
DEAN A. SEEHUSEN, MD, MPH, AND MEGHAN RALEIGH, MD, The National Capital Consortium Family Medicine Residency Program, Fort Belvoir Community Hospital, Fort Belvoir, Virginia
Am Fam Physician. 2014;90(7):481-483
See the full review , clinician summary , consumer summary , and CME activity .
Author disclosure: No relevant financial affiliations.
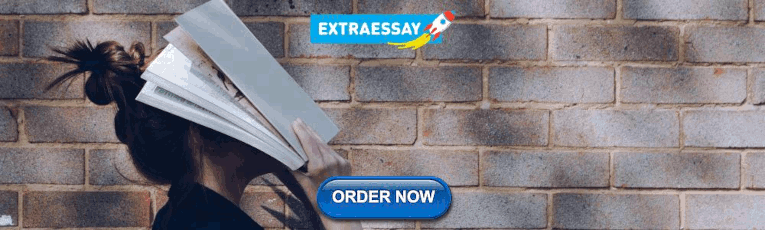
Key Clinical Issue
Does outpatient case management for adults with medical illness and complex care needs improve patient-centered outcomes, quality of care, or resource utilization?
Evidence-Based Answer
Compared with usual care, case management does not reduce mortality in patients with multiple chronic diseases. (Strength of recommendation [SOR]: A, based on consistent, good-quality patient-oriented evidence.) Case management improves patient satisfaction with care for congestive heart failure and cancer, and increases patient perception of care coordination. It improves the quality of care for patients with complex illnesses. (SOR: B, based on inconsistent or limited-quality patient-oriented evidence.) Case management interventions showed mixed results in improving patient quality of life and functional status. For caregivers of patients with dementia, targeted case management programs improve levels of stress, burden, and depression. The effects of case management on health care resource utilization and on costs of care are minimal. (SOR: B, based on inconsistent or limited-quality patient-oriented evidence.)
Practice Pointers
Chronic conditions can become medically complex, and the patient can develop special needs, both of which can quickly overcome the usual capabilities of physicians in the outpatient setting. Case management is one strategy to optimize care for patients with medically complex chronic diseases. 1 The Case Management Society of America defines case management as the collaborative process of assessment, planning, care coordination, evaluation, and advocacy for services to meet patients' comprehensive health needs through communication and available resources to promote quality, cost-effective outcomes. 2 Case management programs vary considerably in their design, goals, personnel, and resources.
This Agency for Healthcare Research and Quality (AHRQ) review focused on non-psychiatric, medically complex care in the outpatient setting. Case management was defined as a “supplemental service, in which a person, usually a nurse or social worker, takes responsibility for coordinating and implementing a patient's care plan, alone or in conjunction with a team of health professionals.” Almost all of the studies evaluated compared case management with usual care. 3
Case management did not reduce mortality in the patients with multiple chronic diseases or in populations with specific diseases. However, it improved disease-specific functioning and quality of life when these measures were specifically targeted by the intervention. Measures of global function and quality of life were not generally improved by case management. 3
Disease-specific health outcomes were inconsistently impacted by case management. For instance, pain was reduced in patients with cancer. While there is heterogeneity in the outcomes for A1C, the results of eight studies taken together suggested that case management improved glucose control. 3 Case management was effective at improving self-management behaviors (e.g., medication compliance). When receipt of a specific health service was an explicit goal, case management was effective at increasing receipt of that health service, but did not improve adherence to guideline-recommended care in general. 3
Overall, case management was associated with improved satisfaction in patients with multiple chronic diseases, congestive heart failure, or cancer, and with increased satisfaction of caregivers of patients with dementia. This increase in satisfaction was mostly because of improved coordination among clinicians. 3 Burden and depression were improved in caregivers of patients with dementia who have case management interventions. 4
Case management did not reduce overall resource utilization or hospitalization rates. It had mixed effects on emergency department use—some studies showed reduced emergency department use in patients who had case management, whereas others showed no effect. There were also variable results on the number of clinic visits for patients with case management services. 3
The setting (outpatient, home health, or integrated health system) did not impact effectiveness, nor did the experience, training, or skills of the case managers studied. Case management interventions may be more successful when protocols or scripts are used, and when the case manager works with a physician or health care team. 3
Although the AHRQ review does not suggest a single proven formula for building an effective case management program, it provides some broad principles. A case management intervention is more likely to work if it has a narrow focus and defined objectives. Case managers should have preintervention training, utilize protocols or scripts, and work closely with clinicians. Whether a case management intervention succeeds seems to depend on numerous factors, many of which will be unique to the individual program. Given this uncertainty, it would be wise to conduct a formal evaluation of effectiveness locally for any new case management program.
editor's note: American Family Physician SOR ratings are different from the Agency for Healthcare Research and Quality Strength of Evidence (SOE) ratings.
The views expressed in this article are those of the authors and do not reflect the policy or position of the U.S. Army Medical Department, Department of the Army, Department of Defense, or the U.S. government.
Sandberg M, Jakobsson U, Midlöv P, Kristensson J. Case management for frail older people–a qualitative study of receivers' and providers' experiences of a complex intervention [published online ahead of print January 10, 2014]. BMC Health Serv Res . http://www.biomedcentral.com/1472-6963/14/14 . Accessed May 22, 2014.
Case Management Society of America. What is a case manager? http://www.cmsa.org/Consumer/FindaCaseManager/WhatisaCaseManager/tabid/276/Default.aspx . Accessed May 21, 2014.
Agency for Healthcare Research and Quality, Effective Health Care Program. Outpatient case management for adults with medical illness and complex care needs. Rockville, Md.: Agency for Healthcare Research and Quality; January 2013. http://effectivehealthcare.ahrq.gov/search-for-guides-reviews-and-reports/?pageaction=displayproduct&productID=733 . Accessed May 21, 2014.
Mittelman MS, Roth DL, Coon DW, Haley WE. Sustained benefit of supportive intervention for depressive symptoms in caregivers of patients with Alzheimer's disease. Am J Psychiatry. 2004;161(5):850-856.
The Agency for Healthcare Research and Quality (AHRQ) conducts the Effective Health Care Program as part of its mission to produce evidence to improve health care and to make sure the evidence is understood and used. A key clinical question based on the AHRQ Effective Health Care Program systematic review of the literature is presented, followed by an evidence-based answer based on the review. AHRQ’s summary is accompanied by an interpretation by an AFP author that will help guide clinicians in making treatment decisions.
This series is coordinated by Joanna Drowos, DO, MPH, MBA, contributing editor. A collection of Implementing AHRQ Effective Health Care Reviews published in AFP is available at https://www.aafp.org/afp/ahrq .
Continue Reading
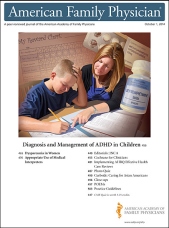
More in AFP
More in pubmed.
Copyright © 2014 by the American Academy of Family Physicians.
This content is owned by the AAFP. A person viewing it online may make one printout of the material and may use that printout only for his or her personal, non-commercial reference. This material may not otherwise be downloaded, copied, printed, stored, transmitted or reproduced in any medium, whether now known or later invented, except as authorized in writing by the AAFP. See permissions for copyright questions and/or permission requests.
Copyright © 2024 American Academy of Family Physicians. All Rights Reserved.
Advertisement
Improvement of outpatient service processes: a case study of the university of Hong Kong-Shenzhen hospital
- Original Paper
- Open access
- Published: 10 November 2023
- Volume 13 , pages 971–985, ( 2023 )
Cite this article
You have full access to this open access article
- Jingsong Chen 1 , 2 &
- Bráulio Alturas ORCID: orcid.org/0000-0003-0142-3737 1
1722 Accesses
Explore all metrics
This work presents a case study of the University of Hong Kong-Shenzhen Hospital (HKU-SZH), which was the first to implement an outpatient appointments registration system. The research question is to determine which factors influence patient satisfaction most.
The study provides an anatomy of the hospital outpatient process through various methods and theories, including a literature review, field research, expert consultation, business process improvement (BPI) theory and information technology, with the aim of identifying the objectives and strategies of the hospital for improving its outpatient process. A quantitative analysis was performed using a questionnaire survey to identify the defects and weaknesses of the current model. The principles, methods and techniques of BPI theory are used to analyse various problems existing in the outpatient process and the extent of their influence. A structural equation model has been established for scientific and quantitative analysis, which can help identify the goals of optimization and measure improvement in the outpatient process and patient satisfaction.
It was determined the source of inefficiency of the current outpatient service process. By means of outpatient process improvement, the study aims to increase the hospital’s efficiency and raise the level of patient satisfaction so that it may enhance its comprehensive competence. In addition, an effective and operable methodology will be generated, which is expected to serve as a reference for other hospitals to improve their operation and management.
Conclusions
It was found that service attitude, service value and waiting time have a significant influence on patient satisfaction.
Similar content being viewed by others
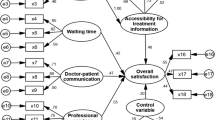
The situation and influencing factors of outpatient satisfaction in large hospitals: Evidence from Henan province, China
Theoretical and Practical Aspects of Outpatient Clinic Optimization
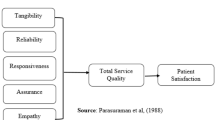
The Effect of Healthcare Service Quality Dimensions on Patient Satisfaction Among Primary Care Settings in Nigeria
Avoid common mistakes on your manuscript.
1 Introduction
Given the increasing competition between major hospitals, patients tend to seek higher quality medical services. In the past, hospitals used to adopt a hierarchical management system driven by power, and a functional management pattern based on division of labour. However, this pattern has been failing to address the need for competition in the socialist economy over time. If a hospital wants to have a sustained competitive edge, it should make an ongoing commitment to raising the level of its medical services. At the same time, it should also foster a service philosophy of “treating patients like customers” and establish a strategic business process to respond to the changing internal and external environment. By renewing operational and management methods and rebuilding outpatient processes, a hospital can build a process management model that serves the interests of its patients.
After 30 years of development, there have been remarkable advances in China’s economy. In addition, people’s living standards and purchasing power have also improved significantly. As people become increasingly rich, they start to attach greater importance to their health. However, with such a large population, coupled with intensifying urbanization, the urban population in China has increased dramatically. These urban residents are in urgent need of high-quality services from hospitals and medical personnel. In China, large and medium-sized hospitals are mainly concentrated in cities and the scale of these hospitals is determined by the registered population of these cities. As a result, medical resources in cities are inadequate and this situation is unlikely to be reversed in the short term. Currently, as the appointment registration model has not yet been widely implemented in large and medium-sized hospitals, large crowds rush to see physicians in the morning. Consequently, patients have to spend longer on registration, payment and drug dispensing, while they have far less time for the critical diagnosis by the doctor. In order to allocate medical resources in a reasonable way and reduce the waiting times to ensure sufficient time for treatment, optimising the outpatient process management model has become inevitable. Introducing the appointment mechanism enables patients to arrange their schedule to see a doctor in advance, which saves unnecessary queuing time and minimizes conflicts between doctors and patients.
Waiting time is a key driver of patient satisfaction, together with medical expertise, specialist availability, hospital location, cost, success rate, clinical care and confidentiality [ 1 ]. Long waiting lists in outpatient clinics are a widely recognized problem [ 2 ]. Physician time is one of the scarcest resources in healthcare and there can be several reasons why a doctor does not spend enough time with patients [ 3 ]. Due to uncertainty in the demand for appointments, it is difficult to provide an exact match between planned physician availability and appointment requests [ 4 ].
Chen (2003) found that currently most domestic hospitals still adopt the traditional outpatient process model [ 5 ], namely: queue for registration → wait to see the doctor → see the doctor (doctor issues a checklist or prescription) → queue to pay fees → queue for examination/tests (or drug dispensing) → further consultation with the doctor (doctor issues a treatment list or prescription) → queue to pay fees → queue for treatment (or drug dispensing). This traditional model is very common in major hospitals, but has many shortcomings, particularly in large, overcrowded hospitals since only a small part of the patient’s time in the hospital is reserved for treatment and this is severely detrimental to the social and economic benefits of the hospital.
Strategic and tactical capacity planning are critical issues that hospitals must face. While these problems have received significant attention, the current queueing-based approaches do not address realistic healthcare constraints such as blocking, transient arrivals, transient capacity assignments and surge capacities [ 6 ].
This study uses BPI Theory, Queuing Theory and System Theory with information technology at its core to analyse the process management model for outpatient appointments in the University of Hong Kong-Shenzhen Hospital (HKU-SZH). As far as we know, these theories have never been combined before to study patient satisfaction in a hospital, hence this is the gap that we intend to fill.
The research question is to know which factors influence patient satisfaction most. A quantitative analysis was performed using a questionnaire survey to identify the defects and weaknesses of the current model. The principles, methods and techniques of BPI theory are used to analyse various problems existing in the outpatient process and the extent of their influence. A structural equation model has been established for scientific and quantitative analysis, which can help identify the goals of optimization and measure improvement in the outpatient process and patient satisfaction. The aim is to maximize the existing resources in outpatient departments, optimize the management model and hospital operation service model, reduce unnecessary waiting time for patients, increase the effective hospital visit rate per unit of time, eliminate the phenomenon of “three longs and one short’, build a social image for hospitals as providers of efficient, convenient and premium medical services, and enable patients to have easy access to medical services. In the literature review section, BPI Theory, Queuing Theory and Six Sigma Theory are reviewed and discussed. These theories served as a conceptual framework for the construction of the questionnaire. In the case study, a questionnaire survey was conducted on the outpatient appointment process management model in HKU-SZH. By establishing a structural equation model for the current outpatient process, the study analyses the influence of factors in the outpatient process on patient satisfaction, so that objective improvement measures can be identified. Thus, three hypotheses were defined:
H1: Service attitude influences patient satisfaction.
H2: Service value influences patient satisfaction.
H3: Waiting time influences patient satisfaction.
2 Literature review
2.1 process management theory.
Process management, as an important management method in modern enterprise management, has been playing an increasing role in enhancing business performance and raising customer satisfaction levels. It has attracted widespread attention from management specialists in business circles. Its successful application in enterprise management has also attracted great attention from hospital management scholars.
In the Taylor and Ford eras, maximization of operational efficiency was the primary target. As a result, the idea of process management gradually developed into a formal management theory. The management master Taylor first proposed the idea of job analysis, followed by the idea of process management, in 1920. In 1980, the idea of quality management became very popular. In 1984, the US-based International Business Corporation, abbreviated as IBM, proposed the Quality Focus Business Process (QFBP) and listed “quality” as the core element of process. In 1988, IBM officially changed QFBP into Business Process Management (BPM). In 1990, the American management theorists Michael Hammer and James Champy first proposed the idea of ‘business process reengineering” in their best-seller Reengineering the Corporation [ 7 ]. This idea fitted the economic development requirements of the new period, since Hammer and Champy both agreed that enterprises should adapt to the changing external environment, gradually establish the customer-oriented operating principle, and reengineer the business processes to suit the external business development. In response to business process reengineering, IBM formally proposed the idea of Process Management (PM).
In 1995, Michael Hammer and Steven Stanton jointly published another book entitled The Reengineering Revolution Handbook [ 8 ], which led to an upsurge in “organizational reengineering” in Western countries. At the time, some scholars maintained that ‘organizational reengineering’ was the latest advance in management science and would definitely bring about a brand-new management revolution with business process re-engineering at its core.
In another study, also conducted in the same hospital (HKU-SZH), apart from assessing resistance related to human factors, it was concluded that rethinking the business process was also important, with the findings suggesting that process reengineering and innovation is a complicated and difficult organizational change [ 9 ].
In the healthcare sector, management scholars have always maintained a positive attitude towards successful management theories and methods in the business world. While findings on the application of management theories such as Business Process Reengineering, Business Process Management, and Process Management have been published in documentary research, empirical studies on other aspects of their application have also appeared in various academic journals. Wong, Doyle and Straus, for example, published an explanation of process management in 1975 [ 10 ].
Process management approaches (such as TQM, lean and six sigma) originally intended for manufacturing settings are increasingly being applied in the healthcare sector [ 11 , 12 ]. In fact, these process management approaches have created an awareness of the operational organizational aspects of care treatments [ 13 ].
2.2 Business process improvement theory
Business Process Improvement (BPI) is a methodology which aims to maintain the competitive edge of an enterprise by constantly perfecting and optimizing the business process. The design and implementation of the process must be continuously improved in order to achieve the best result. BPI means doing the right thing in the right way. Its aim is to increase service time and improve the efficiency of valuable services, while minimizing the time spent on non-value-added services. Usually there are three stages in BPI: firstly, a description of the business process, which includes a clear definition of the business or operation process of the target company through field research in the enterprise; secondly, analysis of the business process, which means looking into the current process of the target company to identify the major causes of existing problems; thirdly, the formulation and implementation of improvement plans, in which redundant or overlapping processes are redesigned and optimized on the basis of the identified problems and their causes.
According to Ding and Sun (2010), most Chinese hospitals follow a serial working mode, which means the work is divided step by step, and only after one step finishes can the next start [ 14 ]. Such a process is rarely value-added since it leads to an unreasonable distribution of medical resources, a low information sharing capacity and low operating efficiency. Zhao (2006) suggests that the effective treatment time of outpatients represents only 10% of their stay in a hospital, as patients have to queue up for treatment and payment. If the percentage can be raised to 30%, then the patients’ stay in hospitals will be cut by two thirds, and if raised to 50%, it will be reduced by four fifths [ 15 ]. However, some hospitals have faced great difficulties in implementing BPI projects, due to their system and structure. Improving patient service satisfaction in these hospitals is particularly difficult. Lin and Yang (2004) insists that Hospital Business Process Management (HBPM) should be applied [ 16 ].
A great deal has been achieved with respect to the use of BPI in the healthcare domain. However, there is still a need for more efficient and effective methods to support and overcome the shortfalls of existing methods. The complex nature of the healthcare domain requires comprehensive BPI that is able to deal with its common problems and challenges [ 17 ].
2.3 Queuing theory
According to Li and Xu (2003), Queuing Theory, or Stochastic Service System Theory, is an important branch of Operations Research [ 18 ]. It studies the probabilistic properties of queuing in various service systems, with a view to achieving optimal service system efficiency. In 1909, Agner Krarup Erlang, a Danish engineer, published The Theory of Probabilities and Telephone Conversations, the first paper on Queuing Theory, which mainly calculates and studies service time and queuing efficiency. Lu (1994) found in his research that Queuing Theory has been constantly improved and enriched by the rapid development of IT technology and has been applied extensively in various sectors such as transportation, health care, shopping and banking [ 19 ]. In the healthcare industry, queuing theory has been implemented mainly through the use of distributed systems, which can be applied to achieve lean and flexible architectures that facilitate healthcare structure interoperability. Systems used in healthcare structures are characterized by proprietary data flow formats and encoding systems, which hinder the possibility of sharing data in a standard format and extracting atomic data for further analysis [ 20 ].
The queuing theory model can be used to measure the service efficiency of different departments in public hospitals, which can improve both service efficiency and patient satisfaction [ 21 ].
2.4 Six sigma theory
According to Liu and Ma (2005), the Greek letter sigma (σ) represents the level of the process or operation. Sometimes the process capability is denoted by Z: for example, Z = 6 means that the process or operation has a process capability of 6σ [ 22 ]. The analysis of process capability usually focuses on whether the level of a process or operation can meet the expectation and to what extent it is met. Usually, Critical to Quality (CTQ) is employed as an indicator for analysis.
Chen (2010) finds in his research that in 2009 the Taizhou Hospital in Zhejiang Province reduced the defect rate from 39.6 to 12.9% by using the Six Sigma Theory management method to define, measure, analyse, improve and control the defect in the dripping speed of outpatient venous transfusion [ 23 ].
Six Sigma in itself does not address the cultural or interpersonal aspects of quality improvement and is limited further as it looks at individual processes rather than taking a system-wide approach. Moreover, poorly developed care processes are inevitably revealed when attempts are made to apply a Six Sigma program in a hospital [ 24 ]. To be effective, Six Sigma is dependent on high quality data and statistical expertise. Front line clinicians must therefore have access to appropriate systems and support (both technical and statistical), so that they can easily collect robust appropriate data for analysis [ 17 ].
2.5 Information technology
In terms of individuals, Information Technology (IT) has changed the way people communicate with each other. As to enterprises, IT has changed the distribution of power within organizations, altered corporate structure and reduced management hierarchies. As a result, corporate structure has become more and more flattening, which puts enterprises in a better position in market competition. IT has opened up space for process improvement, and to some extent, pushed forward process management reform. However, IT, in itself, is not characterized by the thoughts and ideas of “Process Management”. Therefore, debates on medical process arose right when IT was first applied in the medical industry. For example, Shen (2003) proposes several questions, such as “whether information technology should be used to reengineer hospital management or information technology should accommodate hospital management model; and whether information technology should be used to simulate traditional manual work or new ways of work should be redefined.” [ 5 ]. These questions can all be boiled down to the collisions between modern management technologies and traditional management ideas. For instance, the IT-based “One-Card” can integrate all medical processes, including outpatient registration, triage and payment. Using the “One-Card”, patients can finish all the aforementioned processes at one time and significantly reduce the length of waiting; in comparison, outpatient doctor workstation has given rise to the management model where “patients choose doctors”; application of PACS and electronic medical records has reduced the storage time of medical records and accelerated the information transfer of medical records; information system can also further shorten the time required for completion of discharge procedures; besides, internet technologies also make online consultation, booking and registration possible. Therefore, it is believed that full use of IT is necessary for process optimization so as to enable HIS to bring about outpatient services with high quality and efficiency.
2.6 Summary
Table 1 shows the main constructs that result from the theories studied and are the main items related to the hospital environment in the outpatient service, which were used to construct the questionnaire.
3 Informatization in the university of Hong Kong-Shenzhen hospital
Up to now, most of the hardware and software in the University of Hong Kong-Shenzhen Hospital are cutting-edge, thus enabling the hospital to base its clinic services on information technology as far as possible. To reduce manual operations, work that can be done by computers is now finished with the help of software.
Currently, Hong Kong- Shenzhen Hospital possesses seven buildings, a Logistic Service Building with seven floors (for the accommodation of canteen staff, interns and operators on duty), a Science and Education Administration Building with seven floors (for scientific and educational business and various trainings), three General Hospital Buildings with seven floors (the charge standard is governed by government), a VIP Hospital Building with five floors (the charge standard is higher and governed by hospital itself) and an Outpatient and Medical Technology Building with four floors (for outpatients and examination of medical laboratories). The two-floor basements of seven buildings are connected (which are mainly parking lots and a portion of warehouses). The hospital has adopted the internationally accepted Hierarchical Internetworking Model, which consists of access, convergence and core layers. Its buildings are connected by ten-gigabit armored fiber cables, which can be used outdoors. The network speed of computer terminals can reach gigabits. Besides, the whole hospital is covered by wireless network. In the four-floor Outpatient Medical Technology Building and the Hospital Building, wireless nursing, wireless ward rounds of doctors and wireless transmission of vital signs data are realized. Buildings and floors are connected by optical fibers. The Operation Room, Medical Imaging Department and Clinical Laboratory are connected to computer rooms through optical fibers. There are altogether three computer rooms in the hospital. The one on the first basement floor is for external lines and CCTV equipment, while those on the third floor of the Outpatient Medical Technology Building and the fourth floor of the Science and Education Administration Building are used to guarantee the operation of the medical software system in the hospital.
Network in the University of Hong Kong-Shenzhen Hospital is divided into internal and external networks due to network security concerns. The two networks are physically separated, and the Internet/email uses the external network while the medical software operates with the internal network. The hospital medical software structure is as follows (Fig. 1 ):
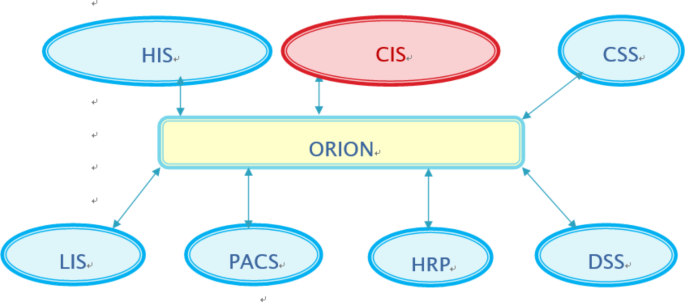
Hospital Medical Software Structure
Adopting HL7 international standards, those seven systems transfer data on the data exchange platform developed by Orion New Zealand Limited. As there is no direct communication among the systems, their interdependence will be reduced to the lowest level. If one system doesn’t function well, the others will not be affected. The hospital needs only to follow the HL7 interface standard when replacing the malfunctioning system and it is the same when some small functional software is connected to the medical software system. The hospital has two main computer rooms: the Main Data Center on the fourth floor of the Science and Education Administration Building, and the Disaster Recovery Center on the third floor of the Outpatient Medical Technology Building (Fig. 2 ).
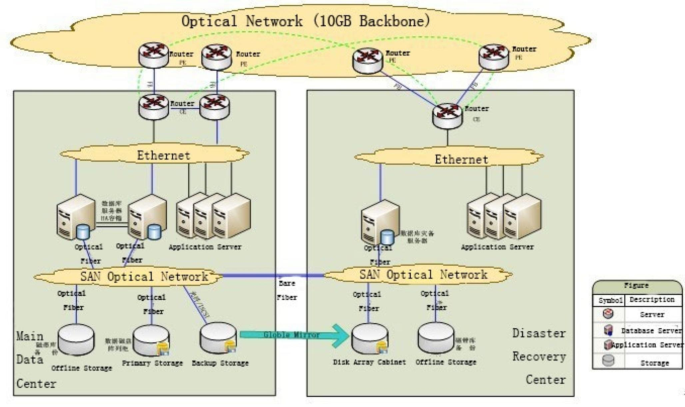
Allocation of Computer Rooms in the Hospital
Currently the hospital has 30 PC servers, 20 of which have been equipped with the VMWARE virtualization software to provide the virtual environment for software operation. Systems such as LIS, PACS, CSS and HRP are installed in PC servers. There are also two IBM minicomputers and two supporting storages, in which HIS and CIS are installed. Besides, the hospital has two blade servers and two supporting storages. Equipped with CTRIX virtualization software, they can provide the installation environment for programs purchased from small companies, including the reproductive system, dental system, central lab system, tumor center system, maternity and child care system, birth certificate management system, knowledge management system, infant anti-theft system and examination management and training system. The blade servers also provide the software testing environment for the hospital.
Additionally, due to the considerable image data, a 300T storage is attached to the PACS system. Each day the hospital produces data of 35G, and it is estimated that the number will reach 80G per day after the hospital is fully opened.
The University of Hong Kong-Shenzhen Hospital has almost completely achieved electronic operation. As the whole treatment process is recorded by electronic medical records, and there are electronic results of various examinations such as biochemical test, imaging examination and pathological examination. Doctors can check the results on computers, and paper records will be abandoned. The hospital is also testing the CA system, an electronic authentication system. According to the plan, the CA system will first of all be applied to PACS and LIS. After it is stabilized, it will be applied to all software systems in the hospital. At that time, the hospital will truly realize electronization while paper-based medical records will be abandoned completely. The CA system has national legal force. It consists of two parts: personal identification and real time data. The system can show clearly who conducts what operation at what time, since all traces of modifications are recorded.
All those measures will significantly enhance the standardized management in the hospital and enable the medical staff to examine various materials in accordance with rules and regulations. It will also promote the development of medical research through data mining.
4.1 Satisfaction measurement for the outpatient service
Based on the literature review, on-the-spot convenience sampling was used to conduct an on-the-spot questionnaire survey that lasted for a month in the hospital. The survey collects basic information, general patient satisfaction with the outpatient process, and scores for each survey item. The structural equation modelling is then established to analyse the effects of basic factors on the degree of patient satisfaction with the outpatient process. In order to ensure that the model can fit the data better, it was optimized and revised and a standardized coefficient path graph was drawn. From an analysis of the direct and indirect effect of key variables on the degree of satisfaction, the current ‘bottleneck problem’ in the hospital’s outpatient process was finally found and reasons for the obstacles in the present outpatient process were summarized.
In order to determine the needs of patients and the status quo of the outpatient business process, a convenience sampling of questionnaires was conducted in April and May 2014 among patients attending the hospital for treatment. This was an on-the-spot questionnaire survey, meaning that the patients anonymously completed a self-administered questionnaire on the premises. Clerks from the IT department gave questionnaires to patients who had finished treatment, who were ready to leave the hospital or who were waiting in the waiting room, injection room or clinical laboratory. The questionnaires were collected when the patients had completed them.
Out of 1,500 questionnaires distributed, 1,132 were collected, representing an effective rate of 88.3%. According to the statistical validity and reliability tests, the survey data is valid.
The questionnaire consists of three parts. The first part deals with basic information, including the respondent’s sex, age, marital status, profession, level of education, place of residence, payment category, and monthly family income, for the purposes of classification and comparison. The second part comprises 24 items, including appointment registration, communication between doctors and patients, medical costs, medical effect, hospital signage, service attitude, hospital environment, hospital hygiene, and waiting time in each phase of the treatment. Based on a survey of variables of satisfaction, it is designed to enable patients to respond to each item and obtain a score for each patient’s attitude, using a cumulating method. The average score of all the patients provides the researchers with an indication of the overall attitude. Each item adopts the Likert-type six-level scale method ranging from negative to positive, in which the numbers 1, 2, 3, 4, 5 and 6 correspond, respectively, to very dissatisfied , not very satisfied , a little dissatisfied , a little satisfied , quite satisfied and very satisfied . Hence, the answer to each item is not confined to “Yes” or “No” but is divided into several types, ranging from “satisfied” to “dissatisfied”, with the medium term representing a neutral attitude. Due to the greater range of options, it can fully embody the differences in patient attitudes. The third part contains open questions which patients need to answer to make suggestions for the hospital’s outpatient work and recommendations for improvement. Table 2 shows the scores for outpatient service items.
A total of 1,322 valid questionnaires were obtained from this survey. On the basis of the statistical validity test and reliability test, the data proved to be valid. After calculating the statistics, the overall degree of patient satisfaction with the hospital is 83.13 points.
The data from the collected questionnaires was introduced via the Epidata platform, issued by EpiData Association from Denmark, and analysed by SPSS 15.0, while AMOS7.0 was used to analyse the variables using structural equation modelling. Statistical approaches such as descriptive statistics analysis and the Wilcoxon rank sum test were also used.
Generally, questionnaires are analysed using the following methods:
4.1.1 Descriptive statistics analysis
The description of the basic information is included, as well as the frequency distribution and percentage analysis, in order to understand the distribution of the sample. Usually, a descriptive analysis of the data is conducted first, to find the inner law, and then a further analysis approach is adopted. Descriptive analysis can be used to analyse all the relevant data of variables in the survey population, mainly including frequency analysis, central tendency analysis, dispersion degree analysis and distribution of data, as well as statistical graphics. Common indicators include mean value, median, mode, variance, and standard deviation.
4.1.2 Cronbach’s reliability coefficient analysis
Reliability refers to the consistency, stability, and dependability of test results. In general, the level of reliability is indicated by inner consistency: the higher the reliability coefficient, the more consistent, stable and dependable the result is. In addition, analysing measured items of variables can shed light on the inner consistency of the measurement dimension. Furthermore, when Cronbach’s is higher than 0.7, its reliability is high, and when it is lower than 0.7, its reliability is low. The lowest acceptable reliability level is 0.5.
4.1.3 Exploratory factor analysis and confirmatory factor analysis
Exploratory factor analysis and confirmatory factor analysis are used to test the convergent validity and discriminant validity of measured items in each dimension. Reliability alone is not sufficient, since a high reliability measurement may turn out to be completely invalid or invalid to some degree. Therefore, a validity test is necessary. Validity refers to whether tools can produce the expected result for which they are designed. The convergent validity test is decided by the capacity of each item and measured concept factors, while the discriminant validity test calculates the correlation index of related concepts according to confirmatory factor analysis, to test whether 95% of the confidence interval of correlation index includes 1.0. If not, it can be regarded as discriminant valid.
4.1.4 Structural equation modelling analysis
Structural equation modelling combines factor analysis with path analysis and incorporates simultaneous equations in econometrics. Therefore, several variables can be dealt with simultaneously. Moreover, measurement errors in independent and dependent variables are allowed and the factor structure and factor relation can be estimated at the same time. A measurement model of greater flexibility is allowed and the fit degree of the overall model can be estimated so that it can be applied to the causal relationship of the overall model. Maximum likelihood (ML) estimation is adopted in the estimation of the model parameter. While testing the fit degree, indicators of preliminary fit criteria, overall model fit and fit of the model’s internal structure are used as criteria. Regarding the overall model fit criteria, chi-square/df value, GFI, RMSR, and RMSEA are used as indicators to study whether the estimated parameters reach a significant level.
Basic information on the patients in the survey is shown in Table 3 . According to data from A9, it was found that 34.3% of the patients had gone to the University of Hong Kong-Shenzhen Hospital for the first time and 65.7% had been there more than twice. Hence, the subjects in the survey are mainly patients who visit the hospital repeatedly and their evaluation of the hospital is therefore stable, with fewer errors in the data. According to the questionnaires, the main reason why the patients chose the hospital was its reputation and the advanced medical skills of the doctors, accounting for 53.8% and 33.7%, respectively, of all reasons given, followed by the simplified treatment process, advanced medical technology and equipment, and good attitude to service, which account for 22.4%, 16.5% and 12.7%, respectively.
4.2 Factors influencing patient satisfaction with the outpatient service
SEM can be divided into two models, namely Measurement Equation and Structural Equation.
(1) Measurement modelling is used to describe the relationship between measurement variables and latent variables. The mathematical expression is as follows.
X = Uxξ + δ.
Y = Uyη + ε.
The definitions for the above-mentioned mathematical expressions are as follows:
ξ represents exogenous latent variables. They serve as independent variables and remain unchanged regardless of influences from other variables in the model.
η represents endogenous latent variables. They change in accordance with changes in other variables in the model.
X represents vectors made up of exogenous measurement variables.
Y represents vectors made up of endogenous measurement variables.
Ux represents the rotated component matrix of exogenous measurement variables in exogenous latent variables.
Uy represents the rotated component matrix of endogenous measurement variables in endogenous latent variables.
δ is the error item, which is caused by exogenous measurement variable X.
ε is the error term, which is caused by endogenous measurement variable Y.
(2) Structural Modelling is used to reflect the relationship between latent variables. The mathematical expression is η = Bη + Γξ + ζ.
B represents the interaction among endogenous latent variables.
Γ represents the effect and influence of exogenous latent variables on endogenous latent variables.
ζ is the error term of the structural equation.
The coefficient matrix composed of B and Γ, as well as the error vector ζ, serve as the link between endogenous latent variables and exogenous latent variables,
In this research, AMOS7.0 (Analysis of Moment Structures) is used to construct structural equation modelling and carry out parameter estimation and relevant test analysis. This software can utilize path graph to customize models and mapping tools to change path graph in order to change models. It can also graphically display parameter estimation and fitting measurement, as well as the degree of freedom at any point in the mapping in the path graph. In this software, the rectangle represents the measurement indicators, the oval represents latent variables that cannot be measured directly, and the circle represents residual error. A double arrow indicates correlation, while a single arrow represents cause and effect. Exogenous latent variables do not have residual errors whereas endogenous latent variables do.
The graphic interface provided by AMOS is used to map out the causal relationship path graph. In mapping, the corresponding factor for every latent variable in the measure indicator is supposed to be 1. In other words, the unit of measurement for latent variables and the corresponding measure indicators are the same. It is also supposed that the default value for the system error of observed variables is 1 and that at least one path coefficient for the measure indicators has 1 as its initial value. Furthermore, AMOS7.0 will automatically set 1 as the value of the path coefficient, which is one indicator of the measure of the same latent variables. (Fig. 3 ).
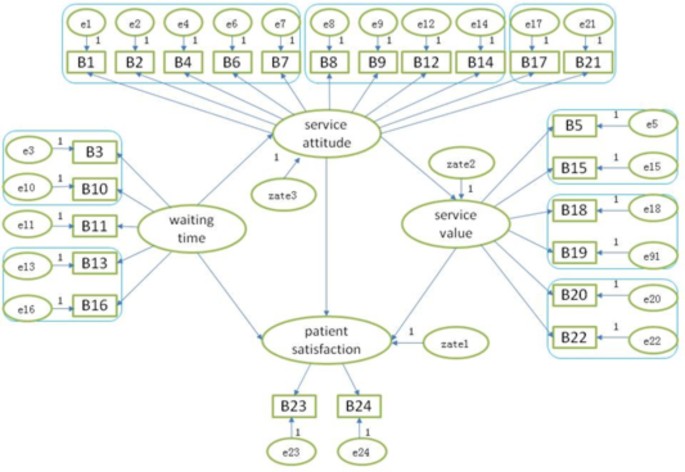
Causal Relationship Path Graph
It is estimated that four latent variables exist in this model, namely, service attitude, service value, waiting time and patient satisfaction. The four variables can be explained and defined as follows:
Service attitude is defined as the outpatient medical staff’s level of patience, conscientiousness and respect for patients.
Service value is defined as the expertise of medical workers, the curative effects of the treatment provided, the rationality of the medical service charge, and hospital hygiene.
Waiting time is defined as the queuing time and organization of patients throughout the outpatient process.
Patient satisfaction is defined as the overall patient satisfaction rate with the outpatient service in the hospital. The satisfaction rate is influenced by the three variables.
24 constructs from B1 to B24 were used to measure the above-mentioned four variables. Among them, the corresponding variables for patient satisfaction are patient loyalty and the overall evaluation score. The variables e1 to e24 represent the measurement errors caused by the measurement variables. The variables from zate1 to zate3 represent the error for endogenous latent variables.
The fitting situation for the model is shown in Table 4 . The indicators of the fitting effect show that the P value which corresponds to the chi-square has a certain statistical significance. The degree of freedom is 1 and the probability value is 0.672, which is higher than the threshold value 0.05. As a result, a null hypothesis is accepted and the model is considered to be appropriate. The ratio of the chi-square to the degree of freedom is more than 2. The values for GFI, AGFI and CFI are all less than 0.9. The value for RMSEA is more than 0.08. Generally speaking, the GFI value should be more than 0.9 while the RMR value and the RMSEA value should be less than 0.05, and the smaller the value, the better. Therefore, this indicates that the fit between data and model is not ideal and this model should be revised. Usually the fitting indicator RMSEA (root mean square error of approximation), which is very sensitive to model errors, is an ideal indicator, particularly when its value is smaller. When its value is less than 0.08, it indicates good fitting. In comparison, it is better for the GFI (goodness of fit index) and AGFI (adjusted goodness of fit index) values to be high. The value of NFI (normed fit index) ranges from 0 to 1. When it surpasses 0.9 the model is acceptable, and the nearer the value is to 1, the better.
In the estimation of regression coefficient, although the regression coefficient P value for wait->satisfaction, attitude->B4, and wait->B3 does not have statistical significance, the other regression coefficient achieves more than 95% in the hypothesis testing of significance. Whether the non-standardized regression coefficient is meaningful is determined by whether the P value is significant (three asterisks indicate that it is significant). Insignificant path needs to be removed in the model revising process afterwards.
5 Results and discussion
The questionnaire and the theoretical model were created on the basis of the theories studied. The results demonstrate that these theories can be useful for the study of patient satisfaction, confirming previous studies. Following an analysis of the theoretical model, the relationship between measured variables and latent variables was found to be clear. After a check on the correction factors, the M.I value between e7 and e15 was found to be the largest, at 15.426. If the correlation between the two variables is strengthened, the chi-square value will decrease to at least 15.426. Therefore, it can be concluded that there is a strong relationship between these two variables. In the causal relationship path diagram, if the correlation of measurement errors between e7 and e15 is strengthened, a revised model can be obtained.
The fitting situation of the revised model is shown in Table 5 , from which it can be seen that the new model is better matched to the data. Furthermore, the revised chi-square value of significance is p = 0.00. The ratio of the chi-square to the degree of freedom is 2.06, slightly larger than 2.0, but the RMSEA value is 0.057, far less than 0.08. The values for GFI, NFI, and CFI are all greater than 0.9, while the values for AGFI and RFI are all less than 0.9. This proves that the revised model matches its data, in comparison to the previous one. The reason why the chi-square value of significance is 0 is probably connected with the abnormal distribution of the data. Following a regression coefficient analysis, it was found that, except for the P value for attitude->B4, which does not have statistical significance, the other regression coefficients are all greater than 95% in the hypothesis testing of significance.
From the standardized coefficients of variables in the output model of AMOS7.0, the correction and degree of correlation between different variables can be found. In this way, it is easier to figure out which factors are the bottleneck that influences patient satisfaction and then help to make efficient improvement plans. In the software, the covariance between two variables can be shown in sample moments. The coefficients between different latent variables are shown in Fig. 4 .
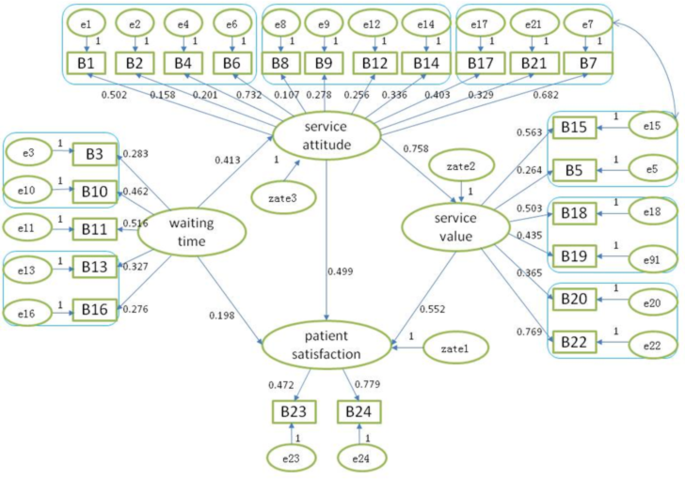
Standardized Coefficient Distribution Map after Revision
5.1 Correlation analysis of latent variables
The coefficient between latent variables means that a change in one variable will cause a change in another variable. This degree of influence is reflected in the correlation coefficient. It can be seen in Fig. 4 that:
The coefficient of influence between service value and patient satisfaction is 0.552. This shows that an addition or improvement of 1% in service value will directly lead to an increase of 0.552% in patient satisfaction.
The direct influence coefficient between service attitude and patient satisfaction is 0.499. The indirect influence coefficient caused by service value is 0.758 × 0.552 = 0.418. Therefore the total influence coefficient is 0.499 + 0.418 = 0.917.
The direct influence coefficient between waiting time and patient satisfaction is 0.198. The indirect influence coefficient caused by service attitude is 0.413 × 0.917 = 0.379. Therefore the total influence coefficient is 0.198 + 0.379 = 0.577.
As a result, the three variables of service attitude, service value and waiting time have a huge influence on patient satisfaction, leading to the verification of the three hypotheses, especially service attitude, given that its coefficient of influence reaches 0.917. Waiting time alone does not have a significant influence on patient satisfaction (its direct influence coefficient is only 0.198). However, if the indirect influence caused by service attitude is also taken into consideration, the influence coefficient will become 0.577, which deserves the attention of the hospital.
The scores for latent variables are shown in Table 6 . It can be seen that patients gave the highest score to service attitude and the next highest score to service value, while giving the lowest score for waiting time. Therefore, reducing the waiting time is the key to making patients more satisfied.
5.2 Analysing the correlation between measurement variables and latent variables
The correlation between “service value” and the price/performance ratio of medical service is the closest, with the correlation coefficient reaching 0.769. The next closest correlation is between inspection fees and therapy effectiveness. Although service value is also related to the general doctors’ medical expertise as well as the hospital environment, comfort and sanitary conditions, the degree of correlation is not significant.
The correlation between service attitude and the physicians’ diagnosis and treatment is the closest, with the correlation coefficient reaching 0.732. The degree of conscientiousness of doctors, namely their work attitude while examining and treating patients, has the second closest correlation with service attitude, with the correlation coefficient reaching 0.682. Furthermore, the patient satisfaction level with the appointment service, pharmacy staff service, hospital cashier service and hospital guidance system have the third closest correlation with service attitude, with each correlation coefficient reaching more than 0.3. The data shows that the attitude of hospital patient guides when answering questions, the patient’s free choice of doctors, the respect for patients when choosing treatment protocols, the protection of patient privacy, and the attitude of the Medical Examination Department personnel are also correlated with service attitude. However, the degree of correlation is not significant, with the correlation coefficients all below 0.3.
Waiting time is most closely related to medical examinations, laboratory tests and queuing for drug dispensing, with the correlation coefficients reaching 0.516, 0.462 and 0.327 respectively. However, waiting time has a weak correlation with queuing and with waiting for triage and payment of fees, with the correlation coefficients both below 0.3. After comparing the values of all correlation coefficients, it can be seen that the waiting time for medical examinations, laboratory tests, and medicine dispensing is relatively long and can significantly influence patient satisfaction. This should be given priority in process optimization. Although patients have to wait for a long time to get treatment, it does not have a huge influence on patient satisfaction, which is probably because patients have the lowest expected value or the highest degree of tolerance towards waiting to be treated.
Given that the normality of the survey data could not be guaranteed, it was decided to conduct the Wilcoxon rank sum test to analyse the influence of some basic factors, such as gender, occupation and age, on outpatient service satisfaction. It was found that factors such as gender, age, payment method and clinical department significantly influence outpatient service satisfaction. The conclusions are as follows:
Male and female patients make quite different comments on service attitude and waiting time: the comments from men are much more positive than those from women.
Age also has a certain influence on the level of satisfaction with service attitude and waiting time. Individuals aged 36 to 45 made the most comments while those aged 46 to 55 produced the most negative ones. Furthermore, older people made more comments on waiting time.
The payment method also has a certain influence on customer loyalty. To be specific, patients with medical insurance that covers part of their medical costs rank first in terms of loyalty, while patients enjoying free treatment or medical insurance that covers all the costs rank second, with those who seek medical treatment at their own expense ranking last.
The clinical department is also a factor that has an influence on waiting time. It was found that the waiting time for treatment in the Gynaecology, Obstetrics and Paediatric departments is much longer than that in other departments. Therefore, the hospital should focus on the Gynaecology, Obstetrics and Paediatric departments when improving its process.
In terms of improving the outpatient hospital service process in the interests of patients, it can be expected that there are mainly three medical service processes which are closely related to the interests of patients, namely departmental treatment, tests and examinations, and diagnosis and treatment. Since the improvement of other processes such as registration, triage for treatment, payment and drug dispensing cannot bring significant benefits to patients, they are defined as non-value-added process. Therefore, the process can be optimized by reducing, integrating, and simplifying the non-value-added processes while ensuring the normal operation of the three above-mentioned processes. The theories studied made it possible to highlight these conclusions.
According to the revised Fig. 4 for standardized coefficient distribution, the waiting time required for different processes has different effects on patient satisfaction, which can be ranked from large to small as follows: examinations (B11), testing (B10), drug dispensing (B13), triage for treatment (B3) and payment (B16). The corresponding coefficients of relationship are 0.516, 0.462, 0.327, 0.283 and 0.276. Hence, reducing the waiting time for examinations, testing and drug dispensing can efficiently improve patient satisfaction.
Following an analysis of the current outpatient service process, the source of inefficiency was determined and can be summarized as follows.
(1) The overly subdivided departments and the lack of medical information sharing result in a poorly-organized and discontinuous outpatient process. Due to the excessive subdivision of functions, patients have to go back and forth between different departments, leading to high patient mobility within the hospital. Currently, some consulting rooms, the pharmacy, examination department, and testing department are even located on different floors. When a doctor asks his or her patient to have a laboratory test, the patient has to leave the consulting room, find the medical laboratory with the test report, and then undergo testing. After the test, the patient has to wait for the result, go back to the consulting room, ask the doctor to prescribe medication and pay for the fee. Only after these processes are completed can the patient receive the medicine. The outpatient process is even more complicated when the patient needs to be transferred to another hospital or needs to visit different departments. Additionally, the lack of information sharing also results in poor coordination and links between different departments. For example, since a doctor knows nothing about supplies of medicine when he or she writes a prescription, the patient may only learn the said medicine is out of stock when they reach the cashier. The patient then has to return to the consultation room to ask the doctor to change the prescription. This situation occurs from time to time in the hospital, not only adding to the flow of patients between the consulting room and cashier, but also affecting the orderliness and continuity of the process.
(2) The unreasonable architectural layout of the outpatient service leads to increased patient mobility and waiting time. In fact, the layout of the medical technology building and departments will directly influence the efficiency of the process. Moreover, the lack of signage in the hospital or the overly small font size of the characters used in signs will further increase patient mobility and waiting time in different service processes. Nurses serving as guides, who know little about the categories of diseases due to their lack of training, cannot provide an efficient service for patients. Furthermore, the location of some departments, such as Orthopaedics and Obstetrics, is badly-designed. The Orthopaedics Department is located on the second floor, while the Obstetrics Department is on the third floor, which presents great difficulties for patients who need to move around.
(3) The medical and health resources are insufficient and unevenly distributed. Although a comprehensive outpatient appointment system has been established in the hospital for the consultation process, there is still not enough medical staff and examination equipment to meet the needs of patients, since the hospital has only been open for a short time. Given the increasing number of outpatients, a further expansion of the hospital is necessary. Moreover, the comparatively uneven distribution of medical resources to some extent leads to uneven patient mobility in different departments. As analysed, the Gynaecology and Obstetrics, the Paediatric departments receive the majority of patients, while there are relatively few dermatologists.
6 Conclusions
This research analyses the current situation regarding the outpatient service process. In addition, patient satisfaction with this hospital was measured by means of a field questionnaire and interviews. In total, 250 patients at the hospital took part in the survey anonymously and patient satisfaction was evaluated using the 6-grade scale. A validity test was also carried out on the reliability coefficient. The analysis of this survey mainly focuses on the basic situation, percentage of overall satisfaction and the scores of all outpatient service items, covering 24 factors, including sex, age, marital status, occupation, degree of education, address, payment category, family monthly income, reservation and registration, communication between doctors and patients, treatment effect, hospital signage, service attitude, hospital environment, hospital hygiene, and waiting time for each process.
In order to determine the specific process that has the greatest impact on patient satisfaction from the quantitative analysis method, which is also the bottleneck for the hospital outpatient process, a structural equation model was used. With this model, the interactive relationship between multiple variables and the relationship between multiple reasons and results, including some unobservable variables (latent variables), can be studied. It was assumed that there were four latent variables in the established model, namely service attitude, service value, waiting time, and patient satisfaction.
Through regression analysis and parameter estimation, the relationship between e7 and e15 was added, and it was found that service attitude, service value and waiting time have a significant influence on patient satisfaction. In particular, the influence coefficient of the service attitude reached 0.917. Meanwhile, the direct and indirect influence of waiting time on patient satisfaction cannot be neglected, since these factors might be the key to improving patient satisfaction.
In this study, the diagnoses and analyses of the HKU-SZH outpatient process were carried out by means of field investigation and measurement of patient satisfaction. Rather than regression analysis, correlation analysis or factor analysis, the measurement is based on a structural equation model, from which the influence of different processes on patient satisfaction can be accurately calculated. This method not only embodies the service philosophy of “putting patients first”, but also serves as an entry point for process reengineering. An in-depth analysis was carried out on deficiencies in the appointment registration system for the all-patients model. Information technology was used to reengineer the HKU-SZH outpatient process so that its medical resources are utilized rationally and efficiently. In this way, the difficulty of accessing medical services was solved to some extent. This empirical study is of practical significance, as it is in line with the Outline National Development Plan for Health Information.
The major limitations and suggestions for further study are as follows:
There is no quantitative analysis to evaluate the effects of process improvement. One method to improve and perfect the process improvement plan would involve devising a scientific measurement scale for patient satisfaction, for a more in-depth investigation of patients and hospital staff. The effects of hospital progress should be evaluated using the Cost-Benefit Analysis Method. Special attention should be paid to the changes in patient satisfaction, especially in the hospital process, before and after process reengineering.
With regard to sharing resources on treatment information, it is necessary to seek help from government departments such as the Shenzhen Health and Family Planning Commission. Only with their help can a medical information-sharing platform be built to ensure treatment information is shared between different hospitals.
Dialogue and negotiations with medical security institutions are recommended, for the purpose of proposing a functional integration of social security cards and co-branded cards. In this way, patients would only need one card for medical services, which is convenient and efficient.
Availability of data and materials
Not applicable.
Code availability
Silas S, Rajsingh EB. A novel patient friendly IT enabled framework for selection of desired healthcare provider. Int J Med Eng Inform. 2019;11:14–40. https://doi.org/10.1504/IJMEI.2019.096890 .
Article Google Scholar
Lewis AK, Taylor NF, Carney PW, Harding KE. Reducing the waitlist of referred patients in a medical specialist outpatient clinic: an observational study. J Health Organ Manag. 2020;35:115–30. https://doi.org/10.1108/JHOM-08-2020-0321 .
Grad JD. Some unhealthy aspects of managed health care. Bus Soc Rev. 1996;53:54.
Google Scholar
Aslani N, Kuzgunkaya O, Vidyarthi N, Terekhov D. A robust optimization model for tactical capacity planning in an outpatient setting. Health Care Manag Sci. 2020;24:26–40. https://doi.org/10.1007/s10729-020-09528-y .
Chen ZH. Optimizing the process of outpatient service through general appointment system. China J Mod Med. 2003;13:142–5.
Curry GL, Moya H, Erraguntla M, Banerjee A. Transient queueing analysis for emergency hospital management. IISE Trans Healthc Syst Eng. 2022;12:36–51. https://doi.org/10.1080/24725579.2021.1933655 .
Hammer M, Champy J. Reengineering the corporation: a manifesto for business revolution. New York, NY, USA: Harper Collins; 1993.
Hammer M, Champy J. Reengineering revolution: a handbook. New York, NY, USA: Harper Collins; 1995.
Xu XP, Ke DG, Deng DN, et al. An innovative medical consultation model in mainland China: Acceptance and perceptions. Int J Health Care Qual Assur. 2019;32:1055–71. https://doi.org/10.1108/IJHCQA-02-2017-0033 .
Wong P, Doyle M, Straus D. Problem solving through process management. J Nurs Adm. 1975;5:37–9. https://doi.org/10.1097/00005110-197501000-00017 .
Kaissi A. Learning from other industries: lessons and challenges for health care organizations. Health Care Manag. 2012;31:65–74. https://doi.org/10.1097/HCM.0b013e318242d399 .
Daultani Y, Chaudhuri A, Kumar S. A decade of lean in healthcare: current state and future directions. Global Bus Rev. 2015;16:1082–99. https://doi.org/10.1177/0972150915604520 .
de Regge M, Gemmel P, Meijboom B. How operations matters in healthcare standardization. Int J Oper Prod Manage. 2019;39:1144–65. https://doi.org/10.1108/IJOPM-03-2019-0227 .
Ding YH, Sun W. Application of business process improvement in hospital management. Soft Sci Health. 2010;5:412–5.
Zhao Q. Business reengineering and its application in hospital operation management. J Qingdao Tech Coll. 2006;4:35–6.
Lin QY, Yang JY. Exploration on hospital process-oriented management. Chin Health Service Manage. 2004;1:26–8.
Ahmed ES, Ahmad MN, Othman SH. Business process improvement methods in healthcare: a comparative study. Int J Health Care Qual Assur. 2019;32:887–908. https://doi.org/10.1108/IJHCQA-07-2017-0116 .
Li J, XU J. Operational research - optimization of Nonlinear System. Beijing, China: Science Press; 2003.
Lu C. Pai Dui Lun. Beijing, China: Beijing University of Posts and Communications Press; 1994.
Muriana C, Gilia G, Mistretta V, et al. A distributed integration system enabling electronic health records: an Italian experience. Int J Med Eng Inform. 2019;11:1–13. https://doi.org/10.1504/IJMEI.2019.096889 .
Zhang L. Application of computer-based queuing theory in public hospital management. J Phys Conf Ser. 2020;1549:1–7. https://doi.org/10.1088/1742-6596/1549/5/052009 .
Liu W, Ma YZ. (2005) Study on the theoretical basis of six sigma quality improvement. J Henan Univ 35.
Chen HX. The practice of reducing the dropping speed of venous transfusion in outpatient service through six sigma management. J Tradit Chin Med. 2010;6:21–3.
Lifvergren S, Gremyr I, Hellström A, et al. Lessons from Sweden’s first large-scale implementation of six Sigma in healthcare. Oper Manage Res. 2010;3:117–28. https://doi.org/10.1007/s12063-010-0038-y .
Download references
Acknowledgements
This work is an outcome of the doctoral thesis of the first author, undertaken at the University Institute of Lisbon (ISCTE-IUL), Portugal, and the Southern Medical University (SMU), Guangzhou, Guangdong, China. It was partially funded by the Portuguese Foundation for Science and Technology (Grant UID/GES/00315/2019) and ISTAR Projects: UIDB/04466/2020 and UIDP/04466/2020.
This work it was partially funded by the Portuguese Foundation for Science and Technology (Grant UID/GES/00315/2019) and ISTAR Projects: UIDB/04466/2020 and UIDP/04466/2020.
Open access funding provided by FCT|FCCN (b-on).
Author information
Authors and affiliations.
Instituto Universitário de Lisboa (ISCTE-IUL), ISTAR-Iscte, Av. das Forças Armadas, 1649-026, Lisboa, Portugal
Jingsong Chen & Bráulio Alturas
School of Health Management, Southern Medical University, Guangzhou, Guangdong, China
Jingsong Chen
You can also search for this author in PubMed Google Scholar
Contributions
Both authors participated in writing and reviewing the manuscript.
Corresponding author
Correspondence to Bráulio Alturas .
Ethics declarations
Conflict of interest.
Both authors declare that they have no competing interests.
Ethics approval
Consent to participate.
Informed consent was obtained from all individual participants included in the study. This study is not a clinical trial.
Consent to publish
Additional information, publisher’s note.
Springer Nature remains neutral with regard to jurisdictional claims in published maps and institutional affiliations.
Rights and permissions
Open Access This article is licensed under a Creative Commons Attribution 4.0 International License, which permits use, sharing, adaptation, distribution and reproduction in any medium or format, as long as you give appropriate credit to the original author(s) and the source, provide a link to the Creative Commons licence, and indicate if changes were made. The images or other third party material in this article are included in the article’s Creative Commons licence, unless indicated otherwise in a credit line to the material. If material is not included in the article’s Creative Commons licence and your intended use is not permitted by statutory regulation or exceeds the permitted use, you will need to obtain permission directly from the copyright holder. To view a copy of this licence, visit http://creativecommons.org/licenses/by/4.0/ .
Reprints and permissions
About this article
Chen, J., Alturas, B. Improvement of outpatient service processes: a case study of the university of Hong Kong-Shenzhen hospital. Health Technol. 13 , 971–985 (2023). https://doi.org/10.1007/s12553-023-00788-6
Download citation
Received : 30 March 2023
Accepted : 18 October 2023
Published : 10 November 2023
Issue Date : November 2023
DOI : https://doi.org/10.1007/s12553-023-00788-6
Share this article
Anyone you share the following link with will be able to read this content:
Sorry, a shareable link is not currently available for this article.
Provided by the Springer Nature SharedIt content-sharing initiative
- Outpatient process
- Business process reengineering
- Information Technology
- Local adaptation
- Find a journal
- Publish with us
- Track your research
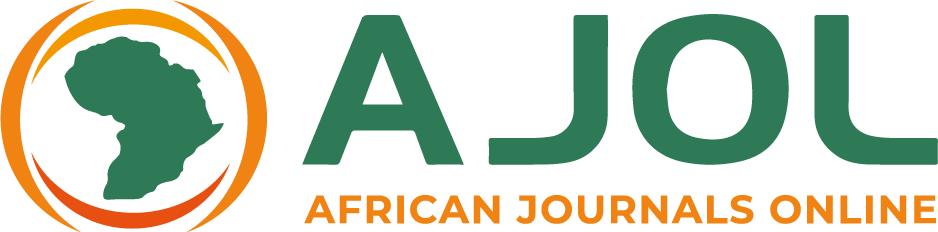
Rwanda Public Health Bulletin Journal / Rwanda Public Health Bulletin / Vol. 5 No. 3 (2024) / Articles (function() { function async_load(){ var s = document.createElement('script'); s.type = 'text/javascript'; s.async = true; var theUrl = 'https://www.journalquality.info/journalquality/ratings/2410-www-ajol-info-rphb'; s.src = theUrl + ( theUrl.indexOf("?") >= 0 ? "&" : "?") + 'ref=' + encodeURIComponent(window.location.href); var embedder = document.getElementById('jpps-embedder-ajol-rphb'); embedder.parentNode.insertBefore(s, embedder); } if (window.attachEvent) window.attachEvent('onload', async_load); else window.addEventListener('load', async_load, false); })();
Article sidebar.
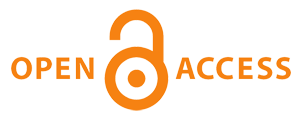
Article Details

This work is licensed under a Creative Commons Attribution-NonCommercial-NoDerivatives 4.0 International License .
Main Article Content
Patient flow analysis in a public hospital: a case of outpatient services at remera rukoma district hospital, emile sebera, celestin hagenimana, flugence rugengamanzi, janvier nahimana, felix kamali, leonille nizeyemaliya, emile twagirumukiza.
INTRODUCTION: Wait times in outpatient services are a major challenge for hospitals. This study at Remera Rukoma District Hospital aimed to analyze patient flow and waiting times in the outpatient department to improve service quality and resource utilization.
METHODS: A cross-sectional study was conducted from November 2023 to January 2024, involving 300 patients. Data was collected by observing patient flow and recording time spent at each touchpoint, then analyzed using SPSS version 25.
RESULTS: The average age of participants was 36.23 years, with a majority being female (55%) and referred patients (82%). Most visits (72.7%) were first-time consultations. Average wait times ranged from 19 minutes at reception to 104 minutes at the pharmacy, with the longest waits observed at the pharmacy.
CONCLUSION: The study highlighted the need for interventions to reduce delays, particularly at the cashier and pharmacy. Recommendations include process improvement, staff training, and regular monitoring of patient flow data to enhance patient satisfaction and healthcare service quality.
AJOL is a Non Profit Organisation that cannot function without donations. AJOL and the millions of African and international researchers who rely on our free services are deeply grateful for your contribution. AJOL is annually audited and was also independently assessed in 2019 by E&Y.
Your donation is guaranteed to directly contribute to Africans sharing their research output with a global readership.
- For annual AJOL Supporter contributions, please view our Supporters page.
Journal Identifiers
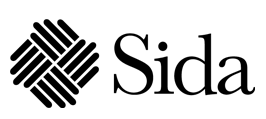

Improving Patient-Reported Outcomes through Enhanced Prescription Ordering and Filling Services
As healthcare shifts toward personalized, outcome-focused care, patient expectations for timely, supportive service are higher than ever. This case study highlights how DATAMARK’s advanced contact center solutions help healthcare providers exceed these expectations, enhancing prescription management, patient communication, and overall care efficiency—ultimately driving better patient-reported outcomes and satisfaction.
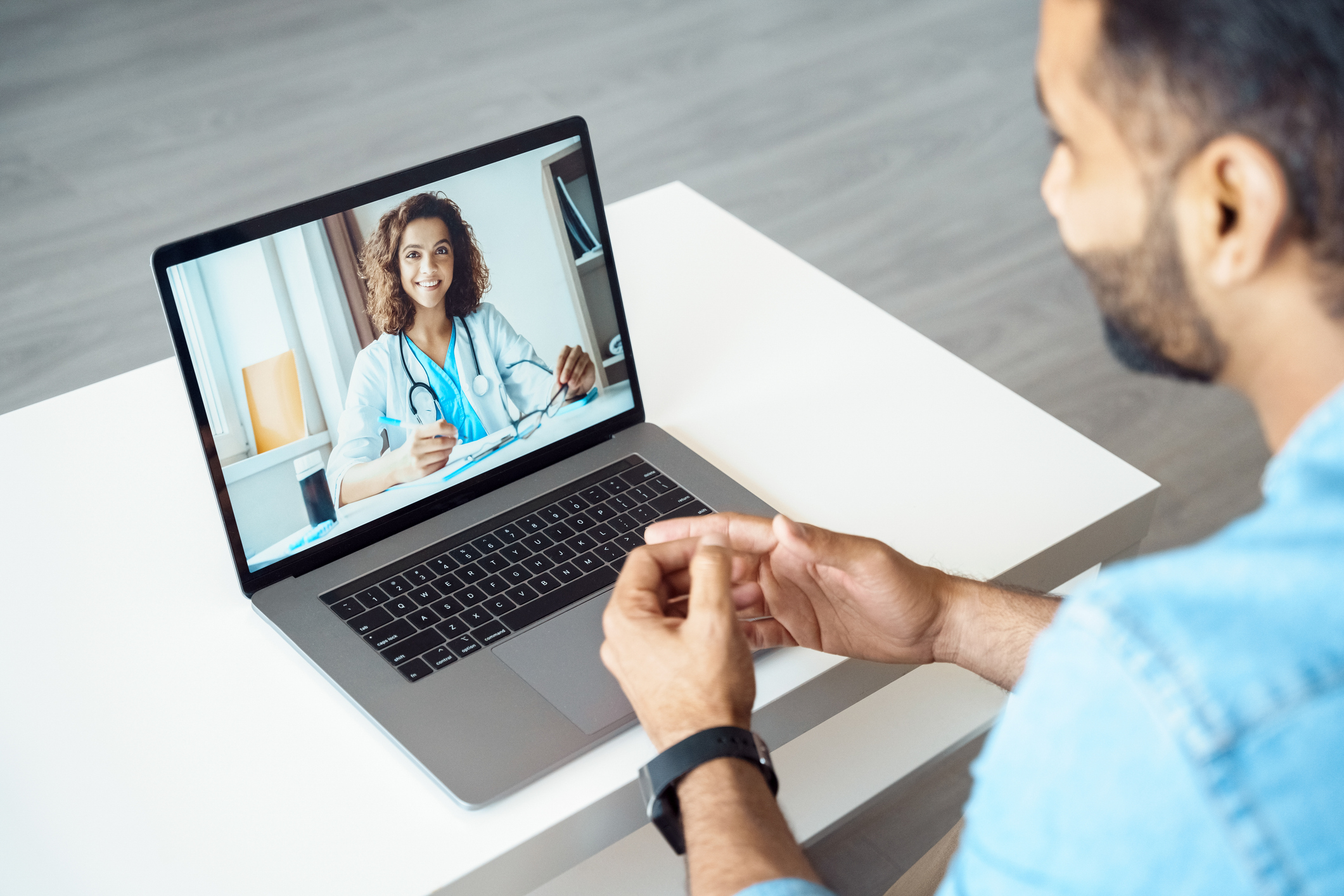
Background: Optimizing Prescription Fulfillment for Improved Patient-Reported Outcomes
A leading healthcare service provider manages prescription ordering and filling through their contact center operations. With the increasing emphasis on Patient-Reported Outcomes (PROs) in healthcare, especially for prescription services, the provider recognized the critical need to implement timely systems to capture and utilize this data. According to new CMS guidelines, providers must begin collecting baseline Patient-Reported Outcome Measures (PROMs) starting July 1, 2024, with outcomes to be publicly reported in 2027. This data provides valuable insights into patients’ health conditions directly from their perspective, covering areas like symptom burden, quality of life, and functional status.
What are Patient-Reported Outcomes (PROs)?
Patient-Reported Outcomes (PROs) refer to reports from patients about their health conditions and treatment outcomes, without interpretation from healthcare providers. These outcomes provide essential insights into symptom burden, quality of life, and functional status, and they are crucial for understanding the effectiveness of healthcare services from the patient’s perspective. Under CMS guidelines, healthcare providers are required to start collecting preoperative and postoperative PRO data for certain procedures beginning July 1, 2024, with the first submission deadline set for September 2025.
Challenge: Enhancing Patient Experience through Contact Center Support
As the healthcare landscape shifts toward more patient-centered care, the healthcare provider faced increasing demand for better management of patient-reported outcomes. Challenges included handling a high volume of patient inquiries about prescription orders, addressing concerns about medication side effects, and ensuring that patients adhered to prescribed treatment plans. Delays, long wait times, and inefficient call routing led to dissatisfaction and negatively impacted the overall patient experience.
DATAMARK Solution: Optimizing Contact Center Services to Improve PROs
Moreover, to address these challenges, DATAMARK partnered with the healthcare provider to enhance their contact center operations. The solution aimed to improve the patient’s experience and support better outcomes through efficient prescription management. Key elements of the solution included:
- Real-Time Prescription Updates using Self-Service IVR: Automated updates provided through a self-service Interactive Voice Response (IVR) system. This system allows patients to check their prescription status at any time without needing to speak to a live agent, which reduces the need for follow-up calls, increases efficiency, and boosts patient satisfaction. The timeliness of these updates plays a crucial role in reducing patient anxiety and improving adherence to medications.
- Predictive Call Routing: Leveraging data from previous interactions, the contact center system routes patients to the most qualified agents based on their needs. This reduces the number of transfers and ensures that concerns are addressed promptly and efficiently. The rules governing predictive routing are continuously updated to ensure patients are connected with agents who are most capable of handling specific queries, leading to faster resolutions and enhanced patient outcomes.
- AI Call Summarization and Keyword Detection: This advanced technology detects adverse events and adverse reactions during calls. AI-driven summarization and keyword detection automatically flag critical information, such as potential side effects or medication-related issues, enabling rapid follow-up and ensuring that patients’ health concerns are addressed swiftly. The timeliness of detecting these events during the calls is critical, as it allows for immediate action to be taken to address patient concerns, thus improving overall patient safety and satisfaction.
Results: Improved Patient Satisfaction and Health Outcomes
The integration of advanced call center technology not only reduced average handle times but also played a crucial role in supporting patient-reported outcomes. By streamlining prescription management and enhancing patient communication, the healthcare provider saw significant improvements in the following areas:
- Increased Medication Adherence: Patients were more likely to adhere to their prescribed medications, leading to better health outcomes.
- Enhanced Patient Satisfaction: With reduced wait times, proactive communication, and real-time prescription updates, patient satisfaction scores improved, reflecting the positive impact on their healthcare experience.
- Improved PRO Collection: By incorporating tools to collect and analyze PROs, the healthcare provider was able to gather valuable insights that helped further refine their contact center services.
In summary, through DATAMARK’s innovative contact center solutions, the healthcare provider successfully enhanced its prescription management operations, improving patient satisfaction and health outcomes. The seamless integration of self-service IVR, predictive call routing, and AI-based tools for adverse event detection contributed to the overall success. Timeliness in rule application and response played a pivotal role in ensuring the efficiency and effectiveness of these solutions, demonstrating the value of patient-centered technologies in the healthcare space.
WANT TO KEEP READING?
More datamark case studies.
We help Fortune 500 companies, large enterprises and government agencies address the greatest challenges to their businesses to increase their profitability as they grow. Explore the case studies below to learn how we’ve helped our customers and how we can help your business, as well.
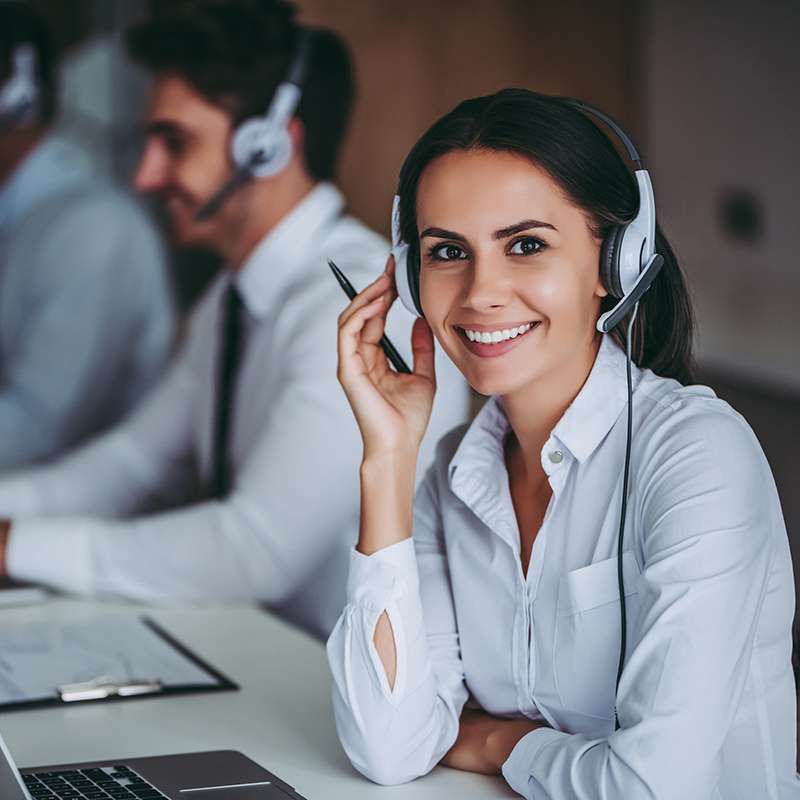
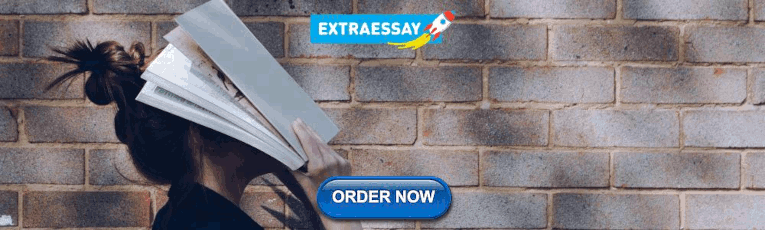
Contact Center Workforce Management Improves Service
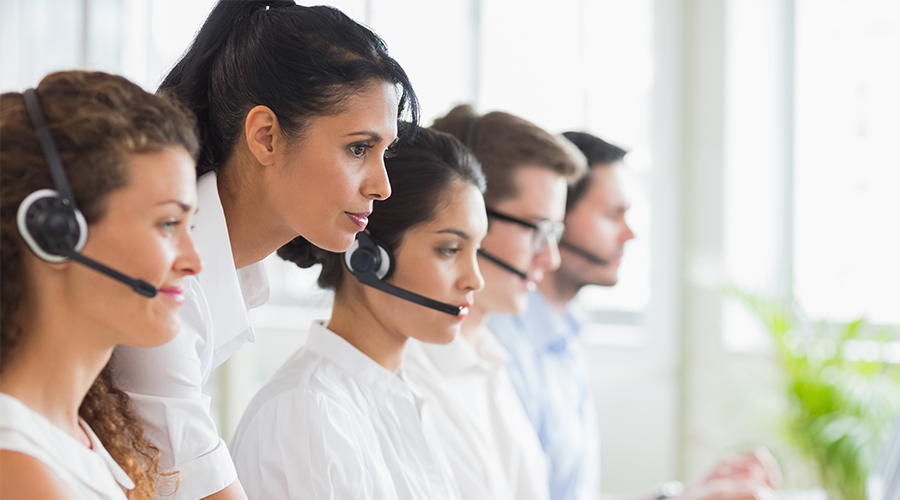
Effective Onboarding Strategies for Contact Centers
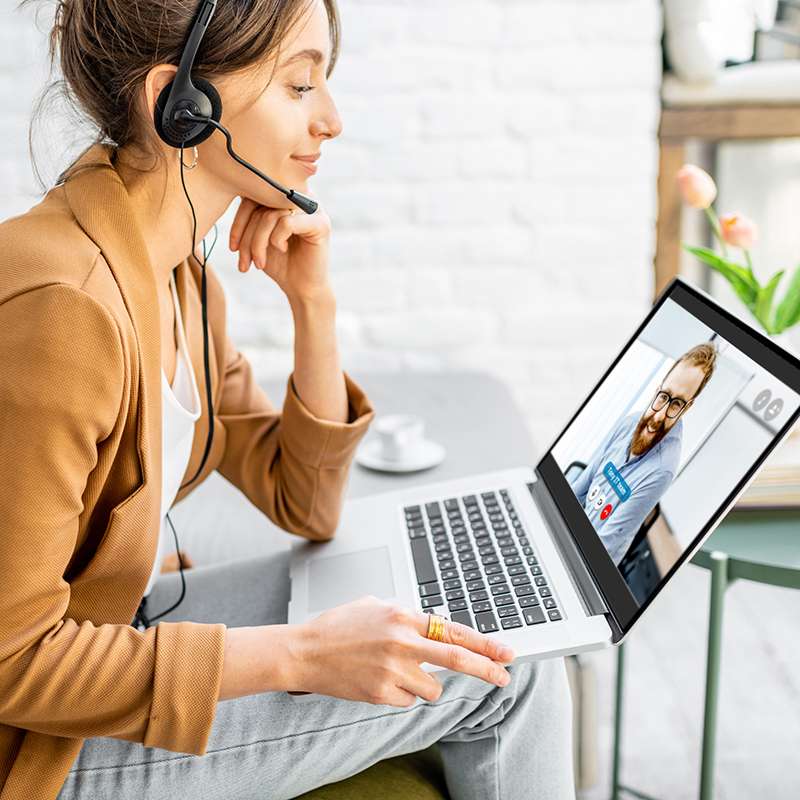
Developing Interpretation Resources at Scale
Let’s stay in touch.
Subscribe to stay informed and updated on the latest Contact Center and BPO insights and strategies shaping the world today.

- Published standards
- Core Information Standard (CIS) update
- Data management in general practice
- Obstetric Anaesthetic Standard
- Standards explained
- Transfers of care
- Palliative and end of life care
- Personalised care and support plan
- Support services
- Patient Portals
- Epilepsy Standard
- Community health services
- Mental health services data sets interventions
- Anaesthetic chart
- Overprescribing
- Information requirements for pharmacy, optometry, dentistry, ambulance and community services
- Community mental health
- Digital medication information assurance
- COVID-19: recording Guidance
- Digital health and care and Covid-19
- Diagnoses recording
- Pharmacogenomic information in clinical practice
- Standardising pathology test results
- Urgent care information flows
- Care home information flows
- Using email in health and social care
- Apps review – Healthily Smart Symptom Checker
- About the scheme
- The standards conformance process
- PRSB partners
- Conformant partners
- Partner events
- Partner news
- Meet the team
- About the PRSB
- The PRSB Board
- The Advisory board
- Coalition for change
- PRSB Professional Network
- Annual report
- Strategy 2023/24
- Latest news
- PRSB monthly newsletter
Case study – Frimley Health
Implementing the outpatient letter standard - frimley health case study.
Outpatient letters are the main method of contact and communication between hospital staff and GPs and are often the sole record of the consultation held by the outpatient department and hospital.
Best practice for most outpatient letters is writing directly to people using services, so that they can better understand what’s been discussed with their healthcare professional, including their treatment and results.
On this page
Lessons learnt.
The PRSB’s Outpatient Letter Standard allows relevant clinical information to be recorded, exchanged and accessed consistently across care settings, and shared with people who use services, in plain English. This results in improving the continuity of care and people’s overall experience of healthcare services.
This case study shows how Frimley Health NHS Foundation Trust worked with two system suppliers, Nuance and Epic , to implement the standard and identifies the benefits that it will have on the efficiency of healthcare services and people’s experience of care.
The Outpatient Letter Standard is one of the standards that could be soon mandated to be implemented by health and care organisations and system suppliers – the case study shows why it makes sense to start the implementation sooner rather than later.

The process

Frimley Health NHS Foundation Trust uses Nuance’s Dragon Medical Once solution, which captures voice-generated content directly into clinical systems. Dragon voice commands can also be used to navigate electronic health records and to insert customised templates, also known as auto-texts.
When Frimley implemented their EPR system supplied by Epic, and they decided it was a great opportunity to utilise the PRSB’s Outpatient Letter Standard, as it’s widely endorsed and would allow them to build the letters using a clear, standardised structure.
Standardisation of information is important as it helps improve interoperability between different systems.
All Frimley’s departments have been involved in the project. The organisation also worked closely with primary care providers, such as GPs, who were supportive of standardising letters and adhering to the PRSB standard. It was reasonably straightforward to build the letter templates to the standard, concentrating on implementing the mandatory fields first.
Almost all letters are transcribed using Dragon Medical One, and Frimley encourages clinicians to use auto-texts and voice commands to make the letter creation more efficient while also making it easier to share standardised templates.
Further work is planned to allow the solution to automate these processes even more.
During the implementation process, Frimley identified that it can be challenging to include some of the fields marked ‘required’, and collection of structured data in the EPR is required, so that recorded data items are brought into the letter.
To make the adoption of Outpatient Letter Standard easier, Frimley is currently working with Epic, to ensure that the PRSB Outpatient Letter Standard becomes an integral part of their UK Foundation System and making the configuration more standardised for new NHS customers.
In the near future, the Frimley team will begin to review the current build to increase the number of optional PRSB fields that might be present in the body of the letter.

The benefits
The Outpatients Letter Standard View the full standard and supporting documentation, examples and other resources.
Why use the standard? In our short film, Dr Ian Logan from The Freeman Hospital, explains how the standard ha s benefited his patients receiving regular kidney dialysis .
Support services Proactive support for our standards and ongoing maintenance and release management of published standards.
An official website of the United States government
Official websites use .gov A .gov website belongs to an official government organization in the United States.
Secure .gov websites use HTTPS A lock ( Lock Locked padlock icon ) or https:// means you've safely connected to the .gov website. Share sensitive information only on official, secure websites.
- Publications
- Account settings
- Advanced Search
- Journal List

Appointment Scheduling Problem in Complexity Systems of the Healthcare Services: A Comprehensive Review
- Author information
- Article notes
- Copyright and License information
Academic Editor: Saeid Jafarzadeh Ghoushchi
Corresponding author.
Received 2021 Nov 26; Revised 2022 Jan 14; Accepted 2022 Jan 25; Collection date 2022.
This is an open access article distributed under the Creative Commons Attribution License, which permits unrestricted use, distribution, and reproduction in any medium, provided the original work is properly cited.
This paper provides a comprehensive review of Appointment Scheduling (AS) in healthcare service while we propose appointment scheduling problems and various applications and solution approaches in healthcare systems. For this purpose, more than 150 scientific papers are critically reviewed. The literature and the articles are categorized based on several problem specifications, i.e., the flow of patients, patient preferences, and random arrival time and service. Several methods have been proposed to shorten the patient waiting time resulting in the shortest idle times in healthcare centers. Among existing modeling such as simulation models, mathematical optimization techniques, Markov chain, and artificial intelligence are the most practical approaches to optimizing or improving patient satisfaction in healthcare centers. In this study, various criteria are selected for structuring the recent literature dealing with outpatient scheduling problems at the strategic, tactical, or operational levels. Based on the review papers, some new overviews, problem settings, and hybrid modeling approaches are highlighted.
1. Introduction
Today, it is widely recognized that a well-designed healthcare process must provide timely and easy access to healthcare facilities for all patients [ 1 ]. Appointment Scheduling (AS) can enhance the utilization of expensive staff and facilities' medical resources while reducing patient wait times. Appointment scheduling aims to build an appointment system that optimizes a specific quality standard in a healthcare application of scheduling tasks under uncertainty. The primary function of healthcare management programs is to minimize patient waiting times in public hospitals and increase patient satisfaction [ 2 ]. Healthcare services coping with a large number of outpatients may have several obstacles to address. For instance, a long waiting period for a treatment negatively impacts the patient's experience and may diminish the quality of care [ 3 ]. In general, healthcare centers such as hospitals and clinics accumulate an increasing number of patients needing their services. Hospitals have to implement quick and effective healthcare facilities to accommodate new patients and keep people patronizing them [ 4 ]. They must successfully identify the bottlenecks, anticipate the effect of diversity on-demand, and compute the optimal capacity distribution [ 5 ]. Healthcare centers are evaluated by recognizing the best methods, applying measurable techniques, and having an obligation to improve. Healthcare clinics use decision support systems to provide low-cost and assessable services to individuals to preserve the care quality of services [ 6 ]. The solutions presented in the literature aim to reduce waiting times by developing decision support systems to manage outpatient clinic services [ 7 ]. Over recent years, healthcare systems have been strained to provide patients with high-quality services despite insufficient funding. One of healthcare's most important issues, ASP, has improved quality and prompt access to health facilities. Time is an essential element in ensuring patient safety and performance, and time is a crucial determinant of patients' satisfaction [ 8 ].
In principle, the purposes of ASPs can be divided into four categories: decreasing service costs, increasing patient satisfaction, reducing waiting time, improving fairness, and reducing costs in healthcare [ 9 ]. One of the central issues in healthcare is fairness, which is a primary concern when scheduling patients and doctors [ 10 ]. Aside from fairness in scheduling, further encouragement is attained through a novel gain framework unique to the division and was not reported previously. Another critical issue on fairness is mending personal scheduling preferences [ 11 ]. The appointment scheduling's main problem is optimizing healthcare resources by improving human resources and medical equipment utilization, leading to the depreciation of the patient waiting times. Several studies have shown that the primary explanation for patient dissatisfaction in outpatient scheduling is often extended waiting time, and fair waiting times are required based on clinical competence [ 12 ]. Simulation models are among the most well-known approaches to investigating random factors' influence on patients' waiting time and doctors' idle time in appointment scheduling [ 13 ]. The optimization model uses a Simulated Annealing method to optimize the patient appointment scheduling mitigating the average service period and whole patient waiting times. According to the obtained result, the entire service time and the patient waiting time have been reduced by about 5% and 38% compared with the current situation, respectively [ 14 , 15 ]. They examined the quality of their solutions via structural results and compared them with heuristic scheduling practices using a discrete event simulation. Some scholars [ 16 , 17 ] applied for advanced work inside the literature to layout models to maximize the variety of patient appointments, minimize affected patient waiting time, and increase patient satisfaction. They also defined the answer set programming to solve the proposed combinatorial optimization problem that exhibited a suitable assessment used in artificial intelligence [ 18 – 20 ]. This paper provides an overview of the no-show problem from the following perspectives: Our contribution in this review study is to assess and examine all scientific work in appointment scheduling from 2000 to 2021, emphasizing complexity techniques. In investigating patient admission scheduling with varied applications, we examine several types of problem descriptions.
Furthermore, we also review the works available in solving other healthcare scheduling, including waiting time, using artificial intelligence, and queuing theory in appointment scheduling. Our review work centered around appointment scheduling in the complexity of healthcare research considering this problem is the most studied healthcare scheduling problem as described, concentrating on various methods used in ASP to decrease waiting time and improve patient satisfaction in healthcare. The remainder of this paper is organized as follows.
Section 2 reviews the existing articles on outpatient scheduling problems and related works. Section 3 presents the broad performance criteria of the present methodologies in appointment scheduling problems. Section 4 discusses various application domains and healthcare application methods, while the patients' choice function has more areas. An affected person chooses a selected provider (which determines carrier fine and health facility revenue), a specific day of the week (carrier delay), and a particular time of appointment (convenience). Finally, the findings and conclusions for future guidance are discussed in Section 5 .
2. Research Methodology
This search aims to uncover papers that seek to determine patients who will turn up for their appointments. As a result, the search is initially limited to articles focusing on the keyword emergency or its synonyms. The comprehensive review is based on the publications related to the issue of appointment scheduling published from 2000 to 2021 in the Web of Science ( WoS ) Core Collection database. As can be seen, the number of recent articles has overgrown. Figure 1 shows the percentage of application domains to the patient and outpatient scheduling problems. As can be seen, most of the existing outpatient appointment scheduling applications are in the field of chemotherapy and radiotherapy. Hence, we recommend a pie chart about different healthcare branches handling the green outpatient scheduling inside a radiotherapy department defined in such a manner to represent different actual-existence situations. The effectiveness of discussed studies is evaluated on randomly generated issues and a real case situation. The outcomes are very encouraging since the developed optimization models can overcome human experts' performance.
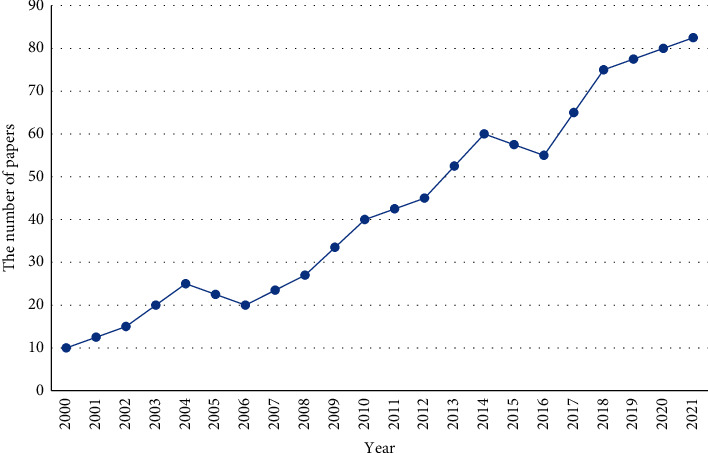
The trend of the published articles in the area of appointment scheduling 2000–2021.
As seen in Figure 1 , appointment scheduling has been discussed in many literature review topics. Generally, the problems are based on highly aggregated information at various times of the year. Nearly half of the contributions are seen in or after 2013, illustrating the increasing topics for researchers in the appointment scheduling program. Since the total number of manuscripts is massive, we restrict manuscripts to those posted in or after 2014 and 2015. The number of papers published in English regarding this issue between 2014 and 2015 is limited. However, the number of articles published after 2015 has risen due to the contribution achieved between researchers and the healthcare sector. They realized that they could benefit from this system's advantages, including better working time for staff and suitable follow-up for ordinary patients with chronic illnesses.
In Figure 2 , we have also considered different publication indexes in appointment scheduling, such as SCI (blue), SJR (orange), IOS (grey), and JCR (yellow). As we can see, the number of papers from 2000–2021 on the SCI and JCR has increased slightly, and it has shown that many authors are believed to publish the article in some well-reputed journals. However, the Appointment scheduling topic is also going viral for many scholars these years as it is essential for healthcare services and management.
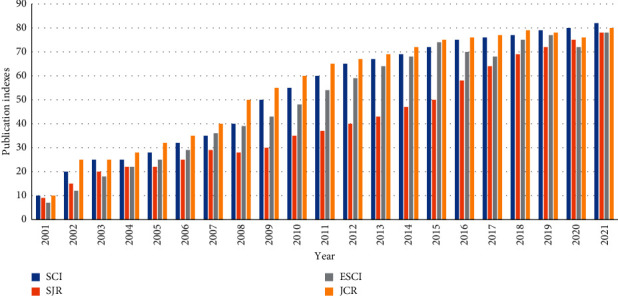
The trend of the publication based on various indexes SCI, JCR, SJR, and IOS in appointment scheduling.
Also, in Figure 3 , author keywords were more likely to define the difficulties and methods. In contrast, keywords plus included general terms like “appointment Systems,” “health care,” “arrival time,” “WTS,” and pleased. VOSviewer is used to display the cooccurrence connection of the network of the 200 keywords. The node's scale indicates the frequency of the keywords, and the thickness of the line indicates the vicinity of the relationship between the three main keywords. Three frequently used terms, “Appointment scheduling,” “optimization,” and “Healthcare,” are highlighted in the center with a more prominent label and a circle. In the graphic, the difference between the two keywords reflects the similarity of the words in terms of the connections. For instance, the keyword “Healthcare” appeared with numerous other terms such as “Systems,” “Optimization,” “admission,” and “arrival time.” As a result, the keyword placement is determined by the number of other keywords that share positive similarities. The cooccurrence map reveals that simulation in appointment scheduling comprises a broad spectrum of issues, including the emergency department, hospital planning network, operation, outpatient capacity planning, appointment scheduling, and resource allocation. The smaller nodes, which are associated with keywords such as “time delivery,” “algorithm,” “fairness,” “discharge,” “delays,” and “performance,” represent the lower cofrequency of these words across the examined papers, despite their tight connection with the Appointment scheduling.
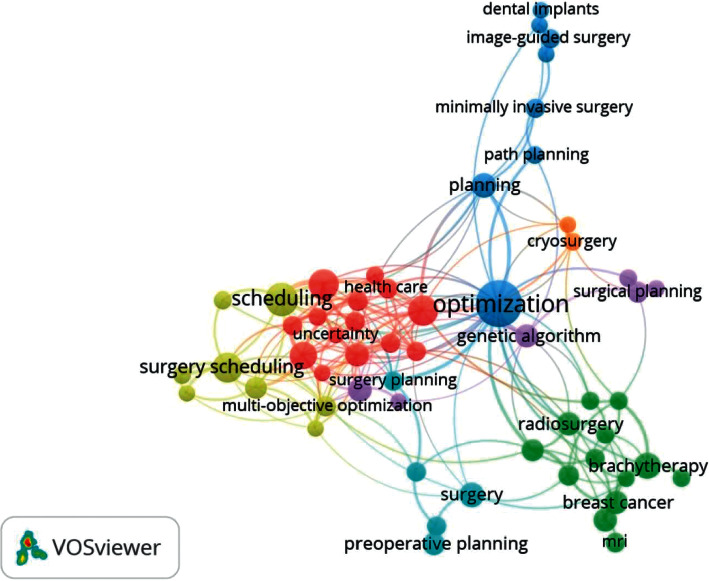
The keywords for the outpatient scheduling problems.
It also depicts the current patients' scheduling core elements in operation research (OR). The number of articles in which the keywords appear to be together, recreating the connection of their different research areas, is used to calculate the strength of the link between two keywords. This contribution has made it possible for researchers in this area to pick up more novel and appealing topics. Table 1 presents a list of outpatient scheduling models and methods' taxonomy. Most of the literature addresses modeling approaches presented earlier in this review paper that considered obtaining stability between patients' wait time and doctors' utilization through a hospital consultation and resources. In reality, direct and indirect waiting time is one of the initial practical factors in appointment scheduling. However, this modeling is difficult for the whole process for many reasons. First, unlike the direct waiting period during which the appointment is stopped is a typical ending; waiting time issues are more realistically modeled as unlimited problems. Second, outpatients are assigned an appropriate appointment time to select several preferred providers in a scheduling problem. Also, ASPs made for a specific doctor are coupled with different days and doctors on an actual day.
Categorizing the various artificial intelligence methods in appointment scheduling 2021–2022.
3. Results and Analysis
There are many techniques in the healthcare research areas. One of the crucial areas is utilizing appointment scheduling. In this section, some methods are analyzed to determine which method is more efficient than the others with their advantages as bases. The admission process is introduced with or without appointment only by the online or call services. The fundamental goal is to minimize access time by assigning part of the resources to patients who call for scheduling on the same day or within a few days [ 31 , 32 ].
3.1. Overlapping Scheduling (OLAS)
The overlapping appointment scheduling (OLAS) model shortens the patient waiting time and the doctor's idle time in an outpatient healthcare hospital with a stochastic service time while maximizing the doctor's utilization and patient satisfaction [ 33 – 35 ]. OLAS model refers to deciding the optimal overlapping periods between the patient appointment and allocated service times. OLAS is usually formulated as an optimization problem to minimize the total cost of patients waiting and doctors' idle time, given the probabilistic distributions for patient flow and the service time [ 36 , 37 ]. This discovery should help improve clinic services and ensure service quality [ 38 ]. Another study at the University Hospital of Egypt [ 39 ] analyzed patients' satisfaction with the quality of services in outpatient clinics, concluding that there is a need for continuous quality improvement and care in the healthcare environment, mainly to satisfy patients. The process of developing an overlap period in clinics with different assumptions is related to the service time distribution, over time, and no-shows [ 40 , 41 ]. OLAS's primary advantages for appointment scheduling are its lack of specific scheduling services, such as alarm and warning of overlapping times. In general, OLAS increases productivity and profit despite the expense of additional staff. Also, some appointment analyses emphasize the importance of the number of operation researches. The OLAS model's objective is to determine the effect of overlapping appointments in a healthcare sector (clinic) setting. Different units are involved in this process [ 42 , 43 ].
3.2. Markovian Scheduling Method (MSM)
The queueing theory has numerous applications in the field of healthcare management. Because they play such a significant role in hospitals, the research of queuing systems has often focused on the busy period and waiting time. A queuing system is typically defined as a patient entering a queue, being served at a service point by a server (doctor), and then exiting the row [ 44 ]. A stochastic process, a type of embedded Markov chain, governs the state-to-state transitions. At the same time, a different probabilistic mechanism determines the time spent in each stage. The transition probabilities are assumed to depend on the current state, and the time spent in each step is considered to depend on the present and following conditions [ 45 , 46 ]. Many researchers use Markovian methods to investigate service scheduling research, such as for ambulance unloading delays, a Markovian queueing model was used [ 47 , 48 ]. Another analysis that used the Markovian model to estimate patient services was the basic Markovian model's waiting time in a hospital using order statistics [ 49 , 50 ]. The Markovian models show that a healthcare condition often depends on the standard sequence of carefully followed steps. These actions can formulate the difficulty, purpose of study, data gathering, concept and validation, and the network model's systems. Markovian chain method for appointment scheduling has conceived a new idea wherein knowledge about that approach depends on one or two booking agents' expertise [ 12 ]. The Markov decision model's different number of sessions and duration determines an optimal policy for a given problem. For instance, the number of semiurgent patients scheduled in a particular week, given the expected demand or the number of appointment scheduling patients, considers walk-in patients' anticipated direction [ 51 , 52 ]. In both cases, it is assumed that the number of this week's patient arrivals is not influenced by the number of patients who arrived last week. We also investigated these two healthcare methods (OLAS) and (MSM) based on how some others considered these in their work.
Based on the investigation in Table 2 , we have shown that most of the papers on appointment scheduling between 2021 and 2022 are applied. Markovian systems other than OLAS as that model have many subsections to use various optimizations methods such as Mixed Linear Integer Programming (MILP), stochastic technique, and queuing theories.
Different methods and objectives in appointment scheduling publications between 2021 and 2022.
The most significant difference between the Markov chain models and other approaches is patients' status during a specific period of time [ 60 , 61 ], called different wait time penalties. A key factor is the order of patient treatment, i.e., first-come-first-serve (FCFS). In the case of a high number of patients requesting the care services, the ordered patients arriving later might be scheduled before those visiting earlier, thus causing the system to increase its “rate of service.” However, enforcing fairness reduces flexibility, which is called different wait time targets. The fairness policy is motivated by expediting early arrivals rather than scheduling late arrivals ahead of them [ 62 , 63 ]. Furthermore, it observed that an individual's waiting times are more variable for the contemporary approach than for the sequential one; this notable feature illustrates the difference in fairness [ 64 ] ( Table 3 ).
The taxonomy of outpatient scheduling models and methods.
3.3. Simulation-Based Complexity of Healthcare
Simulation models are rapidly becoming a well-known approach for dealing with healthcare appointment scheduling concerns and issues. Different simulation methods were investigated in most instances. The complexity of healthcare systems arises from their complex structure, which includes the concepts of queues and flows and social systems and decision making. Modeling complex systems at the personal level rather than the population level may be more beneficial with DES as an operational research technique. Individual entities travel through a succession of discrete events one by one at discrete intervals, among which they must wait in queues because of the limited availability of resources. The simulation approaches for outpatient scheduling problems are categorized mainly in Discrete events, Agent base simulation in healthcare problems, and those are recently widely used on this topic. As reported in the pie chart in Figure 3, most articles use discrete-event simulation (DES) techniques to improve patients' services to reduce the wait time in healthcare centers. As these two methods are mainly considered in most papers, we evaluated their differences in healthcare systems. Discrete event simulation and Agent-based simulation (ABS) have capabilities and limitations. DES and ABS methods are argued to be complementary to each other. Most healthcare systems are based on two major elements; the concept of queues and flows and decision making. DES models can consider the idea of queues and flows, while ABS models can capture human behaviors and decision-making in healthcare systems. A framework for a hybrid model of DES and ABS was proposed to capture both significant elements of healthcare systems.
Also, having those simulation approaches categories for appointment scheduling, discrete-event simulation is a flexible strategy tailored to shape the methods required to notify healthcare scheduling. It allows a broad set of tools than the Markov standard method and enables the development of techniques at a depth suitable to the problem. Its dangers are few and without problems mitigated, bringing our field towards necessities for powerful modes that decision-makers can trust. Most discrete-event simulation has programmed Any Logic or Simulation Arena software to control the clock [ 112 ]. Also, adequately scheduled activities and recognizing the subsequent ones appear to allow for dynamic entities, assigning input variables, and acting technique activities appropriately.
Agent Base Simulation can also model complicated, stochastic, and nonlinear conditions and focus on specific patients. So based on the pie chart in Figure 3 , we will determine these prior years; most of the simulation approaches on ASP have various percentages of each approach. On the other hand, DES varies from ABS in three different ways [ 113 – 116 ]: first, in the method, decision-making actions engage in ABS; second, in its depiction of queuing; and third, in the increased number of tools available to it. Patients that arrive early, late, or on time for their scheduled appointment may be addressed by the hybrid simulation model (HSM) and SO [ 117 – 120 ]. The most notable distinction between the HSM and SO approaches is patients' status at a certain point, referred to as differing wait time penalties. The order of patient care, i.e., FCFS, is important. [ 121 – 123 ]. If many patients need care services, the ordered patients who arrive later may be scheduled ahead of those who come earlier, causing the system to enhance its “rate of service.” This study proposes a combination of ABS [ 124 – 126 ] and nonlinear mixed-integer programming (MIP) to reduce WTS in Ass [ 127 – 129 ]. ABS [ 130 ] updated their concept for broad adoption, and it has been effectively implemented at ten ASs and several hospital units. The machine-learning framework integrates patient information and matching therapies, which detects trends in the simulation platform. As a result, agents offer problematic symptoms to care providers in the form of recurrent patients whose complaints were possibly mistreated in previous visits to appointment scheduling. This ASP study highlights the type of uncertainties: one about the issues involved in the activities, one about the frequency of the tasks, and one about the available resources and employs fuzzy logic to deal with these uncertainties [ 131 , 132 ]. The study divides agents into two types: software and physical. The latter refers to those who can act on their initiative, including everyone from doctors and patients to healthcare workers, nurses, and other hospital personnel.
3.4. Queuing Theory
In theory, the typical queuing problem in appointment scheduling has long been a source of consternation for domestic and international specialists and scholars. Queue theory and accompanying better models have been frequently operated to overcome this challenge. This section adds to the theoretical optimization of queuing problems in hospital management and gives an analysis and decision-making mechanism for enhancing hospital queuing theory and medical service efficiency. The following four essential characteristics are usually used to describe the queuing system.
3.4.1. Patient Arrival Mode
The time it takes for patients to show up at the queuing system is either predictable or unpredictable. The majority of patients in the hospital's queue system arrive randomly. At this moment, the arrival rule of patients entering the procedure is called admission arrival. The focus of queue theory is also on this circumstance.
3.4.2. Service Model
Patient service hours are deterministic or random, and most service hours are random. The probability distribution often describes the time rule of patients receiving services.
3.4.3. Queuing Rules
Healthcare for emergency patients is among the first-come, first-served services. Whenever a patient with a higher priority appears to the system, the patient getting the service must stop and be changed to treat such patients, such as the hospital emergency department for severely ill patients.
3.4.4. Number of Bed Resources
A service system is typically comprised of one or more service stages. Patients' hospital diagnosis frequently necessitates many service phases, such as outpatient visits. After making an appointment, outpatients come to the queue (i.e., the waiting list, arrival time, and idle time) in a first queueing system. When the patient's appointment time arrives, they are withdrawn from the waiting list and placed into a second queueing system. The patient enters the queue at the service facility, receives the accurate service, and then departs the appointment scheduling slot in this separate queueing system. Throughout the present paper, both queueing systems will be referred to as the appointment generating queueing system and the service facility queueing system (see Figure 4 ).
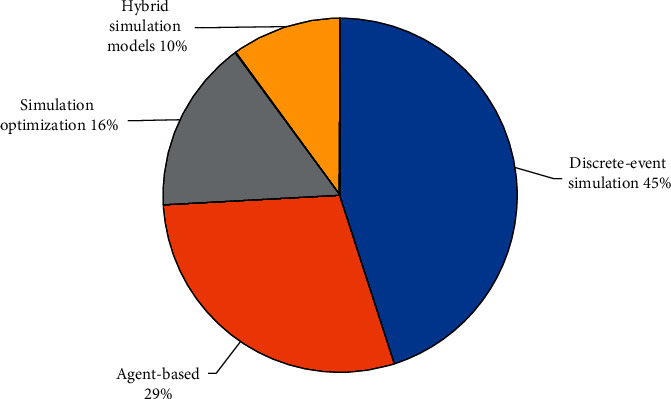
The percentage of different simulation approaches to an outpatient scheduling problem.
The most widely used queuing system is the M/M/s or Erlang delay model for outpatient scheduling waiting time study. This model assumes a single queue with an unlimited waiting room that feeds into s identical servers. It is usually assumed that the patients arrive according to a Poisson process with a constant arrival rate, and the service duration follows an exponential probability distribution. The primary use of the M/M/s method includes only three variables and could be used with little internal data to produce output estimates [ 73 , 133 ]. At the same time, it provided the average arrival rate, the average length of support, and several services. Also, to achieve performance measures such as the probability that arrivals will encounter a significant or average delay, a priority queueing model could be suitable if a facility intends to identify the ability required to assure a centered service level for the best precedence customers [ 134 ]. For example, Queuing analysis is also an essential method in predicting ability needs for potential future situations, including demand rises due to emerging or urgent new illnesses, wanting a physician's care more quickly to prevent extreme scientific consequences [ 135 , 136 ].
3.5. Artificial Intelligence (AI)
Healthcare is one of the research sectors in which Artificial Intelligence (AI) has high potential advantages. Recently, more state-of-the-art AI methods has been addressed through appointment scheduling. In order to improve the efficacy of scientific operations, numerous solutions have been introduced for online systems, appointment/surgical procedure scheduling, medical image analysis, and treatment plan and forecasting of uncommon diseases, and AI is easily carried out in appointment scheduling. The software can significantly affect the ultimate use of resources by considering these various demanding situations in a hospital's everyday working surroundings. AI-based scheduling Machine Learning (ML) models have a significant possible role in improving hospital healthcare services [ 78 ]. ML can maintain even more complicated models that can change several areas simultaneously, as in the postanesthesia treatment unit and surgical centers. Models of AI, which have significant economic consequences, may also restrict another organizational problem [ 137 , 138 ].
Also, any bias against an underrepresented institution in an information set will result in a biased computerized decision. For example, an appointment scheduling software program can make racially discriminatory scheduling decisions. AI programs in healthcare need to avoid such inequalities. AI provides a lead to assuming the fundamentals of AI technologies (machine learning, healthcare) and their proper use in healthcare. It also offers practical support to help decision-makers promote an AI approach that can support its digital healthcare transformation. All investigation outcomes are tracked by AI, which then analyzes patterns to optimize future interactions [ 139 , 140 ]. The AS system optimizes and duplicates the factors that lead to positive results. Each patient engagement is triggered by AI depending on the patient's specific needs. Using AI in the appointment scheduling system can then send out evaluations to patients via e-mail or text message, collecting feedback on the services. The system can then examine this data to identify areas where there is room for development and pass them on to the appropriate doctors. Several hospitals use AI to predict the number of patients to the emergency department two or three days in advance, allowing them to take proactive action in staffing and resource allocation [ 141 – 143 ]. Also, [ 144 ] examined the difficulties and prospects for hospitals to integrate AI into strategic planning and become intelligent systems with feedback-controlled operations and procedures (closed-loop systems). They [ 145 ] presented a model in healthcare scheduling during the COVID-19 outbreak healthcare service. They built on a considerable amount of theory and research on behavioral Internet of Medical Things, big healthcare data analytics, and artificial intelligence-based diagnostic algorithms, Creating a framework for categorizing artificial intelligence. For the sake of the analysis, we separate between care levels (primary, secondary, and tertiary care), planning levels (strategy, operational, and functional), and user groups (doctors, nurses, technicians, patients)
3.5.1. Optimization Methods with AI
This review gives a broad overview of artificial intelligence's role in healthcare. This review does not touch on all healthcare areas that benefit from optimization modeling. However, we have suggested a range of optimization and neural networking applications to healthcare research. These optimization methods have been recently utilized in many optimization methods. However, in healthcare, scheduling is considered more such as convolutional neural networks (CNN), recurrent neural networks (RNN), artificial neural networks (ANN), Ant Colony Optimization (ACO), Genetic Algorithm (GA), Particle Swarm Optimization (PSO), and Whale Optimization Algorithm (WOA).
Based on what we have investigated in Table 1 , we have different methods of artificial intelligence, and as we can see recently, many papers have considered appointment scheduling that utilized PSO and WOA. At the same time, the rest of the areas of the neural network are primarily used in different healthcare areas.
Many scholars have investigated Particle Swarm Optimization (PSO) in their research because this method is a swarm-based intelligent stochastic search technique encouraged in different ways in healthcare scheduling. Consequently, for the versatility of numerical experimentation, PSO has been chiefly applied to address the diverse kinds of optimization problems. The PSO algorithm could be utilized to solve various healthcare scheduling issues. The first entails optimizing the problem's objective function, while the second entails optimizing the cost function of a healthcare system. In different problems, good results are achieved, confirming the PSO method's efficiency over other AI methods. As we can see in Figure 5 , during 2021–2022, many papers, especially in AI and healthcare, have been collected based on PSO with a greater number of publications than other methods in such a healthcare scheduling. Also, we still have some papers from WOA and ACO that form optimization methods that stand behind the PSO methods.
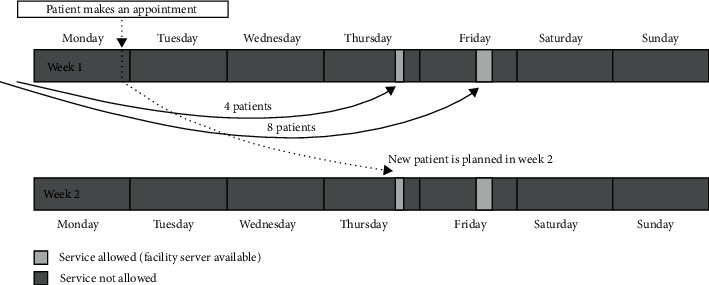
Traditional queueing scheduling of appointments during the week for the patient.
Moreover, the application sets for the outpatient scheduling problem are categorized in Table 1 . The implementations' scope is pervasive, varying from patient scheduling, clinical applications, and medication schedules. In the case of a clinical emergency, it is so significant, for example, for an ambulance to reach the base as fast as possible. The patient waiting time in this situation is an essential indicator of healthcare performance [ 144 , 145 ]. In the case of a scientific emergency, the number of distances is critical for ambulance offerings to reach the site as quickly as possible. In this example, the patient waiting time is a crucial indicator of ambulance system performance. With the upward thrust within the cost of providing first-rate fitness care, hospital and health facility administrators practice price containment by minimizing assets for health care provisions while still striving to provide the best health care for patients. This dilemma is becoming quite prevalent within the health care network, as indicated via the massive frame of literature that analyzes the allocation of scarce health care resources. The fundamental aim is to decrease the operational cost subject to constraints, i.e., maximum vehicle size and maximum waiting time for a patient. It pointed out that the customer waiting time can be reduced by sharing the link between a set of vehicles [ 146 ].
As shown in Table 4 , various review articles have collected essential appointment scheduling applications using simulation, optimization, queuing theory, and artificial intelligence methods. The desired number of keywords in each application is indicated.
Categorizing the application domains for the outpatient scheduling models.
4. Discussion
The scheduling of appointments is a complex combinatorial subject. Since the problem was initially described in its solution, it has allowed patients to be assigned to particular slots or beds in specific relevant departments. At the same time, they allow patients' demands to be addressed to the highest standards possible to ensure that all healthcare limitations are fulfilled. Patients are usually assigned to beds by a centralized admission office, which contacts departments several days ahead of time to ensure effective appointment scheduling. As mentioned in Section 3.1 to 3.5, we have focused on addressing the practices and methods to decrease or resolve appointment scheduling problems. Scholars aspire to continue further investigating the research directions in this field. Appointment scheduling can be accomplished by developing a numerical or simulation model of the booking process, optimizing service resource setup. Many academics have explored the modeling of appointment systems and scheduling algorithms with excellent results. They thoroughly examined the current state of research on associated optimization problems, optimization methods, and models in the healthcare outpatient appointment system [ 148 , 150 ]. If service time follows an exponential distribution, they considered that each patient had a predetermined probability of ASP [ 151 ]. A sequential appointment plan was used to estimate the number of bookings and the scheduled service time to maximize overall service revenue. Customers' priorities are continuously variable in the service system. The literature review discussed above significantly improves the quality of outpatient facilities of the various departments studied in the healthcare clinic. The main contribution is developing a patient-oriented appointment cycle focused on multiple approaches such as scheduling, modeling, and artificial intelligence and fit to improve the efficiency of the outpatient care system. The length of care depends on the patient's characteristics and varies greatly [ 145 ]. Still, we have reviewed numerous papers and several of those models and presented the benefits; nevertheless, determining which one is more efficient is difficult. Many researchers in this field have done simulation work, and we may infer that discrete event simulation has the most benefits and a better concept of solving the constraints. In general, discrete event simulation is a very flexible approach tailored to coordinate the procedures required to implement healthcare scheduling. It also delivers a larger scale than traditional Markov chain optimization, making the model ideal for the problem. Due to different service demands and various priority levels, patient scheduling is complicated. They created [ 152 ] a paradigm that combines stochastic service times into the scheduling problem as a first step in integrating appointment scheduling and advance scheduling. Then, they added to the existing literature by presenting analytical and experimental results for the case of multiclass, multipriority patients with predictable service times.
To settle for the additional waiting time created by appointment scheduling, the provider will approve the service requirement of arrival time. They analyzed [ 153 – 155 ] capacity allocation and appointment scheduling in the presence of arrival time and developed a connect rule dealing with helping to address decisions. Regarding how many slots to reserve for arriving and scheduled patients, the clinic session was given a fixed daily capacity to reduce missed appointments. They developed a finite-state Markov decision model and provided the best acceptable guidelines for determining which types of arriving patients are sufficient [ 149 , 156 , 157 ]. The experimental results show that when the arrival intensity of outpatients does not exceed 20% of the service intensity, accepting all is the best choice. This could be an essential route for future research.
Furthermore, another important research trend is developing a forecasting model to provide new information on the interrelationships of predictors and the conditional probability of forecasting appointment scheduling using machine learning. To examine the probabilistic links between prediction factors in appointment scheduling research, Topuz [ 147 ] built the Bayesian belief network. The predictive models may be linked to the scheduling workflow, and risk assessments can be produced based on various parameters.
4.1. Limitations and Objectives
The study demonstrates that appointment scheduling has shifted significantly from the setting indicated by the operations-research literature. Methods based on system flexibility and variability decrease appear more feasible than quantitative optimization, particularly in high complexity and uncertainty conditions. Also, the complexities of healthcare can describe a dynamic set of operations that interact with each other. Many appointment types, times, and constraints, on the other hand, might increase total system delay because each appointment type and time generates its differential delay and queue. Eventually, minimizing complexity reduces system delays. As a result, appointment scheduling is not without constraints: Our research has certain limitations. First, a healthcare facility with a high patient waiting time enhances the fundamental scheduled gap between patients or decreases the overloading percentage. Second, in our research, the unit cost of patient waiting time, patient dissatisfaction, and physician idle time was set at values based on our consultation with the administration. On the other hand, the goal of an outpatient appointment system is to optimize existing resources, include more doctors in those departments, and reduce the length of stay time waste.
5. Conclusions
This study addressed existing modeling approaches for outpatient appointment scheduling in the healthcare sector. In this regard, about 150 papers are investigated better to understand outpatient appointment scheduling problems in the literature. We considered research literature from 1990 to 2020 according to the WoS database. Then the research status and development trends are summarized by bibliometrics. Based on the statistical reports generated in this study, the reader can observe the growing trend of research interest in recent years (shown in Figure 6 ) due to the increased hospital resources. Despite the abundant literature for outpatient appointment scheduling, there are some opportunities to improve the existing research, including developing the planning models, performance measures, and forecasting skills under different generalized conditions. For instance, more experiments can be structured to improve schedules that are carried out well on this topic. Weak schedule performance (limited performance) is because of high overbooking levels. Understanding the performance dynamics of scheduling systems could lead to developing alternative healthcare access systems. An alternative area of examination is necessary to change the status of overbooking. General public interest in improving healthcare access and service delivery will likely lead to more analysis of the existing approaches. Moreover, mathematics modeling approaches can be further used for multiple providers such as double booking, overtime costs, and to increase efficiency time among visiting doctors.
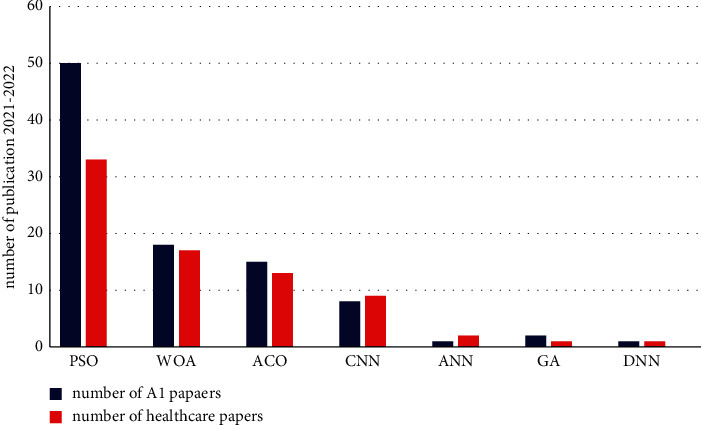
Number of papers in various AI methods in appointment scheduling between 2021 and 2022.
Regarding the effect of uncertain factors on outpatient waiting times, we have mentioned several practical approaches that are well-known methods in this research. Specifically, the Markovian model has difficulty in fairness policy due to the complexity of observing an individual's waiting times and a difference in various fairness factors in healthcare appointment scheduling. However, the OLAS approach is beneficial for this field because of increasing productivity obtained by overlapping time to minimize the total cost of patient waiting time and doctor idle time. It is worth mentioning that discrete-event simulation and other optimization approaches are new trends for future research. Future studies must investigate the scheduled outpatient and walk-in patient with unexpected arrival to disturb the clinic operations.
A further issue that is not often discussed openly is the discrete-event simulation approach used for accurate decision-making in healthcare. Discrete-event simulation may be mainly accurate in healthcare delivery models in place of sickness and screening applications. If a version of healthcare optimization is used, various patients need to be convinced of their benefits and limitations in the healthcare sector. Moreover, other researchers should conduct a more in-depth analysis of walk-in outpatients' effect on the punctuality of the planned arrivals. The method needs more investigation due to the complexity of this problem and could also be expanded further in terms of emergency admissions and intensive care departments. Another field of future research is to formulate the sequencing problems based on individual unpunctuality behaviors. Using a game theory approach, the extension of current models would help account for the unpunctuality between doctors, nurses, and patients. As a research gap, outpatient appointment scheduling problems could be extended to model the multistage health process, i.e., preliminary examination, drug test, and patient preparation or optimizing multiappointment schedules in clinics.
Acknowledgments
The authors gratefully acknowledge the funding supported by the Natural Science Foundation of China (Grant no. 71931008) and Medicine and Engineering Interdisciplinary Research Fund of Shanghai Jiao Tong University (YG2013ZD05).
Data Availability
The datasets used and/or analyzed during the current study are available from the corresponding author on reasonable request.
Conflicts of Interest
The authors declare no conflicts of interest.
- 1. Almomani I., Alsarheed A. Enhancing outpatient clinics management software by reducing patients’ waiting time. Journal of infection and public health . 2016;9(6):734–743. doi: 10.1016/j.jiph.2016.09.005. [ DOI ] [ PubMed ] [ Google Scholar ]
- 2. Shehadeh K. S., Cohn A. E. M., Jiang R. Using stochastic programming to solve an outpatient appointment scheduling problem with random service and arrival times. Naval Research Logistics . 2021;68(1):89–111. doi: 10.1002/nav.21933. [ DOI ] [ Google Scholar ]
- 3. Kuiper A., de Mast J., Mandjes M. The problem of appointment scheduling in outpatient clinics: a multiple case study of clinical practice. Omega . 2021;98 doi: 10.1016/j.omega.2019.102122.102122 [ DOI ] [ Google Scholar ]
- 4. Deceuninck M., Fiems D., De Vuyst S. Outpatient scheduling with unpunctual patients and no-shows. European Journal of Operational Research . 2018;265(1):195–207. doi: 10.1016/j.ejor.2017.07.006. [ DOI ] [ Google Scholar ]
- 5. Zhang J., Dridi M., El Moudni A. A two-level optimization model for elective surgery scheduling with downstream capacity constraints. European Journal of Operational Research . 2019;276(2):602–613. doi: 10.1016/j.ejor.2019.01.036. [ DOI ] [ Google Scholar ]
- 6. Konrad R., Ficarra S., Danko C., Wallace R., Archambeault C. A decision-support approach for provider scheduling in a patient-centered medical home. Journal of Healthcare Management . 2017;62(1):46–59. doi: 10.1097/00115514-201701000-00009. [ DOI ] [ PubMed ] [ Google Scholar ]
- 7. Radman M., Eshghi K. Designing a multi-service healthcare network based on the impact of patients’ flow among medical services. Spectrum . 2018;40(3):637–678. doi: 10.1007/s00291-018-0519-1. [ DOI ] [ Google Scholar ]
- 8. Rezaeiahari M., Khasawneh M. T. An optimization model for scheduling patients in destination medical centers. Operations Research for Health Care . 2017;15:68–81. doi: 10.1016/j.orhc.2017.09.004. [ DOI ] [ Google Scholar ]
- 9. Zhang J., Wang L., Xing L. Large-scale medical examination scheduling technology based on intelligent optimization. Journal of Combinatorial Optimization . 2019;37(1):385–404. doi: 10.1007/s10878-017-0246-6. [ DOI ] [ Google Scholar ]
- 10. Granja C., Almada-Lobo B., Janela F., Seabra J., Mendes A. An optimization based on simulation approach to the patient admission scheduling problem using a linear programing algorithm. Journal of Biomedical Informatics . 2014;52:427–437. doi: 10.1016/j.jbi.2014.08.007. [ DOI ] [ PubMed ] [ Google Scholar ]
- 11. Diamant A., Milner J., Quereshy F. Dynamic patient scheduling for multi-appointment health care programs. Production and Operations Management . 2018;27(1):58–79. doi: 10.1111/poms.12783. [ DOI ] [ Google Scholar ]
- 12. Garaix T., Rostami S., Xie X. Daily outpatient chemotherapy appointment scheduling with random deferrals. Flexible Services and Manufacturing Journal . 2020;32(1):129–153. doi: 10.1007/s10696-018-9326-x. [ DOI ] [ Google Scholar ]
- 13. Guido R., Ielpa G., Conforti D. Scheduling outpatient day service operations for rheumatology diseases. Flexible Services and Manufacturing Journal . 2020;32(1):102–128. doi: 10.1007/s10696-019-09354-7. [ DOI ] [ Google Scholar ]
- 14. Luo L., Zhou Y., Han B. T., Li J. An optimization model to determine appointment scheduling window for an outpatient clinic with patient no-shows. Health Care Management Science . 2019;22(1):68–84. doi: 10.1007/s10729-017-9421-7. [ DOI ] [ PubMed ] [ Google Scholar ]
- 15. Su H., Wan G., Wang S. Online scheduling for outpatient services with heterogeneous patients and physicians. Journal of Combinatorial Optimization . 2019;37(1):123–149. doi: 10.1007/s10878-017-0216-z. [ DOI ] [ Google Scholar ]
- 16. Sun Y., Raghavan U. N., Vaze V., et al. Stochastic programming for outpatient scheduling with flexible inpatient exam accommodation. Health Care Management Science . 2021:1–22. doi: 10.1007/s10729-020-09527-z. [ DOI ] [ PubMed ] [ Google Scholar ]
- 17. Zhuang Z.-Y., Yu V. F. Analyzing the effects of the new labor law on outpatient nurse scheduling with law-fitting modeling and case studies. Expert Systems with Applications . 2021;180:p. 115103. doi: 10.1016/j.eswa.2021.115103. [ DOI ] [ Google Scholar ]
- 18. Shehadeh K. S., Cohn A. E. M., Jiang R. A distributionally robust optimization approach for outpatient colonoscopy scheduling. European Journal of Operational Research . 2020;283(2):549–561. doi: 10.1016/j.ejor.2019.11.039. [ DOI ] [ Google Scholar ]
- 19. Li N., Li X., Forero P. Physician scheduling for outpatient department with nonhomogeneous patient arrival and priority queue. Flexible Services and Manufacturing Journal . 2021:1–37. doi: 10.1007/s10696-021-09414-x. [ DOI ] [ Google Scholar ]
- 20. Benzaid M., Lahrichi N., Rousseau L. M. Chemotherapy appointment scheduling and daily outpatient-nurse assignment. Health Care Management Science . 2020;23(1):34–50. doi: 10.1007/s10729-018-9462-6. [ DOI ] [ PubMed ] [ Google Scholar ]
- 21. Khan M. A., Muhammad K., Sharif M., Akram T., Kadry S. Intelligent fusion-assisted skin lesion localization and classification for smart healthcare. Neural Computing and Applications . 2021;8(15):1–16. doi: 10.1007/s00521-021-06490-w. [ DOI ] [ Google Scholar ]
- 22. Kumari K. K., Babu R. S. R. An efficient modified dragonfly algorithm and whale optimization approach for optimal scheduling of microgrid with islanding constraints. Transactions of the Institute of Measurement and Control . 2021;43(2):421–433. doi: 10.1177/0142331220961657. [ DOI ] [ Google Scholar ]
- 23. Yankai W., Shilong W., Dong L., Chunfeng S., Bo Y. An improved multi-objective whale optimization algorithm for the hybrid flow shop scheduling problem considering device dynamic reconfiguration processes. Expert Systems with Applications . 2021;5(174):114–793. doi: 10.1016/j.eswa.2021.114793. [ DOI ] [ Google Scholar ]
- 24. Pradhan A., Bisoy S. K., Das A. A survey on PSO based meta-heuristic scheduling mechanism in cloud computing environment. Journal of King Saud University-Computer and Information Sciences 10 . 2021;33:1275–1544. doi: 10.1016/j.jksuci.2021.01.003. [ DOI ] [ Google Scholar ]
- 25. Houssein E. H., Mahdy M. A., Shebl D., Manzoor A., Sarkar R., Mohamed W. M. An efficient slime mould algorithm for solving multi-objective optimization problems. Expert Systems with Applications . 2022;5(187):115–870. doi: 10.1016/j.eswa.2021.115870. [ DOI ] [ Google Scholar ]
- 26. Jiang L., Zang X., Dong J., Liang C. A covering traveling salesman problem with profit in the last mile delivery. Optimization Letters . 2022;16(1):375–393. doi: 10.1007/s11590-021-01709-0. [ DOI ] [ PMC free article ] [ PubMed ] [ Google Scholar ]
- 27. Wang X., Zhang M., Chen Y., et al. Risk for Hepatitis B virus reactivation in patients with psoriasis treated with biological agents: a systematic review and meta-analysis. Dermatology and Therapy . 2022;12(8):1–16. doi: 10.1007/s13555-022-00682-5. [ DOI ] [ PMC free article ] [ PubMed ] [ Google Scholar ]
- 28. Bacanin N., Vukobrat N., Zivkovic M., Bezdan T., Strumberger I. Improved Harris Hawks Optimization Adapted for Artificial Neural Network Training. Intelligent and Fuzzy Techniques for Emerging Conditions and Digital Transformation . 2022;18(4):281–289. doi: 10.1007/978-3-030-85577-2_33. [ DOI ] [ Google Scholar ]
- 29. Jiménez-Sánchez A., Mateus D., Kirchhoff S., et al. Curriculum learning for improved femur fracture classification: Scheduling data with prior knowledge and uncertainty. Medical Image Analysis . 2022;7(75):102–273. doi: 10.1016/j.media.2021.102273. [ DOI ] [ PubMed ] [ Google Scholar ]
- 30. Brady B. R., O’Connor P. A., Martz M. P., Grogg T., Nair U. S. Medicaid-Insured Client Characteristics and Quit Outcomes at the Arizona Smokers’ Helpline. The Journal of Behavioral Health Services & Research . 2022;49(1):61–75. doi: 10.1007/s11414-021-09756-2. [ DOI ] [ PubMed ] [ Google Scholar ]
- 31. Zhou S., Yue Q. Sequencing and scheduling appointments for multistage service systems with stochastic service durations and no-shows. International Journal of Production Research . 2021:1–20. doi: 10.1080/00207543.2020.1862431. [ DOI ] [ Google Scholar ]
- 32. Chen P.-S., Chen H. W., Robielos R. A. C., Chen W. Y., De Pedro J. H. B., Archita A. D. Developing a hybrid data-fitting procedure and a case study for patient service time. Journal of the Chinese Institute of Engineers . 2021;44(8):751–761. doi: 10.1080/02533839.2021.1977712. [ DOI ] [ Google Scholar ]
- 33. Hu M., Xu X., Li X., Che T. Managing patients’ no-show behaviour to improve the sustainability of hospital appointment systems: Exploring the conscious and unconscious determinants of no-show behaviour. Journal of Cleaner Production . 2020;269 doi: 10.1016/j.jclepro.2020.122318.122318 [ DOI ] [ Google Scholar ]
- 34. Golmohammadi D. A Decision-Making tool based on historical data for service time prediction in outpatient scheduling. International Journal of Medical Informatics . 2021;156 doi: 10.1016/j.ijmedinf.2021.104591.104591 [ DOI ] [ PubMed ] [ Google Scholar ]
- 35. Torabi E., Cayirli T., Froehle C. M., et al. FASStR: a framework for ensuring high-quality operational metrics in health care. American Journal of Managed Care . 2020;26(6):p. e172. doi: 10.37765/ajmc.2020.43492. [ DOI ] [ PMC free article ] [ PubMed ] [ Google Scholar ]
- 36. Alvarez-Oh H.-J., Balasubramanian H., Koker E., Muriel A. Stochastic appointment scheduling in a team primary care practice with two flexible nurses and two dedicated providers. Service Science . 2018;10(3):241–260. doi: 10.1287/serv.2018.0219. [ DOI ] [ Google Scholar ]
- 37. Lee S. J., Heim G. R., Sriskandarajah C., Zhu Y. Outpatient Appointment block scheduling under patient heterogeneity and patient No-shows. Production and Operations Management . 2018;27(1):28–48. doi: 10.1111/poms.12791. [ DOI ] [ Google Scholar ]
- 38. Habibi M. R. M., Abadi F. M., Tabesh H., Vakili-Arki H., Abu-Hanna A., Eslami S. Evaluation of patient satisfaction of the status of appointment scheduling systems in outpatient clinics: identifying patients’ needs. Journal of Advanced Pharmaceutical Technology & Research . 2018;9(2):p. 51. doi: 10.4103/japtr.JAPTR_134_18. [ DOI ] [ PMC free article ] [ PubMed ] [ Google Scholar ]
- 39. Song J., Bai Y., Wen J. Optimal appointment rule design in an outpatient department. IEEE Transactions on Automation Science and Engineering . 2018;16(1):100–114. [ Google Scholar ]
- 40. Ala A., Alsaadi F. E., Ahmadi M., Mirjalili S. Optimization of an appointment scheduling problem for healthcare systems based on the quality of fairness service using whale optimization algorithm and NSGA-II. Scientific Reports . 2021;11(1):19816–19819. doi: 10.1038/s41598-021-98851-7. [ DOI ] [ PMC free article ] [ PubMed ] [ Google Scholar ]
- 41. Finkelstein S. R., Liu N., Rosenthal D., Poghosyan L. When open access might not work: understanding patient attitudes in appointment scheduling. Health Care Management Review . 2018;43(4):348–358. doi: 10.1097/hmr.0000000000000150. [ DOI ] [ PubMed ] [ Google Scholar ]
- 42. Van de Vrugt N. M., Luen-English S. T., Bastiaansen W. A. P., et al. Integrated scheduling of tasks and gynecologists to improve patient appointment scheduling; a case study. Operations Research for Health Care . 2018;16:10–19. doi: 10.1016/j.orhc.2017.11.002. [ DOI ] [ Google Scholar ]
- 43. Bendavid I., Marmor Y. N., Shnits B. Developing an optimal appointment scheduling for systems with rigid standby time under predetermined quality of service. Flexible Services and Manufacturing Journal . 2018;30(1):54–77. doi: 10.1007/s10696-016-9270-6. [ DOI ] [ Google Scholar ]
- 44. Ramos C., Cataldo A., Ferrer J. C. Appointment and patient scheduling in chemotherapy: a case study in Chilean hospitals. Annals of Operations Research . 2020;286(1):411–439. doi: 10.1007/s10479-018-3085-7. [ DOI ] [ Google Scholar ]
- 45. Bolaji A. L. A., Bamigbola A. F., Shola P. B. Late acceptance hill climbing algorithm for solving patient admission scheduling problem. Knowledge-Based Systems . 2018;145:197–206. doi: 10.1016/j.knosys.2018.01.017. [ DOI ] [ Google Scholar ]
- 46. Lu Y., Xie X., Jiang Z. Dynamic appointment scheduling with wait-dependent abandonment. European Journal of Operational Research . 2018;265(3):975–984. doi: 10.1016/j.ejor.2017.08.026. [ DOI ] [ Google Scholar ]
- 47. Dayarathna V. L., Mismesh H., Nagahisarchoghaei M., Alhumoud A. A discrete event simulation (des) based approach to maximize the patient throughput in outpatient clinic. Engineering Science & Technology Journal . 2019;1(1):1–11. [ Google Scholar ]
- 48. Afshar-Bakeshloo M., Jolai F., Mazinani M., Salehian F. One-for-one period policy and its optimal solution over a finite horizon. International Journal of Operational Research . 2019;35(3):378–396. [ Google Scholar ]
- 49. Lin C. K. Y. Dynamic appointment scheduling with forecasting and priority-specific access time service level standards. Computers & Industrial Engineering . 2019;135:970–986. doi: 10.1016/j.cie.2019.06.049. [ DOI ] [ Google Scholar ]
- 50. Zacharias C., Armony M. Joint panel sizing and appointment scheduling in outpatient care. Management Science . 2017;63(11):3978–3997. doi: 10.1287/mnsc.2016.2532. [ DOI ] [ Google Scholar ]
- 51. Wang C., Wu R., Deng L., Chen Y., Li Y., Wan Y. A bibliometric analysis on No-show research: status, hotspots, trends and outlook. Sustainability . 2020;12(10):p. 3997. doi: 10.3390/su12103997. [ DOI ] [ Google Scholar ]
- 52. Lee H. K., Zhong X., Li J., Musa A. J., Bain P. A. Joint visit in primary care clinics: modeling, analysis, and an application study. IISE Transactions on Healthcare Systems Engineering . 2018;8(2):93–109. doi: 10.1080/24725579.2017.1418766. [ DOI ] [ Google Scholar ]
- 53. Alvarado M., Ntaimo L. Chemotherapy appointment scheduling under uncertainty using mean-risk stochastic integer programming. Health Care Management Science . 2018;21(1):87–104. doi: 10.1007/s10729-016-9380-4. [ DOI ] [ PubMed ] [ Google Scholar ]
- 54. Mueen Z. Developing bulk arrival queuing models with the constant batch policy under uncertainty data using (0-1) variables. International Journal of Nonlinear Analysis and Applications . 2022;13(1):1113–1121. [ Google Scholar ]
- 55. Issabakhsh M., Lee S., Kang H. Scheduling patient appointment in an infusion center: a mixed integer robust optimization approach. Health care management science . 2021;24(1):117–139. doi: 10.1007/s10729-020-09519-z. [ DOI ] [ PubMed ] [ Google Scholar ]
- 56. Zhao J., Wen H. Dynamic planning with reusable healthcare resources: application to appointment scheduling. Flexible Services and Manufacturing Journal . 2021;1(20):156–178. doi: 10.1007/s10696-021-09411-0. [ DOI ] [ Google Scholar ]
- 57. Pan X., Geng N., Xie X. Appointment scheduling and real-time sequencing strategies for patient unpunctuality. European Journal of Operational Research . 2021;295(1):246–260. doi: 10.1016/j.ejor.2021.02.055. [ DOI ] [ Google Scholar ]
- 58. Yu X., Bayram A. Managing capacity for virtual and office appointments in chronic care. Health Care Management Science . 2021;24(4):742–767. doi: 10.1007/s10729-021-09546-4. [ DOI ] [ PMC free article ] [ PubMed ] [ Google Scholar ]
- 59. Kumar R., Soodan B. S., Sharma S. Modelling health care queue management system facing patients’ impatience using queuing theory. Reliability: Theory & Applications . 2021;16(1):61–82. [ Google Scholar ]
- 60. Wang D., Muthuraman K., Morrice D. Coordinated patient appointment scheduling for a multistation healthcare network. Operations Research . 2019;67(3):599–618. doi: 10.1287/opre.2018.1816. [ DOI ] [ Google Scholar ]
- 61. Hesaraki A. F., Dellaert N. P., de Kok T. Generating outpatient chemotherapy appointment templates with balanced flowtime and makespan. European Journal of Operational Research . 2019;275(1):304–318. doi: 10.1016/j.ejor.2018.11.028. [ DOI ] [ Google Scholar ]
- 62. Huang Y. L., Sikder I., Xu G. Optimal override policy for chemotherapy scheduling template via mixed-integer linear programming. Optimization Letters . 2021:1–14. doi: 10.1007/s11590-021-01796-z. [ DOI ] [ Google Scholar ]
- 63. Laan C., van de Vrugt M., Olsman J., Boucherie R. J. Static and dynamic appointment scheduling to improve patient access time. Health systems . 2018;7(2):148–159. doi: 10.1080/20476965.2017.1403675. [ DOI ] [ PMC free article ] [ PubMed ] [ Google Scholar ]
- 64. Klassen K. J., Yoogalingam R. Appointment scheduling in multistage outpatient clinics. Health Care Management Science . 2019;22(2):229–244. doi: 10.1007/s10729-018-9434-x. [ DOI ] [ PubMed ] [ Google Scholar ]
- 65. Loo Y. L., Tang A. Y., Ahmad A., Mustapha A. A generic agent-based dynamic process simulation framework: a self-adaptive modelling approach. Journal of Theoretical and Applied Information Technology . 2017;95(2):340–351. [ Google Scholar ]
- 66. Gill S., Paranjape R. Health Information Systems: Concepts, Methodologies, Tools, and Applications . Hershey, PA, USA: IGI Global; 2010. A review of recent contribution in agent-based health care modeling. [ DOI ] [ Google Scholar ]
- 67. Klassen K. J., Yoogalingam R. Improving performance in outpatient appointment services with a simulation optimization approach. Production and Operations Management . 2009;18(4):447–458. doi: 10.1111/j.1937-5956.2009.01021.x. [ DOI ] [ Google Scholar ]
- 68. Zhang Z., Xie X. Simulation-based optimization for surgery appointment scheduling of multiple operating rooms. IIE Transactions . 2015;47(9):998–1012. doi: 10.1080/0740817x.2014.999900. [ DOI ] [ Google Scholar ]
- 69. Joustra P. E., De Wit J., Struben V. M. D., Overbeek B. J. H., Fockens P., Elkhuizen S. G. Reducing access times for an endoscopy department by an iterative combination of computer simulation and linear programming. Health Care Management Science . 2010;13(1):17–26. doi: 10.1007/s10729-009-9105-z. [ DOI ] [ PMC free article ] [ PubMed ] [ Google Scholar ]
- 70. Kittipittayakorn C., Ying K. C. Using the integration of discrete event and agent-based simulation to enhance outpatient service quality in an orthopedic department. Journal of Healthcare Engineering . 2016;2016 doi: 10.1155/2016/4189206. [ DOI ] [ PMC free article ] [ PubMed ] [ Google Scholar ]
- 71. Ahmed S., Shapiro A., Shapiro E. The Sample Average Approximation Method for Stochastic Programs with Integer Recourse . Submitted for publication; 2002. pp. 1–24. [ Google Scholar ]
- 72. Zonderland M. E., Boucherie R. J., Litvak N., Vleggeert-Lankamp C. L. Planning and scheduling of semi-urgent surgeries. Health Care Management Science . 2010;13(3):256–267. doi: 10.1007/s10729-010-9127-6. [ DOI ] [ PMC free article ] [ PubMed ] [ Google Scholar ]
- 73. Patrick J., Puterman M. L., Queyranne M. Dynamic multi-priority patient scheduling for a diagnostic resource. Operations Research . 2008;56(6):1507–1525. doi: 10.1287/opre.1080.0590. [ DOI ] [ Google Scholar ]
- 74. Lee S., Yih Y. Analysis of an open access scheduling system in outpatient clinics: a simulation study. Simulation Series . 2010;86(8-9):503–518. doi: 10.1177/0037549709358295. [ DOI ] [ Google Scholar ]
- 75. Sevinc S., Sanli U. A., Goker E. Algorithms for scheduling of chemotherapy plans. Computers in Biology and Medicine . 2013;43(12):2103–2109. doi: 10.1016/j.compbiomed.2013.10.012. [ DOI ] [ PubMed ] [ Google Scholar ]
- 76. Lamé G., Jouini O., Stal-Le Cardinal J. Outpatient chemotherapy planning: a literature review with insights from a case study. IIE Transactions on Healthcare Systems Engineering . 2016;6(3):127–139. [ Google Scholar ]
- 77. Romero H. L., Dellaert N. P., Van Der Geer S., Frunt M., Jansen-Vullers M. H., Krekels G. A. M. Admission and capacity planning for the implementation of one-stop-shop in skin cancer treatment using simulation-based optimization. Health Care Management Science . 2013;16(1):75–86. doi: 10.1007/s10729-012-9213-z. [ DOI ] [ PubMed ] [ Google Scholar ]
- 78. Condotta A., Shakhlevich N. V. Scheduling patient appointments via multilevel template: a case study in chemotherapy. Operations Research for Health Care . 2014;3(3):129–144. doi: 10.1016/j.orhc.2014.02.002. [ DOI ] [ Google Scholar ]
- 79. Du G., Liang X., Sun C. Scheduling optimization of home health care service considering patients’ priorities and time windows. Sustainability . 2017;9(2):p. 253. doi: 10.3390/su9020253. [ DOI ] [ Google Scholar ]
- 80. Hussain M. R., Hussain M. E. A new neuroinformatics approach to optimize diagnosis cost in neurology: an operational research tool. International Journal of Online & Biomedical Engineering . 2019;15(6) doi: 10.3991/ijoe.v15i06.10141. [ DOI ] [ Google Scholar ]
- 81. Petrovic D., Morshed M., Petrovic S. Multi-objective genetic algorithms for scheduling of radiotherapy treatments for categorised cancer patients. Expert Systems with Applications . 2011;38(6):6994–7002. doi: 10.1016/j.eswa.2010.12.015. [ DOI ] [ Google Scholar ]
- 82. Bikker I. A., Kortbeek N., van Os R. M., Boucherie R. J. Reducing access times for radiation treatment by aligning the doctor’s schemes. Operations research for health care . 2015;7:111–121. doi: 10.1016/j.orhc.2015.06.005. [ DOI ] [ Google Scholar ]
- 83. Griffiths J. D., Williams J. E., Wood R. M. Scheduling physiotherapy treatment in an inpatient setting. Operations Research for Health Care . 2012;1(4):65–72. doi: 10.1016/j.orhc.2012.08.001. [ DOI ] [ Google Scholar ]
- 84. Alvarado M., Ntaimo L. Chemotherapy appointment scheduling under uncertainty using mean-risk stochastic integer programming. Health Care Management Science . 2018;21(1):87–104. doi: 10.1007/s10729-016-9380-4. [ DOI ] [ PubMed ] [ Google Scholar ]
- 85. Zhu S., Fan W., Liu T., Yang S., Pardalos P. M. Dynamic three-stage operating room scheduling considering patient waiting time and surgical overtime costs. Journal of Combinatorial Optimization . 2020;39(1):185–215. doi: 10.1007/s10878-019-00463-5. [ DOI ] [ Google Scholar ]
- 86. Hahn-Goldberg S., Carter M. W., Beck J. C., Trudeau M., Sousa P., Beattie K. Dynamic optimization of chemotherapy outpatient scheduling with uncertainty. Health Care Management Science . 2014;17(4):379–392. doi: 10.1007/s10729-014-9268-0. [ DOI ] [ PubMed ] [ Google Scholar ]
- 87. Gocgun Y., Puterman M. L. Dynamic scheduling with due dates and time windows: an application to chemotherapy patient appointment booking. Health Care Management Science . 2014;17(1):60–76. doi: 10.1007/s10729-013-9253-z. [ DOI ] [ PubMed ] [ Google Scholar ]
- 88. Robinson L. W., Chen R. R. A comparison of traditional and open-access policies for appointment scheduling. Manufacturing & Service Operations Management . 2010;12(2):330–346. doi: 10.1287/msom.1090.0270. [ DOI ] [ Google Scholar ]
- 89. Riff M. C., Cares J. P., Neveu B. RASON: a new approach to the scheduling radiotherapy problem that considers the current waiting times. Expert Systems with Applications . 2016;64:287–295. doi: 10.1016/j.eswa.2016.07.045. [ DOI ] [ Google Scholar ]
- 90. Castro E., Petrovic S. Combined mathematical programming and heuristics for a radiotherapy pre-treatment scheduling problem. Journal of Scheduling . 2012;15(3):333–346. doi: 10.1007/s10951-011-0239-8. [ DOI ] [ Google Scholar ]
- 91. Turkcan A., Zeng B., Lawley M. Chemotherapy operations planning and scheduling. IIE Transactions on Healthcare Systems Engineering . 2012;2(1):31–49. doi: 10.1080/19488300.2012.665155. [ DOI ] [ Google Scholar ]
- 92. Heshmat M., Eltawil A. Comparison between outpatient appointment scheduling and chemotherapy outpatient appointment scheduling. Egyptian Journal for Engineering Sciences and Technology . 2016;19:326–332. doi: 10.21608/eijest.2016.97138. [ DOI ] [ Google Scholar ]
- 93. Ogulata S. N., Koyuncu M., Karakas E. Personnel and patient scheduling in the high demanded hospital services: a case study in the physiotherapy service. Journal of Medical Systems . 2008;32(3):221–228. doi: 10.1007/s10916-007-9126-4. [ DOI ] [ PubMed ] [ Google Scholar ]
- 94. Villamizar J. R., Coelli F. C., Pereira W. C. A., Almeida R. M. V. R. Discrete-event computer simulation methods in the optimisation of a physiotherapy clinic. Physiotherapy . 2011;97(1):71–77. doi: 10.1016/j.physio.2010.02.009. [ DOI ] [ PubMed ] [ Google Scholar ]
- 95. Schimmelpfeng K., Helber S., Kasper S. Decision support for rehabilitation hospital scheduling. Spectrum . 2012;34(2):461–489. doi: 10.1007/s00291-011-0273-0. [ DOI ] [ Google Scholar ]
- 96. Chien C. F., Huang Y. C., Hu C. H. A hybrid approach of data mining and genetic algorithms for rehabilitation scheduling. International Journal of Manufacturing Technology and Management . 2009;16(1-2):76–100. doi: 10.1504/ijmtm.2009.021505. [ DOI ] [ Google Scholar ]
- 97. Griffiths J. D., Williams J. E., Wood R. M. Modelling activities at a neurological rehabilitation unit. European Journal of Operational Research . 2013;226(2):301–312. doi: 10.1016/j.ejor.2012.10.037. [ DOI ] [ Google Scholar ]
- 98. Huynh N. T., Huang Y. C., Chien C. F. A hybrid genetic algorithm with 2d encoding for the scheduling of rehabilitation patients. Computers & Industrial Engineering . 2018;125:221–231. doi: 10.1016/j.cie.2018.08.030. [ DOI ] [ Google Scholar ]
- 99. Ros-McDonnell L., Szander N., de-la-Fuente-Aragón M. V., Vodopivec R. Scheduling sustainable homecare with urban transport and different skilled nurses using an approximate algorithm. Sustainability . 2019;11(22):p. 6210. doi: 10.3390/su11226210. [ DOI ] [ Google Scholar ]
- 100. Ahmadi-Javid A., Jalali Z., Klassen K. J. Outpatient appointment systems in healthcare: a review of optimization studies. European Journal of Operational Research . 2017;258(1):3–34. doi: 10.1016/j.ejor.2016.06.064. [ DOI ] [ Google Scholar ]
- 101. Conforti D., Guerriero F., Guido R. Non-block scheduling with priority for radiotherapy treatments. European Journal of Operational Research . 2010;201(1):289–296. doi: 10.1016/j.ejor.2009.02.016. [ DOI ] [ Google Scholar ]
- 102. Proctor S., Lehaney B., Reeves C., Khan Z. Modelling patient flow in a radiotherapy department. Insight . 2007;20(3):6–14. doi: 10.1057/ori.2007.15. [ DOI ] [ Google Scholar ]
- 103. Thomsen M. S., Nørrevang O. A model for managing patient booking in a radiotherapy department with differentiated waiting times. Acta Oncologica . 2009;48(2):251–258. doi: 10.1080/02841860802266680. [ DOI ] [ PubMed ] [ Google Scholar ]
- 104. Bouras A., Masmoudi M., Saadani N. E. H., Bahroun Z., Abdeljaouad M. A. Multistage appointment scheduling for outpatient chemotherapy unit: a case study. RAIRO - operations Research . 2021;55(2):589–610. doi: 10.1051/ro/2021025. [ DOI ] [ Google Scholar ]
- 105. Huang Y. L., Bach S. M., Looker S. A. Chemotherapy scheduling template development using an optimization approach. International Journal of Health Care Quality Assurance . 2019 doi: 10.1108/ijhcqa-10-2017-0187. [ DOI ] [ PubMed ] [ Google Scholar ]
- 106. Dodaro C., Galatà G., Grioni A., Maratea M., Mochi M., Porro I. An ASP-based solution to the chemotherapy treatment scheduling problem. Theory and Practice of Logic Programming . 2021;21(6):835–851. doi: 10.1017/s1471068421000363. [ DOI ] [ Google Scholar ]
- 107. Agnetis A., Bianciardi C., Iasparra N. Integrating lean thinking and mathematical optimization: a case study in appointment scheduling of hematological treatments. Operations Research Perspectives . 2019;6 doi: 10.1016/j.orp.2019.100110.100110 [ DOI ] [ Google Scholar ]
- 108. Issabakhsh M., Lee S., Kang H. Scheduling patient appointment in an infusion center: a mixed integer robust optimization approach. Health Care Management Science . 2021;24(1):117–139. doi: 10.1007/s10729-020-09519-z. [ DOI ] [ PubMed ] [ Google Scholar ]
- 109. Dhieb N., Abdulrashid I., Ghazzai H., Massoud Y. Optimized drug regimen and chemotherapy scheduling for cancer treatment using swarm intelligence. Annals of Operations Research . 2021:1–14. doi: 10.1007/s10479-021-04234-6. [ DOI ] [ Google Scholar ]
- 110. Ferreira I., Vasconcelos A. A supervised learning model for medical appointments no-show management. International Journal of Medical Engineering and Informatics . 2022;14(1):90–104. doi: 10.1504/ijmei.2022.119315. [ DOI ] [ Google Scholar ]
- 111. Wu X. D., Khasawneh M. T., Yue D. M., Chu Y. N., Gao Z. T. A simulation study of outpatient scheduling with multiple providers and a single device. International Journal of Computational Intelligence Systems . 2014;7(2):15–25. doi: 10.1080/18756891.2014.947088. [ DOI ] [ Google Scholar ]
- 112. Zhu H., Chen Y., Leung E., Liu X. Outpatient appointment scheduling with unpunctual patients. International Journal of Production Research . 2018;56(5):1982–2002. doi: 10.1080/00207543.2017.1355574. [ DOI ] [ Google Scholar ]
- 113. Fan X., Tang J., Yan C., Guo H., Cao Z. Outpatient appointment scheduling problem considering patient selection behavior: data modeling and simulation optimization. Journal of Combinatorial Optimization . 2019:1–23. doi: 10.1007/s10878-019-00487-x. [ DOI ] [ Google Scholar ]
- 114. Bakker M., Tsui K. L. Dynamic resource allocation for efficient patient scheduling: a data-driven approach. Journal of Systems Science and Systems Engineering . 2017;26(4):448–462. doi: 10.1007/s11518-017-5347-3. [ DOI ] [ Google Scholar ]
- 115. Kritchanchai D., Hoeur S. Simulation modeling for facility allocation of outpatient department. International Journal of Healthcare Management . 2018;11(3):193–201. doi: 10.1080/20479700.2017.1359920. [ DOI ] [ Google Scholar ]
- 116. Li N., Li X., Zhang C., Kong N. Integrated optimization of appointment allocation and access prioritization in patient-centred outpatient scheduling. Computers & Industrial Engineering . 2021;154 doi: 10.1016/j.cie.2021.107125.107125 [ DOI ] [ Google Scholar ]
- 117. Aeenparast A., Tabibi S. J., Shahanaghi K., Aryanejhad M. B. Reducing outpatient waiting time: a simulation modeling approach. Iranian Red Crescent Medical Journal . 2013;15(9):p. 865. doi: 10.5812/ircmj.7908. [ DOI ] [ PMC free article ] [ PubMed ] [ Google Scholar ]
- 118. Creemers S., Lambrecht M. Queueing models for appointment-driven systems. Annals of Operations Research . 2010;178(1):155–172. doi: 10.1007/s10479-009-0646-9. [ DOI ] [ Google Scholar ]
- 119. Green L. V., Savin S. Reducing delays for medical appointments: a queueing approach. Operations Research . 2008;56(6):1526–1538. doi: 10.1287/opre.1080.0575. [ DOI ] [ Google Scholar ]
- 120. Ko J. W., Kim G. G., Yun B. K. A study on the optimal appointment scheduling for the ship maintenance with queueing system with scheduled arrivals. Journal of the Korean Operations Research and Management Science Society . 2013;38(3):13–22. doi: 10.7737/jkorms.2013.38.3.013. [ DOI ] [ Google Scholar ]
- 121. Alvarado M. M., Cotton T. G., Ntaimo L., Pérez E., Carpentier W. R. Modeling and simulation of oncology clinic operations in discrete event system specification. Simulation Series . 2018;94(2):105–121. doi: 10.1177/0037549717708246. [ DOI ] [ Google Scholar ]
- 122. Hribar M. R., Huang A. E., Goldstein I. H., et al. Data-driven scheduling for improving patient efficiency in ophthalmology clinics. Ophthalmology . 2019;126(3):347–354. doi: 10.1016/j.ophtha.2018.10.009. [ DOI ] [ PMC free article ] [ PubMed ] [ Google Scholar ]
- 123. Munavalli J. R., Rao S. V., Srinivasan A., van Merode G. G. An intelligent real-time scheduler for outpatient clinics: a multi-agent system model. Health Informatics Journal . 2020;26(4):2383–2406. doi: 10.1177/1460458220905380. [ DOI ] [ PubMed ] [ Google Scholar ]
- 124. Apornak A., Raissi S., Keramati A., Khalili-Damghani K. A simulation modelling approach to improve waiting time for outpatients of hospital emergency department. International Journal of Knowledge Management in Tourism and Hospitality . 2019;2(2):160–171. doi: 10.1504/ijkmth.2019.107819. [ DOI ] [ Google Scholar ]
- 125. Rahimi Rise Z., Ershadi M. M. An integrated HFMEA simulation-based multi-objective optimization model to improve the performances of hospitals: a case study. Journal of Simulation . 2021:1–22. doi: 10.1080/17477778.2021.2015260. [ DOI ] [ Google Scholar ]
- 126. Morrice D. J., Bard J. F., Koenig K. M. Designing and scheduling a multi-disciplinary integrated practice unit for patient-centered care. Health Systems . 2020;9(4):293–316. doi: 10.1080/20476965.2019.1569481. [ DOI ] [ PMC free article ] [ PubMed ] [ Google Scholar ]
- 127. Chang W. J., Chang Y. H. Design of a patient-centered appointment scheduling with artificial neural network and discrete event simulation. Journal of Service Science and Management . 2018;11(01):p. 71. doi: 10.4236/jssm.2018.111007. [ DOI ] [ Google Scholar ]
- 128. Glowacka K. J., Henry R. M., May J. H. A hybrid data mining/simulation approach for modelling outpatient no-shows in clinic scheduling. Journal of the Operational Research Society . 2009;60(8):1056–1068. doi: 10.1057/jors.2008.177. [ DOI ] [ Google Scholar ]
- 129. Jacobson S. H., Hall S. N., Swisher J. R. Patient Flow . Boston, MA, USA: Springer; 2013. Discrete-event simulation of health care systems; pp. 273–309. [ DOI ] [ Google Scholar ]
- 130. Günal M. M., Pidd M. Discrete event simulation for performance modelling in health care: a review of the literature. Journal of Simulation . 2010;4(1):42–51. doi: 10.1057/jos.2009.25. [ DOI ] [ Google Scholar ]
- 131. Izady N. Appointment capacity planning in specialty clinics: a queueing approach. Operations Research . 2015;63(4):916–930. doi: 10.1287/opre.2015.1391. [ DOI ] [ Google Scholar ]
- 132. Kuiper A., Kemper B., Mandjes M. A computational approach to optimized appointment scheduling. Queueing Systems . 2015;79(1):5–36. doi: 10.1007/s11134-014-9398-6. [ DOI ] [ Google Scholar ]
- 133. Xiang W., Yin J., Lim G. A short-term operating room surgery scheduling problem integrating multiple nurses roster constraints. Artificial Intelligence in Medicine . 2015;63(2):91–106. doi: 10.1016/j.artmed.2014.12.005. [ DOI ] [ PubMed ] [ Google Scholar ]
- 134. Chen M., Decary M. Healthcare Management Forum . No. 1. Vol. 33. Los Angeles, CA, USA: SAGE Publications; 2020. Artificial intelligence in healthcare: an essential guide for health leaders; pp. 10–18. [ DOI ] [ PubMed ] [ Google Scholar ]
- 135. Petrovic D., Morshed M., Petrovic S. Genetic algorithm-based scheduling of radiotherapy treatments for cancer patients . Berlin, Heidelberg: Springer; 2009. pp. 101–105. [ DOI ] [ Google Scholar ]
- 136. Azadeh A., Baghersad M., Farahani M. H., Zarrin M. Semi-online patient scheduling in pathology laboratories. Artificial Intelligence in Medicine . 2015;64(3):217–226. doi: 10.1016/j.artmed.2015.05.001. [ DOI ] [ PubMed ] [ Google Scholar ]
- 137. Krittanawong C. The rise of artificial intelligence and the uncertain future for physicians. European Journal of Internal Medicine . 2018;48:e13–e14. doi: 10.1016/j.ejim.2017.06.017. [ DOI ] [ PubMed ] [ Google Scholar ]
- 138. Nelson A., Herron D., Rees G., Nachev P. Predicting scheduled hospital attendance with artificial intelligence. NPJ digital medicine . 2019;2(1):1–7. doi: 10.1038/s41746-019-0103-3. [ DOI ] [ PMC free article ] [ PubMed ] [ Google Scholar ]
- 139. AlMuhaideb S., Alswailem O., Alsubaie N., Ferwana I., Alnajem A. Prediction of hospital no-show appointments through artificial intelligence algorithms. Annals of Saudi Medicine . 2019;39(6):373–381. doi: 10.5144/0256-4947.2019.373. [ DOI ] [ PMC free article ] [ PubMed ] [ Google Scholar ]
- 140. Wang J., Fung R. Y. Adaptive dynamic programming algorithms for sequential appointment scheduling with patient preferences. Artificial Intelligence in Medicine . 2015;63(1):33–40. doi: 10.1016/j.artmed.2014.12.002. [ DOI ] [ PubMed ] [ Google Scholar ]
- 141. Reuter-Oppermann M., Kühl N. Artificial Intelligence and Data Mining in Healthcare . Berlin, Germany: Springer; 2021. Artificial intelligence for healthcare logistics: an overview and research agenda; pp. 1–22. [ DOI ] [ Google Scholar ]
- 142. Zhang H., Best T. J., Chivu A., Meltzer D. O. Simulation-based optimization to improve hospital patient assignment to physicians and clinical units. Health Care Management Science . 2020;23(1):117–141. doi: 10.1007/s10729-019-09483-3. [ DOI ] [ PubMed ] [ Google Scholar ]
- 143. Singla S. Demand and capacity modelling in healthcare using discrete event simulation. Open Journal of Modelling and Simulation . 2020;8(04):p. 88. doi: 10.4236/ojmsi.2020.84007. [ DOI ] [ Google Scholar ]
- 144. Gupta D., Denton B. Appointment scheduling in health care: Challenges and opportunities. IIE Transactions . 2008;40(9):800–819. doi: 10.1080/07408170802165880. [ DOI ] [ Google Scholar ]
- 145. Koeleman P. M., Koole G. M. Optimal outpatient appointment scheduling with emergency arrivals and general service times. IIE Transactions on Healthcare Systems Engineering . 2012;2(1):14–30. doi: 10.1080/19488300.2012.665154. [ DOI ] [ Google Scholar ]
- 146. Mandelbaum A., Momčilović P., Trichakis N., Kadish S., Leib R., Bunnell C. A. Data-driven appointment-scheduling under uncertainty: the case of an infusion unit in a cancer center. Management Science . 2020;66(1):243–270. doi: 10.1287/mnsc.2018.3218. [ DOI ] [ Google Scholar ]
- 147. Ogulata S. N., Cetik M. O., Koyuncu E., Koyuncu M. A simulation approach for scheduling patients in the department of radiation oncology. Journal of Medical Systems . 2009;33(3):233–239. doi: 10.1007/s10916-008-9184-2. [ DOI ] [ PubMed ] [ Google Scholar ]
- 148. Berg B. P., Denton B. T., Erdogan S. A., Rohleder T., Huschka T. Optimal booking and scheduling in outpatient procedure centers. Computers & Operations Research . 2014;50:24–37. doi: 10.1016/j.cor.2014.04.007. [ DOI ] [ Google Scholar ]
- 149. Chen B. L., Li E. D., Kazunobu Y., Ken K., Shinji N., Miao W. J. Impact of adjustment measures on reducing outpatient waiting time in a community hospital: application of a computer simulation. Chinese Medical Journal . 2010;123(5):574–580. [ PubMed ] [ Google Scholar ]
- 150. Hsieh F. S. A hybrid and scalable multi-agent approach for patient scheduling based on Petri net models. Applied Intelligence . 2017;47(4):1068–1086. doi: 10.1007/s10489-017-0935-y. [ DOI ] [ Google Scholar ]
- 151. Liang B., Turkcan A., Ceyhan M. E., Stuart K. Improvement of chemotherapy patient flow and scheduling in an outpatient oncology clinic. International Journal of Production Research . 2015;53(24):7177–7190. doi: 10.1080/00207543.2014.988891. [ DOI ] [ Google Scholar ]
- 152. Jacobson S. H., Hall S. N., Swisher J. R. Patient Flow: Reducing Delay in Healthcare Delivery . Boston, MA, USA: Springer; 2006. Discrete-event simulation of health care systems; pp. 211–252. [ DOI ] [ Google Scholar ]
- 153. Rau C. L., Tsai P. F. J., Liang S. F. M., et al. Using discrete-event simulation in strategic capacity planning for an outpatient physical therapy service. Health Care Management Science . 2013;16(4):352–365. doi: 10.1007/s10729-013-9234-2. [ DOI ] [ PubMed ] [ Google Scholar ]
- 154. Otten M., Dijkstra S., Leeftink G., et al. Outpatient clinic scheduling with limited waiting area capacity. Journal of the Operational Research Society . 2021:1–22. doi: 10.1080/01605682.2021.1978347. [ DOI ] [ Google Scholar ]
- 155. Lee S., Min D., Ryu J. H., Yih Y. A simulation study of appointment scheduling in outpatient clinics: open access and overbooking. Simulation Series . 2013;89(12):1459–1473. doi: 10.1177/0037549713505332. [ DOI ] [ Google Scholar ]
- 156. Chabouh S., Hammami S., Marcon E., Bouchriha H. Appointment scheduling of inpatients and outpatients in a multistage integrated surgical suite: application to a Tunisian ophthalmology surgery department. Journal of Simulation . 2018;12(1):67–75. doi: 10.1080/17477778.2017.1398288. [ DOI ] [ Google Scholar ]
- 157. Gocgun Y. Simulation-based approximate policy iteration for dynamic patient scheduling for radiation therapy. Health Care Management Science . 2018;21(3):317–325. doi: 10.1007/s10729-016-9388-9. [ DOI ] [ PubMed ] [ Google Scholar ]
Associated Data
This section collects any data citations, data availability statements, or supplementary materials included in this article.
Data Availability Statement
- View on publisher site
- PDF (867.5 KB)
- Collections
Similar articles
Cited by other articles, links to ncbi databases.
- Download .nbib .nbib
- Format: AMA APA MLA NLM
Add to Collections

- 855-263-7215

Our Outpatient Medical Transportation Services
When Sarah turned 50, her doctor recommended a routine colonoscopy. Like many, Sarah felt a mix of anxiety and uncertainty not just about the procedure itself but about the entire process surrounding it. As a busy executive working for a corporation, Sarah’s schedule was always packed, and the idea of arranging transportation for the procedure added another layer of stress.
The Night Before:
The evening before her colonoscopy, Sarah began the necessary preparations. She followed the doctor’s instructions, starting a clear liquid diet and drinking the prescribed laxative solution. The process was uncomfortable, and the frequent trips to the bathroom left her feeling drained and exhausted. Knowing that she would need to be at the surgical center early the next morning added to her anxiety. She worried about feeling weak or light-headed from the lack of food and the effects of the laxative, and the thought of navigating the day alone was daunting.

45-Year-Old Kyle’s Battle: Overcoming Transportation Barriers for a Critical Medical Procedure.
Kyle H from Albuquerque faced a significant hurdle with transportation to and from his scheduled surgery due to living far from family. Amera stepped in, providing a seamless solution by offering transportation services using their nurses as drivers. The result was a smooth and stress-free experience for Kyle, underscoring Amera’s commitment to patient care and convenience.
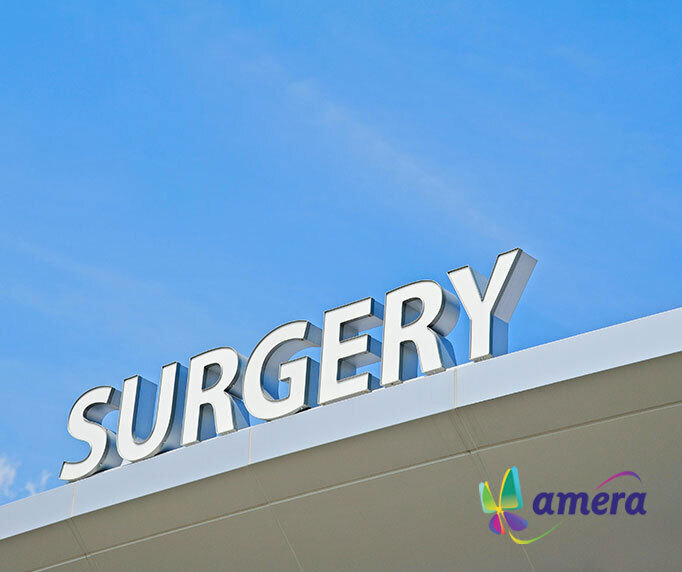
Hospital Transport for Outpatient Services
Outpatient surgery has become more and more the norm for many different procedures. As less invasive techniques are developed, including endoscopy and laser, many patients may find themselves scheduling a day surgery without the downtime or recovery as in the past. With an ambulatory surgery recovery, a patient may go home as soon as the doctor clears them and within a matter of hours. Safer and faster outpatient surgery may make patients feel they don’t need a family member to be there all day when they will be returning home the same day.
Amera’s outpatient transportation service was created to fill the need for safe and reliable transport for minor and ambulatory one-day surgery. No more burdening others with post-surgical transportation needs, trying to find someone willing to sign you out, provide transportation home and make sure you are settled in before you leave.

At Amera, we provide transportation for day surgery or medical procedures.
The different types of centers we provide transportation to commonly include:
- Eye institutes
- Dental surgery centers
- Plastic surgery offices
- Pain injections
- Outpatient surgical centers
- Ambulatory surgery centers
This is not where our, or your list, ends. Amera is available for many different types of outpatient surgery transportation , and our list grows as our business grows. We look forward to helping you the next time you need day medical transportation.
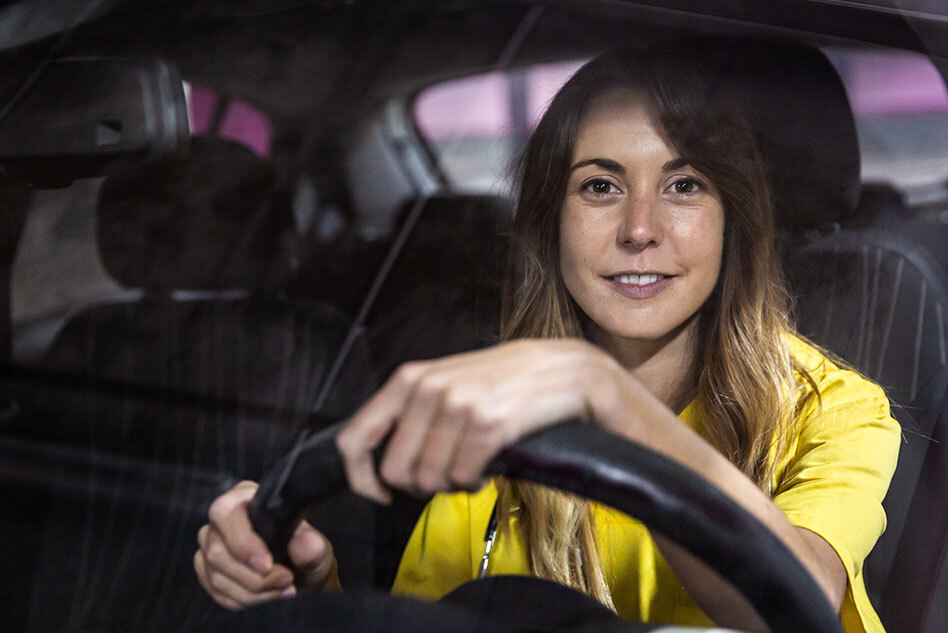
Non-Emergency Medical Transportation
Founded in 2004, Amera has risen quickly to be the largest and fastest-growing provider of medical transportation . Our Mission, Vision, and Values are driven by the same goal: ensuring that lack of medical transportation will never prevent anyone from receiving the medical care they need.
As we grow, we hope to create more partnerships with local, regional, and national medical providers to ensure their patients can get the outpatient services they need. We have extended our central call center to include reservation service in 100 languages for non-emergency medical transportation . We also have chat service, online booking, and same-day transportation service. This is the flexibility offered by a nationwide company growing and constantly adding services to assist our customers better.
Experienced, Trusted and Recognized Nationwide
Our trusted partners.

Our Specialized Care Coordinators are multi-lingual.
Booking hours: mon- sat | 7am to 7pm, express booking available online.
An official website of the United States government
Official websites use .gov A .gov website belongs to an official government organization in the United States.
Secure .gov websites use HTTPS A lock ( Lock Locked padlock icon ) or https:// means you've safely connected to the .gov website. Share sensitive information only on official, secure websites.
- Publications
- Account settings
- Advanced Search
- Journal List
Outpatient Waiting Time in Health Services and Teaching Hospitals: A Case Study in Iran
Rafat mohebbifar, edris hasanpoor, mohammad mohseni, mobin sokhanvar, omid khosravizadeh, haleh mousavi isfahani.
- Author information
- Article notes
- Copyright and License information
Correspondence: Edris Hasanpoor, School of Public Health, Tehran University of Medical Sciences, Tehran, Iran. Tel: 98-937-550-4406. E-mail: [email protected]
Received 2013 Sep 26; Accepted 2013 Oct 24; Issue date 2014 Jan.
This is an open-access article distributed under the terms and conditions of the Creative Commons Attribution license ( http://creativecommons.org/licenses/by/3.0/ ).
Background:
One of the most important indexes of the health care quality is patient’s satisfaction and it takes place only when there is a process based on management. One of these processes in the health care organizations is the appropriate management of the waiting time process. The aim of this study is the systematic analyzing of the outpatient waiting time.
This descriptive cross sectional study conducted in 2011 is an applicable study performed in the educational and health care hospitals of one of the medical universities located in the north west of Iran. Since the distributions of outpatients in all the months were equal, sampling stage was used. 160 outpatients were studied and the data was analyzed by using SPSS software.
Results of the study showed that the waiting time for the outpatients of ophthalmology clinic with an average of 245 minutes for each patient allocated the maximum time among the other clinics for itself. Orthopedic clinic had the minimal waiting time including an average of 77 minutes per patient. The total average waiting time for each patient in the educational hospitals under this study was about 161 minutes.
Conclusion:
by applying some models, we can reduce the waiting time especially in the realm of time and space before the admission to the examination room. Utilizing the models including the one before admission, electronic visit systems via internet, a process model, six sigma model, queuing theory model and FIFO model, are the components of the intervention that reduces the outpatient waiting time.
Keywords: outpatient, quality, queuing theory, FIFO, hospital
1. Introduction
In response to the certain conditions of each period, the health care organizations have observed major changes. These Various changes included the rapid growth of technology costs of the health sector. Increasing complexity of processes along with the increasing competition among the institutions of health care services have changed the opinion of the experts towards the health care systems providing the health services ( Aeinparast, Tabibi, Shahanaghi, & Arianezhad, 2009 ). Nowadays customer care in all the organizations particularly the health care organizations has advanced and progressed and has a specific symbol. One of the comprehensive factors for the patients in the health care organizations is actually the criteria considered for the suitable and desirable treatment which is rapid and suitable. Prompt treatment in hospitals means to minimize the time for getting a health service with an emphasis on the favorable treatment ( Dansky & Miles, 1997 ). All the organizations providing the health services tend to reduce the number of patients admitted in order to reduce the costs and increase the income, in addition providing opportunities for the people has resulted in the increased number of outpatients over the past decade ( Eldabi, Irani, & Paul, 2002 ).
Changes and major challenges in the health sector have led to the changes in the outpatient services showing the appropriate management of these centers ( Vissers, 1998 ). Delays in the access to the medical services are an important issue for the publicly funded health systems ( Schachter, Romann, Djurdev, Levin, & Beaulieu, 2013 ). Effectively managing patient flow in an outpatient unit is a key to achieve operational excellence as well as ensuring clinical quality. It is especially true for an outpatient department in a large hospital as it handles very large volume of patients with a diverse case mix ( Mardiah & Basri, 2013 ). The waiting time for patients is one of the important factors that should be considered in the management and organization of the health care system. Patients waiting time is not the only factor that affects patient satisfaction but it is one of the indexes to evaluate the quality of outpatient services ( Sibbel, Urban, & Saam, 2001 ). One of the most important indexes of the health care quality is patient’s satisfaction and it takes place only when there is a process based on management. One of these processes in the health care organizations is the process of the appropriate management of waiting time ( Boudreaux, d'Autremont, Wood, & Jones, 2004 ).
Outpatient waiting time has two important aspects. One aspect is that the waiting time of the patients referred by the general practitioner who is created from the time of the entry to the hospital for receiving services, creates great costs and can be modified by techniques of reducing the waiting time. The other one is the waiting time from the time of arrival to the hospital till entering to the examination room. The second part of this time is the waiting time which its major part is related to the hospital and comes to the existence in the hospital. And for reducing the waiting time hospitals should approach through basic interventions. Regarding the first domain, policy makers should provide the essential procedures and guidelines, so that the waiting time for health care services is reduced to the minimum.
Clinics outpatient waiting time is the main focus of this research and is considered to be a center for providing the outpatient services. The importance of the outpatient clinic is that it creates the waiting time for patients and this is due to the structural factors and underlying processes of the clinic, such as working process and physical condition of the clinic. The role of management and laborers in the hospital is important for surveying the outpatient waiting time ( Bamgboye & Jarallah, 1994 ). The waiting time of the outpatient in clinics is formed because a patient who seeks a counseling service spends a period of his time in the hospital, instead of working and earning money.
Dansky presented several definitions for the waiting time. One of these definitions refers to the total time that a patient spends to receive a particular service since entering to the hospital till entering the examination room to visit a doctor. Dansky believes that the patient suffering from his own illness and pain should not suffer from other pains ( Leddy, Kaldenberg, & Becker, 2003 ). The main problem related to the outpatient waiting time is obtained from three main factors ( Abdullah, 2005 ):
People (consumers).
Organizations providing health services (suppliers).
Environment.
Nowadays in the hospitals most of the time organizations themselves refer to the second factor which is involved and plays a role in creating waiting time, and there should be greater emphasis on hospitals to reduce the waiting time. In the educational and learning hospitals of universities under this study, time organizations are related to the rate of the entry of outpatients which makes this subject less interesting and it's follow up requires extensive interventions in this field of research. Thus this study aims at the survey of the outpatient waiting time in two educational and learning hospitals affiliated to the University of Ghazvin.
This descriptive cross sectional study is an applicable study. The sampling population of the study in this research includes outpatients visiting the educational and learning hospitals affiliated to the University of Ghazvin. In the beginning in order to ensure the equal distribution of patients in different months of the year, the rate of entry and exit of all the patients in all the clinics under the study in the year 2010 were surveyed. Since the distributions of outpatients in all the months were equal, sampling stage was used.
STAGE 1: Random selection of two health clinics from all the clinics presented in each hospital: Of the clinics presented in two hospitals, urology and orthopedic clinic from hospital A and ophthalmology and dermatology clinic from hospital B was selected.
STAGE 2: randomly selected outpatients were used to collect the sample society from the following sample size formula.
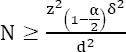
Within a time range of 4 minutes and accuracy of 99% a standard deviation of 18.98 minutes (pilot sample) for 160 persons was obtained. 160 outpatients under the study were divided among four clinics (ophthalmology, dermatology, urology and orthopedics). To ensure the accuracy of the data, the number of outpatients was divided equally during the two weeks and the waiting time was measured on the basis of minutes/second in the presence of a researcher by chronometer.
For gathering the information check lists, we used the researcher certified by the opinion of five teachers. The method for collecting the information was through the presence in hospital and by the observations and interviews performed by the researchers. The data was analyzed by using descriptive mean test, standard deviation, variance and by the software SPSS. The specific variables for measuring the waiting times were evaluated as follows:
Waiting time before admission.
Waiting time in the reception part.
Waiting time in funds part.
Waiting time before examination.
After analyzing the data, the following results were obtained. Out of a total of 160 outpatients, 53% were males and 47% were females. 6/97% of the study population lived in the rural areas of Ghazvin. 11/62% of the residents lived near the suburbs of Qazvin and the remaining 81.41% lived in the Qazvin city itself. The education level of the study population is shown in the table 1 .
Level of education as per degree
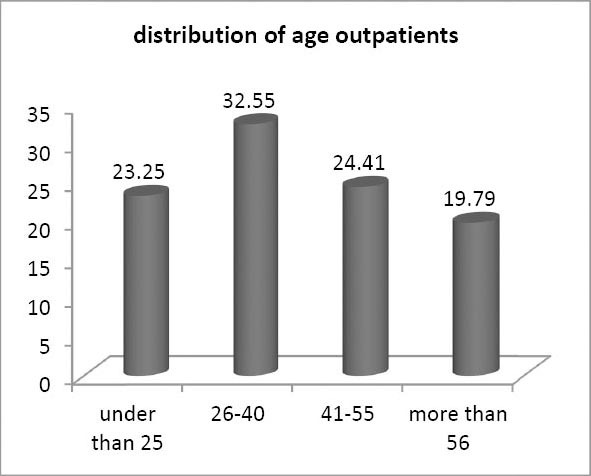
Age distribution of the outpatient under study
In this study, the waiting time for the outpatients of ophthalmology clinic with an average of 245 minutes for each patient allocated the maximum time for itself. This time was 130 minutes (52.84%) in relation to the distance between the cash department and the examination room. 113 minutes (45/93%) belongs to the waiting time of the clinics before the admission. Altogether these two parts created a waiting time of 243 minutes equivalent to 98/78%.
But the minimum waiting time belongs to the orthopedic clinic with an average of 77 minutes allocating to each patient. Out of 77 minutes of waiting time about 65 minutes (83/33%) of it belongs to the distance between the cash department and the examination room. Approximately 10 minutes (12/82%) of the waiting time belongs to the pre admission stage.
The dermatology clinic comparing with the clinics under the study allocated most of the waiting time for the outpatient. The time duration of 216 minutes was allocated for the outpatients and about 86 minutes (46/08%) were related to the pre admission and 59/44% of it belonged to the distance between the cash department and the examination room.
The waiting time of urology clinic was also less than others and the duration of time for each outpatient was 81 minutes. Most of this time belongs to the distance between the cash department and the examination room (85/18%). According to the analytical data obtained from this study, it can be said that most of the waiting time is related to the distance between the cash department and the examination room. 100 minutes from the total waiting time of the outpatient (approximately 161 minutes) that is 61/72% apparently makes this subject clear. However a lot of time was wasted in the department prior to the admission which is about 59 minutes. These two departments accounted for 98/13% of the total waiting time. Therefore the main problem of these two variables under the study can be identified. 81/4% of the epidemiologic study population lived in the city of Qazvin and approximately 66/28% of them had an education level higher than diploma and this strengthens the interventions and models to reduce the waiting time. Table 2 shows the mean total waiting time for each patient and the duration of visits for selected clinics.
Average of waiting time and visiting time for every outpatient
Table 3 shows the average of waiting time and visit time for every outpatient in the selected hospital. This waiting time is unto four domains: pre admission, admission, fund, and before the visit room. Finally, the total of the waiting time has been showed for every outpatient.
Duration of the waiting time for hospitals under study
4. Discussion
On the basis of the entry of the outpatients in the year of this study and equality and acceptance of outpatients in each clinic during all the months, these samples can give us enough information. Our sampling population is enough educated and spends a lot of waiting time in the queues of the hospital to get the health services. The more the educational level and the awareness of the community is, the better health services patients will get, and they can take the advantage of the new acceptance system. However patients only should not be convicted for excessive waiting time. This phenomenon has been converted into a social problem in the developed and developing countries ( Chand, Moskowitz, Norris, Shade, & Willis, 2009 ).
In our study, the waiting time for the outpatients of ophthalmology clinic with an average of 245 minutes for each patient, allocating maximum time for itself was 130 minutes (52.84%) in relation to the distance between the cash department and the examination room. 113 minutes (45/59%) belongs to the waiting time of the clinics before the admission. Altogether these two parts created a waiting time of 243 minutes equivalent to 98/78%. Waiting time in the reception department and the funds department as compared with these two sectors had a significant difference. It should be stated that the waiting time in two reception departments and the funds is acceptable and close to the standards of the hospital ( Aeinparast et al., 2009 ). In the two other departments serious strategies should be considered. But about the minimum waiting time, it can be said that in orthopedic clinic with the lowest waiting time an average of 77 minutes was allocated for per patient. Out of 77 minutes of the waiting time about 65 minutes (83/33%) of it belongs to the distance between the cash department and the examination room. Approximately 10 minutes (12/82%) of the waiting time belongs to the pre admission stage. The dermatology clinic also as compared with the clinics under the study, allocated most of the waiting time for the outpatient. The time duration of 216 minutes was allocated for the outpatients and about 86 minutes (46/08%) were related to the pre admission time and 59/44% of it belongs to the distance between the cash department and the examination room. The waiting time of urology clinic was also less than others and the duration of time for each outpatient was 81 minutes. Most of this time belongs to the distance between the cash department and the examination room (85/18%). According to the analytical data obtained from this study, it can be said that most of the waiting time was related to the distance between the cash department and the examination room. 100 minutes from the total waiting time of the outpatient (approximately 161 minutes) that is 61/72% apparently makes this subject clear. However a lot of time was wasted in the department prior to admission, which is about 59 minutes. These two departments accounted for 98/13% of the total waiting time. Therefore the main problem of these two variables in the study can be identified. 81/4% of the epidemiologic sampling population lived in the city of Qazvin and approximately 66/28% of them had an education level higher than diploma and this strengthens the interventions and models to reduce the waiting time. In the United Kingdom, because of the national health insurance and a wide range of health services this waiting time is less than other countries. Rodney, the statistical consultant in the health research center of England, has estimated the waiting time of outpatients in his studies to be 35 minutes. However this waiting time was allocated for the distance between the admissions of the patient and the examination room for getting the consultation. Rodney considers the referral system effective for reducing the waiting time of the outpatient and effective for the increased duration of visits. He believes that if unnecessary referral of patients is avoided by the family physician, waiting time of patients is reduced and physicians can spend more time for their patients ( Jones, 2000 ).
These results were obtained from the study that Chen performed with the cooperation of medical information management center along with the management of the research centers of Japan in the university hospitals of Guangzhou and China about the visiting time of the physician and waiting time of the outpatient. In this study the visiting time for each patient in the morning was about 42 minutes. This time the duration was less than the afternoon which reached to 25 minutes. And overall average of 33 minutes time duration for each patient is spent by the physicians visit. However the number of patient’s admission in the morning and afternoon shift were less and limited also. Chen relates it to the academic hospitals and believes that in these hospitals the waiting time of patients and the time duration of visit and consultation is more; Which is affected by the education and learning of assistants. In this hospital, the community reservation system is used for the admission of the patients. In this system few days before the arrival of the patient and the patient’s admission, processes of registration are performed. Reservation system has been created from the industrial and commercial systems and the basic foundation of this system has been established in the financial and sales market ( Chen et al., 2010 ).
In a study conducted by Irshad Rahim in the hospitals of Dhaka Bangladesh in the year 2007, total waiting time for each outpatient from the registration process to the entry of the examination room was estimated to be 66 minutes. He stated at the end that this duration of time is very high and the managers of the hospital should undertake appropriate measures to reduce it ( Rahim, Rahman, Talukder, & Anwar, 2007 ).
In the hospitals under the study, the main problem can be identified by the process model. Increased and long waiting time in these hospitals is related to the time before the admission and to the distance between the cash department and the examination room. Approximately 98/13% of the total waiting time for these two departments shows the importance of these two centers. Intervention in these centers of waiting time can lead to the improvement of other sections such as funds department and the reception.
In 1995, Shaw presented in his model that the waiting time for receiving the health services is affected by the demand. He linked these factors to the health care demands of the consumer and stated that if the waiting time of the patient for receiving the health services is too high, The patient can move from the main center to the other private sectors for getting services and even the traditional medicine ( Asefzadeh, 2008 ). Factors within and outside the hospital are involved in generating the waiting time. They are identified by using some techniques such as network diagrams and fish bone diagrams ( Lim & Manaes, 2001 ). In the clinic of hospital B, although the patients would wait for long time before admission, but all of them would be accepted in a short time. It seems that false claim is seen in these patients. These factors need to open people’s attitude towards engineering. However acceptance of increased number of patients could not be ignored. According to the network analysis, four factors were involved in the long waiting time of the patients ( Sharif & Sukeri, 2003 ).
Human Resources: lack of human resources and professional workers in the hospitals such as physicians, licensed nurses and other professional staff.
Equipment and facilities: the type of information system used in hospitals is one of the major factors. Most of the hospitals use the same old admission systems on the same day.
Patients: patients themselves and their attitudes are involved in the increased waiting time.
Registration process.
Despite the long waiting time for outpatients, their visiting time was extremely low. Visiting time of 5 minutes in learning and educational hospitals created a place for challenging the subject. Although the expertise opinion and insight of different specialists in making correct diagnosis is different, but the patient has the right to get a typical expert advice for a standard duration of time ( Helbig, Helbig, Knecht, Kahla-Witzsch, & Gstöttner, 2007 ).
Waiting time leads to the worsening of the patient’s disease which needs consultation with the doctor. The best quality services in health care organizations are the right of every individual. One of the most important tools to improve the quality of health care services is to reduce the long waiting time ( Matthews et al., 1991 ). One of the interventional methods for detecting long waiting time and its resolution is performed by six sigma approaches.
During the last 5 years or so, many leading healthcare institutions have implemented Lean and Six Sigma methodologies with remarkable results in terms of reducing cycle time ( Gijo, Antony, Hernandez, & Scaria, 2013 ). Mohamed Hanafi Abdullah in his study in Malaysia utilized this model to reduce the waiting time. His innovative approach led to the relationship between this model and the approach of the Pareto rule ( Abdullah, 2005 ). And after identification of the axis for creating long waiting time, 80% of the problems related to the long waiting time as the result of the following three factors:
Shortage of physician
Shortage of professional staff
4.1 Types of Models to Reduce the Outpatient Waiting Time
4.1.1 admission model before the acceptance of the patient.
According to this model the patient should not have the waiting time before the admission. In order to implement this method, a reservation system that would function in two ways was used. One of them was admission through telephone. In this method, the patient would contact the hospital by phone and would provide the employees of reception with the basic information. Another reservation system was through the computer. Patients could enter their basic information in the reception department of the outpatient through the internet site of the hospitals. In this case, an officer in the charge of the reception would organize the information. By implementation of this model, when the patients require a consultation, they would wait for less than a minute in the reception department. This time duration for registering the information and ensuring the accuracy of the data was filled up by the patients themselves ( Lee & Yoo, 1996 ). This model can be used in the learning hospitals (university hospitals) with diverse expertise to gain great success. Developing countries with the launch of advertising campaign can draw people’s attention for using these systems.
4.1.2 Electronic Visit System
The best present system to reduce the waiting time is the electronic visit through the web network. This model is obtained from the doctor’s consultant and patient’s relationship. Most of these models are used in hospitals and private clinics. The costs overhead of the clinics have also been reduced by using these systems. The biggest limitation of this system is the lack of patient physician and consultant relationship in the emotional level. Moreover, these systems are mostly used in specialties such as psychiatry. In this case, the physician and patient can meet with each other, if necessary. The experts promote use of this system for family physician and the specialist relationship. This system is expanded and works on three zones, the patient, family physician and specialist. This system works quiet well in the developing countries and particularly in the developed countries with more advanced and extensive medical information systems and new applications ( Taylor, 2006 ).
4.1.3 Queuing Theory
This model suggests that in order to reduce the queues of waiting outpatient, there should be an increase in the patient admission booth and an increase in the number of examination rooms. This model is used when the outpatient number is too high. In addition, sufficient human and physical resources should be present. This model has more functionality in learning hospitals affiliated with the university because of the human funds ( Helbig et al., 2007 ).
4.1.4 Process Model
Perhaps the best model for identifying and solving the problems of outpatient waiting time in the hospitals under the study is the use of a process model. This model presents that the process should be examined to identify the problem ( Willcox et al., 2007 ). We found that from the outpatient's reception till the entry into the examination room, most of the waiting time is related to the distance between the cash department and the examination room and the patient’s reception (98/13%). This model suggests that the factors having more effect on this process should be considered more than other factors and the long term processes should be reduced or eliminated. For example according to this model processes before acceptance of the patient which takes the long waiting time (36/41%) should be eliminated. For this purpose acceptance model can be used before the admission. For example physician specialists can begin sooner visiting their patients, or they can start later or even patients can be admitted later by accepting the patients through simulation model. It should be noted that the faculty physicians in learning hospitals are mostly specialist and should begin counseling immediately after the admission of the first patient. This will lead to the greater utilization of time and will devote more time to the patient during the examination.
4.1.5 FIFO Model (first in first out)
This technique is known as the queue priority and is also compared with the queue of getting bread. It’s not been too long that this model is used. The FIFO technique is a new approach used for the analysis of the waiting time of the outpatients and its reduction. This technique is described as a queue priority. One who is admitted first will be the last person who gets out of the admission queue. This process can be applied to the cash department and the examination room also. Finally the patient admitted first will be the last person exiting from the examination room. This technique is mostly considered for the emergency department as priority plays an important role there. However this technique is used in the entire process from pre admission till the exit of the patient from the examination room ( Ozturk, Di Mascolo, Espinouse, & Gouin, 2010 ).
The specific Time duration for each patient’s visit by doctor is short (5 minutes). This time duration should be at least 15 minutes for each patient. Despite the different diagnostic approach of a physician specialist for the patient and the amount of fee paid by the patient, it is the patient's right to get examined for a longer time. Perhaps one of the reasons behind the frequent visits of patient to the clinic is the shorter time duration which is specified for them. In addition to it, this duration of time can indirectly increase the waiting time of the patients. It can be hypothesized for the visiting time duration and the waiting time of outpatients for the future research.
Time, like human resources and money, is not renewable and it’s not reversible also. It’s the right of the people to make the best use of their time. If the hospitals environment is so pleasant that the waiting time of patient’s is converted from pain to a state of joy and comfort, then it can compensated for the prices paid by the patient ( Helbig et al., 2007 ).
5. Conclusions
According to the present study, The Overall total average waiting time for each patient was about 161 minutes. 161 minutes waiting time is a lot for each outpatient in the hospitals. The models mentioned above can be effective for reducing the waiting time of the outpatient. The adoption of the model before admission and the process model has more functionality in the hospitals under this study. Since the waiting time of the patient was related to pre admission and the distance between the cash department and the examination room, utilization of these models is considered to be a great priority. But in the shorter period of doctor’s visit, there should be much supervision and control in the health care organizations and they should suit the adopted policies.
Acknowledgements
This study has been funded by the School of Health and Para medicals of Qazvin University of Medical Sciences. We are thankful to all the authorities of the clinics for their sincere cooperation.
- Abdullah MH. Study on Outpatients’ Waiting Time in Hospital University Kebangsaan Malaysia (HUKM) Through the Six Sigma Approach1. The Journal of the Department of Statistics, Malaysia. 2005:1. [ Google Scholar ]
- Aeinparast A, Tabibi JT, Shahanaghi K, Arianezhad MB. Estimating outpatient waiting time: a simulation approach. Payesh. 2009;8(4):327–333. [ Google Scholar ]
- Asefzadeh S. Qazvin: hadise-e-emrouz; 2008. Health economic. [ Google Scholar ]
- Bamgboye EA, Jarallah JS. Long-waiting outpatients: target audience for health education. Patient education and counseling. 1994;23(4):49–54. doi: 10.1016/s0738-3991(05)80021-7. http://dx.doi.org/10.1016/0738-3991(1094)90060-90064 . [ DOI ] [ PubMed ] [ Google Scholar ]
- Boudreaux ED, d’Autremont S, Wood K, Jones GN. Predictors of emergency department patient satisfaction: stability over 17 months. Academic emergency medicine. 2004;11(1):51–58. doi: 10.1197/j.aem.2003.06.012. http://dx.doi.org/10.1197/j.aem.2003.1106.1012 . [ DOI ] [ PubMed ] [ Google Scholar ]
- Chand S, Moskowitz H, Norris JB, Shade S, Willis DR. Improving patient flow at an outpatient clinic: study of sources of variability and improvement factors. Health Care Management Science. 2009;12(3):325–340. doi: 10.1007/s10729-008-9094-3. http://dx.doi.org/310.1007/s10729-10008-19094-10723 . [ DOI ] [ PubMed ] [ Google Scholar ]
- Chen B, Li E-d, Yamawuchi K, Kato K, Naganawa S, Miao W-j. Impact of adjustment measures on reducing outpatient waiting time in a community hospital: application of a computer simulation. Chinese Medical Journal (English Edition) 2010;123(5):574. [ PubMed ] [ Google Scholar ]
- Dansky KH, Miles J. Patient satisfaction with ambulatory healthcare services: waiting time and filling time. Hospital & health services administration. 1997;42(2):165. [ PubMed ] [ Google Scholar ]
- Eldabi T, Irani Z, Paul RJ. A proposed approach for modelling health-care systems for understanding. Journal of Management in Medicine. 2002;16(2/3):170–187. doi: 10.1108/02689230210434916. http://dx.doi.org/110.1108/02689230210434916 . [ DOI ] [ PubMed ] [ Google Scholar ]
- Gijo E, Antony J, Hernandez J, Scaria J. Reducing Patient Waiting Time in a Pathology Department Using the Six Sigma Methodology. Leadership in Health Services. 2013;26(4):2–2. [ Google Scholar ]
- Helbig M, Helbig S, Knecht R, Kahla-Witzsch H, Gstöttner W. Quality management: reduced waiting time and enhanced efficiency in a university ear, nose, and throat outpatient department. HNO. 2007;55(1):29. doi: 10.1007/s00106-006-1400-0. [ DOI ] [ PubMed ] [ Google Scholar ]
- Jones R. Outpatient appointments. Feeling a bit peaky. Health Serv J. 2000;110(5732):28–31. [ PubMed ] [ Google Scholar ]
- Leddy KM, Kaldenberg DO, Becker BW. Timeliness in ambulatory care treatment. An examination of patient satisfaction and wait times in medical practices and outpatient test and treatment facilities. J Ambul Care Manage. 2003;26(2):138–149. doi: 10.1097/00004479-200304000-00006. http://dx.doi.org/110.1097/00004479-200304000-200300006 . [ DOI ] [ PubMed ] [ Google Scholar ]
- Lee YJ, Yoo DH. A study on model for decreasing an outpatient waiting time. Journal of Korean Society of Medical Informatics. 1996;2(1):27–39. [ Google Scholar ]
- Lim W, Manaes M. Benchmarking waiting times for clinic services, Kuala Lumpur Hospital. Journal of Quality Improvement. 2001;5(2):32–41. [ Google Scholar ]
- Mardiah FP, Basri MH. The Analysis of Appointment System to Reduce Outpatient Waiting Time at Indonesia's Public Hospital. Human Resource Management Research. 2013;3(1):27–33. [ Google Scholar ]
- Matthews F, Probert C, Battcock T, Frisby S, Chandar M, Mayberry J. Are we wasting time in out-patients departments? Public Health. 1991;105(3):239–242. doi: 10.1016/s0033-3506(05)80113-1. http://dx.doi.org/210.1016/S0033-3506(1005)80113-80111 . [ DOI ] [ PubMed ] [ Google Scholar ]
- Ozturk O, Di Mascolo M, Espinouse M.-L, Gouin A. Paper presented at the 8th International Conference of Modeling and Simulation (MOSIM’ 10); 2010. Minimizing the sum of job completion times for washing operations in hospital sterilization services. [ Google Scholar ]
- Rahim R, Rahman M, Talukder AM, Anwar KH. waiting time of the patients at medical outpatient department of Dhaka medical college hospital. J. med. sci. res. 2007;8:1. [ Google Scholar ]
- Schachter ME, Romann A, Djurdev O, Levin A, Beaulieu M. The British Columbia Nephrologists’ Access Study (BCNAS) – a prospective, health services interventional study to develop waiting time benchmarks and reduce wait times for out-patient nephrology consultations. BMC nephrology. 2013;14(1):1–9. doi: 10.1186/1471-2369-14-182. [ DOI ] [ PMC free article ] [ PubMed ] [ Google Scholar ]
- Sharif JHM, Sukeri S. Study on waiting time at the paediatric dental clinic, Kuala Lumpur Hospital. Journal of Quality Improvement. 2003;7(1):19–23. [ Google Scholar ]
- Sibbel R, Urban C, Saam N. Agent-based modeling and simulation for hospital management. Cooperative agents: applications in the social sciences. 2001;183 [ Google Scholar ]
- Taylor A. electronic visit in East Kent Hospitals NHS. journal of medical sciences. 2006:11. [ Google Scholar ]
- Vissers JH. Health care management modelling: a process perspective. Health Care Management Science. 1998;1(2):77–85. doi: 10.1023/a:1019042518494. http://dx.doi.org/10.1023/A: 1019042518494 . [ DOI ] [ PubMed ] [ Google Scholar ]
- Willcox S, Seddon M, Dunn S, Edwards RT, Pearse J, Tu JV. Measuring and reducing waiting times: a cross-national comparison of strategies. Health Affairs. 2007;26(4):1078–1087. doi: 10.1377/hlthaff.26.4.1078. http://dx.doi.org/1010.1377/hlthaff.1026.1074.1078 . [ DOI ] [ PubMed ] [ Google Scholar ]
- View on publisher site
- PDF (236.5 KB)
- Collections
Similar articles
Cited by other articles, links to ncbi databases.
- Download .nbib .nbib
- Format: AMA APA MLA NLM
Add to Collections
Evaluation of facies heterogeneity in reef carbonate reservoirs: A case study from the oil field, Perm Krai, Central-Eastern Russia
- Dmitriy A. Martyushev Dmitriy A. Martyushev
- PC Polina O. Chalova Polina O. Chalova
- Shadfar Davoodi Shadfar Davoodi
- Umar Ashraf Umar Ashraf
- journal article
- research article
- Published by Elsevier in Geoenergy Science and Engineering
- https://doi.org/10.1016/j.geoen.2023.211814
- FACIES HETEROGENEITY
- WELL LOGGING
- PERMEABILITY
- CARBONATE RESERVOIRS
Funding Information
- Ministry of Education and Science of the Russian Federation (FSNM-2023-0005)
- Tomsk Polytechnic University
This publication has 70 references indexed in Scilit:
- Investigation of pore-type heterogeneity and its inherent genetic mechanisms in deeply buried carbonate reservoirs based on some analytical methods of rock physics Journal of Natural Gas Science and Engineering, 2015
- Quantitative Phase Analysis Based on Rietveld Structure Refinement for Carbonate Rocks Journal of Modern Physics, 2013
- From fractures to flow: A field-based quantitative analysis of an outcropping carbonate reservoir Tectonophysics, 2010
- Oblique normal faulting along the northern edge of the Majella Anticline, central Italy: Inferences on hydrocarbon migration and accumulation Journal of Structural Geology, 2009
- Use of X-ray powder diffraction for quantitative analysis of carbonate rock reservoir samples Powder Technology, 2007
- Pore-Scale Characterization of Carbonates Using X-Ray Microtomography SPE Journal, 2005
- Types of carbonate platforms: a genetic approach Basin Research, 2001
- Loss on ignition as a method for estimating organic and carbonate content in sediments: reproducibility and comparability of results Journal of Paleolimnology, 2001
- Carbonate ramp depositional systems Sedimentary Geology, 1992
- Quantitative mineralogical analysis of carbonate sediments by X‐ray diffraction: a new, automatic method for sediments with low carbonate content Sedimentology, 1982
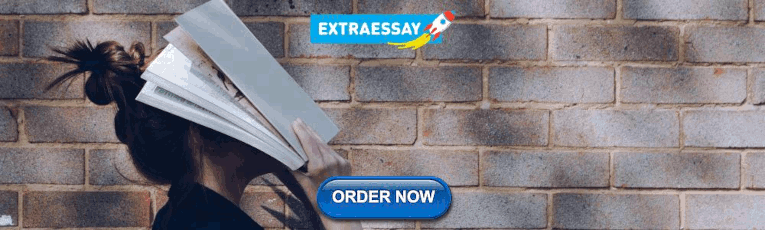
IMAGES
VIDEO
COMMENTS
Although studies of shifted outpatient clinics reported that patient satisfaction was high and waiting times were reduced in some cases, the effects on numbers of referrals to both community and hospital services and on costs were unclear. 19,20 One new study on attachment of a specialist doctor or nurse to primary care teams reported a ...
Sandberg M, Jakobsson U, Midlöv P, Kristensson J. Case management for frail older people-a qualitative study of receivers' and providers' experiences of a complex intervention [published online ...
services to meet the evolving needs of patients and healthcare providers alike. 2. LITERATURE REVIEW Digital transformation has become imperative in modern healthcare systems, revolutionizing how healthcare services are delivered and experienced by patients. In the context of outpatient departments (OPDs), several studies underscore the
nts of financial and human resources to designand create health care experie. The purpose of this paper is to evaluate the patient-centered design of Allegheny Health. us features as well as the workflow design andcomparing the. to the 8 dimensions of patie. tory care center, this paper.
Purpose This work presents a case study of the University of Hong Kong-Shenzhen Hospital (HKU-SZH), which was the first to implement an outpatient appointments registration system. The research question is to determine which factors influence patient satisfaction most. Methods The study provides an anatomy of the hospital outpatient process through various methods and theories, including a ...
INTRODUCTION: Wait times in outpatient services are a major challenge for hospitals. This study at Remera Rukoma District Hospital aimed to analyze patient flow and waiting times in the outpatient department to improve service quality and resource utilization. METHODS: A cross-sectional study was conducted from November 2023 to January 2024, involving 300 patients.
Case studies are appropriate to investigate how or why a phenomenon occurs within a real-life context, 26 ... Outpatient services were provided in groups to maximize staff productivity rather than individually. The nostalgia for the laid-off employees, manageable caseloads, and staff time to focus on health promotion activities were palpable ...
This study examined outpatient services availability from FQHCs and RHCs following hospital closure. Nationally, the number of FQHCs and RHCs in rural and urban areas has increased substantially since 2006. Over the same time period, there was a substantial increase in the number of rural hospitals that closed in the United States This was ...
As healthcare shifts toward personalized, outcome-focused care, patient expectations for timely, supportive service are higher than ever. This case study highlights how DATAMARK's advanced contact center solutions help healthcare providers exceed these expectations, enhancing prescription management, patient communication, and overall care efficiency—ultimately driving better patient ...
The NHS Long Term Plan suggests redesigning outpatient services and removing up to the third of face-to-face outpatient visits by 2024 saving the NHS £1.1 billion. ... Furthermore, it will provide illustrations in the form of case studies from a novel virtual hospital service, Medefer. Medefer is a digitally enabled healthcare solution which ...
This paper focuses on reducing the "patient waiting time" by providing a simulation model of a private hospital in Vietnam in a real case study. The specific unit of analysis is the Outpatient Department (OPD) of this hospital. Gia Khang International Hospital (GIH), the selected hospital in this study, is a new private hospital that ...
This article reports on a case study of outpatient services during the COVID-19 pandemic. Ethical approval for this research was obtained fromthe Health Research Ethics Committee at UNS hospital. The COVID-19 pandemic has changed the outpatient services. The UNS Hospital's management has taken several steps
Implementing the Outpatient Letter Standard - Frimley Health case study Outpatient letters are the main method of contact and communication between hospital staff and GPs and are often the sole record of the consultation held by the outpatient department and hospital. Best practice for most outpatient letters is writing directly to people using services, so […]
The problem of appointment scheduling in outpatient clinics: a multiple case study of clinical practice. Omega . 2021;98 doi: 10.1016/j.omega.2019.102122.102122 ... Karakas E. Personnel and patient scheduling in the high demanded hospital services: a case study in the physiotherapy service. Journal of Medical Systems . 2008;32(3):221-228. doi ...
Case Study Our Outpatient Medical Transportation Services When Sarah turned 50, her doctor recommended a routine colonoscopy. Like many, Sarah felt a mix of anxiety and uncertainty not just about the procedure itself but about the entire process surrounding it. As a busy executive working for a corporation, Sarah's
The aim of this study is the systematic analyzing of the outpatient waiting time. Methods: This descriptive cross sectional study conducted in 2011 is an applicable study performed in the educational and health care hospitals of one of the medical universities located in the north west of Iran.
home Home chevron_right; Publications chevron_right; Evaluation of facies heterogeneity in reef carbonate reservoirs: A case study from the oil field, Perm Krai, Central-Eastern Russia
Download Citation | Pension provision issues: The Perm Krai case study | Subject. This article deals with the issues related to the optimization of the pension system. Objectives. The article aims ...
This study provides new insights into carbonate reef reservoirs' sedimentation and formation processes. It also creates an essential basis for a large-scale and comprehensive analysis of carbonate reef reservoir properties. In this study, a comprehensive classification of rocks was performed using geological, petrophysical, and well-logging data.
Reef deposits of the Upper Devonian oil fields in the north of the Perm Krai (Russia) are a primary hydrocarbon-bearing reservoir rock. This study provides new insights into carbonate reef reservoirs' sedimentation and formation processes. It also creates an essential basis for a large-scale and comprehensive analysis of carbonate reef reservoir properties.