Inorganic Syntheses
The inorganic syntheses series.
There is no rigid definition of what constitutes a suitable synthesis. The major criterion by which syntheses are judged is the potential value to the scientific community. For example, starting materials or intermediates that are useful for synthetic chemistry are appropriate. The synthesis also should represent the best available procedure, and new or improved syntheses are particularly appropriate. Syntheses of compounds that are available commercially at reasonable prices are not acceptable. We do not encourage the submission of compounds that are unreasonably hazardous, and this connection, less dangerous anions generally should be employed in place of perchlorate.
The Inorganic Syntheses series is NOT a repository of primary research data, nor is it the place to report new syntheses. It is expected that all syntheses accepted for publication will have already appeared in the primary, peer-reviewed research literature. Thus those syntheses that are found to be widely used by the scientific community or provide a new synthetic entry to a broad range of compounds are most desired for the series. The series offers authors the chance to lay out intricacies of synthesis and purification in greater detail than possible in the original literature, as well as to provide updates of a tried-and-true synthesis.
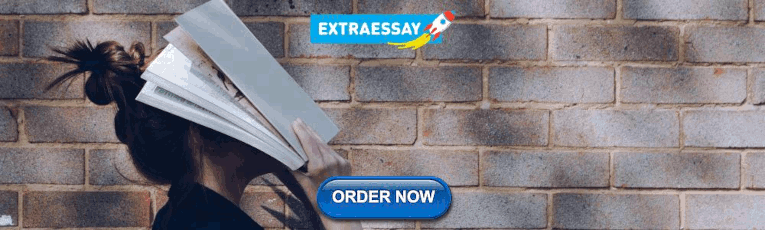
The Inorganic Syntheses Organization
Organization Bylaws (pdf)
Inorganic, Organometallic, and Bioinorganic Synthesis, Spectroscopy, and Catalysis
Inorganic Synthesis PDFs (vol. 1-34)
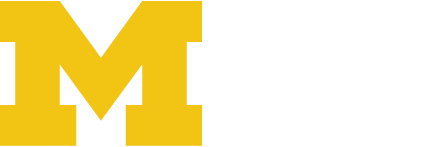
Thank you for visiting nature.com. You are using a browser version with limited support for CSS. To obtain the best experience, we recommend you use a more up to date browser (or turn off compatibility mode in Internet Explorer). In the meantime, to ensure continued support, we are displaying the site without styles and JavaScript.
- View all journals
- My Account Login
- Explore content
- About the journal
- Publish with us
- Sign up for alerts
- Data Descriptor
- Open access
- Published: 25 May 2022
Dataset of solution-based inorganic materials synthesis procedures extracted from the scientific literature
- Zheren Wang 1 , 2 ,
- Olga Kononova ORCID: orcid.org/0000-0001-9267-312X 1 , 2 ,
- Kevin Cruse 1 , 2 ,
- Tanjin He ORCID: orcid.org/0000-0001-8834-8703 1 , 2 ,
- Haoyan Huo ORCID: orcid.org/0000-0003-2227-9121 1 , 2 ,
- Yuxing Fei 1 , 2 ,
- Yan Zeng 2 ,
- Yingzhi Sun ORCID: orcid.org/0000-0003-3559-6863 1 , 2 ,
- Zijian Cai 1 , 2 ,
- Wenhao Sun ORCID: orcid.org/0000-0002-8416-455X 3 &
- Gerbrand Ceder ORCID: orcid.org/0000-0001-9275-3605 1 , 2
Scientific Data volume 9 , Article number: 231 ( 2022 ) Cite this article
6107 Accesses
23 Citations
1 Altmetric
Metrics details
- Cheminformatics
- Computational methods
- Design, synthesis and processing
- Materials science
The development of a materials synthesis route is usually based on heuristics and experience. A possible new approach would be to apply data-driven approaches to learn the patterns of synthesis from past experience and use them to predict the syntheses of novel materials. However, this route is impeded by the lack of a large-scale database of synthesis formulations. In this work, we applied advanced machine learning and natural language processing techniques to construct a dataset of 35,675 solution-based synthesis procedures extracted from the scientific literature. Each procedure contains essential synthesis information including the precursors and target materials, their quantities, and the synthesis actions and corresponding attributes. Every procedure is also augmented with the reaction formula. Through this work, we are making freely available the first large dataset of solution-based inorganic materials synthesis procedures.
Similar content being viewed by others
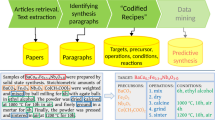
Text-mined dataset of inorganic materials synthesis recipes
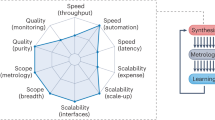
Combinatorial synthesis for AI-driven materials discovery
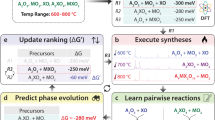
Autonomous and dynamic precursor selection for solid-state materials synthesis
Background & summary.
Big-data-driven approaches have helped to establish a new paradigm of scientific research 1 , 2 , 3 . In materials science specifically, the Materials Genome Initiative (MGI) effort has significantly facilitated and accelerated materials discovery and design by deploying large-scale ab initio computation and building computed databases of structure–property relationships 4 , 5 , 6 . Unlike computational data, the experimentally determined properties and structures of inorganic materials are mainly available in manually curated databases 7 , 8 , 9 , 10 , 11 . Such well-curated experimental databases have led to early machine learning models that address difficult problems in materials research, such as structure prediction 3 . The ability to efficiently design and predict the structure of novel advanced materials with the assistance of computed and experimental databases has shifted the materials innovation challenge toward understanding and determining the synthesis routes for novel materials 12 . In principle, this challenge could also be mastered using data-driven approaches, since only limited predictive theories are available for materials synthesis 13 , 14 , 15 , 16 , 17 , 18 , 19 , 20 , 21 , 22 , 23 . Indeed, in organic chemistry, AI-guided synthesis planning 24 , 25 has already been successfully implemented in certain cases, such as in predicting retrosynthesis 26 and in complex natural product synthesis design 27 . Although datasets for the synthesis of organic materials are widely available 28 , 29 , there is not yet a large-scale database of inorganic synthesis routes, which is needed to train advanced deep-learning models to enable a breakthrough in AI-assisted design and optimization of inorganic materials synthesis.
Scientific publications represent the largest repository of knowledge about materials synthesis and can be used as a reliable source of data. However, human-written descriptions of syntheses require additional levels of interpretation for conversion into a codified, machine-operable format. Additionally, manual extraction of synthesis information is laborious, even for a very limited number of papers 30 , 31 , 32 , 33 . Given these obstacles, an automated information extraction pipeline can accelerate data collection and assist in building structured synthesis procedures from scientific text. Natural language processing (NLP) approaches have been widely developed in the past decade, and various advanced tools for high-quality information extraction from unstructured text are available to researchers.
In materials science, NLP has been used to extract and analyze materials properties 34 , 35 , 36 , applications 37 , 38 , and synthesis conditions for some limited cases 39 . Various NLP tools, including ChemDataExtractor 40 , OSCAR 41 , ChemicalTagger 42 , and others 30 , 43 , 44 , have been developed to extract information from chemical text. Recently, advanced models based on deep convolutional and recurrent neural networks 45 , 46 , 47 , 48 have been proposed to improve the accuracy of chemical data extraction.
Text-mining approaches in materials science have also been used to construct automated pipelines for collecting information about materials synthesis from publications and to build large-scale publicly available datasets from such collected data, including datasets of synthesis formulations for metal oxides 39 , 49 , 50 , germanium-containing zeolites 51 , and perovskites 52 . In recent work, our group has developed a text-mining pipeline to construct the first large-scale dataset of solid-state ceramics synthesis “recipes”, which includes not only the starting materials and final products but also the synthesis actions, their attributes, and balanced chemical-reaction equations 53 .
In the current work, we built a more advanced extraction pipeline (Fig. 1 ) which uses various advanced machine learning and natural language processing techniques to extract precise data for solution-based inorganic materials synthesis procedures from the scientific literature. Solution procedures are considerably more complex than solid state synthesis and require the precise extraction of not only the chemicals involved but also their respective amounts (since they determine concentration in solution). In addition, more complex organic and mixed organic-inorganic compounds are used to solubilize ions or to control solution conditions. By applying the extraction pipeline, we codified 35,675 solution-based inorganic materials synthesis procedures from over 4 million papers. Extracted information includes target material and precursors, their quantities, and the synthesis operations and their attributes. Information about the targets and precursors is then used to build a reaction formula for every synthesis procedure. This dataset is the first large-scale dataset of solution-based synthesis procedures, and provides a foundation to test and verify existing empirical synthesis rules, improve prediction accuracy, and even data-mine new rules to guide synthesis. Also, this codified dataset should pave the way to design optimized synthesis procedures in automated experimentation.
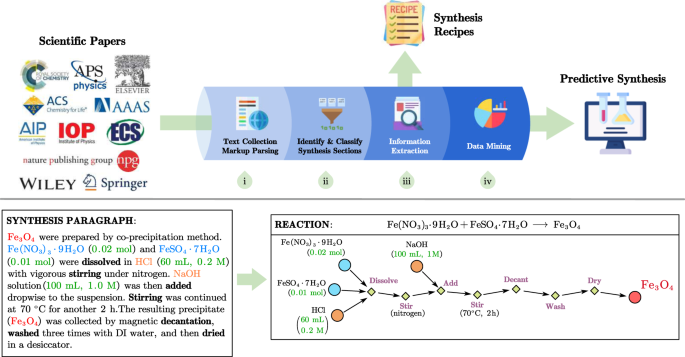
Extraction pipeline and example. Top panel: Schematic representation of the standard text mining pipeline: (i) scrape papers in markup format from the major publishers; (ii) identify and classify synthesis sections; (iii) extract key information including materials, amounts, sequenced operations, and conditions; (iv) store synthesis procedures into the database for future data mining. Bottom panel: Example of a codified procedure extracted from a synthesis paragraph.
Content acquisition
The journal articles used in this work were downloaded with publisher consent from Wiley, Elsevier, the Royal Society of Chemistry, the Electrochemical Society, the American Chemical Society, the American Physical Society, the American Institute of Physics, and Nature Publishing Group. A customized web-scraper, Borges (see Codes Availability section below), was used to automatically download a broad selection of materials-relevant papers published after the year 2000 from publishers’ websites in HTML/XML format. We selected 2000 as the cutoff year as parsing of materials science papers stored as image PDFs (as for most papers published before 2000) introduces a significant number of errors due to the limitations of currently available optical character recognition models on chemistry-containing text 54 , 55 .
To convert the articles from HTML/XML into raw-text files, we developed the LimeSoup toolkit (see Codes Availability section below), which takes into account the specific format standards of various publishers and journals. The full-text and metadata of the articles such as the journal name, article title, abstract, author names, etc., are stored in a MongoDB ( www.mongodb.com ) database collection. To date, we have accumulated 4.06 million articles, which are used for further processing down the pipeline (Fig. 1 ).
Paragraph classification
Paragraphs containing information about solution synthesis (referred to as “synthesis paragraphs” throughout this paper) were identified using a Bidirectional Encoder Representations from Transformers (BERT) model 56 . The model was pre-trained on full-text paragraphs of 2 million papers randomly drawn from our database in a self-supervised way, i.e., by predicting masked words based on their surrounding context. After training the BERT model, we fine-tuned the paragraph classifier using 7,292 paragraphs labeled as either “solid-state synthesis”, “sol-gel precursor synthesis”, “hydrothermal synthesis”, “precipitation synthesis”, or “none of the above”. The resulting F1 score of the paragraph classification is 99.5%, an improvement over the F1 score of 94.6% in our previous work 57 , when evaluated using the same labeled training dataset.
Synthesis procedure extraction
A solution-based synthesis procedure includes the precursors and target materials, their quantities, and the synthesis actions and their attributes, properly sequenced. This is the minimum essential information required to complete a synthesis route. A schematic representation of the procedure is shown in the bottom panel in Fig. 1 . In the sections below, we provide a brief overview of the methods used for each step of the procedure extraction.
Materials entity recognition (MER)
Materials entities in synthesis paragraphs are identified and classified as target , precursor , or other via a two-step sequence-to-sequence model as introduced in our previous work 46 . In the current work, we replaced the original Word2Vec embedding model used previously 58 with a BERT model trained on papers from the materials science domain (see Section “Paragraph classification” above). First, each word token was transformed into a digitized BERT embedding vector. A bi-directional long-short-term memory neural network with a conditional random-field top layer (BiLSTM-CRF) was used to determine whether the token was a materials entity or a regular word, and each materials entity was replaced with the keyword < MAT > before being classified as either a target , precursor , or other material using a second BERT-based BiLSTM-CRF network. In addition to the 834 annotated solid-state synthesis paragraphs from 750 papers used in our previous work 46 , we manually annotated 447 solution-based synthesis paragraphs from 405 papers by labeling each word token as material , target , precursor , or outside . The annotated dataset was split into training, validation, and test sets with a paper-wise ratio of 700:150:305 to train the aforementioned two neural networks.
Extraction of synthesis actions and attributes
We implemented an algorithm which combines a neural network and sentence dependency tree analysis to identify synthesis actions in the text. First, the Word2Vec model from the Gensim library 59 was re-trained on ~400,000 synthesis paragraphs of four synthesis types (see Section “Paragraph classification” above). These word embeddings were used as the input for a recurrent neural network that takes a sentence word-by-word and assigns labels to the verb tokens: not-operation , mixing , heating , cooling , shaping , drying , or purifying . For each obtained synthesis action, we parsed a dependency sub-tree using the SpaCy library 60 to obtain information about the corresponding temperature, time, and environment. To extract the corresponding values of these attributes, we used a rule-based regular expression approach 61 .
Extraction of material quantities
To extract the numerical values of material quantities and assign them to the corresponding materials obtained using the MER model (see Section “MER” above), we applied a rule-based approach to search along the syntax tree 61 . The NLTK library 62 was used to build the syntax trees for each sentence in a paragraph. The words in given sentences are leaf nodes of syntax trees. We then applied an algorithm to cut the syntax tree of each sentence into the largest sub-trees for every material, with each sub-tree having only one material entity: 1. we first identified the materials on leaf nodes; 2. starting from each material, we identified the largest sub-trees, i.e., we traversed the syntax tree upwards until there was more than one material leaf node descending from the same node; 3. the largest sub-tree for a given material was defined as the sub-tree formed by the node and its descendants identified in step 2. Next, we searched for the quantities in each sub-tree given as molarity, concentration, or volume. Finally, we assigned the quantities found to the unique material entity in the sub-tree.
Building reaction formulas
For every synthesis procedure described in a paragraph, we built a chemical formula. Every material entity was converted from a text-string representation into a chemical-data structure using an in-house material parser toolkit (see Codes Availability section below). The data structure included information about the material formula, composition, and ions. We then paired the target with precursors containing at least one element in the target except for hydrogen and oxygen and defined those precursors as “precursor candidates”. Next, we computed the oxidation state change of elements from each “precursor candidate” to the target and determined whether the precursor was oxidized or reduced. If precursors were reduced or oxidized, we also included the corresponding redox agents in the reaction formula. The agents can either be another “precursor candidate” or a commonly used oxidizing or reducing agent from the remaining material entities marked as other or precursor by the MER algorithm (see Section “MER” above).
Dataset generation
The dataset generation followed the protocol displayed in Fig. 1 . We downloaded a total of 4,061,814 papers using web scraping and identified the experimental sections by keyword matching in section headings, with keywords including “experiment”, “synthesis”, “preparation”, and their morphological derivations. ChemDataExtractor 40 was used to split the plain-text paragraphs into sentences and words. After classification (see Section “Paragraph classification” above), 364,076 paragraphs describing solid-state, hydrothermal, sol-gel, and precipitation syntheses were obtained. Among them, 189,553 paragraphs described hydrothermal or precipitation syntheses, which we categorize as solution-based synthesis methods. These paragraphs were further processed to extract the precursors, targets, quantities, and operations with corresponding conditions and to build the reaction formula (Fig. 1 ).
Data Records
The solution-based synthesis dataset is provided as a single JSON file, available at https://doi.org/10.6084/m9.figshare.16583387.v4 63 . There are 20,037 hydrothermal synthesis reactions and 15,638 precipitation synthesis reactions. Each record corresponds to a synthesis procedure extracted from a paragraph and is represented as an individual JSON object. If a paragraph reported the synthesis of several materials, the corresponding reactions were split into separate data records. In addition to the chemical formula, the metadata for each reaction returns the data structure used in our previous work 53 , which includes: DOI of the paper, a snippet of the corresponding synthesis paragraph (50 first and 50 last characters to facilitate its lookup), chemical information about the target and precursor materials used in the reaction, and operations with their corresponding attributes. We also included the materials with their corresponding quantities in the metadata. The details of the data format are given in Table 1
The chemical formula for the reaction is stored as a string ( reaction_string ) as well as in a dictionary containing lists of precursors ( left_side ) and target materials ( right_side ) in the reaction.
The metadata for target materials and precursors used to construct the chemical formula are represented by the following data structure:
material_string : string of material as given in the original paragraph before being parsed into a chemical composition.
material_formula : chemical formula associated with the material (given originally or constructed empirically by parser).
composition : chemical composition of the material derived from its formula. Aside from single-compound materials, we found that a large portion of the materials (predominantly target materials) are composites, mixtures, solid solutions, or alloys written as a sequence of compound-fraction pairs. Therefore, a chemical-composition entity is represented by a list of dictionary entries, where each item is associated with a compound found in the materials formula. The fraction of each compound in the material is given in amount , and its chemical composition (i.e., the elements and stoichiometry) is given in elements . If a material is one compound, the list has only one item and amount = 1.0. If a material is a hydrate, water is added to the composition list with its amount corresponding to the amount of water molecules (if specified).
additives : list of additive elements (i.e., elements used for doping, stabilization, or substitution) resolved from the material string.
elements_vars : lists all variable elements and their corresponding values found in the materials.
amounts_vars : lists all variable element ratios and their corresponding values found in the material formula. The values of each variable are given as a structure with values listing the values of each specific variable and max_value/min_value values if a range is given in the paragraph.
oxygen_deficiency : yes/no attribute that reflects whether a material was synthesized with unspecified oxygen stoichiometry.
mp_id : ID of the lowest-energy polymorph entry in the Materials Project database ( https://materialsproject.org/materialsproject.org ) if the material is found there.
To facilitate querying of the dataset, the targets_string field contains the target material formulas, and the solvents field contains all solvent(s) from matching material entities marked as other by the MER model with a table of common solvents adopted from Common Solvents Used in Organic Chemistry ( https://organicchemistrydata.org/solvents/organicchemistrydata.org/solvents ).
Technical Validation
Extraction completeness and accuracy.
To ensure high accuracy of the dataset, we included only those data that produced complete reaction formulas at the final step of the pipeline. This strategy reduced potential errors in the dataset that may have been caused by composition-parsing failure, incomplete extraction, or incomplete information provided by the text. We applied the extraction pipeline to 189,553 solution-based synthesis paragraphs, 28,749 of which generated a reaction formula, giving an extraction yield of ~15%. To evaluate the source of the loss, we randomly selected and manually checked 100 solution-based synthesis paragraphs that did not produce any reactions. Among those 100 paragraphs, 36 were written with an incomplete list of precursors or targets in the text, such that human experts would not be able to reconstruct the reaction based solely on the information provided in the paragraph. For the remaining 64 paragraphs, the loss was due to: 1. the use of organic precursors with complex groups or complicated notation (e.g., acronyms) that could not be parsed into a chemical composition by our parser or 2. MER misidentification resulting in an incomplete or incorrect list of precursors and (or) target entities such that the reactions could not be built.
To evaluate the quality of the dataset, we had a human expert test 100 randomly pulled entries. The human expert manually extracted the information presented in the procedure, and the results were compared with those extracted by the pipeline. Table 2 presents the accuracy statistics, which include the precision, recall, and F1 scores calculated from the tested entries. For the fields that included reaction, targets, precursors, operations, operation temperatures, time, and atmosphere, the F1 scores were over 90%. The relatively low recall, and hence F1 score, for the extraction of materials quantities can be mainly explained by the MER algorithm missing the corresponding material entity and, thus, the quantities not being assigned. The accuracy of the obtained dataset is comparable to that in our previous work 53 and of other text-mined datasets 49 .
Exploratory data analysis
To test the diversity of the dataset and its coverage of the materials space, we analyzed unique materials (targets and precursors) and reactions. The dataset contains 11,603 unique reactions that include 2,870 unique precursors and 5,416 unique targets. The ten most frequent targets in the dataset and their corresponding precursors are listed in Table 3 . The target list captures materials that have drawn substantial attention in the past two decades: catalysts (ZnO, Fe 2 O 3 , TiO 2 , Fe 3 O 4 , SnO 2 , ZrO 2 , CuO), adsorbents (SiO 2 ), various materials for sensors (ZnO, Fe 2 O 3 , WO 3 ), quantum dots (CdS), and semiconductors (ZnO, TiO 2 , SnO 2 , CdS). Unsurprisingly, these most frequent target materials usually appear in multiple applications, as they possess desirable physical and chemical properties in many scientific and engineering fields.
We use the periodic table representation (Fig. 2 ) to visualize the chemical space covered by the dataset. For each element, the fraction of synthesis procedures containing this element in the target formula is shown with the yellow-to-navy blue gradient framed at the top of each element box. The most data-rich elements are transition metals in the third period, such as Zn, Fe, Ti, Ni, and Co, in accordance with the compounds listed in Table 3 . The next-most prevalent targets are materials with Bi, Sn, Al, W, Mo, Cu, Zr, or Li. The least common elements are rare elements such as Ru, Rh, Hf, Ta, Re, and Ir. The elements Fr, Ra, Tc, and Pm are not present as target materials in the dataset, likely due to their radioactivity. Additionally, we calculated the frequency of co-occurrence of chemical elements and common ions in precursor materials to understand how different ions are brought into solution. In Fig. 2 , the frequencies for each ion are displayed as colored bars. The length of the bar is the fraction of one specific ion paired with the element normalized over all precursors for this element.
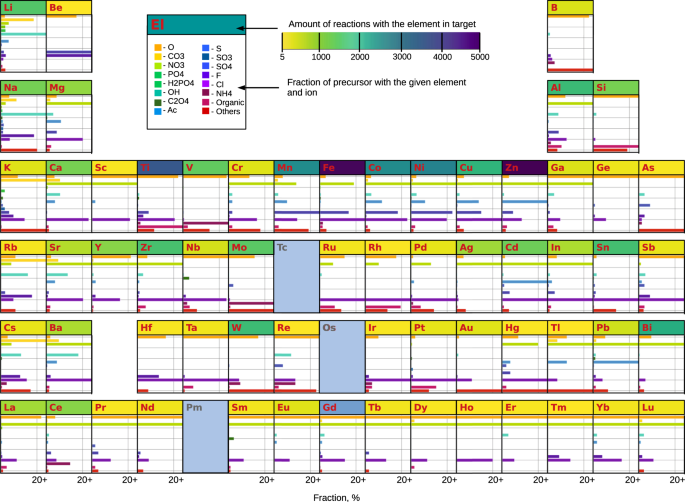
The chemical space covered by the dataset. For each element, the box containing the element name is colored in a yellow-to-navy blue gradient representing the total amount of reactions that produce a target compound containing the element. The bar graph below each element shows the list of ions paired with the element in precursor compounds. The fractions of the precursors (i.e. element + ion) used are shown by the length of the bars. Boxes with no bar graph represent elements occurring in five and fewer targets. “Ac” stands for acetate radical CH 3 COO − in the compound formula.
The commonly used precursors are mainly those that are widely available from companies such as Sigma-Aldrich and Fisher Scientific. For example, Li 2 CO 3 or LiOH for Li and sulfate or chloride for Fe. Inorganic salts, such as nitrates, sulfates, and chlorides, are often used because of their high solubility 64 . Halides are not used for Lanthanide metals because Lanthanide halides are highly hygroscopic, and thus their molar weight is not well defined. We observed that precursors with neighboring elements in the periodic table tend to have similar ions paired. For instance, nitrates, sulfates, and chlorides are commonly used anions for 3rd-period transition metals, whereas the precursors for lanthanides are mostly oxides and nitrates.
We used information about the extracted materials and sequences of synthesis actions to classify the solution-based synthesis procedures into four categories of synthesis protocols (table in Fig. 3 ) according to the following definitions:
solution-mixing with heat treatment step has a final heat treatment step after the precipitate is obtained from the solution;
aqueous solution synthesis has no final heat treatment step after precipitating the compound from the solution and the solvent is water;
non-aqueous solution synthesis has no final heat treatment step after precipitating the compound from solution and the solvent(s) is (are) organic;
aqueous–non-aqueous mixed solution synthesis has no final heat treatment step after precipitating the compound from solution and the solvents are a mixture of water and organic solvent(s).
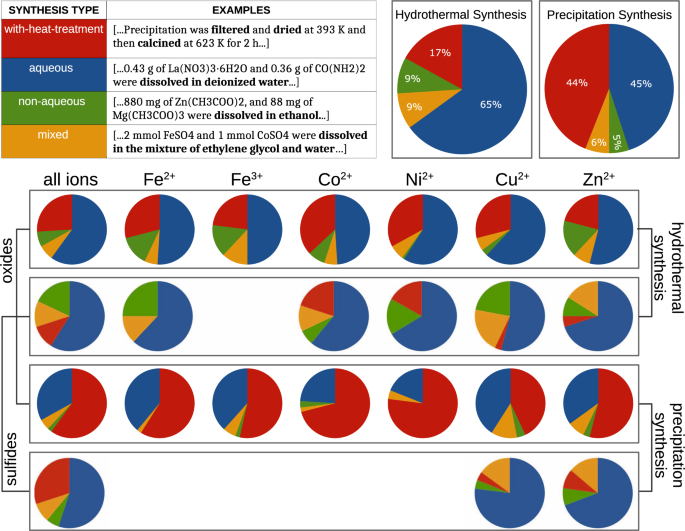
Correspondence between choice of synthesis route and selected types of targets. The top table gives an example of the four synthesis categories defined: with heat treatment step, aqueous, non-aqueous, and mixed. The two pie-charts on the top-right show the fractions of synthesis routes in the hydrothermal and precipitation datasets separately. The four rows of pie charts in the lower half of the figure represent the fractions of the four synthesis routes (given in the table) for all oxides, all sulfides, and individual oxides and sulfides with different oxidation states of data-rich transition metals separately. The first and second rows are results from the hydrothermal dataset. The third and fourth rows are results from the precipitation dataset. Each blank space means that there is not enough data to form a statistic for the corresponding type of target.
The resulting distributions of synthesis protocols over the aforementioned categories are shown in the two pie charts in the top-right corner of Fig. 3 . Note that as solution-based synthesis includes both hydrothermal and precipitation synthesis according to our definition (see Section “Paragraph classification”), we analyzed these synthesis types separately. As observed in the pie charts, only 20% of the procedures in the hydrothermal synthesis subset have a heat treatment step after solution mixing. Among those that do not have heat treatment step, 63% use only water as a solvent, 8% use only organic solvents, and 9% use both water and organic solvents. In contrast, the fractions in the precipitation synthesis subset are 43%, 46%, 5%, and 6%, respectively.
A heat treatment step after solution mixing can be used to dehydrate the targets, decompose the intermediates to produce the final products, change the oxidation state, change the morphology, or improve crystallization 65 , 66 , 67 . To explore this in more detail, we split the targets according to their anion type (oxide, sulfide, etc.) and different oxidation states of several data-rich transition-metal elements. We then computed the distribution of synthesis categories for each of the split subsets. Figure 3 presents the results for the most prevalent subsets of oxides, sulfides, and elements Fe 2+ , Fe 3+ , Co 3+ , Ni 2+ , Cu 2+ , and Zn 2+ . The fraction of procedures with a heat treatment step in precipitation synthesis is larger than that in hydrothermal synthesis. This observation holds for all targets, all oxides, all sulfides, and individual oxides and sulfides with queried oxidation states of transition metals. This finding can also be interpreted as hydrothermal synthesis often being used to obtain final products in a “one-shot” process, without subsequent heat treatment after solution mixing, likely because many compounds can be crystallized as anhydrous powders with controlled size and morphology directly from hydrothermal synthesis. In a standard hydrothermal synthesis procedure, the reaction is performed in an autoclave with autogenic pressure so that it can operate in a wider temperature window, including temperatures above the atmospheric boiling point of the solvent. In contrast, precipitation synthesis is performed under normal pressure. The higher temperature possible in hydrothermal synthesis is associated with enhanced kinetics in chemical transport, nucleation, and crystal growth and thus with a more effective dissolution–recrystallization process, which can help remove defects and improve crystallinity. Furthermore, the physico-chemical properties, such as the viscosity and dielectric constant of water or other solvents, change pronouncedly under conditions of hydrothermal synthesis, affecting the solubility and mobility of species in the solution and eventually facilitating crystallization 68 . Therefore, hydrothermal synthesis does not need a post-synthesis heat treatment as often as precipitation synthesis.
Solution-based synthesis is an important area of materials synthesis 57 and this dataset can help with advancing the science and model building for solution synthesis. Nevertheless, challenges remain in the mining of scientific literature and construction of robust and accurate large-scale datasets. First, the organic precursors with complex radicals commonly used in solution-based synthesis pose a challenge for parsing and extracting chemical information. Constructing reaction formulas becomes problematic when the precursor information is lost. Therefore, these entries are mostly dropped out later in the pipeline. To address this issue, a universal parser that can parse chemical tokens needs to be developed.
Second, our data was extracted from the experimental section in the main body of each paper and does not include any information about the actual synthesis results, e.g., whether the material was synthesized using the reported procedure or which structure was obtained. This problem could be overcome by introducing a model that can parse characterization data (e.g., X-ray diffraction patterns or electron microscopy images) and relate them to the corresponding synthesis conditions, something which, to the best of our knowledge, has not yet been performed. Even though the actual results of a synthesis can be extracted from a paper, there remains the challenge of data interpretation and usage, as the authors usually report only successful and “cherry-picked” experimental results. This introduces significant anthropogenic bias toward “positive” data with little “negative” content in the dataset, thus limiting the tasks for future machine-learning applications 69 , 70 . A promising approach to solve this issue is to incorporate results obtained by autonomous robotic synthesis platforms that can provide a vast amount of “negative” data in a reasonable time frame 71 , 72 .
Finally, solution-based synthesis is advantageous when the control of specimen morphology is required, e.g., when synthesizing noble-metal nanoparticles. However, this dataset does not provide information about the morphology of the synthesized materials, though such information is often contained in characterization or results paragraphs instead of the experimental section. The extraction of morphology and other solution synthesis outcomes is another text-mining challenge in materials science research that requires the development of advanced algorithms and models 30 , which is beyond the scope of the current study.
Usage Notes
The dataset is provided in JSON format as a single file. All major programming languages, such as Python, Matlab, R, and Wolfram Mathematica, can be used to read it. No particular dependency is required.
Because the dataset contains detailed information about chemical formulas as well as the compositions of the target materials and precursors for each procedure, it can be easily used to conduct a literature review by querying desired precursors and (or) targets in different chemical spaces. For example, selecting all TiO 2 synthesized from TiCl 4 allows an exploration of how other synthesis formulations, such as synthesis actions, attributes, and quantities, affect the results. Furthermore, the materials entries in the dataset are supplied with the Materials Project 5 identifiers, thus facilitating the integration of the procedures with the thermochemical data available in the Materials Project 73 , 74 .
In addition, this solution-based synthesis dataset keeps the same data structure as that in the solid-state dataset generated in our previous work 53 . Therefore, it is easy to analyze the procedures from the two datasets.
Despite the dataset being provided as a static snapshot 63 , we intend to update it on a regular basis.
Code availability
The scripts used to classify paragraphs and extract procedures as well as to perform the data analysis are home-written codes which are publicly available at the GitHub repository https://github.com/CederGroupHub/text-mined-solution-synthesis_public with acknowledgement of the current paper.
The underlying libraries used in this project are all open-source:
Tensorflow ( www.tensorflow.org )
Keras ( keras.iokeras.io )
SpaCy ( spacy.iospacy.io ) 60
NLTK ( https://www.nltk.org/ ) 62
gensim ( radimrehurek.comradimrehurek.com ) 59
scikit-learn ( scikit-learn.org ) 75
ChemDataExtractor ( chemdataextractor.org ) 40
Material Parser ( github.com/CederGroupHub/MaterialParser )
Borges ( github.com/CederGroupHub/Borges )
LimeSoup ( github.com/CederGroupHub/LimeSoup ).
Pankratius, V. et al . Computer-aided discovery: Toward scientific insight generation with machine support. IEEE Intelligent Systems 31 , 3–10 (2016).
Article Google Scholar
Tolle, K., Tansley, D. & Hey, A. The fourth paradigm: Data-intensive scientific discovery. Proceedings of the IEEE 99 , 1334–1337 (2011).
Fischer, C. C., Tibbetts, K. J., Morgan, D. & Ceder, G. Predicting crystal structure by merging data mining with quantum mechanics. Nature Materials 5 , 641–646 (2006).
Article ADS CAS PubMed Google Scholar
Holden, J. Materials Genome Initiative for global competitiveness. Tech. Rep., National Science and Technology Council (2011).
Ong, S. P. et al . Python materials genomics (pymatgen): A robust, open-source python library for materials analysis. Computational Materials Science 68 , 314–319 (2013).
Article CAS Google Scholar
Jain, A. et al . Commentary: The materials project: A materials genome approach to accelerating materials innovation. APL Materials 1 , 011002 (2013).
Article ADS CAS Google Scholar
Bergerhoff, G. & Brown, I. D. Crystallographic Databases (F. H. Allen et al. (Hrsg.) Chester, International Union of Crystallography, 1987).
Belsky, A., Hellenbrandt, M., Karen, V. L. & Luksch, P. New developments in the Inorganic Crystal Structure Database (ICSD): accessibility in support of materials research and design. Acta Crystallogr. B 58 , 364–369 (2002).
Article PubMed CAS Google Scholar
Linstrom, P. & Mallard, W. (eds.) NIST Chemistry WebBook, NIST Standard Reference Database Number 69 (National Institute of Standards and Technology, Gaithersburg MD, 20899, 2019).
Blokhin, E. & Villars, P. The PAULING FILE Project and Materials Platform for Data Science: From Big Data Toward Materials Genome , 1–26. Handbook of Materials Modeling (Springer, Cham, 2018).
Villars, P. & Cenzual, K. Pearson’s Crystal Data: Crystal Structure Database for Inorganic Compounds (on DVD) (Release 2018/19). ASM International®, Materials Park, Ohio, USA.
Sumpter, B. G., Vasudevan, R. K., Potok, T. & Kalinin, S. V. A bridge for accelerating materials by design. npj Computational Materials 1 , 15008 (2015).
Bianchini, M. et al . The interplay between thermodynamics and kinetics in the solid-state synthesis of layered oxides. Nature Materials 19 , 1088–1095 (2020).
Kovnir, K. Predictive synthesis. Chemistry of Materials 33 , 4835–4841 (2021).
Kohlmann, H. Looking into the black box of solid-state synthesis. European Journal of Inorganic Chemistry 2019 , 4174–4180 (2019).
Chamorro, J. R. & McQueen, T. M. Progress toward solid state synthesis by design. Accounts of Chemical Research 51 , 2918–2925 (2018).
Article CAS PubMed Google Scholar
Sun, W. et al . The thermodynamic scale of inorganic crystalline metastability. Science Advances 2 , e1600225 (2016).
Article ADS PubMed PubMed Central CAS Google Scholar
Sun, W. et al . A map of the inorganic ternary metal nitrides. Nature Materials 18 , 732–739 (2019).
Aykol, M., Dwaraknath, S. S., Sun, W. & Persson, K. A. Thermodynamic limit for synthesis of metastable inorganic materials. Science Advances 4 , eaaq0148 (2018).
Aykol, M. et al . Network analysis of synthesizable materials discovery. Nature Communications 10 , 2018 (2019).
Aykol, M., Montoya, J. H. & Hummelshøj, J. Rational solid-state synthesis routes for inorganic materials. Journal of the American Chemical Society 143 , 9244–9259 (2021).
Bartel, C. J. et al . Physical descriptor for the gibbs energy of inorganic crystalline solids and temperature-dependent materials chemistry. Nature Communications 9 , 4168 (2018).
McDermott, M. J., Dwaraknath, S. S. & Persson, K. A. A graph-based network for predicting chemical reaction pathways in solid-state materials synthesis. Nature Communications 12 , 3097 (2021).
Article ADS CAS PubMed PubMed Central Google Scholar
Duvenaud, D. K. et al . Convolutional networks on graphs for learning molecular fingerprints. In Cortes, C., Lawrence, N. D., Lee, D. D., Sugiyama, M. & Garnett, R. (eds.) Advances in Neural Information Processing Systems 28 , 2224–2232 (Currant Associates, Inc., 2015).
Ley, S. V., Fitzpatrick, D. E., Ingham, R. J. & Myers, R. M. Organic synthesis: March of the machines. Angew. Chem. Int. Edit. 54 , 3449–3464 (2015).
Segler, M. H. S., Preuss, M. & Waller, M. P. Planning chemical syntheses with deep neural networks and symbolic ai. Nature 555 , 604–610 (2018).
Mikulak-Klucznik, B. et al . Computational planning of the synthesis of complex natural products. Nature 588 , 83–88 (2020).
Goodman, J. Computer software review: Reaxys. J. Chem. Inf. Model. 49 , 2897–2898 (2009).
Kim, S. et al . PubChem 2019 update: improved access to chemical data. Nucleic Acids Res. 47 , D1102–D1109 (2018).
Article PubMed Central Google Scholar
Kononova, O. et al . Opportunities and challenges of text mining in materials research. iScience 24 (2021).
Gaultois, M. et al . Data-driven review of thermoelectric materials: Performance and resource considerations. Chem. Mater. 25 , 2911–2920 (2013).
Ghadbeigi, L., Harada, J. K., Lettiere, B. R. & Sparks, T. D. Performance and resource considerations of Li-ion battery electrode materials. Energy Environ. Sci. 8 , 1640–1650 (2015).
Oh, E. et al . Meta-analysis of cellular toxicity for cadmium-containing quantum dots. Nature Nanotech. 11 , 479 (2016).
Court, C. J. & Cole, J. M. Auto-generated materials database of Curie and Néel temperatures via semi-supervised relationship extraction. Sci. Data 5 , 180111 (2018).
Article CAS PubMed PubMed Central Google Scholar
Court, C. & Cole, J. Magnetic and superconducting phase diagrams and transition temperatures predicted using text mining and machine learning. npj Comput. Mater 6 , 1–9 (2020).
Article ADS Google Scholar
Huang, S. & Cole, J. M. A database of battery materials auto-generated using chemdataextractor. Scientific Data 7 , 260 (2020).
Article PubMed PubMed Central Google Scholar
Onishi, T., Kadohira, T. & Watanabe, I. Relation extraction with weakly supervised learning based on process-structure-property-performance reciprocity. Sci. Technol. Adv. Mater. 19 , 649–659 (2018).
Tshitoyan, V. et al . Unsupervised word embeddings capture latent knowledge from materials science literature. Nature 571 , 95–98 (2019).
Kim, E. et al . Materials synthesis insights from scientific literature via text extraction and machine learning. Chem. Mater 29 , 9436–9444 (2017).
Swain, M. C. & Cole, J. M. ChemDataExtractor: A toolkit for automated extraction of chemical information from the scientific literature. J. Chem. Inf. Model. 56 , 1894–1904 (2016).
Jessop, D. M., Adams, S. E., Willighagen, E. L., Hawizy, L. & Murray-Rust, P. OSCAR4: a flexible architecture for chemical text-mining. J. Cheminformatics 3 , 41 (2011).
Hawizy, L., Jessop, D. M., Adams, N. & Murray-Rust, P. ChemicalTagger: A tool for semantic text-mining in chemistry. J. Cheminformatics 3 , 17 (2011).
Krallinger, M., Rabal, O., Lourenço, A., Oyarzabal, J. & Valencia, A. Information retrieval and text mining technologies for chemistry. Chem. Rev. 117 , 7673–7761 (2017).
Eltyeb, S. & Salim, N. Chemical named entities recognition: A review on approaches and applications. J. Cheminformatics 6 , 17 (2014).
Korvigo, I., Holmatov, M., Zaikovskii, A. & Skoblov, M. Putting hands to rest: efficient deep cnn-rnn architecture for chemical named entity recognition with no hand-crafted rules. J. Cheminform. 10 , 28 (2018).
He, T. et al . Similarity of precursors in solid-state synthesis as text-mined from scientific literature. Chemistry of Materials 32 , 7861–7873 (2020).
Weston, L. et al . Named entity recognition and normalization applied to large-scale information extraction from the materials science literature. J. Chem. Inf. Model. 59 , 3692–3702 (2019).
Kuniyoshi, F., Makino, K., Ozawa, J. & Miwa, M. Annotating and extracting synthesis process of all-solid-state batteries from scientific literature. In Proceedings of The 12th Language Resources and Evaluation Conference , 1941–1950 (European Language Resources Association, Marseille, France, 2020).
Kim, E. et al . Machine-learned and codified synthesis parameters of oxide materials. Sci. Data 4 , 170127 (2017).
Kim, E., Huang, K., Jegelka, S. & Olivetti, E. Virtual screening of inorganic materials synthesis parameters with deep learning. npj Comput. Mater 3 , 53 (2017).
Jensen, Z. et al . A machine learning approach to zeolite synthesis enabled by automatic literature data extraction. ACS Cent. Sci. 5 , 892–899 (2019).
Kim, E. et al . Inorganic materials synthesis planning with literature-trained neural networks. J. Chem. Inf. Model. 60 , 1194–1201 (2020).
Kononova, O. et al . Text-mined dataset of inorganic materials synthesis recipes. Scientific Data 6 , 203 (2019).
Mouchère, H., Zanibbi, R., Garain, U. & Viard-Gaudin, C. Advancing the state of the art for handwritten math recognition: the crohme competitions, 2011–2014. IJDAR 19 , 173–189 (2016).
Mahdavi, M., Zanibbi, R., Mouchère, H., Viard-Gaudin, C. & Garain, U. Icdar 2019 crohme + tfd: Competition on recognition of handwritten mathematical expressions and typeset formula detection. In 2019 International Conference on Document Analysis and Recognition (ICDAR) , 1533–1538 (IEEE, 2019).
Devlin, J., Chang, M.-W., Lee, K. & Toutanova, K. BERT: Pre-training of deep bidirectional transformers for language understanding. In Proceedings of the 2019 Conference of the North American Chapter of the Association for Computational Linguistics: Human Language Technologies, Volume 1 ( Long and Short Papers) , 4171–4186 (Association for Computational Linguistics, Minneapolis, Minnesota, 2019).
Huo, H. et al . Semi-supervised machine-learning classification of materials synthesis procedures. npj Computational Materials 5 , 62 (2019).
Mikolov, T., Sutskever, I., Chen, K., Corrado, G. S. & Dean, J. Distributed representations of words and phrases and their compositionality. In Burges, C. J. C., Bottou, L., Welling, M., Ghahramani, Z. & Weinberger, K. Q. (eds.) Advances in Neural Information Processing Systems 26 , 3111–3119 (Curran Associates, Inc., 2013).
Řehůřek, R. & Sojka, P. Software framework for topic modelling with large corpora. In Proceedings of the LREC 2010 Workshop on New Challenges for NLP Frameworks , 45–50 (ELRA, Valletta, Malta, 2010).
Honnibal, M. & Johnson, M. An improved non-monotonic transition system for dependency parsing. In Proceedings of the 2015 Conference on Empirical Methods in Natural Language Processing , 1373–1378 (Association for Computational Linguistics, Lisbon, Portugal, 2015).
Jurafsky, D. & Martin, J. Speech and Language Processing: An Introduction to Natural Language Processing, Computational Linguistics, and Speech Recognition . Prentice Hall Series in Artificial Intelligence (Pearson Prentice Hall, 2009).
Bird, S., Edward, L. & Ewan, K. Natural Language Processing with Python . (O’Reilly Media Inc, 2009).
Wang, Z. et al . Dataset of solution-based inorganic materials synthesis procedures extracted from the scientific literature. figshare https://doi.org/10.6084/m9.figshare.16583387.v4 (2021).
Wang, D., Xie, T. & Li, Y. Nanocrystals: Solution-based synthesis and applications as nanocatalysts. Nano Research 2 , 30–46 (2009).
Rakhi, R. B., Chen, W., Cha, D. & Alshareef, H. N. Influence of calcination temperature on the morphology and energy storage properties of cobalt oxide nanostructures directly grown over carbon cloth substrates. Materials for Renewable and Sustainable Energy 2 , 17 (2013).
Augustin, M. et al . Manganese oxide phases and morphologies: A study on calcination temperature and atmospheric dependence. Beilstein journal of nanotechnology 6 , 47–59 (2015).
Ellingham, H. Transactions and communications. Journal of the Society of Chemical Industry 63 , 125–160 (1944).
Byrappa, K. & Yoshimura, M. Handbook of Hydrothermal Technology (William Andrew Publishing, Oxford, 2013).
Raccuglia, P. et al . Machine-learning-assisted materials discovery using failed experiments. Nature 533 , 73–76 (2016).
Jia, X. et al . Anthropogenic biases in chemical reaction data hinder exploratory inorganic synthesis. Nature 573 , 251–255 (2019).
Burger, B. et al . A mobile robotic chemist. Nature 583 , 237–241 (2020).
Szymanski, N. J. et al . Toward autonomous design and synthesis of novel inorganic materials. Mater. Horiz. 8 , 2169–2198 (2021).
Persson, K. A., Waldwick, B., Lazic, P. & Ceder, G. Prediction of solid-aqueous equilibria: Scheme to combine first-principles calculations of solids with experimental aqueous states. Phys. Rev. B 85 , 235438 (2012).
Sun, W., Kitchaev, D. A., Kramer, D. & Ceder, G. Non-equilibrium crystallization pathways of manganese oxides in aqueous solution. Nature Communications 10 , 573 (2019).
Pedregosa, F. et al . Scikit-learn: Machine learning in Python. J. Mach. Learn. Res. 12 , 2825–2830 (2011).
MathSciNet MATH Google Scholar
Download references
Acknowledgements
This material is based upon work supported by the National Science Foundation under Grant No. DMR-1922372. We also thank Chris Bartel, and Amalie Trewartha for valuable discussions.
Author information
Authors and affiliations.
Department of Materials Science and Engineering, University of California, Berkeley, CA, 94720, USA
Zheren Wang, Olga Kononova, Kevin Cruse, Tanjin He, Haoyan Huo, Yuxing Fei, Yingzhi Sun, Zijian Cai & Gerbrand Ceder
Materials Sciences Division, Lawrence Berkeley National Laboratory, Berkeley, CA, 94720, USA
Zheren Wang, Olga Kononova, Kevin Cruse, Tanjin He, Haoyan Huo, Yuxing Fei, Yan Zeng, Yingzhi Sun, Zijian Cai & Gerbrand Ceder
Department of Materials Science and Engineering, University of Michigan, Ann Arbor, MI, USA
You can also search for this author in PubMed Google Scholar
Contributions
Z.W. developed the material quantities extraction algorithm, reaction formula algorithm, Materials Parser, operations and conditions extraction algorithms, analyzed the data, and wrote the manuscript. O.K. developed the Materials Parser, operations and conditions extraction algorithms, analyzed the data, and wrote the manuscript. K.C. developed operations and conditions extraction algorithms, manually inspected the dataset, and wrote the manuscript. T.H. developed materials entity recognition algorithm, and manually inspected the dataset. H.H. curated paragraphs databases, and developed the paragraphs classifier. Y.F. developed building reaction formula algorithm and manually inspected the dataset. Y.Z. analyzed the data and manually inspected the dataset. Y.S. analyzed the data and manually inspected the dataset. Z.C. analyzed the data and manually inspected the dataset. W.S. analyzed the data and developed material quantities extraction algorithm. G.C. developed approach, supervised the project, and wrote the manuscript. All authors discussed the results and contributed to the final manuscript.
Corresponding author
Correspondence to Gerbrand Ceder .
Ethics declarations
Competing interests.
The authors declare no competing interests.
Additional information
Publisher’s note Springer Nature remains neutral with regard to jurisdictional claims in published maps and institutional affiliations.
Rights and permissions
Open Access This article is licensed under a Creative Commons Attribution 4.0 International License, which permits use, sharing, adaptation, distribution and reproduction in any medium or format, as long as you give appropriate credit to the original author(s) and the source, provide a link to the Creative Commons license, and indicate if changes were made. The images or other third party material in this article are included in the article’s Creative Commons license, unless indicated otherwise in a credit line to the material. If material is not included in the article’s Creative Commons license and your intended use is not permitted by statutory regulation or exceeds the permitted use, you will need to obtain permission directly from the copyright holder. To view a copy of this license, visit http://creativecommons.org/licenses/by/4.0/ .
Reprints and permissions
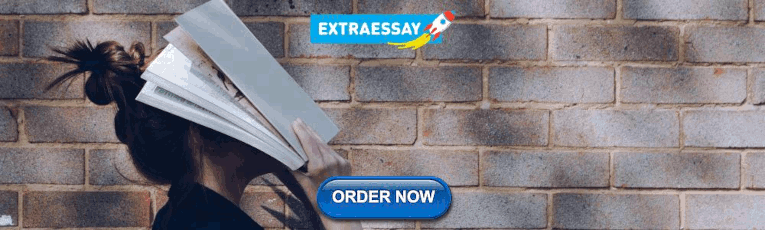
About this article
Cite this article.
Wang, Z., Kononova, O., Cruse, K. et al. Dataset of solution-based inorganic materials synthesis procedures extracted from the scientific literature. Sci Data 9 , 231 (2022). https://doi.org/10.1038/s41597-022-01317-2
Download citation
Received : 07 October 2021
Accepted : 05 April 2022
Published : 25 May 2022
DOI : https://doi.org/10.1038/s41597-022-01317-2
Share this article
Anyone you share the following link with will be able to read this content:
Sorry, a shareable link is not currently available for this article.
Provided by the Springer Nature SharedIt content-sharing initiative
This article is cited by
Structured information extraction from scientific text with large language models.
- John Dagdelen
- Alexander Dunn
- Anubhav Jain
Nature Communications (2024)
Accelerating materials language processing with large language models
- Jaewoong Choi
- Byungju Lee
Communications Materials (2024)
Leveraging language representation for materials exploration and discovery
- Yuxuan Richard Xie
- Elif Ertekin
npj Computational Materials (2024)
Optimal thermodynamic conditions to minimize kinetic by-products in aqueous materials synthesis
- Zheren Wang
- Yingzhi Sun
- Gerbrand Ceder
Nature Synthesis (2024)
Extracting accurate materials data from research papers with conversational language models and prompt engineering
- Maciej P. Polak
- Dane Morgan
Quick links
- Explore articles by subject
- Guide to authors
- Editorial policies
Sign up for the Nature Briefing newsletter — what matters in science, free to your inbox daily.

Synthesis of Inorganic Materials
Cite this chapter.
- Paul J. van der Put 2
784 Accesses
1 Citations
The synthesis of inorganic compounds for use in materials 1,2 is about making solids and this chapter on synthesis describes the preparation of (mainly) nonmolecular solid compounds in solid state reactions, in reactions from liquids, or reactions from the gas phase. The synthesis of inorganic molecules 3 is not much different than that of organic compounds. Some methods of making coordination compounds and organometallics were given in Chapter 3. Their synthesis is not described here because, for the materials technologist, inorganic molecules are used mainly as precursors and not as part of materials.
The place was always stiff with researchers trying to get to the bottom of it all and taking a very long time about it. Douglas Adams , So Long and Thanks for All the Fish
This is a preview of subscription content, log in via an institution to check access.
Access this chapter
- Available as PDF
- Read on any device
- Instant download
- Own it forever
- Compact, lightweight edition
- Dispatched in 3 to 5 business days
- Free shipping worldwide - see info
- Durable hardcover edition
Tax calculation will be finalised at checkout
Purchases are for personal use only
Institutional subscriptions
Unable to display preview. Download preview PDF.
J. W. Evans and L. C. de Jonghe. The Production of Inorganic Materials . Macmillan, New York (1991).
Google Scholar
C.N.R. Rao. Chemical Approaches to the Synthesis of Inorganic Materials . Wiley, New York (1994).
J. D. Woollins (ed). Inorganic Experiments . VCH, Weinheim (1994).
Schwertmann, U. and R. M. Cornell. Iron Oxides in the Laboratory: Preparation and Characterization . VCH, Weinheim (1991).
Y. Arai. Chemistry of Powder Production . Chapman and Hall, London (1996).
Book Google Scholar
K. J. Klabunde. Free Atoms, Clusters, and Nanoscale Particles . Academic, San Diego (1994).
J. J. Moore and H. J. Feng. Combustion synthesis of advanced materials: Part I. Reaction parameters; Part II. Classification, applications, and modeling. Progr. Mater. Sci . 39 , 243, 275 (1995).
Article CAS Google Scholar
T. A. Ring. Fundamentals of Ceramic Powder Processing and Synthesis . Academic, San Diego (1996).
D. L. Smith. Thin-Film Deposition: Principles and Practice . McGraw-Hill, New York (1995).
A. A. Chernov and E. I. Givargizov (eds.). Modern Crystallography, Vol. 3: Crystal Growth . Springer, Berlin (1984).
W. E. Rhine, T. M. Shaw, R. J. Gottschall, and Y. Chen. Synthesis and processing of ceramics: scientific issues. Mat. Res. Soc. Symp. Proc. 249 (1992).
A. K. Varshneya. Fundamentals of Inorganic Glasses . Academic, Boston (1994).
A. Stein, S. W. Keller, and T. E. Mallouk. Turning down the heat: design and mechanism in solid state synthesis. Science 259 , 1558 (1993).
E. Matievic. Preparation and properties of uniform size colloids. Chem. Mater . 5 , 412 (1993).
Article Google Scholar
M. Ozaki. Preparation and Properties of Well-Defined Magnetic Particles. MRS Bull . December 1989, p. 36.
J. R. Backhus, J. H. Harker, and R. K. Sinnet. Coulson and Richardson’s Chemical Engineering , Vols. 1, 2, and 6. Pergamon, Oxford (1990).
W. L. McCabe, J. C. Smith, and P. Harriott. Unit Operations of Chemical Engineering . MacGraw-Hill, New York (1993).
D. Segal. Chemical Synthesis of Advanced Ceramic Materials . Cambridge University Press, Cambridge (1989).
S. C. Deevi (ed.). Manufacturing of covalent ceramics by exothermic reactions. Mater. Res. Soc. Symp. Proc . 327 , 171 (1994).
Y. M. Chiang, J. S. Haggerty, R. P. Messner, and C. Demetry. Reaction-based processing methods for ceramic-matrix composites. Ceram. Bull . 68 , 420 (1989).
CAS Google Scholar
H. Yanagida, K. Koumoto, M. Miyayama, and H. Yamada. The Chemistry of Ceramics . Wiley, Chichester (1996).
G. Mamantov and R. Marassi. Molten Salt Chemistry: An Introduction and Selected Applications . Reidel, Dordrecht (1987).
G. Mamantov and A. I. Popov (eds.). Chemistry of Non-aqueous Solutions: Current Progress . VCH, New York (1994).
T. A. O’Donnell. Superacids and Acidic Melts as Inorganic Chemical Reaction Media . VCH, New York (1993).
R. A. Laudise. Hydrothermal synthesis of crystals. C&EN 28 , 30 (1987).
C. J. Brinker and G. W. Scherer. Sol-Gel Science: The Physics and Chemistry of Sol-Gel Processing . Academic, Boston (1990).
O. A. Gzowski. Gels. J. Mater. Educ . 8 (5), 671 (1986).
L. L. Hench and J. K. West. Chemical Processing of Advanced Materials . Wiley, New York (1992).
R. J. Ayen and P. A. Iacobucci. Metal oxide aerogel preparation by supercritical extraction. Rev. Chem. Engin . 5 , 157 (1988).
L. C. Klein (ed). Sol-Gel Technology of Thin Films, Fibers, Preforms, Electronics, and Specialty Shapes . Noyes, Park Ridge, NJ (1988).
D. L. Smith. Thin Film Deposition . McGraw-Hill, New York (1995).
M. G. Hocking and V. Vasantarasree, and P. S. Skidky. Metallic and Ceramic Coatings . Longman, Harlow (1989), Ch. 4.
A. C. Jones and P. O’Brien. CVD of Compound Semiconductors . VCH, Weinheim (1997).
D. S. Rickerby and A. Matthews (eds.). Advanced Surface Coatings: A Handbook of Surface Engineering . Blackie, Glasgow (1991).
H. K. Pulker, E. Bergmann, and H. M. Gabriel. Wear and Corrosion Resistant Coatings by CVD and PVD . Expert Verlag, Ehningen (1989).
M. Langlet and J. C. Joubert. The pyrosol process or the pyrolysis of an ultrasonically generated aerosol. In C.N.R. Rao (ed.). Chemistry of Advanced Materials . Blackwell’s, London (1992), p. 55.
S. K. Friedlander. Smoke, Dust, and Haze: Fundamentals of Aerosol Behavior . Wiley, New York (1977).
W. C. Hinds. Aerosol Technology: Properties, Behavior, and Measurement of Airborne Particles . Wiley Interscience, New York (1982).
T. Kodas (ed). Aerosols in materials processing. J. Aerosol Science 24 , 273 (1993).
S. Veprek. Application of Low Pressure Plasmas in Materials Science—Especially CVD . Current Topics in Materials Science, Vol. IV. E. Kaldis (ed.). North-Holland, Amsterdam (1980).
Download references
Author information
Authors and affiliations.
Delft University of Technology, Delft, The Netherlands
Paul J. van der Put
You can also search for this author in PubMed Google Scholar
Rights and permissions
Reprints and permissions
Copyright information
© 1998 Springer Science+Business Media New York
About this chapter
van der Put, P.J. (1998). Synthesis of Inorganic Materials. In: The Inorganic Chemistry of Materials. Springer, Boston, MA. https://doi.org/10.1007/978-1-4899-0095-1_8
Download citation
DOI : https://doi.org/10.1007/978-1-4899-0095-1_8
Publisher Name : Springer, Boston, MA
Print ISBN : 978-1-4899-0097-5
Online ISBN : 978-1-4899-0095-1
eBook Packages : Springer Book Archive
Margin Size
- Download Page (PDF)
- Download Full Book (PDF)
- Periodic Table
- Physics Constants
- Scientific Calculator
- Reference & Cite
- Tools expand_more
- Readability
selected template will load here
This action is not available.
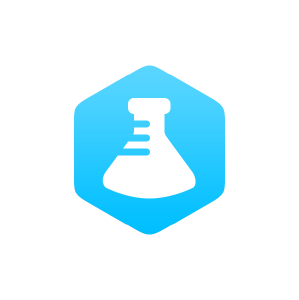
11.3: Types of Inorganic Reactions
- Last updated
- Save as PDF
- Page ID 58842
\( \newcommand{\vecs}[1]{\overset { \scriptstyle \rightharpoonup} {\mathbf{#1}} } \)
\( \newcommand{\vecd}[1]{\overset{-\!-\!\rightharpoonup}{\vphantom{a}\smash {#1}}} \)
\( \newcommand{\id}{\mathrm{id}}\) \( \newcommand{\Span}{\mathrm{span}}\)
( \newcommand{\kernel}{\mathrm{null}\,}\) \( \newcommand{\range}{\mathrm{range}\,}\)
\( \newcommand{\RealPart}{\mathrm{Re}}\) \( \newcommand{\ImaginaryPart}{\mathrm{Im}}\)
\( \newcommand{\Argument}{\mathrm{Arg}}\) \( \newcommand{\norm}[1]{\| #1 \|}\)
\( \newcommand{\inner}[2]{\langle #1, #2 \rangle}\)
\( \newcommand{\Span}{\mathrm{span}}\)
\( \newcommand{\id}{\mathrm{id}}\)
\( \newcommand{\kernel}{\mathrm{null}\,}\)
\( \newcommand{\range}{\mathrm{range}\,}\)
\( \newcommand{\RealPart}{\mathrm{Re}}\)
\( \newcommand{\ImaginaryPart}{\mathrm{Im}}\)
\( \newcommand{\Argument}{\mathrm{Arg}}\)
\( \newcommand{\norm}[1]{\| #1 \|}\)
\( \newcommand{\Span}{\mathrm{span}}\) \( \newcommand{\AA}{\unicode[.8,0]{x212B}}\)
\( \newcommand{\vectorA}[1]{\vec{#1}} % arrow\)
\( \newcommand{\vectorAt}[1]{\vec{\text{#1}}} % arrow\)
\( \newcommand{\vectorB}[1]{\overset { \scriptstyle \rightharpoonup} {\mathbf{#1}} } \)
\( \newcommand{\vectorC}[1]{\textbf{#1}} \)
\( \newcommand{\vectorD}[1]{\overrightarrow{#1}} \)
\( \newcommand{\vectorDt}[1]{\overrightarrow{\text{#1}}} \)
\( \newcommand{\vectE}[1]{\overset{-\!-\!\rightharpoonup}{\vphantom{a}\smash{\mathbf {#1}}}} \)
Learning Outcomes
- Classify a reaction as combination, decomposition, single-replacement, double-replacement, or combustion.
- Determine whether a reaction is an oxidation-reduction reaction.
- Predict the products and balance a combustion reaction.
Many chemical reactions can be classified as one of five basic types. Having a thorough understanding of these types of reactions will be useful for predicting the products of an unknown reaction. The five basic types of chemical reactions are combination, decomposition, single-replacement, double-replacement, and combustion. Analyzing the reactants and products of a given reaction will allow you to place it into one of these categories. Some reactions will fit into more than one category. Identifying a reaction as one of these types does not preclude it from also being an oxidation-reduction (redox) reaction. For example, many combination reactions can also be classified as a redox reaction.
Combination Reactions
A combination reaction , also known as a synthesis reaction , is a reaction in which two or more substances combine to form a single new substance. Combination reactions can also be called synthesis reactions .The general form of a combination reaction is:
\[\ce{A} + \ce{B} \rightarrow \ce{AB}\]
One example of a combination reaction is two elements combining to form a compound. Solid sodium metal reacts with chlorine gas to product solid sodium chloride.
\[2 \ce{Na} \left( s \right) + \ce{Cl_2} \left( g \right) \rightarrow 2 \ce{NaCl} \left( s \right)\]
This reaction can also be classified as a redox reaction due to the changes in oxidation states. Sodium goes from a 0 to +1 oxidation state while chlorine goes from a 0 to a \(-1\) oxidation state.
Notice that in order to write and balance the equation correctly, it is important to remember the seven elements that exist in nature as diatomic molecules (\(\ce{H_2}\), \(\ce{N_2}\), \(\ce{O_2}\), \(\ce{F_2}\), \(\ce{Cl_2}\), \(\ce{Br_2}\), and \(\ce{I_2}\)).
One sort of combination reaction that occurs frequently is the reaction of an element with oxygen to form an oxide. Metals and nonmetals both react readily with oxygen under most conditions. Magnesium reacts rapidly and dramatically when ignited, combining with oxygen from the air to produce a fine powder of magnesium oxide.
\[2 \ce{Mg} \left( s \right) + \ce{O_2} \left( g \right) \rightarrow 2 \ce{MgO} \left( s \right)\]
Decomposition Reactions
A decomposition reaction is a reaction in which a compound breaks down into two or more simpler substances. The general form of a decomposition reaction is:
\[\ce{AB} \rightarrow \ce{A} + \ce{B}\]
Most decomposition reactions require an input of energy in the form of heat, light, or electricity.
Binary compounds are compounds composed of just two elements. The simplest kind of decomposition reaction is when a binary compound decomposes into its elements. Mercury (II) oxide, a red solid, decomposes when heated to produce mercury and oxygen gas.
\[2 \ce{HgO} \left( s \right) \rightarrow 2 \ce{Hg} \left( l \right) + \ce{O_2} \left( g \right)\]
A reaction is also considered to be a decomposition reaction even when one or more of the products is still a compound. A metal carbonate decomposes into a metal oxide and carbon dioxide gas. For example, calcium carbonate decomposes into calcium oxide and carbon dioxide.
\[\ce{CaCO_3} \left( s \right) \rightarrow \ce{CaO} \left( s \right) + \ce{CO_2} \left( g \right)\]
Metal hydroxides decompose on heating to yield metal oxides and water. Sodium hydroxide decomposes to produce sodium oxide and water.
\[2 \ce{NaOH} \left( s \right) \rightarrow \ce{Na_2O} \left( s \right) + \ce{H_2O} \left( g \right)\]
Single-Replacement Reactions
A single-replacement reaction , also known as single-displacement or substitution reaction, is a reaction in which one element replaces a similar element in a compound . The general form of a single-replacement reaction is:
\[\ce{A} + \ce{BC} \rightarrow \ce{AC} + \ce{B}\]
In this general reaction, element \(\ce{A}\) is a metal and replaces element \(\ce{B}\), also a metal, in the compound. When the element that is doing the replacing is a nonmetal, it must replace another nonmetal in a compound, and the general equation becomes:
\[\ce{Y} + \ce{XZ} \rightarrow \ce{XY} + \ce{Z}\]
\(\ce{Y}\) is a nonmetal and replaces the nonmetal \(\ce{Z}\) in the compound with \(\ce{X}\).
Magnesium is a more reactive metal than copper. When a strip of magnesium metal is placed in an aqueous solution of copper (II) nitrate, it replaces the copper. The products of the reaction are aqueous magnesium nitrate and solid copper metal.
\[\ce{Mg} \left( s \right) + \ce{Cu(NO_3)_2} \left( aq \right) \rightarrow \ce{Mg(NO_3)_2} \left( aq \right) + \ce{Cu} \left( s \right)\]
Many metals react easily with acids, and, when they do so, one of the products of the reaction is hydrogen gas. Zinc reacts with hydrochloric acid to produce aqueous zinc chloride and hydrogen (see figure below).
\[\ce{Zn} \left( s \right) + 2 \ce{HCl} \left( aq \right) \rightarrow \ce{ZnCl_2} \left( aq \right) + \ce{H_2} \left( g \right)\]
Double-Replacement Reactions
A double-replacement reaction , also known as double-displacement, is a reaction in which the positive and negative ions of two ionic compounds exchange places to form two new compounds . The general form of a double-replacement reaction is:
\[\ce{AB} + \ce{CD} \rightarrow \ce{AD} + \ce{CB}\]
In this reaction, \(\ce{A}\) and \(\ce{C}\) are positively-charged cations, while \(\ce{B}\) and \(\ce{D}\) are negatively-charged anions. Double-replacement reactions generally occur between substances in aqueous solution. In order for a reaction to occur, one of the products is usually a solid precipitate, a gas, or a molecular compound such as water.
A precipitate forms in a double-replacement reaction when the cations from one of the reactants combine with the anions from the other reactant to form an insoluble ionic compound. When aqueous solutions of potassium iodide and lead (II) nitrate are mixed, the following reaction occurs.
\[2 \ce{KI} \left( aq \right) + \ce{Pb(NO_3)_2} \left( aq \right) \rightarrow 2 \ce{KNO_3} \left( aq \right) + \ce{PbI_2} \left( s \right)\]
Combustion Reactions
A combustion reaction is a reaction in which a substance reacts with oxygen gas, releasing energy in the form of light and heat. Combustion reactions must involve \(\ce{O_2}\) as one reactant. The combustion of hydrogen gas produces water vapor (see figure below).
\[2 \ce{H_2} \left( g \right) + \ce{O_2} \left( g \right) + 2 \ce{H_2O} \left( g \right)\]
Notice that this reaction also qualifies as a combination reaction.
Many combustion reactions occur with a hydrocarbon, a compound made up solely of carbon and hydrogen. The products of the combustion of hydrocarbons are always carbon dioxide and water. Many hydrocarbons are used as fuel because their combustion releases very large amount of heat energy. Propane \(\left( \ce{C_3H_8} \right)\) is a gaseous hydrocarbon that is commonly used as the fuel source in gas grills.
\[\ce{C_3H_8} \left( g \right) + 5 \ce{O_2} \left( g \right) \rightarrow 3 \ce{CO_2} \left( g \right) + 4 \ce{H_2O} \left( g \right)\]
Example \(\PageIndex{1}\)
Ethanol can be used as a fuel source in an alcohol lamp. The formula for ethanol is \(\ce{C_2H_5OH}\). Write the balanced equation for the combustion of ethanol.
Step 1: Plan the problem.
Ethanol and oxygen are the reactants. As with a hydrocarbon, the products of the combustion of an alcohol are carbon dioxide and water.
Step 2: Solve.
Write the skeleton equations: \(\ce{C_2H_5OH} \left( l \right) + \ce{O_2} \left( g \right) \rightarrow \ce{CO_2} \left( g \right) + \ce{H_2O} \left( g \right)\)
Balance the equation.
\[\ce{C_2H_5OH} \left( l \right) + 3 \ce{O_2} \left( g \right) \rightarrow 2 \ce{CO_2} \left( g \right) + 3 \ce{H_2O} \left( g \right)\]
Step 3: Think about your result.
Combustion reactions must have oxygen as a reactant. Note that the water that is produced is in the gas state rather that the liquid state because of the high temperatures that accompany a combustion reaction.
Supplemental Resources
- Simulation of the synthesis of water: www.dit.ncssm/edu/core/Chapte...Synthesis.html
- View the synthesis of calcium oxide at http://www.youtube.com/watch?v-dszSKIM5rqk
- View the reaction between copper and chlorine gas at http://www.youtube.com/watch?v-edLpxdERQZc
- Watch the decomposition of hydrogen peroxide at http://www.youtube.com/watch?v=oX5FyaqNx54
- Watch the decomposition of potassium chlorate at http://www.youtube.com/watch?v=svRIg_kzE68
- A video experiment of hydrogen replacement by calcium can be seen at http://www.youtube.com/watch?v=hjB96do_fRw
- A video experiment of magnesium metal reacting with hydrochloric acid can be viewed at http://www.youtube.com/watch?v=OBdgeJFzSec
- Watch an animation of a double-replacement reaction at http://www.dlt.ncssm.edu/core/Chapte...cidToBase.html
- A video experiment in which a precipitate is formed by reacting sodium chloride with silver nitrate can be seen at http://www.youtube.com/watch?v=eFF3El4mwok
- A video experiment of the double-replacement reaction between copper (II) sulfate and sodium sulfide can be viewed at http://www.youtube.com/watch?v=KkKBDcFfZWo
- A video of the double-replacement reaction between sodium sulfate and barium chloride can be viewed at http://www.youtube.com/watch?v=XaMyfjYLhxU
- View an in-depth explanation of the combustion reaction that occurs when you strike a match at http://www.pbs.org/wgbh/nova/cigarette/onfire.html
- View an exciting video demonstration that confirms the importance of oxygen in a combustion reaction at education.jlab.org/frost/life_candle.html
- View another video demonstration that confirms the importance of oxygen in a combustion reaction at http://education.jlab.org/frost/combustion.html
- Reaction Identification Practice: http://www.sciencegeek.net/Chemistry...tification.htm
Contributors and Attributions
Allison Soult , Ph.D. (Department of Chemistry, University of Kentucky)

- ‹ Prev
- Next ›
Chapter 4: Highly Efficient Rapid Preparation of Inorganic Nanostructured Materials by Microwave Heating
- Published: 17 May 2024
- Special Collection: 2024 eBook Collection Series: Inorganic Materials
- Get permissions
- Cite Icon Cite
F. Chen and Y. Zhu, in Unconventional Green Synthesis of Inorganic Nanomaterials, ed. S. Gross, Royal Society of Chemistry, 2024, vol. 14, ch. 4, pp. 141-251.
Download citation file:
- Ris (Zotero)
- Reference Manager
In recent years, microwave-assisted rapid preparation of inorganic nanostructured materials has attracted much attention due to the incredible increase in reaction rates, high efficiency, and energy saving achieved. The microwave-assisted reaction time can be significantly shortened compared with conventional heating methods. In addition, the effects of microwave irradiation on crystal nucleation and growth, structure, size, morphology, and product yield are also very appealing research topics. In this chapter, we will provide a brief background on microwave chemistry, followed by a detailed discussion of the microwave heating mechanism and microwave effects, for the use of microwave heating to promote the formation and crystallisation of inorganic nanostructured materials. Then, we will outline the unique features of microwave-assisted synthesis and processing. The role of the synthetic parameters will be emphasised and the influences of these experimental parameters on final products will be described. In addition, the apparatus for microwave reactions will be described. Importantly, comparisons between microwave heating and conventional heating will be critically discussed. The major part of this chapter will focus on reviewing the state-of-the-art inorganic nanostructures that are prepared with the help of microwave irradiation. Finally, the challenges and future research directions for microwave-assisted preparation of inorganic nanostructured materials will be outlined.
Institutional access
Digital access.
- Campaigning and outreach
- News and events
- Awards and funding
- Privacy policy
- Journals and databases
- Locations and contacts
- Membership and professional community
- Teaching and learning
- Help and legal
- Cookie policy
- Terms and conditions
- Get Adobe Acrobat Reader
- Registered charity number: 207890
- © Royal Society of Chemistry 2023
This Feature Is Available To Subscribers Only
Sign In or Create an Account
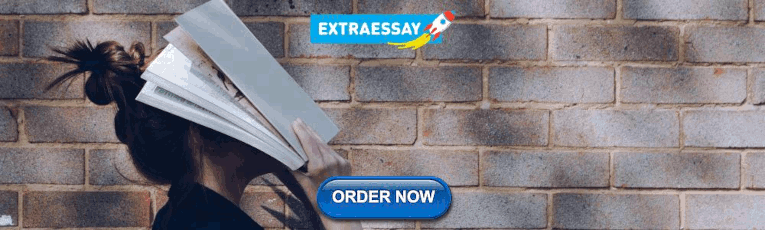
IMAGES
VIDEO
COMMENTS
For anyone working in inorganic chemistry, The Inorganic Syntheses series is the complete and up-to-date review of the area, providing the detailed foolproof information needed by lab chemists on procedures for the preparation of important and timely inorganic compounds.With recent volumes covering the latest hot topics and developing areas such as organometallic chemistry, main group ...
Abstract. High temperature is an important means for inorganic syntheses of novel compounds, materials, and high-temperature phases. In this chapter, we highlight the various important aspects in high-temperature inorganic synthesis, along with their recent progress, such as preparation of rare earth compounds and materials at high temperature, sol-gel process, and precursors in high ...
The volumes in this continuing series provide a compilation of current techniques and ideas in inorganic synthetic chemistry. Includes inorganic polymer syntheses and preparation of important inorganic solids, syntheses used in the development of pharmacologically active inorganic compounds, small-molecule coordination complexes, and related compounds. Also contains valuable information on ...
Book Series: Inorganic Syntheses. Table of Contents. GO TO PART. Free Access. free. Front Matter (Pages: i-xxi) Summary; PDF; Request permissions; CHAPTER One. no. ... SYNTHESIS OF SELECTED TRANSITION METAL AND MAIN GROUP COMPOUNDS WITH SYNTHETIC APPLICATIONS (Pages: 155-204) Hanan E. Abdou, ...
The Inorganic Syntheses Series. The Inorganic Syntheses series is published to provide all users of inorganic substances with detailed and foolproof procedures for the preparation of important and timely compounds. Thus the series is the concern of the entire scientific community. The Editorial Board hopes that all chemists will share in the ...
Inorganic chemistry. The structure of the ionic framework in potassium oxide, K 2 O. Inorganic chemistry deals with synthesis and behavior of inorganic and organometallic compounds. This field covers chemical compounds that are not carbon-based, which are the subjects of organic chemistry.
Predictive synthesis is a grand challenge that would accelerate the discovery of advanced inorganic materials ().The complexity of synthesis mainly originates from the interactions of many design variables, including the diversity of precursor candidates for each element in the target material (oxides, hydroxides, carbonates, etc.), the experimental conditions (temperature, atmosphere, etc ...
The dataset is publicly available and can be used for data mining of various aspects of inorganic materials synthesis. Measurement (s) solid-state synthesis data. Technology Type (s) natural ...
Inorganic Syntheses is a book series which aims to publish "detailed and foolproof" procedures for the synthesis of inorganic compounds. [1] [2] Although this series of books are edited, they usually are referenced like a journal, without mentioning the names of the checkers (referees) or the editor.
The Inorganic Syntheses series is published to provide all users of inorganic substances with detailed and reliable procedures for the preparation of important and timely compounds. Thus the series is the concern of the entire scientific com-munity. The Editorial Board hopes that all chemists will share in the responsibil-
The Inorganic Syntheses series provides all users of inorganic substances with detailed and foolproof procedures for the preparation of important and timely compounds. Includes complete, up-to-date procedures involving important inorganic substances Contains subject, contributor, and formula indexes
The Inorganic Synthesis Series provides all users of inorganic substances with detailed and foolproof procedures for the preparation of important and timely compounds. This new volume includes information on water-solubilizing ligands for organometallics, labile ligand complexes, and the syntheses of cluster compounds and hydrides.
The newest volume in the authoritative Inorganic Syntheses book series provides users of inorganic substances with detailed and foolproof procedures for the preparation of important and timely inorganic and organometallic compounds that can be used in reactions to develop new materials, drug targets, and bio-inspired chemical entities.
Inorganic Synthesis PDFs (vol. 1-34) Volume 1 Download. Volume 2 Download. Volume 3 Download. Volume 4 Download. Volume 5 Download. Volume 6 Download. Volume 7 Download. Volume 8 Download. Volume 9 Download. Volume 10 Download. Volume 11 Download. Volume 12 Download. Volume 13 Download. Volume 14 Download. Volume 15 Download.
The synthesis of inorganic compounds embraces an immense range of techniques and approaches. New organometallic molecules, for example, might demand multi-step organic reactions in the successful production of ligands followed by precision handling and manipulation to form the desired complexes under anaerobic conditions. By contrast ...
No headers. Inorganic chemistry explores the synthesis and behavior of inorganic and organometallic compounds and hence covers all chemical compounds except the myriad organic compounds (carbon-based compounds), which are the subjects of organic chemistry.Inorganic chemistry has applications in every aspect of the chemical industry, including catalysis, materials science, pigments, surfactants ...
In inorganic synthesis, basic IL anions provide the required basic environment of the reactions, while the organic cations may play an important role in the crystal nucleation and growth. Li et al. designed a variety of tetraalkylammonium hydroxide ILs to synthesize metal oxides of various sizes and shapes.
Although datasets for the synthesis of organic materials are widely available 28,29, there is not yet a large-scale database of inorganic synthesis routes, which is needed to train advanced deep ...
chemical synthesis, the construction of complex chemical compounds from simpler ones. It is the process by which many substances important to daily life are obtained. It is applied to all types of chemical compounds, but most syntheses are of organic molecules. Chemists synthesize chemical compounds that occur in nature in order to gain a ...
Ultrahigh pressure inorganic synthesis is a very effective method for synthesizing new inorganic compounds that cannot be obtained under normal conditions. The inorganic compounds synthesized under these high-pressure conditions can have special valences, unique structures, and unprecedented physical and chemical properties. ...
The synthesis of inorganic compounds for use in materials 1,2 is about making solids and this chapter on synthesis describes the preparation of (mainly) nonmolecular solid compounds in solid state reactions, in reactions from liquids, or reactions from the gas phase. The synthesis of inorganic molecules 3 is not much different than that of ...
Inorganic Synthesis: A Manual for Laboratory Experiments. Nikolay Gerasimchuk, Sergiy Tyukhtenko. Cambridge Scholars Publishing, Oct 24, 2019 - Juvenile Nonfiction - 396 pages. This book is designed to develop important practical skills for chemistry majors interested in synthetic chemistry. It will serve to teach students proper techniques for ...
A decomposition reaction is a reaction in which a compound breaks down into two or more simpler substances. The general form of a decomposition reaction is: AB → A + B (11.3.4) Most decomposition reactions require an input of energy in the form of heat, light, or electricity. Binary compounds are compounds composed of just two elements.
The major part of this chapter will focus on reviewing the state-of-the-art inorganic nanostructures that are prepared with the help of microwave irradiation. Finally, the challenges and future research directions for microwave-assisted preparation of inorganic nanostructured materials will be outlined.
M4 could completely convert 200 mM of 1-(4-methoxybenzyl)-3,4,5,6,7,8-hexahydroisoquinoline into (S)-1-(4-methoxybenzyl)-OHIQ in 77% isolated yield, with >99% enantiomeric excess and a high space-time yield of 542 g L -1 day -1, demonstrating a great potential for the synthesis of dextromethorphan intermediate in industrial applications.