Your browser does not support javascript. Some site functionality may not work as expected.
- Images from UW Libraries
- Open Images
- Image Analysis
- Citing Images
- University of Washington Libraries
- Library Guides
- Images Research Guide
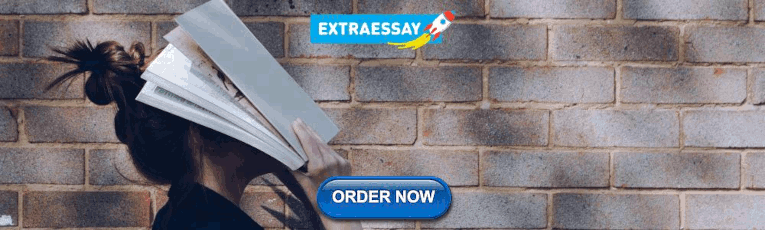
Images Research Guide: Image Analysis
Analyze images.
Content analysis
- What do you see?
- What is the image about?
- Are there people in the image? What are they doing? How are they presented?
- Can the image be looked at different ways?
- How effective is the image as a visual message?
Visual analysis
- How is the image composed? What is in the background, and what is in the foreground?
- What are the most important visual elements in the image? How can you tell?
- How is color used?
- What meanings are conveyed by design choices?
Contextual information
- What information accompanies the image?
- Does the text change how you see the image? How?
- Is the textual information intended to be factual and inform, or is it intended to influence what and how you see?
- What kind of context does the information provide? Does it answer the questions Where, How, Why, and For whom was the image made?
Image source
- Where did you find the image?
- What information does the source provide about the origins of the image?
- Is the source reliable and trustworthy?
- Was the image found in an image database, or was it being used in another context to convey meaning?
Technical quality
- Is the image large enough to suit your purposes?
- Are the color, light, and balance true?
- Is the image a quality digital image, without pixelation or distortion?
- Is the image in a file format you can use?
- Are there copyright or other use restrictions you need to consider?
developed by Denise Hattwig , [email protected]
More Resources
National Archives document analysis worksheets :
- Photographs
- All worksheets
Visual literacy resources :
- Visual Literacy for Libraries: A Practical, Standards-Based Guide (book, 2016) by Brown, Bussert, Hattwig, Medaille ( UW Libraries availability )
- 7 Things You Should Know About... Visual Literacy ( Educause , 2015 )
- Keeping Up With... Visual Literacy (ACRL, 2013)
- Visual Literacy Competency Standards for Higher Education (ACRL, 2011)
- Visual Literacy White Paper (Adobe, 2003)
- Reading Images: an Introduction to Visual Literacy (UNC School of Education)
- Visual Literacy Activities (Oakland Museum of California)
- << Previous: Open Images
- Next: Citing Images >>
- Last Updated: Nov 15, 2023 12:45 PM
- URL: https://guides.lib.uw.edu/newimages

Quick Links:

Visual Methods
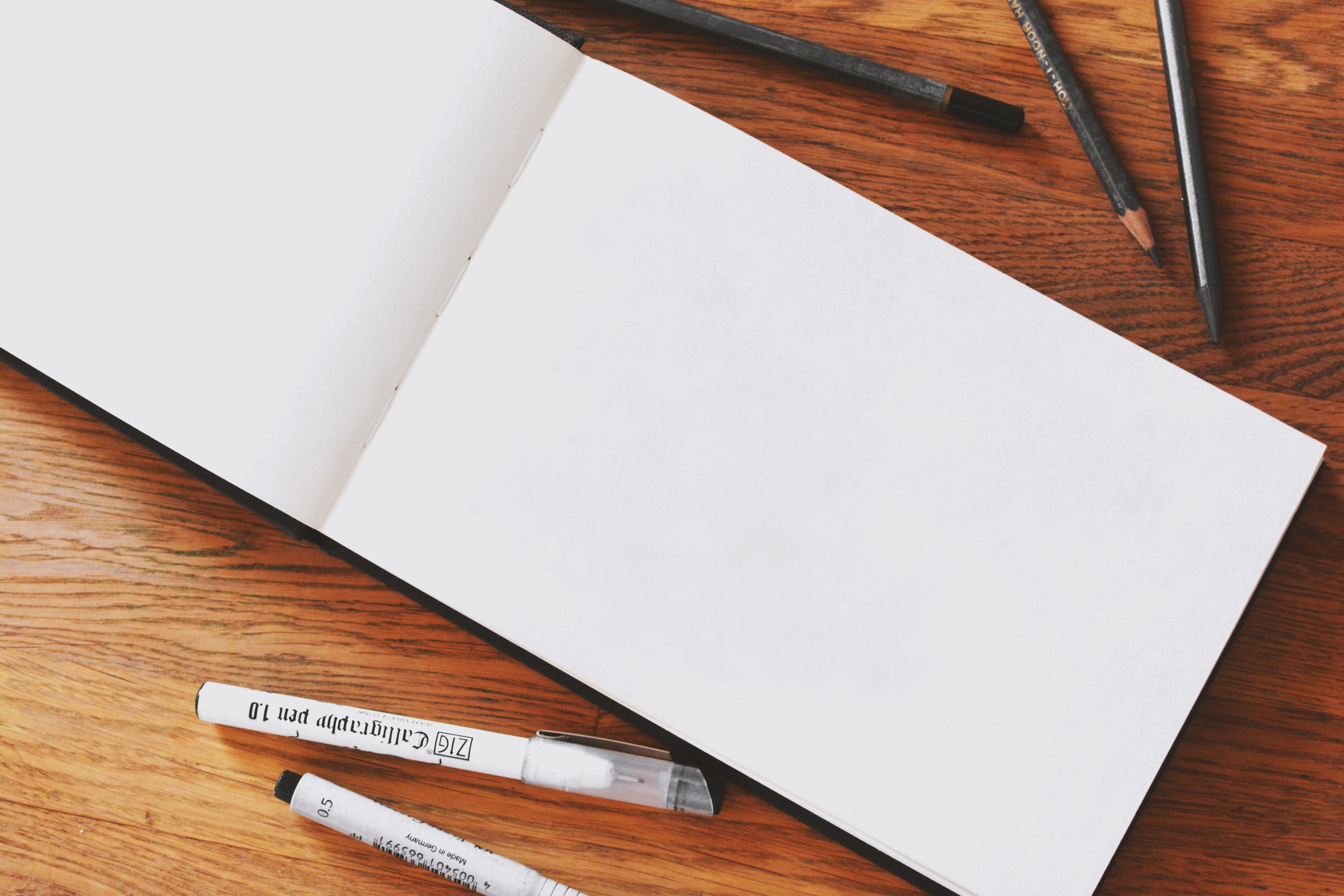
We’re fighting to restore access to 500,000+ books in court this week. Join us!
Internet Archive Audio
- This Just In
- Grateful Dead
- Old Time Radio
- 78 RPMs and Cylinder Recordings
- Audio Books & Poetry
- Computers, Technology and Science
- Music, Arts & Culture
- News & Public Affairs
- Spirituality & Religion
- Radio News Archive
- Flickr Commons
- Occupy Wall Street Flickr
- NASA Images
- Solar System Collection
- Ames Research Center
- All Software
- Old School Emulation
- MS-DOS Games
- Historical Software
- Classic PC Games
- Software Library
- Kodi Archive and Support File
- Vintage Software
- CD-ROM Software
- CD-ROM Software Library
- Software Sites
- Tucows Software Library
- Shareware CD-ROMs
- Software Capsules Compilation
- CD-ROM Images
- ZX Spectrum
- DOOM Level CD

- Smithsonian Libraries
- FEDLINK (US)
- Lincoln Collection
- American Libraries
- Canadian Libraries
- Universal Library
- Project Gutenberg
- Children's Library
- Biodiversity Heritage Library
- Books by Language
- Additional Collections
- Prelinger Archives
- Democracy Now!
- Occupy Wall Street
- TV NSA Clip Library
- Animation & Cartoons
- Arts & Music
- Computers & Technology
- Cultural & Academic Films
- Ephemeral Films
- Sports Videos
- Videogame Videos
- Youth Media
Search the history of over 866 billion web pages on the Internet.
Mobile Apps
- Wayback Machine (iOS)
- Wayback Machine (Android)
Browser Extensions
Archive-it subscription.
- Explore the Collections
- Build Collections
Save Page Now
Capture a web page as it appears now for use as a trusted citation in the future.
Please enter a valid web address
- Donate Donate icon An illustration of a heart shape
Visual methodologies : an introduction to researching with visual materials
Bookreader item preview, share or embed this item, flag this item for.
- Graphic Violence
- Explicit Sexual Content
- Hate Speech
- Misinformation/Disinformation
- Marketing/Phishing/Advertising
- Misleading/Inaccurate/Missing Metadata
![[WorldCat (this item)] [WorldCat (this item)]](https://archive.org/images/worldcat-small.png)
plus-circle Add Review comment Reviews
30 Favorites
DOWNLOAD OPTIONS
No suitable files to display here.
IN COLLECTIONS
Uploaded by station35.cebu on August 2, 2022
SIMILAR ITEMS (based on metadata)
Visual Literacy
- Defining Image/visual resource need
- Finding and accessing images/visual media
- Interpreting and Analyzing Images
- Evaluating Image/visual media sources
- Using Images/visual media effectively
- Visual Literacy Resources
- In the Margins Project
About this Page

This page gives tips and resources for analyzing images and visual media. It also lists resources that discuss analyzing and interpreting images. Please note that there are many ways to analyze images and the tips listed on this page are not the only right way to do this.
Tips and Resources for Analyzing and Interpreting Images
- Tips for Interpreting and Analyzing Images
- Questions to Consider when Interpreting and Analyzing Images
- Resources that Discuss Analyzing and Interpreting Images
- Give yourself time to "take-in" the entire image before you start your research. Make sure you understand what is happening in the image and that you see all of the details. Sometimes it is easy to skip over this step but, it is really important! You can't research an image accurately if you don't understand it.
- Read the Metadata. It is always important to read the information provided for an image. This information will allow you to cite the image probably and start to understand the context of the image.
- Look for the citation information. If the citation information is not provided for the image, it is likely that the resource you are using is not reliable. You always want to be able to cite your images!
Observation
| |
Gaining Context
| |
Finding Meaning
|
- The Art of Seeing Art This links to a page on the Toledo Museum of Art that describes a specific process for analyzing and interpreting images and visual media.
- Analyzing Images (Facing History and Ourselves) This link provides a plan/procedure for analyzing an image. This specific link is geared towards teachers trying to show students how to analyze an image.
- Teaching Students to Critically Read Digital Images: A Visual Literacy Approach Using the DIG Method This is a link to Dana Statton Thompson's article about teaching students to analyze images with a method she created called the DIG method. This link goes to Thompson's article description on Visual Literacy Today. The article title on that page clicks to the article.
- How Do You Look? This link is from the Nasher Museum of Art at Duke University. This link explains what visual literacy is like at Nasher Museum and other visual literacy related resources.
- << Previous: Finding and accessing images/visual media
- Next: Evaluating Image/visual media sources >>
- Last Updated: Jan 16, 2024 10:10 AM
- URL: https://guides.libraries.indiana.edu/Visual_Literacy
Social media
- Instagram for Herman B Wells Library
- Facebook for IU Libraries
Additional resources
Featured databases.
- Resource available to authorized IU Bloomington users (on or off campus) OneSearch@IU
- Resource available to authorized IU Bloomington users (on or off campus) Academic Search (EBSCO)
- Resource available to authorized IU Bloomington users (on or off campus) ERIC (EBSCO)
- Resource available to authorized IU Bloomington users (on or off campus) Nexis Uni
- Resource available without restriction HathiTrust Digital Library
- Databases A-Z
- Resource available to authorized IU Bloomington users (on or off campus) Google Scholar
- Resource available to authorized IU Bloomington users (on or off campus) JSTOR
- Resource available to authorized IU Bloomington users (on or off campus) Web of Science
- Resource available to authorized IU Bloomington users (on or off campus) Scopus
- Resource available to authorized IU Bloomington users (on or off campus) WorldCat
IU Libraries
- Diversity Resources
- About IU Libraries
- Alumni & Friends
- Departments & Staff
- Jobs & Libraries HR
- Intranet (Staff)
- IUL site admin
- Teaching Resources
- Upcoming Events
- On-demand Events
Analyzing Images
- English & Language Arts
- Social Studies
- facebook sharing
- email sharing
Why Analyze Images?
Use this strategy to guide students through a close analysis of an image. By following the steps in this image-analysis procedure, students develop awareness of historical context, develop critical thinking skills, enhance their observation and interpretive skills, and develop conceptual learning techniques. You can use this strategy with any visual media, including a piece of art, photograph, political cartoon, propaganda poster, or video clip.
Save this resource for easy access later.
Lesson plans, how to teach image analysis.
Select an Image
Choose an image that lends itself to deep analysis by students. This analysis strategy works best when the image is one that reflects (intentionally or not) a particular opinion, point of view, or perspective. Visual art, propaganda images, photographs, and political cartoons are good examples of visual media that reflect a perspective.
Lead Students through Analysis
Share the image with students by providing copies or by projecting or displaying it in the classroom. Lead students slowly through the following six steps, pausing between each step to give them significant time for thinking and writing.
Step One: Ask students to look deeply at the picture for a good long time. Have them observe shapes, colors, textures, the position of people and/or objects, etc.
Step Two: Have students write down what they see without making any interpretation about what the picture is trying to say.
Step Three: Ask students: What questions do you have about this picture that you would need to have answered before you can begin to interpret it? Ask as many questions as you have.
Step Four: Have students discuss their questions with two other students in the class to try to find some answers.
Step Five: Given the historical context and subject of the piece, ask students what they think the artist is trying to say (what does the piece mean), and who they think is the intended audience?
Step Six: Discuss your interpretation with the class, and be prepared to support your view by referring to specific elements of the image and what you know about the history of the time.
Discuss the Process
Take a few moments to discuss with students how they experienced this process of analyzing visual media. For many, it may feel uncomfortably slow, but by practicing and discussing this process, students will begin to respond more thoughtfully and critically to the images they encounter every day.
Image Analysis Worksheet
Image analysis procedure, you might also be interested in…, human timeline, introducing a new book, found poems, four corners, gallery walk, iceberg diagrams, café conversations, assigning roles for group work, three good things, unlimited access to learning. more added every month..
Facing History & Ourselves is designed for educators who want to help students explore identity, think critically, grow emotionally, act ethically, and participate in civic life. It’s hard work, so we’ve developed some go-to professional learning opportunities to help you along the way.
Exploring ELA Text Selection with Julia Torres
Working for justice, equity and civic agency in our schools: a conversation with clint smith, centering student voices to build community and agency, inspiration, insights, & ways to get involved.
Introduction to Image Analysis: Understanding the Basics and Applications
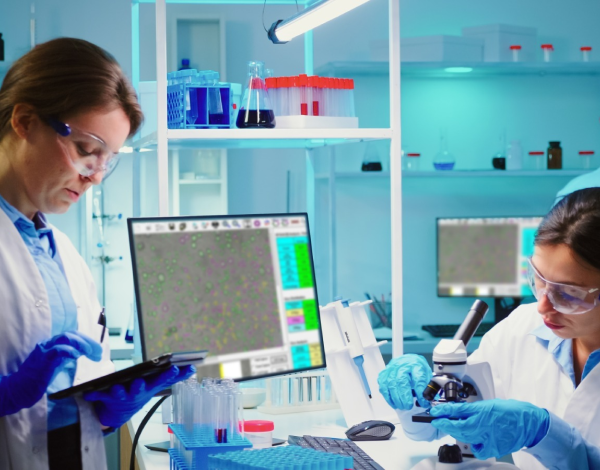
31 Jul 2023
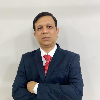
Sandeep Kulkarni
Founder & CEO
Introduction:
Following are some of the basic ideas in image analysis:, image acquisition:, pre-processing:, segmentation:, feature extraction:, object recognition:, image classification:.
Read Also - The Basic Of Image Processing
Image Analysis Applications:
Security and surveillance:, robotics and automation:, agriculture:, pharmaceutical industry:, quality control and inspection:, biometrics:, how has image analysis reshaped the pharmaceutical industry.
Read Also - Image Filtering Techniques in Image Processing
How Has Image Analysis Advanced Scientific Research?
Cellular and molecular biology:, medicine and biomedical research:, neuroscience:, ecology and environmental sciences:, astronomy and astrophysics:, materials science:, social sciences:, how image analysis has made social media platforms more user-friendly, different image analysis software used:, scikit-image:, tensorflow:, cellprofiler:, deeplabcut:.
Read Also - Particle Size Analysis: Importance & Applications
Future of Image Analysis:
Sandeep Kulkarni, Founder & CEO
Sandeep Kulkarni is the founder & CEO of ImageProVision Technology. With over 3 decades of experience behind him, he is your 'go-to' man in the image analysis sector.
Articles, you may also like.
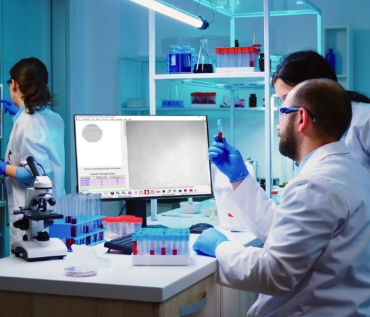
Understanding Image Filtering Techniques in Image Processing
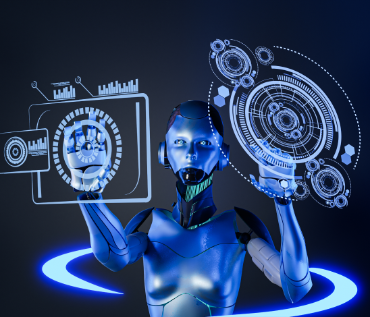
How AI/ML is Used in Particle Analysis Characterization

Beyond Enumeration: ipvMicrobe Colony Counters
Submit your details to schedule your demo..
This site is protected by reCAPTCHA and the Google Privacy Policy and Terms of Service apply.
Thank you for reaching out. We will get back to you soon.
Advertisement
A comprehensive review of image analysis methods for microorganism counting: from classical image processing to deep learning approaches
- Published: 29 September 2021
- Volume 55 , pages 2875–2944, ( 2022 )
Cite this article
- Jiawei Zhang 1 ,
- Chen Li ORCID: orcid.org/0000-0003-1545-8885 1 ,
- Md Mamunur Rahaman 1 ,
- Yudong Yao 1 , 2 ,
- Pingli Ma 1 ,
- Jinghua Zhang 1 , 5 ,
- Xin Zhao 1 , 3 ,
- Tao Jiang 1 , 4 &
- Marcin Grzegorzek 1 , 5
17k Accesses
57 Citations
2 Altmetric
Explore all metrics
Microorganisms such as bacteria and fungi play essential roles in many application fields, like biotechnique, medical technique and industrial domain. Microorganism counting techniques are crucial in microorganism analysis, helping biologists and related researchers quantitatively analyze the microorganisms and calculate their characteristics, such as biomass concentration and biological activity. However, traditional microorganism manual counting methods, such as plate counting method, hemocytometry and turbidimetry, are time-consuming, subjective and need complex operations, which are difficult to be applied in large-scale applications. In order to improve this situation, image analysis is applied for microorganism counting since the 1980s, which consists of digital image processing, image segmentation, image classification and suchlike. Image analysis-based microorganism counting methods are efficient comparing with traditional plate counting methods. In this article, we have studied the development of microorganism counting methods using digital image analysis. Firstly, the microorganisms are grouped as bacteria and other microorganisms. Then, the related articles are summarized based on image segmentation methods. Each part of the article is reviewed by methodologies. Moreover, commonly used image processing methods for microorganism counting are summarized and analyzed to find common technological points. More than 144 papers are outlined in this article. In conclusion, this paper provides new ideas for the future development trend of microorganism counting, and provides systematic suggestions for implementing integrated microorganism counting systems in the future. Researchers in other fields can refer to the techniques analyzed in this paper.
Similar content being viewed by others
U-Net: Convolutional Networks for Biomedical Image Segmentation
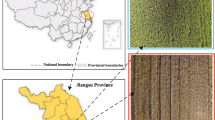
UAV remote sensing detection and target recognition based on SCP-YOLO
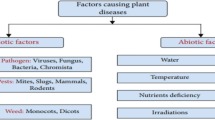
Plant disease detection and classification techniques: a comparative study of the performances
Avoid common mistakes on your manuscript.
1 Introduction
1.1 basic knowledge of microorganisms.
Microorganism is a kind of tiny organism which cannot be observed by naked eyes but can be observed by light microscope or electron microscope (Madigan et al. 1997 ). There are many different types of microorganisms, and the classification standards are various. Generally, microorganisms are composed of bacteria, viruses, fungi and some algae.
Bacteria are unicellular organisms with minimal size, simple structure, lack of nuclei, cytoskeletons, and membranous organelles. It widely distributes in soil and water, and most of them are decomposers at the bottom of the biological chain, such as Escherichia coli . Some bacteria are consumers and producers. For example, sulfur bacteria and iron bacteria are producers. They can use inorganic materials to produce organic substances they need. The rhizobia can consume organic substances produced by the photosynthesis of legumes (Doetsch and Cook 2012 ).
The virus is a kind of microorganism that can spread and infect other organisms. It is small and has a simple structure. It contains only one type of nucleic acid, such as ribonucleic acid(RNA) virus and deoxyribonucleic acid(DNA) virus. It must parasitize in living cells and proliferate in the way of replication. Viruses consist of single and double-stranded RNA virus, single and double-stranded DNA virus (Cui et al. 2019 ). For example, severe acute respiratory syndrome coronavirus 2 (SARS-CoV-2) is a single-stranded RNA virus (Andersen et al. 2020 ).
The fungus is one type of eukaryotic microorganism, including mold, yeast and mushroom, that can produce spores through asexual and sexual reproduction. Tinea pedis is a kind of foot skin disease caused by pathogenic fungi, which is widely spread globally. There are no sebaceous glands between the soles of human feet and toes, so the environment lacking fatty acids and poor air circulation is conducive to the growth of filamentous fungi (Perea et al. 2000 ).
Algae are eukaryotes of the protozoa and most of them are aquatic organisms, which can carry out photosynthesis. Algae can be composed of one or a few cells, or many cells aggregate into tissue-like structures. According to the color, algae can be divided into green algae, brown algae and red algae. Red tide is an abnormal phenomenon in the marine ecosystem. It is caused by the explosive proliferation of red tide algae under specific environmental conditions, which is a signal of marine pollution. During the red tide period, a large number of fish, shrimp, crabs, and shellfish die, causing significant damage to aquatic resources and human health (Kirkpatrick et al. 2004 ).
Some microorganisms are harmful to human beings by causing food decomposition, infect humans and cause diseases, but some microorganisms are beneficial to human beings. Penicillin is an epoch-making discovery in the medical field, which has saved countless lives. Yeast is widely used in industrial fermentation, ethanol production and food production for human beings (Brill 1981 ). Some microorganisms can degrade plastics, treat waste-water, gas, and have great potential in renewable resources (Rizzo et al. 2013 ). There are also many microorganisms in the intestines of healthy people, which can help humans decompose and absorb food and toxic substances. Some microorganisms have adverse effects on the human body and industrial production. For example, the human immunodeficiency virus (HIV) can cause the loss of immune function of patients and cause infection. The disease spreads rapidly, has high mortality and cannot be cured, which has caused a significant threat to world health; SARS-CoV-2 breaks out at the end of December in 2019 (Hui et al. 2020 ). More than 183,000,000 people have been infected worldwide till July 1st, 2021, which becomes a global malignant epidemic (University 2020 ). The SARS-CoV-2 is highly infectious and mainly transmitted through close contact and respiratory droplets. Microorganisms play an essential role in human’s daily life and production. Therefore, beneficial microorganisms should be used wildly, and harmful microorganisms should be prevented.
Microorganism counting is an essential part of microbial research, which is widely used in food and drug safety tests, biomedical tests, and environmental monitoring (Liu et al. 2004 ). At present, there are two main methods for microorganism counting and quantification, one of the methods is manual counting, the other one is computer image analysis counting (Rajapaksha et al. 2019 ). Manual counting mainly includes the plate counting method, hemocytometry and turbidimetry. In the plate counting method, the bacteria are placed in a suitable medium and then wait for them to grow into colonies. After that, the number of colonies is counted through the microscope. The advantage of the plate counting method is that the number of live bacteria can be estimated. However, the operation is complicated, and it takes a period to culture the microorganisms and gets the results. In general, the number of colonies obtained is lower than the actual number of living bacteria because when more than two living bacteria cells stick together, the observed number is still one colony (Balestra and Misaghi 1997 ). In the hemocytometry method, the bacteria are diluted and dropped on a blood cell counting plate, which is then observed under a microscope to calculate the average number of bacteria in each compartment. Finally, the total number of bacteria is estimated. However, the hemocytometry method cannot distinguish the dead bacteria from the live bacteria and can only estimate the total number of bacteria by the average value, which carries out the low accuracy (Sambrook and Russell 2006 ). In the turbidimetry method, a spectrophotometer is applied to measure the optical density of bacterial suspension at a particular wavelength. The cell concentration in bacterial suspension is proportional to the turbidity of bacterial suspension within a specific range, that is, the cell concentration is proportional to the optical density. So, the number of bacteria can be expressed in terms of optical density. However, the turbidimetry method has specific requirements for the wavelength of light in the experimental environment, which should be controlled within the line limit range where the bacterial concentration is proportional to the optical density. Otherwise, the measurement result will have a large error (Dalgaard et al. 1994 ).
It can be seen that the traditional methods can obtain satisfactory counting results under certain conditions, such as when the number of samples is small and the imaging effect is good under the microscope. However, when the sample becomes larger, it is often encountered that the colony is small, the contrast between the colony and the culture medium is not clear, and it is not easy to detect and count with naked eyes. The detection results have the problems like large errors and poor reliability. The sample image contains many particles, and the workload is heavy and dull, which is easy to cause misjudgment. Moreover, the subjectivity of manual counting is common. Even if the same staff member observes the same sample in different periods, different observation results will be obtained (Chien et al. 2007 ). With the development of computer image analysis technology, automatic particle image analysis systems based on image processing and visual analysis can automatically, quickly and objectively count the number of particles contained in the image and extract various characteristic parameters of particles, which significantly reduces the workload and improves the analysis accuracy, so it has been widely used. The image analysis system for microorganism counting can improve counting performance if the quantity of sample is large (Thiran et al. 1994 ). An example image of yeast cells is shown in Fig. 1 . The precise boundaries of microorganisms make it possible to separate and count the number of colonies by image analysis.
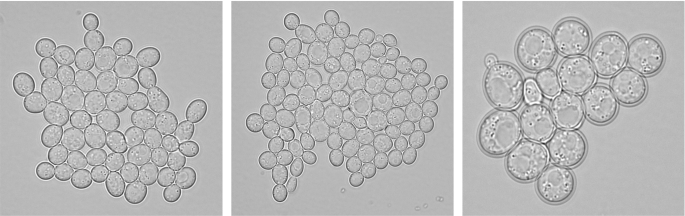
An example of yeast cells image (in (Dietler et al. 2020 ) proposed dataset)
1.2 Motivation of this review
Digital image processing (DIP), also known as computer image processing, refers to converting an image signal into a digital signal and processing it by computer. DIP first appeared in the 1950s, when the electronic computer has developed to a certain level. People can use the computer to process images and improve image quality (Gonzalez et al. 2004 ). The commonly used digital image processing methods include image enhancement, denoising, restoration, coding and compression. DIP has been widely used in many fields. Agricultural and forestry departments understand the growth of plants through remote sensing images, estimate the yield, and monitor the development and management of diseases and insect pests (Amrita and Kaur 2016 ). Through remote sensing image analysis, the water conservancy department can obtain the change of water disaster (Sudiana and Rizkinia 2012 ). The meteorological department is used to analyze the meteorological cloud chart and improve the accuracy of the forecast (Chatterjee and Chaulya 2019 ). The department of national defense, surveys, and mapping use aerial surveys or satellites to obtain regional landform and ground facilities (Feifei et al. 2017 ). The mechanical department can use image processing technology to analyze and identify the metallographic diagram automatically (Privezentsev et al. 2019 ). Medical departments use various digital image analysis technologies to diagnose various diseases automatically (Salvi et al. 2020 ; Madabhushi and Lee 2016 ; Li et al. 2020a ). Because of the flexibility and universality of DIP, there are no complex measurement steps involved, which means it has low learning cost. In the field of microorganism analysis, expensive equipment is usually needed to ensure the accuracy of the measurement. DIP can save this part of this cost (Ekstrom 2012 ). Therefore, DIP has been widely used in microbial counting in many types of research. Its development trend is shown in Fig. 2 , which has shown a good development trend so far.
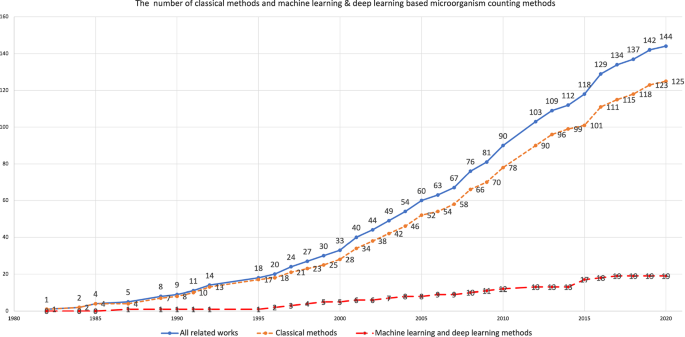
The total number of related works on microorganism counting approaches
As shown in Fig. 2 , the application of digital image processing in the field of microorganism counting has been explored. Since the 1980s, DIP has been applied to microorganism counting. From 1980 to 1995, the application and development of this field is relatively slow, but it is rapidly developed from 1995 to 2010. After 2010, the number of research for microorganism counting increases faster. As for machine learning and deep learning based microorganism counting methods, machine learning is firstly applied in this field in 1987, and then it is slowly developed from 1990 to 1995. Since 1990, microorganism counting methods based on machine learning and deep learning is increasing steadily, and it has a tremendous development since 2015. According to the content of the papers, a possible reason is summed up, that is, the development of the deep learning algorithm can lead to more accurate image segmentation. For example, the segmentation of adherent colonies can lead to more precise microorganism counting.
1.3 Related reviews
The microorganism counting is an essential topic in microbial research, and the relevant works are also relatively abundant. Many researchers have written relevant reviews, which are summarized as follows:
Review Gray et al. ( 2002 ) outlines several image analysis methods for algal cell estimating, and several image segmentation methods based on thresholding, edge tracking and template matching are compared. There are 32 papers summarized, and only three are about the algal counting method. Review Qiu et al. ( 2004 ) describes the development course of bacteria counting and cell size measurement, which contains the classical methods and automated flow analysis technology. More than 33 papers are summarized and 7 of them are about bacteria counting. Review Gracias and McKillip ( 2004 ) describes the use of fluorogenic or chromogenic to classify different species of bacteria and impedance technology for enumeration. There are more than 25 papers are about traditional food bacteria counting methods in total 103 papers. Review Daims and Wagner ( 2007 ) indicates that the difference between microorganism counting and biovolume measurement is whether or not to identify individual objects (cell or cell clusters) in the biomass. There are six papers about automatic cell counting in total 92 papers. Review Barbedo ( 2012a ) describes the object counting methods using digital image processing. The methods are composed of morphological operation, filtering operation, contrast enhancement, transformation, edge detection and image segmentation. They summarized over 130 papers, among them, 29 papers are used for cell counting and 13 papers are about bacteria counting. Review Dazzo and Niccum ( 2015 ) describes the use of CMEIAS for both microorganism counting and biovolume measurement based on image processing. The hierarchical tree classifier and k -Nearest Neighbour classifier are applied for classification. There are 65 papers in total, and more than 30 papers are used for cell counting. Review Li et al. ( 2019a ) describes computer-based microorganism image analysis development and introduces different methods for different microorganism classification. This review is a comprehensive microorganism classification paper. It uses plenty of works of literature for quoting, but there is no significant description for microorganism counting in more than 300 papers in total. Review Puchkov ( 2019 ) describes the main quantitative analysis methods of single bacterial and yeast cells at the cellular and subcellular levels. More than 150 papers are summarized. This review mainly introduces several techniques for scanning, but there is no straightforward application of DIP in microorganism quantification.
Although the reviews above are excellent enough and the descriptions about the current situation of microbial research are objective and detailed. However, there is no targeted research about image analysis based microorganism counting, so it is necessary to do additional research on this aspect. For a clear overview, a histogram (Fig. 3 ) is used to show each of the related survey papers and their contribution to microorganism counting with our proposed studies. Because of the vital role microorganism quantification plays in microbial research, this review focuses on the application of microorganism counting and summarizes each method’s development and prospects. This review has great reference value for microbiological researchers and computer vision researchers. There are more than 136 papers are used for microorganism counting.
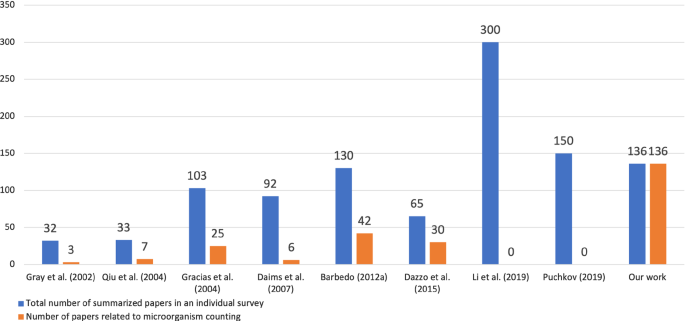
A comparison among recent survey papers. Number of summarized papers in the existing review in comparison to their contribution to image analysis based microorganism counting methods
1.4 Microorganism counting methods
In order to expound the approach of microorganism counting, the organization chart of this review is shown in Fig. 4 . The approach contains five steps: microbiological data acquisition, microscopic image, image pre-processing, microorganism counting methods and evaluation methods.
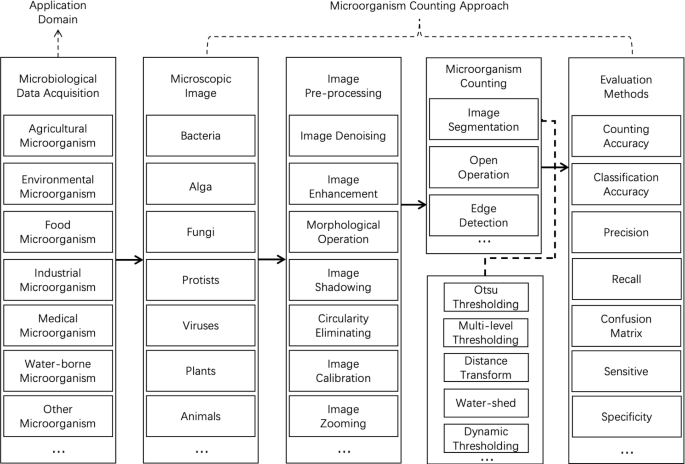
The organisation chart of microorganism counting approaches in this paper
Firstly, according to the different application domains, microorganisms are composed of the following seven categories: agricultural microorganism, environmental microorganism, food microorganism, industrial microorganism, medical microorganism, water-borne microorganism and other microorganisms. Then, the samples are stained and sliced. After that, the microscopic images are captured by the imaging equipment, such as a charge-coupled device (CCD) camera (Gmür et al. 2000 ).
Next, in the pre-processing part, the images are denoised and enhanced to improve the contrast between the object particles and the background. In the process of microorganism microscopic image scanning, the loss of information in the process of electronic transmission and the pretreatment process, such as staining may bring some noise to the microscopic image. In order to improve image quality, image processing can be used to reduce or remove these noises. The main methods to remove noise are wire filter (such as Gaussian filter and Mean filter), median filter and so on (Li et al. 2020b ).
The next step is microorganism counting (Li et al. 2020b ). The objects of the microorganism counting method are separating the adherent colonies and counting. Image segmentation is an essential part of this task, which contains three broad categories: threshold segmentation, edge detection and region extraction. The initial image segmentation method is threshold segmentation, whose core algorithm is the selection of the threshold. At present, there are two main methods, one is based on the iterative method and the other one is Otsu thresholding. For the image with prominent double peaks and deep valley bottom, the iterative method can get satisfactory results quickly, but for images with significant differences in the ratio of target and background, the iterative method cannot segment the target well (Perez and Gonzalez 1987 ). Another standard method is the maximum inter class variance based Otsu method which can achieve good segmentation results for most images (Otsu 1979 ). The advantages of Otsu segmentation are fast and straightforward calculation, not affected by brightness and contrast of images, and most of the segmentation results are satisfactory. Nevertheless, it has limitations such as the sensitivity to noise and cannot support semantic segmentation functions (Xu et al. 2011 ). Edge detection mainly includes gradient and second-order differential operator based methods, Laplace of Gaussian function (LoG) edge detection method and Canny edge detection method. The gradient detection method is the most widely used method among them that usually contains Roberts, Sobel, Prewitt, Kirsch and Robinson (Gonzales and Woods 2002 ). The Watershed method is one of the most popular methods in the region extraction domain, a closed region signature method based on region growth (Levner and Zhang 2007 ). The image segmentation is considered according to the composition of the watershed. The calculation process of the watershed is an iterative labeling process, which has an excellent response to weak edges. However, the watershed algorithm may lead to over-segmentation because of the noise or slight gray-level change of object surface (Strahler 1957 ).
Another critical part of microorganism counting is morphological operations which contain erosion, dilation, open and close. The erosion operation uses structural elements to erode the input image, eliminating the image’s boundary points. It can reduce the size of the object, filter the image interior and eliminate the isolated noise points effectively (Jackway and Deriche 1996 ). Dilation operation is the dual operation of erosion operation. The dilation operation can merge all the background points contacted by the target object into the object, which can increase the target and the shrink holes (Jackway and Deriche 1996 ). The open operation is using the erosion operation firstly and then use the dilation operation. The open operation can eliminate the isolated points in the image, eliminate the burr and connect the two domains so that the outer boundary of the image can be polished by the open operation (Chudasama et al. 2015 ). The close operation is the opposite of the open operation, which means the image is dilated first and then eroded. The close operation can fill small holes, close small cracks, and polish the inner boundary of the image (Chudasama et al. 2015 ).
After image segmentation, the microorganisms need to be classified and counted respectively. Machine learning is widely applied in image classification, which has been developed rapidly. Principle component analysis (PCA) is an unsupervised machine learning algorithm, which is always applied for exploration and dimension reduction of higher dimensional data (Roweis 1998 ). More comprehensible features can be extracted, and valuable information of the sample can be processed faster by using dimension reduction. In addition, dimension reduction can also be applied to visualization and denoising. The primary process of PCA is to map n -dimensional features to k -dimensional features, which are new orthogonal features, also known as principal components. Then the k -dimensional features are re-constructed based on the original n -dimensional features. PCA can increase the sampling density by dropping part of the information, which is helpful for the curse of dimensionality. However, PCA retains the primary information, which is only for the training set, but the primary information is not necessarily meaningful. So the overfitting may be exacerbated by using PCA (Karamizadeh et al. 2013 ). Support vector machine (SVM) is one kind of generalized linear classifier for data classification by using supervised learning (Vishwanathan and Murty 2002 ). The object of SVM learning is to find the separation hyperplane with the most considerable geometric interval, which can divide the training data set correctly. The learning strategy of SVM is to maximize the interval, which can be formalized into a problem to solve the convex quadratic programming. The selection of SVM kernels can make it to be a nonlinear classifier, such as polynomial kernel, RBF kernel, Laplacian kernel and Sigmoid kernel (Han et al. 2012 ).
Artificial neural network (ANN) is a mathematical model of distributed and parallel information processing that imitates animal neural networks’ behavior characteristics. The most commonly used ANN is Multilayer perceptron (MLP), which is a feedforward ANN model (Ghate and Dudul 2010 ). ANN is composed of input layers, hidden layers and output layers, which are fully connected to each other. The structure of ANN is shown in Fig. 5 . ANN is composed of many simple neurons, and each neuron receives input from other neurons. In this way, every neuron restricts and influences each other to achieve nonlinear mapping from input state space to output state space. ANN is a combination of many same simple processing units in parallel. Although the function of each unit is simple, the parallel activities and the ability of information processing are unique. ANN can realize the memory of information through its network structure, and the memory information is stored in the weights between neurons. This makes the network has good fault tolerance and can handle the pattern information processing such as clustering analysis, feature extraction and defect pattern restoration (Zupan 1994 ).
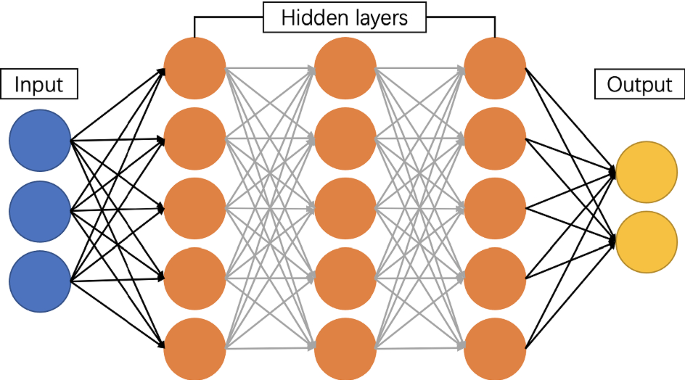
The structure of ANN
Back propagation neural network (BPNN) is a supervised learning, which is developed from ANN (Karsoliya 2012 ). The loss function in BPNN is optimized based on back propagation. In forward-propagating, the data is processed from input layers to output layers. In back propagation, the loss function is transmitted from output layers to input layers, then the weights and biases are optimized based on the gradient descent method. BPNN can carry out the nonlinear mapping from input to output, and can still make the correct mapping for the new non-sample data, which has a specific generalization ability and fault tolerance ability (Dai and MacBeth 1997 ).
Convolutional neural network (CNN) is one kind of feedforward ANN, that is wildly applied in DIP and computer vision (Li et al. 2016 ). The convolutional kernels are applied to scan the whole image and the deep features are then extracted. After pooling, the image can be classified through a fully connected layer. The loss function is minimized with back propagation (Chauhan et al. 2018 ). CNN is developed rapidly after 2010, and it is not only be applied for classification, but segmentation (Unet) and image generation (GAN). VGG-16 is one of the most popular CNN, which is composed of five convolution layers, three pooling layers and three fully connected layers Simonyan and Zisserman ( 2014 ). The structure of VGG-16 is shown in Fig. 6 . Only 3 \(\times\) 3 filters are applied in VGG-16 because the combination of small filters can simulate a larger filter, reducing the parameters and improving the nonlinear ability.
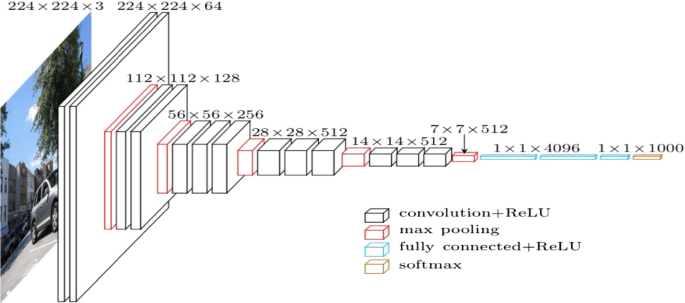
The structure of VGG-16 (in Nash et al. ( 2018 ) Fig. 3)
The last step is system evaluation, which can help researchers systematically perceive the image processing results. The results of the evaluation can help to prompt the accuracy of the system. Counting accuracy is a standard evaluation method in target counting, which is the ratio of the number of detected targets to the ground truth. Generally, the accuracy can only be used to evaluate the global accuracy, because it cannot show whether the detected target and the ground truth target are one-to-one corresponding (Bloem et al. 1995 ). Evaluation of image segmentation and image classification can also reflect the performance of the counting system. True positive (TP), false negative (FN), false positive (FP) and true negative (TN) are four basic metrics in image classification (Zhang et al. 2008b ). Pixel accuracy (PA) is one of the simplest evaluation methods for image segmentation, which means the ratio of the number of correctly classified pixels and the number of whole pixels. The mean pixel accuracy (MPA) is the improved method of PA, which indicates the mean PA of all classes (Zhang et al. 2008b ). Mean intersection over union (MIoU) is the ratio of intersection and union of ground truth and predicted segmentation result. It can be regarded as the mean ratio of TP and the union of TP, FN and FP in the process of image segmentation (Rahman and Wang 2016 ).
1.5 Structure of this review
In this review, a comprehensive overview of microorganism counting using image analysis is presented. The relevant research in the microbial application has been investigated since 1980, and the applications of microorganism counting in different situations are discussed. Furthermore, this paper also summarizes the research motivation and research methods of microorganism counting in the microbial field. The review articles related to this research are also summarized, and the structures of their references are recorded. More than 144 papers are selected from the initial paper dataset and the structure of the systematic review is shown in Fig. 7 . The initial papers are searched from Google Scholar, IEEE, ACM, Nature, Science, Cell, Elsevier, Wiley, Hindawi, IOP, PloS, BMC and Springer, and the keywords contain “microorganism counting”, “bacteria counting”, “cell counting”, “algae counting” and “fungus counting”. Then the duplicate and irrelevant papers are deleted. There are 57 papers about microorganism biovolume counting, which do not conform to this review. Finally, 144 papers are about microorganism counting methods, containing 8 review papers, 82 bacteria counting methods, and 54 other microorganism counting methods.
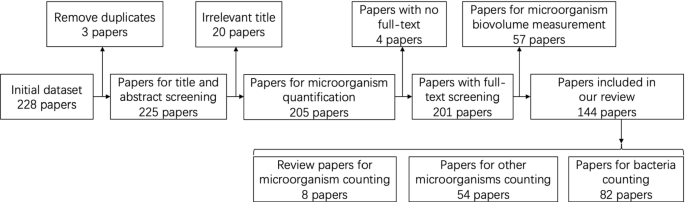
The systematic flow chart of paper selection for our work
The review is structured as follows: In Sect. 2 , the related works of image analysis based bacteria counting are introduced. In Sect. 3 , the related works of other microorganisms counting based on image analysis are introduced. Then in Sect. 4 , the commonly used microorganism counting methods are analyzed, and their different application domains are summarized. Finally, in Sect. 5 this review is concluded by summarizing the whole paper. This review structure can help microbiological workers clearly and quickly understand the development status of this field and obtain the relevant content they need.
2 Bacteria counting methods
Bacteria are one crucial part of the ecosystem because of the cardinal role they play in the carbon and nitrogen cycle, which are closely related to human daily life (Madigan et al. 1997 ). Therefore, bacteria counting has become one of the most important directions in the field of microorganism counting, including the study of bacteria number and colony size. Thus, the bacteria counting methods are summarized in this chapter. Bacteria counting is of great significance in food safety monitoring and industrial safety detection, but manual counting is tedious and redundant work, that is very subjective. Therefore, the research of computer image analysis based bacteria counting is significant. This chapter is structured as follows: the first part summarizes the classic bacteria counting methods. The second part is bacteria counting methods based on machine learning and deep learning. The last part is third-party tools methods.
2.1 Classic counting methods
2.1.1 bacteria counting method based on image enhancement.
In Pettipher and Rodrigues ( 1982 ), Niyazi et al. ( 2007 ), the gray-level contrast is applied for bacteria counting. The contrast is used for counting bacteria and somatic cells of milk (Pettipher and Rodrigues ( 1982 )). In Niyazi et al. ( 2007 ), the maximum size of one colony (defined by the area) and the distribution of the gray color within the colony are further measured beside the gray-level contrast, which has the mistake of less than 3%. The colony counting result is shown in Fig. 8 .
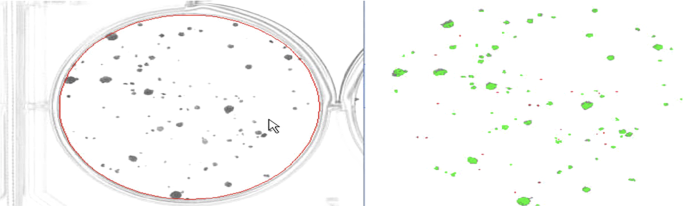
Screenshot of the program. The left panel is the scanned flask and the right panel is the segmented result (in Niyazi et al. ( 2007 ) Fig. 1)
In Shenglang and Yongguang ( 2005 ), histogram equalization is used to enhance the contrast of bacteria images as pre-processing. Then a convolutional filter is used to extract the curvature feature of bacteria and remove the background features. After that, a median filter is used to remove noises. The basis of judging whether there are bacteria in the image is whether the maximum connected number of non-zero gray pixels in the image exceeds a certain threshold. Finally, the number of connected domains is calculated as the number of bacteria in the image. The result shows that the accuracy is more than 95% while counting the number of bacteria.
In Buzalewicz et al. ( 2010 ), optical transforms are used for the determination of bacteria colony number. Because of the scale invariant of Mellin transform, the combination method of Fourier transform and Mellin transform is applied. First, the Fourier transform of the input objects is calculated and the high pass frequency filter is applied to eliminate the zero-order component of the Fourier spectrum. Then the Mellin transform followed by the log-polar transformation is performed. Moreover, the two-dimensional Fourier transform is computed in order to obtain scale and rotation invariance. Finally, the value of the Mellin spectrum is used to evaluate the number of the analyzed object. A good agreement between calculations and manual counting for twelve samples is achieved (the differences range from 1 to 3% and the standard deviation is equal 4.51).
2.1.2 Bacteria counting method based on thresholding
In Masuko et al. ( 1991 ), Trujillo et al. ( 2001 ), Chunhachart and Suksawat ( 2016 ), Gupta et al. ( 2012 ), Sethi and Yadav ( 2012 ), Kaur and Sethi ( 2012 ), Nayak et al. ( 2010 ), Pernthaler et al. ( 1997 ), Sotaquira et al. ( 2009 ), Maretić and Lacković ( 2017 ), Chunhachart and Suksawat ( 2016 ), Kaur and Sethi ( 2012 ), Payasi and Patidar ( 2017 ), global thresholding is applied for bacteria enumeration. The RGB image is firstly converted to YCbCr and Lab color spaces in Sotaquira et al. ( 2009 ). An adaptive median filter (Gupta et al. ( 2012 ), Kaur and Sethi ( 2012 )), a‘flatten filter’ (Trujillo et al. ( 2001 )) or a top-hat algorithm (Pernthaler et al. ( 1997 )) is applied for denoising. Besides, a combination method of Gaussian low-pass filter, simple symmetric moving average filter and median filter is applied for noise removal in Maretić and Lacković ( 2017 ). After thresholding, the morphological operations are applied for image enhancement (Chunhachart and Suksawat ( 2016 ), Kaur and Sethi ( 2012 )), and the detected circles are used for counting the number of colonies that appeared on the selected region. The detection result is shown in Fig. 9 and the average percentage error of 2.13% is obtained in comparison to the counting by the expert. In Payasi and Patidar ( 2017 ), the RGB image is converted to HSI color space image and the image is then segmented based on thresholding. Then the noises are removed, so the labeling and counting of the bacilli in the image will be possible. Afterward, the boundaries of bacteria are detected and stored, and the area and perimeter are calculated. If there is a clump of bacilli, the count of bacilli is increased by the integer, which is closed to the ratio of the area of the clump to the average area of bacilli. The result is shown in Fig. 10 and accuracy of 90% is obtained.
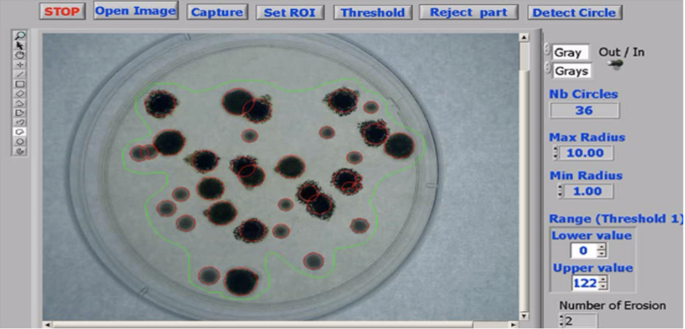
The detection result (in Chunhachart and Suksawat ( 2016 ) Fig. 8)
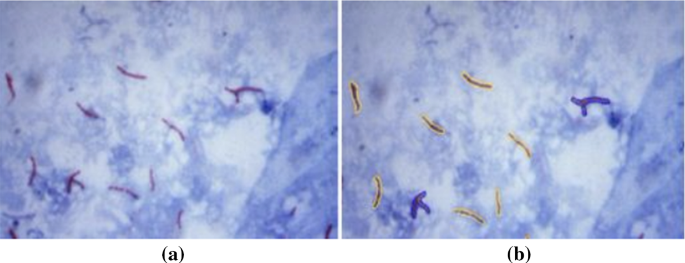
The counting result. a Original image. b Image after shape characterization and segmentation (in Payasi and Patidar ( 2017 ) Fig. 8)
In Shen et al. ( 2010 ); Austerjost et al. ( 2017 ), the iterative local threshold is applied for bacteria colony counting. First, a median filter and contrast enhancement are applied to remove noises and enhance images (Shen et al. ( 2010 )). Then the iterative local threshold method is used for image segmentation. After that, the petri dish edge is removed by detecting the connected region with the maxima white pixel. Finally, the number of bacteria is counted based on eight neighborhoods in Shen et al. ( 2010 ) and the average relative error of 2.5% is obtained. In Austerjost et al. ( 2017 ), after thresholding, the region of interest is examined for objects which will be divided into single colonies and colony clusters by using a classification algorithm based on the previously defined threshold. Afterward, a Hough circle transformation is applied for the segregation of colony clusters into single colonies. The last part of the algorithm is dedicated to finding colonies that could not be detected within the previous steps. For this, the sizes of previously found colonies are compared with other objects found on the plate. If these objects fit into the size range of previously found colonies and have a suitable roundness, they are recognized as a colony. Finally, the detected colonies are all counted with an average accuracy of \(86.76\pm 9.76\%\) .
In Jun ( 2010 ), Clarke et al. ( 2010 ), Marotz et al. ( 2001 ), feng Hu ( 2013 ), Boukouvalas et al. ( 2018 ), Matić et al. ( 2016 ), Siqueira and de Carvalho ( 2017 ), the adaptive threshold is used for bacteria counting. First, a median filter (Jun ( 2010 ), Boukouvalas et al. ( 2018 ), Siqueira and de Carvalho ( 2017 )), a Gamma correction (Matić et al. ( 2016 )) and a Gaussian filter (Clarke et al. ( 2010 )) are used for noise removal. Then the extended minima function is used to find the center of the colonies (Clarke et al. ( 2010 )). Finally, a saturation based adaptive thresholding is applied for image segmentation. The morphological operations such as opening and closing are used for adherence colonies segmentation and image smoothing. The segmentation procedure is shown in Fig. 11 . The results correlate well with the results obtained from manual counting, with a mean difference of less than 3%. Moreover, the distance transform and progressive erosion are applied in feng Hu ( 2013 ) to separate connected colonies into a single one. The counting result of Matić et al. ( 2016 ) is shown in Fig. 12 . In Boukouvalas et al. ( 2018 ), the circular area is detected through Hough transform to obtain only the inner area of the dish and a mask is created for the removal of the unwanted area. Afterward, Gaussian adaptive thresholding is performed for image segmentation because of the different lighting conditions in different areas. Then the histogram of vertical projections of the image is analyzed by varying its rotation angle to align the stripe at a \(90^\circ\) angle. Finally, cross correlation-based granulometry is applied for the determination of the amount of bacteria colonies.
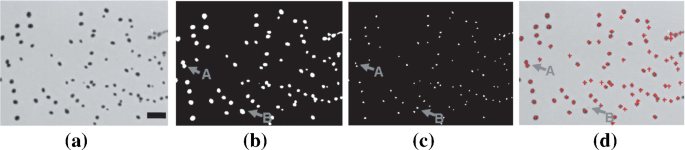
Illustration of the colony counting procedure. a The initial image. b The image after thresholding. c The extended minima of the original image. d The counted colonies (in Clarke et al. ( 2010 ) Fig. 4)
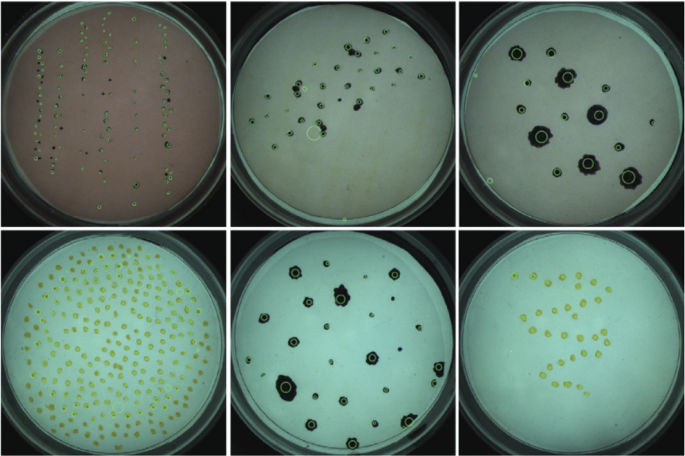
Test result (in Matić et al. ( 2016 ) Fig. 7)
In Zhang et al. ( 2008a ), Alves and Cruvinel ( 2016 ), Sánchez-Femat et al. ( 2016 ), Boukouvalas et al. ( 2019 ), Otsu thresholding is applied for bacteria counting. First, a linear expansion of the histogram (Sánchez-Femat et al. ( 2016 )) and a multi-directional Sobel operation (Boukouvalas et al. ( 2019 )) is applied for image enhancement and edge detection. In Zhang et al. ( 2008a ), the RGB and achromatic are processed, respectively. For RGB images, the Otsu thresholding method is firstly used for segmentation, then the color similarity in HSV (Hue-Saturation-Value) color space is adopted to assist the colony boundaries detection. For achromatic images, the sizes of all objects detected by the Otsu method from the dish/plate region are collected, and the frequency distribution with log base of those size values is generated. Colonies of similar size should occupy the high frequency segment in this distribution, and the frequencies for those massive artifacts should be very low. By this assumption, the large size objects can be removed. Then the hypothesis testing is used to remove minor artifacts which are very similar to the colonies. After Otsu thresholding, a Laplacian filter is applied for edge detection and circular Hough transform is used to detect circular bacteria colonies in Alves and Cruvinel ( 2016 ), Boukouvalas et al. ( 2019 ). The mean error between the proposed method and the manual counting method is less than 10%. In Sánchez-Femat et al. ( 2016 ), Euler’s method is applied for colony counting. The accuracy of 98% is obtained by comparing with the proposed method and manual counting method.
In Zhang and Chen ( 2007 ), Chen and Zhang ( 2008 ), the Otsu and watershed are applied for automatic detection and enumeration of bacteria colonies. First, the contrast-limited adaptive histogram equalization (CLAHE) is used on the converted gray-scale images to enhance the dish/plate contour (Zhang and Chen ( 2007 )). Then, the Otsu threshold is used to detect the dish/plate region and binarize the images automatically. After the morphological operation is used to fill holes, the color similarity values between a pixel and its eight neighbors are calculated and the minimum value is used to detect the object boundaries. Moreover, the watershed algorithm is used for clustered colony separation and the number of viable colonies is counted. The proposed counter performs very well on the blue medium dish/plate, which has average precision, recall, and F-measure values of 0.97, 0.96, and 0.96, respectively in Zhang and Chen ( 2007 ). In Chen and Zhang ( 2008 ), The precision, recall, and F-measure values of the proposed counter are \(0.61\pm 0.29\) , \(0.94\pm 0.06\) , and \(0.69\pm 0.20\) , while the corresponding values of the Clono-Counter are \(0.22\pm 0.25\) , \(1.00\pm 0.00\) , \(0.29\pm 0.31\) , respectively. The segmentation method is shown in Fig. 13 .
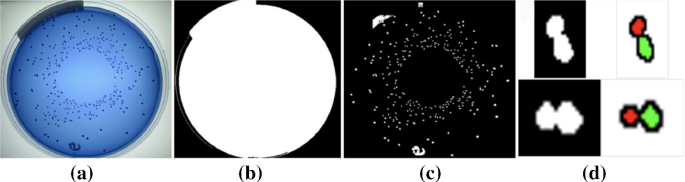
a The original image. b The plate mask. c The colony mask. d Colonies separated from aggregated colony clusters (in Chen and Zhang ( 2008 ) Fig. 2)
2.1.3 Bacteria counting method based on edge detection
In Massana et al. ( 1997 ), Ogawa et al. ( 2003 ), Yamaguchi et al. ( 2004 ), Choudhry ( 2016 ),edge detection is applied for bacteria counting. A Gauss filter (kernel \(5 \times 5\) ), a Laplace filter (kernel \(5\times 5\) ), and a median filter (rank 3) are used for edge detection before thresholding (Massana et al. ( 1997 )), which is shown in Fig. 14 . On the contrast, the Sobel and Laplacine filter are used to detect the edges after thresholding (Yamaguchi et al. ( 2004 )). In Choudhry ( 2016 ), the edge detection system has six major steps. First, the background is subtracted to enhance contrast and reduce the effects caused by uneven illumination. The radius for background subtraction is determined empirically. A starting number can be the average radius of colonies. The next step is sharpening and enhancing the image, which follows by finding the edges. Sobel filter is used in the macro. Then the image is smoothed using Gaussian blur, and converted to black and white. Alternatively, the image can be smoothed by sequential dilate and erode steps. This is followed by the closing of the edges to form a closed circle. Closed objects are filled black using holes filling resulting in images containing black colonies on a white background. To ensure that all colonies are detected, an additional step of closing and filling holes is performed. Here, the size of each pixel is increased, in order to bring the detected edges closer to each other that allows the detection of colonies whose entire edge along the perimeter fails to be otherwise detected. After filling, the size of the pixels is reduced to return the colony size to their original values. After that, denoising and segmentation are applied to remove small particles and separate clustered colonies using thresholding. Finally, the objects are filtered based on size, circularity and measured. Then a new pipeline is developed for the detection of cells and colonies from images. The background is corrected and then the colonies are detected. After that, the parameters are measured and the number of colonies is counted.
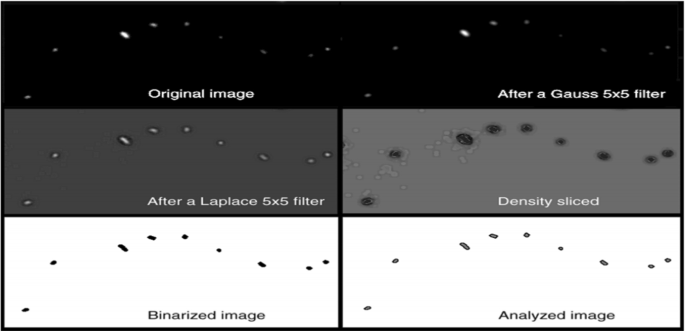
Overview of the whole process of image processing (in Massana et al. ( 1997 ) Fig. 2)
In Barbedo ( 2013 ), five digital processing methods for automatic colony counting are proposed and compared. In the first method, a Gaussian Laplacian filter is applied for edge detection and the connected regions are identified and counted after holes filling. In the second method, the Gaussian Laplacian filter is replaced by the Canny filter. In the third method, three thresholding values are used for image segmentation. In the fourth method, thresholding is used for histogram equalization but not for image segmentation. In the fifth method, the region growing method is applied for segmentation. After that, the concave surface between the connected colonies can be detected to separate the colonies into a single one. Finally, the number of colonies is counted. The accuracy of the first method performs best that obtains the accuracy of 99%.
2.1.4 Bacteria counting method based on watershed
In Ates and Gerek ( 2009 ), Selinummi et al. ( 2005 ), watershed is applied for bacteria counting. In Ates and Gerek ( 2009 ), a median filter is applied first for noise removal and the petri dish boundary is detected and removed. Then the patterns are separated into two groups: colonies and clusters of colonies, based on the classification of circularity ratio. After that, the cluster colonies are segmented based on the watershed (Ates and Gerek ( 2009 )) and marker-controlled watershed(Selinummi et al. ( 2005 )). The watershed segmentation method is shown in Fig. 15 . Finally, the number of actual colonies is estimated as the ratio of cluster area to an average colony area.
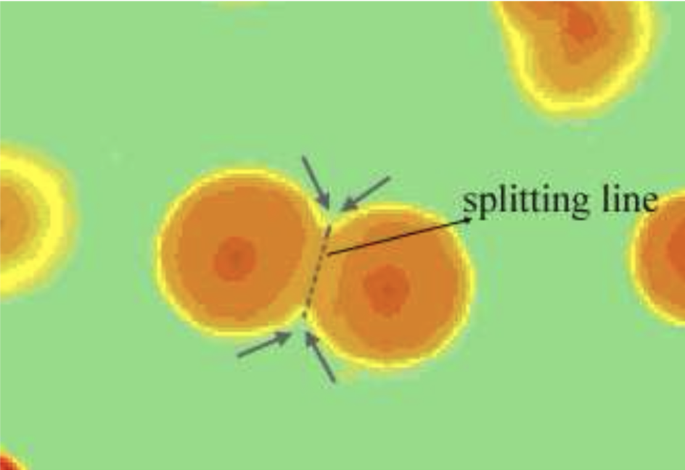
Watershed segmentation for two merged colonies (in Ates and Gerek ( 2009 ) Fig. 5)
In Hong et al. ( 2008 ), Brugger et al. ( 2012 ), Masschelein et al. ( 2012 ), Zhu et al. ( 2018 ), Wong et al. ( 2016 ), Minoi et al. ( 2016 ), Yujie ( 2009 ), Kan ( 2008 ), Fang et al. ( 2008 ), Martinez-Espinosa et al. ( 2016 ), Mukherjee et al. ( 1995 ), the distance transform and watershed are applied for bacteria counting. First, a median filter is used to remove noise and determine the threshold for every single patch in Kan ( 2008 ), Fang et al. ( 2008 ). After that, the iterative threshold (in Fang et al. ( 2008 )), a gray-scale weighted thresholding method (in Hong et al. ( 2008 )), a combination method of distance transform and region growing (Mukherjee et al. ( 1995 )), and Otsu thresholding (in Brugger et al. ( 2012 ), Minoi et al. ( 2016 )) are used to obtain the binary image. Then the objects are detected based on eight neighbor regions in Martinez-Espinosa et al. ( 2016 ). Moreover, an adaptive thresholding method is applied in Brugger et al. ( 2012 ) for secondary binarization to solve the challenges that come from the fact that bacterial strains from the same species may exhibit different colony phenotypes. In Zhu et al. ( 2018 ), image subtraction is carried out to extract the candidate colonies, which are connected to the inner circle of the agar plate and a nonlinear gray transformation is used to enhance the gray-scale. Afterward, a distance transformation is performed on the binarized image and segmentation is done with a watershed transformation. Furthermore, the sharp corners produced by the watershed transformation are removed by using the morphological opening method. After the segmentation algorithm is completed, the Bayes classifier distinguishes the remaining concatenated groups into classes of one, two, three or four containing colonies, and the final colony is counted. Finally, in Masschelein et al. ( 2012 ), Yujie ( 2009 ), the GLCM is extracted and the SVM is applied for classification. The total number of single and clustered colonies is counted with an average relative error of 0.2% in Zhu et al. ( 2018 ). The counting result is shown in Fig. 16 . The processing method of Mukherjee et al. ( 1995 ) is shown in Fig. 17 .
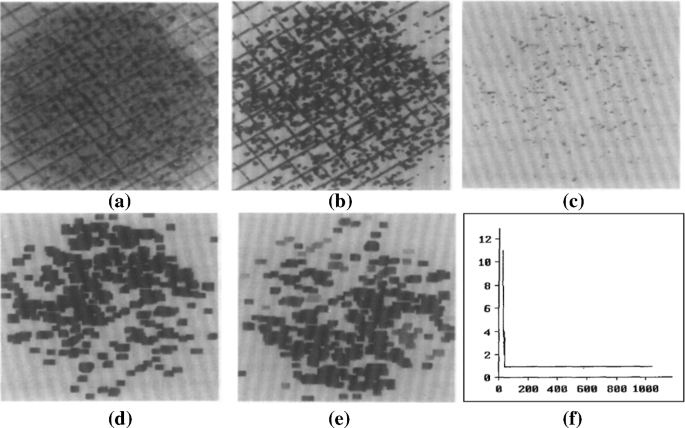
Identified colonies displayed in different colors (in Zhu et al. ( 2018 ) Fig. 11). (Color figure online)

a Original image. b Image after thresholding at gray value 125. c Image after distance transform. d Image after region growing. e Image after component labelling. f Frequency distribution (in Mukherjee et al. ( 1995 ) Fig. 3). (Color figure online)
2.1.5 Bacteria counting method based on color segmentation
In Ogawa et al. ( 2005 ), a distinctive multicolor segmentation algorithm is applied for the accurate and simultaneous differentiation of triple-stained bacteria. The result has the 95% confidence intervals of the regression.
In Schönholzer et al. ( 2002 ), Peitz and van Leeuwen ( 2010 ), Mukti et al. ( 2010 ), RGB images are separated into three channels. The preliminary detection of both bacteria and debris is based on the green channel, and the differentiation between bacteria and debris is based on the processing of the green and blue channels. Then the debris particles are eliminated by the combination of two output images above. Then a Gaussian filter is applied for noise removal and Otsu thresholding is used to roughly separate the data of the relatively dark electrodes from data belonging to the electrode gaps in Peitz and van Leeuwen ( 2010 ). Moreover, the numbers of single and dividing cells and cell agglomerates are determined by a method based on the number of local grey value maxima in Schönholzer et al. ( 2002 ). Finally, cell numbers and cell sizes are calculated based on area and perimeter measurements for each single or dividing cell.
2.2 Machine learning and deep learning counting methods
In Ishii et al. ( 1987 ), Yoon et al. ( 2015 ), Chiang et al. ( 2015 ), principal-component analysis (PCA) is used to separate the biological pattern with the surrounding area. The type of pattern for selection is identified and the objective biological pattern is counted. Moreover, the nearest neighbor searching algorithm is applied to separate touching colonies after PCA in Yoon et al. ( 2015 ), which contains three main steps. First, the local maxima on an absorbance image is found, and a mask image is created in which the locations of the local maxima are marked with 255 and otherwise with 0. Then the local maximal pixels outside the binary segmentation image are masked out by a logical AND operation. Afterward, the clumped blobs are split when the number of local maxima is greater than the number of blobs. The separation result is shown in Fig. 18 . Finally, the image of the bacteria colony is segmented and counted. The accuracy of the colony segmentation and counting algorithm is over 99%. However, in Chiang et al. ( 2015 ), the Otsu thresholding is applied for segmentation after PCA. Then, the distance transform and waster-shed are applied for the division of overlapping colonies. Afterward, the bottom-hat transformation is applied to extract colonies from the rim image. Comparisons show that the proposed system is an effective method with excellent accuracy with a mean value of absolute percentage error of 3.37%.
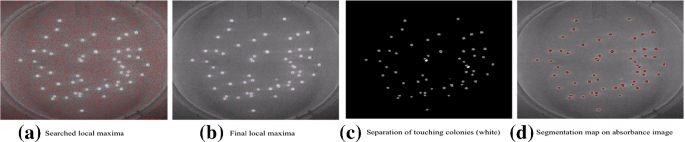
Local absorbance maxima search and separation of touching colonies (in Yoon et al. ( 2015 ) Fig. 14)
In Andreini et al. ( 2015 ), Andreini et al. ( 2016 ), Zhang et al. ( 2010 ), Chen and Zhang ( 2009 ), SVM is applied for bacteria counting and classification. In Andreini et al. ( 2015 , 2016 ), the colonies are segregated from the background by a background removal process based on chromatic information about the specific chromogenic medium used in the culture. Then, a supervised training technique is adopted to obtain a chromatic description of the background and the uncertainty region is obtained as a union of the intersections of some binary masks obtained by imposing a threshold on the probability level of the background and of the infected regions. After that, a mean shift segmentation algorithm is used to associate each image pixel to the corresponding modal density value and a Sobel based edge enhancement is applied to distinguish different classes. Moreover, the considered uncertainty region is divided into two subregions based on the computed thresholding and the histograms of the two sub regions are calculated and compared to establish if a significant separation exists. Finally, SVM is applied for classification and the number of colonies is counted with an accuracy of 99.2%. The segmentation result is shown in Fig. 19 . In Zhang et al. ( 2010 ), Chen and Zhang ( 2009 ), a subtraction operation is applied between the original image and background image to eliminate the background unevenness caused by the light source. Then a median filtering algorithm is applied to smooth the image because it reduces the hot-electron noise and the noise caused by environmental disturbance during image collection, quantify and transmission, and overcomes the blur of the image details created by linear filtering. After that, the gray-level histogram equalization is applied for image enhancement. Then the Otsu thresholding (Zhang et al. ( 2010 )) and watershed (Chen and Zhang ( 2009 )) are used to obtain the binary image. Finally, the shape features are extracted and used for SVM training to identify and count bacteria. It can be seen that the counting results of SVM have a small difference from that of human eye recognition and its relative error is less than 3%, which means that SVM can be used for rod-shaped bacteria counting.The classification results of Chen and Zhang ( 2009 ) is shown in Fig. 20 .
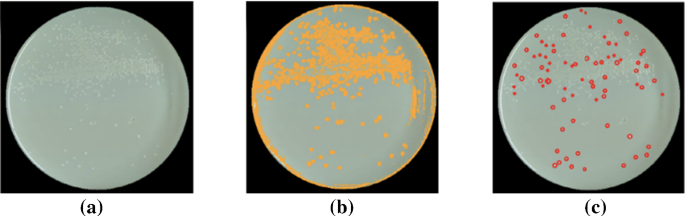
a The original image. b The identified edges within the background. c Candida colonies found on the Petri dish (in Andreini et al. ( 2015 ) Fig. 4)

The classification results for different sizes of the training set (in Chen and Zhang ( 2009 ) Fig. 15)
In Blackburn et al. ( 1998 ), the Marr-Hildreth operator is used for edge detection of bacteria image and threshold is used for image binarization. Then a rank 3 filter is applied to remove pixels that have intensities equivalent to the intensities of amplified background noise. An artificial neural network (ANN) is firstly applied for the classification of bacteria. The ANN is composed of 6 input nodes, 5 intermediate nodes, and 3 output nodes, and then the ANN is activated by using a sigmoid activation function. After training, the images can be analyzed automatically at a rate of 100 images per h. Minimal variation in cell counts between filters is observed (5%) with the filtering procedure used. The bacteria counting procedure is shown in Fig. 21 .
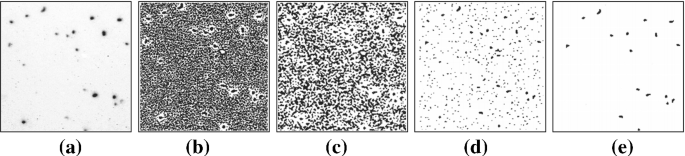
Edge detection procedure. a The original image. b Image after application of the Marr-Hildreth operator with \(3\times 3\) kernel. c Image after application of a rank 3 filter. d Binary image after thresholding. e Image after erosion (in Blackburn et al. ( 1998 ) Fig. 1)
In Shenglang et al. ( 2008 ), Hongwei ( 2012 ), back propagation (BP) neural network and DIP are used for analysis and counting for the microscopic image of bacteria. Median filtering and adaptive filtering are used for denoising and background elimination, and then the iterative algorithm is used for image segmentation in Shenglang et al. ( 2008 ). In Hongwei ( 2012 ), the Otsu thresholding method and the combination of square and circle filter are used for segmentation and edge detection of other microorganisms. After that, morphological operations are applied to smooth the contour of cells and the binary images are obtained. Moreover, the morphology and colorimetry features are extracted and trained in BP neural network for identification and counting. The detection error between the proposed method and the manual counting method is no more than 5%. In Shenglang et al. ( 2008 ), the perimeter, area, shape factor, rectangularity, extension length and gray-scale of the object are input to the BP neural network. Then the neural network is activated by using Sigmoid function, which contains six hidden layers and one output layer. The counting system can analyze the sample in less than 10 minutes, whereas the classical manual counting method takes 48 hours.
In Ferrari et al. ( 2015 , 2017 ); Tamiev et al. ( 2020 ), the convolutional neural network (CNN) is applied for bacterial colony counting. The example of the dataset is shown in Fig. 22 . Then a horizontal flip is performed on the images to double the training dataset and three different artificial color distortions on RGB color space are applied. After that, another transformation is the conversion of the masked dataset in gray-scale color space and seven different values of spatial rescaling before cropping is performed. Then the images are enhanced through normalization concerning the segment orientation (Ferrari et al. ( 2015 )) and contrast limited adaptive histogram equalization (Ferrari et al. ( 2017 )). Finally, CNN is applied for classification and counting that contains five learned layers, four convolutional and one fully connected as shown in Fig. 23 . During the training, the testing accuracy flattens after 15000 iterations. 50,000 iterations have taken approximately 3 hours on an Nvidia Titan Black GPU. The accuracy of 92.8% is obtained. After CNN classification, a watershed algorithm is applied for colony separation in Ferrari et al. ( 2017 ). The testing accuracy increases with the number of training iterations and flattens around 30,000 iterations. 50,000 iterations take approximately one hour on an Nvidia Titan X GPU. The accuracy of 92.1% is obtained after data augmentation. In Tamiev et al. ( 2020 ), a classification-type convolutional neural network (cCNN) is proposed for automatic bacteria classification and counting, and an efficient method for microscope image preprocessing is presented. First, the raw images are segmented with an adaptive binary thresholding method and images with individual cells or cell clusters are cropped. Then the images are trained using cCNN. The network’s output corresponds to the number of cells in given cell clusters and the individual outputs are then added to find the total cell count. The counting accuracy of 86% is obtained. The workflow is shown in Fig. 24 . The result shows a 3.8X increase in processing speed by using an NVIDIA Quadro K620 GPU.
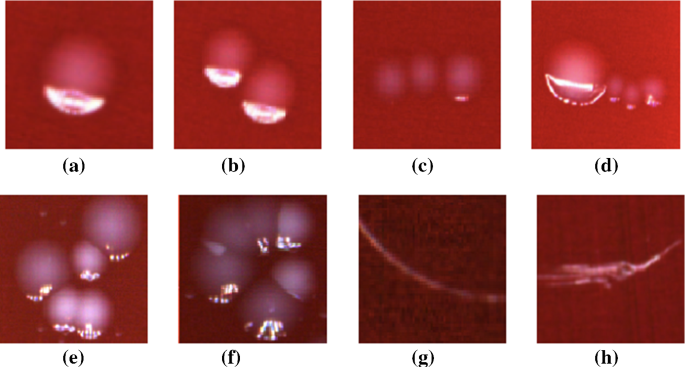
Example of dataset images representing a certain number of colonies, from 1 ( a ) to 6 ( f ), and two example of outliers ( g ) and ( h ) (in Ferrari et al. ( 2015 ) Fig. 2)
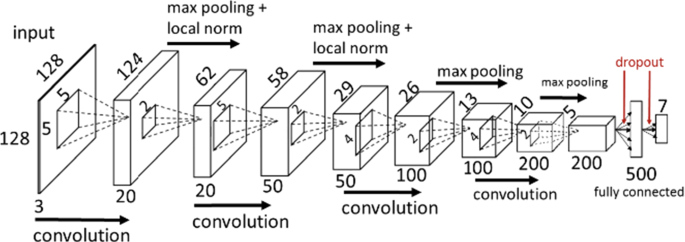
Convolutional Neural Network topology (in Ferrari et al. ( 2015 ) Fig. 3)
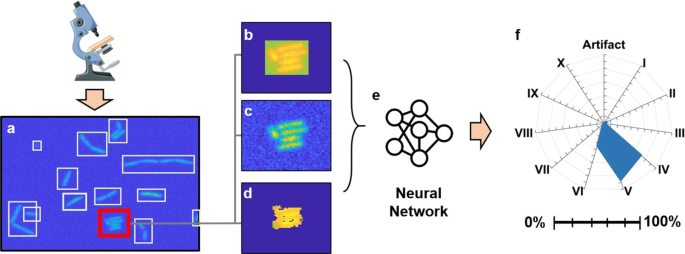
The work flow of image processing. a The image is binarized and annotated manually. b Null Bumper. c Blended. d Masked. e Neural network training. f The input of neural network (in Tamiev et al. ( 2020 ) Fig. 2)
2.3 Third-party tools
In Jung and Lee ( 2016 ), image analysis is used for real-time bacterial counting. First, the time-series high-resolution (HR) images of bacterial microcolonies are reconstructed using sub-pixel sweeping perspective microscopy (SPSM). Then the images are segmented, and the equivalent diameter and number of colonies in each time-lapse image are then calculated. The processed images are shown in Fig. 25 .
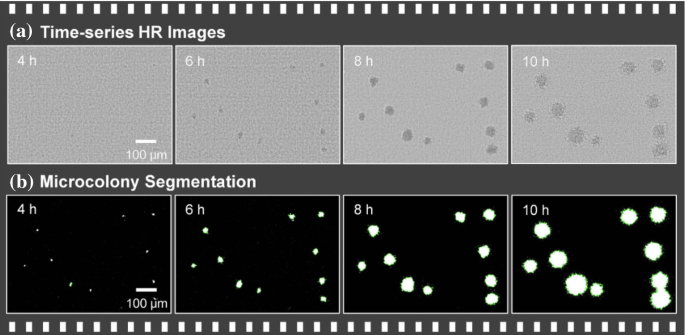
Image processing. a Time-series high-resolution (HR) images. b Following reconstruction of the HR image (in Jung and Lee ( 2016 ) Fig. 3)
In Moller et al. ( 1995 ), Cellstat image analysis program is developed to determine the biovolume of bacteria. They present a method for simultaneous quantitative staining of RNA and DNA using the metachromatic dye AO and quantify the RNA and DNA. The automated image analysis is not biased by the operator, and it allows the analysis of a number of objects, ensuring good statistics. Choosing the right parameters for cell identification makes it possible to discriminate between single cells and clumps of cells. By using a different set of parameters for object recognition, it is possible to detect and measure the intensities of surface-associated microcolonies and single cells on the surface independently. The result of automatic identification of bacteria with Cellstat is shown in Fig. 26 .
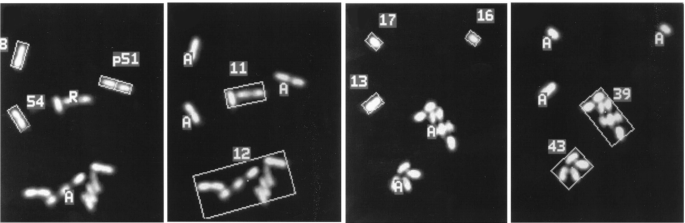
Automatic identification of bacteria with Cellstat. a The cell between 75 and 700 pixels. b The cell between 350 and 700 pixels. c Identification of single cells between 75 and 175 pixels. d Identification of micro colonies on the surface (in Moller et al. ( 1995 ) Fig. 1)
In David and Paul ( 1989 ), ‘Model 2000’ (Image Technology Corporation, Deer Park, New York) image analysis system is used for enumeration and sizing of bacteria, which can detect and enhance each individual cell at the same time. There is no statistical difference in cell counts made manually or by the image analysis system.
In Kildesø and Nielsen ( 1997 ), ‘Kontron Vidas Plus’ (Kontron Elektronik GmbH, Germany) image analyser system is used for airborne microorganisms counting. Gaussian filter is used to remove noises and the edge is derived using Laplace filter. This work has established a possibility of improving exposure assessment of airborne microorganisms through image processing instead of manual counting.
In Shopov et al. ( 2000 ), a program ‘Skidaway Tools’ (Skidaway Institute of Oceanography, 10 Ocean Science Circle, Savannah, USA) is developed based on Marr-Hildreth Gaussian-smoothed Laplacian edge-detection protocol that is proposed in Viles and Sieracki ( 1992 ), with added flat-fielding and edge-strength operators. The alpha-channel is applied in bacteria image segmentation, masking the background and providing a count of the attached bacteria cells.
In Gmür et al. ( 2000 ), ‘IBAS 2.0’ (Kontron Inc., Eching, West Germany) is used to process the images of dental bacteria. A gradient convolution filter is used to process images firstly, then, any white objects below or above an acceptable size range are excluded and the remaining spots are counted automatically after image binary. In Singleton et al. ( 2001 ), ‘IBAS 2.0’ is used for oral microbial quantification. Thresholdiinging is used for images binarization, and the edge-effect rule is used to eliminate the objects of the wrong size. Then the individual bacteria are segmented, and the white spot of images are counted. A close agreement between the automated system and the manual visual counts is observed.
In Nunan et al. ( 2001 ), ‘Zeiss KS300 Imaging System 3.0’ is used for bacteria image processing. The RGB images are decomposed into 3 channels that can be processed separately. Sigma smoothing and top-hat transform are used for edge detection and segmentation in green channel images that can detect all features in the bacteria size range. High pass filter and morphological opening are used to remove autofluorescent objects in red channel images. The top hat transform is used to distinguish bacteria from other objects by detecting the blue halos in blue channel images. The binary images are obtained based on the three-channel images above. The number of cells and other parameters such as area is measured. In Stoderegger and Herndl ( 2005 ), ‘Zeiss KS300’ is used to quantify the natural bacterial community. The binary images are obtained by adjusting the threshold level. Then the images are corrected by excluding or adding cells originally not detected by the channel settings. The individual cell area is determined, and the total area is calculated. Therefore, the number of cells can be obtained.
In Peña et al. ( 2002 ), ‘Image-Pro Plus’ image analysis software (Media Cybernetics, USA) is used for the quantification of bacteria. For aggregates, contour extraction is used for automatic image segmentation. The average equivalent diameter (AED) is used to characterize the size of aggregates, which is used for the automatic elimination of individual cells and debris. A High-Gauss filter is used for image enhancement for individual cells, and a Gaussian filter is used for noise reduction. The roundness value is calculated to select the objects that correspond to individual cells. The binary images based on the two methods above are used for bacteria counting and biovolume measurement.
In O’cleirigh et al. ( 2003 ), ‘Optimas 6.5’ (Media Cybernetics Inc., Silver Spring, MD) image processing system is used to quantify bacteria. Firstly, the low-frequency background noises are isolated and removed, then a combination method of binary erosions to point and dilations within image masks is used to separate cells. The parameters such as cell count and cell volume are measured by using the ‘Optimas’ image processing system. The result shows that the deviation of the experimentally measured density from the known density is 3.2%.
In Putman et al. ( 2005 ), ‘ProtoCOL’ (Version 4.04 from Synoptics Ltd., Cambridge, UK) is used to count the bacteria colonies. When the ‘ProtoCOL’ software is used to process the digital camera image, the count result is highly correlated with the true count but slightly less than the true count.
In Thiel and Blaut ( 2005 ), ‘KS400’ (Carl Zeiss Vision, Hallbergmoos, Germany) is used for automated enumeration of fluorescently labeled bacteria. First, the DAPI images are analyzed to detect single signals at a high spatial resolution. Then, a second analysis system is developed to detect signals with a low signal-to-noise ratio. After, the binary images are obtained by merging the resulting images above. Finally, the third step processes the Cy3 image and thus provides information on the signals that derive from target organisms. The logical ‘AND’ operation of the processed DAPI and Cy3 images ensures that only those signals are counted in both channels. The calculated correlation coefficient of 0.984 indicates that the manual and the automatic counting result are in agreement.
In Wang et al. ( 2007 ), a micro-colony auto counting system ‘MACS’ (Chuo Electric Works, Osaka, Japan) is used for bacteria colony counting. The ‘MACS’ has an automatic scanning stage and blue light emitting diode (LED) as a light source. Micro-colonies are captured using a CCD camera and analyzed using ‘Micro-colony V’ software (version 1.504; Chuo Electric Works). SYBR Green II is used to stain the bacteria images, and the green fluorescence is detected clearly. The stained images and counting results are shown in Fig. 27 .
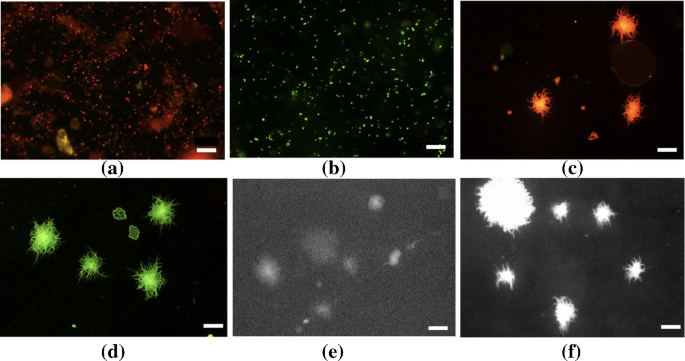
Fluorescence images of single cells and micro-colonies of bacteria. Bacteria in compost are stained with EtBr ( a , c and e ) and SYBR Green II ( b , d and f ). Single bacterial cells in compost suspension before incubation ( a and b ) and micro-colonies developed after incubation on LB medium ( c and d ) observed under blue excitation by epifluorescence microscopy. Micro-colonies are also observed using micro-colony auto counting system ( e and f ) (in Wang et al. ( 2007 ) Fig. 3). (Color figure online)
In Hua et al. ( 2009 ), ‘Davinci’ technology is used for bacteria counting and area calculating. Image enhancement and median filter are applied to remove noises and local binary fitting (LBF) is used for image segmentation. Finally, the connected region is detected as the number of bacteria and the area of the connected region is measured. The average error between the proposed method and the manual counting method is no more than 1.6%.
In Freitas et al. ( 2014 ), the automated enumeration software ‘SigmaScan Pro 5.0’ (Systat Software Inc) is used for the quantification of cells in the biofilm. The intensity thresholding is used for image segmentation. There are no significant differences found using the software thresholding and the manual counting ( \(\hbox {r}>0.05\) ), indicating that the Live/Dead staining is strongly discriminative between bacteria and background, and there is no significant fluorophore bleach effect that could impair the automatic counts.
In Song et al. ( 2018 ), ‘ImageJ’ software version 1.52 (NIH, Bethesda, MD, USA) is applied for automatic bacteria counting. First, the threshold is adjusted to enhance the contrast of the objects of interest. Second, the image is binarized to remove the noise by rendering micro-colony regions with clear boundaries as black and the surrounding background as white. After the binarization, filling-holes processing is conducted to ensure each closed region represents one intact micro-colony. Finally, micro-colony regions above the desired size are outlined, and the number of these regions is automatically counted by ‘ImageJ’ software.
2.4 Summary of image analysis based counting for bacteria
By reviewing the related work of image analysis for bacteria counting and referring to Table 1 , we find that:
Development trend The bacteria counting using image analysis approaches began in the 1980s and developed quickly in the 2010s. This development trend is due to the government and people attach importance to the bacteria problems in recent years, which play essential roles in the food industry and social hygiene. With the development of computer based image analysis technologies, more explorations and higher accuracies will be achieved in the future.
Counting techniques The most frequently used pre-processing methods are the median filter and Gaussian filter, image segmentation methods are thresholding, distance transform and watershed, classifier algorithms are SVMs and ANNs.
3 Other microorganism counting methods
3.1 classic counting methods, 3.1.1 counting methods based on image enhancement.
In Zalewski and Buchholz ( 1996 ), color filtering and contour enhancement are used to separate the yeast cells and compare the automatically detected cell concentration and the traditional cell counting using a counting chamber.
In Barbedo ( 2012b ), the histogram equalization is applied for microorganism counting. First, the images are converted to gray-scale images, and a median smoothing filter is applied for noise removal. Then, the histogram equalization is used for image enhancement, and the enhanced images are submitted to top-hat morphological filtering. Finally, the images are converted to binary images, and the connected regions are counted. The accuracy in correctly identifying the objects is more than 90% and the overall deviation is 8%.
In Dazzo and Gross ( 2013 ), the center for microbial ecology image analysis system (CMEIAS) is developed for understanding microbial ecology at single-cell resolution and spatial scales relevant to the individual microbes and their ecological niches in situ. An optimization method of quadrat size is proposed to reduce the complexity of calculating. Four types of quadrat sizes are proposed, that is \(4\times 4\) , \(6\times 6\) , \(8\times 8\) and \(10 \times 10\) grid. After that, the construction of 2-dimensional scatter plots based on Cartesian coordinates of object centroids can solve serious edge effects when the best possible grid-lattice on the landscape index image still significantly overlaps foreground objects. The index image of the dot map representation derived from the original biofilm landscape image with the optimized grid raster overlay is shown in Fig. 28 .
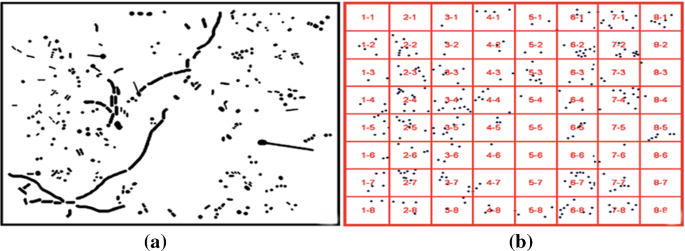
a The original image. b All of the microoragnisms in dot map(in Dazzo and Gross ( 2013 ) Fig. 5)
3.1.2 Counting methods based on thresholding
In Costello and Monk ( 1985 ), Brown et al. ( 1989 ), Dias et al. ( 2003 ), Shijing et al. ( 2012 ), Mazzei et al. ( 2014 ), Cross and Kenerley ( 2004 ), Packer and Thomas ( 1990 ), Tucker et al. ( 1992 ), Zeder et al. ( 2010 ), thresholding is applied for fungi (Cross and Kenerley ( 2004 ), Packer and Thomas ( 1990 )), mycelia (Tucker et al. ( 1992 )), yeast (Costello and Monk ( 1985 )) and protozoan (Brown et al. ( 1989 ), Dias et al. ( 2003 )) counting. In Costello and Monk ( 1985 ), the yeast strains are counted by adjusting the gray-level of the images with the presence of high cell destinies. In Brown et al. ( 1989 ), Dias et al. ( 2003 ), the minimum size of picoplankton and protozoan are set as the filters to remove the noises. Finally, the connected regions are counted as the number of chlorella in Shijing et al. ( 2012 ), and the flood fill algorithm is applied for labeling and tracking in Mazzei et al. ( 2014 ). In Zeder et al. ( 2010 ), the gray-level intensities of pixels determine the threshold. ‘Fixels’ are defined as the primary component of filaments that are can cover small parts of a filament. Once the orientation of the fixel is established, a rectangular field with the same orientation is moved along a line perpendicular to the orientation of the fixel, and the precise placement of the fixel is locally optimized by maximizing the sum of the gray-level intensities in the rectangle. Finally, the filaments are annotated and the lengths of filaments are measured. The accuracy of the method is more than 85%. In Hamid et al. ( 2013 ), thresholding is applied for pus cell counting and feature extraction. The objects that are less than 20 pixels are eliminated after thresholding. Then, the morphological and shape features are extracted for criteria selection. Finally, the single cells and overlap cells are classified and counted, respectively. The identified pus cells are marked on the initial image that is shown in Fig. 29 . It is shown that the reliability of the proposed system is above 80% from the validation results.
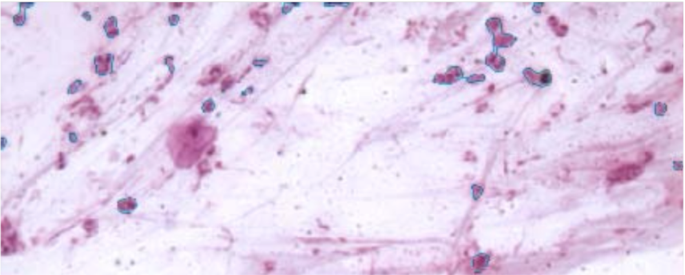
The original image of pus cells (in Hamid et al. ( 2013 ) Fig. 8)
In Jones et al. ( 1992 ), local threshold and morphological transformation are used to calculate spore numbers accurately. Red and blue signals are sampled for pixel segmentation. In all cases, the counts are elevated compared with those obtained by the manual method.
In Robinson et al. ( 1998 ), Xianjiu et al. ( 2012 ), Kim and Cho ( 2013 ), Saur et al. ( 2014 ), Otsu thresholding is applied for the counting of microorganisms. First, the hue, lightness and saturation (HLS) model is applied to transform RGB image into the gray image. Then, the global smoothing (Robinson et al. ( 1998 )) and wavelet shrinkage method (Xianjiu et al. ( 2012 )) are used for noise removal. The wavelet transform is applied in the signal, and the wavelet coefficients are shrunk by thresholding. After that, the inverse wavelet transform is applied. Then, in Robinson et al. ( 1998 ), the binary image consisting of the regional maxima is used as the marker image for the watershed algorithm, and the Sobel filter is used to detect the edge. Finally, Otsu thresholding is used for the accurate estimation of each cell colony area. Total cell number is achieved following identification of cells by application of the shape-independent watershed algorithm. In Xianjiu et al. ( 2012 ), the image dilation is used for image enhancement, and the combination method of Otsu thresholding and the morphological opening is used for image segmentation. Finally, the number of algae is counted based on eight neighborhood regions. The mean accuracy of the proposed method is more than 94% comparing with the manual counting method. In Kim and Cho ( 2013 ), the morphological features are extracted after Otsu thresholding for classification and the number of the object is used for hatching rate measurement. It is shown that the maximum difference is about 19.7%, and the average root-mean squared difference is about 10.9% as the difference between the results using automatic counting (this study) and manual counting is compared. The result of object detection is shown in Fig. 30 . In Saur et al. ( 2014 ), Otsu thresholding is used to quantify moving predators in biofilm. The Otsu thresholding is applied for image binarization, and the noises are removed by filtering. Then the global displacement response and the number of moving objects corresponding to the number of detected individual objects on the processed image are calculated. The calculating results of the two parameters above are shown in Fig. 31 .
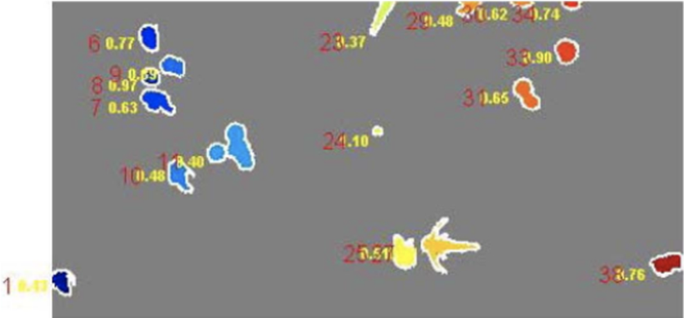
The shape features and boundary tracks of the detected objects (in Kim and Cho ( 2013 ) Fig. 3)
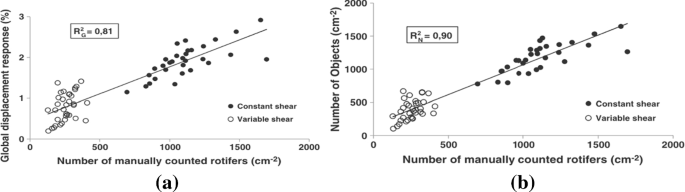
The counting result. a The correlations between the manual counting result and the automatically calculated global displacement response. b The number of objects per \(\hbox {c}m^{2}\) (in Saur et al. ( 2014 ) Fig. 3)
In Song et al. ( 2006 ), the HSI thresholding is used to count the number of algae. A median filter is used to remove noises and reduce the fuzzy edges. Hue-Saturation-Intensity (HSI) threshold is used for image segmentation, and the flood fill method is used to fill the connected region. Area threshold is used to remove debris, and then the images are thinned for central point searching. Finally, the number of algae cells is counted. The accuracy of the method for identifying and counting is more than 90%.
In Zhonglei and Peng ( 2012 ), the histogram thresholding is applied for automatic fungi counting. First, the median filter and linear gray-scale transformation are applied to reduce uneven illumination and noise. Then, the image is segmented based on histogram thresholding. After that, an adaptive smooth filter is used for image enhancement, and morphological operations are applied for smoothing and hole filling. Finally, the connected regions are labeled and counted as the number of fungi to be counted. The average relative error between the proposed method and the manual counting method is 2.56%.
In Sharma ( 2015 ), two methods based on image processing are proposed for microorganisms counting in medical. The first one is based on object recognition, which means the microorganisms’ shape is considered to find out the total number of microbes in the image sample. The histogram equalization is applied for image enhancement that can help to separate the objects from the background. Then the circular hough transformation technique is used to determine the circular objects in the image. The second one is based on thresholding. First, the image is enhanced with histogram equalization and converted to a binary image. Then the Moore neighbor tracing algorithm is applied to detect objects that have close boundaries. Finally, the combination method of thresholding, object recognition and morphological operation is used for counting, and the accuracy of 93% is obtained. The segmentation result is shown in Fig. 32 .

The results after thresholding and morphological operations. a The original image. b Image after thresholding and opening operation. c The identified microorganisms (in Sharma ( 2015 ) Fig. 8)
In Fang et al. ( 2019 ), a multi-threshold image counting method based on improved particle swarm optimization (PSO) is proposed for automatic microbial counting. The two-dimensional maximum entropy algorithm is extended to design the objective function using exponential entropy and an improved PSO algorithm to acquire its maximum value and the best image segmentation effect. Furthermore, the breadth-first search (BFS) algorithm is applied to complete the microorganism marker and counting in the segmented images. Finally, the number of image target segmentation is determined according to the histogram peak searching method. The comparisons of target segmentation results are shown in Fig. 33 .
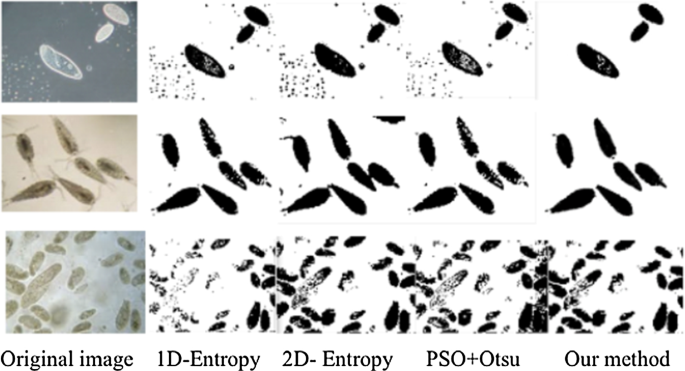
Comparisons of target segmentation effects (in Fang et al. ( 2019 ) Fig. 1)
3.1.3 Counting methods based on edge detection
In Viles and Sieracki ( 1992 ), Sieracki et al. ( 1995 ), the Marr-Hildreth method is used for edge detection and image segmentation of picoplankton (in Viles and Sieracki ( 1992 )) and heterotrophic bacteria (in Sieracki et al. ( 1995 )). Combinations of edge strength and minimum and maximum cell sizes allow the user to count specific cell populations. The images are captured using a charge-coupled device (CCD) imaging system, and the result shows an accurate performance.
In Kocak et al. ( 1999 ), the Snake model is used for low-level interaction, which is a deformable parametric curve with its corresponding energy function, the closed curve with the minimum energy is the target contour. The edge image is produced by subtracting the eroded image from a dilated image. An extermination algorithm is used to examine the image gradient on both sides of the snakelet by computing the directional derivative orthogonal to each of its nodes. The Snake model is used for plankton counting in this research, and the accuracy of 94.12% is achieved. The example of segmentation is shown in Fig. 34 .
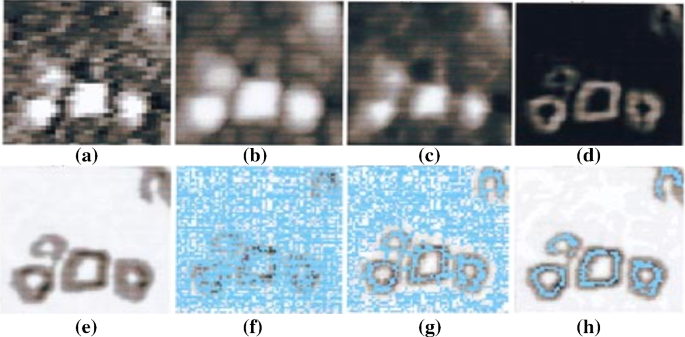
Example of steps in segmentation (In Kocak et al. ( 1999 ) Fig. 12)
In Barber et al. ( 2000 ), the Sobel operator is used to find the edges, and the binary images are obtained using thresholding. A compact Hough transform is used to highlight the centers of circular objects. The local area can be processed to determine a colony boundary, and so the colony area and the colony number can be calculated. An example of colony boundary determination is shown in Fig. 35 .
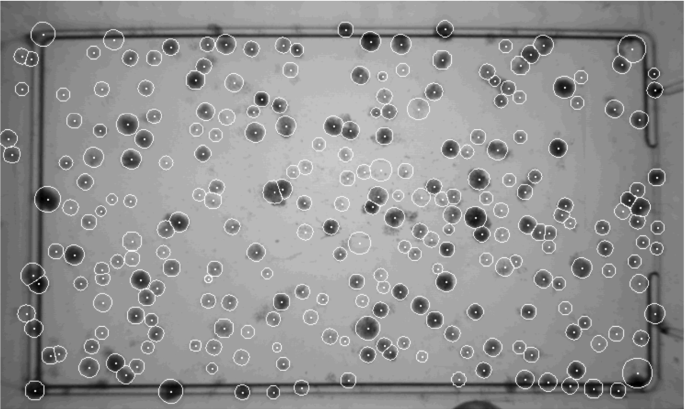
Example of steps in segmentation (In Barber et al. ( 2000 ) Fig. 5)
In Pernthaler et al. ( 2003 ), the gradient transformation is applied for microorganism edge detection. First, a mean background gray level is determined for remapping the image gray values to the total gray value range. Edge detection is performed by using gradient transformation, and a neighborhood median filter is applied to smooth the resulting image. The stained plankton cells are calculated and counted.
In Tsechpenakis et al. ( 2008 ), the probabilities are applied for image segmentation. First, a nonlinear morphological filter, that is, an alternating sequential filter (ASF) is used to preserve the line-type image structures in predefined orientations while filtering random noise. Then the region of animals is segmented based on the probabilities. The isolated pixels or small groups of pixels with probabilities higher than the threshold are eliminated. After that, a nonrigid is used to recover a global transformation that brings the pose of a source shape as close as possible to that of a target shape. The shape estimation of animals is based on the maximum likelihood (ML) approach, and the animal region is extracted based on a probability map. Finally, the number of animals is calculated and compared with the manual counting result.
In Barbedo ( 2012c ), a unified framework for counting agriculture microorganisms is proposed. There are five methods listed for object delineating. In the first method, the Laplacian of Gaussian method is applied for edge detection, then the inner regions are filled, and all connected objects are identified. The only difference between the second method and the first one is that the Canny method is used to detect the edges. In the third method, three different thresholds are applied for image binarization. In the fourth method, contrast is modified by the technique of histogram equalization. In the last method, region growing is applied for segmentation. After object delineating, a decision tree is used for classification. Then the contrast limited adaptive histogram equalization is applied, and the image is morphologically opened using as kernel a disk with a radius of 1% of the image width. Finally, the estimate for the number of objects is calculated based on the number of local maxima.
3.2 Machine learning and deep learning counting methods
In Shabtai et al. ( 1996 ), Embleton et al. ( 2003 ), a neural network is applied for fungus (Shabtai et al. ( 1996 )) and phytoplankton (Embleton et al. ( 2003 )) counting. In Embleton et al. ( 2003 ), the gray-level is used to separate the regions of interest, and then a median filter is used to smooth images. A skeletonize operator is used to separating the filaments and objects. Then the final binary image is used as a mask to take measurements from the original image. Each region in the binary image is given an identifying number, and the size, shape, color and grey level distribution are measured for that region. A neural network is used for the classification and counting of phytoplankton. The automated imaging system takes 75 images for each sample in seven minutes, and the image processing and classification take thirty to forty minutes.
In Benyon et al. ( 1999 ), seven basic features and 17 more complex features are extracted from fungal spores for image analysis. Linear and quadratic discriminant analysis are used for image classification, and then the number of every species of spores is counted based on the results of classification. Genus comparisons using only seven basic features resulted in 98% accuracy.
In Motta et al. ( 2001 ), Akiba and Kakui ( 1997 ), PCA is applied for protozoa (Motta et al. ( 2001 )) and plankton (Akiba and Kakui ( 1997 )) counting and classification. In Motta et al. ( 2001 ), the histogram local equalization is used to enhance the contours of protozoa images, the opening operation and closing operation are used to remove halo. Euclidian Distance Map is used for semi-automated segmentation. A series of erosion and reconstruction are used to eliminate the flocs of the protozoa silhouette. Finally, PCA is used to classify different protozoa, and the number of the various species of protozoa is counted. The main steps of segmentation are shown in Fig. 36 , (a) shows the initial image of protozoa, (b) shows the contour enhancement by histogram local equalization, (c) shows the background suppression by opening (2 iterations) and closing (55 iterations) to remove the halo, (d) shows the semi-automated segmentation based on the Euclidian Distance Map, (e) shows part of the flocs is eliminated by a border-killing routine, (f) shows the hole-filling of the silhouette and semi-automated segmentation based on the Euclidian Distance Map, (g) shows the elimination of flocs by a series of erosion and reconstruction of the protozoa silhouette, (h) shows the localization of flagella and stalk.
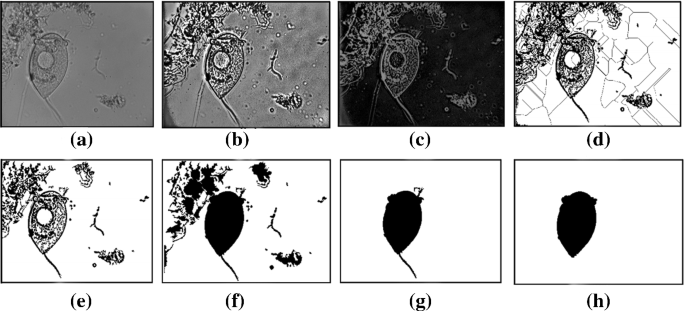
Main steps of segmentation (In Motta et al. ( 2001 ) Fig. 1)
In Grosjean et al. ( 2004 ), the random forest and discriminant vector forest are applied for zooplankton image processing. A threshold is used to eliminate the background and enhance the contrast, then the objects are detected, contoured, and labeled by the image analysis system. The combination method of random forest and discriminant vector forest is used for classification, and the number of each species is counted. The result of object detection is shown in Fig. 37 .
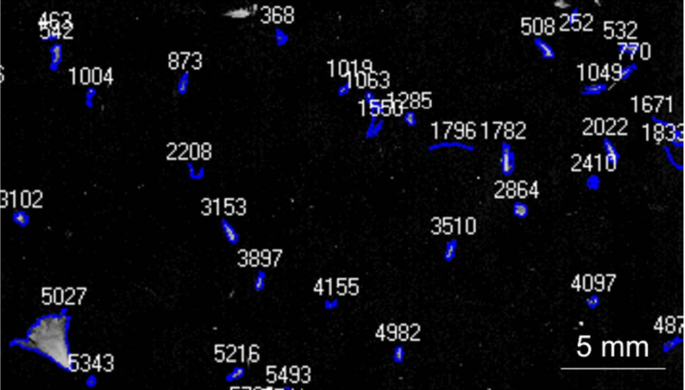
The objects are detected, contoured, and labelled by the image analysis (In Grosjean et al. ( 2004 ) Fig. 3)
In Rong et al. ( 2006 ), BP neural network and image processing are used for classification and counting of zooplankton. Otsu thresholding is used for initial image segmentation, and region growing is used to fill holes. The noises of debris are removed by detecting the particles that are smaller than the set threshold area. Then the features such as gray level co-ocurence matrices (GLCM) and some shape features are measured and used for classification and counting by using a back propagation (BP) neural network. There are 5 nodes in the input layer, 20 nodes in the hidden layer, and 1 node in the output layer, and the Sigmoid function is applied for activation.
In Albaradei et al. ( 2020 ), deep transfer learning is applied for automatic pluripotent stem cell colony counting. First, the RGB image is converted to a binary image by using thresholding. Then, some augmentation techniques are applied to expand the training dataset. The augmentation techniques include color jitter to randomly alter brightness, contrast, saturation, and hue of each image, horizontal/vertical flip, and random rotation. Moreover, the trained SRNetDL model is applied for training. The first 10 layers are frozen to remain the pre-trained network. Then the last 6 layers are fine-tuned. The stochastic gradient descent is applied for optimization. Finally, the number of cell colonies is counted. The overview of transfer learning is shown in Fig. 38 .
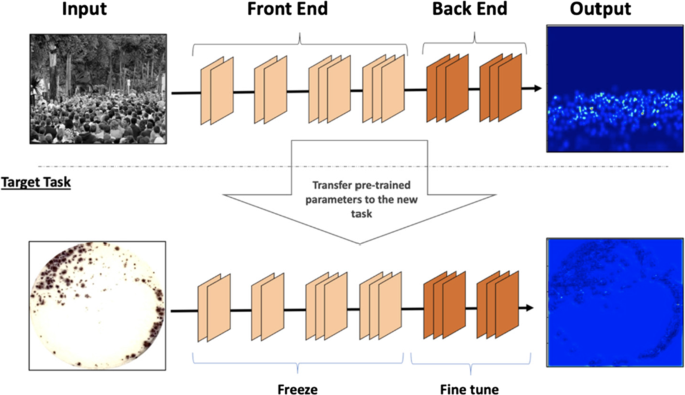
Processing of transfer learning for counting (in Albaradei et al. ( 2020 ) Fig. 2)
3.3 Third-party tools
In Ogawa et al. ( 2012 ), a time-lapse shadow image analysis system is designed for microbial colony counting. First, an agar plate containing many clusters of microbial colonies is trans-illuminated to project their 2-dimensional (2D) shadow images on a color CCD camera. Then the 2D shadow images of every cluster distributed within a 3-mm thick agar layer are captured in focus simultaneously through a multiple focusing system and then converted to 3-dimensional (3D) shadow images. It is possible to determine whether each cluster comprised single or multiple colonies by time-lapse analysis of the 3D shadow images. Finally, the recognized colonies are counted, and the result is compared with the manual counting method, and an excellent value of correlation efficiency is obtained (r = 0.999). The colony detection method is shown in Fig. 39 .
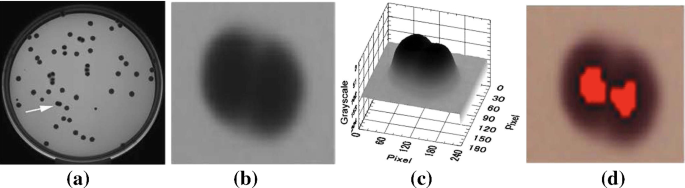
Detection of 2 overlapping colonies of E.coli. a 2D shadow image of agar plate. The arrow points to the cluster of overlapping colonies. b Magnified image. c 3D shadow image. d 2 colonies recognized in the cluster (in Ogawa et al. ( 2012 ) Fig. 6)
In Rolke and Lenz ( 1984 ), ‘Quantimet 720’ image analysis system (Leica Cambridge Ltd., Cambridge, United Kingdom) is used to detect the zooplankton, and then the total number of objects is measured. The detector automatically selects the mean grey-level between image and background within the present range to ensure the optimal detection of the image contours. ‘Quantimet 570’ is developed in 1990 that is used in Bloem et al. ( 1995 ), the images are sharpened with maxima and minimum filter, and then all local maxima values are detected to determine the number of particles. A 5 by 5 convolution filter is used to remove noises, and a skeleton operation is used to separate the particles precisely. The mean differences between the visual and automated method are not significantly different from zero. The first method in Grivet et al. ( 1999 ) is the usage of the ‘Quantimet 570’ image analysis system for scanning and counting the adherent microorganisms. The second method shows the use of thresholding and image skeleton for enumeration. The correlation between the two enumeration methods is highly significant. The example of the second method for counting is shown in Fig. 40 .
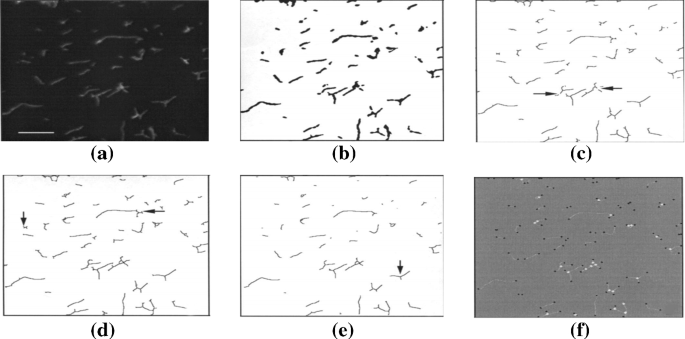
The processing of the actinomyces image. a Original image. b The binary image after a two-step thresholding. c The skeleton selection. d The whole skeleton with branches. e The whole pruned skeleton. f The triple points and end points on the skeletons (in Grivet et al. ( 1999 ) Fig. 3)
In Sieracki et al. ( 1985 ), ‘Artek 810’ image analyzer (Artek Systems Corp., Farmingdale, N.Y.) is used to detect, count and size the picoplankton. The threshold destiny level of detection can be set at 1 to 256 gray-level. Comparisons between visual and image analyzed counts show that none of the mean counts are significantly different at the 95% significance level by the paired t test.
In Estep and MacIntyre ( 1989 ), the ‘Zeus’ image analysis system (Institute of Marine Research, Bergen) is used for algae counting, sizing and identification. Images are enhanced, smoothed, shadowed, and then ‘Zeus’ system is used for automated counting. Comparison counts show no significant difference between the manual method and automatic identification and counting with the image-analysis system.
In Wright et al. ( 1991 ), a constant threshold is used to segment the images instead of an adaptive threshold, and then the Sobel operator is applied to detect the edge of micro plants and animals. Finally, ‘ImageMeasure 5100’ (Microscience, Div., Phoenix Trade, Inc. Seattle, Washington) is used for counting. The developed script reduces the time required to count and measure marine fouling tube worms by at least one order of magnitude over manual counts, with an error of five percent or less.
In Corkidi et al. ( 1998 ), a commercial program ‘IMAGENIA 2000’ (Biocom, Les Ulis, France) is used for image processing and object counting based on the multi-level threshold, and the total number of bright spots over the dark background is counted. The confluent and various sizes image analysis method (COVASIAM) is proposed, which estimates an average of 95.47% ( \(\sigma\) = 8.55%) of the manually counted colonies, while an automated method based on a single-threshold segmentation procedure estimates an average of 76% ( \(\sigma\) = 16.27%) of the manually counted colonies. Fig. 41 h shows the segmentation result of Fig. 41 g, and i shows the superimposed images of Fig. 41 g and (h). COVASIAM gave 135 CFU, representing 97.8% of the manual counts.
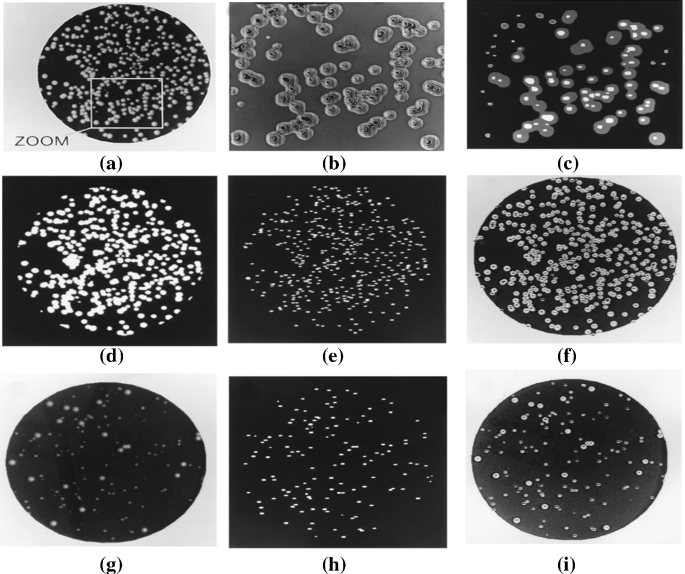
Detection of colony. a The image is digitized and filtered through a binary mask. b Enhanced image. c Resulting image after adding images at T1 and T2 thresholds. d Colony segmentation at a single threshold level of data. e Colony segmentation by using COVASIAM. f Overlap of Fig. 41 a and e. g Digitized image of colony in various size. h Colony segmentation result. i Overlap of Fig. 41 g and h. (In Corkidi et al. ( 1998 ) Fig. 2)
In Rodenacker et al. ( 2001 ), a program ‘IDL’ (Research System Inc., Boulder, USA) is used for image analysis. Thresholding is used to segment the images. The opening operation and closing operation are used to clean the masks and fill holes. A neural network is designed to classify the microorganisms based on shape features that can help count each microorganism species in water. The ‘IDL’ is also used for identification and quantification of phytoplankton in Rodenacker et al. ( 2002 ), and the threshold is used for image segmentation. The segmentation result is shown in Fig. 42 . The morphological and some intensity features are used for identification, and a neural network is designed for classification, then the number of each species is counted.
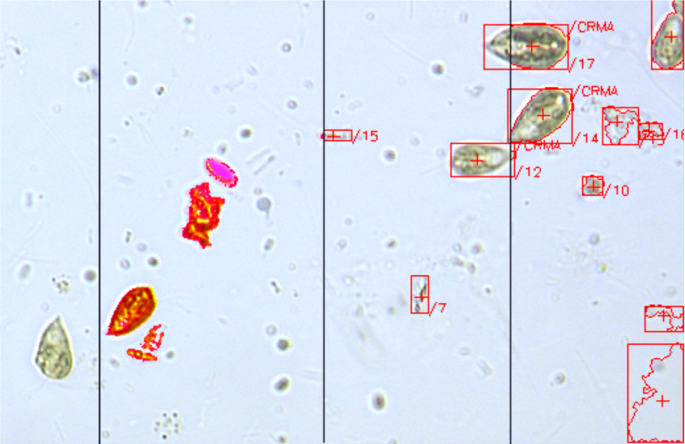
Segmentation of one image, central field with masks, right field with marked featured objects (In Rodenacker et al. ( 2002 ) Fig. 2)
In Sándor et al. ( 2001 ), ‘Quanitmet 570’ computer system (Leica, Cambridge, United Kingdom) is used for image processing. The proportion of the clumps is determined as the mean value of their projected areas, and the mean total hyphal length and the mean number of tips are determined for the freely dispersed mycelia.
In Nishimura et al. ( 2006 ), an automatic cell counting system, ‘Bioplorer’ (BP) (Matsushita Ecology Systems Co. Ltd, Kasugai, Aichi-ken, Japan), is used for the enumeration of yeast cells. BP system is used to exclude yeast cells measuring 5 \(\mu \hbox {m}\) in diameter from the count when cultured yeast. The intensity is used for image segmentation and bright points are counted. In Nishimura et al. ( 2008 ), BP is used for the quantification of eukaryotic and prokaryotic cells. The cells are stained and captured using a CCD camera that can capture photons emitted from bacteria or yeast cells. Bright points are visualized on display and enumerated automatically. The threshold brightness value is optimized for each set of measurements by using BP.
In Eickhorst and Tippkötter ( 2008 ), an image analysis software, ‘AnalySIS’ (Soft Imaging) is used for soil microorganisms counting and detection. First, the images are optimized, such as the contrast of enhancement and gradation. Then the threshold value is set for the color of fluorescent probes. After that, the parameters are set to detect the microorganism pixels and the cell number is automated counted. The ratio of automated to manual counting is 97.7% (± 1.0) for bacteria and 92.2% (± 2.4) for archaea in the investigated paddy soils.
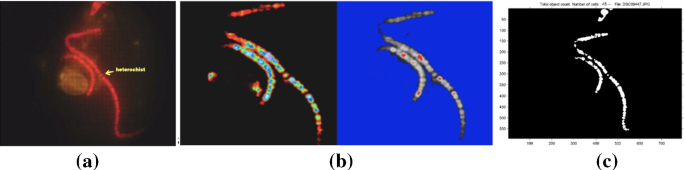
a Digital image of cyanobacteria. b Delimitation A panel edges using Image J software. c The total number of cells in A panel using CellC software (In Ghită et al. ( 2013 ) Fig. 9)
In Ghită et al. ( 2013 ), ‘ImageJ’ is applied for analysis of cyanobacteria from the marine sample. First, the background is separated from the objects based on the intra-class variance threshold method. Then the mathematical morphology operations are used to remove noises produced by specks of staining color in the image. Finally, the clustered objects are separated and counted. The same image is then analyzed with the program ‘CellC’ to count the cells in the filament of cyanobacteria. The result of the proposed method is shown in Fig. 43 . In Stolze et al. ( 2019 ), ‘ImageJ’ is applied for yeast colony counting based on automatic image analysis. First, the RGB image is converted to an 8-bit image, and the thresholding value is adjusted for image binarization. After that, the watershed is applied for splitting merging colonies, and finally, the number of colonies is counted. The proposed method is shown in Fig. 44 .
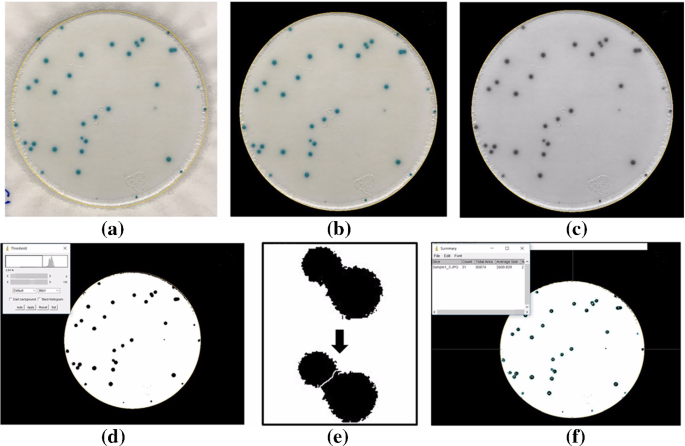
ImageJ automated cell analysis of a Petrifilm image. a Petrifilm colony forming area outlined with the oval ROI tool. b The area outside the ROI is cleared. c Image is converted to 8-bit. d Threshold is set to highlight colonies as black particles. e Merging colonies split by a single pixel line via the Watershed tool. f Particles included in the total count, highlighted and numbered in an overlay on the image (In Stolze et al. ( 2019 ) Fig. 1)
In Bennke et al. ( 2016 ), ‘Automated Cell Measuring and Enumeration tool 2.0’ program is applied for the enumeration of the microbial cell. First, the sample is stained using DAPI and captured using a CCD camera. Then the program is applied for cell determination and enumeration. The parameter values like object area, circularity, mean gray value and signal-to-background ratio are measured. Automated enumeration result is highly correlated with manual counts ( \(r^2>0.9\) ).
3.4 Summary of image analysis based counting for other microorganisms
By reviewing the related work of image analysis for other microorganisms counting and from Table 2 , we find that:
Development trend The counting for other microorganisms using image analysis approaches began in the 1980s and developed in the 2000s. By comparing with the related research on bacteria, the development speed of research about other microorganisms counting is relatively slow, and the research is relatively limited. There are two main reasons to cause this situation, firstly, the structures of bacteria are relatively simple, and most of them are circular, which are more visualized and accessible to evaluated for segmentation results. By contrast, some other microorganisms, such as alga and fungi, are relatively complex in structure and with plenty of hyphae, challenging to be segmented precisely. Secondly, microorganism counting systems are designed but not for one specific type. A dataset is necessary when evaluating systems’ performances, but the number of bacteria datasets is relatively abundant, so the researchers tend to test their systems with bacteria datasets.
Counting techniques The most frequently used pre-processing methods are the medial filter and Gaussian filter, image segmentation methods are thresholding and Otsu thresholding, classifier algorithms are PCA and neural networks.
4 Analysis of image processing based counting methods
The image processing methods based microorganism counting are summarized from Sects. 3 to 4 . It can be seen that the most effective approaches for microorganism image counting are image pre-processing, image segmentation, image classification, connected region detection, and feature extraction. In order to find out the reasons why they are widely used and reveal the potential future direction, in this section, the properties of these methods with their application domains are analyzed and summarized. In order to illustrate the correlation between methods and their applications, some representative works are selected as examples.
4.1 Image pre-processing methods
Because many kinds of microorganisms are colorless, staining methods are necessary to apply before image capture. Different staining methods lead to different color images, so the color feature is not appropriate for automatic microorganism counting. Moreover, due to the illumination and image noise’s inhomogeneity, pre-processing methods should be applied to solve the problems and prepare for image segmentation.
Firstly, to reduce the effect of different colors for image segmentation, the RGB images are usually converted to gray-scale images by adjusting the proportions of red, green, and blue channels. The RGB can also be converted to HSI (Hue-Saturation-Intensity) color space to assist the colony boundaries detection, such as the works in Song et al. ( 2006 ), Nayak et al. ( 2010 ), Payasi and Patidar ( 2017 ). HSI color space can adjust the Intensity but does not change the color type of the original image when processing colored images. Furthermore, it can ultimately reflect the primary attribute of color perception and corresponds to the result of color perception, which is helpful for the following segmentation.
Secondly, the uneven illumination can result in shading and a nonuniform background, which can usually be corrected using background subtraction, linear gray-scale transformation and low pass filtering, such as the works in Choudhry ( 2016 ) and Zhonglei and Peng ( 2012 ) (see Fig. 45 ).
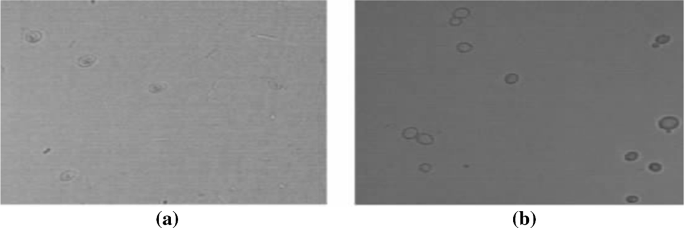
Contrast of the yeast preprocessing image. a Original image. b Filtered image (In Zhonglei and Peng ( 2012 ) Fig. 1)
Thirdly, noise removal is also one of the most necessary parts of pre-processing. Median filter and Gaussian filter are applied for denoising that are easy to approach and perform well in this part. The morphological open and close operations can be used to remove halos that appear while imaging, such as the work in Motta et al. ( 2001 ).
Finally, the contrast of images may not be striking and need to be enhanced for image segmentation. The gray-level histogram equalization is the most acclaimed method that is easy to operate and can enhance the contrast in a global field, such as the work in Zhang et al. ( 2010 ) and the image after enhancement is shown in Fig. 46 . A contrast limited adaptive histogram equalization (CLAHE) is proposed in Ferrari et al. ( 2017 ) for local contrast enhancement, and a linear histogram expansion method is applied in Sánchez-Femat et al. ( 2016 ) that is based on a transformation of the gray levels, a linear distribution of the values that are within the range of 0 to 255 is performed. The performance is shown in Fig. 47 .
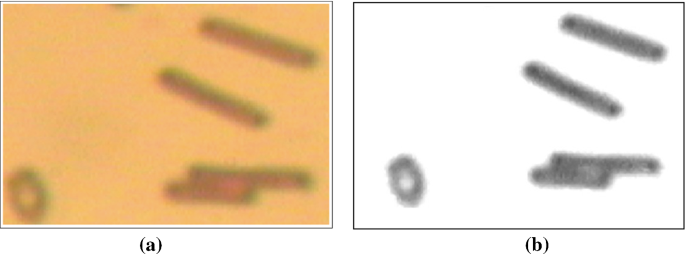
Comparison of original image and enhanced image. a Original image. b Image obtained by histogram equalization (In Zhang et al. ( 2010 ) Fig. 2, Fig. 3)
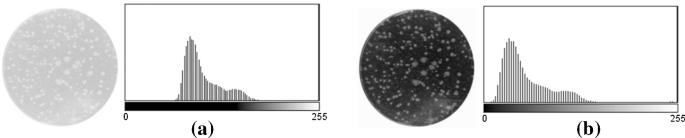
Comparison of original image and enhanced image. a Original image with its gray-level histogram. b Resulting image with its gray-level histogram (In Sánchez-Femat et al. ( 2016 ) Fig. 7, Fig. 8). (Color figure online)
4.2 Image segmentation methods based on thresholding
Image segmentation is the most significant part of microorganism counting methods. The extraction of a region of interest can be regarded as the segmentation of the colony part. Segmentation based on thresholding is the basic technique widely applied for microorganism counting, while many new segmentation methods are proposed to segment the area for counting accurately.
Firstly, the segmentation technique based on thresholding is applied in many works for microorganism counting. Global thresholding is the easiest method for image segmentation when it has strong contrast, and an excellent result can be obtained, such as the works in Gupta et al. ( 2012 ) and Chunhachart and Suksawat ( 2016 ). Most of the segmentation methods are developed from the thresholding method and can improve performance in complex environments.
Secondly, Otsu thresholding is applied in many works such as Zhang and Chen ( 2007 ), Zhang et al. ( 2008a ) and Peitz and van Leeuwen ( 2010 ). The Otsu thresholding is simple and easy to calculate. It can be used to segment the image effectively when the area difference between the target and the background is negligible. Nevertheless, the target and background can not be separated accurately when the gray-scale of the target and the background have a large overlap because the gray-scale distribution is used as the basis of image segmentation. It is also sensitive to noises, so denoising processing is usually applied first. The example of Otsu thresholding based image binarization is shown in Fig. 48 .
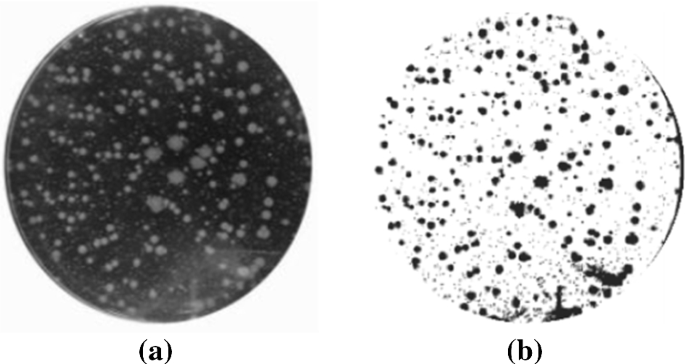
Comparison of gray-scale image and binary image. a Gray-scale image. b Binary image based on Otsu thresholding. (In Sánchez-Femat et al. ( 2016 ) Figs. 8, 9). (Color figure online)
Finally, the segmentation result of the single threshold method is not satisfied when the gray-level of the image may be unevenly distributed, resulting in the influence of illumination. An iterative local threshold method is applied in Shen et al. ( 2010 ), the point with a local maximum threshold is obtained using a Laplacian operator, that is, the initial local thresholds. The microorganism images captured by microscope can be affected by lighting distribution, so the idea of the algorithm is not to calculate the global image threshold, but to calculate the local threshold according to the brightness distribution of different regions of the image, which means different thresholds can be calculated adaptively for different regions of the image. Another method to improve the segmentation performance when traditional thresholding does not work well is the multi-level threshold that is applied in Corkidi et al. ( 1998 ). The performance of multi-level thresholding for segmentation is shown in Fig. 49 . Thresholding based on multi-level method is divided into multi-spatial-level and multi-threshold-level, it can help segment more detailed pieces of information that may be lost using global thresholding.
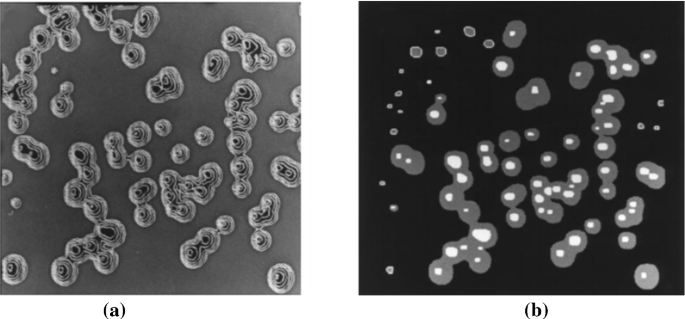
Comparison of gray-scale image and binary image. a Enhanced image. b Multi-level thresholding image (In Corkidi et al. ( 1998 ) Fig. 2)
4.3 Other image segmentation methods
First of all, edge detection is applied in a mass of works that performs well in image segmentation. Sobel operator is a classical method that is easy to be applied, such as the works in Andreini et al. ( 2015 ), Chiang et al. ( 2015 ) and Choudhry ( 2016 ). Laplacian operator is a second order differential operator that is isotropic but sensitive to noises, such as the works in Barbedo ( 2013 ), and Laplacian operator can be combined with Sobel operator that can obtain a better detection result, such as the work in Ogawa et al. ( 2003 ). Canny operator is a multi-stage optimization operator with filtering, enhancement and detection that performs best but is relatively complex to use, such as the works in Matić et al. ( 2016 ) and Barbedo ( 2012c ). Another popular method is Marr-Hildreth operator, that is, a Gaussian filter is applied first for smoothing and a Laplacian filter is applied for image enhancement, such as the works in Viles and Sieracki ( 1992 ) and Blackburn et al. ( 1998 ), that performs well for images with the low signal-to-noise ratio.
Secondly, the method combined with distance transform and watershed is applied in colony segmentation. Distance transform can extract the distance between a non-zero pixel and the nearest zero pixel, that is, the gray-scale value of each pixel in the image is the distance between the pixel and the nearest background pixel. The distance transform is usually applied for image segmentation with watershed, such as the works in Hong et al. ( 2008 ), Yujie ( 2009 ) and Masschelein et al. ( 2012 ). The performance of distance transform and watershed is shown in Fig. 50 .
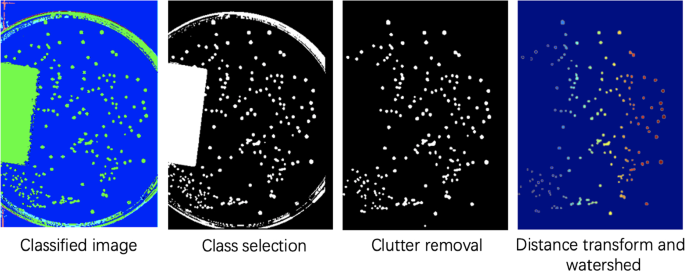
Sample step-sequence for an automated colony counting system (In Masschelein et al. ( 2012 ) Fig. 15)
Thirdly, watershed segmentation is always applied for the separation of connected colonies. The original watershed algorithm performs well in the segmentation process for adherent colonies, such as the works in Zhang et al. ( 2008a ), Ates and Gerek ( 2009 ) and Stolze et al. ( 2019 ). The segmentation method is shown in Figs. 51 and 15 . However, the original watershed may get the results of over-segmentation because of the noise and local discontinuity of the images, the marker-controlled watershed algorithm is applied in Selinummi et al. ( 2005 ). The Hough transformation is applied to extract the object’s marker, and the background is marked and eliminated separately.
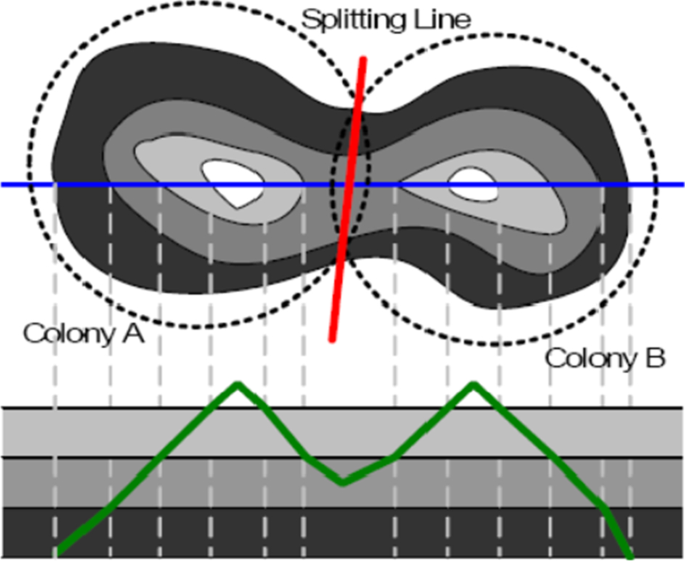
The concept of watershed algorithm (In Zhang et al. ( 2008a ) Fig. 5)
Finally, top-hat transform and bottom-hat transform are morphological operations applied for image segmentation when the illumination is uneven. The top-hat transformation is the difference between the image and the image after the open operation, such as the works in Pernthaler et al. ( 1997 ), Brugger et al. ( 2012 ) and Barbedo ( 2012b ), while the bottom-hat transform is the difference between the image after the close operation and the image, such as the work in Chiang et al. ( 2015 ). The top hat transformation is used for light objects on a dark background, while the bottom hat transformation is used for the opposite.
4.4 Image classification methods
Classification is a necessary operation when the microorganisms need to be counted respectively. Firstly, a decision tree is a supervisor learning that is widely used based on probability analysis. In Barbedo ( 2012c ), a decision tree is applied for the classification of agriculture microorganisms. The decision tree is easy to understand and explain, and can make possible and practical results for large data sources in a relatively short period, but the overfitting problem while classification needs to be solved.
Secondly, support vector machine (SVM) is a kind of linear classifier that classifies data in a binary way according to supervised learning, such as the works in Chen and Zhang ( 2009 ) and Masschelein et al. ( 2012 ). In Yujie ( 2009 ), the shape invariant moment and gray level co-ocurence matrices (GLCM) are extracted for SVM training, and the classification accuracy of bacteria is 99.67%. SVM performs well with small sample and can be trained to solve the problem of high dimensional, but it is sensitive to missing data, and the choice of features has enormous implications for classification results.
Thirdly, artificial neural network (ANN) is a network with self-learning, self-organization, self-adaptation and strong nonlinear function approximation ability, that has strong fault tolerance. In Blackburn et al. ( 1998 ), an ANN is trained for classification and quantification of bacteria, and about 95% of all objects are classified in each image. The classification result is shown in Fig. 52 . ANN has high classification accuracy and strong robustness to noise nerves, but the learning process is unobservable and the output is hard to interpret. Moreover, back propagation (BP) neural network is a multi-layer feedforward network trained by error back propagation, that is the most widely used ANN. In Jun ( 2010 ), BP neural network is applied for bacteria classification, and in Rong et al. ( 2006 ), BP neural network is used for zooplankton classification and counting. BP neural network has strong nonlinear mapping ability and flexible network structure, but the convergence rate is slow. Moreover, it is easy to fall into local minima. Furthermore, the convolutional neural network (CNN) is a feedforward neural network with deep structure and convolution computation representing learning. In Ferrari et al. ( 2015 ), CNN is applied for bacteria colony counting, and the accuracy of 92.8% is obtained. In Tamiev et al. ( 2020 ), a classification-type convolutional neural network (cCNN) is designed for bacteria classification and counting.CNN can automatically extract the features of images and process high-dimensional data quickly, but the pooling layer may lose much valuable information while training.
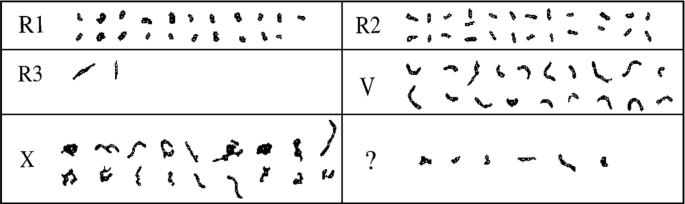
The classification result (In Blackburn et al. ( 1998 ) Fig. 4)
By reviewing all the existing deep learning based microorganism counting methods, the classification can be achieved automatically, but the segmentation part still needs to be adjusted manually. In order to show the development of deep learning and the time for microorganism counting, the training time and counting time of deep learning methods in this review are summarized in Table 3 .
4.5 Analysis of potential methods
Through summarizing the work of image analysis based microorganism counting, it can be found that the accuracy of microorganism counting is continuously improving with the development of computer vision and deep learning technologies, which indicates that computer vision based microorganism counting methods will completely replace the traditional manual counting methods. However, the deep learning methods are mainly applied for microorganism classification, while the microorganism segmentation methods are still adopted the traditional techniques, such as thresholding or watershed, resulting in a huge gap with the state-of-the-art technology. The application of the latest semantic segmentation technology can classify microorganisms at the same time of segmentation, which will be the trend of future development.
According to the existing microorganism counting work, the work of imaging and image analysis are often separated. Therefore, it is difficult to obtain real-time microorganism counting information, leading to time-consuming and workforce waste. BiSeNet is one of the real-time semantic segmentation networks (Yu et al. 2018 ). However, an extra encoding path is applied for spatial information, which is time-consuming. In Fan et al. ( 2021 ), a novel architecture is designed as a Short-Term Dense Concatenate network (STDC network), which is shown in Fig. 53 . Multiple contiguous layers of response maps are connected, and each layer encodes the input image at different scales and in its own field to achieve multi-scale feature representation. Then the Detail Guidance is applied for decoding, which can guide the low-level layers to learn spatial details. Finally, the spatial information and segmentation of deep layers are combined to show the final results. The 71.9% of mIoU is obtained, and the computing speed is 45.2% faster than the original method.
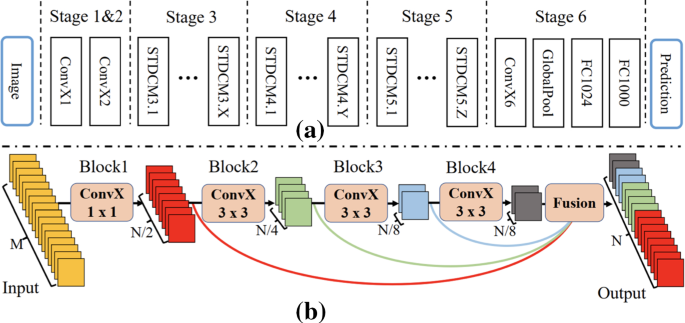
a The original architecture of STDC network. b The proposed architecture of STDC network (In Fan et al. ( 2021 ) Fig. 3)
The work of deep learning based counting methods also provides a new direction for the field. In Yang et al. ( 2020 ), the scale variations of images are solved based on a reverse perspective network, which is shown in Fig. 54 . The reverse perspective networks can reduce the scale variances of images before regression, reducing the complexity of the network. The original is sampled firstly, and then the number of objects can be evaluated by a regression network. The reverse perspective networks can evaluate perspective distortion precisely, which can be correct by uniformly distorting the image. Finally, the images with similar scales are transmitted to the regressor, and 61.2 of mean average error (MAE) is obtained.
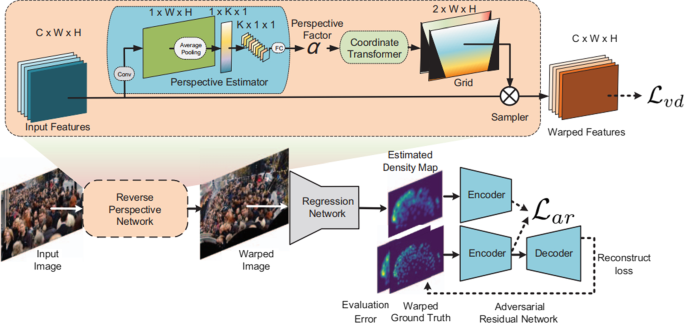
The architecture of the reverse perspective network(In Yang et al. ( 2020 ) Fig. 4)
In Bai et al. ( 2020 ), an adaptive dilated convolution and a novel supervised learning framework is proposed for self-correlation counting works, which is shown in Fig. 55 . In classical counting methods, the models are optimized by comparing the ground truth and predicted image, and the density map is not precise because of the labeling deviation. First, the image is input into the model for feature extraction, and then the density map is output by using six adaptive convolutions. After that, the sample locations are calculated by dilation rates. The result can adapt the scale variation of the images, and the MAE of 66.5 is obtained.
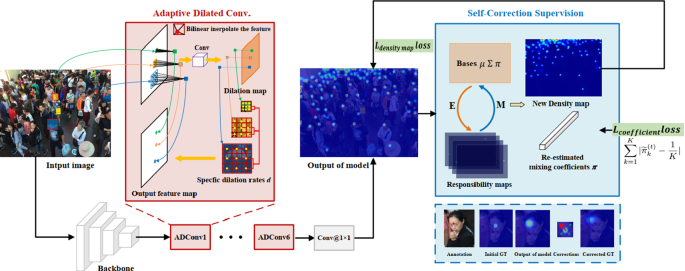
The overview of the adaptive dilated convolution network (In Bai et al. ( 2020 ) Fig. 2)
Deep learning frameworks have quickly become the primary method for analyzing microscopic images. We can infer and predict machine and deep learning based methods will be applied in microorganism counting as well as in other researches such as digital pathology, which is summarized in Salvi et al. ( 2020 ), Madabhushi and Lee ( 2016 ). However, there are two main limitations during the surveying. First, the number of research based on deep learning is limited, proving the vast development potential in this field. Second, most of the deep learning methods are applied for classification but not for segmentation, and the deep learning based segmentation can carry out more precise segmentation results by comparing with classic methods. These two limitations also show the development directions and opportunities in the future. First, traditional manual counting methods will be replaced by deep learning based microorganism counting methods, which cannot only be used for classification, but also for precise segmentation. Second, in the future, the microorganism counting systems will be integrated with sampling, imaging and analyzing systems using deep learning, helping researchers monitor the microorganism timely.
5 Conclusion and future work
In this paper, a comprehensive review of image analysis methods for microorganism counting is proposed. The counting methods are summarized and grouped based on the types of microorganisms, including bacteria counting and other microorganisms counting. Then the methods are separated based on segmentation approaches, such as thresholding methods, edge detection methods, third-party tools and deep learning based methods. By reviewing all the related works, we can find that the classic methods in Sects. 2.1 and 3.1 are developed from the 1980s to 2000s, such as the Otsu thresholding method, watershed algorithm and edge detection methods, which shows a blooming development of digital image processing for microorganism analysis. Since the 2010s, the development of deep learning carries out the microorganism counting results with high accuracy. Furthermore, the development of professional microorganism counting systems is summarized in Sects. 2.3 and 3.3 , such as ‘ImageJ’ and ‘CellC’ show people pay more and more attention to microorganism counting. In summary, the successful development of image analysis based microorganism counting methods shows vast research potential in this field. Moreover, the most frequently used microorganism counting approaches of image preprocessing, image segmentation and image classification are analyzed in Sect. 4 .
The image analysis based microorganism counting methods discussed in this paper can be referred to in other digital image analysis fields. For example, microorganism classification is a significant application field of the microorganism analysis, referring to environmental microorganism classification (Kosov et al. 2018 ), cervical cell classification (Mamunur Rahaman et al. 2021 ), blood cell classification (Su et al. 2014 ), classification for different types of microorganisms (Li et al. 2019a ). Furthermore, the segmentation methods for microorganisms can be referred to by digital image processing workers, such as stem cell segmentation (Huang et al. 2016 ), cancer cell segmentation (Chen et al. 2006 ), environmental microorganism segmentation (Zhang et al. 2021 ). Moreover, microscopic image processing performs an essential role in industrial analysis, such as the monitoring for waste water (Amaral and Ferreira 2005 ), beef carcass evaluation (Cross et al. 1983 ), monitoring of bacteria in milk (Pettipher and Rodrigues 1982 ), monitoring flames in an industrial boiler (Yu and MacGregor 2004 ), softwood lumber grading (Bharati et al. 2003 ) and so on. Finally, the summarized counting methods can also be applied in small object detection, such as sperm counting (Peng et al. 2015 ), crowd counting (Zhang et al. 2015 ) and vehicle counting (Li et al. 2019b ).
In the future, deep learning based microorganism counting methods are promising. Since the COVID-19 broke out in 2019, people pay increasing attention to microorganism analysis. There is still a considerable limitation and opportunity in microorganism research. This review can contribute a lot to the research of microorganism counting for future researchers.
Akiba T, Kakui Y (1997) Development of an in situ zooplankton identification and counting system based on local auto-correlational masks. In: Oceans’ 97. MTS/IEEE conference proceedings, vol 1. IEEE, pp 655–659
Albaradei SA, Napolitano F, Uludag M, Thafar M, Napolitano S, Essack M, Bajic VB, Gao X (2020) Automated counting of colony forming units using deep transfer learning from a model for congested scenes analysis. IEEE Access 8:164340–164346
Article Google Scholar
Alves GM, Cruvinel PE (2016) Customized computer vision and sensor system for colony recognition and live bacteria counting in agriculture. Sensors Transducers 201(6):65
Google Scholar
Amaral A, Ferreira E (2005) Activated sludge monitoring of a wastewater treatment plant using image analysis and partial least squares regression. Anal Chim Acta 544(1–2):246–253
Amrita KL (2016) Image processing techniques on agriculture-a review. Res Cell Int J Eng Sci 22:515–526
Andersen KG, Rambaut A, Lipkin WI, Holmes EC, Garry RF (2020) The proximal origin of SARS-CoV-2. Nat Med 26(4):450–452
Andreini P, Bonechi S, Bianchini M, Mecocci A, Di Massa V (2015) Automatic image analysis and classification for urinary bacteria infection screening. In: International conference on image analysis and processing, Springer, pp 635–646
Andreini P, Bonechi S, Bianchini M, Garzelli A, Mecocci A (2016) Automatic image classification for the urinoculture screening. Comput Biol Med 70:12–22
Ates H, Gerek ON (2009) An image-processing based automated bacteria colony counter. In: 2009 24th international symposium on computer and information sciences, IEEE, pp 18–23
Austerjost J, Marquard D, Raddatz L, Geier D, Becker T, Scheper T, Lindner P, Beutel S (2017) A smart device application for the automated determination of E. coli colonies on agar plates. Eng Life Sci 17(8):959–966
Bai S, He Z, Qiao Y, Hu H, Wu W, Yan J (2020) Adaptive dilated network with self-correction supervision for counting. In: Proceedings of the IEEE/CVF conference on computer vision and pattern recognition, pp 4594–4603
Balestra G, Misaghi I (1997) Increasing the efficiency of the plate counting method for estimating bacterial diversity. J Microbiol Methods 30(2):111–117
Barbedo JGA (2012a) A review on methods for automatic counting of objects in digital images. IEEE Lat Am Trans 10(5):2112–2124
Barbedo JGA (2012b) Method for counting microorganisms and colonies in microscopic images. In: 2012 12th international conference on computational science and its applications, IEEE, pp 83–87
Barbedo JGA (2012c) Unified framework for counting agriculture-related objects in digital images. In: Embrapa Informática Agropecuária-Artigo em anais de congresso (ALICE), In: CONFERENCE ON GRAPHICS, PATTERNS AND IMAGES, 25., 2012, Ouro Preto ..
Barbedo JGA (2013) An algorithm for counting microorganisms in digital images. IEEE Lat Am Trans 11(6):1353–1358
Barber P, Vojnovic B, Kelly J, Mayes C, Boulton P, Woodcock M, Joiner M (2000) An automated colony counter utilising a compact Hough transform. Proc Med Image Underst Anal, MIUA2000:41–44
Bennke CM, Reintjes G, Schattenhofer M, Ellrott A, Wulf J, Zeder M, Fuchs BM (2016) Modification of a high-throughput automatic microbial cell enumeration system for shipboard analyses. Appl Environ Microbiol 82(11):3289–3296
Benyon FH, Jones AS, Tovey ER, Stone G (1999) Differentiation of allergenic fungal spores by image analysis, with application to aerobiological counts. Aerobiologia 15(3):211–223
Bharati M, MacGregor J, Tropper W (2003) Softwood lumber grading through on-line multivariate image analysis techniques. Ind Eng Chem Res 42(21):5345–5353
Blackburn N, Hagström Å, Wikner J, Cuadros-Hansson R, Bjørnsen PK (1998) Rapid determination of bacterial abundance, biovolume, morphology, and growth by neural network-based image analysis. Appl Environ Microbiol 64(9):3246–3255
Bloem J, Veninga M, Shepherd J (1995) Fully automatic determination of soil bacterium numbers, cell volumes, and frequencies of dividing cells by confocal laser scanning microscopy and image analysis. Appl Environ Microbiol 61(3):926–936
Boukouvalas DT, Belan P, Leal CRL, Prates RA, de Araújo SA (2018) Automated colony counter for single plate serial dilution spotting. In: Iberoamerican congress on pattern recognition, Springer, pp 410–418
Boukouvalas DT, Prates RA, Leal CRL, de Araújo SA (2019) Automatic segmentation method for CFU counting in single plate-serial dilution. Chemom Intell Lab Syst 195:103889
Brill WJ (1981) Agricultural microbiology. Sci Am 245(3):198–215
Brown LM, Gargantini I, Brown DJ, Atkinson HJ, Govindarajan J, Vanlerberghe GC (1989) Computer-based image analysis for the automated counting and morphological description of microalgae in culture. J Appl Phycol 1(3):211–225
Brugger SD, Baumberger C, Jost M, Jenni W, Brugger U, Mühlemann K (2012) Automated counting of bacterial colony forming units on agar plates. PLoS ONE 7(3):e33695
Buzalewicz I, Wysocka-Król K, Podbielska H (2010) Image processing guided analysis for estimation of bacteria colonies number by means of optical transforms. Opt Express 18(12):12992–13005
Chatterjee Chaulya (2019) Vision improvement system using image processing technique for adverse weather condition of opencast mines. Int J Min Reclam Environ 33(7):505–516
Chauhan R, Ghanshala KK, Joshi R (2018) Convolutional neural network (CNN) for image detection and recognition. In: 2018 First international conference on secure cyber computing and communication (ICSCCC), IEEE, pp 278–282
Chen WB, Zhang C (2008) Bacteria colony enumeration and classification for clonogenic assay. In: 2008 Tenth IEEE international symposium on multimedia, IEEE, pp 487–488
Chen WB, Zhang C (2009) An automated bacterial colony counting and classification system. Inf Syst Front 11(4):349–368
Chen X, Zhou X, Wong ST (2006) Automated segmentation, classification, and tracking of cancer cell nuclei in time-lapse microscopy. IEEE Trans Biomed Eng 53(4):762–766
Chiang PJ, Tseng MJ, He ZS, Li CH (2015) Automated counting of bacterial colonies by image analysis. J Microbiol Methods 108:74–82
Chien TI, Kao JT, Liu HL, Lin PC, Hong JS, Hsieh HP, Chien MJ (2007) Urine sediment examination: a comparison of automated urinalysis systems and manual microscopy. Clin Chim Acta 384(1–2):28–34
Choudhry P (2016) High-throughput method for automated colony and cell counting by digital image analysis based on edge detection. PLoS ONE 11(2):e0148469
Chudasama D, Patel T, Joshi S, Prajapati GI (2015) Image segmentation using morphological operations. Int J Comput Appl 117(18):16–19
Chunhachart O, Suksawat B (2016) Construction and validation of economic vision system for bacterial colony count. In: 2016 international computer science and engineering conference (ICSEC), IEEE, pp 1–5
Clarke ML, Burton RL, Hill AN, Litorja M, Nahm MH, Hwang J (2010) Low-cost, high-throughput, automated counting of bacterial colonies. Cytometry A 77(8):790–797
Corkidi G, Diaz-Uribe R, Folch-Mallol J, Nieto-Sotelo J (1998) Covasiam: an image analysis method that allows detection of confluent microbial colonies and colonies of various sizes for automated counting. Appl Environ Microbiol 64(4):1400–1404
Costello P, Monk P (1985) Image analysis method for the rapid counting of Saccharomyces cerevisiae cells. Appl Environ Microbiol 49(4):863–866
Cross D, Kenerley C (2004) Modelling the growth of Trichoderma virens with limited sampling of digital images. J Appl Microbiol 97(3):486–494
Cross H, Gilliland D, Durland P, Seideman S (1983) Beef carcass evaluation by use of a video image analysis system. J Anim Sci 57(4):908–917
Cui J, Li F, Shi ZL (2019) Origin and evolution of pathogenic coronaviruses. Nat Rev Microbiol 17(3):181–192
Dai H, MacBeth C (1997) Effects of learning parameters on learning procedure and performance of a BPNN. Neural Netw 10(8):1505–1521
Daims H, Wagner M (2007) Quantification of uncultured microorganisms by fluorescence microscopy and digital image analysis. Appl Microbiol Biotechnol 75(2):237–248
Dalgaard P, Ross T, Kamperman L, Neumeyer K, McMeekin TA (1994) Estimation of bacterial growth rates from turbidimetric and viable count data. Int J Food Microbiol 23(3–4):391–404
David AW, Paul JH (1989) Enumeration and sizing of aquatic bacteria by use of a silicon-intensified target camera linked-image analysis system. J Microbiol Methods 9(4):257–266
Dazzo F, Gross C (2013) CMEIAS Quadrat Maker: a digital software tool to optimize grid dimensions and produce quadrat images for landscape ecology spatial analysis. J Ecosyst Ecography 3(4):136
Dazzo FB, Niccum BC (2015) Use of CMEIAS image analysis software to accurately compute attributes of cell size, morphology, spatial aggregation and color segmentation that signify in situ ecophysiological adaptations in microbial biofilm communities. Computation 3(1):72–98
Dias N, Amaral A, Ferreira E, Lima N (2003) Automated image analysis to improve bead ingestion toxicity test counts in the protozoan Tetrahymena pyriformis. Lett Appl Microbiol 37(3):230–233
Dietler N, Minder M, Gligorovski V, Economou AM, Joly DAHL, Sadeghi A, Chan CHM, Koziński M, Weigert M, Bitbol AF et al (2020) A convolutional neural network segments yeast microscopy images with high accuracy. Nat Commun 11(1):1–8
Doetsch RN, Cook TM (2012) Introduction to bacteria and their ecobiology. Springer Science & Business Media, Berlin
Eickhorst T, Tippkötter R (2008) Improved detection of soil microorganisms using fluorescence in situ hybridization (FISH) and catalyzed reporter deposition (CARD-FISH). Soil Biol Biochem 40(7):1883–1891
Ekstrom MP (2012) Digital image processing techniques, vol 2. Academic Press, Cambridge
Embleton K, Gibson C, Heaney S (2003) Automated counting of phytoplankton by pattern recognition: a comparison with a manual counting method. J Plankton Res 25(6):669–681
Estep KW, MacIntyre F (1989) Counting, sizing, and identification of algae using image analysis. Sarsia 74(4):261–268
Fan M, Lai S, Huang J, Wei X, Chai Z, Luo J, Wei X (2021) Rethinking BiSeNet For Real-time Semantic Segmentation. In: Proceedings of the IEEE/CVF conference on computer vision and pattern recognition, pp 9716–9725
Fang J, Li W, Wang G (2008) Experimental Study for Automatic Colony Counting System Based Onimage Processing. In: International conference on computer and computing technologies in agriculture, Springer, pp 1061–1066
Fang Z, Wenjun C, Zhi W, Xin W (2019) Microorganism image counting based on multi-threshold optimization. In: 2019 IEEE international conference on consumer electronics-Taiwan (ICCE-TW), IEEE, pp 1–2
Feifei X, Zongjian L, Guozhong S (2017) Building texture acquisition and processing based on an unmanned airship low-altitude aerial survey system. Appl Opt 56(27):7648–7655
Ferrari A, Lombardi S, Signoroni A (2015) Bacterial colony counting by convolutional neural networks. In: 2015 37th annual international conference of the IEEE engineering in medicine and biology society (EMBC), IEEE, pp 7458–7461
Ferrari A, Lombardi S, Signoroni A (2017) Bacterial colony counting with convolutional neural networks in digital microbiology imaging. Pattern Recogn 61:629–640
Freitas AI, Vasconcelos C, Vilanova M, Cerca N (2014) Optimization of an automatic counting system for the quantification of Staphylococcus epidermidis cells in biofilms. J Basic Microbiol 54(7):750–757
feng Hu Z (2013) Automated counting and identification of cell colonies based on distance transform and progressive erosion. In: 2013 International conference on advanced computer science and electronics information (ICACSEI 2013), Atlantis Press, pp 139–142
Ghate VN, Dudul SV (2010) Optimal MLP neural network classifier for fault detection of three phase induction motor. Expert Syst Appl 37(4):3468–3481
Ghită S, Sarchizian I, Ardelean II (2013) Microscopic investigation and automated image analysis of hydrocarbon-tolerant marine cyanobacteria mixed populations cultivated in the absence and presence of gasoline or diesel. Int J Biol Biomed Eng 4(7):164–175
Gmür R, Guggenheim B, Giertsen E, Thurnheer T (2000) Automated immunofluorescence for enumeration of selected taxa in supragingival dental plaque. Eur J Oral Sci 108(5):393–402
Gonzalez RC, Woods RE (2008) Digital image processing. Prentice Hall Int 28(4):484–486
Gonzalez RC, Woods RE, Eddins SL (2004) Digital image processing using MATLAB. Pearson Education India
Gracias KS, McKillip JL (2004) A review of conventional detection and enumeration methods for pathogenic bacteria in food. Can J Microbiol 50(11):883–890
Gray A, Young D, Martin N, Glasbey C (2002) Cell identification and sizing using digital image analysis for estimation of cell biomass in High Rate Algal Ponds. J Appl Phycol 14(3):193–204
Grivet M, Morrier JJ, Souchier C, Barsotti O (1999) Automatic enumeration of adherent streptococci or actinomyces on dental alloy by fluorescence image analysis. J Microbiol Methods 38(1–2):33–42
Grosjean P, Picheral M, Warembourg C, Gorsky G (2004) Enumeration, measurement, and identification of net zooplankton samples using the ZOOSCAN digital imaging system. ICES J Mar Sci 61(4):518–525
Gupta S, Kamboj P, Kaushik S (2012) Methodology for automatic bacterial colony counter. Advances in computer science, engineering & applications. Springer, Berlin, pp 559–565
Chapter Google Scholar
Hamid R, Halim NA, Arshad NW, Naim F, Jusof MF, Mohamed Z (2013) Feature extraction of pus cells detection and counting in sputum slide images. In: 2013 Saudi International Electronics, Communications and Photonics Conference, IEEE, pp 1–6
Han S, Qubo C, Meng H (2012) Parameter selection in SVM with RBF kernel function. In: World automation congress 2012, IEEE, pp 1–4
Hong M, Yujie W, Caihong W, Shanrang Y (2008) Study on heterotrophic bacteria colony counting based on image processing method. Control Instrum Chem Ind 35(3):38–41
Hongwei S (2012) The theory of microscopy image treatment and the usage in the detection for animalcule in food. PhD thesis, Jilin University
Hua W, Chunxiao C, Yonghong H, Wenge Y (2009) Research of bacteria ferment controlling system based on davinci technology. Chin J Biomed Eng 28(6):892–898
Huang X, Li C, Shen M, Shirahama K, Nyffeler J, Leist M, Grzegorzek M, Deussen O (2016) Stem cell microscopic image segmentation using supervised normalized cuts. In: 2016 IEEE international conference on image processing (ICIP), IEEE, pp 4140–4144
Hui DS, Azhar EI, Madani TA, Ntoumi F, Kock R, Dar O, Ippolito G, Mchugh TD, Memish ZA, Drosten C et al (2020) The continuing 2019-nCoV epidemic threat of novel coronaviruses to global health–The latest 2019 novel coronavirus outbreak in Wuhan, China. Int J Infect Dis 91:264–266
Ishii T, Adachi R, Omori M, Shimizu U, Irie H (1987) The identification, counting, and measurement of phytoplankton by an image-processing system. ICES J Mar Sci 43(3):253–260
Jackway PT, Deriche M (1996) Scale-space properties of the multiscale morphological dilation-erosion. IEEE Trans Pattern Anal Mach Intell 18(1):38–51
Jones C, Lonergan G, Mainwaring D (1992) The use of image analysis for spore counts of white-rot fungi. Biotechnol Tech 6(5):417–422
Jun D (2010) Rapid detection research for microorganisms in food based on biotechnology and computer vision. PhD thesis, Jilin University
Jung JH, Lee JE (2016) Real-time bacterial microcolony counting using on-chip microscopy. Sci Rep 6(1):1–8
Kan L (2008) Quick quantification system for bacteria number in fresh milk. Master’s thesis, Huazhong University of Science & technology
Karamizadeh S, Abdullah SM, Manaf AA, Zamani M, Hooman A (2013) An overview of principal component analysis. J Signal Inf Process 4(3B):173
Karsoliya S (2012) Approximating number of hidden layer neurons in multiple hidden layer BPNN architecture. Int J Eng Trends Technol 3(6):714–717
Kaur G, Sethi P (2012) A novel methodology for automatic bacterial colony counter. Int J Comput Appl 49(15):21–6
Kildesø J, Nielsen BH (1997) Exposure assessment of airborne microorganisms by fluorescence microscopy and image processing. Ann Occup Hyg 41(2):201–216
Kim S, Cho HY (2013) Automatic estimation of artemia hatching rate using an object discrimination method. Ocean Polar Res 35(3):239–247
Kirkpatrick B, Fleming LE, Squicciarini D, Backer LC, Clark R, Abraham W, Benson J, Cheng YS, Johnson D, Pierce R et al (2004) Literature review of Florida red tide: implications for human health effects. Harmful Algae 3(2):99–115
Kocak DM, da Vitoria Lobo N, Widder EA (1999) Computer vision techniques for quantifying, tracking, and identifying bioluminescent plankton. IEEE J Oceanic Eng 24(1):81–95
Kosov S, Shirahama K, Li C, Grzegorzek M (2018) Environmental microorganism classification using conditional random fields and deep convolutional neural networks. Pattern Recogn 77:248–261
Levner I, Zhang H (2007) Classification-driven watershed segmentation. IEEE Trans Image Process 16(5):1437–1445
Article MathSciNet Google Scholar
Li C, Wang K, Xu N (2019a) A survey for the applications of content-based microscopic image analysis in microorganism classification domains. Artif Intell Rev 51(4):577–646
Li C, Chen H, Li X, Xu N, Hu Z, Xue D, Qi S, Ma H, Zhang L, Sun H (2020a) A review for cervical histopathology image analysis using machine vision approaches. Artif Intell Rev 53(7):4821–4862
Li C, Kulwa F, Zhang J, Li Z, Xu H, Zhao X (2020b) A review of clustering methods in microorganism image analysis. Inf Technol Biomed 1186:13–25
Li W, Li H, Wu Q, Chen X, Ngan KN (2019b) Simultaneously detecting and counting dense vehicles from drone images. IEEE Trans Industr Electron 66(12):9651–9662
Li Y, Hao Z, Lei H (2016) Survey of convolutional neural network. J Comput Appl 36(9):2508–2515
Liu X, Wang S, Sendi L, Caulfield MJ (2004) High-throughput imaging of bacterial colonies grown on filter plates with application to serum bactericidal assays. J Immunol Methods 292(1–2):187–193
Madabhushi A, Lee G (2016) Image analysis and machine learning in digital pathology: challenges and opportunities. Med Image Anal 33:170–175
Madigan MT, Martinko JM, Parker J et al (1997) Brock biology of microorganisms, vol 11. Prentice hall Upper Saddle River, NJ
Mamunur Rahaman M, Li C, Yao Y, Kulwa F, Wu X, Li X, Wang Q (2021) DeepCervix: A Deep Learning-based Framework for the Classification of Cervical Cells Using Hybrid Deep Feature Fusion Techniques. arXiv e-prints pp arXiv–2102
Maretić IS, Lacković I (2017) Automated colony counting based on histogram modeling using gaussian mixture models. In: CMBEBIH 2017, Springer, pp 548–553
Marotz J, Lübbert C, Eisenbeiss W (2001) Effective object recognition for automated counting of colonies in Petri dishes (automated colony counting). Comput Methods Programs Biomed 66(2–3):183–198
Martinez-Espinosa J, Cordova-Fraga T, Vargas-Luna M, Ortiz-Alvarado J, Pablo AR, Cisneros MT, Guzmán-Cabrera R, Aguilar J, Diaz-Medina O (2016) Nondestructive technique for bacterial count based on image processing. Image 12:15
Massana R, Gasol JM, Bjørnsen PK, Blackburn N, Hagstrøm Å, Hietanen S, Hygum BH, Kuparinen J, Pedrós-Alió C (1997) Measurement of bacterial size via image analysis of epifluorescence preparations: description of an inexpensive system and solutions to some of the most common problems. Sci Mar 61(3):397–407
Masschelein B, Robles-Kelly A, Blanch C, Tack N, Simpson-Young B, Lambrechts A (2012) Towards a colony counting system using hyperspectral imaging. In: Imaging, manipulation, and analysis of biomolecules, cells, and tissues x, international society for optics and photonics, vol 8225, p 822510
Masuko M, Hosoi S, Hayakawa T (1991) A novel method for detection and counting of single bacteria in a wide field using an ultra-high-sensitivity TV camera without a microscope. FEMS Microbiol Lett 81(3):287–290
Matić T, Vidović I, Siladi E, Tkalec F (2016) Semi-automatic prototype system for bacterial colony counting. In: 2016 international conference on smart systems and technologies (SST), IEEE, pp 205–210
Mazzei L, Marini S, Craig J, Aguzzi J, Fanelli E, Priede IG (2014) Automated video imaging system for counting deep-sea bioluminescence organisms events. In: 2014 ICPR workshop on computer vision for analysis of underwater imagery, IEEE, pp 57–64
Minoi JL, Chiang TT, Lim T, Yusoff Z, Karim AHA, Zulharnain A (2016) Mobile vision-based automatic counting of bacteria colonies. In: 2016 International conference on information and communication technology (ICICTM), IEEE, pp 41–46
Moller S, Kristensen CS, Poulsen LK, Carstensen JM, Molin S (1995) Bacterial growth on surfaces: automated image analysis for quantification of growth rate-related parameters. Appl Environ Microbiol 61(2):741–748
Motta Md, Pons MN, Vivier H, Amaral A, Ferreira E, Roche N, Mota M (2001) The study of protozoa population in wastewater treatment plants by image analysis. Braz J Chem Eng 18(1):103–111
Mukherjee DP, Pal A, Sarma SE, Majumder DD (1995) Bacterial colony counting using distance transform. Int J Biomed Comput 38(2):131–140
Mukti J, Kale K, Gaikwad A, Kulkarni A, Gadre S (2010) Detection and counting of tuberculosis bacterial cell using image processing. Comput Vis Inf Technol Adv Appl 2010:279
Nash W, Drummond T, Birbilis N (2018) A review of deep learning in the study of materials degradation. npj Mater Degrad 2(1):1–12
Nayak R, Shenoy VP, Galigekere RR (2010) A new algorithm for automatic assessment of the degree of TB-infection using images of ZN-stained sputum smear. In: 2010 international conference on systems in medicine and biology, IEEE, pp 294–299
Nishimura M, Shimakita T, Kamiya E, Tashiro Y, Kogure K (2006) Use of an automatic cell-counting system with LED illumination for enumeration of marine bacteria. Fish Sci 72(4):723–727
Nishimura M, Shimakita T, Matsuzaki T, Tashiro Y, Kogure K (2008) Automatic counting of FISH-labeled microbes by an LED illuminated detecting apparatus. Fish Sci 74(2):405–410
Niyazi M, Niyazi I, Belka C (2007) Counting colonies of clonogenic assays by using densitometric software. Radiat Oncol 2(1):4
Nunan N, Ritz K, Crabb D, Harris K, Wu K, Crawford JW, Young IM (2001) Quantification of the in situ distribution of soil bacteria by large-scale imaging of thin sections of undisturbed soil. FEMS Microbiol Ecol 37(1):67–77
O’cleirigh C, Walsh P, O’cshea D (2003) Morphological quantification of pellets in Streptomyces hygroscopicus var. geldanus fermentation broths using a flatbed scanner. Biotechnol lett 25(19):1677–1683
Ogawa H, Nasu S, Takeshige M, Funabashi H, Saito M, Matsuoka H (2012) Noise-free accurate count of microbial colonies by time-lapse shadow image analysis. J Microbiol Methods 91(3):420–428
Ogawa M, Tani K, Yamaguchi N, Nasu M (2003) Development of multicolour digital image analysis system to enumerate actively respiring bacteria in natural river water. J Appl Microbiol 95(1):120–128
Ogawa M, Tani K, Ochiai A, Yamaguchi N, Nasu M (2005) Multicolour digital image analysis system for identification of bacteria and concurrent assessment of their respiratory activity. J Appl Microbiol 98(5):1101–1106
Otsu N (1979) A threshold selection method from gray-level histograms. IEEE Trans Syst Man Cybern 9(1):62–66
Packer H, Thomas C (1990) Morphological measurements on filamentous microorganisms by fully automatic image analysis. Biotechnol Bioeng 35(9):870–881
Payasi Y, Patidar S (2017) Diagnosis and counting of tuberculosis bacilli using digital image processing. In: 2017 International conference on information, communication, instrumentation and control (ICICIC), IEEE, pp 1–5
Peitz I, van Leeuwen R (2010) Single-cell bacteria growth monitoring by automated DEP-facilitated image analysis. Lab Chip 10(21):2944–2951
Peña C, Reyes C, Larralde-Corona P, Corkidi G, Galindo E (2002) Characterization of Azotobacter vinelandii aggregation in submerged culture by digital image analysis. FEMS Microbiol Lett 207(2):173–177
Peng N, Zou X, Li L (2015) Comparison of different counting chambers using a computer-assisted semen analyzer. Syst Biol Reprod Med 61(5):307–313
Perea S, Ramos MJ, Garau M, Gonzalez A, Noriega AR, del Palacio A (2000) Prevalence and risk factors of tinea unguium and tinea pedis in the general population in spain. J Clin Microbiol 38(9):3226–3230
Perez A, Gonzalez RC (1987) An iterative thresholding algorithm for image segmentation. IEEE Trans Pattern Anal Mach Intell 9(6):742–751
Pernthaler J, Alfreider A, Posch T, Andreatta S, Psenner R (1997) In situ classification and image cytometry of pelagic bacteria from a high mountain lake (gossenkollesee, austria). Appl Environ Microbiol 63(12):4778–4783
Pernthaler J, Pernthaler A, Amann R (2003) Automated enumeration of groups of marine picoplankton after fluorescence in situ hybridization. Appl Environ Microbiol 69(5):2631–2637
Pettipher G, Rodrigues UM (1982) Semi-automated counting of bacteria and somatic cells in milk using epifluorescence microscopy and television image analysis. J Appl Bacteriol 53(3):323–329
Privezentsev D, Zhiznyakov A, Kulkov Y (2019) Analysis of the microhardness of metals using digital metallographic images. Mater Today Proc 11:325–329
Puchkov E (2019) Quantitative Methods for Single-Cell Analysis of Microorganisms. Microbiology 88(1):1–14
Putman M, Burton R, Nahm MH (2005) Simplified method to automatically count bacterial colony forming unit. J Immunol Methods 302(1–2):99–102
Qiu D, Jiao N, Qian L (2004) Advance in measured techniquesof aquatic bacterial counting and cell sizes. J Oceanogr Taiwan Strait 23(3):376–385
Rahman MA, Wang Y (2016) Optimizing intersection-over-union in deep neural networks for image segmentation. In: International symposium on visual computing, Springer, pp 234–244
Rajapaksha P, Elbourne A, Gangadoo S, Brown R, Cozzolino D, Chapman J (2019) A review of methods for the detection of pathogenic microorganisms. Analyst 144(2):396–411
Rizzo L, Manaia C, Merlin C, Schwartz T, Dagot C, Ploy M, Michael I, Fatta-Kassinos D (2013) Urban wastewater treatment plants as hotspots for antibiotic resistant bacteria and genes spread into the environment: a review. Sci Total Environ 447:345–360
Robinson A, Sadr-Kazemi N, Dickason G, Harrison S (1998) Morphological characterisation of yeast colony growth on solid media using image processing. Biotechnol Tech 12(10):763–767
Rodenacker K, Gais P, Jutting U, Hense BA (2001) (Semi-) automatic recognition of microorganisms in water. In: Proceedings 2001 international conference on image processing (Cat. No. 01CH37205), vol 3, IEEE, pp 30–33
Rodenacker K, Gais P, Jütting U, Hense BA (2002) Identification and quantification of phytoplankton by image analysis. GSF-Report 2(02):16–24
Rolke M, Lenz J (1984) Size structure analysis of zooplankton samples by means of an automated image analyzing system. J Plankton Res 6(4):637–645
Rong Y, Rong Z, Song S (2006) Automated classification of zooplankton based on digital image processing. Comput Integr Manuf Syst 23(5):167–170
Roweis S (1998) EM algorithms for PCA and SPCA. Adv Neur Inf Proc Syst 10:626–632
Salvi M, Acharya UR, Molinari F, Meiburger KM (2020) The impact of pre-and post-image processing techniques on deep learning frameworks: A comprehensive review for digital pathology image analysis. Comput Biol Med 128:104129–104129
Sambrook J, Russell DW (2006) Estimation of cell number by hemocytometry counting
Sánchez-Femat E, Cruz-Leija R, Torres-Hernández M, Herrera-Mayorga E (2016) Mobile application for automatic counting of bacterial colonies. In: International conference on software process improvement, Springer, pp 221–230
Sándor E, Szentirmai A, Paul GC, Thomas CR, Pócsi I, Karaffa L (2001) Analysis of the relationship between growth, cephalosporin C production, and fragmentation in Acremonium chrysogenum. Can J Microbiol 47(9):801–806
Saur T, Milferstedt K, Bernet N, Escudié R (2014) An automated method for the quantification of moving predators such as rotifers in biofilms by image analysis. J Microbiol Methods 103:40–43
Schönholzer F, Hahn D, Zarda B, Zeyer J (2002) Automated image analysis and in situ hybridization as tools to study bacterial populations in food resources, gut and cast of Lumbricus terrestris L. J Microbiol Methods 48(1):53–68
Selinummi J, Seppälä J, Yli-Harja O, Puhakka JA (2005) Software for quantification of labeled bacteria from digital microscope images by automated image analysis. Biotechniques 39(6):859–863
Sethi H, Yadav S (2012) Bacterial colony counter: manual vs automatic. Eng Sci Technol 2(1):42–4
Shabtai Y, Ronen M, Mukmenev I, Guterman H (1996) Monitoring micorbial morphogenetic changes in a fermentation process by a self-tuning vision system (STVS). Comput Chem Eng 20:S321–S326
Sharma R (2015) Counting of microorganisms for medical diagnosis using image processing method. Innov Res Sci Technol 1(10):236–241
Shen Wz, Zhao J, Wu Yc, Zheng H (2010) Experimental study for automatic colony counting system based on image processing. In: 2010 International conference on computer application and system modeling (ICCASM 2010), vol 6, IEEE, pp V6–612
Shenglang J, Yongguang Y (2005) The judgment of bacteria in raw milk applying for the matlab image treatment technology. J Dairy Sci Technol 2:61–64
Shenglang J, Yujuan L, Yongguang Y (2008) Rapid detection of total number of bacteria in food using digital micro-image identification technique. Trans Chin Soc Agric Eng 24(4):177–180
Shijing L, Jun C, Xingguo L, Chongwu G (2012) Study on Chlorella automatic counting based on the algae fluorescence excitation effect. Fish Mod 39(5):16–20
Shopov A, Williams SC, Verity PG (2000) Improvements in image analysis and fluorescence microscopy to discriminate and enumerate bacteria and viruses in aquatic samples. Aquat Microb Ecol 22(2):103–110
Sieracki ME, Johnson PW, Sieburth JM (1985) Detection, enumeration, and sizing of planktonic bacteria by image-analyzed epifluorescence microscopy. Appl Environ Microbiol 49(4):799–810
Sieracki ME, Haugen EM, Cucci TL (1995) Overestimation of heterotrophic bacteria in the Sargasso Sea: direct evidence by flow and imaging cytometry. Deep Sea Res Part I 42(8):1399–1409
Simonyan K, Zisserman A (2014) Very deep convolutional networks for large-scale image recognition. arXiv preprint arXiv:14091556
Singleton S, Cahill JG, KeithWatson G, Allison C, Cummins D, Thurnheer T, Guggenheim B, Gmür R (2001) A fully automated microscope bacterial enumeration system for studies of oral microbial ecology. J Immunoassay Immunochem 22(3):253–274
Siqueira AA, de Carvalho PGS (2017) MicroCount: free software for automated microorganism colony counting by computer. IEEE Lat Am Trans 15(10):2006–2011
Song D, Liu H, Dong Q, Bian Z, Wu H, Lei Y (2018) Digital, rapid, accurate, and label-free enumeration of viable microorganisms enabled by custom-built on-glass-slide culturing device and microscopic scanning. Sensors 18(11):3700
Song Q, Yanyou W, Juan C, Xinzheng Z, Xiaofeng Q (2006) Application in algae recognition and counting of microscopic color image processing. J Agric Mech Res 6:199–201
Sotaquira M, Rueda L, Narvaez R (2009) Detection and quantification of bacilli and clusters present in sputum smear samples: a novel algorithm for pulmonary tuberculosis diagnosis. In: 2009 international conference on digital image processing, IEEE, pp 117–121
Stoderegger KE, Herndl GJ (2005) Dynamics in bacterial surface properties of a natural bacterial community in the coastal North Sea during a spring phytoplankton bloom. FEMS Microbiol Ecol 53(2):285–294
Stolze N, Bader C, Henning C, Mastin J, Holmes AE, Sutlief AL (2019) Automated image analysis with ImageJ of yeast colony forming units from cannabis flowers. J Microbiol Methods 164:105681
Strahler AN (1957) Quantitative analysis of watershed geomorphology. EOS Trans Am Geophys Union 38(6):913–920
Su MC, Cheng CY, Wang PC (2014) A neural-network-based approach to white blood cell classification. Sci W J 1:1–9
Sudiana D, Rizkinia M (2012) ALOS/palsar image processing using Dinsar and log ratio for flood early detection in jakarta based on land subsidences. Makara J Technol 15(2):193–200
Tamiev D, Furman PE, Reuel NF (2020) Automated classification of bacterial cell sub-populations with convolutional neural networks. PLoS ONE 15(10):e0241200
Thiel R, Blaut M (2005) An improved method for the automated enumeration of fluorescently labelled bacteria in human faeces. J Microbiol Methods 61(3):369–379
Thiran JP, Becks MO, Macq BM, Mairesse J (1994) Automatic recognition of cancerous cells using mathematical morphology. In: Visualization in Biomedical Computing 1994, International Society for Optics and Photonics, vol 2359, pp 392–401
Trujillo O, Griffis C, Li Y, Slavik M (2001) A machine vision system using immuno-fluorescence microscopy for rapid recognition of Salmonella typhimurium. J Rapid Methods Autom Microbiol 9(2):115–134
Tsechpenakis G, Bianchi L, Metaxas DN, Driscoll M (2008) A novel computational approach for simultaneous tracking and feature extraction of C. elegans populations in fluid environments. IEEE Trans Biomed Eng 55(5):1539–1549
Tucker KG, Kelly T, Delgrazia P, Thomas CR (1992) Fully-automatic measurement of mycelial morphology by image analysis. Biotechnol Prog 8(4):353–359
University JH (2020) Coronavirus covid-19 global cases by the center for systems science and engineering (csse) at johns hopkins university (jhu). Available at: https://coronavirus.jhu.edu/map.html
Viles CL, Sieracki ME (1992) Measurement of marine picoplankton cell size by using a cooled, charge-coupled device camera with image-analyzed fluorescence microscopy. Appl Environ Microbiol 58(2):584–592
Vishwanathan S, Murty MN (2002) SSVM: a simple SVM algorithm. In: Proceedings of the 2002 international joint conference on neural networks. IJCNN’02 (Cat. No. 02CH37290), vol 3, IEEE, pp 2393–2398
Wang X, Yamaguchi N, Someya T, Nasu M (2007) Rapid and automated enumeration of viable bacteria in compost using a micro-colony auto counting system. J Microbiol Methods 71(1):1–6
Wong CF, Joshua Yi Y, Samuel Ken-En G (2016) APD Colony Counter App: Using Watershed algorithm for improved colony counting. Nat Methods Aug 2016:1–3
Wright M, Bakus GJ, Ortiz A, Ormsby B, Barnes DM (1991) Computer image processing and automatic counting and measuring of fouling organisms. Comput Biol Med 21(3):173–180
Xianjiu G, Guosheng Z, Chunyun G (2012) A marine alga counting method based on an image processing technology. J Dalian Fish Univ 27(4):368–372
Xu X, Xu S, Jin L, Song E (2011) Characteristic analysis of Otsu threshold and its applications. Pattern Recogn Lett 32(7):956–961
Yamaguchi N, Ichijo T, Ogawa M, Tani K, Nasu M (2004) Multicolor excitation direct counting of bacteria by fluorescence microscopy with the automated digital image analysis software BACS II. bioimages 12(1):1–7
Yang Y, Li G, Wu Z, Su L, Huang Q, Sebe N (2020) Reverse perspective network for perspective-aware object counting. In: Proceedings of the IEEE/CVF conference on computer vision and pattern recognition, pp 4374–4383
Yoon SC, Lawrence KC, Park B (2015) Automatic counting and classification of bacterial colonies using hyperspectral imaging. Food Bioprocess Technol 8(10):2047–2065
Yu C, Wang J, Peng C, Gao C, Yu G, Sang N (2018) Bisenet: Bilateral segmentation network for real-time semantic segmentation. In: Proceedings of the European conference on computer vision (ECCV), pp 325–341
Yu H, MacGregor JF (2004) Monitoring flames in an industrial boiler using multivariate image analysis. AIChE J 50(7):1474–1483
Yujie W (2009) Detection of industrial microbes based on digital image processing method. Master’s thesis, Northeaste Dianli University
Zalewski K, Buchholz R (1996) Morphological analysis of yeast cells using an automated image processing system. J Biotechnol 48(1–2):43–49
Zeder M, Van den Wyngaert S, Köster O, Felder KM, Pernthaler J (2010) Automated quantification and sizing of unbranched filamentous cyanobacteria by model-based object-oriented image analysis. Appl Environ Microbiol 76(5):1615–1622
Zhang C, Chen WB (2007) An effective and robust method for automatic bacterial colony enumeration. In: International conference on semantic computing (ICSC 2007), IEEE, pp 581–588
Zhang C, Chen WB, Liu WL, Chen CB (2008a) An automated bacterial colony counting system. In: 2008 IEEE International Conference on Sensor Networks, Ubiquitous, and Trustworthy Computing (sutc 2008), IEEE, pp 233–240
Zhang C, Li H, Wang X, Yang X (2015) Cross-scene crowd counting via deep convolutional neural networks. In: Proceedings of the IEEE conference on computer vision and pattern recognition, pp 833–841
Zhang H, Fritts JE, Goldman SA (2008b) Image segmentation evaluation: a survey of unsupervised methods. Comput Vis Image Underst 110(2):260–280
Zhang J, Li C, Kosov S, Grzegorzek M, Shirahama K, Jiang T, Sun C, Li Z, Li H (2021) LCU-Net: A Novel Low-cost U-Net for environmental microorganism image segmentation. Pattern Recognit 115:107885
Zhang R, Zhao S, Jin Z, Yang N, Kang H (2010) Application of SVM in the food bacteria image recognition and count. In: 2010 3rd international congress on image and signal processing, vol 4. IEEE, pp 1819–1823
Zhonglei S, Peng W (2012) Applying digital micro-image processing on rapid detecting the total number of yeast. Sci Technol Food Ind 33(8):105–107
Zhu G, Yan B, Xing M, Tian C (2018) Automated counting of bacterial colonies on agar plates based on images captured at near-infrared light. J Microbiol Methods 153:66–73
Zupan J (1994) Introduction to artificial neural network (ANN) methods: what they are and how to use them. Acta Chim Slov 41:327–327
Download references
Acknowledgements
This work is supported by the “Natural Science Foundation of China” (No. 61806047). We thank Miss Zixian Li and Mr. Guoxian Li for their important discussion.
Author information
Authors and affiliations.
Microscopic Image and Medical Image Analysis Group, College of Medicine and Biological Information Engineering, Northeastern University, Shenyang, 110169, China
Jiawei Zhang, Chen Li, Md Mamunur Rahaman, Yudong Yao, Pingli Ma, Jinghua Zhang, Xin Zhao, Tao Jiang & Marcin Grzegorzek
Department of Electrical and Computer Engineering, Stevens Institute of Technology, Hoboken, NJ, 07030, USA
School of Resources and Civil Engineering, Northeastern University, Shenyang, 110004, China
School of Control Engineering, Chengdu University of Information Technology, Chengdu, 610225, China
Institute of Medical Informatics, University of Luebeck, Luebeck, 23538, Germany
Jinghua Zhang & Marcin Grzegorzek
You can also search for this author in PubMed Google Scholar
Corresponding author
Correspondence to Chen Li .
Additional information
Publisher's note.
Springer Nature remains neutral with regard to jurisdictional claims in published maps and institutional affiliations.
Rights and permissions
Reprints and permissions
About this article
Zhang, J., Li, C., Rahaman, M.M. et al. A comprehensive review of image analysis methods for microorganism counting: from classical image processing to deep learning approaches. Artif Intell Rev 55 , 2875–2944 (2022). https://doi.org/10.1007/s10462-021-10082-4
Download citation
Published : 29 September 2021
Issue Date : April 2022
DOI : https://doi.org/10.1007/s10462-021-10082-4
Share this article
Anyone you share the following link with will be able to read this content:
Sorry, a shareable link is not currently available for this article.
Provided by the Springer Nature SharedIt content-sharing initiative
- Microorganism counting
- Digital image processing
- Microscopic images
- Image analysis
- Image segmentation
- Find a journal
- Publish with us
- Track your research

An official website of the United States government
The .gov means it’s official. Federal government websites often end in .gov or .mil. Before sharing sensitive information, make sure you’re on a federal government site.
The site is secure. The https:// ensures that you are connecting to the official website and that any information you provide is encrypted and transmitted securely.
- Publications
- Account settings
Preview improvements coming to the PMC website in October 2024. Learn More or Try it out now .
- Advanced Search
- Journal List
- J Pathol Inform
Introduction to Digital Image Analysis in Whole-slide Imaging: A White Paper from the Digital Pathology Association
Famke aeffner.
1 Amgen Inc., Amgen Research, Comparative Biology and Safety Sciences, South San Francisco, CA, USA
Mark D. Zarella
2 Department of Pathology and Laboratory Medicine, Drexel University, College of Medicine, Philadelphia, PA, USA
Nathan Buchbinder
3 Proscia, Philadelphia, PA, USA
Marilyn M. Bui
4 Department of Pathology, Moffitt Cancer Center, Tampa, FL, USA
Matthew R. Goodman
5 3scan, San Francisco, CA, USA
Douglas J. Hartman
6 University of Pittsburg Medical Center, Pittsburgh, PA, USA
Giovanni M. Lujan
7 Inform Diagnostics, Irving, TX, USA
Mariam A. Molani
8 Department of Pathology and Microbiology, University of Nebraska Medical Center, Omaha, NE, USA
Anil V. Parwani
9 The Ohio State University Medical Center, Columbus, OH, USA
Kate Lillard
10 Indica Labs, Inc., Corrales, NM, USA
Oliver C. Turner
11 Novartis, Novartis Institutes for BioMedical Research, Preclinical Safety, East Hannover, NJ, USA
Venkata N. P. Vemuri
Ana g. yuil-valdes, douglas bowman.
The advent of whole-slide imaging in digital pathology has brought about the advancement of computer-aided examination of tissue via digital image analysis. Digitized slides can now be easily annotated and analyzed via a variety of algorithms. This study reviews the fundamentals of tissue image analysis and aims to provide pathologists with basic information regarding the features, applications, and general workflow of these new tools. The review gives an overview of the basic categories of software solutions available, potential analysis strategies, technical considerations, and general algorithm readouts. Advantages and limitations of tissue image analysis are discussed, and emerging concepts, such as artificial intelligence and machine learning, are introduced. Finally, examples of how digital image analysis tools are currently being used in diagnostic laboratories, translational research, and drug development are discussed.
I NTRODUCTION
Modern pathology practice is moving toward a digital workflow, cumulating in utilizing computer screens to view scanned histology slides. This process of digitization of glass slides, in combination with the development of specialized software tools to identify and measure events previously observed via a microscope, has brought about the ability for pathologists to utilize digital image analysis on tissue sections. Tissue image analysis, when performed correctly, can result in the generation of tissue-derived readouts that are precise and highly reproducible.
This white paper provides an introduction to the digital analysis of scanned tissue slides and outlines the current state of available software tools as well as their advantages and their limitations. In addition, we provide examples of areas in which these tools are currently utilized to generate data in preclinical and clinical workflows that go beyond the conventional histopathological data provided by manual pathology review.
Image analysis and whole-slide imaging
The term “image analysis” has been reserved for the specific discipline that aims to obtain meaningful information from images in an objective and reproducible manner. The origin of analyzing images with objective tools is almost as old as microscopy itself. It started with measuring and counting when Leeuwenhoek developed a system to measure microscopic objects in the 17 th century.[ 1 ]
Although there were many incremental advances throughout the centuries, image analysis remained largely unchanged until the advent of digital imaging and computerized analysis in the second half of the last century. From the 1970s on, new tools were developed that allowed for easier measurement of cellular or tissue components. In 1980, video cameras became widely available, leading to further improvement of available systems.[ 1 ] Around the year 2000, digital slide scanners became commercially available, and whole-slide imaging (WSI) started to become increasingly common.
The reader is advised to review the Digital Pathology Association's (DPA) white paper on WSI for an introduction to the technical and workflow aspects of digitizing glass slides.[ 2 ] To summarize, the traditional glass histology slide is digitized via a slide scanner and can be viewed on a computer screen or handheld device at a similar resolution as light microscopy. Compared to the general workflow of how tissue sections are prepared and viewed under a microscope, this digital workflow requires additional equipment (e.g., slide scanner, image storage, and digital pathology workstation for viewing), trained personnel, and specific quality control steps (e.g., quality control of scans), all of which require increased information technology and departmental resources.[ 3 ] However, there are multiple advantages of transitioning to a digital workflow, including ease of slides and cases sharing (consulting with other pathologists, or collaborating within interdisciplinary research teams), standardization of teaching, organization of archived digitized slides, and extraction of complex data in a highly reproducible fashion via specialized software.[ 4 ] The pathologist plays a key role both in the process of slide digitization and in the subsequent data generation via the use of appropriate image analysis algorithms.
Whole-slide images of tissue samples are rich in information, some of which was previously only accessible visually by a trained pathologist or biotechnologist whose expertise was based on previous experience and training. Therefore, manual readouts could easily be influenced by inherent cognitive and visual bias.[ 5 ] With a digital image, however, some of this information is amenable to more precise and reproducible extraction, which can reduce or potentially eliminate human bias. In response to this new technology, the market is rapidly expanding with new companies that sell optimized software for extracting relevant digital information from images or that offer the data generation as a service.
Application areas of image analysis
Utilizing digital image analysis tools, the most commonly used categories of measurements that can be extracted from digitized tissue slides are area based, cell based, and measurements pertaining to objects in the tissue aside from cells.[ 4 ]
Area-based measurements include the most basic assessments, for example, quantifying the areas (2-dimensional) of a certain stain (e.g., chemical or immunohistochemistry [IHC] stain), the area of fat vacuoles, or other events present on a slide. Cell-based measurements aim at identifying and enumerating objects, e.g. cells. This identification of individual cells enables subsequent assessment of subcellular compartments. Finally, algorithms can be utilized to assess events or objects present on tissue sections that may not be comprised of individual cells. More detailed descriptions are given in the following sections. When undertaking assessment and quantification of biomarkers, image analysis tools can be of great value to standardize the analysis as well as minimize bias, subjectivity, and variability in the generated data. This includes the application of standard scoring paradigms to IHC-stained sections (e.g., programmed death-ligand 1 [PD-L1] scoring and human epidermal growth factor receptor 2 [HER2] scoring), as well as aid in the quantification of in situ hybridization (ISH) dots. These assessments can further be tuned to limit the quantification of the present biomarker to tissue compartments (e.g., tumor and stroma) and subcellular compartments (membranous, cytoplasmic, nuclear, or combination thereof), to consider variable staining thresholds, and/or to enable more global biomarker data collection that then can be interrogated in postprocessing steps. In addition, digital tissue image analysis tools can be applied not only to routine formalin-fixed, paraffin-embedded tissues but also to frozen sections, whole organ, and embryo mounts.[ 6 , 7 , 8 , 9 ] Similarly, a whole host of staining techniques (and combinations thereof) can be amendable to image analysis, not limited to routine hematoxylin and eosin (H and E) staining, IHC, or ISH labeling (chromogenic and fluorescent).[ 4 , 10 , 11 , 12 , 13 ]
While image analysis tools not only reduce bias introduced by both visual limitations and cognitive traps, they also enable capturing of data from tissue slides that may not be accessible during manual assessment via routine microscopy.[ 5 , 14 ]
Image analysis tools are already widely used in both clinical research and translational research, as well as various disciplines, ranging from oncology and immuno-oncology, to cardiology and hepatology, neurosciences, and many others.[ 8 , 11 , 15 , 16 , 17 , 18 , 19 , 20 ]
T HE B ASICS OF I MAGE A NALYSIS
Cellular analysis.
One of the most fundamental units in a typical histology image is the cell. In H and E-stained slides, in particular, cell nuclei are prominently visible structures. Other cellular compartments are often visible as well and the cell's cytoplasm has its own characteristic staining attributes.[ 21 , 22 , 23 ] Second-order structures formed from arrangements of cells are readily observed in microscopic images and provide important cues that support several scoring and grading systems in cancer.[ 24 , 25 ] A common theme for computational image analysis is to achieve a quantitative representation of cell staining, morphology, and architecture that can ultimately be used to support diagnosis and prediction.[ 4 ] Therefore, demarcating cells or subcellular structures are among the first and most important steps of many image analysis routines.
This specification (enumeration of many objects is possible, cells is only one option) was specifically requested by reviewers. The term “segmentation” represents a process in which an image is spatially parsed into constituent parts that have some significance or utility. Segmentation can be applied to a histology image to delineate tissue compartments such as stroma or epithelium, to distinguish tumor from benign regions, or to identify subcellular structures such as cell nuclei. Cell segmentation generally refers to the process of identifying groups of pixels that represent a cell. Segmentation typically involves two key steps: identification of candidate pixels and demarcation of these pixels into compartments that best approximate the spatial confines of a single cell. This process is often difficult in the presence of adjacent or overlapping cells which can lead to undersegmentation (the representation of more than one cell as a single object).[ 26 ] Methods to avoid undersegmentation may, however, result in oversegmentation (the erroneous division of a single cell into multiple objects) [ Figure 1 ].[ 27 ] A number of segmentation algorithms have been developed to identify cells in histology images. These algorithms typically employ fixed or adaptive thresholding, watershed segmentation, active contour models, template matching with shape priors, or a probabilistic framework and may consist of multiple stages that can incorporate a combination of these strategies.[ 28 , 29 , 30 , 31 , 32 , 33 , 34 , 35 , 36 , 37 , 38 , 39 , 40 , 41 , 42 ] When selecting a segmentation algorithm, users are often faced with the decision to prioritize sensitivity (how likely the algorithm is to capture a weakly stained nucleus or cell), specificity (how well the algorithm rejects artifacts), contour accuracy (how well the algorithm can approximate the exact shape of the nucleus or cell), and the likelihood of under- and oversegmentation. Ground truth defined by manual demarcation of cells by trained observers can help users evaluate the performance of a particular segmentation algorithm.[ 43 ]

Hematoxylin and eosin-stained image analyzed using the “cell detection” algorithm in QuPath (open-source tool). Nuclear segmentation, depicted as red outlines, was fragmented in the rightmost image by setting the noise reduction Gaussian filter σ = 1.0. Conversely, nuclear oversegmentation was achieved by setting σ = 2.5. Black arrows in the left image denote examples of single objects that consisted of multiple nuclei
One of the most common uses of image analysis in pathology is to support the approximation of protein expression as assessed by IHC. For example, for invasive breast cancer, the College of American Pathologists’ (CAP) guidelines instruct pathologists to report the percentage and intensity of cells positively stained for estrogen receptor (ER) and progesterone receptor (PR), which can ultimately guide treatment strategy.[ 44 , 45 ] This procedure can benefit from the aid of quantitative image analysis, which in some cases has been shown to surpass pathologist performance.[ 46 , 47 , 48 ] Quantitative scoring of nuclear stains such as ER and PR requires segmentation of both positive and negative stained cell nuclei. Conventionally, ER and PR expression is parsed according to the intensity of staining. Stained nuclei are categorized into 0 (negative), 1+ (lightly stained), 2+ (intermediately stained), and 3+ (darkly stained) groups that are typically defined by a fixed threshold. The threshold may be applied to the overall intensity of the pixels, a single red, green, and blue channel, or to a single channel following a stain separation procedure.[ 49 ] Once the distribution of 0, 1+, 2+, and 3 +cells is determined, a laboratory may report a measure of intensity either as a simple average (usually rounded) or can use a weighted scoring system such as the H-score that combines information about the intensity of staining with the percentage of cells stained [Equation 1]. Although there is a direct relationship between H-score and the more conventional percent positive metric, H-score may have additional prognostic value not wholly captured by percent positivity.[ 50 , 51 , 52 ]
Equation 1: H =3 × (%3+) +2 × (%2+) + (%1+)
IHC is often used to also detect proteins expressed on the cell membrane or in the cytoplasm. One of the most widely used examples is HER2, a protein expressed at the cell membrane whose expression can directly guide treatment strategy and prognosis in a number of different tissues.[ 53 , 54 , 55 ] In breast tissue, guidelines for interpretation of HER2 expression are fairly well established and have undergone multiple modifications by consensus groups, most recently in 2018 with a set of updated CAP guidelines for scoring and interpretation.[ 56 , 57 , 58 ] The scoring strategy for HER2 combines both staining intensity and the circumferential pattern of staining (stain completeness), both of which are ultimately used to establish a HER2 score. Likewise, PD-L1, a membrane protein whose expression can determine treatment eligibility for immunotherapy, is recommended to be interpreted according to the staining intensity, spatial distribution of staining, and prevalence of staining in the tissue.[ 59 , 60 , 61 ] Image analysis routines can be harnessed to characterize these patterns of staining. The authors believe that quantitative image analysis can enhance the accuracy and reproducibility of interpretation of the staining pattern of various biomarkers.
Cell morphology can also provide important clues to support diagnosis and prediction. A number of classical studies have shown that nuclear area and shape (e.g., circularity and aspect ratio) carry prognostic value independent of other information.[ 62 , 63 , 64 , 65 , 66 , 67 ] Consequently, many groups have developed image analysis procedures to quantitatively measure these features to predict outcomes or to characterize tumors.[ 68 , 69 , 70 , 71 ] Additional cellular features such as H and E staining attributes and texture, nuclear/cytoplasmic ratio, and automated mitotic detection have also been used.[ 72 ]
Color normalization
One of the main obstacles to the development of successful image analysis routines is that stained tissue exhibits significant variability in color, making it difficult to apply simple rules to a dataset with vast differences in staining properties. For example, as noted in the previous sections, staining thresholds can often be an important element to an image analysis algorithm. However, when excessive staining variability is present in a dataset, applying a threshold may produce different results not due to intrinsic properties of the tissue but rather due to different staining or imaging protocols. Other image analysis procedures not based on threshold can also do poorly on image sets with significant color variability.[ 73 ] Figure 2 shows an example of different H and E staining appearances across tissue processed by different institutions, but variation within the same institution is also common.[ 23 , 73 ]

Sample hematoxylin and eosin images obtained from six sources designated on the right depict different color attributes commonly encountered when viewing digital images of slides across laboratories or across imaging modalities
Color normalization is an image processing tool that has been developed to mitigate the effects of color variation by transforming the color properties of an image to align to a single standard, often specified by the user. This serves two purposes: (1) it reduces the overall staining variation within a sample and (2) it forces the color of the image to adhere to a desired color standard which can be useful for visualization. A number of different color normalization approaches have been developed that utilize intensity thresholding, histogram normalization, stain separation, color deconvolution, and structure-based color classification.[ 29 , 49 , 74 , 75 , 76 , 77 , 78 , 79 , 80 , 81 , 82 ] Many of these techniques have demonstrated a reduction in color variability by a factor of 2 or more. However, it is important to detect and assess distortions in the image that may potentially be introduced by these techniques.
T YPICAL A LGORITHM G RAPHICAL U SER I NTERFACE
There are numerous software packages, which are used by pathologists and researchers to step through the workflows described in previous sections.
Beneath the image analysis software are numerous computational algorithms that utilize a variety of parameters as inputs and perform the actual processing and segmentation of the image. Graphical user interfaces (GUIs) essentially expose the “knobs” underlying the algorithms, thus enabling end users who might lack a background in software engineering to interpret the image data and guide the analytic process.
A typical GUI walks the user through a series of steps. As an example, in the following, we describe a two-color chromogenic assay stained with hematoxylin, brown (Ki-67 marker), and red (CD3 T cell marker). The GUI would include a number of user-input parameters such as:
- Color definition – Ability to set the color for each of the stains. In this example, blue for hematoxylin, brown for Ki-67, and red for CD3. Because actual stain colors vary, this GUI could include an eyedropper tool (or a tool with similar functionality) which allows the user to identify an example stain in the image
- Nuclear detection – Many algorithms start with nucleus or cell detection. This GUI may include parameters such as nuclear size, color threshold, roundness, eccentricity, and other parameters to identify individual nuclei while excluding nonnuclear structures
- Marker detection for each biomarker – This GUI would include parameters such as biomarker color (e.g., brown for Ki-67), cellular compartment (nuclear, cytoplasm, and membrane: e.g., nuclear for Ki-67), and intensity threshold to identify the desired stain in the right compartment, while excluding background staining
- Expression categories – This GUI would include values to separate detected cells into negative, low, medium, or high (0, 1+, 2+, or 3+) based on the intensity thresholds. Depending on the use of an algorithm, some of these categories may be locked down to ensure reproducibility of results
- Image analysis outputs – There are a plethora of image features output by algorithms. A GUI may include options to export only a subset of information.
An image analysis workflow is an iterative process where the user is adjusting the algorithm parameters, running the algorithm on a subset of images, and then evaluating the algorithm performance until sufficient algorithm performance is achieved. At times, for example, as part of a clinical trial, certain parameters are locked down after an algorithm has been validated, while others remain adjustable. GUIs often include tools for “fine tuning” the parameters where the user can adjust parameters and view results in real time. These tuning methods may improve accuracy via the establishment of a “human-in-the-loop” step, wherein pathologists contribute to the “ground truth.”
High content feature outputs
As an algorithm identifies cells and cellular compartments, it can extract information from each of these compartments, the individual cell (cellular or object data), as well as the entire population of cells (summary data) in the tissue analyzed. Examples are numerous: an algorithm may determine the cytoplasmic area of each cell, the membrane staining intensity, the number of ISH dots per nucleus, as well as cellular roundness, eccentricity, and many more.[ 4 ] For each of these parameters, the entire population of cells present can be analyzed or summarized. This can be, for example, in the form of plotting the data as a histogram incorporating all data points or a single score for the entire tissue.
Furthermore, objects that are comprised of cells can be interrogated similarly. For example, blood vessels are formed by cells, but when analyzing them via image analysis, one is rarely interested in the data of individual cells, but in the data of individual vascular profiles as well as the entire population of vessels.[ 4 , 83 ] This may include parameters such as major and minor diameter, circumference, and area.
Analog to this, analysis of tissue components that may not be formed by cells can yield similar data. For example, the analysis of vacuoles will result in both population data (e.g., average area of vacuoles, average circumference, range of major diameter), as well as individual vacuole data characterizing each vacuole identified.[ 84 ]
Utilizing the data mentioned above, algorithms can compute various tissue scores. Commonly used manual scoring paradigms range from classifying an entire tissue as positive or negative for a given analyte based on IHC staining, generation of a percent positive stain of a tissue compartment (e.g., percent positive neoplastic cells), scoring paradigms that combine percentage of biomarker-positive cells with the IHC staining intensity, to more complex scoring paradigms such as H-score or AllRed score. A review of these scoring approaches can be found in Aeffner et al. [ 5 ]
Many of the routine manual scoring paradigms commonly used are available in the commercially available software packages. In addition, individual Food and Drug Administration (FDA)-approved image analysis solutions for specific scoring scenarios are available for selecting stains and indications (e.g., HER2 scoring in breast cancer). Due to the wealth of data generated, and the computational power of algorithms, a multitude of new scoring paradigms are possible that go beyond what a pathologist can generate via manual evaluations. These scoring paradigms may be logical expansions of existing ones, e.g., expansions of the H-score into other cellular compartments or combinations thereof.[ 85 ] Others are novel assessments, which, in the case of the Immunoscore ® , have already proven their utility and are actively used in the clinic.[ 86 ]
B EYOND T WO-DIMENSIONAL I MAGE A NALYSIS: S TEREOLOGY AND D IGITAL T HREE-DIMENSIONAL M ODELING
While the most commonly used application of image analysis results in the interrogation of two-dimensional (2D) tissue slides of a three-dimensional (3D) object, this assessment is not always representative of the entire object due to inherent biases.[ 84 ] The two largest sources of bias are sampling bias and geometrical bias.[ 87 ] Stereology is the study of 3D representation from a limited 2D sampling and aims at minimizing bias by utilizing calculations (statistical theory and stochastic geometry) and stringent sampling methods.
In the context of tissue analysis, sampling bias means the probability of staining, sectioning, and imaging the structures of interest. If the subject of the study is sufficiently small, it may be missed if only a few planar sections are examined. For example, 2D sections limit the accuracy of quantification of findings such as chronic allograft vasculopathy. It has been shown that this quantification can be improved by reconstructing the murine coronary system in 3D from 2D digitized slides.[ 88 ] Similarly, geometric bias speaks to the fact that planar sampling may systematically miss structures of interest if a poor or unlucky sampling plane is selected. One of the promises of automated WSI is the ability to increase sampling (both in terms of numbers of slide, as well as of areas samples within a slide) and thus minimize the chances for these systematic biases to affect outcomes. Factors such as tissue shrinkage (which can vary section to section and sample to sample) must also be considered to ensure accurate and representative sampling.[ 89 , 90 ]
Image analysis combined with a stereology approach should be considered when an expected effect size is small or an unbiased estimate is required to compare effects across multiple experimental groups.
Due to the advances of digital image analysis, stereology has recently become more efficient and less cumbersome.[ 87 ] However, it remains a time-intensive methodology that requires to be performed by a skilled stereologist to ensure proper study design, sample preparation, and data interpretation, as well as specially trained histologists for pristine sample preparation.[ 87 ] Furthermore, it requires the usage of a significant amount of tissue, planning, and up-front work. Pilot studies are imperative for successful and accurate data generation.[ 87 ] Hence, stereology is not a methodology that can be applied retrospectively to a sample set.[ 90 ]
A full review of stereology as a methodology is beyond the scope of this manuscript but has been provided elsewhere.[ 87 , 90 , 91 , 92 , 93 , 94 ] Commercial image analysis solutions are available that include stereology modules to aid in application of the stereology method to whole-slide images.
T HE P ATHOLOGIST’S R OLE IN THE I MAGE A NALYSIS W ORKFLOW
Within the general workflow of digital pathology and tissue image analysis, the pathologist plays an important role to ensure the value and quality of generated data. While extensive knowledge on algorithm development and image analysis tools is helpful, it is not a requirement. The value a pathologist brings to this workflow greatly lies in the technical knowledge of tissue handling, fixation, processing, and staining, as well as the specialty expertise in biology, histology, pathology, pathophysiology, biomarker expression, comparative anatomy, etc.[ 4 ]
Before engaging in any image analysis workflow, the quality of the tissue, histology slide, stain, and scan should be confirmed to ensure that it meets a quality standard that will allow collection of meaningful and reproducible data.[ 2 , 4 , 95 , 96 ] The validity of image analysis data can be greatly hampered by performing analysis on low-quality tissue, histology slides, and/or not properly optimized staining results.[ 84 ] Preanalytical variables such as interval between tissue harvest and fixation and total fixation time are often poorly controlled.[ 97 , 98 ] Quality control within a pathology laboratory is addressed later in this publication.
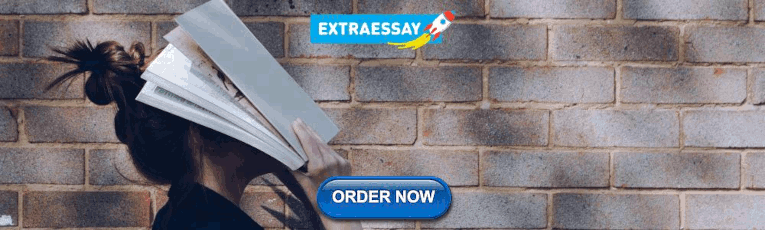
Region of interest selection
Whole-slide images contain a vast amount of information that can be difficult from a computational standpoint to process in their entirety. Furthermore, not every region in the whole-slide image is necessarily informative. Expanses of normal tissue and stromal cells, for example, may even have the effect of “diluting” useful information about the disease state in some image analysis algorithms, an effect that has an analog in molecular diagnostics.[ 99 ] Efforts to confine the image analysis region to one or more smaller regions of interests (ROIs) are therefore often necessary to create an accurate and computationally viable method for tissue image analysis.
Manual versus automated image annotations
Before running any type of image analysis algorithm on a particular slide, image annotations (also known as image masking) of ROIs are usually a prerequisite. Annotation allows the algorithm to focus on a particular area of the slide to perform the analysis. Two main strategies for annotating images can be employed: manual or automated.
Manual annotations often encompass drawing digital lines (inclusion and exclusion annotations) on whole-slide images to mark an ROI for the algorithm.[ 4 ] The algorithm will analyze all tissues included in the inclusion annotation, but omits analysis in that area for tissue regions marked with exclusion annotations. Those exclusions may include tissue structures not deemed for analysis, areas of necrosis, tissue artifacts, and staining artifacts. While manual annotations can be performed by a trained technician, final review and approval by a pathologist is advised.[ 4 ]
More tools have become available to automate the annotation process, especially as part of commercial software packages. In these solutions, the algorithm may come preprogrammed to identify specific features (e.g., mitotic figures and epithelial cells) or can be taught to identify specific features or structures (e.g., lung cancer nodules, glomeruli, and vessels), utilizing machine learning algorithms (discussed later). The accuracy of these automated annotations should nevertheless be checked by a pathologist as well.
Finally, crowdsourcing (as in soliciting help from a large number of individuals via web-based tools) of image annotations has recently been studied and may be an option for specific and large-scale studies.[ 100 ]
In addition to ensuring quality of annotations, the review by a pathologist may also gain valuable insight into the population of the study slides to be analyzed and highlight specific issues or potential pitfalls that may need to be considered during algorithm development.[ 4 ]
Pathologist's role in algorithm development and data review
The pathologist plays a crucial part in the iterative process of algorithm development and the review of generated data and its interpretation.
Whenever the assessment of biomarker expression via staining intensity is part of the objective of an analysis, final algorithm thresholds should be approved by a pathologist before data generation.[ 101 , 102 ] This is often done by reviewing the performance of test algorithms and thresholds on selected smaller areas of the ROI.[ 4 ]
These test algorithms should also be reviewed for other performance criteria. For example, are cells or other desired structures correctly identified and enumerated? Is the target tissue correctly identified and separated from other structures not intended for analysis? Is staining adequately classified (i.e., staining intensity or ISH dots as part of a scoring paradigm)? While it is not necessary for an algorithm to perform with 100% precision and accuracy, a reasonable level of performance should be met that correlates with the general goals of the analysis.[ 103 , 104 ] For example, it may be acceptable for a study which is designed to rank a large number of samples or tissue microarray (TMA) cores by staining to be less accurate, whereas a clinical study to inform treatment decisions or prognosis may be required to meet a more stringent predetermined expectation of accurate classification.[ 105 ] Specific samples that do not meet these predetermined criteria should be failed upon review, which should trigger either reworking of the algorithm, reanalysis, and re-review or exclusion from analysis.[ 4 ] However, depending on the software solution or image analysis toolbox used, the researcher or pathologist's ability to modify algorithms to improve performance may be limited. On the other hand, some commercially available solutions can provide so many options for modification that may be difficult to use or require image analysis experts to be involved in the workflow.[ 84 , 97 ]
Once test algorithms meet desired performance criteria, final analysis can be performed on a study set of slides. A pathologist should review the algorithm performance, typically by viewing the markup images (also known as segmentation overlays), a visual representation of the image segmentation and classification [ Figure 3 ]. Different institutions practice different review strategies, ranging from review of every individual slide and markup to only reviewing a certain percentage of analysis runs. The appropriate extent of analysis review should be informed by the general question interrogated via image analysis and the impact of the data on research, decision-making, and ultimately patients. Only data from algorithms that pass the pathologist's review and the general performance criteria should be included in final reporting.[ 106 ] It is not uncommon that, for a few slides, no suitable analysis solution can be found.

(a) A mouse xenograft tumor sample is stained with hematoxylin and Ki67 (DAB). (b) An enlarged region is shown where nuclei are stained blue and Ki67+ cells are brown. (c) A pathologist-trained random forest classifier is developed to identify tumor (green), stroma (blue), necrosis (red), and glass (gray). (d) The algorithm parameters are fine-tuned with the pathologist's input to optimize the nuclear segmentation and to define intensity thresholds to categorize the expression into four bins: 0+ (blue), 1+ (yellow), 2+ (orange), and 3+ (red)
Final data interpretation should also involve the expertise of a pathologist, but can be performed by a larger team with specialty expertise in various areas relevant to the objectives of the analysis. Due to large amounts of data generated, it is advised to have a biostatistician as part of such team.[ 4 ] Some commercially available algorithm solutions come with data interrogation and plotting tools to aid in this step of the analysis. Similarly, some image analysis service providers offer further statistical analysis and expert interpretation of study data as part of the service package.
Importance of quality control
Although there have been some quality assurance programs instituted for IHC (e.g., United Kingdom National External Quality Assessment System and International Quality Network for Pathology), there are few quality assurance check programs available to users in digital pathology.[ 107 , 108 ] Creating a similar quality stain across different runs of IHC is critical for achieving the optimal value from image analysis. Therefore, a human is still required for quality assurance of digital images before they can be processed by an image analysis algorithm. Inter- and intrarun variability in staining quality can generally be tolerated by pathologists during manual assessment. However, when image analysis tools are used to ask more detailed questions, utilizing the same slides displaying variable staining, results may be greatly influenced by inconsistent staining results. Similarly, technical aspects of the slide digitization, for example, maximum magnification, image compression, and color palette, can have an effect both on the viewed digital image and on the final image analysis results. Tissue heterogeneity can also introduce variability within the image analysis process.[ 84 ]
The validation of an image analysis algorithm for a specific biomarker assay is dependent on a number of other factors related to the histology, staining and scanning quality, and equipment. The level of validation is dependent on the assay's intended use, often referred to as “fit-for-purpose”. Whereas a clinical biomarker assay would require rigorous validation, a research or exploratory assay may require less.
For clinical use of an image analysis algorithm, it is important to know the use cases that the algorithm is intended for and to make sure that the algorithm is adequately validated for those specific tissue conditions, such as frozen tissue, formalin-fixed tissue, B5-fixed tissue, or decalcified tissue. In the future, there are likely to be more commercially available solutions for image analysis in the clinical space. This will be similar to purchasing an analyzer for the clinical pathology laboratory. However, the image analysis algorithms will need to be internally validated by each of the institutions before the algorithms can be used for clinical care. National societies such as the CAP are creating guidelines to facilitate adoption of image analysis into routine pathology workflows.
M ACHINE L EARNING AND A RTIFICIAL I NTELLIGENCE
Speaking the artificial intelligence language.
The field of artificial intelligence (AI) comes with its own broad vocabulary. Many of these terms are used interchangeably or erroneously in common parlance.[ 109 ] While a number of resources exist online that go into great depth in explaining the nuances of the language around AI, there are some concepts at the core of most AI that can serve pathologists as a baseline in understanding the technology:[ 110 ]
- AI – The broadest of these concepts, AI refers to any technology in which machines can perform activities considered “smart” or “human” in nature. This could involve hard coding the machine to perform specific tasks intelligently, machine learning, and other approaches[ 111 ]
- Machine learning – Machine learning is the specific branch of AI in which machines are exposed to a large amount of data with the expectation that these machines establish their own patterns to interpret and act on new data[ 112 ]
- Deep learning – Deep learning is a subset of machine learning. However, while supervised machine learning algorithms require some level of input in training, deep learning leverages artificial neural networks (ANNs) to make its own determination as to whether its interpretation or prediction is correct[ 113 , 114 , 115 ]
- Supervised and unsupervised learning – The goal of machine learning, at its core, is to develop a machine that is capable of going from a set of inputs to a set of outputs. Supervised learning is the application of this concept with a known, defined set of outputs on which the system is assessed, while unsupervised learning has no predefined output set and enables the machine to determine what it defines as the natural patterns present in the data[ 109 , 116 ]
- ANNs – Our own brains operate through a complex network of interconnected neurons. An ANN is a set of layered, connected artificial neurons, with a defined pathway for how data are propagated and moved through the system.[ 117 ] These ANNs provide a means of getting an output that is the result of several, often independent, steps of computation, weighting, and assessment.[ 118 , 119 ]
Brief history and evolution of artificial intelligence
Although AI is a very young field of inquiry, with some of the key contributors still being alive today, it has already been through several hype cycles and deep “AI winters” in its evolution.
Some now propose, however, that in 2018, the “plateau of productivity” has truly been reached and large-scale societal impact will be realized. In 2008, Thomas Fuchs, to some the “founding father of computational pathology”, demonstrated the utility of computational pathology with analysis of TMAs and survival prediction of renal clear cell carcinoma patients.[ 120 ] The advent of graphics processing units (GPUs) in the late 2000s further advanced the field, and in 2012, Dan Ciresan et al. showed a significant improvement on the best performance in the literature for multiple image databases using multicolumn deep neural networks for image classification – the era of convolutional neural networks (CNNs) was born.[ 121 ]
Where are we now?
AI as applied to medical image analysis is still a very young but rapidly burgeoning field. It is <10 years since the advent of GPUs and CNNs, both of which have radically advanced the opportunities in this space. The following are some of the areas in pathology and related fields which either already have seen or are likely to soon see the implementation of AI:
- Radiomics and Pathomics – In other data-rich fields of medicine, AI is already being implemented in health-care practices as a means of addressing workload volume and driving augmentative insights. For example, service providers exist that offer workload easing AI at a fixed per-case price in the field of radiology.[ 122 ] Similar nondiagnostic workflow applications of AI are valuable for pathology. As AI becomes an increasingly relevant part of individual fields of medicine, overlap and cross-specialty application is natural progression for the technology[ 123 , 124 ]
- New Integrative Diagnostics for Oncology: Computational methods have revolutionized the ability to assess the quantitative, spatial, and temporal architecture of tumors. Anant Madabhushi, for example, has pioneered new methods to measure tumor-infiltrating lymphocytes and proposed an image-based risk score, which computes the probability of disease aggressiveness using features mined from medical images for a variety of cancers.[ 120 , 125 ]
- Companion diagnostics – As pharmaceutical companies continue to develop targeted approaches to cancer therapy, there is a growing need for companion diagnostics. Conventionally, these have been biomarker specific. However, AI may serve as a separate approach to correlate patterns in tissue with likelihood or degree of response to therapies[ 113 , 126 ]
- The Camelyon17 was organized by the Diagnostic Image Analysis Group and Department of Pathology of the Radboud University Medical Center (the Netherlands). The purpose of this challenge was to come up with a fully automated method to identify breast cancer metastases in whole-slide images of lymph nodes and to classify each lymph node into one of four stages: (1) cancer negative, (2) isolated tumor cells, (3) micrometastases, or (4) macrometastases. More information including data and results can be found at https://camelyon17.grand-challenge.org/ (last accessed: October 14, 2018).[ 128 ] A similar previous challenge was called Camelyon16, and it is expected that comparable future challenges will be opened up to continue to incentivize and highlight technological advances in this area
- A global initiative to better understand kidney disease, which is using unsupervised learning of whole-slide images, is the nation institutes of health NIH-lead Kidney Precision Medicine Project (KPMP), which has revealed new insights into the morphology of glomerular diseases ( https://www.niddk.nih.gov/research-funding/research-programs/kidney-precision-medicine-project-kpmp ; last accessed: October 3, 2018).
I NCORPORATING I MAGE A NALYSIS INTO P ATHOLOGY W ORKFLOW
Depending on if image analysis tools are used within a clinical setting or as part of a research laboratory in drug development, different aspects need to be considered with regard to how to best integrate image analysis within the laboratory's workflow.
Clinical/diagnostic workflow
The value added by image analysis in pathology is exemplified by biomarkers, mitotic detection, nuclear morphology detection, cancer grading, hematology, and others.[ 72 , 84 , 129 , 130 , 131 , 132 , 133 ] The most frequent application for patient care purpose is a quantitative analysis of IHC study of ER, PR, and HER2 for breast cancer as prognostic and predictive biomarkers.
The key elements to incorporate image analysis in pathology workflow include the quality of the slides (sectioning, staining, and scanning), image quality, ROI selection, algorithm selection, and the expertise of the pathologist who will be able to correlate the image analysis result with the relevant clinical information to make sure it is appropriate for that particular patient/case. Using breast cancer biomarker quantitative image analysis as an example, a few comments on algorithm selection and the role of pathologists are addressed here.
A good quantitative image analysis algorithm produces accurate, precise, and reproducible results. Accuracy defines the degree of correctness or true values of a given laboratory result comparing to a gold standard, which implies freedom of error. Precision defines the degree to which a test provides the same measurement over time.[ 134 ] There are basically three types of algorithm available currently in the diagnostic space: (1) FDA-cleared – FDA has determined that the commercially available product is substantially equivalent to another legally marketed algorithm for the same purpose as safe and effective. A premarket notification is referred as to 510(k);[ 135 ] (2) non-FDA approved commercially available – these products can be FDA registered for investigational use only; and (3) laboratory developed test – the algorithm is designed and used within a single laboratory according to the laboratory's own procedure. When laboratories choose to use quantitative image analysis for clinical purpose, the quantitative image analysis system and algorithm must be validated for diagnostic interpretation. Validation is designed to gather and document evidence that a system or test will consistently produce a result that meets predetermined acceptance criteria. Clinical laboratory improvement amendments and CAP require that laboratories validate the performances of tests to meet the operational needs of the tests.[ 136 ] FDA-cleared quantitative image analysis systems and algorithms have less validation burden than non-FDA-cleared products for the laboratory.
As an example, according to the ASCO/CAP HER2 guideline, quantitative image analysis can be used to achieve consistent interpretation. To further guide pathologists to improve accuracy, precision, and reproducibility in the interpretation of HER2 in breast cancer using image analysis, CAP developed evidence-based recommendations. These include 11 recommendations, 7 of which are based on CAP laboratory accreditation requirements and 4 are based on expert consensus opinions. In summary, quantitative image analysis and procedures must be validated before implementation, followed by regular maintenance and ongoing evaluation of quality control and quality assurance. HER2 analysis performance, interpretation, and reporting should be supervised by pathologists with expertise in image analysis.[ 137 ]
Research and drug development image analysis workflow
Research laboratories performing tissue-based research, especially biomarker discovery, were early adopters of digital pathology due to the valuable and multiparametric outputs offered by quantitative image analysis. It is the pharmaceutical and academic research market which appears to be the majority of users of digital pathology image analysis systems.
High-throughput tissue biomarker screening, particularly in the area of oncology, was bolstered by the introduction of digital pathology image analysis. TMAs, made by transferring cylindrical cores of tissue from paraffin-embedded donor blocks into recipient paraffin, can contain tens to thousands of tissue specimens.[ 138 ] TMA slides are probed using typical IHC or ISH techniques to allow biomarkers to be compared across all specimens simultaneously. TMAs save precious tissue and remove issues associated with slide-to-slide staining variability. TMA workflow tools available within many of today's digital pathology image analysis platforms complement this powerful technology by automating the segmentation and analysis of tissue cores, thus allowing biomarker expression to be scored quickly and objectively. TMAs are used to address a variety of different research questions: to investigate the state of the cancer proteome at different stages of tumorigenesis, to understand biochemical pathways involved in the development of different types of cancer, to identify potential new therapeutic targets, and to screen candidate prognostic or predictive biomarkers.
Within the field of immuno-oncology, researchers have made extensive use of tissue image analysis tools. For example, it has been shown that the number and location of CD8-positive lymphocytes is critical for measuring immune response in PD-L1-positive and PD-L1-negative non-small cell carcinoma samples via analysis with commercial software.[ 139 ] Utilizing TMAs of colonic adenocarcinomas, open-source software demonstrated its ability to analyze IHC staining for CD3, CD8, p53, and PD-L1.[ 140 ] In breast carcinoma TMAs, it was shown that analysis with a commercial software yielded superior results over manual scoring when evaluating the biomarker Ki-67, ER, PR, and HER2.[ 46 ]
While TMAs offer the advantage of being able to screen biomarker expression across large numbers of specimens simultaneously, the small TMA cores may not adequately capture information about tissue and biomarker heterogeneity. It is not uncommon in cancer to find significant regional heterogeneity in tumor biomarker expression within a single biopsy, and in some cases, such as with HER2 expression, this heterogeneity may impact patient outcomes.[ 141 , 142 ] Likewise, in immuno-oncology, lymphocytes may be unevenly distributed within the tumor microenvironment; therefore, a single field of view or biopsy core may not always be representative. Some modern digital pathology image analysis platforms provide tools to help researchers with these “big picture” questions. For example, biomarker heterogeneity can be visualized by creating a spatial plot of cell data and color-coding dots based on the level of biomarker expression [ Figure 4a ]. Researchers can easily identify “hot spots” of high (or low) expression across whole-slide tissues using these “heat map” views. Spatial plots can also be used to quantify the spatial distances between different cell types in the tissue, such as lymphocytes and tumor cells [ Figure 4b ]. In the latter instance, multiple biomarkers may be required to differentiate specific immune and tumor cell phenotypes. In instances where more than two markers are required, fluorescent whole-slide scanning or multispectral imaging platforms are employed in combination with compatible algorithms to facilitate multiplexed analysis.[ 143 ]

Digital pathology image analysis in spatial context reveal biomarker and cell heterogeneity. (a) The inset digital slide with DAB-stained biomarker (brown) was analyzed. Cells identified in the analysis were plotted spatially as a dot plot and each cell “dot” color coded according to the optical density of DAB stain in that cell. Cells in “cooler” colors (blues and greens) have lower stain optical density compared to cells in “warmer” colors (yellow, orange, and red). (b) Tumor cells and immune cells (DAB-positive) identified by image analysis were plotted spatially and analyzed to quantify immune cells within 30 μm of tumor cells (proximal immune cells). Tumor cells are colored blue, proximal immune cells are colored red, and nonproximal immune cells are green. The distance between tumor cells and proximal immune cells are recorded to create a histogram (inset, bottom right) and are connected by nearest neighbor lines in the dot plot
Digital pathology image analysis and related technologies are applicable to areas of research beyond oncology. Scientists interested in metabolic disease states, such as diabetes, may use image analysis to understand the metabolic state of the pancreas in animal models. In this instance, image analysis is used to measure overall expression of metabolic hormones, insulin, glucagon, and/or somatostatin and to quantify the relative number of cells that are secreting these hormones within the pancreatic islets [ Figure 5a ].[ 144 , 145 , 146 ] Changes to islets and pancreatic structure can occur as a result of drug toxicity as well. In this instance, machine learning-based tissue classification approaches are useful for evaluating the relative composition and morphology of the pancreas.[ 146 ] Neuroscientists interested in Alzheimer's disease have adopted digital pathology image analysis and stereological approaches to quantify density and size of beta-amyloid plaques in cortex and hippocampus [ Figure 5b ].[ 147 , 148 , 149 ] In sciatic and optical nerve, digital image analysis is used to calculate axon G-ratios, a measurement of myelination.[ 150 ] Digital pathology image analysis is also ideally suited for screening whole brain tissues for rare, but physiologically relevant events, such as microhemorrhages, which may be missed using conventional microscopy. Large brain sections must be mounted on large format slides, and the development of slide scanners that can handle larger formats has greatly expanded the utility of digital pathology image analysis within the field of neuroscience.

Digital pathology image analysis in the pancreas and brain. (a) Islets stained with antibodies against insulin (red stain) and glucagon (brown stain) in digital slides. Analysis shown bottom left quantifies number of islets that are of islet (orange area in markup) and number of cells that are positive for insulin (red cell markup), glucagon (green cell markup), both (yellow cell markup), or neither marker (white cell markup). (b) Identification of beta-amyloid plaques in brain sections. Slides are probed with antibodies against beta-amyloid (purple) and vessel endothelial marker (brown). Digital image analysis shown bottom right quantifies density, diameter, and area of vessels (red markup) and plaques (green markup), and colocalized area (yellow markup)
Within the drug development pipeline, pharmaceutical researchers utilize many of the same image analysis applications as their academic counterparts during the early phases of drug development. Downstream of discovery, digital image analysis can assist researchers and pathologist in preclinical testing and clinical trials [ Table 1 ]. Some of the quantitative algorithms that emerge from drug development research may transition into the clinic as companion diagnostics, thus closing the circle of technology adoption. While there are only a handful of complementary quantitative algorithms (e.g., HER2), there are no current examples where quantitative algorithms are required to interpret a companion biomarker. Nonetheless, promising findings in the area of immuno-oncology described above suggest that this area of image analysis is poised for significant growth in the coming decade.
Role of image analysis in different phases of drug development
Phase | Image analysis role |
---|---|
Discovery phase | • Quantitative analysis of target and pathway inhibition biomarkers using xenografts and models: Understand how the drug target and pathway biomarkers are regulated by drug concentration and dosing regimen |
• Measurement of target expression and specificity using TMAs and other high-throughput approaches | |
• Correlative analysis (e.g., efficacy) for identification of potential companion or complementary biomarkers based on animal models | |
Preclinical studies | • Animal models and safety assessment (non-GLP): Quantification of hypertrophy, steatosis, fibrosis, and other readouts using traditional image analysis approaches |
• Quantification of organ morphology using machine learning-based tissue classification (e.g., islets in pancreas and glomeruli in kidney) | |
• Additional studies in different animal models to identify potential clinical biomarkers | |
Clinical trials | • Analysis of biomarkers used for patient stratification and prediction of therapeutic response |
• Deep learning approaches to identify classifiers for patient stratification and prediction of therapeutic response |
TMA: Tissue microarray , GLP: Good laboratory practice
A VAILABLE T OOLS: A PPLICATION M ODULES VERSUS D EVELOPMENT E NVIRONMENT
There are numerous tools available to the pathologist and researcher to facilitate the quantitative analysis of histology-based images. These tools can be integrated with a digital pathology image management platform or as a separate software platform. Image analysis platforms are available from both commercial vendors and open-source software providers or as a service from a contract research organization. These service providers may use commercial software or proprietary image analysis tools. In general, there are two different types of image analysis platform tools: prebuilt application modules or development/toolboxes that enable the user to build a custom image analysis pipeline or workflow.
Prebuilt application modules are image analysis algorithms designed to analyze a specific biomarker localization or tissue morphological phenotype. These modules are typically designed for ease of use with a minimum set of adjustable parameters, though they can get quite complex for advanced applications such as multiplex assays. One example of a relatively simple assay is for a single compartment (nuclear) biomarker such as Ki67, a tissue biomarker for cellular proliferation. A nuclear module may include only a few user-adjustable parameters such as color selection (e.g., blue for hematoxylin and brown for DAB IHC stain), nuclear size ( a priori information to help algorithm segment nuclei), and intensity threshold (to identify Ki67-positive cells vs. Ki67-negative cells). The user may also be able to configure which image analysis features are extracted (e.g., number of total cells, positive cells, nuclei area, and nuclei diameter). An example of a more complex image analysis module is an algorithm to measure multiple biomarkers in a single image, i.e., multiplex assays. These are becoming common, especially for immune phenotyping in tissue. This multiplex module would need more user-adjustable parameters to address the segmentation of each individual biomarker. In the case of a multiplex brightfield assay, the user would need to define each color (for example, blue for hematoxylin, brown for CD4, yellow for CD8, and cyan for FoxP3). The user would also need to select the localization of each biomarker (nuclear, cytoplasm, and membrane), thresholds, etc. One can see how the number of user-configurable parameters quickly increases with these multiplex assays. Once configured, the settings can be saved, so that subsequent users can simply load the parameters for testing and running the assay on additional images. These two examples are for cellular localization of biomarkers, but there are numerous other examples of prebuilt image analysis modules such as measuring pancreatic islets, neurons, chromogenic or fluorescent ISH, microvessels, fat vacuoles, and many other applications.
A second approach for image analysis algorithms is the toolbox approach. The software includes a set of tools that are used to build a “script” to analyze each image. The tools are generally organized by different functions: color separation, preprocessing, object segmentation, postprocessing, and filtering. The user would develop the script to include the processing tools required for their specific application. For the Ki67 assay above, a script might include these steps:
- Color separation of blue (hematoxylin) and brown (DAB) to create two image masks (nuclei only and Ki67 only)
- Threshold probability map to identify nuclei or Ki67+ pixels and generate two image masks (nuclei only and Ki67 only)
- Object segmentation algorithm, e.g., watershed algorithm, to segment individual objects from image mask (nuclei and Ki67+ cells)
- Splitting algorithm to split touching nuclei
- Threshold algorithm to distinguish negative and positive Ki67 nuclei
- Postprocessing to remove any objects that are not real nuclei (e.g., based on size and shape)
- Export appropriate image analysis features, e.g., number of total cells and positive cells.
Once a script is developed for a specific assay, the script can be used by other users, so novice users would not necessarily need to know the details of the tools to run the script on their set of images.
There are pros and cons to each approach. The module approach is user-friendly and can often be configured by the novice in an interactive trial-by-error approach with a minimal amount of training. If an available module addresses a user's specific biomarker application, they can typically install and run the image analysis the same day. The toolbox approach requires some expertise to understand what each function does, why you would use each function, and what order to apply each function. The toolbox approach requires more time for training, but can offer more flexibility in optimizing a script to a specific biomarker assay or tissue type. In either case, the approach depends on each user's or group's ability and needs, so it is recommended to spend time to understand tissue image analysis needs and evaluate multiple options to determine which solution is best for each situation.
How to decide between open-source and commercial software
In the past decade, a vast array of digital pathology image analysis software has emerged. Software types differ based on area of application, ease of use, cost, and customer support, among other variables. Open-source software provides a collaborative option for image analysis, whereas commercial software provides more personalized image analysis choices. Each option presents certain advantages and disadvantages [ Table 2 ]. Given the number of open-source and commercial software solutions, it is often difficult to choose which tool is appropriate for a given task.
Pros and cons of open-source versus commercial digital image analysis software
Open source | Commercial | |
---|---|---|
Cost | Free | Moderate to expensive |
Technical support | Limited, provided by email and chat groups | Guaranteed support for customer issues |
License | No software license necessary, and installation and updates administered by user | Software license required; installation and updates administered or supported by vendor |
Viability | Life of software and new development at risk of open-source provider - potential short-term viability | Life of software and new development at risk of vendor - though typically longer-term viability |
Fixing issues | Rapid, collaborative response to troubleshoot malfunctions | Only vendor experts can troubleshoot malfunctions |
Software application training | Limited hands-on training, but online resources often exist | On-site and online training provided by vendor |
Image file compatibility | May be compatible with a variety of image formats | May have limited compatibility |
Analytic software ranges from relatively inexpensive, toolbox-like software for basic tissue color quantification to complex and costly model-based applications for tissue recognition and quantification.[ 151 ] Although current pathology training involves progressive exposure to software, advanced computational skills are not universal. The ideal digital image analysis software should include easy installation, user-friendly instructions and training, optimal speed and capacity, rapid and effective customer support, and an intuitive interface that is easy to use.[ 152 ] Important considerations for choosing digital pathology image analysis software are presented in Table 3 .
Important considerations for choosing digital pathology image analysis software
Consideration | Key question(s) |
---|---|
Application | Will the software be utilized for optimization of clinical/diagnostic workflow, basic research, or research in a regulated environment? |
User-friendliness | Is it easy and convenient to interact with the software interface? |
Training requirement | What level of expertise is required to operate the software? |
What duration of staff training will be required to effectively utilize the software? | |
Performance: Speed and capacity | What is the speed and capacity of the analyzer? |
How long does image processing take for a single image? Can the user increase throughput by utilizing distributed computing? | |
Resources | What additional resources (e.g., technologists, hardware, laboratory footprint, IT infrastructure, etc.,) are required for the optimal function? |
Technical support | Where is the nearest customer support location or time zone? |
In what manner, and how quickly do customer support personnel respond to issues? | |
Licensure | Is a paid license required? |
Per user, per instance, or per laboratory/research unit? | |
File compatibility | In what file format can data be exported? |
Is the software compatible with multiple image file formats from various whole-slide scanners? | |
Including bright-field and/or fluorescent scans? | |
Data storage | What is the average file size of an analysis run and data packages created? |
Cloud storage provided by vendor or own internal storage solution required? | |
Servicing | How are hardware and software malfunctions managed? |
How often are software updates required? | |
Cost-benefit analysis | How much will the image analysis solution cost? |
Can individual modules be acquired based on needs, or will a full package need to be | |
purchased? | |
What is the cost of servicing/updating the software? | |
What are the terms of the warranty? | |
What is the cost of all additional resources required for optimal use of the software? | |
After how much time will use of the analyzer result in a net gain? | |
Will there be enough use cases to justify investment into equipment, infrastructure and staff, or would outsourcing to a service provider be more economical? |
IT: Information technology
Commercial software can be expensive and requires dedicated training. It offers extensive technical support, which helps streamline issues that inevitably arise from frequent use of the software.[ 151 , 152 , 153 , 154 ] An open-source software offers a more cost-effective option for individuals who wish to become familiar with digital analyzers and for those who have infrequent or educational use of image analysis. Ultimately, the successful implementation of digital pathology imaging software will depend on the desired application and other variables. Finally, for infrequent utilization of image analysis, service providers are also available, enabling the usage of this technology without having to invest into own digital workflows, etc.
C ONCLUSION
The combination of image analysis software and pathology expertise provides an opportunity to transform a traditionally qualitative assessment to a quantitative analysis of complex biomarker expression, patterns, and tissue phenotypes. Image analysis and machine learning algorithms can be used to automatically identify tissue compartments of interest, segment individual cells, or anatomical features and categorize these features based on biomarker expression levels and localization. The research scientist and pathologist will continue to be instrumental in both the use and operation of image analysis workflows, which will continue to evolve and transform both preclinical and clinical biomarker research.
A BOUT THE D IGITAL P ATHOLOGY A SSOCIATION
The DPA was founded in 2009 and is a nonprofit organization comprised of pathologists, scientists, technologists, and industry representatives dedicated to advancing the field of digital pathology. The organization's mission is to facilitate education and awareness of digital pathology applications in health care and life sciences. The DPA's committees and task forces are dedicated to enhancing the field. The association collaborates with the FDA on equipment approvals and addressing technology regulations. The members are encouraged to share best practices and promote the use of the technology among colleagues to demonstrate efficiencies and share knowledge and its ultimate benefits to patient care. For more information, visit https://digitalpathologyassociation.org .
Financial support and sponsorship
Conflicts of interest.
There are no conflicts of interest.
Acknowledgments
The authors would like to thank Abbey Norris Kovacinski for her administrative support of this effort. The authors would also like to thank Dr. Navid Farahani for his thoughtful input on sections of this manuscript.
Available FREE in open access from: http://www.jpathinformatics.org/text.asp?2019/10/1/9/253721
R EFERENCES

An official website of the United States government
The .gov means it’s official. Federal government websites often end in .gov or .mil. Before sharing sensitive information, make sure you’re on a federal government site.
The site is secure. The https:// ensures that you are connecting to the official website and that any information you provide is encrypted and transmitted securely.
- Publications
- Account settings
- My Bibliography
- Collections
- Citation manager
Save citation to file
Email citation, add to collections.
- Create a new collection
- Add to an existing collection
Add to My Bibliography
Your saved search, create a file for external citation management software, your rss feed.
- Search in PubMed
- Search in NLM Catalog
- Add to Search
Basic image analysis and manipulation in ImageJ
Affiliation.
- 1 Baylor College of Medicine, Houston, Texas, USA.
- PMID: 23547012
- DOI: 10.1002/0471142727.mb1415s102
Image analysis methods have been developed to provide quantitative assessment of microscopy data. In this unit, basic aspects of image analysis are outlined, including software installation, data import, image processing functions, and analytical tools that can be used to extract information from microscopy data using ImageJ. Step-by-step protocols for analyzing objects in a fluorescence image and extracting information from two-color tissue images collected by bright-field microscopy are included.
PubMed Disclaimer
Similar articles
- Basic Image Analysis and Manipulation in ImageJ/Fiji. Stossi F, Singh PK. Stossi F, et al. Curr Protoc. 2023 Jul;3(7):e849. doi: 10.1002/cpz1.849. Curr Protoc. 2023. PMID: 37498127
- Seeing Is Believing: Quantifying Is Convincing: Computational Image Analysis in Biology. Sbalzarini IF. Sbalzarini IF. Adv Anat Embryol Cell Biol. 2016;219:1-39. doi: 10.1007/978-3-319-28549-8_1. Adv Anat Embryol Cell Biol. 2016. PMID: 27207361 Review.
- Analysis of nuclear organization with TANGO, software for high-throughput quantitative analysis of 3D fluorescence microscopy images. Ollion J, Cochennec J, Loll F, Escudé C, Boudier T. Ollion J, et al. Methods Mol Biol. 2015;1228:203-22. doi: 10.1007/978-1-4939-1680-1_16. Methods Mol Biol. 2015. PMID: 25311132
- Using CellX to quantify intracellular events. Mayer C, Dimopoulos S, Rudolf F, Stelling J. Mayer C, et al. Curr Protoc Mol Biol. 2013;Chapter 14:Unit 14.22.. doi: 10.1002/0471142727.mb1422s101. Curr Protoc Mol Biol. 2013. PMID: 23288460
- Digital autofocus methods for automated microscopy. Shen F, Hodgson L, Hahn K. Shen F, et al. Methods Enzymol. 2006;414:620-32. doi: 10.1016/S0076-6879(06)14032-X. Methods Enzymol. 2006. PMID: 17110214 Review.
- LPA 3 Receptor Phosphorylation Sites: Roles in Signaling and Internalization. Solís KH, Romero-Ávila MT, Rincón-Heredia R, García-Sáinz JA. Solís KH, et al. Int J Mol Sci. 2024 May 18;25(10):5508. doi: 10.3390/ijms25105508. Int J Mol Sci. 2024. PMID: 38791546 Free PMC article.
- DNA methylation analysis of floral parts revealed dynamic changes during the development of homostylous Fagopyrum tataricum and heterostylous F. esculentum flowers. Sala-Cholewa K, Tomasiak A, Nowak K, Piński A, Betekhtin A. Sala-Cholewa K, et al. BMC Plant Biol. 2024 May 23;24(1):448. doi: 10.1186/s12870-024-05162-w. BMC Plant Biol. 2024. PMID: 38783206 Free PMC article.
- Endomucin selectively regulates vascular endothelial growth factor receptor-2 endocytosis through its interaction with AP2. Cano I, Wild M, Gupta U, Chaudhary S, Ng YSE, Saint-Geniez M, D'Amore PA, Hu Z. Cano I, et al. Cell Commun Signal. 2024 Apr 11;22(1):225. doi: 10.1186/s12964-024-01606-w. Cell Commun Signal. 2024. PMID: 38605348 Free PMC article.
- Absence of the Klotho Function Causes Cornea Degeneration with Specific Features Resembling Fuchs Endothelial Corneal Dystrophy and Bullous Keratopathy. Wu CY, Song DF, Chen ZJ, Hu CS, Lin DP, Chang HH. Wu CY, et al. Biology (Basel). 2024 Feb 20;13(3):133. doi: 10.3390/biology13030133. Biology (Basel). 2024. PMID: 38534403 Free PMC article.
- High-Yield Expression and Purification of Scygonadin, an Antimicrobial Peptide, Using the Small Metal-Binding Protein SmbP. Gomez-Lugo JJ, Casillas-Vega NG, Gomez-Loredo A, Balderas-Renteria I, Zarate X. Gomez-Lugo JJ, et al. Microorganisms. 2024 Jan 28;12(2):278. doi: 10.3390/microorganisms12020278. Microorganisms. 2024. PMID: 38399682 Free PMC article.
- Search in MeSH
LinkOut - more resources
Full text sources, other literature sources.
- The Lens - Patent Citations
- scite Smart Citations
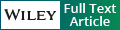
- Citation Manager
NCBI Literature Resources
MeSH PMC Bookshelf Disclaimer
The PubMed wordmark and PubMed logo are registered trademarks of the U.S. Department of Health and Human Services (HHS). Unauthorized use of these marks is strictly prohibited.
- Privacy Policy

Home » Research Methodology – Types, Examples and writing Guide
Research Methodology – Types, Examples and writing Guide
Table of Contents

Research Methodology
Definition:
Research Methodology refers to the systematic and scientific approach used to conduct research, investigate problems, and gather data and information for a specific purpose. It involves the techniques and procedures used to identify, collect , analyze , and interpret data to answer research questions or solve research problems . Moreover, They are philosophical and theoretical frameworks that guide the research process.
Structure of Research Methodology
Research methodology formats can vary depending on the specific requirements of the research project, but the following is a basic example of a structure for a research methodology section:
I. Introduction
- Provide an overview of the research problem and the need for a research methodology section
- Outline the main research questions and objectives
II. Research Design
- Explain the research design chosen and why it is appropriate for the research question(s) and objectives
- Discuss any alternative research designs considered and why they were not chosen
- Describe the research setting and participants (if applicable)
III. Data Collection Methods
- Describe the methods used to collect data (e.g., surveys, interviews, observations)
- Explain how the data collection methods were chosen and why they are appropriate for the research question(s) and objectives
- Detail any procedures or instruments used for data collection
IV. Data Analysis Methods
- Describe the methods used to analyze the data (e.g., statistical analysis, content analysis )
- Explain how the data analysis methods were chosen and why they are appropriate for the research question(s) and objectives
- Detail any procedures or software used for data analysis
V. Ethical Considerations
- Discuss any ethical issues that may arise from the research and how they were addressed
- Explain how informed consent was obtained (if applicable)
- Detail any measures taken to ensure confidentiality and anonymity
VI. Limitations
- Identify any potential limitations of the research methodology and how they may impact the results and conclusions
VII. Conclusion
- Summarize the key aspects of the research methodology section
- Explain how the research methodology addresses the research question(s) and objectives
Research Methodology Types
Types of Research Methodology are as follows:
Quantitative Research Methodology
This is a research methodology that involves the collection and analysis of numerical data using statistical methods. This type of research is often used to study cause-and-effect relationships and to make predictions.
Qualitative Research Methodology
This is a research methodology that involves the collection and analysis of non-numerical data such as words, images, and observations. This type of research is often used to explore complex phenomena, to gain an in-depth understanding of a particular topic, and to generate hypotheses.
Mixed-Methods Research Methodology
This is a research methodology that combines elements of both quantitative and qualitative research. This approach can be particularly useful for studies that aim to explore complex phenomena and to provide a more comprehensive understanding of a particular topic.
Case Study Research Methodology
This is a research methodology that involves in-depth examination of a single case or a small number of cases. Case studies are often used in psychology, sociology, and anthropology to gain a detailed understanding of a particular individual or group.
Action Research Methodology
This is a research methodology that involves a collaborative process between researchers and practitioners to identify and solve real-world problems. Action research is often used in education, healthcare, and social work.
Experimental Research Methodology
This is a research methodology that involves the manipulation of one or more independent variables to observe their effects on a dependent variable. Experimental research is often used to study cause-and-effect relationships and to make predictions.
Survey Research Methodology
This is a research methodology that involves the collection of data from a sample of individuals using questionnaires or interviews. Survey research is often used to study attitudes, opinions, and behaviors.
Grounded Theory Research Methodology
This is a research methodology that involves the development of theories based on the data collected during the research process. Grounded theory is often used in sociology and anthropology to generate theories about social phenomena.
Research Methodology Example
An Example of Research Methodology could be the following:
Research Methodology for Investigating the Effectiveness of Cognitive Behavioral Therapy in Reducing Symptoms of Depression in Adults
Introduction:
The aim of this research is to investigate the effectiveness of cognitive-behavioral therapy (CBT) in reducing symptoms of depression in adults. To achieve this objective, a randomized controlled trial (RCT) will be conducted using a mixed-methods approach.
Research Design:
The study will follow a pre-test and post-test design with two groups: an experimental group receiving CBT and a control group receiving no intervention. The study will also include a qualitative component, in which semi-structured interviews will be conducted with a subset of participants to explore their experiences of receiving CBT.
Participants:
Participants will be recruited from community mental health clinics in the local area. The sample will consist of 100 adults aged 18-65 years old who meet the diagnostic criteria for major depressive disorder. Participants will be randomly assigned to either the experimental group or the control group.
Intervention :
The experimental group will receive 12 weekly sessions of CBT, each lasting 60 minutes. The intervention will be delivered by licensed mental health professionals who have been trained in CBT. The control group will receive no intervention during the study period.
Data Collection:
Quantitative data will be collected through the use of standardized measures such as the Beck Depression Inventory-II (BDI-II) and the Generalized Anxiety Disorder-7 (GAD-7). Data will be collected at baseline, immediately after the intervention, and at a 3-month follow-up. Qualitative data will be collected through semi-structured interviews with a subset of participants from the experimental group. The interviews will be conducted at the end of the intervention period, and will explore participants’ experiences of receiving CBT.
Data Analysis:
Quantitative data will be analyzed using descriptive statistics, t-tests, and mixed-model analyses of variance (ANOVA) to assess the effectiveness of the intervention. Qualitative data will be analyzed using thematic analysis to identify common themes and patterns in participants’ experiences of receiving CBT.
Ethical Considerations:
This study will comply with ethical guidelines for research involving human subjects. Participants will provide informed consent before participating in the study, and their privacy and confidentiality will be protected throughout the study. Any adverse events or reactions will be reported and managed appropriately.
Data Management:
All data collected will be kept confidential and stored securely using password-protected databases. Identifying information will be removed from qualitative data transcripts to ensure participants’ anonymity.
Limitations:
One potential limitation of this study is that it only focuses on one type of psychotherapy, CBT, and may not generalize to other types of therapy or interventions. Another limitation is that the study will only include participants from community mental health clinics, which may not be representative of the general population.
Conclusion:
This research aims to investigate the effectiveness of CBT in reducing symptoms of depression in adults. By using a randomized controlled trial and a mixed-methods approach, the study will provide valuable insights into the mechanisms underlying the relationship between CBT and depression. The results of this study will have important implications for the development of effective treatments for depression in clinical settings.
How to Write Research Methodology
Writing a research methodology involves explaining the methods and techniques you used to conduct research, collect data, and analyze results. It’s an essential section of any research paper or thesis, as it helps readers understand the validity and reliability of your findings. Here are the steps to write a research methodology:
- Start by explaining your research question: Begin the methodology section by restating your research question and explaining why it’s important. This helps readers understand the purpose of your research and the rationale behind your methods.
- Describe your research design: Explain the overall approach you used to conduct research. This could be a qualitative or quantitative research design, experimental or non-experimental, case study or survey, etc. Discuss the advantages and limitations of the chosen design.
- Discuss your sample: Describe the participants or subjects you included in your study. Include details such as their demographics, sampling method, sample size, and any exclusion criteria used.
- Describe your data collection methods : Explain how you collected data from your participants. This could include surveys, interviews, observations, questionnaires, or experiments. Include details on how you obtained informed consent, how you administered the tools, and how you minimized the risk of bias.
- Explain your data analysis techniques: Describe the methods you used to analyze the data you collected. This could include statistical analysis, content analysis, thematic analysis, or discourse analysis. Explain how you dealt with missing data, outliers, and any other issues that arose during the analysis.
- Discuss the validity and reliability of your research : Explain how you ensured the validity and reliability of your study. This could include measures such as triangulation, member checking, peer review, or inter-coder reliability.
- Acknowledge any limitations of your research: Discuss any limitations of your study, including any potential threats to validity or generalizability. This helps readers understand the scope of your findings and how they might apply to other contexts.
- Provide a summary: End the methodology section by summarizing the methods and techniques you used to conduct your research. This provides a clear overview of your research methodology and helps readers understand the process you followed to arrive at your findings.
When to Write Research Methodology
Research methodology is typically written after the research proposal has been approved and before the actual research is conducted. It should be written prior to data collection and analysis, as it provides a clear roadmap for the research project.
The research methodology is an important section of any research paper or thesis, as it describes the methods and procedures that will be used to conduct the research. It should include details about the research design, data collection methods, data analysis techniques, and any ethical considerations.
The methodology should be written in a clear and concise manner, and it should be based on established research practices and standards. It is important to provide enough detail so that the reader can understand how the research was conducted and evaluate the validity of the results.
Applications of Research Methodology
Here are some of the applications of research methodology:
- To identify the research problem: Research methodology is used to identify the research problem, which is the first step in conducting any research.
- To design the research: Research methodology helps in designing the research by selecting the appropriate research method, research design, and sampling technique.
- To collect data: Research methodology provides a systematic approach to collect data from primary and secondary sources.
- To analyze data: Research methodology helps in analyzing the collected data using various statistical and non-statistical techniques.
- To test hypotheses: Research methodology provides a framework for testing hypotheses and drawing conclusions based on the analysis of data.
- To generalize findings: Research methodology helps in generalizing the findings of the research to the target population.
- To develop theories : Research methodology is used to develop new theories and modify existing theories based on the findings of the research.
- To evaluate programs and policies : Research methodology is used to evaluate the effectiveness of programs and policies by collecting data and analyzing it.
- To improve decision-making: Research methodology helps in making informed decisions by providing reliable and valid data.
Purpose of Research Methodology
Research methodology serves several important purposes, including:
- To guide the research process: Research methodology provides a systematic framework for conducting research. It helps researchers to plan their research, define their research questions, and select appropriate methods and techniques for collecting and analyzing data.
- To ensure research quality: Research methodology helps researchers to ensure that their research is rigorous, reliable, and valid. It provides guidelines for minimizing bias and error in data collection and analysis, and for ensuring that research findings are accurate and trustworthy.
- To replicate research: Research methodology provides a clear and detailed account of the research process, making it possible for other researchers to replicate the study and verify its findings.
- To advance knowledge: Research methodology enables researchers to generate new knowledge and to contribute to the body of knowledge in their field. It provides a means for testing hypotheses, exploring new ideas, and discovering new insights.
- To inform decision-making: Research methodology provides evidence-based information that can inform policy and decision-making in a variety of fields, including medicine, public health, education, and business.
Advantages of Research Methodology
Research methodology has several advantages that make it a valuable tool for conducting research in various fields. Here are some of the key advantages of research methodology:
- Systematic and structured approach : Research methodology provides a systematic and structured approach to conducting research, which ensures that the research is conducted in a rigorous and comprehensive manner.
- Objectivity : Research methodology aims to ensure objectivity in the research process, which means that the research findings are based on evidence and not influenced by personal bias or subjective opinions.
- Replicability : Research methodology ensures that research can be replicated by other researchers, which is essential for validating research findings and ensuring their accuracy.
- Reliability : Research methodology aims to ensure that the research findings are reliable, which means that they are consistent and can be depended upon.
- Validity : Research methodology ensures that the research findings are valid, which means that they accurately reflect the research question or hypothesis being tested.
- Efficiency : Research methodology provides a structured and efficient way of conducting research, which helps to save time and resources.
- Flexibility : Research methodology allows researchers to choose the most appropriate research methods and techniques based on the research question, data availability, and other relevant factors.
- Scope for innovation: Research methodology provides scope for innovation and creativity in designing research studies and developing new research techniques.
Research Methodology Vs Research Methods
Research Methodology | Research Methods |
---|---|
Research methodology refers to the philosophical and theoretical frameworks that guide the research process. | refer to the techniques and procedures used to collect and analyze data. |
It is concerned with the underlying principles and assumptions of research. | It is concerned with the practical aspects of research. |
It provides a rationale for why certain research methods are used. | It determines the specific steps that will be taken to conduct research. |
It is broader in scope and involves understanding the overall approach to research. | It is narrower in scope and focuses on specific techniques and tools used in research. |
It is concerned with identifying research questions, defining the research problem, and formulating hypotheses. | It is concerned with collecting data, analyzing data, and interpreting results. |
It is concerned with the validity and reliability of research. | It is concerned with the accuracy and precision of data. |
It is concerned with the ethical considerations of research. | It is concerned with the practical considerations of research. |
About the author
Muhammad Hassan
Researcher, Academic Writer, Web developer
You may also like

Tables in Research Paper – Types, Creating Guide...

Research Topics – Ideas and Examples

Evaluating Research – Process, Examples and...

Research Paper Title – Writing Guide and Example

Research Gap – Types, Examples and How to...

Limitations in Research – Types, Examples and...
Thank you for visiting nature.com. You are using a browser version with limited support for CSS. To obtain the best experience, we recommend you use a more up to date browser (or turn off compatibility mode in Internet Explorer). In the meantime, to ensure continued support, we are displaying the site without styles and JavaScript.
- View all journals
- Explore content
- About the journal
- Publish with us
- Sign up for alerts
- Published: 03 July 2024
Electron holography observation of individual ferrimagnetic lattice planes
- Toshiaki Tanigaki ORCID: orcid.org/0000-0001-9703-9427 1 ,
- Tetsuya Akashi 1 ,
- Takaho Yoshida 1 ,
- Ken Harada 2 ,
- Kazuo Ishizuka 3 ,
- Masahiko Ichimura 1 ,
- Kazutaka Mitsuishi ORCID: orcid.org/0000-0002-9361-4057 4 ,
- Yasuhide Tomioka 5 ,
- Xiuzhen Yu ORCID: orcid.org/0000-0003-3136-7289 2 ,
- Daisuke Shindo 2 , 6 ,
- Yoshinori Tokura ORCID: orcid.org/0000-0002-2732-4983 2 , 7 ,
- Yasukazu Murakami ORCID: orcid.org/0000-0003-0735-5405 8 &
- Hiroyuki Shinada 1
Nature ( 2024 ) Cite this article
Metrics details
- Materials science
- Transmission electron microscopy
Atomic-scale observations of a specific local area would be considerably beneficial when exploring new fundamental materials and devices. The development of hardware-type aberration correction 1 , 2 in electron microscopy has enabled local structural observations with atomic resolution 3 , 4 , 5 as well as chemical and vibration analysis 6 , 7 , 8 . In magnetic imaging, however, atomic-level spin configurations are analysed by electron energy-loss spectroscopy by placing samples in strong magnetic fields 9 , 10 , 11 , which destroy the nature of the magnetic ordering in the samples. Although magnetic-field-free observations can visualize the intrinsic magnetic fields of an antiferromagnet by unit-cell averaging 12 , directly observing the magnetic field of an individual atomic layer of a non-uniform structure is challenging. Here we report that the magnetic fields of an individual lattice plane inside materials with a non-uniform structure can be observed under magnetic-field-free conditions by electron holography with a hardware-type aberration corrector assisted by post-digital aberration correction. The magnetic phases of the net magnetic moments of (111) lattice planes formed by opposite spin orderings between Fe 3+ and Mo 5+ in a ferrimagnetic double-perovskite oxide (Ba 2 FeMoO 6 ) were successfully observed. This result opens the door to direct observations of the magnetic lattice in local areas, such as interfaces and grain boundaries, in many materials and devices.
This is a preview of subscription content, access via your institution
Access options
Access Nature and 54 other Nature Portfolio journals
Get Nature+, our best-value online-access subscription
24,99 € / 30 days
cancel any time
Subscribe to this journal
Receive 51 print issues and online access
185,98 € per year
only 3,65 € per issue
Buy this article
- Purchase on Springer Link
- Instant access to full article PDF
Prices may be subject to local taxes which are calculated during checkout
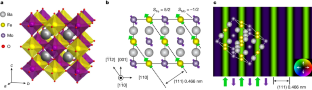
Data availability
The data that support the findings of this study are available from the corresponding author upon request and with the permission of the Japanese government sponsors who funded the work.
Code availability
The code used for the analyses is available from the corresponding author upon request and with the permission of the Japanese government sponsors that funded the work.
Haider, M. et al. Electron microscopy image enhanced. Nature 392 , 768–769 (1998).
Article ADS CAS Google Scholar
Batson, P. E., Dellby, N. & Krivanek, O. L. Sub-ångstrom resolution using aberration corrected electron optics. Nature 418 , 617–620 (2002).
Article ADS CAS PubMed Google Scholar
Jia, C. L., Lentzen, M. & Urban, K. Atomic-resolution imaging of oxygen in perovskite ceramics. Science 299 , 870–873 (2003).
Nellist, P. D. et al. Direct sub-angstrom imaging of a crystal lattice. Science 305 , 1741 (2004).
Article CAS PubMed Google Scholar
Shibata, N. et al. Observation of rare-earth segregation in silicon nitride ceramics at subnanometre dimensions. Nature 428 , 730–733 (2004).
Muller, D. A. et al. Atomic-scale chemical imaging of composition and bonding by aberration-corrected microscopy. Science 319 , 1073–1076 (2008).
Suenaga, K. & Koshino, M. Atom-by-atom spectroscopy at graphene edge. Nature 468 , 1088–1090 (2010).
Hage, F. S., Radtke, G., Kepaptsoglou, D. M., Lazzeri, M. & Ramasse, Q. M. Single-atom vibrational spectroscopy in the scanning transmission electron microscope. Science 367 , 1124–1127 (2020).
Rusz, J. et al. Magnetic measurements with atomic-plane resolution. Nat. Commun. 7 , 12672 (2016).
Article ADS CAS PubMed PubMed Central Google Scholar
Idrobo, J. C. et al. Detecting magnetic ordering with atomic size electron probes. Adv. Struct. Chem. Imaging 2 , 5 (2016).
Article Google Scholar
Wang, Z. et al. Atomic scale imaging of magnetic circular chichroism by achromatic electron microscopy. Nat. Mater. 21 , 221–225 (2018).
Article ADS Google Scholar
Kohno, Y., Seki, T., Findlay, S. D., Ikuhara, Y. & Shibata, N. Real-space visualization of intrinsic magnetic fields of an antiferromagnet. Nature 602 , 234–239 (2022).
Tokura, Y. & Nagaosa, N. Orbital physics in transition-metal oxides. Science 288 , 462–468 (2000).
Brinkman, A. et al. Magnetic effects at the interface between non-magnetic oxides. Nat. Mater. 6 , 493–496 (2007).
Liu, L. et al. Spin-torque switching with the giant spin Hall effect of tantalum. Science 336 , 555–558 (2012).
Lee, J.-S. et al. Titanium d xy ferromagnetism at the LaAlO 3 /SrTiO 3 interface. Nat. Mater. 12 , 703–706 (2013).
Mühlbauer, S. et al. Skyrmion lattice in a chiral magnet. Science 323 , 915–919 (2009).
Article ADS PubMed Google Scholar
Zhu, X. H. et al. Measuring spectroscopy and magnetism of extracted and intracellular magnetosomes using soft X-ray ptychography. Proc. Natl Acad. Sci. USA 113 , E8219–E8227 (2016).
Article CAS PubMed PubMed Central Google Scholar
Wiesendanger, R. et al. Topographic and magnetic-sensitive scanning tunneling microscopy study of magnetite. Science 255 , 583–586 (1992).
Heinze, S. et al. Real-space imaging of two-dimensional antiferromagnetism on the atomic scale. Science 294 , 1488–1495 (2001).
Google Scholar
Kaiser, U., Schwarz, A. & Wiesendanger, R. Magnetic exchange force microscopy with atomic resolution. Nature 446 , 522–525 (2007).
Tanigaki, T. et al. Magnetic field observations in CoFeB/Ta layers with 0.67-nm resolution by electron holography. Sci. Rep. 7 , 16598 (2017).
Article ADS PubMed PubMed Central Google Scholar
Schattschneider, P. et al. Detection of magnetic circular dichroism using a transmission electron microscope. Nature 441 , 486–488 (2006).
Shirota, K., Yonezawa, A., Shibatomi, K. & Yanaka, T. Ferro-magnetic material observation lens system for CTEM with a eucentric goniometer. J. Electron Microsc. 25 , 303–304 (1976).
Harada, K. et al. Real-time observation of vortex lattices in a superconductor by electron microscopy. Nature 360 , 51–53 (1992).
Schofield, M. A., Beleggia, M., Zhu, Y. & Pozzi, G. Characterization of JEOL 2100 F Lorentz-TEM for low-magnification electron holography and magnetic imaging. Ultramicroscopy 108 , 625–634 (2008).
Dunin-Borkowski, R. E. et al. Opportunities for chromatic aberration corrected high-resolution transmission electron microscopy, Lorentz microscopy and electron holography of magnetic minerals. Microsc. Microanal. 18 , 1708–1709 (2012).
Snoeck, E. et al. Off-axial aberration correction using a B-COR for Lorentz and HREM modes. Microsc. Microanal. 20 , 932–933 (2014).
O’Shea, K. J. et al. Nanoscale mapping of the magnetic properties of (111)-oriented La 0.67 Sr 0.33 MnO 3 . Nano Lett. 15 , 5868–5874 (2015).
Gatel, C. et al. Size-specific spin configurations in single iron nanomagnet: from flower to exotic vortices. Nano Lett. 15 , 6952–6957 (2015).
Nagai, T., Kimoto, K., Inoke, K. & Takeguchi, M. Real-space observation of nanoscale magnetic phase separation in dysprosium by aberration-corrected Lorentz microscopy. Phys. Rev. B 96 , 100405 (2017).
Tanigaki, T., Akashi, T., Takahashi, Y., Kawasaki, T. & Shinada, H. Quest for ultimate resolution using coherent electron waves: an aberration-corrected high-voltage electron microscope. Adv. Imaging Electron Phys. 198 , 69–125 (2016).
Shibata, N. et al. Atomic resolution electron microscopy in a magnetic field free environment. Nat. Commun. 10 , 2308 (2019).
Akashi, T. et al. Aberration corrected 1.2-MV cold field-emission transmission electron microscope with a sub-50-pm resolution. Appl. Phys. Lett. 106 , 074101 (2015).
Kobayashi, K.-I., Kimura, T., Sawada, H., Terakura, K. & Tokura, Y. Room-temperature magnetoresistance in an oxide material with an ordered double-perovskite structure. Nature 395 , 677–680 (1998).
Tomioka, Y. et al. Magnetic and electronic properties of a single crystal of ordered double perovskite Sr 2 FeMoO 6 . Phys. Rev. B 61 , 442–427 (2000).
Kim, S. B., Lee, B. W. & Kim, C. S. Neutron and Mössbauer studies of the double perovskite A 2 FeMoO 6 ( A = Sr and Ba). J. Magn. Magn. Mater. 242–245 , 747–750 (2002).
Yu, X. et al. TEM study of the influence of antisite defects on magnetic domain structures in double perovskite Ba 2 FeMoO 6 . J. Electron Microsc. 54 , 61–65 (2005).
Article CAS Google Scholar
Sahnoun, O., Bouhani-Benziane, H., Sahnoun, M. & Driz, M. Magnetic and thermoelectric properties of ordered double perovskite Ba 2 FeMoO 6 . J Alloy. Compd. 714 , 704–708 (2017).
Tamura, T., Kimura, Y. & Takai, Y. Development of a real-time wave field reconstruction TEM system (I): incorporation of an auto focus tracking system. Microscopy 66 , 172–181 (2017).
CAS PubMed Google Scholar
Tonomura, A. Electron-holographic interference microscopy. Adv. Phys. 41 , 59–103 (1992).
Harada, K., Tonomura, A., Togawa, Y., Akashi, T. & Matsuda, T. Double-biprism electron interferometry. Appl. Phys. Lett. 84 , 3229–3231 (2004).
Voelkl, E. & Tang, D. Approaching routine 2π/1000 phase resolution for off-axis type holography. Ultramicroscopy 110 , 447–459 (2010).
Suzuki, T. et al. Improvement of the accuracy of phase observation by modification of phase-shifting electron holography. Ultramicroscopy 118 , 21–25 (2012).
Boureau, V. et al. High-sensitivity mapping of magnetic induction fields with nanometer-scale resolution: comparison of off-axis electron holography and pixelated differential phase contrast. J. Phys. D: Appl. Phys. 54 , 085001 (2021).
Tonomura, A. Electron Holography (Springer, 1999).
Dunin-Borkowski, R. E. et al. Magnetic microstructure of magnetotactic bacteria by electron holography. Science 282 , 1868–1870 (1998).
Murakami, Y., Yoo, J. H., Shindo, D., Atou, T. & Kikuchi, M. Magnetization distribution in the mixed-phase state of hole-doped manganites. Nature 423 , 965–968 (2003).
Kohn, A., Petford-Long, A. K. & Anthony, T. C. Magnetic potential in patterned materials determined using energy-dependent Lorentz phase microscopy. Phys. Rev. B 72 , 014444 (2005).
Genz, F., Niermann, T., Buijsse, B., Freitag, B. & Lehmann, M. Advanced double-biprism holography with atomic resolution. Ultramicroscopy 147 , 33–43 (2014).
Taniguchi, Y., Takai, Y., Ikuta, T. & Shimizu, R. Correction of spherical aberration in HREM image using defocus-modulation image processing. J. Electron Microsc. 41 , 21–29 (1992).
Mayer, R. R., Kirkland, A. I. & Saxton, W. O. A new method for the determination of the wave aberration function for high resolution TEM 1. Measurement of the symmetric aberrations. Ultramicroscopy 92 , 89–109 (2002).
Brigham, E. O. The Fast Fourier Transform (Prentice-Hall, 1974).
Ishizuka, K. & Uyeda, N. A new theoretical and practical approach to the multislice method. Acta Crystallogr. Sect. A: Found. Adv. A33 , 740–749 (1977).
Anisimov, V. I., Zaanen, J. & Andersen, O. K. Band theory and Mott insulators: Hubbard U instead of Stoner I . Phys. Rev. B 44 , 943–954 (1991).
Kresse, G. & Furthmuller, J. Efficient iterative schemes for ab initio total-energy calculations using a plane-wave basis set. Phys. Rev. B 54 , 11169–11186 (1996).
Dunin-Borkowski, R. E., McCartney, M. R., Smith, D. J. & Parkin, S. S. P. Towards quantitative electron holography of magnetic thin films using in situ magnetization reversal. Ultramicroscopy 74 , 61–73 (1998).
Mansuripur, M. Computation of electron-diffraction patterns in Lorentz electron microscopy of thin magnetic films. J. Appl. Phys. 69 , 2455–2464 (1991).
Mansuripur, M. Computation of electron-diffraction patterns in Lorentz electron microscopy of thin magnetic films (abstract). J. Appl. Phys. 69 , 5890 (1991).
Beleggia, M., Fazzini, P. F. & Pozzi, G. A Fourier approach to fields and electron optical phase-shifts calculations. Ultramicroscopy 96 , 93–103 (2003).
Download references
Acknowledgements
This research was supported by a grant from the Japan Society for the Promotion of Science (JSPS) through the ‘Funding Program for World-Leading Innovative R&D on Science and Technology (FIRST Program)’ initiated by the Council for Science, Technology, and Innovation and by a Japan Science and Technology Agency (JST) CREST programme (Grant No. JPMJCR 1664). X.Z.Y. was supported in part by a grant-in-aid for scientific research (A) (Grant No. 19H00660) from JSPS and a JST CREST programme (Grant No. JPMJCR 20T1). Y. Tokura was supported in part by a grant-in-aid for scientific research (S) (Grant No. 23H05431) from JSPS and a JST CREST programme (Grant No. JPMJCR1874).
Author information
Authors and affiliations.
Research & Development Group, Hitachi, Ltd., Hatoyama, Japan
Toshiaki Tanigaki, Tetsuya Akashi, Takaho Yoshida, Masahiko Ichimura & Hiroyuki Shinada
RIKEN Center for Emergent Matter Science (CEMS), Wako, Japan
Ken Harada, Xiuzhen Yu, Daisuke Shindo & Yoshinori Tokura
HREM Research Inc., Matsukazedai, Japan
Kazuo Ishizuka
National Institute for Materials Science, Tsukuba, Japan
Kazutaka Mitsuishi
National Institute of Advanced Industrial Science and Technology (AIST), Tsukuba, Japan
Yasuhide Tomioka
Institute of Multidisciplinary Research for Advanced Materials, Tohoku University, Sendai, Japan
Daisuke Shindo
Department of Applied Physics and Tokyo College, The University of Tokyo, Tokyo, Japan
Yoshinori Tokura
Department of Applied Quantum Physics and Nuclear Engineering, Kyushu University, Fukuoka, Japan
Yasukazu Murakami
You can also search for this author in PubMed Google Scholar
Contributions
T.T., T.A., K.H., Y.M. and H.S. conceived and designed the experiments. T.T., T.A., T.Y. and Y.M. performed the experiments and carried out the analysis. T.T., T.Y., K.I., M.I. and K.M. performed the simulations. Y. Tomioka, X.Z.Y. and Y. Tokura contributed to materials design and synthesized the single crystal. All authors discussed the results and commented on the manuscript.
Corresponding author
Correspondence to Toshiaki Tanigaki .
Ethics declarations
Competing interests.
The authors declare no competing interests.
Peer review
Peer review information.
Nature thanks Xiaoyan Zhong and the other, anonymous, reviewer(s) for their contribution to the peer review of this work. Peer reviewer reports are available.
Additional information
Publisher’s note Springer Nature remains neutral with regard to jurisdictional claims in published maps and institutional affiliations.
Extended data figures and tables
Extended data fig. 1 experimental setup for high-resolution magnetic-field observation and transmission electron microscopic cross-sectional image of the observed sample..
a , Schematic of electron-holography experiment. An 1.2-MeV coherent electron wave passes through the sample (object wave) and vacuum (reference wave). A high-resolution object wave formed by the aberration-corrected imaging system is overlapped with the reference wave by a biprism, and an electron hologram is formed at the image plane, where a direct electron-detection camera is placed. To separate the electrostatic and magnetic phases, pairs of electron holograms with opposite directional magnetizations in the sample are acquired. The magnetization direction in the sample is reversed by using a pulsed magnetic field generated by coils placed near two sides of the sample. b , Schematic of the rectangular sample used to create [ \(\bar{1}\bar{1}\) 2] or [11 \(\bar{2}\) ] directional magnetization along the (111) lattice plane. c , Full view of the cross section. The cross-sectional thin TEM sample of the area observed by electron holography was fabricated after the holography experiment. Carbon is deposited as a protection layer in the sample preparation for cross-sectional observation. d , Enlarged image of the left end of c . The black arrow indicates the observation area subjected to the high-resolution analysis of the magnetic field.
Extended Data Fig. 2 Defocus, astigmatism, and spherical aberration corrections by electron holography.
Reconstructed amplitude ( a ) and phase ( b ) images with spatial frequency up to 1/0.23 nm −1 . c , Fast Fourier transform (FFT) image of the amplitude image with digital defocus of −200 nm. The elongated ring pattern indicates two-fold astigmatism (A1). d , Thon diagram of the amplitude of the reconstructed wave. Minimum amplitude at df = 40 nm (yellow line) indicates that C1 in this reconstructed wave was −40 nm. Aberrations of focus (C1) and A1 values estimated from the Thon diagram are indicated in d . Aberration-corrected amplitude ( e ) and phase ( f ) images. g , FFT image of A1-corrected amplitude image with digital defocus of −200 nm. h , Thon diagram for amplitude of the C1-corrected wave. Experimental defocus (C1) was corrected, and minimum amplitude is shifted to df = 0 nm (red line). The blue pattern is the simulated Thon diagram matched with the aberration-corrected Thon diagram. It indicates the point resolution in the digitally aberration-corrected image is 0.26 nm. i , Thon diagram before spherical aberration correction. Green line shows bending of central black curve due to spherical aberrations (C3: −4.0 mm, C5: 2.14 km). j , Thon diagram after spherical aberration correction.
Extended Data Fig. 3 Aberration-corrected single-phase image and low-resolution magnetic phase.
a , Aberration-corrected phase image. The lattice image of the (111) plane is clearly seen. The scale bar is 2 nm. b , Low-resolution magnetic phase. c , Line profiles of low-resolution magnetic phase of the area indicated by the dashed light-blue rectangle. The phase slope of magnetic phase indicates [ \(\bar{1}\bar{1}\) 2] directional magnetization. A non-magnetic area is clearly visible at the edge of the sample.
Extended Data Fig. 4 Low-resolution simulated magnetic phase.
a , Rectangular model used in the simulation. The shape of the sample in the simulation was set to be similar to the experimental sample. b , Schematics of electron propagations of the object wave ( φ O ) and reference wave ( φ R ). The low-resolution magnetic phase was simulated considering surrounding demagnetizing field outside the sample in the ±4000-nm range in the z direction. c , Phase shift of the reference wave ( φ R ). d , Phase shift of the object wave ( φ O ). e , Observed phase shift ( φ O - φ R ). The background phase slopes (dashed lines) due to the demagnetization fields in φ O and φ R are almost the same, and those effects are subtracted from the hologram observation ( φ O - φ R ) for local high-resolution observation.
Extended Data Fig. 5 Calculated spin distribution, magnetic flux, and electromagnetic phase grating for multislice simulation.
a , Spin distribution per unit cell shown with the projection average. b , Magnetic flux with the [111] directional (in the original unit cell) component per unit cell. c , Magnetic flux with the [ \(\bar{1}\bar{1}\) 2] directional (in the original unit cell) component per unit cell. d , Electrostatic phase grating (conventional). e , Magnetic phase grating with [ \(\bar{1}\bar{1}\) 2] directional magnetization calculated by the Vienna Ab initio simulation package (VASP). The magnetic phase is magnified 10000 to visual inspection. f , Electromagnetic phase grating that is a simple sum of d and e . Please note this is not the electromagnetic phase grating used in the simulation, since the magnetic phase is amplified for visual inspection.
Extended Data Fig. 6 Magnetization properties of bulk Ba 2 FeMoO 6 .
The main graph is the temperature profile of magnetization for a single crystal of Ba 2 FeMoO 6 taken at H// < 111 > = 0.5 T. The inset shows a magnetization hysteresis curve for the same crystal taken at 5 K.
Extended Data Fig. 7 Magnetic-flux distribution in the thin Ba 2 FeMoO 6 sample and atomic arrangements and elemental ordering of Ba 2 FeMoO 6 sample observed in the \([1\bar{1}0]\) direction.
a , TEM image of the thin sample. b , Magnetic flux in the area indicated by the white rectangle in a displayed with the cosine of phase φ M amplified 10 times (cos10 φ M ). Constant flux of h /10 e flows between adjacent contour lines. The white dashed rectangle indicates the area used for evaluating magnetization. c and d , High-angle annular dark-field (HAADF) scanning transmission electron microscopic (STEM) images. e , Elemental mappings of Ba, Fe and Mo obtained by STEM energy-dispersive X-ray spectroscopy. Scale bar is 0.5 nm.
Extended Data Fig. 8 Magnetic-flux distribution in the rectangular-shaped thin Ba 2 FeMoO 6 sample.
a , TEM image of the thin sample with rectangular shape. b , Magnetic flux obtained after applying a pulsed magnetic field of 207 kA/m in the area indicated by the white rectangle in a , as displayed with the cosine of phase φ M amplified 15 times (cos15 φ M ). Constant flux of h /15 e flows between adjacent contour lines.
Extended Data Fig. 9 Procedure of data analysis and obtained data.
a , Flow chart of data analysis. b , Phase \({\varphi }_{\uparrow }\) with + M. c , Phase \({\varphi }_{\downarrow }\) with −M. d , Electrostatic phase. e , Magnetic phase. f , Line profiles of magnetic phase in the [111] direction in the area indicated by the yellow-dashed rectangle in e with different alignment positions for separating electrostatic and magnetic phases. g , Mean squared error of phase obtained from the \({\varphi }_{M}\) background with different alignment positions in the alignment area shown in f (also indicated by the yellow lines in e ). h , Magnetic phase averaged in the (111) lattice plane with 2.5-nm range of the area indicated by the white rectangle in e . i , Magnetic phase when Gaussian blur with σ of 0.4 nm along the lattice plane after the averaging in the (111) lattice plane with 2.5-nm range for e . j , Enlarged image of the area indicated by the white rectangle in i . k , Derivative of magnetic phase i that reflects the in-plane magnetic field. l , Enlarged image of the area indicated by the white rectangle in k . The color wheel in l indicates how color and shade denote magnetic-field directions and strength (i.e., derivatives of magnetic phase range 0-0.06 rad/nm in absolute values). The areas indicated by white squares in j and l correspond to Figs. 2d and g . The white-dashed rectangles in i and l indicate the areas used for evaluating standard deviation. The red-dashed rectangles (W 2.8 nm × H 13.7 nm) in d , i and k show the areas used for evaluating the histograms (Figs. 2e, f and h ) of the peak-to-peak values of electrostatic and magnetic phases and the +peak for the Mo plane and the −peak for the Fe plane of the derivative of the magnetic phase. The scale bar is 2 nm.
Extended Data Fig. 10 Simulations of electrostatic and magnetic phases with sample tilt in the [111] direction.
a , Electrostatic phase shift in the [111] direction without sample tilt β from the orientation of the [1 \(\bar{1}\) 0] zone axis shown in Fig. 3 . Right-side green and light-green lines indicate two digital-focus conditions. Phase shifts at the Fe (Mo) layers are maximized in the thickness range indicated by the light-green (green) lines. b , Magnetic phase shift in the [111] direction without sample tilt from the orientation of the [1 \(\bar{1}\) 0] zone axis shown in Fig. 3 with the same digital focus conditions used in a . c , Electrostatic phase shift in the [111] direction with sample tilt β of 0.121 mrad in the [111] direction from the orientation of the [1 \(\bar{1}\) 0] zone axis shown in Fig. 3 . Phase shifts at the Fe (Mo) layers are maximized with digital focus for the post process for obtaining magnetic phase. Right-side green and light-green lines indicate two digital-focus conditions. Phase shift at the Fe (Mo) layers is maximized in the thickness range indicated by the light-green (green) lines. d , Magnetic phase shift in the [111] direction with sample tilt β of 0.121 mrad from the orientation of the [1 \(\bar{1}\) 0] zone axis shown in Fig. 3 with the same digital focus conditions used in c .
Extended Data Fig. 11 Phase images without applying a 1/0.4 nm −1 filter and obtained data.
a , Phase \({\varphi }_{\uparrow }\) with + M. b , Phase \({\varphi }_{\downarrow }\) with −M. c , Electrostatic phase. d , Magnetic phase. e , Line profile of magnetic phase in the [111] direction (averaging width along lattice plane is 12.3 nm) in the area indicated by white rectangle in d . f , Derivative of magnetic phase profile shown in e . The gray-dashed areas in e and f indicate the areas used for evaluating standard deviation. The scale bar is 2 nm.
Supplementary information
Peer review file, rights and permissions.
Springer Nature or its licensor (e.g. a society or other partner) holds exclusive rights to this article under a publishing agreement with the author(s) or other rightsholder(s); author self-archiving of the accepted manuscript version of this article is solely governed by the terms of such publishing agreement and applicable law.
Reprints and permissions
About this article
Cite this article.
Tanigaki, T., Akashi, T., Yoshida, T. et al. Electron holography observation of individual ferrimagnetic lattice planes. Nature (2024). https://doi.org/10.1038/s41586-024-07673-w
Download citation
Received : 03 October 2020
Accepted : 04 June 2024
Published : 03 July 2024
DOI : https://doi.org/10.1038/s41586-024-07673-w
Share this article
Anyone you share the following link with will be able to read this content:
Sorry, a shareable link is not currently available for this article.
Provided by the Springer Nature SharedIt content-sharing initiative
By submitting a comment you agree to abide by our Terms and Community Guidelines . If you find something abusive or that does not comply with our terms or guidelines please flag it as inappropriate.
Quick links
- Explore articles by subject
- Guide to authors
- Editorial policies
Sign up for the Nature Briefing newsletter — what matters in science, free to your inbox daily.

Information
- Author Services
Initiatives
You are accessing a machine-readable page. In order to be human-readable, please install an RSS reader.
All articles published by MDPI are made immediately available worldwide under an open access license. No special permission is required to reuse all or part of the article published by MDPI, including figures and tables. For articles published under an open access Creative Common CC BY license, any part of the article may be reused without permission provided that the original article is clearly cited. For more information, please refer to https://www.mdpi.com/openaccess .
Feature papers represent the most advanced research with significant potential for high impact in the field. A Feature Paper should be a substantial original Article that involves several techniques or approaches, provides an outlook for future research directions and describes possible research applications.
Feature papers are submitted upon individual invitation or recommendation by the scientific editors and must receive positive feedback from the reviewers.
Editor’s Choice articles are based on recommendations by the scientific editors of MDPI journals from around the world. Editors select a small number of articles recently published in the journal that they believe will be particularly interesting to readers, or important in the respective research area. The aim is to provide a snapshot of some of the most exciting work published in the various research areas of the journal.
Original Submission Date Received: .
- Active Journals
- Find a Journal
- Proceedings Series
- For Authors
- For Reviewers
- For Editors
- For Librarians
- For Publishers
- For Societies
- For Conference Organizers
- Open Access Policy
- Institutional Open Access Program
- Special Issues Guidelines
- Editorial Process
- Research and Publication Ethics
- Article Processing Charges
- Testimonials
- Preprints.org
- SciProfiles
- Encyclopedia
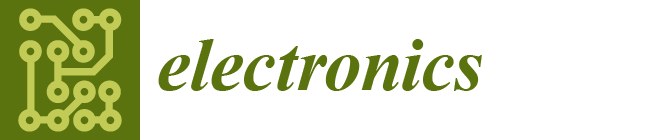
Article Menu
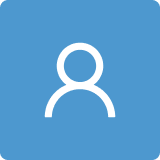
- Subscribe SciFeed
- Recommended Articles
- Google Scholar
- on Google Scholar
- Table of Contents
Find support for a specific problem in the support section of our website.
Please let us know what you think of our products and services.
Visit our dedicated information section to learn more about MDPI.
JSmol Viewer
Matrix separation and poisson multi-bernoulli mixture filtering for extended multi-target tracking with infrared images, 1. introduction, 2. differential low-rank and sparse matrix separation.
Algorithm for solving the differential form of low-rank and sparse matrix separation. |
Data , dictionary , parameters and Step size controller Low rank matrix , sparse matrix Define: Initialize: k = 1, 2, …. (until convergence) where |
3. PMBM for Extended Multi-Target Tracking
3.1. pmbm for state modeling, 3.2. measurement modeling, 3.3. conjugate bayesian filtering, 4. the united framework and complexity analysis, 4.1. detection and filtering framework, 4.2. computational complexity, 5. experiments, 6. conclusions, author contributions, data availability statement, conflicts of interest.
- Tian, F.; Guo, X.; Fu, W. Target Tracking Algorithm Based on Adaptive Strong Tracking Extended Kalman Filter. Electronics 2024 , 13 , 652. [ Google Scholar ] [ CrossRef ]
- Yong, Y.; Kang, J.; Oh, H. Detection-Free Object Tracking for Multiple Occluded Targets in Plenoptic Video. Electronics 2024 , 13 , 590. [ Google Scholar ] [ CrossRef ]
- Yang, S.-Y.; Cheng, H.-Y.; Yu, C.-C. Real-Time Object Detection and Tracking for Unmanned Aerial Vehicles Based on Convolutional Neural Networks. Electronics 2023 , 12 , 4928. [ Google Scholar ] [ CrossRef ]
- Yi, W.; Fang, Z.; Li, W.; Hoseinnezhad, R.; Kong, L. Multi-Frame Track-before-Detect Algorithm for Maneuvering Target Tracking. IEEE Trans. Veh. Technol. 2020 , 69 , 4104–4118. [ Google Scholar ] [ CrossRef ]
- Wan, M.; Gu, G.; Cao, E.; Hu, X.; Qian, W.; Ren, K. In-Frame and Inter-Frame Information Based Infrared Moving Small Target Detection under Complex Cloud Backgrounds. Infrared Phys. Technol. 2016 , 76 , 455–467. [ Google Scholar ] [ CrossRef ]
- Tian, M.; Chen, Z.; Wang, H.; Liu, L. An Intelligent Particle Filter for Infrared Dim Small Target Detection and Tracking. IEEE Trans. Aerosp. Electron. Syst. 2022 , 58 , 5318–5333. [ Google Scholar ] [ CrossRef ]
- Deng, L.; Xu, G.; Zhang, J.; Zhu, H. Entropy-Driven Morphological Top-Hat Transformation for Infrared Small Target Detection. IEEE Trans. Aerosp. Electron. Syst. 2022 , 58 , 962–975. [ Google Scholar ] [ CrossRef ]
- McIntosh, B.; Venkataramanan, S.; Mahalanobis, A. Infrared Target Detection in Cluttered Environments by Maximization of a Target to Clutter Ratio (TCR) Metric Using a Convolutional Neural Network. IEEE Trans. Aerosp. Electron. Syst. 2021 , 57 , 485–496. [ Google Scholar ] [ CrossRef ]
- Zhang, C.; He, Y.; Tang, Q.; Chen, Z.; Mu, T. Infrared Small Target Detection via Interpatch Correlation Enhancement and Joint Local Visual Saliency Prior. IEEE Trans. Geosci. Remote Sens. 2022 , 60 , 5001314. [ Google Scholar ] [ CrossRef ]
- Pang, D.; Ma, P.; Shan, T.; Li, W.; Tao, R.; Ma, Y.; Wang, T. STTM-SFR: Spatial–Temporal Tensor Modeling with Saliency Filter Regularization for Infrared Small Target Detection. IEEE Trans. Geosci. Remote Sens. 2022 , 60 , 5623418. [ Google Scholar ] [ CrossRef ]
- Yao, J.; Hong, D.; Chanussot, J.; Meng, D.; Zhu, X.; Xu, Z. Cross-Attention in Coupled Unmixing Nets for Unsupervised Hyperspectral Super-Resolution. In Computer Vision—ECCV 2020, Proceedings of the 16th European Conference, Glasgow, UK, 23–28 August 2020 ; Vedaldi, A., Bischof, H., Brox, T., Frahm, J.-M., Eds.; Springer International Publishing: Cham, Switzerland, 2020; pp. 208–224. [ Google Scholar ]
- Yao, J.; Cao, X.; Zhao, Q.; Meng, D.; Xu, Z. Robust Subspace Clustering via Penalized Mixture of Gaussians. Neurocomputing 2018 , 278 , 4–11. [ Google Scholar ] [ CrossRef ]
- Ma, D.; Dong, L.; Zhang, M.; Gao, R.; Xu, W. Weighted Adaptive Schatten P-Norm and Transpose Variability Model for Infrared Maritime Small Target Detection. IEEE Trans. Geosci. Remote Sens. 2023 . early access . [ Google Scholar ] [ CrossRef ]
- Luo, Y.; Li, X.; Chen, S.; Xia, C. 4DST-BTMD: An Infrared Small Target Detection Method Based on 4D Data-Sphered Space. IEEE Trans. Geosci. Remote Sens. 2023 , 62 , 5000520. [ Google Scholar ] [ CrossRef ]
- Yao, J.; Hong, D.; Wang, H.; Liu, H.; Chanussot, J. UCSL: Toward Unsupervised Common Subspace Learning for Cross-Modal Image Classification. IEEE Trans. Geosci. Remote Sens. 2023 , 61 , 5514212. [ Google Scholar ] [ CrossRef ]
- Yao, J.; Zhang, B.; Li, C.; Hong, D.; Chanussot, J. Extended Vision Transformer (ExViT) for Land Use and Land Cover Classification: A Multimodal Deep Learning Framework. IEEE Trans. Geosci. Remote Sens. 2023 , 61 , 5514415. [ Google Scholar ] [ CrossRef ]
- Hui, B.; Song, Z.; Fan, H.; Zhong, P. A Dataset for Infrared Detection and Tracking of Dim-Small Aircraft Targets under Ground/Air Background. Sci. Data Bank 2019 , 5 , 291–302. [ Google Scholar ]
- Sprechmann, P.; Bronstein, A.M.; Sapiro, G. Learning Efficient Sparse and Low Rank Models. IEEE Trans. Pattern Anal. Mach. Intell. 2015 , 37 , 1821–1833. [ Google Scholar ] [ CrossRef ] [ PubMed ]
- Mardani, M.; Mateos, G.; Giannakis, G.B. Decentralized Sparsity-Regularized Rank Minimization: Algorithms and Applications. IEEE Trans. Signal Process. 2013 , 61 , 5374–5388. [ Google Scholar ] [ CrossRef ]
- Granström, K.; Fatemi, M.; Svensson, L. Poisson Multi-Bernoulli Mixture Conjugate Prior for Multiple Extended Target Filtering. IEEE Trans. Aerosp. Electron. Syst. 2020 , 56 , 208–225. [ Google Scholar ] [ CrossRef ]
- García-Fernández, Á.F.; Williams, J.L.; Svensson, L.; Xia, Y. A Poisson Multi-Bernoulli Mixture Filter for Coexisting Point and Extended Targets. IEEE Trans. Signal Process. 2021 , 69 , 2600–2610. [ Google Scholar ] [ CrossRef ]
- Du, P.; Hamdulla, A. Infrared Moving Small-Target Detection Using Spatial–Temporal Local Difference Measure. IEEE Geosci. Remote Sens. Lett. 2020 , 17 , 1817–1821. [ Google Scholar ] [ CrossRef ]
- Zhang, P.; Zhang, L.; Wang, X.; Shen, F.; Pu, T.; Fei, C. Edge and Corner Awareness-Based Spatial–Temporal Tensor Model for Infrared Small-Target Detection. IEEE Trans. Geosci. Remote Sens. 2021 , 59 , 10708–10724. [ Google Scholar ] [ CrossRef ]
- Wang, Y.; Chen, X.; Gong, C.; Rao, P. Non-Ellipsoidal Infrared Group/Extended Target Tracking Based on Poisson Multi-Bernoulli Mixture Filter and B-Spline. Remote Sens. 2023 , 15 , 606. [ Google Scholar ] [ CrossRef ]
Click here to enlarge figure
STTM-SFR | WAS N-STTTV | 4DST-BTMD | The Proposed | ||
---|---|---|---|---|---|
BSF | Seq. 1 | 43.54 | 45.33 | 45.74 | |
Seq. 2 | 42.90 | 44.33 | 45.06 | ||
Seq. 3 | 27.62 | 28.44 | 28.23 | ||
Seq. 4 | 35.11 | 36.61 | 37.35 | ||
Seq. 5 | 36.54 | 37.60 | 38.52 | ||
Seq. 6 | 34.05 | 35.11 | 35.53 | ||
SCRG | Seq. 1 | 37.01 | 38.71 | 39.27 | |
Seq. 2 | 36.69 | 37.99 | 38.58 | ||
Seq. 3 | 29.92 | 30.71 | 30.45 | ||
Seq. 4 | 37.70 | 38.03 | 38.62 | ||
Seq. 5 | 38.72 | 39.04 | 39.39 | ||
Seq. 6 | 36.37 | 36.52 | 36.97 |
The statements, opinions and data contained in all publications are solely those of the individual author(s) and contributor(s) and not of MDPI and/or the editor(s). MDPI and/or the editor(s) disclaim responsibility for any injury to people or property resulting from any ideas, methods, instructions or products referred to in the content. |
Share and Cite
Su, J.; Zhou, H.; Yu, Q.; Zhu, J.; Liu, J. Matrix Separation and Poisson Multi-Bernoulli Mixture Filtering for Extended Multi-Target Tracking with Infrared Images. Electronics 2024 , 13 , 2613. https://doi.org/10.3390/electronics13132613
Su J, Zhou H, Yu Q, Zhu J, Liu J. Matrix Separation and Poisson Multi-Bernoulli Mixture Filtering for Extended Multi-Target Tracking with Infrared Images. Electronics . 2024; 13(13):2613. https://doi.org/10.3390/electronics13132613
Su, Jian, Haiyin Zhou, Qi Yu, Jubo Zhu, and Jiying Liu. 2024. "Matrix Separation and Poisson Multi-Bernoulli Mixture Filtering for Extended Multi-Target Tracking with Infrared Images" Electronics 13, no. 13: 2613. https://doi.org/10.3390/electronics13132613
Article Metrics
Further information, mdpi initiatives, follow mdpi.
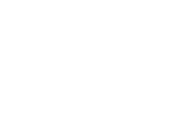
Subscribe to receive issue release notifications and newsletters from MDPI journals

- Advanced Search
Nonlocal Cahn-Hilliard type model for image inpainting
New citation alert added.
This alert has been successfully added and will be sent to:
You will be notified whenever a record that you have chosen has been cited.
To manage your alert preferences, click on the button below.
New Citation Alert!
Please log in to your account
Information & Contributors
Bibliometrics & citations, view options, recommendations, time-fractional cahn–hilliard equation: well-posedness, degeneracy, and numerical solutions.
In this paper, we derive the time-fractional Cahn–Hilliard equation from continuum mixture theory with a modification of Fick's law of diffusion. This model describes the process of phase separation with nonlocal memory effects. We ...
Local discontinuous Galerkin methods for the Cahn-Hilliard type equations
In this paper, we develop local discontinuous Galerkin (LDG) methods for the fourth order nonlinear Cahn-Hilliard equation and system. The energy stability of the LDG methods is proved for the general nonlinear case. Numerical examples for the Cahn-...
Efficient Solvers of Discontinuous Galerkin Discretization for the Cahn---Hilliard Equations
In this paper, we develop and analyze a fast solver for the system of algebraic equations arising from the local discontinuous Galerkin (LDG) discretization and implicit time marching methods to the Cahn---Hilliard (CH) equations with constant and ...
Information
Published in.
Pergamon Press, Inc.
United States
Publication History
Author tags.
- Image inpainting
- Cahn-Hilliard equation
- Nonlocal diffusion operator
- Stationary solution
- Well-posedness
- Convex splitting method
- Research-article
Contributors
Other metrics, bibliometrics, article metrics.
- 0 Total Citations
- 0 Total Downloads
- Downloads (Last 12 months) 0
- Downloads (Last 6 weeks) 0
View options
Login options.
Check if you have access through your login credentials or your institution to get full access on this article.
Full Access
Share this publication link.
Copying failed.
Share on social media
Affiliations, export citations.
- Please download or close your previous search result export first before starting a new bulk export. Preview is not available. By clicking download, a status dialog will open to start the export process. The process may take a few minutes but once it finishes a file will be downloadable from your browser. You may continue to browse the DL while the export process is in progress. Download
- Download citation
- Copy citation
We are preparing your search results for download ...
We will inform you here when the file is ready.
Your file of search results citations is now ready.
Your search export query has expired. Please try again.
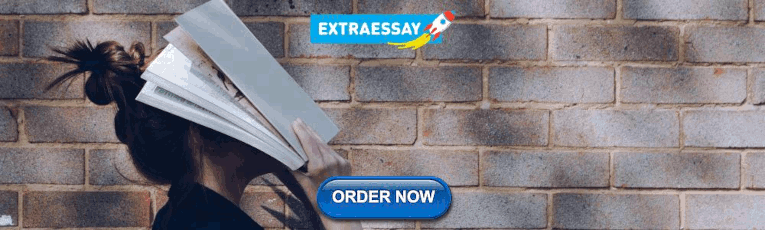
COMMENTS
Visual methodologies are used to understand and interpret images (Barbour, 2014) and include photography, film, video, painting, drawing, collage, sculpture, artwork, graffiti, advertising, and cartoons.Visual methodologies are a new and novel approach to qualitative research derived from traditional ethnography methods used in anthropology and sociology.
Visual analysis is an important step in evaluating an image and understanding its meaning. It is also important to consider textual information provided with the image, the image source and original context of the image, and the technical quality of the image. The following questions can help guide your analysis and evaluation. Content analysis.
Image analysis is used as a fundamental tool for recognizing, differentiating, and. quantifying diverse types of images, including grayscale and color images, multi-. spectral images for a few ...
Image analysis or imagery analysis is the extraction of meaningful information from images; mainly from digital images by means of digital image processing techniques. Image analysis tasks can be as simple as reading bar coded tags or as sophisticated as identifying a person from their face.. Computers are indispensable for the analysis of large amounts of data, for tasks that require complex ...
Sep 21, 2023. by Janet Salmons, PhD, Research Community Manager for Sage Research Methods Community. Researchers using photovoice ask participants to share their observations and experiences using photographs, usually together with written notes. This collection of open-access articles describe analysis of images collected using photovoice or ...
guide for readers on how to start thinking about image analysis, they do not, by themselves, amount to a full methodology. This is why it is recommended for researchers to 'complement' and 'personalise' the present guidelines with the interests and tools offered by more established forms of image analysis.
Visual methodologies are used to understand and interpret images (Barbour, 2014) and include photography, film, video, painting, drawing, collage, sculpture, artwork, graffiti, adver-tising, and cartoons. Visual methodologies are a new and novel approach to qualitative research derived from traditional eth-nography methods used in anthropology ...
While many of the basic image processing and analysis methods described in this chapter are still useful for processing and analyzing two or three-dimensional multispectral and hyperspectral images, it also entails new challenges in handling these types of images. Methods and techniques specific to processing and analyzing multispectral and ...
These images are generated in a range of ways including through participatory activity, those produced by the researcher or using 'found' visual materials (Rose, 2015). Visual data can also be used to elicit discussion or as a focus for analysis. While v isual method s sometimes overlap with both creative methods and arts-based research, ...
"Now in its Fourth Edition, Visual Methodologies: An Introduction to Researching with Visual Methodologies is a bestselling critical guide to the study and analysis of visual culture. Existing chapters have been fully updated to offer a rigorous examination and demonstration of an individual methodology in a clear and structured style.
Give yourself time to "take-in" the entire image before you start your research. Make sure you understand what is happening in the image and that you see all of the details. Sometimes it is easy to skip over this step but, it is really important! You can't research an image accurately if you don't understand it. Read the Metadata.
The approach of this study was a qualitative one by using grounded theory method. The visual images and written text were analyzed by using the descriptive framework proposed by Kress and Leeuwen ...
Abstract. This chapter discusses the development of photography as a research method in social sciences. It describes the different types of photographs used, such as archival photographs and photographs taken by the researcher, and it focuses especially on photographs taken by participants. The uses of different approaches to obtain ...
Choose an image that lends itself to deep analysis by students. This analysis strategy works best when the image is one that reflects (intentionally or not) a particular opinion, point of view, or perspective. Visual art, propaganda images, photographs, and political cartoons are good examples of visual media that reflect a perspective.
Image analysis methods are used to examine the microstructure and characteristics of materials. Images of the materials may be examined by scientists using methods like transmission electron microscopy (TEM) and scanning electron microscopy (SEM). This aids in characterising flaws, comprehending material behaviour, and creating sophisticated ...
In order to improve this situation, image analysis is applied for microorganism counting since the 1980s, which consists of digital image processing, image segmentation, image classification and suchlike. Image analysis-based microorganism counting methods are efficient comparing with traditional plate counting methods.
ASPRS Manual of Remote Se nsing, Fourth Edition, doi: 10.14358/MRS /Chapter7. 631. Chapter 7: Image Processing and Analysis Methods. Sergio Bernardes. Center for Geospat ial Research, Department ...
A full review of stereology as a methodology is beyond the scope of this manuscript but has been provided elsewhere.[87,90,91,92,93,94] Commercial image analysis solutions are available that include stereology modules to aid in application of the stereology method to whole-slide images.
Abstract. Image analysis methods have been developed to provide quantitative assessment of microscopy data. In this unit, basic aspects of image analysis are outlined, including software installation, data import, image processing functions, and analytical tools that can be used to extract information from microscopy data using ImageJ.
Computer image analysis largely involves the fields of computer or machine vision, and medical imaging, and makes heavy use of pattern recognition, digital geometry, and signal processing. It is the quantitative or qualitative characterization of 2D or 3D digital images. Two-dimensional images are usually analyzed in computer vision, whereas 3D ...
Qualitative Research Methodology. This is a research methodology that involves the collection and analysis of non-numerical data such as words, images, and observations. This type of research is often used to explore complex phenomena, to gain an in-depth understanding of a particular topic, and to generate hypotheses.
a, Full view of the electron hologram, showing amorphous regions at the edge of the sample.Scale bar, 5 nm. b, Enlarged image of the area indicated by the white square in a.Spacing of the ...
A newly developed digital image-analysis method was used to characterize the morphological characteristics of coarse aggregates. Regression analyses found that the imaging morphological ...
Multi-target tracking using infrared images is receiving more and more attention. There are many state-of-the-art methods, and the deep learning network and low-rank and sparse matrix separation are two kinds of methods with high accuracy. However, the former suffers from heavy training samples, and the latter requires high-dimensional processing, meaning its computing cost is huge.
A rigorous analysis of the well-posedness of the stationary solution is established using Schauder's fixed point theory. We construct a time stepping scheme based on the convex splitting method with the nonlocal term treated implicitly and the fidelity term treated explicitly.