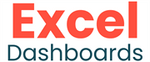
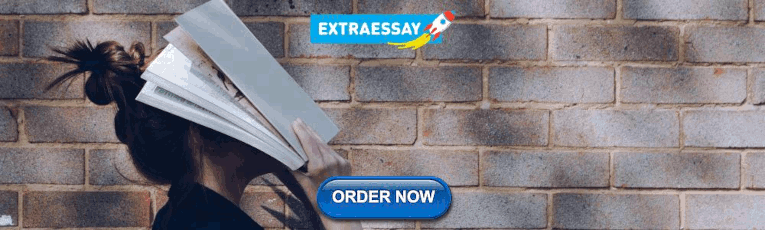
Excel Tutorial: How To Filter Chart In Excel
Introduction.
Filtering charts in Excel is a crucial skill for anyone who works with data and wants to present it in a clear and concise manner. By filtering charts , you can focus on specific data points, highlight trends, and customize the visual representation of your data. In this blog post, we will cover the importance of filtering charts in Excel and provide a step-by-step tutorial on how to do it effectively.
Key Takeaways
- Filtering charts in Excel is essential for presenting data in a clear and concise manner.
- Understanding chart filtering allows you to focus on specific data points and highlight trends.
- Utilizing advanced filter options can enhance the customization of visual representations in Excel.
- Efficient chart filtering practices can streamline data analysis and visualization processes.
- Utilizing chart filtering can greatly enhance the flexibility and versatility of data representation in Excel.
Understanding Chart Filtering
Chart filtering is an essential feature in Excel that allows users to selectively display data on a chart by applying filters based on specific criteria. This functionality helps in customizing the visualization of data to focus on the most relevant information.
Explanation of what chart filtering is
Chart filtering involves the process of manipulating the data displayed on a chart by applying filters to show or hide certain data series, categories, or individual data points. This can be done by directly interacting with the chart or by utilizing the filtering options available in Excel.
Benefits of filtering charts in Excel
Filtering charts in Excel provides several benefits, including:
- Ability to focus on specific data: Chart filtering allows users to focus on specific data points or categories of interest, providing a clearer understanding of the underlying trends and patterns.
- Customized visualization: By selectively displaying data, users can create customized visualizations that effectively communicate the intended message to the audience.
- Improved analysis: Filtering charts helps in conducting more detailed and targeted analysis by isolating the relevant data for closer examination.
Common scenarios where chart filtering is useful
Chart filtering is particularly useful in various scenarios, such as:
- Comparing specific data points: When comparing specific data points, such as sales figures for different regions or product categories, chart filtering allows for a focused comparison without overwhelming the audience with unnecessary details.
- Highlighting trends over time: When visualizing time-series data, chart filtering can be used to highlight specific periods or trends, making it easier to identify patterns and outliers.
- Showing top or bottom performers: In scenarios where the focus is on top or bottom performers, chart filtering helps in highlighting the relevant data while excluding the rest.
Excel Tutorial: How to Filter Chart in Excel
Filtering a chart in Excel allows you to focus on specific data points or categories within your chart, providing a clearer and more targeted visual representation of your data. In this tutorial, we will guide you through the process of applying chart filtering in Excel, using the filter feature to modify chart data, and customizing the filter options to fit specific chart requirements.
Step-by-step guide on how to filter a chart in Excel
- Select the chart: Begin by selecting the chart that you want to filter. This will activate the Chart Tools tab at the top of the Excel window.
- Click on the Filter button: Within the Chart Tools tab, locate and click on the Filter button to open the filter settings for the chart.
- Choose the data to filter: A list of available data series or categories will be displayed. Select the specific data points or categories that you want to filter in the chart.
- Apply the filter: Once you have made your selections, click OK to apply the filter to the chart. The chart will now display only the filtered data.
Using the filter feature in Excel to modify chart data
- Dynamic data modification: The filter feature in Excel allows you to dynamically modify the data displayed in the chart. You can easily add or remove data points or categories from the chart by adjusting the filter settings.
- Interactive charting: Filtering a chart in Excel enables interactive charting, where users can explore different data subsets within the chart by simply adjusting the filter options.
Customizing the filter options to fit specific chart requirements
- Advanced filter criteria: Excel provides advanced filter criteria options, allowing you to customize the filter settings based on specific data conditions, such as value ranges or text-based criteria.
- Multiple filter layers: You can apply multiple filter layers to a chart, filtering data based on different criteria simultaneously to create complex and detailed visualizations.
Using Advanced Filter Options
When working with charts in Excel, it’s important to understand how to use advanced filter options to manipulate and analyze your data effectively. This tutorial will explore the various ways you can use advanced filters to enhance your charts.
Excel offers a range of advanced filter options that allow you to refine and customize the data displayed in your charts. These options include criteria-based filters, date filters, and top/bottom filters.
1. Criteria-based filters:
- Excel allows you to apply multiple criteria to your data, enabling you to filter your charts based on specific conditions.
- By using the advanced filter options, you can create complex filter criteria to isolate the exact data you want to visualize in your charts.
2. Date filters:
- For charts that display time-based data, such as line charts or area charts, you can use date filters to focus on specific time periods.
- Excel’s advanced filter options enable you to easily filter data by date ranges, months, or specific days.
1. Bar chart:
- When working with bar charts, advanced filter options can be used to highlight specific categories or values within the chart.
- You can apply filters to show only certain data points or categories, providing a clearer and more focused representation of your data.
2. Pie chart:
- For pie charts, advanced filters can be used to isolate specific data segments and emphasize key insights.
- By applying filters to your pie chart data, you can emphasize or de-emphasize certain categories, making it easier for stakeholders to interpret the data.
Tips for Efficient Chart Filtering
Filtering charts in Excel can help you analyze and visualize your data more effectively. By following best practices, utilizing keyboard shortcuts, and avoiding common mistakes, you can streamline the process and improve your productivity.
Ensure data is structured properly:
Use named ranges:, clean up unnecessary data:, learn common shortcuts:, customize shortcuts:, over-filtering data:, forgetting to update filters:, ignoring interactive filtering options:, showcasing filtered charts.
When it comes to data visualization, the ability to filter charts in Excel can make a significant impact on the way information is presented and analyzed. In this tutorial, we will explore the various ways filtered charts can be utilized to enhance data visualization and analysis.
Demonstrating the impact of chart filtering on data visualization
Filtered charts allow users to focus on specific data points within a larger dataset, making it easier to identify trends, patterns, and outliers. By demonstrating how filtering affects the visual representation of data, users can gain a better understanding of the impact it has on data visualization.
Using examples to illustrate how filtered charts can enhance analysis
By providing real-life examples of filtered charts in action, users can see how the ability to filter specific data points can lead to more accurate and insightful analysis. These examples will showcase the practical benefits of using filtered charts in Excel.
Highlighting the flexibility and versatility of filtered charts in Excel
Filtered charts are incredibly flexible and versatile , allowing users to customize the display of data in countless ways. By highlighting the various options for filtering charts, users can understand the full extent of the capabilities offered by Excel for data visualization.
In conclusion, chart filtering in Excel plays a crucial role in data visualization and analysis . It allows users to focus on specific data points and gain actionable insights from their charts. I encourage all readers to practice applying chart filters in their Excel worksheets to get a better grasp of its functionality. By utilizing chart filtering effectively , users can improve data representation and make better data-driven decisions for their projects and presentations.
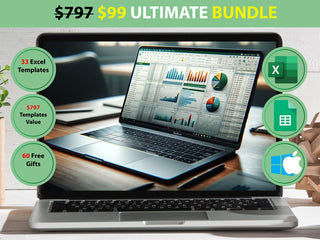
Immediate Download
MAC & PC Compatible
Free Email Support
Related aticles

The Benefits of Excel Dashboards for Data Analysts

Unlock the Power of Real-Time Data Visualization with Excel Dashboards

Unlocking the Potential of Excel's Data Dashboard

Unleashing the Benefits of a Dashboard with Maximum Impact in Excel

Exploring Data Easily and Securely: Essential Features for Excel Dashboards

Unlock the Benefits of Real-Time Dashboard Updates in Excel

Unleashing the Power of Excel Dashboards

Understanding the Benefits and Challenges of Excel Dashboard Design and Development

Leverage Your Data with Excel Dashboards

Crafting the Perfect Dashboard for Excel

An Introduction to Excel Dashboards

How to Create an Effective Excel Dashboard
- Choosing a selection results in a full page refresh.
- Business Essentials
- Leadership & Management
- Credential of Leadership, Impact, and Management in Business (CLIMB)
- Entrepreneurship & Innovation
- Digital Transformation
- Finance & Accounting
- Business in Society
- For Organizations
- Support Portal
- Media Coverage
- Founding Donors
- Leadership Team
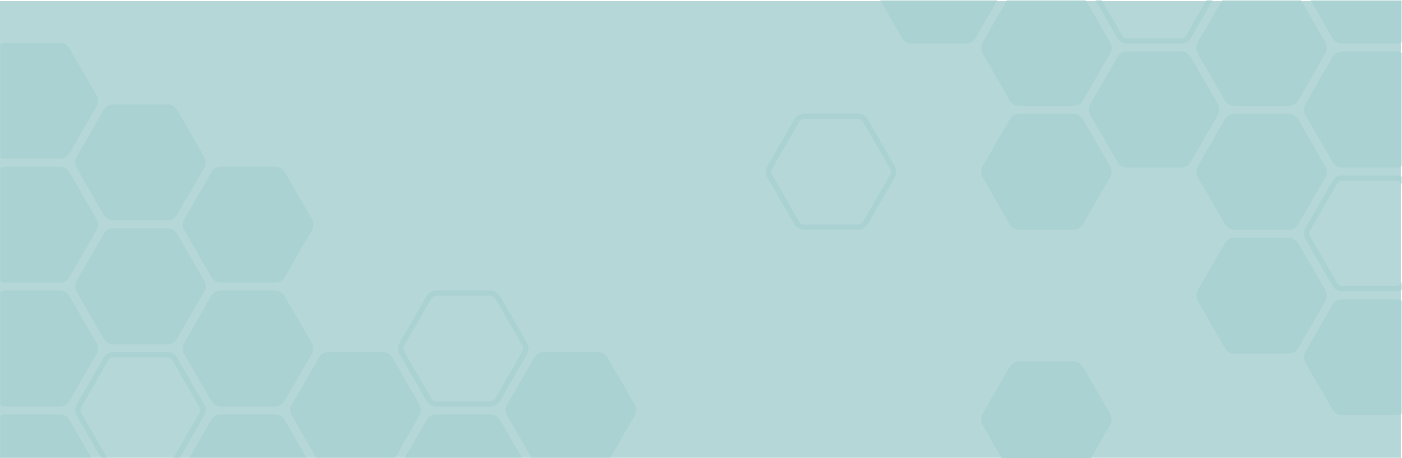
- Harvard Business School →
- HBS Online →
- Business Insights →
Business Insights
Harvard Business School Online's Business Insights Blog provides the career insights you need to achieve your goals and gain confidence in your business skills.
- Career Development
- Communication
- Decision-Making
- Earning Your MBA
- Negotiation
- News & Events
- Productivity
- Staff Spotlight
- Student Profiles
- Work-Life Balance
- AI Essentials for Business
- Alternative Investments
- Business Analytics
- Business Strategy
- Business and Climate Change
- Design Thinking and Innovation
- Digital Marketing Strategy
- Disruptive Strategy
- Economics for Managers
- Entrepreneurship Essentials
- Financial Accounting
- Global Business
- Launching Tech Ventures
- Leadership Principles
- Leadership, Ethics, and Corporate Accountability
- Leading with Finance
- Management Essentials
- Negotiation Mastery
- Organizational Leadership
- Power and Influence for Positive Impact
- Strategy Execution
- Sustainable Business Strategy
- Sustainable Investing
- Winning with Digital Platforms
17 Data Visualization Techniques All Professionals Should Know
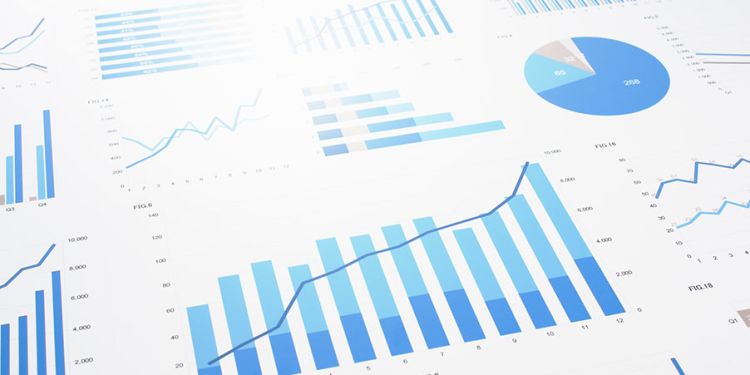
- 17 Sep 2019
There’s a growing demand for business analytics and data expertise in the workforce. But you don’t need to be a professional analyst to benefit from data-related skills.
Becoming skilled at common data visualization techniques can help you reap the rewards of data-driven decision-making , including increased confidence and potential cost savings. Learning how to effectively visualize data could be the first step toward using data analytics and data science to your advantage to add value to your organization.
Several data visualization techniques can help you become more effective in your role. Here are 17 essential data visualization techniques all professionals should know, as well as tips to help you effectively present your data.
Access your free e-book today.
What Is Data Visualization?
Data visualization is the process of creating graphical representations of information. This process helps the presenter communicate data in a way that’s easy for the viewer to interpret and draw conclusions.
There are many different techniques and tools you can leverage to visualize data, so you want to know which ones to use and when. Here are some of the most important data visualization techniques all professionals should know.
Data Visualization Techniques
The type of data visualization technique you leverage will vary based on the type of data you’re working with, in addition to the story you’re telling with your data .
Here are some important data visualization techniques to know:
- Gantt Chart
- Box and Whisker Plot
- Waterfall Chart
- Scatter Plot
- Pictogram Chart
- Highlight Table
- Bullet Graph
- Choropleth Map
- Network Diagram
- Correlation Matrices
1. Pie Chart

Pie charts are one of the most common and basic data visualization techniques, used across a wide range of applications. Pie charts are ideal for illustrating proportions, or part-to-whole comparisons.
Because pie charts are relatively simple and easy to read, they’re best suited for audiences who might be unfamiliar with the information or are only interested in the key takeaways. For viewers who require a more thorough explanation of the data, pie charts fall short in their ability to display complex information.
2. Bar Chart

The classic bar chart , or bar graph, is another common and easy-to-use method of data visualization. In this type of visualization, one axis of the chart shows the categories being compared, and the other, a measured value. The length of the bar indicates how each group measures according to the value.
One drawback is that labeling and clarity can become problematic when there are too many categories included. Like pie charts, they can also be too simple for more complex data sets.
3. Histogram

Unlike bar charts, histograms illustrate the distribution of data over a continuous interval or defined period. These visualizations are helpful in identifying where values are concentrated, as well as where there are gaps or unusual values.
Histograms are especially useful for showing the frequency of a particular occurrence. For instance, if you’d like to show how many clicks your website received each day over the last week, you can use a histogram. From this visualization, you can quickly determine which days your website saw the greatest and fewest number of clicks.
4. Gantt Chart

Gantt charts are particularly common in project management, as they’re useful in illustrating a project timeline or progression of tasks. In this type of chart, tasks to be performed are listed on the vertical axis and time intervals on the horizontal axis. Horizontal bars in the body of the chart represent the duration of each activity.
Utilizing Gantt charts to display timelines can be incredibly helpful, and enable team members to keep track of every aspect of a project. Even if you’re not a project management professional, familiarizing yourself with Gantt charts can help you stay organized.
5. Heat Map
A heat map is a type of visualization used to show differences in data through variations in color. These charts use color to communicate values in a way that makes it easy for the viewer to quickly identify trends. Having a clear legend is necessary in order for a user to successfully read and interpret a heatmap.
There are many possible applications of heat maps. For example, if you want to analyze which time of day a retail store makes the most sales, you can use a heat map that shows the day of the week on the vertical axis and time of day on the horizontal axis. Then, by shading in the matrix with colors that correspond to the number of sales at each time of day, you can identify trends in the data that allow you to determine the exact times your store experiences the most sales.
6. A Box and Whisker Plot

A box and whisker plot , or box plot, provides a visual summary of data through its quartiles. First, a box is drawn from the first quartile to the third of the data set. A line within the box represents the median. “Whiskers,” or lines, are then drawn extending from the box to the minimum (lower extreme) and maximum (upper extreme). Outliers are represented by individual points that are in-line with the whiskers.
This type of chart is helpful in quickly identifying whether or not the data is symmetrical or skewed, as well as providing a visual summary of the data set that can be easily interpreted.
7. Waterfall Chart

A waterfall chart is a visual representation that illustrates how a value changes as it’s influenced by different factors, such as time. The main goal of this chart is to show the viewer how a value has grown or declined over a defined period. For example, waterfall charts are popular for showing spending or earnings over time.
8. Area Chart

An area chart , or area graph, is a variation on a basic line graph in which the area underneath the line is shaded to represent the total value of each data point. When several data series must be compared on the same graph, stacked area charts are used.
This method of data visualization is useful for showing changes in one or more quantities over time, as well as showing how each quantity combines to make up the whole. Stacked area charts are effective in showing part-to-whole comparisons.
9. Scatter Plot

Another technique commonly used to display data is a scatter plot . A scatter plot displays data for two variables as represented by points plotted against the horizontal and vertical axis. This type of data visualization is useful in illustrating the relationships that exist between variables and can be used to identify trends or correlations in data.
Scatter plots are most effective for fairly large data sets, since it’s often easier to identify trends when there are more data points present. Additionally, the closer the data points are grouped together, the stronger the correlation or trend tends to be.
10. Pictogram Chart
Pictogram charts , or pictograph charts, are particularly useful for presenting simple data in a more visual and engaging way. These charts use icons to visualize data, with each icon representing a different value or category. For example, data about time might be represented by icons of clocks or watches. Each icon can correspond to either a single unit or a set number of units (for example, each icon represents 100 units).
In addition to making the data more engaging, pictogram charts are helpful in situations where language or cultural differences might be a barrier to the audience’s understanding of the data.
11. Timeline

Timelines are the most effective way to visualize a sequence of events in chronological order. They’re typically linear, with key events outlined along the axis. Timelines are used to communicate time-related information and display historical data.
Timelines allow you to highlight the most important events that occurred, or need to occur in the future, and make it easy for the viewer to identify any patterns appearing within the selected time period. While timelines are often relatively simple linear visualizations, they can be made more visually appealing by adding images, colors, fonts, and decorative shapes.
12. Highlight Table

A highlight table is a more engaging alternative to traditional tables. By highlighting cells in the table with color, you can make it easier for viewers to quickly spot trends and patterns in the data. These visualizations are useful for comparing categorical data.
Depending on the data visualization tool you’re using, you may be able to add conditional formatting rules to the table that automatically color cells that meet specified conditions. For instance, when using a highlight table to visualize a company’s sales data, you may color cells red if the sales data is below the goal, or green if sales were above the goal. Unlike a heat map, the colors in a highlight table are discrete and represent a single meaning or value.
13. Bullet Graph

A bullet graph is a variation of a bar graph that can act as an alternative to dashboard gauges to represent performance data. The main use for a bullet graph is to inform the viewer of how a business is performing in comparison to benchmarks that are in place for key business metrics.
In a bullet graph, the darker horizontal bar in the middle of the chart represents the actual value, while the vertical line represents a comparative value, or target. If the horizontal bar passes the vertical line, the target for that metric has been surpassed. Additionally, the segmented colored sections behind the horizontal bar represent range scores, such as “poor,” “fair,” or “good.”
14. Choropleth Maps

A choropleth map uses color, shading, and other patterns to visualize numerical values across geographic regions. These visualizations use a progression of color (or shading) on a spectrum to distinguish high values from low.
Choropleth maps allow viewers to see how a variable changes from one region to the next. A potential downside to this type of visualization is that the exact numerical values aren’t easily accessible because the colors represent a range of values. Some data visualization tools, however, allow you to add interactivity to your map so the exact values are accessible.
15. Word Cloud

A word cloud , or tag cloud, is a visual representation of text data in which the size of the word is proportional to its frequency. The more often a specific word appears in a dataset, the larger it appears in the visualization. In addition to size, words often appear bolder or follow a specific color scheme depending on their frequency.
Word clouds are often used on websites and blogs to identify significant keywords and compare differences in textual data between two sources. They are also useful when analyzing qualitative datasets, such as the specific words consumers used to describe a product.
16. Network Diagram

Network diagrams are a type of data visualization that represent relationships between qualitative data points. These visualizations are composed of nodes and links, also called edges. Nodes are singular data points that are connected to other nodes through edges, which show the relationship between multiple nodes.
There are many use cases for network diagrams, including depicting social networks, highlighting the relationships between employees at an organization, or visualizing product sales across geographic regions.
17. Correlation Matrix

A correlation matrix is a table that shows correlation coefficients between variables. Each cell represents the relationship between two variables, and a color scale is used to communicate whether the variables are correlated and to what extent.
Correlation matrices are useful to summarize and find patterns in large data sets. In business, a correlation matrix might be used to analyze how different data points about a specific product might be related, such as price, advertising spend, launch date, etc.
Other Data Visualization Options
While the examples listed above are some of the most commonly used techniques, there are many other ways you can visualize data to become a more effective communicator. Some other data visualization options include:
- Bubble clouds
- Circle views
- Dendrograms
- Dot distribution maps
- Open-high-low-close charts
- Polar areas
- Radial trees
- Ring Charts
- Sankey diagram
- Span charts
- Streamgraphs
- Wedge stack graphs
- Violin plots

Tips For Creating Effective Visualizations
Creating effective data visualizations requires more than just knowing how to choose the best technique for your needs. There are several considerations you should take into account to maximize your effectiveness when it comes to presenting data.
Related : What to Keep in Mind When Creating Data Visualizations in Excel
One of the most important steps is to evaluate your audience. For example, if you’re presenting financial data to a team that works in an unrelated department, you’ll want to choose a fairly simple illustration. On the other hand, if you’re presenting financial data to a team of finance experts, it’s likely you can safely include more complex information.
Another helpful tip is to avoid unnecessary distractions. Although visual elements like animation can be a great way to add interest, they can also distract from the key points the illustration is trying to convey and hinder the viewer’s ability to quickly understand the information.
Finally, be mindful of the colors you utilize, as well as your overall design. While it’s important that your graphs or charts are visually appealing, there are more practical reasons you might choose one color palette over another. For instance, using low contrast colors can make it difficult for your audience to discern differences between data points. Using colors that are too bold, however, can make the illustration overwhelming or distracting for the viewer.
Related : Bad Data Visualization: 5 Examples of Misleading Data
Visuals to Interpret and Share Information
No matter your role or title within an organization, data visualization is a skill that’s important for all professionals. Being able to effectively present complex data through easy-to-understand visual representations is invaluable when it comes to communicating information with members both inside and outside your business.
There’s no shortage in how data visualization can be applied in the real world. Data is playing an increasingly important role in the marketplace today, and data literacy is the first step in understanding how analytics can be used in business.
Are you interested in improving your analytical skills? Learn more about Business Analytics , our eight-week online course that can help you use data to generate insights and tackle business decisions.
This post was updated on January 20, 2022. It was originally published on September 17, 2019.

About the Author
- Skip to main content
- Skip to primary sidebar
- Skip to footer
- QuestionPro

- Solutions Industries Gaming Automotive Sports and events Education Government Travel & Hospitality Financial Services Healthcare Cannabis Technology Use Case NPS+ Communities Audience Contactless surveys Mobile LivePolls Member Experience GDPR Positive People Science 360 Feedback Surveys
- Resources Blog eBooks Survey Templates Case Studies Training Help center

Home Market Research
Data Filtering: What It Is, Uses, Benefits and Example
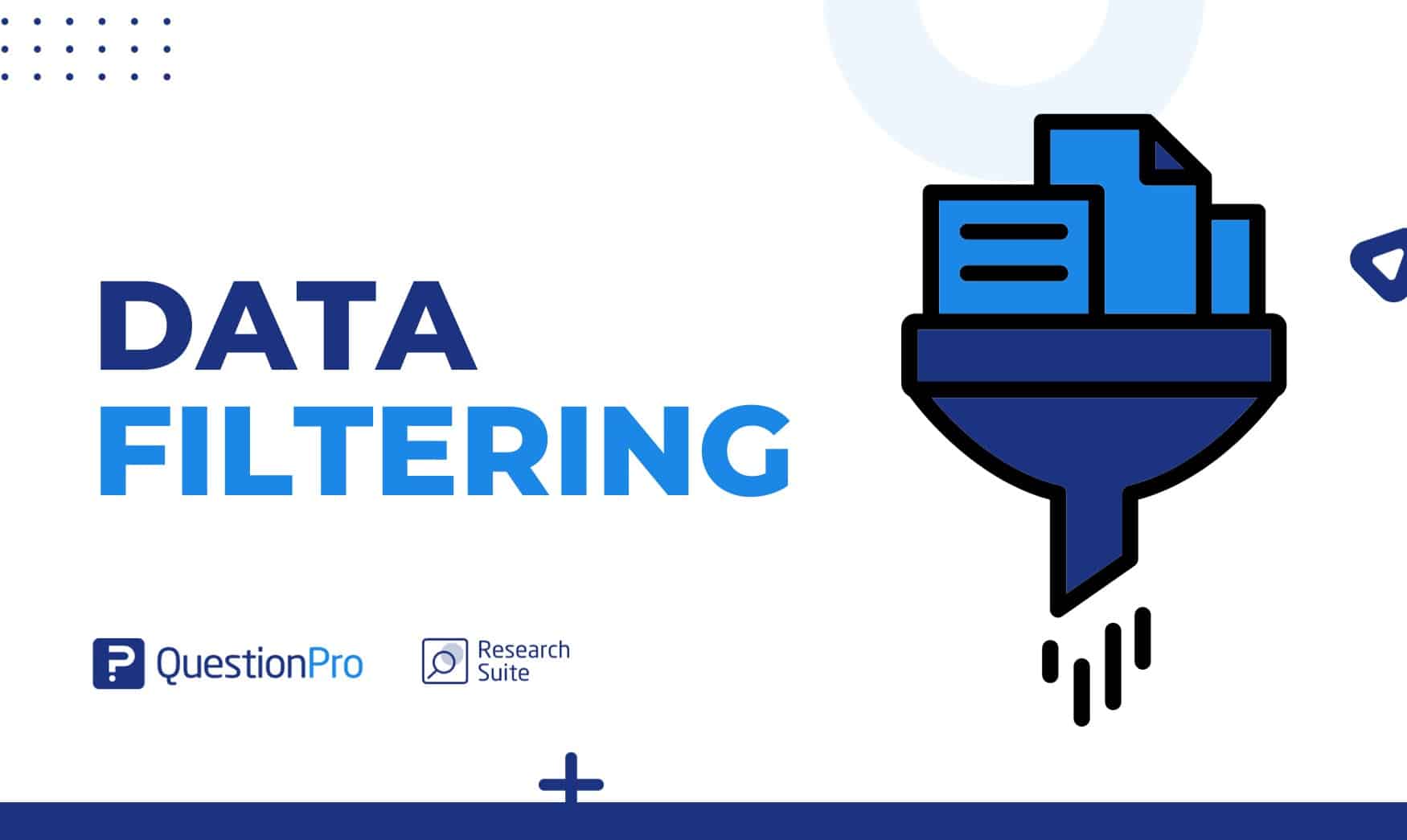
Efficiently navigating through data is crucial in the vast world of information. Data filtering is a key process that helps individuals and organizations extract valuable insights, organize information, and make informed decisions.
Implementing effective filtering strategies in utilizing research is crucial for obtaining accurate and insightful metrics. In this blog post, we’ll explore the essence of data filtering, explore its diverse applications, and highlight the myriad benefits it brings to the table.
What is Data Filtering
Filtering data means choosing or not choosing certain information from a set of data using a set of criteria. This is important for finding important data, getting rid of unnecessary information, and improving the overall quality of the data.
Analyzing data involves finding unusual values by looking at the filtered data to make sure the results are accurate and reliable. Whether you’re working with big sets of data in analytics, databases, or everyday tasks, good filtering can really make your operations more efficient.
How To Do Data Filtering
Data filtering selects and displays a subset of data based on specific criteria. The method for filtering data can vary depending on the context, such as whether you are working with databases, spreadsheets, or programming languages.
To perform data filtering effectively, follow these steps:
1. Define Analysis Criteria
Clearly articulate the specific criteria you aim to analyze. For instance, if the goal is to assess revenue by customer, determine the relevant time period and the specific customers to include in the analysis.
2. Choose Filtering Tools
Select appropriate tools for data filter based on your requirements. Options include SQL queries for database filtering or Excel filters for spreadsheet data. The choice of tools depends on the nature and source of your data.
3. Utilize SQL Queries
Construct SQL queries to filter data based on your defined criteria if working with databases. SQL provides powerful filtering capabilities, allowing you to extract specific subsets of data for analysis.
4. Excel Filters
In spreadsheet applications like Excel, built-in filtering features are used. This allows you to easily sort and display data that meets specific criteria, providing a quick and flexible way to analyze information.
Users can easily refine their search criteria through the intuitive drop-down menu, streamlining data filtering for a more personalized and efficient experience.
5. Specify Time Periods
When filtering data, pay attention to time-related aspects. Specify the time periods relevant to your analysis to ensure accurate and meaningful insights.
6. Employ Multiple Filters
Enhance your analysis by using multiple filters simultaneously. For a comprehensive understanding, filter data based on factors such as time period, customer segment, and product type. This approach helps uncover detailed insights.
7. Explore Data Visualization
Leverage data visualization tools like Tableau or Power BI to create visual representations of your filtered data. These tools facilitate a more intuitive and comprehensive analysis, allowing you to identify trends, patterns, and outliers efficiently.
8. Iterate and Refine
The process of filtering is often iterative. After an initial analysis, assess the results and consider refining your criteria or adjusting filters to gain deeper insights. This iterative approach ensures continuous improvement in the accuracy and relevance of your analysis.
Uses of Data Filtering
It is a versatile technique with various applications across various domains. Here are some key uses of data filtering:
- Excel and Spreadsheet Operations
It is commonly employed in spreadsheet software like Microsoft Excel. Users can filter data rows based on specific conditions, allowing them to view and manipulate only the data that meets certain criteria. This is particularly useful when dealing with large datasets, streamlining the analysis process.
- Data Analysis and Business Intelligence
It plays a crucial role in data analysis and business intelligence. Analysts can focus on subsets of data that are relevant to their research, enabling them to uncover patterns, trends, and insights that might be obscured in a larger dataset.
- Database Management and Queries
In database systems, filtering retrieves specific records that meet certain criteria. This ensures that only relevant data is accessed, reducing processing time and improving overall system performance.
In data base management systems, filtering is integral to crafting SQL queries. By applying filters to SELECT statements, users can retrieve data filters that match specific conditions, avoiding the need to sift through irrelevant information.
- E-commerce and Marketing
For businesses engaged in e-commerce, data filtering aids in targeting specific customer segments. Marketers can leverage this process to tailor campaigns, promotions, and product recommendations based on customer preferences and behaviors.
- Network Security
Filtering is a crucial component of network security and data security, where it is employed to identify and block potentially harmful data or traffic. This helps prevent cyber threats and ensures the integrity of a network.
- Research and Academia
Researchers often sift through vast datasets to identify relevant information for their studies. Data filtering streamlines this process, enabling scholars to focus on the specific data points that are pertinent to their research objectives.
Data filtering offers a multitude of benefits across various industries and organizational functions. Here are key advantages associated with the use of it:
1. Enhanced Decision-Making
By isolating relevant data, decision-makers can make more informed and accurate choices. This is particularly critical in dynamic environments where quick decision-making is essential.
2. Improved Efficiency
Filtering out unnecessary data streamlines processes, reducing the time and resources required for analysis. This efficiency gain is particularly valuable in industries where timely decisions are paramount.
3. Increased Accuracy
Eliminating irrelevant data minimizes the risk of errors and ensures that analyses are based on high-quality, pertinent information.
4. Cost Savings
Efficient data filtering can lead to cost savings by optimizing data source utilization and improving the overall productivity of data-related tasks.
5. Customization and Personalization
Businesses can tailor their offerings and services based on the insights gained through data filters, leading to a more personalized customer experience.
Real-world Examples of Data Filtering
E-commerce product analysis.
In an e-commerce setting, filtering can be used to analyze product sales based on various criteria such as region, time period, or customer demographics. This information helps businesses effectively tailor their marketing strategies to target specific audience segments.
Healthcare Patient Data
Healthcare providers can use filtering to analyze patient records, focusing on specific medical conditions, age groups, or treatment outcomes. This targeted approach can lead to more personalized patient care and improved treatment plans.
Financial Fraud Detection
In the financial sector, data filtering is crucial for detecting fraudulent activities. By setting multiple filters to identify unusual transactions or patterns, financial institutions can quickly pinpoint and investigate potential fraud, safeguarding their customers and assets.
How QuestionPro Filtering Analysis Can Help in Data Filtering
In QuestionPro, filtering analysis means sorting through survey data by using filters during analysis. Filtering helps you concentrate on specific parts of your data, making it easier to get focused and meaningful insights.
Here’s how QuestionPro’s filtering analysis can help in it:
Segmentation of Responses
Filtering allows you to segment and analyze responses based on specific criteria such as demographics, geographic location, or any other filters or relevant variables. This helps in understanding how different groups of respondents perceive or interact with the survey content.
Customized Data Views
You can create customized views of your data by applying filters. For example, analyzing responses from a particular age group and filtering helps in creating a view that only includes data from that specific age range.
Comparative Analysis
Filtering facilitates comparative analysis by enabling you to compare responses across different groups. This is particularly useful when you want to identify patterns or trends that may be specific to certain segments of your audience.
Drilling Down into Specific Issues
If you identify an interesting trend or issue in your overall data, filtering allows you to drill down into specific subsets of responses to gain more detailed insights into the underlying factors contributing to that trend.
Removing Outliers or Irrelevant Data
Filters can be applied to exclude outliers or responses that may not be relevant to your analysis. This ensures that your analysis is focused on the most meaningful and representative data.
Enhancing Data Accuracy
By applying filters, you can enhance the accuracy of your analysis by focusing on responses that meet specific criteria. This can be particularly important when dealing with large datasets where irrelevant or outlier data points might skew results.
Tailoring Reports
When generating reports or exporting data, filtering allows you to tailor the output to include only the information that is most relevant to your research goals. This makes it easier to communicate insights to stakeholders or team members.
In conclusion, data filtering is a cornerstone in the realm of data management, providing a structured approach to handling information. Its applications are diverse, spanning industries and sectors, and its benefits extend from improved decision-making to resource optimization.
As we continue to navigate an increasingly data-driven world, mastering the art of filtering becomes an indispensable skill for individuals and organizations alike.
QuestionPro excels in data filtering, offering a robust platform that empowers users to extract meaningful insights efficiently. With advanced filtering options, it streamlines the analysis process, allowing user defined to sift through large data sets effortlessly.
This capability ensures that decision-makers can focus on relevant information, saving time and enhancing the precision of their decision-making processes. QuestionPro stands as a valuable ally in harnessing the power of filtering for informed decision-making.
LEARN MORE FREE TRIAL
MORE LIKE THIS
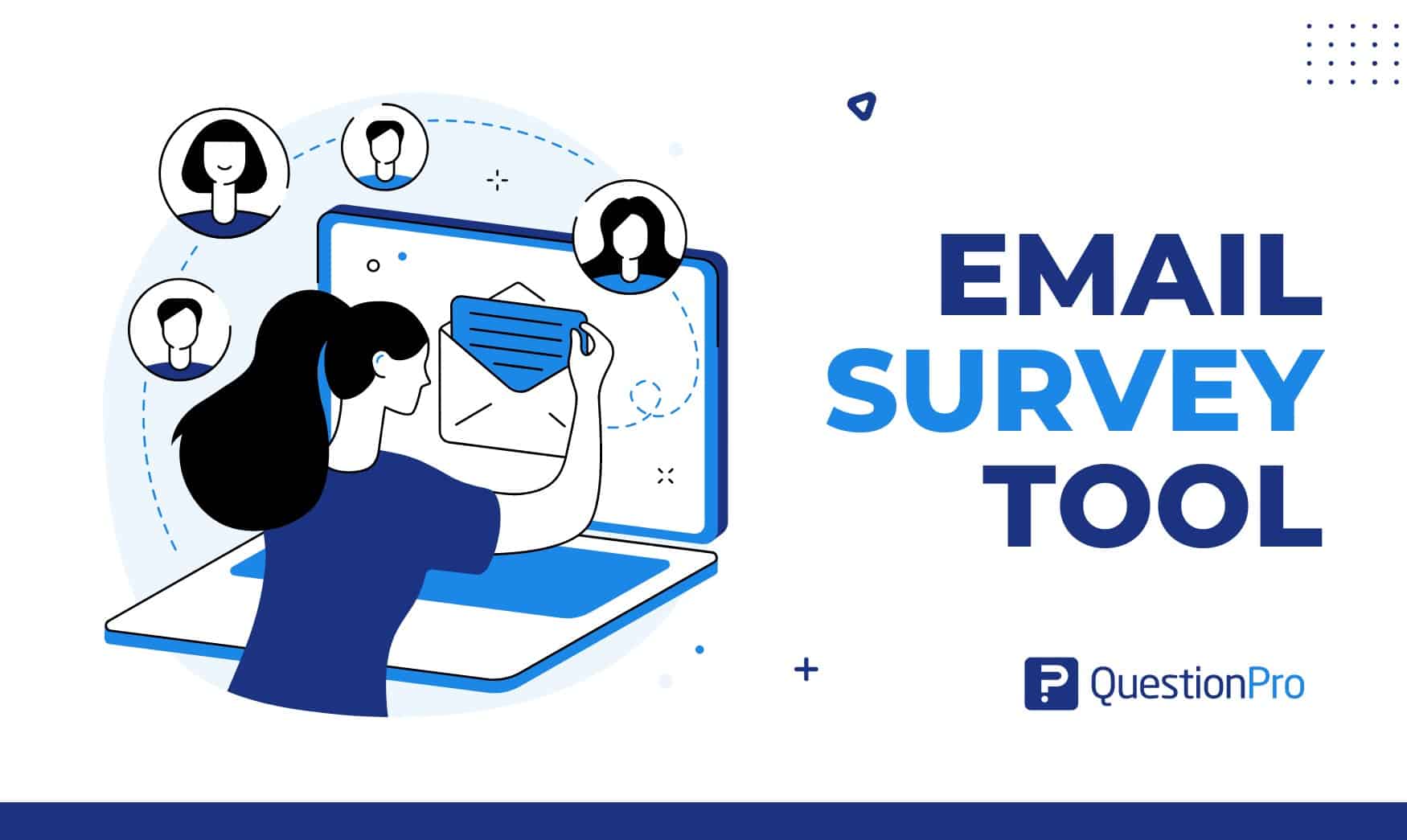
The Best Email Survey Tool to Boost Your Feedback Game
May 7, 2024
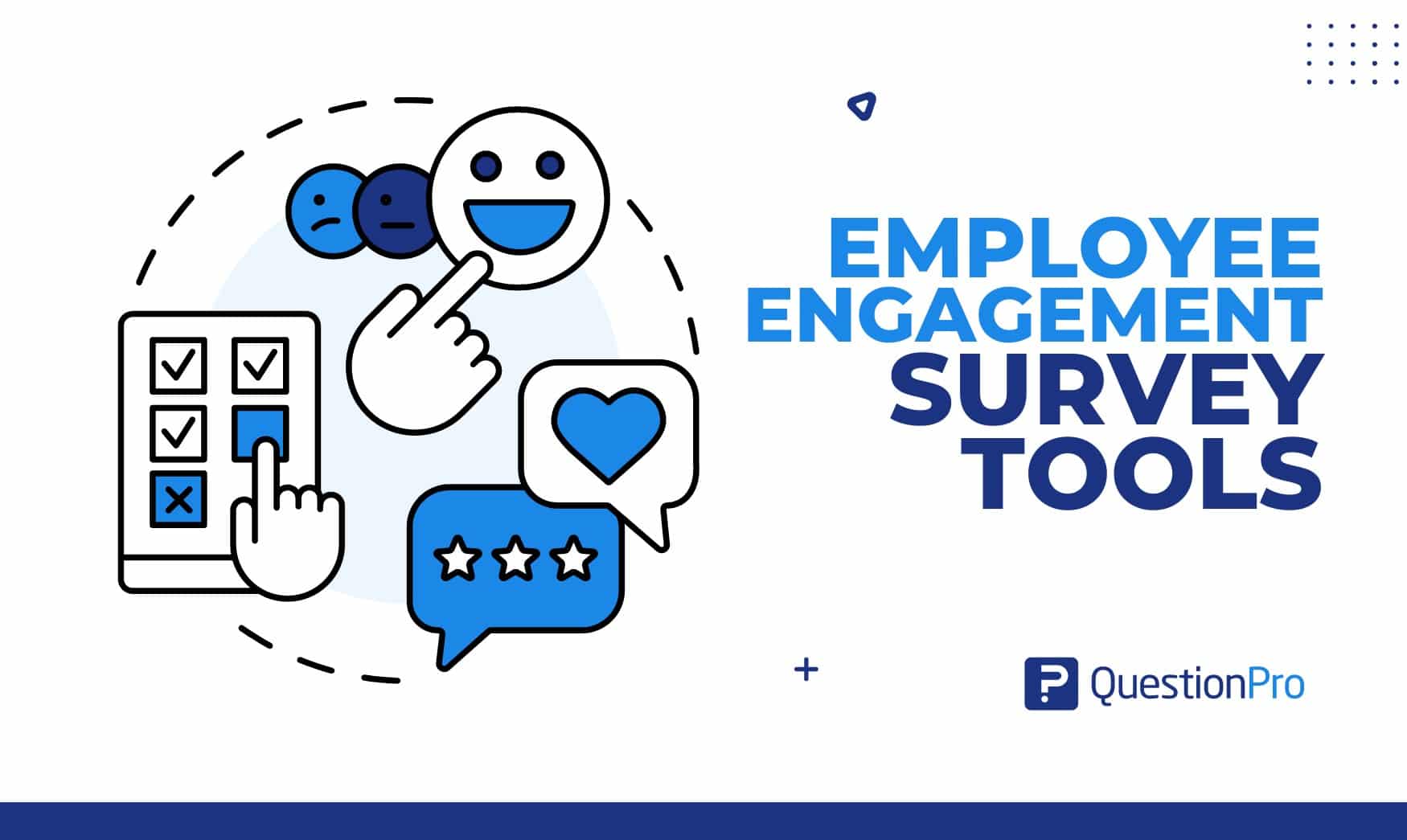
Top 10 Employee Engagement Survey Tools
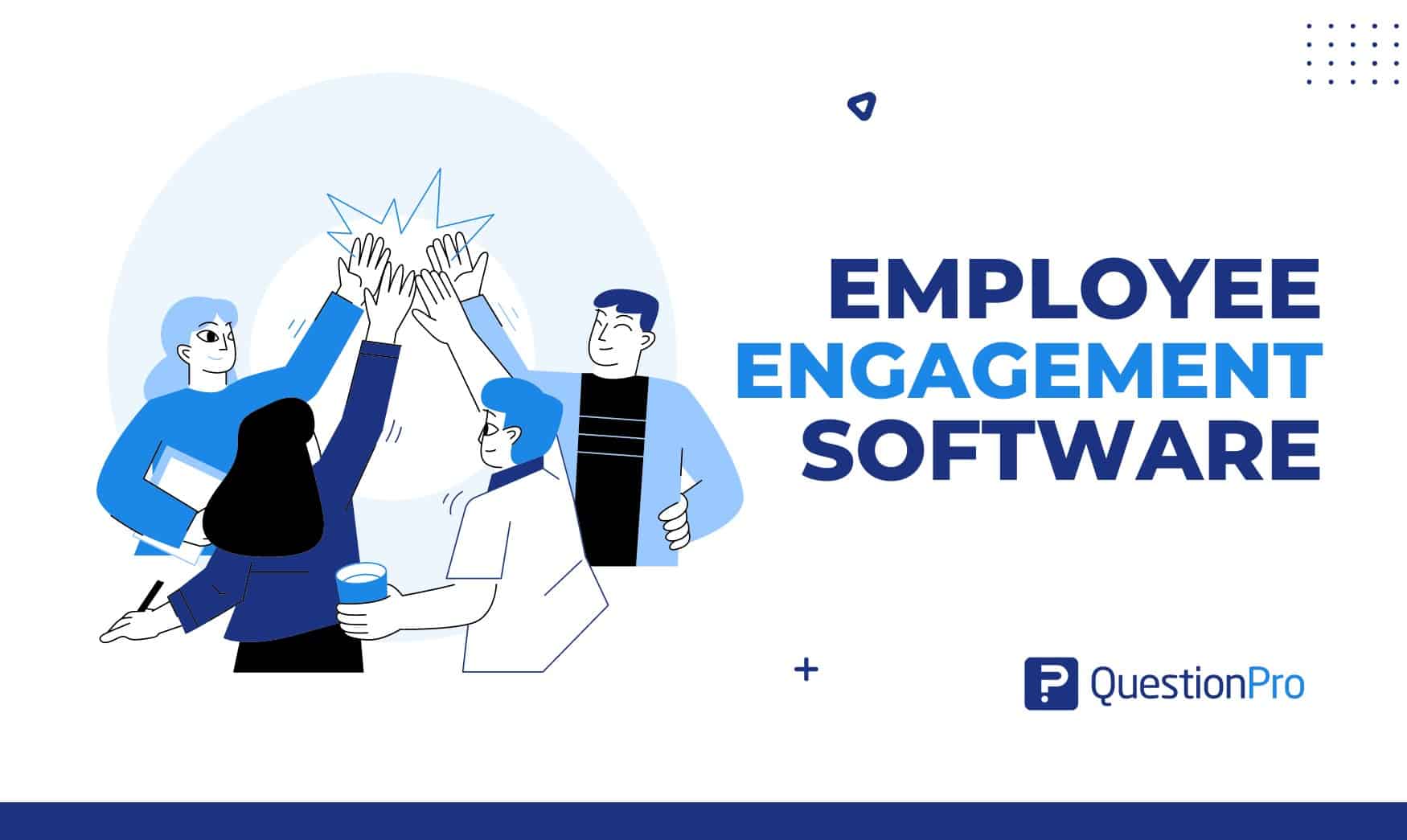
Top 20 Employee Engagement Software Solutions
May 3, 2024
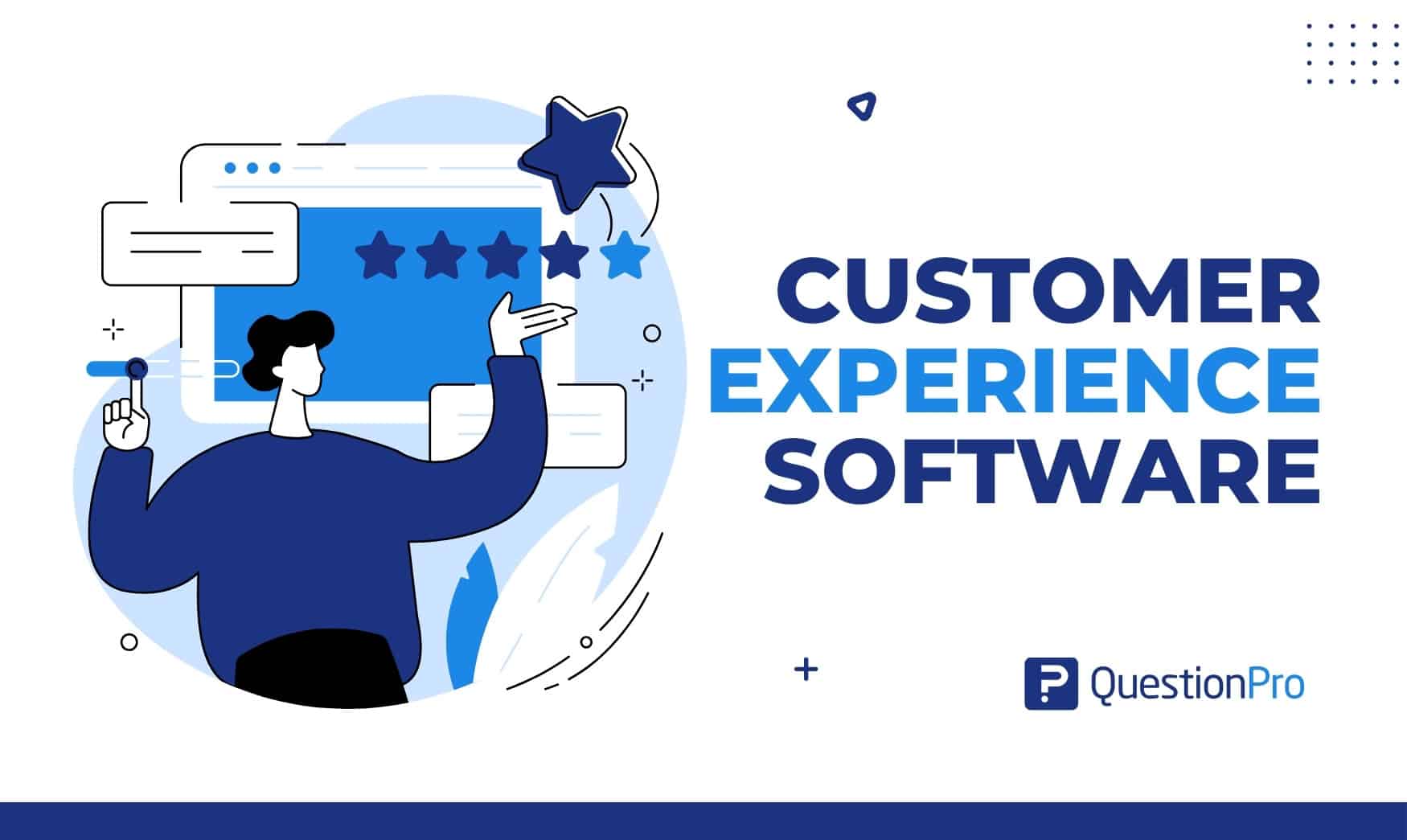
15 Best Customer Experience Software of 2024
May 2, 2024
Other categories
- Academic Research
- Artificial Intelligence
- Assessments
- Brand Awareness
- Case Studies
- Communities
- Consumer Insights
- Customer effort score
- Customer Engagement
- Customer Experience
- Customer Loyalty
- Customer Research
- Customer Satisfaction
- Employee Benefits
- Employee Engagement
- Employee Retention
- Friday Five
- General Data Protection Regulation
- Insights Hub
- Life@QuestionPro
- Market Research
- Mobile diaries
- Mobile Surveys
- New Features
- Online Communities
- Question Types
- Questionnaire
- QuestionPro Products
- Release Notes
- Research Tools and Apps
- Revenue at Risk
- Survey Templates
- Training Tips
- Uncategorized
- Video Learning Series
- What’s Coming Up
- Workforce Intelligence
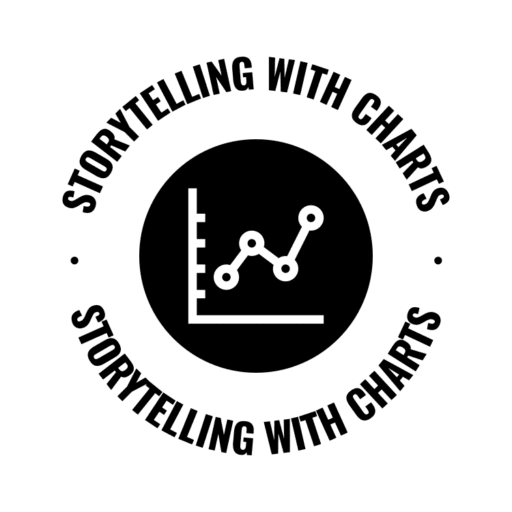
- Chart Guide
- Data Makeover
0 comments
Visualizing Data in Excel: A Comprehensive Guide
By STC
July 15, 2023
Explore the diverse data visualization possibilities in Excel that aid in analyzing and interpreting your data effectively.
Introduction
Welcome to our comprehensive guide on visualizing data in Excel. In this article, we will delve into the world of data visualization and provide you with valuable insights on how to create compelling visual representations of your data using Excel. Whether you are a beginner or an experienced Excel user, this guide will equip you with the knowledge and techniques to effectively communicate your data through visually appealing charts and graphs.
Why Data Visualization Matters
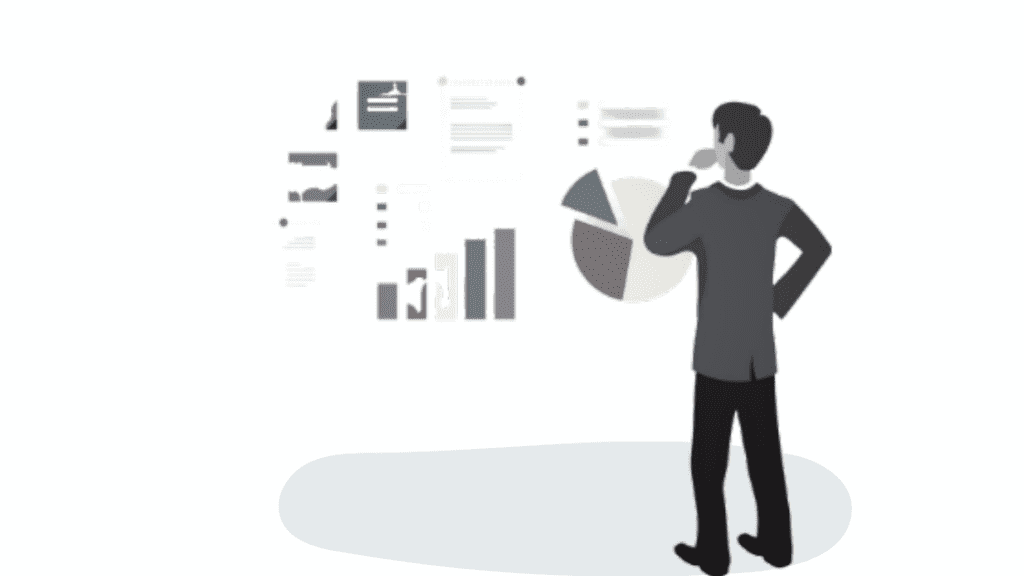
Data visualization is a powerful tool that enables us to make sense of complex datasets. It allows us to identify patterns, trends, and outliers that might not be immediately apparent in raw data. Visualizing data in Excel not only enhances our understanding of the information at hand but also enables us to communicate our findings to others in a clear and concise manner.
Getting Started with Excel Charts
- Selecting the Right Chart Type Choosing the appropriate chart type is crucial for effectively representing your data. Excel offers a wide range of chart options, including bar charts, line charts, pie charts, scatter plots, and more. Consider the nature of your data and the message you want to convey when selecting the most suitable chart type.
- Formatting and Customization Excel provides extensive formatting and customization options to refine the appearance of your charts. From adjusting axis labels to modifying colors and styles, these features allow you to create visually appealing charts that align with your brand or presentation requirements.
- Adding Data Labels and Annotations To enhance the clarity of your visualizations, Excel enables you to add data labels and annotations. These labels provide additional context and make it easier for your audience to interpret the information being presented. You can include axis labels, data point labels, and explanatory text to further enrich your charts.
Advanced-Data Visualization Techniques
- Creating PivotCharts PivotCharts are a powerful feature in Excel that allows you to visualize data from pivot tables. By summarizing and aggregating data, pivot tables provide a comprehensive overview that can be transformed into dynamic and interactive charts. Utilizing PivotCharts enables you to explore and analyze complex datasets with ease.
- Utilizing Advanced Charting Features Excel offers advanced charting features that can take your visualizations to the next level. From trendlines and error bars to 3D charts and sparklines, these tools allow you to add depth and sophistication to your data representations. Experimenting with these features can help you create visually striking charts that captivate your audience.
Best Practices for Effective Data Visualization
To ensure your data visualizations have maximum impact, keep the following best practices in mind:
- Simplify and Declutter Avoid cluttering your charts with excessive information or unnecessary embellishments. Focus on the key message you want to convey and remove any elements that distract from that message. Remember, simplicity is key when it comes to effective data visualization.
- Use Color Strategically Colors can evoke emotions and draw attention to specific areas of your charts. Use color strategically to highlight important data points or to group related information. However, be mindful of accessibility considerations and ensure that your color choices are accessible to individuals with color vision deficiencies.
- Tell a Story with Your Data Data visualization is not just about presenting numbers; it’s about telling a story. Structure your visualizations in a way that guides your audience through a narrative. Start with an introduction, present the main findings, and conclude with a clear takeaway or call to action.
In conclusion, mastering the art of visualizing data in Excel can significantly enhance your ability to analyze and communicate complex information. By selecting the right chart types, utilizing advanced techniques, and following best practices, you can create visually compelling representations that effectively convey your data’s story. We hope this comprehensive guide has provided you with the knowledge and inspiration to create outstanding data visualizations in Excel. Start exploring the power of data visualization today and unlock new insights from your data.
Check StoryTelling with Charts – The Full Story
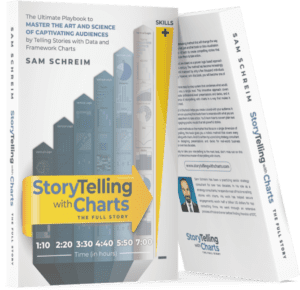
About the author
We are passionate about the power of visual storytelling and believe that charts can convey complex information in a captivating and easily understandable way. Whether you're a data enthusiast, a business professional, or simply curious about the world around you, this page is your gateway to the world of data visualization.
Never miss a good story!
Subscribe to our newsletter to keep up with the latest trends!
Cookie Consent Manager
General information, required cookies, functional cookies, advertising cookies.
We use three kinds of cookies on our websites: required, functional, and advertising. You can choose whether functional and advertising cookies apply. Click on the different cookie categories to find out more about each category and to change the default settings. Privacy Statement
Required cookies are necessary for basic website functionality. Some examples include: session cookies needed to transmit the website, authentication cookies, and security cookies.
Functional cookies enhance functions, performance, and services on the website. Some examples include: cookies used to analyze site traffic, cookies used for market research, and cookies used to display advertising that is not directed to a particular individual.
Advertising cookies track activity across websites in order to understand a viewer’s interests, and direct them specific marketing. Some examples include: cookies used for remarketing, or interest-based advertising.
Back Button Back
- Name cookie name

Andrew Hills (Member) asked a question.
I suspect the answer is that it can't be done, but I'll ask the question anyway.
My users complain that they can't tell what is being filtered and what isn't (Yes, yes, yes, I know. Trust me I've knock some of the plaster off the office wall with my head). I was wondering if there's a way I can visually display the filtered data as something like a tree map or heat map, but where the colour changes depending on if the month is filtered or not. Sort of like automatically highlighting, like this:
But highlighted by the filter.
- Actions & Filters

KALPIT GOYAL (Member)
Can you show your multi-select filter in your dashboard so that everybody knows, which filter is selected. Is it possible in your visual representation, if yes then this will become the solution to your problem.
Andrew Hills (Member)
I mean - I'd love that to be the case, but there was significant pushback when the filter was included. And yes, I know how dumb that is, but I don't get the final say.
I mean if you don't want the check boxes then create a separate sheet with your months and apply the action filter on it, it will reflect the changes on the relevant sheet as well as highlighted the particular months.
Yes I know - this is my fallback position if all else fails. Unfortunately my users have a tendency not to interact with the functionality all that much so I was hoping there was a way of visually displaying the filter another way.
BTW - if the answer is "No there isn't" that's totally fine. I'd be glad to know it can't be done.
According to me above mentioned are the options for you. I hope action filter will derive you to the desired result.
If you feel my solution helps you in anyway then kindly mark it as the helpful and correct answer.
Thanks in advance !!
Related Conversations
Trending topics.
- REST API 51
Introduction to Data Science
Chapter 11 data visualization principles.
We have already provided some rules to follow as we created plots for our examples. Here, we aim to provide some general principles we can use as a guide for effective data visualization. Much of this section is based on a talk by Karl Broman 30 titled “Creating Effective Figures and Tables” 31 and includes some of the figures which were made with code that Karl makes available on his GitHub repository 32 , as well as class notes from Peter Aldhous’ Introduction to Data Visualization course 33 . Following Karl’s approach, we show some examples of plot styles we should avoid, explain how to improve them, and use these as motivation for a list of principles. We compare and contrast plots that follow these principles to those that don’t.
The principles are mostly based on research related to how humans detect patterns and make visual comparisons. The preferred approaches are those that best fit the way our brains process visual information. When deciding on a visualization approach, it is also important to keep our goal in mind. We may be comparing a viewable number of quantities, describing distributions for categories or numeric values, comparing the data from two groups, or describing the relationship between two variables. As a final note, we want to emphasize that for a data scientist it is important to adapt and optimize graphs to the audience. For example, an exploratory plot made for ourselves will be different than a chart intended to communicate a finding to a general audience.
We will be using these libraries:
11.1 Encoding data using visual cues
We start by describing some principles for encoding data. There are several approaches at our disposal including position, aligned lengths, angles, area, brightness, and color hue.
To illustrate how some of these strategies compare, let’s suppose we want to report the results from two hypothetical polls regarding browser preference taken in 2000 and then 2015. For each year, we are simply comparing five quantities – the five percentages. A widely used graphical representation of percentages, popularized by Microsoft Excel, is the pie chart:
Here we are representing quantities with both areas and angles, since both the angle and area of each pie slice are proportional to the quantity the slice represents. This turns out to be a sub-optimal choice since, as demonstrated by perception studies, humans are not good at precisely quantifying angles and are even worse when area is the only available visual cue. The donut chart is an example of a plot that uses only area:
To see how hard it is to quantify angles and area, note that the rankings and all the percentages in the plots above changed from 2000 to 2015. Can you determine the actual percentages and rank the browsers’ popularity? Can you see how the percentages changed from 2000 to 2015? It is not easy to tell from the plot. In fact, the pie R function help file states that:
Pie charts are a very bad way of displaying information. The eye is good at judging linear measures and bad at judging relative areas. A bar chart or dot chart is a preferable way of displaying this type of data.
In this case, simply showing the numbers is not only clearer, but would also save on printing costs if printing a paper copy:
The preferred way to plot these quantities is to use length and position as visual cues, since humans are much better at judging linear measures. The barplot uses this approach by using bars of length proportional to the quantities of interest. By adding horizontal lines at strategically chosen values, in this case at every multiple of 10, we ease the visual burden of quantifying through the position of the top of the bars. Compare and contrast the information we can extract from the two figures.
Notice how much easier it is to see the differences in the barplot. In fact, we can now determine the actual percentages by following a horizontal line to the x-axis.
If for some reason you need to make a pie chart, label each pie slice with its respective percentage so viewers do not have to infer them from the angles or area:
In general, when displaying quantities, position and length are preferred over angles and/or area. Brightness and color are even harder to quantify than angles. But, as we will see later, they are sometimes useful when more than two dimensions must be displayed at once.
11.2 Know when to include 0
When using barplots, it is misinformative not to start the bars at 0. This is because, by using a barplot, we are implying the length is proportional to the quantities being displayed. By avoiding 0, relatively small differences can be made to look much bigger than they actually are. This approach is often used by politicians or media organizations trying to exaggerate a difference. Below is an illustrative example used by Peter Aldhous in this lecture: http://paldhous.github.io/ucb/2016/dataviz/week2.html .
(Source: Fox News, via Media Matters 34 .)
From the plot above, it appears that apprehensions have almost tripled when, in fact, they have only increased by about 16%. Starting the graph at 0 illustrates this clearly:
Here is another example, described in detail in a Flowing Data blog post:
This plot makes a 13% increase look like a five fold change. Here is the appropriate plot:
Finally, here is an extreme example that makes a very small difference of under 2% look like a 10-100 fold change:
(Source: Venezolana de Televisión via Pakistan Today 36 and Diego Mariano.)
Here is the appropriate plot:
When using position rather than length, it is then not necessary to include 0. This is particularly the case when we want to compare differences between groups relative to the within-group variability. Here is an illustrative example showing country average life expectancy stratified across continents in 2012:
Note that in the plot on the left, which includes 0, the space between 0 and 43 adds no information and makes it harder to compare the between and within group variability.
11.3 Do not distort quantities
During President Barack Obama’s 2011 State of the Union Address, the following chart was used to compare the US GDP to the GDP of four competing nations:
Judging by the area of the circles, the US appears to have an economy over five times larger than China’s and over 30 times larger than France’s. However, if we look at the actual numbers, we see that this is not the case. The actual ratios are 2.6 and 5.8 times bigger than China and France, respectively. The reason for this distortion is that the radius, rather than the area, was made to be proportional to the quantity, which implies that the proportion between the areas is squared: 2.6 turns into 6.5 and 5.8 turns into 34.1. Here is a comparison of the circles we get if we make the value proportional to the radius and to the area:
Not surprisingly, ggplot2 defaults to using area rather than radius. Of course, in this case, we really should not be using area at all since we can use position and length:
11.4 Order categories by a meaningful value
When one of the axes is used to show categories, as is done in barplots, the default ggplot2 behavior is to order the categories alphabetically when they are defined by character strings. If they are defined by factors, they are ordered by the factor levels. We rarely want to use alphabetical order. Instead, we should order by a meaningful quantity. In all the cases above, the barplots were ordered by the values being displayed. The exception was the graph showing barplots comparing browsers. In this case, we kept the order the same across the barplots to ease the comparison. Specifically, instead of ordering the browsers separately in the two years, we ordered both years by the average value of 2000 and 2015.
We previously learned how to use the reorder function, which helps us achieve this goal. To appreciate how the right order can help convey a message, suppose we want to create a plot to compare the murder rate across states. We are particularly interested in the most dangerous and safest states. Note the difference when we order alphabetically (the default) versus when we order by the actual rate:
We can make the second plot like this:
The reorder function lets us reorder groups as well. Earlier we saw an example related to income distributions across regions. Here are the two versions plotted against each other:
The first orders the regions alphabetically, while the second orders them by the group’s median.
11.5 Show the data
We have focused on displaying single quantities across categories. We now shift our attention to displaying data, with a focus on comparing groups.
To motivate our first principle, “show the data”, we go back to our artificial example of describing heights to ET, an extraterrestrial. This time let’s assume ET is interested in the difference in heights between males and females. A commonly seen plot used for comparisons between groups, popularized by software such as Microsoft Excel, is the dynamite plot, which shows the average and standard errors (standard errors are defined in a later chapter, but do not confuse them with the standard deviation of the data). The plot looks like this:
The average of each group is represented by the top of each bar and the antennae extend out from the average to the average plus two standard errors. If all ET receives is this plot, he will have little information on what to expect if he meets a group of human males and females. The bars go to 0: does this mean there are tiny humans measuring less than one foot? Are all males taller than the tallest females? Is there a range of heights? ET can’t answer these questions since we have provided almost no information on the height distribution.
This brings us to our first principle: show the data. This simple ggplot2 code already generates a more informative plot than the barplot by simply showing all the data points:
For example, this plot gives us an idea of the range of the data. However, this plot has limitations as well, since we can’t really see all the 238 and 812 points plotted for females and males, respectively, and many points are plotted on top of each other. As we have previously described, visualizing the distribution is much more informative. But before doing this, we point out two ways we can improve a plot showing all the points.
The first is to add jitter , which adds a small random shift to each point. In this case, adding horizontal jitter does not alter the interpretation, since the point heights do not change, but we minimize the number of points that fall on top of each other and, therefore, get a better visual sense of how the data is distributed. A second improvement comes from using alpha blending : making the points somewhat transparent. The more points fall on top of each other, the darker the plot, which also helps us get a sense of how the points are distributed. Here is the same plot with jitter and alpha blending:
Now we start getting a sense that, on average, males are taller than females. We also note dark horizontal bands of points, demonstrating that many report values that are rounded to the nearest integer.
11.6 Ease comparisons
11.6.1 use common axes.
Since there are so many points, it is more effective to show distributions rather than individual points. We therefore show histograms for each group:
However, from this plot it is not immediately obvious that males are, on average, taller than females. We have to look carefully to notice that the x-axis has a higher range of values in the male histogram. An important principle here is to keep the axes the same when comparing data across two plots. Below we see how the comparison becomes easier:
11.6.2 Align plots vertically to see horizontal changes and horizontally to see vertical changes
In these histograms, the visual cue related to decreases or increases in height are shifts to the left or right, respectively: horizontal changes. Aligning the plots vertically helps us see this change when the axes are fixed:
This plot makes it much easier to notice that men are, on average, taller.
If , we want the more compact summary provided by boxplots, we then align them horizontally since, by default, boxplots move up and down with changes in height. Following our show the data principle, we then overlay all the data points:
Now contrast and compare these three plots, based on exactly the same data:
Notice how much more we learn from the two plots on the right. Barplots are useful for showing one number, but not very useful when we want to describe distributions.
11.6.3 Consider transformations
We have motivated the use of the log transformation in cases where the changes are multiplicative. Population size was an example in which we found a log transformation to yield a more informative transformation.
The combination of an incorrectly chosen barplot and a failure to use a log transformation when one is merited can be particularly distorting. As an example, consider this barplot showing the average population sizes for each continent in 2015:
From this plot, one would conclude that countries in Asia are much more populous than in other continents. Following the show the data principle, we quickly notice that this is due to two very large countries, which we assume are India and China:
Using a log transformation here provides a much more informative plot. We compare the original barplot to a boxplot using the log scale transformation for the y-axis:
With the new plot, we realize that countries in Africa actually have a larger median population size than those in Asia.
Other transformations you should consider are the logistic transformation ( logit ), useful to better see fold changes in odds, and the square root transformation ( sqrt ), useful for count data.
11.6.4 Visual cues to be compared should be adjacent
For each continent, let’s compare income in 1970 versus 2010. When comparing income data across regions between 1970 and 2010, we made a figure similar to the one below, but this time we investigate continents rather than regions.
The default in ggplot2 is to order labels alphabetically so the labels with 1970 come before the labels with 2010, making the comparisons challenging because a continent’s distribution in 1970 is visually far from its distribution in 2010. It is much easier to make the comparison between 1970 and 2010 for each continent when the boxplots for that continent are next to each other:
11.6.5 Use color
The comparison becomes even easier to make if we use color to denote the two things we want to compare:
11.7 Think of the color blind
About 10% of the population is color blind. Unfortunately, the default colors used in ggplot2 are not optimal for this group. However, ggplot2 does make it easy to change the color palette used in the plots. An example of how we can use a color blind friendly palette is described here: http://www.cookbook-r.com/Graphs/Colors_(ggplot2)/#a-colorblind-friendly-palette :
There are several resources that can help you select colors, for example this one: http://bconnelly.net/2013/10/creating-colorblind-friendly-figures/ .
11.8 Plots for two variables
In general, you should use scatterplots to visualize the relationship between two variables. In every single instance in which we have examined the relationship between two variables, including total murders versus population size, life expectancy versus fertility rates, and infant mortality versus income, we have used scatterplots. This is the plot we generally recommend. However, there are some exceptions and we describe two alternative plots here: the slope chart and the Bland-Altman plot .
11.8.1 Slope charts
One exception where another type of plot may be more informative is when you are comparing variables of the same type, but at different time points and for a relatively small number of comparisons. For example, comparing life expectancy between 2010 and 2015. In this case, we might recommend a slope chart .
There is no geometry for slope charts in ggplot2 , but we can construct one using geom_line . We need to do some tinkering to add labels. Below is an example comparing 2010 to 2015 for large western countries:
An advantage of the slope chart is that it permits us to quickly get an idea of changes based on the slope of the lines. Although we are using angle as the visual cue, we also have position to determine the exact values. Comparing the improvements is a bit harder with a scatterplot:
In the scatterplot, we have followed the principle use common axes since we are comparing these before and after. However, if we have many points, slope charts stop being useful as it becomes hard to see all the lines.
11.8.2 Bland-Altman plot
Since we are primarily interested in the difference, it makes sense to dedicate one of our axes to it. The Bland-Altman plot, also known as the Tukey mean-difference plot and the MA-plot, shows the difference versus the average:
Here, by simply looking at the y-axis, we quickly see which countries have shown the most improvement. We also get an idea of the overall value from the x-axis.
11.9 Encoding a third variable
An earlier scatterplot showed the relationship between infant survival and average income. Below is a version of this plot that encodes three variables: OPEC membership, region, and population.
We encode categorical variables with color and shape. These shapes can be controlled with shape argument. Below are the shapes available for use in R. For the last five, the color goes inside.
For continuous variables, we can use color, intensity, or size. We now show an example of how we do this with a case study.
When selecting colors to quantify a numeric variable, we choose between two options: sequential and diverging. Sequential colors are suited for data that goes from high to low. High values are clearly distinguished from low values. Here are some examples offered by the package RColorBrewer :
Diverging colors are used to represent values that diverge from a center. We put equal emphasis on both ends of the data range: higher than the center and lower than the center. An example of when we would use a divergent pattern would be if we were to show height in standard deviations away from the average. Here are some examples of divergent patterns:
11.10 Avoid pseudo-three-dimensional plots
The figure below, taken from the scientific literature 38 , shows three variables: dose, drug type and survival. Although your screen/book page is flat and two-dimensional, the plot tries to imitate three dimensions and assigned a dimension to each variable.
Humans are not good at seeing in three dimensions (which explains why it is hard to parallel park) and our limitation is even worse with regard to pseudo-three-dimensions. To see this, try to determine the values of the survival variable in the plot above. Can you tell when the purple ribbon intersects the red one? This is an example in which we can easily use color to represent the categorical variable instead of using a pseudo-3D:
Notice how much easier it is to determine the survival values.
Pseudo-3D is sometimes used completely gratuitously: plots are made to look 3D even when the 3rd dimension does not represent a quantity. This only adds confusion and makes it harder to relay your message. Here are two examples:
11.11 Avoid too many significant digits
By default, statistical software like R returns many significant digits. The default behavior in R is to show 7 significant digits. That many digits often adds no information and the added visual clutter can make it hard for the viewer to understand the message. As an example, here are the per 10,000 disease rates, computed from totals and population in R, for California across the five decades:
We are reporting precision up to 0.00001 cases per 10,000, a very small value in the context of the changes that are occurring across the dates. In this case, two significant figures is more than enough and clearly makes the point that rates are decreasing:
Useful ways to change the number of significant digits or to round numbers are signif and round . You can define the number of significant digits globally by setting options like this: options(digits = 3) .
Another principle related to displaying tables is to place values being compared on columns rather than rows. Note that our table above is easier to read than this one:
11.12 Know your audience
Graphs can be used for 1) our own exploratory data analysis, 2) to convey a message to experts, or 3) to help tell a story to a general audience. Make sure that the intended audience understands each element of the plot.
As a simple example, consider that for your own exploration it may be more useful to log-transform data and then plot it. However, for a general audience that is unfamiliar with converting logged values back to the original measurements, using a log-scale for the axis instead of log-transformed values will be much easier to digest.
11.13 Exercises
For these exercises, we will be using the vaccines data in the dslabs package:
1. Pie charts are appropriate:
- When we want to display percentages.
- When ggplot2 is not available.
- When I am in a bakery.
- Never. Barplots and tables are always better.
2. What is the problem with the plot below:
- The values are wrong. The final vote was 306 to 232.
- The axis does not start at 0. Judging by the length, it appears Trump received 3 times as many votes when, in fact, it was about 30% more.
- The colors should be the same.
- Percentages should be shown as a pie chart.
3. Take a look at the following two plots. They show the same information: 1928 rates of measles across the 50 states.
- They provide the same information, so they are both equally as good.
- The plot on the right is better because it orders the states alphabetically.
- The plot on the right is better because alphabetical order has nothing to do with the disease and by ordering according to actual rate, we quickly see the states with most and least rates.
- Both plots should be a pie chart.
4. To make the plot on the left, we have to reorder the levels of the states’ variables.
Note what happens when we make a barplot:
Define these objects:
Redefine the state object so that the levels are re-ordered. Print the new object state and its levels so you can see that the vector is not re-ordered by the levels.
5. Now with one line of code, define the dat table as done above, but change the use mutate to create a rate variable and re-order the state variable so that the levels are re-ordered by this variable. Then make a barplot using the code above, but for this new dat .
6. Say we are interested in comparing gun homicide rates across regions of the US. We see this plot:
and decide to move to a state in the western region. What is the main problem with this interpretation?
- The categories are ordered alphabetically.
- The graph does not show standarad errors.
- It does not show all the data. We do not see the variability within a region and it’s possible that the safest states are not in the West.
- The Northeast has the lowest average.
7. Make a boxplot of the murder rates defined as
by region, showing all the points and ordering the regions by their median rate.
8. The plots below show three continuous variables.
The line \(x=2\) appears to separate the points. But it is actually not the case, which we can see by plotting the data in a couple of two-dimensional points.
Why is this happening?
- Humans are not good at reading pseudo-3D plots.
- There must be an error in the code.
- The colors confuse us.
- Scatterplots should not be used to compare two variables when we have access to 3.
11.14 Case study: vaccines and infectious diseases
Vaccines have helped save millions of lives. In the 19th century, before herd immunization was achieved through vaccination programs, deaths from infectious diseases, such as smallpox and polio, were common. However, today vaccination programs have become somewhat controversial despite all the scientific evidence for their importance.
The controversy started with a paper 39 published in 1988 and led by Andrew Wakefield claiming there was a link between the administration of the measles, mumps, and rubella (MMR) vaccine and the appearance of autism and bowel disease. Despite much scientific evidence contradicting this finding, sensationalist media reports and fear-mongering from conspiracy theorists led parts of the public into believing that vaccines were harmful. As a result, many parents ceased to vaccinate their children. This dangerous practice can be potentially disastrous given that the Centers for Disease Control (CDC) estimates that vaccinations will prevent more than 21 million hospitalizations and 732,000 deaths among children born in the last 20 years (see Benefits from Immunization during the Vaccines for Children Program Era — United States, 1994-2013, MMWR 40 ). The 1988 paper has since been retracted and Andrew Wakefield was eventually “struck off the UK medical register, with a statement identifying deliberate falsification in the research published in The Lancet, and was thereby barred from practicing medicine in the UK.” (source: Wikipedia 41 ). Yet misconceptions persist, in part due to self-proclaimed activists who continue to disseminate misinformation about vaccines.
Effective communication of data is a strong antidote to misinformation and fear-mongering. Earlier we used an example provided by a Wall Street Journal article 42 showing data related to the impact of vaccines on battling infectious diseases. Here we reconstruct that example.
The data used for these plots were collected, organized, and distributed by the Tycho Project 43 . They include weekly reported counts for seven diseases from 1928 to 2011, from all fifty states. We include the yearly totals in the dslabs package:
We create a temporary object dat that stores only the measles data, includes a per 100,000 rate, orders states by average value of disease and removes Alaska and Hawaii since they only became states in the late 1950s. Note that there is a weeks_reporting column that tells us for how many weeks of the year data was reported. We have to adjust for that value when computing the rate.
We can now easily plot disease rates per year. Here are the measles data from California:
We add a vertical line at 1963 since this is when the vaccine was introduced [Control, Centers for Disease; Prevention (2014). CDC health information for international travel 2014 (the yellow book). p. 250. ISBN 9780199948505].
Now can we show data for all states in one plot? We have three variables to show: year, state, and rate. In the WSJ figure, they use the x-axis for year, the y-axis for state, and color hue to represent rates. However, the color scale they use, which goes from yellow to blue to green to orange to red, can be improved.
In our example, we want to use a sequential palette since there is no meaningful center, just low and high rates.
We use the geometry geom_tile to tile the region with colors representing disease rates. We use a square root transformation to avoid having the really high counts dominate the plot. Notice that missing values are shown in grey. Note that once a disease was pretty much eradicated, some states stopped reporting cases all together. This is why we see so much grey after 1980.
This plot makes a very striking argument for the contribution of vaccines. However, one limitation of this plot is that it uses color to represent quantity, which we earlier explained makes it harder to know exactly how high values are going. Position and lengths are better cues. If we are willing to lose state information, we can make a version of the plot that shows the values with position. We can also show the average for the US, which we compute like this:
Now to make the plot we simply use the geom_line geometry:
In theory, we could use color to represent the categorical value state, but it is hard to pick 50 distinct colors.
11.15 Exercises
Reproduce the image plot we previously made but for smallpox. For this plot, do not include years in which cases were not reported in 10 or more weeks.
Now reproduce the time series plot we previously made, but this time following the instructions of the previous question for smallpox.
For the state of California, make a time series plot showing rates for all diseases. Include only years with 10 or more weeks reporting. Use a different color for each disease.
Now do the same for the rates for the US. Hint: compute the US rate by using summarize: the total divided by total population.
http://kbroman.org/ ↩︎
https://www.biostat.wisc.edu/~kbroman/presentations/graphs2017.pdf ↩︎
https://github.com/kbroman/Talk_Graphs ↩︎
http://paldhous.github.io/ucb/2016/dataviz/index.html ↩︎
http://mediamatters.org/blog/2013/04/05/fox-news-newest-dishonest-chart-immigration-enf/193507 ↩︎
http://flowingdata.com/2012/08/06/fox-news-continues-charting-excellence/ ↩︎
https://www.pakistantoday.com.pk/2018/05/18/whats-at-stake-in-venezuelan-presidential-vote ↩︎
https://www.youtube.com/watch?v=kl2g40GoRxg ↩︎
https://projecteuclid.org/download/pdf_1/euclid.ss/1177010488 ↩︎
http://www.thelancet.com/journals/lancet/article/PIIS0140-6736(97)11096-0/abstract ↩︎
https://www.cdc.gov/mmwr/preview/mmwrhtml/mm6316a4.htm ↩︎
https://en.wikipedia.org/wiki/Andrew_Wakefield ↩︎
http://graphics.wsj.com/infectious-diseases-and-vaccines/ ↩︎
http://www.tycho.pitt.edu/ ↩︎
- Documentation
Interactive Data Visualization
Written by Tereza Seidelova | May 11, 2022

Data visualization is the process of creating a visual representation of information. For centuries, people have been using static data visualizations — the map being among the oldest and most famous examples. Every aspect of data analysis has evolved with technological developments, including data visualization.
Interactive data visualizations have become a common part of most reports and dashboards. They allow users to engage with the data and easily find answers to their specific queries.
What Is an Interactive Data Visualization?
Interactive data visualization, such as insights and dashboards, aims to represent data graphically. Compared to non-interactive visualization, user engagement is needed, such as clicking a button or moving a slider. The core of the visualization is action and reaction, specifically human input and quick visual output.
Non-interactive vs. Interactive Data Visualization
Non-interactive data visualization is static and simple. It can include forms such as graphs, heat maps, and various types of charts (e.g., pie, bar, or line). For example, imagine a chart created in Excel. It is easy to quickly create for simple data queries. In general, non-interactive visualization is more suitable for less complex data stories in which you only perform one or a few queries. It is also the optimal format for printing and sharing reports via email, as the information is static in time and easy to view.
On the other hand, interactive visualizations are perfect for large amounts of data when you have more questions and a trend to investigate. Interactive data visualizations enable you to fluidly answer questions and travel from one visualization to another. It displays data in context, allowing you to easily find answers to your questions or hypotheses.
Interactive visualizations are often used in dashboards and business intelligence (BI) reports. It provides an easy way to understand insights and is more practical and time-saving than using long tables of numbers as in the case of static reports.
Want to see what GoodData can do for you?
Get a guided tour and ask us about GoodData’s features, implementation, and pricing.
Features of Interactive Visualizations
The goal of interactive visualization is to attract users’ attention. When using interactive data visualization, the data you display is all up to you and your curiosity.
The following are typical features of interactive visualizations.
- Filtering allows you to reduce or specify the data that is displayed in the visualization.
- Drilling allows you to move from one visualization to another. It also allows you to send an action from the dashboard.
- Zooming and panning is useful if you want to see a specific detail. You can zoom in and see only a particular part of the visualization without getting distracted or needing to create a new insight.
Let's take a closer look at the features of non-interactive and interactive visualizations. The insights below examine the data from Lenstore’s “ Healthy Lifestyle Cities Report 2021 .” The dataset contains details about components of the lifestyles of some of the world's cities, such as level of happiness, life expectancy, sunshine hours, and annual average working hours.
We will focus on the level of happiness and try to answer the following question: What is the level of happiness in Helsinki? How does the level of happiness in this city compare to other cities?
First, we’ll check the non-interactive data visualization.

You can see that the level of happiness in Helsinki is relatively high, as it is higher than other cities and even higher than the average world's happiness level. You might wonder what causes the difference in levels of happiness and raise another question: Is there any connection between the happiness level and the annual average working hours? If we check the first graph again, we won’t find the necessary data there.
However, if we consult the interactive data visualization below, we will find the answer.
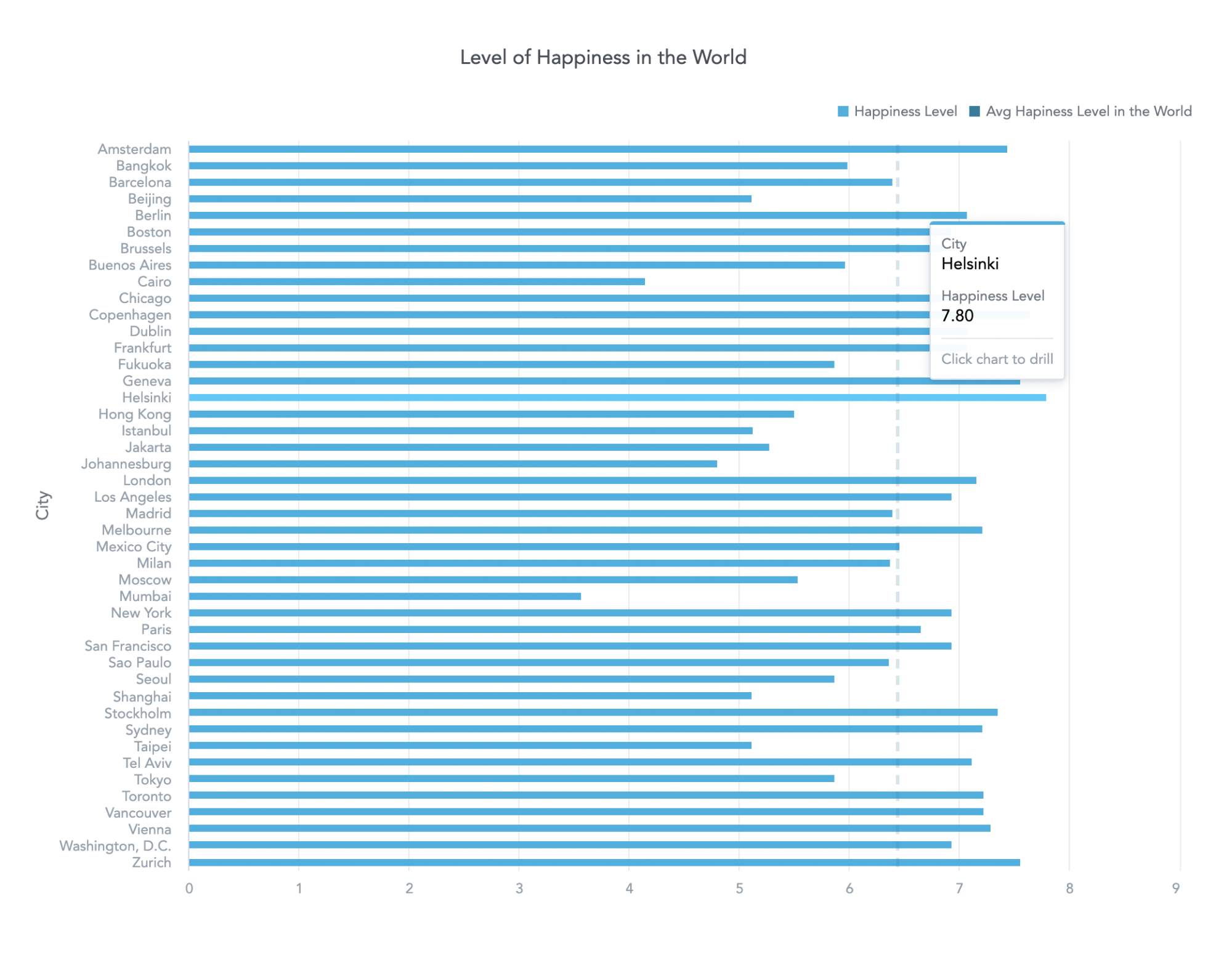
First, you can zoom in and see the data about Helsinki up-close.
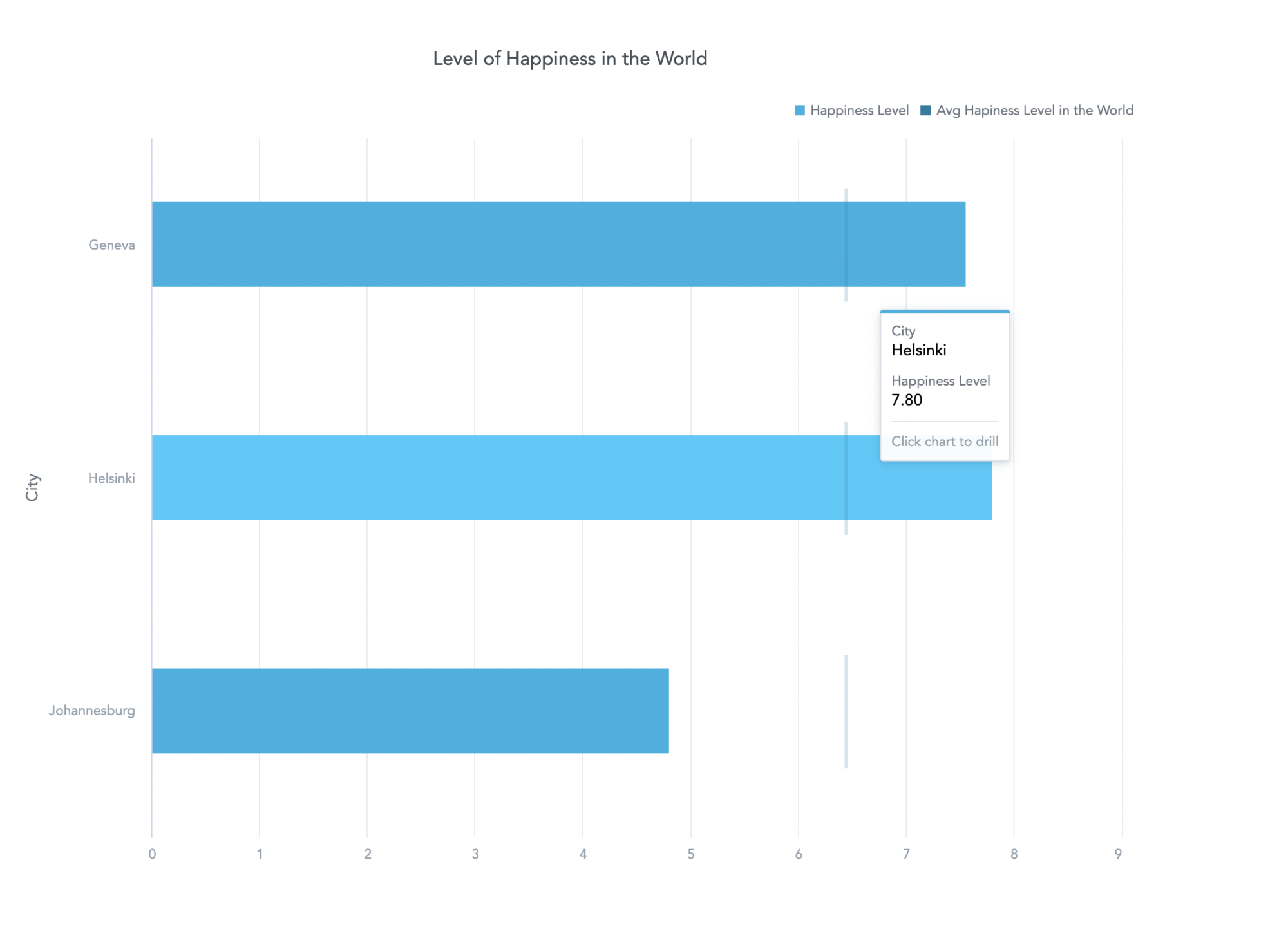
After that, you can drill in and check the annual average working hours in Helsinki and compare them with the world's average. Additionally, you can check the life expectancy in the city and drill into another insight (dashboard).
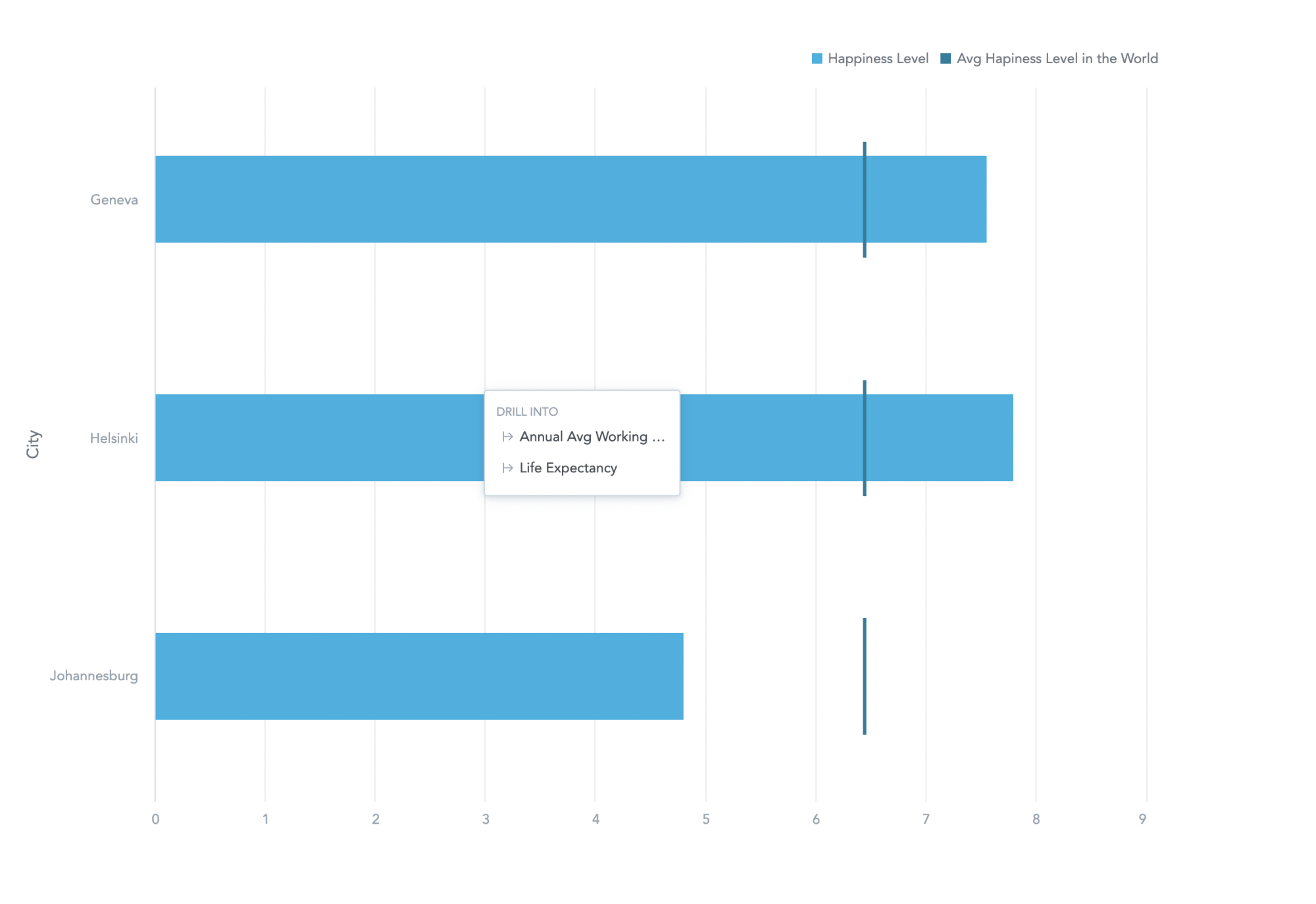
Additionally, you can filter the visualization to compare Helsinki with other European cities only.

Custom Build Applications
In general, there are two possible ways to create interactive data visualizations: End users can use predefined options, including drag-and-drop, while developers can define the business semantics on top of the data so that non-technical people can work with analytics and know what they are dragging and dropping. However, interactivity isn’t limited to dashboard visualizations. It can also apply to building applications on top of data. These applications include various formats of interactivity, such as new visualizations, chatbots, natural language processing, and in-game analytics.
Data is part of many other applications that companies work with and offer to their customers. As such, working with data and its visualizations needs to go beyond the scope of traditional BI tools. The concept of headless BI enables the connection of any application, data platform, or visualization tool to the semantic layer. Compared to traditional BI tools, with headless BI, the semantic model is decoupled from the BI components and exposed as a shared service via APIs and standard interfaces. In simple terms, the analytical backend is separated from the consumption layer so that you can use APIs to access and present data from the semantic layer and visualize them in any application.
Set side-by-side with traditional analytical platforms, in the headless BI platform, everything you build is both human- and machine-readable so you can manage your analytics as a code. Headless BI enables access to data for end users as equally as IT or other technical owners. The platform is flexible and doesn't limit the user. Interactivity can be understood as a way to choose how to display the data.
For instance, chatbot is an application built on top of GoodData Python SDK and Pandas DataFrame. In chatbot, you can manage your data using code in order to create a visualization and interact with it.
Another application built on top of the GoodData platform is Natural Language Query (NLQ) . It allows you to search for information in conversational language. The NLQ server communicates directly with the GoodData semantic layer and is able to create an insight based on your request.
Let's revisit the level of happiness in the world and what can affect it. Now we will focus on the amount of sunshine hours in the city per year. Using NLQ, we can request a column chart displaying the desired information.
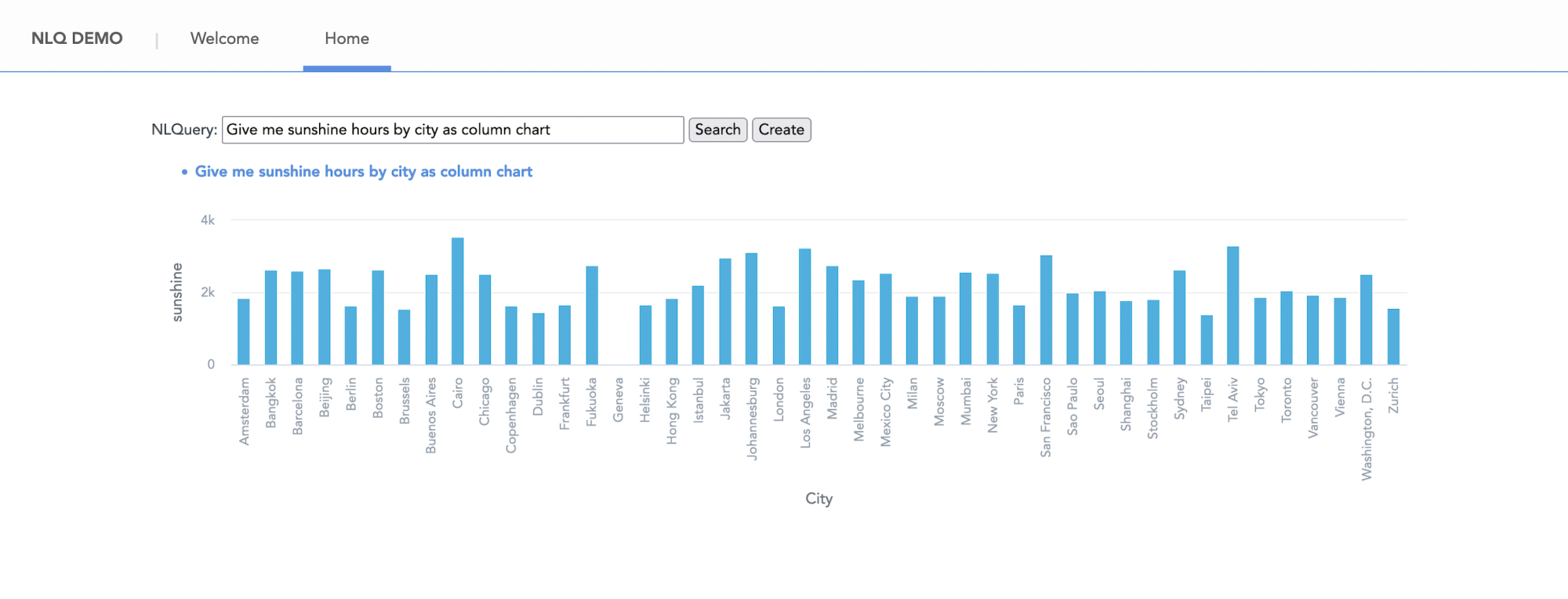
Benefits of Interactive Data Visualizations
Simplify complex data.
Interactive dashboards can represent a complex data story clearly. Incorporated features such as filtering and zooming can help make the data more manageable. Interacting with large datasets and using visualization aspects helps users to quickly understand the story of the data.
With interactive data visualization, you can easily identify trends and relationships between data. Additionally, with the ability to observe how data changes over time, you can identify overlooked cause-and-effect relationships. Hence, you can develop business insights that help assess KPIs' statuses and lead to data-driven decisions.
According to Seyens , half of the human brain is directly or indirectly connected to visual processing. Additionally, at least 65% of people are visual learners. Interactivity based on visualization rather than simple charts enables users to process information easier, as humans naturally interpret visual information better than numbers.
Boost Engagement and Productivity
Interactive insights and dashboards enable you to engage with data in ways that are impossible with non-interactive dashboards. Interacting with data by employing dynamic charts, incorporating shapes, or changing colors can boost users' productivity.
The users get control over what they see, having the opportunity to adjust the visualization according to their needs based on location, age, job, or any other factor. The user becomes an active participant instead of a viewer alone. Diving deeper into the data may raise further questions. However, in comparison to using static visualization, users can find the answers they need without distractions or needing to create a new chart.
Increase Flexibility
A direct connection between the user and the data is a huge benefit. The ability to customize and change the perspective of a visualization depending on what the user needs is the result of personalization of BI. Interactive visualizations offer the flexibility to choose whether to create visualizations directly in the analytics platform or to build your own application on top of the platform. Everything you build is human- and machine-readable, so the data is as approachable for business users as it is for technical users.
If you strive to build a data culture that ingrains data in all processes, products, and people, you need to focus on the interaction of data, not just pure consumption. With GoodData, you can work with predefined interactive dashboards or create your own interactive application and manage your analytics as a code. To get started today, simply request a demo .
Related content

How to Create Metrics in Analytical Designer

Top 7 Must-Have Features for Your Modern BI Tool

What Is a Semantic Data Model?
Subscribe to our newsletter
Get your dose of interesting facts on analytics in your inbox every month.
Subscribe to the GoodData Newsletter
Receive regular updates from our blog, news, and more.
Thank you for subscribing to the GoodData newsletter.
The Ultimate Guide to Data Visualization
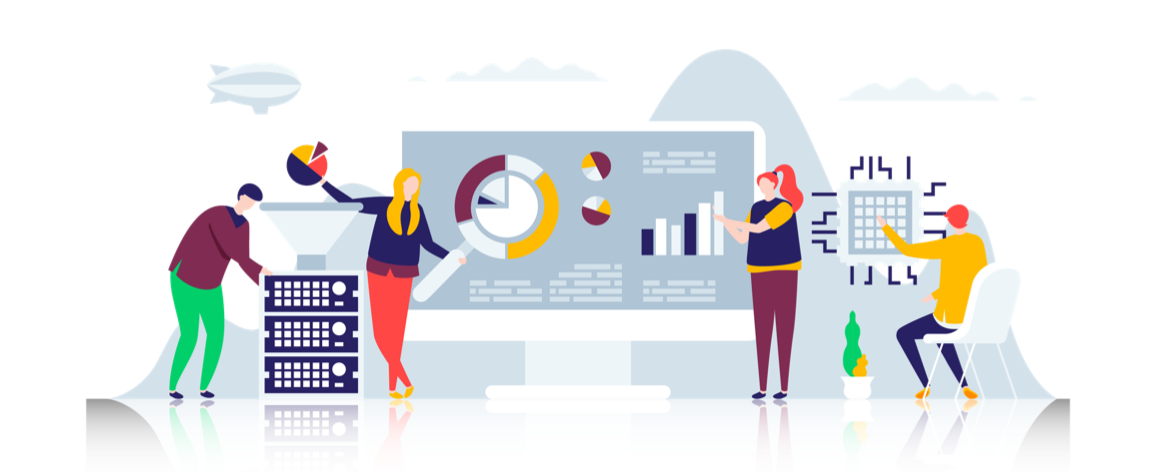
In the world of data, information is power. The ability to take data and transform it into a visual representation that is easy to understand can give you a powerful edge over your competition. In this guide, we will teach you everything you need to know about data visualization. We will discuss what data visualization is, the different types of visuals you can use, how to choose the right type of data visualization for your needs, and how to create effective visuals that communicate your data-driven insights clearly and effectively. And with the recent change by Google from Universal Analytics to Google Analytics 4 (GA4), the rise of understanding how to use a good data visualization tool has increased in importance.
What is data visualization
Data visualization is the process of transforming data into charts and graphs that help to make complex information more easily understood and acted upon. While data visualization can take many different forms, such as charts, graphs, maps, infographics, and diagrams, data visualizations are typically designed to convey a specific message or story clearly and compellingly. Visualization permits true data exploration as well. A good data analyst or data scientist will be able to review data and find connections, correlations, and potential insights.
Through data visualization tools and techniques, data can be presented in a more intuitive way that makes it easier for people to analyze, interpret, and act on information. Expert users can craft stories about events. For instance, Charles Minard mapped Napoleon's invasion of Moscow with an amazingly accurate graph. The map represented the army and the route of the Napoleonic retreat from Moscow and ties that information into the temperature and timescale for a more comprehensive picture of the events.
Whether you are a data scientist looking for new ways to present data insights to your team, or an entrepreneur looking to communicate the key elements of your business model to potential investors, data visualization is an invaluable tool that can help you promote a greater understanding of your data.
Why data visualization is important
Data visualization is important because it helps us to make sense of data. In a world where data is increasingly becoming ubiquitous, the ability to take data and transform it into something easy to understand and act upon is more important than ever before. Data visualization allows us to see relationships, patterns, and trends in key performance indicators that would otherwise be hidden in quantitative data that is presented in a more traditional format, such as a spreadsheet.
Data visualization is also important because it can help us to communicate data-driven insights to others in a way that is easy for them to understand. When data is presented in a visual format, it can be easier for people to see the story that the data is telling, and to understand the implications of that story.
In many cases, data visualization can help us to communicate data-driven insights more effectively than if we were to simply present the data in a tabular format. Oftentimes it is said that a picture tells a thousand words, data visualization creates that picture. When done well it can create the "Aha moment" for business teams, investors, and analysts. It can shape the operational direction of a business.
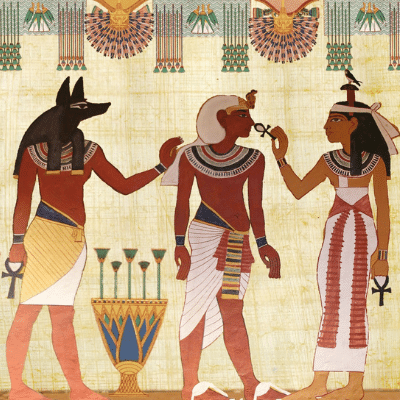
Data Visualization is a Key Tool for a Data Driven Organization
A data-driven organization makes decisions based on data. While all organizations rely on data to some extent, many are unable to comprehend the full scope of their business because there isn't enough information. A data-driven organization can better comprehend company drivers through a robust data discovery and visual discovery process aided by strong data engineering practices .
Data visualization helps data driven organizations by providing a way to see patterns and trends in data. This can help businesses identify opportunities and make better decisions. Data visualization also helps businesses communicate their data more effectively.
By using visuals, businesses can tell a story with their data and make complex data more understandable. In data driven organizations, data visualization is an essential tool for making better decisions and communicating data more effectively.
The History of Data Visualization
Data visualization has a long and varied history, dating back to ancient mathematical diagrams like those found in the ancient Sanskrit treatises. Over time, new tools and technologies allowed scientists and researchers to visualize their data in new and innovative ways, helping them gain valuable new insights into the patterns and trends hidden within their data sets. Today, new advances in digital visualization allow us to create stunning visual representations of our data that can help us make sense of what might otherwise seem like an overwhelming amount of information. Whether analyzing weather patterns or anticipating customer behavior, data visualization provides opportunities for discovery and new insights.
Microsoft Excel Is Not Enough?
Depending on your age, you may remember Lotus 1-2-3 or Quattro Pro, two early spreadsheet applications that were popular in the 1980s and 1990s. These programs allowed users to enter data into cells in a grid, and then to create basic charts and graphs to visualize that data. While these early visualization tools were helpful, they were limited in their ability to create anything other than the most basic visuals.
Microsoft Excel, the most popular spreadsheet application today, has taken data visualization to a new level with its wide array of built-in charting and graphing capabilities. However, even Excel has its limitations when it comes to visual analytics.
Without question, Excel is a great tool for data analysis, but it has its limitations when it comes to data visualization. Excel, like its predecessors, was designed as a spreadsheet application, and while it has some data visualization capabilities, it is not an ideal tool for creating complex data visualizations. Additionally, Excel is not well suited for creating interactive data visualizations that can be explored and interacted with by the user.
Complex Data
The emergence of the internet e-commerce, social graphs, and broader adoption of non-relational databases created an opportunity for a new breed of focused data visualization services. In many cases, data sets were too large or too complex to be effectively represented in a spreadsheet. For these reasons, data visualization experts often use specialized data visualization tools that are designed specifically for creating visual representations of data.
With the introduction of new data visualization platforms, such as Tableau and Qlikview, data analysts and data scientists could more deeply explore their data. These tools allowed users to create more sophisticated visuals, and to interact with data in ways that simply was not possible with a spreadsheet.
Along with Tableau and Qlik, application-specific appliances for data warehousing became wildly popular even in the face of the rising adoption of cloud computing. For instance, Teradata became a nearly $1 billion revenue business selling a data warehouse that helped businesses collect, store, process and analyze data. Teradata was used to create data visualizations that helped businesses understand their data and make better decisions. In time, technologies built to manage massive amounts of internet data became popular.
Technologies such as Hadoop an open-source software framework allowed for the distributed processing of large data sets across clusters of commodity servers. Hadoop was designed to handle data from web applications, and it has become a popular tool for data scientists and data analysts who need to work with large data sets.
Data Visualization is more than just a tool for Big Data
Data visualization is much more than just big data; it is an essential tool for collecting, exploring, analyzing, and interpreting complex data sets. Whether working with millions of records or just a few thousand, being able to visualize data clearly and concisely can be the key to finding insightful trends or identifying potential points of failure. For this reason, it is important to have a strong tool in your arsenal, regardless of the size or complexity of your dataset.
With its intuitive interface and flexible customization options, a good data visualization tool can help you explore your data in new ways and extract deeper meaning from your findings. Some tools even include machine learning capabilities that can automatically uncover patterns and generate predictive models based on big data sets. And by enabling real-time collaboration between team members, these tools also make it easier to work together on complex projects or big data challenges.
Overall, whether you are working with big data or small, having an effective way to visualize your data can be essential for gaining valuable insights and improving decision-making across all aspects of your organization.
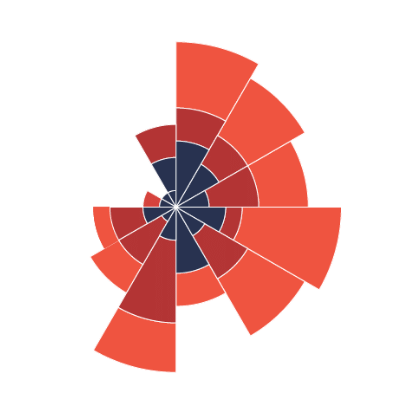
What are some common data visualization techniques?
There are many different data visualization methods that you can use to represent data. Some of the most common methods include charts, graphs, maps, infographics, and diagrams. The best data visualization method for your needs will depend on the type of data you have, the story you want to tell with your data, and your audience. In general, data visualization methods can be divided into two main categories: static and interactive.
Static visualizations are those that are not designed to be interacted with, such as a bar chart or line graph. Interactive data visualizations, on the other hand, are those that allow users to manipulate the data in some way, such as by filtering data points or changing the data visualization type.
Many different techniques can be used to represent data. Some common techniques include:
- Line graphs
- Scatter plots
Each of these techniques has its strengths and is better suited for certain types of data than others. For instance, bar charts are typically used to compare categorical data points, while line graphs are better suited for data that is temporal data values. Scatter plots are often used to visualize the relationship between two numerical data sets, while pie charts are typically used to represent data that is proportions. Histograms can be used to show visual representations for the distribution of data, while heat maps can be used to show the relationship between three data sets.
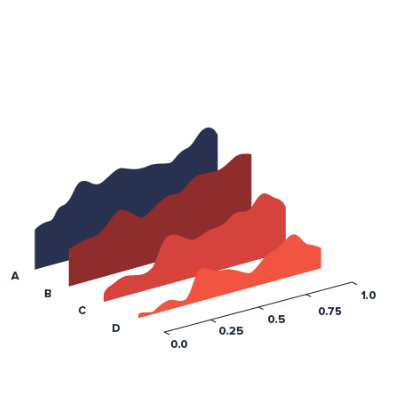
Visualization Methods and Storytelling
While there are many different data visualization methods available, not all of them are equally effective at communicating data-driven insights. When choosing a method, it is important to consider the following:
- The type of data you have: Some visualization methods are better suited for certain types of data than others. For example, line graphs are typically used to visualize data that changes over time, while bar charts are better suited for data that can be divided into categories.
- The story you want to tell: The method you choose should be based on the story you want to tell with your data. For example, if you want to show the relationship between two variables, a scatter plot might be a good data visualization method to use.
- Your audience: The data visualization method you choose should be based on your audience. For example, if you are presenting data to a non-technical audience, you might want to use an infographic or diagram instead of a more complex data visualization method like a heat map.
While there are many different methods available, the best way to learn which data visualization method is right for you is to experiment with different methods and see what works best for your data and your audience. The most important thing is to communicate your data-driven insights in a way that is easy for people to understand.
Most good tools will provide these techniques out of the box. However, some tools may also offer more advanced data visualization techniques that can be used to represent data in more creative ways.
Some common advanced data visualization techniques include:
- Sankey diagrams
- Choropleth maps
- Word clouds
- Spiral graphs
Sankey diagrams are often used to visualize flows of energy or data, while choropleth maps are used to color-code data by geographic region. Word clouds can be used to show the most common words in a data set, while tree maps can be used to show hierarchical data structure. Spiral graphs can be used to visualize data that is cyclical.
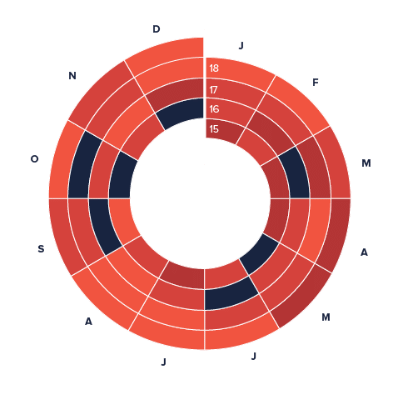
While these techniques are not necessarily appropriate for all data sets, they can be very effective when used appropriately. When choosing a data visualization technique, it is important to consider the type of data you are working with and the message you want to communicate with your data visualization.
What are some common data visualization tools?
There are many different data visualization tools available on the market. In fact, there are so many we have lost count. Some common tools include:
- Tableau (acquired by Salesforce.com)
- Microsoft Power BI
- IBM Watson Analytics
- Looker (acquired by Google)
- Google Data Studio
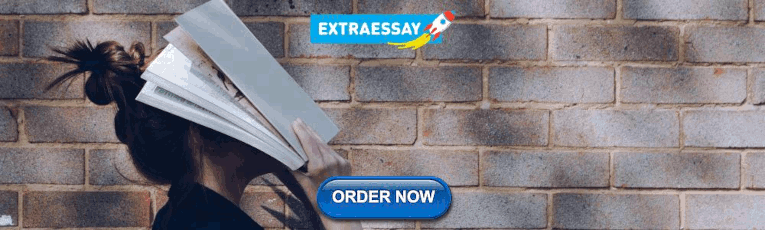
Comparing the Most Popular Tools
Each of these tools has its own strengths.
- Tableau is a very popular platform that has many features and capabilities that make it an excellent choice for businesses of all sizes. One of the key strengths of Tableau is its ability to connect to a wide range of data sources, including databases, spreadsheets, and cloud-based data warehouses. This flexibility makes it easy to integrate Tableau into existing business intelligence infrastructure. Another key strength is Tableau's visual interface, which makes it easy to create interactive dashboards and reports. The drag-and-drop interface is simple to use and requires no programming knowledge, making it ideal for users who are not technical experts. In addition, Tableau's advanced features allow users to perform complex analysis and create sophisticated visualizations. As a result, Tableau is an extremely powerful tool that can help businesses gain insights into their data.
- QlikView is another popular data visualization tool that is used by many organizations. There are many different strengths to using QlikView as a data analysis tool. Perhaps the most significant of these is its ability to handle large and complex datasets with ease. With QlikView, users can easily navigate through huge volumes of data, filtering and visualizing aspects that are relevant to their particular research or project. Additionally, because QlikView operates in real-time on the cloud, it is well suited for monitoring systems that need to provide up-to-date information about changing trends or metrics. Finally, because of its intuitive interface and flexible functionality, Qlikview is easy for users of all levels to learn and use effectively. Similar to Tableau it can be a great tool for a broad array of users from data scientists looking for an advanced tool or a business professionals looking for a simple way to gain insights from their data.
- There are several key strengths to using Microsoft Power BI for data analytics and reporting. First, this tool is extremely versatile, allowing users to create a wide range of charts and graphs to quickly and intuitively visualize different types of data. Power BI integrates seamlessly with a variety of other Microsoft programs like Excel and SharePoint, making it easy to access and combine existing datasets. Finally, its powerful customization features allow users to easily tailor the tool to their specific needs and workflows. Overall, these strengths make Power BI an invaluable tool for organizations looking to gain deeper insights from their data.
- At first glance, Sisense may not seem to be the ideal solution for data analysis and visualization. Compared to many other data analytics platforms, it is less intuitive and offers a more complex interface. However, in reality, these factors become Sisense's greatest strengths when it comes to tackling large datasets. Unlike simpler tools that are limited by their scalability, Sisense can easily handle large volumes of information and process them quickly. In addition, its extensive array of powerful features allows users to customize the platform according to their specific needs and preferences, giving them even more control over large datasets. Overall, for businesses looking for an effective solution for big data analysis and visualization, Sisense is an excellent choice. With its sophisticated capabilities and flexible design, it helps organizations unlock insights from even their largest datasets.
- Looked Data Studio or Google Data Studio is a good visualization tool that has a free from Google. One of the main strengths of Data Studio is its flexibility. businesses can connect to a wide range of data sources, including Google Analytics, AdWords, BigQuery, and PostgreSQL. This allows businesses to create customized dashboards that provide the specific information they need. Additionally, Data Studio provides a variety of templates and tools that businesses can use to create stunning visualizations. GDS provides a range of visual communication assets so that the business can create visuals that effectively communicate their data and possible insights. For a free tool focused on a set of visualization issues, it can be a good solution.
- D3.js is a powerful JavaScript library for manipulating and visualizing data. It is particularly well suited for large data sets, because it can scale to meet the needs of even the most demanding applications. Additionally, D3.js is highly flexible, allowing developers to create custom views and interactions. Finally, D3.js is open source, meaning that it is always improving and evolving as new features are added by the community. In summary, D3.js is an incredibly powerful tool that can be used to create truly stunning visualizations. Since it is open-source, it will require more developer time and should be considered before adoption. As a visualization tool, we at Azumo love it!
Programming Languages
In general, data visualization tools can be divided into two categories: those that require programming and those that do not. The majority of these tools do not require a data analyst to know how to code and can be used by anyone, so long as they can access to the underlying dataset. These include: Tableau, Excel, Google Sheets, Power BI, and SiSense among many many others.
On the other hand, the tools that require programming tend to be more powerful and customizable. These data visualization tools include: D3js, R, and Python (which opens a huge world of possibilities for the data scientist or analyst).
What are some common data visualization challenges?
There are many data visualization challenges that data analysts and data scientists face daily. Some common data visualization challenges include:
- Ensuring that data visualizations are accurate
- Finding the right data visualization tool for the job
- Migrating data between data visualization tools
- Ensuring data visualizations are accessible to all users
- Creating data visualizations that tell a story
Data analysts and data scientists must overcome these challenges to effectively communicate data-driven insights.
Is it easy to migrate between different data visualization tools?
Migrating data between different products and technologies can be difficult. Depending on the data visualization tool you are using, you may need to export your data into a format that can be imported by another data visualization tool. For instance, if you are using Tableau, you may need to export your data into a CSV file for it to be imported by another data visualization tool. Additionally, some data visualization tools may not be able to import data from other data visualization tools. For instance, SiSense is a data visualization tool that can only import data from CSV files. Therefore, if you are using Tableau and want to migrate your data to SiSense, you would need to export your data into a CSV file first.
When between tools we have developed some practical tips based on our understanding and use across all of the tools. When migrating data between data visualization tools, it is important to consider the following:
- The format of the data. Some data visualization tools only accept certain formats (e.g., CSV files). Make sure that the data is in a compatible format before attempting to migrate it.
- The structure of the data. Some data visualization tools have specific requirements for the structure of the data. Make sure that the data is structured correctly before attempting to migrate it.
- The size of the data. Some data visualization tools have limits on the amount of data they can handle. Make sure that the data is within the size limits before attempting to migrate it.
What is a data pipeline and how does it support data visualization
A data pipeline is a series of data processing steps. The data is first ingested into the data platform, and then a series of steps are run to transform the data. Each step in the pipeline delivers an output that is used as the input for the next step. This continues until the pipeline is complete. In some cases, independent steps may be run in parallel. Data pipelines are an essential part of data processing, and they can be used to perform a variety of tasks such as data cleaning, data transformation, and data analysis.
A data pipeline is a set of processes that extract, transform, and load data from one system to another. Data pipelines are commonly used to move data between databases, file systems, and data warehouses. Data pipelines can also be used to process streaming data in real-time.
Data pipelines can help data analysts and data scientists overcome some of these challenges. Data pipelines can be used to ETL data, which stands for extract, transform, and load. ETL is a process in which data is extracted from one system, transformed into a format that can be used by another system, and then loaded into the second system. ETL can be used to migrate data between different data visualization tools. Additionally, ETL can be used to clean and transform data before it is visualized. This can help ensure that data visualizations are accurate.
Data pipelines can also be used to process streaming data in real-time. For instance, if you are tracking the stock market, you may want to create a data visualization that shows how the market is performing over time. Data pipelines can help you do this by ingesting data from the stock market and then processing it in real-time so that it can be visualized.
Data pipelines are an essential part of data processing, and they can be used to perform a variety of tasks such as data ETL, data cleaning, data transformation, and data analysis. Data pipelines can help make sure that data visualizations are accurate and accessible to all users.
The Importance of Data Warehousing
Yes, data warehouses can matter when creating data visualizations for a few reasons. First, data warehouses can provide a single source of truth for data that is used in data visualizations. This is important because it ensures that the data being used in data visualizations is accurate and consistent. Data warehouses can be used to store data from multiple data sources, which can be helpful when creating data visualizations that require data from multiple data sources.
Finally, data warehouses can provide a place to store data that is processed and prepared for use in data visualizations. This can be helpful because it reduces the amount of processing that needs to be done when creating data visualizations. In summary, data warehouses can be helpful when creating data visualizations, but they are not required.
Most tools can connect directly to data sources and do not require a data warehouse. However, data warehouses can provide benefits that make data visualizations more accurate and easier to create.
The Emergence Cloud-Based Data Warehouses like Snowflake
Many enterprises over the last decade have moved new data processing from appliance-based applications like Teradata into the cloud, relying on Amazon's Redshift or Snowflake as two leading examples. While there are some unique differences between Snowflake and Redshift, we sometimes view them as interchangeable as they are both outstanding data warehousing choices for the cloud.
Snowflake is a data warehouse that runs in the cloud. It is designed to handle data from a variety of sources, including data warehouses, data lakes, and streaming data. Snowflake offers many features that make it a good choice for data warehousing, including its ability to scale elastically, its support for semi-structured data, and its data-sharing capabilities.
Snowflake is a good choice for data warehousing for many reasons. However, one of the most important reasons is its ability to scale elastically. This means that Snowflake can scale up or down as needed, without affecting performance. This is a major advantage over data warehouses, like Teradata which ran on-premise, and was expensive to scale.
Another reason why Snowflake is a good choice for data warehousing is its support for semi-structured data. This type of data includes data that is not structured in a traditional way, such as JSON data. JSON data is becoming more and more common, as it is often used to store data from web applications. Snowflake is designed to handle this type of data, which makes it a good choice for data warehousing.
Lastly, Snowflake offers data sharing capabilities that make it a good choice for data warehousing. Data sharing allows multiple users to access the same data at the same time. This is a major advantage over data warehouses that do not offer data sharing, as it can be difficult to coordinate data access when multiple users are involved.
What's Next
Data visualization has come a long way since the early days of spreadsheet applications. Today, data visualization experts have a wide array of tools at their disposal to create stunning visual representations of data. These visuals can help us make sense of what might otherwise seem like an overwhelming amount of information. Whether analyzing weather patterns or anticipating customer behavior, data visualization provides opportunities for discovery and new insights.
Need help with your Data Engineering or Data Analytics project. Connect with Azumo .
Whether we're aware of it or not, computer vision is everywhere in our daily lives. For one, filtered photos are ubiquitous in our social media feeds, news articles, magazines, books—everywhere! Turns out, if you think of images as functions mapping locations in images to pixel values, then filters are just systems that form a new, and preferably enhanced, image from a combination of the original image's pixel values.
Images as Functions
To better understand the inherent properties of images and the technical procedure used to manipulate and process them, we can think of an image, which is comprised of individual pixels, as a function, f . Each pixel also has its own value. For a grayscale image, each pixel would have an intensity between 0 and 255, with 0 being black and 255 being white. f(x,y) would then give the intensity of the image at pixel position (x,y) , assuming it is defined over a rectangle, with a finite range: f: [a,b] x [c,d] → [0, 255].
A color image is just a simple extension of this. f(x,y) is now a vector of three values instead of one. Using an RGB image as an example, the colors are constructed from a combination of Red, Green, and Blue (RGB). Therefore, each pixel of the image has three channels and is represented as a 1x3 vector. Since the three colors have integer values from 0 to 255, there are a total of 256*256*256 = 16,777,216 combinations or color choices.

An image, then, can be represented as a matrix of pixel values.

Image Processing
There are two main types of image processing: image filtering and image warping. Image filtering changes the range (i.e. the pixel values) of an image, so the colors of the image are altered without changing the pixel positions, while image warping changes the domain (i.e. the pixel positions) of an image, where points are mapped to other points without changing the colors.

We will examine more closely image filtering. The goal of using filters is to modify or enhance image properties and/or to extract valuable information from the pictures such as edges, corners, and blobs. Here are some examples of what applying filters can do to make images more visually appealing.

Two commonly implemented filters are the moving average filter and the image segmentation filter.
The moving average filter replaces each pixel with the average pixel value of it and a neighborhood window of adjacent pixels. The effect is a more smooth image with sharp features removed.
If we used a 3x3 neighboring window:

*Often times, applying these filters, as seen with the moving average, blurring, and sharpening filters, will produce unwanted artifacts along the edges of the images. To rid of these artifacts, zero padding, edge value replication, mirror extension, or other methods can be used.
Image segmentation is the partitioning of an image into regions where the pixels have similar attributes, so the image is represented in a more simplified manner, and so we can then identify objects and boundaries more easily. There are multiple ways, which will be discussed in detail in Tutorial 3 , to perform segmentation. Here, we will look at one simple way it can be implemented based on thresholding. In this example, all pixels with an intensity greater than 100 are replaced with a white pixel (intensity 255) and all others are replaced with a black pixel (intensity 0).

2D Convolution
The mathematics for many filters can be expressed in a principal manner using 2D convolution, such as smoothing and sharpening images and detecting edges. Convolution in 2D operates on two images, with one functioning as the input image and the other, called the kernel, serving as a filter. It expresses the amount overlap of one function as it is shifted over another function, as the output image is produced by sliding the kernel over the input image.
For a more formal definition of convolution, click here .
Let's look at some examples:
Shifted right by one pixel:
Blurred (you already saw this above):
Here's a fancier one that is a combination of two filters:
Sharpening filter:
A sharpening filter can be broken down into two steps: It takes a smoothed image, subtracts it from the original image to obtain the "details" of the image, and adds the "details" to the original image.
Step 1: Original - Smoothed = "Details"

Step 2: Original + "Details" = Sharpened

Correlation
While a convolution is a filtering operation, correlation measures the similarity of two signals, comparing them as they are shifted by one another. When two signals match, the correlation result is maximized.
One application is is a vision system for using a hand to remotely control a TV. Template matching, based on correlation, is used to determine the hand position of the user to switch channels, increase or decrease volume, etc.

Edge Detection
In computer vision, edges are sudden discontinuities in an image, which can arise from surface normal, surface color, depth, illumination, or other discontinuities. Edges are important for two main reasons. 1) Most semantic and shape information can be deduced from them, so we can perform object recognition and analyze perspectives and geometry of an image. 2) They are a more compact representation than pixels.
We can pinpoint where edges occur from an image's intensity profile along a row or column of the image. Wherever there is a rapid change in the intensity function indicates an edge, as seen where the function's first derivative has a local extrema.

An image gradient, which is a generalization of the concept of derivative to more than one dimension, points in the direction where intensity increases the most. If the gradient is ∇ f = [ δ f ⁄ δ x , δ f ⁄ δ y ], then the gradient direction would be θ = tan -1 ( δ f ⁄ δ y / δ f ⁄ δ x ), and the edge strength would be the gradient magnitude: ||∇ f || = √ (δ f /δ x ) 2 +(δ f /δ y ) 2 .
However, plotting the pixel intensities often results in noise, making it impossible to identify where an edge is by only taking the first derivative of the function.

If we apply a filter that is a derivative of a Gaussian function, we can eliminate the image noise and effectively locate edges.

Building off of this procedure, we can design an edge detector. The optimal edge detector must be accurate, minimizing the number of false positives and false negatives; have precise localization, pinpointing edges at the positions where they actually occur; and have single response, ensuring that only one edge is found where there only is one edge.

The Canny edge detector is arguably the most commonly used edge detector in the field. It detects edges by:
- Applying the x and y derivatives of a Gaussian filter to the image to eliminate noise, improve localization, and have single response.

- Finding the magnitude and orientation of the gradient at each pixel.
- Performing non-maximum suppression, which thins the edges down to a single pixel in width, since the extracted edge from the gradient after step 2 would be quite blurry and since there can only be one accurate response.

- Thresholding and linking, also known as hysteresis, to create connected edges. The steps are to 1. determine the weak and strong edge pixels by defining a low and a high threshold, respectively, and to 2. link the edge curves with the high threshold first to start with the strong edge pixels, continuing the curves with the low threshold.
Final result:

The Gaussian kernel size, σ, also affects the edges detected. If σ is large, the more obvious, defining edges of the picture are retrieved. Conversely, if σ is small, the finer edges are picked out as well.

To read more about the Canny edge detector, click here .
RANSAC: RANdom SAmple Consensus
Line fitting is important in edge detection since many objects are characterized by straight lines. However, edge detection does not always suffice since there can be extra edge points, muddling up which model would be the best, missing parts of lines, and noise. Thus, Fischler and Bolles developed the RANSAC algorithm, which determines a best fit line given a data set and avoids the effect of outliers by finding inliers. Given a scatterplot and a certain threshold, RANSAC randomly selects a sample of points, counts the number of inliers within the threshold, and repeats this process until the maximum number of inliers is hit.
You can learn more about RANSAC here .
The filter in the apps. Concepts, UX patterns, and design guidelines
What does it mean to filter, the cognitive process behind the interaction, the best practices.
- User Experience
- UX Research

We closely observed and classified the most frequent patterns and best practices

Team Conflux
- The filter as a metaphor
- The most frequent best practices
- Inside the filter concept
- From filter to filters
- The filter and the interaction model
“Regardless of whether a new media designer is working with quantitative data, text, images, video, 3D space or their combinations, she employs the same techniques: copy, cut, paste, search, composite, transform, filter. The existence of such techniques which are not media-specific is another consequence of media status as computer data”
- Lev Manovich, The Language of new media.
Finding information has always been difficult; even today, each of us devotes a lot of time to this activity, on our smartphones or computers. Yet, on the Internet, an infinite amount of information is available to us, apparently so easy to reach: but there are too many.
And then we filter them. The filter metaphor accompanies us on e-commerce apps and sites: it is a widespread need for the user, a widespread function on various platforms.
The filter in the world of interface design is considered a pattern, or a generalizable solution to a recurring problem. We can, therefore, say that the designer community has identified a standard, accepted, consolidated to design the filters, at least in a context like the mobile apps’? Absolutely not.
Very little is needed to verify how homogeneous the solutions at the level of user experience (UX) and user interface (UI) are. Why?
We decided to break it down to analyze it. We closely observed and classified the most frequent patterns and best practices; we have analyzed strengths and weaknesses, noting how different solutions actually seem to respond to different problems. The filter metaphor, apparently unifying and clarifying, hides the complexity of different objectives.

The articulation of the filter concept, based on Norman’s 7-stage model, has allowed us to analyze the phases of interaction between the user and the system in detail and therefore of the filtering operation: at each stage, we associate best practices. By analyzing the micropatterns hidden inside the “filter” pattern, we tried to identify guidelines that adhere to the specific objectives of the people rather than reproduce the filtering function uncritically and standardized.
The filter as a metaphor and as a design pattern
We are surrounded by information, and we are constantly having to select a portion of it: our processing capacity is limited. This selection is often guided by attentional processes, which allow us to shift our attention to some information present in the environment rather than to others; this happens on various perceptive and sensorial levels: we filter visual, auditory information, etc.
Typically, this process is represented with the filter metaphor: like an intelligent filter, it lets only the relevant stimuli pass. It is apparently a “passive” process, a neurophysiological reaction of the brain to external or internal sensory stimuli, influenced by the number of cognitive resources available.
The filter metaphor is also widely used in digital interfaces, where there is a need to represent, above all visually, abstract concepts or functions. When we apply the filter metaphor to an interface, however, we are not referring to the same cognitive process described above. The situation is a bit different for a number of aspects:
- The amount of information on the web that can be accessed is potentially unlimited;
- The type of information accessible is different from an ecological context and constrained by the way it is presented (for example, visually, tactilely, etc.).
These variables (quantity and type of information) condition the way in which we must filter information in the physical world or on a digital interface: these are very different cognitive efforts.Filtering information in a digital environment allows you to selectively and consciously choose the type of information or its salience, based on our interests. At least, we like to think that way.
On the contrary, in an ecological context, this selection is often guided by decisions that are not entirely conscious. But how do we choose to filter and re-filter information? What logic does the user rely on to do an advanced search?
There can be three ways that a filter can work, and to represent them, we can rely on the Boolean logic. From a strictly rational point of view, it is possible to filter information based on the union of two or more criteria (OR), based on the intersection of two or more conditions (AND) or on the basis of the logical operator NOT, or the denial of the conditions (see Figure 1. Logical operators).

Fig. 1 Logical operators
It is good to take into consideration that even if the logical operators appear clear, often in everyday life, the user does not dwell on the difference between the OR and AND approach in linking the filters.
If on an e-commerce site I apply the “dress” – “blue” – “size 48” filters, I expect to find only the blue dresses of size 48. But if I apply the filters “news” – “sport” , will I only find the sports news? Or the double list of news and sports? Is it correct to call it a “filter”?
The standardization of the actions or functions offered to the user contributes to usability, learning, and memorability. The concept of the pattern was devised by Christopher Alexander to symbolize a solution to a recurring problem: a door is a pattern because it can be the solution to the problem of getting out of a building [1]. Similarly, the term pattern has become widespread in the world of interface design, for which the libraries of UX and UI patterns proliferate online. They are not only design guidelines but also the tools to accelerate design decisions based on “what others do.”
Over the years, important features that we find in numerous mobile apps or websites have consolidated into a consolidated grammar, in order to help the designer but also the user.
But to what extent is it useful to adhere to these standard solutions? In particular, in the design of a filter system, a recurring problem, does it make sense to identify ad hoc solutions?
In reality, the various UI patterns and the best practices that are spread in the professional community seem to contradict the same spirit of these “collections”: the high number of libraries of patterns suggests a direction opposite to the general solution to a problem. If it is so difficult to define a “right way” to design a filter, probably the problem lies in the definition of the filter concept: which changes according to the type of content and industry.
UI patterns and the most frequent and best practices
To clarify the filter function in the world of mobile apps, 29 mobile apps, belonging to different content categories, have been taken into consideration. (see Table 1 – Analyzed apps and classification).

Table 1 – Analyzed apps and classification
Subscribe to the Conflux newsletter to stay up to date on the latest UX news
Inside the filter concept. The elements of interaction
For the purpose of our analysis, the filter metaphor has been broken down. Those elements of interaction between the user and the interface that play a role in completing the goal of filtering content have been identified.
1.1 As I access the filter
A first aspect of the problem is the accessibility to the filter functionality. The way in which a function is accessed is the basis of the efficiency of this function. If the user fails to access an important function such as filtering a long list of information, the user experience will be in the minimum, negative and will increase the chances that this user will never return to that app again.
Consequently, the problem of accessibility to the filters can be further broken down.

Figure 2. Various filter representations
1.1.1 Representation of the filter function
One aspect to consider when designing a graphical interface is how to represent a feature. Many guidelines suggest to opt for a combined display or to include icon + label, rather than just the icon or the label alone.
The option to use the icon alone is not advisable: the meaning can be misunderstood. Furthermore, there is a very standard icon for filter functionality, which has achieved popularity with the famous hamburger menu. The most used icons are the funnel (see Booking) or the slider (see Zalando). For example, the slider icon is used by some apps (as in the below example of Cinema Time) to access the settings.

Figure 3 – Cinema Time
1.1.2 Arrangement of access to the filter function
Another aspect to consider when designing a graphical interface is: where should this feature be?
In mobile apps, the filter option is usually on the upper right corner. Over time, users have learned to recognize this behavior and probably expect it in that position: it is located at the top and right in 80% of the analyzed apps.
In other cases, especially for the travel and food apps, it is found on the below part. Typically, apps that place access to the filter feature at the bottom position the filter next to the map. This type of configuration puts the user in a position to filter the information based on their actual geographical location (see Figure 4. TripAdvisor and Open table position the access to the filters at the bottom).
Other apps include the filter in the “search”: when the user performs a search he is asked to fill in or select fields in order to obtain “filtered” results based on the criteria of his interest. Once the user has defined the criteria for his search, he will not be able to access the “filters” again to modify these criteria but will have to start a new search from scratch. In other words, the filter function does not have an autonomous location within the interface but intersects with the search one. This behavior can be found on apps like segugio.it, Immobiliare.it, where a boundless database of options must be tailored to the user’s needs to be “navigable”.

Figure 4. TripAdvisor and Open Table place filter access at the bottom
1.2 From filter to filters. The combination of multiple filter options
1.2.1 Displaying filter options
The way the user views filtering options is another aspect to consider. The two most commonly used modes are: by means of a modal window (pop-up) or on a new page. Most of the analyzed apps (23) opt to display the filters that can be activated on a new page, while the remaining part (6) uses a “pop-up”.
The advantage of using the pop-up is to allow the user to have a simultaneous display between the filtering choices and the feedback on the results. From a cognitive point of view, this choice is considered very appreciable, as the users have direct and punctual feedback between the actions they perform and the effects that these will cause.
The choice of the pop-up, with the consequent co-presence of action and feedback, is particularly frequent in the app of the “shopping” category.

Figure 5. Amazon uses a view with a “modal” window

Figure 6. Skyscanner uses a new screen view
1.2.2 Relationship between “orders” and “filters”
Many sites or mobile apps tend to include sorting functionality within the filter functionality. It is not a particularly correct solution from a logical point of view, as ordering and filtering information are two distinct actions that produce a different result. Ordering means having all the elements of a whole according to a certain criterion. There can be numerous ways of ordering: in alphabetical order, by increasing price, etc.
On the other hand, filtering means displaying only certain elements of a given set. In this case, too, there can be multiple criteria according to which to filter: for example, see all the red clothing items.
Despite this, the inclusion of the order functionality within the filter function is very frequent, and many believe that this mode is appreciated by the user because it would consider the two associated functions. We suggest integrating the two functions, especially when the semantic category on which to filter coincides with the sorting criterion: filtering for a certain price range, it may make sense to sort the results by price.
The analysis performed on the 29 apps showed that:
- 10 apps include the sorting feature under the filter functionality
- 12 apps do not include the sorting feature under the filter functionality
- 7 apps do not have the sorting functionality

Figure 7. Zalando keeps the two features separate

Figure 8. Yoox includes the sort function inside the filter function
1.2.3 Organization of filters in order of importance
Filtering information means choosing some, discarding others. For this reason, the way in which the criteria for choosing this information should follow a hierarchy, that is, it is a good practice to organize them according to the importance and priority they could have for the search. To achieve this hierarchy, it is advisable to place the most important filtering criteria in the first place, so that more attention will be paid to them by the user.
But how to establish the importance of the filter criteria? This decision can be made from two sources: from the designer of the service/app or from the user. Whoever designed the service can define a hierarchy that will then influence the way the user applies the filters, considering that more attention will be paid to the initial parameters, rather than those placed among the last positions. But at the same time, it is true that the users know what they want to filter based on what they need. So the other option is to propose a hierarchy based on the mental model of the users.
In this second approach, the label used for this criterion is very important. Most apps present a “static” list of categories to filter on, which means that for any search the user will do, they will always have to filter by pre-established categories. E-commerce apps like Amazon and eBay adapt the filtering categories and the relative label based on the actions carried out by the user so far, dynamically reconstructing the filter structure according to the position in the navigation process.

Figure 9. Amazon – different filter depending on the search made
1.2.4 Promote important filters
It is considered the best practice to highlight and facilitate access to the most important filters. This is particularly true in the case where the filter functionality allows information to be filtered according to many criteria. The choice of filtering criteria to be promoted must be based on both business and user objectives. The way in which the most important filters can be emphasized are many: they can be displayed separately from the other filters, placed in the highest positions, identified by a different color.

Figure 10. Foursquare- adopts the best practice of promote important filter
1.2.5 Displaying the filters that can be activated
To visualize the activatable filters, we recall the concept of “affordance” (Gibson, 1979) [2]. With the term affordance, Gibson wanted to emphasize that the physical characteristics of the objects invite an action, such as a stone invites to be grasped or a panic bar invites being pushed [3].
Equally the concept of affordance can also be applied to the characteristics of the elements present in an interface: the way in which a key is represented can invite the user to perform an action, like a click.
In our case, a list of criteria on which to filter must call and invite the user to take actions. The filtering criteria can be displayed in a more opaque color (example: just eat), as if they were disabled, and then call up the tap action to enable them; a switch invites the user to move it (example: TripAdvisor), a button invites the user to press, the slider invites the user to select a range (as in the case of Zalando).

Figure 11. Just eat – selection

Figure 12. Tripadvisor -switch

Figure 13. Zalando – button and slider
1.3 I have filtered. And now? The feedback, and any subsequent actions
Applying a filter implies a change in the display of the contents. Consequently, the users will expect to see a change or expect to view only the information and elements that interest them.
All the analyzed apps satisfy this assumption and this confirms the functioning and efficiency of the filter function. 1.3.1 Displaying active filters (is the feedback clear?)
When filters are applied to our search, it is not enough to display only the information we request: we also need to know constantly what kind of information we have filtered. In other words, an interface should always return feedback as a result of the action performed by the user, even more like this, when what you are viewing is only part of a larger whole.
But why should it be so important to provide feedback following user actions? Shouldn’t the users know what action they took? Despite the great abilities we think we possess, such as keeping in mind a large amount of information or doing more things at the same time, we actually have an innate tendency to overestimate our abilities. In fact, multitasking is particularly tiring, and we can’t even keep in mind a large number of contemporary information.
According to Miller’s famous law, the net of the many variables that can influence, we should refer to a number between 5 and 9 information. Consequently, a user who has selected for example 5 filtering criteria, after viewing and reading the details of at least 3 products, he probably will not remember which filters he had selected a few minutes before. And at that point, you won’t know if you had actually correctly selected the price range or brand of the product you are looking for.
It is, therefore, a good choice to provide clear feedback on what is selected; this will also help to lighten the working memory, as well as creating a situation in which the user feels he has everything under control.
Feedback can be given on two levels: immediate feedback on the filter selection (confirming the action of the selection: for which criteria I chose to filter) and permanent feedback on the page where the contents are present (as a filter reminder applied: for which criteria I filtered).
There are many solutions for communicating immediate feedback to the user on the selection made; some apps include a check next to the selected box (Zara), others include a color change (Kayak).
Even the feedback on the content page can be proposed in different ways: some apps show the number of filters that have been applied, close to the filter access mode, as in the case of Kayak.

Figure 14. Zara

Figure 15. Kayak
Other apps, after selecting the filters, highlight the filter label selected on the results page, as in the case of Vivino. Basically, this is the best way to give feedback to the user, very clear and constantly visible.

Figure 16. Vivino -feedback with label

As already indicated, the choice to show the filters in a modal has an advantage in terms of feedback: the pop-up overlaps a part of the content, and many apps like Amazon, eBay and Zara simultaneously show the change of the underlying content on the base of the filters that the user chooses.
1.3.2 Remove the active filters and select new ones
During an advanced search, the users may need to change their search criteria, either because they made mistakes when selecting them, or because they selected too restrictive parameters that did not satisfy the search, or simply because the users have changed their mind. Consequently, for a good UX, the users should be facilitated in removing old filters and selecting new ones.
Some of the analyzed apps require the user to access the filters section again, deselect the old ones, select new ones and confirm their decision; this series of steps requires a greater number of interactions (intended as the number of clicks or taps) and more time to reach the goal. Other apps show the filters that have been selected, allow the user to remove the filter or select new ones in a more immediate way, with fewer interactions and less time to reach the goal. This is the case of Yoox, which allows you to remove every single filter and select new ones, without necessarily having to go back to the page dedicated to the filters.

Figure 18. Yoox
1.4 Permanent filter and customized filter
“Permanent” or “customized” filters are rarely found and difficult to find in apps, and most of those analyzed do not have these options.
They are two concepts that tend to be associated with each other: a customizable filter should also be permanent (in a variable time interval) and vice versa; the permanence of a filter can be considered as a personalization, as it has remained stable on the basis of the choices made by the user.
For this purpose, in this analysis, the apps that use the results of a structured search as a “permanent/customized filter” have been considered.
In fact, Booking, Airbnb or Trivago store search parameters such as the place, dates, and the number of travelers; this does not happen however when you want to do an advanced search and then use the filter functionality.
Zalando, instead, suggests the user a series of filters that have been applied previously, for that type of search/product, recovering a “memory” of a custom filter.

Figure 19. Booking

Figure 20. Air bnb

Figura 21. Trivago
Facile.it, instead, adopts a different strategy: returned users who re-access the app will see the previous search with all the filters applied.

Figure 22. Zalando

Figure 23. Subito.it
The filter and the 7-stage interaction model
The filter functionality can be broken down and analyzed to guide the design decisions more effectively. Following the 7-stage model proposed by Donald Norman, it is possible to break down the problem in terms of the fulfillment of the actions and the evaluation of the consequences on the system.

Norman 7-stage model to guide the design of a filter system
In fact, the feedback cycle begins when the users form a purpose, from which they will subsequently form and plan an intention which they will then concretize with the action proper to their own. Once the action is completed, the users will perceive and interpret the state of the world, which will make them able to assess whether they have achieved their initial purpose or not. In view of this feedback cycle, it is possible to consider some of the aspects of the interface previously exposed as necessary for the successful interaction between the users and the system.
So let’s try to associate Norman’s 7-stage model and how this can guide the design of a filter system.
1. Forming a purpose At this stage, the user will have to focus on the purpose: to perform an advanced search to find exactly what he is looking for, in the shortest time possible. Consequently, he will have to establish the goal of filtering the contents. Already at this preliminary stage, the first problem arises: does the user want to filter content and then do it by relying on the “filter” function or by scrolling and using the “order” function? In fact, these are usually integrated into a single feature.
2. Forming an intention
Once the user has formed a purpose, he proceeds with the formation of an intention, so the user will feel the need to rely on some functionality present in the interface he is facing. To facilitate the formation of the intention, the filter function must be clearly visible and easily accessible to the user; consequently, the way in which the filter is represented is important, where it is located and if there are filters in evidence.
3. Specifying the action
The user will proceed with the planning of the actions he will do to reach his goal: I see the “filter” item at the top right, I click on it, I access the filters, I select those that interest me.
4. Perform the action
The user proceeds with the execution of the actions he had previously planned. Also, in this phase, there are elements of the interface that must be taken into consideration, such as the display of the filters that can be activated and the methods of activating the filtering criteria.
5. Perceiving the state of the world
The user sees that something has changed in his interface, the list of products is different, there are fewer elements, and even some graphics have changed. Providing clear feedback is essential for optimizing the user experience.
6. Interpreting the state of the world
The user, after perceiving that the elements and the contents have changed, will have to understand and interpret whether the selected filters have been applied. Also, in this case, clear and visible feedback supports the interpretation of the state of the world.
7. Evaluating the result
The evaluation phase is very important because, at this stage, the user will be able to tell if he has achieved his purpose or not and if he is satisfied with it. If the user has not achieved his initial purpose or is not satisfied with it, or still understands that he must define a new purpose, it is important to remove the activated filters and select new ones. These actions should be simple and quick.
The work that we did was aimed at analyzing and studying the most widespread solutions for the design of the filter systems, to then be able to define the characteristics and the UI patterns possibly applicable to a multiplicity of contexts.
Defining the international model between the user and the system (in our case, the filter function in mobile apps) has allowed us to suggest UI patterns more precisely, centered on the needs of the user.
Trying to generalize some conclusions, we can say that:
- The icon + label combination appears to be the best choice to avoid misunderstandings since a purely graphic representation mode is not consolidated;
- It is likely that the user expects the filters located at the top right;
- Highlighting the most important filters (promote important filters) can facilitate the planning of the filtering actions;
- The logic according to which the filter works (AND, OR, NOT) should be transparent to the user
- The display of the filters within a modal rather than in a new page allows to apply filters simultaneously and to observe a change of the content in the background;
- Affordance is important: each filtering criterion must invite you to perform an action.
- Immediate feedback is important: the user must know that the system has recognized its action, so it is necessary to foresee it in the selection micro-interactions (ticks, color change, slider, etc.);
- It is advisable to show feedback regarding the activated filters, clearly visible in the interface. The most explicit option is a label that identifies the active filter;
- Highlighting the activated filters allows you to select or deselect them, making the interaction more dynamic, easy and immediate.
[1] Alexander, C. (1977). A pattern language: towns, buildings, construction. Oxford University press. [2] Gibson, J. J. (1977). The theory of affordances. Hilldale, USA, 1, 2. [3] Tale concetto fu portato all’attenzione della comunità dei designer da Norman, che poi ne ha discusso le criticità, e lo ha tendenzialmente sostituito con quello di signifiers.
We also recommend

New Frontiers for UX Design: Designing for New Devices

ChatGPT, Google Bard, and UX Design: Opportunities and Limits
- Customer experience
Contact us for your next UX project!
Surname* Email* Phone * Business*
*Required fields

Thanks for your message
You will receive a reply as soon as possible
Now you'll automatically get email updates from us
We'll get back to you as soon as possible
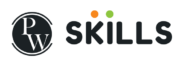
Data Visualization in Data Science: Types, Tools, Best Practices
Data Visualization in Data Science: In the big field of data science, the ability to convert intricate datasets into actionable insights is a fundamental skill. Data visualization is a crucial component, acting as the conduit between raw data and comprehensible insights. In this blog, we’ll talk about data visualization in data science, its types, tools, best practices, and more!
If you want to make an impactful and lucrative career in data science, a Decode Data Science with ML 1.0 could be just what you need!
Table of Contents
What is Data Visualization in Data Science?
At its core, data visualization is the art and science of representing data graphically. By utilizing visual elements like charts, graphs, and maps, it transforms complex datasets into visual formats that are easily interpretable. Beyond aesthetics, effective data visualization tells a story, making data accessible and facilitating informed decision-making.
Data visualization serves as a visual language that allows data scientists to communicate complex ideas to both technical and non-technical stakeholders. Its power lies in simplifying the understanding of large datasets, revealing patterns, trends, and outliers that might be obscured in raw data.
Why Is Data Visualization in Data Science Important?
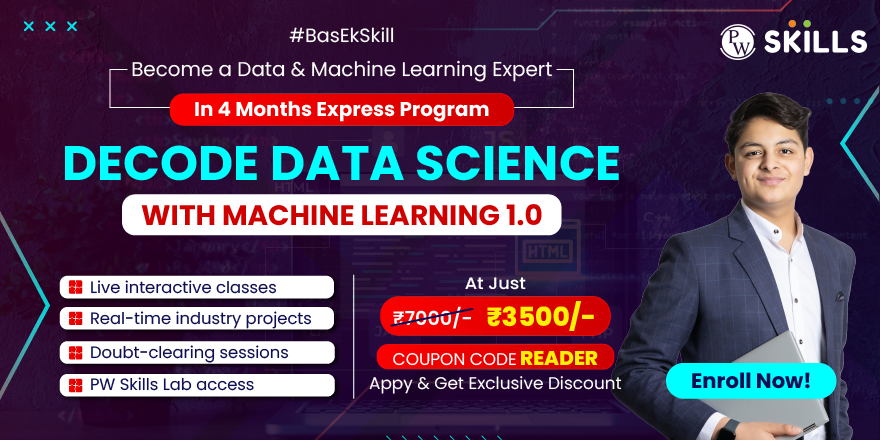
In the intricate landscape of data science, the importance of data visualization transcends mere aesthetics; it plays a pivotal role in shaping the way we understand, interpret, and derive actionable insights from complex datasets. The significance of data visualization is multifaceted and extends to various aspects of the data science workflow.
- Enhancing Data Comprehension for Decision-Making:
- Complexity Simplified: Raw datasets, especially those laden with numerous variables and intricate relationships, can be overwhelming. Data visualization simplifies this complexity, providing a visual roadmap for understanding patterns, trends, and anomalies at a glance.
- Speed of Insight : Visual representations expedite the comprehension process. Decision-makers can swiftly identify key insights, enabling them to make informed choices promptly. This agility is crucial in dynamic environments where quick responses to changing trends are paramount.
- Communicating Insights Effectively:
- Beyond Technical Jargon : In collaborative environments, effective communication between data scientists and stakeholders with varying technical backgrounds is essential. Data visualisations act as a universal language, transcending complex statistical terms and algorithms, and allowing for seamless communication.
- Cross-Functional Collaboration : Visualization facilitates collaboration across diverse teams, ensuring that insights are accessible and understood by all stakeholders. From executives to marketing teams, visualizations break down silos and foster a shared understanding of data-driven insights.
- Detecting Patterns and Trends Efficiently:
- Unveiling Hidden Patterns: Patterns and trends within data are often elusive when buried within rows and columns of spreadsheets. Visualisation brings these patterns to the forefront, making them visually apparent and aiding in the identification of key insights.
- Outlier Detection: Visualization tools excel in highlighting outliers, deviations, or irregularities in datasets that might go unnoticed in raw data. The ability to identify and address outliers is critical for refining models and ensuring data accuracy.
- Improving Data-Driven Storytelling:
- Narrative Impact: Human brains are wired to respond to stories. Data visualization transforms raw numbers into a narrative, making the data more relatable and memorable. It fosters a deeper understanding of the story behind the data, creating a more compelling and impactful narrative.
- Engagement and Advocacy: Well-crafted visualisations can turn data consumers into advocates. When data is presented in an engaging and accessible manner, it becomes a powerful tool for driving decision-makers to take action based on the insights derived.
- Facilitating Exploratory Data Analysis (EDA):
- Interactive Exploration: Data visualization tools often come equipped with interactive features, enabling data scientists to explore data dynamically. This interactivity allows for on-the-fly adjustments, filtering, and drilling down into specific aspects of the data, facilitating a more nuanced understanding during the exploratory phase.
- Hypothesis Generation: Visualisation aids in hypothesis generation by providing an initial visual exploration of the data. Patterns that emerge during EDA guide subsequent analyses and contribute to the formulation of meaningful hypotheses.
Also read: Storytelling with Data: Communicating Insights Effectively
Types of Data Visualization in Data Science
Data visualizations come in various forms, each suited for different types of data and analytical goals. Let’s explore these types in greater detail:
- Charts and Graphs:
Line Charts:
- Ideal for displaying trends and patterns over a continuous interval, such as time.
- Effective in visualizing the progression of numerical data points.
Bar Charts:
- Utilized to compare the quantities of different categories.
- Ideal for showcasing discrete data points and identifying trends across groups.
Pie Charts:
- Depict the proportional distribution of parts of a whole.
- Useful for representing percentages and emphasizing the contribution of individual components.
Scatter Plots:
- Showcase the relationship between two numerical variables.
- Identify correlations, clusters, or outliers in the data.
- Maps and Geospatial Visualizations:
Choropleth Maps:
- Use colour variations to represent data values across different regions.
- Effective for illustrating regional patterns or disparities.
Bubble Maps:
- Integrate size and colour to convey information on a map.
- Useful for highlighting data points with varying magnitudes across geographical locations.
- Visualize the density or intensity of data in a specific geographic area.
- Ideal for representing patterns like population density or temperature variations.
- Infographics:
Combination of Text, Images, and Charts:
- Condense complex information into a visually appealing and easy-to-understand format.
- Ideal for summarising key insights or trends for quick consumption.
Flowcharts:
- Illustrate processes or decision trees in a step-by-step visual format.
- Useful for representing workflows or dependencies within a system.
- Dashboards:
Comprehensive Displays:
- Integrate multiple visualizations and metrics into a single view.
- Provide a holistic understanding of data trends and performance.
Interactive Elements:
- Allow users to customize views, explore specific data points, and gain deeper insights.
- Facilitate real-time decision-making by providing dynamic updates.
Hierarchical Representation:
- Visualize hierarchical data structures with nested rectangles.
- Efficiently represent proportions and relationships within a structured dataset.
Sunburst Charts:
- Display hierarchical data with a radial layout, resembling the concentric circles of a sunburst.
- Ideal for illustrating proportions and relationships within multi level hierarchies.
- Radar Charts:
Multivariate Analysis:
- Display data points on axes emanating from the Centre, forming a polygon.
- Useful for comparing multiple variables across different categories simultaneously.
Spider Charts:
- Similar to radar charts, spider charts represent data in a web-like pattern.
- Effective for showcasing the strengths and weaknesses of different entities across various dimensions.
- Comprehensive displays of multiple visualizations and metrics for a holistic view of data.
Exploring these visualization types empowers data scientists to choose the most suitable format based on the nature of the data and the story they want to convey.
Open Source Visualization Tools
- Matplotlib:
- A widely-used 2D plotting library for Python.
- Provides a variety of chart types, enabling the creation of static, animated, and interactive visualizations.
- Versatile and a staple in the toolkit of many data scientists.
- Built on Matplotlib, Seaborn specializes in statistical data visualization.
- Simplifies the process of creating informative and attractive visualizations.
- Excellent for exploratory data analysis.
- A versatile library supporting interactive visualizations and dashboards.
- Compatible with multiple programming languages, including Python, R, and Julia.
- Ideal for creating dynamic and interactive data visualizations.
- A JavaScript library for producing dynamic, interactive data visualizations in web browsers.
- Provides full control over the visualization process.
- Powerful for creating custom and complex visualizations.
- Tableau Public:
- While not strictly open source, Tableau Public is noteworthy for its accessibility.
- Allows the creation and sharing of interactive charts, dashboards, and reports.
- A free version of Tableau’s data visualization platform with a user-friendly interface.
These open-source visualization tools empower data scientists to transform raw data into meaningful visualizations, fostering a deeper understanding of the underlying patterns and trends.
Also read: What is Data Science Lifecycle, Applications, Prerequisites and Tools
Data Visualization in Data Science Best Practices
Creating effective data visualizations goes beyond choosing the right tool and visualization type. Adopting best practices ensures that your visualizations are not only aesthetically pleasing but also convey accurate and meaningful insights. Here’s a deeper dive into data visualization best practices:
- Design Principles:
- Simplicity: Keep visualisations simple to avoid overwhelming your audience. Eliminate unnecessary details and focus on conveying the main message. Strive for clarity without sacrificing accuracy.
- Consistency: Establish a consistent visual language throughout your visualisations. Use the same colours, fonts, and scales to maintain a cohesive and professional look, enhancing the overall user experience.
- Clarity: Ensure that your visualisation’s message is clear and easily understandable to your target audience. Avoid unnecessary complexity or visual elements that might confuse or distract from the core insights.
- Interactivity:
- Leverage interactivity judiciously to enhance user engagement and exploration. Interactive elements, such as tooltips, filters, and zoom functionalities, can empower users to delve into specific aspects of the data, providing a more personalised and insightful experience.
- Consider the balance between interactivity and simplicity, ensuring that interactive elements enhance rather than complicate the overall user experience.
- Clear labelling is essential for effective data communication. Clearly label axes, data points, and any other relevant elements to provide context and aid interpretation.
- Use concise and informative labels to convey the meaning of each component, making it easy for your audience to understand the key takeaways from the visualization.
- Colour Choice:
- Choose colours purposefully, considering both aesthetics and functionality. Ensure that your colour choices align with the nature of the data and the message you want to convey.
- Consider colour-blindness and accessibility standards, using colour gradients and palettes that are distinguishable by a broad audience.
- Storytelling:
- Construct a narrative around your data to guide viewers through the insights. The story should have a clear beginning, middle, and end, leading the audience through the key points you want to highlight.
- Use annotations, captions, and descriptive titles to articulate the narrative and emphasize critical aspects of the data. A well-told story enhances engagement and understanding.
- Consistent Use of Visualization Types:
- Maintain consistency in the use of visualization types throughout your project or report. Align specific types of visualizations with the nature of the data and the insights you wish to emphasize.
- Avoid unnecessary variation in visualization styles, as consistency helps users become familiar with the representations, making it easier for them to interpret the visualizations.
- Accessibility Considerations:
- Ensure that your visualizations are accessible to a diverse audience. This includes considering factors like font size, colour contrast, and alternative text for users with visual impairments.
- Design visualisations that are inclusive and can be interpreted by individuals with different levels of expertise in the subject matter.
Data Visualization in Data Science Examples
Real-world examples demonstrate the impact of data visualization in solving complex problems and driving decision-making:
- COVID-19 Dashboard:
Global dashboards tracking the spread of COVID-19 showcase the power of data visualization in conveying critical information to the public.
- Financial Trends:
Visualizations of financial data, such as stock market trends and economic indicators, provide insights for investors and policymakers.
- E-commerce Analytics:
Visualizations of customer behavior’s, sales trends, and product performance empower e-commerce businesses to make data-driven decisions.
- Climate Change Data:
Visualizing climate change data helps scientists and policymakers understand patterns and trends, facilitating informed environmental decisions.
Also read: Data Science and Climate Change- Analyzing Environmental Data
Data Visualization Techniques
Effective data visualization involves not only choosing the right type of visualization but also employing various techniques to enhance the clarity and impact of the presented data. Let’s explore some advanced data visualization techniques:
Data Aggregation and Summarization:
- Hierarchical Aggregation: Group data hierarchically to provide a multi-level view. This technique is particularly useful for visualising data with a nested structure, such as organisational hierarchies.
- Temporal Aggregation: Summarise data over time intervals to reveal trends and patterns, especially in time-series data. Aggregating data into days, weeks, or months can simplify complex temporal patterns.
Data Filtering and Drill-Down:
- Interactive Filters: Implementing interactive filters allows users to focus on specific subsets of data. This enhances the relevance of the visualisation and enables users to explore specific scenarios.
- Drill-Down and Drill-Up: Provide users with the ability to drill down into detailed data or drill up to see higher-level summaries. This hierarchical navigation is effective for exploring data at different levels of granularity.
Data Annotation and Storytelling:
- Annotations: Adding annotations to key data points or trends provides context and aids interpretation. Annotations can include text labels, arrows, or shapes that draw attention to specific elements in the visualization.
- Storyboarding: Creating a sequence of visualizations as part of a story or narrative helps guide viewers through the data insights. Each visualization in the sequence builds on the previous one, providing a coherent and logical flow of information.
Comparative Visualizations:
- Small Multiples: Displaying multiple small, similar visualizations side by side allows for easy comparison. This technique is effective for comparing variations across categories or time periods.
- Parallel Coordinates: Suitable for visualising multidimensional data, parallel coordinates represent each data point as a line connecting values on different axes. This technique is useful for identifying patterns and relationships in complex datasets.
Spatial and Geographic Techniques:
- Heatmaps: Using colour gradients to represent the intensity of data values in a matrix. Heatmaps are particularly effective for visualising large datasets with multiple variables.
- Flow Maps: Illustrating the movement of data between geographic locations. Flow maps are valuable for visualizing migration patterns, trade routes, or any data with a spatial component.
Advanced Chart Types:
- Violin Plots: Combining aspects of box plots and kernel density plots, violin plots depict the distribution of data across different categories.
- Radar Charts: Displaying multivariate data on a two-dimensional chart with three or more quantitative variables represented on axes emanating from the centre.
Dynamic and Animated Visualizations:
- Animated Transitions: Using animation to show changes in data over time or in response to user interactions. Animated visualizations can enhance engagement and help convey temporal trends effectively.
Machine Learning-Driven Visualizations:
- Dimensionality Reduction Techniques: Techniques like t-SNE or PCA can be used to reduce high-dimensional data to two or three dimensions for visualization purposes.
- Cluster Visualisations: Using clustering algorithms to group similar data points together and visualising the clusters. This technique aids in identifying patterns and groupings within the data.
Data Visualization Advantages
Data visualization offers a myriad of advantages, each contributing to its pivotal role in the realm of data science:
Enhanced Decision-Making:
- Quick Insights: Visualizations provide a rapid understanding of complex datasets, allowing decision-makers to grasp key trends and patterns at a glance.
- Informed Decision-Making: The visual representation of data facilitates well-informed decisions, especially when dealing with large and intricate datasets.
Improved Communication:
- Cross-Functional Collaboration: Visualization acts as a universal language, bridging the communication gap between technical and non-technical stakeholders. This fosters collaboration and ensures that insights are effectively conveyed to diverse audiences.
- Storytelling: Visualizations turn data into a compelling narrative, making it easier to convey complex information and engage stakeholders in the decision-making process.
Identification of Patterns:
- Swift Pattern Recognition: Visualizations enable the rapid identification of patterns, trends, and outliers that might go unnoticed in raw data. This accelerates the process of drawing meaningful insights from datasets.
- Holistic Understanding: Patterns and relationships become more apparent when presented visually, leading to a holistic understanding of the data’s underlying structure.
Increased Engagement:
- Accessibility: Visualizations make data more accessible to a broader audience, enhancing engagement among stakeholders who may not have a deep understanding of the underlying data.
- Interactive Elements: Incorporating interactive elements in visualizations encourages user engagement, allowing individuals to explore and interact with the data, fostering a deeper connection with the insights.
Time-Efficient Exploration:
- Efficient Data Exploration: Visualization tools enable users to explore and analyse large datasets more efficiently than traditional methods. This accelerates the exploration phase of data analysis, saving valuable time.
- Real-Time Decision Support: Interactive visualizations provide real-time updates, supporting on-the-fly decision-making by allowing users to explore dynamic datasets.
Improved Memory Retention:
- Memorability: Visualizations create a visual imprint that enhances memory retention. Well-designed visualizations make it easier for individuals to recall and share insights with others.
- Educational Value: In educational settings, visualizations aid in the retention of complex concepts by presenting information in a visually engaging manner.
Risk Mitigation:
- Early Detection of Anomalies: Visualizations enable the early detection of anomalies or irregularities in data, allowing organizations to address potential issues before they escalate.
- Scenario Analysis: By visualizing different scenarios, organizations can assess the potential impact of various decisions and identify risks, contributing to more robust risk mitigation strategies.
Facilitates Exploratory Data Analysis (EDA):
- Intuitive Exploration: Visualizations simplify the process of exploratory data analysis, allowing data scientists to intuitively explore relationships and trends within the data.
- Hypothesis Validation: EDA through visualizations aids in validating hypotheses, providing a visual confirmation of patterns and trends observed during the analysis.
Data Visualization Disadvantages
While data visualization is a powerful tool, it is essential to acknowledge and address its potential shortcomings:
Misinterpretation:
- The visual nature of data can sometimes lead to misinterpretation if not presented accurately. Choosing inappropriate chart types, misrepresenting scales, or not providing sufficient context can contribute to misunderstandings.
Biased Representation:
- Visualization choices, such as colour schemes and scale selection, can introduce biases and influence perceptions. It’s crucial to be aware of potential biases and strive for neutrality in visual representations.
Overemphasis on Aesthetics:
- Focusing excessively on creating visually appealing charts might priorities form over function. Aesthetic choices should always serve the goal of conveying information accurately and effectively.
Complexity:
- Creating effective visualizations requires a combination of technical and design skills. Complex datasets may pose challenges in deciding the appropriate visualization methods, leading to either oversimplification or overwhelming complexity.
Data Overload:
- Presenting too much information in a single visualization can overwhelm the audience. It’s important to strike a balance between providing comprehensive insights and avoiding information overload.
Lack of Standardization:
- The absence of standardized conventions for data visualization can sometimes result in confusion. Different interpretations of colour, scale, or symbols can hinder effective communication, especially in a multi-stakeholder environment.
Dependency on Data Quality:
- Data visualizations are only as reliable as the underlying data. Poor data quality, inaccuracies, or missing values can compromise the integrity of visualizations, leading to misguided conclusions.
Tool Dependency:
- Over Reliance on specific tools may limit flexibility. Users should be cautious not to become too dependent on the functionalities of a single tool, especially if it doesn’t cater to all aspects of their data visualization needs.
Accessibility Challenges:
- Visualizations heavily reliant on colour may present challenges for individuals with colour blindness. Ensuring accessibility for all users should be a priority in the design process.
Ethical Considerations:
- The intentional or unintentional manipulation of visualizations to convey a specific narrative raises ethical concerns. Data scientists must priorities transparency and integrity in their visual representations.
Also read: Future of Data Science: Trends to Watch in 2025
In conclusion, data visualization is an indispensable aspect of data science, bridging the gap between raw data and meaningful insights. Understanding its importance, exploring various visualization types, adopting best practices, and leveraging open-source tools are crucial steps toward mastering the art of data visualization.
Kickstart your career in data science with our hands-on, industry-oriented Decode Data Science with ML 1.0 . Get the skills and experience you need to solve real-world problems with data.
How can I ensure my data visualisations are accessible to a diverse audience?
To enhance accessibility, consider incorporating features like alternative text for images, choosing colour palettes with sufficient contrast, and providing text-based descriptions or transcripts for interactive elements. These practices make visualizations inclusive for individuals with diverse needs.
Can data visualisations be utilised for real-time analytics?
Absolutely. Many visualization tools, such as Plotly and Bokeh, support real-time data streaming. Leveraging these capabilities allows data scientists to create dynamic visualizations that update in real-time, enabling a responsive and interactive analytics experience.
How do I address the challenge of visualising unstructured or text data?
When dealing with unstructured or text data, techniques like word clouds, sentiment analysis visualizations, and network graphs can be employed. These methods transform textual information into visually interpretable patterns, providing insights into the underlying content and relationships.
Are there specific considerations for visualising time-series data effectively?
Yes, when visualizing time-series data, consider employing techniques like resampling or aggregating data over meaningful time intervals. This helps in managing data granularity and presenting trends without overwhelming the viewer with excessive detail.
How can I make my data storytelling more engaging through visualization?
Enhance data storytelling by incorporating interactive elements such as tooltips, clickable charts, and animations. These features not only engage the audience but also allow them to explore the data on their terms, fostering a more immersive and impactful storytelling experience.
- What Is Data Science Meaning?
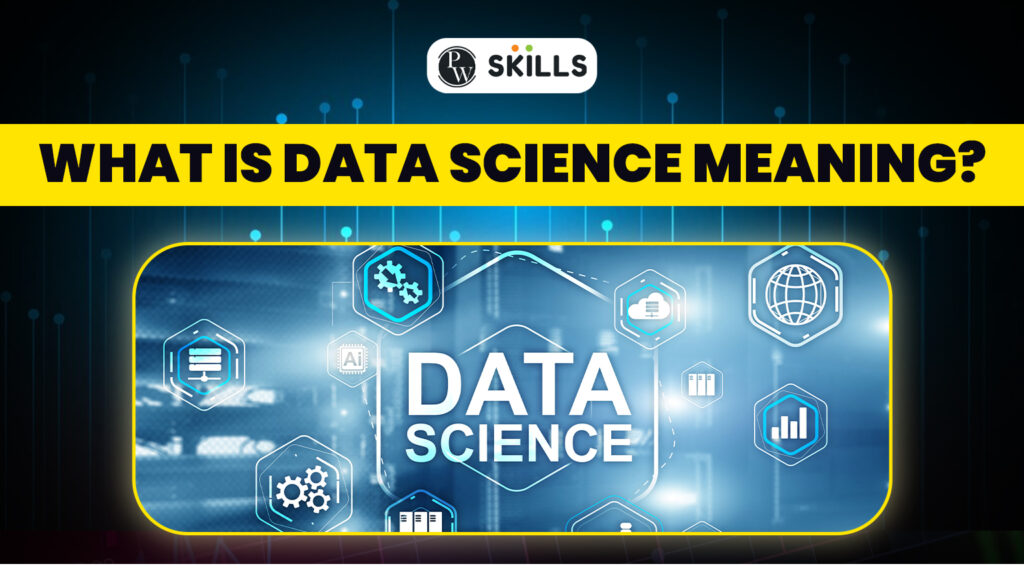
This article will help you to understand Data Science meaning, its advantages, the role of data scientists in data science,…
- What Is Data Science Definition?
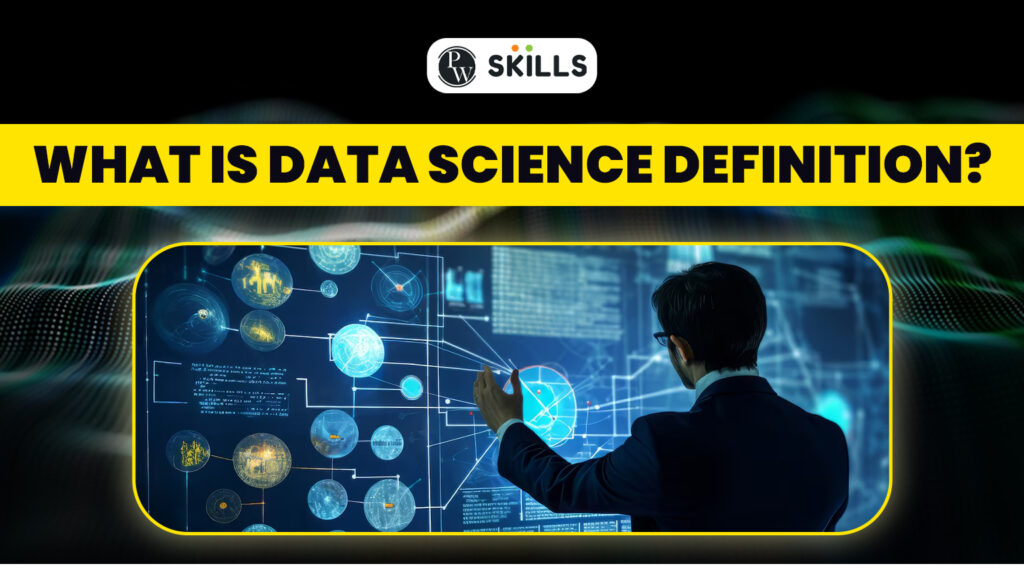
Data science is nothing but a study of data to extract meaningful insights from it, in this article we will…
- What Is Machine Learning Used For?
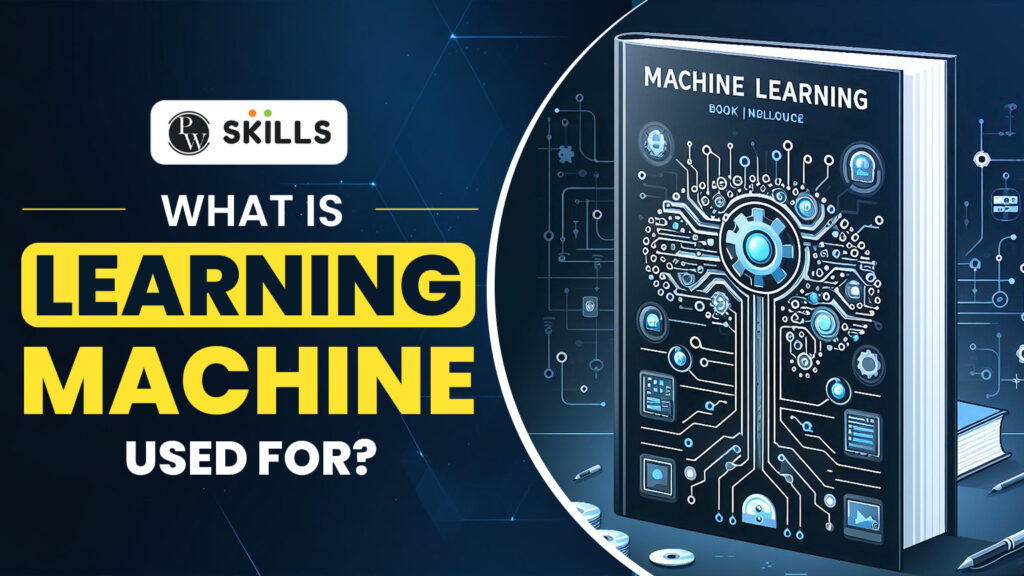
Learn everything you need to know about machine learning, including what machine learning is, its types, uses, and career options…
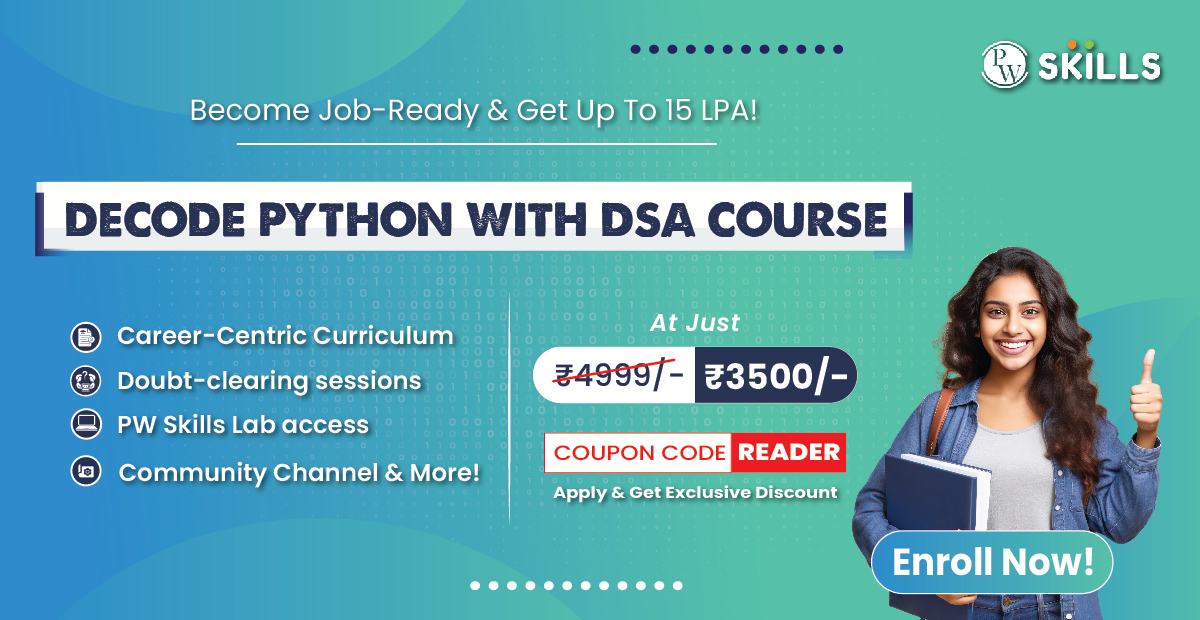
Related Articles
- Data Science Courses: How Do I Choose the Best Data Science Course?
- Top 13 Highest-Paying Data Science Jobs in India
- Top 10 Best Data Science Classes In 2024
- What is AI Data Science?
- What is Linear Regression and Example?
- Decision Tree Algorithm in Machine Learning
- What is the Position of a Data Scientist?
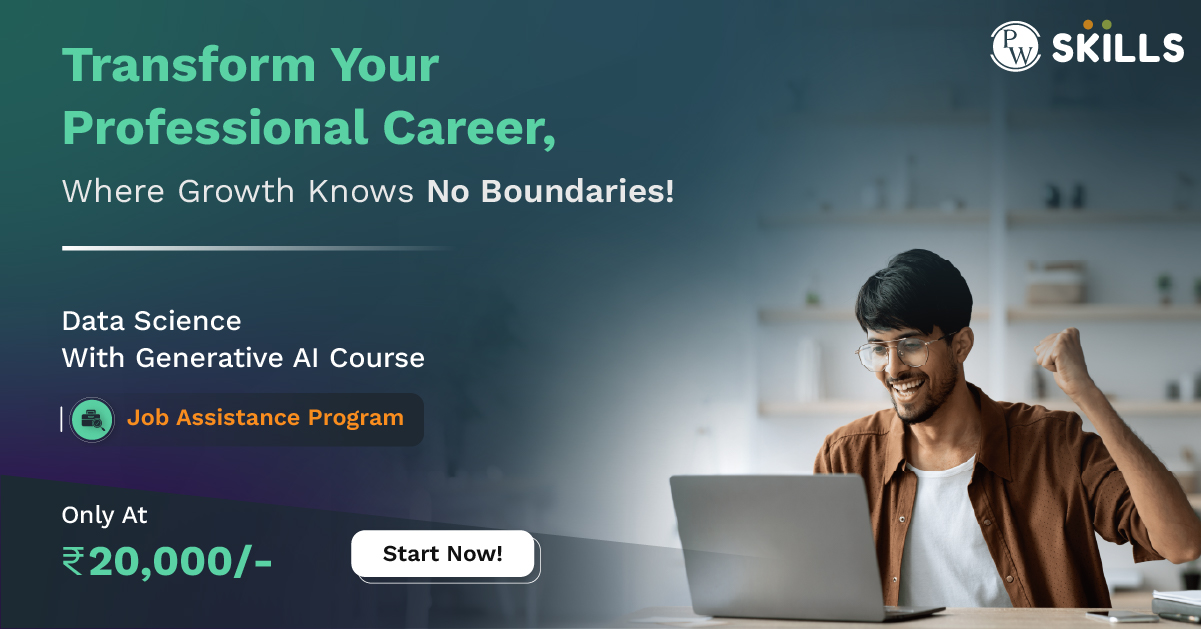
Visualizing Data by Ben Fry
Get full access to Visualizing Data and 60K+ other titles, with a free 10-day trial of O'Reilly.
There are also live events, courses curated by job role, and more.
Chapter 1. The Seven Stages of Visualizing Data
The greatest value of a picture is when it forces us to notice what we never expected to see.
What do the paths that millions of visitors take through a web site look like? How do the 3.1 billion A, C, G, and T letters of the human genome compare to those of the chimp or the mouse? Out of a few hundred thousand files on your computer’s hard disk, which ones are taking up the most space, and how often do you use them? By applying methods from the fields of computer science, statistics, data mining, graphic design, and visualization, we can begin to answer these questions in a meaningful way that also makes the answers accessible to others.
All of the previous questions involve a large quantity of data, which makes it extremely difficult to gain a “big picture” understanding of its meaning. The problem is further compounded by the data’s continually changing nature, which can result from new information being added or older information continuously being refined. This deluge of data necessitates new software-based tools, and its complexity requires extra consideration. Whenever we analyze data, our goal is to highlight its features in order of their importance, reveal patterns, and simultaneously show features that exist across multiple dimensions.
This book shows you how to make use of data as a resource that you might otherwise never tap. You’ll learn basic visualization principles, how to choose the right kind of display for your purposes, and how to provide interactive features that will bring users to your site over and over again. You’ll also learn to program in Processing, a simple but powerful environment that lets you quickly carry out the techniques in this book. You’ll find Processing a good basis for designing interfaces around large data sets, but even if you move to other visualization tools, the ways of thinking presented here will serve you as long as human beings continue to process information the same way they’ve always done.
Why Data Display Requires Planning
Each set of data has particular display needs, and the purpose for which you’re using the data set has just as much of an effect on those needs as the data itself. There are dozens of quick tools for developing graphics in a cookie-cutter fashion in office programs, on the Web, and elsewhere, but complex data sets used for specialized applications require unique treatment. Throughout this book, we’ll discuss how the characteristics of a data set help determine what kind of visualization you’ll use.
Too Much Information
When you hear the term “information overload,” you probably know exactly what it means because it’s something you deal with daily. In Richard Saul Wurman’s book Information Anxiety (Doubleday), he describes how the New York Times on an average Sunday contains more information than a Renaissance-era person had access to in his entire lifetime.
But this is an exciting time. For $300, you can purchase a commodity PC that has thousands of times more computing power than the first computers used to tabulate the U.S. Census. The capability of modern machines is astounding. Performing sophisticated data analysis no longer requires a research laboratory, just a cheap machine and some code. Complex data sets can be accessed, explored, and analyzed by the public in a way that simply was not possible in the past.
The past 10 years have also brought about significant changes in the graphic capabilities of average machines. Driven by the gaming industry, high-end 2D and 3D graphics hardware no longer requires dedicated machines from specific vendors, but can instead be purchased as a $100 add-on card and is standard equipment for any machine costing $700 or more. When not used for gaming, these cards can render extremely sophisticated models with thousands of shapes, and can do so quickly enough to provide smooth, interactive animation. And these prices will only decrease—within a few years’ time, accelerated graphics will be standard equipment on the aforementioned commodity PC.
Data Collection
We’re getting better and better at collecting data, but we lag in what we can do with it. Most of the examples in this book come from freely available data sources on the Internet. Lots of data is out there, but it’s not being used to its greatest potential because it’s not being visualized as well as it could be. (More about this can be found in Chapter 9 , which covers places to find data and how to retrieve it.)
With all the data we’ve collected, we still don’t have many satisfactory answers to the sort of questions that we started with. This is the greatest challenge of our information-rich era: how can these questions be answered quickly, if not instantaneously? We’re getting so good at measuring and recording things, why haven’t we kept up with the methods to understand and communicate this information?
Thinking About Data
We also do very little sophisticated thinking about information itself. When AOL released a data set containing the search queries of millions of users that had been “randomized” to protect the innocent, articles soon appeared about how people could be identified by—and embarrassed by—information regarding their search habits. Even though we can collect this kind of information, we often don’t know quite what it means. Was this a major issue or did it simply embarrass a few AOL users? Similarly, when millions of records of personal data are lost or accessed illegally, what does that mean? With so few people addressing data, our understanding remains quite narrow, boiling down to things like, “My credit card number might be stolen” or “Do I care if anyone sees what I search?”
Data Never Stays the Same
We might be accustomed to thinking about data as fixed values to be analyzed, but data is a moving target. How do we build representations of data that adjust to new values every second, hour, or week? This is a necessity because most data comes from the real world, where there are no absolutes. The temperature changes, the train runs late, or a product launch causes the traffic pattern on a web site to change drastically.
What happens when things start moving? How do we interact with “live” data? How do we unravel data as it changes over time? We might use animation to play back the evolution of a data set, or interaction to control what time span we’re looking at. How can we write code for these situations?
What Is the Question?
As machines have enormously increased the capacity with which we can create (through measurements and sampling) and store data, it becomes easier to disassociate the data from the original reason for collecting it. This leads to an all-too frequent situation: approaching visualization problems with the question, “How can we possibly understand so much data?”
As a contrast, think about subway maps, which are abstracted from the complex shape of the city and are focused on the rider’s goal: to get from one place to the next. Limiting the detail of each shape, turn, and geographical formation reduces this complex data set to answering the rider’s question: “How do I get from point A to point B?”
Harry Beck invented the format now commonly used for subway maps in the 1930s, when he redesigned the map of the London Underground. Inspired by the layout of circuit boards, the map simplified the complicated Tube system to a series of vertical, horizontal, and 45°diagonal lines. While attempting to preserve as much of the relative physical layout as possible, the map shows only the connections between stations, as that is the only information that riders use to decide their paths.
When beginning a visualization project, it’s common to focus on all the data that has been collected so far. The amounts of information might be enormous—people like to brag about how many gigabytes of data they’ve collected and how difficult their visualization problem is. But great information visualization never starts from the standpoint of the data set; it starts with questions. Why was the data collected, what’s interesting about it, and what stories can it tell?
The most important part of understanding data is identifying the question that you want to answer. Rather than thinking about the data that was collected, think about how it will be used and work backward to what was collected. You collect data because you want to know something about it. If you don’t really know why you’re collecting it, you’re just hoarding it. It’s easy to say things like, “I want to know what’s in it,” or “I want to know what it means.” Sure, but what’s meaningful?
The more specific you can make your question, the more specific and clear the visual result will be. When questions have a broad scope, as in “exploratory data analysis” tasks, the answers themselves will be broad and often geared toward those who are themselves versed in the data. John Tukey, who coined the term Exploratory Data Analysis, said “. . . pictures based on exploration of data should force their messages upon us.” [ 1 ] Too many data problems are labeled “exploratory” because the data collected is overwhelming, even though the original purpose was to answer a specific question or achieve specific results.
One of the most important (and least technical) skills in understanding data is asking good questions. An appropriate question shares an interest you have in the data, tries to convey it to others, and is curiosity-oriented rather than math-oriented. Visualizing data is just like any other type of communication: success is defined by your audience’s ability to pick up on, and be excited about, your insight.
Admittedly, you may have a rich set of data to which you want to provide flexible access by not defining your question too narrowly. Even then, your goal should be to highlight key findings. There is a tendency in the visualization field to borrow from the statistics field and separate problems into exploratory and expository , but for the purposes of this book, this distinction is not useful. The same methods and process are used for both.
In short, a proper visualization is a kind of narrative, providing a clear answer to a question without extraneous details. By focusing on the original intent of the question, you can eliminate such details because the question provides a benchmark for what is and is not necessary.
A Combination of Many Disciplines
Given the complexity of data, using it to provide a meaningful solution requires insights from diverse fields: statistics, data mining, graphic design, and information visualization. However, each field has evolved in isolation from the others.
Thus, visual design—the field of mapping data to a visual form—typically does not address how to handle thousands or tens of thousands of items of data. Data mining techniques have such capabilities, but they are disconnected from the means to interact with the data. Software-based information visualization adds building blocks for interacting with and representing various kinds of abstract data, but typically these methods undervalue the aesthetic principles of visual design rather than embrace their strength as a necessary aid to effective communication. Someone approaching a data representation problem (such as a scientist trying to visualize the results of a study involving a few thousand pieces of genetic data) often finds it difficult to choose a representation and wouldn’t even know what tools to use or books to read to begin.
We must reconcile these fields as parts of a single process. Graphic designers can learn the computer science necessary for visualization, and statisticians can communicate their data more effectively by understanding the visual design principles behind data representation. The methods themselves are not new, but their isolation within individual fields has prevented them from being used together. In this book, we use a process that bridges the individual disciplines, placing the focus and consideration on how data is understood rather than on the viewpoint and tools of each individual field.
The process of understanding data begins with a set of numbers and a question. The following steps form a path to the answer:
Obtain the data, whether from a file on a disk or a source over a network.
Provide some structure for the data’s meaning, and order it into categories.
Remove all but the data of interest.
Apply methods from statistics or data mining as a way to discern patterns or place the data in mathematical context.
Choose a basic visual model, such as a bar graph, list, or tree.
Improve the basic representation to make it clearer and more visually engaging.
Add methods for manipulating the data or controlling what features are visible.
Of course, these steps can’t be followed slavishly. You can expect that they’ll be involved at one time or another in projects you develop, but sometimes it will be four of the seven, and at other times all of them.
Part of the problem with the individual approaches to dealing with data is that the separation of fields leads to different people each solving an isolated part of the problem. When this occurs, something is lost at each transition—like a “telephone game” in which each step of the process diminishes aspects of the initial question under consideration. The initial format of the data (determined by how it is acquired and parsed) will often drive how it is considered for filtering or mining. The statistical method used to glean useful information from the data might drive the initial presentation. In other words, the final representation reflects the results of the statistical method rather than a response to the initial question.
Similarly, a graphic designer brought in at the next stage will most often respond to specific problems with the representation provided by the previous steps, rather than focus on the initial question. The visualization step might add a compelling and interactive means to look at the data filtered from the earlier steps, but the display is inflexible because the earlier stages of the process are hidden. Furthermore, practitioners of each of the fields that commonly deal with data problems are often unclear about how to traverse the wider set of methods and arrive at an answer.
This book covers the whole path from data to understanding: the transformation of a jumble of raw numbers into something coherent and useful. The data under consideration might be numbers, lists, or relationships between multiple entities.
It should be kept in mind that the term visualization is often used to describe the art of conveying a physical relationship, such as the subway map mentioned near the start of this chapter. That’s a different kind of analysis and skill from information visualization , where the data is primarily numeric or symbolic (e.g., A, C, G, and T—the letters of genetic code—and additional annotations about them). The primary focus of this book is information visualization: for instance, a series of numbers that describes temperatures in a weather forecast rather than the shape of the cloud cover contributing to them.
To illustrate the seven steps listed in the previous section, and how they contribute to effective information visualization, let’s look at how the process can be applied to understanding a simple data set. In this case, we’ll take the zip code numbering system that the U.S. Postal Service uses. The application is not particularly advanced, but it provides a skeleton for how the process works. ( Chapter 6 contains a full implementation of the project.)
All data problems begin with a question and end with a narrative construct that provides a clear answer. The Zipdecode project (described further in Chapter 6 ) was developed out of a personal interest in the relationship of the zip code numbering system to geographic areas. Living in Boston, I knew that numbers starting with a zero denoted places on the East Coast. Having spent time in San Francisco, I knew the initial numbers for the West Coast were all nines. I grew up in Michigan, where all our codes were four-prefixed. But what sort of area does the second digit specify? Or the third?
The finished application was initially constructed in a few hours as a quick way to take what might be considered a boring data set (a long list of zip codes, towns, and their latitudes and longitudes) and create something engaging for a web audience that explained how the codes related to their geography.
The acquisition step involves obtaining the data. Like many of the other steps, this can be either extremely complicated (i.e., trying to glean useful data from a large system) or very simple (reading a readily available text file).
A copy of the zip code listing can be found on the U.S. Census Bureau web site, as it is frequently used for geographic coding of statistical data. The listing is a freely available file with approximately 42,000 lines, one for each of the codes, a tiny portion of which is shown in Figure 1-1 .
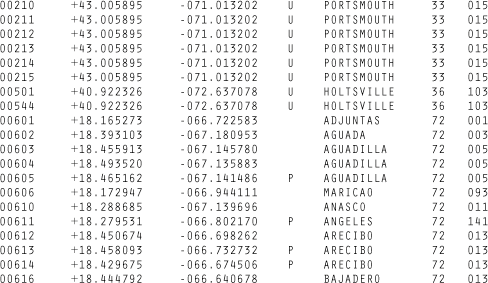
Acquisition concerns how the user downloads your data as well as how you obtained the data in the first place. If the final project will be distributed over the Internet, as you design the application, you have to take into account the time required to download data into the browser. And because data downloaded to the browser is probably part of an even larger data set stored on the server, you may have to structure the data on the server to facilitate retrieval of common subsets.
After you acquire the data, it needs to be parsed—changed into a format that tags each part of the data with its intended use. Each line of the file must be broken along its individual parts; in this case, it must be delimited at each tab character. Then, each piece of data needs to be converted to a useful format. Figure 1-2 shows the layout of each line in the census listing, which we have to understand to parse it and get out of it what we want.
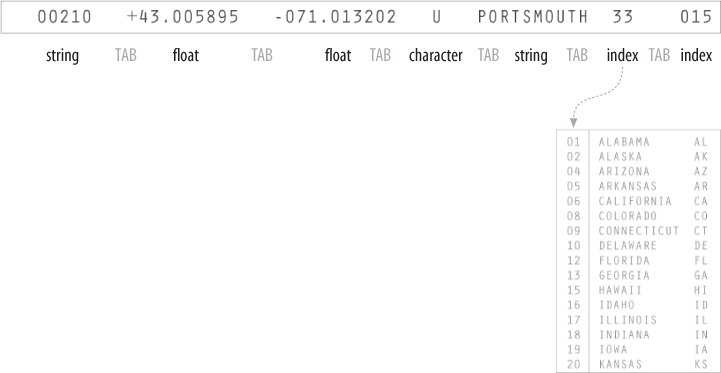
Each field is formatted as a data type that we’ll handle in a conversion program:
A set of characters that forms a word or a sentence. Here, the city or town name is designated as a string. Because the zip codes themselves are not so much numbers as a series of digits (if they were numbers, the code 02139 would be stored as 2139, which is not the same thing), they also might be considered strings.
A number with decimal points (used for the latitudes and longitudes of each location). The name is short for floating point , from programming nomenclature that describes how the numbers are stored in the computer’s memory.
A single letter or other symbol. In this data set, a character sometimes designates special post offices.
A number without a fractional portion, and hence no decimal points (e.g., −14, 0, or 237).
Data (commonly an integer or string) that maps to a location in another table of data. In this case, the index maps numbered codes to the names and two-digit abbreviations of states. This is common in databases, where such an index is used as a pointer into another table, sometimes as a way to compact the data further (e.g., a two-digit code requires less storage than the full name of the state or territory).
With the completion of this step, the data is successfully tagged and consequently more useful to a program that will manipulate or represent it in some way.
The next step involves filtering the data to remove portions not relevant to our use. In this example, for the sake of keeping it simple, we’ll be focusing on the contiguous 48 states, so the records for cities and towns that are not part of those states—Alaska, Hawaii, and territories such as Puerto Rico—are removed. Another project could require significant mathematical work to place the data into a mathematical model or normalize it (convert it to an acceptable range of numbers).
This step involves math, statistics, and data mining. The data in this case receives only a simple treatment: the program must figure out the minimum and maximum values for latitude and longitude by running through the data (as shown in Figure 1-3 ) so that it can be presented on a screen at a proper scale. Most of the time, this step will be far more complicated than a pair of simple math operations.
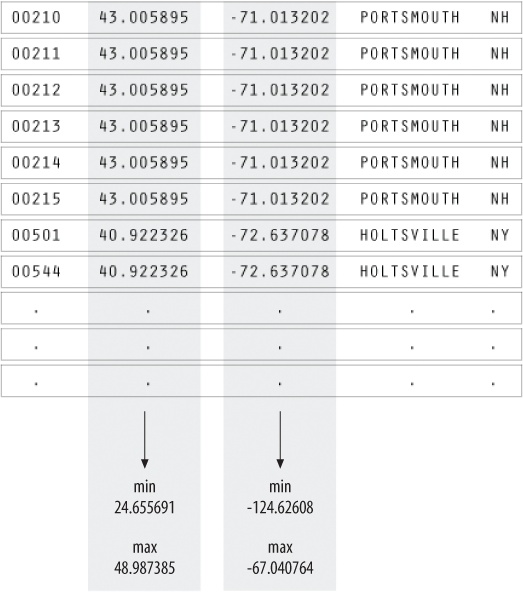
This step determines the basic form that a set of data will take. Some data sets are shown as lists, others are structured like trees, and so forth. In this case, each zip code has a latitude and longitude, so the codes can be mapped as a two-dimensional plot, with the minimum and maximum values for the latitude and longitude used for the start and end of the scale in each dimension. This is illustrated in Figure 1-4 .
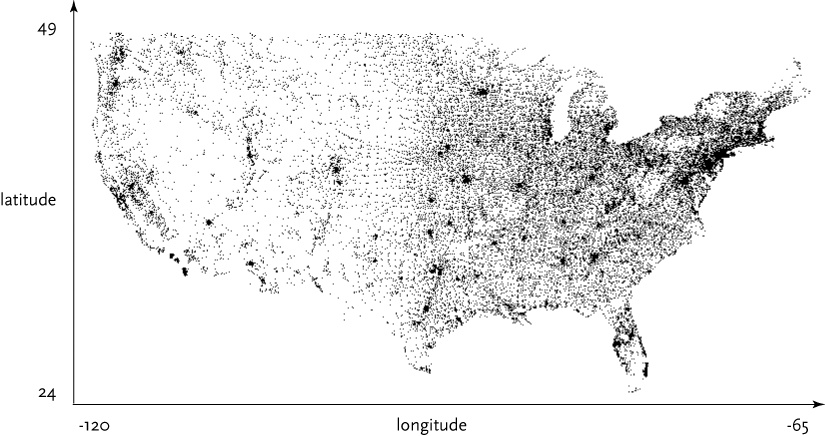
The Represent stage is a linchpin that informs the single most important decision in a visualization project and can make you rethink earlier stages. How you choose to represent the data can influence the very first step (what data you acquire) and the third step (what particular pieces you extract).
In this step, graphic design methods are used to further clarify the representation by calling more attention to particular data (establishing hierarchy) or by changing attributes (such as color) that contribute to readability.
Hierarchy is established in Figure 1-5 , for instance, by coloring the background deep gray and displaying the selected points (all codes beginning with four) in white and the deselected points in medium yellow.
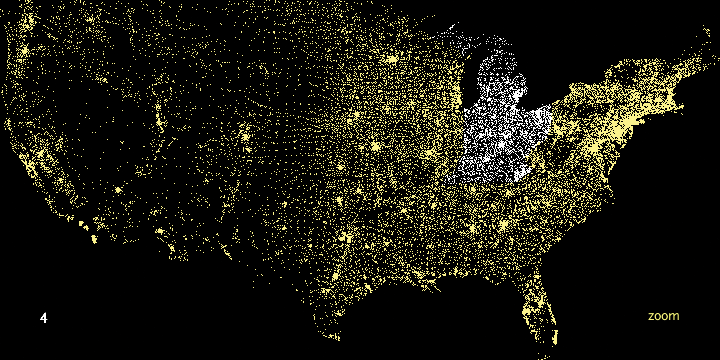
The next stage of the process adds interaction, letting the user control or explore the data. Interaction might cover things like selecting a subset of the data or changing the viewpoint. As another example of a stage affecting an earlier part of the process, this stage can also affect the refinement step, as a change in viewpoint might require the data to be designed differently.
In the Zipdecode project, typing a number selects all zip codes that begin with that number. Figure 1-6 and Figure 1-7 show all the zip codes beginning with zero and nine, respectively.
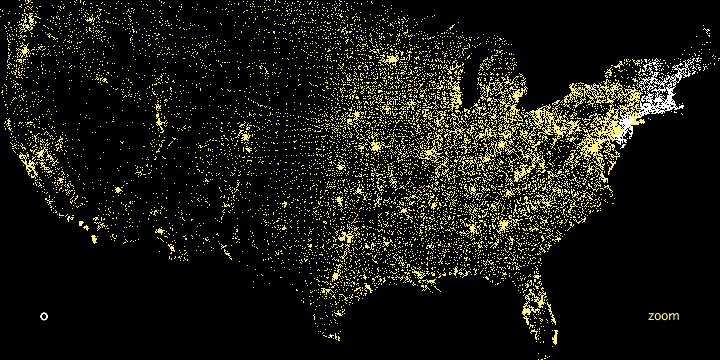
Another enhancement to user interaction (not shown here) enables the users to traverse the display laterally and run through several of the prefixes. After typing part or all of a zip code, holding down the Shift key allows users to replace the last number typed without having to hit the Delete key to back up.
Typing is a very simple form of interaction, but it allows the user to rapidly gain an understanding of the zip code system’s layout. Just contrast this sample application with the difficulty of deducing the same information from a table of zip codes and city names.
The viewer can continue to type digits to see the area covered by each subsequent set of prefixes. Figure 1-8 shows the region highlighted by the two digits 02, Figure 1-9 shows the three digits 021, and Figure 1-10 shows the four digits 0213. Finally, Figure 1-11 shows what you get by entering a full zip code, 02139—a city name pops up on the display.
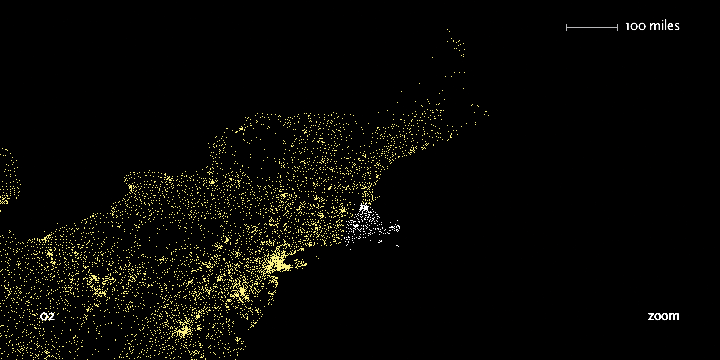
In addition, users can enable a “zoom” feature that draws them closer to each subsequent digit, revealing more detail around the area and showing a constant rate of detail at each level. Because we’ve chosen a map as a representation, we could add more details of state and county boundaries or other geographic features to help viewers associate the “data” space of zip code points with what they know about the local environment.
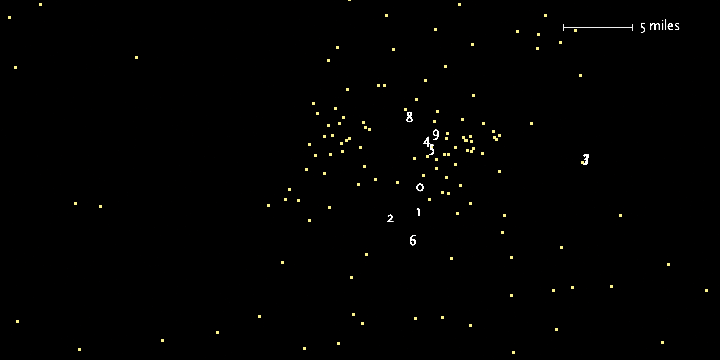
Iteration and Combination
Figure 1-12 shows the stages in order and demonstrates how later decisions commonly reflect on earlier stages. Each step of the process is inextricably linked because of how the steps affect one another. In the Zipdecode application, for instance:
The need for a compact representation on the screen led me to refilter the data to include only the contiguous 48 states.
The representation step affected acquisition because after I developed the application I modified it so it could show data that was downloaded over a slow Internet connection to the browser. My change to the structure of the data allows the points to appear slowly, as they are first read from the data file, employing the data itself as a “progress bar.”
Interaction by typing successive numbers meant that the colors had to be modified in the visual refinement step to show a slow transition as points in the display are added or removed. This helps the user maintain context by preventing the updates on-screen from being too jarring.

The connections between the steps in the process illustrate the importance of the individual or team in addressing the project as a whole. This runs counter to the common fondness for assembly-line style projects, where programmers handle the technical portions, such as acquiring and parsing data, and visual designers are left to choose colors and typefaces. At the intersection of these fields is a more interesting set of properties that demonstrates their strength in combination.
When acquiring data, consider how it can change, whether sporadically (such as once a month) or continuously. This expands the notion of graphic design that’s traditionally focused on solving a specific problem for a specific data set, and instead considers the meta-problem of how to handle a certain kind of data that might be updated in the future.
In the filtering step, data can be filtered in real time, as in the Zipdecode application. During visual refinement, changes to the design can be applied across the entire system. For instance, a color change can be automatically applied to the thousands of elements that require it, rather having to make such a tedious modification by hand. This is the strength of a computational approach, where tedious processes are minimized through automation.
I’ll finish this general introduction to visualization by laying out some ways of thinking about data and its representation that have served me well over many years and many diverse projects. They may seem abstract at first, or of minor importance to the job you’re facing, but I urge you to return and reread them as you practice visualization; they just may help you in later tasks.
Each Project Has Unique Requirements
A visualization should convey the unique properties of the data set it represents. This book is not concerned with providing a handful of ready-made “visualizations” that can be plugged into any data set. Ready-made visualizations can help produce a quick view of your data set, but they’re inflexible commodity items that can be implemented in packaged software. Any bar chart or scatter plot made with Excel will look like a bar chart or scatter plot made with Excel. Packaged solutions can provide only packaged answers, like a pull-string toy that is limited to a handful of canned phrases, such as “Sales show a slight increase in each of the last five years!” Every problem is unique, so capitalize on that uniqueness to solve the problem.
Chapters in this book are divided by types of data, rather than types of display. In other words, we’re not saying, “Here’s how to make a bar graph,” but “Here are several ways to show a correlation.” This gives you a more powerful way to think about maximizing what can be said about the data set in question.
I’m often asked for a library of tools that will automatically make attractive representations of any given data set. But if each data set is different, the point of visualization is to expose that fascinating aspect of the data and make it self-evident. Although readily available representation toolkits are useful starting points, they must be customized during an in-depth study of the task.
Data is often stored in a generic format. For instance, databases used for annotation of genomic data might consist of enormous lists of start and stop positions, but those lists vary in importance depending on the situation in which they’re being used. We don’t view books as long abstract sequences of words, yet when it comes to information, we’re often so taken with the enormity of the information and the low-level abstractions used to store it that the narrative is lost. Unless you stop thinking about databases, everything looks like a table—millions of rows and columns to be stored, queried, and viewed.
In this book, we use a small collection of simple helper classes as starting points. Often, we’ll be targeting the Web as a delivery platform, so the classes are designed to take up minimal time for download and display. But I will also discuss more robust versions of similar tools that can be used for more in-depth work.
This book aims to help you learn to understand data as a tool for human decision-making—how it varies, how it can be used, and how to find what’s unique about your data set. We’ll cover many standard methods of visualization and give you the background necessary for making a decision about what sort of representation is suitable for your data. For each representation, we consider its positive and negative points and focus on customizing it so that it’s best suited to what you’re trying to convey about your data set.
Avoid the All-You-Can-Eat Buffet
Often, less detail will actually convey more information because the inclusion of overly specific details causes the viewer to miss what’s most important or disregard the image entirely because it’s too complex. Use as little data as possible, no matter how precious it seems.
Consider a weather map, with curved bands of temperatures across the country. The designers avoid giving each band a detailed edge (particularly because the data is often fuzzy). Instead, they convey a broader pattern in the data.
Subway maps leave out the details of surface roads because the additional detail adds more complexity to the map than necessary. Before maps were created in Beck’s style, it seemed that knowing street locations was essential to navigating the subway. Instead, individual stations are used as waypoints for direction finding. The important detail is that your target destination is near a particular station. Directions can be given in terms of the last few turns to be taken after you exit the station, or you can consult a map posted at the station that describes the immediate area aboveground.
It’s easy to collect data, and some people become preoccupied with simply accumulating more complex data or data in mass quantities. But more data is not implicitly better, and often serves to confuse the situation. Just because it can be measured doesn’t mean it should. Perhaps making things simple is worth bragging about, but making complex messes is not. Find the smallest amount of data that can still convey something meaningful about the contents of the data set. As with Beck’s underground map, focusing on the question helps define those minimum requirements.
The same holds for the many “dimensions” that are found in data sets. Web site traffic statistics have many dimensions: IP address, date, time of day, page visited, previous page visited, result code, browser, machine type, and so on. While each of these might be examined in turn, they relate to distinct questions. Only a few of the variables are required to answer a typical question, such as “How many people visited page x over the last three months, and how has that figure changed each month?” Avoid trying to show a burdensome multidimensional space that maps too many points of information.
Know Your Audience
Finally, who is your audience? What are their goals when approaching a visualization? What do they stand to learn? Unless it’s accessible to your audience, why are you doing it? Making things simple and clear doesn’t mean assuming that your users are idiots and “dumbing down” the interface for them.
In what way will your audience use the piece? A mapping application used on a mobile device has to be designed with a completely different set of criteria than one used on a desktop computer. Although both applications use maps, they have little to do with each other. The focus of the desktop application may be finding locations and print maps, whereas the focus of the mobile version is actively following the directions to a particular location.
In this chapter, we covered the process for attacking the common modern problems of having too much data and having data that changes. In the next chapter, we’ll discuss Processing, the software tool used to handle data sets in this book.
[ 1 ] * Tukey, John Wilder. Exploratory Data Analysis . Reading, MA: Addison-Wesley, 1977.
Get Visualizing Data now with the O’Reilly learning platform.
O’Reilly members experience books, live events, courses curated by job role, and more from O’Reilly and nearly 200 top publishers.
Don’t leave empty-handed
Get Mark Richards’s Software Architecture Patterns ebook to better understand how to design components—and how they should interact.
It’s yours, free.
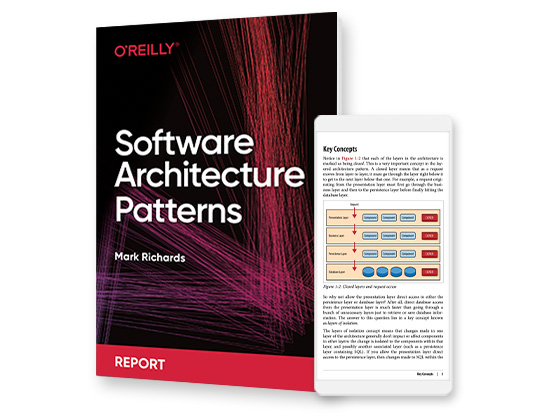
Check it out now on O’Reilly
Dive in for free with a 10-day trial of the O’Reilly learning platform—then explore all the other resources our members count on to build skills and solve problems every day.
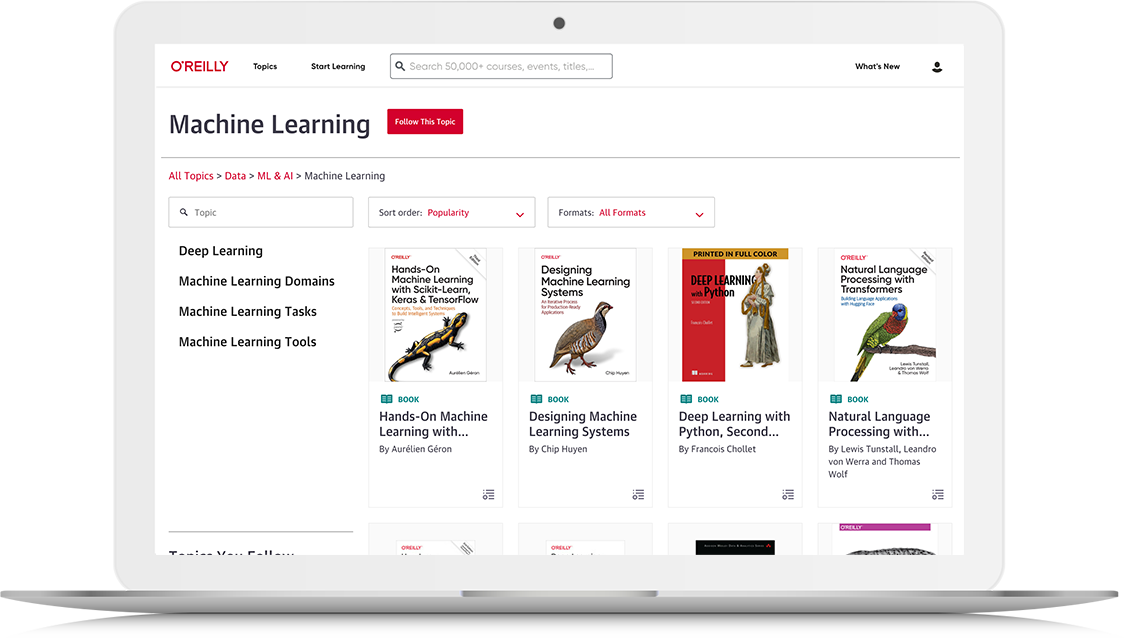
Data Topics
- Data Architecture
- Data Literacy
- Data Science
- Data Strategy
- Data Modeling
- Governance & Quality
- Data Education
- Data Literacy News, Articles, & Education
5 Key Strategies for Making Data Visualization Accessible
“If I can’t picture it, I can’t understand it.” —Albert Einstein Research has found that 65% of the general population are visual learners, meaning they need to see information as images to understand it. The business world confirms this: Visualization is essential in driving success. Take, for instance, data visualization, or, the art of translating data into […]
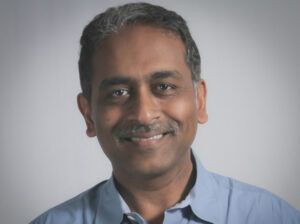
“ If I can’t picture it, I can’t understand it .” —Albert Einstein

Recognizing the essential role of data visualization in business intelligence, it’s evident that accessibility is key to unleashing its potential. Here, I discuss the top five strategies for making data visualization accessible in business:
- Implement Intuitive Visualization Tools
Data visualization tools should be designed for ease of use, involving software that accommodates different levels of expertise and offers features like interactive filtering options and drill-down capabilities. By ensuring that users need little to no technical skill, we democratize the use of data , allowing everyone the opportunity to gain meaningful insights from complex datasets.
- Adopt Strong Design Principles
Develop and adhere to a set of design principles that captivate the audience, including a cohesive color scheme, simple typography, and a thoughtful layout. These elements will improve the readability and impact of data visualizations, making them engaging and easy to interpret.
- Choose Appropriate Visualization Formats
Different visualizations serve different purposes, so the type of visualization should match the type of data being presented. Ensuring a match between the data and its visual representation will enhance clarity and effectiveness. For example, there are bar charts for comparisons, line charts for trends over time, pie charts for proportions, and maps for geographic context. Train team members to recognize which visualizations are best for a particular category of data and the related objectives.
- Storytelling through Data Visualization
Aim to connect emotionally with the audience by making the data meaningful and memorable. Identify a core message within the data, using a logical flow and visual elements like color coding to highlight key features. A compelling visualization will tell a story that guides viewers through the data to uncover insights.
- Facilitate Collaboration and Sharing
Implement collaborative features in data visualization tools and encourage a culture of shared analytics. And this isn’t just for leadership! Practical visualization tools support cooperative efforts in data-driven projects. Features like annotations and shared dashboards can encourage team members to share insights, fostering an environment where diverse and representative perspectives drive decision-making.
As we continue to navigate the ever-evolving landscape of business intelligence, remember that the power of data visualization is not just in the numbers and charts but also in the stories they tell and the decisions they drive. By simplifying complexity with user-friendly tools, enhancing visual appeal, choosing appropriate visualizations, using visuals to tell a data story, and facilitating collaboration, businesses can unlock the full potential of their data. Embracing these strategies democratizes data analytics, ensuring insights are impactful and actionable, enhances decision-making, and fosters a culture of informed data-driven innovation.
- Reviews / Why join our community?
- For companies
- Frequently asked questions
Visual Representation
What is visual representation.
Visual Representation refers to the principles by which markings on a surface are made and interpreted. Designers use representations like typography and illustrations to communicate information, emotions and concepts. Color, imagery, typography and layout are crucial in this communication.
Alan Blackwell, cognition scientist and professor, gives a brief introduction to visual representation:
- Transcript loading…
We can see visual representation throughout human history, from cave drawings to data visualization :
Art uses visual representation to express emotions and abstract ideas.
Financial forecasting graphs condense data and research into a more straightforward format.
Icons on user interfaces (UI) represent different actions users can take.
The color of a notification indicates its nature and meaning.
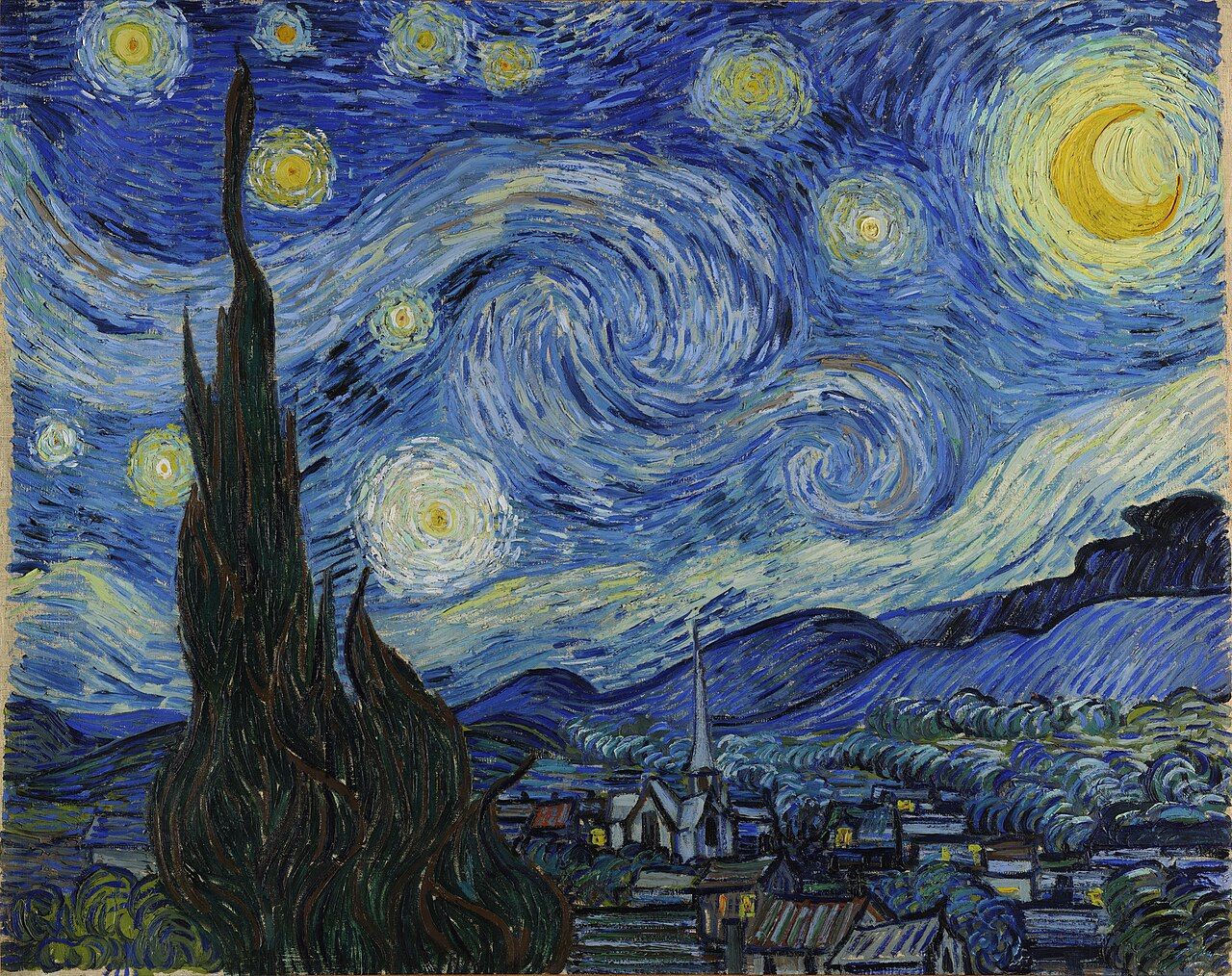
Van Gogh's "The Starry Night" uses visuals to evoke deep emotions, representing an abstract, dreamy night sky. It exemplifies how art can communicate complex feelings and ideas.
© Public domain
Importance of Visual Representation in Design
Designers use visual representation for internal and external use throughout the design process . For example:
Storyboards are illustrations that outline users’ actions and where they perform them.
Sitemaps are diagrams that show the hierarchy and navigation structure of a website.
Wireframes are sketches that bring together elements of a user interface's structure.
Usability reports use graphs and charts to communicate data gathered from usability testing.
User interfaces visually represent information contained in applications and computerized devices.
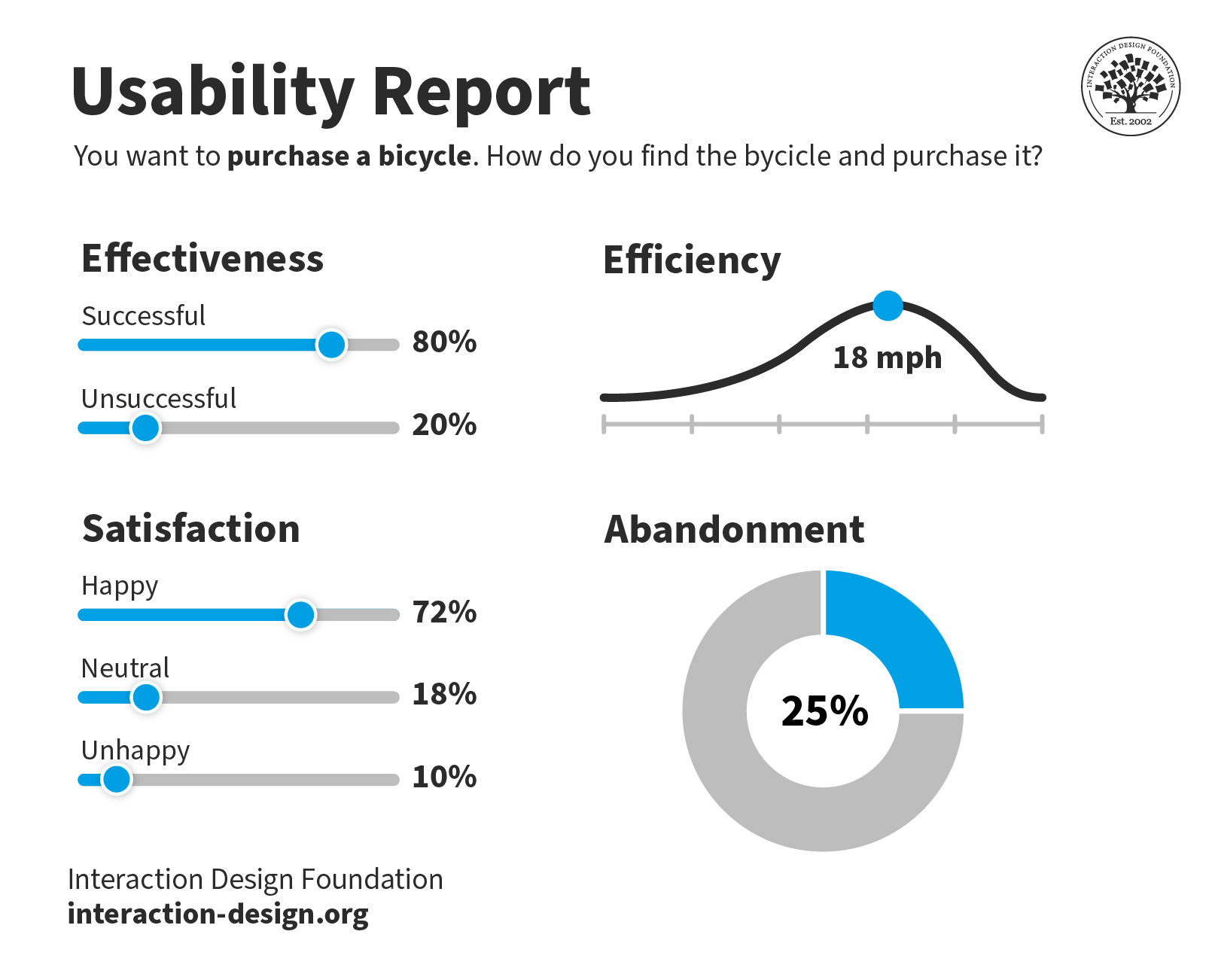
This usability report is straightforward to understand. Yet, the data behind the visualizations could come from thousands of answered surveys.
© Interaction Design Foundation, CC BY-SA 4.0
Visual representation simplifies complex ideas and data and makes them easy to understand. Without these visual aids, designers would struggle to communicate their ideas, findings and products . For example, it would be easier to create a mockup of an e-commerce website interface than to describe it with words.
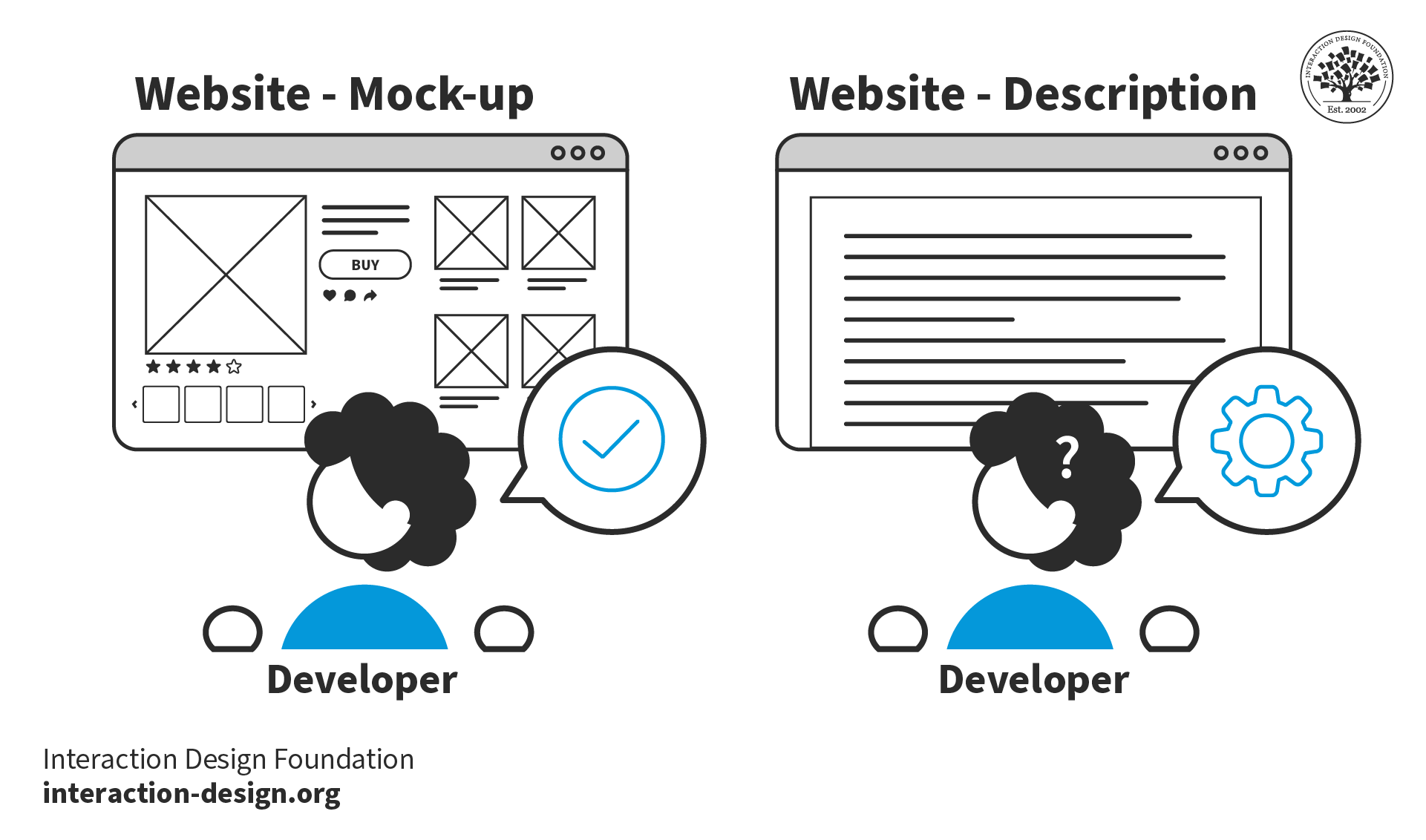
Visual representation simplifies the communication of designs. Without mockups, it would be difficult for developers to reproduce designs using words alone.
Types of Visual Representation
Below are some of the most common forms of visual representation designers use.
Text and Typography
Text represents language and ideas through written characters and symbols. Readers visually perceive and interpret these characters. Typography turns text into a visual form, influencing its perception and interpretation.
We have developed the conventions of typography over centuries , for example, in documents, newspapers and magazines. These conventions include:
Text arranged on a grid brings clarity and structure. Gridded text makes complex information easier to navigate and understand. Tables, columns and other formats help organize content logically and enhance readability.
Contrasting text sizes create a visual hierarchy and draw attention to critical areas. For example, headings use larger text while body copy uses smaller text. This contrast helps readers distinguish between primary and secondary information.
Adequate spacing and paragraphing improve the readability and appearance of the text. These conventions prevent the content from appearing cluttered. Spacing and paragraphing make it easier for the eye to follow and for the brain to process the information.
Balanced image-to-text ratios create engaging layouts. Images break the monotony of text, provide visual relief and illustrate or emphasize points made in the text. A well-planned ratio ensures neither text nor images overwhelm each other. Effective ratios make designs more effective and appealing.
Designers use these conventions because people are familiar with them and better understand text presented in this manner.
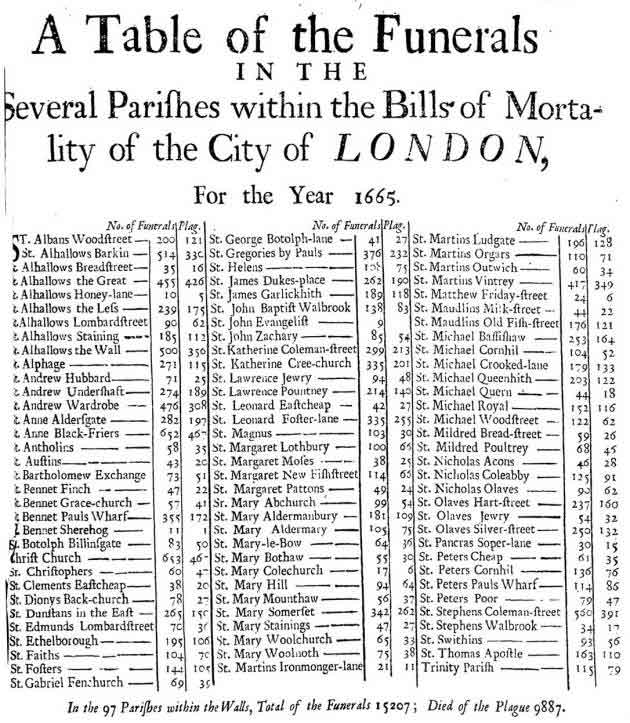
This table of funerals from the plague in London in 1665 uses typographic conventions still used today. For example, the author arranged the information in a table and used contrasting text styling to highlight information in the header.
Illustrations and Drawings
Designers use illustrations and drawings independently or alongside text. An example of illustration used to communicate information is the assembly instructions created by furniture retailer IKEA. If IKEA used text instead of illustrations in their instructions, people would find it harder to assemble the furniture.
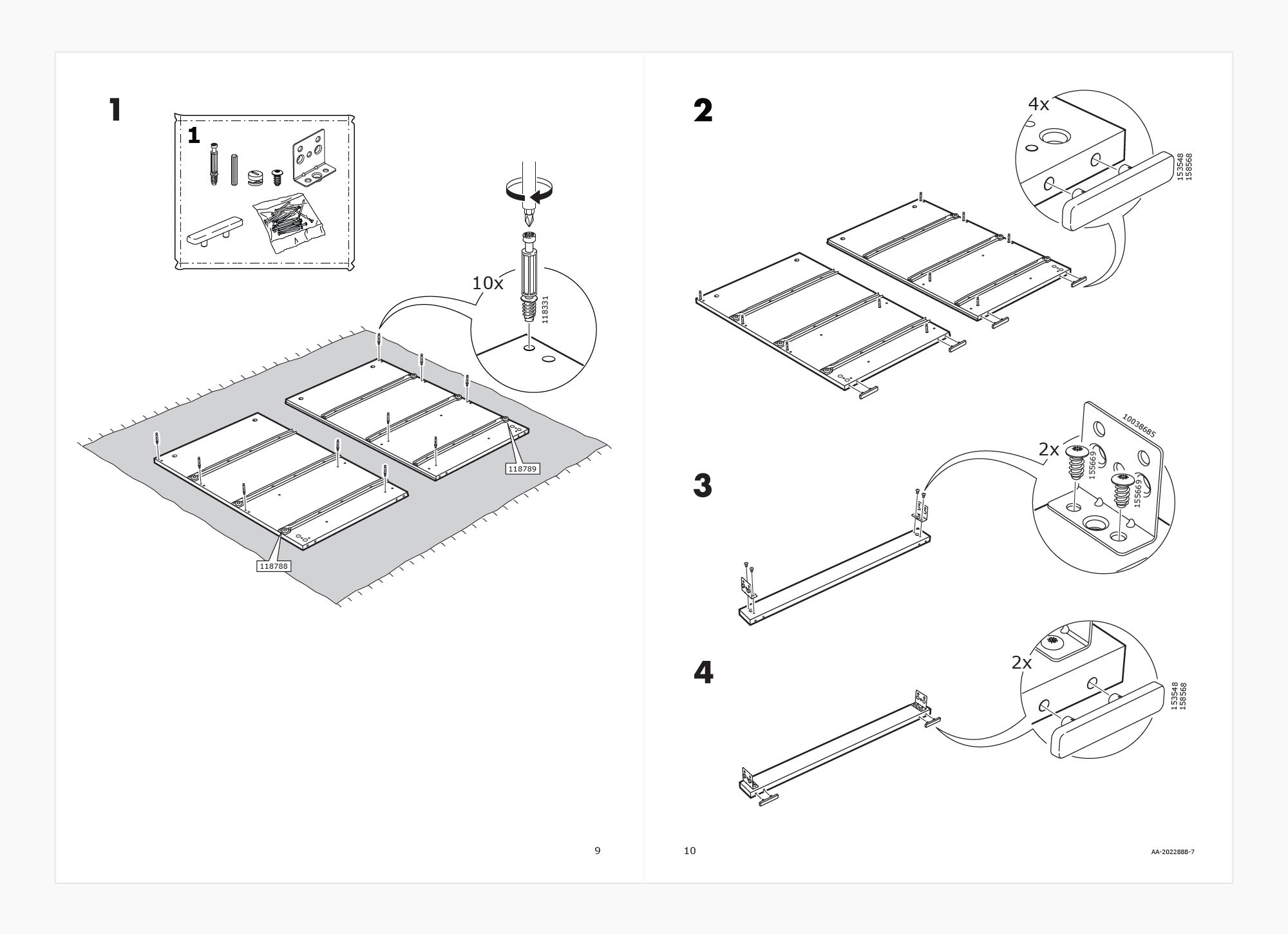
IKEA assembly instructions use illustrations to inform customers how to build their furniture. The only text used is numeric to denote step and part numbers. IKEA communicates this information visually to: 1. Enable simple communication, 2. Ensure their instructions are easy to follow, regardless of the customer’s language.
© IKEA, Fair use
Illustrations and drawings can often convey the core message of a visual representation more effectively than a photograph. They focus on the core message , while a photograph might distract a viewer with additional details (such as who this person is, where they are from, etc.)
For example, in IKEA’s case, photographing a person building a piece of furniture might be complicated. Further, photographs may not be easy to understand in a black-and-white print, leading to higher printing costs. To be useful, the pictures would also need to be larger and would occupy more space on a printed manual, further adding to the costs.
But imagine a girl winking—this is something we can easily photograph.
Ivan Sutherland, creator of the first graphical user interface, used his computer program Sketchpad to draw a winking girl. While not realistic, Sutherland's representation effectively portrays a winking girl. The drawing's abstract, generic elements contrast with the distinct winking eye. The graphical conventions of lines and shapes represent the eyes and mouth. The simplicity of the drawing does not draw attention away from the winking.
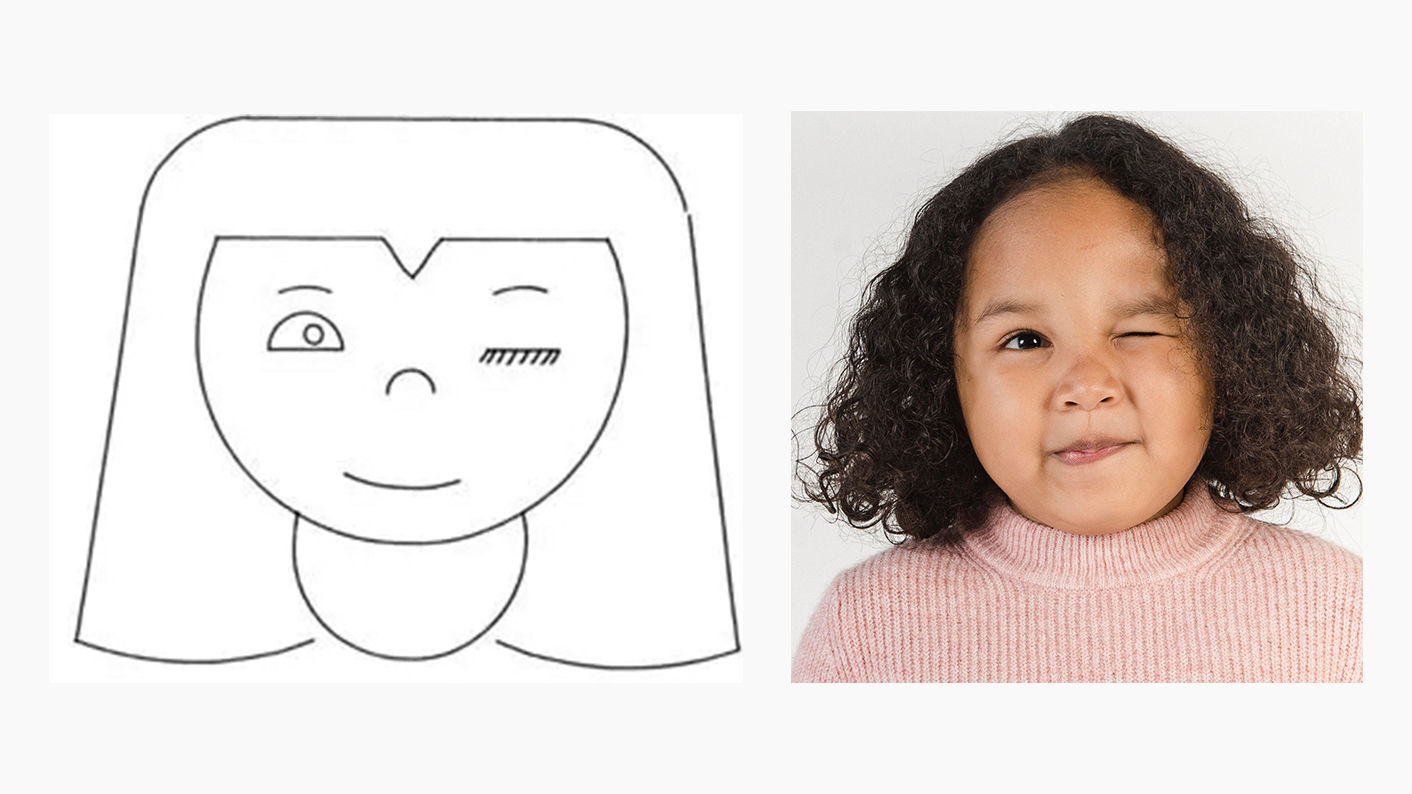
A photo might distract from the focused message compared to Sutherland's representation. In the photo, the other aspects of the image (i.e., the particular person) distract the viewer from this message.
© Ivan Sutherland, CC BY-SA 3.0 and Amina Filkins, Pexels License
Information and Data Visualization
Designers and other stakeholders use data and information visualization across many industries.
Data visualization uses charts and graphs to show raw data in a graphic form. Information visualization goes further, including more context and complex data sets. Information visualization often uses interactive elements to share a deeper understanding.
For example, most computerized devices have a battery level indicator. This is a type of data visualization. IV takes this further by allowing you to click on the battery indicator for further insights. These insights may include the apps that use the most battery and the last time you charged your device.
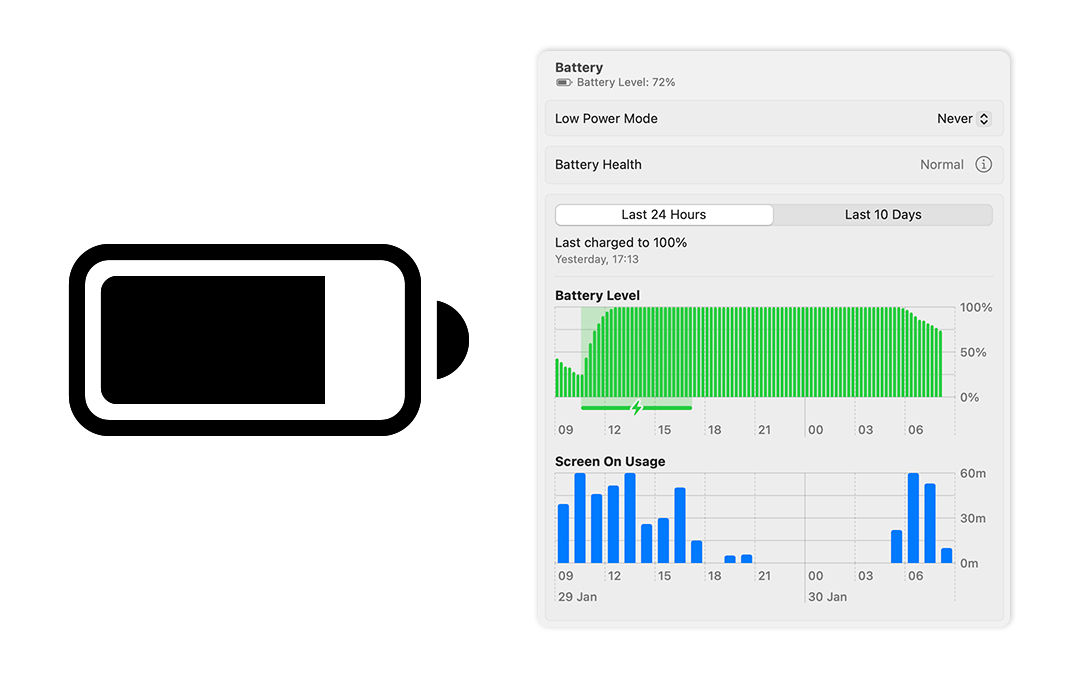
macOS displays a battery icon in the menu bar that visualizes your device’s battery level. This is an example of data visualization. Meanwhile, macOS’s settings tell you battery level over time, screen-on-usage and when you last charged your device. These insights are actionable; users may notice their battery drains at a specific time. This is an example of information visualization.
© Low Battery by Jemis Mali, CC BY-NC-ND 4.0, and Apple, Fair use
Information visualization is not exclusive to numeric data. It encompasses representations like diagrams and maps. For example, Google Maps collates various types of data and information into one interface:
Data Representation: Google Maps transforms complex geographical data into an easily understandable and navigable visual map.
Interactivity: Users can interactively customize views that show traffic, satellite imagery and more in real-time.
Layered Information: Google Maps layers multiple data types (e.g., traffic, weather) over geographical maps for comprehensive visualization.
User-Centered Design : The interface is intuitive and user-friendly, with symbols and colors for straightforward data interpretation.
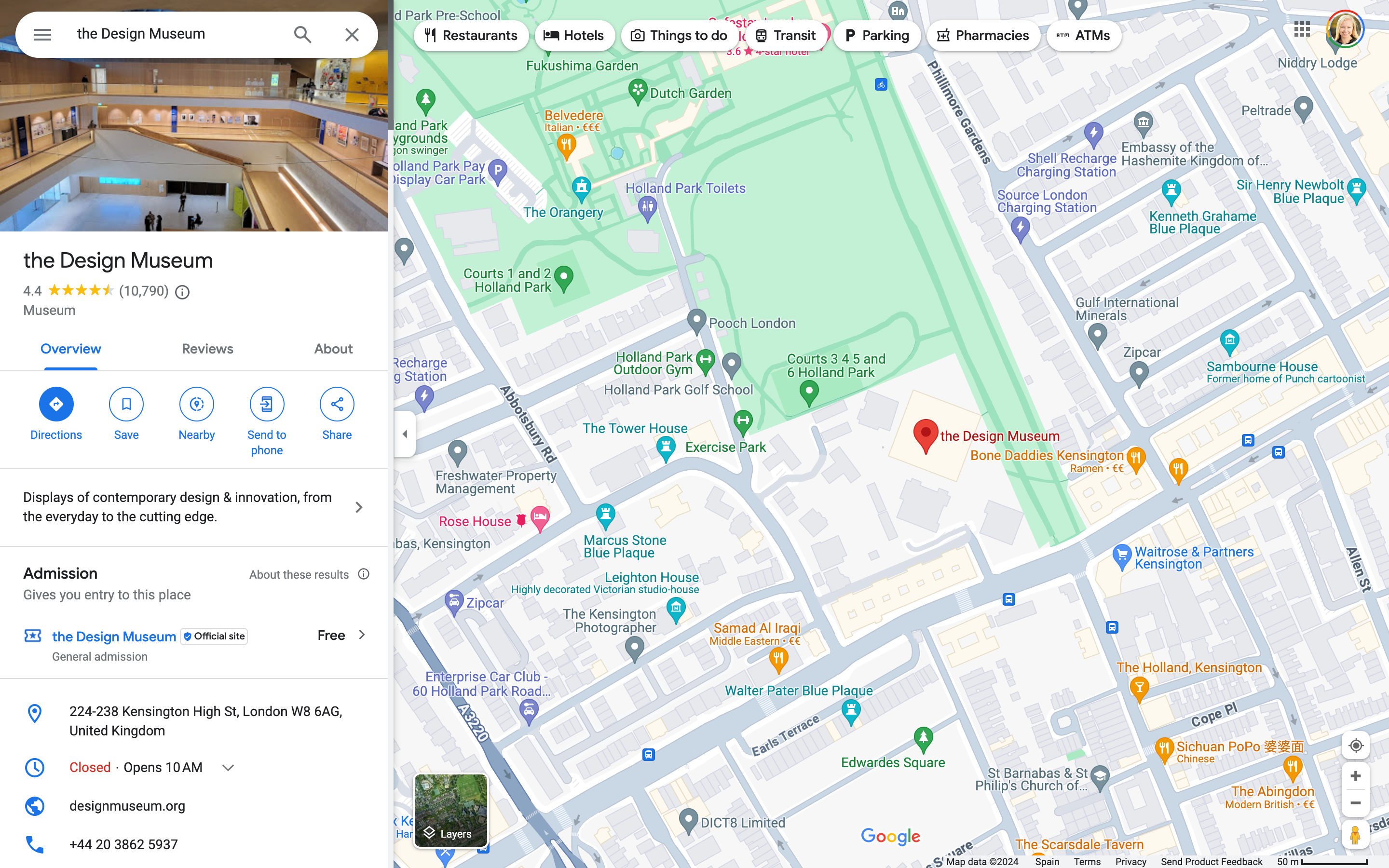
The volume of data contained in one screenshot of Google Maps is massive. However, this information is presented clearly to the user. Google Maps highlights different terrains with colors and local places and businesses with icons and colors. The panel on the left lists the selected location’s profile, which includes an image, rating and contact information.
© Google, Fair use
Symbolic Correspondence
Symbolic correspondence uses universally recognized symbols and signs to convey specific meanings . This method employs widely recognized visual cues for immediate understanding. Symbolic correspondence removes the need for textual explanation.
For instance, a magnifying glass icon in UI design signifies the search function. Similarly, in environmental design, symbols for restrooms, parking and amenities guide visitors effectively.
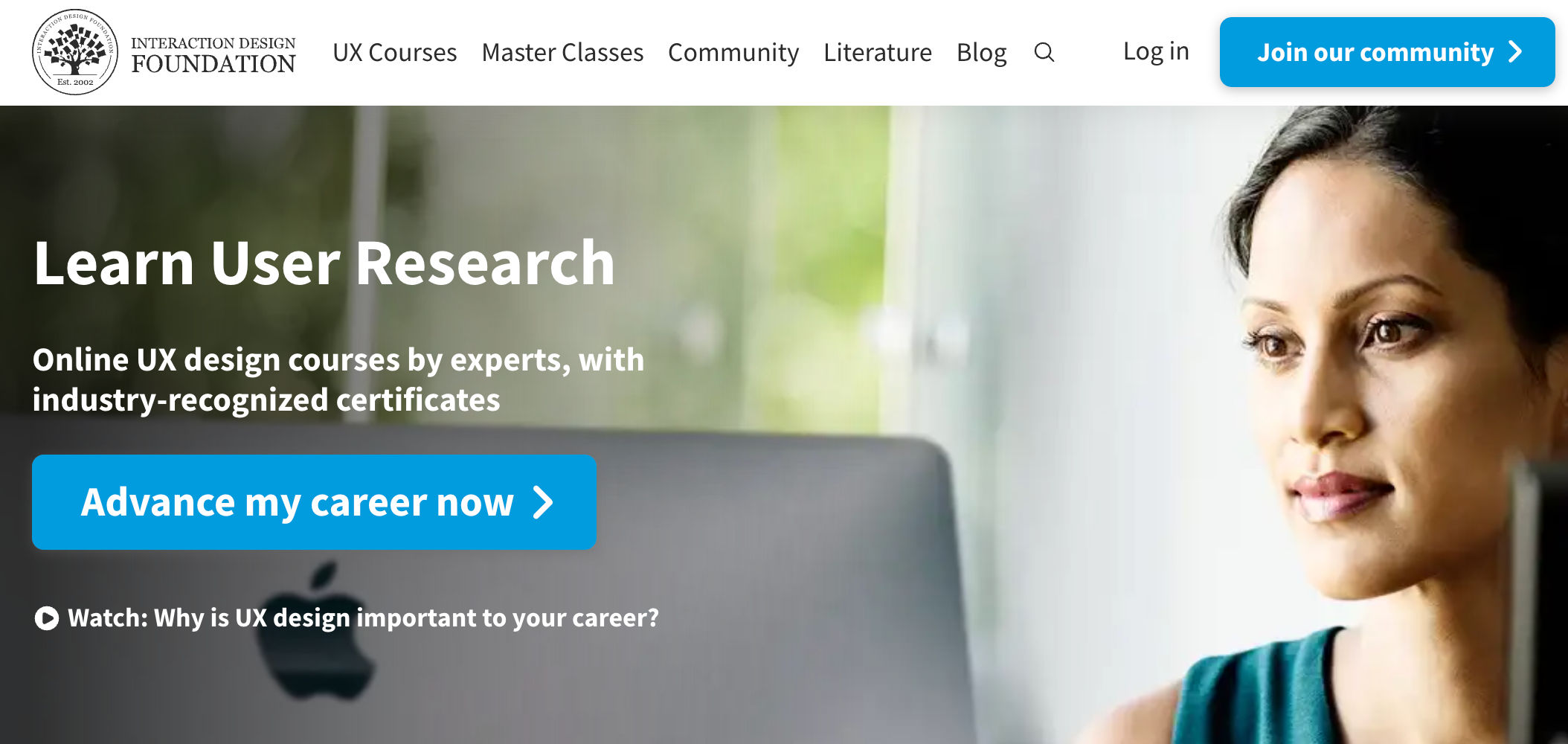
The Interaction Design Foundation (IxDF) website uses the universal magnifying glass symbol to signify the search function. Similarly, the play icon draws attention to a link to watch a video.
How Designers Create Visual Representations
Visual language.
Designers use elements like color , shape and texture to create a communicative visual experience. Designers use these 8 principles:
Size – Larger elements tend to capture users' attention readily.
Color – Users are typically drawn to bright colors over muted shades.
Contrast – Colors with stark contrasts catch the eye more effectively.
Alignment – Unaligned elements are more noticeable than those aligned ones.
Repetition – Similar styles repeated imply a relationship in content.
Proximity – Elements placed near each other appear to be connected.
Whitespace – Elements surrounded by ample space attract the eye.
Texture and Style – Users often notice richer textures before flat designs.
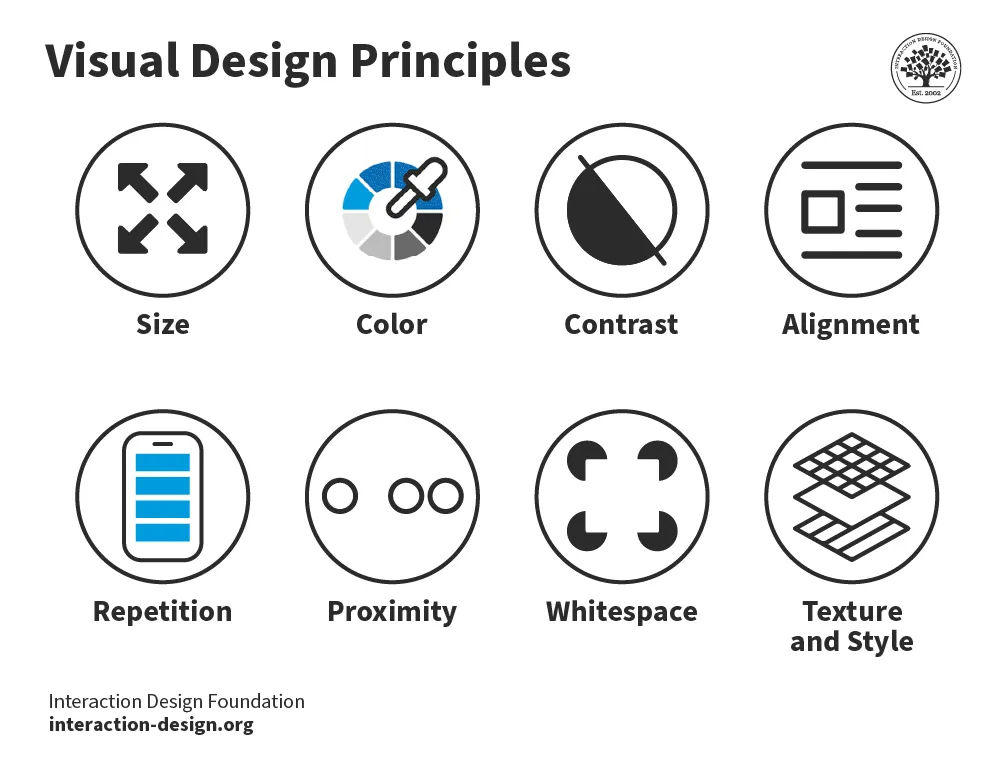
The 8 visual design principles.
In web design , visual hierarchy uses color and repetition to direct the user's attention. Color choice is crucial as it creates contrast between different elements. Repetition helps to organize the design—it uses recurring elements to establish consistency and familiarity.
In this video, Alan Dix, Professor and Expert in Human-Computer Interaction, explains how visual alignment affects how we read and absorb information:
Correspondence Techniques
Designers use correspondence techniques to align visual elements with their conceptual meanings. These techniques include color coding, spatial arrangement and specific imagery. In information visualization, different colors can represent various data sets. This correspondence aids users in quickly identifying trends and relationships .
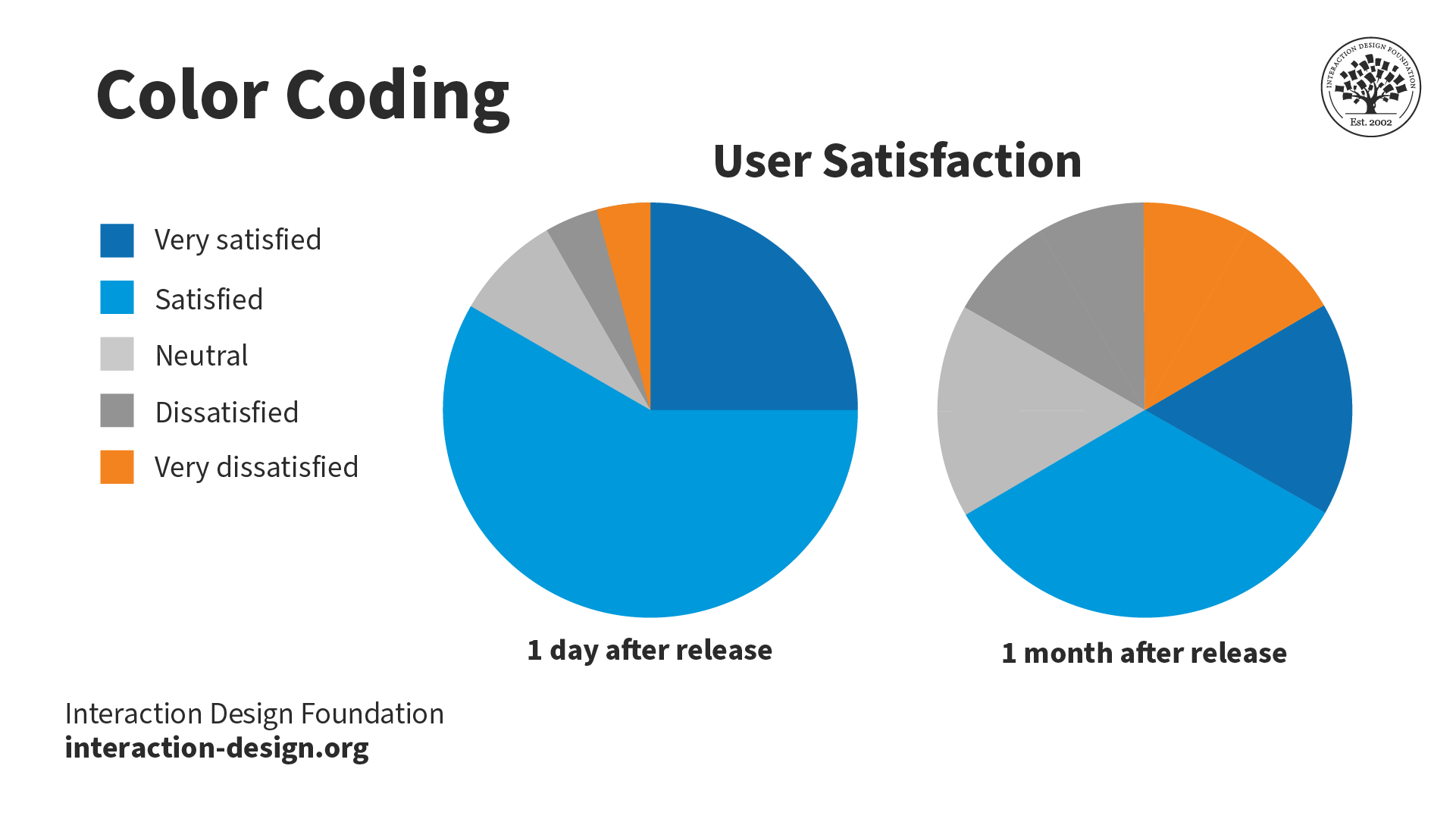
Color coding enables the stakeholder to see the relationship and trend between the two pie charts easily.
In user interface design, correspondence techniques link elements with meaning. An example is color-coding notifications to state their nature. For instance, red for warnings and green for confirmation. These techniques are informative and intuitive and enhance the user experience.
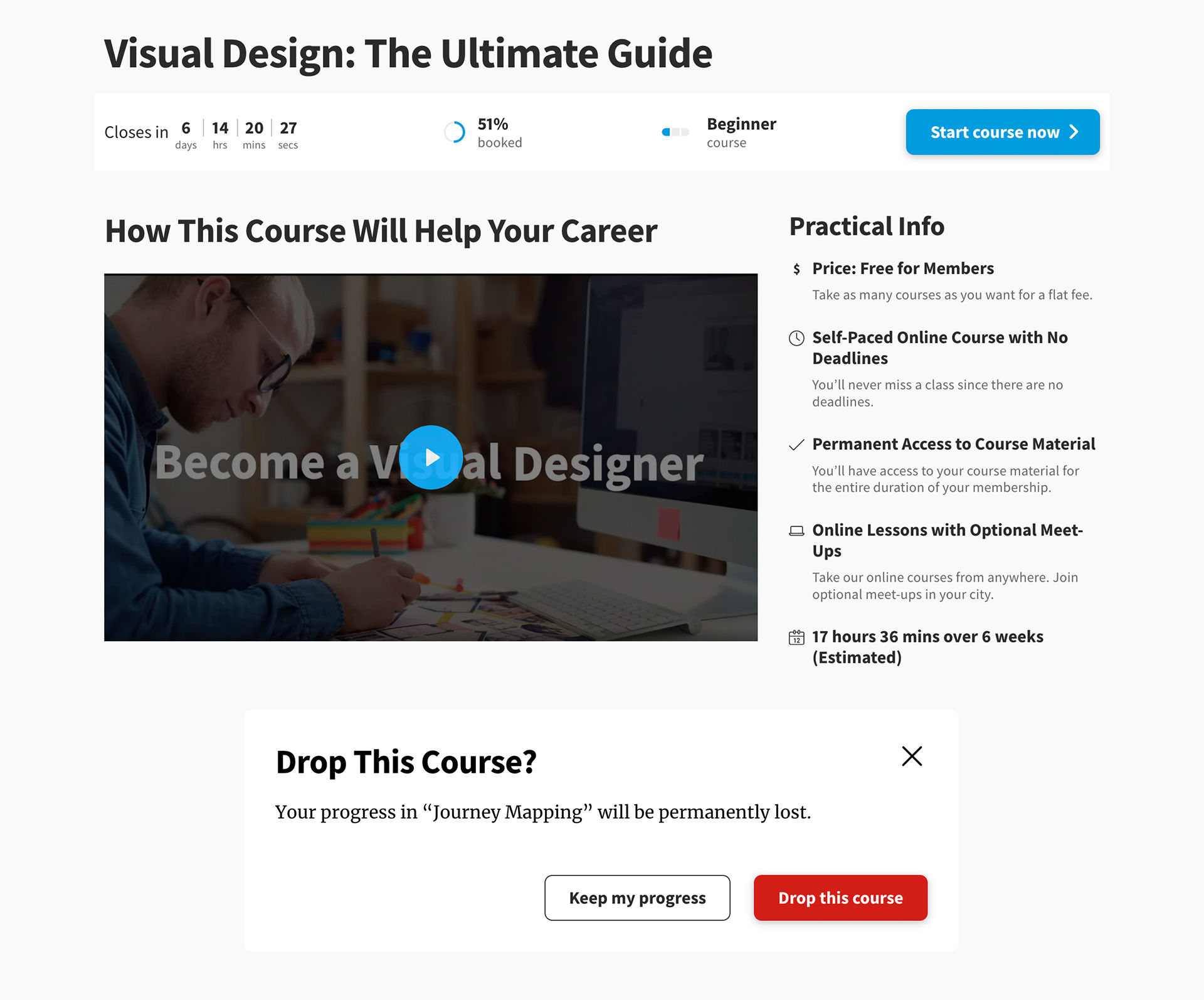
The IxDF website uses blue for call-to-actions (CTAs) and red for warnings. These colors inform the user of the nature of the action of buttons and other interactive elements.
Perception and Interpretation
If visual language is how designers create representations, then visual perception and interpretation are how users receive those representations. Consider a painting—the viewer’s eyes take in colors, shapes and lines, and the brain perceives these visual elements as a painting.
In this video, Alan Dix explains how the interplay of sensation, perception and culture is crucial to understanding visual experiences in design:
Copyright holder: Michael Murphy _ Appearance time: 07:19 - 07:37 _ Link: https://www.youtube.com/watch?v=C67JuZnBBDc
Visual perception principles are essential for creating compelling, engaging visual representations. For example, Gestalt principles explain how we perceive visual information. These rules describe how we group similar items, spot patterns and simplify complex images. Designers apply Gestalt principles to arrange content on websites and other interfaces. This application creates visually appealing and easily understood designs.
In this video, design expert and teacher Mia Cinelli discusses the significance of Gestalt principles in visual design . She introduces fundamental principles, like figure/ground relationships, similarity and proximity.
Interpretation
Everyone's experiences, culture and physical abilities dictate how they interpret visual representations. For this reason, designers carefully consider how users interpret their visual representations. They employ user research and testing to ensure their designs are attractive and functional.
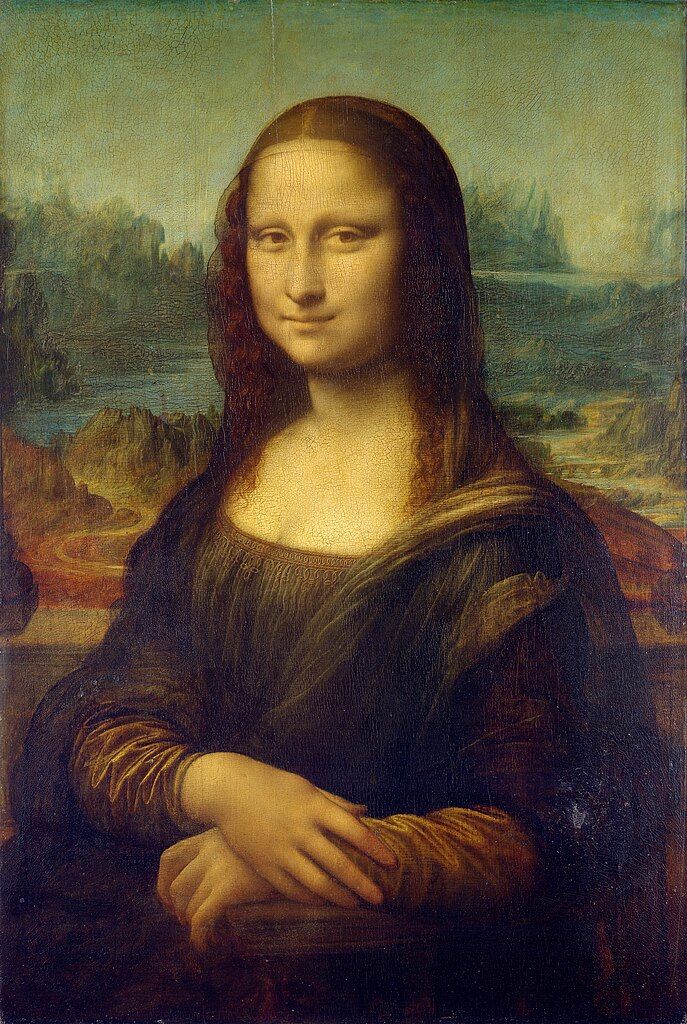
Leonardo da Vinci's "Mona Lisa", is one of the most famous paintings in the world. The piece is renowned for its subject's enigmatic expression. Some interpret her smile as content and serene, while others see it as sad or mischievous. Not everyone interprets this visual representation in the same way.
Color is an excellent example of how one person, compared to another, may interpret a visual element. Take the color red:
In Chinese culture, red symbolizes luck, while in some parts of Africa, it can mean death or illness.
A personal experience may mean a user has a negative or positive connotation with red.
People with protanopia and deuteranopia color blindness cannot distinguish between red and green.
In this video, Joann and Arielle Eckstut, leading color consultants and authors, explain how many factors influence how we perceive and interpret color:
Learn More about Visual Representation
Read Alan Blackwell’s chapter on visual representation from The Encyclopedia of Human-Computer Interaction.
Learn about the F-Shaped Pattern For Reading Web Content from Jakob Nielsen.
Read Smashing Magazine’s article, Visual Design Language: The Building Blocks Of Design .
Take the IxDF’s course, Perception and Memory in HCI and UX .
Questions related to Visual Representation
Some highly cited research on visual representation and related topics includes:
Roland, P. E., & Gulyás, B. (1994). Visual imagery and visual representation. Trends in Neurosciences, 17(7), 281-287. Roland and Gulyás' study explores how the brain creates visual imagination. They look at whether imagining things like objects and scenes uses the same parts of the brain as seeing them does. Their research shows the brain uses certain areas specifically for imagination. These areas are different from the areas used for seeing. This research is essential for understanding how our brain works with vision.
Lurie, N. H., & Mason, C. H. (2007). Visual Representation: Implications for Decision Making. Journal of Marketing, 71(1), 160-177.
This article looks at how visualization tools help in understanding complicated marketing data. It discusses how these tools affect decision-making in marketing. The article gives a detailed method to assess the impact of visuals on the study and combination of vast quantities of marketing data. It explores the benefits and possible biases visuals can bring to marketing choices. These factors make the article an essential resource for researchers and marketing experts. The article suggests using visual tools and detailed analysis together for the best results.
Lohse, G. L., Biolsi, K., Walker, N., & Rueter, H. H. (1994, December). A classification of visual representations. Communications of the ACM, 37(12), 36+.
This publication looks at how visuals help communicate and make information easier to understand. It divides these visuals into six types: graphs, tables, maps, diagrams, networks and icons. The article also looks at different ways these visuals share information effectively.
If you’d like to cite content from the IxDF website , click the ‘cite this article’ button near the top of your screen.
Some recommended books on visual representation and related topics include:
Chaplin, E. (1994). Sociology and Visual Representation (1st ed.) . Routledge.
Chaplin's book describes how visual art analysis has changed from ancient times to today. It shows how photography, post-modernism and feminism have changed how we see art. The book combines words and images in its analysis and looks into real-life social sciences studies.
Mitchell, W. J. T. (1994). Picture Theory. The University of Chicago Press.
Mitchell's book explores the important role and meaning of pictures in the late twentieth century. It discusses the change from focusing on language to focusing on images in cultural studies. The book deeply examines the interaction between images and text in different cultural forms like literature, art and media. This detailed study of how we see and read visual representations has become an essential reference for scholars and professionals.
Koffka, K. (1935). Principles of Gestalt Psychology. Harcourt, Brace & World.
"Principles of Gestalt Psychology" by Koffka, released in 1935, is a critical book in its field. It's known as a foundational work in Gestalt psychology, laying out the basic ideas of the theory and how they apply to how we see and think. Koffka's thorough study of Gestalt psychology's principles has profoundly influenced how we understand human perception. This book has been a significant reference in later research and writings.
A visual representation, like an infographic or chart, uses visual elements to show information or data. These types of visuals make complicated information easier to understand and more user-friendly.
Designers harness visual representations in design and communication. Infographics and charts, for instance, distill data for easier audience comprehension and retention.
For an introduction to designing basic information visualizations, take our course, Information Visualization .
Text is a crucial design and communication element, transforming language visually. Designers use font style, size, color and layout to convey emotions and messages effectively.
Designers utilize text for both literal communication and aesthetic enhancement. Their typography choices significantly impact design aesthetics, user experience and readability.
Designers should always consider text's visual impact in their designs. This consideration includes font choice, placement, color and interaction with other design elements.
In this video, design expert and teacher Mia Cinelli teaches how Gestalt principles apply to typography:
Designers use visual elements in projects to convey information, ideas, and messages. Designers use images, colors, shapes and typography for impactful designs.
In UI/UX design, visual representation is vital. Icons, buttons and colors provide contrast for intuitive, user-friendly website and app interfaces.
Graphic design leverages visual representation to create attention-grabbing marketing materials. Careful color, imagery and layout choices create an emotional connection.
Product design relies on visual representation for prototyping and idea presentation. Designers and stakeholders use visual representations to envision functional, aesthetically pleasing products.
Our brains process visuals 60,000 times faster than text. This fact highlights the crucial role of visual representation in design.
Our course, Visual Design: The Ultimate Guide , teaches you how to use visual design elements and principles in your work effectively.
Visual representation, crucial in UX, facilitates interaction, comprehension and emotion. It combines elements like images and typography for better interfaces.
Effective visuals guide users, highlight features and improve navigation. Icons and color schemes communicate functions and set interaction tones.
UX design research shows visual elements significantly impact emotions. 90% of brain-transmitted information is visual.
To create functional, accessible visuals, designers use color contrast and consistent iconography. These elements improve readability and inclusivity.
An excellent example of visual representation in UX is Apple's iOS interface. iOS combines a clean, minimalist design with intuitive navigation. As a result, the operating system is both visually appealing and user-friendly.
Michal Malewicz, Creative Director and CEO at Hype4, explains why visual skills are important in design:
Learn more about UI design from Michal in our Master Class, Beyond Interfaces: The UI Design Skills You Need to Know .
The fundamental principles of effective visual representation are:
Clarity : Designers convey messages clearly, avoiding clutter.
Simplicity : Embrace simple designs for ease and recall.
Emphasis : Designers highlight key elements distinctively.
Balance : Balance ensures design stability and structure.
Alignment : Designers enhance coherence through alignment.
Contrast : Use contrast for dynamic, distinct designs.
Repetition : Repeating elements unify and guide designs.
Designers practice these principles in their projects. They also analyze successful designs and seek feedback to improve their skills.
Read our topic description of Gestalt principles to learn more about creating effective visual designs. The Gestalt principles explain how humans group elements, recognize patterns and simplify object perception.
Color theory is vital in design, helping designers craft visually appealing and compelling works. Designers understand color interactions, psychological impacts and symbolism. These elements help designers enhance communication and guide attention.
Designers use complementary , analogous and triadic colors for contrast, harmony and balance. Understanding color temperature also plays a crucial role in design perception.
Color symbolism is crucial, as different colors can represent specific emotions and messages. For instance, blue can symbolize trust and calmness, while red can indicate energy and urgency.
Cultural variations significantly influence color perception and symbolism. Designers consider these differences to ensure their designs resonate with diverse audiences.
For actionable insights, designers should:
Experiment with color schemes for effective messaging.
Assess colors' psychological impact on the audience.
Use color contrast to highlight critical elements.
Ensure color choices are accessible to all.
In this video, Joann and Arielle Eckstut, leading color consultants and authors, give their six tips for choosing color:
Learn more about color from Joann and Arielle in our Master Class, How To Use Color Theory To Enhance Your Designs .
Typography and font choice are crucial in design, impacting readability and mood. Designers utilize them for effective communication and expression.
Designers' perception of information varies with font type. Serif fonts can imply formality, while sans-serifs can give a more modern look.
Typography choices by designers influence readability and user experience. Well-spaced, distinct fonts enhance readability, whereas decorative fonts may hinder it.
Designers use typography to evoke emotions and set a design's tone. Choices in font size, style and color affect the emotional impact and message clarity.
Designers use typography to direct attention, create hierarchy and establish rhythm. These benefits help with brand recognition and consistency across mediums.
Read our article to learn how web fonts are critical to the online user experience .
Designers create a balance between simplicity and complexity in their work. They focus on the main messages and highlight important parts. Designers use the principles of visual hierarchy, like size, color and spacing. They also use empty space to make their designs clear and understandable.
The Gestalt law of Prägnanz suggests people naturally simplify complex images. This principle aids in making even intricate information accessible and engaging.
Through iteration and feedback, designers refine visuals. They remove extraneous elements and highlight vital information. Testing with the target audience ensures the design resonates and is comprehensible.
Michal Malewicz explains how to master hierarchy in UI design using the Gestalt rule of proximity:
Literature on Visual Representation
Here’s the entire UX literature on Visual Representation by the Interaction Design Foundation, collated in one place:
Learn more about Visual Representation
Take a deep dive into Visual Representation with our course Perception and Memory in HCI and UX .
How does all of this fit with interaction design and user experience? The simple answer is that most of our understanding of human experience comes from our own experiences and just being ourselves. That might extend to people like us, but it gives us no real grasp of the whole range of human experience and abilities. By considering more closely how humans perceive and interact with our world, we can gain real insights into what designs will work for a broader audience: those younger or older than us, more or less capable, more or less skilled and so on.
“You can design for all the people some of the time, and some of the people all the time, but you cannot design for all the people all the time.“ – William Hudson (with apologies to Abraham Lincoln)
While “design for all of the people all of the time” is an impossible goal, understanding how the human machine operates is essential to getting ever closer. And of course, building solutions for people with a wide range of abilities, including those with accessibility issues, involves knowing how and why some human faculties fail. As our course tutor, Professor Alan Dix, points out, this is not only a moral duty but, in most countries, also a legal obligation.
Portfolio Project
In the “ Build Your Portfolio: Perception and Memory Project ”, you’ll find a series of practical exercises that will give you first-hand experience in applying what we’ll cover. If you want to complete these optional exercises, you’ll create a series of case studies for your portfolio which you can show your future employer or freelance customers.
This in-depth, video-based course is created with the amazing Alan Dix , the co-author of the internationally best-selling textbook Human-Computer Interaction and a superstar in the field of Human-Computer Interaction . Alan is currently a professor and Director of the Computational Foundry at Swansea University.
Gain an Industry-Recognized UX Course Certificate
Use your industry-recognized Course Certificate on your resume , CV , LinkedIn profile or your website.
All open-source articles on Visual Representation
Data visualization for human perception.
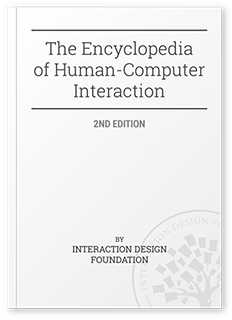
The Key Elements & Principles of Visual Design
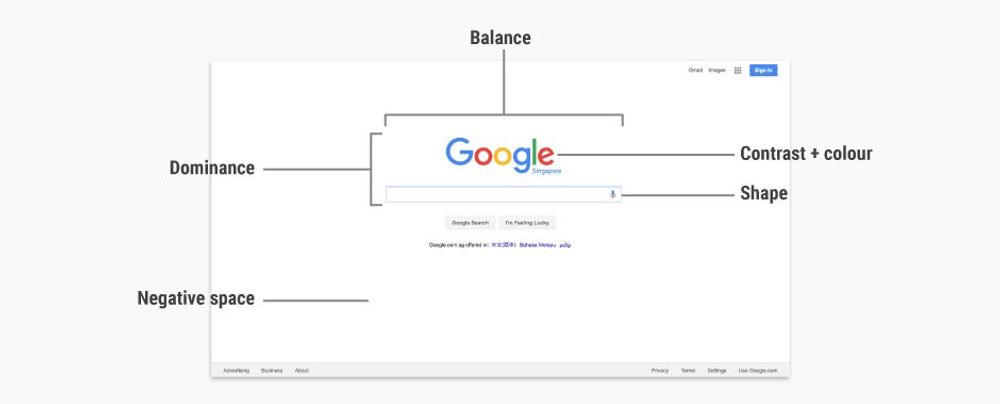
- 1.1k shares
Guidelines for Good Visual Information Representations
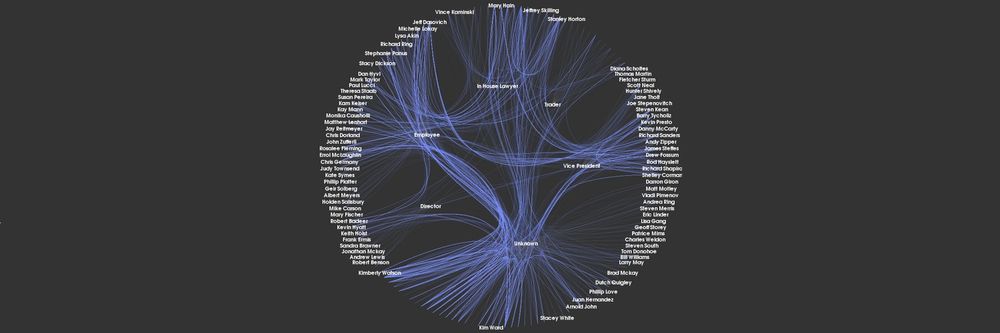
- 4 years ago
Philosophy of Interaction
Information visualization – an introduction to multivariate analysis.
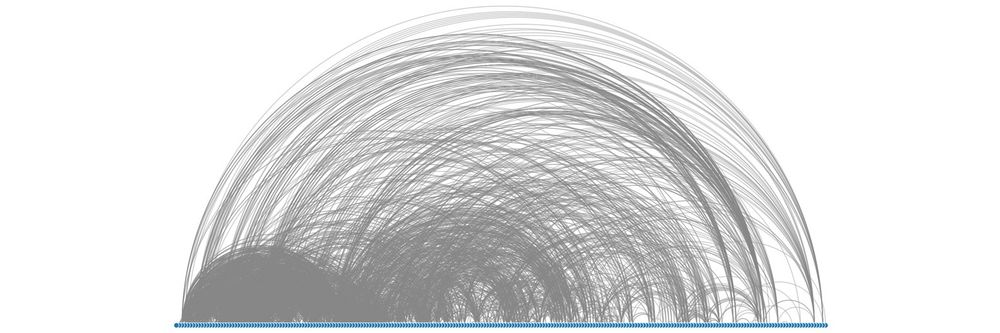
- 8 years ago
Aesthetic Computing
How to represent linear data visually for information visualization.
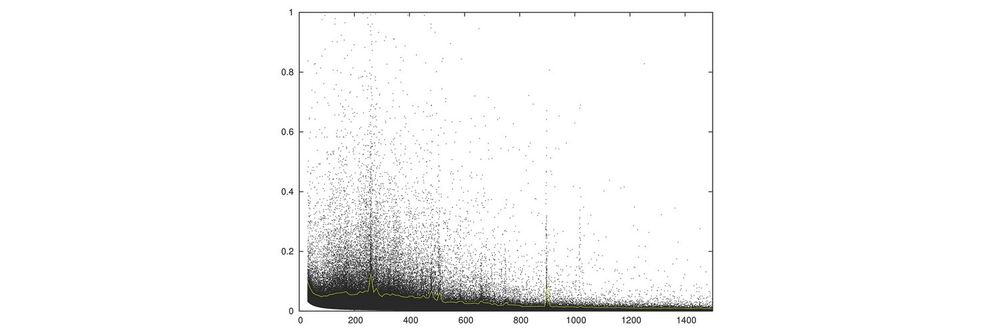
- 5 years ago
Open Access—Link to us!
We believe in Open Access and the democratization of knowledge . Unfortunately, world-class educational materials such as this page are normally hidden behind paywalls or in expensive textbooks.
If you want this to change , cite this page , link to us, or join us to help us democratize design knowledge !
Privacy Settings
Our digital services use necessary tracking technologies, including third-party cookies, for security, functionality, and to uphold user rights. Optional cookies offer enhanced features, and analytics.
Experience the full potential of our site that remembers your preferences and supports secure sign-in.
Governs the storage of data necessary for maintaining website security, user authentication, and fraud prevention mechanisms.
Enhanced Functionality
Saves your settings and preferences, like your location, for a more personalized experience.
Referral Program
We use cookies to enable our referral program, giving you and your friends discounts.
Error Reporting
We share user ID with Bugsnag and NewRelic to help us track errors and fix issues.
Optimize your experience by allowing us to monitor site usage. You’ll enjoy a smoother, more personalized journey without compromising your privacy.
Analytics Storage
Collects anonymous data on how you navigate and interact, helping us make informed improvements.
Differentiates real visitors from automated bots, ensuring accurate usage data and improving your website experience.
Lets us tailor your digital ads to match your interests, making them more relevant and useful to you.
Advertising Storage
Stores information for better-targeted advertising, enhancing your online ad experience.
Personalization Storage
Permits storing data to personalize content and ads across Google services based on user behavior, enhancing overall user experience.
Advertising Personalization
Allows for content and ad personalization across Google services based on user behavior. This consent enhances user experiences.
Enables personalizing ads based on user data and interactions, allowing for more relevant advertising experiences across Google services.
Receive more relevant advertisements by sharing your interests and behavior with our trusted advertising partners.
Enables better ad targeting and measurement on Meta platforms, making ads you see more relevant.
Allows for improved ad effectiveness and measurement through Meta’s Conversions API, ensuring privacy-compliant data sharing.
LinkedIn Insights
Tracks conversions, retargeting, and web analytics for LinkedIn ad campaigns, enhancing ad relevance and performance.
LinkedIn CAPI
Enhances LinkedIn advertising through server-side event tracking, offering more accurate measurement and personalization.
Google Ads Tag
Tracks ad performance and user engagement, helping deliver ads that are most useful to you.
Share Knowledge, Get Respect!
or copy link
Cite according to academic standards
Simply copy and paste the text below into your bibliographic reference list, onto your blog, or anywhere else. You can also just hyperlink to this page.
New to UX Design? We’re Giving You a Free ebook!
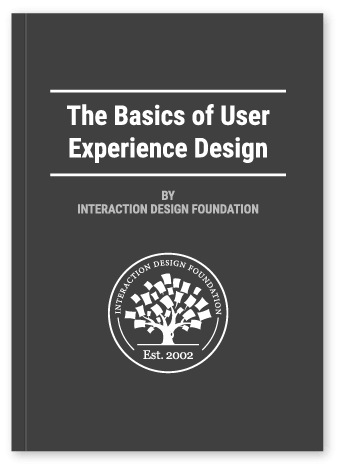
Download our free ebook The Basics of User Experience Design to learn about core concepts of UX design.
In 9 chapters, we’ll cover: conducting user interviews, design thinking, interaction design, mobile UX design, usability, UX research, and many more!

How to Have Fiter in Power BI Affect Multiple Visualizations
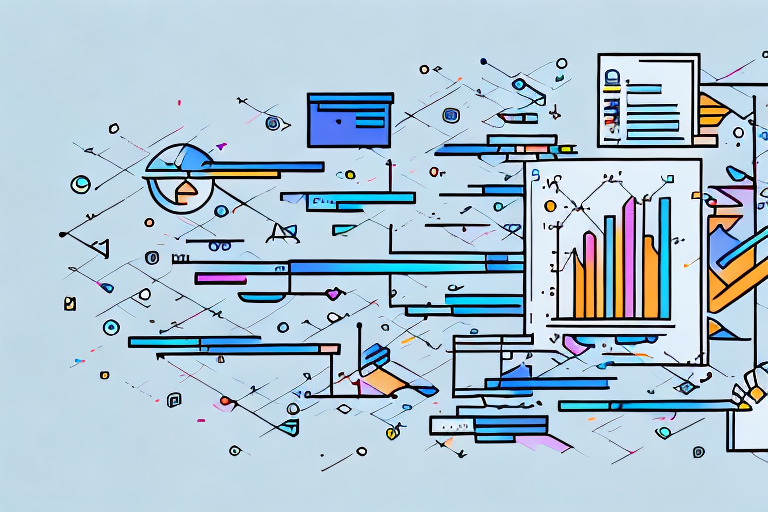
When it comes to data analysis and visualization, Power BI is an incredibly powerful tool that has become the go-to software for many businesses. With its user-friendly interface and extensive range of features, Power BI simplifies the process of creating interactive and informative reports, dashboards, and visualizations. Filtering in Power BI is one of the most important features for data analysis and visualization. Filters allow for isolating subsets of data, which is an essential aspect of reporting.
Table of Contents
Understanding the Basics of Filtering in Power BI
Filters in Power BI work by allowing you to select specific values or data points that you would like to see in your visualizations. Applying filters lets you limit the data in your report to only what is relevant, which can help you draw a more informed conclusion. A filter can be applied to individual visualizations or to an entire page, and it allows users to adjust what data is being analyzed.
It is important to note that filters in Power BI can be applied in various ways, including through slicers, visualizations, and even through the use of natural language queries. Slicers are a type of filter that allows users to interactively filter data by selecting values from a list. Visualizations, on the other hand, allow users to filter data by clicking on specific data points within the visualization. Natural language queries allow users to filter data by typing in a question or statement in plain language, and Power BI will automatically generate a visualization based on the query.
The Importance of Filtering in Power BI
Filtering in Power BI is crucial in making sense of vast amounts of data that would otherwise be too difficult to analyze. By reducing the amount of data visible in a visualization, you can quickly identify trends, patterns, and outliers. Filtering is an efficient way to break down large sets of data into more manageable subsets, which, in turn, can aid in making better business decisions.
Another benefit of filtering in Power BI is that it allows for more personalized and targeted analysis. With the ability to filter by specific criteria, such as time periods, regions, or product categories, you can tailor your analysis to focus on the areas that are most relevant to your business. This can lead to more accurate insights and a better understanding of your data. Additionally, filtering can help to improve the performance of your visualizations by reducing the amount of data that needs to be processed and displayed. Overall, filtering is a powerful tool in Power BI that can greatly enhance your data analysis capabilities.
Exploring Different Types of Filters in Power BI
Power BI has various kinds of filters, each with unique capabilities, depending on your data needs. Some of the most commonly used filters include the basic filters, the advanced filters, and the drill-through filters. Basic filters are the simplest form of filtering in Power BI, which allow you to select a single value or a range of values. Advanced filters provide more complex filtering options, such as filtering based on a condition or a custom formula, and drill-through filters allow you to move from one report to another using the power of filters.
Another type of filter in Power BI is the relative date filter, which allows you to filter data based on a relative time period, such as the last 7 days or the next 30 days. This is useful when you want to analyze data over a specific time frame, without having to manually update the filter every time.
Additionally, Power BI also offers the ability to create custom filters using DAX expressions. This allows you to create complex filters based on multiple conditions and calculations, giving you more control over your data analysis. With the flexibility of Power BI’s filtering options, you can easily customize your reports to meet your specific business needs.
How to Create a Filter in Power BI
Filters in Power BI can be created in several different ways. One of the most straightforward approaches is using the filters pane. To create a filter using the filter pane, select the visualization that you want to filter, and navigate to the filters pane. From there, choose your preferred filter type and set the filter criteria by selecting a column from the available options and setting the relevant values. Another way to create a filter is by using the visual level filtering option, which allows you to apply filters directly to a visualization, regardless of other filters in the report.
You can also create a filter in Power BI by using the advanced filtering option. This option allows you to create complex filters by combining multiple conditions using logical operators such as AND and OR. To use advanced filtering, select the visualization that you want to filter, and navigate to the filters pane. From there, choose the advanced filtering option and set the filter criteria by selecting the relevant columns and setting the conditions using logical operators. This option is particularly useful when you need to create filters that are not possible using the basic filtering options.
The Advantages of Applying Filters to Multiple Visualizations in Power BI
The significant advantage of applying filters to multiple visualizations is that it allows for a more comprehensive and coordinated view of your data. Instead of having to create separate filters for each visualization, you can apply one filter to several visuals simultaneously. This feature saves time and ensures consistency across your reports. It also makes it easier to compare data across different visualizations by having them all filter on the same criterion.
Another advantage of applying filters to multiple visualizations in Power BI is that it enables you to create interactive dashboards. By using filters, you can allow your users to interact with the data and customize their view of the dashboard. This can lead to more engagement and better decision-making. Additionally, filters can be used to highlight specific data points or trends, making it easier for users to identify key insights. Overall, applying filters to multiple visualizations in Power BI is a powerful tool for creating dynamic and interactive reports.
How to Apply a Filter to Multiple Visualizations Simultaneously in Power BI
To apply a filter to multiple visualizations simultaneously in Power BI, you can use the ‘sync slicers’ feature. This feature allows you to synchronize slicers across multiple pages or visuals within the same page. To use sync slicers, navigate to the ‘View’ tab and then to the ‘Sync slicers’ pane. From there, you can select which slicers you want to synchronize and which pages or visuals they should affect. This ensures that when a user interacts with one slicer, it will apply the same filter to all linked visualizations, providing a unified filtering experience across your report.
Tips and Tricks for Efficiently Using Filters in Power BI
Here are some tips to make using filters in Power BI more efficient: Firstly, create a custom hierarchy so that you can filter by multiple levels simultaneously. Secondly, use the drill-through option to focus on specific aspects of your data. Thirdly, create a search box for your filters to ensure an even more efficient search of set data. Lastly, organize your filters into a hierarchy that is intuitive and easy to understand.
Common Mistakes to Avoid When Using Filters in Power BI
One common mistake that new users make when filtering in Power BI is filtering too much data. The goal of filtering is to narrow down data to a single group for analysis, however, narrowing down too much data at too low a level can lead to data isolation, which misses a complete picture. Therefore, it’s better to filter on as high a level as possible to ensure a broader view without excessive loss of data.
Best Practices for Creating Effective Filters in Power BI
The following guidelines will help you to create effective filters in Power BI. Firstly, ensure that your filters contain relevant information specific to your data. Secondly, use the advanced filtering feature only when absolutely necessary. Thirdly, instead of using the same filter for multiple visuals, use individual filter settings for specific visuals. Lastly, keep your filters consistent across all the visuals.
Enhancing Your Data Analysis with Advanced Filtering Techniques in Power BI
Applying advanced filtering techniques can take your data visualization to a new level. Power BI allows you to tap into the Query Editor to utilize these techniques fully. Advanced filtering techniques include Data Modeling, advanced functions, and Query Parameters, among others. Through these features, you can leverage your data by unlocking hidden insights, discovering new correlations and responding proactively to any emerging trends.
How to Troubleshoot Common Issues with Filters in Power BI
When filtering in Power BI, you may encounter common issues such as unable to select values from the filter or failure to apply the filter to the visualization. In most cases, the problem may be caused by the filter data model or issues with the visualizations. To troubleshoot these problems, ensure that the filter model and visualizations are correctly formatted, clear your cache, close and reopen your report, or restart the application.
Improving Your Data Visualization with Dynamic and Interactive Filtering in Power BI
To improve your data visualization, you can enhance it with dynamic and interactive filtering. Dynamic and interactive filtering helps in a comprehensive view of your data analysis. By using dynamic and interactive filters, you can create a more interactive and user-friendly data analysis. Dynamic and interactive filtering can be achieved through the use of various Power BI features such as drill-down, drill-through, tooltips, among others.
Harnessing the Full Potential of Your Data with Advanced Filtering Features in Power BI
By leveraging the advanced filtering features available in Power BI, you can unlock the full potential of your data. Advanced filtering techniques help you get the most out of your data by allowing you to filter by unique values, custom formulas, and even apply calculations to filter data. These advanced filters enable you to delve deep into your data and uncover insights that were previously hidden.
Staying Ahead of the Game: Latest Trends and Developments in Filtering for Power BI
Power BI continues to evolve with new updates and features released regularly. However, as of the current knowledge cutoff in 2023, there is no feature known as “Smart Filter” that automatically creates filters based on detected patterns. Users must continue to apply filters manually or use existing features such as the AI insights to assist with filtering data. It’s important to stay updated with the official Power BI blog or documentation for the latest features and updates.
Overall, creating and applying filters are a crucial aspect of data visualization in Power BI. This feature allows businesses to isolate subsets of data and explore it more comprehensively, leading to better decisions and overall outcomes. Understanding the various types of filters, the best practices for creating, optimizing, and troubleshooting filters, and how they affect the visualizations in your reports is essential for effective data analysis.
By humans, for humans - Best rated articles:
Excel report templates: build better reports faster, top 9 power bi dashboard examples, excel waterfall charts: how to create one that doesn't suck, beyond ai - discover our handpicked bi resources.
Explore Zebra BI's expert-selected resources combining technology and insight for practical, in-depth BI strategies.
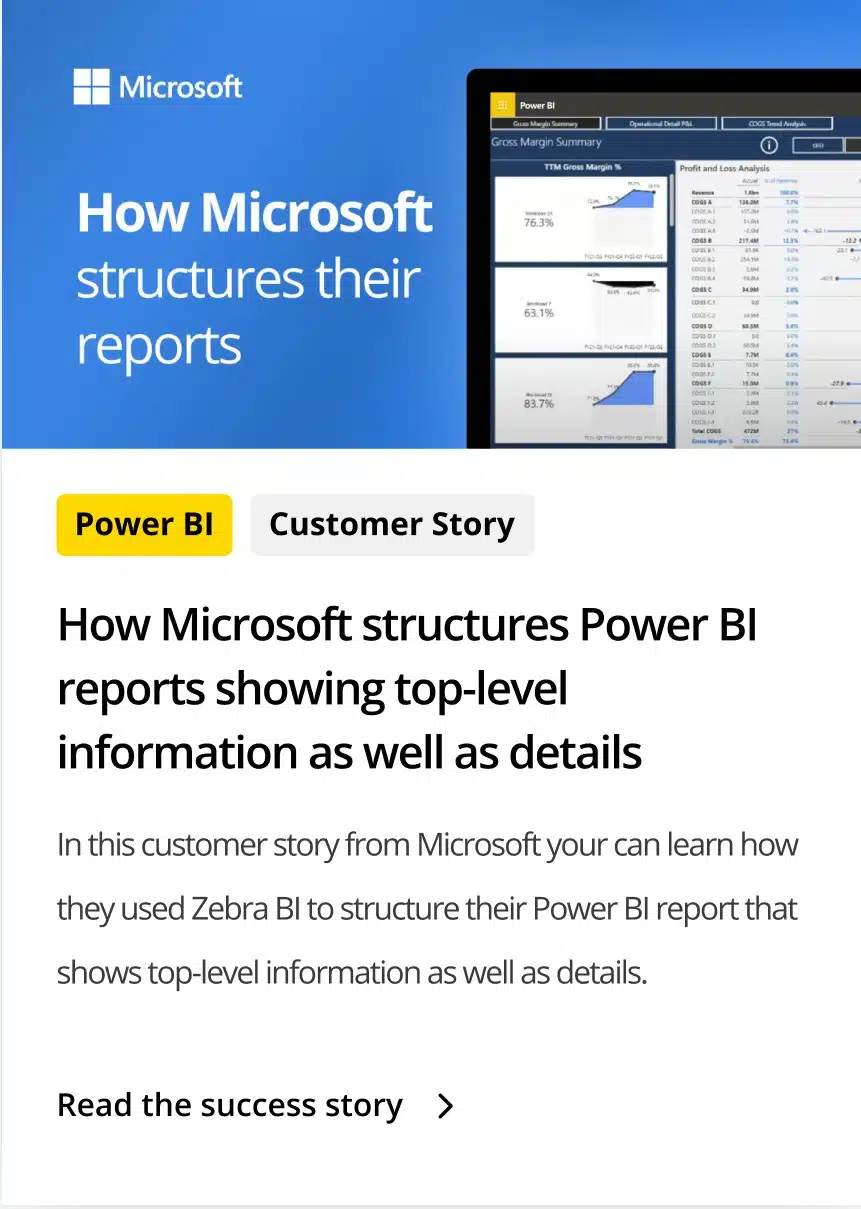
We’ve been experimenting with AI-generated content, and sometimes it gets carried away. Give us a feedback and help us learn and improve! 🤍
Note: This is an experimental AI-generated article. Your help is welcome. Share your feedback with us and help us improve.
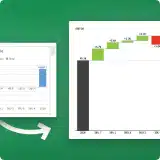

How to Use Advanced Pivot Table in Excel (25 Tips & Techniques)

PivotTable: Basic Things
A PivotTable is a powerful data analysis tool in Microsoft Excel . It allows users to quickly summarize, organize, and gain insights from large datasets. By transforming raw data into a more meaningful and compact format, PivotTables enable efficient analysis without the need for complex formulas or manual data manipulation. They are especially useful when dealing with extensive datasets, providing a user-friendly way to extract valuable information and identify trends, patterns, and outliers. If you’ve mastered the basics of PivotTables, exploring advanced techniques can further enhance your data analysis capabilities.
Basic Components of a PivotTable
- Data Source : The data source serves as the foundation for creating a PivotTable. It originates from the original dataset and should be well-organized, complete with column headers. These headers play a crucial role in defining the fields within the PivotTable.
- To access or hide the Field List, navigate to the “PivotTable Analyze” tab and select or deselect the corresponding option.
- Rows and Columns : In a PivotTable, you can arrange fields from the data source into the “Rows” and “Columns” areas. These selections determine how the data is organized and displayed in the final table.
- Values : The “Values” area contains numerical data that you want to summarize or analyze. You can apply various summary functions (such as sum, count, average, minimum, maximum, etc.) to perform calculations on this data.
- Filters : The “Filters” area allows you to add fields that act as filters. By selecting or deselecting filter options, you can dynamically update the results displayed in the PivotTable.
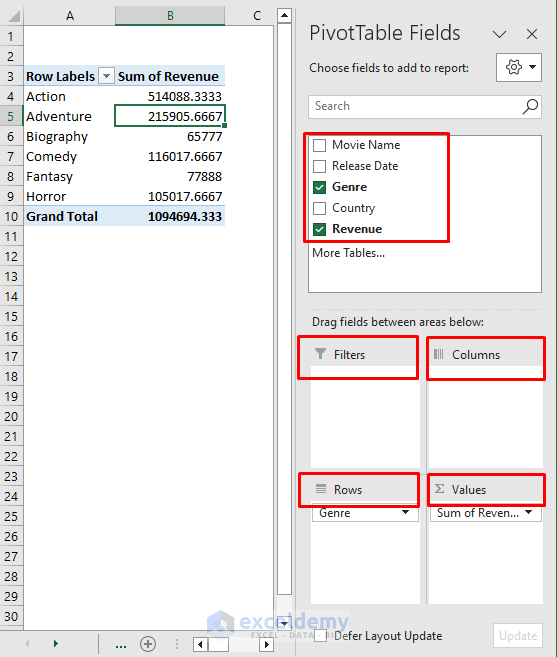
How to Create a Pivot Table in Excel
The below large dataset will be used to create a PivotTable.
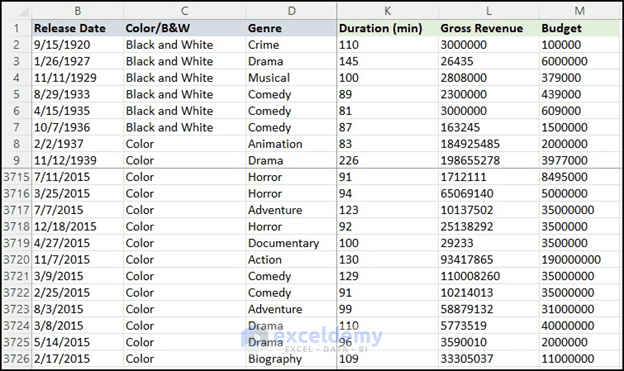
- Begin by opening your Excel workbook containing the dataset you want to analyze.
- Click on any cell within the dataset to ensure it’s selected.
- Navigate to the Insert tab in the Excel ribbon.
- Choose PivotTable and then click on From Table/Range .
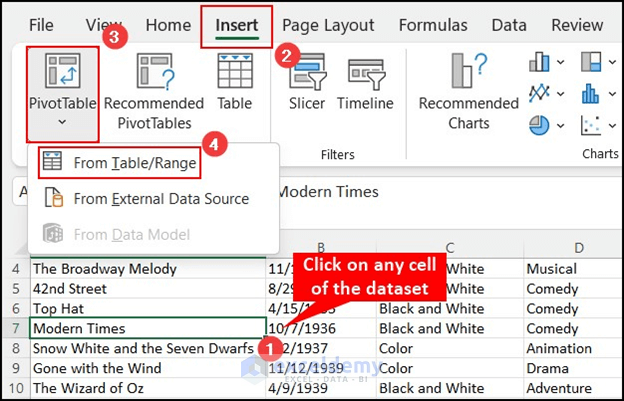
- The PivotTable from table or range dialog box will appear.
- The Table/Range field will automatically be set based on the cell you clicked earlier.
- If you want the PivotTable to appear in a new worksheet , select that option and click OK .
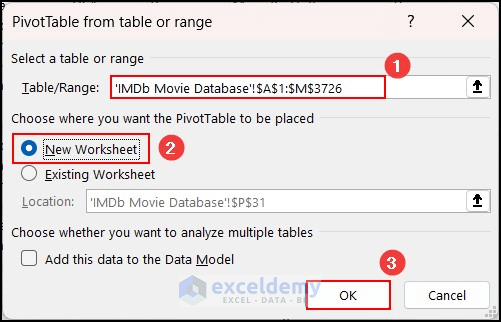
- Now you need to select the fields (columns) from your dataset to include in the PivotTable.
- The Field List will appear on the right side of your screen.
- Rows : Determines how data is organized vertically.
- Columns : Determines how data is organized horizontally.
- Values : Contains the numerical data you want to summarize (e.g., sum, average, count, etc.).
- Filters : Allows you to add fields that act as filters for your PivotTable.
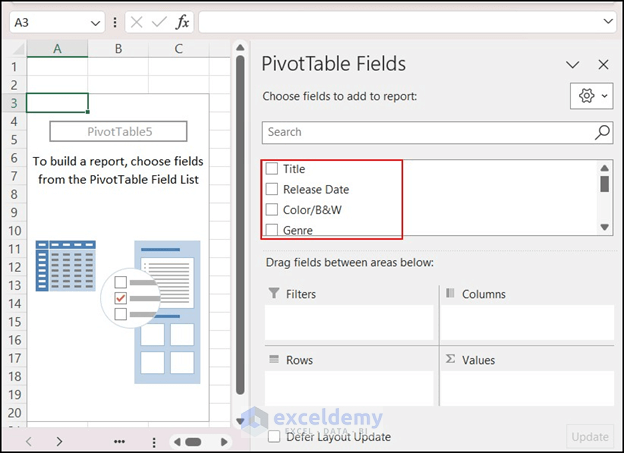
- Rows : Country and Title
- Values : Gross Revenue and Budget
- Filters : Genre
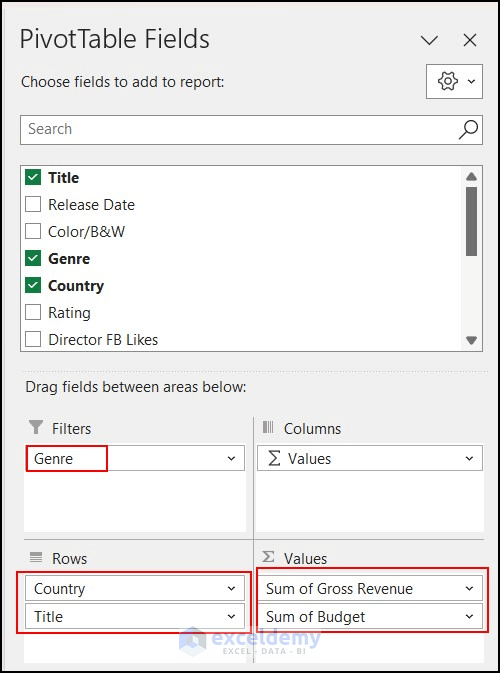
- By arranging these fields, you’ve successfully created a PivotTable that summarizes and analyzes your data.
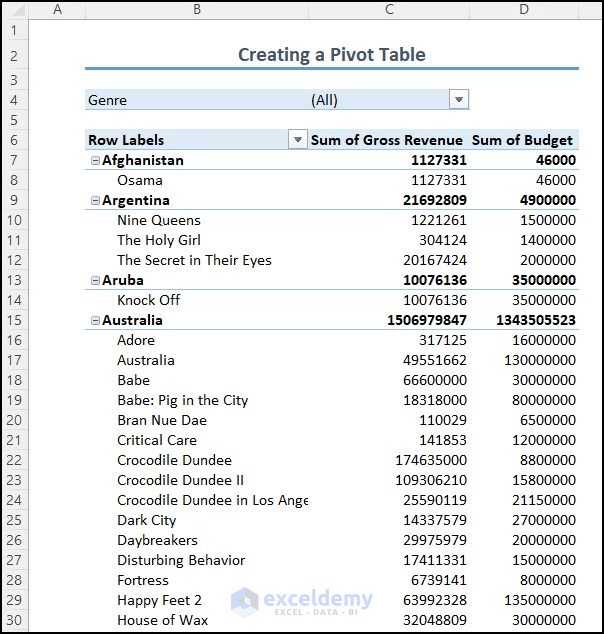
- Press Alt + N + V + T .
- The “PivotTable from table or range” dialog box will appear.
- Follow the same steps as described earlier.
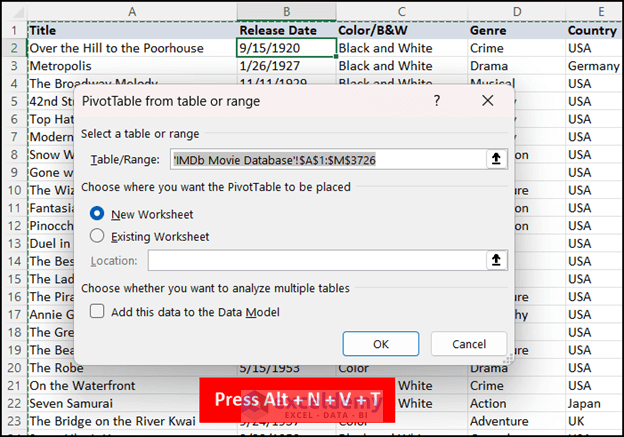
Remember, PivotTables are incredibly versatile and can help you gain valuable insights from your data.
Benefits of Using Advanced Techniques in Excel Pivot Table
Using advanced techniques in Excel Pivot Tables can significantly enhance your data analysis capabilities, making your work more efficient and insightful. Let’s explore the advantages:
- Advanced techniques allow you to perform more complex tasks, such as creating calculated fields, calculated items, and custom formulas. These functionalities enable you to extract deeper insights from your data beyond basic summary functions (e.g., sum, average, count).
- With advanced Pivot Table techniques, you can build sophisticated data models. This includes combining multiple data sources, using Power Query for data shaping and transformation, and establishing relationships between tables.
- Advanced features enable you to create dynamic reports that automatically update when the source data changes. This ensures your analysis remains up-to-date without manual adjustments.
- Slicers and timelines are powerful filtering tools within Pivot Tables. They provide an interactive way to filter data, allowing you to explore different aspects of your dataset effortlessly.
- PivotTables can be used to create charts and graphs. Visualizing your data in this way helps present complex information more intuitively to stakeholders.
- Advanced techniques allow you to explore data at different levels. You can drill down into specific details to gain deeper insights, which is valuable for thorough analysis.
- PivotTables can automatically group date and time data into intervals (e.g., months, quarters, years). This simplifies time-based analysis and provides a clearer view of trends over time.
- As you become proficient with advanced Pivot Table techniques, you’ll save time and effort during data analysis. This efficiency allows you to focus on interpreting results and making informed decisions based on data.
25 Tips & Techniques when using Advanced Pivot Tables
1. use slicers for effortless data filtering.
- Scenario : You have a PivotTable, and you want to filter data quickly with a single click.
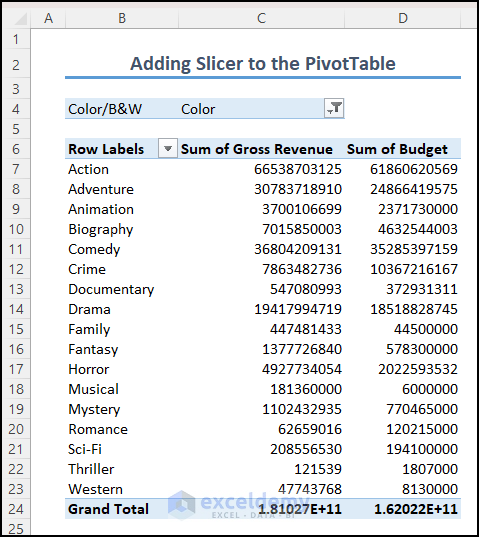
- Click on any cell within your PivotTable .
- Navigate to the Insert tab.
- Select Slicer .
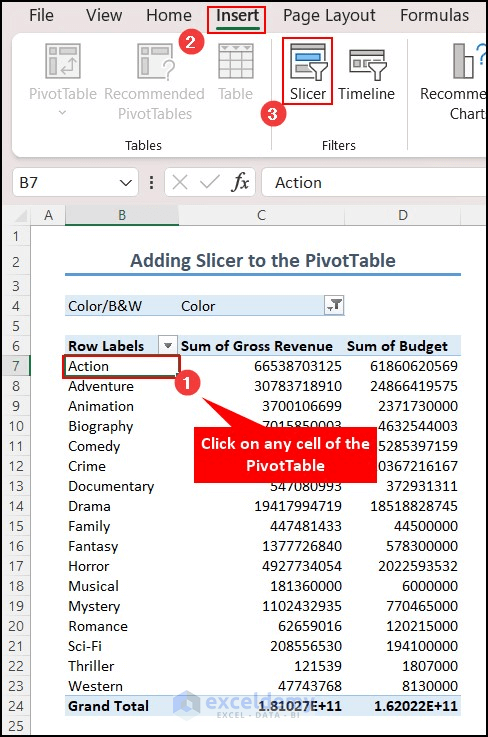
- In the Insert Slicers dialog box, choose the field (e.g., Country ) by which you want to filter your PivotTable .
- Click OK .
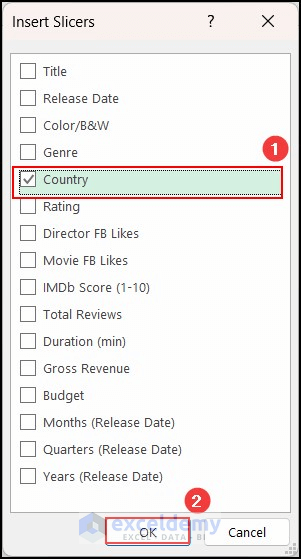
- A slicer will appear next to your PivotTable . You can now select different countries to filter the data.
- The best part? You can select multiple countries simultaneously as filters.

2. Enhance Data Visualization with Timelines
- Scenario : You’re working with a dataset containing movie release dates spanning from 1920 to 2015 . You want to filter data based on release years .
- Select any cell within your PivotTable.
- Go to the Insert tab.
- Choose Timeline .
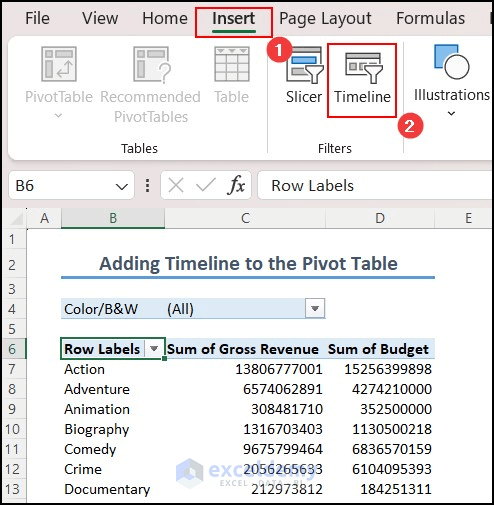
- In the Insert Timelines dialog box, you’ll see the available time-related field (e.g., Release Date ).
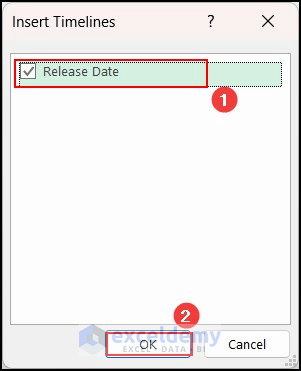
- A timeline will be added to your PivotTable.
- From the dropdown , select the desired time interval (e.g., YEARS ).
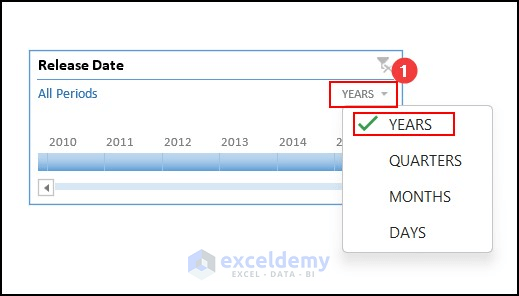
- Now, you can easily filter data by selecting specific years from the timeline.
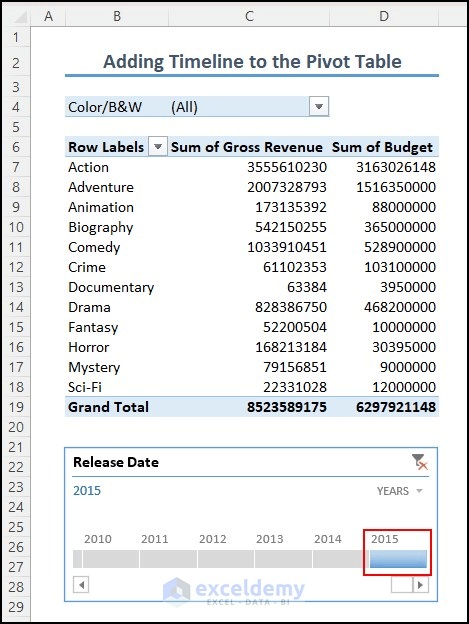
- Bonus: You can even select multiple years, and the PivotTable values will adjust accordingly.
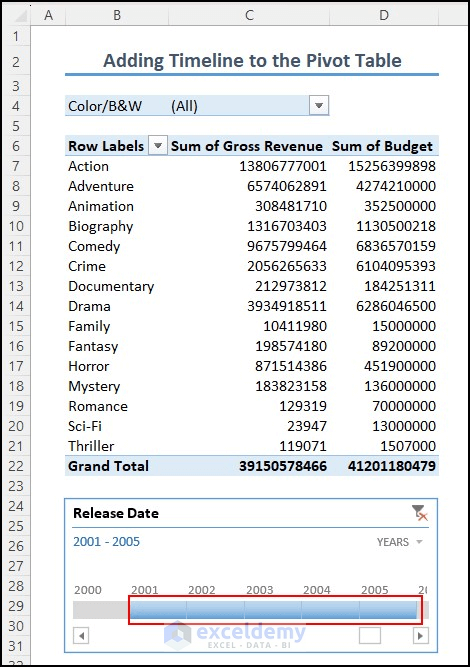
3. Customize Number Format in a PivotTable
Did you know that you can tailor the number format within a PivotTable? It’s a handy feature! Here’s how you can do it:
- Right-click on any cell in the column for which you want to change the number format .
- From the context menu, select Number Format .
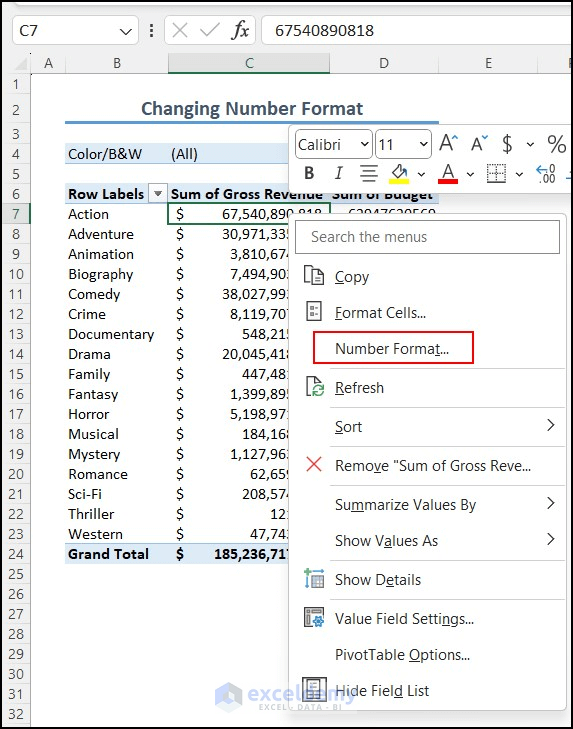
- The Format Cells dialog box will appear.
- Choose an appropriate category (e.g., Accounting ) and set the desired number of decimal places (e.g., 0 ).
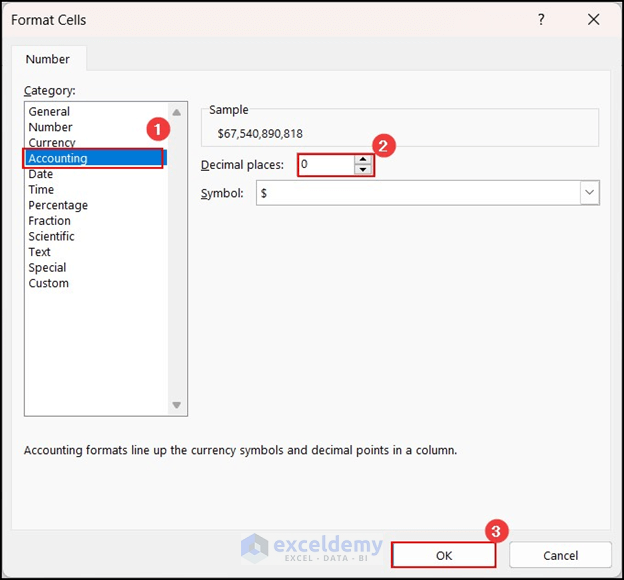
Now you’ll see that the number format has been updated.
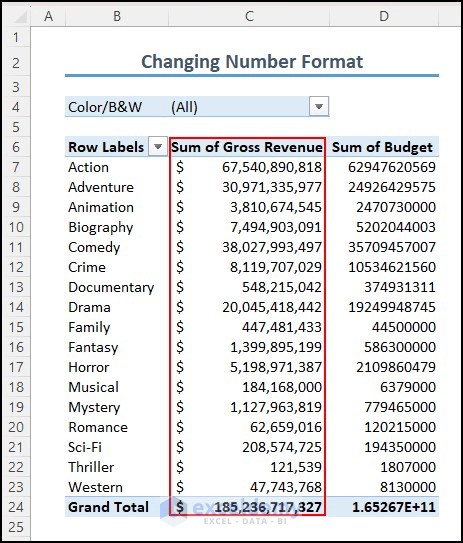
4. Sort Items Using the Context Menu
Sorting items in a PivotTable is essential for better analysis. Follow these steps:
- Right-click on any cell in the column you want to sort.
- Select Sort and then choose More Sort Options .
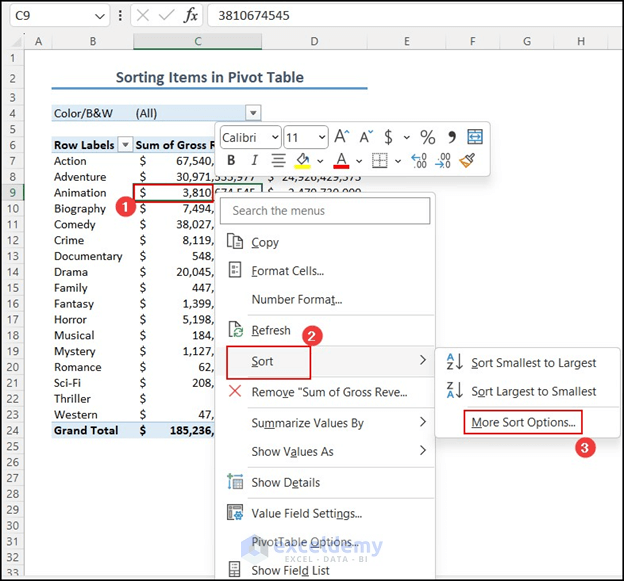
- In the Sort By Value dialog box, specify your sorting preferences (e.g., Smallest to Largest and Top to Bottom ).

Your table will now be sorted based on the sum of the Gross Revenue column.
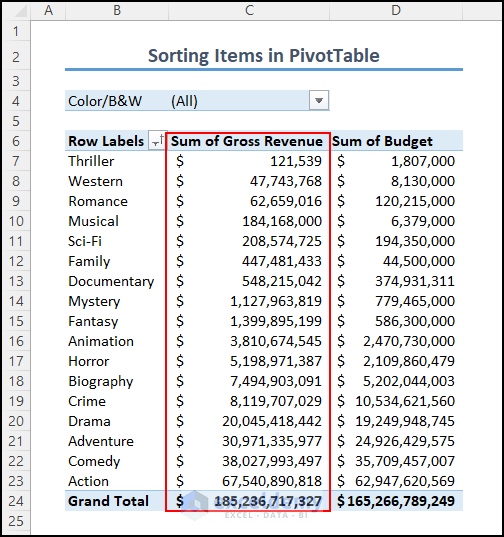
5. Custom Sort Items
Sometimes, you may want to sort PivotTable items according to your own order . Here’s how:
- Create a custom sort order by listing the items in a separate column within the same worksheet .
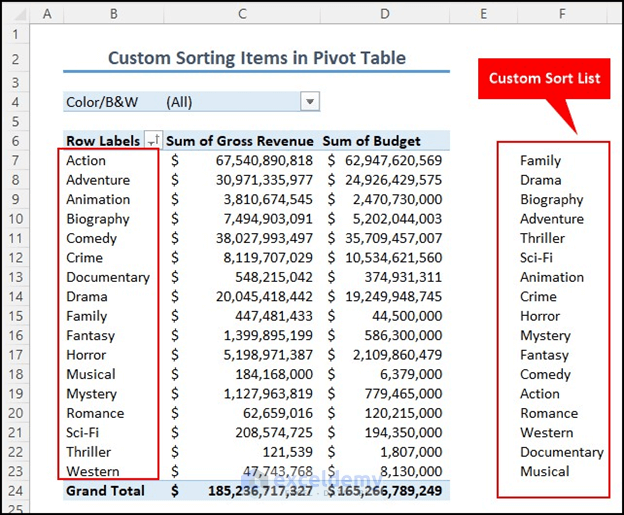
- Click on the File tab.
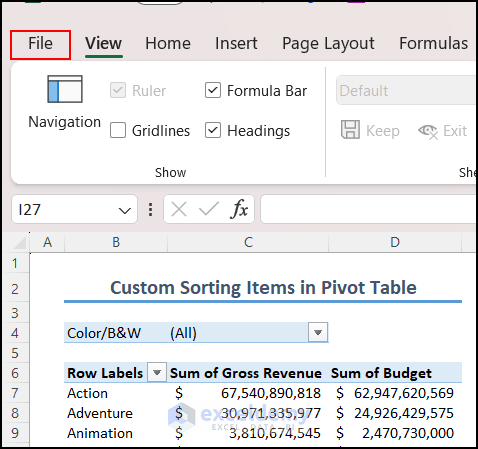
- Go to Options .
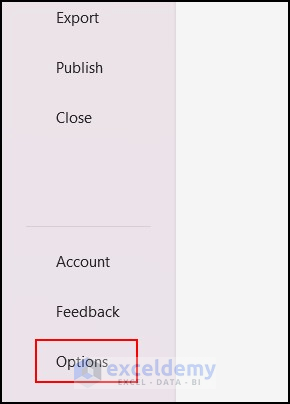
- In the Excel Options dialog box, select Advanced and click on Edit Custom Lists .
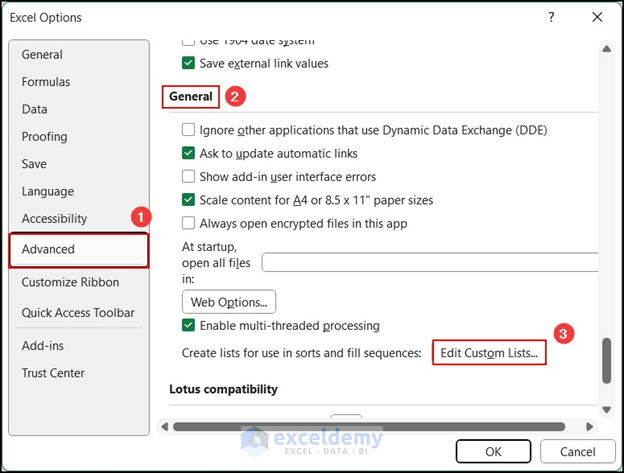
- Specify the cell reference of your custom sort list (or manually enter the items).
- Press Import and then click OK .
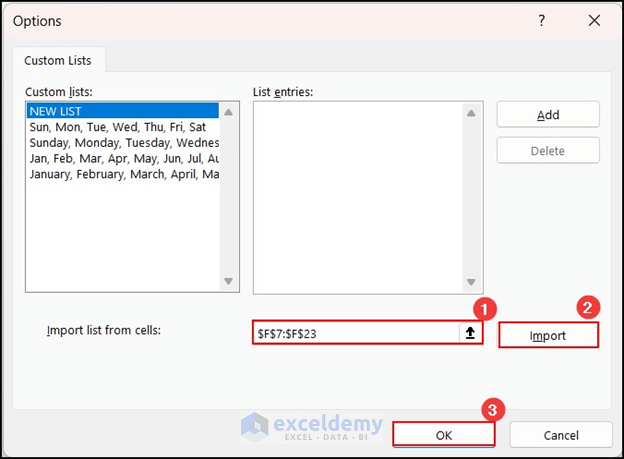
- Press OK when the Excel Options dialog box appears.
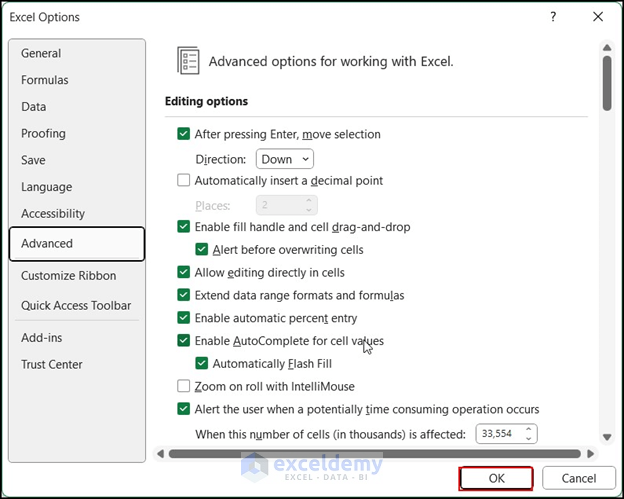
- Refresh the PivotTable by right-clicking on any cell in the column you want to sort.
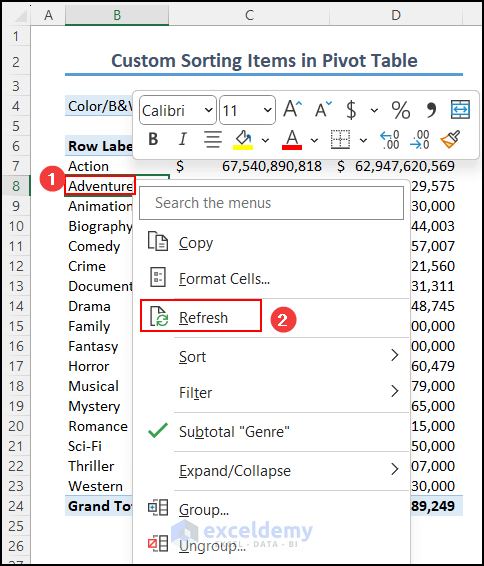
Now the items in the Row Labels column will be custom-sorted according to your preference.
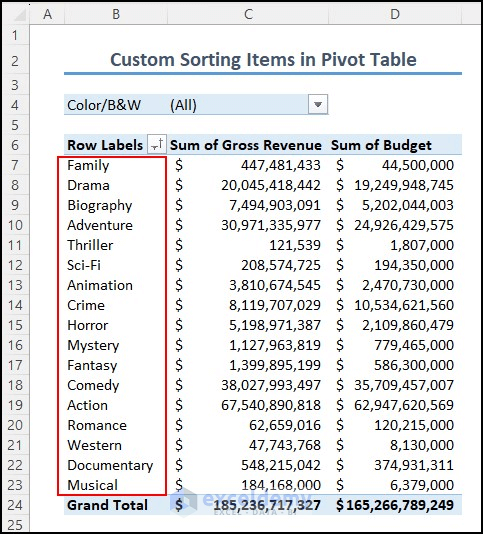
6. Create or Remove a Calculated Field in a PicotTable
Creating a calculated field is an advanced feature in Excel’s PivotTable. It’s a clever technique that allows you to compute various parameters without writing complex formulas. Here’s how you can create or remove a calculated field:
6.1 Create a Calculated Field:
- Click on any cell within the PivotTable.
- Go to the PivotTable Analyze tab.
- Under Calculations , select Fields, Items, & Cells , and then choose Calculated Field .
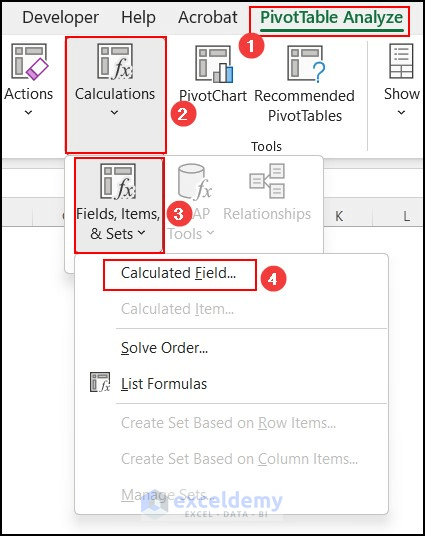
- The Insert Calculated Field dialog box will appear.
- Provide a relevant name for your calculated field (e.g., Gross Profit ).
- From the available fields , select the ones you want to use in your formula and click I nsert Field . For example, you can calculate Gross Profit by subtracting the Budget from the Gross Revenue.
- Review your formula and click OK .
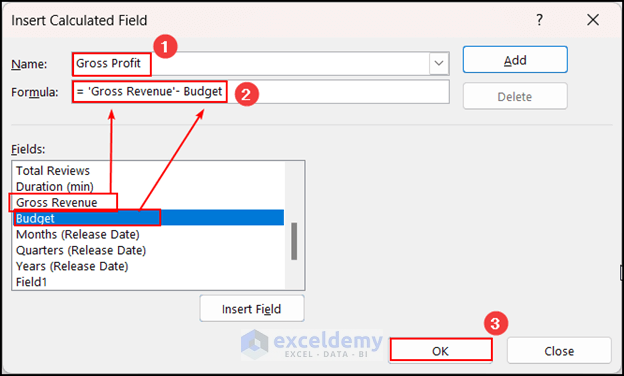
- A new column will be added to your existing PivotTable with the calculated values.
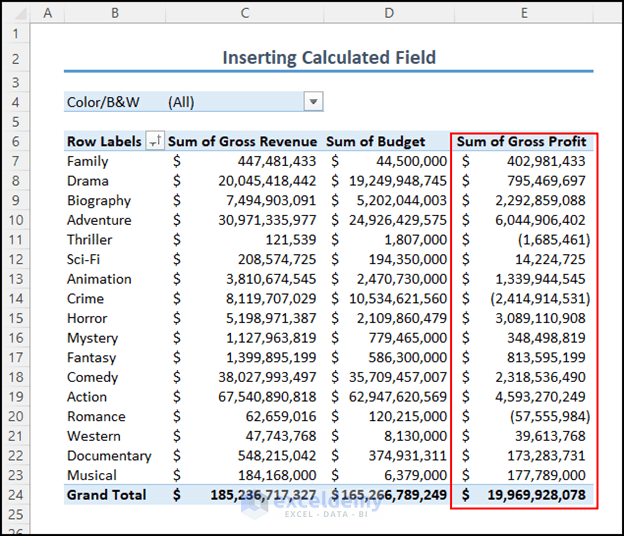
6.2 Remove a Calculated Field
- To remove a calculated field , follow the same steps as when creating one.
- Open the I nsert Calculated Field dialog box.
- Click the dropdown menu and choose the field you want to delete.
- Finally, press Delete and then click OK .
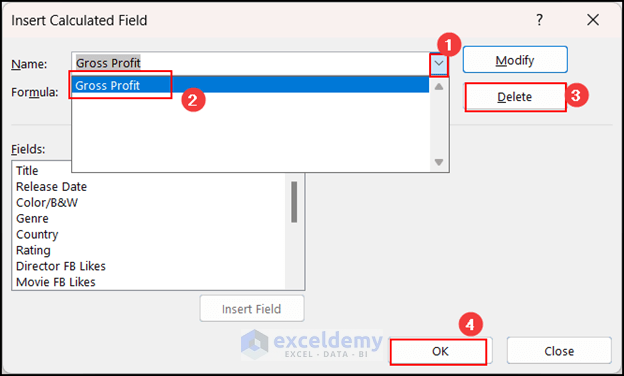
Now you can manage your calculated fields efficiently!
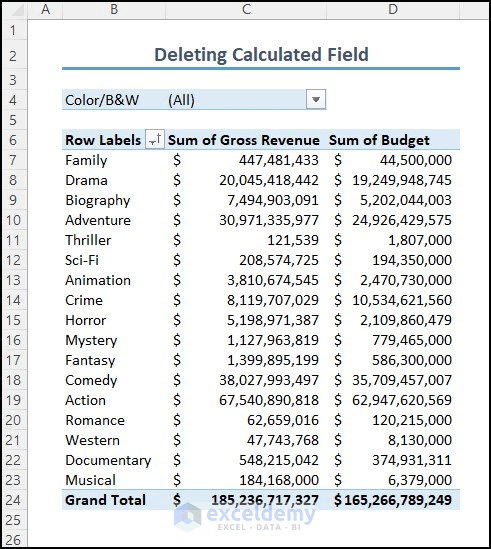
7. Calculate the Difference Between Two Columns
You can easily compute the difference between two columns in a PivotTable without writing any formulas. Follow these quick steps:
- In your dataset, you have Gross Revenue for the years “2014” and “2015.” Let’s calculate the difference in Gross Revenue between these two years.
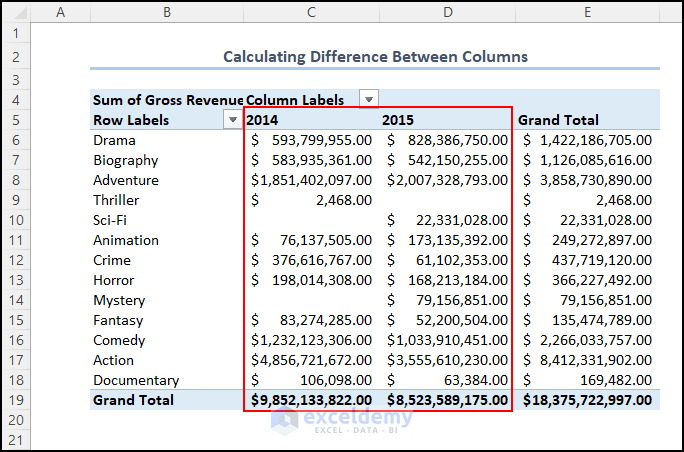
- Go to the Design tab.
- Under Grand Totals , select Off for both Rows and Columns . We don’t need grand totals for this calculation.
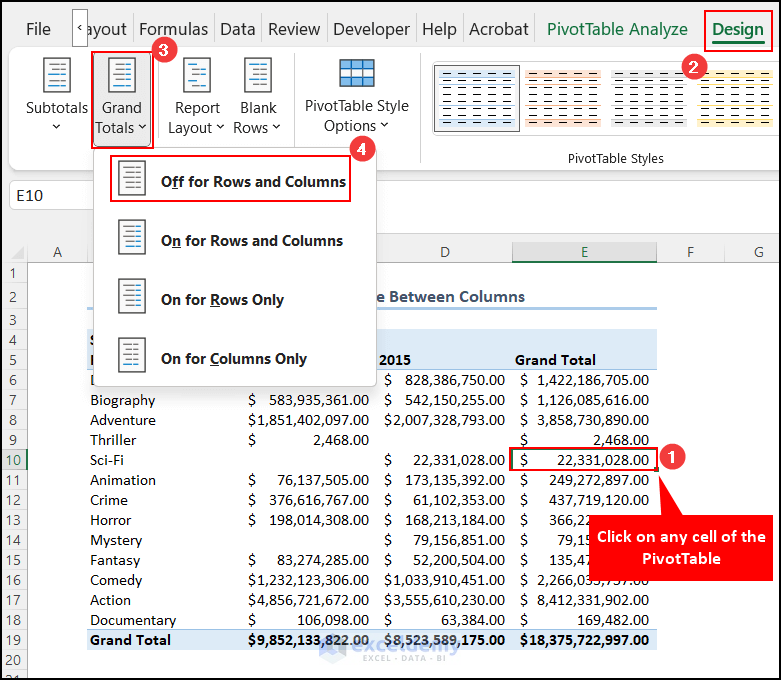
- Now, add the Gross Revenue to the Values area a second time. We’ll use this duplicate field to show the difference.
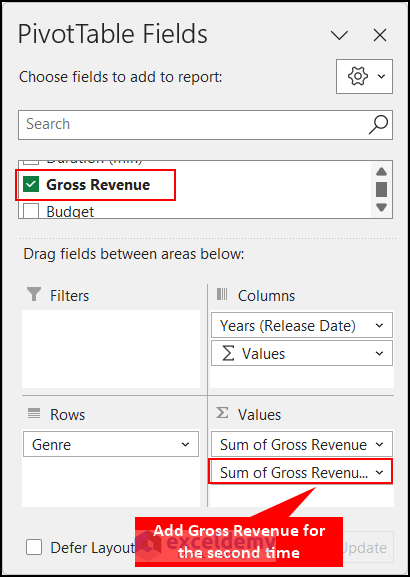
- You’ll see that Gross Revenue has been added a second time.
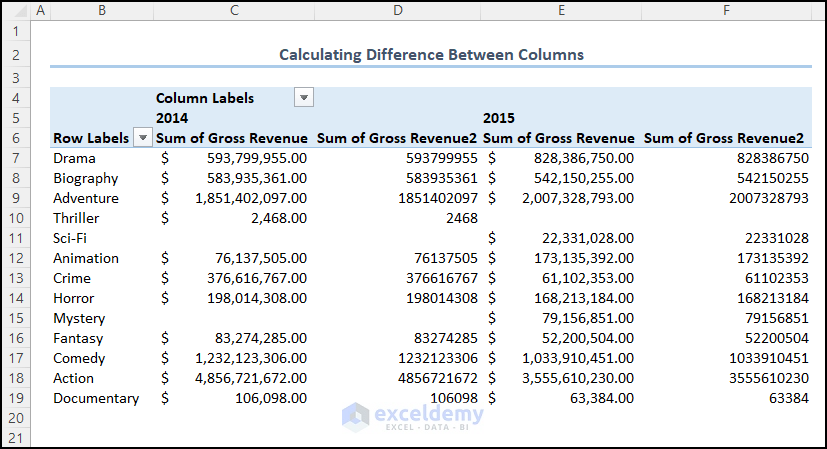
- Right-click on any cell in the newly added “Sum of Gross Revenue2” column.
- Select Show Value As and then choose Difference From… ”
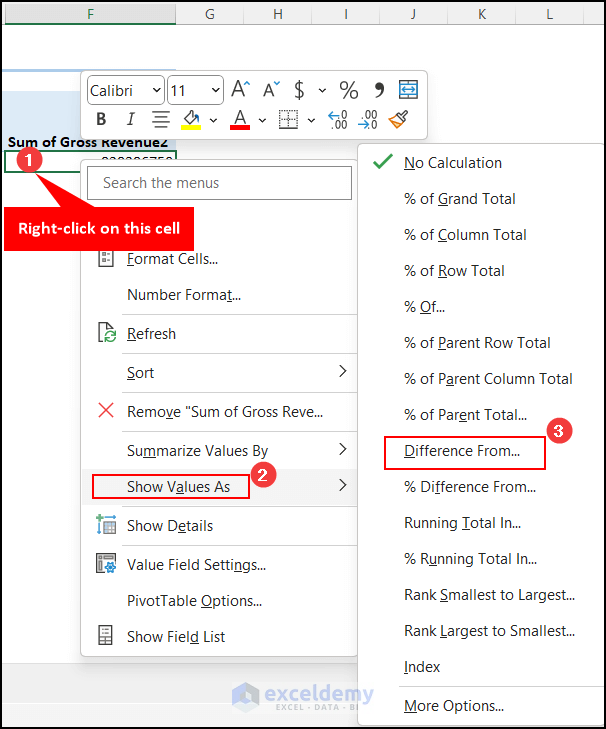
- The Show Values As dialog box will appear. Set Years (Release Date) as the Base Field .
- In the Base Item dropdown, select previous because we want to calculate the difference from the previous column.
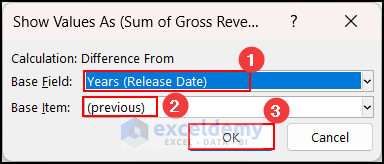
- The difference will now be calculated.
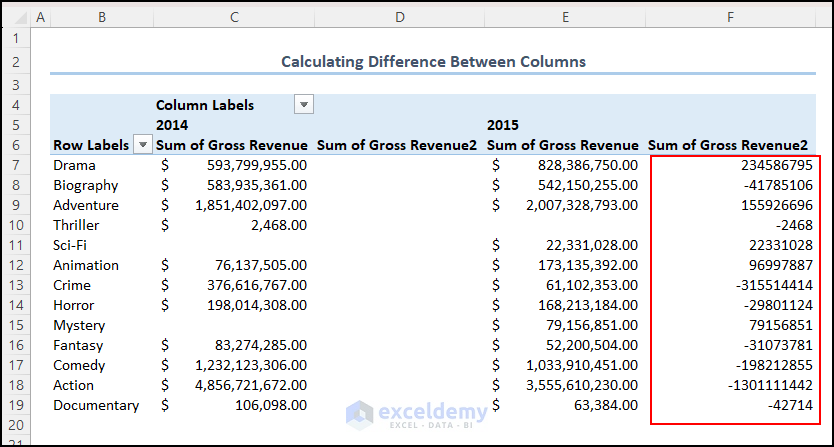
- Finally, edit the name of the column to Difference and hide any unnecessary columns.
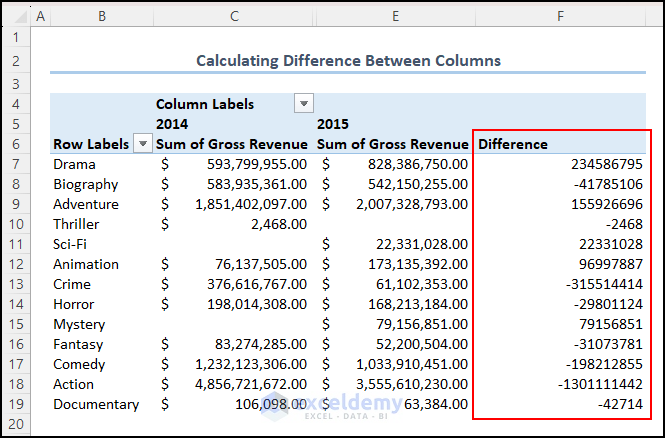
8. Show Percentage of Grand Total
Now let’s determine the Total Reviews as a percentage of the grand total. Follow these steps:
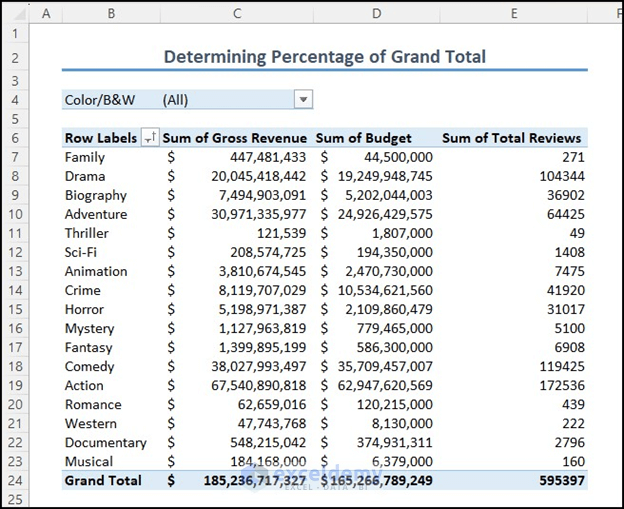
- Right-click on any cell in the column you want to display as a Percentage of the Grand Total .
- Click on Show Values As and then choose % of Grand Total .
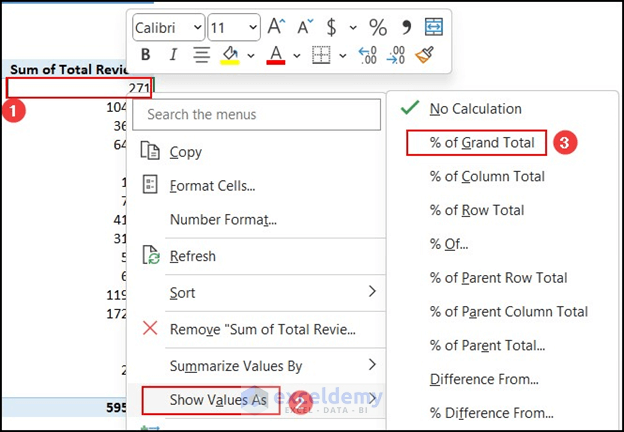
You’ll see that the Sum of Total Reviews is now shown as a percentage of the overall grand total.
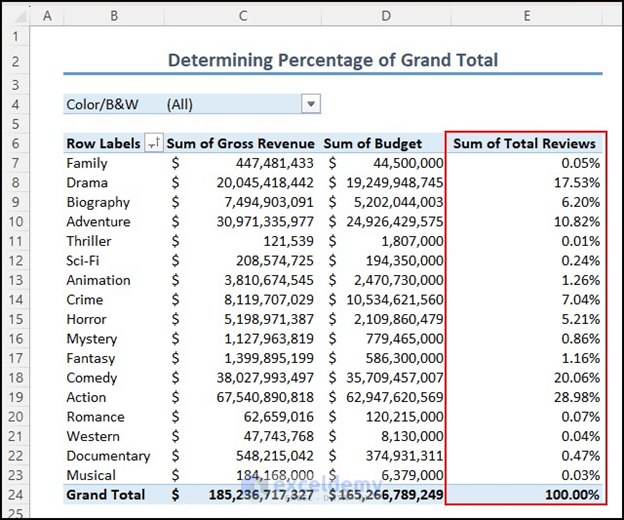
9. Disabling the GETPIVOTDATA Formula
The GETPIVOTDATA function retrieves data from a pivot table by referencing specific values within that table. Unlike regular cell references, it directly extracts data from the source data. Suppose you want to reference a cell value from a PivotTable. For example, you want to display the value of cell D7 in cell E7 by simply writing the formula “ =D7 .” However, after doing this, the GETPIVOTDATA formula still appears in cell E7 . The formula looks like this:
Keeping the GETPIVOTDATA formula can be problematic, especially when creating dynamic dashboards. If it remains active, the data won’t update correctly. Here are some difficulties users face when using GETPIVOTDATA :
- When users frequently change data criteria, GETPIVOTDATA becomes cumbersome. Each time the criteria change, the function must be manually updated.
- If you modify the layout or structure of the PivotTable (e.g., changing the layout), GETPIVOTDATA formulas may break, causing errors in the worksheet.
- GETPIVOTDATA may not work well with calculated fields and items in the PivotTable, leading to incorrect results or errors.
- GETPIVOTDATA often uses hard-coded cell references in the formula. This can be problematic when you want to use cell references or other dynamic formulas.
To avoid these problems, you can turn off the GETPIVOTDATA formula:
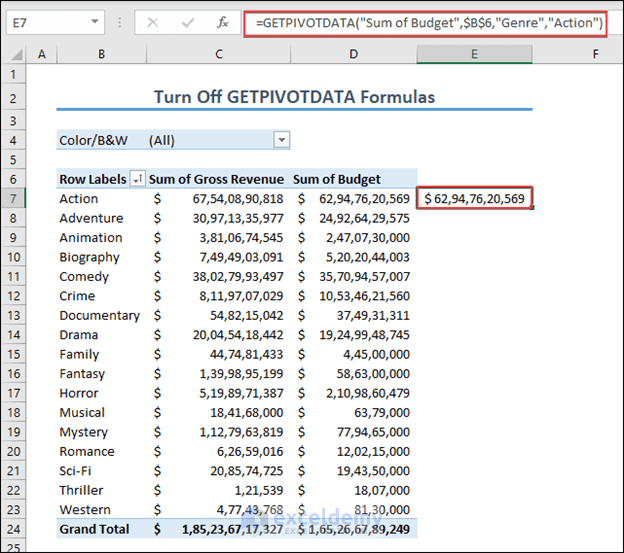
- Click on any cell in the column for which you want to disable GETPIVOTDATA .
- Under Options , click on Generate GetPivotData .
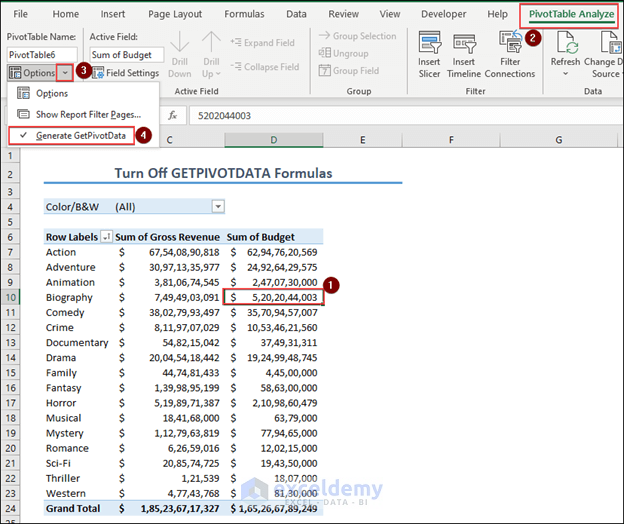
- The checkmark should disappear next to the Generate GetPivotData option.
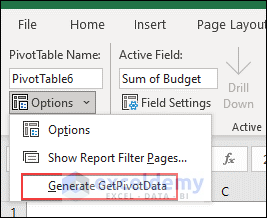
- Now, if you write the formula “ =D7 ” in cell E7 , it will display only the value of cell D7 . This is because you’ve turned off the GETPIVOTDATA option.
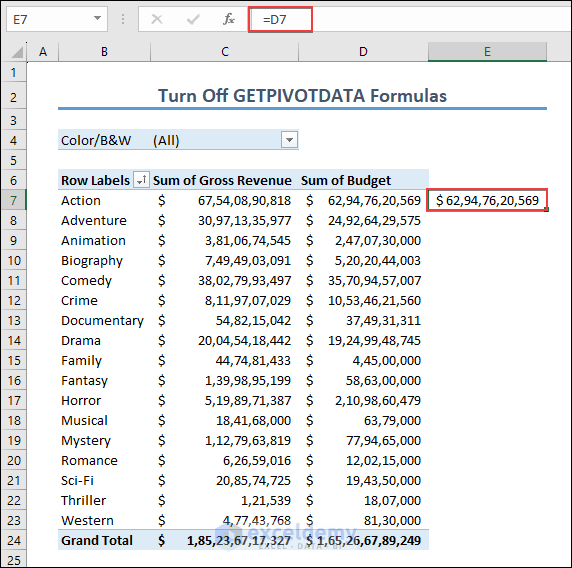
Remember that you can always turn it back on by clicking the Generate GetPivotData option again.
10. Grouping and Ungrouping Items Under a Field
Grouping items in a PivotTable allows you to organize and summarize data effectively. Here’s how you can group and ungroup items:
- First, select the items you want to group together.
- Right-click on the selection.
- From the context menu, choose the Group option.
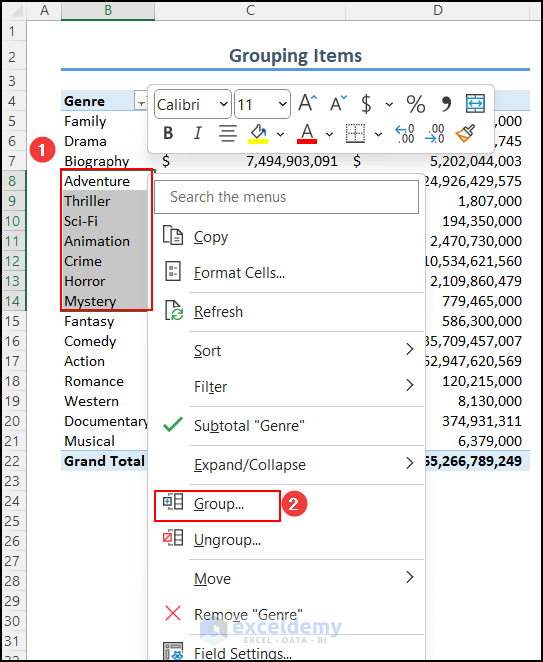
- The selected items will now be grouped under a default name (e.g., Group1 ). You can edit this group name according to your preference.
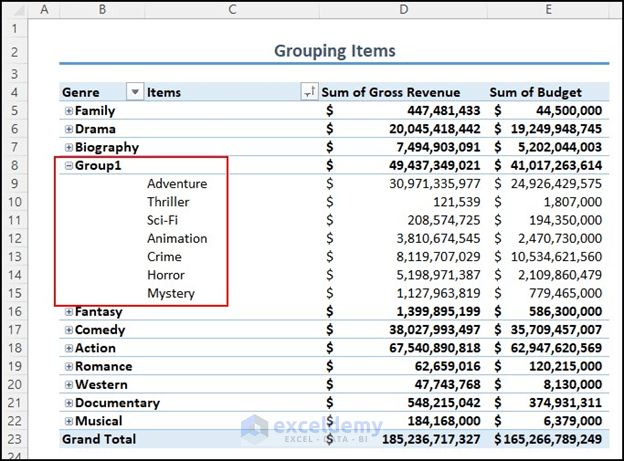
- To ungroup the items, right-click on the group name (e.g., Group1 ).
- Select the Ungroup option.
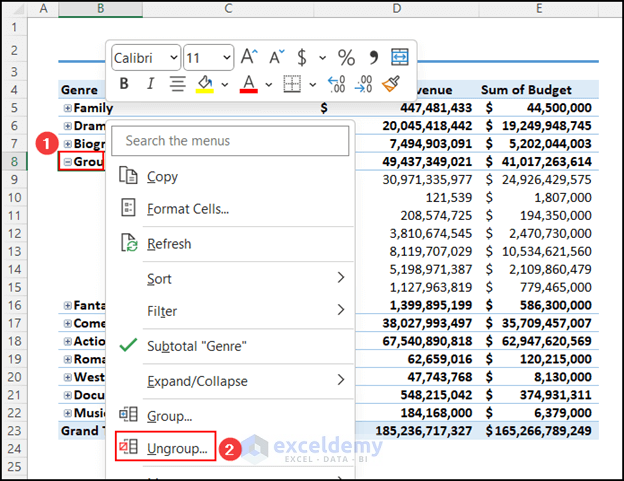
- The items will be ungrouped.
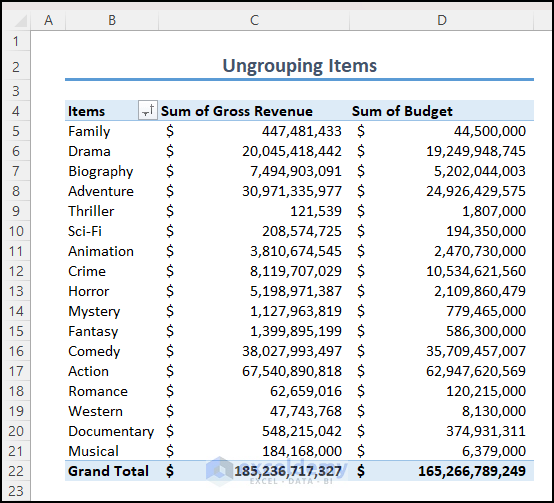
11. Grouping a Date Field
Grouping date fields is useful for analyzing time-based data. Let’s say you have a column called “Released Date” represented by quarters, but you want to group it by months. Follow these steps:
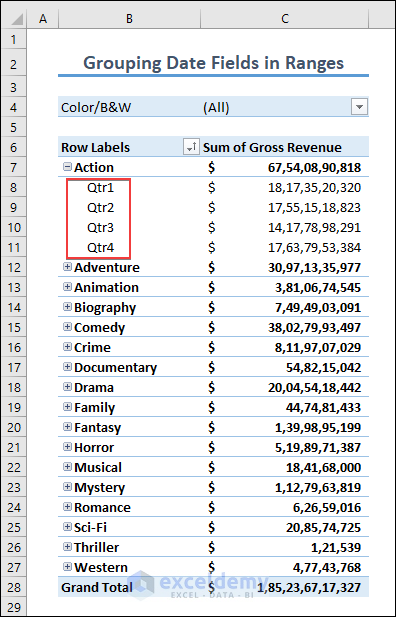
- Right-click on any cell within the Quarters column.
- Select the Group option from the context menu.
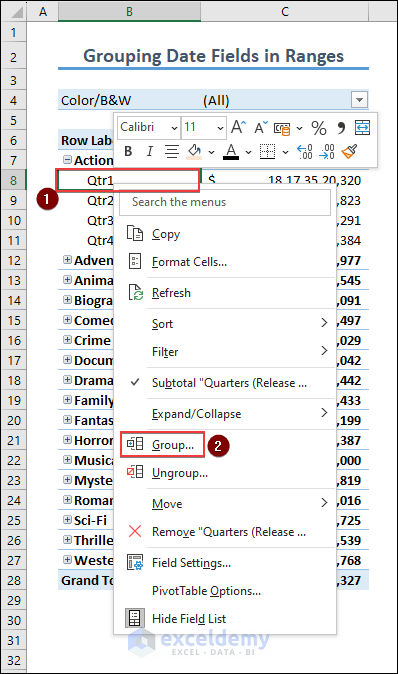
- The Grouping dialog box will open.
- The starting and ending dates will be set automatically based on your dataset, but you can adjust them if needed.
- In the By box, choose your preferred grouping (e.g., Months ).
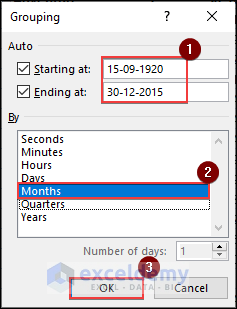
- The dates will now be successfully grouped into Months .
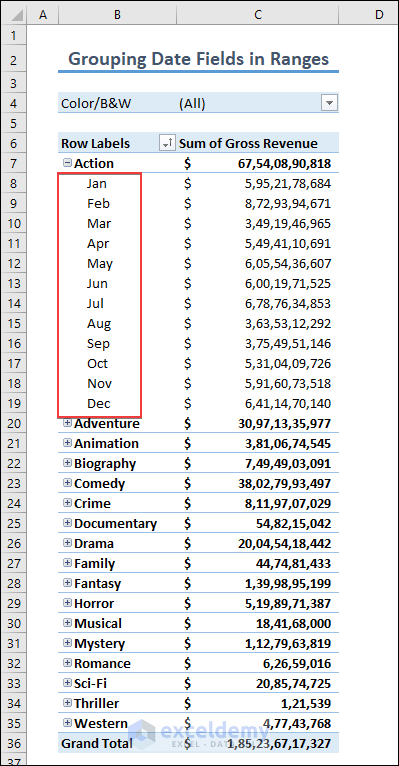
12. Creating a Report Filter
A report filter allows you to filter data in your PivotTable based on specific criteria. Here’s how you can create one:
- The PivotTable Fields pane will appear.
- Drag and drop the field that you want to use as a filter into the Filters area.
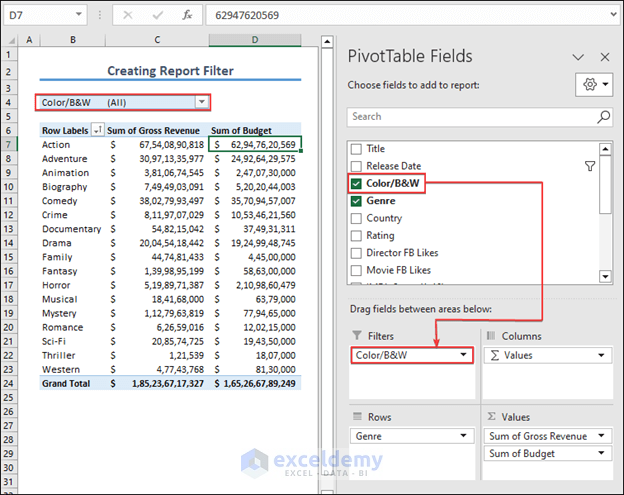
- A filter option will appear just above the table.
- Click on the drop-down arrow.
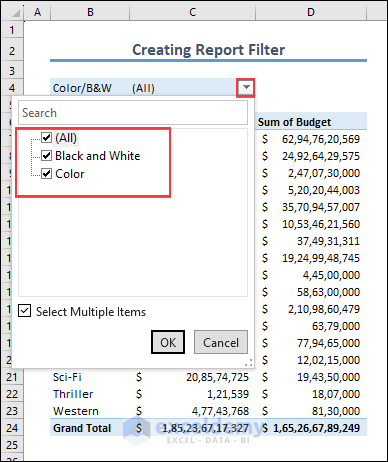
- Select the option you want to see in the PivotTable (e.g., Black and White ) and press OK .
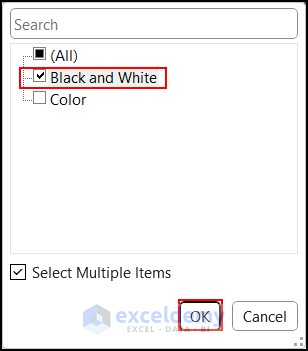
- Now only the values corresponding to your chosen filter will be displayed.
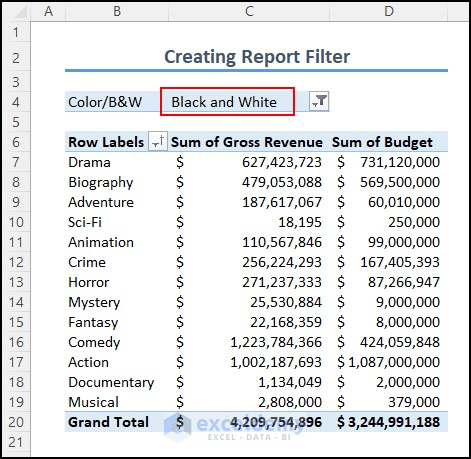
- You can further refine the filter by selecting other options (e.g., Color ).
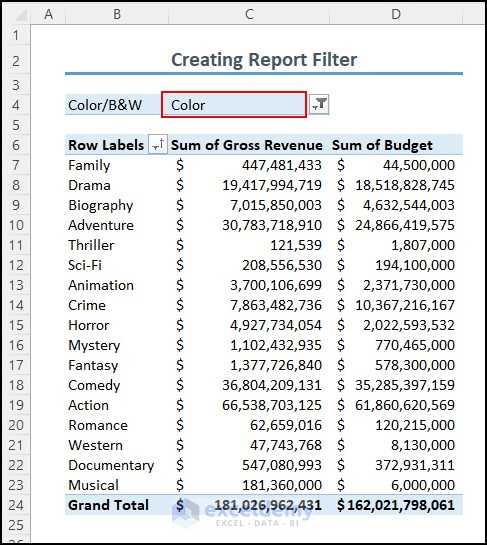
13. Filter Top/Bottom N Values
Suppose you want to filter the top or bottom items based on the sum of gross revenue in your PivotTable.
Follow these steps:
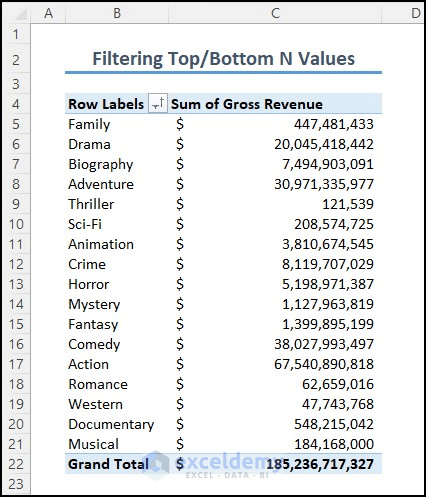
- Click on the drop-down arrow next to the Row Labels .
- Select Value Filters and then choose the Top 10 option.
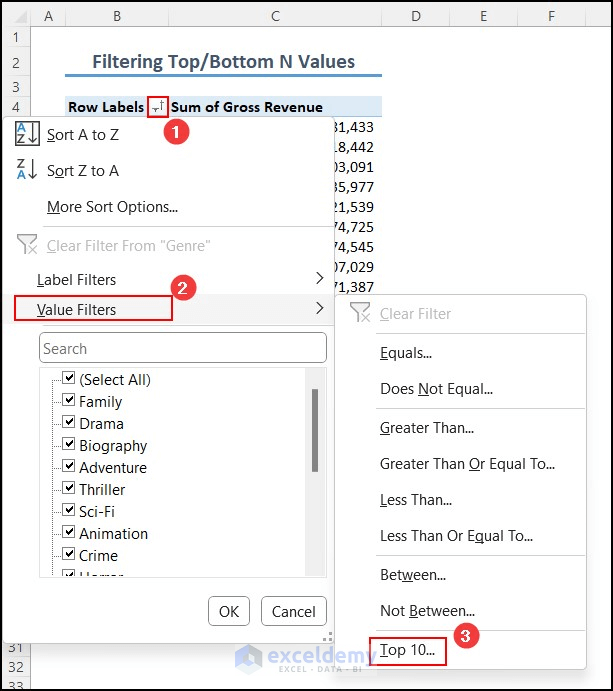
- The Top 10 Filter dialog box will appear.
- From the drop-down, choose Top to show the top values.
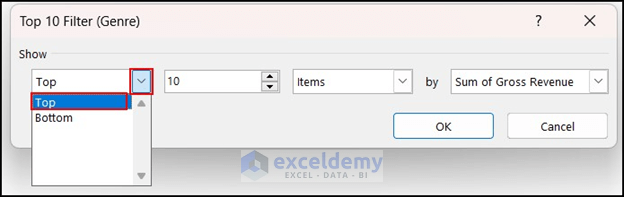
- Select the number of items you want to see. For example, if you want to see the top 12 values, select 12 .
- Under By , choose Sum of Gross Revenue and press OK .
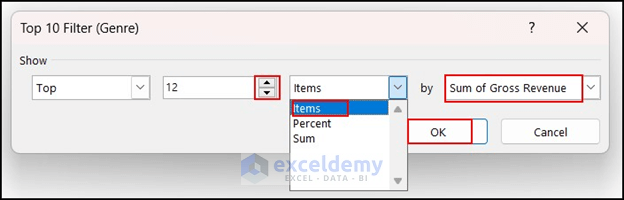
- As a result, you’ll see the top 12 sum of gross revenue values along with their corresponding genres .
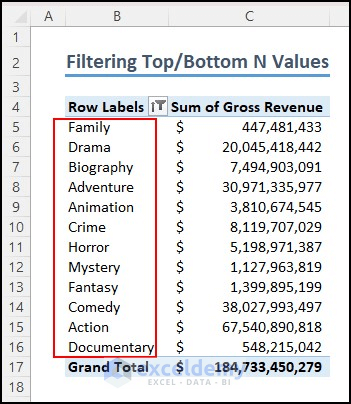
- If you want to show bottom values instead, select Bottom from the dropdown. For example, you can choose the bottom 5 items by sum of gross revenue.
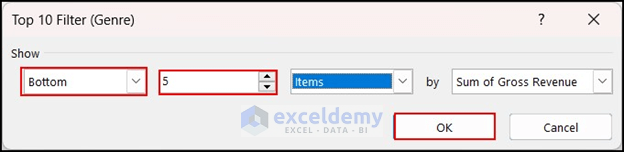
14. Refresh Data
When you update or add any value, the source data of the PivotTable changes. To reflect these changes, you need to refresh the table. Let’s say the sum of duration for the genre Crime in your PivotTable is currently “ 29558 .” If you make changes to any value under this genre, the PivotTable needs to be updated.
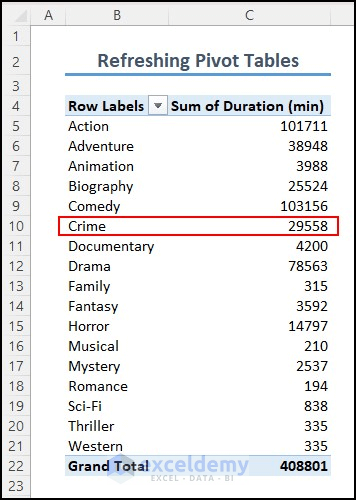
14.1 From the PivotTable Analyze Tab
- Change the value from 110 to 11000 (or any other value) to visualize the change.
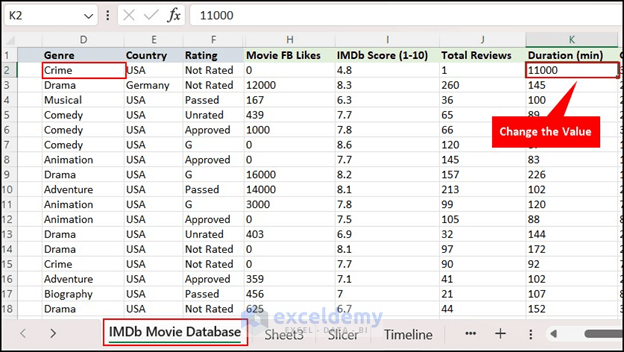
- Click on the PivotTable Analyze tab.
- Select the Refresh command and click on the Refresh option.
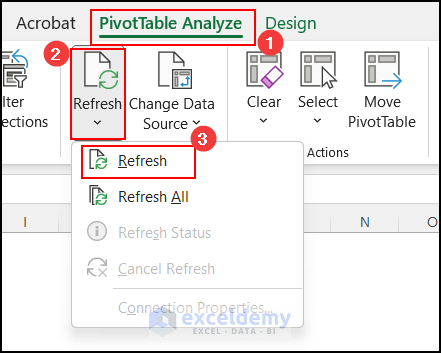
- The sum of duration for the Crime genre will be updated to the new value (e.g., 40448 ).
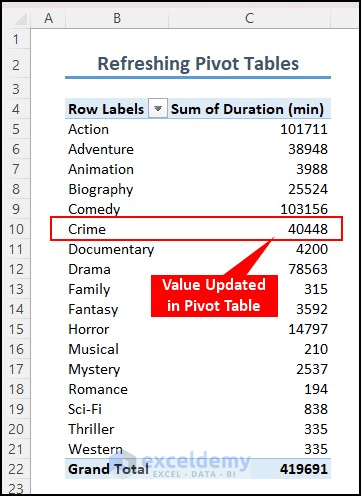
14.2 From PivotTable Options
- Select any cell within the PivotTable.
- Click on the PivotTable Analyze tab.
- Choose the PivotTable dropdown and then click on Options .
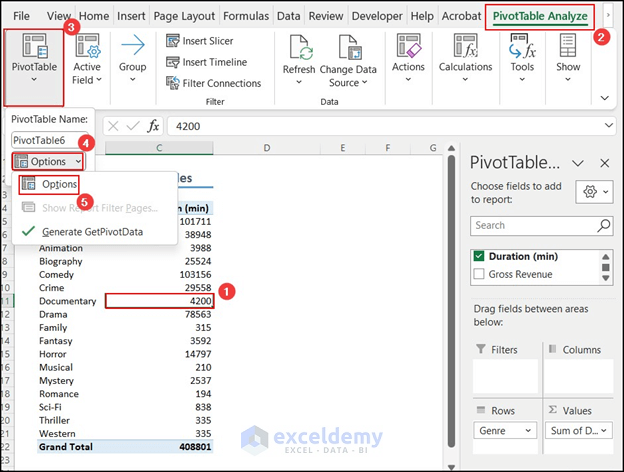
- In the PivotTable Options dialog box, go to the Data section.
- Check the Refresh data when opening the file option.
- Now, the values will automatically refresh every time you open the file.
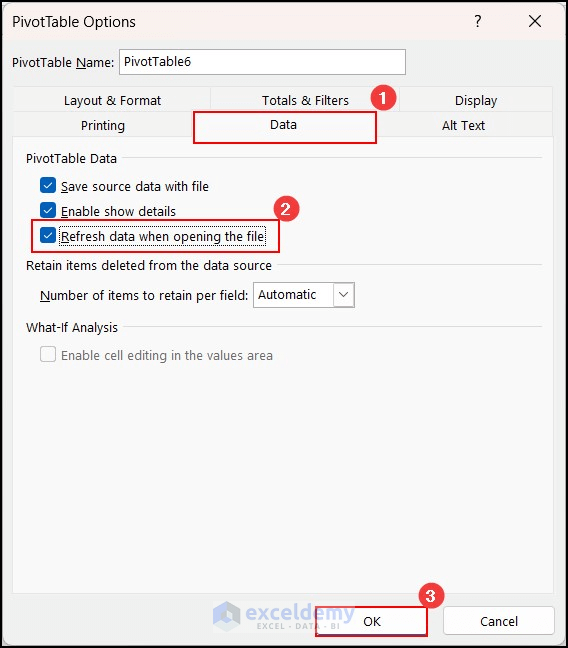
14.3 Refresh Pivot Table When New Column/Row is Added
In your dataset, you can see that the sum of duration for the “Action” movie is “101711.” If you insert new data into the source data of the “Action” movie, the PivotTable should be updated accordingly.
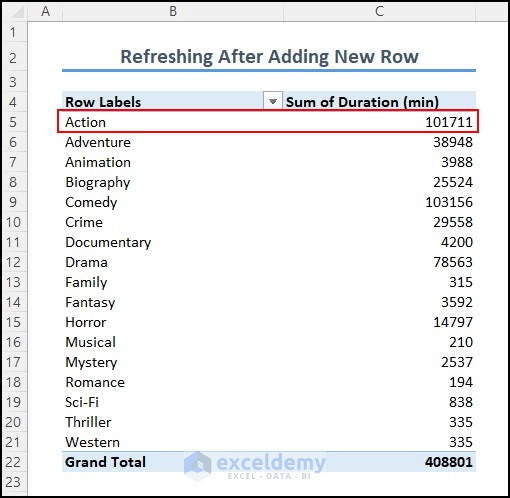
- Insert a new row or column of information into the data source of the PivotTable.
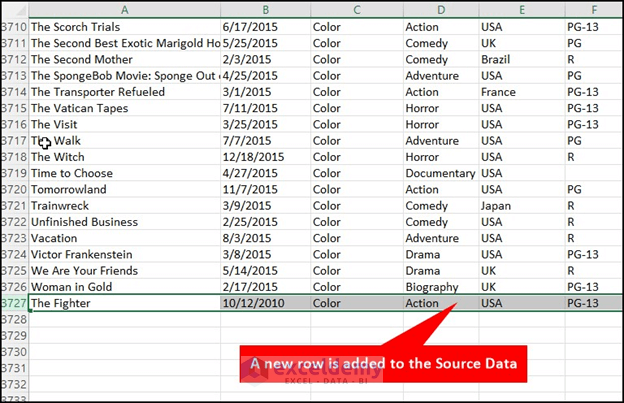
- Right-click on any cell within the PivotTable .
- From the context menu, click on Refresh .
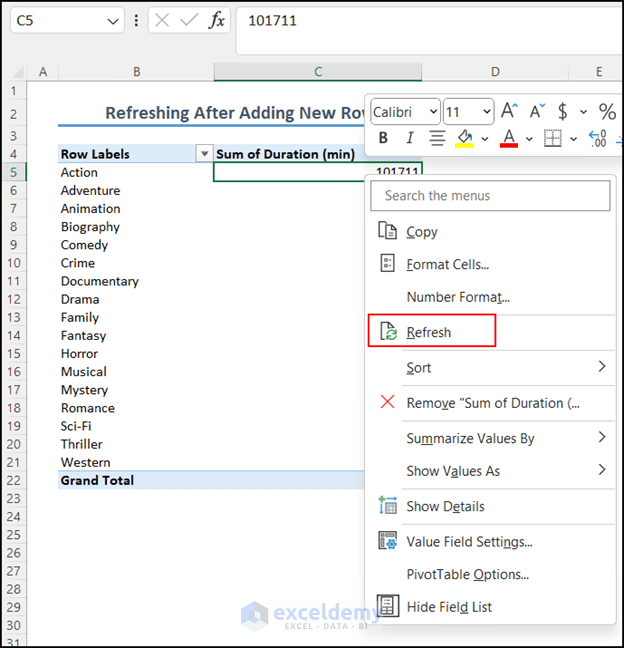
- However, you’ll notice that the PivotTable doesn’t update after clicking “Refresh.” The “Action” movie still shows a duration of “101711” minutes.
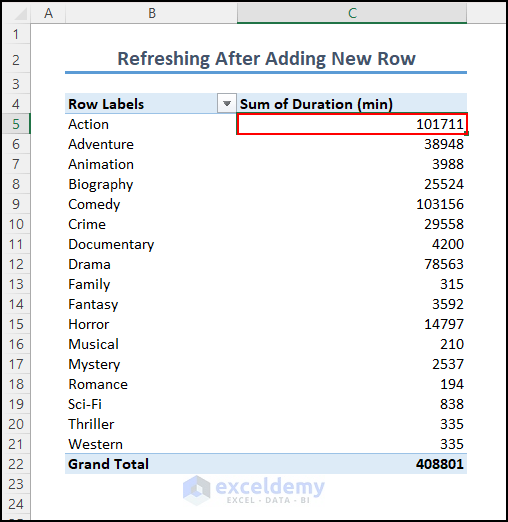
To resolve this issue, follow these additional steps:
- Select the PivotTable Analyze tab.
- Choose Change Data Source and then select Change Data Source again.
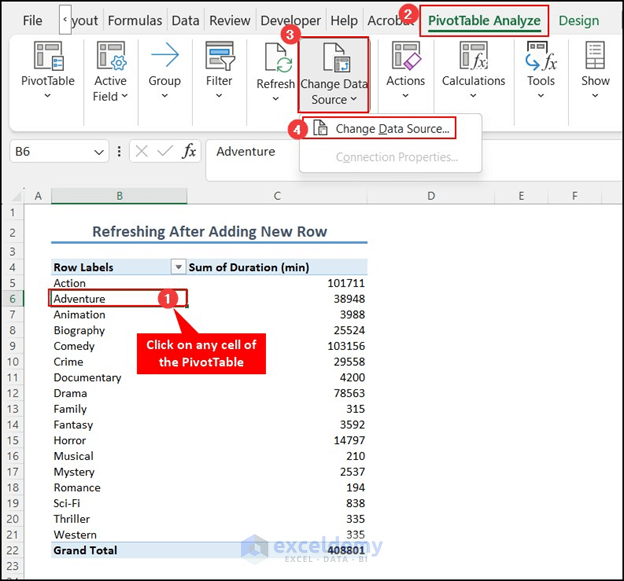
- The Move PivotTable dialog box will appear.
- This time, select the entire table, including the newly added row, in the “Table/Range” box.
- Finally, click OK .
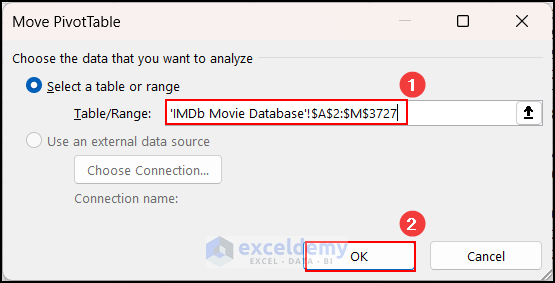
- As a result, you’ll see that the data is now updated in the PivotTable.

15. Hide/Unhide Subtotals
In a PivotTable, subtotals are typically shown. However, there are situations where you might need to hide these subtotals. Follow these steps:
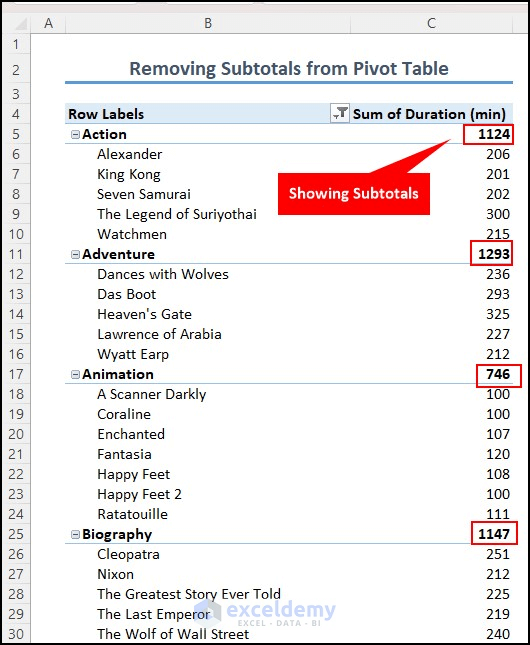
- First, click on any cell within the Sum of Duration column.
- Then, select the Design tab.
- Click on Subtotals and choose the option Do not Show Subtotals .
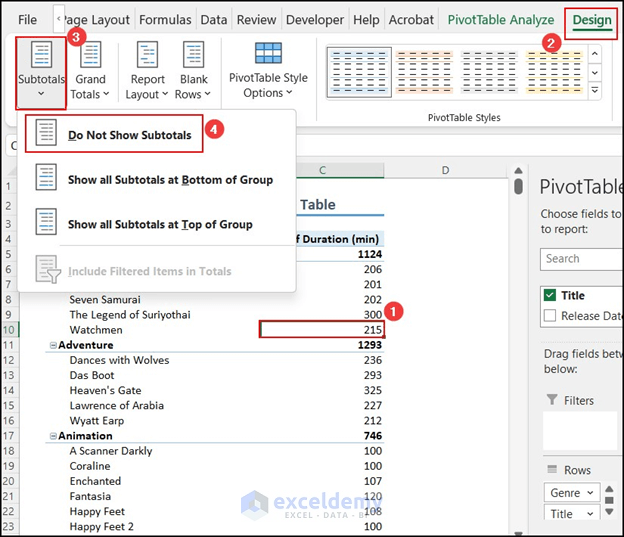
- As a result, the subtotals will be hidden.
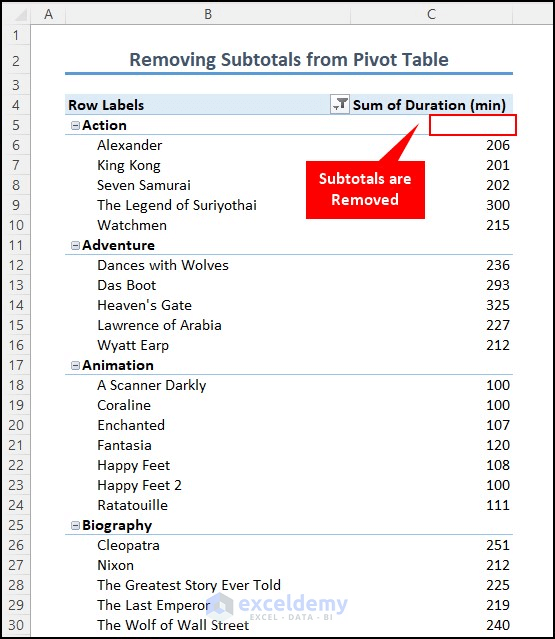
- If you want to Unhide them, select the option Show all Subtotals at Top of Group under Subtotals .
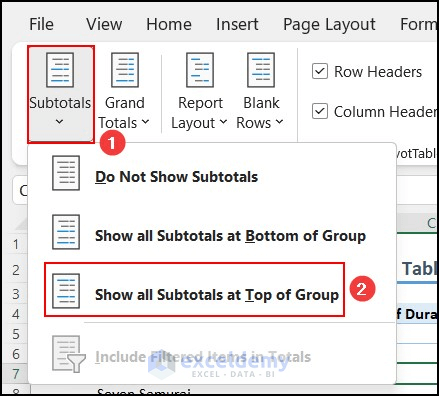
16. Delete Source Data and Restore It with a Double-click
Sometimes, to reduce file size, you may need to delete the source data of a PivotTable. Fortunately, deleting the source data won’t affect the table itself. Here’s how to do it:
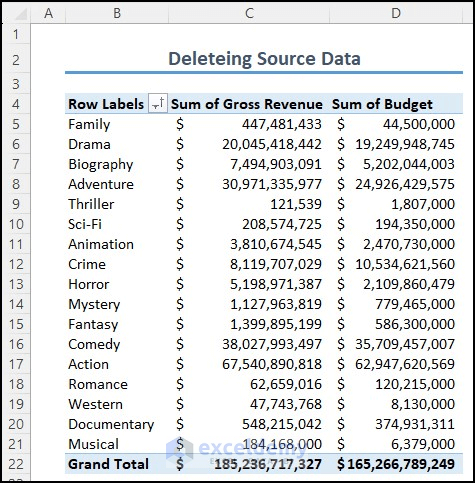
- Right-click on the sheet where the source data is stored.
- Select the Delete option to remove it.
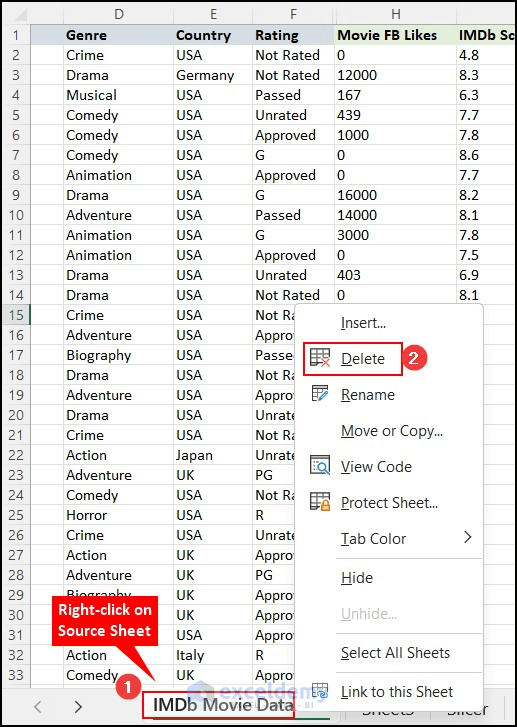
- If you want to restore the source data, right-click on any cell within the PivotTable.
- Choose Show Details .
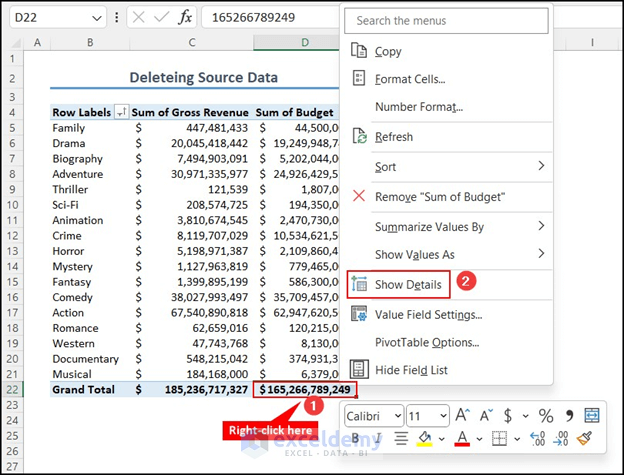
- The data will be restored in a table form.
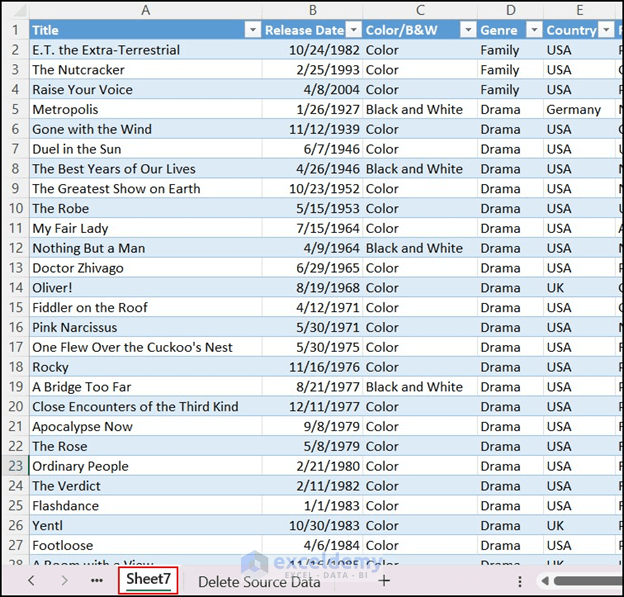
- Alternatively, you can double-click on the output of the Grand Total cell to restore the source data.
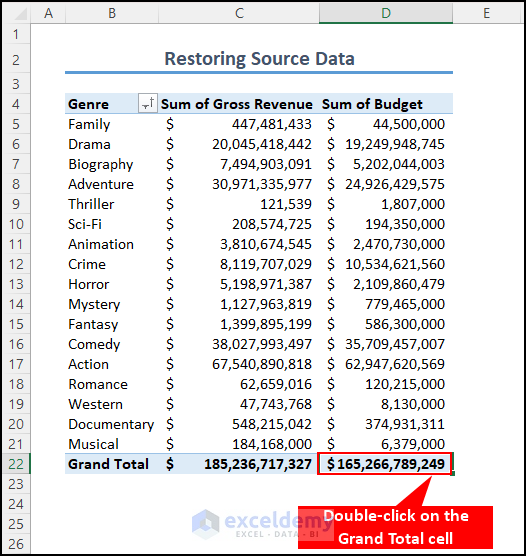
17. Drill Down Pivot Table
Drilling down in a PivotTable is a useful feature to show detailed information from a summarized table. Follow these steps:
- Initially, double-click on the item you want to drill down into.
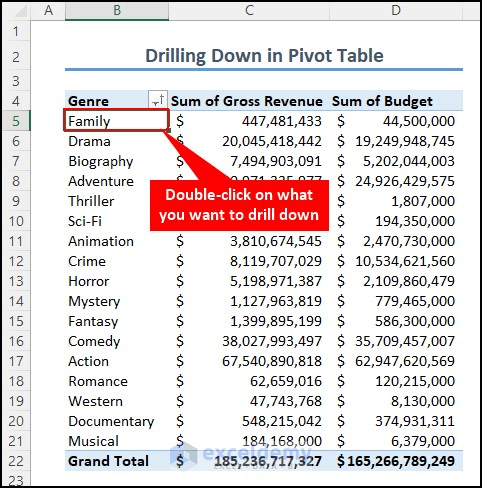
- The Show Detail dialog box will appear.
- Choose the field that contains the detail you want to see. For example, if you want to see details by country, select Country .
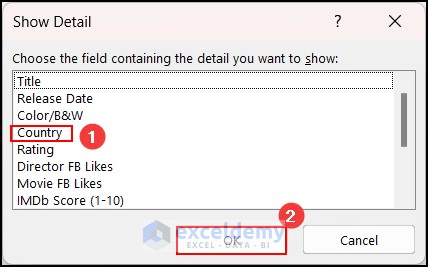
- The items will now have a plus ( + ) sign next to them.
- Double-click on any item to drill down further.
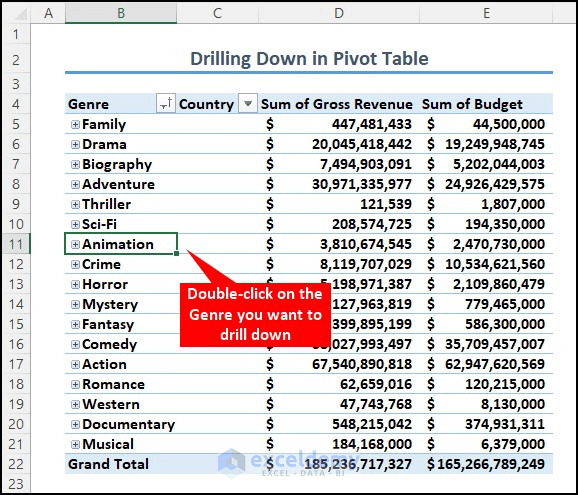
- It will show the names of countries that have released movies in the Animation genre, along with their corresponding values.
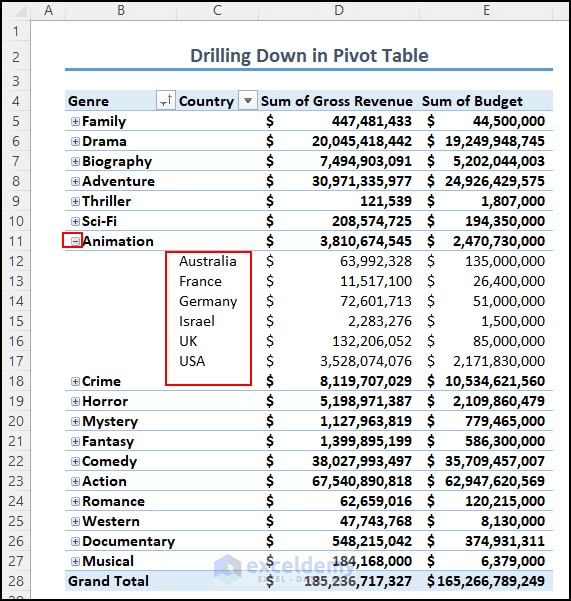
18. Create Different Styles in Pivot Table
- Start by clicking on any cell within the PivotTable.
- Next, select the Design tab.
- Click on the drop-down icon for PivotTable Styles .
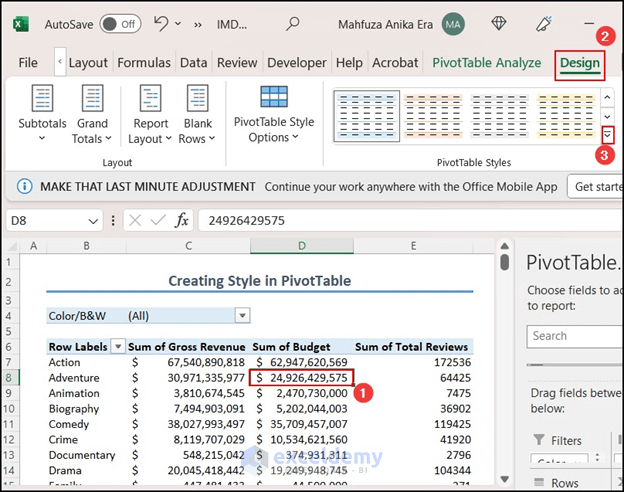
- Now, choose New PivotTable Style…
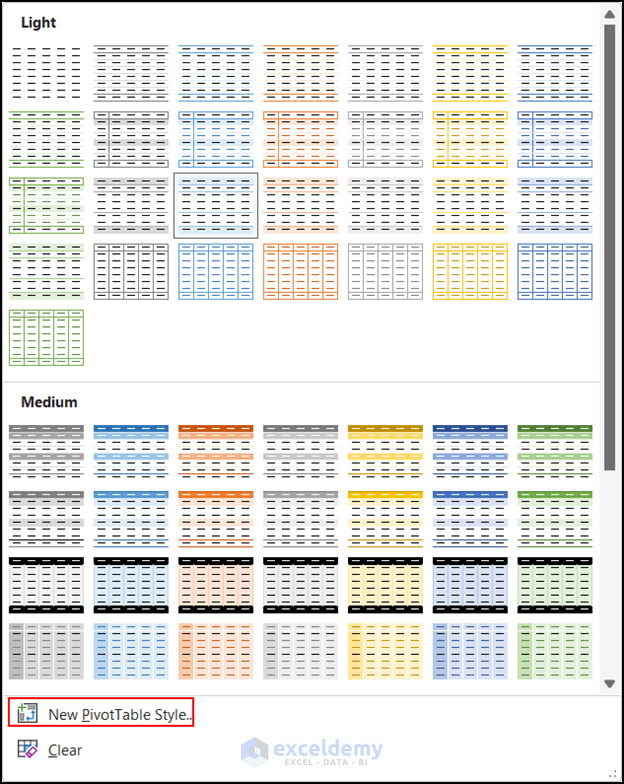
- The New PivotTable Style dialog box will appear.
- Give your custom PivotTable style a name.
- Select the element you want to format from the Table Element options. For example, I’ve chosen the Header Row .
- Click on Format .
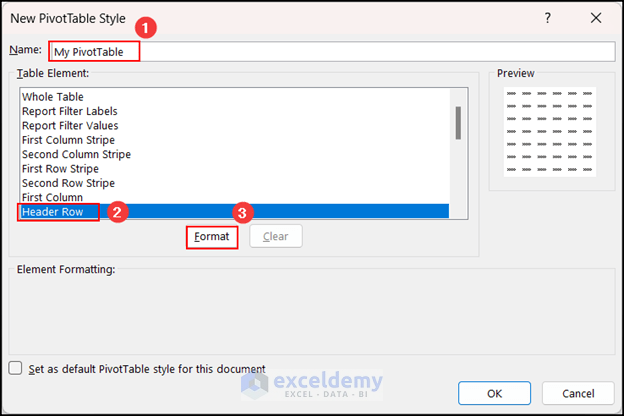
- Customize the cell formatting according to your preference. In my case, I’ve changed the Fill color.
- Check the Sample and press OK .
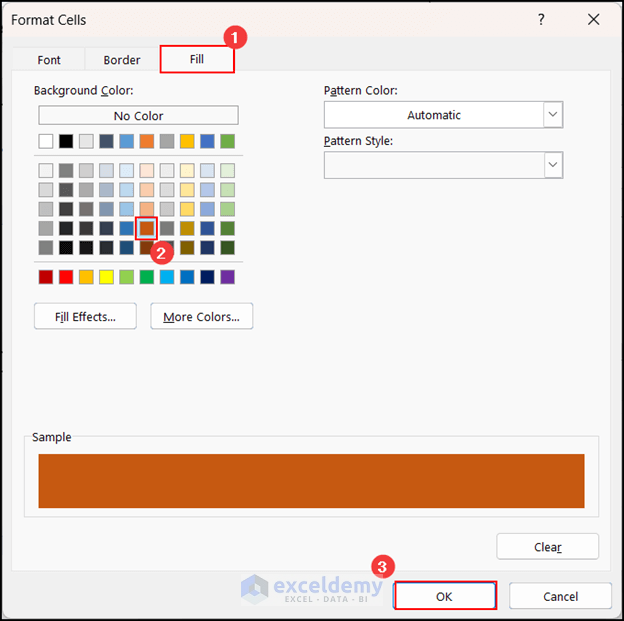
- After reviewing the Preview , click OK .
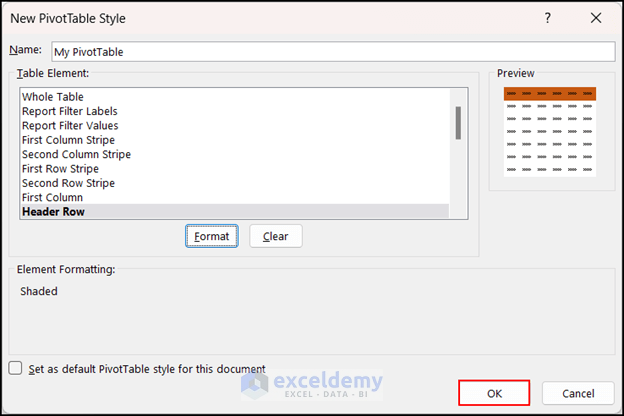
- You’ll now see your created PivotTable style listed in the PivotTable Styles command as Custom .
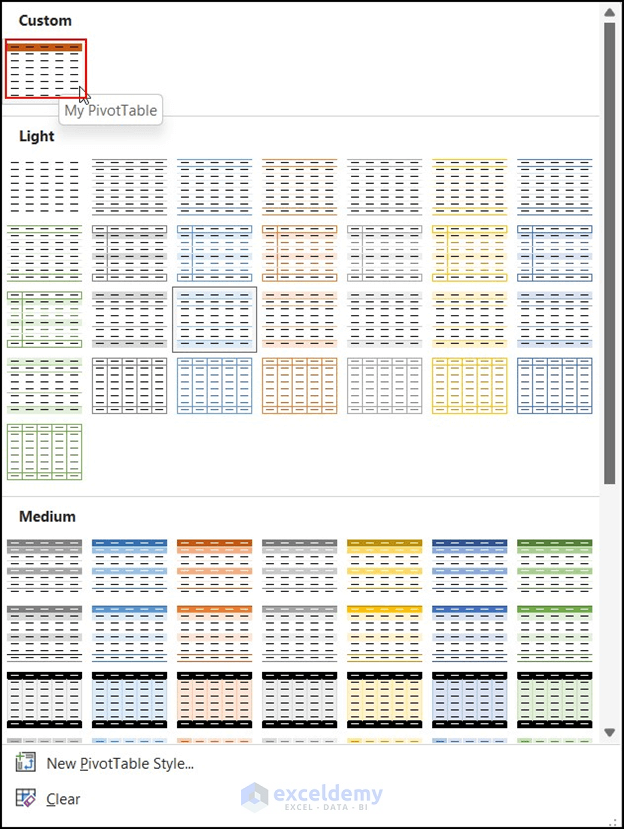
19. Change Layout of Pivot Table
- Click on Report Layout and choose the layout you want to display. I’ve selected Show in Compact Form .
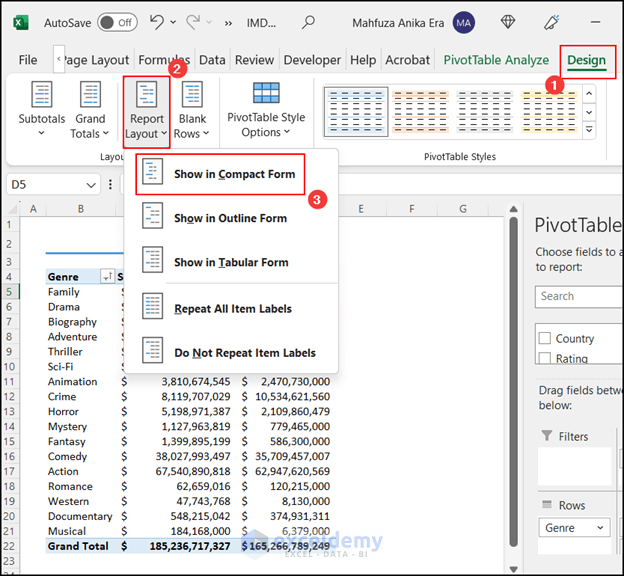
- The PivotTable will now appear in the Compact Form layout.
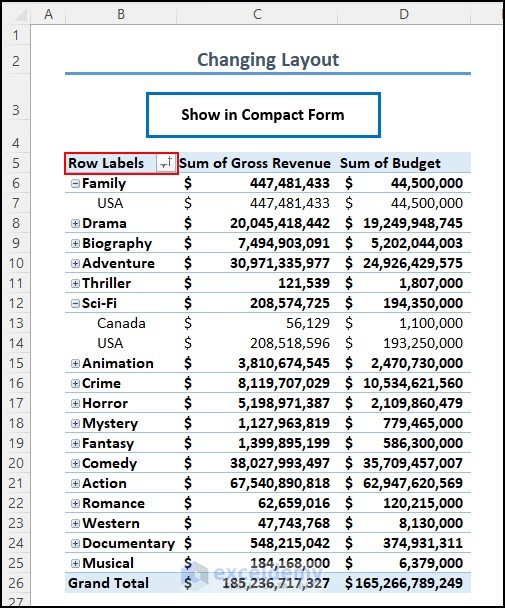
- If you choose Show in Tabular Form , it will look different.
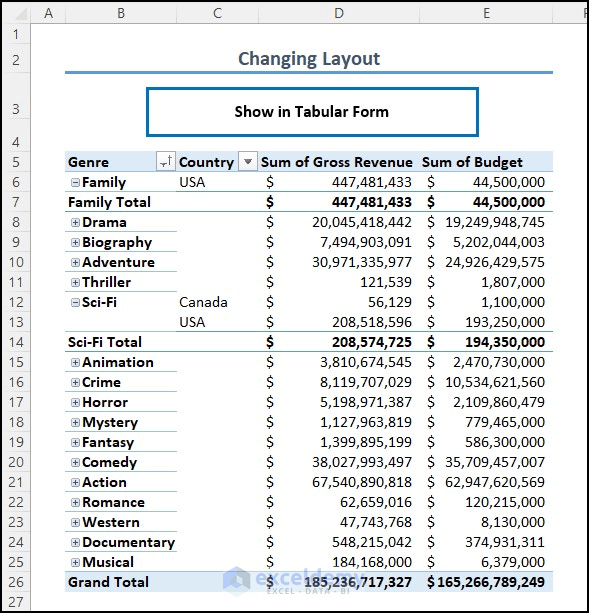
- Similarly, selecting Show in Outline Form will produce a different output.
- Choose a layout that best suits your table.
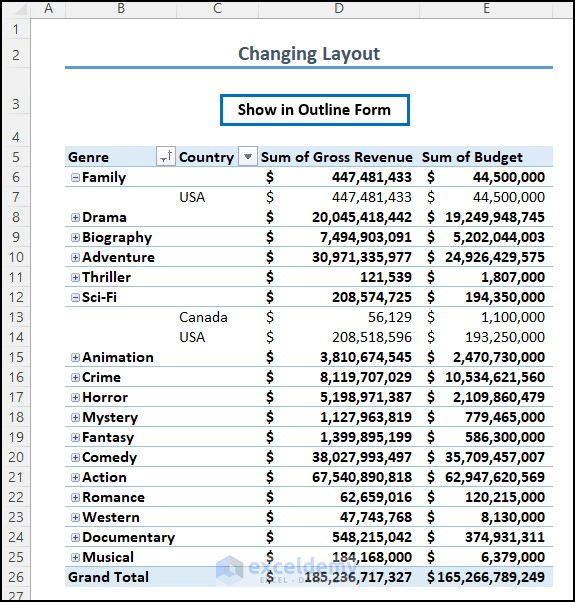
20. Restrict Column Width Change after Refresh
In a PivotTable, adjusting column widths according to your needs is common. However, after refreshing the table, the column widths automatically adjust to autofit the content. Unfortunately, this can sometimes affect the overall appearance of your table. To prevent this:
- In the following image, I’ve increased the column width to improve readability.
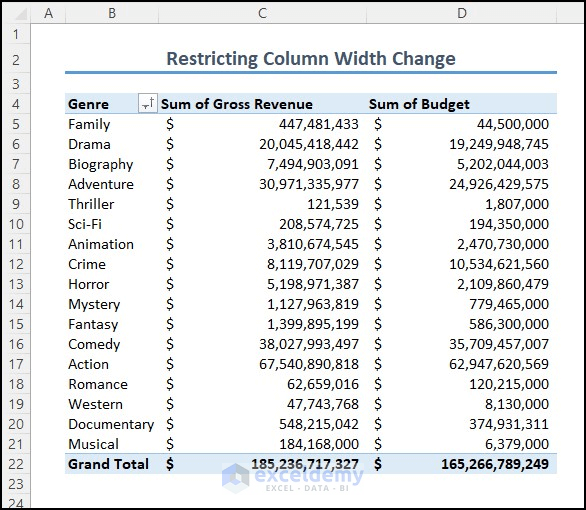
- Now, if I click on the Refresh button, the columns will autofit again.
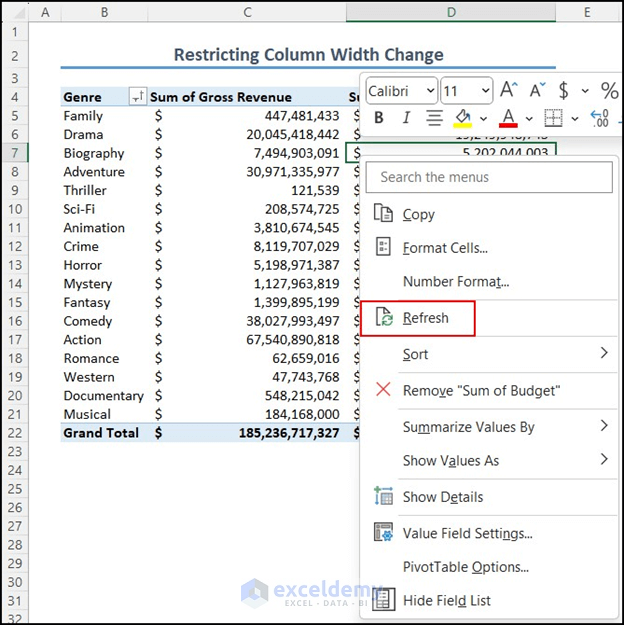
- To restrict column width changes after refresh, right-click on any cell within the PivotTable.
- Click on PivotTable Options.

- In the PivotTable Options dialog box, select the Layout & Format option.
- Uncheck the box that says Autofit column widths on update .
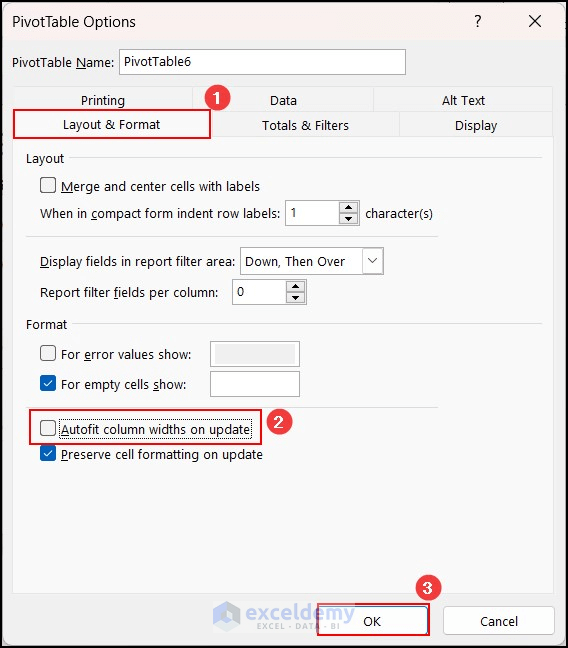
21. Display Items with No Data
In a PivotTable, some items may have no data associated with them. By default, the PivotTable hides the field names for these data-less items. However, you can display them using the following steps:

- In your dataset, there are hidden items that lack data.
- Right-click on any cell within the PivotTable.
- Click on Field Settings .
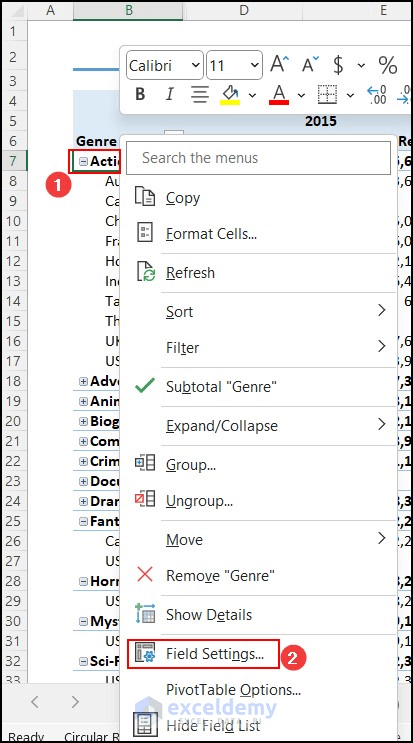
- The Field Settings dialog box will open.
- Select the Layout & Print option and then click on Show items with no data .
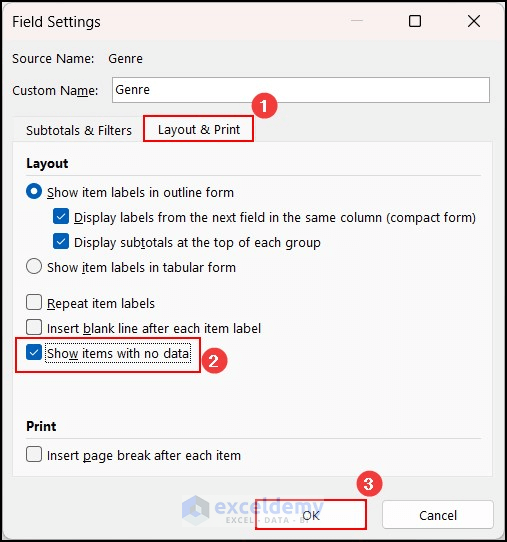
- As a result, the items that previously had no data will now be displayed.
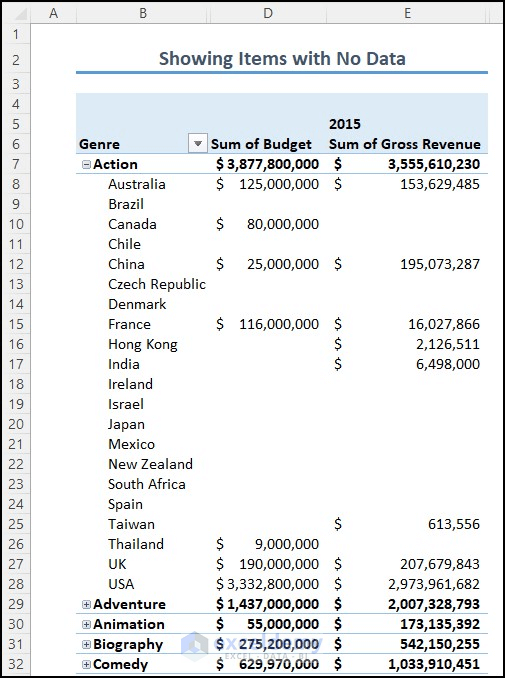
22. Substitute Blank Cells in Pivot Table
In a PivotTable, you can replace any blank cell with a value. If you want to provide additional information about these blank cells, follow this technique:
- Consider the dataset where there are many blank cells. For example, a country didn’t release any movies under the “Action” genre.
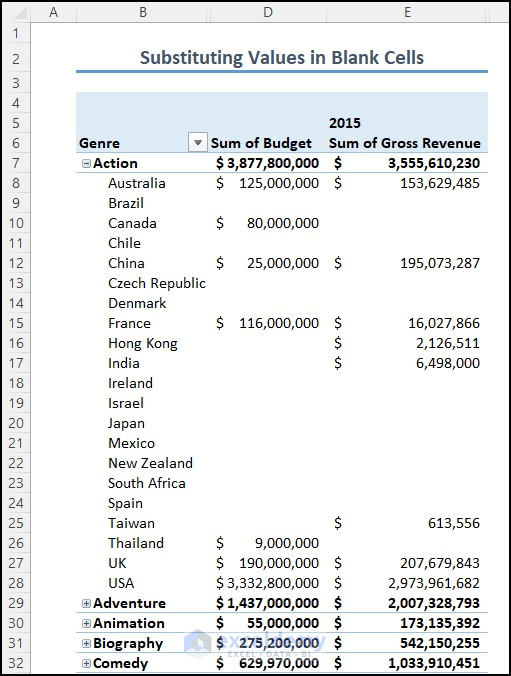
- Then, select PivotTable Options from the context menu.
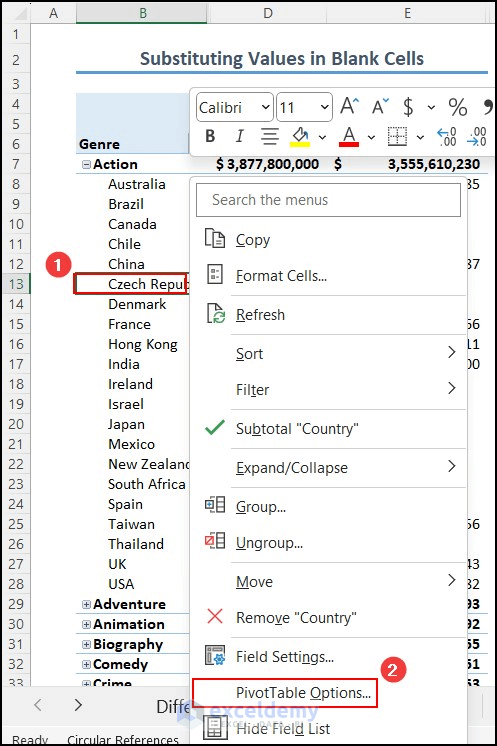
- In the PivotTable Options dialog box, click on Layout & Format .
- Write the text you want to substitute for the blank values in the For empty cells show box (e.g., “No Release”).
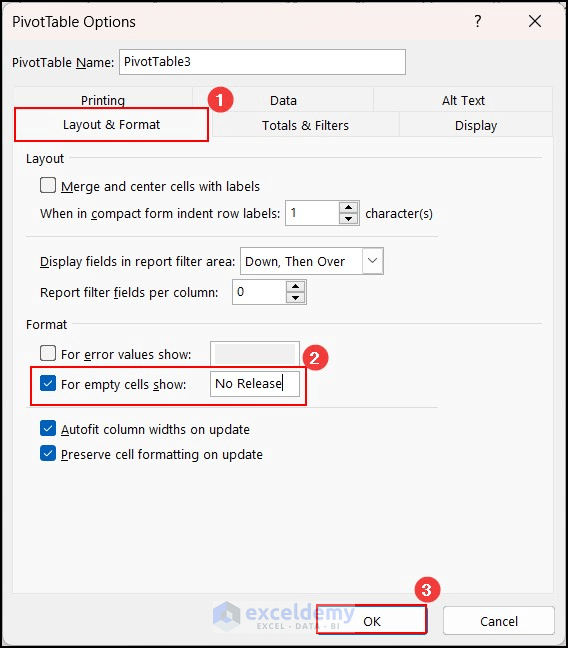
- The blank cells will now be substituted with the specified values.
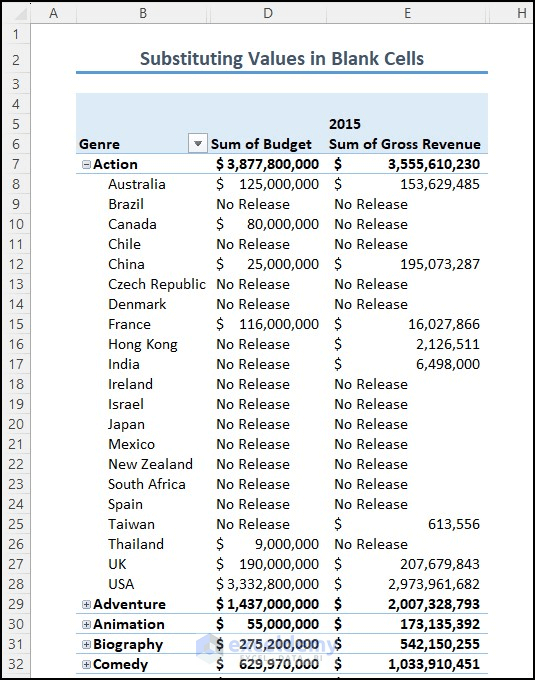
23. Attach Data Bars in Pivot Table
You can enhance your PivotTable by adding data bars. These bars provide a visual representation of data and make the table more attractive and easier to understand. Follow these steps:
- Then, select the Home tab.
- Go to Conditional Formatting and click on Data Bars , then choose More Rules…
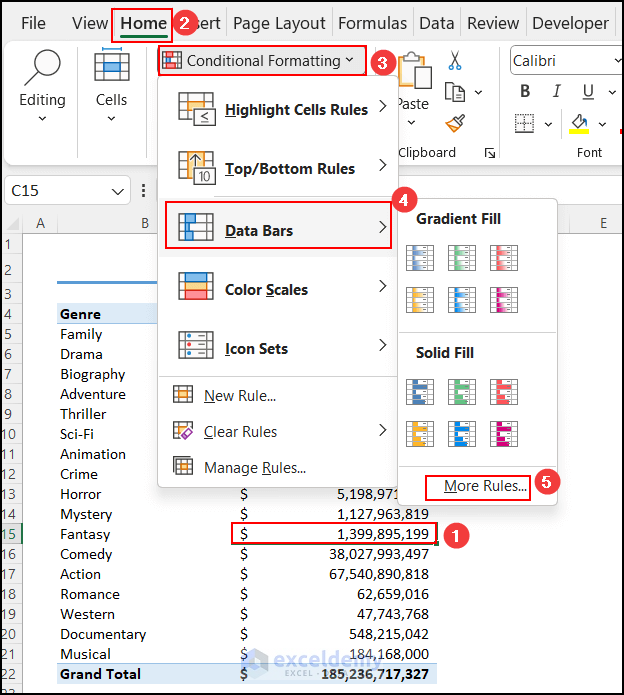
- The New Formatting Rule dialog box will appear.
- Select All cells showing ‘Sum of Gross Revenue’ values .
- Click on the Show Bar Only option if you want to display only the bars.
- Choose a color and check the preview.

- As a result, data bars will be added to the selected cells.
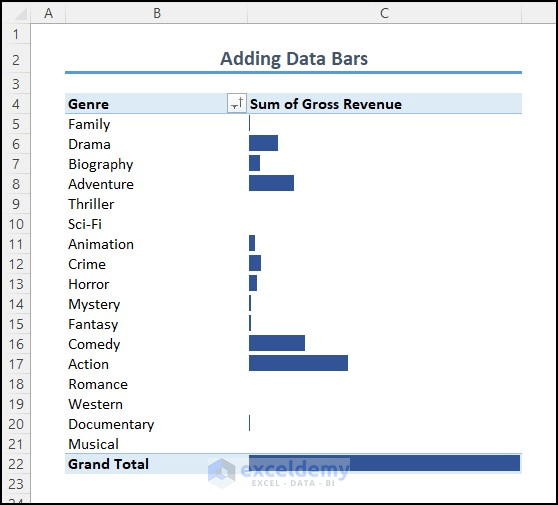
24. Create a Pivot Chart
Adding a Pivot Chart to your PivotTable can enhance the readability of your worksheet. Follow these steps to create a Pivot Chart from a Pivot Table:
- Go to the Insert tab.
- Click on PivotChart and select the desired chart type.
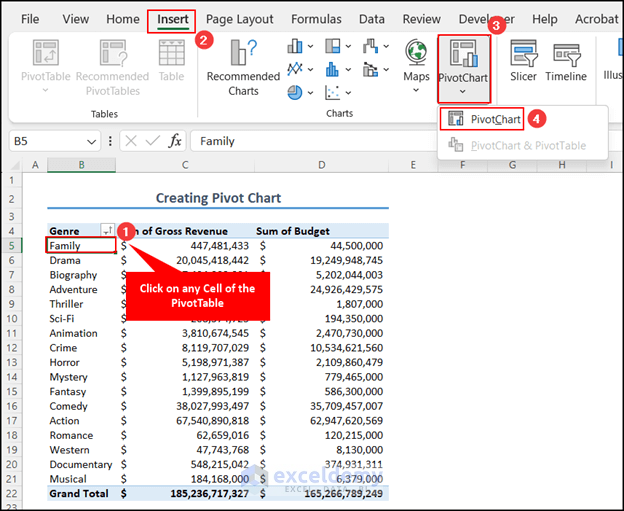
- The Insert Chart dialog box will appear.
- Choose the chart type you want (for example, Pie chart ).
- Click OK to insert the Pivot Chart.
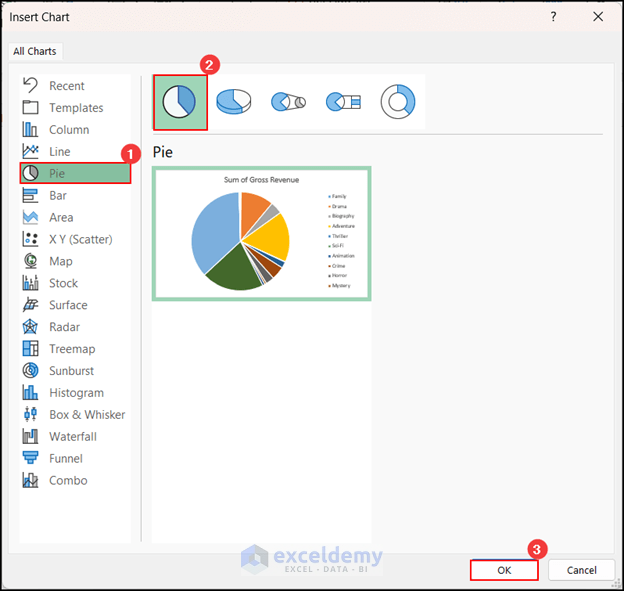
- A Pivot Chart is inserted.
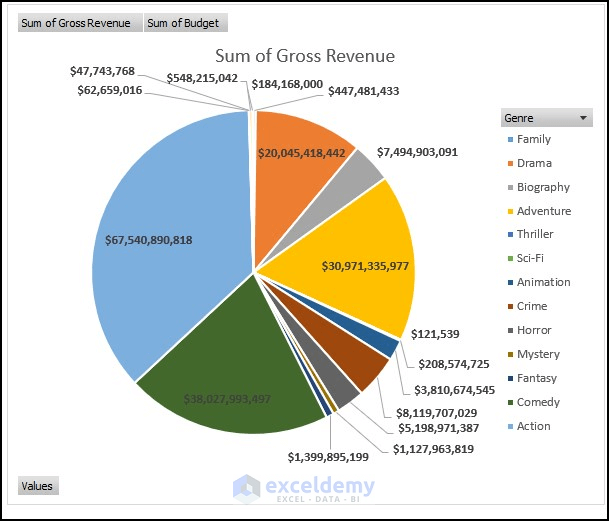
25. Create Multiple Pivot Tables
Suppose you have a dataset with two types of movies: Black and White and Color . You want to create separate PivotTables for each movie type. Here’s how you can do it:
- Click on Show and then select Field List .
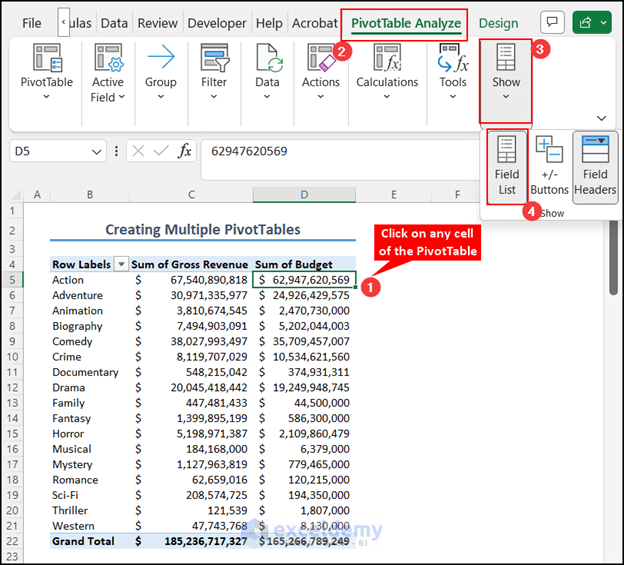
- The PivotTable Fields pane will open.
- Drag the field (e.g., Color/B&W ) to the Filters area.
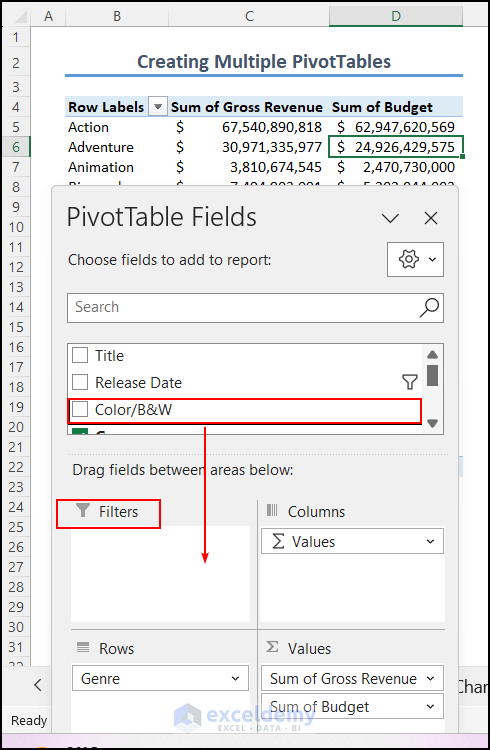
- This will insert a filter option into the existing PivotTable.

- The existing PivotTable is in a sheet named Multiple Pivot Tables .
- Now create two separate PivotTables based on the filter options:
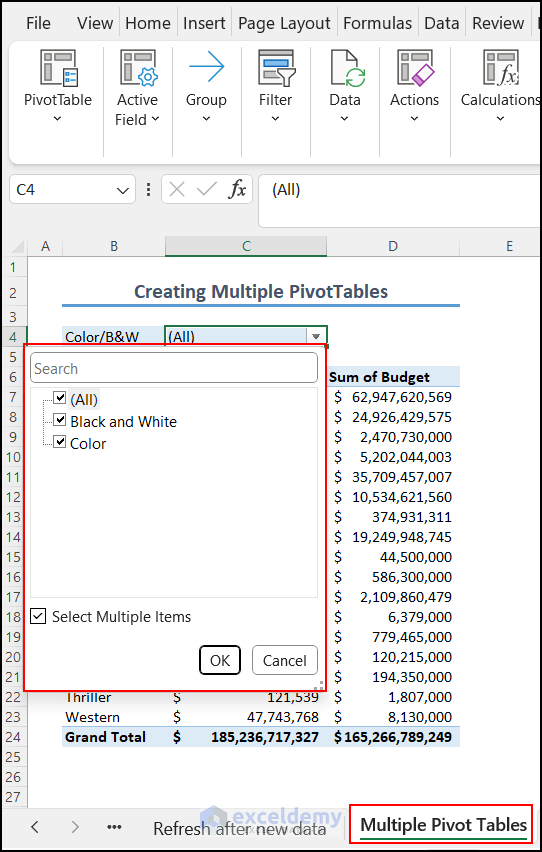
- Go to PivotTable Analyze > PivotTable > Options .
- Click on Show Report Filter Pages…
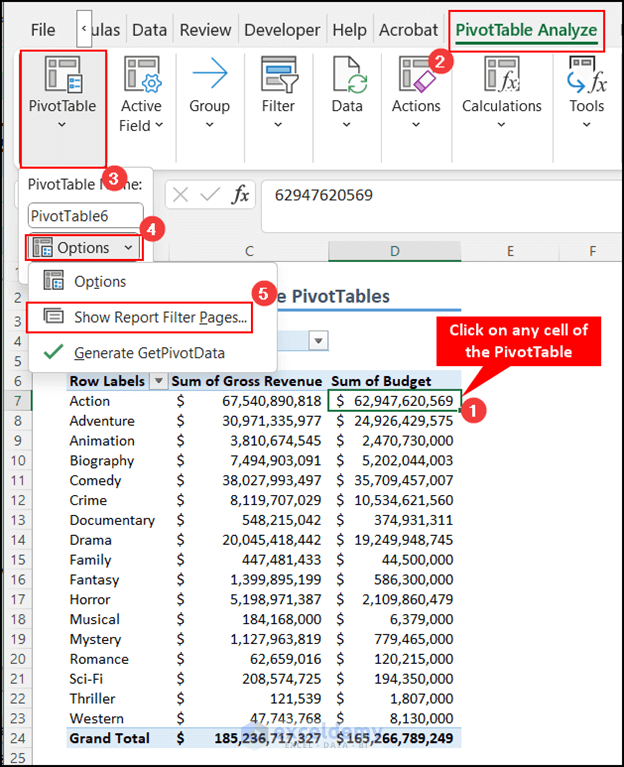
- Select the filter item from the Show Report Filter Pages dialog box.

- You can see another two PivotTables have been inserted based on the filter options. The name of the worksheets is based on the filter options.

Apply Keyboard Shortcuts to Enhance Productivity with Pivot Table
Using keyboard shortcuts in Excel is always helpful. Here are some PivotTable-related keyboard shortcuts that can save you time and effort:
Things to Remember
- Select the Appropriate Data Range: When creating a PivotTable, make sure to choose the relevant data range. Avoid including unnecessary rows or columns.
- Regularly Refresh Your PivotTable: If your data source changes or updates frequently, remember to refresh your PivotTable to reflect the latest data.
- Use Clear and Descriptive Field Names: When working with a large dataset, use field names that are easy to understand and describe the data accurately.
- Choose the Right Summary Function: Depending on the type of data you want to analyze (e.g., numeric values, counts, averages), select the appropriate summary function for your PivotTable.
Frequently Asked Questions
1. What’s the differences between a pivot table and a pivot chart?
- A Pivot Table is a data analysis tool that summarizes and aggregates data based on specific criteria (rows, columns, values).
- A Pivot Chart is a graphical representation of the data within a Pivot Table. It helps visualize trends and patterns by converting data into different types of graphs (e.g., bar charts, line charts, pie charts).
2. Are there any limitations to advanced pivot tables?
While advanced Pivot Tables are powerful, they may face limitations:
- Handling very large datasets could impact performance.
- Complex calculations might slow down processing.
- Customizations may be less flexible compared to specialized data analysis tools. Remember these tips and insights to make the most of your PivotTable experience!
Download Practice Workbook
You can download the practice workbook from here:
What is ExcelDemy?

Mahfuza Anika Era graduated from the Bangladesh University of Engineering and Technology in Civil Engineering. She has been with ExcelDemy for almost a year, where he has written nearly 30 articles and reviewed many. She has also worked on the ExcelDemy Forum and solved 50+ user problems. Currently, she is working as a team leader for ExcelDemy. Her role is to guide his team to write reader-friendly content. Her interests are Advanced Excel, Data Analysis, Charts & Dashboards,... Read Full Bio
Leave a reply Cancel reply
ExcelDemy is a place where you can learn Excel, and get solutions to your Excel & Excel VBA-related problems, Data Analysis with Excel, etc. We provide tips, how to guide, provide online training, and also provide Excel solutions to your business problems.
Contact | Privacy Policy | TOS
- User Reviews
- List of Services
- Service Pricing
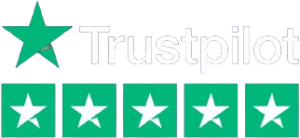
- Create Basic Excel Pivot Tables
- Excel Formulas and Functions
- Excel Charts and SmartArt Graphics
- Advanced Excel Training
- Data Analysis Excel for Beginners

Advanced Excel Exercises with Solutions PDF

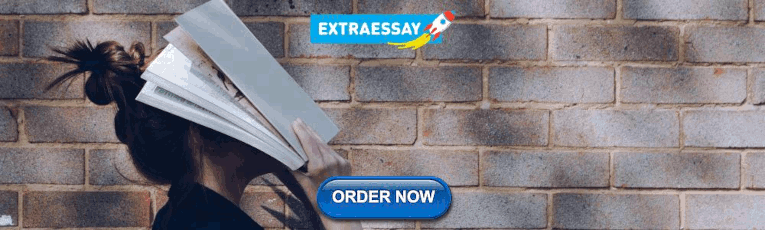
IMAGES
VIDEO
COMMENTS
A slicer is a visual representation of filtering options. You can display multiple slicers and filter the table by multiple values from each. ... options in the other slicer may become unavailable—indicating that there are no data available for that option under the current filtering conditions. Converting Tables to Ranges. Although Excel ...
C. Utilizing filter options for different chart types (e.g., bar chart, pie chart) 1. Bar chart: ... By demonstrating how filtering affects the visual representation of data, users can gain a better understanding of the impact it has on data visualization. Using examples to illustrate how filtered charts can enhance analysis.
Here are some important data visualization techniques to know: 1. Pie Chart. Pie charts are one of the most common and basic data visualization techniques, used across a wide range of applications. Pie charts are ideal for illustrating proportions, or part-to-whole comparisons.
Leverage data visualization tools like Tableau or Power BI to create visual representations of your filtered data. These tools facilitate a more intuitive and comprehensive analysis, allowing you to identify trends, patterns, and outliers efficiently. ... With advanced filtering options, it streamlines the analysis process, allowing user ...
Choosing the appropriate chart type is crucial for effectively representing your data. Excel offers a wide range of chart options, including bar charts, line charts, pie charts, scatter plots, and more. Consider the nature of your data and the message you want to convey when selecting the most suitable chart type. Formatting and Customization
Andrew Hills (Member) asked a question. Visual Representations of filters. Hi All, I suspect the answer is that it can't be done, but I'll ask the question anyway. My users complain that they can't tell what is being filtered and what isn't (Yes, yes, yes, I know. Trust me I've knock some of the plaster off the office wall with my head).
Chapter 11. Data visualization principles. We have already provided some rules to follow as we created plots for our examples. Here, we aim to provide some general principles we can use as a guide for effective data visualization. Much of this section is based on a talk by Karl Broman 30 titled "Creating Effective Figures and Tables" 31 and ...
Interactive Data Visualization. Data visualization is the process of creating a visual representation of information. For centuries, people have been using static data visualizations — the map being among the oldest and most famous examples. Every aspect of data analysis has evolved with technological developments, including data visualization.
Data visualization has come a long way since the early days of spreadsheet applications. Today, data visualization experts have a wide array of tools at their disposal to create stunning visual representations of data. These visuals can help us make sense of what might otherwise seem like an overwhelming amount of information.
Edges are important for two main reasons. 1) Most semantic and shape information can be deduced from them, so we can perform object recognition and analyze perspectives and geometry of an image. 2) They are a more compact representation than pixels. We can pinpoint where edges occur from an image's intensity profile along a row or column of the ...
1.1.1 Representation of the filter function . One aspect to consider when designing a graphical interface is how to represent a feature. Many guidelines suggest to opt for a combined display or to include icon + label, rather than just the icon or the label alone. The option to use the icon alone is not advisable: the meaning can be misunderstood.
At its core, data visualization is the art and science of representing data graphically. By utilizing visual elements like charts, graphs, and maps, it transforms complex datasets into visual formats that are easily interpretable. Beyond aesthetics, effective data visualization tells a story, making data accessible and facilitating informed ...
A single-option label doesn't always provide enough context. Sometimes it's necessary to provide additional information for each option. A select card allows you to add context to each option so that users can choose the right one. The large area of the card allows users to make selections easier. It also makes the cue of the selected card ...
This prevents skewing the visual representation and ensures a fair and accurate portrayal of data. ... In an insurance claims management system, robust data aggregation, and filtering options can greatly enhance the effectiveness of data visualization. Data Aggregation: The system could aggregate claims data at various levels, such as by type ...
Basic visual representation of zip code data. ... In the filtering step, data can be filtered in real time, as in the Zipdecode application. During visual refinement, changes to the design can be applied across the entire system. For instance, a color change can be automatically applied to the thousands of elements that require it, rather ...
Here, I discuss the top five strategies for making data visualization accessible in business: Implement Intuitive Visualization Tools. Data visualization tools should be designed for ease of use, involving software that accommodates different levels of expertise and offers features like interactive filtering options and drill-down capabilities.
Common Filter Options in Power BI Visuals. 1. Basic Filters. Column Filters: Users can filter data by selecting specific values within a column, limiting the visual to display only the chosen data points. Relative Date Filters: This option allows users to filter data based on relative time periods, such as the last 7 days or the next month.
Visual Representation refers to the principles by which markings on a surface are made and interpreted. Designers use representations like typography and illustrations to communicate information, emotions and concepts. Color, imagery, typography and layout are crucial in this communication. Alan Blackwell, cognition scientist and professor ...
From there, choose your preferred filter type and set the filter criteria by selecting a column from the available options and setting the relevant values. Another way to create a filter is by using the visual level filtering option, which allows you to apply filters directly to a visualization, regardless of other filters in the report.
The Mass Filter Visual takes interactivity and data exploration to the next level by allowing users to filter multiple selections in one go by copying and pasting multiple records.
Select Table/Range Option. To begin, select any cell of the dataset. Then open the Insert Tab >> select PivotTable >> click on From Table/Range. PivotTable from table or range dialog box will open up. The Table/Range will be automatically set as you clicked the cell of the dataset previously.
This paper proposes a novel infrared and visible image fusion method based on domain transform filtering (DTF) and sparse representation (SR). First, infrared and visible images are decomposed using a low-pass filter into base and detail layers, respectively. An SR-based rule is designed to fuse the detail layers.
Such approaches can also produce visual representations at an efficient size, which are essential for fast place recognition. Nevertheless, most metric learning approaches only consider the pairwise similarity while ignoring the internal relationship among the images of the entire set. ... When filtering is applied to Gardens Point (Fig. 14 (b ...
A technology based on Kalman filtering method combined with multi-channel gain training reinforcement learning for uncalibrated camera visual servo tasks is proposed in this paper. First, a dynamic system with state variables formed from the elements of the image Jacobian matrix is constructed to describe the mapping relationship between two ...