The effects of online simulation-based collaborative problem-solving on students’ problem-solving, communication and collaboration attitudes
- Open access
- Published: 18 March 2024
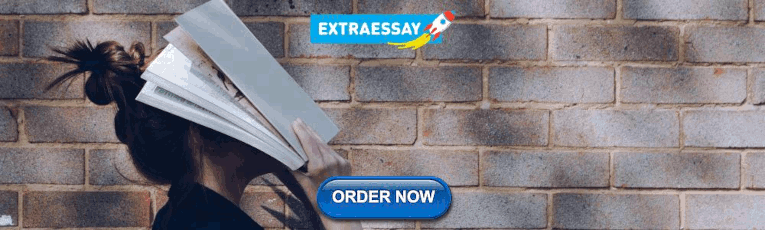
Cite this article
You have full access to this open access article
- Meng-Jun Chen 1 ,
- Hsiao-Ching She ORCID: orcid.org/0000-0002-5316-4426 1 &
- Pei-Yi Tsai 1
557 Accesses
Explore all metrics
Despite national curricula and instructional reforms calling for collaborative problem-solving skills (CPS), however, there is an absence of a theory-laden model showing how to effectively construct CPS for science learning. We therefore developed and validated a simulation-based CPS model that exploits its constructs, sequences, and causal relationships, and evaluating its effectiveness on students’ problem-solving. Over the span of a two-week physics science course, 57 ninth-grade students were recruited from two intact middle school classes to engage in this online simulation-based collaborative problem-solving (CPS) program. This program consisted of nine electrochemistry problem-solving lessons spread across four class sessions, each lasting 45 min. Results indicated that the simulation-based CPS model was validated and proven to contribute to effective problem-solving by linking PS solution proposing, peer communication, implementing PS solutions with simulation, and providing evidence-based explanations. The simulation-based CPS model successfully improved the performance of both high- and low-achieving students. With the support and presence of high-achievers, low-achievers’ collaboration attitude was boosted, which lead them to achieve similar learning success.
Similar content being viewed by others
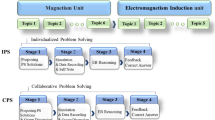
Can CPS better prepare 8th graders for problem-solving in electromagnetism and bridging the gap between high- and low-achievers than IPS?
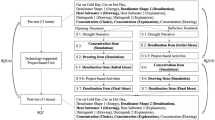
Comparing Simulation Sequencing in a Chemistry Online-Supported Project-Based Learning Unit
Promoting Students’ Writing Skills in Science through an Educational Simulation: The GlobalEd 2 Project
Avoid common mistakes on your manuscript.
1 Introduction
Collaborative problem-solving (CPS) has become increasingly recognized as a powerful tool for helping students solve complex scientific problems collaboratively, thus national curriculums and instructional reforms across many nations (Binkley et al., 2012 ; Darling-Hammond & McLaughlin, 2011 ) have incorporated CPS skills. OECD defines CPS as an individual’s ability to share and integrate their existing knowledge and perspectives with others when solving problems together (OECD, 2013 ). Collaborative learning offers students the opportunity to construct a shared understanding of knowledge and meaning-making of the content (Fischer et al., 2013 ). Understanding chemistry concepts can be challenging because they require understanding three representational levels, macroscopic, microscopic, and symbolic (Johnstone, 1993 ). Electrochemistry is one of the most complex topics in the study of chemistry (Supasorn et al., 2014 ). The primary reason that electrochemistry is considered one of the difficult topics both at the high school and undergraduate levels is that most processes involve the microscopic level (molecular level) that cannot be observed directly (Rahayu et al., 2022 ), or involve the complex nature and too many concepts (Akram et al., 2014 ). Individuals may find it challenging to grasp microscopic concepts and solve complex problems on their own. The inclusion of CPS skills in educational and professional settings has the potential to equip individuals with the necessary skills and tools to tackle complex problems and thrive in the twenty-first century (Griffin & Care, 2014 ). Low academic achievement may hinder students’ school learning and future careers (Al-Zoubi & Younes, 2015 ). Cook et al. ( 2008 ) reported that students’ academic achievement and prior knowledge are critical for predicting their knowledge construction and comprehension. Other studies suggested that low-achieving students can be as proficient at problem-solving skills as high-achieving students with appropriate instruction (Ben‐David & Zohar, 2009 ; Grimberg & Hand, 2009 ). While science instruction and curriculum reforms have been widespread, a theory-laden model on how to build an effective simulation-based CPS for science learning is still lacking. The purpose of this study is therefore to develop and validate a simulation-based CPS model and investigate its effectiveness in promoting students' learning of science and minimizing the achievement gap between low- and high-achievers.
2 Theoretical frameworks
Studies of CPS have found that it improves student problem-solving competency (Malik et al., 2019 ), engagement (Unal & Cakir, 2021 ) and content knowledge (Harskamp & Ding, 2007 ). Garrison ( 1991 ) decomposed the problem-solving process into problem identification, problem description, problem exploration, applicability, and integration. In some studies, the PS process is divided into problem representation, solutions search, and solutions implementation (Bransford & Schwartz, 1999 ; Newell & Simon, 1972 ), or meeting the problem, analyzing problems and issues, discovering, and reporting, and presenting and evaluating solutions (Chua et al., 2016 ). Another study delineated physics problem-solving as identifying known conceptions, providing possible solutions, evaluating solutions, implementing solutions, and providing evidence-based explanations (Cheng et al., 2017 ). Considering that the literatures above share the PS components of proposing problem solutions, implementing solutions, and providing evidence-based explanations, we incorporate them into our CPS model.
Collaborative learning improves the acquisition and retention of knowledge and helps students solve problems (García-Valcárcel et al., 2014 ). Science is a process in which scientific knowledge is socially constructed, and in which discursive activity is central to the science process (Driver et al., 2000 ). Duran ( 2014 ) noted that communication helps obtain information or new ideas that can help understand a problem better and working together to develop effective solutions to complex problems. Dialogues and the discussions of ideas encourage students’ thinking and learning (Faranda & Clarke, 2004 ). CPS provides students with a communication platform to reconstruct their knowledge and thinking, filling gaps in their understanding and formulating strategies that can collaboratively tackle complex issues (Fawcett & Garton, 2005 ). Studies of group work found that a critical relationship existed between providing explanations and achievement (Howe & Tolmie, 2003 ; Veenman & Spaans, 2005 ). Moreover, explaining to others can enhance learning since the explainer can reorganize and clarify the material, recognize misconceptions, fill in the gaps in their understanding, internalize and acquire new strategies and knowledge, and develop new perspectives and understanding (Saxe et al., 2002 ). The groups failed to make progress or seemed to be functioning ineffectively when no group member could answer the question, exhibited problems communicating, and worked without allowing true dialogue (Johnson & Johnson, 2009 ). Communication is a key component of collaboration, which enables students to solve problems together.
Computer simulation has been recognized as a promising tool for supporting CPS activities during scientific learning (Andrews-Todd & Forsyth, 2020 ; Ceberio et al., 2016 ). The simulation can provide opportunities for students to test the invisible and abstract phenomena in the real world and integrate multiple perspectives from their team members, which ultimately aids their understanding of scientific concepts (Akpınar, 2014 ; Lu & Lin, 2017 ). Simulations can reveal invisible, abstract, and microscopic phenomena that are difficult to view in the real world (Chou et al., 2022 ; Sinensis et al., 2019 ), and thus help students construct knowledge by observing concrete simulated phenomena (Saab et al., 2012 ). Simulations offer a unique opportunity to engage students in interactive, hands-on learning experiences that can support their learning of science (Rutten et al., 2012 ). Providing learners with simulations can help them gain a deeper understanding of complex concepts and microscopic phenomena.
3 Hypotheses development and research model for simulation-based CPS
As identified in the literature, communicating with one’s partner, proposing solutions to a problem, implementing those solutions with simulation, and developing evidence-based explanations are essential for CPS. However, their constructs, sequences, and causal relationships remain unclear. Based on the theoretical frameworks above, we have proposed the constructs and causal relationships among these elements that govern our research hypothesis in Fig. 1 . We hypothesize that including communication among group members may lead to the development of PS solutions, which further influence the implementation PS solutions with simulations and evidence-based explanations, and thereby contributing to their problem-solving performance. The following hypotheses were proposed to validate its effectiveness using the partial least squares structural equation model (PLS-SEM).
H1. Communication dialogues between students have a significant positive effect on their PS solution generation.
H2. PS solutions proposed by students have a significant positive effect on their implementation of PS solutions with simulations.
H3. PS solutions proposed by students have a significant positive effect on their ability to make evidence-based explanations of the results.
H4. Implementing PS solutions with simulation has a significant effect on their ability to provide evidence-based explanations.
H5. Evidence-based explanations provided by students have a significant impact on their problem-solving performance.
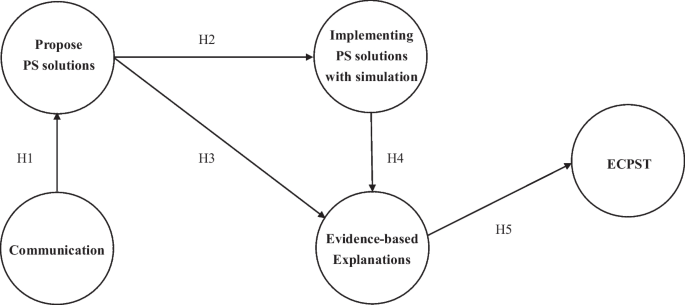
Proposed model construct for simulation-based CPS learning
4 Research questions
This study aims to determine whether our validated simulation-based CPS model can enhance students’ electrochemistry problem-solving abilities and benefit students of varying achievement levels through online collaboration. Therefore, the following four research questions are proposed as guidelines: (1) whether high- and low-achievers would significantly improve their performance on the electrochemical problem-solving test (ECPST) after learning;(2) whether high- and low-achievers would significantly improve their performance in proposing problem-solving (PS) solutions after peer communication; (3) whether high- and low-achievers would engage in a different amount of supportive dialogues, including giving support, requesting support, and reminding; and (4) whether high- and low-achievers differ in their attitudes toward collaborations after completing the online electrochemistry CPS learning.
5.1 Subjects and procedures
Over the span of a two-week physics science course, a total of 57 ninth grade students from a middle school two intact classes were recruited to participate in this online simulation-based collaborative problem-solving (CPS) program. To prove and validate the effectiveness of this simulation-base CPS program, thus we designed an entire electrochemistry unit with nine electrochemistry problem-solving lessons spread across four class sessions, each lasting 45 min. The nine electrochemistry problem-solving lessons comprised five on galvanic cells and four on electrolytic cells (Fig. 2 ). Each simulation-based CPS lesson was designed with four components: communication with partners, proposing PS solutions, implementing PS solutions with simulations, and making evidence-based reasoning. During four class sessions over two weeks, high- and low-achievers were anonymously paired up heterogeneously without knowing the identities of their partners. It is to ensure that social status does not negatively impact the ability to engage in communication dialogues, problem-solving, and collaborations.
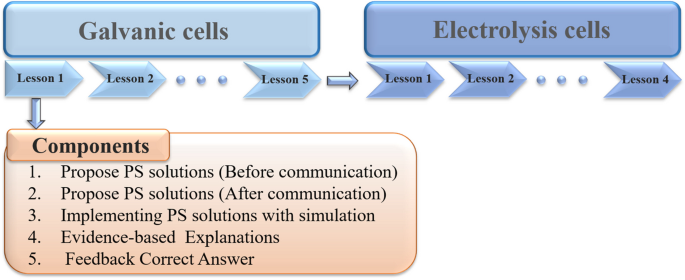
The design of online simulation-based CPS learning
Students were classified into high- and low-achievers based on their school science achievements. We used median school science achievement scores, with a threshold of 80 points, to classify students into high and low achievers. Students with school science achievement scores ≥ 80 points were classified as high achievers, and those with scores < 80 points were classified as low achievers. Heterogeneous groups were formed, each comprising one high- and one low-achiever. One week before and after online electrochemical collaborative problem-solving (CPS) program, all students were administered the electrochemical problem-solving test (ECPST). During the online learning, students’ online problem-solving processes were collected and recorded in MySQL database, including their problem-solving (PS) solutions, implementation PS solutions with simulations, evidence-based explanations, and communication dialogues.
5.2 The development of online electrochemistry collaborative problem-solving (CPS) learning activities
The electrochemistry CPS project was developed based on the national standards for 9th grade chemistry curriculum. A panel of three scientists designed the electrochemistry problem-solving content, including a science education professor, a Ph.D. candidate in science education with three years of middle school science teaching experience, and an experienced middle school science teacher. To create the online electrochemistry CPS program, Unity 3D technologies were used to develop simulations and experiments, the photon network was used to build multi-person collaborations, and a MySQL database was utilized to collect data.
Nine problem-solving lessons were designed: five on the topic of galvanic cells and four on the topic of electrolytic cells. Each CPS lesson required the students to communicate with their partners, propose PS solutions, implement PS solutions with simulation, and provide evidence-based explanations (Fig. 2 ). The five lessons on galvanic cells covered identifying electrode pairs to generate electric currents, finding electrolyte solutions to produce electric currents, finding salt bridge solutions to generate a current, identifying the electronic flow between electrodes, and identifying the movement of ions in the electrolyte solutions. The four lessons on electrolysis cells covered identifying electrolyte solutions, identifying how the electronic flow affects the anode and cathode in electrolysis, finding electrolyte solutions to produce gases during electrolysis at particular electrodes, and finding electrode pairs for copper sulfate electrolysis without changing their colors.
During the CPS process, each student must propose at least two PS solutions (Fig. 3 A). Upon submitting their proposed PS solutions, they were required to communicate with their partners for revising and modifying their proposals as needed (Fig. 3 B). Once their PS solutions have been finalized, they needed to implement their PS solutions with their teammates by running simulations in rotation, and their simulation screens would be automatically shared. During the simulation, they were able to test their proposed PS solutions and observe the changes of macroscopic (color change, electrochemical reaction product, etc.) and microscopic phenomena (ions, electrons, etc.) (Fig. 3 C). By implementing their PS solutions with 3D simulation, they were able to validate whether their PS solutions were feasible and workable. Students had to record the simulation results. Students were also required to provide evidence-based explanations to assess their physics understanding after completing these problem-solving processes (Fig. 3 D & E). The feedback with the correct answer was given after completing the evidence-based explanations (Fig. 3 F).
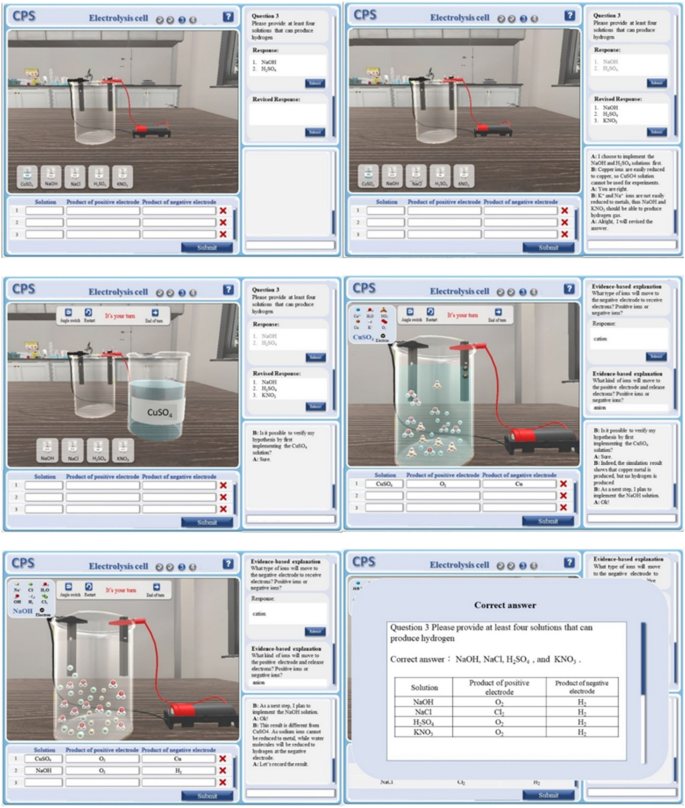
Screen shots for online simulated-based CPS learning platform
5.3 Electrochemical problem-solving test (ECPST)
The ECPST is an open-ended diagnostic instrument designed to measure students’ electrochemical problem-solving performance before and after the intervention. The same panel of three developed the ECPST to ensure the questions were properly constructed and relevant to an online electrochemical problem-solving program. It consists of three galvanic cells and three electrolytic cells which required students to propose three viable solutions to each question and explain the reasons for their proposed PS solutions. Each correct solution was worth 2–4 points, depending on how many subcomponents were required. Students were awarded two points for a correct response, one point for a partially-correct response, and zero point for an incorrect response. A maximum achievable cumulative score was 64 points. Two raters scored students’ ECPST results based on the coding system, and the inter-rater reliability was 0.916.
5.4 Attitudes toward collaborations
PISA 2015 designed eight items of the attitudes toward collaboration questionnaire, including two indices of cooperation that reflected students’ valuing of relationships and teamwork (OECD, 2013 ). The four statements that comprised the index of valuing relationships were related to altruistic interactions which the student engages in collaborative activities not for their own benefit: “I am a good listener”; “I enjoy seeing my classmates be successful”; “I take into account what others are interested in”; and “I enjoy considering different perspectives.” By contrast, three of the four statements that comprised the index of valuing teamwork were related to what teamwork produces as opposed to working alone: “I prefer working as part of a team to working alone”; “I find that teams make better decisions than individuals”; and “I find that teamwork raises my own efficiency.”
5.5 Analyses of the online problem-solving processes and communication dialogues
Students’ online problem-solving processes were analyzed: communicating with partners, proposing the PS solution, implementing PS solutions with simulations, making evidence-based reasoning. In the PS solutions, the student who proposed each correct solution would earn a point. In the evidence-based explanations, two points for a correct response, one for a partially correct response, and zero for an incorrect response. The coding system for students’ implementation of PS solutions with simulations results assigned one point when they correctly reported their simulation results and one point for running accurate simulation. The inter-rater reliability of these three rubrics for PS solution, implementing PS solutions with simulations, and making evidence-based explanations were 0.963, 0.966, and 0.927, respectively. Students’ online discussion dialogues were analyzed with a coding system, which included giving support, requesting support, and reminding partners of the three categories; and the inter-rater reliability was 0.913.
5.6 PLS-SEM model
Hair et al. ( 2022 ) advocated that partial least squares structural equation modeling (PLS-SEM) is appropriate for analyzing small sample sizes and validating theoretical frameworks. PLS is increasingly used in education for developing exploratory models (Barclay et al., 1995 ). The PLS-SEM comprises two components, the measurement, and the structural model (Henseler et al., 2009 ).
The measurement model assesses indicator reliability using outer loading, where the value should exceed 0.50. The Cronbach’s α and the composite reliability (CR) are measures of internal consistency and both should be greater than 0.60. The average variance extracted (AVE) assesses convergence validity, which should be greater than 0.50 (Fornell & Larcker, 1981 ; Hair et al., 2022 ). To assess the discriminant validity of the PLS-SEM model, two commonly used criteria are the Fornell-Larcker criterion and the heterotrait-monotrait ratio (HTMT). According to the Fornell-Larcker criterion, the square root of each construct’s diagonal AVE must be greater than the correlation between that construct with all the other constructs. The HTMT determines whether the correlation between the two constructs is less than 0.90 (Henseler et al., 2015 ). Accordingly, we used the PLS-SEM methodology to examine hypotheses 1 through 5, as previously stated.
The structure model obtains various coefficients for evaluating the research hypothesis formulated (Henseler & Chin, 2010 ). To calculate the goodness of fit of the structural model when using PLS-SEM, the standardized root mean residual (SRMR) was used. An SRMR value of less than 0.10, or equal to 0.08, indicates a good fit in the PLS-SEM model, according to Ringle et al. ( 2015 ). However, PLS-SEM is still in its early stages and may not always be applicable. As a result, reporting these criteria should be exercised with caution. In addition, the path coefficient and of determination (R 2 ) coefficient were reported, and all statistical analyses were performed using SmartPLS 4.
6.1 PLS-SEM model
6.1.1 measurement model.
Table 1 presents the convergent validity and reliability of the proposed constructs of the model. There was satisfactory reliability among each of these indicators, with the loadings ranging from 0.82 to 0.97. The Cronbach’s alpha coefficients were all above 0.71, indicating adequate reliability. The CR indices were above 0.87, confirming each construct’s internal reliability. According to the convergent validity, the average variance extracted (AVE) ranged from 0.77 to 0.88. It reveals that the indicators account for more than 77% of the variance of each construct.
The discriminant validity was also assessed using the Fornell-Larcker criterion. Based on the results, the square root of each construct’s AVE was greater than the correlation between that construct and all others. Further, the HTMT of the correlations was below 0.90, thus confirming discriminant validity (Table 2 ).
6.1.2 Structural model
Evaluation of the structural model involved assessing the significance level of the relationships between constructs and the prediction quality of each construct. An evaluation of the path coefficient of the structural model using PLS-SEM appears in Fig. 4 . The SRMR value of the structural model was 0.095, which is less than 0.10, indicating a good fit in the PLS-SEM model, based on Ringle et al.’s ( 2015 ) recommendation. However, the criteria for model fit in PLS-SEM are still in the early stages of research and may not always be applicable (Ringle et al., 2015 ). Table 3 summarizes five hypotheses supported by the proposed structural model to show the direct effect between constructs. Among proposing PS solutions, communication, implementing PS solutions with simulation, evidence-based explanations, and ECPST, the R 2 values ranged between 0.18 and 0.48, indicating small to moderate predictability. The f 2 values for communication → proposed PS solutions, proposed PS solutions → evidence-based explanations, proposed PS solutions → implementing PS solutions with simulation, implementing PS solutions with simulation → evidence-based explanations, and evidence-based explanations → ECPST were 0.26, 0.14, 0.21, 0.15, 0.92, respectively.
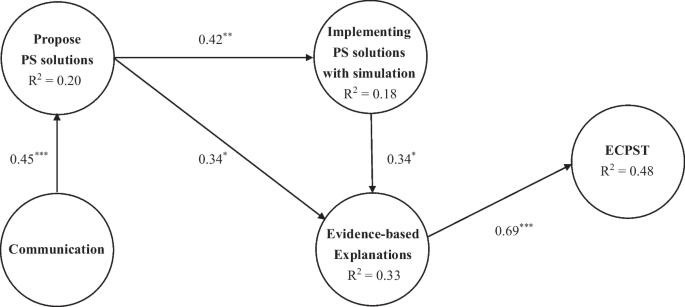
Path coefficient of the model ( *** p < 0.001, ** p < 0.01, * p < 0.05)
Furthermore, the results of the indirect effect are presented in Table 4 , where it can be observed that three paths demonstrated statistical significance. The results indicated that significant indirect effects exist in communication → proposed PS solutions → evidence-based explanations, communication → proposed PS solutions → implementing PS solutions with simulation, and proposed PS solutions → evidence-based explanations → ECPST. However, since communication can predict the evidence-based explanations (β = 0.217, p < 0.001) and implementing PS solutions with simulation (β = 0.189, p < 0.005), and proposed PS solutions can predict ECPST (β = 0.333, p < 0.001), therefore, the indirect effect for these paths were partially mediated.
6.2 The effectiveness of simulation-based CPS model on low- and high-achievers’ problem-solving
6.2.1 electrochemical problem-solving test (ecpst).
To answer the first research question, this study used the one-factor repeated measure ANOVA to examine whether high- and low-achievers would significantly improve their performance on the electrochemical problem-solving test (ECPST) after learning (Table 5 ). The results indicated that the ECPST performance improved significantly from the pretest to the posttest (F = 172.94, p < 0.001), and achievement level also significantly affected performance (F = 21.94, p < 0.001). Based on a simple main effect analysis, both high-achievers (F = 63.77, p < 0.001) and low-achievers (F = 136.66, p < 0.000) made significant progress from pretest to posttest (Table 6 ). As for achievement levels, high-achievers scored significantly higher than low-achievers in the pretest (F = 16.16, p < 0.001) and posttest (F = 13.38, p < 0.01) ECPST.
6.2.2 Online PS solutions
To answer the second research question, this study used a one-factor repeated measure ANOVA to examine whether high- and low-achievers would significantly improve their performance in proposing problem-solving (PS) solutions after peer communication (Table 7 ). The students’ PS solution performance improved significantly from before to after peer communication (F = 12.30, p < 0.01), as well as their achievement levels (F = 9.73, p < 0.01). This study found a significant interaction between the achievement levels and the PS solutions before and after peer communication (F = 4.65, p < 0.05). Therefore, the simple main effect proceeded accordingly (Table 8 ). Based on the simple main effect analysis, only low-achievers (F = 16.36, p < 0.001) made significant progress on students’ PS solution performance from before to after peer communication. A significantly higher PS solution performance was only observed in high-achievers before peer communication than in the low-achievers (F = 15.52, p < 0.001). After peer communication, high- and low-achievers did not significantly differ in their performance in providing PS solutions (F = 3.97, p = 0.051).
6.2.3 Online communication dialogues
To answer the third research question, this study used the one-factor multivariate analysis of variance (MANOVA) to determine whether high- and low-achievers would engage in a different amount of supportive dialogues, including giving support, requesting support, and reminding (Table 9 ). According to the results, high-achievers allocated significantly more dialogues to giving support than low-achievers (F = 5.97, p < 0.05). However, high- and low- achievers, however, allocated similar amounts of requesting support and reminding dialogues (F = 0.01, p = 0.941 and F = 0.042, p = 0.839).
6.3 Attitudes toward collaborations and their association with online PS solutions
To answer the last research question, we used a one-factor analysis of covariance (ANCOVA) to examine whether high- and low-achievers differ in their attitudes toward collaborations after completing the online electrochemistry CPS learning (Table 10 ). Results show that the low-achievers’ attitudes toward collaboration after learning were significantly higher than those of the high-achievers (F = 4.05, p < 0.05) when the effects of collaboration attitudes before learning were controlled. Also, the low-achievers’ value of teamwork was significantly higher than that of high-achievers (F = 7.12, p < 0.05).
The scatter plot illustrated that most low-achievers’ PS solutions performance had moved upward from the lower right part of the plot after peer communication when the effects of collaboration attitudes were controlled (Fig. 5 ). However, most of the high-achievers’ PS solutions performance remain unchanged. In other words, students with low-achievement levels scored much higher on PS solutions after peer communication when their collaboration attitudes were controlled. However, high-achievers did not differ much in their PS solution performance after peer communication. Similar scatter plot patterns were also found for teamwork value and PS solution performance when the effects of teamwork value were controlled (Fig. 6 ). After peer communication, the association between high-achievers’ post-collaboration attitudes with their PS solution performance became more negative, while low-achievers’ collaboration attitudes did not change much. We found the same association pattern for the teamwork value with PS solutions.
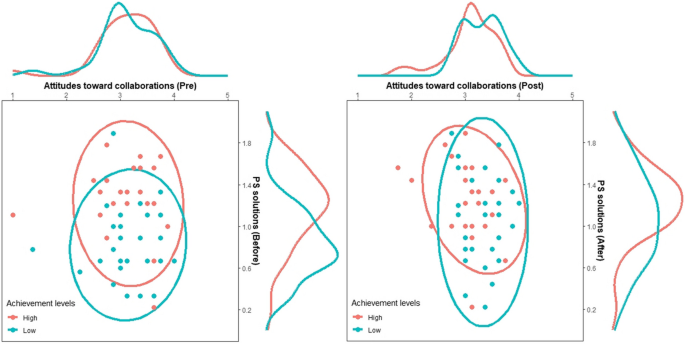
Scatter plot and marginal distribution displayed the relationship between high- and low-achievers’ attitudes toward collaborations and their online PS solution performance before and after collaboration
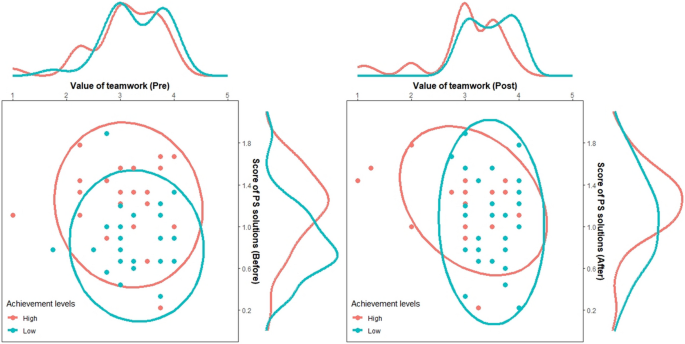
Scatter plot and marginal distribution displayed the relationship between high- and low-achievers’ value of teamwork and their online PS solution performance before and after collaboration
7 Discussion
In the present study, we established an empirical proved theory-laden model of online simulation-based CPS for learning science effectively. Using PLS-SEM, we examined the influence of casual relationships between proposing PS solutions, peer communication, implementing solutions with simulation, making evidence-based explanations, and overall problem-solving performance. In summary, our proposed research model achieved an impressive predictive level and supported our five hypotheses. According to previous studies, having a human who can communicate effectively with others in addition to solving problems in the real world makes them more competitive (Bender, 2012 ; Erozkan, 2013 ). The students required communication skills to explain a valid conclusion based on the evidence of science in problem-solving (Yusuf & Adeoye, 2012 ). They support our finding that communication directly influences proposing PS solutions and indirectly influences implementing PS solutions with simulation and making evidence-based explanations. Previous studies reported that computer simulations are an effective tool for supporting the use of CPS for scientific learning (Andrews-Todd & Forsyth, 2020 ; Ceberio et al., 2016 ). Additionally, by integrating simulations with CPS instruction, students gain a better understanding of abstract concepts (Sinensis et al., 2019 ) and CPS skills (Lin et al., 2018 ). Our findings indicated that simulations directly influence students' evidence-based explanations and contribute to their effective problem-solving, which supports above literatures.
The present study demonstrated that using simulation-based CPS effectively leverages both low- and high-achievers to achieve great success in their problem-solving performance. Regarding online CPS learning process, only low-achievers made significant improvement in their scores of PS solution after peer communication, whereas high-achievers did not. High- and low-achievers' online PS solution scores differ significantly before peer communication, but not after. As a result of peer communication, the low-achievers advanced and achieved the same PS solution score levels as high-achievers. No study has reported similar findings, despite an extensive review of the literature. A deeper investigation into this question revealed interesting findings. According to the communication dialogues between students, high achievers gave significantly more support than low achievers. Andrews-Todd and Forsyth ( 2020 ) suggested that collaborative problem-solving groups with at least one member with high cognitive skills lead to enhanced learning performance. It helps explain when high-achievers offer more support to the low-achievers, they are more likely to significantly improve the PS solutions performance of the latter. It implies that the presence and support of high-achievers play a significant role in improving the problem-solving performance of low-achievers. Our results indicated that communication has a direct influence on students’ proposal of PS solutions, which supports why low-achievers significantly improved their PS solution scores. This highlights the unique contribution of our simulation-based CPS model in enhancing low-achievers’ online PS solutions performance through communication and collaboration.
Regarding attitudes toward collaborations, low-achievers perceived a significantly higher collaboration attitudes and its subscale of teamwork value after learning compared to high-achievers. A fascinating pattern derived from the scatter plot and marginal distribution showed that most low-achievers had scored much higher on PS solutions performance after peer communication, when the effects of collaboration attitudes were controlled. However, high-achievers did not differ much in their PS solutions scores after peer communication. The subscale of teamwork value followed a similar pattern. Earlier studies reported that students who have experienced online collaborative learning learned more than they would have individually (Hernández-Selles et al., 2019 ; Ku et al., 2013 ). The OECD reported that disadvantaged students in most countries and economies value teamwork more than advantaged students (OECD, 2013 ), similar to our case. These studies lead us to conclude that the use of theory-laden simulation-based CPS model effectively enhances low-achievers’ collaboration attitudes and their teamwork value, which contributes to their PS solution performance.
This study has shown that online simulation-based CPS models that feature communication, PS solutions, simulation implementation, and evidence-based explanations effectively enhance students’ problem-solving performance. Some potential implications and practical applications are provided below. Firstly, it is highly recommended that future applications of CPS in classroom or online learning include these four components. These four components are crucial not only to provide the opportunity for students to communicate and collaborate but also to enhance their generation of problem-solving solutions, which further impacts their implementation of PS solutions and evidence-based explanations and ultimately leads to greater problem-solving success. Secondly, future applications of CPS in classroom or online learning should group students heterogeneously to minimize the gaps between high and low achievers. Both high and low achievers showed statistically significant improvements in electrochemistry problem-solving using this simulation-based CPS. After peer communication and collaboration in which high achievers offered more support to low achievers, the low achievers improved and achieved the same PS solution score as the high achievers. Therefore, the low achievers developed a more positive attitude toward collaboration and teamwork than the high achievers. Consequently, it is imperative to include members of varying cognitive abilities and achievement levels when forming CPS groups. This practice can reduce the disparities among group members and improve the learning performance of low achievers. Thirdly, students should be given opportunities to visualize microscopic-level phenomena through simulation or animation when solving science problems because scientific concepts are inherent in many micro-level phenomena. It is vital to leverage visual tools such as images, animations, and simulations during this process. The use of simulations, especially, provides a microscopic view of phenomena and allows users to actively manipulate variables and interact with them. Ultimately, we hope that our study will provide insight into the future of simulation-based CPS in all aspects of science learning and problem-solving.
Availability of data and materials
The data that support the findings of this study are available from the corresponding author upon reasonable request.
Akpınar, E. (2014). The use of interactive computer animations based on POE as a presentation tool in primary science teaching. Journal of Science Education and Technology, 23 (4), 527–537.
Article ADS Google Scholar
Akram, M., Surif, J., & Ali, M. (2014). Conceptual difficulties of secondary school students in electrochemistry. Asian Social Science, 10 , 276–281.
Article Google Scholar
Al-Zoubi, S. M., & Younes, M. B. (2015). Low academic achievement: Causes and results. Theory and Practice in Language Studies, 5 , 2262–2268.
Andrews-Todd, J., & Forsyth, C. M. (2020). Exploring social and cognitive dimensions of collaborative problem solving in an open online simulation-based task. Computers in Human Behavior, 104 , 105759.
Barclay, D., Thompson, R., & Higgins, C. (1995). The partial least squares (PLS) approach to causal modeling: Personal computer use as an illustration. Technology Studies, 2 , 285–309.
Ben-David, A., & Zohar, A. (2009). Contribution of meta-strategic knowledge to scientific inquiry learning. International Journal of Science Education, 31 (12), 1657–1682.
Bender, T. (2012). Discussion-based online teaching to enhance student learning: Theory, practice and assessment . Stylus Publishing, LLC.
Google Scholar
Binkley, M., Erstad, O., Herman, J., Raizen, S., Ripley, M., Miller-Ricci, M., & Rumble, M. (2012). Defining Twenty-First Century Skills. In P. Griffin, B. McGaw, & E. Care (Eds.), Assessment and Teaching of 21st Century Skills (pp. 17–66). Springer.
Chapter Google Scholar
Bransford, J. D., & Schwartz, D. L. (1999). Rethinking transfer: A simple proposal with multiple implications. Review of Research in Education, 24 , 61–100.
Ceberio, M., Almudí, J. M., & Franco, Á. (2016). Design and application of interactive simulations in problem-solving in University-Level Physics Education. Journal of Science Education and Technology, 25 (4), 590–609.
Cheng, S.-C., She, H.-C., & Huang, L.-Y. (2017). The impact of problem-solving instruction on middle school students’ physical science learning: Interplays of knowledge, reasoning, and problem solving. Eurasia Journal of Mathematics, Science and Technology Education , 14 (3), 731–743. https://doi.org/10.12973/ejmste/80902
Chou, R.-J., Liang, C.-P., Huang, L.-y., & She, H.-C. (2022). The impacts of online skeuomorphic physics inquiry–based learning with and without simulation on 8th graders’ scientific inquiry performance. Journal of Science Education and Technology , 31 , 357–371. https://doi.org/10.1007/s10956-022-09960-5
Chua, B. L., Tan, O. S., & Liu, W. C. (2016). Journey into the problem-solving process: Cognitive functions in a PBL environment. Innovations in Education and Teaching International, 53 (2), 191–202.
Cook, M., Wiebe, E. N., & Carter, G. (2008). The influence of prior knowledge on viewing and interpreting graphics with macroscopic and molecular representations. Science Education, 92 (5), 848–867.
Darling-Hammond, L., & McLaughlin, M. W. (2011). Policies that support professional development in an Era of Reform. Phi Delta Kappan, 92 (6), 81–92.
Driver, R., Newton, P., & Osborne, J. (2000). Establishing the norms of scientific argumentation in classrooms. Science Education, 84 (3), 287–312.
Duran, M. (2014). A study on 7th Grade Students’ Inquiry and Communication Competencies. Procedia - Social and Behavioral Sciences, 116 , 4511–4516.
Erozkan, A. (2013). The effect of communication skills and interpersonal problem solving skills on social self-efficacy. Kuram Ve Uygulamada Egitim Bilimleri, 13 , 739–745.
Faranda, W. T., & Clarke, I. (2004). Student observations of outstanding teaching: Implications for marketing educators. Journal of Marketing Education, 26 (3), 271–281.
Fawcett, L. M., & Garton, A. F. (2005). The effect of peer collaboration on children’s problem-solving ability. British Journal of Educational Psychology, 75 (2), 157–169.
Article PubMed Google Scholar
Fischer, F., Kollar, I., Stegmann, K., & Wecker, C. (2013). Toward a script theory of guidance in computer-supported collaborative learning. Educational Psychologist, 48 (1), 56–66.
Article PubMed PubMed Central Google Scholar
Fornell, C., & Larcker, D. F. (1981). Evaluating structural equation models with unobservable variables and measurement error. Journal of Marketing Research, 18 (1), 39–50.
García-Valcárcel, A., Basilotta Gómez-Pablos, V., & López García, C. (2014). ICT in collaborative learning in the classrooms of primary and secondary education. Comunicar, 21 , 65–74.
Garrison, D. (1991). Critical thinking and adult education: A conceptual model for developing critical thinking in adult learners. International Journal of Lifelong Education, 10 , 287–303.
Griffin, P., & Care, E. (2014). Assessment and teaching of 21st century skills: Methods and approach . Springer.
Grimberg, B. I., & Hand, B. M. (2009). Cognitive pathways: Analysis of students’ written texts for science understanding. International Journal of Science Education, 31 , 503–521.
Hair, J. F., Hult, G. T. M., Ringle, C. M., & Sarstedt, M. (2022). A primer on partial least squares structural equation modeling (PLS-SEM) (3rd ed.). Sage publications.
Harskamp, E., & Ding, N. (2007). Structured collaboration versus individual learning in solving physics problems. International Journal of Science Education, 28 (14), 1669–1688.
Henseler, J., & Chin, W. W. (2010). A comparison of approaches for the analysis of interaction effects between latent variables using partial least squares path modeling. Structural Equation Modeling, 17 , 82–109.
Article MathSciNet Google Scholar
Henseler, J., Ringle, C. M., & Sarstedt, M. (2015). A new criterion for assessing discriminant validity in variance-based structural equation modeling. Journal of the Academy of Marketing Science, 43 (1), 115–135.
Henseler, J., Ringle, C. M., & Sinkovics, R. R. (2009). The use of partial least squares path modeling in international marketing. In R. R. Sinkovics & P. N. Ghauri (Eds.), New Challenges to International Marketing (Vol. 20, pp. 277–319). Emerald Group Publishing Limited.
Hernández, N., Muñoz Carril, P., & Gonzalez-Sanmamed, M. (2019). Computer-supported collaborative learning: An analysis of the relationship between interaction, emotional support and online collaborative tools. Computers & Education, 138 , 1–12. https://doi.org/10.1016/j.compedu.2019.04.012
Howe, C., & Tolmie, A. (2003). Group work in primary school science: Discussion, consensus and guidance from experts. International Journal of Educational Research, 39 (1), 51–72.
Johnson, D. W., & Johnson, R. T. (2009). An educational psychology success story: Social interdependence theory and cooperative learning. Educational Researcher, 38 (5), 365–379.
Johnstone, A. H. (1993). The development of chemistry teaching: A changing response to changing demand. Journal of Chemical Education, 70 (9), 701.
Ku, H.-Y., Tseng, H., & Akarasriworn, C. (2013). Collaboration factors, teamwork satisfaction, and student attitudes toward online collaborative learning. Computers in Human Behavior, 29 , 922–929.
Lin, K.-Y., Yu, K.-C., Hsiao, H. S., Chang, Y.-S., & Chien, Y.-H. (2018). Effects of web-based versus classroom-based STEM learning environments on the development of collaborative problem-solving skills in junior high school students. International Journal of Technology and Design Education, 30 (1), 21–34.
Lu, H.-K., & Lin, P.-C. (2017). A study of the impact of collaborative problem-solving strategies on students’ performance of simulation-based learning — A case of network basic concepts course. International Journal of Information and Education Technology, 7 (5), 361–366.
Malik, A., Minan Chusni, M., & Yanti. (2019). Enhancing student’s problem-solving ability through Collaborative Problem Solving (CPS) on simple harmonic motion concept. Journal of Physics: Conference Series, 1175 , 012179.
Newell, A., & Simon, H. (1972). Human problem solving. Englewood Cliffs, NJ: Prentice-Hall.
Organisation for Economic Co-operation and Development (OECD) (2013). PISA 2015 collaborative problem solving frameworks. Paris, France: PISA, OECD Publishing. Retrieved from http://www.oecd.org/pisa/pisaproducts/pisa2015draftframeworks.htm
Rahayu, J., Solihatin, E., & Rusmono, R. (2022). The development of online module to improve chemistry learning outcomes in high schools. International Journal of Education, Information Technology, and Others, 5 (3), 31–46.
Ringle, C., Da Silva, D., & Bido, D. (2015). Structural equation modeling with the SmartPLS. Bido, D., da Silva, D., & Ringle, C. (2014). Structural Equation Modeling with the Smartpls. Brazilian Journal of Marketing, 13 (2).
Rutten, N., van Joolingen, W. R., & van der Veen, J. T. (2012). The learning effects of computer simulations in science education. Computers & Education, 58 (1), 136–153.
Saab, N., van Joolingen, W., & Van Hout-Wolters, B. (2012). Support of the collaborative inquiry learning process: Influence of support on task and team regulation. Metacognition and Learning, 7 , 7–23.
Saxe, R., Guberman, S. R., & Gearheart, B. (2002). Peer interaction and the development of mathematical understandings: A new framework for research and educational practice . In H. Daniels (Ed.), Charting the Agenda (pp. 137–174). Routledge.
Sinensis, A. R., Firman, H., Hamidah, I., & Muslim, M. (2019). Reconstruction of collaborative problem solving based learning in thermodynamics with the aid of interactive simulation and derivative games. Journal of Physics: Conference Series, 1157 , 032042.
Supasorn, S., Khattiyavong, P., Jarujamrus, P., & Promarak, V. (2014). Small-scale inquiry-based experiments to enhance high school students' Conceptual understanding of electrochemistry. International Proceedings of Economics Development and Research, 81 , 85–91.
Unal, E., & Cakir, H. (2021). The effect of technology-supported collaborative problem solving method on students’ achievement and engagement. Education and Information Technologies, 26 (4), 4127–4150.
Veenman, M. V. J., & Spaans, M. A. (2005). Relation between intellectual and metacognitive skills: Age and task differences. Learning and Individual Differences, 15 (2), 159–176.
Yusuf, F. A., & Adeoye, E. A. (2012). Developing critical thinking and communication skills in students: Implications for practice in education. African research review, 6 (1), 311–324.
Download references
Acknowledgements
We acknowledge the financial support that we have received from our Ministry of Science and Technology (MOST), Grand number MOST 107-2511-H-009 -003 -MY3
Open Access funding enabled and organized by National Yang Ming Chiao Tung University.
Author information
Authors and affiliations.
Institute of Education, National Yang Ming Chiao Tung University, 1001, University Road, Hsinchu City, 30010, Taiwan, Republic of China
Meng-Jun Chen, Hsiao-Ching She & Pei-Yi Tsai
You can also search for this author in PubMed Google Scholar
Corresponding author
Correspondence to Hsiao-Ching She .
Ethics declarations
Conflict of interest.
The authors have no conflict of interest to disclose.
Additional information
Publisher's note.
Springer Nature remains neutral with regard to jurisdictional claims in published maps and institutional affiliations.
Rights and permissions
Open Access This article is licensed under a Creative Commons Attribution 4.0 International License, which permits use, sharing, adaptation, distribution and reproduction in any medium or format, as long as you give appropriate credit to the original author(s) and the source, provide a link to the Creative Commons licence, and indicate if changes were made. The images or other third party material in this article are included in the article's Creative Commons licence, unless indicated otherwise in a credit line to the material. If material is not included in the article's Creative Commons licence and your intended use is not permitted by statutory regulation or exceeds the permitted use, you will need to obtain permission directly from the copyright holder. To view a copy of this licence, visit http://creativecommons.org/licenses/by/4.0/ .
Reprints and permissions
About this article
Chen, MJ., She, HC. & Tsai, PY. The effects of online simulation-based collaborative problem-solving on students’ problem-solving, communication and collaboration attitudes. Educ Inf Technol (2024). https://doi.org/10.1007/s10639-024-12609-y
Download citation
Received : 30 August 2023
Accepted : 28 February 2024
Published : 18 March 2024
DOI : https://doi.org/10.1007/s10639-024-12609-y
Share this article
Anyone you share the following link with will be able to read this content:
Sorry, a shareable link is not currently available for this article.
Provided by the Springer Nature SharedIt content-sharing initiative
- Computer simulation
- Collaborative problem-solving
- Peer communication
- Collaboration attitudes
- High-vs. low-achievers
- Find a journal
- Publish with us
- Track your research
What Is a Computer Simulation and How Does It Work? A Deep Dive.

Computer simulations are programs that run various mathematical scenarios to determine the potential scope or impact that a particular scenario could have.
For example, simulations help car manufacturers to virtually crash test their new lines of vehicles. Instead of physically crashing dozens of new cars, researchers run simulations to see all possible scenarios that could occur to both the vehicle and passengers in a multitude of accidents. These simulations determine if the car is safe enough to drive.
The idea is that computer simulations allow researchers to replicate possible real-world events — ranging from the spread of infectious diseases to impending hurricanes — so we can save time and money planning for the future.
What Is a Computer Simulation?
Put simply, computer simulations are computer programs that model a real-life scenario or product and test many possible outcomes against it.
“If it’s too large-scale, too expensive or too risky to work with the real system itself — that’s why we use computer simulation,” Barry Nelson, a professor of engineering at Northwestern University, said. “Simulation allows you to create data or systems that are conceptual, that people want to build, want to consider or want to change. I sometimes say that simulation is data analytics for systems that don’t yet exist.”
Computer Simulation Definition
A computer simulation uses mathematical equations to model possible real-world scenarios, products or settings and create various responses to them. It works by duplicating the real-life model and its functions, and once the simulation is up and running, the simulation creates a record of what is being modeled and its responses which is translated into data.
Although computer simulations are typically used to test potential real-world scenarios, they can be more theoretical too. In 2016, scientists at Argonne National Laboratory near Chicago, Illinois concluded that it would take only a couple of months for zombies to overrun the city and wipe out its population.
Fortunately, we now have “the knowledge to develop an actionable program to train the population to both better defend themselves against zombies and also take offensive actions that are the most effective,” Chick Macal, an Argonne senior systems engineer, told Built In. Phew.
Zombies aren’t real, but infectious diseases are. Macal and his co-researchers wanted to predict how more plausible infectious diseases might spread, and to determine the most effective methods of intervention and policy action. Their research relied on what’s called agent-based computer modeling and simulation. This method has allowed researchers in all types of academic disciplines and commercial industries to figure out how things (equipment, viruses, etc.) would function or act in certain environments without having to physically replicate those conditions. In the case of Macal and his cohorts, that means no humans living — or undead — were harmed in the course of their work.
Macal’s colleague, computational scientist Jonathan Ozik, described this part of their work as the “computational discovery of effective interventions,” and it’s especially good at working with a particular population of people. An added benefit, he said, is that “we can do these experiments without worrying about the cost of experiments or even ethical and privacy considerations,” because the populations they study are synthetic, mathematical representations, not the real thing.
Read Next: What Is Quantum Computing?
How Do Computer Simulations Work?
Computer simulation is a step-by-step process in which a computer simulation program is modeled after a real-world system (a system can be a car, a building or even a tumor). In order to replicate the system and possible outcomes, the simulation uses mathematical equations to create an algorithm that defines the system’s state, or the combination of different possible variables.
If you’re simulating a car crash, for example, the simulation’s algorithm can be used to test what would happen if there was a storm during the crash against what happens when the weather is more mild.
The simulation calculates the system’s state at a given time (t), then it moves to t+1 and so on. Once the simulation is complete, the sequence of variables are saved as large datasets, which can then be translated into a visualization.
“We’re not interested in simply extrapolating into the future,” Macal said. “We’re interested in looking at all the uncertainties as well as different parameters that characterize the model, and doing thousands or millions of simulations of all the different possibilities and trying to understand which interventions would be most robust. And this is where high-performance computing comes in.”
Thanks to the robust data-crunching powers of supercomputers , simulation is more advanced than ever — and evolving at a rapid pace.
The computational resources at their disposal, Ozik said, allow researchers “to fully explore the behaviors that these models can exhibit rather than just applying ad hoc approaches to find certain interesting behaviors that might reflect some aspect of reality.”
Which is to say, the simulations are much broader, and therefore even more realistic — at least from a hypothetical perspective.
Computer Simulations in the Real World
Plenty of simulations are done with far less computing power than Argonne possesses. Alison Bridger, department chair of meteorology and climate science at San Jose State University in California, said on-site cluster computers are strong enough to run the climate simulation models she builds. Cloud computing services like those offered by Amazon (AWS) and Microsoft (Azure) are gradually gaining a foothold in the space as well.
Along with nuclear physics, meteorology was one of the first disciplines to make use of computer simulation after World War II. And climate modeling, Bridger said, “is like a close cousin of weather forecasting. Back in the 1960s, people used early weather forecasting models to predict the climate. Before you can predict the weather, you have to be able to properly reproduce it with your model.”
Bridger’s work employs a widely used “local scale” model called WRF, which stands for Weather, Research and Forecasting and can produce “reasonably good simulations of weather on the scale of, say, Northern Illinois — so Chicago up to Green Bay and down into the central part of the state. It will forecast things like high and low temperatures, rain and so forth. And it’s typically only run to simulate 24, 48 or 72 hours of weather.”
In further explaining her process, Bridger employs the imagery of a cube centered over Chicago that’s roughly a kilometer east-west by a kilometer north-south. The goal is to predict the temperature in the cube’s center and extrapolate that reading to the entire thing. There are also, in her telling, additional cubes surrounding the initial one “stacked up all the way to the top of the atmosphere” whose future temperatures will be predicted in various time increments — in an hour, in 12 hours, in one day, in three days and so on.
Next, temperature-affecting variables are added to the mix, such as amount of sunshine, cloud cover, natural disasters like wildfires and manmade pollution. It’s then a matter of applying the laws of physics to determine a variety of weather-related events: rising and falling temperatures, amount of wind and rain.
Computer simulations can be used for much more than climate and weather predictions.
6 Examples of Computer Simulations
Whether scientists want to better understand healthcare responses or even explore blackholes, computer simulation allows for important research opportunities. Here are six that stand out:
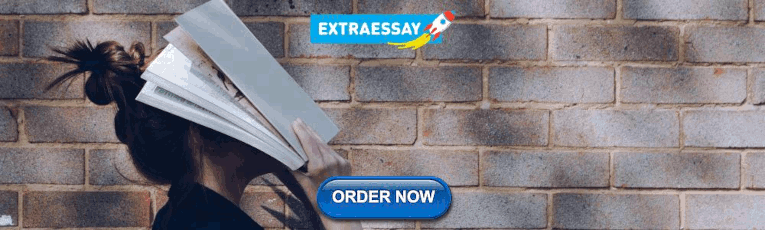
1. Responding To Pandemics
Along with Ozik and their fellow researcher Nick Collier, Macal also worked on a modeling and simulation project that determined what might happen if the deadly Ebola virus — which initially spread through West Africa in 2013 through 2016, with devastating effects — would impact the U.S. population. Part of that process involved visiting Chicago hospitals to learn about Ebola-related procedures, then incorporating those procedures into their models.
2. Improving Cancer Treatment
Other Argonne scientists have used modeling and simulation to improve cancer treatment through predictive medicine, finding out how various patients and tumors respond to different drugs.
And one 2019 study found positive results in simulating breast cancer tumors. For the study, researchers built a computer simulation that modeled tumors from four different patients under 12-week therapy treatments. After two of the simulated tumors didn’t respond to treatment, they concluded that more frequent, lower doses of chemotherapy could reduce a low proliferative tumor, while lower doses of antiangiogenic agents helped poorly perfused tumors respond to drug treatment better.
3. Predicting Health Code Violations
In Chicago, the city’s Department of Public Health uses computer modeling and simulation to predict where critical violations might pop up first. Those restaurants are then bumped to the top of a 15,000-establishment list that’s overseen by only three dozen inspectors. And apparently it’s working: One simulation yielded 14 percent more violations, which ideally means earlier inspection and a lower chance of patrons getting sick from poorly refrigerated fish.
4. Understanding Our Relationship with Religion and Crisis
Computer simulation is being used in interesting ways at the University of Boston. Wesley Wildman, a professor of philosophy, theology and ethics, uses computer simulation to study — as he put it in an article for The Conversation — “how religion interacts with complex human minds, including in processes such as managing reactions to terrifying events.”
In order to do so, he and his team designed a world and filled it with computer-controlled characters, or “agents,” that are “programmed to follow rules and tendencies identified in humans through psychological experiments, ethnographic observation and social analysis.”
Then they observed what happened when their agents were tested against real-world examples like a massive earthquake that struck Christchurch, New Zealand in 2011.
“The better our agents mimic the behavior of real humans in those sorts of circumstances,” Wildman said, “the more closely aligned the model is with reality, and the more comfortable we are saying humans are likely to behave the way the agents did in new and unexplored situations.”
5. Researching Earthquakes
In Germany, a team at the Leibniz Supercomputing Centre performed earthquake simulations using the devastating Indian Ocean earthquake of 2004, which spurred a massive tsunami, as their point of origin.
According to Professor Michael Bader of Germany’s Institut für Informatik, they wanted to “better understand the entire process of why some earthquakes and resulting tsunamis are so much bigger than others. Sometimes we see relatively small tsunamis when earthquakes are large, or surprisingly large tsunamis connected with relatively small earthquakes. Simulation is one of the tools to get insight into these events.”
But it’s far from perfect. New York Times reporter Sheri Fink detailed how a Seattle-based disaster response startup called One Concern developed an earthquake simulation that failed to include many densely populated commercial structures in its test-runs “because damage calculations relied largely on residential census data.” The potential real-world result of this faulty predictive model: Rescuers might not have known the location of many victims in need.
6. Exploring Black Holes
In 2022, researchers built a black hole simulation by modeling a single-file chain of atoms to create the event horizon of a black hole. This led to the team observing Hawking radiation, the hypothetical theory that particles formed by the edge of a black hole create temperatures that are inversely proportional to a black hole’s mass.
Although the research is still in its early stages, this could potentially help scientists understand and resolve the differences between the general theory of relativity and quantum mechanics.
Uses of Computer Simulation in Different Industries
In the past 75 years, computer modeling and simulation has evolved from a primarily scientific tool to something industry has embraced for the purposes of optimization and profitability.
“Industry is embracing simulation at a faster rate than ever before and connecting it to what I would call data analytics for things like scheduling and supply chain management ,” Macal said. “Industry is trying to simulate everything they do because they realize it’s cheaper and quicker than actually building a prototype system.”
When Northwestern’s Nelson spoke with Built In, he had recently returned from the annual Applied Probability Conference. There, the simulation applications discussed included but weren’t limited to the following: aviation modeling, cybersecurity , environmental sustainability and risk, financial risk management, healthcare, logistics , supply chain and transportation, semiconductor manufacturing, military applications, networking communications, project management and construction.
“Frequently, companies that use simulation want to optimize system performance in some sense,” Nelson said, using a car company that wants to build a new assembly plant or decide what vehicles to bring to market as an example.
“So optimization is a key to lots of business in industry, but optimal solutions are often brittle,” he continued. “By which I mean, if small issues about the assumptions or the modeling approximations you made are wrong, then suddenly something that appeared to be optimal in your model can be catastrophically bad.”
Nelson added: “When people build mathematical and computer models, even though the model may have been built from data, they treat it as if the model is correct and therefore the solution that [results] is optimal. What we try to do is continue to incorporate in the model the uncertainty that was created when we built it.”
The financial crisis of 2008, Nelson said, is one instance where model risk was detrimentally downplayed.
“The financial industry uses a tremendous number of very sophisticated mathematical computer modeling [methods],” he said. “And it’s quite clear that the correlations among various financial instruments and securities and so on were kind of ignored, so we got cascading failures .”
Such cautionary tales, however, don’t mean that those who create the mathematical and computer models on which simulations are based must strive for perfection, because no model is perfect and models drive progress. Demanding perfection, Nelson said, “would paralyze us. But as we start to make more life-critical decisions based on models, then it does become more important to account for risks.”
Related Reading: 17 High-Performance Computing Applications and Examples
The Future of Computer Simulations
Imagine this: It’s years from now and someone you know has been diagnosed with a cancerous tumor. But instead of immediately bombarding them with radiation and highly toxic chemotherapy drugs and hoping for the best, doctors instead perform tests from which they create a mathematical, virtual twin of that person’s malignant growth. The digital replica is then subjected to computational interventions in the form of millions or even billions of simulations that quickly determine the most effective form of treatment.
It’s less fantastical than it sounds.
“Recent developments in cancer-specific ‘big data’ and experimental technologies, coupled with advances in data analysis and high-performance computational capabilities, are creating unprecedented opportunities to advance understanding of cancer at greater and more precise scales,” the National Cancer Institute reported .
Other revolutionary developments with far-reaching impact are already being implemented.
As Los Alamos National Laboratory physicist Justin Smith told Science Daily , “we can now model materials and molecular dynamics billions of times faster compared to conventional quantum methods, while retaining the same level of accuracy.”
That’s good news for drug developers, whose researchers study molecular movement in order to see what’s suitable for use in pharmaceutical manufacturing, as well as patients who are all too often caught up in a detrimental guessing game when it comes to treatment.
Penn State researchers working in tandem with colleagues at the University of Almeria in Spain developed “a computer model that can help forecasters recognize potential severe storms more quickly and accurately.” As Steve Wistar, a senior forensic meteorologist at AccuWeather, explained, the tool could lead to better forecasts because he and his fellow forecasters will have “a snapshot of the most complete look of the atmosphere.”
And so, while we may or may not be living in a computer-simulated world , the world is being transformed by computer simulation. As computers get faster and research methods more refined, there’s no telling where it might lead.
Mudi Yang, a cosmos-simulating high school senior from Nashville, put it eloquently when he said , “Computer simulations gave us the ability to create virtual worlds, and those virtual worlds allowed us to better understand our real one.”
Great Companies Need Great People. That's Where We Come In.
InstructionalDesign.org
Home » Learning Theories » General Problem Solver (A. Newell & H. Simon)
General Problem Solver (A. Newell & H. Simon)
The General Problem Solver (GPS) was a theory of human problem solving stated in the form of a simulation program (Ernst & Newell, 1969; Newell & Simon, 1972). This program and the associated theoretical framework had a significant impact on the subsequent direction of cognitive psychology. It also introduced the use of productions as a method for specifying cognitive models.
The theoretical framework was information processing and attempted to explain all behavior as a function of memory operations, control processes and rules. The methodology for testing the theory involved developing a computer simulation and then comparing the results of the simulation with human behavior in a given task. Such comparisons also made use of protocol analysis (Ericsson & Simon, 1984) in which the verbal reports of a person solving a task are used as indicators of cognitive processes.
GPS was intended to provide a core set of processes that could be used to solve a variety of different types of problems. The critical step in solving a problem with GPS is the definition of the problem space in terms of the goal to be achieved and the transformation rules. Using a means-end-analysis approach, GPS would divide the overall goal into subgoals and attempt to solve each of those. Some of the basic solution rules include: (1) transform one object into another, (2) reduce the different between two objects, and (3) apply an operator to an object. One of the key elements need by GPS to solve problems was an operator-difference table that specified what transformations were possible.
Application
While GPS was intended to be a general problem-solver, it could only be applied to “well-defined” problems such as proving theorems in logic or geometry, word puzzles and chess. However, GPS was the basis other theoretical work by Newell et al. such as SOAR and GOMS . Newell (1990) provides a summary of how this work evolved.
Here is a trace of GPS solving the logic problem to transform L1= R*(-P => Q) into L2=(Q \/ P)*R (Newell & Simon, 1972, p420):
Goal 1: Transform L1 into LO Goal 2: Reduce difference between L1 and L0 Goal 3: Apply R1 to L1 Goal 4: Transform L1 into condition (R1) Produce L2: (-P => Q) *R Goal 5: Transform L2 into L0 Goal 6: Reduce difference between left(L2) and left(L0) Goal 7: Apply R5 to left(L2) Goal 8: Transform left(L2) into condition(R5) Goal 9: Reduce difference between left(L2) and condition(R5) Rejected: No easier than Goal 6 Goal 10: Apply R6 to left(L2) Goal 11: Transform left(L2) into condition(R5) Produce L3: (P \/ Q) *R Goal 12: Transform L3 into L0 Goal 13: Reduce difference between left(L3) and left(L0) Goal 14: Apply R1 to left(L3) Goal 15: Transform left(L3) into condition(R1) Produce L4: (Q \/ P)*R Goal 16: Transform L4 into L0 Identical, QED
- Problem-solving behavior involves means-ends-analysis, i.e., breaking a problem down into subcomponents (subgoals) and solving each of those.
- Ericsson, K. & Simon, H. (1984). Protocol Analysis. Cambridge, MA: MIT Press.
- Ernst, G. & Newell, A. (1969). GPS: A Case Study in Generality and Problem Solving. New York: Academic Press.
- Newell, A. (1990). Unified Theories of Cognition. Cambridge, MA: Harvard University Press.
- Newell, A. & Simon, H. (1972). Human Problem Solving. Englewood Cliffs, NJ: Prentice-Hall.
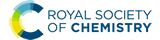
Using computer simulations in chemistry problem solving
Spyridon Avramiotis ab and Georgios Tsaparlis * c a Program of Graduate Studies “Chemistry Education and New Educational Technologies” (DiCheNET), Department of Chemistry, University of Athens, GR-157 71, Athens, Greece b Model Experimental Lyceum, Ionidios School of Piraeus, GR-185 35, Piraeus, Greece. E-mail: [email protected] c Department of Chemistry, University of Ioannina, GR-451 10, Ioannina, Greece. E-mail: [email protected]
First published on 7th May 2013
This study is concerned with the effects of computer simulations of two novel chemistry problems on the problem solving ability of students. A control–experimental group, equalized by pair groups ( n Exp = n Ctrl = 78), research design was used. The students had no previous experience of chemical practical work. Student progress was checked twice, once 15 minutes after they had started looking for a solution, before the experimental group was exposed to the simulation, and again after completion of the test. The 15 minutes check confirmed the equivalence of the two groups. The findings both verified the difficulty of the problems, and indicated improved mean achievement of the experimental group (students who were shown the problem simulations), in comparison to the control group (students who solved the problem in the traditional way). Most students assumed that the major benefit of the simulations was to help them with the proper application of the equations. The effects of scientific reasoning/developmental level and of disembedding ability were also examined. The performance level for formal reasoners was found to be higher than that for transitional reasoners and that for transitional reasoners higher than for concrete ones. Field independent students were found to outperform field intermediate ones, and field intermediate students were found to outperform field dependent ones. Finally, in most cases the experimental group outperformed the control group at all levels of the above two cognitive factors.
Introduction
Problem solving.
Central and crucial for problem solving are also the number and quality of available relative operative schemata in long-term memory. Piaget considered a schema as an internal structure or representation, and operations as the ways in which we manipulate schemata. The operative schemata entering a problem constitute the logical structure of the problem. According to Niaz and Robinson (1992) (see also Tsaparlis et al. , 1998 ), the logical structure of a problem represents the degree to which it requires formal operational reasoning.
At this point, it is necessary to distinguish between what have been termed ‘problems’ and ‘exercises’. Exercises can be carried out relatively easily by many students, as they require only the application of well-known and practiced procedures ( algorithms ) for their solution. The skills that are necessary for this are as a rule lower-order cognitive skills (LOCS). On the other hand, a real/novel problem requires that the solver must be able to use higher-order cognitive skills (HOCS) ( Zoller, 1993 ; Zoller and Tsaparlis, 1997 ). Note that the degree to which a problem is a real problem or merely an exercise will depend on both the student’s background and the teaching ( Niaz, 1995 ). Thus, a problem that requires HOCS for some students may require LOCS for others in a different context.
A thorough classification of problem types has been made by Johnstone (1993, 2001) . In this work, we are interested in real/novel problems. Such problems, requiring both the development of appropriate strategies and HOCS, prove very difficult for inexperienced students. A number of researchers ( Simon and Simon, 1978 ; Larkin and Reif, 1979 ; Reif, 1981, 1983 ) have studied the differences between expert and novice problem solvers. The basic differences were: (a) the comprehensive and complete schemata of the experts, in contrast to the sketchy ones of the novices; and (b) the extra step of the qualitative analysis undertaken by the experts, before they move into detailed and quantitative means of solution. According to Reif (1983) , a ‘basic description’ of a problem is the essential first stage in problem solving:
“The manner in which a problem is initially described is crucially important since it can determine whether the subsequent solution of the problem is easy or difficult – or even impossible. The crucial role of the initial description of a problem is, however, easily overlooked because it is a preliminary step which experts usually do rapidly and automatically without much conscious awareness. A model of effective problem solving must thus, in particular, specify explicitly procedures for generating a useful initial description of any problem … The basic description summarizes the information specified and to be found, introduces useful symbols, and expresses available information in various symbolic forms ( e.g. in verbal statements as well as in diagrams)” (pp. 949–950).
Experience, or a lack of experience, on the part of secondary students with realistic chemical and physicochemical systems, such as those involved in chemical problems is also relevant to this work.
Problem solving and practical work
Problem solving ability can be enhanced by associated laboratory activities. Interestingly, Kerr (1963, cited in Johnstone and Al-Shuaili, 2001) listed among the aims for practical work that it should provide training in problem solving and Roth (1994) found that physics problem solving at the upper secondary level was improved by means of practical work. Bowen and Phelps (1997) reported that demonstrations tend to improve the problem-solving capabilities of the students because they help them switch between various forms of representing problems dealing with chemical phenomena (for instance, symbolic and macroscopic). Deese et al. (2000) found that demonstration assessments promote critical thinking and a deeper conceptual understanding of important chemical principles.
In previously published work ( Kampourakis and Tsaparlis, 2003 ), a laboratory/practical activity, involving the well-known ammonia-fountain experiment, was used in order to find out if it could contribute to the solution of a demanding chemistry problem on the gas laws (this is the same as problem 1 in the present study). Furthermore, the extent to which the practical activity, together with a follow-up discussion/interpretation in the classroom, could contribute to the improvement of the problem-solving ability of the students was assessed. The subjects were from tenth and eleventh grade (16–17 year olds). It was found that students from the experimental groups achieved higher scores than those obtained by students from the control groups. The differences, although not large, were in many cases, statistically significant. Mean achievement was, however, low, and only a small proportion of the students considered that the practical activity had been relevant/useful to the solution of the problem. It was concluded that many students lacked a good understanding of the concepts that relate to the ideal-gas equation. In addition, as is the case with most Greek students, the students had no previous experience in working with chemicals and carrying out, or even watching, experiments. It seems possible that observing the chemical experiment so dominated their attention that little opportunity was left for any mental processing of what was going on and why. In addition, the experiment chosen involved many details (including the production of ammonia gas from the reaction of ammonium chloride with sodium hydroxide ), and involved many physics and chemistry concepts. It therefore seems likely that an overload of students’ working memory took place, preventing significant improvement in their ability to solve the problem.
The present study
The central research question being investigated ( Research question 1) is as follows: Does watching a simulation on a computer screen while attempting to solve a problem have an effect on students’ ability to solve the problem? In addition, the study offers the opportunity to investigate further two important factors that are known to have an important effect on students’ problem solving performance:
(2) Is the ability of students to solve problems related to their scientific reasoning/developmental level?
(3) Is disembedding ability (degree of field dependence/independence) connected to students’ ability in problem solving?
Computer simulations in science education
Richard E. Mayer’s cognitive theory of multimedia learning, which is based on the fact that paying attention to several tasks simultaneously will result in a portion of the working memory not being available for learning ( Mayer, 1997, 2001 ; Mayer and Moreno, 1998 ), is of relevance to this study. Mayer’s theory discusses a number of design principles for efficient multimedia instruction. The modality principle states that materials which present both verbal and graphical information should present the verbal information in an auditory format, and not as written text ( Moreno and Mayer, 1999 ). On the other hand, according to the split attention effect, students learn better from animation and narration rather than from animation, narration, and on-screen text ( Mayer, 2001 ). Other principles include the spatial contiguity principle – “Students learn better when corresponding words and pictures are presented near rather than far from each other on the page or screen”; the temporal contiguity principle – “Students learn better when corresponding words and pictures are presented simultaneously rather than successively”; the coherence principle – “Students learn better when extraneous material is excluded rather than included”; and the individual differences principle – “Design effects are stronger for low-knowledge learners than for high knowledge learners, and for high-spatial learners rather than for low-spatial learners”.
Virtual interactive experiments performed on the computer appear to be a powerful tool ( Lajoie, 1993 ; Josephsen and Kristensen, 2006 ). Research shows that a computer-based learning environment can reduce the time required for performing a task, and at the same time reduce the cognitive load. Careful design and instructions can then contribute to enhanced student learning ( Lajoie, 1993 ; Lajoi et al. , 1998, 2001 ; van Bruggen et al. , 2002 ; Josephsen and Kristensen, 2006 ).
According to Oakes and Rengarajan [2002 , cited in Akaygun and Jones (2013) ], an animation is a multimedia presentation that is rich in graphics and sound, but not in interactivity, while a simulation is defined as an interactive and explorative representation. The authors maintain that it is not always possible to re-create in a simulation an accurate real-world environment; further, the more sophisticated a simulation, the more accurately it represents and describes the target phenomenon.
Computer simulations have been used in a variety of teaching situations especially as a substitute for, or complement to, the chemistry laboratory ( Butler and Griffin, 1979 ; Akaygun and Jones, 2013 ). Early laboratory applications involved simulations of macroscopic laboratory procedures. Later simulations extended the emphasis to representing phenomena at the atomic and molecular level, as well as to simulating large and expensive laboratory equipment. Although animations and simulations are different in terms of their level of interactivity, both have been used as effective tools for chemistry instruction. For instance, a number of studies have found that students who received instruction that included computer animations of chemical processes at the molecular level were better able to comprehend chemistry concepts involving the particulate level of matter than those who did not ( Williamson and Abraham, 1995 ; Sanger and Greenbowe, 1997a, 1997b ; Burke et al. , 1998 ; Sanger et al. , 2000 ; Ardac and Akaygun, 2004, 2005 ).
A main feature of simulations is their dynamic information and character, which increases the information-processing demand, and thus may not contribute to improved learning in comparison with static pictures ( Rieber, 1990 ; Lewalter, 2003 ; Lowe, 2003 ). Lewalter maintains that dynamic visuals may reduce the load of cognitive processing by supporting the construction of a mental model, but they may cause higher cognitive load because of their transitory nature. Focusing on one type of presentation component may result in missing information from a different presentation component, because of the split attention effect. This effect occurs not only when one has to attend to multiple presentation stimuli (such as combinations of picture and text), but also when attending to a single presentation that includes temporal changes (as is the case in an animation or a simulation). Control of the variables in a dynamic visual can reduce the split attention effect.
The effect of scientific reasoning and disembedding ability on problem solving
In a study on non-algorithmic quantitative physical chemistry problems that have many of the features of novel/realistic problems ( Tsaparlis, 2005 ), it was reported that functional mental capacity and disembedding ability played important roles and were definitely more significant than either scientific reasoning or working memory capacity. Note that the psychometric test for measuring mental capacity (the figural intersection test) involves disembedding ability in addition to information processing.
The effects of scientific reasoning and disembedding ability have also been examined in the area of acid–base equilibria, and were found to be important for student performance, with the latter ability clearly having the larger effect ( Demerouti et al. , 2004 ). Developmental level was connected with conceptual understanding and application, but less so where complex conceptual situations and/or chemical calculations were involved. Disembedding ability was important in situations requiring demanding conceptual understanding, and where this is combined with chemical calculations. Overton and Potter (2011) investigated students' success in solving, and their attitudes towards, context-rich open-ended problems in chemistry, and compared these to algorithmic problems. They found a positive correlation between algorithmic problem solving scores and mental capacity (as measured with the figural intersection test), while scores in open-ended problem solving correlated with both mental capacity and disembedding ability. St Clair-Thompson et al. (2012) compared further algorithmic and open-ended problems with respect to mental capacity and working memory capacity; for the algorithmic problems working memory was reported to be the best predictor, while for open-ended problems both working memory capacity and mental capacity were important. On the other hand, BouJaoude et al. (2004) found that among a number of cognitive variables (learning orientation, developmental level, mental capacity, but not including disembedding ability), developmental level had the highest power to predict student performance in conceptual problems.
In the second year, 120 tenth-year students, coming from a different urban upper secondary school also in the Piraeus district (school B) participated. These students were also divided into four classes, and 74 students who had been equalized by pairs were selected. These 74 students formed the two study groups, I B and II B , each with n = 37. One group of school B acted as EG and the other as CG. Because of lack of instructional time, only problem 2 was used in the second year of the study.
The first author was a teacher in both schools. A chemistry teacher acted as the second teacher in school A, while a physicist took on this role in school B. All three were experienced teachers, with the first two holding postgraduate degrees in chemistry education. In both schools and for both problems, each teacher taught one EG and one CG of students.
All students in both schools had the same experience in working with chemicals in the lab, and all had attended the same computer simulations. Matching by pairs of the two groups for each year of the study was carried out on the basis of the following parameters: (i) mean achievement in three tests plus a final in-term exam in the chemistry course; (ii) scientific reasoning (developmental level) of the students (see below). In addition each pair of students had similar mean achievement in two final in-term exams in the physics course; Table 1 contains details about this matching by pairs. Statistical comparisons using a Student t -test for independent samples and Pearson correlations demonstrate the equivalence of the groups.
The testing procedure
The problems.
Problem 1 : A vessel contained gaseous ammonia ( NH 3 ) at a pressure p 1 = 2 atm, and a temperature of 27 °C. Part of the ammonia gas was transferred to a container containing water , where it dissolved completely to produce 2 L of a 0.1 M aqueous ammonia solution. If the pressure in the vessel is reduced to 1.18 atm, find the volume of the vessel.
The logical structure of problem 1 involves two main schemata: the ideal gas equation and concentration of aqueous solutions (molarity). The problem caused various difficulties to the students. In particular, they failed to connect the fall in the gas pressure with the ammonia solution that was formed. To make the problem as close as possible to a real problem (and not a traditional exercise), no further comments about the problem were provided, and the value of the ideal gas constant was not given, so the students needed to know or be able to calculate the value of the ideal gas constant in the proper units.
Most successful solvers applied the ideal gas equation twice, for both the initial and the final state of the ammonia gas in the flask: p 1 V = n 1 RT (1) and p 2 V = n 2 RT (2). From these two equations, they arrived at the relationship n 1 / p 1 = n 2 / p 2 (3). If n s is the number of moles of ammonia dissolved in water , then n 2 = n 1 − n s . Substituting into eqn (3) provides solution of the resulting equation for n 1 . Finally, substitution of the value for n 1 into eqn (1) leads to the calculation of V . A much smaller proportion of students did not use the ideal gas equation, but instead started with the equation p 1 / p 2 = n 1 / n 2 (at constant V and T ), and went on as mentioned above. A number of students (11 out of 41, 26.8%) made (eventually successful or unsuccessful) use of the difference between the two given pressures in their solution, which led to the relationship p 1 − p 2 = n s RT / V . This alternative method of solution is easier and faster, so the students who employed it demonstrated a good conceptual understanding of the problem. Note that the existence of a pressure gauge in the experimental setting is likely to have contributed substantially to the idea to subtract the two pressures, a fact that was admitted by the students who employed this method.
The marking scheme for problem 1 is given in Appendix 1 ( Table 6 ). Partial marks were allocated to the various steps in the solution procedure as follows: 10.0 + 2.5 = 12.5 marks for calculation of moles of ammonia gas dissolved in water ; 11.25 marks each for eqn (1) and (2); 2.5 marks each for conversion of degrees Celsius to degrees Kelvin and for knowing or estimating the value of R ; 20.0 marks for the relationship n 2 = n 1 − n s ; 22.5 marks for the algebraic manipulations that lead to a final expression for V ; 10.0 marks for intermediate numerical calculations; finally, an additional 7.5 marks for the correct numerical result with proper units. Four experienced chemistry teachers independently marked fifteen randomly selected papers, according to the agreed marking scheme. The Pearson correlation coefficients between the four markers varied between 0.94 and 0.99.
Problem 2: A closed vessel with a volume of 4 L, at a temperature of 207 °C, contains a mixture of sulfur (S) and precisely the quantity of oxygen gas (O 2 ) required for complete combustion . The vessel is supplied with a piston and an exhaust gas valve. The mixture is ignited so that all the sulfur is burnt and all of the oxygen is consumed [S(s) + O 2 (g) → SO 2 (g)]. By pressing the piston, all the combustion gas produced is transferred into a beaker containing 0.5 L cold water , where part of the gas is dissolved to give a solution with a concentration of 0.96 mol L −1 . The remaining gas is collected in an inverted test-tube, and was found to occupy a volume of 0.448 L at STP. Calculate the pressure in the closed vessel before igniting the mixture and compressing the piston.
Here again the value of the ideal gas constant was not supplied for the same reason as for problem 1. On the other hand, so as not to further increase the complexity of the problem, we included in it the relevant chemical equation. To solve this problem one has to consider the moles n 1 of SO 2 that were dissolved in water and the moles n 2 that were collected in the test-tube. The total amount of SO 2 is then n 1 + n 2 . Next, the moles of O 2 which were present in the vessel before combustion have to be calculated from the reaction stoichiometry. Finally, the ideal-gas equation has to be applied to calculate the initial pressure in the vessel.
Problem 2 is more demanding than problem 1, having a more complex logical structure. In addition to the schemata of the solvation and the ideal-gas equation, it involves the extra schema of the stoichiometry of the chemical reaction ( combustion of sulfur). A further complication arises from the fact that part of the gas produced is dissolved in water and the remainder is collected separately.
The marking scheme for problem 2 is given in Appendix 1 ( Table 7 ). All student papers were marked by the first author, who was a chemistry teacher in school A and one of the two teachers in school B. Validity and reliability of the marking schemes was judged by having 40 papers (20 for each group, EG and CG) marked by another teacher chemist. The correlation between the two markings was high ( r = 0.96).
The simulations
The same procedure and construction perspective was adopted for the simulations of both problems. Three screens were created; the one that appears first is the simulation of the experimental set-up, while the other two screens play supporting roles: one is descriptive/explanatory about the equipment and its connection with the problem; and the other provides functional help. When execution of the problem is initiated, the main page, which shows the experimental set up, appears. Navigation is horizontal, that is, there is ability to switch between the three screens: Description of the experiment; Restart the experiment; Help, by using three “buttons”, which are always present and active. Further details about the simulations are provided in Appendix 2.
Psychometric measures
Disembedding ability was assessed by means of the Hidden Figures Test, which is a 20 minute test that was devised and calibrated by El-Banna (1987) , and is similar to the Group Embedded Figures Test, GEFT, devised by Witkin ( Witkin et al. , 1971 ; Witkin, 1978 ). The two groups from school A were NOT matched in terms of this ability: in group I A there were 21 field dependent, 14 field intermediate, and 6 field independent students while in group II A there were 15 field dependent, 14 field intermediate, and 12 field independent students. On the other hand, the two groups from school B were matched, with each group having 6 field dependent, 20 field intermediate, and 11 field independent students.
Achievement in solving the problems
The following conclusions can be drawn from the data: in all cases, performance was low and below a 50% value. Achievement by the EG was higher for both problems. There were statistically significant differences in favor of the EG for the following cases: school A for problem 1 and sum of schools A for problem 1 and B for problem 2.
Achievement of school A was much higher for problem 2 (especially for the CG), and this can be attributed to the experience these students gained, both from solving problem 1 and from their practice with the simulation program. The considerable improvement displayed by the CG from school A on problem 2 (showing only a very small and statistically insignificant inferior achievement to that for the corresponding EG from school A) might be attributed to the fact that the CG students for problem 2 acted as the EG for problem 1, so they had previously experienced the simulation program for problem 1. While for problem 1 there is a statistically significant difference, in the case of problem 2 only for school B was the difference substantial and near statistical significance.
An important finding relates to the number of successful solvers, both in each problem, and with regard to the effect of the simulations. We identified students who achieved a mark over 80% in each problem as successful solvers. From a total of 41 students, there were 6 successful solvers of problems 1 (14.6%), of which 5 belonged to the EG and 1 to the CG. Also, from a total of 78 students, there were 23 successful solvers of problem 2 (29.3%), 11 belonged to the EG and 12 to the CG. Recall the effect of practice on the achievement of the CG from school A on problem 2.
It is of particular interest to examine the improvement of mean achievement from 15 minutes until the end of testing (see Tables 2 and 3 ). The highest positive effect of the simulation was noted in the case of school B for problem 2, in which case the EG improved its mean achievement from 15 minutes until the end of testing by 4.2 times (8.65 → 36.4), while the CG improved by only 2.5 times (10.5 → 26.4). On the other hand, the group from school A that acted as EG for problem 1 improved its mean achievement by about a factor of 2 (16.3 → 32.1) for problem 1, but much less (only 1.3 times) for problem 2 (29.4 → 38.8) when it acted as CG. This can be attributed to two factors: this group knew that as CG they were not going to watch a simulation, hence they worked steadily during the first 15 minutes, thus advancing their solution further (29.4), and, as a result, their further improvement by the end of time (38.8) was not particularly impressive. However, the fact that their overall achievement (38.8) is comparable to that of the corresponding EG (40.6) is impressive; their experience of working with the simulation for problem 1 could well have played a role. Finally, the group from school A that acted as CG for problem 1 improved its mean achievement by about a factor of 2 (13.4 → 23.1) for problem 1, but much more (3.6 times) for problem 2 (11.4 → 40.6) when it acted as EG. It is apparent that all groups got better with more time, but using the simulation was far more effective in most cases (except for the case of school A for problem 2). Note that all the above changes of score from time 15 minutes until the end of testing are statistically significant ( p < 0.001), as judged by using the t test for dependent samples.
Turning to some qualitative aspects of the use of the simulations, discussions with the students after the intervention showed that most students initially assumed that the simulations did not help them in the solution of the problems but were useful in helping with the proper application of the equations. Further discussion revealed some interesting aspects of the students’ actions and attitudes, with several of them admitting that through the simulations they “cleared out something (in their minds)”. We quote below some of the most useful comments that were collected:
• Using the computer did not disturb them, but on the contrary they found it interesting .
• The instructions were clear .
• They had no problem using the quick instructions of the software, and they did not need practice .
• They did not assume that they were helped with the final solution of the problems .
• In the simulation of problem 1, the pressure gauge helped them realise that pressure changed .
• They received an overall help from the simulation of problem 2 because it was long and it was difficult for them to keep in mind the procedure .
• Some students protested that they had never before solved a similar problem before taking the test with problem 1.
Effect of scientific reasoning on the effectiveness of the simulation
Effect of disembedding ability on the effectiveness of the simulation, conclusion and answers to the research questions.
Research question 1 . Does watching a simulation on a computer screen while attempting to solve a problem have an effect on students’ ability to solve the problem?
Our results showed improved mean achievement for the EG, that is for the students who used/viewed the problem simulations, in comparison to the CG, who solved the problem in the traditional way by thinking and writing on paper. This was more evident when the results for the two schools were combined (school A for problem 1 and school B for problem 2) to give a larger sample (sum A + B).
Achievement levels at school A were much higher for problem 2 (especially for the CG), and this can be attributed both to the experience these students gained from solving problem 1 and from their practice with the simulation program. The considerable improvement in problem 2 for the CG at school A might be attributed to the fact that the CG for problem 2 had previously acted as the EG for problem 1, so it had experienced the benefits of the simulation program for problem 1. An additional fact that supports the above speculation is that in the interlude between the two teaching periods when the two problems were administered to the students of school A, the solution to problem 1 was presented and relevant discussions were carried out by both the EG and the CG. We assume that the model solution and the related discussion must have contributed greatly to the improved results of the students from school A in problem 2.
We repeat that the equivalence of the two groups of students (EG and CG) was established by a proper composition that was based on their achievement in chemistry and physics in-term exams, as well as in the Lawson test of scientific reasoning. In addition, the equivalence was checked by comparing achievement, during the first 15 minutes of the test for each of the two problems of this study, where the performance of the two groups was found to be similar.
A deeper analysis of the students’ solution procedures showed that marks were low irrespective of the use or non-use of the simulations. This suggests that there is a mental ‘jump’ from the solution of the component subproblems to the synthesis and solution of the whole problem. It appears that the effect of the simulations for the relevant experimental set-ups did not really lead to the final solution of the problems. The simulations rather appeared to help with the solution of the subproblems or component steps in a problem. Therefore, the overall performance on the problem improved, because after viewing the simulations more students were successful in using the equations, relationships, and data appropriately. Examples of students attempting to solve exercises or problems by using equations, relationships, and data unsuccessfully or combining them at random (or by using incorrect equations and relationships) are well-known both to experienced teachers and in the problem solving literature. According to our marking schemes (see Appendix 1), a total mark of 30–40% could be achieved by a student who just knew the proper equations and relationships, and applied them correctly. In particular, the handling of volumes (volume of the vessel, volume of water , volume of the gas) proved difficult. It is at this point that the simulations appeared particularly helpful.
Research question 2 . Is the ability of students to solve problems related to their scientific reasoning/developmental level?
As mentioned earlier, research has shown that the developmental level of students is the most consistent predictor of success when dealing with significant changes in the logical structure of chemistry problems ( Niaz and Robinson, 1992 ; Tsaparlis et al. , 1997). Our results indicate that the two problems of this study (especially problem 2) had a rich logical structure (see comments after the two problems). With one exception, formal students performed better than transitional students, and transitional students better than concrete ones, with the differences between EG and CG being highest in the case of the formal students. In most cases, the EG students performed better than the CG students at all levels of logical thinking, but, due to the small sample sizes, the differences are not statistically significant except in the case of formal students in the sum A (problem 1) + B (problem 2).
Research question 3 . Is disembedding ability (degree of field dependence/independence) connected to students’ ability in problem solving?
It is known from the literature that in the solving of realistic/novice problems, disembedding ability plays an important and dominant role ( Demerouti et al. , 2004 ; Tsaparlis, 2005 ; Overton and Potter, 2011 ). Our results indicate that both problems used in this study have features of novelty/were real problems for our students. With some exceptions, field independent students outperformed field intermediate students, and field intermediate students outperformed field dependent ones. Also, with one exception, the EG students performed better than the CG students at all levels of disembedding ability. However, due to small sample sizes, the differences are not statistically significant.
Final comments
Our previous study examined the effect of a laboratory/practical activity involving the ammonia-fountain experiment on the solution of problem 1 ( Kampourakis and Tsaparlis, 2003 ). While both studies were carried out with tenth-grade general education Greek students (around 16 years old), the previous study also involved some eleventh-grade students (around 17 years old), who were following a stream of studies that included advanced chemistry among its main subjects. The tenth-grade students of the experimental group of this previous study had a mean achievement of 18.6% in problem 1, while the corresponding eleventh-grade students achieved a mean score of 37.0%. The second score is about the same as the marks achieved by the EG students in the present study. The difference for tenth grade students between the present and the previous study could be attributed, in part, to the fact that chemistry was taught as a one-period per week course in the previous study, whereas the teaching time had been doubled in the present study. Finally, the students involved in the present study came from an urban region of Piraeus, while those from the previous study came from a semi-urban region in north-western Greece.
It follows from a comparison of the two studies, that we cannot with any certainty assert that the simulations are more effective than, and hence preferable to, real practical activities. However, we would point out that actual experiments often involve extra, background information that is not relevant to a particular problem. This may prevent students from paying attention to key stimuli relevant to the problem, by causing an overload of the working memory [ Kempa and Ward, 1988 ; Johnstone and Letton, 1990 – see the relevant discussion in Kampourakis and Tsaparlis (2003) ]. It is also important to appreciate that simulations are, usually, safer, faster, more economical and easier to perform and repeat than real experiments. A good understanding of the relevant theory is of course very important for problem solving. “Students who lack the requisite theoretical framework will not know where to look, or how to look, in order to make observations appropriate to the task in hand, or how to interpret what they see. Consequently, much of the activity will be unproductive” ( Johnstone and Al-Shuaili, 2001 ). “Knowing what to observe, knowing how to observe it, observing it and describing the observations are all theory-dependent and therefore fallible and biased” ( Hodson, 1986 ). Last but not least, let us not forget that an important first step in problem solving in science (after reading the problem) is to make a drawing of the problem situation ( Mettes et al. , 1980 ; Reif, 1981, 1983 ; Genya, 1983 ). Simulations are capable of providing a better picture of a problem than is possible with a simple drawing.
Appendix 1. Marking schemes for the two problems
Appendix 2. further details about the simulations, simulation of problem 1.
As can be seen from Fig. 2 , the experimental set up consists of a vessel that contains gaseous ammonia . The vessel is supplied with a pressure gauge that initially shows a reading of 2 atm. The vessel is also supplied with a thermometer that shows a reading of 27 °C. From the vessel, a tube, supplied with a stopcock, joins the vessel to a beaker filled with water . Over the beaker there is a dropper that contains phenolphthalein indicator . This allows the student to check for the presence of base ( ammonia ) in the beaker by introducing a few drops of the indicator . The students can turn on the stopcock by moving the cursor over it and by left clicking. When the stopcock is turned on, the pressure gauge shows a rapid fall in pressure. The student can turn off the stopcock and stop the flow of ammonia gas. Eventually the pressure falls to 1 atm. Some bubbles appear at the end of the tube inside the water making the flow of gas perceptible. Ammonia molecules in constant motion are shown within the tube (when it is turned on) as well as in the solution. The student is free to choose his/her actions; for instance, if he/she chooses to drop indicator before passing ammonia gas, there will be no color change in the solution. In this case, however, if ammonia is passed after that, the solution will immediately turn red.
Simulation of problem 2
A tube which starts with a stopcock and ends in a beaker filled with water emerges from the vessel. In the beaker there is also an inverted graduated tube filled with water . This tube is able to collect the sulfur dioxide gas that is not dissolved in the water. The vessel carries a switch that can ignite the mixture.
As in the case of the simulation for problem 1, the ‘Description of the experiment’ button provides a user guide, directing the simulation in an ordered manner: first they ignite the mixture and see the video, then they turn on the stopcock, and finally they push the piston. The ‘Help’ feature is also present.
The student can start and watch a video, showing the combustion of sulfur. Note that the students had previously observed a demonstration of the combustion of sulfur, the production of sulfur dioxide and its dissolution in water . After combustion is complete, the student can transfer all the produced gas into the beaker containing water by pushing the piston. In the beaker, part of the gas is dissolved in the water, and part is collected in the inverted tube. While this occurs, gas bubbles appear within the inverted tube replacing water . Sulfur dioxide molecules appear in constant motion in the solution.
Fig. 3 shows a series of three shots, in which the piston is gradually pushed, so that the produced gas is transferred into the beaker containing water and the inversed test tube.
Acknowledgements
- Akaygun S. and Jones L. L., (2013), Dynamic visualizations: tools for understanding particulate nature of matter, in Tsaparlis G. and Sevian H. (ed.), Concepts of matter in science education , vol. 19, Innovations in Science Education and Technology, Springer, ISBN 978-94-007-5913-8.
- Ardac D. and Akaygun S., (2004), Effectiveness of multimedia-based instruction that emphasizes molecular representations on students’ understanding of chemical change, J. Res. Sci. Teach. , 40 , 317–337.
- Ardac D. and Akaygun S., (2005), Using static and dynamic visuals to represent chemical change at molecular level, Int. J. Sci. Educ. , 27 , 1269–1298.
- Baddeley A. D., (1992), Working memory, Science , 255 , 556–559.
- Bannert M., (2002), Managing cognitive load – recent trends in cognitive load theory, Learn. Instruct. , 12 , 139–146.
- BouJaoude S., Salloum S. and Abd-El-Khalick F., (2004), Relationships between selective cognitive variables and students’ ability to solve chemistry problems, Int. J. Sci. Educ. , 26 , 63–84.
- Bowen C. W. and Phelps A. J., (1997), Demonstration-based cooperative testing in general chemistry: a broader assessment-of-learning technique, J. Chem. Educ ., 74 , 715–719.
- Burke K., Greenbowe T. and Windschitl M., (1998), Developing and using conceptual computer animations for chemistry instruction, J. Chem. Educ. , 75 , 1658–1661.
- Butler W. M. and Griffin H. C., (1979), Simulations in the general chemistry laboratory with microcomputers, J. Chem. Educ. , 56 , 543.
- Cohen L. and Holliday M., (1982), Statistics for social scientists , London: Harper & Row.
- Deese W., Ramsey L. L., Walczyk J. and Eddy D., (2000), Using demonstration assessments to improve learning, J. Chem. Educ. , 77 , 1511–1516.
- Demerouti M., Kousathana M. and Tsaparlis G., (2004), Acid–base equilibria, part II, effect of developmental level and disembedding ability on students’ conceptual understanding and problem-solving ability, Chem. Educator , 9 , 132–137.
- Domin D. S., (1999a), Review of laboratory instructional styles, J. Chem. Educ. , 76 , 543–547.
- Domin D. S., (1999b), A content analysis of general chemistry laboratory manuals for evidence of higher-order cognitive tasks, J. Chem. Educ. , 76 , 109–112.
- El-Banna H. A. A. M., (1987), The development of a predictive theory of science education based upon information processing hypothesis , PhD thesis, University of Glasgow, UK.
- Genya J., (1983), Improving student’s problem-solving skills – a methodological approach for a preparatory chemistry course, J. Chem. Educ. , 60 , 478–482.
- Hodson D., (1986), The nature of scientific observation, Sch. Sci. Rev. , 68 (242), 17–29.
- Johnstone A. H., (1993), Introduction, in Wood C. and Sleet R. (ed.), Creative problem solving in chemistry , London: The Royal Society of Chemistry, pp. iv–vi.
- Johnstone A. H., (2001). Can problem solving be taught? Univ. Chem. Educ ., 5 , 69–73.
- Johnstone A. H. and Al-Shuaili A., (2001), Learning in laboratory: some thoughts from the literature, Univ. Chem. Educ ., 5 , 42–51.
- Johnstone A. H. and El-Banna H., (1986), Capacities, demands and processes – a predictive model for science education, J. Educ. Chem. , 23 , 80–84.
- Johnstone A. H., Hogg W. and Ziane M., (1993), A working memory model applied to physics problem solving, Int. J. Sci. Educ. , 15 , 663–672.
- Johnstone A. H. and Letton K. M., (1990), Investigating undergraduate laboratory work, Educ. Chem. , 27 (1), 9–11.
- Josephsen J. and Kristensen A. K., (2006), Simulation of laboratory assignments to support students’ learning of introductory inorganic chemistry, Chem. Educ. Res. Pract. , 7 , 266–279.
- Kampourakis C. and Tsaparlis G., (2003), A study of the effect of a practical activity on problem solving in chemistry, Chem. Educ. Res. Pract ., 4 , 319–333.
- Kautz C. H., Lovrude M. E., Herron P. R. L. and McDermott L. C., (1999), Research on student understanding of the ideal gas law, Proceedings, 2nd International Conference of the European Science Education Research Association ( ESERA ) vol. 1, Germany: Kiel, pp. 83–85.
- Kempa R. F. and Ward J. E., (1988), Observational thresholds in school chemistry, Int. J. Sci. Educ ., 10 , 275–284.
- Kerr J. F., (1963), Practical work in school science: an account of an inquiry into the nature and purpose of practical work in school science teaching in England and Wales , Leicester: Leicester University Press.
- Lajoie S. P., (1993), Computer environments as cognitive tools for enhancing learning, in Lajoie S. P. and Derry S. J. (ed.), Computers as cognitive tools , New Jersey: Lawrence Erlbaum Hillsdale, pp. 261–288.
- Lajoie S. P., Azevedo R. and Fleiszer D. M., (1998), Cognitive tools for assessment and learning in a high information flow environment, J. Educ. Comp. Res. , 18 , 205–235.
- Lajoie S. P., Lavigne M. C., Guerrera C. and Munsie S. D., (2001), Constructing knowledge in the context of BioWorld, Instr. Sci. , 29 , 155–186.
- Larkin J. H. and Reif F., (1979), Understanding and teaching problem solving in physics, Eur. J. Sci. Educ. , 1 , 191–203.
- Lawson A. E., (1978), Development and validation of the classroom test of formal reasoning, J. Res. Sci. Teach. , 15 , 11–24.
- Lewalter D., (2003). Cognitive strategies for learning from static and dynamic visuals. Learn. Instruct. , 13 , 177–189.
- Lowe R. K., (2003). Animation and learning: selective processing of information in dynamic graphics, Learn. Instruct. , 13 , 157–176.
- Mayer R. E., (1997). Multimedia learning: are we asking the right questions?, Educ. Psychol. , 32 , 1–19.
- Mayer R. E., (2001). Multimedia learning , New York: Cambridge University Press.
- Mayer R. E. and Moreno R., (1998), A split-attention effect in multimedia learning: evidence for dual information processing systems in working memory. J. Educ. Psychol. , 90 , 312–320.
- Mettes C. T. C. W., Pilot A., Roossing H. J. and Kramers-Pal H., (1980), Teaching and learning problem solving in science, Part I, General strategy, J. Chem. Educ. , 57 , 882–885.
- Miller G. A., (1956), The magical number seven plus or minus two: some limits on our capacity for processing information, Psych. Rev ., 63 , 81–97.
- Moreno R. and Mayer R., (1999), Cognitive principles of multimedia learning: the role of modality and contiguity. J. Educ. Psychol. , 91 , 358–368.
- Niaz M., (1991), Correlates of formal operational reasoning: a neo-Piagetian analysis, J. Res. Sci. Teach. , 28 , 19–40.
- Niaz M., (1995), Relationship between student performance on conceptual and computational problems of chemical equilibrium, Int. J. Sci. Educ. , 17 , 343–355.
- Niaz M., de Nunez S. G. and de Pineda R. I., (2000), Academic performance of high school students as a function of mental capacity, cognitive style, mobility-fixity dimension, and creativity, J. Creat. Behav. , 34 , 18–29.
- Niaz M. and Robinson W. R., (1992), Manipulation of logical structure of chemistry problems and its effect on student performance, J. Res. Sci. Teach. , 29 , 211–226.
- Oakes K. and Rengarajan R., (2002), Practice makes perfect – E-learning – simulation in training, http://www.questia.com/library/1G1-94174474/practice-makes-perfect-e-learning, accessed 17 March 2013.
- Overton T. L. and Potter N. M., (2011), Investigating students' success in solving and attitudes towards context-rich open-ended problems in chemistry, Chem. Educ. Res. Pract. , 12 , 294–302.
- Reif F., (1981), Teaching problem solving – a scientific approach, Phys. Teach. , 19 , 329–363.
- Reif F., (1983), How can chemists teach problem solving? Suggestions derived from studies of cognitive processes, J. Chem. Educ. , 60 , 948–953.
- Rieber L. P., (1990). Using computer animated graphics in science instruction with children, J. Educ. Psych. , 82 , 135–140.
- Roth W.-M., (1988), Short-term memory and problem solving in physics education , Ontario Canada: Dept. of Science, Appleby College Oakville.
- Roth W.-M., (1994), Experimenting in a constructivist high school physics laboratory, J. Res. Sci. Teach. , 31 ,197–223.
- Sanger M. and Greenbowe T., (1997a), Common student misconceptions in electrochemistry: galvanic, electrolytic, and concentration cells, J. Res. Sci. Teach. , 34 , 377–398.
- Sanger M. and Greenbowe T., (1997b), Students’ misconceptions in electrochemistry: current flow in electrolyte solutions and the salt bridge, J. Chem. Educ. , 74 , 819–823.
- Sanger M., Phelps A. and Fienhold J., (2000), Using a computer animation to improve students' conceptual understanding of a can-crushing demonstration, J. Chem. Educ. , 77 , 1517–1520.
- Simon H. A., (1974), How big is a chunk? Science , 183 , 482–488.
- Simon D. P. and Simon H. A., (1978), Individual differences in solving physics problems, in Siegler R. S. (ed.), Childrens’ thinking: what develops? Hillstate NJ: Lawrence Erlbaum Associates, pp. 325–348.
- Stamovlasis D. and Tsaparlis G., (2001), Application of complexity theory to an information processing model in science education, Nonlinear Dynamics Psychol. Life Sci. , 5 , 267–286.
- Stamovlasis D. and Tsaparlis G., (2003), A complexity theory model in science education problem solving: random walks for working memory and mental capacity, Nonlinear Dynamics Psychol. Life Sci. , 7 , 221–244.
- Stamovlasis D. and Tsaparlis G., (2012), Applying catastrophe theory to an information-processing model of problem solving in science education, Sci. Educ. , 96 , 392–410.
- St Clair-Thompson H., Overton T. and Bugler M., (2012), Mental capacity and working memory in chemistry: algorithmic versus open-ended problem solving, Chem. Educ. Res. Pract. , 13 , 484–489.
- Sweller J., (1988), Cognitive load during problem solving: effects on learning, Cogn. Sci. , 12 , 257–285.
- Sweller J. and Chandler P., (1991), Cognitive load theory and the format of instruction, Cogn. Instr. , 8 , 292–332.
- Tsaparlis G., (1998), Dimensional analysis and predictive models in problem solving, Int. J. Sci. Educ. , 20 , 335–350.
- Tsaparlis G., (2005), Non-algorithmic quantitative problem solving in university physical chemistry: a correlation study of the role of selective cognitive variables, Res. Sci. Tech. Educ. , 23 , 125–148.
- Tsaparlis G., (2009), Learning at the macro level: the role of practical work, in Gilbert J. K. and Treagust D. F. (ed.), Multiple representations in chemical education , Milton Keynes: Springer, pp. 109–136.
- Tsaparlis G. and Angelopoulos V., (2000), A model of problem-solving: its operation, validity, and usefulness in the case of organic-synthesis problems, Sci. Educ. , 84 , 151–153.
- Tsaparlis G., Kousathana M. and Niaz M., (1998), Molecular-equilibrium problems: manipulation of logical structure and of M- demand, and their effect on student performance, Sci. Educ. , 82 , 437–454.
- van Bruggen J. M., Kirschner P. A. A. and Jochems W., (2002), External representation of argumentation in CSCL and the management of cognitive load, Learn. Instruct. , 12 , 121–138.
- Williamson V. M. and Abraham M. R., (1995), The effects of computer animation on the particulate mental models of college chemistry students, J. Res. Sci. Teach. , 32 , 521–534.
- Witkin H. A., (1978), Cognitive styles in personal and cultural adaptation , Worcester, MA: Clark University Press.
- Witkin H. A., Oltman P. K., Raskin E. and Karp S. A., (1971), A manual for the embedded figures test , Palo Alto: Consulting Psychologists Press.
- Zoller U., (1993), Are lecture and learning compatible? Maybe for LOCS: unlikely for HOCS, J. Chem. Educ. , 70 , 195–197.
- Zoller U. and Tsaparlis G., (1997), HOCS and LOCS students: the case of chemistry, Res. Sci. Educ. , 27 , 117–130.

- Table of Contents
- Random Entry
- Chronological
- Editorial Information
- About the SEP
- Editorial Board
- How to Cite the SEP
- Special Characters
- Advanced Tools
- Support the SEP
- PDFs for SEP Friends
- Make a Donation
- SEPIA for Libraries
- Entry Contents
Bibliography
Academic tools.
- Friends PDF Preview
- Author and Citation Info
- Back to Top
Computer Simulations in Science
Computer simulation was pioneered as a scientific tool in meteorology and nuclear physics in the period directly following World War II, and since then has become indispensable in a growing number of disciplines. The list of sciences that make extensive use of computer simulation has grown to include astrophysics, particle physics, materials science, engineering, fluid mechanics, climate science, evolutionary biology, ecology, economics, decision theory, medicine, sociology, epidemiology, and many others. There are even a few disciplines, such as chaos theory and complexity theory, whose very existence has emerged alongside the development of the computational models they study.
After a slow start, philosophers of science have begun to devote more attention to the role of computer simulation in science. Several areas of philosophical interest in computer simulation have emerged: What is the structure of the epistemology of computer simulation? What is the relationship between computer simulation and experiment? Does computer simulation raise issues for the philosophy of science that are not fully covered by recent work on models more generally? What does computer simulation teach us about emergence? About the structure of scientific theories? About the role (if any) of fictions in scientific modeling?
1.1 A Narrow definition
1.2 a broad definition, 1.3 an alternative point of view, 2.1 equation-based simulations, 2.2 agent-based simulations, 2.3 multiscale simulations, 2.4 monte carlo simulations, 3. purposes of simulation, 4.1 novel features of eocs, 4.2 eocs and the epistemology of experiment, 4.3 verification and validation, 4.4 eocs and epistemic entitlement, 4.5 pragmatic approaches to eocs, 5. simulation and experiment, 6. computer simulation and the structure of scientific theories, 7. emergence, 8. fictions, other internet resources, related entries, 1. what is computer simulation.
No single definition of computer simulation is appropriate. In the first place, the term is used in both a narrow and a broad sense. In the second place, one might want to understand the term from more than one point of view.
In its narrowest sense, a computer simulation is a program that is run on a computer and that uses step-by-step methods to explore the approximate behavior of a mathematical model. Usually this is a model of a real-world system (although the system in question might be an imaginary or hypothetical one). Such a computer program is a computer simulation model . One run of the program on the computer is a computer simulation of the system. The algorithm takes as its input a specification of the system’s state (the value of all of its variables) at some time t. It then calculates the system’s state at time t+1. From the values characterizing that second state, it then calculates the system’s state at time t+2, and so on. When run on a computer, the algorithm thus produces a numerical picture of the evolution of the system’s state, as it is conceptualized in the model.
This sequence of values for the model variables can be saved as a large collection of “data” and is often viewed on a computer screen using methods of visualization. Often, but certainly not always, the methods of visualization are designed to mimic the output of some scientific instrument—so that the simulation appears to be measuring a system of interest.
Sometimes the step-by-step methods of computer simulation are used because the model of interest contains continuous (differential) equations (which specify continuous rates of change in time) that cannot be solved analytically—either in principle or perhaps only in practice. This underwrites the spirit of the following definition given by Paul Humphreys: “any computer-implemented method for exploring the properties of mathematical models where analytic methods are not available” (1991, 500). But even as a narrow definition, this one should be read carefully, and not be taken to suggest that simulations are only used when there are analytically unsolvable equations in the model. Computer simulations are often used either because the original model itself contains discrete equations—which can be directly implemented in an algorithm suitable for simulation—or because the original model consists of something better described as rules of evolution than as equations .
In the former case, when equations are being “discretized” (the turning of equations that describe continuous rates of change into discrete equations), it should be emphasized that, although it is common to speak of simulations “solving” those equations, a discretization can at best only find something which approximates the solution of continuous equations, to some desired degree of accuracy. Finally, when speaking of “a computer simulation” in the narrowest sense, we should be speaking of a particular implementation of the algorithm on a particular digital computer, written in a particular language, using a particular compiler, etc. There are cases in which different results can be obtained as a result of variations in any of these particulars.
More broadly, we can think of computer simulation as a comprehensive method for studying systems. In this broader sense of the term, it refers to an entire process. This process includes choosing a model; finding a way of implementing that model in a form that can be run on a computer; calculating the output of the algorithm; and visualizing and studying the resultant data. The method includes this entire process—used to make inferences about the target system that one tries to model—as well as the procedures used to sanction those inferences. This is more or less the definition of computer simulation studies in Winsberg 2003 (111). “Successful simulation studies do more than compute numbers. They make use of a variety of techniques to draw inferences from these numbers. Simulations make creative use of calculational techniques that can only be motivated extra-mathematically and extra-theoretically. As such, unlike simple computations that can be carried out on a computer, the results of simulations are not automatically reliable. Much effort and expertise goes into deciding which simulation results are reliable and which are not.” When philosophers of science write about computer simulation, and make claims about what epistemological or methodological properties “computer simulations” have, they usually mean the term to be understood in this broad sense of a computer simulation study.
Both of the above definitions take computer simulation to be fundamentally about using a computer to solve, or to approximately solve, the mathematical equations of a model that is meant to represent some system—either real or hypothetical. Another approach is to try to define “simulation” independently of the notion of computer simulation, and then to define “computer simulation” compositionally: as a simulation that is carried out by a programmed digital computer. On this approach, a simulation is any system that is believed, or hoped, to have dynamical behavior that is similar enough to some other system such that the former can be studied to learn about the latter.
For example, if we study some object because we believe it is sufficiently dynamically similar to a basin of fluid for us to learn about basins of fluid by studying the it, then it provides a simulation of basins of fluid. This is in line with the definition of simulation we find in Hartmann: it is something that “imitates one process by another process. In this definition the term ‘process’ refers solely to some object or system whose state changes in time” (1996, 83). Hughes (1999) objected that Hartmann’s definition ruled out simulations that imitate a system’s structure rather than its dynamics. Humphreys revised his definition of simulation to accord with the remarks of Hartmann and Hughes as follows:
System S provides a core simulation of an object or process B just in case S is a concrete computational device that produces, via a temporal process, solutions to a computational model … that correctly represents B, either dynamically or statically. If in addition the computational model used by S correctly represents the structure of the real system R, then S provides a core simulation of system R with respect to B. (2004, p. 110)
(Note that Humphreys is here defining computer simulation, not simulation generally, but he is doing it in the spirit of defining a compositional term.) It should be noted that Humphreys’ definitions make simulation out to be a success term, and that seems unfortunate. A better definition would be one that, like the one in the last section, included a word like “believed” or “hoped” to address this issue.
In most philosophical discussions of computer simulation, the more useful concept is the one defined in 1.2. The exception is when it is explicitly the goal of the discussion to understand computer simulation as an example of simulation more generally (see section 5). Examples of simulations that are not computer simulations include the famous physical model of the San Francisco Bay (Huggins & Schultz 1973). This is a working hydraulic scale model of the San Francisco Bay and Sacramento-San Joaquin River Delta System built in the 1950s by the Army Corps of engineers to study possible engineering interventions in the Bay. Another nice example, which is discussed extensively in (Dardashti et al., 2015, 2019) is the use of acoustic “dumb holes” made out of Bose-Einstein condensates to study the behavior of Black Holes. Physicist Bill Unruh noted that in certain fluids, something akin to a black hole would arise if there were regions of the fluid that were moving so fast that waves would have to move faster than the speed of sound (something they cannot do) in order to escape from them (Unruh 1981). Such regions would in effect have sonic event horizons. Unruh called such a physical setup a “dumb hole” (“dumb” as in “mute”) and proposed that it could be studied in order to learn things we do not know about black holes. For some time, this proposal was viewed as nothing more than a clever idea, but physicists have recently come to realize that, using Bose-Einstein condensates, they can actually build and study dumb holes in the laboratory. It is clear why we should think of such a setup as a simulation: the dumb hole simulates the black hole. Instead of finding a computer program to simulate the black holes, physicists find a fluid dynamical setup for which they believe they have a good model and for which that model has fundamental mathematical similarities to the model of the systems of interest. They observe the behavior of the fluid setup in the laboratory in order to make inferences about the black holes. The point, then, of the definitions of simulation in this section is to try to understand in what sense computer simulation and these sorts of activities are species of the same genus. We might then be in a better situation to understand why a simulation in the sense of 1.3 which happens to be run on a computer overlaps with a simulation in the sense of 1.2. We will come back to this in section 5.
Barberousse et al. (2009), however, have been critical of this analogy. They point out that computer simulations do not work the way Unruh’s simulation works. It is not the case that the computer as a material object and the target system follow the same differential equations. A good reference about simulations that are not computer simulations is Trenholme 1994.
2. Types of Computer Simulations
Two types of computer simulation are often distinguished: equation-based simulations and agent-based (or individual-based ) simulations . Computer Simulations of both types are used for three different general sorts of purposes: prediction (both pointwise and global/qualitative), understanding, and exploratory or heuristic purposes.
Equation-based simulations are most commonly used in the physical sciences and other sciences where there is governing theory that can guide the construction of mathematical models based on differential equations. I use the term “equation based” here to refer to simulations based on the kinds of global equations we associate with physical theories—as opposed to “rules of evolution” (which are discussed in the next section.) Equation based simulations can either be particle-based, where there are n many discrete bodies and a set of differential equations governing their interaction, or they can be field-based, where there is a set of equations governing the time evolution of a continuous medium or field. An example of the former is a simulation of galaxy formation, in which the gravitational interaction between a finite collection of discrete bodies is discretized in time and space. An example of the latter is the simulation of a fluid, such as a meteorological system like a severe storm. Here the system is treated as a continuous medium—a fluid—and a field representing its distribution of the relevant variables in space is discretized in space and then updated in discrete intervals of time.
Agent-based simulations are most common in the social and behavioral sciences, though we also find them in such disciplines as artificial life, epidemiology, ecology, and any discipline in which the networked interaction of many individuals is being studied. Agent-based simulations are similar to particle-based simulations in that they represent the behavior of n-many discrete individuals. But unlike equation-particle-based simulations, there are no global differential equations that govern the motions of the individuals. Rather, in agent-based simulations, the behavior of the individuals is dictated by their own local rules
To give one example: a famous and groundbreaking agent-based simulation was Thomas Schelling’s (1971) model of “segregation.” The agents in his simulation were individuals who “lived” on a chessboard. The individuals were divided into two groups in the society (e.g. two different races, boys and girls, smokers and non-smokers, etc.) Each square on the board represented a house, with at most one person per house. An individual is happy if he/she has a certain percent of neighbors of his/her own group. Happy agents stay where they are, unhappy agents move to free locations. Schelling found that the board quickly evolved into a strongly segregated location pattern if the agents’ “happiness rules” were specified so that segregation was heavily favored. Surprisingly, however, he also found that initially integrated boards tipped into full segregation even if the agents’ happiness rules expressed only a mild preference for having neighbors of their own type.
In section 2.1 we discussed equation-based models that are based on particle methods and those that are based on field methods. But some simulation models are hybrids of different kinds of modeling methods. Multiscale simulation models, in particular, couple together modeling elements from different scales of description. A good example of this would be a model that simulates the dynamics of bulk matter by treating the material as a field undergoing stress and strain at a relatively coarse level of description, but which zooms into particular regions of the material where important small scale effects are taking place, and models those smaller regions with relatively more fine-grained modeling methods. Such methods might rely on molecular dynamics, or quantum mechanics, or both—each of which is a more fine-grained description of matter than is offered by treating the material as a field. Multiscale simulation methods can be further broken down into serial multiscale and parallel multiscale methods. The more traditional method is serial multi-scale modeling. The idea here is to choose a region, simulate it at the lower level of description, summarize the results into a set of parameters digestible by the higher level model, and pass them up to into the part of the algorithm calculating at the higher level.
Serial multiscale methods are not effective when the different scales are strongly coupled together. When the different scales interact strongly to produce the observed behavior, what is required is an approach that simulates each region simultaneously. This is called parallel multiscale modeling. Parallel multiscale modeling is the foundation of a nearly ubiquitous simulation method: so called “sub-grid” modeling. Sub-grid modeling refers to the representation of important small-scale physical processes that occur at length-scales that cannot be adequately resolved on the grid size of a particular simulation. (Remember that many simulations discretize continuous equations, so they have a relatively arbitrary finite “grid size.”) In the study of turbulence in fluids, for example, a common practical strategy for calculation is to account for the missing small-scale vortices (or eddies ) that fall inside the grid cells. This is done by adding to the large-scale motion an eddy viscosity that characterizes the transport and dissipation of energy in the smaller-scale flow—or any such feature that occurs at too small a scale to be captured by the grid.
In climate science and kindred disciplines, sub-grid modeling is called “parameterization.” This, again, refers to the method of replacing processes—ones that are too small-scale or complex to be physically represented in the model— by a more simple mathematical description. This is as opposed to other processes—e.g., large-scale flow of the atmosphere—that are calculated at the grid level in accordance with the basic theory. It is called “parameterization” because various non-physical parameters are needed to drive the highly approximative algorithms that compute the sub-grid values. Examples of parameterization in climate simulations include the descent rate of raindrops, the rate of atmospheric radiative transfer, and the rate of cloud formation. For example, the average cloudiness over a 100 km 2 grid box is not cleanly related to the average humidity over the box. Nonetheless, as the average humidity increases, average cloudiness will also increase—hence there could be a parameter linking average cloudiness to average humidity inside a grid box. Even though modern-day parameterizations of cloud formation are more sophisticated than this, the basic idea is well illustrated by the example. The use of sub-grid modeling methods in simulation has important consequences for understanding the structure of the epistemology of simulation. This will be discussed in greater detail in section 4.
Sub-grid modelling methods can be contrasted with another kind of parallel multiscale model where the sub-grid algorithms are more theoretically principled, but are motivated by a theory at a different level of description. In the example of the simulation of bulk matter mentioned above, for example, the algorithm driving the smaller level of description is not built by the seat-of-the-pants. The algorithm driving the smaller level is actually more theoretically principled than the higher level in the sense that the physics is more fundamental: quantum mechanics or molecular dynamics vs. continuum mechanics. These kinds of multiscale models, in other words, cobble together the resources of theories at different levels of description. So they provide for interesting examples that provoke our thinking about intertheoretic relationships, and that challenge the widely-held view that an inconsistent set of laws can have no models.
In the scientific literature, there is another large class of computer simulations called Monte Carlo (MC) Simulations. MC simulations are computer algorithms that use randomness to calculate the properties of a mathematical model and where the randomness of the algorithm is not a feature of the target model. A nice example is the use of a random algorithm to calculate the value of π. If you draw a unit square on a piece of paper and inscribe a circle in it, and then randomly drop a collection of objects inside the square, the proportion of objects that land in the circle would be roughly equal to π/4. A computer simulation that simulated a procedure like that would be called a MC simulation for calculating π.
Many philosophers of science have deviated from ordinary scientific language here and have shied away from thinking of MC simulations as genuine simulations. Grüne-Yanoff and Weirich (2010) offer the following reasoning: “The Monte Carlo approach does not have a mimetic purpose: It imitates the deterministic system not in order to serve as a surrogate that is investigated in its stead but only in order to offer an alternative computation of the deterministic system’s properties” (p.30). This shows that MC simulations do not fit any of the above definitions aptly. On the other hand, the divide between philosophers and ordinary language can perhaps be squared by noting that MC simulations simulate an imaginary process that might be used for calculating something relevant to studying some other process. Suppose I am modeling a planetary orbit and for my calculation I need to know the value of π. If I do the MC simulation mentioned in the last paragraph, I am simulating the process of randomly dropping objects into a square, but what I am modeling is a planetary orbit. This is the sense in which MC simulations are simulations, but they are not simulations of the systems they are being used to study. However, as Beisbart and Norton (2012) point out, some MC simulations (viz. those that use MC techniques to solve stochastic dynamical equations referring to a physical system) are in fact simulations of the systems they study.
There are three general categories of purposes to which computer simulations can be put. Simulations can be used for heuristic purposes, for the purpose of predicting data that we do not have, and for generating understanding of data that we do already have.
Under the category of heuristic models, simulations can be further subdivided into those used to communicate knowledge to others, and those used to represent information to ourselves. When Watson and Crick played with tin plates and wire, they were doing the latter at first, and the former when they showed the results to others. When the army corps built the model of the San Francisco Bay to convince the voting population that a particular intervention was dangerous, they were using it for this kind of heuristic purpose. Computer simulations can be used for both of these kinds of purposes—to explore features of possible representational structures; or to communicate knowledge to others. For example: computer simulations of natural processes, such as bacterial reproduction, tectonic shifting, chemical reactions, and evolution have all been used in classroom settings to help students visualize hidden structure in phenomena and processes that are impractical, impossible, or costly to illustrate in a “wet” laboratory setting.
Another broad class of purposes to which computer simulations can be put is in telling us about how we should expect some system in the real world to behave under a particular set of circumstances. Loosely speaking: computer simulation can be used for prediction. We can use models to predict the future, or to retrodict the past; we can use them to make precise predictions or loose and general ones. With regard to the relative precision of the predictions we make with simulations, we can be slightly more fine-grained in our taxonomy. There are a) Point predictions: Where will the planet Mars be on October 21st, 2300? b) “Qualitative” or global or systemic predictions: Is the orbit of this planet stable? What scaling law emerges in these kinds of systems? What is the fractal dimension of the attractor for systems of this kind? and c) Range predictions: It is 66% likely that the global mean surface temperature will increase by between 2–5 degrees C by the year 2100; it is “highly likely” that sea level will rise by at least two feet; it is “implausible” that the thermohaline will shut down in the next 50 years.
Finally, simulations can be used to understand systems and their behavior. If we already have data telling us how some system behaves, we can use computer simulation to answer questions about how these events could possibly have occurred; or about how those events actually did occur.
When thinking about the topic of the next section, the epistemology of computer simulations, we should also keep in mind that the procedures needed to sanction the results of simulations will often depend, in large part, on which of the above kind of purpose or purposes the simulation will be put to.
4. The Epistemology of Computer Simulations
As computer simulation methods have gained importance in more and more disciplines, the issue of their trustworthiness for generating new knowledge has grown, especially when simulations are expected to be counted as epistemic peers with experiments and traditional analytic theoretical methods. The relevant question is always whether or not the results of a particular computer simulation are accurate enough for their intended purpose. If a simulation is being used to forecast weather, does it predict the variables we are interested in to a degree of accuracy that is sufficient to meet the needs of its consumers? If a simulation of the atmosphere above a Midwestern plain is being used to understand the structure of a severe thunderstorm, do we have confidence that the structures in the flow—the ones that will play an explanatory role in our account of why the storm sometimes splits in two, or why it sometimes forms tornados—are being depicted accurately enough to support our confidence in the explanation? If a simulation is being used in engineering and design, are the predictions made by the simulation reliable enough to sanction a particular choice of design parameters, or to sanction our belief that a particular design of airplane wing will function? Assuming that the answer to these questions is sometimes “yes”, i.e. that these kinds of inferences are at least sometimes justified, the central philosophical question is: what justifies them? More generally, how can the claim that a simulation is good enough for its intended purpose be evaluated? These are the central questions of the epistemology of computer simulation (EOCS).
Given that confirmation theory is one of the traditional topics in philosophy of science, it might seem obvious that the latter would have the resources to begin to approach these questions. Winsberg (1999), however, argued that when it comes to topics related to the credentialing of knowledge claims, philosophy of science has traditionally concerned itself with the justification of theories, not their application. Most simulation, on the other hand, to the extent that it makes use of the theory, tends to make use of the well-established theory. EOCS, in other words, is rarely about testing the basic theories that may go into the simulation, and most often about establishing the credibility of the hypotheses that are, in part, the result of applications of those theories.
Winsberg (2001) argued that, unlike the epistemological issues that take center stage in traditional confirmation theory, an adequate EOCS must meet three conditions. In particular it must take account of the fact that the knowledge produced by computer simulations is the result of inferences that are downward , motley , and autonomous .
Downward . EOCS must reflect the fact that in a large number of cases, accepted scientific theories are the starting point for the construction of computer simulation models and play an important role in the justification of inferences from simulation results to conclusions about real-world target systems. The word “downward” was meant to signal the fact that, unlike most scientific inferences that have traditionally interested philosophers, which move up from observation instances to theories, here we have inferences that are drawn (in part) from high theory, down to particular features of phenomena. Motley . EOCS must take into account that simulation results nevertheless typically depend not just on theory but on many other model ingredients and resources as well, including parameterizations (discussed above), numerical solution methods, mathematical tricks, approximations and idealizations, outright fictions, ad hoc assumptions, function libraries, compilers and computer hardware, and perhaps most importantly, the blood, sweat, and tears of much trial and error. Autonomous . EOCS must take into account the autonomy of the knowledge produced by simulation in the sense that the knowledge produced by simulation cannot be sanctioned entirely by comparison with observation. Simulations are usually employed to study phenomena where data are sparse. In these circumstances, simulations are meant to replace experiments and observations as sources of data about the world because the relevant experiments or observations are out of reach, for principled, practical, or ethical reasons.
Parker (2013) has made the point that the usefulness of these conditions is somewhat compromised by the fact that it is overly focused on simulation in the physical sciences, and other disciplines where simulation is theory-driven and equation-based. This seems correct. In the social and behavioral sciences, and other disciplines where agent-based simulation (see 2.2) are more the norm, and where models are built in the absence of established and quantitative theories, EOCS probably ought to be characterized in other terms.
For instance, some social scientists who use agent-based simulation pursue a methodology in which social phenomena (for example an observed pattern like segregation) are explained, or accounted for, by generating similar looking phenomena in their simulations (Epstein and Axtell 1996; Epstein 1999). But this raises its own sorts of epistemological questions. What exactly has been accomplished, what kind of knowledge has been acquired, when an observed social phenomenon is more or less reproduced by an agent-based simulation? Does this count as an explanation of the phenomenon? A possible explanation? (see e.g., Grüne-Yanoff 2007). Giuseppe Primiero (2019) argues that there is a whole domain of “artificial sciences” built around agent-based and multi-agent system based simulations, and that it requires its own epistemology--one where validation cannot be defined by comparison with an existing real-world system, but must be defined vis a vis an intended system.
It is also fair to say, as Parker does (2013), that the conditions outlined above pay insufficient attention to the various and differing purposes for which simulations are used (as discussed in 2.4). If we are using a simulation to make detailed quantitative predictions about the future behavior of a target system, the epistemology of such inferences might require more stringent standards than those that are involved when the inferences being made are about the general, qualitative behavior of a whole class of systems. Indeed, it is also fair to say that much more work could be done in classifying the kinds of purposes to which computer simulations are put and the constraints those purposes place on the structure of their epistemology.
Frigg and Reiss (2009) argued that none of these three conditions are new to computer simulation. They argued that ordinary ‘paper and pencil’ modeling incorporate these features. Indeed, they argued that computer simulation could not possibly raise new epistemological issues because the epistemological issues could be cleanly divided into the question of the appropriateness of the model underlying the simulation, which is an issue that is identical to the epistemological issues that arise in ordinary modeling, and the question of the correctness of the solution to the model equations delivered by the simulation, which is a mathematical question, and not one related to the epistemology of science. On the first point, Winsberg (2009b) replied that it was the simultaneous confluence of all three features that was new to simulation. We will return to the second point in section 4.3
Some of the work on the EOCS has developed analogies between computer simulation in order to draw on recent work in the epistemology of experiment, particularly the work of Allan Franklin; see the entry on experiments in physics .
In his work on the epistemology of experiment, Franklin (1986, 1989) identified a number of strategies that experimenters use to increase rational confidence in their results. Weissart (1997) and Parker (2008a) argued for various forms of analogy between these strategies and a number of strategies available to simulationists to sanction their results. The most detailed analysis of these relationships is to be found in Parker 2008a, where she also uses these analogies to highlight weaknesses in current approaches to simulation model evaluation.
Winsberg (2003) also makes use of Ian Hacking’s (1983, 1988, 1992) work on the philosophy of experiment. One of Hacking’s central insights about experiment is captured in his slogan that experiments have a life of their own’ (1992: 306). Hacking intended to convey two things with this slogan. The first was a reaction against the unstable picture of science that comes, for example, from Kuhn. Hacking (1992) suggests that experimental results can remain stable even in the face of dramatic changes in the other parts of sciences. The second, related, point he intended to convey was that ‘experiments are organic, develop, change, and yet retain a certain long-term development which makes us talk about repeating and replicating experiments’ (1992: 307). Some of the techniques that simulationists use to construct their models get credentialed in much the same way that Hacking says that instruments and experimental procedures and methods do; the credentials develop over an extended period of time and become deeply tradition-bound. In Hacking’s language, the techniques and sets of assumptions that simulationists use become ‘self-vindicating’. Perhaps a better expression would be that they carry their own credentials. This provides a response to the problem posed in 4.1, of understanding how simulation could have a viable epistemology despite the motley and autonomous nature of its inferences.
Drawing inspiration from another philosopher of experiment (Mayo 1996), Parker (2008b) suggests a remedy to some of the shortcomings in current approaches to simulation model evaluation. In this work, Parker suggests that Mayo’s error-statistical approach for understanding the traditional experiment—which makes use of the notion of a “severe test”—could shed light on the epistemology of simulation. The central question of the epistemology of simulation from an error-statistical perspective becomes, ‘What warrants our taking a computer simulation to be a severe test of some hypothesis about the natural world? That is, what warrants our concluding that the simulation would be unlikely to give the results that it in fact gave, if the hypothesis of interest were false (2008b, 380)? Parker believes that too much of what passes for simulation model evaluation lacks rigor and structure because it:
consists in little more than side-by-side comparisons of simulation output and observational data, with little or no explicit argumentation concerning what, if anything, these comparisons indicate about the capacity of the model to provide evidence for specific scientific hypotheses of interest. (2008b, 381)
Drawing explicitly upon Mayo’s (1996) work, she argues that what the epistemology of simulation ought to be doing, instead, is offering some account of the ‘canonical errors’ that can arise, as well as strategies for probing for their presence.
Practitioners of simulation, particularly in engineering contexts, in weapons testing, and in climate science, tend to conceptualize the EOCS in terms of verification and validation . Verification is said to be the process of determining whether the output of the simulation approximates the true solutions to the differential equations of the original model. Validation , on the other hand, is said to be the process of determining whether the chosen model is a good enough representation of the real-world system for the purpose of the simulation. The literature on verification and validation from engineers and scientists is enormous and it is beginning to receive some attention from philosophers.
Verification can be divided into solution verification and code verification. The former verifies that the output of the intended algorithm approximates the true solutions to the differential equations of the original model. The latter verifies that the code, as written, carries out the intended algorithm. Code verification has been mostly ignored by philosophers of science; probably because it has been seen as more of a problem in computer science than in empirical science—perhaps a mistake. Part of solution verification consists in comparing computed output with analytic solutions (so called “benchmark solutions”). Though this method can of course help to make case for the results of a computer simulation, it is by itself inadequate , since simulations are often used precisely because analytic solution is unavailable for regions of solution space that are of interest. Other indirect techniques are available: the most important of which is probably checking to see whether and at what rate computed output converges to a stable solution as the time and spatial resolution of the discretization grid gets finer.
The principal strategy of validation involves comparing model output with observable data. Again, of course, this strategy is limited in most cases, where simulations are being run because observable data are sparse. But complex strategies can be employed, including comparing the output of subsystems of a simulation to relevant experiments (Parker, 2013; Oberkampf and Roy 2010).
The concepts of verification and validation has drawn some criticism from philosophers. Oreskes et al. 1994, a very widely-cited article, was mostly critical of the terminology, arguing that “validity,” in particular, is a property that only applies to logical arguments, and that hence the term, when applied to models, might lead to overconfidence.
Winsberg (2010, 2018, p.155) has argued that the conceptual division between verification and validation can be misleading, if it is taken to suggest that there is one set of methods which can, by itself, show that we have solved the equations right, and that there is another set of methods, which can, by itself, show that we’ve got the right equations. He also argued that it is misleading to think that the epistemology of simulation is cleanly divided into an empirical part (verification) and a mathematical (and computer science) part (validation.) But this misleading idea often follows discussion of verification and validation. We find this both in the work of practitioners and philosophers.
Here is the standard line from a practitioner, Roy: “Verification deals with mathematics and addresses the correctness of the numerical solution to a given model. Validation, on the other hand, deals with physics and addresses the appropriateness of the model in reproducing experimental data. Verification can be thought of as solving the chosen equations correctly, while validation is choosing the correct equations in the first place” (Roy 2005).
Some philosophers have put this distinction to work in arguments about the philosophical novelty of simulation. We first raised this issue in section 4.1, where Frigg and Reiss argued that simulation could have no epistemologically novel features, since it contained two distinct components: a component that is identical to the epistemology of ordinary modeling, and a component that is entirely mathematical. “We should distinguish two different notions of reliability here, answering two different questions. First, are the solutions that the computer provides close enough to the actual (but unavailable) solutions to be useful?…this is a purely mathematical question and falls within the class of problems we have just mentioned. So, there is nothing new here from a philosophical point of view and the question is indeed one of number crunching. Second, do the computational models that are the basis of the simulations represent the target system correctly? That is, are the simulation results externally valid? This is a serious question, but one that is independent of the first problem, and one that equally arises in connection with models that do not involve intractable mathematics and ordinary experiments” (Frigg and Reiss 2009).
But verification and validation are not, strictly speaking, so cleanly separable. That is because most methods of validation, by themselves, are much too weak to establish the validity of a simulation. And most model equations chosen for simulation are not in any straightforward sense “the right equations”; they are not the model equations we would choose in an ideal world. We have good reason to think, in other words, that there are model equations out there that enjoy better empirical support, in the abstract. The equations we choose often reflect a compromise between what we think best describes the phenomena and computational tractability. So the equations that are chosen are rarely well “validated” on their own. If we want to understand why simulation results are taken to be credible, we have to look at the epistemology of simulation as an integrated whole, not as cleanly divided into verification and validation—each of which, on its own, would look inadequate to the task.
So one point is that verification and validation are not independently-successful and separable activities. But the other point is that there are not two independent entities onto which these activities can be directed: a model chosen to discretized, and a method for discretizing it. Once one recognizes that the equations to be “solved” are sometimes chosen so as to cancel out discretization errors, etc. (Lenhard 2007 has a very nice example of this involving the Arakawa operator), this later distinction gets harder to maintain. So success is achieved in simulation with a kind of back-and-forth, trial-and-error, piecemeal adjustment between model and method of calculation. And when this is the case, it is hard even to know what it means to say that a simulation is separately verified and validated.
No one has argued that V&V isn’t a useful distinction, but rather that scientists shouldn’t overinflate a pragmatically useful distinction into a clean methodological dictate that misrepresents the messiness of their own practice. Collaterally, Frigg and Reiss’s argument for the absence of epistemological novelty in simulation fails for just this reason. It is not “a purely mathematical question” whether the solutions that the computer provides close enough to the actual (but unavailable) solutions to be useful. At least not in this respect: it is not a question that can be answered, as a pragmatic matter, entirely using mathematical methods. And hence it is an empirical/epistemological issue that does not arise in ordinary modeling.
A major strand of ordinary (outside of the philosophy of science) epistemology is to emphasize the degree to which it is a condition for the possibility of knowledge that we rely on our senses and the testimony of other people in a way that we cannot ourselves justify. According to Tyler Burge (1993,1998), belief in the results of these two processes are warranted but not justified. Rather, according to Burge, we are entitled to these beliefs. “[w]e are entitled to rely, other things equal, on perception, memory, deductive and inductive reasoning, and on…the word of others” (1993, p. 458). Beliefs in which a believer is entitled are those that are unsupported by evidence available to the believer, but which the believer is nevertheless warranted in believing.
Some work in EOCS has developed analogies between computer simulation and the kinds of knowledge producing practices Burge associates with entitlement . (See especially Barberousse and Vorms, 2014, and Beisbart, 2017.) This is, in some ways, a natural outgrowth of Burge’s arguments that we view computer assisted proofs in this way (1998). Computer simulations are extremely complex, often the result of the epistemic labor of a diverse set of scientists and other experts, and perhaps most importantly, epistemically opaque (Humphreys, 2004). Because of these features, Beisbart argues that it is reasonable to treat computer simulations in the same way that we treat our senses and the testimony of others: simply as things that can be trusted on the assumption that everything is working smoothly. (Beisbart, 2017).
Symons and Alvarado (2019) argue that there is a fundamental problem with this approach to EOCS and it has to do with a feature of computer-aided proof that was crucial to Burge’s original account: that of a being a ‘transparent conveyor’. “It is very important to note, for example, that Burge’s account of content preservation and transparent conveying requires that the recipient already has reason not to doubt the source” (p. 13). But Symons and Alvarado point to many of the properties of computer simulations (drawing from Winsberg 2010 and Ruphy 2015) in virtue of which they fail to have these properties. Lenhard and Küster 2019 is also relevant here, as they argue that there are many features of computer simulation that make them difficult to reproduce and that therefore undermine some of the stability that would be required for them to be transparent conveyors. For these reasons and others having to do with many of the features discussed in 4.2 and 4.3, Symons and Alvarado argue that it is implausible that we should view computer simulation as a basic epistemic practice on a par with sense perception, memory, testimony, or the like.
Another approach to EOCS is to ground it in the practical aspects of the craft of modeling and simulation. According to this view, in other words, the best account we can give of the reasons we have for believing the results of computer simulation studies is to have trust in the practical skills and craft of the modelers that use them. A good example of this kind of account is (Hubig and Kaminski, 2017). The epistemological goal of this kind of work is to identify the locus of our trust in simulations in practical aspects of the craft of modeling and simulation, rather than in any features of the models themselves. (Resch et al, 2017) argue that a good part of the reason we should trust simulations is not because of the simulations themselves, but because of the interpretive artistry of those who employ their art and skill to interpret simulation outputs. Symons and Alvarado (2019) are also critical of this approach, arguing that “Part of the task of the epistemology of computer simulation is to explain the difference between the contemporary scientist’s position in relation to epistemically opaque computer simulations..” (p.7) and the believers in a mechanical oracle’s relation to their oracles. Pragmatic and epistemic considerations, according to Symons and Alvarado, co-exist, and they are not possible competitors for the correct explanation of our trust in simulations--the epistemic reasons are ultimate what explain and ground the pragmatic ones.
Working scientists sometimes describe simulation studies in experimental terms. The connection between simulation and experiment probably goes back as far as von Neumann, who, when advocating very early on for the use of computers in physics, noted that many difficult experiments had to be conducted merely to determine facts that ought, in principle, to be derivable from theory. Once von Neumann’s vision became a reality, and some of these experiments began to be replaced by simulations, it became somewhat natural to view them as versions of experiment. A representative passage can be found in a popular book on simulation:
A simulation that accurately mimics a complex phenomenon contains a wealth of information about that phenomenon. Variables such as temperature, pressure, humidity, and wind velocity are evaluated at thousands of points by the supercomputer as it simulates the development of a storm, for example. Such data, which far exceed anything that could be gained from launching a fleet of weather balloons, reveals intimate details of what is going on in the storm cloud. (Kaufmann and Smarr 1993, 4)
The idea of “in silico” experiments becomes even more plausible when a simulation study is designed to learn what happens to a system as a result of various possible interventions: What would happen to the global climate if x amount of carbon were added to the atmosphere? What will happen to this airplane wing if it is subjected to such-and-such strain? How would traffic patterns change if an onramp is added at this location?
Philosophers, consequently, have begun to consider in what sense, if any, computer simulations are like experiments and in what sense they differ. A related issue is the question of when a process that fundamentally involves computer simulation can counts as measurement (Parker, 2017) A number of views have emerged in the literature centered around defending and criticizing two theses:
The identity thesis . Computer simulation studies are literally instances of experiments. The epistemological dependence thesis . The identity thesis would (if it were true) be a good reason (weak version), or the best reason (stronger version), or the only reason (strongest version; it is a necessary condition) to believe that simulations can provide warrants for belief in the hypotheses that they support. A consequence of the strongest version is that only if the identity thesis is true is there reason to believe that simulations can confer warrant for believing in hypotheses.
The central idea behind the epistemological dependence thesis is that experiments are the canonical entities that play a central role in warranting our belief in scientific hypotheses, and that therefore the degree to which we ought to think that simulations can also play a role in warranting such beliefs depends on the extent to which they can be identified as a kind of experiment.
One can find philosophers arguing for the identity thesis as early as Humphreys 1995 and Hughes 1999. And there is at least implicit support for (the stronger) version of the epistemological dependence thesis in Hughes. The earliest explicit argument in favor of the epistemological dependence thesis, however, is in Norton and Suppe 2001. According to Norton and Suppe, simulations can warrant belief precisely because they literally are experiments. They have a detailed story to tell about in what sense they are experiments, and how this is all supposed to work. According to Norton and Suppe, a valid simulation is one in which certain formal relations (what they call ‘realization’) hold between a base model, the modeled physical system itself, and the computer running the algorithm. When the proper conditions are met, ‘a simulation can be used as an instrument for probing or detecting real world phenomena. Empirical data about real phenomena are produced under conditions of experimental control’ (p. 73).
One problem with this story is that the formal conditions that they set out are much too strict. It is unlikely that there are very many real examples of computer simulations that meet their strict standards. Simulation is almost always a far more idealizing and approximating enterprise. So, if simulations are experiments, it is probably not in the way that Norton and Suppe imagined.
More generally, the identity thesis has drawn fire from other quarters.
Gilbert and Troitzsch argued that “[t]he major difference is that while in an experiment, one is controlling the actual object of interest (for example, in a chemistry experiment, the chemicals under investigation), in a simulation one is experimenting with a model rather than the phenomenon itself.” (Gilbert and Troitzsch 1999, 13). But this doesn’t seem right. Many (Guala 2002, 2008, Morgan 2003, Parker 2009a, Winsberg 2009a) have pointed to problems with the claim. If Gilbert and Troitzsch mean that simulationists manipulate models in the sense of abstract objects, then the claim is difficult to understand—how do we manipulate an abstract entity? If, on the other hand, they simply mean to point to the fact that the physical object that simulationists manipulate—a digital computer—is not the actual object of interest, then it is not clear why this differs from ordinary experiments.
It is false that real experiments always manipulate exactly their targets of interest. In fact, in both real experiments and simulations, there is a complex relationship between what is manipulated in the investigation on the one hand, and the real-world systems that are the targets of the investigation on the other. In cases of both experiment and simulation, therefore, it takes an argument of some substance to establish the ‘external validity’ of the investigation – to establish that what is learned about the system being manipulated is applicable to the system of interest. Mendel, for example, manipulated pea plants, but he was interested in learning about the phenomenon of heritability generally. The idea of a model organism in biology makes this idea perspicuous. We experiment on Caenorhabditis elegans because we are interested in understanding how organism in general use genes to control development and genealogy. We experiment on Drosophila melanogaster , because it provides a useful model of mutations and genetic inheritance. But the idea is not limited to biology. Galileo experimented with inclined planes because he was interested in how objects fall and how they would behave in the absence of interfering forces—phenomena that the inclined plane experiments did not even actually instantiate.
Of course, this view about experiments is not uncontested. It is true that, quite often, experimentalists infer something about a system distinct from the system they interfere with. However, it is not clear whether this inference is proper part of the original experiment. Peschard (2010) mounts a criticism along these lines, and hence can be seen as a defender of Gilbert and Troitzsch. Peschard argues that the fundamental assumption of their critics—that in experimentation, just as in simulation, what is manipulated is a system standing in for a target system—is confused. It confuses, Peschard argues, the epistemic target of an experiment with its epistemic motivation. She argues that while the epistemic motivation for doing experiments on C. elegans might be quite far-reaching, the proper epistemic target for any such experiment is the worm itself. In a simulation, according to Peschard, however, the epistemic target is never the digital computer itself. Thus, simulation is distinct from experiment, according to her, in that its epistemic target (as opposed to merely its epistemic motivation) is distinct from the object being manipulated. Roush (2017) can also be seen as a defender of the Gilbert and Troitzsch line, but Roush appeals to sameness of natural kinds as the crucial feature that separates experiments and simulations. Other opponents of the identity thesis include Giere (2009) and Beisbart and Norton (2012, Other Internet Resources).
It is not clear how to adjudicate this dispute, and it seems to revolve primarily around a difference of emphasis. One can emphasize the difference between experiment and simulation, following Gilbert and Troitzsch and Peschard, by insisting that experiments teach us first about their epistemic targets and only secondarily allow inferences to the behavior of other systems. (I.e., experiments on worms teach us, in the first instance, about worms, and only secondarily allow us to make inferences about genetic control more generally.) This would make them conceptually different from computer simulations, which are not thought to teach us, in the first instance, about the behavior of computers, and only in the second instance about storms, or galaxies, or whatever.
Or one can emphasize similarity in the opposite way. One can emphasize the degree to which experimental targets are always chosen as surrogates for what’s really of interest. Morrison, 2009 is probably the most forceful defender of emphasizing this aspect of the similarity of experiment and simulation. She argues that most experimental practice, and indeed most measurement practice, involve the same kinds of modeling practices as simulations. In any case, pace Peschard, nothing but a debate about nomenclature—and maybe an appeal to the ordinary language use of scientists; not always the most compelling kind of argument—would prevent us from saying that the epistemic target of a storm simulation is the computer, and that the storm is merely the epistemic motivation for studying the computer.
Be that as it may, many philosophers of simulation, including those discussed in this section, have chosen the latter path—partly as a way of drawing attention to ways in which the message lurking behind Gilbert and Troitzsch’s quoted claim paints an overly simplistic picture of experiment. It does seem overly simplistic to paint a picture according to which experiment gets a direct grip on the world, whereas simulation’s situation is exactly opposite . And this is the picture one seems to get from the Gilber and Troitzsch quotation. Peschard’s more sophisticated picture involving a distinction between epistemic targets and epistemic motivations goes a long way towards smoothing over those concerns without pushing us into the territory of thinking that simulation and experiment are exactly the same , in this regard.
Still, despite rejecting Gilbert and Troitzsch’s characterization of the difference between simulation and experiment, Guala and Morgan both reject the identity thesis. Drawing on the work of Simon (1969), Guala argues that simulations differ fundamentally from experiments in that the object of manipulation in an experiment bears a material similarity to the target of interest, but in a simulation, the similarity between object and target is merely formal. Interestingly, while Morgan accepts this argument against the identity thesis, she seems to hold to a version of the epistemological dependency thesis . She argues, in other words, that the difference between experiments and simulations identified by Guala implies that simulations are epistemologically inferior to real experiments – that they have intrinsically less power to warrant belief in hypotheses about the real world because they are not experiments.
A defense of the epistemic power of simulations against Morgan’s (2002) argument could come in the form of a defense of the identity thesis, or in the form of a rejection of the epistemological dependency thesis. On the former front, there seem to be two problems with Guala’s (2002) argument against the identity thesis. The first is that the notion of material similarity here is too weak, and the second is that the notion of mere formal similarity is too vague, to do the required work. Consider, for example, the fact that it is not uncommon, in the engineering sciences, to use simulation methods to study the behavior of systems fabricated out of silicon. The engineer wants to learn about the properties of different design possibilities for a silicon device, so she develops a computational model of the device and runs a simulation of its behavior on a digital computer. There are deep material similarities between, and some of the same material causes are at work in, the central processor of the computer and the silicon device being studied. On Guala’s line of reasoning, this should mark this as an example of a real experiment, but that seems wrong. The peculiarities of this example illustrate the problem rather starkly, but the problem is in fact quite general: any two systems bear some material similarities to each other and some differences.
On the flip side, the idea that the existence of a formal similarity between two material entities could mark anything interesting is conceptually confused. Given any two sufficiently complex entities, there are many ways in which they are formally identical, not to mention similar. There are also ways in which they are formally completely different. Now, we can speak loosely, and say that two things bear a formal similarity, but what we really mean is that our best formal representations of the two entities have formal similarities. In any case, there appear to be good grounds for rejecting both the Gilbert and Troitzsch and the Morgan and Guala grounds for distinguishing experiments and simulations.
Returning to the defense of the epistemic power of simulations, there are also grounds for rejecting the epistemological dependence thesis. As Parker (2009a) points out, in both experiment and simulation, we can have relevant similarities between computer simulations and target systems, and that’s what matters. When the relevant background knowledge is in place, a simulation can provide more reliable knowledge of a system than an experiment. A computer simulation of the solar system, based on our most sophisticated models of celestial dynamics, will produce better representations of the planets’ orbits than any experiment.
Parke (2014) argues against the epistemological dependency thesis by undermining two premises that she believes support it: first, that experiments generate greater inferential power than simulations, and second, that simulations cannot surprise us in the same way that experiments can. The argument that simulations cannot surprise us comes from Morgan (2005). Pace Morgan, Parke argues that simulationists are often surprised by their simulations, both because they are not computationally omniscient, and because they are not always the sole creators of the models and code they use. She argues, moreover, that ‘[d]ifferences in researcher’s epistemic states, alone, seem like the wrong grounds for tracking a distinction between experiment and simulation’ (258). Adrian Curry (2017) defends Morgan’s original intuition by making two friendly amendments. He argues that the distinction Morgan was really after was between two different kinds of surprise, and in particular to what the source of surprise is: surprise due to bringing out theoretical knowledge into contact with the world are distinctive of experiment. He also more carefully defines surprise in a non-psychological way such that it is a “quality the attainment of which constitutes genuine epistemic progress” (p. 640).
Paul Humphreys (2004) has argued that computer simulations have profound implications for our understanding of the structure of theories; he argues that they reveal inadequacies with both the semantic and syntactic views of scientific theories. This claim has drawn sharp fire from Roman Frigg and Julian Reiss (2009). Frigg and Reiss argue that whether a model admits of analytic solution or not has no bearing on how it relates to the world. They use the example of the double pendulum to show this. Whether or not the pendulum’s inner fulcrum is held fixed (a fact which will determine whether the relevant model is analytically solvable) has no bearing on the semantics of the elements of the model. From this, they conclude that the semantics of a model, or how it relates to the world, is unaffected by whether or not the model is analytically solvable.
This was not responsive, however, to the most charitable reading of what Humphreys was pointing at. The syntactic and semantic views of theories, after all, were not just accounts of how our abstract scientific representations relate to the world. More particularly, they were not stories about the relation between particular models and the world, but rather about the relation between theories and the world, and the role, if any, that models played in that relation.
They were also stories that had a lot to say about where the philosophically interesting action is when it comes to scientific theorizing. The syntactic view suggested that scientific practice could be adequately rationally reconstructed by thinking of theories as axiomatic systems, and, more importantly, that logical deduction was a useful regulative ideal for thinking about how inferences from theory to the world are drawn. The syntactic view also, by omission, made if fairly clear that modeling played, if anything, only a heuristic role in science. (This was a feature of the syntactic view of theories that Frederick Suppe, one of its most ardent critics, often railed against.) Theories themselves had nothing to do with models, and theories could be compared directly to the world, without any important role for modeling to play.
The semantic view of theories, on the other hand, did emphasize an important role for models, but it also urged that theories were non-linguistic entities. It urged philosophers not to be distracted by the contingencies of the particular form of linguistic expression a theory might be found in, say, a particular textbook.
Computer simulations, however, do seem to illustrate that both of these themes were misguided. It was profoundly wrong to think that logical deduction was the right tool for rationally reconstructing the process of theory application. Computer simulations show that there are methods of theory application that vastly outstrip the inferential power of logical deduction. The space of solutions, for example, that is available via logical deduction from the theory of fluids is microscopic compared with the space of applications that can be explored via computer simulation. On the flip side, computer simulations seem to reveal that, as Humphreys (2004) has urged, syntax matters. It was wrong, it turns out, to suggest, as the semantic view did, that the particular linguistic form in which a scientific theory is expressed is philosophically uninteresting. The syntax of the theory’s expression will have a deep effect on what inferences can be drawn from it, what kinds of idealizations will work well with it, etc. Humphreys put the point as follows: “the specific syntactic representation used is often crucial to the solvability of the theory’s equations” (Humphreys 2009, p.620). The theory of fluids can be used to emphasize this point: whether we express that theory in Eulerian or Lagrangian form will deeply affect what, in practice, we can calculate and how; it will affect what idealizations, approximations, and calculational techniques will be effective and reliable in which circumstances. So the epistemology of computer simulation needs to be sensitive to the particular syntactic formulation of a theory, and how well that particular formulation has been credentialed. Hence, it does seem right to emphasize, as Humphreys (2004) did, that computer simulations have revealed inadequacies with both the syntactic and semantic theories.
Paul Humphreys (2004) and Mark Bedau (1997, 2011) have argued that philosophers interested in the topic of emergence can learn a great deal by looking at computer simulation. Philosophers interested in this topic should consult the entry on emergent properties , where the contributions of all these philosophers have been discussed.
The connection between emergence and simulation was perhaps best articulated by Bedau in his (2011). Bedau argued that any conception of emergence must meet the twin hallmarks of explaining how the whole depends on its parts and how the whole is independent of its parts. He argues that philosophers often focus on what he calls “strong” emergence, which posits brute downward causation that is irreducible in principle. But he argues that this is a mistake. He focuses instead on what he calls “weak” emergence, which allows for reducibility of wholes to parts in principle but not in practice . Systems that produce emergent properties are mere mechanisms, but the mechanisms are very complex (they have very many independently interacting parts). As a result, there is no way to figure out exactly what will happen given a specific set of initial and boundary conditions, except to “crawl the causal web”. It is here that the connection to computer simulation arises. Weakly emergent properties are characteristic of complex systems in nature. And it is also characteristic of complex computer simulations that there is no way to predict what they will do except to let them run. Weak emergence explains, according to Bedau, why computer simulations play a central role in the science of complex systems. The best way to understand and predict how real complex systems behave is to simulate them by crawling the micro-causal web, and see what happens.
Models of course involve idealizations. But it has been argued that some kinds of idealization, which play an especially prominent role in the kinds of modeling involved in computer simulation, are special—to the point that they deserve the title of “fiction.” This section will discuss attempts to define fictions and explore their role in computer simulation.
There are two different lines of thinking about the role of fictions in science. According to one, all models are fictions. This line of thinking is motivated by considering the role, for example, of “the ideal pendulum” in science. Scientists, it is argued, often make claims about these sorts of entities (e.g., “the ideal pendulum has a period proportional to the square-root of its length”) but they are nowhere to be found in the real world; hence they must be fictional entities. This line of argument about fictional entities in science does not connect up in any special way with computer simulation—readers interested in this topic should consult the entry on -->scientific representation --> [forthcoming].
Another line of thinking about fictions is concerned with the question of what sorts of representations in science ought to be regarded as fictional. Here, the concern is not so much about the ontology of scientific model entities, but about the representational character of various postulated model entities. Here, Winsberg (2009c) has argued that fictions do have a special connection to computer simulations. Or rather, that some computer simulations contain elements that best typify what we might call fictional representations in science, even if those representations are not uniquely present in simulations.
He notes that the first conception of a fiction—mentioned above—which makes “any representation that contradicts reality a fiction” (p. 179), doesn’t correspond to our ordinary use of the term: a rough map is not fiction. He then proposes an alternative definition: nonfiction is offered as a “good enough” guide to some part of the world (p. 181); fiction is not. But the definition needs to be refined. Take the fable of the grasshopper and the ant. Although the fable offers lessons about how the world is, it is still fiction because it is “a useful guide to the way the world is in some general sense” rather than a specific guide to the way a part of the world is, its “prima facie representational target”, a singing grasshopper and toiling ant. Nonfictions, on the other hand, “point to a certain part of the world” and are a guide to that part of the world (p. 181).
These kinds of fictional components of models are paradigmatically exemplified in certain computer simulations. Two of his examples are the “silogen atom” and “artificial viscosity.” Silogen atoms appear in certain nanomechanical models of cracks in silicon—a species of the kind of multiscale models that blend quantum mechanics and molecular mechanics mentioned in section 2.3. The silogen containing models of crack propagation in silicon work by describing the crack itself using quantum mechanics and the region immediately surrounding the crack using classical molecular dynamics. To bring together the modeling frameworks in the two regions, the boundary gets treated as if it contains ‘silogen’ atoms, which have a mixture of the properties of silicon and those of hydrogen. Silogen atoms are fictions. They are not offered as even a ‘good enough’ description of the atoms at the boundary—their prima facie representational targets. But they are used so that the overall model can be hoped to get things right. Thus the overall model is not a fiction, but one of its components is. Artificial viscosity is a similar sort of example. Fluids with abrupt shocks are difficult to model on a computational grid because the abrupt shock hides inside a single grid cell, and cannot be resolved by such an algorithm. Artificial viscosity is a technique that pretends that the fluid is highly viscous—a fiction—right were the shock is, so that he shock becomes less abrupt, and blurs over several grid cells. Getting the viscosity, and hence the thickness of the shock, wrong, helps to get the overall model to work “well enough.” Again, the overall model of the fluid is not a fiction, it is a reliable enough guide to the behavior of the fluid. But the component called artificial viscosity is a fiction—it is not being used to reliably model the shock. It is being incorporated into a larger modeling framework so as to make that larger framework, “reliable enough.”
This account has drawn two sorts of criticisms. Toon (2010) has argued that this definition of a fiction is too narrow. He gives examples of historical fictions like I, Claudius , and Schindler’s Ark , which he argues are fictions, despite the fact that “they are offered as ‘good enough’ guides to those people, places and events in certain respects and we are entitled to take them as such.” (p. 286–7). Toon, presumably, supports a broader conception of the role of fictions in science, then, according to which they do not play a particularly prominent or heightened role in computer simulation.
Gordon Purves (forthcoming) argues that there are examples of fictions in computational models (his example is so-called “imaginary cracks”), and elsewhere, that do not meet the strict requirements discussed above. Unlike Toon, however, he also wants to delineate fictional modeling elements from the non-fictional ones. His principal criticism is of the criterion of fictionhood in terms of social norms of use—and Purves argues that we ought to be able to settle whether or not some piece of modeling is a fiction in the absence of such norms. Thus, he wants to find an intrinsic characterization of a scientific fiction. His proposal takes as constitutive of model fictions that they fail to have the characteristic that Laymon (1985) called “piecewise improvability” (PI). PI is a characteristic of many models that are idealizations; it says that as you de-idealize, your model becomes more and more accurate. But as you de-idealize a silogen atom, you do not get a more and more accurate simulation of a silicon crack. But Purves takes this failure of PI to be constitutive of a fiction, rather than merely symptomatic of them.
- Barberousse, A., and P. Ludwig, 2009. “Models as Fictions,” in Fictions in Science. Philosophical Essays in Modeling and Idealizations , London: Routledge, 56–73.
- Barberousse, A., and Vorms, M. 2014. “About the warrants of computer-based empirical knowledge,” Synthese , 191(15): 3595–3620.
- Bedau, M.A., 2011. “Weak emergence and computer simulation,” in P. Humphreys and C. Imbert (eds.), Models, Simulations, and Representations , New York: Routledge, 91–114.
- –––, 1997. “Weak Emergence,” Noûs (Supplement 11), 31: 375–399.
- Beisbart, C. and J. Norton, 2012. “Why Monte Carlo Simulations are Inferences and not Experiments,” in International Studies in Philosophy of Science , 26: 403–422.
- Beisbart, C., 2017. “Advancing knowledge through computer simulations? A socratic exercise,” in M. Resch, A. Kaminski, & P. Gehring (eds.), The Science and Art of Simulation (Volume I), Cham: Springer, pp. 153–174./
- Burge, T., 1993. “Content preservation,” The Philosophical Review , 102(4): 457–488.
- –––, 1998. “Computer proof, apriori knowledge, and other minds: The sixth philosophical perspectives lecture,” Noûs, , 32(S12): 1–37.
- Currie, Adrian, 2018. “The argument from surprise,” Canadian Journal of Philosophy , 48(5): 639–661
- Dardashti, R., Thebault, K., and Winsberg, E., 2015. “Confirmation via analogue simulation: what dumb holes could tell us about gravity,” in British Journal for the Philosophy of Science , 68(1): 55–89
- Dardashti, R., Hartmann, S., Thebault, K., and Winsberg, E., 2019. “Hawking radiation and analogue experiments: A Bayesian analysis,” in Studies in History and Philosophy of Modern Physics , 67: 1–11.
- Epstein, J., and R. Axtell, 1996. Growing artificial societies: Social science from the bottom-up , Cambridge, MA: MIT Press.
- Epstein, J., 1999. “Agent-based computational models and generative social science,” Complexity , 4(5): 41–57.
- Franklin, A., 1996. The Neglect of Experiment , Cambridge: Cambridge University Press.
- –––, 1989. “The Epistemology of Experiment,” The Uses of Experiment , D. Gooding, T. Pinch and S. Schaffer (eds.), Cambridge: Cambridge University Press, 437–60.
- Frigg, R., and J. Reiss, 2009. “The philosophy of simulation: Hot new issues or same old stew,” Synthese , 169: 593–613.
- Giere, R. N., 2009. “Is Computer Simulation Changing the Face of Experimentation?,” Philosophical Studies , 143: 59–62
- Gilbert, N., and K. Troitzsch, 1999. Simulation for the Social Scientist , Philadelphia, PA: Open University Press.
- Grüne-Yanoff, T., 2007. “Bounded Rationality,” Philosophy Compass , 2(3): 534–563.
- Grüne-Yanoff, T. and Weirich, P., 2010. “Philosophy of Simulation,” Simulation and Gaming: An Interdisciplinary Journal , 41(1): 1–31.
- Guala, F., 2002. “Models, Simulations, and Experiments,” Model-Based Reasoning: Science, Technology, Values , L. Magnani and N. Nersessian (eds.), New York: Kluwer, 59–74.
- –––, 2008. “Paradigmatic Experiments: The Ultimatum Game from Testing to Measurement Device,” Philosophy of Science , 75: 658–669.
- Hacking, I., 1983. Representing and Intervening: Introductory Topics in the Philosophy of Natural Science , Cambridge: Cambridge University Press.
- –––, 1988. “On the Stability of the Laboratory Sciences,” The Journal of Philosophy , 85: 507–15.
- –––, 1992. “Do Thought Experiments have a Life of Their Own?” PSA (Volume 2), A. Fine, M. Forbes and K. Okruhlik (eds.), East Lansing: The Philosophy of Science Association, 302–10.
- Hartmann, S., 1996. “The World as a Process: Simulations in the Natural and Social Sciences,” in R. Hegselmann, et al. (eds.), Modelling and Simulation in the Social Sciences from the Philosophy of Science Point of View , Dordrecht: Kluwer, 77–100.
- Hubig, C, & Kaminski, A., 2017. “Outlines of a pragmatic theory of truth and error in computer simulation,” in M. Resch, A. Kaminski, & P. Gehring (eds.), The Science and Art of Simulation (Volume I), Cham: Springer, pp. 121–136.
- Hughes, R., 1999. “The Ising Model, Computer Simulation, and Universal Physics,” in M. Morgan and M. Morrison (eds.), Models as Mediators , Cambridge: Cambridge University Press.
- Huggins, E. M.,and E. A. Schultz, 1967. “San Francisco bay in a warehouse,” Journal of the Institute of Environmental Sciences and Technology , 10(5): 9–16.
- Humphreys, P., 1990. “Computer Simulation,” in A. Fine, M. Forbes, and L. Wessels (eds.), PSA 1990 (Volume 2), East Lansing, MI: The Philosophy of Science Association, 497–506.
- –––, 1995. “Computational science and scientific method,” in Minds and Machines , 5(1): 499–512.
- –––, 2004. Extending ourselves: Computational science, empiricism, and scientific method , New York: Oxford University Press.
- –––, 2009. “The philosophical novelty of computer simulation methods,” Synthese , 169: 615–626.
- Kaufmann, W. J., and L. L. Smarr, 1993. Supercomputing and the Transformation of Science , New York: Scientific American Library.
- Laymon, R., 1985. “Idealizations and the testing of theories by experimentation,” in Observation, Experiment and Hypothesis in Modern Physical Science , P. Achinstein and O. Hannaway (eds.), Cambridge, MA: MIT Press, 147–73.
- Lenhard, J., 2007. “Computer simulation: The cooperation between experimenting and modeling,” Philosophy of Science , 74: 176–94.
- –––, 2019. Calculated Surprises: A Philosophy of Computer Simulation , Oxford: Oxford University Press
- Lenhard, J. & Küster, U., 2019. Minds & Machines . 29: 19.
- Morgan, M., 2003. “Experiments without material intervention: Model experiments, virtual experiments and virtually experiments,” in The Philosophy of Scientific Experimentation , H. Radder (ed.), Pittsburgh, PA: University of Pittsburgh Press, 216–35.
- Morrison, M., 2012. “Models, measurement and computer simulation: The changing face of experimentation,” Philosophical Studies , 143: 33–57.
- Norton, S., and F. Suppe, 2001. “Why atmospheric modeling is good science,” in Changing the Atmosphere: Expert Knowledge and Environmental Governance , C. Miller and P. Edwards (eds.), Cambridge, MA: MIT Press, 88–133.
- Oberkampf, W. and C. Roy, 2010. Verification and Validation in Scientific Computing , Cambridge: Cambridge University Press.
- Oreskes, N., with K. Shrader-Frechette and K. Belitz, 1994. “Verification, Validation and Confirmation of Numerical Models in the Earth Sciences,” Science , 263(5147): 641–646.
- Parke, E., 2014. “Experiments, Simulations, and Epistemic Privilege,” Philosophy of Science , 81(4): 516–36.
- Parker, W., 2008a. “Franklin, Holmes and the Epistemology of Computer Simulation,” International Studies in the Philosophy of Science , 22(2): 165–83.
- –––, 2008b. “Computer Simulation through an Error-Statistical Lens,” Synthese , 163(3): 371–84.
- –––, 2009a. “Does Matter Really Matter? Computer Simulations, Experiments and Materiality,” Synthese , 169(3): 483–96.
- –––, 2013. “Computer Simulation,” in S. Psillos and M. Curd (eds.), The Routledge Companion to Philosophy of Science , 2nd Edition, London: Routledge.
- –––, 2017. “Computer Simulation, Measurement, and Data Assimilation,” British Journal for the Philosophy of Science , 68(1): 273–304.
- Peschard, I., 2010. “Modeling and Experimenting,” in P. Humphreys and C. Imbert (eds), Models, Simulations, and Representations , London: Routledge, 42–61.
- Primiero, G., 2019. “A Minimalist Epistemology for Agent-Based Simulations in the Artificial Sciences,” Minds and Machines , 29(1): 127–148.
- Purves, G.M., forthcoming. “Finding truth in fictions: identifying non-fictions in imaginary cracks,” Synthese .
- Resch, M. M., Kaminski, A., & Gehring, P. (eds.), 2017. The science and art of simulation I: Exploring-understanding-knowing , Berlin: Springer.
- Roush, S., 2015. “The epistemic superiority of experiment to simulation,” Synthese , 169: 1–24.
- Roy, S., 2005. “Recent advances in numerical methods for fluid dynamics and heat transfer,” Journal of Fluid Engineering , 127(4): 629–30.
- Ruphy, S., 2015. “Computer simulations: A new mode of scientific inquiry?” in S. O. Hansen (ed.), The Role of Technology in Science: Philosophical Perspectives , Dordrecht: Springer, pp. 131–149
- Schelling, T. C., 1971. “Dynamic Models of Segregation,” Journal of Mathematical Sociology , 1: 143–186.
- Simon, H., 1969. The Sciences of the Artificial , Boston, MA: MIT Press.
- Symons, J., & Alvarado, R., 2019. “Epistemic Entitlements and the Practice of Computer Simulation,” Minds and Machines , 29(1): 37–60.
- Toon, A., 2010. “Novel Approaches to Models,” Metascience , 19(2): 285–288.
- Trenholme R., 1994. “Analog Simulation,” Philosophy of Science , 61: 115–131.
- Unruh, W. G., 1981. “Experimental black-hole evaporation?” Physical Review Letters , 46(21): 1351–53.
- Winsberg, E., 2018. Philosophy and Climate Science , Cambridge: Cambridge University Press
- –––, 2010. Science in the Age of Computer Simulation , Chicago: The University of Chicago Press.
- –––, 2009a. “A Tale of Two Methods,” Synthese , 169(3): 575–92
- –––, 2009b. “Computer Simulation and the Philosophy of Science,” Philosophy Compass , 4/5: 835–845.
- –––, 2009c. “A Function for Fictions: Expanding the scope of science,” in Fictions in Science: Philosophical Essays on Modeling and Idealization , M. Suarez (ed.), London: Routledge.
- –––, 2006. “Handshaking Your Way to the Top: Inconsistency and falsification in intertheoretic reduction,” Philosophy of Science , 73: 582–594.
- –––, 2003. “Simulated Experiments: Methodology for a Virtual World,” Philosophy of Science , 70: 105–125.
- –––, 2001. “Simulations, Models, and Theories: Complex Physical Systems and their Representations,” Philosophy of Science , 68: S442–S454.
- –––, 1999. “Sanctioning Models: The Epistemology of Simulation,” Science in Context , 12(3): 275–92.
How to cite this entry . Preview the PDF version of this entry at the Friends of the SEP Society . Look up topics and thinkers related to this entry at the Internet Philosophy Ontology Project (InPhO). Enhanced bibliography for this entry at PhilPapers , with links to its database.
- Phys.org – computer simulations .
- Computer simulation , at sciencedaily.com.
- IPPC – Intergovernmental Panel on Climate Change .
biology: experiment in | computation: in physical systems | computer science, philosophy of | computing: modern history of | emergent properties | models in science | physics: experiment in | science: theory and observation in | scientific representation | scientific theories: structure of
Copyright © 2019 by Eric Winsberg < winsberg @ usf . edu >
- Accessibility
Support SEP
Mirror sites.
View this site from another server:
- Info about mirror sites
The Stanford Encyclopedia of Philosophy is copyright © 2023 by The Metaphysics Research Lab , Department of Philosophy, Stanford University
Library of Congress Catalog Data: ISSN 1095-5054
Help | Advanced Search
Computer Science > Computation and Language
Title: comm: collaborative multi-agent, multi-reasoning-path prompting for complex problem solving.
Abstract: Large Language Models (LLMs) have shown great ability in solving traditional natural language tasks and elementary reasoning tasks with appropriate prompting techniques. However, their ability is still limited in solving complicated science problems. In this work, we aim to push the upper bound of the reasoning capability of LLMs by proposing a collaborative multi-agent, multi-reasoning-path (CoMM) prompting framework. Specifically, we prompt LLMs to play different roles in a problem-solving team, and encourage different role-play agents to collaboratively solve the target task. In particular, we discover that applying different reasoning paths for different roles is an effective strategy to implement few-shot prompting approaches in the multi-agent scenarios. Empirical results demonstrate the effectiveness of the proposed methods on two college-level science problems over competitive baselines. Our further analysis shows the necessity of prompting LLMs to play different roles or experts independently. We release the code at: this https URL
Submission history
Access paper:.
- HTML (experimental)
- Other Formats

References & Citations
- Google Scholar
- Semantic Scholar
BibTeX formatted citation

Bibliographic and Citation Tools
Code, data and media associated with this article, recommenders and search tools.
- Institution
arXivLabs: experimental projects with community collaborators
arXivLabs is a framework that allows collaborators to develop and share new arXiv features directly on our website.
Both individuals and organizations that work with arXivLabs have embraced and accepted our values of openness, community, excellence, and user data privacy. arXiv is committed to these values and only works with partners that adhere to them.
Have an idea for a project that will add value for arXiv's community? Learn more about arXivLabs .
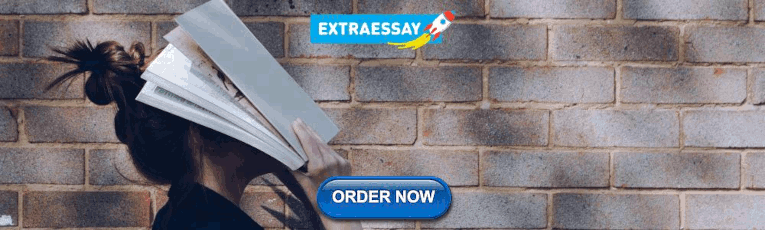
IMAGES
VIDEO
COMMENTS
Computer simulation (alternately computer modeling) is the study and practice of creating models of actual processes on the computer.Computer simulation can be used in biology, physics, economics, chemistry, engineering, and other scientific fields: it is often more economical, faster, or otherwise preferable to study a simulation of a process of interest, rather than studying the process itself.
In recent years, interactive computer simulations have been progressively integrated in the teaching of the sciences and have contributed significant improvements in the teaching-learning process. Practicing problem-solving is a key factor in science and engineering education. The aim of this study was to design simulation-based problem-solving teaching materials and assess their ...
Problem-Based Learning Combined with Computer Simulation on Students' Problem-Solving and Creative Thinking Skills. International Journal of Instruction, 14(3), 519-534. ... combined with simulation on students' problem-solving and creative thinking skills. By employing a quasi-experimental design, this study used essay and ...
An authoritative guide to computer simulation grounded in a multi-disciplinary approach for solving complex problems. Simulation and Computational Red Teaming for Problem Solving offers a review of computer simulation that is grounded in a multi-disciplinary approach.The authors present the theoretical foundations of simulation and modeling paradigms from the perspective of an analyst.
and problem solving through computer simulation: to what extent we now have theories for these phenomena, and what the content of these theories is. Since we want to talk about these substantive matters, we shall simply make the following assertions, which are validated by existing computer programs.
This special issue collects four historical case studies that focus on exemplary instances of what are today regarded as computer simulations: mathematical problem-solving with the ENIAC computer (Electronic Numerical Integrator and Computer) in the 1940s; the introduction of Monte Carlo simulations in particle physics of the 1960s; the development of the Paris-Durham shock model in ...
Despite national curricula and instructional reforms calling for collaborative problem-solving skills (CPS), however, there is an absence of a theory-laden model showing how to effectively construct CPS for science learning. We therefore developed and validated a simulation-based CPS model that exploits its constructs, sequences, and causal relationships, and evaluating its effectiveness on ...
An authoritative guide to computer simulation grounded in a multi-disciplinary approach for solving complex problems. Simulation and Computational Red Teaming for Problem Solving offers a review of computer simulation that is grounded in a multi-disciplinary approach.The authors present the theoretical foundations of simulation and modeling paradigms from the perspective of an analyst.
The second half was (a) a computer simulation or (b) traditional enrichment and application exercises. Following the last day of instruction, students were tested on basic facts, concepts, and health problem-solving skills. Posttest results indicated significant differences on basic facts and concepts that were reinforced by the simulation (p ...
Computer simulation is a step-by-step process in which a computer simulation program is modeled after a real-world system (a system can be a car, a building or even a tumor). In order to replicate the system and possible outcomes, the simulation uses mathematical equations to create an algorithm that defines the system's state, or the ...
This setup provides a computational environment in which simulations can be started and analyzed in the same notebook. A key learning activity is a project in which students tackle a given task over a period of approximately 2 months in a small group. Our experience is that the self-paced problem-solving nature of the project work -- combined ...
Computer Simulation of Human Thinking: A theory of problem solving expressed as a computer program permits simulation of thinking processes. Allen Newell and Herbert A. Simon Authors Info & Affiliations. Science. 22 Dec 1961. Vol 134, Issue 3495. pp. 2011-2017. DOI: 10.1126/science.134.3495.2011.
Computer Simulation of Human Thinking A theory of problem solving expressed as a computer program permits simulation of thinking processes. Allen Newell and Herbert A. Simon The path of scientific investigation in any field of knowledge records a response to two opposing pulls. On the one side, a powerful attraction is ex-erted by "good ...
The General Problem Solver (GPS) was a theory of human problem solving stated in the form of a simulation program (Ernst & Newell, 1969; Newell & Simon, 1972). This program and the associated theoretical framework had a significant impact on the subsequent direction of cognitive psychology. It also introduced the use of productions as a method ...
Achievement in solving the problems Table 3 shows the achievement of the EGs and CGs for schools A and B, respectively, as well as for the sum of schools A and B, but with school A entering in the latter sum only through problem 1 (the reason will become clear below). Recall that school A did both problems, while school B tackled only problem 2. The table also contains data for the statistical ...
Teaching Problem Solving. one way to enhance these kinds of cognitive skills is through educational. simulations. Simulations are thought to increase student participation (Boocock & Schild, 1968; Farran, 1968; Stembler, 1975) and allow low-. achieving students much-needed practice in applying what they've learned.
Mental simulation is the process of consciously enacting a sequence of events. Mental simulation plays an important role in phenomena such as problem solving, judgment, decision making, and planning. The Recognition-Primed Decision model explains how people can make decisions without having to compare options.
Due to this, the problem-solving capacity is found to be derived from the connection between these nodes []. As a result, numerous approaches of structuring mind have been observed to be simulating, starting from creation of artificial neurons to depicting mind as a collection of symbols, rules and plans. ... ACT-R is a computer simulation or ...
In recent years, interactive computer simulations have been progressively integrated in the teaching of the sciences and have contributed significant improvements in the teaching-learning process. Practicing problem-solving is a key factor in science and engineering education. The aim of this study was to design simulation-based problem-solving teaching materials and assess their effectiveness ...
Problem-solving. Perhaps the most important set of skills is a clear understanding of the problem you're trying to solve. Anyone can write a line of code. ... Computer simulation and modeling tools like MATLAB are evolving every day. New technologies will enable us to do more, with less effort. The key for any employee is to continuously stay ...
Computer simulation was pioneered as a scientific tool in meteorology and nuclear physics in the period directly following World War II, and since then has become indispensable in a growing number of disciplines. The list of sciences that make extensive use of computer simulation has grown to include astrophysics, particle physics, materials ...
In review, a simulation program is a computer model of a real-world phenomenon. These simulation programs can help people visualize a real-world problem to find possible solutions to the problem.
SOME PERSPECTIVES ON COMPUTER SIMULATION We are all familiar with the many information-processing, problem-solving uses of digital computers. But the symbol-manipulating abilities of a computer suit it to other duties as well. For example, computer programs have been developed that prove theorems in mathematics and symbolic logic, recognize
Recent advancements in large language models (LLMs) have showcased their exceptional abilities across various tasks, such as code generation, problem-solving and reasoning. Existing benchmarks evaluate tasks in isolation, yet the extent to which LLMs can understand prose-style tasks, identify the underlying problems, and then generate appropriate code solutions is still unexplored. Addressing ...
Addressing the challenge of automated geometry math problem-solving in artificial intelligence (AI) involves understanding multi-modal information and mathematics. Current methods struggle with accurately interpreting geometry diagrams, which hinders effective problem-solving. To tackle this issue, we present the Geometry problem sOlver with natural Language Description (GOLD) model. GOLD ...
The P vs NP question is a problem in mathematics and computer science, but that does not mean it will be confined there. May 03, 2024 05:30 am | Updated 05:30 am IST
View a PDF of the paper titled Finite volume simulation of a semi-linear Neumann problem (Keller-Segel model) on rectangular domains, by Nardjess Benoudina and 2 other authors. View PDF HTML (experimental) Abstract: In this study, the finite volume method is implemented for solving the problem of the semilinear equation: $-d \delta u+ u=u^q (d ...
Solving the Graph Burning Problem for Large Graphs. We propose an exact algorithm for the Graph Burning Problem ( GBP ), an NP-hard optimization problem that models the spread of influence on social networks. Given a graph G with vertex set V, the objective is to find a sequence of k vertices in V, namely, v1,v2, …,vk, such that k is minimum ...
Large Language Models (LLMs) have shown great ability in solving traditional natural language tasks and elementary reasoning tasks with appropriate prompting techniques. However, their ability is still limited in solving complicated science problems. In this work, we aim to push the upper bound of the reasoning capability of LLMs by proposing a collaborative multi-agent, multi-reasoning-path ...