Information Retrieval: Recent Advances and Beyond
Ieee account.
- Change Username/Password
- Update Address
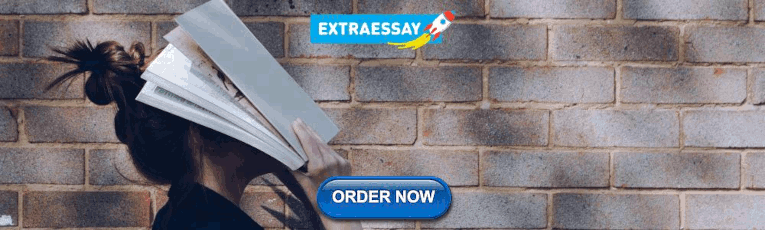
Purchase Details
- Payment Options
- Order History
- View Purchased Documents
Profile Information
- Communications Preferences
- Profession and Education
- Technical Interests
- US & Canada: +1 800 678 4333
- Worldwide: +1 732 981 0060
- Contact & Support
- About IEEE Xplore
- Accessibility
- Terms of Use
- Nondiscrimination Policy
- Privacy & Opting Out of Cookies
A not-for-profit organization, IEEE is the world's largest technical professional organization dedicated to advancing technology for the benefit of humanity. © Copyright 2024 IEEE - All rights reserved. Use of this web site signifies your agreement to the terms and conditions.
Thank you for visiting nature.com. You are using a browser version with limited support for CSS. To obtain the best experience, we recommend you use a more up to date browser (or turn off compatibility mode in Internet Explorer). In the meantime, to ensure continued support, we are displaying the site without styles and JavaScript.
- View all journals
- Explore content
- About the journal
- Publish with us
- Sign up for alerts
- Published: 30 April 2002
An introduction to information retrieval: applications in genomics
- P M Nadkarni 1
The Pharmacogenomics Journal volume 2 , pages 96–102 ( 2002 ) Cite this article
1215 Accesses
7 Citations
Metrics details
Information retrieval (IR) is the field of computer science that deals with the processing of documents containing free text, so that they can be rapidly retrieved based on keywords specified in a user's query. IR technology is the basis of Web-based search engines, and plays a vital role in biomedical research, because it is the foundation of software that supports literature search. Documents can be indexed by both the words they contain, as well as the concepts that can be matched to domain-specific thesauri; concept matching, however, poses several practical difficulties that make it unsuitable for use by itself. This article provides an introduction to IR and summarizes various applications of IR and related technologies to genomics.
This is a preview of subscription content, access via your institution
Access options
Subscribe to this journal
Receive 6 print issues and online access
251,40 € per year
only 41,90 € per issue
Buy this article
- Purchase on Springer Link
- Instant access to full article PDF
Prices may be subject to local taxes which are calculated during checkout
Similar content being viewed by others
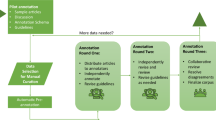
NLM-Chem, a new resource for chemical entity recognition in PubMed full text literature
Rezarta Islamaj, Robert Leaman, … Zhiyong Lu
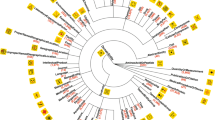
NERO: a biomedical named-entity (recognition) ontology with a large, annotated corpus reveals meaningful associations through text embedding
Kanix Wang, Robert Stevens, … Andrey Rzhetsky
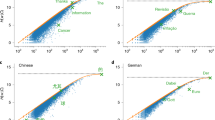
A universal information theoretic approach to the identification of stopwords
Martin Gerlach, Hanyu Shi & Luís A. Nunes Amaral
Salton G . Automatic Text Processing: the transformation, analysis, and retrieval of information by computer Addison-Wesley: Reading, MA 1989
Google Scholar
Van Rijsbergen CJ . Information Retrieval Butterworths: London, UK 1979
Baeza-Yates R, Ribeiro-Neto B . Modern Information Retrieval Addison-Wesley Longman: Harlow, UK 1999
Witten IH, Moffat A, Bell TC . Managing Gigabytes Morgan Kaufman: San Francisco, CA 1999
Porter MF . An algorithm for suffix stripping Program 1980 14 : 130–137
Article Google Scholar
Harman D . How effective is suffixing? J Am Soc Inform Sci 1991 42 : 7–15
Xu J, Croft WB . Corpus-based stemming using co-occurrence of word variants ACM Trans Inform Syst 1979 16 : 61–81
Nadkarni PM, Chen RS, Brandt CA . UMLS concept indexing for production databases: a feasibility study J Am Med Inform Assoc 2001 8 : 80–91
Article CAS Google Scholar
Elkin PL, Cimino JJ, Lowe HJ, Aronow DB, Payne TH, Pincett PS et al . Mapping to MESH: the art of trapping MESH equivalence from within narrative text In Proc Symposium on Computer Applications in Medical Care 1988 pp 185–190
Aronson A, Rindflesch T, Browne A . Exploiting a large thesaurus for information retrieval In Proceedings of the RIAO 1994 pp 197–216
Aronson AR, Rindflesch TC . Query expansion using the UMLS Metathesaurus In Proceedings/AMIA Annual Fall Symposium 1997 pp 485–489
Rindflesch TC, Aronson AR . Ambiguity resolution while mapping free text to the UMLS Metathesaurus In Proceedings–the Annual Symposium on Computer Applications in Medical Care 1994 pp 240–244
Masys D . Linking microarray data to the literature (Editorial) Nature Genet 2001 27 : 9–10
Mutalik P, Deshpande A, Nadkarni P . Use of general-purpose negation detection to augment concept indexing of medical documents: a quantitative study using the UMLS J Am Med Inform Assoc 2001 8 : 598–609
Williams JH, Perriens MP . Automated full text indexing and searching systems In IBM Information Systems Symposium Washington, DC 1968 pp 335–350
Sparck Jones K . A statistical interpretation of term specificity and its application in retrieval J Documentation 1972 28 : 11–21
Sparck-Jones K, Walter S, Robertson SE . Information retrieval: development and comparative experiments (Part I) Inform Proc Manage 2000 36 : 779–808
Sparck-Jones K, Walter S, Robertson SE . Information retrieval: development and comparative experiments (Part 2) Inform Proc Manage 2000 36 : 809–840
Google Inc Google: Technology Overview 2001
Marshall E . Medline searches turn up cases of suspected plagiarism (News) Science 1998 279 : 473–474
OMIM. Online Mendelian Inheritance in Man In: McKusick–Nathans Institute for Genetic Medicine, Johns Hopkins University (Baltimore, MD) and National Center for Biotechnology Information, National Library of Medicine (Bethesda, MD) 2001
Ley K, Brewer K, Moton A . A web-based research tool for functional genomics of the microcirculation: the leukocyte adhesion cascade Microcirculation 1999 6 : 259–265
Achard F, Vayssix G, Dessen P, Barillot E . Virgil database for rich links (1999 update) Nucl Acids Res 1999 27 : 113–114
Rebhan M, Chalifa-Casp iV, Prilusky J, Lancet D . GeneCards: a novel functional genomics compendium with automated data mining and query reformulation support Bioinformatics 1998 14 : 656–664
Wu S, Manber U . Fast text searching allowing errors Commun ACM 1992 35 : 83–91
Masys D, Welsh J, Lynn Fink J, MG, Klacansky I, Corbeil J . Use of keyword hierarchies to interpret gene expression patterns Bioinformatics 2001 17 : 319–326
National Center for Biotechnology Information. PubMed help 2001
Tanabe L, Scherf U, Smith L, Lee J, Hunter L, Weinstein J . MedMiner: an Internet text-mining tool for biomedical information, with application to gene expression profiling Biotechniques 1999 27 : 1210–1217
Rindflesch T, Hunter L, Aronson A . Mining molecular binding terminology from biomedical text In AMIA Fall Symposium 1999 pp 127–31
Rindflesch T, Tanabe L, Weinstein J, Hunter L . EDGAR: extraction of drugs, genes and relations from the biomedical literature In Pacific Symposium on Biocomputing, Honolulu, Hawaii 2000 pp 517–528
Swanson D, Smalheiser N . An interactive system for finding complementary literatures: a stimulus to scientific discovery Artif Intell 1997 91 : 183–203
Finn R . Program uncovers hidden connections in the literature The Scientist 1998 12 : www.the-scientist.com
Swanson D . Migraine and magnesium: eleven neglected connections Perspect Biol Med 1988 31 : 526–557
Download references
Acknowledgements
The author thanks Cynthia Brandt, MD, and John Fisk, MD, of the Yale Center for Medical Informatics, and the anonymous reviewers for feedback on the article. The author is supported by grants U01 ES10867–02 from the National Institute of Environmental Health Sciences, R01 LM06843–02 from the National Library of Medicine and U01 CA78266–04 from the National Cancer Institute.
Author information
Authors and affiliations.
Center for Medical Informatics, Yale University School of Medicine, New Haven, Connecticut, USA
P M Nadkarni
You can also search for this author in PubMed Google Scholar
Corresponding author
Correspondence to P M Nadkarni .
Rights and permissions
Reprints and permissions
About this article
Cite this article.
Nadkarni, P. An introduction to information retrieval: applications in genomics. Pharmacogenomics J 2 , 96–102 (2002). https://doi.org/10.1038/sj.tpj.6500084
Download citation
Received : 24 October 2001
Revised : 24 November 2001
Accepted : 26 November 2001
Published : 30 April 2002
Issue Date : February 2002
DOI : https://doi.org/10.1038/sj.tpj.6500084
Share this article
Anyone you share the following link with will be able to read this content:
Sorry, a shareable link is not currently available for this article.
Provided by the Springer Nature SharedIt content-sharing initiative
- information retrieval
- full-text indexing
- text processing
This article is cited by
Diagnosis of rare diseases: a scoping review of clinical decision support systems.
- Jannik Schaaf
- Martin Sedlmayr
- Holger Storf
Orphanet Journal of Rare Diseases (2020)
Quick links
- Explore articles by subject
- Guide to authors
- Editorial policies


An official website of the United States government
The .gov means it’s official. Federal government websites often end in .gov or .mil. Before sharing sensitive information, make sure you’re on a federal government site.
The site is secure. The https:// ensures that you are connecting to the official website and that any information you provide is encrypted and transmitted securely.
- Publications
- Account settings
Preview improvements coming to the PMC website in October 2024. Learn More or Try it out now .
- Advanced Search
- Journal List
- J Med Libr Assoc
- v.107(2); 2019 Apr
Errors in search strategies used in systematic reviews and their effects on information retrieval
Associated data.
Errors in search strategies negatively affect the quality and validity of systematic reviews. The primary objective of this study was to evaluate searches performed in MEDLINE/PubMed to identify errors and determine their effects on information retrieval.
A PubMed search was conducted using the systematic review filter to identify articles that were published in January of 2018. Systematic reviews or meta-analyses were selected from a systematic search for literature containing reproducible and explicit search strategies in MEDLINE/PubMed. Data were extracted from these studies related to ten types of errors and to the terms and phrases search modes.
The study included 137 systematic reviews in which the number of search strategies containing some type of error was very high (92.7%). Errors that affected recall were the most frequent (78.1%), and the most common search errors involved missing terms in both natural language and controlled language and those related to Medical Subject Headings (MeSH) search terms and the non-retrieval of their more specific terms.
Conclusions
To improve the quality of searches and avoid errors, it is essential to plan the search strategy carefully, which includes consulting the MeSH database to identify the concepts and choose all appropriate terms, both descriptors and synonyms, and combining search techniques in the free-text and controlled-language fields, truncating the terms appropriately to retrieve all their variants.
INTRODUCTION
The search for information is a basic component of systematic reviews [ 1 , 2 ]. The objective of the search is to retrieve all publications that are potentially relevant to the object of study to minimize bias in forming conclusions [ 3 ]. To achieve this goal, it is essential to search multiple databases using a comprehensive search strategy that is free from errors.
Search results are evaluated primarily by two measures: recall and precision. The ideal result has high rates of both recall and precision, although this goal is difficult to achieve due to the tradeoff relationship that exists between the two [ 4 ]. Hence, it is necessary to find an equilibrium between them. Obtaining a high recall rate [ 3 , 5 ] with a reasonable level of precision that minimizes the time and resources necessary to examine the retrieved records is a priority in systematic reviews.
Although the literature indicates that directly measuring whether a search for information has retrieved all the relevant records is impossible [ 6 ] and that certain factors external to the search engine can prevent finding all pertinent records (e.g., incorrect indexing [ 7 ], the lack of standardization of article abstracts or inconsistent terminology [ 8 ]), an error-free strategy can increase the recall of relevant studies and, hence, the quality of a review [ 9 , 10 ].
Excellent literature is available on constructing a good search strategy, ranging from classic books in the field of documentation science [ 11 – 14 ] to specific manuals on systematic reviews [ 1 , 3 , 15 ]. To improve search quality, the following approaches have been proposed: (a) the participation of librarians and information professionals in teams who, as search experts, develop strategic reviews [ 1 , 16 – 19 ], an approach that has been associated with higher quality search strategies [ 20 , 21 ] and fewer errors [ 22 ], and (b) peer review using the standardized Peer Review of Electronic Search Strategies (PRESS) instrument [ 23 – 25 ].
Although numerous articles have been published regarding the quality of search strategies in systematic reviews, almost all of them have focused on determining whether these reports included complete and precise information to allow them to be reproduced [ 26 – 33 ]. Of reviews in the Cochrane Library, the authors found one study by Sampson and McGowan that evaluated the errors in search strategies using MEDLINE (Ovid) [ 9 ].
MEDLINE is the medical database that is most frequently used in systematic reviews to find information [ 34 , 35 ] and is accessible via different interfaces. Various studies have confirmed that PubMed is the interface that authors most frequently use [ 36 , 37 ]. This interface has advantages such as free access, slightly greater sensitivity than MEDLINE-Ovid in searches for systematic reviews [ 38 ], status as the most current database [ 20 ], and information from sources other than MEDLINE, such as online books and articles from life sciences journals, making PubMed the preferred option for conducting literature searches. However, to date, no studies have evaluated search errors in studies using MEDLINE/PubMed. Given that such errors are related to the characteristics and functionalities of the database and its retrieval language syntax, we propose to fill this gap in the literature in this study. Our main objective is to evaluate the search strategies of systematic reviews in PubMed to identify errors, analyze their impact on information retrieval, and propose solutions.
Identification of studies
MEDLINE/PubMed was searched on February 1, 2018, using the systematic review thematic filter as a search phrase [ 39 ]. The following search statement was used with no language limitation:
Systematic [sb] Filters: Free full text; Publication date from 2018/01/01
Selection criteria
Three inclusion criteria were defined and applied in successive phases:
- The included articles were required to be systematic reviews, systematic review protocols, or meta-analyses that included a systematic literature database search. All other types of articles were excluded, including those that applied meta-analysis techniques using numerical data obtained from data banks rather than through information searches in bibliographic databases.
- Only articles that used MEDLINE/PubMed for their searches were included. All publications that did not search this database or that searched MEDLINE but from which the interface could not be determined were excluded.
- The included articles were required to include a search strategy that had been described in sufficient detail to be reproducible, and the strategy must have been explicitly described in the full article text or in supplementary files stored in MEDLINE/PubMed. Articles that did not thoroughly describe their search strategies or that pertained to other databases were excluded. Also excluded were those strategies that simply mentioned the search concepts or simply presented a sequence of search terms, whether combined with Boolean operators or not (without parentheses), indicating that such searches had been applied to all the consulted databases, but without a specific syntax or an expressed declaration that the search had been conducted through PubMed.
The three authors independently examined the abstracts and full texts of the articles selected for inclusion. Discrepancies were resolved by consensus.
Data extraction
For each review, the search strategy obtained from the methods section and/or online supplementary files was downloaded. Based on prior studies [ 9 , 23 , 25 ] and our knowledge and experience, the errors that affected recall or precision were selected. Then, the meaning of some of these errors was adapted to the PubMed syntax, resulting in the following list of errors for evaluation:
- incorrect use of Boolean operators (e.g., using AND instead of OR or vice versa)
- lack of parentheses (e.g., unmatched parentheses or inappropriately combined terms due to missing parentheses)
- lack of morphological variations of the terms (e.g., not truncated, truncated but with too much specificity, or syntax errors in truncation)
- missing Medical Subject Headings (MeSH) terms (e.g., where adequate descriptors for various concepts existed in the controlled vocabulary but were not present in the search strategy)
- MeSH terms not searched in the [mesh] field (e.g., where MeSH terms are included in the strategy but are searched only in the free-text fields)
- nonexplosion of MeSH terms (e.g., where records containing more specific terms were not retrieved); no errors were considered when the MeSH terms were deliberately not exploded [mesh:noexp] or were only searched in the title fields because we assumed that the authors sought a high precision rate; however, if authors searched without field tags, truncating the terms or in [all] [tw] tags, we assumed that they wanted to achieve a high recall rate and it was considered an error
- MeSH terms not searched in free-text fields (e.g., records containing the search language terms were not retrieved from free-text fields)
- missing synonyms
- repetition of morphological variations of the terms
- term redundancy (e.g., when a phrase exists that already contains a term is searched, but the term is also searched as an OR term, or when an already included field is searched in all of the OR fields [all fields])
Information was also gathered regarding the search method that had been used for:
- phrases: double quotes, truncation, field codes, and automatic mapping
- individual terms: double quotes, truncation, field codes, and automatic mapping
To evaluate missing MeSH terms and synonyms, we consulted the MeSH controlled vocabulary. This vocabulary contains three types of terms, organized hierarchically: descriptors (main headings), qualifiers (subheadings), and supplementary concepts (supplementary concept records). We confirmed whether each term in the search strategy existed or was a MeSH term and if so, whether more specific terms and synonyms existed that appeared below the Entry Terms entry.
Process and data analysis
A statistical analysis of the data was conducted using SPSS version 22 to obtain the frequency and percentages for each type of error.
To analyze the impact of each error type on recall, we selected a strategy from the studied reviews. A search was then performed in PubMed, first using only the fragment containing the error and then with the corrected fragment. The number of records retrieved was noted in each case. These strategies were performed on April 25, 2018.
Our initial search retrieved 677 records. In the first phase, 159 records that were not systematic reviews were excluded: despite using the Systematic Review filter, 25 of the results consisted of data corrections, letters to the editor, editorials, and retractions; 132 were narrative reviews, controlled trials, prospective and retrospective studies, case series, and surveys; and 2 did not offer full text access. In the second phase, 165 records where MEDLINE/PubMed was not used for the search were excluded. In the third phase, all the reviews for which a specific search strategy in MEDLINE/PubMed could not be identified were excluded. Of these, 11 pertained to another database, 126 mentioned only the concepts and terms, and 79 listed only the terms combined with Boolean operators, but the strategy used in MEDLINE/PubMed was not specified. Finally, 137 systematic reviews were selected that contained a complete, reproducible search strategy for MEDLINE/PubMed ( Figure 1 ).

Flow chart of included studies
Search errors
Of the search strategies, 92.7% contained some type of error. To facilitate their presentation, the errors were grouped into 2 categories: those that affect recall and those that do not, with the former occurring more frequently (78.1%) than the latter (59.9%). Table 1 presents the frequency of the different types of errors.
Frequency and types of errors in MEDLINE/PubMed search strategies

The errors that affect recall occur for two main reasons: (1) missing terms (synonyms, morphological variations, and MeSH terms) and (2) the search mode for the descriptors. Search mode errors occur because the descriptors are not searched in the [mesh] field, either explicitly or through automatic mapping; because they are not exploded (and, thus, their more specific terms are not retrieved) when searching in the text words [tw] field; because automatic mapping is disabled by truncating descriptors or enclosing MeSH phrases in double quotes; or because terms are not searched in free-text fields such as the title and abstract. During the search evaluation process, we identified additional types of errors, such as failures in the analysis of concepts.
The most frequent search errors that did not affect recall involve repetitions of morphological variations of words despite truncation and term redundancies. Neither of these errors affects information retrieval negatively with respect to either recall or precision.
Errors due to incorrect searches for a phrase lower the precision of the results by either directly combining the two terms using the AND operator or truncating the first term in a phrase formed by two or more terms separated by spaces, which disables automatic phrase search. In the latter case, PubMed combines the terms with the AND operator. Strategies that contain the Boolean operators OR and AND also yield less precision, as does failing to enclose terms in parentheses that belong to the same concept, because these strategies result in records being retrieved that do not contain all the searched concepts.
Although errors resulting from the incorrect use of Boolean operators can affect both recall and precision, of those found in our study, one has no effect when the terms are combined with the AND operator, and the other affects precision because it utilizes the OR and AND operators together without a term between them (possibly a transcription error). In this case, the second operator (the correct one) is ignored.
Truncation was used in 63 searches (46.0%). In about half of these (22.6%), variations of the terms were repeated. One-third had missing variations such that within the same search strategy, some terms were truncated, whereas others were not, and some strategies used truncation but included repeated variations.
Search modes
There are two ways to search terms in PubMed: without field tags, which activates automatic term mapping, or with field tags.
The number of strategies that used field tags for all the terms was greater (46.7%) than the number of strategies in which all terms appeared without tags (21.2%) ( Table 2 ). Note that searching all fields [all] is equivalent to automatic mapping, unless the terms are truncated or enclosed in double quotes, because they turn off automatic mapping. Of the strategies that use only field tags, the most frequent are those that combine searching in the MeSH field and in the title/abstract. Those strategies that searched only in the title and abstract fields are candidates for reduced recall when the terms are descriptors, as are those that search only MeSH fields, because they will fail to retrieve records that contain the search terms in the title and abstract fields.
Search strategies that use field codes and/or automatic mapping

Phrase searches
Phrases are searched to retrieve records that contain adjacent terms in the order indicated; this approach ensures the precision of the results. The most common approach is to use field tags. The option to search for a phrase with the terms separated by spaces is not suitable because PubMed processes them with automatic mapping, retrieving not only all records that contain the phrase (if it recognizes it as one), but also those that contain both terms but not as a phrase (combined with AND), causing noise in the results due to contextual ambiguity ( Table 3 ).
Ways to search for phrases and individual terms

Searches for individual terms
Failure to use field tags can affect precision because PubMed may substitute the search terms with others that have different meanings; hence, it is advisable to ensure that mapping is performed in the manner desired ( Table 3 ).
Effects of errors on information retrieval and solutions
The tables that show the effects of errors on information retrieval are included in the supplemental appendix . Both tables include specific examples of the different error types identified in search strategies and corresponding solutions. To better differentiate between the results, the numbers of records retrieved in PubMed are shown separately for the case of errors that affect recall ( Table 4, supplemental appendix ) and those that do not affect recall ( Table 5, supplemental appendix ).
The results of this study reveal that the percentage of search strategies that contain various types of errors is quite high (92.7%) and that 78.1% of these errors affect recall. Therefore, these errors can influence the conclusions of systematic reviews.
We found only one study (Sampson and McGowan [ 9 ]) that identified errors in search strategies. This study differed from ours in ways that should be considered when comparing them: their strategies were carried out in MEDLINE but on a different platform (Ovid), and their descriptions and number of errors did not completely agree with the results of our study. For example, our study did not include errors such as whether the search strategy was adapted to other databases, errors whose determination was subjective (term relevance), or any errors specific to the Ovid interface. Meanwhile, Sampson and McGowan did not include some errors that were analyzed in this study, such as a lack of synonyms or searches for descriptors in free-text fields.
Despite these differences, the percentages of strategies that contain at least 1 error were very similar in both studies (92.7% vs. 90.5%). We found fewer errors due to inappropriate use of Boolean operators (1.5% vs. 19.0%); however, we found more frequent errors due to missing term variations (48.2% vs. 20.6%) and redundancy (34.3% vs. 12.7%). One plausible reason was that the reviews that Sampson and McGowan analyzed were published in the Cochrane Database of Systematic Reviews, which is considered the gold standard for evidence-based practice [ 40 ]. Consequently, one might expect fewer errors in these reviews than in the reviews in our study, which had been published in any free full text journal.
Many of the errors that we found revealed a lack of knowledge regarding the principles of information retrieval and/or the specific characteristics of searching in the PubMed database. While supplemental Tables 4 and 5 describe specific solutions for each error, different aspects that influence the correct design of a search strategy and that, therefore, constitute general solutions for avoiding errors are presented below.
Search strategy design always begins with an analysis of the main concepts and the choice of terms to use for each concept. Incorrect identification of the concepts is a serious error that affects search success. The MeSH database is a very useful tool for identifying concepts and choosing appropriate terms. It is recommended that controlled vocabulary and natural language terms be used [ 25 ], regardless of whether they are synonyms or alternative terms, along with their variations and different possible sequences within a phrase [ 41 ].
All possible variations of the terms must be considered. Truncation can be used to avoid having to explicitly include all possible variants in the strategy. In PubMed, the symbol for truncation is the asterisk (*), and its effect is to retrieve all the words that contain the root (the part of the word preceding the asterisk), thus increasing recall.
Familiarity with some of the aspects of the correct use of truncation in PubMed is required:
- Only the end of a word can be truncated. Truncation to the left or within a word is not allowed.
- Descriptors cannot be truncated when searching in the [mesh] field. When descriptors are truncated, variations are not retrieved, because only the exact descriptor will be searched. For example, hypertens* [mesh] is equivalent to searching for hypertension [mesh].
- When searching for a phrase, only the last term should be truncated. If a prior term is truncated, the entry will not be searched as a phrase; instead, PubMed will search all variations of that term linked with AND to the next term.
- Truncating a term or phrase enclosed in double quotes has no effect. PubMed will retrieve all the records that contain the exact character string located before the asterisk but will not retrieve records with any of its variations.
- Truncation disables automatic mapping.
After selecting the terms, it is necessary to use search techniques with controlled language and free text. Previous studies show that the best results are obtained by combining the techniques of free text and controlled language search [ 40 ]. A search conducted exclusively with the latter can miss relevant information due to indexing failures (not all the main concepts addressed in the articles appear as descriptors) and due to the possible lack of suitable descriptors for representing a concept. Additionally, this loss is greater in PubMed because most current records do not yet have MeSH terms assigned; hence, these records would not be retrieved.
The search with controlled language consists of searching for descriptors in the [mesh] field, and it offers two advantages regarding information retrieval:
- It improves recall. When the indexing is consistent, using a single term to search for a concept favors retrieval of all documents that address that concept without having to use any synonym because PubMed explodes the term by default—that is, it retrieves all the more specific terms located below it in the hierarchical MeSH structure.
- It improves precision. When a term is present in the [mesh] field, it means that the concept represented by that term is addressed in a significant way in the article—much more so than if the term appeared only in the abstract.
The free-text search involves searching for natural language terms in text fields such as the title and abstract [ 22 ].
It is also important to be knowledgeable regarding the principles of information retrieval in order to avoid committing basic errors and to apply these principles to the particular characteristics of the search language of the database used. Among these, the following should be noted:
- Terms related to the same concept should be linked by the OR operator, whereas terms referring to distinct concepts should be linked with AND. When several terms are separated by spaces, PubMed processes them through automatic mapping, translating the query into phrases and/or terms combined correctly with the Boolean operators.
- The Boolean operators are processed from left to right, and the AND operator takes precedence over the OR operator. When Boolean operators with different precedence (OR, AND) exist in the same search strategy, it is important to ensure that the search order process is the desired one. Otherwise, using parentheses allows prioritization of the operations that must be executed first. For the strategy to be executed correctly, it is advisable to enclose terms that pertain to the same concept in parentheses.
- When a concept is formed by two or more terms, it is appropriate to search the terms as a phrase. The procedure for performing phrase searches in PubMed includes the following steps: (a) truncating the final term, (b) joining terms with a hyphen, (c) enclosing the phrase in double quotes, and (d) using a field tag. Failure to search as a phrase and instead combining the terms with the AND operator introduces noise due to contextual ambiguity, which increases recall but reduces precision.
An efficient search strategy design requires knowledge of the differences and similarities between both modes and between the different fields, including how such characteristics affect the recall and precision of the results. Field tags enable users to take advantage of certain search features in PubMed, such as searching for phrases and controlling the fields in which terms appear. Which field to use depends on the search objectives. In a search where a high recall rate is desirable, terms that are descriptors should be searched for in the [mesh] field and in the title and abstract fields [tiab]; terms that are not descriptors should be searched for in the title and abstract fields [tiab] and in the authors’ keywords [ot].
The fields [all] and [tw] should be used with caution with certain terms, because they can introduce noise when they appear, for example, in non-thematic fields such as author affiliation. Meanwhile, it should be taken into account that MeSH terms searched in the [tw] field are not exploded and do not retrieve more specific terms (lower recall), while terms searched in the [all] field are processed automatically unless truncated or enclosed in double quotes, in which case they are searched in all fields. However, because automatic mapping is disabled, if the terms are MeSH terms, they will not be exploded.
Failing to use field tags transfers control of the search process to PubMed’s automatic mapping, which can sometimes cause significant noise, whether due to mapping to inadequate MeSH terms and/or combining the terms in a phrase with the AND operator.
Limitations
The study included only systematic reviews published during a single month. Nevertheless, we believe that this sample is sufficiently large to demonstrate many of the search strategy errors that occur when using PubMed.
The specific impact of the errors identified in search strategies for information retrieval was demonstrated through examples that were obtained from existing systematic reviews, and solutions were proposed based on the characteristics of PubMed. The main limitation is that the overall impact on the final result of the entire search strategy is not measured here, as doing so would have required knowing exactly what information the authors were seeking and, in many cases, modifying the complete strategy to present an error-free version, which would have made this article excessively long.
Search error identification was based on the strategies described in the selected reviews; however, it was possible that in some cases such error might have been introduced during transcription or that searchers might have utilized a version that was modified or adapted.
The effects of errors on information retrieval were demonstrated in this article using examples of search strategies on concrete topics, and this impact might be larger or smaller on other topics, according to the number of publications in the database.
Another limitation was that only systematic reviews of free full-text articles in PubMed were evaluated. Although this selection criterion did not influence the study’s main objective of identifying error types, it might have influenced the number and percentage of search strategies that generated errors because Cochrane reviews or reviews published in journals with stricter criteria were not evaluated.
CONCLUSIONS
The importance of information searches in systematic reviews is frequently discussed in the literature. Despite this, our study reveals that the number of search strategies that contain errors is very high and that the majority of these errors affect recall. Such errors occur primarily due to the failure to use synonyms or truncations to retrieve the different morphological variants of terms. Other frequent error types (although to a lesser extent) involve missing MeSH terms and failure to retrieve more specific terms through nonexplosion.
We recommend the following measures to improve the quality of PubMed search strategies:
- Consult the controlled MeSH vocabulary: Doing so will help to identify concepts and to select adequate terms, which are two key steps for achieving success in searching.
- Combine the techniques of controlled vocabulary and free-text searches: To avoid losing current relevant information, MeSH terms should be searched in both the [mesh] field and in the title and abstract free-text fields [tiab]. Terms that are not descriptors should be searched in the [tiab] and author keyword [ot] fields.
- It is preferable to search terms and phrases using field tags rather than allowing PubMed to process the search through automatic mapping, because the more specific approach avoids PubMed mapping searches to inappropriate terms, possibly causing noise.
Terms must be truncated to retrieve all possible variations; however, it is important to consider that: (a) individual terms are truncated when they are searched in free-text fields; (b) MeSH terms are not truncated when searched in the [mesh] field; and (c) when a phrase is searched, only the last term should be truncated.
SUPPLEMENTAL FILE
- Open access
- Published: 10 April 2024
Remote sensing image information extraction based on Compensated Fuzzy Neural Network and big data analytics
- Rui Sun 1 , 2 ,
- Zhengyin Zhang 3 ,
- Yajun Liu 4 ,
- Xiaohang Niu 1 &
- Jie Yuan 5
BMC Medical Imaging volume 24 , Article number: 86 ( 2024 ) Cite this article
108 Accesses
Metrics details
Medical imaging AI systems and big data analytics have attracted much attention from researchers of industry and academia. The application of medical imaging AI systems and big data analytics play an important role in the technology of content based remote sensing (CBRS) development. Environmental data, information, and analysis have been produced promptly using remote sensing (RS). The method for creating a useful digital map from an image data set is called image information extraction. Image information extraction depends on target recognition (shape and color). For low-level image attributes like texture, Classifier-based Retrieval(CR) techniques are ineffective since they categorize the input images and only return images from the determined classes of RS. The issues mentioned earlier cannot be handled by the existing expertise based on a keyword/metadata remote sensing data service model. To get over these restrictions, Fuzzy Class Membership-based Image Extraction (FCMIE), a technology developed for Content-Based Remote Sensing (CBRS), is suggested. The compensation fuzzy neural network (CFNN) is used to calculate the category label and fuzzy category membership of the query image. Use a basic and balanced weighted distance metric. Feature information extraction (FIE) enhances remote sensing image processing and autonomous information retrieval of visual content based on time-frequency meaning, such as color, texture and shape attributes of images. Hierarchical nested structure and cyclic similarity measure produce faster queries when searching. The experiment’s findings indicate that applying the proposed model can have favorable outcomes for assessment measures, including Ratio of Coverage, average means precision, recall, and efficiency retrieval that are attained more effectively than the existing CR model. In the areas of feature tracking, climate forecasting, background noise reduction, and simulating nonlinear functional behaviors, CFNN has a wide range of RS applications. The proposed method CFNN-FCMIE achieves a minimum range of 4–5% for all three feature vectors, sample mean and comparison precision-recall ratio, which gives better results than the existing classifier-based retrieval model. This work provides an important reference for medical imaging artificial intelligence system and big data analysis.
Peer Review reports
Introduction
It is important to investigate medical imaging AI systems and big data analytics. With the rapid development of remote sensing technology, remote sensing images, as typical applications of medical imaging AI systems and big data analytics, play a huge role in environmental monitoring, economic investigation, natural disaster prediction, environmental degradation monitoring, etc. Several efforts have been made to develop an effective method for information extraction operations in RS. The availability of many high-resolution (HR) photos provides a benefit for more accurate information extraction by creating sophisticated categorization methods. The process of RS classification is complicated and takes various elements into account. First, more information on the relative usefulness of various forms is required to better assist the user in making judgments regarding image classification. Utilizing Content-Based Image Extraction (CBIE) in CBRS. CBIE is a method that searches a database of images using visual content. CBIE uses optical characteristics like color and texture to describe pictures. CBIE is a subset of imaging, and computer vision derives many approaches from those disciplines. In the first place, neural networks are data-driven self-adaptive methods that can change based on the data without requiring user-defined or distributional form specifications for the underlying model. When remote sensing data is provided in digital format, neural networks, a computer technology, must be used for digital processing and analysis. Fuzzy concepts and neural network technologies are interacting and combining due to development. There is a technical problem with the typical neural network. For instance, the ideas are unsure about how to choose a fuzzy membership function, fuzzy logic reasoning, and imprecise optimized calculation. Due to the neural network’s incredibly effective and precise classification capabilities, both recall rate and retrieval time have been markedly enhanced.
A Compensated Fuzzy Neural Network (CFNN) offered as a solution to this issue follows the integration of fuzzy theory with the neural network. It has compensating fuzzification capabilities and quick study calculation capabilities. CFNN is a hybrid system that combines neural networks and balanced fuzzy logic. It can strengthen the image stability of the process and increase the fault tolerance of the extraction process. Agriculture, temperature, water bodies, and many more fields use remote sensing as a key means of earth monitoring without direct physical contact [ 1 ]. Due to the difficulty in managing data queries due to the sheer volume of data from several sources, current data organization, storage, and management solutions cannot satisfy application requirements [ 2 ]. Massive RS photos are essential for many applications, particularly combustion RS images, as they capture the planet’s surface enhancement, spatial guidance, disaster relief, and more. Early on, RS information is one type of pricey and in-demand resource [ 3 ]. The job of RS-Image Retrieval (RS-IR), which seeks to find a group of objects that are comparable to a given query image, is crucial in remote sensing applications [ 4 ]. One of the fundamental problems is usually the segmentation of remote-sensing images with imbalanced samples [ 5 ]. The handling of elevated RS for high-resolution images has become difficult due to the rapid development of their volume and resolution.
The customary content-based Remote-Sensing Image Extraction (CBRSIE) technologies [ 6 ]. A significant result is Content-Based Image Extraction (CBIE), which tries to analyze photographs using features of an image comparable to the query image the user submitted. With this method, an image’s description comprises dynamically retrieved visual elements, including hue, structure, and shape [ 7 ]. It is only necessary to normalize one channel when using a grayscale image. However, if normalizing a RedGreenBlue(RGB 3 channels), we should use the same standards for each channel [ 8 ]. Unorganized image entity information and structured metadata comprise the majority of remote sensing data. Due to the peculiarities of huge geographic data realization, the ability to aggregate all entity data is governed by several factors, including the access mechanism, available storage space, labor costs, etc. [ 9 ]. Structure extraction from remote sensing pictures can be done in several ways, including extracting features based on prior knowledge of things like strong edges, shape designs, roof colors, shadows, etc. [ 10 ]. The CCFN can carry out compensated fuzzy reasoning and features a fleet self-study algorithm [ 11 ]. With the number of images expanding rapidly, Content-Based Image Extraction (CBIE) has garnered more attention [ 12 ]. Depending on the IF-THEN implication, a fuzzy logic system concludes. Based on the study’s findings, control rules are developed concurrently, and class labels are constructed. The produced model’s functionality is evaluated for accuracy [ 13 ].
The planned work’s contribution is as follows:
A unique model is recommended for image information extraction from an RS field using the CBIE technique called FMCIE to handle low-level images like texture from the RS sector.
Integrated proposed FMCIE with CFNN model is used to compute the query image’s class label and fuzzy class membership.
Feature Information Extraction and Hierarchically nested structures are analyzed to get similar recurrent images to form a query database. The outcomes reduce the retrieval speed of pictures from the RS field.
It shows the ratio of coverage, average mean precision, and recall. Comparison of the proposed work is better than other existing Classifier based Retrieval methods.
The planned work is structured as follows: Categorization of earlier knowledge describing the relationship between RS and CFNN for efficient Retrieval of images and its similarities using various methods are discussed in Sect. 2. To extract similar images from the stored dataset of the RS field, Sect. 3 introduces the CFNN and suggests an FCMIE model for the system. The experimental findings for CBIR using FMCIE models are presented in Sect. 4, which also provides the best precision, recall, and coverage ratio. Compared to traditional Classifier-based models, average Mean Precision (AMP) from RS pictures. The conclusion and the potential for future improvement in the RS image analysis using Compensated Fuzzy Neural Networks are discussed in Sect. 5.
Related work
Shao et al. (2019) suggested a method to simultaneously distinguish light clouds and quasi-components in RS photos using a Multiresolution Functionalities Convolutional Neural Network (MF-CNN) [ 14 ]. In terms of proper identification rate, the self-contrast approach performs the worst, and the majority of values across the board for cloud detection are less than 0.75, rates more favorable in terms of the good detection rate.
Tang et al. (2018) suggested the Features Extraction method and which is created using the Bag-Of-Words (BOW) paradigm and deep learning technology [ 15 ]. The learning method is divided into two steps: building features and learning picture descriptors. Over 50 countries are represented in the photographs, all taken from Google Earth. The original range of each image is 256 for 256, and the pixel ranges from 29 to 0.3 m.
Ghrabat et al. (2019) elaborated an innovative SVM-based Convolutional Neural Network(SVMB-CNN) is utilized to classify the characteristics after they have been optimized using a modified evolutionary approach [ 16 ]. The undesirable data in the dataset are removed using a Gaussian filtering approach. The experimental outcomes of the Corel 1K dataset’s precision, recall, and retrieval rate, which has respective values of 99, 97, and 95% better results, are obtained from our proposed model.
Desai et al. (2021) proposed a Convolution for Neural-Networks (CNN) based Support Vector Machines (SVM) are utilized to provide an effective deep learning framework for quick picture retrieval [ 17 ]. The suggested architecture uses SVM for classification and CNN to extract features. The outcome of this proposed work is: For the confusion matrix, the category with the highest accuracy rate (95%) and genuine positive sign (45%) is Animals, whereas the class with the lowest accuracy rate (60%) and real positive value (30) is Hills.
Sezavar et al. (2019) defined a novel search technique called the Modified Grasshopper Optimization Algorithm (MGOA) suggested to solve the modeled problem and effectively locate related photos [ 18 ]. It is noted that the proposed strategy achieves the best P(0.5) and P(1) scores at 93 and 81%, respectively. The proposed approach yields the best results, whose vector size is above 1024, whereas the proposed approach that performs better as a feature vector that is 70 bytes in size.
Ghrabat et al. (2019) proposed the Multiple Ant Colony Optimization (MACO) method to locate pertinent characteristics, and it is used with all of the features [ 19 ]. The relevant factors are used for the Greedy learning of the Deep Boltzmann Machine classifier (GD-BM). Compared to current methods like the a priori classification algorithm, the GD-BM offers a 28% improvement in accuracy. The system now only takes about 3.75 ms for each image, which is better than the current system’s time usage.
Guanglong et al. (2020) presented an online cognitive angular position error compensation method that utilizes incremental learning to decrease joint angle error [ 20 ]. This method predicts and updates the compensation in real-time using the Self-Feedback Incremental- FNN (SFIFN). The efficiency of the suggested method for correcting joint angle inaccuracy is demonstrated. The proposed model decreased the inaccuracy by around 0.02 degrees compared to the direct compensation method.
Zhang, Jin, et al. (2021) proposed a compensation fuzzy neural network technique that incorporates the fuzzy compensation algorithm and recurrent neural network for detecting and recognizing table tennis’s technical and strategic indicators [ 5 ]. The information and prediction outcomes show that the model’s accuracy rises with increasing the number of input coordinates. The acceptable error range in a practical application is less than 40 mm when the input data length is 30 mm.
A novel loss function-based picture segmentation technique built on ConvNet was created and tested by Chen et al. (2021) [ 21 ] to provide a more accurate quantitative evaluation of bone metastases because it can measure activity in overlapped structures. The suggested quasi-Convolution model, trained using models formulated, produced Coefficients of Dice Similarity (CDS) of 0:70 and 0:80 for tumor and bones segmentation in Quantitative analysis of Bone based Single-Photon Emission in Computed Tomography QBSPECT/CT.
Chen et al. (2021) suggested a method based on fuzzy neural networks that examine the factors contributing to music instruction’s eligibility in universities and colleges [ 22 ]. The rate of low teaching quality indicated by 30 polytechnic institutions and 30 universities using the fuzzy control method for incident detection ratio in music training effectiveness of 81.7% of colleges and universities are comprehensive.
Ai et al. (2019) elaborated a technique for the automatic control of the antimony flotation process, and an adaptive fuzzy neural network control strategy based on data was created [ 23 ]. The LSTM (Long ShortTerm Memory) network and the Radial Base Function Neural Network (RBFNN)are integrated into the data-driven model. The outcome of our strategy reduced the standard deviation by 0.1809 and 0.2589 sample images compared to the other two control methods.
Ye et al. (2018) proposed CNN fundamental features and a weighted range-based retrieval approach [ 24 ]. Before extracting the pre-trained network is initially perfectly alright to use some scanned copy from the objective data set before CNN features and labels the images in the retrieved collected data. When there are more than ten training images, our methods produce better outcomes than the alternatives. With the suggested methods, the standard deviation of the search sequence number is 0.04 lowest.
Summary of this session: The work included image information extraction in the remote sensing field, and integration with the fuzzy neural network is assessed and compiled. This literature survey refers to efficient and improved methods Content-Based Image Retrieval (CBIR) techniques and QBSPECT/CT, LSTM, CNN, Fuzzy logic, and Classifier based Retrieval algorithms for mitigating efficient image retrieval by various researchers and authors. Also, did a literature survey to find published models for image handling and management in addition to feature extraction strategies and evaluation metrics. Comparison of platforms like CR, SFIFN, GA, MGOA, and another BOW-based CNN, including effective image information extraction.
Proposed work
From medical imaging AI systems, the major challenge with a Content-Based Image Extraction system (CBIE) is to retrieve the image information features from the RS that accurately represent the contents of images in a database. A thorough analysis of the image feature information performance is necessary for this extraction. Colour and texture feature extraction is part of CBIE. The color histogram, color correlogram, and color moment are frequently used color properties that are compared. Image information extraction effectiveness increases when texture and color features are integrated. Also mentioned are the similarity measurement criteria used to find matches and retrieve the correctness of the images in an RS field. Feature Information Extraction describes utilizing a computer to collect visual data and determine if each object’s pixels are included in an extracting feature. The edge characteristic, analysis of the corner feature’s present location, color highlight, texture showcase, and edge function are some of the picture features employed in several feature extraction methods in this study. A unique model is recommended for image information extraction from an RS field using the CBIR technique called FMCIE to handle low-level images like texture from the RS sector. Integrated proposed FMCIE with CFNN model is used to compute the query image’s class label and fuzzy class membership. Feature Information Extraction and Hierarchically nested structures are analyzed to get similar recurrent photos to form a query database. The outcomes reduce the retrieval speed of images from the RS field. It shows the ratio of coverage, Average Mean Precision, and recall Comparison of the proposed work is better than other existing Classifier-based retrieval methods.
In Fig. 1 , the suggested model for the CBRS technique of image information extraction is depicted clearly with the CFNN-FMCIE method of information extraction process from RS. Initially, the freely available dataset is downloaded, and the image is taken from a stored database of RS. Then the query image is considered as input for the proposed model. The algorithm steps and formulas are explained clearly in that module. After that measure, the distance metric of the image is calculated with the necessary equation. FIE is used to extract information on image attributes like color, shape, and texture; the region is analyzed. The output is forwarded for hierarchical arrangement structures, and similarity measurement is taken for those images stored in a database. The experimental outcomes show the information attributes extracted from them and the retrieval ratio of coverage, Average Mean Precision, and recall of the proposed method are better than conventional models.
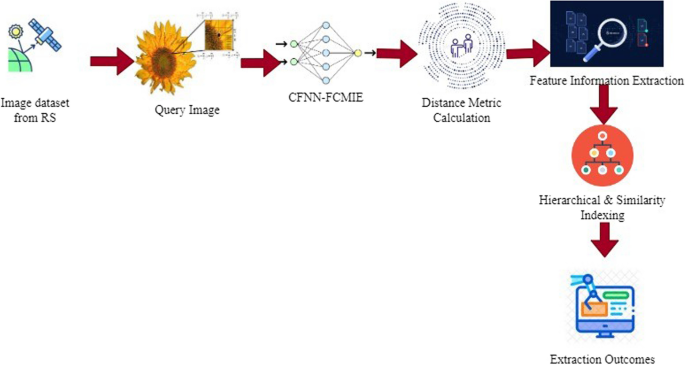
CFNN - FCMIE method for image information extraction from RS with medical imaging AI systems and big data analytics
CFNN for fuzzy class membership-based image extraction and big data analytics
The proposed FCMIE method can extract the image information using the convolution method. The data is a high-dimensional matrix with the corresponding width and length for the 3D image. It consists of two steps: Retrieval based on the class label and fuzzy class membership-based image extraction (FCMIE) of the query picture value. Secondly, weighted distance is measured when the search space is restricted to the classes. Multiple labeled image datasets and the related feature set are used to train a multi-layered fuzzy class neural network with one hidden layer. Statistical texture features are extracted using the wavelet transform. These feature sets are utilized to calculate the query image’s class membership value and the feature space distance between the query and database images. Maximum nonlinear separations between various made in the feature space are the objective of learning. The neural network requires 𝑑 =20 cells at the input nodes (one cluster at the input layer is utilized as a biassed input) and c (number of output variable) nodes at the hidden layers to categorize feature maps of dimensions ten into the numeric count of classes. The buried layer of the network makes use of “ \(2d+1\) ” neurons. A 4-fold cross-validation approach is used to evaluate the image information extraction and retrieval speed performance.
FCMIE value of the image of \(X\) having feature vector \(\overrightarrow{f}\left(X\right)\) For the particular class, j is calculated by the equation
Where \({0}_{j}\left(\overrightarrow{f}\left(X\right)\right)\) represents the output layer called \({j}^{th}\) neuron for the input value \(\overrightarrow{f}\left(X\right)\) . \({\mu }_{j}\left(\overrightarrow{f}\left(X\right)\right)\) Represents the fuzzy class membership of image \(X\) to the particular class outcome \(j\) . The outcome of FCMIE must satisfy the following constraints. Mean is represented by \({\mu }_{j}\) . The output variable is represented by \(c.\)
The suggested method FCMIE in Fig. 2 uses a trained neural network to automatically determine the fuzzy Membership of the query image from the given feature set. The feature set mapping is not necessary for this. The suggested method minimizes the number of categories for learning by not requiring manual regrouping of perceptually related textures from distinct classes.
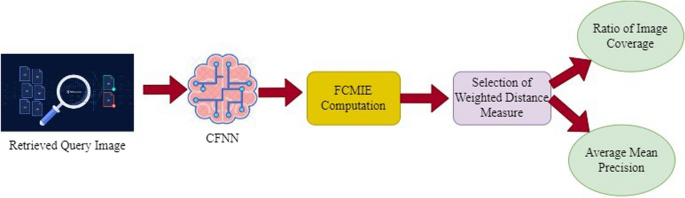
Class Membership-based FCMIE method image information extraction
FCMIE Pseudocode for Image Information Extraction from RS.
Resize all images in each database, feed them to CFNN, and start training.
Save essential information features of images
Initialize FCMIE
If \({o}_{j}\left(\overrightarrow{f}\left(X\right)\right)\le\,t\)
Extract query features from a stored database
Distribute the images information randomly
Use class Measure for Weighted Distance else
Use Distance metric simple calculation, \(d\left(X,{Y}_{j}\right)\) Endif.
Evaluating metrics performance is one of the most important steps in content-based RS image extraction. A wide range of system performance measurement techniques is applied. The performance techniques, recall, and Average Mean Precision was employed.
Measure for weighted distance
The FCMIE, as mentioned earlier, method of image extraction then needs to take weighted distance measures when the space is restricted to the classes proposed by the Classifier; the classifier-based retrieval strategy provides 90% retrieval performance for each valid classification. However, the technique utterly fails to function in the event of misclassification. A weighted distance metric is suggested to consider retrieving efficiency in real and misclassification scenarios. For each proper classification Classifier, the goal is to apply a minimal penalty to all images within the same class and a comparatively bigger penalty to all the other category images in the database. When retrieving database images, the same sentence is applied to all of the images for each misclassification. Then the proposed weighted distance between two images in the feature vectors from the RS field is calculated by the equation
Where \(Z\) represents the constant number that should not be negative. \({\mu }_{j}\left(\overrightarrow{f}\left(X\right)\right)\) Represents the fuzzy class membership of image \(X\) to the particular class outcome \(j\) . \(d(X,{Y}_{j})\) represents the distance between the \(X\,and\,{Y}_{j}\)
Ratio of coverage
The above metrics and weighted distance measures for statistical feature image vectors are analyzed. The approaches most frequently used to evaluate the efficacy of retrieval models have been recalling, precision, and recall precision. In particular, the user cannot calculate the recall index until they have viewed every relevant image, which is only achievable through a thorough search. The rate of uptake that can be used for RS image information extraction was utilized as the performance parameter in this study because the user could assess the effectiveness of the information extraction search progress. According to the equation below, this ratio of coverage can be determined.
Where \(R\) is the count of overall related pictures in the stored component, \({n}_{{R}_{i}}\) Is the count of related pictures in the first \(10i\) pictures. When the outcome of the first condition \((10i\le R )\) , the ratio of coverage is equal to the precision; when the ranges of \((10i>R),\) the percentage of capacity is similar to the recall. In this equation, the value of \(i\) is taken as {1, 2, 3, 4, 5, 10, 20}.
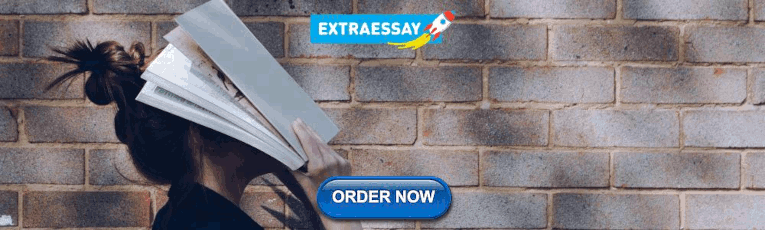
Average mean precision
After calculating the Ratio of Coverage, the performance measure needs to calculate another metric named Average Mean Precision. The single-value metrics of precision and re-call-precision are based on the entire collection of photos supplied by the information retrieval. It is advisable to monitor the presentation range of the getting images for algorithms that produce a rated image sequence. The more relevant photos are ranked higher in the average precision index. The precisions calculated for each appropriate illustration in the ranking sequence are averaged to get this index. The mean precision scores for each search make up the average precision for a group of inquiries. It is determined as
where \(rk\) is the rank of an image, \({I}_{r}\) represents the count of related pictures obtained, \({I}_{s}\) Denotes the count of relevant source pictures obtained, \({\rho }_{rk}\) Is the priority part in the relevant pictures that are obtained, and \({\rho }_{s}\) Is the rank number in the real related pictures that are obtained?
Features information extraction (FIE)
After identifying the ratio of coverage and Average Mean Precision of a retrieved image by the above-mentioned weighted distance measure, then need to calculate Features Information Extraction (FIE) is the process of extracting characteristics from an image, such as its color, texture, shape, and edges. A combination of features is necessary to obtain reliable retrieval results; a single factor does not provide accurate results. The pre-processing and feature extraction stages can be used to categorize the tasks carried out by CBIE. Noise is removed during the pre-processing step, and specific object properties important for understanding the image are enhanced. Segmentation of the image is also used to distinguish objects from the backdrop of the picture. Shape, color, texture, and other features are employed to describe the contents of the image during the feature extraction stage. Moments, scatter plots, and automated manner are a few approaches that can be used to achieve the color aspect. It is possible to implement the texture aspect via vector quantization or transforms. At this stage, highly similar image information extraction is also carried out. The following formulation can be used to measure similarity. The recall and precision of the system’s retrieval performance can be evaluated. Accuracy examines the system’s ability to recover only the relevant models, whereas recall evaluates the system’s capacity to retrieve all applicable models.
Distance metric based on weighted depth includes general structure of residual network, domain adaptation, inter-class adaptation of subdomain, intra-class adaptation of subdomain and filtering mechanism (DF). On the premise that the source domain and the target domain contain the same category, the residual network is used to learn and classify the invariant features of the samples in the source domain and the target domain. The loss is used to dynamically adjust the domain adaptation to increase the credibility of the pseudo-label and ensure that the samples are not too close to distinguish. On this basis, the intra-class and inter-class adaptation of subdomains are adopted to reduce the deviation of cross-domain conditional distribution, increase the inter-class distance of different categories and improve the classification accuracy.
Feature Information Extraction aims to divide the picture’s elements into various groups. Often, these subgroups consist of a single point, smooth curves, or a region. A variety of features typically describes the image. These features can be categorized using various criteria, including feature points, line features, and regional characteristics, depending on how they are represented in the image data. The depth recovery method relies on the scaling-composite scaling factor range of the picture to retrieve the desired data from the concept based on the attribute of the process and the information extraction of the targeted image in all domains. As shown by the following expression,
create Multiple blurred images using a synthesis weighting data model.
Where \(\sqrt{s}\) Is the image time-frequency composite’s normalized factor a weighting distance metric.
Map just one function to the continuous evening image of the moment aggregate scaled 0 and then execute a period composites heavy transform 2D function \(y\left(t\right)\) of the velocity and rhythm shift \(a\) and \(b\) , as illustrated above.
changing the source picture’s attribute \(f\left(t\right)\) By rephrasing the sentence to produce a variable’s time scale and time shift \(a\,and\,b\) .
Create a multi-frame period composite weighting signal form for the fuzzy image.
With the condition of \(rect\left(t\right)=1\) and \(\left|t\right|\le \frac{1}{2}\)
Signal of the period aggregate scaled inter fuzzy picture modulation law is a hyperbolic function.
Where \({f}_{0}\) represents the frequency of the central arithmetic value.
Therefore, the time-frequency composites weighting algorithm can better achieve the extraction technique of image features than the conventional time domain.
From Fig. 3 , the analysis of image information attributes stored in Low-level feature representation, which provides the basic details, is created by knowledge specialists and is frequently constructed by the channel colors or shape signals of data. RS photography consists of various features than original photos. The feature part is the basic building block despite being one of the most basic features, the spectral quality. It represents the reflectivity of the relevant regions for the environment factor by coding the important details.

The framework of CFNN-based FIE of image retrieved from RS
The color feature is the visual element that is most frequently employed in picture retrieval. The color feature is reasonably resistant to background issues. In a 3D image, every aspect can be used as a point. Color spaces that are frequently used are RGB. The R, G, denotes a color, and B is in the RGB color space, where R means the brightness of the red component, G indicates the strength of the green piece, and B is the sharpness of the blue part. The HSV color model characterizes colors according to their brightness and hues (Luminance). This model presents the link between colors in a more understandable manner. A color model describes a reference frame and a space inside it where every other color is represented by just one point. The below formula can be used to convert a pixel’s RGB representation into its HSV values:
Value is represented as \(V\) , which defines the strength of the color. \(S\) represents the saturation levels of the colors presented in the image. Users’ linguistically based queries can be answered using the Color moment. The color histogram is a common color feature employed in numerous picture retrieval systems. The color histogram is resistant to rotation and slow changes in the angle of vision, occlusion, perspective axis, and size.
FIE-Texture
Another aspect of a picture that is used in computer vision and pattern recognition is texture. A recurring pattern of one or more parts in various relative spatial places creates texture, described as the surface structure. The repetition includes regional changes in the elements’ scale, position, or other geometrical and visual characteristics. The capacity to match texture similarity is frequently helpful in identifying between portions of photos with similar hues. Alternative techniques for retrieving textures include using Haar wave-lets transforms. The comparative brightness of particular pixel pairs from each image. Calculations can be made to determine their degree of difference, hardness, positional precision, consistency, regularity, directional cues, and unpredictableness.
Haar-based wave-lets transformation
Wavelet transforms offer a multiresolution method for classifying and analyzing textures. A function is represented by the wavelet transform as a combination of a wavelet family of fundamental operations. A two-dimensional image’s wavelet change calculation also uses recursive filtering and subsampling as part of its multiresolution method. The idea is divided into four frequency sub-bands at each level: LL, LH, HL, and HH, where \(L\) stands for low frequency and \(H\) for high frequency.
The first part of the \(X\) -element array is used to record the average, and the second half is used to store the coefficients. The standards serve as the data source for the wavelet calculation’s subsequent phase. \(i\) defines the individual element of the resolution presented in the image, and \(a\) represents the feature vector of an image queried. The data set’s odd and even elements can be used to produce an average and a wavelet coefficient using the Haar equations.
Hierarchical layered & similarity indexing system with medical imaging AI systems and big data analytics
From the previously mentioned FIE, the color and texture attributes of the image are calculated. Image delineation is only one factor in a CBIE’s effectiveness; feature indexing and a similarity measurement matrix are also crucial for facilitating query execution. Generally, a feature index refers to an organizational database framework that facilitates quick Retrieval. To address the problems with knowledge discovery on a large-scale dataset, it is still possible to get data from small data sets by comparing the striking similarities between such a seek and each photo in the dataset. The database indexing that has been introduced intends to organize and structure the picture database into a straightforward but efficient type of data groupings and hierarchies. This study’s methodology is substantially different. Based on the mean frequencies of the cluster centers, data groups at higher layers reflect one or more groups at a lower layer in hierarchically layered data clusters. The first layer of image clusters is generated based on feature representations calculated from the Neural Network model. Data cluster groups are formed by combining the related data points using a partition-based clustering approach in CFNN, even if the idea of optimizing Retrieval by constructing hierarchical structures has been considered.
In Fig. 4 above, the hierarchical and similarity indexing system for image information extraction is organized based on the below-mentioned equations. The relative localized density concerning the query picture, defined by an appropriate kernel range between the present individual frame and all of the other images within the cluster, measures the level of similarity between both the query image and images inside each collection:
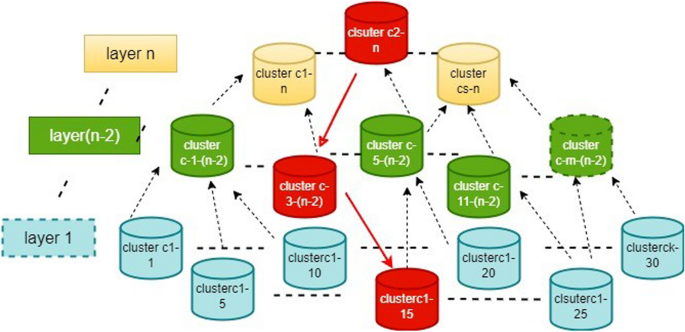
The Hierarchical Layered & Similarity Indexing System with medical imaging AI systems and big data analytics
Where \({M}^{c}\) Are the associated images count with the cluster \({c}^{n}\) , the distance between the image queried and the real picture of the particular \({c}^{th}\) cluster is represented by \({{d}_{ij}}^{C}.\) Total number of images extracted in the \({c}^{th}\) cluster is denoted by N. A hierarchical nesting can utilize a variety of distance measurements, including Euclidean and Cosine distances. By using a kernel of the Cauchy type to specify the local density \({D}_{c}^{i}\) . It is demonstrable that the Cauchy kind kernel can be calculated but asymptotically leads to Gaussian.
Where \(F=\{{f}_{1},{f}_{2},{f}_{3},\dots .{f}_{2048}\}\) is the feature vector. The Mean Value of a similar image is represented by \({\mu }_{i}\) . Scalar product is mentioned by the \({X}_{i}\) Need to be updated recursively.
Concerning the query image, the cluster with the highest local density \({D}^{c}\) . It is most likely to include related images.
In Eq. 19 above, \({C}_{i}^{*}\) Represents the overall city block distance of the particular cluster in a group of related images. The query image is compared to every shot in the powerful team at the lowest layer in the last step. The significance score is calculated using the City Block distance for distance-based grading. The scores they earned are then used to decide the order of the photographs. The relevant and query images are more comparable when the City Block distance is shorter and vice versa.
Experimental results
Since Synthetic Aperture Radar (SAR) imaging systems are not impacted by weather and can thus be utilized both at night and throughout the day., Remote Sensing (RS) of the environment research. It has examined their advantages for various land and maritime applications. It is necessary to test and validate image processing methods on actual and fake images when designing them for synthetic aperture radar applications. The design and development of algorithms to cope with SAR data are supported by benchmark databases from https://ieee-dataport.org/ . As a result, it offers and finances experiments for regional, national, and international geoscience and remote sensing contests [ 25 ]. The technique is shown to restore synthetic aperture radar with good accuracy. Use domain-specific datasets to train and test neural network models to optimize them for CBIE. The question of whether such networks can be employed as an overall image feature extractor is uncertain. Focusing on new optimization techniques for Synthetic Aperture Radar (SAR) images.
Pritpal Singh et al. proposed a new algorithm based on the mixture of FFQOA and CNN in 2023, which is called FFQoA ConnectTwo. In this algorithm, FFQOA searches for the optimal weights associated with layers by simultaneously achieving the minimum classification error. The application of FFQOAconNetwork in image classification is demonstrated by using benchmark data sets. The empirical analysis shows that compared with other algorithms, FFQOAconNetwork can effectively solve the MOOP problem [ 26 ]. The main purpose of FFQOAK proposed by Pritpal Singh et al. in 2021 is to segment the CT scan image of the chest, so that the infected area can be accurately detected. The proposed method is verified by using different chest CT scan images of COVID-19 patients [ 27 , 28 ].
Simultaneous parameter estimation and model selection are used to illustrate how the model selection process works on textured and untextured areas of the image; the flying image was chosen. In contrast to the previous examples, the attributes of more than one model are no longer dominant in the reconstructed image. Instead, a greater diversity of visual elements can be successfully described and rebuilt using many models. The findings of the studies examine how various factors impact retrieval efficiency.
The report provides a list of assessment methods and is available to the public statistics. To facilitate assessing the RS features extracted quantitatively, the coverage- ratio and Average Mean Precision values obtained after the remote sensing image database underwent 180 trials with the following settings: with the I ranges from \(\{\text{1,2},\text{3,4},\text{5,10,20}\}\) samples.
As shown in Fig. 5 , with the FIE methods’ help, the image information extraction outcomes using the Color and texture attribute in the Average Mean precision-to-recall ratio are calculated from Eq. 6 . Precision is inversely correlated with recall. Precision tells us how well our CFNN model predicts a specific image feature information FIE-color and FIE-texture. The horizontal x-axis recall measure gives quantitative information about our CFNN model’s ability to detect a particular feature, like the color or texture of an image. Modeling of moment aggregate scaled information for several hazy images is obtained from Eq. 7 . CFNN-based FIE - Color images feature vector characteristics are analyzed from Eqs. 12 & 13 . At the same time, the FIE-Texture information of queried images is calculated from Eq. 14 .
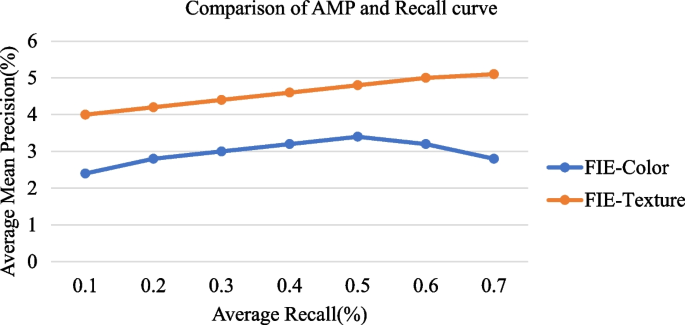
Difference between the AMP and recall graph for CFNN-based FIE
Figure 6 shows the fastest retrieval performance attained using the suggested hierarchical indexing strategy based on the CFNN method. Compared to conventional Classifier-based Retrieval of image information extraction, Content-Based Remote Sensing integrated with CFNN achieves a fast retrieval speed of image information from a database.
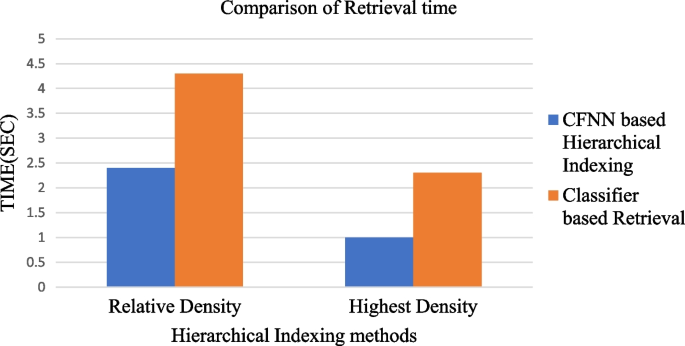
Comparison of retrieval time using Hierarchical Indexing System based on CFNN with CR
The method Relative Local Density value of an image is retrieved from Eqs. 15 and 17 . Similarly, the Highest Local Density method accesses image information from Eqs. 18 & 19 . As a result, computing similarity for every image in the stored database requires additional time. The conventional CR method poses a larger retrieval time than our proposed CFNN integrated with a hierarchical indexing system. The proposed method takes only 1 s to retrieve image information from the queried database.
From the above Fig. 7 , the Comparison between CFNN-FCMIE and CR based on Distance Metric for an Image Information Extraction is depicted from the freely available RS image dataset mentioned in [ 25 ]. The Distance Metric identified from the number of image samples retrieved from the database is analyzed by Eqs. 4 and 5 . CFNN-FCMIE method shows the least distance weight matrix for an image sample collected. At the same time, existing Classifier-based Retrieval consumes a large amount of weighted distance.
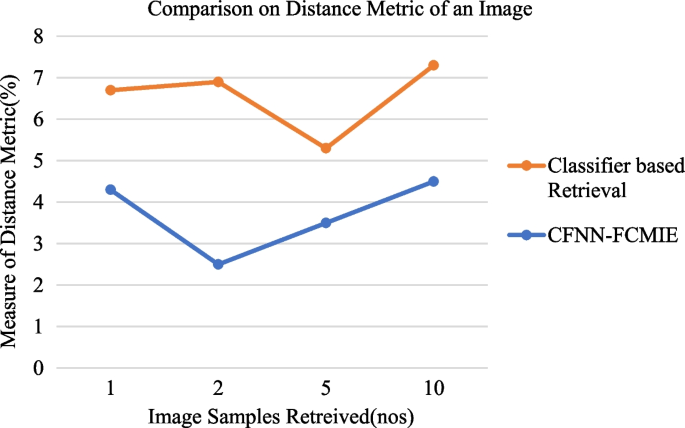
Comparison between CFNN-FCMIE and CR based on distance metric for image information
The below-depicted Fig. 8 represents the Comparison between the Evaluation metrics of sample image information extraction based on the Membership function with various input variables of an image retrieved from [ 25 ]. The horizontal values represent the evaluation metrics like the feature vector of an image sample, the sample mean representation, and the precision-recall value of the information retrieved. These values are calculated from Eqs. 1 and 2 .
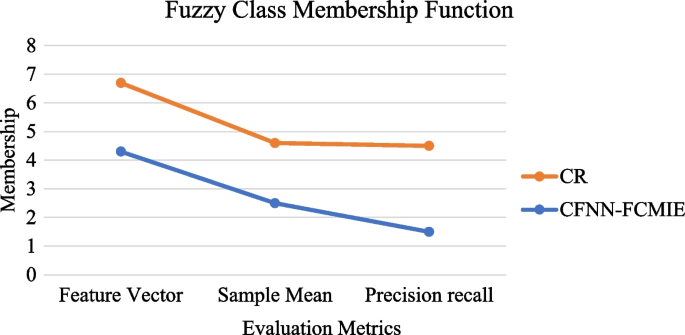
Comparison between the Evaluation metrics of sample image information extraction based on Membership
In Fig. 8 above, the conventional CR method poses the highest functional output ranges, approximately 7% of all three metrics, which is unsuitable for efficient image information extraction. The performance of the Compensated Fuzzy Neural Network(CFNN) used to extract visual information is correlated with the graph structure of the membership function. After image information extraction during training, the functional lines are displayed by the membership functions of the input variables a and b. The graphic shows how the input variable’s membership function’s center and width have altered due to image information extraction. The functional membership values are changed for each parameter based on the three different evaluation metrics. The proposed method achieves a minimal value range of 4–5% for all three metrics compared to what has been acquired from Eq. 3 .
Medical imaging AI systems and big data analytics have attracted much attention from researchers of industry and academia. The application of medical imaging AI systems and big data analytics play an important role in the technology of content based remote sensing (CBRS) development. For image information retrieval in CBIE, many color and texture aspects are investigated. Remote sensing can considerably improve retrieval performance regarding coverage ratio and Average Mean Precision. For image retrieval in CBIE, many color and texture aspects are analyzed. The proposed method CFNN-FCMIE achieves a minimal value range of 4–5% for all three feature vectors, the sample means, and the precision-recall rate of Comparison, which gives better results than the existing Classifier based Retrieval model. The strength of the CFNN-FCMIE method improves the retrieval performance of image information effectively, and a hierarchical structure was used in the model’s construction; hence it is scalable. So, a dynamic image dataset can be hierarchically handled via feature indexing, and integration is straightforward. The future enhancement is to retain the computational effectiveness of the querying process while preserving multi-dimensional and highly discriminative image representations produced by the CFNN model integrated with the RS field. The work serves an important reference to medical imaging AI systems and big data analytics. Accuracy is inversely proportional to recall. Accuracy tells us the effect of CFNN model in predicting five colors and five textures of specific image feature information. The retrieval time of CFNN integrated with hierarchical indexing system is shortened to 1 s. The method in this paper achieves a minimum range of 4–5% for all three metrics.
Availability of data and materials
The figures used to support the findings of this study are included in the article.
Ansper A. Retrieval of chlorophyll a from Sentinel-2 MSI data for the European Union water framework directive reporting purposes. Remote Sens. 2018;11(1):64.
Article Google Scholar
Huang K, Li G, Wang J. Rapid retrieval strategy for massive remote sensing metadata based on GeoHash coding. Remote Sens Lett. 2018;9(11):1070–8.
Li Y, Ma J, Zhang Y. Image retrieval from remote sensing big data: a survey. Inform Fusion. 2021;67:94–115.
Song W, Gao Z, Dian R, Ghamisi P, Zhang Y, Jón AB. Asymmetric hash code learning for remote sensingimage retrieval. IEEE Trans Geosci Remote Sens. 2022;60:1–14.
CAS Google Scholar
Zhang J. Automatic detection method of technical and tactical indicators for table tennis based on trajectory prediction using compensation fuzzy neural network. Comput Intell Neurosci. 2021;2021:3155357.
PubMed PubMed Central Google Scholar
Tang X, Yang Y, Ma J, Cheung Y-M, Liu C, Liu F, Zhang X, Jiao L. Meta-hashing for remote sensing image retrieval. IEEE Trans Geosci Remote Sens. 2021;60:1–19.
Google Scholar
Ma C, Xia W, Chen F, Liu J, Dai Q, Jiang L, Duan J, Liu W. A content-based remote sensing image change information retrieval model. ISPRS Int J Geo-Information. 2017;6(10):310.
Naaz MDM. An enhanced approach for extraction of text from an image using fuzzy logic[J]. 2020.
Wu H, Fu K. a management of remote sensing big data base on standard metadata file and database management system. Int Arch Photogrammetry Remote Sensing Spatial Inform Sci. 2020;42:653–7.
Guo M, Liu H, Xu Y, Huang Y. Building extraction based on U-Net with an attention block and multiple losses. Remote Sens. 2020;12(9):1400.
Shiu Y, et al. Deep neural networks for automated detection of marine mammal species. J Sci Rep. 2020;10(1):1–12 The neural network has adaptive and self-learning capabilities that can accurately approximate nonlinear systems.
Liu C, Ma J, Tang X, Liu F, Zhang X, Licheng Jiao. Deep hash learning for remote sensing image retrieval. IEEE Trans Geosci Remote Sens. 2020;59(4):3420–43.
Muhamediyeva DT. Building and training a fuzzy neural model of data mining tasks. J Phys Confer Ser. 2022;2182(1):012024. IOP Publishing.
Shao Z, Pan Y, Diao C, Cai J. Cloud detection in remote sensing images based on multiscale features-convolutional neural network. IEEE Trans Geosci Remote Sens. 2019;57(6):4062–76.
Tang X, Zhang X, Liu F, Jiao L. Unsupervised deep feature learning for remotesensing image retrieval. Remote Sens. 2018;10:8.
Ghrabat MJ, Ma G, Maolood IY, Alresheedi SS, Abduljabbar ZA. An effective image retrieval based on optimized genetic algorithm utilized a novel SVM-based convolutional neural network classifier. Human-centric Comput Inform Sci. 2019;9(1):1–29.
Desai P, Pujari J, Sujatha C, Kamble A, Anusha Kambli. Hybrid Approach for Content-based image Retrieval using VGG16 Layered Architecture and SVM: an application of Deep Learning. SN Comput Sci. 2021;2(3):1–9.
Sezavar A, Farsi H, Mohamadzadeh S. A modified grasshopper optimization algorithm combined with CNN for content-based image retrieval. Int J Eng. 2019;32(7):924–30.
Ghrabat MJ, Ma G, Abduljabbar ZA, Al Sibahee MA, Jassim SJ. Greedy learning of deep Boltzmann machine (GDBM) ‘s variance and search algorithm for efficient image retrieval. IEEE Access. 2019;7:169142–59.
Du G, Liang Y, Gao BY, Otaibi SA, Li D. A cognitive, joint angle compensation system based on a self-feedback fuzzy neural network with incremental learning. IEEE Trans Industr Inf. 2020;17(4):2928–37.
Chen J, Li Y, Luna LP, Hyun W, Chung SP, Rowe Y, Du LB, Solnes, Eric C. Frey. Learning fuzzy clustering for SPECT/CT segmentation via convolutional neural networks. Med Phys. 2021;48(7):3860–77.
Article PubMed Google Scholar
Chen X. Compensated fuzzy neural network-based music teaching ability assessment model. Comput Intell Neurosci. 2021;2021:3865190.
Article PubMed PubMed Central Google Scholar
Ai M, Xie Y, Xie S, Li F. Data-driven-based adaptive fuzzy neural network control for the antimony flotation plant. J Franklin Inst. 2019;356(12):5944–60.
Ye F, Xiao H, Zhao X, Dong M, Luo W, Min W. Remote sensing image retrieval using convolutional neural network features and weighted distance. IEEE Geosci Remote Sens Lett. 2018;15(10):1535–9.
Nobre R, Rodrigues A, Rosa R, Medeiros F, Feitosa R, Estevão A, Barros A. GRSS SAR/PolSAR DATABASE. https://doi.org/10.21227/H28W2J .
Singh P, Muchahari MK. Solving multi-objective optimization problem of convolutional neural network using fast forward quantum optimization algorithm: Application in digital image classification. Adv Eng Software. 2023;176:103370. ISSN 0965–9978.
Singh P, Bose SS. A quantum-clustering optimization method for COVID-19 CT scan image segmentation. Expert Syst Appl. 2021;185:115637. ISSN 0957–4174.
Singh P, Bose SS. Ambiguous D-means fusion clustering algorithm based on ambiguous set theory: special application in clustering of CT scan images of COVID-19. Knowl Based Syst. 2021;231:107432. ISSN 0950–7051.
Download references
Acknowledgements
The authors would like to show sincere thanks to those techniques who have contributed to this research.
This work was not supported by any funds.
Author information
Authors and affiliations.
Yellow River Conservancy Technical Institute, Kaifeng, Henan, 475001, China
Rui Sun & Xiaohang Niu
China University of Mining and Technology, Xuzhou, China
Guangdong Nuclear Industry Geology Bureau Surveying and Mapping Institute, Guangzhou, Guangdong, 510800, China
Zhengyin Zhang
POWERCHINA HARBOUR Co.,LTD, Tianjin, 300450, China
School of Information Engineering, Minzu University of China, Beijing, 100081, China
You can also search for this author in PubMed Google Scholar
Contributions
Rui Sun and Zhengyin Zhang contributed to supervision. Yajun Liu and Xiaohang Niu contributed to validation data curation, project administration, software, supervision, validation, and visualization. Jie Yuan contributed to formal analysis. Shekhar Boers contributed to investigation. Rui Sun contributed to writing—review and editing. All authors have read and agreed to the published version of the manuscript.
Corresponding author
Correspondence to Zhengyin Zhang .
Ethics declarations
Ethics approval and consent to participate, consent for publication, competing interests.
The authors declare no competing interests.
Additional information
Publisher’s note.
Springer Nature remains neutral with regard to jurisdictional claims in published maps and institutional affiliations.
Rights and permissions
Open Access This article is licensed under a Creative Commons Attribution 4.0 International License, which permits use, sharing, adaptation, distribution and reproduction in any medium or format, as long as you give appropriate credit to the original author(s) and the source, provide a link to the Creative Commons licence, and indicate if changes were made. The images or other third party material in this article are included in the article's Creative Commons licence, unless indicated otherwise in a credit line to the material. If material is not included in the article's Creative Commons licence and your intended use is not permitted by statutory regulation or exceeds the permitted use, you will need to obtain permission directly from the copyright holder. To view a copy of this licence, visit http://creativecommons.org/licenses/by/4.0/ . The Creative Commons Public Domain Dedication waiver ( http://creativecommons.org/publicdomain/zero/1.0/ ) applies to the data made available in this article, unless otherwise stated in a credit line to the data.
Reprints and permissions
About this article
Cite this article.
Sun, R., Zhang, Z., Liu, Y. et al. Remote sensing image information extraction based on Compensated Fuzzy Neural Network and big data analytics. BMC Med Imaging 24 , 86 (2024). https://doi.org/10.1186/s12880-024-01266-9
Download citation
Received : 08 January 2024
Accepted : 30 March 2024
Published : 10 April 2024
DOI : https://doi.org/10.1186/s12880-024-01266-9
Share this article
Anyone you share the following link with will be able to read this content:
Sorry, a shareable link is not currently available for this article.
Provided by the Springer Nature SharedIt content-sharing initiative
- Information retrieval
- Feature information extraction
- Remote sensing
- Fuzzy logic
- Medical imaging AI systems
- Big data analytics
BMC Medical Imaging
ISSN: 1471-2342
- General enquiries: [email protected]
Information retrieval: a view from the Chinese IR community
- Review Article
- Published: 29 September 2020
- Volume 15 , article number 151601 , ( 2021 )
Cite this article
- Zhumin Chen 1 ,
- Xueqi Cheng 2 ,
- Shoubin Dong 3 ,
- Zhicheng Dou 4 ,
- Jiafeng Guo 2 ,
- Xuanjing Huang 5 ,
- Yanyan Lan 2 ,
- Chenliang Li 6 ,
- Tie-Yan Liu 8 ,
- Yiqun Liu 9 ,
- Bing Qin 10 ,
- Mingwen Wang 11 ,
- Jirong Wen 4 ,
- Min Zhang 9 ,
- Peng Zhang 12 &
- Qi Zhang 5
182 Accesses
9 Citations
Explore all metrics
During a two-day strategic workshop in February 2018, 22 information retrieval researchers met to discuss the future challenges and opportunities within the field. The outcome is a list of potential research directions, project ideas, and challenges. This report describes the major conclusions we have obtained during the workshop. A key result is that we need to open our mind to embrace a broader IR field by rethink the definition of information, retrieval, user, system, and evaluation of IR. By providing detailed discussions on these topics, this report is expected to inspire our IR researchers in both academia and industry, and help the future growth of the IR research community.
This is a preview of subscription content, log in via an institution to check access.
Access this article
Price includes VAT (Russian Federation)
Instant access to the full article PDF.
Rent this article via DeepDyve
Institutional subscriptions
Similar content being viewed by others
Industry Day Overview
Building a Common Framework for IIR Evaluation
2AIRTC: The Amharic Adhoc Information Retrieval Test Collection
Bush V. As we may think. The Atlantic Monthly, 1945, 176(1): 101–108
Google Scholar
Clarke C. From the chair… ACM SIGIR Forum, 2016, 50(1): 1
Zobel J, Moffat A. Inverted files for text search engines. ACM Computing Surveys (CSUR), 2006, 38(2): 6
Article Google Scholar
Salton G, Wong A, Yang C S. A vector space model for automatic indexing. Communications of the ACM, 1975, 18(11): 613–620
Robertson S, Zaragoza H. The probabilistic relevance framework: Bm25 and beyond. Foundations and Trends® in Information Retrieval, 2009, 3(4): 333–389
Lv Y, Zhai C. Positional language models for information retrieval. In: Proceedings of the 32nd International ACM SIGIR Conference on Research and Development in Information Retrieval. 2009, 299–306
Zhai C, Lafferty J. A study of smoothing methods for language models applied to ad hoc information retrieval. ACM SIGIR Forum, 2017, 51(2): 268–276
Page L, Brin S, Motwani R, Winograd T. The pagerank citation ranking: bringing order to the web. Technical Report, Stanford InfoLab, 1999
Kleinberg J M. Authoritative sources in a hyperlinked environment. Journal of the ACM, 1999, 46(5): 604–632
Article MathSciNet Google Scholar
Chen C P, Zhang C Y. Data-intensive applications, challenges, techniques and technologies: a survey on big data. Information Sciences, 2014, 275: 314–347
Sanderson M, Croft W B. The history of information retrieval research. Proceedings of the IEEE, 2012, 100 (Special Centennial Issue): 1444–1451
Chaudhuri S, Dayal U. An overview of data warehousing and olap technology. ACM Sigmod Record, 1997, 26(1): 65–74
Borlund P. The IIR evaluation model: a framework for evaluation of interactive information retrieval systems. Information Research, 2003, 8(3): 289–291
Hinton G, Deng L, Yu D, Dahl G, Mohamed A R, Jaitly N, Senior A, Vanhoucke V, Nguyen P, Kingsbury B. Deep neural networks for acoustic modeling in speech recognition. IEEE Signal Processing Magazine, 2012, 29(6): 82–97
LeCun Y, Bengio Y. Convolutional networks for images, speech, and time series. The Handbook of Brain Theory and Neural Networks, 1995, 3361(10): 1995
Socher R, Huang E H, Pennin J, Manning C D, Ng A Y. Dynamic pooling and unfolding recursive autoencoders for paraphrase detection. In: Proceedings of Advances in Neural Information Processing Systems. 2011, 801–809
Craswell N, Croft W B, Guo J, Mitra B, de Rijke M. Neu-IR: the SIGIR 2016 workshop on neural information retrieval. In: Proceedings of the 39th International ACM SIGIR Conference on Research and Development in Information Retrieval. 2016, 1245–1246
Craswell N, Croft W B, de Rijke M, Guo J, Mitra B. SIGIR 2017 workshop on neural information retrieval (Neu-Ir’17). In: Proceedings of the 40th International ACM SIGIR Conference on Research and Development in Information Retrieval. 2017, 1431–1432
Goodfellow I, Pouget-Abadie J, Mirza M, Xu B, Warde-Farley D, Ozair S, Courville A, Bengio Y. Generative adversarial nets. In: Proceedings of Advances in Neural Information Processing Systems. 2014, 2672–2680
Mnih V, Kavukcuoglu K, Silver D, Rusu A A, Veness J, Bellemare M G, Graves A, Riedmiller M, Fidjeland A K, Ostrovski G, Petersen S, Beattie C, Sadik A, Antonoglou I, King H, Kumaran D, Wierstra D, Legg S, Hassabis D. Human-level control through deep reinforcement learning. Nature, 2015, 518(7540): 529–533
Silver D, Schrittwieser J, Simonyan K, Antonoglou I, Huang A, Guez A, Hubert T, Baker L, Lai M, Bolton A, Chen Y, Lillicrap T, Hui F, Sifre L, Driessche G V D, Graepel T, Hassabis D. Mastering the game of go without human knowledge. Nature, 2017, 550(7676): 354
Wang J, Yu L, Zhang W, Gong Y, Xu Y, Wang B, Zhang P, Zhang D. Irgan: a minimax game forunifying generative and discriminative information retrieval models. In: Proceedings of the 40th International ACM SIGIR Conference on Research and Development in Information Retrieval. 2017, 515–524
Agichtein E, Brill E, Dumais S. Improving web search ranking by incorporating user behavior information. In: Proceedings of the 29th Annual International ACM SIGIR Conference on Research and Development in Information Retrieval. 2006, 19–26
Granka L A, Joachims T, Gay G. Eye-tracking analysis of user behavior in www search. In: Proceedings of the 27th Annual International ACM SIGIR Conference on Research and Development in Information Retrieval. 2004, 478–479
Morris M R, Teevan J, Panovich K. What do people ask their social networks, and why?: a survey study of status message q&a behavior. In: Proceedings of the SIGCHI Conference on Human Factors in Computing Systems. 2010, 1739–1748
Croft W B, Cronen-Townsend S, Lavrenko V. Relevance feedback and personalization: a language modeling perspective. In: Proceedings of the 2nd DELOS Network of Excellence Workshop on Personalisation and Recommender Systems in Digital Libraries. 2001
Thomee B, Lew M S. Interactive search in image retrieval: a survey. International Journal of Multimedia Information Retrieval, 2012, 1(2): 71–86
Said A, Jain B J, Narr S, Plumbaum T. Users and noise: the magic barrier of recommender systems. In: Proceedings of International Conference on User Modeling, Adaptation, and Personalization. 2012, 237–248
Swan M. Blockchain: Blueprint for a New Economy. O’Reilly Media, Inc., 2015
Akyildiz I F, Akan Ö B, Chen C, Fang J, Su W. Interplanetary internet: state-of-the-art and research challenges. Computer Networks, 2003, 43(2): 75–112
Lavanya B M. Blockchain technology beyond bitcoin: an overview. International Journal of Computer Science and Mobile Applications, 2018, 6(1): 76–80
Seebacher S, Schüritz R. Blockchain technology as an enabler of service systems: a structured literature review. In: Proceedings of International Conference on Exploring Services Science. 2017, 12–23
Croft W B, Metzler D, Strohman T. Search Engines: Information Retrieval in Practice. Addison-Wesley Reading, 2010
Voorhees E M, Harman D K. TREC: Experiment and Evaluation in Information Retrieval. Cambridge: MIT Press, 2005
Kelly D. Methods for evaluating interactive information retrieval systems with users. Foundations and Trends®R in Information Retrieval, 2009, 3(1–2): 1–224
Ellis D. Theory and explanation in information retrieval research. Journal of Information Science, 1984, 8(1): 25–38
Vakkari P, Järvelin K. Explanation in information seeking and retrieval. New Directions in Cognitive Information Retrieval, 2006, 19: 113–138
Singh J, Anand A. EXS: explainable search using local model agnostic interpretability. In: Proceedings of the 12th ACM International Conference on Web Search and Data Mining. 2019, 770–773
Luo G, Tang C, Yang H, Wei X. Medsearch: a specialized search engine for medical information retrieval. In: Proceedings of the 17th ACM Conference on Information and Knowledge Management. 2008, 143–152
Huang P S, He X, Gao J, Deng L, Acero A, Heck L. Learning deep structured semantic models for Web search using clickthrough data. In: Proceedings of the 22nd ACM International Conference on Information & Knowledge Management. 2013, 2333–2338
Guo J, Fan Y, Ai Q, Croft W B. A deep relevance matching model for ad-hoc retrieval. In: Proceedings of the 25th ACM International on Conference on Information and Knowledge Management. 2016, 55–64
Zhang Y, Rahman M M, Braylan A, Dang B, Chang H L, Kim H, Mc-Namara Q, Angert A, Banner E, Khetan V, McDonnell T, Nguyen A T, Xu D, Wallace B C, Leasey M. Neural information retrieval: a literature review. 2016, arXiv preprint arXiv:1611.06792
Mitra B, Craswell N. Neural models for information retrieval. 2017, arXiv preprint arXiv:1705.01509
Guo J, Fan Y, Pang L, Yang L, Ai Q, Zamani H, Wu C, Croft W B, Cheng X. A deep look into neural ranking models for information retrieval. 2019, arXiv preprint arXiv:1903.06902
Sharma D, Kumar S, Kholia C. Multi-modal information retrieval system. US Patent 7,054,818, 2006
Lee D, Park J, Ahn J H. On the explanation of factors affecting ecommerce adoption. In: Proceedings of the International Conference on Information Systems. 2001, 109–120
Jamali M, Ester M. A matrix factorization technique with trust propagation for recommendation in social networks. In: Proceedings of the 4th ACM Conference on Recommender Systems. 2010, 135–142
Callison-Burch C. Fast, cheap, and creative: evaluating translation quality using amazon’s mechanical turk. In: Proceedings of the 2009 Conference on Empirical Methods in Natural Language Processing. 2009, 286–295
Gubbi J, Buyya R, Marusic S, Palaniswami M. Internet of Things (IoT): a vision, architectural elements, and future directions. Future Generation Computer Systems, 2013, 29(7): 1645–1660
Abadi M, Barham P, Chen J, Chen Z, Davis A, Dean J, Devin M, Ghemawat S, Irving G, Isard M, Kudlur M, Levenberg J, Monga R, Moore S, Murray D G, Steiner B, Tucker P, Vasudevan V, Warden P, Wicke M, Yu Y, Zheng X. Tensorflow: a system for large-scale machine learning. In: Proceedings of the 12th USENIX Symposium on Operating Systems Design and Implementation. 2016, 265–283
Jia Y, Shelhamer E, Donahue J, Karayev S, Long J, Girshick R, Guadarrama S, Darrell T. Caffe: convolutional architecture for fast feature embedding. In: Proceedings of the 22nd ACM International Conference on Multimedia. 2014, 675–678
Paszke A, Gross S, Chintala S, Chanan G. Pytorch: tensors and dynamic neural networks in python with strong GPU acceleration. 2017
McCandless M, Hatcher E, Gospodnetic O. Lucene in Action: Covers Apache Lucene 3.0. Greenwich, CT: Manning Publications Co., 2010
Download references
Acknowledgements
We would like to thank Chinese Information Processing Society of China, CAS Key Laboratory of Network Data Science and Technology, Institute of Computing Technology, Chinese Academy of Sciences, and ACM SIGIR Beijing Chapter for suporting the strategic workshop. Thank Professor Bo Zhang (Tsinghua University) and Ming Zhou (Microsoft Research Asia) for contributing the keynotes and valuable discussions in the workshop.
Author information
Authors and affiliations.
School of Computer Science and Technology, Shandong University, Jinan, 250100, China
Zhumin Chen & Jun Ma
Institute of Computing Technology, Chinese Academy of Sciences, Beijing, 100190, China
Xueqi Cheng, Jiafeng Guo & Yanyan Lan
School of Computer Science and Engineering, South China University of Technology, Guangzhou, 510006, China
Shoubin Dong
School of Information, Renmin University of China, Beijing, 100872, China
Zhicheng Dou, Jirong Wen & Jun Xu
School of Computer Science, Fudan University, Shanghai, 200433, China
Xuanjing Huang & Qi Zhang
School of Cyber Science and Engineering, Wuhan University, Wuhan, 430072, China
Chenliang Li
School of Big Data, Shanxi University, Taiyuan, 200433, China
Microsoft Research Asia, Beijing, 100080, China
Tie-Yan Liu
Department of Computer Science and Technology, Tsinghua University, Beijing, 100084, China
Yiqun Liu & Min Zhang
School of Computer Science and Technology, Harbin Institute of Technology, Harbin, 150001, China
School of Computer Information and Engineering, Jiangxi Normal University, Nanchang, 330022, China
Mingwen Wang
School of Computer Science and Technology, Tianjin University, Tianjin, 300072, China
You can also search for this author in PubMed Google Scholar
Corresponding authors
Correspondence to Jiafeng Guo , Yanyan Lan or Yiqun Liu .
Additional information
Zhumin Chen is an associate professor in School of Computer Science and Technology, Shandong University, China. His research interests include information retrieval and natural language processing. His research is supported by the Natural Science Fund of China, Key Science and Technology Innovation Project of Shandong Province, etc.
Xueqi Cheng is a full professor and vice director of the Institute of Computing Technology, Chinese Academy of Sciences (CAS), and the director of the CAS Key Laboratory of Network Data Science and Technology, China. His research areas include Web search and data mining, data science, and social media analytics. He is the general secretary of CCF Committee on Big Data, the vice-chair of CIPS Committee on Information Retrieval, the general co-chair of SIGIR’20 and WSDM’15. He is the principal investigator of more than 10 major research projects, funded by NSFC and MOST. He was awarded the NSFC Distinguished Youth Scientist (2014), the National Prize for Progress in Science and Technology (2012), the China Youth Science and Technology Award (2011).
Shoubin Dong received the PhD degree in electronic engineering from the University of Science and Technology of China (USTC), China in 1994. She was a visiting scholar at the School of Computer Science and Language Technology Institute, Carnegie Mellon University (CMU), Pittsburgh, USA from 2001 to 2002. She is now a professor with the School of Computer Science and Engineering, South China University of Technology (SCUT), China. Her research interests include information retrieval, natural language processing, high-performance computing, etc.
Zhicheng Dou is currently a professor at School of Information, Renmin University of China, China. He received his PhD and BS degrees in computer science and technology from the Nankai University, China in 2008 and 2003, respectively. He worked at Microsoft Research Asia from July 2008 to September 2014. His current research interests are information retrieval, natural language processing, and big data analytics.
Jiafeng Guo is a professor in Institute of Computing Technology, Chinese Academy of Sciences, and University of Chinese Academy of Sciences, China. He has worked on a number of topics related to web search and data mining. His current research is focused on representation learning and neural models for information retrieval and filtering. He has published more than 80 papers in several top conferences/journals. His work on IR has received the Best Paper Award in ACM CIKM (2011), Best Student Paper Award in ACM SIGIR (2012) and Best Full Paper Runner-up Award in ACM CIKM (2017). Moreover, he has served as the PC member for the prestigious conferences including SIGIR, WWW, KDD, WSDM, and ACL, and the associate editor of TOIS.
Xuanjing Huang is a professor of the School of Computer Science, Fudan University, China. Her research interest includes natural language processing, information retrieval, artificial intelligence, deep learning and data intensive computing. She has published more than 100 papers in major conferences including ACL, SIGIR, IJCAI, AAAI, NIPS, ICML, CIKM, EMNLP, WSDM, and COLING. In the research community, she served as the PC Co-Chair of CCL 2019, NLPCC 2017, CCL 2016, SMP 2015, and SMP 2014, the organizer of WSDM 2015, competition chair of CIKM 2014, tutorial chair of COLING 2010, SPC or PC member of past WSDM, SIGIR, WWW, CIKM, ACL, IJCAI, KDD, EMNLP, COLING, and many other conferences.
Yanyan Lan is a professor in Institute of Computing Technology, Chinese Academy of Sciences, China. She leads a research group working on Big Data and Machine Learning. Her current research interests include machine learning, information retrieval and natural language processing. From April 2018 to March 2019, she acted as a visiting scholar in the department of statistics, UC Berkeley. She has published over 70 papers on top conferences including ICML, NIPS, SIGIR, WWW, etc. Her paper entitled “Top-k Learning to Rank: Labeling, Ranking, and Evaluation” has won the Best Student Paper Award of SIGIR 2012, and the paper entitled “Learning Visual Features from Snapshots for Web Search” has won the Best Paper RunnerUp Award of CIKM2017.
Chenliang Li received PhD from Nanyang Technological University, Singapore in 2013. Currently, he is an associate professor at School of Cyber Science and Engineering, Wuhan University, China. His research interests include information retrieval, text/web mining, data mining and natural language processing. He is a co-recipient of Best Student Paper Award Honorable Mention in ACM SIGIR 2016, and serves as an editorial board member of JASIST and IPM.
Ru Li, Professor, PhD Supervisor. She is the Vicedean of the School of Computer and Information Technology, and the School of Big Data of Shanxi University, the standing council member of Chinese Information Processing Society (CIPS), the committee member of Computational Linguistics, Information Retrieval, and Language and Knowledge Computing of CIPS. Her research interests include Chinese information processing and information retrieval. She has published more than 70 papers in both international and national important academic journals and conferences, including in the IEEE Transactions on Knowledge and Data Engineering, the Annual Meeting of the Association for Computational Linguistics, and the International Conference on Computational Linguistics, and so on. She has won three Second Prize for scientific and technological progress in Shanxi.
Tie-Yan Liu, assistant managing director of Microsoft Research Asia, fellow of the IEEE, and distinguished member of the ACM. He is an adjunct professor at Carnegie Mellon University (CMU) and Tsinghua University. His research interest includes learning to rank, deep learning, reinforcement learning, and distributed learning. He has served as general chair, program committee chair, local chair, or area chair for a dozen of international conferences including WWW/WebConf, SIGIR, KDD, ICML, NIPS, IJCAI, AAAI, ACL, ICTIR, as well as associate editor of ACM Transactions on Information Systems, ACM Transactions on the Web, and Neurocomputing.
Yiqun Liu is professor and Department co-Chair at the Department of Computer Science and Technology in Tsinghua University, China. His major research interests are in Web search, user behavior analysis, and natural language processing. He also works as a visiting research professor of National University of Singapore and a visiting professor of National Institute of Informatics (NII) of Japan, as well as a member of Tiangong AI Research Center which is founded by Tsinghua and Sogou Inc.
Jun Ma received his BSc, MSc, and PhD degrees from Shandong University in China, Ibaraki University and Kyushu University in Japan, respectively. He is a full professor at Shandong University. He was a senior researcher in the Department of Computer Science at Ibaraki University in 1994 and German GMD and Fraunhofer from the year 1999 to 2003. His research interests include information retrieval, Web data mining, recommendation systems, and machine learning. He has published more than 150 papers in the international journals and conferences, including SIGIR, WWW, MM, TOIS, and TKDE. He is a member of the ACM and IEEE.
Bing Qin, a professor and doctoral supervisor of the School of Computer Science and Technology, at Harbin Institute of Technology, China. Her main research directions are natural language processing, information extraction, text mining, sentiment analysis, etc. She has published more than 80 papers in the several international top journals and conferences such as ACL, COLING, EMNLP, IEEE TKDE, IEEE TASLP, etc. She has leaded over several the National Natural Science Foundations of China, as well as the key research and development projects of the National Science and Technology Ministry. She was awarded the first prize of Qian Weichang Chinese Information Processing Science and Technology Award by the Chinese Information Processing Society and the second prize of Heilongjiang Province Technical Invention.
Mingwen Wang is currently a professor of Jiangxi Normal University, China. He received the BS (1985) and MS (1988) degrees in mathematics from Jiangxi Normal University, China, and the PhD (2000) degree in computer science from Shanghai Jiaotong University, China. His research interests include information retrieval, natural language processing, and machine learning.
Jirong Wen is a professor and the dean of School of Information, Renmin University of China (RUC), China. He is also the Executive Dean of Gaoling School of Artificial Intelligence, and Director of Beijing Key Laboratory of Big Data Research. He received his PhD degree in 1999 from the Institute of Computing Technology, the Chinese Academy of Science, China. His main research interests include information retrieval, data mining and machine learning. He worked at Microsoft Research Asia (MSRA) for 14 years and once was the group manager of the Web Search and Mining Group.
Jun Xu is a professor with the School of Information, Renmin University of China, China. His research interests include learning to rank and semantic matching in search. He has published more than 50 papers in international conferences (e.g., SIGIR, WSDM) and journals (e.g., ACM TOIS, IEEE TKDE). He has won the Best Paper Award in AIRS (2010), Best Paper Runner-up in CIKM (2017), and Test of Time Award Honorable Mention in SIGIR (2019). He is serving as SPC for SIGIR, WWW, AAAI, and ACML, editorial board member for JASIST, and associate editor for ACM TIST.
Min Zhang is a tenured associate professor in the Department of Computer Science & Technology, Tsinghua University, China, specializes in Web search and recommendation, and user modeling. She is the vice director of Intelligent Technology & Systems lab at CS Dept., and vice director of Intelligent Information Acquisition, AI Institute, Tsinghua University. She also serves as ACM SIGIR Executive Committee Member, Associate Editor for the ACM Transaction of Information Systems (TOIS), Web Mining and Content Analysis Track Chair of theWebConf 2020, Short Paper Chair of SIGIR 2018, Program Chair of WSDM 2017, etc. She also owns 12 patents.
Peng Zhang is an associate professor and Vice Dean of School of Computer Science and Technology, College of Computing and Intelligence, Tianjin University, China. He obtained his PhD at Robert Gordon University, United Kingdom in 2013. His research is focused on the tensor space language models, explainable neural network design and quantum theory inspired language models. He has published papers on refereed journals such as IEEE TNN, IEEE TKDE, ACM TIST, ACM TALIP, JASIST, IP&M, and on top conferences such as NeurIPS, SIGIR, ACL, AAAI, IJCAI, CIKM, WWW, and EMNLP. He won ECIR 2011 Best Poster Award and SIGIR 2017 Best Paper Award Honorable Mention.
Qi Zhang received the PhD degree in computer science from Fudan University, China. He is a professor of computer science at Fudan University, China. His research interests include natural language processingand information retrieval.
Electronic Supplementary Material
Rights and permissions.
Reprints and permissions
About this article
Chen, Z., Cheng, X., Dong, S. et al. Information retrieval: a view from the Chinese IR community. Front. Comput. Sci. 15 , 151601 (2021). https://doi.org/10.1007/s11704-020-9159-0
Download citation
Received : 12 March 2019
Accepted : 21 July 2019
Published : 29 September 2020
DOI : https://doi.org/10.1007/s11704-020-9159-0
Share this article
Anyone you share the following link with will be able to read this content:
Sorry, a shareable link is not currently available for this article.
Provided by the Springer Nature SharedIt content-sharing initiative
- information retrieval
- redefinition
- information
- scope of retrieval
- retrieval models
- system architecture
- Find a journal
- Publish with us
- Track your research

Maintenance work is planned for Wednesday 1st May 2024 from 9:00am to 11:00am (BST).
During this time, the performance of our website may be affected - searches may run slowly and some pages may be temporarily unavailable. If this happens, please try refreshing your web browser or try waiting two to three minutes before trying again.
We apologise for any inconvenience this might cause and thank you for your patience.
Materials Horizons
High-entropy materials for thermoelectric applications: towards performance and reliability.
High-entropy materials (HEMs), including alloys, ceramics and other entropy-stabilized compounds, have attracted considerable attention in different application fields. This is due to their intrinsically unique concept and properties, such as innovative chemical composition, structural characteristics, and correspondingly improved functional properties. By establishing an environment with different chemical compositions, HEMs present unparalleled prospects for novel materials possessing superior attributes when contrasted with conventional counterparts. Notably, there has been a pronounced emphasis on investigating HEMs, especially in energy-related fields such as thermoelectric (TE). In this review, we started with the basic definitions of TE fundamentals, the existing thermoelectric materials (TEMs), and the strategies adopted for their improvement. Moreover, we introduced HEMs, summarized the core effects of high-entropy (HE), and emphasized how HE will open new avenues for designing high-entropy thermoelectric materials (HETEMs) with promising performance and high reliability. Through selecting and analyzing recent scientific publications, this review outlines recent scientific breakthroughs and the associated challenges in the field of HEMs for TE applications. Finally, we classified the different types of HETEMs based on their structure and properties and discussed their recent advances in literature.
- This article is part of the themed collection: Recent Review Articles
Article information
Download citation, permissions.

N. OUELDNA, N. Sabi, H. Aziam, V. Trabadelo and H. Ben youcef, Mater. Horiz. , 2024, Accepted Manuscript , DOI: 10.1039/D3MH02181E
To request permission to reproduce material from this article, please go to the Copyright Clearance Center request page .
If you are an author contributing to an RSC publication, you do not need to request permission provided correct acknowledgement is given.
If you are the author of this article, you do not need to request permission to reproduce figures and diagrams provided correct acknowledgement is given. If you want to reproduce the whole article in a third-party publication (excluding your thesis/dissertation for which permission is not required) please go to the Copyright Clearance Center request page .
Read more about how to correctly acknowledge RSC content .
Social activity
Search articles by author.
This article has not yet been cited.
Advertisements
Britain decided not to list genomics as critical infrastructure, deputy PM says
- Medium Text
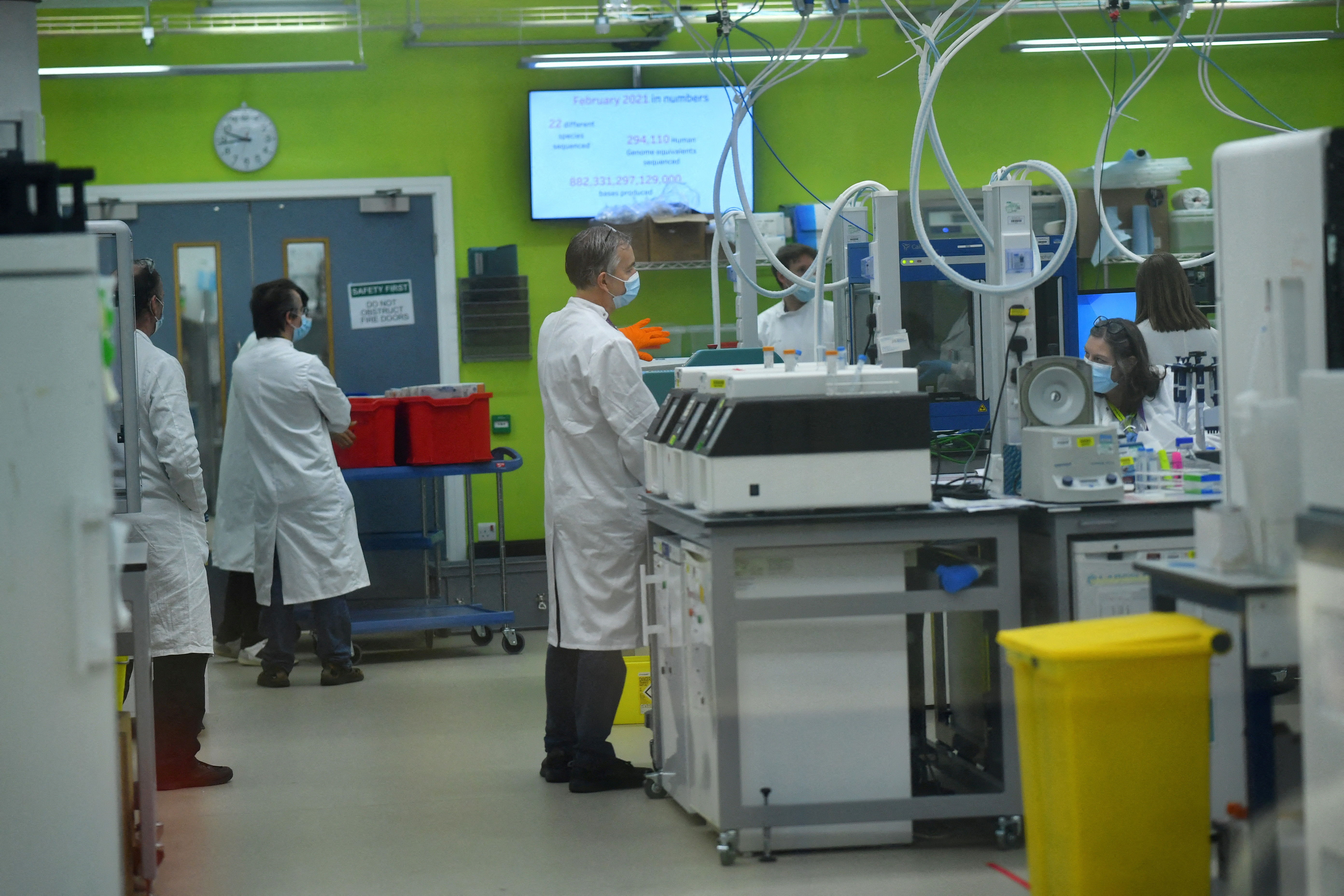
Coming soon: Get the latest news and expert analysis about the state of the global economy with Reuters Econ World. Sign up here.
Reporting by Alistair Smout; Editing by Sharon Singleton
Our Standards: The Thomson Reuters Trust Principles. New Tab , opens new tab
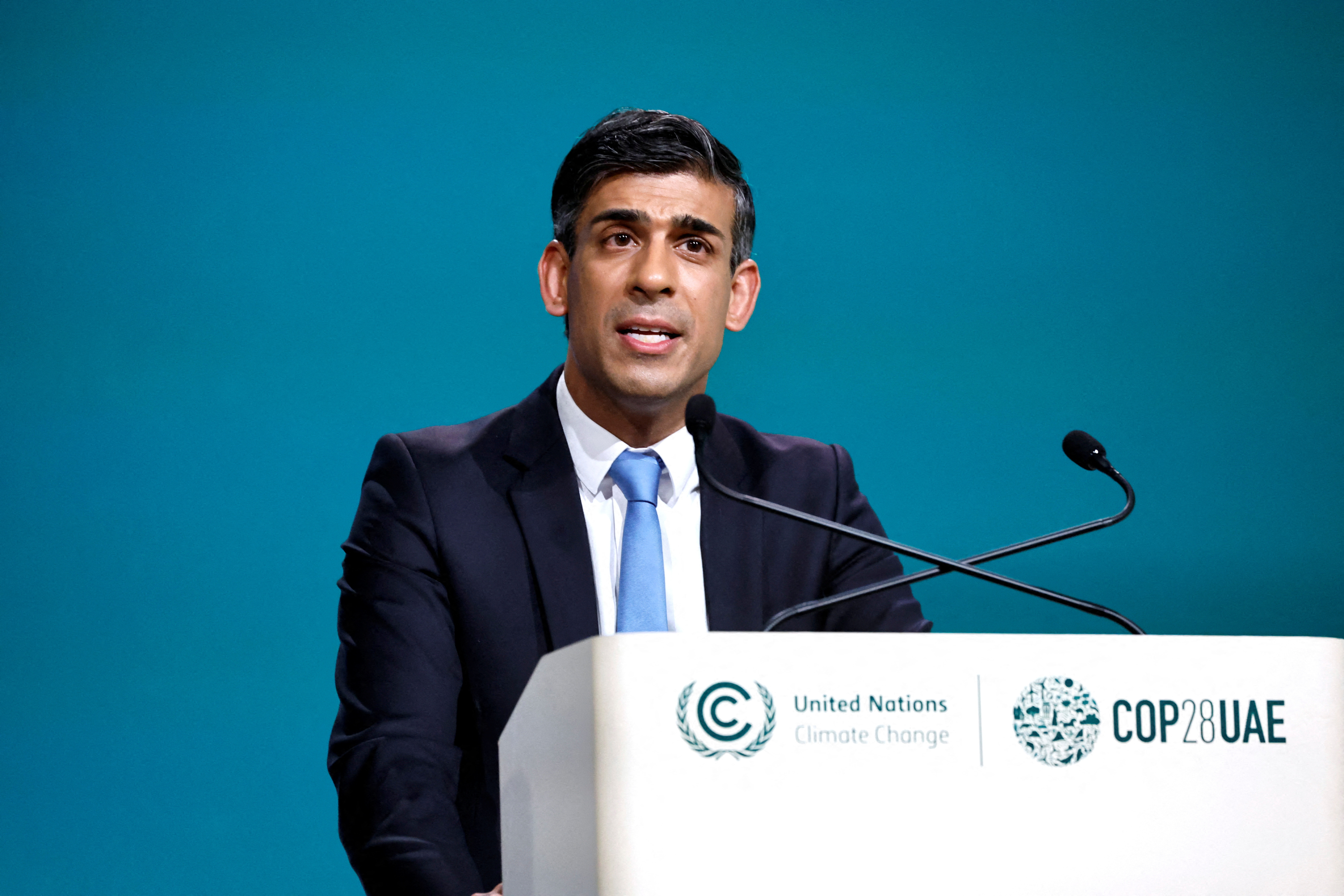
World Chevron
At least two people are dead and 14 others injured in a shooting at a block party in Memphis, Tennessee, on Saturday, according to police.
South Korea on Sunday protested Japanese Prime Minister Fumio Kishida's offering to Tokyo's Yasukuni Shrine with "deep disappointment" and urged Japanese leaders to show repentance for the country's wartime past.
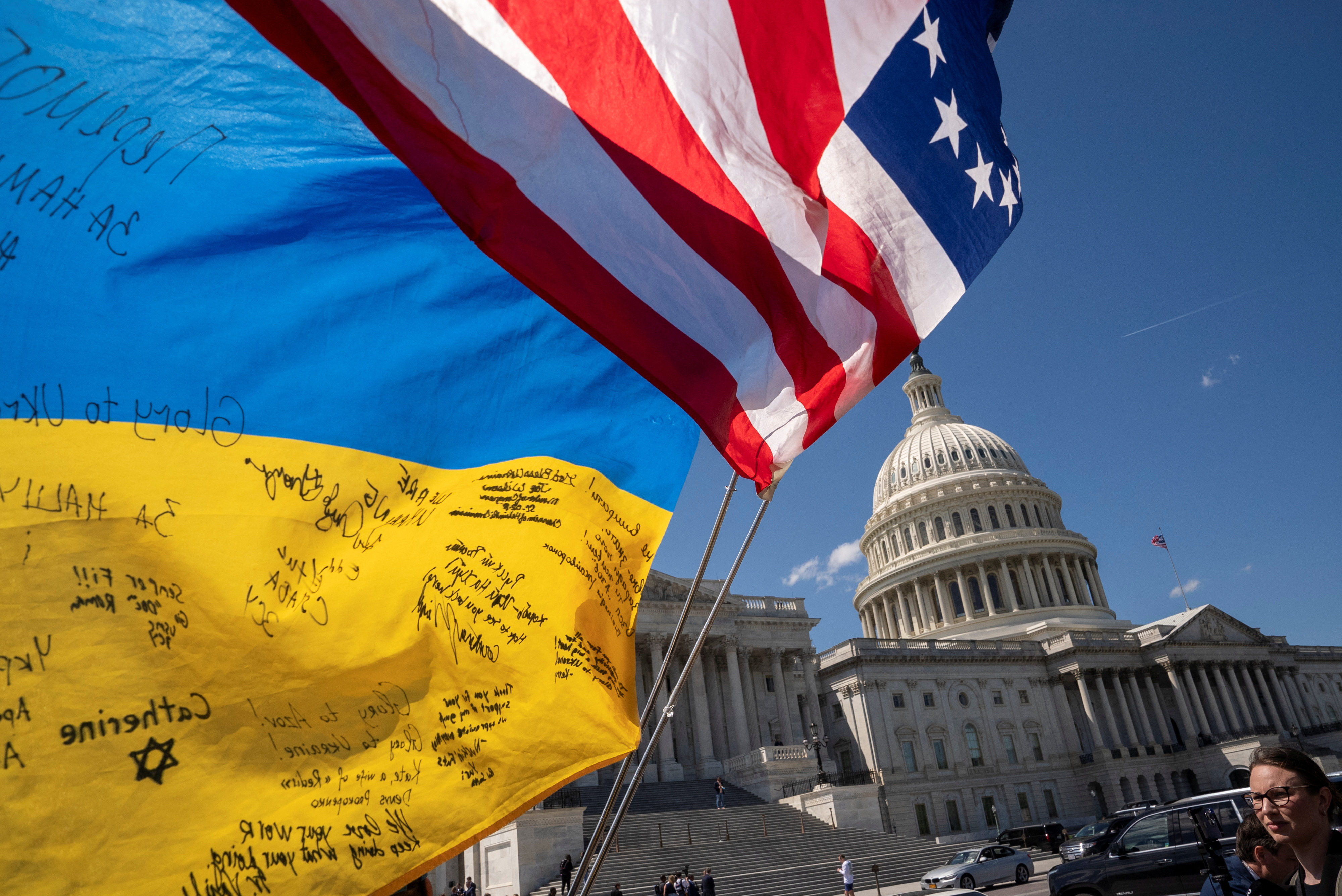
Two Japanese navy helicopters crashed into the sea during a training exercise, killing at least one of the eight crew members on board, the defence minister said on Sunday.
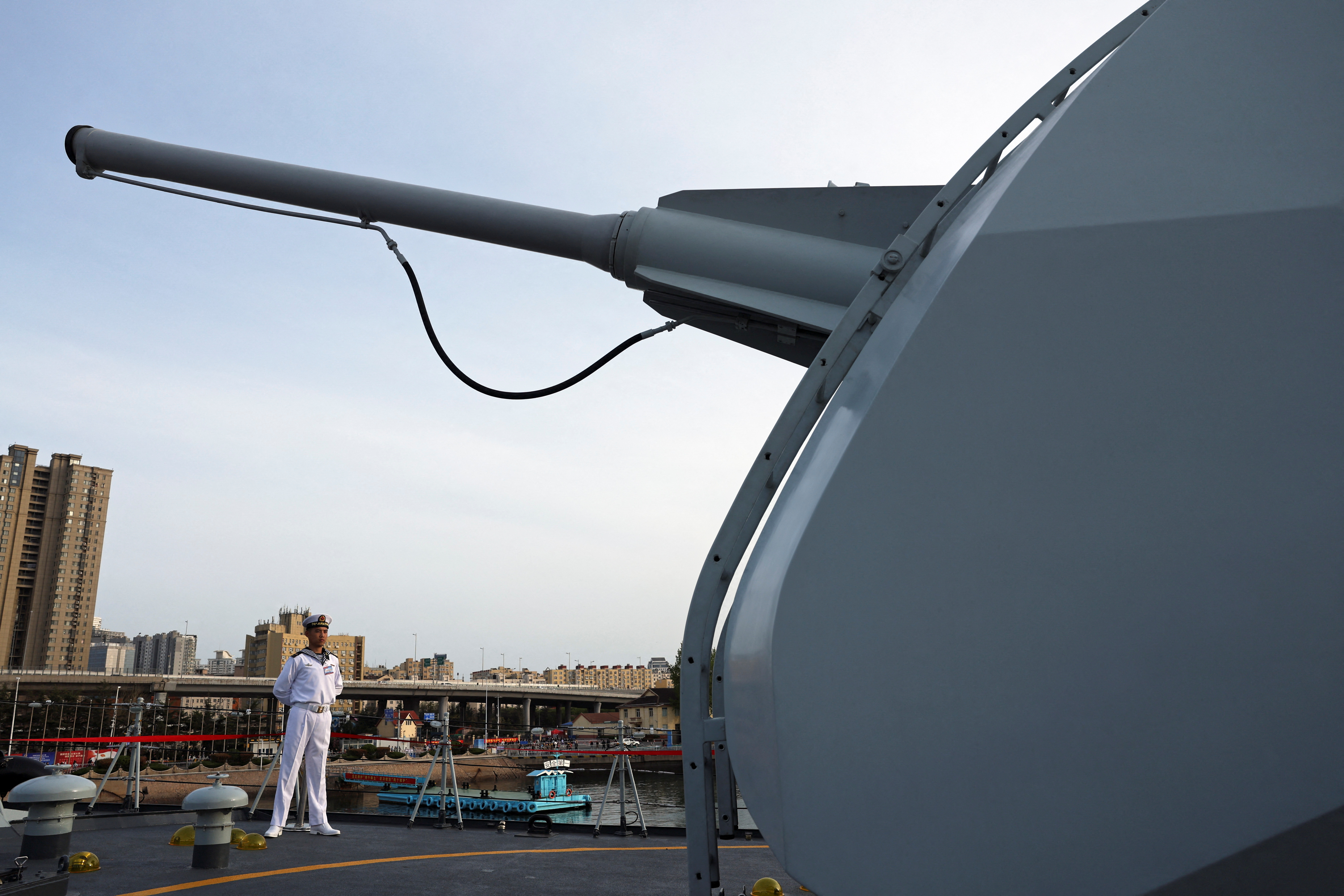
- Share full article
Advertisement
Supported by
Tesla Will Recall Cybertruck in Latest Setback
A federal auto safety agency said the accelerator pedal on the pickup truck, sales of which began in late 2023, could become stuck, increasing the risk of accidents.

By J. Edward Moreno
Tesla has agreed to recall nearly 4,000 of its Cybertruck pickups to fix an accelerator pedal that can get stuck, raising the risk of crashes, a federal safety agency said on Friday.
The defect could cause the vehicle to accelerate unintentionally, the National Highway Traffic Safety Administration said in a notice posted on its website . Tesla started selling the Cybertruck, its first pickup truck, in November after many delays.
The recall is yet another setback for Tesla, the largest electric vehicle manufacturer in the United States. The company has been losing market share to emerging competitors and reported this month that its sales in the first three months of the year fell from the same period a year earlier — the first time that has happened since the start of the pandemic.
Tesla’s recent troubles have unnerved investors, and the company’s stock has fallen roughly 40 percent so far this year.
The federal safety agency said all 3,878 Cybertrucks on U.S. roads produced from Nov. 13 to April 4 have the defect, which it said was caused by soap being used as lubricant during assembly at Tesla’s factory in Austin, Texas. The residual soap “reduced the retention of the pad to the pedal,” the agency said.
Tesla first received a customer complaint on March 31, and by last Friday it had completed its assessment and voluntarily recalled the affected vehicles, the notice said.
The agency said Tesla was not aware that the defect had caused any crashes, injuries or deaths. Some owners of the Cybertruck in recent days have posted videos and photographs on social media describing the defect and saying they were able to stop the vehicle by pressing down on the brake pedal.
Tesla will replace or repair the accelerator pedal on Cybertrucks free of charge, the safety agency said.
The company has faced several recalls in the past year. In February, it recalled more than two million vehicles because the font size on a warnings lights panel was too small. In December, the company recalled more than two million vehicles to change its Autopilot software to provide more prominent alerts that remind drivers to keep their hands on the wheel when using the system, which can perform certain driving functions.
Tesla did not immediately respond to a request for comment. Elon Musk, the company’s chief executive, told workers this week that the company would cut 10 percent of its work force . On the same day, two senior executives announced that they were leaving the company.
The electric carmaker has struggled to maintain its recent rapid growth as more established automakers have started making and selling battery-powered cars, and demand for those vehicles has slowed. Tesla has been slow to respond to that competition; the Cybertruck was its first new model since 2020, but its unusual angular design and starting price of more than $80,000 are expected to limit its appeal and sales.
Tesla’s market share in the United States was 51 percent in the first quarter, a drop from 62 percent at the start of 2023, according to Kelley Blue Book.
J. Edward Moreno is a business reporter at The Times. More about J. Edward Moreno
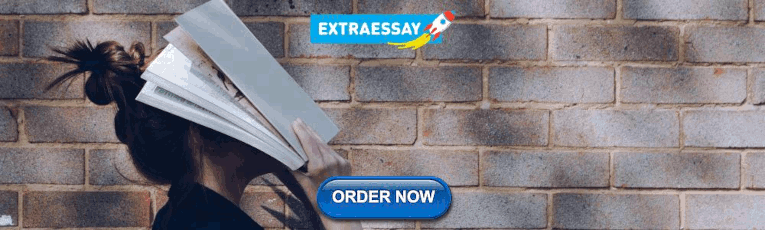
IMAGES
VIDEO
COMMENTS
This paper is divided into three different sections. The first section gives a brief overview of the information retrieval system. The second section describes the information search process, it presents all the phases of text pro- ∗ Corresponding author. Tel.: +212641513301 ; fax: +0-000-000-0000.
Information Retrieval: Recent Advances and Beyond. In this paper, we provide a detailed overview of the models used for information retrieval in the first and second stages of the typical processing chain. We discuss the current state-of-the-art models, including methods based on terms, semantic retrieval, and neural.
a valuable resource for researchers, practitioners, and newcomers to the information retriev al domain, fostering knowledge growth, innov ation, and the development of nov el ideas and techniques ...
Classical term-based retrieval, also known as Boolean retrieval, is a traditional ap-proach to information retrieval that matches the terms in a query to the terms in a document [21]. It is simple, fast, and easy to implement but has limitations such as the inability to handle synonyms, polysemy, and context [22,23].
Abstract. With the growth of electronic data and the expansion of the World Wide Web (WWW), many classic existing retrieval models and systems ignore information about the actual user and search context. Due to the constraints imposed by this fact, context has received more attention in the information retrieval (IR) literature and its ...
This paper provides an extensive and thorough overview of the models and techniques utilized in the first and second stages of the typical information retrieval processing chain. Our discussion encompasses the current state-of-the-art models, covering a wide range of methods and approaches in the field of information retrieval. We delve into the historical development of these models, analyze ...
Retrieving information from memory influences memory in complex ways. In this Review, Roediger and Abel describe positive and negative effects of three facets of memory retrieval and the influence ...
Unlike information retrieval, knowledge extraction directly taps into a publication's content to extract and categorize data. The construction of structured data that can be saved into a schematized database and processed automatically from unstructured data (e.g., a simple text document) is a vast research field.
This article presents a historical overview of 40 years of IIR evaluation studies using the method of systematic review. A total of 2,791 journal and conference units were manually examined and 127 articles were selected for analysis in this study, based on predefined inclusion and exclusion criteria.
Jan 2022. Yi-Hsuan Chuang. Ja-Hwung Su. Ding-Hong Han. Chih-Chi Wang. Request PDF | A Review on recent research in information retrieval | In this paper, we present a survey of modeling and ...
Information retrieval (IR) is the field of computer science that deals with the processing of documents containing free text, so that they can be rapidly retrieved based on keywords specified in a ...
Clinical information retrieval (IR) plays a vital role in modern healthcare by facilitating efficient access and analysis of medical literature for clinicians and researchers. This scoping review aims to offer a comprehensive overview of the current state of clinical IR research and identify gaps and potential opportunities for future studies in this field. The main objective was to assess and ...
The objective of this article is to conduct a comprehensive review of cross-modal retrieval which incorporates image and text modalities, the main concerns of which are different from previous surveys and reviews. So, the motivation behind this review article is: 1. Lack of a full-fledged review article on image and text modalities. 2.
The Information Retrieval user experience has remained largely unchanged since its inception for computers and mobile devices alike. However, recent developments in Virtual Reality hardware (pioneered by Oculus Rift in 2013) could introduce a new environment for Information Retrieval. This paper reports the results of a Scoping Literature Review (PRISMA-ScR) by rigorously examining the entire ...
Organized activities related to the storage, location, search, and retrieval of information. | Explore the latest full-text research PDFs, articles, conference papers, preprints and more on ...
A PubMed search was conducted using the systematic review filter to identify articles that were published in January of 2018. ... It is also important to be knowledgeable regarding the principles of information retrieval in order to avoid committing basic errors and to apply these principles to the particular characteristics of the search ...
In this chapter we review methods and studies of information retrieval in the ordinary (nonfuzzy) sense, leaving consideration of fuzzy retrieval for later chapters. The topics covered in the present chapter are: 1. An example of a document database and a prototypical information retrieval system are introduced.
Abstract. A digital library enables users to interact effectively with information distributed across a network. These network information systems support search and display of items from organized collections. In the historical evolution of digital libraries, the mechanisms for retrieval of scientific literature have been particularly important.
Background: Clinical information retrieval (IR) plays a vital role in modern healthcare by facilitating efficient access and analysis of medical literature for clinicians and researchers.This scoping review aims to offer a comprehensive overview of the current state of clinical IR research and identify gaps and potential opportunities for future studies in this field.
Information Retrieval Methods Group Systematic Review Checklist. Title: Instructions: This checklist is designed to aid you in organizing the evaluation of the information retrieval activities for a review and to make explicit the criteria to be use during the evaluation. Each section of the checklist requires the evaluation of a specific activity.
Medical imaging AI systems and big data analytics have attracted much attention from researchers of industry and academia. The application of medical imaging AI systems and big data analytics play an important role in the technology of content based remote sensing (CBRS) development. Environmental data, information, and analysis have been produced promptly using remote sensing (RS). The method ...
Abstract. During a two-day strategic workshop in February 2018, 22 information retrieval researchers met to discuss the future challenges and opportunities within the field. The outcome is a list of potential research directions, project ideas, and challenges. This report describes the major conclusions we have obtained during the workshop.
The increase in satellite instruments sounding the atmosphere will increase the frequency of several instruments simultaneously measuring either the same vertical profile or vertical profiles related to nearby geo-locations, and users will consult fused products rather than individual measurements. Therefore, the retrieval products should be optimized for use in data fusion operations, rather ...
Meta A.I. is powered by LLaMA 3, the company's newest A.I. technology for generating prose, conducting conversations and creating images. Video by Meta. "With LLaMA 3, Meta A.I. will now be ...
This paper discusses the use of Structural Equation Modeling (SEM) in providing an in-depth explanation of evaluation results and an explanation of failures and successes of a system; in particular, we focus on the case of evaluation of Information Retrieval systems. 1.
The Sixth Extinction: An Unnatural History, by Elizabeth Kolbert. Kolbert's Pulitzer Prize-winning investigation into the mass extinction of living species takes place on "the front lines of ...
Abstract and Figures. Background: Clinical information retrieval (IR) plays a vital role in modern healthcare by facilitating efficient access and analysis of medical literature for clinicians and ...
High-entropy materials (HEMs), including alloys, ceramics and other entropy-stabilized compounds, have attracted considerable attention in different application fields. This is due to their intrinsically unique concept and properties, such as innovative chemical composition, structural characteristics, and c Recent Review Articles
Britain has decided not to designate genomics as critical national infrastructure, Deputy Prime Minister Oliver Dowden said on Thursday, adding a review of the issue had concluded that the current ...
By J. Edward Moreno. April 19, 2024. Tesla has agreed to recall nearly 4,000 of its Cybertruck pickups to fix an accelerator pedal that can get stuck, raising the risk of crashes, a federal safety ...