- Privacy Policy

Home » Factor Analysis – Steps, Methods and Examples
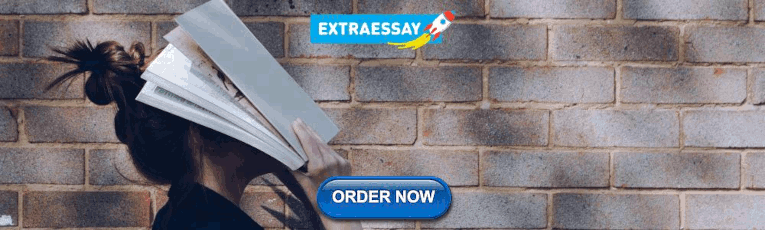
Factor Analysis – Steps, Methods and Examples
Table of Contents

Factor Analysis
Definition:
Factor analysis is a statistical technique that is used to identify the underlying structure of a relatively large set of variables and to explain these variables in terms of a smaller number of common underlying factors. It helps to investigate the latent relationships between observed variables.
Factor Analysis Steps
Here are the general steps involved in conducting a factor analysis:
1. Define the Research Objective:
Clearly specify the purpose of the factor analysis. Determine what you aim to achieve or understand through the analysis.
2. Data Collection:
Gather the data on the variables of interest. These variables should be measurable and related to the research objective. Ensure that you have a sufficient sample size for reliable results.
3. Assess Data Suitability:
Examine the suitability of the data for factor analysis. Check for the following aspects:
- Sample size: Ensure that you have an adequate sample size to perform factor analysis reliably.
- Missing values: Handle missing data appropriately, either by imputation or exclusion.
- Variable characteristics: Verify that the variables are continuous or at least ordinal in nature. Categorical variables may require different analysis techniques.
- Linearity: Assess whether the relationships among variables are linear.
4. Determine the Factor Analysis Technique:
There are different types of factor analysis techniques available, such as exploratory factor analysis (EFA) and confirmatory factor analysis (CFA). Choose the appropriate technique based on your research objective and the nature of the data.
5. Perform Factor Analysis:
a. Exploratory Factor Analysis (EFA):
- Extract factors: Use factor extraction methods (e.g., principal component analysis or common factor analysis) to identify the initial set of factors.
- Determine the number of factors: Decide on the number of factors to retain based on statistical criteria (e.g., eigenvalues, scree plot) and theoretical considerations.
- Rotate factors: Apply factor rotation techniques (e.g., varimax, oblique) to simplify the factor structure and make it more interpretable.
- Interpret factors: Analyze the factor loadings (correlations between variables and factors) to interpret the meaning of each factor.
- Determine factor reliability: Assess the internal consistency or reliability of the factors using measures like Cronbach’s alpha.
- Report results: Document the factor loadings, rotated component matrix, communalities, and any other relevant information.
b. Confirmatory Factor Analysis (CFA):
- Formulate a theoretical model: Specify the hypothesized relationships among variables and factors based on prior knowledge or theoretical considerations.
- Define measurement model: Establish how each variable is related to the underlying factors by assigning factor loadings in the model.
- Test the model: Use statistical techniques like maximum likelihood estimation or structural equation modeling to assess the goodness-of-fit between the observed data and the hypothesized model.
- Modify the model: If the initial model does not fit the data adequately, revise the model by adding or removing paths, allowing for correlated errors, or other modifications to improve model fit.
- Report results: Present the final measurement model, parameter estimates, fit indices (e.g., chi-square, RMSEA, CFI), and any modifications made.
6. Interpret and Validate the Factors:
Once you have identified the factors, interpret them based on the factor loadings, theoretical understanding, and research objectives. Validate the factors by examining their relationships with external criteria or by conducting further analyses if necessary.
Types of Factor Analysis
Types of Factor Analysis are as follows:
Exploratory Factor Analysis (EFA)
EFA is used to explore the underlying structure of a set of observed variables without any preconceived assumptions about the number or nature of the factors. It aims to discover the number of factors and how the observed variables are related to those factors. EFA does not impose any restrictions on the factor structure and allows for cross-loadings of variables on multiple factors.
Confirmatory Factor Analysis (CFA)
CFA is used to test a pre-specified factor structure based on theoretical or conceptual assumptions. It aims to confirm whether the observed variables measure the latent factors as intended. CFA tests the fit of a hypothesized model and assesses how well the observed variables are associated with the expected factors. It is often used for validating measurement instruments or evaluating theoretical models.
Principal Component Analysis (PCA)
PCA is a dimensionality reduction technique that can be considered a form of factor analysis, although it has some differences. PCA aims to explain the maximum amount of variance in the observed variables using a smaller number of uncorrelated components. Unlike traditional factor analysis, PCA does not assume that the observed variables are caused by underlying factors but focuses solely on accounting for variance.
Common Factor Analysis
It assumes that the observed variables are influenced by common factors and unique factors (specific to each variable). It attempts to estimate the common factor structure by extracting the shared variance among the variables while also considering the unique variance of each variable.
Hierarchical Factor Analysis
Hierarchical factor analysis involves multiple levels of factors. It explores both higher-order and lower-order factors, aiming to capture the complex relationships among variables. Higher-order factors are based on the relationships among lower-order factors, which are in turn based on the relationships among observed variables.
Factor Analysis Formulas
Factor Analysis is a statistical method used to describe variability among observed, correlated variables in terms of a potentially lower number of unobserved variables called factors.
Here are some of the essential formulas and calculations used in factor analysis:
Correlation Matrix :
The first step in factor analysis is to create a correlation matrix, which calculates the correlation coefficients between pairs of variables.
Correlation coefficient (Pearson’s r) between variables X and Y is calculated as:
r(X,Y) = Σ[(xi – x̄)(yi – ȳ)] / [n-1] σx σy
where: xi, yi are the data points, x̄, ȳ are the means of X and Y respectively, σx, σy are the standard deviations of X and Y respectively, n is the number of data points.
Extraction of Factors :
The extraction of factors from the correlation matrix is typically done by methods such as Principal Component Analysis (PCA) or other similar methods.
The formula used in PCA to calculate the principal components (factors) involves finding the eigenvalues and eigenvectors of the correlation matrix.
Let’s denote the correlation matrix as R. If λ is an eigenvalue of R, and v is the corresponding eigenvector, they satisfy the equation: Rv = λv
Factor Loadings :
Factor loadings are the correlations between the original variables and the factors. They can be calculated as the eigenvectors normalized by the square roots of their corresponding eigenvalues.
Communality and Specific Variance :
Communality of a variable is the proportion of variance in that variable explained by the factors. It can be calculated as the sum of squared factor loadings for that variable across all factors.
The specific variance of a variable is the proportion of variance in that variable not explained by the factors, and it’s calculated as 1 – Communality.
Factor Rotation : Factor rotation, such as Varimax or Promax, is used to make the output more interpretable. It doesn’t change the underlying relationships but affects the loadings of the variables on the factors.
For example, in the Varimax rotation, the objective is to minimize the variance of the squared loadings of a factor (column) on all the variables (rows) in a factor matrix, which leads to more high and low loadings, making the factor easier to interpret.
Examples of Factor Analysis
Here are some real-time examples of factor analysis:
- Psychological Research: In a study examining personality traits, researchers may use factor analysis to identify the underlying dimensions of personality by analyzing responses to various questionnaires or surveys. Factors such as extroversion, neuroticism, and conscientiousness can be derived from the analysis.
- Market Research: In marketing, factor analysis can be used to understand consumers’ preferences and behaviors. For instance, by analyzing survey data related to product features, pricing, and brand perception, researchers can identify factors such as price sensitivity, brand loyalty, and product quality that influence consumer decision-making.
- Finance and Economics: Factor analysis is widely used in portfolio management and asset pricing models. By analyzing historical market data, factors such as market returns, interest rates, inflation rates, and other economic indicators can be identified. These factors help in understanding and predicting investment returns and risk.
- Social Sciences: Factor analysis is employed in social sciences to explore underlying constructs in complex datasets. For example, in education research, factor analysis can be used to identify dimensions such as academic achievement, socio-economic status, and parental involvement that contribute to student success.
- Health Sciences: In medical research, factor analysis can be utilized to identify underlying factors related to health conditions, symptom clusters, or treatment outcomes. For instance, in a study on mental health, factor analysis can be used to identify underlying factors contributing to depression, anxiety, and stress.
- Customer Satisfaction Surveys: Factor analysis can help businesses understand the key drivers of customer satisfaction. By analyzing survey responses related to various aspects of product or service experience, factors such as product quality, customer service, and pricing can be identified, enabling businesses to focus on areas that impact customer satisfaction the most.
Factor analysis in Research Example
Here’s an example of how factor analysis might be used in research:
Let’s say a psychologist is interested in the factors that contribute to overall wellbeing. They conduct a survey with 1000 participants, asking them to respond to 50 different questions relating to various aspects of their lives, including social relationships, physical health, mental health, job satisfaction, financial security, personal growth, and leisure activities.
Given the broad scope of these questions, the psychologist decides to use factor analysis to identify underlying factors that could explain the correlations among responses.
After conducting the factor analysis, the psychologist finds that the responses can be grouped into five factors:
- Physical Wellbeing : Includes variables related to physical health, exercise, and diet.
- Mental Wellbeing : Includes variables related to mental health, stress levels, and emotional balance.
- Social Wellbeing : Includes variables related to social relationships, community involvement, and support from friends and family.
- Professional Wellbeing : Includes variables related to job satisfaction, work-life balance, and career development.
- Financial Wellbeing : Includes variables related to financial security, savings, and income.
By reducing the 50 individual questions to five underlying factors, the psychologist can more effectively analyze the data and draw conclusions about the major aspects of life that contribute to overall wellbeing.
In this way, factor analysis helps researchers understand complex relationships among many variables by grouping them into a smaller number of factors, simplifying the data analysis process, and facilitating the identification of patterns or structures within the data.
When to Use Factor Analysis
Here are some circumstances in which you might want to use factor analysis:
- Data Reduction : If you have a large set of variables, you can use factor analysis to reduce them to a smaller set of factors. This helps in simplifying the data and making it easier to analyze.
- Identification of Underlying Structures : Factor analysis can be used to identify underlying structures in a dataset that are not immediately apparent. This can help you understand complex relationships between variables.
- Validation of Constructs : Factor analysis can be used to confirm whether a scale or measure truly reflects the construct it’s meant to measure. If all the items in a scale load highly on a single factor, that supports the construct validity of the scale.
- Generating Hypotheses : By revealing the underlying structure of your variables, factor analysis can help to generate hypotheses for future research.
- Survey Analysis : If you have a survey with many questions, factor analysis can help determine if there are underlying factors that explain response patterns.
Applications of Factor Analysis
Factor Analysis has a wide range of applications across various fields. Here are some of them:
- Psychology : It’s often used in psychology to identify the underlying factors that explain different patterns of correlations among mental abilities. For instance, factor analysis has been used to identify personality traits (like the Big Five personality traits), intelligence structures (like Spearman’s g), or to validate the constructs of different psychological tests.
- Market Research : In this field, factor analysis is used to identify the factors that influence purchasing behavior. By understanding these factors, businesses can tailor their products and marketing strategies to meet the needs of different customer groups.
- Healthcare : In healthcare, factor analysis is used in a similar way to psychology, identifying underlying factors that might influence health outcomes. For instance, it could be used to identify lifestyle or behavioral factors that influence the risk of developing certain diseases.
- Sociology : Sociologists use factor analysis to understand the structure of attitudes, beliefs, and behaviors in populations. For example, factor analysis might be used to understand the factors that contribute to social inequality.
- Finance and Economics : In finance, factor analysis is used to identify the factors that drive financial markets or economic behavior. For instance, factor analysis can help understand the factors that influence stock prices or economic growth.
- Education : In education, factor analysis is used to identify the factors that influence academic performance or attitudes towards learning. This could help in developing more effective teaching strategies.
- Survey Analysis : Factor analysis is often used in survey research to reduce the number of items or to identify the underlying structure of the data.
- Environment : In environmental studies, factor analysis can be used to identify the major sources of environmental pollution by analyzing the data on pollutants.
Advantages of Factor Analysis
Advantages of Factor Analysis are as follows:
- Data Reduction : Factor analysis can simplify a large dataset by reducing the number of variables. This helps make the data easier to manage and analyze.
- Structure Identification : It can identify underlying structures or patterns in a dataset that are not immediately apparent. This can provide insights into complex relationships between variables.
- Construct Validation : Factor analysis can be used to validate whether a scale or measure accurately reflects the construct it’s intended to measure. This is important for ensuring the reliability and validity of measurement tools.
- Hypothesis Generation : By revealing the underlying structure of your variables, factor analysis can help generate hypotheses for future research.
- Versatility : Factor analysis can be used in various fields, including psychology, market research, healthcare, sociology, finance, education, and environmental studies.
Disadvantages of Factor Analysis
Disadvantages of Factor Analysis are as follows:
- Subjectivity : The interpretation of the factors can sometimes be subjective, depending on how the data is perceived. Different researchers might interpret the factors differently, which can lead to different conclusions.
- Assumptions : Factor analysis assumes that there’s some underlying structure in the dataset and that all variables are related. If these assumptions do not hold, factor analysis might not be the best tool for your analysis.
- Large Sample Size Required : Factor analysis generally requires a large sample size to produce reliable results. This can be a limitation in studies where data collection is challenging or expensive.
- Correlation, not Causation : Factor analysis identifies correlational relationships, not causal ones. It cannot prove that changes in one variable cause changes in another.
- Complexity : The statistical concepts behind factor analysis can be difficult to understand and require expertise to implement correctly. Misuse or misunderstanding of the method can lead to incorrect conclusions.
About the author
Muhammad Hassan
Researcher, Academic Writer, Web developer
You may also like

Cluster Analysis – Types, Methods and Examples

Discriminant Analysis – Methods, Types and...

MANOVA (Multivariate Analysis of Variance) –...

Documentary Analysis – Methods, Applications and...

ANOVA (Analysis of variance) – Formulas, Types...

Graphical Methods – Types, Examples and Guide

An official website of the United States government
The .gov means it’s official. Federal government websites often end in .gov or .mil. Before sharing sensitive information, make sure you’re on a federal government site.
The site is secure. The https:// ensures that you are connecting to the official website and that any information you provide is encrypted and transmitted securely.
- Publications
- Account settings
Preview improvements coming to the PMC website in October 2024. Learn More or Try it out now .
- Advanced Search
- Journal List
- CBE Life Sci Educ
- v.18(1); Spring 2019
One Size Doesn’t Fit All: Using Factor Analysis to Gather Validity Evidence When Using Surveys in Your Research
† Department of Science and Mathematics Education, Umeå University, 901 87 Umeå, Sweden
‡ Department of Biological Sciences, Florida International University, Miami, FL 33199
Christopher Runyon
§ Department of Educational Psychology, University of Texas at Austin, Austin, TX 78712
ǁ National Board of Medical Examiners, Philadelphia, PA 19104
Associated Data
Across all sciences, the quality of measurements is important. Survey measurements are only appropriate for use when researchers have validity evidence within their particular context. Yet, this step is frequently skipped or is not reported in educational research. This article briefly reviews the aspects of validity that researchers should consider when using surveys. It then focuses on factor analysis, a statistical method that can be used to collect an important type of validity evidence. Factor analysis helps researchers explore or confirm the relationships between survey items and identify the total number of dimensions represented on the survey. The essential steps to conduct and interpret a factor analysis are described. This use of factor analysis is illustrated throughout by a validation of Diekman and colleagues’ goal endorsement instrument for use with first-year undergraduate science, technology, engineering, and mathematics students. We provide example data, annotated code, and output for analyses in R, an open-source programming language and software environment for statistical computing. For education researchers using surveys, understanding the theoretical and statistical underpinnings of survey validity is fundamental for implementing rigorous education research.
THE USE OF SURVEYS IN BIOLOGY EDUCATION RESEARCH
Surveys and achievement tests are common tools used in biology education research to measure students’ attitudes, feelings, and knowledge. In the early days of biology education research, researchers designed their own surveys (also referred to as “measurement instruments” 1 ) to obtain information about students. Generally, each question on these instruments asked about something different and did not involve extensive use of measures of validity to ensure that researchers were, in fact, measuring what they intended to measure ( Armbruster et al. , 2009 ; Rissing and Cogan, 2009 ; Eddy and Hogan, 2014 ). In recent years, researchers have begun adopting existing measurement instruments. This shift may be due to researchers’ increased recognition of the amount of work that is necessary to create and validate survey instruments (cf. Andrews et al. , 2017 ; Wachsmuth et al. , 2017 ; Wiggins et al. , 2017 ). While this shift is a methodological advancement, as a community of researchers we still have room to grow. As biology education researchers who use surveys, we need to understand both the theoretical and statistical underpinnings of validity to appropriately employ instruments within our contexts. As a community, biology education researchers need to move beyond simply adopting a “validated” instrument to establishing the validity of the scores produced by the instrument for a researcher’s intended interpretation and use. This will allow education researchers to produce more rigorous and replicable science. In this primer, we walk the reader through important validity aspects to consider and report when using surveys in their specific context.
Measuring Variables That Are Not Directly Observable
Some variables measured in education studies are directly observable. For example, the percent of international students in a class or the amount of time students spend on a specific task can both be directly observed by the researcher. Other variables that researchers may want to measure are not directly observable, such as students’ attitudes, feelings, and knowledge. The measurement of unobservable variables is what we focus on in this primer. To study these unobservable variables, researchers collect several related observable variables (responses to survey items) and use them to make inferences about the unobservable variable, termed “latent variable” or “construct” 2 in the measurement literature. For example, when assessing students’ knowledge of evolution, it is intuitive that a single item (i.e., a test question) would not be sufficient to make judgments about the entirety of students’ evolution knowledge. Instead, students’ scores from several items measuring different aspects of evolution are combined into a sum score. The measurement of attitudes and feelings (e.g., students’ goals, students’ interest in biology) is no different. For example, say a researcher wanted to understand the degree to which students embrace goals focused on improving themselves, agentic goals , as will be seen in our illustrating example in this primer. Instead of asking students one question about how important it is for them to improve themselves, an instrument was created to include a number of items that focus on slightly different aspects of improving the self. The observed responses to these survey items can then be combined to represent the construct agentic goal endorsement . To combine a number of items to represent one construct, the researcher must provide evidence that these items truly represent the same construct. In this paper, we provide an overview of the evidence necessary to have confidence in using a survey instrument for one’s specific purpose and go into depth for one type of statistical evidence for validity: factor analysis.
The aims of this article are 1) to briefly review the theoretical background for instrument validation and 2) to provide a step-by-step description of how to use factor analysis to gather evidence about the number and nature of constructs in an instrument. We begin with a brief theoretical background about validity and constructs to situate factor analysis in the larger context of instrument validation. Next, we discuss coefficient alpha, a statistic currently used, and often misused, in educational research as evidence for validity. The remainder of the article explores the statistical method of factor analysis. We describe what factor analysis is, when it is appropriate to use it, what we can learn from it, and the essential steps in conducting it. An evaluation of the number and nature of constructs in the Diekman et al. (2010) goal-endorsement instrument when used with first-year undergraduate science, technology, engineering, and mathematics (STEM) students is provided to illustrate the steps involved in conducting a factor analysis and how to report it in a paper (see Boxes 1 – 7 ). The illustrating example comes from a unique data collection and analysis made by the authors of this article. Data, annotated code, and output from the analyses run in R (an open-source programming language and software environment for statistical computing; R Core Team, 2016 ) for this example are included in the Supplemental Material.
BOX 1. How to describe the purpose (abbreviated), instrument, and sample for publication illustrated with the goal-endorsement example
Defining the construct and intended use of the instrument.
The aim of this study was to analyze the internal structure of the goal-endorsement instrument described by Diekman et al. (2010) for use with incoming first-year university STEM students. The objective is to use the knowledge gained through the survey to design STEM curricula that might leverage the goals students perceive as most important to increase student interest in their STEM classes.
The theoretical framework leading to the development of this survey has a long and well-established history. In 1966, Bakan (1966) originally proposed that two orientations could be used to characterize the human experience: agentic (orientation to the self) and communal (orientation to others). Agentic goals can thus be seen as goals focusing on improving the self or one’s own circumstances. Communal goals are goals focusing on helping others and one’s community and being part of a community. Gender socialization theory contributed to our understanding of who holds these goals most strongly: women are socialized to desire and assume more communal roles, while males assume more agentic roles ( Eagly et al. , 2000 ; Prentice and Carranza, 2002 ; Su et al. , 2009 ).
This framework and survey were first used in the context of STEM education by Diekman et al. (2010) . They found these two goal orientations to be predictive of women’s attrition from STEM, particularly when they perceive STEM careers to be at odds with the communal goals important to them. Current research in this area has expanded beyond the focus on gender differences and has recognized that all humans value communal goals to some degree and that there is also variation in importance placed on communal goals by racial and ethnic groups ( Smith et al. , 2014 ), social class (Stephens et al. , 2012), and college generation status ( Allen et al. , 2015 ). The majority of this work has been done with the general population of undergraduates. Our proposed use of the survey is to explore the variation in goals among different groups in a STEM-exclusive sample.
The instrument
The goal-endorsement survey described by Diekman et al. , (2010) aims to measure how others-focused (communal) versus self-focused (agentic) students are. The instrument asks students to rate “how important each of the following kinds of goals [is] to you personally” on a scale of 1 (not at all important) to 7 (very important). The original measurement instrument has 23 items that have been reported as two factors: agentic (14 items) and communal (nine items) goals (see Table 2 for a listing of the items). The survey has been used many times in different contexts and has been shown to be predictive in ways hypothesized by theory. Diekman et al. (2010) briefly report on an EFA supporting the proposed two-factor structure of the instrument with a sample of undergraduates from introductory psychology courses.
Data collection and participants
The questionnaire was distributed in Fall 2015 and 2016 to entering first-year undergraduate students in STEM fields (biology, biochemistry, physics, chemistry, math, and computer science) at a large southern U.S. R1 university. Students took the questionnaire in the weeks before their first Fall semester. In total, 796 students (70% women) completed the questionnaire. Fifteen percent of the students were first-generation students, and 24% came from underrepresented minorities.
Sample size
In our study, the total sample size was 796 students. Considering the number of factors (two) and the relatively large number of items per factor (nine and 14), the sample size was deemed more than sufficient to perform factor analysis ( Gagne and Hancock, 2006 ; Wolf et al. , 2013 ).
BOX 7. Writing conclusions from factor analysis for publication using the goal-endorsement example
Conclusions.
The results from the factor analysis did not confirm the proposed two-factor goal-endorsement scale for use with college STEM majors. Instead, our results indicated five subscales: prestige, autonomy, competency, service, and connection ( Table 4 ). The five-factor solution aligned with Diekman et al. ’s (2010) original two-factor scale, because communal items did not mix with agentic items. Our sample did, however, allows us to further refine the solution for the original two scales. Finer parsing of the agentic and communal scales may help identify important differences between students and allow researchers to better understand factors contributing to retention in STEM majors. In addition, with items related to autonomy and competency moved to their own scales, the refined prestige scale focusing on factors like power, recognition, and status may be a more direct contrast to the service scale. Additional evidence in support of this refinement include that the five-factor solution better distinguishes the service scale and the prestige scale (factor correlation = 0.21) than the two-factor solution (factor correlation between agentic and communal factors = 0.35). Further, retention may be significantly correlated to prestige but not to autonomy. Alternatively, differences between genders may exist for the service scale but not the connection scale.
Proposed five-factor solution. Items within each factor are ordered by highest to lowest factor loadings
On the basis of the result of this factor analysis, we recommend using the five-factor solution for interpreting the results of the current data set, but interpret the connection and competency scales with some caution, for reasons summarized in the next section.
Limitations and future studies
The proposed five-factor solution needs additional work. In particular, both the competency and connection scales need further development. Only two items represented connection, and this is not adequate to represent the full aspect of this construct, especially to make it clearly distinct from the construct of service. The competency scale included only three items, coefficient alpha was 0.66, and factor loadings for the scale were low (<0.40) for demonstrating skills or competency.
Another limitation of this study is that the sample consisted of 70% women, an overrepresentation of women for a typical undergraduate STEM population. Further studies should confirm whether the suggested dimensionality holds in a more representative sample. Future studies should also test whether the instrument has the same structure with STEM students from different backgrounds (i.e., measurement invariance should be investigated). The work presented here only establishes the dimensionality of the survey. We recommend the collection of other types of validity evidence, such as evidence based on content or relationships to other variables, to further strengthen our confidence that the scores from this survey represent STEM students’ goal orientation.
WHAT IS VALIDITY?
The quality of measurements is important to all sciences. Although different terms are used in different disciplines, the underlining principles and problems are the same across disciplines. For example, in physics, the terms “accuracy” and “precision” are commonly used to describe how confident researchers should be in their measurements. In the discourse about survey quality, validity and reliability are the key concepts for measurement quality. Roughly, validity refers to whether an instrument actually measures what it is designed to measure and reliability is the consistency of the instrument’s measurements.
In this section, we will briefly outline what validity is and the many types of validity evidence. Reliability, and its relation to validity, will be discussed in The Misuse of Coefficient Alpha . Before getting into the details, we need to emphasize a critical concept about validity that is often overlooked: validity is not a characteristic of an instrument, but rather a characteristic of the use of an instrument in a particular context. Anytime an instrument is used in a new context, at least some measures of its validity must be established for that specific context.
Validity Is Not a Property of the Instrument
The concept of validity within educational measurements has been acknowledged and discussed for a long time (e.g., Cronbach and Meehl, 1955 ; Messick, 1995 ; Cizek, 2016 ; Kane, 2016 ; Slaney, 2017 ). According to the latest Standards for Educational and Psychological Testing published by the American Educational Research Association (AERA), American Psychology Association (APA) & National Council on Measurement in Education (NCME) in 2014:
Validity refers to the degree of which evidence and theory support the interpretations of the test score for the proposed use. ( AERA, APA, and NCME, 2014 , p. 11)
Thus, validity is not a property of the measurement instrument but rather refers to its proposed interpretation and use. Validity must be considered each time an instrument is used ( Kane, 2016 ). An instrument may be validated for a certain population and purpose, but that does not mean it will work across all populations and for all purposes. For example, a validation of Diekman’s goal-endorsement instrument (Diekman et al., 2010) as a reasonable measure of university students’ goal endorsement does not automatically validate the use of the instrument for measuring 6-year-olds’ goal endorsement. Similarly, a test validated for one purpose, such as being a reasonable measure of sixth-grade mathematical achievement, does not automatically validate it for use with other purposes, such as placement and advancement decisions ( Kane, 2016 ). The validation of a survey may also be time sensitive, as cultures continually change. Using a survey from the 1980s about the use of technology would be employing a dated view of what is meant by “technology” today.
Types of Validity Evidence
Validation is a continuous and iterative process of collecting many different types of evidence to support that researchers are measuring what they aim to measure. The latest Standards for Educational and Psychological Testing describes many types of validity evidence to consider when validating an instrument for a particular purpose ( AERA, APA, and NCME, 2014 , chap. 1). These types of evidence and illustrative examples are summarized in Table 1 . For example, one important aspect to consider is whether the individual items that make up the survey are interpreted by the respondents in the way intended by the researcher. Researchers must also consider whether the individual items constitute a good representation of the construct and whether the items collectively represent all the important aspects of that construct. Looking at our illustrative example ( Box 1 and Table 2 ), we could ask whether items 15–23 (i.e., helping others, serving humanity, serving community, working with people, connection with others, attending to others, caring for others, intimacy, and spiritual rewards) in the goal-endorsement instrument constitute a good representation of the construct “helping others and one’s community”? Yet another type of validity evidence involves demonstrating that the scores obtained for a construct on an instrument of interest correlate to other measures of the same or closely related constructs.
Types of validity evidence to consider when validating an instrument according to the Standards for Educational and Psychological Testing ( AERA, APA, and NCME, 2014 )
a Many of the example considerations are in reference to the elements in the Diekman et al. (2010) instrument; we provide these only as motivating examples and encourage readers to apply the example within their own work.
b If and how to include consequences of testing as a measure of validity is highly debated in educational and psychological measurement (see Mehrens, 1997 ; Lissitz and Samuelsen, 2007 ; Borsboom et al. , 2004 ; Cizek, 2016 ; Kane, 2016 ). We chose to present the view of validity as described in the latest Standards for Educational and Psychological Testing ( AERA, APA, and NCME, 2014 ).
Items included in the Diekman et al. (2010) goal-endorsement instrument a
a Items 1–14 originally represented the agentic scale, and items 15–23 represented the communal scale. Standardized pattern coefficients from the initial EFA for the three-, four-, and five-factor solutions are reported in columns 3–14. For clarity, pattern coefficients <0.2 are not shown.
The use of existing surveys usually allows the collection of less validity evidence than the creation and use of a new survey. Specifically, if previous studies collected validity evidence for the use of the survey for a similar purpose and with a similar population as the intended research, researchers can then reference that validity evidence and present less of their own. It is important to note that, even if a survey has a long history of established use, this alone does not provide adequate validity evidence for it being an appropriate measurement instrument. It is worth researchers’ time to go through the published uses of the survey and identify all the different types of validity evidence that have been collected. They can then identify the additional evidence they want to collect to feel confident applying the instrument for their intended interpretation and use. For a more detailed description of different types of validity evidence and a pedagogical description of the process of instrument validation, see Reeves and Marbach-Ad (2016) and Andrews et al. (2017) .
In this article, we will focus on the third type of validity evidence listed in Table 1 , evidence based on internal structure. Investigating the internal structure of an instrument is crucial in order to be confident that you can combine several related items to represent a specific construct. We will describe an empirical tool to gather information about the internal relationships between items in a measurement instrument: factor analysis. On its own, factor analysis is not sufficient to establish the validity of the use of an instrument in a researcher’s context and for their purpose. However, when factor analysis is combined with other validity evidence, it can increase a researcher’s confidence that they are invoking the theoretical frameworks used in the development of the instrument: that is, the researcher is correctly interpreting the results as representing the construct the instrument purports to measure.
INSTRUMENT SCOPE: ONE OR SEVERAL CONSTRUCTS?
As described in Measuring Variables That Are Not Directly Observable , a construct cannot be directly measured. Instead, different aspects of a construct are represented by different individual items. The foundational assumption in instrument development is that the construct is what drives respondents to answer similarly on all these items. Thus, it is reasonable to distill the responses on all these items into one single score and make inferences about the construct. Measurement instruments can be used to measure a single construct, several distinct constructs, or even make finer distinctions within a construct. The number of intended constructs or aspects of a construct to be measured are referred to as an instrument’s dimensionality .
Unidimensional Scales
An instrument that aims to measure one underlying construct is a unidimensional scale. To interpret a set of items as if they measure the same construct, one must have both theoretical and empirical evidence that the items function as intended; that they do, indeed represent a single construct. If a researcher takes a single value (such as the mean) to represent a set of responses to a group of items that are unrelated to one another theoretically (e.g., I like biology, I enjoy doing dissection, I know how to write a biology lab report), the resulting value would be difficult to interpret at best, if not meaningless. While all of these items are related to biology, they do not represent a specific, common construct. Obviously, taking the mean response from these three items as a measure of interest in biology would be highly problematic. For example, one could be interested in biology but dislike dissection, and one’s laboratory writing skills are likely influenced by aspects other than interest in biology. Even when a set of items theoretically seem to measure the same construct, the researcher must empirically show that students demonstrate a coherent response pattern over the set of items to validate their use to measure the construct. If students do not demonstrate a coherent response, this indicates that the items are not functioning as intended and they may not all measure the same construct. Thus, the single value used to represent the construct from that group of items would contain very little information about the intended construct.
Multidimensional Scales
An instrument that is constructed to measure several related constructs or several different aspects of a construct is called a multidimensional scale. For example, the Diekman et al. (2010) goal-endorsement instrument (see items in Box 1 and Table 2 ) we use in this article is a multidimensional scale: it theoretically aims to measure two different aspects of student goal endorsement. To be able to separate the results into two subscales, one must test that the items measure distinctly different constructs. It is important to note that whether a set of items represents different constructs can differ depending on the intended populations, which is why collecting evidence on the researcher’s own population is so critical. Wigfield and Eccles (1992) illustrate this concept in a study of children of different ages. Children in early or middle elementary school did not seem to distinguish between their perceptions of interest, importance, and usefulness of mathematics, while older children did appear to differentiate between these constructs. Thus, while it is meaningful to discuss interest, importance, and usefulness as distinct constructs for older children, is it not meaningful to do so with younger children.
In summary, before using a survey, one has to have gathered all the appropriate validity evidence for the proposed interpretations and use. When measuring a construct, one important step in this validation procedure is to explicitly describe and empirically analyze the assumed dimensionality of the survey.
THE MISUSE OF COEFFICIENT ALPHA: UNDERSTANDING THE DIFFERENCE BETWEEN RELIABILITY AND VALIDITY
In many biology educational research papers, researchers only provide coefficient alpha (also called Cronbach’s alpha) as evidence of validity. For example, in Eddy et al. (2015) , the researchers describe the alpha of two scales on a survey and no other evidence of validity or dimensionality. This usage is widely agreed to be a misuse of coefficient alpha ( Green and Yang, 2009 ). To understand why this is the case, we have to understand how validity and reliability differ and what specifically coefficient alpha measures.
Reliability is about consistency when a testing procedure is repeated ( AERA, APA, and NCME, 2014 ). For example, assuming that students do not change their goal endorsement, do repeated measurements of students’ goal endorsement using Diekman’s goal-endorsement instrument give consistent results? Theoretically, reliability can be defined as the ratio between the true variance in the construct among the participating respondents (the latent, unobserved variance the researcher aims to interpret) and the observed variance as measured by the measurement instrument ( Crocker and Algina, 2008 ). The observed variance for an item is a combination of the true variance and measurement error. Measurement error is the extent that responses are affected by factors other than the construct of interest ( Fowler, 2014 ). For example, ideally, students’ responses to Diekman’s goal-endorsement instrument would only be affected by their actual goal endorsement. But students’ responses may also be affected by things unrelated to the construct of goal endorsement. For instance, responses on communal goals items may be influenced by social desirability, students’ desire to answer in a way that they think others would want them to. Students’ responses on items may also depend on external circumstances while they were completing the survey, such as time of the day or the noise level in their environment when they were taking the survey. While it is impossible to avoid measurement error completely, minimizing measurement error increases the ratio between the true and the observed variance, which increases the likelihood that the instrument will yield similar results over repeated use.
Unfortunately, a construct cannot, by definition, be directly measured; the true score variance is unknown. Thus, reliability itself cannot be directly measured and must be estimated. One way to estimate reliability is to distribute the instrument to the same group of students multiple times and analyze how similar the responses of the same students are over time. Often it is not desirable or practically feasible to distribute the same instrument multiple times. Coefficient alpha provides a means to estimate reliability for an instrument based on a single distribution. 3 Simply put, coefficient alpha is the correlation of an instrument to itself ( Tavakol and Dennick, 2011 ). Calculation of coefficient alpha is based on the assumption that all items in a scale measure the same construct. If the average correlation among items on a scale is high, then the scale is said to be reliable.
The use and misuse of coefficient alpha as an estimate of reliability has been extensively discussed by researchers (e.g., Green and Yang, 2009 ; Sijtsma, 2009 ; Raykov and Marcoulides, 2017 ; McNeish, 2018 ). It is outside the scope of this article to fully explain and take a stand among these arguments. Although coefficient alpha may be a good estimator of reliability under certain circumstances, it has limitations. We will further elaborate on two limitations that are most pertinent within the context of instrument validation.
Limitation 1: Coefficient Alpha Is about Reliability, Not Validity
A high coefficient alpha does not prove that researchers are measuring what they intended to measure, only that they measured the same thing consistently. In other words, coefficient alpha is an estimation of reliability. Reliability and validity complement each other: for valid interpretations to be made using an instrument, the reliability of that instrument must be high. However, if the test is invalid, then reliability does not matter. Thus, high reliability is necessary, but not sufficient, to make valid interpretations from scores resulting from instrument administration. Consider this analogy using observable phenomena: a calibrated scale might produce consistent values for the weight of a student and thus the measure is reliable, but using this score to make interpretations about the students’ height would be completely invalid. Similarly, a survey’s coefficient alpha could be high, but the survey instrument could still not be measuring what the researcher intended it to measure.
Limitation 2: Coefficient Alpha Does Not Provide Evidence of Dimensionality of the Scale
Coefficient alpha does not provide evidence for whether the instrument measures one or several underlying constructs ( Schmitt, 1996 ; Sijtsma, 2009 ; Yang and Green, 2011 ). Schmitt (1996) provides two illustrative examples of why a high coefficient alpha should not be taken as a proof of a unidimensional instrument. He shows that a six-item instrument, in which all items have equal correlations to one another (unidimensional instrument), could yield the same coefficient alpha as a six-item instrument with item correlations clearly showing a two-dimensional pattern (i.e., an instrument with item correlation of 0.5 across all items has the same coefficient alpha as an instrument with item correlations of 0.8 between some items and items correlations of 0.3 between other items). Thus, as Yang and Green (2011) conclude, “A scale can be unidimensional and have a low or a high coefficient alpha; a scale can be multidimensional and have a low or a high coefficient alpha” (p. 380).
In conclusion, reporting only coefficient alpha is not sufficient evidence 1) to make valid interpretations of the scores from an instrument or 2) to prove that a set of items measure only one underlying construct (unidimensionality). We encourage readers interested in learning more about reliability to read chapters 7–9 in Bandalos (2018) . In the following section, we describe another statistical tool, factor analysis, which actually tests the dimensionality among a set of items.
FACTOR ANALYSIS: EVIDENCE OF DIMENSIONALITY AMONG A SET OF ITEMS
Factor analysis is a statistical technique that analyzes the relationships between a set of survey items to determine whether the participant’s responses on different subsets of items relate more closely to one another than to other subsets, that is, it is an analysis of the dimensionality among the items ( Raykov and Marcoulides, 2008 ; Leandre et al. , 2012; Tabachnick and Fidell, 2013 ; Kline, 2016 ; Bandalos, 2018 ). This technique was explicitly developed to better elucidate the dimensionality underpinning sets of achievement test items ( Mulaik, 1987 ). Speaking in terms of constructs, factor analysis can be used to analyze whether it is likely that a certain set of items together measure a predefined construct (collecting validity evidence relating to internal structure; Table 1 ). Factor analysis can broadly be divided into exploratory factor analysis (EFA) and confirmatory factor analysis (CFA).
Exploratory Factor Analysis
EFA can be used to explore patterns underlying a data set. As such, EFA can elucidate how different items and constructs relate to one another and help develop new theories. EFA is suitable during early stages of instrument development. By using EFA, the researcher can identify items that do not empirically belong to the intended construct and that should be removed from the survey. Further, EFA can be used to explore the dimensionality of the instrument. Sometimes EFA is conflated with principal component analysis (PCA; Leandre et al. , 2012). PCA and EFA differ from each other in several fundamental ways. EFA is a statistical technique that should be used to identify plausible underlying constructs for a set of items. In EFA, the variance the items share is assumed to represent the construct and the nonshared variance is assumed to represent measurement errors. PCA is a data reduction technique that does not assume an underlying construct. PCA reduces a number of observed variables to a smaller number of components that account for the most variance in the observed variables. In PCA, all variance is considered, that is, it assumes no measurement errors. Within educational research, PCA may be useful when measuring multiple observable variables, for example, when creating an index from a checklist of different behaviors. For readers interested in reading more about the distinction between EFA and PCA and why EFA is the most suitable for exploring constructs, see Leandre et al. (2012) or Raykov and Marcoulides (2008) .
Confirmatory Factor Analysis
CFA is used to confirm a previously stated theoretical model. Essentially, when using CFA, the researcher is testing whether the data collected supports a hypothesized model. CFA is suitable when the theoretical constructs are well understood and clearly articulated and the validity evidence on the internal structure of the scale (the relationship between the items) has already been obtained in similar contexts. The researcher can then specify the relationship between the item and the construct and use CFA to confirm the hypothesized number of constructs, the relationship between the constructs, and the relationship between the constructs and the items. CFA may be appropriate when a researcher is using a preexisting survey that has an established structure with a similar population of respondents.
A Brief Technical Description of Factor Analysis
Mathematically, factor analysis involves the analysis of the variances and covariances among the items. The shared variance among items is assumed to represent the construct. In factor analysis, the constructs (the shared variances) are commonly referred to as factors. Nonshared variance is considered error variance. During an EFA, the covariances among all items are analyzed together, and items sharing a substantial amount of variance are collapsed into a factor. During a CFA the shared variance among items that are prespecified to measure the same underlying construct is extracted. Figure 1 illustrates EFA and CFA on an instrument consisting of eight observable variables (items) aiming to measure two constructs (factors): F1 and F2. In EFA, no a priori assumption of which items represent which factors is necessary: the EFA determines these relationships. In CFA, the shared variance of items 1–4 are specified by the researcher to represent F1, and the shared variance of items 5–8 are specified to represent F2. Even further, part of what CFA tests is that items 1–4 do not represent F2, and items 5–8 do not represent F1. For both EFA and CFA, nonshared variance is considered error variance.

Conceptual illustration of EFA and CFA. Observed variables (items 1–8) by squares, and constructs (factors F1 and F2) are represented by ovals. Factor loading/pattern coefficients representing the effect of the factor on the item (i.e., the unique correlation between the factor and the item) are represented by arrows. σ j , variance for factor j ; E i , unique error variance for item i . The factor loading for one item on each factor is set to 1 to give the factors an interpretable scale.
Figures illustrating the relationships between items and factors (such as Figure 1 ) are interpreted as follows. The double-headed arrow between the factors represents the correlation between the two factors (factor correlations). Each one-directional arrow between the factors and the items represents the unique correlation between the factor and the item (called “pattern coefficient” in EFA and “factor loading” in CFA). The pattern coefficients and factor loadings are similar to regression coefficients in a multiple regression. For example, consider the self-promotion item on Diekman’s goal-endorsement instrument. The factor loading/pattern coefficient for this item tells the researcher how much of the average respondent’s answer on this item is due to his or her general interest in agentic goals versus something unique about that item (error variance). For readers interested in more mathematical details about factor analysis, we recommend Kline (2016) , Tabachnick and Fidell (2013) , or Yong and Pearce (2013) .
Should EFA and CFA Be Applied on the Same Sample?
If a researcher decides that EFA is the best approach for analyzing the data, the results from the EFA should ideally be confirmed with a CFA before using the measurement instrument for research. This confirmation should never be conducted on the same sample as the initial EFA. Doing so does not provide generalizable information, as the CFA will be (essentially) repeating many of the relationships that were established through the EFA. Additionally, there could be something nuanced about the way the particular sample responds to items that might not be found in a second sample. For these reasons (among others), it is best practice to perform an EFA and CFA on independent samples. If a researcher has a large enough sample size, this can be done by randomly dividing the initial sample into two independent groups. It is also not uncommon for a researcher using an existing survey to decide that a CFA is suitable to start with but then discover that the data do not fit to the theoretical model specified. In this case, it is completely justified and recommended to conduct a second round of analyses starting with an EFA on half of the initial sample followed by a CFA on the other half of the sample ( Bandalos and Finney, 2010 ).
FACTOR ANALYSIS STEP BY STEP
In this section, we 1) describe the important considerations when preparing to perform a factor analysis, 2) introduce the essential analytical decisions made during an analysis, and 3) discuss how to interpret the outputs from factor analyses. We illustrate each step with real data using factor analysis to analyze the dimensionality of a goal-endorsement instrument ( Diekman et al. , 2010 ). Further, annotated code and output for running and analyzing EFA and CFA in R are provided as Supplemental Material (R syntax and Section 2) along with sample data.
Before delving into the technical details, we would like to be clear that conducting a factor analysis involves many decisions. There are no golden rules to follow to make these decisions. Instead, the researcher must make holistic judgments based on his or her specific context and available theoretical and empirical information. Factor analysis requires collecting evidence to build an argument to support a suggested instrument structure. The more time a researcher spends with the data investigating the effect of different possible decisions, the more confident they will be in finalizing the survey structure. As always, it is critical that a researcher’s decisions are guided by previously collected evidence and empirical information and not by a priori assumptions that the researcher wishes to support.
Defining the Construct and Intended Use of the Instrument
An essential prerequisite when selecting (or developing) and analyzing an instrument is to explicitly define the intended purpose and use of the instrument. Further, the theoretical construct or constructs that one aims to measure should be clearly defined, and the current general understanding of the construct should be described. The next step is to confirm a good alignment between the construct of interest and the instrument selected to measure it, that is, that the items on the instrument actually represent what one aims to measure (evidence based on content; Table 1 ). For a researcher to be able to use CFA for validation, an instrument must include at least four items in total. A multidimensional scale should have at least three but preferably five or more items for each theorized subscale. In very special cases, two items can be acceptable for a subscale ( Yong and Pearce, 2013 ; Kline, 2016 ). 4 For an abbreviated example of how to write up this type of validity for a manuscript using a survey instrument, see Box 1 .
Sample Size
The appropriate sample size needed for factor analysis is a multifaceted question. Larger sample sizes are generally better, as they will enhance the accuracy of all estimates and increase statistical power ( Gagne and Hancock, 2006 ). Early guidelines on sample sizes for factor analysis were general in their nature, such as a minimum sample size of 100 or 200 (e.g., see Boomsma, 1982 ; Gorsuch, 1983 ; Comrey and Lee, 1992 ). Although it is very tempting to adopt such general guidelines, caution must be taken, as they might lead to underestimating or overestimating the sample size needed ( Worthington and Whittaker, 2006 ; Tabachnick and Fidell, 2013 ; Wolf et al. , 2013 ).
The sample size needed depends on many elements, including number of factors, number of items per factor, size of factor loadings or pattern coefficients, correlations between factors, missing values in the data, reliability of the measurements, and the expected effect size of the parameters of interest ( Gagne and Hancock, 2006 ; Worthington and Whittaker, 2006 ; Wolf et al. , 2013 ). Wolf et al. (2013) showed that a sufficient sample size for a one-factor CFA with eight items and factor loadings of 0.8 could be as low as 30 respondents. For a two-factor CFA with three or four items per scale and factor loadings of 0.5, a sample size of ∼450 respondents is needed. For EFA, Leandre et al. (2012) recommend that “under moderately” good conditions (communalities 5 of 0.40–0.70 and at least three items for each factor), a sample of at least 200 should be sufficient, while under poor conditions (communalities lower than 0.40 and some factors with only two items for each factor), a sample size of at least 400 is needed. Thus, when deciding on an appropriate sample size, one should consider the unique properties of the actual survey. The articles written by Wolf et al. (2013) and Gagne and Hancock (2006) provide a good starting point for such considerations. See Box 1 for an example of how to discuss sample size decisions in a manuscript.
In some cases, it may be implausible to have the large sample sizes necessary to obtain stable estimates from an EFA or a CFA. Often researchers must work with data that have already been collected or are using a study design that simply does not include a large number of respondents. In these circumstances, it is strongly recommended that one use a measurement instrument that has already been validated for use in a similar population for a similar purpose. In addition to considering and analyzing other relevant types of validity evidence (see Table 1 ), the researchers should report on validity evidence based on internal structure from other studies and describe the context of those studies relative to their own context. The researchers should also acknowledge in the methods and limitation sections that they could not run dimensionality checks on their sample. Further, researchers can also analyze a correlation matrix 6 of the responses to the survey items from their own data collection to get a sense of how the items may relate to one another in their context. This correlation matrix may be reported to help provide preliminary validity evidence based on internal structure.
Properties of the Data
As with any statistical analysis, before performing a factor analysis the researcher must investigate whether the data meet the assumptions for the proposed analysis. Section 1 of the Supplemental Material provides a summary of what a researcher should check for in the data for the purposes of meeting the assumptions of a factor analysis and an illustration applied to the example data. These include analyses of missing values, outliers, factorability, normality, linearity, and multicollinearity. Box 3 provides an example of how to report these analyses in a manuscript.
BOX 3. How to interpret and report CFA output for publication using the goal-endorsement example, initial CFA
Descriptive statistics.
No items were missing more than 1.3% of their values, and this missingness was random (Little’s MCAR test: chi-square = 677.719, df = 625, p = 0.075 implemented with the BaylorEdPsych package; Beaujean, 2012 ). Mean values for the items ranged from 4.1 to 6.3. Most items had a skewness and kurtosis below |1.0|, and all items had a skewness below |2.0| and kurtosis below |4.0|. Mardia’s multivariate normality test (implemented with the psych package; Revelle 2017 ) showed significant multivariate skewness and kurtosis values. Intra-subscale correlations ranged from 0.02 to 0.73, and the lowest tolerance value was 0.36.
Interpreting output from the initial two-factor CFA
Results from the initial two-factor CFA indicated that, in our population, the data did not support the model specified. The chi-square test of model fit was significant (χ 2 = 1549, df = 229, p < 0.00), but this test is known to be sensitive to minor model misspecification with large sample sizes ( n = 796). However, additional model fit indices also indicated that the data did not support the model specified. SRMR was 0.079, suggesting good fit, but CFI was 0.818, and RMSEA was 0.084. Thus, the hypothesized model was not empirically supported by the data.
To better understand this model misspecification, we explored the factor loadings, correlational residuals, original interitem correlation matrix, and modification indices. Several factor loadings were well below 0.7, indicating that the factors did not explain these items well. Analysis of correlational residuals did not point out any special item-pair correlation as especially problematic; rather, several correlational residuals were residuals greater than |0.10|. Consequently, the poor model fit did not seem to be primarily caused by a few ill-fitting items. A reinvestigation of the interitem correlation matrix made when analyzing the factorability of the data (see the Supplemental Material, Section 1) suggested the presence of more than two factors. This was most pronounced for the agentic scale, for which some items had a relatively high correlation to one another and lower correlations to other items in that scale. Inspection of the modification indices suggested adding correlations between, for example, the items achievement and mastery. Together, these patterns indicate that the data might be better represented by more than two factors.
Analytic Considerations for CFA
Once the data are screened to determine their properties, several analytical decisions must be made. Because there are some differences in analytical decisions and outputs for EFA and CFA, we will discuss EFA and CFA in separate sections. We will start with CFA, as most researchers adopting an existing instrument will use this method first and may not ever need to perform an EFA. See Box 2 for how to report analytical considerations for a CFA in a manuscript.
BOX 2. What to report in the methods of a publication for a CFA using the goal-endorsement example
We chose to start with a CFA to confirm a two-factor solution, because 1) the theoretical framework underlying the instrument is well understood and articulated and 2) Diekman et al. (2010) performed an EFA on a similar population to ours that supported the two-factor solution. If the assumed factor model was confirmed, then we could confidently combine the items into two sum scores and interpret the data as representing both an agentic and a communal factor. CFA was run using the R package lavaan ( Rosseel, 2012 ).
Selecting an estimator
In consideration of the ordinal and nonnormal nature of the data, the robust maximum-likelihood estimation (MLR) was used to extract the variances from the data. Full-information maximum likelihood in the estimation procedure was used to handle the missing data.
Specifying a two-factor CFA
To confirm the factor structure proposed by Diekman et al. (2010) , we specified a two-factor CFA, with items 1–14 representing the agentic scale and items 15–23 representing the communal factor ( Table 2 ). Correlation between the two factors was allowed. For identification purposes, the factor loading for one item on each factor was set to 1. The number of variances and covariances in the data was 276 (23(23 + 1)/2), which was larger than the number of parameter estimates (one factor correlation, 23 error terms, 21 factor loadings, and variances for each factor). Thus, the model was overidentified.
Selecting model fit indices and setting cutoff values
Multiple fit indices (chi-square value from robust MLR [MLR χ 2 ]; comparative fit index [CFI]; the root-mean-square error of approximation [RMSEA]; and the standardized root-mean-square residual [SRMR]) were consulted to evaluate model fit. The fit indices were chosen to represent an absolute, a parsimony-adjusted, and an incremental fit index. Consistent with the recommendations by Hu and Bentler (1999) , the following criteria were used to evaluate the adequacy of the models: CFI > 0.95, SRMR < 0.08, and RMSEA < 0.06. Coefficient alpha was computed based on the model results and used to assess reliability. Values > 0.70 were considered acceptable.
Selecting an Estimator.
When performing a CFA, a researcher must choose a statistical method for extracting the variance from the data. There are several different methods available, including unweighted least squares, generalized least squares, maximum likelihood, robust maximum likelihood, principal axis factoring, alpha factoring, and image factoring. Each of these methods has its strengths and weaknesses. Kline (2016) and Tabachnick and Fidell (2013) provide a useful discussion of several of these methods and when best to apply each one. In general, because data from surveys are often on an ordinal level (e.g., data from Likert scales) and sometimes slightly nonnormally distributed, estimators robust against nonnormality, such as maximum-likelihood estimation with robust standard errors (MLR) or weighted least-squares estimation (WLS), are often suitable for performing CFA. Whether or not MLR or WLS is most suitable depends partly on the number of response options for the survey items. MLR work best when data can be considered continuous. In most cases, scales with seven response options work well for this purpose, whereas scales with five response options are questionably continuous. MLR is still often used in estimation for five response options, but with four or fewer response options, WLS is better ( Finney and DiStefano, 2006 ). The decision regarding the number of response options to include in a survey should not be driven by these considerations. Rather, the number of response options and properties of the data should drive the selection of the CFA estimator. Although more response options for an item allow researchers to model it as continuous, respondents may not be able to meaningfully differentiate between the different response options. Fewer response options usually offer less ambiguity, but usually result in less variation in the response. For example, if students are provided with 10 options to indicate their level agreement with a given item, it is possible that not all of the response options may be used. In such a case, fewer response options may better capture the latent distribution of possible responses to an item.
Specifying the Model.
The purpose of a CFA is to test whether the data collected with an instrument support the hypothesized model. Using theory and previous validations of the instrument, the researcher specifies how the different items and factors relate to one another (see Figure 1 for an example model). For a CFA, the number of parameters that the researcher aims to estimate (e.g., error terms, variances, correlations and factor loadings) must be less than or equal to the number of possible variances and covariances among the items ( Kline, 2016 ). For a CFA, a simple equation tells you the number of possible variances and covariances: p ( p + 1)/2, where p = number of items. If the number of parameters to estimate is more than the number of possible variances and covariances among the items, the CFA is called “underidentified” and will not provide interpretable results. When the number of parameters to be estimated equals the number of covariances and variances among the items, the model is deemed “just identified” and will result in perfect fit of the data to the model, regardless of the true relationship between the items. To test whether the data fit the theoretical model, the number of parameters that are being estimated needs to be less than the number of variances and covariances observed in the data. In this case, the model is “overidentified.” For the example CFA in Figure 1 , the number of possible variances and covariances is 8(8 + 1)/2 = 36, and the number of parameters to estimate is 17 (one factor correlation, eight error terms, six factor loadings, and variances for each of the two factors 7 ), thus the model is overidentified.
Choosing Appropriate Model Fit Indices.
The true splendor of CFA is that so-called model fit indices have been developed to help researchers understand whether the data support the hypothesized theoretical model. 8 The closest statistic to an omnibus test of model fit is the model chi-square test. The null hypothesis for the chi-square test is that there is no difference between the hypothesized model and the observed relationships within the data. Several researchers argue that this is an unrealistic hypothesis ( Hu and Bentler, 1999 ; Tabachnick and Fidell, 2013 ). A close approximation of the data to the model is more realistic than a perfect model fit. Further, the model chi-square test is very sensitive to sample size (the chi-square statistic tends to increase with an increase in sample size, all other considerations constant; Kline, 2016). Thus, while large sample sizes provide good statistical power, the null hypothesis that the factor model and the data do not differ from each other may be rejected although the difference is actually quite small. Given these concerns, it is important to consider the result of the chi-square test in conjunction with multiple other model fit indices.
Many model fit indices have been developed that quantify the degree of fit between the model and the data. That is, the values provided by these indices are not intended to make binary (fit vs. no fit) judgments about model fit. These model fit indices can be divided into absolute, parsimony-adjusted, and incremental fit indices ( Bandalos and Finney, 2010 ). Because each type of index has its strengths and weaknesses (e.g., sensitivity to sample size, model complexity, or misspecified factor correlations), using at least two different types of fit indices is recommended ( Hu and Bentler, 1999 ; Tabachnick and Fidell, 2013 ). The researcher should decide a priori which model fit indices to use and the cutoff values that will be considered a good enough indicator of model fit to the data. Hu and Bentler (1999) recommend using one of the relative fix indices such as comparative fit index (CFI) with a cutoff of >0.95 in combination with standardized root-mean-square residual (SRMR; absolute fit indices, good model < 0.08) or root-mean-square error of approximation (RMSEA; parsimony-adjusted fit indices, good model < 0.06) as indicators for good fit. Some researchers, including Hu and Bentler (1999) , caution against using these cutoff values as golden rules because it might lead to incorrect rejection of acceptable models ( Marsh et al. , 2004 ; Perry et al. , 2015 ).
Interpreting the Outputs from CFA
After making all the suggested analytical decisions, a researcher is now ready to apply a CFA to the data. Model fit indices that the researcher a priori decided to use are the first element of the output that should be interpreted from a CFA. If these indices suggest that the data do not fit the specified model, then the researcher does not have empirical support for using the hypothesized survey structure. This is exactly what happened when we initially ran a CFA on Diekman’s goal-endorsement instrument example (see Box 3 ). In this case, focus should shift to understanding the source of the model misfit. For example, one should ask whether there are any items that do not seem to correlate with their specified latent factor, whether any correlations seem to be missing, or whether some items on a factor group together more strongly than other items on that same factor. These questions can be answered by analyzing factor loadings, correlation residuals, and modification indices. In the following sections, we describe these in more detail. See Boxes 3 , 6 , and 7 for examples of how to discuss and present output from a CFA in a paper.
BOX 6. How to interpret and report CFA output for publication using the goal-endorsement example, second CFA
Based on the results from the EFAs, a second CFA was specified using the five-factor model with 20 items (excluding 4: mastery, 10: competition, and 22: intimacy). The specified five-factor CFA demonstrated appropriate model fit (χ 2 = 266, df = 160, p < 0.00, CFI = 0.959, RMSEA = 0.046, and SRMR = 0.050). Factor loadings were close to or above 0.70 for all but three items ( Figure 2 ), meaning that, for most items, around 50% of the variance in the items was explained ( R 2 ≈ 0.5) by the theorized factor. This means that the factors explained most of the items well. Factor correlations were highest between the service and connection factors (0.76) and the autonomy and competency (0.67) factors. The lowest factor correlation found was between the prestige and service factors (0.21). Coefficient alpha values for the subscales were 0.81, 0.77, 0.66, 0.87, and 0.77 for prestige, autonomy, competency, service, and connection, respectively.

Results from the final five-factor CFA model. Survey items (for items descriptions see Table 3 ) are represented by squares and factors are represented by ovals. The numbers below the double-headed arrows represent correlations between the factors; the numbers by the one-directional arrows between the factors and the items represent standardized factor loadings. Small arrows indicate error terms. *, p < 0.01; p < 0.001 for all other estimates.
Factor Loadings.
As mentioned in Brief Technical Description of Factor Analysis , factor loadings represent how much of the respondent’s response to an item is due to the factor. When a construct is measured using a set of items, the assumption is that each item measures a slightly different aspect of the construct and that the common variance among them is the best possible representation of the construct. High, but not too high, factor loadings for these items are preferred. If several items have high standardized factor loadings 9 (e.g., above 0.9), this suggests that they share a lot of variance, which indicates that these items may be too similar and thus do not contribute unique information ( Clark and Watson, 1995 ). On the other hand, if an item has a low factor loading on its focal factor, it means that item shares no or little variance with the other items that theoretically belong to the same focal factor and thus its contribution to the factor is low. Including items with low factor loadings when combining the scores from several items into a single score (sum, average, or common variance) will introduce bias into the results. 10 There is, however, no clear rule for when an item has a factor loading that is too low to be included. Bandalos and Finney (2010) argue that, because the items are specifically chosen to indicate a factor, one would hope that the variability explained in the item by the factor would be high (at least 50%). Squaring the standardized factor loadings provides the amount of variability explained in the item by the factor ( R 2 ), indicating that it is desirable to have standardized factor loadings of at least 0.7 ( R 2 = 0.7 2 = ∼50%). However, the acceptable strength of the factor loading depends on the theoretically assumed relationship between the item and the factor. Some items might be more theoretically distant from the factor and therefore have lower factor loadings, but still comprise an essential part of the factor. This reinforces the idea that there are no hard and fast rules in factor analysis. Even if an item does not reach the suggested level for factor loading, if a researcher can argue from a theoretical basis for its inclusion, then it could be included.
Correlation Residuals.
As mentioned before, CFA is used to confirm a previously stated theoretical model. In CFA, the collected data are used to evaluate the accuracy of the proposed model by comparing the discrepancy between what the theoretical model implies (e.g., a two-factor model in the Diekman et al. [2010] example) and what is observed in the actual data. Correlation residuals represent the differences between the observed correlations in the data and the correlations implied by the CFA ( Bandalos and Finney, 2010 ). Local areas of misfit can be identified by inspecting correlational residuals. Correlation residuals greater than |0.10| are indicative of a specific item-pair relationship that is poorly reproduced by the model ( Kline, 2016 ). This guideline may be too low when working with small sample sizes and too large when working with large samples sizes and, as with all other fit indices, should only be used as one element among many to understand model fit.
Modification Indices.
Most statistical software used for CFA provides modification indices that can easily be viewed by the user. Modification indices propose a series of possible additions to the model and estimate the amount the model’s chi-square value would decrease if the suggested parameter were added (recall that a lower chi-square value indicates better model fit). For example, if an item strongly correlates with two factors but is constrained to only correlate with one, the modification index associated with adding a relationship to the second factor would indicate how much the chi-square model fit is expected to improve with the addition of this factor loading. In short, modification indices can be used to better understand which items or relationships might be driving the poor model fit.
If (and only if) theoretically justified, a suggested relationship can be added or problematic items can be removed during a CFA. However, caution should be taken before adding or removing any parameters ( Bandalos and Finney, 2010 ). As Bandalos and Finney (2010) state, “Researchers must keep in mind that the purpose of conducting a CFA study is to gain a better understanding of the underlying structure of the variables, not to force models to fit” (p. 112). If post hoc changes to the model are made, the analysis becomes more explorative in nature, and thus tenuous. The modified model should ideally be confirmed with a new data set to avoid championing a model that has an artificially good model fit.
Best practice if the model does not fit (as noted in Factor Analysis ) is to split the data and conduct a second round of analyses starting with an EFA using half of the sample and then conducting a CFA with the other half ( Bandalos and Finney, 2010 ). To see an example of how to write up this secondary CFA analysis, see Boxes 6 and 7 of the goal-endorsement example.
When the Model Fit Is Good.
When model fit indices indicate that the hypothesized model is a plausible explanation of the relationships between the items in the data, factor loadings and the correlation between the latent variables in the model (so-called factor correlations) can be interpreted and a better understanding of the construct can be gained. It is also now appropriate to calculate and report the coefficient alpha, omega, or any other index of reliability for each of the subscales. The researcher can more confidently use the results from the instrument to make conclusions about the intended constructs based on combined scale scores (given that other relevant validity evidence presented in Table 1 also supports the intended interpretations). If a researcher has used CFA to examine the dimensionality of the items and finds that the scale functions as intended, this information should be noted in the methods section of the research manuscript when describing the measurement instruments used in the study. At the very least, the researcher should report the estimator and fit indices that were used and accompanying values for the fit indices. If the scale has been adapted in some way, or if it is being empirically examined for the first time, all of the factor loadings and factor correlations should also be reported so future researchers can compare their values with these original estimates. These could be reported as a standalone instrument validation paper or in the methods section of a study using that instrument.
Analytical Considerations for EFA
If a researcher’s data do not fit the model proposed in the CFA, then using the items as indicators of the hypothesized construct is not sensible. If the researcher wants to continue to use the existing items, it is prudent to investigate this misfit to better understand the relationships between the items. This calls for the use of an EFA, where the relationships between variables and factors are not predetermined (i.e., a model is not specified a priori) but are instead allowed to emerge from the data. As mentioned before, EFA could also be the first choice for a researcher if the instrument is in an early stage of development. We outline the steps for conducting an EFA in the following sections. See Box 4 for a description of how to describe analytical considerations for an EFA in the methods section.
BOX 4. What to report in the methods of a publication for an EFA using the goal-endorsement example
Because the results from the initial CFA indicated that the data did not support a two-factor solution, we proceeded with an EFA to explore the factor structure of the data. The original sample was randomly divided into equal-sized parts, and EFA was performed on half of the sample ( n = 398) to determine the dimensionality of the goal-endorsement scale and detect possible problematic items. This was followed by a CFA ( n = 398) to confirm the result gained from the EFA. EFA and CFA were run using the R package lavaan ( Rosseel, 2012 ).
Selecting an estimator for the EFA
Considering the ordinal and nonnormal nature of the data, a principal axis factor estimator was used to extract the variances from the data. Only cases with complete items were used in the EFA.
Factor rotation
Due to the fact that theory and the preceding CFA indicated that the different subscales are correlated, quartimin rotation (an oblique rotation) was chosen for the EFA.
Determining the number of factors
Visual inspection of the scree plot, parallel analysis (PA) based on eigenvalues from the principal components and factor analysis in combination with theoretical considerations were used to decide on the appropriate number of factors to retain. PA was implemented with the psych package ( Revelle, 2017 ).
Just as with CFA, the first step in an EFA is selecting a statistical method to use to extract the variances from the data. The considerations for the selection of this estimator are similar to those for CFA (see Selecting an Estimator ). One of the most commonly used methods for extracting variance when conducting an EFA on ordinal data with slight nonnormality is principal axis factoring (Leandre et al. , 2012). If the items in one’s instrument have fewer than five response options, WLS can be considered.
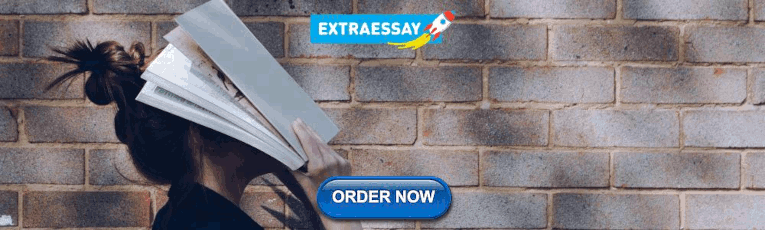
Factor Rotation.
Factor rotation is a technical step to make the final output from the model easier to interpret (see Bandalos, 2018 , pp. 327–334, for more details). The main decision for the researcher to make here is whether the rotation should be orthogonal or oblique ( Raykov and Marcoulides, 2008 ; Leandre et al. , 2012; Bandalos, 2018 ). Orthogonal means that the factors are uncorrelated to one another in the model. Oblique allows the factors to correlate to one another. In educational studies, factors are likely to correlate to one another; thus oblique rotation should be chosen unless a strong hypothesis for uncorrelated factors exists (Leandre et al. , 2012). Orthogonal and oblique are actually families of rotations, so once the larger choice of family is made, a specific rotation method must be chosen. The specific rotation method within the oblique category that is chosen does not generally have a strong effect on the results ( Bandalos and Finney, 2010 ). However, the researcher should always provide information about which rotation method was used ( Bandalos and Finney, 2010 ).
Determining the Number of Factors.
After selecting the methods for estimation and rotation, researchers must determine how many factors to extract for EFA. This step is recognized as the greatest challenge of an EFA, and the issue has generated a large amount of debate (e.g., Cattell, 1966 ; Crawford et al. , 2010 ; Leandre et al. , 2012). Commonly used methods are to retain all factors with an eigenvalue >1 or to use a scree plot. Eigenvalues are roughly a measure of the amount of information contained in a factor, so factors with higher eigenvalues are the most useful for understanding the data. A scree plot is a plot of eigenvalues versus number of factors. Scree plots allow researchers to visually estimate the number of factors that are informative by considering the shape of the plot (see the annotated output in the Supplemental Material, Section 2, for an example of a scree plot). These two methods are considered heuristic, and many researchers recommend also using parallel analysis (PA) or the minimum average partial correlation test to determine the appropriate number of factors ( Ledesma and Valero-Mora, 2007 ; Leandre et al. , 2012; Tabachnick and Fidell, 2013 ). In addition, several statistics that mathematically analyze the shape of the scree plot have been developed in an effort to provide a nonvisual method of determining the number of factors ( Ruscio and Roche, 2012 ; Raiche et al. , 2013).
We recommend using a number of these indices, as well as theoretical considerations, to determine the number of factors to retain. The results of all of the various methods discussed provide plausible solutions that can all be explored to evaluate the best solution. When these indices are in agreement, this provides more evidence of a clear factor structure in the data. To make each factor interpretable, it is of outmost importance that the number and nature of factors retained make theoretical sense (see Box 5 for a discussion on how many factors to retain). Further, the intended use for the survey should also be considered. For example, say a researcher is interested in studying two distinct populations of students. If the empirical and theoretical evidence supports both a two-factor and a three-factor solution, but the three-factor solution provides a clearer distinction between two populations of interest, then the researcher might choose the three-factor solution (see Box 7 ).
BOX 5. How to interpret and report EFA output for publication using the goal-endorsement example
Initial efas.
Parallel analysis based on eigenvalues from the principal components and factor analysis indicated three components and five factors. The scree plot indicated an initial leveling out at four factors and a second leveling out at six factors.
We started by running a three-factor model and then increased the number of factors by one until we had run all models ranging from three to six factors. The pattern matrices were then examined in detail with a special focus on whether the factors made theoretical sense (see Table 2 for pattern matrices for the three-, four-, and five-factor models). The three-factor solution consisted of one factor with high factor loadings for the items representing communal goals (explaining 17% of the variance in the data). The items originally representing agentic goals were split into two factors. One factor included items that theoretically could be described as prestige (explaining 12% of the variance in the data) and the other items related to autonomy and competency (explaining 11% of the variance in the data). The total variance explained by the three-factor model was 41%. In the four-factor solution, the autonomy and competency items were split into two different factors. In the five-factor solution, three items from the original communal goals scale (working with people, connection to others, and intimacy) contributed most to the additional factor. In total, 48% of the variance was explained by the five-factor model. For a six-factor solution, the sixth factor included only one item with pattern loadings greater than 0.40, and thus a six-factor solution was deemed to be inappropriate.
In conclusion, the communal scale might represent one underlying construct as suggested by previous research or it might be split into two subscales represented by items related to 1) serving others and 2) connection. Our data did not support a single agentic factor. Instead, these items seemed to fit on two or three subscales: prestige, autonomy, and possibly competency. Because all the suggested solutions (three-, four-, and five-factor solutions) included a number of poorly fitting items, we decided to remove items and run a second set of EFAs before proceeding to the CFA.
Second round of EFAs
On the basis of the results from the initial EFAs, we first continued with a three-factor solution, removing items with low pattern coefficients (<0.40; 10: success, 14: competition, and 22: intimacy, to begin with; Table 2 ). When these variables were removed in a stepwise manner, additional items now showed low pattern coefficients (<0.40) and/or low communalities in the new EFA solutions. The new items showing low pattern coefficients were items belonging to their own factors in the five-factor EFA (i.e., items representing competency and connection). Not until all items from these two scales were removed was a stable three-factor solution achieved with pattern coefficients >0.40. Thus, to achieve a three-factor solution, including only items with pattern coefficients >0.40, we had to drop 30% of the items and, consequently, extensively narrow the content validity of the scale.
To further explore a five-factor solution, we decided, on the basis of the empirical results and the theoretical meaning of the items, to stepwise remove items 4 (mastery), 14 (competition), and 22 (intimacy). We used an inclusive pattern coefficient cutoff (<0.40) for this initial round of validation, because we wanted to keep as many items as possible from the original scale. If some items continue to show pattern coefficients below 0.5 over repeated data collections, researchers should reconsider whether these items should be kept in the scale. The new 20-item five-factor solution resulted in theoretically the same factors as for the first five-factor EFA, but now all pattern coefficients but one were above 0.50 on the primary factor and below 0.20 on the other factors ( Table 3 ). In total, 52% of the variance in the data was explained.
Standardized pattern coefficients for the Diekman et al. (2010) goal-endorsement instrument from the second EFA for the five-factor solutions a
a For clarity, pattern coefficients <0.2 are not shown.
In conclusion, the initial CFA, as well as the EFA analysis, indicated that the two-dimensional scale previously suggested was not supported in our sample. The EFA analysis mainly indicated a three- or a five-factor solution. To achieve a good three-factor solution, we had to exclude 30% of the original items. The final three factors were labeled “prestige,” “autonomy,” and “service.” Both the empirical data and theoretical consideration suggested two additional factors: a competency factor and a connection factor. We continued with this five-factor solution, as it allowed us to retain more of the original items and made theoretical sense, as the five factors were just a further parsing of the original agentic and communal scales.
Interpreting Output from EFA
The aim of EFA is to gain a better understanding of underlying patterns in the data, investigate dimensionality, and identify potentially problematic items. In addition to the results from parallel analysis or other methods used to estimate the number of factors, other informative measures include pattern coefficients and communalities. These outputs from an EFA will be discussed in this section. See Box 5 for an example of how to write up the output from an EFA.
Pattern Coefficients and Communalities.
Pattern coefficients and communalities are parameters describing the relationship between the items and the factors. They help researchers understand the meaning of the factors and identify items that do not empirically appear to belong to their theorized factor.
Pattern coefficients closely correspond to factor loadings in CFA, and they are commonly the focal output from an EFA (Leandre et al. , 2012). Pattern coefficients represent the impact each factor has on an item after controlling for the impact of all the other factors on that item. A high pattern coefficient suggests that the item is well explained by a particular factor. However, as with CFA, there is no clear rule as to when an item has a pattern coefficient too low to be considered part of a particular factor. Guidelines for minimum pattern coefficient values range from 0.40 to 0.70. In other words, all items with pattern coefficients equal to or higher than the chosen cutoff value can be considered “good” items and should be kept in the survey ( Matsunaga, 2010 ).
It is also important to consider the magnitude of any cross-loadings. Cross-loading describes the situation in which an item seems to be influenced by more than one factor in the model. Cross-loading is indicated when an item has high pattern coefficients for multiple factors. Using that item is problematic when creating a summed/mean score for a factor, as responses to that item are not uniquely driven by its hypothesized factor, but instead by additional measured factors. Cross-loadings higher than 0.20 or 0.30 are usually considered to be problematic ( Matsunaga, 2010 ), especially if the item does not have a particularly strong loading on a focal factor.
Communality represents the percentage of the variance in responses on an item accounted for by all factors in the proposed model. Communalities are similar to R 2 in CFA (see Factor Loadings ). However, in CFA, the variance in an item is only explained by one factor, while in EFA, the variance in one item can be explained by several factors. Low communality for an item means that the variance in the item is not well explained by any part of the model, and thus that item could be a subject for elimination.
We emphasize that, even if pattern coefficients or communalities indicate that an item might be subject for elimination, it is important to consider the alignment between the item and hypothesized construct before actually eliminating the item. The items in a scale are presumably chosen for some theoretical reason, and eliminating any items can cause a decrease in content validity ( Bandalos and Finney, 2010 ). If any item is removed, the EFA should be rerun to ensure that the original factor structure persists. This can be done on the same data set, as EFA is exploratory in nature.
Interpreting the Final Solution.
Once the factors and the items make empirical and theoretical sense, the factor solution can be interpreted, and suitable names for the factors should be chosen (see Box 5 for a discussion of the output from an EFA). Important sources of information for this include: the amount variance explained by the whole solution and the factors, factor correlations, pattern coefficients, communality values, and the underlying theory. Because the names of the factors will be used to communicate the results, it is crucial that the names reflect the meaning of the underlying items. Because the item responses are manifestations of the constructs, different sets of items representing a construct will, accordingly, lead to slightly different nuanced interpretations of that construct. Once a plausible solution has been identified by an EFA, it is important to note that stronger support for the solution can be obtained by testing the hypothesized model using a CFA on a new sample.
CONCLUDING REMARKS
In this article, we have discussed the need for understanding the validity evidence available for an existing survey before its use in discipline-based educational research. We emphasized that validity is not a property of the measurement instrument itself but is instead a property of the instrument’s use. Thus, each time a researcher decides to use an instrument, they have to consider to what degree evidence and theory support the intended interpretations and use of the instrument. A researcher should always review the different kinds of validity evidence described by AERA, APA, and NCME (2014 ; Table 1 ) before using an instrument and should identify the evidence they need to feel confident when employing the instrument for an intended use. When using several related items to measure an underlying construct, one important validity aspect to consider is whether a set of items can confidently be combined to represent that construct. In this paper, we have shown how factor analysis (both exploratory and confirmatory) can be used to investigate that.
We recognize that the information presented herein may seem daunting and a potential barrier to carrying out important, substantive, educational research. We appreciate this sentiment and have experienced those fears ourselves, but we feel that properly understanding procedures for vetting instruments before their use is essential for robust and replicable research. To reiterate, at issue here is the confidence and trust one can have in one’s own research, both after its initial completion and in future studies that will rely on the replicability of results. Again, we can use an analogy for the measurement of unobservable phenomena: one would not expect an uncalibrated and calibrated scale to produce the same values for the weight of a rock. This does not mean that the uncalibrated scale will necessarily produce invalid measurements, only that one’s confidence in its ability to do so should be tempered by the knowledge that it has not yet been calibrated. Research conducted using uncalibrated or biased instruments, regardless of discipline, is at risk of inferring conclusions that are incorrect. The researcher may make the appropriate inferences given the values provided by the instrument, but if the instrument itself is invalid for the proposed use, then the inferences drawn are also invalid. Our aim in presenting these methods is to strengthen the research conducted in biology education and continue to improve the quality of biology education in higher education.
Supplementary Material
Acknowledgments.
We are indebted to Ashely Rowland, Melissa McCartney, Matthew Kararo, Julie Charbonnier, and Marie Janelle Tacloban for their comments on earlier versions of this article. The research reported in this paper was supported by awards from the National Science Foundation (NSF DUE 1534195 and 1711082). This research was conducted under approved IRB 2015-06-0055, University of Texas at Austin.
1 In this article, we will use the terms “surveys,” “measurement instrument,” and “instrument” interchangeably. We will, however, put the most emphasis on the term “measurement instrument,” because it conveys the importance of considering the quality of the measurement resulting from the instrument’s use.
2 “Latent variables” and “constructs” both refer to phenomena that are not directly observable. Examples could include a student’s goals, the strength of his or her interest in biology, or his or her tolerance of failure. The term “latent variable” is commonly used when discussing these phenomena from a measurement point of view, while “construct” is a more general term used when discussing these phenomena from a theoretical perspective. In this article, we will use the term “construct” only when referring to phenomena that are not directly observable.
3 In addition to coefficient alpha, there are a number of other reliability estimates available. We refer interested readers to Bandalos (2018) , Sijtsma (2009) , and Crocker and Algina (2008) .
4 This is partly due to identification issues (see Specifying the Model ).
5 In EFA, communalities describe how much of the variance in an item is explained by the factor. For more information about communalities, see Interpreting Output from EFA .
6 For a description of a correlation matrix, see the Supplemental Material, Sections 1 and 2.
7 It is necessary to set the metric to interpret factor loadings and variances in a CFA model. This is commonly done by either 1) choosing one of the factor loadings and fixing it to 1 (this is done for each factor in the model) or 2) by fixing the variance of the latent factors to 1. We have chosen the former approach for this example.
8 For some software and estimation methods, model fit indices are also provided for EFA. In a similar way as for CFA, these model fit indices can be used to evaluate the fit of the data to the model.
9 When using CFA, the default setting in most software is to provide factor loadings in the original metric of the items, such that the results are covariances between the items and the factor. Because these values are unstandardized, it is sometimes hard to interpret these relationships. For this reason, it is common to standardize factor loadings and other model relationships (e.g., correlations between latent factors), which puts them in the more familiar correlation format that is bounded by −1 and +1.
10 When distilling the responses of several items into a single score, one is implicitly assuming that all of the items measure the underlying construct equally well (usually without measurement error) and are of equal theoretical importance. Fully discussing the nuances of how to create a single score from a set of items is beyond the scope of this paper, but we would be remiss if we did not at least mention it and encourage the reader to seek more information, such as DiStefano et al . (2009 ).
- Allen J. M., Muragishi G. A., Smith J. L., Thoman D. B., Brown E. R. (2015). To grab and to hold: Cultivating communal goals to overcome cultural and structural barriers in first-generation college students’ science interest . Translational Issues in Psychological Science , ( 4 ), 331. [ PMC free article ] [ PubMed ] [ Google Scholar ]
- American Educational Research Association, American Psychological Association, and National Council for Measurement in Education (AERA, APA, and NCME). (2014). Standards for educational and psychological testing . Washington, DC. [ Google Scholar ]
- Andrews S. E., Runyon C., Aikens M. L. (2017). The math–biology values instrument: Development of a tool to measure life science majors’ task values of using math in the context of biology . CBE—Life Sciences Education , ( 3 ), ar45. [ PMC free article ] [ PubMed ] [ Google Scholar ]
- Armbruster P., Patel M., Johnson E., Weiss M. (2009). Active learning and student-centered pedagogy improve student attitudes and performance in introductory biology . CBE—Life Sciences Education , ( 3 ), 203–213. [ PMC free article ] [ PubMed ] [ Google Scholar ]
- Bakan D. (1966). The duality of human existence: An essay on psychology and religion . Oxford, UK: Rand McNally. [ Google Scholar ]
- Bandalos D. L. (2018). Measurement theory and applications for the social sciences . New York: Guilford. [ Google Scholar ]
- Bandalos D. L., Finney S. J. (2010). Factor analysis. Exploratory and confirmatory . In Hancock G. R., Mueller R. O. (Eds.), The reviewer’s guide to quantitative methods in the social science (pp. 93–114). New York: Routledge. [ Google Scholar ]
- Beaujean A. A. (2012). BaylorEdPsych: R package for Baylor University educational psychology quantitative courses . Retrieved from https://CRAN.R-project.org/package=BaylorEdPsych
- Boomsma A. (1982). Robustness of LISREL against small sample sizes in factor analysis models . In Joreskog K. G., Wold H. (Eds.), Systems under indirect observation: Causality, structure, prediction (Part 1, pp. 149–173). Amsterdam, Netherlands: North Holland. [ Google Scholar ]
- Borsboom D., Mellenbergh G. J., van Heerden J. (2004). The concept of validity . Psychological Review , ( 4 ), 1061–1071. [ PubMed ] [ Google Scholar ]
- Cattell R. B. (1966). The scree test for the number of factors . Multivariate Behavioral Research , ( 2 ), 245–276. [ PubMed ] [ Google Scholar ]
- Cizek G. J. (2016). Validating test score meaning and defending test score use: Different aims, different methods . Assessment in Education: Principles, Policy & Practice , ( 2 ), 212–225. 10.1080/0969594X.2015.1063479 [ CrossRef ] [ Google Scholar ]
- Clark L. A., Watson D. (1995). Constructing validity: Basic issues in objective scale development . Psychological Assessment , ( 3 ), 309–319. [ Google Scholar ]
- Comrey A. L., Lee H. B. (1992). A first course in factor analysis (2nd ed.). Hillsdale, NJ: Erlbaum. [ Google Scholar ]
- Crawford A.V., Green S.B., Levy R., Lo W-J., Scott L., Svetina D., Thompson M.S. (2010). Evaluation of parallel analysis methods for determining the number of factors . Educational and Psychological Measurement , ( 6 ), 885–901. [ Google Scholar ]
- Crocker L., Algina J. (2008). Introduction to classical and modern test theory . Mason, OH: Cengage Learning. [ Google Scholar ]
- Cronbach L. J., Meehl P. E. (1955). Construct validity in psychological tests . Psychological Bulletin , ( 4 ), 281–302. [ PubMed ] [ Google Scholar ]
- Diekman A. B., Brown E. R., Johnston A. M., Clark E. K. (2010). Seeking congruity between goals and roles: A new look at why women opt out of science, technology, engineering, and mathematics careers . Psychological Science , ( 8 ), 1051–1057. [ PubMed ] [ Google Scholar ]
- DiStefano C., Zhu M., Mindrila D. (2009). Understanding and using factor scores: Considerations for the applied researcher . Practical Assessment, Research & Evaluation , ( 20 ), 1–11. [ Google Scholar ]
- Eagly A. H., Wood W., Diekman A. (2000). Social role theory of sex differences and similarities: A current appraisal . In Eckes T., Trautner H. M. (Eds.), The developmental social psychology of gender (pp. 123–174). Mahwah, NJ: Erlbaum. [ Google Scholar ]
- Eddy S. L., Brownell S. E., Thummaphan P., Lan M. C., Wenderoth M. P. (2015). Caution, student experience may vary: Social identities impact a student’s experience in peer discussions . CBE—Life Sciences Education , ( 4 ), ar45. [ PMC free article ] [ PubMed ] [ Google Scholar ]
- Eddy S. L., Hogan K. A. (2014). Getting under the hood: How and for whom does increasing course structure work? . CBE—Life Sciences Education , ( 3 ), 453–468. [ PMC free article ] [ PubMed ] [ Google Scholar ]
- Finney S. J., DiStefano C. (2006). Nonnormal and categorical data in structural equation modeling . In Hancock G. R., Mueller R. O. (Eds.), A second course in structural equation modeling (pp. 269–314). Greenwich, CT: Information Age. [ Google Scholar ]
- Fowler F. J. (2014). Survey research methods . Los Angeles: Sage. [ Google Scholar ]
- Gagne P., Hancock G. R. (2006). Measurement model quality, sample size, and solution propriety in confirmatory factor models . Multivariate Behavioral Research , ( 1 ), 65–83. [ PubMed ] [ Google Scholar ]
- Gorsuch R. L. (1983). Factor analysis (2nd ed.). Hillsdale, NJ: Erlbaum. [ Google Scholar ]
- Green S. B., Yang Y. (2009). Commentary on coefficient alpha: A cautionary tale . Psychometrika , ( 1 ), 121–135. [ Google Scholar ]
- Hu L., Bentler P. (1999). Cutoff criteria for fit indexes in covariance structure analysis: Conventional criteria versus new alternatives . Structural Equation Modeling: A Multidisciplinary Journal , ( 1 ), 1–55. [ Google Scholar ]
- Kane M. T. (2016). Explicating validity . Assessment in Education: Principles, Policy & Practice , ( 2 ), 198–211. 10.1080/0969594X.2015.1060192 [ CrossRef ] [ Google Scholar ]
- Kline R. B. (2016). Principles and practise of structural equation modeling (4th ed.). New York: Guilford. [ Google Scholar ]
- Leandre R., Fabrigar L. R., Wegener D. T. (2012). Exploratory factor analysis . Oxford, UK: Oxford University Press. [ Google Scholar ]
- Ledesma R.D., Valero-Mora P. (2007). Determining the number of factors to retain in EFA: An easy-to-use computer program for carrying out parallel analysis . Practical Assessment, Research & Evaluation , ( 2 ) [ Google Scholar ]
- Lissitz R. W., Samuelsen K. (2007). A suggested change in terminology and emphasis regarding validity and education . Educational Researcher , ( 8 ), 437–448. [ Google Scholar ]
- Marsh H.W., Hau K-T, Wen Z. (2004). In search of golden rules: Comment on hypothesis-testing approaches to setting cutoff values for fit indexes and dangers in overgeneralizing Hu and Bentler’s (1999) findings . Structural Equation Modeling: A Multidisciplinary Journal , ( 3 ), 320–341, 10.1207/s15328007sem1103_2 [ CrossRef ] [ Google Scholar ]
- Matsunaga M. (2010). How to factor analyze your data right: Do’s, don’t and how-to’s . International Journal of Psychological Research , Retrieved February 24, 2019, from www.redalyc.org/html/2990/ 299023509007/ [ Google Scholar ]
- McNeish D. (2018). Thanks coefficient alpha, we’ll take it from here . Psychological Methods , ( 3 ), 412–433. 10.1037/met0000144 [ PubMed ] [ CrossRef ] [ Google Scholar ]
- Mehrens W. A. (1997). The consequences of consequential validity . Educational Measurement: Issues and Practise , ( 2 ), 16–18. [ Google Scholar ]
- Messick S. (1995). Validity of psychological-assessment—Validation of inferences from person’s responses and performances as scientific inquiry into score meaning . American Psychologist , ( 9 ), 741–749. [ Google Scholar ]
- Mulaik S. A. (1987). A brief history of the philosophical foundations of exploratory factor analysis . Journal of Multivariate Behavioral Research , ( 3 ), 267–305. 10.1207/s15327906mbr2203_3 [ PubMed ] [ CrossRef ] [ Google Scholar ]
- Perry J. L., Nicholls A. R, Clough P. J., Crust L. (2015). Assessing model fit: Caveats and recommendations for confirmatory factor analysis and exploratory structural equation modeling . Measurement in Physical Education and Exercise Science , ( 1 ), 12–21. [ Google Scholar ]
- Prentice D. A., Carranza E. (2002). What women and men should be, shouldn’t be, are allowed to be, and don’t have to be: The contents of prescriptive gender stereotypes . Psychology of Women Quarterly , ( 4 ), 269–281. [ Google Scholar ]
- Raîche G., Walls T., Magis, D.Riopel, M.Blais, J.-G., (2013). Non-graphical solutions for Cattell’s scree test . Methodology , , 23–29. 10.1027/1614-2241/a000051 [ CrossRef ] [ Google Scholar ]
- Raykov T., Marcoulides G. A. (2008). An introduction to applied multivariate analysis . New York: Routledge. [ Google Scholar ]
- Raykov T., Marcoulides G. A. (2017). Thanks coefficient alpha, We still need you! Educational and Psychological Measurement . 10.1177/0013164417725127 [ PMC free article ] [ PubMed ] [ CrossRef ] [ Google Scholar ]
- R Core Team. (2016). R: A language and environment for statistical computing . Vienna, Austria: R Foundation for Statistical Computing; Retrieved February 24, 2019, from www.R-project.org [ Google Scholar ]
- Reeves T. D., Marbach-Ad G. (2016). Contemporary test validity in theory and practice: A primer for discipline-based education researchers . CBE—Life Sciences Education , ( 1 ), rm1. [ PMC free article ] [ PubMed ] [ Google Scholar ]
- Revelle W. (2017). psych: Procedures for Personality and Psychological Research, Northwestern University, Evanston, Illinois, USA . Retrieved February 24, 2019, from https://CRAN.R-project.org/package=psychVersion=1.7.8
- Rissing S. W., Cogan J. G. (2009). Can an inquiry approach improve college student learning in a teaching laboratory ? CBE—Life Sciences Education , ( 1 ), 55–61. [ PMC free article ] [ PubMed ] [ Google Scholar ]
- Rosseel Y. (2012). lavaan: An R Package for Structural Equation Modeling . Journal of Statistical Software , ( 2 ), 1–36. [ Google Scholar ]
- Ruscio J., Roche B. (2012). Determining the number of factors to retain in an exploratory factor analysis using comparison data of known factorial structure . Psychological Assessment , ( 2 ), 282. [ PubMed ] [ Google Scholar ]
- Schmitt N. (1996). Uses and abuses of coefficient alpha . Psychological Assessment , ( 4 ), 350–353. [ Google Scholar ]
- Sijtsma K. (2009). On the use, the misuse, and the very limited usefulness of Cronbach’s alpha . Psychometrika , ( 1 ), 107. [ PMC free article ] [ PubMed ] [ Google Scholar ]
- Slaney K. (2017). Construct validity: Developments and debates . In Validating psychological constructs: Historical, Philosophical, and Practical Dimensions (pp. 83–109) (Palgrave studies in the theory and history of psychology). London: Palgrave Macmillan. [ Google Scholar ]
- Smith J. L., Cech E., Metz A., Huntoon M., Moyer C. (2014). Giving back or giving up: Native American student experiences in science and engineering . Cultural Diversity and Ethnic Minority Psychology , ( 3 ), 413. [ PubMed ] [ Google Scholar ]
- Stephens N. M., Fryberg S. A., Markus H. R., Johnson C. S., Covarrubias R. (2012). Unseen disadvantage: How American universities’ focus on independence undermines the academic performance of first-generation college students . Journal of Personality and Social Psychology , ( 6 ), 1178–1197. Retrieved from 10.1037/a0027143 [ PubMed ] [ CrossRef ] [ Google Scholar ]
- Su R., Rounds J., Armstrong P. I. (2009). Men and things, women and people: A meta-analysis of sex differences in interests . Psychological Bulletin , ( 6 ), 859. [ PubMed ] [ Google Scholar ]
- Tabachnick B. G., Fidell L. S. (2013). Using multivariate statistics (6th ed). Boston: Pearson. [ Google Scholar ]
- Tavakol M., Dennick R. (2011). Making sense of Cronbach’s alpha . International Journal of Medical Education , , 53–55. Retrieved February 24, 2019, from 10.5116/ijme.4dfb.8dfd [ PMC free article ] [ PubMed ] [ CrossRef ] [ Google Scholar ]
- Wachsmuth L. P., Runyon C. R., Drake J. M., Dolan E. L. (2017). Do biology students really hate math? Empirical insights into undergraduate life science majors’ emotions about mathematics . CBE—Life Sciences Education , ( 3 ), ar49. [ PMC free article ] [ PubMed ] [ Google Scholar ]
- Wigfield A., Eccles J. S. (1992). The development of achievement task values: A theoretical analysis . Developmental Review , ( 3 ), 265–310. 10.1016/0273-2297(92)90011-p [ CrossRef ] [ Google Scholar ]
- Wiggins B. L., Eddy S. L., Wener-Fligner L., Freisem K., Grunspan D. Z., Theobald E. J., Crowe A. J. (2017). ASPECT: A survey to assess student perspective of engagement in an active-learning classroom . CBE—Life Sciences Education , ( 2 ), ar32. [ PMC free article ] [ PubMed ] [ Google Scholar ]
- Wolf E. J., Harrington K. M., Clark S. L., Miller M. W. (2013). Sample size requirements for structural equation models: An evaluation of power, bias, and solution propriety . Educational and Psychological Measurement , ( 6 ), 913–934. [ PMC free article ] [ PubMed ] [ Google Scholar ]
- Worthington R. L., Whittaker T. A. (2006). Scale development research: A content analysis and recommendations for best practices . The Counseling Psychologist , ( 6 ), 806–838. [ Google Scholar ]
- Yang Y., Green S. B. (2011). Coefficient alpha: A reliability coefficient for the 21st century ? Journal of Psychoeducational Assessment , ( 4 ), 377–392. [ Google Scholar ]
- Yong A. G., Pearce S. (2013). A beginner’s guide to factor analysis: Focusing on exploratory factor analysis . Tutorials in Quantitative Methods for Psychology , ( 2 ), 79–94. [ Google Scholar ]
Confirmatory Factor Analysis and Structural Equation Modeling
Cite this chapter.
- Aek Phakiti 5
7865 Accesses
11 Citations
This chapter explains the core principles of confirmatory factor analysis (CFA) and structural equation modeling (SEM) that can be used in applied linguistics research. CFA and SEM are multivariate statistical techniques researchers use to test a hypothesis or theory. This chapter provides essential guidelines for not only how to read CFA and SEM reports but also how to perform CFA. CFA differs from exploratory factor analysis in many ways (e.g., statistical assumptions and procedures, assessment of model fit and methods for extracting factors). Researchers employ SEM to evaluate or test among observed variables and latent variables. In this chapter, EQS Program is used to illustrate how to perform CFA.
This is a preview of subscription content, log in via an institution to check access.
Access this chapter
- Available as PDF
- Read on any device
- Instant download
- Own it forever
- Available as EPUB and PDF
- Compact, lightweight edition
- Dispatched in 3 to 5 business days
- Free shipping worldwide - see info
- Durable hardcover edition
Tax calculation will be finalised at checkout
Purchases are for personal use only
Institutional subscriptions
Akaike, H. (1987). Factor analysis and AIC. Psychometrika, 52 (3), 317–332.
Article Google Scholar
Bentler, P. M. (1985–2018). EQS Version 6 for Windows [Computer software] . Encino, CA: Multivariate Software.
Google Scholar
Bentler, P. M. (1990). Comparative fit indexes in structural models. Psychological Bulletin, 107 (2), 238–246.
Bentler, P. M. (2006). EQS structural equation program manual . Encino, CA: Multivariate Software.
Bentler, P. M., & Bonett, D. G. (1980). Significance tests and goodness-of-fit in the analysis of covariance structures. Psychological Bulletin, 88 (3), 588–606.
Bentler, P. M., & Wu, E. J. C. (2006). EQS for Windows user’s guide . Encino, CA: Multivariate Software.
Bohrnstedt, G. W., & Carter, T. M. (1971). Robustness in regression analysis. In H. L. Costner (Ed.), Sociological methodology (pp. 118–146). San Fransisco: Jossey-Bass.
Bozdogan, H. (1987). Model selection and Akaike’s information criteria (AIC): The general theory and its analytical extensions. Psychometrika, 52 (3), 345–370.
Brown, T. A. (2015). Confirmatory factor analysis for applied research (2nd ed.). New York and London: Guilford Press.
Byrne, B. M. (2006). Structural equation modeling with EQS and EQS/Windows: Basic concepts, applications, and programming . New York and London: Psychology Press.
Cliff, N. (1983). Some cautions concerning the application of causal modeling methods. Multivariate Behavioral Research, 18 (1), 115–126.
Cohen, J. (1992). A power primer. Psychological Bulletin, 112 (1), 155–159.
Grant, R., MacDonald, R., Phakiti, A., & Cook, H. (2014). The importance of writing in mathematics: Quantitative analysis of U.S. English learners’ academic language proficiency and mathematics achievement. In E. Stracke (Ed.), Intersections: Applied linguistics as a meeting place (pp. 208–232). Newcastle upon Tyne). London: Cambridge Scholars Publishing.
Hancock, G. R., & Schoonen, R. (2015). Structural equation modelling: Possibilities for language learning researchers. Language Learning, 65 (S1), 160–184.
In’nami, Y., & Koizumi, R. (2010). Can structural equation models in second language testing and learning research be successfully replicated? International Journal of Testing, 10 , 262–273.
Jöreskog, K. G. (1971). Simultaneous factor analysis in several populations. Psychometrika, 57 , 239–257.
Kaplan, D. (1995). Statistical power in structural equation modeling. In R. H. Hoyle (Ed.), Structural equation modeling: Concepts, issues, and applications (pp. 100–117). Thousand Oaks: SAGE.
Kline, R. B. (2016). Principles and practice of structural equation modeling (4th ed.). New York and London: The Guilford Press.
Ockey, G. J. (2014). Exploratory factor analysis and structural equation modeling. In A. J. Kunnan (Ed.), The Companion to language assessment (pp. 1224–1444). Oxford: John Wiley & Sons.
Ockey, G. J., & Choi, I. (2015). Structural equation modeling reporting practices for language assessment. Language Assessment Quarterly, 12 (3), 305–319.
Pearl, J. (2000). Causality: Models, reasoning, and inference . Cambridge: Cambridge University Press.
Pedhazur, E. J., & Schmelkin, L. P. (1992). Measurement, design, and analysis: An integrated approach . Hillsdale, NJ: Holt, Rinehart and Winston.
Phakiti, A. (2008). Strategic competence as a fourth-order factor model: A structural equation modeling approach. Language Assessment Quarterly, 5 (1), 20–42.
Plonsky, L. (2013). Study quality in SLA: An assessment of designs, analyses, and reporting practices in quantitative L2 research. Studies in Second Language Acquisition, 35 (4), 655–687.
Plonsky, L., & Oswald, F. L. (2014). How big is ‘big’? Interpreting effect sizes in L2 research. Language Learning, 64 (4), 878–912.
Rubenfeld, S., & Clément, R. (2012). Intercultural conflict and mediation: An intergroup perspective. Language Learning, 62 (4), 1205–1230.
Schoonen, R. (2015). Structural equation modelling in L2 research. In L. Plonsky (Ed.), Advancing quantitative methods in second language research (pp. 213–242). New York: Routledge.
Chapter Google Scholar
Schumacker, R. E., & Lomax, R. G. (2016). A beginner’s guide to structural equation modeling (4th ed.). New York and London: Routledge.
Thompson, B. (2000). Ten commandments of structural equation modeling. In L. G. Grimm & P. R. Yarnold (Eds.), Reading and understanding more multivariate statistics (pp. 261–283). Washington: American Psychological Association.
Ullman, J. B. (1996). Structural equation modeling. In B. G. Tabachnick & L. S. Fidell (Eds.), Using multivariate statistics (3rd ed., pp. 709–811). New York: Harper Collins College Publishers.
Vandergrift, L., & Baker, S. (2015). Learner variables in second language listening comprehension: An exploratory path analysis. Language Learning, 65 (2), 390–416.
Weston, R., & Gore, P. A. (2006). A brief guide to structural equation modeling. The Counselling Psychologist, 34 (5), 719–751.
Wheaton, B., Muthen, B., Alwin, D. F., & Summers, G. (1977). Assessing reliability and stability in panel models. Sociological Methodology, 8 , 84–136.
Winke, P. (2014). Testing hypotheses about language learning using structural equation modeling. Annual Review of Applied Linguistics, 34 , 102–122.
Download references
Author information
Authors and affiliations.
Sydney School of Education and Social Work, The University of Sydney, Sydney, NSW, Australia
Aek Phakiti
You can also search for this author in PubMed Google Scholar
Corresponding author
Correspondence to Aek Phakiti .
Editor information
Editors and affiliations.
Sydney School of Education and Social Work, University of Sydney, Sydney, NSW, Australia
Department of Linguistics, Germanic, Slavic, Asian and African Languages, Michigan State University, East Lansing, MI, USA
Peter De Costa
Applied Linguistics, Northern Arizona University, Flagstaff, AZ, USA
Luke Plonsky
School of Education, UNSW Sydney, Sydney, NSW, Australia
Sue Starfield
Copyright information
© 2018 The Author(s)
About this chapter
Phakiti, A. (2018). Confirmatory Factor Analysis and Structural Equation Modeling. In: Phakiti, A., De Costa, P., Plonsky, L., Starfield, S. (eds) The Palgrave Handbook of Applied Linguistics Research Methodology. Palgrave Macmillan, London. https://doi.org/10.1057/978-1-137-59900-1_21
Download citation
DOI : https://doi.org/10.1057/978-1-137-59900-1_21
Publisher Name : Palgrave Macmillan, London
Print ISBN : 978-1-137-59899-8
Online ISBN : 978-1-137-59900-1
eBook Packages : Social Sciences Social Sciences (R0)
Share this chapter
Anyone you share the following link with will be able to read this content:
Sorry, a shareable link is not currently available for this article.
Provided by the Springer Nature SharedIt content-sharing initiative
- Publish with us
Policies and ethics
- Find a journal
- Track your research
- Skip to primary navigation
- Skip to main content
- Skip to primary sidebar
Institute for Digital Research and Education
A Practical Introduction to Factor Analysis: Exploratory Factor Analysis
This seminar is the first part of a two-part seminar that introduces central concepts in factor analysis. Part 1 focuses on exploratory factor analysis (EFA). Although the implementation is in SPSS, the ideas carry over to any software program. Part 2 introduces confirmatory factor analysis (CFA). Please refer to A Practical Introduction to Factor Analysis: Confirmatory Factor Analysis .
I. Exploratory Factor Analysis
- Motivating example: The SAQ
- Pearson correlation formula
Partitioning the variance in factor analysis
- principal components analysis
- principal axis factoring
- maximum likelihood
Simple Structure
- Orthogonal rotation (Varimax)
- Oblique (Direct Oblimin)
- Generating factor scores
Back to Launch Page
Introduction.
Suppose you are conducting a survey and you want to know whether the items in the survey have similar patterns of responses, do these items “hang together” to create a construct? The basic assumption of factor analysis is that for a collection of observed variables there are a set of underlying variables called factors (smaller than the observed variables), that can explain the interrelationships among those variables. Let’s say you conduct a survey and collect responses about people’s anxiety about using SPSS. Do all these items actually measure what we call “SPSS Anxiety”?

Motivating Example: The SAQ (SPSS Anxiety Questionnaire)
Let’s proceed with our hypothetical example of the survey which Andy Field terms the SPSS Anxiety Questionnaire. For simplicity, we will use the so-called “ SAQ-8 ” which consists of the first eight items in the SAQ . Click on the preceding hyperlinks to download the SPSS version of both files. The SAQ-8 consists of the following questions:
- Statistics makes me cry
- My friends will think I’m stupid for not being able to cope with SPSS
- Standard deviations excite me
- I dream that Pearson is attacking me with correlation coefficients
- I don’t understand statistics
- I have little experience of computers
- All computers hate me
- I have never been good at mathematics
Pearson Correlation of the SAQ-8
Let’s get the table of correlations in SPSS Analyze – Correlate – Bivariate:
From this table we can see that most items have some correlation with each other ranging from \(r=-0.382\) for Items 3 and 7 to \(r=.514\) for Items 6 and 7. Due to relatively high correlations among items, this would be a good candidate for factor analysis. Recall that the goal of factor analysis is to model the interrelationships between items with fewer (latent) variables. These interrelationships can be broken up into multiple components
Since the goal of factor analysis is to model the interrelationships among items, we focus primarily on the variance and covariance rather than the mean. Factor analysis assumes that variance can be partitioned into two types of variance, common and unique
- Communality (also called \(h^2\)) is a definition of common variance that ranges between \(0 \) and \(1\). Values closer to 1 suggest that extracted factors explain more of the variance of an individual item.
- Specific variance : is variance that is specific to a particular item (e.g., Item 4 “All computers hate me” may have variance that is attributable to anxiety about computers in addition to anxiety about SPSS).
- Error variance: comes from errors of measurement and basically anything unexplained by common or specific variance (e.g., the person got a call from her babysitter that her two-year old son ate her favorite lipstick).
The figure below shows how these concepts are related:
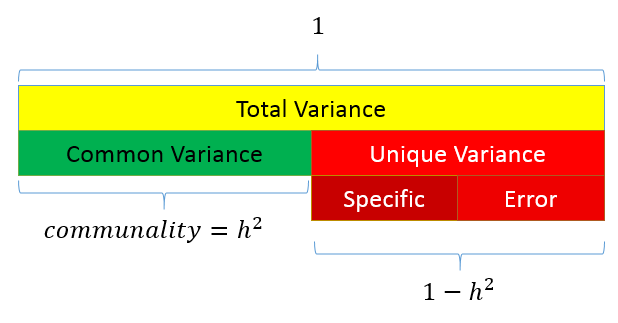
Performing Factor Analysis
As a data analyst, the goal of a factor analysis is to reduce the number of variables to explain and to interpret the results. This can be accomplished in two steps:
- factor extraction
- factor rotation
Factor extraction involves making a choice about the type of model as well the number of factors to extract. Factor rotation comes after the factors are extracted, with the goal of achieving simple structure in order to improve interpretability.
Extracting Factors
There are two approaches to factor extraction which stems from different approaches to variance partitioning: a) principal components analysis and b) common factor analysis.
Principal Components Analysis
Unlike factor analysis, principal components analysis or PCA makes the assumption that there is no unique variance, the total variance is equal to common variance. Recall that variance can be partitioned into common and unique variance. If there is no unique variance then common variance takes up total variance (see figure below). Additionally, if the total variance is 1, then the common variance is equal to the communality.
Running a PCA with 8 components in SPSS
The goal of a PCA is to replicate the correlation matrix using a set of components that are fewer in number and linear combinations of the original set of items. Although the following analysis defeats the purpose of doing a PCA we will begin by extracting as many components as possible as a teaching exercise and so that we can decide on the optimal number of components to extract later.
First go to Analyze – Dimension Reduction – Factor. Move all the observed variables over the Variables: box to be analyze.

Under Extraction – Method, pick Principal components and make sure to Analyze the Correlation matrix. We also request the Unrotated factor solution and the Scree plot. Under Extract, choose Fixed number of factors, and under Factor to extract enter 8. We also bumped up the Maximum Iterations of Convergence to 100.
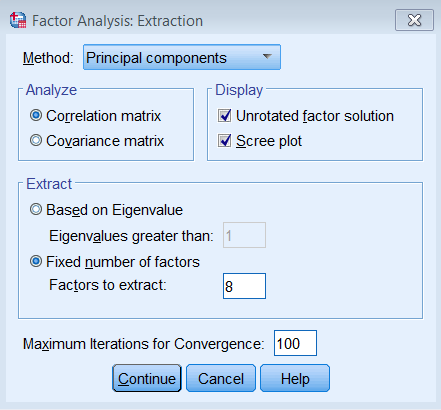
The equivalent SPSS syntax is shown below:
Eigenvalues and Eigenvectors
Before we get into the SPSS output, let’s understand a few things about eigenvalues and eigenvectors.
Eigenvalues represent the total amount of variance that can be explained by a given principal component. They can be positive or negative in theory, but in practice they explain variance which is always positive.
- If eigenvalues are greater than zero, then it’s a good sign.
- Since variance cannot be negative, negative eigenvalues imply the model is ill-conditioned.
- Eigenvalues close to zero imply there is item multicollinearity, since all the variance can be taken up by the first component.
Eigenvalues are also the sum of squared component loadings across all items for each component, which represent the amount of variance in each item that can be explained by the principal component.
Eigenvectors represent a weight for each eigenvalue. The eigenvector times the square root of the eigenvalue gives the component loadings which can be interpreted as the correlation of each item with the principal component. For this particular PCA of the SAQ-8, the eigenvector associated with Item 1 on the first component is \(0.377\), and the eigenvalue of Item 1 is \(3.057\). We can calculate the first component as
$$(0.377)\sqrt{3.057}= 0.659.$$
In this case, we can say that the correlation of the first item with the first component is \(0.659\). Let’s now move on to the component matrix.
Component Matrix
The components can be interpreted as the correlation of each item with the component. Each item has a loading corresponding to each of the 8 components. For example, Item 1 is correlated \(0.659\) with the first component, \(0.136\) with the second component and \(-0.398\) with the third, and so on.
The square of each loading represents the proportion of variance (think of it as an \(R^2\) statistic) explained by a particular component. For Item 1, \((0.659)^2=0.434\) or \(43.4\%\) of its variance is explained by the first component. Subsequently, \((0.136)^2 = 0.018\) or \(1.8\%\) of the variance in Item 1 is explained by the second component. The total variance explained by both components is thus \(43.4\%+1.8\%=45.2\%\). If you keep going on adding the squared loadings cumulatively down the components, you find that it sums to 1 or 100%. This is also known as the communality , and in a PCA the communality for each item is equal to the total variance.
Summing the squared component loadings across the components (columns) gives you the communality estimates for each item, and summing each squared loading down the items (rows) gives you the eigenvalue for each component. For example, to obtain the first eigenvalue we calculate:
$$(0.659)^2 + (-.300)^2 – (-0.653)^2 + (0.720)^2 + (0.650)^2 + (0.572)^2 + (0.718)^2 + (0.568)^2 = 3.057$$
You will get eight eigenvalues for eight components, which leads us to the next table.
Total Variance Explained in the 8-component PCA
Recall that the eigenvalue represents the total amount of variance that can be explained by a given principal component. Starting from the first component, each subsequent component is obtained from partialling out the previous component. Therefore the first component explains the most variance, and the last component explains the least. Looking at the Total Variance Explained table, you will get the total variance explained by each component. For example, Component 1 is \(3.057\), or \((3.057/8)\% = 38.21\%\) of the total variance. Because we extracted the same number of components as the number of items, the Initial Eigenvalues column is the same as the Extraction Sums of Squared Loadings column.
Choosing the number of components to extract
Since the goal of running a PCA is to reduce our set of variables down, it would useful to have a criterion for selecting the optimal number of components that are of course smaller than the total number of items. One criterion is the choose components that have eigenvalues greater than 1. Under the Total Variance Explained table, we see the first two components have an eigenvalue greater than 1. This can be confirmed by the Scree Plot which plots the eigenvalue (total variance explained) by the component number. Recall that we checked the Scree Plot option under Extraction – Display, so the scree plot should be produced automatically.

The first component will always have the highest total variance and the last component will always have the least, but where do we see the largest drop? If you look at Component 2, you will see an “elbow” joint. This is the marking point where it’s perhaps not too beneficial to continue further component extraction. There are some conflicting definitions of the interpretation of the scree plot but some say to take the number of components to the left of the the “elbow”. Following this criteria we would pick only one component. A more subjective interpretation of the scree plots suggests that any number of components between 1 and 4 would be plausible and further corroborative evidence would be helpful.
Some criteria say that the total variance explained by all components should be between 70% to 80% variance, which in this case would mean about four to five components. The authors of the book say that this may be untenable for social science research where extracted factors usually explain only 50% to 60%. Picking the number of components is a bit of an art and requires input from the whole research team. Let’s suppose we talked to the principal investigator and she believes that the two component solution makes sense for the study, so we will proceed with the analysis.
Running a PCA with 2 components in SPSS
Running the two component PCA is just as easy as running the 8 component solution. The only difference is under Fixed number of factors – Factors to extract you enter 2.
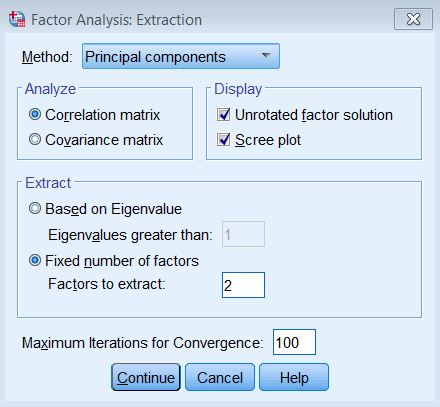
We will focus the differences in the output between the eight and two-component solution. Under Total Variance Explained, we see that the Initial Eigenvalues no longer equals the Extraction Sums of Squared Loadings. The main difference is that there are only two rows of eigenvalues, and the cumulative percent variance goes up to \(51.54\%\).
Similarly, you will see that the Component Matrix has the same loadings as the eight-component solution but instead of eight columns it’s now two columns.
Again, we interpret Item 1 as having a correlation of 0.659 with Component 1. From glancing at the solution, we see that Item 4 has the highest correlation with Component 1 and Item 2 the lowest. Similarly, we see that Item 2 has the highest correlation with Component 2 and Item 7 the lowest.
Quick check:
True or False
- The elements of the Component Matrix are correlations of the item with each component.
- The sum of the squared eigenvalues is the proportion of variance under Total Variance Explained.
- The Component Matrix can be thought of as correlations and the Total Variance Explained table can be thought of as \(R^2\).
1.T, 2.F (sum of squared loadings), 3. T
Communalities of the 2-component PCA
The communality is the sum of the squared component loadings up to the number of components you extract. In the SPSS output you will see a table of communalities.
Since PCA is an iterative estimation process, it starts with 1 as an initial estimate of the communality (since this is the total variance across all 8 components), and then proceeds with the analysis until a final communality extracted. Notice that the Extraction column is smaller Initial column because we only extracted two components. As an exercise, let’s manually calculate the first communality from the Component Matrix. The first ordered pair is \((0.659,0.136)\) which represents the correlation of the first item with Component 1 and Component 2. Recall that squaring the loadings and summing down the components (columns) gives us the communality:
$$h^2_1 = (0.659)^2 + (0.136)^2 = 0.453$$
Going back to the Communalities table, if you sum down all 8 items (rows) of the Extraction column, you get \(4.123\). If you go back to the Total Variance Explained table and summed the first two eigenvalues you also get \(3.057+1.067=4.124\). Is that surprising? Basically it’s saying that the summing the communalities across all items is the same as summing the eigenvalues across all components.
1. In a PCA, when would the communality for the Initial column be equal to the Extraction column?
Answer : When you run an 8-component PCA.
- The eigenvalue represents the communality for each item.
- For a single component, the sum of squared component loadings across all items represents the eigenvalue for that component.
- The sum of eigenvalues for all the components is the total variance.
- The sum of the communalities down the components is equal to the sum of eigenvalues down the items.
1. F, the eigenvalue is the total communality across all items for a single component, 2. T, 3. T, 4. F (you can only sum communalities across items, and sum eigenvalues across components, but if you do that they are equal).
Common Factor Analysis
The partitioning of variance differentiates a principal components analysis from what we call common factor analysis. Both methods try to reduce the dimensionality of the dataset down to fewer unobserved variables, but whereas PCA assumes that there common variances takes up all of total variance, common factor analysis assumes that total variance can be partitioned into common and unique variance. It is usually more reasonable to assume that you have not measured your set of items perfectly. The unobserved or latent variable that makes up common variance is called a factor , hence the name factor analysis. The other main difference between PCA and factor analysis lies in the goal of your analysis. If your goal is to simply reduce your variable list down into a linear combination of smaller components then PCA is the way to go. However, if you believe there is some latent construct that defines the interrelationship among items, then factor analysis may be more appropriate. In this case, we assume that there is a construct called SPSS Anxiety that explains why you see a correlation among all the items on the SAQ-8, we acknowledge however that SPSS Anxiety cannot explain all the shared variance among items in the SAQ, so we model the unique variance as well. Based on the results of the PCA, we will start with a two factor extraction.
Running a Common Factor Analysis with 2 factors in SPSS
To run a factor analysis, use the same steps as running a PCA (Analyze – Dimension Reduction – Factor) except under Method choose Principal axis factoring. Note that we continue to set Maximum Iterations for Convergence at 100 and we will see why later.
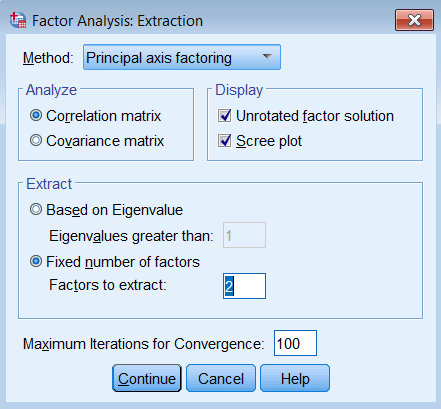
Pasting the syntax into the SPSS Syntax Editor we get:
Note the main difference is under /EXTRACTION we list PAF for Principal Axis Factoring instead of PC for Principal Components. We will get three tables of output, Communalities, Total Variance Explained and Factor Matrix. Let’s go over each of these and compare them to the PCA output.
Communalities of the 2-factor PAF
The most striking difference between this communalities table and the one from the PCA is that the initial extraction is no longer one. Recall that for a PCA, we assume the total variance is completely taken up by the common variance or communality, and therefore we pick 1 as our best initial guess. What principal axis factoring does is instead of guessing 1 as the initial communality, it chooses the squared multiple correlation coefficient \(R^2\). To see this in action for Item 1 run a linear regression where Item 1 is the dependent variable and Items 2 -8 are independent variables. Go to Analyze – Regression – Linear and enter q01 under Dependent and q02 to q08 under Independent(s).
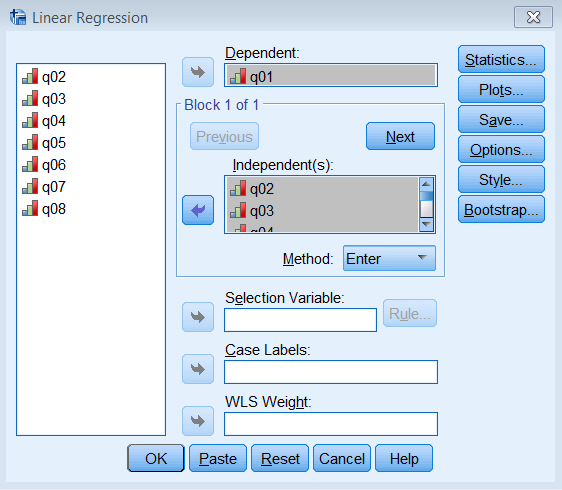
Pasting the syntax into the Syntax Editor gives us:
The output we obtain from this analysis is
Note that 0.293 (highlighted in red) matches the initial communality estimate for Item 1. We can do eight more linear regressions in order to get all eight communality estimates but SPSS already does that for us. Like PCA, factor analysis also uses an iterative estimation process to obtain the final estimates under the Extraction column. Finally, summing all the rows of the extraction column, and we get 3.00. This represents the total common variance shared among all items for a two factor solution.
Total Variance Explained (2-factor PAF)
The next table we will look at is Total Variance Explained. Comparing this to the table from the PCA we notice that the Initial Eigenvalues are exactly the same and includes 8 rows for each “factor”. In fact, SPSS simply borrows the information from the PCA analysis for use in the factor analysis and the factors are actually components in the Initial Eigenvalues column. The main difference now is in the Extraction Sums of Squares Loadings. We notice that each corresponding row in the Extraction column is lower than the Initial column. This is expected because we assume that total variance can be partitioned into common and unique variance, which means the common variance explained will be lower. Factor 1 explains 31.38% of the variance whereas Factor 2 explains 6.24% of the variance. Just as in PCA the more factors you extract, the less variance explained by each successive factor.
A subtle note that may be easily overlooked is that when SPSS plots the scree plot or the Eigenvalues greater than 1 criteria (Analyze – Dimension Reduction – Factor – Extraction), it bases it off the Initial and not the Extraction solution. This is important because the criteria here assumes no unique variance as in PCA, which means that this is the total variance explained not accounting for specific or measurement error. Note that in the Extraction of Sums Squared Loadings column the second factor has an eigenvalue that is less than 1 but is still retained because the Initial value is 1.067. If you want to use this criteria for the common variance explained you would need to modify the criteria yourself.
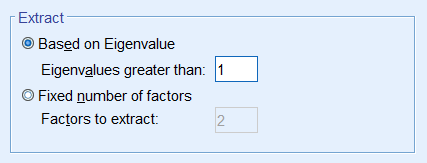
- In theory, when would the percent of variance in the Initial column ever equal the Extraction column?
- True or False, in SPSS when you use the Principal Axis Factor method the scree plot uses the final factor analysis solution to plot the eigenvalues.
Answers: 1. When there is no unique variance (PCA assumes this whereas common factor analysis does not, so this is in theory and not in practice), 2. F, it uses the initial PCA solution and the eigenvalues assume no unique variance.
Factor Matrix (2-factor PAF)
First note the annotation that 79 iterations were required. If we had simply used the default 25 iterations in SPSS, we would not have obtained an optimal solution. This is why in practice it’s always good to increase the maximum number of iterations. Now let’s get into the table itself. The elements of the Factor Matrix table are called loadings and represent the correlation of each item with the corresponding factor. Just as in PCA, squaring each loading and summing down the items (rows) gives the total variance explained by each factor. Note that they are no longer called eigenvalues as in PCA. Let’s calculate this for Factor 1:
$$(0.588)^2 + (-0.227)^2 + (-0.557)^2 + (0.652)^2 + (0.560)^2 + (0.498)^2 + (0.771)^2 + (0.470)^2 = 2.51$$
This number matches the first row under the Extraction column of the Total Variance Explained table. We can repeat this for Factor 2 and get matching results for the second row. Additionally, we can get the communality estimates by summing the squared loadings across the factors (columns) for each item. For example, for Item 1:
$$(0.588)^2 + (-0.303)^2 = 0.437$$
Note that these results match the value of the Communalities table for Item 1 under the Extraction column. This means that the sum of squared loadings across factors represents the communality estimates for each item.
The relationship between the three tables
To see the relationships among the three tables let’s first start from the Factor Matrix (or Component Matrix in PCA). We will use the term factor to represent components in PCA as well. These elements represent the correlation of the item with each factor. Now, square each element to obtain squared loadings or the proportion of variance explained by each factor for each item. Summing the squared loadings across factors you get the proportion of variance explained by all factors in the model. This is known as common variance or communality, hence the result is the Communalities table. Going back to the Factor Matrix, if you square the loadings and sum down the items you get Sums of Squared Loadings (in PAF) or eigenvalues (in PCA) for each factor. These now become elements of the Total Variance Explained table. Summing down the rows (i.e., summing down the factors) under the Extraction column we get \(2.511 + 0.499 = 3.01\) or the total (common) variance explained. In words, this is the total (common) variance explained by the two factor solution for all eight items. Equivalently, since the Communalities table represents the total common variance explained by both factors for each item, summing down the items in the Communalities table also gives you the total (common) variance explained, in this case
$$ (0.437)^2 + (0.052)^2 + (0.319)^2 + (0.460)^2 + (0.344)^2 + (0.309)^2 + (0.851)^2 + (0.236)^2 = 3.01$$
which is the same result we obtained from the Total Variance Explained table. Here is a table that that may help clarify what we’ve talked about:
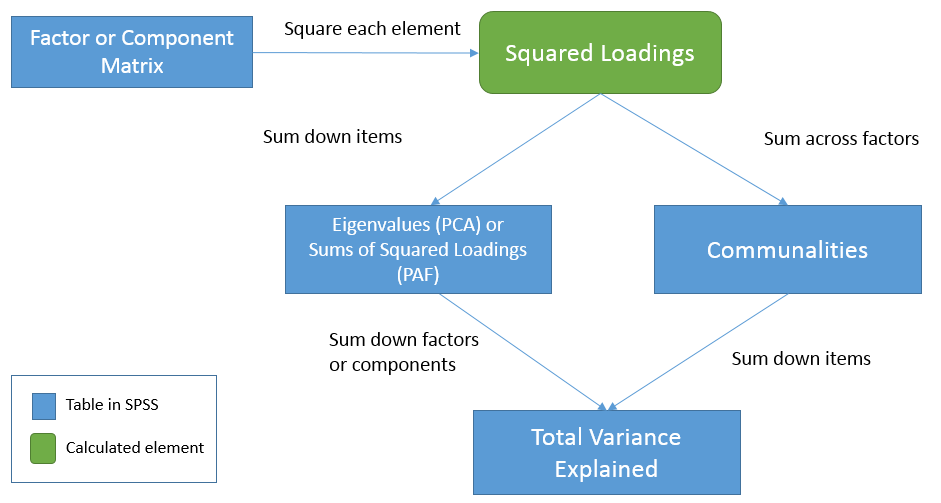
In summary:
- Squaring the elements in the Factor Matrix gives you the squared loadings
- Summing the squared loadings of the Factor Matrix across the factors gives you the communality estimates for each item in the Extraction column of the Communalities table.
- Summing the squared loadings of the Factor Matrix down the items gives you the Sums of Squared Loadings (PAF) or eigenvalue (PCA) for each factor across all items.
- Summing the eigenvalues or Sums of Squared Loadings in the Total Variance Explained table gives you the total common variance explained.
- Summing down all items of the Communalities table is the same as summing the eigenvalues or Sums of Squared Loadings down all factors under the Extraction column of the Total Variance Explained table.
True or False (the following assumes a two-factor Principal Axis Factor solution with 8 items)
- The elements of the Factor Matrix represent correlations of each item with a factor.
- Each squared element of Item 1 in the Factor Matrix represents the communality.
- Summing the squared elements of the Factor Matrix down all 8 items within Factor 1 equals the first Sums of Squared Loading under the Extraction column of Total Variance Explained table.
- Summing down all 8 items in the Extraction column of the Communalities table gives us the total common variance explained by both factors.
- The total common variance explained is obtained by summing all Sums of Squared Loadings of the Initial column of the Total Variance Explained table
- The total Sums of Squared Loadings in the Extraction column under the Total Variance Explained table represents the total variance which consists of total common variance plus unique variance.
- In common factor analysis, the sum of squared loadings is the eigenvalue.
Answers: 1. T, 2. F, the sum of the squared elements across both factors, 3. T, 4. T, 5. F, sum all eigenvalues from the Extraction column of the Total Variance Explained table, 6. F, the total Sums of Squared Loadings represents only the total common variance excluding unique variance, 7. F, eigenvalues are only applicable for PCA.
Maximum Likelihood Estimation (2-factor ML)
Since this is a non-technical introduction to factor analysis, we won’t go into detail about the differences between Principal Axis Factoring (PAF) and Maximum Likelihood (ML). The main concept to know is that ML also assumes a common factor analysis using the \(R^2\) to obtain initial estimates of the communalities, but uses a different iterative process to obtain the extraction solution. To run a factor analysis using maximum likelihood estimation under Analyze – Dimension Reduction – Factor – Extraction – Method choose Maximum Likelihood.
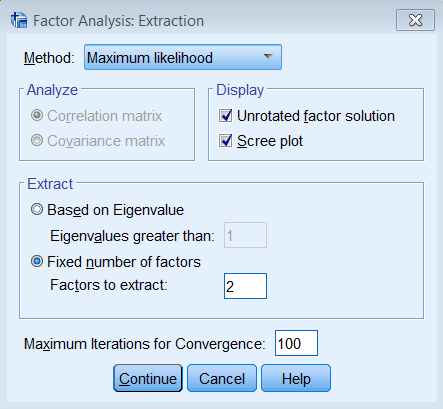
Although the initial communalities are the same between PAF and ML, the final extraction loadings will be different, which means you will have different Communalities, Total Variance Explained, and Factor Matrix tables (although Initial columns will overlap). The other main difference is that you will obtain a Goodness-of-fit Test table, which gives you a absolute test of model fit. Non-significant values suggest a good fitting model. Here the p -value is less than 0.05 so we reject the two-factor model.
In practice, you would obtain chi-square values for multiple factor analysis runs, which we tabulate below from 1 to 8 factors. The table shows the number of factors extracted (or attempted to extract) as well as the chi-square, degrees of freedom, p-value and iterations needed to converge. Note that as you increase the number of factors, the chi-square value and degrees of freedom decreases but the iterations needed and p-value increases. Practically, you want to make sure the number of iterations you specify exceeds the iterations needed. Additionally, NS means no solution and N/A means not applicable. In SPSS, no solution is obtained when you run 5 to 7 factors because the degrees of freedom is negative (which cannot happen). For the eight factor solution, it is not even applicable in SPSS because it will spew out a warning that “You cannot request as many factors as variables with any extraction method except PC. The number of factors will be reduced by one.” This means that if you try to extract an eight factor solution for the SAQ-8, it will default back to the 7 factor solution. Now that we understand the table, let’s see if we can find the threshold at which the absolute fit indicates a good fitting model. It looks like here that the p -value becomes non-significant at a 3 factor solution. Note that differs from the eigenvalues greater than 1 criteria which chose 2 factors and using Percent of Variance explained you would choose 4-5 factors. We talk to the Principal Investigator and at this point, we still prefer the two-factor solution. Note that there is no “right” answer in picking the best factor model, only what makes sense for your theory. We will talk about interpreting the factor loadings when we talk about factor rotation to further guide us in choosing the correct number of factors.
- The Initial column of the Communalities table for the Principal Axis Factoring and the Maximum Likelihood method are the same given the same analysis.
- Since they are both factor analysis methods, Principal Axis Factoring and the Maximum Likelihood method will result in the same Factor Matrix.
- In SPSS, both Principal Axis Factoring and Maximum Likelihood methods give chi-square goodness of fit tests.
- You can extract as many factors as there are items as when using ML or PAF.
- When looking at the Goodness-of-fit Test table, a p -value less than 0.05 means the model is a good fitting model.
- In the Goodness-of-fit Test table, the lower the degrees of freedom the more factors you are fitting.
Answers: 1. T, 2. F, the two use the same starting communalities but a different estimation process to obtain extraction loadings, 3. F, only Maximum Likelihood gives you chi-square values, 4. F, you can extract as many components as items in PCA, but SPSS will only extract up to the total number of items minus 1, 5. F, greater than 0.05, 6. T, we are taking away degrees of freedom but extracting more factors.
Comparing Common Factor Analysis versus Principal Components
As we mentioned before, the main difference between common factor analysis and principal components is that factor analysis assumes total variance can be partitioned into common and unique variance, whereas principal components assumes common variance takes up all of total variance (i.e., no unique variance). For both methods, when you assume total variance is 1, the common variance becomes the communality. The communality is unique to each item, so if you have 8 items, you will obtain 8 communalities; and it represents the common variance explained by the factors or components. However in the case of principal components, the communality is the total variance of each item, and summing all 8 communalities gives you the total variance across all items. In contrast, common factor analysis assumes that the communality is a portion of the total variance, so that summing up the communalities represents the total common variance and not the total variance. In summary, for PCA, total common variance is equal to total variance explained , which in turn is equal to the total variance, but in common factor analysis, total common variance is equal to total variance explained but does not equal total variance.
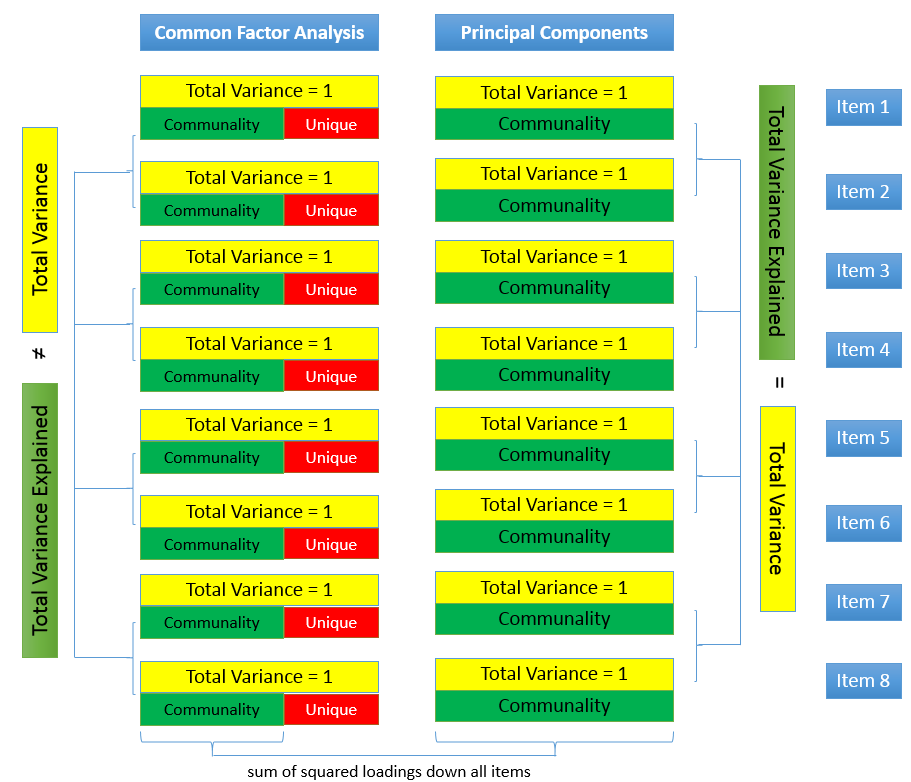
The following applies to the SAQ-8 when theoretically extracting 8 components or factors for 8 items:
- For each item, when the total variance is 1, the common variance becomes the communality.
- In principal components, each communality represents the total variance across all 8 items.
- In common factor analysis, the communality represents the common variance for each item.
- The communality is unique to each factor or component.
- For both PCA and common factor analysis, the sum of the communalities represent the total variance explained.
- For PCA, the total variance explained equals the total variance, but for common factor analysis it does not.
Answers: 1. T, 2. F, the total variance for each item, 3. T, 4. F, communality is unique to each item (shared across components or factors), 5. T, 6. T.
Rotation Methods
After deciding on the number of factors to extract and with analysis model to use, the next step is to interpret the factor loadings. Factor rotations help us interpret factor loadings. There are two general types of rotations, orthogonal and oblique.
- orthogonal rotation assume factors are independent or uncorrelated with each other
- oblique rotation factors are not independent and are correlated
The goal of factor rotation is to improve the interpretability of the factor solution by reaching simple structure.
Simple structure
Without rotation, the first factor is the most general factor onto which most items load and explains the largest amount of variance. This may not be desired in all cases. Suppose you wanted to know how well a set of items load on each factor; simple structure helps us to achieve this.
The definition of simple structure is that in a factor loading matrix:
- Each row should contain at least one zero.
- For m factors, each column should have at least m zeroes (e.g., three factors, at least 3 zeroes per factor).
For every pair of factors (columns),
- there should be several items for which entries approach zero in one column but large loadings on the other.
- a large proportion of items should have entries approaching zero.
- only a small number of items have two non-zero entries.
The following table is an example of simple structure with three factors:
Let’s go down the checklist to criteria to see why it satisfies simple structure:
- each row contains at least one zero (exactly two in each row)
- each column contains at least three zeros (since there are three factors)
- for every pair of factors, most items have zero on one factor and non-zeros on the other factor (e.g., looking at Factors 1 and 2, Items 1 through 6 satisfy this requirement)
- for every pair of factors, all items have zero entries
- for every pair of factors, none of the items have two non-zero entries
An easier criteria from Pedhazur and Schemlkin (1991) states that
- each item has high loadings on one factor only
- each factor has high loadings for only some of the items.
For the following factor matrix, explain why it does not conform to simple structure using both the conventional and Pedhazur test.
Solution: Using the conventional test, although Criteria 1 and 2 are satisfied (each row has at least one zero, each column has at least three zeroes), Criteria 3 fails because for Factors 2 and 3, only 3/8 rows have 0 on one factor and non-zero on the other. Additionally, for Factors 2 and 3, only Items 5 through 7 have non-zero loadings or 3/8 rows have non-zero coefficients (fails Criteria 4 and 5 simultaneously). Using the Pedhazur method, Items 1, 2, 5, 6, and 7 have high loadings on two factors (fails first criteria) and Factor 3 has high loadings on a majority or 5/8 items (fails second criteria).
Orthogonal Rotation (2 factor PAF)
We know that the goal of factor rotation is to rotate the factor matrix so that it can approach simple structure in order to improve interpretability. Orthogonal rotation assumes that the factors are not correlated. The benefit of doing an orthogonal rotation is that loadings are simple correlations of items with factors, and standardized solutions can estimate unique contribution of each factor. The most common type of orthogonal rotation is Varimax rotation. We will walk through how to do this in SPSS.
Running a two-factor solution (PAF) with Varimax rotation in SPSS
The steps to running a two-factor Principal Axis Factoring is the same as before (Analyze – Dimension Reduction – Factor – Extraction), except that under Rotation – Method we check Varimax. Make sure under Display to check Rotated Solution and Loading plot(s), and under Maximum Iterations for Convergence enter 100.

Pasting the syntax into the SPSS editor you obtain:
Let’s first talk about what tables are the same or different from running a PAF with no rotation. First, we know that the unrotated factor matrix (Factor Matrix table) should be the same. Additionally, since the common variance explained by both factors should be the same, the Communalities table should be the same. The main difference is that we ran a rotation, so we should get the rotated solution (Rotated Factor Matrix) as well as the transformation used to obtain the rotation (Factor Transformation Matrix). Finally, although the total variance explained by all factors stays the same, the total variance explained by each factor will be different.
Rotated Factor Matrix (2-factor PAF Varimax)
The Rotated Factor Matrix table tells us what the factor loadings look like after rotation (in this case Varimax). Kaiser normalization is a method to obtain stability of solutions across samples. After rotation, the loadings are rescaled back to the proper size. This means that equal weight is given to all items when performing the rotation. The only drawback is if the communality is low for a particular item, Kaiser normalization will weight these items equally with items with high communality. As such, Kaiser normalization is preferred when communalities are high across all items. You can turn off Kaiser normalization by specifying
Here is what the Varimax rotated loadings look like without Kaiser normalization. Compared to the rotated factor matrix with Kaiser normalization the patterns look similar if you flip Factors 1 and 2; this may be an artifact of the rescaling. Another possible reasoning for the stark differences may be due to the low communalities for Item 2 (0.052) and Item 8 (0.236). Kaiser normalization weights these items equally with the other high communality items.
Interpreting the factor loadings (2-factor PAF Varimax)
In the table above, the absolute loadings that are higher than 0.4 are highlighted in blue for Factor 1 and in red for Factor 2. We can see that Items 6 and 7 load highly onto Factor 1 and Items 1, 3, 4, 5, and 8 load highly onto Factor 2. Item 2 does not seem to load highly on any factor. Looking more closely at Item 6 “My friends are better at statistics than me” and Item 7 “Computers are useful only for playing games”, we don’t see a clear construct that defines the two. Item 2, “I don’t understand statistics” may be too general an item and isn’t captured by SPSS Anxiety. It’s debatable at this point whether to retain a two-factor or one-factor solution, at the very minimum we should see if Item 2 is a candidate for deletion.
Factor Transformation Matrix and Factor Loading Plot (2-factor PAF Varimax)
The Factor Transformation Matrix tells us how the Factor Matrix was rotated. In SPSS, you will see a matrix with two rows and two columns because we have two factors.
How do we interpret this matrix? Well, we can see it as the way to move from the Factor Matrix to the Rotated Factor Matrix. From the Factor Matrix we know that the loading of Item 1 on Factor 1 is \(0.588\) and the loading of Item 1 on Factor 2 is \(-0.303\), which gives us the pair \((0.588,-0.303)\); but in the Rotated Factor Matrix the new pair is \((0.646,0.139)\). How do we obtain this new transformed pair of values? We can do what’s called matrix multiplication. The steps are essentially to start with one column of the Factor Transformation matrix, view it as another ordered pair and multiply matching ordered pairs. To get the first element, we can multiply the ordered pair in the Factor Matrix \((0.588,-0.303)\) with the matching ordered pair \((0.773,-0.635)\) in the first column of the Factor Transformation Matrix.
$$(0.588)(0.773)+(-0.303)(-0.635)=0.455+0.192=0.647.$$
To get the second element, we can multiply the ordered pair in the Factor Matrix \((0.588,-0.303)\) with the matching ordered pair \((0.773,-0.635)\) from the second column of the Factor Transformation Matrix:
$$(0.588)(0.635)+(-0.303)(0.773)=0.373-0.234=0.139.$$
Voila! We have obtained the new transformed pair with some rounding error. The figure below summarizes the steps we used to perform the transformation

The Factor Transformation Matrix can also tell us angle of rotation if we take the inverse cosine of the diagonal element. In this case, the angle of rotation is \(cos^{-1}(0.773) =39.4 ^{\circ}\). In the factor loading plot, you can see what that angle of rotation looks like, starting from \(0^{\circ}\) rotating up in a counterclockwise direction by \(39.4^{\circ}\). Notice here that the newly rotated x and y-axis are still at \(90^{\circ}\) angles from one another, hence the name orthogonal (a non-orthogonal or oblique rotation means that the new axis is no longer \(90^{\circ}\) apart. The points do not move in relation to the axis but rotate with it.
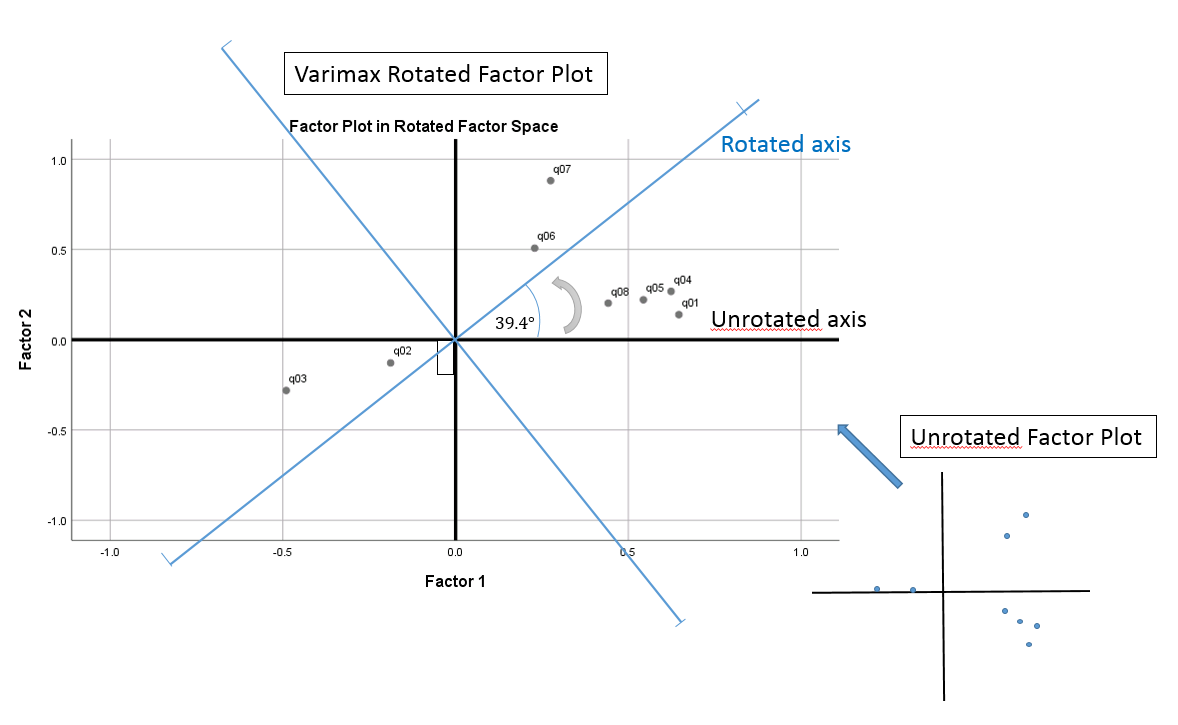
Total Variance Explained (2-factor PAF Varimax)
The Total Variance Explained table contains the same columns as the PAF solution with no rotation, but adds another set of columns called “Rotation Sums of Squared Loadings”. This makes sense because if our rotated Factor Matrix is different, the square of the loadings should be different, and hence the Sum of Squared loadings will be different for each factor. However, if you sum the Sums of Squared Loadings across all factors for the Rotation solution,
$$ 1.701 + 1.309 = 3.01$$
and for the unrotated solution,
$$ 2.511 + 0.499 = 3.01,$$
you will see that the two sums are the same. This is because rotation does not change the total common variance. Looking at the Rotation Sums of Squared Loadings for Factor 1, it still has the largest total variance, but now that shared variance is split more evenly.
Other Orthogonal Rotations
Varimax rotation is the most popular but one among other orthogonal rotations. The benefit of Varimax rotation is that it maximizes the variances of the loadings within the factors while maximizing differences between high and low loadings on a particular factor. Higher loadings are made higher while lower loadings are made lower. This makes Varimax rotation good for achieving simple structure but not as good for detecting an overall factor because it splits up variance of major factors among lesser ones. Quartimax may be a better choice for detecting an overall factor. It maximizes the squared loadings so that each item loads most strongly onto a single factor.
Here is the output of the Total Variance Explained table juxtaposed side-by-side for Varimax versus Quartimax rotation.
You will see that whereas Varimax distributes the variances evenly across both factors, Quartimax tries to consolidate more variance into the first factor.
Equamax is a hybrid of Varimax and Quartimax, but because of this may behave erratically and according to Pett et al. (2003), is not generally recommended.
Oblique Rotation
In oblique rotation, the factors are no longer orthogonal to each other (x and y axes are not \(90^{\circ}\) angles to each other). Like orthogonal rotation, the goal is rotation of the reference axes about the origin to achieve a simpler and more meaningful factor solution compared to the unrotated solution. In oblique rotation, you will see three unique tables in the SPSS output:
- factor pattern matrix contains partial standardized regression coefficients of each item with a particular factor
- factor structure matrix contains simple zero order correlations of each item with a particular factor
- factor correlation matrix is a matrix of intercorrelations among factors
Suppose the Principal Investigator hypothesizes that the two factors are correlated, and wishes to test this assumption. Let’s proceed with one of the most common types of oblique rotations in SPSS, Direct Oblimin.
Running a two-factor solution (PAF) with Direct Quartimin rotation in SPSS
The steps to running a Direct Oblimin is the same as before (Analyze – Dimension Reduction – Factor – Extraction), except that under Rotation – Method we check Direct Oblimin. The other parameter we have to put in is delta , which defaults to zero. Technically, when delta = 0, this is known as Direct Quartimin. Larger positive values for delta increases the correlation among factors. However, in general you don’t want the correlations to be too high or else there is no reason to split your factors up. In fact, SPSS caps the delta value at 0.8 (the cap for negative values is -9999). Negative delta factors may lead to orthogonal factor solutions. For the purposes of this analysis, we will leave our delta = 0 and do a Direct Quartimin analysis.
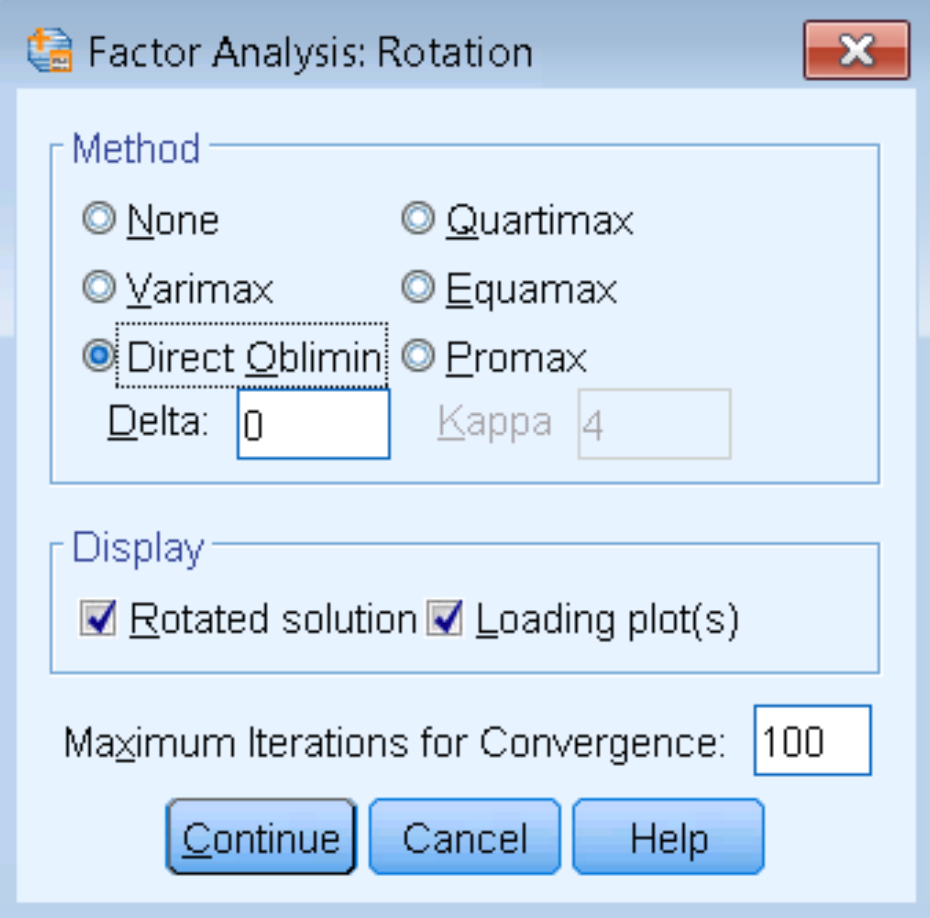
All the questions below pertain to Direct Oblimin in SPSS.
- When selecting Direct Oblimin, delta = 0 is actually Direct Quartimin.
- Smaller delta values will increase the correlations among factors.
- You typically want your delta values to be as high as possible.
Answers: 1. T, 2. F, larger delta values, 3. F, delta leads to higher factor correlations, in general you don’t want factors to be too highly correlated
Factor Pattern Matrix (2-factor PAF Direct Quartimin)
The factor pattern matrix represent partial standardized regression coefficients of each item with a particular factor. For example, \(0.740\) is the effect of Factor 1 on Item 1 controlling for Factor 2 and \(-0.137\) is the effect of Factor 2 on Item 1 controlling for Factor 1. Just as in orthogonal rotation, the square of the loadings represent the contribution of the factor to the variance of the item, but excluding the overlap between correlated factors. Factor 1 uniquely contributes \((0.740)^2=0.405=40.5\%\) of the variance in Item 1 (controlling for Factor 2 ), and Factor 2 uniquely contributes \((-0.137)^2=0.019=1.9%\) of the variance in Item 1 (controlling for Factor 1).
Factor Structure Matrix (2-factor PAF Direct Quartimin)
The factor structure matrix represent the simple zero-order correlations of the items with each factor (it’s as if you ran a simple regression of a single factor on the outcome). For example, \(0.653\) is the simple correlation of Factor 1 on Item 1 and \(0.333\) is the simple correlation of Factor 2 on Item 1. The more correlated the factors, the more difference between pattern and structure matrix and the more difficult to interpret the factor loadings. From this we can see that Items 1, 3, 4, 5, and 8 load highly onto Factor 1 and Items 6, and 7 load highly onto Factor 2. Item 2 doesn’t seem to load well on either factor.
Additionally, we can look at the variance explained by each factor not controlling for the other factors. For example, Factor 1 contributes \((0.653)^2=0.426=42.6\%\) of the variance in Item 1, and Factor 2 contributes \((0.333)^2=0.11=11.0%\) of the variance in Item 1. Notice that the contribution in variance of Factor 2 is higher \(11\%\) vs. \(1.9\%\) because in the Pattern Matrix we controlled for the effect of Factor 1, whereas in the Structure Matrix we did not.
Factor Correlation Matrix (2-factor PAF Direct Quartimin)
Recall that the more correlated the factors, the more difference between pattern and structure matrix and the more difficult to interpret the factor loadings. In our case, Factor 1 and Factor 2 are pretty highly correlated, which is why there is such a big difference between the factor pattern and factor structure matrices.
Factor plot
The difference between an orthogonal versus oblique rotation is that the factors in an oblique rotation are correlated. This means not only must we account for the angle of axis rotation \(\theta\), we have to account for the angle of correlation \(\phi\). The angle of axis rotation is defined as the angle between the rotated and unrotated axes (blue and black axes). From the Factor Correlation Matrix, we know that the correlation is \(0.636\), so the angle of correlation is \(cos^{-1}(0.636) = 50.5^{\circ}\), which is the angle between the two rotated axes (blue x and blue y-axis). The sum of rotations \(\theta\) and \(\phi\) is the total angle rotation. We are not given the angle of axis rotation, so we only know that the total angle rotation is \(\theta + \phi = \theta + 50.5^{\circ}\).
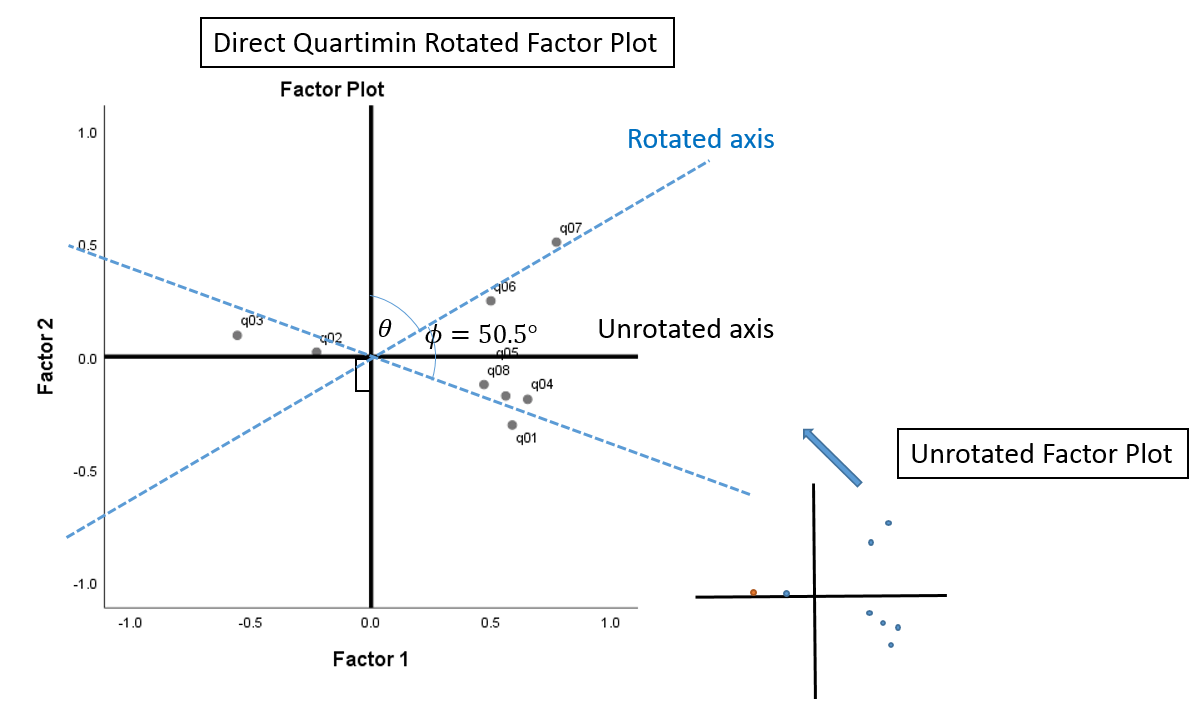
Relationship between the Pattern and Structure Matrix
The structure matrix is in fact a derivative of the pattern matrix. If you multiply the pattern matrix by the factor correlation matrix, you will get back the factor structure matrix. Let’s take the example of the ordered pair \((0.740,-0.137)\) from the Pattern Matrix, which represents the partial correlation of Item 1 with Factors 1 and 2 respectively. Performing matrix multiplication for the first column of the Factor Correlation Matrix we get
$$ (0.740)(1) + (-0.137)(0.636) = 0.740 – 0.087 =0.652.$$
Similarly, we multiple the ordered factor pair with the second column of the Factor Correlation Matrix to get:
$$ (0.740)(0.636) + (-0.137)(1) = 0.471 -0.137 =0.333 $$
Looking at the first row of the Structure Matrix we get \((0.653,0.333)\) which matches our calculation! This neat fact can be depicted with the following figure:

As a quick aside, suppose that the factors are orthogonal, which means that the factor correlations are 1′ s on the diagonal and zeros on the off-diagonal, a quick calculation with the ordered pair \((0.740,-0.137)\)
$$ (0.740)(1) + (-0.137)(0) = 0.740$$
and similarly,
$$ (0.740)(0) + (-0.137)(1) = -0.137$$
and you get back the same ordered pair. This is called multiplying by the identity matrix (think of it as multiplying \(2*1 = 2\)).
- Without changing your data or model, how would you make the factor pattern matrices and factor structure matrices more aligned with each other?
- True or False, When you decrease delta, the pattern and structure matrix will become closer to each other.
Answers: 1. Decrease the delta values so that the correlation between factors approaches zero. 2. T, the correlations will become more orthogonal and hence the pattern and structure matrix will be closer.
Total Variance Explained (2-factor PAF Direct Quartimin)
The column Extraction Sums of Squared Loadings is the same as the unrotated solution, but we have an additional column known as Rotation Sums of Squared Loadings. SPSS says itself that “when factors are correlated, sums of squared loadings cannot be added to obtain total variance”. You will note that compared to the Extraction Sums of Squared Loadings, the Rotation Sums of Squared Loadings is only slightly lower for Factor 1 but much higher for Factor 2. This is because unlike orthogonal rotation, this is no longer the unique contribution of Factor 1 and Factor 2. How do we obtain the Rotation Sums of Squared Loadings? SPSS squares the Structure Matrix and sums down the items.
As a demonstration, let’s obtain the loadings from the Structure Matrix for Factor 1
$$ (0.653)^2 + (-0.222)^2 + (-0.559)^2 + (0.678)^2 + (0.587)^2 + (0.398)^2 + (0.577)^2 + (0.485)^2 = 2.318.$$
Note that \(2.318\) matches the Rotation Sums of Squared Loadings for the first factor. This means that the Rotation Sums of Squared Loadings represent the non- unique contribution of each factor to total common variance, and summing these squared loadings for all factors can lead to estimates that are greater than total variance.
Interpreting the factor loadings (2-factor PAF Direct Quartimin)
Finally, let’s conclude by interpreting the factors loadings more carefully. Let’s compare the Pattern Matrix and Structure Matrix tables side-by-side. First we highlight absolute loadings that are higher than 0.4 in blue for Factor 1 and in red for Factor 2. We see that the absolute loadings in the Pattern Matrix are in general higher in Factor 1 compared to the Structure Matrix and lower for Factor 2. This makes sense because the Pattern Matrix partials out the effect of the other factor. Looking at the Pattern Matrix, Items 1, 3, 4, 5, and 8 load highly on Factor 1, and Items 6 and 7 load highly on Factor 2. Looking at the Structure Matrix, Items 1, 3, 4, 5, 7 and 8 are highly loaded onto Factor 1 and Items 3, 4, and 7 load highly onto Factor 2. Item 2 doesn’t seem to load on any factor. The results of the two matrices are somewhat inconsistent but can be explained by the fact that in the Structure Matrix Items 3, 4 and 7 seem to load onto both factors evenly but not in the Pattern Matrix. For this particular analysis, it seems to make more sense to interpret the Pattern Matrix because it’s clear that Factor 1 contributes uniquely to most items in the SAQ-8 and Factor 2 contributes common variance only to two items (Items 6 and 7). There is an argument here that perhaps Item 2 can be eliminated from our survey and to consolidate the factors into one SPSS Anxiety factor. We talk to the Principal Investigator and we think it’s feasible to accept SPSS Anxiety as the single factor explaining the common variance in all the items, but we choose to remove Item 2, so that the SAQ-8 is now the SAQ-7.
- In oblique rotation, an element of a factor pattern matrix is the unique contribution of the factor to the item whereas an element in the factor structure matrix is the non- unique contribution to the factor to an item.
- In the Total Variance Explained table, the Rotation Sum of Squared Loadings represent the unique contribution of each factor to total common variance.
- The Pattern Matrix can be obtained by multiplying the Structure Matrix with the Factor Correlation Matrix
- If the factors are orthogonal, then the Pattern Matrix equals the Structure Matrix
- In oblique rotations, the sum of squared loadings for each item across all factors is equal to the communality (in the SPSS Communalities table) for that item.
Answers: 1. T, 2. F, represent the non -unique contribution (which means the total sum of squares can be greater than the total communality), 3. F, the Structure Matrix is obtained by multiplying the Pattern Matrix with the Factor Correlation Matrix, 4. T, it’s like multiplying a number by 1, you get the same number back, 5. F, this is true only for orthogonal rotations, the SPSS Communalities table in rotated factor solutions is based off of the unrotated solution, not the rotated solution.
As a special note, did we really achieve simple structure? Although rotation helps us achieve simple structure, if the interrelationships do not hold itself up to simple structure, we can only modify our model. In this case we chose to remove Item 2 from our model.
Promax Rotation
Promax rotation begins with Varimax (orthgonal) rotation, and uses Kappa to raise the power of the loadings. Promax really reduces the small loadings. Promax also runs faster than Varimax, and in our example Promax took 3 iterations while Direct Quartimin (Direct Oblimin with Delta =0) took 5 iterations.
- Varimax, Quartimax and Equamax are three types of orthogonal rotation and Direct Oblimin, Direct Quartimin and Promax are three types of oblique rotations.
Answers: 1. T.
Generating Factor Scores
Suppose the Principal Investigator is happy with the final factor analysis which was the two-factor Direct Quartimin solution. She has a hypothesis that SPSS Anxiety and Attribution Bias predict student scores on an introductory statistics course, so would like to use the factor scores as a predictor in this new regression analysis. Since a factor is by nature unobserved, we need to first predict or generate plausible factor scores. In SPSS, there are three methods to factor score generation, Regression, Bartlett, and Anderson-Rubin.
Generating factor scores using the Regression Method in SPSS
In order to generate factor scores, run the same factor analysis model but click on Factor Scores (Analyze – Dimension Reduction – Factor – Factor Scores). Then check Save as variables, pick the Method and optionally check Display factor score coefficient matrix.
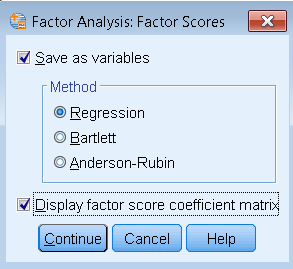
The code pasted in the SPSS Syntax Editor looksl like this:
Here we picked the Regression approach after fitting our two-factor Direct Quartimin solution. After generating the factor scores, SPSS will add two extra variables to the end of your variable list, which you can view via Data View. The figure below shows what this looks like for the first 5 participants, which SPSS calls FAC1_1 and FAC2_1 for the first and second factors. These are now ready to be entered in another analysis as predictors.
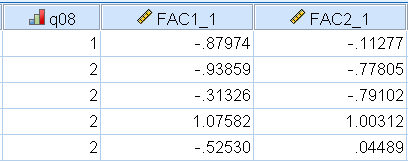
For those who want to understand how the scores are generated, we can refer to the Factor Score Coefficient Matrix. These are essentially the regression weights that SPSS uses to generate the scores. We know that the ordered pair of scores for the first participant is \(-0.880, -0.113\). We also know that the 8 scores for the first participant are \(2, 1, 4, 2, 2, 2, 3, 1\). However, what SPSS uses is actually the standardized scores, which can be easily obtained in SPSS by using Analyze – Descriptive Statistics – Descriptives – Save standardized values as variables. The standardized scores obtained are: \(-0.452, -0.733, 1.32, -0.829, -0.749, -0.2025, 0.069, -1.42\). Using the Factor Score Coefficient matrix, we multiply the participant scores by the coefficient matrix for each column. For the first factor:
$$ \begin{eqnarray} &(0.284) (-0.452) + (-0.048)-0.733) + (-0.171)(1.32) + (0.274)(-0.829) \\ &+ (0.197)(-0.749) +(0.048)(-0.2025) + (0.174) (0.069) + (0.133)(-1.42) \\ &= -0.880, \end{eqnarray} $$
which matches FAC1_1 for the first participant. You can continue this same procedure for the second factor to obtain FAC2_1.
The second table is the Factor Score Covariance Matrix,
This table can be interpreted as the covariance matrix of the factor scores, however it would only be equal to the raw covariance if the factors are orthogonal. For example, if we obtained the raw covariance matrix of the factor scores we would get
You will notice that these values are much lower. Let’s compare the same two tables but for Varimax rotation:
If you compare these elements to the Covariance table below, you will notice they are the same.
Note with the Bartlett and Anderson-Rubin methods you will not obtain the Factor Score Covariance matrix.
Regression, Bartlett and Anderson-Rubin compared
Among the three methods, each has its pluses and minuses. The regression method maximizes the correlation (and hence validity) between the factor scores and the underlying factor but the scores can be somewhat biased. This means even if you have an orthogonal solution, you can still have correlated factor scores. For Bartlett’s method, the factor scores highly correlate with its own factor and not with others, and they are an unbiased estimate of the true factor score. Unbiased scores means that with repeated sampling of the factor scores, the average of the scores is equal to the average of the true factor score. The Anderson-Rubin method perfectly scales the factor scores so that the factor scores are uncorrelated with other factors and uncorrelated with other factor scores . Since Anderson-Rubin scores impose a correlation of zero between factor scores, it is not the best option to choose for oblique rotations. Additionally, Anderson-Rubin scores are biased.
In summary, if you do an orthogonal rotation, you can pick any of the the three methods. For orthogonal rotations, use Bartlett if you want unbiased scores, use the regression method if you want to maximize validity and use Anderson-Rubin if you want the factor scores themselves to be uncorrelated with other factor scores. If you do oblique rotations, it’s preferable to stick with the Regression method. Do not use Anderson-Rubin for oblique rotations.
- If you want the highest correlation of the factor score with the corresponding factor (i.e., highest validity), choose the regression method.
- Bartlett scores are unbiased whereas Regression and Anderson-Rubin scores are biased.
- Anderson-Rubin is appropriate for orthogonal but not for oblique rotation because factor scores will be uncorrelated with other factor scores.
Answers: 1. T, 2. T, 3. T
Your Name (required)
Your Email (must be a valid email for us to receive the report!)
Comment/Error Report (required)
How to cite this page
- © 2021 UC REGENTS
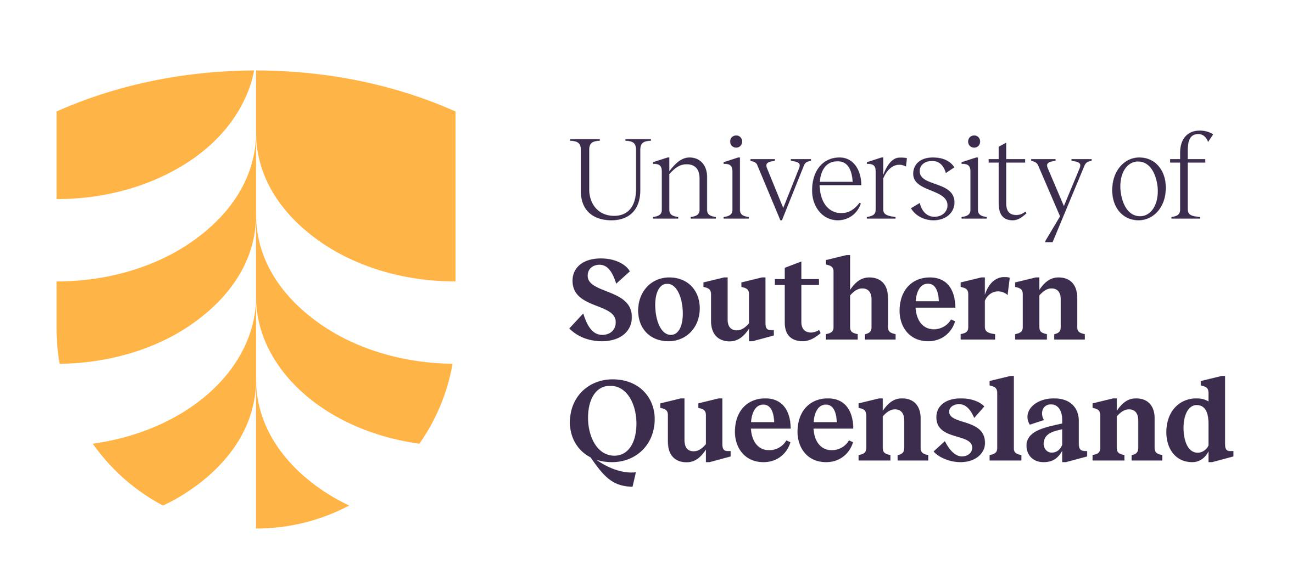
Want to create or adapt books like this? Learn more about how Pressbooks supports open publishing practices.
Section 8.6: EFA Write Up
Learning Objectives
At the end of this section you should be able to answer the following questions:
- What are some common elements of a Results Section in an EFA write up?
- What are some common Tables included in an EFA write up?
Guide (only) to Writing the Results section
A brief guide for the result write-up is: What analysis was conducted and for what purpose (include extraction method, number of items, and number of participants or sample size, including a test of sampling adequacy). What were the outcomes from data screening. You should present results from the analysis (not describe the analysis), i.e. answer the research questions:
- The criterion for determining the number of components to extract
- Method of rotation
- Cut-off used for retaining items for interpretation
- Appraise the solution (e.g. are there distinct components or are there many items with cross pattern coefficients?)
- Describe the components and name the components
- Estimate the internal consistency of each component
- A full example write-up will be provided.
Guide to Table/s to be Included
For the tables you should include:
A summary of eigenvalue, the total variance accounted by each component, and the cumulative percentage of total variance accounted by the four components.
Also the items, pattern coefficients (in descending order), and communalities (indicate before rotation since they are values from the output) of the items.
See the EFA Example Write-Up .
Statistics for Research Students Copyright © 2022 by University of Southern Queensland is licensed under a Creative Commons Attribution 4.0 International License , except where otherwise noted.
Share This Book

APA Reporting SPSS Factor Analysis
Introduction, creating apa tables - the easy way, table i - factor loadings & communalities, table ii - total variance explained, table iii - factor correlations.
Creating APA style tables from SPSS factor analysis output can be cumbersome. This tutorial therefore points out some tips, tricks & pitfalls. We'll use the results of SPSS Factor Analysis - Intermediate Tutorial .
All analyses are based on 20-career-ambitions-pca.sav (partly shown below).
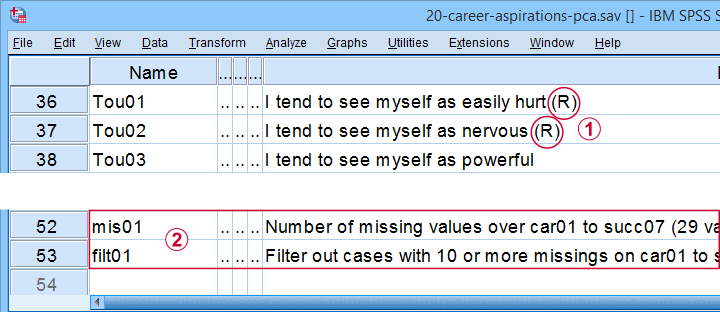
After opening these data, you can replicate the final analyses by running the SPSS syntax below.
For a wide variety of analyses, the easiest way to create APA style tables from SPSS output is usually to
- adjust your analyses in SPSS so the output is as close as possible to the desired end results. Changing table layouts (which variables/statistics go into which rows/columns?) is also best done here.
- copy-paste one or more tables into Excel or Googlesheets. This is the easiest way to set decimal places, fonts, alignment, borders and more;
- copy-paste your table(s) from Excel into WORD . Perhaps adjust the table widths with “autofit”, and you'll often have a perfect end result.
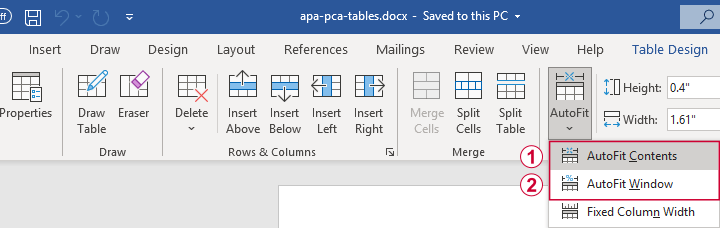
The figure below shows an APA style table combining factor loadings and communalities for our example analysis.
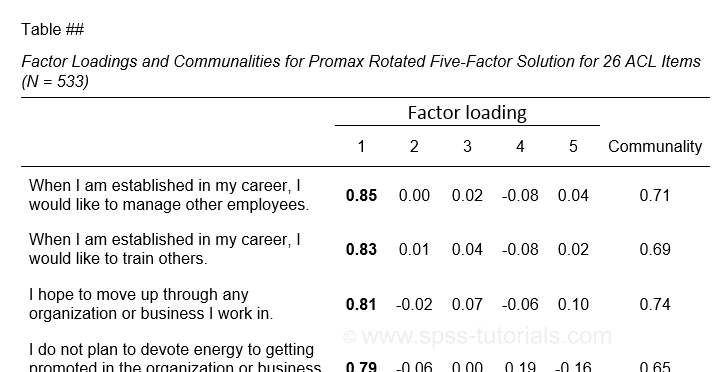
If you take a good look at the SPSS output, you'll see that you cannot simply copy-paste these tables for combining them in Excel. This is because the factor loadings (pattern matrix) table follows a different variable order than the communalities table. Since the latter follows the variable order as specified in your syntax, the easiest fix for this is to
- make sure that only variable names (not labels) are shown in the output;
- copy-paste the correctly sorted pattern matrix into Excel;
- copy-paste the variable names into the FACTOR syntax and rerun it.
Tip: try and replace the line breaks between variable names by spaces as shown below.
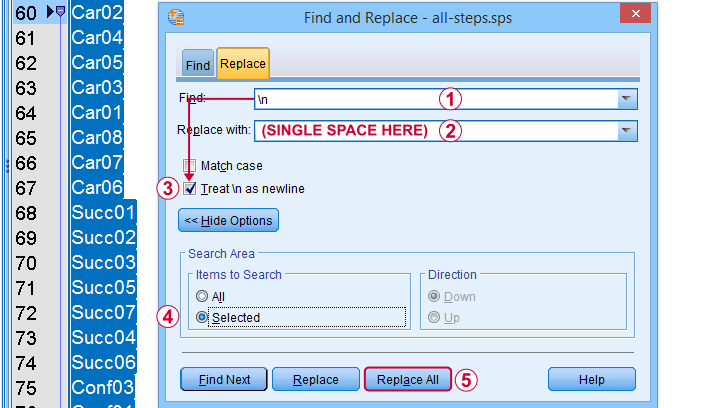
Also, you probably want to see only variable labels (not names) from now on. And -finally- we no longer want to hide any small absolute factor loadings shown below.
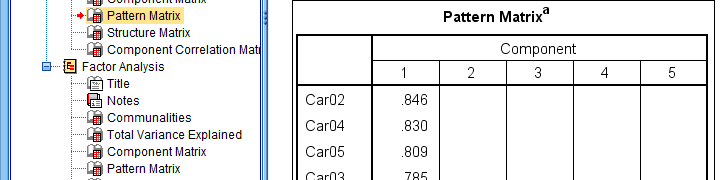
The syntax below does all that and thus creates output that is ideal for creating APA style tables.
You can now safely combine the communalities and pattern matrix tables and make some final adjustments. The end result is shown in this Googlesheet , partly shown below.
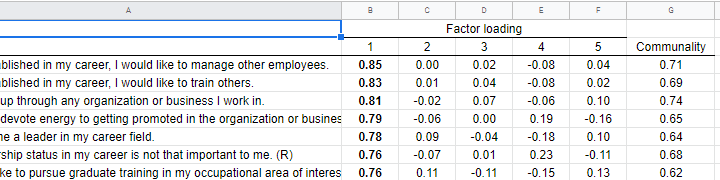
Since decimal places, fonts, alignment and borders have all been set, this table is now perfect for its final copy-paste into WORD.
The screenshot below shows how to report the Eigenvalues table in APA style.
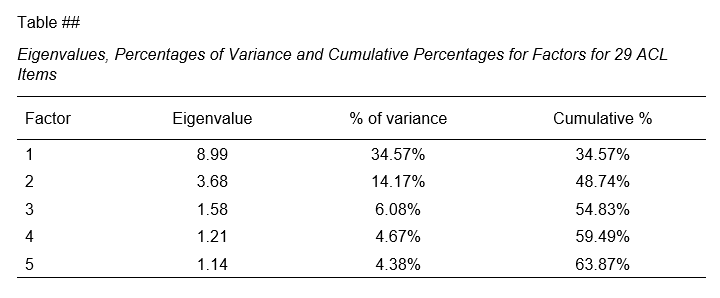
The corresponding SPSS output table comes fairly close to this. However, an annoying problem are the missing percent signs.
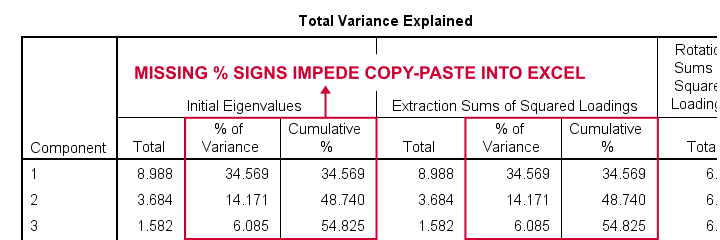
If we copy-paste into Excel and set a percentage format, 34.57 is converted into 3,457%. This is because Excel interprets these numbers as proportions rather than percentage points as SPSS does. The easiest fix is setting a percent format for these columns in SPSS before copy-pasting into Excel.
The OUTPUT MODIFY example below does just that for all Eigenvalues tables in the output window.
After this tiny fix, you can copy-paste this table from SPSS into Excel. We can now easily make some final adjustments (including the removal of some rows and columns) and copy-paste this table into WORD.
If you used an oblique factor rotation, you'll probably want to report the correlations among your factors. The figure below shows an APA style factor correlations table.
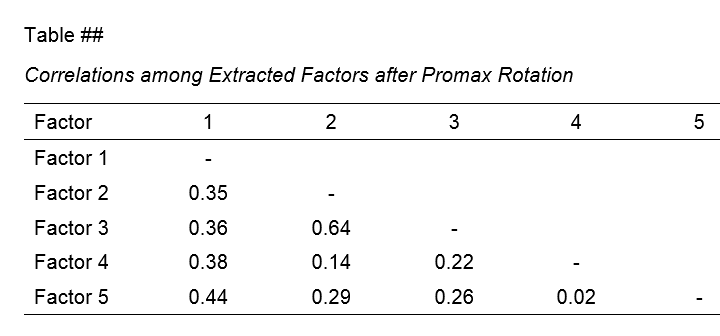
The corresponding SPSS output table (shown below) is pretty different from what we need.
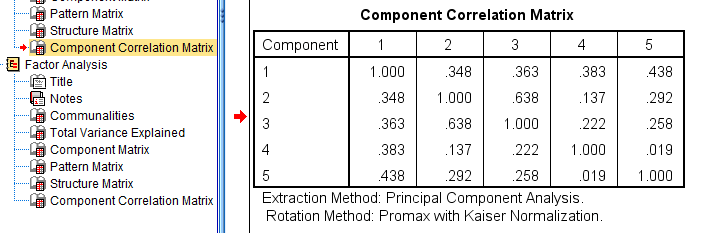
Adjusting this table manually is pretty doable. However, I personally prefer to use an SPSS Python script for doing so.
You can download my script from LAST-FACTOR-CORRELATION-TABLE-TO-APA.sps . This script is best run from an INSERT command as shown below.
I highly recommend trying this script but it does make some assumptions:
- the above syntax assumes the script is located in D:\DOWNLOADS so you probably need to change that;
- the script assumes that you've the SPSS Python3.x essentials properly installed (usually the case for recent SPSS versions);
- the script assumes that no SPLIT FILE is in effect.
If you've any trouble or requests regarding my script, feel free to contact me and I'll see what I can do.
Final Notes
Right, so these are the basic routines I follow for creating APA style factor analysis tables. I hope you'll find them helpful.
If you've any feedback, please throw me a comment below.
Thanks for reading!
Tell us what you think!
This tutorial has 2 comments:.
By Iris on July 15th, 2022
Hi, quick question. Where can you find the sig levels from the correlation table? Or do you just have to report it without them?
By Ruben Geert van den Berg on July 16th, 2022
They're not usually reported.
You could obtain them by saving the factor scores as new variables and running correlations over them as covered in SPSS Correlation Analysis .
However, this only works if you use listwise exclusion of missing values which is a bad idea for the data used in this tutorial.
With pairwise exclusion (used here) the correlations from FACTOR may differ from those from CORRELATIONS due to missing values on the created factor score variables.
Hope that helps!
SPSS tutorials
Privacy Overview
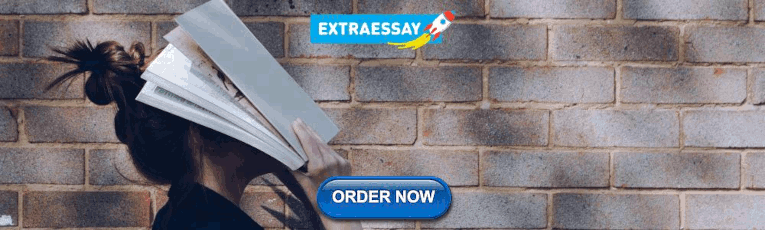
IMAGES
VIDEO
COMMENTS
Exploratory factor analysis (EFA) is one of a family of multivariate statistical methods that attempts to identify the smallest number of hypothetical constructs (also known as factors, dimensions, latent variables, synthetic variables, or internal attributes) that can parsimoniously explain the covariation observed among a set of measured variables (also called observed variables, manifest ...
Factor analysis (FA) allows us to simplify a set of complex variables or items using statistical procedures to explore the underlying dimensions that explain the relationships between the multiple variables/items. For example, to explore inter-item relationships for a 20-item instrument, a basic analysis would produce 400 correlations; it is ...
factor loadings and symptom commonalties from the exploratory factor analysis (efa) of the 37 presence, severity, and secondary crs symptom at baseline. the efa was fit using ordinary least squares and an oblimin rotation (number of patients = 3535). loadings less than 0.3 were omitted for readability. communalities represent the fraction of each
The scree plot below relates to the factor analysis example later in this post. The graph displays the Eigenvalues by the number of factors. Eigenvalues relate to the amount of explained variance. The scree plot shows the bend in the curve occurring at factor 6. Consequently, we need to extract five factors.
Factor Analysis Steps. Here are the general steps involved in conducting a factor analysis: 1. Define the Research Objective: Clearly specify the purpose of the factor analysis. Determine what you aim to achieve or understand through the analysis. 2. Data Collection: Gather the data on the variables of interest.
Why Factor Analysis? 1. Testing of theory ! Explain covariation among multiple observed variables by ! Mapping variables to latent constructs (called "factors") 2. Understanding the structure underlying a set of measures ! Gain insight to dimensions ! Construct validation (e.g., convergent validity)
As with any statistical analysis, before performing a factor analysis the researcher must investigate whether the data meet the assumptions for the proposed analysis. Section 1 of the Supplemental Material provides a summary of what a researcher should check for in the data for the purposes of meeting the assumptions of a factor analysis and an ...
As can be seen, it consists of seven main steps: reliable measurements, correlation matrix, factor analysis versus principal component analysis, the number of factors to be retained, factor rotation, and use and interpretation of the results. Below, these steps will be discussed one at a time. 2.2.1. Measurements.
1 Introduction. Factor analysis is a significant instrument which is utilized in development, refinement, and evaluation of tests, scales, and measures (Williams, Brown et al. 2010). Exploratory factor analysis (EFA) is widely used and broadly applied statistical approach in information system, social science, education and psychology.
Exploratory factor analysis. Exploratory Factor Analysis (EFA) is a statistical method used to describe variability among observed, correlated variables. The goal of performing exploratory factor analysis is to search for some unobserved variables called factors (Rui Sarmento & Costa, 2017).
Higher-order factor analysis is testable in CFA. Phakiti (), for example, employed CFA to test a fourth-order factor model of strategic competence based on four five-point Likert-type scale questionnaires (two trait and two state) collected over two occasions (two-month interval; N = 561).Based on this study, strategic competence was confirmed as a higher-order factor that governs both ...
Purpose. This seminar is the first part of a two-part seminar that introduces central concepts in factor analysis. Part 1 focuses on exploratory factor analysis (EFA). Although the implementation is in SPSS, the ideas carry over to any software program. Part 2 introduces confirmatory factor analysis (CFA).
Exploratory factor analysis is an inductive method to produce the common cause principle from a set of items (Haig 2005; Williams et al. 2010). This study performed EFA to capture common factors ...
1. An Overview of Factor Analysis Characteristics of Factor Analysis Exploratory Vs. Confirmatory Factor Analysis Assumptions of Exploratory Factor Analysis Historical Developments of Factor Analysis Uses of Factor Analysis in Health Care Research Decision-Making Process in Exploratory Factor Analysis 2. Designing and Testing the Instrument Types of Measurement and Frameworks The Use of Latent ...
Guide (only) to Writing the Results section. A brief guide for the result write-up is: What analysis was conducted and for what purpose (include extraction method, number of items, and number of participants or sample size, including a test of sampling adequacy). What were the outcomes from data screening.
On the Interpretation of Factor Analysis. J. Scott Armstrong. Sloan School, MIT, now at. The Wharton School, University of Pennsylvania. Peer Soelberg. University of Wisconson, Milwaukee. Abstract ...
5.3 EXPLORATORY FACTOR ANALYSIS 109 5.3.1 Total Variances Explained 109 5.3.2 Rotated Factor Scores and Factor Correlation Results 112 5.3.2.1 Factor 1: Psychological Adjustment 114 5.3.2.2 Factor 2: Self-actualisation 116 5.3.2.3 Factor 3: Stress Management 117 5.4 SUMMARY 118 CHAPTER 6: CONCLUSIONS, LIMITATIONS AND RECOMMENDATIONS 119
Confirmatory Factor Analysis (CFA) is a particular form of factor analysis, most commonly used in social. research. In confirmatory factor anal ysis, the researcher first develops a hypothesis ...
This article reports results of a confirmatory factor analysis performed to cross-validate the factor structure . of the Educators' Attitudes Toward Educational Research Scale. The original scale had been developed by the author and revised based on the results of an exploratory factor analysis. In the present study, the revised sca-
factor analysis (EFA) provided evidence for the four-factor solution for the 26-item ISSQ accounting for 48.65% of the shared variance. Additionally, the ISSQ was found to have adequate internal consistency, a Cronbach alpha of .85 for the overall instrument and subscale alphas ranging from .72 to .81.
the sample variances of the standardized loadings for each factor summed over the m factors. procedure (marked in blue): "rotate," asks for factor rotation and we specified the Varimax rotation of our … Using Varimax Rotation To perform factor analysis with varimax rotation: Open the ' …
Creating APA style tables from SPSS factor analysis output can be cumbersome. This tutorial therefore points out some tips, tricks & pitfalls. We'll use the results of SPSS Factor Analysis - Intermediate Tutorial. All analyses are based on 20-career-ambitions-pca.sav (partly shown below). Note that some items were reversed and therefore had ...
The application of factor analysis for questionnaire evaluation provides very valuable inputs to the decision makers to focus on few important factors rather than a large number of parameters ...
Graduate Thesis Or Dissertation ... An R-technique factor analysis was utilized to identify common professional competencies. A three factor solution generated 68 competencies that had factor loadings of ± .45 or higher. Factors identified were: 1. Factor I, Administration, was a general factor with four subfactors.
Factor analysis is a way to look at many pieces of information and find groups among them. These groups are called "factors". Factor analysis is used to see if many things are related or similar. For example, if a person is asked many questions, factor analysis could be used to see if some of the answers are similar.. This method is used in many fields.
Investment Thesis. The Schwab U.S. Small-Cap ETF (NYSEARCA:SCHA) ranks well in categories like assets under management, expenses, liquidity, and diversification. ... SCHA Factor Analysis.