; Irrigation; Agriculture and Environment
- Q16 - R&D; Agricultural Technology; Biofuels; Agricultural Extension Services
- Q17 - Agriculture in International Trade
- Q18 - Agricultural Policy; Food Policy
- Browse content in Q2 - Renewable Resources and Conservation
- Q23 - Forestry
- Q28 - Government Policy
- Browse content in Q3 - Nonrenewable Resources and Conservation
- Q32 - Exhaustible Resources and Economic Development
- Q33 - Resource Booms
- Browse content in Q4 - Energy
- Q41 - Demand and Supply; Prices
- Q48 - Government Policy
- Browse content in Q5 - Environmental Economics
- Q51 - Valuation of Environmental Effects
- Q52 - Pollution Control Adoption Costs; Distributional Effects; Employment Effects
- Q53 - Air Pollution; Water Pollution; Noise; Hazardous Waste; Solid Waste; Recycling
- Q54 - Climate; Natural Disasters; Global Warming
- Q55 - Technological Innovation
- Q56 - Environment and Development; Environment and Trade; Sustainability; Environmental Accounts and Accounting; Environmental Equity; Population Growth
- Q58 - Government Policy
- Browse content in R - Urban, Rural, Regional, Real Estate, and Transportation Economics
- Browse content in R1 - General Regional Economics
- R10 - General
- R11 - Regional Economic Activity: Growth, Development, Environmental Issues, and Changes
- R12 - Size and Spatial Distributions of Regional Economic Activity
- R13 - General Equilibrium and Welfare Economic Analysis of Regional Economies
- R15 - Econometric and Input-Output Models; Other Models
- Browse content in R2 - Household Analysis
- R21 - Housing Demand
- R23 - Regional Migration; Regional Labor Markets; Population; Neighborhood Characteristics
- Browse content in R3 - Real Estate Markets, Spatial Production Analysis, and Firm Location
- R30 - General
- R31 - Housing Supply and Markets
- Browse content in R4 - Transportation Economics
- R41 - Transportation: Demand, Supply, and Congestion; Travel Time; Safety and Accidents; Transportation Noise
- Browse content in R5 - Regional Government Analysis
- R52 - Land Use and Other Regulations
- R58 - Regional Development Planning and Policy
- Browse content in Z - Other Special Topics
- Browse content in Z1 - Cultural Economics; Economic Sociology; Economic Anthropology
- Z10 - General
- Z12 - Religion
- Z13 - Economic Sociology; Economic Anthropology; Social and Economic Stratification
- Z19 - Other
- Browse content in Z2 - Sports Economics
- Z20 - General
- Author Guidelines
- Submission Site
- Open Access
- Self-Archiving Policy
- About The Economic Journal
- About the Royal Economic Society
- Editorial Board
- Advertising and Corporate Services
- Journals on Oxford Academic
- Books on Oxford Academic

Article Contents
1. why is rising obesity a problem, 2. what determines food choices, 3. what can governments do to reduce obesity, 4. final comments, obesity, poverty and public policy.
- Article contents
- Figures & tables
- Supplementary Data
Rachel Griffith, Obesity, Poverty and Public Policy, The Economic Journal , Volume 132, Issue 644, May 2022, Pages 1235–1258, https://doi.org/10.1093/ej/ueac013
- Permissions Icon Permissions
Obesity rates in the United Kingdom, and around the world, are high and rising. They are higher, and rising faster, amongst people growing up and living in deprivation. These patterns raise potential concerns about both market failures and equity. There is much that policy can do to address these concerns. However, policy can also do harm if it is poorly targeted or has unintended consequences. In order to design effective policies we need an understanding of who we are trying to target, and for what reasons. This paper provides an overview of some of the evidence, and some recent policy initiatives.
Obesity rates in the UK, and around the world, are high and rising. They are higher, and rising faster, amongst people growing up and living in deprivation. Rising obesity is a concern because it suggests that there are potential market failures that are leading people to make suboptimal choices about the foods they eat and the activities they engage in. These choices are potentially suboptimal in the sense that they may lead to higher than anticipated costs for the person themselves in the future and for wider society. Even if markets are functioning well, obesity may also potentially be a concern for equity reasons. If some children, for example those from disadvantaged backgrounds, are not able to access sufficient nourishment for healthy development, then there might be a role for policy intervention to provide greater equality of opportunity by ensuring access to a nutritious diet.
This paper provides an overview of the main evidence (and lack of evidence) on why obesity is an issue of public policy concern, what are some of the factors that might be driving rising obesity and its association with deprivation, and where policy might be most effective at improving welfare. There is much that public policy can do in terms of changing market signals, such as relative prices, and changing the choice environment to encourage people to make choices that better align with their own long-term interests. However, policy can also do harm if it is poorly targeted or has unintended consequences. In order to design effective policies we need an understanding of who we are trying to target, and for what reasons.
Obesity has risen dramatically in recent years in the UK and around the world. 1 Obesity is defined using the ‘body mass index’ (BMI), which is the ratio of weight to height squared (kilograms per |${\rm metre}^2$| ). BMI is a simple summary statistic used by medical professionals as an indicator of whether an individual is overweight (or underweight) and how overweight they are. An adult is obese when their BMI is over 30, they are morbid or severely obese when their BMI is over 40. BMI is not a perfect indicator, nor is it the only indicator that medical professionals care about. 2 For example, excess fat around the waist is another indicator. However, BMI is relatively easy to measure and track across time and locations, it is correlated with other measures, and it is seen as useful as a broad and relatively easy to measure indicator.
In England in 2018 nearly one in three adults was obese, and around one in twenty-five were morbidly obese. The rate of obesity in adults has doubled since 1993, shown in Figure 1 . Obesity rates are higher in more deprived areas (see Table 5 of NHSDigital, 2019 ). The statistics show similar trends in Scotland, Wales and Northern Ireland and other parts of the world.

Adult Obesity Rate in England.
Notes: Obese is defined as a BMI over 30; morbid or severely obese is defined as a BMI over 40.
Source . Table 6 of NHSDigital ( 2019 ).
Obesity in children is also high, for example, around one in five 10–11 year olds in England were obese in 2019. Worryingly, children are becoming obese at younger ages and are staying obese into adulthood (Johnson et al ., 2015 ). Obesity is more prevalent in more deprived areas, with children living in the most deprived regions being nearly twice as likely to be obese as those living in the least deprived regions. If we focus on children that are severely obese, the rate in the most deprived regions is over four times the least deprived areas (NHSDigital, 2020 ). 3
The gap in obesity rates between children growing up in the least and most deprived areas has widened over the last decade, as shown in Figure 2 . Panel (a) shows that in 2006 the gap was 8.5 percentage points; by 2019, it had grown to 13.3. Panel (b) shows that, for severely obese children, the gap between the share of children in the least and most deprived areas grew from 3.1 percentage points in 2006 to 5.3 in 2019.

Child Obesity Rate in England, by Deprivation.
Notes: Location is measured by the postcode of the child’s school. The dashed lines show 95% confidence intervals. See footnote 3 for definitions of the least and most deprived regions. Details on how obesity in children is measured is available in NHS ( 2011 ).
Source . Tables 13(a) and 14(b) of NHSDigital ( 2020 ).
Rising obesity is a concern because it suggests that there might be market failures that are leading people to make suboptimal choices. These market failures could arise if people do not fully account for the costs that obesity imposes on wider society, and on themselves, in the future when they make consumption choices. While there are many good papers that try to estimate the extent of these social costs, 4 the magnitude and nature of these costs (and in particular how they vary across different people), and what market failures are causing them, is still not fully understood. 5 However, policy-makers (and many others) believe that these costs are large, particularly amongst children, and especially amongst those growing up in deprivation.
Even if markets are functioning well, obesity may also potentially be a concern for equity reasons. Ensuring that all children, including those from disadvantaged backgrounds, are well nourished seems a corner stone of the provision of equality of opportunity. It is well established that child nutrition has important impacts on later life outcomes (see, among others, Currie, 2009 ; Almond et al ., 2018 and Lundborg et al ., 2021 ). Higher and growing rates of obesity amongst children from disadvantaged backgrounds might indicate that these children are not able to access sufficient nourishment, and suggest a role for policy intervention to provide greater equality of opportunity.
Obesity is associated with, and potentially causes, a number of adverse health, social and economic outcomes. Obesity arises due to a caloric imbalance (too many calories consumed relative to expended) leading to excess weight. It is also associated with, and might be an indicator of, a potentially unhealthy balance of nutrients, for example, a diet with too many sugars and carbohydrates. Obesity can also be associated with food insecurity (the inability to regularly access a healthy diet) if, when people do have the resources and ability to obtain food, they choose low-cost calorie-dense foods with a low nutritional value.
The main medical concern about excess weight is that it indicates an excess of fat (too much bone or muscle is not a problem). Excess fat is thought to increase an individual’s risk factor for a number of diseases, including metabolic syndrome, high blood pressure, atherosclerosis, heart disease, diabetes, high blood cholesterol, cancers and sleep disorders (NIH, 2021 ). The increased risk of these diseases likely increases costs to the healthcare system, both through an increase in the prevalence or severity of these diseases, and also because the costs of treating obese patients can be higher than normal weight individuals. Hospital admissions either directly attributable to obesity, or where obesity was a factor, are more prevalent amongst individuals from more deprived areas (NHS, 2020 ).
Obesity in childhood can have significant impacts on physical and psychological health (Sahoo et al ., 2015 ). The widening gap in obesity rates between children growing up in the least and most deprived areas raises the concern that obesity, and associated poor nutrition, may be important drivers of long-term inequalities. There is no strong causal evidence on the impact of obesity and poor nutrition on outcomes, but Public Health England (PHE) and the Centers for Disease Control and Prevention (CDC) in the United States highlight being obese as at least correlated with long-term harms in children, for example, through increased school absences and behavioural problems. We do not have good evidence on whether these effects are all driven by poor health, which feeds through to poor social and educational outcomes, or whether other factors are also at play. But at least amongst public health officials there is a concern that as well as affecting health, childhood obesity can have potentially important consequences for children’s long-term social and economic outcomes. 6 Economists have formalised the costs and related effects that fall on the person themselves in the future as ‘internalities’ . 7 For children, they are likely too young to understand the long-term consequences of eating an unhealthy diet, and so it is not factored into their decision-making, and for some children at least, their parents may also not fully account for these effects either.
Obesity is the result of an imbalance in energy consumed and energy expended. A common question is whether it matters what type of calories you eat, or is it only calories (net of energy expended on activities) that matter? Many governments give advice on the ‘optimal’ combination of foods; 8 however, the evidence seems to suggest that many different combinations of foods can yield healthy outcomes. 9 Recently, attention has focused on processed foods as leading to poor health outcomes, rather than foods containing any particular macro nutrients. 10
Excess consumption of some types of foods is also associated, and possibly causally so, with specific diseases. For example, high consumption of foods that have a lot of ‘free sugars’ (sugars added in manufacturing) can cause insulin resistance, which can cause diabetes (Ludwig, 2002 ; Kalra and Gupta, 2014 ; Imamura et al ., 2015 ). High consumption of salt can harden your arteries, leading to high blood pressure and cardiovascular disease (Trieu et al ., 2015 ).
Excess sugar consumption has been a particular target of policy-makers around the world. To see one reason why, consider Figure 3 . The horizontal axis shows age, and the vertical access shows grams of added sugar per day. Added sugar does not include naturally occurring sugars, for example in fruit or milk. The red dashed line is the UK government’s recommended maximum daily consumption based on medical advice. The solid black line shows the mean daily consumption reported in the National Diet and Nutrition Survey (NDNS); the dashed black lines show 95% confidence intervals. The NDNS is a continuous, cross-sectional survey. It is designed to collect detailed, quantitative information on food consumption, nutrient intake and nutritional status of the general population aged 1.5 years and over. The survey covers a representative sample of around 1,000 people per year. Respondents are asked to record consumption of all foods over two days. What is clear from panel (a) is that consumption is way above the recommended maximum at all ages, but particularly at younger ages. Panels (b)–(d) show that in fact almost all young children consume more than the medically recommended amounts of added sugar.

Sugar Consumption by Age.
Source . Panel (a) is Figure 1 , panels (b)–(d) are Figure 2 of Griffith et al . ( 2020 ), using National Diet and Nutrition Survey (NDNS).
Another common question is—if weight gain results from eating more calories than you burn in activity, is it only calories that matter, or does increasing activity through exercise lead to weight loss? In principle yes, but the relationship between exercise and weight loss is complicated. Exercise is good for you for all sorts of reasons, but some evidence suggests that on its own it might not lead to a lot of weight loss. This is partly because you would have to increase the amount of exercise you do by quite a lot, and also because the body responds in complicated ways that might mitigate some of the effects of increasing exercise on weight loss (see, for example, Prentice and Jebb, 2004 and Jebb, 2015 ). On the other hand, the analysis in Griffith et al . (2016a ) suggests that a reduction in the strenuousness of daily life may be at least partially responsible for the increase in obesity in adults over the 1980s and 1990s in the UK.
In this section we discuss some of the important factors that determine food choices. If markets are functioning well then consumers’ choices will be determined by market prices, income and the attributes of consumption that yield (positive or negative) utility. For markets to function well requires that consumers have good information about these attributes and about the utility they generate, and that consumers can and do act on this information appropriately; it also requires that consumers can access the foods they want to buy and that prices reflect costs.
We highlight some of the possible reasons that people might be making suboptimal choices, due to market failures or resource constraints. This is important because in order to design good policy we need to understand why some people are making bad choices. In the next section we consider how some specific policies might encourage people to make better choices, or to otherwise mitigate the negative consequences of their suboptimal choices, and whether they might also have other unintended consequences.
2.1. Food Prices
The price of foods is obviously an important determinant of consumers’ choices, and many policies aim to change relatives prices of different food products or food groups in order to incentivise producers and consumers to account for the excess social costs of consumption. In this section we highlight some of the main recent trends in food prices.
2.1.1. Price levels
From the 1980s until the mid-2000s, food prices have fallen in OECD countries; see OECD ( 2020 ). In the UK, this was particularly the case (Griffith et al ., 2015 ). The reduction in food prices benefited poorer households, for whom foods represent a significant share of their budget, and a much higher proportion than for richer households. While access to cheaper food could have contributed to people eating more, increasing the overall price of food seems unlikely to be an effective way to reduce obesity or improve diet quality. It will hit the poorest hardest, and the increase would likely have to be very large to have an appreciable impact. Food prices in the UK increased dramatically in the mid-2000s due to the depreciation of the sterling, though fell back below the OECD average reasonably quickly, but now look likely to rise again due to increased trade costs due to Brexit. There is so far no indication that these large price rises are having a positive impact on health or reducing obesity.
2.1.2. Relative prices
Changing the relative prices of different foods is a policy that many governments are pursuing, for example by introducing taxes on sugar sweetened beverages. The National Food Strategy (Dimbleby, 2021 ) has recommended expanding this to a more general tax on added sugar.
How do the prices of different food products and food groups vary with the healthiness of that product? This is not a simple question to answer. One common approach is to show that the average price per calorie of more healthy products is higher than that of less healthy products. 11
However, this comparison of prices misses the key point. Why do some foods cost more than others? The price of a product depends on the interaction of supply and demand factors. If something costs more to make or grow then this will typically be reflected in a higher price. However, if there are social costs to the consumption of some foods—that is, if the costs of production do not fully reflect the costs to society of that product being consumed—then the price might be ‘too low’, in the sense that there may be a benefit (in terms of higher social welfare) if government intervened to raise the price above the market price. It is the existence of these social costs that provide a rationale for taxes on unhealthy foods, such as sugary drinks. The appropriate level of these taxes does not depend on the differences in price between healthy and unhealthy products, but on the magnitude of the social costs that are associated with the consumption of unhealthy foods.
Another reason why the price of two products that cost the same to produce might differ is if firms have market power that enables them to mark prices up above marginal cost. If one product is much more popular, and has fewer substitutes, than another, then the firm can markup the price by more. Processed foods are typically produced and sold in more concentrated markets with more advertising, so if anything, we would expect the price of these products to be marked up above marginal costs by more than products where producers have less market power.
If healthy foods are more expensive to produce, there may also be equity reasons to provide targeted subsidies to low-income households to reduce the costs of healthy foods. For example, Healthy Start Vouchers and Free School Meals (discussed further in Subsection 3.4 ) do that in the UK.
2.1.3. Time use and prices
Some foods take time to prepare, and both the technology of food product and the opportunity cost of time can affect the costs of doing this. Households may increase their time spent searching for lower prices or in home production in order to reduce the costs of consumption at some points in time (Stigler, 1961 ; Becker, 1965 ; Aguiar and Hurst, 2007 ). They may also change the composition of their shopping basket (e.g., switching from a preferred brand to a cheaper generic product) to maintain its nutritional quality for a given cost.
Several papers study the ways that households reduced the prices they paid in response to the adverse shocks to incomes and food prices over the 2007–8 recession. Unlike during previous recessions in the UK the amount that households spent on food did not keep pace with rising food prices, and this led some to infer a substantial reduction in the size and nutritional quality of households’ food baskets (see, for example, Lock et al ., 2009 ; Taylor-Robinson et al ., 2013 ), with similar concerns in the United States (US Department of Agriculture, 2010 ; US Department of Agriculture, 2013 ). Griffith et al . ( 2016b ) showed that in the UK households were able to exploit various mechanisms to smooth, or ‘insure’, the quantity and nutritional quality of their food basket in the face of these adverse shocks. Evidence from the United States suggests that, as economic conditions worsened, households spent more time shopping and thus paid lower prices (Kaplan and Menzio, 2015 ), increased their use of sales, switched to generic products (Nevo and Wong, 2019 ) and switched to low-price retailers (Coibion et al ., 2014 ).
The costs of making and eating nutritious foods is not just the money spent on buying the ingredients, but also the time spent in preparation. Griffith et al . ( 2022 ) showed that over the last several decades the share of the food budget that goes on ingredients fell, while the share on processed foods increased. This is surprising because they also showed that the market prices of ingredients declined most. The distinction between ingredients and prepared foods is particularly relevant due to the recent attention on processed foods as leading to poor health outcomes, discussed above.
Griffith et al . ( 2022 ) documented that time spent on food management, which includes shopping and cooking, declined between 1974 and 2000; Cutler et al . ( 2003 ) showed the same is true in the United States. Mean hours on food management have fallen, with women reducing time spent and men increasing time spent on these activities, but not by enough to compensate for the reduction by women. Women are spending more time in the labour market; labour force participation has increased, hours worked conditional on participation have increased and wage offers have increased. Putting these together, Griffith et al . ( 2022 ) constructed a shadow price of a home cooked meal. The shadow price reflects both the costs of purchasing the ingredients and the time needed to prepare it for consumption, where the cost of time is estimated and has increased due to outside labour market opportunities for women. Figure 4 shows that, while market prices have fallen, the shadow price—the cost of home cooked food—has increased.

Market and Shadow Prices of Foods.
Notes : The shadow price incorporates the observed wage for labour market participants, and the maximum of the estimated market wage or the estimated reservation wage for non-participants.
Source . Figure 4.2 of Griffith et al . ( 2022 ).
2.2. Income
There is clearly a strong correlation between deprivation and obesity (see Figure 2 for example), and more generally there are strong intergenerational correlations in health and income (see, for example, Case et al ., 2002 ). However, convincingly identifying the causal impacts of income on obesity and nutrition in a developed countries context remains a challenge.
A large and growing literature suggests that even relatively mild negative economic shocks in childhood can have long lasting negative impacts, although these are heterogeneous (see the survey in Almond et al ., 2018 ). For example, Hoynes et al . ( 2016 ) used the roll out of the Food Stamp Program in the United States in the 1960s and early 1970s to show that access to food stamps in childhood leads to a significant reduction in the incidence of metabolic syndrome (conditions that include obesity, high blood pressure, heart disease and diabetes) and, for women, an increase in economic self-sufficiency. However, a literature that looks at the short-run impacts of economic shocks suggests that diet quality is either not affected by, or is improved by, adverse economic conditions, 12 and Adda et al . ( 2009 ) showed that permanent income shocks have little effect on a range of health outcomes.
Another way that income and deprivation might affect the nutritional quality of individuals’ diets is through the availability of healthy foods. Many papers have documented that healthy foods are less available, or cost more, in lower income neighbourhoods—what is referred to as ‘food deserts’. 13
One important question, on which there is still limited evidence, is what is the direction of causation in this observed relationship. The food offering in any location is a result of supply and demand factors. Is the supply of healthy foods driven by restrictions to supply, or by differences in demand preferences by consumers in those locations? Allcott et al . ( 2019a ) provided evidence for the United States that it is largely differences in preferences, and not supply constraints. The answer to this is important for policy design; either response might merit policy intervention, but the effective policy will differ. Even where differences in the food offering are driven by differences in the market demand curve, it might be that individuals within a market with a restricted offering have preferences that differ from the mean, and they are affected by supply constraints.
In the next section we discuss some of the ways that income might interact with other factors to affect the way that people make decisions, and that might lead to market failures and suboptimal outcomes.
2.3. Information, Cognition, Self-Control and Advertising
In addition to prices and incomes economists have long studied the importance of information, and the ways that information is processed, in determining consumer choices (see, for example, Stigler, 1961 ; Nelson, 1970 ; Loewenstein et al ., 2014 ), and the role of information in promoting healthier food choices (see, for example, Schofield and Mullainathan, 2008 ; Wisdom et al ., 2010 ; Reutskaja et al ., 2011 ).
There is a long history of government policies that aim at providing information and education, for example, on the safety benefits of wearing seat belts and the health consequences of smoking. There have been many information campaigns on food and nutrition; in the UK these have included the Eatwell Guide, the five-a-day campaign, Change4life and nutrient labelling regulations, amongst many others.
Information campaigns will be most effective where people want, but lack, information. One important reason that some campaigns might not be that successful is if people already have the information they need (people probably already know that vegetables are good for them). However, work by behavioural economists suggests that people do not always fully pay attention to the information they have when making decisions (Bordalo et al ., 2013 ), for example, some people may group products into categories in order to reduce ‘cognitive overload’ (Mullainathan et al ., 2008 ). Work by Sendhil Mullainathan and colleagues 14 has looked at the impact of poverty on cognition. The poor often behave in less capable ways, which can perpetuate them staying in poverty. This body of work argues that poverty directly impedes cognitive function, because poverty-related concerns consume mental resources, leaving less for other tasks. The fact of being poor means that you have to cope not only with a shortfall of money, but also with many other calls on cognitive resources. This view suggests that the poor are less capable not because of inherent traits, but because the very context of poverty imposes a load of concerns on people that impedes cognitive capacity.
Another reason that people might not fully take account of all of the information available to them is that they might succumb to temptation due to self-control problems. Read and Van Leeuwen ( 1998 ) and Sadoff et al . ( 2020 ) provided some of the most direct evidence (based on experiments in the field) of self-control problems in diet. Cherchye et al . ( 2017 ) showed that, as well as some people eating a healthier diet than others, there is considerable variation in the quality of most individuals’ diets over time that cannot be explained by standard factors such as prices and incomes, and which is likely to be at least partially driven by self-control problems in food choice.
An extensive psychological literature shows that individual choice behaviour varies with context and time, and that individuals sometimes use self-regulation and behaviour modification in an attempt to mitigate these influences (see the references and discussion in Rabin, 1998 and DellaVigna, 2009 ). For example, experimental evidence suggests that individuals may be willing to impose (sometimes costly) commitments on themselves. 15 New Years’ resolutions to eat a more healthy diet are an example of a common form of self-regulation and behaviour modification with regards to diet (Dai et al ., 2014 ; 2015 ).
Figure 5 shows an example of these fluctuations in diet quality over the calendar year. Panel (a) shows variation in the nutritional quality of food purchased by a large sample of UK households. 16 This suggests a clear ‘reset’ in January of each year to a healthier diet, with a decline over the year. Panel (b) shows the same trend in Google searches for the term ‘healthy foods’.

Variation in Diet Quality.
Source . Figures 1(b) and 2(a) in Cherchye et al . ( 2017 ).
Cherchye et al . ( 2017 ) used information on individuals’ stated preferences and attitudes to investigate whether greater fluctuations in the share of calories from healthy food reflect impulsive behaviour. Their findings suggest that fluctuations are larger for individuals who state that they are more impulsive (e.g., spend money without thinking). They relate their findings to the literature that finds empirical evidence of considerable within-individual variation in choice behaviour in other settings, 17 as well as in grocery purchases using alternative identification strategies. 18 They formalise this behaviour in a two-selves model of food purchasing behaviour in the spirit of this literature, in which individuals’ food choices are the outcome of an intra-personal bargaining process between a healthy and an unhealthy self. 19
What affects might advertising have on food choices? In the economics literature advertising is modelled as either informative (it gives consumers information about a characteristic of the product) or distortionary (it gives consumers misleading information, or distracts them from information they have). 20 Informative advertising will improve the choices that consumers make, while distortionary advertising will lead to worse choices. Another important distinction for our purposes here is whether the impact of advertising is to expand the market, or whether it is largely rivalrous, leading to shifts in market share between firms within a market. If advertising expands a market then it is more likely to have adverse impacts on nutrition (if the products being advertised are less nutritious), whereas if advertising largely leads consumers to switch between products that have similar nutrient value (e.g., between Coca Cola and Pepsi) then its impact on nutrition will likely be smaller. We return to discuss this further in Subsection 3.3 .
Advertising might amplify problems of temptation and self-control; the products that are advertised most heavily are also those that are the least healthy (see, for example, the figure on UK advertising expenditure by food group in Abi-Rafeh et al ., 2021 ). Experimental evidence shows that children exposed to food advertising ate more and were more likely to be obese. 21 Advertisers can frame a consumers’ view of a product using a desirable product category, or transfer desirable attributes from other products in the same category in the consumers’ mind. For example, in the context of food advertising, a kind of chewing gum can be viewed as healthy by ‘coarse’ thinking consumers if it is advertised as low-fat (Schofield and Mullainathan, 2008 ). This may be particularly true for people living in poverty who have a lot of other things to worry about and so experience cognitive overload (Mani et al ., 2013 ).
Griffith et al . ( 2018a ) attempted to measure exposure of consumers to food advertising in the UK, and estimated that households in the lowest income quartile see something like 20% more adverts for unhealthy foods than households in the highest income quartile; this is because they watch more TV, and they watch at a time and watch TV shows on which these adverts are more likely to be shown.
Governments are considering, and have implemented, a large range of policies that change relative prices, alter the choice environment, provide information and education to consumers, incentivise firms to reformulate, encourage a more active lifestyle and more. Policies that are aimed at correcting market failures should reduce externalities (costs imposed on wider society) and internalities (costs imposed on the person themselves in the future), while minimising any unintended adverse consequences. Policies that are aimed at alleviating equity concerns should be well targeted and minimise deadweight costs.
Designing and implementing policies that meet these ambitions is difficult. 22 That does not mean that it is not worth trying, but it is important to recognise that policies can (inadvertently) do harm as well as good. For example, poorly designed taxes might fail to improve outcomes if people with high externalities or internalities do not respond, yet could impose additional costs on exactly those people it is intended to help.
3.1. Corrective Taxes
Corrective taxes are a common approach to tackle externalities. 23 Increasing the overall price of food seems unlikely to be an effective way to reduce obesity. It will hit the poorest hardest, and the increase would likely have to be very large to have an appreciable impact. Instead, corrective taxes generally aim to change relative prices , i.e., to increase the price of less healthy foods relative to more healthy foods.
To date, one of the most popular corrective taxes aimed at reducing obesity and improving nutrition is taxes on sugary soft drinks. 24 The UK introduced the Soft Drinks Industry Levy in 2018, and Dimbleby ( 2021 ) is recommending broader taxes on added sugar and salt in the UK. Griffith et al . ( 2020 ) reviewed twenty-seven studies of taxes in eleven jurisdictions—all studies find that taxes lead to increased prices—pass-through is lower in smaller jurisdictions; in settings like the UK, taxes are fully passed through to prices. Most studies find that taxes led to substantial reductions in purchases of soda. Allcott et al . ( 2019b ) provided further discussion of the evidence.
One key ingredient to understanding whether soda taxes are effective is to know whether they lead to reductions in consumption in those individuals who generate the largest externalities and internalities. Unfortunately, we do not have good estimates of the scale or distribution of externalities and internalities; this is a key piece of missing evidence. Policy-makers in the UK and elsewhere have targeted some specific groups more than others, including the young, poor and heavy sugar consumers. One question is whether these groups are responsive to taxes. If they are, and if policy-makers are right that they suffer higher internalities, then they gain in the long run due to reduced internalities, which compensates them for the loss from higher prices. However, if they are not responsive to taxes then they do not benefit from reduction in internalities, and they are made worse off because they pay higher prices.
Dubois et al . ( 2020 ) used UK data to study how well targeted taxes on sugary drinks are, and in doing so tackle a number of methodological challenges. It is important to capture heterogeneity in preferences and in responses across people, and in order to study how well targeted the policy is, to be able to relate this heterogeneity to demographics of interest. They focused on the young, poor and heavy sugar consumers because policy-makers have focused on these groups, for which they believe consumption leads to high internalities. Dubois et al . ( 2020 ) exploited longitudinal data and relaxed some of the parametric assumptions imposed by traditional methods for estimating demand in differentiated product markets. Their results show that high sugar consumers would be less responsive to a tax than low sugar consumers, but that the young are more responsive than the old, so this form of tax is well targeted in one dimension, but not the other.
O’Connell and Smith ( 2020 ) considered the design of taxes on sugar-sweetened beverages, accounting for the fact that firms have market power, showing how optimal policy depends on the relative size of price-cost margins among externality generating goods and alternative products, and the degree of consumer switching across these product sets. They showed that taking these factors into account can substantially increase the welfare improvements achieved by these taxes.
3.2. Incentivising Reformulation
Consumer information campaigns, such as those to promote greater consumption of fruit and vegetables (Stables et al ., 2002 ; Capacci and Mazzocchi, 2011 ) and reduce salt consumption (PHE, 2020b ), have been a favoured policy of governments. However, changing the behaviour of a large number of consumers can be challenging, for many of the reasons discussed above, and strong evidence on their effectiveness has been limited. Because of this, many governments have focused instead on encouraging and incentivising firms to reformulate (see, for example, Vagnoni and Prpa, 2021 ).
Griffith et al . ( 2017 ) showed that following a large public health campaign in the UK resulted in a decline in dietary salt intake but that this was entirely attributable to product reformulation; consumer switching between products worked in the opposite direction and led to a slight increase in the salt intensity of grocery products purchased.
When the UK soft drinks industry levy was introduced, an explicit aim was to encourage reformulation. The tax has two rates. Products that contain between 5–8 g of sugar per 100 mL are taxed at the rate of 18p per litre of drink, and those that contains 8 g of sugar per 100 mL or more are taxed at 24p per litre of drink. Because the tax is based on volume, not directly on sugar, the tax rate within a band declines in sugar intensity; see the dashed line in Figure 6 . The idea behind this design was to give producers incentives to reformulate to just below 8 g and just below 5 g. These points were chosen with detailed knowledge of the industry, and the technological feasibility of reformulation.

Reformulation Following the SDIL.
Notes: The dashed line shows the tax per gram of sugar under the UK Soft Drinks Industry Levy (SDIL), which was introduced on April 6, 2018. The bars are based on the Kantar (FMCG) Purchase Panel (Take Home) 2016–9 (Kantar UK, 2020 ). The figure was created in Stata using three lines of code: ‘replace sugars=sugars/100’ to make the variable gram of sugar per 100 g, ‘collapse (mean) sugars,by(rf prodcode)’ to make the data at the product (rather than transaction) level, and ‘twoway histogram sugars if (lowsugarcaloriefat==‘Regular’ |$|$| lowsugarcaloriefat==‘Standard’) & sugars |$\lt $| =20, width(0.25) lc(black) fc(black) frac |$||$| line taxpersugar sugars if (lowsugarcaloriefat==‘Regular’ |$|$| lowsugarcaloriefat==‘Standard’) & sugars |$\lt $| =20, lc(black) lp(dash) lw(thick) yaxis(2) legend(off)’ to draw the figure.
The different panels in Figure 6 show the evolution of the distribution of soft drinks available in the market by sugar intensity. Prior to the introduction of the tax (panels (a) and (b)) there was a mass point of products with around 10 g of sugar per 100 g; this is approximately the sugar intensity of a standard can of Coca Cola. After the tax (panels (c) and (d)) we see a shift towards lower sugar intensity, with a pronounced shifting to reformulate below 5 g per 100 g, the lower tax threshold, and by 2019 we see considerable bunching just below this point. 25
This result is somewhat surprising, as standard models do not suggest that the optimal tax design is tiered in this way. Nonetheless, it seems in this case that the introduction of the tax was at least associated with reformulation. However, more work is needed to understand whether this design was what caused the reformulation. What would a more standard linear corrective tax on sugar have achieved? If, for some reason, this banded design was more effective, what does it require in terms of information about the technology of production to know where to position the bands if it was to be extended to other products.
3.3. Changing the Choice Environment
A large number of policies aim to change the choice environment in which consumers make decisions, by altering the products that consumers perceive to be in their choice set, removing temptation and changing the way that information is presented. These types of policies (sometimes called ‘nudge’ policies) are attractive because they are often low cost to the policy-maker and might be less regressive than taxes (Farhi and Gabaix, 2020 ).
Regulations specify how nutritional information is presented to consumers (for example, through simpler front-of-package labelling 26 and standards of measurement), how and when products can be advertised (for example, the UK bans online advertising of products that are high in fat, sugar or salt (DCMS and DHSC, 2021 ), where products can be sold (for example, fast food outlets are restricted near schools, 27 and sugary treats are discouraged from being placed near the check out counter), amongst others.
Above we raised the possibility that advertising distorts choices, and we saw that unhealthy foods, and particularly very sugary products, are the most advertised. Dubois et al . ( 2018 ) studied the impact of banning advertising for junk food (using the market for crisps, or potato chips, as an example). They modelled consumer choice and firm behaviour, in a model where firms compete in prices and advertising. They showed that advertising affects the choices that consumers make, and affects firms’ strategic behaviours. However, in order to interpret the welfare impacts of this ban, we have to take a stance on whether advertising is informative of distortionary. 28 Dubois et al . ( 2018 ) did not have a strategy for identifying whether advertising for crisps is informative or persuasive, so they calculated the welfare impact of banning advertising in both situations.
Subjectively looking at adverts for junk food, which show sports stars and models eating crisps, it seems likely that they distract people from characteristics of the product that people do not like (for example, price and the bad health consequences of eating crisps), and lead people to choose to buy more junk food (than they would in the absence of adverts). In the case where adverts are persuasive and distort consumers’ decision-making the impact of banning junk food adverts is to lead consumers to pay more attention to the unattractive characteristics (price and unhealthiness). Because firms can no longer compete in advertising, and because consumers pay more attention to prices, price competition increases, and this leads prices to fall. So while banning persuasive advertising reduces purchases of junk foods, it also leads to a reduction in prices, which partially mitigates that impact.
In 2007 adverts for food and drink that are high in fat, salt or sugar (HFSS)—junk foods—were banned from children’s TV in the UK (see Section 8 of Conway, 2021 ). This led to a reduction in the number of adverts for HFSS that children viewed, but firms' response to the advertising restrictions partially mitigated this (Ofcom, 2010 ). Firms adapted their advertising strategies in a number of ways, such as shifting the timing of adverts to avoid showing them during children’s programs, and changed the nature of the adverts they showed. Despite the ban, most adverts that children see on TV are for junk foods (Griffith et al ., 2018a ), and because of this the UK government is currently legislating to extend restrictions to adverts for high in fat, salt or sugar (see Griffith et al ., 2019 and DHSC and DCMS, 2021 ).
3.4. Cash and In-Kind Benefits
Above we have cited evidence that poor nutrition is clearly associated with poverty, and argued that it is likely that this at least partially represents a causal relationship (although conclusive scientific evidence on this is still lacking). For example, it may be that poverty impedes cognitive functioning. Even in the absence of market failures associated with poverty, it might be that households living in poverty might not be able to obtain as nutritious of a diet as households with higher incomes. Society might take the view, particularly for children, that this is not the level of inequality we prefer, and want policies that improve the diet quality of children growing up in poverty.
Child poverty in the UK increased from 2011–2 to 2016–7, the first increase sustained over such a substantial period since the 1990s (Bourquin et al ., 2020 ). Out-of-work households are more likely to be in poverty—about 60% are in poverty, with the poverty rate in working households more like 20%. However, the share of households who are workless is reasonably low in the UK, or at least it was prior to the pandemic, so more children living in poverty are living in households with at least some work. We do not yet know the full impact of the COVID-19 pandemic, but it looks likely to be worse in households in poverty, and it may increase worklessness and poverty amongst some groups. The UK government introduced increases in benefits to help people through the pandemic; however, these were temporary (Waters and Wernham, 2021 ), and there have been large real-term cuts in the generosity of out-of-work benefits over the decade before the pandemic (Bourquin et al ., 2020 ). 29
The main policies in the UK that target children in poverty and/or child nutrition include cash benefits (such as the child credit component of universal credit), and in-kind and conditional benefits, such as Free School Meals and Healthy Start Vouchers.
Reforms to universal credit are the most direct way to lift households out of income poverty. A household on benefits currently gets around £3,000 per year for an extra child. However, increasing this would be expensive, and possibly not that well targeted at the poorest children or at improving nutrition. The value of Free School Meals and Healthy Start Vouchers is much lower, but they are targeted at out-of-work families or families with very low earnings. That means that boosting these benefits would benefit, on average, the very poorest households, compared to say raising Universal Credit standard allowances or the child elements.
Healthy Start Vouchers have been shown to be effective at improving nutritional outcomes (see, for example, Griffith et al ., 2018b ); however, take-up varies across these benefits. Figure 7 shows that it is lower for Healthy Start Vouchers, and has declined in recent years. Addressing this would need to be a priority to make this a more effective policy. Healthy Start vouchers are also available for low-income pregnant women before they give birth to their child; this is one of the few benefits that is available to (low-income) pregnant women, a time that is thought to be important for later life health outcomes (see, among others, Case et al ., 2002 ; Currie 2009 ; Almond and Currie, 2011 ; Almond et al ., 2018 ).

Take-Up Rates of Different Child-Related Benefits, 2011–8.
Notes: The decline in take-up of child benefit is related to the introduction of the high income child benefit charge in 2013.
Source . Figure 2 of Augsburg et al . ( 2021 ) from Crawley and Dodds ( 2018 ), HMRC ( 2019 ; 2020 ) and Holford and Rabe ( 2020 ).
Free School Meals provide food directly to children, so are likely to be particularly effective if we think that an important problem is that parents are able or do not provide sufficient nutritional foods (for any of the reasons discussed above). There may be challenges in expanding Free School Meals depending how it was expanded. For example, in April 2020 the government introduced the COVID Summer Food Fund, which aimed to provide Free School Meals to children when they were not in school; however, half of children eligible for free school meals were not able to access this programme (Crawford et al ., 2020 ). Children who attended school were almost six times more likely to get a free school meal than children who did not. Families of children who had a free school meal were more likely to use a food bank than families who could not. Augsburg et al . ( 2021 ) provided further discussion of these policies.
There is growing concern about the impacts that arise from people making suboptimal choices regarding food consumption. Market failures related to information, cognition and a lack of self-control potentially lead to high costs on wider society and on the person themselves in the future. In addition, obesity and poor nutrition seem likely to be important constraints on the opportunities of children, particularly those growing up in deprivation, raising equity concerns.
There is much that policy can potentially do in terms of changing market signals, such as relative prices, and policies that change the choice environment to encourage people to make choices that better align with their own long-term interests. But to design these policies well we need a better understanding of who we are trying to target, and for what reasons.
We do not know enough about the magnitude and distributions of the market failures: what are the major externalities and who generates most of them, and what are the unanticipated future costs that are caused by obesity and poor nutrition and how do the vary across people? There is a growing body of evidence on these issues, but much of it is still anecdotal or based on correlations. A better evidence base on this is not simply of academic interest, it is essential to design a well-targeted policy. Poorly targeted and poorly designed policies can do harm to the individuals they are aiming to help.
Many of these policies will also interact in important ways. For example, soda taxes increase the prices of sugary drinks and reduce consumption through the price channel, but taxes may also change firms’ other strategic choices, such as advertising. Advertising itself can shape demand, affecting price elasticities, and has long-term effects on demand, leading to dynamic considerations. Careful consideration needs to be given to designing policies that are robust to these concerns. More work needs to be done to understand these interactions, and to understand how dynamic firm and consumer responses affect our evaluation of how effective and well targeted different policies will be.
It is also important to remember that there are potential equity as well as efficiency concerns. If poverty is an important factor driving the growth in obesity then it is also important to look at policies that directly lift people out of deprivation. The long-term decline (until recent rises) in food prices has had important welfare benefits for low-income households. Policies that lead to increased prices without improving nutritional outcomes will have adverse consequences for these households.
This paper draws heavily on joint work with a number of people who I have had the privilege to work with, in particular Pierre Dubois, Martin O’Connell and Kate Smith. I gratefully acknowledge financial support from the European Research Council (ERC) under ERC-2015-AdG-694822, the Economic and Social Research Council (ESRC) under the Centre for the Microeconomic Analysis of Public Policy (CPP), grant number RES-544-28-0001. Data supplied by TNS UK Limited. The use of TNS UK Ltd. data in this work does not imply the endorsement of TNS UK Ltd. in relation to the interpretation or analysis of the data. All errors and omissions remain the responsibility of the author.
This paper was originally delivered as the Past President’s Address at the RES 2021 Annual Conference.
See, for example, Ritchie and Roser ( 2017 ), NHS ( 2020 ), WHO ( 2021 ).
See Harvard School of Public Policy ( 2012 ) for a discussion of why BMI is used, and NHS ( 2019 ).
These statistics are based on the index of multiple deprivation (IMD), which is the official measure of relative deprivation for small areas (lower super output areas) in England. IMD deciles are calculated by ranking the 32,844 small areas in England from most deprived to least deprived and dividing them into ten equal groups. The most deprived line in the figure shows the mean for children living in the 10% of most deprived small areas nationally (decile 1), the least deprived are those living in the 10% of least deprived small areas nationally (decile 10). Further details are available at: https://www.gov.uk/government/statistics/english-indices-of-deprivation-2019 .
I will not attempt to survey this literature here, but see, for example, Bhattacharya and Sood ( 2011 ) and Allcott et al . ( 2019b ), both of whom discuss this issue in the US context.
See the useful articulation of what compelling evidence for suboptimal choices would look like in Bernheim and Taubinsky ( 2018 ).
See the summary in CDC ( 2021 ) and the references therein, including Vaidya ( 2006 ), Lloyd et al . ( 2012 ), Narang and Mathew ( 2012 ), Cote et al . ( 2013 ), Halfon et al . ( 2013 ), Mohanan et al . ( 2014 ), Pollock ( 2015 ), Morrison et al . ( 2015 ), Lundborg et al . ( 2021 ). See also PHE ( 2020a ).
See, for example, https://en.wikipedia.org/wiki/Internality and Herrnstein et al . ( 1993 ).
For example, many countries publish dietary reference intakes (Trumbo et al ., 2002 or public guidance such as the UK Eatwell Guide ( https://www.nhs.uk/live-well/eat-well/the-eatwell-guide/ ).
See, Venn ( 2020 ), and the systematic literature review in Fogelholm et al . ( 2012 ), which suggests that the combination of macro nutrients is not important for weight loss.
See, for example, Monteiro et al . ( 2019 ), WHO ( 2020 ).
One problem with this approach is that on the vertical axis (price per calorie) calories appear as the denominator and the measure on the horizontal axis is increasing in calorie density. This creates a mechanical relationship in the two variables. An alternative way to measure the cost of a product is price per kilogram. However, weight is often not a particularly useful unit of comparison across different food products.
Some examples include the following. Studying variation over time across US states, Ruhm (2000) showed that diets become less healthy and obesity increases when the economic situation improves. Dehejia and Lleras-Muney (2004) found that babies conceived in recessions have a lower probability of bad outcomes, such as low birth weight, congenital malformations and post-neonatal mortality. Griffith et al . ( 2016b ) and the papers cited above showed that, when households experienced negative income shocks over the 2007–8 recession, they were largely able to maintain the quality of their diet by adjusting shopping effort (searching out products on sale, visiting more stores to find cheaper offers) and compromising on non-nutritional characteristics (e.g., switching from branded to store brand products, buying in bulk).
This literature is mainly from the United States—see the references in Allcott et al . ( 2019a )—however, it has also been put forward by public health researchers in the UK—see, for example, https://www.sheffield.ac.uk/social-sciences/news/12-million-living-uk-food-deserts-studys-shows .
See, among others, Banerjee and Mullainathan ( 2010 ), Shah et al . ( 2012 ; 2018 ), Mani et al . ( 2013 ; 2020 ), Schilbach et al . ( 2016 ).
See Read and Van Leeuwen ( 1998 ), Read et al . ( 1999 ), Trope and Fishbach ( 2000 ), Ariely and Wertenbroch ( 2002 ) and Gilbert et al . ( 2002 ).
The figure is based on data on households’ shopping baskets. Each food product is categorised based on the Nutritional Profile Model (NPM). The NPM is the measure used in the UK to categorise foods for regulatory purposes (DHSC, 2011 ). It combines measures of ‘unhealthy’ characteristics (energy, saturated fat, sugars and sodium) and ‘healthy’ characteristics (fruit, vegetable and nut content, fibre and protein) into a single index. Products are assigned a score between −15 and 30: a higher NPS indicates a less healthy food product. For example, fruits and vegetables mostly have NPS scores less than zero, while chocolate bars, sweets and crisps tend to have NPS scores that are above 5.
See Oster and Morton ( 2005 ), Ashraf et al . ( 2006 ), DellaVigna and Malmendier ( 2006 ), Bucciol ( 2012 ) and Hinnosaar ( 2016 ).
See Shapiro ( 2005 ), Milkman et al . ( 2010 ) and Sadoff et al . ( 2020 ).
The model draws on insights from the literature on collective household models; see Chiappori ( 1988 ; 1992 ), Browning and Chiappori ( 1998 ), Chiappori and Ekeland ( 2009 ), Dunbar et al . ( 2013 ) and Browning et al . ( 2013 ).
Bagwell ( 2007 ) provided a comprehensive discussion about the impact of advertising on consumer choice.
See a review of the epidemiology and public health literatures in Boyland et al . ( 2016 ), Norman et al . ( 2018 ) and Russell et al . ( 2019 ) showed that children exposed to TV adverts for less healthy foods consume more food in the immediate period after watching them; Boyland et al . ( 2016 ) and Norman et al . ( 2018 ) showed that exposure to advertising for less healthy foods also influences food preferences and purchasing patterns.
One indicator of this difficulty is the fact that there is a large industry of diet and exercise programs on which many people spend a lot of time and money, with limited success.
Subsidies for healthy foods is another potential policy; see, for example, DHSC ( 2021 ). Price floors are another policy that has been advocated by the World Health Organisation; see the evaluation of the introduction of a price floor on alcohol in Scotland in Griffith et al . ( 2022 ).
As of April 2021, over fifty jurisdictions had implemented taxes on sugary soft drinks (GFRP, 2021 ).
Dickson et al . ( 2021 ) showed evidence of reformulation in response to the UK sugar tax include; Barahona et al . ( 2020 ) showed evidence that breakfast cereal producers in Chile reformulated in response to the introduction of new labelling regulations.
A number of studies show that front-of-package labels are effective in shifting consumption towards healthier products; see, among others, Rudd Center for Food Policy & Obesity ( 2008 ), Allais et al . ( 2015 ), Barahona et al . ( 2020 ), Fichera and von Hinke ( 2020 ).
Currie et al . ( 2010 ) showed that proximity to fast food outlets increase the probability of gaining weight amongst US teenagers.
These cuts were partly due to relatively high inflation combined with the cash-terms freeze to many benefits claimed by workless households, as well as to reductions in generosity due to the introduction of universal credit. The temporary increases only unwind these cuts by a small proportion.
Abi-Rafeh R. , Dubois P. , Griffith R. , O’Connell M. ( 2021 ). ‘ What is the likely impact of advertising restrictions on obesity? ’, https://www.economicsobservatory.com .
Adda J. , Banks J. , von Gaudecker H.M. ( 2009 ). ‘ The impact of income shocks on health: Evidence from cohort data ’, Journal of the European Economic Association , vol. 7 ( 6 ), pp. 1361 – 99 .
Google Scholar
Aguiar M. , Hurst E. ( 2007 ). ‘ Life-cycle prices and production ’, American Economic Review , vol. 97 ( 5 ), pp. 1533 – 59 .
Allais O. , Etilé F. , Lecocq S. ( 2015 ). ‘ Mandatory labels, taxes and market forces: An empirical evaluation of fat policies ’, Journal of Health Economics , vol. 43 , pp. 27 – 44 .
Allcott H. , Diamond R. , Dube J.P. , Handbury J. , Rahkovsky I. , Schnell M. ( 2019a ). ‘ Food deserts and the causes of nutritional inequality ’, Quarterly Journal of Economics , vol. 134 ( 4 ), pp. 1793 – 844 .
Allcott H. , Lockwood B.B. , Taubinsky D. ( 2019b ). ‘ Should we tax soda? An overview of theory and evidence ’, Journal of Economic Perspectives , vol. 33 ( 2 ), pp. 202 – 27 .
Almond D. , Currie J. ( 2011 ). ‘ Killing me softly: The fetal origins hypothesis ’, Journal of Economic Perspectives , vol. 25 ( 3 ), pp. 153 – 72 .
Almond D. , Currie J. , Duque V. ( 2018 ). ‘ Childhood circumstances and adult outcomes: Act II ’, Journal of Economic Literature , vol. 56 ( 4 ), pp. 1360 – 446 .
Ariely D. , Wertenbroch K. ( 2002 ). ‘ Procrastination, deadlines, and performance: Self-control by precommitment ’, Psychological Science , vol. 13 ( 3 ), pp. 219 – 24 .
Ashraf N. , Karlan D. , Yin W. ( 2006 ). ‘ Tying odysseus to the mast: Evidence from a commitment savings product in the Philippines ’, The Quarterly Journal of Economics , vol. 121 ( 2 ), pp. 635 – 72 .
Augsburg B. , Cribb J. , Griffith R. , Scott-Reillly F. ( 2021 ). ‘ How can policy reduce food poverty among children? ’, https://www.economicsobservatory.com/how-can-policy-reduce-food-poverty-among-children .
Bagwell K. ( 2007 ). ‘ The economic analysis of advertising ’, in ( Armstrong M. , Porter R. , eds.), Handbook of Industrial Organization , pp. 1701 – 844 ., Amsterdam : North-Holland .
Google Preview
Banerjee A. , Mullainathan S. ( 2010 ). ‘ The shape of temptation: Implications for the economic lives of the poor ’, Working paper 15973, National Bureau of Economic Research .
Barahona N. , Otero C. , Otero S. , Kim J. ( 2020 ). ‘ Equilibrium effects of food labeling policies ’, Working paper, Social Science Research Network .
Becker G.S. ( 1965 ). ‘ A theory of the allocation of time ’, Economic Journal , vol. 75 ( 299 ), pp. 493 – 517 .
Bernheim B.D. , Taubinsky D. ( 2018 ). ‘ Behavioral public economics ’, in ( Bernheim B.D. , DellaVigna S. and Laibson D. , eds.), Handbook of Behavioral Economics , pp. 381 – 516 ., New York : Elsevier .
Bhattacharya J. , Sood N. ( 2011 ). ‘ Who pays for obesity? ’, Journal of Economic Perspectives , vol. 25 ( 1 ), pp. 139 – 58 .
Bordalo P. , Gennaioli N. , Shleifer A. ( 2013 ). ‘ Salience and consumer choice ’, Journal of Political Economy , vol. 121 ( 5 ), pp. 803 – 43 .
Bourquin P. , Joyce R. , Keiller A.N. ( 2020 ). ‘ Living standards, poverty and inequality in the UK: 2020 ’, IFS Report, https://ifs.org.uk/publications/14901 .
Boyland E.J. , Nolan S. , Kelly B. , Tudur-Smith C. , Jones A. , Halford J.C. , Robinson E. ( 2016 ). ‘ Advertising as a cue to consume: A systematic review and meta-analysis of the effects of acute exposure to unhealthy food and nonalcoholic beverage advertising on intake in children and adults ’, The American Journal of Clinical Nutrition , vol. 103 ( 2 ), pp. 519 – 33 .
Browning M. , Chiappori P.A. ( 1998 ). ‘ Efficient intra-household allocations: A general characterization and empirical tests ’, Econometrica , vol. 66 ( 6 ), pp. 1241 – 78 .
Browning M. , Chiappori P.A. , Lewbel A. ( 2013 ). ‘ Estimating consumption economies of scale, adult equivalence scales, and household bargaining power ’, Review of Economic Studies , vol. 80 ( 4 ), pp. 1267 – 303 .
Bucciol A. ( 2012 ). ‘ Measuring self-control problems: A structural estimation ’, Journal of the European Economic Association , vol. 10 ( 5 ), pp. 1084 – 115 .
Capacci S. , Mazzocchi M. ( 2011 ). ‘ Five-a-day, a price to pay: An evaluation of the UK program impact accounting for market forces ’, Journal of Health Economics , vol. 30 ( 1 ), pp. 87 – 98 .
Case A. , Lubotsky D. , Paxson C. ( 2002 ). ‘ Economic status and health in childhood: The origins of the gradient ’, American Economic Review , vol. 92 ( 5 ), pp. 1308 – 34 .
CDC . ( 2021 ). ‘ Causes and consequences of childhood obesity ’, https://www.cdc.gov/obesity/childhood/causes.html .
Cherchye L. , De Rock B. , Griffith R. , O’Connell M. , Vermeulen F. ( 2017 ). ‘ A new year a new you? Heterogeneity and self-control in food purchases ’, European Economic Review , vol. 127 , pp. 1 – 19 .
Chiappori P.A. ( 1988 ). ‘ Rational household labor supply ’, Econometrica , vol. 56 ( 1 ), pp. 63 – 90 .
Chiappori P.A. ( 1992 ). ‘ Collective labor supply and welfare ’, Journal of Political Economy , vol. 100 ( 3 ), pp. 437 – 67 .
Chiappori P.A. , Ekeland I. ( 2009 ). ‘ The microeconomics of efficient group behavior: Identification ’, Econometrica , vol. 77 ( 3 ), pp. 763 – 99 .
Coibion O. , Gorodnichenko Y. , Hong G.H. ( 2014 ). ‘ The cyclicality of sales, regular and effective prices: Business cycle and policy implications ’, American Economic Review , vol. 105 ( 3 ), pp. 993 – 1029 .
Conway L. ( 2021 ). ‘ Advertising to children ’, House of Commons Library Research Briefing .
Cote A.T. , Harris K.C. , Panagiotopoulos C. , Sandor G.G.S. , Devlin A.M. ( 2013 ). ‘ Childhood obesity and cardiovascular dysfunction ’, Journal of the American College of Cardiology , vol. 62 ( 15 ), pp. 1309 – 19 .
Crawford C. , Greaves E. , Rabe B. ( 2020 ). ‘ What difference will the COVID summer food fund make to children’s lives? ’, https://www.economicsobservatory.com .
Crawley D.H. , Dodds R. ( 2018 ). ‘ The UK healthy start scheme. What happened? What next? ’, First Steps Nutrition Trust , p. 85 , report published by The First Steps Nutrition Trust: London .
Currie J. ( 2009 ). ‘ Healthy, wealthy, and wise: Socioeconomic status, poor health in childhood, and human capital development ’, Journal of Economic Literature , vol. 47 ( 1 ), pp. 87 – 122 .
Currie J. , DellaVigna S. , Moretti E. , Pathania V. ( 2010 ). ‘ The effect of fast food restaurants on obesity and weight gain ’, American Economic Journal: Economic Policy , vol. 2 ( 3 ), pp. 32 – 63 .
Cutler D. , Glaeser E. , Shapiro J. ( 2003 ). ‘ Why have Americans become more obese? ’, The Journal of Economic Perspectives , vol. 17 ( 3 ), pp. 93 – 118 .
Dai H. , Milkman K.L. , Riis J. ( 2014 ). ‘ The fresh start effect: Temporal landmarks motivate aspirational behavior ’, Management Science , vol. 60 ( 10 ), pp. 2563 – 82 .
Dai H. , Milkman K.L. , Riis J. ( 2015 ). ‘ Put your imperfections behind you: Temporal landmarks spur goal initiation when they signal new beginnings ’, Psychological Science , vol. 26 ( 12 ), pp. 1927 – 36 .
DCMS and DHSC . ( 2021 ). ‘ Introducing a total online advertising restriction for products high in fat, sugar and salt (HFSS) ’, https://www.gov.uk/government/consultations/total-restriction-of-online-advertising-for-products-high-in-fat-sugar-and-salt-hfss/introducing-a-total-online-advertising-restriction-for-products-high-in-fat-sugar-and-salt-hfss .
DellaVigna S. ( 2009 ). ‘ Psychology and economics: Evidence from the field ’, Journal of Economic Literature , vol. 47 ( 2 ), pp. 315 – 72 .
DellaVigna S. , Malmendier U. ( 2006 ). ‘ Paying not to go to the gym ’, American Economic Review , vol. 96 ( 3 ), pp. 694 – 719 .
DHSC . ( 2011 ). ‘ The nutrient profiling model ’, https://www.gov.uk/government/publications/the-nutrient-profiling-model .
DHSC . ( 2021 ). ‘ New pilot to help people exercise more and eat better ’, https://www.gov.uk/government/news/new-pilot-to-help-people-exercise-more-and-eat-better .
DHSC and DCMS . ( 2021 ). ‘ Further advertising restrictions for products high in fat, salt and sugar ’, https://www.gov.uk/government/consultations/further-advertising-restrictions-for-products-high-in-fat-salt-and-sugar .
Dickson A. , Gehrsitz M. , Kemp J. ( 2021 ). ‘ Does a spoonful of sugar levy help the calories go down? An analysis of the UK soft drinks industry levy ’, Discussion Paper 14528, Institute of Labor Economics .
Dimbleby H. ( 2021 ). ‘ The national food strategy ’, https://www.nationalfoodstrategy.org/ .
Dubois P. , Griffith R. , O’Connell M. ( 2018 ). ‘ The effects of banning advertising in junk food markets ’, Review of Economic Studies , vol. 1 ( 1 ), pp. 396 – 436 .
Dubois P. , Griffith R. , O’Connell M. ( 2020 ). ‘ How well targeted are soda taxes? ’, American Economic Review , vol. 110 ( 11 ), pp. 3661 – 704 .
Dunbar G.R. , Lewbel A. , Pendakur K. ( 2013 ). ‘ Children’s resources in collective households: Identification, estimation, and an application to child poverty in Malawi ’, American Economic Review , vol. 103 ( 1 ), pp. 438 – 71 .
Farhi E. , Gabaix X. ( 2020 ). ‘ Optimal taxation with behavioral agents ’, American Economic Review , vol. 110 ( 1 ), pp. 298 – 336 .
Fichera E. , von Hinke S. ( 2020 ). ‘ The response to nutritional labels: Evidence from a quasi-experiment ’, Journal of Health Economics , vol. 72 , pp. 1 – 17 .
Fogelholm M. , Anderssen S. , Gunnarsdottir I. , Lahti-Koski M. ( 2012 ). ‘ Dietary macronutrients and food consumption as determinants of long-term weight change in adult populations: A systematic literature review ’, Food & Nutrition Research , vol. 56 , p. 19103 .
GFRP . ( 2021 ). ‘ Sugary drink taxes around the world ’, The Global Food Research Program, University of North Carolina, https://globalfoodresearchprogram.org/wp-content/uploads/2021/04/SSB_sugary_drink_taxes_maps.pdf .
Gilbert D.T. , Gill M.J. , Wilson T.D. ( 2002 ). ‘ The future is now: Temporal correction in affective forecasting ’, Organizational Behavior and Human Decision Processes , vol. 88 ( 1 ), pp. 430 – 44 .
Griffith R. , Jin W.M. , Lechene V. ( 2022 ). ‘ The decline of home cooked food ’, Fiscal Studies , Forthcoming .
Griffith R. , Lluberas R. , Luhrmann M. ( 2016a ). ‘ Gluttony and sloth? Calories, labour market activity and the rise of obesity ’, Journal of the European Economic Association , vol. 14 ( 6 ), pp. 1253 – 86 .
Griffith R. , O’Connell M. , Smith K. ( 2015 ). ‘ Relative prices, consumer preferences, and the demand for food ’, Oxford Review of Economic Policy , vol. 31 ( 1 ), pp. 116 – 30 .
Griffith R. , O’Connell M. , Smith K. ( 2016b ). ‘ Shopping around: How households adjusted food spending over the great recession ’, Economica , vol. 83 ( 330 ), pp. 247 – 80 .
Griffith R. , O’Connell M. , Smith K. ( 2017 ). ‘ The importance of product reformulation versus consumer choice in improving diet quality ’, Economica , vol. 84 ( 333 ), pp. 34 – 53 .
Griffith R. , O’Connell M. , Smith K. , Stroud R. ( 2018a ). ‘ Children’s exposure to TV advertising of food and drink ’, https://ifs.org.uk/publications/13019 .
Griffith R. , O’Connell M. , Smith K. , Stroud R. ( 2019 ). ‘ The potential impacts of banning television advertising of HFSS food and drink before the watershed ’, https://ifs.org.uk/publications/14132 .
Griffith R. , O’Connell M. , Smith K. , Stroud R. ( 2020 ). ‘ What’s on the menu? Policies to reduce young people’s sugar consumption ’, Fiscal Studies , vol. 41 ( 1 ), pp. 165 – 97 .
Griffith R. , von Hinke S. , Smith S. ( 2018b ). ‘ Getting a healthy start: The effectiveness of targeted benefits for improving dietary choices ’, Journal of Health Economics , vol. 58 , pp. 176 – 87 .
Griffith R. , O'Connell M. , Smith K. ( 2022 ). ‘ Price floors and externality correction ’, Economic Journal , Forthcoming .
Halfon N. , Larson K. , Slusser W. ( 2013 ). ‘ Associations between obesity and comorbid mental health, developmental, and physical health conditions in a nationally representative sample of US children aged 10 to 17 ’, Academic Pediatrics , vol. 13 ( 1 ), pp. 6 – 13 .
Harvard School of Public Policy . ( 2012 ). ‘ Why use BMI? ’, https://www.hsph.harvard.edu/obesity-prevention-source/obesity-definition/obesity-definition-full-story/ .
Herrnstein R.J. , Loewenstein G.F. , Prelec D. , Vaughan W. ( 1993 ). ‘ Utility maximization and melioration: Internalities in individual choice ’, Journal of Behavioral Decision Making , vol. 6 ( 3 ), pp. 149 – 85 .
Hinnosaar M. ( 2016 ). ‘ Time inconsistency and alcohol sales restrictions ’, European Economic Review , vol. 87 , pp. 108 – 31 .
HMRC . ( 2019 ). ‘ Child benefit, child tax credit (CTC) and working tax credit (WTC) take-up rates 2017 to 2018 ’, https://www.gov.uk/government/statistics/child-benefit-child-tax-credit-ctc-and-working-tax-credit-wtc-take-up-rates-2017-to-2018 .
HMRC . ( 2020 ). ‘ Child benefit statistics: Annual release, August 2020 main commentary ’, https://www.gov.uk/government/statistics/child-benefit-statistics-annual-release-august-2020/child-benefit-statistics-annual-release-august-2020-main-commentary .
Holford A. , Rabe B. ( 2020 ). ‘ Impact of the universal infant free school meal policy ’, Report by ISER: Essex, Institute for Social and Economic Research .
Hoynes H. , Schanzenbach D.W. , Almond D. ( 2016 ). ‘ Long-run impacts of childhood access to the safety net ’, American Economic Review , vol. 106 ( 4 ), pp. 903 – 34 .
Imamura F. , O’Connor L. , Ye Z. , Mursu J. , Hayashino Y. , Bhupathiraju S.N. , Forouhi N.G. ( 2015 ). ‘ Consumption of sugar sweetened beverages, artificially sweetened beverages, and fruit juice and incidence of type 2 diabetes: Systematic review, meta-analysis, and estimation of population attributable fraction ’, BMJ , vol. 351 , h3576 .
Jebb S. ( 2015 ). ‘ Healthy body, healthy mind ’, https://www.ox.ac.uk/research/research-in-conversation/healthy-body-healthy-mind/susan-jebb .
Johnson W. , Li L. , Kuh D. , Hardy R. ( 2015 ). ‘ How has the age-related process of overweight or obesity development changed over time? Co-ordinated analyses of individual participant data from five United Kingdom Birth Cohorts ’, PloS Medicine , vol. 12 ( 5 ), e1001828 .
Kalra S. , Gupta Y. ( 2014 ). ‘ Free sugars: The less the better ’, The Lancet Diabetes & Endocrinology , vol. 2 ( 6 ), p. 452 .
Kantar UK . ( 2020 ). ‘ Kantar FMCG purchase panel ’, https://www.kantarworldpanel.com/global/Sectors/FMCG .
Kaplan G. , Menzio G. ( 2015 ). ‘ The morphology of price dispersion ’, International Economic Review , vol. 56 ( 4 ), pp. 1165 – 206 .
Lloyd L.J. , Langley-Evans S.C. , McMullen S. ( 2012 ). ‘ Childhood obesity and risk of the adult metabolic syndrome: A systematic review ’, International Journal of Obesity (2005) , vol. 36 ( 1 ), pp. 1 – 11 .
Lock K. , Stuckler D. , Charlesworth K. , McKee M. ( 2009 ). ‘ Potential causes and health effects of rising global food prices ’, British Medical Journal , vol. 339 .
Loewenstein G. , Sunstein C.R. , Golman R. ( 2014 ). ‘ Disclosure: Psychology changes everything ’, Annual Review of Economics , vol. 6 ( 1 ), pp. 391 – 419 .
Ludwig D.S. ( 2002 ). ‘ The glycemic index: Physiological mechanisms relating to obesity, diabetes, and cardiovascular disease ’, JAMA , vol. 287 ( 18 ), pp. 2414 – 23 .
Lundborg P. , Rooth D.O. , Alex-Petersen J. ( 2021 ). ‘ Long-term effects of childhood nutrition: Evidence from a school lunch reform ’, The Review of Economic Studies , vol. 89 ( 2 ), rdab028 .
Mani A. , Mullainathan S. , Shafir E. , Zhao J. ( 2013 ). ‘ Poverty impedes cognitive function ’, Science , vol. 341 ( 6149 ), pp. 976 – 80 .
Mani A. , Mullainathan S. , Shafir E. , Zhao J. ( 2020 ). ‘ Scarcity and cognitive function around payday: A conceptual and empirical analysis ’, Journal of the Association for Consumer Research , vol. 5 ( 4 ), pp. 365 – 76 .
Milkman K.L. , Rogers T. , Bazerman M.H. ( 2010 ). ‘ I’ll have the ice cream soon and the vegetables later: A study of online grocery purchases and order lead time ’, Marketing Letters , vol. 21 ( 1 ), pp. 17 – 35 .
Mohanan S. , Tapp H. , McWilliams A. , Dulin M. ( 2014 ). ‘ Obesity and asthma: Pathophysiology and implications for diagnosis and management in primary care ’, Experimental Biology and Medicine (Maywood) , vol. 239 ( 11 ), pp. 1531 – 40 .
Monteiro C.A. , Cannon G. , Lawrence M. , da Costa Louzada M.L. , Machado P.P. ( 2019 ). ‘ Ultra-processed foods, diet quality, and health using the NOVA classification system ’, FAO Report .
Morrison K.M. , Shin S. , Tarnopolsky M. , Taylor V.H. ( 2015 ). ‘ Association of depression & health related quality of life with body composition in children and youth with obesity ’, Journal of Affective Disorders , vol. 172 , pp. 18 – 23 .
Mullainathan S. , Schwartzstein J. , Shleifer A. ( 2008 ). ‘ Coarse thinking and persuasion ’, The Quarterly Journal of Economics , vol. 123 ( 2 ), pp. 577 – 619 .
Narang I. , Mathew J.L. ( 2012 ). ‘ Childhood obesity and obstructive sleep apnea ’, Journal of Nutrition and Metabolism , vol. 2012 , p. 134202 .
Nelson P. ( 1970 ). ‘ Information and consumer behavior ’, Journal of Political Economy , vol. 78 ( 2 ), pp. 311 – 29 .
Nevo A. , Wong A. ( 2019 ). ‘ The elasticity of substitution between time and market goods: Evidence from the great recession ’, International Economic Review , vol. 60 ( 1 ), pp. 25 – 51 .
NHS . ( 2011 ). ‘ A simple guid to classifying body mass index in children ’, https://webarchive.nationalarchives.gov.uk/ukgwa/20170110173352mp_/http://www.noo.org.uk/uploads/doc/vid_11762_classifyingBMIinchildren.pdf .
NHS . ( 2019 ). ‘ Obesity ’, https://www.nhs.uk/conditions/obesity/ .
NHS . ( 2020 ). ‘ Statistics on obesity, physical activity and diet, England, 2020 ’, https://digital.nhs.uk/data-and-information/publications/statistical/statistics-on-obesity-physical-activity-and-diet/england-2020 .
NHSDigital . ( 2019 ). ‘ Health survey for England ’, http://digital.nhs.uk/pubs/hse2018 .
NHSDigital . ( 2020 ). ‘ National child measurement programme, England 2019/20 school year ’, https://digital.nhs.uk/data-and-information/publications/statistical/national-child-measurement-programme/2019-20-school-year .
NIH . ( 2021 ). ‘ What are overweight and obesity? ’, https://www.nhlbi.nih.gov/health/overweight-and-obesity (last accessed: 6 April 2022) .
Norman J. , Kelly B. , McMahon A.T. , Boyland E. , Baur L.A. , Chapman K. , King L. , Hughes C. , Bauman A. ( 2018 ). ‘ Sustained impact of energy-dense TV and online food advertising on children’s dietary intake: A within-subject, randomised, crossover, counter-balanced trial ’, International Journal of Behavioral Nutrition and Physical Activity , vol. 15 ( 1 ), p. 37 .
O’Connell M. , Smith K. ( 2020 ). ‘ Corrective tax design and market power ’, CEPR DP 14582.
OECD . ( 2020 ). ‘ Consumer price indices (CPIs)—complete database: Consumer prices—annual inflation ’, https://data.oecd.org/price/inflation-cpi.htm .
Ofcom . ( 2010 ). ‘ HFSS advertising restrictions: Final review ’, https://www.ofcom.org.uk/__data/assets/pdf_file/0024/31857/hfss-review-final.pdf .
Oster S.M. , Morton F.M.S. ( 2005 ). ‘ Behavioral biases meet the market: The case of magazine subscription prices ’, The BE Journal of Economic Analysis & Policy , vol. 5 ( 1 ).
PHE . ( 2020a ). ‘ Childhood obesity: Applying all our health ’, https://www.gov.uk/government/publications/childhood-obesity-applying-all-our-health/childhood-obesity-applying-all-our-health .
PHE . ( 2020b ). ‘ Salt reduction: Targets for 2024 ’, https://www.gov.uk/government/publications/salt-reduction-targets-for-2024 .
Pollock N.K. ( 2015 ). ‘ Childhood obesity, bone development, and cardiometabolic risk factors ’, Molecular and Cellular Endocrinology , vol. 410 , pp. 52 – 63 .
Prentice A. , Jebb S. ( 2004 ). ‘ Energy intake/physical activity interactions in the homeostasis of body weight regulation ’, Nutrition Reviews , vol. 62 ( s2 ), pp. S98 – 104 .
Rabin M. ( 1998 ). ‘ Psychology and economics ’, Journal of Economic Literature , vol. 36 ( 1 ), pp. 11 – 46 .
Read D. , Loewenstein G. , Kalyanaraman S. ( 1999 ). ‘ Mixing virtue and vice: Combining the immediacy effect and the diversification heuristic ’, Journal of Behavioral Decision Making , vol. 12 ( 4 ), pp. 257 – 73 .
Read D. , Van Leeuwen B. ( 1998 ). ‘ Predicting hunger: The effects of appetite and delay on choice ’, Organizational Behavior and Human Decision Processes , vol. 76 ( 2 ), pp. 189 – 205 .
Reutskaja E. , Nagel R. , Camerer C.F. , Rangel A. ( 2011 ). ‘ Search dynamics in consumer choice under time pressure: An eye-tracking study ’, American Economic Review , vol. 101 ( 2 ), pp. 900 – 26 .
Ritchie H. , Roser M. ( 2017 ). ‘ Obesity ’, www.ourworldindata.org/obesity .
Rudd Center for Food Policy & Obesity . ( 2008 ). ‘ Rudd menu labeling final report ’, https://oyc.yale.edu/sites/default/files/RuddMenuLabelingReport2008.pdf (last accessed: 6 April 2022) .
Russell S.J. , Croker H. , Viner R.M. ( 2019 ). ‘ The effect of screen advertising on children’s dietary intake: A systematic review and meta-analysis ’, Obesity Reviews , vol. 20 ( 4 ), pp. 554 – 68 .
Sadoff S. , Samek A. , Sprenger C. ( 2020 ). ‘ Dynamic inconsistency in food choice: Experimental evidence from two food deserts ’, The Review of Economic Studies , vol. 87 ( 4 ), pp. 1954 – 88 .
Sahoo K. , Sahoo B. , Choudhury A.K. , Sofi N.Y. , Kumar R. , Bhadoria A.S. ( 2015 ). ‘ Childhood obesity: Causes and consequences ’, Journal of Family Medicine and Primary Care , vol. 4 ( 2 ), pp. 187 – 92 .
Schilbach F. , Schofield H. , Mullainathan S. ( 2016 ). ‘ The psychological lives of the poor ’, American Economic Review , vol. 106 ( 5 ), pp. 435 – 40 .
Schofield H. , Mullainathan S. ( 2008 ). ‘ The psychology of nutrition messages ’, in ( Helmchen L. , Kaestner R. , Lo Sasso A. , eds.), Beyond Health Insurance: Public Policy to Improve Health , pp. 145 – 72 ., London : Emerald Group Publishing Limited .
Shah A.K. , Mullainathan S. , Shafir E. ( 2012 ). ‘ Some consequences of having too little ’, Science , vol. 338 ( 6107 ), pp. 682 – 85 .
Shah A.K. , Zhao J. , Mullainathan S. , Shafir E. ( 2018 ). ‘ Money in the mental lives of the poor ’, Social Cognition , vol. 36 ( 1 ), pp. 4 – 19 .
Shapiro J.M. ( 2005 ). ‘ Is there a daily discount rate? Evidence from the food stamp nutrition cycle ’, Journal of Public Economics , vol. 89 ( 2–3 ), pp. 303 – 25 .
Stables G. , Subar A. , Patterson B. , Dodd K. , Heimendinger J. , Duyn M.V. , Nebeling L. ( 2002 ). ‘ Changes in vegetables and fruit consmption and awareness among US adults: Results of the 1991 and 1997 5 a day for better health program surveys ’, Journal of the American Dietetic Association , vol. 102 , pp. 809 – 17 .
Stigler G.J. ( 1961 ). ‘ The economics of information ’, Journal of Political Economy , vol. 69 ( 3 ), pp. 213 – 25 .
Taylor-Robinson D. , Rougeaux E. , Harrison D. , Whitehead M. , Ben Barr , Pearce A. ( 2013 ). ‘ The rise of food poverty in the UK ’, British Medical Journal , vol. 347 .
Trieu K. , Neal B. , Hawkes C. , Dunford E. , Campbell N. , Rodriguez-Fernandez R. , Legetic B. , McLaren L. , Barberio A. , Webster J. ( 2015 ). ‘ Salt reduction initiatives around the world—a systematic review of progress towards the global target ’, PloS One , vol. 10 ( 7 ), e0130247 .
Trope Y. , Fishbach A. ( 2000 ). ‘ Counteractive self-control in overcoming temptation ’, Journal of Personality and Social Psychology , vol. 79 ( 4 ), pp. 493 – 506 .
Trumbo P. , Schlicker S. , Yates A.A. , Poos M. ; The National Food and Nutrition Board of the Institute of Medicine Academies . ( 2002 ). ‘ Dietary reference intakes for energy, carbohydrate, fiber, fat, fatty acids, cholesterol, protein and amino acids ’, Journal of the American Dietetic Association , vol. 102 ( 11 ), pp. 1621 – 30 .
US Department of Agriculture . ( 2010 ). ‘ Food expenditures and diet quality among low-income households and individuals ’, Report by James Mabli and Laura Castner and James Ohls and Mary Kay Fox and Mary Kay Crepinsek and Elizabeth Condon, https://mathematica.org/publications/food-expenditures-and-diet-quality-among-lowincome-households-and-individuals-summary (last accessed: 6 April 2022) .
US Department of Agriculture . ( 2013 ). ‘ Household food security in the United States in 2012 ’, Economic Research Service Report 155, by Coleman-Jensen, Alisha, Mark Nord and Anita Singh, United States Department of Agriculture .
Vagnoni C. , Prpa E. ( 2021 ). ‘ Food and drink reformulation to reduce fat, sugar and salt ’, https://post.parliament.uk/research-briefings/post-pn-0638/ .
Vaidya V. ( 2006 ). ‘ Psychosocial aspects of obesity ’, Advances in Psychosomatic Medicine , vol. 27 , pp. 73 – 85 .
Venn B.J. ( 2020 ). ‘ Macronutrients and human health for the 21st century ’, Nutrients , vol. 12 ( 8 ), p. 2363 .
Waters T. , Wernham T. ( 2021 ). ‘ The expiry of the universal credit uplift: Impacts and policy options ’, https://ifs.org.uk/publications/15528 .
WHO . ( 2020 ). ‘ Healthy diet ’, https://www.who.int/news-room/fact-sheets/detail/healthy-diet .
WHO . ( 2021 ). ‘ Obesity ’, https://www.who.int/westernpacific/health-topics/obesity .
Wisdom J. , Downs J.S. , Loewenstein G. ( 2010 ). ‘ Promoting healthy choices: Information versus convenience ’, American Economic Journal: Applied Economics , vol. 2 ( 2 ), pp. 164 – 78 .
Email alerts
Citing articles via.
- Recommend to your Librarian
Affiliations
- Online ISSN 1468-0297
- Print ISSN 0013-0133
- Copyright © 2024 Royal Economic Society
- About Oxford Academic
- Publish journals with us
- University press partners
- What we publish
- New features
- Open access
- Institutional account management
- Rights and permissions
- Get help with access
- Accessibility
- Advertising
- Media enquiries
- Oxford University Press
- Oxford Languages
- University of Oxford
Oxford University Press is a department of the University of Oxford. It furthers the University's objective of excellence in research, scholarship, and education by publishing worldwide
- Copyright © 2024 Oxford University Press
- Cookie settings
- Cookie policy
- Privacy policy
- Legal notice
This Feature Is Available To Subscribers Only
Sign In or Create an Account
This PDF is available to Subscribers Only
For full access to this pdf, sign in to an existing account, or purchase an annual subscription.
Correlation Between Poverty and Obesity Essay
Introduction, obesity in the uk, obesity in the us, is there any correlation between poverty and obesity, list of references.
Over the last few years, there has been a concern about the rising cases of obesity in the United Kingdom (UK). It is worrying that obesity may soon become an epidemic in the country. In the UK and other Western nations, the poor are now, for the first time in history, proportionately the most obese in the population groups.
There is the need to address this concern because as opposed to the usual perception that obesity varies by gender, age, and income among other factors, economic deprivation is now believed to be causing the disease. The changing lifestyles that link obesity to income seem to be on the rise.
The present discussion is complicated because of the fact that there are other several factors that might cause obesity, which is unrelated to poverty levels. However, latest research on the intricate links between obesity and poverty in the United States (US) shows uniformity for women and children from low socio-economic status (SES).
This paper discusses the correlation between obesity and poverty in the UK but also refers to situation in the US. Although the title suggests a link between poverty and obesity, it has not been proved and this essay is an attempt to examine many factors that contribute to impacts of poverty .
The present research seeks to achieve this through assessing the suggestion that today in the Western nations; the poor are now, for the first time in history, proportionately the most obese in the population groups. The paper will also attempt to establish whether there is a correlation between obesity and poverty as well as provide a summary of the crucial issues identified in the discussion.
It is notable that the proportion of the UK population with obesity has escalated significantly between 1993 and 2009. The increase has been 13 percent for males in 1993 to 22 percent in 2009. Conversely, the population proportion for females has escalated from 16 percent in 1993 to 24 percent in 2009 (The Poverty Site, 2011).
Furthermore, 22 percent of the working population presently has obesity. This percentage is significantly higher when compared with the case in 1993 when only 14 percent of the working population lived with obesity (The Poverty Site 2011). It is also notable that both men and women have similar chances of developing obesity.
It is important to point out that there is no apparent correlation between obesity and income. This is because the population groups with the lowest incidences of obesity include poor males and rich females. Furthermore, there is no clear correlation between fatness and social class (The Poverty Site, 2011). There is a general feeling among experts that the obesity problem in the country is not worse and the trend may be flattening out.
The obesity problem in the US is significantly different from the situation in the UK. America apparently has the highest obesity rates. This is because one – third proportion of the population has obesity. In addition, another one – third of the population is overweight (Levine, 2011).
As opposed to the situation in the UK, obesity problem in the US is likely to worsen. Child obesity is also a major predicament in the US There are some environmental influences, which influence obesity.
There is a consensus that varying household’s socio-economic level influences differing obesity incident levels. This is because the socio-economic levels that determine food security, defined by Zizza, Duffy and Gerrior (2008) as “limited or uncertain availability of nutritionally adequate and safe food or limited or uncertain ability to acquire acceptable foods in socially acceptable ways” is fluctuating significantly.
Notably, obesity incidences among women escalate with increasing levels of poverty (Levine, 2011). However, the level of poverty among men causes diverse obesity incidences, which form according to the varying occupations held. It is apparent that high poverty levels among women escalate their chances of developing obesity (Levine, 2011).
There is a considerable correlation between food deficiency and obesity for males and females in Britain. The correlation is observable when the prevalence levels for obesity are studied across economically deprived populations. It is notable that fatness prevalence levels escalate or stagnates at similar rates when compared against deprivation (Levine, 2011).
In England, 30 percent of females and 25 percent of males described as the most economically poor in the society are obese. The figures changes to 19 percent for females and 22 percent for males among wealthy people. Notably, the changes in the figures do not show large differences pertaining to the strength of the correlation between obesity and economic deprivation (Department of Health, 2011).
Arguing that economic deprivation causes obesity becomes more confusing when studying the same across the population of children. The boys who have the lowest chances of developing obesity are slightly the poor ones (Department of Health, 2011). The trend changes slightly among girls because those who develop obesity are within the median income levels (Levine, 2011). Generally, children from economically deprived households frequently experience challenges with nutrition. This has undesirable influence on the youngsters’ mental health and well-being, which in the long-term may become a contributing factor to obesity.
Lobstein, Baur and Uauy (2004), also support this concern by arguing that in the industrialized nations such as the UK and the US youngsters in economically deprived groups are at utmost danger of developing obesity. It is notable that introducing overweight into the analysis process complicates the understanding on the relationship.
In Britain, people have continued adding weight while their food purchase rates have been declining over the decades. Therefore, it can be said that the changing lifestyle and eating behaviors may be causing obesity. This also has a link with the peoples’ engagement with physical activities (Franklin, Jones, Love et al., 2012).
It indicates that people are engaging in less physical activity sessions because of the changes brought about by globalization and technological advancement. These developments make people to enjoy sedentary life in the high and low-income households (Lobstein, Baur and Uauy, 2004).
There are studies linking sedentary lifestyles such as working with the technological materials, including playing video games and watching television for several hours to obesity. Therefore, while discussing the correlation between economic deprivation and obesity there is the need to study the sedentary lifestyles adopted by people in high and low-income areas (Zizza, Duffy and Gerrior, 2008).
The nutritional value of the food that people consume has a significant impact on obesity development. This is particularly in reference to the amount of fat and its nutritional value in the food consumed. The income earned by households influences their food intake. It is noteworthy that the differences in food consumption reveal no major gaps under varying income groups (Franklin et al., 2012).
For example, looking at two different scenarios involving an individual from the least economically sustainable household and another one from the richest household, their calories consumption does not show major differences Franklin et al., 2012).
However, the people who are not very poor and not very rich may consume more calories than the poorest mad richest individuals may. This indicates that there is a weak correlation between economic deprivation and obesity (Lobstein, Baur and Uauy, 2004). Furthermore, this brings up additional concerns such as changing lifestyles that makes people to avoid engaging in physical activities.
The claim for the changing lifestyle is strengthened by the notion that readily processed high-calorie foodstuffs are cheaper for the low-income earners (Levine, 2011).
It is notable that even supermarkets located in the neighborhoods of economically deprived individuals normally stock more unhealthy foods. Therefore, the economically deprived households will prefer the cheaper and unhealthy foods, which are high in calorie, thereby increasing their chances for developing obesity (Lobstein, Baur and Uauy, 2004).
In the US, the issues may be similar as pointed out by Levine (2011). A study conducted by Levine (2011) to establish the link between “poverty and obesity in the US” generated helpful findings. The study raised the issue that Americans living in the poorest neighborhoods were more likely to develop obesity (Kaiser, Smith and Allison, 2012).
The study indicates that people living in low-income areas are underprivileged in terms of access to food. Furthermore, the economically deprived individuals lack access to clean and fresh foodstuff. They also have challenges of accessing sufficient food (Cassell & Gleaves, 2006).
The relationship with the obesity emerges from the fact that the economically deprived household’s lives in hunger and cannon easily access reasonably cheaper and healthier foodstuff (Kaiser, Smith and Allison, 2012). In this case, hunger together with poor accessibility to healthy food, cannot be the only factors that affect obesity.
The study concluded that much as economic deprivation is thought to have links with obesity, it is important to study the level of sedentariness of the poor people (Lobstein, Baur and Uauy, 2004). This is because the study also noted that the most economically deprived individuals also had the highest levels of sedentariness.
The economically deprived in the population may have sedentary lifestyles because of different reasons. The poor people living in low-income neighborhoods may fear to engage in physical activities outdoors because of the high crime rates (Kaiser, Smith and Allison, 2012). In addition, the economically deprived people may not have access to parks and sports venues (Kaiser, Smith and Allison, 2012).
This is because the relevant institutions sometimes fail to provide the facilities in such areas. The notion that engaging in physical activities requires certain sportswear may also hinder the economically deprived people from taking part in the physical activities since they cannot afford the appropriate sportswear (Levine, 2011).
A study by Drewnowski (2009), affirmed that obesity and economic deprivation are linked. The author argued that incidences of obesity followed economic status gradient. He noted that the highest rates of obesity were observable among the poor in the US.
He also pointed out the fact that the UK Department of Health (2011) reported that in the low economic status populations, more women than men developed obesity. The author also indicates that environmentally low-income neighborhoods had the highest incidences of obesity (Drewnowski, 2009). The author concludes that obesity and food uncertainty showed a relationship.
With the ongoing discussions, what remains unfamiliar is whether reversing economic deprivation situation among the poor populations would also overturn sedentary lifestyles. However, unclear the relationship between economic deprivation and obesity remain it would be too expensive to disregard the possible links (Levine, 2011). This paper reflects on the statement that in the Western nations the poor are now, for the first time in history, proportionately the most obese in the population groups and affirms that it is considerably true (Drewnowski, 2009).
In summary, this paper has established that there is a considerable correlation between economic deprivation and obesity in both the UK and the US. The paper has also established that two aspects of economic deprivation among the poor people lead to obesity. The individual household low economic status and environmentally the poor neighborhoods have links with obesity.
The economically deprived households and individuals lack access to nutritionally adequate and healthy foodstuffs. Therefore, they rely on cheaper, readily packed foods, which are high in calorie. The paper also identified that both the UK and the US have different levels of obesity. Whereas obesity problems are beginning in the UK, one-third of the American population already suffers obesity while another one third is overweight.
This paper has also made considerable discussion regarding the role of sedentary lifestyle among the rich and the economically deprived. Throughout the discussions, it emerged, that poverty alone may not cause obesity among the economically deprived populations. The paper concludes by affirming that there is a considerable correlation between obesity and economic deprivation.
Cassell, D. K., & Gleaves, D. H. 2006, The encyclopedia of obesity and eating disorders , Facts on File, New York.
Department of Health 2011, Equality Analysis: A call to action on obesity in England . Web.
Drewnowski, A. 2009, “Obesity, diets, and social inequalities”, Nutrition Reviews , Vol. 67, pp. 36–39, Willey Online Library. Web.
Franklin, B., Jones, A., Love, D., Puckett, S., Macklin, J., and White – Means, S. 2012, “Exploring Mediators of Food Insecurity and Obesity: A Review of Recent Literature”, Journal of Community Health , Vol. 37, No. 1, pp. 253 – 264, National Institute of Health. Web.
Kaiser, K. A., Smith, D. L., and Allison, D. B. 2012, “Conjectures on some curious connections among social status, calorie restriction, hunger, fatness, and longevity”, Annals of the New York Academy of Sciences , Vol. 1264, pp. 1–12, Willey Online Library. Web.
Levine, J. 2011, “Poverty and Obesity in the US”, Diabetes , Vol. 60, No. 11, pp. 2667-2668. Web.
Lobstein, T., Baur, L., and Uauy, R 2004, “Obesity in children and young people: a crisis in public health”, Obesity Reviews , Vol. 5, pp. 4–85, Willey Online Library. Web.
The Poverty Site. 2011, Obesity . Web.
Zizza, C. A., Duffy, P. A., and Gerrior, S. A 2008,” Food Insecurity Is not Associated With Lower Energy Intakes” Obesity , Vol. 16, pp. 1908–1913, Willey Online Library. Web.
- The Issue of Chronic Sleep Deprivation
- Sleep Deprivation Impacts on College Students
- Sleep Deprivation and Learning at University
- Sugar and Its Role in Overweight/Obesity Development or Treatment
- Importance of Red Wine to Human Health
- The Weight Loss Science and the Recommended Procedures
- Benefits of Vegetarianism
- Problem of Obesity in Modern Society
- Chicago (A-D)
- Chicago (N-B)
IvyPanda. (2019, April 13). Correlation Between Poverty and Obesity. https://ivypanda.com/essays/correlation-between-poverty-and-obesity/
"Correlation Between Poverty and Obesity." IvyPanda , 13 Apr. 2019, ivypanda.com/essays/correlation-between-poverty-and-obesity/.
IvyPanda . (2019) 'Correlation Between Poverty and Obesity'. 13 April.
IvyPanda . 2019. "Correlation Between Poverty and Obesity." April 13, 2019. https://ivypanda.com/essays/correlation-between-poverty-and-obesity/.
1. IvyPanda . "Correlation Between Poverty and Obesity." April 13, 2019. https://ivypanda.com/essays/correlation-between-poverty-and-obesity/.
Bibliography
IvyPanda . "Correlation Between Poverty and Obesity." April 13, 2019. https://ivypanda.com/essays/correlation-between-poverty-and-obesity/.
Loading metrics
Open Access
Perspective
Perspectives are commissioned from an expert and discuss the clinical practice or public health implications of a published study. The original publication must be freely available online.
See all article types »
Addressing socioeconomic inequalities in obesity: Democratising access to resources for achieving and maintaining a healthy weight
* E-mail: [email protected]
Affiliation Centre for Diet and Activity Research, MRC Epidemiology Unit, University of Cambridge, Cambridge, United Kingdom

Published: July 28, 2020
- https://doi.org/10.1371/journal.pmed.1003243
- Reader Comments
Citation: Adams J (2020) Addressing socioeconomic inequalities in obesity: Democratising access to resources for achieving and maintaining a healthy weight. PLoS Med 17(7): e1003243. https://doi.org/10.1371/journal.pmed.1003243
Copyright: © 2020 Jean Adams. This is an open access article distributed under the terms of the Creative Commons Attribution License , which permits unrestricted use, distribution, and reproduction in any medium, provided the original author and source are credited.
Funding: JA is funded by the Centre for Diet and Activity Research (CEDAR), a UK Clinical Research Collaboration (UKCRC) Public Health Research Centre of Excellence. Funding from the British Heart Foundation, Cancer Research UK, Economic and Social Research Council, Medical Research Council, the National Institute for Health Research, and the Wellcome Trust, under the auspices of the UK Clinical Research Collaboration, is gratefully acknowledged. The funders had no role in study design, data collection and analysis, decision to publish, or preparation of the manuscript.
Competing interests: I have read the journal’s policy and the authors of this manuscript have the following competing interests: I am a member of the PLOS Medicine editorial board.
In low-income countries, overweight and obesity are more common in more socioeconomically affluent groups [ 1 ]. This pattern flattens and then reverses as country-level income increases. In high-income countries, those living in less affluent circumstances are more likely to experience overweight and obesity. For example, in England, adults living in the most deprived fifth of neighbourhoods are almost twice as likely to be living with obesity (where the prevalence of obesity is 36%) as those living in the least deprived fifth (where the prevalence of obesity is 20%) [ 2 ]. These socioeconomic inequalities in unhealthy body weight manifest early in life, with an obvious relationship seen between neighbourhood deprivation and the experience of overweight or obesity in 4- to 5-year-old children in England [ 3 ]. As more countries experience epidemiological transitions, this inverse association between socioeconomic position and prevalence of unhealthy weight is becoming more common [ 1 ].
The standard energy balance explanation of unhealthy body weight proposes that weight gain occurs, and unhealthy weight is maintained, when energy intake is greater than energy expenditure. The problem of obesity becomes easily framed within this explanation as one of quantity and personal gluttony and laziness: either energy intake is too high, energy expenditure is too low, or both. Applied to the specific case of socioeconomic inequalities in obesity, this framing leads to the proposal that these personal failings are more common in less affluent groups. The obvious solution that can flow is one of personal restraint and discipline, particularly for those living in less affluent circumstances.
A closer look at socioeconomic differences in both dietary and physical activity patterns reveals that these differences may not simply be ones of quantity. Important socioeconomic differences in the quality of both diet and physical activity are becoming clear. For example, there is little evidence of socioeconomic differences in British children’s achievement of international recommendations for 60 minutes of moderate- to vigorous-intensity physical activity per day. But more affluent children do accumulate more of the vigorous-intensity activity that is particularly associated with body weight than their less affluent counterparts, and this appears to be via more participation in organised sport [ 4 ]. Similarly, there is little evidence that total dietary energy varies consistently across socioeconomic groups in the United Kingdom, but dietary quality does. Those living in more affluent households eat more fruit and vegetables than those living in less affluent homes, drink fewer sugar-sweetened beverages, and are more likely to consume diets associated with lower cardiovascular risk [ 5 , 6 ].
These findings suggest that we cannot explain socioeconomic inequalities in unhealthy body weight as due to differences in gluttony and laziness, nor view the solution as one of greater personal restraint and discipline. Doing so would be both untrue and unhelpful. Instead, the question becomes one of why there are consistent differences in the quality of diet and physical activity that people living in different circumstances have access to.
Socioeconomic position is often measured in terms of education, income, occupational social class, or neighbourhood circumstances. But the concept captures more than any of these indicators alone. It is about access to resources in their widest sense—certainly financial resources, but also social, physical, cognitive, and other resources.
Recent changes in food practices associated with COVID-19 restrictions highlight how these practices are related to the social and physical resources that people have access to. In April 2020, when most UK schools, restaurants, cafes, and workplaces were closed, and government advice was to ‘stay at home’, half of UK adults reported that they were eating more home-cooked food and less takeaway and fast food than normal [ 7 ]. This suggests that longer-term declines in home food preparation [ 8 ] may have more to do with changes in predictable time spent at home and the availability of alternative sources of food rather than any widespread loss of cooking skills. And in more ‘normal’ times, these social and physical resources are distinctly socioeconomically patterned. People living in less affluent circumstances are less likely to have predictable working hours, and takeaway outlets are more common in less affluent neighbourhoods [ 9 ].
Access to financial resources is a key component of socioeconomic position. Although it is often assumed that absolute destitution is rare in high-income countries, the visit by the United Nations’ Special Rapporteur on Extreme Poverty and Human Rights to the UK in 2019 highlighted this is not the case. His report opens with the estimate that 1.5 million people in the UK were unable to afford basic necessities in 2017 [ 10 ].
Recent, but pre-COVID-19, data from the UK indicate that one-fifth to one-quarter of adults experienced food insecurity (i.e., limited or uncertain access to adequate and safe food due to financial constraints) in the previous 12 months [ 11 , 12 ]. Thus, each year, 20%–25% of adults in the UK worry about being able to afford food or skip meals because they cannot afford to buy food. Although it may seem superficially paradoxical, in high-income countries, food insecurity is consistently associated with obesity and poorer dietary quality, particularly in women [ 13 ]. This reflects known differences in food prices—healthier foods and diets tend to be more expensive [ 14 ]—meaning that under conditions of financial constraint, people turn first to lower-quality, less healthy diets, before sacrificing on absolute energy quantity. The finding of a consistent association between food insecurity and unhealthy body weight further undermines the assumption that obesity is a problem of personal excess and laziness.
Financial constraints may similarly act as a barrier to the organised sports that tend to make up the vigorous physical activity that is most associated with body weight. Many such sports require clothing and equipment to be bought and classes or other facilities to be paid for. Here, too, social and physical resources are important, with less affluent families reporting a lack of time to support their children doing these activities and less actual or perceived access to appropriate facilities [ 15 ].
Viewing obesity as a problem of quality, rather than quantity, and understanding socioeconomic position in terms of access to a wide variety of resources lead to the conclusion that socioeconomic inequalities in obesity are due to differential access to the resources required to access high-quality diets and physical activity. Rather than admonishments to the ‘poor’ to eat more prudently or exercise more frequently, the solution to socioeconomic inequalities in obesity presented by this framing is to provide everyone with access to adequate resources to achieve and maintain a healthy body weight. Reshaping fiscal, social, and physical environments to make it easier to access healthier practices—via, for example, planning restrictions on hot food takeaway outlets, taxes on less healthy foods, and subsidies on children’s access to sport—is likely to help. However, the most powerful way to ensure that everyone has adequate access to the resources required to achieve and maintain a healthy weight may be through stronger welfare and employment policies, including higher minimum wages, working hour mandates, and universal basic income [ 16 ].
- View Article
- PubMed/NCBI
- Google Scholar
- 2. Lifestyles Team at NHS Digital. Health Survey for England 2018. London: NHS Digital, part of the Government Statistical Service; 2019 Dec 3 [cited 2020 May 29]. Available from: https://digital.nhs.uk/data-and-information/publications/statistical/health-survey-for-england/2018/summary .
- 3. Lifestyles Team at NHS Digital. National Child Measurement Programme, England 2018/19 School Year. London: NHS Digital, part of the Government Statistical Service; 2019 Oct 13 [cited 2020 May 29]. Available from: https://digital.nhs.uk/data-and-information/publications/statistical/national-child-measurement-programme/2018-19-school-year/final-page .
- 5. Bates B, Collins D, Cox L, Nicholson S, Page P, Roberts C, et al. National Diet and Nutrition Survey Years 1 to 9 of the Rolling Programme (2008/2009–2016/2017): Time trend and income analyses. London: Public Health England; 2019.
- 7. Obesity Health Alliance. Briefing: How are COVID-19 measures affecting the food environment? London: Obesity Health Alliance; 2020 [cited 2020 May 29]. http://obesityhealthalliance.org.uk/wp-content/uploads/2020/05/OHA-polling-data-summary-final.pdf .
- 10. Special Rapporteur on extreme poverty and human rights. Visit to the United Kingdom of Great Britain and Northern Ireland. New York: United Nations General Assembly; 2019.
Socioeconomic Factors: Poverty and Obesity
- First Online: 21 August 2021
Cite this chapter
- Tahereh Alavi Hojjat 2
1219 Accesses
2 Altmetric
The modern relationship between obesity and poverty is nuanced and complex. Being poor in one of the poorest countries in the world may be associated with scarce nutrition and emaciation, while being poor in a developed country may mean a higher risk of obesity. In this chapter, we will explain variations in poverty (depending upon geography, social stratification, and economic development), and will identify how socioeconomic groups with low personal capital, low health capital, and low social capital have higher obesity rates compared with socioeconomic groups with higher endowments of capital. We will support the argument that while government programs do help the poor, poverty remains high due to a sharp increase in inequality of economic outcomes.
This is a preview of subscription content, log in via an institution to check access.
Access this chapter
- Available as EPUB and PDF
- Read on any device
- Instant download
- Own it forever
- Compact, lightweight edition
- Dispatched in 3 to 5 business days
- Free shipping worldwide - see info
- Durable hardcover edition
Tax calculation will be finalised at checkout
Purchases are for personal use only
Institutional subscriptions
Webb Hooper M, Nápoles AM, Pérez-Stable EJ. COVID-19 and racial/ethnic disparities. JAMA. 2020;323(24):2466–7. https://doi.org/10.1001/jama.2020.8598 .
Article CAS PubMed Google Scholar
Meyer BD, Sullivan JX. Consumption and income inequality and the great recession. Am Econ Rev. 2013;103(3):178–83. https://doi.org/10.1257/aer.103.3.178 .
Article Google Scholar
Meyer BD, Sullivan JX. Further results on measuring the well-being of the poor using income and consumption. J Hum Resour. 2003;38S:1180–220.
Haveman R, Wolff EN. The concept and measurement of asset poverty: levels, trends and composition for the U. S., 1983-2001. J Econ Inequal. 2004;2(2):145.
Danzinger S. The mismeasure of poverty. The New York Times. 2013, Sept 18; p. A21.
Google Scholar
Heggeness ML, Hokayem C. Life on the edge: living near poverty in the United States, 1966–2011. U.S. Census Bureau SEHSD Working Paper 2013–02, Washington, DC, 2013.
Semega J, Kollar M, Creamer J, Mohanty A. Income and poverty in the United States: 2018 (Report Number P60–266). [Internet]. 2019; United States Census Bureau. Available from: https://www.census.gov/library/publications/2019/demo/p60-266.html#:~:text=The%20official%20poverty%20rate%20in,14.8%20percent%20to%2011.8%20percent/ .
Larrimore J, Mortenson J, Splinter D. Presence and persistence of poverty in U.S. tax data, NBER Working Paper No. 26966, [Internet]; National Bureau of Economic Research, 2020.; Available from: https://www.nber.org/papers/w26966/ .
Kharas H, DiNucci CD, Hamel K, Tong B. To move the needle on ending extreme poverty, focus on rural areas. [Internet]. 21 February 2020; Available from: https://www.brookings.edu/blog/future-development/2020/02/21/to-move-the-needle-on-ending-extreme-poverty-focus-on-rural-areas/?/ . Accessed on 23 Feb 2020.
Kharas H. Future development: who gained from global growth last decade-and who will benefit by 2030? [Internet]. 2020; Available from: https://www.brookings.edu/blog/future-development/2020/01/16/who-gained-from-global-growth-last-decade-and-who-will-benefit-by-2030/ . Accessed on Jan 2020.
Zumbrun J. World news: extreme poverty rises, World Bank say-pandemic tips about 100 million people into category of those living on less than $ 1.90 a day. Wall Street J. 2020:A. 8.
Aaronson D, Hu L, Rajan A. Did Covid-19 disproportionately affect mothers’ labor market activity? Chicago Fed Letter, 2021, Jan, No. 2021–450. https://doi.org/10.21033/cfl-2021-450 .
U.S. Department of Agriculture (USDA). 2012 Census highlights: farm economics. ACH12–2, May 2014. See also environmental working group, farm subsidy database [Internet]. (n.d). Available from: https://farm.ewg.org
Schanzenbach DW. The Hamilton Project: Strengthening SNAP for a more food-secure, healthy America, [Internet]. 2013; Brookings Institution, Available from: https://www.hamiltonproject.org/assets/legacy/files/downloads_and_links/THP_SchDiscPaper_pages_Final.pdf/ . Accessed on 10 Dec 2019.
USDA Food Expenditures. [Internet]. 2015.; Available from: https://www.ers.usda.gov/data-products/food-expenditure-series/ .
Ray R. Why are Blacks dying at higher rates from COVID-19? [Internet]. 9 April 2020; Available from: https://www.brookings.edu/blog/fixgov/2020/04/09/why-are-blacks-dying-at-higher-rates-from-covid-19 . Accessed on 10 April 2020.
Piketty T, Saez E. Income inequality in the United States, 1913-1998. Q J Econ. 2003;113(1):1–39.
Piketty T, Saez E, Zucman G. Distributional national accounts: methods and estimates for the United States. Q J Econ. 2018;133(2):553–609.
Mishel L, Wolfe J. CEO compensation has grown 940% since 1978, Economic Policy Institute [Internet]. 14 August 2019; Available from: https://www.epi.org/publication/ceo-compensation-2018/ .
Wolff EN. Household wealth trend in the United States, 1962 To 2016: Has middle class wealth recovered? Working Paper 24085, National Bureau of Economics Research. 2017; Available from: http://www.nber.org/papers/w24085 /.
Wolff EN. Wealth taxation in the United States, Working paper 26544 [Internet]. 2019; National Bureau of Economic Research, Available from: http://www.nber.org/papers/w26544/ .
Watson PW. Real wage growth is actually falling. [Internet], 2018.; Available from: https://www.forbes.com/sites/patrickwwatson/2018/09/25/real-wage-growth-is-actually-falling/?sh=6ed53fbb7284 /.
Sawhill I, Karpilow, Q. Raising the minimum wage and redesigning the EITC [Internet], Center on Children and Families, Brookings, January 30, 2014; Available from: https://www.brookings.edu/wp-content/uploads/2016/06/30-Raising-Minimum-Wage-Redesigning-EITC-sawhill.pdf . Accessed on 19 Dec 2020.
Aaronson D, Hu L, Rajan A. How much did minimum wage drive real wage growth during the late 2020? [Internet]. 2020. Chicago Fed Letter, No. 435. Federal Reserve Bank of Chicago, Available from: https://www.chicagofed.org/publications/chicago-fed-letter/2020/435/ .
Wilkinson RG, Pickett K. The spirit level: why more equal societies almost always do better. London: Allen Lane; 2009.
Wilkinson RG, Pickett K. Why greater equality makes societies stronger: the spirit level. New York: Bloomsbury Press; 2009.
Gordon RJ. The rise and fall of American growth: the U.S. standard of living since the civil war. Princeton/Oxford: Princeton University Press; 2016.
Book Google Scholar
Brynjolfsson E, McAfee A. The second machine age: work, progress, and prosperity in a time of brilliant technologies. New York: W.W. Norton; 2014.
Sawhill I. The forgotten Americans: an economic agenda for a divided nation. New Haven/London: Yale University Press; 2018.
Goldin C, Katz LF. The race between education and technology: The evolution of U.S. educational wage differentials, 1890 to 2005, [Internet]. NBER Working Paper No. 12984. 2007.; Available from: http://www.nber.org/papers/w12984/ .
Poverty: not always with us, Briefing [Internet]. The Economist , June 1, 2013, Available from: https://www.economist.com/briefing/2013/06/01/not-always-with-us /.
The World Health Organization. Obesity and inequalities: Guidance for addressing inequities in overweight and obesity [Internet]. 2000; Available from: https://www.euro.who.int/__data/assets/pdf_file/0003/247638/obesity-090514.pdf /.
Sobal J, Stunkard AJ. Socioeconomic status and obesity: a review of the literature. Psychol Bull. 1989;105(2):260–75.
Article CAS Google Scholar
Hajat A, Kaufman JS, Rose KM, Siddiqi A, Thomas JC. Do the wealthy have a health advantage? Cardiovascular disease risk factors and wealth. Soc Sci Med. 2010;71(11):1935–42.
Australian Bureau of Statistics. Australian health survey: updated results, 2011–2012. 2012. ABS cat. no. 4364.0.55.003. Canberra: Australian Bureau of Statistics.
Monteiro CA, Moura EC, Conde WL, Popkin BM. Socioeconomic status and obesity in adult populations of developing countries: a review. Bull World Health Organ. 2004;82(12):940–6.
PubMed Google Scholar
Dinsa GD, Goryakin Y, Fumagalli E, Suhrcke M. Obesity and socioeconomic status in developing countries: a systematic review. Obes Rev. 2012;13:1067–79. https://doi.org/10.1111/j.1467-789X.2012.01017.x .
Article CAS PubMed PubMed Central Google Scholar
Au N, Johnston DW. Too much of a good thing? Exploring the impact of wealth on weight. Health Econ. 2014;24:1403–21.
Cutler DM, Lleras-Muney A. Understanding differences in health behaviors by education. J Health Econ. 2010;29(1):1–28.
Fuchs VR. Time preference and health: an exploratory study. In economic aspects of health. Chicago: University of Chicago Press; 1982. p. 93–120.
Cawley J. An instrumental variables approach to measuring the effect of body weight on employment disability. Health Serv Res. 2000;35(5):1159–79.
CAS PubMed PubMed Central Google Scholar
Puhl RM, Brownell KD. Bias, discrimination, and obesity. Obesity. 2001;9(12):788–805.
Ogden CL, Fakhouri TH, Carroll MD, et al. Prevalence of obesity among adults, by household income and education — United States, 2011–2014. MMWR Morb Mortal Wkly Rep. 2017;66:1369–73. https://doi.org/10.15585/mmwr.mm6650a1 . Accessed on 6 April 2021.
National Survey of Children’s Heath. State of childhood obesity. 2020, Oct. Available from https://stateofchildhoodobesity.org/children1017/ .
Centers for Disease Control and Prevention. Adult obesity prevalence maps. 2021. Available from https://www.cdc.gov/obesity/data/prevalence-maps.html . Accessed on 7 April 2021.
Blisard N, Stewart H, Jolliffe D. Low-income households’ expenditures on fruits and vegetables. (Report No. 833), [Internet]. 2004. Available from: http://www.ers.usda.gov/publications/aer-agricultural-economic-report/aer833.aspx /. Accessed on 1 May 2004.
Tomer JF. What causes obesity? And why has it grown so much? Challenge. 2011;54(4):22.
Henderson LJ. Obesity, poverty and diversity: theoretical and strategic challenges. In: Zoltan J, Acs Z, Lyles A, editors. Obesity, business, and public policy. Cheltenham, UK: Edward Elgar; 2007. p. 57–75.
Morland K, Wing S, Roux AD, Poole G. Neighborhood characteristics associated with the location of food stores and food service places. Am J Prevent Med. 2002;22:23–9.
Tomer JF. Intangible capital: its contribution to economic growth, well-being and rationality. Cheltenham, UK: Edward Elgar; 2008.
Hobbes T. Leviathan. Oxford, UK: Oxford University Press; 1998.
Subramanian SV, Kawachi I. Income inequality and health: what have we learned so far? Epidemiol Rev. 2004;26(1):78–91.
Centers for Disease Control and Prevention. CDC health disparities and inequalities report, morbidity and mortality. Weekly Report (MMWR). 2011;60(Supplement)
Lakdawalla D, Philipson T.National bureau of economics: Working paper , Report No. 8946 [Internet]; 2002.; Available from: http://www.nber.org/papers/w8946/ . Accessed on 1 Jan 2011.
Braveman P. Health disparities and health equality: concepts and measurement. Ann Rev Public Health. 2014;27:167–94.
Satel S, Marmor TR. Does inequality make you sick? [Internet]. 16 July 2001; The Weekly Standard, Available from: https://www.aei.org/articles/does-inequality-make-you-sick/ .
Reeve VR, Sawhill I. A new contract with the middle class. Washington, DC: Brookings; 2020.
Jargowsky PA. Take the money and run: economic segregation in U.S. metropolitan areas. Am Sociol Rev. 1996;61(6):984–8.
Collin V, Vegas E. Unequally disconnected: Access to online learning in the U.S [Internet]. June 22, 2020.; Available from: https://www.brookings.edu/blog/education-plus-development/2020/06/22/unequally-disconnected-access-to-online-learning-in-the-us/ . Accessed on 24 June 2020.
Sinclair C, Reeve R. Class Notes: unequal internet access, employment at older ages, and more [Internet], Brookings, 22 April 2020; Available from: https://www.brookings.edu/blog/up-front/2020/04/22/class-notes-unequal-internet-access-employment-at-older-ages-and-more/ . Accessed on 18 Dec 2020.
Center for Global Development, COVID: School closures and education system, [Internet]. 2020.; Available from: https://www.cgdev.org/topics/covid-education /.
Download references
Author information
Authors and affiliations.
Center Valley, PA, USA
Tahereh Alavi Hojjat
You can also search for this author in PubMed Google Scholar
Corresponding author
Correspondence to Tahereh Alavi Hojjat .
Rights and permissions
Reprints and permissions
Copyright information
© 2021 The Author(s), under exclusive license to Springer Nature Switzerland AG
About this chapter
Hojjat, T.A. (2021). Socioeconomic Factors: Poverty and Obesity. In: The Economics of Obesity. Springer, Cham. https://doi.org/10.1007/978-3-030-78487-4_5
Download citation
DOI : https://doi.org/10.1007/978-3-030-78487-4_5
Published : 21 August 2021
Publisher Name : Springer, Cham
Print ISBN : 978-3-030-78486-7
Online ISBN : 978-3-030-78487-4
eBook Packages : Medicine Medicine (R0)
Share this chapter
Anyone you share the following link with will be able to read this content:
Sorry, a shareable link is not currently available for this article.
Provided by the Springer Nature SharedIt content-sharing initiative
- Publish with us
Policies and ethics
- Find a journal
- Track your research
Why Poverty Leads to Obesity and Life-Long Problems
- Health Care
Connect with the author
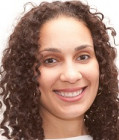
The United States finds itself in the midst of an “obesity epidemic,” as many news outlets and public agencies have proclaimed. For good reasons, researchers and public health experts are especially concerned about obesity among children and adolescents. Over the last three decades obesity has grown almost three-fold among youngsters. Obesity is a risk for all groups of Americans, but what is often left unsaid is the special vulnerability of the most disadvantaged groups. Obesity is especially rampant among Americans with the lowest levels of education and the highest poverty rates. Given the increasing economic insecurity facing many in our nation today, it is important to understand why and how poverty heightens the rise of obesity among youth. Only if we understand the causes at work can we effectively design strategies to reduce this major health risk to already vulnerable people. Why the Risk of Obesity is Greater for the Poor Eating right and engaging in regular physical activity are decisions all individuals in America can make to lower the risk of obesity and associated health problems. But of course the choices made by children and adolescents are strongly affected by the family and community environments in which they live. Impoverished living conditions matter especially for the young, because they throw up many barriers to engaging in healthy behaviors. Poor families have limited food budgets and choices, and must often stretch supplies toward the end of the month, before another check or allocation of Food Stamps arrives. This leads to unhealthy behaviors in several ways:
- Families choose high-fat foods dense with energy – foods such as sugars, cereals, potatoes and processed meat products – because these foods are more affordable and last longer than fresh vegetables and fruits and lean meats and fish.
- Poor families often live in disadvantaged neighborhoods where healthy foods are hard to find. Instead of large supermarkets, poor neighborhoods have a disproportionate number of fast food chains and small food stores providing cheap, high-fat foods.
- Economic insecurity – such as trouble paying bills or rent – leads to stress, and people often cope by eating high-fat, sugary foods.
Options for regular physical activity can also be restricted for poor people:
- Families cannot usually afford to pay for organized children’s activities outside of school – and schools in impoverished areas are less likely to run sports or physical activity programs than schools with more resources.
- Due to inflexible work schedules, lack of transportation, or unmet needs for child care, poor parents, especially single mothers, may find it hard to support extra activities for their children. Leaving kids in front of the TV is often all stressed poor parents can manage.
- In many poor neighborhoods, parks, playgrounds, trails, and free public gyms are often not available or safe. Neighborhoods may be crime-ridden, and there may be no nearby indoor places for play or exercise. Ironically, parental efforts to keep kids safe and indoors may increase encourage sedentary behaviors such as watching TV and playing video games.
The Long-Term Downsides of Youthful Obesity Compared to those who are not poor, adolescents in impoverished families and communities are more likely to be obese – or become overweight in young adulthood. And obesity in young adulthood is not usually temporary. It is a kind of lifelong sentence to ill health and socioeconomic disadvantages.
- Obese young adults are a high risk for ongoing health problems such as cardiovascular disease, depression and certain cancers.
- Being overweight in young adulthood is associated with unemployment, limits on educational attainment and lower levels of income.
- Because the bad consequences are long-lasting and recurrent, obesity needs to be prevented or reversed for poor youngsters. Policymakers are looking for the best ways to do just that.
What Can be Done? To effectively prevent and reverse obesity among especially vulnerable poor people, we have to think of the issues in more than just individual terms. We must look for ways to begin to improve the social and physical environments in which low-income people live. Interventions in neighborhoods and community institutions can make a difference.
- For example, New York City is sponsoring a “Green Carts” program that uses mobile food carts to offer fresh produce in certain poor neighborhoods.
- New neighborhood resources are also needed to help people connect with one another for safe, organized activities conducive to good physical and mental health.
- Schools and Head Start programs can be key players too, because youngsters spend so much time in them and they are valued community institutions. Research shows that incorporating physical activities and good nutrition into school and pre-school offerings can make a real difference in the fight against childhood and youth obesity.
Investing in a diverse set of physical activities and nutritional programs in neighborhoods and educational institutions may be the best way to reduce the risk of obesity among poor youngsters. But such efforts need reliable funding from governments and charities to boost and leverage valuable programs in neighborhood centers and schools serving disadvantaged Americans.
Related Content
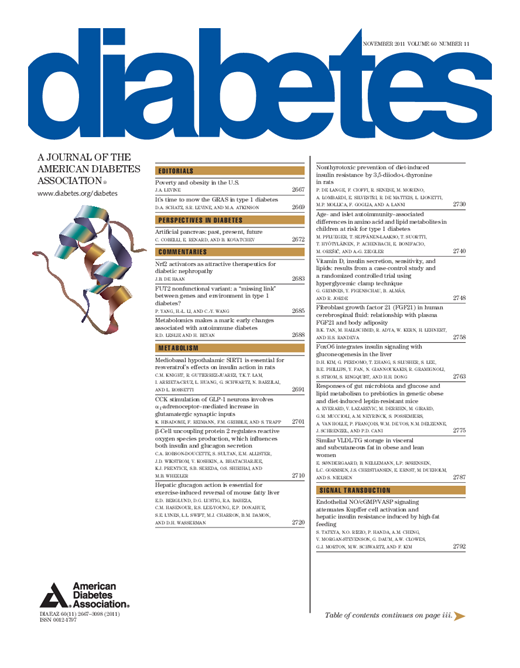
- Next Article
Article Information
Poverty and obesity in the u.s..
The opinions are those of the author alone and not of the American Diabetes Association or the Mayo Clinic.
- Split-Screen
- Article contents
- Figures & tables
- Supplementary Data
- Peer Review
- Open the PDF for in another window
- Cite Icon Cite
- Get Permissions
James A. Levine; Poverty and Obesity in the U.S.. Diabetes 1 November 2011; 60 (11): 2667–2668. https://doi.org/10.2337/db11-1118
Download citation file:
- Ris (Zotero)
- Reference Manager
High-income countries have greater rates of obesity than middle- and low-income countries ( 1 ). Countries that develop wealth also develop obesity; for instance, with economic growth in China and India, obesity rates have increased by several-fold ( 1 ). The international trend is that greater obesity tracks with greater wealth ( 2 , 3 ).
The U.S. is one of the wealthiest countries in the world and accordingly has high obesity rates; one-third of the population has obesity plus another third is overweight. The situation is predicted to worsen; rising childhood obesity rates forewarn of worsening statistics ( 4 ). While it is agreed that both individual factors such as genetic susceptibility and behavior are important in life-long weight gain, evidence is ill-defined with respect to the nature of the environmental influences that impact obesity ( 5 ).
In 2010, 15.1% of Americans lived in poverty based upon family income census data ( 6 ). With the economic downturn, the number of people in the U.S. living in poverty rose to 46 million people—the greatest number in more than 50 years ( 6 ).
Are poverty and obesity associated? Poverty rates and obesity were reviewed across 3,139 counties in the U.S. ( 2 , 6 ). In contrast to international trends, people in America who live in the most poverty-dense counties are those most prone to obesity ( Fig. 1A ). Counties with poverty rates of >35% have obesity rates 145% greater than wealthy counties.
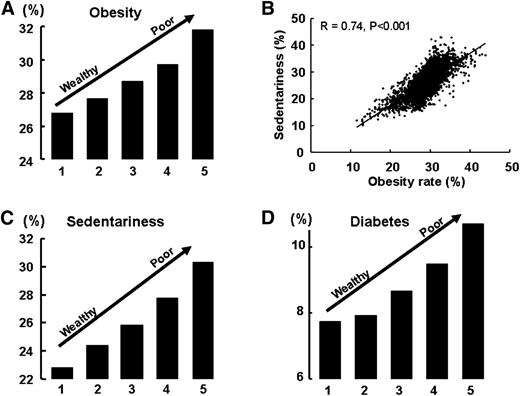
Data from 3,139 counties in the U.S. Quintiles are cohorts of counties ranked by the percentage of people living with poverty. Quintile 1, the wealthiest quintile, includes 630 U.S. counties with a mean county poverty rate of 8.2% (median household income, $56,259). Quintile 5, the poorest quintile, includes 629 counties with a mean poverty rate of 25% (median household income, $32,679). A : County age-adjusted obesity rates by poverty quintile. B : County obesity rates vs. county leisure-time sedentary rates (sedentary adults are those who report no physical activity or exercise other than at their regular job). C : County sedentary rates. D : Age-adjusted diabetes rate by poverty quintile.
How is poverty linked to obesity? It has been suggested that individuals who live in impoverished regions have poor access to fresh food. Poverty-dense areas are oftentimes called “food deserts,” implying diminished access to fresh food ( 7 ). However, 43% of households with incomes below the poverty line ($21,756) are food insecure (uncertain of having, or unable to acquire, sufficient food) ( 7 ). Accordingly, 14% of U.S. counties have more than 1 in 5 individuals use the Supplemental Nutrition Assistance Program. The county-wide utility of the program, as expected, correlates with county-wide poverty rates ( r = 0.81) ( 7 ). Thus, in many poverty-dense regions, people are in hunger and unable to access affordable healthy food, even when funds avail. The double-edged sword of hunger and poor availability of healthy food is, however, unlikely to be the only reason as to why obesity tracks with poverty.
There is evidence of the association between sedentariness, poor health, obesity, diabetes, other metabolic diseases, and premature death ( 8 ). Sedentary individuals move 2 h per day less than active individuals and expend less energy, and they are thereby prone to obesity, chronic metabolic disease, and cardiovascular death ( 9 ). More than half of county-to-county variance in obesity can be accounted for by variance in sedentariness ( Fig. 1B ). Overall, the poorest counties have the greatest sedentariness ( Fig. 1C ) and obesity.
Several reasons may explain why people living in poor counties are less active. One reason may be that violence tracks with poverty, thereby preventing people from being active out-of-doors. Similarly, parks and sports facilities are less available to people living in poor counties ( 5 ), and people who live in poverty-dense regions may be less able to afford gym membership, sports clothing, and/or exercise equipment. There are multiple individual and environmental reasons to explain why poverty-dense counties may be more sedentary and bear greater obesity burdens. What is unknown is whether reversing poverty would reverse sedentariness and obesity. It is an urgent matter to address—both rates of childhood obesity and poverty are concomitantly on the rise ( 1 , 2 ).
The link between obesity, inactivity, and poverty may be too costly to ignore because obesity-associated chronic disease already accounts for 70% of U.S. health costs. For instance, counties with greatest rates of poverty have greatest diabetes rates too ( Fig. 1D ). In 2009, 27% of people living in the U.S. with annual household outcomes below $25,000 were uninsured (no private or government health insurance). This cohort represents, 15,483,000 people, ∼5 million with obesity and ∼1 million with diabetes ( 10 ). With expanded health care provision in the U.S., the potential incremental health care costs of diabetes alone for these individuals approximates $9 billion/year, or $9,000 per new diabetes patient/year ( 11 ). There are, however, additional economic factors that may impact the cost-return equation, for instance, 1 ) potential savings associated with diabetes prevention, 2 ) the opportunity to develop and deliver high-quality and low-cost diabetes care to poverty-dense communities, 3 ) the health cost savings associated with the prevention of diabetes complications in patients with diabetes, and 4 ) the potential lost tax revenues associated with disability ( 12 ). Add these figures to the health care costs of other chronic obesity-associated diagnoses such as hypertension, hyperlipidemia, sleep disorders, arthritis, cardiovascular disease, and asthma and the projected health care costs of poverty increase.
Halting U.S. diabesity epidemic and curtailing its health cost may necessitate addressing poverty.
No potential conflicts of interest relevant to this article were reported.
Email alerts
- Online ISSN 1939-327X
- Print ISSN 0012-1797
- Diabetes Care
- Clinical Diabetes
- Diabetes Spectrum
- Standards of Medical Care in Diabetes
- Scientific Sessions Abstracts
- BMJ Open Diabetes Research & Care
- ShopDiabetes.org
- ADA Professional Books
Clinical Compendia
- Clinical Compendia Home
- Latest News
- DiabetesPro SmartBrief
- Special Collections
- DiabetesPro®
- Diabetes Food Hub™
- Insulin Affordability
- Know Diabetes By Heart™
- About the ADA
- Journal Policies
- For Reviewers
- Advertising in ADA Journals
- Reprints and Permission for Reuse
- Copyright Notice/Public Access Policy
- ADA Professional Membership
- ADA Member Directory
- Diabetes.org
- X (Twitter)
- Cookie Policy
- Accessibility
- Terms & Conditions
- Get Adobe Acrobat Reader
- © Copyright American Diabetes Association
This Feature Is Available To Subscribers Only
Sign In or Create an Account
Racial and Ethnic Disparities in Adult Obesity in the United States: CDC’s Tracking to Inform State and Local Action
ESSAY — Volume 16 — April 11, 2019
Ruth Petersen, MD, MPH 1 ; Liping Pan, MD, MPH 1 ; Heidi M. Blanck, PhD 1 ( View author affiliations )
Suggested citation for this article: Petersen R, Pan L, Blanck HM. Racial and Ethnic Disparities in Adult Obesity in the United States: CDC’s Tracking to Inform State and Local Action. Prev Chronic Dis 2019;16:180579. DOI: http://dx.doi.org/10.5888/pcd16.180579 .
PEER REVIEWED
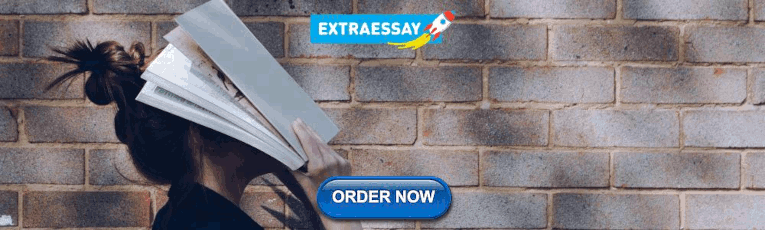
What Causes These Disparities?
What is dnpao doing to address these disparities, what’s next, acknowledgments, author information.
The Centers for Disease Control and Prevention (CDC) plays a key role in tracking data on the burden of obesity and its related racial and ethnic disparities to provide information that can highlight areas where state and local actions are most needed. Until further innovations allow for measured data on height and weight to be available for all states, self-reported data are the best source for understanding where the burden of obesity is highest among different populations. This understanding is critical given that the prevalence of obesity is increasing among adults in the United States (1). As such, obesity continues to put a strain on overall health status, health care costs, productivity, and the capacity for deployment and readiness of military personnel. Adults with obesity often have multiple-organ system complications from the condition and, as a result, are more at risk for heart disease, stroke, type 2 diabetes, and multiple types of cancers (2). The estimated annual medical cost of obesity in the United States was $147 billion in 2008 (3). Compared with spending for someone of normal weight, medical spending for a person with obesity was $1,429 higher (42% higher) per year (3). Adult obesity decreases productivity, and the cost of lost productivity is between $3.4 and $6.4 billion per year (4). Adult obesity also increases the risk of workplace injuries (2). Obesity among young adults limits the eligibility for many to serve in our military, given the weight standards for recruitment that nearly 1 in 4 young adults are not able to meet (5).
Among many other factors, the risk of adult obesity is greater among adults who had obesity as children, and racial and ethnic disparities exist by the age of 2 (6). If nothing else is done in the United States beyond what is being done now, simulated growth trajectories that model today’s children show that over half (59% of today’s toddlers and 57% of children aged 2 to 19) will have obesity at age 35 (7). Early feeding patterns, including how babies are fed and how caregivers use food in response to an infant’s mood, affect acute growth, future eating patterns, and the risk of obesity (8). Similarly, family and caregiver modeling of healthy behaviors, food offerings, and active playtime, as well as characteristics of neighborhoods such as walkability and traffic volume, may affect children’s nutrition and physical activity habits (9,10).
As sectors come together to reduce the obesity epidemic, we are aware how challenging success will be due to factors such as 1) the contributing risk factors of genetic and biological attributes; 2) individual behaviors (parenting styles, dietary patterns, physical activity levels, medication use, sleep, stress management); and 3) community and societal factors that influence individual, family, and collective access to healthy, affordable foods and beverages; access to safe and convenient places for physical activity; and exposure to the marketing of unhealthy products (2).
By using self-reported data of height and weight from the Behavioral Risk Factor Surveillance System, CDC’s Division of Nutrition, Physical Activity, and Obesity (DNPAO) has published state-specific obesity maps since 1999. Obesity is defined as a body mass index (a person’s weight in kilograms divided by the square of height in meters) of 30.0 or higher. These maps have shown the growing epidemic that has affected our nation from coast to coast. Although the data collection methods changed in 2011, which somewhat limits our ability to assess trends, the 2017 data continue to show that obesity prevalence among adults remains high across the country (Figure 1). The state-specific prevalence ranges from a low of 22.6% in Colorado to a high of 38.1% in West Virginia (11).
Figure 1. Prevalence of self-reported obesity among US adults, by state and territory, Behavioral Risk Factor Surveillance System (BRFSS), 2017. Obesity was defined as a body mass index of 30 or higher based on self-reported weight in kilograms divided by the square of the height in meters. Prevalence estimates reflect changes in BRFSS methods that started in 2011. These estimates should not be compared to prevalence estimates before 2011. No area had a prevalence of <20%, and all had sufficient data to determine prevalence. [A tabular version of this figure is also available.]
For the past 4 years, CDC has published more detailed state and territorial maps that combine 3 years of data to create stable estimates of self-reported adult obesity by race/ethnicity. These maps help demonstrate the geographic and racial/ethnic disparities in obesity burden. Although the previously released overall state-specific maps demonstrate where obesity may be influencing health, health care costs, well-being, and productivity across states and regions, the racial and ethnic maps for 2015 through 2017 illustrate that the negative effects are disproportionately burdensome for particular populations. Combined data for 2015 through 2017 allowed for assessment by major racial/ethnic categories and found that non-Hispanic black adults had the highest prevalence of obesity (38.4%) overall, followed by Hispanic adults (32.6%) and non-Hispanic white adults (28.6%). To identify areas of highest burden, we used a cut point of 35%. We chose this cut point because it was a somewhat natural breaking point in the data and roughly reflected areas with the highest burden. By using this cut point, we found that overall, 31 states and the District of Columbia had an obesity prevalence of 35% or higher among non-Hispanic black adults; 8 states had an obesity prevalence of 35% or higher among Hispanic adults; and only 1 state had an obesity prevalence of 35% or higher among non-Hispanic white adults (Figure 2).
Figure 2. Prevalence of self-reported obesity among non-Hispanic white, non-Hispanic black, and Hispanic adults, by state and territory, Behavioral Risk Factor Surveillance System, 2015–2017. Obesity was defined as a body mass index of 30 or higher based on self-reported weight in kilograms divided by the square of the height in meters. Prevalence estimates reflect changes in BRFSS methods that started in 2011. These estimates should not be compared to prevalence estimates before 2011. Areas are indicated as having insufficient data if they had a sample size of less than 50 or a relative standard error (dividing the standard error by the prevalence) of 30% or more. [A tabular version of this figure is also available.]
Although the exact causes of these differences in obesity are not all known, they likely in part reflect differences in social and economic advantage related to race or ethnicity (12). This concept aligns with other, more general statements about health disparities explaining that disparities are “closely linked with social, economic, and/or environmental disadvantage” and show the effect where groups of people “have systematically experienced greater social and/or economic obstacles to health . . . based on their racial or ethnic group” (13). Underlying risks that may help explain disparities in obesity prevalence among non-Hispanic black and the Hispanic populations could include lower high school graduation rates, higher rates of unemployment, higher levels of food insecurity, greater access to poor quality foods, less access to convenient places for physical activity, targeted marketing of unhealthy foods, and poor access to health care or referrals to convenient community organizations that aid family-management or self-management resources (14–17).
From a large number of high-quality applicants, in 2018 DNPAO competitively funded 16 state health departments (or a similar entity), 15 land grant colleges and universities, and 31 community-focused grantees to work over the course of 5 years with multiple sectors and coalitions to prioritize and implement best practices to increase healthy eating and active living to prevent obesity and other chronic diseases. With technical assistance from DNPAO public health specialists and subject matter experts, grantees use a menu of evidence-based strategies and performance metrics to develop their implementation plan, work plan, and evaluation process. To obtain the largest public health impact from limited resources, grantees are asked to focus their work on populations that have the greatest disparities and needs. Strategies for DNPAO grantees include establishing healthy nutrition standards in settings such as workplaces, hospitals, early care and education (ECE), after-school and recreational programs, and faith-based organizations; working with food vendors, distributors, and producers to increase procurement and sales of healthier foods; improving programs and systems at the state and local level to increase access to healthier food; and implementing community planning and transportation plans that support safe and accessible physical activity by connecting sidewalks, paths, bike routes, public transit with homes, ECE, schools, parks and recreation centers, and other everyday destinations.
As an example of reaching vulnerable individuals, state health department grantees may focus obesity prevention efforts at a state level by targeting early obesity risk through system changes in the ECE setting through state licensing, state subsidy, or state quality rating systems. States may pair these efforts with promoting the use of food reimbursement programs for meals that meet minimum nutritional standards among centers serving low-income children. In addition, state health departments may work to set a standard for implementation of food service guidelines so other government entities, work sites, park and recreation centers, and hospitals can follow that example and obtain the needed technical assistance for spreading implementation. State health department grantees may also work across sectors (such as the transportation and community planners) to improve environmental supports for physical activity through the implementation of master plans and land-use interventions. These efforts to increase access to safe and convenient places for physical activity are generally targeted to geographical areas with the highest burden of obesity and chronic disease. Such efforts can include connecting neighborhoods with sidewalks, paths, bike routes, and public transit that lead to local schools, parks and recreation centers, and local businesses.
DNPAO manages 2 additional public health practice programs that have had success in reducing the risk factors for obesity in populations with the greatest disparities. These programs include the Racial and Ethnic Approaches to Community Health (REACH) program and the High Obesity Program (HOP). The REACH program focuses on improving health for racial and ethnic groups with the highest disease burden. Obesity reduction among the black population is often a key goal for REACH recipients. For example, from 2008 through 2012, 14 REACH grantees implemented strategies to address disparities in obesity among black populations. These strategies included expanding healthy food choices in grocery stores, creating neighborhood farmers markets, implementing Complete Street policies, and improving walkability and safety of neighborhood streets. The prevalence of obesity decreased about 1 percentage point in these REACH communities, but not in the comparison populations during the same time (18).
Land grant universities in states where counties have more than a 40% prevalence of adult obesity are eligible to apply for HOP. These grantees work in predominantly rural areas where residents may have less access to healthy foods and fewer opportunities to be physically active, which may increase their risk of obesity (19–21). HOP grantees use the same menu of DNPAO evidence-based strategies to improve nutrition and physical activity to reduce obesity and other chronic diseases; however, they might tailor their implementation plan given the rural nature of their target population with the highest risk of obesity. Examples include work at the Texas AgriLife Extension (Texas A&M University), which established a farmers market at a local community center to help increase access to fresh produce. Since the creation of this market, more than 800 community members purchased over 12,000 pounds of fresh fruits and vegetables. Another example is the work of the extension staff in Ouachita County (University of Arkansas) at a low-income housing complex to improve access to physical activity for residents with limited mobility. They identified a walking path and developed signs to indicate how many laps equaled a half-mile. Eighty-four percent of residents now walk regularly and use the path at least 1 or 2 times a week (22).
Implementing approaches that take into account racial and ethnic disparities is critical to addressing the high burden of obesity and its many negative consequences. Although a population-based approach is needed to increase availability and access to healthy foods and beverages and safe and convenient places for physical activity for all Americans, targeted approaches are needed to address the risks that drive the disparities. Such an approach will mean taking into account food insecurity, safe drinking water, and cultural nutrition and physical activity patterns as well as environmental and policy contexts that influence the risk. Efforts may need to include more attention to upstream determinants of health or attributes of the communities where the populations with the highest burden live. The findings linking neighborhood features to one’s health status illustrate how a community can influence risk of many chronic health conditions, including obesity. For example, a study of neighborhoods in 3 US metropolitan regions (San Diego, Seattle, and Baltimore) from 2009 to 2010 assessed pedestrian environment features for walkability factors (eg, density). The study found that “across all three regions, low-income neighborhoods and neighborhoods with a high proportion of racial/ethnic minorities had poorer aesthetics and social elements (eg, graffiti, broken windows, litter) than neighborhoods with higher median income or fewer racial/ethnic minorities” (20). Likewise, if marketing of unhealthy products and/or fast-food establishments are unequally distributed across a community or are clustered near schools, communities may consider addressing this issue paired with improving healthy offerings (16,23,24). For individuals from the groups with the largest disparities, it is also important to focus attention on enhancing access to and reimbursement for quality health care services for growth assessment and obesity screening, and for persons with obesity and disease risk, appropriate referral to evidence-based healthy weight or prediabetes management programs and other treatment modalities (25,26).
In isolation, DNPAO resources, equivalent to $0.31 investment per American per year, will not be able to prevent obesity among at-risk Americans nor reduce the racial and ethnic disparities in the national burden of obesity. In addition to public health, many partners are needed, including policy makers, state and local organizations, business and community leaders, ECE, schools, industry, federal agencies, health care systems and providers, payers, faith-based organizations, community planners, food growers and distributors, families, and individuals. Using combined approaches, these partners should strive to best improve the ability to prevent obesity and its consequences for those with the burden. Such multisector partnerships can create positive changes at the community level to promote healthy eating and active living in areas where individuals may be at risk for obesity because of where they live and work. These focus areas could include making it easier for families with children to buy healthy, affordable foods and beverages near their homes; helping to provide access to safe, free drinking water in places such as community parks, recreation areas, child care centers, and schools; helping local schools open up gyms, playgrounds, and sports fields during nonschool hours so more children can safely play; increasing the number of safe and accessible sidewalks and bike paths to schools, parks and everyday destinations; and helping schools and ECE providers use best practices for improving nutrition and increasing physical activity. Demonstrated success in these approaches would be reductions in the disparities in upstream indicators (ie, improved community and behavioral determinants of health) and reductions in the obesity burden that is evident in CDC’s childhood obesity data and the maps above.
DNPAO is committed to supporting efforts to reduce racial and ethnic disparities in obesity by continuing to share what is working through partners and grantees, to develop tools that aid community engagement and the implementation of evidenced-based interventions, and to track obesity and its risk factors. Each sector and organization has a role to play in being part of the solution. To reduce the current disparities that exist in the burden of obesity, all parts of society need to relentlessly and intentionally work to address the causes of these disparities to help give all a fair chance at health.
No financial support was received for this work. The findings and conclusions of this report are those of the authors and do not necessarily reflect the official position of CDC.
Corresponding Author: Ruth Petersen, MD, MPH, National Center for Chronic Disease Prevention and Health Promotion, Centers for Disease Control and Prevention, 4770 Buford Hwy, MS S107-5, Atlanta, GA 30341-3717. Telephone: 770-488-6001. Email: [email protected] .
Author Affiliations: 1 National Center for Chronic Disease Prevention and Health Promotion, Centers for Disease Control and Prevention, Atlanta, Georgia.
- Hales CM, Carroll MD, Fryar CD, Ogden CL. Prevalence of obesity among adults and youth: United States, 2015–2016. NCHS Data Brief 2017;(288):1–8. PubMed
- Centers for Disease Control and Prevention. Adult obesity causes and consequences. https://www.cdc.gov/obesity/adult/causes.html. Accessed September 14, 2018.
- Finkelstein EA, Trogdon JG, Cohen JW, Dietz W. Annual medical spending attributable to obesity: payer-and service-specific estimates. Health Aff (Millwood) 2009;28(5):w822–31. CrossRef PubMed
- Trogdon JG, Finkelstein EA, Hylands T, Dellea PS, Kamal-Bahl SJ. Indirect costs of obesity: a review of the current literature. Obes Rev 2008;9(5):489–500. CrossRef PubMed
- Mission Readiness. Too fat to fight: retired military leaders want junk food out of America’s schools. http://cdn.missionreadiness.org/MR_Too_Fat_to_Fight-1.pdf. Accessed September 14, 2018.
- Pan L, Freedman DS, Sharma AJ, Castellanos-Brown K, Park S, Smith RB, et al. Trends in obesity among participants aged 2–4 years in the Special Supplemental Nutrition Program for Women, Infants, and Children — United States, 2000–2014. MMWR Morb Mortal Wkly Rep 2016;65(45):1256–60. CrossRef PubMed
- Ward ZJ, Long MW, Resch SC, Giles CM, Cradock AL, Gortmaker SL. Simulation of growth trajectories of childhood obesity into adulthood. N Engl J Med 2017;377(22):2145–53. CrossRef PubMed
- Healthy Eating Research. Feeding guidelines for infants and young toddlers: a responsive parenting approach feeding. https://healthyeatingresearch.org/research/feeding-guidelines-for-infants-and-young-toddlers-a-responsive-parenting-approach/. Accessed September 14, 2018.
- Davison KK, Lawson HA, Coatsworth JD. The Family-centered Action Model of Intervention Layout and Implementation (FAMILI): the example of childhood obesity. Health Promot Pract 2012;13(4):454–61. CrossRef PubMed
- Ding D, Sallis JF, Kerr J, Lee S, Rosenberg DE. Neighborhood environment and physical activity among youth: a review. Am J Prev Med 2011;41(4):442–55. CrossRef PubMed
- Centers for Disease Control and Prevention. Adult obesity prevalence maps. https://www.cdc.gov/obesity/data/prevalence-maps.html. Accessed September 23, 2018.
- Krueger PM, Reither EN. Mind the gap: race/ethnic and socioeconomic disparities in obesity. Curr Diab Rep 2015;15(11):95. CrossRef PubMed
- US Department of Health and Human Services. National stakeholder strategy for achieving health equity. https://www.minorityhealth.hhs.gov/npa/files/Plans/NSS/CompleteNSS.pdf. Accessed September 12, 2018.
- Romieu I, Dossus L, Barquera S, Blottière HM, Franks PW, Gunter M, et al. Energy balance and obesity: what are the main drivers? Cancer Causes Control 2017;28(3):247–58. CrossRef PubMed
- University of Wisconsin Population Health Institute. County health rankings key findings 2018. http://www.countyhealthrankings.org/explore-health-rankings/rankings-reports/2018-county-health-rankings-key-findings-report. Accessed September 12, 2018.
- University of Connecticut Rudd Center for Food Policy and Obesity. Increasing disparities in unhealthy food advertising targeted to Hispanic and black youth. http://uconnruddcenter.org/files/Pdfs/TargetedMarketingReport2019.pdf. Accessed January 19, 2019.
- US Department of Agriculture, Economic Research Service. Interactive charts and highlights. 2018. https://www.ers.usda.gov/topics/food-nutrition-assistance/food-security-in-the-us/interactive-charts-and-highlights/. Accessed September 12, 2018.
- Liao Y, Siegel PZ, Garraza LG, Xu Y, Yin S, Scardaville M, et al. Reduced prevalence of obesity in 14 disadvantaged black communities in the United States: a successful 4-year place-based participatory intervention. Am J Public Health 2016;106(8):1442–8. CrossRef PubMed
- National Advisory Committee on Rural Health and Human Services. Social determinants of health: National Advisory Committee on Rural Health and Human Services Policy Brief, January 2017. https://www.hrsa.gov/advisorycommittees/rural/publications/nac_brief_social_determinants_health.pdf. Accessed September 14, 2018.
- Thornton CM, Conway TL, Cain KL, Gavand KA, Saelens BE, Frank LD, et al. Disparities in pedestrian streetscape environments by income and race/ethnicity. SSM Popul Health 2016;2:206–16. CrossRef PubMed
- Grimm KA, Moore LV, Scanlon KS; Centers for Disease Control and Prevention. Access to healthier food retailers — United States, 2011. MMWR Suppl 2013;62(3):20–6. PubMed
- Centers for Disease Control and Prevention. State and local programs. High Obesity Program (2014–2018). https://www.cdc.gov/nccdphp/dnpao/state-local-programs/hop-1809/past-program.html. Accessed January 22, 2019.
- Kwate NO, Yau CY, Loh JM, Williams D. Inequality in obesigenic environments: fast food density in New York City. Health Place 2009;15(1):364–73. CrossRef PubMed
- Kwate NO, Loh JM. Separate and unequal: the influence of neighborhood and school characteristics on spatial proximity between fast food and schools. Prev Med 2010;51(2):153–6. CrossRef PubMed
- Grossman DC, Bibbins-Domingo K, Curry SJ, Barry MJ, Davidson KW, Doubeni CA, et al. Screening for obesity in children and adolescents: US Preventive Services Task Force recommendation statement. JAMA 2017;317(23):2417–26. CrossRef PubMed
- Centers for Disease Control and Prevention. Childhood Obesity Research Demonstration (CORD) 1.0. https://www.cdc.gov/obesity/strategies/healthcare/cord1.html. Accessed January 8. 2019.
The opinions expressed by authors contributing to this journal do not necessarily reflect the opinions of the U.S. Department of Health and Human Services, the Public Health Service, the Centers for Disease Control and Prevention, or the authors’ affiliated institutions.
Exit Notification / Disclaimer Policy
- The Centers for Disease Control and Prevention (CDC) cannot attest to the accuracy of a non-federal website.
- Linking to a non-federal website does not constitute an endorsement by CDC or any of its employees of the sponsors or the information and products presented on the website.
- You will be subject to the destination website's privacy policy when you follow the link.
- CDC is not responsible for Section 508 compliance (accessibility) on other federal or private website.
- Free Samples
- Premium Essays
- Editing Services Editing Proofreading Rewriting
- Extra Tools Essay Topic Generator Thesis Generator Citation Generator GPA Calculator Study Guides Donate Paper
- Essay Writing Help
- About Us About Us Testimonials FAQ
- Studentshare
- Relationship between poverty and obesity
Relationship between poverty and obesity - Essay Example
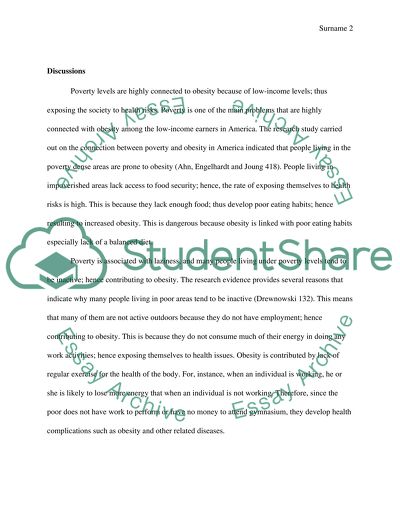
- Subject: Literature
- Type: Essay
- Level: College
- Pages: 7 (1750 words)
- Downloads: 3
- Author: izaiahschmeler
Extract of sample "Relationship between poverty and obesity"
- The Relationship Between Host Community And Guest Tourism
- Cited: 0 times
- Copy Citation Citation is copied Copy Citation Citation is copied Copy Citation Citation is copied
CHECK THESE SAMPLES OF Relationship between poverty and obesity
The concerns of obesity in children and young people: physical activity and sedentary behaviours, the correlation between obesity and poverty, obesity and health reform, does poverty make people obese, race, place, and obesity, the cause of obesity can be found in an obese society, correlation between obesity and poverty, obesity, poverty and diabetes.
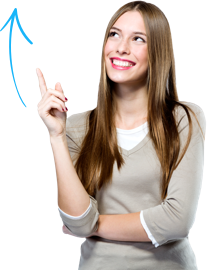
- TERMS & CONDITIONS
- PRIVACY POLICY
- COOKIES POLICY
- ConscienHealth
- Advocacy Organizations
- Affiliates & Advocates
- Service Partners
- Publications
- LATEST NEWS

Phones, Social Media, Mental Health, and Obesity
Skimming the headlines, it would be easy to think that the combination of mobile phones and social media are responsible for quite a range of our current ills – including mental health and obesity. If you want to dig deeper, you can find a whole tome on the subject from Jonathan Haidt. He has woven quite a compelling narrative. Phones have stolen the joy of play from childhood, he says. They’ve created an Anxious Generation .
A string of anecdotes and correlations might make a compelling narrative. But it does not add up to compelling evidence. It is not at all clear exactly what lies at the root of important problems we face with mental and physical health.
The reality of how we interact with technology is more complex than popular narratives suggest. How we use technology can be more important than the technology itself.
Phones and Adolescent Mental Health
Writing in the Atlantic, developmental psychologist Candice Odgers suggests that Haidt is overstating his case:
“Since 2008, I have studied 10-to-15-year-olds using their mobile phones, with the goal of testing how a wide range of their daily experiences, including their digital-technology use, influences their mental health. My colleagues and I have repeatedly failed to find compelling support for the claim that digital-technology use is a major contributor to adolescent depression and other mental-health symptoms.
“Many other researchers have found the same.”
The challenge of understanding causality requires more than a good narrative.
Phones and Obesity
Researchers have found suggestions that there is a relationship between excessive smartphone use and obesity. But causality is not so clear in this case, either. A recent study in BMC Public Health sums up the state of knowledge on this:
“Results indicate that excessive screen time on smartphones does have complex associations with youth health. Further investigation with more robust study designs is needed to inform smartphone-specific screen time guidelines for youth.”
So yes, more research is needed for a genuine understanding of causality in the relationships among health, phones, and social media. Moral panic is not adequate for solving complex problems.
Click here for access to Odgers’ essay in the Atlantic and here for the study phones and obesity in BMC Public Health.
Waiting with a Phone, photograph by Ted Kyle / ConscienHealth
Subscribe by email to follow the accumulating evidence and observations that shape our view of health, obesity, and policy.
Your email:
May 26, 2024
3 Responses to “Phones, Social Media, Mental Health, and Obesity”
May 26, 2024 at 7:37 am, Elizabeth Guckenheimer said:
Adolescents are distressed about climate change, school shootings, wars, poverty, student debt, stripping away of reproductive rights, homelessness, silencing, global epidemics, inequality and injustice, and many more real issues including political violence and the rhetoric of cruelty. Blaming cell phones is a distraction and, in my view, an excuse not to fix the deep problems that we, the adults have created and all too often, turn our backs on. My advice to the expert adults is to stop talking and start listening. What sound like mental health problems may resolve into real concerns about the present and the future. And after you have listened, take action.
May 26, 2024 at 7:39 am, Joe Gitchell said:
Drat, Ted! Now I’m going to have to read the Odgers article properly!
I am very fond of Jon Haidt and found his essay on The Atlantic compelling (TBC, his narrative is a lot more complicated/sophisticated than “it’s the smartphones”)–but that shouldn’t have me turn off my curiosity, right??!!
Good on the Atlantic to offer readers both takes, I’d say.
Oh, and I do have “feelings” when it comes to the journal, BMC Public Health (see below). Don’t get me started on that unless you have a lot of time to kill!
Haidt in The Atlantic: https://www.theatlantic.com/technology/archive/2024/03/teen-childhood-smartphone-use-mental-health-effects/677722/
Insight in to Joe’s BMC Public Health frustration: https://retractionwatch.com/2023/10/04/authors-file-complaint-with-publisher-as-journal-retracts-vaping-paper/
May 26, 2024 at 7:42 am, Ted said:
Yes, Joe, BMC Public Health publishes a lot of dubious stuff. And Jonathan Haidt is one smart cookie.
I started writing this post with the thought that phones and social media are clearly problematic. But I could not come up with compelling evidence for that. So the best answer I can offer is, “It’s complicated.”
Leave a Reply
Search this blog.
- access to care
- anti-obesity medicines
- bariatric surgery
- childhood obesity
- evidence based medicine
- food industry
- food policy
- health disparities
- health economics
- health insurance
- health outcomes
- health reporting
- Obesity Action Coalition
- obesity care
- obesity causes
- obesity prevention
- obesity statistics
- obesity treatment
- physical activity
- public health
- scientific integrity
- scientific rigor
- semaglutide
- The Obesity Society
- weight bias
- weight discrimination
- weight loss
- weight stigma
- February 2024
- January 2024
- December 2023
- November 2023
- October 2023
- September 2023
- August 2023
- February 2023
- January 2023
- December 2022
- November 2022
- October 2022
- September 2022
- August 2022
- February 2022
- January 2022
- December 2021
- November 2021
- October 2021
- September 2021
- August 2021
- February 2021
- January 2021
- December 2020
- November 2020
- October 2020
- September 2020
- August 2020
- February 2020
- January 2020
- December 2019
- November 2019
- October 2019
- September 2019
- August 2019
- February 2019
- January 2019
- December 2018
- November 2018
- October 2018
- September 2018
- August 2018
- February 2018
- January 2018
- December 2017
- November 2017
- October 2017
- September 2017
- August 2017
- February 2017
- January 2017
- December 2016
- November 2016
- October 2016
- September 2016
- August 2016
- February 2016
- January 2016
- December 2015
- November 2015
- October 2015
- September 2015
- August 2015
- February 2015
- January 2015
- December 2014
- November 2014
- October 2014
- September 2014
- August 2014
- February 2014
- January 2014
- December 2013
- November 2013
- October 2013
- September 2013
- August 2013
- February 2013
- January 2013
- December 2012
- November 2012
- October 2012
- September 2012
- August 2012
- February 2012
- January 2012
- October 2011
- February 2011
- January 2011
- December 2010
- October 2010
- September 2010
- October 2009
- August 2009
- Consumer Trends
- Food & Nutrition
- Food Industry
- Health & Obesity
- Health Policy
- Scientific Meetings & Publications

outstanding advocacy
Recognized by The Obesity Society & Obesity Action Coalition
©2009-2013 ConscienHealth. All rights reserved. | Website Design by Mariela Antunes | Hosting by DTS
- Share full article

How Free School Meals Went Mainstream
Over the past decade, many more schools started to offer free meals to all children, regardless of family income.
More than 21 million American children now attend schools that offer free meals regardless of family income — a tenfold increase from 2010. Credit... Will Warasila for The New York Times
Supported by

By Susan Shain
- May 21, 2024
Kurt Marthaller, who oversees school food programs in Butte, Mont., faces many cafeteria-related challenges: children skipping the lunch line because they fear being judged, parents fuming about surprise bills they can’t afford, unpaid meal debts of $70,000 districtwide.
But at nearly half of Mr. Marthaller’s schools, these concerns have vanished. At those schools, all students get free breakfast and lunch, regardless of their family’s income. At one school, West Elementary, children grab milk cartons, cereal bars and bananas from folding tables on their way to class, with almost 80 percent of students eating breakfast there each school day.
“We’ve done a lot of good things to feed kids here in Butte,” Mr. Marthaller said. But introducing universal free meals, he added, was “probably the best thing we ever did.”
Advocates for free school meals have pushed for them to be offered to every student for a long time, but saw significant progress in the last decade and a half. Their first big win came quietly, in 2010, when Congress passed an under-the-radar policy called the community eligibility provision , which made it easier for schools to serve free meals to all. Then, during the Covid-19 pandemic, the federal government let every public school student eat for free, rapidly transforming the nation’s thinking around school meals.
Eight states have passed their own universal free meal legislation since the federal largesse ended in 2022. Dozens more have introduced similar bills or have one in the works. A surge of additional schools — nearly 7,000 — have signed up for the community eligibility program that West Elementary participates in: As of the 2022-23 school year, roughly four in 10 public schools were enrolled.
In total, more than 21 million American children now attend schools that offer free meals to all — a tenfold increase from 2010. “Schools did not want to go back to charging some kids,” said Crystal FitzSimons, the director of child nutrition programs and policy at the nonprofit Food Research and Action Center. “They saw the huge benefits of providing free meals to all students: supporting families, supporting kids, changing the culture of the cafeteria.”

A tale of two lunches
From above, Butte looks as if it were carved out of a mountain range with an ice cream scoop. Once known as “ the richest hill on Earth ” for its copper mines, Butte was one of the largest cities west of the Mississippi in its heyday. Today it has approximately 35,000 residents, many of whom have been there for generations.
Amber Moore lives on the east side of town, in a blue house with a view of Our Lady of the Rockies, a 90-foot-tall mountaintop statue of the Virgin Mary. A stay-at-home mom, she lives with her husband, Jake, a telecommunications technician, and their five children, four cats and two dogs.
The Moores’ house is zoned for Whittier Elementary School, which, unlike West, does not participate in community eligibility and does not have universal free meals. So five nights a week, Ms. Moore clears off a patch of her kitchen counter and sets out five lunchboxes. In goes the SunnyD, the cheese stick, the ham-and-cheese sandwich, the Lay’s, the clementine and the fruit snacks. Ms. Moore uses three loaves of bread each week just on lunches. Add breakfast to the equation and she spends about $250 per month on the two meals.
“That’s like a power bill,” she said. “It’s not a small amount.” That bill was eliminated during the pandemic. For those two years, Ms. Moore’s children ate breakfast and lunch at school every day. Then, like the majority of schools around the country, Whittier returned to charging for meals in August 2022. And Ms. Moore returned to packing lunches.
Though low-income students at all American public schools technically qualify for free and reduced-price meals, one-third of eligible students do not participate, according to a Food Research and Action Center estimate. One reason is stigma: Because the school-provided meal, often called “hot lunch,” has long been viewed as a form of welfare , eating it can be a painful marker of poverty.
Parents may also fail to complete the requisite paperwork because they have volatile incomes, face language barriers or are embarrassed about their finances. (As Mr. Marthaller put it, “I think it’s a pride thing.”) Others may be struggling but ineligible: To receive free or reduced-price meals, a family of four must earn less than $55,500 per year . When meals are free to all, advocates say, these obstacles are eliminated.
The Moores don’t qualify for reduced-price meals: Mr. Moore’s income puts them over the limit by $465 a month. “It’s one of those frustrating things,” Ms. Moore said. “I’m sure a lot of parents are in that middle area where it’s like, well, shoot.”
‘Their brains are fired up’
The push for a national school lunch program initially came during the Great Depression , when children were hungry and farmers had surpluses to sell. In the 1960s, school breakfast was added. School meals have since become the nation’s second-largest food safety net , after food stamps.
As childhood obesity rates soared, however, the lunch program was criticized as a contributing factor. In 2010, the first lady, Michelle Obama, who made childhood obesity a signature issue, pressed for the passage of the Healthy, Hunger-Free Kids Act, which led school cafeterias to serve more fruits, vegetables and whole grains and less salt, sugar and unhealthy fats. Policymakers also saw it as an opportunity to feed more hungry children. So, without much fanfare, they tucked the community eligibility provision, or C.E.P., inside the bill.
Under the C.E.P., offering universal free meals became less cumbersome: If 40 percent of a school or district’s students qualify for programs like food stamps or Head Start or are homeless, migrants or in foster care, it can serve free meals to everyone . It does not need to collect individual applications; it simply applies for the program and is qualified for the next four years.
Even the C.E.P.’s architects have been surprised by its impact. “I certainly did not foresee that a little more than 10 years later, 20 million kids would be enrolled in schools that were doing C.E.P.,” said Cindy Long, administrator of the Food and Nutrition Service of the Agriculture Department, who helped design the 2010 act.
The benefits of universal free meals are myriad, experts say. Most crucially, more children eat , helping to combat hunger in a country where 17 percent of households with children experience food insecurity. They also eat more healthful food . When students are well fed, they learn better: Some research suggests that schoolwide free meals can improve test scores , attendance and behavior . Such programs also help schools, by lessening paperwork, and parents, by reducing food expenses .
Like most people, Amanda Denny, a fourth-grade teacher at West Elementary, had never heard of the C.E.P. But she has seen the difference that universal school meals can make. “In my classroom, when those kids do eat breakfast, they are ready to start their day,” she said. “Their brains are fired up, and they’re ready to learn.”
Last October, the threshold to qualify for the C.E.P. was lowered , making more schools and districts eligible. The Moores’ school, Whittier, is now eligible, as are most other schools in Butte. But because of how the federal government calculates reimbursements for school meals, only schools with high populations of needy students break even using the C.E.P.; the rest usually lose money by participating . Advocates have been pushing for higher reimbursement rates so more schools can afford the program.
But in one draft federal budget, House Republicans proposed ending the C.E.P. altogether, arguing that public funds shouldn’t pay for wealthy children to eat lunch . Jonathan Butcher, an education researcher at the Heritage Foundation, believes school lunch aid has ballooned far beyond its original intent. He would like to see the provision repealed.
“They’re not just saying, ‘How can we better get food to kids that need it? They’re saying: ‘Eh, let’s not bother with the details. Let’s just give it to everybody’,” Mr. Butcher said. “That’s not being respectful to taxpayers, nor is it advancing the idea that we should improve a very wasteful school lunch program.”
Most of the states that have passed their own free school meal legislation did so with bipartisan support. To pay for the programs, California, Maine, Minnesota, New Mexico, Vermont and Michigan tapped general revenue or education funds ; Massachusetts and Colorado raised taxes on their highest earners. (In Colorado, the program has been so popular that it is facing a $56 million funding shortfall this year.)
Ms. FitzSimons, of the Food Research and Action Center, believes food is just as integral to public education as transportation and books, which are typically offered to students at no charge. “We spend billions of dollars on funding for education,” she said. “If kids are sitting in class unable to learn because they’re hungry, because their stomachs are growling, then we’re wasting our money.”
At West Elementary, a stuffed bison head presides over the cafeteria. There is no cash register, and at lunchtime, children whiz through the line, grabbing trays of applesauce and teriyaki-doused “steakettes.” They plop down next to friends eating peanut-butter-and-jelly sandwiches from colorful lunchboxes.
Ryder is a third grader who wants to be a YouTuber or a police officer when he grows up (and, he said, “if that doesn’t work out, NASA”). He was shocked to learn that children at other schools have to pay for lunch. “That’s mean,” he said. His friend Louis agreed: “That is cold.”
Things were different for Kaylee Rabson, a fifth-generation Butte resident whose son attends West. “When we were younger, it was definitely very separated,” she said. “Like, if you went to hot lunch, you were kind of embarrassed.” Now, all her son’s friends eat the school lunch — at least when pizza or walking tacos (ground beef, veggies and cheese in a Doritos bag) are on the menu.
“It’s ‘I eat hot lunch because it sounds good, not because I need to.’ It really has erased the stigma,” Ms. Rabson said. “They’re just there having lunch together.”
What are your experiences with free or reduced-price lunch?
This story was published by The New York Times’s Headway team in partnership with High Country News.
The Headway initiative is funded through grants from the Ford Foundation, the William and Flora Hewlett Foundation and the Stavros Niarchos Foundation (SNF), with Rockefeller Philanthropy Advisors serving as a fiscal sponsor. The Woodcock Foundation is a funder of Headway’s public square. Funders have no control over the selection or focus of stories or the editing process and do not review stories before publication. The Times retains full editorial control of the Headway initiative.
Susan Shain is a reporting fellow for Headway, a section of The Times that explores the world's challenges through the lens of progress. More about Susan Shain
Advertisement

An official website of the United States government
The .gov means it’s official. Federal government websites often end in .gov or .mil. Before sharing sensitive information, make sure you’re on a federal government site.
The site is secure. The https:// ensures that you are connecting to the official website and that any information you provide is encrypted and transmitted securely.
- Publications
- Account settings
Preview improvements coming to the PMC website in October 2024. Learn More or Try it out now .
- Advanced Search
- Journal List
- HHS Author Manuscripts

The Epidemiology of Obesity: A Big Picture
The epidemic of overweight and obesity presents a major challenge to chronic disease prevention and health across the life course around the world. Fueled by economic growth, industrialization, mechanized transport, urbanization, an increasingly sedentary lifestyle, and a nutritional transition to processed foods and high calorie diets over the last 30 years, many countries have witnessed the prevalence of obesity in its citizens double, and even quadruple. Rising prevalence of childhood obesity, in particular, forebodes a staggering burden of disease in individuals and healthcare systems in the decades to come. A complex, multifactorial disease, with genetic, behavioral, socioeconomic, and environmental origins, obesity raises risk of debilitating morbidity and mortality. Relying primarily on epidemiologic evidence published within the last decade, this non-exhaustive review discusses the extent of the obesity epidemic, its risk factors—known and novel—, sequelae, and economic impact across the globe.
1. Introduction
Obesity is a complex, multifactorial, and largely preventable disease ( 1 ), affecting, along with overweight, over a third of the world’s population today ( 2 , 3 ). If secular trends continue, by 2030 an estimated 38% of the world’s adult population will be overweight and another 20% will be obese ( 4 ). In the USA, the most dire projections based on earlier secular trends point to over 85% of adults being overweight or obese by 2030 ( 5 ). While growth trends in overall obesity in most developed countries seem to have leveled off ( 2 ), morbid obesity in many of these countries continues to climb, including among children. In addition, obesity prevalence in developing countries continues to trend upwards toward US levels.
Obesity is typically defined quite simply as excess body weight for height, but this simple definition belies an etiologically complex phenotype primarily associated with excess adiposity, or body fatness, that can manifest metabolically and not just in terms of body size ( 6 ). Obesity greatly increases risk of chronic disease morbidity—namely disability, depression, type 2 diabetes, cardiovascular disease, certain cancers—and mortality. Childhood obesity results in the same conditions, with premature onset, or with greater likelihood in adulthood ( 6 ). Thus, the economic and psychosocial costs of obesity alone, as well as when coupled with these comorbidities and sequealae, are striking.
In this article, we outline the prevalence and trends of obesity, then review the myriad risk factors to which a preventive eye must be turned, and finally present the costs of obesity in terms of its morbidity, mortality, and economic burden.
2. Classification of Body Weight in Adults
The current most widely used criteria for classifying obesity is the body mass index (BMI; body weight in kilograms, divided by height in meters squared, Table 1 ), which ranges from underweight or wasting (<18.5 kg/m 2 ) to severe or morbid obesity (≥40 kg/m 2 ). In both clinical and research settings, waist circumference, a measure of abdominal adiposity, has become an increasingly important and discriminating measure of overweight/obesity ( 7 ). Abdominal adiposity is thought to be primarily visceral, metabolically active fat surrounding the organs, and is associated with metabolic dysregulation, predisposing individuals to cardiovascular disease and related conditions ( 8 ). Per internationally used guidelines of metabolic syndrome—a cluster of dysmetabolic conditions that predispose individuals to cardiovascular disease of which abdominal adiposity is one component—a waist circumference resulting in increased cardiovascular risk is defined as ≥94 cm in European men, and ≥80 cm in European women, with different cut points recommended in other races and ethnicities (e.g., ≥90 and ≥80 cm in men and women, respectively, in South Asians, Chinese, and Japanese) ( 8 , 9 ).
Common Classifications of Body Weight in Adults and Children
Abbreviations used: BMI, body mass index; IOTF, International Obesity Task Force; SD, standard deviation; WHO, World Health Organization; WH weight-for-height; Z, z score.
3. Classification of Body Weight in Children
In children, body weight classifications ( Table 1 ) differ from those of adults because body composition varies greatly as a child develops, and further varies between boys and girls primarily owing to differences in sexual development and maturation. The World Health Organization (WHO) Child Growth Standards are the most widely currently used classification system of weight and height status for children from birth to 5 years old, based on data from children in six regions across the globe born and raised in optimal conditions ( 10 ). In 2007, the WHO published updated growth references combining the 1977 National Center for Health Statistics (NCHS)/WHO growth reference and the 2006 WHO Child Growth Standards to create the most recent BMI-for-age references for individuals aged 5–19 years ( 11 ). Thus, the latest WHO guidelines are designed to represent relatively seamless standards and references from birth all the way into late adolescence/early adulthood.
In the USA, the Centers for Disease Control and Prevention (CDC) currently use the 2000 CDC growth references based on 1963–1994 US children’s data, to determine age- and sex-specific BMI percentiles for children aged 2–19 years ( 12 ). Overweight is defined in US children as age- and sex-specific BMI ≥85th and <95th percentile, while obesity is ≥95th percentile ( 13 ). Cut points for severe obesity in childhood have been proposed in recognition of the alarming growing prevalence of this extreme condition, defined as the 99th BMI percentile ( 13 ) or 120% of the 95th percentile ( 14 ). For US children <2 years old, the CDC currently uses the 2006 WHO Child Growth Standards, described above ( 15 ).
4. Prevalence and Trends
4.1. adult obesity—us and europe.
The first indications that obesity was taking on epidemic proportions originated in the USA and Europe. With few restrictions on access to or availability of food, the prevalence of overweight and obesity in the USA climbed virtually unmitigated over the last 50 years. Today, those who are overweight (BMI 25–<30 kg/m 2 ) or obese (BMI ≥30 kg/m 2 ) in the USA eclipse two-fold the numbers of those who are normal weight ( 16 ). In US adults, 1960–1994 trends showed that while levels of overweight hovered at approximately 31% over the time period, in contrast, age-adjusted obesity jumped from 13 to 23%, bringing the crude prevalence of overweight or obesity to 55% of the American population ( 17 ). Unfortunately, 1994 did not represent the endpoint of the upward trend, as the following decade saw adult obesity rise from 23 to 32% by 2003–2004 ( 16 ). In the last 10 years, national estimates of obesity seem to indicate that the steady upward trend of obesity in Americans has leveled off at a prevalence of about 35% ( 16 ) ( Figure 1 ), perhaps having reached some “Malthusian” obesity limit. However, certain subpopulations are faring worse than others, as 2011–2012 obesity rates in Hispanics and non-Hispanic blacks were 43 and 48%, respectively, pointing to a disproportionate burden in differing racial/ethnic and/or socioeconomic status (SES) groups. Gender also plays a role, with women being disproportionately affected by extreme obesity (classes 2–3, BMI ≥35 kg/m 2 ) than men, regardless of age or race/ethnicity ( 16 ).

Trends in age-adjusted prevalence of overweight, obesity, and extreme obesity in US adults, aged 20–74 years, 1960–2012. Trends in prevalence of overweight as BMI 25–<30 kg/m 2 (circles), and upward trends in obesity as BMI ≥30 kg/m 2 (squares), and extreme obesity as BMI ≥40 kg/m 2 (diamonds) in adult males (closed points) and females (open points). The figure is based on data from NHES I (1960–1962), NHANES I (1971–1974), NHANES II (1976–1980), NHANES III (1988–1994), and NHANES (1999–2000, 2001–2002, 2003–2004, 2005–2006, 2007–2008, 2009–2010, 2011–2012). Data derived are derived from Ogden, et al ., and Fryar, et al . ( 16 , 141 ). BMI, body mass index; NHANES, National Health and Nutrition Examination Survey; NHES, National Health Examination Survey.
Meanwhile, in Europe, longitudinal data (1992–1998 to 1998–2005) from participants in five countries involved in the European Prospective Investigation into Cancer and Nutrition (EPIC) study (Italy, the United Kingdom, the Netherlands, Germany, and Denmark), indicate that adult obesity increased modestly from 13 to 17% in that time period ( 18 ). However, were such linear trends were to continue, the overall obesity prevalence in these populations could reach 30% by 2015, paralleling US rates. A more conservative projection suggests a prevalence of just 20% obesity in these populations by 2015, if public awareness and public health measures take hold ( 18 ).
European studies including populations beyond EPIC indicate there is considerable disparity in overweight/obesity between European countries. A systematic review of national and regional surveys conducted between 1990 and 2008 points to obesity rates as low as 4.0 and 6.2% in French men and women, respectively (regional survey, 1994–1996), and as high as 30.0 and 32.0% in Czech men and women, respectively (national survey, 2002–2005) ( 19 ). Regional trends within Europe are apparent, with southern Italy and southern Spain, and Eastern European countries showing higher prevalence of obesity than countries in Western and Northern Europe ( 19 ). As in the USA, these data suggest that socioeconomic disparities and relatively recent/ongoing economic transitions are playing a considerable role in apparent differences across and within countries with respect to obesity risk.
4.2. Child Obesity—USA and Europe
US children may be faring better than their adult counterparts in some ways ( 16 ), potentially offsetting earlier dire predictions of rampant obesity by 2030 ( 5 ). In national surveys, levels of overweight in children, as in adults, seem to have leveled off (or even declined) at approximately 30% of US children aged 2–19 years ( 16 , 20 ). However, this belies a potentially disturbing long-term trend in the rising prevalence of extreme obesity (equivalent to adult class 2 obesity and higher, BMI ≥35 kg/m 2 ). Since 1999–2000, the prevalence of class 2 obesity in children (BMI ≥120% of the 95th percentile) has risen from 3.8 to 5.9% and class 3 obesity (BMI ≥140% of the 95th percentile) has doubled from 0.9 to 2.1%, the latter category jumping 30% since 2009–2010 alone ( 20 ). Again, as in their adult counterparts, certain sub-populations appear to be faring worse than others, notably Hispanic girls and Black boys, in whom overweight, obesity, and class 2 obesity have increased significantly ( 20 ).
Childhood obesity prevalence varies considerably between and within countries as well. Relatively recent estimates based on 2007–2008 data of children aged 6–9-years collected in 12 European countries as a part of the WHO European Childhood Obesity Surveillance Initiative observed overweight/obesity (BMI z score >+1 standard deviation [SD]) prevalence of 19.3–49.0% of boys and 18.4–42.5% of girls, while obesity (BMI z score >+2 SD) affected 6.0–26.6% of boys and 4.6–17.3% of girls. Researchers continued to observe the trend of north-south and west-east gradients evident in adults, with the highest levels of overweight in southern European countries ( 21 ).
4.3. Obesity Beyond North America and Europe
The data discussed above focus on the USA and European countries, many with robust national health surveillance programs. While historical data tends to be scarcer outside of these regions, an alarming picture has emerged over the last decades in low- and middle-income countries around the globe, complicated by rapidly changing socioeconomic environments. While country-specific trends are not discussed in this article, regional and national estimates of long-term changes in child (<20 years old) and adult (>20 years old) overweight and obesity have increased in nearly all countries and regions since 1980 ( Figure 2 ) ( 2 , 3 ). While the USA still may boast the largest absolute numbers of overweight and obese individuals, several other nations exceed the USA in terms of overall prevalence and, moreover, the rate of growth in certain countries is disheartening. For example, the prevalence of overweight and obesity in nationally representative Mexican adults was estimated to be 71.3% overweight/obese, with overweight at 38.8% and obesity at 32.4% ( 22 ). This prevalence represents an increase of 15% since 2000, placing this population among the most rapidly accelerating in terms of obesity prevalence over the last decade. Further, while rates of overweight remained relatively stable since 2000 at approximately 38% overall, extreme obesity (class 3, BMI ≥40 kg/m 2 ) increased by an estimated 76.5% from 2000 to 2012. These trends are also evident in countries outside of the Americas. In China, for example, between 1993 and 2009, overweight (BMI 25 to <27.5 kg/m 2 ) doubled in men (8 to 17%) and increased from 11 to 14% in women. Meanwhile, obesity (BMI ≥27.5 kg/m 2 ) nearly quadrupled in men, from 3 to 11%, and doubled in women, from 5 to 10%. Chinese children are faring as badly as their adult counterparts: overweight/obesity doubled from 6 to 13% in children aged 6–17 years over the same time period, suggesting that the obesity epidemic will continue to deepen in this country ( 23 ).

Prevalence of overweight and obesity in adults aged ≥20 years by global region, 1980–2008. From left to right, each column represents the estimated regional prevalence of overweight and obesity for 1980, 1985, 1990, 1995, 2000, 2005, and 2008. For a given region, a dark gray column indicates the lowest estimated prevalence in the trend, while the highest estimated prevalence is indicated by a black column. As is evident, the vast majority of regions demonstrate the lowest estimated prevalence of overweight and obesity in 1980, and the highest in 2008, demonstrating the global reach of obesity. The scale shows 25, 50, and 100% prevalence columns, for reference. Asterisks denotes high income. Data are sourced from Stevens, et al . ( 3 ).
5. Risk Factors for Obesity
Currently, our greatest gap in knowledge is not regarding the numbers of risk factors, nor in their independent impact on risk, but rather in how they interact with one another—their confluence—to produce today’s aptly if unfortunately named “globesity” epidemic. Obesity arises as the result of an energy imbalance between calories consumed and the calories expended, creating an energy surplus and a state of positive energy balance resulting in excess body weight. This energy imbalance is partially a result of profound social and economic changes at levels well beyond the control of any single individual. These “obesogenic” changes—economic growth, growing availability of abundant, inexpensive, and often nutrient-poor food, industrialization, mechanized transportation, urbanization—have been occurring in high-income countries since the early 20th century, and today these forces are accelerating in low- and middle-income countries. And yet, not all of us living in obesogenic environments experience the same growth in our waistlines. Hereditary factors—genetics, family history, racial/ethnic differences—and our particular socioeconomic and sociocultural milieus have been shown to affect risk of obesity ( Table 2 ) even in ostensibly similar obesogenic environments. So while body weight regulation is and should be viewed as a complex interaction between environmental, socioeconomic, and genetic factors, ultimately, personal behaviors in response to these conditions continue to play a dominant role in preventing obesity. Importantly, apart from genetics, every risk factor discussed below is modifiable .
Risk Factors, Comorbidities, and Sequelae of Obesity
5.1. Genetics of Obesity
To date, over 60 relatively common genetic markers 1 have been implicated in elevated susceptibility to obesity ( 24 , 25 ); however, the 32 most common genetic variants are thought to account for <1.5% of the overall inter-individual variation in BMI ( 24 ). When these 32 “top” genetic hits are combined into a genetic risk of obesity score, those with the highest genetic risk (i.e., carriers of over 38 risk alleles), have just a 2.7 kg/m 2 higher BMI on average than those with a low genetic risk. This translates into about a 15-lb (7-kg) weight difference between two 5’3” (160 cm) individuals with high versus low genetic risk ( 24 ). Although genetics undoubtedly play a role, this relatively small difference in BMI, coupled with the dramatic rise in obesity over the last half century in developed and developing nations alike point to obesity risk factors beyond genetics. A concomitant and rich area of research has therefore evolved investigating gene-environment interaction based on the idea that underlying genetic risk predisposes individuals to particularly adverse (or beneficial) effects of behavioral or environmental exposures such as diet and exercise, a concept scientifically popularized in, for example, the “thrifty gene” hypothesis ( 26 ). In many ways, these types of gene-environment interactions are playing out in population research: for example, a variant in FTO (rs9939609)—the strongest obesity susceptibility locus—increases odds of obesity in risk allele carriers by an estimated 23% per allele; however, this risk is modified by physical activity in adults ( 27 , 28 ) and children ( 29 ), among other factors. Nevertheless, these types of interactions have so far been investigated in relatively few genetic risk loci out of millions, and with just a handful of environmental factors, raising important questions of how to aggregate this complexity for public health and ultimately personalized medicine.
In addition, parental diet, lifestyle, and other exposures have been implicated in subsequent offspring obesity risk, including famine exposure ( 30 ), parental obesity ( 31 – 33 ), smoking ( 34 ), endocrine-disrupting and other chemicals ( 35 , 36 ), and weight gain during gestation and gestational diabetes ( 33 , 37 ). These and other studies point to lasting effects of fetal programming that via differing mechanisms, likely epigenetic, result in substantial repercussions in life course health, with implications across the socioeconomic/food availability spectrum. Careful management of diet and lifestyle in pre- and perinatal periods could exert a considerable impact on the obesity epidemic for generations to come ( 37 ).
5.2. Individual Behaviors
5.2.1. diet.
In the decades preceding the 21st century, the vast majority of research on obesity risk factors focused on individual-level, largely modifiable behaviors. The role of diet and physical activity in mitigating obesity risk and reducing prevalent obesity have received the most attention, and with good reason: 15% of deaths in 2000 in the USA were attributable to excess weight, owing to poor diet and physical inactivity ( 38 ). Caloric intake and expenditure needed for weight maintenance or healthy growth has historically taken center stage ( 39 ), and caloric restriction remains today a primary focus of most popular and clinical weight-management and weight-loss approaches.
Beyond overall caloric intake to regulate body weight, a tremendous amount of research has attempted to resolve the roles of diet quality and dietary patterns, including those specifying combinations of macronutrients ( 40 ). Evidence from clinical trials have almost universally shown that caloric restriction, regardless of dietary pattern, is associated with better weight outcomes ( 40 ). Although the metabolic nuances and relative merits of the differing dietary patterns for various comorbid conditions are still being investigated, the evidence seems to suggest that merely adhering to a diet—nearly irrespective of what type of healthy diet it is—has an impact on weight loss/control ( 41 – 43 ).
For long-term maintenance of healthy weight, evidence from observational cohorts indicate that diets that are considered “healthier” lead to better long-term weight maintenance, or at least mitigate weight gain typically associated with aging through middle age. For example, research in US health professionals pointed to averaged 4-year weight gain throughout middle age as being strongly associated with increasing intake of potato chips and potatoes, sugar-sweetened beverages, and processed and unprocessed red meats, but inversely associated with the intake of vegetables, fruits, whole grains, nuts, and yogurt ( 44 ). Specific food groups, such as sugar-sweetened beverages, have received considerable attention largely because added sugar consumption (primarily as sugar-sweetened beverages) has been rising concomitantly with prevalent obesity ( 45 ). Indeed, the weight of the evidence about the role of sugar-sweetened beverages in obesity ( 46 , 47 ) is a strong impetus for public health interventions and policies, such as limiting advertising on these beverages as in Mexico ( 48 ), attempts to limit beverage sizes permitted for sale as in New York City ( 49 ), taxation, eliminating sale in schools, etc.
5.2.2. Physical Activity, Sedentary Behaviors, and Sleep
Personal behaviors beyond diet (physical activity, sleep, sedentary and screen time, and stress) have also been independently associated with weight change and maintenance in adulthood. Combined with diet, these elements have synergistic and likely cumulative effects on an individual’s ability to maintain or obtain a healthy body weight over the life course. Recently reviewed evidence from randomized trials and observational studies support 2008 US recommendations for weight management ( 50 ), consistently showing that in general, 150–250 minutes per week of moderate intensity activity is required to prevent weight gain, or aid in weight loss when accompanied by dietary restriction ( 51 ). Activity (>250 minutes per week) is associated with weight loss and weight maintenance after weight loss ( 51 ). Leisure-time activities involving sitting, but which are not truly restful behaviors, such as getting <6 or >8 hours of sleep in adults and adolescents ( 44 , 52 – 55 ) or <10–11 hours of sleep in children ( 52 ), television viewing or screen time ( 44 , 56 , 57 ), and other leisure-time sitting ( 58 ) are also associated with weight gain.
5.3. Socioeconomic Risk Factors: Income and Education
Income has had a shifting role in obesity risk over the last century. As late as the mid-20th century, the USA and Europe could link wealth directly with obesity—the wealthier an individual, the more likely to be overweight. Over the last few decades, however, perhaps owing to the abundance of cheap and highly available food, coupled with changing sociocultural norms, this link has flipped. Today, wealth in the USA tends to be inversely correlated with obesity, and it is those who are at or below the level of poverty who appear to have the highest rates of obesity ( 59 ). Indeed, in US cities where the homeless are surveyed, the prevalence of overweight and obesity parallels that of non-homeless populations, contrary to our typical beliefs about thinness accompanying food insecurity or homelessness ( 60 , 61 ).
More broadly, across 11 Organisation for Economic Co-Operation and Development (OECD) countries, SES, whether defined by household income or occupation-based social class, showed an inverse relationship with obesity: women, in particular, had consistently higher prevalence of overweight/obesity the less affluent they were ( 62 ). In men, too, those in low income strata tended to have higher prevalence of obesity, but the gradient for overweight reversed in about half of the countries surveyed. That is, in some countries, poverty was associated with more prevalent overweight than wealth, but in others, lower income was associated with more favorable weight status. The differences between sexes in terms of income status and obesity, in particular the trend reversal in men, may be in part due to low-paying jobs typically involving more physically demanding work performed by men more than by women ( 62 ). Adding complexity to this picture is the role of education: in the 11 OECD countries discussed above, education showed a strong inverse relationship with overweight/obesity, particularly in women, who had consistently higher prevalence of overweight/obesity the less educated they were ( 62 ).
As wealth rises in low- and middle-income countries, it is expected for poverty-obesity patterns to begin more closely mimicking those of high-income countries. Evidence of this transition is already accumulating. In explorations of the role of education and wealth in women and weight status in four middle-income countries (Colombia, Peru, Jordan, and Egypt), authors observed a significant interaction between education and wealth: in women with little or no education, higher income was associated with 9–40% higher odds of obesity, while in those with higher levels of education, the association with income was either not present (Egypt, Peru) or associated with 14–16% lower odds of obesity (Jordan, Colombia) ( 63 ). This suggests that in currently transitioning economies, education may offset the apparently negative effects of increasing purchasing power in emerging obesogenic environments. However, the protective effect of education has yet to be seen in the poorer countries, such as India, Nigeria, and Benin, where both education and wealth were directly associated with increased odds of obesity ( 63 ).This is perhaps unsurprising, as obesity was relatively rare at <6.0% of women in these countries, and >50% of women had little or no education.
The glimmer of hope, then, is that in the context of a paradigm of diseases of affluence, in which the transition to wealth seem to invariably lead to higher obesity and thus greater chronic disease burden, higher education levels may yet offset some of the frightening challenges that lay before us.
5.4. Environmental 2 Risk Factors
5.4.1. the built environment.
Research on the built environment tends to focus on a few measurable characteristics of neighborhoods as they relate to weight status, while holding sociodemographic and other person-level characteristics constant. Such neighborhood characteristics range from more concrete factors (e.g., fast food restaurants, supermarkets, parks, transportation, etc.) to more variably scored factors (e.g., walkability, neighborhood healthiness). Most studies of the built environment have been cross-sectional, tending to focus on one or two characteristics; thus, findings on the relative importance or effects of given characteristics on obesity have been inconsistent ( 66 – 72 ), revealing the fundamental challenge of teasing out whether neighborhood characteristics play a causal role in weight status, or whether health-minded folks inhabit health-friendly areas to begin with (residential selection bias) ( 73 ). However, the emerging picture points to the primacy of diet-related built environments over those associated with physical activity. While presence of neighborhood physical activity or recreational spaces has been associated with increased physical activity levels or energy expenditure ( 71 , 72 ), healthy food environments, characterized by availability of produce or presence of supermarkets over convenience stores or fast food restaurants, play a potentially more important role ( 68 , 70 , 74 , 75 ).
Research on the causality of the built environment as obesity-inducing or health-promoting is critical for municipalities and public health authorities to justify potentially costly improvements to public spaces and/or zoning regulations. There is an unmet need for standardized measures, definitions, and criteria, established residential and occupational geographic radii relevant to health, and research methodologies that can take into account the complexity of something as seemingly simple as a neighborhood.
5.4.2. Environmental “Pathogens”: Viruses, Microbiomes, and Social Networks
Growing evidence from animal and human studies indicates that obesity may be attributable to infection, or that obesity itself may be a contagion. Infectious agents include viruses, the trillions of microbiota inhabiting the human gut, and, of course, obese humans as infectious agents themselves.
Although several viruses have been identified as potentially having a causal role in obesity ( 76 ), Ad-36 is among the most studied, being causally associated with adiposity in animals. Studies in diverse human populations generally support greater Ad-36 viral loads as probably causal of obesity in both children and adults ( 76 – 79 ), with links to other metabolic traits ( 77 , 79 ).
Ground-breaking research in the last decade has emerged on the role of trillions of gut bacteria—the human microbiome—in relation to obesity, energy metabolism, and carbohydrate and lipid digestion, opening promising therapeutic avenues for obesity and disease ( 80 ). Two primary phyla of bacteria differ in their proportions in lean vs. obese populations; these proportions change as obese individuals lose weight and correlate highly with the percentage of body weight lost ( 81 ). Broad and sometimes dramatic changes in microbiome populations have been catalogued following gastric bypass surgery ( 80 ), and in both the short- ( 82 , 83 ) and long-term ( 81 , 83 ) in response to changes in dietary composition ( 80 ). Research in mice indicates that increased adiposity is a transmissible trait via microbiome transplantation ( 84 ), and has prompted similar experimental fecal transplantation research in humans for the promotion of weight loss ( 85 ). In addition, other research has examined the feeding of pre- and probiotics as therapeutic modalities designed to manipulate the gut microbiome; these strategies also show promise for a range of conditions ( 85 ).
Finally, the importance of social networks—real and virtual—in obesity is a fascinating, relatively new area of research that capitalizes on known characteristics of infectious disease transmission. In a landmark 2007 study examining the spread of obesity due to social ties using 32-year prospective data from the Framingham Heart Study, Christakis and Fowler ( 86 ) showed that an individual’s chances of becoming obese increased by 57% if he or she had a friend who became obese in a given 4-year interval. This was a stronger risk ratio than that observed between pairs of adult siblings or even between spouses. Conversely, it may be possible to capitalize on the social contagion of obesity in the reverse direction, that is, in the promotion of healthy weight and behavior. Intervention studies of weight loss often include a social-relational component, although the evidence supporting any single approach or its efficacy is relatively scarce ( 87 ). In theory, a supportive network, community, or coaching relationship is supposed to improve weight loss; despite a lack of strong evidence, it is a key component of many popular commercial (e.g., Weight Watchers), trial/intervention, and online approaches.
6. Costs of Obesity: Co-Morbidities, Mortality, and Economic Burden
Obesity is associated with concomitant or increased risk of nearly every chronic condition, from diabetes, to dyslipidemia, to poor mental health. Its impacts on risk of stroke and cardiovascular disease, certain cancers, and osteoarthritis are significant.
6.1. Overall Mortality
In the year 2000 in the USA, 15% of deaths were attributable to excess weight, owing to poor diet and physical inactivity ( 38 ). Overweight/obesity in middle age shortens life expectancy by an estimated 4–7 years ( 88 ). Many long-term cohort studies, as well as three recent major syntheses of pooled data from established cohorts ( 89 – 91 ), which adequately accounted for history of smoking and chronic disease status, unequivocally show that overweight and obesity over the life course is associated with excess risk of total mortality, death from cardiovascular disease, diabetes, cancer, or accidental death ( 89 – 97 ).
Some studies suggest that excess body weight may be protective against mortality from certain chronic conditions—resulting in a so-called “obesity paradox.” However, most studies that have shown an obesity paradox, or no association between obesity and mortality, have been conducted in groups of older (>65) or elderly patients or in those with chronic conditions, or have inadequately accounted for smoking. Indeed, the role of excess adiposity in old age is unclear. While the protective effects of overweight in specific instances of diseased older populations may be real, these observations are fraught with methodological problems, especially reverse causation, and belie the limitations of generalizing excess adiposity’s supposed benefits to younger populations over the life course, not least because excess body weight leads to higher disease incidence to begin with ( 7 ).
6.2. Diabetes
Excess weight and diabetes are so tightly linked that the American Diabetes Association recommends physicians test for type 2 diabetes and assess risk of future diabetes in asymptomatic people ≥45 years old simply if they are overweight/obese, and regardless of age if they are severely obese ( 98 ). Overweight raises risk of developing type 2 diabetes by a factor of three, and obesity by a factor of seven, compared to normal weight ( 99 ). Excess weight in childhood and in young adulthood, and weight gain through early to mid-adulthood are strong risk factors for diabetes ( 100 – 102 ). While not every overweight/obese individual has diabetes, some 80% of those with diabetes are overweight/obese ( 103 ). Obesity itself raises diabetes risk even in the absence of other metabolic dysregulation (insulin resistance, poor glycemic control, hypertension, dyslipidemia). While metabolically healthy obese individuals are estimated to have half the risk of their metabolically unhealthy counterparts, they still have four times the risk of those who are normal weight and metabolically healthy ( 104 ).
6.3. Heart and Vascular Diseases
Ischemic heart disease and stroke are the leading causes of death in the USA and globally ( 105 ). Excess body weight is a well-known risk factor for heart disease and ischemic stroke, including their typical antecedents—dyslipidemia and hypertension. Recent studies have consistently shown that benign obesity appears to be a myth ( 106 – 108 ); overweight clearly adds to risk of heart disease and stroke beyond its implications for hypertension, dyslipidemia, and dysglycemia.
Given childhood obesity rates, research has lately focused on the role of obesity in early life and subsequent adulthood disease. Obesity in childhood or adolescence has been associated with twofold or higher risk of adult hypertension, coronary heart disease, and stroke ( 100 ). A recent study pooling data from four child cohorts (aged 11 years at baseline with average 23-year follow-up), observed that, compared with individuals who were normal weight in childhood and non-obese as adults, those who were normal weight or overweight but became obese as adults, or who were obese and stayed obese into adulthood, had considerably higher risk of high-risk dyslipidemia, hypertension, and higher carotid intima-media thickness. Notably, those individuals who were overweight/obese as children, but non-obese as adults, had similar risk profiles to those individuals who were never obese, indicating that the potential health effects of childhood obesity can be offset by weight loss prior to or while entering into adulthood ( 109 ).
6.4. Cancer
An estimated 6% of all cancers (4% in men, 7% in women) diagnosed in 2007 were attributable to obesity ( 110 ). Beyond being a major risk factor for diabetes, which itself is a risk factor for most cancers, obesity has long been understood to be associated with increased risk of esophageal, colon, pancreatic, postmenopausal breast, endometrial, and renal cancers ( 111 ). More recently, evidence has accumulated that overweight and/or obesity raise risk of cancers of the gallbladder ( 112 ), liver ( 113 ), ovaries (epithelial) ( 114 ), and advanced cancer of the prostate ( 115 ), as well as leukemia ( 116 ).
6.5. Trauma and Infection
A study in Pennsylvania (USA) trauma centers (2000–2009) showed that in-hospital mortality and risk of major complications of surgery were increased in obese patients as compared to non-obese patients. Severely obese patients had upwards of 30% increased risk of mortality from their trauma than non-obese patients, and double the risk of major complications. Severely obese females also had more than double the risk of developing wound complications, and quadruple the risk of developing decubitus ulcers ( 117 ). A recent meta-analysis of obesity in trauma care concluded that obesity was associated with 45% increased odds of mortality, longer stays in the intensive care unit, and higher rates of complications, and tended to associate with longer durations of mechanical ventilation and longer stays in the hospital overall, compared to non-obese patients, despite equivalent injury severity ( 118 ).
While elevated risk of chronic disease is a seemingly obvious consequence of obesity, increasing attention is being given to increased risk of infection and infectious disease in obesity, including surgical-site, intensive care unit (ICU)-acquired catheter, blood, nosocomial, urinary tract, and cellulitis and other skin infections ( 119 ), community-acquired infections, and poorer recovery outcomes owing to higher risk of influenza, pneumonia, bacteremia, and sepsis ( 119 ). Impaired immunological response may be an underlying mechanism; recent research has demonstrated lower vaccine efficacy and serological response to vaccination in the obese. For example, a recent study estimated an eightfold increase in the odds of non-responsiveness to hepatitis-B vaccination in obese versus normal-weight women ( 120 ).
The consequences of a global obesity epidemic may not merely be greater chronic and infectious disease burden for the obese, but also a greater global burden of infectious disease owing to obesity. Greater infectious disease vigilance may be required in populations with high levels of overweight/obesity, and there is a clear need for better clinical practice guidelines (e.g., use and dosage of antimicrobials, vaccines, other pharmaceuticals) for obese individuals.
6.6. Mental Health
The role of weight in mental health faces causal challenges, but what is clear is that obesity and adiposity are associated with anatomical as well as functional changes in the human brain. Studies in older populations have shown that BMI is inversely correlated with brain volume, and that obese older adults, compared to normal weight counterparts, show atrophy in the frontal lobes, anterior cingulate gyrus, hippocampus, and thalamus ( 121 ). In addition, obesity in children and adolescents (aged >9 years) has been associated with smaller orbitofrontal cortex gray matter volume, along with poorer performance in certain domains of executive function (e.g., inhibitory control) ( 122 ). Being overweight in midlife increases risk of Alzheimer's disease, vascular dementia, or any type of dementia by 35, 33, and 26%, respectively; even higher risk is observed for obesity ( 123 ). Importantly, physical activity, even among overweight individuals, may stave off poor mental functioning: moderately active or highly active adult overweight Finns did not have significantly increased risk of poor mental functioning at a 7-year follow-up compared to those who were normal weight and highly active, but inactive and overweight patients presented a nearly 40% increased risk of poor mental functioning ( 124 ). Thus, exercise may play an important mediating role in the relationship between excess body weight and age-related cognitive decline.
6.7. Economic Burden of Obesity
In the USA, recent estimates indicate that obese men are thought to incur an additional US$1,152 per year in medical spending, particularly due to hospitalizations and prescription drugs, compared to their non-obese counterparts, while obese women incur over double that of obese men, an additional US$3,613 per year in medical spending (year 2005 values). Extrapolating these costs to the national level, authors estimate some US$190 billion per year of healthcare spending, approximately 21% of US healthcare expenditures, is due to treating obesity and obesity-related conditions ( 125 ).
Total hospital costs account for a part of this: another author group studied non-bariatric, non-obstetric hospital procedures for obese patients, finding they were US$648 higher (year 2009 values) per capita than for non-obese patients. The estimated national hospital expenditures for the largest volume surgical procedures was US$160 million higher per year for obese than for their non-obese counterparts ( 126 ).
Employers bear a substantial brunt of obesity-related costs in the USA. Data from the Human Capital Management Services Research Reference Database (2001–2012) on employees and their dependents was used to compare medical, drug, sick leave, short-term disability, and workers’ compensation costs as well as absent days across three BMI strata: <27, ≥27–<30, and ≥30 kg/m 2 . Each of the costs was incrementally higher in ascending BMI categories. For example, total annual costs and total days absent in the highest vs. lowest BMI strata were US$6,313 versus US$4,258 (year 2012 values), and 7.5 versus 4.5 days. In addition, productivity was lowest in the obese group ( 127 ).
Finally, lifetime direct incremental medical costs of obesity in childhood in the USA were estimated to range from US$12,660 to US$19,630 (year 2012 values) for an obese 10-year old compared to a normal-weight 10-year old, if expected weight gain through adulthood among the normal weight child occurs ( 128 ). If normal weight children were to not continue on the typical weight gain trajectory into overweight/obesity, estimated incremental medical costs for today’s 10-year old obese child ranges between US$16,310 and US$39,080. Putting these figures into perspective, multiplying the lifetime medical cost estimate of US$19,000 by the number of obese 10-year-olds today generates a total direct medical cost of obesity of roughly US$14 billion for this 10-year old age group alone. In terms of big picture savings, the upper estimate of US$39,000 per case represents two years of public college tuition for that child ( 128 ).
In Europe, a 2008 review of 13 studies in ten Western European countries estimated the obesity-related healthcare burden had a relatively conservative upper limit of €10.4 billion annually (in Germany, in 1995 € equivalent), and ranging between <0.1 to 0.61% of each country’s gross domestic product (GDP). The review relied on study data from as early as the 1980s in the Netherlands, through 2002 in most of the remaining countries surveyed ( 129 ). A more recent review focused on 19 studies published in 2007–2010 in eight Western European countries (predominantly Germany, Denmark, and the United Kingdom). Excess health care costs of obesity or derivations of excess health care costs by comparisons of mean costs between normal weight and obese individuals in seven of the reviewed studies were between €117 and €1,873 per person (based on the € valuation given in each study year). Excess costs increased particularly due to severe obesity. Approximately 23% of medication costs and 6.9% of out-of-pocket costs were attributable to overweight or obesity. Health economic models estimated that 2.1–4.7% of total health care costs and 2.8% of total hospital costs were due to overweight and obesity. Total (direct and indirect) costs were generally unchanged from the 2008 estimate of the earlier review, accounting for 0.47–0.61% of GDP in these countries ( 130 ).
In the context of the Brazilian Unified Health System (i.e., public hospitals), estimated direct costs of diseases related to overweight/obesity in outpatient and inpatient care based on 2008–2010 data were US$2.1 billion annually (year 2010 values), 68.4% of which was attributable to hospitalizations, and the remainder due to ambulatory procedures ( 131 ). The largest costs of outpatient and inpatient care in both sexes were due to cardiovascular disease (US$747 million) followed by overweight- and obesity-related neoplasms (US$299.8 million), asthma (US$34 million), type 2 diabetes (US$3.7 million), and osteoarthritis (US$3.9 million). Authors estimated that these direct costs were a considerable underestimate of the true burden of overweight/obesity in Brazil, which would include private health care expenditures, as well as indirect costs due to lost productivity, premature death, and home care ( 131 ).
Given the predicted rise in obesity in Brazil, coronary heart disease, stroke, hypertension, cancers, osteoarthritis, and diabetes are projected to at least double by 2050, with concomitant doubling in health care costs, from US$5.8 billion in 2010 to US$10.1 billion per year—totaling US$330 billion over 40 years (year 2010 values). It is estimated that a 5% reduction in mean BMI across the population could save Brazil some US$57 billion over that time frame ( 132 ). A similar analytic approach that substituted Mexican prevalence and trends for the Brazilian ones estimated 2010 costs of obesity at US$806 million (year 2000 values), which were projected to increase to US$1.7 billion by 2050, at which point a mere 1% reduction in BMI prevalence in Mexico could save an estimated US$85 million per year ( 133 ).
Of course, none of these estimates include dollars spent on the weight-loss industry, which is estimated to be over US$60 billion dollars in 2014 in the USA alone ( 134 ), and includes non-prescription drugs and supplements, diet plans, gym memberships, workout videos, and an endless stream of money-making schemes.
7. Touching on Solutions, and Some Conclusions
Obesity is a major contributor to preventable disease and death across the globe, and poses a nearly unprecedented challenge not just to those tasked with addressing it at the public health level, or at the healthcare provider level, but to each of us as individuals, for none of us are immune. Increasing ease of life, owing to reduced physical labor and automated transportation, an increasingly sedentary lifestyle, and liberal access to calorie-dense food, driven by dramatic economic growth in many parts of the world in the last century, have turned a once rare disease of the affluent into one of the most common diseases—increasingly of the poor. That barely one in three people in the USA today are normal weight portends, quite simply, an astounding and frightening future. Significant reductions in public health and healthcare expenditures could occur around the world if we were able to stem the tide of childhood obesity trends, and if young and middle-aged overweight and obese adults lost approximately10% of their body weight, as recommended for a considerably reduced risk of debilitating chronic conditions ( 135 ).
Obesity is complex. Although its risk factors are myriad and compounding, there is an urgent need for deeper understanding of the way risk factors interact with each other, and the potential solutions to the epidemic are as multi-leveled and complex as its causes. There are calls for applying systems-level ( 136 ) and systems epidemiology ( 137 ) approaches to this and related nutrition and metabolic diseases, approaches which attempt to comprehensively address biological, behavioral, and environmental contributors to disease as well as their intricate feedback loops. Additional research on solutions to this epidemic would include, for example, examining the relative cost/benefit to individuals and populations of individual versus systemic policies and/or interventions, concurrently or independently, particularly when individuals and communities must decide between approaches given limited resources, and moreover, with the currently limited evidence in the case of broad industry, agricultural, or public health policies. For example, we could attempt to limit national production and import of sugar-sweetened beverages, tax sugar-sweetened beverages, or restrict fast food restaurant zoning. These largely political acts seem relatively inexpensive, but may have economic impacts in communities and regions beyond what we currently understand. We may push for the increasing medicalization of obesity, including developing an obesity vaccine. While such a “cure” may someday arise, the medicalization of a condition typically improves its treatment rather than its prevention, and prevention is key in the case of obesity. However, preventing and remediating obesity in children and adults—e.g., via health and wellness incorporation into curricula at every educational level from kindergarten through medical school—requires vast resources allocated to educators, as well as earlier diagnosis and treatment of overweight (education, counseling, drug treatment, etc.). Given these resource costs, perhaps greater attention should be given to pregnancy, a condition which is already highly medicalized and which may be an ideal preventive avenue for the provision of nutrition education and intensive monitoring of weight gain, to ensure that children have the most optimal start with respect to their future obesity risk. Clearly, no single approach is optimal, but with limited resources, an evidence base supporting one or more approaches or their combination is needed, as is tenacity and perhaps some audacity by local government and public health authorities in testing some of these approaches within their populations. However, an epidemic of this magnitude needs, quite simply, more resources. One of the reasons why the American Medical Association opted to declare obesity a “disease” was to give obesity the label it needs for greater allocation of resources for research, prevention, and treatment ( 1 ).
Despite the many unknowns, we can be cautiously optimistic about our ability to address the obesity epidemic. Indeed, we have relatively successfully faced similarly daunting public health challenges before: smoking, to name just one. While tobacco can loosely be thought of as a single product, and our food culture is infinitely more complex, as a case study in how to approach obesity, it provides numerous lessons in multi-level solutions to a major health threat in terms of both mitigation and prevention. We began by developing an understanding of smoking’s epidemiological impact and the healthcare costs borne by society, uncovered its biological basis, learned about and applied behavior change, and initiated and carried out vast public health, public policy, political, and economic strategies that ultimately affected whole environments as well as sociocultural norms.
It took over half a century to achieve the immense success we have with regard to smoking in the USA and still we are not yet tobacco-free ( 138 ); other parts of the world continue to wrestle with it to a greater degree. It has only been a couple decades since we first deeply appreciated that obesity was epidemic. We clearly still have a long way to go.
Key Points for Decision Makers
- In 2013, an estimated one in three adults worldwide was overweight or obese, and adult obesity exceeded 50% in several countries around the globe. While the prevalence of adult obesity in the developed world seems to have stabilized, the prevalence of obesity in children and adolescents globally, as well as adults obesity in developing countries, is still increasing. In addition, some developed countries continue to observe increasing prevalence of extreme classes of obesity.
- Overweight and obesity—defined as excess body weight for height—have genetic, behavioral, socioeconomic, and environmental origins.
- Obesity increases risk of major chronic diseases, including heart disease, diabetes, depression, and many cancers, as well as premature death.
- Estimates of annual healthcare costs attributable to obesity are US$190 billion per year in the USA, approximately 21% of US healthcare expenditures.
- Given its complexity, the obesity epidemic requires multilevel and integrated solutions, from individual intervention, to broad food policy, industry, and agriculture initiatives.
Acknowledgements
The authors declare no conflict of interest. AH is supported by an American Diabetes Association Mentor-Based Postdoctoral Fellowship award. FH is supported by NIH grants DK51158, HL60712, P30 DK46200, and U54CA155626. The authors broadly thank the researchers in this field for their consistent and tireless work in illuminating the etiology, sequelae, and solutions to this complex condition.
1 See also http://www.genome.gov/gwastudies/
2 We do not review the impact of food, agriculture, trade, and nutrition policy on obesity in the present paper, but refer interested readers to a recent review ( 64 ). Further, we do not address the body of growing evidence on the role of environmental pollutants–“obesogens”–in obesity, specifically those known as endocrine-disrupting chemicals. We refer readers to recent reviews on the topic ( 35 , 36 , 65 ).
Author Contributions
AH wrote the first draft of the paper. AH and FH contributed to writing, revised, and edited the paper. AH is the final guarantor of this work and takes full responsibility for its contents. Both authors read and approved the final manuscript.
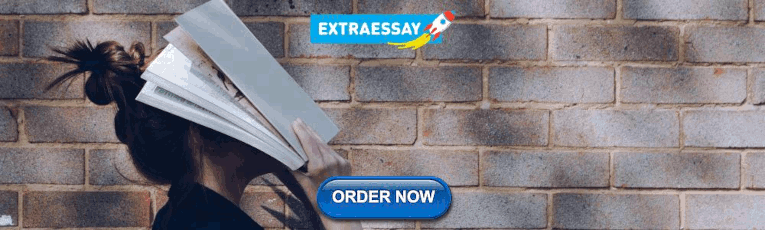
IMAGES
VIDEO
COMMENTS
The evidence for social and environmental factors that contribute to obesity are often underappreciated. Obesity prevalence is significantly associated with sex, racial ethnic identity, and socioeconomic status, which creates complex relationships between each of these characteristics. Food availability remains an important factor associated with obesity that relates to differences in ...
Among women, obesity was indeed most prevalent at lower income levels: 42% of women living in households with income below 130% of the poverty level were obese, compared with 29% of women in households at or above 350% of poverty. But the correlation was significant only for white women, though the trend was similar for all racial and ethnic ...
Obesity in children is also high, for example, around one in five 10-11 year olds in England were obese in 2019. Worryingly, children are becoming obese at younger ages and are staying obese into adulthood (Johnson et al., 2015).Obesity is more prevalent in more deprived areas, with children living in the most deprived regions being nearly twice as likely to be obese as those living in the ...
Poverty rates and obesity were reviewed across 3,139 counties in the U.S. (2,6). In contrast to international trends, people in America who live in the most poverty-dense counties are those most prone to obesity (Fig. 1A). Counties with poverty rates of >35% have obesity rates 145% greater than wealthy counties.
Obesity and poverty statistics. Research suggests that there are higher rates of obesity in under-resourced states in the United States. States with more than 35% of people living in poverty had a ...
poverty and obesity. At first glance, one would intuitively think that obesity and poverty couldn't be linked. One assumes that if a person is obese, they must have enough to eat. But this is simply not true. In the U.S. today, poverty is the leading cause of obesity. The main reason I will examine for this connection is income and
Obesity in the UK. It is notable that the proportion of the UK population with obesity has escalated significantly between 1993 and 2009. The increase has been 13 percent for males in 1993 to 22 percent in 2009. Conversely, the population proportion for females has escalated from 16 percent in 1993 to 24 percent in 2009 (The Poverty Site, 2011).
The problem of obesity becomes easily framed within this explanation as one of quantity and personal gluttony and laziness: either energy intake is too high, energy expenditure is too low, or both. Applied to the specific case of socioeconomic inequalities in obesity, this framing leads to the proposal that these personal failings are more ...
Abstract. The modern relationship between obesity and poverty is nuanced and complex. Being poor in one of the poorest countries in the world may be associated with scarce nutrition and emaciation, while being poor in a developed country may mean a higher risk of obesity. In this chapter, we will explain variations in poverty (depending upon ...
Obese young adults are a high risk for ongoing health problems such as cardiovascular disease, depression and certain cancers. Being overweight in young adulthood is associated with unemployment, limits on educational attainment and lower levels of income. Because the bad consequences are long-lasting and recurrent, obesity needs to be ...
Introduction. Obesity prevalence has tripled since the 1970s. Currently, it has reached epidemic proportions throughout the globe and become a significant cause of morbidity and mortality ( WHO, 2020).It is a major risk factor in the development of the leading causes of global deaths, such as diabetes, cardiovascular diseases and cancer ( Al-Goblan et al., 2014; De Pergola & Silvestris, 2013 ...
Adults living in poverty are at greater risk for a number of health issues, such as diabetes,51 heart disease and stroke,52, 53 obesity (primarily among women),54 depression,55 disability,56 poor oral health,57 and premature mortality.58 Those living in poverty also have higher rates of physical
Are poverty and obesity associated? Poverty rates and obesity were reviewed across 3,139 counties in the U.S. (2,6). In contrast to international trends, people in America who live in the most poverty-dense counties are those most prone to obesity . Counties with poverty rates of >35% have obesity rates 145% greater than wealthy counties.
THREE ESSAYS ON OBESITY, POVERTY, AND THE LABOR MARKET Maximilian David Schmeiser, Ph.D. Cornell University 2008 This dissertation broadly examines the economic and health-related consequences of individual behaviors, and their interaction with government programs. It is divided into three distinct chapters.
ESSAY — Volume 16 — April 11, 2019 Print. ... Obesity reduction among the black population is often a key goal for REACH recipients. For example, from 2008 through 2012, 14 REACH grantees implemented strategies to address disparities in obesity among black populations. These strategies included expanding healthy food choices in grocery ...
The official poverty rate in 2010 was 15.1 percent; up from 14.3 percent in 2009; this was the third consecutive annual increase in the poverty rate (Census Bureau, 2011). One might assume that obesity and poverty are not associated, but this is not accurate. The correlation between poverty and obesity can be …show more content… 102).
1004 Words. 5 Pages. Open Document. Poverty and obesity do not make us think of the same people, but in some ways this is not true. In some instances poverty does cause obesity because the people in poverty are not able to buy the more expensive and healthy foods. By not being able to buy these healthy foods they are forced to either go hungry ...
Professor (Jennifer James) Devry University. 4 December 2011. First Draft. Connection between poverty and obesity It is said that obesity can be caused by not knowing how to combat and prevent this common disease before it begins in poverty stricken areas. Additionally the availability of nutritious food choices and the means to have safe ...
Obesity is a person having a higher BMI than normal for your height and age, which can have many health consequences while growing up and during adulthood. The definition of poverty is the condition or state of having little to no money, means of support or goods; condition of being poor. Poverty results in starvation and other problems.
The U.S. Census has documented the degree to which poorer children are affected by obesity rates: • Poor children have higher rates of obesity (around 20% of all poor children) than do non-poor children (around 15%). • African-American children (21%) and Mexican-American children (23%) have higher rates of obesity than non-Hispanic white ...
PDF | Obesity rates in the UK, and around the world, are high and rising. ... Obesity, Poverty and Public Policy. February 2022; The Economic Journal 132(644) ... While there are many good papers ...
Conclusion Although environmental, behavioral, psychological, social and other economic factors contribute to obesity, poverty levels are causally associated to obesity to a larger extent. Poverty levels are highly connected to obesity because of low-income levels; thus exposing the society to health risks.
Click here for access to Odgers' essay in the Atlantic and here for the study phones and obesity in BMC Public Health. Waiting with a Phone, photograph by Ted Kyle / ConscienHealth ... Adolescents are distressed about climate change, school shootings, wars, poverty, student debt, stripping away of reproductive rights, homelessness, silencing ...
In 2010, the first lady, Michelle Obama, who made childhood obesity a signature issue, pressed for the passage of the Healthy, Hunger-Free Kids Act, which led school cafeterias to serve more ...
1. Introduction. Obesity is a complex, multifactorial, and largely preventable disease (), affecting, along with overweight, over a third of the world's population today (2,3).If secular trends continue, by 2030 an estimated 38% of the world's adult population will be overweight and another 20% will be obese ().In the USA, the most dire projections based on earlier secular trends point to ...
Saving Poverty From Obesity. Brent Royeton. DeVry University. Saving Poverty From Obesity. Obesity is a problem that most people would assume is caused by laziness and poor lifestyle choices. Rarely would anyone think that poverty could be a contributing factor to obesity. A large question often asked is "How can our impoverished communities ...