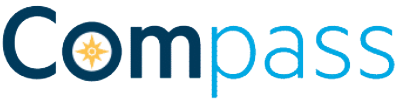
The Compass for SBC
Helping you Implement Effective Social and Behavior Change Projects
How-To-Guide
How to Conduct a Program Analysis
Home > How to Guides > How to Conduct a Program Analysis
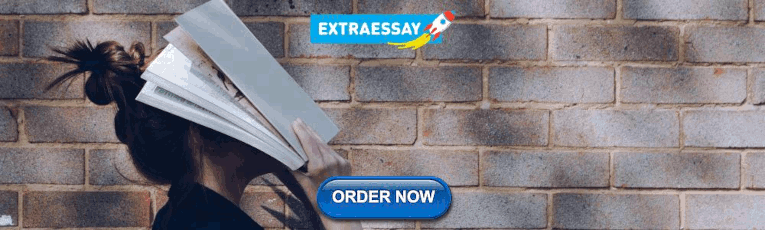
Introduction
__________________________________________________________
Click here to access this Guide in Arabic – مراجعة هذا الدليل باللغة العربية، انقر هنا
Click here to access this Guide in Portuguese – Guias em Português
A program analysis is the process of looking at an organization’s intended social and behavior change communication (SBCC) program and then identifying enabling and limiting factors to implementing it successfully.
A program analysis is used as a planning tool when the program is under development and helps the program team explore the following factors that may enhance or constrain the planned SBCC program and then utilizes the findings for program design:
- Human and Physical Resources
- Political Environment and Trends
- Programmatic and Management Structure
- Community and Client Dynamics
- Technological Resources
- Financial Resources and Funding
A program analysis is only as good as its information. Research and accurate data are vital to identifying key issues relevant to the success of a program.
Why Conduct a Program Analysis?
A program analysis helps the team understand key constraints that must be addressed for a program to be successful. SBCC programs risk failure if fundamental limiting factors, weaknesses and threats are not addressed. Findings from the program analysis inform the design of the program/campaign strategy and identify clear actions to be taken to enhance the potential for success.
Who Should Conduct a Program Analysis?
A small, focused team should conduct the program analysis . Members should include research, health/social service and program and communication staff. When feasible, it can be a good idea to include stakeholders from outside the program to participate and bring a neutral perspective to the process.
When Should a Program Analysis Be Conducted?
Conduct a program analysis after completing the situation analysis and audience analysis . Information collected during these analyses will inform the program analysis. Use the findings to develop the SBCC strategy.
Estimated Time Needed
Completing a program analysis can take up to two weeks. Consider the size of the project, scope of the literature review, how much data is available and easily accessible, and whether additional stakeholder or audience input is needed. Allow for additional time to fill in any gaps that may exist.
Learning Objectives
After completing the activities in the program analysis guide, the team will understand:
- The opportunities that exist to improve the design or implementation of the SBCC strategy.
- Which challenges to address before or as part of implementing the SBCC strategy.
Prerequisites
- Situation Analysis
- Audience Analysis
Step 1: Decide on a Framework
There are many ways to assess an organization’s ability to design and implement a program, including a full organization/communication capacity assessment, Force Field Analysis, Theory of Constraints and SWOT (Strengths, Weaknesses, Opportunities and Threats) analysis (see Resources for more information). If an organization has a program that has already begun, it may rely on recent program assessments or evaluations if available.
Step 2: Implement Chosen Framework
This guide outlines the Force Field Analysis framework, which is a relatively quick and simple way to analyze a program and its potential for success. Force Field Analysis assists a program team in identifying what factors – both internal and external – can help them reach the shared vision/change desired (forces for change) and what factors might keep them from reaching the vision (forces against change). The graphic below illustrates the concepts behind implementing the Force Field Analysis framework.
Step 3: Describe Proposed Change
Using the Force Field Analysis template (see Force Field Analysis Template ), in the center box, describe the change the program hopes to accomplish, which should be based on the shared vision established during the situation analysis . The shared vision may need to be condensed to create a concise version of the change the program desires.
Step 4: Brainstorm Forces for Change
Brainstorm all the forces, both internal and external, that might help the program achieve the desired change. See the table below for questions to help identify forces for change and examples of such forces:
Be sure to consider all the financial, human, technological and non-material resources the program can take advantage of. List each force for change in an arrow on the left side of the template (see Force Field Analysis Template ).
Step 5: Brainstorm Forces against Change
Next, brainstorm all of the forces, both internal and external, that might prevent the program from achieving the desired change. See the table below for questions to help identify forces against change and examples of such forces:
List each force against change in an arrow on the right side of the (see Force Field Analysis Template ).
Step 6: Score Each Force
For each force listed, assign a score that represents how much influence that force has on the desired change. The team can decide on the scale, but one way is to score each force from 1 to 5, with 1 being weak influence and 5 being strong influence. The team can add up the scores for each side (for and against) to determine whether forces for change are greater than forces against change. There does not need to be an equal number of forces for each side. For example:
Step 7: Consider Appropriate Actions
Examine the completed Force Field Analysis sheet and ask what actions the program team might take to increase the potential for successful change. Ask what the program could do to strengthen the forces that support change and weaken the forces that oppose change. What weaknesses should the program address? What strengths should it build on to enhance successful change? Typically, the program should focus on the forces with the strongest influence. These actions then make up the strategy the program develops. A successful SBCC strategy addresses the key constraints to reaching the vision and capitalizes on core opportunities and assets.
Step 8: Use the Analysis Findings
Whatever template the team uses, the program analysis should highlight actions for inclusion in the strategy that will help the program reach the shared vision defined during the situation analysis . Using the results from the analysis, determine how the program can:
- Eliminate or weaken the factors that stand in the way of reaching the vision.
- Enhance the factors that support reaching the vision.
- Build on key strengths and utilize available assets.
- Mobilize neutral forces to help the program achieve the vision.
- Minimize key weaknesses and avoid threats.
- Capitalize on key opportunities.
Use the results of the program analysis when designing the SBCC strategy, messages, interventions and monitoring and evaluation plans.
Repeat or revisit the analysis as needed to check assumptions, incorporate new information or as the program or environment changes.
Force Field Analysis Template
PMTCT Communication Interventions SWOT Analysis
Using SWOT for a Neighborhood Development Project
External Resources
https://www.urbanreproductivehealth.org/sites/mle/files/ar03jan-11.pdf
Tips & Recommendations
- Avoid writing a long list of influencing internal and external factors. Prioritize the list and include only those that are likely to have a major impact on the shared vision.
- Try to be honest and objective in identifying both positives and negatives. Overestimating abilities or underestimating challenges can negatively impact the program, strategy and health situation.
- If outsiders cannot participate in the analysis, try to look at the program’s challenges and opportunities as an outsider (in addition to looking at them as insiders).
- Really think through and analyze the enabling and limiting factors – listing them is not sufficient.
Lessons Learned
- Conducting a program analysis helps avoid unhappy surprises and capitalize on advantages and opportunities.
- A program analysis is most beneficial if it is used to support the vision and address the problem defined during the situation analysis and incorporate the information learned from the audience analysis .
Resources and References
SWOT Analysis: Strengths, Weaknesses, Opportunities, and Threats
The Theory of Constraints (TOC)
SBCC Mapping Tool for Assessment
Writing a Communication Strategy for Development Programmes: A Guideline for Programme Managers and Communication Officers
SWOT Analysis
SBCC Capacity Assessment Tools
- O’Sullivan, G.A., Yonkler, J.A., Morgan, W., and Merritt, A.P. A Field Guide to Designing a Health Communication Strategy, Baltimore, MD: Johns Hopkins Bloomberg School of Public Health/Center for Communication Programs , March 2003.
- UNICEF. Writing a Communication Strategy .
- Mind Tools. Force Field Analysis: Analyzing the Pressures For and Against Change .
- Business Dictionary
Banner Photo: © 2011 A.M. Ahad, Courtesy of Photoshare
ABOUT HOW TO GUIDES
SBC How-to Guides are short guides that provide step-by-step instructions on how to perform core social and behavior change tasks. From formative research through monitoring and evaluation, these guides cover each step of the SBC process, offer useful hints, and include important resources and references.
Share this Article
Program Analysis (Winter Semester 2020/21)
Quick facts.
This course introduces the principles and practice of automatically analyzing large software systems. The course provides an overview of program analysis and then covers three topics in more detail: Static analysis, which analyzes the source code of a program, dynamic analysis, which reasons about the runtime behavior of a program, and test generation, which creates inputs to run programs. In addition to lectures, students will deepen their understanding through a practical course project (implement a program analysis based on an existing framework) and homework assignments. Besides academic achievements, the course will help students to improve their programming skills by learning about common sources of mistakes and about techniques to find them.
This is a preliminary schedule and may be subject to change. "L" stands for lecture, "P" stands for project, and "E" stands for exercise. Lectures will be distributed via YouTube and have a recommended time window to watch them (but you can watch them any time). Events with a precise date happen on that date. Events printed in italics happen synchronously via Webex and will not be recorded, i.e., you should plan to attend them at the given time. Events printed in bold are deadlines (strict).
Course Project
The course project is about implementing a static taint analysis for JavaScript based on the general data flow analysis framework. More details will be published during the semester.
News and Events
4/2024: LintQ, our static analysis framework for checking quantum programs, will be presented at FSE'24.
3/2024: Introducing RepairAgent, the first autonomous, LLM-based agent for automated program repair .
2/2024: Michael is joining UC Berkeley for a sabbatical.
2/2024: Luca has successfully defended his PhD thesis on "Supporting Software Evolution via Search and Prediction" and is starting a post-doc at USI Lugano.
1/2024: DyPyBench , a novel benchmark of executable Python code, will be presented at FSE'24.
12/2023: Our paper on repairing static type errors in Python will be presented at ICSE'24.
12/2023: ACM SIGSOFT Distinguished Paper Award for our work on LExecutor .
10/2023: Our work on universal fuzzing with large language models will be presented at ICSE'24.
9/2023: Daniel receives the infos award for the best PhD thesis of the year 2022 in our Computer Science Department . Congrats!
8/2023: Our empirical study of workflows of GitHub Actions will be presented at ICSE'24.
8/2023: Group retreat at Obermarchtal.
7/2023: Our work on LExecutor will be presented at FSE'23. Congrats to Beatriz on the first top-tier paper during her PhD!
7/2023: Two awards at ISSTA'23: An ACM SIGSOFT Distinguished Paper Award for bimodal taint analysis and an ACM SIGSOFT Distinguished Artifact Award for our work on WebAssembly call graphs .
7/2023: Co-organizing two Dagstuhl seminars in 2024: Code Search (April) and Automated Programming and Program Repair (October).
6/2023: Thanks to Prem Devanbu for the amazing time we had during his five-month visit of our lab .
5/2023: Interested in call graphs and/or WebAssembly? Check out our new ISSTA'23 paper .
5/2023: Luca is heading out for an internship at Uber. Have fun!
4/2023: Beatriz is joining the group as a PhD student.
2/2023: Prem Devanbu is joining the lab for five months with a Humboldt Research Award . Welcome!
2/2023: Fun and inspiring Dagstuhl seminar on Programming Language Processing .
1/2023: Our paper on bimodal taint analysis will be presented at ISSTA'23.
12/2022: Four papers accepted at ICSE'23. Congrats to everyone involved!
12/2022: Michael gets recognized as an ACM Distinguished Member .
11/2022: Our work on DiffSearch ( paper , tool ) will appear in IEEE TSE.
10/2022: Islem wins the ACM Student Research Competition at ASE'22 for his work on detecting inconsistencies in if-condition-raise statements.
10/2022: Our ASE'22 paper on CrystalBLEU has been selected for an ACM SIGSOFT Distinguished Paper Award .
10/2022: Our survey of code search techniques will appear in ACM CSUR.
10/2022: Huimin Hu is joining the group as a PhD student. Welcome!
9/2022: Our paper on type annotations in Python is receiving an ACM SIGSOFT Distinguished Paper Award at FSE'22.
9/2022: Michael will serve as PC Chair of ISSTA 2024 .
7/2022: Our work on CrystalBLEU , a novel metric for measuring the similarity of code, will be presented at ASE'22. Congrats to Aryaz!
7/2022: Daniel successfully (with summa cum laude) defends his PhD on program analysis for WebAssembly. Congrats!
6/2022: Three papers accepted at FSE'22, on Python type annotations , on the first dynamic analysis framework for Python , and on neural code editing to generate vulnerabilities .
5/2022: Luca wins the 2nd prize in the ACM Student Research Competition at ICSE'22 for his work on DiffSearch . Congrats!
5/2022: Looking for particular kinds of code changes, e.g., to build a dataset? Check out DiffSearch , our scalable and precise search engine for code changes.
5/2022: Group retreat at Schloss Hornberg.
3/2022: Our paper on recovering precise types in WebAssembly binaries will appear at PLDI'22.
3/2022: Our paper on bugs in quantum computing platforms will appear at OOPSLA'22.
2/2022: Interview with Deutschlandfunk (one of three German national radio stations) on neural software analysis.
12/2021: Our papers on learning name-value inconsistencies from runtime behavior and on test generation for asynchronous JavaScript APIs will appear at ICSE'22.
11/2021: Our paper on obfuscating JavaScript by opportunistically translating it to WebAssembly has been accepted at S&P'22.
11/2021: Stuttgart is forming a new ELLIS unit , with Michael as one of its fellows.
10/2021: Two new papers on bugs in quantum computing platforms and fuzzing WebAssembly
9/2021: Islem is joining the group as a PhD student. Welcome!
7/2021: Distinguished Artifact Award for our ISSTA'21 paper on finding JSON schema-related bugs .
7/2021: Our paper on comparing neural models of code and developers has been accepted at ASE'21.
7/2021: Our SemSeed paper is receiving an ACM SIGSOFT Distinguished Paper Award at FSE'21.
6/2021: Our work on learning to make compiler optimizations more effective will be presented at MAPS'21.
5/2021: Our paper on semantic bug seeding will appear at FSE'21.
5/2021: Jibesh has successfully defended his PhD thesis on Analyzing Code Corpora to Improve the Correctness and Reliability of Programs .
5/2021: Our work on Mir , an RWX permission model for Node.js packages, has been accepted to CCS'21.
4/2021: Our papers on finding JSON schema-related bugs and on continuous test suite failure prediction have been accepted at ISSTA'21.
4/2021: Our article on neural software analysis is going to appear in the Communications of the ACM.
1/2021: Our work on WasmBench, a study and benchmark involving thousands of WebAssembly binaries, will be presented at The Web Conference (WWW) 2021.
12/2020: Organizing a Dagstuhl seminar on learning-based program analysis (together with Baishakhi , Eran , and Charles ), to be held in January 2022.
12/2020: Our paper on IdBench, a benchmark for semantic representations of identifiers , will be presented at ICSE'21.
12/2020: Andrew successfully defends his PhD thesis on " Learning to Find Bugs in Programs and their Documentation "
12/2020: Matteo Paltenghi is joining the group as as PhD student. Welcome!
11/2020: New review article that gives an overview of neural software analysis .
11/2020: Check out our work on an RWX permission model for Node.js packages .
10/2020: New paper on application-level caching to appear in IEEE Software.
10/2020: Aryaz Eghbali is joining the group as a PhD student. Welcome!
9/2020: Martin Torp is joining as a visiting PhD student from Aarhus University. Welcome!
7/2020: Our study of string-related software bugs will appear at ASE 2020.
6/2020: Our work on WebAssembly (in)security will be presented at USENIX Security 2020.
5/2020: Our paper on TypeWriter will appear at FSE'20.
4/2020: Our paper on bug localization on millions of files will appear at ISSTA'20.
4/2020: From PhD to faculty: Cristian has accepted a tenure-track faculty position at CISPA . Congrats!
3/2020: Cristian has successfully defended his PhD thesis on JavaScript security and privacy. Congrats!
3/2020: JetBrains ships IDE plugins that implement DeepBugs for JavaScript and Python . Great to see our ideas used in the wild!
- Home (current)
- Course Info
- Paper Commentaries
Assignments
- Discussion board
- Assignment dropbox
- Anonymous feedback
Welcome to CSE 501!
Administrative information, course information.
501 is a class that focuses on the techniques that can be used to understand programs. These techniques serve as the foundations of a diversity of tools, from compilers to program analyzers, bug finders, malware detectors, etc. It is not a class that focuses on building a classical compiler from start to finish. Instead, the goal of the class is to supply students with a toolset that they can use for their own research, whenever they need to build tools that can understand programs.
The class is a project-based one where students come up with their project ideas that they work on throughout the quarter. We will have a few initial assignments to familarize students with available tools for analyzing programs. More information about the project will be provided later in the quarter.
We will discuss classical and recent research papers during each class meeting. We expect you to have read the paper before each meeting and be ready to participate in the discussion.
Prerequisites
There are no formal prerequisites for the course. However, we expect you to have an understanding of data structures and mathematical logic, and know how to program. You do not have to have already taken a compiler or a programming languages class. However, undergraduates are recommended to do so.
Optional references
Assignments: 30% Project: 50% Paper commentaries and class participation: 20% As this is a graduate class, the class will be A-centered, but students are not guaranteed a grade of A.
Paper reading and commentaries (with writing suggestions from Prof. Ernst)
We will discuss 1-2 papers during each lecture. To help you prepare, you will write a one-paragraph commentary on each paper, and submit it at least 24 hours before the class meets. We will post all summaries before class online. The commentary should reflect your understanding and analysis of the issues raised by the paper, and should also help direct (both your and others') preparation for in-class discussion.
One suggestion for the format for the commentary is to critique the paper, listing the following three points: its biggest contribution (and, briefly, why that result was not already obvious), its biggest mistake (in motivation, methodology, algorithm, data analysis, conclusions, or some other area), and the biggest question that it raises (or the most interesting and important follow-on work that it suggests). Another acceptable format is to summarize the paper, describing its thesis, approach, and conclusions, and stating why it is significant. The commentary should also list questions that you have about the paper, such as about technical points or connections to related work.
We encourage you to use the commentaries to ask questions or comment on those posted by others. If you have a question, it is likely that many other people have the same question but are too shy (or vain, or insecure) to ask it; they will appreciate your raising the point. However, do come to class prepared: carefully read the paper and get as much as you can out of it on your own. Doing so will make the class time that much more productive.
You will have access to all the other students’ submissions. Please read them, because reading the other summaries is a good way for you to get perspective. You can see what you missed (or what other people missed), and whether you agree with their take on the key ideas. It will help to make the class sessions more productive.
Application paper presentations
- Motivate the paper: what is problem being tackled, and why is it important?
- What has been previously done (if any) to solve the problem? Why is it not solved?
- What is the proposed solution? How is it described in the paper? Does it leverage existing techniques or invent new ones? How are they significant?
- What are the limitations of the techniques proposed? Did the authors address them?
- How was the proposed solution evaluated? Was it sufficient?
- Does the paper open up new areas of research? Does it solve the problem for once and for all? What will happen if the proposed solution is widely adopted (if not so already)?
- The Paper Revieweing Process
- Reviewing technical papers
- Cormode, How NOT to review a paper: the tools and techniques of the adversarial reviewer," SIGMOD Record, 37:4, 2008.
Paper summaries
- HW1: Dataflow analysis
- HW2: Axiomatic semantics
Security Research: Program Analysis Meets Security
- First Online: 05 November 2023
Cite this chapter
- Padmanabhan Krishnan 8
Part of the book series: Lecture Notes in Computer Science ((LNCS,volume 13490))
Included in the following conference series:
- International Colloquium on Theoretical Aspects of Computing
90 Accesses
In this paper we present the key features of some of the security analysis tools developed at Oracle, Labs. These include Parfait, a static analyser, Affogato a dynamic analysis based on run-time instrumentation of Node.js applications and Gelato a dynamic analysis tool that inspects only the client-side code written in JavaScript. We show the how these tools can be integrated at different phases of the software development life-cycle. This paper is based on the presentation at the ICTAC school in 2021.
This is a preview of subscription content, log in via an institution to check access.
Access this chapter
- Available as EPUB and PDF
- Read on any device
- Instant download
- Own it forever
- Compact, lightweight edition
- Dispatched in 3 to 5 business days
- Free shipping worldwide - see info
Tax calculation will be finalised at checkout
Purchases are for personal use only
Institutional subscriptions
Cifuentes, C.: Towards intelligent application security (invited talk). In: SOAP (2021)
Google Scholar
Cifuentes, C., Keynes, N., Li, L., Hawes, N., Valdiviezo, M.: Transitioning Parfait into a development tool. IEEE Secur. Privacy 10 (3), 16–23 (2012)
Officer, D.D.C.I.: DoD enterprise devsecops reference design. Tech. rep, Department of Defense (2019)
Gauthier, F., Hassanshahi, B., Jordan, A.: AFFOGATO: runtime detection of injection attacks for node.js. In: Companion Proceedings for the ISSTA/ECOOP Workshops, pp. 94–99. ACM (2018)
Gauthier, F., Hassanshahi, B., Selwyn-Smith, B., Mai, T.N., Schlüter, M., Williams, M.: Experience: Model-Based, Feedback-Driven, Greybox Web Fuzzing with BackREST. In: European Conference on Object-Oriented Programming, ECOOP. LIPIcs, vol. 222, pp. 29:1–29:30. Schloss Dagstuhl - Leibniz-Zentrum für Informatik (2022)
Gauthier, F., Keynes, N., Allen, N., Corney, D., Krishnan, P.: Scalable static analysis to detect security vulnerabilities: Challenges and solutions. In: IEEE SecDev (2018)
Hassanshahi, B., Lee, H., Krishnan, P.: Gelato: Feedback-driven and guided security analysis of client-side web applications. In: SANER (2022)
Jordan, A., Gauthier, F., Hassanshahi, B., Zhao, D.: SAFE-PDF: robust detection of javascript PDF malware using abstract interpretation. Tech. rep, CoRR (2018)
Krishnan, P., Lu, Y., Raghavendra, K.R.: Detecting unauthorised information flows using points-to analysis. Engineering and Technology Reference (2016)
Krishnan, P., O’Donoghue, R., Allen, N., Lu, Y.: Commit-time incremental analysis. In: SOAP. ACM (2019)
Lattner, C., Adve, V.: The LLVM compiler framework and infrastructure tutorial. In: LCPC: Mini Workshop on Compiler Research Infrastructures (2004)
Nielson, F., Nielson, H.R., Hankin, C.: Principles of Program Analysis. Springer, 2 edn. (2005)
Park, J., Ryou, Y., Park, J., Ryu, S.: Analysis of Javascript web applications using SAFE 2.0. In: ICSE, pp. 59–62. IEEE Computer Society (2017)
Rajapakse, R.N., Zahedi, M., Babar, M.A., Shen, H.: Challenges and solutions when adopting devsecops: A systematic review. Information and Software Technology 141 (2022)
Download references
Acknowledgements
This paper is reporting the work done by the entire team at Oracle Labs, Australia over many years. The author is thankful for their support in developing this paper.
Author information
Authors and affiliations.
Oracle Labs, Brisbane, QLD, 4000, Australia
Padmanabhan Krishnan
You can also search for this author in PubMed Google Scholar
Corresponding author
Correspondence to Padmanabhan Krishnan .
Editor information
Editors and affiliations.
Nazarbayev University, Astana, Kazakhstan
Antonio Cerone
Rights and permissions
Reprints and permissions
Copyright information
© 2023 The Author(s), under exclusive licence to Springer Nature Switzerland AG
About this chapter
Krishnan, P. (2023). Security Research: Program Analysis Meets Security. In: Cerone, A. (eds) Formal Methods for an Informal World. ICTAC 2021. Lecture Notes in Computer Science, vol 13490. Springer, Cham. https://doi.org/10.1007/978-3-031-43678-9_6
Download citation
DOI : https://doi.org/10.1007/978-3-031-43678-9_6
Published : 05 November 2023
Publisher Name : Springer, Cham
Print ISBN : 978-3-031-43677-2
Online ISBN : 978-3-031-43678-9
eBook Packages : Computer Science Computer Science (R0)
Share this chapter
Anyone you share the following link with will be able to read this content:
Sorry, a shareable link is not currently available for this article.
Provided by the Springer Nature SharedIt content-sharing initiative
- Publish with us
Policies and ethics
- Find a journal
- Track your research
- Skip to main content
- Skip to primary sidebar
- Skip to footer
- QuestionPro

- Solutions Industries Gaming Automotive Sports and events Education Government Travel & Hospitality Financial Services Healthcare Cannabis Technology Use Case NPS+ Communities Audience Contactless surveys Mobile LivePolls Member Experience GDPR Positive People Science 360 Feedback Surveys
- Resources Blog eBooks Survey Templates Case Studies Training Help center

Home Market Research
Data Analysis in Research: Types & Methods
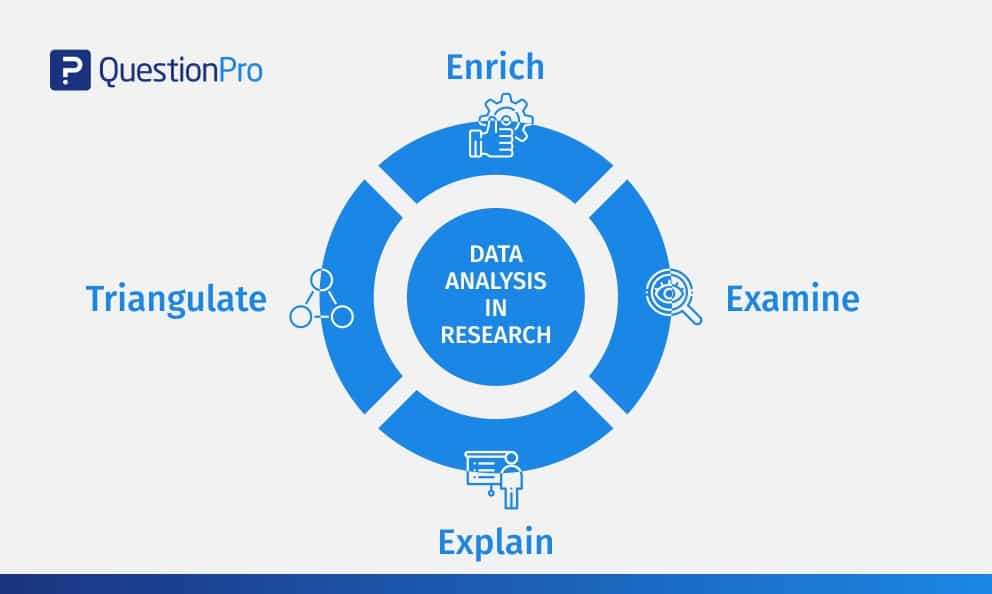
Content Index
Why analyze data in research?
Types of data in research, finding patterns in the qualitative data, methods used for data analysis in qualitative research, preparing data for analysis, methods used for data analysis in quantitative research, considerations in research data analysis, what is data analysis in research.
Definition of research in data analysis: According to LeCompte and Schensul, research data analysis is a process used by researchers to reduce data to a story and interpret it to derive insights. The data analysis process helps reduce a large chunk of data into smaller fragments, which makes sense.
Three essential things occur during the data analysis process — the first is data organization . Summarization and categorization together contribute to becoming the second known method used for data reduction. It helps find patterns and themes in the data for easy identification and linking. The third and last way is data analysis – researchers do it in both top-down and bottom-up fashion.
LEARN ABOUT: Research Process Steps
On the other hand, Marshall and Rossman describe data analysis as a messy, ambiguous, and time-consuming but creative and fascinating process through which a mass of collected data is brought to order, structure and meaning.
We can say that “the data analysis and data interpretation is a process representing the application of deductive and inductive logic to the research and data analysis.”
Researchers rely heavily on data as they have a story to tell or research problems to solve. It starts with a question, and data is nothing but an answer to that question. But, what if there is no question to ask? Well! It is possible to explore data even without a problem – we call it ‘Data Mining’, which often reveals some interesting patterns within the data that are worth exploring.
Irrelevant to the type of data researchers explore, their mission and audiences’ vision guide them to find the patterns to shape the story they want to tell. One of the essential things expected from researchers while analyzing data is to stay open and remain unbiased toward unexpected patterns, expressions, and results. Remember, sometimes, data analysis tells the most unforeseen yet exciting stories that were not expected when initiating data analysis. Therefore, rely on the data you have at hand and enjoy the journey of exploratory research.
Create a Free Account
Every kind of data has a rare quality of describing things after assigning a specific value to it. For analysis, you need to organize these values, processed and presented in a given context, to make it useful. Data can be in different forms; here are the primary data types.
- Qualitative data: When the data presented has words and descriptions, then we call it qualitative data . Although you can observe this data, it is subjective and harder to analyze data in research, especially for comparison. Example: Quality data represents everything describing taste, experience, texture, or an opinion that is considered quality data. This type of data is usually collected through focus groups, personal qualitative interviews , qualitative observation or using open-ended questions in surveys.
- Quantitative data: Any data expressed in numbers of numerical figures are called quantitative data . This type of data can be distinguished into categories, grouped, measured, calculated, or ranked. Example: questions such as age, rank, cost, length, weight, scores, etc. everything comes under this type of data. You can present such data in graphical format, charts, or apply statistical analysis methods to this data. The (Outcomes Measurement Systems) OMS questionnaires in surveys are a significant source of collecting numeric data.
- Categorical data: It is data presented in groups. However, an item included in the categorical data cannot belong to more than one group. Example: A person responding to a survey by telling his living style, marital status, smoking habit, or drinking habit comes under the categorical data. A chi-square test is a standard method used to analyze this data.
Learn More : Examples of Qualitative Data in Education
Data analysis in qualitative research
Data analysis and qualitative data research work a little differently from the numerical data as the quality data is made up of words, descriptions, images, objects, and sometimes symbols. Getting insight from such complicated information is a complicated process. Hence it is typically used for exploratory research and data analysis .
Although there are several ways to find patterns in the textual information, a word-based method is the most relied and widely used global technique for research and data analysis. Notably, the data analysis process in qualitative research is manual. Here the researchers usually read the available data and find repetitive or commonly used words.
For example, while studying data collected from African countries to understand the most pressing issues people face, researchers might find “food” and “hunger” are the most commonly used words and will highlight them for further analysis.
LEARN ABOUT: Level of Analysis
The keyword context is another widely used word-based technique. In this method, the researcher tries to understand the concept by analyzing the context in which the participants use a particular keyword.
For example , researchers conducting research and data analysis for studying the concept of ‘diabetes’ amongst respondents might analyze the context of when and how the respondent has used or referred to the word ‘diabetes.’
The scrutiny-based technique is also one of the highly recommended text analysis methods used to identify a quality data pattern. Compare and contrast is the widely used method under this technique to differentiate how a specific text is similar or different from each other.
For example: To find out the “importance of resident doctor in a company,” the collected data is divided into people who think it is necessary to hire a resident doctor and those who think it is unnecessary. Compare and contrast is the best method that can be used to analyze the polls having single-answer questions types .
Metaphors can be used to reduce the data pile and find patterns in it so that it becomes easier to connect data with theory.
Variable Partitioning is another technique used to split variables so that researchers can find more coherent descriptions and explanations from the enormous data.
LEARN ABOUT: Qualitative Research Questions and Questionnaires
There are several techniques to analyze the data in qualitative research, but here are some commonly used methods,
- Content Analysis: It is widely accepted and the most frequently employed technique for data analysis in research methodology. It can be used to analyze the documented information from text, images, and sometimes from the physical items. It depends on the research questions to predict when and where to use this method.
- Narrative Analysis: This method is used to analyze content gathered from various sources such as personal interviews, field observation, and surveys . The majority of times, stories, or opinions shared by people are focused on finding answers to the research questions.
- Discourse Analysis: Similar to narrative analysis, discourse analysis is used to analyze the interactions with people. Nevertheless, this particular method considers the social context under which or within which the communication between the researcher and respondent takes place. In addition to that, discourse analysis also focuses on the lifestyle and day-to-day environment while deriving any conclusion.
- Grounded Theory: When you want to explain why a particular phenomenon happened, then using grounded theory for analyzing quality data is the best resort. Grounded theory is applied to study data about the host of similar cases occurring in different settings. When researchers are using this method, they might alter explanations or produce new ones until they arrive at some conclusion.
LEARN ABOUT: 12 Best Tools for Researchers
Data analysis in quantitative research
The first stage in research and data analysis is to make it for the analysis so that the nominal data can be converted into something meaningful. Data preparation consists of the below phases.
Phase I: Data Validation
Data validation is done to understand if the collected data sample is per the pre-set standards, or it is a biased data sample again divided into four different stages
- Fraud: To ensure an actual human being records each response to the survey or the questionnaire
- Screening: To make sure each participant or respondent is selected or chosen in compliance with the research criteria
- Procedure: To ensure ethical standards were maintained while collecting the data sample
- Completeness: To ensure that the respondent has answered all the questions in an online survey. Else, the interviewer had asked all the questions devised in the questionnaire.
Phase II: Data Editing
More often, an extensive research data sample comes loaded with errors. Respondents sometimes fill in some fields incorrectly or sometimes skip them accidentally. Data editing is a process wherein the researchers have to confirm that the provided data is free of such errors. They need to conduct necessary checks and outlier checks to edit the raw edit and make it ready for analysis.
Phase III: Data Coding
Out of all three, this is the most critical phase of data preparation associated with grouping and assigning values to the survey responses . If a survey is completed with a 1000 sample size, the researcher will create an age bracket to distinguish the respondents based on their age. Thus, it becomes easier to analyze small data buckets rather than deal with the massive data pile.
LEARN ABOUT: Steps in Qualitative Research
After the data is prepared for analysis, researchers are open to using different research and data analysis methods to derive meaningful insights. For sure, statistical analysis plans are the most favored to analyze numerical data. In statistical analysis, distinguishing between categorical data and numerical data is essential, as categorical data involves distinct categories or labels, while numerical data consists of measurable quantities. The method is again classified into two groups. First, ‘Descriptive Statistics’ used to describe data. Second, ‘Inferential statistics’ that helps in comparing the data .
Descriptive statistics
This method is used to describe the basic features of versatile types of data in research. It presents the data in such a meaningful way that pattern in the data starts making sense. Nevertheless, the descriptive analysis does not go beyond making conclusions. The conclusions are again based on the hypothesis researchers have formulated so far. Here are a few major types of descriptive analysis methods.
Measures of Frequency
- Count, Percent, Frequency
- It is used to denote home often a particular event occurs.
- Researchers use it when they want to showcase how often a response is given.
Measures of Central Tendency
- Mean, Median, Mode
- The method is widely used to demonstrate distribution by various points.
- Researchers use this method when they want to showcase the most commonly or averagely indicated response.
Measures of Dispersion or Variation
- Range, Variance, Standard deviation
- Here the field equals high/low points.
- Variance standard deviation = difference between the observed score and mean
- It is used to identify the spread of scores by stating intervals.
- Researchers use this method to showcase data spread out. It helps them identify the depth until which the data is spread out that it directly affects the mean.
Measures of Position
- Percentile ranks, Quartile ranks
- It relies on standardized scores helping researchers to identify the relationship between different scores.
- It is often used when researchers want to compare scores with the average count.
For quantitative research use of descriptive analysis often give absolute numbers, but the in-depth analysis is never sufficient to demonstrate the rationale behind those numbers. Nevertheless, it is necessary to think of the best method for research and data analysis suiting your survey questionnaire and what story researchers want to tell. For example, the mean is the best way to demonstrate the students’ average scores in schools. It is better to rely on the descriptive statistics when the researchers intend to keep the research or outcome limited to the provided sample without generalizing it. For example, when you want to compare average voting done in two different cities, differential statistics are enough.
Descriptive analysis is also called a ‘univariate analysis’ since it is commonly used to analyze a single variable.
Inferential statistics
Inferential statistics are used to make predictions about a larger population after research and data analysis of the representing population’s collected sample. For example, you can ask some odd 100 audiences at a movie theater if they like the movie they are watching. Researchers then use inferential statistics on the collected sample to reason that about 80-90% of people like the movie.
Here are two significant areas of inferential statistics.
- Estimating parameters: It takes statistics from the sample research data and demonstrates something about the population parameter.
- Hypothesis test: I t’s about sampling research data to answer the survey research questions. For example, researchers might be interested to understand if the new shade of lipstick recently launched is good or not, or if the multivitamin capsules help children to perform better at games.
These are sophisticated analysis methods used to showcase the relationship between different variables instead of describing a single variable. It is often used when researchers want something beyond absolute numbers to understand the relationship between variables.
Here are some of the commonly used methods for data analysis in research.
- Correlation: When researchers are not conducting experimental research or quasi-experimental research wherein the researchers are interested to understand the relationship between two or more variables, they opt for correlational research methods.
- Cross-tabulation: Also called contingency tables, cross-tabulation is used to analyze the relationship between multiple variables. Suppose provided data has age and gender categories presented in rows and columns. A two-dimensional cross-tabulation helps for seamless data analysis and research by showing the number of males and females in each age category.
- Regression analysis: For understanding the strong relationship between two variables, researchers do not look beyond the primary and commonly used regression analysis method, which is also a type of predictive analysis used. In this method, you have an essential factor called the dependent variable. You also have multiple independent variables in regression analysis. You undertake efforts to find out the impact of independent variables on the dependent variable. The values of both independent and dependent variables are assumed as being ascertained in an error-free random manner.
- Frequency tables: The statistical procedure is used for testing the degree to which two or more vary or differ in an experiment. A considerable degree of variation means research findings were significant. In many contexts, ANOVA testing and variance analysis are similar.
- Analysis of variance: The statistical procedure is used for testing the degree to which two or more vary or differ in an experiment. A considerable degree of variation means research findings were significant. In many contexts, ANOVA testing and variance analysis are similar.
- Researchers must have the necessary research skills to analyze and manipulation the data , Getting trained to demonstrate a high standard of research practice. Ideally, researchers must possess more than a basic understanding of the rationale of selecting one statistical method over the other to obtain better data insights.
- Usually, research and data analytics projects differ by scientific discipline; therefore, getting statistical advice at the beginning of analysis helps design a survey questionnaire, select data collection methods , and choose samples.
LEARN ABOUT: Best Data Collection Tools
- The primary aim of data research and analysis is to derive ultimate insights that are unbiased. Any mistake in or keeping a biased mind to collect data, selecting an analysis method, or choosing audience sample il to draw a biased inference.
- Irrelevant to the sophistication used in research data and analysis is enough to rectify the poorly defined objective outcome measurements. It does not matter if the design is at fault or intentions are not clear, but lack of clarity might mislead readers, so avoid the practice.
- The motive behind data analysis in research is to present accurate and reliable data. As far as possible, avoid statistical errors, and find a way to deal with everyday challenges like outliers, missing data, data altering, data mining , or developing graphical representation.
LEARN MORE: Descriptive Research vs Correlational Research The sheer amount of data generated daily is frightening. Especially when data analysis has taken center stage. in 2018. In last year, the total data supply amounted to 2.8 trillion gigabytes. Hence, it is clear that the enterprises willing to survive in the hypercompetitive world must possess an excellent capability to analyze complex research data, derive actionable insights, and adapt to the new market needs.
LEARN ABOUT: Average Order Value
QuestionPro is an online survey platform that empowers organizations in data analysis and research and provides them a medium to collect data by creating appealing surveys.
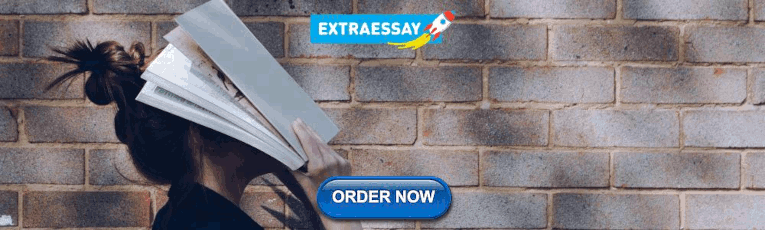
MORE LIKE THIS
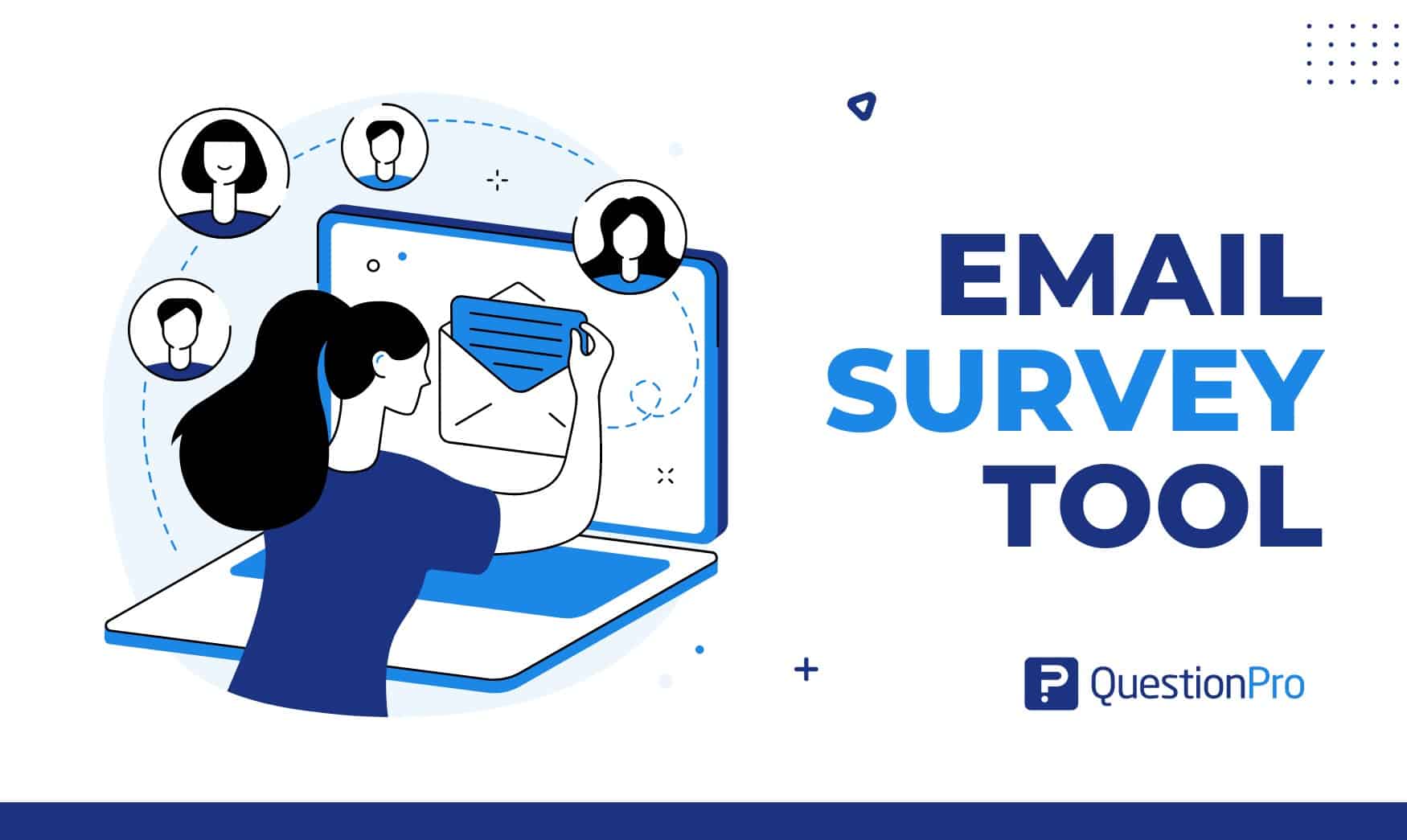
The Best Email Survey Tool to Boost Your Feedback Game
May 7, 2024
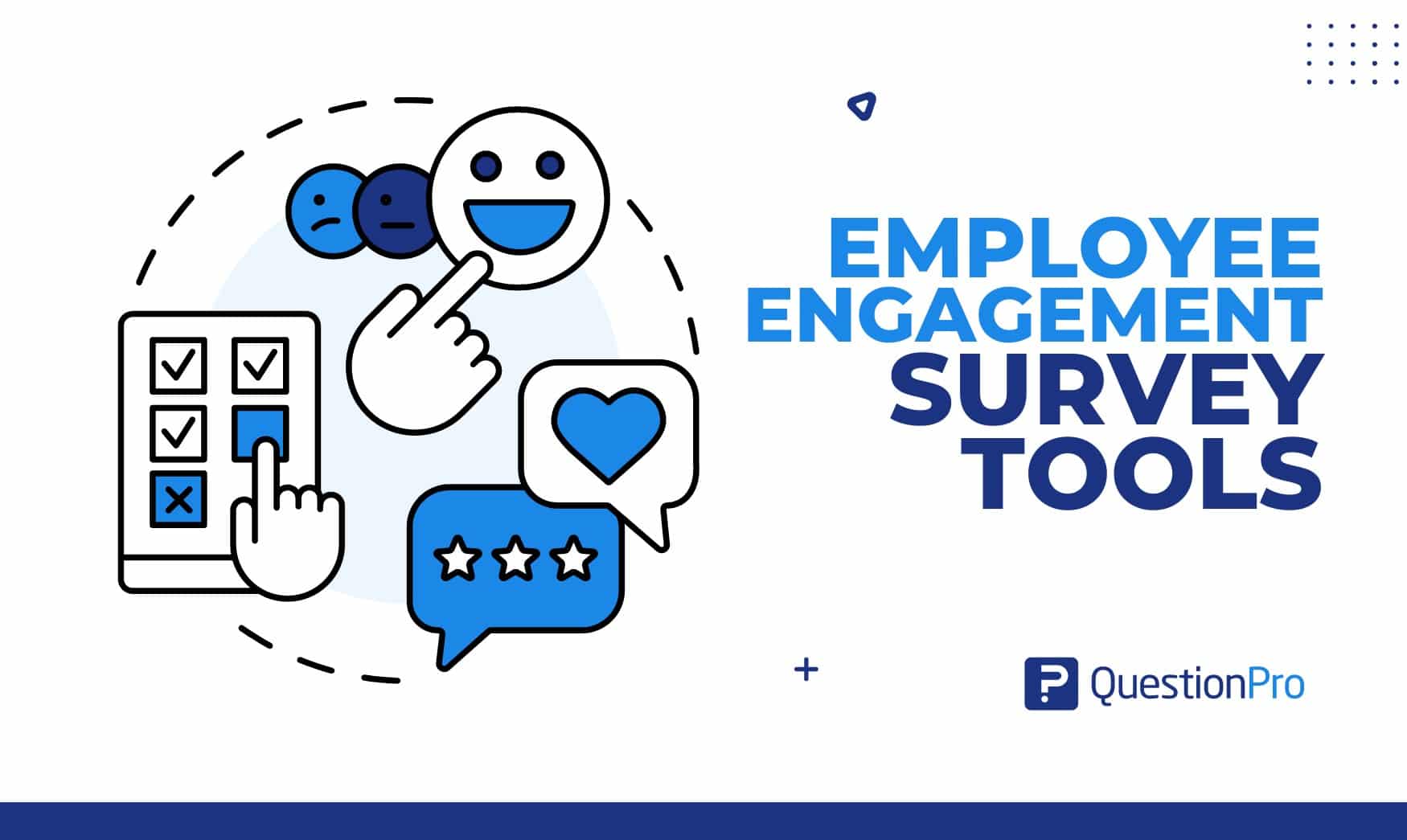
Top 10 Employee Engagement Survey Tools
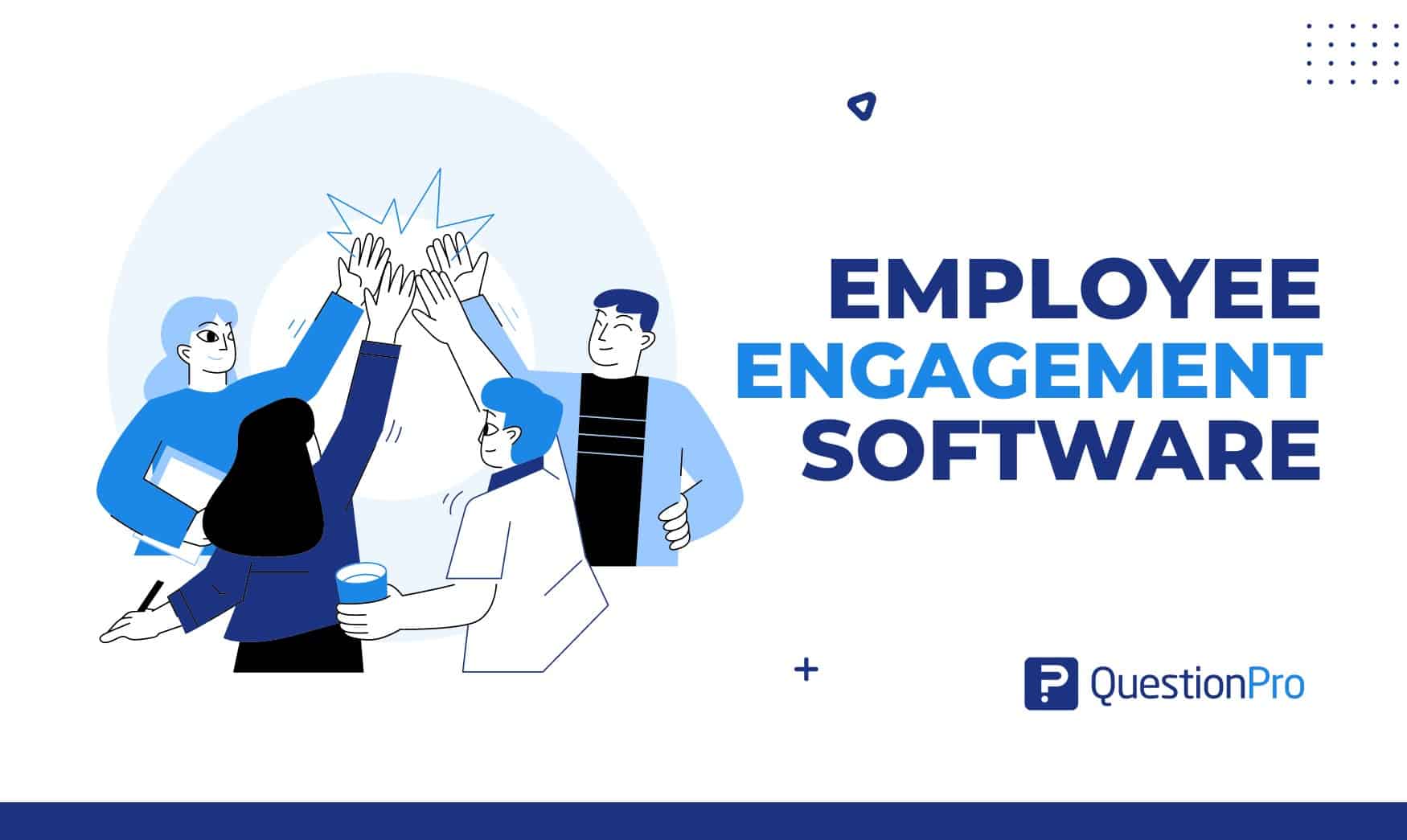
Top 20 Employee Engagement Software Solutions
May 3, 2024
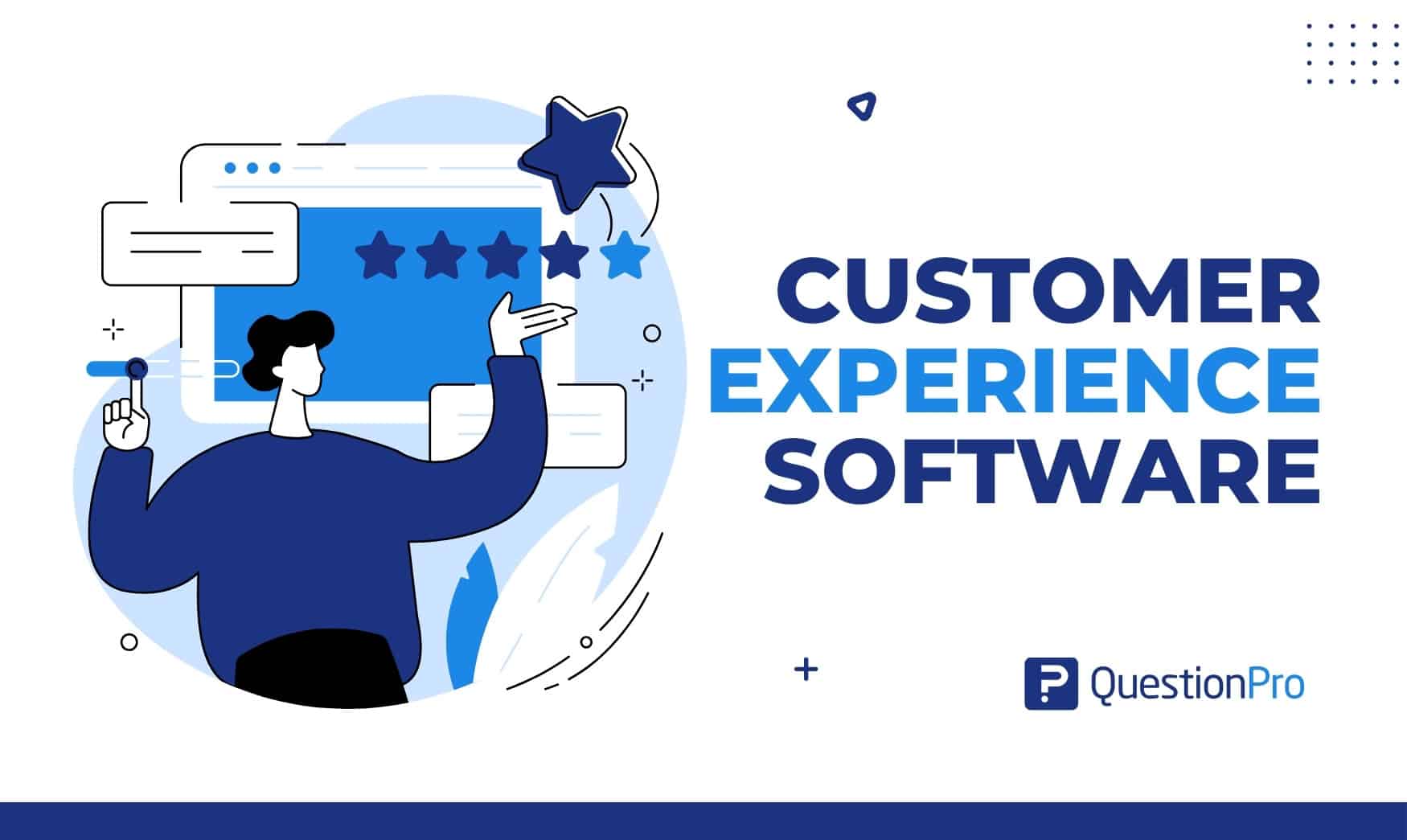
15 Best Customer Experience Software of 2024
May 2, 2024
Other categories
- Academic Research
- Artificial Intelligence
- Assessments
- Brand Awareness
- Case Studies
- Communities
- Consumer Insights
- Customer effort score
- Customer Engagement
- Customer Experience
- Customer Loyalty
- Customer Research
- Customer Satisfaction
- Employee Benefits
- Employee Engagement
- Employee Retention
- Friday Five
- General Data Protection Regulation
- Insights Hub
- Life@QuestionPro
- Market Research
- Mobile diaries
- Mobile Surveys
- New Features
- Online Communities
- Question Types
- Questionnaire
- QuestionPro Products
- Release Notes
- Research Tools and Apps
- Revenue at Risk
- Survey Templates
- Training Tips
- Uncategorized
- Video Learning Series
- What’s Coming Up
- Workforce Intelligence
Skip to content
- Our Faculty
Educational Programs
News & events, current projects, testing effects of cash transfers on biological aging and risk for alzheimer's disease.
The primary objectives of this project are to assess the effects of a cash transfer program on the prevention of biological aging and dementia risk in younger and older individuals. Additionally, we aim to investigate the association between biological aging and cognitive function in this population. The results of this research could hold substantial implications for public health by revealing the causal and long-term health consequences of socioeconomic interventions on health. Moreover, it will offer fresh perspectives on the levels of biological aging and Alzheimer’s Disease risk within the context of lower- and middle-income countries. (National Institute on Aging R01AG087158)
Genomic Analysis of the CALERIE Trial to Generate New Knowledge for Geroscience .
We are conducting genomic analysis of blood, muscle, and adipose tissue from the first-ever randomized controlled trial of caloric restriction in healthy, non-obese adults. We are studying how caloric restriction, which is proven to increase healthy lifespan in many animal models, may affect biological aging in humans. (National Institute on Aging R01AG061378)
Genetic Analysis of the Dutch Hunger Winter Families Study to Boost Rigor and Robustness for Testing In-Utero Famine Effects on Aging-Related Health Conditions and Biological Aging .
The graying global population makes interventions to extend healthy lifespan a public heath priority. Health insults during the perinatal period are linked with risk for aging-related health conditions, including obesity, type 2 diabetes, and cardio-metabolic disease. If these associations are causal, interventions to prevent perinatal insults and to reverse their biological damage could delay disease onset and prolong healthspan, the length of time a person remains healthy. (National Institute on Aging R01AG066887)
The MyGoals for Healthy Aging Multi-Center Randomized Controlled Trial
MyGoals for Healthy Aging is a randomized controlled trial testing if a novel anti-poverty program slows biological aging in highly disadvantaged public housing recipients, including by inducing changes in risk factors for Alzheimer's disease/Alzheimer's disease-related dementias. (National Institute on Aging R01AG073402)
Life-course Sociogenomic Analysis of Social Inequalities in Aging
Life-course social science links early-life social disadvantage with adverse outcomes in aging. A gap in knowledge is how social disadvantage is biologically embedded, leading to social inequalities. A hypothesis is that social disadvantage actually hastens aging. While everyone ages chronologically at the same rate, biological changes with aging may proceed faster for some than others. These changes are thought to be a root cause of disease/disability and an intervention target to extend healthy lifespan. (Russel Sage Foundation BioSS Grant 1810-08987 )
Developing Epigenetic Measurements to Forecast Long-Term Benefits of Early-Life Interventions
Early-life adversity a major driver of health and social problems across the life-course. Several interventions, including home-visitor programs, show potential to protect young children from lifelong damage arising from early-adversity. These interventions increase parental nurturing and prevent some early-life stress exposures. In the short- to medium-term, they benefit families and children. But longer-term outcomes vary substantially across children. (Jacobs Foundation Young Scholars Award; CIFAR CF-0249-CP22-034)
Root out friction in every digital experience, super-charge conversion rates, and optimize digital self-service
Uncover insights from any interaction, deliver AI-powered agent coaching, and reduce cost to serve
Increase revenue and loyalty with real-time insights and recommendations delivered to teams on the ground
Know how your people feel and empower managers to improve employee engagement, productivity, and retention
Take action in the moments that matter most along the employee journey and drive bottom line growth
Whatever they’re are saying, wherever they’re saying it, know exactly what’s going on with your people
Get faster, richer insights with qual and quant tools that make powerful market research available to everyone
Run concept tests, pricing studies, prototyping + more with fast, powerful studies designed by UX research experts
Track your brand performance 24/7 and act quickly to respond to opportunities and challenges in your market
Explore the platform powering Experience Management
- Free Account
- For Digital
- For Customer Care
- For Human Resources
- For Researchers
- Financial Services
- All Industries
Popular Use Cases
- Customer Experience
- Employee Experience
- Net Promoter Score
- Voice of Customer
- Customer Success Hub
- Product Documentation
- Training & Certification
- XM Institute
- Popular Resources
- Customer Stories
- Artificial Intelligence
- Market Research
- Partnerships
- Marketplace
The annual gathering of the experience leaders at the world’s iconic brands building breakthrough business results, live in Salt Lake City.
- English/AU & NZ
- Español/Europa
- Español/América Latina
- Português Brasileiro
- REQUEST DEMO
Online Survey Software
Discover what your customers and employees are really thinking.
Survey software gets answers to your most important customer, employee, marketing and product questions. It can handle everything from simple customer feedback questionnaires to detailed research projects for the world’s biggest brands.
Buy Online Free Trial
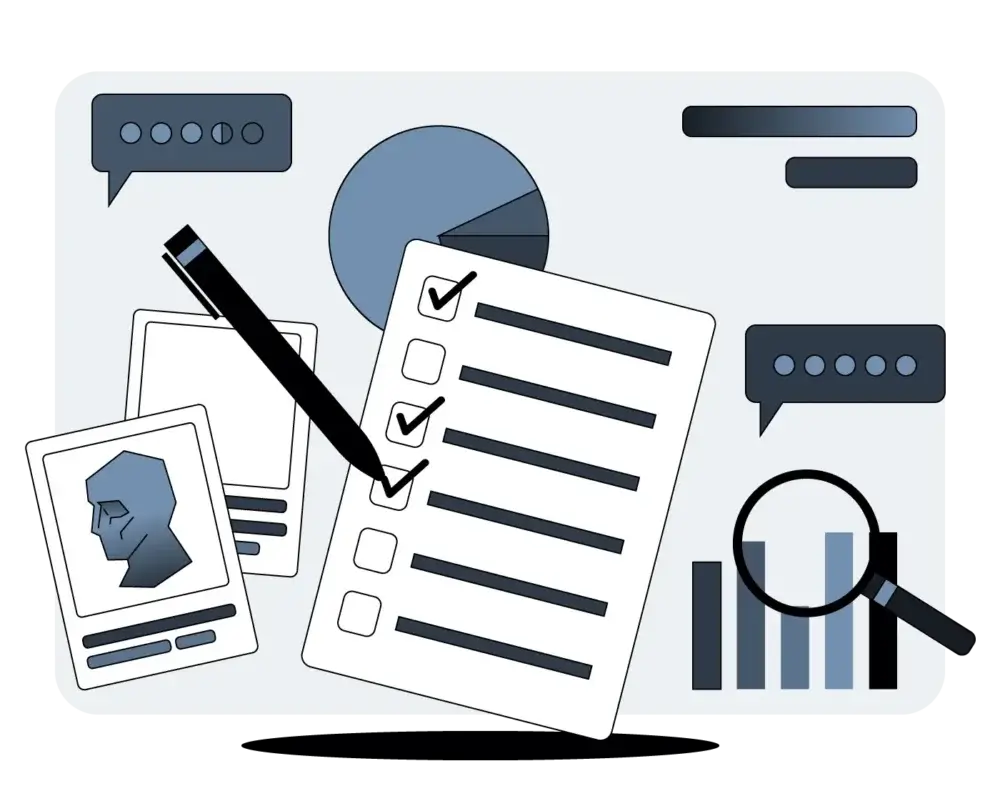
Today's reality—sound familiar?
2.6x more success could have been realized in marketing campaigns with better research & insights., 23% of organizations don’t have a clear market research strategy in place., 13% of marketing spend is wasted for reasons that could have been addressed through better market research., with online survey software you can:.
- Eliminate manual data collection
- Get real-time, actionable insights
- Reach more people, faster and easier
- Get better, more honest responses
- Create professional surveys without any experience
Ready to take your market research to the next level?
Answers and insights from your audience, wherever they are.
Wherever you need to gather data, survey software can help. From a simple survey link you can paste anywhere, to advanced integrations with your CRM, to email, social, website, QR code, SMS and offline surveys, we’ll help you reach your target respondents, no matter where they are.
Drag-and-drop simplicity for even the most advanced surveys
Choose from 23 question types (including video/audio responses) and use advanced logic, branching, quotas, API integrations into Zendesk and email triggers to build and launch your project. It’s all done in an intuitive drag-and-drop software interface that makes even the most sophisticated surveys easy to create, launch and analyze.
Next-level survey reports and dashboards
Make better decisions with advanced reports and dashboards you can share in seconds. Choose from over 30 different graph types, share reports online, or export survey data to popular formats like CSV, TSV, Excel, SPSS and more.
Built-in intelligence with every type of survey
Leverage advanced analysis, including video feedback summarization powered by generative AI, crosstabs, and statistical analysis tools. Automatically review survey design to ensure methodology best practices, response quality, and compliance with internal policies and PII.
You’re in good company
Qualtrics has helped us bring some exciting new products to life, and ensured that we’re communicating the benefits in a way that resonates
Qualtrics enabled us to break silos that previously existed, helping us share customer insights across the group and reach our goals quicker
Survey software FAQs
A survey is a method of gathering information using relevant questions from a sample of people with the aim of understanding populations as a whole. Surveys provide a critical source of data and insights for everyone engaged in the information economy, from businesses to media, to government and academics.
Survey software is a tool used to design, send and analyze surveys online. It’s the primary method of collecting feedback at scale whether that’s a simple questionnaire or a detailed study such as customer or employee feedback as part of a more structured experience management program. Cloud-based survey technology has revolutionized the ability to get data, quickly, from a large number of respondents by automating the process of sending out surveys across a variety of channels from websites and mobile to apps, email and even chatbots.
Surveys provide quick, quantitative data on a wide audience’s opinions, preferences, and experiences. They are cost-effective, easy to administer, and can reach a large population. They also allow for anonymity, increasing the chance of honest responses, and their standardized format makes it easy to aggregate and analyze data for clear insights into trends and patterns.
To create a survey , define the objectives, choose target participants, design clear and concise questions, select a survey tool or platform, and ensure the layout is logical. Test the survey, distribute it, and collect responses. Remember to keep it as brief as possible while gathering the necessary information.
To write survey questions , be clear and specific to avoid confusion. Use simple, unbiased language, and opt for closed-ended questions for easier analysis. Ensure questions are relevant to your objectives, and avoid leading or loaded questions that could influence answers. Pretest your questions to catch any issues and revise as needed for clarity and objectivity.
Now used by more than 18,000+ brands, and supporting more than 1.3 billion surveys a year, Qualtrics empowers organizations to gather invaluable customer insights and take immediate, game-changing action – with zero coding required. The Qualtrics survey tool makes it easy to get answers to your most important marketing, branding, customer, and product questions, with easy-to-use tools that can handle everything from simple customer feedback questionnaires to detailed research projects.
Qualtrics Strategic Research pricing is based on interactions including number of survey responses and minutes of video feedback. Our special online pricing offer starts at $420 per month and can be purchased here . Alternatively, you can get started with a free account with basic functionality, or get 30 days access to advanced features with a free trial .
Yes, we offer a free account option with basic survey functionality.
You might also like
7 Tips for Writing Great Questions
The Qualtrics Hand Book Of Question Design
Qualitative research design handbook
2024 Research Trends Report
How AI will Reinvent the Market Research Industry
Quantitative and qualitative research design
Request Demo
Ready to learn more about Qualtrics?
Study Shows How Higher Education Supports Asian American, Native Hawaiian, and Pacific Islander Students Through Culturally Relevant Courses, Programs, and Research
Analysis of minority-serving institutions on the East and West Coasts demonstrates layered processes to build students’ capacities
The model minority myth paints a picture of Asian Americans as a monolithic group with unparalleled success in academics. A new NYU study unpacks this myth, exploring the needs of Asian American, Native Hawaiian, and Pacific Islander students and how higher education institutions support these populations.
In 2007, Congress established a federal designation for higher education institutions that enroll at least 10 percent of undergraduate Asian American, Native Hawaiian, and Pacific Islander (AA&NHPI) students, and who enroll a significant proportion of students from low socioeconomic backgrounds. This designation as an Asian American and Native American Pacific Islander Serving Institution (AANAPISI) was among one of the newest categories of minority-serving institutions that receive federal funding to advance educational equity and support for ethnic and racial minorities.
In a two-site case study, Mike Hoa Nguyen , assistant professor of education at NYU Steinhardt, collected data from interviews, internal and public university documents, and observations of activities, courses, and meetings to determine the process in which AANAPISI programs expand students’ capacities through culturally relevant coursework, mentorship, research, and civic engagement. His findings are published in The Review of Higher Education .
“AANAPISIs demonstrate a federal commitment to supporting the unique educational needs of AA&NHPI students, which are too often obscured by the model minority myth,” said Nguyen. “This myth dangerously asserts that Asian American students, and Native Hawaiian and Pacific Islander students by association, are universally successful and unparalleled in their academic achievements. AANAPISIs play a major role in addressing this problem, and in doing so, provide critical resources to uplift the students they serve. This study documents the process in which these colleges and universities engage in this important work.”
Nguyen's study centered on a large, public community college on the West Coast and a large, urban, regional public university on the East Coast. Nguyen’s findings related to the experiences of students in these programs.
He uncovered a five-tiered process that the two institutions use to build opportunities for learning, practice, and engagement:
AA&NHPI Focused Coursework At both institutions, courses focused on these populations are offered through the institutions’ Asian American Studies programs, where students are exposed to concepts connected to their racial and ethnic identities. One student shared her experience with a course, Asian Women in the United States, “Through my experience with that class I learned…for the first time, issues that affected my community. Specifically, me as an Asian American woman, specifically Vietnamese American…”
Teaching and Mentoring Students who had previously taken AA&NHPI coursework provided tutoring and mentoring to support new students with classwork, programs, books, and scholarship applications. According to one mentor, “Cambodian Americans fall through the cracks, we’re just not in higher ed…It’s not a supportive space for us…[the AANAPISI faculty] understand…from their own community work, from being on campus, and [from] teaching for so long that…when they find students who fit these demographics it makes sense for them to mentor them.”
Advanced AA&NHPI Focused Coursework After serving as mentors, students often take more advanced courses focused on theoretical, historical, and contemporary issues regarding the AA&NHPI experience to continue their academics while gaining tools to make larger contributions toward their communities.
Academic and Research Development Students who complete advanced coursework are provided opportunities to engage in academic projects and research with faculty and staff, presenting research at conferences or publishing in peer-reviewed journals.
Professional and Community Experience The final step in the process offers opportunities for students to engage in community-based projects, internships, and employment with partner organizations, government offices, or other schools. A student shared that his research experience led to the creation of a Vietnamese American organizing and training program. “[Researchers] found out that Vietnamese Americans in [the neighborhood] don't participate in civics or politics…they basically feel disenfranchised, like their vote doesn’t matter…So, the research showed that there needs to be an organization to help push and provide opportunities to talk about politics in a Vietnamese American progressive context…”
“AANAPISIs are the backbone for AA&NHPI students in higher education. These institutions account for six percent of all colleges and universities, yet enroll over 40 percent of all AA&NHPI undergraduates,” said Nguyen. “This study offers new understandings of the critical role that AANAPISIs play to expand educational opportunity and enrich learning experiences—which can be adopted beyond AANAPISIs and for other students—as well as inform the work of policymakers as they seek new solutions to refine and regulate the administration of minority-serving institutions.”
Funding for this study was provided by the UCLA Institute of American Cultures and the UCLA Asian American Studies Center.
Press Contact
You are using an outdated browser. Upgrade your browser today or install Google Chrome Frame to better experience this site.
- Professional learning
Teach. Learn. Grow.
Teach. learn. grow. the education blog.
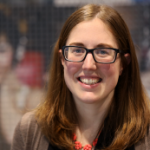
Summer learning loss: What we know and what we’re learning

Concerns about students losing ground academically during summer break go back at least a century, with early evidence suggesting that summer contributed to large disparities in students’ outcomes. This narrative spurred expansion of a variety of summer programs and interventions aimed at stemming summer learning loss.
However, in the last five years, there has been a spirited debate about two long-standing questions about students’ summers: 1) the degree to which test scores actually drop during the summer and 2) the degree to which summer break contributes to educational inequities. A new layer to this conversation is the response to the learning disruptions caused by the COVID-19 pandemic. School leaders and policymakers have used the summer break as a potential time for academic recovery. Summer programs have emerged as one of the most popular recovery strategies offered by school districts, with an estimated $5.8 billion of ESSER funds expected to be spent on summer programs by September 2024.
With more focus on the impact of summer on students’ learning and the potential to extend the school year, it is essential for educators, policymakers, and families to have an up-to-date understanding of the impact of summer breaks on students’ learning patterns. In this post, we aim to highlight what is known about summer learning loss by quickly summarizing recent research and posing some questions that remain unanswered about the role of summers on students’ learning.
Students’ test scores flatten or drop during the summer
While our initial understanding of summer learning loss dates back to studies conducted in the 70s and 80s , a recent collection of studies in the last six years provides a fresh look at students’ learning across summers using four modern assessments ( ECLS-K direct cognitive tests , MAP® Growth™, Star, and i-Ready) with large national (though not typically nationally representative) samples. See “School’s out: The role of summers in understanding achievement disparities,” “When does inequality grow? School, summer, and achievement gaps,” “Evidence of ‘summer learning loss’ on the i-Ready diagnostic assessment,” “Findings on summer learning loss often fail to replicate, even in recent data,” and “Inequality in reading and math skills forms mainly before kindergarten: A replication, and partial correction, of ‘Are schools the great equalizer?’”
Figure 1 compares the test score patterns across four different studies. Three important patterns stand out:
- On average, test scores flatten or drop during the summer , with larger drops typically in math than reading.
- Studies using test scores from ECLS-K:2011 show that student learning slows down but does not drop over the summers after kindergarten and first grade. However, research using interim and diagnostic assessments ( MAP Growth , Star, and i-Ready ) has found far larger summer drops across a range of grade levels.
- Given the sizable differences in the magnitude of test score drops across tests, it remains uncertain whether summer slide should be considered a trivial issue or a serious educational challenge.
Figure 1. Comparison of summer slide estimates across datasets
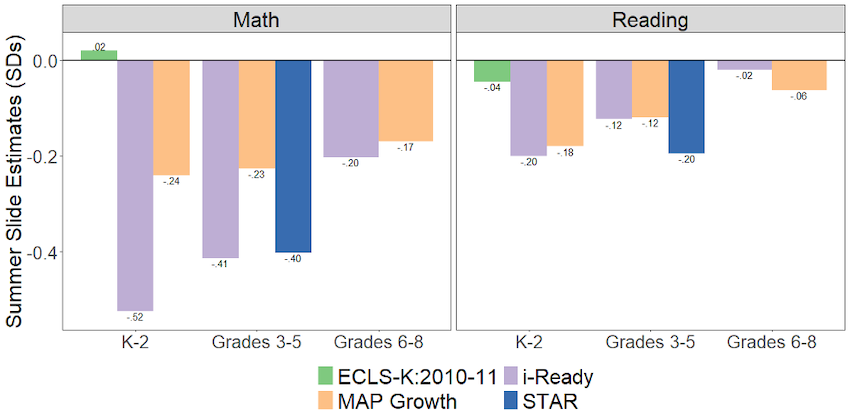
Note: All estimates are reported as the total average summer test score change in standard deviation (SD) units relative to the prior spring test score. Whenever possible, we report the estimate that adjusted scores for time in school prior/after testing in the fall and spring. Sources: Author calculations based on data reported in ECLS-K:20210-11 , MAP Growth , i-Ready , and Star .
Who is most likely to show summer learning loss.
While all three diagnostic assessments show some degree of summer slide in grades 3–8 on average, the research community lacks consensus about whether summers disproportionately impact certain students. Paul von Hippel and colleagues have pointed out that whether and how much summers contribute to educational inequalities (across students of different income levels, races, ethnicities, and genders) depends on the test used to study students’ learning patterns. Nonetheless, we can present a few key patterns from this line of research:
- Learning rates are more variable during the summer than during the school year. See “School’s out: The role of summers in understanding achievement disparities,” “When does inequality grow? School, summer, and achievement gaps,” and “Inequality in reading and math skills forms mainly before kindergarten: A replication, and partial correction, of ‘Are schools the great equalizer?’”
- Gaps between students attending low- and high-poverty schools do not consistently widen during the summer. See “Is summer learning loss real, and does it widen test score gaps by family income?” and “Is summer learning loss real?”
- Test score differences between Black and white students hold steady or narrow during the summer. See “Do test score gaps grow before, during, or between the school years? Measurement artifacts and what we know in spite of them” and “When does inequality grow? School, summer, and achievement gaps,” though results can be sensitive to the metric and test used. See also “Black-white summer learning gaps: Interpreting the variability of estimates across representations” and “Findings on summer learning loss often fail to replicate, even in recent data.”
- The field cannot really explain why differences in students’ summer learning occur. See “Rethinking summer slide: The more you gain, the more you lose” and “Inequality in reading and math skills forms mainly before kindergarten: A replication, and partial correction, of ‘Are schools the great equalizer?’”
Planning effective summer programming
It is clear across recent studies that summer is a particularly variable time for students. Summer break is also increasingly a time in which districts are offering a range of academic offerings.
During summer 2022, an estimated 90% of school districts offered summer programs with an academic focus. However, evidence on the effectiveness of academic summer programs during and after the COVID-19 pandemic is limited. One study of eight summer programs in summer 2022 found a small positive impact on math test scores (0.03 SD), but not on reading. The improvements in math were largely driven by elementary students compared to middle schoolers. However, the effectiveness of these programs remained consistent across student groups, including race/ethnicity, poverty, and English learner status.
It is crucial to recognize the challenges associated with scaling up summer programs. In the districts studied, only 13% of students participated in the summer programs , which only lasted for an average of three to four weeks. Prior research indicates that for summer programs to yield measurable academic benefits, they should run at least five weeks with at least three hours of instruction a day. Additionally, getting students to regularly attend summer programs remains a significant hurdle. To address this issue, districts should actively recruit families to participate and offer a mix of academic instruction and engaging extracurricular activities. By adopting these strategies, districts can maximize the effectiveness of their summer programs and better support student learning during the break.
If you’re interested in learning more about effective summer programs, we encourage you to read the following:
- “Effective summer programming: What educators and policymakers should know”
- “Investing in successful summer programs: A review of evidence under the Every Student Succeeds Act”
- “Analysis: Summer learning is more popular than ever. How to make sure your district’s program is effective”
- “The impact of summer learning programs on low-income children’s mathematics achievement: A meta-analysis”
- “The effects of summer reading on low-income children’s literacy achievement from kindergarten to grade 8: A meta-analysis of classroom and home interventions”
Recommended for you
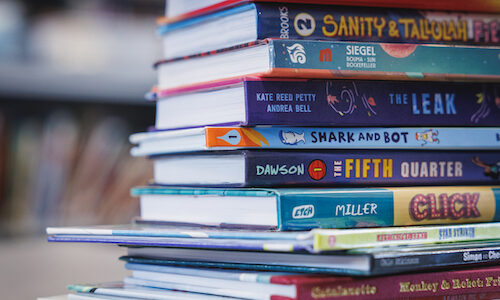
From the pages of Literacy Today : How to boost reading achievement using dyslexia research
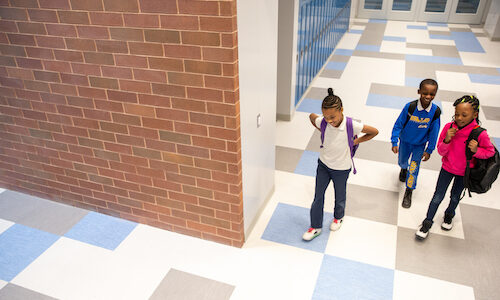
NWEA research snapshot: Insights on academic recovery strategies
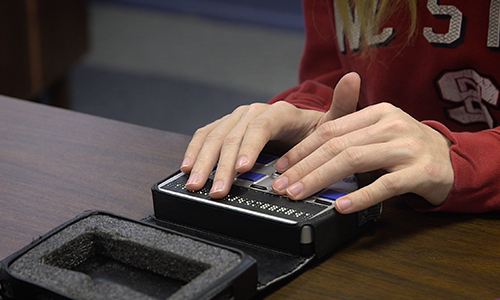
How AI can improve digital accessibility in math
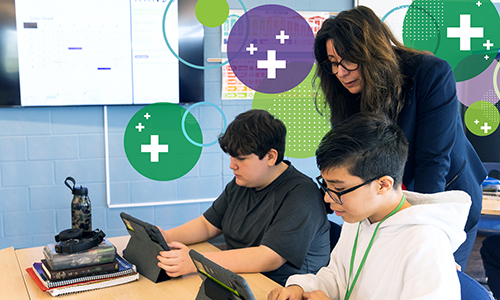
White Paper
Worth the investment: Why research and innovation matter for improving outcomes for all kids
Making sound, student-focused decisions amid constant change can feel impossible. Partnering with a research-based assessment provider can help.
Read the white paper
STAY CURRENT by subscribing to our newsletter
You are now signed up to receive our newsletter containing the latest news, blogs, and resources from nwea..
- Open access
- Published: 08 May 2024
Does health voucher intervention increase antenatal consultations and skilled birth attendances in Cameroon? Results from an interrupted time series analysis
- Isidore Sieleunou ORCID: orcid.org/0000-0001-7264-4540 1 , 2 &
- Roland Pascal Enok Bonong ORCID: orcid.org/0000-0002-9552-5365 2
BMC Health Services Research volume 24 , Article number: 602 ( 2024 ) Cite this article
Metrics details
Limited access to health services during the antenatal period and during childbirth, due to financial barriers, is an obstacle to reducing maternal and child mortality. To improve the use of health services in the three regions of Cameroon, which have the worst reproductive, maternal, neonatal, child and adolescent health indicators, a health voucher project aiming to reduce financial barriers has been progressively implemented since 2015 in these three regions. Our research aimed to assess the impact of the voucher scheme on first antenatal consultation (ANC) and skilled birth attendance (SBA).
Routine aggregated data by month over the period January 2013 to May 2018 for each of the 33 and 37 health facilities included in the study sample were used to measure the effect of the voucher project on the first ANC and SBA, respectively. We estimated changes attributable to the intervention in terms of the levels of outcome indicators immediately after the start of the project and over time using an interrupted time series regression. A meta-analysis was used to obtain the overall estimates.
Overall, the voucher project contributed to an immediate and statistically significant increase, one month after the start of the project, in the monthly number of ANCs (by 26%) and the monthly number of SBAs (by 57%). Compared to the period before the start of the project, a statistically significant monthly increase was observed during the project implementation for SBAs but not for the first ANCs. The results at the level of health facilities (HFs) were mixed. Some HFs experienced an improvement, while others were faced with the status quo or a decrease.
Conclusions
Unlike SBAs, the voucher project in Cameroon had mixed results in improving first ANCs. These limited effects were likely the consequence of poor design and implementation challenges.
Peer Review reports
Reducing maternal, newborn, and child mortality is one of the world's top public health priorities. The third of the seventeen Sustainable Development Goals (SDGs) reflects the international commitment to improving maternal and child health. By 2030, the goals include reducing the global maternal mortality ratio to less than 70 per 100,000 live births, neonatal mortality to 12 per 1,000 live births at most, and under-five mortality to less than 25 per 1,000 live births [ 1 ].
However, despite considerable improvements in recent decades, maternal mortality has remained a major public health concern globally, with more than 295,000 maternal deaths in 2017 and sub-Saharan Africa (SSA) alone accounting for approximately 66% of this global picture [ 2 ]. On the other hand, despite dramatic reductions in child mortality over the last 30 years, the global burden of child deaths has remained immense, with a total of 5.2 million under-five deaths in 2019, representing an average of 14,000 deaths every day [ 3 ].
While from 2000 to 2017, the global maternal mortality ratio (MMR) decreased by 38% [ 2 ], Cameroon's MMR skyrocketed from 511 in 1998 to 782 in 2011 before declining to 467 in 2018 [ 4 ].
A priority toward ending preventable maternal and child deaths is to improve access to and use of quality health services and qualified nurses at birth [ 5 , 6 ]. One of the basic elements is the presence of pregnant women at antenatal consultations. Previous studies have shown that performing prenatal consultations reduces the risk of neonatal mortality [ 7 , 8 ].
However, women in developing countries encounter significant barriers to accessing conventional health services, including poor education, physical and financial barriers, and limited voice and decision-making power [ 9 , 10 ]. The poor quality of available health services offers a further disincentive [ 6 ]. This translates to only half of parturient women receiving skilled assistance at delivery and many fewer receiving postpartum cares [ 6 ].
In Cameroon, the country’s comparatively slow reduction in maternal and child mortalities is likely due to insufficient coverage of reproductive, maternal, neonatal, child and adolescent health (RMNCAH) services; for instance, in 2018, an estimated 65% of women in Cameroon attended at least four antenatal consultations (ANC) visits, 69% gave birth with the assistance of qualified personnel, and 59% received postnatal care (PNC) [ 11 ]. In addition, these general estimates hide enormous disparities. Overall, 65% of the pregnant women who attended the four ANCs included more than 79% of those in urban areas but only 52% of those in rural areas. Moreover, while this rate was 91% in the richest quintile, only one-third (37%) of the poorest pregnant women attended the four ANCs [ 11 ].
The complexity of barriers to accessing care in developing countries indicates that any solution to improving maternal health service utilization must be comprehensive and address both supply- and demand-side health system constraints. This is particularly important in a context such as Cameroon where household out-of-pocket (OOP) spending was the single largest source of financing for the health sector, at 71 percent of total health spending in 2017, well above the WHO benchmark of 15-20 percent, and exceeding the average for SSA (33 percent) and countries of similar income such as Kenya (24 percent) and Ghana (40 percent) [ 12 ].
As ability to pay remains an important determinant of women’s access to healthcare, many countries have sought to improve coverage of maternal services by reducing financial barriers to seeking services [ 13 , 14 ]. Strategies implemented at the country level include national health insurance and user fee removals/exemptions, and at the subnational level, community-based health insurance, health vouchers and conditional cash transfers [ 15 ].
Given that limited access to emergency obstetric and neonatal care (EmONC) is a major contributor to high maternal mortality [ 16 ], increasing pregnant women's use of health facilities for assisted delivery could help reduce maternal and new born morbidity and mortality, as previous studies have indicated [ 17 , 18 ].
In recent years, there has been growing interest in the use of vouchers and other innovative financing mechanisms to increase access to EmONCs for low-income women [ 19 , 20 , 21 , 22 , 23 , 24 , 25 ]. By providing a financial or in-kind reward conditioned on the achievement of agreed-upon performance goals, vouchers are described as a promising holistic approach to foster the use of cost-effective services by the poor and other disadvantaged populations [ 22 ].
Vouchers can act on the demand side, the supply side, or both sides. Demand-side incentives encourage service use not only by reducing the financial burden but also by offering women a choice of providers and informing them of the benefits of using maternal health services. Supply-side incentives aim to improve the quality and responsiveness of service delivery.
To date, findings from the few assessments of reproductive health voucher programs suggest that, if implemented well, they have the potential to improve both assisted and facility-based deliveries [ 19 , 20 , 22 , 24 , 26 ]. Yet, there is a paucity of evidence based on rigorous evaluation studies, making it challenging to draw consistent conclusions about the impact of voucher initiatives and to make subsequent policy recommendations.
The current study evaluated a pilot voucher program in Cameroon, a country where approximately 39% of all deliveries took place at home at the time of the program’s inception [ 27 ]. The research aimed to assess the impact of the voucher scheme on first antenatal consultation and skilled birth attendance (SBA). In the following, we present a brief description of the Cameroon voucher program. We then present our data and methods, followed by the results. We end with a discussion of the study’s results, as well as the implications of these findings.
Voucher program in Cameroon
Results from the 2014 Multiple Indicator Cluster Survey (MICS) indicate an enormous disparity in health outcomes among Cameroon's ten regions, with the three northern regions (Adamawa, North, Far North) bearing the brunt of the disease burden [ 27 ]. For example, while the Far North and North regions represented 27.5% of the total population of children under five years in 2014, both regions accounted for 63% of the total excess mortality during the same period [ 27 ]. In addition, while 65% of women nationwide gave birth with the help of qualified personnel, only 29%, 36% and 53% in the Far North, North and Adamawa regions, respectively, gave birth in the same conditions. Moreover, these three regions featuring the lowest frequencies of ANCs and assisted deliveries, were home to more than 60% of the country’s poorest population [ 28 ].
Initiated in 2015, the voucher programme is a government programme, supported with funding from German and French partners, that aims to reduce financial barriers to maternal and neonatal care in the three northern regions of Cameroon.
Under the project, (poor) women can purchase subsidized vouchers for 6000 FCFA (≈$11), a co-payment of 10% of the actual cost of the service package estimated at 60,000 FCFA (approximately USD109), that covered the cost of a benefit package including services for pregnant women and their new-borns up to 42 days after delivery. In addition, beneficiaries are provided with transportation from their house to the nearest health facility and transportation from health centers to referral hospitals. Health facilities offering services for the voucher scheme are compensated for extra costs incurred. All pregnant women living within the 3 northern regions of Cameroon were eligible for the programme. To be included in the programme, health facilities are required to meet minimum quality standards based on national guidelines for the provision of maternal care. Women can redeem vouchers at any participating facility, and the contracted facilities submit claims to be reimbursed at standard rates for each service provided.
At its inception, the programme implementation was outsourced to the ‘Centre International de Développement et de Recherche’ (CIDR), an international organization. Since November 2018, the management of the scheme has been transferred to a national entity: the Regional Funds for Health Promotion (RFHP). A transfer protocol signed between the ministry of public health (MPH) and CIDR made provisions for the training of the RFHP personnel to take over the implementation.
Study design, data source and study sample
To achieve the study objectives, we used a quasi-experimental study design. Specifically, for each health facility (HF) that was enrolled in the health voucher project, the potential effect of the project was measured using an analysis of interrupted time series [ 29 , 30 , 31 , 32 ]. This method compares changes in the indicators of interest before and after the start of the intervention. It is based on the fundamental assumption that, in the absence of intervention, the trend of the interest indicator remains unchanged over time [ 31 ]. It is desirable to have at least 12 observation points for the indicator or variable of interest before and after the start of the intervention, respectively [ 29 ].
We used secondary data from the monitoring and evaluation system database populated by the three regional implementing agencies of the health voucher project, let by the CIDR-CARE prior to the transfer of the project to the RFHP that began in 2018. These databases were updated quarterly by trained research assistants after monthly data collection from the registries of all health facilities enrolled in the project. Data quality control was carried out jointly by the team from the MPH in charge of monitoring project implementation and by the project team. The data used in this study are monthly aggregates of the variables of interest over the period from January 2013 to May 2018 (i.e., 65 months of observation).
The database contains information on 42 health facilities (HFs) enrolled in the health voucher project, spread across three regions: 12 HFs in the Adamawa region, 15 in the North region and 14 in the Far North region. These HFs were sequentially enrolled in the health voucher project and not at the same time. In the Adamawa region, activities started in 9 HFs in May 2015 and in 3 HFs in March 2016. In the North region, the implementation of activities started in May 2015 in one HF, in June 2015 in 5 HFs and in July 2016 in 9 HFs. For the Far North region, the intervention started in 4 HFs in June 2015, in 3 HFs in March 2016 and in 7 HFs in July 2016. For the analysis of each outcome, HFs included in the sample were those with at least 90% data completeness over the selected period. Thus, the sample sizes for analysis of the outcomes associated with the first antenatal consultation and assisted deliveries were 33 and 37, respectively.
Study variables
Two dependent variables were considered for this evaluation: (i) the monthly number of first ANC visits in each HF and (ii) the monthly number of SBA in each HF.
Covariables
X it : a time-dependent dichotomous variable that takes the value 0 for the months before the start of the health voucher project in HF i and 1 after the start of the project.
T t : time variable measured in months, with values ranging from 0 (January 2013) to 64 (May 2018).
X it *(T t -θ i ): interaction variable between the variables X it and T t centered on the value corresponding to the month of project start in HF i (θ i ).
Statistical analysis
Descriptive analysis.
To explore the outcomes, we used descriptive statistics (mean, median, standard deviation, interquartile range, absolute frequency, relative frequency) and trend curves.
Statistical modeling
For each HF and for each outcome, the estimation of the effects of the health voucher project was carried out using a negative binomial regression.
Since both outcomes are count variables, the choice of negative binomial regression instead of Poisson regression, which is the classic model for this type of variable, was considered to overcome the violation of the fundamental assumption underlying Poisson regression, which states that the mean is equal to the variance. Let Y it be the value of the considered outcome observed in HF i at time t. Y it follows a Poisson distribution with parameter μ it (Y it ~Poisson (μ it )). The general equation of the model used is shown below:
The other parameters of the model are described below.
β 0 = intercept (value of the dependent variable at month 1 of follow-up);
β 1 = slope of the outcome trajectory before the start of the health voucher project;
β 2 = change in the level of outcome at the end of the first month of implementation of the health voucher project;
β 3 = difference between the slope of the outcome trajectory after and before the start of the health voucher project;
variable γ it is the term that differentiates Poisson regression from negative binomial regression. In other words, e γit follows a gamma distribution with mean 1 and variance α (e γit ~ gamma (1/α, α)), with α being the overdispersion parameter.
The coefficient β 2 assesses the immediate effect of the project and β 3 assesses the effect of the project over time.
The graphs used to explore the evolution of outcomes over time highlighted the presence of seasonality. Thus, 11 dichotomous variables were considered in the different models. Equation ( 1 ) becomes log (μ it ) = β 0 + β 1 T t + β 2 X it + β 3 X it *(T t -θ i ) + ɸ 1 February + ɸ 2 March + ɸ 3 April + ɸ 4 May + ɸ 5 June + ɸ 6 July + ɸ 7 August + ɸ 8 September + ɸ 9 October + ɸ 10 November + ɸ 11 December + γ it .
The variables February, March … December take the value 1 if the observation relates to this month and 0 otherwise. The month of January was considered a reference.
Because the project did not start at the same time in all HFs, to obtain estimates representing the overall situation, a meta-analysis was used [ 33 ]. Thus, the pooled estimates and their confidence intervals were obtained by combining the regression coefficients of each HF using the inverse variance method. Random effects models were used to consider the strong heterogeneity highlighted by the statistics I 2 =100*(Q-df)/Q (with Q the statistics of Cochran's Q-test of heterogeneity and df the number of degrees of freedom corresponding here to the number of HFs minus one). The values 0%, 25%, 50% and 75% of the I 2 statistics represent the following levels of heterogeneity: absent, weak, moderate, and strong, respectively [ 33 , 34 ]. The incidence-rate ratio (IRR) for each HF per month as well as the aggregate estimates were graphically represented using a "forest plot". The analysis was stratified by region.
The statistical significance threshold used for interpreting the results was 5%. All the statistical analyses were performed with Stata/SE software version 14.2.
Descriptive statistics
The results in Table 1 show that the overall level of data completeness is 98.9% for the monthly number of first ANC visits and 99.3% for the monthly number of SBAs. In all regions, better data completeness was observed in the post-start period of the intervention. For the descriptive statistics of the two variables of interest, overall, the average (respectively the median) of the monthly number of first ANC visits was 58.6 (respectively 50.0). For the monthly number of SBAs, the mean and median were 52.3 and 31.0, respectively. The observed differences between the means and medians illustrate the asymmetry of the distributions of these variables. We also found that the means and medians of these two variables appeared to be greater during the implementation period of the project than during the period prior to the intervention.
Furthermore, Fig. 1 shows that there was an increasing trend over time for the monthly average of the first ANC and the monthly average of the SBA. It also emerged that the positive slope was more abrupt for SBA.
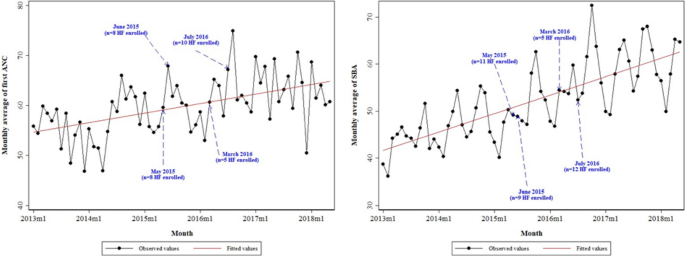
Evolution of the monthly averages of the number of first ANC visits and SBAs in the selected Health facilities between January 2013 and May 2018
Effects of the health voucher project
First antenatal consultation (anc).
Table 2 and Figure S 3 displays contrasting results. Overall, at the end of the first month of implementation of the project, controlling for other variables, a statistically significant increase of nearly 26% in the monthly number of first ANCs was observed in the 33 HFs considered in the study sample (IRR = 1.258 [95% CI: 1.075, 1.472]). A similar increase was recorded in the North region but was not statistically significant (IRR = 1.246 [95% CI: 0.976, 1.591]). In the Adamawa region, the increase was nearly 73% (IRR = 1.726 [95% CI: 1.117, 2.668]). Conversely, in the Far North region, a nonsignificant reduction of 0.2% was noted (IRR = 0.998 [95% CI: 0.882, 1.129]). These overall results hid disparities across facilities. In the Adamawa region, out of 10 HFs, there was a statistically significant increase in the monthly number of first ANCs at the end of the first month of project implementation in five HFs and a statistically significant decrease in one HF. In the Far North region, of the 10 HFs, a statistically significant increase was recorded in two HFs, and a statistically significant reduction was recorded in one HF. In the North region, of the 13 HFs, six exhibited a statistically significant increase in the aforementioned indicator and one exhibited a statistically significant decrease.
Moreover, regarding the difference between the slope of the trajectory of the first ANC after and before the start of the project, Table 2 and Figure S 4 does not show statistically significant results, either overall or by region. However, in one HF in the Adamawa region, a statistically significant increase in the slope of the trajectory of the first ANC was observed during the project implementation period compared to the situation prior to the intervention. Conversely, a statistically significant decrease was recorded in one HF. In the Far North region, no HF exhibited a statistically significant increase, but a statistically significant decrease was observed in two HFs. In the North region, two HFs exhibited a statistically significant increase, and five HFs exhibited a statistically significant decrease.
Skilled birth attendance (SBA)
Table 2 and Figure S 7 shows that by the end of the first month of implementation of the project, a statistically significant increase of nearly 57% in the monthly number of SBAs was recorded in the 37 HFs selected in the study sample, controlling for other variables (IRR = 1.566 [95% CI: 1.358, 1.806]).
A statistically significant increase in this indicator was also observed in each of the three regions. However, there were disparities between HFs. In the Adamawa region, out of 13 health facilities, there was a statistically significant increase in the monthly number of assisted deliveries at the end of the first month of project implementation in nine HFs and a statistically significant decrease in one HF. In the Far North region, of the 11 HFs, a statistically significant increase was recorded in eight HFs and a statistically significant decrease was recorded in two HFs. In the North region, of the 13 HFs, seven recorded a statistically significant increase and one a statistically significant decrease in the indicator of interest.
In addition, Table 2 and Figure S 8 indicates that, overall, the intervention had a positive effect on SBAs (IRR = 1.009 [95% CI: 1.002, 1.016]). A similar finding is observed in the three regions, with the Far North region being the only region that was statistically significant. When considering the analysis of HFs, the results are mixed. In the Adamawa region, a positive and statistically significant result was recorded for four HFs while a negative and statistically significant result was observed for three HFs. In the Far North region, statistically significant results were recorded for five HFs and all these results were positive. In the North region, two HFs recorded a positive result and three recorded a negative result.
The high values of the I 2 statistics reveal that a very large proportion of the total observed variance is due to a real difference in effect measures between HFs (Figure S 1 to S 8 ).
Our study explored the effect of the Health voucher Project on the use of health services. Overall, a statistically significant increase was observed in the number of first ANCs at the end of the first month of project implementation (success). However, this improvement was not sustained over time, with less than 10% of all HFs (3/33) experiencing an increase in ANCs.
For the SBAs, there was a statistically significant increase at the end of the first month of project implementation, with a sustained pattern over time. When looking at the individual HFs, 2/3 (65%) recorded success at the end of the first month of implementation, while 30% experienced overall improvement during the project implementation compared to the period before the start of the project.
These findings suggest that between the pre-intervention/roll-out and full implementation phases, the Cameroon voucher programme modestly increased the use of facility for ANC and SBA, consistent with previously reported results from evaluations of maternal health voucher programmes from other LMICs [ 21 , 35 , 36 , 37 , 38 ].
Our results therefore indicate that in a country such as Cameroon, where progress toward universal health coverage is still to be achieved [ 39 ], reducing financial risk by providing subsidies to offset the costs of receiving RMNCAH services may be a good cost-effective intervention to improve service utilization.
Pregnant women were more likely to use the voucher system for SBAs than for the first ANC visits. One explanation could be the late attendance of pregnant women at health facilities, as more than 70% of pregnant women in these three regions are reported to have their first contact with a health facility after the first trimester of pregnancy [ 27 ], or the late acquisition of vouchers. In-depth discussions with health care providers and direct beneficiaries are needed to better understand the realities underlying these trends.
The decrease in first ANC and SBA over time in some HFs could be explained by the increasing expansion of service coverage, with the opening of new health facilities that were not yet included in the project and that were used by some pregnant women. On the other hand, the context of growing insecurity linked to Boko Haram and other rebel groups in neighboring countries could also constitute a barrier to the use of health facilities in these regions.
It is also important to note that the voucher program is conceptually designed to target the poorest populations. In Cameroon, however, the project covers all women of reproductive age in the intervention areas, regardless of socioeconomic status. We suspect that the contribution of the 6,000 FCFA ($11 US) remains a major barrier to the use of health services for the poorest women, especially since the project covers mostly urban areas, raising the question of program equity as reported elsewhere [ 13 , 14 , 16 ]. This challenge was also highlighted in an unpublished qualitative study.
Focusing on strategies that prioritize the poorest women and strengthen community engagement can ensure equity and achieve sustainable results over time. For example, in Bangladesh and Cambodia, the voucher programme focused on those most in need and reimbursed care givers in facilities to motivate them [ 40 , 41 ]. Moreover, both countries have successfully partnered with recipient communities to improve the targeting of the poor [ 40 , 41 , 42 ].
In addition to stimulating demand, voucher schemes are often proposed as a way to improve the quality of care, as is the case in Cameroon, where health facility accreditation mechanisms are used, alongside the performance-based financing scheme implemented nationwide. However, experiences show that providers may find reimbursement rates to be unattractive and engage in practices such as providing inconsistent quality of care or ‘skimming’ programme users who require minimal intervention. Moreover, as reported in other voucher programs, the most significant problem faced by the voucher scheme in Cameroon was the delay in paying for health facilities, which led to staff demotivation and mistrust between the managers of the scheme and the beneficiaries [ 41 ] and suggested a need for greater attention to issues related to implementation in such a program [ 26 ].
This study helps to extend the body of knowledge generated by previous research on health voucher programmes in LMICs. However, in interpreting our findings, the strengths and limitations of the study design should be considered.
First, most studies on voucher programmes to date have examined the immediate or shorter-term impact of the intervention on service utilization [ 21 ]. Our study examined the immediate to longer-term effects of the intervention and used a quasi-experimental design, known as a reliable approach, to provide robust estimates of the effect of an intervention when a randomized controlled trial cannot be conducted or when a control group is lacking [ 29 , 31 ]. Unlike in cross-sectional observational studies, interrupted time series analysis allows us to estimate the dynamics of change driven by the intervention, controlling for secular changes that might have occurred in the absence of the intervention [ 29 , 43 ]. This approach thus makes it possible to observe whether the intervention has an immediate or delayed, sudden or gradual effect and whether this effect persists or is temporary. Furthermore, there is no real consensus on the number of observation points needed to use the interrupted time series method. However, the statistical power increases with the number of time points [ 30 ]. Some authors recommend 12 observation points before and after the start of the intervention [ 29 ]. In our study, only one HF had 10 observation points before the start of the project, and the others had observation points ranging from 14 and 42. During the project implementation period, the number of observations varied between 23 and 37.
At the time of the study, 81 facilities had already enrolled in the voucher project. We limited ourselves to 33 HFs for the first ANC and to 37 HFs for the SBA analysis because the data prior to the project were either unavailable or insufficient. Therefore, the results presented in this study may be a fragmented view of the project’s effect. In addition, analysis that could provide insight into the RMNCAH continuum of care was not possible due to the limited quality of data (high frequency of missing data) for some key indicators, such as the fourth ANC and postnatal consultation, as reported with other voucher programs [ 22 , 44 , 45 , 46 ].
In identifying the impact of an intervention, it is important that there are no exogenous factors influencing the results. During the implementation of the voucher program in Cameroon, there were no closures of health facilities that could have an impact on the two selected indicators. Population growth naturally leads to an increase in the number of pregnant women in absolute terms, and consequently to an increase in the number of SBAs. Because demographic data were only available for each health district and not for each health facility, estimates of expected populations or pregnant women were not included into the various negative binomial regression models as a control variable. As a result, the estimates obtained may be biased.
It is also important to point out that due to its fragility, the northern part of the country is a convergence zone of several programs and projects, including those of health. Therefore, other interventions may have also contributed to the achievement of these outcome levels. One of the most important programmes is the National Multi-sector Program to Combat Maternal, Newborn and Child Mortality, which was created in 2013.
Finally, we would like to underline that the fidelity of the program's implementation was hampered by deviations, leading for instance to extending the intervention to all women of childbearing age. At present, the program is more akin to an obstetric risk insurance system, as described for example in Mauritania [ 47 ].
This study provided important insight into the Cameroon voucher scheme. The intervention had a significant early effect on the first ANC and SBA but failed to effectively sustain these results over time for the first indicator. These mixed effects were likely the consequence of poor design and implementation challenges, including the fact that the programme did not include specific equity measures to facilitate uptake by the poorest people. This suggests that for a complex intervention such as a voucher, it is critical to properly implement practice strategies that can sustain the long-term impact of the programme.
Availability of data and materials
The data that support the findings of this study are available from the Ministry of Public Health (MPH) of Cameroon, but restrictions apply due to the terms of our contract with the MPH, and so, data are not publicly available. The corresponding author should be contacted for the process to request data access.
Abbreviations
- Antenatal consultation
Centre International de Développement et de Recherche
Emergency obstetric and neonatal care
Communauté financière africaine
Health facility
Low- and middle-income country
Maternal mortality ratio
Multiple Indicator Cluster Survey
Ministry of public health
Out-of-pocket
Post-natal care
Regional Funds for Health Promotion
Reproductive, maternal, neonatal, child and adolescent health
- Skilled birth attendance
Sustainable Development Goals
Sub Saharan Africa
United States dollar
World health organization
Nations Unies. Développement durable. 2021. Objectif de Développement Durable - Santé et Bien-Être pour tous. 2021. Available at: https://www.un.org/sustainabledevelopment/fr/health/ . Accessed 3 June 2023.
WHO, UNICEF, UNFPA, World Bank Group, United Nations Population Division. Trends in maternal mortality: 2000 to 2017. Geneva, Switzerland: World Health Organization; 2019. p. 119. Available at: http://www.who.int/reproductivehealth/publications/maternal-mortality-2000-2017/en/ . Accessed 10 May 2023.
UN Inter-agency Group for Child Mortality Estimation. Levels & Trends in Child Mortality. New York, NY 10017 États-Unis: United Nations Children’s Fund; 2020 p. 56. Available at: https://www.un.org/development/desa/pd/sites/www.un.org.development.desa.pd/files/unpd_2020_levels-and-trends-in-child-mortality-igme-.pdf . Accessed 2 Jul 2023.
Institut National de la Statistique (INS). Enquête Démographique et de Santé et à Indicateurs Multiples EDS-MICS 2011. 2011. Available at: https://dhsprogram.com/pubs/pdf/fr260/fr260.pdf . Accessed 2 Feb 2023.
Donnay F. Maternal survival in developing countries: what has been done, what can be achieved in the next decade. Int J Gynecol Obstet. 2000;70(1):89–97. https://doi.org/10.1016/S0020-7292(00)00236-8 .
Article CAS Google Scholar
Singh S, Darroch JE, Ashford LS, Vlassoff M. Adding It Up: The costs and Benefits of Investing in family Planning and maternal and new born health. GUTTMACHER INSTITUTE; 2009. Available at: https://www.guttmacher.org/sites/default/files/pdfs/pubs/AddingItUp2009.pdf . Accessed 20 April 2023.
Tekelab T, Chojenta C, Smith R, Loxton D. The impact of antenatal care on neonatal mortality in sub-Saharan Africa: a systematic review and meta-analysis. PLOS One. 2019;14(9):e0222566. https://doi.org/10.1371/journal.pone.0222566 .
Article CAS PubMed PubMed Central Google Scholar
Wondemagegn AT, Alebel A, Tesema C, Abie W. The effect of antenatal care follow-up on neonatal health outcomes: a systematic review and meta-analysis. Public Health Rev. 2018;39(1):33. https://doi.org/10.1186/s40985-018-0110-y .
Article PubMed PubMed Central Google Scholar
Matsuoka S, Aiga H, Rasmey LC, Rathavy T, Okitsu A. Perceived barriers to utilization of maternal health services in rural Cambodia. Health Policy. 2010;95(2):255–63. https://doi.org/10.1016/j.healthpol.2009.12.011 .
Article PubMed Google Scholar
Sharma S, Smith S, Sonneveldt E, Pine M, Dayaratna V, Sanders R. Formal and Informal Fees for Maternal Health Care Services in Five Countries. USAID; 2005. Available at: http://www.policyproject.com/pubs/workingpapers/WPS16.pdf . Accessed 30 Jan 2023.
Institut National de la Statistique (INS) and IFC. Enquête Démographique et de Santé EDS 2018. INS et IFC 2020. Available at: https://dhsprogram.com/what-we-do/survey/survey-display-511.cfm . Accessed 4 Jan 2023.
WHO. World Health Organization. 2020. Global Health Expenditure Database. Available at: https://apps.who.int/nha/database/Home/Index/en . Accessed 13 Feb 2023.
Gabrysch S, Campbell OM. Still too far to walk: Literature review of the determinants of delivery service use. BMC Pregnancy Childbirth. 2009;11(9):34. https://doi.org/10.1186/1471-2393-9-34 .
Article Google Scholar
Dzakpasu S, Powell-Jackson T, Campbell OMR. Impact of user fees on maternal health service utilization and related health outcomes: a systematic review. Health Policy Plan. 2014;29(2):137–50. https://doi.org/10.1093/heapol/czs142 .
Ensor T, Ronoh J. Effective financing of maternal health services: a review of the literature. Health Policy. 2005;75(1):49–58. https://doi.org/10.1016/j.healthpol.2005.02.002 .
Richard F, Witter S, de Brouwere V. Innovative approaches to reducing financial barriers to obstetric care in low-income countries. Am J Public Health. 2010;100(10):1845–52. https://doi.org/10.2105/AJPH.2009.179689 .
World Health Organization. Making pregnancy safer: the critical role of the skilled attendant: a joint statement by WHO, ICM and FIGO. Geneva: WHO; 2004. 24 p. Available at: https://apps.who.int/iris/bitstream/handle/10665/42955/9241591692.pdf?sequence=1&isAllowed=y . Accessed 15 April 2023.
Baral YR, Lyons K, Skinner J, van Teijlingen ER. Determinants of skilled birth attendants for delivery in Nepal. Kathmandu Univ Med J. 2010;8(3):325–32. https://doi.org/10.3126/kumj.v8i3.6223 .
Bellows NM, Bellows BW, Warren C. Systematic review: the use of vouchers for reproductive health services in developing countries: systematic review. Trop Med Int Health TM IH. 2011;16(1):84–96. https://doi.org/10.1111/j.1365-3156.2010.02667.x .
Brody CM, Bellows N, Campbell M, Potts M. The impact of vouchers on the use and quality of health care in developing countries: a systematic review. Glob Public Health. 2013;8(4):363–88. https://doi.org/10.1080/17441692.2012.759254 .
Hunter BM, Harrison S, Portela A, Bick D. The effects of cash transfers and vouchers on the use and quality of maternity care services: a systematic review. PLOS One. 2017;12(3):e0173068. https://doi.org/10.1371/journal.pone.0173068 .
Nguyen HTH, Hatt L, Islam M, Sloan NL, Chowdhury J, Schmidt JO, et al. Encouraging maternal health service utilization: an evaluation of the Bangladesh voucher program. Soc Sci Med. 2012;74(7):989–96. https://doi.org/10.1016/j.socscimed.2011.11.030 .
Azmat SK, Ali M, Rahman MdM. Assessing the sustainability of two independent voucher-based family planning programs in Pakistan: a 24-months post-intervention evaluation. Contracept Reprod Med. 2023;8(1):43. https://doi.org/10.1186/s40834-023-00244-w .
Nandi A, Charters TJ, Quamruzzaman A, Strumpf EC, Kaufman JS, Heymann J, et al. Health care services use, stillbirth, and neonatal and infant survival following implementation of the Maternal Health Voucher Scheme in Bangladesh: A difference-in-differences analysis of Bangladesh Demographic and Health Survey data, 2000 to 2016. PLOS Med. 2022;19(8):e1004022. https://doi.org/10.1371/journal.pmed.1004022 .
Sultana N, Hossain A, Das H, Pallikadavath S, Koeryaman M, Rahman M, et al. Is the maternal health voucher scheme associated with increasing routine immunization coverage? Experience from Bangladesh. Front Public Health. 2023;2(11):963162. https://doi.org/10.3389/fpubh.2023.963162 .
Hunter BM, Murray SF. Demand-side financing for maternal and newborn health: what do we know about factors that affect implementation of cash transfers and voucher programmes? BMC Pregnancy Childbirth. 2017;17(1):262. https://doi.org/10.1186/s12884-017-1445-y .
Institut National de la Statistique. Cameroon - Enquête par Grappes à Indicateurs Multiples 2014. Yaoundé, Cameroun: Institut National de la Statistique; 2015; p. 504. Available at: https://mics-surveys-prod.s3.amazonaws.com/MICS5/West%20and%20Central%20Africa/Cameroon/2014/Final/Cameroon%202014%20MICS_French.pdf . Accessed 22 Jan 2023
Institut National de la Statistique. Annuaire statistique 2017. 2018. Available at: http://www.statistics-cameroon.org/news.php?id=513 . Accessed May 10, 2023.
Wagner AK, Soumerai SB, Zhang F, Ross-Degnan D. Segmented regression analysis of interrupted time series studies in medication use research. J Clin Pharm Ther. 2002;27(4):299–309. https://doi.org/10.1046/j.1365-2710.2002.00430.x .
Article CAS PubMed Google Scholar
Bernal JL, Cummins S, Gasparrini A. Interrupted time series regression for the evaluation of public health interventions: a tutorial. Int J Epidemiol. 2017;46(1):348–55. https://doi.org/10.1093/ije/dyw098 .
Linden A. ITSA: Stata module to perform interrupted time series analysis for single and multiple groups. Statistical Software Components. Boston College Department of Economics; 2021. Available at: https://ideas.repec.org/c/boc/bocode/s457793.html . Accessed 21Oct 2023.
Ramsay CR, Matowe L, Grilli R, Grimshaw JM, Thomas RE. Interrupted time series designs in health technology assessment: Lessons from two systematic reviews of behavior change strategies. Int J Technol Assess Health Care. 2003;19(4):613–23. https://doi.org/10.1017/S0266462303000576 .
Gebski V, Ellingson K, Edwards J, Jernigan J, Kleinbaum D. Modelling interrupted time series to evaluate prevention and control of infection in healthcare. Epidemiol Infect. 2012;140(12):2131–41. https://doi.org/10.1017/S0950268812000179 .
Borenstein M, Hedges LV, Higgins J, Rothstein HR. Introduction to Meta-Analysis. 2nd ed. Hoboken: Wiley; 2021. p. 500.
Book Google Scholar
Obare F., Warren C., Abuya T., Askew I., Bellows B. Assessing the population-level impact of vouchers on access to health facility delivery for women in Kenya. Soc Sci Med. 2014;183–9. https://doi.org/10.1016/j.socscimed.2013.12.007 .
Obare F, Warren C, Kanya L, Abuya T, Bellows B. Community-level effect of the reproductive health vouchers program on out-of-pocket spending on family planning and safe motherhood services in Kenya. BMC Health Serv Res. 2015;15(1):343. https://doi.org/10.1186/s12913-015-1000-3 .
Bellows B, Kyobutungi C, Mutua MK, Warren C, Ezeh A. Increase in facility-based deliveries associated with a maternal health voucher programme in informal settlements in Nairobi Kenya. Health Policy Plan. 2013;28(2):134–42. https://doi.org/10.1093/heapol/czs030 .
Amendah DD, Mutua MK, Kyobutungi C, Buliva E, Bellows B. Reproductive health voucher program and facility based delivery in informal settlements in nairobi: a longitudinal analysis. PLOS One. 2013;8(11):e80582. https://doi.org/10.1371/journal.pone.0080582 .
Sieleunou I, Tamga DM, Maabo Tankwa J, Aseh Munteh P, Longang Tchatchouang EV. Strategic health purchasing progress mapping in cameroon: a scoping review. Health Syst Reform. 2021;10(7):1. https://doi.org/10.1080/23288604.2021.1909311 .
Ir P, Horemans D, Souk N, Van Damme W. Using targeted vouchers and health equity funds to improve access to skilled birth attendants for poor women: a case study in three rural health districts in Cambodia. BMC Pregnancy Childbirth. 2010;10(1):1. https://doi.org/10.1186/1471-2393-10-1 .
Ahmed S, Khan MM. A maternal health voucher scheme: what have we learned from the demand-side financing scheme in Bangladesh? Health Policy Plan. 2011;26(1):25–32. https://doi.org/10.1093/heapol/czq015 .
Ridde V, Yaogo M, Kafando Y, Sanfo O, Coulibaly N, Nitiema PA, et al. A community-based targeting approach to exempt the worst-off from user fees in Burkina Faso. J Epidemiol Community Health. 2010;64(01):10–5. https://doi.org/10.1136/jech.2008.086793 .
Eccles M, Grimshaw J, Campbell M, Ramsay C. Research designs for studies evaluating the effectiveness of change and improvement strategies. BMJ Qual Saf. 2003;12(1):47–52. https://doi.org/10.1136/qhc.12.1.47 .
Ahmed S, Khan MM. Is demand-side financing equity enhancing? Lessons from a maternal health voucher scheme in Bangladesh. Soc Sci Med. 2011;72(10):1704–10. https://doi.org/10.1016/j.socscimed.2011.03.031 .
Agha S. Impact of a maternal health voucher scheme on institutional delivery among low income women in Pakistan. Reprod Health. 2011;8(1):10. https://doi.org/10.1186/1742-4755-8-10 .
Van de Poel E, Flores G, Ir P, O’Donnell O, Van Doorslaer E. Can vouchers deliver? An evaluation of subsidies for maternal health care in Cambodia. Bull World Health Organ. 2014;92(5):331–9. https://doi.org/10.2471/BLT.13.129122 .
Philibert A, Ravit M, Ridde V, Dossa I, Bonnet E, Bedecarrats F, Dumont A. Maternal and neonatal health impact of obstetrical risk insurance scheme in Mauritania: a quasi experimental before-and-after study. Health Policy Plan. 2017;32(3):405–17. https://doi.org/10.1093/heapol/czw142 .
Download references
Acknowledgements
We would like to thank Dr Bassirou Bouba and Dr Okala from the voucher project, Dr Yumo Habakkuk and Bashirou Ndindumouh from Research for Development International, Dr Denise Tamga from the Worlb Bank Office, and Dr Aubin Baleba from UNFPA. We are much indebted to the SPARC team for continuously reviewed our work and provided valuable comments. Finally, the authors would also like to acknowledge the work of the anonymous reviewers who provided us with extremely helpful comments and feedback.
This work was supported by the Bill & Melinda Gates Foundation [Grant number: OPP1179622].
Author information
Authors and affiliations.
The Global Financing Facility (GFF), Dakar, Senegal
Isidore Sieleunou
Research for Development International, 30883, Yaoundé, Cameroon
Isidore Sieleunou & Roland Pascal Enok Bonong
You can also search for this author in PubMed Google Scholar
Contributions
IS and RPEB conceived and designed the study. RPEB managed the data, including quality control, provided statistical advice on study design and analyzed the data. IS drafted the manuscript, and all authors contributed substantially to its revision. All authors agreed to the final approval of the version to be published. All authors agreed to be accountable for all aspects of the work.
Corresponding author
Correspondence to Isidore Sieleunou .
Ethics declarations
Ethics approval and consent to participate.
Ethical approval for the study was obtained from the Cameroon National Ethics Committee for Human Health Research (CNECHHR) (N0 2020/07/1274/CE/CNERSH/SP). Administrative authorization was granted by the Cameroonian Ministry of Health (D30-607/N/MINSANTE/SG/DROS/CRSPE/BBM, N0 631-32-20). All methods were performed in accordance with the relevant guidelines and regulations. The CNECHHR waived the need for participants’ informed consent in this retrospective study because the data used were fully anonymised and aggregated.
Consent for publication
Not applicable.
Competing interests
The authors declare no competing interests.
Additional information
Publisher’s note.
Springer Nature remains neutral with regard to jurisdictional claims in published maps and institutional affiliations.
Supplementary Information
Supplementary material 1., rights and permissions.
Open Access This article is licensed under a Creative Commons Attribution 4.0 International License, which permits use, sharing, adaptation, distribution and reproduction in any medium or format, as long as you give appropriate credit to the original author(s) and the source, provide a link to the Creative Commons licence, and indicate if changes were made. The images or other third party material in this article are included in the article's Creative Commons licence, unless indicated otherwise in a credit line to the material. If material is not included in the article's Creative Commons licence and your intended use is not permitted by statutory regulation or exceeds the permitted use, you will need to obtain permission directly from the copyright holder. To view a copy of this licence, visit http://creativecommons.org/licenses/by/4.0/ . The Creative Commons Public Domain Dedication waiver ( http://creativecommons.org/publicdomain/zero/1.0/ ) applies to the data made available in this article, unless otherwise stated in a credit line to the data.
Reprints and permissions
About this article
Cite this article.
Sieleunou, I., Enok Bonong, R.P. Does health voucher intervention increase antenatal consultations and skilled birth attendances in Cameroon? Results from an interrupted time series analysis. BMC Health Serv Res 24 , 602 (2024). https://doi.org/10.1186/s12913-024-10962-9
Download citation
Received : 27 December 2023
Accepted : 08 April 2024
Published : 08 May 2024
DOI : https://doi.org/10.1186/s12913-024-10962-9
Share this article
Anyone you share the following link with will be able to read this content:
Sorry, a shareable link is not currently available for this article.
Provided by the Springer Nature SharedIt content-sharing initiative
- Results-based financing
- Health financing
BMC Health Services Research
ISSN: 1472-6963
- General enquiries: [email protected]
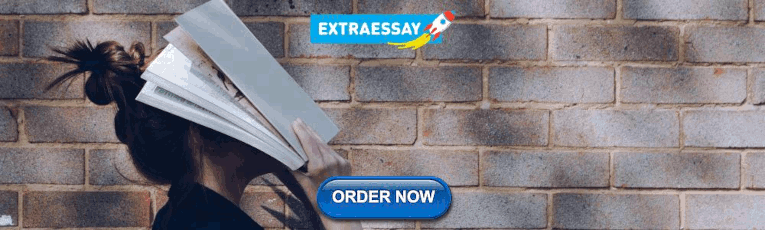
IMAGES
VIDEO
COMMENTS
A program analysis is only as good as its information. Research and accurate data are vital to identifying key issues relevant to the success of a program. Why Conduct a Program Analysis? A program analysis helps the team understand key constraints that must be addressed for a program to be successful. SBCC programs risk failure if fundamental ...
Program analysis concerns static techniques for computing reliable approximate information about the dynamic behaviour of programs. Applications include compilers (for code improvement), software validation (for detecting errors in algorithms or breaches of security) and transformations between data representation (for solving problems such as the Y2K problem).
We assert that by understanding the above, the program analysis research community can focus on analyzers that are most amenable to real world use. For researchers, our ndings also provide a view into today's industrial realities with respect to program analysis. 2. SURVEY In an e ort to understand developers' perspectives on pro-
In computer science, program analysis is the process of automatically analyzing the behavior of computer programs regarding a property such as correctness, robustness, safety and liveness. Program analysis focuses on two major areas: program optimization and program correctness.The first focuses on improving the program's performance while reducing the resource usage while the latter focuses ...
•Program analysis: automatic reasoning about programs •Foundations, formalism, techniques, applications, implementations •Aims: •Understand relative strengths and weaknesses of different analyses •Understand some current challenges in program analysis •Research project: •Advance the state-of-the-art in program analysis; OR
• Involved with program analysis for ~10 years. • PhD from UC Berkeley, Masters from IIT Bombay (India) • Worked at IBM Research, Microsoft Research, and Samsung Research America • Advising PhD students at CMU's Institute for Software Research • Developed tools for improving developer productivity, finding input-validation software
Functional Programming. learn about lambda calculus, Haskell, and OCaml. learn to make formal arguments about program behavior. Type Theory. learn how to design and reason about type systems. use type-based analysis to find synchronization errors, avoid information leaks and manage your memory efficiently. Axiomatic Semantics/Program Logics.
Algebraic Program Analysis. Algebraic Program Analysis. Zachary Kincaid1(B), Thomas Reps2(B , and John Cyphert2(B) 1Princeton University, Princeton, NJ 08540, USA [email protected]. 2University of Wisconsin, Madison, WI 53706, USA [email protected], [email protected] Abstract. This paper is a tutorial on algebraic program analysis.
skills learned in the course to a capstone research project that involves designing, implement-ing, and evaluating a novel program analysis. Overall program analysis is an area with deep mathematical foundations that is also very practically useful. I hope you will also find it to be fun!
skills learned in the course to a capstone research project that involves designing, implement-ing, and evaluating a novel program analysis. Overall program analysis is an area with deep mathematical foundations that is also very practically useful. I hope you will also find it to be fun!
The course provides an overview of program analysis and then covers three topics in more detail: Static analysis, which analyzes the source code of a program, dynamic analysis, which reasons about the runtime behavior of a program, and test generation, which creates inputs to run programs. In addition to lectures, students will deepen their ...
501 is a graduate level, project based class in programming languages implementation. This quarter, we will study techniques and algorithms that can be used to understand programs. We will also look into applications of these techniques across a wide-variety of fields, from classical program optimization to security and utilizing novel hardware.
The term "evaluation framework" and other associated terms are common in the practice and the discipline of program evaluation. These terms correspond to a variety of meanings across contexts and organizations and, thus, tend to lack overall consistency.
This paper describes how a number of program-analysis problems can be solved by transforming them to graph-reachability problems. Some of the program-analysis problems that are amenable to this treatment include program slicing, certain dataflow-analysis problems, one version of the problem of approximating the possible "shapes" that heap ...
The framework presented distinguishes program evaluation from research and encourages health professionals to apply an evaluative lens in order that value judgements about the merit, worth, and significance of programs can be made. ... In general terms, the data collection methods and analysis used in program evaluation are the same as those ...
How does program evaluation answer questions about whether a program works, or how to improve it. Basically, program evaluations systematically collect and analyze data about program activities and outcomes. The purpose of this guide is to briefly describe the methods used in the systematic collection and use of data.
Program evaluation - An assessment using systematic data collection and analysis of one or more programs, policies, and organizations intended to assess their effectiveness and efficiency. Performance measurement - The ongoing monitoring and reporting of a program's accomplishments and progress, particularly towards its pre-established goals.
Parfait [2, 6] is a static analysis tool that focuses on high precision (90% true positive reports) and scalable (approximately 10 min per million lines of code on a standard developer laptop).This is possible because it focuses only on vulnerabilities that matter (e.g., SQL injection, cross-site scripting, XXE). These vulnerabilities are identified by the owner of the codebase.
Definition of research in data analysis: According to LeCompte and Schensul, research data analysis is a process used by researchers to reduce data to a story and interpret it to derive insights. The data analysis process helps reduce a large chunk of data into smaller fragments, which makes sense. Three essential things occur during the data ...
Many of the master's program faculty are associates of the Population Studies and Training Center (PSTC) at Brown, an interdisciplinary social science research and graduate training center. The Spatial Analysis courses in this program are taught by experts affiliated with the Spatial Structures in the Social Sciences (S4) Initiative.
U.S. Geological Survey Data Citation Analysis, 2016-2022. In 2022, publication and data linkages were evaluated using two methods in an effort to understand how a data citation workflow has been implemented by the U.S. Geological Survey (USGS) since the 2016 USGS instructional memorandum, Public Access to Results of Federally Funded Research at ...
Research & Analysis. Evidence-based analysis and rigorous evaluation are critical tools to promote effective policies and strong management in the Federal nutrition assistance programs. FNS works continuously to achieve its mission by setting goals and utilizing evidence, measurement, and learning to improve results of programs and the ...
The Science Synthesis Analysis, and Research (SSAR) Program provides analysis and synthesis of scientific data and information, interdisciplinary research to improve understanding of Earth system changes, and preservation of scientific data and samples and library collections.
DAN GOLDHABER, PhD, is the director of the National Center for Analysis of Longitudinal Data in Education Research (CALDER), vice president at American Institutes for Research, and the director of the Center for Education Data and Research (CEDR) at the University of Washington, 3876 Bridge Way North, Suite 201, Seattle, WA 98103; [email protected].
Learners who complete the seven courses in this certificate program should be equipped to apply for entry-level jobs as UX designers. By the end of this course, you will be able to: - Plan a UX research study, including the project background, research goals, research questions, Key Performance Indicators, methodology, participants, and script.
The results of this research could hold substantial implications for public health by revealing the causal and long-term health consequences of socioeconomic interventions on health. Moreover, it will offer fresh perspectives on the levels of biological aging and Alzheimer's Disease risk within the context of lower- and middle-income countries.
Leverage advanced analysis, including video feedback summarization powered by generative AI, crosstabs, and statistical analysis tools. ... or a detailed study such as customer or employee feedback as part of a more structured experience management program. Cloud-based survey technology has revolutionized the ability to get data, quickly, from ...
Analysis of minority-serving institutions on the East and West Coasts demonstrates layered processes to build students' capacities. ... Programs, and Research. Apr 30, 2024. Apr 30, 2024. Education and Social Sciences Steinhardt School of Culture, Education, and Human Development. New York.
How to make sure your district's program is effective" "The impact of summer learning programs on low-income children's mathematics achievement: A meta-analysis" "The effects of summer reading on low-income children's literacy achievement from kindergarten to grade 8: A meta-analysis of classroom and home interventions"
Study design, data source and study sample. To achieve the study objectives, we used a quasi-experimental study design. Specifically, for each health facility (HF) that was enrolled in the health voucher project, the potential effect of the project was measured using an analysis of interrupted time series [29,30,31,32].This method compares changes in the indicators of interest before and after ...