
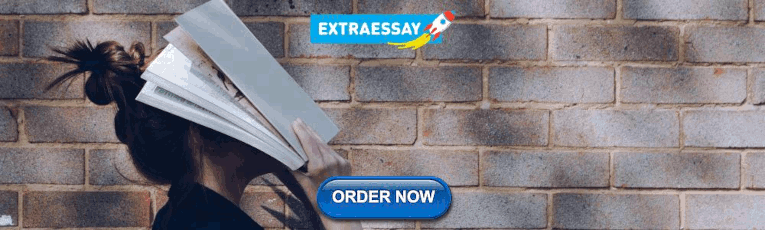
UK Biobank Research Analysis Platform
The uk biobank research analysis platform.
The UK Biobank Research Analysis Platform enables researchers working with UK Biobank's large-scale biomedical database and research resource, to access it in the cloud from anywhere in the world. It has been designed to accommodate the vast and increasing scale of the UK Biobank resource.
The Research Analysis Platform (RAP), enabled by DNAnexus technology and powered by Amazon Web Services (AWS), exponentially increases the scale and accessibility of the world’s most comprehensive biomedical database for researchers around the world to advance understanding of human disease.
If you already have a RAP account, or wish to set one up click the link below to access the platform.
The UK Biobank RAP
Who can use it?
The UK Biobank Research Analysis Platform (RAP) is available to all UK Biobank approved researchers, that are collaborating on an approved in progress project.
How can I access it?
To access the platform you will need to have an existing UK Biobank AMS account with an approved UK Biobank access application ID.
You can access the RAP user guide from the useful links on this page. The user guide, which includes a video demonstrating key platform functionality, will continue to be updated as will our Frequently Asked Questions.
The UK Biobank dataset is held in the platform at no cost to researchers. There are costs associated with compute, data storage in support of analyses in the platform and egress of permitted data; more information can be found in our useful links.
On sign-up to the Platform, you will receive £40 credit (sufficient, for example, to run around 100 hours of analyses, including example GWAS and PRS analyses using genotype data) towards the cost of any compute or data storage used. Once the free credit has been consumed, researchers will need to provide billing details to perform subsequent analyses.
Useful links
Sign up to the UK Biobank RAP
Join the conversation
RAP user guide
Frequently Asked Questions
View related news release
300,000 participant exomes now accessible for approved researchers
Financial support to increase accessibility
UK Biobank can provide financial support with the application fee for researchers, including early career researchers, in countries classed as low-and-middle-income by UK Biobank. AWS Credits are also available to early career researchers anywhere in the world to offset the cost of compute analysis and storage in the Research Analysis Platform (RAP). If researchers meet criteria for both funds then they can benefit from both. Find out more about both programmes below.
Find out more about financial support
Join the online community
An online community forum has been established for those using the platform to support the research community and provide advice on how best to use the new platform.
Ask questions, share experiences and request support from the teams at UK Biobank and DNAnexus. It is our intention that the platform evolves and enables broad and diverse health research, so log on and share your knowledge with others performing their analyses in the cloud.
To find out more about this community and to sign up for updates on the RAP, please click below.
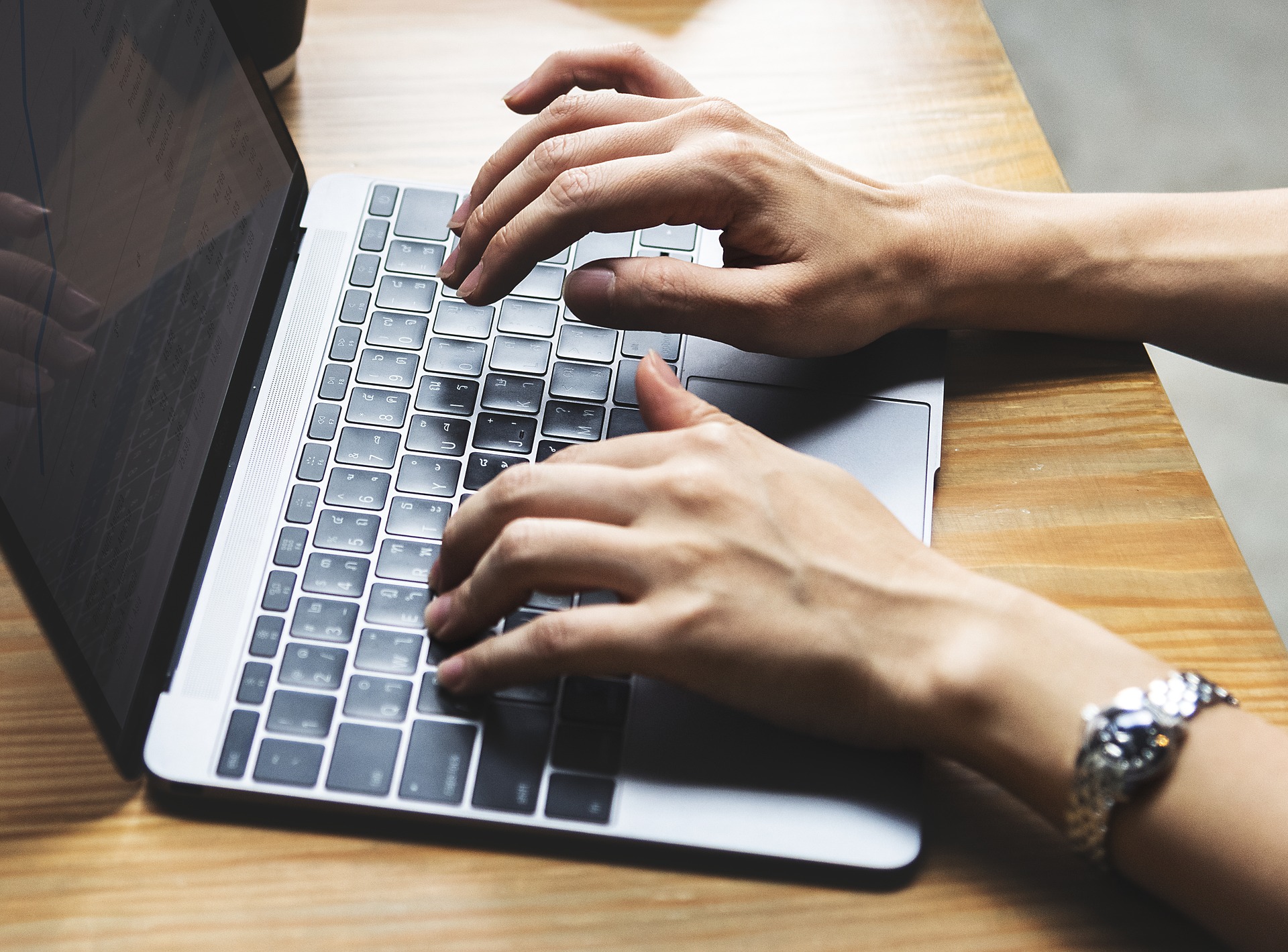
The UK Biobank Research Analysis Platform takes the fantastic data generated by the UK Biobank project and removes the few barriers to entry.
By making all of the data available on the cloud through DNAnexus, I can readily scale my computing needs based on my current analysis. The support team recruited by DNAnexus to assist researchers in using this cloud resource has been incredibly responsive. I look forward to using and watching the Research Analysis Platform grow over the coming years.
Eugene Gardner, MRC Epidemiology Unit, University of Cambridge
Last updated April 10 th 2024
© UK Biobank Limited 2024 | Registered in England and Wales with company number 4978912. Registered as a charity in England and Wales (number 1101332) and in Scotland (number SC039230). Registered office Units 1-2 Spectrum Way, Stockport, Cheshire, SK3 0SA.
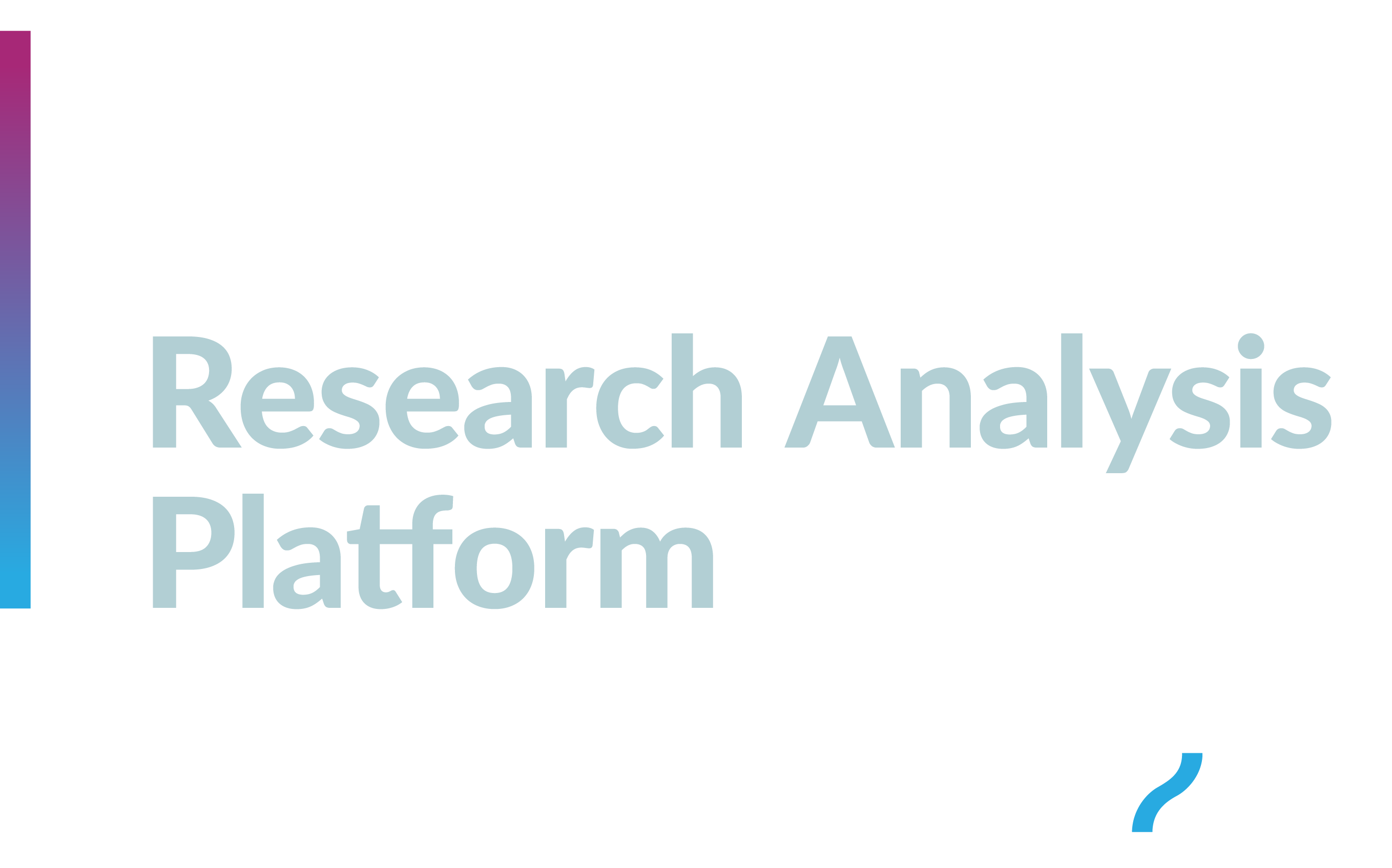
Gain access to comprehensive biomedical data at a scale the world has never seen.
At a glance, discover the uk biobank research analysis platform.
The UK Biobank RAP, enabled by DNAnexus, is an all-in-one platform: secure, compliant cloud infrastructure + tools + UK Biobank data
UK Biobank is a large-scale biomedical database and research resource, containing in-depth genetic and health information from half a million UK participants, globally accessible to approved researchers undertaking vital research into the most common and life-threatening diseases. The database is regularly augmented with additional data & researchers from around the world needed to be able to securely access the growing dataset.
Key Benefits
Cloud based.
Cloud-based infrastructure allows democratization of access to the data
Scalability
A platform built to process the scale and complexity of UK Biobank multi-omics and clinical data with ease
Reduce risks with a purpose-built platform designed to proactively manage local and regional security and compliance requirements
High performance environment allows for faster analysis without incurring high compute costs
Benefits of the Platform
Securely conduct diverse set of analyses on large scale genetic, imaging, lifestyle, and health record data on a leading cloud research analysis platform.
Work within an operating system designed to support end-to-end high performance computing utilizing robust and cutting edge tools/features. Reduce operating costs while having access to high performance infrastructure with minimal maintenance costs. Scale complex multi-omics projects through cloud computing without having to pay for idle infrastructure or scramble to increase infrastructure during high workloads.
Platform Credits Program
Apply now to receive funding towards your work on the UK Biobank RAP.
The UK Biobank Platform Credits Program is courtesy of AWS. The program is available to all early career researchers and those researchers from low- and low-middle income countries to explore the RAP in detail, develop and test tools and methods, and undertake analysis to support their research project. Credits can be used to cover costs of compute and storage above £40 credits provided by DNAnexus.
Registration is free and takes less than 2 minutes!
Get Started Today
We make it easy to get started analysing data in the cloud. Sign up for the UK Biobank RAP & connect your UK Biobank AMS account and you're ready to start performing your analysis.
If you need help getting started, visit our Quick Start Guide or the UK Biobank Community Forum .
Scientific Publications
Plasma proteomic signature predicts myeloid neoplasm risk, population estimates of ovarian cancer risk in a cohort of patients with bladder cancer, joint exposure to ambient air pollutants, genetic risk, and ischemic stroke: a prospective analysis in uk biobank, identification of phenomic data in the pathogenesis of cancers of the gastrointestinal (gi) tract in the uk biobank, prospective study design and data analysis in uk biobank, uk biobank subjects carrying protein truncating variants in herc1 are not at substantially increased risk of minor psychiatric disorders, proteome-wide mendelian randomization identifies causal plasma proteins in lung cancer, comprehensive whole-genome analyses of the uk biobank reveal significant sex differences in both genotype missingness and allele frequency on the x chromosome, share tools & tutorials with the ukb-rap community.
Join the community forum, a collaborative space where researchers can ask questions, share research tools/publication, and support their peers about the use of UK Biobank Research Analysis Platform’s data, products, and services.
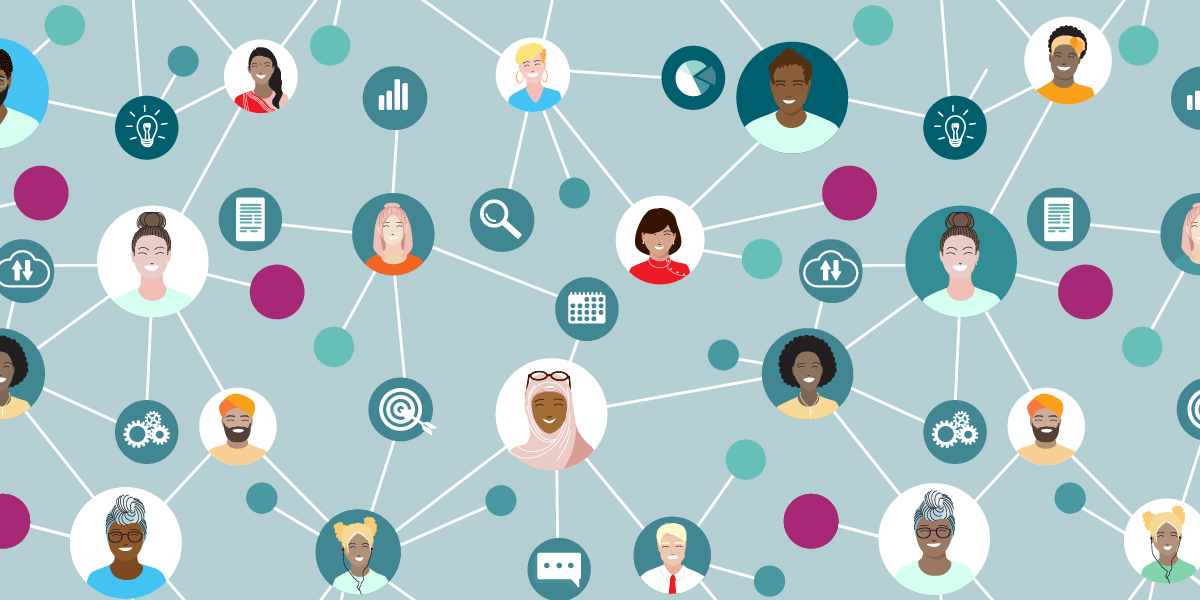
Frequently Asked Questions
What is the uk biobank rap.
Answer: The UK Biobank Research Analysis Platform is a cloud-based platform providing a research environment that allows researchers to access UK Biobank data without the need to download large data files. It provides access to storage and compute resources that allow researchers to undertake their analyses within the platform. Read the RAP user guide for more information.
How much does it cost to use the UK Biobank RAP?
What data is available on the uk biobank rap.
All data is available within the RAP, but access to data (and the ability to download data) depends on the UK Biobank Tier Access Fee paid. There are also certain restrictions on downloading from the RAP. Please see the linked table for further information.
Can I import data onto the UK Biobank RAP?
Yes, as long as the data imported into the RAP is to enable research in line with your research project.
There is no limit on the size of data (save that the cost of data storage to the researcher will increase according to the amount of data stored in the RAP) or the type of data that can be imported into the RAP. Data must be used in line with the UK Biobank’s Material Transfer Agreement and the RAP terms and conditions.
What software and pipelines are available on the UK Biobank RAP?
The RAP has an extensive tool library to cover your needs for genomic analysis, statistical analysis, image processing and much more. You can find more information and a list of tools in the DNAnexus Documentation.
Can I bring my own workflows onto the UK Biobank RAP?
In many cases the RAP allows you to use apps and workflows you’ve developed in another environment. You can find more detailed information on how to do so in our Documentation and strategies in our Creating Workflows webinar.
Can I receive support getting started on the UK Biobank RAP?
Once signed up you h ave access to the DNAnexus Community, a forum where you can access helpful guides and strategies and post your own questions. You can find topics dedicated to getting started on the Platform and on specific topics related to working on RAP. DNAnexus and UK Biobank team members also monitor the forum and will be able to answer your questions. You can find the Community here.
Are there any funding support programs available on the UK Biobank platform?
Yes. Through the UK Biobank Platform Credits Program
The UK Biobank Platform Credits Program is a courtesy of AWS . The program is available to early career researchers or researchers from low to low-middle income countries and is designed to allow researchers to explore the RAP in detail, develop and test tools and methods, and undertake analysis to support their research project. Credits can be used to cover costs of compute and storage above £40 credits provided by DNAnexus.
UK Biobank defines early career researchers as “ an individual within an academic institution within four years of the award of their PhD or equivalent professional training, or within four years of starting their first academic appointment (full-time or part-time), excluding career breaks)”. Early career researchers also include those bona fide students eligible for reduced Access fees.
Researchers in low and middle income countries eligible for reduced Access Fees will al so be able to apply for this program.
Learn more.
"UK Biobank's platform will make data more accessible to researchers. Free computing for researchers working in resource-poor settings and for young scientists is a fantastic way of increasing the use of UK Biobank's amazing resource."
DR MARK EFFINGHAM Deputy CEO / UK Biobank
"This platform will democratise access, helping to unleash the imaginations of the world's best scientific minds - wherever they are - to make discoveries that improve human health."
PROFESSOR SIR RORY COLLINS Principal Investigator / UK Biobank
"We enthusiastically support the foundational UK Biobank project as it breaks new ground in the advancement of disease research through the integration of deep healthcare data with genomics and advanced tools."
RICHARD DALY CEO / DNAnexus
Apply for Access
Become an approved uk biobank researcher today to explore the world's largest biomedical database all in one place through the uk biobank research analysis platform..
Cookies on this website
We use cookies to ensure that we give you the best experience on our website. If you click 'Accept all cookies' we'll assume that you are happy to receive all cookies and you won't see this message again. If you click 'Reject all non-essential cookies' only necessary cookies providing core functionality such as security, network management, and accessibility will be enabled. Click 'Find out more' for information on how to change your cookie settings.
- Accessibility
- Tools and Facilities
UK Biobank (UKB) Research Analysis Platform (RAP)
UKB is a large-scale biomedical database and research resource, containing in-depth genetic and health information from 0.5 million UK participants, including sociodemographics, environmental factors, lifestyle factors, blood biochemistry assays, health outcome linkage, multimodal imaging, genotypes, metabolomics, wearable device derived data, proteomics, whole exome sequencing, whole genome sequencing, etc. The total size of UK Biobank resources is expected to exceed 20 Petabytes in 2023. The UKB RAP, enabled by DNAnexus technology and powered by Amazon Web Services, has been designed to accommodate the rapid growth of the resource and to enable more researchers across the world to access these data without limitations of transferring, collating, storing, and accessing data at this scale. It brings the researchers to the data, making access and compute more widely available.
Availability
- provides access to UKB data to all researchers with approved UKB projects.
- provides multiple ways to interact with the platform: (1) web browser, (2) executables, command line interface, and programming languages, (3) batch jobs, and (4) building your own tools.
- provides built-in tool repository with apps and workflows for bioinformatics, genetics, and statistical analysis
- supports multiple commonly used analytic languages: R, bash, Python, Stata.
- provides customised computing resources: Spark cluster support on multiple instances; machine learning and GPU instances; HAIL and other distributed frameworks.
Data storage and analysis may occur fees.
We offer (1) platform exploration credits of £40 for all RAP users, and (2) UKB Platform Credits Programme for early career researchers and researchers from low-middle income countries.
https://ukbiobank.dnanexus.com
Contact Dr Qi Feng
For more information please visit:
- online documentation https://dnanexus.gitbook.io/uk-biobank-rap
- helpdesk https://dnanexus.gitbook.io/uk-biobank-rap/technical-support
- online training https://dnanexus.gitbook.io/uk-biobank-rap/getting-started/research-analysis-platform-training-webinars
- community portal https://community.dnanexus.com/s/
April 10, 2024 DNAnexus
Announcing Additional Support for the UK Biobank Research Analysis Platform
It’s been over two years since the launch of the UK Biobank Research Analysis Platform (UKB-RAP) and we've learned a lot about what researchers need and the diverse needs of this user community. To address this diversity, DNAnexus is launching an official support model that is multi-pronged and will serve the different needs of this growing community.
Starting on April 10, our new support model will pair our standard support with new paid service packages. Our standard support will continue to enable users to send email inquiries about billing, administrative issues, and report platform performance issues and bugs. You can also view tutorials and webinars and post questions on the UK Biobank Community Forum .
However, for users who need a bit more support for their customized workflows or want 1:1 expert guidance from the DNAnexus team, we are launching new service packages that will be available in addition to our existing standard support. These new customized and flexible service packages are made up of tickets that users redeem to get the additional help they may need for managing specific queries, troubleshooting a custom applet, or receiving scientific guidance. Service packages are available in bundles of 5, 20, 50 or 100 service tickets. You can find more information on our new service packages and purchase them via the order form here. Service packages require a DNAnexus Billing Portal account. If you need to create a Billing Account, follow these instructions.
Please note that support questions that require our experts to directly access and/or process UK Biobank data at your direction will require more discussion with DNAnexus. View our FAQ for more details and feel free to contact us at [email protected] about your inquiry.
For all UKB-RAP users that have open/ongoing queries that our DNAnexus team has been helping with, we assure you that we will continue to work with you to resolve your open ticket even once these changes have taken effect. If you have additional questions about the transition, please email [email protected] .
[1] For more information on guidelines for DNAnexus acting as a Third Party Data Processor, please reference the UK Biobank MTA .
Announcing Enhanced Nextflow Support
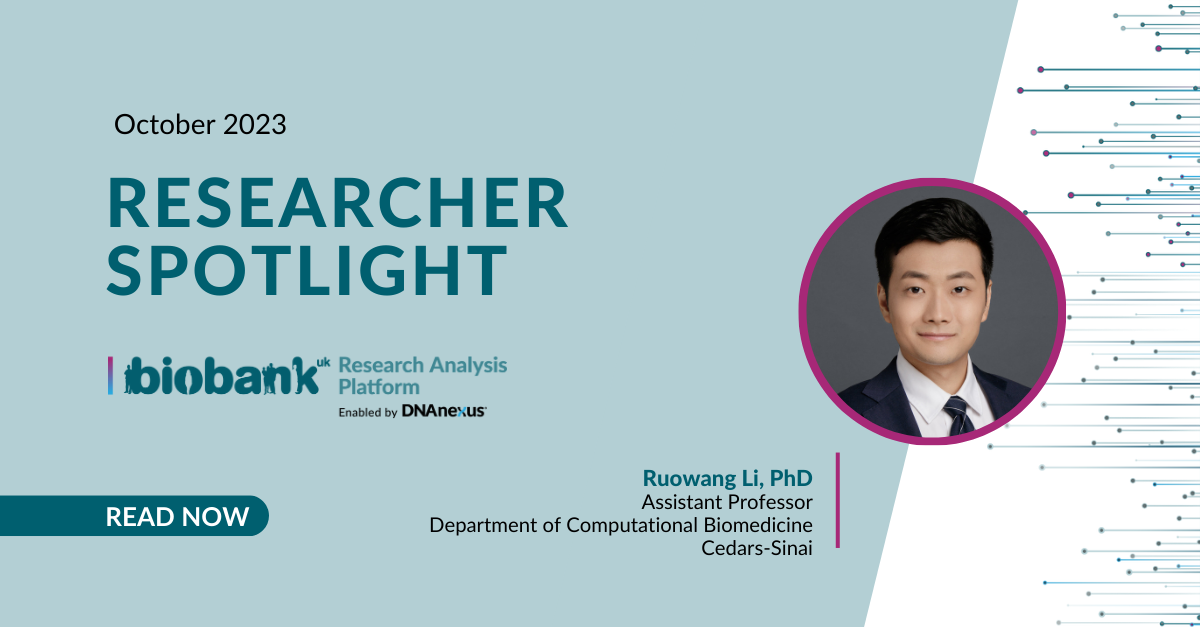
UK Biobank RAP Researcher Spotlight: October 2023
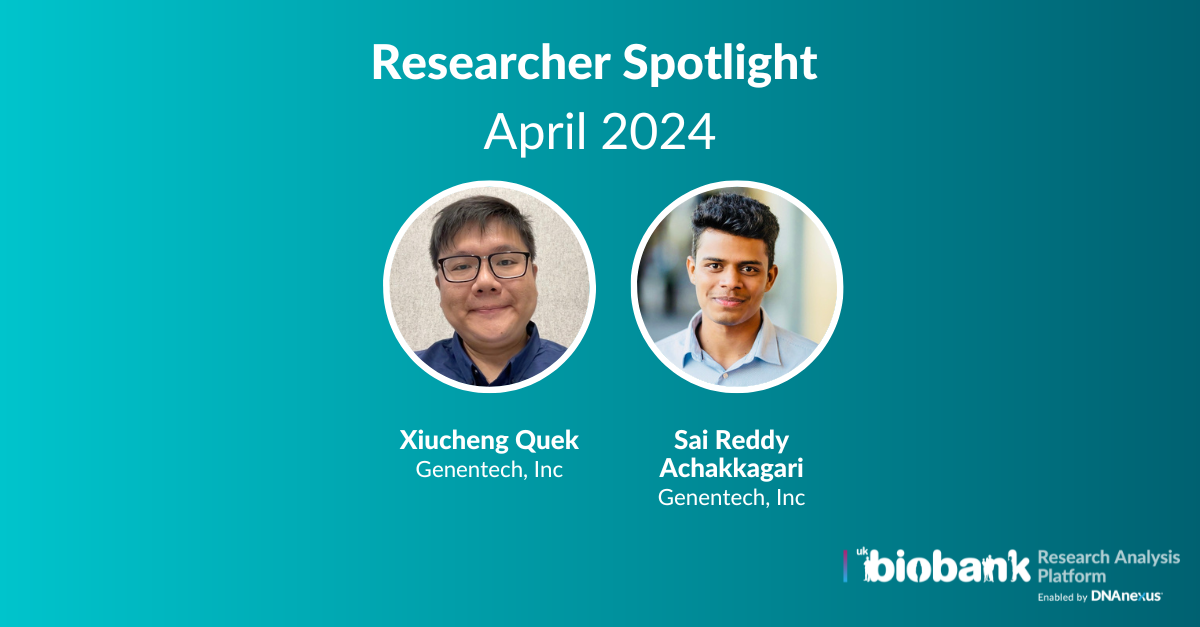
UK Biobank RAP Researcher Spotlight: April 2024
About dnanexus.
DNAnexus the leader in biomedical informatics and data management, has created the global network for genomics and other biomedical data, operating in 33 countries including North America, Europe, China, Australia, South America, and Africa. The secure, scalable, and collaborative DNAnexus Platform helps thousands of researchers across a spectrum of industries — biopharmaceutical, bioagricultural, sequencing services, clinical diagnostics, government, and research consortia — accelerate their genomics programs.
The DNAnexus team is made up of experts in computational biology and cloud computing who work with organizations to tackle some of the most exciting opportunities in human health, making it easier—and in many cases feasible—to work with genomic data. With DNAnexus, organizations can stay a step ahead in leveraging genomics to achieve their goals. The future of human health is in genomics. DNAnexus brings it all together.
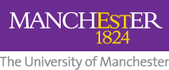
Research IT
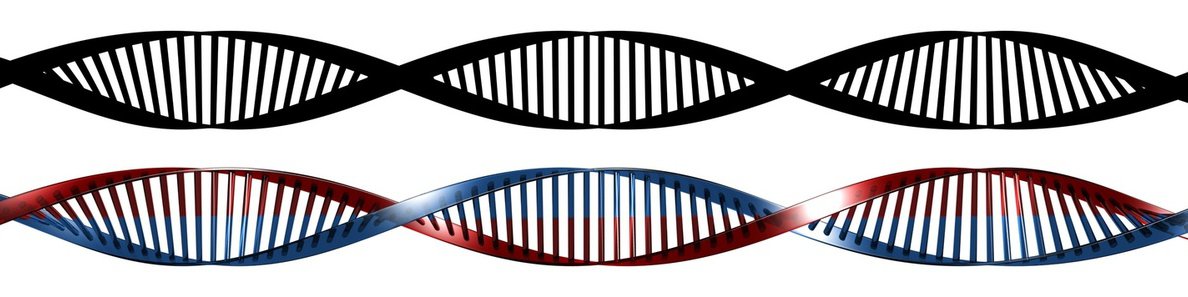
UoM UK Biobank Users Wrap Up for 23/24
The last UoM UK Biobank Users meeting of the academic year recently took place but there is still plenty of activity going on over the summer!
The last meeting of the UoM UK Biobank Users took place on the 15th of May and featured an extended presentation on the strengths and limitations of the UK Biobank datasets from Prof Martin Rutter from the School of Medical Sciences.
It was great to have Martin along and to introduce him to the group especially as he will take up his position as Deputy Chief Scientist at UK Biobank in June 2024. Martins slides “Impacts of disrupted sleep and circadian rhythm: clinical and biological insights from the UK Biobank” are now available on the group space on CaDiR .
The group meetings may have finished for the summer but activities are already being planned for the first meeting of the new academic year where we will be pleased to welcome back speakers from the UK Biobank itself. We’re also pleased to welcome William Lloyd from the Division of Informatics, Imaging and Data Sciences to the group organisers. Will is starting a joint role with the University and the UK Biobank in the summer so is an excellent addition to the team!
Keep an eye on the user space on Teams for online activity over the summer. We have been approached by the UK Biobank to help gather feedback on various items such as the Research Analysis Platform (RAP) and their website. We'll post requests for input in the group CaDiR space.
Also remember that you can still ask questions about the use of UK Biobank, using the Research Application Platform (RAP), clarification on how to apply for access etc as well as information about UK Biobank related events and much more on the dedicated Teams space !

An official website of the United States government
The .gov means it’s official. Federal government websites often end in .gov or .mil. Before sharing sensitive information, make sure you’re on a federal government site.
The site is secure. The https:// ensures that you are connecting to the official website and that any information you provide is encrypted and transmitted securely.
- Publications
- Account settings
Preview improvements coming to the PMC website in October 2024. Learn More or Try it out now .
- Advanced Search
- Journal List
- v.8(6); 2024 Jun
- PMC11114472

Bone health, cardiovascular disease, and imaging outcomes in UK Biobank: a causal analysis
Dorina-gabriela condurache.
NIHR Barts Biomedical Research Centre, William Harvey Research Institute, Centre for Advanced Cardiovascular Imaging, Queen Mary University of London, Charterhouse Square, London, EC1M 6BQ, England, United Kingdom
Barts Heart Centre, St Bartholomew’s Hospital, Barts Health National Health Service (NHS) Trust, West Smithfield, London EC1A 7BE, England, United Kingdom
Stefania D’Angelo
MRC Lifecourse Epidemiology Centre, University of Southampton, Tremona Road, Southampton SO16 6YD, England,United Kingdom
Ahmed M Salih
Department of Population Health Sciences, University of Leicester, Leicester LE1 7RH, England, United Kingdom
Department of Computer Science, Faculty of Science, University of Zakho, Zakho 42002, Kurdistan Region, Iraq
Liliana Szabo
Semmelweis University, Heart and Vascular Centre, Budapest, Hungary
Celeste McCracken
Division of Cardiovascular Medicine, Radcliffe Department of Medicine, National Institute for Health Research Oxford Biomedical Research Centre, University of Oxford, Oxford University Hospitals NHS Foundation Trust, Oxford OX3 9DU, England, United Kingdom
Adil Mahmood
Elizabeth m curtis.
NIHR Southampton Biomedical Research Centre, University of Southampton and University Hospital Southampton NHS Foundation Trust, Southampton SO16 6YD, England, United Kingdom
Andre Altmann
Department of Medical Physics and Biomedical Engineering, Centre for Medical Image Computing (CMIC), University College London, London WC1E 6BT, England, United Kingdom
Steffen E Petersen
Health Data Research UK, British Heart Foundation Data Science Centre, London NW1 2BE, England, United Kingdom
Nicholas C Harvey
Zahra raisi-estabragh, associated data.
This project was carried out under UK Biobank Access Application 3593. UK Biobank will make the data available to all bona fide researchers for all types of health-related research that is in the public interest, without preferential or exclusive access for any persons. All researchers will be subject to the same application process and approval criteria as specified by UK Biobank. For more details on the access procedure, see the UK Biobank website: http://www.ukbiobank.ac.uk/register-apply .
This study examined the association of estimated heel bone mineral density (eBMD, derived from quantitative ultrasound) with: (1) prevalent and incident cardiovascular diseases (CVDs: ischemic heart disease (IHD), myocardial infarction (MI), heart failure (HF), non-ischemic cardiomyopathy (NICM), arrhythmia), (2) mortality (all-cause, CVD, IHD), and (3) cardiovascular magnetic resonance (CMR) measures of left ventricular and atrial structure and function and aortic distensibility, in the UK Biobank. Clinical outcomes were ascertained using health record linkage over 12.3 yr of prospective follow-up. Two-sample Mendelian randomization (MR) was conducted to assess causal associations between BMD and CMR metrics using genetic instrumental variables identified from published genome-wide association studies. The analysis included 485 257 participants (55% women, mean age 56.5 ± 8.1 yr). Higher heel eBMD was associated with lower odds of all prevalent CVDs considered. The greatest magnitude of effect was seen in association with HF and NICM, where 1-SD increase in eBMD was associated with 15% lower odds of HF and 16% lower odds of NICM. Association between eBMD and incident IHD and MI was non-significant; the strongest relationship was with incident HF (SHR: 0.90 [95% CI, 0.89–0.92]). Higher eBMD was associated with a decreased risk in all-cause, CVD, and IHD mortality, in the fully adjusted model. Higher eBMD was associated with greater aortic distensibility; associations with other CMR metrics were null. Higher heel eBMD is linked to reduced risk of a range of prevalent and incident CVD and mortality outcomes. Although observational analyses suggest associations between higher eBMD and greater aortic compliance, MR analysis did not support a causal relationship between genetically predicted BMD and CMR phenotypes. These findings support the notion that bone-cardiovascular associations reflect shared risk factors/mechanisms rather than direct causal pathways.
Graphical Abstract

Introduction
Cardiovascular diseases (CVDs) are the leading cause of mortality and a major contributor to disability worldwide. 1 Osteoporotic bone fractures affect 1 in 2 women and 1 in 5 men over 50 yr, resulting in substantial long-term disability and reduced survival. 2
Emerging epidemiological evidence suggests an association between osteoporosis and CVD outcomes. 3-8 For instance, a recent prospective cohort study from the UK Biobank 3 found that osteoporosis was strongly associated with cardiovascular mortality in men, with data suggesting a more than 2-fold increased risk of heart failure (HF) and coronary artery disease in those with osteoporosis. 4
Osteoporosis and CVDs have a number of shared risk factors such as older age, sedentary lifestyle, tobacco use, excess alcohol intake, premature menopause, and vitamin D deficiency. 8 Recently, an increasing body of biological and epidemiological evidence has provided support for a link between the 2 conditions beyond age and shared risk factors. It is suggested that a common pathogenic mechanism, including inflammation and imbalance in mineral metabolism, is implicated in their pathogenesis. 8 , 9 Although associations between BMD and CVDs have been reported, there is unclear evidence regarding direct causal pathways between the two.
We present the most comprehensive evaluation of the relationship between bone and cardiovascular health in the UK Biobank. The aims of the present study were to explore the relationships of: (1) estimated heel bone mineral density (eBMD) with prevalent and incident CVDs and mortality events; (2) eBMD with cardiovascular magnetic resonance (CMR) measures of cardiac structure and function; (3) genetically predicted BMD with 58 CMR phenotypes using 2-sample Mendelian Randomization (MR) analysis.
To our knowledge, this is the first large-scale population-based study to examine the causal associations between BMD and cardiovascular health through detailed CMR phenotyping and MR analysis.
The utilization of CMR provides a highly sensitive and nuanced view of cardiovascular status capturing both clinically manifest diseases and pre-clinical cardiac alterations. This granularity enables a more precise assessment of the cardiac implications of BMD variations. By integrating the 2-sample MR analysis, our study seeks to provide a more definitive assessment of the causal effects of BMD on cardiovascular health, addressing a gap in the current understanding of these complex interrelations.
Materials and methods
Setting and study population.
The UK Biobank includes over half a million individuals from across the United Kingdom (UK), aged 40–69 yr old at recruitment, which occurred over a 4-yr period between 2006 and 2010. Baseline assessment included a series of detailed questionnaires, face-to-face interviews, physical measures, and blood sampling. 10 The UK Biobank Imaging Study, which includes CMR, is underway and aims to scan 100 000 of the original participants. 11 Linkages to national health data, such as Hospital Episode Statistics (HES) and Office for National Statistics death registration data, permit prospective tracking of incident health events for all UK Biobank participants.
Ascertainment of exposure
Heel eBMD was derived for all participants from QUS measurement of the calcaneus, using a Sahara Clinical Bone Sonometer (Hologic Corporation) according to a standardized protocol. 12 The Sahara system measures the speed of sound (SOS, in m/s) and the broadband ultrasonic attenuation (BUA, in dB/MHz), which are used to estimate BMD (in g/cm 2 ) per the manufacturer’s software. eBMD (in g/cm 2 ) was derived as a linear combination of SOS and BUA (ie, eBMD = 0.002592 * (BUA + SOS) − 3.687). Vox software was used to automatically collect data from the sonometer (denoted direct input). In cases where direct input failed, quantitative ultrasound (QUS) outcomes were manually keyed into Vox by the attending healthcare technician or nurse (ie, manual input). QUS parameters are good predictors of fragility fractures and correlate reliably with BMD measured by DXA. 13-16
Ascertainment of clinical and mortality outcomes
The following prevalent and incident CVDs were included: ischemic heart disease (IHD), myocardial infarction (MI), HF, cardiomyopathies, atrial fibrillation (AF). Baseline date was the date each participant was recruited into the UK Biobank, from which their susceptibility to the events of interest was measured. Prevalent events were conditions present at baseline. Incident events were those occurring for the first time after baseline. Mortality outcomes (all-cause mortality, CVD mortality, IHD mortality) were defined according to the primary cause of death ascertained from death registration data. Individuals with record of the outcome of interest at baseline were excluded from the incident analyses for that condition. Diseases were defined based on a combination of UK Biobank baseline assessment records and HES International Classification of Disease codes (code set: Table SS1 ).
Ascertainment of covariates
Covariates were selected based on their potential role as true confounders, determined from reported relationships with the exposure and outcome from published literature and biological plausibility. Age at baseline was used for models of prevalent and incident outcomes. Townsend deprivation index, a socio-economic measure of deprivation, was calculated prior to participants joining the UK Biobank based on area of residence. Educational level, alcohol intake frequency (daily or almost daily, 3–4 times per wk never, 1–2 times per wk, 1–3 times per mo, special occasions only, and never), smoking status (never smoker and current smoker), and physical activity (ascertained as duration of moderate physical activity [min/d)) were derived from self-report. BMI was calculated from height and weight measures taken at UK Biobank assessment. Diabetes, hypertension, and hypercholesterolemia status, at imaging, were defined based on self-report of the condition in UK Biobank assessments, self-reported of use of medication for the condition, or relevant ICD code in linked HES records ( Table SS1 ).
CMR image acquisition and analysis
CMR examinations were performed on 1.5 Tesla scanners (MAGNETOM Aera, Syngo Platform VD13A, Siemens Healthcare) in dedicated imaging units in accordance with predefined protocols. 17 Images were analyzed using automated pipelines. 18 The following CMR phenotypes were considered: left ventricular (LV) wall thickness (WT), LV mass (LVM), LV end-diastolic volume (LVEDV), LVM to LVEDV ratio, LV stroke volume (LVSV), LV ejection fraction, LV global functional index, LV global longitudinal strain, left atrial maximum volume, left atrial ejection fraction, right ventricle end-diastolic volume (RVEDV), right ventricle stroke volume, right ventricle ejection fraction (RVEF), aortic distensibility (AoD).
Alterations of CMR-derived metrics have known widely described significance in their relation to disease and prognosis. There is a large body of literature describing such relationships in clinical and population cohorts. Importantly, CMR may detect subclinical cardiovascular alterations before disease occurrence. For instance, greater LV mass has been highlighted as a poor prognostic marker across many studies, 18 , 19 LV global longitudinal strain has been linked to poorer prognosis across a number of cohorts, 20 , 21 and arterial stiffness (as indicated by lower aortic compliance) has a well-established linked to greater IHD risk. 22 , 23
Mendelian randomization
Two-sample MR was conducted to assess causal association between genetically predicted BMD and CMR metrics. We reviewed existing literature to identify genome-wide association studies (GWAS) capturing BMD (exposure) and CMR phenotypes (outcome). We ensured comparability of the exposure and outcome GWAS populations and that there was no overlap in cohorts between the two. Notably, the eBMD GWAS was not used due to the complete sample overlap, which can bias the MR estimates. From the identified GWAS studies, we selected suitable genetic instruments required for a 2-sample MR analysis.
Genome-wide association studies BMD (exposure)
Medina-Gomez et al. 24 conduced a meta-analysis GWAS for total body (TB) BMD, including a total of 66 628 individuals from 30 cohorts across Europe, Australia, and America, comprising mostly individuals from European ancestry (86%).
GWAS cardiac function and structure (outcomes)
A total of 58 CMR measures of cardiac function and structure from 7 studies were considered. All studies used the UK Biobank to calculate the cardiac measures and conduct the GWAS. There were differences in sample size and quality control criteria across studies. Due to these variations, we have limited the analysis to metrics that were included in more than one study. For additional information regarding the studied included, refer to Table SS2 in the Supplemental material.
Selection of instrumental variables
The instrumental variables were selected from the BMD GWAS including the result of GWAS when all individuals were considered. We chose variants that passed the GWAS standard P -value threshold ( P < 5 × 10 −8 ). Then, we applied linkage disequilibrium (LD) clumping to choose independent variants. GWAS summary statistics were extracted from variants that passed both the GWAS P -value threshold and LD clumping (windows size = 10 000, r 2 threshold = 0.001, population = European). We employed SNPs, with a comprehensive list and detailed information on each variant in Table SS3 . The SNPs were derived from TB BMD and were all present in the outcome; however, 4 SNPs (rs11995824, rs2553773, rs447911, and rs780096) were excluded due to palindromicity, leaving 81 SNPs in the analysis. The scope of our association analysis did not extend to the establishment of minor allele frequency (MAF) ranges or imputation quality thresholds for SNP inclusion; however, comprehensive MAF and imputation quality data can be found in the study by Medina-Gomez et al. 24
For the same set of variants, GWAS summary statistics for cardiac metrics were extracted. Thereafter , 2-sample MR was conducted to assess the causal association between BMD (Non-UK Biobank) as exposure and the 58 cardiac function and structure metrics (from UK Biobank) as outcome. Inverse variance weighted (IVW) method was used as the main analysis, while MR-Egger, weighted median, and weighted mode were used as complementary sensitivity analyses to detect direct and horizontal pleiotropy. Estimates of pleiotropy (Egger intercept) and heterogeneity are found in Table SS4 . The analysis was conducted using the R package TwoSampleMR. 25
Statistical analysis
Statistical analysis was performed using RStudio V.4.1.0 ( https://www.R-project.org/ ) and Stata V.17. 26 Baseline characteristics are presented as number (percentage) for categorical variables, mean (SD) for normally distributed continuous variables, and median (IQR) for non-normally distributed continuous variables. Logistic regression and competing risk regression were used to estimate association of heel eBMD with prevalent and incident CVDs, respectively. The results are reported as odds ratios (ORs) and sub-distribution HRs (SHR) per 1-SD increment of eBMD and 95% CIs. The censor date was September 30, 2021, providing an average prospective follow-up of 12.3 yr. Current analysis does not account for multiple testing. The application will attenuate some of the already weak associations. This is in keeping with the notion that the relationship between BMD and CVD outcomes is small.
We estimated association of baseline heel eBMD with mortality outcomes using Cox regression models and the results are reported as hazard ratio (HRs) per 1-SD increment of BMD. In participants with CMR data available, we used multivariable linear regression to estimate the associations of heel eBMD with selected cardiovascular phenotypes. Associations of eBMD with CMR metrics are reported as SD change in CMR measure per 1-SD increment in eBMD. To allow comparison of the magnitude of effects across CMR metrics, we report standardized beta-coefficients with corresponding 95% CI.
We created 3 models with different layers of adjustment. Model 1 was adjusted for age and sex; model 2 was adjusted for model 1 variables plus diabetes, hypertension, high cholesterol, smoking status, alcohol consumption frequency, physical activity, Townsend deprivation score, education. Our fully adjusted model, model 3, was adjusted for model 2 variables plus BMI. To examine potential sex differential relationships, we report P -values for sex interaction terms (sex × eBMD) in fully adjusted models (model 3) and present sex stratified analyses for each outcome.
Baseline characteristics
Baseline eBMD was available for 485 257 participants. At the time of recruitment, their mean age was 56.5 ± 8.1 yr and 54.5% of the participants were women. Mean eBMD was 0.55 (SD 0.14) g/cm 2 . Baseline participant characteristics are summarized in Table 1 .
Baseline characteristics of men and women with heel eBMD measured at baseline.
Counts variables are presented as number (percentage), continuous variables as mean (SD) or median (IQR) based on distribution.
Within the whole sample, the most common prevalent CVDs were IHD, MI, and AF with rates of 4.9% ( n = 23 699), 2.3% ( n = 11 201), and 0.5% ( n = 2474), respectively ( Table 1 ). The least common prevalent CVDs were HF ( n = 2180, 0.5%) and non-ischemic cardiomyopathy (NICM) ( n = 821, 0.2%). The most common incident diseases were IHD ( n = 32 408, 6.7%) and arrhythmia ( n = 23 149, 4.8%). There were 10 106 (2.1%) incident MIs and 13 957 (2.9%) incident cases each of HF. Over a follow-up period of 12.3 yr, we observed 35 950 (7.4%) deaths; of these, 13 073 were attributed to CVD and 4146 to IHD ( Table 1 ). CMR data were available for 25 320 participants. CMR phenotypes are presented in Table 1 .
Association of eBMD with prevalent disease
Within the entire cohort, higher heel eBMD was associated with decreased odds of all prevalent CVDs considered ( Table 2 ). The greatest magnitude of effect was with HF and NICM, where 1-SD increase in eBMD was associated with 15% lower odds of HF (OR: 0.85 [95% CI, 0.81–0.90]) and 16% lower odds of NICM (OR: 0.84 [95% CI, 0.78–0.81] in the fully adjusted models. Higher eBMD was also associated with reduced odds of arrhythmia, MI, and IHD, but with very small effect sizes (HR: 0.95 to 0.97).
Associations between baseline heel eBMD a and prevalent CVDs a .
Results are reported as odds ratios (ORs) per 1-SD increment of eBMD. Model 1 is adjusted for age and sex. Model 2 is adjusted for age, sex, diabetes, hypertension, high cholesterol, smoking status, BMI, alcohol intake frequency, physical activity, Townsend score, and educational level. Model 3: Model 2 + BMI.
In sex-stratified analyses, higher eBMD appeared to show a greater protective effect in women than men across all prevalent CVDs considered ( Table SS5 ). We observed significant sex interaction in association with prevalent MI and arrhythmia outcomes ( Table SS5 ). The inverse associations of eBMD with prevalent MI had greater magnitude of effect in women (OR: 0.9; 95% CI, 0.87–0.96) that men (OR: 0.97; 95% CI, 0.95–0.97). In associations between eBMD and prevalent arrhythmia, the relationship attenuated to null in men, but remained statistically significant in women (OR:0.88; 95% CI, 0.81–0.96).
Association of eBMD with incident CVD and mortality events
In fully adjusted models, higher eBMD was associated with lower risk of incident HF (SHR: 0.90 [95% CI, 0.89–0.92]), NICM (SHR: 0.95 [95% CI, 0.91–0.99]), and AF (SHR: 0.95 [95% CI, 0.94–0.97]) in the whole cohort ( Table 3 ). Associations between eBMD and incident IHD and MI were statistically non-significant ( Figure 1 ). Higher eBMD was consistently associated with a decreased risk of all-cause (HR: 0.87 [95% CI, 0.86–0.88]), CVD (HR: 0.85 [95% CI, 0.46–0.87]), and IHD (HR: 0.88 [95% CI, 0.45–0.91]) mortality, after adjusting for all relevant covariates (model 3). Detailed results of the multivariable Cox proportional hazard regression analyses are reported in Figure 2 .
Associations between baseline heel eBMD a and incident CVDs a .
Results are reported as sub-distribution hazard ratios (SHR) per 1-SD increment of eBMD obtained from Fine and Gray competing risks model. Model 1 is adjusted for age and sex. Model 2 is adjusted for age, sex, diabetes, hypertension, high cholesterol, smoking status, alcohol intake frequency, physical activity, Townsend score, and educational level. Model 3: Model 2 + BMI.

Associations between baseline heel eBMD and incident CVDs ( n = 399 297). Footnote: Estimates are from model 3 adjusted for age, sex, diabetes, hypertension, high cholesterol, smoking status, BMI, alcohol intake frequency, physical activity, Townsend score, educational level, and BMI. The x -axis represents the sub-distribution hazard ratios (SHR) per 1-SD increment of eBMD obtained from Fine and Gray competing risk model. The y -axis lists incident cardiovascular diseases: IHD, MI, cardiomyopathies, HF, and AF.

Association between baseline heel BMD and mortality events ( n = 399 297). Footnote: Estimates are from model 3 adjusted for age, sex, diabetes, hypertension, high cholesterol, smoking status, BMI, alcohol intake frequency, physical activity, Townsend score, educational level and BMI. The x -axis represents the hazard ratios (HR) obtained with Cox proportional hazard model. The y -axis lists mortality events.
There was evidence of significant sex-specific associations between eBMD and all incident CVDs considered ( Figure 3 , Table SS6 ). Interaction terms with sex and eBMD, in fully adjusted models, were statistically significant for all incident CVDs included in our analysis. Higher eBMD appears to have a more protective relationship in women across all incident CVDs. Notably, in sex-stratified analyses, higher eBMD is associated with significantly lower risk of incident IHD (OR: 0.95; 95% CI, 0.93–0.98) and incident cardiomyopathies (OR: 0.88; 95% CI, 0.82–0.94) in women, while in men these relationships appeared statistically non-significant.

Sex-specific associations of heel eBMD with incident CVDs (SHR) and mortality events (HR): detailed estimates in Table SS6 and Table SS7 and model 3 . Footnote: Estimates are from model 3 adjusted for age, sex, diabetes, hypertension, high cholesterol, smoking status, BMI, alcohol intake frequency, physical activity, Townsend score, educational level, and BMI. The x -axis represents the hazard ratios (HR) and sub-distribution hazard ratio (SHR). The y -axis lists incident CVDs and mortality events.
In the relationships with mortality events, the sex interaction term was statistically significant in relation to all-cause mortality ( Figure 3 , Table SS7 ). In sex-stratified analyses, higher eBMD was associated with significantly lower risk of all mortality outcomes (all-cause, CVD, IHD) in both men and women.
Association of heel eBMD with CMR metrics
Higher heel eBMD was associated with greater AoD in fully adjusted linear regression models (β: 0.02 [95% CI, 0.009–0.04]). Associations with other CMR metrics were non-significant in fully adjusted models ( Table 4 ).
Association between baseline heel eBMD and CMR outcomes (exposure and outcomes are in SD).
Results are standardized beta coefficients (β) obtained with linear regression model. Model 1 is adjusted for age and sex. Model 2 is adjusted for age, sex, diabetes, hypertension, high cholesterol, smoking status, alcohol intake frequency, physical activity, Townsend score, and educational level. Model 3: Model 2 + BMI.
MR analysis
The set of instrumental variables included 81 variants representing genetically predicted BMD, after applying GWAS P -value threshold and LD clumping. The results of the main analysis ( Table SS4 ) indicate that 14 metrics (mostly from RV [eg, smaller RVEDV and RVESV] and 2 metrics from LV [Max LV and LVSV]) are significantly (IVW P -value <.05) influenced by BMD. However, the results of the sensitivity analysis did not confirm support for these association ( P -value > .05), indicating a violation of the method’s assumptions (eg, direct or horizontal pleiotropy). Accordingly, this analysis did not provide evidence to support a potential causal relationship between BMD and CMR-derived cardiac function and structure measures.
Summary of findings
We present the largest and most comprehensive evaluation of the relationship between bone and CV health in the UK Biobank. In this population-based cohort of 485 257 individuals, we examined the relationship between eBMD with prevalent and incident health outcomes, mortality (both all-cause and attributable to CVD and IHD), and CMR phenotypes. Although there were modest inverse associations between eBMD and CVDs events, the most significant associations were observed with mortality outcomes. Higher eBMD was associated with greater AoD; that is, better bone health was associated with better vascular health. However, associations with other CMR metrics were null. Furthermore, MR analysis did not support a causal link between genetically predicted BMD and a wide range of CMR phenotypes. Although there is an evident relationship between bone and CV health, our results indicate that this is most likely due to shared risk factors and common underlying biological processes rather than a direct causal effect. These findings provide insight into mechanistic pathways and inform long-term care and risk stratification considerations.
Interpretation in the context of existing evidence
Our study found that individuals with higher heel eBMD were associated with a reduced risk of both prevalent and incident CVDs. This observation finds resonance with an established body of literature, which posits a relationship between BMD and CVD risk. 3-8 , 27 For instance, a European epidemiological study suggests a 23% reduction in the risk of incident HF for every 1-SD increase in BMD. 28 A systematic review and meta-analysis of 11 studies reported that individuals with low BMD had a 33% higher CVD risk. 29 Although the available literature is consistent with our findings of links between BMD and CVDs, the notably larger effect sizes in these reports compared to our analysis likely reflect greater residual confounding, compared to our models, which included extensive confounder adjustment.
Sex-stratified sub-analysis revealed significant sex-specific associations between baseline heel eBMD and the incidence of various CVD outcomes. Higher eBMD was more protective against incident CVDs in women compared to men, particularly for IHD, HF, and arrhythmia. This is in contrast to the HUNT study, 30 which showed a small protective association of BMD on MI and AF in men but not in the female population. Conversely, the research conducted by Yang et al. 4 showed no gender difference between BMD, and the risk of CVD was observed in the sex-specific stratified analysis. Moreover, Gao et al. 31 found that lower BMD was linked with higher risk of HF in older Black women and White men. The appreciation of sex differential relationships can be challenging, as this requires a much greater level of statistical powered. Sex-stratified analyses with CVD outcomes are prone to be differentially powered across men and women with propensity toward being underpowered in women who tend to have fewer events. Our analysis, including a large number of men and women and with over 12 yr prospective follow-up, had opportunity to capture adequate CVD events across both sexes, enhancing our ability to reliably detect sex-specific associations of eBMD. Furthermore, although reports in the literature are mixed, the greater influence of eBMD on cardiovascular health in women observed in our study is biologically consistent, particularly given the influence of menopause on both bone and cardiovascular health.
Associations of heel eBMD with mortality outcomes exhibit a larger effect size and demonstrate consistently statistically significant results across the different mortality outcomes considered, despite the previously described smaller associations with prevalent and incident IHD, respectively. This observation aligns with a growing body of evidence from observational studies over the past decade that has highlighted potential links between heel BMD and mortality events. 32-34
The results of the main analysis confirm the association of better bone quality (higher eBMD) with better arterial health as reflected by higher AoD. In line with a recent study conducted in the same population, 35 our findings not only replicate the observed inverse relationship between bone quality and arterial compliance as measured by CMR but also extend beyond, by examining a wider range of clinical outcomes, CMR parameters, and causal relationships using MR analysis.
To our knowledge this is the first large-scale population-based study to examine the causal associations between genetically predicted BMD and CMR phenotypes using 2-sample MR analysis. The findings do not support a causal link between genetically predicted BMD and CMR metrics, indicating that previously described observational relationships may be influenced by residual confounding rather than direct causality. Our study not only provides pivotal insights into the complex interplay between bone and cardiovascular health but also paves the way for future research to delve into the causality between genetically predicted BMD and cardiovascular outcomes. This will further enhance our understanding and inform both screening strategies and therapeutic interventions.
Limitations
As the age range in UK Biobank was limited to 40 to 69 yr at recruitment, our results may not be applicable to individuals outside this age window. There is significant healthy and wealthy volunteer selection in the UK Biobank, which may limit generalizability of our findings. 36 , 37 The exposure of interest (eBMD) was derived from QUS of the heel; however, DXA is the reference standard for assessment of BMD and diagnosis of osteoporosis in current guidelines. 38 , 39 The UK Biobank imaging substudy includes DXA as part of its imaging protocol; however, the number of participants and duration of follow-up is currently limited for this subset. Thus, for the present analysis, eBMD was selected as the exposure of interest as it provided substantially greater statistical power of the order of many magnitudes. In future, studies with DXA may be considered as more data become available and more outcomes accrue.
Higher BMD is linked to reduced risk of prevalent and incident CVD and mortality across a range of outcomes. Observational analyses further suggest associations between higher eBMD and better vascular health, as reflected by greater aortic compliance. MR does not support a causal relationship between BMD and cardiovascular structure and function across an extensive range of metrics. These findings support the notion that bone-cardiovascular associations reflect shared risk factors/mechanisms rather than direct causal pathways.
Supplementary Material
Supplemental_material_clean_version_ziae058, acknowledgments.
This study was conducted using the UK Biobank resource under access application 3593. We would like to thank all the UK Biobank participants, staff involved with planning, collection, and analysis.
Contributor Information
Dorina-Gabriela Condurache, NIHR Barts Biomedical Research Centre, William Harvey Research Institute, Centre for Advanced Cardiovascular Imaging, Queen Mary University of London, Charterhouse Square, London, EC1M 6BQ, England, United Kingdom. Barts Heart Centre, St Bartholomew’s Hospital, Barts Health National Health Service (NHS) Trust, West Smithfield, London EC1A 7BE, England, United Kingdom.
Stefania D’Angelo, MRC Lifecourse Epidemiology Centre, University of Southampton, Tremona Road, Southampton SO16 6YD, England,United Kingdom.
Ahmed M Salih, NIHR Barts Biomedical Research Centre, William Harvey Research Institute, Centre for Advanced Cardiovascular Imaging, Queen Mary University of London, Charterhouse Square, London, EC1M 6BQ, England, United Kingdom. Department of Population Health Sciences, University of Leicester, Leicester LE1 7RH, England, United Kingdom. Department of Computer Science, Faculty of Science, University of Zakho, Zakho 42002, Kurdistan Region, Iraq.
Liliana Szabo, NIHR Barts Biomedical Research Centre, William Harvey Research Institute, Centre for Advanced Cardiovascular Imaging, Queen Mary University of London, Charterhouse Square, London, EC1M 6BQ, England, United Kingdom. Barts Heart Centre, St Bartholomew’s Hospital, Barts Health National Health Service (NHS) Trust, West Smithfield, London EC1A 7BE, England, United Kingdom. Semmelweis University, Heart and Vascular Centre, Budapest, Hungary.
Celeste McCracken, Division of Cardiovascular Medicine, Radcliffe Department of Medicine, National Institute for Health Research Oxford Biomedical Research Centre, University of Oxford, Oxford University Hospitals NHS Foundation Trust, Oxford OX3 9DU, England, United Kingdom.
Adil Mahmood, NIHR Barts Biomedical Research Centre, William Harvey Research Institute, Centre for Advanced Cardiovascular Imaging, Queen Mary University of London, Charterhouse Square, London, EC1M 6BQ, England, United Kingdom. Barts Heart Centre, St Bartholomew’s Hospital, Barts Health National Health Service (NHS) Trust, West Smithfield, London EC1A 7BE, England, United Kingdom.
Elizabeth M Curtis, MRC Lifecourse Epidemiology Centre, University of Southampton, Tremona Road, Southampton SO16 6YD, England,United Kingdom. NIHR Southampton Biomedical Research Centre, University of Southampton and University Hospital Southampton NHS Foundation Trust, Southampton SO16 6YD, England, United Kingdom.
Andre Altmann, Department of Medical Physics and Biomedical Engineering, Centre for Medical Image Computing (CMIC), University College London, London WC1E 6BT, England, United Kingdom.
Steffen E Petersen, NIHR Barts Biomedical Research Centre, William Harvey Research Institute, Centre for Advanced Cardiovascular Imaging, Queen Mary University of London, Charterhouse Square, London, EC1M 6BQ, England, United Kingdom. Barts Heart Centre, St Bartholomew’s Hospital, Barts Health National Health Service (NHS) Trust, West Smithfield, London EC1A 7BE, England, United Kingdom. Health Data Research UK, British Heart Foundation Data Science Centre, London NW1 2BE, England, United Kingdom.
Nicholas C Harvey, MRC Lifecourse Epidemiology Centre, University of Southampton, Tremona Road, Southampton SO16 6YD, England,United Kingdom. NIHR Southampton Biomedical Research Centre, University of Southampton and University Hospital Southampton NHS Foundation Trust, Southampton SO16 6YD, England, United Kingdom.
Zahra Raisi-Estabragh, NIHR Barts Biomedical Research Centre, William Harvey Research Institute, Centre for Advanced Cardiovascular Imaging, Queen Mary University of London, Charterhouse Square, London, EC1M 6BQ, England, United Kingdom. Barts Heart Centre, St Bartholomew’s Hospital, Barts Health National Health Service (NHS) Trust, West Smithfield, London EC1A 7BE, England, United Kingdom.
Author contributions
Zahra Raisi-Estabragh and Nicholas C. Harvey conceptualized the idea and designed the statistical analysis plan. Stefania D’Angelo led the statistical analysis. Ahmed M. Salih conducted the MR analysis. Andre Altmann provided expert advice on the MR analysis. Dorina-Gabriela Condurache interpreted the results and wrote the original manuscript. Liliana Szabo and Celeste McCracken provided advice on the study design and statistical analysis. Adil Mahmood, Andre Altmann, Elizabeth M. Curtis, and Steffen E. Petersen provided input on data analysis and interpretation of results and reviewed subsequent drafts. Zahra Raisi-Estabragh and Nicholas C. Harvey provided overall supervision. Zahra Raisi-Estabragh is the guarantor of the work. All co-authors reviewed the manuscript, provided critical review of the work, and approved the final version. Dorina-Gabriela Condurache and Stefania D’Angelo are joint first authors. Nicholas C. Harvey and Zahra Raisi-Estabragh are joint senior authors.
Dorina-Gabriela Condurache (Investigation, Writing—original draft, Writing—review & editing), Stefania D’Angelo (Data curation, Formal analysis), Ahmed Salih (Data curation, Formal analysis), Liliana Szabo (Formal analysis, Methodology), Celeste McCracken (Formal analysis, Methodology), Adil Mahmood (Investigation, Writing—review & editing), Elizabeth M. Curtis (Investigation, Writing—review & editing), Andre Altmann (Formal analysis, Methodology), Steffen E. Petersen (Investigation, Writing—review & editing), Nicholas C. Harvey (Conceptualization, Investigation, Methodology, Supervision, Writing—review & editing), and Zahra Raisi-Estabragh (Conceptualization, Investigation, Methodology, Supervision, Writing—review & editing)
D.G.C. was supported by the Barts Charity (G-002530). A.S. was supported by a British Heart Foundation project grant (PG/21/10619). C.M. is supported by the Oxford National Institute for Health and Care Research (NIHR) Biomedical Research Centre (IS-BRC-1215-20008). L.S. was supported by the Barts Charity (G-002389). A.M. recognizes the NIHR Integrated Academic Training program which supports his Academic Clinical Fellowship post. N.C.H., E.M.C., and S.D. are supported by the UK Medical Research Council (MRC) [MC_PC_21003; MC_PC_21001], and NIHR Southampton Biomedical Research Centre, University of Southampton and University Hospital Southampton NHS Foundation Trust, UK. Z.R.E. recognizes the NIHR Integrated Academic Training program which supports her Academic Clinical Lectureship post. Z.R.E. was supported by British Heart Foundation Clinical Research Training Fellowship No. FS/17/81/33318. This work acknowledges the support of the National Institute for Health and Care Research Barts Biomedical Research Centre (NIHR203330); a delivery partnership of Barts Health NHS Trust, Queen Mary University of London, St George’s University Hospitals NHS Foundation Trust, and St George’s University of London. The funders of the study had no role in study design, data collection, data analysis, data interpretation, or decision to publish. The authors were not precluded from accessing data in the study, and they accept responsibility to submit for publication.
Conflicts of interest
S.E.P. provides consultancy to Cardiovascular Imaging Inc., Calgary, Alberta, Canada. All other authors declare no conflicts of interest.
Data availability
Ethical approval.
This study complies with the Declaration of Helsinki; the work was covered by the ethical approval for UK Biobank studies from the NHS National Research Ethics Service on June 17, 2011 (Ref 11/NW/0382) and extended on June 18, 2021(Ref 21/NW/0157) with written informed consent obtained from all participants. Individuals who withdrew consent after recruitment are not included in the analysis.
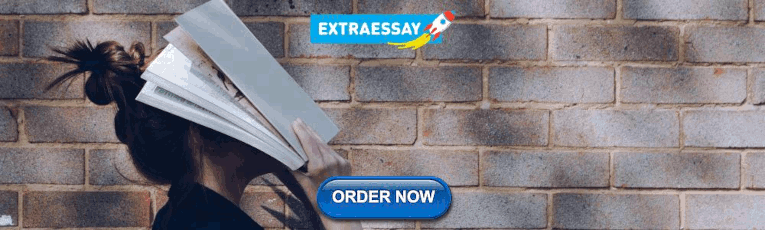
Association between socioeconomic deprivation and bone health status in the UK biobank cohort participants
- Original Article
- Open access
- Published: 28 May 2024
Cite this article
You have full access to this open access article
- Mafruha Mahmud ORCID: orcid.org/0000-0002-2090-2621 1 ,
- David John Muscatello ORCID: orcid.org/0000-0002-2391-4396 1 ,
- Md Bayzidur Rahman 2 , 5 , 6 &
- Nicholas John Osborne ORCID: orcid.org/0000-0002-6700-2284 1 , 3 , 4
256 Accesses
3 Altmetric
Explore all metrics
The effect of deprivation on total bone health status has not been well defined. We examined the relationship between socioeconomic deprivation and poor bone health and falls and we found a significant association. The finding could be beneficial for current public health strategies to minimise disparities in bone health.
Socioeconomic deprivation is associated with many illnesses including increased fracture incidence in older people. However, the effect of deprivation on total bone health status has not been well defined. To examine the relationship between socioeconomic deprivation and poor bone health and falls, we conducted a cross-sectional study using baseline measures from the United Kingdom (UK) Biobank cohort comprising 502,682 participants aged 40–69 years at recruitment during 2006–2010.
We examined four outcomes: 1) low bone mineral density/osteopenia, 2) fall in last year, 3) fracture in the last five years, and 4) fracture from a simple fall in the last five years. To measure socioeconomic deprivation, we used the Townsend index of the participant’s residential postcode.
At baseline, 29% of participants had low bone density (T-score of heel < -1 standard deviation), 20% reported a fall in the previous year, and 10% reported a fracture in the previous five years. Among participants experiencing a fracture, 60% reported the cause as a simple fall. In the multivariable logistic regression model after controlling for other covariates, the odds of a fall, fracture in the last five years, fractures from simple fall, and osteopenia were respectively 1.46 times (95% confidence interval [CI] 1.42–1.49), 1.26 times (95% CI 1.22–1.30), 1.31 times (95% CI 1.26–1.36) and 1.16 times (95% CI 1.13–1.19) higher for the most deprived compared with the least deprived quantile.
Socioeconomic deprivation was significantly associated with poor bone health and falls. This research could be beneficial to minimise social disparities in bone health.
Similar content being viewed by others
The social gradient of fractures at any skeletal site in men and women: data from the geelong osteoporosis study fracture grid.
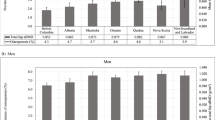
Geographic variation in bone mineral density and prevalent fractures in the Canadian longitudinal study on aging
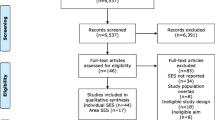
Socio-economic inequalities in fragility fracture incidence: a systematic review and meta-analysis of 61 observational studies
Avoid common mistakes on your manuscript.
Introduction
Low bone mineral density is common in older people and is a risk factor for osteoporotic fractures [ 1 ]. In the year 2000, an estimated nine million fractures occurred worldwide due to osteoporosis [ 2 ]. Known risk factors for sustaining osteoporotic fractures as well as falls include increasing age, female sex, Black ethnicity, geographical location, latitude, lack of physical activity, deficiency of calcium and vitamin D [ 3 , 4 ]. The prevalence of osteoporosis and consequent incidence of fractures and falls has been increasing rapidly, along with the cost of treatment [ 5 ]. In the United States (US), the mean cost of hospitalisation in 2004 for an injurious fall in a person aged 65 years or older was US$ 17,483 [ 4 ]. The estimated cost of the incident and past fragility fractures (fracture from a simple fall such as from standing height or lower) in Europe was €37 billion in 2010 [ 6 ]. In the UK, during 2003–2013, the mean length of hospital stays for patients aged over 60 years with hip fracture was 20 days, which was highly correlated with the higher cost of hospitalisation [ 7 ].
Socioeconomic status (SES) can be defined as a relative term by which the social and economic situation of a community or person within the community can be described. Generally, proxy measures are used in place of precise estimations of socioeconomic status. Proxies include household income, educational attainment, employment status, homeownership, and difficulty accessing resources [ 8 , 9 ]. However, the significance of these measures can vary across different populations or geographical locations [ 10 ].
It is well established that socioeconomic deprivation is associated with poor health [ 11 ]. Deprivation is associated with increased incidence and prevalence of many chronic illnesses, including cardiovascular diseases [ 12 ], asthma [ 13 ], cancer [ 14 ], and diabetes [ 15 ]. It is also associated with increased fracture incidence in older people [ 16 ]. A recent UK study which ran over the 14 years from 2001 reported a high burden of hip fracture incidence in men in the northeast region of the UK, where deprivation is more prominent [ 17 ]. The effect of deprivation on total bone health status has, however, not been well defined. Inconsistent findings characterise the association between material deprivation and bone health: some studies, for example, find a strong association between poor bone health and deprivation, while the results of others have been inconclusive [ 18 , 19 , 20 ]. A systematic review published in 2011 reported a lack of evidence supporting an association between SES and bone mineral density [ 21 ]. Availability of multiple composite deprivation indices, variation in these measures across countries, and use of inappropriate indices may produce inaccurate or varying results [ 20 , 22 ]. In the UK, multiple composite indices are applied to define socioeconomic disadvantage, such as the Townsend deprivation index, the Index of Multiple Deprivation (IMD), the Carstairs index, and the Jarman underprivileged area index [ 23 ]. IMD which derived from administrative data has been used by the UK Government to measure deprivation. However, according to the UK Data Service, the Townsend Deprivation Index measure which is based on census data, has been widely used in research for health, education, and crime to establish whether relationships exist with deprivation. In a recent study (2017) Sanah Yousaf from the UK data service reported that unlike Townsend scores, the IMD scores are relative and based on administrative data for small areas (neighbourhoods) in England, but not validated for other parts of the UK. The Carstairs index is similar to the Townsend index, except that the unemployment component only considers males. In contrast, the Townsend measure is gender inclusive [ 23 ].
It is crucial to identify and mitigate risk factors for developing osteoporosis as well as address its consequences, including fractures and falls [ 5 ]. To examine the importance of socioeconomic deprivation as a risk factor for poor bone health status and falls, a cross-sectional study of the UK Biobank cohort using their baseline data was undertaken.
A cross-sectional study was conducted using baseline data from UK Biobank cohort participants. The details of the data collection, including the methods used, are described elsewhere [ 24 ]. Briefly, the UK Biobank is a UK-wide cohort study that recruited 502,682 participants (both men and women) during 2006–2010. The participants were middle-aged and older persons (40–69 years-old at recruitment). On recruitment, each participant was invited to one of 22 assessment centres distributed across Great Britain. Characteristics such as age, sex, body mass index, current residential address, ethnicity, lifestyle factors, and medication history were collected using touchscreen questionnaires, oral interviews, and physical measures. Northern Ireland is not included in the Biobank population. Participants gave informed consent before joining the UK Biobank study; additional information on consent and privacy can be found on the Biobank website [ 25 ].
The outcome variables were defined by four bone health status measures: 1) low heel bone mineral density at baseline(osteopenia), 2) bone fracture in the last 5 years, 3) fracture in the last 5 years due to a simple fall, and 4) fall in the last year. Low bone mineral density was determined by the T-score of the person’s heel ultrasound. The T-score represents the difference, measured in standard deviations between the observed bone density and the expected value for a healthy young adult of same sex. A T-score of -1 SD or below is deemed low bone mineral density [ 26 ]. Fracture in the last 5 years was based on a self-reported response to the touch screen questionnaire: "Have you fractured any bones in the last 5 years?”. Participants who responded with a positive answer were then asked: "Did the fracture result from a simple fall (for example, from standing height)?" which we refer to as “fracture from a simple fall in the last 5 years”. “Fall in the last year” was the response to: “In the last year, have you had any falls?".
The main study factor is socioeconomic deprivation. We used the Townsend deprivation index data of the UK Biobank, which is a proxy measure of the socioeconomic deprivation status of each participant at the area level. Among deprivation variables available throughout UK, the Townsend index data was selected as the most suitable because it applies to the whole of the UK, unlike other UK deprivation indices that consider only England or unemployment in males. The Townsend index data are aggregate area-based values of material deprivation from census data based on four indicators: the percentage unemployed among economically active 16–74-year-olds, regardless of gender; the percentage of households that are overcrowded; the percentage of households that do not own a vehicle; and the percentage of households that are rented or otherwise not owned [ 23 ]. Overcrowding is assessed by the census variable ‘persons per room’ where households with more occupants than available rooms are classified as overcrowded [ 23 ]. The Townsend index for the period just before participants joined the study was available in the Biobank database. It was assigned from national census output areas that included participants’ residential postcode. Townsend scores include both positive and negative values, and a value of zero represents the mean deprivation level of all UK census areas. Increasingly negative values represent lower deprivation levels while increasing positive values represent higher deprivation [ 23 , 27 ] and the index has the national population mean of 0 . We categorised the index into 5 quantiles.
Several covariates identified from existing literature and available in the UK Biobank were included in our statistical model. These were age, sex, body mass index, ethnicity, smoking and alcohol intake, oily fish consumption, and vitamin D supplementation, as well as days per week of moderate and vigorous physical activity [ 28 ]. Age was divided into 4 groups: less than 45, 45 to 54, 55 to 64, and 65 years or more. Sex was divided into 2 groups: female and male. Similarly, BMI was categorised as one of 4 groups: underweight (< 18.5 kg/m 2 ), average (18.5–24.9 kg/m 2 ), overweight (25–29.9 kg/m 2 ) and obese (≥ 30 kg/m 2 ). We categorised ethnicity into two subgroups from available multiple ethnic group: White and non-White. Smoking status was defined by never, current, and previous smoker. Consumption of alcohol was divided into 3 categories: never/special occasion/1–3 times a month, 1–4 times a week, and daily. To collect information about oily fish consumption, participants were asked the question, “How often do you eat oily fish? (e.g., sardines, salmon, mackerel, herring)”. Information about vitamin D supplementation was obtained from the question related to vitamin and mineral supplementation taken on a regular basis. We categorised two Biobank variables for physical activity: number of days per week of moderate and of vigorous physical activity lasting at least ten minutes. These were sorted into 3 groups: less than 2 days, 2–4 days, and more than 4 days per week. Vigorous physical activity was any activity causing sweating or hard breathing, such as fast cycling, aerobics, heavy lifting. Moderate activity included, for example, carrying light loads, or cycling at a normal pace, but not walking. The details of the questionnaires can be found in the UK Biobank website.
Statistical analysis
For descriptive analysis, the distribution (mean, standard deviation, median, frequency) and p values were calculated for each of the explanatory variables with each outcome. Univariable logistic regression models were fitted to select variables for the base models using a cut-off P-value of < 0.25. We adopted the backward elimination method (cut-off p-values were < 0.05) to come up with the final model after adjusting for potential confounders for all four outcomes separately. Deprivation, as the main exposure variable, was forced to remain in the model regardless of p value.
To check the trends of odds ratios across deprivation quantiles, logistic regression models were fitted using the midpoints of the deprivation quantiles as a continuous variable. Scatter plots were created to visualise trends, with the vertical axis denoting the log of the odds, and the horizontal axis indicating midpoints of the quantiles. All the statistical analyses were conducted using SAS 9.4 software.
Heel ultrasound was performed on 321,823 participants, of which 29% (94,448) had low bone density. A fall in the last year was reported by 20% (99,090/501585) of participants, and 10% (47,462/498700) reported a fracture in the last five years. Of fractures in the last five years, more than half (27,826 or 6% of total participants) were due to a simple fall. The mean and standard deviations of the Townsend deprivation index of the participants were -1.29 ± 3.09 and the population was skewed towards negative scores consistent with less deprivation than the overall UK population that has a mean score of 0 (Fig. 1 ).
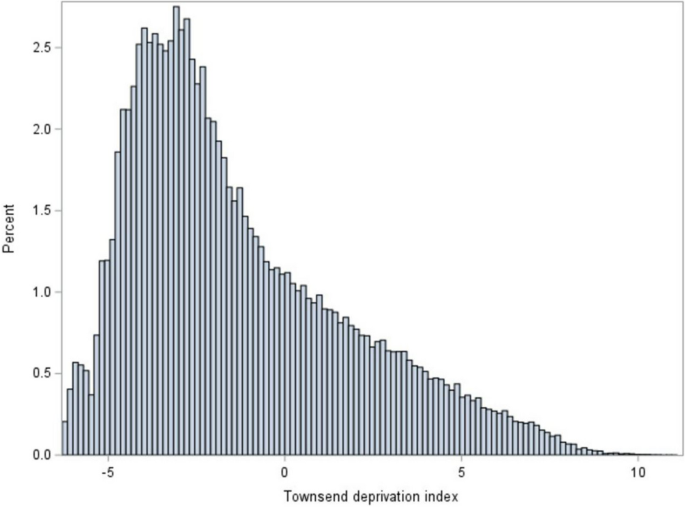
Histogram of the Townsend deprivation index of the 501,740 Biobank cohort participants at recruitment
The distribution of bone health outcomes by each explanatory variable that describe the participant was examined (Supplementary information 1 for more details). There was very little difference in the proportion with low bone density across the deprivation categories. Compared with participants without low bone density, those with low bone density had a similar distribution of deprivation status of their area of residence, were more frequently female (66% versus 50%), aged 55 years or older (age 55–64: 47% versus 41%; age ≥ 65: 22% versus 17%), had average (37% versus 29%) or underweight (1% versus 0.5%) BMI categories, and the lowest frequency of vigorous physical activity (< 2 days/week: 60% versus 53%).
Compared with participants who had not fallen in the last year, those who had fallen were more frequently a resident in the most deprived area category (24% versus 19%), female (63% versus 52%), obese (30% versus 23%), and had the lowest frequency of physical activity (< 2 days/week: 58% versus 53%). Compared with participants who had a fracture in the last 5 years, those without a fracture were more frequently a resident of the most deprived area category (23% versus 20%), and female (59% versus 54%). In addition, fractures from simple falls in the last 5 years were more frequent in participants from the most deprived areas compared to people who had not had a fracture from a simple fall in the last 5 years (23% versus 20%).
Deprivation and bone health outcomes
Unadjusted and adjusted associations between deprivation and all four bone health outcomes were shown in Table 1 . After adjusting for other covariates, the odds of low bone density in the most deprived group were 1.16 times (95% CI 1.13–1.19) higher than the least deprived group. The other covariates independently associated with higher odds of low bone density were older age (compared to < 45 years), female sex (compared to male), White ethnicity (compared to non-White), underweight BMI (compared to average BMI), ever smoking (compared to never) and having less than 2 days of vigorous physical activity (compared to more than 4 days). More details can be found in SI 4 .
After adjusting for other covariates, being in the most deprived quantile was associated with 46% (AOR = 1.46 95% CI 1.42–1.49, p < 0.0001) higher odds of a fall in the last year compared to the least deprived quantile. Other factors that were independently associated with falls in the last year are shown in Table 2 .
Fractures in the last five years and fractures from the simple fall
The adjusted odds of fractures in the last five years were 26% (95% CI 22%-30%) higher for people who were in the most deprived quantile than for the least deprived (Table 1 ). Other associated factors that were related to fractures in the last five years were shown in Table 3 .
Similarly, the odds of fracture from a simple fall were 31% higher (95% CI 26%-36%) in the most deprived, compared to the least deprived, group (Table 1 ). For other risk factors, a dose–response relationship was observed between age and fracture from simple fall where odds of fracture were 89% higher than those in age group 65 and more (aOR 1.89; 95% CI 1.79–2.00), 60% higher among age group 55–64 years (aOR 1.61; 95% CI 1.53–1.70), and 12% higher in the age group 45–54 years (aOR 1.12; 95% CI 1.06–1.18) compared to those aged under 45 years. However, little or no evidence was found for the association between fracture in the last five years and age group 55–64 years (aOR 1.00; 95% CI 0.96–1.03) and 65 years or more (aOR 1.01; 95% CI 0.98–1.05). Additionally, females were almost twice as likely to have higher odds of sustaining a fracture from a simple fall compared to males (aOR 2.06; 95% CI 2.00–2.12; p < 0.0001) and low BMI was associated with higher odds of sustaining a fracture from a simple fall, the underweight individuals having the highest odds (OR 1.38; 95% CI 1.24–1.53) compared to the participants with average BMI. Moreover, 2–4 days of moderate or vigorous physical activity was more likely to be protective than more than 4 days (aOR 0.94; 95% CI 0.91–0.97 and aOR 0.86; 95% CI 0.83–0.90, respectively). Details can be found in SI 5 .
The p-values from the logistic regression trend test for deprivation against all four bone health outcomes were < 0.0001. All outcomes show robust evidence for the presence of positive linear trends between deprivation and poor bone health (Figs. 2 , 3 and SI 2 , 3 ).
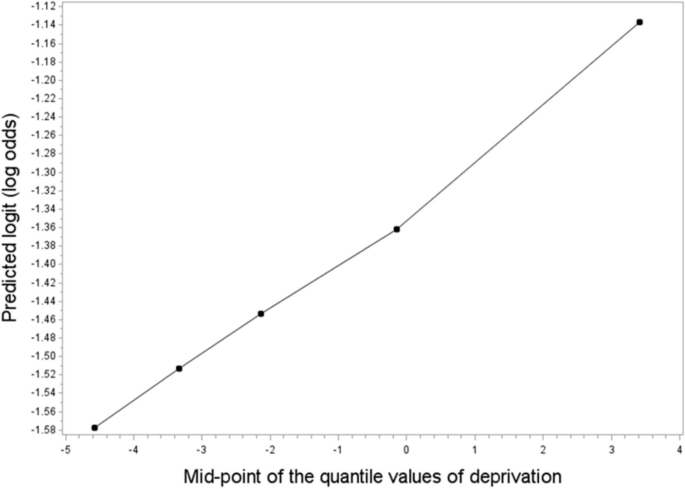
Scatter plot of the test for linear trend between the logarithm of the odds of fall in the last year and deprivation (unadjusted estimate)
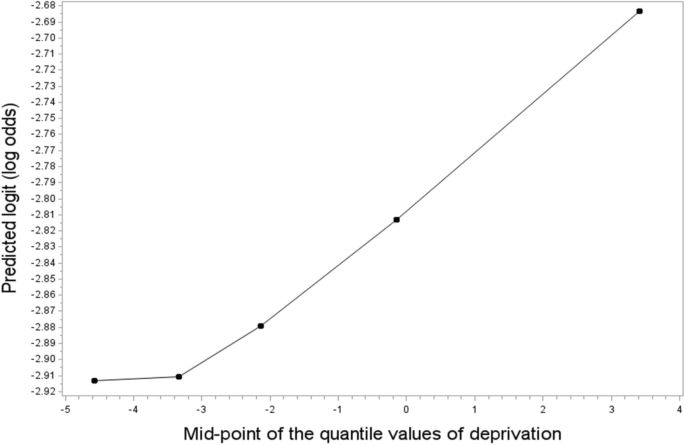
Scatter plot of the test for linear trend between the logarithm of the odds of fracture from a simple fall in the last five years and deprivation (unadjusted estimate)
This study examined the relationship between socioeconomic deprivation and four measures of bone health status including low bone mineral density, falls, and fractures, among the UK Biobank participants. We used the Townsend deprivation index as a proxy measure of deprivation. The participant population was skewed towards lower deprivation compared with the national population mean. We found that the odds of falls, fracture from a simple fall, and low bone mineral density were independently and positively associated with greater socioeconomic deprivation. The presence of a positive trend towards improving bone health with declining deprivation suggests a dose–response relationship.
The main strength of our study was the large sample size. To our knowledge, this is the first study to investigate the relationship between low bone mineral density, falls, and deprivation in such a large population in the UK. Additionally, we used Townsend deprivation index which is a very useful and validated tool [ 11 ] to measure socioeconomic deprivation across all over the UK.
Our result was consistent with a recent UK prospective study of 333 hospital patients with radiographically confirmed fractures of the distal radius that identified a positive ecological relationship between deprivation, measured by the index of multiple deprivation, and risk of fall [ 29 ]. However, while that study found no association between very low bone density and deprivation, the current study found a significant association. Differences in the methodological approach used to define low bone density could possibly explain the different results; for example, Johnson and colleagues used the Fracture Risk Assessment Tool (FRAX) whereas we used measured bone density T-score to define low bone density. Our finding also aligned with the findings of a systematic review on SES and bone mineral density in adults conducted by Brennan el al (2011). In the review, there was an increased risk of low bone mineral density found after exposure to measures related to SES including low educational levels. However, they reported that limited evidence exists for other parameters of SES such as income and unemployment in both genders [ 21 ]. In that context, our study has added significant value to address the notable research gap as the Townsend deprivation index includes unemployment status in both male and female. Also, ownership of car and housing information have played an important proxy measure for household earning and capital. Our results also supported the findings from a Canadian population-based study of more than 1.2 million subjects where lower wage earners had nearly double the risk of osteoporotic fracture risk compared to higher wage earners [ 30 ]. A similar result was found in the Geelong Osteoporosis study in Australia, where lower SES was associated with increased fracture incidence compared to higher SES [ 31 ].
Our study also confirmed that the risk of falls and low bone density rises with increasing age, female sex, and White ethnicity. This is consistent with the findings from other studies [ 4 , 30 ]. Increasing age is related to low bone density and osteoporosis which causes muscle weakness, spinal deformities or reduced postural control leading to higher risk of falls [ 32 ]. This is especially evident in women with osteoporosis after menopause due to estrogen decline [ 32 ]. Ethnicity or race have also previously been associated with falls and fractures [ 4 , 33 ]. In the UK, the fracture rate was higher in White populations, where the risk of fragility fracture was 4.7 times higher in White than in Black women aged 18 years and over [ 33 ]. Smoking is also a recognised risk factor where non-smokers and previous smokers were less likely to have a fracture than current smokers [ 4 , 34 ].
We found that more frequent alcohol consumption was associated with improved bone health. This result is unsurprising because alcohol stimulates calcitonin secretion, which causes the new bone formation and resorption of bone [ 35 , 36 ]. By contrast, Qiao et al. (2020) conducted a cross-sectional study with meta-analysis of 8475 participants (18–79 years) in China using data from the Henan Rural Cohort Study which found that moderate/heavy drinking in women increased the risk of osteoporosis, but no evidence was found for osteopenia either in women or men [ 8 ]. One reason for this conflicting result might be that most of the participants in our study were moderate alcohol drinkers, which might have a protective role. Another reason could be the age range of participants; the Biobank study recruited middle and older age groups, while the Chinese study included participants aged 18–79 years. Further study of the role of alcohol in bone health is required.
BMI is an important risk factor where underweight individuals are identified as being at higher risk of developing osteopenia or osteoporosis whereas overweight or obesity is protective compared to those of average weight [ 8 ]. The relationship between obesity and bone mineral density is quite complex and multifactorial that includes mechanical, hormonal and inflammatory factors [ 37 ]. One of the plausible explanations is increased mechanical loading and muscle strains are associated with increased body mass which directly affects bone geometry and modelling. Other factors include oestrogen, adipocytokines (leptin, adiponectin, resistin), ghrelin and cytokines (IL-6 and TNF-α). These metabolic factors are associated with maintaining skeletal homeostasis either by stimulating osteoblast formation or inhibiting osteoclastic activity except IL6 and TNF-α which promote osteoclastogenesis [ 37 ]. On the other hand, obesity has also been associated with the risk of fracture [ 36 ]. Consistent with this result, we found that despite higher BMI was related to lower odds of osteopenia, it also contributed to the increased odds of fracture compared to average weight. Also, obese participants had around twice the odds of a fall than those with an average BMI in our study. Similar result was found in a study involving 5681 community-dwelling individuals aged 65 years or over where the risk of falling was higher in people with obesity compared to those in the normal BMI category [ 38 ]. We also found that underweight individuals had nearly twice the risk of a fall than people with average BMI. This finding is also consistent with other study where low BMI or underweight was associated with increased falls risk when compared to normal weight individuals [ 39 ]. BMI abnormalities, including both high and low BMI in the elderly, correlate with other comorbidities, such as arthritis, diabetes, stroke, high blood pressure, and particular medication, eventually leading to falls and injurious falls [ 38 , 40 ]. Another probable reason might be that low BMI is related to low bone mass, which causes osteoporosis to develop and results in falls and fractures. In contrast, other studies have reported no association between underweight and risk of falls and fracture [ 41 , 42 ]. The inconsistency might be the omission of SES and physical activity level in their study. Mitchell et al. (2014) attributed to lack of physical activity, chronic diseases like diabetes, hypertension, osteoporosis, anxiety, depression, and some medications such as sleeping pills, tranquillisers, and anti-depressants as factors responsible for falls among older obese adults [ 38 ]. A meta-analysis of 22 prospective cohort studies reported an inverse association between physical activity and fracture risk [ 43 ], finding that physical activity was protective of and contributed to increase in skeletal muscle and neuromuscular function, hence, decreased fracture risk. Our study also supported with the finding that frequent physical activity decreased the odds of falls and fractures. However, when compared to more than 4 days, 2–4 days per week of moderate to vigorous physical activity (10 min or more) was protective against odds of fall and fracture from a simple fall. A possible explanation is that with more frequent occasions of physical exercise comes a higher risk of injury or falls.
There were several limitations to our study. First, a ‘healthy volunteer’ selection bias exists in this cohort. Assessing the representativeness of UK Biobank participants, Fry et al. (2017) found them to be generally healthier, less socially deprived, and to have a lower prevalence of obesity, smoking, and daily alcohol consumption than the general population. Nonetheless, the authors suggested that due to its large and heterogeneous sample the cohort is suitable for studying associations between exposures and outcomes but is not appropriate for population-based estimates of disease prevalence [ 44 ]. On the other hand, a study using the UK Biobank found a similar prevalence of musculoskeletal pain among the participants to that reported in population-based epidemiological studies in the UK [ 45 ]. Second, the available data did not include the volume of alcohol consumed by participants. However, 143,667 participants responded to the question regarding the amount of alcohol they consumed on a typical drinking day, which could be a proxy for the volume of alcohol consumed. Among those respondents, nearly 80% reported a maximum intake of 4 units of alcohol. From this response, we can assume that most drank alcohol moderately, which might have a protective role. Thirdly, in our study only bone related covariates were considered for the outcome fall in the last year. However, there are non-bone-related risk factors such as vertigo, Parkinson disease, antiepileptic drug use, visual impairment, and reduced physical functioning that could be considered in future studies.
Finally, in our study, we used the Townsend deprivation index as an ecological measure, rather than as an individual level measure, which may not reflect the individual’s situation [ 11 ]. According to Lee and colleagues, the area-level approach only helps to understand health inequalities in a relatively small number of people. Most families living in an underprivileged area were not underprivileged; only a minority of households were counted as ‘poor’ [ 11 ]. However, validation and reliability analyses were done to measure the validity and reliability of ten deprivation indices, including the Townsend deprivation index, one of the most reliable and valid area-based measures of deprivation [ 11 ]. According to Gordon [ 11 ], the census-based deprivation indices are extremely important because they provide key information and resource has been allocated according to that in both local government and health. Lee et al. [ 46 ] examined the reliability of 10 census-based deprivation indexes using classical test theory that comprises 1% sample of households (215,789 households) in Britain. In the analysis the correlation between the “True” Deprivation Score and the Index Score for the Townsend deprivation index was 0.65. They also conducted a validation test (Spearman’s Rank Correlation) using three validating variables including standardized illness ratio (illness), standardized mortality ratio (SMR 0–64), and the estimated average weekly earnings (mean earn) by ten deprivation indexes for the 10,500 electoral wards in Britain. They found that Spearman’s Rank Correlation Coefficient by Townsend deprivation index for SMR 0–64 was -0.71, standardized illness ratio was 0.76, and estimated average weekly earnings was 0.63. Based on these result Gordon concluded that the Townsend deprivation index is reasonably valid on all three criteria and reasonably reliable [ 11 ].
In conclusion, socioeconomic deprivation was significantly associated with poor bone health status. The research finding could be used to influence current public health guidelines to minimise social disparities in bone health. Investigating the causal pathway between deprivation and poor bone health to identify modifiable risk factors would be beneficial for the prevention of bone fragility and its consequences. A public health approach that targets disadvantaged groups such as promoting healthy lifestyle, a nutritious diet, tobacco control, reduced alcohol intake, regular physical activity, and bone health education including awareness-raising campaigns featuring peoples’ real-life experiences of consequences of poor bone health, could help to reduce the disability burden in the community.
Data availability
To obtain data used in this study, researchers would need to apply for access at the UK Biobank.
Morrison A, Fan T, Sen SS, Weisenfluh L (2013) Epidemiology of falls and osteoporotic fractures: a systematic review. Clin Econ Outcomes Res CEOR 5:9–18. https://doi.org/10.2147/CEOR.S38721
Article Google Scholar
The International Osteoporosis Foundation (2014) The global burden of osteoporosis: a factsheet. Available from: www.iofbonehealth.org/sites/default/files/media/PDFs/Fact%20Sheets/2014-factsheet-osteoporosis-A4.pdf . Accessed 3 Sept 2020
Kim T, Choi SD, Xiong S (2020) Epidemiology of fall and its socioeconomic risk factors in community-dwelling Korean elderly. PLoS ONE 15(6):e0234787. https://doi.org/10.1371/journal.pone.0234787
Article CAS PubMed PubMed Central Google Scholar
The World Health Organization, Ageing & Life Course Unit (2008) WHO global report on falls prevention in older age. Available from: https://www.who.int/ageing/publications/Falls_prevention7March.pdf . Accessed 2 Oct 2021
Reginster JY, Burlet N (2006) Osteoporosis: a still increasing prevalence. Bone 38(2 Suppl 1):S4-9. https://doi.org/10.1016/j.bone.2005.11.024
Article PubMed Google Scholar
Hernlund E, Svedbom A, Kanis JA et al (2013) Osteoporosis in the European Union: medical management, epidemiology and economic burden. Arch Osteoporos 8(1–2):136. https://doi.org/10.1007/s11657-013-0136-1
Leal J, Gray AM, Javaid MK et al (2016) Impact of hip fracture on hospital care costs: a population-based study. Osteoporos Int 27(2):549–558. https://doi.org/10.1007/s00198-015-3277-9
Article CAS PubMed Google Scholar
Qiao D, Liu X, Wang C et al (2020) Gender-specific prevalence and influencing factors of osteopenia and osteoporosis in Chinese rural population: the Henan Rural Cohort Study. BMJ Open 10:e028593. https://doi.org/10.1136/bmjopen-2018-028593
Article PubMed PubMed Central Google Scholar
ABS (2011) Measures of socioeconomic status. Australian Bureau of Statistics. Available from: https://www.abs.gov.au/ausstats/[email protected]/mf/1244.0.55.001 . Accessed 24 Sept 2019
Baker EH (2014) Socioeconomic status, definition. In: Cockerham WC, Dingwall R, Quah S (eds) The Wiley Blackwell encyclopedia of health, illness, behaviour, and society. https://doi.org/10.1002/9781118410868.wbehibs395
Gordon D (2003) Area-based deprivation measures: a UK perspective. In: Kawachi I, Berkman LF (eds) Neighbourhoods and health. Oxford University Press, pp 179–207
Barakat K, Stevenson S, Wilkinson P, Suliman A, Ranjadayalan K, Timmis AD (2001) Socioeconomic differentials in recurrent ischemia and mortality after acute myocardial infarction. Heart 85(4):390. https://doi.org/10.1136/heart.85.4.390
Litonjua AA, Carey VJ, Weiss ST, Gold DR (1999) Race, socioeconomic factors, and area of residence are associated with asthma prevalence. Paediatr Pulmonol 28(6):394–401. https://doi.org/10.1002/(sici)1099-0496(199912)28:6%3c394::aid-ppul2%3e3.0.co;2-6
Article CAS Google Scholar
Hole DJ, McArdle CS (2002) Impact of socioeconomic deprivation on outcome after surgery for colorectal cancer. Br J Surg 89(5):586–590. https://doi.org/10.1046/j.1365-2168.2002.02073.x
Evans JM, Newton RW, Ruta DA, MacDonald TM, Morris AD (2000) Socioeconomic status, obesity and prevalence of Type 1 and Type 2 diabetes mellitus. Diabet Med 17(6):478–480
Aitken SA, Duckworth AD, Clement N D, McQueen MM (2013) The relationship between social deprivation and the incidence of adult fractures. J Bone Joint Surge 95(6). https://doi.org/10.2106/JBJS.K.00631
Bhimjiyani A, Neuburger J, Jones T, Ben-Shlomo Y, Gregson CL (2018) Inequalities in hip fracture incidence are greatest in the North of England: regional analysis of the effects of social deprivation on hip fracture incidence across England. Public Health 162:25–31. https://doi.org/10.1016/j.puhe.2018.05.002
Quah C, Boulton C, Moran C (2011) The influence of socio-economic status on the incidence, outcome, and mortality of fractures of the hip. J Bone Joint Surg 93(6):801–805. https://doi.org/10.1302/0301-620X.93B6.24936
Holmberg T, Möller S, Rubin KH et al (2019) Socio-economic status and risk of osteoporotic fractures and the use of DXA scans: data from the Danish population-based ROSE study. Osteoporos Int 30(2):343–353. https://doi.org/10.1007/s00198-018-4768-2
Icks A, Haastert B, Rosenbauer J et al (2009) Hip fractures and area level socioeconomic conditions: a population-based study. BMC Public Health 9(1):114. https://doi.org/10.1186/1471-2458-9-114
Brennan SL, Pasco JA, Urquhart DM, Oldenburg B, Wang Y, Wluka AE (2011) Association between socioeconomic status and bone mineral density in adults: a systematic review. Osteoporos Int 22(2):517–527. https://doi.org/10.1007/s00198-010-1261-y
Temam S, Varraso R, Le Moual N et al (2017) Ability of ecological deprivation indices to measure social inequalities in a French cohort. BMC Public Health 17(1):956. https://doi.org/10.1186/s12889-017-4967-3
Yousaf S, Bonsall A (2017) UK Townsend deprivation scores from 2011 census data. Colchester, UK: UK Data Service
Allen N, Sudlow C, Pell J et al (2012) UK Biobank: Current status and what it means for epidemiology. Health Policy and Technology 1(3):123–126. https://doi.org/10.1016/j.hlpt.2012.07.003
The UK Biobank (2021) Available from: https://www.ukbiobank.ac.uk/explore-your-participation/basis-of-your-participation . Accessed 2 Oct 2021
Kanis JA (2002) Diagnosis of osteoporosis and assessment of fracture risk. The Lancet 359(9321):1929–1936. https://doi.org/10.1016/S0140-6736(02)08761-5
Townsend P (1987) Deprivation. J Soc Policy 16(2):125–146
Lin LY, Smeeth L, Langan S, Warren-Gash C (2021) Distribution of vitamin D status in the UK: a cross-sectional analysis of UK Biobank. BMJ Open 11(1):e038503. https://doi.org/10.1136/bmjopen-2020-038503
Johnson NA, Dias JJ (2019) The effect of social deprivation on fragility fracture of the distal radius. Injury 50(6):1232–1236. https://doi.org/10.1016/j.injury.2019.04.025
Brennan SL, Yan L, Lix LM, Morin SN, Majumdar SR, Leslie WD (2015) Sex- and age-specific associations between income and incident major osteoporotic fractures in Canadian men and women: a population-based analysis. Osteoporos Int 26:59–65. https://doi.org/10.1007/s00198-014-2914-z
Brennan SL, Holloway KL, Pasco JA et al (2015) The social gradient of fractures at any skeletal site in men and women: data from the Geelong Osteoporosis Study Fracture Grid. Osteoporos Int 26(4):1351–1359. https://doi.org/10.1007/s00198-014-3004-y
Meyer F, Konig HH, Hajek A (2019) Osteoporosis, fear of falling, and restrictions in daily living. Evidence from a nationally representative sample of community-dwelling older adults. Front Endocrinol (Lausanne) 10:646. https://doi.org/10.3389/fendo.2019.00646
Curtis EM, van der Velde R, Harvey NC et al (2016) Epidemiology of fractures in the United Kingdom 1988–2012: variation with age, sex, geography, ethnicity and socioeconomic status. Bone 87:19–26. https://doi.org/10.1016/j.bone.2016.03.006
Kanis JA, Johnell O, Tenenhouse A et al (2005) Smoking and fracture risk: a meta-analysis. Osteoporos Int 16(2):155–162. https://doi.org/10.1007/s00198-004-1640-3
Jugdaohsingh R, O’Connell MA, Sripanyakorn S, Powell JJ (2006) Moderate alcohol consumption and increased bone mineral density: potential ethanol and non-ethanol mechanisms. Proc Nutr Soc 65(3):291–310. https://doi.org/10.1079/pns2006508
Fini M, Salamanna F, Giavaresi G et al (2012) Role of obesity, alcohol and smoking on bone health. Front Biosci (Elite Ed) 4:2586–2606. https://doi.org/10.2741/e575
Gkastaris K, Goulis DG, Potoupnis M, Anastasilakis AD, Kapetanos G (2020) Obesity, osteoporosis and bone metabolism. J Musculoskelet Neuronal Interact 20(3):372–381
CAS PubMed PubMed Central Google Scholar
Mitchell RJ, Lord SR, Harvey LA, Close JC (2014) Associations between obesity and overweight and fall risk, health status and quality of life in older people. Aust N Z J Public Health 38(1):13–18. https://doi.org/10.1111/1753-6405.12152
Ogliari G, Ryg J, Andersen-Ranberg K, Scheel-Hincke LL, Masud T (2021) Association between body mass index and falls in community-dwelling men and women: a prospective, multinational study in the Survey of Health, Ageing and Retirement in Europe (SHARE). Eur Geriatr Med 837–849. https://doi.org/10.1007/s41999-021-00485-5 .
Ylitalo KR, Karvonen-Gutierrez CA (2016) Body mass index, falls, and injurious falls among U.S.adults: Findings from the 2014 Behavioural Risk Factor Surveillance System. Prev Med 91:217–223. https://doi.org/10.1016/j.ypmed.2016.08.044
Xiang BY, Huang W, Zhou GQ, Hu N, Chen H, Chen C (2017) Body mass index and the risk of low bone mass–related fractures in women compared with men: A PRISMA-compliant meta-analysis of prospective cohort studies. Medicine 96(12):e5290. https://doi.org/10.1097/MD.0000000000005290
Himes CL, Reynolds SL (2012) Effect of obesity on falls, injury, and disability. J Am Geriatr Soc 60(1):124–129. https://doi.org/10.1111/j.1532-5415.2011.03767.x
Qu X, Zhang X, Dai K et al (2014) Association between physical activity and risk of fracture. J Bone Miner Res 29(1):202–211. https://doi.org/10.1002/jbmr.2019
Fry A, Littlejohns TJ, Allen NE (2017) Comparison of socio-demographic and health-related characteristics of UK Biobank participants with those of the general population. Am J Epidemiol 186(9):1026–1034. https://doi.org/10.1093/aje/kwx246
Macfarlane GJ, Beasley M, Smith BH, Jones GT, Macfarlane TV (2015) Can large surveys conducted on highly selected populations provide valid information on the epidemiology of common health conditions? An analysis of UK Biobank data on musculoskeletal pain. Br J Pain 9(4):203–212. https://doi.org/10.1177/2049463715569806
Lee P, Murie A, Gordon D (1995) Area measures of deprivation: a study of current methods and best practices in the identification of poor areas in Great Britain: University of Birmingham, Centre for Urban and Regional Studies
Download references
Acknowledgements
This research has been conducted using the UK Biobank Resource under Application Number 15700. The authors would like to thank all participants and staff of the UK Biobank for their support. The authors also extend their gratitude to Dr. Mark Cherrie for his invaluable contribution to the study. Mafruha Mahmud was supported by an Australian Government Research Training Program (RTP) Scholarship. David Muscatello was supported by an NHMRC Investigator Grant (APP1194109). The contents of the published material are solely the responsibility of the Administering Institution, a Participating Institution or individual authors and do not reflect the views of the NHMRC.
Open Access funding enabled and organized by CAUL and its Member Institutions. Funding was obtained from The MED MI Platform (Medical and Environmental Data—a Mashup Infrastructure) MR/K019341/1 is funded in part by the UK Medical Research Council (MRC) and the UK Natural Environment Research Council (NERC) and by the European Regional Development Fund Programme 2007 to 2013 and European Social Fund Convergence Programme for Cornwall and the Isles of Scilly to the University of Exeter Medical School.
Author information
Authors and affiliations.
School of Population Health, University of New South Wales, Sydney, Australia
Mafruha Mahmud, David John Muscatello & Nicholas John Osborne
Australian Institute of Health Innovation, Macquarie University, Sydney, Australia
Md Bayzidur Rahman
School of Public Health, The University of Queensland, Herston, QLD, 4006, Australia
Nicholas John Osborne
European Centre for Environment and Human Health, University of Exeter, Truro, TR1 3HD, UK
Kirby Institute, UNSW, Kensington, Australia
The School of Medicine, The University of Notre Dame, Sydney, Australia
You can also search for this author in PubMed Google Scholar
Corresponding author
Correspondence to Mafruha Mahmud .
Ethics declarations
Ethical approval.
“All procedures performed in studies involving human participants were in accordance with the ethical standards of the institutional and/or national research committee and with the 1964 Helsinki declaration and its later amendments or comparable ethical standards.”
Approval was obtained from the UNSW Human Research Ethics Committee (Approval number HC17854) and the UK Biobank (Application number 15700).
Informed consent
Informed consent was obtained by the UK Biobank from all individual participants included in the study. Details can be found in the following website: https://www.ukbiobank.ac.uk/media/05ldg1ez/consent-form-uk-biobank.pdf
Conflicts of interest/Competing interests
Mafruha Mahmud, David John Muscatello, Bayzidur Rahman, and Nicholas J. Osborne declare that they have no conflict of interest.
Additional information
Publisher's note.
Springer Nature remains neutral with regard to jurisdictional claims in published maps and institutional affiliations.
Supplementary Information
Below is the link to the electronic supplementary material.
Supplementary file1 (DOCX 826 KB)
Rights and permissions.
Open Access This article is licensed under a Creative Commons Attribution-NonCommercial 4.0 International License, which permits any non-commercial use, sharing, adaptation, distribution and reproduction in any medium or format, as long as you give appropriate credit to the original author(s) and the source, provide a link to the Creative Commons licence, and indicate if changes were made. The images or other third party material in this article are included in the article's Creative Commons licence, unless indicated otherwise in a credit line to the material. If material is not included in the article's Creative Commons licence and your intended use is not permitted by statutory regulation or exceeds the permitted use, you will need to obtain permission directly from the copyright holder. To view a copy of this licence, visit http://creativecommons.org/licenses/by-nc/4.0/ .
Reprints and permissions
About this article
Mahmud, M., Muscatello, D.J., Rahman, M.B. et al. Association between socioeconomic deprivation and bone health status in the UK biobank cohort participants. Osteoporos Int (2024). https://doi.org/10.1007/s00198-024-07115-3
Download citation
Received : 27 September 2023
Accepted : 27 April 2024
Published : 28 May 2024
DOI : https://doi.org/10.1007/s00198-024-07115-3
Share this article
Anyone you share the following link with will be able to read this content:
Sorry, a shareable link is not currently available for this article.
Provided by the Springer Nature SharedIt content-sharing initiative
- Deprivation
- Low bone mineral density
- Find a journal
- Publish with us
- Track your research
Combining biomedical data from breast cancer patients could lead to ‘groundbreaking discoveries’ in fight against disease
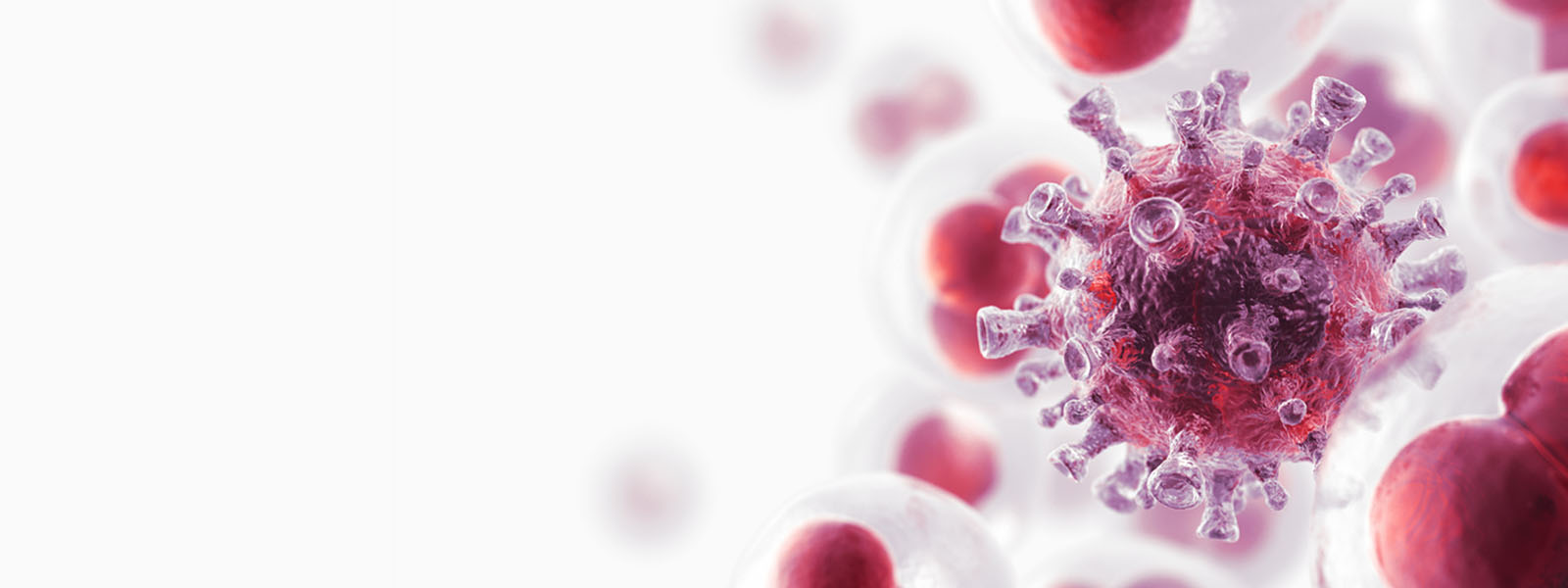
Human plasma proteomic profiles indicative of cardiorespiratory fitness
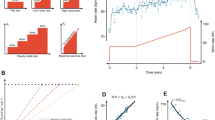
Cardiorespiratory fitness assessment using risk-stratified exercise testing and dose–response relationships with disease outcomes
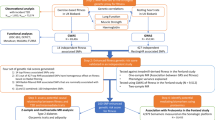
Causal associations between cardiorespiratory fitness and type 2 diabetes
CRF is a powerful prognostic marker linked to greater health, quality of life and longevity across the life course 1 , 2 , 3 , 4 , 5 , 6 . Measuring CRF is an important component of clinical care in several disease conditions 3 , 7 and is often considered an essential health metric on par with clinical vital signs 6 . Nevertheless, widespread clinical assessment of CRF for risk stratification and health promotion has been limited by test availability, cost and factors (for example, musculoskeletal) that may limit the ability to perform maximum effort exercise. An alternative approach—easily accessible, training-responsive biomarkers of CRF—may address these limitations and enable discovery of pharmacological targets that mimic effects of exercise. Exercise is accompanied by widespread changes in the human metabolic state, spanning pathways of tissue regeneration and fibrosis, muscle structure, mitochondrial dysfunction, insulin resistance and inflammation 8 , 9 , 10 , 11 , 12 . While molecular surrogates of CRF and training responses are associated with clinical prognosis 8 , 10 , 13 , most studies have been across a single population with limited follow-up and outcomes and have demonstrated effect sizes that are not significantly additive over standard risk factors.
Here, we performed an international population-based study of 14,145 individuals with CRF measures spanning four different population-based observational cohorts (the Coronary Artery Risk Development in Young Adults (CARDIA) study; the Fenland Study; the Baltimore Longitudinal Study of Aging (BLSA); and the Health, Risk Factors, Exercise Training and Genetics (HERITAGE) family sutdy) with diverse modes of CRF assessment to define and validate a proteomic signature of CRF. Leveraging data from around 22,000 participants from the UK Biobank (UKB), we tested the association of a proteomic signature of CRF with a broad array of clinical outcomes (death, cardiovascular, metabolic, malignancy, neurological) and examined the interaction with polygenic risk. In HERITAGE, we evaluated whether a 20-week exercise training program modified a proteomic signature of CRF. To our knowledge, this study provides the largest, most comprehensive human population-based proteomic study of CRF, demonstrating its broad functional and clinical relevance to human disease with a path for clinical translation.
Characteristics of study samples
Our initial sample to establish relations of the circulating proteome with CRF included participants from CARDIA. The CARDIA sample consisted of 2,238 individuals with a median age 51 years (56% female, 43% Black; Table 1 ). CARDIA participants were generally overweight (median body mass index (BMI) 29 kg m −2 ) with a modest prevalence of diabetes (14%) and treated hypertension (26%). We did not observe any important differences between our CARDIA derivation (70%) and validation (30%) subsets (split randomly, balanced on exercise treadmill test (ETT) time). We validated our findings in three external cohorts: Fenland 14 ; BLSA 15 ; and HERITAGE 10 . These cohorts spanned early to older adulthood with a wide range of BMI and comorbidity (Supplementary Table 1a ). A subsample of the UKB ( N = 21,988; median age 58 years, 54% female, 93% white; Supplementary Table 1b ) with available proteomics was used to test the association of the CRF proteome with a broad array of outcomes. The method of CRF assessment differed across cohorts ( Methods ), which—in conjunction with cohort-specific differences (for example, age)—contributed to differences in CRF distributions.
Development of a proteomic CRF score
We sought to develop an integrative score of CRF to leverage the multiorgan and diverse drivers of CRF. Using penalized regression (least absolute shrinkage and selection operator (LASSO)) across the assayed proteome, we developed a proteomic CRF score in the CARDIA derivation subset, using ETT time as the CRF measure, and validated it across approximately 12,500 participants across four samples (Fig. 1 ). We achieved a >95% reduction in proteomic space (272 aptamers selected from 7,230 candidates) with good calibration in both the CARDIA derivation (Spearmanʼs ρ = 0.79) and validation subsets (Spearmanʼs ρ = 0.67; Fig. 2 ), comparable with previously published metabolomic 13 or proteomic instruments 16 . We observed mechanistically plausible directionality for many of the proteins of the highest effect sizes (Table 2 ), including proteins implicated in innate immunity and inflammation (C5a 17 , 18 ), atherosclerosis (AGER 19 , RGMB 19 ), neuronal survival and growth (CDNF 20 , LSAMP 21 ), cell physiology (TNR—migration, adhesion, differentiation; DUSP13—differentiation, proliferation), oxidative stress (MRM1 22 ), energy expenditure and substrate fuel utilization (OLFM2 23 , FABP4 24 , FABP3 25 , HNF4A 26 , GLYATL2), adiposity (LEP, CA6 27 ), peripheral muscle responses to exercise (MB 28 , ATF6 29 ) and autophagy (GLIPR2 30 ).
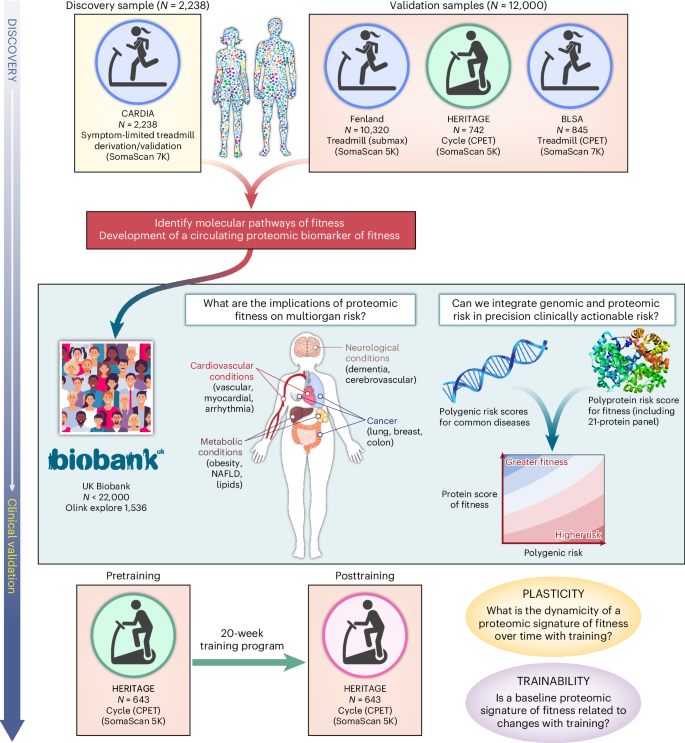
We developed and validated a circulating proteomic signature of CRF across four cohorts and various exercise modalities. In the UKB, we examined the relationship a proteomic CRF signature with a broad range of clinical endpoints and examined its interaction with polygenic risk. In HERITAGE, we examined the association of the proteomic CRF signature with response to exercise training and correlated changes in signature with changes in CRF. NAFLD, nonalcoholic fatty liver disease.
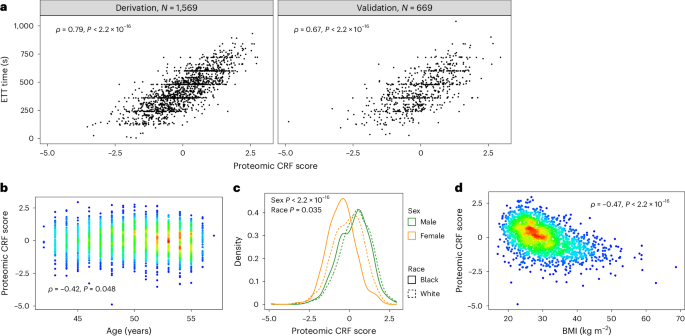
a , Correlations between the proteomic CRF score and CRF (defined by ETT time) in CARDIA across derivation (left) and validation (right) samples. b – d , Correlations of the proteomic CRF score with age ( b ), sex and race ( c ) and BMI ( d ). Colors on scatter plots represent density of overlapping observations, with red being the most dense and blue the least dense. P values in a , b and d are from Spearman rank correlation tests. P values in c are from linear regression modeling of the proteomic CRF score as a function of sex and race. All P values are from two-sided tests.
After recalibration to shared proteins across each of our validation samples (Fenland, HERITAGE, BLSA; Supplementary Tables 3 – 5 and Methods ), we observed differences in fit against measured CRF, most likely owing to heterogeneity in methods for assessment of CRF (Extended Data Fig. 1 ). The best validation fits were observed in HERITAGE ( ρ = 0.71) and BLSA ( ρ = 0.68), where CRF was assessed by symptom-limited peak exercise testing with directly measured gas exchange (peak VO 2 ). The weakest validation fit was observed in Fenland ( ρ = 0.35), where CRF was estimated from heartrate response to submaximal exercise with extrapolation to age-predicted maximal heartrate. We observed consistent differences in the proteomic CRF score by sex (men higher) and inverse associations with age and BMI (Extended Data Figs. 1 and 2 ), consistent with the general epidemiology of CRF 14 .
Relations of a proteomic CRF score with clinical outcomes
Given the multicohort replication of the proteomic CRF score and its biological plausibility, we next sought to test its clinical relevance. We identified a sample of 21,988 UKB participants with proteomic data (Olink Explore 1536) and with survival data for a wide array of outcomes (Supplementary Table 1b ). Over a median follow-up of 13.7 years (25th–75th percentile, 13.0–14.5 years), 2,394 deaths occurred (other outcomes reported in Supplementary Table 7 ). Per each 1 s.d. higher CRF proteome score, we observed a near 50% lower hazard of all-cause mortality (hazard ratio (HR) = 0.53, 95% confidence interval (CI) 0.50–0.56; P < 0.0001) and cause-specific mortality (Fig. 3a ; all HRs and 95% CIs in Supplementary Table 7 ), robust to adjustment for standard clinical risk factors and bioimpedance-based measured fat mass. In addition to censoring at other causes of death for models for cause-specific mortality, we observed similar results using Fine–Gray competing risk models (Supplementary Table 8 ). Strikingly, we observed a consistent and strong protective association of a greater proteomic CRF score for cardiovascular, metabolic and neurological outcomes (but not with most cancers). Moreover, the proteomic CRF score improved risk prediction beyond standard risk factors, with improved discrimination and reclassification across nearly every endpoint (for example, all-cause mortality: C -index 0.75 to 0.77, P < 0.001; cardiovascular mortality: C -index 0.79 to 0.82, P < 0.001; Fig. 3a ). Reclassification was substantial, with a near 30–40% net reclassification beyond clinical risk factors for most conditions across several systems.
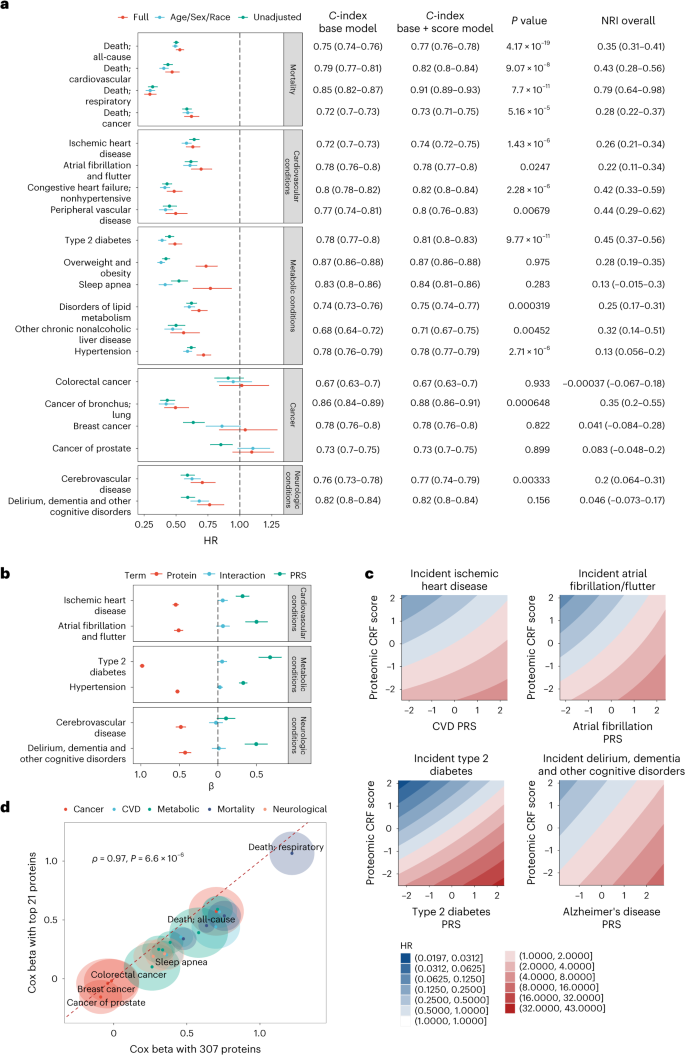
a , Forest plot of Cox model results with proteomic score as the main predictor, grouped by outcome category. The ‘full’ adjustment model includes adjustment for age, sex, race, BMI, systolic blood pressure, diabetes, Townsend deprivation index, smoking, alcohol and LDL. Error bars, 95% CI. The adjoining table reports the C -index for Cox models without proteomic score (Base) and with the score (Score). Base models include age, sex, race, BMI, systolic blood pressure, diabetes, Townsend deprivation index, smoking, alcohol and LDL. Reported P value is from comparison testing of C-indices by z distribution (two-sided) without correct for multiple comparison. b , Cox beta coefficients from models including an interaction between the protein score of CRF and PRSs of the indicated conditions or diseases. Error bars, 95% CI. c , Contour map of the model predicted HR across the range of protein score of fitness and PRSs. The referent hazard was set at the median of the protein score and median of the PRS. Values reported and visualized are from point estimates and 95% CI. d , Comparison of Cox model coefficients from a parsimonious 21-protein panel and the full 307-protein panel. The halo represents the 95% CI around the model coefficient. P value is from two-sided Spearman rank correlation test. For visualization, we reversed the sign of the beta coefficients. Full data on sample sizes, model estimates and results of statistical testing may be found in Supplementary Tables 7 and 13 .
To evaluate whether the strong associations with clinical outcomes were confounded by proteomic markers of disease in the CARDIA cohort from which the proteomic CRF score was derived, we conducted a sensitivity analysis by deriving the proteomic CRF from a subset of the CARDIA study cohort that excluded participants with a history of cardiovascular disease (CVD—myocardial infarction, stroke, heart failure, carotid artery disease, peripheral artery disease), diabetes and hypertension. This proteomic CRF score was then translated for use in the UKB in the same manner, and we observed directionally consistent results as our primary analysis with slightly decreased effect sizes (Supplementary Tables 9 – 12 ).
Integration of a proteomic CRF score and polygenic risk
Previous reports have highlighted the complementary impact of polygenic risk and lifestyle in human disease 31 , 32 , 33 , 34 . Given the centrality of CRF as an integrative measure of human health, we next explored interaction between the proteomic CRF score and polygenic risk of common diseases (Fig. 3b and Supplementary Table 13 ). We constructed models for six conditions with established polygenic risk scores (PRS) within the UKB, as a function of the proteomic CRF score, a corresponding PRS and their multiplicative interaction with adjustments for age, sex, race and four principal components of genetic ancestry. While several PRS-by-proteomic CRF score interactions reached weak statistical significance (including CVD and type 2 diabetes), the effect sizes were marginal. Overall, we observed a substantial and additive effect between the proteomic CRF score and each PRS on the corresponding disease outcome, with highest hazards of disease observed among those participants with the lowest proteomic CRF score (corresponding to poor CRF) and high genetic risk (Fig. 3c ). For most conditions, the standardized estimates for the proteomic CRF score were on the order of (or higher than) those for PRS (for example, diabetes: HR proteome = 0.37, 95% CI 0.35–0.40; HR PRS = 1.97, 95% CI 1.83–2.12).
Association of a parsimonious proteomic CRF score with clinical risk
Even with regularization in regression, one main limitation in most multivariable proteomic approaches is the lack of sufficient reduction in molecular dimension to permit clinical translation 16 (for example, 307 proteins in our recalibrated proteomic CRF score used in UKB). To address the feasibility of clinical translation, we constructed an ‘abbreviated’ score including coefficients from the top 21 most important proteins (ranked by absolute value of the LASSO beta coefficient). We selected 21 proteins since Olink currently offers 21-plex absolute quantification panels. In CARDIA, this abbreviated 21-protein score was correlated with CRF ( ρ = 0.71). In UKB, we observed consistent effect sizes for nearly all outcomes between the recalibrated proteomic CRF score (307 proteins) and the abbreviated 21-protein score, albeit with generally slightly lower effect sizes for the abbreviated CRF score (Fig. 3d and Supplementary Table 7 ). These results support plausibility of translation of these results as a biomarker panel of CRF that can be measured at the scale necessary to offer clinical utility.
Dynamicity of the proteomic CRF score with training
To leverage the human proteome for CRF assessment, it is critical to evaluate its potential for modification through intervention. After a 20-week exercise training program in HERITAGE 35 , we observed an increase in the recalibrated (nonabbreviated) proteomic CRF score (paired t -test, 0.14; 95% CI, 0.11–0.18; P = 2.5 × 10 −15 ), which was correlated with a change in peak VO 2 (Extended Data Fig. 3 ). In regression modeling, we found that a change in the recalibrated proteomic CRF score was associated with a change in peak VO 2 (1 s.d. increase in recalibrated proteomic CRF score ≈ 0.84 ± 0.25 ml kg −1 min −1 increase in peak VO 2 ; P = 8.5 × 10 −4 ), independent of age, sex, race, BMI, pretraining peak VO 2 and pretraining recalibrated proteomic CRF score. There were no differences in the response to changes in the proteomic CRF score with training by sex ( P = 0.62). Additionally, we examined whether the pretraining proteomic CRF score was associated with the VO 2 response to training, and observed that a higher recalibrated proteomic CRF score was associated with a greater increase in peak VO 2 with training, independent of age, sex and race (0.59 ± 0.17 ml kg −1 min −1 increase per 1 s.d. increase in recalibrated proteomic CRF score; P = 6.4 × 10 −4 ), with mitigation of the association when further adjusted for BMI (0.30 ± 0.17 ml kg −1 min −1 increase per 1 s.d. increase in recalibrated proteomic CRF score; P = 0.08). Constituents of the proteomic CRF score that exhibited significant changes with 20-week training in HERITAGE 36 were correlated with an array of metabolic, vascular and myocardial phenotypes in CARDIA (Fig. 4 and Supplementary Table 14 ). Several of these proteins exhibit clinical and molecular plausibility, with reduction in adiposity (LEP), lipid metabolism (RARRES2), regulation of bone morphogenic protein pathways (RGMB) and mitigation of ischemia-reperfusion injury (CDNF 37 ) among others. Many were not related to cardiometabolic phenotypes in CARDIA, suggesting potential new mechanisms of benefit.
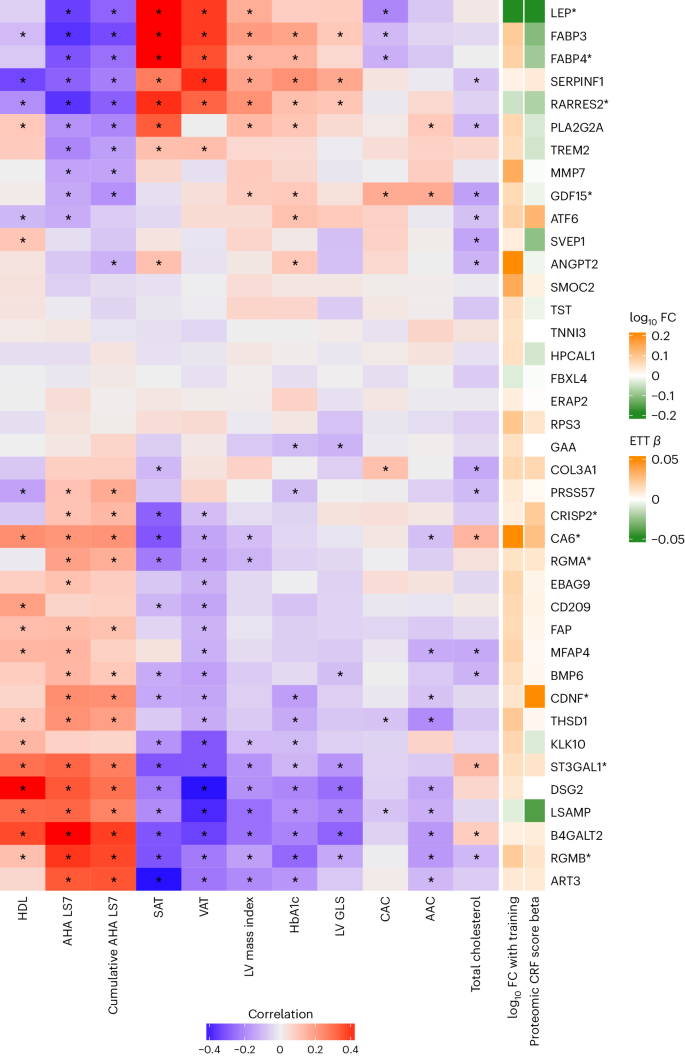
Heatmap of Pearson correlations between individual proteins and cardiometabolic risk factors and disease in CARDIA using the CARDIA validation sample ( N = 589–669). Proteins visualized are included in the proteomic CRF score and change after a 20-week exercise intervention in HERITAGE (false discovery rate < 5%). Proteins marked with an asterisk are included in the abbreviated 21-protein score. Cells marked with an asterisk indicate Pearson correlations with false discovery rate < 5%. AAC, abdominal aorta calcification; AHA LS7, American Heart Association Life Simple 7; CAC, coronary artery calcification; DBP, diastolic blood pressure; eGFR, estimated glomerular filtration rate; FC, fold change; GLS, global longitudinal strain; HbA1c, hemoglobin A1c; HDL, high density lipoprotein; LV, left ventricular; PA, physical activity; SAT, subcutaneous adipose tissue; SBP, systolic blood pressure; VAT, visceral adipose tissue.
The notion that tissue-specific, exercise-responsive biomolecules (‘exerkines’ 35 , 38 ) mirror the metabolic benefits of physical exercise has prompted various efforts to catalog these biomolecular changes 8 , 10 , 11 , 13 , 16 , 39 . Several studies have highlighted acute metabolic changes during physical exercise that are linked to important physiological processes such as insulin resistance, inflammation and metabolic health across a wide array of mediators (for example, metabolites 8 , 11 , 39 , 40 , proteins 10 , 16 and transcripts 11 , 41 ), some of which overlap in association with total habitual physical activity 12 . While all biomolecule types offer relevant insights as functional biomarkers of CRF, the proteome can rapidly capture functional information (a ‘cause’ and ‘effect’ of CRF), broad cellular processes (with direct pathway implication) and application to a clinical setting as a quantifiable blood-based surrogate of CRF.
Here, we studied a diverse group of 14,145 individuals with varied modes of CRF assessment to characterize the circulating proteomic architecture of CRF. Beginning in a sample of 2,238 middle-aged Black and white adults in the CARDIA study, we successfully developed and validated a broad-based proteomic signature of CRF (‘proteomic CRF score’) using symptom-limited treadmill exercise test that displayed a consistent relation across submaximal treadmill exams in 10,320 individuals in the UK (Fenland, estimated maximal VO 2 ) and maximal cardiopulmonary exercise tests (CPETs) in 1,587 individuals in the USA (BLSA, treadmill VO 2 ; HERITAGE, cycle VO 2 ). Proteins included in the proteomic CRF score specified pathways canonically implicated in CRF biology across several systems, including inflammation and hemostasis, muscle and adipose physiology, pathways of energy and fuel metabolism, oxidative stress and neuronal survival, among others. In 21,988 UKB participants, we observed two key findings of clinical relevance. First, the proteomic CRF score was strongly, independently associated with a range of metabolic, cardiovascular and neurological clinical outcomes, many displaying significant prognostic improvement over standard risk factors (via reclassification and discrimination metrics). Second, these associations appeared to be additive to polygenic risk, suggesting a role for multiomic evaluation in clinical risk assessment. These prognostic relations were maintained using an abbreviated 21-protein panel (the largest currently available for direct absolute protein quantification with Olink). The proteomic CRF score was also dynamic with a 20-week exercise training program, and was associated with response to training. To our knowledge, these data provide the largest report to date establishing a biologically plausible, population-based proteomic biomarker of CRF across a diverse setting, linking these measures to phenotypes and precision medicine risk assessment approaches (including human genetics) longitudinally.
Although other studies have demonstrated the ability of broad circulating proteomics to predict diverse health outcomes 16 , the highest priority protein targets are likely to differ for each outcome, presenting challenges for developing unifying lifestyle or pharmacological approaches for broad risk modification or health promotion. In line with established relations of greater CRF itself with protection from a wide array of adverse cardiovascular 2 , 42 , respiratory 43 , oncological 44 and neurocognitive outcomes 45 , we observed a proteomic signature trained on CRF (‘proteomic CRF score’) was associated with diverse clinical outcomes in a large sample of around 22,000 UKB participants (an order of magnitude larger than previous studies 16 ). Beyond merely establishing a statistical association, the proteomic CRF score offered significant improvement in risk reclassification and discrimination across several conditions (for example, all-cause death, cardiovascular death, diabetes), suggesting its potential to augment clinical risk prediction. Moreover, in line with previous work demonstrating lack of strong interaction between genetics and lifestyle 31 , proteomic and genetic risk were complementary, with the highest clinical risks observed for those individuals with both high proteomic and genomic risk and a lowered risk for those individuals with high proteomic CRF across genetic risk. A critical finding was that these associations were robust to increased parsimony via an abbreviated 21-protein proteomic CRF score, laying groundwork for future studies of clinical translation. In this context, a proteomic CRF score may have clinical utility as a surrogate of CRF to extend its applicability to resource-limited settings, older adults or individuals with contraindications to exercise or musculoskeletal disabilities (with impaired achievement of peak exercise) in whom direct CRF assessment is challenging.
Given modifiability of CRF with lifestyle interventions (for example, physical activity 46 )—a critical test for any precision biomarker of CRF lies in modifiability with training. After a 20-week exercise training program within HERITAGE, we observed a modest but significant relation between changes in the proteomic CRF score with training and the peak VO 2 , with a 1 s.d. increase in proteomic score corresponding to an increase in peak VO 2 of nearly 1 ml kg −1 min −1 (approximately 20% of the mean effect of training in HERITAGE). While HERITAGE is a healthy group (and effect sizes in a clinical population probably vary), 1 ml kg −1 min −1 is considered a ‘clinically actionable’ effect size in CVD 47 : in the HF-ACTION trial, an increase in peak VO 2 of approximately 0.9 ml kg −1 min −1 was associated with a ~5% lower risk of mortality 48 . This effect size is greater than the median 3-month increase in peak VO 2 observed among HF-ACTION participants randomized to exercise intervention (0.6 ml kg −1 min −1 ), but is on par with effects of diet and exercise within a trial of participants with HFpEF 49 . Moreover, we observed an association between pretraining proteomic score and changes in peak VO 2 with training. These findings contribute new contributory evidence on the plasticity of the proteomic CRF biomarker, supporting broad, ongoing efforts to develop multiomic biomarkers of CRF with divergent exercise and training regimens toward personalization of exercise training responses 50 .
The innovation of our approach is contextualized by a rich history of approaches targeting CRF prediction to ease clinical translation. Indeed, previous work to develop nonexercise prediction models of CRF has spanned physical activity questionnaires 51 , 52 , 53 , 54 , 55 , 56 , 57 , 58 , 59 , 60 , resting heartrate 53 , 58 , 60 , BMI/body composition 51 , 52 , 53 , 54 , 55 , 56 , 57 , 58 , 59 , 60 , 61 , 62 , 63 , genetics 64 , proteomics 16 , metabolomics 13 and activity monitor data 61 , 62 , 63 , 65 . However, most previous studies have been conducted in healthy or trained individuals and lack a demonstration of strong relations with to multisystem clinical outcomes. The current approach represents a notable advance, merging populations at higher metabolic risk (mirroring the advancing prevalence of cardiometabolic diseases worldwide), modes of exercise, a broad proteomic space, with several validation samples incorporating human genetics (UKB), subclinical phenotypes (CARDIA) and exercise training response (HERITAGE). As precision medicine approaches advance, incorporation of several methods (for example, wearable activity monitor plus ‘omics’) to refine clinically translatable estimates of CRF are likely to improve on any single method.
While biological plausibility and reproducibility of previous smaller studies suggest external validity, several important limitations of this work merit discussions. CRF assessments were not standardized across cohorts, which were themselves variable by age, geography, race and time epoch, although this heterogeneity may also be viewed as a strength since it highlights the robustness of our approach through successful crossvalidation. In addition, there was an interval of around 5 years between the proteomic and CRF assessment in CARDIA, which may have introduced additional variability in our estimates. However, replication of our multivariable proteomic CRF score across three additional studies (Fenland, HERITAGE and BLSA), and demonstration of its modifiability with exercise training (HERITAGE) testifies to the transportability of this approach. Although our study was limited in representation of older adults, the prognostic utility of proteomics independent of age, sex and race are a testament to potential clinical relevance. The proteomic platform utilized in the derivation samples was aptamer-based (SomaScan), which has some limitations in terms of specificity on per-protein level 66 . Nonetheless, we validated the clinical associations of these signatures in a different platform (Olink) in a broader set of individuals (UKB). The assessment of outcomes in UKB was administrative, with potential attendant misclassification and ascertainment biases, which we would anticipate leading to a bias toward null association. Additional forthcoming consortium-level studies across a wider range of exercise types will be important tools to study for potential sex-specific differences and may help clarify proteomic effects from changes in metabolic or lifestyle factors and CRF 50 .
In summary, we define, characterize, and validate a CRF-related proteome across four studies including approximately 14,000 individuals, spanning age, sex, race, geography and type of CRF assessment. CRF-related proteins demonstrated biological plausibility (including consistency with previous studies) and identified individuals with high risk of adverse clinical events across a wide array of organ systems in around 22,000 individuals. Proteomic risk appeared additive to polygenic risk and was maintained down to a clinically actionable proteomic panel. These results suggest the potential for population-based proteomics to provide a biologically relevant, clinically actionable molecular barometer of CRF with clinical potential.
Population-based cohorts
Coronary artery risk development in young adults.
The CARDIA study is a prospective, population-based, cohort study designed to study risk factors for cardiovascular disease development through the lifecourse. The original study commenced in 1985–1986 across four US field centers (Birmingham, AL; Chicago, IL; Minneapolis, MN and Oakland, CA) to study risk factor development throughout young adulthood to midlife, as previously described 72 , 73 , 74 , 75 . For this study, we included 2,238 individuals with circulating proteomics (SomaScan) at Year 25 (2010–2011) and ETT time for CRF at year 20 (2005–2006). We intentionally did not refine the CARDIA study population based on reason for stopping ETT or thresholds signifying maximal effort (for example, 85% maximum predicted heartrate) to preserve a maximal sample size and include participants who stopped early for several reasons that may reflect heightened clinical risk. Characterization of demographic, clinical and exercise test data were used as previously published 76 , 77 . Specifically, CVD was defined as a history of myocardial infarction, heart failure, stroke, carotid artery disease and peripheral artery disease. Participants provided written informed consent and approval to use deidentified data from CARDIA for this study was provided by the Institutional Review Board (IRB) at Vanderbilt University Medical Center (IRB no. 211402).
The Fenland Study is a population-based cohort study of 12,435 participants (born between 1950 and 1975) recruited from general practices in Cambridgeshire, UK, from January 2005 to April 2015 78 . Exclusion criteria were known diabetes, pregnancy or lactation, inability to walk unaided for a minimum of 10 min, psychosis or terminal illness. Our analytic sample included 5,473 women and 4,847 men with available CRF testing, proteomic and clinical data who attended one of three study sites (Cambridge, Ely or Wisbech). The study was approved by the Cambridge Local Research Ethics Committee (NRES Committee, East of England Cambridge Central, reference no. 04/Q0108/19). All participants provided written informed consent for blood sample measurements, exercise testing and other assessments beyond the baseline examination.
Baltimore Longitudinal Study of Aging
The BLSA is a prospective, longitudinal cohort study commenced in 1958 to study age-related conditions 15 , 79 . Our analytic sample included 845 participants who had undergone CPETs and had circulating plasma proteins quantified at the same time. Demographic and exercise data were defined as previously published 80 . The BLSA study protocol was approved by the Internal Review Board of the Intramural Research Program of the National Institutes of Health (protocol no. 03AG0325) and all participants provided written informed consent at each visit.
Health, Risk Factors, Exercise Training and Genetics study
HERITAGE is a study of the genetic and nongenetic contributors to biological responses to aerobic exercise training 81 . Participants were recruited as family units with African or European descent at five centers in the USA and Canada between 1992 and 1997, as described 81 . Participants had to be healthy without cardiometabolic disease but with a sedentary lifestyle for the 3 months preceding enrollment. We included published association data from 742 participants with directly measured maximal aerobic capacity (peak VO 2 ) before exercise training and circulating proteomics 10 . Proteomic changes after a 20-week training period were also included 36 . All participants provided written informed consent. The IRB at Beth Israel Deaconess Medical Center approved this study (IRB no. 2016P000186).
The UKB is a population-based study of >500,000 participants aged 40–69 years when recruited between 2006 and 2010 across the UK. UKB was constructed to enable large-scale scientific discoveries of human health 82 . Recently, the study coordinators released proteomics data using the Olink Explore 1536 panel on approximately 52,000 UKB participants. Our analytic sample included 21,988 participants without missing values for the proteins used to calculate a proteomic score of CRF. Approval for UKB access is under proposal no. 57492.
To maximize external validity and generalizability across broad populations, we selected CARDIA as the discovery cohort to develop a proteomic score of CRF, despite 5-year differences between proteomic and CRF assessments. Unlike Fenland and HERITAGE, which excluded participants with prevalent cardiometabolic disease, CARDIA is a population-based study inclusive of prevalent conditions. While BLSA and UKB included participants with prevalent cardiometabolic disease, the number of participants with both CRF and proteomic data is less than half of that in CARDIA. Additional considerations that guided our selection of CARDIA include its broad proteomic coverage (7k SomaScan versus 5k SomaScan in HERITAGE, Fenland and Olink Explore 1536 in UKB), and use of a symptom-limited maximal stress test (Fenland and UKB impute peak VO 2 data from submaximal tests).
CRF assessment
CRF was assessed in CARDIA, BLSA, Fenland and HERITAGE according to cohort-specific protocols. In CARDIA, a symptom-limited ETT (modified Balke protocol) was performed as previously described 76 , 83 , 84 . Each test consisted of a maximum 18 min, with changes in treadmill speed or grade every 2 min with a maximum workload of 19 metabolic equivalents of task (METs) (for example, 5.6 miles per hour and 25% incline). Participants were excluded from ETT if they had cardiovascular or pulmonary diseases, musculoskeletal diseases worsened by exercise, uncontrolled metabolic or infectious disease, severe rest hypertension (systolic over 200 mmHg or diastolic over 110 mmHg), electrocardiographic features of ischemic heart disease or arrhythmia, pregnancy or at the discretion of exercise personnel. CRF was estimated as the duration of time a participant was able to walk/run on the treadmill. We did not exclude participants based on submaximal or early test conclusion in CARDIA.
In Fenland, CRF was assessed using a submaximal treadmill test (with imputation to maximal effort as described, methods taken from ref. 14 with attribution provided by this statement) to generate estimated maximal oxygen consumption (peak VO 2 ) per kilogram of total body mass. Participants exercised for up to 21 min while treadmill speed and incline increased across four stages. Exercise heartrate response was recorded using a combined heartrate and movement sensor (Actiheart; CamNtech) 85 . The test ended if one of the following criteria were satisfied: (1) levelling-off of heartrate (<3 beats per min (bpm)) despite an increase in workrate; (2) reaching 90% of the participant’s age-predicted maximal heartrate 86 ; (3) exercising above 80% of age-predicted maximal heartrate for over 2 min; (4) reaching a respiratory exchange ratio (RER) of 1.1; (5) participant desire to stop; (6) participant indication of angina, light-headedness or nausea; or (7) failure of the testing equipment. Gas exchange measurements were sometimes unavailable for various reasons (for example, participants declining to wear a gas analysis mask, mask fit issues during exercise, system errors) that could be correlated with health-related factors. To mitigate biases that would emerge from the exclusion of participants lacking gas exchange data, and to maintain a standardized approach in estimating peak VO 2 across the study, we opted to extrapolate the workrate-to-heartrate relationship to age-predicted maximal heartrate. Peak VO 2 was estimated by extrapolating the linear relationship between heartrate and treadmill workrate 87 to age-predicted maximal heartrate 86 , adding an estimate of resting energy expenditure, and then converting the resultant workrate value to VO 2 (ml O 2 min −1 kg −1 ) using a caloric equivalent for oxygen of 20.35 J ml O 2 −1 .
In HERITAGE, CRF was measured using a cycle ergometer with metabolic cart gas exchange measures with VO 2 averaged over 20 s intervals, as described 10 . CRF was defined as the peak VO 2 and exercise peak was determined from at least one of the following: RER >1.1, a plateau in VO 2 (<100 ml min −1 change in the last three measures), or a maximal heartrate within 10 bpm of the age-predicted maximum. After baseline CRF assessment, HERITAGE participants underwent supervised exercise training three times per week for 20 weeks 10 . CRF assessment was then repeated after completion of the training protocol.
In BLSA, CRF was measured using a symptom-limited treadmill exercise test with metabolic cart gas exchange measures using a modified Balke protocol with VO 2 averaged over 30 s intervals 80 . Exercise testing ended after self-reported exhaustion or health- and/or safety-related stopping criteria occurred. To ensure that the maximal VO 2 was achieved, the analysis was limited to participants with an RER ≥ 1. Of the 845 participants included in our study, 133 (15%) had RER between 1 and 1.1. Of these participants, 119 (89%) either reached >85% of their age-predicted maximum heartrate (calculated as 220 − age) or rated their exertion during the treadmill test as 17 or great on a 20-point Borg perceived exertion scale.
Proteomic quantification in CARDIA was performed using aptamer-based technology (Somalogic). Overall, 7,524 circulating aptamers were quantified. A total of 68 participants had more than one measurement of plasma proteins (at the same visit), and their protein data was averaged. We excluded nonhuman proteins ( N = 233) and proteins with a coefficient of variation >20% ( N = 61). Using principal component analysis on a matrix of the log-transformed, and scaled proteomic data, we checked visually for batch effects and participant outliers by plotting the first two principal components against each other. No batch effects were detected, and no participant outliers were identified (Supplementary Fig. 1 ). Fenland (5k aptamer platform), HERITAGE (5k aptamer platform) and BLSA (7k aptamer platform) also used SomaScan proteomics technology with methods described previously 10 , 16 , 88 , 89 . The UKB quantified circulating proteins using the Olink Explore 1536 panel 90 , and we excluded proteins where >40% of measurements were below the limit of detection ( N = 130) or were missing in >20% of participants ( N = 3). Of note, as noted above, HERITAGE data was used as published; the remainder of cohorts were analyzed as part of this work.
Statistical methods
Construction and validation of a proteomic score of crf (‘crf proteome’).
To explore the multidimensionality of the CRF proteome, we used LASSO regression within a linear modeling framework to develop a multivariable signature of CRF. For the purposes of analysis, the CARDIA cohort was split into a 70% derivation and 30% validation sample balanced on ETT time. The LASSO model was constructed in the CARDIA derivation sample with CRF (ETT time) as the outcome. Adjustments for age, sex, race and BMI were included as unpenalized factors (forced in regression models) with the entire proteome included as penalized factors for selection. Proteins were log-transformed, and proteins and CRF were standardized (mean 0, variance 1) for modeling. Crossvalidation was used for model hyperparameter optimization. Each CARDIA participant’s proteomic CRF score was defined as a linear combination of each protein concentration by the respective model coefficient. We excluded age, sex, race, BMI and intercept coefficients in the score calculation, such that each protein coefficient was conditioned on these covariates (to reduce dependence of the final score on these covariates). Protein scores were standardized (mean 0, variance 1) for downstream analyses.
External cohort validation of the CRF proteome
To test the external validity of the CRF proteome across additional cohorts with different proteomic coverages, we employed a recalibration approach. Our recalibration effort used a LASSO model in CARDIA, where the original score (as above) was the dependent variable and all overlapping proteins were included as independent variables. This approach generated coefficients in CARDIA that could be applied to Fenland, HERITAGE and UKB. It was not needed in BLSA, where the platform was the same as CARDIA. Recalibration accuracy (based on correlation between the original score and the recalibrated scores in CARDIA) was excellent (HERITAGE score, Pearson r = 0.98; Fenland score, Pearson r = 0.99; UKB score, Pearson r = 0.93).
Relation of the CRF proteome with clinical outcomes and its interaction with polygenic risk
Finally, we performed survival analysis in UKB to estimate the prospective association of the CRF proteome with a broad array of outcomes. Death and death category (cardiovascular death, cancer death, respiratory death) were defined by using death registry data (UKB Data Field 40000) and the International Classification of Disease tenth revision (ICD10) code provided for primary cause of death (UKB Data Field 40001). Mappings for ICD10 data to death category were informed by previous work 91 . The censor dates for death data (and other outcome data) were determined for each participant using the location of initial assessment (UKB Data Field 54) and the region-specific censor dates provided by the UKB. Survival analysis with death outcomes were censored on 30 November 2022 for all alive participants. Survival analysis with incident disease outcomes (for example, chronic obstructive pulmonary disease) were censored on 31 October 2022 for participants in England ( N = 19,768), 31 July 2021 for participants in Scotland ( N = 1,356), and 28 February 2018 for participants in Wales ( N = 864) without events or the death date. Other outcomes in UKB were defined by ICD10 diagnosis codes. To group the ICD10 codes into relevant phenotypes, we used the PheWAS package to generate Phecodes, which represent a composite phenotypes comprised of several related ICD10 codes 92 . For each Phecode, we generated a case, control and excluded status for each participant. Participants with an ‘excluded’ status for a given Phecode were those who had a confounding ICD10 code. This confounding code would not qualify the participant as a case but would disqualify them as being a control. To determine the date of onset for each phenotype, source ICD10 codes were mapped individually to Phecodes, and the date of the earliest qualifying ICD10 code was selected. Prevalent cases were excluded from incident disease models, with prevalent cases being defined as those with a Phecode before their assessment visit, a self-reported diagnosis (UKB Data Field 20002), or a physician diagnosis (UKB Data Fields 2453, 2443, 6150). Details for model phecodes and the corresponding exclusion criteria are listed in the Supplementary Table 7 .
Models were constructed using standard Cox regression with the proteomic CRF score as the predictor and the following nested adjustments: (1) unadjusted; (2) age, sex, race; (3) age, sex, race, Townsend deprivation index, body mass index, diabetes, smoking status, alcohol use, systolic blood pressure, low-density lipoprotein (LDL); (4) age, sex, race, Townsend deprivation index, body mass index, diabetes, smoking status, alcohol use, systolic blood pressure, LDL, fat mass as measured by bioimpedance (UKB Data Field 23101). We compared survival models using the maximal set of adjustments with and without the proteomic CRF score to examine differences in C-statistics and net reclassification index (NRI; calculated at the 75th percentile for NRI for events). Our primary analysis for cause-specific death used a ‘cause-specific’ approach where participants without the event of interest (for example, CVD death) are censored at the time of last known vital status or time of death from another cause (for example, cancer death). This approach was complemented using a competing risk framework with a Fine–Gray model with separate models for each of the three modes of death analyzed (for example, CVD, cancer, respiratory). For incident disease models, participants who did not experience the event were censored at the region-specific censor date or the date of death.
To examine potential complementarity of the CRF proteome with polygenic risk of diseases associated with CRF, we used Cox regression models with proteomic CRF score and standard polygenic risk score (UKB Fields 26206, 26212, 26223, 26244, 26248, 26285 (ref. 93 )) as independent variables (with an interaction term between the two) with adjustments for age, sex, race and four principal components of genetic ancestry (UKB Field 26201).
To examine the potential for clinical translation, we examined performance of a 21-protein score (the maximum number of proteins in an absolute quantification Olink panel currently available) with the recalibrated protein score (307 proteins) in standard Cox models in UKB and compared beta coefficients on the two versions of the CRF proteome. The 21 proteins selected were the top 21 proteins from the recalibrated 307-protein score LASSO model, ranked by the absolute value of the beta coefficients.
Dynamicity of CRF proteome with exercise training
Finally, to examine the modifiability of the proteomic CRF score with exercise training and how it tracks with changes in peak VO 2 , in HERITAGE we used paired t -tests and regression models for change in peak VO 2 as a function of change in proteomic CRF score with adjustments for age, sex, race, BMI, pretraining peak VO 2 and pretraining proteomic CRF score. To test whether the proteomic CRF score was associated with the response to exercise training, we used a model of posttraining peak VO 2 as a function of pretraining proteomic CRF score adjusted for baseline peak VO 2 , age, sex, race and BMI.
Analyses were conducted with R v.4 or later. All P values reported are from two-sided tests.
Reporting summary
Further information on research design is available in the Nature Portfolio Reporting Summary linked to this article.
Data availability
Data for this study are publicly available via the CARDIA coordinating center ( www.cardia.dopm.uab.edu ), the Fenland Study coordinating center ( https://www.mrc-epid.cam.ac.uk/research/data-sharing/ ), published data from HERITAGE 10 , 35 and the UKB ( https://www.ukbiobank.ac.uk ). Participants did not consent to unrestricted data sharing at the time of study conduct for BLSA. Data from BLSA may be obtained via application to the BLSA coordinating center ( https://www.blsa.nih.gov ).
Code availability
Statistical code for the analyses can be found at https://github.com/asperry125/CRF-Proteomics .
Shah, R. V. et al. Association of fitness in young adulthood with survival and cardiovascular risk: the Coronary Artery Risk Development in Young Adults (CARDIA) study. JAMA Intern. Med. 176 , 87–95 (2016).
Article PubMed PubMed Central Google Scholar
Kodama, S. et al. Cardiorespiratory fitness as a quantitative predictor of all-cause mortality and cardiovascular events in healthy men and women: a meta-analysis. JAMA 301 , 2024–2035 (2009).
Article CAS PubMed Google Scholar
Mancini, D. M. et al. Value of peak exercise oxygen consumption for optimal timing of cardiac transplantation in ambulatory patients with heart failure. Circulation 83 , 778–786 (1991).
Sandvik, L. et al. Physical fitness as a predictor of mortality among healthy, middle-aged Norwegian men. N. Engl. J. Med. 328 , 533–537 (1993).
Wei, M. et al. Relationship between low cardiorespiratory fitness and mortality in normal-weight, overweight, and obese men. JAMA 282 , 1547–1553 (1999).
Ross, R. et al. Importance of assessing cardiorespiratory fitness in clinical practice: a case for fitness as a clinical vital sign. A scientific statement from the American Heart Association. Circulation 134 , e653–e699 (2016).
Article PubMed Google Scholar
Balady, G. J. et al. Clinician’s guide to cardiopulmonary exercise testing in adults: a scientific statement from the American Heart Association. Circulation 122 , 191–225 (2010).
Nayor, M. et al. Metabolic architecture of acute exercise response in middle-aged adults in the community. Circulation 142 , 1905–1924 (2020).
Article CAS PubMed PubMed Central Google Scholar
Robbins, J. M. et al. Association of dimethylguanidino valeric acid with partial resistance to metabolic health benefits of regular exercise. JAMA Cardiol. 4 , 636–643 (2019).
Robbins, J. M. et al. Human plasma proteomic profiles indicative of cardiorespiratory fitness. Nat. Metab. 3 , 786–797 (2021).
Contrepois, K. et al. Molecular choreography of acute exercise. Cell 181 , 1112–1130.e1116 (2020).
Nayor, M. et al. Integrative analysis of circulating metabolite levels that correlate with physical activity and cardiorespiratory fitness. Circ. Genom. Precis Med 15 , e003592 (2022).
Shah, R. V. et al. Blood-based fingerprint of cardiorespiratory fitness and long-term health outcomes in young adulthood. J. Am. Heart Assoc. 11 , e026670 (2022).
Gonzales, T. I. et al. Descriptive epidemiology of cardiorespiratory fitness in UK adults: the Fenland Study. Med. Sci. Sports Exerc. 55 , 507–516 (2023).
Shock, N. W. et al. Normal Human Aging: The Baltimore Longitudinal Study of Aging NIH publication 84-2450 (National Institutes of Health, 1984).
Williams, S. A. et al. Plasma protein patterns as comprehensive indicators of health. Nat. Med. 25 , 1851–1857 (2019).
Klos, A. et al. The role of the anaphylatoxins in health and disease. Mol. Immunol. 46 , 2753–2766 (2009).
Camus, G. et al. Anaphylatoxin C5a production during short-term submaximal dynamic exercise in man. Int. J. Sports Med. 15 , 32–35 (1994).
Yang, F. et al. Proteomic insights into the associations between obesity, lifestyle factors, and coronary artery disease. BMC Med 21 , 485 (2023).
Huttunen, H. J. & Saarma, M. CDNF protein therapy in Parkinson’s disease. Cell Transplant. 28 , 349–366 (2019).
Pimenta, A. F. et al. The limbic system-associated membrane protein is an Ig superfamily member that mediates selective neuronal growth and axon targeting. Neuron 15 , 287–297 (1995).
Knupp, J., Arvan, P. & Chang, A. Increased mitochondrial respiration promotes survival from endoplasmic reticulum stress. Cell Death Differ. 26 , 487–501 (2019).
Gonzalez-Garcia, I. et al. Olfactomedin 2 deficiency protects against diet-induced obesity. Metabolism 129 , 155122 (2022).
Numao, S., Uchida, R., Kurosaki, T. & Nakagaichi, M. Differences in circulating fatty acid-binding protein 4 concentration in the venous and capillary blood immediately after acute exercise. J. Physiol. Anthropol. 40 , 5 (2021).
Li, B., Syed, M. H., Khan, H., Singh, K. K. & Qadura, M. The role of fatty acid binding protein 3 in cardiovascular diseases. Biomedicines 10 , 2283 (2022).
Huck, I., Morris, E. M., Thyfault, J. & Apte, U. Hepatocyte-specific hepatocyte nuclear factor 4 alpha (HNF4) deletion decreases resting energy expenditure by disrupting lipid and carbohydrate homeostasis. Gene Expr. 20 , 157–168 (2021).
Carayol, J. et al. Protein quantitative trait locus study in obesity during weight-loss identifies a leptin regulator. Nat. Commun. 8 , 2084 (2017).
Roxin, L. E., Hedin, G. & Venge, P. Muscle cell leakage of myoglobin after long-term exercise and relation to the individual performances. Int. J. Sports Med. 7 , 259–263 (1986).
Wu, J. et al. The unfolded protein response mediates adaptation to exercise in skeletal muscle through a PGC-1alpha/ATF6alpha complex. Cell Metab. 13 , 160–169 (2011).
Zhao, Y. et al. GLIPR2 is a negative regulator of autophagy and the BECN1-ATG14-containing phosphatidylinositol 3-kinase complex. Autophagy 17 , 2891–2904 (2021).
Khera, A. V. et al. Genetic risk, adherence to a healthy lifestyle, and coronary disease. N. Engl. J. Med. 375 , 2349–2358 (2016).
Rutten-Jacobs, L. C. et al. Genetic risk, incident stroke, and the benefits of adhering to a healthy lifestyle: cohort study of 306 473 UK Biobank participants. Br. Med. J. 363 , k4168 (2018).
Article Google Scholar
Al Ajmi, K., Lophatananon, A., Mekli, K., Ollier, W. & Muir, K. R. Association of nongenetic factors with breast cancer risk in genetically predisposed groups of women in the UK Biobank cohort. JAMA Netw. Open 3 , e203760 (2020).
Lourida, I. et al. Association of lifestyle and genetic risk with incidence of dementia. JAMA 322 , 430–437 (2019).
Robbins, J. M. & Gerszten, R. E. Exercise, exerkines, and cardiometabolic health: from individual players to a team sport. J. Clin. Invest. 133 , e168121 (2023).
Robbins, J. M. et al. Plasma proteomic changes in response to exercise training are associated with cardiorespiratory fitness adaptations. JCI Insight 8 , e165867 (2023).
Maciel, L. et al. New cardiomyokine reduces myocardial ischemia/reperfusion injury by PI3K-AKT pathway via a putative KDEL-receptor binding. J. Am. Heart Assoc. 10 , e019685 (2021).
Chow, L. S. et al. Exerkines in health, resilience and disease. Nat. Rev. Endocrinol. 18 , 273–289 (2022).
Lewis, G. D. et al. Metabolic signatures of exercise in human plasma. Sci. Transl. Med. 2 , 33ra37 (2010).
Stanford, K. I. et al. 12,13-diHOME: an exercise-induced lipokine that increases skeletal muscle fatty acid uptake. Cell Metab. 27 , 1111–1120.e1113 (2018).
Shah, R. et al. Small RNA-seq during acute maximal exercise reveal RNAs involved in vascular inflammation and cardiometabolic health. Am. J. Physiol. Heart Circ. Physiol. 13 , H1162–H1167 (2017).
Clausen, J. S. R., Marott, J. L., Holtermann, A., Gyntelberg, F. & Jensen, M. T. Midlife cardiorespiratory fitness and the long-term risk of mortality: 46 years of follow-up. J. Am. Coll. Cardiol. 72 , 987–995 (2018).
Hansen, G. M. et al. Midlife cardiorespiratory fitness and the long-term risk of chronic obstructive pulmonary disease. Thorax 74 , 843–848 (2019).
Ekblom-Bak, E. et al. Association between cardiorespiratory fitness and cancer incidence and cancer-specific mortality of colon, lung, and prostate cancer among Swedish men. JAMA Netw. Open 6 , e2321102 (2023).
Wu, C. H. et al. Cardiorespiratory fitness is associated with sustained neurocognitive function during a prolonged inhibitory control task in young adults: an ERP study. Psychophysiology 59 , e14086 (2022).
Nayor, M. et al. Physical activity and fitness in the community: the Framingham Heart Study. Eur. Heart J. 42 , 4565–4575 (2021).
Lewis, G. D. et al. Developments in exercise capacity assessment in heart failure clinical trials and the rationale for the design of METEORIC-HF. Circ. Heart Fail. 15 , e008970 (2022).
Swank, A. M. et al. Modest increase in peak VO 2 is related to better clinical outcomes in chronic heart failure patients: results from heart failure and a controlled trial to investigate outcomes of exercise training. Circ. Heart Fail. 5 , 579–585 (2012).
Kitzman, D. W. et al. Effect of caloric restriction or aerobic exercise training on peak oxygen consumption and quality of life in obese older patients with heart failure with preserved ejection fraction: a randomized clinical trial. JAMA 315 , 36–46 (2016).
Sanford, J. A. et al. Molecular transducers of physical activity consortium (MoTrPAC): mapping the dynamic responses to exercise. Cell 181 , 1464–1474 (2020).
Jackson, A. S. et al. Prediction of functional aerobic capacity without exercise testing. Med. Sci. Sports Exerc. 22 , 863–870 (1990).
Heil, D. P., Freedson, P. S., Ahlquist, L. E., Price, J. & Rippe, J. M. Nonexercise regression models to estimate peak oxygen consumption. Med. Sci. Sports Exerc. 27 , 599–606 (1995).
Whaley, M. H., Kaminsky, L. A., Dwyer, G. B. & Getchell, L. H. Failure of predicted VO2peak to discriminate physical fitness in epidemiological studies. Med. Sci. Sports Exerc. 27 , 85–91 (1995).
George, J. D., Stone, W. J. & Burkett, L. N. Non-exercise VO2max estimation for physically active college students. Med. Sci. Sports Exerc. 29 , 415–423 (1997).
Matthews, C. E., Heil, D. P., Freedson, P. S. & Pastides, H. Classification of cardiorespiratory fitness without exercise testing. Med. Sci. Sports Exerc. 31 , 486–493 (1999).
Malek, M. H., Housh, T. J., Berger, D. E., Coburn, J. W. & Beck, T. W. A new nonexercise-based VO 2max equation for aerobically trained females. Med. Sci. Sports Exerc. 36 , 1804–1810 (2004).
Malek, M. H., Housh, T. J., Berger, D. E., Coburn, J. W. & Beck, T. W. A new non-exercise-based Vo2max prediction equation for aerobically trained men. J. Strength Cond. Res. 19 , 559–565 (2005).
PubMed Google Scholar
Jurca, R. et al. Assessing cardiorespiratory fitness without performing exercise testing. Am. J. Prev. Med. 29 , 185–193 (2005).
Bradshaw, D. I. et al. An accurate VO2max nonexercise regression model for 18-65-year-old adults. Res. Q. Exerc. Sport 76 , 426–432 (2005).
Nes, B. M. et al. Estimating V·O 2peak from a nonexercise prediction model: the HUNT Study, Norway. Med. Sci. Sports Exerc. 43 , 2024–2030 (2011).
Cao, Z. B. et al. Prediction of VO2max with daily step counts for Japanese adult women. Eur. J. Appl. Physiol. 105 , 289–296 (2009).
Cao, Z. B. et al. Predicting VO2max with an objectively measured physical activity in Japanese women. Med. Sci. Sports Exerc. 42 , 179–186 (2010).
Cao, Z. B., Miyatake, N., Higuchi, M., Miyachi, M. & Tabata, I. Predicting VO 2max with an objectively measured physical activity in Japanese men. Eur. J. Appl. Physiol. 109 , 465–472 (2010).
Cai, L. et al. Causal associations between cardiorespiratory fitness and type 2 diabetes. Nat. Commun. 14 , 3904 (2023).
Spathis, D. et al. Longitudinal cardio-respiratory fitness prediction through wearables in free-living environments. NPJ Digit. Med. 5 , 176 (2022).
Katz, D. H. et al. Proteomic profiling platforms head to head: leveraging genetics and clinical traits to compare aptamer- and antibody-based methods. Sci. Adv. 8 , eabm5164 (2022).
da Silva, W. A. B. et al. Physical exercise increases the production of tyrosine hydroxylase and CDNF in the spinal cord of a Parkinson’s disease mouse model. Neurosci. Lett. 760 , 136089 (2021).
Graham, J. R. et al. Serine protease HTRA1 antagonizes transforming growth factor-beta signaling by cleaving its receptors and loss of HTRA1 in vivo enhances bone formation. PLoS ONE 8 , e74094 (2013).
Lee, J. et al. EWSR1, a multifunctional protein, regulates cellular function and aging via genetic and epigenetic pathways. Biochim. Biophys. Acta, Mol. Basis Dis. 1865 , 1938–1945 (2019).
Jung, I. H. et al. SVEP1 is a human coronary artery disease locus that promotes atherosclerosis. Sci. Transl. Med. 13 , eabe0357 (2021).
Nakamura, R. et al. Serum fatty acid-binding protein 4 (FABP4) concentration is associated with insulin resistance in peripheral tissues, a clinical study. PLoS ONE 12 , e0179737 (2017).
Wagenknecht, L. E. et al. Cigarette smoking behavior is strongly related to educational status: the CARDIA study. Prev. Med. 19 , 158–169 (1990).
Dyer, A. R. et al. Alcohol intake and blood pressure in young adults: the CARDIA Study. J. Clin. Epidemiol. 43 , 1–13 (1990).
Bild, D. E. et al. Physical activity in young black and white women. The CARDIA Study. Ann. Epidemiol. 3 , 636–644 (1993).
Sidney, S. et al. Comparison of two methods of assessing physical activity in the Coronary Artery Risk Development in Young Adults (CARDIA) Study. Am. J. Epidemiol. 133 , 1231–1245 (1991).
Sidney, S. et al. Symptom-limited graded treadmill exercise testing in young adults in the CARDIA study. Med. Sci. Sports Exerc. 24 , 177–183 (1992).
Pettee Gabriel, K. et al. Factors associated with age-related declines in cardiorespiratory fitness from early adulthood through midlife: CARDIA. Med. Sci. Sports Exerc. 54 , 1147–1154 (2022).
Lindsay, T. et al. Descriptive epidemiology of physical activity energy expenditure in UK adults (the Fenland study). Int J. Behav. Nutr. Phys. Act. 16 , 126 (2019).
Ferrucci, L. The Baltimore Longitudinal Study of Aging (BLSA): a 50-year-long journey and plans for the future. J. Gerontol. A Biol. Sci. Med. Sci. 63 , 1416–1419 (2008).
Simonsick, E. M., Fan, E. & Fleg, J. L. Estimating cardiorespiratory fitness in well-functioning older adults: treadmill validation of the long distance corridor walk. J. Am. Geriatr. Soc. 54 , 127–132 (2006).
Bouchard, C. et al. The HERITAGE family study. Aims, design, and measurement protocol. Med. Sci. Sports Exerc. 27 , 721–729 (1995).
Protocol for a Large-Scale Prospective Epidemiological Resource (UK Biobank, 2006); www.ukbiobank.ac.uk/media/gnkeyh2q/study-rationale.pdf
Carnethon, M. R. et al. Association of 20-year changes in cardiorespiratory fitness with incident type 2 diabetes: the coronary artery risk development in young adults (CARDIA) fitness study. Diabetes Care 32 , 1284–1288 (2009).
Balke, B. & Ware, R. W. An experimental study of physical fitness of Air Force personnel. US Armed Forces Med. J. 10 , 675–688 (1959).
CAS Google Scholar
Brage, S., Brage, N., Franks, P. W., Ekelund, U. & Wareham, N. J. Reliability and validity of the combined heart rate and movement sensor Actiheart. Eur. J. Clin. Nutr. 59 , 561–570 (2005).
Tanaka, H., Monahan, K. D. & Seals, D. R. Age-predicted maximal heart rate revisited. J. Am. Coll. Cardiol. 37 , 153–156 (2001).
Brage, S. et al. Hierarchy of individual calibration levels for heart rate and accelerometry to measure physical activity. J. Appl. Physiol. (1985) 103 , 682–692 (2007).
Pietzner, M. et al. Synergistic insights into human health from aptamer- and antibody-based proteomic profiling. Nat. Commun. 12 , 6822 (2021).
Candia, J., Daya, G. N., Tanaka, T., Ferrucci, L. & Walker, K. A. Assessment of variability in the plasma 7k SomaScan proteomics assay. Sci. Rep. 12 , 17147 (2022).
Sun, B. B. et al. Plasma proteomic associations with genetics and health in the UK Biobank. Nature 622 , 329–338 (2023).
Gonzales, T. I. et al. Cardiorespiratory fitness assessment using risk-stratified exercise testing and dose-response relationships with disease outcomes. Sci. Rep. 11 , 15315 (2021).
Wu, P. et al. Mapping ICD-10 and ICD-10-CM codes to phecodes: workflow development and initial evaluation. JMIR Med. Inf. 7 , e14325 (2019).
Thompson, D. J. et al. UK Biobank release and systematic evaluation of optimised polygenic risk scores for 53 diseases and quantitative traits. Preprint at medRxiv https://doi.org/10.1101/2022.06.16.22276246 (2022).
Download references
Acknowledgements
A.S.P. is supported by the AHA (20SFRN35120123). J.M.R. is supported by the National Institutes of Health (NIH) (K23HL150327). R.V.S. is supported by grants from the American Heart Association (AHA) and NIH. M.N. is supported by NIH (R01HL156975, R01HL131029) and by a Career Investment Award from the Department of Medicine, Boston University School of Medicine. R.E.G. and M.A.S. were funded by R01NR019628. T.T., K.A.W., and L.F. are supported by the National Institute on Aging’s Intramural Research Program. P.R. is supported by the John S. LaDue Memorial Fellowship at Harvard Medical School. Q.S.W. is supported by the NIH (R01HL140074). M.Y.M. was supported by the NIH (K23HL171855). B.C. is supported by an Early Career Investigator Grant from the American Lung Association. The BLSA study was funded by the National Institute on Aging’s Intramural Research Program. Proteomics in CARDIA were funded by a grant to R.K. (R01HL122477). CARDIA is conducted and supported by the National Heart, Lung, and Blood Institute (NHLBI) in collaboration with the University of Alabama at Birmingham (75N92023D00002 and 75N92023D00005), Northwestern University (75N92023D00004), University of Minnesota (75N92023D00006) and Kaiser Foundation Research Institute (75N92023D00003). This manuscript has been reviewed by CARDIA for scientific content. Exercise testing in CARDIA was funded by a grant to S.S. and B. Sternfeld (R01HL078972). The Fenland Study is funded by the UK Medical Research Council, with proteomic assessment funded by Somalogic; Investigators T.G., N.J.W. and S.B. received support from the UK Medical Research Council (MC_UU_00006/1, MC_UU_00006/4) as well as the National Institute for Health and Care Research Cambridge Biomedical Research Centre (IS-BRC-1215-20014). The HERITAGE study was supported by several grants from the NHLBI (R01HL45670, R01HL47317, R01HL47321, R01HL47323 and R01HL47327).
Author information
These authors contributed equally: Andrew S. Perry, Eric Farber-Eger, Tomas Gonzales, Toshiko Tanaka, Jeremy M. Robbins.
These authors jointly supervised this work: Robert E. Gerszten, Soren Brage, Quinn S. Wells, Matthew Nayor, Ravi V. Shah.
Authors and Affiliations
Vanderbilt Translational and Clinical Cardiovascular Research Center, Vanderbilt University School of Medicine, Nashville, TN, USA
Andrew S. Perry, Eric Farber-Eger, Quinn S. Wells & Ravi V. Shah
MRC Epidemiology Unit, University of Cambridge, Cambridge, UK
Tomas Gonzales, Nick Wareham & Soren Brage
Longtidudinal Studies Section, Translational Gerontology Branch, National Institute on Aging, NIH, Baltimore, MD, USA
Toshiko Tanaka & Luigi Ferrucci
Cardiovascular Institute, Beth Israel Deaconess Medical Center, Harvard Medical School, Boston, MA, USA
Jeremy M. Robbins, Shuliang Deng, Jacob L. Barber, Prashant Rao, Michael Y. Mi & Robert E. Gerszten
Department of Medicine, University of Michigan, Ann Arbor, MI, USA
Venkatesh L. Murthy
Department of Biostatistics, Vanderbilt University Medical Center, Nashville, TN, USA
Lindsey K. Stolze, Shilin Zhao & Shi Huang
Department of Preventive Medicine, Northwestern University Feinberg School of Medicine, Chicago, IL, USA
Laura A. Colangelo, Lifang Hou & Donald M. Lloyd-Jones
Multimodal Imaging of Neurodegenerative Disease (MIND) Unit, National Institute on Aging, NIH, Baltimore, MD, USA
Keenan A. Walker
Division of Cancer Epidemiology and Genetics, National Cancer Institute, Rockville, MD, USA
Eleanor L. Watts
Department of Epidemiology, University of Alabama at Birmingham, Birmingham, AL, USA
Kelley Pettee Gabriel & Bjoern Hornikel
Kaiser Permanente, Oakland, CA, USA
Stephen Sidney
Cardiology Division, Massachusetts General Hospital, Boston, MA, USA
Nicholas Houstis & Gregory D. Lewis
Division of Pulmonary, Critical Care, and Sleep Medicine, Department of Medicine, University of California Davis, Sacramento, CA, USA
Gabrielle Y. Liu
Department of Laboratory Medicine and Pathology, University of Minnesota, Minnesota, MN, USA
Bharat Thyagarajan
Department of Medicine, Northwestern University Feinberg School of Medicine, Chicago, IL, USA
Sadiya S. Khan
Division of Pulmonary and Critical Care Medicine, Department of Medicine, Brigham and Women’s Hospital, Boston, MA, USA
Bina Choi & George Washko
Division of Pulmonary and Critical Care Medicine, Department of Medicine, Northwestern University Feinberg School of Medicine, Chicago, IL, USA
Ravi Kalhan
Human Genomic Laboratory, Pennington Biomedical Research Center, Baton Rouge, LA, USA
Claude Bouchard
Department of Exercise Science, University of South Carolina Columbia, Columbia, SC, USA
Mark A. Sarzynski
Sections of Cardiovascular Medicine and Preventive Medicine and Epidemiology, Department of Medicine, Boston University School of Medicine, Boston, MA, USA
Matthew Nayor
You can also search for this author in PubMed Google Scholar
Contributions
A.S.P., T.G., S.B., M.N. and R.V.S. contributed to the conceptualization. Analyses in CARDIA were performed by A.S.P., L.A.C. and R.V.S. Analyses in Fenland were performed by T.G. and S.B. Analyses in BLSA were performed by T.T. and K.A.W. Analyses in UKB were performed by A.S.P., E.F.-E., S.H., Q.S.W. and R.V.S. Analyses in HERITAGE were performed by J.M.R., S.D. and R.E.G. A.S.P., T.G., E.F.-E., T.T., J.M.R., V.L.M., L.K.S., S.Z., S.H., L.A.C., S.D., L.H., D.M.L.-J., K.A.W., L.F., E.L.W., J.L.B., P.R., M.Y.M., K.P.G., B.H., S.S., N.H., G.D.L., G.Y.L., B.T., S.S.K., G.W., B.C., R.K., N.W., C.B., M.A.S., R.E.G., S.B., Q.S.W., M.N. and R.V.S., contributed to data acquisition, data analysis or interpretation of data. A.S.P. and R.V.S. drafted the initial manuscript. All authors contributed to critical revisions and approval of the final manuscript.
Corresponding author
Correspondence to Ravi V. Shah .
Ethics declarations
Competing interests.
R.V.S. and A.S.P. have applied for a patent related to the findings in this manuscript. R.V.S. is supported in part by grants from the National Institutes of Health and the American Heart Association. In the past 12 months, R.V.S. has served for a consultant for Amgen and Cytokinetics. R.V.S. is a co-inventor on a patent for ex-RNAs signatures of cardiac remodeling and a pending patent on proteomic signatures of fitness and lung and liver diseases. V.L.M. has received grant support from Siemens Healthineers, NIDDK, NIA, NHLBI and AHA. V.L.M. has received other research support from NIVA Medical Imaging Solutions. V.L.M. owns stock in Eli Lilly, Johnson & Johnson, Merck, Bristo-Myers Squibb, Pfizer and stock options in Ionetix. V.L.M. has received research grants and speaking honoraria from Quart Medical. G.D.L. has hospital-based research agreements with from National Institutes of Health R01-HL 151841, R01-HL131029, R01-HL159514, U01HL160278, American Heart Association 15GPSGC-24800006 and SFRN for research involving exercise omics, and has received consulting fees from American Regent, Amgen, Cytokinetics, Boehringer Ingelheim, and Edwards and has received royalties from UpToDate for scientific content authorship related to exercise physiology. M.N. has received speaking honoraria from Cytokinetics. The remaining authors declare no competing interests.
Peer review
Peer review information.
Nature Medicine thanks Jonatan Ruiz, Jason Gill and Lili Niu for their contribution to the peer review of this work. Primary Handling Editor: Michael Basson, in collaboration with the Nature Medicine team.
Additional information
Publisher’s note Springer Nature remains neutral with regard to jurisdictional claims in published maps and institutional affiliations.
Extended data
Extended data fig. 1 relationship of a protein score of fitness with vo 2 max, age, sex, race and bmi in 3 validation cohorts..
The proteomic CRF score was scaled (mean 0, variance 1) in BLSA and HERITAGE cohorts. Colors on scatter plots represent density of overlapping observations with red being the most dense and blue the least dense. P values on panels showing the relationship of the proteomic CRF score with sex and race are from linear regression models of the proteomic CRF score as a function of sex and race. All other panels report P values from Spearman rank correlation tests. P values below 2.2 × 10 –16 are reported as p < 2.2e-16.
Extended Data Fig. 2 Relations of a protein score of fitness with age, sex, race and BMI in UK Biobank.
Colors on scatter plots represent density of overlapping observations with red being the most dense and blue the least dense. P values on panels showing the relationship of the proteomic CRF score with sex and race are from linear regression models of the proteomic CRF score as a function of sex and race. All other panels report P values from Spearman rank correlation tests. P values below 2.2 × 10 –16 are reported as p < 2.2e-16.
Extended Data Fig. 3 Correlation of change in proteomic CRF score with change in peak VO 2 with exercise training in HERITAGE.
After a 20-week exercise training program in HERITAGE, we observed correlation between changes in the proteomic CRF score with changes in peak VO 2 , which were replicated in regression models. P value is from two sided Spearman rank correlation test.
Supplementary information
Supplementary information.
Supplemental Fig. 1.
Reporting Summary
Supplemental tables 1–14.
Supplemental Tables 1–14.
Rights and permissions
Open Access This article is licensed under a Creative Commons Attribution 4.0 International License, which permits use, sharing, adaptation, distribution and reproduction in any medium or format, as long as you give appropriate credit to the original author(s) and the source, provide a link to the Creative Commons licence, and indicate if changes were made. The images or other third party material in this article are included in the article’s Creative Commons licence, unless indicated otherwise in a credit line to the material. If material is not included in the article’s Creative Commons licence and your intended use is not permitted by statutory regulation or exceeds the permitted use, you will need to obtain permission directly from the copyright holder. To view a copy of this licence, visit http://creativecommons.org/licenses/by/4.0/ .
Reprints and permissions
About this article
Cite this article.
Perry, A.S., Farber-Eger, E., Gonzales, T. et al. Proteomic analysis of cardiorespiratory fitness for prediction of mortality and multisystem disease risks. Nat Med (2024). https://doi.org/10.1038/s41591-024-03039-x
Download citation
Received : 18 October 2023
Accepted : 30 April 2024
Published : 04 June 2024
DOI : https://doi.org/10.1038/s41591-024-03039-x
Share this article
Anyone you share the following link with will be able to read this content:
Sorry, a shareable link is not currently available for this article.
Provided by the Springer Nature SharedIt content-sharing initiative
Quick links
- Explore articles by subject
- Guide to authors
- Editorial policies
Sign up for the Nature Briefing newsletter — what matters in science, free to your inbox daily.

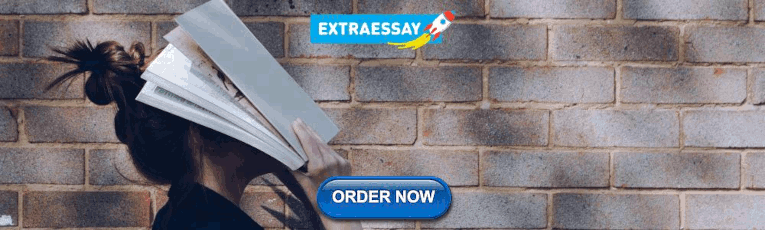
IMAGES
VIDEO
COMMENTS
The UK Biobank Research Analysis Platform takes the fantastic data generated by the UK Biobank project and removes the few barriers to entry. By making all of the data available on the cloud through DNAnexus, I can readily scale my computing needs based on my current analysis. The support team recruited by DNAnexus to assist researchers in ...
Experience the global network for scientific collaboration and accelerated discovery
The UK Biobank Research Analysis Platform is an informatics platform that provides access to, and analysis of, UK Biobank data by its registered researcher community. Read the announcement. See the DNAnexus website for more information on the Research Analysis Platform, including details on applying for access. Next 500k WGS FAQ.
Note that as a rule, Research Analysis Platform access and sharing restrictions are related to UK Biobank access applications. All Research Analysis Platform projects are linked to a UK Biobank-approved access application. To access a project, a user must be named as a Principal Investigator or collaborator, on the linked access application ...
On the Research Analysis Platform, you'll be able to work with UK Biobank data from data-fields associated with your approved access application. To access this data, start by creating a project and having the data dispensed to this project. Data dispensal can take over an hour, or even longer, in some cases. You can monitor the process by ...
Abstract. UK Biobank is a large-scale prospective study with deep phenotyping and genomic data. Its open-access policy allows researchers worldwide, from academia or industry, to perform health ...
MOUNTAIN VIEW, Calif. — Sept. 28, 2023 — DNAnexus, Inc., the leading provider of cloud-based genomic and biomedical data access and companion analysis software, today announced that the DNAnexus-enabled UK Biobank Research Analysis Platform (UKB-RAP) community has grown to more than 5,000 users around the world. The UKB-RAP was designed to allow researchers to access and analyze the ...
The UK Biobank Platform Credits Program is courtesy of AWS. The program is available to all early career researchers and those researchers from low- and low-middle income countries to explore the RAP in detail, develop and test tools and methods, and undertake analysis to support their research project. Credits can be used to cover costs of compute and storage above £40 credits provided by ...
That's why we partnered with UK Biobank to build a scalable, secure, and collaborative Research Analysis Platform to accelerate the speed and scale of health-related research. Enabled by DNAnexus technology and powered by Amazon Web Services (AWS), RAP provides approved researchers with the ability to access and analyze the 11 petabytes of ...
An introduction to using the Research Analysis Platform to explore and analyze the uniquely rich UK Biobank dataset. Get details on how to:-Use the Platform ...
This series of short tutorials introduces you to the UKB-RAP and highlights key features of the platform (Cohort Browser, JupyterLab, RStudio) to help you ge...
Leveraging the power and scalability of the cutting-edge, cloud-native DNAnexus Platform, the Research Analysis Platform enables researchers easily and quick...
Here's how: Navigate to the Research Analysis Platform. Click Create an Account. Fill out the Create New Account form, then click Create Account. Note that in selecting a username, you don't need to use the same username you use on the UK Biobank AMS. You'll receive an email with a link to click, to activate your account.
UK Biobank (UKB) Research Analysis Platform (RAP) UKB is a large-scale biomedical database and research resource, containing in-depth genetic and health information from 0.5 million UK participants, including sociodemographics, environmental factors, lifestyle factors, blood biochemistry assays, health outcome linkage, multimodal imaging ...
Online support within the UK Biobank Research Analysis Platform supports experienced and new users alike, providing a springboard for improving workflow and sharing best practice for analysis of uniquely large data sets. "The UK Biobank RAP provided a reliable and transparent service, ideal to develop, test and benchmark my scientific software.
It's been over two years since the launch of the UK Biobank Research Analysis Platform (UKB-RAP) and we've learned a lot about what researchers need and the diverse needs of this user community. To address this diversity, DNAnexus is launching an official support model that is multi-pronged and will serve the different needs of this growing ...
Will is starting a joint role with the University and the UK Biobank in the summer so is an excellent addition to the team! Keep an eye on the user space on Teams for online activity over the summer. We have been approached by the UK Biobank to help gather feedback on various items such as the Research Analysis Platform (RAP) and their website.
This project was carried out under UK Biobank Access Application 3593. UK Biobank will make the data available to all bona fide researchers for all types of health-related research that is in the public interest, without preferential or exclusive access for any persons. ... The analysis included 485 257 participants (55% women, mean age 56.5 ...
An analysis of UK Biobank data on musculoskeletal pain. Br J Pain 9(4):203-212. ... Funding was obtained from The MED MI Platform (Medical and Environmental Data—a Mashup Infrastructure) MR/K019341/1 is funded in part by the UK Medical Research Council (MRC) and the UK Natural Environment Research Council (NERC) and by the European Regional ...
On the Research Analysis Platform Projects screen, click the New Project button. The New Project wizard will open in a modal window. In the Project Name field, enter a name for your project. In the Application ID field, enter the number of the approved UK Biobank access application from which you'll draw the data to be used in this project.
The researchers reviewed studies from the past five years that used UK Biobank data to research breast cancer. Genetic data. Their results, published in the Computational and Structural Biotechnology Journal, found 125 studies, with 76 focusing on genetic data, and only two studies looking at protein and metabolic data. None used all types of ...
Data on testosterone and SHBG: Summary statistics for the association of rs1229984 with SD increments in the concentrations of hormones and SHBG were obtained from a publicly available GWAS of all women, regardless of menopausal status, from the UK Biobank, extracted from the OpenGWAS platform 37 (data set used for total testosterone: ieu-b ...
When you create a project on the UK Biobank Research Analysis Platform, the system dispenses the data corresponding to the data-fields listed in the access application associated with the project. Bulk data-fields are dispensed as files. See Bulk Data Files below for more. Tabular data-fields and linked health data are placed into a Spark SQL ...
In a cohort of around 22,000 individuals in the UK Biobank, a proteomic CRF score was associated with a reduced risk of all-cause mortality (unadjusted hazard ratio 0.50 (95% confidence interval 0 ...