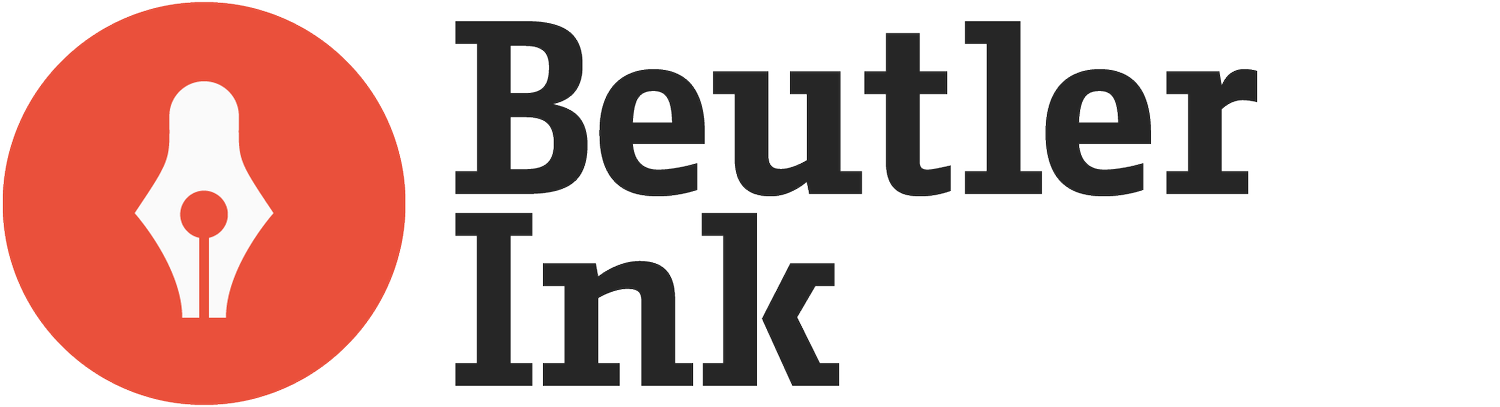
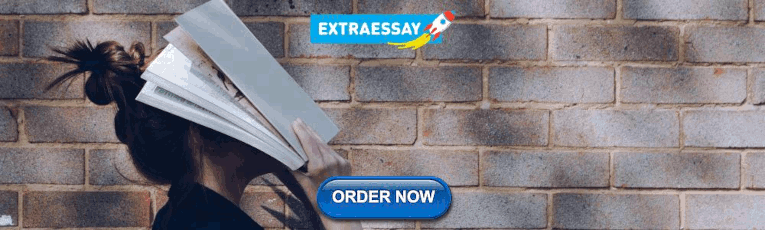
Data Viz 101: Everything you need to know about line graphs
Hello friends, and welcome back to data viz 101, beutler ink's introductory seminar on the wonderful world of data visualization..
In our last post, we continued with our deep dive on data viz history by looking at the work of Florence Nightingale and John Snow, who both used data visualizations to address health crises (poor medical care for troops in Crimea and cholera outbreaks in London, respectively). Their work underscores the real value of presenting data visually—to help viewers see trends, gather insights, and understand complex stories.
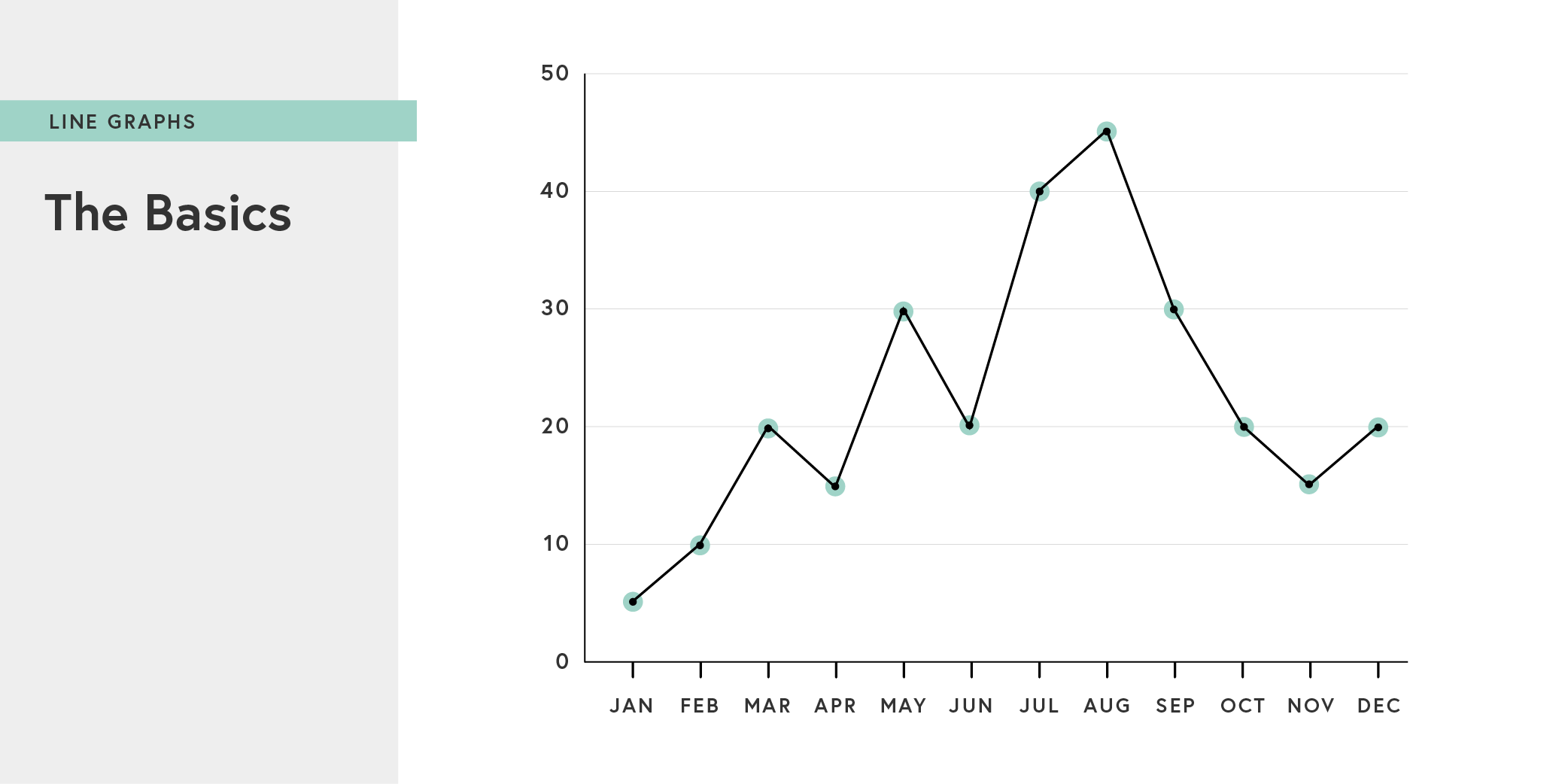
You don't need fancy 3D models or interactive microsites to convey this information—you can use something as simple as line graph. A line graph (or line chart) is a form of data visualization in which data points are aligned along an X-Y axis, and then connected by straight line segments. Line charts are one of the oldest forms of data visualization, as our previous post about William Playfair makes clear.
Line graphs are especially effective at tracking changes over time by using the Y axis to represent intervals of time (often days, months, or years). Line graphs can be used to track multiple data sets on the same chart to see any correlations. So whether you’re tracking increasing national debt levels (as Playfair did) or the important internet trends (like you'd see in one of Mary Meeker's slide decks), simple line graphs prove an accurate and easy-to-follow depiction of data.
Best practices
Even the most basic versions of line graphs (like the ones Excel can generate for you) are generally effective, but there are some considerations to keep in mind when you're setting the data parameters.
First, context is always critical. Include all relevant data points, even if that makes a "trend" look less significant.
Second, you should generally try to show the zero baseline of the y-axis. Without it, your data isn’t grounded, and your visual language will depict something different than the data itself.
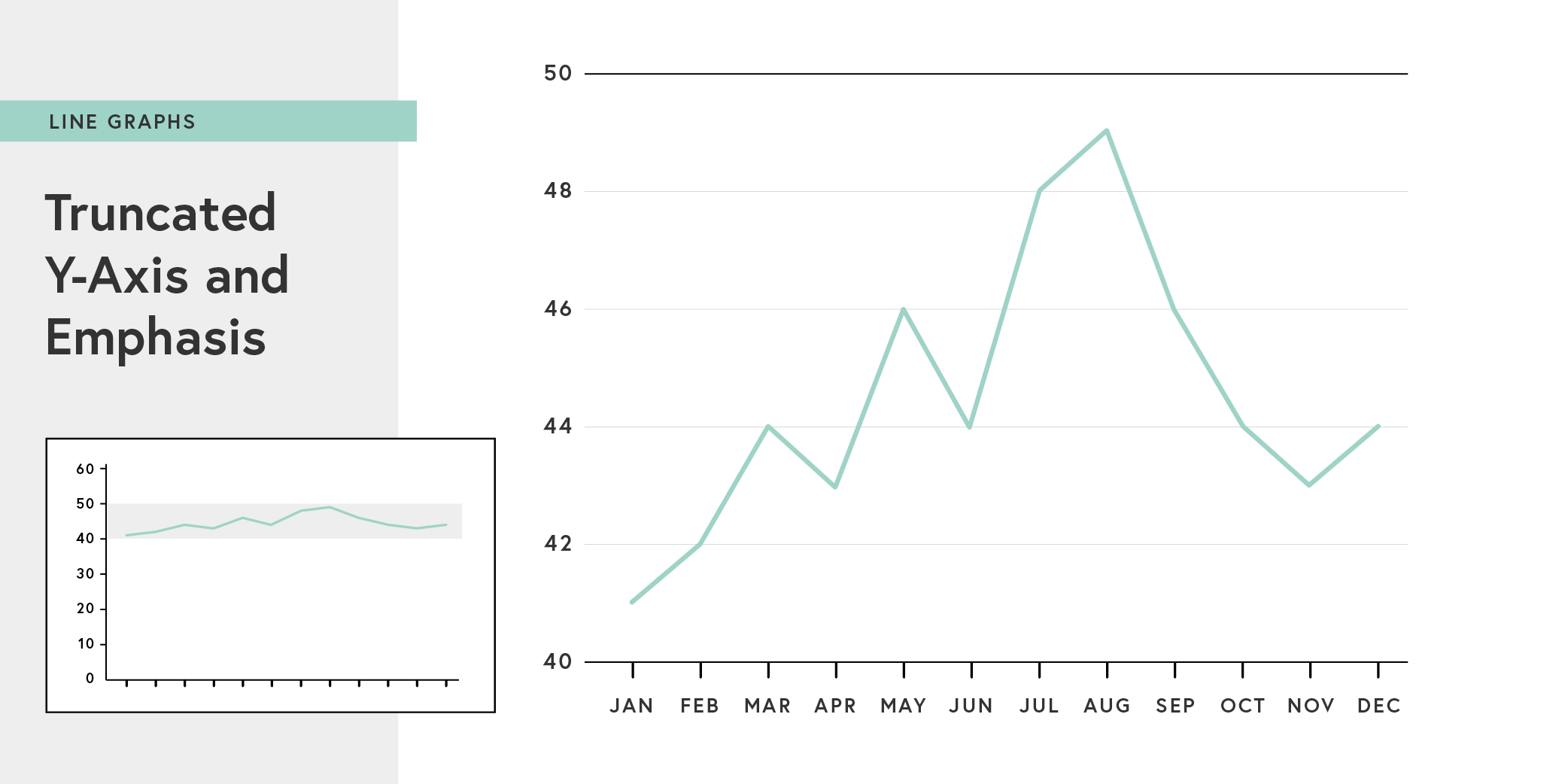
Due to space limitations, however, you might want to zoom in to highlight changes that are significant to the dataset, even if they don’t appear pronounced. In that case, you can highlight variation—while still showing zero baseline—by using an inset with the full data range.
Data Labeling
Finally, data labeling is very important with line charts, as the lines will often intersect and overlap. Here, the data categories are easy to decipher when the labels are attached to the lines, rather than depicted using a key on the bottom.
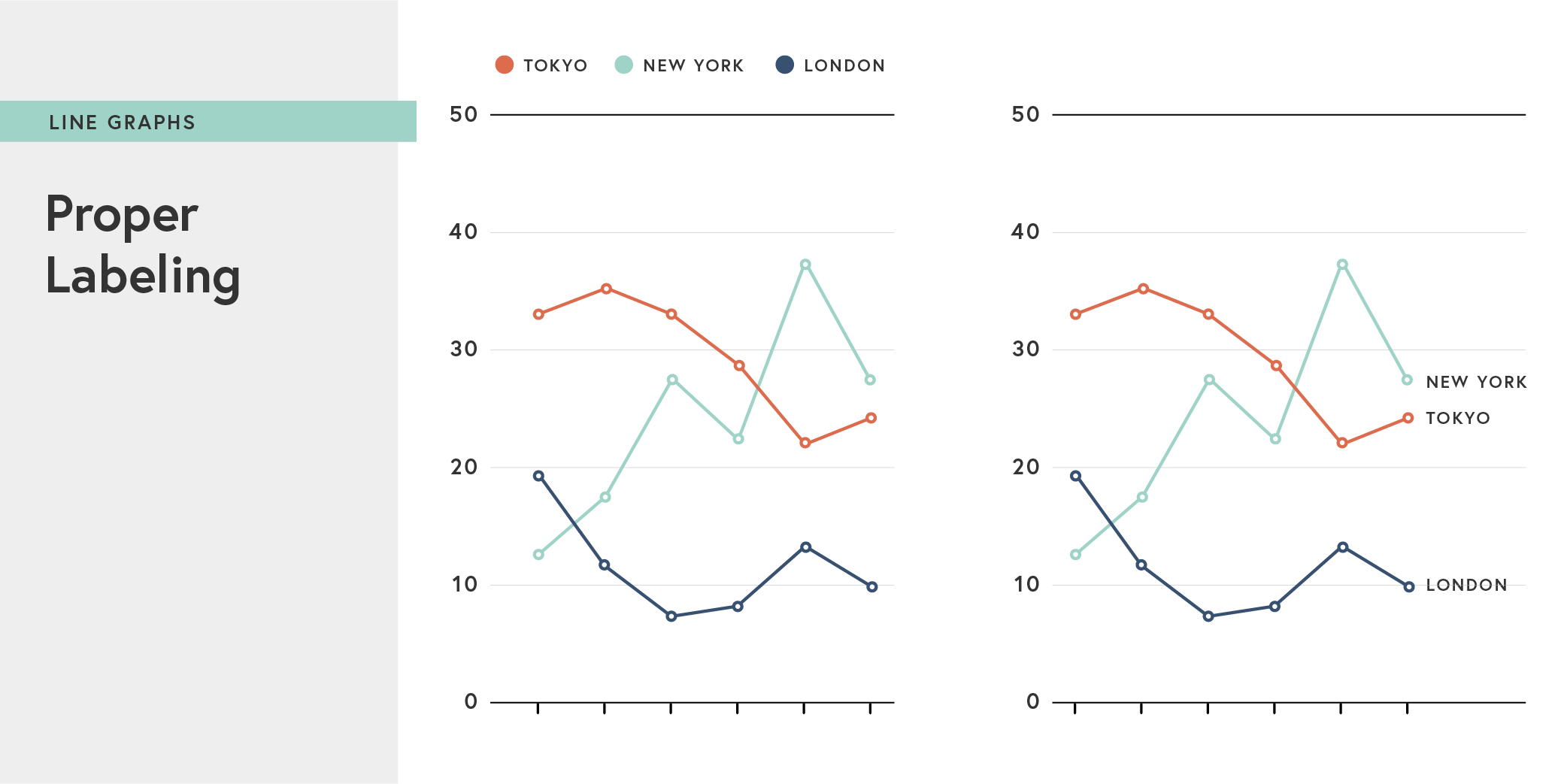
Remember, the only goal of data visualization is to clearly present accurate information. Or at least it should be. Be mindful of design choices that might, even unintentionally, obscure this goal.
Swipe Right for RFPs
Data viz 101: florence nightingale, john snow, and prescriptive visualizations.
A Complete Guide To The Power Of Line Graphs With Examples On When To Use Them
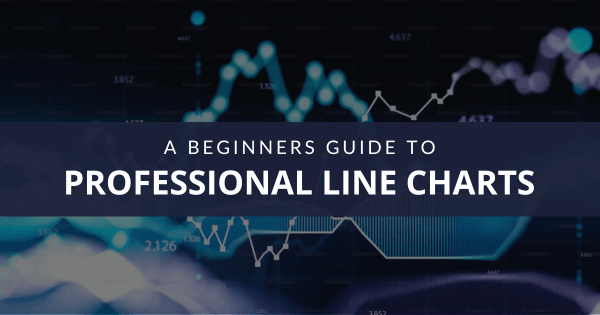
Table of Contents
1) What Is A Line Graph?
2) Line Graphs Benefits & Limitations
3) When To Use A Line Graph
4) Types Of Line Charts
5) Tips To Make A Line Graph
6) Line Chart Examples
Graphs and charts have been a part of our lives for many years now. Every day, we encounter graphical representations of data in our jobs and also in the news or advertisements. That is because visuals make it easier to convey and understand critical information, breaching the knowledge gap between audiences across industries.
That being said, for data visualizations to be successful and avoid misleading your audience, it is necessary to understand the different use cases for each visual. At datapine, we are committed to making data and analytics a friendlier practice, and that starts with understanding the role each visualization plays in your data storytelling process.
That is why we are putting together a series of blog posts that do a deep dive into different types of graphs and charts to help understand what each of them can do for your analytical efforts. We have already covered bar charts , column charts , spider charts , area charts , and gauge charts ; today is the turn of line charts. So, buckle up and enjoy the journey to understanding the value of this powerful visual.
What Is A Line Graph?
A line graph (or line chart) is a data visualization type used to observe how various data points, connected by straight lines, change over time. It is often used to identify and interpret trends, patterns, and relationships in continuous data.

Just like other types of graphs and charts , line graphs are composed of a vertical and a horizontal axis. In this case, the vertical axis (y) represents the values of the data points, and the horizontal one (x) represents the time across which the data points are plotted. The straight lines that unite the data points make it possible for the users to analyze how the values changed over time, making line graphs popular visualizations to identify trends and patterns, observe growth or declines, and spot outliers, among other things.
Although they look simple, line graphs are complex visuals that can efficiently convey various kinds of data. Some of the most common ones include time series data that record values at a specific time interval, such as stock prices or monthly website traffic; continuous data that shows an uninterrupted flow of values, such as population growth over the years; and sequential data, which shows values that have a sequential order, such as manufacturing stages.
Benefits & Limitations Of Line Graphs
As you will learn throughout this guide, line graphs offer a range of advantages that can significantly improve how you interpret and interact with your data. That being said, they also have some limitations or challenges that you should be aware of, and below, we will discuss both.
Benefits:
Starting with benefits, line graphs are versatile visuals that enable you to simplify complex data sets into a highly digestible format. They are especially useful when dealing with large data sets as they lower cognitive overload by highlighting relevant trends that otherwise remain hidden in a sea of raw information. This also makes them easier to interpret and friendlier for non-technical users.
Another great benefit of this visual is their ability to communicate stories compellingly through the progression of data points. For example, a line graph showing quarterly profits with an upward trend tells the growth story of a company. This makes them highly engaging visuals for projects or presentations.
Going a step further, line graphs can also help users predict future scenarios by analyzing historical trends. For example, you can use a line diagram to show historical sales volume and identify seasonal effects to plan your production and marketing strategies ahead of time.
These are just a few of the multiple benefits of professional line graphs. Now, let’s discuss some of the limitations. Understanding some of these challenges or limitations can help you ensure your data is accurately represented.
Limitations:
While line graphs stand out when highlighting trends and relationships between data sets, their effectiveness diminishes when it comes to plotting and analyzing more complex trends, such as showcasing how multiple variables interact in a multivariate scenario. In that case, it is better to use more complex visuals like a bubble chart.
Another point that can be considered a limitation is that line graphs are only valuable for representing continuous or sequential data. They become less valuable when plotting categorical or discrete data, which is better suited for bar or pie graphs. For example, if you are trying to represent the number of animals at the ten largest Zoos in the country, then a line chart is not the best choice as there is no valuable reason to connect the data points with a line.
What Are Line Charts Used For (& When Should You Avoid Them)
Now that you have a better understanding of what line graphs are and how they are constructed, let’s discuss in which context you should use them and in which ones you should stay away from them.
- Tracking changes over time : As mentioned earlier, the most popular use case for line graphs is to track changes over time. The fluctuations on the lines make it easy to identify trends and extract valuable conclusions about how the data has changed in a day, week, month, year, or more.
- Plot multiple data series: Line charts can also be used to plot multiple data sets that are represented by multiple individual lines. Doing so allows users to get a deeper understanding of how the different variables change over time and how each affects the other.
- To represent continuous data sets : These visuals also prove valuable when providing an uninterrupted flow of continuous data and how it evolves over an extended period. An example of this can be temperature fluctuations across the day, which are constantly changing with no interruptions.
- To show small changes in data : The versatility of line graphs allows users to format the chart to show very small changes in the data. By zooming into the information, you can spot smaller trends and changes that might be relevant to the analysis.
After reading the limitations portion of the blog, you probably have a sense of when not to use a line chart. As a reminder, we will quickly go through it again below.
- You should avoid using line charts when plotting data that is not continuous or sequential.
- Line diagrams also become less effective when using fractional or decimal values as they are harder to plot.
- You should also know these graphs can become overcrowded when plotting too many lines. We recommend sticking to a maximum of 5 lines to keep the design clean and understandable. More on this later in the post.
Types Of Line Graphs
Just like other chart types we covered in this series, line graphs have variations. There are four line graph types that we will discuss today. Each uses the same principles we have discussed up to this point, with some variations that make them suitable for different analytical scenarios. Let’s dive into them!
- Simple line graph
This is the simplest version of the line chart. As seen in the image below, it only tracks one dependent variable and how it changes over time. It is a simple chart that doesn’t provide deeper insights rather than upward or downward fluctuations in the data. That is not to say it can’t prove valuable for specific scenarios. The simple line chart can provide context and uncover relevant trends, especially when visualized with other charts in a professional financial dashboard .
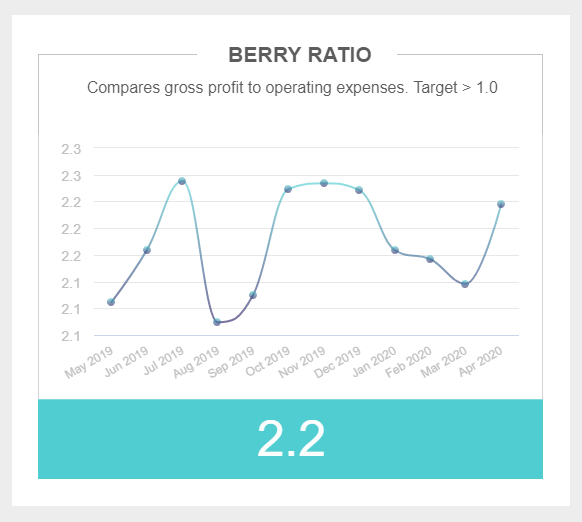
- Multiple line graph
As its name suggests, the multiple line graph plots various dependent variables against a single independent time variable. The variables are often represented with different colors to make it easier for the user to compare them and extract conclusions from the data. Unlike the simple line chart, this type offers a more complex experience that allows for a deeper analysis and exploration of the data. The different lines can be compared with each other to extract valuable conclusions.

**click to enlarge**
- Combined line graph
While this is not a type of line chart per se, it is a variation that has become increasingly popular amongst analysts. What we define as a “combined line graph” is basically the act of combining a line chart with another type of chart to get an advanced view of data. In the image below, we see a combination of a line and a bar chart with two y-axis, one for each chart. While each of these variables can work independently, when put together in this insightful graph, they tell a complete story that allows decision-makers to dig a bit deeper and extract valuable insights.

Mixing two chart types into one is an invaluable analytical feature that can boost your decision-making process. datapine's professional online data analysis tool allows you to generate advanced charts like the one above in just a few seconds.
- Sparkline chart
Last but not least, we have the sparkline chart. This particular variation, which is very popular in finance, is built with multiple small line charts that are mainly used to show changes over a period of time. Due to the small size of the lines, there are no labels on the line charts. However, the statistics on the side provide the necessary information to understand the starting and ending values.
The image below shows a sparkline chart comparing up-sell and cross-sell rates for different sales agents. With that information, we can identify which agents are most successful and replicate their strategies with other agents to ensure success across the board. Likewise, we can identify struggling agents and provide them with help or additional training.
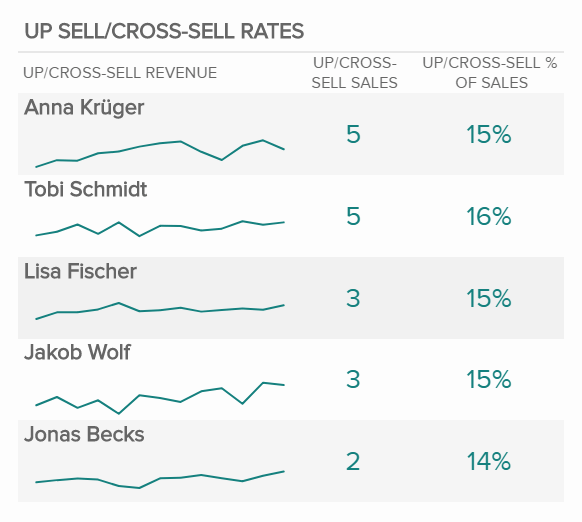
Tips To Create A Line Chart
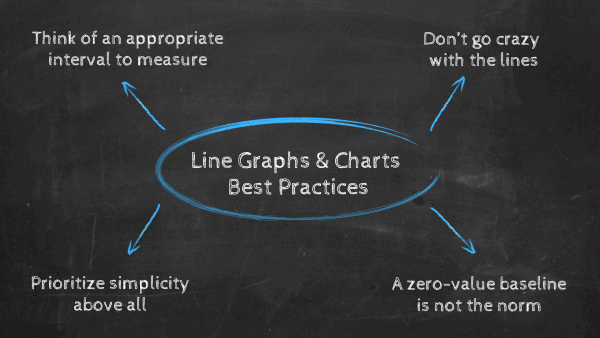
Now that you know the theoretical part of this visual, it is time to think about how to make a line chart. Just like any type of visuals, line graphs are constructed following some key considerations to prevent them from being misleading or too overcrowded. Following these best practices and tips will help you ensure you are building a successful visual.
- Think of an appropriate interval to measure
The first best practice you should follow is to carefully pick the time interval or bin size in which you will plot your values. If you are using time as your measurement, ensure you are not using an interval that is too wide, as this can hide valuable trends. Likewise, if you are using an interval that is too small, it may only show insignificant fluctuations. Therefore, it is essential to consider the time interval based on the goal of the analysis and the nature of the data. In some cases, analysts and designers might plot two lines, one for the overall trend and a second for a more detailed data view with a smaller time interval. This way, you get a complete picture of the situation.
- Don’t go crazy with the lines
If you ask anyone about a key design principle of data visualizations, it is to avoid overcrowding them at all costs. While some visuals have the space to fit in multiple variables, like bar charts, most of them can only fit a couple before becoming messy and more challenging to understand. This is the exact case of line graphs. As mentioned earlier, we recommend you stick to five or fewer lines per graph, as adding more can look like a tangle of lines that is impossible to analyze.
It is important to mention that this is not a mandatory rule. If there is enough space between the lines, you can still add as many as you want. So, it would be best always to use your judgment during the design stage.
- A zero-value baseline is not the norm
If you have ever read one of our data visualization posts, then you might be aware of the zero baseline rule. This rule says that your charts should be plotted using a zero baseline to prevent the data from being misleading to the eye of the audience. Well, believe it or not, this rule does not apply to line charts. This is because the main goal of this visual is to highlight changes over time; therefore, the size of the values is not as relevant. Hence, when plotting your graph, you can start your vertical axes at the value that will highlight the trends and changes most efficiently.
That being said, if you are trying to represent a frequency distribution using a line diagram, then your baseline should always start at zero.
- Simplicity above all
This is more of a general tip, but it applies to any kind of visual you ever design. We are talking about simplicity. When designing your graph with a professional line graph maker, you should keep every aspect of the chart as simple as possible. Stick to a couple of colors that are not too strong or bright, avoid using 3D lines or effects, and use a plain, thin line to avoid deviating the audience's attention from the data. When it comes to labels, use precise language, considering the level of knowledge your audience has. Lastly, avoid using grid lines at the background of your chart unless it is truly necessary. With all these considerations in mind, you are set to generate a stunning visual.
Line Graphs Examples & Templates
As you learned from the previous section, creating a line chart involves some key considerations and best practices to follow to be successful. These powerful visuals prove to be highly valuable in a number of business areas due to their engaging and user-friendly nature. To help you keep putting their value into perspective, below, we will show five examples of line charts generated with a professional line graph maker.

Our first line graph template tracks the revenue per sales representative, with each colored line representing a different sales agent. What makes this sample so valuable is that it offers a dotted red line as a target value, which allows the chart user to clearly understand at a glance which sales reps are underperforming and which ones are overperforming. The information on the chart is also compared to the previous period to understand if there has been any improvement. Having this information at hand in a professional line chart for sales can help managers spot inefficiencies and offer underperforming agents some additional training and assistance.

The following line chart template is a sparkline format, and it tracks one of the most important KPIs for a marketing dashboard : the cost per acquisition. It basically measures how much it costs the business to acquire a new customer. In this case, it is divided by channel and tracked weekly. Since we are only interested in learning which channel is the most profitable to acquire customers, the sparkline format is a valuable way to quickly understand how each channel performs compared to the others.
- Manufacturing
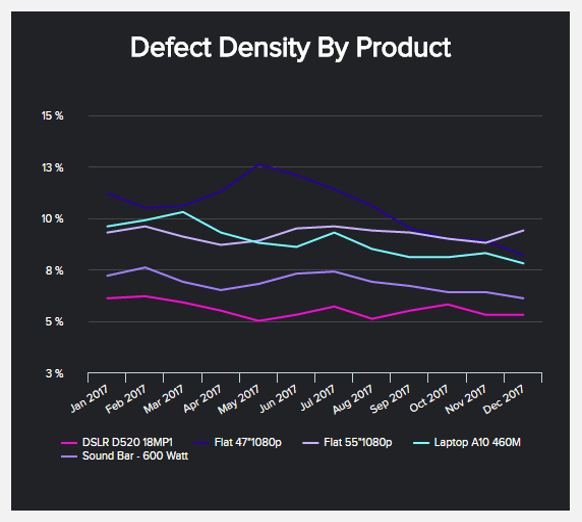
Our next line graph sample tracks the defect density by product every month of the year. Many manufacturing KPIs could benefit from this type of visual as decision-makers in this industry need an agile view of their data to ensure operations are running as expected. Here, we can observe that there weren’t such big spikes in the defect density except for the product Flat 47”1080p, which saw a significant spike during April and July. Luckily, the defect density of this product decreased continuously throughout the rest of the year. However, it is still the second highest of all products; therefore, it should be looked into more detail.
- Customer Service
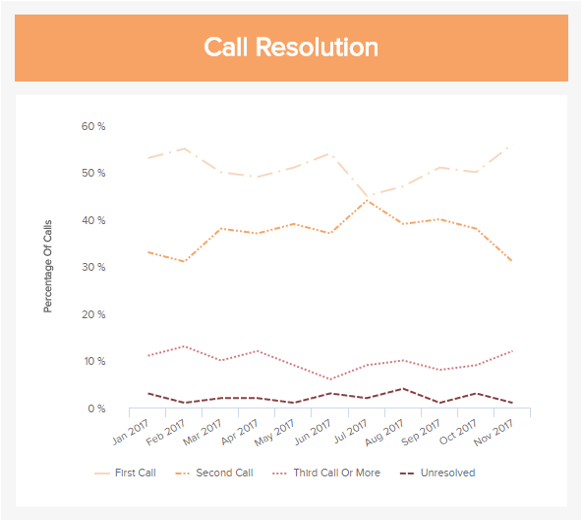
The first call resolution is one of the most important customer service KPIs to track, and it is also a perfect example of a line chart format with multiple variables. The KPI is tracked monthly over 11 months and against the percentage of calls that get solved on the first, second, and third try, as well as the unresolved ones. Looking at the chart above, we can see that over 50% of the calls during the year were resolved in the first call, which is a positive result. However, we can also see that the percentage of unresolved calls increased since April, reaching its highest point by November. This means something is going wrong in the service department that needs to be fixed to ensure that the percentage starts to decrease again.

Last but not least, in our list of line chart samples, we have an example of the simple line chart format tracking a single variable, the inventory-to-sales ratio. Essentially, this most insightful of logistics KPIs tracks the ratio between the available inventory for sale and the actual quantity sold. In this case, the KPI is tracked every three days and shows a lot of fluctuations, which makes it harder to extract deeper conclusions on its own. However, when visualized together with other relevant KPIs like the inventory turnover or the carrying cost of inventory in a professional logistics dashboard , decision-makers can get a complete picture of the situation and ensure efficient inventory management across the board.
If you want to see more examples like the ones above, check out our library with over 350+ KPI examples for different industries and functions.
Key Takeaways Of Line Charts
As we learned from this insightful guide, line graphs are powerful visualizations that help businesses identify trends, patterns, and relationships in continuous data. The best part is that this versatile visual can be used in different formats for basic and more advanced analytical scenarios, making it a great tool to boost a company’s data-driven efforts.
The bottom line is there is no better visual than line charts when analyzing how data changes over time. With the right tools and data visualization techniques , anyone without the need for prior analytical knowledge can start using line charts to visualize their most relevant KPIs and inform their strategies.
As you saw earlier in our list of line graph examples, these visuals are valuable in a number of industries and functions. So, if you are ready to apply all your knowledge and start generating the best line charts, then try our professional online data visualization tool for a 14-day free trial today!
Data Visualization: Line Graph
Learn how to use a line chart and when, its advantages and disadvantages and which of our apps to use to make it.
Available in:
By Edda Viviana Forero Triana. Published: August 16, 2021.
A line chart is a graphical representation of information that changes continuously over time. In the image that opens this post, we see “markers” of data points connected by straight lines that delimit the start, duration, and end of a variable on the time axis. Line charts are used in different fields for different purposes. They are instrumental when creating a graphical representation of changes in values overtime is necessary. For example, in finance, a particular company in the stock market uses this chart to see how specific company security develops over a defined time.
How to create a line chart?
Line charts consist of two axes ( Cartesian grid) : the x-axis (horizontal) and the y-axis (vertical), which is graphically called (x,y). The y-axis has a quantitative value, while the x-axis has a category scale. A tip is to use independent variables, such as dates, on the X-axis and dependent numeric variables on the Y-axis.
If you want to try creating your line charts, Datasketch offers two simple apps: STATIC SIMPLE CHARTS and SIMPLE CHARTS .
What types of variables can you use?
Line charts are suitable for representing quantitative data and can also be used for ordinal qualitative data . They show the change in the value of the data through their ordered values for a criterion.
A line chart is generally constructed by marking points that correspond to each data value ordered according to a criterion and then joining these by lines.
Important: the X-axis value does not need to be time, but you can use any data that behaves as a function concerning the variable on the X-axis.
What is its main purpose?
Its main purpose is to show data values changes and help in predictive data analysis (visualize trends). A graph showing quantitative values over a given time interval is more effective than bar charts in visualizing change over a defined time-space.
Limitations?
Line charts have some limitations. For example, they tend to lose clarity when there are too many data points . However, they are also easy to manipulate visually to achieve specific effects. For example, the degree of apparent change can be manipulated visually by adjusting the range of data points on the axes.
Source: https://gizmodo.com/how-to-lie-with-data-visualization-1563576606
When to use line charts?
When changes are minor, it is better to use line charts rather than bar charts. Line charts can also compare changes over the same period for more than one group.
If you want to learn more about data visualization tools and need to create a simple and easy visualization of your data, we invite you to visit our free tools. https://www.datasketch.co/dashboard/apps
- GRÁFICA DE LÍNEAS ¿Qué son las gráficas de líneas? ¿Para qué tipo de variables se usan? (es)
- Gráfico de líneas (es)
- Line Graph - Learn about this chart and tools to create it
- Line Graph Definition
Line graphs, also called line charts, are used to represent quantitative data collected over a specific subject and a specific time interval. All the data points are connected by a line. Data points represent the observations that are collected on a survey or research. The line graph has an x-axis and a y-axis.
The world today is becoming more and more information-oriented. Every part of our lives utilizes data in one form or another. The tabular representation of data is an ideal way of presenting them systematically. When these numerical figures are represented graphically in the form of a bar graph or a line graph, they become more noticeable and easily understandable, leaving a long-lasting effect on the mind of the observer. Let us understand line graph in detail in the following sections.
What is a Line Graph?
Line graph gives a graphical representation of the changes that had occurred over a given period of time. Line graph has a horizontal axis called the x-axis and a vertical axis called the y-axis. The x-axis usually has a time period over which we would like to measure the quantity of a specific thing or an item in the y-axis. Line graph helps to analyze the trend of whether the quantity in the y-axis is increasing or decreasing over a period of time. Line graph gives a clear picture of an increasing or a decreasing trend.
Line Graph Definition
A line graph is a type of chart or graph that is used to show information that changes over time. A line graph can be plotted using several points connected by straight lines.
Line Graph Example
The line graph shown above represents the sale of bicycles by a bicycle company from the month of January till June. Here, the x-axis represents the time interval and the y-axis represents the number of bicycles sold each month. The dark point on the graph denotes a data point. A data point on a line graph represents the quantity or a number that matches a particular time in the x-axis. In the example shown, the number of bicycles sold in the month of January is 50. Similarly, in the month of February 30 bicycles were sold. We can interpret this data for each month using the data point. The line segment connecting these individual data points gives a picture of whether the sale of bicycles is in an increasing or decreasing trend.
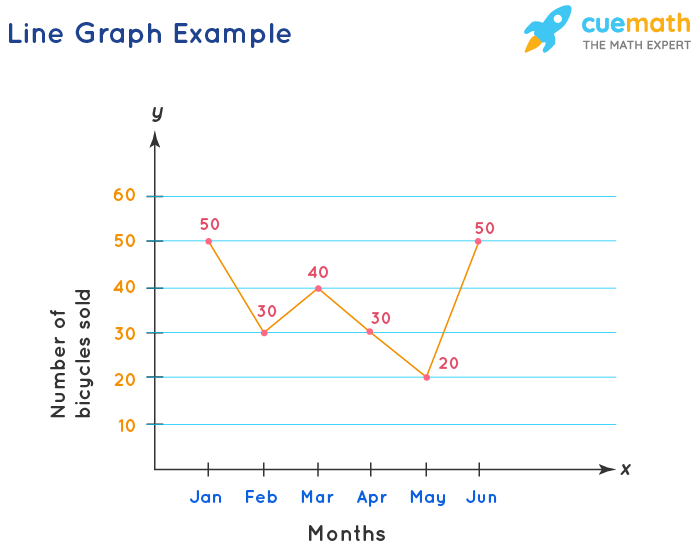
A few key takeaways from line graph are as follows:
- A line graph is a graph that is used to display change over time as a series of data points connected by straight line segments on two axes.
- A line graph is also called a line chart. It helps to determine the relationship between two sets of values, with one data set always being dependent on the other data set.
- They are helpful to demonstrate information on factors and patterns. Line diagrams can make expectations about the consequences of information not yet recorded.
- The slope of the line is the most important observation in this case. The slope represents how steep a line is. It helps in comparing the magnitude of change between any two consecutive points on the graph. For example: The steeper the slope, the greater is the change in magnitude between two consecutive points.
Parts of a Line Graph
Line graph consists of a horizontal x-axis and a vertical y-axis. Most line graphs only deal with positive number values, so these axes typically intersect near the bottom of the y-axis and the left end of the x-axis. The point at which the axes intersect is always (0,0). Each axis is labeled with a data type. For example, the x-axis could be days, weeks, quarters, or years, while the y-axis shows revenue in dollars. Data points are plotted and connected by a line in a "dot-to-dot" fashion.
Let's understand the various parts of a line graph.
- Title: The title of the graph tells us what the graph is all about, i.e., what information is depicted by the graph.
- Labels: The horizontal axis across the bottom and the vertical label along the side tell us what kinds of data are being shown.
- Scales: The horizontal scale across the bottom and the vertical scale along the side tell us how much or how many.
- Points: The points or dots on the graph represent the (x,y) coordinates or ordered pairs. More than one data line can be present in a line graph. Here, data on the horizontal axis is the independent variable, and data on the y-axis is the dependent variable.
- Lines: Straight lines connecting the points give estimated values between the points.
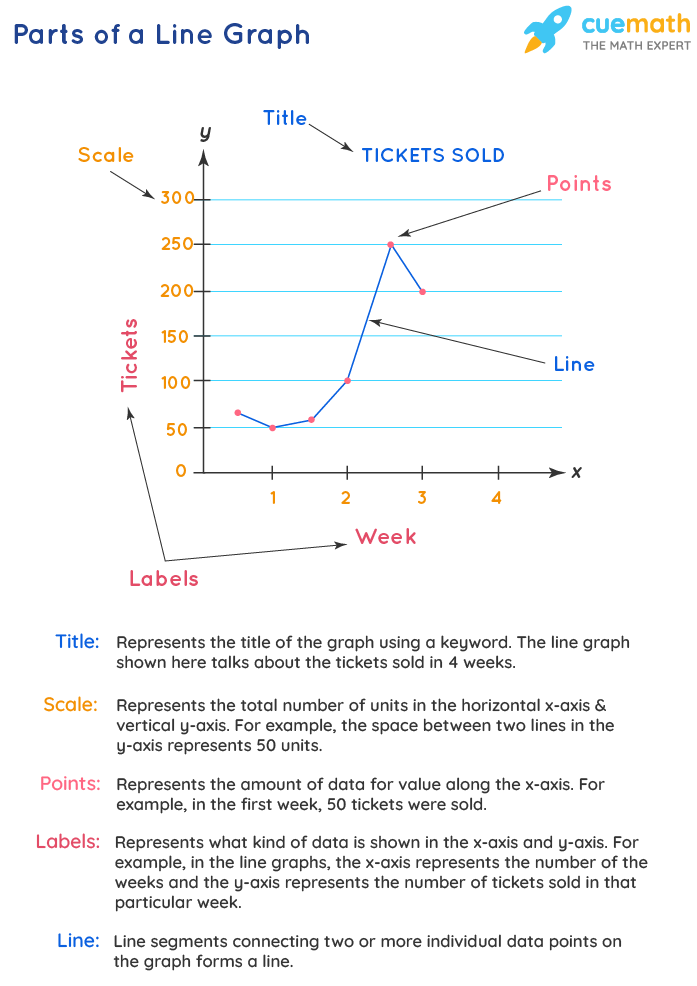
While comparing data sets, the line graph is only useful if the x and y axes follow the same scales. The x-axis is also known as the independent axis because its values do not depend on anything. For example, time is always placed on the x-axis since it continues to change regardless of anything else. The y-axis is also known as the dependent axis because its values depend on variables on the x-axis: at this time, the company had these many sales. The result is that the straight line always progresses in a horizontal fashion and for each x value, there is a unique value of y. For example, the company cannot have two different value sales at the same time.
Reading a Line Graph
Consider the following steps to read and interpret a line graph:
- Look at the title.
- See the labeling of axes.
- Check out the emerging patterns to understand the trend.
- See the data values to get exact figures.
Observe the line graph shown below. Let us read it and list out the key observations from the line graph.
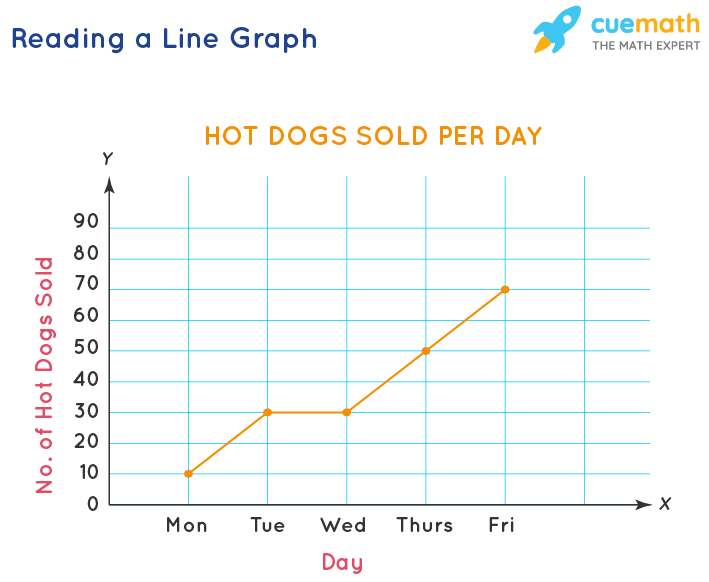
- This graph provides information about the daily sale of hotdogs every day of the week.
- The line graph has an increasing slope, which means that the sale of hot dogs has increased starting from Monday. We can clearly see that there were 40 more hot dogs sold on Thursday than on Monday. (50 were sold on Thursday and 10 were sold on Monday, so the difference is 50 - 10 = 40)
- One other important observation is that the sale remains the same on Tuesday and Wednesday.
Plotting a Line Graph
The following steps can be used to create a line graph.
- Create a table: Draw the x- and y-axes on a page. On the top of the page, place a title that briefly describes the purpose of the chart.
- Label each axis: If time is one of the factors, it should go along the horizontal (x) axis. The other numeric values, i.e., the dependent variables measured should be placed along the vertical (y) axis. Each axis should be labeled with the name of the numeric system as well as the measurements being used. For example, you may label the x-axis with independent variables like hours or months, indicating that each number written on the axis is the number of hours or months. Divide each axis evenly into applicable increments.
- Add data: Data for a line graph is usually presented in a two-column table corresponding to the x- and y-axes. Once you've added your data, your line graph will automatically reflect its values.
- Create a key: If you are comparing multiple items, you'll want to create a key that identifies what each line is by its color.
Important Notes on Line Graph:
Here are some key points to remember while creating line graph.
- Choose the specific title showing the exact information.
- Take variable values in short terms to understand them easily.
- Do not mess with too many lines over the graph. The maximum should be four lines to facilitate comparisons.
Kinds of Data in a Line Graph
Line charts can display continuous data over time, set against a common scale, and are therefore ideal for showing trends in data at equal intervals or over time. As a general rule, use a line chart when your data includes non-numeric (category) data. For example, a shopkeeper wants to analyze the growth of his business over a period of one year. So, the time factor, i.e., the names of months would be considered on the horizontal x-axis whereas the vertical y-axis will be used to depict the numerical values, i.e., the monthly profit.
Line Graph Advantages
The advantages of using line graph are as follows.
- It is beneficial for showing changes and trends over different time periods.
- It is also helpful to show small changes that are difficult to measure in other graphs.
- Line graph is common and effective charts because they are simple, easy to understand, and efficient.
- It is useful to highlight anomalies within and across data series.
- More than one line may be plotted on the same axis as a form of comparison.
Line Graph Disadvantages
Here are some limitations of using line graph.
- Plotting too many lines over the graph makes it cluttered and confusing to read.
- A wide range of data is challenging to plot over a line graph.
- They are only ideal for representing data that have numerical values and total figures such as values of total rainfall in a month.
- If consistent scales on the axis aren't used, it might lead to the data of a line graph appearing inaccurate.
- Also, line graph is inconvenient if you have to plot fractions or decimal numbers.
Line Graph vs Scatter Plot
Observe the two graphs shown below and notice the difference between the two,
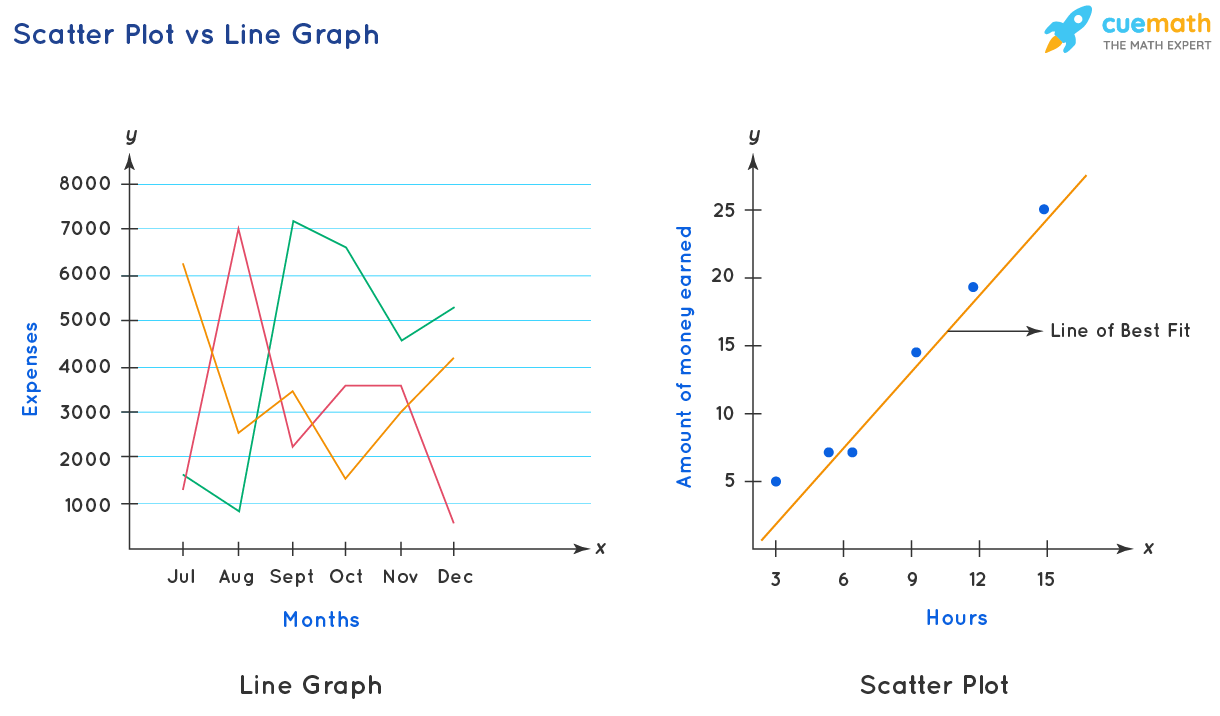
Line graph and scatter plot are two different tools. Both the tools have data points that are marked with small circles. A line graph connects every data point with the next data point with a line segment, whereas a scatter plot does not connect individual data points. We can know the trend of growth by looking at the line in a line graph that connects the data points, whereas in a scatter plot a line of regression or the 'line of best fit is drawn which depicts the relationship between two different sets of data along the x-axis and the y-axis. The key difference between a line graph and a scatter plot is that line graph gives how quantitatively the data along the y-axis increases with respect to a given time period, whereas, in a scatter plot, we can see how the data represented in the y-axis changes with increase in the value of data in the x-axis. Graph A is a line graph whereas Graph B is called the scattered graph or scatter plot. These two types of charts record data information on both X and Y axes. The table shown below lists the key differences between a line graph and a scatter plot.
Related Topics:
Check out some interesting articles related to a line graph.
- Linear Graph
- Absolute Value Graph
- Line Graph Calculator
Line Graph Solved Examples
Example 1: A bookshop made a line graph of the number of books it sold each week during a certain period. Based on the information provided in the above line graph, find how many fewer books were sold in week 8 than in week 7.
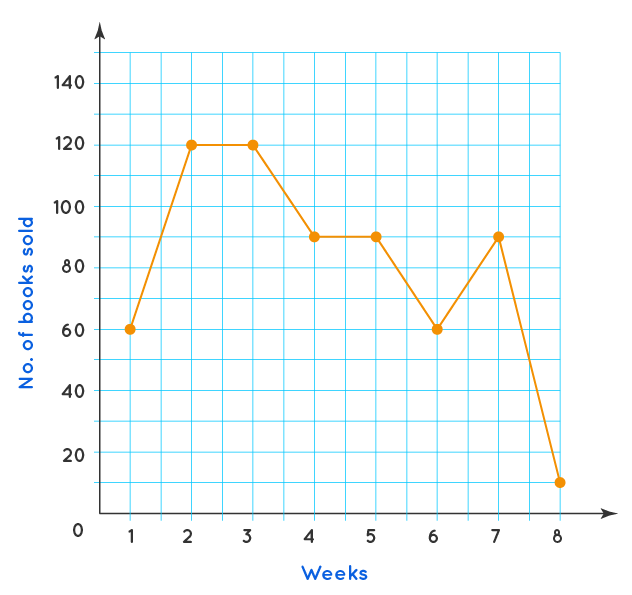
Number of books sold in week 7 = 90 Number of books sold in week 8 = 10 So, the difference = 80 Therefore, there were 80 fewer books sold in week 8 as compared to week 7.
Example 2: The temperature of a city from 5 a.m. to 8 p.m. on a day was recorded in the form of a line graph as shown below. Study the graph and answer the following questions. a) At which time of the day was the temperature 40° F? b) What was the maximum recorded temperature?
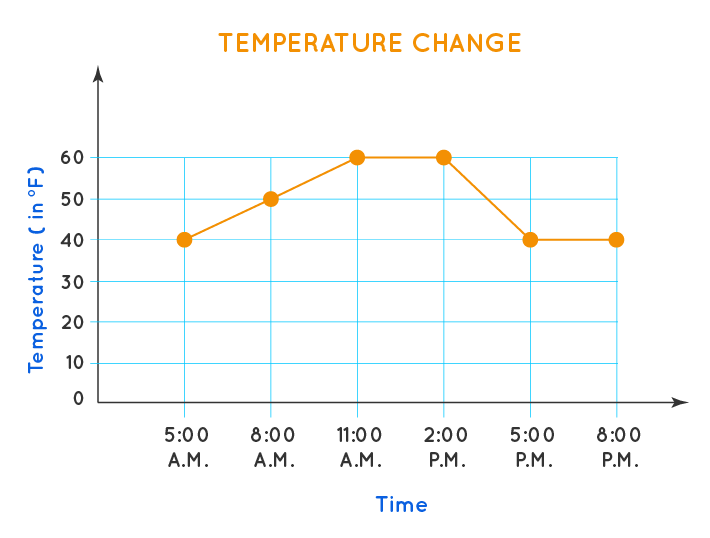
a) The temperature was 40° F at 5 a.m. and from 5 p.m. to 8 p.m. b) The maximum recorded temperature was 60° F.
Example 3: Study the following graph to answer the given questions: Production of two companies A & B over the years is as shown in the figure. For company A, what is the percent decrease in production from 1994 to 1995?
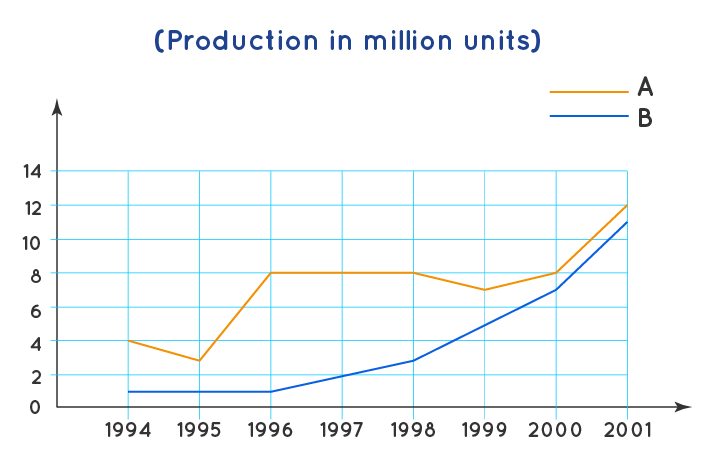
Company A's production in 1994 = 4 million units; Company A's production in 1995 = 3 million units; Difference in production 1994 to 1995 for company A = 4 - 3 =1; Original production = 4
Using the formula :
% Decrease = (Decrease in production/Original production) × 100
Required percentage decrease = \( \dfrac{1}{4} \times 100 = 25\% \) Therefore, there was a decrease of 25%.
go to slide go to slide go to slide
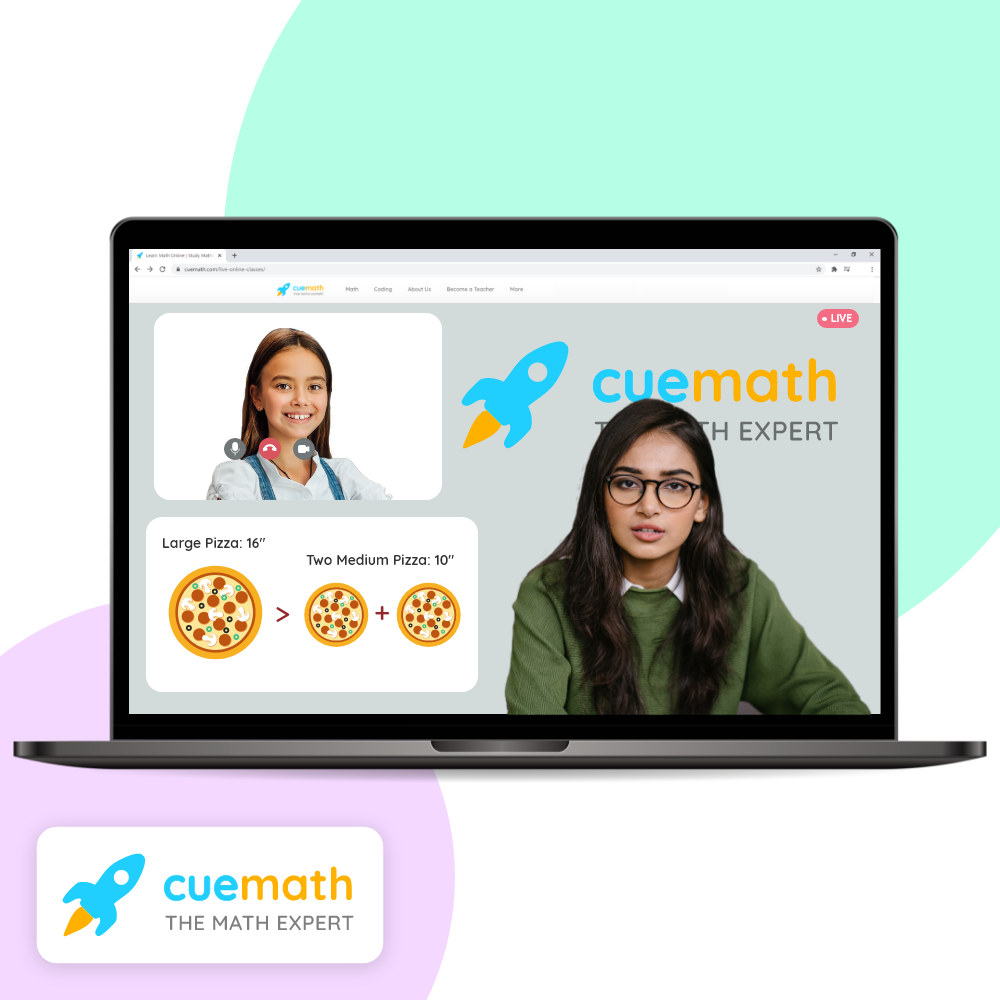
Book a Free Trial Class
Line Graph Practice Questions
go to slide go to slide
FAQs on Line Graph
A line graph is a tool used in statistics to analyze the trend of data that changes over a specified interval of time in a coordinate plane . Here the time and data are represented in an x-and-y-axis . It is also called a line chart. The x-axis or the horizontal axis usually has the time; and the data that changes with respect to the time is present in the vertical axis or the y-axis. Data obtained for every interval of time is called a 'data point'. It is represented using a small circle. An example of a line graph would be to record the temperature of a city for all the days of a week to analyze the increasing or decreasing trend.
What is a Data Point in a Line Graph?
The point of intersection on the line graph that matches a time on the x-axis to a data value on the y-axis is called a data point. The data points in a line graph are represented using small circles or dots. They are connected by line segments to determine the growth trend.
What are the Parts of a Line Graph?
The important parts of a line graph are as follows.
- Title: Represents what information is depicted by the graph.
- Labels: The names given to the horizontal x-axis and the vertical y-axis that tell us about the kinds of data that are being shown.
- Scales: The total number of units or quantity along the horizontal and vertical axis. For example, if we are measuring the sale of a number of cups of coffee for every hour in a day, then in the x-axis we can have hour 1, hour 2, hour 3, and so on. In the y-axis, we can have 10, 20, 30, and so on. In the y-axis, the scale is an interval of 10 units, since the number increases by 10 consecutively.
- Lines: Individual line segment connecting two adjacent data points form a line in a line graph.
What are the Steps in Creating a Line Graph?
The steps in creating a line graph are as follows.
- Give a title to the line graph.
- Draw the x-axis and y-axis on the page and label them. The x-axis is an independent variable which is usually a time period and the y-axis is a dependent variable which is usually a quantity. For example, you may label the x-axis with independent variables like hours or months, indicating that each number written on the axis is the number of hours or months. Divide each axis evenly into applicable increments.
- Add data to the line graph by creating a two-column table with values and plot the data points and join them by line segments.
- If multiple line items are being compared, lines with different colors should be drawn to avoid confusion and a key should be created to clearly distinguish each one of them.
What is a Straight Line Graph?
A straight-line graph is a linear graph involving two variables. Its general form is y = mx + c, where 'x' and 'y' are the variables, 'm' is the slope pf graph and 'c' is constant which is the value of 'y' when x = 0.
What are the Advantages of a Line Graph?
A line graph is a tool to do trend analysis on a subject over a period of time. There are some advantages of using a line graph. They are as follows.
- A line graph shows the increase or decrease in trends over different intervals of time, be it a week, or for a number of months or years.
- It helps in detecting small changes that are difficult to measure in other graphs, as we have line segments connecting every 2 data points.
- It is simple and easy to read and interpret.
- It is helpful in highlighting anomalies within and across data series.
- Comparison of data can be done by plotting more than one line. This is done to effectively compare more than one set of similar types of data for varying periods of time.
What are the Disadvantages of a Line Graph?
Though a line graph is easily readable and easy to use, there are some disadvantages too in using them. They are as follows.
- Too many lines in a line graph make it unreadable and cluttered.
- Only a limited range of data can be plotted to make the best use of a line graph.
- Inconsistent scales across the axes would lead to inaccurate data.
- They are not useful when it comes to plot fractions and decimals.
What are the Differences Between a Line Graph and a Scatter Plot?
- Line graph is useful when there is a need to analyze continuous data over a period of time, whereas, scatter plots work best when comparing large numbers of data points without regard to time.
- Line graph has only time periods across the x-axis and any quantitative data along the y-axis, whereas, scatter plots have quantitative data along both the x-axis and y-axis.
- Line graph uses line segments to join the individual data points, whereas, in a scatter plot , data points are not joined here, but a 'line of best fit' is drawn to know the relationship between the data along the x-axis and the y-axis.
- Line graph is useful to analyze the trends of a small amount of data, whereas, scatter plots are used to explore the patterns of large amounts of data .
What is a Double Line Graph?
It is a line graph representation of two sets of information on a single graph. It is generally used for the purpose of comparing the outcomes, information, or events. Usually, the lines are denoted by different colors to have clarity of the data.
How Many Lines Can be Present in a Line Graph?
There can be more than one line in a line graph as it helps to compare the data. It is advisable to use different colors to avoid confusion and errors in interpretation. Avoid using more than 4-5 lines simultaneously as the lines may become an unreadable tangle and you may have a hard time interpreting them.
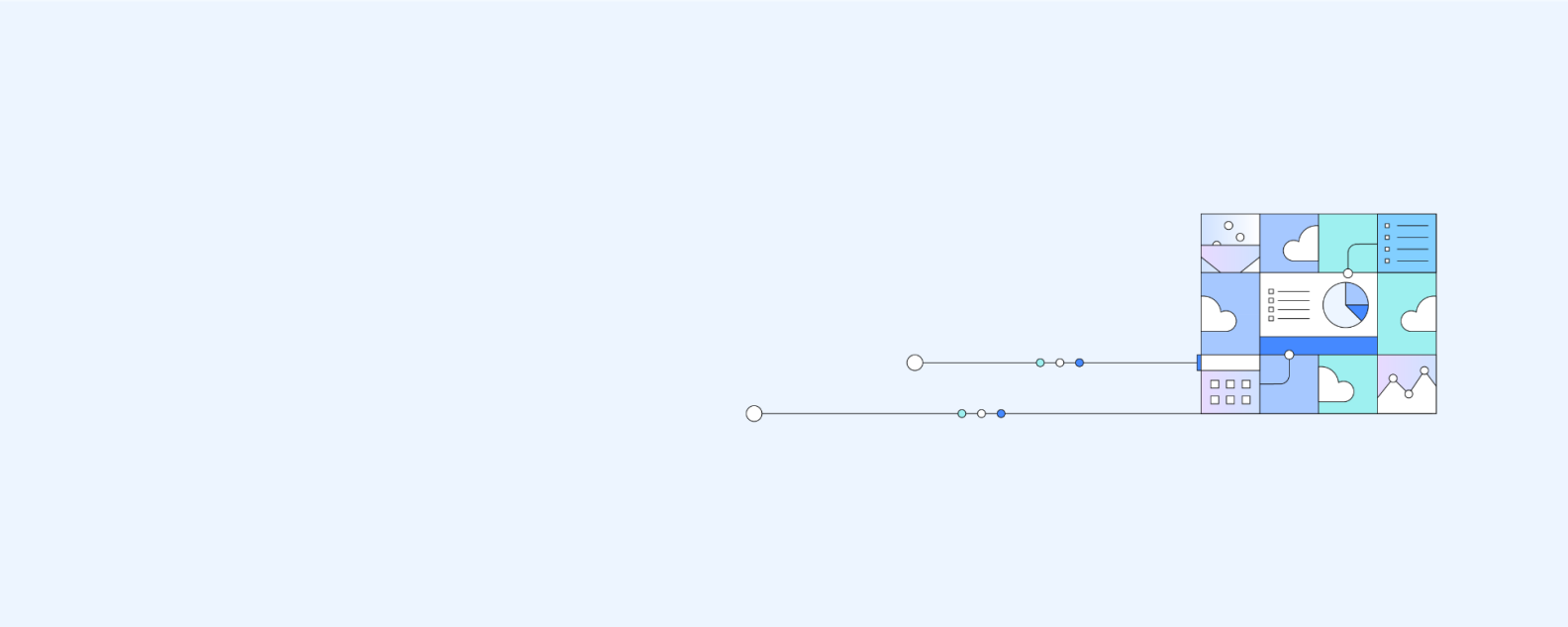
Data visualization is the representation of data through use of common graphics, such as charts, plots, infographics and even animations. These visual displays of information communicate complex data relationships and data-driven insights in a way that is easy to understand.
Data visualization can be utilized for a variety of purposes, and it’s important to note that is not only reserved for use by data teams. Management also leverages it to convey organizational structure and hierarchy while data analysts and data scientists use it to discover and explain patterns and trends. Harvard Business Review (link resides outside ibm.com) categorizes data visualization into four key purposes: idea generation, idea illustration, visual discovery, and everyday dataviz. We’ll delve deeper into these below:
Idea generation
Data visualization is commonly used to spur idea generation across teams. They are frequently leveraged during brainstorming or Design Thinking sessions at the start of a project by supporting the collection of different perspectives and highlighting the common concerns of the collective. While these visualizations are usually unpolished and unrefined, they help set the foundation within the project to ensure that the team is aligned on the problem that they’re looking to address for key stakeholders.
Idea illustration
Data visualization for idea illustration assists in conveying an idea, such as a tactic or process. It is commonly used in learning settings, such as tutorials, certification courses, centers of excellence, but it can also be used to represent organization structures or processes, facilitating communication between the right individuals for specific tasks. Project managers frequently use Gantt charts and waterfall charts to illustrate workflows . Data modeling also uses abstraction to represent and better understand data flow within an enterprise’s information system, making it easier for developers, business analysts, data architects, and others to understand the relationships in a database or data warehouse.
Visual discovery
Visual discovery and every day data viz are more closely aligned with data teams. While visual discovery helps data analysts, data scientists, and other data professionals identify patterns and trends within a dataset, every day data viz supports the subsequent storytelling after a new insight has been found.
Data visualization
Data visualization is a critical step in the data science process, helping teams and individuals convey data more effectively to colleagues and decision makers. Teams that manage reporting systems typically leverage defined template views to monitor performance. However, data visualization isn’t limited to performance dashboards. For example, while text mining an analyst may use a word cloud to to capture key concepts, trends, and hidden relationships within this unstructured data. Alternatively, they may utilize a graph structure to illustrate relationships between entities in a knowledge graph. There are a number of ways to represent different types of data, and it’s important to remember that it is a skillset that should extend beyond your core analytics team.
Use this model selection framework to choose the most appropriate model while balancing your performance requirements with cost, risks and deployment needs.
Register for the ebook on generative AI
The earliest form of data visualization can be traced back the Egyptians in the pre-17th century, largely used to assist in navigation. As time progressed, people leveraged data visualizations for broader applications, such as in economic, social, health disciplines. Perhaps most notably, Edward Tufte published The Visual Display of Quantitative Information (link resides outside ibm.com), which illustrated that individuals could utilize data visualization to present data in a more effective manner. His book continues to stand the test of time, especially as companies turn to dashboards to report their performance metrics in real-time. Dashboards are effective data visualization tools for tracking and visualizing data from multiple data sources, providing visibility into the effects of specific behaviors by a team or an adjacent one on performance. Dashboards include common visualization techniques, such as:
- Tables: This consists of rows and columns used to compare variables. Tables can show a great deal of information in a structured way, but they can also overwhelm users that are simply looking for high-level trends.
- Pie charts and stacked bar charts: These graphs are divided into sections that represent parts of a whole. They provide a simple way to organize data and compare the size of each component to one other.
- Line charts and area charts: These visuals show change in one or more quantities by plotting a series of data points over time and are frequently used within predictive analytics. Line graphs utilize lines to demonstrate these changes while area charts connect data points with line segments, stacking variables on top of one another and using color to distinguish between variables.
- Histograms: This graph plots a distribution of numbers using a bar chart (with no spaces between the bars), representing the quantity of data that falls within a particular range. This visual makes it easy for an end user to identify outliers within a given dataset.
- Scatter plots: These visuals are beneficial in reveling the relationship between two variables, and they are commonly used within regression data analysis. However, these can sometimes be confused with bubble charts, which are used to visualize three variables via the x-axis, the y-axis, and the size of the bubble.
- Heat maps: These graphical representation displays are helpful in visualizing behavioral data by location. This can be a location on a map, or even a webpage.
- Tree maps, which display hierarchical data as a set of nested shapes, typically rectangles. Treemaps are great for comparing the proportions between categories via their area size.
Access to data visualization tools has never been easier. Open source libraries, such as D3.js, provide a way for analysts to present data in an interactive way, allowing them to engage a broader audience with new data. Some of the most popular open source visualization libraries include:
- D3.js: It is a front-end JavaScript library for producing dynamic, interactive data visualizations in web browsers. D3.js (link resides outside ibm.com) uses HTML, CSS, and SVG to create visual representations of data that can be viewed on any browser. It also provides features for interactions and animations.
- ECharts: A powerful charting and visualization library that offers an easy way to add intuitive, interactive, and highly customizable charts to products, research papers, presentations, etc. Echarts (link resides outside ibm.com) is based in JavaScript and ZRender, a lightweight canvas library.
- Vega: Vega (link resides outside ibm.com) defines itself as “visualization grammar,” providing support to customize visualizations across large datasets which are accessible from the web.
- deck.gl: It is part of Uber's open source visualization framework suite. deck.gl (link resides outside ibm.com) is a framework, which is used for exploratory data analysis on big data. It helps build high-performance GPU-powered visualization on the web.
With so many data visualization tools readily available, there has also been a rise in ineffective information visualization. Visual communication should be simple and deliberate to ensure that your data visualization helps your target audience arrive at your intended insight or conclusion. The following best practices can help ensure your data visualization is useful and clear:
Set the context: It’s important to provide general background information to ground the audience around why this particular data point is important. For example, if e-mail open rates were underperforming, we may want to illustrate how a company’s open rate compares to the overall industry, demonstrating that the company has a problem within this marketing channel. To drive an action, the audience needs to understand how current performance compares to something tangible, like a goal, benchmark, or other key performance indicators (KPIs).
Know your audience(s): Think about who your visualization is designed for and then make sure your data visualization fits their needs. What is that person trying to accomplish? What kind of questions do they care about? Does your visualization address their concerns? You’ll want the data that you provide to motivate people to act within their scope of their role. If you’re unsure if the visualization is clear, present it to one or two people within your target audience to get feedback, allowing you to make additional edits prior to a large presentation.
Choose an effective visual: Specific visuals are designed for specific types of datasets. For instance, scatter plots display the relationship between two variables well, while line graphs display time series data well. Ensure that the visual actually assists the audience in understanding your main takeaway. Misalignment of charts and data can result in the opposite, confusing your audience further versus providing clarity.
Keep it simple: Data visualization tools can make it easy to add all sorts of information to your visual. However, just because you can, it doesn’t mean that you should! In data visualization, you want to be very deliberate about the additional information that you add to focus user attention. For example, do you need data labels on every bar in your bar chart? Perhaps you only need one or two to help illustrate your point. Do you need a variety of colors to communicate your idea? Are you using colors that are accessible to a wide range of audiences (e.g. accounting for color blind audiences)? Design your data visualization for maximum impact by eliminating information that may distract your target audience.
An AI-infused integrated planning solution that helps you transcend the limits of manual planning.
Build, run and manage AI models. Prepare data and build models on any cloud using open source code or visual modeling. Predict and optimize your outcomes.
Unlock the value of enterprise data and build an insight-driven organization that delivers business advantage with IBM Consulting.
Your trusted Watson co-pilot for smarter analytics and confident decisions.
Use features within IBM Watson® Studio that help you visualize and gain insights into your data, then cleanse and transform your data to build high-quality predictive models.
Data Refinery makes it easy to explore, prepare, and deliver data that people across your organization can trust.
Learn how to use Apache Superset (a modern, enterprise-ready business intelligence web application) with Netezza database to uncover the story behind the data.
Predict outcomes with flexible AI-infused forecasting and analyze what-if scenarios in real-time. IBM Planning Analytics is an integrated business planning solution that turns raw data into actionable insights. Deploy as you need, on-premises or on cloud.
What is a Line Chart?
Line charts are graphical representations that succinctly illustrate trends and patterns in numerical data. For quantitative analysis, line charts excel in portraying continuous data over a specific period. This enables swift and comprehensive interpretation for businesses.
Line charts are far more than mere visual aids. They are a dynamic conduit for grasping the essence of data's evolution. Through a sequence of plotted points interconnected by lines, line charts capture the progression of a variable over time or another continuous interval.
These charts provide a concise representation of data dynamics, showcasing fluctuations, trends, and anomalies that may be concealed within raw data. To understand line charts, you need to identify and understand their foundational components.
Defining Line Chart
A line chart, also known as a line graph or curve chart, is a graphical representation used to display data points connected by straight lines. This type of chart is particularly useful for visualizing trends, changes, and relationships in data over a continuous interval, often time. Each data point on the chart represents a value associated with a specific category or time point. The connecting lines between these points allow viewers to discern patterns and variations in the data with ease.
Origins and Evolution
The roots of line charts can be traced back to the 18th century when they were first used by mathematicians and scientists to illustrate various mathematical functions. However, the modern concept of line charts gained prominence in the 19th century, notably with the works of renowned statisticians like William Playfair. His innovations in data visualization laid the foundation for the charts we recognize today. With the advent of computers and advanced software, line charts have become an indispensable tool for analysts, researchers, and decision-makers across diverse fields.
The Time-Tested Utility
Line charts excel in depicting changes over time, making them an ideal choice for tracking trends and identifying patterns in data sequences. By organizing data points along an x-axis (horizontal) representing time and a y-axis (vertical) indicating the data values, line charts provide a clear narrative of how variables evolve. This temporal perspective facilitates the identification of growth, decline, fluctuations, and potential outliers within datasets. Whether it's stock market trends, weather patterns, or population dynamics, line charts unveil stories hidden within the data's temporal dimension.
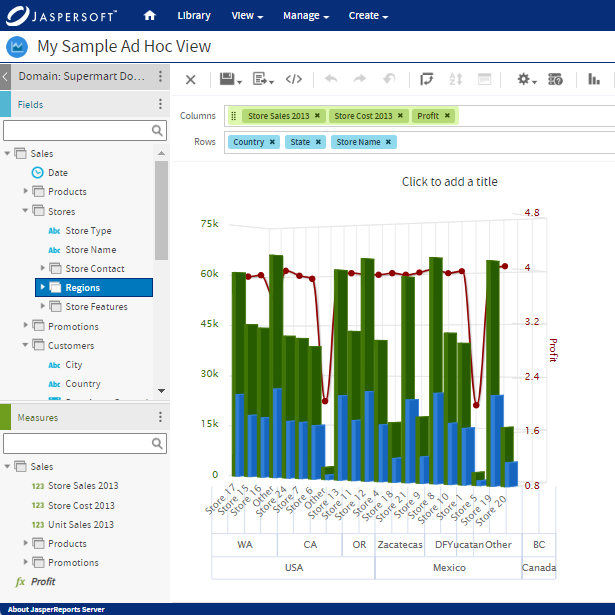
Types of Data That Can Be Displayed Using Line Charts
As you embark on this exploration of line charts, each data point connected by a line carries the potential to unveil narratives about change, growth, and fluctuations in the data. From their origins in mathematics and statistics to their present role in data-driven decision-making, line charts offer a unique lens through which to comprehend the dynamic nature of information.
Line charts are a versatile tool for visualizing various types of data that exhibit trends, changes, and relationships over time. Here are several types of data that are well-suited for representation using line charts.
Time Series Data
Line charts are particularly effective for showcasing time series data, where data points are collected and recorded at regular intervals over time. This type of data reveals trends, patterns, and seasonality, making it perfect for line charts. Examples include stock prices, temperature variations, sales figures, and website traffic data. By plotting these data points chronologically, line charts provide an immediate visual grasp of how values change over specific time periods.
Continuous Data
Continuous data is characterized by a smooth and uninterrupted flow of values. Line charts work well to display such data, enabling the visualization of gradual changes or fluctuations. These charts allow viewers to identify subtle shifts or trends within datasets. For instance, a line chart can effectively display population growth over years, where the data is continuously changing without abrupt interruptions.
Sequential Data
When data points are related in a sequential order, a line chart can help depict how values progress over the sequence. This type of data is often used to show processes or events that unfold over time. Line charts can illustrate step-by-step progressions, such as a project's timeline, educational attainment levels, or manufacturing stages.
Comparison of Multiple Data Sets
Line charts can also compare multiple data sets with different trends, revealing how their patterns diverge or converge. This is useful when you want to understand how different variables change in relation to each other. For example, a line chart could compare the revenue growth of different product categories over a specific period.
Fluctuations & Trends
Line charts are excellent for highlighting fluctuations and trends in data, even when they are not strictly related to time. These fluctuations could represent changes in any measurable quantity, such as monthly rainfall amounts, quarterly exam scores, or annual inflation rates. By displaying these fluctuations as a continuous line, the chart makes it easy to identify the overall trend while observing variations.
The versatility of line charts extends to various data types, offering an effective way to present insights from diverse fields. From time-based trends to continuous data flows, these charts have the power to make complex data understandable, uncovering valuable insights that inform decisions and strategies.
Continuous Variables & Line Charts
Line charts are especially suitable for visualizing continuous variables, which are numerical values that can take any value within a specific range. These variables often involve measurements that can be broken down into infinitely small increments. Line charts showcase the smooth progression of such variables, capturing their gradual changes and trends. For example, if you're plotting the temperature over the course of a day, the temperature is a continuous variable as it can change in infinitely small increments throughout the day. Line charts help reveal patterns and fluctuations in these variables, making them ideal for illustrating the relationships between different continuous measurements.
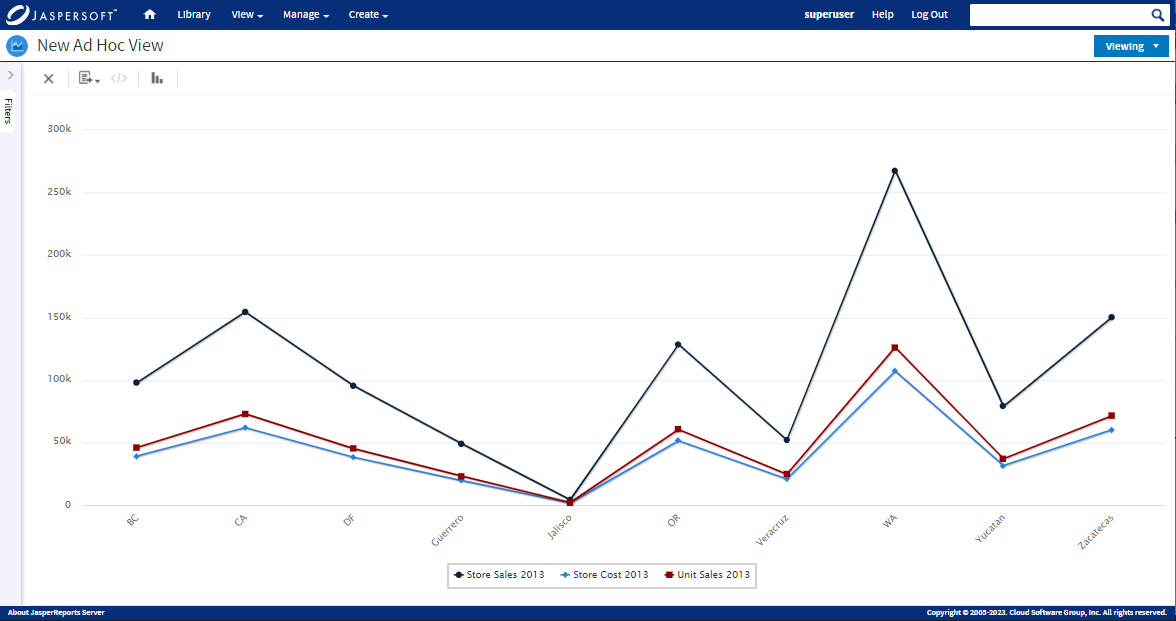
Examples of Data Sets & Line Chart Visualization
Here are some examples of data sets that are commonly depicted using line charts:
- Stock Prices: Line charts are frequently used to visualize stock prices over time. Each data point represents the closing price of a stock on a specific day. By connecting these data points with lines, a line chart provides a clear depiction of a stock's price movements and trends, aiding investors in identifying patterns and making informed decisions.
- Temperature Fluctuations: Temperature data, whether recorded hourly, daily, or monthly, can be effectively visualized using line charts. The chart displays how temperatures rise and fall, revealing seasonal changes, daily patterns, and anomalies.
- Population Growth: Line charts can depict population growth over decades or centuries. The chart illustrates how a population increases or decreases over time, helping demographers, sociologists, and policymakers understand demographic trends.
- Financial Metrics: Various financial metrics, such as revenue, profit, or expenses, can be visualized with line charts. These charts reveal trends and fluctuations in financial data, providing insights into the financial health of a company or organization.
- Economic Indicators: Economic indicators like GDP (Gross Domestic Product), inflation rates, and unemployment rates can be effectively represented using line charts. These charts offer a concise way to show the performance of an economy over time.
- Social Media Engagement: Line charts are often used to visualize metrics related to social media engagement, such as the number of likes, shares, and comments over time. This helps marketers and content creators understand the impact of their online content.
In essence, line charts offer a versatile solution for visualizing a wide range of continuous data. They simplify complex information, making it accessible and understandable, whether it's stock market trends, weather patterns, or demographic shifts.
Reading Line Charts
Line charts are effective tools for visualizing trends, patterns, and anomalies in data over time. Interpreting a line chart involves analyzing the plotted lines, identifying trends, and extracting insights.
Analyzing Patterns & Trends
Line charts allow you to spot trends in data easily. When the line slopes upward, it signifies an increasing trend. Conversely, a downward-sloping line indicates a decreasing trend.
Inflection Points, Peaks, & Valleys
Inflection points, where the trend changes direction, are essential to identify. Peaks represent the highest points on the line, indicating the peak values. Valleys, on the other hand, are the lowest points, representing the lowest values. These points offer insights into potential turning points in data trends.
Understanding line charts enables you to extract valuable insights from data trends, aiding decision-making and providing a comprehensive view of how values evolve over time.
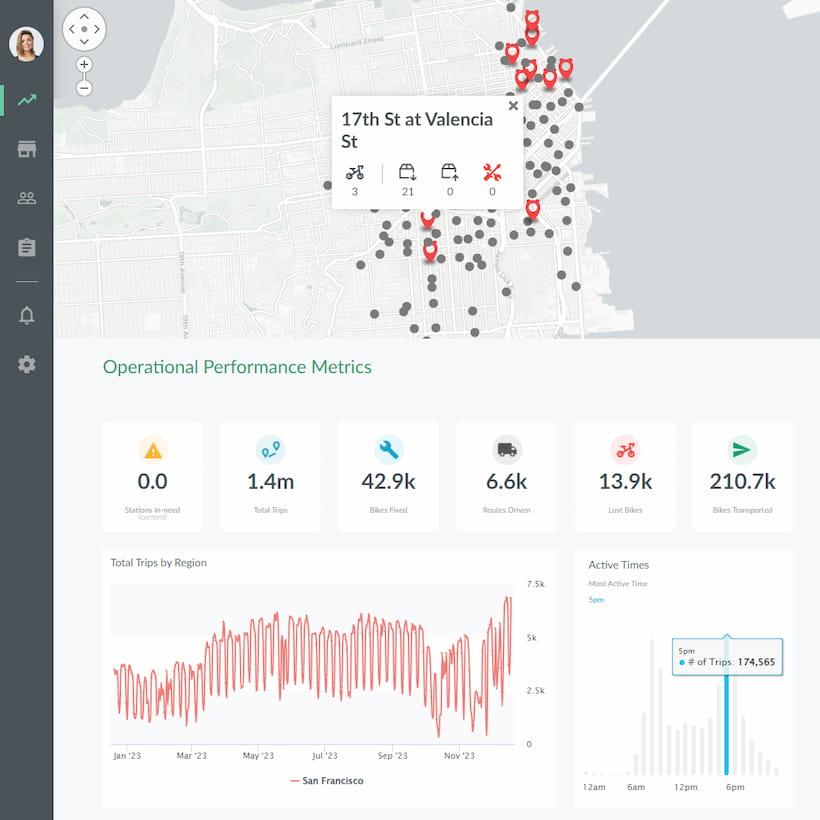
Advantages of Line Charts
Line charts offer a range of distinct advantages that contribute to their popularity and effectiveness in visualizing data trends. These advantages make them an invaluable tool for analysts, researchers, and decision-makers seeking to understand and communicate complex data. Here's an in-depth exploration of these advantages:
Simplification of Complex Data
Line charts excel in simplifying intricate data sets. By transforming data points into a sequence of connected dots, these charts condense elaborate information into a clear visual representation. This simplification is especially valuable when dealing with large datasets, as it enables viewers to focus on overarching trends while minimizing cognitive overload. Complex data can be quickly grasped and interpreted.
Enhanced Visibility of Trends
Line charts are unparalleled in their ability to illuminate trends over time. The continuous lines that connect data points provide an immediate overview of how values change across different time intervals. This dynamic representation not only reveals patterns and fluctuations but also showcases the trajectory of data points. This feature is particularly useful for detecting gradual changes and seasonality that might be obscured in raw data.
Effective Communication of Narratives
Line charts are not just visual representations; they tell compelling data-driven stories. They bring data to life, visually illustrating progressions, changes, and trends. This makes them an invaluable tool for communicating insights in presentations, reports , or discussions. For instance, a line chart depicting the upward trajectory of quarterly profits effectively communicates the growth story of a business.
Comparative Analysis
Line charts enable both the visualization and comparison of multiple trends simultaneously. By plotting multiple lines representing different variables on the same chart, viewers can easily discern correlations, relationships, and disparities. This capability enriches data analysis by offering deeper insights into how various data sets interact and influence each other.
Predictive Insights
Line charts are not only descriptive but also predictive tools. By analyzing historical trends, analysts can extrapolate potential future patterns. For instance, studying a line chart displaying past sales figures might reveal consistent spikes during holiday seasons, facilitating informed predictions and strategic planning.
Ease of Interpretation
The innate simplicity of line charts contributes to their widespread appeal. They are straightforward and easy to understand, making them accessible to a diverse range of audiences. Whether the audience comprises data experts or individuals with limited analytical experience, line charts facilitate quick and meaningful insights. This ease of interpretation enhances effective communication of data-driven findings.
Visualizing Continuous Data
Line charts are particularly effective in visualizing continuous data, such as time-series information. As time progresses, line charts offer an uninterrupted visual representation of how values evolve, making them ideal for tracking phenomena that unfold over extended periods. This continuous depiction aids in understanding the patterns and trends that emerge over time.
Limitations & Considerations
While line charts are a versatile and powerful tool for visualizing trends, it's important to be aware of their limitations and consider certain factors when using them. Understanding these limitations and considerations helps ensure accurate and meaningful data representation.
Limited Data Relationships Beyond Trends
Line charts excel in depicting trends over time. However, their effectiveness diminishes when it comes to illustrating complex data relationships or correlations that extend beyond simple trends. For instance, if you need to showcase how multiple variables interact in a multivariate scenario, a different chart type, such as a scatter plot or a bubble chart, might be more suitable.
Data Nature & Suitability
Consider the nature of your data before opting for a line chart. Line charts are most effective for displaying continuous data, such as time-series information or sequences. If your data is categorical or discrete, using a bar chart or a pie chart might better capture its essence. Always choose a chart type that aligns with the inherent nature of your data to ensure accurate representation.
Contextual Clarity
Context is key when interpreting line charts. Failing to provide adequate context can lead to misinterpretation. Make sure to label your axes clearly, provide relevant units, and include descriptive titles. Additionally, adding annotations or explanatory notes can help viewers understand sudden spikes, dips, or anomalies in the data. Contextual clarity ensures that your audience grasps the implications of the trends they are observing.
Avoiding Overcrowding
When plotting numerous data series on a single line chart, the risk of overcrowding arises. Too many lines can lead to a cluttered and confusing visualization, making it difficult to discern individual trends. In such cases, consider using multiple line charts or grouping related data series to maintain visual clarity.
Misleading Visual Perception
Line charts rely on visual perception to convey information. However, this can sometimes lead to misinterpretation. Viewers might perceive a change in slope as a more significant change than it actually is. For example, a minor change in sales might appear drastic due to the visual representation. To address this, consider using a logarithmic scale if your data spans multiple orders of magnitude.
Selecting Appropriate Intervals
When working with time-series data, selecting the appropriate time intervals for the x-axis is crucial. Choosing intervals that are too large can oversimplify trends, while intervals that are too small might overwhelm viewers with data points. Finding the right balance ensures that your line chart effectively captures the patterns you want to showcase.
While line charts are an invaluable tool for depicting trends over time, understanding their limitations and considering the nature of your data is essential for accurate and meaningful representation. By choosing the right chart type, providing proper context, and being mindful of potential pitfalls, you can harness the power of line charts to effectively communicate insights and patterns within your data.
In real-world applications, line charts prove invaluable for deciphering trends, patterns, and changes over time. They simplify complex data into clear visual narratives. This allows line charts to empower professionals to make informed decisions, develop strategies, and gain deeper insights into their respective domains.
Line Charts with Jaspersoft
Related resources, jaspersoft in action: embedded bi demo.
See everything Jaspersoft has to offer – from creating beautiful data visualizations and dashboards to embedding them into your application.
On-demand demo (22:28)
Creating Addictive Dashboards
Learn how to build dashboards that your users will love. Turn your data into interactive, visually engaging metrics that can be embedded into your web application.
On-demand webinar (56:17)
Ready to give it a spin?
Start your 30-day trial now.
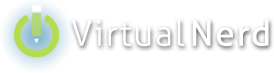
What is a Line Graph?
Want to see a line graph? Check out this tutorial! It introduces you to these neat graphs:
- define ling graph
Background Tutorials
Points and lines.
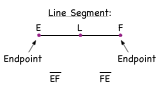
What is a Line Segment?
A math term can really tell you a lot about the thing it's describing. Take the term line segment. A line segment is just part of a line! In this tutorial, learn about line segments, how to name them, and what the midpoint of a line segment is!
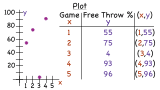
How Do You Graph a Relation From a Table?
When you have a relation given as a table of x-values and y-values, it can sometimes be helpful to graph those points in order to get a visual representation of the relation. This tutorial will show you how to take values from a table and plot them on the coordinate plane!
Further Exploration
Graphs and displaying data.
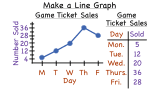
How Do You Make a Line Graph?
- Terms of Use
Graph Viz 101: visual representation of graphs
Back to blog
Graph Viz 101 is a series of posts to teach the basics of graph visualization, written by Sébastien Heymann in collaboration with Bénédicte Le Grand of Université de Paris 1. This is our third post, please discuss it below!
In this blog post we provide a short introduction to the most common ways to represent graphs: matrices and node-link diagrams.
What makes graph data particular is the key importance of relationships. Observing and navigating in this context calls for the development of suitable visualization and interaction techniques in conjunction with storage and data mining solutions. Graphs have therefore received a large attention from Information Visualization researchers, which has led to multiple methods and techniques for their representation and exploration. Usually, representations of graphs are projections of the topology on two or three dimensional spaces using algorithms that calculate nodes coordinates. These algorithms are called layouts . We present here two classical representations: matrix-based representations, and representations with dots and lines on which we will more specifically focus in GraphViz 101.
Matrix-Based Representations
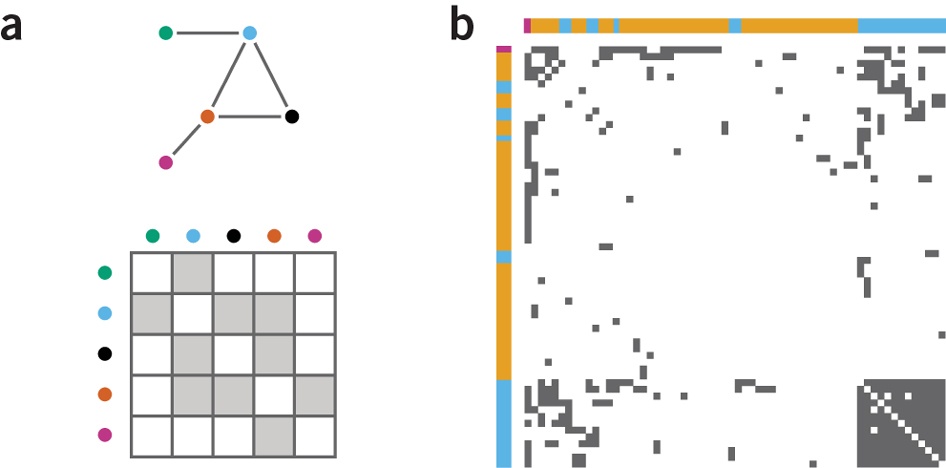
Introduced in ( Bertin 1967 ), matrix-based representations rely on the adjacency matrix, i.e. a Boolean matrix whose rows and columns represent the nodes of the graph. For each link between two nodes, the cell at the intersection of the corresponding row and column contains the value “true”. Otherwise, it is set to “false”. It is possible to replace the Boolean values by those links’ attributes to add more information to the representation.
Matrix-based representations can be “reordered” through successive permutations of its rows and columns to reveal interesting patterns in the graph structure. One of the main advantages of this representation is to avoid occlusion problems encountered using the representation with dots and lines, which we will see in the following post. Matrices are efficient to perform basic tasks like identifying the most connected node, a link between two nodes, or a common neighbor of two nodes. However they perform poorly on more complex tasks such as finding a path between two nodes, even in small matrices ( Ghoniem 2004 ). Such drawbacks may be the reason why they remain underused compared to representations with dots and lines.
Representations with Dots and Lines
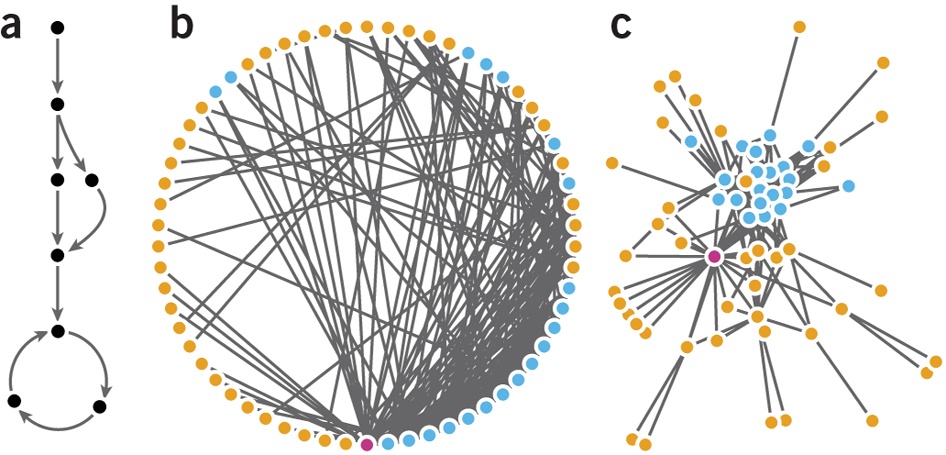
These representations rely on “graph drawing”, which is the art and science of making node-link diagrams using layout algorithms. These diagrams represent nodes as dots and edges as line segments (or curves). A significant majority of graph visualization software implement such representations: in 2007, ( Henry 2007 ) referenced 54 (out of 55) node-link based systems in the Social Network Analysis Repository , and 49 (out of 52) on the Visual Complexity website.
Force-directed algorithms are the most common layouts. They are usually described as spring embedders ( Kobourov 2013 ) due to the way the forces are computed: roughly speaking, connected nodes tend to be closer, while disconnected nodes tend to be more distant. More precisely, force-directed layouts compute repulsive forces between all nodes, but also attractive forces among linked nodes. Forces are calculated and applied on each node at each layout iteration to update its position until the algorithm has converged to a stable position of nodes.
All force-directed algorithms rely on a formula for the attraction force and one another for the repulsion force. The “spring-electric” layout proposed in ( Eades 1984 ), for instance, is a simulation inspired by real life. It uses the repulsion formula of electrically charged particles ( Fr = k/d2 ) and the attraction formula of springs ( Fa = -k.d ) involving the geometric distance d between two nodes. The pseudo-code is given as follows:
Fruchterman and Rheingold (Fruchterman 1991) have created an efficient algorithm using different forces (attraction Fa = d2/k and repulsion Fr = -k2/d , with k adjusting the scaling of the graph). Moreover, recent software like Gephi draw the visualization at each iteration, thus providing real-time feedback to users. When layouts are implemented with no stopping condition, users can tweak the layout parameters in real-time until they decide to stop its execution. Interaction while calculating layout is usually made technically possible by using multi-threading processing, and by using the GPU for rendering the visualization. The goal is to avoid the layout algorithm being perceived as a “black box” by the analyst (although no scientific study has been performed yet to verify this belief), and to accelerate the testing of the layout parameters to obtain an aesthetically good visualization.
The targeted visualization of force-directed layouts is a rough mapping between the distances in the projection space and the distances in the graph topology . The goal is to enable a visual interpretation of the topology using the spatial positions of nodes. When a “good” layout is applied, the resulting image hastens the understanding of the network structure by revealing visual patterns. The readability of graphical representations can be defined by the relative ease with which users finds the information they are looking for. Alternative definitions include the potential to make sense of the data, the familiarity to users, and aesthetic criteria; readability is subjective because the result should be visually appealing and depend on the analysis task. However, some metrics are available to compare layouts, such as the number of occlusions, the uniformity of edge lengths, and the number of edge crossings. A more detailed introduction to this topic can be found in ( Tamassia 2013 ).
Other kinds of representation exist, but you should be able to cover most of your needs using matrix-based representations and nodelink diagrams. As choosing a representation may also depend on the analysis task to perform, ( Henry 2007 ) provides the following comparison guide, see the table below.
In the next post we will go deeper in the visual language of node-link diagrams.
Don’t miss out the Graph Viz 101 series! Subscribe to the email alerts below (you can unsubscribe any time), or follow us on your favorite social network: Twitter , LinkedIn , Facebook . Help us spread it to see everyone making better and useful graph visualizations!
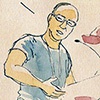
A spotlight on graph technology directly in your inbox.
Jira Software
Project and issue tracking
Content collaboration
Jira Service Management
High-velocity ITSM
Visual project management
- View all products
Marketplace
Connect thousands of apps and integrations for all your Atlassian products
Developer Experience Platform
Jira Product Discovery
Prioritization and roadmapping
You might find helpful
Cloud Product Roadmap
Atlassian Migration Program
Work Management
Manage projects and align goals across all teams to achieve deliverables
IT Service Management
Enable dev, IT ops, and business teams to deliver great service at high velocity
Agile & DevOps
Run a world-class agile software organization from discovery to delivery and operations
BY TEAM SIZE
Small Business
BY TEAM FUNCTION
Software Development
BY INDUSTRY
Telecommunications
Professional Services
What's new
Atlassian together.
Get Atlassian work management products in one convenient package for enterprise teams.
Atlassian Trust & Security
Customer Case Studies
Atlassian University
Atlassian Playbook
Product Documentation
Developer Resources
Atlassian Community
Atlassian Support
Enterprise Services
Partner Support
Purchasing & Licensing
Work Life Blog
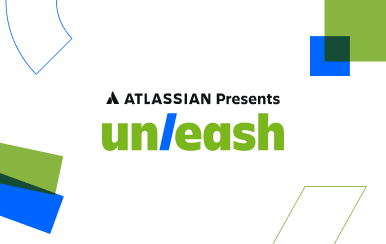
Atlassian Presents: Unleash
Product updates, hands-on training, and technical demos – catch all that and more at our biggest agile & DevOps event.
- Atlassian.com
How to choose the right data visualization
Posted by: mike yi, mel restori.
Data visualizations are a vital component of a data analysis, as they have the capability of summarizing large amounts of data efficiently in a graphical format. There are many chart types available , each with its own strengths and use cases. One of the trickiest parts of the analysis process is choosing the right way to represent your data using one of these visualizations.
In this article, we will approach the task of choosing a data visualization based on the type of task that you want to perform.
Common roles for data visualization include:
- showing change over time
- showing a part-to-whole composition
- looking at how data is distributed
- comparing values between groups
- observing relationships between variables
looking at geographical data
The types of variables you are analyzing and the audience for the visualization can also affect which chart will work best within each role. Certain visualizations can also be used for multiple purposes depending on these factors.
Charts for showing change over time
One of the most common applications for visualizing data is to see the change in value for a variable across time. These charts usually have time on the horizontal axis, moving from left to right, with the variable of interest’s values on the vertical axis. There are multiple ways of encoding these values:
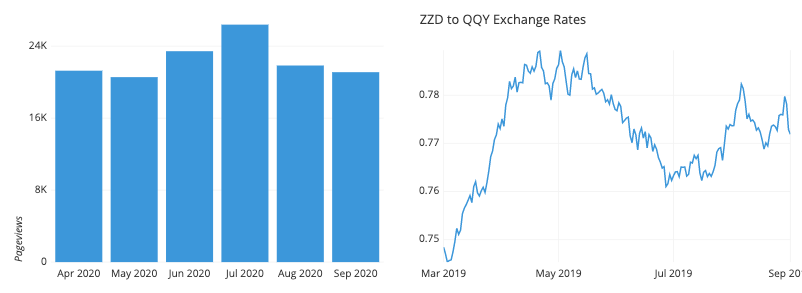
- Bar charts encode value by the heights of bars from a baseline.
- Line charts encode value by the vertical positions of points connected by line segments. This is useful when a baseline is not meaningful, or if the number of bars would be overwhelming to plot.
- A box plot can be useful when a distribution of values need to be plotted for each time period; each set of box and whiskers can show where the most common data values lie.
There are a number of specialist chart types for the financial domain, like the candlestick chart or Kagi chart.
Charts for showing part-to-whole composition
Sometimes, we need to know not just a total, but the components that comprise that total. While other charts like a standard bar chart can be used to compare the values of the components, the following charts put the part-to-whole decomposition at the forefront:

- The pie chart and cousin donut chart represent the whole with a circle, divided by slices into parts.
- A stacked bar chart modifies a bar chart by dividing each bar into multiple sub-bars, showing a part-to-whole composition within each primary bar.
- Similarly, a stacked area chart modifies the line chart by using shading under the line to divide the total into sub-group values.
A host of other more intricate chart types have also been developed to show hierarchical relationships. These include the Marimekko plot and treemap.
Charts for looking at how data is distributed
One important use for visualizations is to show how data points’ values are distributed. This is particularly useful during the exploration process, when trying to build an understanding of the properties of data features.
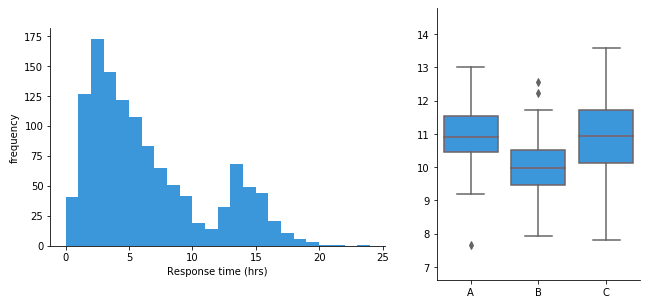
- Bar charts are used when a variable is qualitative and takes a number of discrete values.
- A histogram is used when a variable is quantitative, taking numeric values.
- Alternatively, a density curve can be used in place of a histogram, as a smoothed estimate of the underlying distribution.
- A violin plot compares numeric value distributions between groups by plotting a density curve for each group.
The box plot is another way of comparing distributions between groups, but with a summary of statistics rather than an estimated distributional shape.
Charts for comparing values between groups
Another very common application for a data visualization is to compare values between distinct groups. This is frequently combined with other roles for data visualization, like showing change over time, or looking at how data is distributed.

- A bar chart compares values between groups by assigning a bar to each group.
- A dot plot can be used similarly, except with value indicated by point positions instead of bar lengths. This is like a line chart with the line segments removed, eliminating the ‘connection’ between sequential points. Also like a line chart, a dot plot is useful when including a vertical baseline would not be meaningful.
- A line chart can be used to compare values between groups across time by plotting one line per group.
- A grouped bar chart allows for comparison of data across two different grouping variables by plotting multiple bars at each location, not just one.
- Violin plots and box plots are used to compare data distributions between groups.
- A funnel chart is a specialist chart for showing how quantities move through a process, like tracking how many visitors get from being shown an ad to eventually making a purchase.
- Bullet charts are another specialist chart for comparing a true value to one or more benchmarks.
One sub-category of charts comes from the comparison of values between groups for multiple attributes. Examples of these charts include the parallel coordinates plot (and its special case the slope plot), and the dumbbell plot.
Charts for observing relationships between variables
Another task that shows up in data exploration is understanding the relationship between data features. The chart types below can be used to plot two or more variables against one another to observe trends and patterns between them.
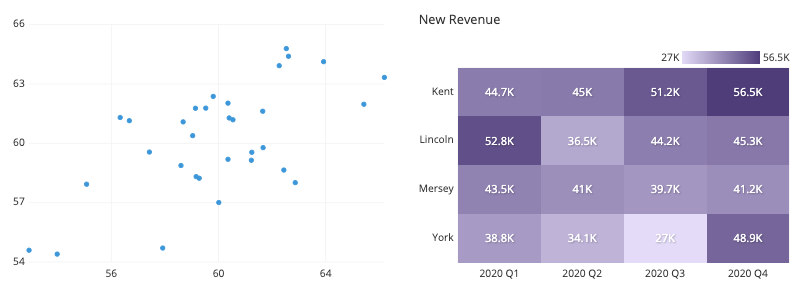
- The scatter plot is the standard way of showing the relationship between two variables.
- Scatter plots can also be expanded to additional variables by adding color, shape, or size to each point as indicators, as in a bubble chart .
- When a third variable represents time, points in a scatter plot can be connected with line segments, generating a connected scatter plot .
- Another alternative for a temporal third-variable is a dual-axis plot , such as plotting a line chart and bar chart with a shared horizontal axis.
- When one or both variables being compared are not numeric, a heatmap can show the relationship between groups. Heatmaps can also be used for purely numeric data, like in a 2-d histogram or 2-d density curve.
Charts for looking at geographical data
Sometimes, data includes geographical data like latitude and longitude or regions like country or state. While plotting this data might just be extending an existing visualization onto a map background (e.g. plotting points like in a scatter plot on top of a map), there are other chart types that take the mapping domain into account. Two of these are highlighted below:
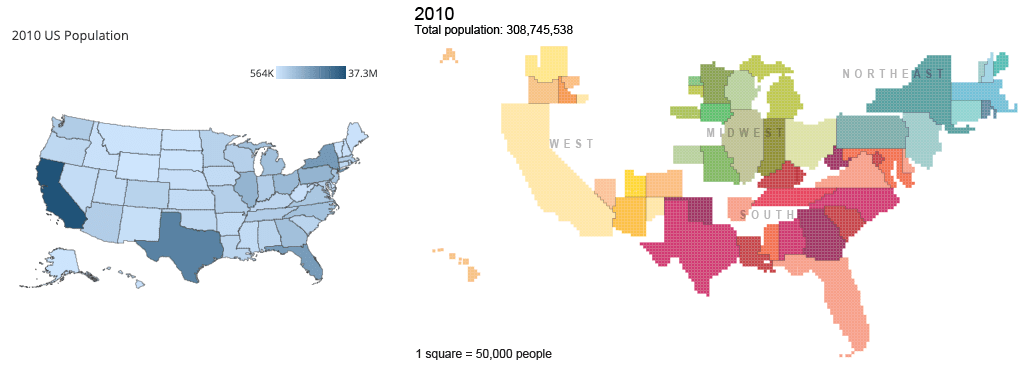
Right: Cartogram of US Population from census.gov
- A choropleth is like a heatmap that colors in geopolitical regions rather than a strict grid.
- Cartograms take a different approach by using the size of each region to encode value. This approach necessitates some distortion in shapes and topology.
Closing thoughts
Choosing the right chart for the job depends on the kinds of variables that you are looking at and what you want to get out of them. The above is only a general guideline: it is possible that breaking out of the standard modes will help you gain additional insights. Experiment with not just different chart types, but also how the variables are encoded in each chart. It’s also good to keep in mind that you aren’t limited to showing everything in just one plot. Often it is better to keep each individual plot as simple and clear as possible, and instead use multiple plots to make comparisons, show trends, and demonstrate relationships between multiple variables.

35 Data Visualization Types to Master the Art of Data
Ready to unlock the power of your data? Brush up on data visualization types that will level-up the information you’re sharing!
Data visualization is all about figuring out how to present data in a way that’s not only visually appealing but also, and more importantly, gets a point across in the most effective way possible.
The problem with relying solely on raw data or basic tables is that they can be confusing, overwhelming, and lack context. Data without clear visualization can miscommunicate information and lead to poor decision-making.
It was business altering when I discovered the various tools and resources for effective data visualization. I especially appreciate how they help transform abstract numbers into tangible visuals, making it easier for everyone – from analysts to stakeholders – to understand complex datasets.
In this post, we’re going to look at the most popular yet effective data visualization types. We’re going to dive deep into each type, illustrating their uses, strengths, and limitations, and offering you a roadmap to transform your data into compelling stories.
So, grab your favorite drink (coffee, I’m thinking), and let’s dive into the many data visualization types!
Categorical Data Visualizations
Categorical data visualizations are an excellent tool for comparing different categories or segments within your dataset. These data visualization types are easy to understand, making them a popular choice for many data analysts.
Think of them as dealing with non-numerical or grouped data, where values fall into a specific category. These are often used to showcase comparisons, distributions, and relationships in a dataset, giving you the power to reveal patterns, trends, and insights that may be otherwise obscured in raw data.
If you’re the type of person who struggles to make sense of seemingly random data points, or if you’re a data enthusiast who loves uncovering hidden trends and insights, you may find this category of data visualization extremely beneficial.
#1: Bar Chart – Making Comparisons Effortless
The bar chart, often also known as a column chart, is a staple in the toolbox of data visualization types. Serving as a simple and effective tool, bar charts facilitate the comparison of data across categories. With one axis dedicated to numerical values and the other representing various categories or subjects under scrutiny, the bar chart brings your data to life.

Whether you choose to orient your bars vertically or horizontally depends largely on the nature of your data. Vertical bar charts place numerical values on the y-axis, offering a quick glance at the size differences, while horizontal bar charts, with numerical values on the x-axis, provide more space for lengthy category labels.
With a bar chart, you can:
- Transform complex datasets into easily understandable visuals.
- Visualize comparisons between different categories.
- Communicate detailed data trends effectively.
An essential tip for leveraging the power of bar charts is considering the complexity of your category labels. If your qualitative data features long or descriptive names, opt for a horizontal bar chart. For an extra layer of creativity, consider using color-coding systems, 3D bars, animated effects, or even photographic backgrounds. Alternatively, a stacked bar chart can illustrate part-to-whole relationships within your categories.
#2: Pie Chart – Showcasing Parts of a Whole
The pie chart ranks high among commonly used data visualizations types, given its simplicity and clarity when demonstrating parts of a whole. The entire circle represents the total, while each individual slice corresponds to a proportion of this total.
Pie charts are ideal for datasets with no more than five or six parts, as this keeps each slice visible and distinguishable. With more than this, slices may become too thin, and with similar values, discerning differences can become challenging. Successful pie charts use contrasting yet harmonious colors, ensuring each slice is visually distinct.

With a pie chart, you can:
- Transform intricate data into easily digestible reports.
- Create clear visualizations of proportional relationships.
- Enhance communication through visual aids.
If your data contains more than six segments, a bar chart could be a more suitable alternative, maintaining the clarity and simplicity of a pie chart while accommodating larger datasets.
#3: Bullet Graph – Compact Data Storytelling
While not as widely recognized as bar or pie charts, bullet graphs pack a punch when it comes to presenting a wealth of information in a compact space. Bullet graphs excel in demonstrating performance against a goal or comparable metric, offering a rich, concise display of key metrics without overwhelming your audience.
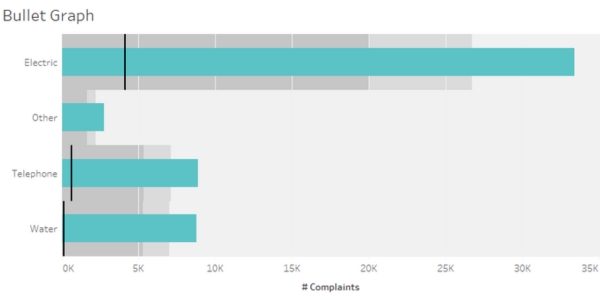
Bullet graphs can help you:
- Present performance data relative to a set target or benchmark.
- Highlight measures, drawing attention to whether they fall within an acceptable range.
- Display multiple measures in a confined space, perfect for dashboard presentations.
Remember, the key to successfully using bullet graphs is to provide clear context. A bullet graph comparing current sales to a set target, with color-coded ranges indicating performance levels, can effectively convey a lot of information at a glance. However, they may not be suitable when your data demands a different context or when illustrating data over time.
Ready to get hands-on with these data visualization types? Check out our A to Z list of data visualization tools .
Hierarchical Data Visualizations Types: Revealing Order and Structure
Hierarchical data visualization techniques are invaluable when you’re dealing with data that’s organized into some sort of hierarchy, whether that be nested categories, familial relationships, organizational structures, or rankings. They help to bring out the order and structure inherent in the data, making it easier to understand and interpret. Here, we will delve into three common types: Tree Diagrams, Treemaps, and Sunburst Charts.
#4: Tree Diagrams – Simplifying Complex Structures
The tree diagram, also known as a hierarchical tree, is a visualization tool that clearly delineates hierarchical relationships within your data. This structure comprises ‘nodes’ and ‘edges’, with each node representing a data point and each edge representing the connection between these points.

Using a tree diagram , you can:
- Visualize intricate hierarchical data in a straightforward, logical manner.
- Clarify relationships and connections between various data points.
- Create an easy-to-follow map of data lineage or processes.
One crucial point to note when using tree diagrams is to maintain a logical and straightforward layout. Overcomplication can quickly lead to confusion. Remember that the main aim is to present a hierarchical relationship in the most comprehensible way.
#5: Treemaps – Depicting Hierarchies and Proportions
Treemaps take a slightly different approach to representing hierarchical data. Instead of focusing solely on the hierarchy, they simultaneously demonstrate proportions within the hierarchy through varying sizes of rectangles. Each rectangle represents a data point, with its size proportional to a particular dimension of the data.

Treemaps allow you to:
- Represent hierarchical relationships and proportions simultaneously.
- Accommodate large amounts of data within a confined space.
- Highlight significant data points through size and color variation.
While treemaps can be incredibly insightful, they may not be suitable if your data set involves too many small, similarly sized categories, which may make the map hard to read and interpret.
#6: Sunburst Charts – Circular Representation of Hierarchies
Sunburst charts, also known as radial treemaps, present hierarchical data in a circular format, making them particularly useful for displaying data that wraps around at the end-points (like hours in a day or months in a year). Each layer of the circle represents a level in the hierarchy, with the innermost layer being the top of the hierarchy.

With a sunburst chart , you can:
- Visualize complex hierarchical structures in a unique, engaging manner.
- Demonstrate a full cycle of data effectively.
- Highlight the proportion of different elements at each hierarchical level.
Keep in mind that while sunburst charts can provide a visually appealing way to present hierarchies, they might not be the best choice for data with many hierarchical levels, as the chart may become crowded and difficult to interpret.
Interested in strategies to enhance your data visualizations? We cover this and more in our in-depth guide on Data Visualization Basics .
Multidimensional Data Visualization Types
Sometimes, your data isn’t as simple as comparing two variables, or understanding hierarchical structures. You may be dealing with complex datasets where you need to understand relationships across multiple dimensions. For these situations, you can leverage multidimensional data visualizations such as Scatter Plots, Bubble Charts, and Radar/Spider Charts.
#7: Scatter Plots – Uncovering Correlations
A scatter plot, also known as a scatter chart or scattergram, is a type of visualization that uses dots to represent the values obtained for two different variables – one plotted along the x-axis and the other along the y-axis. This type of chart can be used to display and compare numeric values, such as scientific, statistical, and engineering data.
By using a scatter plot, you can:
- Identify types of correlation between variables, if any.
- Spot any unusual observations in your dataset.
- Forecast trends by using lines of best fit.
While scatter plots can be effective at demonstrating relationships, it’s important to remember that correlation doesn’t always mean causation. Also, scatter plots may not be as effective when dealing with categorical data visualization types.
#8: Bubble Charts – Adding a Third Dimension
A bubble chart is a variation of a scatter plot. Like scatter plots, they display data across two axes, but they add a third dimension, represented by the size of the dots or ‘bubbles’. This third dimension allows you to incorporate even more data into your analysis.

With a bubble chart , you can:
- Display three dimensions of data effectively.
- Show connections and differences in a dataset that would be difficult to express otherwise.
- Highlight significant data points through size variation.
Remember that while bubble charts can be visually engaging and informative, too many bubbles or bubbles that are too similar in size can lead to confusion, so it might not make the best data visualization types. Be careful about the scale of your bubbles – disproportionate sizes can distort data interpretation.
#9: Radar/Spider Charts – Comparing Multivariate Data
Radar or spider charts are a unique way of showing multiple data points in a two-dimensional chart, making them useful for comparing multivariate data to and can really pique interest when thinking about data visualization types.
Each variable is given its own axis, all of which are radially distributed around a central point. Data points are plotted along these axes and connected to form a polygon.

Radar/Spider charts allow you to:
- Compare multiple quantitative variables.
- Understand the strengths and weaknesses of different variables.
- Visualize multivariate data in a compact format.
However, these charts can become messy and hard to read when there are too many variables, or the values are too similar. Also, the area covered by the polygon can sometimes give a misleading impression if the values are not evenly distributed.
Ready to step up your data visualization game? Discover how to take your skills to the next level in our comprehensive guide on Data Visualization Basics .
Sequential Data Visualizations: Tracking Change Over Time
Data that is collected over time holds a unique place in data analysis. Time-series data, or sequential data, has its own set of visualization tools which are effective in showing trends, fluctuations, and patterns over a period.
Tracking metrics and KPIs over time is an excellent way to see trends.
It helps to be able to look at the same data from different perspectives at the same time and see how they fit together. Stephen Few via Tableau Blog
#10: Line Graphs – Highlighting Trends and Fluctuations
A line graph, or line chart, is a powerful tool for showing continuous data, typically over time. It comprises points connected by line segments, with the x-axis often representing time and the y-axis the quantitative variable.
Line graphs enable you to:
- Visualize trends and fluctuations in data over time.
- Compare changes in the same variable across different groups.
- Forecast future trends using historical data.
Line graphs are flexible and straightforward, but they can become cluttered if there are too many lines or time points. Also, they may not effectively represent data where values fluctuate drastically.
#11: Area Charts – Quantifying Changes Over Time
Area charts are similar to line graphs, but with the area below the line filled in. This can be beneficial when you want to demonstrate how a quantity has changed over time, particularly when you want to show the contribution of different components to a total.
With an area chart, you can:
- Visualize the magnitude of trends over time.
- Display the part-to-whole relationships.
- Highlight the total across a trend.
Despite their advantages, area charts can be hard to read if there are too many categories or if the categories overlap significantly.
#12: Stream Graphs – Displaying Density Over Time
A stream graph, also known as a theme river, is a type of stacked area graph which is displaced around a central axis, resulting in a flowing, organic shape. Stream graphs are used to display high-volume datasets, showing the changes in data over time.
Stream graphs allow you to:
- Visualize large sets of sequential data.
- Display the density of data flow over time.
- Highlight anomalies and major events within a dataset.
Stream graphs can be very visually appealing, but they might not be the best choice when precision is key, as it can be difficult to discern the exact values represented.
#13: Gantt Charts – Visualizing Project Timelines
Gantt Charts are an essential tool in project management and are used to illustrate a project schedule. It allows for the representation of the duration of tasks against the progression of time. A Gantt chart is a type of bar chart that shows both the scheduled and completed work over a period.

Using a Gantt chart , you can:
- Plan and schedule projects of all sizes.
- Set realistic timeframes for project completion.
- Monitor progress and stay on track with your plan.
While Gantt Charts are excellent for planning and tracking progress, they can become overly complex for large projects with many tasks or dependencies. In such cases, it’s crucial to maintain and update the chart regularly to reflect the true status of the project.
Geospatial Data Visualizations: Mapping Your Data
When your data is tied to specific geographical locations, traditional graphs and charts may not suffice. This is where geospatial visualizations come in. These data visualization types, such as Maps, Choropleth Maps, and Cartograms, allow you to represent data in relation to real-world locations.
Plus. Who doesn’t love a good map for context?
#14: Maps – Plotting Geographical Data
Maps are one of the most traditional forms of data visualization, providing a straightforward method of representing geographical data. This could be as simple as plotting the location of specific events or as complex as showing data variations across different regions.

With a map , you can:
- Display the geographic distribution of data.
- Identify regional patterns and trends.
- Highlight areas of interest or concern.
While maps are a powerful tool for geospatial data visualization, they may not be as effective when comparing quantities across regions, due to size and proximity variations.
#15: Choropleth Maps – Showing Regional Variations
A Choropleth map uses differing shades or colors to represent statistical data on a predefined geographic area, such as countries, states, or counties. The color intensity represents the quantity of the variable of interest, helping to visualize how this variable changes across the map.
Choropleth maps allow you to:
- Display divided geographic areas that are colored or patterned in relation to a data variable.
- Visualize how a measurement varies across a geographic area.
- Identify regional patterns and variations.
Keep in mind that choropleth maps can sometimes be misleading, as they give equal visual weight to each region, regardless of their size or the number of data points in each region.
#16: Cartograms – Distorting Reality for Clarity
Cartograms are a type of map in which some variable (like population or GDP) is substituted for land area or distance. The geometry or space of the map is distorted to convey the information of this alternate variable.
Cartograms help you to:
- Represent a specific variable more effectively by sizing regions accordingly.
- Compare variables independently from the geographical size of regions.
- Highlight discrepancies in data relative to geographic size.
Remember, though cartograms can provide a powerful representation of data, they can also distort the perception of geographical space, potentially causing confusion.
#17: Heat Maps – Showcasing Density and Intensity
Heat Maps is one of the powerful data visualization types used to represent complex data sets through color gradations. They’re often used to display how a particular quantity or frequency varies across different areas of the map.
For instance, a heat map can show the concentration of population in a region or the intensity of traffic at different times of the day.
With a heat map, you can:
- Represent complex data in an understandable way.
- Identify hotspots or areas with high concentration or activity.
- Spot correlations and patterns in large data sets.
However, heat maps may not be effective when used with data sets with few variations or when individual data points need to be distinct.
#18: Dot Distribution Maps – Representing Location and Frequency
Dot Distribution Maps, also known as dot density maps, are a type of thematic map that uses a dot symbol to show the presence of a feature or phenomenon. They’re used to visualize the geographical distribution of a particular attribute, such as population density in different regions.
Using a dot distribution map, you can:
- Depict spatial patterns or the geographical distribution of a particular phenomenon.
- Indicate the presence or frequency of an occurrence.
- Provide a visual representation of raw data.
Remember, the interpretation of dot distribution maps can be somewhat subjective, and they may not provide a clear picture of the data if the dots are too close together, overlapping, or too spread out.
#19: Parallel Coordinates – Multidimensional Analysis
Parallel Coordinates are an exceptional type of visualization used to plot individual data elements across multiple dimensions. Each data attribute has its parallel vertical axis, and values are plotted as points on each axis, connected by line segments. This visualization type is particularly useful when dealing with multivariate data.
When you use parallel coordinates, you can:
- Explore and analyze multidimensional numerical data.
- Detect correlations, outliers, and trends across multiple dimensions.
- Compare multiple variables without losing sight of individual data points.
However, parallel coordinates may not be as effective when dealing with large data sets due to overplotting. They also require a bit of learning to interpret accurately.
#20: Matrix Plots – Complex Comparisons Simplified
Matrix Plots or Matrix Charts provide a grid-like visual representation of data. Each cell in the grid represents a specific value, often using color to denote this value. It’s a great way to visualize large amounts of data and understand the correlation between different variables.
With a matrix plot, you can:
- Represent complex and large data in a simplified and concise manner.
- Compare multiple variables at once.
- Spot patterns and correlations quickly.
Keep in mind that matrix plots can be less intuitive to understand at first glance and may not be suitable when you want to emphasize individual data points.
#21: Radar Charts – Multivariate Observations
Radar Charts, also known as Spider Charts, use a circular display with several different quantitative axes starting from the same point for a detailed view of data. Each variable has its axis, and the data points are connected, forming a polygon. Radar charts are best used when you want to observe which variables have similar values or if there are any outliers amongst them.
Using radar charts, you can:
- Understand the pattern of each individual data series.
- Highlight similarities or differences between different groups.
Remember, radar charts can become cluttered and hard to read when used with many variables or categories. Additionally, they can distort data perception when the axes aren’t uniformly scaled.
#22: Word Clouds – Textual Emphasis
Word Clouds, also known as tag clouds, depict textual data where the size of each word represents its frequency or importance in a body of text. They are a fun and visually appealing way to highlight popular or high-impact words, with larger-sized words indicating higher frequency or importance.
With Word Clouds, you can:
- Visualize textual data, emphasizing popular or recurring themes.
- Analyze and present customer feedback, social media sentiment, or keyword research.
- Create visually engaging presentations of textual content.
However, keep in mind that Word Clouds are best used for illustrative purposes rather than deep analysis, as they lack precise quantitative values.
#23: Highlight Tables – Focus on Categories
Highlight Tables take data tables a step further by adding color to represent values, helping you focus on specific categories. The color intensity reflects the value in the cell, offering an at-a-glance overview of the data.
Using Highlight Tables, you can:
- Add an extra layer of detail to a basic table.
- Bring focus to high or low values in a large dataset.
- Easily compare categorical data.
Remember that while highlight tables are useful for bringing attention to specific data points, they can become overwhelming and difficult to interpret if they’re too complex or have too many categories.
#24: Bubble Clouds – Multidimensional Textual Visualization
Bubble Clouds, sometimes called Circle Packing or Bubble Charts, visualize data hierarchically as a cluster of circles. The size and color of each circle can represent additional variables. Bubble Clouds can present numeric, categorical, or textual data and are helpful when the data has many layers of categorization.
With Bubble Clouds, you can:
- Represent multilayered or hierarchical data.
- Compare and contrast different categories and subcategories.
- Add visual interest to complex datasets.
Keep in mind, however, that like with many other visually intense plots, Bubble Clouds can be challenging to understand and interpret if overused or if they include too many categories or subcategories.
Unique and Complex Data Visualizations
These data visualization types are less common but can provide unique insights when used correctly. They often display more complex data structures or more specific types of data and are best used when simpler visualizations fall short.
#25: Streamgraphs – Show Volume Over Time
Streamgraphs are stacked area charts with smooth, flowing shapes, used to visualize changes in data over time. The aesthetic appeal of streamgraphs often makes them a popular choice for public data visualizations.
With Streamgraphs, you can:
- Display high-volume data over time in a visually engaging way.
- Showcase patterns and trends in large datasets.
- Highlight the magnitude of change between different categories over time.
However, Streamgraphs can be harder to read and interpret than basic line or bar charts due to their flowing shapes, so it’s essential to consider your audience’s data literacy.
#26: Waterfall Charts – Bridge the Gap
Waterfall charts are a form of data visualization that helps demonstrate how an initial value is affected by subsequent positive and negative values. It effectively showcases the cumulative effect of sequential data, providing a ‘bridge’ from one data point to the next, hence the name “waterfall.”
With Waterfall Charts, you can:
- Visualize the cumulative effect of sequential positive and negative values.
- Show how an initial value is adjusted to a final value.
- Depict the incremental changes in a metric over time or between categories.
Keep in mind that Waterfall Charts can become complex and hard to interpret if they contain too many categories or steps.
#27: Chord Diagrams – Visualizing Inter-Relationships
Chord Diagrams are circular charts used to display the inter-relationships between data in a matrix. The data points are arranged around a circle with the relationships depicted as arcs connecting the data points.
With Chord Diagrams, you can:
- Represent complex inter-relationships between different data points.
- Visualize network structures or flow data.
- Present multidimensional data in a single plot.
Chord Diagrams are complex and require a higher degree of data literacy to interpret correctly. Therefore, it’s advisable to use them when your audience has a good understanding of the data and the relationships being represented.
#33: Heatmaps – Visualize Magnitude of Phenomena
Heatmaps are data visualizations that use color-coding to represent different values of data. Heatmaps are excellent tools for displaying large amounts of data and showing variance across multiple variables, helping to visualize complex data sets.
With Heatmaps, you can:
- Visualize large amounts of data in a compact space.
- Display variations across multiple variables.
- Understand complex data sets intuitively through color differentiation.
However, Heatmaps can become hard to interpret when there are too many categories or if the color differentiation isn’t clear.
#34: Dot Distribution Maps – Geographical Representation of Data
Dot Distribution Maps are used to show the geographical distribution of phenomena. Each dot represents a specific quantity of the phenomena at a particular location. They are most effective when you want to show density or distribution over a geographic area.
With Dot Distribution Maps, you can:
- Show geographic distribution of a single category or multiple categories.
- Highlight density or concentration in specific areas.
- Represent large datasets on a geographical layout.
Dot Distribution Maps can become confusing when there are too many dots or categories, so it’s essential to use them judiciously.
#35: Bubble Clouds – Multi-Dimensional Visualizations
Bubble clouds are similar to scatter plots but with an additional dimension represented by the size of the bubbles. The X and Y axes represent two dimensions, while the size (and sometimes color) of the bubbles represent additional dimensions.
- Visualize multi-dimensional data in a single plot.
- Show relationships and disparities between data points.
- Highlight the significance of specific data points using the bubble size.
Bubble Clouds can become complex if there are too many bubbles or if the bubbles overlap, making it hard to interpret the data accurately.
Remember, while adding more types of visualizations to your list can make it comprehensive, the key is to help your reader understand when and how to use each type effectively.
The Art of Choosing the Right Visualization: Concluding Thoughts
Navigating the vast landscape of data visualizations can initially seem like a daunting task, but with the right understanding and tools, it transforms into an exciting journey. Remember, data visualizations are a powerful medium to convey complex information in an easily digestible and engaging way. However, the effectiveness of your visualization hinges on choosing the right type.
When deciding which visualization to use, here are some fundamental aspects to consider:
1. The Nature of Your Data: The type and structure of your data are key determinants in your choice of visualization. Numerical data might be best served by bar or line charts, while geographical data can be presented as a map. Categorical data, on the other hand, might warrant a pie chart or a treemap.
2. The Message You Want to Convey: What’s the story you want to tell with your data? Are you highlighting a trend, comparing items, or showing a relationship? The goal of your communication heavily influences your choice.
3. The Audience: Consider who will be interpreting your visualization. What’s their level of data literacy? Are they familiar with more complex visualizations or should you stick to the basics? Tailoring your visualization to your audience ensures your data story is received as intended.
4. Simplicity vs. Complexity: While some visualizations can depict complex, multi-dimensional data, simplicity often leads to better understanding. If a simpler visualization can tell the same story, it might be the better choice.
5. Trial and Experimentation: Don’t be afraid to experiment with different visualizations. Often, it’s not until you see your data in several visual forms that the most effective one becomes apparent.
In conclusion, the art of data visualization lies in striking the balance between aesthetic appeal and functional communication. The right visualization accentuates your data’s story, driving insight and aiding decision-making. Each type of data visualization has its strengths and appropriate uses, so choose wisely and let your data shine. And always remember, the ultimate aim of data visualization is not just to make data look pretty, but to make it meaningful and accessible for everyone.
If you’re intrigued by the possibilities of data visualization, learn about the key skills you need to master in our essential guide on Data Visualization Basics .
Become a Better Analyst in Just 5 Minutes a Week
☕Data Brewed is your go-to resource to level-up your data analytics and visualization game.
Actionable tips & curated discoveries to stay ahead of the curve.
Unsubscribe at anytime
You’re in!
✨ Get ready for actionable tips and curated discoveries delivered right to your inbox.
Analyze, Visualize, Thrive!
I'm Renee, the creator behind Coffee Break Data, where my MS in Data Analytics fuels my drive to simplify the complex. I'm here to boost your data confidence, one insightful—and caffeinated—moment at a time.
Similar Posts
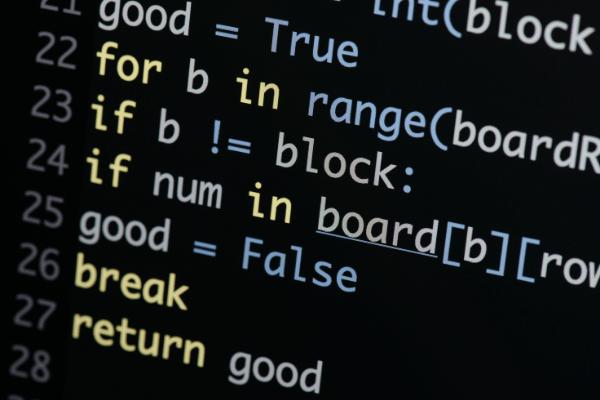
Does Tableau Need Coding?
Tableau has become a huge player in the data space because it has powerful features. You’ll find plenty of great looking dashboards to…
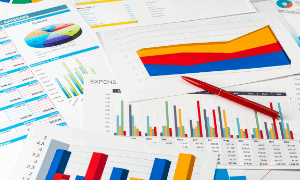
How Easy Is It To Learn Data Visualization?
Yay! I’m so excited that you’re here thinking about learning data visualization! If you’re willing to put the effort in, you will be…
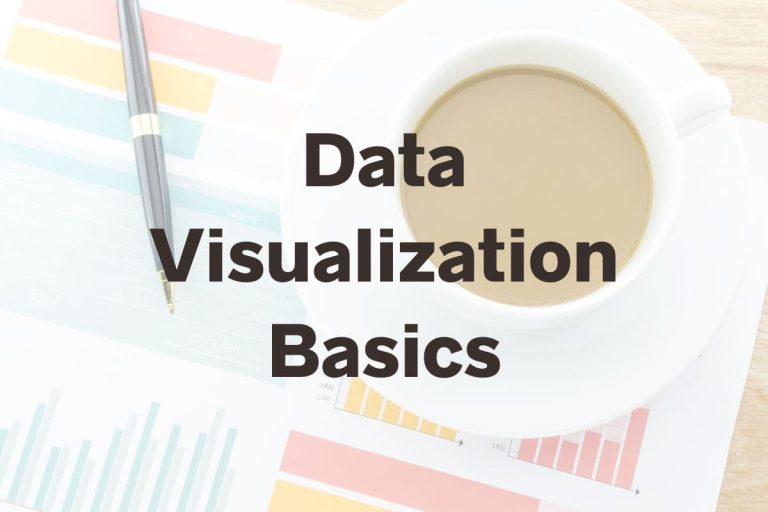
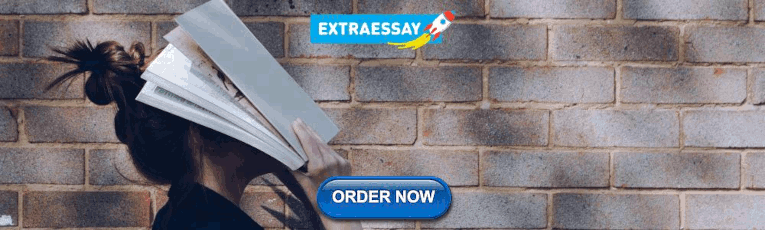
Uncover Insights Faster: Mastering Data Visualization Basics
Mastering the data visualization basics paves the way for impactful presentations & facilitate data-driven decision making with the right strategies.
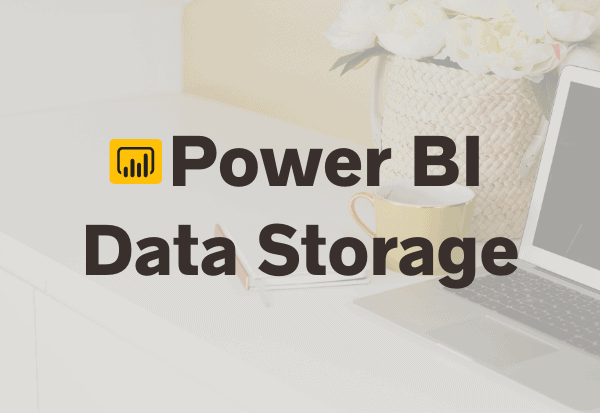
This Is How Data Is Stored In Power BI
Knowing where data is stored helps you stay on top of your data’s security. Data tools make analyzing data so much more simple,…
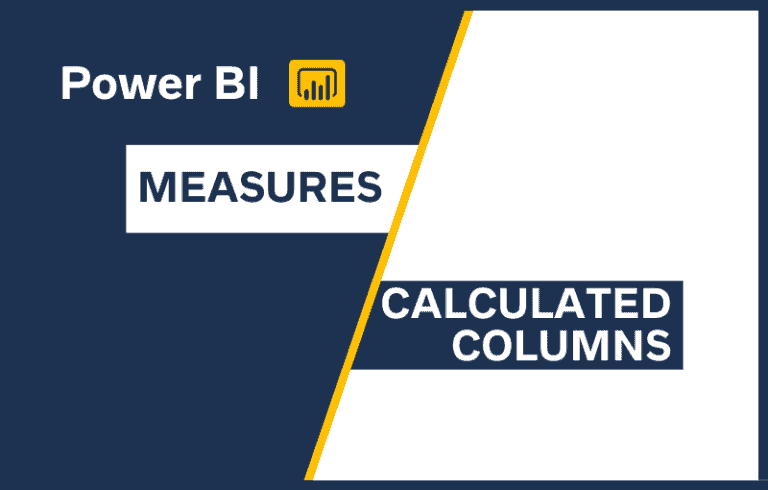
Power BI Measures vs Calculated Columns – Why is it so hard?!
When first learning Power BI, it can be difficult to figure out when to create a new measure versus when to create a…
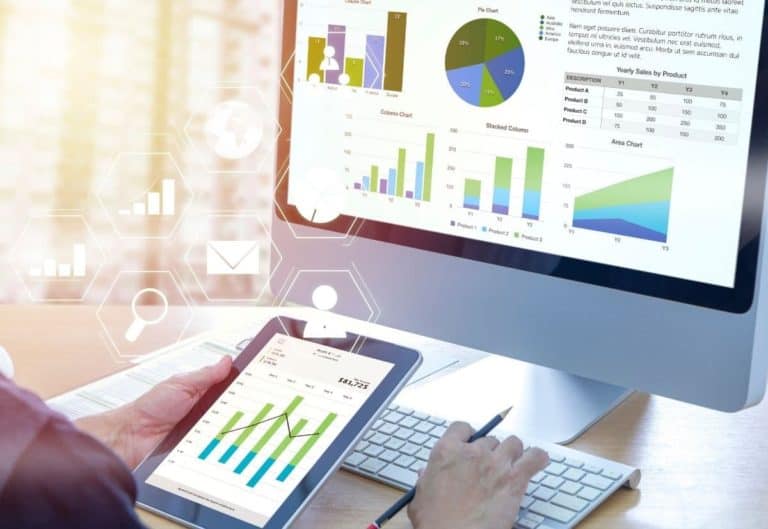
22 FREE Visualization Tools to Try Today
Software can be expensive, and data visualization software is no exception. Microsoft’s Power BI appears free until you realize the levels of their…
Leave a Reply Cancel reply
You must be logged in to post a comment.

Line Segment – Definition, Formula, Examples
What is line segment, real life examples of line segment, how to measure a line segment.
- Solved Examples
- Practice Problems
- Frequently Asked Questions
A line segment is a line section that can link two points .
Let us understand the line segment with the help of the diagrams below:
This is a line! It has no endpoints and extends endlessly in both directions.
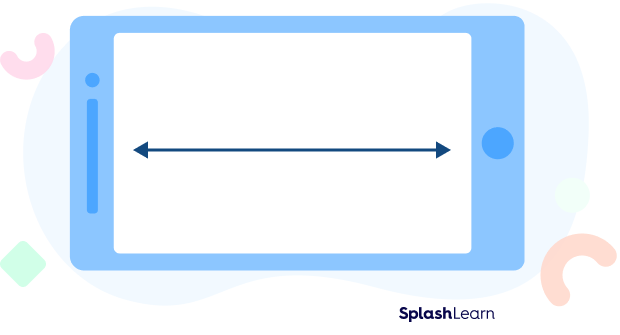
If you mark two points A and B on it and pick this segment separately, it becomes a line segment.
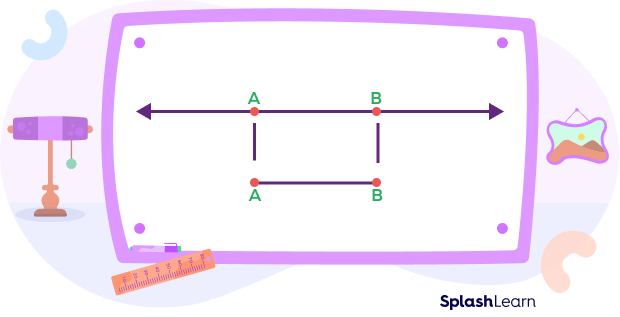
This line segment has two endpoints A and B whose length is fixed. The length of this line segment is the distance between its endpoints A and B.
So, a line segment is a piece or part of a line having two endpoints. Unlike a line, a line segment has a definite length.
The length of a line segment can be measured either in metric units such as millimeters , centimeters , or customary units like feet or inches .
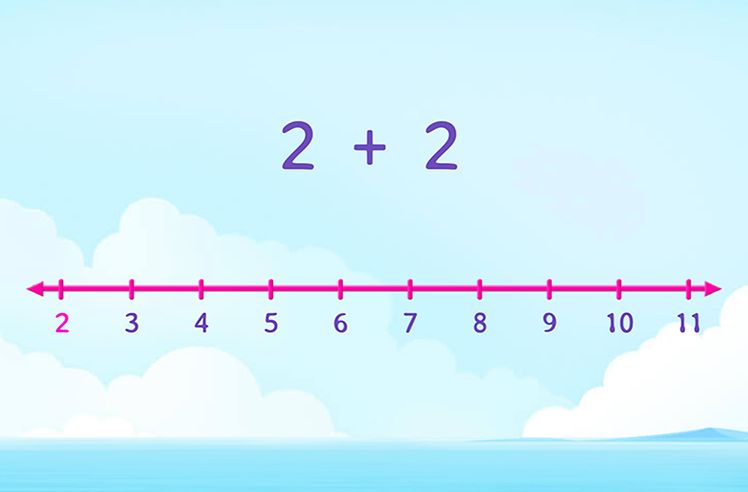
Symbol of Line
A line segment is usually represented by the bar symbol ( — ) on top of the endpoints. Say, a line segment has endpoints P and Q, it can be denoted by $\overline{PQ}$.
Related Worksheets
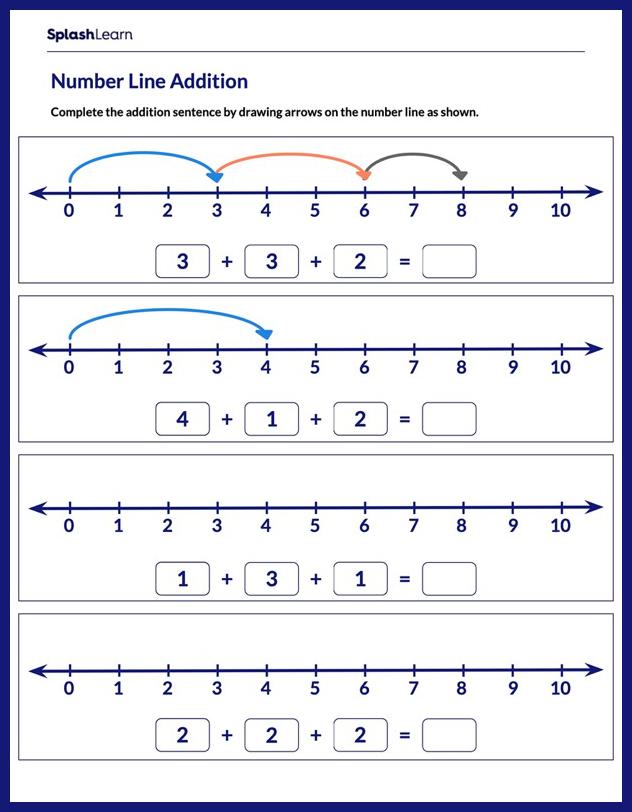
- Edges of table.
- Side of a square or triangle
- Matchstick.
- Edge of a ruler .
When you have two line segments, you can tell whether a line segment is longer or shorter than the other just by looking at them. We can see that the line segment CD is longer than AB. However, we cannot always rely on observation to find the length of a line segment.
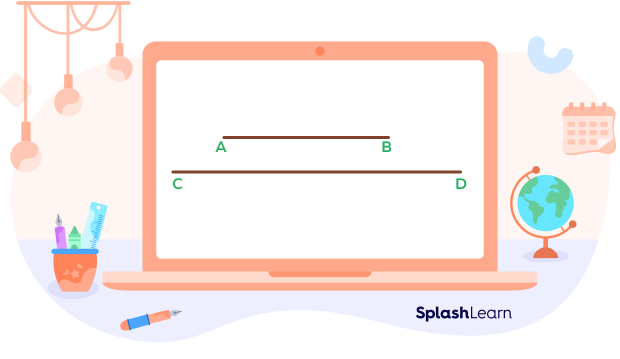
Follow the given steps to measure the length of a line segment:
Step 1: Pick up a scale to measure the length of a line segment. Generally, smaller line segments are measured using a centimeter scale.
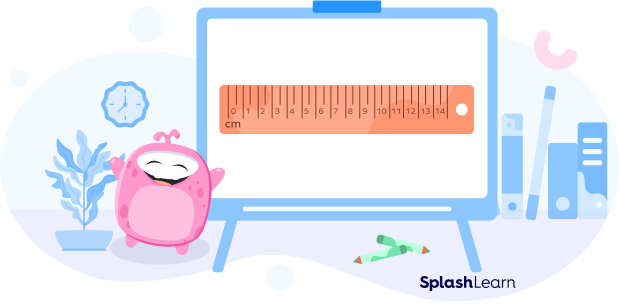
Step 2: Identify the line segment you want to measure.
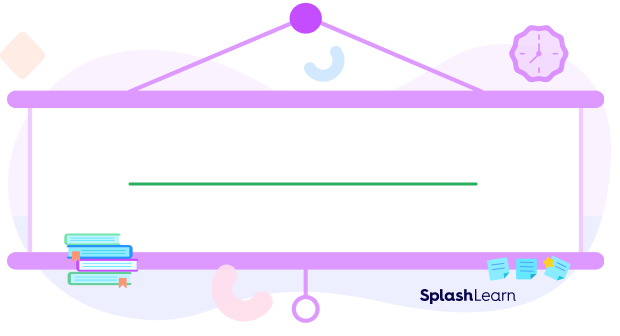
Step 3: Place the zero marking of the ruler at the starting point of the line segment.
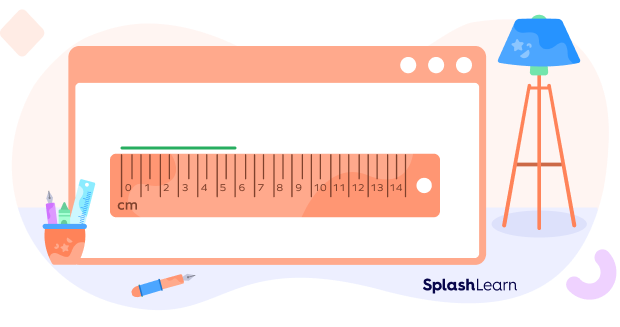
Step 4: Read the number on the scale where the line segment ends. In this case, it is 5. So, the length of the given line segment is 5 cm.
Drawing a Line Segment Using Ruler and Compass
Suppose we need to draw a line segment of length 5 cm. We will follow the given steps:
- Step 1: Draw a line of any length. Mark a point A on the line, which is the starting point of the line segment.
- Step 2: Using a ruler, place the pointer of the compass 5 cm apart from the pencil’s lead.
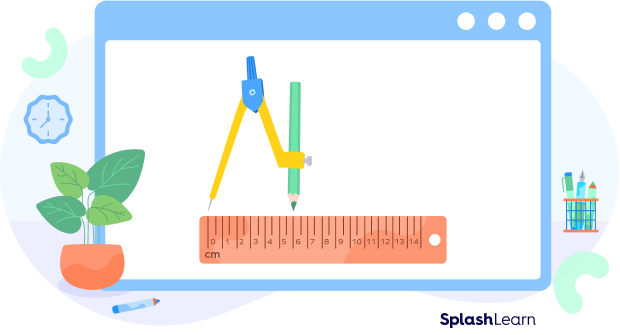
- Step 3: Place the pointer of the compass at A and mark an arc on the line with the pencil point.
- Step 4: Mark the point where the arc and the line intersect as B.
- Step 5: AB is the required line segment of length 5 cm.
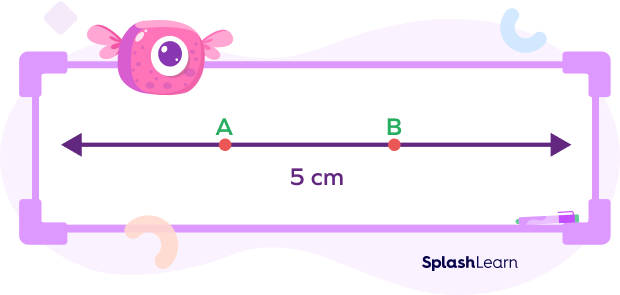
Fun Facts About Line Segments
Two words, line and segment, combine to form a line segment. The word line originates from Latin Segmentum, which means strip, a piece cut off, or a segment of earth, while segment comes from Latin Segmentum, which means strip, a piece cut off, or a segment of earth.
Solved Examples on Line Segments
Example 1: Write the name of given line segments.

$\overline{PQ}$ , $\overline{XY}$
Example 2: Write all possible line segments in the given figure.

$\overline{UV}$, $\overline{VW}$ , $\overline{UW}$
Example 3: Count the number of line segments in the given figure.
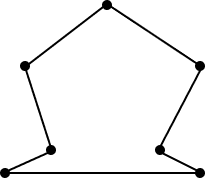
The given figure is made of 7 line segments.
Practice Problems on Line Segments
Line segment.
Attend this Quiz & Test your knowledge.
Determine which of the following has two endpoints:
Which of these represent a line segment, a rectangle has ______ sides., which of these is the unit of line segment..
If you want to learn more about Line Segments in fun and new ways or want to study similar math concepts like ratios, fractions, etc. easily, visit SplashLearn for some interesting Q&A and solved examples. What’s more, SplashLearn offers you to sign up for free now!
Frequently Asked Questions on Line Segments
What is the difference between a line and line segment?
A line segment has endpoints, whereas a line can be extended on both ends indefinitely.
Is there a difference between a line segment and a ray?
Yes. A ray and a line segment are different. A ray has one endpoint, while a line segment has two. One end of the ray extends indefinitely, whereas, in a line segment, the endpoints are always defined and fixed.
How do you define the midpoint of a line segment?
The midpoint of a line segment is the point in the middle of the line segment that divides it into two equal sections.
What is line segment congruence?
If two figures are the same size and shape, they are congruent. As a result, any two line segments with the same length can be deemed congruent.
RELATED POSTS
- Edges in Math – Definition With Examples
- Like and Unlike Algebraic Terms – Definition, Facts, Examples, FAQs
- Perimeter of a Rhombus – Definition, Formula, Examples, Facts, FAQs
- Coprime Numbers: Definition, Steps, List, Properties, Examples
- International Place Value Chart – Solved Examples, Facts, FAQs
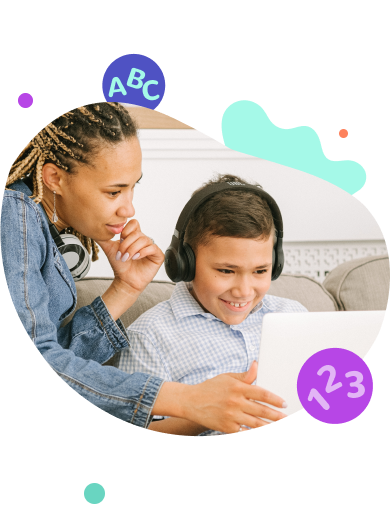
Math & ELA | PreK To Grade 5
Kids see fun., you see real learning outcomes..
Make study-time fun with 14,000+ games & activities, 450+ lesson plans, and more—free forever.
Parents, Try for Free Teachers, Use for Free
10 Data Analytics Charts: Types and Use Cases [+ Data Visualization Examples]
15 min read
![a visual representation of data using line segments 10 Data Analytics Charts: Types and Use Cases [+ Data Visualization Examples] cover](https://blog-static.userpilot.com/blog/wp-content/uploads/2023/11/10-data-analytics-charts-types-and-use-case-plus-data-visualization-examples_30e02eb570780026d686c273cf755177_2000.png)
You can’t do anything with data without data analytics charts.
They help you visualize product analytics data , make sense of it, and come up with the right decisions for your business.
However, there are many types of data charts, and learning when to use each can become confusing (and sometimes a matter of preference). So, in this guide, we’ll go through each one, explain their varieties, and discuss when are the best instances to use them.
- Bar charts illustrate categorical data with horizontal bars where the lengths of the bars are proportional to the values they represent. Its types include simple bar charts, grouped bar charts, and stacked bar charts.
- Column charts display information in vertical bars where the lengths of the bars correspond to the magnitudes they represent. There are clustered column charts and stacked column charts.
- Line charts, as the name suggests, use lines to connect individual data values and map a graph. It can be represented as a single-line chart or multiline chart.
- Funnel charts illustrate the progressive reduction of data as it passes through multiple phases, which is helpful for mapping the sales funnel or the customer journey. It can be either vertical or horizontal.
- Pie charts reflect the proportional representation of different categories within a whole. They are called “pie” charts because of their round shape, which is divided into “slices” or sectors representing different segments. It can be a basic pie, an exploded pie chart, or a doughnut chart.
- Heatmaps use color-coded systems to represent the magnitude of data points across a web page. It can track user’s eyes, clicks, mouse movements, scrolling patterns, rage clicks, and overall engagement with your app.
- Cohort tables are a versatile tool to visualize and track the behavior of different user groups—or cohorts—over time. And they can either be time-based or behavioral, depending on the metric you’re comparing.
- A dot chart, or dot plot, places individual data points on a simple scale without any adjustments. It can vary between basic dot charts, Cleveland dot charts, and quantile dot charts.
- Bubble charts use circles or “bubbles” to represent the magnitude of a data point, helping you to visualize three dimensions of data in one plot. It can either be in 3D or scattered.
- A stacked area chart is a multilayered graphic that accumulates data points from multiple categories to create a cumulative effect, with each layer representing a different category. It can be illustrated as a basic stacked chart or as a 100% stacked chart to represent percentages.
- Since you’ll need a product analytics tool to visualize your data like this, why not book a Userpilot demo ?
#1 Bar charts
Bar charts illustrate categorical data with horizontal bars where the lengths of the bars are proportional to the values they represent. It makes comparing different categories trivially easy, quick, and efficient for data comparison (and decision-making).
Types of bar chart
There are many ways to organize data in bar charts, here are three types:
- Simple Bar Chart : The most simple type of chart for comparing different groups. Each bar represents a single category, and their length corresponds to its value.
- Grouped Bar Chart : This is used to compare the values of different sub-groups of your main categories. It displays the sub-categories by groups for easy comparison. For example, to compare how different teams performed at different tasks.
- Stacked Bar Chart : Stacked bar charts “stack” sub-group bars on top of each other, making it simple to see the contribution of each sub-group to a total value. It’s useful when surveying, for example, companies from different industries and comparing how many respondents from each industry chose a specific response.
When to use bar charts
Bar charts are especially useful for:
- Visually comparing the quantities or values of different categories.
- Displaying the distribution of data within sub-groups and categories.
- Comparing values across a single dimension and multiple categories.
- Studying the performance of different segments in your sample.
Bar chart example
An example of a bar chat use case could be to showcase your product’s daily usage and compare the popularity of your main features—understanding the percentage of users who are engaging with your product.

#2 Column charts
Column charts are just like bar charts, with the important difference being that they display information in vertical bars. Here, the lengths of the bars correspond to the magnitudes they represent as well. It can be used to compare different data sets and track changes in values over time, as well as spot trends across time and data groups.
Types of column charts
These types of column charts follow the same pattern as the bar charts, and they include:
- Clustered Column Chart. Which is ideal for comparing different categories of data points across multiple groups. It makes it easier to interpret how different segments perform against each other. And it works better with medium-sized datasets, so it doesn’t clutter the chart with too many sub-categories.
- Stacked Column Chart. It stacks data vertically within a column to display the total sum between categories while also comparing their values across other categories. It’s useful when you have multiple categories that contribute to a total sum (like total sales broken down by product) and need to visualize these proportions and highlight individual contributions.
When to use column charts
Column charts are used for different purposes, including:
- Comparing the values of segmented groups, as well as dates, months, quarters, etc.
- Showcasing trends over time, especially when focusing on time intervals (months, quarters, years, etc.) with no specific continuity.
- Ranking or ordering the categories based on the magnitude of their values.
- Comparing the performance of different segments across time or other categories.
- Denoting the frequency of events or occurrences.
Column chart example
If you need to compare your product’s main feature usage over time, for example, you can use a stacked column chart to display the number of daily active users who engage with these features each day.
This way, you can spot patterns or trends you can leverage to prepare marketing initiatives such as sending feature update announcements.

#3 Line charts
Line charts, as the name suggests, use lines to connect individual data points. It’s useful to monitor the variability of a value over a period of time. They can clearly illustrate trends in data at equal intervals, making them a go-to model for showcasing progress and forecasting future trends.
Types of line graph
The different types of line graphs are pretty simple:
- Basic Line Chart : This chart type displays data points connected by one straight line, representing a single variable’s evolution over a certain period. It excels in showcasing trends and patterns of only one metric.
- Multiline Chart : It showcases correlations and trends as well but between multiple variables. Each line represents a different parameter, making it possible to compare multiple data sets concurrently.
When to use line charts
Line charts are especially useful for:
- Tracing trends and patterns in data over a continuous dimension (most commonly, time).
- Comparing trends and patterns between different categories or variables.
- Prove a relationship and correlation between two variables.
- Displaying cumulative effects over time.
Line chart example
Let’s say you need to track product usage by device in order to optimize your app’s compatibility and prioritize the most used devices. A multiline chart can easily show you the trends and changes in product usage for every device so you can compare and see what optimizations are worth implementing.

#4 Funnel charts
Funnel charts illustrate the progressive reduction of data as it passes through multiple phases. Essentially, these charts lay out a process that begins at 100% and follows a smaller percentage after each stage so you can visualize, for example, how many leads passed through a sales process. They’re helpful for visualizing the customer journey or sales process, analyzing each step, and identifying potential areas of friction that can be improved.
Funnel chart types
There are two different types of funnel charts:
- Vertical Funnel Chart : This chart type displays data in a vertical direction, starting from the top and narrowing down to the bottom. Ideal for illustrating a process that has consequential steps, it’s especially handy for visualizing sales or conversion processes where you start with a large initial group that gradually dwindles down to a final result.
- Horizontal Funnel Chart : Quite similar to its vertical counterpart but displayed horizontally, this chart is perfect for scenarios where horizontal space is more than vertical, like presentations and infographics. It offers the same functionality of illustrating processes with sequential steps, often used for depicting pathways such as website visitor paths, till the user reaches the final action point.
When to use funnel charts
Funnel charts are primarily used to perform funnel analysis , which in itself can help you do many important tasks, such as:
- Laying out your marketing funnel to check how each stage is performing.
- Representing the stages of your sales pipeline and see how your leads have progressed through it.
- Displaying the conversion funnel across multiple channels.
- Visualizing how users progress through the user journey, spot points of friction, and optimize the customer experience.
Funnel chart example
In SaaS, you can perform funnel analysis to visualize the user journey . This allows you to identify stages where users are facing the most friction and dropping off the most often—providing improvement opportunities for the product experience.

#5 Pie charts
Pie charts reflect the proportional representation of different categories within a whole. They are called ‘pie’ charts because of their round shape, which is divided into “slices” or sectors representing different segments.
Each slice of the pie corresponds to a category from your data set, and the size of each slice is proportional to the percentage of the whole that category represents, allowing you to instantly understand the relationship between the part and the whole.
Types of pie chart
Although there are many types of pie charts, these are the most used varieties you’ll end up using:
- Basic pie charts : The simple, circular charts display data percentages in the form of slices, where each slice represents a unique segment from a whole sample.
- Exploded pie charts : These are variations of the basic pie chart where one or more slices are separated from the main body of the chart. They work great for highlighting certain categories or emphasizing distinctions between parts of the data being analyzed.
- Doughnut charts : These are similar to basic pie charts but with a blank center, giving them a doughnut-like appearance. The empty center offers a space for additional information, such as overall data figures or the sample size.
When to use pie charts
Pie charts are useful for comparing data from a handful of segments, as well as percentages. Just beware that too many slices can clutter the chart and make it hard to discern.
Pie chart example
A great use case for pie charts is to watch feature frequency broken down by plans. This way, you can find out which type of users are more likely to engage with specific features.

#6 Heatmaps
Heatmaps use color-coded systems to represent the magnitude of data points across a web page. They’re designed to convert complex statistical data into an intuitive color spectrum.
Said spectrum helps you identify scrolling trends, click patterns, or popular movements on a website so you can determine which elements draw the most attention and action from users.
Types of heatmap
Although there are many ways to make heatmaps, most heatmaps tools will offer these types of graphics:
- Eye Tracking Heatmaps : It tracks users’ eye movements across the interface, showcasing where they gaze, pause, and blink. It provides insights into visual patterns and user attention so you can improve the interface designs to build more engagement.
- Click Heatmaps : These maps visualize where users click or tap the most (and therefore show what elements draw the most attention). They’re often color-coded, with hot spots showing popular areas and cold spots showing areas with fewer interactions.
- Movement Heatmaps : They capture cursor or finger movement across the screen, where yellow or red areas indicate higher levels of activity. This way, you can see the mouse movements users take when navigating your product or website.
- Scroll Heatmaps : These maps show how far down users scroll, typically showing warmer colors at the top while gradually fading out as you move down the page. This way, you can assess the effectiveness of your page length, the placement of key content, and overall user engagement.
- Engagement Heatmaps : They measure which sections of your interface are visually engaging to users, offering insights on design aesthetics and content relevance to improve user experience.
When to use heatmaps
Heatmaps are especially helpful for tracking navigational data within an app or website, allowing you to:
- Display data density to identify concentrations or patterns within a specific data set.
- Represent user engagement on a website or application.
- Optimize your website or app to incentivize specific user behavior.
Heatmap example
One way to leverage heatmaps is to analyze feature engagement. For example, you could use a tool like Userpilot to track how many interactions users have with your app features.
This way, you can see which buttons are most clicked, and optimize your feature’s interface to drive more product stickiness .

#7 Cohort tables
Cohort tables are a versatile tool to visualize and track the behavior of different user groups—or cohorts—over time. Here, the columns usually represent user groups (who, for example, signed up on different periods), while the rows represent time periods.
This chart lets you compare data from multiple groups across a timeline you can use to keep track of user engagement, retention, and churn rates .
Types of cohort table
There are two common types of cohort tables:
- Time-based Cohort Tables : It groups customers by specific time frames (like the month they made their first purchase) and lists their performance across multiple periods of time (days, weeks, months.) It can be useful for comparing, for example, onboarding completion between users who signed up before and after a specific update.
- Behavioral Cohort Tables : It groups customers based on an action performed during a specific frame (users who completed primary onboarding the last month) and correlates it with other behavioral data such as engagement, retention, or conversions. This way, you can come up with personalized product strategies for cohorts that seem to be disengaged.
When to use cohort tables
Cohort tables are quite useful for:
- Tracking the performance of multiple cohorts (retention, engagement, milestones, etc) over time.
- Identifying cohorts that are disengaging or require special attention.
- Analyze the receptivity of a new feature you just launched and see how long it takes for different cohorts to adopt it over time.
Cohort table example
A great instance to use cohort tables is to track retention rates over time, as it allows you to watch the percentage of users from each cohort who come back to use a specific feature over time.
This way, you can see which cohorts are disengaging too fast and understand why.

#8 Dot charts
A dot chart, or dot plot, places individual data points on a simple scale without any adjustments. It helps you visualize individual data points to pin down trends, detect patterns, or find outliers within various datasets.
Its precision makes it easier to interpret statistical data that can’t be accurately visualized with a line chart.
Dot chart types
- Basic Dot Charts : They’re useful to present grouped or clustered data points, where each dot represents an individual data point. It can provide a simple, clean visualization of sample data without any adjustment.
- Cleveland Dot Charts : It emphasizes the relative position of data points along a common horizontal (or vertical) line, making it easier to compare different data points and observe trends within your dataset. It’s very similar to a bar chart but uses dots instead.
- Quantile Dot Charts (Wilkinson chart) : This chart uses dots to display density. It can effectively illustrate the variation or distribution across different categories, magnitudes, or time periods—making it useful for large data sets.
When to use dot charts
Dot charts are very versatile and can be used for many purposes, including:
- Highlighting individual data points in small datasets, hence allowing you to place special emphasis on each specific observation.
- Organize, analyze, and visualize larger sets of data.
- Visualizing patterns, trends, or clusters within multiple segments of data.
- Illustrate the variability and dispersion of data, helping you spot specific patterns or correlations within the data.
Dot chart example
One way to use dot charts is to track feature interactions among active users and see, for instance, the popularity of each feature according to the number of interactions it has from users.
This way, you can see which features are mostly used by loyal customers and try to replicate their behavior in the rest of your user base to drive customer loyalty .

#9 Bubble charts
Bubble charts help you to visualize three dimensions of data in one plot. They function like scatter plots but use circles or “bubbles” to represent the magnitude of a data point.
Essentially, the location of the bubble along both the x and y-axes represents two dimensions, while the bubble’s size measures the third dimension.
These charts work great for displaying relationships between three variables without having to rely on 3D graphics.
Bubble chart types
There are two ways to visualize bubble charts:
- 3D Bubble Charts : It illustrates the bubbles in 3D to visually represent the three dimensions of data. Bubbles can vary in size and color based on the data they represent, making them useful for complex multi-variable analyses and identifying relationships between three or more variables.
- Scatter Plot Bubble Charts : These charts work as dot plots but with bubbles that represent a third dimension. It helps highlight relationships between multiple variables and works great for forecasting trends, identifying potential outliers, or shaking out correlations within your data set.
When to use bubble charts
Overall, bubble charts are an excellent tool to visualize data where multiple variables can represent a correlation, making it good for:
- Find out the relationship between multiple business metrics to find out which numbers lead to business success.
- Visualizing the correlation between in-app behaviors such as time spent on the page and engagement.
- Plot feature requests to get a visual representation of priorities based on parameters like user demand, development time, and potential impact.
- Comparing the risk/reward ratio of multiple strategies to make more informed decisions.
Bubble chart example
A great instance of a bubble chart use case is for studies that require statistical depth. The following example (by Hubspot) shows how the gender and age of a user can have an effect on their time spent online.
And as the graphic shows, it seems like females tend to spend more time online as they grow up.

#10 Stacked area charts
A stacked area chart is a multilayered graphic that accumulates data points from multiple categories to create a cumulative effect, with each layer representing a different category.
It uses the “area” beneath the lines to represent volume, making it useful when you need to display how individual categories contribute to the whole over time while maintaining the total and proportions of each category intact.
Stacked area chart types
Stacked area charts are often represented in two ways:
- Basic Stacked Area Chart : The regular visual that layers series of data upon each other, filling in the space below the lines to clearly show the cumulative value of multiple data series over time. It can be used for understanding the proportions of categories contributing to total values.
- 100% Stacked Area Chart : This chart type also visualizes the cumulative trends of multiple data series. However, it normalizes the data to represent each category as a percentage of a whole (100%). It provides a more comparative perspective, allowing easy identification of not just the total trends but the relative contributions of each category over time.
When to use stacked area charts
Stacked area charts are an excellent visualization tool for:
- Visualizing the contribution of multiple categories of data over time to check their performance.
- Spot changes or shifts in the composition of data over time to point out trends and patterns.
- Compare the performance or contribution of each category over time and see which ones make more impact.
- Illustrate the relative proportions of different components within a total, whether by a sum or a percentage.
Stacked area chart example
An example of how to use stacked area charts is to observe the cumulative effect of users with different plans on their engagement with a specific activity and track it over time.
This way, despite seeing the same number of engagements in the last few days, you can discern if the majority of it is coming from freemium or paid users (and if it has changed recently).

There are data analytics charts for every context and pool of data.
Once you have enough experience using them, you’ll be able to collect more insights from the same data, perform data-driven strategies, and unlock product growth potential for your company.
And since you’ll need a product analytics tool for this, why not book a Userpilot demo ? Here, you’ll be able to actively process user data and implement product strategies that lead to more SaaS growth—all with a low budget and without having to code!
Leave a comment Cancel reply
Save my name, email, and website in this browser for the next time I comment.
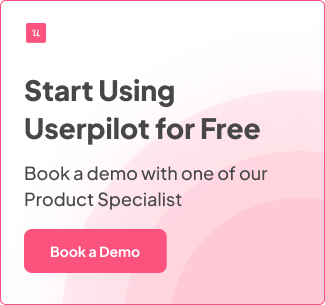
Get The Insights!
The fastest way to learn about Product Growth,Management & Trends.
The coolest way to learn about Product Growth, Management & Trends. Delivered fresh to your inbox, weekly.

The fastest way to learn about Product Growth, Management & Trends.
You might also be interested in ...
13 best customer tracking software options for your product, pendo resource center: limitations and better alternatives.
Adina Timar
UserGuiding vs Whatfix: Which is Better for Interactive User Guides?
Aazar Ali Shad
Home Blog Design Understanding Data Presentations (Guide + Examples)
Understanding Data Presentations (Guide + Examples)
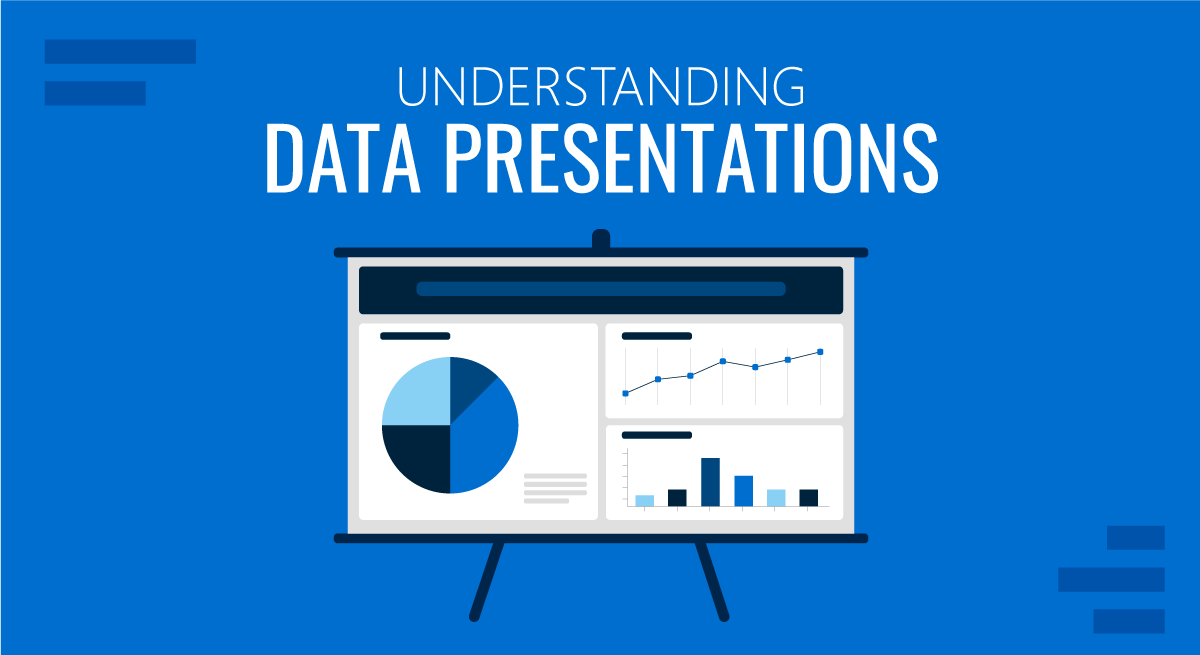
In this age of overwhelming information, the skill to effectively convey data has become extremely valuable. Initiating a discussion on data presentation types involves thoughtful consideration of the nature of your data and the message you aim to convey. Different types of visualizations serve distinct purposes. Whether you’re dealing with how to develop a report or simply trying to communicate complex information, how you present data influences how well your audience understands and engages with it. This extensive guide leads you through the different ways of data presentation.
Table of Contents
What is a Data Presentation?
What should a data presentation include, line graphs, treemap chart, scatter plot, how to choose a data presentation type, recommended data presentation templates, common mistakes done in data presentation.
A data presentation is a slide deck that aims to disclose quantitative information to an audience through the use of visual formats and narrative techniques derived from data analysis, making complex data understandable and actionable. This process requires a series of tools, such as charts, graphs, tables, infographics, dashboards, and so on, supported by concise textual explanations to improve understanding and boost retention rate.
Data presentations require us to cull data in a format that allows the presenter to highlight trends, patterns, and insights so that the audience can act upon the shared information. In a few words, the goal of data presentations is to enable viewers to grasp complicated concepts or trends quickly, facilitating informed decision-making or deeper analysis.
Data presentations go beyond the mere usage of graphical elements. Seasoned presenters encompass visuals with the art of data storytelling , so the speech skillfully connects the points through a narrative that resonates with the audience. Depending on the purpose – inspire, persuade, inform, support decision-making processes, etc. – is the data presentation format that is better suited to help us in this journey.
To nail your upcoming data presentation, ensure to count with the following elements:
- Clear Objectives: Understand the intent of your presentation before selecting the graphical layout and metaphors to make content easier to grasp.
- Engaging introduction: Use a powerful hook from the get-go. For instance, you can ask a big question or present a problem that your data will answer. Take a look at our guide on how to start a presentation for tips & insights.
- Structured Narrative: Your data presentation must tell a coherent story. This means a beginning where you present the context, a middle section in which you present the data, and an ending that uses a call-to-action. Check our guide on presentation structure for further information.
- Visual Elements: These are the charts, graphs, and other elements of visual communication we ought to use to present data. This article will cover one by one the different types of data representation methods we can use, and provide further guidance on choosing between them.
- Insights and Analysis: This is not just showcasing a graph and letting people get an idea about it. A proper data presentation includes the interpretation of that data, the reason why it’s included, and why it matters to your research.
- Conclusion & CTA: Ending your presentation with a call to action is necessary. Whether you intend to wow your audience into acquiring your services, inspire them to change the world, or whatever the purpose of your presentation, there must be a stage in which you convey all that you shared and show the path to staying in touch. Plan ahead whether you want to use a thank-you slide, a video presentation, or which method is apt and tailored to the kind of presentation you deliver.
- Q&A Session: After your speech is concluded, allocate 3-5 minutes for the audience to raise any questions about the information you disclosed. This is an extra chance to establish your authority on the topic. Check our guide on questions and answer sessions in presentations here.
Bar charts are a graphical representation of data using rectangular bars to show quantities or frequencies in an established category. They make it easy for readers to spot patterns or trends. Bar charts can be horizontal or vertical, although the vertical format is commonly known as a column chart. They display categorical, discrete, or continuous variables grouped in class intervals [1] . They include an axis and a set of labeled bars horizontally or vertically. These bars represent the frequencies of variable values or the values themselves. Numbers on the y-axis of a vertical bar chart or the x-axis of a horizontal bar chart are called the scale.
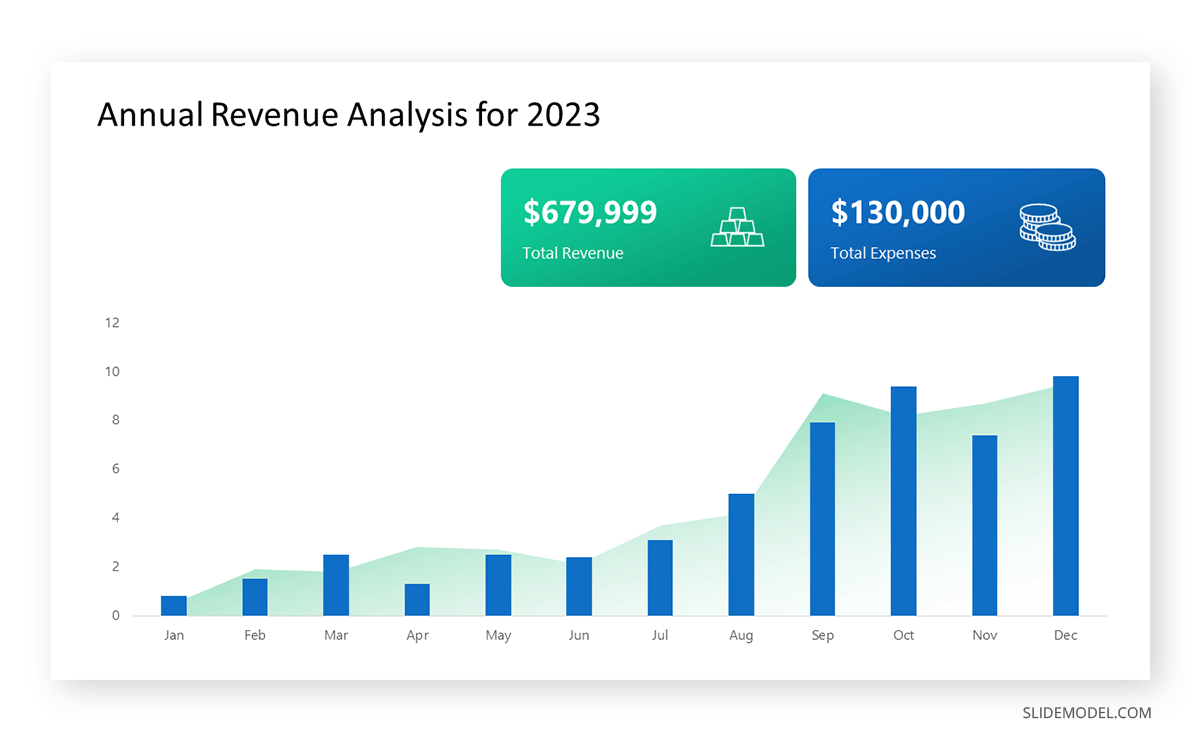
Real-Life Application of Bar Charts
Let’s say a sales manager is presenting sales to their audience. Using a bar chart, he follows these steps.
Step 1: Selecting Data
The first step is to identify the specific data you will present to your audience.
The sales manager has highlighted these products for the presentation.
- Product A: Men’s Shoes
- Product B: Women’s Apparel
- Product C: Electronics
- Product D: Home Decor
Step 2: Choosing Orientation
Opt for a vertical layout for simplicity. Vertical bar charts help compare different categories in case there are not too many categories [1] . They can also help show different trends. A vertical bar chart is used where each bar represents one of the four chosen products. After plotting the data, it is seen that the height of each bar directly represents the sales performance of the respective product.
It is visible that the tallest bar (Electronics – Product C) is showing the highest sales. However, the shorter bars (Women’s Apparel – Product B and Home Decor – Product D) need attention. It indicates areas that require further analysis or strategies for improvement.
Step 3: Colorful Insights
Different colors are used to differentiate each product. It is essential to show a color-coded chart where the audience can distinguish between products.
- Men’s Shoes (Product A): Yellow
- Women’s Apparel (Product B): Orange
- Electronics (Product C): Violet
- Home Decor (Product D): Blue
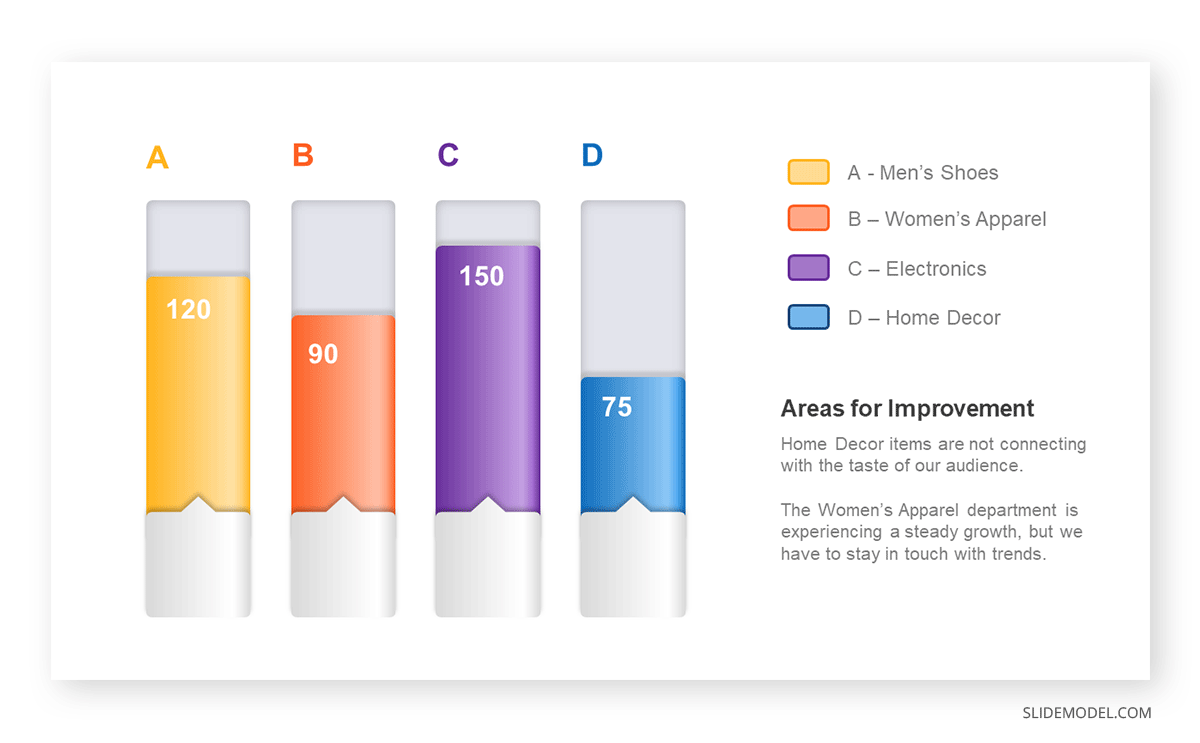
Bar charts are straightforward and easily understandable for presenting data. They are versatile when comparing products or any categorical data [2] . Bar charts adapt seamlessly to retail scenarios. Despite that, bar charts have a few shortcomings. They cannot illustrate data trends over time. Besides, overloading the chart with numerous products can lead to visual clutter, diminishing its effectiveness.
For more information, check our collection of bar chart templates for PowerPoint .
Line graphs help illustrate data trends, progressions, or fluctuations by connecting a series of data points called ‘markers’ with straight line segments. This provides a straightforward representation of how values change [5] . Their versatility makes them invaluable for scenarios requiring a visual understanding of continuous data. In addition, line graphs are also useful for comparing multiple datasets over the same timeline. Using multiple line graphs allows us to compare more than one data set. They simplify complex information so the audience can quickly grasp the ups and downs of values. From tracking stock prices to analyzing experimental results, you can use line graphs to show how data changes over a continuous timeline. They show trends with simplicity and clarity.
Real-life Application of Line Graphs
To understand line graphs thoroughly, we will use a real case. Imagine you’re a financial analyst presenting a tech company’s monthly sales for a licensed product over the past year. Investors want insights into sales behavior by month, how market trends may have influenced sales performance and reception to the new pricing strategy. To present data via a line graph, you will complete these steps.
First, you need to gather the data. In this case, your data will be the sales numbers. For example:
- January: $45,000
- February: $55,000
- March: $45,000
- April: $60,000
- May: $ 70,000
- June: $65,000
- July: $62,000
- August: $68,000
- September: $81,000
- October: $76,000
- November: $87,000
- December: $91,000
After choosing the data, the next step is to select the orientation. Like bar charts, you can use vertical or horizontal line graphs. However, we want to keep this simple, so we will keep the timeline (x-axis) horizontal while the sales numbers (y-axis) vertical.
Step 3: Connecting Trends
After adding the data to your preferred software, you will plot a line graph. In the graph, each month’s sales are represented by data points connected by a line.
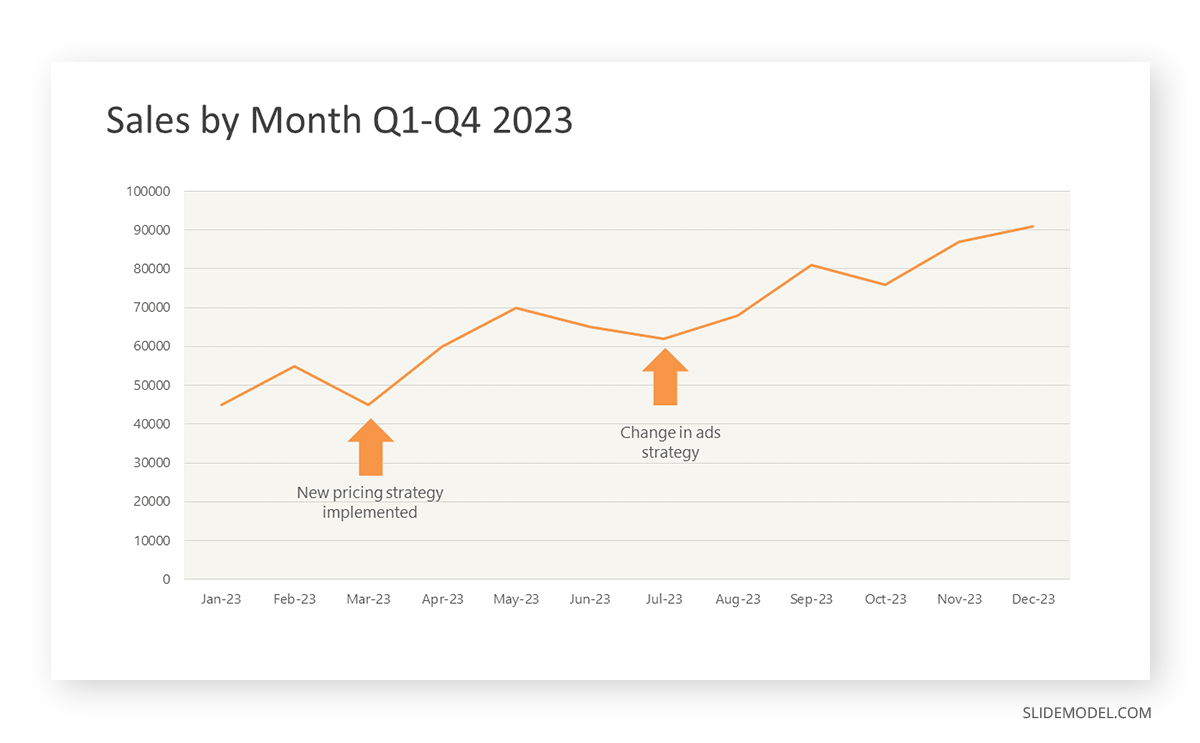
Step 4: Adding Clarity with Color
If there are multiple lines, you can also add colors to highlight each one, making it easier to follow.
Line graphs excel at visually presenting trends over time. These presentation aids identify patterns, like upward or downward trends. However, too many data points can clutter the graph, making it harder to interpret. Line graphs work best with continuous data but are not suitable for categories.
For more information, check our collection of line chart templates for PowerPoint and our article about how to make a presentation graph .
A data dashboard is a visual tool for analyzing information. Different graphs, charts, and tables are consolidated in a layout to showcase the information required to achieve one or more objectives. Dashboards help quickly see Key Performance Indicators (KPIs). You don’t make new visuals in the dashboard; instead, you use it to display visuals you’ve already made in worksheets [3] .
Keeping the number of visuals on a dashboard to three or four is recommended. Adding too many can make it hard to see the main points [4]. Dashboards can be used for business analytics to analyze sales, revenue, and marketing metrics at a time. They are also used in the manufacturing industry, as they allow users to grasp the entire production scenario at the moment while tracking the core KPIs for each line.
Real-Life Application of a Dashboard
Consider a project manager presenting a software development project’s progress to a tech company’s leadership team. He follows the following steps.
Step 1: Defining Key Metrics
To effectively communicate the project’s status, identify key metrics such as completion status, budget, and bug resolution rates. Then, choose measurable metrics aligned with project objectives.
Step 2: Choosing Visualization Widgets
After finalizing the data, presentation aids that align with each metric are selected. For this project, the project manager chooses a progress bar for the completion status and uses bar charts for budget allocation. Likewise, he implements line charts for bug resolution rates.
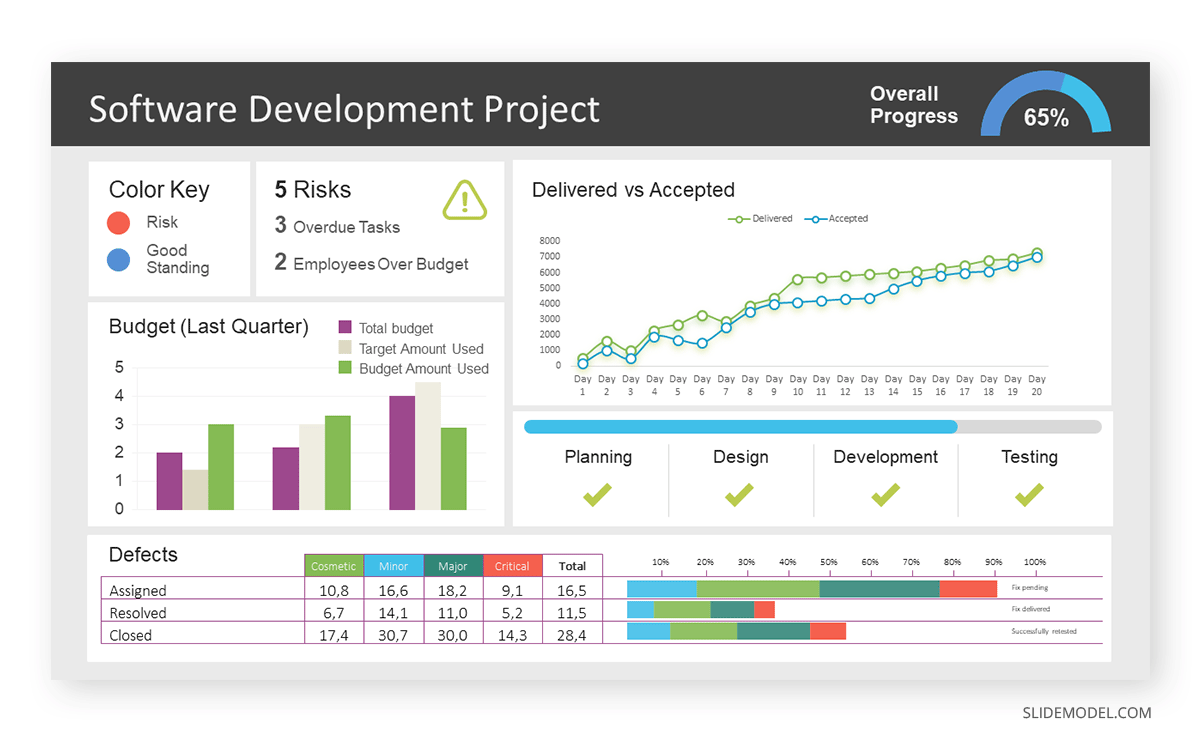
Step 3: Dashboard Layout
Key metrics are prominently placed in the dashboard for easy visibility, and the manager ensures that it appears clean and organized.
Dashboards provide a comprehensive view of key project metrics. Users can interact with data, customize views, and drill down for detailed analysis. However, creating an effective dashboard requires careful planning to avoid clutter. Besides, dashboards rely on the availability and accuracy of underlying data sources.
For more information, check our article on how to design a dashboard presentation , and discover our collection of dashboard PowerPoint templates .
Treemap charts represent hierarchical data structured in a series of nested rectangles [6] . As each branch of the ‘tree’ is given a rectangle, smaller tiles can be seen representing sub-branches, meaning elements on a lower hierarchical level than the parent rectangle. Each one of those rectangular nodes is built by representing an area proportional to the specified data dimension.
Treemaps are useful for visualizing large datasets in compact space. It is easy to identify patterns, such as which categories are dominant. Common applications of the treemap chart are seen in the IT industry, such as resource allocation, disk space management, website analytics, etc. Also, they can be used in multiple industries like healthcare data analysis, market share across different product categories, or even in finance to visualize portfolios.
Real-Life Application of a Treemap Chart
Let’s consider a financial scenario where a financial team wants to represent the budget allocation of a company. There is a hierarchy in the process, so it is helpful to use a treemap chart. In the chart, the top-level rectangle could represent the total budget, and it would be subdivided into smaller rectangles, each denoting a specific department. Further subdivisions within these smaller rectangles might represent individual projects or cost categories.
Step 1: Define Your Data Hierarchy
While presenting data on the budget allocation, start by outlining the hierarchical structure. The sequence will be like the overall budget at the top, followed by departments, projects within each department, and finally, individual cost categories for each project.
- Top-level rectangle: Total Budget
- Second-level rectangles: Departments (Engineering, Marketing, Sales)
- Third-level rectangles: Projects within each department
- Fourth-level rectangles: Cost categories for each project (Personnel, Marketing Expenses, Equipment)
Step 2: Choose a Suitable Tool
It’s time to select a data visualization tool supporting Treemaps. Popular choices include Tableau, Microsoft Power BI, PowerPoint, or even coding with libraries like D3.js. It is vital to ensure that the chosen tool provides customization options for colors, labels, and hierarchical structures.
Here, the team uses PowerPoint for this guide because of its user-friendly interface and robust Treemap capabilities.
Step 3: Make a Treemap Chart with PowerPoint
After opening the PowerPoint presentation, they chose “SmartArt” to form the chart. The SmartArt Graphic window has a “Hierarchy” category on the left. Here, you will see multiple options. You can choose any layout that resembles a Treemap. The “Table Hierarchy” or “Organization Chart” options can be adapted. The team selects the Table Hierarchy as it looks close to a Treemap.
Step 5: Input Your Data
After that, a new window will open with a basic structure. They add the data one by one by clicking on the text boxes. They start with the top-level rectangle, representing the total budget.
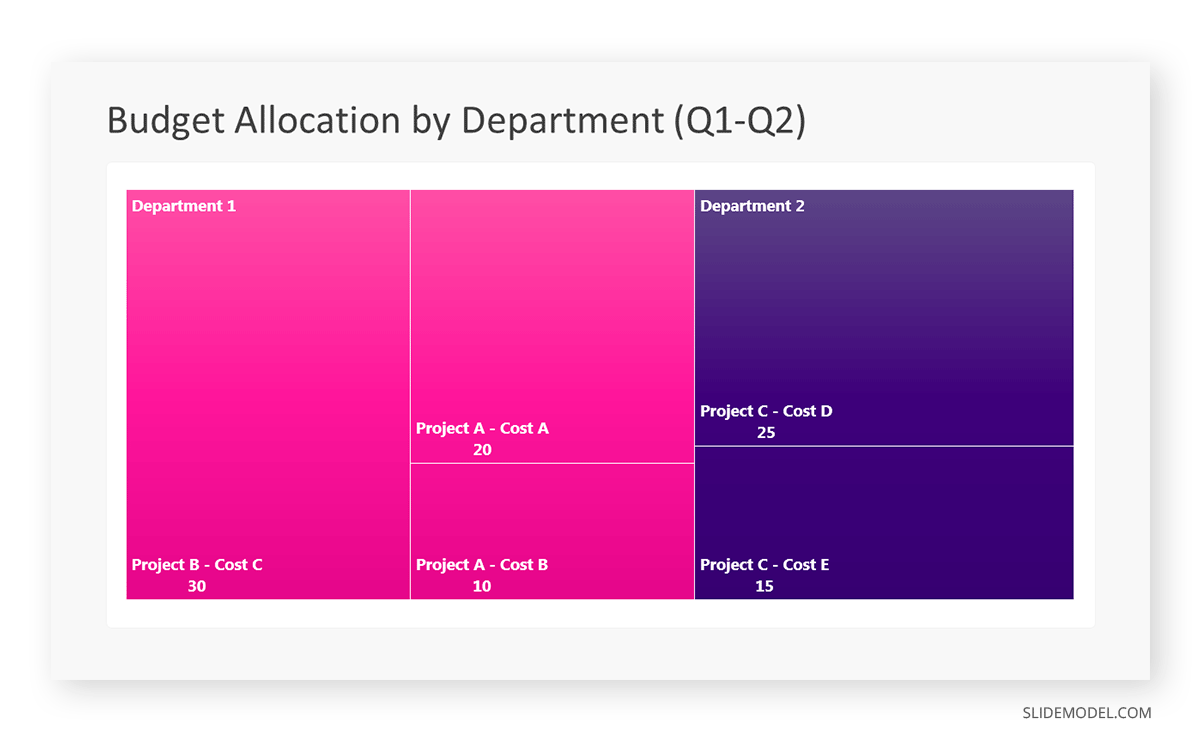
Step 6: Customize the Treemap
By clicking on each shape, they customize its color, size, and label. At the same time, they can adjust the font size, style, and color of labels by using the options in the “Format” tab in PowerPoint. Using different colors for each level enhances the visual difference.
Treemaps excel at illustrating hierarchical structures. These charts make it easy to understand relationships and dependencies. They efficiently use space, compactly displaying a large amount of data, reducing the need for excessive scrolling or navigation. Additionally, using colors enhances the understanding of data by representing different variables or categories.
In some cases, treemaps might become complex, especially with deep hierarchies. It becomes challenging for some users to interpret the chart. At the same time, displaying detailed information within each rectangle might be constrained by space. It potentially limits the amount of data that can be shown clearly. Without proper labeling and color coding, there’s a risk of misinterpretation.
A heatmap is a data visualization tool that uses color coding to represent values across a two-dimensional surface. In these, colors replace numbers to indicate the magnitude of each cell. This color-shaded matrix display is valuable for summarizing and understanding data sets with a glance [7] . The intensity of the color corresponds to the value it represents, making it easy to identify patterns, trends, and variations in the data.
As a tool, heatmaps help businesses analyze website interactions, revealing user behavior patterns and preferences to enhance overall user experience. In addition, companies use heatmaps to assess content engagement, identifying popular sections and areas of improvement for more effective communication. They excel at highlighting patterns and trends in large datasets, making it easy to identify areas of interest.
We can implement heatmaps to express multiple data types, such as numerical values, percentages, or even categorical data. Heatmaps help us easily spot areas with lots of activity, making them helpful in figuring out clusters [8] . When making these maps, it is important to pick colors carefully. The colors need to show the differences between groups or levels of something. And it is good to use colors that people with colorblindness can easily see.
Check our detailed guide on how to create a heatmap here. Also discover our collection of heatmap PowerPoint templates .
Pie charts are circular statistical graphics divided into slices to illustrate numerical proportions. Each slice represents a proportionate part of the whole, making it easy to visualize the contribution of each component to the total.
The size of the pie charts is influenced by the value of data points within each pie. The total of all data points in a pie determines its size. The pie with the highest data points appears as the largest, whereas the others are proportionally smaller. However, you can present all pies of the same size if proportional representation is not required [9] . Sometimes, pie charts are difficult to read, or additional information is required. A variation of this tool can be used instead, known as the donut chart , which has the same structure but a blank center, creating a ring shape. Presenters can add extra information, and the ring shape helps to declutter the graph.
Pie charts are used in business to show percentage distribution, compare relative sizes of categories, or present straightforward data sets where visualizing ratios is essential.
Real-Life Application of Pie Charts
Consider a scenario where you want to represent the distribution of the data. Each slice of the pie chart would represent a different category, and the size of each slice would indicate the percentage of the total portion allocated to that category.
Step 1: Define Your Data Structure
Imagine you are presenting the distribution of a project budget among different expense categories.
- Column A: Expense Categories (Personnel, Equipment, Marketing, Miscellaneous)
- Column B: Budget Amounts ($40,000, $30,000, $20,000, $10,000) Column B represents the values of your categories in Column A.
Step 2: Insert a Pie Chart
Using any of the accessible tools, you can create a pie chart. The most convenient tools for forming a pie chart in a presentation are presentation tools such as PowerPoint or Google Slides. You will notice that the pie chart assigns each expense category a percentage of the total budget by dividing it by the total budget.
For instance:
- Personnel: $40,000 / ($40,000 + $30,000 + $20,000 + $10,000) = 40%
- Equipment: $30,000 / ($40,000 + $30,000 + $20,000 + $10,000) = 30%
- Marketing: $20,000 / ($40,000 + $30,000 + $20,000 + $10,000) = 20%
- Miscellaneous: $10,000 / ($40,000 + $30,000 + $20,000 + $10,000) = 10%
You can make a chart out of this or just pull out the pie chart from the data.
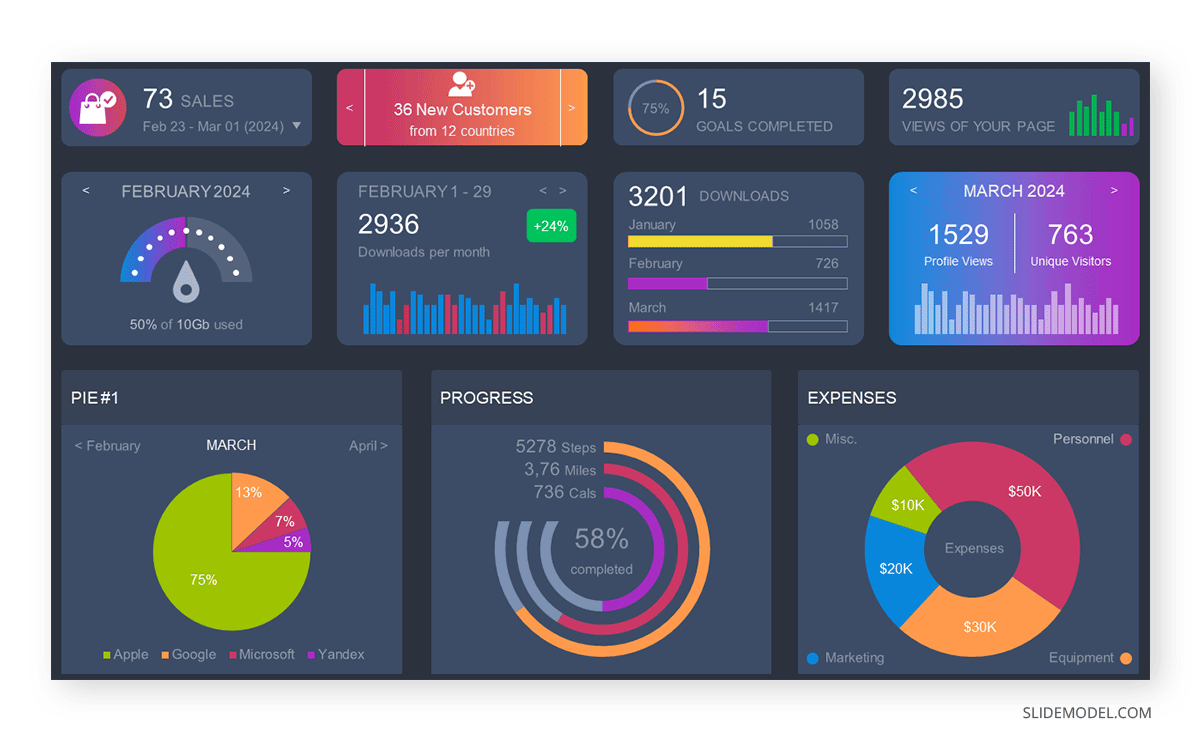
3D pie charts and 3D donut charts are quite popular among the audience. They stand out as visual elements in any presentation slide, so let’s take a look at how our pie chart example would look in 3D pie chart format.
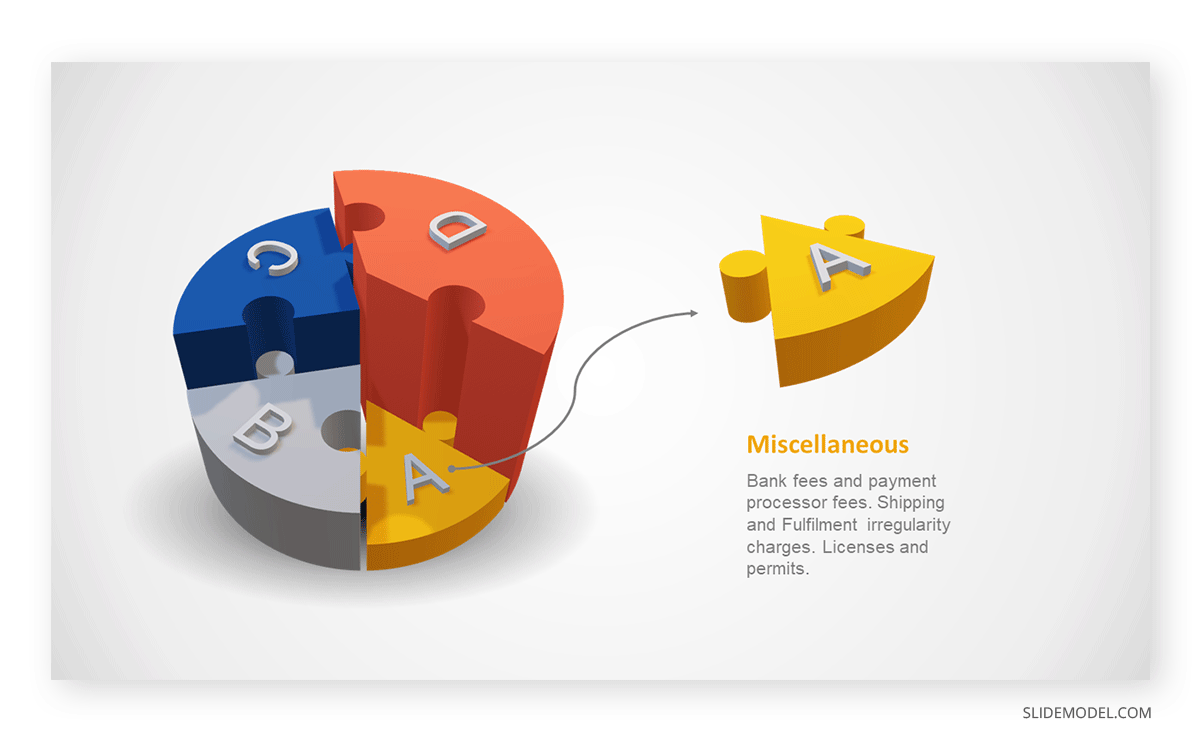
Step 03: Results Interpretation
The pie chart visually illustrates the distribution of the project budget among different expense categories. Personnel constitutes the largest portion at 40%, followed by equipment at 30%, marketing at 20%, and miscellaneous at 10%. This breakdown provides a clear overview of where the project funds are allocated, which helps in informed decision-making and resource management. It is evident that personnel are a significant investment, emphasizing their importance in the overall project budget.
Pie charts provide a straightforward way to represent proportions and percentages. They are easy to understand, even for individuals with limited data analysis experience. These charts work well for small datasets with a limited number of categories.
However, a pie chart can become cluttered and less effective in situations with many categories. Accurate interpretation may be challenging, especially when dealing with slight differences in slice sizes. In addition, these charts are static and do not effectively convey trends over time.
For more information, check our collection of pie chart templates for PowerPoint .
Histograms present the distribution of numerical variables. Unlike a bar chart that records each unique response separately, histograms organize numeric responses into bins and show the frequency of reactions within each bin [10] . The x-axis of a histogram shows the range of values for a numeric variable. At the same time, the y-axis indicates the relative frequencies (percentage of the total counts) for that range of values.
Whenever you want to understand the distribution of your data, check which values are more common, or identify outliers, histograms are your go-to. Think of them as a spotlight on the story your data is telling. A histogram can provide a quick and insightful overview if you’re curious about exam scores, sales figures, or any numerical data distribution.
Real-Life Application of a Histogram
In the histogram data analysis presentation example, imagine an instructor analyzing a class’s grades to identify the most common score range. A histogram could effectively display the distribution. It will show whether most students scored in the average range or if there are significant outliers.
Step 1: Gather Data
He begins by gathering the data. The scores of each student in class are gathered to analyze exam scores.
After arranging the scores in ascending order, bin ranges are set.
Step 2: Define Bins
Bins are like categories that group similar values. Think of them as buckets that organize your data. The presenter decides how wide each bin should be based on the range of the values. For instance, the instructor sets the bin ranges based on score intervals: 60-69, 70-79, 80-89, and 90-100.
Step 3: Count Frequency
Now, he counts how many data points fall into each bin. This step is crucial because it tells you how often specific ranges of values occur. The result is the frequency distribution, showing the occurrences of each group.
Here, the instructor counts the number of students in each category.
- 60-69: 1 student (Kate)
- 70-79: 4 students (David, Emma, Grace, Jack)
- 80-89: 7 students (Alice, Bob, Frank, Isabel, Liam, Mia, Noah)
- 90-100: 3 students (Clara, Henry, Olivia)
Step 4: Create the Histogram
It’s time to turn the data into a visual representation. Draw a bar for each bin on a graph. The width of the bar should correspond to the range of the bin, and the height should correspond to the frequency. To make your histogram understandable, label the X and Y axes.
In this case, the X-axis should represent the bins (e.g., test score ranges), and the Y-axis represents the frequency.
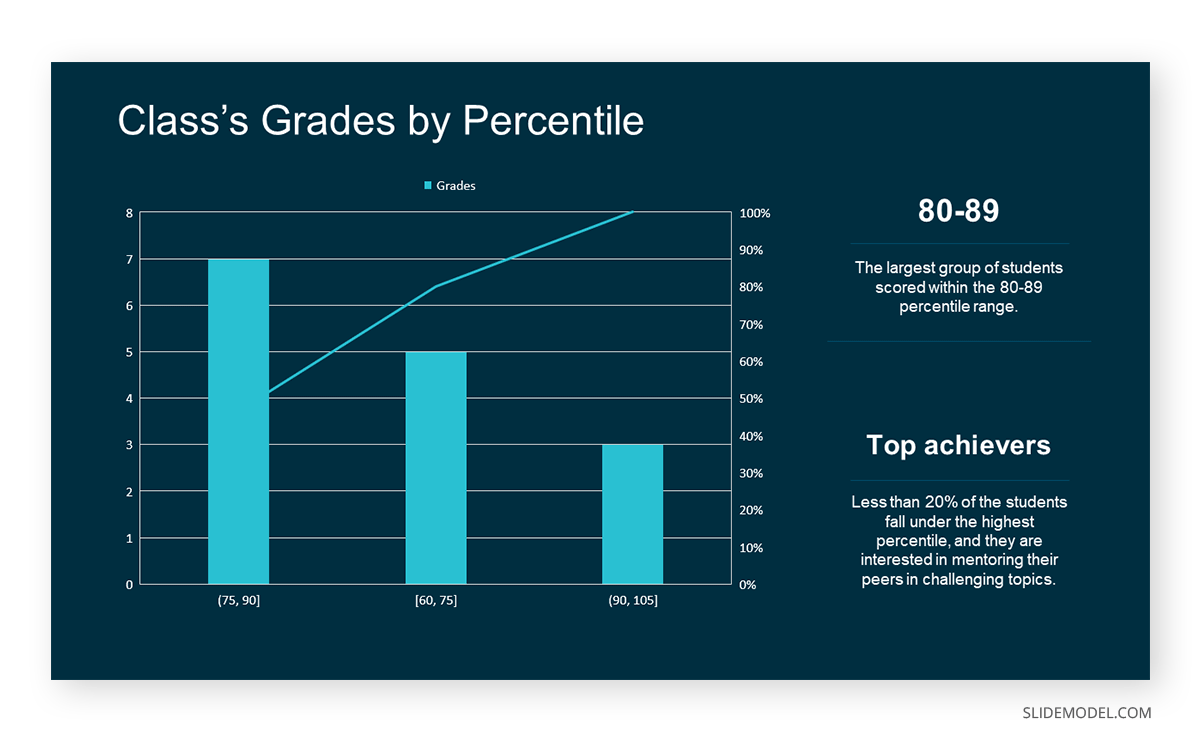
The histogram of the class grades reveals insightful patterns in the distribution. Most students, with seven students, fall within the 80-89 score range. The histogram provides a clear visualization of the class’s performance. It showcases a concentration of grades in the upper-middle range with few outliers at both ends. This analysis helps in understanding the overall academic standing of the class. It also identifies the areas for potential improvement or recognition.
Thus, histograms provide a clear visual representation of data distribution. They are easy to interpret, even for those without a statistical background. They apply to various types of data, including continuous and discrete variables. One weak point is that histograms do not capture detailed patterns in students’ data, with seven compared to other visualization methods.
A scatter plot is a graphical representation of the relationship between two variables. It consists of individual data points on a two-dimensional plane. This plane plots one variable on the x-axis and the other on the y-axis. Each point represents a unique observation. It visualizes patterns, trends, or correlations between the two variables.
Scatter plots are also effective in revealing the strength and direction of relationships. They identify outliers and assess the overall distribution of data points. The points’ dispersion and clustering reflect the relationship’s nature, whether it is positive, negative, or lacks a discernible pattern. In business, scatter plots assess relationships between variables such as marketing cost and sales revenue. They help present data correlations and decision-making.
Real-Life Application of Scatter Plot
A group of scientists is conducting a study on the relationship between daily hours of screen time and sleep quality. After reviewing the data, they managed to create this table to help them build a scatter plot graph:
In the provided example, the x-axis represents Daily Hours of Screen Time, and the y-axis represents the Sleep Quality Rating.
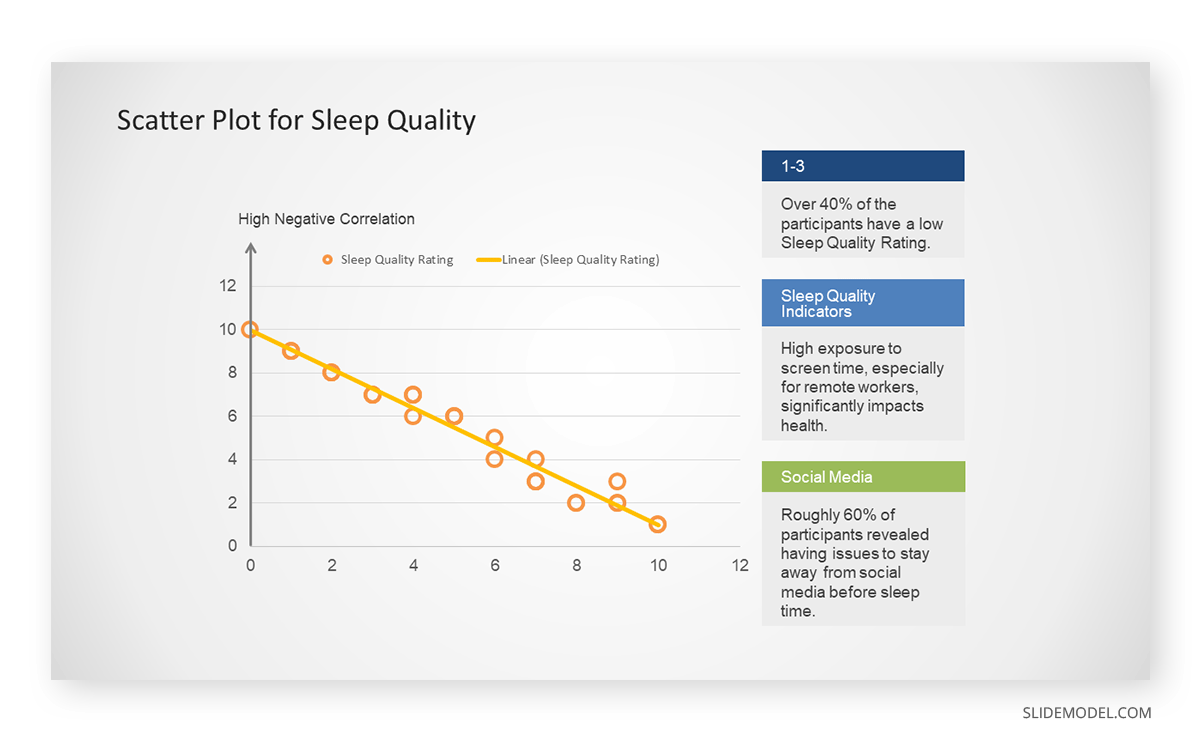
The scientists observe a negative correlation between the amount of screen time and the quality of sleep. This is consistent with their hypothesis that blue light, especially before bedtime, has a significant impact on sleep quality and metabolic processes.
There are a few things to remember when using a scatter plot. Even when a scatter diagram indicates a relationship, it doesn’t mean one variable affects the other. A third factor can influence both variables. The more the plot resembles a straight line, the stronger the relationship is perceived [11] . If it suggests no ties, the observed pattern might be due to random fluctuations in data. When the scatter diagram depicts no correlation, whether the data might be stratified is worth considering.
Choosing the appropriate data presentation type is crucial when making a presentation . Understanding the nature of your data and the message you intend to convey will guide this selection process. For instance, when showcasing quantitative relationships, scatter plots become instrumental in revealing correlations between variables. If the focus is on emphasizing parts of a whole, pie charts offer a concise display of proportions. Histograms, on the other hand, prove valuable for illustrating distributions and frequency patterns.
Bar charts provide a clear visual comparison of different categories. Likewise, line charts excel in showcasing trends over time, while tables are ideal for detailed data examination. Starting a presentation on data presentation types involves evaluating the specific information you want to communicate and selecting the format that aligns with your message. This ensures clarity and resonance with your audience from the beginning of your presentation.
1. Fact Sheet Dashboard for Data Presentation
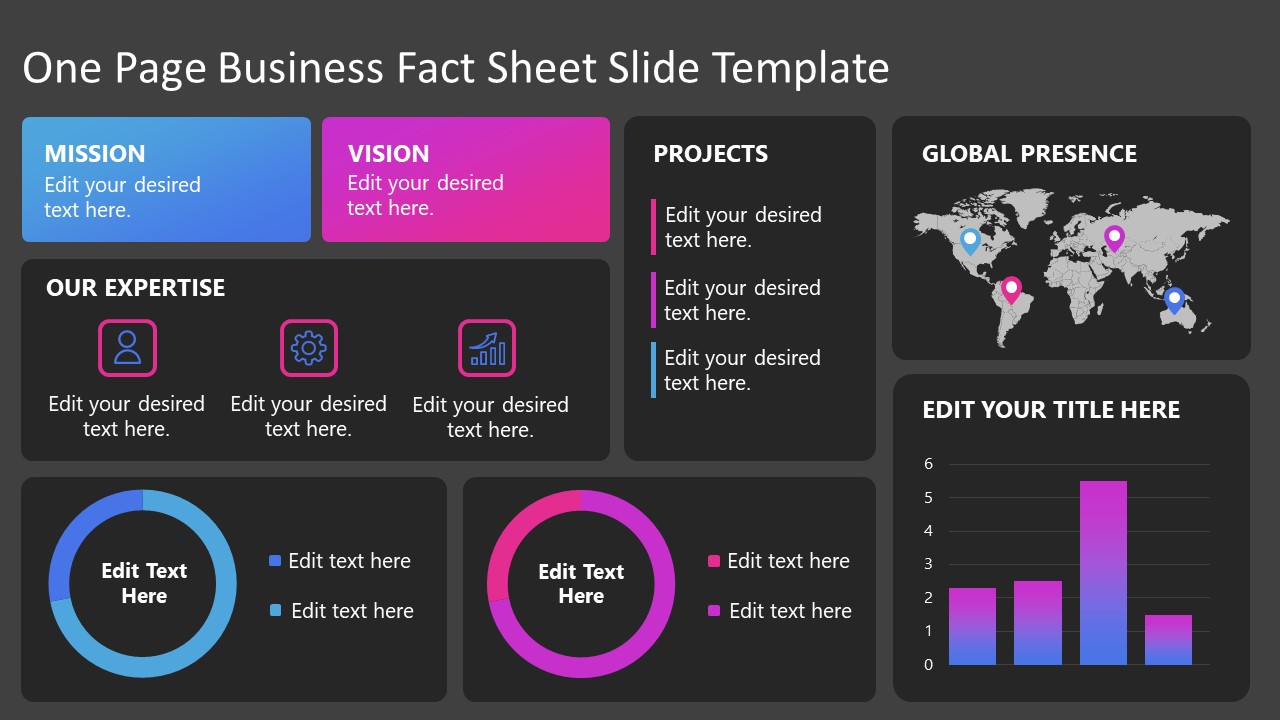
Convey all the data you need to present in this one-pager format, an ideal solution tailored for users looking for presentation aids. Global maps, donut chats, column graphs, and text neatly arranged in a clean layout presented in light and dark themes.
Use This Template
2. 3D Column Chart Infographic PPT Template
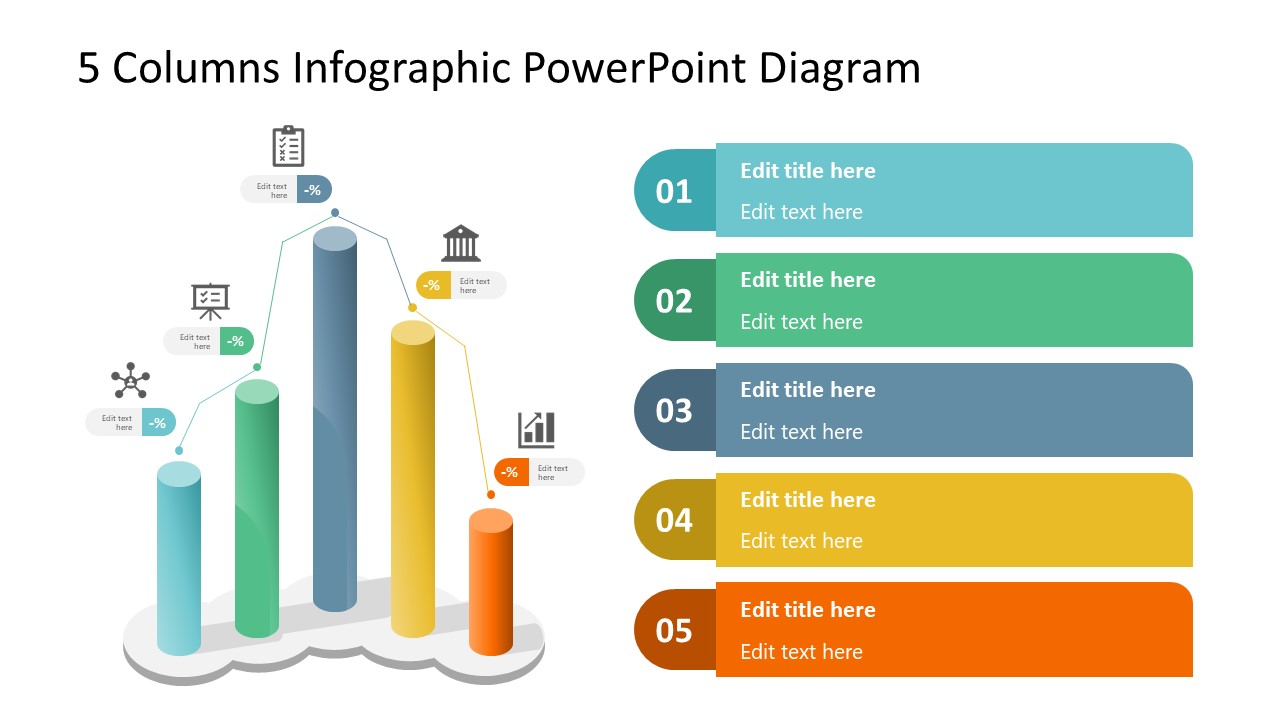
Represent column charts in a highly visual 3D format with this PPT template. A creative way to present data, this template is entirely editable, and we can craft either a one-page infographic or a series of slides explaining what we intend to disclose point by point.
3. Data Circles Infographic PowerPoint Template
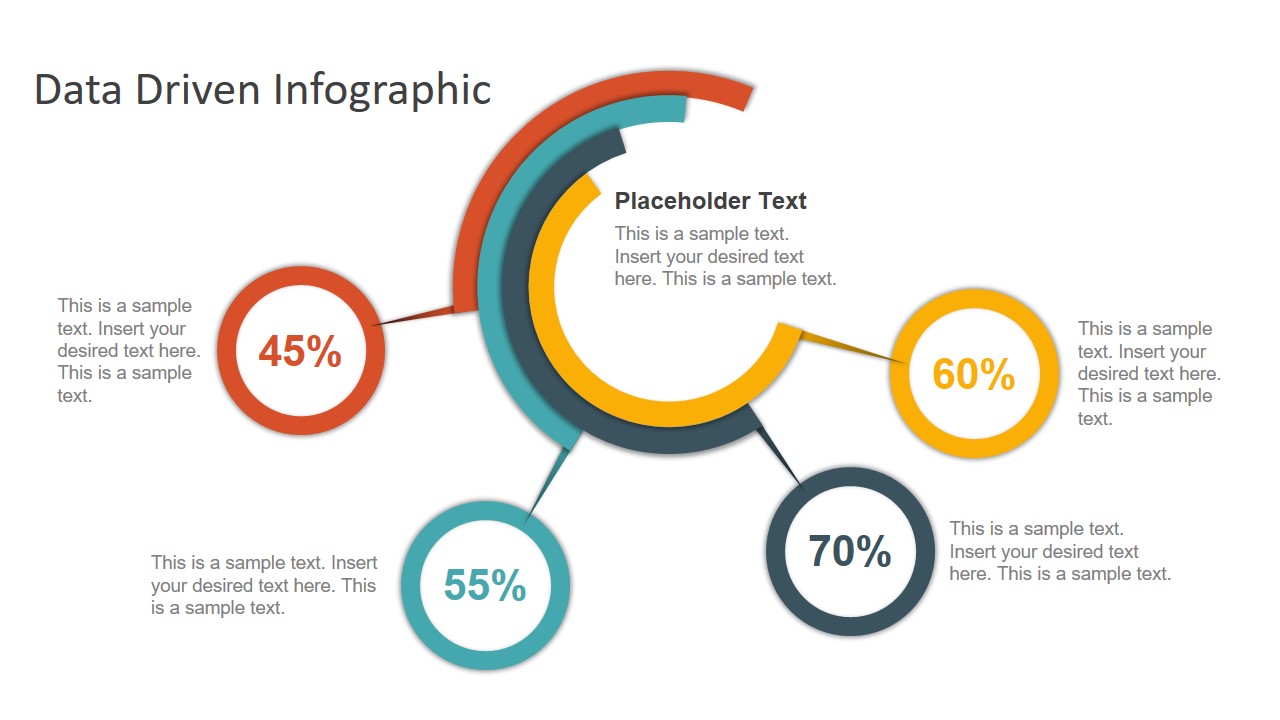
An alternative to the pie chart and donut chart diagrams, this template features a series of curved shapes with bubble callouts as ways of presenting data. Expand the information for each arch in the text placeholder areas.
4. Colorful Metrics Dashboard for Data Presentation
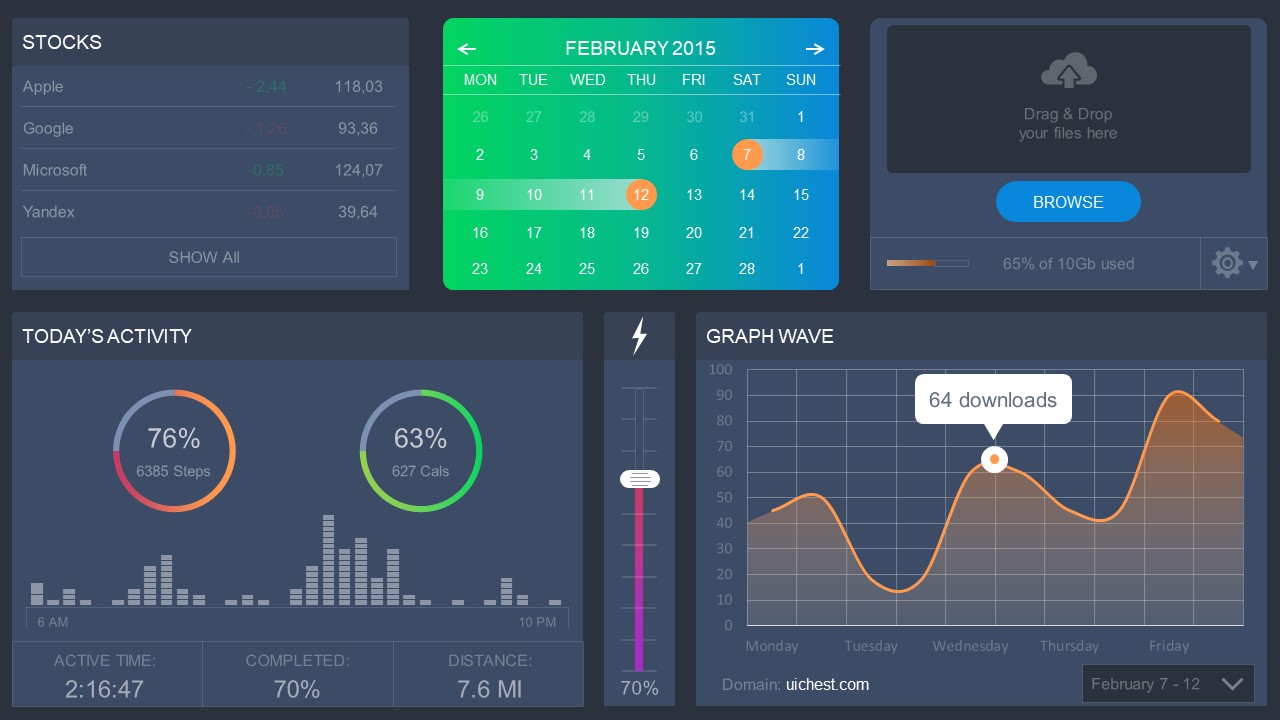
This versatile dashboard template helps us in the presentation of the data by offering several graphs and methods to convert numbers into graphics. Implement it for e-commerce projects, financial projections, project development, and more.
5. Animated Data Presentation Tools for PowerPoint & Google Slides
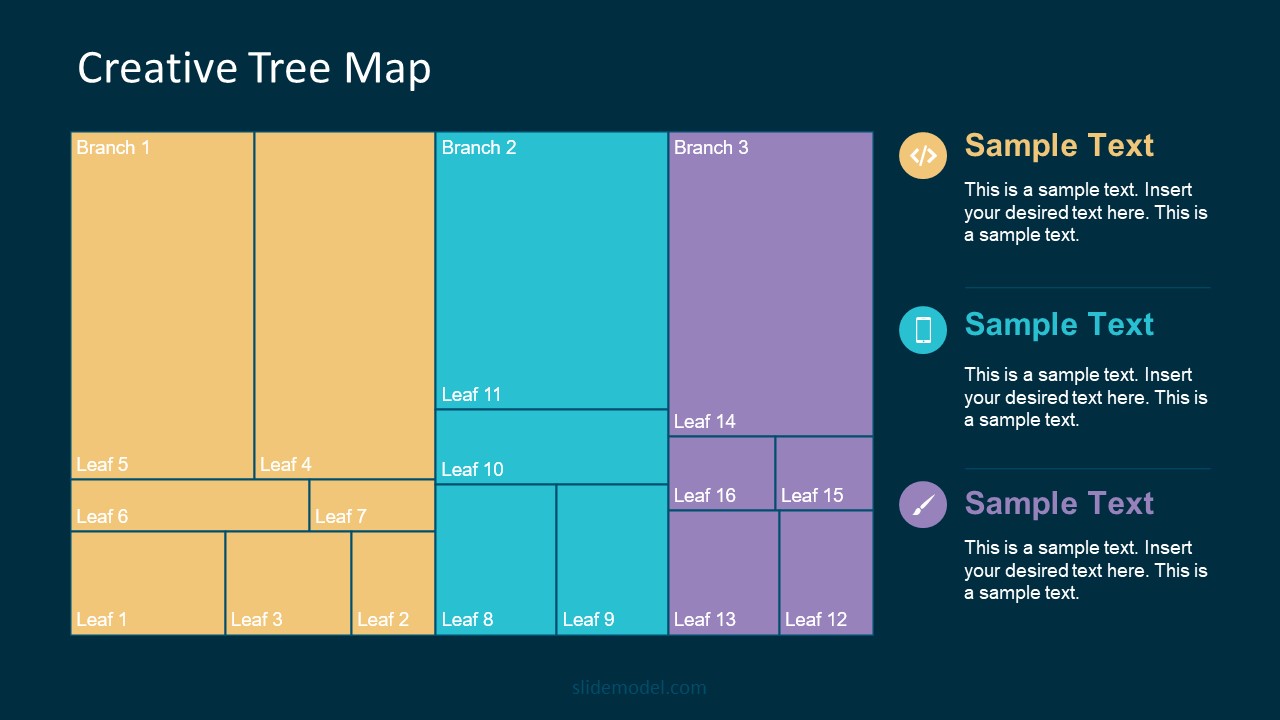
A slide deck filled with most of the tools mentioned in this article, from bar charts, column charts, treemap graphs, pie charts, histogram, etc. Animated effects make each slide look dynamic when sharing data with stakeholders.
6. Statistics Waffle Charts PPT Template for Data Presentations
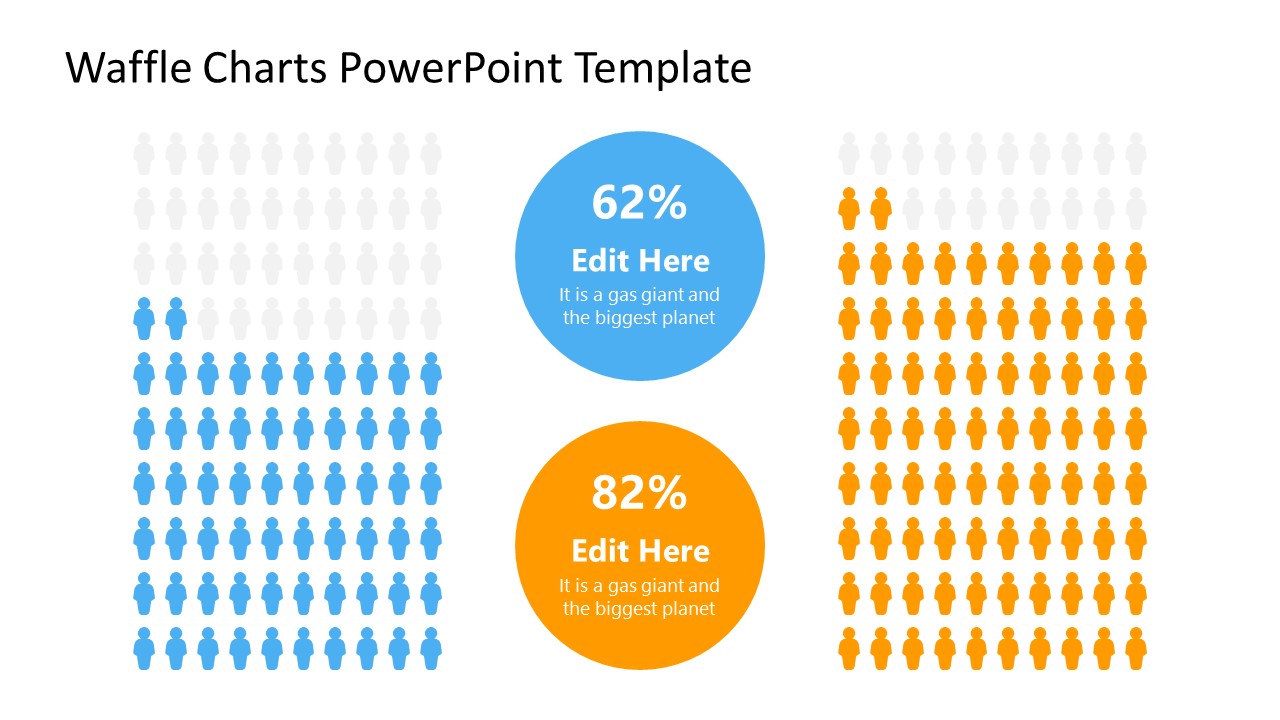
This PPT template helps us how to present data beyond the typical pie chart representation. It is widely used for demographics, so it’s a great fit for marketing teams, data science professionals, HR personnel, and more.
7. Data Presentation Dashboard Template for Google Slides
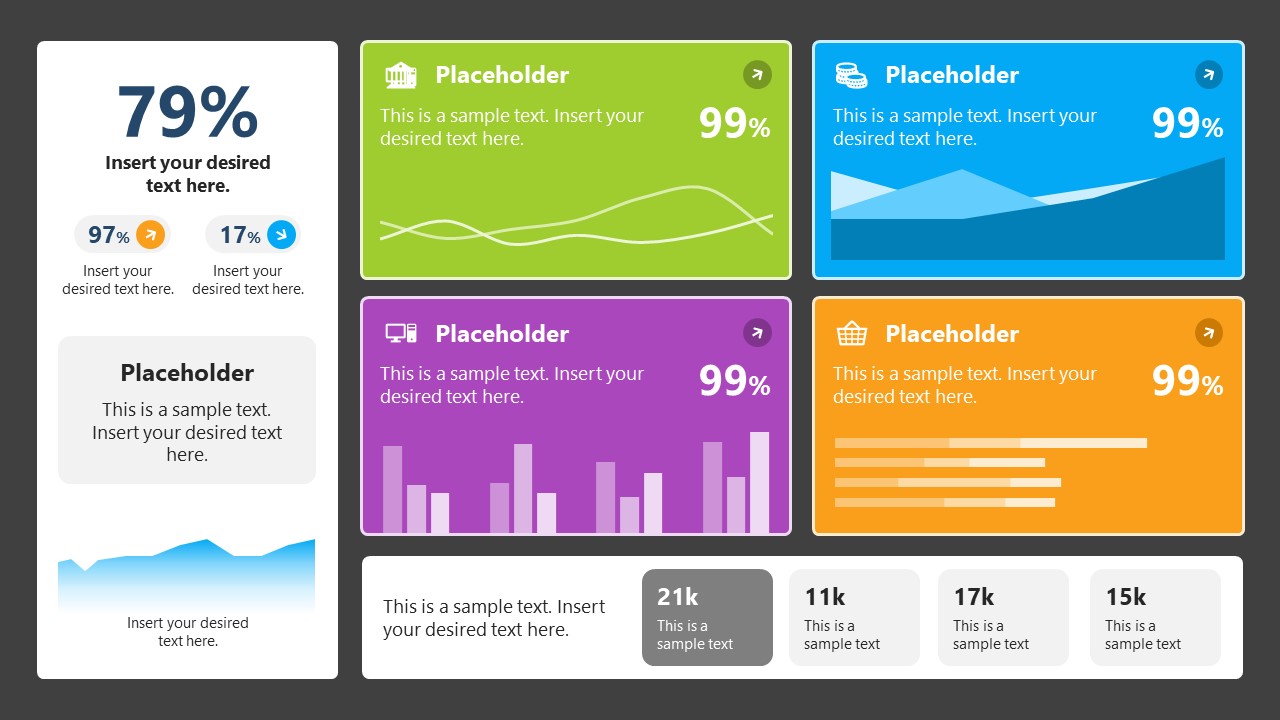
A compendium of tools in dashboard format featuring line graphs, bar charts, column charts, and neatly arranged placeholder text areas.
8. Weather Dashboard for Data Presentation
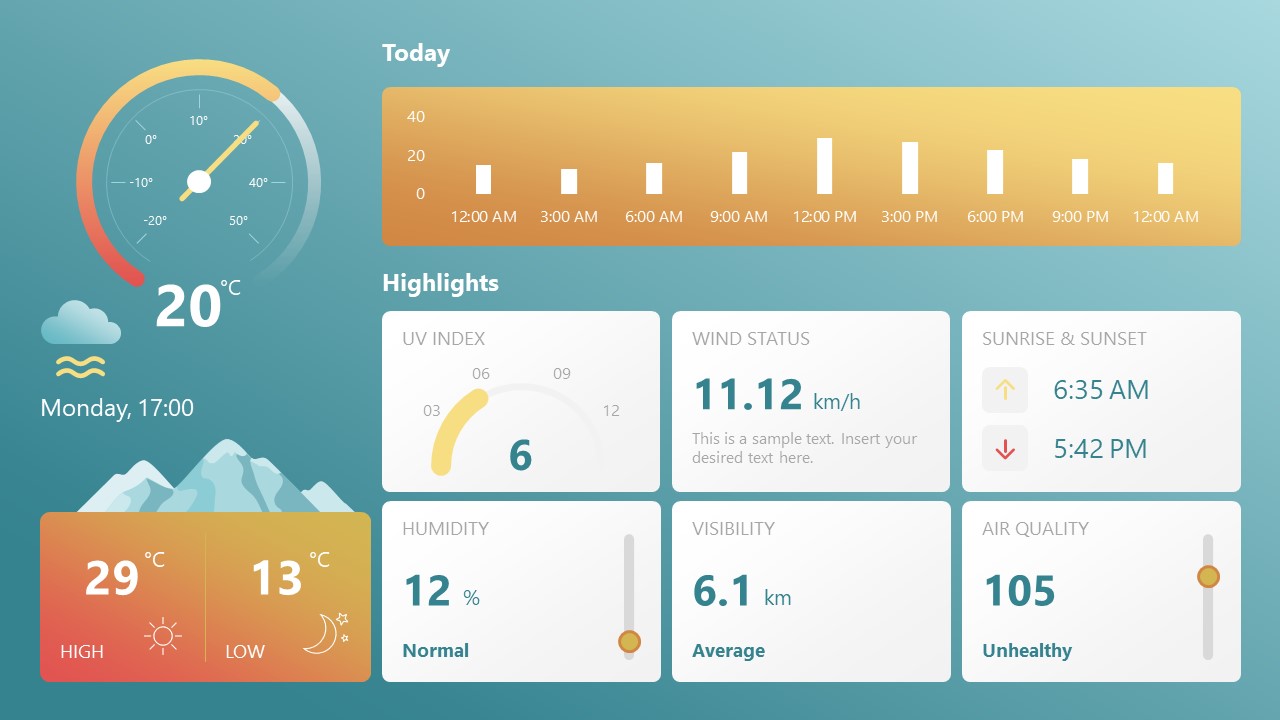
Share weather data for agricultural presentation topics, environmental studies, or any kind of presentation that requires a highly visual layout for weather forecasting on a single day. Two color themes are available.
9. Social Media Marketing Dashboard Data Presentation Template
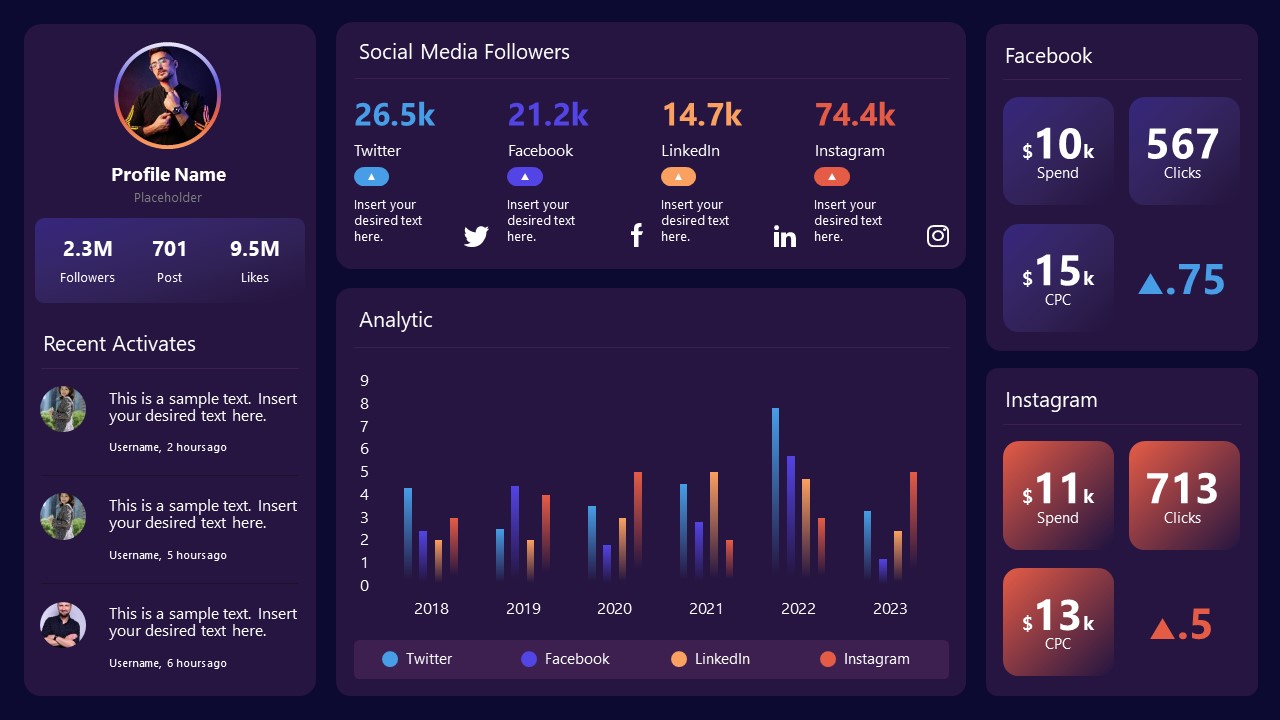
Intended for marketing professionals, this dashboard template for data presentation is a tool for presenting data analytics from social media channels. Two slide layouts featuring line graphs and column charts.
10. Project Management Summary Dashboard Template
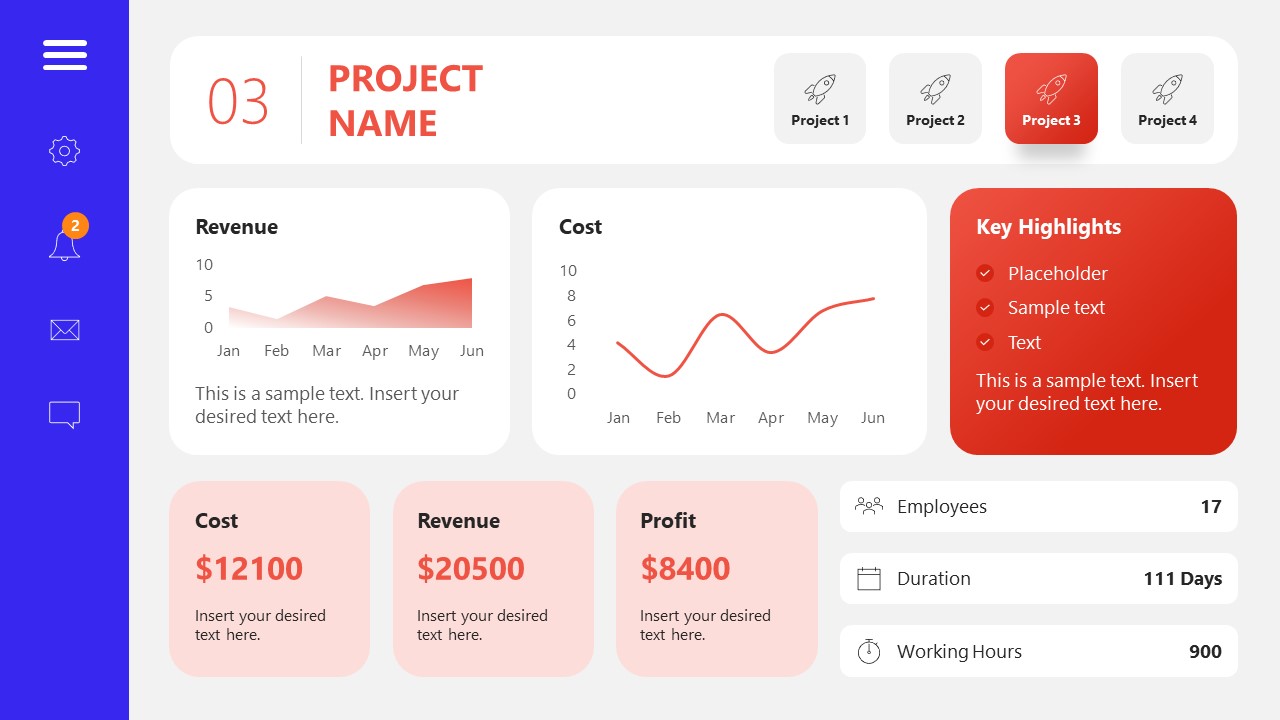
A tool crafted for project managers to deliver highly visual reports on a project’s completion, the profits it delivered for the company, and expenses/time required to execute it. 4 different color layouts are available.
11. Profit & Loss Dashboard for PowerPoint and Google Slides
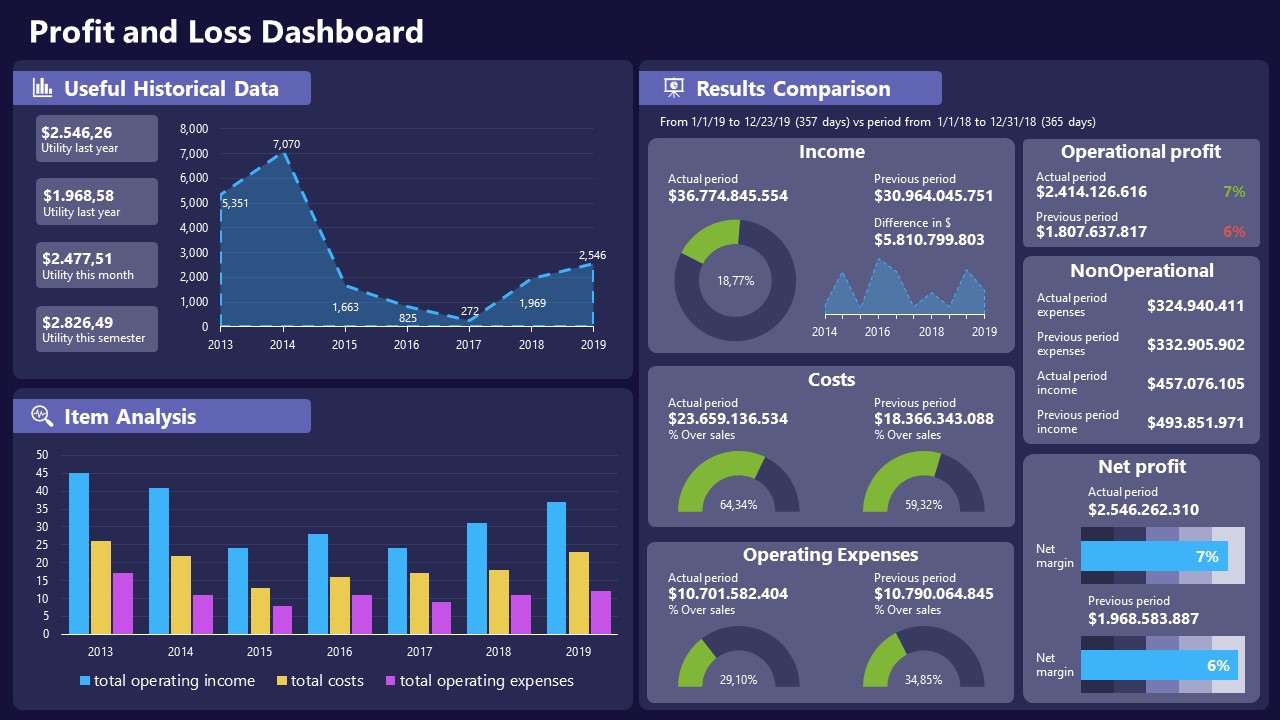
A must-have for finance professionals. This typical profit & loss dashboard includes progress bars, donut charts, column charts, line graphs, and everything that’s required to deliver a comprehensive report about a company’s financial situation.
Overwhelming visuals
One of the mistakes related to using data-presenting methods is including too much data or using overly complex visualizations. They can confuse the audience and dilute the key message.
Inappropriate chart types
Choosing the wrong type of chart for the data at hand can lead to misinterpretation. For example, using a pie chart for data that doesn’t represent parts of a whole is not right.
Lack of context
Failing to provide context or sufficient labeling can make it challenging for the audience to understand the significance of the presented data.
Inconsistency in design
Using inconsistent design elements and color schemes across different visualizations can create confusion and visual disarray.
Failure to provide details
Simply presenting raw data without offering clear insights or takeaways can leave the audience without a meaningful conclusion.
Lack of focus
Not having a clear focus on the key message or main takeaway can result in a presentation that lacks a central theme.
Visual accessibility issues
Overlooking the visual accessibility of charts and graphs can exclude certain audience members who may have difficulty interpreting visual information.
In order to avoid these mistakes in data presentation, presenters can benefit from using presentation templates . These templates provide a structured framework. They ensure consistency, clarity, and an aesthetically pleasing design, enhancing data communication’s overall impact.
Understanding and choosing data presentation types are pivotal in effective communication. Each method serves a unique purpose, so selecting the appropriate one depends on the nature of the data and the message to be conveyed. The diverse array of presentation types offers versatility in visually representing information, from bar charts showing values to pie charts illustrating proportions.
Using the proper method enhances clarity, engages the audience, and ensures that data sets are not just presented but comprehensively understood. By appreciating the strengths and limitations of different presentation types, communicators can tailor their approach to convey information accurately, developing a deeper connection between data and audience understanding.
[1] Government of Canada, S.C. (2021) 5 Data Visualization 5.2 Bar Chart , 5.2 Bar chart . https://www150.statcan.gc.ca/n1/edu/power-pouvoir/ch9/bargraph-diagrammeabarres/5214818-eng.htm
[2] Kosslyn, S.M., 1989. Understanding charts and graphs. Applied cognitive psychology, 3(3), pp.185-225. https://apps.dtic.mil/sti/pdfs/ADA183409.pdf
[3] Creating a Dashboard . https://it.tufts.edu/book/export/html/1870
[4] https://www.goldenwestcollege.edu/research/data-and-more/data-dashboards/index.html
[5] https://www.mit.edu/course/21/21.guide/grf-line.htm
[6] Jadeja, M. and Shah, K., 2015, January. Tree-Map: A Visualization Tool for Large Data. In GSB@ SIGIR (pp. 9-13). https://ceur-ws.org/Vol-1393/gsb15proceedings.pdf#page=15
[7] Heat Maps and Quilt Plots. https://www.publichealth.columbia.edu/research/population-health-methods/heat-maps-and-quilt-plots
[8] EIU QGIS WORKSHOP. https://www.eiu.edu/qgisworkshop/heatmaps.php
[9] About Pie Charts. https://www.mit.edu/~mbarker/formula1/f1help/11-ch-c8.htm
[10] Histograms. https://sites.utexas.edu/sos/guided/descriptive/numericaldd/descriptiven2/histogram/ [11] https://asq.org/quality-resources/scatter-diagram
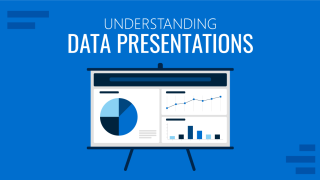
Like this article? Please share
Data Analysis, Data Science, Data Visualization Filed under Design
Related Articles
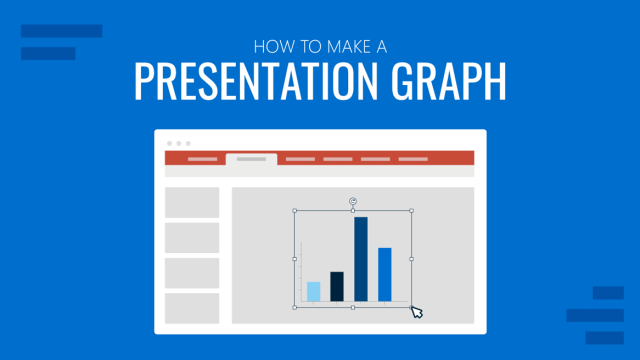
Filed under Design • March 27th, 2024
How to Make a Presentation Graph
Detailed step-by-step instructions to master the art of how to make a presentation graph in PowerPoint and Google Slides. Check it out!
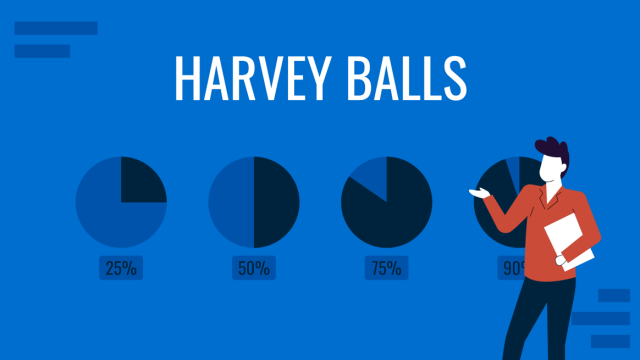
Filed under Presentation Ideas • January 6th, 2024
All About Using Harvey Balls
Among the many tools in the arsenal of the modern presenter, Harvey Balls have a special place. In this article we will tell you all about using Harvey Balls.
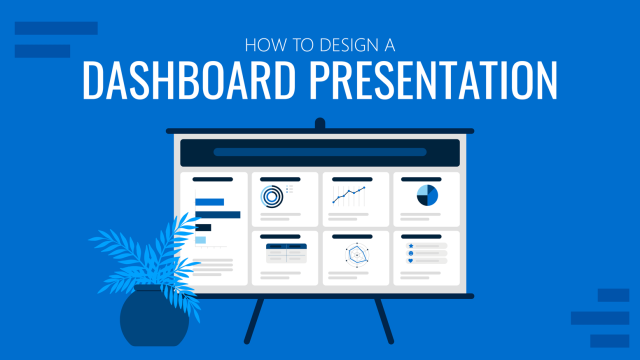
Filed under Business • December 8th, 2023
How to Design a Dashboard Presentation: A Step-by-Step Guide
Take a step further in your professional presentation skills by learning what a dashboard presentation is and how to properly design one in PowerPoint. A detailed step-by-step guide is here!
Leave a Reply
Code With C
The Way to Programming
- C Tutorials
- Java Tutorials
- Python Tutorials
- PHP Tutorials
- Java Projects
Understanding Line Segments in Coding
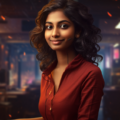
Hey there, fellow coding enthusiasts! Today, I’m diving into the fascinating world of line segments in programming 🤓. Buckle up, because we’re about to embark on a journey filled with definitions, properties, implementations, challenges, and best practices related to line segments in coding.
Exploring Line Segments
Line segments are like the unsung heroes of geometry, those little straight paths that connect two points but don’t go on forever like regular lines. Let’s break it down:
Definition of Line Segments
So, imagine you have two points, and you draw a straight line connecting them. That, my friends, is a line segment! It’s like a tiny road that has a clear start and end point. No infinite exploration here, just a direct path from A to B 🚶♂️.
Properties of Line Segments
Now, line segments come with some unique qualities. They have a fixed length, unlike lines that keep stretching till infinity. You can measure them precisely with rulers, no guesswork involved! And remember, a line segment is the shortest distance between two points—straight as an arrow! 🏹
Implementing Line Segments in Code
Time to bring these mathematical marvels into the realm of programming! Let’s see how we can work with line segments in our code:
Representation of Line Segments in Programming
In code, we often represent line segments using structures or classes. These structures store the coordinates of the two endpoints, making it easy for us to manipulate and perform operations on them. It’s like storing the GPS coordinates of the starting and ending points of your journey 🗺️.
Operations on Line Segments
Now, what can we do with these line segments? Plenty! We can calculate the length of a line segment, find intersections with other line segments, or even determine if two line segments are parallel. It’s like having a Swiss Army knife for geometry problems—versatile and handy! 🔪
Applications of Line Segments in Coding
Line segments aren’t just theoretical concepts; they have real-world applications in coding too! Let’s explore where you might encounter them:
Geometric Algorithms involving Line Segments
From computational geometry to computer graphics, line segments play a crucial role in various algorithms. Think about how they help in collision detection, shape recognition, or even pathfinding in games. Line segments are like the building blocks of complex geometric solutions! 🎮
Real-life Examples of Line Segments in Programs
In everyday programming, line segments sneak into unexpected places. They could be used to represent roads on a map, boundaries in image processing, or even in designing user interfaces with precise alignments. Line segments are the silent heroes making our digital lives easier! 💻
Challenges in Working with Line Segments
Ah, the inevitable hurdles that come with dealing with line segments in code. Let’s face these challenges head-on:
Common Errors when Dealing with Line Segments
One common mistake is forgetting to handle degenerate cases, like when two line segments are parallel or overlapping. These scenarios can lead to incorrect results if not properly addressed. Remember, even in geometry, exceptions are the rule! 🤯
Strategies to Overcome Line Segment Programming Challenges
To master line segment programming, we need to embrace defensive coding practices. Always validate input, handle edge cases diligently, and test extensively. Trust me, a little extra caution can save you from countless debugging nightmares! 🕵️♀️
Best Practices for Handling Line Segments
Now, let’s wrap up with some golden rules for shining bright while working with line segments in your code:
Efficient Ways to Handle Line Segments in Code
Opt for efficient algorithms when dealing with line segments. Whether it’s calculating intersections or checking for collinearity, choose methods that balance speed and accuracy. Efficiency is the name of the game! ⏱️
Tips for Optimizing Line Segment Algorithms
Don’t settle for average—optimize your line segment algorithms for peak performance . Look for opportunities to reduce redundant calculations, use data structures wisely, and stay updated on the latest optimization techniques. Keep those algorithms lean and mean! 💪
And there you have it, a lively journey through the world of line segments in coding! Remember, line segments may seem straightforward, but their applications and implications are far-reaching and profound. Embrace them, master them, and let these tiny paths guide you to geometric greatness! Thanks for joining me on this adventure! 🚀
Overall, understanding line segments in coding is like following a treasure map—full of twists, turns, and hidden gems. So, go forth, code wizards, and conquer those line segments with confidence and flair! Until next time, happy coding and may your lines always be straight and your segments always connected! ✨👩💻🔗
Program Code – Understanding Line Segments in Coding
### Code Output:
### Code Explanation:
The code snippet encapsulates the concept of a line segment in a class named LineSegment , which is defined by two endpoints (points). Each point is represented as a tuple of x and y coordinates.
- Class Initialization ( __init__ ): The class is initialized with two points ( point1 and point2 ), which are stored as attributes of the instance.
- Length Method ( length ): This method computes the length of the line segment using the distance formula derived from the Pythagorean theorem. It calculates the square root of the sum of the squares of the differences in x and y coordinates between the two endpoints.
- Midpoint Method ( midpoint ): This method calculates the midpoint of the line segment. The midpoint is the average of the x coordinates and the y coordinates of the endpoints, respectively.
- Representation Method ( __repr__ ): This method returns a string representation of the line segment, showing both endpoints. This is helpful for debugging and displaying the line segment.
The if __name__ == '__main__': block demonstrates an example usage of the LineSegment class. It creates a line segment with endpoints at (1, 2) and (4, 6), and it prints the line segment itself, its length, and its midpoint. The calculations conform to basic geometric principles, showcasing how the code accurately models line segments with simple Python constructs.
Frequently Asked Questions: Understanding Line Segments in Coding
What is a line segment in coding.
A line segment in coding refers to a straight path between two points in a coordinate system . It consists of all the points on the line between the two endpoints.
How are line segments represented in programming languages?
In programming languages , line segments are typically represented by storing the coordinates of the two endpoints of the segment. This allows for calculations related to length, slope, and other properties of the line segment.
Can line segments have negative coordinates?
Yes, line segments can have negative coordinates. In a Cartesian coordinate system, points can lie in any of the four quadrants, allowing for negative x and y values.
Are line segments directional in coding?
No, line segments are not inherently directional in coding. They are defined by their two endpoints and represent a connection between those points without a specified direction.
How are line segments used in computer graphics?
In computer graphics, line segments are fundamental for drawing shapes and objects on the screen. They are often used to create geometric figures, outlines, and patterns.
What algorithms are commonly used to work with line segments?
Algorithms like Bresenham’s line algorithm and the Midpoint line algorithm are frequently used to render and manipulate line segments efficiently in computer graphics.
Can line segments intersect with each other?
Yes, line segments can intersect with each other. When two line segments share a common point, they are said to intersect. Determining if and where line segments intersect is a common problem in computational geometry.
How can line segments be styled or customized in programming?
Line segments can be styled or customized by changing parameters such as color, thickness, transparency, and line style. These visual properties can enhance the appearance of line segments in graphical applications.
Are there any libraries or modules specifically designed for working with line segments in coding?
There are libraries and modules in programming languages like Python (e.g., matplotlib, pygame) that offer functionalities to work with line segments efficiently for tasks such as drawing, manipulation, and analysis.
What are some practical applications of working with line segments in coding?
Practical applications of line segments in coding include computer-aided design (CAD), image processing, games development, geographical information systems (GIS), and more.
Hope these FAQs help clear up any doubts you have about line segments in coding! 🚀
You Might Also Like
Binary decision diagrams: simplifying complex decision processes, optimizing data search in binary search trees, binary search tree: structure, operations, and applications, binary tree search: navigating trees for efficient data retrieval, searching in a binary search tree: algorithms and efficiency.
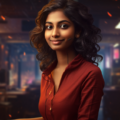
Leave a Reply Cancel reply
Your email address will not be published. Required fields are marked *
Latest Posts
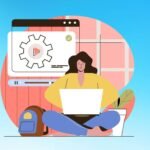
Top Machine Learning Projects in Python with Source Code for Your Next Project
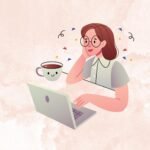
Machine Learning Projects for Final Year with Source Code: A Comprehensive Guide to Success
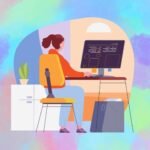
Top 10 Machine Learning Projects for Students to Excel in Machine Learning Project
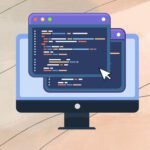
Top Machine Learning Projects for Students: A Compilation of Exciting ML Projects to Boost Your Skills in 2022 Project
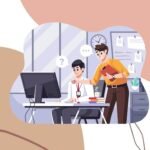
Top Machine Learning Projects on GitHub for Deep Learning Enthusiasts – Dive into Exciting Project Ideas Now!
Privacy overview.
Sign in to your account
Username or Email Address
Remember Me

- Why MicroStrategy
- Customer Stories

- MicroStrategy ONE
- AI & Augmented
- Workstation
- HyperIntelligence
- Multi-Cloud
- Embedded Analytics
- Innovations

- Financial Services

- Community & Support
- Partner Network
- Documentation

- About MicroStrategy
- Investor Relations

- Lightning for Corporations

- Get started
KB81670: Creating visual representation of data using the Line Chart
- Line Chart: Square
- Line Chart: Circle
- Line Chart: Ring
- Attributes: 1 or more
- Metrics: 1 or more
- Attached to this article is a Dossier (.mstr) that contains a demo of the Line Chart in action.
- To view this dossier with the visualization, download the .mstr file attached and deploy it in MicroStrategy Web.
- To open the demo visualization in MicroStrategy Web, navigate to MicroStrategy Web and click the "Upload MicroStrategy file" option to upload the file .mstr file.
- Dec 4, 2017
- Mar 21, 2024
Nothing here yet?
Log in to post to this feed.
ATTACHMENTS
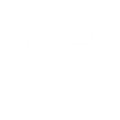
Feb 23, 2024 256 KB png
Feb 23, 2024 964 KB zip
Related Articles
- Number of Views 10.39K
- Number of Views 1.08K
- Number of Views 3.49K
- Number of Views 5.07K
- Number of Views 1.39K
Trending Articles
- How to use Guacamole to copy and paste text or files in MicroStrategy on AWS
- All new Download site is now available!
- How to increase the Java Heap size in Tomcat Application Server
- KB35109: "Error type: Odbc error. Odbc operation attempted: SQLExecDirect. [HYT00:0: on SQLHANDLE] [MicroStrategy][ODBC Or…
- KB485225: “KrbException: Cannot locate default realm” error in MicroStrategy Web logs using Kerberos Integrated Authentica…
© 2023 Copyright MicroStrategy Incorporated. All Rights Reserved.
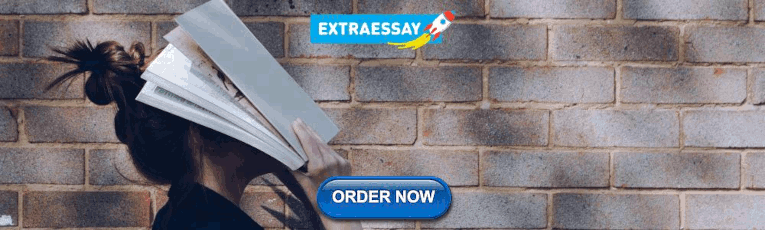
IMAGES
VIDEO
COMMENTS
In this code snippet, x and y lists are defined as before, and then a line plot is created using the plt.plot() function with customized settings. The line color is set to green using the color parameter, and the line style is set to dashed using the linestyle parameter. The linewidth parameter is set to 2 to make the line thicker.. Markers are added to each data point using the marker ...
A line graph (or line chart) is a form of data visualization in which data points are aligned along an X-Y axis, and then connected by straight line segments. Line charts are one of the oldest forms of data visualization, as our previous post about William Playfair makes clear. Line graphs are especially effective at tracking changes over time ...
A line chart or line graph is a type of chart which displays information as a series of data points called 'markers' connected by straight line segments. It is a basic type of chart common in many fields. Line charts are most often used to visualize data that changes over time. If you have a line chart, you can change the look of the data ...
A line chart is a type of chart that provides a visual representation of data in the form of points that are connected in a straight line. A line chart is one of the simplest methods to understand any financial data and trading data. Let us discuss more a line chart, the types, advantages and disadvantages, and solve a few examples as well.
A line graph (or line chart) is a data visualization type used to observe how various data points, connected by straight lines, change over time. It is often used to identify and interpret trends, patterns, and relationships in continuous data. Just like other types of graphs and charts, line graphs are composed of a vertical and a horizontal axis.
The Power of Good Data Visualization. Data visualization involves the use of graphical representations of data, such as graphs, charts, and maps. Compared to descriptive statistics or tables, visuals provide a more effective way to analyze data, including identifying patterns, distributions, and correlations and spotting outliers in complex ...
A line chart is a graphical representation of information that changes continuously over time. In the image that opens this post, we see "markers" of data points connected by straight lines that delimit the start, duration, and end of a variable on the time axis. Line charts are used in different fields for different purposes.
Engineers and economists use this format because it facilitates views of relationships between two sets of data: The line segments' slope and direction reflect their ratios and changes in polarity. ... solving the example problems in Figure 19 requires that they see the underlying link between the visual and verbal representations of the ...
Line graphs are used to represent quantitative data collected over a specific subject and a specific time interval. All the data points are connected by a line. Data points represent the observations that are collected on a survey or research. Learn about a line graph, its parts, reading and creating them, advantages and disadvantages along with solved examples.
Data visualization is the representation of data through use of common graphics, such as charts, plots, infographics and even animations. These visual displays of information communicate complex data relationships and data-driven insights in a way that is easy to understand. Data visualization can be utilized for a variety of purposes, and it ...
A line chart, also known as a line graph or curve chart, is a graphical representation used to display data points connected by straight lines. This type of chart is particularly useful for visualizing trends, changes, and relationships in data over a continuous interval, often time. Each data point on the chart represents a value associated ...
What is a Line Segment? A math term can really tell you a lot about the thing it's describing. Take the term line segment. A line segment is just part of a line! In this tutorial, learn about line segments, how to name them, and what the midpoint of a line segment is!
Area plot is like a line chart in terms of how data values are plotted on the chart and connected using line segments. In an area plot, however, the area between the line segments and the x-axis ...
These diagrams represent nodes as dots and edges as line segments (or curves). A significant majority of graph visualization software implement such representations: in 2007, referenced 54 (out of 55) node-link based systems in the Social Network Analysis Repository, and 49 (out of 52) on the Visual Complexity website.
In this article, we will approach the task of choosing a data visualization based on the type of task that you want to perform. Common roles for data visualization include: showing change over time. showing a part-to-whole composition. looking at how data is distributed. comparing values between groups.
Terms in this set (37) a visual representation of data using horizontal or vertical bars whose lengths are related to the frequency of the data. bar graph. a visual representation of data in which a circle is divided into sectors, each of which represents a percent of the whole data set. circle graph. facts, statistics, and pieces of information.
When deciding which visualization to use, here are some fundamental aspects to consider: 1. The Nature of Your Data: The type and structure of your data are key determinants in your choice of visualization. Numerical data might be best served by bar or line charts, while geographical data can be presented as a map.
Step 1: Draw a line of any length. Mark a point A on the line, which is the starting point of the line segment. Step 2: Using a ruler, place the pointer of the compass 5 cm apart from the pencil's lead. Step 3: Place the pointer of the compass at A and mark an arc on the line with the pencil point. Step 4: Mark the point where the arc and the ...
Line charts, as the name suggests, use lines to connect individual data values and map a graph. It can be represented as a single-line chart or multiline chart. ... Visualizing patterns, trends, or clusters within multiple segments of data. ... Plot feature requests to get a visual representation of priorities based on parameters like user ...
Using multiple line graphs allows us to compare more than one data set. They simplify complex information so the audience can quickly grasp the ups and downs of values. From tracking stock prices to analyzing experimental results, you can use line graphs to show how data changes over a continuous timeline. They show trends with simplicity and ...
Terms in this set (37) bar graph. a visual representation of data using horizontal or vertical bars whose lengths are related to the frequency of the data. circle graph. a visual representation of data in which a circle is divided into sectors, each of which represents a percent of the whole data set. data.
Representation of Line Segments in Programming. In code, we often represent line segments using structures or classes. These structures store the coordinates of the two endpoints, making it easy for us to manipulate and perform operations on them. ... Look for opportunities to reduce redundant calculations, use data structures wisely, and stay ...
A line chart or line graph is a type of chart which displays information as a series of data points called 'markers' connected by straight line segments. It is a basic type of chart common in many fields. Line charts are most often used to visualize data that changes over time. If you have a line chart, you can change the look of the data ...