Browse Econ Literature
- Working papers
- Software components
- Book chapters
- JEL classification
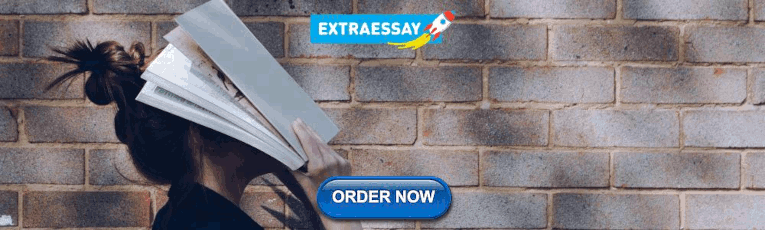
More features
- Subscribe to new research
RePEc Biblio
Author registration.
- Economics Virtual Seminar Calendar NEW!
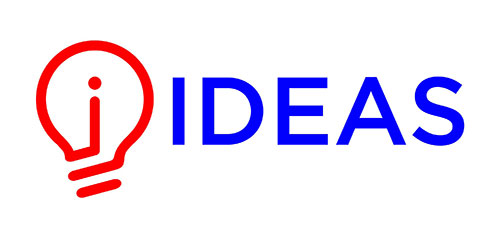
Methods Of Portfolio Management - A Review Of Literature -
- Author & abstract
- 11 References
- Most related
- Related works & more
Corrections
(Faculty of Business, Babes-Bolyai University, Cluj-Napoca, Romania)
Suggested Citation
Download full text from publisher, references listed on ideas.
Follow serials, authors, keywords & more
Public profiles for Economics researchers
Various research rankings in Economics
RePEc Genealogy
Who was a student of whom, using RePEc
Curated articles & papers on economics topics
Upload your paper to be listed on RePEc and IDEAS
New papers by email
Subscribe to new additions to RePEc
EconAcademics
Blog aggregator for economics research
Cases of plagiarism in Economics
About RePEc
Initiative for open bibliographies in Economics
News about RePEc
Questions about IDEAS and RePEc
RePEc volunteers
Participating archives
Publishers indexing in RePEc
Privacy statement
Found an error or omission?
Opportunities to help RePEc
Get papers listed
Have your research listed on RePEc
Open a RePEc archive
Have your institution's/publisher's output listed on RePEc
Get RePEc data
Use data assembled by RePEc
Patent portfolio management: literature review and a proposed model
Affiliation.
- 1 a Production Engineering Department, Polytechnic School , University of São Paulo , São Paulo , Brazil.
- PMID: 29718747
- DOI: 10.1080/13543776.2018.1472238
Introduction: Patents and patent portfolios are gaining attention in the last decades, from the called 'pro-patent era' to the recent billionaire transactions involving patent portfolios. The field is growing in importance, both theoretically and practically and despite having substantial literature on new product development portfolio management, we have not found an article relating this theory to patent portfolios.
Areas covered: The paper develops a systematic literature review on patent portfolio management to organize the evolution and tendencies of patent portfolio management, highlighting distinctive features of patent portfolio management. Interview with IP manager of three life sciences companies, including a leading multinational group provided relevant information about patent portfolio management.
Expert opinion: Based on the systematic literature review on portfolio management, more specifically, on new product development portfolio theory, and interview the paper proposes the paper proposes a reference model to manage patent portfolios. The model comprises four stages aligned with the three goals of the NPD portfolio management: 1 - Linking strategy of the Company's NPD Portfolio to Patent Portfolio; 2 - Balancing the portfolio in buckets; 3 - Patent Valuation (maximizing valuation); 4 - Regularly reviewing the patent portfolio.
Keywords: Patent portfolio management; intellectual property; patent strategy; patenting; portfolio management.
Publication types
- Drug Industry / economics
- Drug Industry / legislation & jurisprudence*
- Intellectual Property*
- Models, Theoretical
- Patents as Topic / legislation & jurisprudence*
What we know about the low-risk anomaly: a literature review
- Open access
- Published: 28 April 2023
- Volume 37 , pages 297–324, ( 2023 )
Cite this article
You have full access to this open access article
- Joshua Traut ORCID: orcid.org/0000-0003-1627-1297 1
5303 Accesses
6 Altmetric
Explore all metrics
It is well documented that less risky assets tend to outperform their riskier counterparts across asset classes. This paper provides a structured summary of the current state of literature regarding this so-called low-risk anomaly. It provides an overview of empirical findings across implementation methodologies and asset classes. Furthermore, it presents the most prevailing causes, which are namely exposure to other factors, coskewness risk, investor constraints, behavioral biases, and agency problems. The paper concludes that despite some critiques there are good reasons to believe that the low-risk anomaly can be evaluated as an investment factor. It also identifies that more research is required to disentangle the proposed causes to fully understand the big picture of the anomaly with certainty.
Similar content being viewed by others
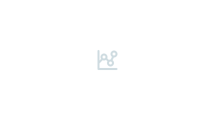
ESG Integration and the Investment Management Process: Fundamental Investing Reinvented
Emiel van Duuren, Auke Plantinga & Bert Scholtens
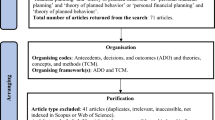
Financial planning behaviour: a systematic literature review and new theory development
Kingsley Hung Khai Yeo, Weng Marc Lim & Kwang-Jing Yii
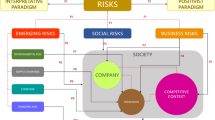
Flexibility and Resilience in Corporate Decision Making: A New Sustainability-Based Risk Management System in Uncertain Times
Davide Settembre-Blundo, Rocío González-Sánchez, … Fernando E. García-Muiña
Avoid common mistakes on your manuscript.
1 Introduction
Factor investing has gained increasing traction in academia and thousands of papers have been published with regard to this strain of literature (Jensen et al. 2022 ; Harvey 2017 ). Pioneering works developed investment factors that are based on the findings that small (big) firms, firms with low (high) book-to-equity ratios, and firms showing positive (negative) return momentum outperform (underperform) the market (Fama and French 1992 ; Basu 1977 ; Carhart 1997 ; Jegadeesh and Titman 1993 |). In a similar fashion, a persistent outperformance was discovered for low-risk assets in many asset classes [e.g. Frazzini and Pedersen ( 2014 )]. Unlike for the above-mentioned factors, the respective measure to capture low-risk assets is not agreed on, resulting in a variety of proposed low-risk measures. Because the outperformance of low-risk assets directly violates the common assumption that taking risks is rewarded with returns in financial markets, the low-risk investment effect is also called low-risk anomaly. To date, multiple causes for the anomaly have been identified, but there is still no consensus about which rationale prevails. The goal of this paper is to provide a comprehensive overview of the current state of research that examines the empirical identification of the low-risk anomaly across asset classes as well as the causes for its existence.
This is not the first time that the literature covering the low-risk anomaly is reviewed in a stand-alone paper. However, current reviews either focus solely on the causes of the low-risk anomaly (Blitz et al. 2014 ) or are strongly tilted towards equities (Blitz et al. 2020 ). The contribution of this paper is thus threefold. First, it summarizes current findings of the low-risk anomaly across asset classes, markets and methods. Second, it provides an overview of the most employed empirical methods to capture the low-risk anomaly. Thus, rather than just showing the findings of current research, it also gives insights into how those findings were generated. Third, it includes studies that neglect the existence of the low-risk anomaly and therefore provides a balanced overview of the current state of research.
The reading proceeds as follows. Section 2 provides a short historical background on the development of the anomaly. Section 3 collects empirical evidence in various asset classes. Section 4 lists and explains proposed causes for the anomaly. Section 5 discusses the current state of research and provides a general conclusion of the findings.
2 Background
The capital asset pricing model (CAPM) revolutionized the finance literature as it replaced the thought that an asset’s risk is its own volatility with the idea that the relevant measure of risk is how an asset covaries with the market. The CAPM is based on the work of Markowitz ( 1952 ) who defines risk as the variance of returns. He finds that diversification among asset reduces risk and hence increases the risk-return profile of an investment. Idiosyncratic risk can be eliminated and only systematic risk—the market risk of an investment—remains. The effect of diversification is illustrated in Fig. 1 .
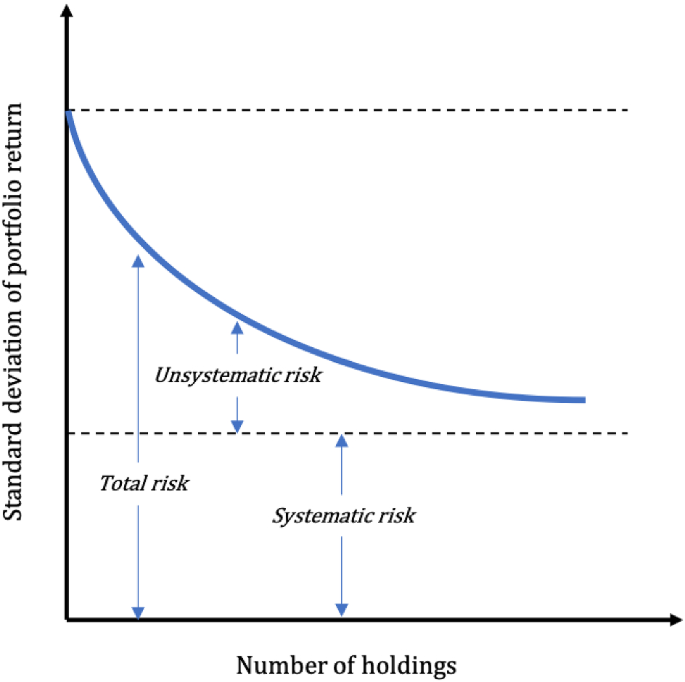
Diversification benefits. This figure is based on Markowitz ( 1952 ) and shows that with an increasing number of portfolio holdings the standard deviation of portfolio returns can be reduced. This is due to a reduction of idiosyncratic risk which vanishes as a result of diversification
The idea of the CAPM was first developed by Jack Treynor in a private manuscript (Treynor 1961 ) but was published independently by Sharpe ( 1964 ), Lintner ( 1965 ) and Mossin ( 1966 ). The model is based on Markowitz’s assumption that investors are risk-averse and seek portfolios that provide the highest return at a certain level of risk. An optimal portfolio offers the minimum risk-return ratio at the chosen risk level. As unsystematic risk can be diversified away, systematic risk was thought to be the only variable that mattered to investors. Based on this, the CAPM formula describes the relationship between risk and return for an asset i as
The return of an asset i ( \({\dot{r}}_i\) ) is defined as the risk-free rate ( \(r_f\) ) plus the asset’s market sensitivity ( \(\beta _{i,m}\) ) times the excess return of the market ( \({\dot{r}}_m-r_f\) ). Hence, the only variable controllable by investors to set portfolio returns is their investment’s exposure to the market. The dots indicate absolute returns in Eq. ( 1 ). We can also rewrite Eq. ( 1 ) in a shorter form for the asset’s excess return as
Here, returns without a dot represent excess returns. This notation will be kept throughout the paper. After its publication the CAPM was criticized by a large body of literature because the linear relation between market exposure and returns could not be proven empirically with certainty. Among the early studies, while some confirm that the relation exists (Fama and MacBeth 1973 ), others find that it is much flatter than implied by the CAPM (Black et al. 1972 ; Friend and Blume 1970 ; Miller and Scholes 1972 ) and some even find a negative relationship between risk and return (Haugen and Heins 1975 ). Doubts about the CAPM were further fostered as more later studies could not confirm the linear relationship between market risk and return (Haugen and Baker 1991 , 1996 ; Fama and French 1992 ; Frazzini and Pedersen 2014 ). Similarly, other studies find that risky stocks (in terms of idiosyncratic volatility) underperform their low-risk counterparts (Ang et al. 2006 , 2009 ; Clarke et al. 2010 ). Another body of literature that examines the empirical performance of the theoretical minimum-variance portfolio comes to a related conclusion. These studies find that while the minimum-variance portfolio achieves a significant reduction of volatility, it also delivers comparable or even higher average returns than the market portfolio (Clarke et al. 2006 , 2011 ; Haugen and Baker 1991 ; Jagannathan and Ma 2003 ).
All of the above studies document the so-called “low-risk anomaly”. As different concepts to measure an asset’s risk are applied in the literature, the investment style that captures the anomaly was given various names such as defensive, low-risk, low-volatility, or quality factor. I will call all of these related factors low-risk factors. An overview of the different low-risk factors that were implemented across asset classes is provided in the next section.
3 Evidence in markets
Like most factors, low-risk factors are most researched in equity markets. Regardless, there is a considerable literature that took the concept from equity markets and adapted it to other asset classes. Given this inequality of available literature, the equity section is more extensive than the others.
3.1 Equities
In equity markets three different measures to asses a stock’s riskiness are assessed by literature. These three measures are a stock’s market beta, its idiosyncratic volatility (IV), and it’s total volatility of returns. Relatedly, there also exists a strain of literature that examines the minimum variance portfolio. In this section I will first review the studies that focus mostly on confirming one of the three measures empirically and the mean variance portfolio, before I review studies that compare multiple concepts.
3.1.1 Market beta
As one of the first who detect the low-risk anomaly, in their empirical assessment Black et al. ( 1972 ) not only find that the CAPM does not hold but also propose a new model that captures the flatter linear relationship between systematic risk and return. According to this model an asset’s return is defined as
In addition to the market risk Black et al. ( 1972 ) introduce a second factor return ( \(r_\beta \) ) that captures the flatter relationship between market risk and returns. They call this factor “beta factor” because its coefficient is a function of the asset’s beta. They show empirically that in the US \(r_\beta \) is generally positive. This means that stocks with a low beta earn positive CAPM excess returns whereas high-beta stocks underperform the predictions made by CAPM.
Black ( 1993 ) replicates the study of Black et al. ( 1972 ) and empirically confirms their suggestion that \(r_\beta \) is positive in the US. In doing so, he sorts stocks based on beta and builds ten portfolios—the 10% of stocks with the largest beta in the first portfolio, and so on. He then takes the excess returns from the ten portfolios and weights them by ( \(1-\beta _j\) ), where \(\beta _j\) represents portfolio j ’s beta. The resulting portfolio goes long low-beta stocks and short their high-beta counterparts. Black’s empirical tests show that this beta-neutral portfolio earns positive statistically significant excess returns over the sample period between 1931 and 1991. He concludes that the beta factor indeed earns a positive risk-premium.
Frazzini and Pedersen ( 2014 ) further confirm the existence of a risk factor related to an asset’s beta. They estimate market beta of security i according to the following formula
where \({\hat{\sigma }}_i\) and \({\hat{\sigma }}_m\) are the one-year rolling standard deviations of asset i and the market portfolio m and \({\hat{\rho }}_{im}\) is the five-year rolling correlation between the two. They separate time horizons because correlations appear to move more slowly than volatilities (De Santis and Gerard 1997 ). In 20 developed equity markets they rank stocks on estimated betas every month and form simple portfolios that are long low-beta stocks and short high-beta stocks. Within long and short legs of the portfolios stocks are weighted by ranked betas so stocks with low/high betas have higher weightings in the long/short side of the portfolio. Both legs of the portfolio are then rescaled to have a beta of one, thus making the aggregated portfolio beta neutral. Over the sample period 19 out of the 20 betting against beta (BAB) country portfolios earn positive excess returns of which eleven are statistically significant at the 5% level. Han et al. ( 2020 ) apply the same factor construction methodology to Chinese A-shares (a region not covered by Frazzini and Pedersens’s study) and show that the BAB factor also earns a statistically significant alpha in this region.
Asness et al. ( 2014 ) also follow the methodology of Frazzini and Pedersen to investigate BAB portfolios in the US, Japan, Europe ex-UK, and UK. They deviate from the previous methodology in that they use BARRA risk models Footnote 1 to estimate volatilities and correlations in Eq. ( 3 ). To form country portfolios they calculate z-scores based on a stocks beta rank. They use these z-scores as standard weights for the long-short portfolio construction as z-scores add up to zero. Then they volatility-adjust the long and short side of the portfolio to have equal volatilities and scale the final portfolio to have a volatility of 10%. Each month they create such low-risk long-short portfolios in each region and aggregate them to a global low-risk portfolio with the following weights: US, 50%; Japan, 16.7%; Europe ex-UK, 16.7%; and UK, 16.7%. Their resulting portfolio earns positive excess returns that have an average Sharpe ratio of 0.61 in the sample period from 1990 to 2013.
3.1.2 Idiosyncratic volatility
Ang et al. ( 2006 ) take a different angle at quantifying the low-risk anomaly. Given the empirical failure of the CAPM and the ubiquity of the three-factor model by Fama and French ( 1992 ) (FF3) they quantify a firms riskiness as its residual volatility relative to the FF3 model. More precisely, they define a stock’s idiosyncratic risk as \(\sqrt{var(\epsilon _i)}\) resulting from the following equation
where SMB , and HML are referring to the size and value factor of the FF3 model. Ang et al. ( 2006 ) compute monthly IVs for U.S. stocks with the above regression formulas using daily return data. They sort stocks into quintile portfolios based on their IV, for which they calculate value-weighted returns and rebalance them every month. Over their sample period from 1963 to 2000 they report statistically significant negative CAPM and FF3 alphas for a portfolio that goes long high-risk stocks and shorts their low-risk counterparts. Ang et al. ( 2009 ) reinforce the findings in a subsequent study within which they apply the same methodology to a larger sample of 23 developed countries. Again they find that high-risk stocks tend to earn significantly lower returns than low-risk stocks. A more recent study by Dimson et al. ( 2017 ) further confirms the out-of-sample validity of the results. They estimate a stock’s idiosyncratic risk as the three-month volatility of \(\epsilon _i\) in Eq. ( 4 ). In their two samples covering US and UK stock markets low-risk stocks earned average annual returns of 10.9% and 11.6% each year while high-risk stocks earned 4.1% and 4.2% respectively.
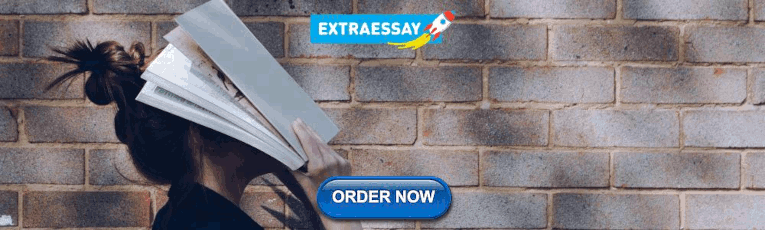
3.1.3 Total return volatility
Arguably the simplest measure to quantify riskiness of stocks is employed by Blitz and van Vliet ( 2007 ) for large cap stocks in the FTSE World Developed index between 1985 and 2006. They construct monthly equally-weighted decile portfolios within which they rank stocks on their past three-year volatility of weekly returns. Across those volatility-sorted portfolios excess returns rise accompanied with a fall in volatilities, leading to a monotonic decrease in Sharpe ratios from 0.72 to 0.05 starting at the low-volatility portfolio.
Following Blitz and van Vielt’s research on developed markets, Blitz et al. ( 2013 ) reinforce the findings by expanding the methodology to emerging markets. They sort stocks covered in the S &P/IFC Investable Emerging Markets Index based on historical three-year volatility of weekly returns. Their quintile country-neutral portfolios that have the lowest volatility significantly outperform the counterparts exhibiting the highest volatility. Joshipura and Joshipura ( 2016 , 2019 ) use the same sorting methodology for the Indian stock markets and confirm the finding that low-volatility stocks generate outperformance
Baker and Haugen ( 2012 ) extend the study to 21 developed countries and 12 emerging markets. Every month they build decile portfolios based on a stock’s return volatility over the past 24 months using monthly return data. In their sample period from 1990 to 2011 they find that in every market the lowest risk decile portfolio earns higher returns than the portfolio holding the riskiest stocks.
3.1.4 Minimum variance portfolio
The minimum variance methodology differs from the above examined methods in that it does not attempt to separate risky stocks from its less risky counterparts. Instead, it attempts to form a portfolio that has minimum total variance. In doing so, risky stocks may be added to the portfolio if they reduce total risk, for example if they are negatively covary with other stocks.
Haugen and Baker ( 1991 ) investigate the performance of the minimum variance portfolio versus the market portfolio between 1972 and 1989. They use the largest 1000 US stocks to quarterly form the minimum variance portfolio based on the historic covariance matrix over the last 24 months. They also impose constraints to ensure diversification and abandon short-selling. In their sample period, the minimum variance portfolio has a lower volatility and earns higher returns than the Wilshire 5000 index that proxies the market portfolio. Further evidence that the minimum variance portfolio generates outperformance—even when reallocated less regularly is gathered by Jagannathan and Ma ( 2003 ). Similar to Haugen and Baker ( 1991 ) they build the minimum variance portfolio imposing diversification and short-selling constraints for 500 randomly chosen stocks traded on NYSE and AMEX. They reallocate the portfolio on a yearly basis and show that it earns much higher returns at lower variation than the market portfolio. Clarke et al. ( 2006 ) extend the study of Haugen and Baker ( 1991 ) to cover the time horizon from 1968 to 2005 and to use additional covariance structuring methodologies. More precisely, they use principal components following Connor and Korajczyk ( 1988 ) and Bayesian shrinkage following Ledoit and Wolf ( 2004 ). Based on monthly (daily) returns of the largest 1000 stocks in the US they estimate two structured covariance matrices for the past 60 months (250 days) and compose the minimum variance portfolio imposing short-selling and diversification constraints. For both structuring methodologies they find that minimum variance portfolios have about 75% of the realized risk of the general market, but earn similar returns. They replicate their results using the same methodology in Clarke et al. ( 2011 ) from 1968 to 2009 and find that the minimum variance portfolio outperforms the market regarding both, risk and return. Chen et al. ( 2018 ) investigate minimum variance portfolios calculated from daily returns over the past two years in the Chinese A-shares market and find that these portfolios strongly outperform the market regarding risk and return.
3.1.5 Comparison of measures
With multiple measures employed to capture the low-risk factor, the question which one does it best automatically arises. This question was investigated by multiple studies though only few investigate all four above presented methods.
Blitz and van Vliet ( 2007 ) compare the performance of their proposed measure of total return volatility to market beta. Same as for return volatility, they rank stocks based on three-year historical regional betas. Though they find the same pattern in portfolio returns—that lower risk portfolios outperform—this pattern is more irregular and less strong for portfolios sorted beta than it is for those sorted on total return volatility. Baker et al. ( 2011 ) perform the same comparison and sort stocks on five-year volatility and beta into quintile portfolios. They find that low risk portfolios consistently outperform from 1968 to 2008 in the US and also report that the effect of volatility is larger than that of beta.
Soe ( 2012 ) also compares two of the proposed methodologies—low-volatility and minimum-volatility strategies. They find that the two approaches exhibit very similar performance characteristics. De Carvalho et al. ( 2012 ) confirm the similarity of low-risk factor strategies. They investigate five popular low risk portfolios and find that they generally load on low-beta and low-volatility factors. Furthermore, they find that the performance of these portfolios is relatively similar. Scherer ( 2011 ) and Chow et al. ( 2014 ) come to the same conclusions in their studies on widely used low-risk strategies.
Walkshäusl ( 2014 ) compares the low-volatility, the low-beta, and the minimum volatility strategy for the MSCI universe of developed and emerging markets between 2001 and 2011. Regarding low-volatility and low-beta he follows Baker et al. ( 2011 ) and builds portfolios based on the historic five-year measure of the respective style. The minimum volatility portfolio is proxied by MSCI minimum volatility indices. Walkshäusl compares the performance of the quintile portfolios with the minimum betas/volatilities with the minimum variance portfolio. Parts of his analysis are visualized in Fig. 2 . He finds that though strategies generate higher returns than the market benchmark, these differences are not statistically significant. Also, between strategies return differences are relatively small. Standard deviations of the three strategies are substantially lower than that of the market and statistically significant for all style portfolios. Again, differences between strategies tend to be small.
Comparable to the pattern in equity markets, in bond markets literature finds that low-risk bonds tend to outperform. Most literature defines specific factors for government bonds and corporate bonds separately. Because of this, the two asset classes will be examined separately in this section. In general, the most promising risk-adjusted returns in both asset classes can be earned with bonds that are characterized by short maturities and high credit ratings (Ilmanen et al. 2004 ).
3.2.1 Government bonds
Pilotte and Sterbenz ( 2006 ) study Treynor and Sharpe ratios for US Treasury securities between 1959 and 1997. They find that both ratios are highest for short-term bills and decrease as maturity rises. Same as for equities, Frazzini and Pedersen ( 2014 ) use beta to determine the riskiness of a bond. They define beta as the sensitivity of the bond to an equally-weighted portfolio of all Treasuries used in the analysis and build seven beta ranked portfolios. They find that between 1952 and 2012 low-beta Treasuries earn positive alphas that turn negative for their high-beta counterparts. Also, Sharpe ratios increase from low-beta to high-beta portfolios and a long-short of the lowest and highest portfolio delivers the highest significant Sharpe ratio of 0.81. It has to be noted that though they rank Treasuries based on beta, this is empirically equivalent to ranking on duration or maturity. Hence, they confirm the results of Pilotte and Sterbenz ( 2006 ). Brooks et al. ( 2018 ) take a similar route and employ effective duration as the measure for government bond risk. They sort bonds into three portfolio buckets based on duration across 13 developed markets. A long-short portfolio that buys (sells) the most (least) favorable bucket earns an average return of 1.04% p.a. The same methodology is used by Kothe et al. ( 2021 ) in a later analysis who report significant excess returns of 0.77% p.a. for this strategy. Focusing on 20 non-US developed bond markets Durham ( 2016 ) confirms the return generation of low-risk government bond strategies. He uses the methodology of Frazzini and Pedersen ( 2014 ) but performs minor tweaks such as taking daily instead of monthly data for the portfolio construction. Over his sample period from 1962 to 2013 he reports an average excess return of 25 bps per month for the BAB long-short portfolio.
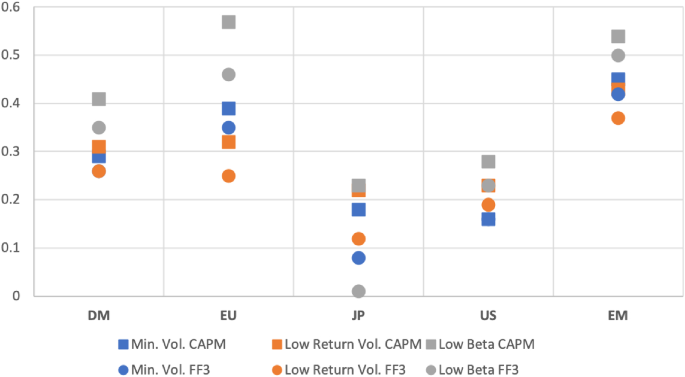
Monthly CAPM and FF3 alphas of low-risk investment strategies in different markets. This illustration is based on the results reported in Walkshäusl ( 2014 ). It shows CAPM and FF3 alphas for low-volatility, low-beta, and minimum volatility strategies in developed markets (DM), the European Union (EU), Japan (JP), the US, and emerging markets (EM). Strategies are based on the MSCI universe between 2001 and 2011. Low-volatility and low-beta returns are represented by the quintile of stocks that have the lowest respective measure each month. The minimum volatility strategy is proxied by MSCI minimum volatility indices
Few studies extend analyzed assets beyond developed markets. Zaremba and Czapkiewicz ( 2017 ) perform a broad market study across 25 developed and emerging markets. They take modified duration as the relevant low-risk measure to build long-short portfolios after sorting bonds into tercile buckets. They report that this strategy earns average significant returns of 0.27% p.a. across all markets.
3.2.2 Corporate Bonds
The methodologies used for corporate bonds are similar to those for government bonds. Derwall et al. ( 2009 ) find that low-maturity corporate bonds outperform their long-maturity counterparts in the US. Aussenegg et al. ( 2015 ) report the same finding in European corporate bond markets. Using the same methodology as for their government bond analysis, Frazzini and Pedersen ( 2014 ) build long-short portfolios for US corporate bonds based on their sensitivity to an equally-weighted portfolio of all corporate bonds used in the analysis. They report that alphas and Sharpe ratios of corporate bond portfolios increase for portfolios that hold more low-risk assets.
To enhance the robustness of low-risk corporate bond strategies, a strain of literature developed that extends these strategies with fundamental measures of risk. This more comprehensive approach is used by Brooks et al. ( 2018 ) who focus on public companies in 13 developed markets and Israel et al. ( 2018 ) who solely cover the US market. Similar to the strategies above, low duration is one criterion characterizing a low-risk security. Additionally, market leverage computed as
and gross profitability computed as
are taken as measures to identify low-risk corporate bonds. Within both studies, assets are broken down into quintiles according to the aggregated low-risk factor. From the least to the most favorable quintile, returns rise while volatility either decreases or remains at the same level. The best risk-return ratio is achieved when a long-short portfolio of the first and the last quintile is built.
A more simplified approach that incorporates fundamental measures is employed by Houweling and van Zundert ( 2017 ) who use maturity and rating to define a low-risk portfolio for the US corporate bond market. They construct two asset pools, one for investment-grade and one for high-yield bonds, arguing that the assets in those pools differ substantially in terms of market participants and other market characteristics. Within both pools, they favor short-dated, high-rated bonds and create a long-short portfolio that buys the top 10% of the most favorable bonds and sells the bottom 10% of investible assets. In both markets, significant excess returns can be earned with this strategy.
De Carvalho et al. ( 2014 ) test five risk metrics in developed investment-grade corporate bond markets that are denominated in USD, EUR, GBP, and JPY between 1997 and 2012. The risk metrics are duration-times-yield (or yield elasticity), modified duration, yield to maturity, duration-times-spread, and option-adjusted spread. Based on these measures they form quintile portfolios and find that duration-times-yield sorted portfolios show the biggest differences in Sharpe ratios between the lowest and the highest portfolio irrespective of the underlying currency. Also, alphas for low-risk portfolios are positive and turn negative for the corresponding high-risk portfolios for four out of the five risk measures used in the analysis.
Lastly, Chung et al. ( 2019 ) run a comprehensive analysis of low-risk investing in corporate bond markets and find that bonds that have more exposure to aggregate market volatility earn lower returns—even when adjusted for bond characteristics such as ratings and maturities. In contrast to the equity literature, they also find that bonds with a higher IV over the past six months calculated similarly to Eq. ( 4 ) but with different factors have higher returns. However, they also document that bonds with high stock return volatility have lower expected returns.
The low-risk anomaly is apparent in almost every market. This section only presents a small extract of the existing literature, primarily to show how widespread the outperformance of low-risk assets is in different markets.
In addition to stocks and bonds Frazzini and Pedersen ( 2014 ) apply their BAB factor to credit indices, equity indices, commodities and foreign exchange. Following the same methodology as discussed in Sect. 3.1 , they form portfolios sorted on beta and document that their long-short BAB portfolios generate positive excess returns. Frazzini and Pedersen ( 2022 ) further investigate the low-risk anomaly in options and levered ETFs. They find that the higher the leverage, the lower the risk-adjusted return of such products. They further demonstrate that BAB factor portfolios earn large and statistically significant abnormal returns with Sharpe ratios above one. Similarly, Cao and Han ( 2013 ) find that returns of delta-hedged options decrease with an increase in the IV of the underlying stock.
The low-risk anomaly does not solely hold for investments of all kind but also for investment professionals. Jordan and Riley ( 2015 ) document that the low-risk anomaly is also present in the cross section of mutual fund returns, meaning that funds with low return volatility tend to outperform their peers.
In more fragmented financial markets, the pattern can also be observed. Eraker and Ready ( 2015 ) find that very risky over-the-counter stocks have very poor average returns. Similarly, Moskowitz and Vissing-Jørgensen ( 2002 ) find that despite being more risky, private equity stocks do not deliver higher returns than publicly traded stocks, making them unattractive from a risk-return standpoint. Relatedly, Adhami et al. ( 2023 ) observe an inverse relationship between risk and return in crowdlending markets.
Lastly, a very comprehensive study by Falkenstein ( 2010 ) detects the low-risk effect in 20 asset classes. This study does not solely focus on common financial assets such as equities and bonds but also incorporates more exotic fields such as movie production, lotteries, and sports bets.
3.4 Replicability and robustness
In the presence of multiple factors that claim to capture the low-risk anomaly, a discussion developed whether it makes sense to claim significance for factors that are almost identical. Harvey ( 2017 ) addresses this issue and states that too much attention is drawn on p-values. He claims that since significant results are more likely to be published, there is a strong incentive for p-hacking and data manipulation in factor research. Harvey et al. ( 2016 ) share this view and argue that t-stat boundaries to assess significance should be much higher for factors. They show that there is a publication bias, meaning that new factors are more likely to be published than replication studies of existing ones. Furthermore, they demonstrate that the performance of published factors tends to degrade after publication, which as they argue is an indicator for data-mining and p-hacking in the factor literature. In a very comprehensive study Jensen et al. ( 2022 ) address this critique. They replicate 153 factors that they cluster into 13 themes in 93 countries and assess their replicability. The theme “Low risk” is found to have a replication rate of 100% in the US, developed ex. US, and emerging equity markets. Other studies deliver contrasting results. One study replicates 452 anomalies across different time horizons and shows that for low-risk equity factors low-risk stocks outperform their high-risk peers but in most settings they don’t pass the 5% significance hurdle (Hou et al. 2020 ). Pyun ( 2021 ) similarly finds that the IV premium significantly decreased after the seminal paper by Ang et al. ( 2006 ) was published. Though all of these studies find a premium, the contrary results for its significance indicate that a critical view of the anomaly may be worthwhile.
Regarding robustness, many of the studies that examine the low-risk anomaly perform robustness tests with respect to other common risk factors and macroeconomic variables. For example Frazzini and Pedersen ( 2014 ) calculate alphas of their BAB factor to FF3 factors and momentum and find that BAB alphas remain significant. Another example is the study composed by Ang et al. ( 2009 ) who control for the FF3 factors, momentum, volume, and liquidity. They also find, that their excess returns cannot be explained by the control variables.
Based on the findings of Baker et al. ( 2011 ) who report that the low-risk effect is stronger for smaller stocks than for larger stocks, low-risk factors have been criticized as many anomalies are known to be concentrated in small-cap stocks and therefore difficult to exploit. For example, Novy-Marx and Velikov ( 2022 ) criticize the methodology behind the BAB factor because high parts of its premium are driven short selling highly illiquid micro caps. Other scholars do not support those claims and show that for example, the IV factor is especially robust when penny stocks are excluded (Chen et al. 2020 ). Relatedly, Auer and Schuhmacher ( 2015 ) show that the low-risk anomaly is strongly present among the largest, most liquid US stocks.
Other studies deliver more support for low-risk factors and show that their excess returns do not stem from industry or country bets (Asness et al. 2014 ; Baker et al. 2014 ). Exposure to interest rate risk as a possible explanation was examined by De Franco et al. ( 2017 ) who conclude that, although low-risk stocks are significant exposed to interest rate risk, this only explains a very small part of their outperformance.
However, there are also more critical voices in literature. Cederburg and O’Doherty ( 2016 ) investigate the performance of beta sorted portfolios. They find that properly accounting for time-series variation of portfolio risk properly explains the low-risk anomaly. In other words, taking into account that beta is not constant over time, vanishes the anomaly. In their analysis they use a conditional CAPM, following Boguth et al. ( 2011 ), within which beta is modeled as a function of lagged state variables, a procedure also known as standard instrumental variable method. Another more critical study attributes the IV effect to liquidity. It shows that when taking quote-midpoint returns the IV effect vanishes and attributes the anomaly to bounces in trade prices (Han et al. 2015 ).
4 Proposed causes
Even though a lot of empirical evidence exists that supports the existence of a low-risk anomaly, no consensus about the rationales behind it is reached. There are rational and irrational theories why low-risk factors generate excess returns. First, I will cover the rational explanations such as coskewness risk, exposure to other factors, and investor constraints before I will elaborate on studies delivering behavioral explanations. Though I attempt so clearly separate between proposed causes, note that there are strong interrelations and the categorization here is only done to provide some structure.
4.1 Exposure to other factors
In the presence of multiple factors that attempt to measure the low-risk anomaly, there are a number of studies that investigate the interrelations of different low-risk factors. In his comparison of low risk strategies Walkshäusl ( 2014 ) regresses the returns of minimum variance, low-beta, and minimum total return volatility strategies in different regions on the returns of the same strategy in developed markets. He finds that all strategies in developed markets share a significant common return component that is not shared with emerging markets. Asness et al. ( 2019 ) dig deeper into this discovery and directly disentangle the two driving components of the BAB strategy, volatility and correlation. They find that both, volatility and correlation contribute equally to the performance of the BAB factor. In a similar fashion Chen and Petkova ( 2012 ) decompose the IV factor into exposure to market variance and exposure to market correlation. They find that only variance is priced and has a negative premium. Further they show that the differences in performance between high (low) IV factor portfolios have positive (negative) loadings with respect to innovations in average stock variance. Because of the negative premium such portfolios earn lower (higher) expected returns. In his study Scherer ( 2011 ) investigates the performance of minimum-variance portfolios. He finds that when controlling for low-beta, residual return volatility as well as the market return factors, 79% of the variance in returns of minimum variance portfolios can be explained. He infers that outperformance by minimum variance portfolios is subsumed by other low-risk factors.
Beyond low-risk factors there are also other studies suggesting that low-risk factors have exposure to other factors and thus generate outperformance. In his influential paper Fu ( 2009 ) finds that high returns of high volatility stocks are largely explainable by the short-term return reversal of small cap, high-volatility stocks. In other words, stocks with high risk have high contemporaneous returns that tend to reverse in the following month. This claim is supported by the finding of Huang et al. ( 2010 ) who report that return reversals can explain the low-risk anomaly. However, other studies document that the results in Fu ( 2009 ) are prone to look-ahead bias and thus not useful to overturn the findings of low-risk premia (Park et al. 2020 ; Fink et al. 2012 ; Guo et al. 2014 ). Size is another common factor that is claimed to render the low-risk anomaly insignificant. Riskier stocks tend to be small while low-risk stocks are most likely large caps. Creating buckets with equal market capitalization share in the portfolio sorts based on IV causes all buckets to generate the same returns, thus eliminating the low-risk effect (Bali and Cakici 2008 ). These findings are further confirmed for other low-risk strategies by Hou et al. ( 2020 ) and Pyun ( 2021 ). Novy-Marx ( 2016 ) challenges low-risk strategies in an integrated approach by controlling for size, profitability and value. In line with the studies mentioned in Sect. 3.4 , when controlling for the FF3 factors, he confirms that low-risk strategies generate alpha. However, he shows that this outperformance is generated because low-risk strategies short unprofitable small growth firms. Hence, the low-risk anomaly can be explained by combining value, size and profitability factors. Fama and French ( 2016 ) further support the finding and argue that low-beta returns can be explained by their profitability and investment factors. These finding is also bolstered by the theory of Johnson ( 2004 ) who shows that leverage ratios of firms (usually a measure for profitability) can explain the low-risk anomaly. Generally, when comparing common profitability factors [e.g. Asness et al. ( 2018 )] to low-risk factors some commonalities can be identified—especially in corporate bond markets. For example, gross profitability and leverage, both profitability factors, are used as variables to identify low-risk corporate bonds. The inclusion of such fundamental values also led to a discussion whether profitability factors are related to value factors (Novy-Marx 2013 ).
4.2 Skewness risk
Another popular explanation for the low-risk anomaly is that it compensates investors for skewness risk. There are two sides of this theory that are going to be covered separately here. While this section focuses on the rational explanations of the skewness risk premium, the behavioral side of the premium is covered later in Sect. 4.4 under “Preference for Lotteries”.
The reasoning to attribute the low-risk anomaly to skewness is pretty straight forward. Most asset pricing models do not reach beyond the second moment of returns (e.g. covariance in the CAPM) and hence skewness is not included in most pricing kernels. The fact that skewness of returns can change the expected value of asset returns is thus ignored and traditional pricing models diverge from empirically observed prices. The studies of Rubinstein ( 1973 ) and Kraus and Litzenberger ( 1976 ) suggest that the empirical failure of the CAPM may be attributable to ignoring the effect of skewness in asset returns. More precisely, their findings indicate that investors demand compensation for accepting negatively skewed returns. Harvey and Siddique ( 2000 ) build upon this idea and develop an asset pricing model that incorporates skewness. They extend the CAPM by an additional term which makes their pricing function
where the additional term \(\beta _{i,s}\) represents the sensitivity of stock i to the squared market return ( \(r_{m}^2\) ) and thus measures the stock’s coskewness with the market. Harvey and Siddique ( 2000 ) test their model empirically and find that the model indeed helps to explain the cross-section of equity returns. Schneider et al. ( 2020 ) take this enhanced asset pricing model and directly relate it to low-risk anomalies. First, they show that the residual returns of long-short portfolios based on beta and IV are associated with negative skewness. To further allocate the performance of low-risk strategies to negative skewness, they build factors based on ex-ante skewness. In doing so, they extract implied skewness from option prices following Schneider and Trojani ( 2015 ) and build four factors of implied skewness that earn positive excess returns with which they control for skew exposure in low-risk strategies. Their results show that controlling for each of their four factors significantly reduces negative coskewness of residual returns and largely eliminates alphas of low-risk strategies built on beta and IV. The reductions of alpha are so pronounced that the remaining alpha of the low-risk strategies becomes statistically insignificant.
Boyer et al. ( 2010 ) also investigate the relation of implied skewness and low-risk strategies. They follow Chen et al. ( 2001 ) using firm-level variables to predict idiosyncratic skewness of stocks. The results of their analysis indicate that idiosyncratic volatility is a strong predictor of idiosyncratic skewness. When they control for their skew measure in IV factors they find that excess returns become much lower and statistically insignificant. Relatedly, Bali et al. ( 2020 ) argue that idiosyncratic skewness of returns can be used to measure firms’ growth options. With their skewness-based measure they reason that investors prefer stocks with a large number of embedded growth opportunities. They show that when controlling for their measure of firms’ growth options the IV anomaly can be strongly reduced. Their line of reasoning is supported by other studies that also find that the low-risk anomaly vanishes when controlling for growth options (Bhamra and Shim 2017 ; Barinov and Chabakauri 2021 ).
4.3 Investor constraints
Literature has found multiple investor constraints that are thought to cause the low-risk anomaly. I split those causes into borrowing/leverage constraints and short-selling constraints.
4.3.1 Borrowing/leverage constraints
The reasoning behind this theory is pretty straight forward. If agents are constraint regarding their leverage, the points on the capital market line that lie above the tangency portfolio are out of reach for those agents. The only way they can earn higher returns is to buy riskier assets that are located further up on the efficient frontier. These constrained agents bid up prices of riskier assets and cause the low-risk anomaly. This theory is illustrated in Fig. 3 where the dotted line represents the theoretical security market line and the dashed line shows the expected security market line with no leverage available for agents. The red semicircles indicate some agent’s utility function.
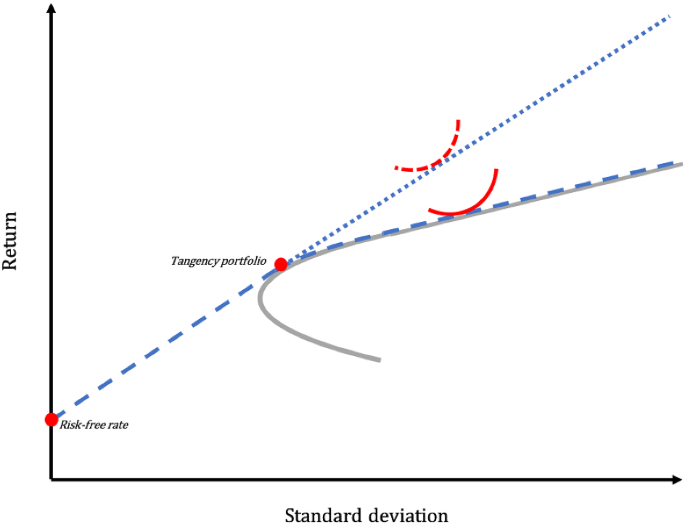
Security Market Line with no Leverage. This figure illustrates the arguments made by Frazzini and Pedersen ( 2014 ). It shows the efficient frontier of an investment universe (grey line) and the respective capital market lines for investors wo can or cannot use leverage (blue lines). The figure shows that an unconstrained investor can reach investments on the capital market line above the tangency portfolio by using leverage (dotted blue line). The investor who cannot use leverage falls back on the efficient frontier above the tangency portfolio (dashed blue line). Above the tangency portfolio his capital market line is less steep than that of the unconstrained investor. The dashed blue line thus represents the capital market line for investors who cannot use leverage. The red utility curves demonstrate that the unconstrained investor (dashed red line) can invest into more favorable portfolios above the tangency portfolio than the investor facing leverage constraints (solid red line). (Colour figure online)
Contemporaneous to the first empirical findings that detected the low risk anomaly Black ( 1972 ) and Brennan ( 1971 ) showed in their theoretical works that a restriction on investor borrowing reduces the slope of the capital market line. This extension of the CAPM brings it closer to the empirical findings of that time. When Black ( 1993 ) revisited the low-risk anomaly, he also claimed that borrowing restrictions enforced by law (like margin rules, bankruptcy laws, and tax rules) are the reason why the anomaly exists.
Frazzini and Pedersen ( 2014 ) lay out convincing arguments for leverage constraints being the cause for the low-risk anomaly. They predict that if a funding liquidity shock occurs, required returns on their BAB factor rise, leading to losses in the current BAB portfolio. This is expected because agents may need to de-lever their current BAB portfolios or stretch further to buy high-beta assets. Furthermore, they reason that when a liquidity shock occurs, all prices drop simultaneously and betas converge towards one. They are able to bolster their theory empirically as they show that their BAB factor performs worse when funding constraints are higher and also confirm that betas converge when funding conditions are less uncertain. In doing so, they take the TED spread and its volatility as a proxy for funding conditions and funding uncertainty. In addition to the, empirical support of their theory, they further show that investors who are constrained in leverage, such as mutual funds (because they have to hold cash to meet redemptions) hold portfolios with betas above one on while unconstrained firms like leveraged buyout funds hold assets with betas below one. Jylhä ( 2018 ) adds on to the findings of Frazzini and Pedersen ( 2014 ) and further confirms their theory empirically. He measures investor’s leverage constraints using the minimum level of margin required when purchasing stock on credit that is set by the Federal Reserve. Consistent with the theory he finds that when margin requirements increase, the security market line significantly flattens, making the BAB factor more profitable. Boguth and Simutin ( 2018 ) take a different route to the problem. They invert the argument and take the average market beta of mutual funds (who are assumed to face leverage restrictions) as a proxy for investor’s leverage constraints. They show that this proxy predicts returns of the BAB portfolio, strengthening the argument for a connection between leverage constraints and abnormal low-risk returns.
Adrian et al. ( 2014 ) also approach the explanation from another angle as they construct an intermediary stochastic discount factor based on theory covering shocks to leverage of security brokers. They find that this leverage factor correlates strongly with BAB portfolios and explains the cross-section of returns sorted on betas. In their decomposition of the BAB factor Asness et al. ( 2019 ) also test the leverage constraint theory. They show that the BAB factor can be predicted by margin debt held by customers at NYSE member organizations relative to the market capitalization of those NYSE firms, a measure for severity of leverage constraints. In their study focusing on leveraged options and ETFs Frazzini and Pedersen ( 2022 ) show that BAB factors also work in those products. The findings are related to the theory that leverage constraints cause the anomaly because the they show that investors are willing to pay a premium for securities with embedded leverage, and intermediaries who meet this demand need to be compensated for their costs and risk. Lastly, Asness et al. ( 2012 ) lay out the theory in a portfolio allocation problem between bonds and stocks. They also reason that leverage constraints lead investors to allocate portfolio suboptimally from a theoretical perspective.
4.3.2 Short-selling constraints
The theory behind the reasoning why short-selling constraints cause the low-risk anomaly is closely related to behavioral reasons that are covered hereafter. In contrast to the leverage constraint theory, it does not explain the low-risk anomaly as a whole but rather gives insights into why risky stocks are overpriced.
Generally, this literature assumes that there is divergence of opinion about the fair price of an asset. The scarcity of short-selling can thus cause a price to rise above the aggregate valuation of investors. Pessimistic investors are unable to express their view on the development of the asset in trades and thus prices are generally set by optimists. This effect of short-selling constraints on prices is highest when difference of opinion is high. Hence, there must be a negative correlation between risk-adjusted returns and dispersion of beliefs. This theoretical outline and the effect of short-selling constraints is pioneered by Miller ( 1977 ). Similar asset pricing predictions are outlined in other theoretical papers such as those by Duffie et al. ( 2002 ), Morris ( 1996 ), Chen et al. ( 2002 ) and Hong and Sraer ( 2016 ).
The claims made by the theories above have been challenged and confirmed by empirical studies. Dieter et al. ( 2002 ) use the standard deviation in analysts’ forecasts about future earnings to proxy for divergence of opinion among investors. They show that stocks with a wide range of opinions will earn lower returns than otherwise similar stocks. These results are confirmed by other studies who follow their methodology to identify controversial stocks (Hong and Sraer 2016 ; Gebhardt et al. 2001 ).
Figlewski ( 1981 ) takes a different route and sorts constituents of the S &P 500 index into decile portfolios based on short sale interest relative to the total amount of outstanding shares for each stock. According to his reasoning, high short interest indicates high divergence of opinion. He finds that with increasing short interest, the performance of the decile portfolios gets worse. Thus, he confirms that stocks with more controversial information are overpriced and consequently earn lower returns. Chen et al. ( 2002 ) criticize the assumption that short interest can be used to proxy divergence of opinion and instead take breath of ownership as a proxy. They define breath of ownership as the number of investors who have long positions in a particular stock. Low breath means high divergence of opinion because many investors do not express their pessimistic view due to short-selling constraints. Using mutual fund data they find support for their hypothesis and show that stocks with the lowest breath in ownership underperform their peers.
Danielsen and Sorescu ( 2001 ) tackle the problem from a different angle. They argue that with the introduction of options, short-selling constraints on the underlying stock are mitigated. In their empirical analysis they show that option introductions are accompanied with a contemporaneous increase in short interest of that stock and a price decline. This finding also supports the hypothesis that short-selling constraints cause overpricing of assets.
A more narrow study is provided by Stambaugh et al. ( 2015 ) who investigate the IV factor and allocate the low-risk anomaly to arbitrage risk. According to them stocks with high IV have the highest arbitrage risk. At the same IV level, this effect should be seen more dramatically in overpriced stocks as due to short-selling constraints more investors could arbitrage away mispricing in underpriced stocks. Stambaugh et al. ( 2015 ) investigate their claims and measure a stock’s mispricing via 11 return anomalies that survive adjustment to the FF3 model. Given this proxy for arbitrage, they show that as predicted, the low-risk anomaly is significantly negative (positive) among the most overpriced (underpriced) stocks, and the negative effect among the overpriced stocks is significantly stronger. Further they show that the negative effect among overpriced stocks is stronger for stocks less easily shorted, as proxied by low institutional ownership. Liu et al. ( 2018 ) expand the approach of Stambaugh et al. ( 2015 ) and attempt to explain the low-risk anomaly based on beta. They show that the anomaly is only present in the most overpriced segment of stocks. Further they show that when controlling for IV, the anomaly vanishes.
4.4 Investor behavior
In the area of finance there is a large strain of literature focusing on investors’ behavior. Numerous behavioral biases can be related to irrational pricing and thus be argued to cause the low-risk anomaly. Within this section, I will present two of the most commonly referred two biases, which are overconfidence and preference for lotteries of investors. Regardless, these two behavioral biases are certainly not an exhaustive list for behavioral explanations of the low-risk anomaly.
4.4.1 Overconfidence
Overconfidence is a common phenomenon that is not only omnipresent in finance [e.g. Fischhoff et al. ( 1977 ), Kahneman and Tversky ( 1973 )]. Generally, overconfidence refers to the fact that most individuals think they can perform a certain task better than average. In finance this imposes that investors put too much emphasis on their predictions. Furthermore, they are likely to be active in more volatile stocks because in this segment the highest returns can be earned and an active investor can demonstrate his skill the most. Both of the above lead to an overpricing of risky stocks (Blitz et al. 2014 ). Overconfidence is also closely related to short-selling constraints. Similarly, the phenomenon is most common when the extent of disagreement among investors is high. As argued before, this is especially the case for high-volatility assets (Cornell 2009 ; Baker et al. 2011 ). The claims are bolstered empirically in various studies. The findings are that, on average, self-directed individual investors do trade suboptimally, lowering their expected returns through excessive trading (Odean 1999 ; Barber and Odean 2000 , 2001 , 2002 ).
A related area of study focuses on attention-grabbing stocks. This literature argues that investors are likely to buy stocks that grab their attention. In a sense, they overestimate their knowledge about such stocks, which is why this literature can be related to overconfidence. The study of Barber and Odean ( 2008 ) focuses on attention-grabbing stocks and argues that high-risk stocks are more likely to grab attention (e.g. because of extreme price movements or news coverage). This causes a strong upward pressure on prices of such stocks, which flattens the risk return relationship postulated by the CAPM. The claim that investors are likely to buy stocks that are more attention grabbing is supported by the findings of Grullon et al. ( 2004 ). They document that firms with high advertising spending have a larger number of individual and institutional investors.
Viewing overconfidence of investors more broadly, it can also be interpreted as investor sentiment. Baker and Wurgler ( 2006 ) measure investor sentiment via aggregating six proxies (e.g. share turnover) that they orthogonalize to several macroeconomic conditions. They find that after times of low sentiment, small and risky stocks tend to earn excessive returns. This indicates that as sentiment improves, investors bid up prices of risky stocks, which is in line with the theories outlined above. Further, Stambaugh et al. ( 2015 ) use the methodology of Baker and Wurgler ( 2006 ) to investigate the IV factor. They show that the negative (positive) returns of overpriced high-volatility (underpriced low-volatility) stocks is significantly stronger when investor sentiment is high (low). In other words, strong investor sentiment increases the magnitude of the low-risk anomaly.
4.4.2 Preference for lotteries
While it is already shown in Sect. 4.2 that coskewness risk is an explanatory variable for the low-risk anomaly, there is also a strain of literature, that focuses more on the behavioral aspect of investors’ preference for coskewness.
Kumar ( 2009 ) documents that some individual investors prefer stocks with lottery-like payoffs. Such stocks are characterized by a low price with high volatility and skewness. In other words, they offer a small chance for high returns in the short term at low costs. Barberis and Huang ( 2008 ) model this preference with the cumulative prospect theory of Tversky and Kahneman ( 1992 ). Cumulative prospect theory is a modified version of the prospect theory by Kahneman and Tversky ( 1979 ) within which individuals evaluate risks using a utility function that is defined over gains and losses. Barberis and Huang ( 2008 ) demonstrate that with their model a lottery-like security with positively skewed returns can be overpriced by investors, thus earning negative average excess returns. They attribute this to investors preference for lotteries and argue that rational investors cannot arbitrage away this irrationality. Brunnermeier et al. ( 2007 ) also provide a theoretical model that captures investors’ preference for lottery-like stocks. Building on the model of Brunnermeier and Parker ( 2005 ) within which investors care about expected future utility flows and are happier if they overestimate the probabilities of states of the world in which their investments pay off well. Hence, this theory is also very closely related to the above-discussed issue of overconfidence. With their model Brunnermeier et al. ( 2007 ) show that in equilibrium investors make suboptimal decisions and favor lottery-like stocks.
Kumar et al. ( 2011 ) confirm the existence of lottery-preferences by controlling for investor holding of lottery-like stocks in geographic regions where different attitudes towards gambling are prevalent due to different religions. Han and Kumar ( 2013 ) provide further support for the lottery-preference theory empirically. They find that stocks with high retail trade proportion have lottery-like features and earn low alphas. Bali et al. ( 2011 ) directly test the proposal that lottery preferences cause low-risk anomalies. They create decile stock portfolios based on their maximum daily return over the past month. They find that stocks with the highest past maximum daily returns significantly underperform those with the lowest past maximum daily return. Though similar in construction, they show that when controlling for their factor that selects stocks based on past maximum daily returns the low-risk anomaly measured by IV diminishes. In summary, they attribute their finding to investors willingness to overpay for stocks that may exhibit extreme positive returns. Bali et al. ( 2017 ) use the same framework to explain the return anomaly of stocks sorted on beta. In their analysis they conclude that the beta anomaly is a manifestation of the effect of lottery demand on stock returns. More empirical support for this claim is gathered by Asness et al. ( 2019 ) who decompose the BAB factor into its correlation and variance components. They proxy lottery demand with a factor portfolio that is built as a long-short portfolio based on maximum return divided by volatility. In doing so, they confirm that parts of the low-risk factor returns can be attributed to lottery demand. The preference for lottery-like payoffs is also documented by Moskowitz and Vasudevan ( 2022 ) in sports betting markets. The authors argue that since betting markets are not prone to systematic risk, only the behavioral preference of lottery like payoffs can explain the existence of the low-risk anomaly in betting and financial markets. A more comprehensive overview of lottery preferences with more examples is provided by Ilmanen ( 2012 ).
4.5 Agency problems
Same as for behavioral biases, the literature on agency problems is relatively wide and many issues can be related to the low-risk anomaly. Again, I will only present the most commonly referred to explanations, benchmarks and option-like reward systems.
4.5.1 Benchmarks
Benchmarks are argued to cause the low-risk anomaly because relative performance becomes more important than absolute performance in presence of a benchmark. Sensoy ( 2009 ) reports that in the US 94.6% of mutual funds are benchmarked to some popular index in the US. In an effort to beat benchmarks, fund managers tend to increase their exposure to stocks with a beta above one because most of them cannot take on leverage directly. Doing so, they can beat their benchmark, while aiming to maintain relatively low tracking errors. As a result, riskier stocks are overpriced and earn lower returns (Christoffersen and Simutin 2017 ; Baker et al. 2011 ). Cornell ( 2009 ) develops a model that incorporates this idea. He finds that in particular, a benchmark makes institutional investment managers less likely to exploit the low-volatility anomaly. Karceski ( 2002 ) takes the opposite view on the problem and focuses on the mutual fund investor. He shows that investors evaluate performance of mutual funds cross-sectionally and care more about the outperformance of funds in bull markets than about their underperformance in bear markets. As a result, fund managers care the most about outperforming their peers in bull markets. This can most easily be done via increasing the risk of the fund’s investments, causing overpricing of risky assets.
4.5.2 Option-like reward system
Another agency issue that incentivizes mutual fund managers to strive for riskier stocks is their reward system. Baker et al. ( 2011 ) argue that as most fund managers’ remuneration is directly linked to the fund’s performance, they have an incentive to increase risk. Hsu et al. ( 2013 ) make a similar argument for analysts. They state that analysts inflate earnings forecasts more aggressively for volatile stocks, in part because the inflation is more difficult for investors to detect. They do so because they have a similar incentive to stand out, just as fund managers. To make a career analysts also have to identify the highest-performing stocks and outperform their peers.
Evidence for the claims can be found in empirical studies. It is documented that mutual funds are tilted toward smaller stocks with higher volatility and average betas above one. In other words, they have consistent negative exposure toward the low-risk anomaly (Sias 1996 ; Frazzini and Pedersen 2014 ; Beveratos et al. 2017 ; Ang et al. 2017 ). Relatedly, Agarwal et al. ( 2022 ) find that smaller, younger mutual funds with poor recent performance own more risky stocks, arguably to attract more capital, which ultimately increases fee earnings and thus manager remuneration. Furthermore, a lab-in-the-field experiment by Kirchler et al. ( 2018 ) with a large group of investment professionals reveals that ranking and tournament incentives (that are typically present in the mutual fund industry) drive risk-taking of participants.
5 Conclusion
Before the paper can be concluded it makes sense to recapitulate on the claims made by Harvey et al. ( 2016 ) and Harvey ( 2017 ). From those the question arises whether the low-risk anomaly can be classified as an investment factor or may actually be a false positive - a non-existent factor that was is falsely believed to be relevant. Though concerns about the ever growing factor zoo are rightfully made, in light of the above review, it is rather unlikely that the low-risk anomaly is a false positive.
First, unlike other proposed risk-factors that may be exposed to p-hacking, the low-risk anomaly did not evolve from a search of potential alpha-generating factors. Instead, it was discovered from research that performed empirical studies on the predictions of the CAPM. The development of investment strategies that outperform was not the incentive of these early studies, making the storyline of the low-risk anomaly more convincing. Second, as outlined in Sect. 3 , there are multiple measures for low-risk factors that were tested across different asset classes, samples, time horizons and markets. The critique of a publication bias and degradation of performance are rightfully made but demand further investigation as results differ among studies. Third, many studies show that the effect is robust to widely accepted investment factors and other macroeconomic variables that drive asset returns. Fourth, as outlined above in Sect. 4 there are various clear economic and behavioral rationales for the findings of the empirical applications of which most are likely to persist in the future. Overall, while it is hard to deny the low-risk anomaly as an investment factor, it still can be questioned whether all its implementation methodologies have a raison d’être. The presented literature shows that most of them have exposure to the same risks and are almost indistinguishable.
Though, they strengthen the argument that the low-risk anomaly can be interpreted as a risk factor, the large number of potential explanations raises the question, which of them are the actual cause of the low-risk effect. While there are studies that attempt to disentangle the effect into its different components (Asness et al. 2019 ) more such work is required to identify the primary causes with certainty. The main problem with this task is that many proposed causes are interrelated and may result in the same phenomenon. In their literature review Blitz et al. ( 2014 ) suggest promising future research directions and methods that would help to further clarify the current root-cause problem.
Overall, it can be concluded that low-risk factors in asset returns are a widely researched area of finance. Unlike other factors, various implementation methodologies and causes have been identified by current literature. This paper provides a structured overview of current findings focusing on models and methods employed. It shows that the factor is predominantly measured via market beta, idiosyncratic volatility, total return volatility or the minimum variance portfolio in equity markets. In bond markets, it is implemented by filtering for low maturity and creditworthy bonds. The proposed causes for the anomaly include exposure to other factors, coskewness risk, investor constraints, behavioral biases, and agency problems. The low-risk anomaly as such appears to be pretty robust given its empirical evidence in various markets despite some critical voices regarding its replication and independence from other investment factors. Regarding its causes however, it still has to be validated which one of the proposed reasons prevails the most. Future research in this direction is faced with the intricate task to disentangle the different roots of the problem but could help to further understand what ultimately causes the low risk anomaly.
They use a variety of BARRA risk models depending on the availability and robustness throughout time for the different equity markets covered in the analysis. BARRA risk models are multi-factor models based on the concept of (Rosenberg 1974 ) that are used to forecast a security’s risk.
Adhami, S., Gianfrate, G., Johan, S.A.: Risks and returns in crowdlending. Eurasian. Bus. Rev. 13 , 309–340 (2023)
Adrian, T., Etula, E., Muir, T.: Financial intermediaries and the cross-section of asset returns. J. Financ. 69 (6), 2557–2596 (2014)
Article Google Scholar
Agarwal, V., Jiang, L., Wen, Q.: Why do mutual funds hold lottery stocks? J. Financ. Quant. Anal. 57 (3), 825–856 (2022)
Ang, A., Hodrick, R.J., Xing, Y., Zhang, X.: The cross-section of volatility and expected returns. J. Financ. 61 (1), 259–299 (2006)
Ang, A., Hodrick, R.J., Xing, Y., Zhang, X.: High idiosyncratic volatility and low returns: international and further U.S evidence. J. Financ. Econ. 91 (1), 1–23 (2009)
Ang, A., Madhavan, A., Sobczyk, A.: Crowding, capacity, and valuation of minimum volatility strategies. J. Index Invest. 7 (4), 41–50 (2017)
Asness, C.S., Frazzini, A., Gormsen, N.J., Pedersen, L.H.: Betting against correlation: testing theories of the low-risk effect. J. Financ. Econ. 135 (3), 629–652 (2019)
Asness, C.S., Frazzini, A., Pedersen, L.H.: Leverage aversion and risk parity. Financ. Anal. J. 68 (1), 47–59 (2012)
Asness, C.S., Frazzini, A., Pedersen, L.H.: Low-risk investing without industry bets. Financ. Anal. J. 70 (4), 24–41 (2014)
Asness, C.S., Frazzini, A., Pedersen, L.H.: Quality minus junk. Rev. Acc. Stud. 24 , 34–112 (2018)
Asness, C.S., Ilmanen, A., Israel, R., Moskowitz, T.J.: Investing with style. J. Invest. Manag. 13 (1), 27–63 (2014)
Google Scholar
Auer, B.R., Schuhmacher, F.: Liquid betting against beta in dow jones industrial average stocks. Financ. Anal. J. 71 (6), 30–43 (2015)
Aussenegg, W., Götz, L., Jelic, R.M.: Common factors in the performance of European corporate bonds—evidence before and after financial crisis. Eur. Financ. Manag. 21 (2), 265–308 (2015)
Baker, M., Bradley, B., Taliaferro, R.: The low-risk anomaly: a decomposition into micro and macro effects. Financ. Anal. J. 70 (2), 43–58 (2014)
Baker, M., Bradley, B., Wurgler, J.: Benchmarks as limits to arbitrage: understanding the low volatility anomaly. Financ. Anal. J. 67 (1), 40–54 (2011)
Baker, M., Wurgler, J.: Investor sentiment and the cross-section of stock returns. J. Financ. 61 (4), 1645–1680 (2006)
Baker, N.L., Haugen, R.A.: Low risk stocks outperform within all observable markets of the world. SSRN Electron. J. (2012)
Bali, T.G., Brown, S.J., Murray, S., Tang, Y.: A lottery-demand-based explanation of the beta anomaly. J. Financ. Quant. Anal. 52 (6), 2369–2397 (2017)
Bali, T.G., Cakici, N.: Idiosyncratic volatility and the cross section of expected returns. J. Financ. Quant. Anal. 43 (1), 29–58 (2008)
Bali, T.G., Cakici, N., Whitelaw, R.F.: Maxing out: stocks as lotteries and the cross-section of expected returns. J. Financ. Econ. 99 (2), 427–446 (2011)
Bali, T.G., Del Viva, L., Lambertides, N., Trigeorgis, L.: Growth options and related stock market anomalies: profitability, distress, lotteryness, and volatility. J. Financ. Quant. Anal. 55 (7), 2150–2180 (2020)
Barber, B.M., Odean, T.: Trading is hazardous to your wealth: the common stock investment performance of individual investors. J. Financ. 55 (2), 773–806 (2000)
Barber, B.M., Odean, T.: Boys will be boys: gender, overconfidence, and common stock investment. Q. J. Econ. 116 (1), 261–292 (2001)
Barber, B.M., Odean, T.: Online investors : do the slow die first? Rev. Financ. Stud. 15 (2), 455–487 (2002)
Barber, B.M., Odean, T.: All that glitters: the effect of attention and news on the buying behavior of individual and institutional investors. Rev. Financ. Stud. 21 (2), 785–818 (2008)
Barberis, N., Huang, M.: Stocks as lotteries: the implications of probability weighting for security prices. Am. Econ. Rev. 98 (5), 2066–2100 (2008)
Barinov, A., Chabakauri, G.: Idiosyncratic volatility, growth options, and the cross-section of returns. SSRN Electron. J. (2021)
Basu, S.: Investment performance of common stocks in relation to their price-earnings ratios : a test of the efficient market hypothesis. J. Financ. 32 (3), 663–682 (1977)
Beveratos, A., Bouchaud, J.-P., Ciliberti, S., Laloux, L., Lempérière, Y., Potters, M., Simon, G.: Deconstructing the low-vol anomaly. J. Portf. Manag. 44 (1), 91–103 (2017)
Bhamra, H.S., Shim, K.H.: Stochastic idiosyncratic cash flow risk and real options: implications for stock returns. J. Econ. Theory 168 , 400–431 (2017)
Black, F.: Capital market equilibrium with restricted borrowing. J. Bus. 45 (3), 444–455 (1972)
Black, F.: Beta and return: announcements of the ‘death of beta’ seem premature. J. Portf. Manag. 20 (1), 8–18 (1993)
Black, F., Jensen, M., Scholes, M.: The capital asset pricing model: some empirical tests. In: Jensen, M. (ed.) Studies in the Theory of Capital Markets, pp. 79–121. Praeger (1972)
Blitz, D., Falkenstein, E., van Vliet, P.: Explanations for the volatility effect: an overview based on the CAPM assumptions. J. Portf. Manag. 40 (3), 61–76 (2014)
Blitz, D., Pang, J., van Vliet, P.: The volatility effect in emerging markets. Emerg. Mark. Rev. 16 (1), 31–45 (2013)
Blitz, D., van Vliet, P.: The volatility effect. J. Portf. Manag. 34 (1), 102–113 (2007)
Blitz, D., van Vliet, P., Baltussen, G.: The volatility effect revisited. J. Portf. Manag. 46 (2), 45–63 (2020)
Boguth, O., Carlson, M., Fisher, A., Simutin, M.: Conditional risk and performance evaluation: volatility timing, overconditioning, and new estimates of momentum alphas. J. Financ. Econ. 102 (2), 363–389 (2011)
Boguth, O., Simutin, M.: Leverage constraints and asset prices: insights from mutual fund risk taking. J. Financ. Econ. 127 (2), 325–341 (2018)
Boyer, B., Mitton, T., Vorkink, K.: Expected idiosyncratic skewness. Rev. Financ. Stud. 23 (1), 170–202 (2010)
Brennan, M.J.: Capital market equilibrium with divergent borrowing and lending rates. J. Financ. Quant. Anal. 6 (5), 1197–1205 (1971)
Brooks, J., Palhares, D., Richardson, S.: Style investing in fixed income. J. Portf. Manag. 44 (4), 127–139 (2018)
Brunnermeier, M.K., Gollier, C., Parker, J.A.: Optimal beliefs, asset prices, and the preference for skewed returns. Am. Econ. Rev. 97 (2), 159–165 (2007)
Brunnermeier, M.K., Parker, J.A.: Optimal expectations. Am. Econ. Rev. 95 (4), 1092–1118 (2005)
Cao, J., Han, B.: Cross section of option returns and idiosyncratic stock volatility. J. Financ. Econ. 108 (1), 231–249 (2013)
Carhart, M.M.: On persistence in mutual fund performance. J. Financ. 52 (1), 57–82 (1997)
Cederburg, S., O’Doherty, M.S.: Does it pay to bet against beta? On the conditional performance of the beta anomaly. J. Financ. 71 (2), 737–774 (2016)
Chen, A., Pong, E., Wang, Y.: Accessing the china a-shares market via minimum-variance investing. J. Portf. Manag. 45 (1), 106–117 (2018)
Chen, J., Hong, H., Stein, J.C.: Forecasting crashes: trading volume, past returns, and conditional skewness in stock prices. J. Financ. Econ. 61 (3), 345–381 (2001)
Chen, J., Hong, H., Stein, J.C.: Breadth of ownership and stock returns. J. Financ. Econ. 66 (2–3), 171–205 (2002)
Chen, L.H., Jiang, G.J., Xu, D.D., Yao, T.: Dissecting the idiosyncratic volatility anomaly. J. Empir. Financ. 59 , 193–209 (2020)
Chen, Z., Petkova, R.: Does idiosyncratic volatility proxy for risk exposure? Rev. Financ. Stud. 25 (9), 2745–2787 (2012)
Chow, T.-M., Hsu, J.C., Kuo, L.-L., Li, F.: A study of low-volatility. J. Portf. Manag. 40 (4), 89–105 (2014)
Christoffersen, S.E.K., Simutin, M.: On the demand for high-beta stocks: evidence from mutual funds. Rev. Financ. Stud. 30 (8), 2596–2620 (2017)
Chung, K.H., Wang, J., Wu, C.: Volatility and the cross-section of corporate bond returns. J. Financ. Econ. 133 (2), 397–417 (2019)
Clarke, R., de Silva, H., Thorley, S.: Minimum-variance portfolios in the U.S. equity market. J. Portf. Manag. 33 (1), 10–12 (2006)
Clarke, R., de Silva, H., Thorley, S.: Know your VMS exposure. J. Portf. Manag. 36 (2), 52–59 (2010)
Clarke, R., de Silva, H., Thorley, S.: Minimum-variance portfolio composition. J. Portf. Manag. 37 (2), 31–45 (2011)
Connor, G., Korajczyk, R.A.: Risk and return in equilibrium APT: application of a new test methodology. J. Financ. Econ. 21 (2), 255–289 (1988)
Cornell, B.: The pricing of volatility and skewness: a new interpretation. J. Invest. 18 (3), 27–30 (2009)
Danielsen, B.R., Sorescu, S.M.: Why do option introductions depress stock prices? A study of diminishing short sale constraints. J. Financ. Quant. Anal. 36 (4), 451–484 (2001)
De Carvalho, R.L., Dugnolle, P., Xiao, L., Moulin, P.: Low-risk anomalies in global fixed income: evidence from major broad markets. J. Fixed Income 23 (4), 51–70 (2014)
De Carvalho, R.L., Lu, X., Moulin, P.: Demystifying equity risk—based strategies: a simple. J. Portf. Manag. 38 (3), 56–70 (2012)
De Franco, C., Monnier, B., Rulik, K.: Interest rate exposure of volatility portfolios. J. Index Invest. 8 (2), 53–67 (2017)
De Santis, G., Gerard, B.: International asset pricing and portfolio diversification with time-varying risk. J. Financ. 52 , 1881–1912 (1997)
Derwall, J., Huij, J., de Zwart, G. J.: The Short-Term Corporate Bond Anomaly. SSRN Electronic Journal. (2009)
Dieter, K.B., Malloy, J.C., Scherbina, A.: Differences of opinion and the cross section of stock returns. J. Financ. 57 (5), 2113–2141 (2002)
Dimson, E., Marsh, P., Staunton, M.: Factor-based investing: the long-term evidence. J. Portf. Manag. 43 (5), 15–37 (2017)
Duffie, D., Gârleanu, N., Pedersen, L.H.: Securities lending, shorting, and pricing. J. Financ. Econ. 66 (2–3), 307–339 (2002)
Durham, J.B.: Betting against beta with bonds: Worry or love the steepener? Financ. Anal. J. 72 (6), 57–85 (2016)
Eraker, B., Ready, M.: Do investors overpay for stocks with lotterylike payoffs? An examination of the returns of OTC stocks. J. Financ. Econ. 115 (3), 486–504 (2015)
Falkenstein, E.G.: Risk and return in general: theory and evidence. SSRN Electron. J. (2010)
Fama, E.F., French, K.R.: The cross-section of expected stock returns. J. Financ. 47 (2), 427–465 (1992)
Fama, E.F., French, K.R.: Dissecting anomalies with a five-factor model. Rev. Financ. Stud. 29 (1), 69–103 (2016)
Fama, E.F., MacBeth, J.: Risk, return and equilibrium: empirical tests. J. Polit. Econ. 81 (3), 637–697 (1973)
Figlewski, S.: The informational effects of restrictions on short sales: some empirical evidence. J. Financ. Quant. Anal. 16 (4), 463–476 (1981)
Fink, J.D., Fink, K.E., He, H.: Expected idiosyncratic volatility measures and expected returns. Financ. Manage. 41 (3), 519–553 (2012)
Fischhoff, B., Slovic, P., Lichtenstein, S.: Knowing with certainty: the appropriateness of extreme confidence. J. Exp. Psychol. 3 (4), 552–564 (1977)
Frazzini, A., Pedersen, L.H.: Betting against beta. J. Financ. Econ. 111 (1), 1–25 (2014)
Frazzini, A., Pedersen, L.H.: Embedded leverage. Rev. Asset Pric. Stud. 12 (1), 1–52 (2022)
Friend, I., Blume, M.: Measurement of portfolio performance under uncertainty. Am. Econ. Rev. 60 (4), 561–575 (1970)
Fu, F.: Idiosyncratic risk and the cross-section of expected stock returns. J. Financ. Econ. 91 (1), 24–37 (2009)
Gebhardt, W.R., Lee, C.M., Swaminathan, B.: Toward an implied cost of capital. J. Account. Res. 39 (1), 135–176 (2001)
Grullon, G., Kanatas, G., Weston, J.P.: Advertising, breadth of ownership, and liquidity. Rev. Financ. Stud. 17 (2), 439–461 (2004)
Guo, H., Kassa, H., Ferguson, M.F.: On the relation between EGARCH idiosyncratic volatility and expected stock returns. J. Financ. Quant. Anal. 49 (1), 271–296 (2014)
Han, B., Kumar, A.: Speculative retail trading and asset prices. J. Financ. Quant. Anal. 48 (2), 377–404 (2013)
Han, X., Li, K., Li, Y.: Investor overconfidence and the security market line: new evidence from China. J. Econ. Dyn. Control 117 , 103961 (2020)
Han, Y., Hu, T., Lesmond, D.A.: Liquidity biases and the pricing of cross-sectional idiosyncratic volatility around the world. J. Financ. Quant. Anal. 50 (6), 1269–1292 (2015)
Harvey, C.R.: Presidential address: the scientific outlook in financial economics. J. Financ. 72 (4), 1399–1440 (2017)
Harvey, C.R., Liu, Y., Zhu, H.: ... and the cross-section of expected returns. Rev. Financ. Stud. 29 (1), 5–68 (2016)
Harvey, C.R., Siddique, A.: Conditional skewness in asset pricing tests. J. Financ. 55 (3), 1263–1295 (2000)
Haugen, R.A., Baker, N.L.: The efficient market inefficiency of capitalization-weighted stock portfolios. J. Portf. Manag. 17 (3), 35–40 (1991)
Haugen, R.A., Baker, N.L.: Commonality in the determinants of expected stock returns. J. Financ. Econ. 41 (3), 401–439 (1996)
Haugen, R.A., Heins, A.J.: Risk and the rate of return on financial assets: some old wine in new bottles. J. Financ. Quant. Anal. 10 (5), 775–784 (1975)
Hong, H., Sraer, D.A.: Speculative betas. J. Financ. 71 (5), 2095–2144 (2016)
Hou, K., Xue, C., Zhang, L.: Replicating anomalies. Rev. Financ. Stud. 33 (5), 2019–2133 (2020)
Houweling, P., van Zundert, J.: Factor investing in the corporate bond market. Financ. Anal. J. 73 (2), 100–115 (2017)
Hsu, J.C., Kudoh, H., Yamada, T.: When sell-side analysts meet high-volatility stocks: an alternative explanation for the low-volatility puzzle. J. Invest. Manag. 11 (2), 28–46 (2013)
Huang, W., Liu, Q., Rhee, S.G., Zhang, L.: Return reversals, idiosyncratic risk, and expected returns. Rev. Financ. Stud. 23 (1), 147–168 (2010)
Ilmanen, A.: Do financial markets reward buying or seliing insurance and lottery tickets? Financ. Anal. J. 68 (5), 2012 (2012)
Ilmanen, A., Byrne, R., Gunasekera, H., Minikin, R.: Which risks have been best rewarded? J. Portf. Manag. 30 (2), 53–57 (2004)
Israel, R., Palhares, D., Richardson, S.A.: Common factors in corporate bond returns. J. Invest. Manag. 16 (2), 17–46 (2018)
Jagannathan, R., Ma, T.: Risk reduction in large portfolios: why imposing the wrong constraints helps. J. Financ. 58 (4), 1651–1683 (2003)
Jegadeesh, N., Titman, S.: Returns to buying winners and selling losers: implications for stock market efficiency. J. Financ. 48 (1), 65–91 (1993)
Jensen, T.I., Kelly, B.T., Pedersen, L.H.: Is there a replication crisis in finance? NBER Working Paper Series, w28432 (2022)
Johnson, T.C.: Forecast dispersion and the cross section of expected returns. J. Financ. 59 (5), 1957–1978 (2004)
Jordan, B.D., Riley, T.B.: Volatility and mutual fund manager skill. J. Financ. Econ. 118 (2), 289–298 (2015)
Joshipura, N., Joshipura, M.: The volatility effect: evidence from Indian markets. Appl. Financ. Lett. 5 (1), 12–17 (2016)
Joshipura, N., Joshipura, M.: The volatility effect: recent evidence from Indian markets. Theor. Econ. Lett. 09 (06), 2152–2164 (2019)
Jylhä, P.: Margin requirements and the security market line. J. Financ. 73 (3), 1281–1321 (2018)
Kahneman, D., Tversky, A.: On the psychology of prediction. Psychol. Rev. 80 (4), 237–251 (1973)
Kahneman, D., Tversky, A.: Prospect theory: an analysis of decision under risk. Econometrica 47 (2), 263–291 (1979)
Karceski, J.: Returns-chasing behavior, mutual funds, and beta’s death. J. Financ. Quant. Anal. 37 (4), 559–594 (2002)
Kirchler, M., Lindner, F., Weitzel, U.: Rankings and risk-taking in the finance industry. J. Financ. 73 (5), 2271–2302 (2018)
Kothe, J., Lohre, H., Rother, C.: Rates factors and global asset allocation. J. Fixed Income 30 (3), 6–25 (2021)
Kraus, A., Litzenberger, R.H.: Skewness preference and the valuation of risk assets. J. Financ. 31 (4), 1085–1100 (1976)
Kumar, A.: Who gambles in the stock market? J. Financ. 64 (4), 1889–1933 (2009)
Kumar, A., Page, J.K., Spalt, O.G.: Religious beliefs, gambling attitudes, and financial market outcomes. J. Financ. Econ. 102 (3), 671–708 (2011)
Ledoit, O., Wolf, M.: Honey, I shrunk the sample covariance matrix. J. Portf. Manag. 30 (4), 110–119 (2004)
Lintner, J.: The valuation of risk assets and the selection of risky investments in stock portfolios and capital budgets. Rev. Econ. Stat. 47 (1), 13–37 (1965)
Liu, J., Stambaugh, R.F., Yuan, Y.: Absolving beta of volatility’s effects. J. Financ. Econ. 128 (1), 1–15 (2018)
Markowitz, H.: Portfolio selection. J. Financ. 7 (1), 77–91 (1952)
Miller, E.M.: Risk, uncertainty, and divergence of opinion. J. Financ. 32 (4), 1151–1168 (1977)
Miller, E.M., Scholes, M.: Rates of return in relation to risk: a reexamination of some recent findings. In: Jensen, M. (ed.) Studies in the Theory of Capital Markets, pp. 47–78. Praeger (1972)
Morris, S.: Speculative investor behavior and learning. Q. J. Econ. 111 (4), 1111–1133 (1996)
Moskowitz, T.J., Vasudevan, K.: Betting without beta. SSRN Electron. J. (2022)
Moskowitz, T.J., Vissing-Jørgensen, A.: The returns to entrepreneurial investment: a private equity premium puzzle? Am. Econ. Rev. 92 (4), 745–778 (2002)
Mossin, J.: Equilibrium in a capital asset market. Econometrica 34 (4), 768–783 (1966)
Novy-Marx, R.: The other side of value: the gross profitability premium. J. Financ. Econ. 108 (1), 1–28 (2013)
Novy-Marx, R.: Understanding defensive equity. Natl. Bureau Econ. Res. 20591, 3 (2016)
Novy-Marx, R., Velikov, M.: Betting against betting against beta. J. Financ. Econ. 143 (1), 80–106 (2022)
Odean, T.: Do investors trade too much? Adva. Behav. Econ. 89 (5), 1279–1298 (1999)
Park, S.G., Wei, K.C.J., Zhang, L.: The Fu (2009) positive relation between idiosyncratic volatility and expected returns is due to look-ahead. Crit. Financ. Rev. (forthcoming) (2020)
Pilotte, E.A., Sterbenz, F.P.: Sharpe and treynor ratios on treasury bonds. J. Bus. 79 (1), 149–180 (2006)
Pyun, C.: Documenting the post-2000 decline in the idiosyncratic volatility effect. Crit. Financ. Rev. 10 (3), 419–427 (2021)
Rosenberg, B.: Extra-market components of covariance in security returns. J. Financ. 9 (2), 263–274 (1974)
Rubinstein, M.: The fundamental theorem of parameter-preference security valuation. J. Financ. Quant. Anal. 8 (1), 61–69 (1973)
Scherer, B.: A note on the returns from minimum variance investing. J. Empir. Financ. 18 (4), 652–660 (2011)
Schneider, P. G., Trojani, F.: Fear Trading. Swiss Finance Institute Research Paper. 15–03 (2015)
Schneider, P.G., Wagner, C., Zechner, J.: Low-risk anomalies? J. Financ. 75 (5), 2673–2718 (2020)
Sensoy, B.: Performance evaluation and self- designated benchmark indexes in the mutual fund management industry. J. Financ. Econ. 92 (1), 25–39 (2009)
Sharpe, W.F.: Capital asset prices: a theory of market equilibrium under conditions of risk. J. Financ. 19 (3), 425–442 (1964)
Sias, R.W.: Volatility and the institutional investor. Financ. Anal. J. 52 (2), 13–20 (1996)
Soe, A.M.: Low-volatility portfolio construction: ranking versus optimization. J. Portf. Manag. 2 (3), 63–73 (2012)
Stambaugh, R.F., Yu, J., Yuan, Y.U.: Arbitrage asymmetry and the idiosyncratic volatility puzzle. J. Financ. 70 (5), 1903–1948 (2015)
Treynor, J.L.: Market value, time, and risk (unpublished manuscript) (1961)
Tversky, A., Kahneman, D.: Advances in prospect theory: cumulative representation of uncertainty. Springer 5 (4), 297–323 (1992)
Walkshäusl, C.: International low-risk investing. J. Portf. Manag. 41 (1), 45–56 (2014)
Zaremba, A., Czapkiewicz, A.: The cross section of international government bond returns. Econ. Model. 66 , 171–183 (2017)
Download references
Acknowledgements
I am grateful to an anonymous referee as well as Manuel Ammann, Markus Schmid, Kevin Schneider and participants at the “Topics in Asset Management” Ph.D. seminar at the University of St. Gallen for their comments on an earlier version of this paper.
Open access funding provided by University of St.Gallen
Author information
Authors and affiliations.
Swiss Institute of Banking and Finance, University of St. Gallen, Unterer Graben 21, 9000, St. Gallen, Switzerland
Joshua Traut
You can also search for this author in PubMed Google Scholar
Corresponding author
Correspondence to Joshua Traut .
Additional information
Publisher's note.
Springer Nature remains neutral with regard to jurisdictional claims in published maps and institutional affiliations.
Rights and permissions
Open Access This article is licensed under a Creative Commons Attribution 4.0 International License, which permits use, sharing, adaptation, distribution and reproduction in any medium or format, as long as you give appropriate credit to the original author(s) and the source, provide a link to the Creative Commons licence, and indicate if changes were made. The images or other third party material in this article are included in the article’s Creative Commons licence, unless indicated otherwise in a credit line to the material. If material is not included in the article’s Creative Commons licence and your intended use is not permitted by statutory regulation or exceeds the permitted use, you will need to obtain permission directly from the copyright holder. To view a copy of this licence, visit http://creativecommons.org/licenses/by/4.0/ .
Reprints and permissions
About this article
Traut, J. What we know about the low-risk anomaly: a literature review. Financ Mark Portf Manag 37 , 297–324 (2023). https://doi.org/10.1007/s11408-023-00427-0
Download citation
Accepted : 26 January 2023
Published : 28 April 2023
Issue Date : September 2023
DOI : https://doi.org/10.1007/s11408-023-00427-0
Share this article
Anyone you share the following link with will be able to read this content:
Sorry, a shareable link is not currently available for this article.
Provided by the Springer Nature SharedIt content-sharing initiative
- Factor investing
- Asset pricing
- Style investing
JEL Classification
- Find a journal
- Publish with us
- Track your research
Page Unavailable
Unfortunately, the page you requested is temporarily unavailable.
Please try again later or call 1-800-522-7297 between the hours of 8:30AM – 7:00PM EST
Akamai Reference Number: 18.24fc733e.1713142265.eae7d10
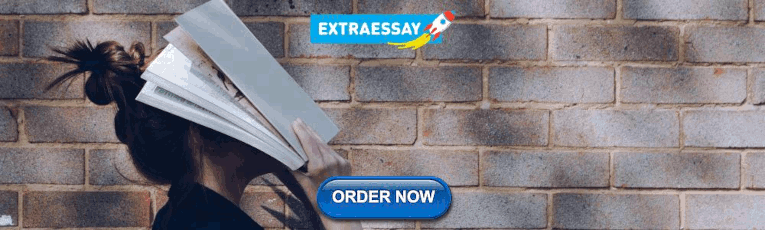
IMAGES
VIDEO
COMMENTS
Sustainability integration in project portfolio management helps shape strategic, organizational, and project-based contexts. The authors conducted a structured literature review from 2000 to 2021 and developed a novel integrative framework presenting a holistic view highlighting three substantive research themes: sustainability mindset, sustainability assessment, and sustainability ...
The portfolio review rates these dimensions across multiple portfolios or groupings of projects, and it identifies key dependencies among them and tracks portfolios against key baselines. ... The general management literature on portfolio management is closely aligned with strategic management and may be a good starting point for public sector ...
literature in this research field, identifying the main relevant aspects of project portfolio management in the public sector. METHODOLOGY The literature review is not based only in a single methodology, it can be done using different methodologies as bibliometric, content analysis and semantic analysis. Randolph (2009)
Frey T, Buxmann P (2012) IT project portfolio management-a structured literature review. Gutiérrez E (2014) Managing ambiguity when evaluating and selecting new ideas in project portfolio management. Int J Innov Technol Manag 11(5):1. Article Google Scholar Henderson BD (1970) The product portfolio.
Some studies suggest project portfolio management (PPM) as a dynamic capability, that is, ... The paper is structured as follows. A literature review summarises the relevance of context to PPM, the choice of viewing project portfolios as organisations, and dominant approach to theorising about the context in PPM literature. ...
Keywords Innovation Portfolio management · Services · Digital services · Digitalization · Servitization · Literature review · State of the art * Theresa Eckert [email protected]‑chemnitz.de Stefan Hüsig [email protected]‑chemnitz.de 1 Technical University of Chemnitz, Thüringer Weg 7, 09126 Chemnitz, Germany
Section 4 identifies the main challenges of managing portfolios from a literature review; ... Therefore, effective portfolio management needs to achieve consensus toward a common goal, which requires organizational processes (rather than ad hoc decisions): (1) strategy (firm's strategic orientation and aspirations); (2) organization ...
Project Portfolio Risk Management (PPRM) has been identified as a relevant area regarding project portfolio success. This paper reports on a structured literature review of PPRM.
Definitions for project portfolio management are analyzed to provide a context for project portfolio management, followed by a literature review of five theories to examine the relevance and relatedness of these theories to project portfolio panagement (PfM). The theoretical foundation for each theory is discussed and the key elements that ...
The review covers the literature on decision m aking, and organisational and portfolio management published between 1860 and 2016, with Google scholar used to retrieve the
integrative framework, literature review, project portfolio management, sustainability, sustainability integration, sustainable mindset Introduction Advances in incorporating sustainability goals and principles into the organizational strategy are of significance for projects, project portfolios, and longer-term organizational learning and
Project portfolio management (PPM) has a central position in both the business literature and companies' management practices. Although PPM has been practiced for decades and the theoretical contributions in the field have grown, overviews of the state-of-the-art of PPM research and its processes are scarce. Therefore, this paper analyses the PPM literature to identify relevant PPM concepts ...
However, these methods were born to be applied at team level and not at project portfolio level, where the complexity of managing multiple agile projects and teams leads to several challenges of resource management, priorities and governance model of the project portfolio. The systematic literature review performed in this research aims to ...
Pardalos et al. [ 140] have initially provided an extensive review on the use of optimization models for portfolio selection. A series of excellent literature reviews in the field of portfolio selection with multiple criteria have, also, been developed by Aouni et al. [ 9 ], Zopounidis and Doumpos [ 230 ], Patari et al. [ 142] and Jayasekara et ...
The key challenges of PPM are identified, a new framework for classifying PPM MCDM related methods is proposed and a literature review of applications of MCDm methods to PPM is presented. Project portfolio management (PPM) has become a key element of large organisations' service delivery due to the close attention inherently paid to numerous issues in the discipline of project management.
Downloadable! In recent years, a growing body of literature in portfolio management has devoted a great deal of attention for this subject. The theoretical foundation to portfolio management was offered by Harry Markowitz at the beginning of the 1950s. The limitations of the original Markowitz model have stimulated the occurrence of extended or modified models - two of the best known (and ...
Expert opinion: Based on the systematic literature review on portfolio management, more specifically, on new product development portfolio theory, and interview the paper proposes the paper proposes a reference model to manage patent portfolios. The model comprises four stages aligned with the three goals of the NPD portfolio management: 1 ...
Summer internship project report on a study on portfolio management at sharekhan limited,. Information technology portfolio management literature review, framework, and research issues. Portfolio management process for the city of espoo. A project report on portfolio management a case study of india infoline finance d by sanjay gupta issuu.
Patents as Topic / legislation & jurisprudence*. Based on the systematic literature review on portfolio management, more specifically, on new product development portfolio theory, and interview the paper proposes the paper proposes a reference model to manage patent portfolios. The model comprises four stages aligned with the three goals of the ...
portfolio management literature was not sufficiently mature for a formal systematic review. A search of public administration and policy journals in JSTOR returned 63 mentions of "portfolio management" in articles from 1978 to 2019. However, term used in different ways; the meaning and uses of the term were not consistent
The study undertakes a comprehensive integrative review of the literature, focusing on the quantitative aspects through a bibliometric analysis, and qualitative aspects through a systematic approach. ... Dr. Shubham Singhania is currently working as an Assistant Professor (Finance and Accounting) at Jaipuria Institute of Management, Jaipur. He ...
Relatedly, there also exists a strain of literature that examines the minimum variance portfolio. In this section I will first review the studies that focus mostly on confirming one of the three measures empirically and the mean variance portfolio, before I review studies that compare multiple concepts. 3.1.1 Market beta
Fidelity Institutional Asset Management (FIAM) investment management services and products are managed by the Fidelity Investments companies of FIAM LLC, a U.S. registered investment adviser, or Fidelity Institutional Asset Management Trust Company, a New Hampshire trust company. FIAM products and services may be presented by FDC LLC, a non ...