
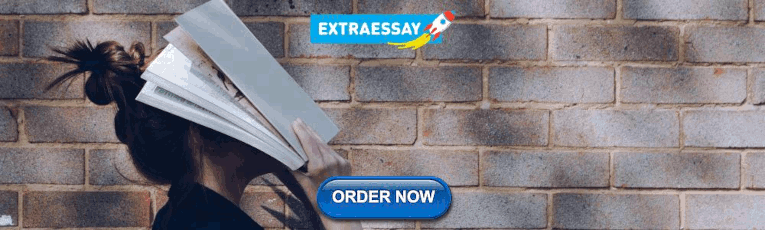
Research Design 101
Everything You Need To Get Started (With Examples)
By: Derek Jansen (MBA) | Reviewers: Eunice Rautenbach (DTech) & Kerryn Warren (PhD) | April 2023
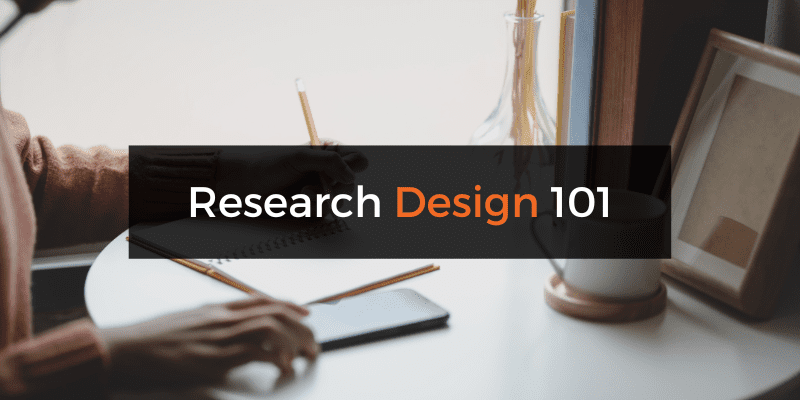
Navigating the world of research can be daunting, especially if you’re a first-time researcher. One concept you’re bound to run into fairly early in your research journey is that of “ research design ”. Here, we’ll guide you through the basics using practical examples , so that you can approach your research with confidence.
Overview: Research Design 101
What is research design.
- Research design types for quantitative studies
- Video explainer : quantitative research design
- Research design types for qualitative studies
- Video explainer : qualitative research design
- How to choose a research design
- Key takeaways
Research design refers to the overall plan, structure or strategy that guides a research project , from its conception to the final data analysis. A good research design serves as the blueprint for how you, as the researcher, will collect and analyse data while ensuring consistency, reliability and validity throughout your study.
Understanding different types of research designs is essential as helps ensure that your approach is suitable given your research aims, objectives and questions , as well as the resources you have available to you. Without a clear big-picture view of how you’ll design your research, you run the risk of potentially making misaligned choices in terms of your methodology – especially your sampling , data collection and data analysis decisions.
The problem with defining research design…
One of the reasons students struggle with a clear definition of research design is because the term is used very loosely across the internet, and even within academia.
Some sources claim that the three research design types are qualitative, quantitative and mixed methods , which isn’t quite accurate (these just refer to the type of data that you’ll collect and analyse). Other sources state that research design refers to the sum of all your design choices, suggesting it’s more like a research methodology . Others run off on other less common tangents. No wonder there’s confusion!
In this article, we’ll clear up the confusion. We’ll explain the most common research design types for both qualitative and quantitative research projects, whether that is for a full dissertation or thesis, or a smaller research paper or article.
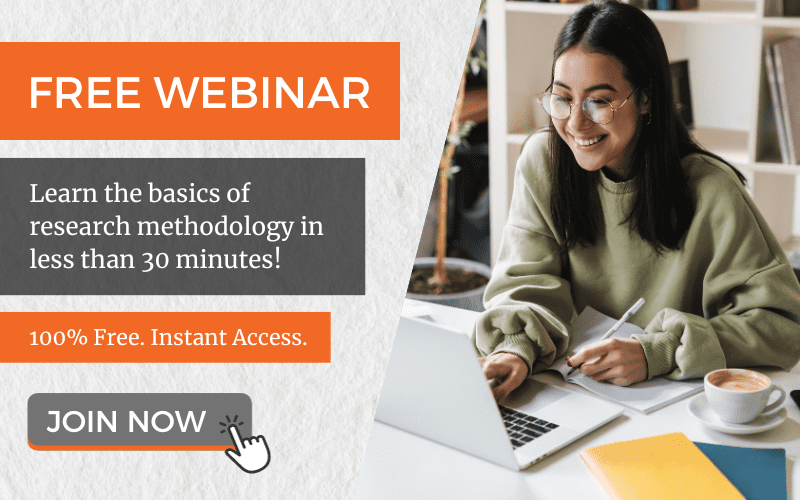
Research Design: Quantitative Studies
Quantitative research involves collecting and analysing data in a numerical form. Broadly speaking, there are four types of quantitative research designs: descriptive , correlational , experimental , and quasi-experimental .
Descriptive Research Design
As the name suggests, descriptive research design focuses on describing existing conditions, behaviours, or characteristics by systematically gathering information without manipulating any variables. In other words, there is no intervention on the researcher’s part – only data collection.
For example, if you’re studying smartphone addiction among adolescents in your community, you could deploy a survey to a sample of teens asking them to rate their agreement with certain statements that relate to smartphone addiction. The collected data would then provide insight regarding how widespread the issue may be – in other words, it would describe the situation.
The key defining attribute of this type of research design is that it purely describes the situation . In other words, descriptive research design does not explore potential relationships between different variables or the causes that may underlie those relationships. Therefore, descriptive research is useful for generating insight into a research problem by describing its characteristics . By doing so, it can provide valuable insights and is often used as a precursor to other research design types.
Correlational Research Design
Correlational design is a popular choice for researchers aiming to identify and measure the relationship between two or more variables without manipulating them . In other words, this type of research design is useful when you want to know whether a change in one thing tends to be accompanied by a change in another thing.
For example, if you wanted to explore the relationship between exercise frequency and overall health, you could use a correlational design to help you achieve this. In this case, you might gather data on participants’ exercise habits, as well as records of their health indicators like blood pressure, heart rate, or body mass index. Thereafter, you’d use a statistical test to assess whether there’s a relationship between the two variables (exercise frequency and health).
As you can see, correlational research design is useful when you want to explore potential relationships between variables that cannot be manipulated or controlled for ethical, practical, or logistical reasons. It is particularly helpful in terms of developing predictions , and given that it doesn’t involve the manipulation of variables, it can be implemented at a large scale more easily than experimental designs (which will look at next).
That said, it’s important to keep in mind that correlational research design has limitations – most notably that it cannot be used to establish causality . In other words, correlation does not equal causation . To establish causality, you’ll need to move into the realm of experimental design, coming up next…
Need a helping hand?
Experimental Research Design
Experimental research design is used to determine if there is a causal relationship between two or more variables . With this type of research design, you, as the researcher, manipulate one variable (the independent variable) while controlling others (dependent variables). Doing so allows you to observe the effect of the former on the latter and draw conclusions about potential causality.
For example, if you wanted to measure if/how different types of fertiliser affect plant growth, you could set up several groups of plants, with each group receiving a different type of fertiliser, as well as one with no fertiliser at all. You could then measure how much each plant group grew (on average) over time and compare the results from the different groups to see which fertiliser was most effective.
Overall, experimental research design provides researchers with a powerful way to identify and measure causal relationships (and the direction of causality) between variables. However, developing a rigorous experimental design can be challenging as it’s not always easy to control all the variables in a study. This often results in smaller sample sizes , which can reduce the statistical power and generalisability of the results.
Moreover, experimental research design requires random assignment . This means that the researcher needs to assign participants to different groups or conditions in a way that each participant has an equal chance of being assigned to any group (note that this is not the same as random sampling ). Doing so helps reduce the potential for bias and confounding variables . This need for random assignment can lead to ethics-related issues . For example, withholding a potentially beneficial medical treatment from a control group may be considered unethical in certain situations.
Quasi-Experimental Research Design
Quasi-experimental research design is used when the research aims involve identifying causal relations , but one cannot (or doesn’t want to) randomly assign participants to different groups (for practical or ethical reasons). Instead, with a quasi-experimental research design, the researcher relies on existing groups or pre-existing conditions to form groups for comparison.
For example, if you were studying the effects of a new teaching method on student achievement in a particular school district, you may be unable to randomly assign students to either group and instead have to choose classes or schools that already use different teaching methods. This way, you still achieve separate groups, without having to assign participants to specific groups yourself.
Naturally, quasi-experimental research designs have limitations when compared to experimental designs. Given that participant assignment is not random, it’s more difficult to confidently establish causality between variables, and, as a researcher, you have less control over other variables that may impact findings.
All that said, quasi-experimental designs can still be valuable in research contexts where random assignment is not possible and can often be undertaken on a much larger scale than experimental research, thus increasing the statistical power of the results. What’s important is that you, as the researcher, understand the limitations of the design and conduct your quasi-experiment as rigorously as possible, paying careful attention to any potential confounding variables .
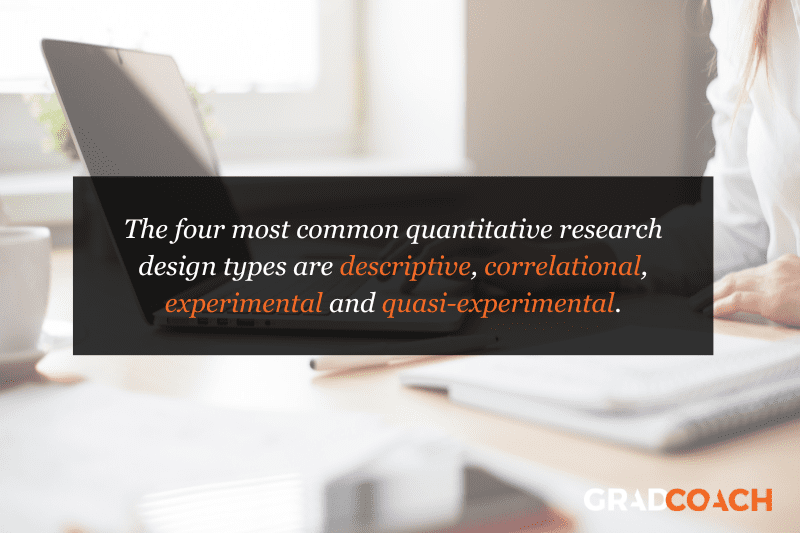
Research Design: Qualitative Studies
There are many different research design types when it comes to qualitative studies, but here we’ll narrow our focus to explore the “Big 4”. Specifically, we’ll look at phenomenological design, grounded theory design, ethnographic design, and case study design.
Phenomenological Research Design
Phenomenological design involves exploring the meaning of lived experiences and how they are perceived by individuals. This type of research design seeks to understand people’s perspectives , emotions, and behaviours in specific situations. Here, the aim for researchers is to uncover the essence of human experience without making any assumptions or imposing preconceived ideas on their subjects.
For example, you could adopt a phenomenological design to study why cancer survivors have such varied perceptions of their lives after overcoming their disease. This could be achieved by interviewing survivors and then analysing the data using a qualitative analysis method such as thematic analysis to identify commonalities and differences.
Phenomenological research design typically involves in-depth interviews or open-ended questionnaires to collect rich, detailed data about participants’ subjective experiences. This richness is one of the key strengths of phenomenological research design but, naturally, it also has limitations. These include potential biases in data collection and interpretation and the lack of generalisability of findings to broader populations.
Grounded Theory Research Design
Grounded theory (also referred to as “GT”) aims to develop theories by continuously and iteratively analysing and comparing data collected from a relatively large number of participants in a study. It takes an inductive (bottom-up) approach, with a focus on letting the data “speak for itself”, without being influenced by preexisting theories or the researcher’s preconceptions.
As an example, let’s assume your research aims involved understanding how people cope with chronic pain from a specific medical condition, with a view to developing a theory around this. In this case, grounded theory design would allow you to explore this concept thoroughly without preconceptions about what coping mechanisms might exist. You may find that some patients prefer cognitive-behavioural therapy (CBT) while others prefer to rely on herbal remedies. Based on multiple, iterative rounds of analysis, you could then develop a theory in this regard, derived directly from the data (as opposed to other preexisting theories and models).
Grounded theory typically involves collecting data through interviews or observations and then analysing it to identify patterns and themes that emerge from the data. These emerging ideas are then validated by collecting more data until a saturation point is reached (i.e., no new information can be squeezed from the data). From that base, a theory can then be developed .
As you can see, grounded theory is ideally suited to studies where the research aims involve theory generation , especially in under-researched areas. Keep in mind though that this type of research design can be quite time-intensive , given the need for multiple rounds of data collection and analysis.
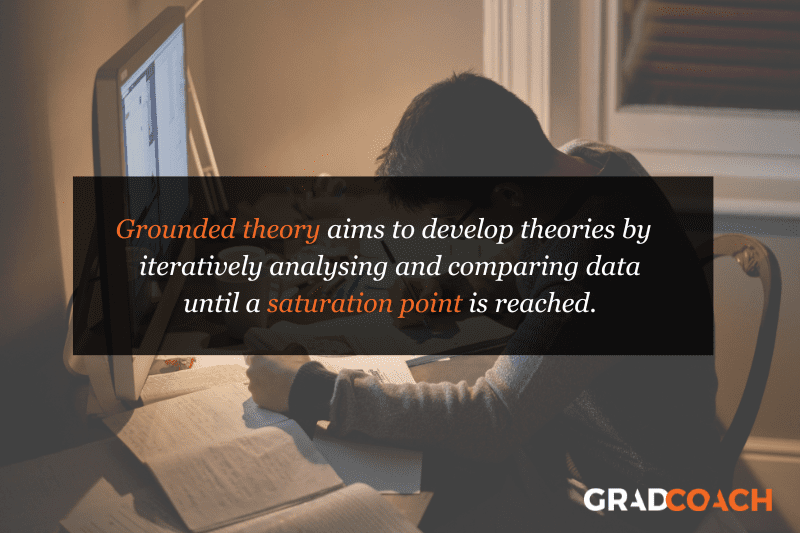
Ethnographic Research Design
Ethnographic design involves observing and studying a culture-sharing group of people in their natural setting to gain insight into their behaviours, beliefs, and values. The focus here is on observing participants in their natural environment (as opposed to a controlled environment). This typically involves the researcher spending an extended period of time with the participants in their environment, carefully observing and taking field notes .
All of this is not to say that ethnographic research design relies purely on observation. On the contrary, this design typically also involves in-depth interviews to explore participants’ views, beliefs, etc. However, unobtrusive observation is a core component of the ethnographic approach.
As an example, an ethnographer may study how different communities celebrate traditional festivals or how individuals from different generations interact with technology differently. This may involve a lengthy period of observation, combined with in-depth interviews to further explore specific areas of interest that emerge as a result of the observations that the researcher has made.
As you can probably imagine, ethnographic research design has the ability to provide rich, contextually embedded insights into the socio-cultural dynamics of human behaviour within a natural, uncontrived setting. Naturally, however, it does come with its own set of challenges, including researcher bias (since the researcher can become quite immersed in the group), participant confidentiality and, predictably, ethical complexities . All of these need to be carefully managed if you choose to adopt this type of research design.
Case Study Design
With case study research design, you, as the researcher, investigate a single individual (or a single group of individuals) to gain an in-depth understanding of their experiences, behaviours or outcomes. Unlike other research designs that are aimed at larger sample sizes, case studies offer a deep dive into the specific circumstances surrounding a person, group of people, event or phenomenon, generally within a bounded setting or context .
As an example, a case study design could be used to explore the factors influencing the success of a specific small business. This would involve diving deeply into the organisation to explore and understand what makes it tick – from marketing to HR to finance. In terms of data collection, this could include interviews with staff and management, review of policy documents and financial statements, surveying customers, etc.
While the above example is focused squarely on one organisation, it’s worth noting that case study research designs can have different variation s, including single-case, multiple-case and longitudinal designs. As you can see in the example, a single-case design involves intensely examining a single entity to understand its unique characteristics and complexities. Conversely, in a multiple-case design , multiple cases are compared and contrasted to identify patterns and commonalities. Lastly, in a longitudinal case design , a single case or multiple cases are studied over an extended period of time to understand how factors develop over time.
As you can see, a case study research design is particularly useful where a deep and contextualised understanding of a specific phenomenon or issue is desired. However, this strength is also its weakness. In other words, you can’t generalise the findings from a case study to the broader population. So, keep this in mind if you’re considering going the case study route.
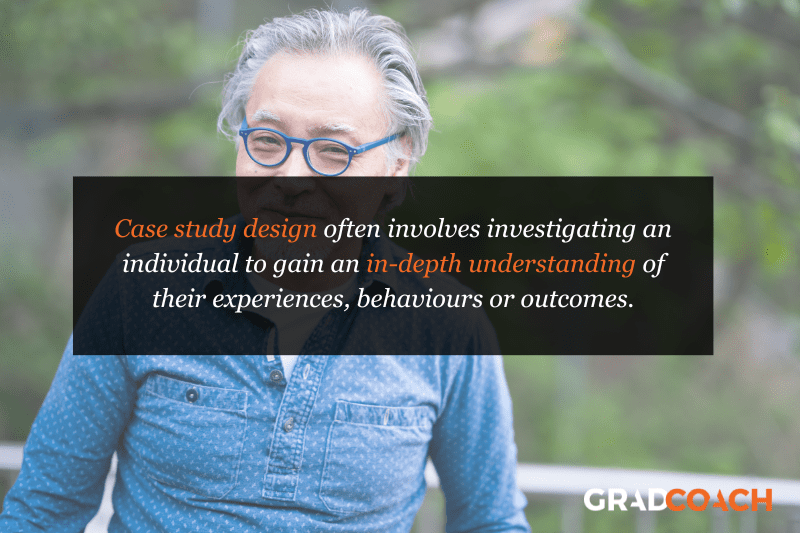
How To Choose A Research Design
Having worked through all of these potential research designs, you’d be forgiven for feeling a little overwhelmed and wondering, “ But how do I decide which research design to use? ”. While we could write an entire post covering that alone, here are a few factors to consider that will help you choose a suitable research design for your study.
Data type: The first determining factor is naturally the type of data you plan to be collecting – i.e., qualitative or quantitative. This may sound obvious, but we have to be clear about this – don’t try to use a quantitative research design on qualitative data (or vice versa)!
Research aim(s) and question(s): As with all methodological decisions, your research aim and research questions will heavily influence your research design. For example, if your research aims involve developing a theory from qualitative data, grounded theory would be a strong option. Similarly, if your research aims involve identifying and measuring relationships between variables, one of the experimental designs would likely be a better option.
Time: It’s essential that you consider any time constraints you have, as this will impact the type of research design you can choose. For example, if you’ve only got a month to complete your project, a lengthy design such as ethnography wouldn’t be a good fit.
Resources: Take into account the resources realistically available to you, as these need to factor into your research design choice. For example, if you require highly specialised lab equipment to execute an experimental design, you need to be sure that you’ll have access to that before you make a decision.
Keep in mind that when it comes to research, it’s important to manage your risks and play as conservatively as possible. If your entire project relies on you achieving a huge sample, having access to niche equipment or holding interviews with very difficult-to-reach participants, you’re creating risks that could kill your project. So, be sure to think through your choices carefully and make sure that you have backup plans for any existential risks. Remember that a relatively simple methodology executed well generally will typically earn better marks than a highly-complex methodology executed poorly.
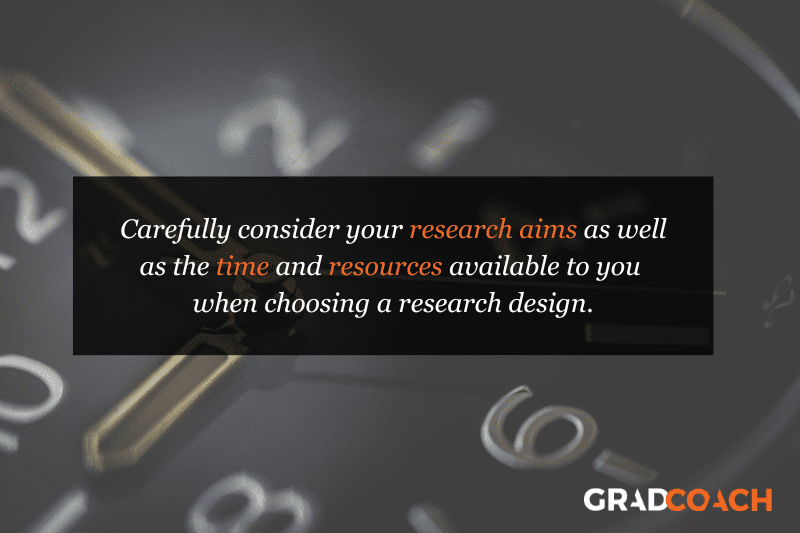
Recap: Key Takeaways
We’ve covered a lot of ground here. Let’s recap by looking at the key takeaways:
- Research design refers to the overall plan, structure or strategy that guides a research project, from its conception to the final analysis of data.
- Research designs for quantitative studies include descriptive , correlational , experimental and quasi-experimenta l designs.
- Research designs for qualitative studies include phenomenological , grounded theory , ethnographic and case study designs.
- When choosing a research design, you need to consider a variety of factors, including the type of data you’ll be working with, your research aims and questions, your time and the resources available to you.
If you need a helping hand with your research design (or any other aspect of your research), check out our private coaching services .
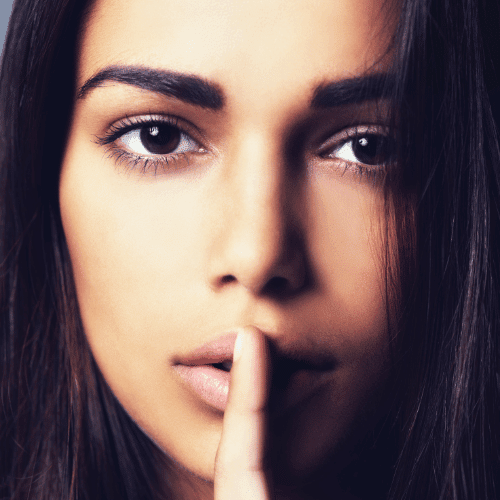
Psst... there’s more!
This post was based on one of our popular Research Bootcamps . If you're working on a research project, you'll definitely want to check this out ...
You Might Also Like:
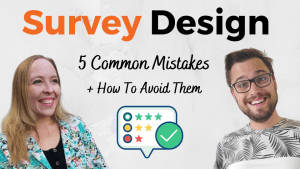
Is there any blog article explaining more on Case study research design? Is there a Case study write-up template? Thank you.
Thanks this was quite valuable to clarify such an important concept.
Thanks for this simplified explanations. it is quite very helpful.
This was really helpful. thanks
Thank you for your explanation. I think case study research design and the use of secondary data in researches needs to be talked about more in your videos and articles because there a lot of case studies research design tailored projects out there.
Please is there any template for a case study research design whose data type is a secondary data on your repository?
This post is very clear, comprehensive and has been very helpful to me. It has cleared the confusion I had in regard to research design and methodology.
This post is helpful, easy to understand, and deconstructs what a research design is. Thanks
how to cite this page
Thank you very much for the post. It is wonderful and has cleared many worries in my mind regarding research designs. I really appreciate .
Submit a Comment Cancel reply
Your email address will not be published. Required fields are marked *
Save my name, email, and website in this browser for the next time I comment.
- Print Friendly
Have a language expert improve your writing
Run a free plagiarism check in 10 minutes, automatically generate references for free.
- Knowledge Base
- Methodology
Research Design | Step-by-Step Guide with Examples
Published on 5 May 2022 by Shona McCombes . Revised on 20 March 2023.
A research design is a strategy for answering your research question using empirical data. Creating a research design means making decisions about:
- Your overall aims and approach
- The type of research design you’ll use
- Your sampling methods or criteria for selecting subjects
- Your data collection methods
- The procedures you’ll follow to collect data
- Your data analysis methods
A well-planned research design helps ensure that your methods match your research aims and that you use the right kind of analysis for your data.
Table of contents
Step 1: consider your aims and approach, step 2: choose a type of research design, step 3: identify your population and sampling method, step 4: choose your data collection methods, step 5: plan your data collection procedures, step 6: decide on your data analysis strategies, frequently asked questions.
- Introduction
Before you can start designing your research, you should already have a clear idea of the research question you want to investigate.
There are many different ways you could go about answering this question. Your research design choices should be driven by your aims and priorities – start by thinking carefully about what you want to achieve.
The first choice you need to make is whether you’ll take a qualitative or quantitative approach.
Qualitative research designs tend to be more flexible and inductive , allowing you to adjust your approach based on what you find throughout the research process.
Quantitative research designs tend to be more fixed and deductive , with variables and hypotheses clearly defined in advance of data collection.
It’s also possible to use a mixed methods design that integrates aspects of both approaches. By combining qualitative and quantitative insights, you can gain a more complete picture of the problem you’re studying and strengthen the credibility of your conclusions.
Practical and ethical considerations when designing research
As well as scientific considerations, you need to think practically when designing your research. If your research involves people or animals, you also need to consider research ethics .
- How much time do you have to collect data and write up the research?
- Will you be able to gain access to the data you need (e.g., by travelling to a specific location or contacting specific people)?
- Do you have the necessary research skills (e.g., statistical analysis or interview techniques)?
- Will you need ethical approval ?
At each stage of the research design process, make sure that your choices are practically feasible.
Prevent plagiarism, run a free check.
Within both qualitative and quantitative approaches, there are several types of research design to choose from. Each type provides a framework for the overall shape of your research.
Types of quantitative research designs
Quantitative designs can be split into four main types. Experimental and quasi-experimental designs allow you to test cause-and-effect relationships, while descriptive and correlational designs allow you to measure variables and describe relationships between them.
With descriptive and correlational designs, you can get a clear picture of characteristics, trends, and relationships as they exist in the real world. However, you can’t draw conclusions about cause and effect (because correlation doesn’t imply causation ).
Experiments are the strongest way to test cause-and-effect relationships without the risk of other variables influencing the results. However, their controlled conditions may not always reflect how things work in the real world. They’re often also more difficult and expensive to implement.
Types of qualitative research designs
Qualitative designs are less strictly defined. This approach is about gaining a rich, detailed understanding of a specific context or phenomenon, and you can often be more creative and flexible in designing your research.
The table below shows some common types of qualitative design. They often have similar approaches in terms of data collection, but focus on different aspects when analysing the data.
Your research design should clearly define who or what your research will focus on, and how you’ll go about choosing your participants or subjects.
In research, a population is the entire group that you want to draw conclusions about, while a sample is the smaller group of individuals you’ll actually collect data from.
Defining the population
A population can be made up of anything you want to study – plants, animals, organisations, texts, countries, etc. In the social sciences, it most often refers to a group of people.
For example, will you focus on people from a specific demographic, region, or background? Are you interested in people with a certain job or medical condition, or users of a particular product?
The more precisely you define your population, the easier it will be to gather a representative sample.
Sampling methods
Even with a narrowly defined population, it’s rarely possible to collect data from every individual. Instead, you’ll collect data from a sample.
To select a sample, there are two main approaches: probability sampling and non-probability sampling . The sampling method you use affects how confidently you can generalise your results to the population as a whole.
Probability sampling is the most statistically valid option, but it’s often difficult to achieve unless you’re dealing with a very small and accessible population.
For practical reasons, many studies use non-probability sampling, but it’s important to be aware of the limitations and carefully consider potential biases. You should always make an effort to gather a sample that’s as representative as possible of the population.
Case selection in qualitative research
In some types of qualitative designs, sampling may not be relevant.
For example, in an ethnography or a case study, your aim is to deeply understand a specific context, not to generalise to a population. Instead of sampling, you may simply aim to collect as much data as possible about the context you are studying.
In these types of design, you still have to carefully consider your choice of case or community. You should have a clear rationale for why this particular case is suitable for answering your research question.
For example, you might choose a case study that reveals an unusual or neglected aspect of your research problem, or you might choose several very similar or very different cases in order to compare them.
Data collection methods are ways of directly measuring variables and gathering information. They allow you to gain first-hand knowledge and original insights into your research problem.
You can choose just one data collection method, or use several methods in the same study.
Survey methods
Surveys allow you to collect data about opinions, behaviours, experiences, and characteristics by asking people directly. There are two main survey methods to choose from: questionnaires and interviews.
Observation methods
Observations allow you to collect data unobtrusively, observing characteristics, behaviours, or social interactions without relying on self-reporting.
Observations may be conducted in real time, taking notes as you observe, or you might make audiovisual recordings for later analysis. They can be qualitative or quantitative.
Other methods of data collection
There are many other ways you might collect data depending on your field and topic.
If you’re not sure which methods will work best for your research design, try reading some papers in your field to see what data collection methods they used.
Secondary data
If you don’t have the time or resources to collect data from the population you’re interested in, you can also choose to use secondary data that other researchers already collected – for example, datasets from government surveys or previous studies on your topic.
With this raw data, you can do your own analysis to answer new research questions that weren’t addressed by the original study.
Using secondary data can expand the scope of your research, as you may be able to access much larger and more varied samples than you could collect yourself.
However, it also means you don’t have any control over which variables to measure or how to measure them, so the conclusions you can draw may be limited.
As well as deciding on your methods, you need to plan exactly how you’ll use these methods to collect data that’s consistent, accurate, and unbiased.
Planning systematic procedures is especially important in quantitative research, where you need to precisely define your variables and ensure your measurements are reliable and valid.
Operationalisation
Some variables, like height or age, are easily measured. But often you’ll be dealing with more abstract concepts, like satisfaction, anxiety, or competence. Operationalisation means turning these fuzzy ideas into measurable indicators.
If you’re using observations , which events or actions will you count?
If you’re using surveys , which questions will you ask and what range of responses will be offered?
You may also choose to use or adapt existing materials designed to measure the concept you’re interested in – for example, questionnaires or inventories whose reliability and validity has already been established.
Reliability and validity
Reliability means your results can be consistently reproduced , while validity means that you’re actually measuring the concept you’re interested in.
For valid and reliable results, your measurement materials should be thoroughly researched and carefully designed. Plan your procedures to make sure you carry out the same steps in the same way for each participant.
If you’re developing a new questionnaire or other instrument to measure a specific concept, running a pilot study allows you to check its validity and reliability in advance.
Sampling procedures
As well as choosing an appropriate sampling method, you need a concrete plan for how you’ll actually contact and recruit your selected sample.
That means making decisions about things like:
- How many participants do you need for an adequate sample size?
- What inclusion and exclusion criteria will you use to identify eligible participants?
- How will you contact your sample – by mail, online, by phone, or in person?
If you’re using a probability sampling method, it’s important that everyone who is randomly selected actually participates in the study. How will you ensure a high response rate?
If you’re using a non-probability method, how will you avoid bias and ensure a representative sample?
Data management
It’s also important to create a data management plan for organising and storing your data.
Will you need to transcribe interviews or perform data entry for observations? You should anonymise and safeguard any sensitive data, and make sure it’s backed up regularly.
Keeping your data well organised will save time when it comes to analysing them. It can also help other researchers validate and add to your findings.
On their own, raw data can’t answer your research question. The last step of designing your research is planning how you’ll analyse the data.
Quantitative data analysis
In quantitative research, you’ll most likely use some form of statistical analysis . With statistics, you can summarise your sample data, make estimates, and test hypotheses.
Using descriptive statistics , you can summarise your sample data in terms of:
- The distribution of the data (e.g., the frequency of each score on a test)
- The central tendency of the data (e.g., the mean to describe the average score)
- The variability of the data (e.g., the standard deviation to describe how spread out the scores are)
The specific calculations you can do depend on the level of measurement of your variables.
Using inferential statistics , you can:
- Make estimates about the population based on your sample data.
- Test hypotheses about a relationship between variables.
Regression and correlation tests look for associations between two or more variables, while comparison tests (such as t tests and ANOVAs ) look for differences in the outcomes of different groups.
Your choice of statistical test depends on various aspects of your research design, including the types of variables you’re dealing with and the distribution of your data.
Qualitative data analysis
In qualitative research, your data will usually be very dense with information and ideas. Instead of summing it up in numbers, you’ll need to comb through the data in detail, interpret its meanings, identify patterns, and extract the parts that are most relevant to your research question.
Two of the most common approaches to doing this are thematic analysis and discourse analysis .
There are many other ways of analysing qualitative data depending on the aims of your research. To get a sense of potential approaches, try reading some qualitative research papers in your field.
A sample is a subset of individuals from a larger population. Sampling means selecting the group that you will actually collect data from in your research.
For example, if you are researching the opinions of students in your university, you could survey a sample of 100 students.
Statistical sampling allows you to test a hypothesis about the characteristics of a population. There are various sampling methods you can use to ensure that your sample is representative of the population as a whole.
Operationalisation means turning abstract conceptual ideas into measurable observations.
For example, the concept of social anxiety isn’t directly observable, but it can be operationally defined in terms of self-rating scores, behavioural avoidance of crowded places, or physical anxiety symptoms in social situations.
Before collecting data , it’s important to consider how you will operationalise the variables that you want to measure.
The research methods you use depend on the type of data you need to answer your research question .
- If you want to measure something or test a hypothesis , use quantitative methods . If you want to explore ideas, thoughts, and meanings, use qualitative methods .
- If you want to analyse a large amount of readily available data, use secondary data. If you want data specific to your purposes with control over how they are generated, collect primary data.
- If you want to establish cause-and-effect relationships between variables , use experimental methods. If you want to understand the characteristics of a research subject, use descriptive methods.
Cite this Scribbr article
If you want to cite this source, you can copy and paste the citation or click the ‘Cite this Scribbr article’ button to automatically add the citation to our free Reference Generator.
McCombes, S. (2023, March 20). Research Design | Step-by-Step Guide with Examples. Scribbr. Retrieved 22 April 2024, from https://www.scribbr.co.uk/research-methods/research-design/
Is this article helpful?
Shona McCombes

Skills for Learning : Research Skills
Data analysis is an ongoing process that should occur throughout your research project. Suitable data-analysis methods must be selected when you write your research proposal. The nature of your data (i.e. quantitative or qualitative) will be influenced by your research design and purpose. The data will also influence the analysis methods selected.
We run interactive workshops to help you develop skills related to doing research, such as data analysis, writing literature reviews and preparing for dissertations. Find out more on the Skills for Learning Workshops page.
We have online academic skills modules within MyBeckett for all levels of university study. These modules will help your academic development and support your success at LBU. You can work through the modules at your own pace, revisiting them as required. Find out more from our FAQ What academic skills modules are available?
Quantitative data analysis
Broadly speaking, 'statistics' refers to methods, tools and techniques used to collect, organise and interpret data. The goal of statistics is to gain understanding from data. Therefore, you need to know how to:
- Produce data – for example, by handing out a questionnaire or doing an experiment.
- Organise, summarise, present and analyse data.
- Draw valid conclusions from findings.
There are a number of statistical methods you can use to analyse data. Choosing an appropriate statistical method should follow naturally, however, from your research design. Therefore, you should think about data analysis at the early stages of your study design. You may need to consult a statistician for help with this.
Tips for working with statistical data
- Plan so that the data you get has a good chance of successfully tackling the research problem. This will involve reading literature on your subject, as well as on what makes a good study.
- To reach useful conclusions, you need to reduce uncertainties or 'noise'. Thus, you will need a sufficiently large data sample. A large sample will improve precision. However, this must be balanced against the 'costs' (time and money) of collection.
- Consider the logistics. Will there be problems in obtaining sufficient high-quality data? Think about accuracy, trustworthiness and completeness.
- Statistics are based on random samples. Consider whether your sample will be suited to this sort of analysis. Might there be biases to think about?
- How will you deal with missing values (any data that is not recorded for some reason)? These can result from gaps in a record or whole records being missed out.
- When analysing data, start by looking at each variable separately. Conduct initial/exploratory data analysis using graphical displays. Do this before looking at variables in conjunction or anything more complicated. This process can help locate errors in the data and also gives you a 'feel' for the data.
- Look out for patterns of 'missingness'. They are likely to alert you if there’s a problem. If the 'missingness' is not random, then it will have an impact on the results.
- Be vigilant and think through what you are doing at all times. Think critically. Statistics are not just mathematical tricks that a computer sorts out. Rather, analysing statistical data is a process that the human mind must interpret!
Top tips! Try inventing or generating the sort of data you might get and see if you can analyse it. Make sure that your process works before gathering actual data. Think what the output of an analytic procedure will look like before doing it for real.
(Note: it is actually difficult to generate realistic data. There are fraud-detection methods in place to identify data that has been fabricated. So, remember to get rid of your practice data before analysing the real stuff!)
Statistical software packages
Software packages can be used to analyse and present data. The most widely used ones are SPSS and NVivo.
SPSS is a statistical-analysis and data-management package for quantitative data analysis. Click on ‘ How do I install SPSS? ’ to learn how to download SPSS to your personal device. SPSS can perform a wide variety of statistical procedures. Some examples are:
- Data management (i.e. creating subsets of data or transforming data).
- Summarising, describing or presenting data (i.e. mean, median and frequency).
- Looking at the distribution of data (i.e. standard deviation).
- Comparing groups for significant differences using parametric (i.e. t-test) and non-parametric (i.e. Chi-square) tests.
- Identifying significant relationships between variables (i.e. correlation).
NVivo can be used for qualitative data analysis. It is suitable for use with a wide range of methodologies. Click on ‘ How do I access NVivo ’ to learn how to download NVivo to your personal device. NVivo supports grounded theory, survey data, case studies, focus groups, phenomenology, field research and action research.
- Process data such as interview transcripts, literature or media extracts, and historical documents.
- Code data on screen and explore all coding and documents interactively.
- Rearrange, restructure, extend and edit text, coding and coding relationships.
- Search imported text for words, phrases or patterns, and automatically code the results.
Qualitative data analysis
Miles and Huberman (1994) point out that there are diverse approaches to qualitative research and analysis. They suggest, however, that it is possible to identify 'a fairly classic set of analytic moves arranged in sequence'. This involves:
- Affixing codes to a set of field notes drawn from observation or interviews.
- Noting reflections or other remarks in the margins.
- Sorting/sifting through these materials to identify: a) similar phrases, relationships between variables, patterns and themes and b) distinct differences between subgroups and common sequences.
- Isolating these patterns/processes and commonalties/differences. Then, taking them out to the field in the next wave of data collection.
- Highlighting generalisations and relating them to your original research themes.
- Taking the generalisations and analysing them in relation to theoretical perspectives.
(Miles and Huberman, 1994.)
Patterns and generalisations are usually arrived at through a process of analytic induction (see above points 5 and 6). Qualitative analysis rarely involves statistical analysis of relationships between variables. Qualitative analysis aims to gain in-depth understanding of concepts, opinions or experiences.
Presenting information
There are a number of different ways of presenting and communicating information. The particular format you use is dependent upon the type of data generated from the methods you have employed.
Here are some appropriate ways of presenting information for different types of data:
Bar charts: These may be useful for comparing relative sizes. However, they tend to use a large amount of ink to display a relatively small amount of information. Consider a simple line chart as an alternative.
Pie charts: These have the benefit of indicating that the data must add up to 100%. However, they make it difficult for viewers to distinguish relative sizes, especially if two slices have a difference of less than 10%.
Other examples of presenting data in graphical form include line charts and scatter plots .
Qualitative data is more likely to be presented in text form. For example, using quotations from interviews or field diaries.
- Plan ahead, thinking carefully about how you will analyse and present your data.
- Think through possible restrictions to resources you may encounter and plan accordingly.
- Find out about the different IT packages available for analysing your data and select the most appropriate.
- If necessary, allow time to attend an introductory course on a particular computer package. You can book SPSS and NVivo workshops via MyHub .
- Code your data appropriately, assigning conceptual or numerical codes as suitable.
- Organise your data so it can be analysed and presented easily.
- Choose the most suitable way of presenting your information, according to the type of data collected. This will allow your information to be understood and interpreted better.
Primary, secondary and tertiary sources
Information sources are sometimes categorised as primary, secondary or tertiary sources depending on whether or not they are ‘original’ materials or data. For some research projects, you may need to use primary sources as well as secondary or tertiary sources. However the distinction between primary and secondary sources is not always clear and depends on the context. For example, a newspaper article might usually be categorised as a secondary source. But it could also be regarded as a primary source if it were an article giving a first-hand account of a historical event written close to the time it occurred.
- Primary sources
- Secondary sources
- Tertiary sources
- Grey literature
Primary sources are original sources of information that provide first-hand accounts of what is being experienced or researched. They enable you to get as close to the actual event or research as possible. They are useful for getting the most contemporary information about a topic.
Examples include diary entries, newspaper articles, census data, journal articles with original reports of research, letters, email or other correspondence, original manuscripts and archives, interviews, research data and reports, statistics, autobiographies, exhibitions, films, and artists' writings.
Some information will be available on an Open Access basis, freely accessible online. However, many academic sources are paywalled, and you may need to login as a Leeds Beckett student to access them. Where Leeds Beckett does not have access to a source, you can use our Request It! Service .
Secondary sources interpret, evaluate or analyse primary sources. They're useful for providing background information on a topic, or for looking back at an event from a current perspective. The majority of your literature searching will probably be done to find secondary sources on your topic.
Examples include journal articles which review or interpret original findings, popular magazine articles commenting on more serious research, textbooks and biographies.
The term tertiary sources isn't used a great deal. There's overlap between what might be considered a secondary source and a tertiary source. One definition is that a tertiary source brings together secondary sources.
Examples include almanacs, fact books, bibliographies, dictionaries and encyclopaedias, directories, indexes and abstracts. They can be useful for introductory information or an overview of a topic in the early stages of research.
Depending on your subject of study, grey literature may be another source you need to use. Grey literature includes technical or research reports, theses and dissertations, conference papers, government documents, white papers, and so on.
Artificial intelligence tools
Before using any generative artificial intelligence or paraphrasing tools in your assessments, you should check if this is permitted on your course.
If their use is permitted on your course, you must acknowledge any use of generative artificial intelligence tools such as ChatGPT or paraphrasing tools (e.g., Grammarly, Quillbot, etc.), even if you have only used them to generate ideas for your assessments or for proofreading.
- Academic Integrity Module in MyBeckett
- Assignment Calculator
- Building on Feedback
- Disability Advice
- Essay X-ray tool
- International Students' Academic Introduction
- Manchester Academic Phrasebank
- Quote, Unquote
- Skills and Subject Suppor t
- Turnitin Grammar Checker
{{You can add more boxes below for links specific to this page [this note will not appear on user pages] }}
- Research Methods Checklist
- Sampling Checklist
Skills for Learning FAQs

0113 812 1000
- University Disclaimer
- Accessibility
- Skip to main content
- Skip to primary sidebar
- Skip to footer
- QuestionPro

- Solutions Industries Gaming Automotive Sports and events Education Government Travel & Hospitality Financial Services Healthcare Cannabis Technology Use Case NPS+ Communities Audience Contactless surveys Mobile LivePolls Member Experience GDPR Positive People Science 360 Feedback Surveys
- Resources Blog eBooks Survey Templates Case Studies Training Help center

Home Market Research
Data Analysis in Research: Types & Methods
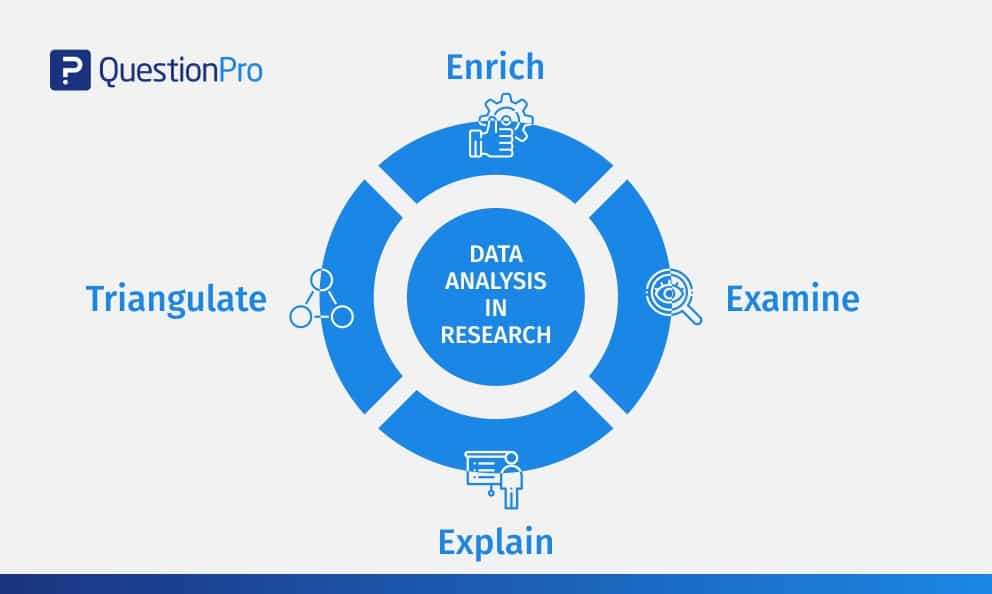
Content Index
Why analyze data in research?
Types of data in research, finding patterns in the qualitative data, methods used for data analysis in qualitative research, preparing data for analysis, methods used for data analysis in quantitative research, considerations in research data analysis, what is data analysis in research.
Definition of research in data analysis: According to LeCompte and Schensul, research data analysis is a process used by researchers to reduce data to a story and interpret it to derive insights. The data analysis process helps reduce a large chunk of data into smaller fragments, which makes sense.
Three essential things occur during the data analysis process — the first is data organization . Summarization and categorization together contribute to becoming the second known method used for data reduction. It helps find patterns and themes in the data for easy identification and linking. The third and last way is data analysis – researchers do it in both top-down and bottom-up fashion.
LEARN ABOUT: Research Process Steps
On the other hand, Marshall and Rossman describe data analysis as a messy, ambiguous, and time-consuming but creative and fascinating process through which a mass of collected data is brought to order, structure and meaning.
We can say that “the data analysis and data interpretation is a process representing the application of deductive and inductive logic to the research and data analysis.”
Researchers rely heavily on data as they have a story to tell or research problems to solve. It starts with a question, and data is nothing but an answer to that question. But, what if there is no question to ask? Well! It is possible to explore data even without a problem – we call it ‘Data Mining’, which often reveals some interesting patterns within the data that are worth exploring.
Irrelevant to the type of data researchers explore, their mission and audiences’ vision guide them to find the patterns to shape the story they want to tell. One of the essential things expected from researchers while analyzing data is to stay open and remain unbiased toward unexpected patterns, expressions, and results. Remember, sometimes, data analysis tells the most unforeseen yet exciting stories that were not expected when initiating data analysis. Therefore, rely on the data you have at hand and enjoy the journey of exploratory research.
Create a Free Account
Every kind of data has a rare quality of describing things after assigning a specific value to it. For analysis, you need to organize these values, processed and presented in a given context, to make it useful. Data can be in different forms; here are the primary data types.
- Qualitative data: When the data presented has words and descriptions, then we call it qualitative data . Although you can observe this data, it is subjective and harder to analyze data in research, especially for comparison. Example: Quality data represents everything describing taste, experience, texture, or an opinion that is considered quality data. This type of data is usually collected through focus groups, personal qualitative interviews , qualitative observation or using open-ended questions in surveys.
- Quantitative data: Any data expressed in numbers of numerical figures are called quantitative data . This type of data can be distinguished into categories, grouped, measured, calculated, or ranked. Example: questions such as age, rank, cost, length, weight, scores, etc. everything comes under this type of data. You can present such data in graphical format, charts, or apply statistical analysis methods to this data. The (Outcomes Measurement Systems) OMS questionnaires in surveys are a significant source of collecting numeric data.
- Categorical data: It is data presented in groups. However, an item included in the categorical data cannot belong to more than one group. Example: A person responding to a survey by telling his living style, marital status, smoking habit, or drinking habit comes under the categorical data. A chi-square test is a standard method used to analyze this data.
Learn More : Examples of Qualitative Data in Education
Data analysis in qualitative research
Data analysis and qualitative data research work a little differently from the numerical data as the quality data is made up of words, descriptions, images, objects, and sometimes symbols. Getting insight from such complicated information is a complicated process. Hence it is typically used for exploratory research and data analysis .
Although there are several ways to find patterns in the textual information, a word-based method is the most relied and widely used global technique for research and data analysis. Notably, the data analysis process in qualitative research is manual. Here the researchers usually read the available data and find repetitive or commonly used words.
For example, while studying data collected from African countries to understand the most pressing issues people face, researchers might find “food” and “hunger” are the most commonly used words and will highlight them for further analysis.
LEARN ABOUT: Level of Analysis
The keyword context is another widely used word-based technique. In this method, the researcher tries to understand the concept by analyzing the context in which the participants use a particular keyword.
For example , researchers conducting research and data analysis for studying the concept of ‘diabetes’ amongst respondents might analyze the context of when and how the respondent has used or referred to the word ‘diabetes.’
The scrutiny-based technique is also one of the highly recommended text analysis methods used to identify a quality data pattern. Compare and contrast is the widely used method under this technique to differentiate how a specific text is similar or different from each other.
For example: To find out the “importance of resident doctor in a company,” the collected data is divided into people who think it is necessary to hire a resident doctor and those who think it is unnecessary. Compare and contrast is the best method that can be used to analyze the polls having single-answer questions types .
Metaphors can be used to reduce the data pile and find patterns in it so that it becomes easier to connect data with theory.
Variable Partitioning is another technique used to split variables so that researchers can find more coherent descriptions and explanations from the enormous data.
LEARN ABOUT: Qualitative Research Questions and Questionnaires
There are several techniques to analyze the data in qualitative research, but here are some commonly used methods,
- Content Analysis: It is widely accepted and the most frequently employed technique for data analysis in research methodology. It can be used to analyze the documented information from text, images, and sometimes from the physical items. It depends on the research questions to predict when and where to use this method.
- Narrative Analysis: This method is used to analyze content gathered from various sources such as personal interviews, field observation, and surveys . The majority of times, stories, or opinions shared by people are focused on finding answers to the research questions.
- Discourse Analysis: Similar to narrative analysis, discourse analysis is used to analyze the interactions with people. Nevertheless, this particular method considers the social context under which or within which the communication between the researcher and respondent takes place. In addition to that, discourse analysis also focuses on the lifestyle and day-to-day environment while deriving any conclusion.
- Grounded Theory: When you want to explain why a particular phenomenon happened, then using grounded theory for analyzing quality data is the best resort. Grounded theory is applied to study data about the host of similar cases occurring in different settings. When researchers are using this method, they might alter explanations or produce new ones until they arrive at some conclusion.
LEARN ABOUT: 12 Best Tools for Researchers
Data analysis in quantitative research
The first stage in research and data analysis is to make it for the analysis so that the nominal data can be converted into something meaningful. Data preparation consists of the below phases.
Phase I: Data Validation
Data validation is done to understand if the collected data sample is per the pre-set standards, or it is a biased data sample again divided into four different stages
- Fraud: To ensure an actual human being records each response to the survey or the questionnaire
- Screening: To make sure each participant or respondent is selected or chosen in compliance with the research criteria
- Procedure: To ensure ethical standards were maintained while collecting the data sample
- Completeness: To ensure that the respondent has answered all the questions in an online survey. Else, the interviewer had asked all the questions devised in the questionnaire.
Phase II: Data Editing
More often, an extensive research data sample comes loaded with errors. Respondents sometimes fill in some fields incorrectly or sometimes skip them accidentally. Data editing is a process wherein the researchers have to confirm that the provided data is free of such errors. They need to conduct necessary checks and outlier checks to edit the raw edit and make it ready for analysis.
Phase III: Data Coding
Out of all three, this is the most critical phase of data preparation associated with grouping and assigning values to the survey responses . If a survey is completed with a 1000 sample size, the researcher will create an age bracket to distinguish the respondents based on their age. Thus, it becomes easier to analyze small data buckets rather than deal with the massive data pile.
LEARN ABOUT: Steps in Qualitative Research
After the data is prepared for analysis, researchers are open to using different research and data analysis methods to derive meaningful insights. For sure, statistical analysis plans are the most favored to analyze numerical data. In statistical analysis, distinguishing between categorical data and numerical data is essential, as categorical data involves distinct categories or labels, while numerical data consists of measurable quantities. The method is again classified into two groups. First, ‘Descriptive Statistics’ used to describe data. Second, ‘Inferential statistics’ that helps in comparing the data .
Descriptive statistics
This method is used to describe the basic features of versatile types of data in research. It presents the data in such a meaningful way that pattern in the data starts making sense. Nevertheless, the descriptive analysis does not go beyond making conclusions. The conclusions are again based on the hypothesis researchers have formulated so far. Here are a few major types of descriptive analysis methods.
Measures of Frequency
- Count, Percent, Frequency
- It is used to denote home often a particular event occurs.
- Researchers use it when they want to showcase how often a response is given.
Measures of Central Tendency
- Mean, Median, Mode
- The method is widely used to demonstrate distribution by various points.
- Researchers use this method when they want to showcase the most commonly or averagely indicated response.
Measures of Dispersion or Variation
- Range, Variance, Standard deviation
- Here the field equals high/low points.
- Variance standard deviation = difference between the observed score and mean
- It is used to identify the spread of scores by stating intervals.
- Researchers use this method to showcase data spread out. It helps them identify the depth until which the data is spread out that it directly affects the mean.
Measures of Position
- Percentile ranks, Quartile ranks
- It relies on standardized scores helping researchers to identify the relationship between different scores.
- It is often used when researchers want to compare scores with the average count.
For quantitative research use of descriptive analysis often give absolute numbers, but the in-depth analysis is never sufficient to demonstrate the rationale behind those numbers. Nevertheless, it is necessary to think of the best method for research and data analysis suiting your survey questionnaire and what story researchers want to tell. For example, the mean is the best way to demonstrate the students’ average scores in schools. It is better to rely on the descriptive statistics when the researchers intend to keep the research or outcome limited to the provided sample without generalizing it. For example, when you want to compare average voting done in two different cities, differential statistics are enough.
Descriptive analysis is also called a ‘univariate analysis’ since it is commonly used to analyze a single variable.
Inferential statistics
Inferential statistics are used to make predictions about a larger population after research and data analysis of the representing population’s collected sample. For example, you can ask some odd 100 audiences at a movie theater if they like the movie they are watching. Researchers then use inferential statistics on the collected sample to reason that about 80-90% of people like the movie.
Here are two significant areas of inferential statistics.
- Estimating parameters: It takes statistics from the sample research data and demonstrates something about the population parameter.
- Hypothesis test: I t’s about sampling research data to answer the survey research questions. For example, researchers might be interested to understand if the new shade of lipstick recently launched is good or not, or if the multivitamin capsules help children to perform better at games.
These are sophisticated analysis methods used to showcase the relationship between different variables instead of describing a single variable. It is often used when researchers want something beyond absolute numbers to understand the relationship between variables.
Here are some of the commonly used methods for data analysis in research.
- Correlation: When researchers are not conducting experimental research or quasi-experimental research wherein the researchers are interested to understand the relationship between two or more variables, they opt for correlational research methods.
- Cross-tabulation: Also called contingency tables, cross-tabulation is used to analyze the relationship between multiple variables. Suppose provided data has age and gender categories presented in rows and columns. A two-dimensional cross-tabulation helps for seamless data analysis and research by showing the number of males and females in each age category.
- Regression analysis: For understanding the strong relationship between two variables, researchers do not look beyond the primary and commonly used regression analysis method, which is also a type of predictive analysis used. In this method, you have an essential factor called the dependent variable. You also have multiple independent variables in regression analysis. You undertake efforts to find out the impact of independent variables on the dependent variable. The values of both independent and dependent variables are assumed as being ascertained in an error-free random manner.
- Frequency tables: The statistical procedure is used for testing the degree to which two or more vary or differ in an experiment. A considerable degree of variation means research findings were significant. In many contexts, ANOVA testing and variance analysis are similar.
- Analysis of variance: The statistical procedure is used for testing the degree to which two or more vary or differ in an experiment. A considerable degree of variation means research findings were significant. In many contexts, ANOVA testing and variance analysis are similar.
- Researchers must have the necessary research skills to analyze and manipulation the data , Getting trained to demonstrate a high standard of research practice. Ideally, researchers must possess more than a basic understanding of the rationale of selecting one statistical method over the other to obtain better data insights.
- Usually, research and data analytics projects differ by scientific discipline; therefore, getting statistical advice at the beginning of analysis helps design a survey questionnaire, select data collection methods , and choose samples.
LEARN ABOUT: Best Data Collection Tools
- The primary aim of data research and analysis is to derive ultimate insights that are unbiased. Any mistake in or keeping a biased mind to collect data, selecting an analysis method, or choosing audience sample il to draw a biased inference.
- Irrelevant to the sophistication used in research data and analysis is enough to rectify the poorly defined objective outcome measurements. It does not matter if the design is at fault or intentions are not clear, but lack of clarity might mislead readers, so avoid the practice.
- The motive behind data analysis in research is to present accurate and reliable data. As far as possible, avoid statistical errors, and find a way to deal with everyday challenges like outliers, missing data, data altering, data mining , or developing graphical representation.
LEARN MORE: Descriptive Research vs Correlational Research The sheer amount of data generated daily is frightening. Especially when data analysis has taken center stage. in 2018. In last year, the total data supply amounted to 2.8 trillion gigabytes. Hence, it is clear that the enterprises willing to survive in the hypercompetitive world must possess an excellent capability to analyze complex research data, derive actionable insights, and adapt to the new market needs.
LEARN ABOUT: Average Order Value
QuestionPro is an online survey platform that empowers organizations in data analysis and research and provides them a medium to collect data by creating appealing surveys.
MORE LIKE THIS
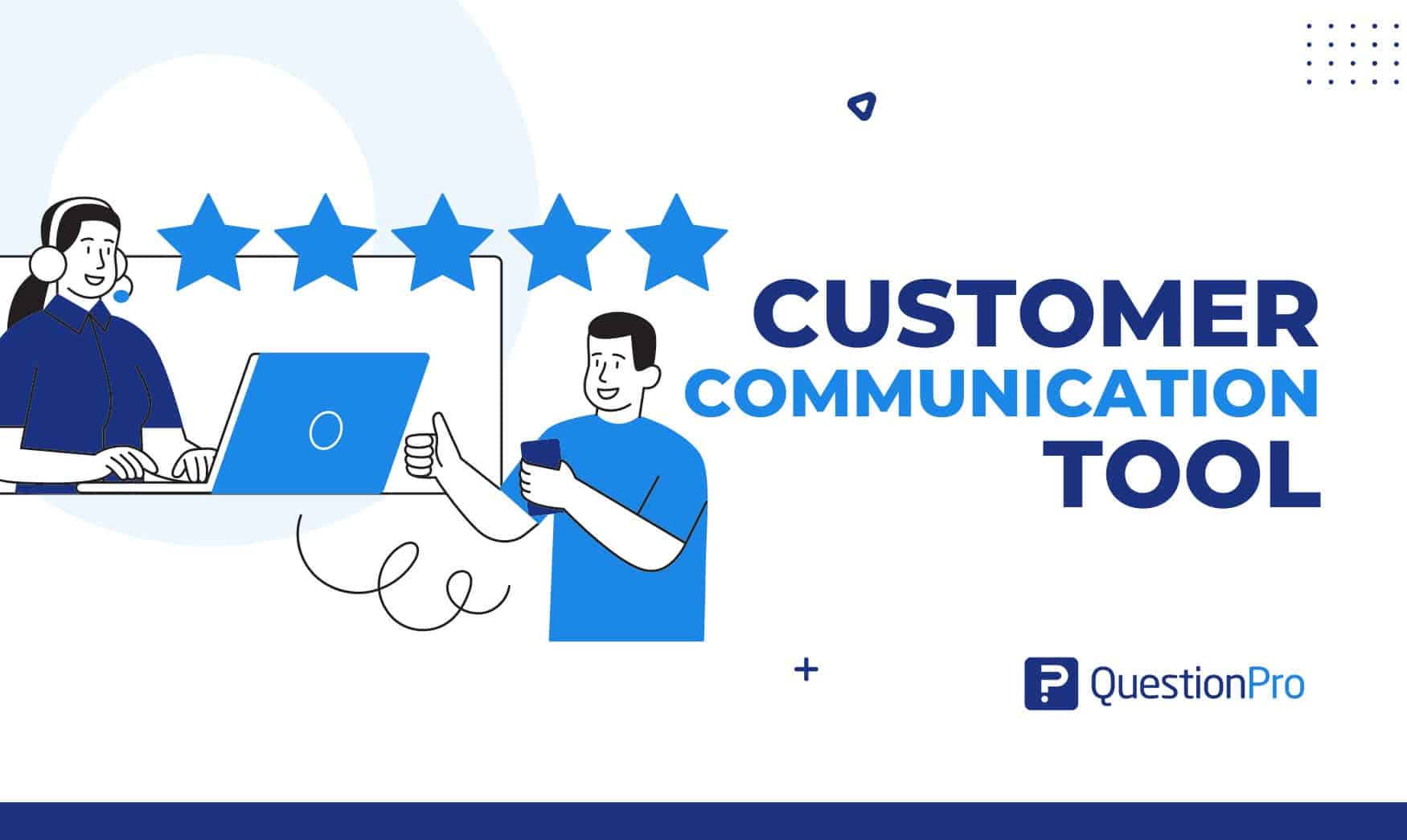
Customer Communication Tool: Types, Methods, Uses, & Tools
Apr 23, 2024
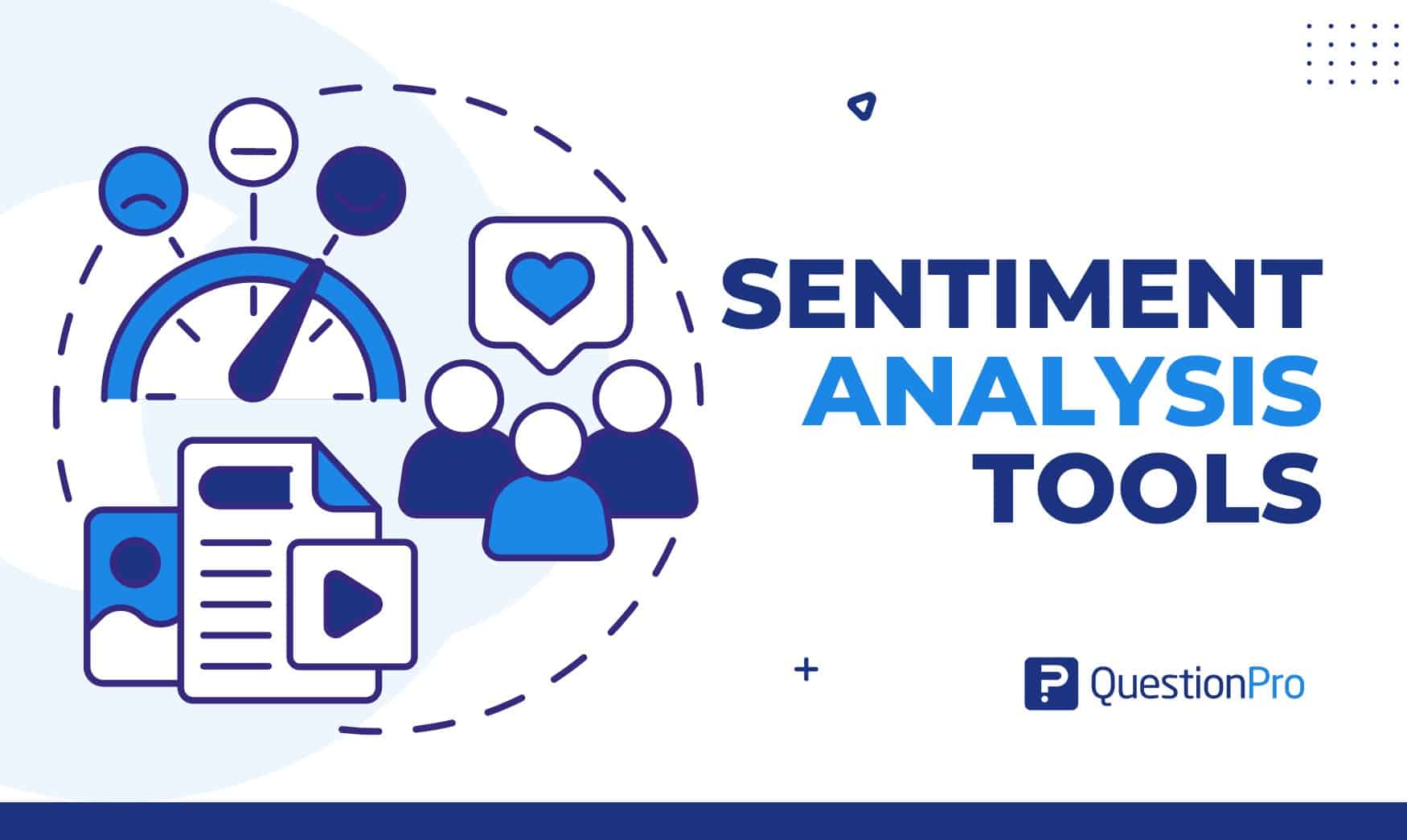
Top 12 Sentiment Analysis Tools for Understanding Emotions
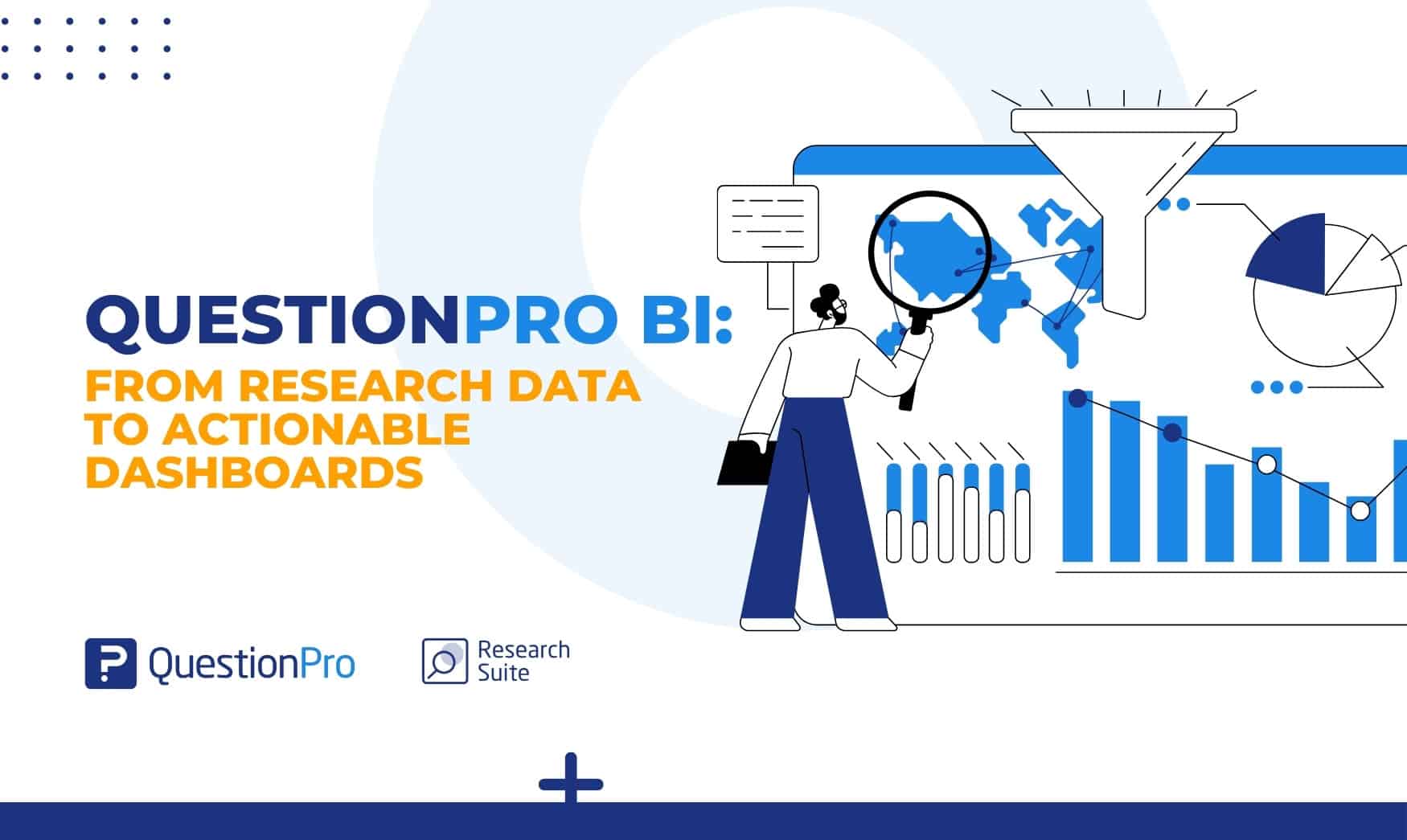
QuestionPro BI: From Research Data to Actionable Dashboards
Apr 22, 2024
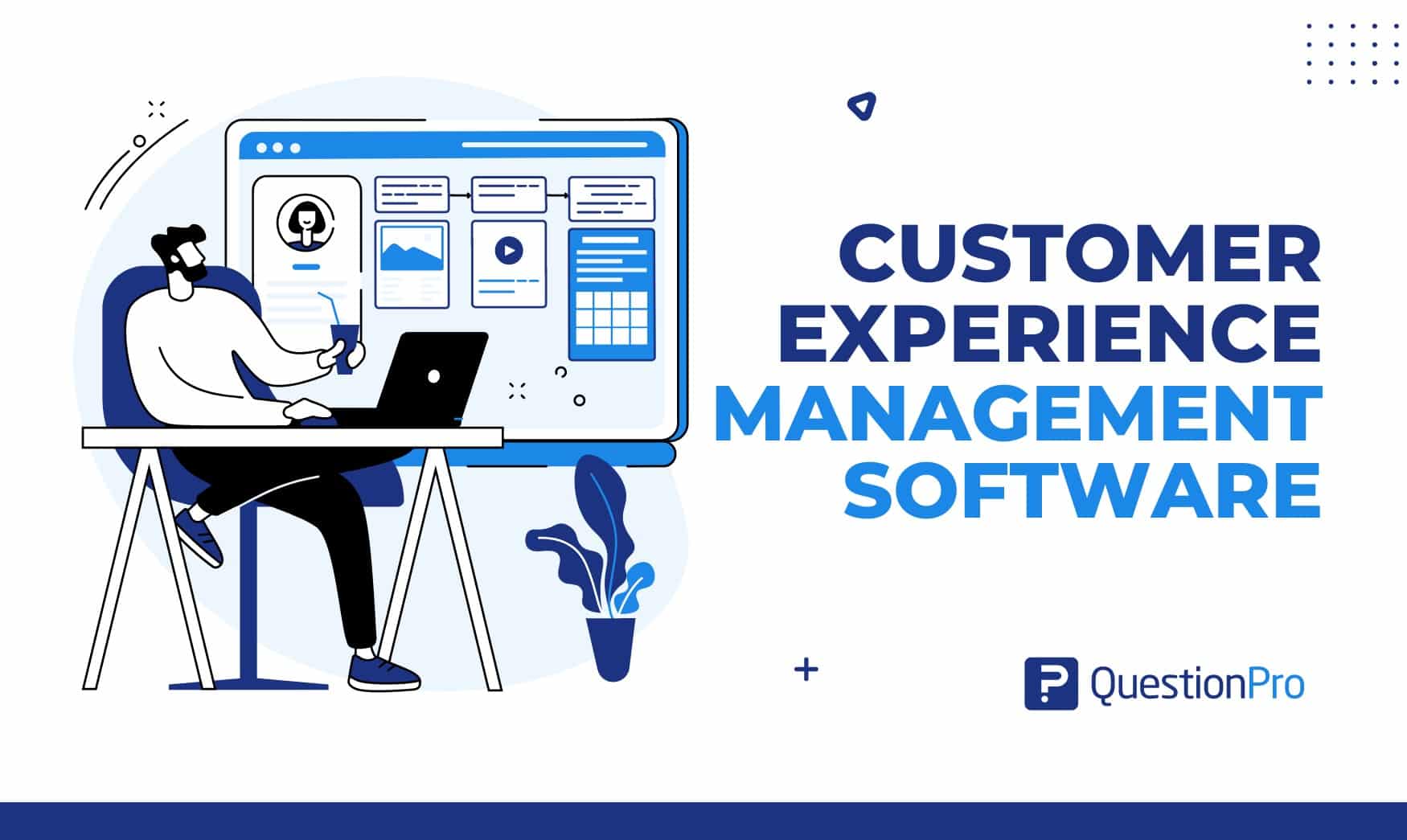
21 Best Customer Experience Management Software in 2024
Other categories.
- Academic Research
- Artificial Intelligence
- Assessments
- Brand Awareness
- Case Studies
- Communities
- Consumer Insights
- Customer effort score
- Customer Engagement
- Customer Experience
- Customer Loyalty
- Customer Research
- Customer Satisfaction
- Employee Benefits
- Employee Engagement
- Employee Retention
- Friday Five
- General Data Protection Regulation
- Insights Hub
- Life@QuestionPro
- Market Research
- Mobile diaries
- Mobile Surveys
- New Features
- Online Communities
- Question Types
- Questionnaire
- QuestionPro Products
- Release Notes
- Research Tools and Apps
- Revenue at Risk
- Survey Templates
- Training Tips
- Uncategorized
- Video Learning Series
- What’s Coming Up
- Workforce Intelligence
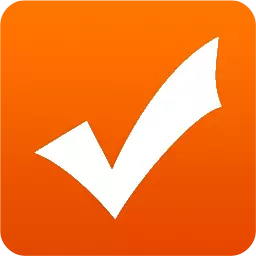
What is Project Analysis and Why it is Important?
By Aastha Shaw Jan 25, 2022
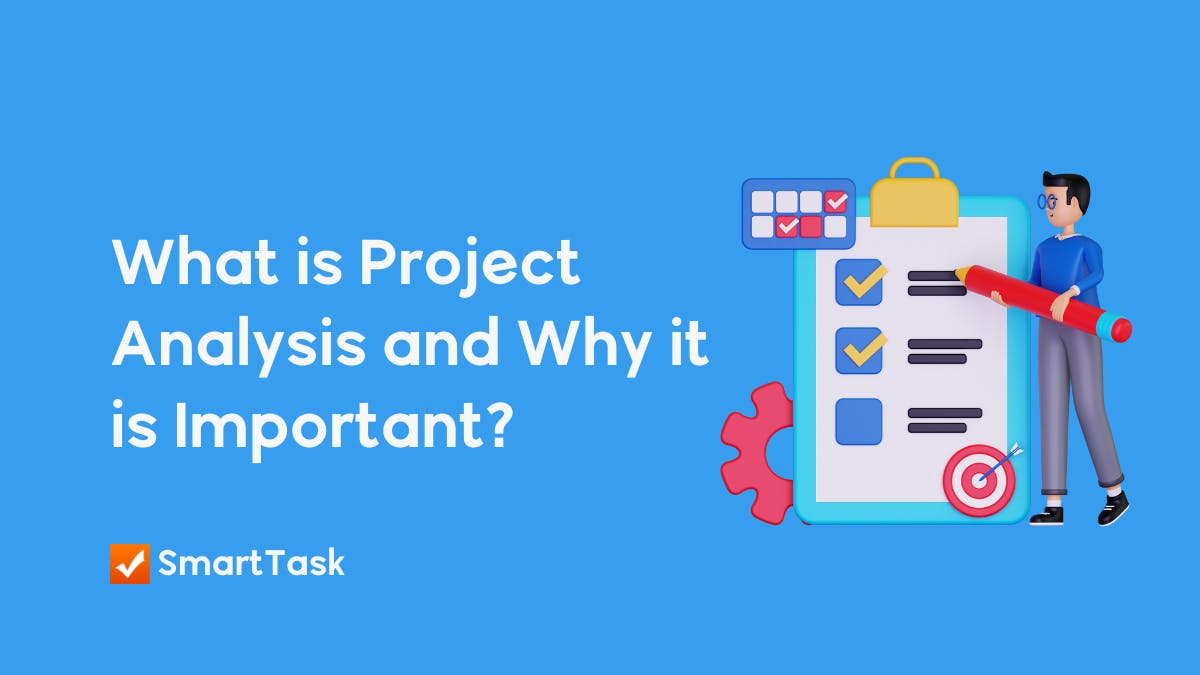
What do you do to check if a project is going on track?
You analyze it!
Consistent project analysis helps you make the right choices at the right time, leading you towards a more successful outcome and the highest possible ROI.
Here we will talk about project analysis, its importance, the different types of project analysis, and lastly, how you can implement it using the right tools.
What is Project Analysis?
When executing a project , you need to analyze it periodically. Failing to do so, would mean unexpected challenges, overlooked critical information, or flaws in the work process that manifest as the project unfolds.
This is why you need project analysis.
It is assessing every expense or problem related to a project before working on it and evaluating the outcome once the work is done.
Analyze your projects with SmartTask
Importance of project analysis.
A study conducted last year stated that over two-thirds of all projects were not completed on time and went over the budget.
What separates the failed two-thirds of the projects from the successful one-third?
Regular analysis.
Project analysis lets you see the present problems and prepare for and avoid future problems. This ensures smooth project execution and timely project delivery.
For efficient project analysis, it is equally important to have the right tool that will help you monitor and analyze your project from its initiation to completion.
Types of project analysis:
A. ongoing project risk analysis.
What happens if your key teammate gets injured during a project and needs to take a month off? Or your equipment malfunctions which stop the work, but the labor charges keep adding up? Or a natural calamity takes place?
All of these can affect the project timeline and cost.
Risk analysis ensures that the least number of surprises occur during your project. It helps you predict the uncertainties in the projects and minimize the occurrence and impact of these uncertainties.
How to do project risk analysis?
1. Define Critical Path:
Each project consists of dependent tasks that rely on one or more tasks to be performed in a particular order for their completion.
This is where understanding the longest chain of dependencies or the project's critical path becomes very important. Any delay in the critical path would ultimately lead to missed deadlines.
You can use project management software that helps you map your project plan and highlight its critical path. (In the image below, you would see the critical path highlighted in red)
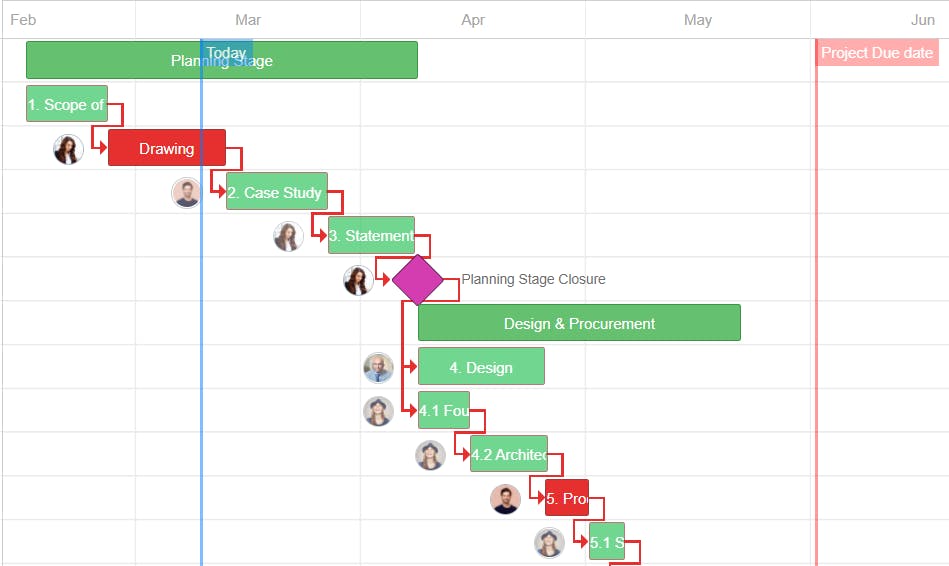
2. Streamline communication channels:
You don't want to be spending most of your time coordinating with the management, sales team, clients, and vendors. So, it's essential to keep communication and information flowing in one tool.
Establishing a good communication channel instead of checking on everyone for updates will help you better track task progress and save time. With a proper communication channel in place, task assignees can keep you updated in real-time and let you know about any delays, problems, or requirements easily.
Thus, it is critical to have a project management tool that allows real-time collaboration & communication via Chat, Task Comments, and Video conferencing.
3. Regularly monitor risks:
Once you have defined the project's critical path and streamlined the communication channel, you need to focus on how each task is panning out.
You would not want to be unaware of situations such as delays in the shipment of raw material on which a lot of your project tasks depend.
This is why we recommend tracking and analyzing the project's progress every week. (In SmartTask, there's a News Feed view that would allow you to share the project status with the team and for team members to share their thoughts on the status update.)
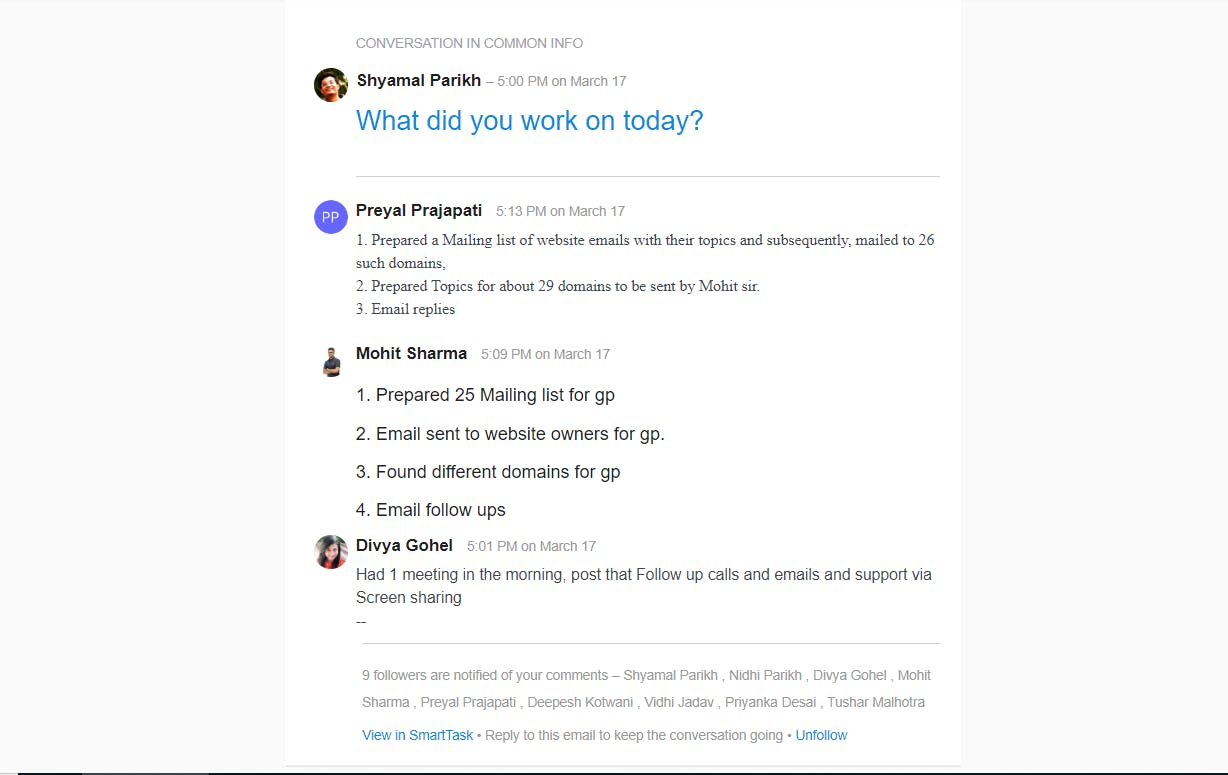
Say today is Saturday, and you are going through the last week's progress. You identified that one procurement item might become a bottleneck and affect the critical path.
This will help you immediately take necessary actions and save your project from facing major drawbacks.
Monitor your projects with SmartTask
Free for Unlimited Users, Tasks, & Projects
4. Determine their impact on the project:
Every risk has an impact, some more than others.
You can evaluate the impact of the risks by looking at:
- How much will the task be delayed?
- Would it affect the critical path?
If the delay does affect the critical path, can we have the procurement team expedite the delivery? Or is it a lost cause?
5. Prepare a contingency plan to treat those risks:
A contingency plan helps you to be prepared for future challenges and reduce the biggest risks to manageable levels. It is a course of action that enables you to deal with a situation that might happen.
In the above example, we tried to expedite the raw material delivery; however, it is clear now that the item won't be delivered on time.
Now, as a project manager, it's time to re-evaluate the project plan and see if you can save the project from overshooting the timeline.
There are two ways to deal with this:
- Allocating additional resources to tasks dependent on raw material shipments and attempting to complete tasks ahead of schedule. - This would speed up your work and allow you to get back on track.
- Executing dependent processes in parallel. - Some tasks can be executed parallelly, but we avoid doing this to be safe and complete the tasks with perfection. - But with good planning and required resources, you can execute dependent tasks parallelly to complete the project on time and with the same quality.
Your decision would be based on the trade-offs you are ready to make. So, it's wise to your team's and management's feedback to get better insights and make the best decisions.
6. Regularly update the team on the project’s progress:
It’s important to keep everyone updated on the project progress and all the important decisions taken. With SmartTask’s News Feed feature you can update your teammates and project stakeholders about everything related to your project in real-time.
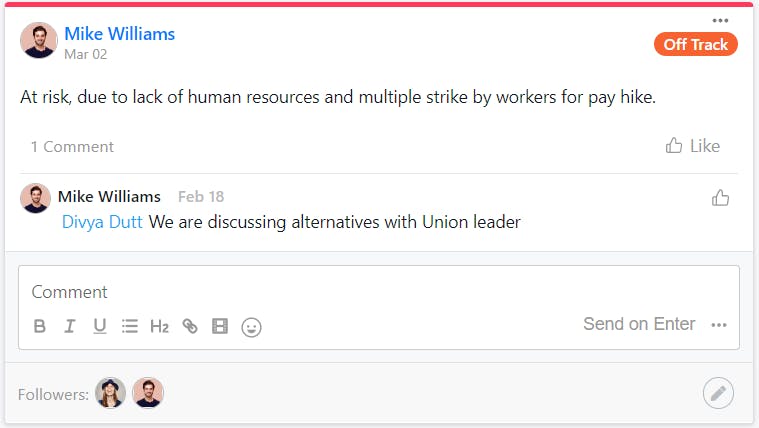
On the feed update itself, they can share their feedback, mention others, and share important files.
With SmartTask, you can record all your project history in one place and easily access them when needed. It also helps you track your projects to identify any potential problems and bottlenecks so that you can deal with them on time.
B. Project Cost Analysis
Suppose you land a software project. You roughly forecast the project timeline, resources, budget, etc., and reach a tentative project cost estimate at the project proposal time.
But moving forward without a thorough project cost analysis leads to budget overruns, missed deadlines, and a miserably failed project.
Therefore it's critical to conduct a proper project cost analysis to develop a strategic plan to avoid repetitive cost overruns and save your project from sinking.
How to do project cost analysis?
1. Determine the project goal:
Before you start working on project costing, your team needs to have a clear idea of the final goal and requirements of the project.
This is where a clearly defined client requirement document helps.
The client's requirement document will help your team divide the project into milestones and adequately define all the resources needed for timely project delivery.
2. Draw up a project plan:
Once the milestones are set, utilize project management software to map out tasks within those milestones with their delivery timelines and expectations.
You can use task dependencies to better map out the project plan and provide that clear understanding of the critical path to your team.
Lastly, assign each task to a suitable person. A good collaboration software would notify each team member when a new task is assigned.
3. Set Time Estimates:
One of the most crucial cost factors of any project is the resources needed to accomplish this project.
Make sure that the time to be spent on a task is set as a Time estimate. (Note: Time estimates or efforts needed to accomplish the task and task timeline are independent of each other.)
4. Define Resource Cost:
A good resource management software allows you to define the cost per hour and Billing rate for each resource.
So, along with the fixed cost, assignee, time estimates, and efforts needed to accomplish each task in the project, you also get a clear idea of the cost per resource. And in turn, you can set and track the total cost of the project better.
5. Set a Factor of Safety:
While you understand the project's total cost, it's essential to consider contingency funds if things don't go as planned.
Government compliances, taxes, client and vendor delays may delay the project and increase the cost of delivery.
It's prudent to consider all these unknown factors and add them to the project cost as a Factor of Safety.
While each project is unique and Factor of Safety(FOS) percentages can be different, we recommend not having less than 20% as FOS.
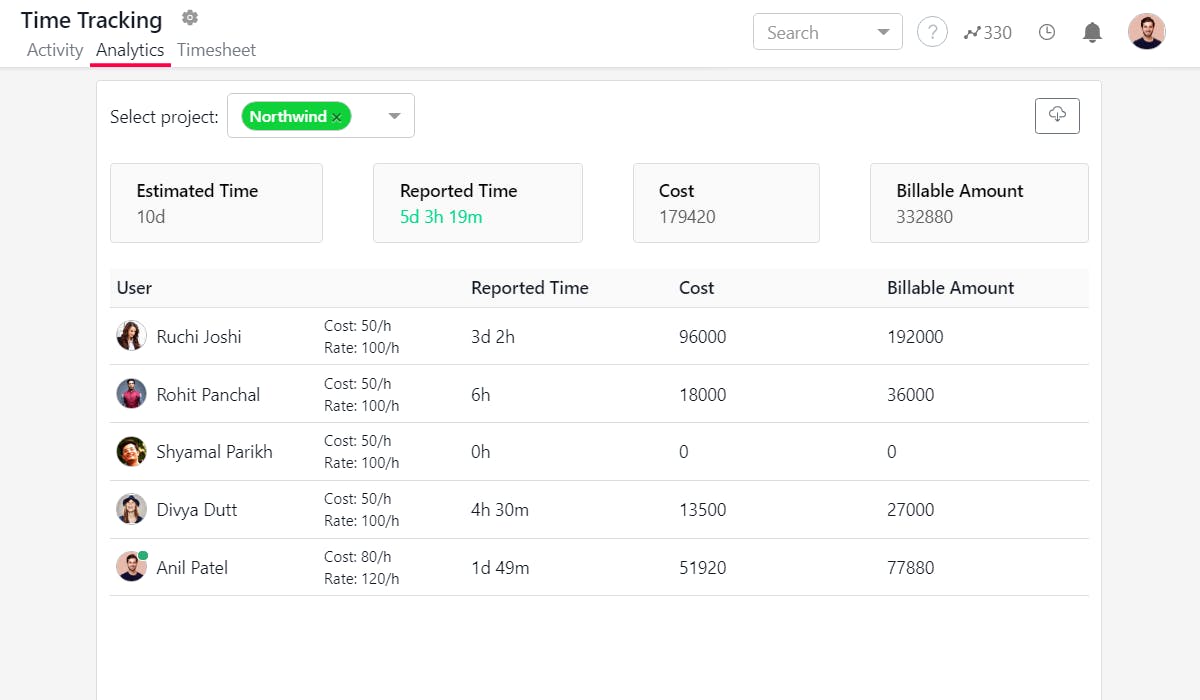
Track your project's cost with ease
Try SmartTask - Free for Unlimited Users
C. Workload Analysis
Suppose your team has two software engineers who are working on a project. You divide the tasks equally amongst them.
After they start working, you see that while Engineer A has completed his tasks, Engineer B has not started his work.
But when dwelled deeper, it is seen that Engineer A didn't have much on his plate, whereas Engineer B was overloaded with tasks from more than 3 projects, causing the delay.
To avoid such situations, you need a Workload analysis tool that would forecast each team member's workload, whether they are overloaded or under-utilized.
How to do workload analysis?
1. Define effort required in each project:
As noted in the "Project cost analysis" section, define the time effort required to accomplish each task in the project.
Depending on the task's timeline and the effort required, estimate each project member's number of hours blocked.
2. Group projects to understand the holistic picture:
Since a team member may be involved in multiple projects , it's important to group all these projects. This will give you a better understanding of their total effort and responsibilities across different projects.
Here's what the workload would look like. As you can see, the red color indicates overloading.
A workload view helps you track what your team members are working on what and also enables you to reassign the task from one member to another if required.
3. Balance workload among the team :
If your team is continuously overburdened and stressed out due to extra work, it might result in a breakdown soon. Thus it's important to distribute the workload among your team members evenly.
Once you identify resource overloading, here's how you can handle it:
- Unloading the task - By re-allocating the task from one person to another.
- Extending the task's timeline- To lower the effort required per day.
Want to save your team from burnout?
D. process analysis.
Sometimes a particular process can be complicated and unnecessarily lengthy, leading to poor project performance.
Process analysis helps you analyze and improve the process with inefficiencies that can affect your bottom line.
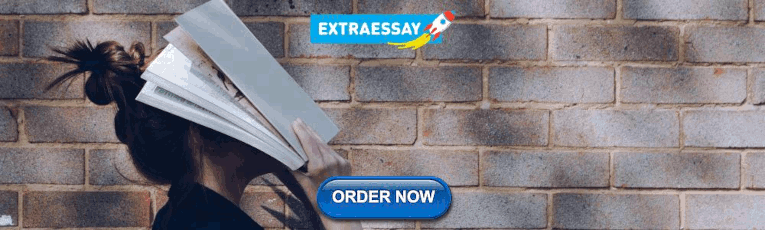
How to do process analysis?
1. Define what you want to analyze:
Say, if you are running the same type of project, again and again, it is normal that you might fix a template and follow the same process for similar future projects.
This often leaves out the opportunity to dwell and improve on the process as you keep following the same template again and again.
You need to quit this cycle and identify where your processes need revamping.
2. Collect all information about the process:
Collect as much information from all the past projects as possible on the selected process..
Identify the stakeholders and all the people involved in the process.
Gather data on how they tackle the process, what they do, when they do it, how often, what tools they use, what procedures they currently follow, and more.
3. Analyze the process:
Now looking at the collected information and the existing process template, perform a thorough analysis to figure out problems like;
- What are the most critical aspects of the project?
- What are the most time-consuming aspects?
- Is there anything causing delays?
- Which tasks get delayed regularly?
- Is it possible to speed up the process?
- Are there any steps that can be automated or eliminated?
- What are the most prevalent complaints from people involved in the process?
- In which areas are human errors most likely to occur?
This is where having project management software with portfolio view can make your task way easier.
With a portfolio, you can group all the similar projects together and get all the insights like due dates, delays, assignees, progress, and more at once.
Don’t forget to get feedback from important stakeholders and people involved to ensure that you are not missing out on anything.
4. Make changes for improvement:
It’s time to envision how the process can be improved in future and eventually change the project template to reflect those improvements.
These improvements can be addition or removal of tasks, automating repetitive taks, change in timelines, or effort required for each task, change in team, and so on...
Suppose your goal is to shorten the process cycle. Then you need to develop solutions like automating your process where possible and reduce manual labor to save time.
Also, make sure to convey any changes to the stakeholders involved and monitor the process regularly.
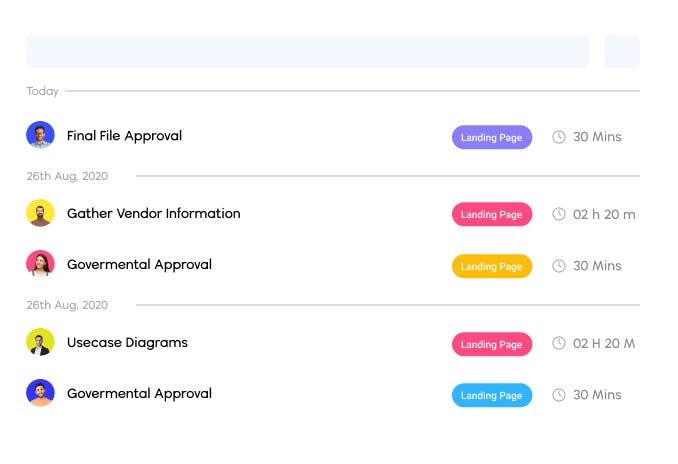
Keep in mind that business process analysis is a continuous process. You must examine the processes regularly and improve them to keep the process error-free.
Here’s a detailed video on how to do process analysis:
To conclude…
Project analysis is critical for companies and project managers to make their projects more successful and sustainable.
While it’s evident that problems and challenges will come your way, you can keep things under control with the right tool and approach.
There are many project management tools in the market, but SmartTask checks all the boxes for analyzing and managing your projects efficiently.
So be smart and get SmartTask to make assessing your projects easier and deliver better.
Want help with Project Analysis? Book a Free Consultation
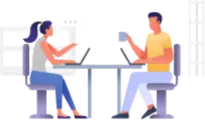
All in One - Work Management Tool
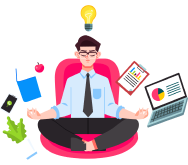
Free Forever

SmartTask is the best online collaboration tool to manage your team's progress.
- Task Management
- Project Management
- Integrations
- Asana Alternative
- Trello Alternative
- Clickup Alternative
- Monday.com Alternative
- Smartsheet Alternative
- Basecamp Alternative
- Wrike Alternative
- Plutio Alternative
- NiftyPM Alternative
- Become an Affiliate
- Privacy Policy
- Terms of Service
- GDPR Compliant


An official website of the United States government
The .gov means it’s official. Federal government websites often end in .gov or .mil. Before sharing sensitive information, make sure you’re on a federal government site.
The site is secure. The https:// ensures that you are connecting to the official website and that any information you provide is encrypted and transmitted securely.
- Publications
- Account settings
Preview improvements coming to the PMC website in October 2024. Learn More or Try it out now .
- Advanced Search
- Journal List
- Int J Environ Res Public Health

Research Project Evaluation—Learnings from the PATHWAYS Project Experience
Aleksander galas.
1 Epidemiology and Preventive Medicine, Jagiellonian University Medical College, 31-034 Krakow, Poland; [email protected] (A.G.); [email protected] (A.P.)
Aleksandra Pilat
Matilde leonardi.
2 Fondazione IRCCS, Neurological Institute Carlo Besta, 20-133 Milano, Italy; [email protected]
Beata Tobiasz-Adamczyk
Background: Every research project faces challenges regarding how to achieve its goals in a timely and effective manner. The purpose of this paper is to present a project evaluation methodology gathered during the implementation of the Participation to Healthy Workplaces and Inclusive Strategies in the Work Sector (the EU PATHWAYS Project). The PATHWAYS project involved multiple countries and multi-cultural aspects of re/integrating chronically ill patients into labor markets in different countries. This paper describes key project’s evaluation issues including: (1) purposes, (2) advisability, (3) tools, (4) implementation, and (5) possible benefits and presents the advantages of a continuous monitoring. Methods: Project evaluation tool to assess structure and resources, process, management and communication, achievements, and outcomes. The project used a mixed evaluation approach and included Strengths (S), Weaknesses (W), Opportunities (O), and Threats (SWOT) analysis. Results: A methodology for longitudinal EU projects’ evaluation is described. The evaluation process allowed to highlight strengths and weaknesses and highlighted good coordination and communication between project partners as well as some key issues such as: the need for a shared glossary covering areas investigated by the project, problematic issues related to the involvement of stakeholders from outside the project, and issues with timing. Numerical SWOT analysis showed improvement in project performance over time. The proportion of participating project partners in the evaluation varied from 100% to 83.3%. Conclusions: There is a need for the implementation of a structured evaluation process in multidisciplinary projects involving different stakeholders in diverse socio-environmental and political conditions. Based on the PATHWAYS experience, a clear monitoring methodology is suggested as essential in every multidisciplinary research projects.
1. Introduction
Over the last few decades, a strong discussion on the role of the evaluation process in research has developed, especially in interdisciplinary or multidimensional research [ 1 , 2 , 3 , 4 , 5 ]. Despite existing concepts and definitions, the importance of the role of evaluation is often underestimated. These dismissive attitudes towards the evaluation process, along with a lack of real knowledge in this area, demonstrate why we need research evaluation and how research evaluation can improve the quality of research. Having firm definitions of ‘evaluation’ can link the purpose of research, general questions associated with methodological issues, expected results, and the implementation of results to specific strategies or practices.
Attention paid to projects’ evaluation shows two concurrent lines of thought in this area. The first is strongly associated with total quality management practices and operational performance; the second focuses on the evaluation processes needed for public health research and interventions [ 6 , 7 ].
The design and implementation of process’ evaluations in fields different from public health have been described as multidimensional. According to Baranowski and Stables, process evaluation consists of eleven components: recruitment (potential participants for corresponding parts of the program); maintenance (keeping participants involved in the program and data collection); context (an aspect of environment of intervention); resources (the materials necessary to attain project goals); implementation (the extent to which the program is implemented as designed); reach (the extent to which contacts are received by the targeted group); barriers (problems encountered in reaching participants); exposure (the extent to which participants view or read material); initial use (the extent to which a participant conducts activities specified in the materials); continued use (the extent to which a participant continues to do any of the activities); contamination (the extent to which participants receive interventions from outside the program and the extent to which the control group receives the treatment) [ 8 ].
There are two main factors shaping the evaluation process. These are: (1) what is evaluated (whether the evaluation process revolves around project itself or the outcomes which are external to the project), and (2) who is an evaluator (whether an evaluator is internal or external to the project team and program). Although there are several existing gaps in current knowledge about the evaluation process of external outcomes, the use of a formal evaluation process of a research project itself is very rare.
To define a clear evaluation and monitoring methodology we performed different steps. The purpose of this article is to present experiences from the project evaluation process implemented in the Participation to Healthy Workplaces and Inclusive Strategies in the Work Sector (the EU PATHWAYS project. The manuscript describes key project evaluation issues as: (1) purposes, (2) advisability, (3) tools, (4) implementation, and (5) possible benefits. The PATHWAYS project can be understood as a specific case study—presented through a multidimensional approach—and based on the experience associated with general evaluation, we can develop patterns of good practices which can be used in other projects.
1.1. Theoretical Framework
The first step has been the clear definition of what is an evaluation strategy or methodology . The term evaluation is defined by the Cambridge Dictionary as the process of judging something’s quality, importance, or value, or a report that includes this information [ 9 ] or in a similar way by the Oxford Dictionary as the making of a judgment about the amount, number, or value of something [ 10 ]; assessment and in the activity, it is frequently understood as associated with the end rather than with the process. Stufflebeam, in its monograph, defines evaluation as a study designed and conducted to assist some audience to assess an object’s merit and worth. Considering this definition, there are four categories of evaluation approaches: (1) pseudo-evaluation; (2) questions and/or methods-oriented evaluation; (3) improvement/accountability evaluation; (4) social agenda/advocacy evaluation [ 11 ].
In brief, considering Stufflebeam’s classification, pseudo-evaluations promote invalid or incomplete findings. This happens when findings are selectively released or falsified. There are two pseudo-evaluation types proposed by Stufflebeam: (1) public relations-inspired studies (studies which do not seek truth but gather information to solicit positive impressions of program), and (2) politically controlled studies (studies which seek the truth but inappropriately control the release of findings to right-to-know audiences).
The questions and/or methods-oriented approach uses rather narrow questions, which are oriented on operational objectives of the project. Questions oriented uses specific questions, which are of interest by accountability requirements or an expert’s opinions of what is important, while method oriented evaluations favor the technical qualities of program/process. The general concept of these two is that it is better to ask a few pointed questions well to get information on program merit and worth [ 11 ]. In this group, one may find the following evaluation types: (a) objectives-based studies: typically focus on whether the program objectives have been achieved through an internal perspective (by project executors); (b) accountability, particularly payment by results studies: stress the importance of obtaining an external, impartial perspective; (c) objective testing program: uses standardized, multiple-choice, norm-referenced tests; (d) outcome evaluation as value-added assessment: a recurrent evaluation linked with hierarchical gain score analysis; (e) performance testing: incorporates the assessment of performance (by written or spoken answers, or psychomotor presentations) and skills; (f) experimental studies: program evaluators perform a controlled experiment and contrast the outcomes observed; (g) management information system: provide information needed for managers to conduct their programs; (h) benefit-cost analysis approach: mainly sets of quantitative procedures to assess the full cost of a program and its returns; (i) clarification hearing: an evaluation of a trial in which role-playing evaluators competitively implement both a damning prosecution of a program—arguing that it failed, and a defense of the program—and arguing that it succeeded. Next, a judge hears arguments within the framework of a jury trial and controls the proceedings according to advance agreements on rules of evidence and trial procedures; (j) case study evaluation: focused, in-depth description, analysis, and synthesis of a particular program; (k) criticism and connoisseurship: certain experts in a given area do in-depth analysis and evaluation that could not be done in other way; (l) program theory-based evaluation: based on the theory beginning with another validated theory of how programs of a certain type within similar settings operate to produce outcomes (e.g., Health Believe Model, Predisposing, Reinforcing and Enabling Constructs in Educational Diagnosis and Evaluation and Policy, Regulatory, and Organizational Constructs in Educational and Environmental Development - thus so called PRECEDE-PROCEED model proposed by L. W. Green or Stage of Change Theory by Prochaska); (m) mixed method studies: include different qualitative and quantitative methods.
The third group of methods considered in evaluation theory are improvement/accountability-oriented evaluation approaches. Among these, there are the following: (a) decision/accountability oriented studies: emphasizes that evaluation should be used proactively to help improve a program and retroactively to assess its merit and worth; (b) consumer-oriented studies: wherein the evaluator is a surrogate consumer who draws direct conclusions about the evaluated program; (c) accreditation/certification approach: an accreditation study to verify whether certification requirements have been/are fulfilled.
Finally, a social agenda/advocacy evaluation approach focuses on the assessment of difference, which is/was intended to be the effect of the program evaluation. The evaluation process in this type of approach works in a loop, starting with an independent evaluator who provides counsel and advice towards understanding, judging and improving programs as evaluations to serve the client’s needs. In this group, there are: (a) client-centered studies (or responsive evaluation): evaluators work with, and for, the support of diverse client groups; (b) constructivist evaluation: evaluators are authorized and expected to maneuver the evaluation to emancipate and empower involved and affected disenfranchised people; (c) deliberative democratic evaluation: evaluators work within an explicit democratic framework and uphold democratic principles in reaching defensible conclusions; (d) utilization-focused evaluation: explicitly geared to ensure that program evaluations make an impact.
1.2. Implementation of the Evaluation Process in the EU PATHWAYS Project
The idea to involve the evaluation process as an integrated goal of the PATHWAYS project was determined by several factors relating to the main goal of the project, defined as a special intervention to existing attitudes to occupational mobility and work activity reintegration of people of working age, suffering from specific chronic conditions into the labor market in 12 European Countries. Participating countries had different cultural and social backgrounds and different pervasive attitudes towards people suffering from chronic conditions.
The components of evaluation processes previously discussed proved helpful when planning the PATHWAYS evaluation, especially in relation to different aspects of environmental contexts. The PATHWAYS project focused on chronic conditions including: mental health issues, neurological diseases, metabolic disorders, musculoskeletal disorders, respiratory diseases, cardiovascular diseases, and persons with cancer. Within this group, the project found a hierarchy of patients and social and medical statuses defined by the nature of their health conditions.
According to the project’s monitoring and evaluation plan, the evaluation process followed specific challenges defined by the project’s broad and specific goals and monitored the progress of implementing key components by assessing the effectiveness of consecutive steps and identifying conditions supporting the contextual effectiveness. Another significant aim of the evaluation component on the PATHWAYS project was to recognize the value and effectiveness of using a purposely developed methodology—consisting of a wide set of quantitative and qualitative methods. The triangulation of methods was very useful and provided the opportunity to develop a multidimensional approach to the project [ 12 ].
From the theoretical framework, special attention was paid to the explanation of medical, cultural, social and institutional barriers influencing the chance of employment of chronically ill persons in relation to the characteristics of the participating countries.
Levels of satisfaction with project participation, as well as with expected or achieved results and coping with challenges on local–community levels and macro-social levels, were another source of evaluation.
In the PATHWAYS project, the evaluation was implemented for an unusual purpose. This quasi-experimental design was developed to assess different aspects of the multidimensional project that used a variety of methods (systematic review of literature, content analysis of existing documents, acts, data and reports, surveys on different country-levels, deep interviews) in the different phases of the 3 years. The evaluation monitored each stage of the project and focused on process implementation, with the goal of improving every step of the project. The evaluation process allowed to perform critical assessments and deep analysis of benefits and shortages of the specific phase of the project.
The purpose of the evaluation was to monitor the main steps of the Project, including the expectations associated with a multidimensional, methodological approach used by PATHWAYS partners, as well as improving communication between partners, from different professional and methodological backgrounds involved in the project in all its phases, so as to avoid errors in understanding the specific steps as well as the main goals.
2. Materials and Methods
The paper describes methodology and results gathered during the implementation of Work Package 3, Evaluation of the Participation to Healthy Workplaces and Inclusive Strategies in the Work Sector (the PATHWAYS) project. The work package was intended to keep internal control over the run of the project to achieve timely fulfillment of tasks, milestones, and purpose by all project partners.
2.1. Participants
The project consortium involved 12 partners from 10 different European countries. There were academics (representing cross-disciplinary research including socio-environmental determinants of health, clinicians), institutions actively working for the integration of people with chronic and mental health problems and disability, educational bodies (working in the area of disability and focusing on inclusive education), national health institutes (for rehabilitation of patients with functional and workplace impairments), an institution for inter-professional rehabilitation at a country level (coordinating medical, social, educational, pre-vocational and vocational rehabilitation), a company providing patient-centered services (in neurorehabilitation). All the partners represented vast knowledge and high-level expertise in the area of interest and all agreed with the World Health Organization’s (WHO) International Classification of Functioning, Disability and Health-ICF and of the biopsychosocial model of health and functioning. The consortium was created based on the following criteria:
- vision, mission, and activities in the area of project purposes,
- high level of experience in the area (supported by publications) and in doing research (being involved in international projects, collaboration with the coordinator and/or other partners in the past),
- being able to get broad geographical, cultural and socio-political representation from EU countries,
- represent different stakeholder type in the area.
2.2. Project Evaluation Tool
The tool development process involved the following steps:
- (1) Review definitions of ‘evaluation’ and adopt one which consorts best with the reality of public health research area;
- (2) Review evaluation approaches and decide on the content which should be applicable in the public health research;
- (3) Create items to be used in the evaluation tool;
- (4) Decide on implementation timing.
According to the PATHWAYS project protocol, an evaluation tool for the internal project evaluation was required to collect information about: (1) structure and resources; (2) process, management and communication; (3) achievements and/or outcomes and (4) SWOT analysis. A mixed methods approach was chosen. The specific evaluation process purpose and approach are presented in Table 1 .
Evaluation purposes and approaches adopted for the purpose in the PATHWAYS project.
* Open ended questions are not counted here.
The tool was prepared following different steps. In the paragraph to assess structure and resources, there were questions about the number of partners, professional competences, assigned roles, human, financial and time resources, defined activities and tasks, and the communication plan. The second paragraph, process, management and communication, collected information about the coordination process, consensus level, quality of communication among coordinators, work package leaders, and partners, whether project was carried out according to the plan, involvement of target groups, usefulness of developed materials, and any difficulties in the project realization. Finally, the paragraph achievements and outcomes gathered information about project specific activities such as public-awareness raising, stakeholder participation and involvement, whether planned outcomes (e.g., milestones) were achieved, dissemination activities, and opinions on whether project outcomes met the needs of the target groups. Additionally, it was decided to implement SWOT analysis as a part of the evaluation process. SWOT analysis derives its name from the evaluation of Strengths (S), Weaknesses (W), Opportunities (O), and Threats (T) faced by a company, industry or, in this case, project consortium. SWOT analysis comes from the business world and was developed in the 1960s at Harvard Business School as a tool for improving management strategies among companies, institutions, or organization [ 13 , 14 ]. However, in recent years, SWOT analysis has been adapted in the context of research to improve programs or projects.
For a better understanding of SWOT analysis, it is important to highlight the internal features of Strengths and Weaknesses, which are considered controllable. Strengths refers to work inside the project such as capabilities and competences of partners, whereas weaknesses refers to aspects, which needs improvement, such as resources. Conversely, Opportunities and Threats are considered outside factors and uncontrollable [ 15 ]. Opportunities are maximized to fit the organization’s values and resources and threats are the factors that the organization is not well equipped to deal with [ 9 ].
The PATHWAYS project members participated in SWOT analyses every three months. They answered four open questions about strengths, weaknesses, opportunities, and threats identified in evaluated period (last three months). They were then asked to assess those items on 10-point scale. The sample included results from nine evaluated periods from partners from ten different countries.
The tool for the internal evaluation of the PATHWAYS project is presented in Appendix A .
2.3. Tool Implementation and Data Collection
The PATHWAYS on-going evaluation took place at three-month intervals. It consisted of on-line surveys, and every partner assigned a representative who was expected to have good knowledge on the progress of project’s progress. The structure and resources were assessed only twice, at the beginning (3rd month) and at the end (36th month) of the project. The process, management, and communication questions, as well as SWOT analysis questions, were asked every three months. The achievements and outcomes questions started after the first year of implementation (i.e., after 15th month), and some of items in this paragraph, (results achieved, whether project outcomes meet the needs of the target groups and published regular publications), were only implemented at the end of the project (36th month).
2.4. Evaluation Team
The evaluation team was created from professionals with different backgrounds and extensive experience in research methodology, sociology, social research methods and public health.
The project started in 2015 and was carried out for 36 months. There were 12 partners in the PATHWAYS project, representing Austria, Belgium, Czech Republic, Germany, Greece, Italy, Norway, Poland, Slovenia and Spain and a European Organization. The on-line questionnaire was sent to all partners one week after the specified period ended and project partners had at least 2 weeks to fill in/answer the survey. Eleven rounds of the survey were performed.
The participation rate in the consecutive evaluation surveys was 11 (91.7%), 12 (100%), 12 (100%), 11 (91.7%), 10 (83.3%), 11 (91.7%), 11 (91.7%), 10 (83.3%), and 11 (91.7%) till the project end. Overall, it rarely covered the whole group, which may have resulted from a lack of coercive mechanisms at a project level to answer project evaluation questions.
3.1. Evaluation Results Considering Structure and Resources (3rd Month Only)
A total of 11 out of 12 project partners participated in the first evaluation survey. The structure and resources of the project were not assessed by the project coordinator and as such, the results in represent the opinions of the other 10 participating partners. The majority of respondents rated the project consortium as having at least adequate professional competencies. In total eight to nine project partners found human, financial and time resources ‘just right’ and the communication plan ‘clear’. More concerns were observed regarding the clarity of tasks, what is expected from each partner, and how specific project activities should be or were assigned.
3.2. Evaluation Results Considering Process, Management and Communication
The opinions about project coordination, communication processes (with coordinator, between WP leaders, and between individual partners/researchers) were assessed as ‘good’ and ‘very good’, along the whole period. There were some issues, however, when it came to the realization of specific goals, deliverables, or milestones of the project.
Given the broad scope of the project and participating partner countries, we created a glossary to unify the common terms used in the project. It was a challenge, as during the project implementation there were several discussions and inconsistencies in the concepts provided ( Figure 1 ).

Partners’ opinions about the consensus around terms (shared glossary) in the project consortium across evaluation waves (W1—after 3-month realization period, and at 3-month intervals thereafter).
Other issues, which appeared during project implementation, were recruitment of, involvement with, and cooperation with stakeholders. There was a range of groups to be contacted and investigated during the project including individual patients suffering from chronic conditions, patients’ advocacy groups and national governmental organizations, policy makers, employers, and international organizations. It was found that during the project, the interest and the involvement level of the aforementioned groups was quite low and difficult to achieve, which led to some delays in project implementation ( Figure 2 ). This was the main cause of smaller percentages of “what was expected to be done in designated periods of project realization time”. The issue was monitored and eliminated by intensification of activities in this area ( Figure 3 ).

Partners’ reports on whether the project had been carried out according to the plan ( a ) and the experience of any problems in the process of project realization ( b ) (W1—after 3-month realization period, and at 3-month intervals thereafter).

Partners’ reports on an approximate estimation (in percent) of the project plan implementation (what has been done according to the plan) ( a ) and the involvement of target groups (W1—after 3-month realization period, and at 3-month intervals thereafter) ( b ).
3.3. Evaluation Results Considering Achievements and Outcomes
The evaluation process was prepared to monitor project milestones and deliverables. One of the PATHWAYS project goals was to raise public awareness surrounding the reintegration of chronically ill people into the labor market. This was assessed subjectively by cooperating partners and only half (six) felt they achieved complete success on that measure. The evaluation process monitored planned outcomes according to: (1) determination of strategies for awareness rising activities, (2) assessment of employment-related needs, and (3) development of guidelines (which were planned by the project). The majority of partners completely fulfilled this task. Furthermore, the dissemination process was also carried out according to the plan.
3.4. Evaluation Results from SWOT
3.4.1. strengths.
Amongst the key issues identified across all nine evaluated periods ( Figure 4 ), the “strong consortium” was highlighted as the most important strength of the PATHWAYS project. The most common arguments for this assessment were the coordinator’s experience in international projects, involvement of interdisciplinary experts who could guarantee a holistic approach to the subject, and a highly motivated team. This was followed by the uniqueness of the topic. Project implementers pointed to the relevance of the analyzed issues, which are consistent with social needs. They also highlighted that this topic concerned an unexplored area in employment policy. The interdisciplinary and international approach was also emphasized. According to the project implementers, the international approach allowed mapping of vocational and prevocational processes among patients with chronic conditions and disability throughout Europe. The interdisciplinary approach, on the other hand, enabled researchers to create a holistic framework that stimulates innovation by thinking across boundaries of particular disciplines—especially as the PATHWAYS project brings together health scientists from diverse fields (physicians, psychologists, medical sociologists, etc.) from ten European countries. This interdisciplinary approach is also supported by the methodology, which is based on a mixed-method approach (qualitative and quantitative data). The involvement of an advocacy group was another strength identified by the project implementers. It was stressed that the involvement of different types of stakeholders increased validity and social triangulation. It was also assumed that it would allow for the integration of relevant stakeholders. The last strength, the usefulness of results, was identified only in the last two evaluation waves, when the first results had been measured.

SWOT Analysis—a summary of main issues reported by PATHWAYS project partners.
3.4.2. Weaknesses
The survey respondents agreed that the main weaknesses of the project were time and human resources. The subject of the PATHWAYS project turned out to be very broad, and therefore the implementers pointed to the insufficient human resources and inadequate time for the implementation of individual tasks, as well as the project overall. This was related to the broad categories of chronic diseases chosen for analysis in the project. On one hand, the implementers complained about the insufficient number of chronic diseases taken into account in the project. On the other hand, they admitted that it was not possible to cover all chronic diseases in details. The scope of the project was reported as another weakness. In the successive waves of evaluation, the implementers more often pointed out that it was hard to cover all relevant topics.
Nevertheless, some of the major weaknesses reported during the project evaluation were methodological problems. Respondents pointed to problems with the implementation of tasks on a regular basis. For example, survey respondents highlighted the need for more open questions in the survey that the questionnaire was too long or too complicated, that the tools were not adjusted for relevancy in the national context, etc. Another issue was that the working language was English, but all tools or survey questionnaire needed to be translated into different languages and this issue was not always considered by the Commission in terms of timing and resources. This issue could provide useful for further projects, as well as for future collaborations.
The difficulties of involving stakeholders were reported, especially during tasks, which required their active commitment, like participation in in-depth interviews or online questionnaires. Interestingly, the international approach was considered both strength and weakness of the project. The implementers highlighted the complexity of making comparisons between health care and/or social care in different countries. The budget was also identified as a weakness by the project implementers. More funds obtained from the partners could have helped PATHWAYS enhance dissemination and stakeholders’ participation.
3.4.3. Opportunities
A list of seven issues within the opportunities category reflects the positive outlook of survey respondents from the beginning of the project to its final stage. Social utility was ranked as the top opportunity. The implementers emphasized that the project could fill a gap between the existing solutions and the real needs of people with chronic diseases and mental disorders. The implementers also highlighted the role of future recommendations, which would consist of proposed solutions for professionals, employees, employers, and politicians. These advantages are strongly associated with increasing awareness of employment situations of people with chronic diseases in Europe and the relevance of the problem. Alignment with policies, strategies, and stakeholders’ interests were also identified as opportunities. The topic is actively discussed on the European and national level, and labor market and employment issues are increasingly emphasized in the public discourse. What is more relevant is that the European Commission considers the issue crucial, and the results of the project are in line with its requests for the future. The implementers also observed increasing interest from the stakeholders, which is very important for the future of the project. Without doubt, the social network of project implementers provides a huge opportunity for the sustainability of results and the implementation of recommendations.
3.4.4. Threats
Insufficient response from stakeholders was the top perceived threat selected by survey respondents. The implementers indicated that insufficient involvement of stakeholders resulted in low response rates in the research phase, which posed a huge threat for the project. The interdisciplinary nature of the PATHWAYS project was highlighted as a potential threat due to differences in technical terminology and different systems of regulating the employment of persons with reduced work capacity in each country, as well as many differences in the legislation process. Insufficient funding and lack of existing data were identified as the last two threats.
One novel aspect of the evaluation process in the PATHWAYS project was a numerical SWOT analysis. Participants were asked to score strengths, weaknesses, opportunities, and threats from 0 (meaning the lack of/no strengths, weaknesses) to 10 (meaning a lot of ... several ... strengths, weaknesses). This concept enabled us to get a subjective score of how partners perceive the PATHWAYS project itself and the performance of the project, as well as how that perception changes over time. Data showed an increase in both strengths and opportunities and a decrease in weaknesses and threats over the course of project implementation ( Figure 5 ).

Numerical SWOT, combined, over a period of 36 months of project realization (W1—after 3-month realization period, and at 3-month intervals thereafter).
4. Discussion
The need for project evaluation was born from an industry facing challenges regarding how to achieve market goals in more efficient way. Nowadays, every process, including research project implementation, faces questions regarding its effectiveness and efficiency.
The challenge of a research project evaluation is that the majority of research projects are described as unique, although we believe several projects face similar issues and challenges as those observed in the PATHWAYS project.
The main objectives of the PATHWAYS Project were (a) to identify integration and re-integration strategies that are available in Europe and beyond for individuals with chronic diseases and mental disorders experiencing work-related problems (such as unemployment, absenteeism, reduced productivity, stigmatization), (b) to determine their effectiveness, (c) to assess the specific employment-related needs of those people, and (d) to develop guidelines supporting the implementation of effective strategies of professional integration and reintegration. The broad area of investigation, partial knowledge in the field, diversity of determinants across European Union countries, and involvement with stakeholders representing different groups caused several challenges in the project, including:
- problem : uncovered, challenging, demanding (how to encourage stakeholders to participate, share experiences),
- diversity : different European regions; different determinants: political, social, cultural; different public health and welfare systems; differences in law regulations; different employment policies and issues in the system,
- multidimensionality of research: some quantitative, qualitative studies including focus groups, opinions from professionals, small surveys in target groups (workers with chronic conditions).
The challenges to the project consequently led to several key issues, which should be taken, into account during project realization:
- partners : with their own expertise and interests; different expectations; different views on what is more important to focused on and highlighted;
- issues associated with unification : between different countries with different systems (law, work-related and welfare definitions, disability classification, others);
- coordination : as multidimensionality of the project may have caused some research activities by partners to move in a wrong direction (data, knowledge which is not needed for the project purposes), a lack of project vision in (some) partners might postpone activities through misunderstanding;
- exchange of information : multidimensionality, the fact that different tasks were accomplished by different centers and obstacles to data collection required good communication methods and smooth exchange of information.
Identified Issues and Implemented Solutions
There were several issues identified through the semi-internal evaluation process performed during the project. Those, which might be more relevant for the project realization, are mentioned in the Table 2 .
Issues identified by the evaluation process and solutions implemented.
The PATHWAYS project included diverse partners representing different areas of expertise and activity (considering broad aspect of chronic diseases, decline in functioning and of disability, and its role in a labor market) in different countries and social security systems, which caused a challenge when developing a common language to achieve effective communication and better understanding of facts and circumstances in different countries. The implementation of continuous project process monitoring, and proper adjustment, enabled the team to overcome these challenges.
The evaluation tool has several benefits. First, it covers all key areas of the research project including structure and available resources, the run of the process, quality and timing of management and communication, as well as project achievements and outcomes. Continuous evaluation of all of these areas provides in-depth knowledge about project performance. Second, the implementation of SWOT tool provided opportunities to share out good and bad experiences by all project partners, and the use of a numerical version of SWOT provided a good picture about inter-relations strengths—weaknesses and opportunities—threats in the project and showed the changes in their intensity over time. Additionally, numerical SWOT may verify whether perception of a project improves over time (as was observed in the PATHWAYS project) showing an increase in strengths and opportunities and a decrease in weaknesses and threats. Third, the intervals in which partners were ‘screened’ by the evaluation questionnaire seems to be appropriate, as it was not very demanding but frequent enough to diagnose on-time some issues in the project process.
The experiences with the evaluation also revealed some limitations. There were no coercive mechanisms for participation in the evaluation questionnaires, which may have caused a less than 100% response rate in some screening surveys. Practically, that was not a problem in the PATHWAYS project. Theoretically, however, this might lead to unrevealed problems, as partners experiencing troubles might not report them. Another point is asking about quality of the consortium to the project coordinator, which has no great value (the consortium is created by the coordinator in the best achievable way and it is hard to expect other comments especially at the beginning of the project). Regarding the tool itself, the question Could you give us approximate estimation (in percent) of the project plan realization (what has been done according to the plan)? was expected to collect information about the project partners collecting data on what has been done out of what should be done during each evaluation period, meaning that 100% was what should be done in 3-month time in our project. This question, however, was slightly confusing at the beginning, as it was interpreted as percentage of all tasks and activities planned for the whole duration of the project. Additionally, this question only works provided that precise, clear plans on the type and timing of tasks were allocated to the project partners. Lastly, there were some questions with very low variability in answer types across evaluation surveys (mainly about coordination and communication). Our opinion is that if the project runs/performs in a smooth manner, one may think such questions useless, but in more complicated projects, these questions may reveal potential causes of troubles.
5. Conclusions
The PATHWAYS project experience shows a need for the implementation of structured evaluation processes in multidisciplinary projects involving different stakeholders in diverse socio-environmental and political conditions. Based on the PATHWAYS experience, a clear monitoring methodology is suggested as essential in every project and we suggest the following steps while doing multidisciplinary research:
- Define area/s of interest (decision maker level/s; providers; beneficiaries: direct, indirect),
- Identify 2–3 possible partners for each area (chain sampling easier, more knowledge about; check for publications),
- Prepare a research plan (propose, ask for supportive information, clarify, negotiate),
- Create a cross-partner groups of experts,
- Prepare a communication strategy (communication channels, responsible individuals, timing),
- Prepare a glossary covering all the important issues covered by the research project,
- Monitor the project process and timing, identify concerns, troubles, causes of delays,
- Prepare for the next steps in advance, inform project partners about the upcoming activities,
- Summarize, show good practices, successful strategies (during project realization, to achieve better project performance).
Acknowledgments
The current study was part of the PATHWAYS project, that has received funding from the European Union’s Health Program (2014–2020) Grant agreement no. 663474.
The evaluation questionnaire developed for the PATHWAYS Project.
SWOT analysis:
What are strengths and weaknesses of the project? (list, please)
What are threats and opportunities? (list, please)
Visual SWOT:
Please, rate the project on the following continua:
How would you rate:
(no strengths) 0 1 2 3 4 5 6 7 8 9 10 (a lot of strengths, very strong)
(no weaknesses) 0 1 2 3 4 5 6 7 8 9 10 (a lot of weaknesses, very weak)
(no risks) 0 1 2 3 4 5 6 7 8 9 10 (several risks, inability to accomplish the task(s))
(no opportunities) 0 1 2 3 4 5 6 7 8 9 10 (project has a lot of opportunities)
Author Contributions
A.G., A.P., B.T.-A. and M.L. conceived and designed the concept; A.G., A.P., B.T.-A. finalized evaluation questionnaire and participated in data collection; A.G. analyzed the data; all authors contributed to writing the manuscript. All authors agreed on the content of the manuscript.
Conflicts of Interest
The authors declare no conflict of interest.
Thank you for visiting nature.com. You are using a browser version with limited support for CSS. To obtain the best experience, we recommend you use a more up to date browser (or turn off compatibility mode in Internet Explorer). In the meantime, to ensure continued support, we are displaying the site without styles and JavaScript.
- View all journals
- My Account Login
- Explore content
- About the journal
- Publish with us
- Sign up for alerts
- Open access
- Published: 22 April 2024
A method for managing scientific research project resource conflicts and predicting risks using BP neural networks
- Xuying Dong 1 &
- Wanlin Qiu 1
Scientific Reports volume 14 , Article number: 9238 ( 2024 ) Cite this article
Metrics details
- Computer science
- Engineering
This study begins by considering the resource-sharing characteristics of scientific research projects to address the issues of resource misalignment and conflict in scientific research project management. It comprehensively evaluates the tangible and intangible resources required during project execution and establishes a resource conflict risk index system. Subsequently, a resource conflict risk management model for scientific research projects is developed using Back Propagation (BP) neural networks. This model incorporates the Dropout regularization technique to enhance the generalization capacity of the BP neural network. Leveraging the BP neural network’s non-linear fitting capabilities, it captures the intricate relationship between project resource demand and supply. Additionally, the model employs self-learning to continuously adapt to new scenarios based on historical data, enabling more precise resource conflict risk assessments. Finally, the model’s performance is analyzed. The results reveal that risks in scientific research project management primarily fall into six categories: material, equipment, personnel, financial, time, and organizational factors. This study’s model algorithm exhibits the highest accuracy in predicting time-related risks, achieving 97.21%, surpassing convolutional neural network algorithms. Furthermore, the Root Mean Squared Error of the model algorithm remains stable at approximately 0.03, regardless of the number of hidden layer neurons, demonstrating excellent fitting capabilities. The developed BP neural network risk prediction framework in this study, while not directly influencing resource utilization efficiency or mitigating resource conflicts, aims to offer robust data support for research project managers when making decisions on resource allocation. The framework provides valuable insights through sensitivity analysis of organizational risks and other factors, with their relative importance reaching up to 20%. Further research should focus on defining specific strategies for various risk factors to effectively enhance resource utilization efficiency and manage resource conflicts.
Similar content being viewed by others
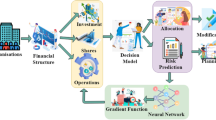
Optimized backpropagation neural network for risk prediction in corporate financial management
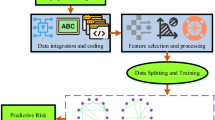
A case study on the relationship between risk assessment of scientific research projects and related factors under the Naive Bayesian algorithm
Xuying Dong & Wanlin Qiu
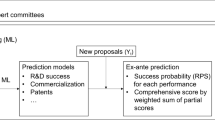
Prediction of SMEs’ R&D performances by machine learning for project selection
Hyoung Sun Yoo, Ye Lim Jung & Seung-Pyo Jun
Introduction
In the twenty-first century, driven by rapid technological innovation and a substantial increase in research funding, the number of scientific research projects has experienced exponential growth. These projects, serving as pivotal drivers of scientific and technological advancement, encompass a wide array of domains, including natural sciences, engineering, medicine, and social sciences, among others. This extensive spectrum attracts participation from diverse researchers and institutions 1 , 2 . However, this burgeoning landscape of scientific research projects brings forth a set of accompanying challenges and predicaments. Foremost among these challenges is the persistent issue of resource scarcity and the diversity of project requirements. This quandary poses a formidable obstacle to the management and execution of scientific research initiatives. It not only impacts the project’s quality and efficiency but can also cast a shadow on an organization’s reputation and the output of its research endeavors 3 , 4 , 5 . For instance, when two university research projects concurrently require the use of a specific instrument with limited availability or in need of maintenance, it may result in both projects being unable to proceed as planned, leading to resource conflicts. Similarly, competition for research funding from the same source can introduce conflicts in resource allocation decisions by the approval authority. These issues are widespread in research projects, and surveys indicate that project delays or budget overruns due to improper resource allocation are common in scientific research. For example, a study on research projects funded by the National Institutes of Health in the United States revealed that approximately 30% of projects faced delays due to improper resource allocation. In Europe, statistics from the European Union’s Framework Programme for Science and Innovation indicate that resource conflicts have impeded about 20% of transnational collaborative research projects from achieving their established research objectives on time. Furthermore, scientific research projects encompass a spectrum of resource requirements essential for their seamless progression, including but not limited to materials, equipment, skilled personnel, adequate funding, and time 6 . The predicament arises when multiple research initiatives necessitate identical or analogous resources simultaneously, creating a challenge for organizations to provide equitable support during peak demand periods. To mitigate the risks associated with resource conflicts, organizations must continually administer their resource allocation and strike a harmonious equilibrium between resource requisites and their availability 7 .
The Back Propagation (BP) neural network, as a prominent deep learning algorithm, boasts exceptional data processing capabilities. Notably, neural networks possess the capacity to swiftly process extensive datasets and extract intricate mapping relationships within data, rendering them versatile tools employed across various domains, including project evaluation, risk assessment, and cost prediction 8 , 9 . Scientific research project management constitutes a dynamic process. As projects advance and environmental factors evolve, the risk landscape may undergo continuous transformation 10 . The BP neural network’s inherent self-learning ability empowers it to iteratively update its model based on fresh data, enabling seamless adaptation to new circumstances and changes, thereby preserving the model’s real-time relevance 11 . In conclusion, this approach is poised to enhance project management efficiency and quality, mitigate risks, and foster the potential for the successful realization of scientific research projects.
The primary objective of this study is to formulate an evaluation and risk prediction framework for scientific research project management utilizing the BP neural network. This framework aims to address the issues associated with resource discrepancies and conflicts within the realm of scientific research project management. This study addresses the primary inquiry: What types of resource conflict risks exist in scientific research project management? An extensive literature review and empirical data analysis are conducted to answer this question, identifying six main risk categories: materials, equipment, personnel, finance, time, and organizational factors. A comprehensive resource conflict risk index system is constructed based on these categories. To quantitatively assess the importance of different resource conflict risk factors, the Analytic Hierarchy Process (AHP) is employed. This method allowed for the quantification of the influence of each risk factor objectively and accurately by constructing judgment matrices and calculating the weights of each factor. Subsequently, exploration is conducted into the utilization of BP neural networks to construct a resource conflict risk management model for scientific research projects. A BP neural network model is developed incorporating Dropout technology to capture complex correlations between project resource demand and supply. This model self-learns to adapt to new scenarios in historical data, thereby improving prediction accuracy. Research project data is collected from several universities in Xi’an from September 2021 to March 2023 to validate the effectiveness and accuracy of the proposed model. This data is utilized to train and test the model, and its performance is compared with other advanced algorithms such as CNN and BiLSTM. The evaluation is based on two key metrics: accuracy and root mean square error (RMSE), demonstrating excellent fitting ability and prediction accuracy.
The innovation introduced in this study is rooted in the recognition that the proliferation of scientific research initiatives can precipitate resource conflicts and competition, potentially leading to adverse outcomes such as project failure or resource inefficiency. This study harnesses a multi-layer BP neural network as its central computational tool, concomitantly incorporating the establishment of a resource conflict risk index system. This comprehensive model for evaluating and predicting the risks in scientific research project management takes into account both the resource conflict risk index system and the intrinsic characteristics of the BP neural network. This combined approach serves to enhance the efficiency of managing scientific research projects, curtail resource wastage, mitigate the risk of resource conflicts, and ultimately furnish robust support for the enduring success of scientific research endeavors.
Related work
Current research landscape in scientific research project management.
Scientific research projects hold a pivotal role in advancing scientific and technological frontiers, fostering knowledge generation, and driving innovation. Effective project management in this context ensures the timely delivery, adherence to budgetary constraints, and attainment of predefined quality standards. Numerous scholars have contributed to the body of knowledge concerning scientific research project management. Significant risks in scientific research project management include improper resource allocation, time delays, budget overruns, and collaboration challenges. For instance, concerning time management, Khiat 12 illustrated that insufficient project planning or external factors often hinder project deadlines. Regarding financial management, Gao 13 highlighted the lack of transparency in fund allocation and unreasonable budgeting, leading to unnecessary research cost overruns. Previous studies have predominantly concentrated on developing diverse methodologies and tools to identify and assess potential risks in scientific research projects. For instance, quantitative models have been employed by researchers like Jeong et al. 14 to evaluate project failure probabilities and devise corresponding risk mitigation strategies. Concurrently, Matel et al. 15 utilized artificial intelligence (AI), including neural networks and machine learning, to conduct comprehensive analyses of project data and predict potential issues throughout project progression.
The preceding studies offer essential groundwork and insights for the scientific research project management discussed in this study. They illuminate key risks encountered in scientific research project management, including inadequate resource allocation, time constraints, budgetary overruns, and collaboration hurdles. These risks are pervasive in scientific research project management, directly impacting project execution efficiency and outcomes. Moreover, these studies furnish empirical data and case studies, elucidating the underlying causes and mechanisms of these risks. For example, the research conducted by Khiat and Gao offers a nuanced understanding of risk factors, enriching the comprehension of the challenges in scientific research project management. Additionally, these studies introduce diverse methods and tools for identifying and evaluating potential risks in scientific research projects. For instance, the works of Jeong et al. and Matel et al. utilize quantitative models and artificial intelligence techniques to comprehensively analyze project data and forecast potential issues in project advancement. These methodologies and tools serve as valuable resources for constructing the research framework and methodologies in this study. Despite the commendable strides made in employing multidisciplinary approaches to address the challenges posed by scientific research project management, the issues related to resource allocation conflicts and quality assurance during project implementation remain fertile ground for future exploration and active investigation.
Application of BP neural network in project risk and resource management
BP neural networks are renowned for their non-linear fitting and self-learning capabilities, rendering them invaluable for discerning intricate relationships and patterns in project management. Their applications span diverse areas, including resource allocation, risk assessment, schedule forecasting, cost estimation, and more, culminating in heightened efficiency and precision within project management practices. Numerous scholars have ventured into the realm of BP neural network applications within project management. Zhang et al. 16 introduced a real-time network attack detection method underpinned by deep belief networks and support vector machines. Their findings underscore the method’s potential for bolstering network security risk management, extending novel data security safeguards to scientific research project management. Gong et al. 17 devised an AI-driven human resources management system. This system autonomously evaluates employee performance and needs, proffering intelligent managerial recommendations. Bai et al. 18 harnessed BP neural networks to tackle the intricate challenge of selecting service providers for project management portfolios. Leveraging neural networks, they prognosticate the performance of diverse service providers, lending support to project management decision-making. Sivakumar et al. 19 harnessed BP neural networks to prognosticate the prioritization of production facilities in the bus body manufacturing sector. Their work serves as an illustrative testament to the potential of neural networks in the production and resource allocation facets of scientific project management. Liu et al. 20 undertook an analysis of the influential factors and early warning signs pertaining to construction workers’ safety conditions. This investigation underscores the profound implications of neural networks in safety management within the context of engineering and construction project management. Li et al. 21 harnessed optimized BP neural networks to anticipate risks in the financial management arena of listed companies. Their outcomes underscore the utility of neural networks in financial management, providing an exemplar of a risk assessment tool for scientific research project management.
The comprehensive analysis of the aforementioned studies reveals that BP neural networks exhibit substantial capabilities in scrutinizing historical project data, discerning intricate resource demand–supply dynamics, and offering valuable insights for project management decisions and optimizations. These applications underscore the potential of BP neural networks as indispensable tools within the project management domain. Nonetheless, several challenges persist, particularly concerning the real-time adaptability of BP neural networks and their capacity to cater to dynamic project management requisites.
Research in the field of scientific research project resource management and risk prediction
Within the realm of scientific research project resource management and risk prediction, various studies by notable scholars warrant attention. Jehi et al. 22 employed statistical models for risk prediction but overlooked the intricate resource conflict relationships within scientific research projects. Efficient project resource management and accurate risk prediction are pivotal for ensuring smooth project execution and attaining desired outcomes. Asamoah et al. elucidated that scientific research projects necessitate both tangible and intangible resources 23 , encompassing materials, equipment, personnel, funding, and time. The judicious allocation and optimal utilization of these resources significantly influence project progress and outcomes. Misallocation of resources can lead to setbacks such as project delays and budget overruns. Meanwhile, Zwikael et al. identified organizational culture, awareness, support, rewards, and incentive programs as key drivers impacting the effective management of scientific research project benefits 24 . These risks can profoundly affect project advancement and outcomes, underscoring the importance of accurate prediction and adept management. Farooq et al. advocated for scientific project management, emphasizing the need for enhanced risk management strategies and management efficacy to foster sustainable enterprise development 25 .
In conclusion, studies on project resource management and risk prediction encompass diverse facets, including resource allocation, risk assessment, and model development. These efforts offer essential theoretical and methodological underpinnings for the effective execution of scientific research endeavors. Given the ongoing expansion and growing complexity of scientific projects, further research on resource management and risk prediction is imperative to navigate increasingly intricate circumstances.
A comprehensive review of methods employed in scientific research project management and risk assessment reveals a predominant focus on quantitative analysis, qualitative research, and the integration of AI techniques. In particular, the utilization of BP neural networks, as demonstrated in studies such as Sivakumar et al., Liu et al., and Li et al., underscores their capacity to furnish real-time data analysis and decision-making support for project managers. However, it remains evident that challenges persist in harnessing the full potential of BP neural networks in terms of real-time adaptability and resource allocation within the multifaceted landscape of dynamic project management. Hence, this study accentuates the existing methodological challenges associated with resource conflict resolution, risk management, and overall scientific research project management. Through the optimization and refinement of BP neural network applications in risk assessment, this study strives to furnish organizations with effective decision-making tools. Ultimately, the insights gleaned from this study aim to serve as a valuable reference for scientific research project managers as they navigate the complexities of project risk management.
Prediction method for scientific research project management risks based on the BP neural network
Analysis of the construction of a scientific research project management risk system.
Scientific research project management constitutes a specialized discipline encompassing the planning, organization, execution, and oversight of scientific research endeavors. Its primary objective is to facilitate the effective attainment of research objectives and anticipated outcomes. The overarching aim of scientific research project management is to optimize resource allocation, schedule planning, and risk mitigation, thereby ensuring the successful culmination of research projects 26 , 27 . A visual representation of the fundamental task processes integral to scientific research project management is depicted in Fig. 1 .
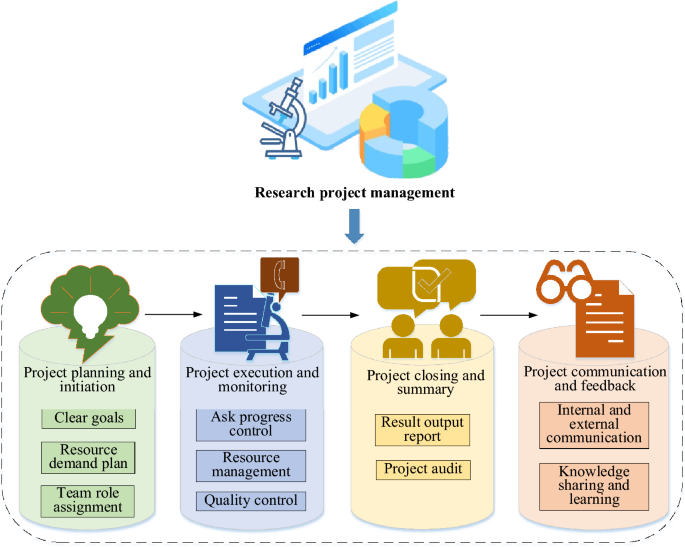
Schematic representation of key scientific research project management tasks.
Scientific research project management, as illustrated in Fig. 1 , constitutes an essential framework to ensure the efficient and organized execution of scientific research endeavors. It encompasses four core phases: project planning and initiation, project execution and monitoring, project closure and summarization, and project communication and feedback 28 . The meticulous determination of project requisites is of particular significance, encompassing financial resources, personnel, equipment, materials, and more. Failure to ensure the effective utilization and judicious allocation of these resources during project management may introduce the risk of hindrances in the smooth progress and achievement of the research project’s envisioned objectives.
Ongoing scientific research projects necessitate an array of resources, encompassing both tangible assets such as materials, equipment, and funds, and intangible elements like time, personnel expertise, and organizational support 29 , 30 . These resources are intricately interwoven within scientific research projects and collectively influence project success. However, when confronted with limited total resources, resource conflicts can arise when multiple projects vie for the utilization of the same resources. Consequently, this study has devised a resource conflict risk index system tailored for the management of scientific research projects. This system stratifies risks according to the categories of resources implicated in the project implementation process, as depicted in Fig. 2 . In this study, ensuring the representativeness and comprehensiveness of risk assessment for resource conflicts in scientific research project management is pivotal. A multifaceted and systematic approach is adopted to define risk categories. A comprehensive literature review initially identifies common resource conflicts in scientific research project management. Subsequently, through interviews and surveys with industry research project managers, firsthand information on specific challenges and risk factors encountered during project execution is collected. Additionally, referencing international standards and best practices ensures the authority and applicability of risk classification. The outcome of these efforts is illustrated in Fig. 2 , showcasing a meticulously designed resource conflict risk index system. It encompasses six major categories: equipment risk, material risk, personnel risk, financial risk, time risk, and organizational risk, further subdivided into 17 specific sub-items. Acknowledging the complexity and diversity of research projects, it is recognized that, despite efforts made, other potential risks may not be included in the current model. A dynamic iterative approach is proposed to address this challenge, integrate additional risk factors, and continuously optimize the model. Specific steps are outlined to enhance the model’s capabilities. Firstly, establishing a monitoring system to regularly collect user feedback and industry updates allows the prompt discovery and incorporation of new risk factors. Simultaneously, closely monitoring the latest research findings in the domestic and international scientific research project management field ensures the continuous integration of new discoveries from academia. Additionally, a dedicated team conducts regular in-depth reviews of the existing risk index system, adding, deleting, or adjusting the weights of risk factors as needed based on actual requirements. This process enables the model to better adapt to the current project management environment and future trends. Secondly, utilizing the newly integrated dataset to cross-validate the model ensures that the newly added risk factors are appropriately assessed and predicted. By comparing the performance of different versions of the model, a more accurate measurement of the effects of optimization is achieved. Finally, research project managers are encouraged to provide real-time feedback, including the model’s performance in actual applications, overlooked risk points, and improvement suggestions, enhancing the model’s usability and reliability. These methods aim to construct a more refined, flexible, and adaptable scientific research project risk assessment model that continuously evolves to meet changing needs. Through continuous optimization and improvement, this model is believed to more effectively assist project managers in making risk-based decisions and promote the success rate of scientific research projects.
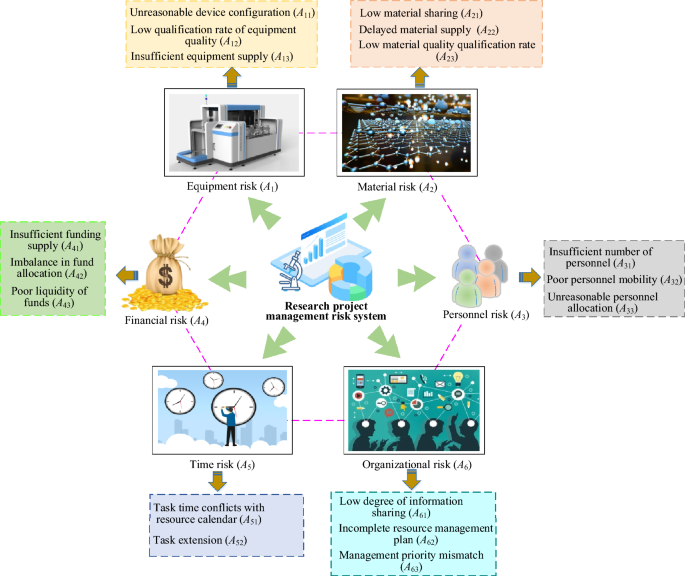
Resource conflict risk indicator system for scientific research project management.
As depicted in Fig. 2 , this risk system underscores the significance of material quality and timely supply in project execution. The establishment of this resource conflict risk indicator system forms a fundamental basis for subsequent model development and risk forecasting, empowering project managers to gain comprehensive insights into and effectively manage resource conflict risks.
Weight analysis process using APH for the risk indicator system
The AHP is primarily employed for the comprehensive analysis of multifaceted problem systems, involving the segmentation of interrelated factors into hierarchical levels. It subsequently facilitates objective assessments at each tier. This method typically deconstructs problems into a tripartite structure comprising the following levels: the objective layer (highest), the criteria layer (intermediate), and the indicator layer (fundamental) 31 , 32 . In this context, the objective layer pertains to the project’s resource conflict risk, which represents the core challenge addressed by this structural model. The criteria layer provides an initial decomposition of the objective layer and establishes the foundational logical framework for third-level indicators. The indicator layer encompasses risk factors, specifically, the potential triggers for resource conflict risks. The weight analysis process, employing the AHP for the risk indicator system, is delineated in Fig. 3 .
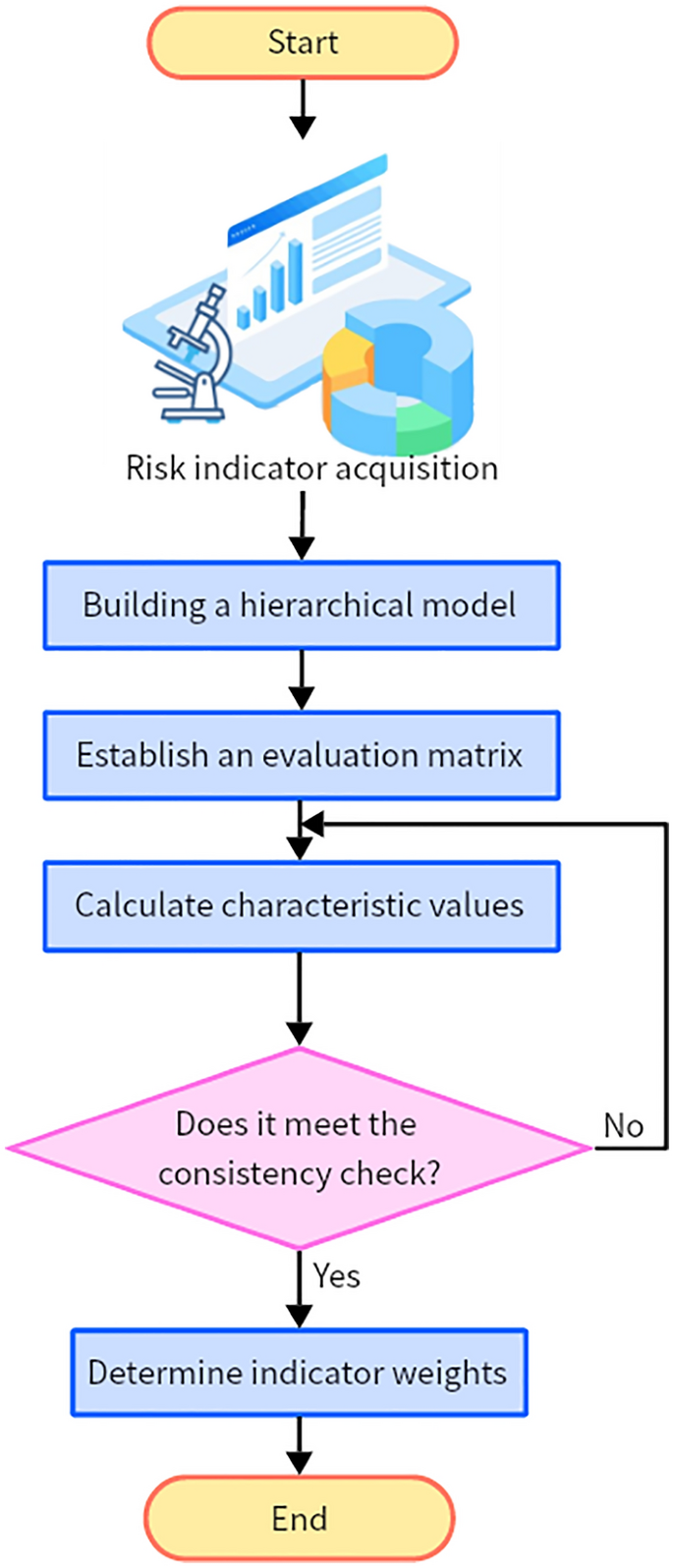
Weight analysis process of applying the hierarchical analysis method to the risk indicator system.
In Fig. 3 , the application of the AHP to the weight analysis of the scientific research project management risk indicator system follows a general procedure: sequentially defining individual problems, creating a hierarchical structural model, constructing pairwise comparison matrices, performing hierarchical ranking calculations and consistency tests, and finally, selecting evaluation criteria systematically for assessment.
The initial step involves breaking down the intricate problem into distinct components, creating a hierarchical structure model comprising the target layer, criterion layer, and indicator layer.
In this phase, the assessment of relative importance between elements leads to the formation of a pairwise comparison judgment matrix, denoted as matrix A , as depicted in Eq. ( 1 ).
In Eq. ( 1 ), \(a_{ij} > 0\) , \(a_{ji} = 1/a_{ij}\) , and \(a_{ii} = 1\) .
The AHP calculations are performed following the classic methodology proposed by Rehman 33 . The process begins by computing the product M i of the elements within each row, as illustrated in Eq. ( 2 ).
The next step involves calculating the n -th root of M i , as described in Eq. ( 3 ).
Next, the process involves normalizing \(W = \left[ {W_{1} ,W_{2} , \cdots ,W_{n} } \right]^{T}\) , as shown in Eq. ( 4 ).
Finally, the maximum eigenvalue \(\lambda_{\max }\) is calculated via Eq. ( 5 ).
The calculation of weights and the consistency test of the judgment matrix involve the use of the eigenvalue method to calculate the weight vector of the judgment matrix. This is demonstrated in Eq. ( 6 ).
In Eq. ( 6 ), \(\lambda_{\max }\) denotes the maximum characteristic root of A , Q signifies the eigenvector, and the weight vector is obtained by normalizing Q.
Continuing with the consistency testing, the weight vector must undergo evaluation for consistency. To initiate this evaluation, calculate the Consistency Index ( C.I. ) using Eq. ( 7 ).
Next, it is imperative to determine the corresponding average Random Consistency Index ( R.I. ). Subsequently, the Consistency Ratio ( C.R. ) is computed using the formula presented in Eq. ( 8 ).
If the calculated C.R. is less than 0.1, it indicates that the judgment matrix meets the prescribed consistency criteria, and the assigned weight values for each indicator are considered valid. However, if the calculated C.R. equals or exceeds 0.1, this signals the need for adjustments to the judgment matrix. To address this, the matrix is re-evaluated, and consistency checks are repeatedly performed until the matrix achieves the required level of consistency.
Analyzing the resource conflict risk management model for scientific research projects based on the BP neural network
This section focuses on predicting and evaluating the potential occurrence of various risk factors within scientific research projects. The objective is to facilitate the selection of appropriate response strategies aimed at minimizing losses stemming from risks associated with scientific research endeavors. Resource management within scientific research projects is a complex undertaking, with resource conflict risks influenced by a multitude of factors. Furthermore, as projects evolve, the risk landscape undergoes dynamic changes. In contrast to conventional statistical models, BP neural networks offer distinctive advantages. They employ a combination of forward signal propagation and reverse error-adjustment learning techniques, showcasing exceptional self-learning capabilities, distributed knowledge storage, and associative memory functions 34 . The BP neural network model, rooted in the backpropagation algorithm, evolved from the necessity to simulate biological neural systems and meet the demands of machine learning. Originating in the 1980s, it became a prominent deep learning model, continually iterating and adjusting connection weights to minimize the error between output and target. This learning mechanism allows the BP neural network to adapt to complex non-linear relationships, showcasing robust approximation and generalization capabilities. Over time, enhanced computer hardware and algorithm optimization led to widespread application and development of the BP neural network model. Algorithmically, various improvements, including the momentum method, adaptive learning rate, and regularization, were introduced to boost training speed and generalization ability, addressing challenges such as susceptibility to local minima in traditional BP algorithms. The advent of deep learning saw the integration of the BP neural network into deeper structures like ResNet and CNN, enabling it to handle more intricate tasks and data. The model’s applicability expanded across diverse domains, including image and speech recognition, natural language processing, financial forecasting, and medical diagnosis, yielding breakthrough results. Moreover, technological advancements like big data and cloud computing have enhanced the training and application efficiency of the BP neural network model, presenting new avenues for development. In conclusion, the evolution of the BP neural network model stems from algorithmic refinements, structural enhancements, and broadened applications, providing potent tools for addressing diverse practical challenges. The data transmission process of the BP neural network is illustrated in Fig. 4 .
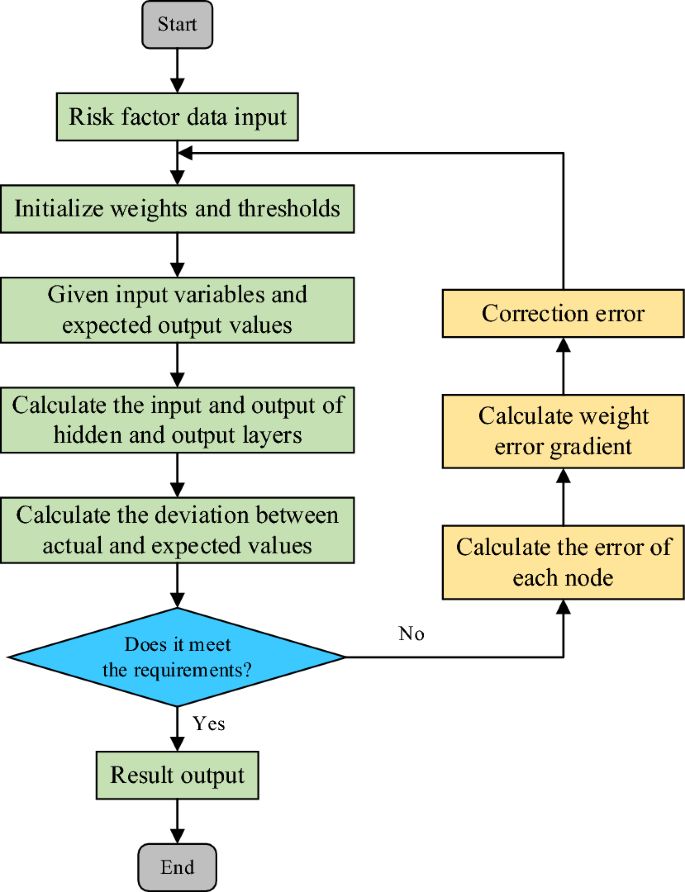
Data transmission flow chart of the BP neural network.
Figure 4 illustrates the data transmission process in the BP neural network, highlighting forward propagation, which entails processing and transmitting received data information. This unidirectional propagation begins at the input layer, traverses through the hidden layers, and culminates in the output layer to yield the network’s overall output. Let the received input data be denoted as X = ( x 1 , x 2 …, x n ), with ‘ n ’ signifying the number of neurons in the input layer. The connections between the input layer and the hidden layer initially possess randomized weight values. This citation is derived from Liu et al.’s recommendation 35 to prevent premature convergence to local minima during the training process. Representing the weight of the connection between the i -th neuron in the input layer and the j -th neuron in the hidden layer as W ij . The notation follows Narkhede et al.’s study 36 , which offers a comprehensive explanation of neural network fundamentals and operational principles. The information received by the hidden layer is expressed in Eq. ( 9 ).
In Eq. ( 9 ), i represents the number assigned to neurons in the input layer, ‘ j ’ pertains to the number of neurons in the hidden layer, and A = ( a 1 , a 2 …, a m ) symbolizes the input variables received by the hidden layer. Upon receiving these variables, the hidden layer neuron transforms them into the output value of the hidden layer using the activation function. The methodology in this section draws from the research by Narengbam et al. 37 on activation functions in deep learning models. Specifically, the treatment of the output layer mirrors that of the hidden layers, and the computation of output layer neurons adheres to the methodology outlined in the cited literature.
In Eq. ( 10 ), Y = ( y 1 , y 2 …, y m ) represents the output variables of the hidden layer. The computation method for the input and output values of the output layer parallels that of the hidden layer. The weight denoted as v jk signifies the connection between the j -th neuron in the hidden layer and the k -th neuron in the output layer. The information received by the output layer is described in Eq. ( 11 ).
The output value of the output layer neurons, once activated by the activation function, is expressed in Eq. ( 12 ).
At this juncture, the output value O denoted as \(O = \left( {o_{1} ,o_{2} , \cdots ,o_{z} } \right)\) is obtained, signifying the conclusion of the forward propagation process.
In the backpropagation process, the loss function J quantifies the error between the neural network’s output value and the true value (referring to the definition and application of the loss function in neural network optimization as articulated by Özden et al. 38 ), as illustrated in Eq. ( 13 ).
During the neural network’s training process, the weight, denoted as W , and the bias vector, denoted as b , play essential roles. The gradient descent method is employed to optimize the neural network (derived from Kumar et al.’s 39 analysis of the effectiveness of optimization algorithms in deep learning training). Each iteration within the gradient descent method updates the parameters W and b as per Eqs. ( 14 ) and ( 15 ).
where α represents the learning rate. The crucial step involves computing derivatives using backpropagation, employing the BP algorithm to calculate \(\frac{\partial }{{\partial W_{ij}^{\left( l \right)} }}J\left( {W,b;x,y} \right)\) and \(\frac{\partial }{{\partial b_{i}^{\left( l \right)} }}J\left( {W,b;x,y} \right)\) . These two components represent the derivatives of the cost function J ( W , b ; x , y ) for a single sample ( x , y ). Once this derivative is computed, deriving the derivatives of the overall cost function J ( W , b ; x , y ) becomes relatively straightforward. The calculated results are presented in Eqs. ( 16 ) and ( 17 ).
This study aims to develop a resource conflict risk management model tailored to predict and assess the resource conflict risks inherent in scientific research projects during execution. Resource conflicts arise from competition for limited resources like equipment, funding, and personnel among multiple projects. If unaddressed, these conflicts can significantly impede project progress and outcomes. The model’s specific objectives are to analyze project-related information (e.g., project scale, duration, funding, personnel allocation) to predict potential conflict points in resource allocation, enabling project managers to proactively mitigate or avoid conflicts and optimize resource utilization effectively. To achieve these objectives, we employ a BP neural network approach for model construction, chosen for its superior non-linear mapping capability and self-learning characteristics, enabling it to learn from extensive historical project data and identify complex resource conflict risk patterns. The model construction entails key steps: Data preprocessing involves cleaning and normalizing collected project data to meet model input requirements. Feature selection entails choosing highly correlated feature variables associated with resource conflict risks as model inputs based on expert knowledge and data analysis results. Model training and validation involve training the BP neural network with labeled historical project data and evaluating and optimizing model performance through techniques like cross-validation. Through these methods, the developed model accurately predicts resource conflict risks in scientific research project management, providing decision support for project managers to enhance resource utilization efficiency and foster successful project completion.
While the BP neural network possesses robust learning and non-linear fitting capabilities, inadequate training data can lead to suboptimal fitting. In some cases, the network may only excel at learning from a limited dataset, generating a mapping function (typically represented as a weight vector) that closely matches the training dataset. Consequently, it may struggle to generalize well to new data, exhibiting insufficient generalization abilities. This scenario is known as overfitting. To mitigate overfitting, this study introduces the Dropout regularization method 40 when applying the BP neural network to scientific research project risk management. The Dropout method involves freezing nodes within the input and hidden layers. It is particularly useful when specific neuron correlations in the input layer hinder continuous error convergence during training. The node freezing rate should strike a balance—not too low, as it would have an insignificant impact on the neural network, and not too high, which could lead to underfitting. Therefore, this study sets the node freezing rate for the Dropout regularization method at 50%. By incorporating the Dropout method into the BP neural network, the network topology used for managing resource conflict risks in scientific research projects, based on the BP neural network, is depicted in Fig. 5 .
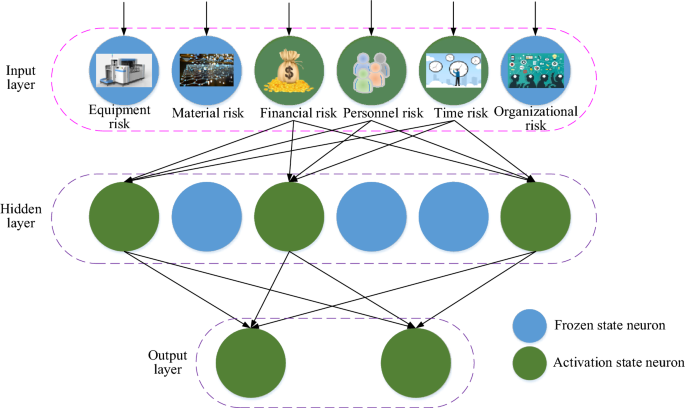
Network topology based on the BP neural network applied to the resource conflict risk management model for scientific research projects.
As depicted in Fig. 5 , this model incorporates a novel approach. During each training iteration, a randomly selected set of neurons, encompassing those associated with equipment, materials, and organizational risk factors, is temporarily frozen. These frozen neurons do not participate in either the forward propagation calculations or the subsequent backpropagation error adjustments within the current training cycle. The weights connecting these neurons to others retain their previous states or revert to their initial values from the last training update. As the next training iteration commences, the neurons previously frozen are unfrozen, and a new batch of neurons is randomly chosen for freezing. This iterative process effectively bolsters the BP neural network’s ability to generalize from limited data, particularly when addressing resource conflict risk management in research projects.
The integration of the Dropout method into the BP neural network introduces further opportunities for optimization. Adjustments to the network’s depth, the number of neurons, and the choice of activation functions within the risk prediction model can be made. The specific optimization procedure for the BP neural network is outlined in Fig. 6 .
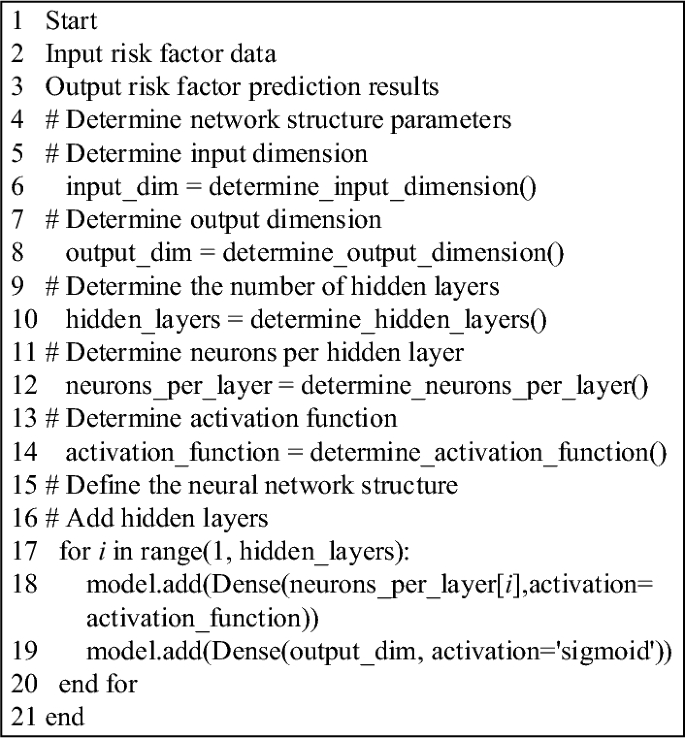
Flowchart presenting the pseudocode algorithm for optimizing the BP neural network.
Experimental evaluation
To assess the performance of the resource conflict risk management model developed in this study, a BP neural network was constructed utilizing the ‘newff’ function within MATLAB. Python was employed for data preprocessing and algorithm implementation. The training of the BP neural network involved configuring parameters for net.trainFcn and net. trainParam following network initialization. Training iterations continued until the error met the predefined performance criterion. The dataset utilized in this study consisted of research project information spanning all universities in Xi’an, China, from September 2021 to March 2023. In comprehensively evaluating the performance of the resource conflict risk management model developed in this study, the scope and objectives of data collection are first determined, focusing primarily on scientific research projects at major universities in the Xi’an area. Data sources included publicly available project records, official website information, and pertinent research project databases. The utilization of web scraping techniques facilitates automated data collection, encompassing details such as project names, principal investigators, start and completion dates, funding particulars, research areas, and participating personnel. Rigorous anonymization and encryption measures are implemented to uphold information security. Subsequently, to enhance understanding of the data characteristics, exploratory data analysis is conducted on the cleaned dataset. This involves calculating descriptive statistics, conducting distribution tests, and performing correlation analysis. Such steps aid in identifying the most influential feature variables for the predictive model. Given that raw data often contain missing values, outliers, or inconsistencies, comprehensive data cleaning is executed, which includes imputation of missing values, removal of outlier data, and standardization of data formats. To safeguard individual privacy, sensitive information such as project leader names undergoes anonymization and encryption. Concerning the application of the AHP in this study, this method is employed to ascertain the relative weights of various risk factors (including materials, equipment, funding, time, personnel skills, and organizational support). The operational process involves establishing a pairwise comparison judgment matrix based on expert assessments and historical data analysis. Each element in the matrix reflects the importance of one risk factor relative to another. The weights of each risk factor are determined by calculating the maximum eigenvalue of the judgment matrix and its corresponding eigenvector. Consistency indices and random consistency ratios are used to verify the consistency of the judgment matrix, deeming the derived weights acceptable only when the random consistency ratio is below 0.1. Using these meticulously assigned weighted risk factors throughout the model evaluation process, resource conflict risk prediction is conducted via the BP neural network using data collected from actual scientific research projects.
Subsequently, rigorous data anonymization procedures were applied, including de-identification, data anonymization, and encryption of sensitive information. The data preprocessing workflow encompassed comprehensive data cleaning to rectify missing or outlier data points. Ultimately, data from 8,175 research projects were amassed and segregated into training and testing subsets, with an 80% to 20% partition ratio.
To assess the performance of the model developed in this study, an initial step involved employing the AHP to evaluate the weights assigned to each factor, including materials, equipment, funds, time, personnel skills, and organization. Subsequently, the algorithm presented in this study was combined with the Convolutional Neural Network (CNN) 41 , Bidirectional Long Short-Term Memory (BiLSTM) 42 , and comparative experiments were conducted in alignment with recent studies conducted by Liu et al. and Li et al. The evaluation primarily relied on accuracy and RMSE as key metrics, precisely measuring model prediction accuracy. Additionally, the Garson sensitivity analysis method was employed to assess the sensitivity of risk factors across various algorithms.
Results and discussions
Analysis of weights and sensitivity results of different factors.
The analysis of weights and sensitivities for various factors is depicted in Figs. 7 and 8 .
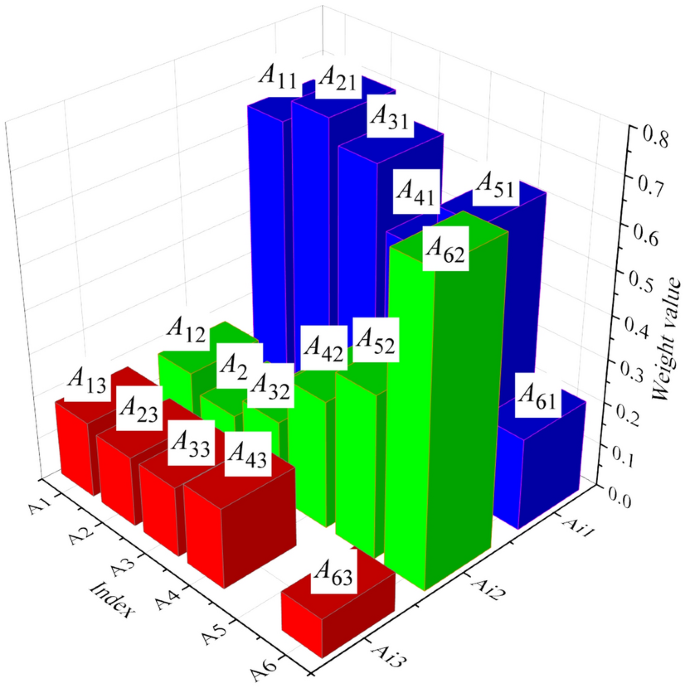
Weight results of different factors.
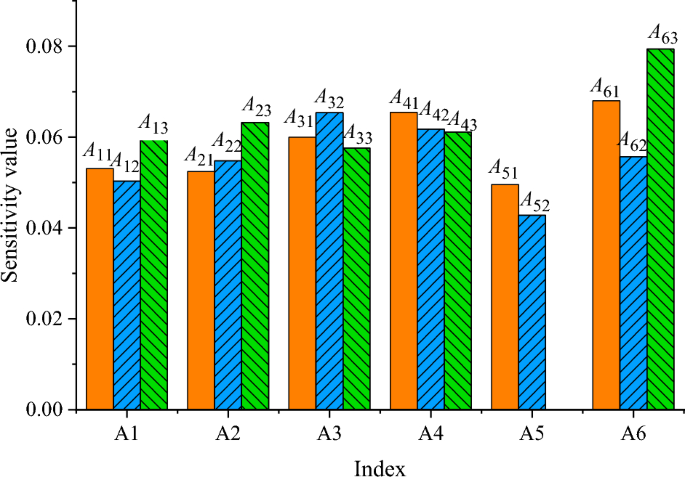
Sensitivity results of different factors.
Figure 7 highlights the various risk factors present in scientific research project management, including materials, equipment, funds, time, personnel skills, and organization. A more in-depth examination of the weight of sub-indicators within each factor reveals that A 21 holds the highest weight value, at 0.705, while A 63 carries the smallest weight value. Consequently, the application of the AHP in this study enables a clear representation of the significance of each influencing factor. This, in turn, facilitates a more targeted and informed decision-making process, allowing for decisions that align better with the actual circumstances and desired outcomes.
Figure 8 reveals notable variations in the sensitivity of each risk factor to the model’s output variables. Organizational risk emerges as the most influential factor on the comprehensive risk value, accounting for a relative importance of 20.31%. Following closely are financial risk at 18.84%, personnel risk at 18.30%, material risk at 17.04%, equipment risk at 16.29%, and time risk at 9.24%. A more detailed scrutiny of the sensitivity of individual sub-indicators within each factor uncovers that A52 exhibits the lowest sensitivity, standing at 4.28%, while A63 records the highest sensitivity, reaching 7.84%.
Model performance comparison results under different algorithms
In-depth analysis encompassed evaluating the accuracy and RMSE outcomes of distinct algorithms across diverse indicators, as depicted in Figs. 9 and 10 .
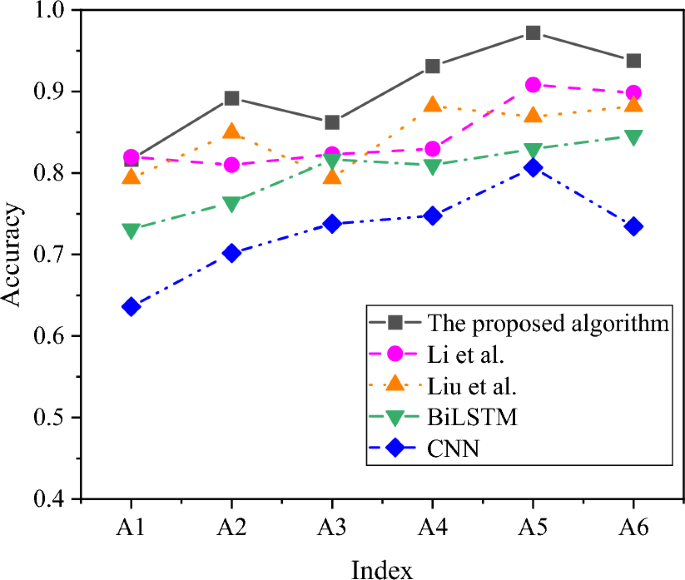
Visual representation of accuracy results achieved by different algorithms across various factors.
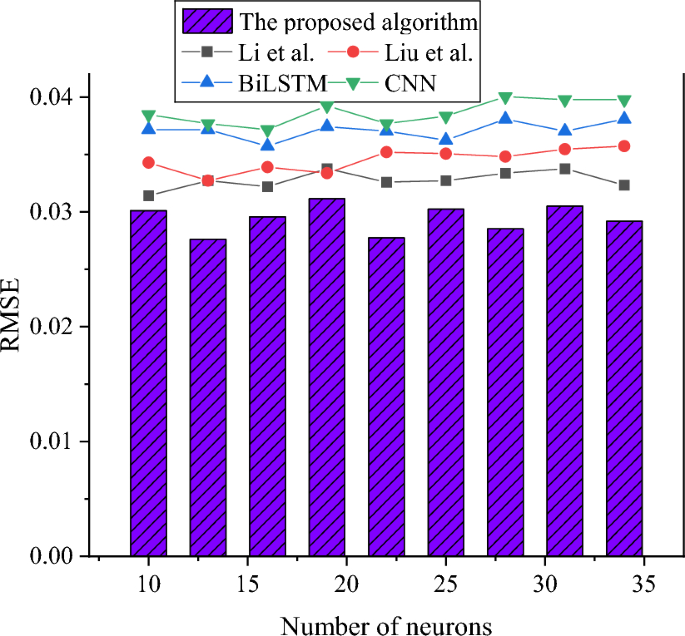
RMSE comparison results of each algorithm under different numbers of neurons.
Figure 9 illustrates that the accuracy of various algorithms remains relatively stable across different index factors. Notably, the risk prediction accuracy achieved by the algorithm proposed in this study outperforms other model algorithms across various factors. The highest risk prediction accuracy is observed in the time factor, reaching an impressive 97.21%, while the equipment factor yields the lowest prediction accuracy, hovering around 80%. Upon further comparison of risk prediction accuracy across algorithms, it becomes evident that the model algorithms proposed in this study outperform Li et al.’s model algorithm and Liu et al.’s model algorithm. Additionally, the proposed model algorithm surpasses BiLSTM and CNN. Consequently, this study’s model algorithm effectively identifies risk factors in the management of scientific research projects.
Figure 10 presents the RMSE results of each algorithm, and it is evident that increasing the number of hidden layer neurons does not significantly alter the RMSE values. Specifically, the RMSE of the model algorithm introduced in this study consistently remains around 0.03. In contrast, other model algorithms yield RMSE values exceeding 0.031, indicating higher errors compared to the model proposed in this study. When arranging the RMSE results in ascending order, it becomes apparent that the order is as follows: the model algorithm introduced in this study has the lowest RMSE, followed by Li et al.’s proposed model algorithm, Liu et al.’s proposed model algorithm, BiLSTM, and CNN. Therefore, the research model demonstrates effective risk prediction in scientific research project management, characterized by lower identification errors and superior fitting capabilities.
This study established a resource conflict risk index system for scientific research project management and introduced a BP neural network as a risk prediction model. Leveraging its non-linear fitting and self-learning capabilities, the model effectively captured intricate resource demand and supply dynamics, enabling a more precise assessment of resource conflict risks. The performance evaluation revealed the model’s strength in predicting time-related risks, achieving an accuracy rate of 97.21% with an RMSE consistently around 0.03, indicating strong fitting capabilities. The developed BP neural network model in this study effectively predicts resource conflict risks in scientific research project management, serving as a valuable decision support tool for risk assessment. However, certain limitations are acknowledged in this research. Firstly, the dataset is derived from universities in a specific region (Xi’an), and although sizable, it may not comprehensively represent all types of scientific research projects. Future endeavors could involve incorporating more diverse and extensive data sources to enhance the model’s universality and robustness. Secondly, despite the notable advantages of BP neural networks in addressing non-linear problems, the selection of appropriate network structures and parameter settings remains a challenge. Subsequent work could focus on further enhancing the network’s performance through the exploration of additional optimization algorithms. In terms of future research directions, the following points are proposed: Firstly, considering the integration of various machine learning and deep learning technologies to obtain more comprehensive risk prediction results. Secondly, exploring the application of the model in scientific research projects of different scales and types to validate and broaden its applicability. Lastly, investigating the integration of the model into a real-time project management system can provide project managers with dynamic risk monitoring and warning services.
Data availability
All data generated or analysed during this study are included in this published article [and its supplementary information files].
Ren, S. et al. The emerging driving force of inclusive green growth: Does digital economy agglomeration work?. Bus. Strateg. Environ. 31 (4), 1656–1678 (2022).
Article Google Scholar
Wang, W., Hu, Y. & Lu, Y. Driving forces of China’s provincial bilateral carbon emissions and the redefinition of corresponding responsibilities. Sci. Total Environ. 857 , 159404 (2023).
Article ADS CAS PubMed Google Scholar
Do, S. T., Nguyen, V. T. & Likhitruangsilp, V. RSIAM risk profile for managing risk factors of international construction joint ventures. Int. J. Constr. Manag. 23 (7), 1148–1162 (2023).
Google Scholar
Nguyen, H. D., Do, Q. N. H. & Macchion, L. Influence of practitioners’ characteristics on risk assessment in Green Building projects in emerging economies: A case of Vietnam. Eng. Constr. Archit. Manag. 30 (2), 833–852 (2023).
Shayan, S., Pyung Kim, K. & Tam, V. W. Y. Critical success factor analysis for effective risk management at the execution stage of a construction project. Int. J. Constr. Manag. 22 (3), 379–386 (2022).
Alam, I., Sarwar, N. & Noreen, I. Statistical analysis of software development models by six-pointed star framework. PLoS ONE 17 (4), e0264420 (2022).
Article CAS PubMed PubMed Central Google Scholar
Pham, H. T. et al. Supply chain risk management research in construction: A systematic review. Int. J. Constr. Manag. 23 (11), 1945–1955 (2023).
Zhao, Y. et al. Predicting delays in prefabricated projects: SD-BP neural network to define effects of risk disruption. Eng. Constr. Archit. Manag. 29 (4), 1753–1776 (2022).
Zhang, X. et al. Application of grey feed forward back propagation-neural network model based on wavelet denoising to predict the residual settlement of goafs. PLoS ONE 18 (5), e0281471 (2023).
Article PubMed PubMed Central Google Scholar
El Khatib, M., Al Mulla, A. & Al, K. W. The role of blockchain in E-governance and decision-making in project and program management. Adv. Internet Things 12 (3), 88–109 (2022).
Ujong, J. A., Mbadike, E. M. & Alaneme, G. U. Prediction of cost and duration of building construction using artificial neural network. Asian J. Civil Eng. 23 (7), 1117–1139 (2022).
Khiat, H. Using automated time management enablers to improve self-regulated learning. Act. Learn. High. Educ. 23 (1), 3–15 (2022).
Gao, J. Analysis of enterprise financial accounting information management from the perspective of big data. Int. J. Sci. Res. 11 (5), 1272–1276 (2022).
Jeong, J. & Jeong, J. Quantitative risk evaluation of fatal incidents in construction based on frequency and probability analysis. J. Manag. Eng. 38 (2), 04021089 (2022).
Matel, E. et al. An artificial neural network approach for cost estimation of engineering services. Int. J. Constr. Manag. 22 (7), 1274–1287 (2022).
Zhang, H. et al. A real-time and ubiquitous network attack detection based on deep belief network and support vector machine. IEEE/CAA J. Autom. Sin. 7 (3), 790–799 (2020).
Gong, Y. et al. Design and interactive performance of human resource management system based on artificial intelligence. PLoS ONE 17 (1), e0262398 (2022).
Bai, L. et al. Service provider portfolio selection for project management using a BP neural network. Ann. Oper. Res. 308 , 41–62 (2022).
Article MathSciNet Google Scholar
Sivakumar, A. et al. Prediction of production facility priorities using Back Propagation Neural Network for bus body building industries: A post pandemic research article. Qual. Quant. 57 (1), 561–585 (2023).
Article CAS PubMed Google Scholar
Liu, N. et al. Influencing factors and prewarning of unsafe status of construction workers based on BP neural network. Appl. Sci. 13 (6), 4026 (2023).
Article CAS Google Scholar
Li, X., Wang, J. & Yang, C. Risk prediction in financial management of listed companies based on optimized BP neural network under digital economy. Neural Comput. Appl. 35 (3), 2045–2058 (2023).
Jehi, L. et al. Individualizing risk prediction for positive coronavirus disease 2019 testing: Results from 11,672 patients. Chest 158 (4), 1364–1375 (2020).
Asamoah, R. O. et al. Identifying intangible resources to enhance profitability strategies of Small-Medium Scale Construction Firms (SMSCFs) in developing countries. Int. J. Construct. Manag. 22 (11), 2207–2214 (2022).
Zwikael, O. & Huemann, M. Project benefits management: Making an impact on organizations and society through projects and programs. Int. J. Project Manag. 41 (8), 102538 (2023).
Farooq, R. A review of knowledge management research in the past three decades: A bibliometric analysis. VINE J. Inf. Knowl. Manag. Syst. 54 (2), 339–378 (2024).
Bergevin, M. D. et al. Cache a Killer: Cache Valley virus seropositivity and associated farm management risk factors in sheep in Ontario, Canada. PLoS ONE 18 (8), e0290443 (2023).
Huang, G., Lee, S. M. & Clinciu, D. L. Competitive advantages of organizational project management maturity: A quantitative descriptive study in Australia. PLoS ONE 18 (6), e0287225 (2023).
Yesica, R. et al. Project management office manager’s competences: Systematic literature review. Int. J. Project Organ. Manag. 15 (2), 253–278 (2023).
Yu, C. & Hsiao, Y. C. IT project management resource: Identifying your project’s common goals. Int. J. Inf. Technol. Project Manag. 13 (1), 1–15 (2022).
Qu, S. et al. The performance evaluation of management mode of small water resources projects. PLoS ONE 18 (4), e0282357 (2023).
Wu, Z. et al. Urban flood risk assessment in Zhengzhou, China, based on a D-number-improved analytic hierarchy process and a self-organizing map algorithm. Remote Sens. 14 (19), 4777 (2022).
Article ADS Google Scholar
Lin, C. L., Fan, C. L. & Chen, B. K. Hybrid analytic hierarchy process-artificial neural network model for predicting the major risks and quality of Taiwanese construction projects. Appl. Sci. 12 (15), 7790 (2022).
Rehman, A. et al. Multi-hazard susceptibility assessment using the analytical hierarchy process and frequency ratio techniques in the Northwest Himalayas, Pakistan. Remote Sens. 14 (3), 554 (2022).
Liu, J. et al. Developing a hybrid algorithm based on an equilibrium optimizer and an improved backpropagation neural network for fault warning. Processes 11 (6), 1813 (2023).
Narkhede, M. V., Bartakke, P. P. & Sutaone, M. S. A review on weight initialization strategies for neural networks. Artif. Intell. Rev. 55 (1), 291–322 (2022).
Narengbam, L. & Dey, S. Harris hawk optimization trained artificial neural network for anomaly based intrusion detection system. Concurr. Comput. Pract. Exp. 35 (23), e7771 (2023).
Özden, A. & İşeri, İ. COOT optimization algorithm on training artificial neural networks. Knowl. Inf. Syst. 65 (8), 3353–3383 (2023).
Kumar, G., Singh, U. P. & Jain, S. Swarm intelligence based hybrid neural network approach for stock price forecasting. Comput. Econ. 60 (3), 991–1039 (2022).
Zhao, Y. Application of BP neural network algorithm in visualization system of sports training management. Soft Comput. 27 (10), 6845–6854 (2023).
Nketiah, E. A. et al. Recurrent neural network modeling of multivariate time series and its application in temperature forecasting. PLoS ONE 18 (5), e0285713 (2023).
Kumar, T. A. et al. A novel CNN gap layer for growth prediction of palm tree plantlings. PLoS ONE 18 (8), e0289963 (2023).
Liu, J. et al. Research on reservoir porosity prediction method based on bidirectional longshort-term memory neural network. Prog. Geophys. 37 (5), 1993–2000 (2022).
Download references
Author information
Authors and affiliations.
Institute of Policy Studies, Lingnan University, Tuen Mun, 999077, Hong Kong
Xuying Dong & Wanlin Qiu
You can also search for this author in PubMed Google Scholar
Contributions
Dong Xuying and Qiu Wanlin studied the specific situation of BP neural network, and combined with the experience of scientific research project management, Dong Xuying designed a scientific research project management evaluation and risk prediction method based on BP neural network. At the same time, Qiu Wanlin collected and analyzed the experimental data in this paper according to the actual situation. Dong Xuying and Qiu Wanlin wrote the first draft together.
Corresponding author
Correspondence to Wanlin Qiu .
Ethics declarations
Competing interests.
The authors declare no competing interests.
Additional information
Publisher's note.
Springer Nature remains neutral with regard to jurisdictional claims in published maps and institutional affiliations.
Supplementary Information
Supplementary information., rights and permissions.
Open Access This article is licensed under a Creative Commons Attribution 4.0 International License, which permits use, sharing, adaptation, distribution and reproduction in any medium or format, as long as you give appropriate credit to the original author(s) and the source, provide a link to the Creative Commons licence, and indicate if changes were made. The images or other third party material in this article are included in the article's Creative Commons licence, unless indicated otherwise in a credit line to the material. If material is not included in the article's Creative Commons licence and your intended use is not permitted by statutory regulation or exceeds the permitted use, you will need to obtain permission directly from the copyright holder. To view a copy of this licence, visit http://creativecommons.org/licenses/by/4.0/ .
Reprints and permissions
About this article
Cite this article.
Dong, X., Qiu, W. A method for managing scientific research project resource conflicts and predicting risks using BP neural networks. Sci Rep 14 , 9238 (2024). https://doi.org/10.1038/s41598-024-59911-w
Download citation
Received : 26 September 2023
Accepted : 16 April 2024
Published : 22 April 2024
DOI : https://doi.org/10.1038/s41598-024-59911-w
Share this article
Anyone you share the following link with will be able to read this content:
Sorry, a shareable link is not currently available for this article.
Provided by the Springer Nature SharedIt content-sharing initiative
- BP neural network
- Scientific research project management
- Regularization
- Resource conflict risk
- Deep learning
By submitting a comment you agree to abide by our Terms and Community Guidelines . If you find something abusive or that does not comply with our terms or guidelines please flag it as inappropriate.
Quick links
- Explore articles by subject
- Guide to authors
- Editorial policies
Sign up for the Nature Briefing: AI and Robotics newsletter — what matters in AI and robotics research, free to your inbox weekly.

Certification of the total element mass fractions in UME EnvCRM 03 soil sample via a joint research project
- Practitioner's Report
- Published: 23 April 2024
Cite this article
- Alper Isleyen 1 ,
- Suleyman Z. Can 1 ,
- Oktay Cankur 1 ,
- Murat Tunc 1 ,
- Jochen Vogl 2 ,
- Maren Koenig 2 ,
- Milena Horvat 3 ,
- Radojko Jacimovic 3 ,
- Tea Zuliani 3 ,
- Vesna Fajon 3 ,
- Aida Jotanovic 4 ,
- Luka Gaževic 5 ,
- Milena Milosevic 5 ,
- Maria Ochsenkuehn–Petropoulou 6 ,
- Fotis Tsopelas 6 ,
- Theopisti Lymberopoulou 6 ,
- Lamprini-Areti Tsakanika 6 ,
- Olga Serifi 6 ,
- Klaus M. Ochsenkuehn 6 ,
- Ewa Bulska 7 ,
- Anna Tomiak 7 ,
- Eliza Kurek 7 ,
- Zehra Cakılbahçe 1 ,
- Gokhan Aktas 1 ,
- Hatice Altuntas 1 ,
- Elif Basaran 1 ,
- Barıs Kısacık 1 &
- Zeynep Gumus 1
Soil certified reference material (CRM), UME EnvCRM 03 was produced by a collaborative approach among national metrology institutes, designated institutes and university research laboratories within the scope of the EMPIR project: Matrix Reference Materials for Environmental Analysis. This paper presents the sampling and processing methodology, homogeneity, stability, characterization campaign, the assignment of property values and their associated uncertainties in compliance with ISO 17034:2016. The material processing methodology involves blending a natural soil sample with a contaminated soil sample obtained by spiking elemental solutions for 8 elements (Cd, Co, Cu, Hg, Ni, Pb, Sb and Zn) to reach the level of warning risk monitoring values specified for metals and metalloids of soils in Europe. Comparative homogeneity and stability test data were obtained by two different institutes, ensuring the reliability and back up of the data. The certified values and associated expanded uncertainties for the total mass fractions of thirteen elements (As, Cd, Co, Cr, Cu, Fe, Hg, Mn, Ni, Pb, Sb, V and Zn) are established. The developed CRM can be used for the development and validation of measurement procedures for the determination of the total mass fractions of elements in soil and also for quality control/assurance purposes. The developed CRM is the first example of a soil material originating from Türkiye.
This is a preview of subscription content, log in via an institution to check access.
Access this article
Price includes VAT (Russian Federation)
Instant access to the full article PDF.
Rent this article via DeepDyve
Institutional subscriptions
Matrix reference materials for environmental analysis EURAMET project page. https://www.euramet.org/research-innovation/search-research-projects/details/project/matrix-reference-materials-for-environmental-analysis
ISO 17034 (2016) General requirements for the competence of reference materials producers
Vassileva E, Azemard S, Mandjukov P (2017) Certification for trace elements and methyl mercury mass fractions in IAEA-456 marine sediment sample Accred. Qual Assur 23:29–37
Google Scholar
Mackey EA, Christopher SJ, Lindstrom RM, Long SE, Marlow AF, Murphy KE, Paul RL, Popelka-Filcoff RS, Rabb SA, Sieber JR, Spatz RO, Tomlin BE, Wood LJ, Yu LL, Zeisler R, Yen JH, Wilson SA, Adams MG, Brown ZA, Lamothe PL, Taggart JE, Jones C, Nebelsick J (2010) NIST special publication 260–172, certification of three NIST renewal soil standard reference materials for element content: SRM 2709a San Joaquin Soil, SRM 2710a Montana Soil I, and SRM 2711a Montana Soil II
Birgersson-Liebich A, Venelinov T, Santoro A, Held A (2010) Certification report, the certification of the mass fraction of the total content and the aqua regia extractable content of As, Cd, Co, Cr, Cu, Mn, Ni, Pb and Zn in Loam soil certified reference material ERM ® -CC141
Scharf H, Lück D, Bremser W (2006) Bericht zur Zertifizierung der gesamtgehalte und der mit königswasser extrahierbaren gehalte der elemente As, Cd, Co, Cr, Cu, Hg, Mn, Ni, Pb und Zn in einer bodenprobe Zertifiziertes Referenzmaterial BAM-U110
Griepink B, Muntau H, Vercoutere K (1994) Final report, certification of the total contents (mass fractions) of Cd, Co, Cu, Pb, Mn, Hg and Ni and the aqua regia soluble contents (mass fractions) of Cd, Pb, Ni and Zn in a light sandy soil CRM 142R
Semenkov IN, Koroleva TV (2019) International environmental legislation on the content of chemical elements in soils: guidelines and schemes. Eurasian Soil Sci 52(10):1289–1297
Article CAS Google Scholar
Carlon C (Ed.) (2007) Derivation methods of soil screening values in Europe. A review and evaluation of national procedures towards harmonization. European Commission Joint Research Centre, Ispra
Karaca A, Türkmen C, Arcak S, Haktanır K, Topçuoğlu B, Yıldız H (2009) The determination of the effect of Cayirhan coal-fired power plant emission on heavy metals and sulphur contents of regional soils. Ankara Üniversitesi Çevrebilimleri Dergisi 1(1):25–41
Article Google Scholar
Lamberty A, Schimmel H, Pauwels J (1998) The study of the stability of reference materials by isochronous measurements. Fres J Anal Chem 360:359–361
ISO Guide 35 (2017) Reference materials — guidance for characterization and assessment of homogeneity and stability
Linsinger TPJ, Pauwels J, Van der Veen AMH, Schimmel H, Lamberty A (2001) Homogeneity and stability of reference materials. Accred Qual Assur 6:20–25
Certificate of the Reference Material UME EnvCRM 03-Soil. https://rm.ume.tubitak.gov.tr/sertifika/ume_crm_envcrm03_certificate.pdf
International vocabulary of metrology - basic and general concepts and associated terms, 3rd ed (VIM 3). Available from https://www.bipm.org or as ISO/IEC guide 99-12:2007
ISO/TC 334 Position Paper (2023) The need for assessment of commutability of reference materials. https://committee.iso.org/files/live/sites/tc334/files/ISO-TC334_Commutability_document_2023-03.pdf
Download references
Acknowledgements
The work of this study is part of the project 14RPT03-EnvCRM, which was funded within the framework of the EMPIR. The EMPIR initiative is co-funded by the European Union’s Horizon 2020 research and innovation programme and the EMPIR Participating States. Authors thank to TUBITAK UME intern trainees Esma Eroğlu, Büşra Bıyıklı, Onur Uygun, H. Merve Kırbaş for their dedicated work during the processing of the soil material and Doğan Meriç for the logistics and supply of the soil material. We dedicate this article to the memory of Prof. Osman Yavuz Ataman, a doyen of analytical chemistry, who encouraged and directed us in producing reference materials.
European Metrology Programme for Innovation and Research, 14RPT03-EnvCRM.
Author information
Authors and affiliations.
TÜBİTAK UME-Ulusal Metroloji Enstitüsü, Kocaeli, Türkiye
Alper Isleyen, Suleyman Z. Can, Oktay Cankur, Murat Tunc, Zehra Cakılbahçe, Gokhan Aktas, Hatice Altuntas, Elif Basaran, Barıs Kısacık & Zeynep Gumus
BAM-Bundesanstalt für Materialforschung und –prüfung, Berlin, Germany
Jochen Vogl & Maren Koenig
IJS-Institute Jozef Stefan, Ljubljana, Slovenia
Milena Horvat, Radojko Jacimovic, Tea Zuliani & Vesna Fajon
IMBIH- Institute of Metrology, Sarajevo, Bosnia and Herzegovina
Aida Jotanovic
Directorate of Measures and Precious Metals, MoE-DMDM- Ministry of Economy, Beograde, Serbia
Luka Gaževic & Milena Milosevic
NTUA-National Technical University of Athens, Athens, Greece
Maria Ochsenkuehn–Petropoulou, Fotis Tsopelas, Theopisti Lymberopoulou, Lamprini-Areti Tsakanika, Olga Serifi & Klaus M. Ochsenkuehn
UWAR-University of Warsaw, Warsaw, Poland
Ewa Bulska, Anna Tomiak & Eliza Kurek
You can also search for this author in PubMed Google Scholar
Contributions
A.I. wrote the main manuscript text. AI., Z.C., G.A., H.A., E.B., B.K., Z.G. contributed to the material processing of the soil CRM. S.Z.C., O.C., M.T., J.V., M.K., M.H., R.J., T.Z., V.F., A.J., L.G., M.M., M.O-P., F.T., T.L., L-A.T., O.S.,K.M.O., E.B., A.T., E.K. contributed to the analysis and data evaluation. All authors reviewed the manuscript.
Corresponding author
Correspondence to Alper Isleyen .
Ethics declarations
Conflict of interest.
The authors declare no competing interests.
Additional information
Publisher's note.
Springer Nature remains neutral with regard to jurisdictional claims in published maps and institutional affiliations.
Supplementary Information
Below is the link to the electronic supplementary material.
Supplementary file1 (DOCX 2407 KB)
Rights and permissions.
Reprints and permissions
About this article
Isleyen, A., Can, S.Z., Cankur, O. et al. Certification of the total element mass fractions in UME EnvCRM 03 soil sample via a joint research project. Accred Qual Assur (2024). https://doi.org/10.1007/s00769-024-01597-8
Download citation
Received : 26 December 2023
Accepted : 02 April 2024
Published : 23 April 2024
DOI : https://doi.org/10.1007/s00769-024-01597-8
Share this article
Anyone you share the following link with will be able to read this content:
Sorry, a shareable link is not currently available for this article.
Provided by the Springer Nature SharedIt content-sharing initiative
- Total element content
- Certification
- Environmental pollution monitoring
- Find a journal
- Publish with us
- Track your research
Numbers, Facts and Trends Shaping Your World
Read our research on:
Full Topic List
Regions & Countries
- Publications
- Our Methods
- Short Reads
- Tools & Resources
Read Our Research On:
Audiences are declining for traditional news media in the U.S. – with some exceptions
A declining share of U.S. adults are following the news closely, according to recent Pew Research Center surveys. And audiences are shrinking for several older types of news media – such as local TV stations, most newspapers and public radio – even as they grow for newer platforms like podcasts, as well as for a few specific media brands.
Pew Research Center has long tracked trends in the news industry. In addition to asking survey questions about Americans’ news consumption habits , our State of the News Media project uses several other data sources to look at various aspects of the industry, including audience size, revenue and other metrics.
The data in this analysis comes from a variety of sources as part of Pew Research Center’s State of the News Media fact sheets. The fact sheets use a range of methodologies to study the health of the U.S. news industry, including analysis of industry data and direct reporting to solicit information unavailable elsewhere. All sources are cited in chart and graphic notes or within the text of the fact sheets. Read the methodology .
Pew Research Center is a subsidiary of The Pew Charitable Trusts, its primary funder. This is the latest report in Pew Research Center’s ongoing investigation of the state of news, information and journalism in the digital age, a research program funded by The Pew Charitable Trusts, with generous support from the John S. and James L. Knight Foundation.
The latest data shows a complex picture. Here are some of our key findings:
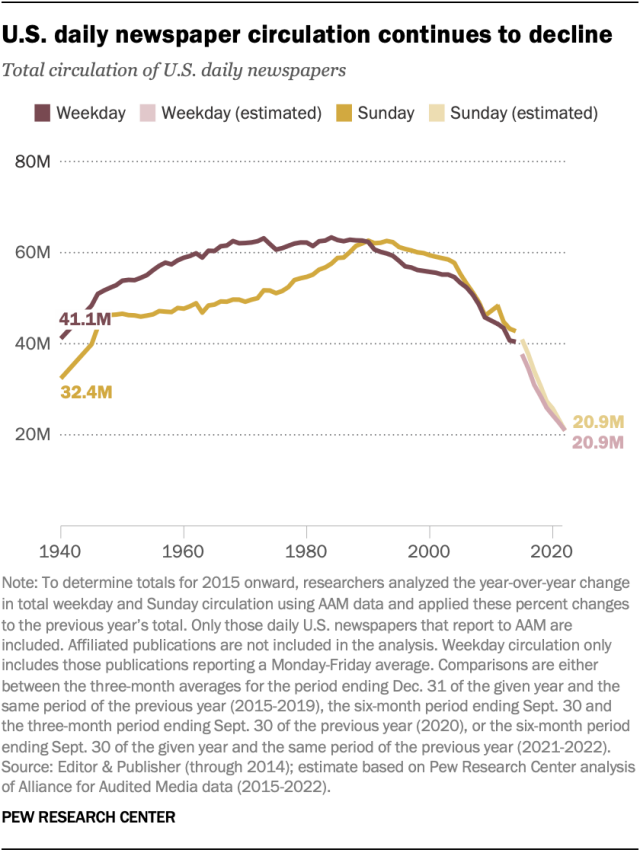
- For the most part, daily newspaper circulation nationwide – counting digital subscriptions and print circulation – continues to decline, falling to just under 21 million in 2022, according to projections using data from the Alliance for Audited Media (AAM). Weekday circulation is down 8% from the previous year and 32% from five years prior, when it was over 30 million. Out of 136 papers included in this analysis, 120 experienced declines in weekday circulation in 2022.
- While most newspapers in the United States are struggling, some of the biggest brands are experiencing digital growth. AAM data does not include all digital circulation to three of the nation’s most prominent newspapers : The New York Times, The Wall Street Journal and The Washington Post. But while all three are experiencing declines in their print subscriptions, other available data suggests substantial increases in digital subscriptions for The New York Times and The Wall Street Journal. (Similar data is not available for The Washington Post.) For example, The New York Times saw a 32% increase in digital-only subscriptions in 2022, surpassing 10 million subscribers and continuing years of growth, according to filings with the U.S. Securities and Exchange Commission (SEC). There are many reasons this data is not directly comparable with the AAM data, including the fact that some digital subscriptions to The New York Times do not include news and are limited to other products like cooking and games. Still, these brands are bucking the overall trend.
- Overall, digital traffic to newspapers’ websites is declining. The average monthly number of unique visitors to the websites of the country’s top 50 newspapers (based on circulation, and including The New York Times, The Wall Street Journal and The Washington Post) declined 20% to under 9 million in the fourth quarter of 2022, down from over 11 million in the same period in 2021, according to Comscore data. The length of the average visit to these sites is also falling – to just under a minute and a half in the last quarter of 2022.
- Traffic to top digital news websites is not picking up the slack. Overall, traffic to the most visited news websites – those with at least 10 million unique visitors per month in the fourth quarter of a given year – has declined over the past two years. The average number of monthly unique visitors to these sites was 3% lower in October-December 2022 than in the same period in 2021, following a 13% drop the year before that, according to Comscore. The length of the average visit to these sites is getting shorter, too. (These sites can include newspapers’ websites, such as that of The New York Times, as well as other digital news sites like those of CNN, Fox News or Axios.)
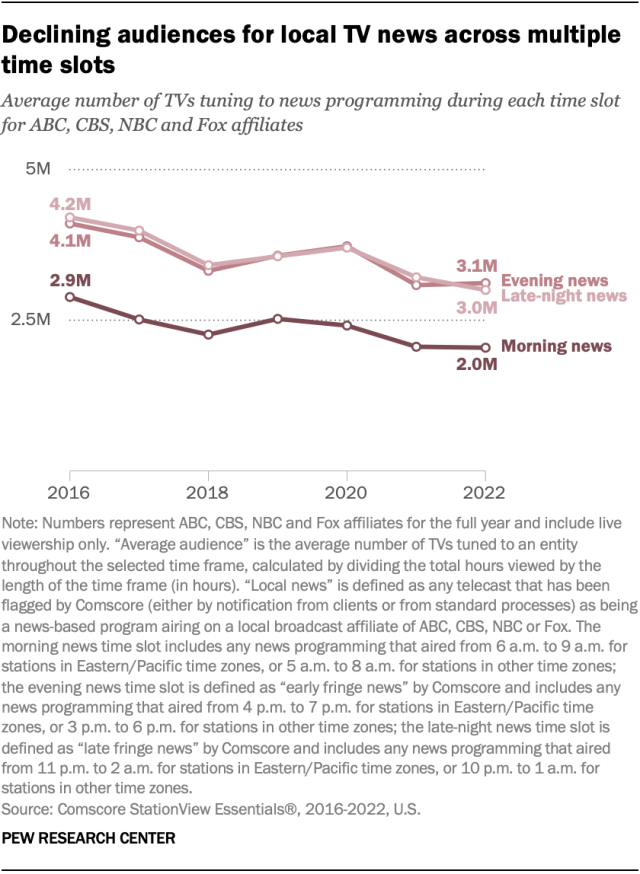
- Across several years of data, there has been a drop in audiences for local TV news , affecting morning, evening and late-night time slots alike. For example, the average number of TVs tuning into ABC, CBS, NBC and Fox affiliates for the evening news was just over 3 million in 2022, down from just over 4 million in 2016.
- Audience trends are a little more mixed when it comes to TV news on cable and network stations. Prime-time and daytime audiences for CNN, Fox News and MSNBC all grew in 2020, the first year of the COVID-19 pandemic , before declining in 2021. Fox News’ audiences ticked back up in 2022 , while the audiences for the other two channels continued to decline.
- Audiences for news programming on ABC, CBS and NBC have been relatively stable in recent years, with some variation depending on the time slot. Audiences for evening news are up slightly since 2016 on all three networks, but they are modestly down for morning news.
- The story is mixed when it comes to audio, too. The share of Americans who listen to terrestrial radio has declined in recent years, as has listenership on NPR and PRX . But there has been a clear rise in audiences for podcasts and other types of online audio . Although podcasts often are not news-related, about two-thirds of U.S. podcast listeners say they hear news discussed on the podcasts they listen to.
Economic trends in the news industry
Declines in audience don’t necessarily mean declines in revenue, with some industries faring better than others in 2022. The newspaper industry and network television, for example, saw losses in advertising revenue, while local TV revenue followed typical patterns associated with election years.
Here are some data points on how these media sectors are faring economically, based on data from filings with the SEC, industry tracking companies and other sources:
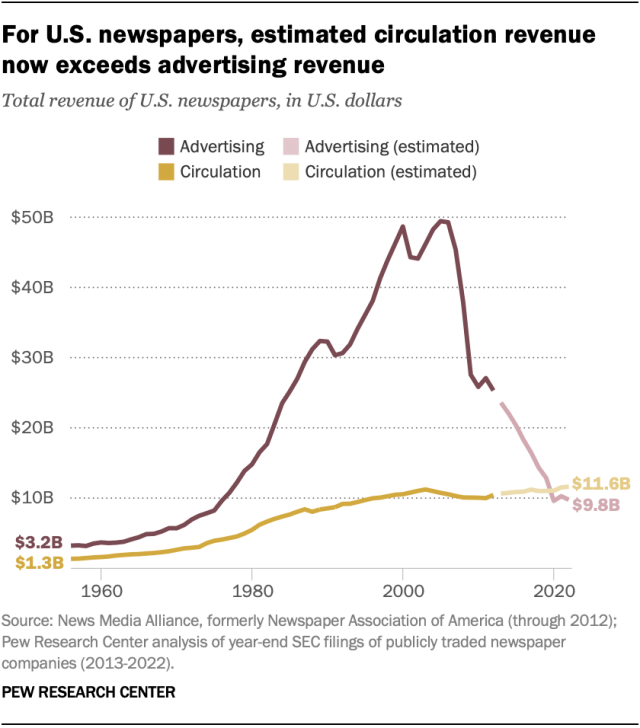
- Advertising revenue for newspapers has continued to decline steadily. In 2020, projections of circulation revenue surpassed advertising revenue for the first time since at least 1956 (the first year for which data is available), and that pattern has held steady in recent years. The makeup of advertising revenue is changing as well: Nearly half of newspaper companies’ advertising revenue (48%) came from digital advertising in 2022, up from 19% in 2012.
- For local television , advertising revenue has remained roughly stable on the whole, bolstered by increased digital advertising and consistent spikes in political advertising revenue during election years. Political advertising, in particular, has grown over the past decade, increasing from roughly $600 million in the 2012 presidential election year to about $1.9 billion in 2022 (a midterm election year). Local TV stations also have seen increasing revenue from retransmission fees, paid by cable and satellite systems to carry local channels.
- Local public radio station revenue has increased, from roughly $1.1 billion in 2020 to about $1.2 billion in 2021. Revenue for NPR, specifically, increased 8% between 2021 and 2022.
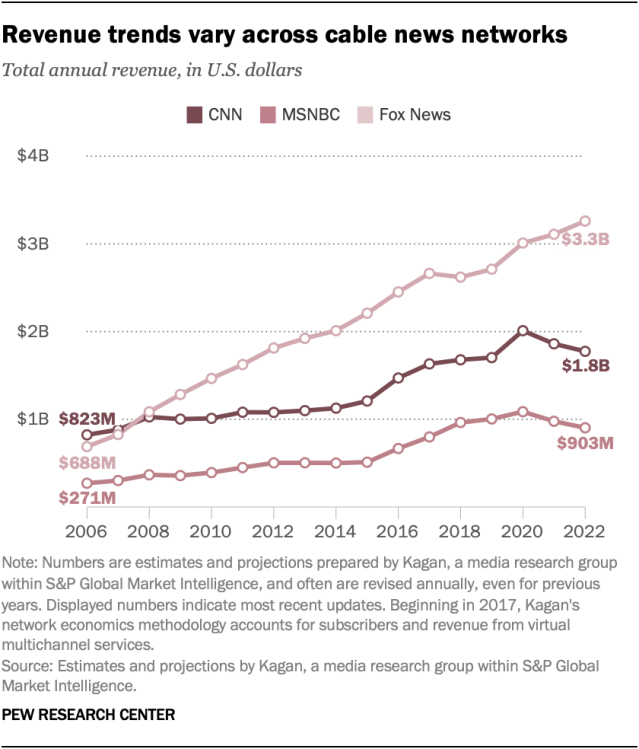
- Revenue trends for cable TV news vary depending on the network. Among the three major cable news networks, Fox News led in both total revenue and net advertising revenue, which increased from 2020 to 2022. CNN and MSNBC saw slight declines over the same period.
- Advertising revenue has generally declined for ABC, CBS and NBC news programming since 2020, in both the morning and evening time slots.
- Digital News Landscape
- Media & Society
- Media Industry
- News Platforms & Sources
- Social Media & the News
- State of the News Media (Project)
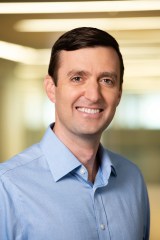
Michael Lipka is an associate director focusing on news and information research at Pew Research Center
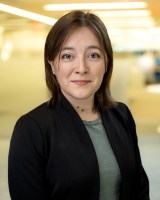
Elisa Shearer is a senior researcher focusing on news and information research at Pew Research Center
How Hispanic Americans Get Their News
Many americans find value in getting news on social media, but concerns about inaccuracy have risen, 5 facts about how americans use facebook, two decades after its launch, social media and news fact sheet, more americans are getting news on tiktok, bucking the trend seen on most other social media sites, most popular.
1615 L St. NW, Suite 800 Washington, DC 20036 USA (+1) 202-419-4300 | Main (+1) 202-857-8562 | Fax (+1) 202-419-4372 | Media Inquiries
Research Topics
- Age & Generations
- Coronavirus (COVID-19)
- Economy & Work
- Family & Relationships
- Gender & LGBTQ
- Immigration & Migration
- International Affairs
- Internet & Technology
- Methodological Research
- News Habits & Media
- Non-U.S. Governments
- Other Topics
- Politics & Policy
- Race & Ethnicity
- Email Newsletters
ABOUT PEW RESEARCH CENTER Pew Research Center is a nonpartisan fact tank that informs the public about the issues, attitudes and trends shaping the world. It conducts public opinion polling, demographic research, media content analysis and other empirical social science research. Pew Research Center does not take policy positions. It is a subsidiary of The Pew Charitable Trusts .
Copyright 2024 Pew Research Center
Terms & Conditions
Privacy Policy
Cookie Settings
Reprints, Permissions & Use Policy
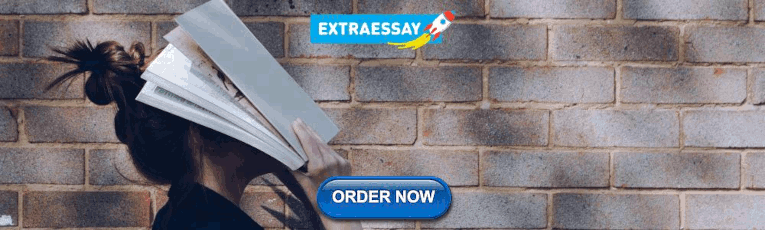
IMAGES
VIDEO
COMMENTS
A research aim is a broad statement indicating the general purpose of your research project. It should appear in your introduction at the end of your problem statement, before your research objectives. Research objectives are more specific than your research aim. They indicate the specific ways you'll address the overarching aim.
Definition: Research Project is a planned and systematic investigation into a specific area of interest or problem, with the goal of generating new knowledge, insights, or solutions. It typically involves identifying a research question or hypothesis, designing a study to test it, collecting and analyzing data, and drawing conclusions based on ...
Research methods are specific procedures for collecting and analyzing data. Developing your research methods is an integral part of your research design. When planning your methods, there are two key decisions you will make. First, decide how you will collect data. Your methods depend on what type of data you need to answer your research question:
Step 1: Consider your aims and approach. Step 2: Choose a type of research design. Step 3: Identify your population and sampling method. Step 4: Choose your data collection methods. Step 5: Plan your data collection procedures. Step 6: Decide on your data analysis strategies. Other interesting articles.
Research design refers to the overall plan, structure or strategy that guides a research project, from its conception to the final analysis of data. Research designs for quantitative studies include descriptive, correlational, experimental and quasi-experimenta l designs. Research designs for qualitative studies include phenomenological ...
A research project for students is an extended essay that presents a question or statement for analysis and evaluation. During a research project, you will present your own ideas and research on a subject alongside analysing existing knowledge. How to write a research report The next section covers the research project steps necessary to ...
Table of contents. Step 1: Consider your aims and approach. Step 2: Choose a type of research design. Step 3: Identify your population and sampling method. Step 4: Choose your data collection methods. Step 5: Plan your data collection procedures. Step 6: Decide on your data analysis strategies.
Overview. Data analysis is an ongoing process that should occur throughout your research project. Suitable data-analysis methods must be selected when you write your research proposal. The nature of your data (i.e. quantitative or qualitative) will be influenced by your research design and purpose. The data will also influence the analysis ...
Learn how to analyze and interpret your data with SAGE Research Methods, a comprehensive online resource for researchers of all levels.
INTRODUCTION. A clean, well-thought-out proposal forms the backbone for the research itself and hence becomes the most important step in the process of conduct of research.[] The objective of preparing a research proposal would be to obtain approvals from various committees including ethics committee [details under 'Research methodology II' section [Table 1] in this issue of IJA) and to ...
Definition of research in data analysis: According to LeCompte and Schensul, research data analysis is a process used by researchers to reduce data to a story and interpret it to derive insights. The data analysis process helps reduce a large chunk of data into smaller fragments, which makes sense. Three essential things occur during the data ...
Introduction. Statistical analysis is necessary for any research project seeking to make quantitative conclusions. The following is a primer for research-based statistical analysis. It is intended to be a high-level overview of appropriate statistical testing, while not diving too deep into any specific methodology.
Step 4: Create a research design. The research design is a practical framework for answering your research questions. It involves making decisions about the type of data you need, the methods you'll use to collect and analyze it, and the location and timescale of your research. There are often many possible paths you can take to answering ...
data analysis, the process of systematically collecting, cleaning, transforming, describing, modeling, and interpreting data, generally employing statistical techniques. Data analysis is an important part of both scientific research and business, where demand has grown in recent years for data-driven decision making.Data analysis techniques are used to gain useful insights from datasets, which ...
You analyze it! Consistent project analysis helps you make the right choices at the right time, leading you towards a more successful outcome and the highest possible ROI. Here we will talk about project analysis, its importance, the different types of project analysis, and lastly, how you can implement it using the right tools.
An effective analysis can be valuable for making informed decisions based on data and research. Writing an analysis can help you build support around a particular idea, cause or project. Knowing how to write one is a valuable skill for any career. In this article, you will learn what an analysis is, why it's an important tool to use in ...
Data Analytics Projects for Beginners. As a beginner, you need to focus on importing, cleaning, manipulating, and visualizing the data. Data Importing: learn to import the data using SQL, Python, R, or web scraping. Data Cleaning: use various Python and R libraries to clean and process the data.
Data Analysis. Definition: Data analysis refers to the process of inspecting, cleaning, transforming, and modeling data with the goal of discovering useful information, drawing conclusions, and supporting decision-making. It involves applying various statistical and computational techniques to interpret and derive insights from large datasets.
According to the PATHWAYS project protocol, an evaluation tool for the internal project evaluation was required to collect information about: (1) structure and resources; (2) process, management and communication; (3) achievements and/or outcomes and (4) SWOT analysis. A mixed methods approach was chosen.
Scientific research project management, as illustrated in Fig. 1, constitutes an essential framework to ensure the efficient and organized execution of scientific research endeavors.It encompasses ...
DATA PRESENTATION, ANALYSIS AND INTERPRETATION. 4.0 Introduction. This chapter is concerned with data pres entation, of the findings obtained through the study. The. findings are presented in ...
Step 1: Write your hypotheses and plan your research design. To collect valid data for statistical analysis, you first need to specify your hypotheses and plan out your research design. Writing statistical hypotheses. The goal of research is often to investigate a relationship between variables within a population. You start with a prediction ...
Soil certified reference material (CRM), UME EnvCRM 03 was produced by a collaborative approach among national metrology institutes, designated institutes and university research laboratories within the scope of the EMPIR project: Matrix Reference Materials for Environmental Analysis. This paper presents the sampling and processing methodology, homogeneity, stability, characterization campaign ...
Furthermore, Ogunsanmi's proficiency in utilizing Research Electronic Data Capture (REDCap) for project development and data collection and analysis in randomized controlled trials underscores ...
WHO 2024 data call aims to collect data on antifungal agents in the preclinical development pipeline using the survey link below. The 2024 preclinical data analyses will feed into the WHO antifungal pipeline analysis and data will be available for all stakeholders including existing and future funders of R&D, through the WHO Global Health R&D ...
What is a research project? A research project is an academic, scientific, or professional undertaking to answer a research question.Research projects can take many forms, such as qualitative or quantitative, descriptive, longitudinal, experimental, or correlational.What kind of research approach you choose will depend on your topic.
The data in this analysis comes from a variety of sources as part of Pew Research Center's State of the News Media fact sheets. The fact sheets use a range of methodologies to study the health of the U.S. news industry, including analysis of industry data and direct reporting to solicit information unavailable elsewhere.
The most logical way to structure quantitative results is to frame them around your research questions or hypotheses. For each question or hypothesis, share: A reminder of the type of analysis you used (e.g., a two-sample t test or simple linear regression). A more detailed description of your analysis should go in your methodology section.