
An official website of the United States government
- Special Topics
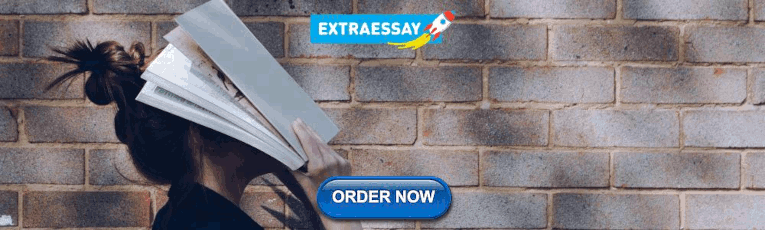
Digital Economy
BEA is developing tools to better capture the effects of fast-changing technologies on the U.S. economy and on global supply chains. The project seeks to calculate the digital economy's contribution to U.S. GDP, improve measures of high-tech goods and services, and offer a more complete picture of international trade. Other goals are to advance research for digital goods and services, the sharing economy, and free digital content.
- Current release: December 6, 2023
- Next release: Data covering 2021 expected in late 2022
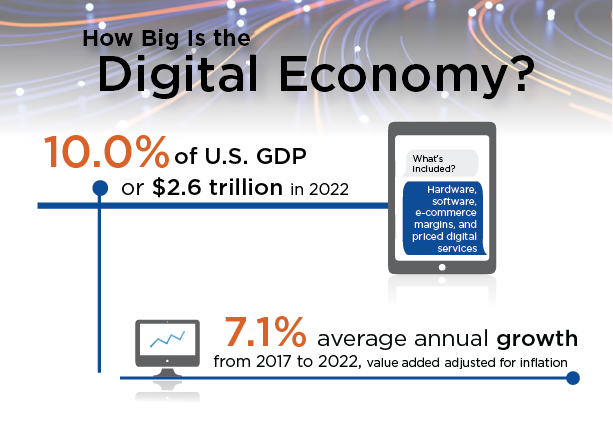
Digital Economy Satellite Account
BEA includes in its definition of the digital economy four major types of goods and services:
- Infrastructure, or the basic physical materials and organizational arrangements that support the existence and use of computer networks and the digital economy, primarily information and communications technology (ICT) goods and services.
- E-commerce, or the remote sale of goods and services over computer networks.
- Priced digital services, or services related to computing and communication that are performed for a fee charged to the consumer.
- Federal nondefense digital services represents the annual budget for federal nondefense government agencies whose services are directly related to supporting the digital economy.
BEA is continuing to explore data and methodology to expand the coverage of the digital economy estimates. For more information on the goods and service currently included in the BEA estimates, please see the latest article " U.S. Digital Economy: New and Revised Estimates, 2017–2022 ."
Please email all comments to [email protected]
- U.S. Digital Economy: New and Revised Estimates, 2017–2022 Survey of Current Business , December 6, 2023
- Data | 2017–2022 XLSX
- Infographic | How Big Is the Digital Economy in 2022 PDF and image PNG
Articles and Blog Posts
- Introducing Consumer Durable Digital Services into the BEA Digital Economy Satellite Account by Benjamin Bridgman, Tina Highfill, and Jon Samuels | June 2023
- Measuring Digital Intermediation Services: Experimental Estimates of Gross Output for Rideshare, Travel Services, and Food/Grocery Delivery Service Platforms by Tina Highfill and Brian Quistorff | October 2023
- Research Spotlight Measuring the Digital Economy Survey of Current Business, May 2019
Frequently Asked Questions (FAQs)
- How do the initial digital economy measures compare with industry economic impact reports and BEA satellite accounts?
- Can the initial digital economy estimates show how much the digital economy contributed to the overall economy as a percent of GDP?
- What is gross output by industry and how does it differ from gross domestic product (or value added) by industry?
- What is a satellite account?
- How is the digital economy captured in the core statistics currently produced by the Bureau of Economic Analysis (BEA)?
- How does BEA define the digital economy?
- What is missing from the digital economy estimates?
- How are free social media, email, and other ad-supported Internet services included in the BEA digital economy estimates?
Previously Published Estimates
- New and Revised Statistics of the U.S. Digital Economy, 2005–2021 PDF
- Data | 2005–2021 XLSX
- Infographic | How Big Is the Digital Economy in 2021 PDF and image PNG
- New and Updated Digital Economy Statistics—May 2022 PDF
- Data | 2005–2020 XLSX
- Infographic | How Big Is the Digital Economy in 2020 PDF and image PNG
- Updated Digital Economy Estimates – June 2021 PDF
- Data | 2005–2019 XLSX
- Infographic | How Big Is the Digital Economy in 2019 PDF and image PNG
- New Digital Economy Estimates—August 2020 PDF
- Data | 2005–2018 XLSX
- Infographic | How Big Is the Digital Economy in 2018 PDF and image PNG
- An Update Incorporating Data from the 2018 Comprehensive Update of the Industry Economic Accounts—April 2019 PDF
- Data | 1997–2017 XLSX
- Defining and Measuring the Digital Economy BEA Working Paper, March 2018
- Data | 2005–2016 XLSX
- Infographic | How Big is the Digital Economy in 2017 PDF and image PNG
International Trade in ICT and Potentially ICT-Enabled Services
BEA's statistics on trade in information and communications technology (ICT) and potentially ICT-enabled services complement its standard presentation of international trade in services statistics by examining the extent to which ICT may be used to facilitate trade in services. ICT services are those used to facilitate information processing and communication; potentially ICT-enabled services are services that can predominantly be delivered remotely over ICT networks. BEA measures potentially ICT-enabled services rather than ICT-enabled services themselves because for many types of services the actual mode of delivery is unknown.
- Interactive data: International Services Tables
- Trade in Services in 2021 and Services Supplied Through Affiliates in 2020 Survey of Current Business October 2022 - includes a section on ICT and potentially ICT-enabled services.
- Trends in U.S. Trade in Information and Communications Technology (ICT) Services and in ICT-Enabled Services PDF Alexis Grimm - Survey of Current Business May 2016
- Paper: Trends in Digitally-Enabled Trade in Services PDF
- Blog: Trade in Digitally Enabled Services Shows Strong Growth
- Previously Published Estimates XLS Country estimates in these files use a slightly different definition of ICT and potentially ICT-enabled services than in the interactive data.
Price Measurement of High-Tech Goods and Services
BEA is consistently working toward improving price measurement, especially for high-tech goods and services which frequently experience changing characteristics, improved quality, and price changes relative to other goods and services.
Research Publications and Working Papers
- Implications of Consumer Heterogeneity on Price Measures for Technology Goods by Adam Hale Shapiro and Ana Aizcorbe | August 2010
- Why Are Semiconductor Price Indexes Falling So Fast?: Industry Estimates and Implications for Productivity Measurement by Ana Aizcorbe | September 2005
- Differences in Hedonic and Matched-Model Price Indexes: Do the Weights Matter? by Ana Aizcorbe and Yvon Pho | September 2005
- Price Deflators for High Technology Goods and the New Buyer Problem March 2005
Data as an Asset
- Capitalizing Data: Case Studies of Tax Forms and Individual Credit Reports PDF by Rachel Soloveichik | June 2023
- Valuing the U.S. Data Economy Using Machine Learning and Online Job Postings PDF by José Bayoán Santiago Calderón and Dylan G. Rassier | August 2022
- Presentation: Valuing Stocks and Flows of Data Assets for the U.S. Business Sector PDF BEA Advisory Committee Meeting | May 2022
- Treatment of Data in National Accounts PDF by Dylan G. Rassier, Robert J. Kornfeld, and Erich H. Strassner | May 2019
- Value of Data: There's No Such Thing as a Free Lunch in the Digital Economy by Wendy C.Y. Li, Makoto Nirei, and Kazufumi Yamana | February 2019
Other Research and Information
Research papers.
- Marketing, Other Intangibles, and Output Growth in 61 United States Industries by Leo Sveikauskas, Rachel Soloveichik, Corby Garner, Peter B. Meyer, James Bessen, and Matthew Russell
- Measuring the Cost of Open Source Software Innovation on GitHub by J Bayoán Santiago Calderón , Carol Robbins , Ledia Guci , Gizem Korkmaz , and Brandon L. Kramer | July 2022
- Is Productivity on Vacation? The Impact of the Digital Economy on the Value of Leisure PDF by Benjamin Bridgman | February 2018
- Measuring the "Free" Digital Economy within the GDP and Productivity Accounts PDF by Leonard Nakamura, Jon Samuels, and Rachel Soloveichik | October 2017
- Valuing 'Free' Media in GDP: An Experimental Approach PDF by Leonard Nakamura, Jon Samuels and Rachel Soloveichik | June 2016
Of Interest
- OECD Handbook on Compiling Digital Supply and Use Tables , November 2023
- Videos: Measuring the Digital Economy from the Fifth IMF Statistical Forum, November 2017
- Podcast: GDP and the Value of "Free" featuring BEA's Rachel Soloveichik, with The Economic Statistics Centre of Excellence, July 2017
What is the Digital Economy Satellite Account?
Measures the digital economy's contribution to U.S. GDP, improves measures of high-tech goods and services, and offers a more complete picture of international trade. Includes valuing digital-enabling infrastructure, e-commerce transactions, and digital media.
What's a Satellite Account?
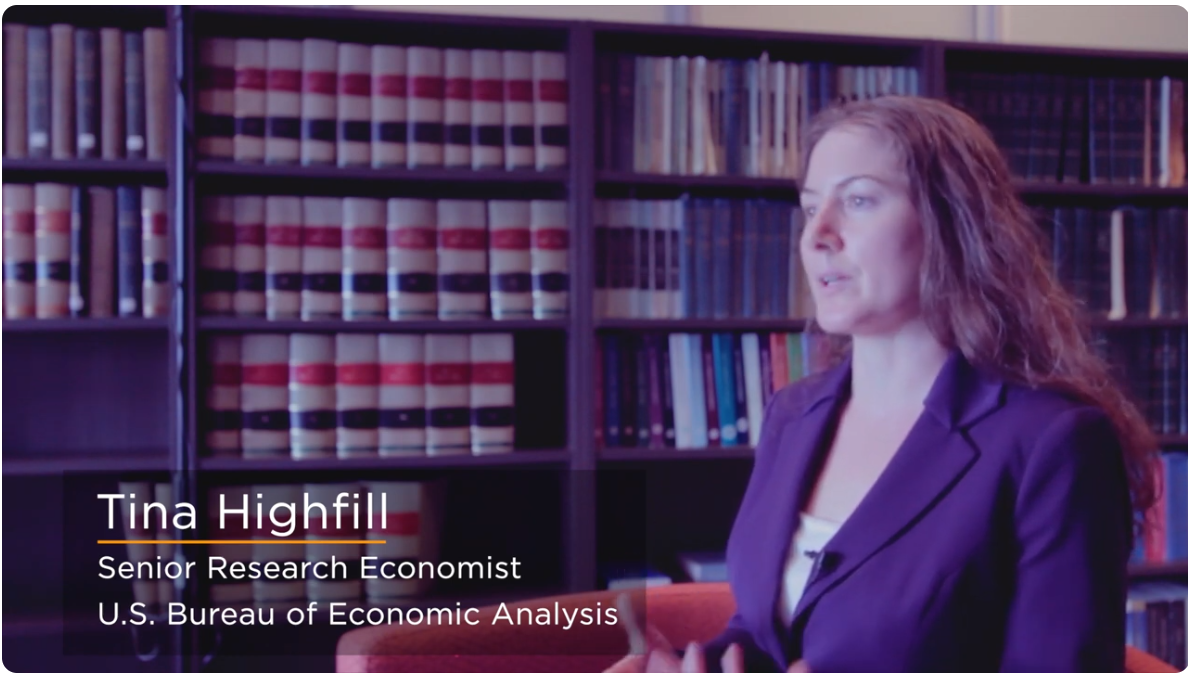
Stay on top of updates...
- Digital Economy Tina Highfill (301) 278-9266 [email protected]
We pursue a deeper understanding of the digital economy and its impact on the future of work and society.
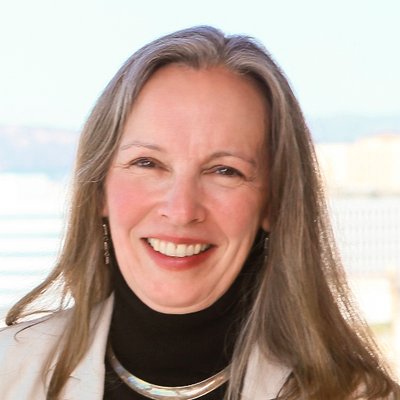
WATCH : SEMINAR SERIES
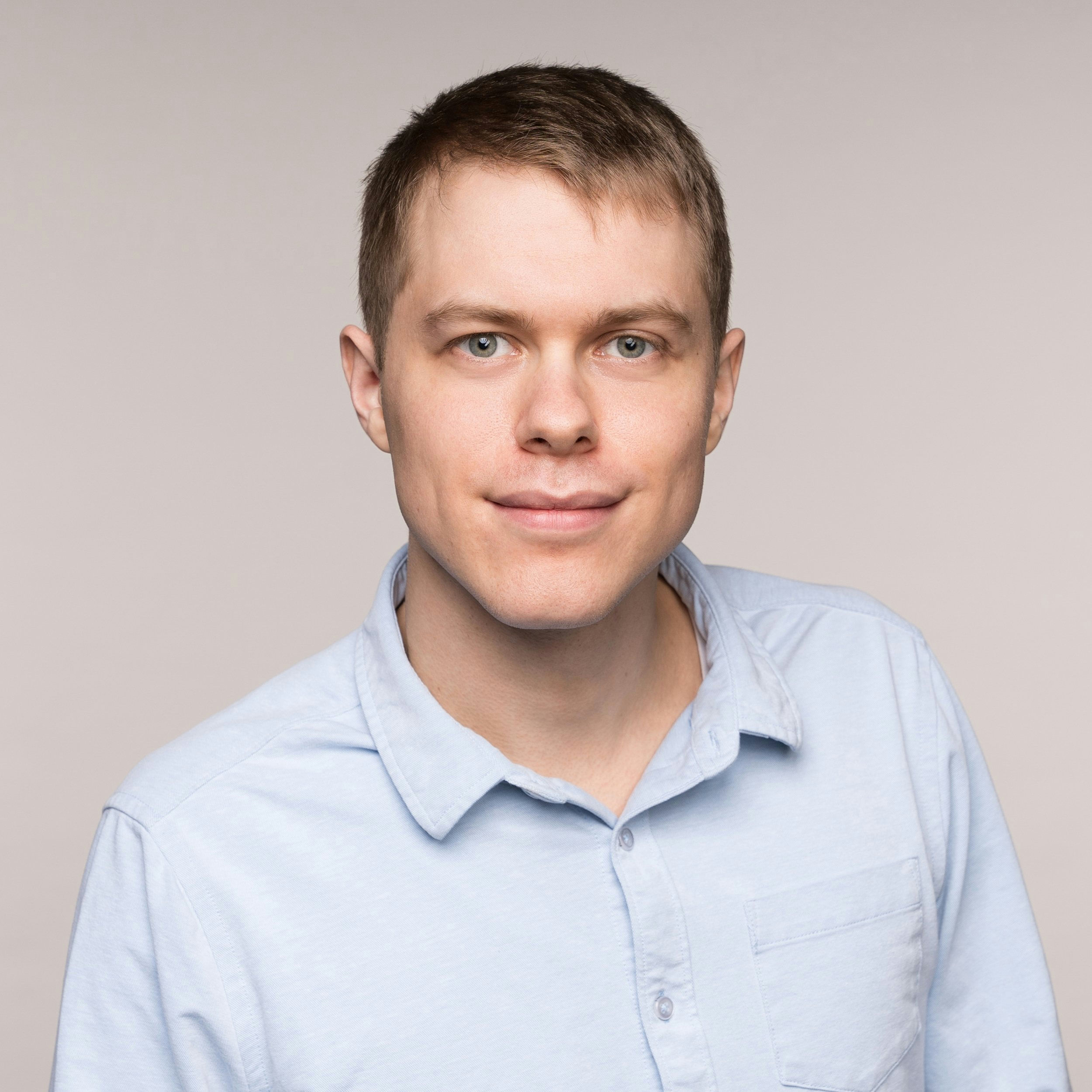
REGISTER : SEMINAR SERIES
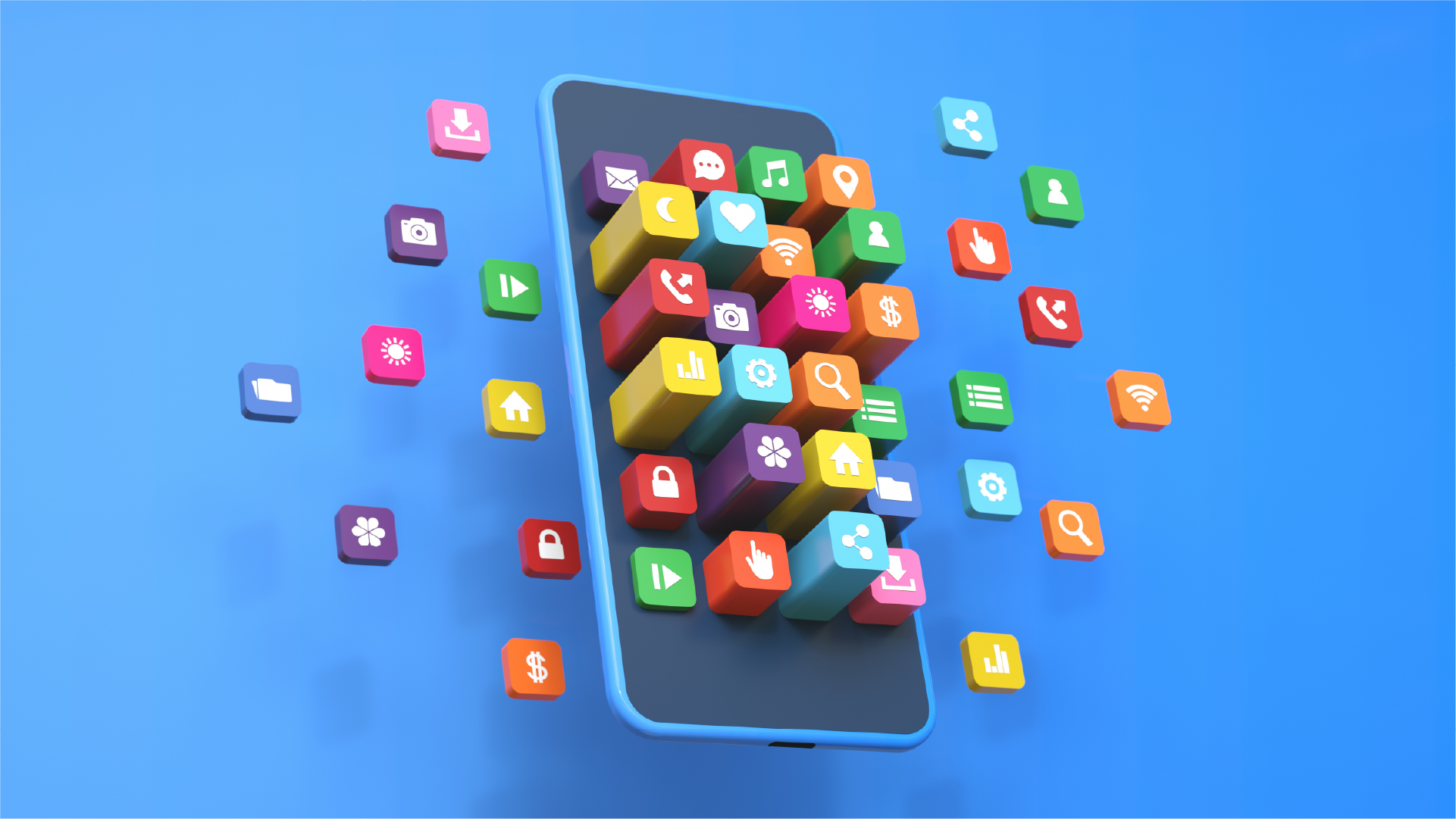
Our research is helping companies, policymakers, students, and professionals rise to the challenges and opportunities created by the digital economy.
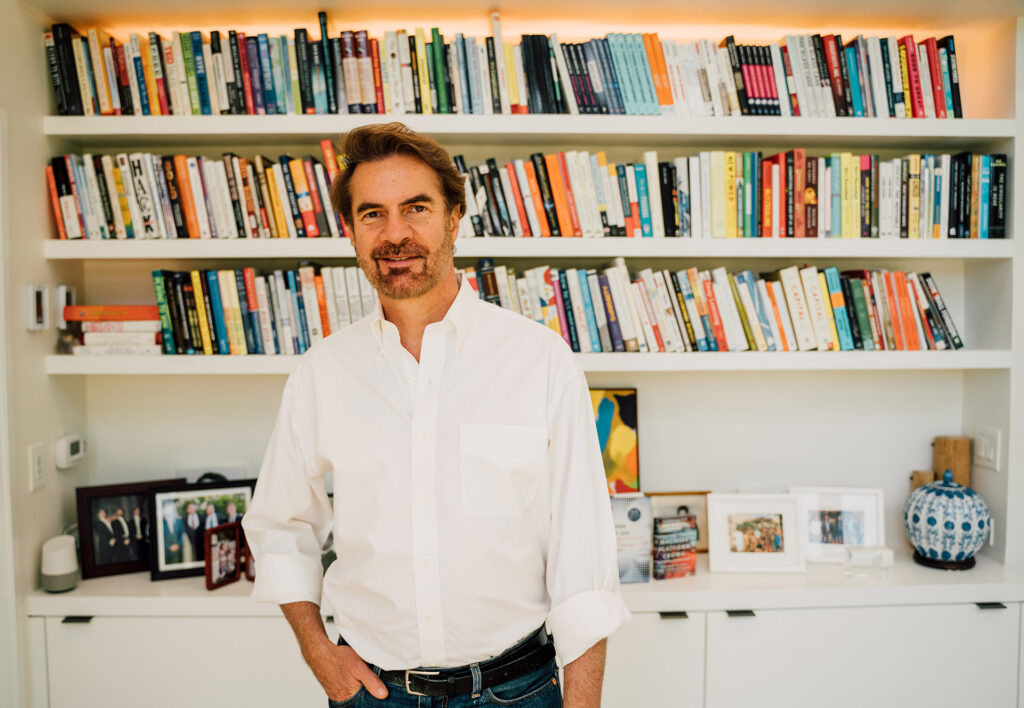
Leading the way
The Lab is directed by Erik Brynjolfsson , whose research focuses on the economic implications of digital technologies and artificial intelligence. One of the most cited authors on the economics of information, Brynjolfsson was among the first researchers to measure productivity contributions of IT and the complementary role of organizational capital and other intangibles.
PUBLICATIONS
Recent papers + articles
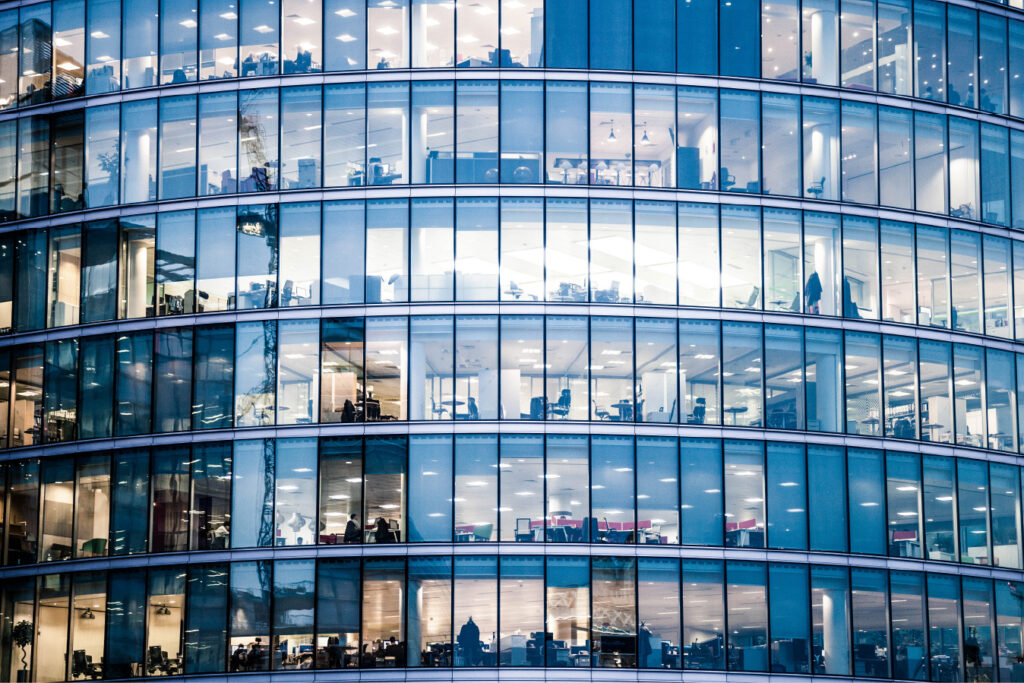
AI Adoption in America: Who, What, and Where
A team of experts, including researchers from Stanford Digital Economy Lab, studied the early adoption and diffusion of five AI-related technologies—automated-guided vehicles, machine learning, machine vision, natural language processing, and voice recognition—as documented in the 2018 Annual Business Survey of 850,000 firms across the United States. Discover what they learned. READ WORKING PAPER
The Evolution of Working from Home
Nicholas Bloom is among a group of researchers tracking the evolution of remote work, which was exacerbated by the Covid-19 pandemic. READ WORKING PAPER
Generative AI at Work
In a first-of-its-kind study, researchers examined how introducing generative AI into the workflow at a call center of nearly 5,000 workers boosted productivity. READ WORKING PAPER
Our mission is to advance our collective understanding of the digital economy in order to build a tech-driven economy that benefits everyone.
Views on AI are often divided between the doomers and the evangelists. Here Stanford Professor @erikbryn talks with @tejparikh90 on the huge potential and the risks. He says AI could double average US productivity growth. https://t.co/le2WPglALy — Tony Tassell (@TonyTassell) January 31, 2024
- Stanford Home
- Maps & Directions
- Search Stanford
- Emergency Info
- Terms of Use
- Non-Discrimination
- Accessibility
Click through the PLOS taxonomy to find articles in your field.
For more information about PLOS Subject Areas, click here .
Loading metrics
Open Access
Peer-reviewed
Research Article
Study on the effect of digital economy on high-quality economic development in China
Roles Data curation
Affiliation School of Economics and Management, Chongqing University of Posts and Telecommunications, Chongqing, China
Roles Conceptualization
* E-mail: [email protected]

Roles Formal analysis
Roles Methodology
- Wei Zhang,
- Siqi Zhao,
- Xiaoyu Wan,
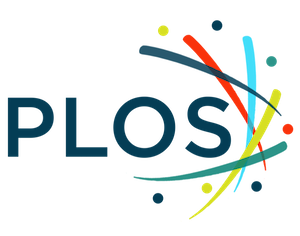
- Published: September 21, 2021
- https://doi.org/10.1371/journal.pone.0257365
- Peer Review
- Reader Comments
At present, the digital economy, which takes information technology and data as the key elements, is booming and has become an important force in promoting the economic growth of various countries. In order to explore the current dynamic trend of China’s digital economy development and the impact of the digital economy on the high-quality economic development, this paper measures the digital economic development index of 30 cities in China from the three dimensions of digital infrastructure, digital industry, and digital integration, uses panel data of 30 cities in China from 2015 to 2019 to construct an econometric model for empirical analysis, and verifies the mediating effect of technological progress between the digital economy and high-quality economic development. The results show that (1) The development level of China’s digital economy is increasing year by year, that the growth of digital infrastructure is obvious, and that the development of the digital industry is relatively slow. (2) Digital infrastructure, digital industry and digital integration all have significant positive effects on regional total factor productivity, and the influence coefficients are 0.2452, 0.0773 and 0.3458 respectively. (3) Regarding the transmission mechanism from the digital economy to the high-quality economic development, the study finds that the mediating effect of technological progress is 0.1527, of which the mediating effect of technological progress in the eastern, northeast, central and western regions is 1.70%, 9.25%, 28.89% and 21.22% respectively. (4) From the perspective of spatial distribution, the development level of the digital economy in the eastern region is much higher than that in other non-eastern regions, and the development of digital economy in the eastern region has a higher marginal contribution rate to the improvement of the total factor productivity. This study can provide a theoretical basis and practical support for the government to formulate policies for the development of the digital economy.
Citation: Zhang W, Zhao S, Wan X, Yao Y (2021) Study on the effect of digital economy on high-quality economic development in China. PLoS ONE 16(9): e0257365. https://doi.org/10.1371/journal.pone.0257365
Editor: László Vasa, Szechenyi Istvan University: Szechenyi Istvan Egyetem, HUNGARY
Received: April 18, 2021; Accepted: August 29, 2021; Published: September 21, 2021
Copyright: © 2021 Zhang et al. This is an open access article distributed under the terms of the Creative Commons Attribution License , which permits unrestricted use, distribution, and reproduction in any medium, provided the original author and source are credited.
Data Availability: The data underlying the results presented in the study are available from ( http://www.stats.gov.cn/ ).The data results in the manuscript and the data sets in the supporting information are all collected by authors.
Funding: This work was supported by the Project of Humanities and Social Sciences Ministry of Education in China [18YJC790224], the Ministry of Education Layout Foundation of Humanities and Social Sciences [19XJA630004], the Planning Project of Chongqing Social Science [2016BS057], the talent Introduction Project of Chongqing University of Posts and Telecommunications [A2016-04, K2015-128]. The funders play a guiding and supporting role in our study, and they had no role in study design, data collection and analysis, decision to publish, or preparation of the manuscript.
Competing interests: No authors have competing interests
1 Introduction
China’s fourteenth five-year plan for national economic and social development proposes to build a new development pattern of domestic and international dual cycles. Accelerating the construction of a new development pattern with the domestic big cycle as the main body and the domestic and international double cycles mutually promoting each other is an important strategic choice for connecting domestic and international markets, promoting high-quality development of an open economy, and adapting to new changes in the global economy. The digital economy, dominated by a new generation of information technology, has become an important path for efficiently meeting market demand and smoothing the domestic and international dual cycles, and will become an important driving force and breakthrough point for boosting the new development pattern of dual cycles. In recent years, the digital economy has grown rapidly in China. As a new economic form that leads the future, it has unprecedentedly reconstructed a new picture of economic and social development and is a new variable for improving economic quality and efficiency. According to the data of the 2019 Digital Economy Report released by the United Nations International Trade, based on the report’s definition of the digital economy, the scale of the digital economy accounts for between 4.5% and 15.5% of the world’s gross domestic product (GDP). In terms of the added value of information and communication technology, the United States and China account for 40% of the world’s total added value, and the development of the digital economy has become a common choice for major global powers and regions to reshape global competitiveness. According to the most recent “White Paper on the Development of China’s Digital Economy (2021)” released by the China Academy of Information and Communications Technology, the proportion of China’s digital economy in GDP has increased year by year from 2005 to 2020, from 14.2% to 38.6%.
At present, a unified standard has not yet been setup for the academic definition of the concept of the digital economy. In the academic field, in 1996, Tapscott, an American IT consulting expert, first put forward the concept of a digital economy in the report “Digital Economy: Opportunities and Risks in the Era of Network Intelligence”. The concept’s main feature is the digital flow and transmission of information over the network [ 1 ]. In 1998, in the government report “Emerging Digital Economy” released by the US Department of Commerce, the term digital economy was included for the first time, and the concept of the digital economy was gradually recognized by governments and scholars worldwide. Since then, the related research on the digital economy has begun to rise, the concept of the digital economy has been continuously enriched and deepened in this process, and the research category of the digital economy has been constantly improved. There are two main points of view to define the digital economy in a narrow sense. One is that the digital economy is divided into two parts, namely, ICT services and manufacturing, which are summed up as the digital economy, and the other part comprises retail, the platform economy and the sharing economy, which are mainly supported by ICT and cannot be distinguished by official industry codes. Maglio believes that the digital economy consists of four parts: Internet infrastructure, e-commerce, the digital delivery of goods and services, and the retail sales of tangible goods. Meisenberg believes that the digital economy has three main components: the e-business infrastructure, e-business and e-commerce. In recent years, many studies have further defined related products or industries on the basis of defining the components of the digital economy. Bukht divides the digital economy into three levels: the first layer is the core layer, which is the digital (IT/ICT) domain, including hardware manufacturing, software and IT consulting, information services, and telecommunications. The second layer is the narrow caliber, which is the narrow digital economy, including e-commerce, digital services, and platform economy. The third layer is the wide caliber, that is, the broad sense of digital economy, including e-commerce, Industry 4.0, precision agriculture, and the algorithm economy [ 2 ]. With the development digital technology, including emerging digital technology and the resulting new industries and new business types, the connotation of the digital economy has been gradually enriched. As a historical category, in the process of the interaction of technology, organization and institution in the economic system, the digital economy is a macro emergence, which is based on the high coordination of human economic activities and the continuous optimization of the new production organization mode shaped by the high coordination of human economic activities and an interaction based on technology [ 3 , 4 ]. With the in-depth advancement of supply-side structural reforms, digital technology has flourished and rapidly innovated, and has penetrated widely into other economic fields. Chinese scholars generally divide the digital economy into digital industrialization and industrial digitization. Digital industrialization is equivalent to the traditional information industry, including electronic and communication equipment manufacturing, Internet-related services, software and information technology services in the national economic industry classification. Due to the continuous integration of information technology and other industrial sectors of the national economy, the added value of the digital economy is generated in traditional industries. This portion represents the part of the digitalization of the industry.
At present, the research on the domestic digital economy focuses on the discussion of the development strategy of China’s digital economy and its comparison with other countries (regions). Based on the basic connotation of the digital economy, with the deepening of digitalization, scholars believe that the combination of digital technology and traditional industries can achieve the following: realize the green and rapid development of the GDP [ 5 , 6 ]; realize the transformation of the consumption structure [ 7 ]; improve the quality of human capital [ 8 , 9 ]; drive the industrial economy from being labor-intensive to becoming technology-intensive; consolidate infrastructure construction [ 10 – 12 ]; make full and effective use of data resources [ 13 , 14 ]; strengthen technological innovation; deepen integrated applications; and create a relaxed environment to better develop the digital economy. China should strengthen its advantages in areas such as 5G, in global digital competition, further strengthen the research and development of key core technologies and develop the whole industry chain. Other scholars believe that in the development of China’s digital economy there are some problems, such as unbalanced, inadequate and uncoordinated development. These problems are mainly concentrated in the areas comprising the digital infrastructure, the degree of upgrading of the digital industry, information network security, and the deep integration of digital technology penetration and traditional industries. There is a phenomenon of digital poverty accumulation in space [ 15 ]. At present, China’s economy is in a period of transitional development and slow growth, and the development of the economy depends more on the quality and efficiency of economic growth than on quantity and speed [ 16 ]. The quantitative indicators of economic growth, such as gross domestic product and national income, are no longer the only focus of the government, as the focus has gradually shifted from quantity to quality in order to promote the construction of resource-conserving and environmentally friendly societies [ 17 ]. Starting with the economic growth theory of classical economics, the primary concern of economists has always been the quantity rather than the quality of economy’s growth. With the help of the Moran index and a spatial econometric model, Bai et al. pointed out that there are significant spatial spillover benefits in China’s economic growth and that the economic growth of a province depends on both local input and neighbouring regional input [ 18 ]. To provide a basis for a clear understanding of the source of economy growth, other scholars use various econometric models to explore the specific effects of driving factors such as education, political uncertainty, foreign direct investment, human capital, banking globalization and information and communication technology, on the quantitative indicators of economic growth [ 19 – 21 ]. Developing an evaluation index system of high-quality economic development, Qi constructs an economy growth quality measurement system covering scale, performance, structure and coordination [ 22 ]. Based on the matrix method, Frolov constructs a regional economic growth quality evaluation system that combines the average annual productivity growth rate and per capita development index [ 23 ]. Regarding the research on the relationship between the digital economy and high-quality economic development, more scholars have studied the impact of the digital economy on high-quality economic development from the perspectives of big data empowerment [ 24 ], the integration of the digital economy and the real economy [ 25 ], shared economy [ 26 ], digital finance [ 27 ], and policy supply systems [ 28 ]. Generally, the digital economy is in line with the new development concept of innovation, coordination, green, openness and sharing, and in China is becoming a major strategic development direction, which will effectively promote the high-quality development of China’s economy.
From the above research results, it can be found that the research contents of scholars in the field of digital economy are relatively extensive, involving the connotation, characteristics, impact effects, index system construction and evaluation and other aspects. However, at present, there are few empirical studies on the promotion of high-quality economic development by digital economy, and few literatures use panel data to study the dynamic changes of the development of digital economy in China, which leads to the limitations of the evaluation of the index system and the lack of continuity in the observation of the development of digital economy. On the one hand, on the basis of previous studies on the index system of the digital economy, this paper selects the core dimensions and measurement indicators of the digital economy, and tries to take the development of the interprovincial digital economy in China from 2015 to 2019 as the research object in order to evaluate the overall changes and regional differences in the development of China’s digital economy. On the other hand, on the basis of the evaluation index of the digital economy, an econometric model is constructed to study the effect of the digital economy on high-quality economic development, and technological progress is introduced as an intermediary variable between the two aspects, developing thereby a more objective study of the influence mechanism of the digital economy on high-quality economic development and an analysis from a new perspective. Additionally, this paper also examines the interaction between the digital economy and interprovincial factors to study the regional heterogeneity of the digital economy in promoting high-quality economic development. Moreover, this study deeply explains that the impact of the digital economy on high-quality economic development in the eastern region has a higher marginal contribution rate. Thus, our findings have good policy reference value for China in efforts to accelerate the development of the digital economy, narrow the regional “digital economic gap” and promote high-quality economic development.
2 Theoretical analysis and research hypothesis
This paper discusses the direct impact of the digital economy on high-quality economic development at both the macro and micro levels. From a macro perspective, the digital economy affects economic development by affecting production input and output efficiency, which is reflected in the increase in factor input, the improvement of factor allocation efficiency, and the increase in total factor productivity brought about by technological progress and technological spillover [ 29 ]. For developing countries, digitization is considered to be the main driving force of economy growth. It improves capital and labor productivity, reduces transaction costs, and promotes the integration of countries into the global market system [ 30 , 31 ]. For the developed countries, the impact of the digital economy on the quality of economic development is mainly reflected in promoting sustainable development [ 32 ] and improving enterprise agility [ 8 ]. The digital economy was the main driving force of economic growth in the United States from 2004 to 2012 [ 33 ]. The independent R&D investment and technological progress of its production sector had a significant positive impact on total factor productivity (TFP) growth [ 34 ]. Informatization plays a significant role in promoting TFP growth in 12 major countries of the OECD [ 35 ]. The data from 2009 to 2018 in the 15 advanced economies of the European Union show that national and industry digital policies can significantly promote economic growth [ 8 ]. In addition, the increase in the productivity of digital assets has brought about a sharp drop in the employment rate in the large-scale production system [ 36 ], which also has created a long-term and stabilizing effect on the development of technological innovation [ 37 ]. From a micro point of view, digital manufacturing technology improves the competitiveness of enterprises and improves the performance of the company [ 38 ], effectively promotes the development of the electronic government [ 39 ], and helps enterprises to realize the business model of a circular economy [ 40 ]. Generally, despite the rapid development of information technology and digital technology, the digital economy represented by information data and cloud computing has not played its greatest role in economic development [ 41 ].
In the era of the digital economy, according to the theory of comparative advantage and first-mover advantage, because of its high technology, high permeability and high growth, for its development, the digital economy depends on platforms, intelligence and ecology to create opportunities for regional economic growth, technological progress and digital infrastructure construction. Therefore, areas with a sound infrastructure and in which the digital economy is developed will have comparative advantages and first-mover advantages, including a high agglomeration of the digital industry, the application of digital technology, digital ecosystems and so on. First-mover advantages include the improvement of the digital infrastructure, human capital accumulation and so on, which will form the driving force for the high-quality development of the regional economy. Based on the above analysis, the research hypothesis H1 is proposed:
- H1: The development of the digital economy is conducive to the improvement of total factor productivity, which directly promotes high-quality economic development. Compared with regions with a poor level of digital economy development, regions with a better foundation can benefit from the development of the digital economy.
Total factor productivity refers to the residual value excluding the contribution rate of all tangible production factors in economic growth. Technological progress is an important contribution factor of this residual value, and information technology is a significant attribute of the digital economy. According to the endogenous growth theory, endogenous R&D and innovation are the core elements that drive economic growth and technological progress [ 42 ]. The advancement of digital technology is regarded as not only an extension of the production possibility boundary, but also an extension of the innovation possibility boundary [ 29 ]. Therefore, the digital economy can improve total factor productivity by promoting technological progress. On the one hand, in the era of the digital economy, the cost structure of the production and operation of enterprises has changed, forming a structure of high fixed cost and low marginal cost. Network externalities gradually enlarge this cost structure, which gradually reduces the average cost of production and operation of enterprises, and produces economies of scale. On the other hand, in the era of a digital economy, enterprises pay more attention to the diversified production of products or services. Enterprises accumulate user data through multilateral platforms and then in the process of developing other products or services, import users of the original platform, reducing operating costs. At the same time, the growth of the digital economy also enables the parallel development of multiple business models to achieve economies of scope. Additionally, information technology can enable the effective integration of the information of both market supply and demand in the same space and period of time on the platform; information technology can be used to improve the matching efficiency of supply and demand and can simplify the information redundancy in the operation of the economic system, reducing market transaction costs and improving the operation efficiency of the economic system. Finally, the development of information technology transforms traditional industries. New generation information technologies, such as big data, cloud computing and artificial intelligence, are applied to the production, operation, circulation and consumption of traditional industries, with data as the key production factors. The digital division of labour, enable the realization of an industrial ecology of knowledge sharing and factor coordination, and significantly improves the development efficiency of traditional industries. Therefore, this article proposes hypothesis H2:
- H2: The development of the digital economy can indirectly have a positive impact on total factor productivity by promoting technological progress.
3 The measurement of digital economy in China
3.1 the measuring index for interprovincial digital economy in china.
The digital economy originated from the fifth technological revolution and its leading technology is information and communications technology. Different from the economies emerging from the previous four technological revolutions, the digital economy presents three typical characteristics. First, data have become the core factors of production and in the supply of the traditional factors of production, resources with data elements as the core are effectively allocated; in addition, the dimension and volume of the supply of data elements is constantly enriched so that data permeates all aspects of economy and social development, reflecting the integration of the development of the digital economy. Second, the digital infrastructure has become a supporting facility, and the new generation of information technology represented by big data, artificial intelligence, mobile Internet and cloud computing has become the leading technology in the development of the digital economy, advancing the information revolution into the era of flexibility, sharing and high-performance computing [ 43 ]. In addition, the infrastructure including the communications infrastructure, the Internet of Things terminals, cloud computing resources, etc. has adapted to technological development and entered the fast lane of development. Thirdly, the digital economy has become the main driving force of economic development in this era. With the advantages of having special netizens, China will gradually release its economic growth momentum [ 44 ], and its contribution rate to economic growth will increase steadily.
Combined with the above analysis of the essential attributes and characteristics of the digital economy, referring to digital economy reports and white papers issued by authoritative research institutions in China, and following the principles of the timeliness, representativeness, comparability and data availability, this paper holds that the development of the digital economy refers to the use of a new generation of information technology and information infrastructure to infiltrate all aspects of economic and social development; moreover, this development also refers to the undertaking of a series of activities to promote the development of traditional industries and the optimization of the economic structure. Therefore, this paper is constructing an index system to measure the development of China’s interprovincial digital economy from three dimensions: the digital infrastructure, the digital industry and the digital fusion effect.
As shown in Table 1 , for measuring the development of the digital economy, this paper designs an index system, which includes 3 second-level indicators and 12 third-level indicators, and evaluates the development level of China’s interprovincial digital economy. To make the statistical indicators of different regions comparable in the time section, this paper uses the proportional index as much as possible. Additionally, the development effect of digital economy integration is influenced by many factors, which cannot be measured by a single indicator. Therefore, this article draws on the online government index and the digital life index in the China Interprovincial Information Society Index developed by the Ministry of Industry and Information Technology and the National Information Center’s China Interprovincial Information Society Index. These three indicators are all structured from second-hand data, and other indicators are based on information from the National Bureau of Statistics and the statistical yearbooks of various provinces and cities. For details of the data standardization and confirmation process, please refer to S1 Appendix .
- PPT PowerPoint slide
- PNG larger image
- TIFF original image
https://doi.org/10.1371/journal.pone.0257365.t001
- Digital economic infrastructure sub-index. This part of the index is mainly used to measure the development level of the interprovincial digital economic infrastructure, which is an important prerequisite for the regional development of the digital economy. This paper mainly investigates two aspects: the mobile Internet and the Internet ports. The mobile Internet development level is characterized by mobile phone penetration and mobile Internet per capita access traffic. The Internet port development level is explained by the number of Internet bandwidth access ports and enterprise-owned websites.
- Digital economic industrial development sub-index. This part of the index is mainly used to do the following: measure the industrial scale, enterprises and investment development status of the interprovincial digital economy; explain the core industrial scale of the digital economy with the added value of information technology services and electronic equipment manufacturing industry; explain through the number of enterprises the development level of the regional economy related enterprises and explain through ICT investment the regional digital economic investment.
- Digital Economy Integration and Application sub-index. This part of the index is mainly used to measure the development level of interprovincial digital economy integration. China’s consumer Internet is one of the core components of the digital economy; therefore, the regional e-commerce sales volume is used to measure the development of the regional “digital economy + service industry”. The level of industrial digitization cannot be measured by a single index; therefore, this paper draws lessons from the integration development index of the Ministry of Industry and Information Technology to measure it. In addition, the concepts “digital economy + government affairs” and “digital economy + people’s livelihood” are two important aspects of the development of digital fusion. Therefore, to measure the development level of digital economy integration, we draw lessons from the online government index and the digital living index in the information society index issued by the National Information Center.
3.2 The present situation of digital economy in China
The changes in China’s interprovincial digital economic development index and in its three sub-indices from 2015 to 2019 are shown in Fig 1 . During the sample period, the overall development level of China’s digital economy showed an upward trend year by year, and the growth trend was relatively stable, rising from 43.39 points in 2015 to 59.31 points in 2019 with an increase of 36.69% and an average annual growth rate of 8.13%. The development level of the digital economy has improved significantly. Regarding the sub-dimensional indices with an average annual growth rate of 12.45%, the digital economic infrastructure sub-index rose from 40.84 in 2015 to 65.29 in 2019, an increase of 59.87%. Since the 13th Five-Year Plan, from the construction of broadband China to the “speed-up and fee reduction” of the telecommunications industry, China has made great efforts to develop its digital infrastructure and to promote China’s digital infrastructure construction in an orderly manner and from a global perspective, effectively bridging the regional digital infrastructure gap. The digital economic industrial development sub-index rose from 46.72 in 2015 to 50.39 in 2019, reflecting an increase of 7.87% and an average annual growth rate of only 1.91%. On the whole, the digital industry has not significantly improved the development of the digital economy in China. The reason for this is strongly correlated with China’s digital economy’s industrial distribution, which is characterized by a concentration of most of the digital core industries in the eastern developed areas. This industrial concentration has led to the lack of resource elements for the development of the digital industry in other central and western regions, which has had a certain impact on the overall development of China’s digital industry. The digital economy integration and application sub-index rose from 43.63 in 2015 to 59.95 in 2019, representing an increase of 37.38 percent and an average annual growth rate of 8.26 percent. Since the 13th Five-Year Plan, the state has vigorously promoted the integration of industrialization and information paid attention to the development of e-government, promoted the development of national governance in the direction of digitalization and intelligence and used digital technology in an orderly manner to improve the efficiency of traditional economic and social operations throughout the country.
https://doi.org/10.1371/journal.pone.0257365.g001
The contribution in terms of importance to the development of China’s digital economy from 2015 to 2019 is in the following order: the digital infrastructure, digital integration and application, and the development of the digital industry, with contribution rates of 163.18%, 101.88% and 21.45%, respectively. From 2015 to 2019, the development of China’s digital economy depended more on the construction of the digital infrastructure, and the effect of digital integration also played a certain role in economic and social development. However, the pulling effect of the digital industry on the development of the digital economy has not been shown. One of the possible reasons is the vigorous development of the consumer Internet based on its usage by Chinese netizens, which has also promoted the construction of the digital infrastructure. However, the development of the digital industry represented by the growth of the industrial Internet on the supply side lags behind relatively and the mutually beneficial promotion of industrial development has not yet developed between the regions. Therefore, how to develop the digital economy industry in the future is an important issue on which to focus to promote the development of the digital economy.
3.3 The pattern of the interprovincial digital economy in China
As seen from Table 2 below, in the regional pattern of the development of China’s digital economy, the eastern region is developed and the non-eastern regions are underdeveloped. In 2019, the average comprehensive index of China’s digital economic development was 59.31, and 11 provinces and cities were above the average, including 9 eastern provinces and cities except Hebei as well as Chongqing and Sichuan, while the other 19 provinces and cities were below average. According to the standard of China’s National Bureau of Statistics, China’s provinces and cities are divided into four regions: the east, the northeast, the central and the west. In the eastern region, with the comprehensive index of the digital economy reaching more than 80 in 2019, Beijing, Guangzhou, Jiangsu and Shanghai are in the first echelon; the second echelon includes Jiangsu and Zhejiang, and the third echelon comprises Tianjin, Hebei, Fujian and Hainan. In the three northeastern provinces, due to the development model of partial industrialization and the lack of digital resource elements, the overall development level of the digital economy is relatively balanced, but the overall development is in the middle and lower levels. In the central region, in the level of development, the digital economy of Anhui and Guizhou is the leader and is more balanced as a whole. In the western region, the provinces and cities represented by Sichuan and Chongqing have a better level of development, while Yunnan, Guangxi, Xinjiang and Gansu have a relatively low level of development.
https://doi.org/10.1371/journal.pone.0257365.t002
On the one hand, due to the advantages of a digital infrastructure and a digital resource endowment, the comprehensive index of the digital economy in the developed areas was at a high level in 2015, and there was relatively limited improvement of the index by 2019. In the provinces and cities represented by Guizhou, Inner Mongolia, Chongqing and Guangxi, the increase in the digital economic index was much higher than that of other regions during the sample study period. Guizhou promoted the construction of a big data comprehensive experimental area and vigorously developed the big data industry. Chongqing combined the advantages of its own traditional industrial manufacturing base and vigorously developed a digital transformation of manufacturing. These areas combined national policy arrangements and their own industrial advantages and resource endowments to improve the development level of the digital economy. In addition, the provinces and cities represented by Yunnan, Liaoning and Jiangxi demonstrated a relatively low increase in the comprehensive index of digital economic development during the sample period, and the development of the digital economy in these underdeveloped areas started relatively late. A backward infrastructure and a lack of human resources has further limited the development of the digital economy, which not only had a certain impact on the current economic and social development but also widened the "digital economic gap" between regions. In the 14th Five-Year Plan, the issue of how to improve the development level of the digital economy both systematically and comprehensively in underdeveloped areas will become an important topic.
On the other hand, from the perspective of China’s regional digital economic development, the variances of China’s digital economy development index from 2015 to 2019 are 199.14, 184.88, 178.41, 170.40 and 165.58, respectively, which shows that the “digital economic gap” among China’s provinces as a whole is constantly narrowing. This narrowing reflects the state’s policy support for promoting the balanced regional development of the digital economy in recent years, including strengthening the regional cooperation and exploring the model of regional coordinated development of the digital economy. Among other initiatives, speeding up the construction and promotion of the digital economy demonstration zone, at the same time, has also played a large part in the region’s digital economy development based on the regions own resource endowment and industrial structure. In 2019, the variance of the digital economic development index of the eastern, central, northeast and western regions was 119.26, 14.61, 8.81 and 53.30, respectively. Although the development of the digital economy in the eastern region is better, the “digital divide” in the region is significantly larger than that in other regions, which is mainly reflected in the fact that the development level of the digital economy in provinces and cities such as Beijing, Shanghai and Guangzhou are much higher than that in other regions. In the future, how to replicate and spread the digital economy development experience of developed regions to other regions is one of the effective ways to solve the “digital economy gap” among provinces in China.
Table 3 below divides the club classification of the development of China’s interprovincial digital economy into four levels, in which the highly developed and moderately developed areas of the digital economy are all above the national average. The other 19 regions, except Hebei are concentrated in the northeast, western and central provinces and cities, reflecting the fact that the development level of China’s interprovincial digital economy shows significant regional heterogeneity and an obvious “Matthew effect” [ 45 ].
https://doi.org/10.1371/journal.pone.0257365.t003
4 Model construction
4.1 variable definition, (1) dependent variable..
Among them, capital investment K is measured by using the internationally accepted perpetual inventory method to estimate the physical capital stock of each province and city in the sample period. The specific formula is K t = I t +(1− δ ) K t −1 , and the estimation of the physical capital stock at the beginning of the period is K 0 = I 0 /(g+δ). Taking into account that the base period is set earlier, the initial capital stock estimation will have less impact on the later capital stock estimation. This article sets the base period in 2010. I t is the investment amount in year t , expressed by the total fixed asset investment of the whole society, and is converted to 2010 constant prices RMB by the price index; δ is the fixed asset depreciation rate. This article refers to the practice of most scholars to calculate the capital stock of all provinces and cities across the country and sets δ = 9.6%. In the calculation of total factor productivity, different documents have certain differences in the calculation of the value of capital output elasticity a . In the process of calculating the total factor productivity of various provinces and cities, this paper studies the value of capital output elasticity a as 0.6 [ 49 ]. The statistical description of the measured data is shown in Table 3 (TFP). In addition, the capital output elasticity a is set to 0.5 and 0.7 for the robustness test.
(2) Independent variable.
The digital economic index of the core independent variable is calculated by a total of 12 indicators from three dimensions: the infrastructure sub-index, the digital industry sub-index and the digital fusion sub-index. The indicators that reflect the level of technological progress among provinces in China are calculated comprehensively by two indicators, namely, regional patent application authorizations and regional technology market turnover, and the data come from the National Bureau of Statistics. Since the process of index standardization and weighting is similar to the process of measuring digital economic index, we will not repeat it here.
The definitions of relevant variables in this paper are shown in Table 4 , and the descriptive statistics of the variables are shown in Table 5 .
https://doi.org/10.1371/journal.pone.0257365.t004
https://doi.org/10.1371/journal.pone.0257365.t005
4.2 Research methodology
(1) direct effects model..
In model (2), TFP it represents the province’s total factor productivity, DEI it represents the level of digital economy development, and the indicator data come from the previous measurements of the digital economy index. According to the previous analysis, the development of the digital economy can increase total factor productivity by promoting technological progress. Regarding control variables, consistent with existing research practices [ 50 ], technological progress (TP), level of technology development (TD), level of financial development (FD), level of industrial structure (IS), level of opening up (OPEN) and level of financial R&D investment (RD) are added. i represents the province, t represents the year, β 0 represents the intercept term, and μ it represents the random error term.
Using the general benchmark model, this paper tests the impact of the three dimensions of the digital economic index, namely, the basic sub-index (INF), the industrial sub-index (DIND) and the integration sub-index (FUSE), on total factor productivity. The model setting is similar to model (2).
(2) Mediating effect model.
Compared with those in model (2), the controls in this model do not include technical progress indicators, and the definition of other variables is the same as that in model (2).
Regarding whether there is a mediating effect and the extent of the mediating effect, the test process of the mediating effect is as follows: if the coefficient α is significant and both η and λ are significant, the mediating effect is significant; if the coefficient α is not significant or both η and λ are not significant, there is no mediating effect. If the coefficient α is significant, both η and λ are significant, and the coefficient θ is less than the coefficient α , then technological progress is part of the mediation variable, in which case, the mediation effect accounts for ηλ / α . If the coefficient α is significant, both η and λ are significant, but θ is not significant, then there is a complete mediation effect; that is, the effect of the digital economy in improving total factor productivity must be fully exerted through the mediation effect.
5 Results and discussion
5.1 direct effect test.
This paper uses the panel data from 30 provinces and cities in China from 2015 to 2019 for estimation. Since both the LR test and Wald test show that the random disturbance term in the total benchmark model has heteroscedasticity between groups, the Wooldridge test shows that the disturbance term has the first order within the group. Relatedly, this article adopts feasible generalized least squares (FGLS) to estimate the parameters to overcome the influence of heteroscedasticity and autocorrelation and adopts the OLS and GEE (generalized estimating equation) methods to test the robustness. In the OLS model estimation, the time effect and individual effect of the regional sample are controlled. The OLS model estimation in the following is the same. The regression results of the general benchmark regression model and each sub-dimension index are shown in Table 6 (This paper uses Excel software for data statistics and Stata 15.0 software for regression analysis).
https://doi.org/10.1371/journal.pone.0257365.t006
As shown in Table 6 , column (1) shows the FGLS estimation result of the general benchmark model. The digital economy has a significant positive effect on total factor productivity, with an impact coefficient of 0.4551, and is significant at the level of 1%, which indicates that in China’s digital economy, every one percentage point increase in the level of development can directly increase the total factor productivity by 0.4551 percentage points, which proves that the digital economy plays an important role in increasing the total factor productivity. In another core variable, the influence coefficient of technological progress on the improvement of total factor productivity reaches 0.1527, which is also significant at the level of 1%, which can indicate that technological progress has a certain effect on improving China’s interprovincial total factor productivity but its influence coefficient is obviously low. This shows that the development of the digital economy and the improvement of the level of technological progress both have a certain positive impact on the improvement of regional total factor productivity, but the impact of the digital economy is significantly greater than that of technological progress. This shows that the development of the digital economy generates more advantages than just the advantage of raising the level of technological progress and has a deeper and broader connotation and meaning. Regarding the other control variables, the coefficients of influence on total factor productivity in descending order are opening up, financial development, technology development, industrial structure and financial subsidies. The influence coefficient of financial subsidies on total factor productivity is significantly negative. Appropriate government financial subsidies can improve the performance of enterprises, thereby improving the operating efficiency of the entire economic system, while excessive subsidies have a negative impact on corporate performance [ 51 ]. In addition, incidents of collusion between local governments and enterprises are common in China and fiscal subsidies based on political connections can distort the effective allocation of scarce resources in the entire society [ 52 ]. The empirical data in this article show that at this stage, fiscal subsidies have not produced good effects and have even produced certain side effects. Therefore, as subsidies are currently not efficient, it is important to understand how to combine the information technology characteristics of the digital economy to change the previous extensive and rent-seeking financial subsidy model and to provide "precise subsidies" to enterprises with high R&D efficiency to achieve "making full use of money". In addition, columns (5) and (6) display the GEE and OLS regression results of the general benchmark model, respectively. The results show that the digital economy and technological progress play a significant role in improving total factor productivity and both are significant at the 0.01 level. The significance of other control variables is basically consistent with the result of FGLS estimation, which shows that the FGLS estimation of the general benchmark model in this paper has good stability.
The regression results of the effects of the infrastructure sub-index, industrial sub-index and integration sub-index on total factor productivity are shown in columns (2) to (4) of Table 6 . The influence coefficients are 0.2452, 0.0773 and 0.3458. The industrial sub-index is significant at the 5% level, and the infrastructure sub-index and integration sub-index are significant at the 1% level. The influence coefficients ranked from large to small are the integration sub-index, the infrastructure sub-index, and the industry sub-index. Combining the influence coefficient of each sub-index reveals that at present, the role of China’s digital economy in improving total factor productivity is mainly reflected in the integration effect, mainly demonstrated in the rapid development of China’s consumer Internet and e-commerce, and the supporting information infrastructure has also been improved. However, as the core component of the digital economy, the role of the digital industry in promoting total factor productivity is not as good as that of the former two, and the development of basic innovation and information technology depends on the high-quality development of the digital industry. Compared with the digital infrastructure and digital integration and application, the digital industry is a driving force for the development of the digital economy. Therefore, for areas with a good digital infrastructure, it is necessary to focus on the development of the digital industry to realize the transformation of the industrial structure and improve total factor productivity in the future.
5.2 Mediating effect test
The above analysis confirms that the digital economic index and its fractal index play a significant role in improving total factor productivity. Consistent with the previous theoretical analysis, technological progress is an important basis for giving full play to the role of the digital economy in improving total factor productivity, and the continuous improvement of the development level of the digital economy has also had a certain impact on technological progress. Therefore, it is necessary to identify the internal mechanism of the development of the digital economy in improving total factor productivity from the perspective of technological progress. On the one hand, the digital economy is a new form of economic development that occurs after the era of the industrial economy and that is different from the previous economic development model. The digital economy has significant technical effects, scale effects and multilateral effects. Through the use of intelligent technology to improve the previous production, manufacturing, circulation, transaction and other links, compared with previous economic forms, the digital economy generates higher production efficiency. On the other hand, the digital economy is a technology-economic paradigm [ 53 ]. The rapid development of information technology lays a good foundation for the digital economy. The development of a new generation of information technology represented by big data, cloud computing, the Internet of Things and artificial intelligence is a strong support mechanism that gives full play to the efficiency of the digital economy. Therefore, does technological progress play a significant role in the process of facilitating the ability of the digital economy to improve total factor productivity? What is the degree of contribution? Next, this paper uses the stepwise regression estimation method to carry out an in-depth study of these problems.
In testing the comprehensive effect of the digital economy on the improvement of total factor productivity, the intermediary variables of technological progress are excluded. Table 7 , columns (1)~(3) shows the results of the FGLS, GEE and OLS estimation of the effect of the digital economy on total factor productivity. The elasticity coefficients of the digital economy to the improvement of total factor productivity based on the regression results of FGLS, GEE and OLS are 0.5335, 0.3548 and 0.5133, respectively, which are all significant at the level of 0.01. Moreover, in the estimation of the FGLS method for eliminating heteroscedasticity and autocorrelation among variables, the regression coefficient is higher than that of the other two estimation methods, indicating that endogeneity problems will underestimate the comprehensive effects. Therefore, this paper mainly uses FGLS as the main estimation method. Because the influence coefficient of the digital economic index in the comprehensive effect is significantly positive, which accords with the preliminary test step of the intermediary effect, this paper argues according to the existence of the intermediary effect, that is, the assertion that the comprehensive effect includes direct effect and indirect effect, and then analyses the intermediary effect of technological progress.
https://doi.org/10.1371/journal.pone.0257365.t007
Table 7 , columns (4)~(6) calculate the effect of the digital economy on the level of regional technological progress. The regression coefficients of FGLS, GEE and OLS are 0.5133, 0.1676 and 0.5335, respectively, and they all pass the significance test of 0.01. It can be seen that the influence of the development of the digital economy on the level of technological progress in the region is robust, and the results show that the development of the digital economy has a significant positive effect on improving the level of technological progress in the region.
In order to examine the influence mechanism by which the digital economy improves total factor productivity, to test whether technological progress is a complete intermediary variable and whether there are direct effects in its influence process, model (4) brings the direct and indirect effects of digital economic development into the same model for analysis and still uses FGLS, GEE and OLS methods to estimate parameters. As shown in the results of (1), (5) and (6) of Table 6 , the estimated coefficients of technological progress are 0.1527, 0.3132 and 0.1527, respectively, and the regression coefficient is still significant. It can be concluded that the intermediary effect of technological progress is significant. The results of Table 7 (1) show that the total effect of the digital economy on the improvement of total factor productivity is 0.5335, and those of Table 7 (4) show that the effect of the digital economy on the improvement of regional technological progress is 0.5133. The proportion of the intermediary effect of technological progress in the total effect is 14.69%, which shows that technological progress has a certain transmission effect between digital economy and total factor productivity.
5.3 Regional heterogeneity analysis
Existing academic studies and related reports have pointed out that the development level of the digital economy in the eastern region is significantly higher than that in the central and western regions, but there is no practical explanation for the impact of regional heterogeneity on high-quality economic development. Therefore, to study whether there is a significant difference in the effect of the digital economy on high-quality economic development between the eastern region and the non-eastern regions, this paper first adds the interprovincial digital economic development index and the interaction term DEI × dum between the eastern region and the non-eastern region through the use of formula ( 6 ), in which dum = 1 represents the eastern region and dum = 0 represents the non-eastern region (according to China’s National Bureau of Statistics, the eastern region includes 10 provinces and cities, including Beijing, Tianjin, Hebei, Shanghai, Jiangsu, Zhejiang, Fujian, Shandong, Guangdong and Hainan, while the rest of the northeast, central and western regions are divided into the non-eastern regions), and the parameters are estimated.
In addition to studying the effect of the comprehensive index of the digital economy, this article will continue to study the interaction effects between the three sub-dimensions of the digital infrastructure, the digital industry, and digital integration and the regions. The empirical model setting is similar to formula ( 6 ) and will not be repeated here.
The results of (1), (5) and (6) of Table 8 estimate the interaction terms between the digital economy index and the regions by the FGLS, GEE and OLS methods, and the influence coefficients are 0.0968, 0.0619 and 0.0968, respectively; the results are all significant at the level of 1%. This result has good robustness, indicating that the effect of the digital economy on the promotion of total factor productivity is more significant in the eastern region. Due to the early start of the development of the digital economy in the eastern region and because the digital infrastructure and resource elements in the eastern region are more perfect than those in the non-eastern regions, the eastern region has a certain first-mover advantage and comparative advantage. Because the non-eastern regions lag behind the eastern region in the stage of economic development, the lag of the development of the digital economy further limits its positive effect on the improvement of total factor productivity. According to the three sub-indices of the digital economy index, the regression results of the infrastructure sub-index, the industrial sub-index, the integration sub-index with the regional interaction items are 0.0677, -0.4376 and -0.2336, respectively. This paper uses the “digital access gap”, “digital industry gap” and “digital application gap” to represent these three interactive items. The influence coefficient of the digital access gap is the largest, that of the digital application gap is the second largest, and that of the digital industry gap is the smallest. On the one hand, there is no strict regional boundary for digital applications in China. Due to Internet platform products or services available throughout the country, enterprises and individuals in all regions can access digital application products or services. The "digital divide" is more reflected in the “digital access gap”, which once again verifies that the eastern region, due to its more perfect digital infrastructure, has greater advantages in the development of the digital economy in improving total factor productivity. According to the 45th Statistical report on the Development of Internet Networks in China, regarding download speeds in the eastern, central and western regions, the average download rate of 4G mobile broadband users in the eastern region reached 24.60 megabytes at the end of 2019, while the central region and the western region were lower by 0.93 Mbit/s and 1.58 megabytes, respectively, showing an obvious digital infrastructure gap. On the other hand, there is also a significant difference in the impact of the “digital industry gap” on the promotion of total factor productivity between the eastern and non-eastern regions. Regarding the progress of industrial development, the eastern region has always been at the forefront and ahead of the non-eastern regions, and digital transformation is the only way to build a modern industrial system. According to the 2018 report on the Digital Development of Chinese Enterprises released by the International Data Company (IDC), China’s retail, entertainment, financial and other consumer enterprises have experienced a high degree of digital transformation. While most of these enterprises are concentrated in the eastern region, the degree of digitization of manufacturing and resource industries is relatively low, and most of the manufacturing industries with successful digital transformation are concentrated in the eastern region. On the other hand, the enterprises with digital transformation demonstrated by individual cases and in some local areas are mostly concentrated in the non-eastern regions, resulting in a significant "digital industry gap" between the eastern and non-eastern regions.
https://doi.org/10.1371/journal.pone.0257365.t008
The above analysis shows that in eastern China, the impact of digital economic development on the improvement of total factor productivity is more significant than that in non-eastern regions. In view of the great differences in economic development and digital economy development in different regions of China, this paper divides the samples into eastern, northeastern, central and western regions to examine the impact of digital economy development in different regions on the improvement of total factor productivity. In addition, technological progress may play a more significant intermediary role in different regions.
As shown in Table 9 , the influence coefficients of the digital economy index on total factor productivity in the eastern, northeastern, central and western regions are 0.6170, 0.3398, 0.1267 and 0.3688, respectively, and they are all significant at the 1% level. These results show that the development of the digital economy has a significant impact on the improvement of total factor productivity, and it also proves the robustness of the previous results. In addition, the influence coefficient of the digital economic index in the eastern region is significantly larger than that in the other three regions, which also proves that the better level of economic development in the eastern region is an important factor in the higher level of digital economic development in that region. The influence coefficients of technological progress on total factor productivity in the eastern, northeast, central and western regions are 0.0193, 0.1272, 0.8072 and 0.2621, respectively. The influence coefficient of technological progress on total factor productivity in the central region is slightly higher than that in the other three regions. One of the possible explanations is that the marginal impact of technological progress on the improvement of total factor productivity in the central region was greater than that in the other regions during the sample period. The reason and mechanism of this need to be further studied.
https://doi.org/10.1371/journal.pone.0257365.t009
As shown in Table 10 , the influence coefficients of the digital economy index on technological progress in the eastern, northeast, central and western regions are 0.5425, 0.2472, 0.4531 and 0.2986, respectively, and they are all significant at the 1% level. These results show that the development of the digital economy has a significant impact on improving the level of technological progress, but the influence coefficient in the eastern region is significantly greater than that in other regions. This shows that the benign mutual promotion mechanism between digital economic development and technological progress in the eastern region is better than that in the other three regions. In addition, in the eastern, northeastern, central and western regions, the intermediary effects of technological progress in the influence mechanism promoting the digital economy to improve total factor productivity are 1.70%, 9.25%, 28.89% and 21.22%, respectively. However, at the overall level of China, the intermediary effect of technological progress in promoting the improvement of total factor productivity in the digital economy is 31.31%. It can be seen that the mediating effect of technological progress is more significant in the central and western regions. From another perspective, it shows that the central and western regions, which are at the middle level of digital economy development, are more important to improve the level of technological progress in the process of achieving high-quality economic development through the development of digital economy. In the future, how to effectively deal with the imbalance of regional economic development aggravated by the “digital economic gap” is the issue that must be addressed to realize the balanced development of China’s economy.
https://doi.org/10.1371/journal.pone.0257365.t010
5.4 Robustness test
To verify the stability of the results, this paper assumes that the core parameters of total factor productivity are a = 0.5 and a = 0.7; the entropy method and equality method are used to calculate the digital economic development index, and the robustness test from the above four aspects is conducted. Table 11 shows the results of the four tests, and the regression results are basically consistent with the estimated results of the previous general benchmark model, indicating that the conclusions of this paper are robust.
https://doi.org/10.1371/journal.pone.0257365.t011
5.5 Endogenous test
The benchmark regression results initially verified the promotion effect of the digital economy on total factor productivity, but there may be endogenous problems in the process of model causality identification. One source of endogeneity is reverse causality, that is, while the development of the digital economy promotes the high-quality development of the regional economy, the high-quality development of the regional economy is in turn promoting the development of the digital economy. In response to this problem, this article refers to the research [ 54 ], and uses the one-period lag of DEI as an independent variable for regression. Three methods of FGLS, GEE, and OLS were used to endogeneity testing. And the regression results are shown in Table 12 . The influence coefficients of the digital economy index on total factor productivity are 0.4499, 0.3048, and 0.4499 respectively, and they are all significant at the 1% level. This result is very robust. The regression results show that after considering the possible endogenous problems of the model, the development of the digital economy still has a significant positive role in promoting total factor productivity, which further supports the above conclusions. The instrumental variable estimation results in this paper are valid, and the robustness of the research hypothesis is further supported.
https://doi.org/10.1371/journal.pone.0257365.t012
6 Conclusions
As China’s economic development has entered a new normal status, the digital economy has become one of the important ways to drive high-quality economic development. Academic circles have not comprehensively discussed the effect and mechanism in the impact of the digital economy on high-quality economic development. For this reason, this paper empirically examines the effect of the digital economy on high-quality economic development, demonstrates its transmission mechanism from the perspective of technological progress, and draws the following main conclusions.
From 2015 to 2019, the development level of China’s digital economy had been showing an increasing trend year by year, in which the increase in the sub-index of the digital infrastructure is more obvious. Besides, the growth trend from the digital fusion effect has been basically consistent with the development level of the digital economy, but the development of digital industry has been relatively slow. Therefore, it is necessary to vigorously develop the digital industry. Attention should not only be paid to the development of the consumer Internet but a focus should also be placed on the digital transformation of the industrial Internet and the real economy; the digital industry must be gradually released to lead the digital economy and high-quality economic development. Firstly, the construction of the digital infrastructure should be consolidated, the deep integration of new infrastructure, such as 5G, big data, the Internet of Things, artificial intelligence and industrial networks, should be actively promoted, and traditional enterprises should be supported to carry out sustainable digital infrastructure construction and transformation. Secondly, China should deeply cultivate the development of the industrial Internet and promote the construction of a modern industrial system. It is necessary to establish a perfect cross-boundary capacity and mechanism of the industrial Internet and to create a good environment for cooperation between physical enterprises and Internet enterprises by breaking down various industrial, regional and operational barriers. A technical system, standards and norms, business models and competition rules must be established and adapted in an integrated development.
The development level of the digital economy in eastern China is much higher than that in other non-eastern regions, and the development of the digital economy in Beijing, Guangdong, Shanghai, Jiangsu and Zhejiang is in the first echelon of the country. In addition, in the eastern region, the marginal contribution of the digital economy to the improvement of total factor productivity is significantly higher than that in the non-eastern region, which has much to do with the first-mover advantage and comparative advantage in the development of digital economy in the eastern region. From the perspective of sub-dimensions, regarding the role of regional heterogeneity in the effects of the digital economy’s promotion of high-quality economic development, the influence coefficient of the digital infrastructure sub-index is the largest, followed by the industrial sub-index and finally the integration sub-index. This shows that there is an obvious “digital access gap” between the eastern region and the non-eastern region, while the “digital industry gap” is the smallest among regions. It is necessary to narrow the “digital economy gap” between regions. While the country is expanding the size of the digital economy as a whole, all regions should develop a digital economy industry according to local conditions and should highlight the comparative advantages of the digital economy industry in each region. Firstly, on the basis of ensuring the key links and core enterprises of the digital economy industrial chain in the eastern region, China should promote the gradient transfer of digital economic production capacity in developed areas to the central, western and northeastern regions in an orderly manner. The digital economic development experience of developed regions should be replicated and spread to other regions. Secondly, the government’s governance system should be improved, and the modernization level of national digital governance should be enhanced. The empirical results of this paper show that financial R&D subsidies have no significant positive effect on the improvement of technological progress and the development level of the digital economy and even affect the market competition mechanism and lead to inefficiency. Therefore, it is necessary to clarify the position of the government in leading the development of the digital economy, to draw a clear distinction between the market and enterprises, to formulate a negative control list of financial R&D investment, and to accurately subsidize basic and nonmarket R&D. The financial subsidies that affect market competition should be strictly controlled.
The comprehensive index of the digital economy, the sub-index of digital infrastructure, the sub-index of the digital industry and the sub-index of digital fusion all have a significant positive impact on regional total factor productivity, which confirms the importance of developing a digital economy in order to achieve high-quality economic development. The influence coefficients of the three sub-indices ranked from large to small are as follows: the integration sub-index, the infrastructure sub-index and the industry sub-index. In addition, technological progress plays an important role in the influence mechanism of the digital economy on high-quality economic development, and its intermediary effect can explain approximately 1/3 of the total effect. In this regard, it is necessary to highlight the role of technological progress, attach importance to technological innovation at the bottom of the digital layer, and to facilitate sustainable innovation and development. First, Break through the core technological bottleneck with a new strategy of open thinking and comprehensive endogenous capabilities for independent innovation, and achieve a dynamic balance between core independent control and opening up. Second, based on the strategy of expanding domestic demand, China should highlight the social requirements for the development of digital core technology, break the institutional and institutional obstacles that hinder the circulation of talent, capital, and other innovative elements in the country, and rely on high-tech enterprises. China should further promote the integration of industry, university and research and solve the transformation from “science” to “technology” and then to “application”.
Supporting information
https://doi.org/10.1371/journal.pone.0257365.s001
S1 Appendix. The process of data standardization and weight determination.
https://doi.org/10.1371/journal.pone.0257365.s002
- View Article
- Google Scholar
Thank you for visiting nature.com. You are using a browser version with limited support for CSS. To obtain the best experience, we recommend you use a more up to date browser (or turn off compatibility mode in Internet Explorer). In the meantime, to ensure continued support, we are displaying the site without styles and JavaScript.
- View all journals
- My Account Login
- Explore content
- About the journal
- Publish with us
- Sign up for alerts
- Open access
- Published: 11 September 2023
Research on the influence of digital finance on the economic efficiency of energy industry in the background of artificial intelligence
- Qiao He 1 &
- Ying Xue 2
Scientific Reports volume 13 , Article number: 14984 ( 2023 ) Cite this article
2188 Accesses
1 Citations
Metrics details
- Environmental sciences
- Environmental social sciences
- Solid Earth sciences
China's economic growth has reached a new plateau. It is no longer appropriate to use the old economic growth model, which relied on labor, land resources, mineral resources, and other economic considerations. Under the background of artificial intelligence, high-quality economic development is an inevitable trend. A new financial paradigm called "digital finance" integrates financial services with information technologies. Digital financial technology is thought to be a crucial foundation for fostering high-quality and sustainable economic and social development since it may offer more economic entities reduced cost of capital and more realistic financial service skills than in traditional financial models. In the era of artificial intelligence, how to reasonably release the momentum of digital finance for China's sustained economic growth has become a hot topic of discussion at this stage. This paper studies the impact of digital finance on the economic efficiency of the energy industry in the context of artificial intelligence. Relevant metrics were also calculated. The findings revealed that: The benchmark regression result of digital finance on the efficiency of the green economy was 0.4685 before adding the main restrictions; the benchmark regression result of digital finance on the efficiency of the green economy was 0.2243 after adding the main constraints. As a result, data finance had a favorable impact on the effectiveness of the green economy.
Similar content being viewed by others
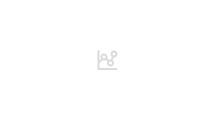
The impact of artificial intelligence on employment: the role of virtual agglomeration
Yang Shen & Xiuwu Zhang
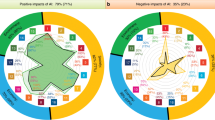
The role of artificial intelligence in achieving the Sustainable Development Goals
Ricardo Vinuesa, Hossein Azizpour, … Francesco Fuso Nerini
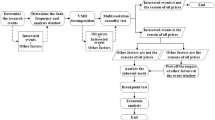
The impact of Russia–Ukraine war on crude oil prices: an EMC framework
Qi Zhang, Yi Hu, … Shouyang Wang
Introduction
The coordinated growth of the real economy and the digital economy takes the conventional "reverse integration" path, with the financial sector serving as the first example of its transformation and development characteristics in the tertiary sector. Digital finance generally refers to the use of electronic information technology by traditional financial institutions and online businesses to carry out new financial services like payment, project investment, and equity financing. The limitations on time and space between product transactions and financial services have been eliminated by the quick development of digital finance. In the era of artificial intelligence, what is the role of the rise and application of digital finance in the critical period of innovation in promoting strategic planning. Whether it can fill the shortcomings of the traditional financial system and improve the efficiency of urban green economic development and the financial market department's strong support and promotion of innovation and manufacturing, and better support the economy of the energy industry, requires further review and debate. Digital financial technology can provide more accurate Market trend forecast and energy price forecast by processing large-scale data and applying advanced data analysis technology. This will help energy companies make more intelligent decisions, optimize resource allocation, reduce production and operating costs, and thus improve Economic efficiency. The application of digital financial technology can improve the Economic efficiency of the energy industry, improve the efficiency of resource utilization, reduce waste and promote the sustainable development of the energy industry.
Literature review
Numerous professionals and academics have always focused their research on strategies to increase the effectiveness of the urban green economy. In order to create a model that could be used for urban green economy planning, Liu T enhanced the conventional algorithm and merged the principle of machine learning algorithm. Efficiency indicators for the green economy were evaluated in terms of input, anticipated output, and unexpected production. Comparison and analysis were done on the green efficiency determined using the relaxation value calculation model. The study's findings demonstrated that the model could be used in the design phase of urban green planning and that it had specific effects 1 . China's green economic efficiency and green total factor productivity were assessed and examined by Gao X. Furthermore, the shortcomings of conventional clustering techniques in high-dimensional data clustering were highlighted by outlining the properties of high-dimensional data. A sampling and residual squared-based density peak clustering technique was put forth. The experimental comparison on the data set revealed that in terms of time complexity and clustering outcomes, the modified algorithm outperformed the delayed procedure call approach 2 . Sarcheshmeh M examined the performance of urban green space in terms of social and economic indices in the Mashhad metropolitan region. 15 social questions and 5 economic questions from the research questionnaire were tested and examined using the SPSS22 program. The findings demonstrated that there was no appreciable impact on the management effectiveness of the urban green space sector in the city of Mashhad. From the perspectives of citizens and managers, several features of the social index were rated as desirable 3 . In order to examine the dynamic changes in the economic effectiveness of urban land use in South Korea at the regional level and to determine whether it would be feasible to implement the green belt policy, Yongrok C used the ecological efficiency measurement model. In order to increase the economic benefits of urban land use and execute sustainable green space management, more performance-oriented policy solutions were advocated 4 . These studies do have some impact on increasing the effectiveness of urban green economy and urban planning, but digital finance has received far too little attention. The market for digital finance is quickly taking over with the pace of the new economic system. The city's long-term development would have an effect on how effective the urban green economy is.
There are more research on the direct or indirect effects of digital finance on economic growth than there are on the effect of digital finance on the effectiveness of urban green economies. Based on the database for the growth of digital financial inclusion and the China Family Panel Studies, Xie W investigated the relationship between coastal rural residents' entrepreneurship and the development of China Family Panel Studies (CFPS). The empirical findings indicated that a crucial factor in encouraging rural entrepreneurship was the thorough development of digital financial inclusion. The monetary capital index and the payment index both significantly boosted rural inhabitants' entrepreneurial activity. The study also discovered that the effects of digital financial inclusion on rural residents' entrepreneurship exhibited signs of geographical variation 5 . In the context of economic digitization and the development direction of contemporary financial technology legal supervision, Barykin S determined the function of digital finance in the financial system. By adding new features of digital assets, the digital financial cube might be expanded to match the level of openness of industrial firms in the future Industry 4.0 technological framework 6 . The long-term causal impacts of digital financial inclusion on economic growth in sub-Saharan Africa were investigated by Thaddeus K J. The study made use of quarterly data from 2011 to 2017 and a sample of 22 sub-Saharan African nations. The findings indicated a long-term causal link between digital financial inclusion and economic growth in sub-Saharan Africa, with the causal relationship running one way from economic growth to inclusion in the latter 7 . Rastogi S set out to investigate how unified payment interface affects financial inclusion, economic development, and financial literacy of the underprivileged in India. He discovered that financial literacy was being impacted. Financial stability and trust both served as partial moderators of the significant associations between digital financial inclusion and economic development as well as the significant link between financial literacy and financial inclusion. This fostered financial inclusion and economic growth for the underprivileged in addition to supporting financial literacy 8 . Lin Boqiang uses the non radial direction distance function to build green Economic efficiency indicators that can evaluate cities at prefecture level and above in China under the super efficiency framework, and further empirically studies the impact of economic agglomeration on green Economic efficiency. To solve the endogenous problem caused by reverse causality between economic agglomeration and green Economic efficiency 9 .
The perfect combination of digital technology and financial services has created a new financial service model. With the help of intelligent digital technology, digital finance can provide lower capital cost and faster service mode for the real economy, provide financial services with "high efficiency, convenience and sustainable commercial services" for the energy industry, and complete the unification of objectivity and precision of financial services. This paper discusses the influence of digital finance on the economic efficiency of the energy industry under the background of artificial intelligence, and aims to provide theoretical guidance for the improvement of the green economic efficiency in the energy industry.
The influence mechanism of digital finance on the economic efficiency of the energy industry
New energy technologies include solar power generation, water energy, wind energy, tidal energy, sea surface temperature difference energy, wave energy, firewood, peat soil, biochemical material energy conversion, geothermal energy, tar sand, etc. At this stage, it is generally recognized that new energy and renewable resources are based on the development trend of new technology application, and gradually change the development and utilization of renewable resources. The traditional fossil energy resources with environmental pollution problems and limited total amount should be replaced by new energy sources that will not be limited by the total amount and the utilization of the recycling system. The key development areas include solar power generation, tidal energy, hydrogen energy and wind energy.
The new energy industry is the exploration, development and utilization of new energy. It uses social methods to achieve effective utilization and popularization, including the whole process of scientific research, industrial utilization, production, manufacturing and operation. It is a high-tech that commercializes solar power generation, wind energy, bioenergy, etc. From the perspective of the characteristics of the industrial chain, the new energy industry is to replace the new industries with strategic status represented by fossil energy, and has extremely important obligations in replacing fossil energy, promoting economic growth, protecting the environment, and building a harmonious society; From the perspective of the whole industry chain, the new energy industry can be divided into energy supply, product research and development, investment and manufacturing, transportation and trading.
The Corona Virus Disease 2019 pandemic has had a major impact on the traditional financial services provided by financial institutions, but it has also accelerated the digital transformation of these services. According to the statistics and analysis of the China Asset Appraisal Association, during the epidemic period, the average service item replacement rate of online banking reached 96%. Despite the epidemic's considerable effects on small and micro businesses and traditional financial "long-tail clients", However, under the background of the intelligent era, the development speed of digital banking is enough to solve the problems of these groups. Through "zero contact" to provide them with low-cost, convenient and fast service projects, especially the contact-free loan has become an important means to help the sustainable development of the energy industry 10 .
The development of digital finance requires a complete institutional system, and the institutional system of digital finance is the financial ecosystem, which is composed of the main body of the ecosystem and the financial ecological environment. The close combination of the two can produce a regular financial ecosystem with internal logic and self-improvement. Judging from the current overall situation of China's financial institution management system, it has basically formed a large digital financial service ecological chain dominated by banking, Internet banking, non-bank finance, and large and medium-sized financial high-tech companies with electronic payment system, integrity management system, legal norms as infrastructure and institutional guarantee, which is dominated by the "one committee, one bank, two committees and one bureau" supervisory agency 11 , 12 . A schematic representation of the structure of the digital financial ecosystem is given in Fig. 1 .
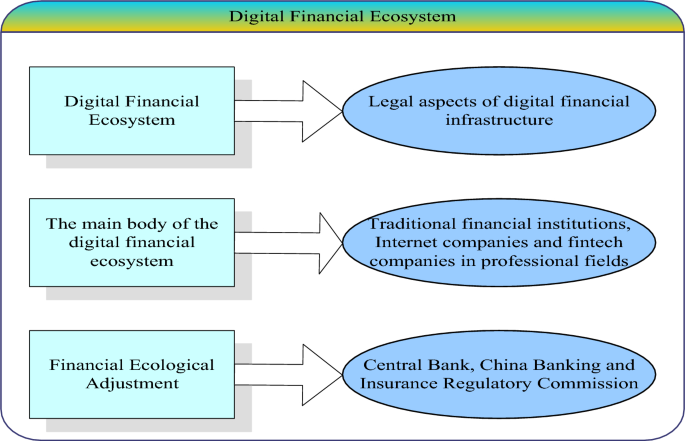
Digital financial ecosystem.
At this point, a significant trend is the close integration of digital technology with finance. In the era of artificial intelligence, digital technology is playing a unique and important role in modern finance. The following points mostly highlight the benefits of digital finance: Firstly, by increasing financing channels, the threshold for financial services has been lowered; secondly, by greatly reducing service prices, comprehensive financial services have achieved sustainable development; thirdly, the personalized financial services can better meet the various requirements of different users; the fourth is to help reduce information asymmetry and provide new risk management methods 13 .
According to different levels of financial functions, digital finance can be divided into three categories: basic functions, leading functions and derivative functions. Figure 2 shows the mechanism of digital finance on the efficiency of urban green development. There are three behavioral paths for the above three functions. The first is digital finance → intermediary services → inclusive utility. Digital finance uses digital information technology to manufacture and expand the role of finance. The network effect of digital technology expands the boundaries of traditional financial services and reduces the service cost of traditional finance. The scale and economic characteristics of digital finance reduce the entry threshold and related costs for innovative enterprises. At the same time, by relying on digital technology, the ability to obtain data and analyze information has been greatly improved and the information asymmetry and the cost of credit intermediary companies have been reduced, and the credit environment has been optimized. After building a three-dimensional credit image based on enterprise big data and cloud technology, sporadic enterprises and start-up companies that are difficult to obtain the support of traditional credit services would obtain a high probability of credit. In order to increase the effectiveness of the urban green economy, the development of digital finance would also help traditional finance change and grow. It would also make full use of the complementary roles that traditional finance and digital finance play in advancing economic growth. Therefore, digital finance will promote the development of traditional finance, and will promote the economic development of the energy industry, and achieve the effect of improving the economic efficiency of the energy industry 14 , 15 .
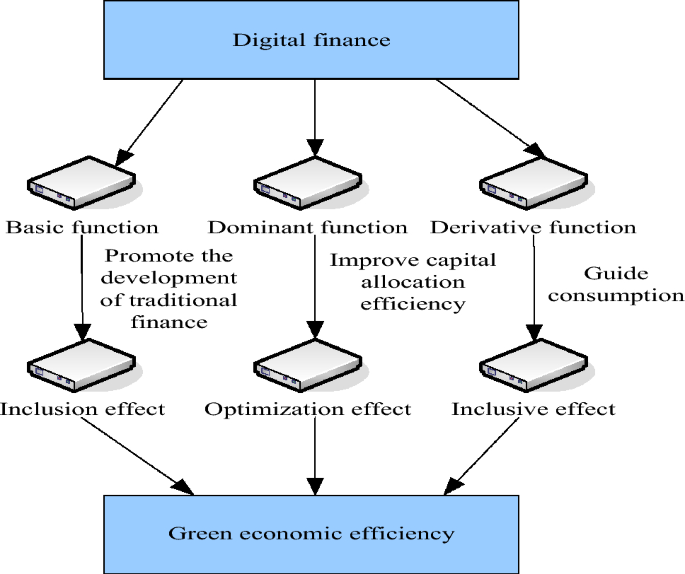
The impact of digital finance on how well urban green development is carried out.
The second is digital finance → resource allocation service → upgrade utility. Resource allocation service is the core role of finance and an excellent way to correctly guide use value. On the one hand, the birth of digital finance has promoted competition among financial formats and enhanced the charm of folk capital and the financial system, and improved the efficiency and capability of capital allocation. The use of artificial intelligence and electronic information technology can better match the investment needs and financing needs, reduce the financing pressure of the energy industry, and make the capital used more efficiently and quickly for innovation. On the other hand, the circulation of capital factor commodities has been improved. For a long time, in the factor market, the government department has the dominance and dominance of the vast majority of manufacturing factors, and there may be behaviors such as abuse of power. In addition, the popularity of local protectionism and the emergence of administrative systems have resulted in serious market segmentation. The inconsistency and segmentation of the elements of the sales market make some enterprises, especially state-owned enterprises, lose the driving force of "self-innovation". This harms the development of the urban green economy's efficiency. To provide enough financial factors for the supply-side structure's green development, Digital finance enables the energy industry to overcome regional barriers and enhance the environment for the free flow of capital. Therefore, by enhancing and upgrading the efficiency of regional capital element allocation, data finance can achieve the effect of boosting the efficiency of urban green economy 16 .
The third is digital finance → redistribution of finance → inclusive utility. The rapid development of inclusive finance, on the one hand, helps low-income people get rid of poverty and become rich, which improves the level of per capita consumption and promotes economic transformation and upgrading; on the other hand, with the expansion of the number of netizens and network coverage and the rapid rise of e-commerce and Internet consumer finance, the consumption structure of urban residents has also gradually changed. The demand-side consumption capacity and consumption structure have been upgraded, and the energy industry has increased its demand for high-quality products. This has prompted the energy industry to expand the scope of its technology investment and product development efforts, and to encourage the growth of a local green economy. Therefore, digital financing encourages the energy industry to expand technology investment and product research and development, which has the effect of improving the efficiency of urban green economy 17 .
The energy industry is an indispensable part of economic development. Digital finance provides loans to small and medium-sized energy enterprises to meet the financing needs of small and medium-sized energy enterprises, thus stimulating regional economic growth. However, these small and medium-sized energy enterprises are struggling with financial problems and high financing costs. Only a small number of enterprises can apply for loans from financial institutions through official channels, and other enterprises are under pressure of capital loans. The growth of financial inclusion through digital means has reduced borrowing costs and simplified processes. By providing special loans to such enterprises to help them improve their financing and risk management capabilities, it will help improve their profitability and ultimately improve China's economic growth rate 18 , 19 .
If the capital supply cannot keep up, there will be a lock-in effect, and it is imperative to get rid of this inefficient equilibrium state. The basic strategy is to provide specific capital elements for the energy industry, so the assistance of participating banks is essential, and micro loans for small and medium-sized energy industries can help them achieve higher output. Continuous investment in capital and technology will reduce marginal costs, which will have an impact on increasing output and income 20 , 21 . As shown in Fig. 3 , the structure of micro credit's anti lock support effect.
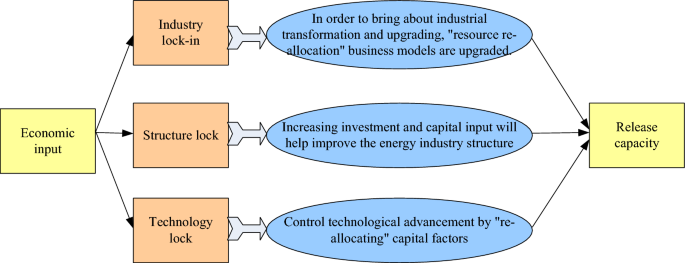
Anti-lock-in support effect structure diagram of microfinance.
This paper discusses the impact of digital finance on the economic efficiency of the energy industry in the context of artificial intelligence. The calculation formula of some indicators related to the measurement of the economic efficiency of the energy industry is as follows:
\(T\) -set of control variables; \({GTFP}_{au}\) -Green economic efficiency of energy industry; \({df}_{au}\) -digital finance; \({df2}_{au}\) -square term of digital finance; \({\omega }_{au}\) -disturbance term; \({\theta }_{a}\) -time fixed effect
\({m}_{au}{^\prime}\) -a collection of independent variables; \({\mathrm{g}}_{\mathrm{au}}\) -threshold variables
\(distrk\) -degree of capital misallocation
\({\mathrm{lngdp}}_{\mathrm{au}}\) -degree of capital distortion
\({MP}_{au}\) -margin of capital
\(\mathrm{d}\) - \(\mathrm{d}\) kinds of inputs; L-L kinds of expected outputs; J-J kinds of undesired outputs; \(\upgamma \) -green total factor productivity efficiency value.
Restrictions:
Let the formulas be:
\({\mathrm{cap}}_{\mathrm{au}}\) -fixed capital stock of the whole society; \({\propto }_{\mathrm{a}}\) -capital depreciation rate
\({\mathrm{cap}}_{\mathrm{a},0}\) -cap initial capital stock; \({\mathrm{o}}_{\mathrm{a}}\) -cap average annual growth rate.
Empirical study on the impact of digital finance on economic efficiency of energy industry
In order to explore the impact of digital finance on the economic efficiency of the energy industry in the context of artificial intelligence, we calculated some indicators of the economic efficiency development level of the energy industry 22 , 23 . Kao (1999) Panel data cointegration test uses the correlation information between individuals to decompose Panel data into inter individual mean and intra individual changes. If the inter individual mean is non-stationary and the residual term is stationary, then the existence of cointegration can be verified. The results are as follows:
As shown in Fig. 4 , the change index of green economic efficiency development of energy industry in some cities of China from 2010 to 2020. We selected 20 cities in China for data analysis. The standard deviation is used to measure the Statistical dispersion of a group of data. The larger the standard deviation, the higher the volatility of the data. The average is the average of the green Economic efficiency development index. From the average and median, the average development level of green economic efficiency of these energy industries has increased from 0.1782 in 2012 to 0.3891 in 2020, and the median has also increased from 0.1342 in 2012 to 0.3247 in 2020. Both are rising year by year. From these two indicators, the green economic efficiency level of the energy industry shows a trend of doubling, this also means that the green economy development level of the energy industry has made a qualitative leap. The coefficient of variation did not change significantly from 2010 to 2020, with a value of 0.5687 in 2010 and 0.5682 in 2020. From the perspective of range and coefficient of variation, the range describes the difference between the highest level and the lowest level. In 2012, the range value of green economic efficiency of the energy industry was 0.4213, while in 2020, the range value of green economic efficiency of the energy industry was 0.8925, which also shows an increasing trend year by year. This means that the gap between the development levels of green economy of the energy industry is increasing year by year, while the difference between the extreme values from 2018 to 2020 shows a trend of slowing growth, this also shows that we are also increasing the level of green economy development in economically backward energy industries. It can be seen from the figure that the coefficient of variation of the green economic efficiency of the energy industry fluctuates, but it does not change much, and even shows a downward trend, which also shows that the green development level of the energy industry does not show a development trend of two-level differentiation.
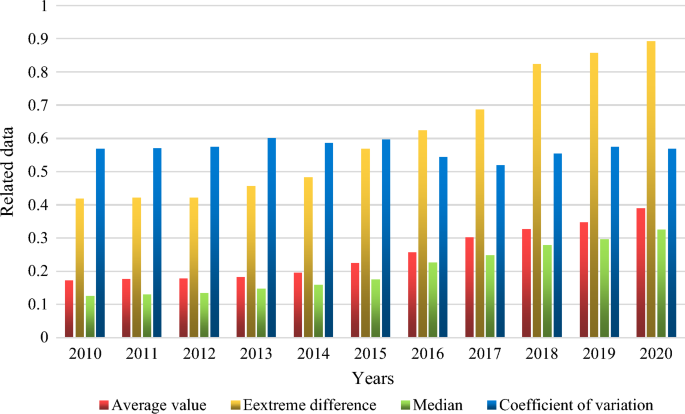
Change index of green economic efficiency development of energy industry in some cities.
Regression analysis is conducted with or without control variables to examine the robustness of digital finance on the effectiveness of green economy in the energy industry 24 . The regression results of the efficiency standards of green economy and digital finance in the energy industry are shown in Fig. 5 . Where, A represents the result of basic regression without major control factors, and B represents the result of benchmark regression including major control components. It can be seen that before adding the main restrictions, the benchmark regression result of digital finance on the effectiveness of green economy is 0.4685. After the main limiting factors are included, the benchmark regression result of the effectiveness of digital finance on the green economy is 0.2243. Therefore, data finance has a beneficial impact on the effectiveness of the green economy. The green development level of the energy industry does not show a trend of two-stage differentiation, and the benchmark regression results slightly decrease after adding limiting factors. Digital finance will affect the green development level of the energy industry.
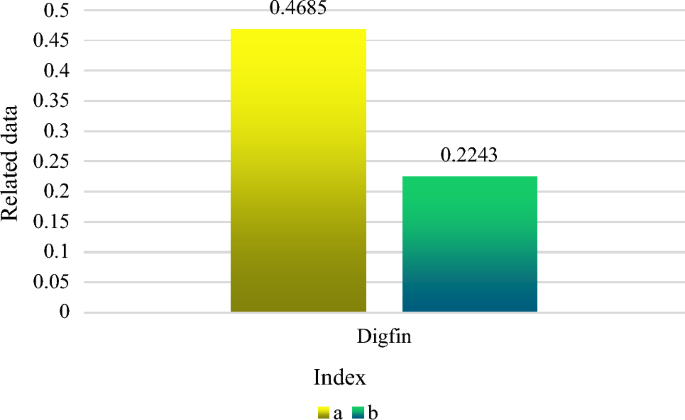
Digital finance and green economy efficiency benchmark regression results.
The benchmark regression coefficient results of the influence of pertinent variables on green economic efficiency are shown in Table 1 . It is clear that the benchmark regression coefficients for improving industrial structure, economic development level, and income from both the public sector and higher education are all positive and pass the 5% significance level test. This demonstrates how investing in financial education, upgrading the industrial structure, and the degree of economic development all help the green economy grow and become more efficient. Despite being positive, the benchmark regression coefficient of environmental legislation on green economic efficiency fails the test of significance. The expense of reducing environmental pollution has perhaps increased, which forces businesses to implement relevant technology advancements. The benchmark regression coefficient for openness to the effectiveness of the green economy is negative, and thus failed the significance threshold test. This may be because the entry of foreign high-tech has raised pressure on environmental governance by bringing about not only economic development but also an industrial chain that produces a lot of pollution and uses a lot of energy.
Choosing cross-sectional analysis with fixed effects rather than random effects means that there are fixed differences between individuals, and the impact of these differences on variables is constant. This fixed effects model assumes that individual specific factors have a significant impact on the observed variables, and these factors are fixed during the observation period.
The computation of the conduction effect is shown in Fig. 6 . They are digital finance-green economy development efficiency, digital finance-scientific and technical innovation-green economy efficiency, and digital finance-green economy efficiency as a whole. The conduction line of direct effect is digital finance-green economy efficiency. It can be seen that the computed value of the direct relationship between digital finance and green economic efficiency is 0.1698, indicating that the growth of urban green economic efficiency would be directly impacted by the development of digital finance. The calculated indirect effect value is 0.0413, which suggests that digital finance can boost technological innovation to make cities more environmentally friendly by saving energy and lowering consumption and pollution. The level of green economic growth can be raised while industrial upgrading is encouraged. The total effect of digital finance on the effectiveness of green economy in the energy industry is the sum of its direct effect and indirect effect, of which the intermediary effect accounts for 19.56% of the total effect.
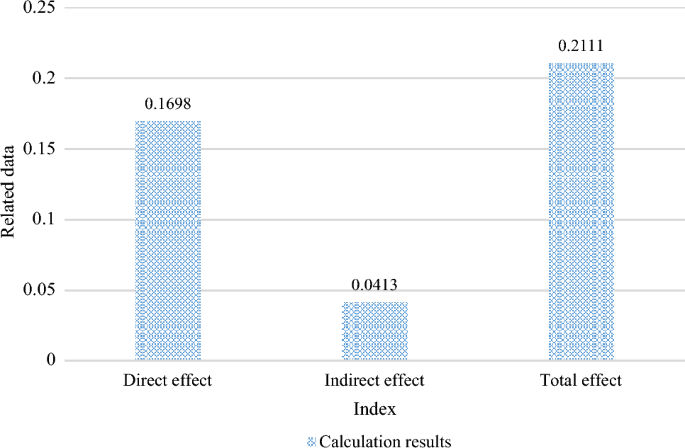
Conduction effect calculation results.
The panel quantile estimation can assess the effect of digital finance on it under each quantile based on the distribution of green economy efficiency levels. The efficiency of the green economy and digital finance are shown in Fig. 7 as the panel quantile regression findings. It is can be seen that for the five quantiles, the estimated coefficient of digital finance climbs as the quantile increases from 0.3042 for the 10% quantile to 0.4276 for the 90% quantile. The increase in the favorable effect is 0.1234, and the significance threshold is 1%. In other words, digital finance has a good effect on the effectiveness of the green economy, and the promotion effect would get stronger as the quantile value rises. This does not help digital finance increase the efficiency of the green economy. However, as the green economy expands and digital infrastructure continues to advance, the beneficial role that digital finance plays in fostering the growth of the green economy would only grow.
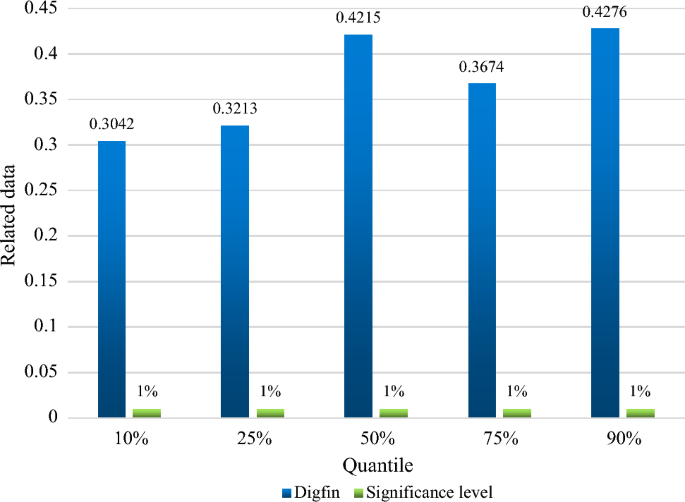
Panel quantile regression results of digital finance and green economy efficiency.
In the panel Quantile regression analysis data of digital finance and green Economic efficiency, the estimation coefficient of digital finance is constantly improving, and the significance threshold has always been 1%, so the rise of quantile value will make the promotion of green Economic efficiency stronger.
Conclusions
This paper analyzes the impact of digital finance on the green economic efficiency of energy industry in the context of artificial intelligence, and evaluates the green economic performance of energy industry in some cities from 2010 to 2020. The empirical research results show that the rapid development of digital finance will significantly improve the efficiency of green economy in the energy industry, and show diversity with the change of city size and industrial development level. Digital finance has the synergistic effect of independent innovation and ecological compensation. Through independent innovation and environmental security management, we can jointly improve the efficiency of green economy. Based on this paper, the following suggestions are put forward: encourage financial institutions, insurance and other traditional finance to transform to digital, use data technology to safeguard the traditional financial system, and accelerate the construction of intelligent facilities in various regions; Give full play to the coordinating role of the financial technology service management system in the introduction of innovation policies, patent applications and other aspects. Accelerate the cooperation between the government and the digital financial platform, and give full play to the aggregation effect of financial markets and policies on independent innovation. Make full use of the ecological compensation effect of digital finance on production units, promote financial innovation through joint development of digital finance, and promote the growth of small and medium-sized enterprises in the upstream and downstream of the green industrial chain and supply chain. The government can formulate policies to encourage energy companies to adopt digital financial technologies, such as blockchain, Big data analysis and artificial intelligence, to improve efficiency and reduce costs. For example, the government can provide tax or subsidy incentives to encourage enterprises to invest in the research and application of digital technology. At the same time, it is necessary to prevent losses caused by excessive economic leverage, so that data finance can better provide energy for the urban real economy.
Data availability
Datasets generated and/or analyzed during the current study are available from the corresponding author on request.
Liu, T., Xin, B. & Wu, F. Urban green economic planning based on improved genetic algorithm and machine learning. J. Intell. Fuzzy Syst. 40 (4), 7309–7322 (2021).
Article Google Scholar
Gao, X. Urban green economic development indicators based on spatial clustering algorithm and blockchain. J. Intell. Fuzzy Syst. 40 (3), 1–12 (2020).
Google Scholar
Sarcheshmeh, M., Khakpoor, B. A. & Shokuhi, M. A. Analysis of economic and social indicators in optimizing the performance of urban green space management (a study of Mashhad metropolis). Geoj. Tour. Geosites 32 (4), 1370–1375 (2020).
Yongrok, C. & Na, W. The economic efficiency of urban land use with a sequential slack-based model in Korea. Sustainability 9 (1), 79–79 (2017).
Xie, W., Wang, T. & Zhao, X. Does digital inclusive finance promote coastal rural entrepreneurship?. J. Coastal Res. 103 (sp1), 240–240 (2020).
Barykin, S. & Shamina, L. The logistics approach to perspectives for the digital technologies in Russia. IOP Conf. Ser. Mater. Sci. Eng. 918 (1), 012187–012196 (2020).
Thaddeus, K. J., Chi, A. N. & Manasseh, C. O. Digital financial inclusion and economic growth: Evidence from Sub-Saharan Africa (2011–2017). Int. J. Bus. Manag. 8 (4), 212–226 (2020).
Rastogi, S., Panse, C. & Sharma, A. Unified Payment Interface (UPI): A digital innovation and its impact on financial inclusion and economic development. Univ. J. Account. Financ. 9 (3), 518–530 (2021).
Boqiang, L. & Ruipeng, T. China’s economic agglomeration and green Economic efficiency. Econ. Res. 54 (2), 119–132 (2019).
Monteiro, L., Cristina, R. & Sciubba, E. Water and energy efficiency assessment in urban green spaces. Energies 14 (17), 5490–5490 (2021).
Gwak, J. H., Bo, K. L. & Lee, W. K. Optimal location selection for the installation of urban green roofs considering honeybee habitats along with socio-economic and environmental effects. J. Environ. Manag. 189 (15), 125–133 (2017).
Zhou, L., Zhou, C. & Che, L. Spatio-temporal evolution and influencing factors of urban green development efficiency in China. J. Geog. Sci. 30 (5), 724–742 (2020).
Fu, J., Xiao, G. & Wu, C. Urban green transformation in Northeast China: A comparative study with Jiangsu, Zhejiang and Guangdong provinces. J. Clean. Prod. 273 (3), 122551–122551 (2020).
Vargas-Hernandez, J. G. & Pallagst, K. Urban green innovation: Public interest, territory democratization and institutional design. Int. J. Green Comput. 11 (1), 57–73 (2020).
Zanizdra, M. Y., Harkushenko, O. M. & Vishnevsky, V. Digital and green economy: Common grounds and contradictions. Sci. Innov. 17 (3), 14–27 (2021).
Delcart, L., Neacsu, N. & Oncioiu, I. Regions and cities as stimulators towards green and digital economy. Int. J. Innov. Digit. Econ. 9 (4), 1–10 (2018).
Bongomin, G., Yourougou, P. & Munene, J. C. Digital financial innovations in the twenty-first century: Do transaction tax exemptions promote mobile money services for financial inclusion in developing countries?. J. Econ. Admin. Sci. 36 (3), 185–203 (2019).
Wang, Z., Jin, W. & Dong, Y. Hierarchical life-cycle design of reinforced concrete structures incorporating durability, economic efficiency and green objectives. Eng. Struct. 157 (15), 119–131 (2018).
Sha, R., Li, J. & Ge, T. How do price distortions of fossil energy sources affect China’s green economic efficiency?. Energy 2021 (1), 121017–121017 (2021).
Ren, Y., Wang, C. & Xu, L. Spatial spillover effect of producer services agglomeration on green economic efficiency: Empirical research based on spatial econometric model. J. Intell. Fuzzy Syst. 37 (5), 6389–6402 (2019).
Li, Q. Regional technological innovation and green economic efficiency based on DEA model and fuzzy evaluation. J. Intell. Fuzzy Syst. 37 (3), 1–11 (2019).
Zhang, D., Chen, L. & Yang, Y. Assessing the green economic efficiency of municipalities and provinces in China with a Meta-US-SBM model. Paper Asia 2 (2), 159–162 (2019).
Batrancea, L. M., Pop, M. C., Rathnaswamy, M. M., Batrancea, I. & Rus, M.-I. An empirical investigation on the transition process toward a green economy. Sustainability 13 (23), 13151 (2021).
Aivaz, K. A., Munteanu, I. F., Stan, M. I., Stan, M.-I. & Chiriac, A. A multivariate analysis of the links between transport noncompliance and financial uncertainty in times of COVID-19 pandemics and war. Sustainability 14 (16), 10040 (2022).
Download references
Research on identification and regulation of performance window dressing of China Securities Investment Funds (2023-JC-YB-618), Shaanxi 2023 Natural Science Foundation research project. Research on the motivation, risk and effect of hedging based on empirical data of Chinese listed companies (105–45119026).
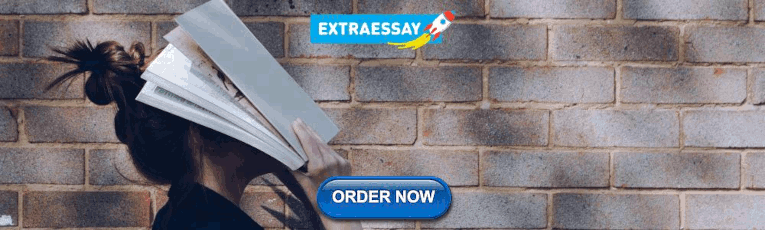
Author information
Authors and affiliations.
School of Economics and Management, Xi’an University of Technology, Xi’an, 710000, Shaanxi, China
School of Finance, Shanghai Lixin University of Accounting and Finance, Shanghai, 201209, China
You can also search for this author in PubMed Google Scholar
Contributions
Q.H., Y.X. wrote the main manuscript text.
Corresponding author
Correspondence to Ying Xue .
Ethics declarations
Competing interests.
The authors declare no competing interests.
Additional information
Publisher's note.
Springer Nature remains neutral with regard to jurisdictional claims in published maps and institutional affiliations.
Rights and permissions
Open Access This article is licensed under a Creative Commons Attribution 4.0 International License, which permits use, sharing, adaptation, distribution and reproduction in any medium or format, as long as you give appropriate credit to the original author(s) and the source, provide a link to the Creative Commons licence, and indicate if changes were made. The images or other third party material in this article are included in the article's Creative Commons licence, unless indicated otherwise in a credit line to the material. If material is not included in the article's Creative Commons licence and your intended use is not permitted by statutory regulation or exceeds the permitted use, you will need to obtain permission directly from the copyright holder. To view a copy of this licence, visit http://creativecommons.org/licenses/by/4.0/ .
Reprints and permissions
About this article
Cite this article.
He, Q., Xue, Y. Research on the influence of digital finance on the economic efficiency of energy industry in the background of artificial intelligence. Sci Rep 13 , 14984 (2023). https://doi.org/10.1038/s41598-023-42309-5
Download citation
Received : 28 February 2023
Accepted : 08 September 2023
Published : 11 September 2023
DOI : https://doi.org/10.1038/s41598-023-42309-5
Share this article
Anyone you share the following link with will be able to read this content:
Sorry, a shareable link is not currently available for this article.
Provided by the Springer Nature SharedIt content-sharing initiative
This article is cited by
Research on the coupling mechanism and influencing factors of digital economy and green technology innovation in chinese urban agglomerations.
- Xuesi Zhong
Scientific Reports (2024)
By submitting a comment you agree to abide by our Terms and Community Guidelines . If you find something abusive or that does not comply with our terms or guidelines please flag it as inappropriate.
Quick links
- Explore articles by subject
- Guide to authors
- Editorial policies
Sign up for the Nature Briefing: Anthropocene newsletter — what matters in anthropocene research, free to your inbox weekly.

Read our research on: Gun Policy | International Conflict | Election 2024
Regions & Countries
Shared, collaborative and on demand: the new digital economy, the sharing economy and on-demand services are weaving their way into the lives of (some) americans, raising difficult issues around jobs, regulation and the potential emergence of a new digital divide.
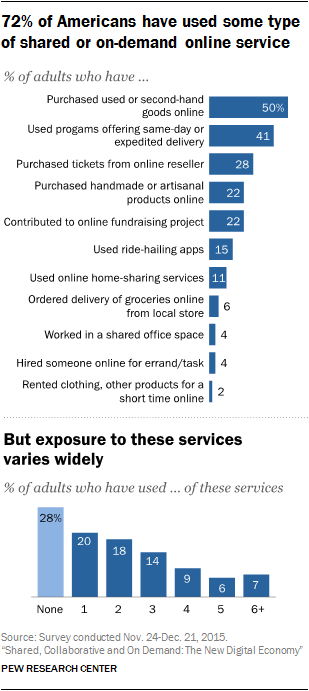
A national Pew Research Center survey of 4,787 American adults – its first-ever comprehensive study of the scope and impact of the shared, collaborative and on-demand economy – finds that usage of these platforms varies widely across the population. In total, 72% of American adults have used at least one of 11 different shared and on-demand services. And some incorporate a relatively wide variety of these services into their daily lives: Around one-in-five Americans have used four or more of these services, and 7% have used six or more.
At the same time, around one-quarter of Americans (28%) say they have not used any major shared or on-demand platforms, and many are wholly unfamiliar with the tools and vocabulary of the new digital economy. For instance, 15% of Americans have used ride-hailing apps like Uber or Lyft, but twice as many have never heard of these apps before. Similarly, 11% of Americans have used home-sharing platforms like Airbnb or VRBO, but roughly half have never heard of home-sharing sites. In addition:
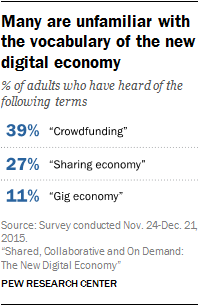
- 73% are not familiar with the term “sharing economy.”
- 89% are not familiar with the term “gig economy.”
Each of these individual platforms has its own unique user base. Still, exposure to these shared, collaborative and on-demand services at a broad level is heavily concentrated among certain demographic cohorts. In particular:
College graduates – 39% of college graduates have used four or more of these services, compared with just 8% of those with a high school degree or less. At the same time, around one-quarter of college graduates have used none (11%) or only one (15%) of these services.
Those with relatively high household incomes – 41% of Americans with an annual household income of $100,000 or more have used four or more of these services, three times the proportion among households earning less than $30,000 annually.
Those under the age of 45 – Exposure to these shared and on-demand services begins to drop off rapidly starting at around age 45. Around one-third of those ages 18-44 have used four or more of these services, and relatively few in this age range have no exposure at all to these services. By contrast, 44% of Americans ages 50 and older (and 56% of those ages 65 and older) have not used any of these 11 platforms.
A number of these services – though by no means all of them – are offered primarily in and around urban population centers. And urban and suburban residents are around twice as likely as those living in rural areas to use four or more of these services. At the same time, around one-quarter of urban and suburban dwellers have not used any of the platforms measured in this survey.
This report offers a detailed examination of three different services that exemplify the shared, collaborative and on-demand economy: ride-hailing apps, home-sharing platforms and crowdfunding services. Key findings about each of these three services are discussed in more detail below.
Ride-hailing apps (used by 15% of American adults)
Ride-hailing users’ attitudes toward these services are strongly positive; users are in near-universal agreement that ride-hailing saves them time and stress, and that these services offer good jobs for people who prioritize flexible working hours.
When presented with a number of attributes that might describe ride-hailing apps, users respond especially strongly to two in particular: 86% of ride-hailing users feel that these services save their users time and stress, while 80% feel that they offer good jobs for those who want flexible working hours. Users also broadly agree that these services could be a good transportation option for older adults with limited mobility, and that they are less expensive than traditional taxi services.
Ride-hailing users are more divided on questions such as whether or not these services simply help serve people who already have plenty of transit options available to them, and whether they are a good way to transport unaccompanied minors. But relatively few (11%) think that these services collect too much personal information about their users. And a sizable majority of users feel that these services use drivers that they feel safe riding with (70% feel that this describes these services well, while just 5% disagree).
Frequent ride-hailing users are less likely than other Americans to own a car – but also rely heavily on a range of other transit options
Some 3% of American adults use ride-hailing apps on a daily or weekly basis, and around two-thirds of these regular ride-hailing users indicate that they own a car or regularly drive a personal vehicle. Although this means that a majority of these regular ride-hailing users are car owners/drivers, they are significantly less likely to own or drive a car than either occasional ride-hailing users or Americans who do not use ride-hailing at all.
Yet although they are less dependent on personal vehicle ownership, these regular ride-hailing users rely heavily on a wide range of personal transportation options that go well beyond ride-hailing alone – such as taking public transit, walking or riding a bike, or even using traditional taxi services. To be sure, this trend carries a strong geographic component: For Americans who live far from urban centers, a personal vehicle might be the only available option to get from one place to another. But for those with the option of doing so, ride-hailing apps can serve as one element among many in a network of transportation options that can help reduce users’ reliance on personal vehicle ownership.
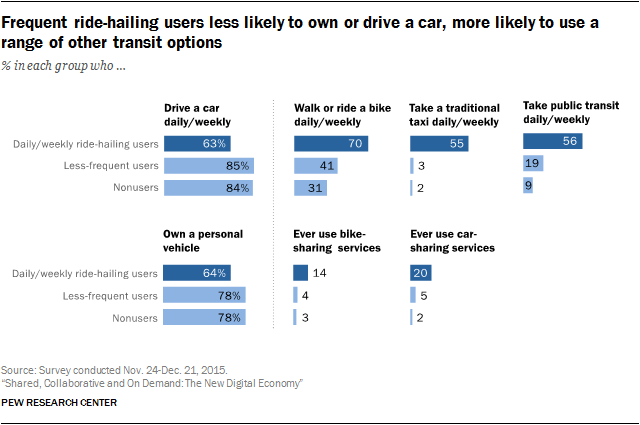
Ride-hailing users are closely following the debate over the best way to regulate ride-hailing apps – and are extremely reluctant to apply legacy taxi regulations to these new services
Just 15% of Americans have used ride-hailing apps themselves, but nearly half of all adults (48%) have heard at least something about the debate happening in cities across the country over how best to regulate these services. Ride-hailing users themselves are following this issue especially closely: Fully 85% of ride-hailing users have heard at least something about this debate, and 39% indicate that they have heard “a lot” about it.
When it comes to their views on how to best regulate these services, the public at large is relatively split. Among Americans who have heard of this issue, 42% feel that these services should not be required to follow the same rules and regulations as existing taxi companies when it comes to things like pricing, insurance or disability access; 35% think that they should have to follow these rules, while the remaining 23% are not sure how they feel.
But although the engaged segment of the general public is relatively divided, ride-hailing users themselves tend to favor the notion (by a two-to-one ratio) that these services should not have to follow the same rules and regulations as legacy taxi operators. Among ride-hailing users who have heard of this debate, 57% believe that these services should not be required to follow the existing regulations that are in place for incumbent providers, while just 27% believe that existing rules and regulations should in fact apply to these new market entrants.
Are they contractors or employees? Employers or platforms? Users have varying views about the relationship between ride-hailing apps and ride-hailing drivers
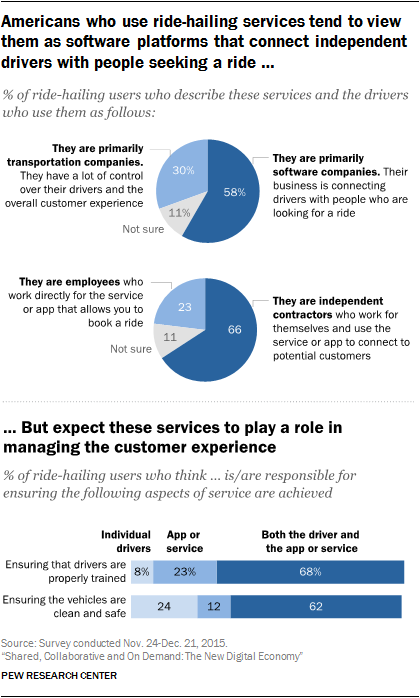
At the same time, ride-hailing users place varying degrees of responsibility on drivers and the services themselves when it comes to managing various aspects of the day-to-day customer experience:
- 68% of ride-hailing users believe that both drivers and the services themselves should be responsible for making sure that drivers are properly trained. One-quarter (23%) believe this is the sole responsibility of the services, while 8% think it is the responsibility of each individual driver.
- 62% of users believe that both drivers and services should be responsible for making sure that the vehicles that customers request are clean and safe. Some 12% think this is the responsibility of the services alone, while 24% think it is up to individual drivers to ensure this.
Ride-hailing apps are discussed in detail in Chapter 2 of this report.
Home-sharing platforms (used by 11% of adults)
Around one-in-ten Americans have used a home-sharing site such as Airbnb or HomeAway to stay in someone’s home for a period of time – and these services appeal to a relatively broad age spectrum in comparison with ride-hailing apps. Americans ages 35-44 are nearly twice as likely as those ages 18-24 to have used home-sharing services (16% vs. 9%), and the median age of home-sharing users in the United States is 42 – nearly a decade older than the median ride-hailing user.
Users view these services as a good option for groups traveling together and a good way for homeowners to make extra income; at the same time, many users view these services as best for adventurous travelers, and around one-in-five think of them as risky to use
When presented with several attributes that might define home-sharing services, users respond especially strongly to the notion that these services are a good option for families or people who travel as a group (87% of users feel that this describes home-sharing sites well), and that they are a good way for homeowners to earn extra money (85%). At the same time, many users of these sites view home-sharing as something that is perhaps not for everyone. Roughly half of users (53%) say these services are best-suited for adventurous travelers; 42% say the properties on these sites are not always as appealing as they seem online; and around one-in-five (18%) say they are generally risky to use.
37% of home-sharing users have used these services to stay in a shared space in someone’s home
Some 37% of home-sharing users report that they have used these services to reserve a single room or other type of shared space in someone’s home (this works out to 4% of all American adults). However, many of these users express some level of reservation about doing so. Some 48% of those who have stayed in this type of shared lodging say they worry about staying with someone they have never met before, and 66% of these users feel that home-sharing services are best for adventurous travelers. In addition, these users are twice as likely to have had a bad experience using home-sharing compared with other users.
As was true of ride-hailing, the structure of home-sharing services is difficult for many users to place into the context of the traditional hospitality industry
Much like ride-hailing apps in the context of the employer/worker relationship, home-sharing services are structured much differently from traditional hotels – and consumers are not always clear on the precise nature of this new arrangement. Some 58% of home-sharing users indicate that they see these services as software companies whose business is simply connecting people with a spare room or empty house with others who are looking for a place to stay. At the same time, 26% view them as hospitality companies that vouch for the quality of the properties they list and have a good deal of control over the customer experience.
And as was also true in the case of ride-hailing, home-sharing users place varying levels of responsibility on homeowners and the services themselves when it comes to managing specific aspects of the day-to-day user experience:
- 67% of home-sharing users believe that both individual homeowners and the services themselves should be responsible for making sure that properties are described accurately. Some 8% think it is the sole responsibility of the app or service that people use to book their stay, while one-quarter (23%) believe it is the sole responsibility of individual homeowners.
- 57% of users believe that both homeowners and services should be responsible for resolving payment issues between hosts and guests. Some 31% think this is the responsibility of the services alone, while 11% think it is the responsibility of individual homeowners.
- 53% of users believe that both homeowners and services should be responsible for addressing problems that might come up during someone’s stay. One-in-ten (10%) believe that this is the sole responsibility of the app or service, while 35% believe it is the responsibility of the homeowner alone.
Most home-sharing users are not following the debate over the legal and regulatory status of these services particularly closely; those who are following this issue tend to feel that these services should be legal, and that owners should not have to pay hotel or lodging taxes
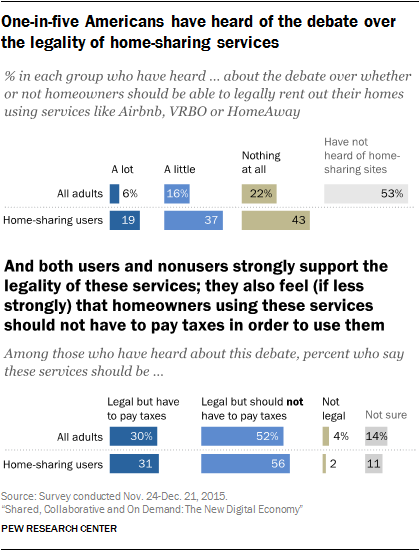
But although home-sharing users have not been following the legal debate over these services with nearly the same intensity as their ride-hailing counterparts, those home-sharing users who have been following this issue tend to feel that these services should be both legal and tax-free for owners. Among home-sharing users who are aware of the legal debate over these services, 56% believe that these services should be legal, and that owners should not have to pay any local hotel or lodging taxes; just 31% believe that owners should be able to legally rent out these services but should have to pay taxes for the privilege of doing so.
Home-sharing sites are discussed in more detail in Chapter 3 .
Crowdfunding sites (used by 22% of adults)
Around one-in-five American adults have contributed to an online fundraising project on a site like Kickstarter or GoFundMe, and 3% of Americans have created their own fundraising project on one of these sites.
Most crowdfunding donors have made relatively modest contributions to a handful of projects
The overwhelming majority of crowdfunding users have contributed to a handful of projects: Some 87% of donors say they have contributed to a total of five projects or fewer on these sites. And while 62% of donors have given $50 at most to an individual project, relatively large donations are not uncommon: 21% of these donors have given between $51 and $100 to an individual project, 14% have given between $101 and $500, and 3% have donated more than $500 at one time.
Contributions to help an individual in need – often a friend or family member – are the most common type of crowdfunding donation
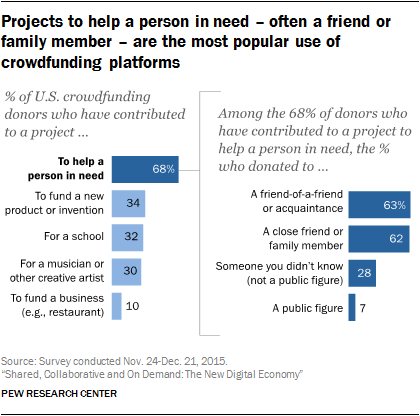
- 34% of crowdfunding donors have contributed to a project to fund a new product or invention.
- 32% have contributed to a project for a school.
- 30% to a project for a musician or other creative artist.
- 10% to a project for a new restaurant or other type of business.
Contributions to help someone in need are more often directed toward a person who is already known by the donor, as opposed to a stranger or a public figure. Among those who have contributed to a crowdsourced project to help someone in need, 63% say they have given to help a friend of a friend or an acquaintance, while 62% have contributed to help a close friend or member of their family. By contrast, just 7% of these donors have given to a campaign to assist a public figure, while 28% have given to help someone who was not a public figure but whom they did not know personally.
Men and women take a different approach to crowdfunding: Women are more likely to contribute to help someone in need, while men are more likely to fund new products or inventions
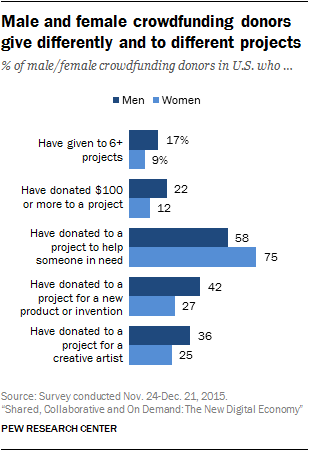
Crowdfunding sites are discussed in more detail in Chapter 4 .
Other notable findings
- Men and women are equally likely to use most shared and on-demand platforms, but women are twice as likely as men to buy handmade or artisanal goods online: 29% of women have done this, compared with 15% of men.
- A cohort of young adults uses ride-hailing apps with great intensity: 7% of all 18- to 29-year-olds (and 10% of 18- to 29-year-olds living in urban areas) indicate that they use ride-hailing on a daily or weekly basis.
- 27% of Americans are aware of ride-hailing apps and indicate that they live in an area where these services are offered, but have not yet tried ride-hailing themselves.
- Users’ views on how best to regulate ride-hailing and home-sharing services stand in contrast to their overall political leanings. Even users of these services who are politically liberal tend to support the notion that they should be legal and tax-free (in the case of home-sharing), or that they should be free to operate outside of existing regulatory structures (in the case of ride-hailing).
- Men and women tend to perceive and experience these services somewhat differently when it comes to the issue of user safety – though these differences are not always dramatic or consistent. For instance, women are more likely than men to express safety concerns about the drivers used by ride-hailing services, and women are also more likely to report having a bad experience using a home-sharing site. At the same time, a substantial majority of female ride-hailing users indicate that these services do indeed use drivers that they feel safe riding with. And female home-sharing users are no more likely than men to view these services as risky to use.
- 2% of Americans have contributed to an online fundraising project to help someone in need primarily because they felt socially obligated to do so.
- 6% of women ages 18-49 have started their own online fundraising project, double the figure for the population as a whole.
- To a greater extent than many of the services discussed in this report, ride-hailing apps are primarily available in and around metro areas. ↩
Sign up for our Internet, Science and Tech newsletter
New findings, delivered monthly
Report Materials
Table of contents, ride-hailing services are seen by minorities as a benefit to areas underserved by taxis, sharing and on-demand services attract a small but active group of ‘super users’, q&a: the impact and evolution of the sharing economy, how americans define the sharing economy, americans and the new digital economy: 8 key findings, most popular.
About Pew Research Center Pew Research Center is a nonpartisan fact tank that informs the public about the issues, attitudes and trends shaping the world. It conducts public opinion polling, demographic research, media content analysis and other empirical social science research. Pew Research Center does not take policy positions. It is a subsidiary of The Pew Charitable Trusts .
By clicking “Accept All Cookies,” you agree to the storing of cookies on your device to enhance site navigation and analyze site usage.
READ ABOUT OUR ACTION-PACKED YEAR! 2023 ANNUAL REPORT NOW AVAILABLE
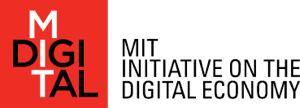
Search the site
The ide newsletter.
Get the latest from MIT IDE delivered weekly to your inbox.
MIT Initiative on the Digital Economy
MIT Sloan School of Management
245 First St, Room E94-1521
Cambridge, MA 02142-1347
617-452-3216
- share on facebook
- share on twitter
- share on linkedin
- share by email
- IDE Members Only
2024 MIT IDE Annual Conference
The mit initiative on the digital economy’s annual conference returns in a new format for 2024.
May 20 - 24, 2024
All Day EDT
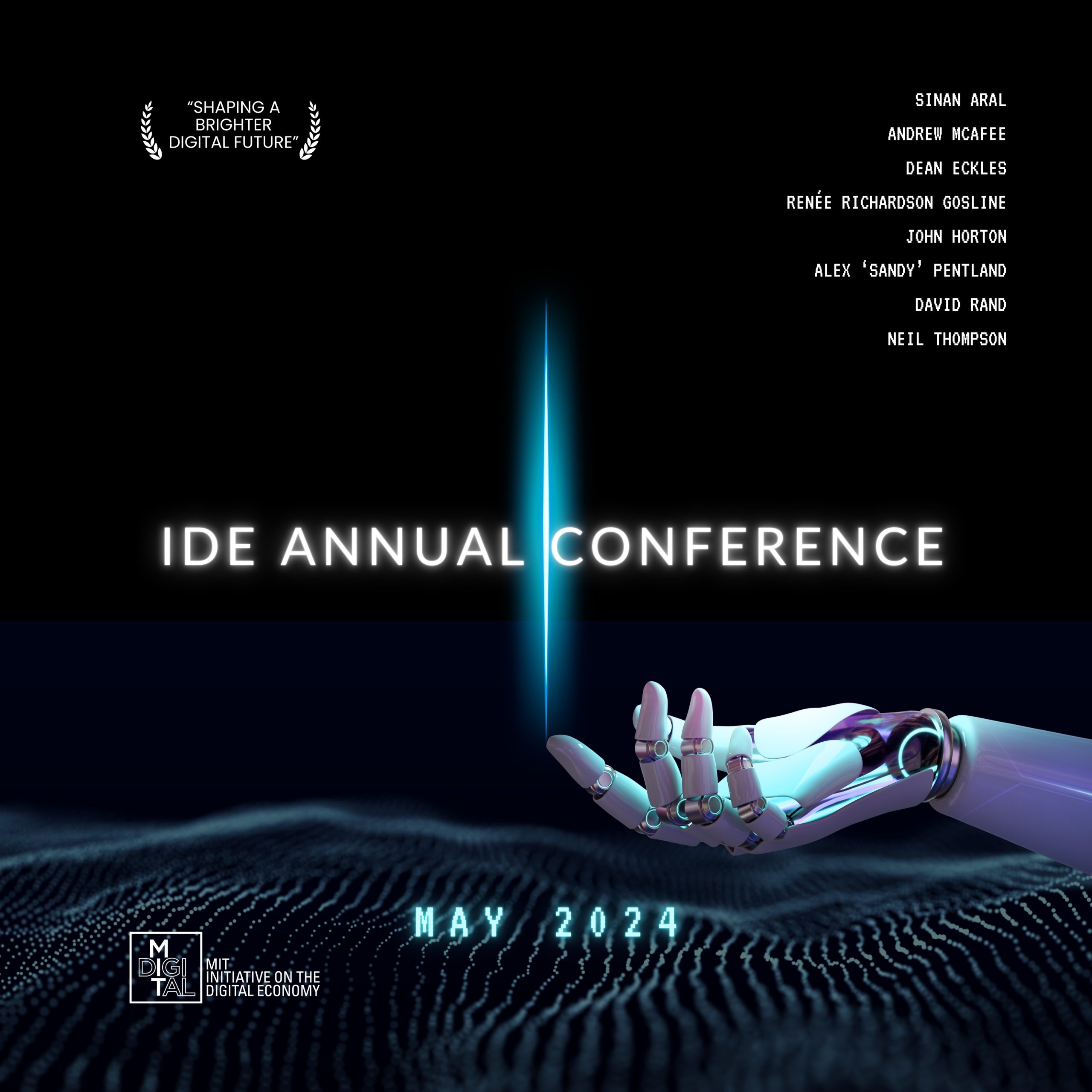
Join us for a special online event taking place the week of May 20. Over the course of a week, tune in to a limited series that shares our most recent research - and how new technologies are shaping a brighter digital future. In each episode, IDE researchers will dig into their latest research to examine questions like:
- Are accuracy prompts stemming misinformation?
- What does the end of Moore’s Law mean for AI?
- How can firms benefit by increasing friction points?
- When will Quantum Computing have real-world applications?
- Are distributed organizations a near-term reality?
Learn about these topics and more at the 2024 IDE Annual Conference
This event is open to IDE members and stakeholders only. To register, please refer to your invitation from IDE or contact our Associate Director, Albert Scerbo: [email protected]
ORIGINAL RESEARCH article
Energy efficiency in chinese cities in the era of digital economy--empirical study and improvement mechanisms provisionally accepted.
- 1 Institute of Statistics and Applied Mathematics, Anhui University of Finance and Economics, China
The final, formatted version of the article will be published soon.
There is an urgent need to improve energy efficiency in the face of the twin challenges of a fragile energy supply and "dual-carbon" targets. New production methods driven by digital technologies have attracted much attention because of their positive impacts on green and low carbon development, raising questions about whether the digital economy can improve energy efficiency through factor reorganization and information flow upgrading. In this study, 285 cities in China from 2010 to 2019 are studied, and the non expected output SBM model combined with the common frontier Malmquist index is used to measure energy efficiency, and the relationship between the digital economy and energy efficiency is explored. The findings show that the development of the digital economy can effectively improve energy efficiency, and this conclusion holds even when endogeneity is controlled using the two stage least squares (2SLS) method of selecting instrumental variables. There is heterogeneity in the digital economy on energy efficiency, mainly in terms of resource endowment, city size and regional differences. In addition, the digital economy has spatial relevance on energy efficiency, affecting the energy efficiency of neighboring regions through spatial spillover effects. Overall, this study provides a useful reference for digital economy empowerment to improve energy technology efficiency, highlighting the potential role of digital economy in promoting sustainable energy development.
Keywords: digital economy, energy efficiency, Digital empowerment, Spatial spillovers, Malmquist index
Received: 23 Jan 2024; Accepted: 15 Apr 2024.
Copyright: © 2024 Wu, Wang and Zhang. This is an open-access article distributed under the terms of the Creative Commons Attribution License (CC BY) . The use, distribution or reproduction in other forums is permitted, provided the original author(s) or licensor are credited and that the original publication in this journal is cited, in accordance with accepted academic practice. No use, distribution or reproduction is permitted which does not comply with these terms.
* Correspondence: Mr. Wenshuai Wu, Institute of Statistics and Applied Mathematics, Anhui University of Finance and Economics, Bengbu, Anhui Province, China
People also looked at
Postdoctoral Research: Applying AI in Environment and Sustainability
How to apply.
Please include the materials listed below in a single pdf with your application:
- Detailed CV
- Statement describing the candidate's qualifications and interest in the position. In particular the candidate's interest in applying their computational training and skills to problems and issues in the environment and sustainability domain (3 pages)
- Brief statement describing interest in, experience with, and commitment to diversity, equity, and inclusiveness (one-half page)
- Undergraduate and graduate transcripts
- Names and contact information for three (3) references
Review of applications will begin immediately and will continue until the position is filled. Questions about the position can be addressed to Prof. Bill Currie ( [email protected] ). If you are having issues uploading your PDF, please contact Joshua Wright( [email protected] , SEAS HR Business Partner).
The School for Environment and Sustainability (SEAS) at the University of Michigan seeks applications for a postdoctoral position to apply artificial intelligence (AI) approaches to problems and issues in the domain of Environment and Sustainability. The candidate will be advised and mentored by Bill Currie, a Professor in SEAS. Currie uses computer modeling to understand the functioning of ecosystems & landscapes and the impacts of human activities on the environment. He is an elected fellow of American Association for the Advancement of Science (AAAS) for his work on modeling the biogeochemistry and ecology of forests, watersheds, and wetlands. From a perspective of understanding human-environment systems, he collaborates with others across a range of fields from wildlife conservation to economics, civil engineering, and urban planning. Currie also currently serves in administrative roles as Associate Dean for Research and Engagement in SEAS and Co-Director of the Schmidt AI in Science Postdoctoral Fellowship Program in MIDAS (Michigan Institute for Data Science).
Research topics will be developed collaboratively between the candidate and Dr. Currie. These could include grounding (strengthening the ability of AI systems to understand the concepts they reason and operate within the environmental domain), alignment (researching how well AI systems represent objective truths or societal expectations in the environmental domain), knowledge modeling, automated research workflows, or other topics. The candidate will have opportunities for significant training and skills development through MIDAS (Michigan Institute for Data Science) programs including boot camps and symposiums and will be expected to engage in these activities. The candidate will be part of a vibrant postdoctoral community both in MIDAS and in SEAS, including the Institute for Global Change Biology, the Center for Sustainable Systems, the Sustainable Future Hub, and others.
This postdoctoral position will be initially hired for a 12-month period with the potential of renewal for an additional 12 months. Renewal will be based on demonstration of academic productivity and meeting other expectations of the position. Compensation includes the standard benefits package for postdoctoral researchers at U-M.
Mission Statement
The School for Environment and Sustainability ( https://seas.umich.edu/ ) is a collaborative and interdisciplinary school. Our mission includes contributing to the protection of environmental resources and the achievement of a sustainable society. We accomplish this by generating and sharing knowledge, contributing to policy and engaging managers and stakeholders. The University of Michigan is a top-ranked public university with excellence in research and teaching. Ann Arbor, home to the University of Michigan, is a town known for arts, culture, parks and restaurants.
SEAS is committed to creating and maintaining an inclusive and equitable environment that respects diverse experiences, promotes generous listening and communications, and discourages and restoratively responds to acts of discrimination, harassment, or injustice. Our commitment to diversity, equity and inclusion is deeply rooted in our values for a sustainable and just society.
Why Work at Michigan?
In addition to a career filled with purpose and opportunity, The University of Michigan offers a competitive salary and comprehensive benefits package to help you stay well, protect yourself and your family and plan for a secure future. Benefits include:
- Generous time off
- Many choices of comprehensive health insurance
- Life insurance
- Long-term disability coverage
- Flexible spending accounts for healthcare and dependent care expenses
Required Qualifications*
- PhD in data science, statistics, computer science, AI or a related field.
- Experience using computational data science or AI approaches. This may include machine learning, artificial neural nets, knowledge modeling, Bayesian modeling, or other approaches.
- Strong communication skills.
- Ability to work both as part of a team and independently.
- Experience in preparing research findings for conference presentations and refereed journal articles.
- Experience leading the development of manuscripts publication and must show evidence of success in publishing refereed journal articles.
- An interest in applying AI methods and approaches in the environment and sustainability domain.
Additional Information
Salary will be determined based on experience.
The candidate will be expected to be in-person on campus at least 50% of the time. Remote and flexible work agreements may be made to support partial off-site work at a remote location.
U-M EEO/AA Statement
The University of Michigan is an equal opportunity/affirmative action employer.
Enhancing University Performance Evaluation through Digital Technology: A Deep Learning Approach for Sustainable Development
- Published: 17 April 2024
Cite this article
- Shuyan Xu 1 &
- Siufong Sze 1
In the era of sustainable development, the evaluation of higher education institutions’ performance has gained paramount importance. This paper presents a novel approach to university performance evaluation by harnessing the power of digital technology, specifically deep learning techniques. Building upon the foundation of sustainable development theory and the experiences of developed countries, we propose a multi-classification model for university performance evaluation, offering both technical methods and theoretical underpinning for educational reform. Our research focuses on the integration of digital technology, including artificial intelligence, deep learning, and data mining, into the assessment of university performance. We employ a multi-layer restricted Boltzmann machines (RBMs) feature extraction approach coupled with the SoftMax classifier to enhance the accuracy of university performance predictions. The paper provides a comprehensive description of the model’s architecture, the forward propagation process, and the solution methodology. Comparative experiments are conducted to evaluate various feature extraction methods, highlighting the superior feature expression capabilities of RBMs over traditional approaches. The results demonstrate that our proposed model surpasses the SoftMax classifier and Deep Belief Networks (DBN) in terms of prediction accuracy, average accuracy, and average recall rate, indicating its practical significance in performance evaluation. While our study offers valuable insights and advancements in university performance assessment, we acknowledge the need for further exploration of potential trade-offs, computational complexity, model interpretability, and generalization performance. These aspects warrant continued investigation to refine and optimize our approach for the benefit of the knowledge economy, innovation, entrepreneurship, and society at large.
This is a preview of subscription content, log in via an institution to check access.
Access this article
Price includes VAT (Russian Federation)
Instant access to the full article PDF.
Rent this article via DeepDyve
Institutional subscriptions
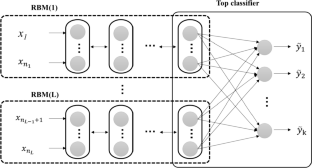
Data Availability
The data can be obtained according to the requirements.
Altbach, P., & de Wit, H. (2020). Postpandemic outlook for higher education is bleakest for the poorest. International Higher Education, 102 , 3–5.
Google Scholar
Anju, P., Raj, A. A. B., & Shekhar, C. (2020). Pulse Doppler processing-A novel digital technique. 2020 4th International Conference on Intelligent Computing and Control Systems (ICICCS) (pp. 1089–1095). IEEE.
Chapter Google Scholar
Armytage, W. H. G. (2018). Thoughts after Robbins (pp. 77–100). Routledge: The New University.
Auranen, O., & Nieminen, M. (2010). University research funding and publication performance-An international comparison. Research Policy, 39 (6), 822–834.
Article Google Scholar
Daraio, C., Bonaccorsi, A., & Simar, L. (2015). Rankings and university performance: A conditional multidimensional approach. European Journal of Operational Research, 244 (3), 918–930.
De, W. A. (2017). Strategic performance management: A managerial and behavioral approach . Bloomsbury Publishing.
Ferrell, B. R., Twaddle, M. L., Melnick, A., & Diane, E. (2018). National consensus project clinical practice guidelines for quality palliative care guidelines. Journal of Palliative Medicine, 21 (12), 1684–1689.
Geiger, T., & Pivovarova, M. (2018). The effects of working conditions on teacher retention. Teachers and Teaching, 24 (6), 604–625.
Gibb, A. (2000). Small and medium enterprise development: Borrowing from elsewhere? A research and development agenda. Journal of Small Business and Enterprise Development, 7 (3), 199–211.
Hallinger, P., & Chatpinyakoop, C. (2019). A bibliometric review of research on higher education for sustainable development, 1998–2018. Sustainability, 11 (8), 2401.
Holmberg, J., & Sandbrook, R. (2019). Sustainable development: What is to be done? Policies for a small planet (pp. 19–38). Routledge.
Joanicjusz, N., & Jonas, S. (2014). Application of DEA method in efficiency evaluation of public higher education institutions. Technological and Economic development of Economy, 20 (1), 25–44.
Johnes, J., & Li, Y. (2008). Measuring the research performance of Chinese higher education institutions using data envelopment analyses. China Economic Review, 19 , 679–696.
Kempkes, G., & Pohl, C. (2010). The efficiency of German universities–Some evidence from nonparametric and parametric methods. Applied Economics, 42 (16), 2063–2079.
Khosla, R., Miranda, N. D., Trotter, P. A., Mazzone, A., Renaldi, R., McElroy, C., Cohen, F., Jani, A., Perera-Salazar, R., & McCulloch, M. (2021). Cooling for Sustainable Development. Nature Sustainability, 4 (3), 201–208.
Lăzăroiu, G. (2017). Is there an absence of capability in sustainable development in universities? Educational Philosophy and Theory, 49 (14), 1305–1308.
Linton, G., & Klinton, M. (2019). University entrepreneurship education: A design thinking approach to learning. Journal of Innovation and Entrepreneurship, 8 (1), 1–11.
Mone, E. M., & London, M. (2018). Employee engagement through effective performance management: A practical guide for managers . Routledge.
Book Google Scholar
Nazarko, J., & Šaparauskas, J. (2014). Application of DEA method in efficiency evaluation of public higher education institutions. Technological and Economic Development of Economy, 20 (1), 25–44.
O’Flaherty, J., & Liddy, M. (2018). The impact of development education and education for sustainable development interventions: A synthesis of the research. Environmental Education Research, 24 (7), 1031–1049.
Pietrzak, M., Pietrzak, P., & Baran, J. (2016). Efficiency assessment of public higher education with the application of data envelopment analysis: The evidence from Poland. Online Journal of Applied Knowledge Management (OJAKM), 4 (2), 59–73.
Purwanti, Y. (2021). The influence of digital marketing & innovation on the school performance. Turkish Journal of Computer and Mathematics Education (TURCOMAT), 12 (7), 118–127.
Reznichenko, S. M., Takhumova, O. V., Zaitseva, N. A., Larionova, A. A., & Dashkova, E. V. (2018). Methodological aspects of assessing factors affecting the sustainable development of the region. Modern Journal of Language Teaching Methods, 8 (11), 69–79.
Sagarra, M., Mar-Molinero, C., & Agasisti, T. (2017). Exploring the efficiency of Mexican universities: Integrating data envelopment analysis and multidimensional scaling. Omega, 67 , 123–133.
Sarmah, R., & Aayushi, S. (2022). Effectiveness of social advertisement campaigns for societal improvement. International Journal of Social Ecology and Sustainable Development (IJSESD), 13 (1), 1–19.
Silvestre, B. S., & Ţîrcă, D. M. (2019). Innovations for sustainable development: Moving toward a sustainable future. Journal of Cleaner Production, 208 , 325–332.
Sum, N., & Bob, J. (2013). Competitiveness, the knowledge-based economy and higher education. Journal of the Knowledge Economy, 4 , 24–44.
Tariq, A., Badir, Y., & Chonglerttham, S. (2019). Green innovation and performance: Moderation analyses from Thailand. European Journal of Innovation Management, 22 (3), 446–467.
Van, D. W., Bouckaert, G., & Halligan, J. (2015). 2015 . Performance management in the public sector.
Wood, D. J. (2010). Measuring corporate social performance: A review. International Journal of Management Reviews, 12 (1), 50–84.
Download references
Author information
Authors and affiliations.
Minnan University of Science and Technology, Quanzhou, 362700, Fujian, China
Shuyan Xu & Siufong Sze
You can also search for this author in PubMed Google Scholar
Contributions
SX was responsible for researching the conceptual design and interpretation of the results. SS was responsible for data collection and analysis and project management. All authors reviewed the results and approved the final version of the manuscript.
Corresponding author
Correspondence to Shuyan Xu .
Ethics declarations
Ethical approval.
This article does not contain any studies with human participants or animals performed by any of the authors.
Informed Consent
The authors declare that all the authors have informed consent.
Conflict of Interest
The authors declare no competing interests.
Additional information
Publisher's note.
Springer Nature remains neutral with regard to jurisdictional claims in published maps and institutional affiliations.
Rights and permissions
Springer Nature or its licensor (e.g. a society or other partner) holds exclusive rights to this article under a publishing agreement with the author(s) or other rightsholder(s); author self-archiving of the accepted manuscript version of this article is solely governed by the terms of such publishing agreement and applicable law.
Reprints and permissions
About this article
Xu, S., Sze, S. Enhancing University Performance Evaluation through Digital Technology: A Deep Learning Approach for Sustainable Development. J Knowl Econ (2024). https://doi.org/10.1007/s13132-024-01928-7
Download citation
Received : 05 January 2024
Accepted : 22 March 2024
Published : 17 April 2024
DOI : https://doi.org/10.1007/s13132-024-01928-7
Share this article
Anyone you share the following link with will be able to read this content:
Sorry, a shareable link is not currently available for this article.
Provided by the Springer Nature SharedIt content-sharing initiative
- University performance evaluation
- Sustainable development
- Digital technology
- Deep learning
- Higher education
- Knowledge economy
- Find a journal
- Publish with us
- Track your research
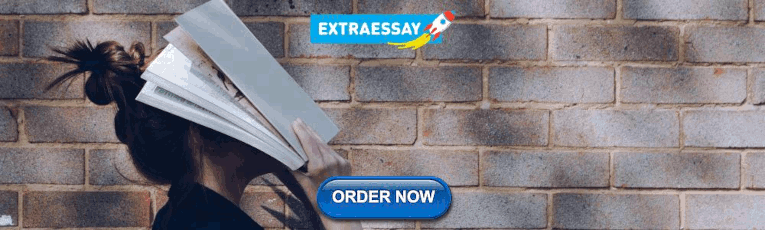
IMAGES
VIDEO
COMMENTS
Abstract. Research on the digital economy continues to develop but is limited to one country and/or field. From a bibliometric retrospective review, this study purposes to visually research mapping and research trends in the field of the digital economy on an international scale. This study used bibliometric techniques with secondary data from ...
Split digital economy research in the realm of sustainability into three streams (That is, CE, DE, and EIE). (Thomas and Autio, 2020) Digital economy [100] 1994-2021: Philosophical and metaphysical: The philosophical variability of digital economy concepts is mostly due to distinctions in the tool for data collection and environmental effects.
Generally, a combination of reference cases, primary research, and analysis is used to conduct research on the digital economy, with the data being used to achieve the desired results. In the realm of the digital economy, tourism is a crucial area that can significantly impact economic growth. The use of digital technologies in this field, such ...
The MIT Initiative on the Digital Economy is a team of visionary thought leaders exploring solutions that will shape our destiny in a digital world. The IDE examines how the rapidly advancing digital economy is changing how we work, interact, and live. We seek out the best practices that will impact the way businesses and people adapt in a ...
The project seeks to calculate the digital economy's contribution to U.S. GDP, improve measures of high-tech goods and services, and offer a more complete picture of international trade. Other goals are to advance research for digital goods and services, the sharing economy, and free digital content. Current release: December 6, 2023
AI Adoption in America: Who, What, and Where. A team of experts, including researchers from Stanford Digital Economy Lab, studied the early adoption and diffusion of five AI-related technologies—automated-guided vehicles, machine learning, machine vision, natural language processing, and voice recognition—as documented in the 2018 Annual Business Survey of 850,000 firms across the United ...
The year 2015 saw trends in digital economy research shift toward industry, but it was not a very significant change. In 2016, the trend in digital economy research was on the internet and information systems, and for the first time, more attention was given to the "digital" component of the digital economy than the "economic" component.
Our research and publications provide authoritative and evidence-based analysis on trade and development and policy recommendations to help countries tackle today's biggest challenges. Featured. ... The data-driven digital economy is characterized by large imbalances: Only 20 per cent of people in least developed countries use the Internet ...
Although the effect of the digital economy in promoting high-quality economic development is increasing day by day, research analysing this mechanism from the spatial perspective is very scarce. This study measures the level of the digital economy and high-quality economic development based on the panel data of 31 provinces in China from 2013 to 2020. On this basis, the direct, spillover, and ...
At present, the digital economy, which takes information technology and data as the key elements, is booming and has become an important force in promoting the economic growth of various countries. In order to explore the current dynamic trend of China's digital economy development and the impact of the digital economy on the high-quality economic development, this paper measures the digital ...
As an emerging economic form, the digital economy is a crucial force in promoting high-quality economic development, resolving regional development incoherence, and improving the level of urban resilience. In this paper, the urban resilience indicator system is composed of four dimensions: social resilience, economic resilience, infrastructure resilience, and ecological resilience.
Research on the digital economy. Tapscott. first proposed the concept of a digital economySince then, the connotation of the digital economy has been deepening. Based on the existing research, we systematically examine the role of digital infrastructure, digital industrialization, and industrial digitization in the digital economic system and the coupling mechanism between different subjects.
Transformation of the economy involves several strategic approaches to digital development: strategy of inertial digital development; catch-up digital development strategy; leadership strategy in the digital market. Inertial digital development strategy implies the absence of large-scale efforts aimed at digital and innovative development, low ...
Significant advancements have been made in studying innovation within the digital economy over the past 20 years. Research on innovation and the digital economy is crucial since it changes all facets of human existence, including business models and entrepreneurial trends. Research regarding innovation in the digital economy has experienced growth over time. However, only a small number of ...
1. Introduction. The boom of the digital economy has cultivated a new impetus of economic development in the past decade (Li et al., 2020), and the scale of the digital economy in dominant economies reached 32.6 trillion USD in 2020, representing a nominal annual growth rate of 3%.Specifically, the United States and European Union have relied on leading technological innovation to establish ...
MIT IDE research lead, Neil Thompson, co-authored a paper estimating human worker displacement when AI is introduced for computer vision tasks. The study found the impact to be less dramatic than others have reported. ... MIT Initiative on the Digital Economy. MIT Sloan School of Management. 245 First St, Room E94-1521. Cambridge, MA 02142-1347 ...
The research shows that: First, digital economy and industrial green development typically exhibit a pattern of fluctuation and rise, but the overall level is relatively low, and the difference between provinces is clear, with a distribution trend of high east and low west. Second, digital economy has an overall favorable impact on industrial GTFP.
The efficiency of the green economy and digital finance are shown in Fig. 7 as the panel quantile regression findings. It is can be seen that for the five quantiles, the estimated coefficient of ...
A national Pew Research Center survey of 4,787 American adults - its first-ever comprehensive study of the scope and impact of the shared, collaborative and on-demand economy - finds that usage of these platforms varies widely across the population. In total, 72% of American adults have used at least one of 11 different shared and on-demand ...
The gradual recovery of the economy has positioned the digital economy as a vital force in driving global economic growth. However, the sustainability of this emerging economic sector is being tested by unexpected systemic shocks. There is a scarcity of research on the factors influencing the sustainable development of the digital economy.
The MIT Initiative on the Digital Economy's Annual Conference returns in a new format for 2024! May 20 - 24, 2024. All Day EDT. Join us for a special online event taking place the week of May 20. Over the course of a week, tune in to a limited series that shares our most recent research - and how new technologies are shaping a brighter ...
The digital economy can alleviate financing constraints to provide vitality for enterprise innovation. The digital economy has a more significant role in promoting the innovation of non-state-owned enterprises and large enterprises, and has a greater role in driving innovation for enterprises in the eastern and central regions.
The ongoing advancement of the digital economy has accelerated the transformation of both new and traditional forms of kinetic energy in economic development (X. Zhang 2019).Moreover, substantial shifts have transpired in the allocation and organization of corporate innovation resources (Wang et al. 2020).Business growth has experienced rapid acceleration due to innovations in business models ...
1. Introduction. Digital economy, a brand-new economic form recently, originates from networked intelligence, which can be related back to the 1990s (Tapscott, 2014).It is not clearly defined until the G20 leaders' Hangzhou Summit in September 2016 that the digital economy, with information technology as the core and modern network as the carrier, comes from a series of economic activities ...
Research on the path of digital economy promoting high-quality development of the health and wellness industry [J]. Journal of Panzhihua University: Comprehensive Edition, 2023, 40 (1): 10. [2] Ouyang Zhouhui. Research on the Integration and Development of Digital Economy and Elderly Care Service
There is an urgent need to improve energy efficiency in the face of the twin challenges of a fragile energy supply and "dual-carbon" targets. New production methods driven by digital technologies have attracted much attention because of their positive impacts on green and low carbon development, raising questions about whether the digital economy can improve energy efficiency through factor ...
The booming digital economy has made data an important resource (Iansiti, 2021). In 2017, The Economist argued that "the world's most valuable resource is no longer oil, but data". So, the data market and data ecosystem have become an emerging research area of the digital economy.
The School for Environment and Sustainability (SEAS) at the University of Michigan seeks applications for a postdoctoral position to apply artificial intelligence (AI) approaches to problems and issues in the domain of Environment and Sustainability. The candidate will be advised and mentored by Bill Currie, a Professor in SEAS.
In the era of sustainable development, the evaluation of higher education institutions' performance has gained paramount importance. This paper presents a novel approach to university performance evaluation by harnessing the power of digital technology, specifically deep learning techniques. Building upon the foundation of sustainable development theory and the experiences of developed ...
Here, C ds t represents the integration level of the digital economy and the real economy in period t, u d t denotes the development level of the digital economy within period t, and u s t represents the development level of the real economy within the same time span. It is worth noting that in cases where both the digital economy and real economy exhibit relatively modest development levels ...