How to Write Limitations of the Study (with examples)
This blog emphasizes the importance of recognizing and effectively writing about limitations in research. It discusses the types of limitations, their significance, and provides guidelines for writing about them, highlighting their role in advancing scholarly research.
Updated on August 24, 2023

No matter how well thought out, every research endeavor encounters challenges. There is simply no way to predict all possible variances throughout the process.
These uncharted boundaries and abrupt constraints are known as limitations in research . Identifying and acknowledging limitations is crucial for conducting rigorous studies. Limitations provide context and shed light on gaps in the prevailing inquiry and literature.
This article explores the importance of recognizing limitations and discusses how to write them effectively. By interpreting limitations in research and considering prevalent examples, we aim to reframe the perception from shameful mistakes to respectable revelations.
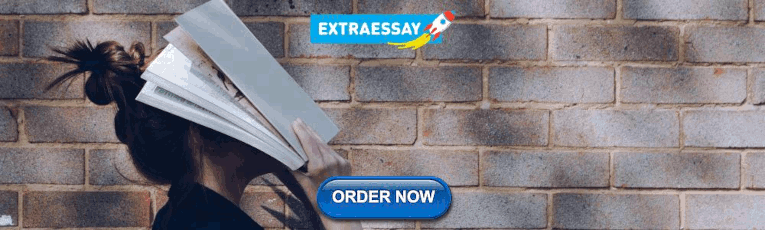
What are limitations in research?
In the clearest terms, research limitations are the practical or theoretical shortcomings of a study that are often outside of the researcher’s control . While these weaknesses limit the generalizability of a study’s conclusions, they also present a foundation for future research.
Sometimes limitations arise from tangible circumstances like time and funding constraints, or equipment and participant availability. Other times the rationale is more obscure and buried within the research design. Common types of limitations and their ramifications include:
- Theoretical: limits the scope, depth, or applicability of a study.
- Methodological: limits the quality, quantity, or diversity of the data.
- Empirical: limits the representativeness, validity, or reliability of the data.
- Analytical: limits the accuracy, completeness, or significance of the findings.
- Ethical: limits the access, consent, or confidentiality of the data.
Regardless of how, when, or why they arise, limitations are a natural part of the research process and should never be ignored . Like all other aspects, they are vital in their own purpose.
Why is identifying limitations important?
Whether to seek acceptance or avoid struggle, humans often instinctively hide flaws and mistakes. Merging this thought process into research by attempting to hide limitations, however, is a bad idea. It has the potential to negate the validity of outcomes and damage the reputation of scholars.
By identifying and addressing limitations throughout a project, researchers strengthen their arguments and curtail the chance of peer censure based on overlooked mistakes. Pointing out these flaws shows an understanding of variable limits and a scrupulous research process.
Showing awareness of and taking responsibility for a project’s boundaries and challenges validates the integrity and transparency of a researcher. It further demonstrates the researchers understand the applicable literature and have thoroughly evaluated their chosen research methods.
Presenting limitations also benefits the readers by providing context for research findings. It guides them to interpret the project’s conclusions only within the scope of very specific conditions. By allowing for an appropriate generalization of the findings that is accurately confined by research boundaries and is not too broad, limitations boost a study’s credibility .
Limitations are true assets to the research process. They highlight opportunities for future research. When researchers identify the limitations of their particular approach to a study question, they enable precise transferability and improve chances for reproducibility.
Simply stating a project’s limitations is not adequate for spurring further research, though. To spark the interest of other researchers, these acknowledgements must come with thorough explanations regarding how the limitations affected the current study and how they can potentially be overcome with amended methods.
How to write limitations
Typically, the information about a study’s limitations is situated either at the beginning of the discussion section to provide context for readers or at the conclusion of the discussion section to acknowledge the need for further research. However, it varies depending upon the target journal or publication guidelines.
Don’t hide your limitations
It is also important to not bury a limitation in the body of the paper unless it has a unique connection to a topic in that section. If so, it needs to be reiterated with the other limitations or at the conclusion of the discussion section. Wherever it is included in the manuscript, ensure that the limitations section is prominently positioned and clearly introduced.
While maintaining transparency by disclosing limitations means taking a comprehensive approach, it is not necessary to discuss everything that could have potentially gone wrong during the research study. If there is no commitment to investigation in the introduction, it is unnecessary to consider the issue a limitation to the research. Wholly consider the term ‘limitations’ and ask, “Did it significantly change or limit the possible outcomes?” Then, qualify the occurrence as either a limitation to include in the current manuscript or as an idea to note for other projects.
Writing limitations
Once the limitations are concretely identified and it is decided where they will be included in the paper, researchers are ready for the writing task. Including only what is pertinent, keeping explanations detailed but concise, and employing the following guidelines is key for crafting valuable limitations:
1) Identify and describe the limitations : Clearly introduce the limitation by classifying its form and specifying its origin. For example:
- An unintentional bias encountered during data collection
- An intentional use of unplanned post-hoc data analysis
2) Explain the implications : Describe how the limitation potentially influences the study’s findings and how the validity and generalizability are subsequently impacted. Provide examples and evidence to support claims of the limitations’ effects without making excuses or exaggerating their impact. Overall, be transparent and objective in presenting the limitations, without undermining the significance of the research.
3) Provide alternative approaches for future studies : Offer specific suggestions for potential improvements or avenues for further investigation. Demonstrate a proactive approach by encouraging future research that addresses the identified gaps and, therefore, expands the knowledge base.
Whether presenting limitations as an individual section within the manuscript or as a subtopic in the discussion area, authors should use clear headings and straightforward language to facilitate readability. There is no need to complicate limitations with jargon, computations, or complex datasets.
Examples of common limitations
Limitations are generally grouped into two categories , methodology and research process .
Methodology limitations
Methodology may include limitations due to:
- Sample size
- Lack of available or reliable data
- Lack of prior research studies on the topic
- Measure used to collect the data
- Self-reported data

The researcher is addressing how the large sample size requires a reassessment of the measures used to collect and analyze the data.
Research process limitations
Limitations during the research process may arise from:
- Access to information
- Longitudinal effects
- Cultural and other biases
- Language fluency
- Time constraints

The author is pointing out that the model’s estimates are based on potentially biased observational studies.
Final thoughts
Successfully proving theories and touting great achievements are only two very narrow goals of scholarly research. The true passion and greatest efforts of researchers comes more in the form of confronting assumptions and exploring the obscure.
In many ways, recognizing and sharing the limitations of a research study both allows for and encourages this type of discovery that continuously pushes research forward. By using limitations to provide a transparent account of the project's boundaries and to contextualize the findings, researchers pave the way for even more robust and impactful research in the future.
Charla Viera, MS
See our "Privacy Policy"
Ensure your structure and ideas are consistent and clearly communicated
Pair your Premium Editing with our add-on service Presubmission Review for an overall assessment of your manuscript.
- Privacy Policy

Home » Limitations in Research – Types, Examples and Writing Guide
Limitations in Research – Types, Examples and Writing Guide
Table of Contents

Limitations in Research
Limitations in research refer to the factors that may affect the results, conclusions , and generalizability of a study. These limitations can arise from various sources, such as the design of the study, the sampling methods used, the measurement tools employed, and the limitations of the data analysis techniques.
Types of Limitations in Research
Types of Limitations in Research are as follows:
Sample Size Limitations
This refers to the size of the group of people or subjects that are being studied. If the sample size is too small, then the results may not be representative of the population being studied. This can lead to a lack of generalizability of the results.
Time Limitations
Time limitations can be a constraint on the research process . This could mean that the study is unable to be conducted for a long enough period of time to observe the long-term effects of an intervention, or to collect enough data to draw accurate conclusions.
Selection Bias
This refers to a type of bias that can occur when the selection of participants in a study is not random. This can lead to a biased sample that is not representative of the population being studied.
Confounding Variables
Confounding variables are factors that can influence the outcome of a study, but are not being measured or controlled for. These can lead to inaccurate conclusions or a lack of clarity in the results.
Measurement Error
This refers to inaccuracies in the measurement of variables, such as using a faulty instrument or scale. This can lead to inaccurate results or a lack of validity in the study.
Ethical Limitations
Ethical limitations refer to the ethical constraints placed on research studies. For example, certain studies may not be allowed to be conducted due to ethical concerns, such as studies that involve harm to participants.
Examples of Limitations in Research
Some Examples of Limitations in Research are as follows:
Research Title: “The Effectiveness of Machine Learning Algorithms in Predicting Customer Behavior”
Limitations:
- The study only considered a limited number of machine learning algorithms and did not explore the effectiveness of other algorithms.
- The study used a specific dataset, which may not be representative of all customer behaviors or demographics.
- The study did not consider the potential ethical implications of using machine learning algorithms in predicting customer behavior.
Research Title: “The Impact of Online Learning on Student Performance in Computer Science Courses”
- The study was conducted during the COVID-19 pandemic, which may have affected the results due to the unique circumstances of remote learning.
- The study only included students from a single university, which may limit the generalizability of the findings to other institutions.
- The study did not consider the impact of individual differences, such as prior knowledge or motivation, on student performance in online learning environments.
Research Title: “The Effect of Gamification on User Engagement in Mobile Health Applications”
- The study only tested a specific gamification strategy and did not explore the effectiveness of other gamification techniques.
- The study relied on self-reported measures of user engagement, which may be subject to social desirability bias or measurement errors.
- The study only included a specific demographic group (e.g., young adults) and may not be generalizable to other populations with different preferences or needs.
How to Write Limitations in Research
When writing about the limitations of a research study, it is important to be honest and clear about the potential weaknesses of your work. Here are some tips for writing about limitations in research:
- Identify the limitations: Start by identifying the potential limitations of your research. These may include sample size, selection bias, measurement error, or other issues that could affect the validity and reliability of your findings.
- Be honest and objective: When describing the limitations of your research, be honest and objective. Do not try to minimize or downplay the limitations, but also do not exaggerate them. Be clear and concise in your description of the limitations.
- Provide context: It is important to provide context for the limitations of your research. For example, if your sample size was small, explain why this was the case and how it may have affected your results. Providing context can help readers understand the limitations in a broader context.
- Discuss implications : Discuss the implications of the limitations for your research findings. For example, if there was a selection bias in your sample, explain how this may have affected the generalizability of your findings. This can help readers understand the limitations in terms of their impact on the overall validity of your research.
- Provide suggestions for future research : Finally, provide suggestions for future research that can address the limitations of your study. This can help readers understand how your research fits into the broader field and can provide a roadmap for future studies.
Purpose of Limitations in Research
There are several purposes of limitations in research. Here are some of the most important ones:
- To acknowledge the boundaries of the study : Limitations help to define the scope of the research project and set realistic expectations for the findings. They can help to clarify what the study is not intended to address.
- To identify potential sources of bias: Limitations can help researchers identify potential sources of bias in their research design, data collection, or analysis. This can help to improve the validity and reliability of the findings.
- To provide opportunities for future research: Limitations can highlight areas for future research and suggest avenues for further exploration. This can help to advance knowledge in a particular field.
- To demonstrate transparency and accountability: By acknowledging the limitations of their research, researchers can demonstrate transparency and accountability to their readers, peers, and funders. This can help to build trust and credibility in the research community.
- To encourage critical thinking: Limitations can encourage readers to critically evaluate the study’s findings and consider alternative explanations or interpretations. This can help to promote a more nuanced and sophisticated understanding of the topic under investigation.
When to Write Limitations in Research
Limitations should be included in research when they help to provide a more complete understanding of the study’s results and implications. A limitation is any factor that could potentially impact the accuracy, reliability, or generalizability of the study’s findings.
It is important to identify and discuss limitations in research because doing so helps to ensure that the results are interpreted appropriately and that any conclusions drawn are supported by the available evidence. Limitations can also suggest areas for future research, highlight potential biases or confounding factors that may have affected the results, and provide context for the study’s findings.
Generally, limitations should be discussed in the conclusion section of a research paper or thesis, although they may also be mentioned in other sections, such as the introduction or methods. The specific limitations that are discussed will depend on the nature of the study, the research question being investigated, and the data that was collected.
Examples of limitations that might be discussed in research include sample size limitations, data collection methods, the validity and reliability of measures used, and potential biases or confounding factors that could have affected the results. It is important to note that limitations should not be used as a justification for poor research design or methodology, but rather as a way to enhance the understanding and interpretation of the study’s findings.
Importance of Limitations in Research
Here are some reasons why limitations are important in research:
- Enhances the credibility of research: Limitations highlight the potential weaknesses and threats to validity, which helps readers to understand the scope and boundaries of the study. This improves the credibility of research by acknowledging its limitations and providing a clear picture of what can and cannot be concluded from the study.
- Facilitates replication: By highlighting the limitations, researchers can provide detailed information about the study’s methodology, data collection, and analysis. This information helps other researchers to replicate the study and test the validity of the findings, which enhances the reliability of research.
- Guides future research : Limitations provide insights into areas for future research by identifying gaps or areas that require further investigation. This can help researchers to design more comprehensive and effective studies that build on existing knowledge.
- Provides a balanced view: Limitations help to provide a balanced view of the research by highlighting both strengths and weaknesses. This ensures that readers have a clear understanding of the study’s limitations and can make informed decisions about the generalizability and applicability of the findings.
Advantages of Limitations in Research
Here are some potential advantages of limitations in research:
- Focus : Limitations can help researchers focus their study on a specific area or population, which can make the research more relevant and useful.
- Realism : Limitations can make a study more realistic by reflecting the practical constraints and challenges of conducting research in the real world.
- Innovation : Limitations can spur researchers to be more innovative and creative in their research design and methodology, as they search for ways to work around the limitations.
- Rigor : Limitations can actually increase the rigor and credibility of a study, as researchers are forced to carefully consider the potential sources of bias and error, and address them to the best of their abilities.
- Generalizability : Limitations can actually improve the generalizability of a study by ensuring that it is not overly focused on a specific sample or situation, and that the results can be applied more broadly.
About the author
Muhammad Hassan
Researcher, Academic Writer, Web developer
You may also like

How to Cite Research Paper – All Formats and...

Data Collection – Methods Types and Examples

Delimitations in Research – Types, Examples and...

Research Paper Format – Types, Examples and...

Research Process – Steps, Examples and Tips

Research Design – Types, Methods and Examples
- USC Libraries
- Research Guides
Organizing Your Social Sciences Research Paper
- Limitations of the Study
- Purpose of Guide
- Design Flaws to Avoid
- Independent and Dependent Variables
- Glossary of Research Terms
- Reading Research Effectively
- Narrowing a Topic Idea
- Broadening a Topic Idea
- Extending the Timeliness of a Topic Idea
- Academic Writing Style
- Applying Critical Thinking
- Choosing a Title
- Making an Outline
- Paragraph Development
- Research Process Video Series
- Executive Summary
- The C.A.R.S. Model
- Background Information
- The Research Problem/Question
- Theoretical Framework
- Citation Tracking
- Content Alert Services
- Evaluating Sources
- Primary Sources
- Secondary Sources
- Tiertiary Sources
- Scholarly vs. Popular Publications
- Qualitative Methods
- Quantitative Methods
- Insiderness
- Using Non-Textual Elements
- Common Grammar Mistakes
- Writing Concisely
- Avoiding Plagiarism
- Footnotes or Endnotes?
- Further Readings
- Generative AI and Writing
- USC Libraries Tutorials and Other Guides
- Bibliography
The limitations of the study are those characteristics of design or methodology that impacted or influenced the interpretation of the findings from your research. Study limitations are the constraints placed on the ability to generalize from the results, to further describe applications to practice, and/or related to the utility of findings that are the result of the ways in which you initially chose to design the study or the method used to establish internal and external validity or the result of unanticipated challenges that emerged during the study.
Price, James H. and Judy Murnan. “Research Limitations and the Necessity of Reporting Them.” American Journal of Health Education 35 (2004): 66-67; Theofanidis, Dimitrios and Antigoni Fountouki. "Limitations and Delimitations in the Research Process." Perioperative Nursing 7 (September-December 2018): 155-163. .
Importance of...
Always acknowledge a study's limitations. It is far better that you identify and acknowledge your study’s limitations than to have them pointed out by your professor and have your grade lowered because you appeared to have ignored them or didn't realize they existed.
Keep in mind that acknowledgment of a study's limitations is an opportunity to make suggestions for further research. If you do connect your study's limitations to suggestions for further research, be sure to explain the ways in which these unanswered questions may become more focused because of your study.
Acknowledgment of a study's limitations also provides you with opportunities to demonstrate that you have thought critically about the research problem, understood the relevant literature published about it, and correctly assessed the methods chosen for studying the problem. A key objective of the research process is not only discovering new knowledge but also to confront assumptions and explore what we don't know.
Claiming limitations is a subjective process because you must evaluate the impact of those limitations . Don't just list key weaknesses and the magnitude of a study's limitations. To do so diminishes the validity of your research because it leaves the reader wondering whether, or in what ways, limitation(s) in your study may have impacted the results and conclusions. Limitations require a critical, overall appraisal and interpretation of their impact. You should answer the question: do these problems with errors, methods, validity, etc. eventually matter and, if so, to what extent?
Price, James H. and Judy Murnan. “Research Limitations and the Necessity of Reporting Them.” American Journal of Health Education 35 (2004): 66-67; Structure: How to Structure the Research Limitations Section of Your Dissertation. Dissertations and Theses: An Online Textbook. Laerd.com.
Descriptions of Possible Limitations
All studies have limitations . However, it is important that you restrict your discussion to limitations related to the research problem under investigation. For example, if a meta-analysis of existing literature is not a stated purpose of your research, it should not be discussed as a limitation. Do not apologize for not addressing issues that you did not promise to investigate in the introduction of your paper.
Here are examples of limitations related to methodology and the research process you may need to describe and discuss how they possibly impacted your results. Note that descriptions of limitations should be stated in the past tense because they were discovered after you completed your research.
Possible Methodological Limitations
- Sample size -- the number of the units of analysis you use in your study is dictated by the type of research problem you are investigating. Note that, if your sample size is too small, it will be difficult to find significant relationships from the data, as statistical tests normally require a larger sample size to ensure a representative distribution of the population and to be considered representative of groups of people to whom results will be generalized or transferred. Note that sample size is generally less relevant in qualitative research if explained in the context of the research problem.
- Lack of available and/or reliable data -- a lack of data or of reliable data will likely require you to limit the scope of your analysis, the size of your sample, or it can be a significant obstacle in finding a trend and a meaningful relationship. You need to not only describe these limitations but provide cogent reasons why you believe data is missing or is unreliable. However, don’t just throw up your hands in frustration; use this as an opportunity to describe a need for future research based on designing a different method for gathering data.
- Lack of prior research studies on the topic -- citing prior research studies forms the basis of your literature review and helps lay a foundation for understanding the research problem you are investigating. Depending on the currency or scope of your research topic, there may be little, if any, prior research on your topic. Before assuming this to be true, though, consult with a librarian! In cases when a librarian has confirmed that there is little or no prior research, you may be required to develop an entirely new research typology [for example, using an exploratory rather than an explanatory research design ]. Note again that discovering a limitation can serve as an important opportunity to identify new gaps in the literature and to describe the need for further research.
- Measure used to collect the data -- sometimes it is the case that, after completing your interpretation of the findings, you discover that the way in which you gathered data inhibited your ability to conduct a thorough analysis of the results. For example, you regret not including a specific question in a survey that, in retrospect, could have helped address a particular issue that emerged later in the study. Acknowledge the deficiency by stating a need for future researchers to revise the specific method for gathering data.
- Self-reported data -- whether you are relying on pre-existing data or you are conducting a qualitative research study and gathering the data yourself, self-reported data is limited by the fact that it rarely can be independently verified. In other words, you have to the accuracy of what people say, whether in interviews, focus groups, or on questionnaires, at face value. However, self-reported data can contain several potential sources of bias that you should be alert to and note as limitations. These biases become apparent if they are incongruent with data from other sources. These are: (1) selective memory [remembering or not remembering experiences or events that occurred at some point in the past]; (2) telescoping [recalling events that occurred at one time as if they occurred at another time]; (3) attribution [the act of attributing positive events and outcomes to one's own agency, but attributing negative events and outcomes to external forces]; and, (4) exaggeration [the act of representing outcomes or embellishing events as more significant than is actually suggested from other data].
Possible Limitations of the Researcher
- Access -- if your study depends on having access to people, organizations, data, or documents and, for whatever reason, access is denied or limited in some way, the reasons for this needs to be described. Also, include an explanation why being denied or limited access did not prevent you from following through on your study.
- Longitudinal effects -- unlike your professor, who can literally devote years [even a lifetime] to studying a single topic, the time available to investigate a research problem and to measure change or stability over time is constrained by the due date of your assignment. Be sure to choose a research problem that does not require an excessive amount of time to complete the literature review, apply the methodology, and gather and interpret the results. If you're unsure whether you can complete your research within the confines of the assignment's due date, talk to your professor.
- Cultural and other type of bias -- we all have biases, whether we are conscience of them or not. Bias is when a person, place, event, or thing is viewed or shown in a consistently inaccurate way. Bias is usually negative, though one can have a positive bias as well, especially if that bias reflects your reliance on research that only support your hypothesis. When proof-reading your paper, be especially critical in reviewing how you have stated a problem, selected the data to be studied, what may have been omitted, the manner in which you have ordered events, people, or places, how you have chosen to represent a person, place, or thing, to name a phenomenon, or to use possible words with a positive or negative connotation. NOTE : If you detect bias in prior research, it must be acknowledged and you should explain what measures were taken to avoid perpetuating that bias. For example, if a previous study only used boys to examine how music education supports effective math skills, describe how your research expands the study to include girls.
- Fluency in a language -- if your research focuses , for example, on measuring the perceived value of after-school tutoring among Mexican-American ESL [English as a Second Language] students and you are not fluent in Spanish, you are limited in being able to read and interpret Spanish language research studies on the topic or to speak with these students in their primary language. This deficiency should be acknowledged.
Aguinis, Hermam and Jeffrey R. Edwards. “Methodological Wishes for the Next Decade and How to Make Wishes Come True.” Journal of Management Studies 51 (January 2014): 143-174; Brutus, Stéphane et al. "Self-Reported Limitations and Future Directions in Scholarly Reports: Analysis and Recommendations." Journal of Management 39 (January 2013): 48-75; Senunyeme, Emmanuel K. Business Research Methods. Powerpoint Presentation. Regent University of Science and Technology; ter Riet, Gerben et al. “All That Glitters Isn't Gold: A Survey on Acknowledgment of Limitations in Biomedical Studies.” PLOS One 8 (November 2013): 1-6.
Structure and Writing Style
Information about the limitations of your study are generally placed either at the beginning of the discussion section of your paper so the reader knows and understands the limitations before reading the rest of your analysis of the findings, or, the limitations are outlined at the conclusion of the discussion section as an acknowledgement of the need for further study. Statements about a study's limitations should not be buried in the body [middle] of the discussion section unless a limitation is specific to something covered in that part of the paper. If this is the case, though, the limitation should be reiterated at the conclusion of the section.
If you determine that your study is seriously flawed due to important limitations , such as, an inability to acquire critical data, consider reframing it as an exploratory study intended to lay the groundwork for a more complete research study in the future. Be sure, though, to specifically explain the ways that these flaws can be successfully overcome in a new study.
But, do not use this as an excuse for not developing a thorough research paper! Review the tab in this guide for developing a research topic . If serious limitations exist, it generally indicates a likelihood that your research problem is too narrowly defined or that the issue or event under study is too recent and, thus, very little research has been written about it. If serious limitations do emerge, consult with your professor about possible ways to overcome them or how to revise your study.
When discussing the limitations of your research, be sure to:
- Describe each limitation in detailed but concise terms;
- Explain why each limitation exists;
- Provide the reasons why each limitation could not be overcome using the method(s) chosen to acquire or gather the data [cite to other studies that had similar problems when possible];
- Assess the impact of each limitation in relation to the overall findings and conclusions of your study; and,
- If appropriate, describe how these limitations could point to the need for further research.
Remember that the method you chose may be the source of a significant limitation that has emerged during your interpretation of the results [for example, you didn't interview a group of people that you later wish you had]. If this is the case, don't panic. Acknowledge it, and explain how applying a different or more robust methodology might address the research problem more effectively in a future study. A underlying goal of scholarly research is not only to show what works, but to demonstrate what doesn't work or what needs further clarification.
Aguinis, Hermam and Jeffrey R. Edwards. “Methodological Wishes for the Next Decade and How to Make Wishes Come True.” Journal of Management Studies 51 (January 2014): 143-174; Brutus, Stéphane et al. "Self-Reported Limitations and Future Directions in Scholarly Reports: Analysis and Recommendations." Journal of Management 39 (January 2013): 48-75; Ioannidis, John P.A. "Limitations are not Properly Acknowledged in the Scientific Literature." Journal of Clinical Epidemiology 60 (2007): 324-329; Pasek, Josh. Writing the Empirical Social Science Research Paper: A Guide for the Perplexed. January 24, 2012. Academia.edu; Structure: How to Structure the Research Limitations Section of Your Dissertation. Dissertations and Theses: An Online Textbook. Laerd.com; What Is an Academic Paper? Institute for Writing Rhetoric. Dartmouth College; Writing the Experimental Report: Methods, Results, and Discussion. The Writing Lab and The OWL. Purdue University.
Writing Tip
Don't Inflate the Importance of Your Findings!
After all the hard work and long hours devoted to writing your research paper, it is easy to get carried away with attributing unwarranted importance to what you’ve done. We all want our academic work to be viewed as excellent and worthy of a good grade, but it is important that you understand and openly acknowledge the limitations of your study. Inflating the importance of your study's findings could be perceived by your readers as an attempt hide its flaws or encourage a biased interpretation of the results. A small measure of humility goes a long way!
Another Writing Tip
Negative Results are Not a Limitation!
Negative evidence refers to findings that unexpectedly challenge rather than support your hypothesis. If you didn't get the results you anticipated, it may mean your hypothesis was incorrect and needs to be reformulated. Or, perhaps you have stumbled onto something unexpected that warrants further study. Moreover, the absence of an effect may be very telling in many situations, particularly in experimental research designs. In any case, your results may very well be of importance to others even though they did not support your hypothesis. Do not fall into the trap of thinking that results contrary to what you expected is a limitation to your study. If you carried out the research well, they are simply your results and only require additional interpretation.
Lewis, George H. and Jonathan F. Lewis. “The Dog in the Night-Time: Negative Evidence in Social Research.” The British Journal of Sociology 31 (December 1980): 544-558.
Yet Another Writing Tip
Sample Size Limitations in Qualitative Research
Sample sizes are typically smaller in qualitative research because, as the study goes on, acquiring more data does not necessarily lead to more information. This is because one occurrence of a piece of data, or a code, is all that is necessary to ensure that it becomes part of the analysis framework. However, it remains true that sample sizes that are too small cannot adequately support claims of having achieved valid conclusions and sample sizes that are too large do not permit the deep, naturalistic, and inductive analysis that defines qualitative inquiry. Determining adequate sample size in qualitative research is ultimately a matter of judgment and experience in evaluating the quality of the information collected against the uses to which it will be applied and the particular research method and purposeful sampling strategy employed. If the sample size is found to be a limitation, it may reflect your judgment about the methodological technique chosen [e.g., single life history study versus focus group interviews] rather than the number of respondents used.
Boddy, Clive Roland. "Sample Size for Qualitative Research." Qualitative Market Research: An International Journal 19 (2016): 426-432; Huberman, A. Michael and Matthew B. Miles. "Data Management and Analysis Methods." In Handbook of Qualitative Research . Norman K. Denzin and Yvonna S. Lincoln, eds. (Thousand Oaks, CA: Sage, 1994), pp. 428-444; Blaikie, Norman. "Confounding Issues Related to Determining Sample Size in Qualitative Research." International Journal of Social Research Methodology 21 (2018): 635-641; Oppong, Steward Harrison. "The Problem of Sampling in qualitative Research." Asian Journal of Management Sciences and Education 2 (2013): 202-210.
- << Previous: 8. The Discussion
- Next: 9. The Conclusion >>
- Last Updated: May 9, 2024 11:05 AM
- URL: https://libguides.usc.edu/writingguide
How to present limitations in research
Last updated
30 January 2024
Reviewed by
Limitations don’t invalidate or diminish your results, but it’s best to acknowledge them. This will enable you to address any questions your study failed to answer because of them.
In this guide, learn how to recognize, present, and overcome limitations in research.
- What is a research limitation?
Research limitations are weaknesses in your research design or execution that may have impacted outcomes and conclusions. Uncovering limitations doesn’t necessarily indicate poor research design—it just means you encountered challenges you couldn’t have anticipated that limited your research efforts.
Does basic research have limitations?
Basic research aims to provide more information about your research topic. It requires the same standard research methodology and data collection efforts as any other research type, and it can also have limitations.
- Common research limitations
Researchers encounter common limitations when embarking on a study. Limitations can occur in relation to the methods you apply or the research process you design. They could also be connected to you as the researcher.
Methodology limitations
Not having access to data or reliable information can impact the methods used to facilitate your research. A lack of data or reliability may limit the parameters of your study area and the extent of your exploration.
Your sample size may also be affected because you won’t have any direction on how big or small it should be and who or what you should include. Having too few participants won’t adequately represent the population or groups of people needed to draw meaningful conclusions.
Research process limitations
The study’s design can impose constraints on the process. For example, as you’re conducting the research, issues may arise that don’t conform to the data collection methodology you developed. You may not realize until well into the process that you should have incorporated more specific questions or comprehensive experiments to generate the data you need to have confidence in your results.
Constraints on resources can also have an impact. Being limited on participants or participation incentives may limit your sample sizes. Insufficient tools, equipment, and materials to conduct a thorough study may also be a factor.
Common researcher limitations
Here are some of the common researcher limitations you may encounter:
Time: some research areas require multi-year longitudinal approaches, but you might not be able to dedicate that much time. Imagine you want to measure how much memory a person loses as they age. This may involve conducting multiple tests on a sample of participants over 20–30 years, which may be impossible.
Bias: researchers can consciously or unconsciously apply bias to their research. Biases can contribute to relying on research sources and methodologies that will only support your beliefs about the research you’re embarking on. You might also omit relevant issues or participants from the scope of your study because of your biases.
Limited access to data : you may need to pay to access specific databases or journals that would be helpful to your research process. You might also need to gain information from certain people or organizations but have limited access to them. These cases require readjusting your process and explaining why your findings are still reliable.
- Why is it important to identify limitations?
Identifying limitations adds credibility to research and provides a deeper understanding of how you arrived at your conclusions.
Constraints may have prevented you from collecting specific data or information you hoped would prove or disprove your hypothesis or provide a more comprehensive understanding of your research topic.
However, identifying the limitations contributing to your conclusions can inspire further research efforts that help gather more substantial information and data.
- Where to put limitations in a research paper
A research paper is broken up into different sections that appear in the following order:
Introduction
Methodology
The discussion portion of your paper explores your findings and puts them in the context of the overall research. Either place research limitations at the beginning of the discussion section before the analysis of your findings or at the end of the section to indicate that further research needs to be pursued.
What not to include in the limitations section
Evidence that doesn’t support your hypothesis is not a limitation, so you shouldn’t include it in the limitation section. Don’t just list limitations and their degree of severity without further explanation.
- How to present limitations
You’ll want to present the limitations of your study in a way that doesn’t diminish the validity of your research and leave the reader wondering if your results and conclusions have been compromised.
Include only the limitations that directly relate to and impact how you addressed your research questions. Following a specific format enables the reader to develop an understanding of the weaknesses within the context of your findings without doubting the quality and integrity of your research.
Identify the limitations specific to your study
You don’t have to identify every possible limitation that might have occurred during your research process. Only identify those that may have influenced the quality of your findings and your ability to answer your research question.
Explain study limitations in detail
This explanation should be the most significant portion of your limitation section.
Link each limitation with an interpretation and appraisal of their impact on the study. You’ll have to evaluate and explain whether the error, method, or validity issues influenced the study’s outcome and how.
Propose a direction for future studies and present alternatives
In this section, suggest how researchers can avoid the pitfalls you experienced during your research process.
If an issue with methodology was a limitation, propose alternate methods that may help with a smoother and more conclusive research project. Discuss the pros and cons of your alternate recommendation.
Describe steps taken to minimize each limitation
You probably took steps to try to address or mitigate limitations when you noticed them throughout the course of your research project. Describe these steps in the limitation section.
- Limitation example
“Approaches like stem cell transplantation and vaccination in AD [Alzheimer’s disease] work on a cellular or molecular level in the laboratory. However, translation into clinical settings will remain a challenge for the next decade.”
The authors are saying that even though these methods showed promise in helping people with memory loss when conducted in the lab (in other words, using animal studies), more studies are needed. These may be controlled clinical trials, for example.
However, the short life span of stem cells outside the lab and the vaccination’s severe inflammatory side effects are limitations. Researchers won’t be able to conduct clinical trials until these issues are overcome.
- How to overcome limitations in research
You’ve already started on the road to overcoming limitations in research by acknowledging that they exist. However, you need to ensure readers don’t mistake weaknesses for errors within your research design.
To do this, you’ll need to justify and explain your rationale for the methods, research design, and analysis tools you chose and how you noticed they may have presented limitations.
Your readers need to know that even when limitations presented themselves, you followed best practices and the ethical standards of your field. You didn’t violate any rules and regulations during your research process.
You’ll also want to reinforce the validity of your conclusions and results with multiple sources, methods, and perspectives. This prevents readers from assuming your findings were derived from a single or biased source.
- Learning and improving starts with limitations in research
Dealing with limitations with transparency and integrity helps identify areas for future improvements and developments. It’s a learning process, providing valuable insights into how you can improve methodologies, expand sample sizes, or explore alternate approaches to further support the validity of your findings.
Editor’s picks
Last updated: 11 January 2024
Last updated: 15 January 2024
Last updated: 25 November 2023
Last updated: 12 May 2023
Last updated: 30 April 2024
Last updated: 18 May 2023
Last updated: 10 April 2023
Latest articles
Related topics, .css-je19u9{-webkit-align-items:flex-end;-webkit-box-align:flex-end;-ms-flex-align:flex-end;align-items:flex-end;display:-webkit-box;display:-webkit-flex;display:-ms-flexbox;display:flex;-webkit-flex-direction:row;-ms-flex-direction:row;flex-direction:row;-webkit-box-flex-wrap:wrap;-webkit-flex-wrap:wrap;-ms-flex-wrap:wrap;flex-wrap:wrap;-webkit-box-pack:center;-ms-flex-pack:center;-webkit-justify-content:center;justify-content:center;row-gap:0;text-align:center;max-width:671px;}@media (max-width: 1079px){.css-je19u9{max-width:400px;}.css-je19u9>span{white-space:pre;}}@media (max-width: 799px){.css-je19u9{max-width:400px;}.css-je19u9>span{white-space:pre;}} decide what to .css-1kiodld{max-height:56px;display:-webkit-box;display:-webkit-flex;display:-ms-flexbox;display:flex;-webkit-align-items:center;-webkit-box-align:center;-ms-flex-align:center;align-items:center;}@media (max-width: 1079px){.css-1kiodld{display:none;}} build next, decide what to build next.

Users report unexpectedly high data usage, especially during streaming sessions.

Users find it hard to navigate from the home page to relevant playlists in the app.

It would be great to have a sleep timer feature, especially for bedtime listening.

I need better filters to find the songs or artists I’m looking for.
Log in or sign up
Get started for free

Organizing Academic Research Papers: Limitations of the Study
- Purpose of Guide
- Design Flaws to Avoid
- Glossary of Research Terms
- Narrowing a Topic Idea
- Broadening a Topic Idea
- Extending the Timeliness of a Topic Idea
- Academic Writing Style
- Choosing a Title
- Making an Outline
- Paragraph Development
- Executive Summary
- Background Information
- The Research Problem/Question
- Theoretical Framework
- Citation Tracking
- Content Alert Services
- Evaluating Sources
- Primary Sources
- Secondary Sources
- Tertiary Sources
- What Is Scholarly vs. Popular?
- Qualitative Methods
- Quantitative Methods
- Using Non-Textual Elements
- Limitations of the Study
- Common Grammar Mistakes
- Avoiding Plagiarism
- Footnotes or Endnotes?
- Further Readings
- Annotated Bibliography
- Dealing with Nervousness
- Using Visual Aids
- Grading Someone Else's Paper
- How to Manage Group Projects
- Multiple Book Review Essay
- Reviewing Collected Essays
- About Informed Consent
- Writing Field Notes
- Writing a Policy Memo
- Writing a Research Proposal
- Acknowledgements
The limitations of the study are those characteristics of design or methodology that impacted or influenced the application or interpretation of the results of your study. They are the constraints on generalizability and utility of findings that are the result of the ways in which you chose to design the study and/or the method used to establish internal and external validity.
Importance of...
Always acknowledge a study's limitations. It is far better for you to identify and acknowledge your study’s limitations than to have them pointed out by your professor and be graded down because you appear to have ignored them.
Keep in mind that acknowledgement of a study's limitations is an opportunity to make suggestions for further research. If you do connect your study's limitations to suggestions for further research, be sure to explain the ways in which these unanswered questions may become more focused because of your study.
Acknowledgement of a study's limitations also provides you with an opportunity to demonstrate to your professor that you have thought critically about the research problem, understood the relevant literature published about it, and correctly assessed the methods chosen for studying the problem. A key objective of the research process is not only discovering new knowledge but also to confront assumptions and explore what we don't know.
Claiming limitiations is a subjective process because you must evaluate the impact of those limitations . Don't just list key weaknesses and the magnitude of a study's limitations. To do so diminishes the validity of your research because it leaves the reader wondering whether, or in what ways, limitation(s) in your study may have impacted the findings and conclusions. Limitations require a critical, overall appraisal and interpretation of their impact. You should answer the question: do these problems with errors, methods, validity, etc. eventually matter and, if so, to what extent?
Structure: How to Structure the Research Limitations Section of Your Dissertation . Dissertations and Theses: An Online Textbook. Laerd.com.
Descriptions of Possible Limitations
All studies have limitations . However, it is important that you restrict your discussion to limitations related to the research problem under investigation. For example, if a meta-analysis of existing literature is not a stated purpose of your research, it should not be discussed as a limitation. Do not apologize for not addressing issues that you did not promise to investigate in your paper.
Here are examples of limitations you may need to describe and to discuss how they possibly impacted your findings. Descriptions of limitations should be stated in the past tense.
Possible Methodological Limitations
- Sample size -- the number of the units of analysis you use in your study is dictated by the type of research problem you are investigating. Note that, if your sample size is too small, it will be difficult to find significant relationships from the data, as statistical tests normally require a larger sample size to ensure a representative distribution of the population and to be considered representative of groups of people to whom results will be generalized or transferred.
- Lack of available and/or reliable data -- a lack of data or of reliable data will likely require you to limit the scope of your analysis, the size of your sample, or it can be a significant obstacle in finding a trend and a meaningful relationship. You need to not only describe these limitations but to offer reasons why you believe data is missing or is unreliable. However, don’t just throw up your hands in frustration; use this as an opportunity to describe the need for future research.
- Lack of prior research studies on the topic -- citing prior research studies forms the basis of your literature review and helps lay a foundation for understanding the research problem you are investigating. Depending on the currency or scope of your research topic, there may be little, if any, prior research on your topic. Before assuming this to be true, consult with a librarian! In cases when a librarian has confirmed that there is a lack of prior research, you may be required to develop an entirely new research typology [for example, using an exploratory rather than an explanatory research design]. Note that this limitation can serve as an important opportunity to describe the need for further research.
- Measure used to collect the data -- sometimes it is the case that, after completing your interpretation of the findings, you discover that the way in which you gathered data inhibited your ability to conduct a thorough analysis of the results. For example, you regret not including a specific question in a survey that, in retrospect, could have helped address a particular issue that emerged later in the study. Acknowledge the deficiency by stating a need in future research to revise the specific method for gathering data.
- Self-reported data -- whether you are relying on pre-existing self-reported data or you are conducting a qualitative research study and gathering the data yourself, self-reported data is limited by the fact that it rarely can be independently verified. In other words, you have to take what people say, whether in interviews, focus groups, or on questionnaires, at face value. However, self-reported data contain several potential sources of bias that should be noted as limitations: (1) selective memory (remembering or not remembering experiences or events that occurred at some point in the past); (2) telescoping [recalling events that occurred at one time as if they occurred at another time]; (3) attribution [the act of attributing positive events and outcomes to one's own agency but attributing negative events and outcomes to external forces]; and, (4) exaggeration [the act of representing outcomes or embellishing events as more significant than is actually suggested from other data].
Possible Limitations of the Researcher
- Access -- if your study depends on having access to people, organizations, or documents and, for whatever reason, access is denied or otherwise limited, the reasons for this need to be described.
- Longitudinal effects -- unlike your professor, who can literally devote years [even a lifetime] to studying a single research problem, the time available to investigate a research problem and to measure change or stability within a sample is constrained by the due date of your assignment. Be sure to choose a topic that does not require an excessive amount of time to complete the literature review, apply the methodology, and gather and interpret the results. If you're unsure, talk to your professor.
- Cultural and other type of bias -- we all have biases, whether we are conscience of them or not. Bias is when a person, place, or thing is viewed or shown in a consistently inaccurate way. It is usually negative, though one can have a positive bias as well. When proof-reading your paper, be especially critical in reviewing how you have stated a problem, selected the data to be studied, what may have been omitted, the manner in which you have ordered events, people, or places and how you have chosen to represent a person, place, or thing, to name a phenomenon, or to use possible words with a positive or negative connotation. Note that if you detect bias in prior research, it must be acknowledged and you should explain what measures were taken to avoid perpetuating bias.
- Fluency in a language -- if your research focuses on measuring the perceived value of after-school tutoring among Mexican-American ESL [English as a Second Language] students, for example, and you are not fluent in Spanish, you are limited in being able to read and interpret Spanish language research studies on the topic. This deficiency should be acknowledged.
Brutus, Stéphane et al. Self-Reported Limitations and Future Directions in Scholarly Reports: Analysis and Recommendations. Journal of Management 39 (January 2013): 48-75; Senunyeme, Emmanuel K. Business Research Methods . Powerpoint Presentation. Regent University of Science and Technology.
Structure and Writing Style
Information about the limitations of your study are generally placed either at the beginning of the discussion section of your paper so the reader knows and understands the limitations before reading the rest of your analysis of the findings, or, the limitations are outlined at the conclusion of the discussion section as an acknowledgement of the need for further study. Statements about a study's limitations should not be buried in the body [middle] of the discussion section unless a limitation is specific to something covered in that part of the paper. If this is the case, though, the limitation should be reiterated at the conclusion of the section.
If you determine that your study is seriously flawed due to important limitations , such as, an inability to acquire critical data, consider reframing it as a pilot study intended to lay the groundwork for a more complete research study in the future. Be sure, though, to specifically explain the ways that these flaws can be successfully overcome in later studies.
But, do not use this as an excuse for not developing a thorough research paper! Review the tab in this guide for developing a research topic . If serious limitations exist, it generally indicates a likelihood that your research problem is too narrowly defined or that the issue or event under study is too recent and, thus, very little research has been written about it. If serious limitations do emerge, consult with your professor about possible ways to overcome them or how to reframe your study.
When discussing the limitations of your research, be sure to:
- Describe each limitation in detailed but concise terms;
- Explain why each limitation exists;
- Provide the reasons why each limitation could not be overcome using the method(s) chosen to gather the data [cite to other studies that had similar problems when possible];
- Assess the impact of each limitation in relation to the overall findings and conclusions of your study; and,
- If appropriate, describe how these limitations could point to the need for further research.
Remember that the method you chose may be the source of a significant limitation that has emerged during your interpretation of the results [for example, you didn't ask a particular question in a survey that you later wish you had]. If this is the case, don't panic. Acknowledge it, and explain how applying a different or more robust methodology might address the research problem more effectively in any future study. A underlying goal of scholarly research is not only to prove what works, but to demonstrate what doesn't work or what needs further clarification.
Brutus, Stéphane et al. Self-Reported Limitations and Future Directions in Scholarly Reports: Analysis and Recommendations. Journal of Management 39 (January 2013): 48-75; Ioannidis, John P.A. Limitations are not Properly Acknowledged in the Scientific Literature. Journal of Clinical Epidemiology 60 (2007): 324-329; Pasek, Josh. Writing the Empirical Social Science Research Paper: A Guide for the Perplexed . January 24, 2012. Academia.edu; Structure: How to Structure the Research Limitations Section of Your Dissertation . Dissertations and Theses: An Online Textbook. Laerd.com; What Is an Academic Paper? Institute for Writing Rhetoric. Dartmouth College; Writing the Experimental Report: Methods, Results, and Discussion. The Writing Lab and The OWL. Purdue University.
Writing Tip
Don't Inflate the Importance of Your Findings! After all the hard work and long hours devoted to writing your research paper, it is easy to get carried away with attributing unwarranted importance to what you’ve done. We all want our academic work to be viewed as excellent and worthy of a good grade, but it is important that you understand and openly acknowledge the limitiations of your study. Inflating of the importance of your study's findings in an attempt hide its flaws is a big turn off to your readers. A measure of humility goes a long way!
Another Writing Tip
Negative Results are Not a Limitation!
Negative evidence refers to findings that unexpectedly challenge rather than support your hypothesis. If you didn't get the results you anticipated, it may mean your hypothesis was incorrect and needs to be reformulated, or, perhaps you have stumbled onto something unexpected that warrants further study. Moreover, the absence of an effect may be very telling in many situations, particularly in experimental research designs. In any case, your results may be of importance to others even though they did not support your hypothesis. Do not fall into the trap of thinking that results contrary to what you expected is a limitation to your study. If you carried out the research well, they are simply your results and only require additional interpretation.
Yet Another Writing Tip
A Note about Sample Size Limitations in Qualitative Research
Sample sizes are typically smaller in qualitative research because, as the study goes on, acquiring more data does not necessarily lead to more information. This is because one occurrence of a piece of data, or a code, is all that is necessary to ensure that it becomes part of the analysis framework. However, it remains true that sample sizes that are too small cannot adequately support claims of having achieved valid conclusions and sample sizes that are too large do not permit the deep, naturalistic, and inductive analysis that defines qualitative inquiry. Determining adequate sample size in qualitative research is ultimately a matter of judgment and experience in evaluating the quality of the information collected against the uses to which it will be applied and the particular research method and purposeful sampling strategy employed. If the sample size is found to be a limitation, it may reflect your judgement about the methodological technique chosen [e.g., single life history study versus focus group interviews] rather than the number of respondents used.
Huberman, A. Michael and Matthew B. Miles. Data Management and Analysis Methods. In Handbook of Qualitative Research. Norman K. Denzin and Yvonna S. Lincoln, eds. (Thousand Oaks, CA: Sage, 1994), pp. 428-444.
- << Previous: 8. The Discussion
- Next: 9. The Conclusion >>
- Last Updated: Jul 18, 2023 11:58 AM
- URL: https://library.sacredheart.edu/c.php?g=29803
- QuickSearch
- Library Catalog
- Databases A-Z
- Publication Finder
- Course Reserves
- Citation Linker
- Digital Commons
- Our Website
Research Support
- Ask a Librarian
- Appointments
- Interlibrary Loan (ILL)
- Research Guides
- Databases by Subject
- Citation Help
Using the Library
- Reserve a Group Study Room
- Renew Books
- Honors Study Rooms
- Off-Campus Access
- Library Policies
- Library Technology
User Information
- Grad Students
- Online Students
- COVID-19 Updates
- Staff Directory
- News & Announcements
- Library Newsletter
My Accounts
- Interlibrary Loan
- Staff Site Login

FIND US ON

21 Research Limitations Examples
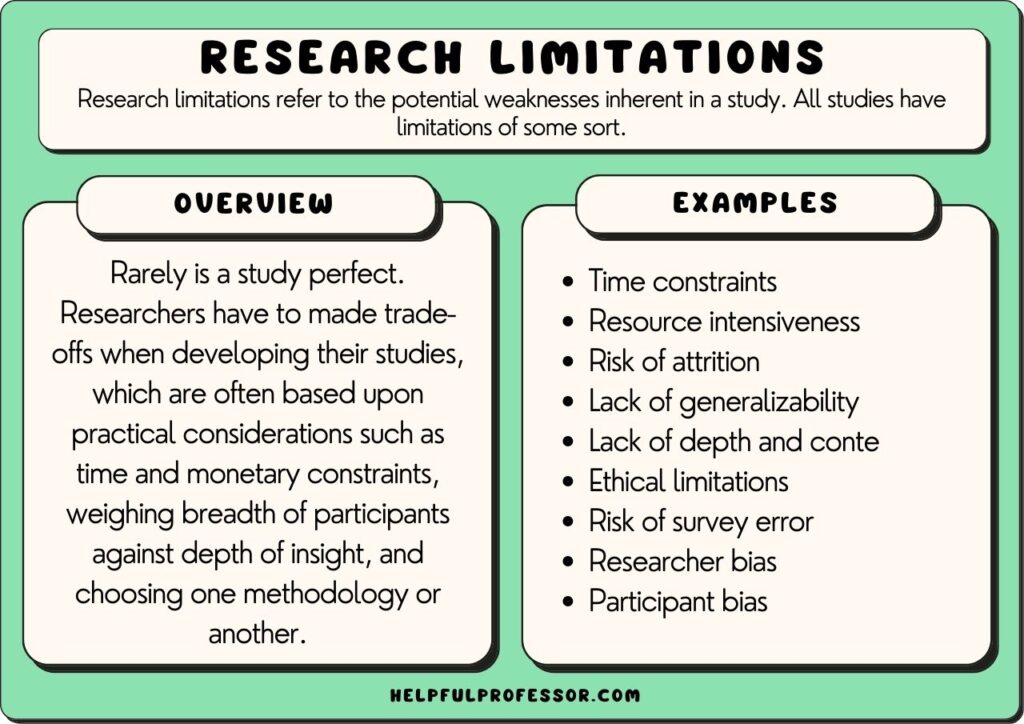
Research limitations refer to the potential weaknesses inherent in a study. All studies have limitations of some sort, meaning declaring limitations doesn’t necessarily need to be a bad thing, so long as your declaration of limitations is well thought-out and explained.
Rarely is a study perfect. Researchers have to make trade-offs when developing their studies, which are often based upon practical considerations such as time and monetary constraints, weighing the breadth of participants against the depth of insight, and choosing one methodology or another.
In research, studies can have limitations such as limited scope, researcher subjectivity, and lack of available research tools.
Acknowledging the limitations of your study should be seen as a strength. It demonstrates your willingness for transparency, humility, and submission to the scientific method and can bolster the integrity of the study. It can also inform future research direction.
Typically, scholars will explore the limitations of their study in either their methodology section, their conclusion section, or both.
Research Limitations Examples
Qualitative and quantitative research offer different perspectives and methods in exploring phenomena, each with its own strengths and limitations. So, I’ve split the limitations examples sections into qualitative and quantitative below.
Qualitative Research Limitations
Qualitative research seeks to understand phenomena in-depth and in context. It focuses on the ‘why’ and ‘how’ questions.
It’s often used to explore new or complex issues, and it provides rich, detailed insights into participants’ experiences, behaviors, and attitudes. However, these strengths also create certain limitations, as explained below.
1. Subjectivity
Qualitative research often requires the researcher to interpret subjective data. One researcher may examine a text and identify different themes or concepts as more dominant than others.
Close qualitative readings of texts are necessarily subjective – and while this may be a limitation, qualitative researchers argue this is the best way to deeply understand everything in context.
Suggested Solution and Response: To minimize subjectivity bias, you could consider cross-checking your own readings of themes and data against other scholars’ readings and interpretations. This may involve giving the raw data to a supervisor or colleague and asking them to code the data separately, then coming together to compare and contrast results.
2. Researcher Bias
The concept of researcher bias is related to, but slightly different from, subjectivity.
Researcher bias refers to the perspectives and opinions you bring with you when doing your research.
For example, a researcher who is explicitly of a certain philosophical or political persuasion may bring that persuasion to bear when interpreting data.
In many scholarly traditions, we will attempt to minimize researcher bias through the utilization of clear procedures that are set out in advance or through the use of statistical analysis tools.
However, in other traditions, such as in postmodern feminist research , declaration of bias is expected, and acknowledgment of bias is seen as a positive because, in those traditions, it is believed that bias cannot be eliminated from research, so instead, it is a matter of integrity to present it upfront.
Suggested Solution and Response: Acknowledge the potential for researcher bias and, depending on your theoretical framework , accept this, or identify procedures you have taken to seek a closer approximation to objectivity in your coding and analysis.
3. Generalizability
If you’re struggling to find a limitation to discuss in your own qualitative research study, then this one is for you: all qualitative research, of all persuasions and perspectives, cannot be generalized.
This is a core feature that sets qualitative data and quantitative data apart.
The point of qualitative data is to select case studies and similarly small corpora and dig deep through in-depth analysis and thick description of data.
Often, this will also mean that you have a non-randomized sample size.
While this is a positive – you’re going to get some really deep, contextualized, interesting insights – it also means that the findings may not be generalizable to a larger population that may not be representative of the small group of people in your study.
Suggested Solution and Response: Suggest future studies that take a quantitative approach to the question.
4. The Hawthorne Effect
The Hawthorne effect refers to the phenomenon where research participants change their ‘observed behavior’ when they’re aware that they are being observed.
This effect was first identified by Elton Mayo who conducted studies of the effects of various factors ton workers’ productivity. He noticed that no matter what he did – turning up the lights, turning down the lights, etc. – there was an increase in worker outputs compared to prior to the study taking place.
Mayo realized that the mere act of observing the workers made them work harder – his observation was what was changing behavior.
So, if you’re looking for a potential limitation to name for your observational research study , highlight the possible impact of the Hawthorne effect (and how you could reduce your footprint or visibility in order to decrease its likelihood).
Suggested Solution and Response: Highlight ways you have attempted to reduce your footprint while in the field, and guarantee anonymity to your research participants.
5. Replicability
Quantitative research has a great benefit in that the studies are replicable – a researcher can get a similar sample size, duplicate the variables, and re-test a study. But you can’t do that in qualitative research.
Qualitative research relies heavily on context – a specific case study or specific variables that make a certain instance worthy of analysis. As a result, it’s often difficult to re-enter the same setting with the same variables and repeat the study.
Furthermore, the individual researcher’s interpretation is more influential in qualitative research, meaning even if a new researcher enters an environment and makes observations, their observations may be different because subjectivity comes into play much more. This doesn’t make the research bad necessarily (great insights can be made in qualitative research), but it certainly does demonstrate a weakness of qualitative research.
6. Limited Scope
“Limited scope” is perhaps one of the most common limitations listed by researchers – and while this is often a catch-all way of saying, “well, I’m not studying that in this study”, it’s also a valid point.
No study can explore everything related to a topic. At some point, we have to make decisions about what’s included in the study and what is excluded from the study.
So, you could say that a limitation of your study is that it doesn’t look at an extra variable or concept that’s certainly worthy of study but will have to be explored in your next project because this project has a clearly and narrowly defined goal.
Suggested Solution and Response: Be clear about what’s in and out of the study when writing your research question.
7. Time Constraints
This is also a catch-all claim you can make about your research project: that you would have included more people in the study, looked at more variables, and so on. But you’ve got to submit this thing by the end of next semester! You’ve got time constraints.
And time constraints are a recognized reality in all research.
But this means you’ll need to explain how time has limited your decisions. As with “limited scope”, this may mean that you had to study a smaller group of subjects, limit the amount of time you spent in the field, and so forth.
Suggested Solution and Response: Suggest future studies that will build on your current work, possibly as a PhD project.
8. Resource Intensiveness
Qualitative research can be expensive due to the cost of transcription, the involvement of trained researchers, and potential travel for interviews or observations.
So, resource intensiveness is similar to the time constraints concept. If you don’t have the funds, you have to make decisions about which tools to use, which statistical software to employ, and how many research assistants you can dedicate to the study.
Suggested Solution and Response: Suggest future studies that will gain more funding on the back of this ‘ exploratory study ‘.
9. Coding Difficulties
Data analysis in qualitative research often involves coding, which can be subjective and complex, especially when dealing with ambiguous or contradicting data.
After naming this as a limitation in your research, it’s important to explain how you’ve attempted to address this. Some ways to ‘limit the limitation’ include:
- Triangulation: Have 2 other researchers code the data as well and cross-check your results with theirs to identify outliers that may need to be re-examined, debated with the other researchers, or removed altogether.
- Procedure: Use a clear coding procedure to demonstrate reliability in your coding process. I personally use the thematic network analysis method outlined in this academic article by Attride-Stirling (2001).
Suggested Solution and Response: Triangulate your coding findings with colleagues, and follow a thematic network analysis procedure.
10. Risk of Non-Responsiveness
There is always a risk in research that research participants will be unwilling or uncomfortable sharing their genuine thoughts and feelings in the study.
This is particularly true when you’re conducting research on sensitive topics, politicized topics, or topics where the participant is expressing vulnerability .
This is similar to the Hawthorne effect (aka participant bias), where participants change their behaviors in your presence; but it goes a step further, where participants actively hide their true thoughts and feelings from you.
Suggested Solution and Response: One way to manage this is to try to include a wider group of people with the expectation that there will be non-responsiveness from some participants.
11. Risk of Attrition
Attrition refers to the process of losing research participants throughout the study.
This occurs most commonly in longitudinal studies , where a researcher must return to conduct their analysis over spaced periods of time, often over a period of years.
Things happen to people over time – they move overseas, their life experiences change, they get sick, change their minds, and even die. The more time that passes, the greater the risk of attrition.
Suggested Solution and Response: One way to manage this is to try to include a wider group of people with the expectation that there will be attrition over time.
12. Difficulty in Maintaining Confidentiality and Anonymity
Given the detailed nature of qualitative data , ensuring participant anonymity can be challenging.
If you have a sensitive topic in a specific case study, even anonymizing research participants sometimes isn’t enough. People might be able to induce who you’re talking about.
Sometimes, this will mean you have to exclude some interesting data that you collected from your final report. Confidentiality and anonymity come before your findings in research ethics – and this is a necessary limiting factor.
Suggested Solution and Response: Highlight the efforts you have taken to anonymize data, and accept that confidentiality and accountability place extremely important constraints on academic research.
13. Difficulty in Finding Research Participants
A study that looks at a very specific phenomenon or even a specific set of cases within a phenomenon means that the pool of potential research participants can be very low.
Compile on top of this the fact that many people you approach may choose not to participate, and you could end up with a very small corpus of subjects to explore. This may limit your ability to make complete findings, even in a quantitative sense.
You may need to therefore limit your research question and objectives to something more realistic.
Suggested Solution and Response: Highlight that this is going to limit the study’s generalizability significantly.
14. Ethical Limitations
Ethical limitations refer to the things you cannot do based on ethical concerns identified either by yourself or your institution’s ethics review board.
This might include threats to the physical or psychological well-being of your research subjects, the potential of releasing data that could harm a person’s reputation, and so on.
Furthermore, even if your study follows all expected standards of ethics, you still, as an ethical researcher, need to allow a research participant to pull out at any point in time, after which you cannot use their data, which demonstrates an overlap between ethical constraints and participant attrition.
Suggested Solution and Response: Highlight that these ethical limitations are inevitable but important to sustain the integrity of the research.
For more on Qualitative Research, Explore my Qualitative Research Guide
Quantitative Research Limitations
Quantitative research focuses on quantifiable data and statistical, mathematical, or computational techniques. It’s often used to test hypotheses, assess relationships and causality, and generalize findings across larger populations.
Quantitative research is widely respected for its ability to provide reliable, measurable, and generalizable data (if done well!). Its structured methodology has strengths over qualitative research, such as the fact it allows for replication of the study, which underpins the validity of the research.
However, this approach is not without it limitations, explained below.
1. Over-Simplification
Quantitative research is powerful because it allows you to measure and analyze data in a systematic and standardized way. However, one of its limitations is that it can sometimes simplify complex phenomena or situations.
In other words, it might miss the subtleties or nuances of the research subject.
For example, if you’re studying why people choose a particular diet, a quantitative study might identify factors like age, income, or health status. But it might miss other aspects, such as cultural influences or personal beliefs, that can also significantly impact dietary choices.
When writing about this limitation, you can say that your quantitative approach, while providing precise measurements and comparisons, may not capture the full complexity of your subjects of study.
Suggested Solution and Response: Suggest a follow-up case study using the same research participants in order to gain additional context and depth.
2. Lack of Context
Another potential issue with quantitative research is that it often focuses on numbers and statistics at the expense of context or qualitative information.
Let’s say you’re studying the effect of classroom size on student performance. You might find that students in smaller classes generally perform better. However, this doesn’t take into account other variables, like teaching style , student motivation, or family support.
When describing this limitation, you might say, “Although our research provides important insights into the relationship between class size and student performance, it does not incorporate the impact of other potentially influential variables. Future research could benefit from a mixed-methods approach that combines quantitative analysis with qualitative insights.”
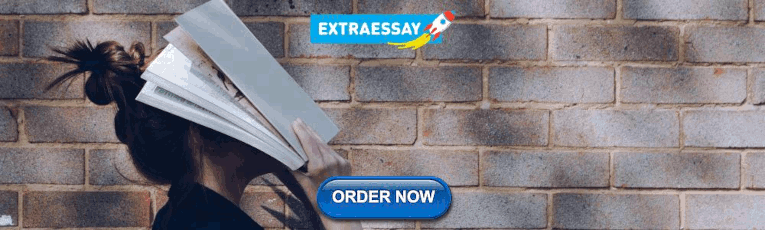
3. Applicability to Real-World Settings
Oftentimes, experimental research takes place in controlled environments to limit the influence of outside factors.
This control is great for isolation and understanding the specific phenomenon but can limit the applicability or “external validity” of the research to real-world settings.
For example, if you conduct a lab experiment to see how sleep deprivation impacts cognitive performance, the sterile, controlled lab environment might not reflect real-world conditions where people are dealing with multiple stressors.
Therefore, when explaining the limitations of your quantitative study in your methodology section, you could state:
“While our findings provide valuable information about [topic], the controlled conditions of the experiment may not accurately represent real-world scenarios where extraneous variables will exist. As such, the direct applicability of our results to broader contexts may be limited.”
Suggested Solution and Response: Suggest future studies that will engage in real-world observational research, such as ethnographic research.
4. Limited Flexibility
Once a quantitative study is underway, it can be challenging to make changes to it. This is because, unlike in grounded research, you’re putting in place your study in advance, and you can’t make changes part-way through.
Your study design, data collection methods, and analysis techniques need to be decided upon before you start collecting data.
For example, if you are conducting a survey on the impact of social media on teenage mental health, and halfway through, you realize that you should have included a question about their screen time, it’s generally too late to add it.
When discussing this limitation, you could write something like, “The structured nature of our quantitative approach allows for consistent data collection and analysis but also limits our flexibility to adapt and modify the research process in response to emerging insights and ideas.”
Suggested Solution and Response: Suggest future studies that will use mixed-methods or qualitative research methods to gain additional depth of insight.
5. Risk of Survey Error
Surveys are a common tool in quantitative research, but they carry risks of error.
There can be measurement errors (if a question is misunderstood), coverage errors (if some groups aren’t adequately represented), non-response errors (if certain people don’t respond), and sampling errors (if your sample isn’t representative of the population).
For instance, if you’re surveying college students about their study habits , but only daytime students respond because you conduct the survey during the day, your results will be skewed.
In discussing this limitation, you might say, “Despite our best efforts to develop a comprehensive survey, there remains a risk of survey error, including measurement, coverage, non-response, and sampling errors. These could potentially impact the reliability and generalizability of our findings.”
Suggested Solution and Response: Suggest future studies that will use other survey tools to compare and contrast results.
6. Limited Ability to Probe Answers
With quantitative research, you typically can’t ask follow-up questions or delve deeper into participants’ responses like you could in a qualitative interview.
For instance, imagine you are surveying 500 students about study habits in a questionnaire. A respondent might indicate that they study for two hours each night. You might want to follow up by asking them to elaborate on what those study sessions involve or how effective they feel their habits are.
However, quantitative research generally disallows this in the way a qualitative semi-structured interview could.
When discussing this limitation, you might write, “Given the structured nature of our survey, our ability to probe deeper into individual responses is limited. This means we may not fully understand the context or reasoning behind the responses, potentially limiting the depth of our findings.”
Suggested Solution and Response: Suggest future studies that engage in mixed-method or qualitative methodologies to address the issue from another angle.
7. Reliance on Instruments for Data Collection
In quantitative research, the collection of data heavily relies on instruments like questionnaires, surveys, or machines.
The limitation here is that the data you get is only as good as the instrument you’re using. If the instrument isn’t designed or calibrated well, your data can be flawed.
For instance, if you’re using a questionnaire to study customer satisfaction and the questions are vague, confusing, or biased, the responses may not accurately reflect the customers’ true feelings.
When discussing this limitation, you could say, “Our study depends on the use of questionnaires for data collection. Although we have put significant effort into designing and testing the instrument, it’s possible that inaccuracies or misunderstandings could potentially affect the validity of the data collected.”
Suggested Solution and Response: Suggest future studies that will use different instruments but examine the same variables to triangulate results.
8. Time and Resource Constraints (Specific to Quantitative Research)
Quantitative research can be time-consuming and resource-intensive, especially when dealing with large samples.
It often involves systematic sampling, rigorous design, and sometimes complex statistical analysis.
If resources and time are limited, it can restrict the scale of your research, the techniques you can employ, or the extent of your data analysis.
For example, you may want to conduct a nationwide survey on public opinion about a certain policy. However, due to limited resources, you might only be able to survey people in one city.
When writing about this limitation, you could say, “Given the scope of our research and the resources available, we are limited to conducting our survey within one city, which may not fully represent the nationwide public opinion. Hence, the generalizability of the results may be limited.”
Suggested Solution and Response: Suggest future studies that will have more funding or longer timeframes.
How to Discuss Your Research Limitations
1. in your research proposal and methodology section.
In the research proposal, which will become the methodology section of your dissertation, I would recommend taking the four following steps, in order:
- Be Explicit about your Scope – If you limit the scope of your study in your research question, aims, and objectives, then you can set yourself up well later in the methodology to say that certain questions are “outside the scope of the study.” For example, you may identify the fact that the study doesn’t address a certain variable, but you can follow up by stating that the research question is specifically focused on the variable that you are examining, so this limitation would need to be looked at in future studies.
- Acknowledge the Limitation – Acknowledging the limitations of your study demonstrates reflexivity and humility and can make your research more reliable and valid. It also pre-empts questions the people grading your paper may have, so instead of them down-grading you for your limitations; they will congratulate you on explaining the limitations and how you have addressed them!
- Explain your Decisions – You may have chosen your approach (despite its limitations) for a very specific reason. This might be because your approach remains, on balance, the best one to answer your research question. Or, it might be because of time and monetary constraints that are outside of your control.
- Highlight the Strengths of your Approach – Conclude your limitations section by strongly demonstrating that, despite limitations, you’ve worked hard to minimize the effects of the limitations and that you have chosen your specific approach and methodology because it’s also got some terrific strengths. Name the strengths.
Overall, you’ll want to acknowledge your own limitations but also explain that the limitations don’t detract from the value of your study as it stands.
2. In the Conclusion Section or Chapter
In the conclusion of your study, it is generally expected that you return to a discussion of the study’s limitations. Here, I recommend the following steps:
- Acknowledge issues faced – After completing your study, you will be increasingly aware of issues you may have faced that, if you re-did the study, you may have addressed earlier in order to avoid those issues. Acknowledge these issues as limitations, and frame them as recommendations for subsequent studies.
- Suggest further research – Scholarly research aims to fill gaps in the current literature and knowledge. Having established your expertise through your study, suggest lines of inquiry for future researchers. You could state that your study had certain limitations, and “future studies” can address those limitations.
- Suggest a mixed methods approach – Qualitative and quantitative research each have pros and cons. So, note those ‘cons’ of your approach, then say the next study should approach the topic using the opposite methodology or could approach it using a mixed-methods approach that could achieve the benefits of quantitative studies with the nuanced insights of associated qualitative insights as part of an in-study case-study.
Overall, be clear about both your limitations and how those limitations can inform future studies.
In sum, each type of research method has its own strengths and limitations. Qualitative research excels in exploring depth, context, and complexity, while quantitative research excels in examining breadth, generalizability, and quantifiable measures. Despite their individual limitations, each method contributes unique and valuable insights, and researchers often use them together to provide a more comprehensive understanding of the phenomenon being studied.
Attride-Stirling, J. (2001). Thematic networks: an analytic tool for qualitative research. Qualitative research , 1 (3), 385-405. ( Source )
Atkinson, P., Delamont, S., Cernat, A., Sakshaug, J., & Williams, R. A. (2021). SAGE research methods foundations . London: Sage Publications.
Clark, T., Foster, L., Bryman, A., & Sloan, L. (2021). Bryman’s social research methods . Oxford: Oxford University Press.
Köhler, T., Smith, A., & Bhakoo, V. (2022). Templates in qualitative research methods: Origins, limitations, and new directions. Organizational Research Methods , 25 (2), 183-210. ( Source )
Lenger, A. (2019). The rejection of qualitative research methods in economics. Journal of Economic Issues , 53 (4), 946-965. ( Source )
Taherdoost, H. (2022). What are different research approaches? Comprehensive review of qualitative, quantitative, and mixed method research, their applications, types, and limitations. Journal of Management Science & Engineering Research , 5 (1), 53-63. ( Source )
Walliman, N. (2021). Research methods: The basics . New York: Routledge.
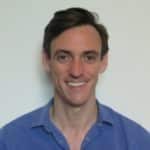
Chris Drew (PhD)
Dr. Chris Drew is the founder of the Helpful Professor. He holds a PhD in education and has published over 20 articles in scholarly journals. He is the former editor of the Journal of Learning Development in Higher Education. [Image Descriptor: Photo of Chris]
- Chris Drew (PhD) https://helpfulprofessor.com/author/chris-drew-phd/ Social-Emotional Learning (Definition, Examples, Pros & Cons)
- Chris Drew (PhD) https://helpfulprofessor.com/author/chris-drew-phd/ What is Educational Psychology?
- Chris Drew (PhD) https://helpfulprofessor.com/author/chris-drew-phd/ What is IQ? (Intelligence Quotient)
- Chris Drew (PhD) https://helpfulprofessor.com/author/chris-drew-phd/ 5 Top Tips for Succeeding at University
Leave a Comment Cancel Reply
Your email address will not be published. Required fields are marked *
- Affiliate Program

- UNITED STATES
- 台灣 (TAIWAN)
- TÜRKIYE (TURKEY)
- Academic Editing Services
- - Research Paper
- - Journal Manuscript
- - Dissertation
- - College & University Assignments
- Admissions Editing Services
- - Application Essay
- - Personal Statement
- - Recommendation Letter
- - Cover Letter
- - CV/Resume
- Business Editing Services
- - Business Documents
- - Report & Brochure
- - Website & Blog
- Writer Editing Services
- - Script & Screenplay
- Our Editors
- Client Reviews
- Editing & Proofreading Prices
- Wordvice Points
- Partner Discount
- Plagiarism Checker
APA Citation Generator
MLA Citation Generator
Chicago Citation Generator
Vancouver Citation Generator
- - APA Style
- - MLA Style
- - Chicago Style
- - Vancouver Style
- Writing & Editing Guide
- Academic Resources
- Admissions Resources
How to Present the Limitations of the Study Examples
What are the limitations of a study?
The limitations of a study are the elements of methodology or study design that impact the interpretation of your research results. The limitations essentially detail any flaws or shortcomings in your study. Study limitations can exist due to constraints on research design, methodology, materials, etc., and these factors may impact the findings of your study. However, researchers are often reluctant to discuss the limitations of their study in their papers, feeling that bringing up limitations may undermine its research value in the eyes of readers and reviewers.
In spite of the impact it might have (and perhaps because of it) you should clearly acknowledge any limitations in your research paper in order to show readers—whether journal editors, other researchers, or the general public—that you are aware of these limitations and to explain how they affect the conclusions that can be drawn from the research.
In this article, we provide some guidelines for writing about research limitations, show examples of some frequently seen study limitations, and recommend techniques for presenting this information. And after you have finished drafting and have received manuscript editing for your work, you still might want to follow this up with academic editing before submitting your work to your target journal.
Why do I need to include limitations of research in my paper?
Although limitations address the potential weaknesses of a study, writing about them toward the end of your paper actually strengthens your study by identifying any problems before other researchers or reviewers find them.
Furthermore, pointing out study limitations shows that you’ve considered the impact of research weakness thoroughly and have an in-depth understanding of your research topic. Since all studies face limitations, being honest and detailing these limitations will impress researchers and reviewers more than ignoring them.
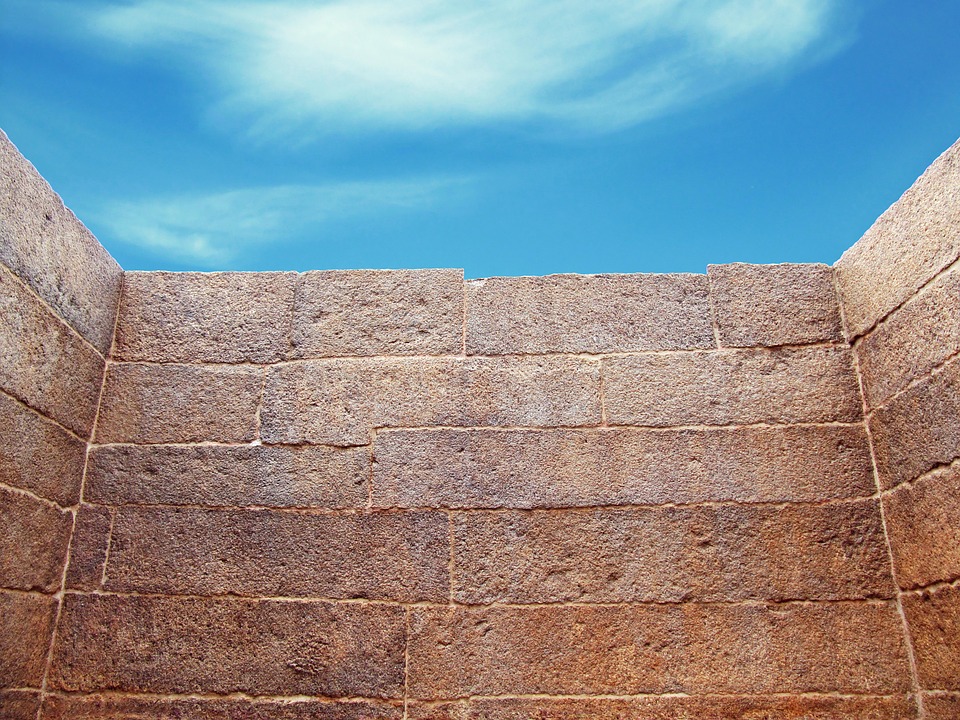
Where should I put the limitations of the study in my paper?
Some limitations might be evident to researchers before the start of the study, while others might become clear while you are conducting the research. Whether these limitations are anticipated or not, and whether they are due to research design or to methodology, they should be clearly identified and discussed in the discussion section —the final section of your paper. Most journals now require you to include a discussion of potential limitations of your work, and many journals now ask you to place this “limitations section” at the very end of your article.
Some journals ask you to also discuss the strengths of your work in this section, and some allow you to freely choose where to include that information in your discussion section—make sure to always check the author instructions of your target journal before you finalize a manuscript and submit it for peer review .
Limitations of the Study Examples
There are several reasons why limitations of research might exist. The two main categories of limitations are those that result from the methodology and those that result from issues with the researcher(s).
Common Methodological Limitations of Studies
Limitations of research due to methodological problems can be addressed by clearly and directly identifying the potential problem and suggesting ways in which this could have been addressed—and SHOULD be addressed in future studies. The following are some major potential methodological issues that can impact the conclusions researchers can draw from the research.
Issues with research samples and selection
Sampling errors occur when a probability sampling method is used to select a sample, but that sample does not reflect the general population or appropriate population concerned. This results in limitations of your study known as “sample bias” or “selection bias.”
For example, if you conducted a survey to obtain your research results, your samples (participants) were asked to respond to the survey questions. However, you might have had limited ability to gain access to the appropriate type or geographic scope of participants. In this case, the people who responded to your survey questions may not truly be a random sample.
Insufficient sample size for statistical measurements
When conducting a study, it is important to have a sufficient sample size in order to draw valid conclusions. The larger the sample, the more precise your results will be. If your sample size is too small, it will be difficult to identify significant relationships in the data.
Normally, statistical tests require a larger sample size to ensure that the sample is considered representative of a population and that the statistical result can be generalized to a larger population. It is a good idea to understand how to choose an appropriate sample size before you conduct your research by using scientific calculation tools—in fact, many journals now require such estimation to be included in every manuscript that is sent out for review.
Lack of previous research studies on the topic
Citing and referencing prior research studies constitutes the basis of the literature review for your thesis or study, and these prior studies provide the theoretical foundations for the research question you are investigating. However, depending on the scope of your research topic, prior research studies that are relevant to your thesis might be limited.
When there is very little or no prior research on a specific topic, you may need to develop an entirely new research typology. In this case, discovering a limitation can be considered an important opportunity to identify literature gaps and to present the need for further development in the area of study.
Methods/instruments/techniques used to collect the data
After you complete your analysis of the research findings (in the discussion section), you might realize that the manner in which you have collected the data or the ways in which you have measured variables has limited your ability to conduct a thorough analysis of the results.
For example, you might realize that you should have addressed your survey questions from another viable perspective, or that you were not able to include an important question in the survey. In these cases, you should acknowledge the deficiency or deficiencies by stating a need for future researchers to revise their specific methods for collecting data that includes these missing elements.
Common Limitations of the Researcher(s)
Study limitations that arise from situations relating to the researcher or researchers (whether the direct fault of the individuals or not) should also be addressed and dealt with, and remedies to decrease these limitations—both hypothetically in your study, and practically in future studies—should be proposed.
Limited access to data
If your research involved surveying certain people or organizations, you might have faced the problem of having limited access to these respondents. Due to this limited access, you might need to redesign or restructure your research in a different way. In this case, explain the reasons for limited access and be sure that your finding is still reliable and valid despite this limitation.
Time constraints
Just as students have deadlines to turn in their class papers, academic researchers might also have to meet deadlines for submitting a manuscript to a journal or face other time constraints related to their research (e.g., participants are only available during a certain period; funding runs out; collaborators move to a new institution). The time available to study a research problem and to measure change over time might be constrained by such practical issues. If time constraints negatively impacted your study in any way, acknowledge this impact by mentioning a need for a future study (e.g., a longitudinal study) to answer this research problem.
Conflicts arising from cultural bias and other personal issues
Researchers might hold biased views due to their cultural backgrounds or perspectives of certain phenomena, and this can affect a study’s legitimacy. Also, it is possible that researchers will have biases toward data and results that only support their hypotheses or arguments. In order to avoid these problems, the author(s) of a study should examine whether the way the research problem was stated and the data-gathering process was carried out appropriately.
Steps for Organizing Your Study Limitations Section
When you discuss the limitations of your study, don’t simply list and describe your limitations—explain how these limitations have influenced your research findings. There might be multiple limitations in your study, but you only need to point out and explain those that directly relate to and impact how you address your research questions.
We suggest that you divide your limitations section into three steps: (1) identify the study limitations; (2) explain how they impact your study in detail; and (3) propose a direction for future studies and present alternatives. By following this sequence when discussing your study’s limitations, you will be able to clearly demonstrate your study’s weakness without undermining the quality and integrity of your research.
Step 1. Identify the limitation(s) of the study
- This part should comprise around 10%-20% of your discussion of study limitations.
The first step is to identify the particular limitation(s) that affected your study. There are many possible limitations of research that can affect your study, but you don’t need to write a long review of all possible study limitations. A 200-500 word critique is an appropriate length for a research limitations section. In the beginning of this section, identify what limitations your study has faced and how important these limitations are.
You only need to identify limitations that had the greatest potential impact on: (1) the quality of your findings, and (2) your ability to answer your research question.

Step 2. Explain these study limitations in detail
- This part should comprise around 60-70% of your discussion of limitations.
After identifying your research limitations, it’s time to explain the nature of the limitations and how they potentially impacted your study. For example, when you conduct quantitative research, a lack of probability sampling is an important issue that you should mention. On the other hand, when you conduct qualitative research, the inability to generalize the research findings could be an issue that deserves mention.
Explain the role these limitations played on the results and implications of the research and justify the choice you made in using this “limiting” methodology or other action in your research. Also, make sure that these limitations didn’t undermine the quality of your dissertation .
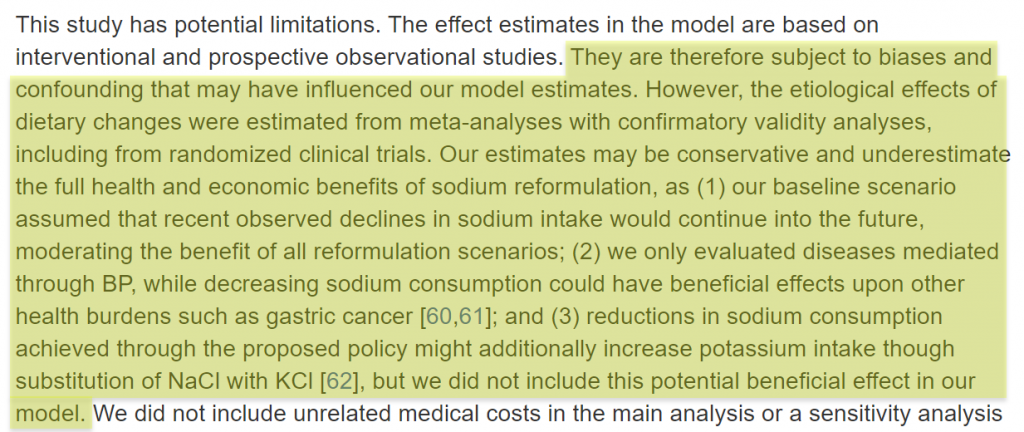
Step 3. Propose a direction for future studies and present alternatives (optional)
- This part should comprise around 10-20% of your discussion of limitations.
After acknowledging the limitations of the research, you need to discuss some possible ways to overcome these limitations in future studies. One way to do this is to present alternative methodologies and ways to avoid issues with, or “fill in the gaps of” the limitations of this study you have presented. Discuss both the pros and cons of these alternatives and clearly explain why researchers should choose these approaches.
Make sure you are current on approaches used by prior studies and the impacts they have had on their findings. Cite review articles or scientific bodies that have recommended these approaches and why. This might be evidence in support of the approach you chose, or it might be the reason you consider your choices to be included as limitations. This process can act as a justification for your approach and a defense of your decision to take it while acknowledging the feasibility of other approaches.
P hrases and Tips for Introducing Your Study Limitations in the Discussion Section
The following phrases are frequently used to introduce the limitations of the study:
- “There may be some possible limitations in this study.”
- “The findings of this study have to be seen in light of some limitations.”
- “The first is the…The second limitation concerns the…”
- “The empirical results reported herein should be considered in the light of some limitations.”
- “This research, however, is subject to several limitations.”
- “The primary limitation to the generalization of these results is…”
- “Nonetheless, these results must be interpreted with caution and a number of limitations should be borne in mind.”
- “As with the majority of studies, the design of the current study is subject to limitations.”
- “There are two major limitations in this study that could be addressed in future research. First, the study focused on …. Second ….”
For more articles on research writing and the journal submissions and publication process, visit Wordvice’s Academic Resources page.
And be sure to receive professional English editing and proofreading services , including paper editing services , for your journal manuscript before submitting it to journal editors.
Wordvice Resources
Proofreading & Editing Guide
Writing the Results Section for a Research Paper
How to Write a Literature Review
Research Writing Tips: How to Draft a Powerful Discussion Section
How to Captivate Journal Readers with a Strong Introduction
Tips That Will Make Your Abstract a Success!
APA In-Text Citation Guide for Research Writing
Additional Resources
- Diving Deeper into Limitations and Delimitations (PhD student)
- Organizing Your Social Sciences Research Paper: Limitations of the Study (USC Library)
- Research Limitations (Research Methodology)
- How to Present Limitations and Alternatives (UMASS)
Article References
Pearson-Stuttard, J., Kypridemos, C., Collins, B., Mozaffarian, D., Huang, Y., Bandosz, P.,…Micha, R. (2018). Estimating the health and economic effects of the proposed US Food and Drug Administration voluntary sodium reformulation: Microsimulation cost-effectiveness analysis. PLOS. https://journals.plos.org/plosmedicine/article?id=10.1371/journal.pmed.1002551
Xu, W.L, Pedersen, N.L., Keller, L., Kalpouzos, G., Wang, H.X., Graff, C,. Fratiglioni, L. (2015). HHEX_23 AA Genotype Exacerbates Effect of Diabetes on Dementia and Alzheimer Disease: A Population-Based Longitudinal Study. PLOS. Retrieved from https://journals.plos.org/plosmedicine/article?id=10.1371/journal.pmed.1001853
Advertisement
How to conduct systematic literature reviews in management research: a guide in 6 steps and 14 decisions
- Review Paper
- Open access
- Published: 12 May 2023
- Volume 17 , pages 1899–1933, ( 2023 )
Cite this article
You have full access to this open access article
- Philipp C. Sauer ORCID: orcid.org/0000-0002-1823-0723 1 &
- Stefan Seuring ORCID: orcid.org/0000-0003-4204-9948 2
23k Accesses
43 Citations
6 Altmetric
Explore all metrics
Systematic literature reviews (SLRs) have become a standard tool in many fields of management research but are often considerably less stringently presented than other pieces of research. The resulting lack of replicability of the research and conclusions has spurred a vital debate on the SLR process, but related guidance is scattered across a number of core references and is overly centered on the design and conduct of the SLR, while failing to guide researchers in crafting and presenting their findings in an impactful way. This paper offers an integrative review of the widely applied and most recent SLR guidelines in the management domain. The paper adopts a well-established six-step SLR process and refines it by sub-dividing the steps into 14 distinct decisions: (1) from the research question, via (2) characteristics of the primary studies, (3) to retrieving a sample of relevant literature, which is then (4) selected and (5) synthesized so that, finally (6), the results can be reported. Guided by these steps and decisions, prior SLR guidelines are critically reviewed, gaps are identified, and a synthesis is offered. This synthesis elaborates mainly on the gaps while pointing the reader toward the available guidelines. The paper thereby avoids reproducing existing guidance but critically enriches it. The 6 steps and 14 decisions provide methodological, theoretical, and practical guidelines along the SLR process, exemplifying them via best-practice examples and revealing their temporal sequence and main interrelations. The paper guides researchers in the process of designing, executing, and publishing a theory-based and impact-oriented SLR.
Similar content being viewed by others
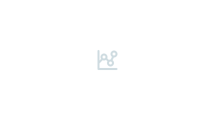
What is Qualitative in Qualitative Research
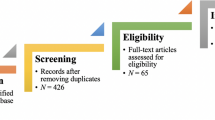
Criteria for Good Qualitative Research: A Comprehensive Review
Reporting reliability, convergent and discriminant validity with structural equation modeling: a review and best-practice recommendations.
Avoid common mistakes on your manuscript.
1 Introduction
The application of systematic or structured literature reviews (SLRs) has developed into an established approach in the management domain (Kraus et al. 2020 ), with 90% of management-related SLRs published within the last 10 years (Clark et al. 2021 ). Such reviews help to condense knowledge in the field and point to future research directions, thereby enabling theory development (Fink 2010 ; Koufteros et al. 2018 ). SLRs have become an established method by now (e.g., Durach et al. 2017 ; Koufteros et al. 2018 ). However, many SLR authors struggle to efficiently synthesize and apply review protocols and justify their decisions throughout the review process (Paul et al. 2021 ) since only a few studies address and explain the respective research process and the decisions to be taken in this process. Moreover, the available guidelines do not form a coherent body of literature but focus on the different details of an SLR, while a comprehensive and detailed SLR process model is lacking. For example, Seuring and Gold ( 2012 ) provide some insights into the overall process, focusing on content analysis for data analysis without covering the practicalities of the research process in detail. Similarly, Durach et al. ( 2017 ) address SLRs from a paradigmatic perspective, offering a more foundational view covering ontological and epistemological positions. Durach et al. ( 2017 ) emphasize the philosophy of science foundations of an SLR. Although somewhat similar guidelines for SLRs might be found in the wider body of literature (Denyer and Tranfield 2009 ; Fink 2010 ; Snyder 2019 ), they often take a particular focus and are less geared toward explaining and reflecting on the single choices being made during the research process. The current body of SLR guidelines leaves it to the reader to find the right links among the guidelines and to justify their inconsistencies. This is critical since a vast number of SLRs are conducted by early-stage researchers who likely struggle to synthesize the existing guidance and best practices (Fisch and Block 2018 ; Kraus et al. 2020 ), leading to the frustration of authors, reviewers, editors, and readers alike.
Filling these gaps is critical in our eyes since researchers conducting literature reviews form the foundation of any kind of further analysis to position their research into the respective field (Fink 2010 ). So-called “systematic literature reviews” (e.g., Davis and Crombie 2001 ; Denyer and Tranfield 2009 ; Durach et al. 2017 ) or “structured literature reviews” (e.g., Koufteros et al. 2018 ; Miemczyk et al. 2012 ) differ from nonsystematic literature reviews in that the analysis of a certain body of literature becomes a means in itself (Kraus et al. 2020 ; Seuring et al. 2021 ). Although two different terms are used for this approach, the related studies refer to the same core methodological references that are also cited in this paper. Therefore, we see them as identical and abbreviate them as SLR.
There are several guidelines on such reviews already, which have been developed outside the management area (e.g. Fink 2010 ) or with a particular focus on one management domain (e.g., Kraus et al. 2020 ). SLRs aim at capturing the content of the field at a point in time but should also aim at informing future research (Denyer and Tranfield 2009 ), making follow-up research more efficient and productive (Kraus et al. 2021 ). Such standalone literature reviews would and should also prepare subsequent empirical or modeling research, but usually, they require far more effort and time (Fisch and Block 2018 ; Lim et al. 2022 ). To achieve this preparation, SLRs can essentially a) describe the state of the literature, b) test a hypothesis based on the available literature, c) extend the literature, and d) critique the literature (Xiao and Watson 2019 ). Beyond guiding the next incremental step in research, SLRs “may challenge established assumptions and norms of a given field or topic, recognize critical problems and factual errors, and stimulate future scientific conversations around that topic” (Kraus et al. 2022 , p. 2578). Moreover, they have the power to answer research questions that are beyond the scope of individual empirical or modeling studies (Snyder 2019 ) and to build, elaborate, and test theories beyond this single study scope (Seuring et al. 2021 ). These contributions of an SLR may be highly influential and therefore underline the need for high-quality planning, execution, and reporting of their process and details.
Regardless of the individual aims of standalone SLRs, their numbers have exponentially risen in the last two decades (Kraus et al. 2022 ) and almost all PhD or large research project proposals in the management domain include such a standalone SLR to build a solid foundation for their subsequent work packages. Standalone SLRs have thus become a key part of management research (Kraus et al. 2021 ; Seuring et al. 2021 ), which is also underlined by the fact that there are journals and special issues exclusively accepting standalone SLRs (Kraus et al. 2022 ; Lim et al. 2022 ).
However, SLRs require a commitment that is often comparable to an additional research process or project. Hence, SLRs should not be taken as a quick solution, as a simplistic, descriptive approach would usually not yield a publishable paper (see also Denyer and Tranfield 2009 ; Kraus et al. 2020 ).
Furthermore, as with other research techniques, SLRs are based on the rigorous application of rules and procedures, as well as on ensuring the validity and reliability of the method (Fisch and Block 2018 ; Seuring et al. 2021 ). In effect, there is a need to ensure “the same level of rigour to reviewing research evidence as should be used in producing that research evidence in the first place” (Davis and Crombie 2001 , p.1). This rigor holds for all steps of the research process, such as establishing the research question, collecting data, analyzing it, and making sense of the findings (Durach et al. 2017 ; Fink 2010 ; Seuring and Gold 2012 ). However, there is a high degree of diversity where some would be justified, while some papers do not report the full details of the research process. This lack of detail contrasts with an SLR’s aim of creating a valid map of the currently available research in the reviewed field, as critical information on the review’s completeness and potential reviewer biases cannot be judged by the reader or reviewer. This further impedes later replications or extensions of such reviews, which could provide longitudinal evidence of the development of a field (Denyer and Tranfield 2009 ; Durach et al. 2017 ). Against this observation, this paper addresses the following question:
Which decisions need to be made in an SLR process, and what practical guidelines can be put forward for making these decisions?
Answering this question, the key contributions of this paper are fourfold: (1) identifying the gaps in existing SLR guidelines, (2) refining the SLR process model by Durach et al. ( 2017 ) through 14 decisions, (3) synthesizing and enriching guidelines for these decisions, exemplifying the key decisions by means of best practice SLRs, and (4) presenting and discussing a refined SLR process model.
In some cases, we point to examples from operations and supply chain management. However, they illustrate the purposes discussed in the respective sections. We carefully checked that the arguments held for all fields of management-related research, and multiple examples from other fields of management were also included.
2 Identification of the need for an enriched process model, including a set of sequential decisions and their interrelations
In line with the exponential increase in SLR papers (Kraus et al. 2022 ), multiple SLR guidelines have recently been published. Since 2020, we have found a total of 10 papers offering guidelines on SLRs and other reviews for the field of management in general or some of its sub-fields. These guidelines are of double interest to this paper since we aim to complement them to fill the gap identified in the introduction while minimizing the doubling of efforts. Table 1 lists the 10 most recent guidelines and highlights their characteristics, research objectives, contributions, and how our paper aims to complement these previous contributions.
The sheer number and diversity of guideline papers, as well as the relevance expressed in them, underline the need for a comprehensive and exhaustive process model. At the same time, the guidelines take specific foci on, for example, updating earlier guidelines to new technological potentials (Kraus et al. 2020 ), clarifying the foundational elements of SLRs (Kraus et al. 2022 ) and proposing a review protocol (Paul et al. 2021 ) or the application and development of theory in SLRs (Seuring et al. 2021 ). Each of these foci fills an entire paper, while the authors acknowledge that much more needs to be considered in an SLR. Working through these most recent guidelines, it becomes obvious that the common paper formats in the management domain create a tension for guideline papers between elaborating on a) the SLR process and b) the details, options, and potentials of individual process steps.
Our analysis in Table 1 evidences that there are a number of rich contributions on aspect b), while the aspect a) of SLR process models has not received the same attention despite the substantial confusion of authors toward them (Paul et al. 2021 ). In fact, only two of the most recent guidelines approach SLR process models. First, Kraus et al. ( 2020 ) incrementally extended the 20-year-old Tranfield et al. ( 2003 ) three-stage model into four stages. A little later, Paul et al. ( 2021 ) proposed a three-stage (including six sub-stages) SPAR-4-SLR review protocol. It integrates the PRISMA reporting items (Moher et al. 2009 ; Page et al. 2021 ) that originate from clinical research to define 14 actions stating what items an SLR in management needs to report for reasons of validity, reliability, and replicability. Almost naturally, these 14 reporting-oriented actions mainly relate to the first SLR stage of “assembling the literature,” which accounts for nine of the 14 actions. Since this protocol is published in a special issue editorial, its presentation and elaboration are somewhat limited by the already mentioned word count limit. Nevertheless, the SPAR-4-SLR protocol provides a very useful checklist for researchers that enables them to include all data required to document the SLR and to avoid confusion from editors, reviewers, and readers regarding SLR characteristics.
Beyond Table 1 , Durach et al. ( 2017 ) synthesized six common SLR “steps” that differ only marginally in the delimitation of one step to another from the sub-stages of the previously mentioned SLR processes. In addition, Snyder ( 2019 ) proposed a process comprising four “phases” that take more of a bird’s perspective in addressing (1) design, (2) conduct, (3) analysis, and (4) structuring and writing the review. Moreover, Xiao and Watson ( 2019 ) proposed only three “stages” of (1) planning, (2) conducting, and (3) reporting the review that combines the previously mentioned conduct and the analysis and defines eight steps within them. Much in line with the other process models, the final reporting stage contains only one of the eight steps, leaving the reader somewhat alone in how to effectively craft a manuscript that contributes to the further development of the field.
In effect, the mentioned SLR processes differ only marginally, while the systematic nature of actions in the SPAR-4-SLR protocol (Paul et al. 2021 ) can be seen as a reporting must-have within any of the mentioned SLR processes. The similarity of the SLR processes is, however, also evident in the fact that they leave open how the SLR analysis can be executed, enriched, and reflected to make a contribution to the reviewed field. In contrast, this aspect is richly described in the other guidelines that do not offer an SLR process, leading us again toward the tension for guideline papers between elaborating on a) the SLR process and b) the details, options, and potentials of each process step.
To help (prospective) SLR authors successfully navigate this tension of existing guidelines, it is thus the ambition of this paper to adopt a comprehensive SLR process model along which an SLR project can be planned, executed, and written up in a coherent way. To enable this coherence, 14 distinct decisions are defined, reflected, and interlinked, which have to be taken across the different steps of the SLR process. At the same time, our process model aims to actively direct researchers to the best practices, tips, and guidance that previous guidelines have provided for individual decisions. We aim to achieve this by means of an integrative review of the relevant SLR guidelines, as outlined in the following section.
3 Methodology: an integrative literature review of guidelines for systematic literature reviews in management
It might seem intuitive to contribute to the debate on the “gold standard” of systematic literature reviews (Davis et al. 2014 ) by conducting a systematic review ourselves. However, there are different types of reviews aiming for distinctive contributions. Snyder ( 2019 ) distinguished between a) systematic, b) semi-systematic, and c) integrative (or critical) reviews, which aim for i) (mostly quantitative) synthesis and comparison of prior (primary) evidence, ii) an overview of the development of a field over time, and iii) a critique and synthesis of prior perspectives to reconceptualize or advance them. Each review team needs to position itself in such a typology of reviews to define the aims and scope of the review. To do so and structure the related research process, we adopted the four generic steps for an (integrative) literature review by Snyder ( 2019 )—(1) design, (2) conduct, (3) analysis, and (4) structuring and writing the review—on which we report in the remainder of this section. Since the last step is a very practical one that, for example, asks, “Is the contribution of the review clearly communicated?” (Snyder 2019 ), we will focus on the presentation of the method applied to the initial three steps:
(1) Regarding the design, we see the need for this study emerging from our experience in reviewing SLR manuscripts, supervising PhD students who, almost by default, need to prepare an SLR, and recurring discussions on certain decisions in the process of both. These discussions regularly left some blank or blurry spaces (see Table 1 ) that induced substantial uncertainty regarding critical decisions in the SLR process (Paul et al. 2021 ). To address this gap, we aim to synthesize prior guidance and critically enrich it, thus adopting an integrative approach for reviewing existing SLR guidance in the management domain (Snyder 2019 ).
(2) To conduct the review, we started collecting the literature that provided guidance on the individual SLR parts. We built on a sample of 13 regularly cited or very recent papers in the management domain. We started with core articles that we successfully used to publish SLRs in top-tier OSCM journals, such as Tranfield et al. ( 2003 ) and Durach et al. ( 2017 ), and we checked their references and papers that cited these publications. The search focus was defined by the following criteria: the articles needed to a) provide original methodological guidance for SLRs by providing new aspects of the guideline or synthesizing existing ones into more valid guidelines and b) focus on the management domain. Building on the nature of a critical or integrative review that does not require a full or representative sample (Snyder 2019 ), we limited the sample to the papers displayed in Table 2 that built the core of the currently applied SLR guidelines. In effect, we found 11 technical papers and two SLRs of SLRs (Carter and Washispack 2018 ; Seuring and Gold 2012 ). From the latter, we mainly analyzed the discussion and conclusion parts that explicitly developed guidance on conducting SLRs.
(3) For analyzing these papers, we first adopted the six-step SLR process proposed by Durach et al. ( 2017 , p.70), which they define as applicable to any “field, discipline or philosophical perspective”. The contrast between the six-step SLR process used for the analysis and the four-step process applied by ourselves may seem surprising but is justified by the use of an integrative approach. This approach differs mainly in retrieving and selecting pertinent literature that is key to SLRs and thus needs to be part of the analysis framework.
While deductively coding the sample papers against Durach et al.’s ( 2017 ), guidance in the six steps, we inductively built a set of 14 decisions presented in the right columns of Table 2 that are required to be made in any SLR. These decisions built a second and more detailed level of analysis, for which the single guidelines were coded as giving low, medium, or high levels of detail (see Table 3 ), which helped us identify the gaps in the current guidance papers and led our way in presenting, critically discussing, and enriching the literature. In effect, we see that almost all guidelines touch on the same issues and try to give a comprehensive overview. However, this results in multiple guidelines that all lack the space to go into detail, while only a few guidelines focus on filling a gap in the process. It is our ambition with this analysis to identify the gaps in the guidelines, thereby identifying a precise need for refinement, and to offer a first step into this refinement. Adopting advice from the literature sample, the coding was conducted by the entire author team (Snyder 2019 ; Tranfield et al. 2003 ) including discursive alignments of interpretation (Seuring and Gold 2012 ). This enabled a certain reliability and validity of the analysis by reducing the within-study and expectancy bias (Durach et al. 2017 ), while the replicability was supported by reporting the review sample and the coding results in Table 3 (Carter and Washispack 2018 ).
(4) For the writing of the review, we only pointed to the unusual structure of presenting the method without a theory section and then the findings in the following section. However, this was motivated by the nature of the integrative review so that the review findings at the same time represent the “state of the art,” “literature review,” or “conceptualization” sections of a paper.
4 Findings of the integrative review: presentation, critical discussion, and enrichment of prior guidance
4.1 the overall research process for a systematic literature review.
Even within our sample of only 13 guidelines, there are four distinct suggestions for structuring the SLR process. One of the earliest SLR process models was proposed by Tranfield et al. ( 2003 ) encompassing the three stages of (1) planning the review, (2) conducting a review, and (3) reporting and dissemination. Snyder ( 2019 ) proposed four steps employed in this study: (1) design, (2) conduct, (3) analysis, and (4) structuring and writing the review. Borrowing from content analysis guidelines, Seuring and Gold ( 2012 ) defined four steps: (1) material collection, (2) descriptive analysis, (3) category selection, and (4) material evaluation. Most recently Kraus et al. ( 2020 ) proposed four steps: (1) planning the review, (2) identifying and evaluating studies, (3) extracting and synthesizing data, and (4) disseminating the review findings. Most comprehensively, Durach et al. ( 2017 ) condensed prior process models into their generic six steps for an SLR. Adding the review of the process models by Snyder ( 2019 ) and Seuring and Gold ( 2012 ) to Durach et al.’s ( 2017 ) SLR process review of four papers, we support their conclusion of the general applicability of the six steps defined. Consequently, these six steps form the backbone of our coding scheme, as shown in the left column of Table 2 and described in the middle column.
As stated in Sect. 3 , we synthesized the review papers against these six steps but experienced that the papers were taking substantially different foci by providing rich details for some steps while largely bypassing others. To capture this heterogeneity and better operationalize the SLR process, we inductively introduced the right column, identifying 14 decisions to be made. These decisions are all elaborated in the reviewed papers but to substantially different extents, as the detailed coding results in Table 3 underline.
Mapping Table 3 for potential gaps in the existing guidelines, we found six decisions on which we found only low- to medium-level details, while high-detail elaboration was missing. These six decisions, which are illustrated in Fig. 1 , belong to three steps: 1: defining the research question, 5: synthesizing the literature, and 6: reporting the results. This result underscores our critique of currently unbalanced guidance that is, on the one hand, detailed on determining the required characteristics of primary studies (step 2), retrieving a sample of potentially relevant literature (step 3), and selecting the pertinent literature (step 4). On the other hand, authors, especially PhD students, are left without substantial guidance on the steps critical to publication. Instead, they are called “to go one step further … and derive meaningful conclusions” (Fisch and Block 2018 , p. 105) without further operationalizations on how this can be achieved; for example, how “meet the editor” conference sessions regularly cause frustration among PhDs when editors call for “new,” “bold,” and “relevant” research. Filling the gaps in the six decisions with best practice examples and practical experience is the main focus of this study’s contribution. The other eight decisions are synthesized with references to the guidelines that are most helpful and relevant for the respective step in our eyes.
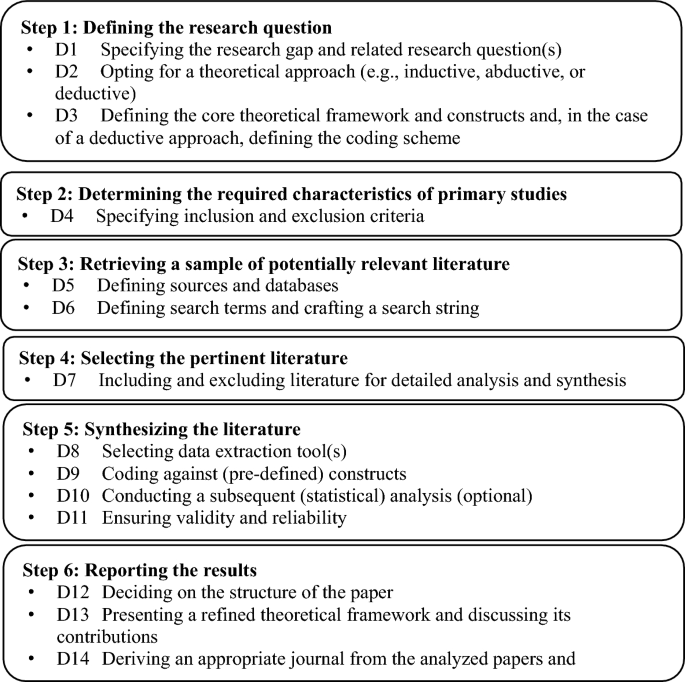
The 6 steps and 14 decisions of the SLR process
4.2 Step 1: defining the research question
When initiating a research project, researchers make three key decisions.
Decision 1 considers the essential tasks of establishing a relevant and timely research question, but despite the importance of the decision, which determines large parts of further decisions (Snyder 2019 ; Tranfield et al. 2003 ), we only find scattered guidance in the literature. Hence, how can a research topic be specified to allow a strong literature review that is neither too narrow nor too broad? The latter is the danger in meta-reviews (i.e., reviews of reviews) (Aguinis et al. 2020 ; Carter and Washispack 2018 ; Kache and Seuring 2014 ). In this respect, even though the method would be robust, the findings would not be novel. In line with Carter and Washispack ( 2018 ), there should always be room for new reviews, yet over time, they must move from a descriptive overview of a field further into depth and provide detailed analyses of constructs. Clark et al. ( 2021 ) provided a detailed but very specific reflection on how they crafted a research question for an SLR and that revisiting the research question multiple times throughout the SLR process helps to coherently and efficiently move forward with the research. More generically, Kraus et al. ( 2020 ) listed six key contributions of an SLR that can guide the definition of the research question. Finally, Snyder ( 2019 ) suggested moving into more detail from existing SLRs and specified two main avenues for crafting an SLR research question that are either investigating the relationship among multiple effects, the effect of (a) specific variable(s), or mapping the evidence regarding a certain research area. For the latter, we see three possible alternative approaches, starting with a focus on certain industries. Examples are analyses of the food industry (Beske et al. 2014 ), retailing (Wiese et al. 2012 ), mining and minerals (Sauer and Seuring 2017 ), or perishable product supply chains (Lusiantoro et al. 2018 ) and traceability at the example of the apparel industry (Garcia-Torres et al. 2019 ). A second opportunity would be to assess the status of research in a geographical area that composes an interesting context from a research perspective, such as sustainable supply chain management (SSCM) in Latin America (Fritz and Silva 2018 ), yet this has to be justified explicitly, avoiding the fact that geographical focus is taken as the reason per se (e.g., Crane et al. 2016 ). A third variant addresses emerging issues, such as SCM, in a base-of-the-pyramid setting (Khalid and Seuring 2019 ) and the use of blockchain technology (Wang et al. 2019 ) or digital transformation (Hanelt et al. 2021 ). These approaches limit the reviewed field to enable a more contextualized analysis in which the novelty, continued relevance, or unjustified underrepresentation of the context can be used to specify a research gap and related research question(s). This also impacts the following decisions, as shown below.
Decision 2 concerns the option for a theoretical approach (i.e., the adoption of an inductive, abductive, or deductive approach) to theory building through the literature review. The review of previous guidance on this delivers an interesting observation. On the one hand, there are early elaborations on systematic reviews, realist synthesis, meta-synthesis, and meta-analysis by Tranfield et al. ( 2003 ) that are borrowing from the origins of systematic reviews in medical research. On the other hand, recent management-related guidelines largely neglect details of related decisions, but point out that SLRs are a suitable tool for theory building (Kraus et al. 2020 ). Seuring et al. ( 2021 ) set out to fill this gap and provided substantial guidance on how to use theory in SLRs to advance the field. To date, the option for a theoretical approach is only rarely made explicit, leaving the reader often puzzled about how advancement in theory has been crafted and impeding a review’s replicability (Seuring et al. 2021 ). Many papers still leave related choices in the dark (e.g., Rhaiem and Amara 2021 ; Rojas-Córdova et al. 2022 ) and move directly from the introduction to the method section.
In Decision 3, researchers need to adopt a theoretical framework (Durach et al. 2017 ) or at least a theoretical starting point, depending on the most appropriate theoretical approach (Seuring et al. 2021 ). Here, we find substantial guidance by Durach et al. ( 2017 ) that underlines the value of adopting a theoretical lens to investigate SCM phenomena and the literature. Moreover, the choice of a theoretical anchor enables a consistent definition and operationalization of constructs that are used to analyze the reviewed literature (Durach et al. 2017 ; Seuring et al. 2021 ). Hence, providing some upfront definitions is beneficial, clarifying what key terminology would be used in the subsequent paper, such as Devece et al. ( 2019 ) introduce their terminology on coopetition. Adding a practical hint beyond the elaborations of prior guidance papers for taking up established constructs in a deductive analysis (decision 2), there would be the question of whether these can yield interesting findings.
Here, it would be relevant to specify what kind of analysis is aimed for the SLR, where three approaches might be distinguished (i.e., bibliometric analysis, meta-analysis, and content analysis–based studies). Briefly distinguishing them, the core difference would be how many papers can be analyzed employing the respective method. Bibliometric analysis (Donthu et al. 2021 ) usually relies on the use of software, such as Biblioshiny, allowing the creation of figures on citations and co-citations. These figures enable the interpretation of large datasets in which several hundred papers can be analyzed in an automated manner. This allows for distinguishing among different research clusters, thereby following a more inductive approach. This would be contrasted by meta-analysis (e.g., Leuschner et al. 2013 ), where often a comparatively smaller number of papers is analyzed (86 in the respective case) but with a high number of observations (more than 17,000). The aim is to test for statistically significant correlations among single constructs, which requires that the related constructs and items be precisely defined (i.e., a clearly deductive approach to the analysis).
Content analysis is the third instrument frequently applied to data analysis, where an inductive or deductive approach might be taken (Seuring et al. 2021 ). Content-based analysis (see decision 9 in Sect. 4.6 ; Seuring and Gold 2012 ) is a labor-intensive step and can hardly be changed ex post. This also implies that only a certain number of papers might be analyzed (see Decision 6 in Sect. 4.5 ). It is advisable to adopt a wider set of constructs for the analysis stemming even from multiple established frameworks since it is difficult to predict which constructs and items might yield interesting insights. Hence, coding a more comprehensive set of items and dropping some in the process is less problematic than starting an analysis all over again for additional constructs and items. However, in the process of content analysis, such an iterative process might be required to improve the meaningfulness of the data and findings (Seuring and Gold 2012 ). A recent example of such an approach can be found in Khalid and Seuring ( 2019 ), building on the conceptual frameworks for SSCM of Carter and Rogers ( 2008 ), Seuring and Müller ( 2008 ), and Pagell and Wu ( 2009 ). This allows for an in-depth analysis of how SSCM constructs are inherently referred to in base-of-the-pyramid-related research. The core criticism and limitation of such an approach is the random and subjectively biased selection of frameworks for the purpose of analysis.
Beyond the aforementioned SLR methods, some reviews, similar to the one used here, apply a critical review approach. This is, however, nonsystematic, and not an SLR; thus, it is beyond the scope of this paper. Interested readers can nevertheless find some guidance on critical reviews in the available literature (e.g., Kraus et al. 2022 ; Snyder 2019 ).
4.3 Step 2: determining the required characteristics of primary studies
After setting the stage for the review, it is essential to determine which literature is to be reviewed in Decision 4. This topic is discussed by almost all existing guidelines and will thus only briefly be discussed here. Durach et al. ( 2017 ) elaborated in great detail on defining strict inclusion and exclusion criteria that need to be aligned with the chosen theoretical framework. The relevant units of analysis need to be specified (often a single paper, but other approaches might be possible) along with suitable research methods, particularly if exclusively empirical studies are reviewed or if other methods are applied. Beyond that, they elaborated on potential quality criteria that should be applied. The same is considered by a number of guidelines that especially draw on medical research, in which systematic reviews aim to pool prior studies to infer findings from their total population. Here, it is essential to ensure the exclusion of poor-quality evidence that would lower the quality of the review findings (Mulrow 1987 ; Tranfield et al. 2003 ). This could be ensured by, for example, only taking papers from journals listed on the Web of Science or Scopus or journals listed in quartile 1 of Scimago ( https://www.scimagojr.com/ ), a database providing citation and reference data for journals.
The selection of relevant publication years should again follow the purpose of the study defined in Step 1. As such, there might be a justified interest in the wide coverage of publication years if a historical perspective is taken. Alternatively, more contemporary developments or the analysis of very recent issues can justify the selection of very few years of publication (e.g., Kraus et al. 2022 ). Again, it is hard to specify a certain time period covered, but if developments of a field should be analyzed, a five-year period might be a typical lower threshold. On current topics, there is often a trend of rising publishing numbers. This scenario implies the rising relevance of a topic; however, this should be treated with caution. The total number of papers published per annum has increased substantially in recent years, which might account for the recently heightened number of papers on a certain topic.
4.4 Step 3: retrieving a sample of potentially relevant literature
After defining the required characteristics of the literature to be reviewed, the literature needs to be retrieved based on two decisions. Decision 5 concerns suitable literature sources and databases that need to be defined. Turning to Web of Science or Scopus would be two typical options found in many of the examples mentioned already (see also detailed guidance by Paul and Criado ( 2020 ) as well as Paul et al. ( 2021 )). These databases aggregate many management journals, and a typical argument for turning to the Web of Science database is the inclusion of impact factors, as they indicate a certain minimum quality of the journal (Sauer and Seuring 2017 ). Additionally, Google Scholar is increasingly mentioned as a usable search engine, often providing higher numbers of search results than the mentioned databases (e.g., Pearce 2018 ). These results often entail duplicates of articles from multiple sources or versions of the same article, as well as articles in predatory journals (Paul et al. 2021 ). Therefore, we concur with Paul et al. ( 2021 ) who underline the quality assurance mechanisms in Web of Science and Scopus, making them preferred databases for the literature search. From a practical perspective, it needs to be mentioned that SLRs in management mainly rely on databases that are not free to use. Against this limitation, Pearce ( 2018 ) provided a list of 20 search engines that are free of charge and elaborated on their advantages and disadvantages. Due to the individual limitations of the databases, it is advisable to use a combination of them (Kraus et al. 2020 , 2022 ) and build a consolidated sample by screening the papers found for duplicates, as regularly done in SLRs.
This decision also includes the choice of the types of literature to be analyzed. Typically, journal papers are selected, ensuring that the collected papers are peer-reviewed and have thus undergone an academic quality management process. Meanwhile, conference papers are usually avoided since they are often less mature and not checked for quality (e.g., Seuring et al. 2021 ). Nevertheless, for emerging topics, it might be too restrictive to consider only peer-reviewed journal articles and limit the literature to only a few references. Analyzing such rapidly emerging topics is relevant for timely and impact-oriented research and might justify the selection of different sources. Kraus et al. ( 2020 ) provided a discussion on the use of gray literature (i.e., nonacademic sources), and Sauer ( 2021 ) provided an example of a review of sustainability standards from a management perspective to derive implications for their application by managers on the one hand and for enhancing their applicability on the other hand.
Another popular way to limit the review sample is the restriction to a certain list of journals (Kraus et al. 2020 ; Snyder 2019 ). While this is sometimes favored by highly ranked journals, Carter and Washispack ( 2018 ), for example, found that many pertinent papers are not necessarily published in journals within the field. Webster and Watson ( 2002 ) quite tellingly cited a reviewer labeling the selection of top journals as an unjustified excuse for investigating the full body of relevant literature. Both aforementioned guidelines thus discourage the restriction to particular journals, a guidance that we fully support.
However, there is an argument to be made supporting the exclusion of certain lower-ranked journals. This can be done, for example, by using Scimago Journal quartiles ( www.scimagojr.com , last accessed 13. of April 2023) and restricting it to journals in the first quartile (e.g., Yavaprabhas et al. 2022 ). Other papers (e.g., Kraus et al. 2021 ; Rojas-Córdova et al. 2022 ) use certain journal quality lists to limit their sample. However, we argue for a careful check by the authors against the topic reviewed regarding what would be included and excluded.
Decision 6 entails the definition of search terms and a search string to be applied in the database just chosen. The search terms should reflect the aims of the review and the exclusion criteria that might be derived from the unit of analysis and the theoretical framework (Durach et al. 2017 ; Snyder 2019 ). Overall, two approaches to keywords can be observed. First, some guides suggest using synonyms of the key terms of interest (e.g., Durach et al. 2017 ; Kraus et al. 2020 ) in order to build a wide baseline sample that will be condensed in the next step. This is, of course, especially helpful if multiple terms delimitate a field together or different synonymous terms are used in parallel in different fields or journals. Empirical journals in supply chain management, for example, use the term “multiple supplier tiers ” (e.g., Tachizawa and Wong 2014 ), while modeling journals in the same field label this as “multiple supplier echelons ” (e.g., Brandenburg and Rebs 2015 ). Second, in some cases, single keywords are appropriate for capturing a central aspect or construct of a field if the single keyword has a global meaning tying this field together. This approach is especially relevant to the study of relatively broad terms, such as “social media” (Lim and Rasul 2022 ). However, this might result in very high numbers of publications found and therefore requires a purposeful combination with other search criteria, such as specific journals (Kraus et al. 2021 ; Lim et al. 2021 ), publication dates, article types, research methods, or the combination with keywords covering domains to which the search is aimed to be specified.
Since SLRs are often required to move into detail or review the intersections of relevant fields, we recommend building groups of keywords (single terms or multiple synonyms) for each field to be connected that are coupled via Boolean operators. To determine when a point of saturation for a keyword group is reached, one could monitor the increase in papers found in a database when adding another synonym. Once the increase is significantly decreasing or even zeroing, saturation is reached (Sauer and Seuring 2017 ). The keywords themselves can be derived from the list of keywords of influential publications in the field, while attention should be paid to potential synonyms in neighboring fields (Carter and Washispack 2018 ; Durach et al. 2017 ; Kraus et al. 2020 ).
4.5 Step 4: selecting the pertinent literature
The inclusion and exclusion criteria (Decision 6) are typically applied in Decision 7 in a two-stage process, first on the title, abstract, and keywords of an article before secondly applying them to the full text of the remaining articles (see also Kraus et al. 2020 ; Snyder 2019 ). Beyond this, Durach et al. ( 2017 ) underlined that the pertinence of the publication regarding units of analysis and the theoretical framework needs to be critically evaluated in this step to avoid bias in the review analysis. Moreover, Carter and Washispack ( 2018 ) requested the publication of the included and excluded sources to ensure the replicability of Steps 3 and 4. This can easily be done as an online supplement to an eventually published review article.
Nevertheless, the question remains: How many papers justify a literature review? While it is hard to specify how many papers comprise a body of literature, there might be certain thresholds for which Kraus et al. ( 2020 ) provide a useful discussion. As a rough guide, more than 50 papers would usually make a sound starting point (see also Paul and Criado 2020 ), while there are SLRs on emergent topics, such as multitier supply chain management, where 39 studies were included (Tachizawa and Wong 2014 ). An SLR on “learning from innovation failures” builds on 36 papers (Rhaiem and Amara 2021 ), which we would see as the lower threshold. However, such a low number should be an exception, and anything lower would certainly trigger the following question: Why is a review needed? Meanwhile, there are also limits on how many papers should be reviewed. While there are cases with 191 (Seuring and Müller 2008 ), 235 (Rojas-Córdova et al. 2022 ), or up to nearly 400 papers reviewed (Spens and Kovács 2006 ), these can be regarded as upper thresholds. Over time, similar topics seem to address larger datasets.
4.6 Step 5: synthesizing the literature
Before synthesizing the literature, Decision 8 considers the selection of a data extraction tool for which we found surprisingly little guidance. Some guidance is given on the use of cloud storage to enable remote team work (Clark et al. 2021 ). Beyond this, we found that SLRs have often been compiled with marked and commented PDFs or printed papers that were accompanied by tables (Kraus et al. 2020 ) or Excel sheets (see also the process tips by Clark et al. 2021 ). This sheet tabulated the single codes derived from the theoretical framework (Decision 3) and the single papers to be reviewed (Decision 7) by crossing out individual cells, signaling the representation of a particular code in a particular paper. While the frequency distribution of the codes is easily compiled from this data tool, the related content needs to be looked at in the papers in a tedious back-and-forth process. Beyond that, we would strongly recommend using data analysis software, such as MAXQDA or NVivo. Such programs enable the import of literature in PDF format and the automatic or manual coding of text passages, their comparison, and tabulation. Moreover, there is a permanent and editable reference of the coded text to a code. This enables a very quick compilation of content summaries or statistics for single codes and the identification of qualitative and quantitative links between codes and papers.
All the mentioned data extraction or data processing tools require a license and therefore are not free of cost. While many researchers may benefit from national or institutional subscriptions to these services, others may not. As a potential alternative, Pearce ( 2018 ) proposed a set of free open-source software (FOSS), including an elaboration on how they can be combined to perform an SLR. He also highlighted that both free and proprietary solutions have advantages and disadvantages that are worthwhile for those who do not have the required tools provided by their employers or other institutions they are members of. The same may apply to the literature databases used for the literature acquisition in Decision 5 (Pearce 2018 ).
Moreover, there is a link to Step 1, Decision 3, where bibliometric reviews and meta-analyses were mentioned. These methods, which are alternatives to content analysis–based approaches, have specific demands, so specific tools would be appropriate, such as the Biblioshiny software or VOSviewer. As we will point out for all decisions, there is a high degree of interdependence among the steps and decisions made.
Decision 9 looks at conducting the data analysis, such as coding against (pre-defined) constructs, in SLRs that rely, in most cases, on content analysis. Seuring and Gold ( 2012 ) elaborated in detail on its characteristics and application in SLRs. As this paper also explains the process of qualitative content analysis in detail, repetition is avoided here, but a summary is offered. Since different ways exist to conduct a content analysis, it is even more important to explain and justify, for example, the choice of an inductive or deductive approach (see Decision 2). In several cases, analytic variables are applied on the go, so there is no theory-based introduction of related constructs. However, to ensure the validity and replicability of the review (see Decision 11), it is necessary to explicitly define all the variables and codes used to analyze and synthesize the reviewed material (Durach et al. 2017 ; Seuring and Gold 2012 ). To build a valid framework as the SLR outcome, it is vital to ensure that the constructs used for the data analysis are sufficiently defined, mutually exclusive, and comprehensively exhaustive. For meta-analysis, the predefined constructs and items would demand quantitative coding so that the resulting data could be analyzed using statistical software tools such as SPSS or R (e.g., Xiao and Watson 2019 ). Pointing to bibliometric analysis again, the respective software would be used for data analysis, yielding different figures and paper clusters, which would then require interpretation (e.g., Donthu et al. 2021 ; Xiao and Watson 2019 ).
Decision 10, on conducting subsequent statistical analysis, considers follow-up analysis of the coding results. Again, this is linked to the chosen SLR method, and a bibliographic analysis will require a different statistical analysis than a content analysis–based SLR (e.g., Lim et al. 2022 ; Xiao and Watson 2019 ). Beyond the use of content analysis and the qualitative interpretation of its results, applying contingency analysis offers the opportunity to quantitatively assess the links among constructs and items. It provides insights into which items are correlated with each other without implying causality. Thus, the interpretation of the findings must explain the causality behind the correlations between the constructs and the items. This must be based on sound reasoning and linking the findings to theoretical arguments. For SLRs, there have recently been two kinds of applications of contingency analysis, differentiated by unit of analysis. De Lima et al. ( 2021 ) used the entire paper as the unit of analysis, deriving correlations on two constructs that were used together in one paper. This is, of course, subject to critique as to whether the constructs really represent correlated content. Moving a level deeper, Tröster and Hiete ( 2018 ) used single-text passages on one aspect, argument, or thought as the unit of analysis. Such an approach is immune against the critique raised before and can yield more valid statistical support for thematic analysis. Another recent methodological contribution employing the same contingency analysis–based approach was made by Siems et al. ( 2021 ). Their analysis employs constructs from SSCM and dynamic capabilities. Employing four subsets of data (i.e., two time periods each in the food and automotive industries), they showed that the method allows distinguishing among time frames as well as among industries.
However, the unit of analysis must be precisely explained so that the reader can comprehend it. Both examples use contingency analysis to identify under-researched topics and develop them into research directions whose formulation represents the particular aim of an SLR (Paul and Criado 2020 ; Snyder 2019 ). Other statistical tools might also be applied, such as cluster analysis. Interestingly, Brandenburg and Rebs ( 2015 ) applied both contingency and cluster analyses. However, the authors stated that the contingency analysis did not yield usable results, so they opted for cluster analysis. In effect, Brandenburg and Rebs ( 2015 ) added analytical depth to their analysis of model types in SSCM by clustering them against the main analytical categories of content analysis. In any case, the application of statistical tools needs to fit the study purpose (Decision 1) and the literature sample (Decision 7), just as in their more conventional applications (e.g., in empirical research processes).
Decision 11 regards the additional consideration of validity and reliability criteria and emphasizes the need for explaining and justifying the single steps of the research process (Seuring and Gold 2012 ), much in line with other examples of research (Davis and Crombie 2001 ). This is critical to underlining the quality of the review but is often neglected in many submitted manuscripts. In our review, we find rich guidance on this decision, to which we want to guide readers (see Table 3 ). In particular, Durach et al. ( 2017 ) provide an entire section of biases and what needs to be considered and reported on them. Moreover, Snyder ( 2019 ) regularly reflects on these issues in her elaborations. This rich guidance elaborates on how to ensure the quality of the individual steps of the review process, such as sampling, study inclusion and exclusion, coding, synthesizing, and more practical issues, including team composition and teamwork organization, which are discussed in some guidelines (e.g., Clark et al. 2021 ; Kraus et al. 2020 ). We only want to underline that the potential biases are, of course, to be seen in conjunction with Decisions 2, 3, 4, 5, 6, 7, 9, and 10. These decisions and the elaboration by Durach et al. ( 2017 ) should provide ample points of reflection that, however, many SLR manuscripts fail to address.
4.7 Step 6: reporting the results
In the final step, there are three decisions on which there is surprisingly little guidance, although reviews often fail in this critical part of the process (Kraus et al. 2020 ). The reviewed guidelines discuss the presentation almost exclusively, while almost no guidance is given on the overall paper structure or the key content to be reported.
Consequently, the first choice to be made in Decision 12 is regarding the paper structure. We suggest following the five-step logic of typical research papers (see also Fisch and Block 2018 ) and explaining only a few points in which a difference from other papers is seen.
(1) Introduction: While the introduction would follow a conventional logic of problem statement, research question, contribution, and outline of the paper (see also Webster and Watson 2002 ), the next parts might depend on the theoretical choices made in Decision 2.
(2) Literature review section: If deductive logic is taken, the paper usually has a conventional flow. After the introduction, the literature review section covers the theoretical background and the choice of constructs and variables for the analysis (De Lima et al. 2021 ; Dieste et al. 2022 ). To avoid confusion in this section with the literature review, its labeling can also be closer to the reviewed object.
If an inductive approach is applied, it might be challenging to present the theoretical basis up front, as the codes emerge only from analyzing the material. In this case, the theory section might be rather short, concentrating on defining the core concepts or terms used, for example, in the keyword-based search for papers. The latter approach is exemplified by the study at hand, which presents a short review of the available literature in the introduction and the first part of the findings. However, we do not perform a systematic but integrative review, which allows for more freedom and creativity (Snyder 2019 ).
(3) Method section: This section should cover the steps and follow the logic presented in this paper or any of the reviewed guidelines so that the choices made during the research process are transparently disclosed (Denyer and Tranfield 2009 ; Paul et al. 2021 ; Xiao and Watson 2019 ). In particular, the search for papers and their selection requires a sound explanation of each step taken, including the provision of reasons for the delimitation of the final paper sample. A stage that is often not covered in sufficient detail is data analysis (Seuring and Gold 2012 ). This also needs to be outlined so that the reader can comprehend how sense has been made of the material collected. Overall, the demands for SLR papers are similar to case studies, survey papers, or almost any piece of empirical research; thus, each step of the research process needs to be comprehensively described, including Decisions 4–10. This comprehensiveness must also include addressing measures for validity and reliability (see Decision 11) or other suitable measures of rigor in the research process since they are a critical issue in literature reviews (Durach et al. 2017 ). In particular, inductively conducted reviews are prone to subjective influences and thus require sound reporting of design choices and their justification.
(4) Findings: The findings typically start with a descriptive analysis of the literature covered, such as journals, distribution across years, or (empirical) methods applied (Tranfield et al. 2003 ). For modeling-related reviews, classifying papers against the approach chosen is a standard approach, but this can often also serve as an analytic category that provides detailed insights. The descriptive analysis should be kept short since a paper only presenting descriptive findings will not be of great interest to other researchers due to the missing contribution (Snyder 2019 ). Nevertheless, there are opportunities to provide interesting findings in the descriptive analysis. Beyond a mere description of the distributions of the single results, such as the distribution of methods used in the sample, authors should combine analytical categories to derive more detailed insights (see also Tranfield et al. 2003 ). The distribution of methods used might well be combined with the years of publication to identify and characterize different phases in the development of a field of research or its maturity. Moreover, there could be value in the analysis of theories applied in the review sample (e.g., Touboulic and Walker 2015 ; Zhu et al. 2022 ) and in reflecting on the interplay of different qualitative and quantitative methods in spurring the theoretical development of the reviewed field. This could yield detailed insights into methodological as well as theoretical gaps, and we would suggest explicitly linking the findings of such analyses to the research directions that an SLR typically provides. This link could help make the research directions much more tangible by giving researchers a clear indication of how to follow up on the findings, as, for example, done by Maestrini et al. ( 2017 ) or Dieste et al. ( 2022 ). In contrast to the mentioned examples of an actionable research agenda, a typical weakness of premature SLR manuscripts is that they ask rather superficially for more research in the different aspects they reviewed but remain silent about how exactly this can be achieved.
We would thus like to encourage future SLR authors to systematically investigate the potential to combine two categories of descriptive analysis to move this section of the findings to a higher level of quality, interest, and relevance. The same can, of course, be done with the thematic findings, which comprise the second part of this section.
Moving into the thematic analysis, we have already reached Decision 13 on the presentation of the refined theoretical framework and the discussion of its contents. A first step might present the frequencies of the codes or constructs applied in the analysis. This allows the reader to understand which topics are relevant. If a rather small body of literature is analyzed, tables providing evidence on which paper has been coded for which construct might be helpful in improving the transparency of the research process. Tables or other forms of visualization might help to organize the many codes soundly (see also Durach et al. 2017 ; Paul and Criado 2020 ; Webster and Watson 2002 ). These findings might then lead to interpretation, for which it is necessary to extract meaning from the body of literature and present it accordingly (Snyder 2019 ). To do so, it might seem needless to say that the researchers should refer back to Decisions 1, 2, and 3 taken in Step 1 and their justifications. These typically identify the research gap to be filled, but after the lengthy process of the SLR, the authors often fail to step back from the coding results and put them into a larger perspective against the research gap defined in Decision 1 (see also Clark et al. 2021 ). To support this, it is certainly helpful to illustrate the findings in a figure or graph presenting the links among the constructs and items and adding causal reasoning to this (Durach et al. 2017 ; Paul and Criado 2020 ), such as the three figures by Seuring and Müller ( 2008 ) or other examples by De Lima et al. ( 2021 ) or Tipu ( 2022 ). This presentation should condense arguments made in the assessed literature but should also chart the course for future research. It will be these parts of the paper that are decisive for a strong SLR paper.
Moreover, some guidelines define the most fruitful way of synthesizing the findings as concept-centric synthesis (Clark et al. 2021 ; Fisch and Block 2018 ; Webster and Watson 2002 ). As presented in the previous sentence, the presentation of the review findings is centered on the content or concept of “concept-centric synthesis.” It is accompanied by a reference to all or the most relevant literature in which the concept is evident. Contrastingly, Webster and Watson ( 2002 ) found that author-centric synthesis discusses individual papers and what they have done and found (just like this sentence here). They added that this approach fails to synthesize larger samples. We want to note that we used the latter approach in some places in this paper. However, this aims to actively refer the reader to these studies, as they stand out from our relatively small sample. Beyond this, we want to link back to Decision 3, the selection of a theoretical framework and constructs. These constructs, or the parts of a framework, can also serve to structure the findings section by using them as headlines for subsections (Seuring et al. 2021 ).
Last but not least, there might even be cases where core findings and relationships might be opposed, and alternative perspectives could be presented. This would certainly be challenging to argue for but worthwhile to do in order to drive the reviewed field forward. A related example is the paper by Zhu et al. ( 2022 ), who challenged the current debate at the intersection of blockchain applications and supply chain management and pointed to the limited use of theoretical foundations for related analysis.
(5) Discussion and Conclusion: The discussion needs to explain the contribution the paper makes to the extant literature, that is, which previous findings or hypotheses are supported or contradicted and which aspects of the findings are particularly interesting for the future development of the reviewed field. This is in line with the content required in the discussion sections of any other paper type. A typical structure might point to the contribution and put it into perspective with already existing research. Further, limitations should be addressed on both the theoretical and methodological sides. This elaboration of the limitations can be coupled with the considerations of the validity and reliability of the study in Decision 11. The implications for future research are a core aim of an SLR (Clark et al. 2021 ; Mulrow 1987 ; Snyder 2019 ) and should be addressed in a further part of the discussion section. Recently, a growing number of literature reviews have also provided research questions for future research that provide a very concrete and actionable output of the SLR (e.g. Dieste et al. 2022 ; Maestrini et al. 2017 ). Moreover, we would like to reiterate our call to clearly link the research implications to the SLR findings, which helps the authors craft more tangible research directions and helps the reader to follow the authors’ interpretation. Literature review papers are usually not strongly positioned toward managerial implications, but even these implications might be included.
As a kind of normal demand, the conclusion should provide an answer to the research question put forward in the introduction, thereby closing the cycle of arguments made in the paper.
Although all the works seem to be done when the paper is written and the contribution is fleshed out, there is still one major decision to be made. Decision 14 concerns the identification of an appropriate journal for submission. Despite the popularity of the SLR method, a rising number of journals explicitly limit the number of SLRs published by them. Moreover, there are only two guidelines elaborating on this decision, underlining the need for the following considerations.
Although it might seem most attractive to submit the paper to the highest-ranking journal for the reviewed topic, we argue for two critical and review-related decisions to be made during the research process that influence whether the paper fits a certain outlet:
The theoretical foundation of the SLR (Decision 3) usually relates to certain journals in which it is published or discussed. If a deductive approach was taken, the journals in which the foundational papers were published might be suitable since the review potentially contributes to the further validation or refinement of the frameworks. Overall, we need to keep in mind that a paper needs to be added to a discussion in the journal, and this can be based on the theoretical framework or the reviewed papers, as shown below.
Appropriate journals for publication can be derived from the analyzed journal papers (Decision 7) (see also Paul and Criado 2020 ). This allows for an easy link to the theoretical debate in the respective journal by submitting it. This choice is identifiable in most of the papers mentioned in this paper and is often illustrated in the descriptive analysis.
If the journal chosen for the submission was neither related to the theoretical foundation nor overly represented in the body of literature analyzed, an explicit justification in the paper itself might be needed. Alternatively, an explanation might be provided in the letter to the editor when submitting the paper. If such a statement is not presented, the likelihood of it being transferred into the review process and passing it is rather low. Finally, we want to refer readers interested in the specificities of the publication-related review process of SLRs to Webster and Watson ( 2002 ), who elaborated on this for Management Information Systems Quarterly.
5 Discussion and conclusion
Critically reviewing the currently available SLR guidelines in the management domain, this paper synthesizes 14 key decisions to be made and reported across the SLR research process. Guidelines are presented for each decision, including tasks that assist in making sound choices to complete the research process and make meaningful contributions. Applying these guidelines should improve the rigor and robustness of many review papers and thus enhance their contributions. Moreover, some practical hints and best-practice examples are provided on issues that unexperienced authors regularly struggle to present in a manuscript (Fisch and Block 2018 ) and thus frustrate reviewers, readers, editors, and authors alike.
Strikingly, the review of prior guidelines reported in Table 3 revealed their focus on the technical details that need to be reported in any SLR. Consequently, our discipline has come a long way in crafting search strings, inclusion, and exclusion criteria, and elaborating on the validity and reliability of an SLR. Nevertheless, we left critical areas underdeveloped, such as the identification of relevant research gaps and questions, data extraction tools, analysis of the findings, and a meaningful and interesting reporting of the results. Our study contributes to filling these gaps by providing operationalized guidance to SLR authors, especially early-stage researchers who craft SLRs at the outset of their research journeys. At the same time, we need to underline that our paper is, of course, not the only useful reference for SLR authors. Instead, the readers are invited to find more guidance on the many aspects to consider in an SLR in the references we provide within the single decisions, as well as in Tables 1 and 2 . The tables also identify the strongholds of other guidelines that our paper does not want to replace but connect and extend at selected occasions, especially in SLR Steps 5 and 6.
The findings regularly underline the interconnection of the 14 decisions identified and discussed in this paper. We thus support Tranfield et al. ( 2003 ) who requested a flexible approach to the SLR while clearly reporting all design decisions and reflecting their impacts. In line with the guidance synthesized in this review, and especially Durach et al. ( 2017 ), we also present a refined framework in Figs. 1 and 2 . It specifically refines the original six-step SLR process by Durach et al. ( 2017 ) in three ways:
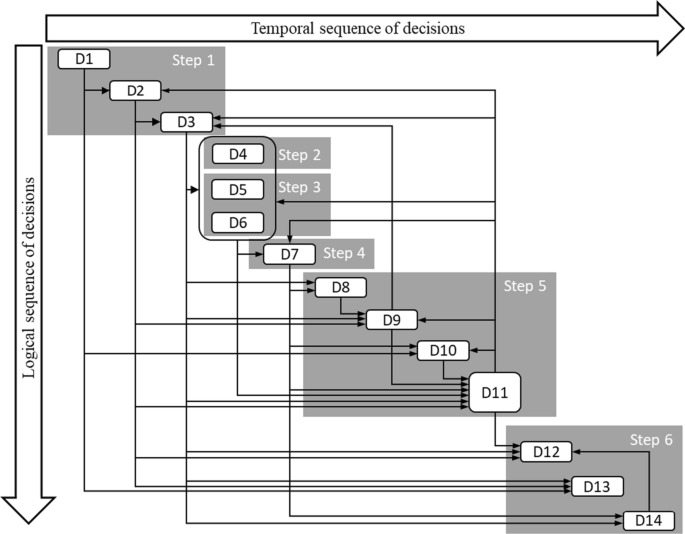
Enriched six-step process including the core interrelations of the 14 decisions
First, we subdivided the six steps into 14 decisions to enhance the operationalization of the process and enable closer guidance (see Fig. 1 ). Second, we added a temporal sequence to Fig. 2 by positioning the decisions from left to right according to this temporal sequence. This is based on systematically reflecting on the need to finish one decision before the following. If this need is evident, the following decision moves to the right; if not, the decisions are positioned below each other. Turning to Fig. 2 , it becomes evident that Step 2, “determining the required characteristics of primary studies,” and Step 3, “retrieving a sample of potentially relevant literature,” including their Decisions 4–6, can be conducted in an iterative manner. While this contrasts with the strict division of the six steps by Durach et al. ( 2017 ), it supports other guidance that suggests running pilot studies to iteratively define the literature sample, its sources, and characteristics (Snyder 2019 ; Tranfield et al. 2003 ; Xiao and Watson 2019 ). While this insight might suggest merging Steps 2 and 3, we refrain from this superficial change and building yet another SLR process model. Instead, we prefer to add detail and depth to Durach et al.’s ( 2017 ) model.
(Decisions: D1: specifying the research gap and related research question, D2: opting for a theoretical approach, D3: defining the core theoretical framework and constructs, D4: specifying inclusion and exclusion criteria, D5: defining sources and databases, D6: defining search terms and crafting a search string, D7: including and excluding literature for detailed analysis and synthesis, D8: selecting data extraction tool(s), D9: coding against (pre-defined) constructs, D10: conducting a subsequent (statistical) analysis (optional), D11: ensuring validity and reliability, D12: deciding on the structure of the paper, D13: presenting a refined theoretical framework and discussing its contents, and D14: deriving an appropriate journal from the analyzed papers).
This is also done through the third refinement, which underlines which previous or later decisions need to be considered within each single decision. Such a consideration moves beyond the mere temporal sequence of steps and decisions that does not reflect the full complexity of the SLR process. Instead, its focus is on the need to align, for example, the conduct of the data analysis (Decision 9) with the theoretical approach (Decision 2) and consequently ensure that the chosen theoretical framework and the constructs (Decision 3) are sufficiently defined for the data analysis (i.e., mutually exclusive and comprehensively exhaustive). The mentioned interrelations are displayed in Fig. 2 by means of directed arrows from one decision to another. The underlying explanations can be found in the earlier paper sections by searching for the individual decisions in the text on the impacted decisions. Overall, it is unsurprising to see that the vast majority of interrelations are directed from the earlier to the later steps and decisions (displayed through arrows below the diagonal of decisions), while only a few interrelations are inverse.
Combining the first refinement of the original framework (defining the 14 decisions) and the third refinement (revealing the main interrelations among the decisions) underlines the contribution of this study in two main ways. First, the centrality of ensuring validity and reliability (Decision 11) is underlined. It becomes evident that considerations of validity and reliability are central to the overall SLR process since all steps before the writing of the paper need to be revisited in iterative cycles through Decision 11. Any lack of related considerations will most likely lead to reviewer critique, putting the SLR publication at risk. On the positive side of this centrality, we also found substantial guidance on this issue. In contrast, as evidenced in Table 3 , there is a lack of prior guidance on Decisions 1, 8, 10, 12, 13, and 14, which this study is helping to fill. At the same time, these underexplained decisions are influenced by 14 of the 44 (32%) incoming arrows in Fig. 2 and influence the other decisions in 6 of the 44 (14%) instances. These interrelations among decisions to be considered when crafting an SLR were scattered across prior guidelines, lacked in-depth elaborations, and were hardly explicitly related to each other. Thus, we hope that our study and the refined SLR process model will help enhance the quality and contribution of future SLRs.
Data availablity
The data generated during this research is summarized in Table 3 and the analyzed papers are publicly available. They are clearly identified in Table 3 and the reference list.
Aguinis H, Ramani RS, Alabduljader N (2020) Best-practice recommendations for producers, evaluators, and users of methodological literature reviews. Organ Res Methods. https://doi.org/10.1177/1094428120943281
Article Google Scholar
Beske P, Land A, Seuring S (2014) Sustainable supply chain management practices and dynamic capabilities in the food industry: a critical analysis of the literature. Int J Prod Econ 152:131–143. https://doi.org/10.1016/j.ijpe.2013.12.026
Brandenburg M, Rebs T (2015) Sustainable supply chain management: a modeling perspective. Ann Oper Res 229:213–252. https://doi.org/10.1007/s10479-015-1853-1
Carter CR, Rogers DS (2008) A framework of sustainable supply chain management: moving toward new theory. Int Jnl Phys Dist Logist Manage 38:360–387. https://doi.org/10.1108/09600030810882816
Carter CR, Washispack S (2018) Mapping the path forward for sustainable supply chain management: a review of reviews. J Bus Logist 39:242–247. https://doi.org/10.1111/jbl.12196
Clark WR, Clark LA, Raffo DM, Williams RI (2021) Extending fisch and block’s (2018) tips for a systematic review in management and business literature. Manag Rev Q 71:215–231. https://doi.org/10.1007/s11301-020-00184-8
Crane A, Henriques I, Husted BW, Matten D (2016) What constitutes a theoretical contribution in the business and society field? Bus Soc 55:783–791. https://doi.org/10.1177/0007650316651343
Davis J, Mengersen K, Bennett S, Mazerolle L (2014) Viewing systematic reviews and meta-analysis in social research through different lenses. Springerplus 3:511. https://doi.org/10.1186/2193-1801-3-511
Davis HTO, Crombie IK (2001) What is asystematicreview? http://vivrolfe.com/ProfDoc/Assets/Davis%20What%20is%20a%20systematic%20review.pdf . Accessed 22 February 2019
De Lima FA, Seuring S, Sauer PC (2021) A systematic literature review exploring uncertainty management and sustainability outcomes in circular supply chains. Int J Prod Res. https://doi.org/10.1080/00207543.2021.1976859
Denyer D, Tranfield D (2009) Producing a systematic review. In: Buchanan DA, Bryman A (eds) The Sage handbook of organizational research methods. Sage Publications Ltd, Thousand Oaks, CA, pp 671–689
Google Scholar
Devece C, Ribeiro-Soriano DE, Palacios-Marqués D (2019) Coopetition as the new trend in inter-firm alliances: literature review and research patterns. Rev Manag Sci 13:207–226. https://doi.org/10.1007/s11846-017-0245-0
Dieste M, Sauer PC, Orzes G (2022) Organizational tensions in industry 4.0 implementation: a paradox theory approach. Int J Prod Econ 251:108532. https://doi.org/10.1016/j.ijpe.2022.108532
Donthu N, Kumar S, Mukherjee D, Pandey N, Lim WM (2021) How to conduct a bibliometric analysis: an overview and guidelines. J Bus Res 133:285–296. https://doi.org/10.1016/j.jbusres.2021.04.070
Durach CF, Kembro J, Wieland A (2017) A new paradigm for systematic literature reviews in supply chain management. J Supply Chain Manag 53:67–85. https://doi.org/10.1111/jscm.12145
Fink A (2010) Conducting research literature reviews: from the internet to paper, 3rd edn. SAGE, Los Angeles
Fisch C, Block J (2018) Six tips for your (systematic) literature review in business and management research. Manag Rev Q 68:103–106. https://doi.org/10.1007/s11301-018-0142-x
Fritz MMC, Silva ME (2018) Exploring supply chain sustainability research in Latin America. Int Jnl Phys Dist Logist Manag 48:818–841. https://doi.org/10.1108/IJPDLM-01-2017-0023
Garcia-Torres S, Albareda L, Rey-Garcia M, Seuring S (2019) Traceability for sustainability: literature review and conceptual framework. Supp Chain Manag 24:85–106. https://doi.org/10.1108/SCM-04-2018-0152
Hanelt A, Bohnsack R, Marz D, Antunes Marante C (2021) A systematic review of the literature on digital transformation: insights and implications for strategy and organizational change. J Manag Stud 58:1159–1197. https://doi.org/10.1111/joms.12639
Kache F, Seuring S (2014) Linking collaboration and integration to risk and performance in supply chains via a review of literature reviews. Supp Chain Mnagmnt 19:664–682. https://doi.org/10.1108/SCM-12-2013-0478
Khalid RU, Seuring S (2019) Analyzing base-of-the-pyramid research from a (sustainable) supply chain perspective. J Bus Ethics 155:663–686. https://doi.org/10.1007/s10551-017-3474-x
Koufteros X, Mackelprang A, Hazen B, Huo B (2018) Structured literature reviews on strategic issues in SCM and logistics: part 2. Int Jnl Phys Dist Logist Manage 48:742–744. https://doi.org/10.1108/IJPDLM-09-2018-363
Kraus S, Breier M, Dasí-Rodríguez S (2020) The art of crafting a systematic literature review in entrepreneurship research. Int Entrep Manag J 16:1023–1042. https://doi.org/10.1007/s11365-020-00635-4
Kraus S, Mahto RV, Walsh ST (2021) The importance of literature reviews in small business and entrepreneurship research. J Small Bus Manag. https://doi.org/10.1080/00472778.2021.1955128
Kraus S, Breier M, Lim WM, Dabić M, Kumar S, Kanbach D, Mukherjee D, Corvello V, Piñeiro-Chousa J, Liguori E, Palacios-Marqués D, Schiavone F, Ferraris A, Fernandes C, Ferreira JJ (2022) Literature reviews as independent studies: guidelines for academic practice. Rev Manag Sci 16:2577–2595. https://doi.org/10.1007/s11846-022-00588-8
Leuschner R, Rogers DS, Charvet FF (2013) A meta-analysis of supply chain integration and firm performance. J Supply Chain Manag 49:34–57. https://doi.org/10.1111/jscm.12013
Lim WM, Rasul T (2022) Customer engagement and social media: revisiting the past to inform the future. J Bus Res 148:325–342. https://doi.org/10.1016/j.jbusres.2022.04.068
Lim WM, Yap S-F, Makkar M (2021) Home sharing in marketing and tourism at a tipping point: what do we know, how do we know, and where should we be heading? J Bus Res 122:534–566. https://doi.org/10.1016/j.jbusres.2020.08.051
Lim WM, Kumar S, Ali F (2022) Advancing knowledge through literature reviews: ‘what’, ‘why’, and ‘how to contribute.’ Serv Ind J 42:481–513. https://doi.org/10.1080/02642069.2022.2047941
Lusiantoro L, Yates N, Mena C, Varga L (2018) A refined framework of information sharing in perishable product supply chains. Int J Phys Distrib Logist Manag 48:254–283. https://doi.org/10.1108/IJPDLM-08-2017-0250
Maestrini V, Luzzini D, Maccarrone P, Caniato F (2017) Supply chain performance measurement systems: a systematic review and research agenda. Int J Prod Econ 183:299–315. https://doi.org/10.1016/j.ijpe.2016.11.005
Miemczyk J, Johnsen TE, Macquet M (2012) Sustainable purchasing and supply management: a structured literature review of definitions and measures at the dyad, chain and network levels. Supp Chain Mnagmnt 17:478–496. https://doi.org/10.1108/13598541211258564
Moher D, Liberati A, Tetzlaff J, Altman DG (2009) Preferred reporting items for systematic reviews and meta-analyses: the PRISMA statement. PLoS Med 6:e1000097. https://doi.org/10.1371/journal.pmed.1000097
Mukherjee D, Lim WM, Kumar S, Donthu N (2022) Guidelines for advancing theory and practice through bibliometric research. J Bus Res 148:101–115. https://doi.org/10.1016/j.jbusres.2022.04.042
Mulrow CD (1987) The medical review article: state of the science. Ann Intern Med 106:485–488. https://doi.org/10.7326/0003-4819-106-3-485
Page MJ, McKenzie JE, Bossuyt PM, Boutron I, Hoffmann TC, Mulrow CD, Shamseer L, Tetzlaff JM, Akl EA, Brennan SE, Chou R, Glanville J, Grimshaw JM, Hróbjartsson A, Lalu MM, Li T, Loder EW, Mayo-Wilson E, McDonald S, McGuinness LA, Stewart LA, Thomas J, Tricco AC, Welch VA, Whiting P, Moher D (2021) The PRISMA 2020 statement: an updated guideline for reporting systematic reviews. J Clin Epidemiol 134:178–189. https://doi.org/10.1016/j.jclinepi.2021.03.001
Pagell M, Wu Z (2009) Building a more complete theory of sustainable supply chain management using case studies of 10 exemplars. J Supply Chain Manag 45:37–56. https://doi.org/10.1111/j.1745-493X.2009.03162.x
Paul J, Criado AR (2020) The art of writing literature review: What do we know and what do we need to know? Int Bus Rev 29:101717. https://doi.org/10.1016/j.ibusrev.2020.101717
Paul J, Lim WM, O’Cass A, Hao AW, Bresciani S (2021) Scientific procedures and rationales for systematic literature reviews (SPAR-4-SLR). Int J Consum Stud. https://doi.org/10.1111/ijcs.12695
Pearce JM (2018) How to perform a literature review with free and open source software. Pract Assess Res Eval 23:1–13
Rhaiem K, Amara N (2021) Learning from innovation failures: a systematic review of the literature and research agenda. Rev Manag Sci 15:189–234. https://doi.org/10.1007/s11846-019-00339-2
Rojas-Córdova C, Williamson AJ, Pertuze JA, Calvo G (2022) Why one strategy does not fit all: a systematic review on exploration–exploitation in different organizational archetypes. Rev Manag Sci. https://doi.org/10.1007/s11846-022-00577-x
Sauer PC (2021) The complementing role of sustainability standards in managing international and multi-tiered mineral supply chains. Resour Conserv Recycl 174:105747. https://doi.org/10.1016/j.resconrec.2021.105747
Sauer PC, Seuring S (2017) Sustainable supply chain management for minerals. J Clean Prod 151:235–249. https://doi.org/10.1016/j.jclepro.2017.03.049
Seuring S, Gold S (2012) Conducting content-analysis based literature reviews in supply chain management. Supp Chain Mnagmnt 17:544–555. https://doi.org/10.1108/13598541211258609
Seuring S, Müller M (2008) From a literature review to a conceptual framework for sustainable supply chain management. J Clean Prod 16:1699–1710. https://doi.org/10.1016/j.jclepro.2008.04.020
Seuring S, Yawar SA, Land A, Khalid RU, Sauer PC (2021) The application of theory in literature reviews: illustrated with examples from supply chain management. Int J Oper Prod Manag 41:1–20. https://doi.org/10.1108/IJOPM-04-2020-0247
Siems E, Land A, Seuring S (2021) Dynamic capabilities in sustainable supply chain management: an inter-temporal comparison of the food and automotive industries. Int J Prod Econ 236:108128. https://doi.org/10.1016/j.ijpe.2021.108128
Snyder H (2019) Literature review as a research methodology: an overview and guidelines. J Bus Res 104:333–339. https://doi.org/10.1016/j.jbusres.2019.07.039
Spens KM, Kovács G (2006) A content analysis of research approaches in logistics research. Int Jnl Phys Dist Logist Manage 36:374–390. https://doi.org/10.1108/09600030610676259
Tachizawa EM, Wong CY (2014) Towards a theory of multi-tier sustainable supply chains: a systematic literature review. Supp Chain Mnagmnt 19:643–663. https://doi.org/10.1108/SCM-02-2014-0070
Tipu SAA (2022) Organizational change for environmental, social, and financial sustainability: a systematic literature review. Rev Manag Sci 16:1697–1742. https://doi.org/10.1007/s11846-021-00494-5
Touboulic A, Walker H (2015) Theories in sustainable supply chain management: a structured literature review. Int Jnl Phys Dist Logist Manage 45:16–42. https://doi.org/10.1108/IJPDLM-05-2013-0106
Tranfield D, Denyer D, Smart P (2003) Towards a methodology for developing evidence-informed management knowledge by means of systematic review. Br J Manag 14:207–222. https://doi.org/10.1111/1467-8551.00375
Tröster R, Hiete M (2018) Success of voluntary sustainability certification schemes: a comprehensive review. J Clean Prod 196:1034–1043. https://doi.org/10.1016/j.jclepro.2018.05.240
Wang Y, Han JH, Beynon-Davies P (2019) Understanding blockchain technology for future supply chains: a systematic literature review and research agenda. Supp Chain Mnagmnt 24:62–84. https://doi.org/10.1108/SCM-03-2018-0148
Webster J, Watson RT (2002) Analyzing the past to prepare for the future: writing a literature review. MIS Q 26:xiii–xxiii
Wiese A, Kellner J, Lietke B, Toporowski W, Zielke S (2012) Sustainability in retailing: a summative content analysis. Int J Retail Distrib Manag 40:318–335. https://doi.org/10.1108/09590551211211792
Xiao Y, Watson M (2019) Guidance on conducting a systematic literature review. J Plan Educ Res 39:93–112. https://doi.org/10.1177/0739456X17723971
Yavaprabhas K, Pournader M, Seuring S (2022) Blockchain as the “trust-building machine” for supply chain management. Ann Oper Res. https://doi.org/10.1007/s10479-022-04868-0
Zhu Q, Bai C, Sarkis J (2022) Blockchain technology and supply chains: the paradox of the atheoretical research discourse. Transp Res Part E Logist Transp Rev 164:102824. https://doi.org/10.1016/j.tre.2022.102824
Download references
Open Access funding enabled and organized by Projekt DEAL.
Author information
Authors and affiliations.
EM Strasbourg Business School, Université de Strasbourg, HuManiS UR 7308, 67000, Strasbourg, France
Philipp C. Sauer
Chair of Supply Chain Management, Faculty of Economics and Management, The University of Kassel, Kassel, Germany
Stefan Seuring
You can also search for this author in PubMed Google Scholar
Contributions
The article is based on the idea and extensive experience of SS. The literature search and data analysis has mainly been performed by PCS and supported by SS before the paper manuscript has been written and revised in a common effort of both authors.
Corresponding author
Correspondence to Stefan Seuring .
Ethics declarations
Conflict of interest.
The authors have no competing interests to declare that are relevant to the content of this article.
Additional information
Publisher's note.
Springer Nature remains neutral with regard to jurisdictional claims in published maps and institutional affiliations.
Rights and permissions
Open Access This article is licensed under a Creative Commons Attribution 4.0 International License, which permits use, sharing, adaptation, distribution and reproduction in any medium or format, as long as you give appropriate credit to the original author(s) and the source, provide a link to the Creative Commons licence, and indicate if changes were made. The images or other third party material in this article are included in the article's Creative Commons licence, unless indicated otherwise in a credit line to the material. If material is not included in the article's Creative Commons licence and your intended use is not permitted by statutory regulation or exceeds the permitted use, you will need to obtain permission directly from the copyright holder. To view a copy of this licence, visit http://creativecommons.org/licenses/by/4.0/ .
Reprints and permissions
About this article
Sauer, P.C., Seuring, S. How to conduct systematic literature reviews in management research: a guide in 6 steps and 14 decisions. Rev Manag Sci 17 , 1899–1933 (2023). https://doi.org/10.1007/s11846-023-00668-3
Download citation
Received : 29 September 2022
Accepted : 17 April 2023
Published : 12 May 2023
Issue Date : July 2023
DOI : https://doi.org/10.1007/s11846-023-00668-3
Share this article
Anyone you share the following link with will be able to read this content:
Sorry, a shareable link is not currently available for this article.
Provided by the Springer Nature SharedIt content-sharing initiative
- Methodology
- Replicability
- Research process
- Structured literature review
- Systematic literature review
JEL Classification
- Find a journal
- Publish with us
- Track your research

An official website of the United States government
The .gov means it's official. Federal government websites often end in .gov or .mil. Before sharing sensitive information, make sure you're on a federal government site.
The site is secure. The https:// ensures that you are connecting to the official website and that any information you provide is encrypted and transmitted securely.
- Publications
- Account settings
- Browse Titles
NCBI Bookshelf. A service of the National Library of Medicine, National Institutes of Health.
O’Haire C, McPheeters M, Nakamoto E, et al. Engaging Stakeholders To Identify and Prioritize Future Research Needs [Internet]. Rockville (MD): Agency for Healthcare Research and Quality (US); 2011 Jun. (Methods Future Research Needs Reports, No. 4.)

Engaging Stakeholders To Identify and Prioritize Future Research Needs [Internet].
Appendix g strengths and limitations of stakeholder engagement methods.
View in own window
References: Appendix G
- Cite this Page O’Haire C, McPheeters M, Nakamoto E, et al. Engaging Stakeholders To Identify and Prioritize Future Research Needs [Internet]. Rockville (MD): Agency for Healthcare Research and Quality (US); 2011 Jun. (Methods Future Research Needs Reports, No. 4.) Appendix G, Strengths and Limitations of Stakeholder Engagement Methods.
- PDF version of this title (571K)
Other titles in this collection
- AHRQ Methods Future Research Needs Series
Related information
- PMC PubMed Central citations
- PubMed Links to PubMed
Recent Activity
- Strengths and Limitations of Stakeholder Engagement Methods - Engaging Stakehold... Strengths and Limitations of Stakeholder Engagement Methods - Engaging Stakeholders To Identify and Prioritize Future Research Needs
Your browsing activity is empty.
Activity recording is turned off.
Turn recording back on
Connect with NLM
National Library of Medicine 8600 Rockville Pike Bethesda, MD 20894
Web Policies FOIA HHS Vulnerability Disclosure
Help Accessibility Careers

Understanding the Limitations of Research Ethics in Management Studies
Table of Contents
As researchers, we must adhere to the highest ethical standards to ensure the integrity of our work. However, it’s important to understand the limitations of research ethics to avoid any unintentional negative consequences. In this blog, we’ll explore the limitations of research ethics in management studies and how to address them.
What are Research Ethics?
Research ethics are a set of moral principles that guide researchers on how to conduct research in an ethical manner. These principles include informed consent, confidentiality, protection of participants from harm, and the right to withdraw from the study.
Limitations of Research Ethics
Despite the importance of research ethics, there are limitations that can affect the validity and generalizability of the research findings. Some of the limitations of research ethics in management studies include:
1. Lack of diversity in the sample population
The sample population used in research studies is often limited to a specific demographic, which may not be representative of the larger population. This can result in biased findings and limit the generalizability of the research.
2. Ethnocentrism
Researchers may unknowingly impose their cultural beliefs and values on the research participants, leading to biased data collection and analysis.
3. Difficulty in obtaining informed consent
In some cases, obtaining informed consent from participants can be challenging. For example, if the participants are minors or have cognitive impairments, they may not be able to provide informed consent, which can limit the study’s validity.
4. Limited access to participants
Some populations may be difficult to access, such as incarcerated individuals or those with certain medical conditions, which can limit the generalizability of the research findings.
5. Limited funding
Research studies often require significant funding, which can limit the sample size or scope of the study. This can impact the validity and generalizability of the research.
Addressing the Limitations
To address the limitations of research ethics, researchers can take several steps. For example, they can:
- Ensure a diverse sample population that is representative of the larger population.
- Take measures to avoid ethnocentrism, such as working with a diverse team and being mindful of personal biases.
- Use alternative methods for obtaining informed consent, such as proxy consent for participants who are unable to provide informed consent.
- Work with community organizations or advocacy groups to gain access to hard-to-reach populations.
- Look for alternative sources of funding or collaborate with other researchers to increase the scope of the study.
Research ethics are crucial to ensure the integrity of our work, but it’s important to understand their limitations. By acknowledging and addressing these limitations, we can increase the validity and generalizability of our research findings. As researchers, it’s our responsibility to continuously strive for ethical and high-quality research.
How useful was this post?
Click on a star to rate it!
Average rating 0 / 5. Vote count: 0
No votes so far! Be the first to rate this post.
We are sorry that this post was not useful for you! 😔
Let us improve this post!
Tell us how we can improve this post?
Research Methodology for Management Decisions
1 Research Methodology: An Overview
- Meaning of Research
- Research Methodology
- Research Method
- Business Research Method
- Types of Research
- Importance of business research
- Role of research in important areas
2 Steps for Research Process
- Research process
- Define research problems
- Research Problem as Hypothesis Testing
- Extensive literature review in research
- Development of working hypothesis
- Preparing the research design
- Collecting the data
- Analysis of data
- Preparation of the report or the thesis
3 Research Designs
- Functions and Goals of Research Design
- Characteristics of a Good Design
- Different Types of Research Designs
- Exploratory Research Design
- Descriptive Research Design
- Experimental Research Design
- Types of Experimental Designs
4 Methods and Techniques of Data Collection
- Primary and Secondary Data
- Methods of Collecting Primary Data
- Merits and Demerits of Different Methods of Collecting Primary Data
- Designing a Questionnaire
- Pretesting a Questionnaire
- Editing of Primary Data
- Technique of Interview
- Collection of Secondary Data
- Scrutiny of Secondary Data
5 Attitude Measurement and Scales
- Attitudes, Attributes and Beliefs
- Issues in Attitude Measurement
- Scaling of Attitudes
- Deterministic Attitude Measurement Models: The Guttman Scale
- Thurstone’s Equal-Appearing Interval Scale
- The Semantic Differential Scale
- Summative Models: The Likert Scale
- The Q-Sort Technique
- Multidimensional Scaling
- Selection of an Appropriate Attitude Measurement Scale
- Limitations of Attitude Measurement Scales
6 Questionnaire Designing
- Introductory decisions
- Contents of the questionnaire
- Format of the questionnaire
- Steps involved in the questionnaire
- Structure and Design of Questionnaire
- Management of Fieldwork
- Ambiguities in the Questionnaire Methods
7 Sampling and Sampling Design
- Advantage of Sampling Over Census
- Simple Random Sampling
- Sampling Frame
- Probabilistic As pects of Sampling
- Stratified Random Sampling
- Other Methods of Sampling
- Sampling Design
- Non-Probability Sampling Methods
8 Data Processing
- Editing of Data
- Coding of Data
- Classification of Data
- Statistical Series
- Tables as Data Presentation Devices
- Graphical Presentation of Data
9 Statistical Analysis and Interpretation of Data: Nonparametric Tests
- One Sample Tests
- Two Sample Tests
- K Sample Tests
10 Multivariate Analysis of Data
- Regression Analysis
- Discriminant Analysis
- Factor Analysis
11 Ethics in Research
- Principles of research ethics
- Advantages of research ethics
- Limitations of the research ethics
- Steps involved in ethics
- What are research misconducts?
12 Substance of Reports
- Research Proposal
- Categories of Report
- Reviewing the Draft
13 Formats of Reports
- Parts of a Report
- Cover and Title Page
- Introductory Pages
- Reference Section
- Typing Instructions
- Copy Reading
- Proof Reading
14 Presentation of a Report
- Communication Dimensions
- Presentation Package
- Audio-Visual Aids
- Presenter’s Poise
Click through the PLOS taxonomy to find articles in your field.
For more information about PLOS Subject Areas, click here .
Loading metrics
Open Access
Peer-reviewed
Research Article
Re-use of research data in the social sciences. Use and users of digital data archive
Contributed equally to this work with: Elina Late, Michael Ochsner
Roles Conceptualization, Data curation, Formal analysis, Investigation, Methodology, Project administration, Resources, Validation, Visualization, Writing – original draft, Writing – review & editing
* E-mail: [email protected]
Affiliation Faculty of Information Technology and Communication Sciences, Tampere University, Tampere, Finland

Roles Conceptualization, Data curation, Formal analysis, Investigation, Methodology, Project administration, Validation, Visualization, Writing – original draft, Writing – review & editing
Affiliation Swiss Centre of Expertise in the Social Sciences, University of Lausanne, Lausanne, Switzerland
- Elina Late,
- Michael Ochsner
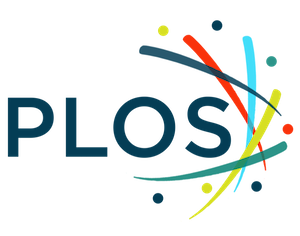
- Published: May 10, 2024
- https://doi.org/10.1371/journal.pone.0303190
- Reader Comments
The aim of this paper is to investigate the re-use of research data deposited in digital data archive in the social sciences. The study examines the quantity, type, and purpose of data downloads by analyzing enriched user log data collected from Swiss data archive. The findings show that quantitative datasets are downloaded increasingly from the digital archive and that downloads focus heavily on a small share of the datasets. The most frequently downloaded datasets are survey datasets collected by research organizations offering possibilities for longitudinal studies. Users typically download only one dataset, but a group of heavy downloaders form a remarkable share of all downloads. The main user group downloading data from the archive are students who use the data in their studies. Furthermore, datasets downloaded for research purposes often, but not always, serve to be used in scholarly publications. Enriched log data from data archives offer an interesting macro level perspective on the use and users of the services and help understanding the increasing role of repositories in the social sciences. The study provides insights into the potential of collecting and using log data for studying and evaluating data archive use.
Citation: Late E, Ochsner M (2024) Re-use of research data in the social sciences. Use and users of digital data archive. PLoS ONE 19(5): e0303190. https://doi.org/10.1371/journal.pone.0303190
Editor: Hong Qin, University of Tennessee at Chattanooga, UNITED STATES
Received: April 19, 2023; Accepted: April 19, 2024; Published: May 10, 2024
Copyright: © 2024 Late, Ochsner. This is an open access article distributed under the terms of the Creative Commons Attribution License , which permits unrestricted use, distribution, and reproduction in any medium, provided the original author and source are credited.
Data Availability: All relevant data are within the manuscript and its Supporting Information files.
Funding: This research was partially funded by Academy of Finland ( https://www.aka.fi/en/ ) grant 351247 (EL) and benefitted from a Short Term Scientific Mission of the COST Action CA 15137 ‘European Network for Research Evaluation in the SSH (ENRESSH)’, supported by European Cooperation in Science and Technology ( https://www.cost.eu/ ) (EL, MO). The funders had no role in study design, data collection and analysis, decision to publish, or preparation of the manuscript.
Competing interests: The authors have declared that no competing interests exist.
Introduction
In the context of the Open Science agenda and the Responsible Research and Innovation movement, nations and organizations have put a lot of effort in building research infrastructures for supporting scholars in open science practice. Research data archives (also referred as data repositories) are part of the infrastructure and their aim is to capture and share digital research datasets. Archiving digital research data aims for improving the quality of research, and for economical savings assuming that data once archived will be useful and used by others [ 1 ]. Interest in facilitating data sharing and re-use is high, which is evident in funding agencies’, research organizations’, publishers’ and archives’ efforts in drafting policies regulating data sharing and management [ 2 ]. Also, data openness and sharing are increasingly important factors in the evaluation of impact, concerning both research infrastructures and scholars [ 3 , 4 ].
In the social sciences there is a long tradition in re-using time-series datasets such as those by the World Bank or OECD. However, in the era of open science, data sharing has widened its use to individual scholars uploading their data, which most likely form most of the contents in the digital data archives. Yet, despite the massive financial and intellectual investments, it is still unclear how extensively, by whom and for what purposes research datasets are downloaded from the archives [ 5 ]. The proposed benefits of open data will be materialised only fully if the available data are used or re-used by others [ 5 ]. Also, the importance of creating quantitative metrics for evaluating the impact of research infrastructures is widely recognized [ 6 ].
Will it be possible to realise the optimistic promises of open responsible science when the social sciences go digital? While open research data and data infrastructures have drawn a lot of attention, is there a demand for open data, do differences in re-use exist across types of data, how broad is the base of potential users and where is potential to develop and what service portfolios to be developed? Answering to these questions is vital to understand the evolving knowledge creating practices, the impact of open data and the development of open science and its implementation in research practice. Additionally, this information is important for the archives to better understand the potential needs of their user base. Most of the earlier work has based on self-reported data re-use and focused especially on the experiences and needs of scholars [e.g. 7 – 12 ]. However, before data citation practices are fully formalized in social sciences, log data and number of downloads are useful to measure the frequency of data re-use [ 5 , 13 , 14 ]. Also, Khan, Thelwall and Kousha [ 12 ] call for more comprehensive disciplinary information about repository uptake for enhancing sustainable data sharing.
By now, only very few studies relying on user log data gathered from the social science archives exist. For example, Borgman and colleagues analysed user log data to identify data re-use in the Dutch interdisciplinary data archive DANS [ 5 ] using number of downloads and users. Focusing on data re-use in the social sciences, Late and Kekäläinen [ 15 ] studied the use of the Finnish research data archive in more detail based on enriched log data. Applying their methodology using enriched log data we study the use of Swiss data repository, FORSbase, that archives both qualitative and quantitative social science research data. Our study supplements the findings by Late and Kekäläinen [ 15 ] by providing comparative evidence from another context. We investigate whether there is a demand for open data in the social sciences and address the following research questions:
- How many times and by how many users are datasets downloaded from the FORSbase?
- What type of datasets are downloaded from the archive most often?
- What roles do the users of the archive represent?
- For what purposes are datasets downloaded?
The article is structured as follows. First, we present related literature concerning open data, data archives and data re-use in the social sciences. We will then describe the research setting and present the results, which will be discussed before being put into the policy and research practice context to draw conclusions.
Research data and data archives in the social sciences
The European Commission [ 16 ] defines research infrastructures as “facilities that provide resources and services for research communities to conduct research and foster innovation” (p. 1). Research data archives are thus part of the infrastructure supporting and enabling open science by storing, managing, and disseminating research data by public (or private) funding without a fee for the users. Although, studies have shown that many scholars rely on their personal data storage for sharing data [ 12 , 17 ], there is a long-standing tradition of using and providing open research data and having large data repositories in the social sciences [ 18 ]. International organisations like the World Bank, International Monetary Fund, Freedom House, the OECD or EUROSTAT have provided valuable data for social scientists for decades just as well as national public statistical offices [see e.g., 19 – 21 ]. Furthermore, for more specific data, national and international data infrastructures, such as the General Social Survey in the US since 1972, the World Values Survey, the European Values Study, the International Social Survey Programme, or Inter-university Consortium of Political and Social (ICPSR), have been offering rich datasets in open access to social scientists [see, e.g., 22 , 23 ]. Also, individual scholars or teams generated and shared data, such as the Democracy Index [ 24 ], the Polity Project [ 25 ], or the World Inequality Database [ 26 ]. Social science data archives providing a hub for sources for secondary analyses have been established in the 1960ies in the US as well as in Europe [ 18 , 27 ] and for example, the CESSDA, Consortium of European social science data archives, exists since 1976 [ 27 ]. Established data archives, provide support and curation for long-term data preservation for the entire data life cycle and tools for data search [ 28 , 29 ].
However, while especially international comparative quantitative social science has this long-standing tradition, in other sub-fields like psychology it has been usual that data and measurement instruments were part of a business model and available in closed access. Qualitative social science does not look back on a similar tradition of sharing data even though in 1994, the Qualitative Data Archival Resource Center has been established at the University of Exeter to foster re-use of qualitative data [ 30 ]. However, this policy-based request has resulted in a heated debate whether it is ethical to share qualitative data because data are potentially sensitive [ 30 , 31 ]. The shift to open science in the STEM fields has changed the attention of policy makers and put pressures on those sub-fields in the social sciences where open data sharing has not yet been part of the tradition and, at the same time, opened new opportunities and increased reputation of the shared existing data infrastructures.
Research data has thus been seen as theory-laden concept with a long history [ 5 ]. Data can take different forms in different disciplines and a particular combination of interests, abilities and accessibility determine what is identified as data in each instance [ 32 ]. Borgman [ 33 ] defines data as “entities used as evidence of phenomena for the purposes of research or scholarship” (p. 25). Data are not seen only as by-products of research but as research outputs, valuable commodities, and public objects [ 1 ]. Data in the social sciences can remain relevant for analysis for a long time as societal developments and historical perspectives can offer new opportunities of, and approaches to, analysis of historical data to researchers.
Re-use of research data in social sciences
Open access to research data is an essential aspect in open science because, among others, it facilitates the verification of given results and enhances the effectiveness of research by the re-use of data. However, also negative aspects of data re-use have been identified, such as narrowing the scope and increasing the bias of research [ 34 , 35 ] and leading to injustice in work division, i.e., when data collectors document and share their data, others may take just advantage of the work accomplished by others, as data stewardship is not acknowledged yet [ 36 ]. Furthermore, not all kinds of data can be opened due to data protection and ethical principles [ 37 ]. This is a frequent issue in the social sciences and earlier studies have claimed relatively low levels of data sharing and re-use [ 38 – 41 ]. However, some data are frequently re-used in the social sciences as for example the open data published by the European Social Survey led to at least 5000 scientific English language publications between 2003 and 2020 [ 42 ].
The whole concept of data re-use needs to be understood far more deeply. Re-use of data can mean for example re-using data to reproduce research, to re-use data independently or to integrate data with other data [ 12 , 33 ]. Re-using a dataset in its original form can be difficult, even if adequate documentation and tools are available, since much must be understood about why the data were collected and why various decisions about data collection, cleaning, and analysis were made [ 33 , 43 , 44 ]. Combining datasets is far more challenging, as extensive information must be known about each dataset if they are to be interpreted and trusted sufficiently to draw conclusions [ 45 ].
By now several studies have analysed scholars needs, experiences and perceptions of data re-use relying on surveys and interviews [ 7 – 12 , 46 , 47 ]. In a recent survey [ 12 ] almost half of the respondents representing social sciences reported re-using data. However, there was some variation between research fields. Data re-use was more frequent by experienced scholars and by those sharing their data. When selecting data for re-use, scholars consider proper documentation, openness, information on usability of data, availability of data in a universal standard format and evidence that the dataset has an associated publication as important factors [ 12 ].
Social scientists re-using data value data that are comprehensive, easy to obtain, easy to manipulate, and credible [ 46 ]. Identified obstacles for data re-use are, for example, barriers to access, lacking interoperability and lack of support [ 47 ]. Faniel, Frank and Yakel [ 9 ] identified ICPSR’s data users’ information needs in 12 contexts relating to how data was originally produced, about the repository it has been archived and about the previous re-use of data. They argue that scholars representing different disciplines have distinct differences in the types of information desires, that should be considered in service development. For example, information about missing data was important for the social scientists. Studies focusing on data re-use by novice scholars emphasize the importance of details about the data collection and coding procedures and peer support for data use [ 8 ]. Re-using data may contribute to the knowledge creating skills of junior scholars and foster them to socialize to their disciplinary communities [ 48 ].
Studies have also focused on how data is searched [ 49 , 50 ] and witnessed scholars struggling with finding datasets to re-use [ 12 , 51 ]. Most typically, data is found from relevant papers, conducting web searches, and searching from disciplinary and interdisciplinary data archives [ 12 ]. Recently, Lafia, Million and Hemphill [ 52 ] studied data search basing their analysis on usage data from ICPSR website. They identified three user paths for navigating the website: direct, orienting, and scenic. Direct and scenic paths targeted dataset retrieval, as orienting paths aimed gathering contextual information. They argue that data archives should support both direct and serendipitous data discovery.
Only a few studies have investigated the use of data archives in the social sciences relying on log data. Borgman and colleagues studied the use of the Danish Data Archiving and Networked Services (DANS) using transaction logs, documentation, and interviews, and showed that communities of data infrastructures can be amorphous and evolve considerably over time [ 5 ]. They argue that trust plays an important role in the re-use of a dataset collected by someone else and the reputation of the hosting archive and organizations responsible for the curation process are important elements in trust creation.
Late and Kekäläinen [ 15 ] studied the use and users of the Finnish research data archive for social sciences by analysing user log data between 2015 and 2018. According to their study, most datasets were downloaded at least once during the time frame and a clear majority of the downloaded data were quantitative. They discovered that the datasets from the archive were downloaded most often for the purposes of studying or master’s or bachelor’s theses. One fifth of the downloading’s were made for research purposes. Similarly, Bishop’s and Kuula-Luumi’s study [ 53 ] about the re-use of qualitative datasets showed that data was downloaded for studying, master’s theses, teaching and research, indicating that data re-use is even less prevalent for qualitative studies. According to Late and Kekäläinen [ 15 ] the most typical downloaded dataset was survey data. The Finnish research data archive was most often used by social scientists from Finnish universities. However, there were users from other European countries and even from outside Europe and other organizations. Borgman and colleagues [ 5 ] argue that user behaviour tends to correlate with existing data practices in a field, and archives tend to be tailored accordingly. However, the results by Late and Kekäläinen [ 15 ] showed that users of the archive for social sciences data represented all major disciplines. Thus, data practices in several fields must be considered when developing the services.
Research setting
The context: forsbase.
The research data archive investigated in this study is FORSbase. FORS is the Swiss Centre of Expertise in the Social Sciences that offers data and consulting services in social sciences, conducts national and international surveys, and offers data and research information services to researchers and academic institutions [ 54 ]. FORSbase was the archive for research projects and research data in the social sciences in Switzerland managed by FORS. It was established in 1992 and was replaced in December 2021 by SWISSUbase ( https://www.swissubase.ch/ ) based at the same institution and issued in collaboration with several partners that includes the functions of FORSbase but serves as the national data repository across disciplines in Switzerland.
Research data from FORSbase and SWISSUbase can be accessed from the online catalogue ( https://forsbase.unil.ch/ and https://www.swissubase.ch/ ). The catalogue is available in English, German and French. Datasets are downloadable free of charge, but users are required to register before downloading datasets. The database has a special structure: It is centred around research projects. Each project can have several datasets and each dataset can have different versions, while only the latest available version is downloadable.
The FORSbase and SWISSUbase data services follow FAIR data principles [ 1 ] and have obtained the official certification of CoreTrustSeal. The CoreTrustSeal is a community based non-profit organization promoting sustainable and trustworthy data infrastructures. FORSbase is a member of CESSDA. The change from FORSbase to SWISSUbase does not have any impact on our analysis and its conclusions because the FORSbase service is integrated in SWISSUbase. The main difference is that the services have been upscaled to accommodate research data and projects from other disciplines (and transdisciplinary research).
Data collection and analyses
The study is based on quantitative user log data that was collected from FORSbase for the time window from 29.2.2016 to 9.2.2020. This time window represents the full user data available for FORSbase since its rebuild in 2016 until the time of the start of our project. The log data contains information about the number of downloads and downloaded datasets. The data is enriched with a) project information data collected from the database and b) data coming from the registration survey that users have to fill in when downloading data. The project information entails information about the archived datasets such as the dataset type. Registration survey data entails information about the users including their role and purpose of data use. Each time a person downloads data from FORSbase, this information is collected.
The data is structured as follows (see Fig 1 ): the main unit is a download; downloads are cross-nested across datasets and users (a user downloading a dataset creates thus a unique download). Each download also points to the version of the dataset that has been downloaded. The raw number of observations (downloads) in the data was 6661. Removing downloads from the dataset made for testing purposes by the FORSbase team resulted in 6656 observations.
- PPT PowerPoint slide
- PNG larger image
- TIFF original image
https://doi.org/10.1371/journal.pone.0303190.g001
The process continued with variable selection and coding. Nine variables analysed in this study are presented in Table 1 along with the research question(s) the variable is used to address. Information for variables 1 to 4 is collected automatically whereas information for variable 5 is constructed in two steps, the name being drawn automatically from the database and then assigned a type of dataset manually from the project information data in the FORSbase online catalogue. Information for variables 6 to 9 is asked from the users in a survey format during registration and when downloading the data.
https://doi.org/10.1371/journal.pone.0303190.t001
To identify how many times and by how many users are datasets downloaded from FORSbase (RQ1), we analyse the download date, user id, and dataset id ( Table 1 , variables 1–3). Concerning the number of downloads, we analyse the full number and share of downloaded datasets and unique user-dataset downloads ( Table 1 , variable 3) to control for downloading dataset updates and to exclude duplicate downloads. By analysing the unique dataset downloads we can identify whether the same user downloaded the same dataset twice or two versions of it. Concerning the number of users, we analyse the average number of downloads for the registered users and the active users ( Table 1 , variable 2). Registered users are those who have registered to FORSbase for archiving and downloading data. The number of registered users was asked from the archive personnel in time of the data collection in 2020. Active users are those who downloaded data during the time window of the data collection. Each user is identified in the data with a unique user ID number automatically provided by the system during registration ( Table 1 , variable 2).
To identify what type of datasets are downloaded from the archive most often (RQ2) we use the id of the dataset , the type of dataset (quantitative or qualitative data) and the name of the dataset ( Table 1 , variables 3, 4, 5). The name of the downloaded dataset ( Table 1 , variable 5) was also used to study the 10 most downloaded datasets in more detail. For these datasets, information (i.e., descriptive details) were traced from the FORSbase online catalogue.
To analyse what roles do the users of the archive represent (RQ3), we use the role of the downloading user ( Table 1 ). Originally, users were provided a list of 11 roles from which they selected the most suitable one. For the analyses, some categories were combined to form a shorter list of seven different roles (i.e., student, doctoral student, lecturer/post doc, professor, other research/project manager, teacher, and non-academic).
Finally, to identify for what purposes datasets are downloaded (RQ4), we use information on the use purpose of the data , the research description and whether a publication is expected ( Table 1 , variables 7,8, 9). When users were downloading datasets from FORSbase, they were asked whether the dataset was downloaded either for research or for teaching purposes ( Table 1 , variable 7). Although these categories did not serve well for the students downloading datasets for their course work, they were forced to choose between the two options. Therefore, for the means of this study, a new use purpose type “studying” was constructed manually in two steps. First, all the users that identified themselves as students were identified from the data ( Table 1 , variable 6). In the second step, the coding was assigned by thoroughly reading the research descriptions ( Table 1 , variable 8) written by the students to find out the purpose of the download. Based on these descriptions we also categorised the sub-type of studying purpose if possible (e.g., bachelor theses, master’s theses). However, the research description was asked only for those downloads where the users were indicating research ( Table 1 , variable 7) as the purpose for the download. Consequently, this information is missing for the downloads where users indicated teaching as purpose. Obviously, this applies also to students who had selected teaching as use purpose. These were categorised as studying as we assume that students do not teach yet but chose teaching as there was no option for studying. Downloading data for doctoral dissertation were categorized as “research” purpose.
Variable nine ( Table 1 ) was used to study the purpose of research use of the dataset by asking whether the user was expecting a publication resulting from the downloaded dataset. This information was asked only for those downloading data for research purposes. Thus, this information is missing for the downloads the users indicated teaching as the purpose.
For the analyses step the data were gathered into one dataset and analysed with Stata 16. Given that we analyse full data, we do not apply inferential statistics. Whenever we are interested in differences between groups, we apply bootstrapped 95 per cent stability intervals to indicate the precision of the estimates. Differences were then tested also using bootstrapping procedures either with regression models (numbers of downloads per user group) or tests on the equality of proportions [ 55 ] for the intention to publish across user groups.
Number of dataset downloads
In February 2020, at the time of our data collection, FORSbase had 6628 registered users. The archive contained 725 datasets, the majority of which were quantitative. Within the time window that covers 49 months, a total of 6656 downloads were made from FORSbase ( Table 2 ). This results in an average 136 downloads per month or 5 downloads per day. When excluding incomplete months from 2016 and 2020 in our dataset, we cover a total of 6593 downloads over 47 months, leading to a mean of 140 downloads per month ( range = 40–286, median = 122). From 2017 to 2018, the number of downloads increased by 18 per cent and from 2018 to 2019 by 16 per cent. The downloads per months show a high volatility as can be seen from Fig 2 that shows the downloads per month for fully covered months, i.e., March 2016 to January 2020, and a smoothed moving average. The figure makes visible an increase of downloads over time with a tendency to stabilise. Note that March, April and October, November show the highest downloads while July and August show the lowest downloads, reflecting semester beginnings for highs and semester break for lows.
Smoothed moving average is calculated using weights as suggested in [ 56 ].
https://doi.org/10.1371/journal.pone.0303190.g002
https://doi.org/10.1371/journal.pone.0303190.t002
Of the 725 datasets archived in FORSbase, 470 datasets were downloaded at least once representing 65 per cent of all archived datasets. One fifth of the downloaded datasets were downloaded once and 13 per cent twice. Consequently, 67 per cent were downloaded three times or more (see Table 3 ). Datasets, however, can be updated and new versions are released. Users are informed so that they can download the new version. This leads to the fact that some datasets are downloaded more often than others. Additionally, users can download the same dataset twice (e.g., on two different workstations). To control for updates and to have a measure that reflects better the number of times a dataset is used (as opposed to downloaded), we identified duplicates, i.e., if the same user downloaded the same dataset twice or two versions of it. This was counted as one unique user-dataset download (see Table 3 , columns on the right). Both measures are somewhat imperfect because, on the one hand, regarding the full count measure, a dataset that is published quickly and corrected afterwards will score more downloads than one that is not updated. On the other hand, regarding the corrected measure, it might be that a same user downloads the same data multiple times for different persons, e.g., as teacher and student (a situation that is not compliant to the user agreement) or for different uses. Additionally, it is not clearly defined by the database what a “version” is. It is usually an update of the same dataset, but it could also be used to have a dataset updated with new waves while another dataset would create a new dataset for each new wave added. We did our best to control for the later and try to treat a study (and each wave) as a dataset if archived separately.
https://doi.org/10.1371/journal.pone.0303190.t003
Table 4 shows that the main download statistics between the two measures differ only slightly. The mean amounts to 9 downloads per data (8 if only unique user-dataset downloads are counted), but the distribution is highly skewed with a first quartile of 0 downloads, a median of 2 downloads and a third quartile of 6 downloads irrespective of how to count dataset-downloads.
https://doi.org/10.1371/journal.pone.0303190.t004
Type of most downloaded datasets
FORSbase allows the archiving of both quantitative and qualitative data. Qualitative data can be archived since 2017 only. From the 725 datasets, only 15 datasets were archived as qualitative datasets, which corresponds to 2 per cent. Of the 470 datasets that were downloaded at least once, 5 were qualitative datasets (1%). On the level of downloads, the vast majority (98%) of the downloads concerned quantitative datasets. Qualitative datasets were downloaded only 15 times (13 times if we consider only unique user-dataset downloads). Two of which were downloaded once, two twice and one nine times (7 times if only unique user-dataset downloads are counted).
Ten datasets were downloaded more than 100 times (see Table 5 ). Downloads for these 10 datasets represent almost 40 per cent of all downloads from FORSbase in the given time window. FORS was the collector of eight out of the ten most downloaded datasets. The other two datasets were collected by Swiss universities. The most downloaded datasets were all quantitative and either cumulative datasets or single year issues of longitudinal (cross-sectional or panel) surveys collected at regular intervals. Those surveys can be considered social sciences data infrastructures of national or even international importance and are designed for secondary data analysis.
https://doi.org/10.1371/journal.pone.0303190.t005
The most downloaded dataset, SHP Data Waves 1–19, is the Swiss annual household panel study based on a random sample of private households in Switzerland, interviewing all household members mainly by telephone. SHP is provided free of charge from FORSbase for the scientific community [ 57 ]. Other datasets are related with Swiss elections or popular votes (datasets 2, 3, 4, 5, 6, 9) or with education and civil society (datasets 7, 10).
The fact that the share of the ten most downloaded datasets decreases slightly if duplicates and versions of the same dataset are excluded ( Table 5 “Percentage of total downloads” vs. “Percentage of unique user-dataset downloads”) shows that the most downloaded datasets are updated more often than the other datasets. However, the ranking of the most downloaded datasets does not change substantially showing that duplicates and versions spread quite evenly across those highly downloaded datasets. The bootstrapped 95%-stability intervals (see Table 5 , column 3 in brackets) show that the ranking consists of four parts: A clear leader (dataset 1) and a clear second place (dataset 2) followed by a middle part (datasets 3 to 8) and studies 9 and 10 form the fourth group.
Users of the archive
During the examined time window, 2281 unique users downloaded data from FORSbase. These users are called as “active users” in Table 6 . In February 2020, there were 6628 registered users in FORSbase. Thus, only a third of the registered users downloaded a dataset during the time window (note that to upload data, one needs to register as a user). One half of the active users downloaded only one dataset during the given time period ( Table 6 , column on the righthand side). One fifth downloaded two datasets and 28 per cent downloaded three or more datasets. There was a group of heavy users downloading more than 5 datasets (5% of the registered users and 13% of the active users). At the end of the scale, one user downloaded 149 datasets during the time window. The group of 306 users downloading at least five datasets combined more than half (51.7%) of all the downloads during the time window. On average, considering all registered users, one user downloaded one dataset, while considering only active users, a user downloaded 2.9 datasets.
https://doi.org/10.1371/journal.pone.0303190.t006
Looking at unique user-dataset downloads ( Table 7 ), 58 per cent of the active users downloaded only one unique dataset whereas 21 per cent downloaded two and 22 per cent three or more. The group of heavy users (5+ downloaded datasets) amounts to 4 per cent of all registered users and 11 per cent of the active users. The person who downloaded most datasets downloaded 140 unique datasets. If only unique user-dataset downloads are considered, the average is 0.9 downloads per registered user and 2.6 downloads per active user.
https://doi.org/10.1371/journal.pone.0303190.t007
A clear majority of users downloaded only quantitative datasets (99%), 8 users downloaded both quantitative and qualitative data and 4 users only qualitative data.
Regarding the role of users, the majority of the downloads were made by users registered as students, while doctoral students, lecturers/postdocs and professors and other researchers were downloading less, and teachers and non-academics the least ( Table 8 ).
https://doi.org/10.1371/journal.pone.0303190.t008
Regarding download frequency across user groups, students were more likely to download many datasets compared to scholars, teachers, and non-academics (see Fig 3 ). Note that using bootstrapped regression, only the difference between students and scholars, teachers and non-academics were significant. If one takes only unique user-dataset downloads into account, students downloaded significantly more unique datasets than all other groups except for non-academics (as the latter have a large variability). However, the user roles are not clear-cut entities as the same person can indicate a different role for each download. This means that for unique user-dataset downloads only the first role is retained.
Average number of Downloads per user group with bootstrapped 95% stability intervals using 1000 resamples on the basis of a) all downloads and b) only unique user-dataset downloads.
https://doi.org/10.1371/journal.pone.0303190.g003
Purpose of the downloads
The majority of the downloads were made for studying purposes (see Table 9 ). Of those downloading data for study purposes, at least 13 per cent (n = 497) downloaded the dataset for a bachelor’s thesis and at least 12 per cent (n = 452) for master’s thesis (combining 14.3% of all downloads used for a BA or MA thesis). However, these numbers represent minima because not all users did describe their purpose of download in such detail and the users not describing the purpose in detail might have used the data for a thesis as well.
https://doi.org/10.1371/journal.pone.0303190.t009
Almost 40 per cent of the downloads served research purposes. Out of downloads used for research, at least 5 per cent download data for doctoral thesis (2% of the total downloads). However, the real share of downloading data for doctoral theses is probably much higher since more than 14 per cent of the users were registered as doctoral students.
Finally, only 3 per cent of the downloads served teaching purposes. This is surprising given that the biggest user group are students, and one would expect that it is the teachers who inform students about the dataset(s) used in the courses. However, users can only indicate one purpose for the download but can of course use it for many purposes after download. Also, it might mean that some teachers invite students to download the data themselves, while others download it and distribute the data to the students–which would mean that even more users would be students as the data covers only those students who downloaded the data themselves.
Users downloading datasets were also asked if they expect to write publications using the downloaded dataset. This was asked only if they were indicating that they were using the data for research and not teaching. Also, the question has a high share of non-response (463 or 7% of those who indicated research as the use of the download). Of those who replied to the question, a large majority (77.4%) did not expect to publish and just over one fifth expected to do so. Those downloading the dataset for research purposes were most likely to expect to write a publication (43%). Expectedly, professors, lecturers/postdoctoral researchers, and doctoral students expected publication more often compared to students ( Table 10 ). Indeed, professors, lecturers/post-docs and, more unexpectedly, non-academics have a similar percentage intending to publish as the bootstrapped differences are not significant. All other groups do differ significantly from these three groups and between each other. The relationship between role and intention to publish is quite strong with a Cramér’s V of 0.43.
https://doi.org/10.1371/journal.pone.0303190.t010
This study investigated whether there is a demand for open data in the social sciences by examining the use and users of a research data archive. It continued a discussion started by Late and Kekäläinen [ 15 ] studying the use of social science research data archives based on user log data. The results show that there is a demand for research data as datasets have been downloaded frequently from the FORSbase, i.e., on average 145 downloads per month. As in Finland [ 15 ], the number of downloads has increased in Switzerland from 2016 to 2019. During the time window of the study, a large majority (65%) of the datasets archived in FORSbase were downloaded at least for once. The share of downloaded datasets was similar with the Finnish results (70%) [ 15 ].
An overwhelming majority of the downloaded datasets are quantitative. The number of archived qualitative datasets in FORSbase is very low, which explains the low numbers in the downloads. Earlier studies have discussed the obstacles of data sharing and re-use in social sciences [ 38 – 40 , 58 ]. Our results suggest that there might be strong differences in the habit of downloading open data from repositories across different specialisations: in qualitative social sciences, data sharing seems to be far less prominent than in quantitative social sciences. There is little evidence about the re-use of qualitative datasets and further studies are needed to understand the potential and pitfalls of open data policies for qualitative studies [ 53 , 58 ]. The lack of data sharing, and re-use has certainly several reasons but ethical issues play an important role [ 59 ].
In this study, from the 725 archived datasets, the ten most frequently downloaded ones were investigated in more detail. Each of these datasets was downloaded more than 100 times, the most popular being downloaded more than 600 times. The downloads of these ten datasets amounts to almost 40 per cent of all downloads from the archive, which indicates that, similar to publications [ 60 ], a small share of datasets gains most of the attention. The same phenomenon was observed by Late and Kekäläinen [ 15 ]. The most frequently downloaded datasets share a few properties: all of them are longitudinal or time-series survey data collected not by individual scholars or research groups but by organizations or consortia such as FORS. Also, those datasets are local survey projects and the analysed archive, FORSbase, is the main source for obtaining this data. International longitudinal or time-series datasets were not among the ten most downloaded, even though local versions of these datasets would be available in the archive. Researchers interested in those cross-national datasets are more likely to download the datasets containing data from several countries from the international repository. Again, these results are in line with the study of Late and Kekäläinen [ 15 ]. In Finland, most downloaded datasets were local and national surveys. However, in the Finnish archive, the most downloaded datasets also included large international statistics collected by a single scholar. Qualitative datasets were also more often downloaded from the Finnish archive compared to the Swiss archive.
The fact that the most downloaded datasets were collected by prestigious and well-known organizations is in line with the argument raised in earlier studies [ 5 , 9 ] that scholars’ trust in data is essential for the data re-use. However, what is considered as trustworthy may differ between disciplines. For the social scientists, reputation along with data selection and cleaning process play an important role in trust creation [ 61 ]. Systematic documentation and providing high quality paradata (i.e. data about the data) is valued by the data users [ 8 , 9 , 12 , 62 ]. Other factors influencing the users’ trust in the data archives are recommendations, frequency of use, past experiences, and perceptions of the role of the archive [ 10 ]. However, frequently downloaded datasets are probably more well-known and thus, more visible for the users. Data findability is another critical point for data re-use that should be supported better [ 12 , 52 ]. Furthermore, archives can increase their own visibility and prestige by archiving high quality and well-known datasets by establishing collection strategies and profiling for certain topics and data types to gain competitive advantage and reputation. However, the value of non-used (or non-downloaded) datasets cannot be overlooked, since they may become valuable in the future as needs are difficult to predict (i.e. delayed recognition in science [ 63 ]).
Earlier studies have not investigated the number of users of the data archives although it can be considered as an is important metric for evaluating the impact of archives. Our results show that FORSbase was used by more than 2000 unique users as one third of the registered users downloaded data from FORSbase. Most of them downloaded only one dataset. However, there was a smaller group of heavy users of the archive downloading several datasets and forming a remarkable share of all downloads. This might be an indication of field specific differences; in some fields of social sciences data can and is re-used more often. Also, it might indicate personal differences between the users. Users that have found datasets useful come back for downloading more relevant data or new versions of the datasets. Indeed, other studies have shown that scholars sharing their data are also more active re-users of data shared by others [ 12 ]. Our results show, however, that not all registered users download data which might indicate that some users of FORSbase use it for archiving, not data retrieval. Late and Kekäläinen [ 15 ] showed that users represented several countries, disciplines, and organisations. Our data did not allow for such analyses.
Earlier research has focused mainly on scholars’ data sharing and re-use practices and shown experienced scholars being the most active data re-users [ 12 ]. Yet, our findings confirm the results by Late and Kekäläinen’s [ 15 ] that students form the largest user group for the data archive. Students as a special user group should be taken into special consideration by data archives and service providers since there is a great potential in this user group as future data users and providers. Re-using data is important for developing knowledge creation skills and in socializing into the discipline [ 48 ]. Novice users have specific needs for data re-use and are influenced by experiences of their mentors [ 8 ]. Therefore, data archives need to pay special attention when thinking what services could be offered especially for the students and what guidance students need. More research, for example on the data management skills of students, is certainly needed. This is not only relevant for students who want to become future academics, but data becomes an important part of many professions in a digitalised society and skills in data use, management, archiving, and documenting will be relevant competences students need to learn. Also, scholars wish training for data management skills [ 64 ]. The role of data archives along with data managers and libraries have been identified as central in fostering such skills [ 17 ].
Only three per cent of the downloads served teaching purposes. However, studies by Late and Kekäläinen [ 15 ] and Bishop and Kuula-Luumi [ 53 ] show higher share of downloads for teaching purposes from Finnish and UK archives. There might be several reasons for the difference. However, users of FORSbase can only indicate one use purpose per download, while they could use the data for several purposes. Researchers can download a dataset for a research project and then use this project and the dataset in teaching without re-downloading the data and register it as a purpose for teaching. Also, they may ask the students to download the data, for example, in a research methods seminar. The high share of students among the users suggests that teaching is a frequent use of the datasets downloaded from FORSbase. However, an important question for future research is what data re-use means in teaching. Is it rather to teach research methods or also to replicate studies and foster the idea of responsible research already in teaching? Familiarizing students with the open research infrastructures might be an effective way to promote open science ideals.
More than one third of the downloads were made for research purposes. The share of research use was lower in the study by Late and Kekäläinen [ 15 ] covering only on fifth of the total use. In the Swiss archive, about half of the downloads for research were expected to result in a publication. Professors, lecturers, and post-doctoral scholars were most likely to plan to use the dataset for a publication. However, there is little evidence about how often re-used data are actually utilized in publications and for what purposes data are used for [ 65 ]. Unfortunately, no further information is available from our data that shows other research purposes than publications. Regarding Responsible Research and Innovation, it would be interesting to follow how often data is re-used for validation or replication purposes rather than publication.
Regarding the policy demand for open science and open data, the valorisation of data sharing becomes relevant. Data stewardship is not yet a relevant aspect in academic career development, which might hinder the motivation to share and document data sufficiently [ 36 , 39 ]. However, European guidelines for responsible research assessment have already included data and data sharing as research outputs and activities to be recognized in the evaluation [ 66 ]. Therefore, further efforts should be made to study how (and how often) re-used datasets are cited in publications and how archives guide users to cite data. Data citation practices in social sciences are still evolving since citations are shown to be often incomplete or erroneous [ 15 , 67 – 69 ]. Not all re-used research data are cited, at least not in a formal way [ 15 ]. Developing more formal data citation practices would enable a quantitative evaluation of the impact of data re-use. The challenge is to get scholars to cite data in a systematic way [ 70 ]. This would also serve the need to provide quantitative metrics for evaluating the impact of research infrastructures [ 6 ]. User log data can provide information concerning the number of downloaded data, but for evaluating the impact on research, further studies are needed exploiting, for example, bibliometric methods.
Practical implications and limitations of the study
The results provide several practical implications for utilizing user log data for evaluating digital data archive use and as a source of research data. First, it would be important for the archives to define clearly what a data “version” is and to separate updates from new waves that comprise a new dataset. As new versions and updates of the datasets influence user behaviour and the number of downloads and thus, should be taken into consideration when user log data is used in archive evaluation or in research. The most frequently downloaded datasets are characterised by various versions and are updated more often than datasets provided by individual scholars. In our study we decided to analyse both, the full number of downloads and unique downloads to recognize the share of duplicates. The differences were not significant yet existed. Further, our results provide implications for collecting user log data. For example, information collection should cover all kinds of users and use types. In the case of FORSbase, for example, “studying” as a data re-use purpose was not provided. This underlines the importance of user studies for the service providers to truly know who their clients are. Given the relevance of replicational and open research data in science policy and the lack of knowledge on open research data practices, it is also advisable to archives to collect meaningful log data to be able to supplement ethical considerations with empirical evidence on data re-use.
This study comes with limitations: making conclusions about data re-use based on user log data is somewhat unreliable since it is likely that not all downloaded datasets are used, or some are used for many times or for other purposes than expected. Generalizing findings across organizations may be challenging because download metrics may be contingent on the specific characteristics of the data archive or related organisations [ 4 ]. For example, datasets can be used as course material possibly leading to hundreds of data downloads [ 15 ]. Additionally, log-data cannot provide qualitative insights into the data re-use (e.g., why a dataset was selected and how it was used). Still, user log data can give useful insights into re-use of research data and the users of data archives on the macro level beyond self-reported data re-use and from the point of view of the archive [ 5 ]. Our findings show that data is downloaded for various purposes and by various user groups from the archive. Thus, studying data re-use based for example on citations captures only part of the data re-use. Results of this study will give grounds for future studies in this respect. In addition, we analysed log data only from one archive. However, as our results are in line with a similar study conducted in Finland [ 15 ], we believe the results can be generalised to similar national social science data archives. Future research will show how the frequency of data downloads will develop as open data practices establish in the social sciences.
Conclusions
This study contributes to our understanding of the utilization of digital data archives in the realm of social sciences. The findings indicate the demand for social science data, as evidenced by the increasing number of data downloads from a Swiss data archive. However, it is noteworthy that as majority of the archived datasets were downloaded at least once, a limited set of longitudinal and time-series survey datasets compiled by organizations rather than individual scholars gained substantial share of the downloads. Since the case archive primarily specializes in housing quantitative data, the re-use of qualitative data was marginal. Among the users, students constituted a significant proportion who accessed the archive to acquire data for their educational purposes. Nonetheless, the user base encompassed individuals from diverse roles, including experienced and novice scholars and non-academics. As the findings are in line with previous research [ 15 ] it is likely to find similar patterns across data archives specialised for the social sciences. The increasing availability of digital datasets for the re-use may create new data practices within social sciences.
Enriched log data capturing the use of the digital data archive provide a macro level understanding about the re-use of the data from singular archive. To obtain more comprehensive insights into data re-use and evolving data practices within social sciences, future research applying quantitative and qualitative approaches is needed. A future research agenda on data re-use would include comparative studies of different archives (which would preclude some previous agreement on the collection of meta-data between archives), studies into the (epistemological and empirical) meanings and definitions of re-use of research data in social sciences and into the trade-offs between collecting new data versus re-using existing data. A very important issue is the data citation practices in social sciences. For further developing the research infrastructures, user studies are needed to address how users interact with the infrastructures, what obstacles they face and what support they desire.
Supporting information
https://doi.org/10.1371/journal.pone.0303190.s001
Acknowledgments
We thank Dr. Jaana Kekäläinen for her valuable comments for the manuscript.
- View Article
- PubMed/NCBI
- Google Scholar
- 3. Coalition for advancing research assessment (CoARA). The Agreement on Reforming Research Assessment. 2022 Jul 20 [cited 2024 Jan 2]. Available from: https://coara.eu/agreement/the-commitments/
- 14. Ingwersen P. Scientific datasets: informetric characteristics and social utility metrics for biodiversity data sources. In: Chen C, Larsen R, editors. Library and Information Sciences: Trends and Research. Cham: Springer Nature; 2014. p. 107–117.
- 16. European Commission. European Research Infrastructures;2021 [Internet] [cited Feb 5 2024]. Available from: https://ec.europa.eu/info/research-and-innovation/strategy/strategy-2020-2024/our-digital-future/european-research-infrastructures_en
- 19. Freedom House. Freedom in the world: the annual survey of political rights and civil liberties. 1978. Freedom House.
- 20. International Labour Organisation. Cost of social security. 1997. International Labour Organisation.
- 21. Oba J. Social sciences databases in OECD countries. An overview. In: OECD editor. Social sciences for a digital world. Building infrastructure and databases for the future. Paris: OECD Publishing, 2000. p. 29–74.
- 23. Smith TW. Who, What, When, Where, and why: An Analysis of Usage of the General Social Survey, 1972–2000. GSS Project Report No. 22. National Opinion Research Center; 2000 Jul. https://gss.norc.org/Documents/reports/project-reports/PR22.pdf
- 26. UNU-WIDER [Internet]. World Income Inequality Database V1. 0. 2000. United Nations University Helsinki. Available from: https://www.wider.unu.edu/project/wiid-%E2%80%93-world-income-inequality-database
- 27. Mauer R. Das GESIS Datenarchiv für Sozialwissenschaften. Scivero. In: Altenhöner R. & Oellers editors C. Langzeitarchivierung von Forschungsdaten Standards und disziplinspezifische Lösungen, 2012.p. 197–215.
- 30. Corti L. Qualitative Data Archival Resource Centre, University of Essex, UK. In Forum Qualitative Sozialforschung/Forum: Qualitative Social Research. 2000;1(3). https://doi.org/10.17169/fqs-1.3.1048
- 33. Borgman CL. Big data, little data, no data: Scholarship in the networked world. MIT press; 2015.
- 42. European Social Survey. European Research Infrastructure Consortium Annual Activity Report 01 June 2020 to 31 May 2021. European Social Survey. Available from: https://www.europeansocialsurvey.org/sites/default/files/2023-06/ESS_ERIC_annual_activity_report_2020-2021.pdf
- 52. Lafia S, Million AJ, Hemphill L. Direct, Orienting, and Scenic Paths: How Users Navigate Search in a Research Data Archive. Proceedings of the 2023 Conference on Human Information Interaction and Retrieval 2023 Mar 19; Austin, USA. New York; ACM, 2023. https://doi.org/10.1145/3576840.3578275
- 54. forscenter.ch [Internet] FORS; 2021 [cited 2021 Jun 4]. Available from: https://forscenter.ch/
- 55. Acock AC. A Gentle Introduction to Stata. 6th ed. 2018. Stata Press, College Station TX.
- 57. forscenter.ch [Internet] Swiss household panel, 2021 [cited 2021 Jun 4] Available from: https://forscenter.ch/projects/swiss-household-panel/
- 60. Merton RK. The sociology of science: Theoretical and empirical investigations. 1973. University of Chicago press, Chicago.
- 66. scienceeurope.org [Internet] Science Europe, The Agreement on Reforming Research Assessment. 2022. [cited 2023 March 30]. Available from: https://www.scienceeurope.org/media/y41ks1wh/20220720-rra-agreement.pdf
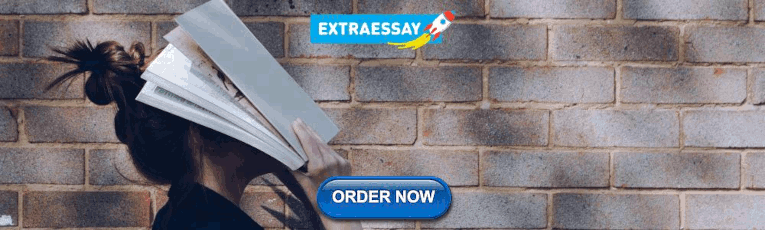
IMAGES
VIDEO
COMMENTS
Common types of limitations and their ramifications include: Theoretical: limits the scope, depth, or applicability of a study. Methodological: limits the quality, quantity, or diversity of the data. Empirical: limits the representativeness, validity, or reliability of the data. Analytical: limits the accuracy, completeness, or significance of ...
Limitations in Research. Limitations in research refer to the factors that may affect the results, conclusions, and generalizability of a study.These limitations can arise from various sources, such as the design of the study, the sampling methods used, the measurement tools employed, and the limitations of the data analysis techniques.
Possible Limitations of the Researcher. Access-- if your study depends on having access to people, organizations, data, or documents and, for whatever reason, access is denied or limited in some way, the reasons for this needs to be described.Also, include an explanation why being denied or limited access did not prevent you from following through on your study.
A common and long-established practice of leading management journals is that they require that authors make a theoretical contribution (Boer et al., 2015).Rabetino et al. (2020) note that such contributions are based on diverse ontological, epistemological, and methodological assumptions; embrace disparate conceptual approaches (behavioral, institutional, evolutionary, etc.); and seek to ...
Experimental research designs are important because they minimize threats to internal validity. Internal validity is the confidence a researcher has that a change (whether naturally occurring or due to manipulation) in the independent variable causes the observed change in the dependent variable. Although there are a number of confounding variables that may threaten internal validity, the most ...
Here's an example of a limitation explained in a research paper about the different options and emerging solutions for delaying memory decline. These statements appeared in the first two sentences of the discussion section: "Approaches like stem cell transplantation and vaccination in AD [Alzheimer's disease] work on a cellular or molecular level in the laboratory.
Brutus, Stéphane et al. Self-Reported Limitations and Future Directions in Scholarly Reports: Analysis and Recommendations. Journal of Management 39 (January 2013): 48-75; Ioannidis, John P.A. Limitations are not Properly Acknowledged in the Scientific Literature. Journal of Clinical Epidemiology 60 (2007): 324-329; Pasek, Josh.
This study also has certain limitations. First, we have not completely examined the causes that can hinder or enable integration between the performance management system and the risk management system, and we have not exhaustively examined the industry's possible effect on risk-performance integration. Second, we have not examined, with a ...
Limitations generally fall into some common categories, and in a sense we can make a checklist for authors here. Price and Murnan ( 2004) gave an excellent and detailed summary of possible research limitations in their editorial for the American Journal of Health Education. They discussed limitations affecting internal and external validity ...
build reviewers' trust in you and your research, discussing every drawback, no matter how small, can give the impression that the study is irreparably flawed. For each limitation you identify, provide a sentence that refutes the limitation or that provides information to counterbalance or otherwise minimize the limitation's perceived impact.
tion is the essence of using models as research tools. However, there is the all-important question whether quantification is pos-sible or even desirable in all the fields of research. This is particularly relevant whenever humans are involved as is the case for management science. Is it pos-
21 Research Limitations Examples. By Chris Drew (PhD) / November 19, 2023. Research limitations refer to the potential weaknesses inherent in a study. All studies have limitations of some sort, meaning declaring limitations doesn't necessarily need to be a bad thing, so long as your declaration of limitations is well thought-out and explained.
Step 1. Identify the limitation (s) of the study. This part should comprise around 10%-20% of your discussion of study limitations. The first step is to identify the particular limitation (s) that affected your study. There are many possible limitations of research that can affect your study, but you don't need to write a long review of all ...
Abstract. Study limitations represent weaknesses within a research design that may influence outcomes and conclusions of the research. Researchers have an obligation to the academic community to present complete and honest limitations of a presented study. Too often, authors use generic descriptions to describe study limitations.
Limitations put medical research articles at risk. The accumulation of limitations (variables having additional limitation components) are gaps and flaws diluting the probability of validity. There is currently no assessment method for evaluating the effect(s) of limitations on research outcomes other than awareness that there is an effect.
Discussing the research methods used can shed light on generalizability limitations. For example, if your study employed a qualitative approach, the depth and detail provided might come at the ...
The application of systematic or structured literature reviews (SLRs) has developed into an established approach in the management domain (Kraus et al. 2020), with 90% of management-related SLRs published within the last 10 years (Clark et al. 2021).Such reviews help to condense knowledge in the field and point to future research directions, thereby enabling theory development (Fink 2010 ...
referred to as OR, is a discipline that employs mathematical and analytical metho ds to solve complex. decision-making problems. The synergy between Operations Research and management is pivotal ...
Wright D, Corner J, Hopkinson J, et al. Listening to the views of people affected by cancer about cancer research: an example of participatory research in setting the cancer research agenda. Health Expect. 2006; 9 (1):3-12. [PMC free article: PMC5060319] [PubMed: 16436157]
Despite the importance of research ethics, there are limitations that can affect the validity and generalizability of the research findings. Some of the limitations of research ethics in management studies include: 1. Lack of diversity in the sample population. The sample population used in research studies is often limited to a specific ...
Social policy research often uses and/or generates a huge amount of research data. This poses two problems that have gained increasing prominence in recent social science debates: the quality of research data and, as a means of improving it, enhancing data transparency (i.e. the free availability of the relevant original research data). 1 In order to improve one's research, how can a ...
conference, or a published research paper in an academic journal. "Limitations of Research". is a section in the standard research report (the research report is usually divided into the ...
Top management team (TMT) heterogeneity-performance research using demographic indicators has contributed to strategic management by showing that top managers do indeed "matter" to firm outcomes. The authors argue, however, that limitations inherent in demographics-based TMT studies preclude their use in specifying how top managers influence their firms. This is an elemental problem because ...
Understand research design and the process of research design. Formulate a research problem and state it as a hypothesis. 1.3 MEANING OF RESEARCH Research is a process to discover new knowledge to find answers to a question. The word research has two parts re (again) and search (find) which denote that we are taking up an
Naturally, our study has limitations. Because of our focus on rich, detailed insights from a few senior employees, our findings revolve around large-scale agile in the aerospace industry. ... In R. Lee, O. Ormandjieva, A. Abran, & C. Constantinides (Eds.), Software engineering research, management and applications 2010 (Vol. 296, pp. 241-255 ...
The aim of this paper is to investigate the re-use of research data deposited in digital data archive in the social sciences. The study examines the quantity, type, and purpose of data downloads by analyzing enriched user log data collected from Swiss data archive. The findings show that quantitative datasets are downloaded increasingly from the digital archive and that downloads focus heavily ...
Long-term straw mulching was known to change soil nutrient content, aggregate distribution and extracellular enzyme activities. However, the impact of long-term straw mulching on microbial nutrient limitations and carbon-use efficiency (CUE st) within aggregates remains unclear.To fill the gap, we conducted a 10-year field experiment in a semi-arid region and used an ecoenzymatic stoichiometry ...