
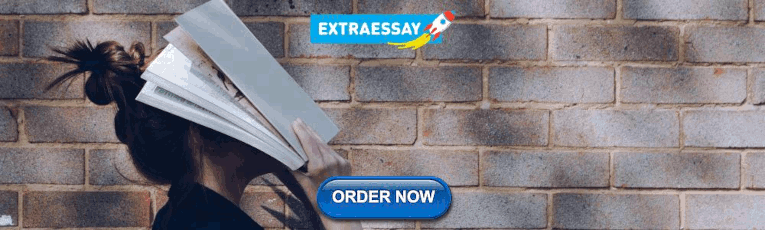
How to Perform Case Study Using Excel Data Analysis

In the latest Excel 365, performing any case study for business or research, it’s quite easier and smarter. It includes more exclusive features which give our required case studies a better way. I hope, you will learn the easiest way to perform a case study using Excel data analysis with some clear illustrations.
What Is Data Analysis in Excel?
The Analyze Data tool in Excel 365 is a powerful tool to manage our data by writing our queries directly in a search box. So we’ll be able to ask anything about our data without using any complex commands or formulas. Along with that, we can get very enriched and vivid visual illustrations or patterns of our data. In the earlier versions of Excel, the tool is named Data Analysis .
How Data Analysis Helps in Excel
- It can search according to the queries through our natural language.
- Provides high-level graphical illustrations and patterns.
- Easy to get the Pivot Tables and Pivot Charts.
- Faster steps save time.
- The interest of Fields can be changed easily.
How to Use Excel Data Analysis for Case Study
Now let’s see how to apply the Analyze Data tool to perform a data analysis case study. But first, get introduced to our dataset that represents some categories’ yearly sales and profits of a company.
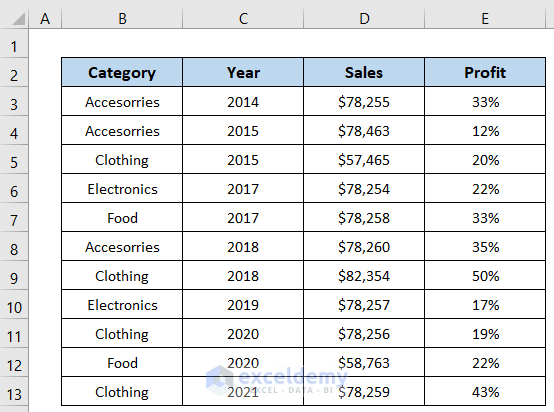
Read More: How to Install Data Analysis in Excel
With Default Analyze Data Option
First, we’ll see the default analyses that Excel makes automatically. Excel shows the most commonly used analyzes by default.
- Click any data from the dataset.
- Next, click as follows: Home > Analyze Data .
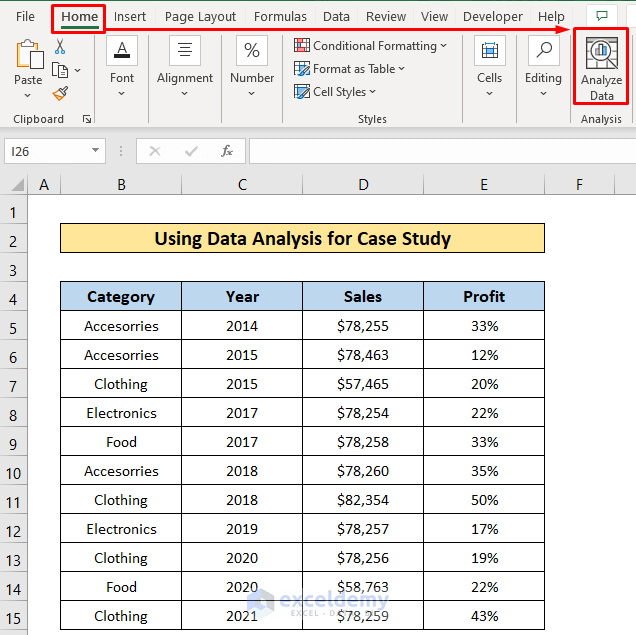
Soon after, you will get an Analyze Data field on the right side of your Excel window. Where you will see different kinds of cases like- Pivot Tables and Pivot Charts .
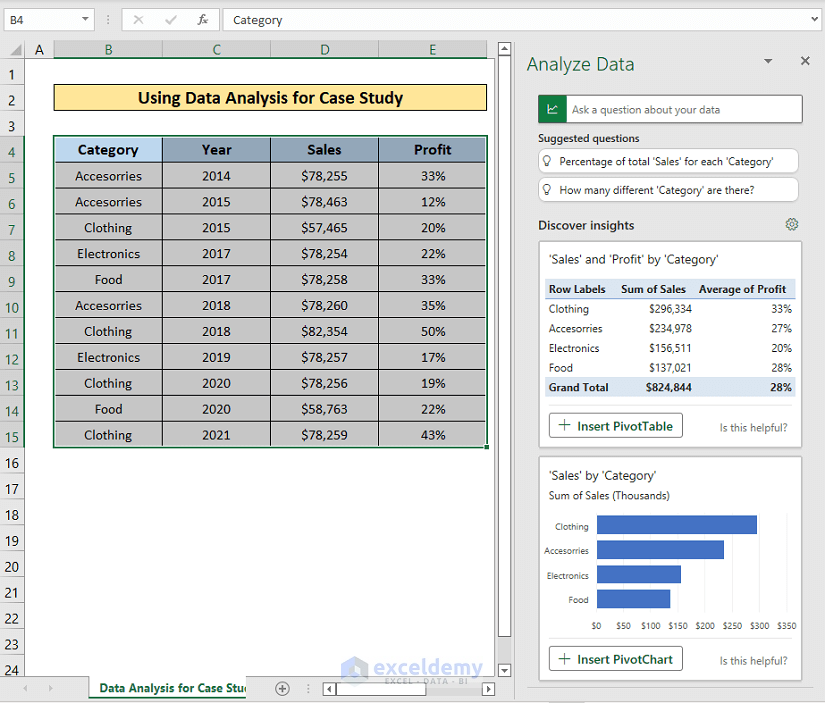
- Look, there is a sample Pivot Table of Sales and Profit by Category. Click on Insert Pivot Table .
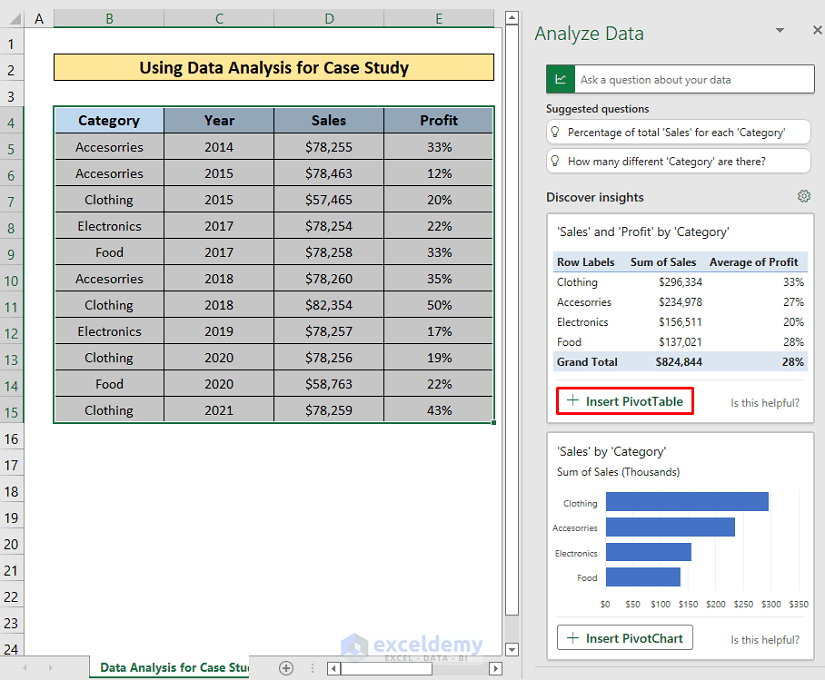
Now see, the Pivot Table is inserted in a new sheet.
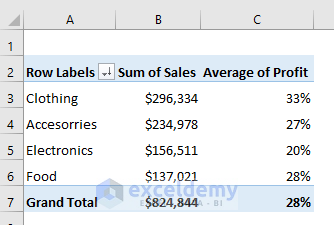
- Click on Insert Pivot Chart from the Sales by Category section then you will get the Pivot Chart in a new sheet.
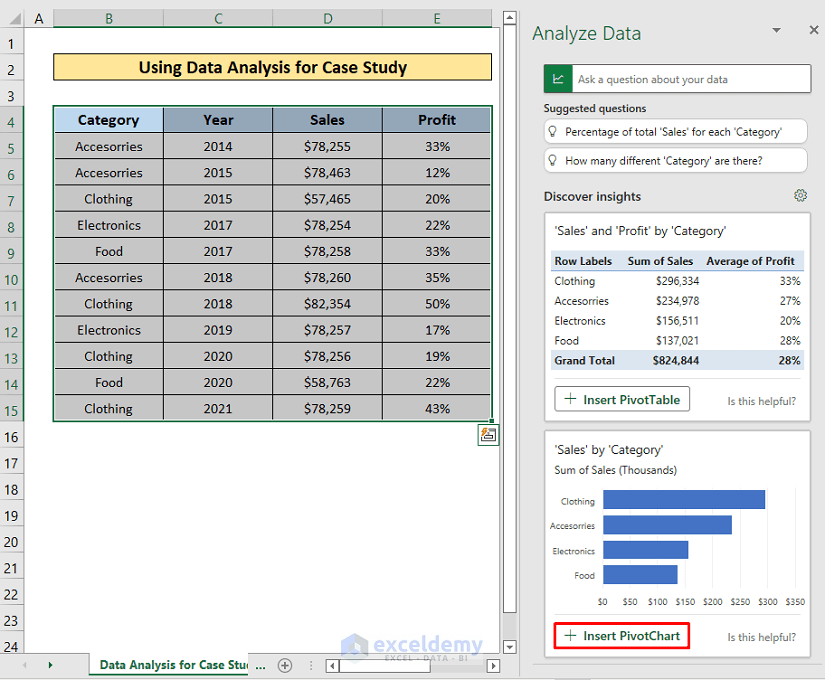
Here’s the chart.
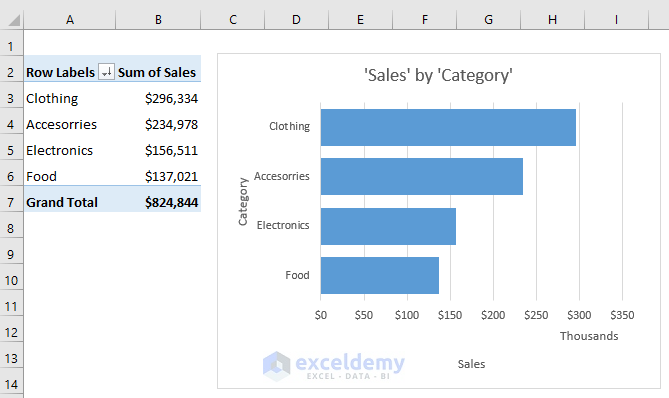
- Scroll down more and Excel will show you more possible Pivot Tables and Charts .
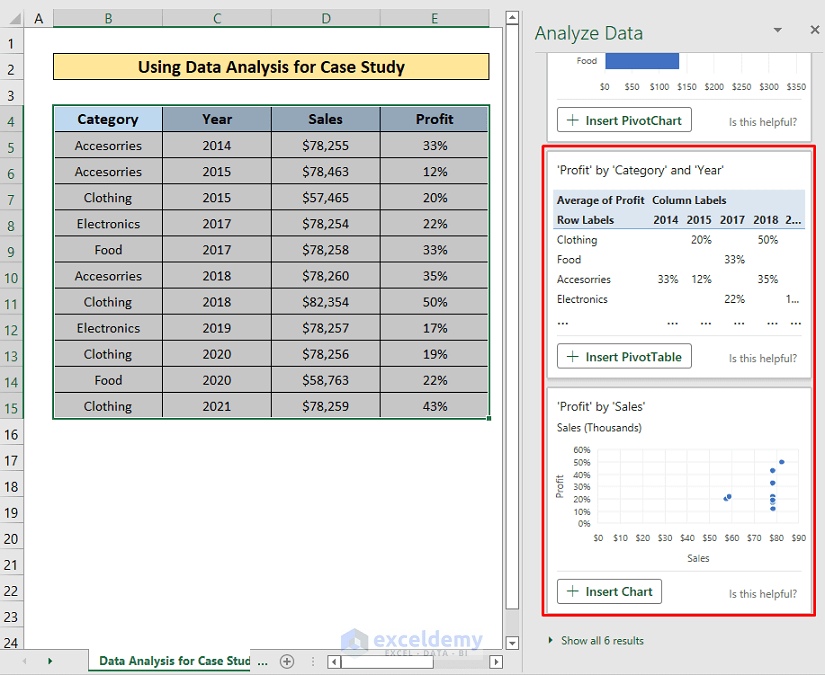
Feel free to use them if you prefer.
Read More: How to Analyze Data in Excel Using Pivot Tables
Analyze by Inserting Queries
Here, we’ll learn how to analyze data by inserting queries in the ‘ Ask a question about your data ’ box.
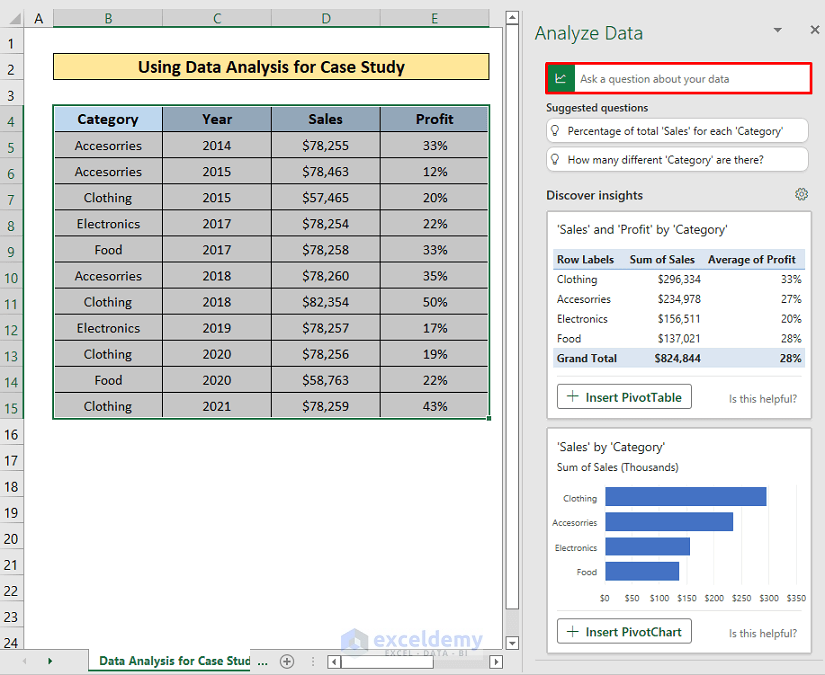
- When you will click on the question box, it will show some default questions. Click one of them and it will show the answer according to the question. See, I clicked Total ‘Sales’ of ‘Accessories’ over ‘Year’ .
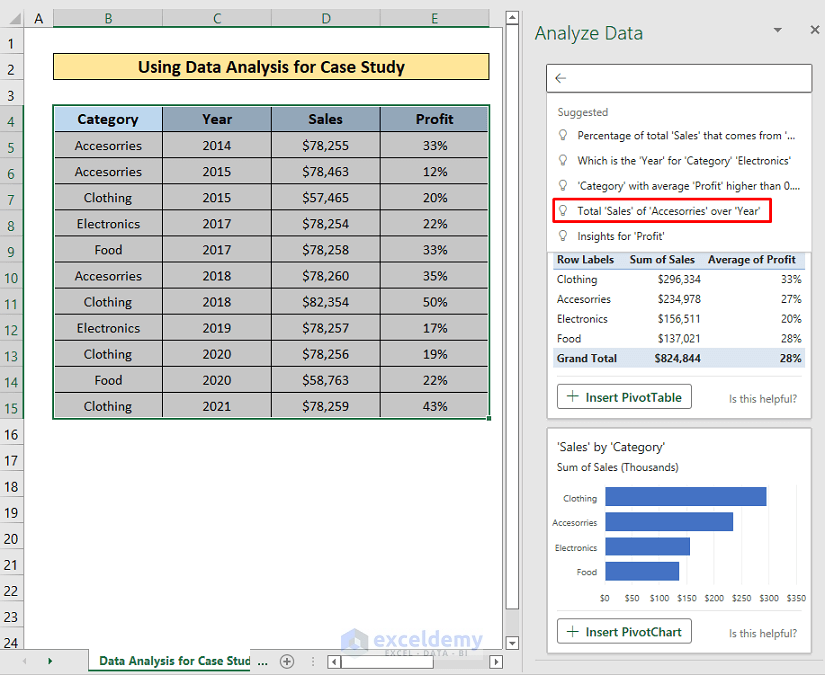
It’s the answer from Excel.
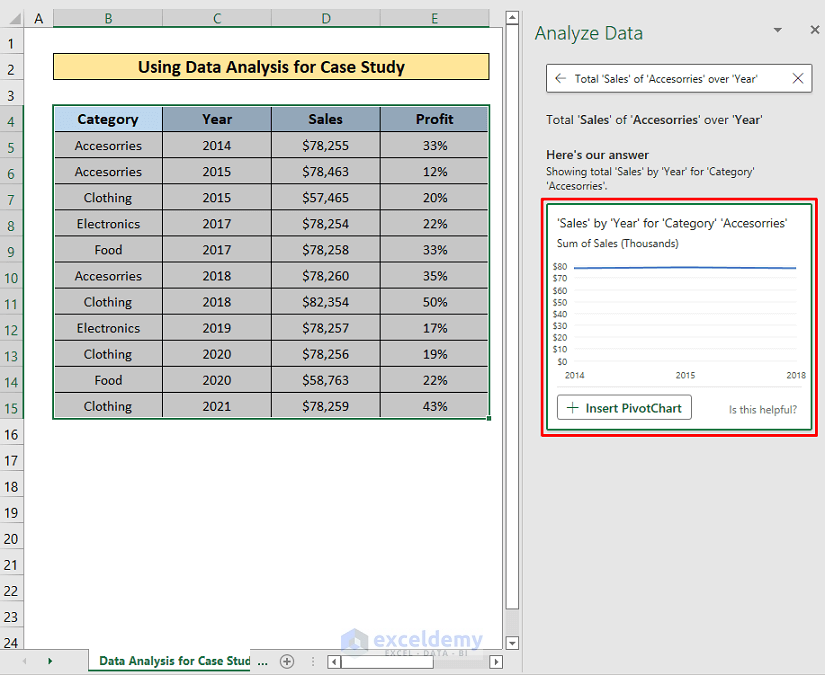
- Or you can write your question. I asked- Profit chart according to Year .
- After that, hit the ENTER button .
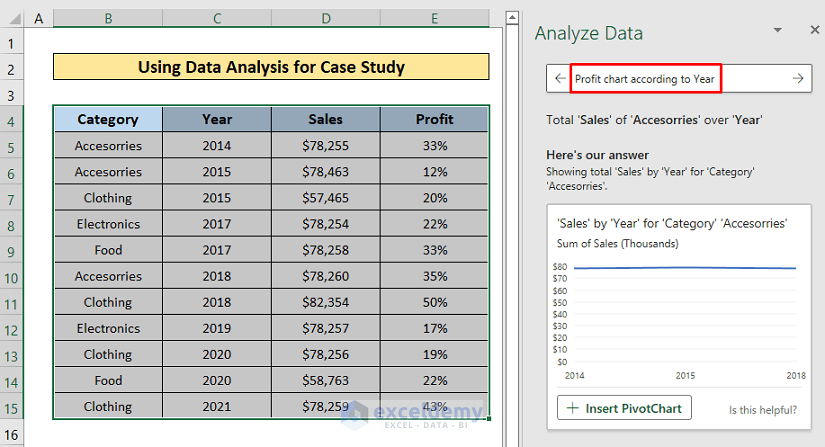
- Now you see, it’s showing the chart of profit by year. Click on Insert PivotChart .
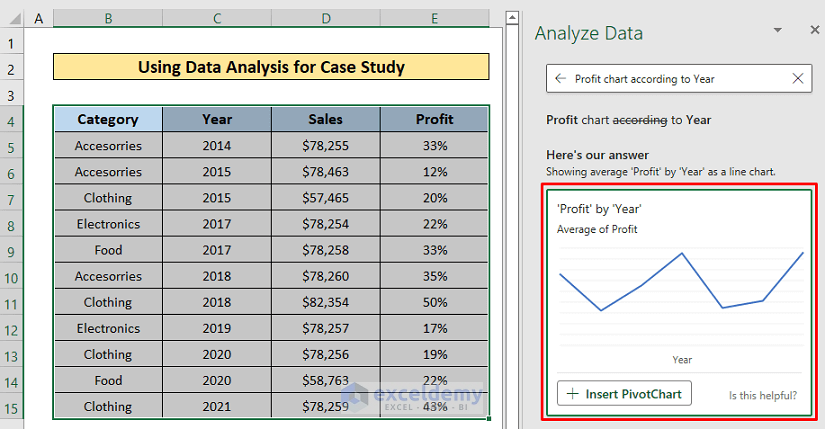
Soon after, a new sheet will open up with the PivotChart .
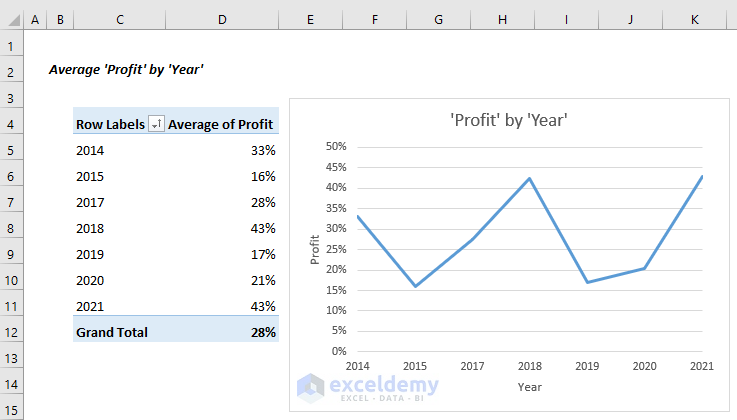
- Also, there is a Setting icon in the Discover insights part, click it and a dialog box will open up to select the customized fields of interest.
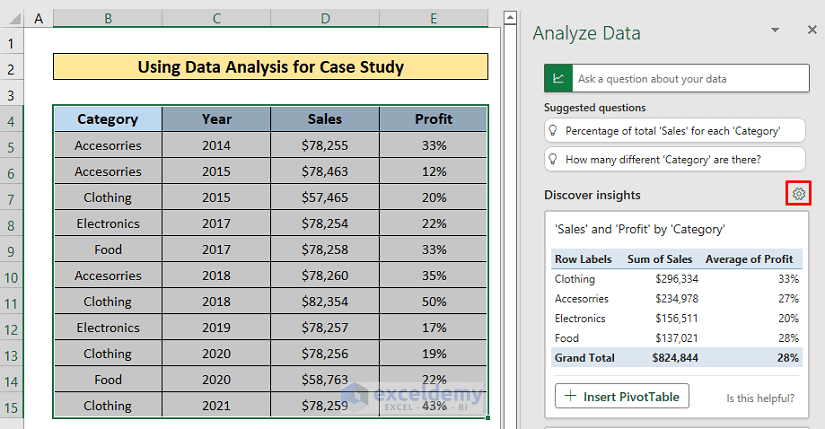
- Mark your desired fields from here. I marked Category and Profit.
- Finally, just click Update .
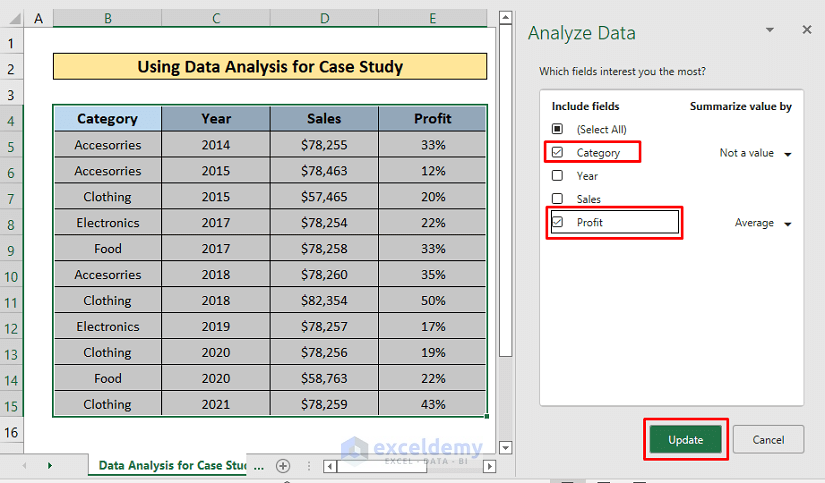
Now it is showing the answers only about Category and Profit.
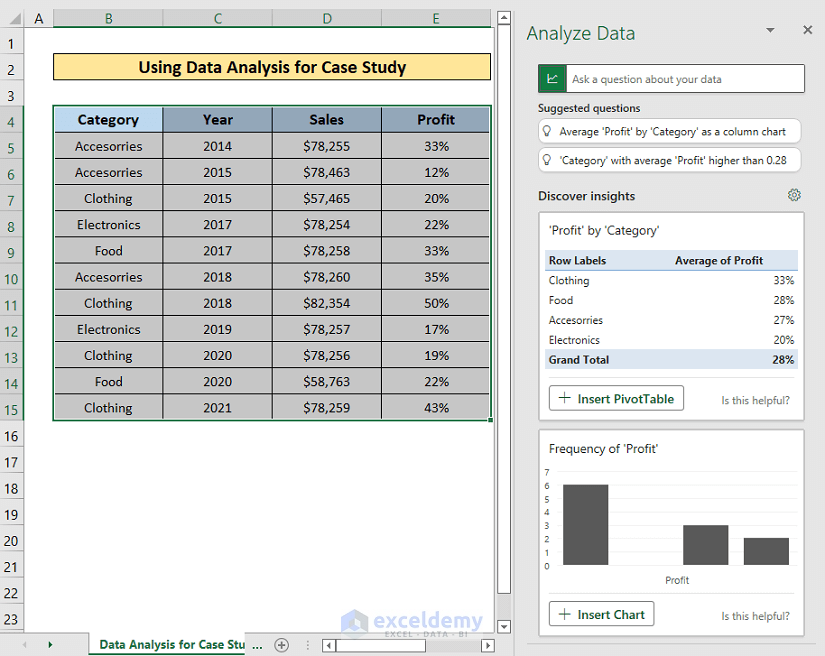
Things to Remember
- The Analyze Data tool is only available in the latest Excel 365. But in the earlier versions, it is named Data Analysis ToolPak and available as Add-ins by default.
Download Practice Workbook
You can download the free Excel workbook from here and practice on your own.
I hope the procedures described above will be good enough to perform a case study using Excel Data Analysis. Feel free to ask any question in the comment section and please give me feedback.
Related Articles
- How to Use Data Analysis Toolpak in Excel
- How to Enter Data for Analysis in Excel
- How to Make Histogram Using Analysis ToolPak
- [Fixed!] Data Analysis Not Showing in Excel
<< Go Back to Data Analysis with Excel | Learn Excel
What is ExcelDemy?
Tags: Data Analysis with Excel

Md. Sourov Hossain Mithun, an Excel and VBA Content Developer at Softeko's ExcelDemy project, joined in October 2021. Holding a Naval Architecture & Marine Engineering degree from BUET, Mithun showcases expertise during his two-year tenure. With over 200 published articles on Excel topics, he earned a promotion to Team Leader, excelling in leading diverse teams. Mithun's passion extends to Advanced Excel, Excel VBA, Data Analysis, and Python programming, contributing significantly to the innovative and dynamic environment of ExcelDemy... Read Full Bio
Leave a reply Cancel reply
ExcelDemy is a place where you can learn Excel, and get solutions to your Excel & Excel VBA-related problems, Data Analysis with Excel, etc. We provide tips, how to guide, provide online training, and also provide Excel solutions to your business problems.
Contact | Privacy Policy | TOS
- User Reviews
- List of Services
- Service Pricing
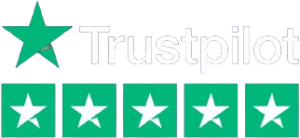
- Create Basic Excel Pivot Tables
- Excel Formulas and Functions
- Excel Charts and SmartArt Graphics
- Advanced Excel Training
- Data Analysis Excel for Beginners

Advanced Excel Exercises with Solutions PDF

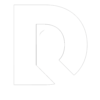
Table of Contents
Data Analysis Using Excel Case Study
Data analysis is an essential skill in today’s business world. As organizations deal with increasing amounts of data, it becomes crucial for professionals to make sense of this information and derive useful insights. Excel is a powerful and versatile tool that can assist in analyzing and presenting data effectively, particularly through the use of case studies.
A case study is a detailed examination of a specific situation or problem in order to better understand the complexities involved. By using Excel for data analysis, individuals can explore and analyze the data related to the case study in a comprehensive and structured manner. Excel offers various tools and functionalities, such as PivotTables, slicers, and data visualization features, which allow users to assess patterns, trends, and relationships within the data.
Applying these techniques for data analysis in Excel case studies enables professionals to make well-informed business decisions and communicate their findings effectively. By leveraging the capabilities of Excel in conjunction with case studies, individuals can unlock valuable insights that drive organizational success and contribute to an enhanced understanding of the overall data landscape.
Excel Basics for Data Analysis
Dataset preparation.
When working with Excel, the first step in data analysis is dataset preparation . This process involves setting up the data in a structured format, with clearly defined headers and cells. To start, you must import or enter your data into an Excel spreadsheet, ensuring that each record is represented by a row and each variable by a column. Headers should be placed in the top row and provide descriptive labels for each column. Proper organization of your dataset helps to ensure accurate analysis and interpretation .
For example, suppose you have a dataset that contains the following information:
In this dataset, the headers are “Year,” “Category,” “Sales,” and “Profit.” Each row represents a record, and the cells contain the corresponding data.
Data Cleaning
The next step in data analysis using Excel is data cleaning . Data cleaning is the process of identifying and correcting errors, inconsistencies, and inaccuracies in your dataset. Common data cleaning tasks include:
- Removing duplicate records,
- Filling in missing values,
- Correcting data entry errors,
- Standardizing and formatting variable names and values.
To perform data cleaning in Excel, you can use various functions and tools:
- Remove duplicates: To remove duplicate records, select your dataset and navigate to the Data tab. Click the “Remove Duplicates” button and select the columns to be used for identifying duplicate rows.
- Fill in missing values: Use Excel functions such as VLOOKUP , HLOOKUP , and INDEX-MATCH to fill in missing values based on other data in your dataset. You can also use the IFERROR function to handle errors when looking up values.
- Correct data entry errors: Use Excel’s “Find and Replace” tool (Ctrl + F) to search for and correct errors in your dataset. You may need to perform this multiple times for different errors.
- Standardize and format variable names and values: Use Excel functions such as UPPER , LOWER , PROPER , and TRIM to standardize text data. Format numerical values using the Number Format options in the Home tab.
By ensuring your dataset is clean and well-organized, you can confidently move forward with more advanced data analysis tasks in Excel.
Powerful Excel Functions
Excel is a versatile tool when it comes to data analysis. There are many powerful functions that can help you perform complex calculations and analysis easily. In this section, we will explore some of the top functions in three categories: Text Functions, Date Functions, and Lookup Functions.
Text Functions
Text Functions are crucial when working with large sets of data containing text. These functions help in cleaning, extracting, and modifying text data. Some key text functions include:
- LEFT : Extracts a specified number of characters from the beginning of a text string.
- RIGHT : Extracts a specified number of characters from the end of a text string.
- MID : Extracts a specified number of characters from a text string, starting at a specified position.
- TRIM : Removes extra spaces from text, leaving a single space between words and no space at the beginning or end of the text.
- CONCATENATE : Joins multiple text strings into one single string.
- FIND : Locates the position of a specific character or text string within another text string.
Date Functions
Date Functions are essential for dealing with dates and times in data analysis. These functions help in calculating the difference between dates, extracting parts of a date, and performing various date-related calculations. Some notable date functions include:
- TODAY : Returns the current date.
- NOW : Returns the current date and time.
- DATEDIF : Calculates the difference between two dates in days, months, or years.
- DATE : Creates a date by combining individual day, month, and year values.
- WEEKDAY : Returns the day of the week corresponding to a specific date, as an integer between 1 (Sunday) and 7 (Saturday).
- EOMONTH : Returns the last day of the month for a given date.
Lookup Functions
Lookup Functions are powerful tools used to search and retrieve data from a specific range or table in Excel. These functions can save time and effort when working with large datasets. Some essential lookup functions include:
- VLOOKUP : Searches for a specific value in the first column of a range and returns a corresponding value from a specified column.
- HLOOKUP : Searches for a specific value in the first row of a range and returns a corresponding value from a specified row.
- INDEX : Returns a value from a specific cell within a range, using row and column numbers.
- MATCH : Searches for a specific value in a range and returns its relative position within that range.
- XLOOKUP : Performs a lookup by searching for a specific value in a range or table and returning a corresponding value from another column or row (available only in Excel 365 and Excel 2019).
These powerful Excel functions can help make the process of data analysis more efficient and accurate. In combination with appropriate formatting, tables, and other visual aids, these functions can greatly enhance your ability to process and understand large datasets.
Related Article: Excel Functions for Data Analysts.
Data Exploration and Visualization
In the process of data analysis using Excel, data exploration and visualization play essential roles in revealing patterns, trends, and relationships within the data. This section will cover two primary techniques for data visualization in Excel: Charts and Trends, and Pivot Tables and Pivot Charts.
Charts and Trends
Charts in Excel are a highly effective method of uncovering patterns and relationships within the dataset. There are various types of charts available in Excel that cater to different use cases, such as bar charts, line charts, and scatter plots. These chart types can be customized to suit the needs of the analysis and to emphasize specific trends or patterns.
Trends in the data can be identified with the help of charts, and Excel offers trend lines functionalities to visualize these trends more clearly. By applying a trend line, one can easily identify the overall direction (positive or negative) of the dataset and make predictions based on this information. Additionally, Excel offers built-in formatting options that can help emphasize certain data points or highlight particular trends for easier interpretation.
Pivot Tables and Pivot Charts
Pivot Tables are another powerful data analysis feature in Excel. They allow the user to summarize, reorganize, and filter data by dragging and dropping columns into different areas. This enables the user to analyze data across multiple dimensions, revealing hidden insights and patterns.
To complement Pivot Tables, Excel also offers Pivot Charts, which allow users to create dynamic visualizations derived from the Pivot Table data. Pivot Charts offer the same chart types as regular Excel charts but with the added capability to update the chart when the Pivot Table data is altered. This makes Pivot Charts ideal for creating interactive and easily updatable visualizations.
Overall, incorporating these techniques into the data analysis process can enhance understanding and unveil valuable insights from the dataset. When using Excel for data analysis, data exploration and visualization with Charts and Trends, as well as Pivot Tables and Pivot Charts, can provide a comprehensive and insightful overview of the data in question.
Case Study: Covid-19 Data Analysis
Data collection and cleaning.
The Covid-19 pandemic has generated vast amounts of data, requiring researchers and analysts to collect, clean, and organize data sets to gain valuable insights. Several sources, such as the World Health Organization and Johns Hopkins University , provide updated information on confirmed cases, recoveries, and deaths.
Data collection starts with gathering raw data from various sources. These data sets may have inconsistencies, missing values, or discrepancies, which need to be addressed to ensure accurate analysis. Data cleaning is a critical step in this process, involving tasks such as removing duplicates, filling in missing values, and correcting errors.
Exploratory Data Analysis
Once the data is clean and organized, exploratory data analysis (EDA) can be conducted using tools like Excel. EDA helps analysts understand the data, identify patterns, and generate hypotheses for further investigation.
Some useful techniques in conducting EDA in Excel include:
- Pivot Tables : These allow users to summarize and reorganize data quickly, providing aggregated views of the data.
- Charts and Graphs : Visual representations of data, such as bar charts or line graphs, can display trends, correlations, or patterns more clearly than raw numbers.
- Descriptive Statistics : Excel’s built-in functions allow easy calculation of measures such as mean, median, and standard deviation, providing a preliminary statistical analysis of the data.
In the context of Covid-19 data, EDA can help reveal important information about the pandemic’s progression. For example, analysts can:
- Compare infection rates across countries or regions
- Monitor changes in case numbers over time
- Evaluate the effectiveness of public health interventions and policies
The insights gained from exploratory data analysis can guide further research, inform decision-making, and contribute to a better understanding of the pandemic’s impact on public health.
Case Study: Stock Market Data Analysis
Data collection and preparation.
The first step in the stock market data analysis case study is collecting and preparing the data. This process involves gathering historical stock prices, trading volumes, and other relevant financial metrics from reliable sources. The data can be cleaned and organized in Excel, removing any errors or inconsistencies. It’s essential to verify the collected data’s accuracy to ensure the analysis’s validity.
After preparing the financial data, the next step is to compute essential measures and ratios. These may include:
- Price-to-Earnings (P/E) Ratio
- Dividends Yield
- Total Return
- Moving Averages
Calculating these ratios and measures provides a general overview of a company’s performance in the stock market, which can be further analyzed with Excel tools.
Profit and Loss Analysis
In this stage of the case study, profit and loss analysis is conducted to assess the stock’s performance. Using Excel PivotTables, we can summarize the data to identify trends or patterns in the stock market. For instance, we can analyze the historical profits and losses of multiple stocks during a specific state or market condition.
Analyzing profit and loss data can also be done with natural language capabilities in Excel. This feature allows us to ask questions about the dataset, and Excel will produce relevant results. For example, we could pose a question like “Which stocks had the highest profit margins in the last quarter?” or “What is the average loss for the technology sector?”
After exploring the profits and losses of the stocks, we can gain insights into which stocks or sectors are more profitable or risky. This information can help potential investors make informed decisions about their investment strategies. Additionally, the insights from the case study can serve as a reference point for future stock market analyses.
Remember, this case study only serves as an example of how to conduct stock market data analysis using Excel. By adapting and expanding on these techniques, one can harness the power of Excel to explore various aspects of financial markets and derive valuable insights.
Case Study: San Diego Burrito Ratings
Data gathering and cleaning.
The main objective of this case study is to evaluate and analyze the various factors that contribute to the ratings of San Diego burritos. The data used in this analysis is collected from different sources, which include customer reviews and ratings from Yelp, along with other relevant information about burrito sales and geographical distribution. The raw data is then compiled and cleaned to ensure that it is consistent and free from any discrepancies or errors. This process involves standardizing the fields and records, as well as filtering out any irrelevant information. The cleaned data is then organized into a structured format, which is suitable for further analysis using Excel PivotTables and Charts.
Use of Pivot Tables and Charts
After cleaning and organizing the data, Excel PivotTables are utilized to analyze the regional distribution of San Diego burrito ratings. By categorizing the data based on regions, such as East and West, it becomes convenient to identify the ratings and sales trends across these regions. The organized data is then sorted based on the ratings and popularity of burrito establishments within specific densely populated areas.
Using Pivot Charts, a graphical representation of the data is created to provide a clear and comprehensive visual of the ratings distribution in different regions of San Diego. It becomes easier to discern patterns and trends, allowing for the development of informed conclusions on the factors influencing the popularity and success of burrito establishments.
Throughout the analysis, various parameters are investigated, which include the relationship between ratings and sales, the potential impact of particular fields on popularity, and the apparent differences between densely populated regions in terms of burrito preferences. By utilizing PivotTables and Charts confidently, it is possible to draw insights and conclusions that can help optimize marketing strategies, guide customer preferences, and influence the overall success of burrito establishments across San Diego.
Case Study: Shark Attack Records Analysis
Data collection and pre-processing.
In this case study, the primary focus is on the analysis of shark attack records recorded between 1900 and 2016, consisting of just under 5,300 records or observations. To begin the analysis, the data needs to be collected from a reliable source and pre-processed to ensure its accuracy and relevance.
Data pre-processing is an essential step to prepare the dataset for analysis. It involves checking for missing values, outliers, and inconsistencies in the data. Additionally, it may also require converting the data into a suitable format, such as categorizing dates or splitting location information into separate columns (latitude and longitude).
Identifying Trends and Patterns
Once the dataset has been pre-processed, it’s time to dive into the analysis using Microsoft Excel. Excel offers a fast and central way to analyze data and search for trends and patterns within shark attack records. One powerful tool for this purpose is Excel’s PivotTables, which allows users to easily aggregate and summarize data.
Some possible trends and patterns that can be identified through the analysis of shark attack records include:
- Temporal Trends: Analyzing the frequency of shark attacks over time to identify any patterns in the occurrence of attacks, such as seasonality or specific years with higher attack rates.
- Geographical Patterns: Identifying areas with a higher concentration of shark attacks, which can provide insights into hotspots and potentially dangerous locations.
- Victim Demographics: Examining the demographics of shark attack victims, such as age, gender, and activity type, to determine if certain groups are more prone to attacks.
- Species Involved: Investigating the types of shark species responsible for attacks and their relative frequency in the dataset.
By utilizing Excel’s data analysis tools and PivotTables, researchers can confidently and clearly identify trends and patterns in the shark attack records, providing valuable insights into shark behavior and risk factors associated with shark attacks. This analysis can be helpful in understanding and managing the risks associated with shark encounters for both public safety and conservation efforts.
Related Article: How to Solve Data Analysis Real World Problems.
Additional Resources and Exercises
Kaggle and data analysis courses.
Kaggle is a popular platform that offers data science competitions, datasets, and courses to help you improve your data analysis skills in Excel. The courses are designed for various skill levels, and they cover essential concepts like PivotTables and data visualization. The comprehensive exercises and practical case studies provide a real-world context for mastering data analysis techniques.
The course reviews on Kaggle are usually quite positive, with many users appreciating the knowledgeable instructors and engaging content. If you’re looking to become a data analyst or enhance your existing skills, exploring the data analysis courses on Kaggle is a great starting point.
Power Query in Excel
Power Query is a powerful data analysis tool in Excel that enables you to import, transform, and combine data from various sources. This feature is particularly useful when working with large datasets or preparing data for analysis. There are numerous resources available to learn how to use Power Query effectively.
To practice using Power Query, consider working on exercises that focus on data cleansing, data transformation, and data integration. As you progress, you will gain a deeper understanding of the various Power Query functionalities and become more confident in your data analysis abilities.
In conclusion, engaging with additional resources like Kaggle courses and Power Query exercises will help you hone your Excel data analysis skills and enable you to tackle complex case studies with ease.
Frequently Asked Questions
How can excel be used for effective case study analysis.
Excel is a versatile tool that can be utilized for effective case study analysis. By organizing and transforming data into easily digestible formats, users can better identify trends, patterns, and insights within their data sets. Excel also offers various functions and tools, such as pivot tables, data tables, and data visualization, which enable users to analyze case study data more efficiently and uncover valuable information.
Which Excel functions are most useful for data analysis in case studies?
There are numerous Excel functions that can be highly useful for data analysis in case studies. These include:
- VLOOKUP, which allows users to search for specific information in large data sets
- INDEX-MATCH, a more advanced alternative to VLOOKUP that’s capable of handling more complex data structures
- IF, which helps in making conditional statements and decisions in data analysis
- AVERAGE, MAX, MIN, and COUNT for basic data aggregation
- SUMIFS and COUNTIFS, which allow users to perform conditional aggregation based on predefined criteria
What are some examples of data analysis projects using Excel?
Many different projects can benefit from data analysis using Excel, such as financial analysis, market research, sales performance tracking, and customer behavior analysis. Businesses across industries are known to use Excel for evaluating their case studies and forming data-driven decisions based on their insights.
How can Excel pivot tables aid in analyzing case study data?
Pivot tables in Excel are powerful, enabling users to summarize and analyze large data sets quickly and efficiently. They allow users to group and filter data based on different dimensions, making it much easier to identify trends, patterns, and relationships within the data. Additionally, pivot tables provide user-friendly drag-and-drop functionalities, allowing for easy customization and requiring minimal Excel proficiency.
In which industries is Excel data analysis most commonly applied in case studies?
Excel data analysis is widely used across various industries for case studies, including:
- Finance and banking, for analyzing investment portfolios, risk management, and financial performance
- Healthcare, for patient data analysis and identifying patterns in disease occurrence
- Marketing and sales, to analyze customer data and product performance
- Retail, for inventory management and sales forecasting
- Manufacturing, to evaluate the efficiency and improve production processes
What steps should be followed for a successful data analysis process in Excel?
A successful data analysis process in Excel typically involves the following steps:
- Data collection: Gather relevant data from various sources and consolidate it in Excel.
- Data cleaning and preprocessing: Remove any errors, duplicate records, or missing values in the data, and reformat it as necessary.
- Data exploration: Familiarize with the data, identify patterns, and spot trends through descriptive analysis and visualization techniques.
- Data analysis: Use relevant functions, formulas, and tools such as pivot tables to analyze the data and extract valuable insights.
- Data visualization: Create charts, graphs, or dashboard reports to effectively visualize the findings for improved understanding and decision-making.
What you should know:
- Our Mission is to Help you to Become a Professional Data Analyst.
- This Website is a Home for Data Analysts. Get our latest in-depth Data Analysis and Artificial Intelligence Lessons and Updates in your Inbox.
Tech Writer | Data Analyst | Digital Creator
Get Our Professional Data Analyst Roadmap for free
You may also like
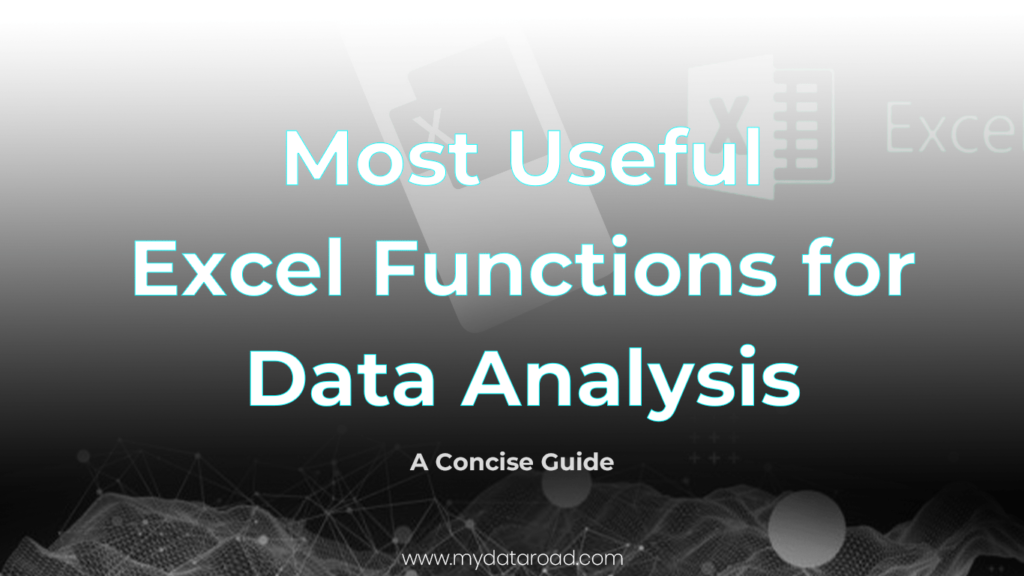
Analyze Data in Excel
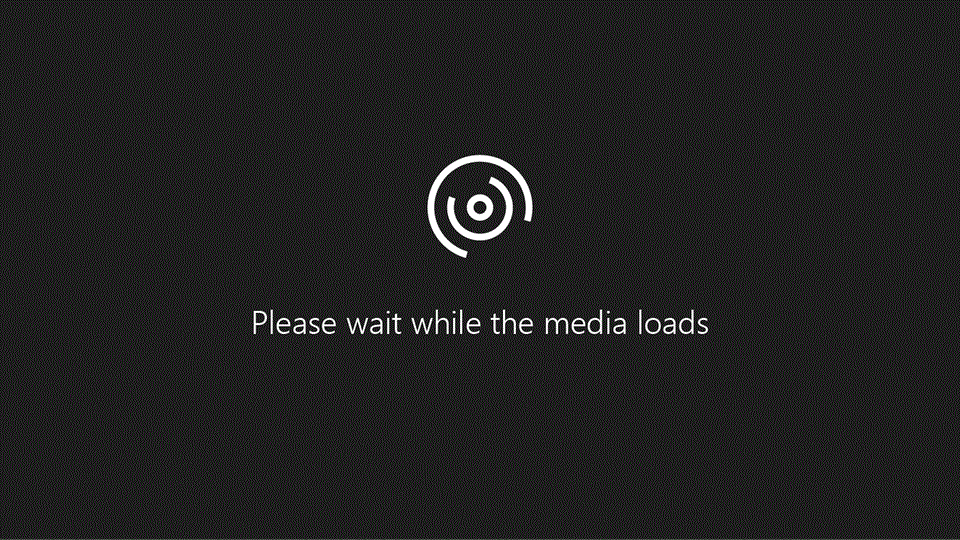
Analyze Data in Excel empowers you to understand your data through natural language queries that allow you to ask questions about your data without having to write complicated formulas. In addition, Analyze Data provides high-level visual summaries, trends, and patterns.
Have a question? We can answer it!
Simply select a cell in a data range > select the Analyze Data button on the Home tab. Analyze Data in Excel will analyze your data, and return interesting visuals about it in a task pane.
If you're interested in more specific information, you can enter a question in the query box at the top of the pane, and press Enter . Analyze Data will provide answers with visuals such as tables, charts or PivotTables that can then be inserted into the workbook.
If you are interested in exploring your data, or just want to know what is possible, Analyze Data also provides personalized suggested questions which you can access by selecting on the query box.
Try Suggested Questions
Just ask your question
Select the text box at the top of the Analyze Data pane, and you'll see a list of suggestions based on your data.

You can also enter a specific question about your data.

Analyze Data is available to Microsoft 365 subscribers in English, French, Spanish, German, Simplified Chinese, and Japanese. If you are a Microsoft 365 subscriber, make sure you have the latest version of Office . To learn more about the different update channels for Office, see: Overview of update channels for Microsoft 365 apps .
The Natural Language Queries functionality in Analyze Data is being made available to customers on a gradual basis. It may not be available in all countries or regions at this time.
Get specific with Analyze Data
If you do not have a question in mind, in addition to Natural Language, Analyze Data analyzes and provides high-level visual summaries, trends, and patterns.
You can save time and get a more focused analysis by selecting only the fields you want to see. When you choose fields and how to summarize them, Analyze Data excludes other available data - speeding up the process and presenting fewer, more targeted suggestions. For example, you might only want to see the sum of sales by year. Or you could ask Analyze Data to display average sales by year.
Select Which fields interest you the most?
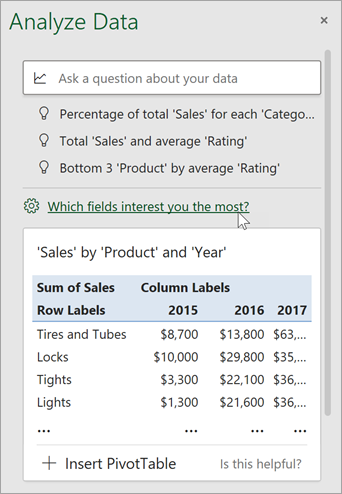
Select the fields and how to summarize their data.

Analyze Data offers fewer, more targeted suggestions.

Note: The Not a value option in the field list refers to fields that are not normally summed or averaged. For example, you wouldn't sum the years displayed, but you might sum the values of the years displayed. If used with another field that is summed or averaged, Not a value works like a row label, but if used by itself, Not a value counts unique values of the selected field.
Analyze Data works best with clean, tabular data.
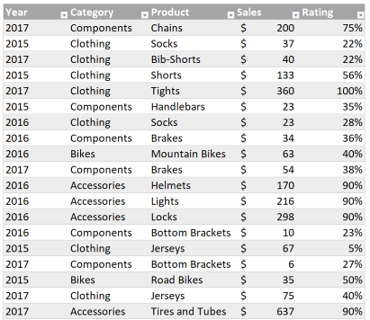
Here are some tips for getting the most out of Analyze Data:
Analyze Data works best with data that's formatted as an Excel table . To create an Excel table, click anywhere in your data and then press Ctrl+T .
Make sure you have good headers for the columns. Headers should be a single row of unique, non-blank labels for each column. Avoid double rows of headers, merged cells, etc.
If you have complicated, or nested data, you can use Power Query to convert tables with cross-tabs, or multiple rows of headers.
Didn't get Analyze Data? It's probably us, not you.
Here are some reasons why Analyze Data may not work on your data:
Analyze Data doesn't currently support analyzing datasets over 1.5 million cells. There is currently no workaround for this. In the meantime, you can filter your data, then copy it to another location to run Analyze Data on it.
String dates like "2017-01-01" will be analyzed as if they are text strings. As a workaround, create a new column that uses the DATE or DATEVALUE functions, and format it as a date.
Analyze Data won't work when Excel is in compatibility mode (i.e. when the file is in .xls format). In the meantime, save your file as an .xlsx, .xlsm, or .xlsb file.
Merged cells can also be hard to understand. If you're trying to center data, like a report header, then as a workaround, remove all merged cells, then format the cells using Center Across Selection. Press Ctrl+1 , then go to Alignment > Horizontal > Center Across Selection .
Analyze Data can't analyze data when Excel is in compatibility mode (i.e. when the file is in .xls format). In the meantime, save your file as an .xlsx, .xlsm, or xslb file.
Analyze Data works best with data that's formatted as an Excel table . To create an Excel table, click anywhere in your data and then click Home > Tables > Format as Table .
We're always improving Analyze Data
Even if you don't have any of the above conditions, we may not find a recommendation. That's because we are looking for a specific set of insight classes, and the service doesn't always find something. We are continually working to expand the analysis types that the service supports.
Here is the current list that is available:
Rank : Ranks and highlights the item that is significantly larger than the rest of the items.
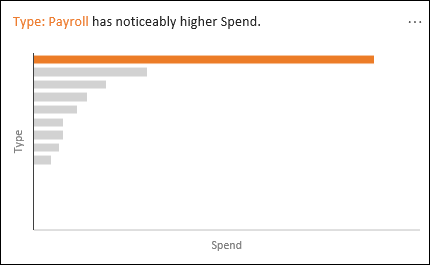
Trend : Highlights when there is a steady trend pattern over a time series of data.
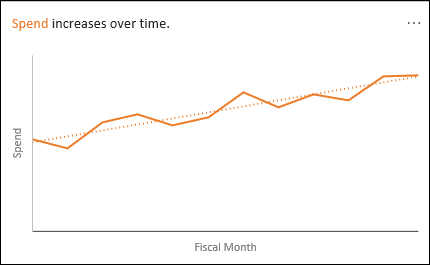
Outlier : Highlights outliers in time series.
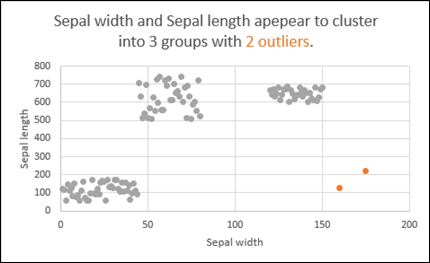
Majority : Finds cases where a majority of a total value can be attributed to a single factor.
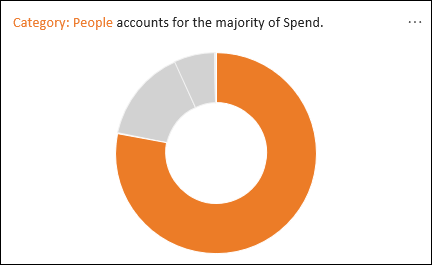
If you don't get any results, please send us feedback by going to File > Feedback .
Microsoft Privacy Policy
Because Analyze Data analyzes your data with artificial intelligence services, you might be concerned about your data security. You can read the Microsoft privacy statement for more details.
Licensing information for Analyze Data
Analyze Data uses material from third-parties. If you'd like to read the details, see Licensing information for Analyze Data .
Need more help?
You can always ask an expert in the Excel Tech Community or get support in Communities .

Want more options?
Explore subscription benefits, browse training courses, learn how to secure your device, and more.

Microsoft 365 subscription benefits
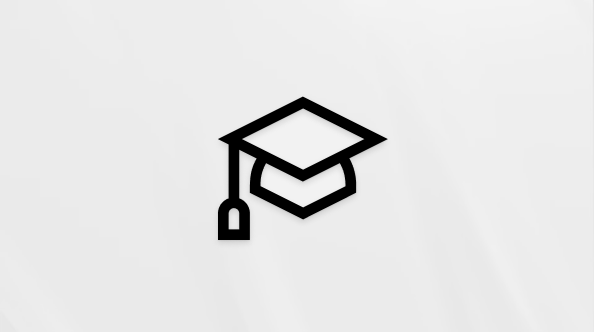
Microsoft 365 training
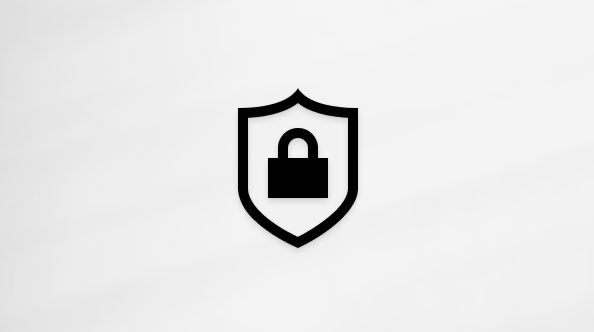
Microsoft security

Accessibility center
Communities help you ask and answer questions, give feedback, and hear from experts with rich knowledge.
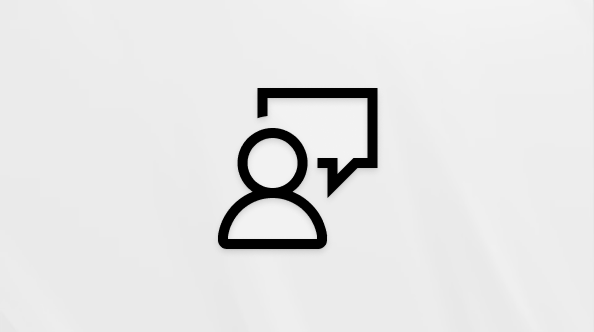
Ask the Microsoft Community
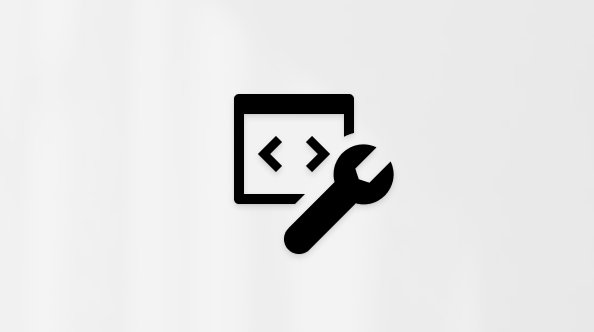
Microsoft Tech Community

Windows Insiders
Microsoft 365 Insiders
Was this information helpful?
Thank you for your feedback.

Supply Chain Analysis: Data Analysis Case Study Using Excel
A supply chain analysis project using microsoft excel and microsoft power bi..

Supply chain analytics plays a crucial role in driving data-driven decision-making across industries, including manufacturing, retail, healthcare, and logistics. In this report, I presented an analysis of a Fashion and Beauty startup’s supply chain data, specifically focused on the movement of makeup products. By collecting, analyzing, and interpreting this dataset, I aim to gain insights that can inform strategic decisions and optimize supply chain operations.
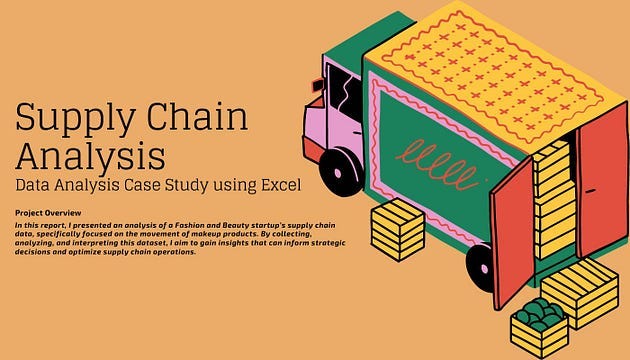
Dataset Overview
The dataset comprises various features related to the fashion and makeup product supply chain. These features provide valuable information for understanding the flow of products from suppliers to customers. The following features include:
Product Type
Availability
Number of products sold
Revenue generated
Customer demographics
Stock levels
Order quantities
Shipping times
Shipping carriers
Shipping costs
Supplier name
Production volumes
Manufacturing lead time
Manufacturing costs
Inspection results
Defect rates
Transportation modes
Supply Chain Analysis using Excel
The supply chain represents a network of interconnected processes involved in the production and delivery of goods to customers. Analyzing the various components of a supply chain is crucial for identifying opportunities to enhance its effectiveness and generate greater value for customers. In this report, I conducted a supply chain analysis using Excel and gave valuable insights into optimizing supply chain operations.
Dataset Source
To perform a supply chain analysis on this company, it is crucial to gather data related to various stages of the supply chain. This includes information on sourcing, manufacturing, transportation, inventory management, sales, and customer demographics.
Fortunately, I have come across this excellent dataset that provides comprehensive data about the supply chain of a Fashion and Beauty startup.
In the following section, I will guide you through the process I use to conduct a supply chain analysis using Excel.
Dataset from Github
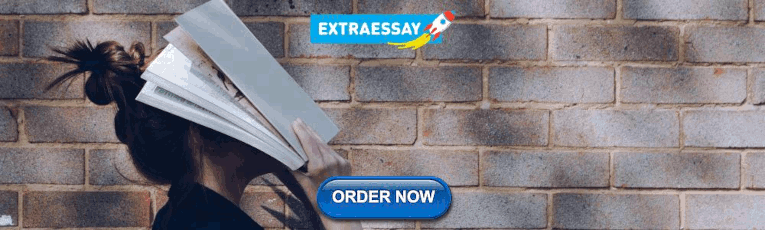
Dataset Analysis using Excel
The first step is to import the file into Excel.
After importing the file, the next task is to remove any duplicate entries. To do this, I selected all the data by clicking on cell A1 and then pressed Ctrl+A. Then click on the “Data” tab and choose “Remove Duplicates” under the “Data Tools” section. Once duplicates are removed, the next step is to filter the data to identify any spelling errors or unwanted names. To do this, I can click on the “Filter” tool under the “Sort & Filter” section. While filtering, I noticed that some entries under the “Customer Demographics” category are labelled as “Unknown” instead of specific demographics like Male, Female or Non-Binary. Since I won’t be using this data, I proceeded with the existing information. At this stage, the data cleaning process is considered complete, and I have moved on to the next steps.
To facilitate further analysis, I converted the data into a table. I achieved this by selecting the entire data range (Ctrl+A) and then pressing Ctrl+T to convert it into a table. The next step is to create a pivot table. Navigated to the “Insert” tab and select the “PivotTable” tool. Choose the location for the pivot table (such as a new worksheet) and specify the data range (Table 1 or the relevant table name). Once the pivot table was created, I formatted the numbers to display as whole numbers and removed any decimal places for better readability.
A. Now, I’m ready to analyze the data and provide insights based on the following requirements or objectives.
I begin the analysis of the Supply Chain by examining the correlation between product prices and the corresponding revenue they generate.
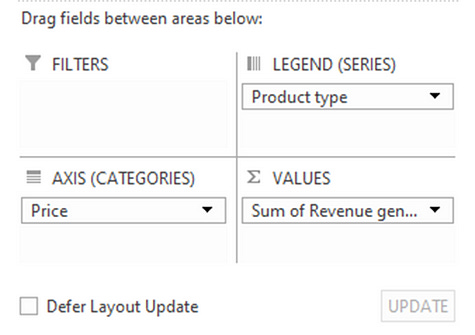
Therefore, the company generates a higher revenue from skincare products, and there is a positive relationship between the price of skincare products and the revenue they generate.
B. Now, I examined the sales based on different product types.
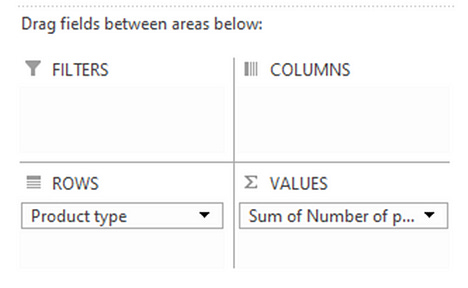
Skincare products account for 45% of the company’s business, while haircare products contribute to 29.5% of the revenue, and cosmetics make up 25.5% of the total sales.
C. Now, I analyzed the total revenue generated from shipping carriers.
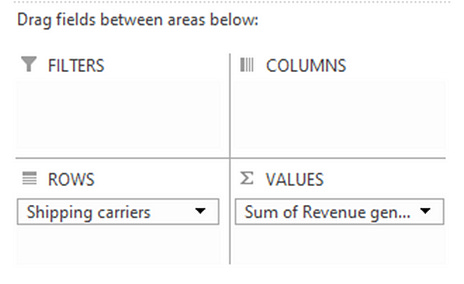
The company utilizes three shipping carriers for transportation, and among them, Carrier B contributes significantly to the company’s revenue generation.
D. Now, I examined the average lead time and average manufacturing costs for all products of the company.
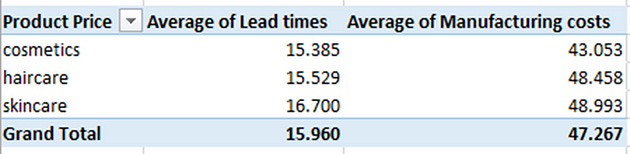
Analyzing SKUs
In the dataset, there is a column labelled SKUs, which stands for Stock Keeping Units. SKUs are unique codes assigned to products to facilitate inventory management and tracking. They serve as a means to identify and differentiate individual items, ensuring accurate monitoring of stock levels. For instance, in a toy store with a diverse range of toys, each toy would be assigned a unique SKU as a secret number known only to the store, enabling efficient inventory control.
A. Now, I analyzed the revenue generated by each SKU.
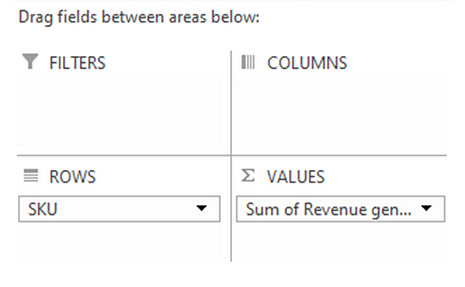
Another column in the dataset is labelled Stock levels. Stock levels indicate the quantity of products available in a store or business’s inventory at a given time
B. Now, I examined the stock levels of each SKU.
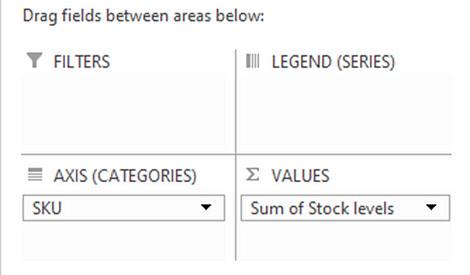
C. Now, I analyzed the order quantity of each SKU.
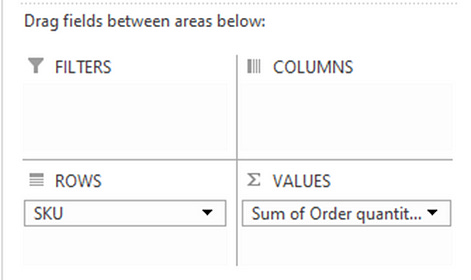
Cost Analysis
A. Now, I analyzed the shipping costs associated with different carriers.
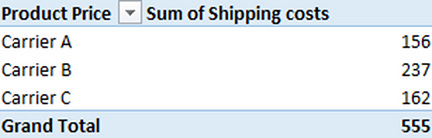
The above visualizations revealed that Carrier B contributes significantly to the company’s revenue. However, it is also the most expensive carrier among the three options.
B. Now, I examined the distribution of costs by transportation mode.
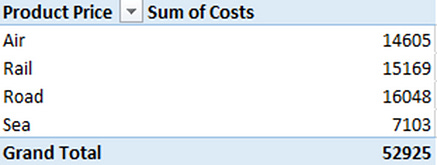
The company allocates a larger portion of its transportation expenses to the Road and Rail modes for the transportation of goods.
Analyzing Defect Rate
The defect rate in the supply chain refers to the percentage of products that are found to have issues or are damaged after being shipped.
A. Now, I analyzed the average defect rate across all product types.
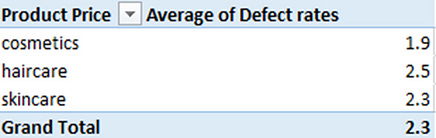
Haircare products have a higher defect rate compared to other product types.
B. Now, I examined the defect rates based on the mode of transportation.
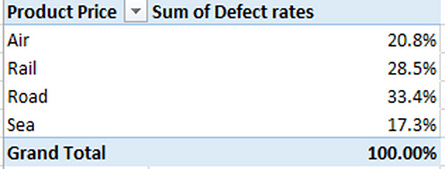
Road transportation exhibits a higher defect rate, while Air transportation demonstrates the lowest defect rate. This showcases how Excel programming language can be utilized to analyze a company’s supply chain.
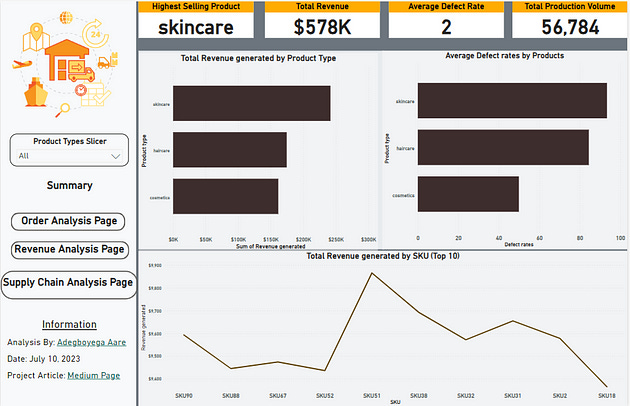
I successfully created a dashboard using Power BI that presents the insights mentioned earlier. The dashboard effectively highlights essential revenue-related information, including:
1. Revenue generated from each supplier. 2. Revenue generated from each location. 3. Revenue generated from each company product. 4. Total products delivered by each carrier.
These insightful visualizations offer valuable data-driven perspectives, enabling informed decision-making and strategic planning.
Supply Chain Analysis involves examining different aspects of a supply chain to identify areas for improvement and enhance the overall efficiency of the supply chain, ultimately delivering greater value to customers. This report provided an overview of conducting a supply chain analysis using Excel, highlighting key steps and techniques and revenue generated.
Thank you for reading, for more guides like this follow me on;
Twitter || LinkedIn
Buy Me a Coffee
If you find this article insightful you can support me by buying me a coffee (Click HERE ).
Remember to like, share, comment and subscribe.

Ready for more?
Cyclistic Case Study Using Spreadsheets, SQL and Tableau
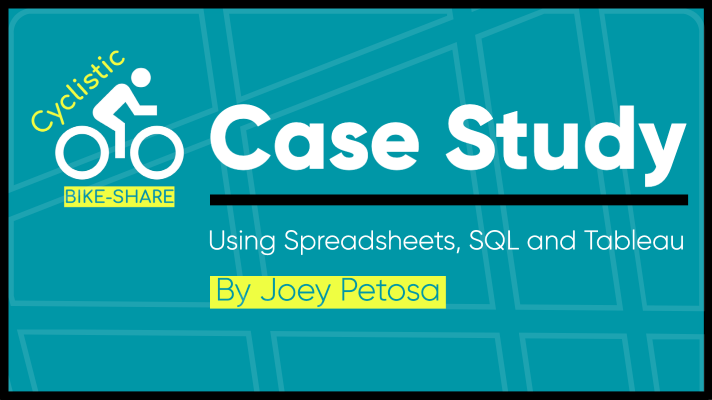
In this case study, I analyze historical data from a Chicago based bike-share company in order to identify trends in how their customers use bikes differently. The main tools I use are spreadsheets, SQL and Tableau. Here are the highlights:
Tableau Dashboard: Cyclistic Bikeshare in Chicago
Slides: Where Rubber Meets Road in Converting Casual Riders to Cyclistic Members
GitHub: Cyclistic Case Study Repository
A more in-depth breakdown of the case study scenario is included below, followed by my full report.
Cyclistic is a bike-share company based in Chicago with two types of customers. Customers who purchase single-ride or full-day passes are known as casual riders , while those who purchase annual memberships are known as members . Cyclistic’s financial analysts have concluded that annual members are much more profitable than casual riders. The director of marketing believes the company’s future success depends on maximizing the number of annual memberships.
The marketing analytics team wants to understand how casual riders and annual members use Cyclistic bikes differently. From these insights, the team will design a new marketing strategy to convert casual riders into annual members. The primary stakeholders for this project include Cyclistic’s director of marketing and the Cyclistic executive team. The Cyclistic marketing analytics team are secondary stakeholders.
Defining the problem
The main problem for the director of marketing and marketing analytics team is this: Design marketing strategies aimed at converting Cyclistic’s casual riders into annual members. There are three questions that will guide this future marketing program. For my scope on this project, I will anlyze the first question:
1) How do annual members and casual riders use Cyclistic bikes differently? 2) Why would casual riders buy Cyclistic annual memberships? 3) How can Cyclistic use digital media to influence casual riders to become members?
By looking at the data, we will be able to first get a broad sense of certain patterns that are occurring in the two different groups. Understanding the differences will provide more accurate customer profiles for each group. These insights will help the marketing analytics team design high quality targeted marketing for converting casual riders into members. For the Cyclistic executive team, these insights will help Cyclistic maximize the number of annual members and will fuel future growth for the company.
Business task
Analyze historical bike trip data to identify trends in how annual members and casual riders use Cyclistic bikes differently. #
Data sources
We’ll be using Cyclistic’s historical bike trip data from the last 12 months, which is publicly available here . The data is made available by Motivate International Inc. under this license . The data is stored in spreadsheets. There are 12 .CSV files total:
It is structured data, organized in rows (records) and columns (fields). Each record represents one trip, and each trip has a unique field that identifies it: ride_id . Each trip is anonymized and includes the following fields:
Bike station data that is made publicly available by the city of Chicago will also be used. It can be downloaded here . In terms of bias and credibility, both data sources we are using ROCCC:
Reliable and original: this is public data that contains accurate, complete and unbiased info on Cyclistic’s historical bike trips. It can be used to explore how different customer types are using Cyclistic bikes.
Comprehensive and current: these sources contain all the data needed to understand the different ways members and casual riders use Cyclistic bikes. The data is from the past 12 months. It is current and relevant to the task at hand. This is important because the usefulness of data decreases as time passes.
Cited: these sources are publicly available data provided by Cyclistic and the City of Chicago. Governmental agency data and vetted public data are typically good sources of data.
Data cleaning and manipulation
Microsoft excel: initial data cleaning and manipulation.
Our next step is making sure the data is stored appropriately and prepared for analysis. After downloading all 12 zip files and unzipping them, I housed the files in a temporary folder on my desktop. I also created subfolders for the .CSV files and the .XLS files so that I have a copy of the original data. Then, I launched Excel, opened each file, and chose to Save As an Excel Workbook file. For each .XLS file, I did the following:
- Formatted as custom DATETIME
- Format > Cells > Custom > yyyy-mm-dd h:mm:ss
- Calculated the length of each ride by subtracting the column started_at from the column ended_at (example: =D2-C2 )
- Formatted as TIME
- Format > Cells > Time > HH:MM:SS (37:30:55)
- Calculated the date of each ride started using the DATE command (example: =DATE(YEAR(C2),MONTH(C2),DAY(C2)) )
- Format > Cells > Date > YYYY-MM-DD
- Entered the month of each ride and formatted as number (example: January: =1 )
- Format > Cells > Number
- Entered the year of each ride and formatted as general
- Format > Cells > General > YYYY
- Calculated the start time of each ride using the started_at column
- Calculated the end time of each ride using the ended_at column
- Calculated the day of the week that each ride started using the WEEKDAY command (example: =WEEKDAY(C2,1) )
- Formatted as a NUMBER with no decimals
- Format > Cells > Number (no decimals) > 1,2,3,4,5,6,7
- Note: 1 = Sunday and 7 = Saturday
After making these updates, I saved each .XLS file as a new .CSV file.
BigQuery: further data cleaning and manipulation via SQL
Since these datasets are so large, it makes sense to move our analysis to a tool that is better suited for handling large datasets. I chose to use SQL via BigQuery .
In order to continue processing the data in BigQuery, I created a bucket in Google Cloud Storage to upload all 12 files. I then created a project in BigQuery and uploaded these files as datasets. I’ve provided my initial cleaning and transformation SQL queries here for reference: initial_setup_query.sql
The results from the COUNT DISTINCT query for each table are very interesting. We can see that the three summer months have the highest trip counts, followed by alternating spring and fall months before ending with winter months:
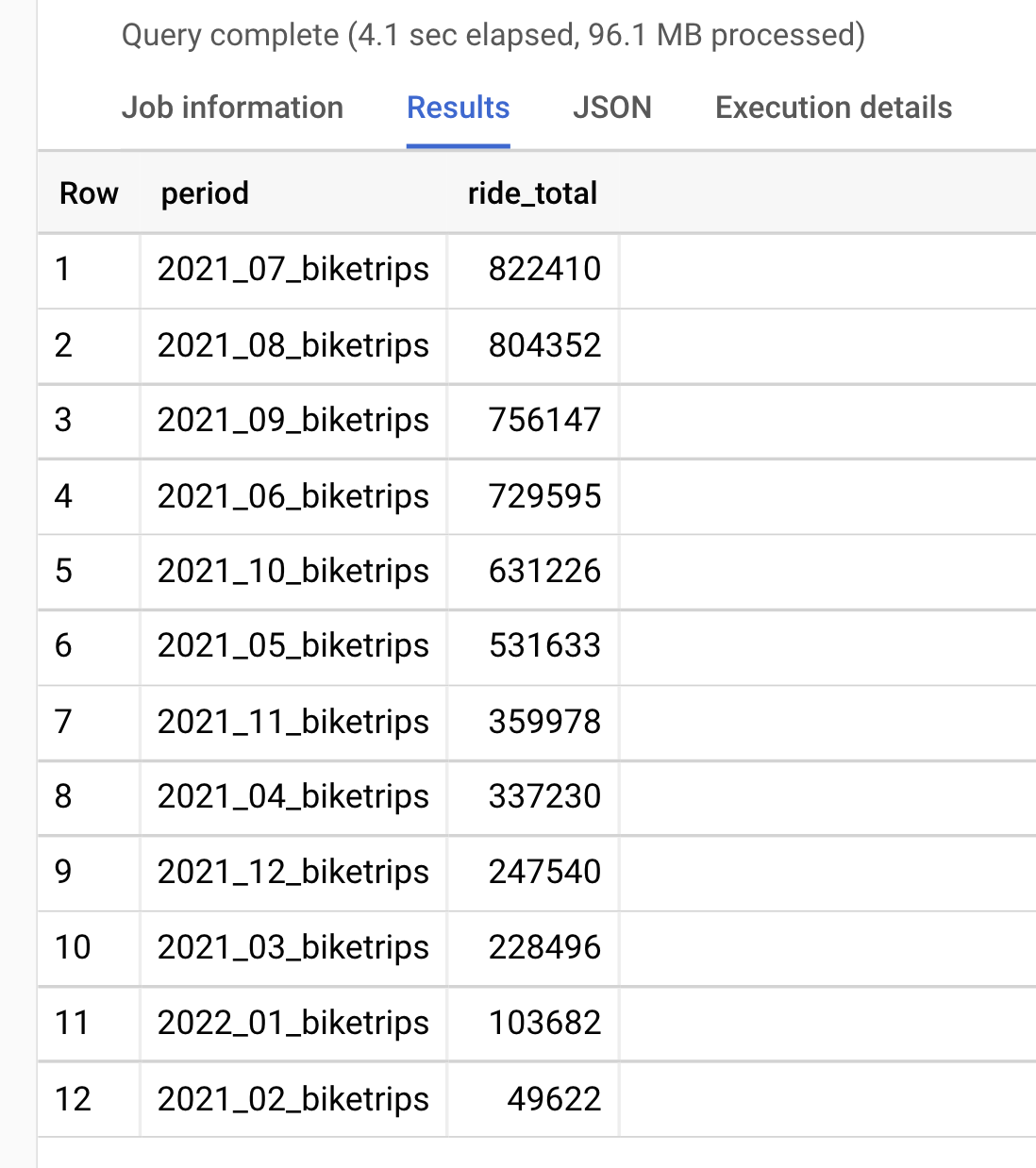
Create quarterly tables
In order to perform analysis by season, let’s combine these tables. We’ll create Q1, Q2, Q3 and Q4 tables for analysis. We’ll have two Q1 tables– one for 20221 and one for 2022 – since we have FEB/MAR data from 2021 and JAN data from 2022:
- Table 1) 2021_Q1 -> FEB(02), MAR(03)
- Table 2) 2021_Q2 -> APR(04), MAY(05), JUN(06)
- Table 3) 2021_Q3 -> JUL(07), AUG(08), SEP(09)
- Table 4) 2021_Q4 -> OCT(10), NOV(11), DEC(12)
- Table 5) 2022_Q1 -> JAN(01)
We’ll first create 2021_Q2 and then repeat for the remaining four tables:
Clean and transform day of week
Some additional data cleaning is needed on the new table. First, we’ll update the format for day_of_week from FLOAT to STRING . Then, we’ll change the values from numbers to their corresponding day names (i.e. 1 = Sunday, 7 = Saturday. We’ll start with 2021_Q1 and repeat for the remaining four tables:
Delete old tables
Now that we have our tables organized into quarters, we can delete the original monthly tables from BigQuery. We no longer need the monthly tables since the data is available in the quarter tables. Also, it costs money to store these datasets in BigQuery.
Analysis #1: Exploratory
2021_q1 - quarterly data exploration.
We’ll select a few columns from 2021_Q1 to preview in a temporary table. This will help give us an idea of potential trends and relationships to explore further:
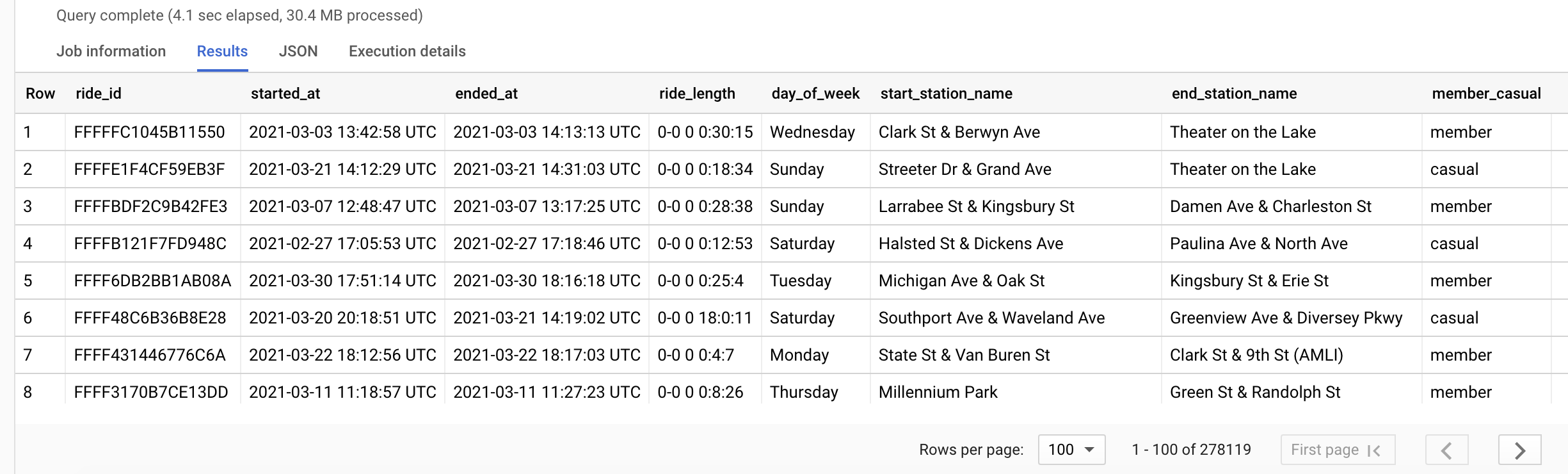
The above query returned 278,119 rows. That is the number of recorded trips we have data for in this quarter. Let’s dive deeper into those trip totals.
Total trips
We’ll create total columns for overall, annual members and casual riders. We’ll also calculate percentages of overall total for both types:
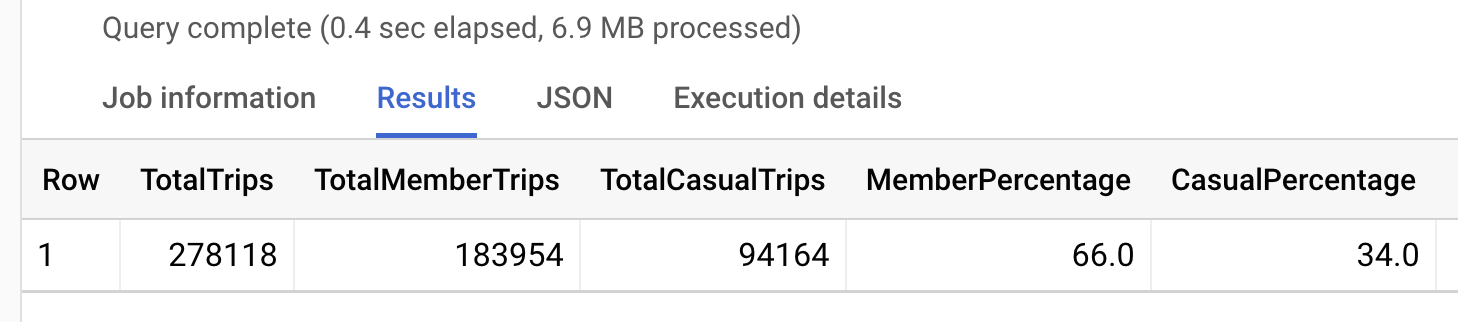
Of the 278,118 total trips in 2021_Q1, 66% were from annual members while 34% were from casual riders.
Average ride lengths
How does average ride_length differ for these groups?
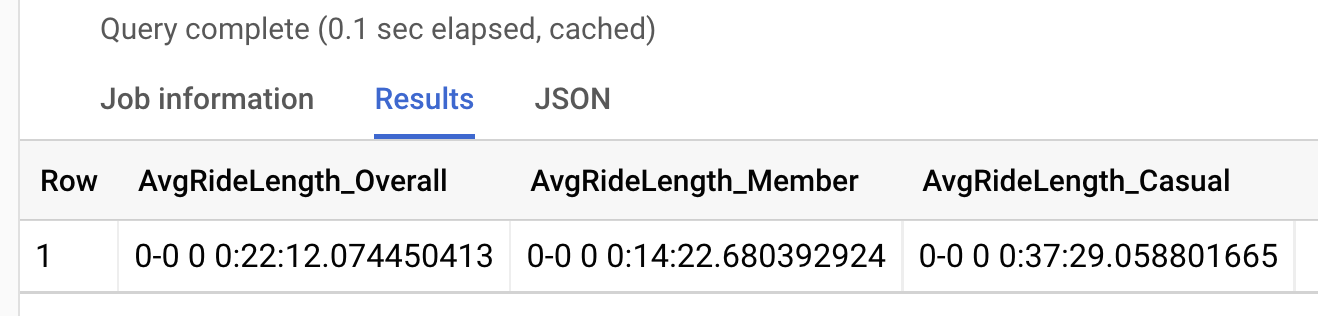
We can see that casual riders average about 23 more minutes per ride. That seems like a pretty big difference. What influence are outliers having on these averages? Let’s investigate.
Max ride lengths
We’ll look at the maximum values for ride_length to see if anything extreme is influencing the casual rider average:
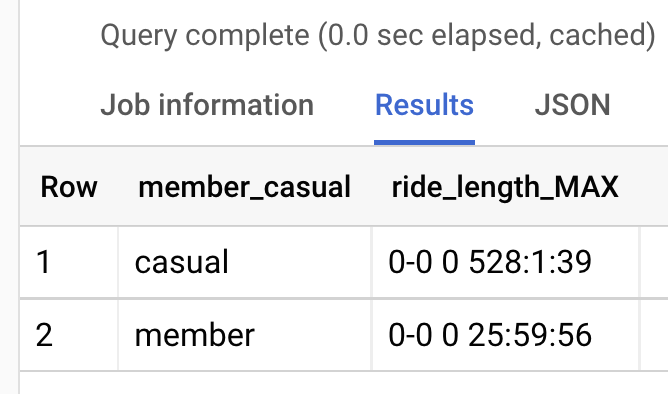
As we suspected, the casual riders average ride_length was significantly impacted by at least one outlier. The longest trip duration for casual riders was 528 hours, or 22 days. Meanwhile, the longest for annual was about 26 hours.
Let’s take a look at the top 100 highest ride_length values for casual riders to confirm there is more than one outlier affecting the average:
Median ride lengths
Since there are more than a few outliers impacting the average, we’re going to use median instead of average. Median will be more accurate for our analysis:
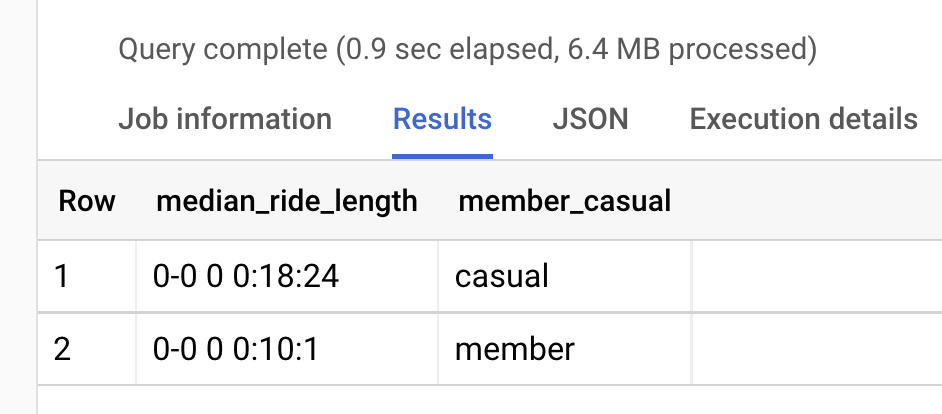
Now we see a much closer number, with 18 minutes for casual riders and 10 minutes for annual members.
Busiest day for rides
Let’s see which day has the most rides for annual members and casual riders:
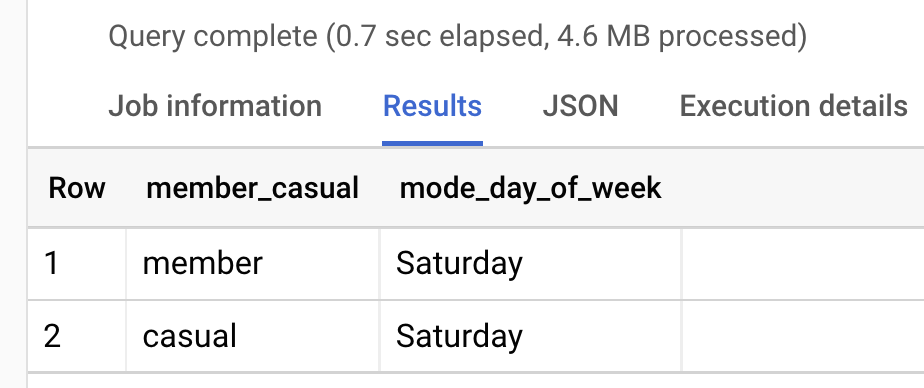
Unsurprisingly, Saturday is the most popular day for both annual members and casual riders.
Median ride length per day
Let’s look at the median ride lengths per day for both annual members and casual riders. Since Saturday is the most popular overall, do we think it will also have the highest median ride length?
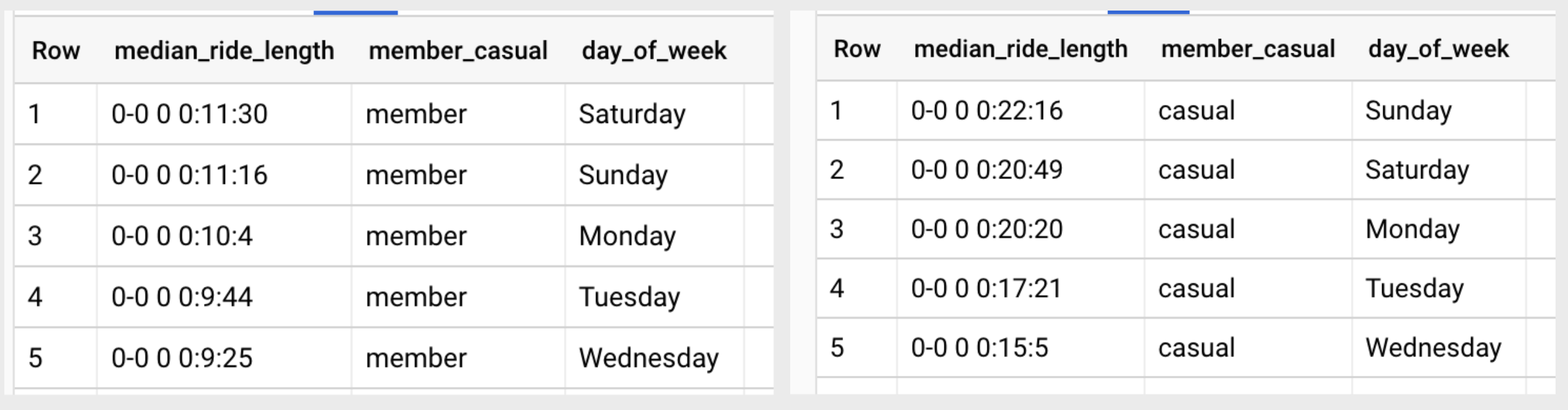
Very interesting! The median ride length for casual riders on the top five days (SUN, SAT, MON, TUE, WED) is nearly double the amount for annual members on their top five days (SAT, SUN, MON, TUE, WED).
Total rides per day
Let’s look at total rides per day. We’ll create columns for overall total, annual members and casual riders:
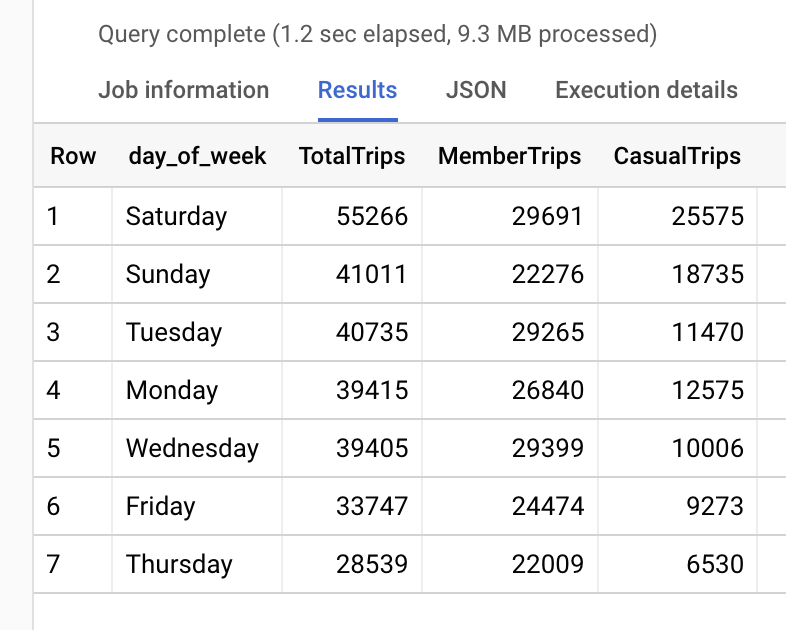
Start stations
Next, we’ll look at the most popular start stations for trips. We’ll again include columns for overall, annual member and casual rider totals per start station:
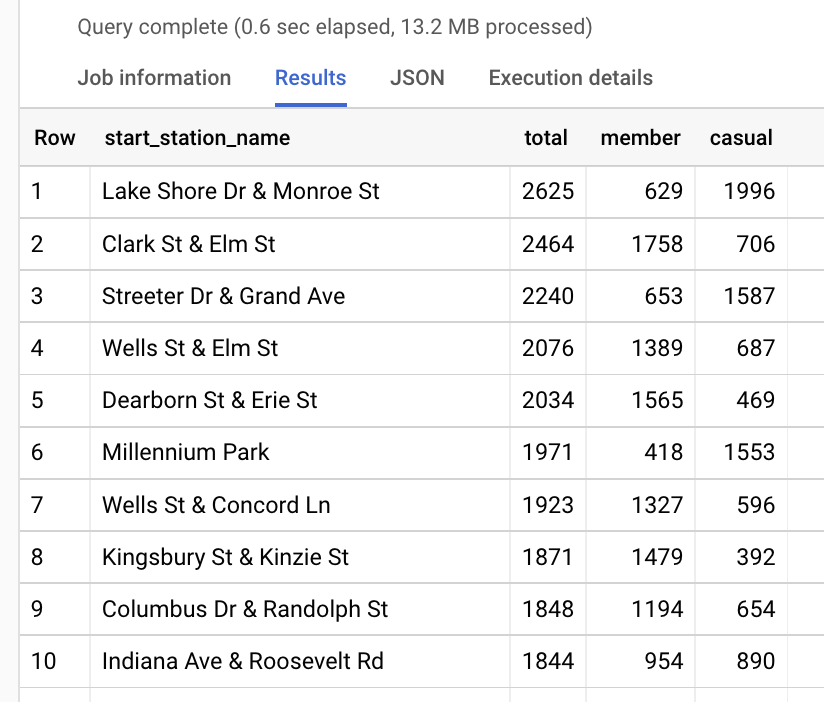
We can begin to see some interesting patterns in the start station data. It looks like casual riders and annual members tend to favor different regions for beginning their trips. By updating the ORDER BY function to sort by casual DESC and member DESC in two separate queries, we can compare the top ten start stations for both:
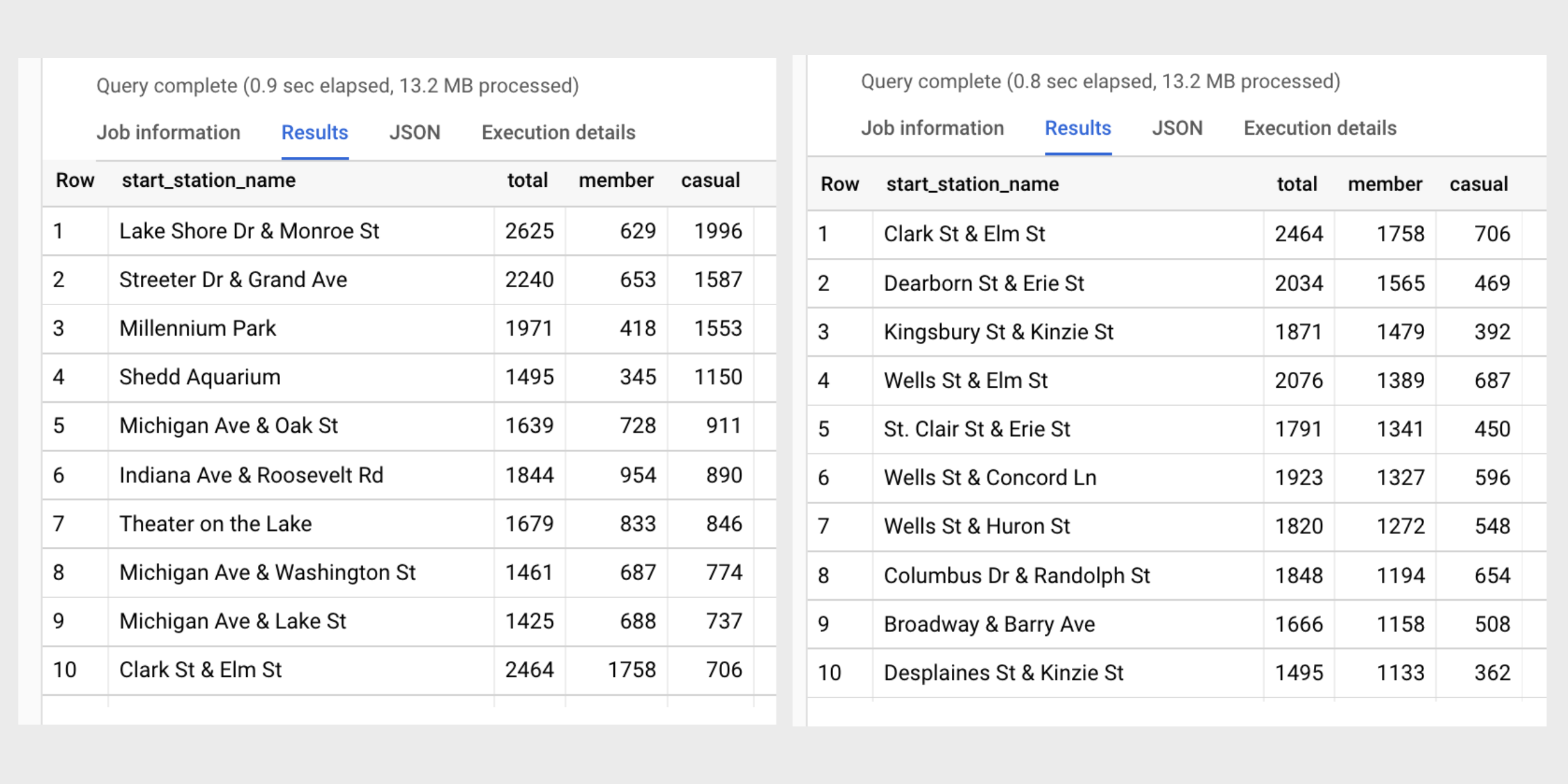
Wow! There is only one start station that cracks the top ten for both lists. The Clark St & Elm St start station is ranked #1 for annual members and #10 for casual riders. The casual riders seem to favor stations near the water like Lake Shore Dr & Monroe St and Streeter Dr & Grand Ave , while annual members frequent start stations in the River North neighborhood like Dearborn St & Erie St and Kingsbury St & Kinzie St .
An initial hypothesis for casual riders could be that they tend to favor start stations near the water and close to tourist attractions because they use bikes for weekend entertainment. An initial hypothesis for annual members could be that they tend to favor start stations in downtown, retail areas because they are using bikes for their work commutes and shopping trips.
Quarterly data exploration (cont.)
Instead of walking through each quarter like we’ve done for 2021_Q1, I will instead provide links to the full SQL files. The queries used are similar to the ones above:
- analysis_2021_Q1.sql
- analysis_2021_Q2.sql
- analysis_2021_Q3.sql
- analysis_2021_Q4.sql
- analysis_2022_Q1.sql
I’ll included some high-level quarterly analysis notes in the next section.
Analysis #2: Summary
Full_year - trends, relationships and insights.
In order to analyze all twelve months together, we’ll combine the five quarterly tables into one table. The queries used to accomplish this are included here for reference. I’ve also provided the SQL file used for full year analysis: analysis_full_year.sql .
For a summary and overall visualization of my full year analysis, please visit the Tableau Public dashboard I created here: Tableau Dashboard: Cyclistic Bikeshare in Chicago . I will also highlight some of the interesting trends and relationships I discovered below.
Annual Members vs Casual Riders
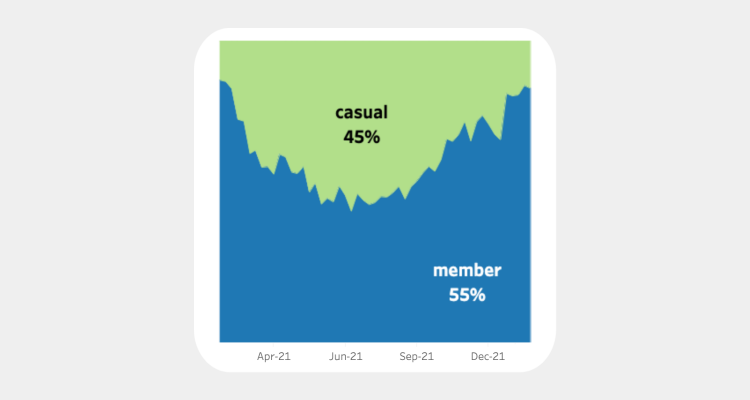
Seasonal trends
Summer vs winter.
The busiest time of year for overall bike trips is Q3– July, August and September. This makes sense because these months are mainly summer time. Bike riding is better suited for warmer weather, which is also why we see a major drop-off in total rides during the winter months of Q1– January, February and March.
Annual members outnumbered casual riders in every quarter except Q3. Interestingly, the annual members nearly doubled the casual ridership in Q1 and Q4 while only slightly edging them out in Q2.
Median ride length
We can see that casual riders consistently have longer rides than annual members.
Day of week
Which days of the week have the highest number of rides for casual riders vs annual members? Let’s look at the mode for each quarter and for the full year:

Casual riders were extremely consistent, with Saturday revealing itself as their preferred day of week for each quarter and across the full year. Meanwhile, the annual members looked to favor the middle of the week for their bike use. The most popular day for them acrosss the full year was Wednesday . Let’s see how the total rides for each day stack up for both groups:
How about median ride length per day of week for both groups?
A few fascinating insights from the above chart:
U-shape pattern Sunday and Saturday are favored by both groups for longer rides, while ride duration decreases towards the middle of the week before increasing again on Friday. This results in a u-shape trend for both groups in the above chart, although it is much more dramatic for casual riders.
Range differences For annual members, difference between their longest day and their shortest day is 1 minute and 44 seconds. For casual riders, difference is 4 minute and 57 seconds. That is a 185.58% increase in difference for casual riders.
Annual members: day-to-day consistency The annual members may have shorter ride lengths when compared to casual riders, but they are extremely consistent with their bike use day-over-day.
Casual riders: weekend warriors The daily median ride length for casual riders is consistently higher than that of annual members. The range of their ride length duration varies at a greater amount than that of annual members. Sundays and Saturdays stand out as their longest ride days.
Do members and casual riders have different preferences for bike type? Are classic bikes more popular than electric bikes?
We can see that classic bikes are favored by both groups. Let’s look at the percentages of bike type use within each group:
Looking at the above, we might ask what exactly is a docked bike and why are only casual riders using them?
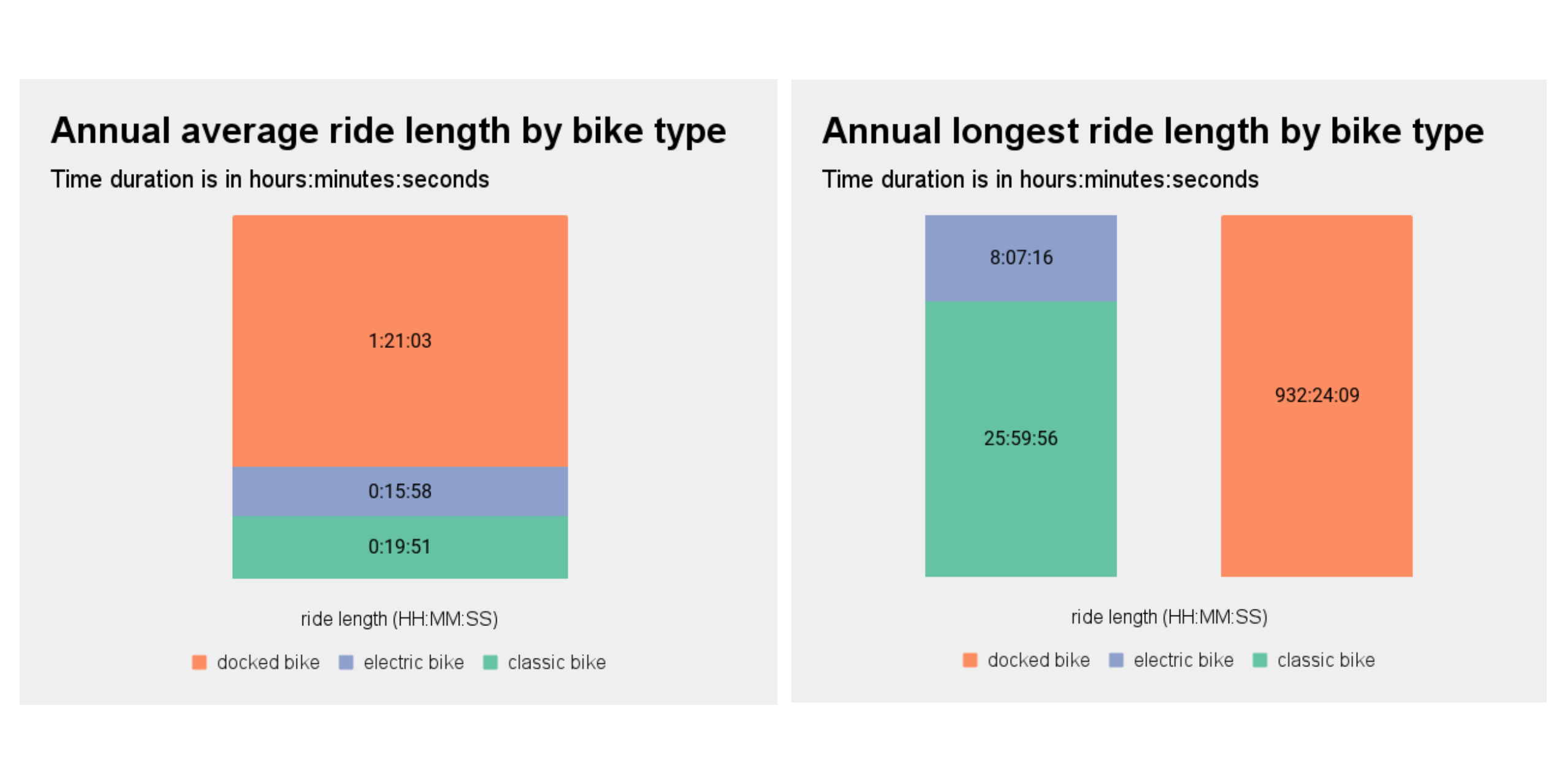
We can now see from the above charts that docked bikes are the culprit for the outliers affecting our ride length averages from earlier in our analysis. This is something we should discuss with our team further and address.
Start and end station use
In the Tableau Dashboard I created, which is again available here , there is a worksheet that allows the exploration of start and end station use by members, casual riders and combined overall rides. The snapshot below is from the overall view. While interacting with the dashboard, we can see that casual riders have a higher max than annual members. Annual members have a lower max, but we can see more colors represented across the member map versus the consistent coloring across the casual map. This tells us that rides by members are more distributed across stations while rides by casual riders are more top heavy in that a huge chunk are happening at the same few stations.
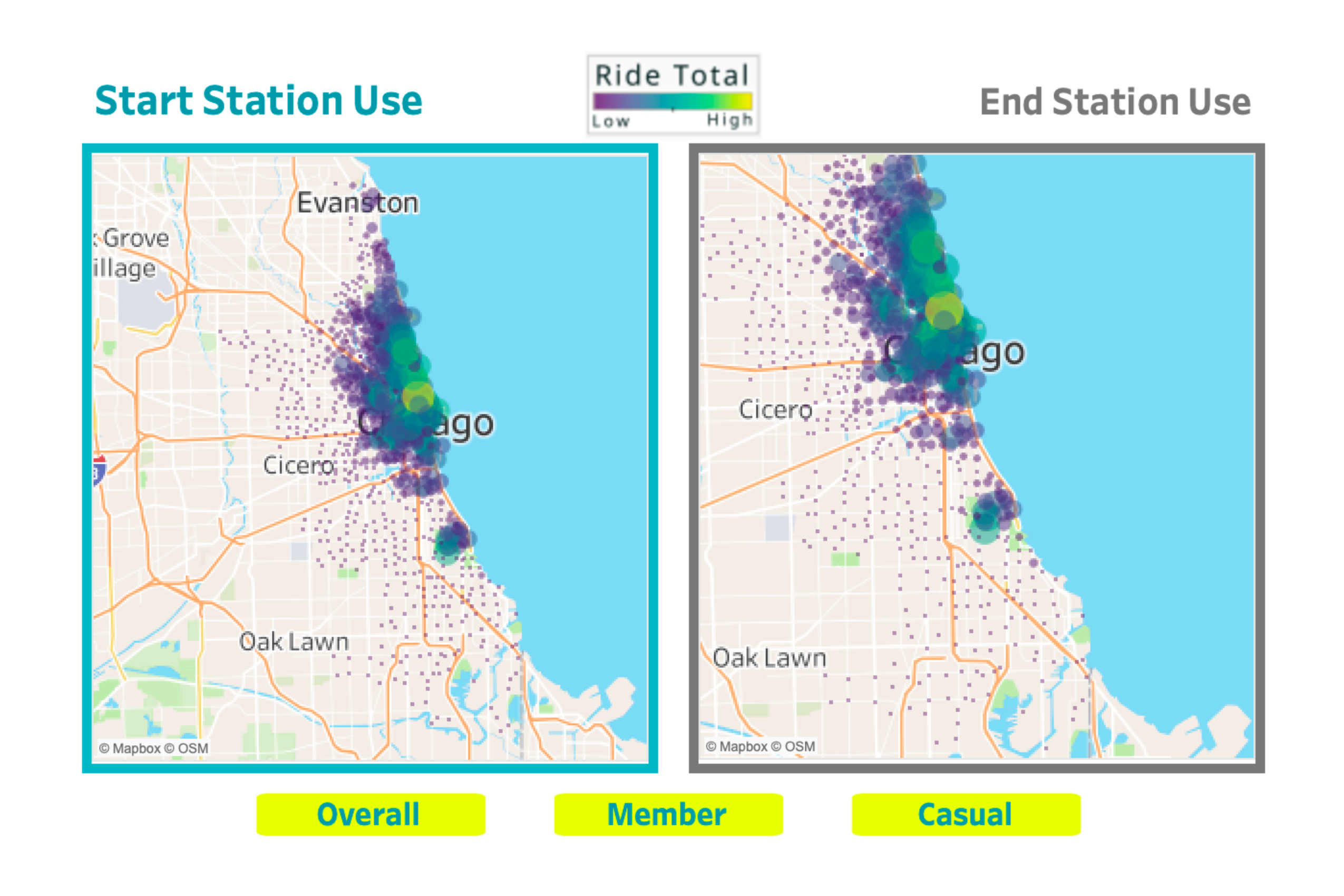
Stakeholder presentation and dashboard
I’ve provided links below for my dashboard and shareholder presentation, which includes the following:
- A summary of my analysis
- Supporting visualizations and key findings
- Three recommendations based on my analysis
Presentation: Where Rubber Meets Road in Converting Casual Riders to Cyclistic Members

Data Analytics Case Study Guide 2024
by Sam McKay, CFA | Data Analytics
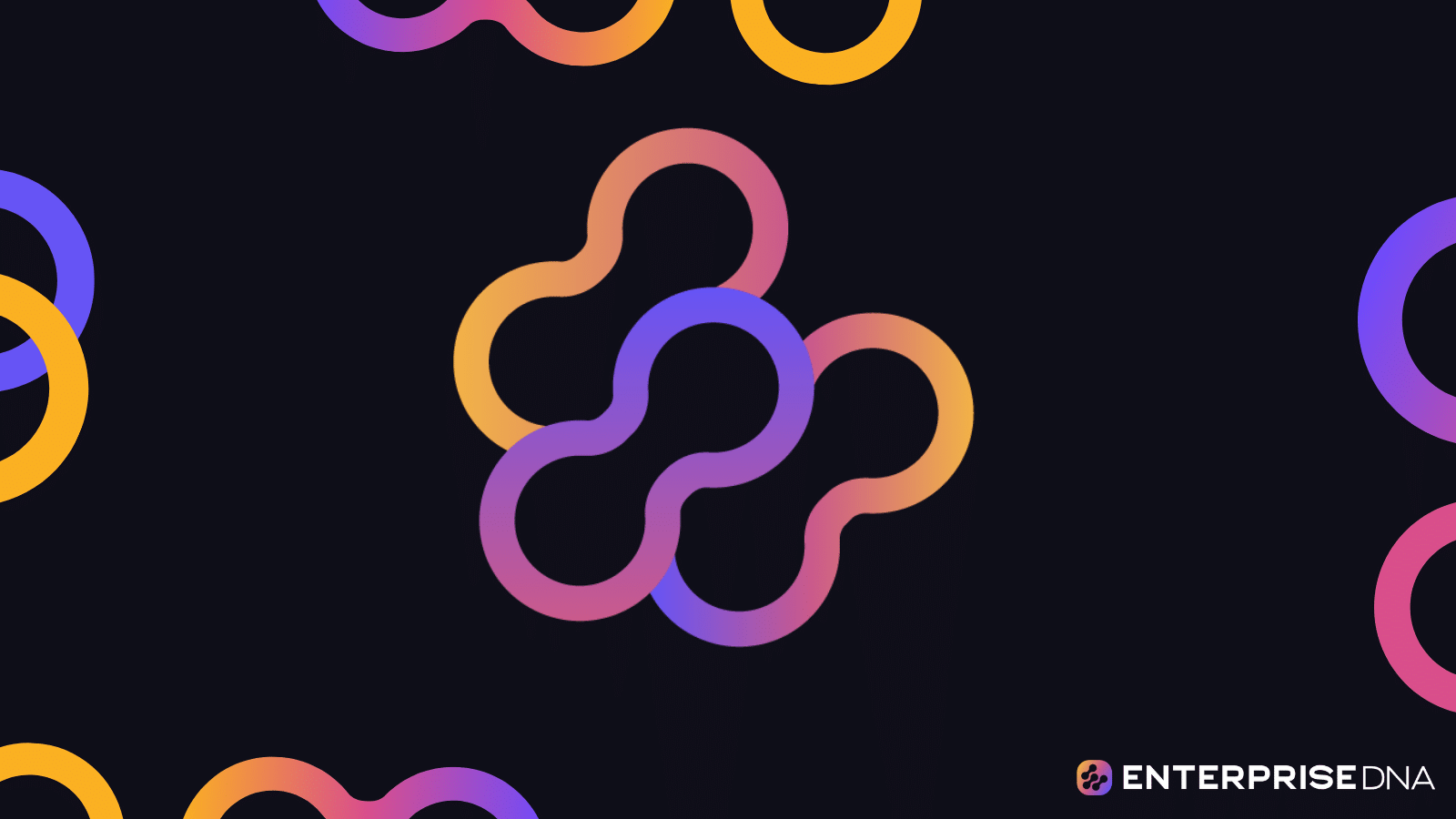
Data analytics case studies reveal how businesses harness data for informed decisions and growth.
For aspiring data professionals, mastering the case study process will enhance your skills and increase your career prospects.
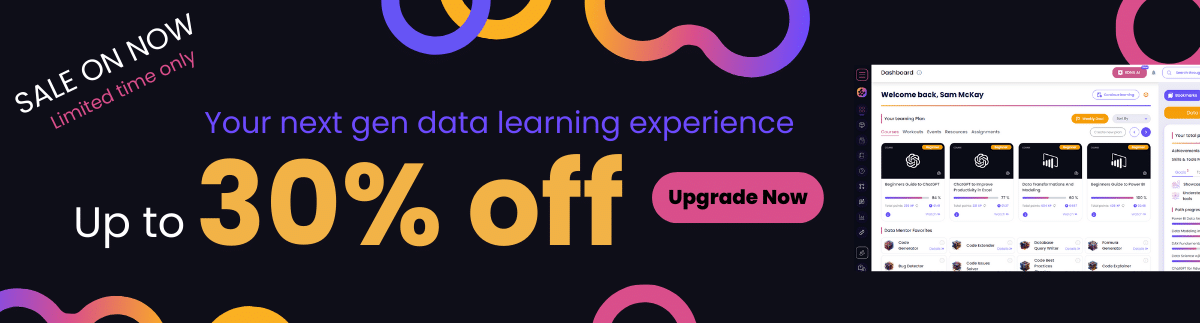
So, how do you approach a case study?
Use these steps to process a data analytics case study:
Understand the Problem: Grasp the core problem or question addressed in the case study.
Collect Relevant Data: Gather data from diverse sources, ensuring accuracy and completeness.
Apply Analytical Techniques: Use appropriate methods aligned with the problem statement.
Visualize Insights: Utilize visual aids to showcase patterns and key findings.
Derive Actionable Insights: Focus on deriving meaningful actions from the analysis.
This article will give you detailed steps to navigate a case study effectively and understand how it works in real-world situations.
By the end of the article, you will be better equipped to approach a data analytics case study, strengthening your analytical prowess and practical application skills.
Let’s dive in!
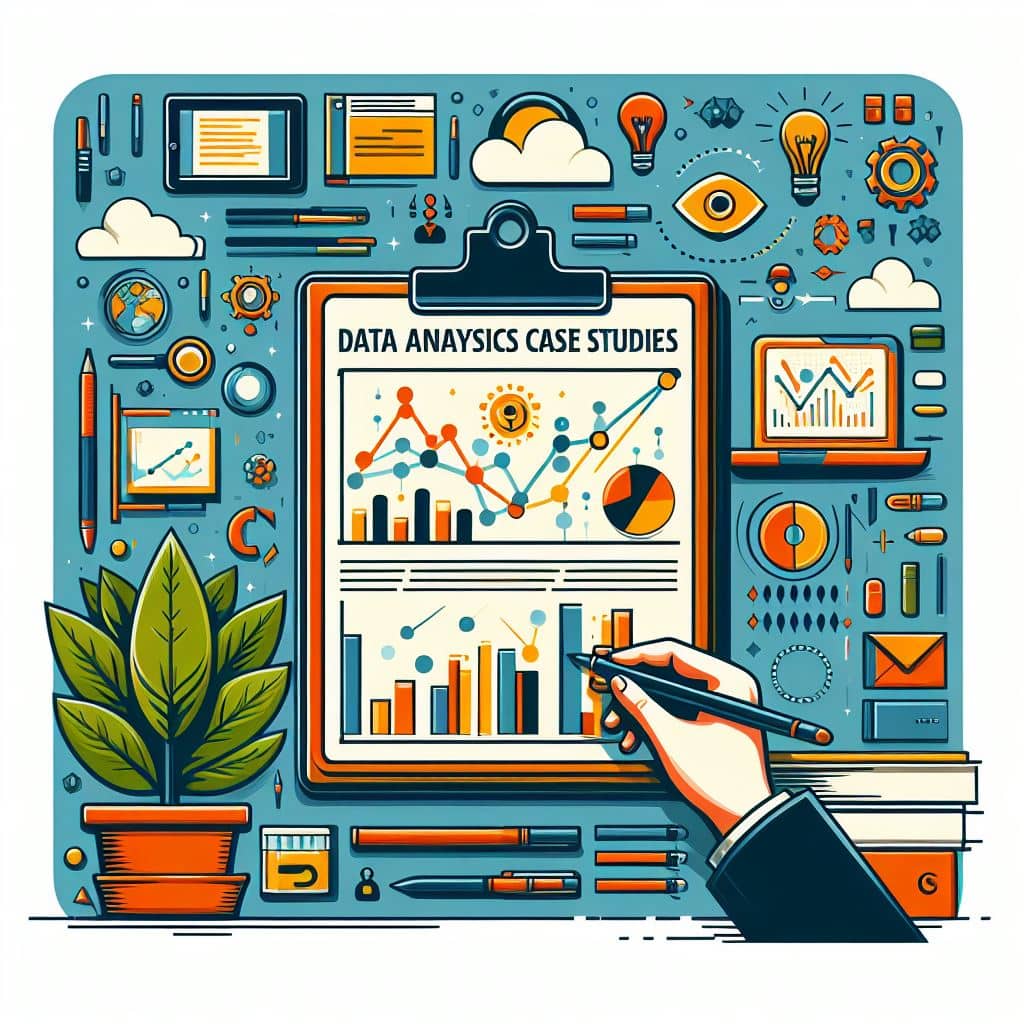
Table of Contents
What is a Data Analytics Case Study?
A data analytics case study is a real or hypothetical scenario where analytics techniques are applied to solve a specific problem or explore a particular question.
It’s a practical approach that uses data analytics methods, assisting in deciphering data for meaningful insights. This structured method helps individuals or organizations make sense of data effectively.
Additionally, it’s a way to learn by doing, where there’s no single right or wrong answer in how you analyze the data.
So, what are the components of a case study?
Key Components of a Data Analytics Case Study
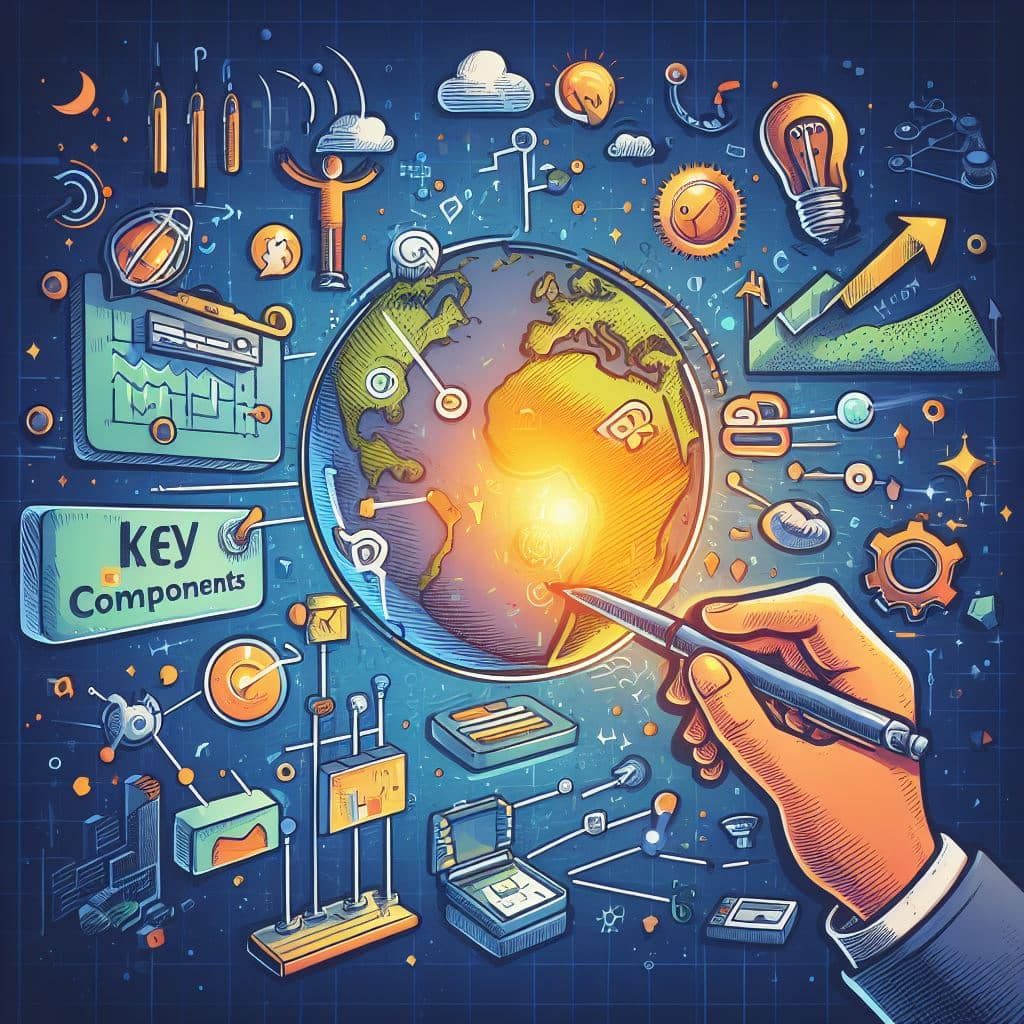
A data analytics case study comprises essential elements that structure the analytical journey:
Problem Context: A case study begins with a defined problem or question. It provides the context for the data analysis , setting the stage for exploration and investigation.
Data Collection and Sources: It involves gathering relevant data from various sources , ensuring data accuracy, completeness, and relevance to the problem at hand.
Analysis Techniques: Case studies employ different analytical methods, such as statistical analysis, machine learning algorithms, or visualization tools, to derive meaningful conclusions from the collected data.
Insights and Recommendations: The ultimate goal is to extract actionable insights from the analyzed data, offering recommendations or solutions that address the initial problem or question.
Now that you have a better understanding of what a data analytics case study is, let’s talk about why we need and use them.
Why Case Studies are Integral to Data Analytics
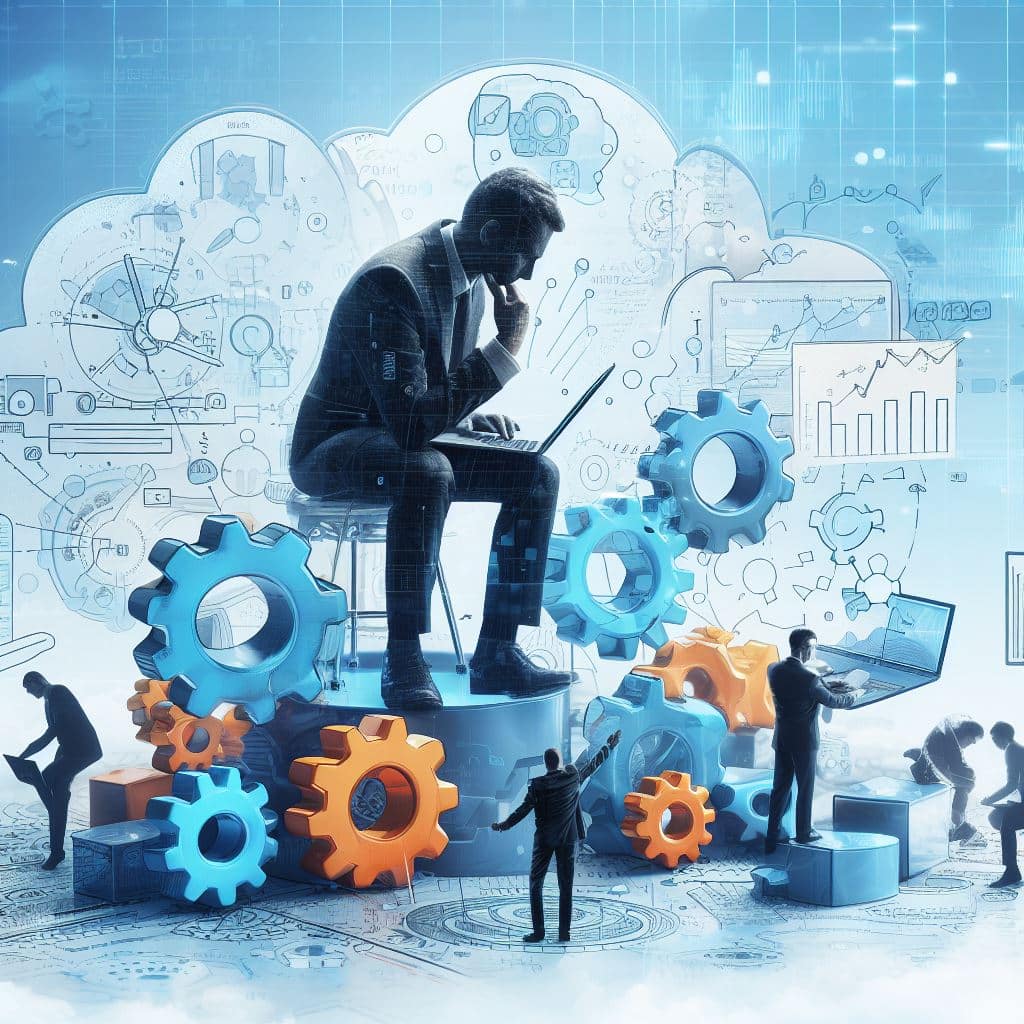
Case studies serve as invaluable tools in the realm of data analytics, offering multifaceted benefits that bolster an analyst’s proficiency and impact:
Real-Life Insights and Skill Enhancement: Examining case studies provides practical, real-life examples that expand knowledge and refine skills. These examples offer insights into diverse scenarios, aiding in a data analyst’s growth and expertise development.
Validation and Refinement of Analyses: Case studies demonstrate the effectiveness of data-driven decisions across industries, providing validation for analytical approaches. They showcase how organizations benefit from data analytics. Also, this helps in refining one’s own methodologies
Showcasing Data Impact on Business Outcomes: These studies show how data analytics directly affects business results, like increasing revenue, reducing costs, or delivering other measurable advantages. Understanding these impacts helps articulate the value of data analytics to stakeholders and decision-makers.
Learning from Successes and Failures: By exploring a case study, analysts glean insights from others’ successes and failures, acquiring new strategies and best practices. This learning experience facilitates professional growth and the adoption of innovative approaches within their own data analytics work.
Including case studies in a data analyst’s toolkit helps gain more knowledge, improve skills, and understand how data analytics affects different industries.
Using these real-life examples boosts confidence and success, guiding analysts to make better and more impactful decisions in their organizations.
But not all case studies are the same.
Let’s talk about the different types.
Types of Data Analytics Case Studies
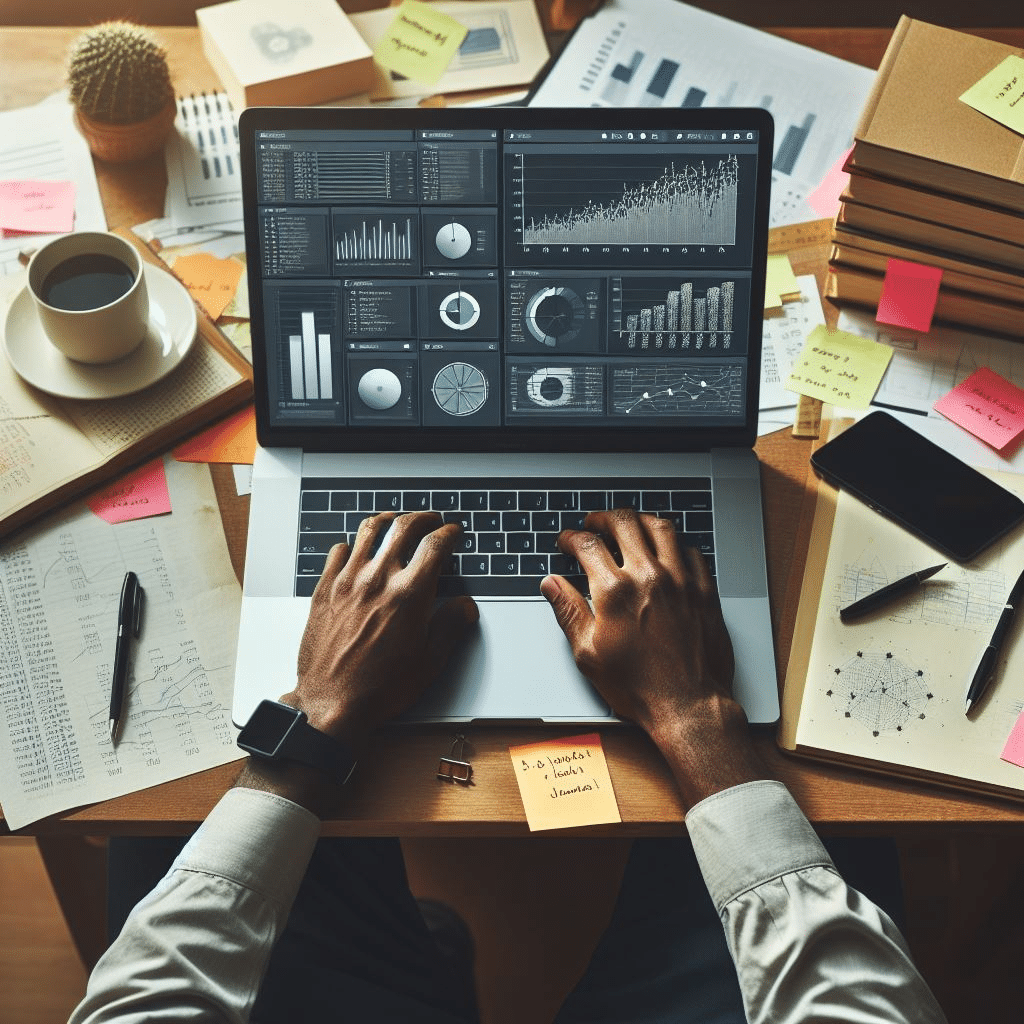
Data analytics encompasses various approaches tailored to different analytical goals:
Exploratory Case Study: These involve delving into new datasets to uncover hidden patterns and relationships, often without a predefined hypothesis. They aim to gain insights and generate hypotheses for further investigation.
Predictive Case Study: These utilize historical data to forecast future trends, behaviors, or outcomes. By applying predictive models, they help anticipate potential scenarios or developments.
Diagnostic Case Study: This type focuses on understanding the root causes or reasons behind specific events or trends observed in the data. It digs deep into the data to provide explanations for occurrences.
Prescriptive Case Study: This case study goes beyond analytics; it provides actionable recommendations or strategies derived from the analyzed data. They guide decision-making processes by suggesting optimal courses of action based on insights gained.
Each type has a specific role in using data to find important insights, helping in decision-making, and solving problems in various situations.
Regardless of the type of case study you encounter, here are some steps to help you process them.
Roadmap to Handling a Data Analysis Case Study
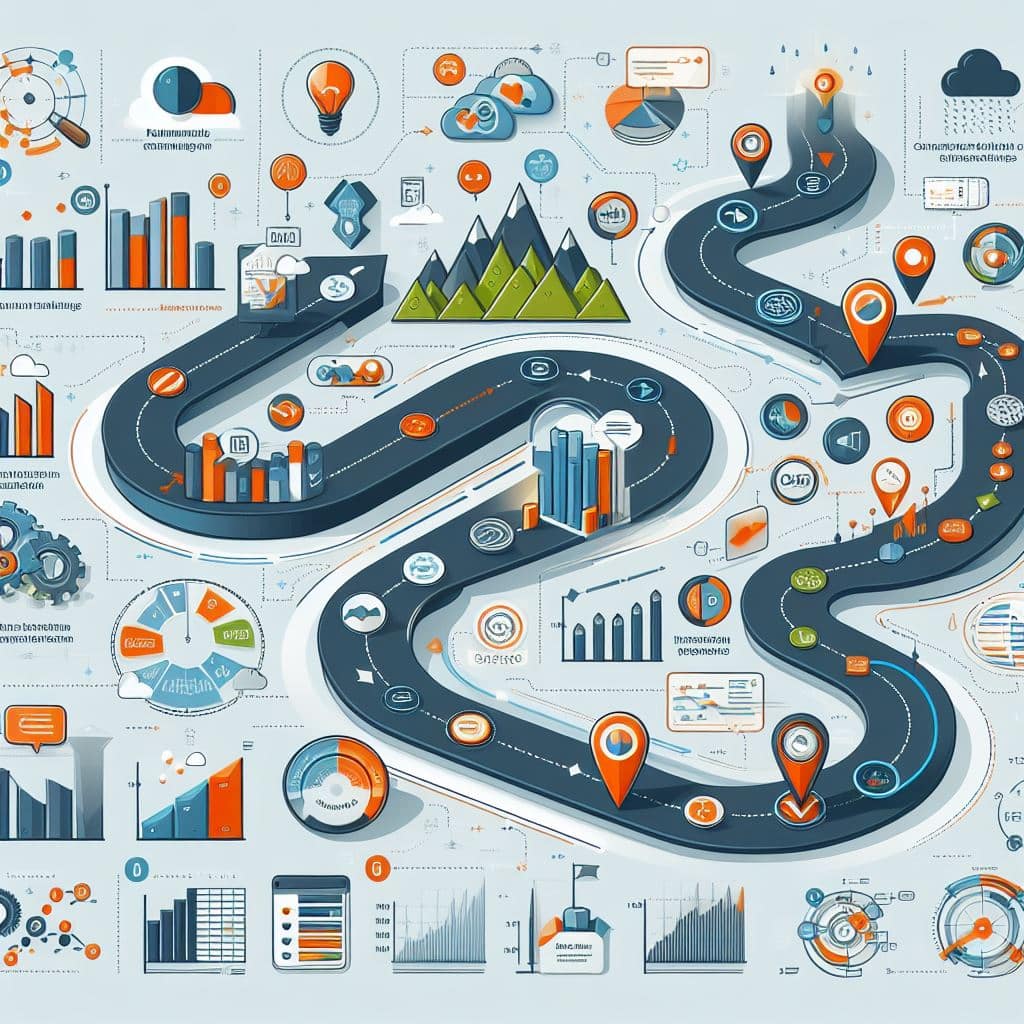
Embarking on a data analytics case study requires a systematic approach, step-by-step, to derive valuable insights effectively.
Here are the steps to help you through the process:
Step 1: Understanding the Case Study Context: Immerse yourself in the intricacies of the case study. Delve into the industry context, understanding its nuances, challenges, and opportunities.
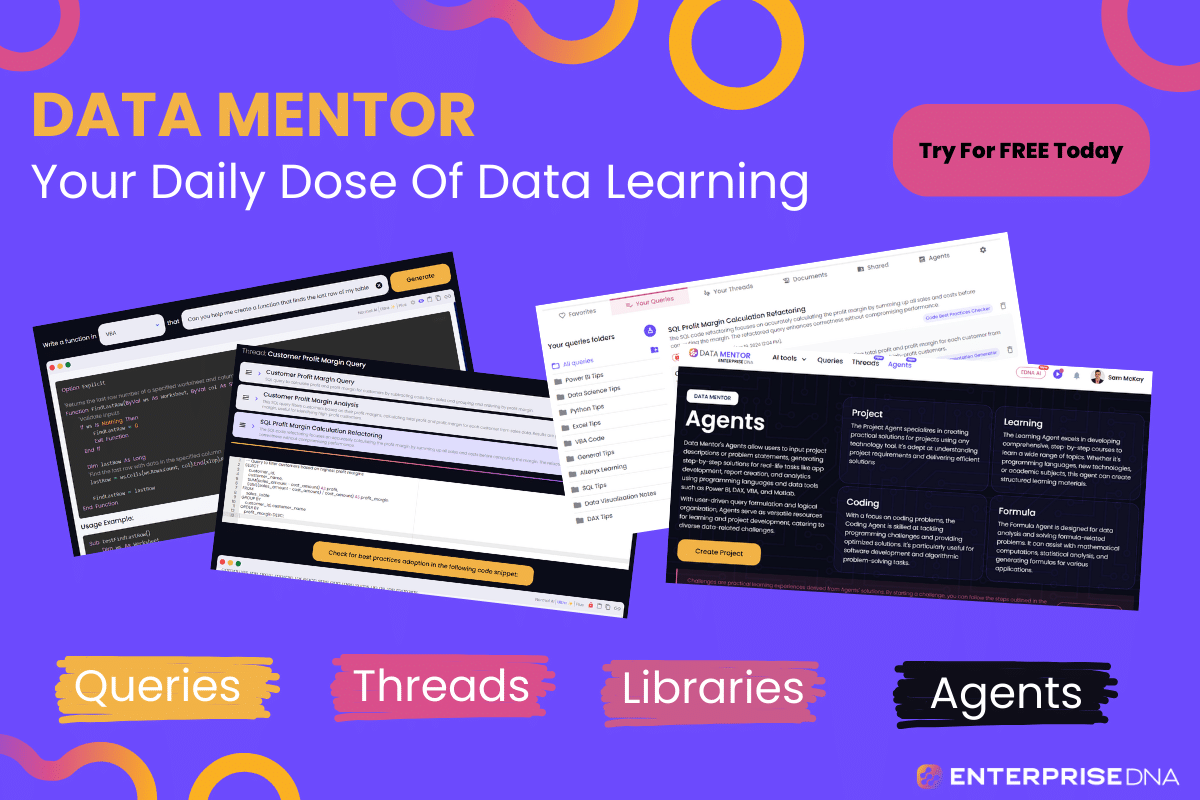
Identify the central problem or question the study aims to address. Clarify the objectives and expected outcomes, ensuring a clear understanding before diving into data analytics.
Step 2: Data Collection and Validation: Gather data from diverse sources relevant to the case study. Prioritize accuracy, completeness, and reliability during data collection. Conduct thorough validation processes to rectify inconsistencies, ensuring high-quality and trustworthy data for subsequent analysis.
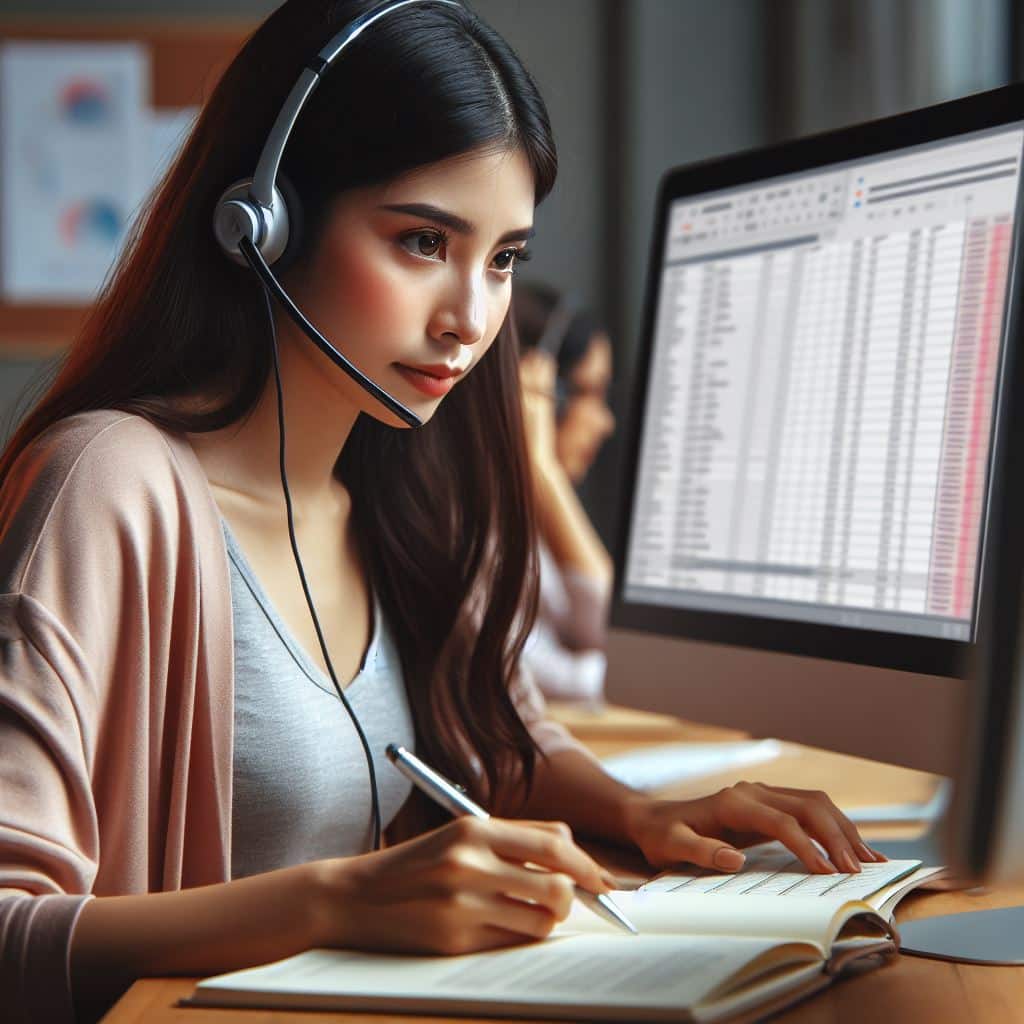
Step 3: Problem Definition and Scope: Define the problem statement precisely. Articulate the objectives and limitations that shape the scope of your analysis. Identify influential variables and constraints, providing a focused framework to guide your exploration.
Step 4: Exploratory Data Analysis (EDA): Leverage exploratory techniques to gain initial insights. Visualize data distributions, patterns, and correlations, fostering a deeper understanding of the dataset. These explorations serve as a foundation for more nuanced analysis.
Step 5: Data Preprocessing and Transformation: Cleanse and preprocess the data to eliminate noise, handle missing values, and ensure consistency. Transform data formats or scales as required, preparing the dataset for further analysis.
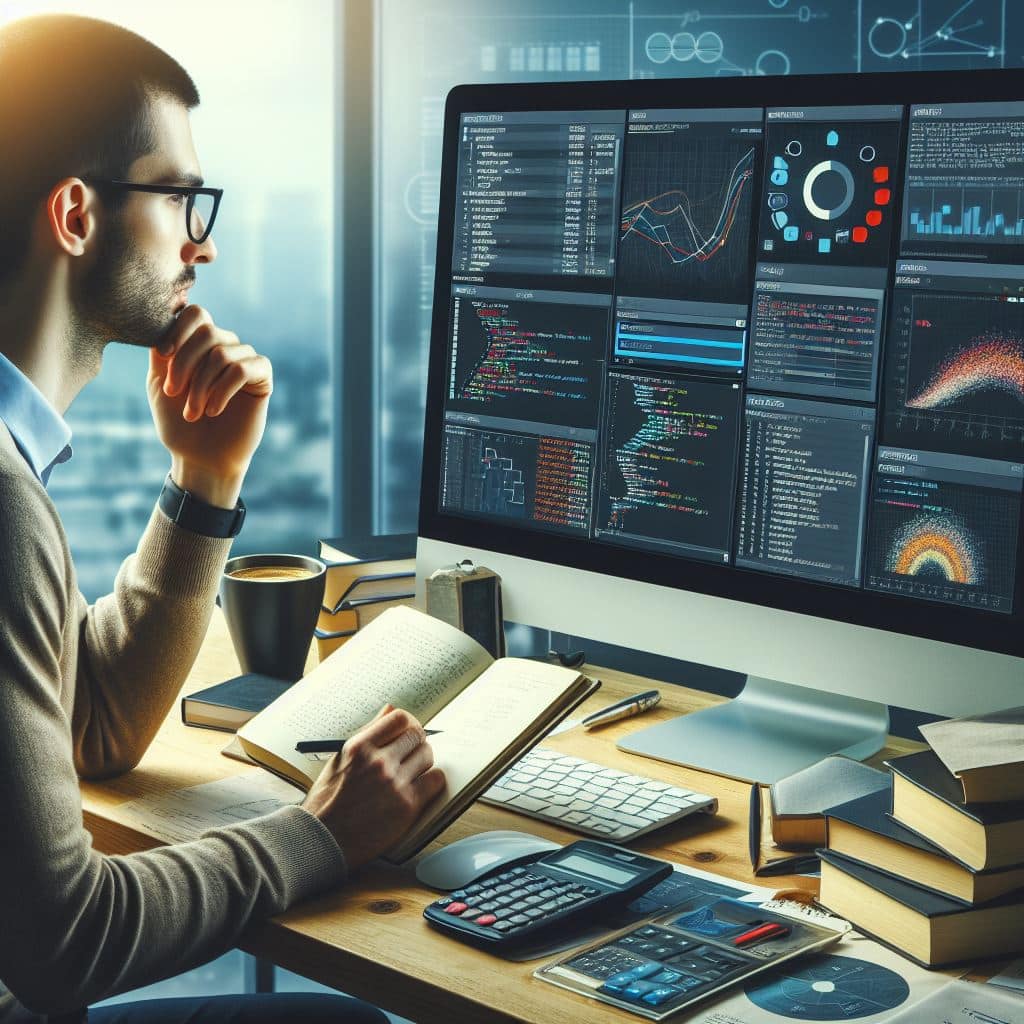
Step 6: Data Modeling and Method Selection: Select analytical models aligning with the case study’s problem, employing statistical techniques, machine learning algorithms, or tailored predictive models.
In this phase, it’s important to develop data modeling skills. This helps create visuals of complex systems using organized data, which helps solve business problems more effectively.
Understand key data modeling concepts, utilize essential tools like SQL for database interaction, and practice building models from real-world scenarios.
Furthermore, strengthen data cleaning skills for accurate datasets, and stay updated with industry trends to ensure relevance.
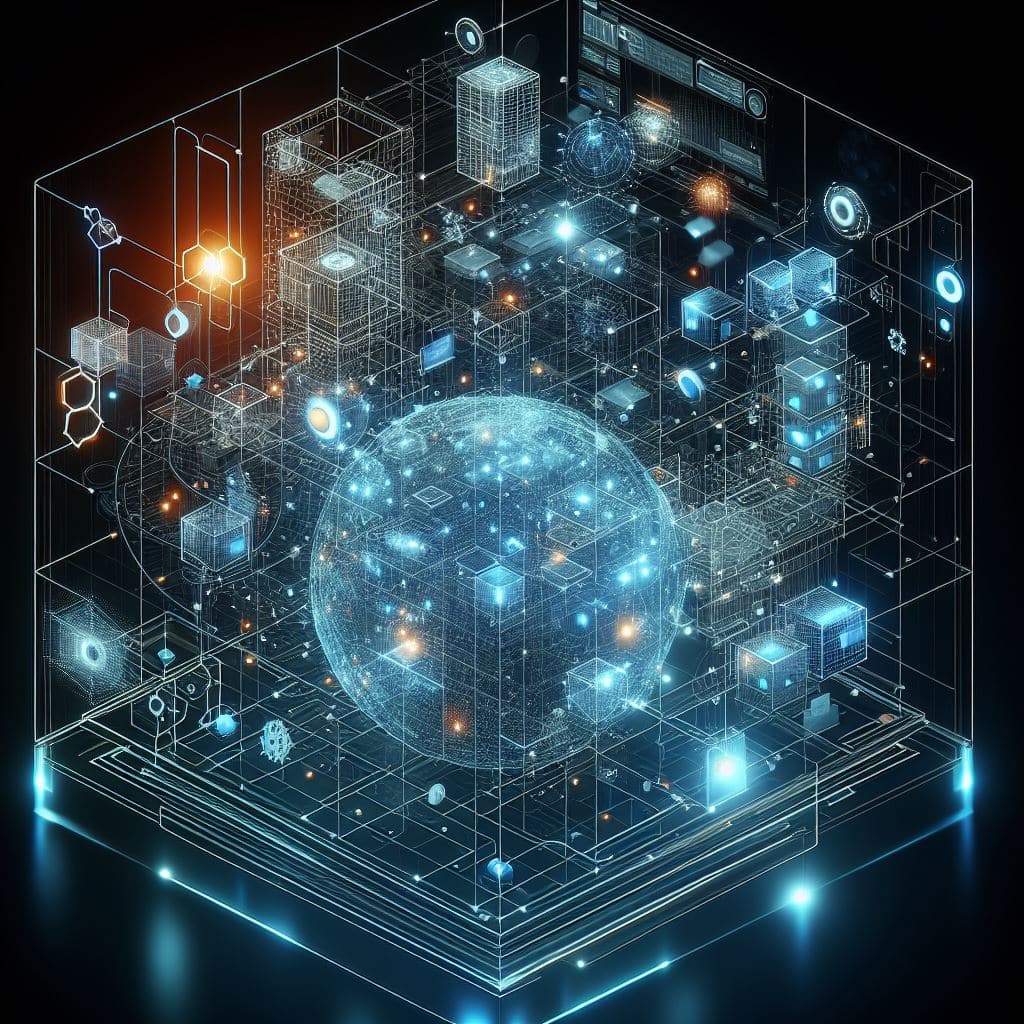
Step 7: Model Evaluation and Refinement: Evaluate the performance of applied models rigorously. Iterate and refine models to enhance accuracy and reliability, ensuring alignment with the objectives and expected outcomes.
Step 8: Deriving Insights and Recommendations: Extract actionable insights from the analyzed data. Develop well-structured recommendations or solutions based on the insights uncovered, addressing the core problem or question effectively.
Step 9: Communicating Results Effectively: Present findings, insights, and recommendations clearly and concisely. Utilize visualizations and storytelling techniques to convey complex information compellingly, ensuring comprehension by stakeholders.
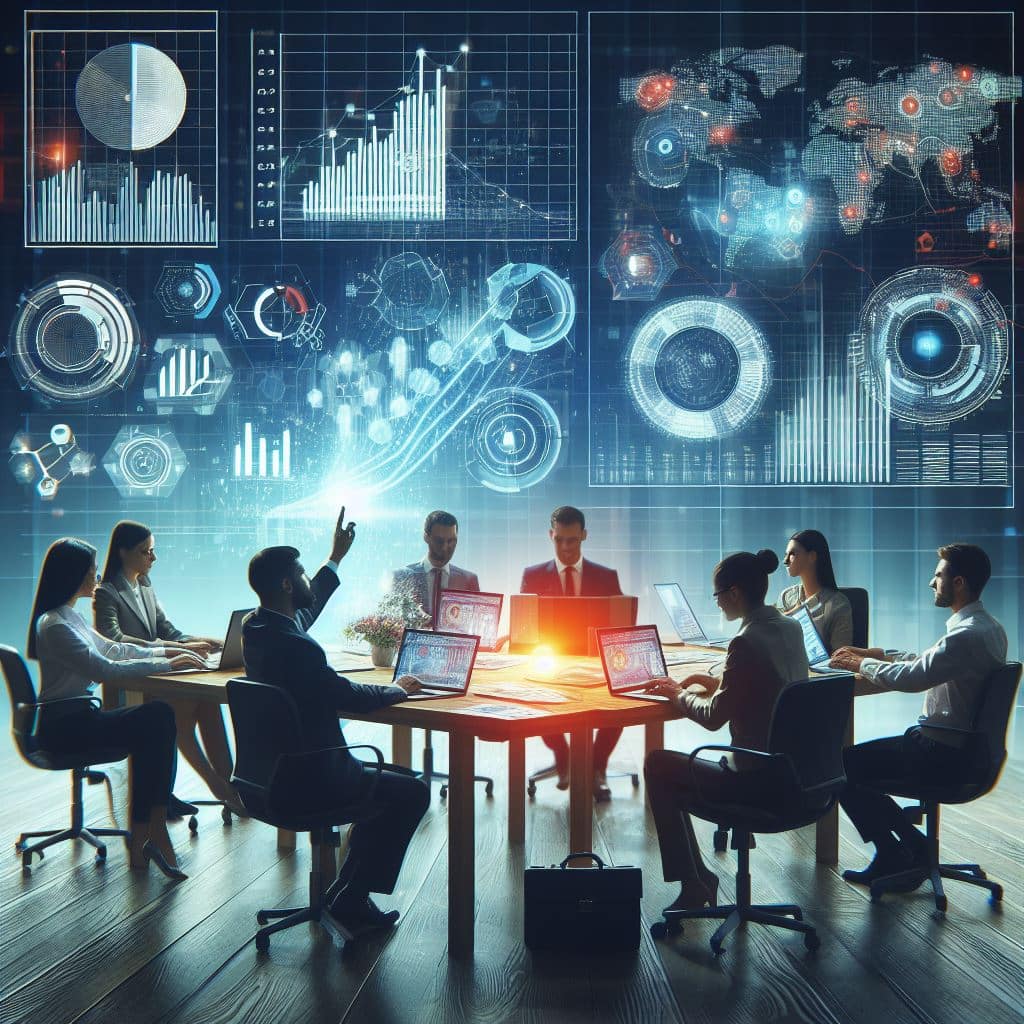
Step 10: Reflection and Iteration: Reflect on the entire analysis process and outcomes. Identify potential improvements and lessons learned. Embrace an iterative approach, refining methodologies for continuous enhancement and future analyses.
This step-by-step roadmap provides a structured framework for thorough and effective handling of a data analytics case study.
Now, after handling data analytics comes a crucial step; presenting the case study.
Presenting Your Data Analytics Case Study
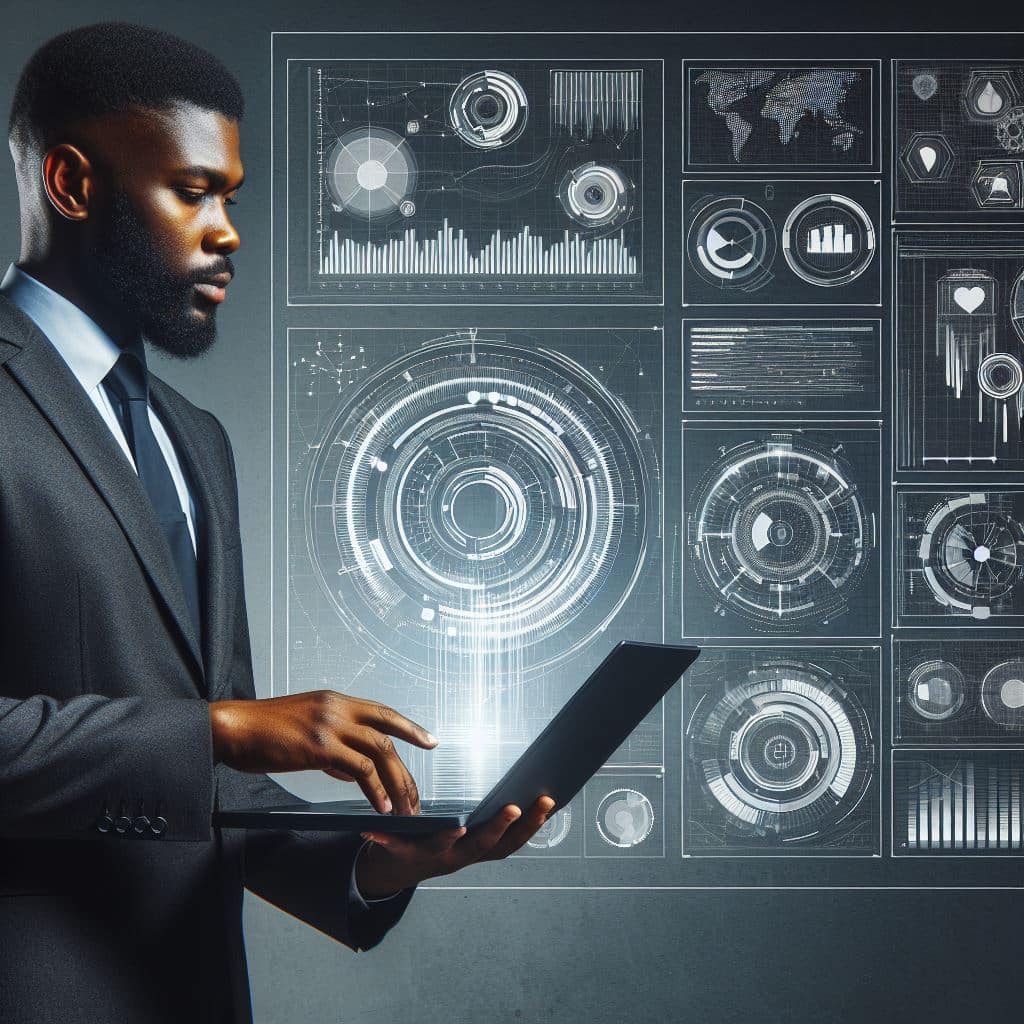
Presenting a data analytics case study is a vital part of the process. When presenting your case study, clarity and organization are paramount.
To achieve this, follow these key steps:
Structuring Your Case Study: Start by outlining relevant and accurate main points. Ensure these points align with the problem addressed and the methodologies used in your analysis.
Crafting a Narrative with Data: Start with a brief overview of the issue, then explain your method and steps, covering data collection, cleaning, stats, and advanced modeling.
Visual Representation for Clarity: Utilize various visual aids—tables, graphs, and charts—to illustrate patterns, trends, and insights. Ensure these visuals are easy to comprehend and seamlessly support your narrative.
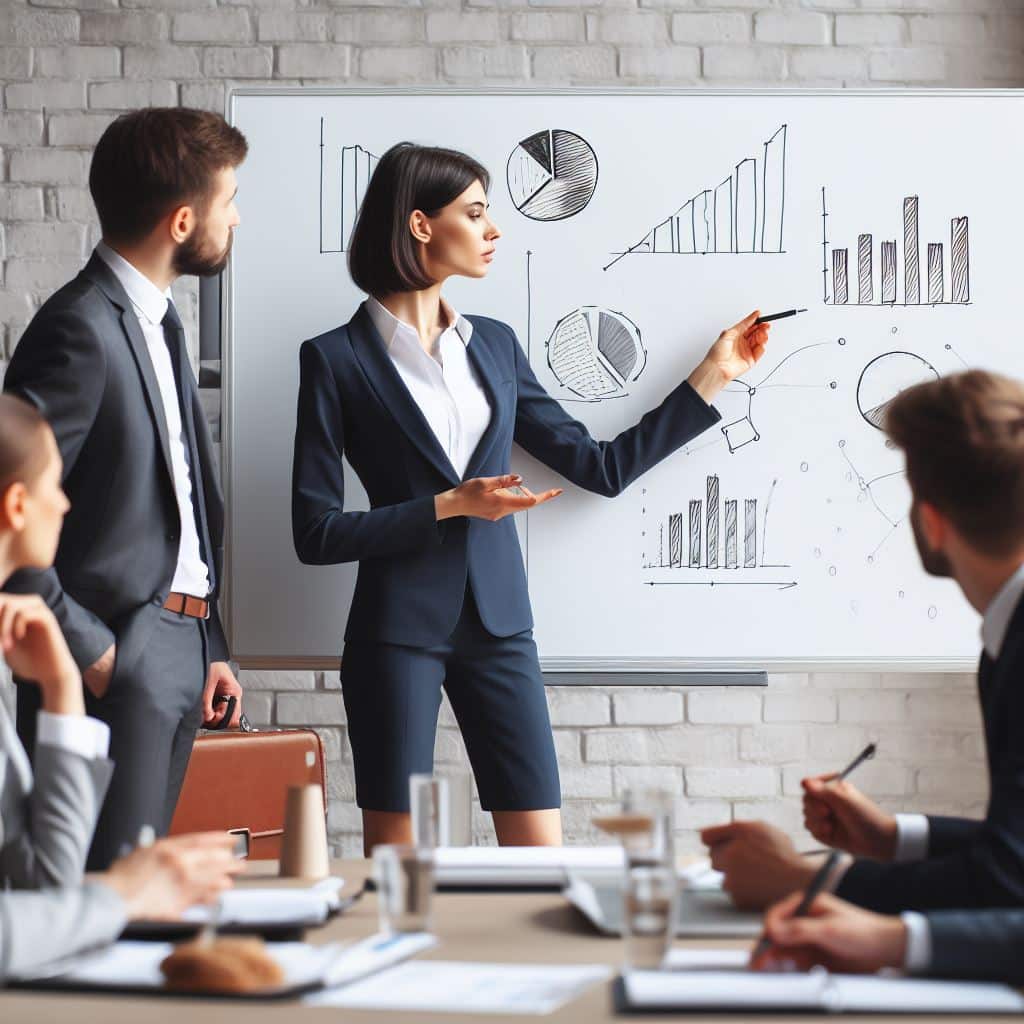
Highlighting Key Information: Use bullet points to emphasize essential information, maintaining clarity and allowing the audience to grasp key takeaways effortlessly. Bold key terms or phrases to draw attention and reinforce important points.
Addressing Audience Queries: Anticipate and be ready to answer audience questions regarding methods, assumptions, and results. Demonstrating a profound understanding of your analysis instills confidence in your work.
Integrity and Confidence in Delivery: Maintain a neutral tone and avoid exaggerated claims about findings. Present your case study with integrity, clarity, and confidence to ensure the audience appreciates and comprehends the significance of your work.
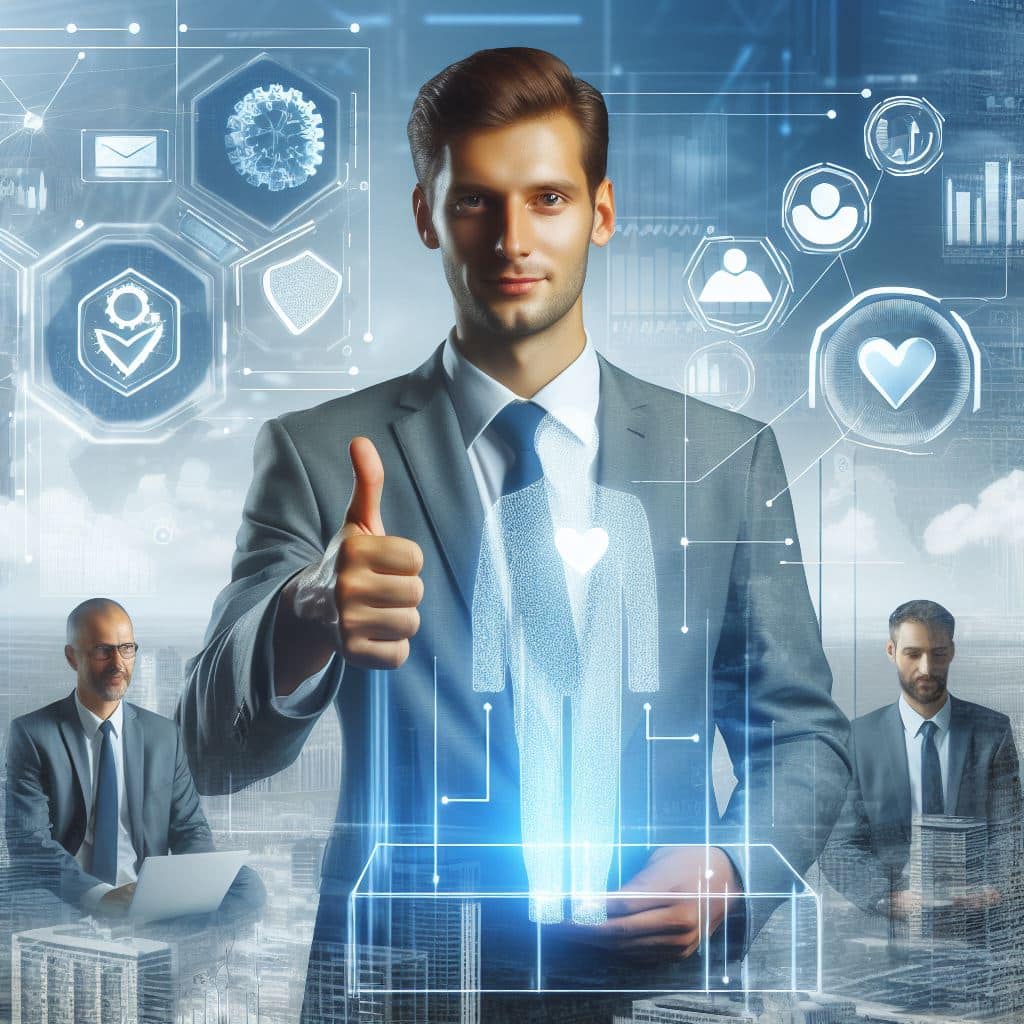
By organizing your presentation well, telling a clear story through your analysis, and using visuals wisely, you can effectively share your data analytics case study.
This method helps people understand better, stay engaged, and draw valuable conclusions from your work.
We hope by now, you are feeling very confident processing a case study. But with any process, there are challenges you may encounter.
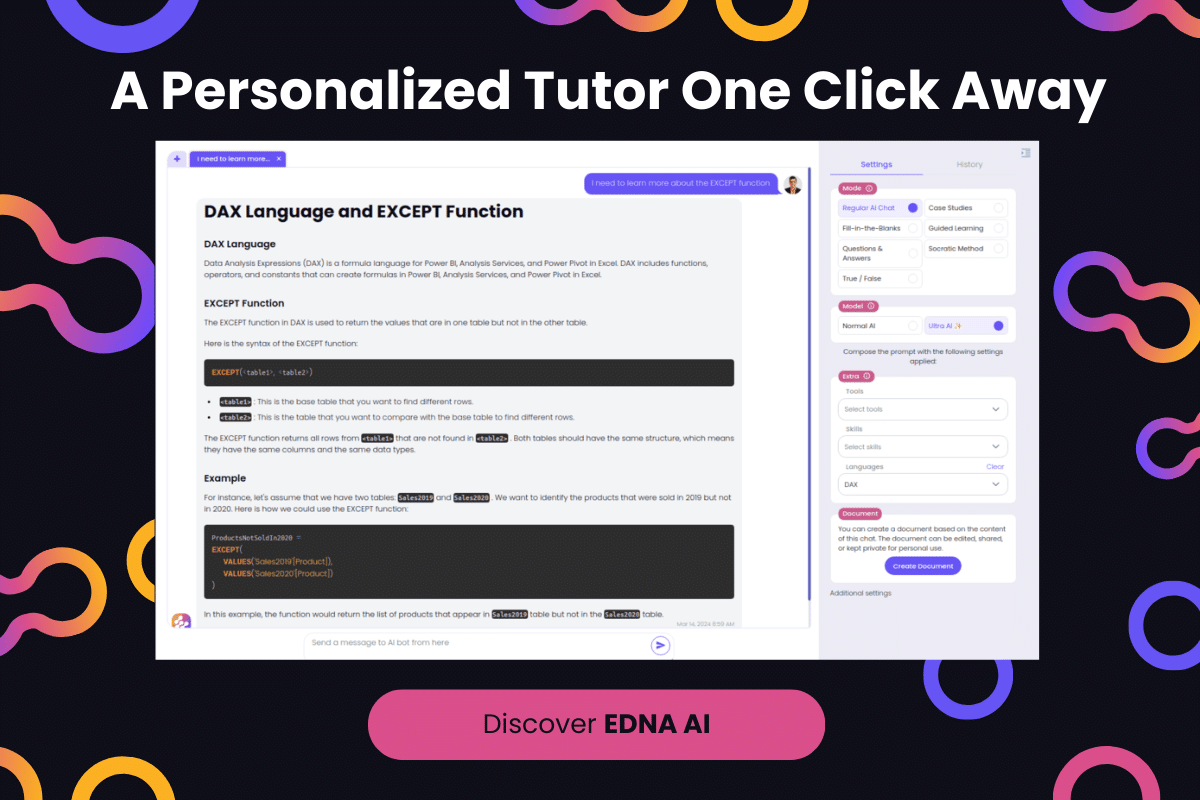
Key Challenges in Data Analytics Case Studies
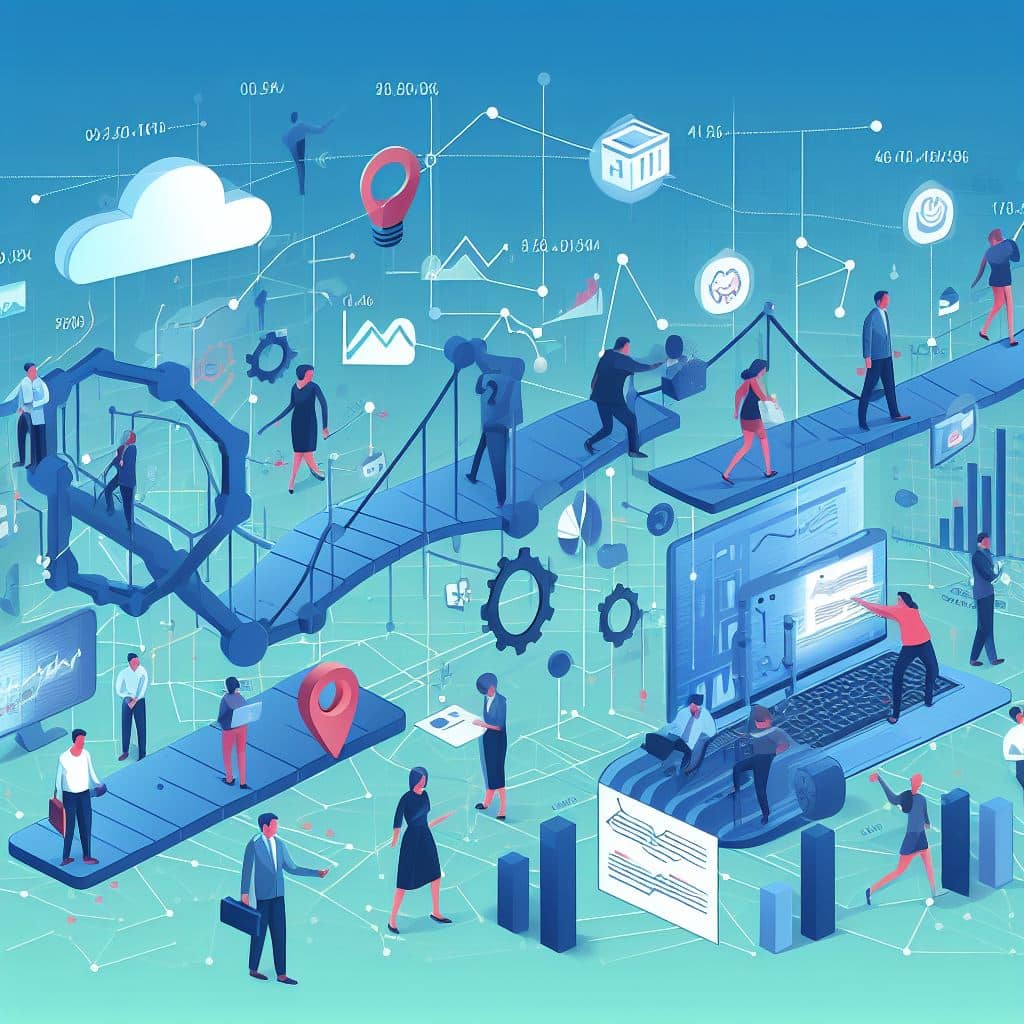
A data analytics case study can present various hurdles that necessitate strategic approaches for successful navigation:
Challenge 1: Data Quality and Consistency
Challenge: Inconsistent or poor-quality data can impede analysis, leading to erroneous insights and flawed conclusions.
Solution: Implement rigorous data validation processes, ensuring accuracy, completeness, and reliability. Employ data cleansing techniques to rectify inconsistencies and enhance overall data quality.
Challenge 2: Complexity and Scale of Data
Challenge: Managing vast volumes of data with diverse formats and complexities poses analytical challenges.
Solution: Utilize scalable data processing frameworks and tools capable of handling diverse data types. Implement efficient data storage and retrieval systems to manage large-scale datasets effectively.
Challenge 3: Interpretation and Contextual Understanding
Challenge: Interpreting data without contextual understanding or domain expertise can lead to misinterpretations.
Solution: Collaborate with domain experts to contextualize data and derive relevant insights. Invest in understanding the nuances of the industry or domain under analysis to ensure accurate interpretations.
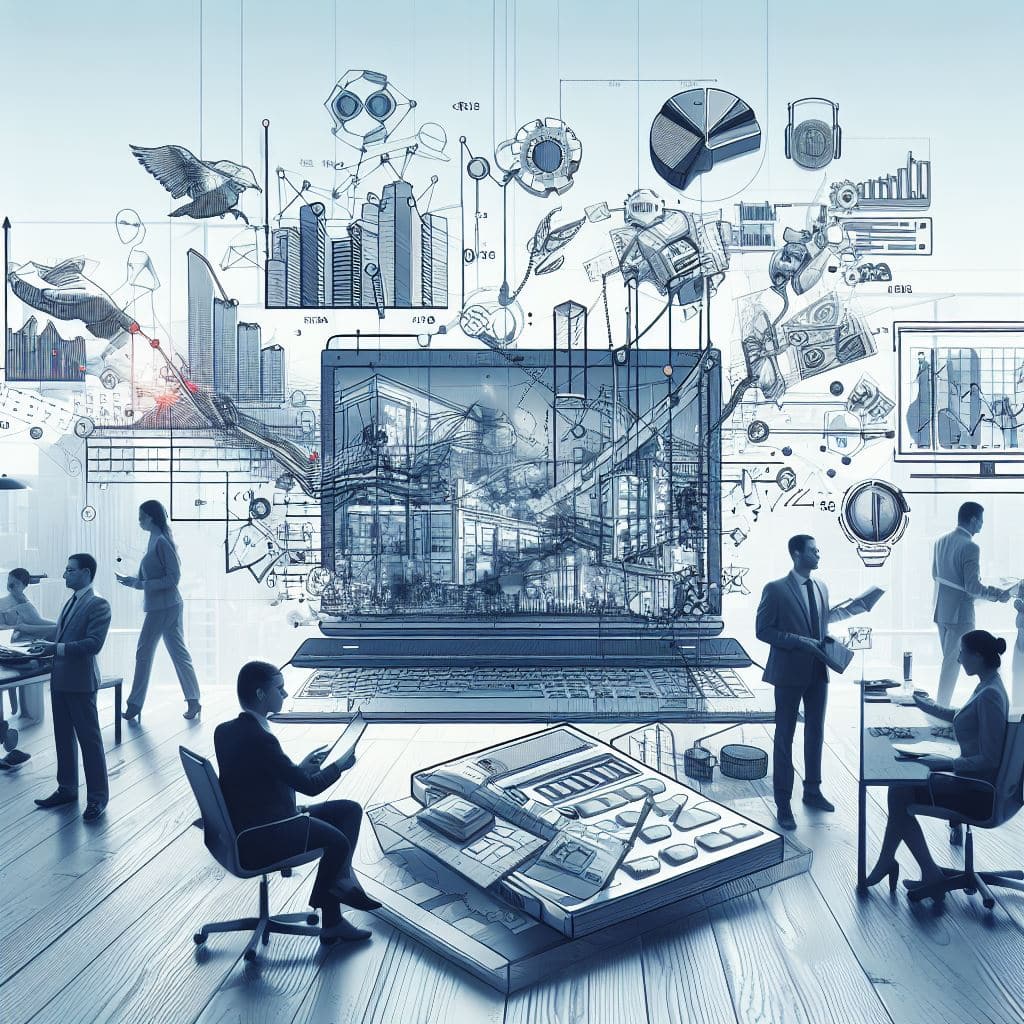
Challenge 4: Privacy and Ethical Concerns
Challenge: Balancing data access for analysis while respecting privacy and ethical boundaries poses a challenge.
Solution: Implement robust data governance frameworks that prioritize data privacy and ethical considerations. Ensure compliance with regulatory standards and ethical guidelines throughout the analysis process.
Challenge 5: Resource Limitations and Time Constraints
Challenge: Limited resources and time constraints hinder comprehensive analysis and exhaustive data exploration.
Solution: Prioritize key objectives and allocate resources efficiently. Employ agile methodologies to iteratively analyze and derive insights, focusing on the most impactful aspects within the given timeframe.
Recognizing these challenges is key; it helps data analysts adopt proactive strategies to mitigate obstacles. This enhances the effectiveness and reliability of insights derived from a data analytics case study.
Now, let’s talk about the best software tools you should use when working with case studies.
Top 5 Software Tools for Case Studies
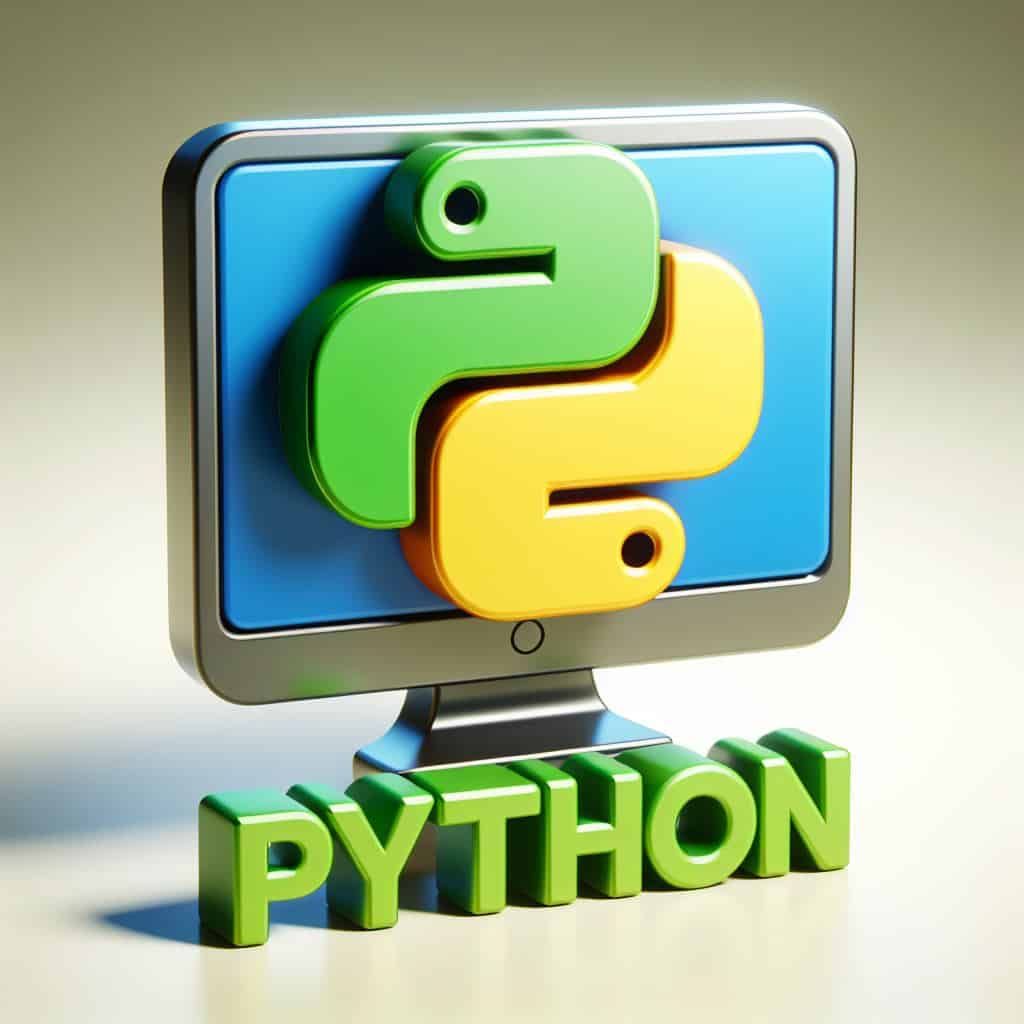
In the realm of case studies within data analytics, leveraging the right software tools is essential.
Here are some top-notch options:
Tableau : Renowned for its data visualization prowess, Tableau transforms raw data into interactive, visually compelling representations, ideal for presenting insights within a case study.
Python and R Libraries: These flexible programming languages provide many tools for handling data, doing statistics, and working with machine learning, meeting various needs in case studies.
Microsoft Excel : A staple tool for data analytics, Excel provides a user-friendly interface for basic analytics, making it useful for initial data exploration in a case study.
SQL Databases : Structured Query Language (SQL) databases assist in managing and querying large datasets, essential for organizing case study data effectively.
Statistical Software (e.g., SPSS , SAS ): Specialized statistical software enables in-depth statistical analysis, aiding in deriving precise insights from case study data.
Choosing the best mix of these tools, tailored to each case study’s needs, greatly boosts analytical abilities and results in data analytics.
Final Thoughts
Case studies in data analytics are helpful guides. They give real-world insights, improve skills, and show how data-driven decisions work.
Using case studies helps analysts learn, be creative, and make essential decisions confidently in their data work.
Check out our latest clip below to further your learning!
Frequently Asked Questions
What are the key steps to analyzing a data analytics case study.
When analyzing a case study, you should follow these steps:
Clarify the problem : Ensure you thoroughly understand the problem statement and the scope of the analysis.
Make assumptions : Define your assumptions to establish a feasible framework for analyzing the case.
Gather context : Acquire relevant information and context to support your analysis.
Analyze the data : Perform calculations, create visualizations, and conduct statistical analysis on the data.
Provide insights : Draw conclusions and develop actionable insights based on your analysis.
How can you effectively interpret results during a data scientist case study job interview?
During your next data science interview, interpret case study results succinctly and clearly. Utilize visual aids and numerical data to bolster your explanations, ensuring comprehension.
Frame the results in an audience-friendly manner, emphasizing relevance. Concentrate on deriving insights and actionable steps from the outcomes.
How do you showcase your data analyst skills in a project?
To demonstrate your skills effectively, consider these essential steps. Begin by selecting a problem that allows you to exhibit your capacity to handle real-world challenges through analysis.
Methodically document each phase, encompassing data cleaning, visualization, statistical analysis, and the interpretation of findings.
Utilize descriptive analysis techniques and effectively communicate your insights using clear visual aids and straightforward language. Ensure your project code is well-structured, with detailed comments and documentation, showcasing your proficiency in handling data in an organized manner.
Lastly, emphasize your expertise in SQL queries, programming languages, and various analytics tools throughout the project. These steps collectively highlight your competence and proficiency as a skilled data analyst, demonstrating your capabilities within the project.
Can you provide an example of a successful data analytics project using key metrics?
A prime illustration is utilizing analytics in healthcare to forecast hospital readmissions. Analysts leverage electronic health records, patient demographics, and clinical data to identify high-risk individuals.
Implementing preventive measures based on these key metrics helps curtail readmission rates, enhancing patient outcomes and cutting healthcare expenses.
This demonstrates how data analytics, driven by metrics, effectively tackles real-world challenges, yielding impactful solutions.
Why would a company invest in data analytics?
Companies invest in data analytics to gain valuable insights, enabling informed decision-making and strategic planning. This investment helps optimize operations, understand customer behavior, and stay competitive in their industry.
Ultimately, leveraging data analytics empowers companies to make smarter, data-driven choices, leading to enhanced efficiency, innovation, and growth.
Related Posts
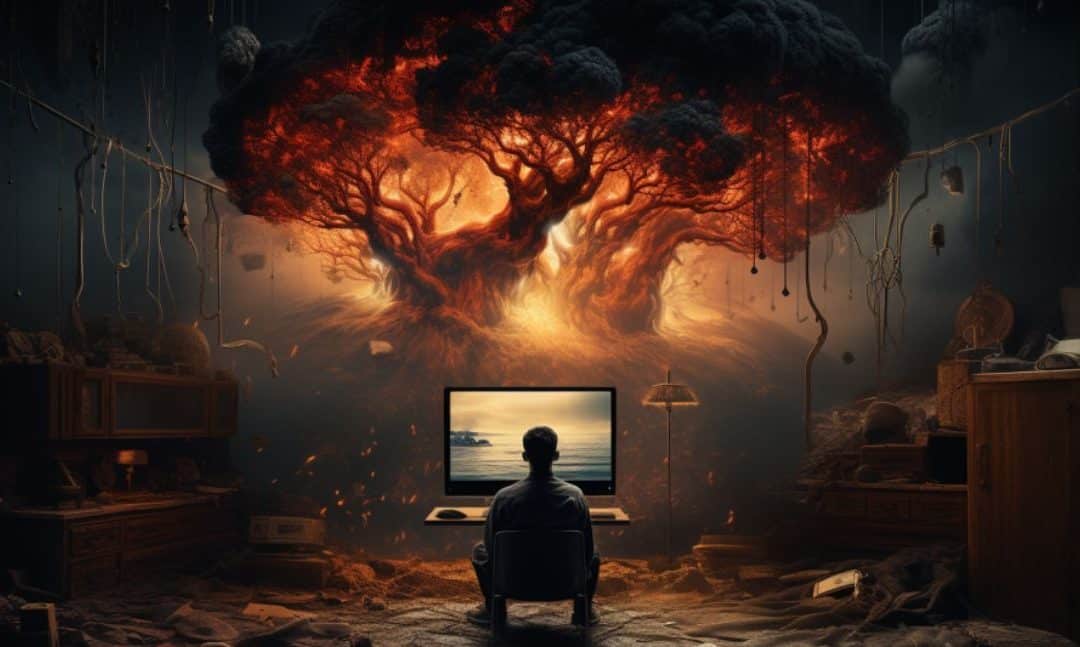
4 Types of Data Analytics: Explained
Data Analytics
In a world full of data, data analytics is the heart and soul of an operation. It's what transforms raw...
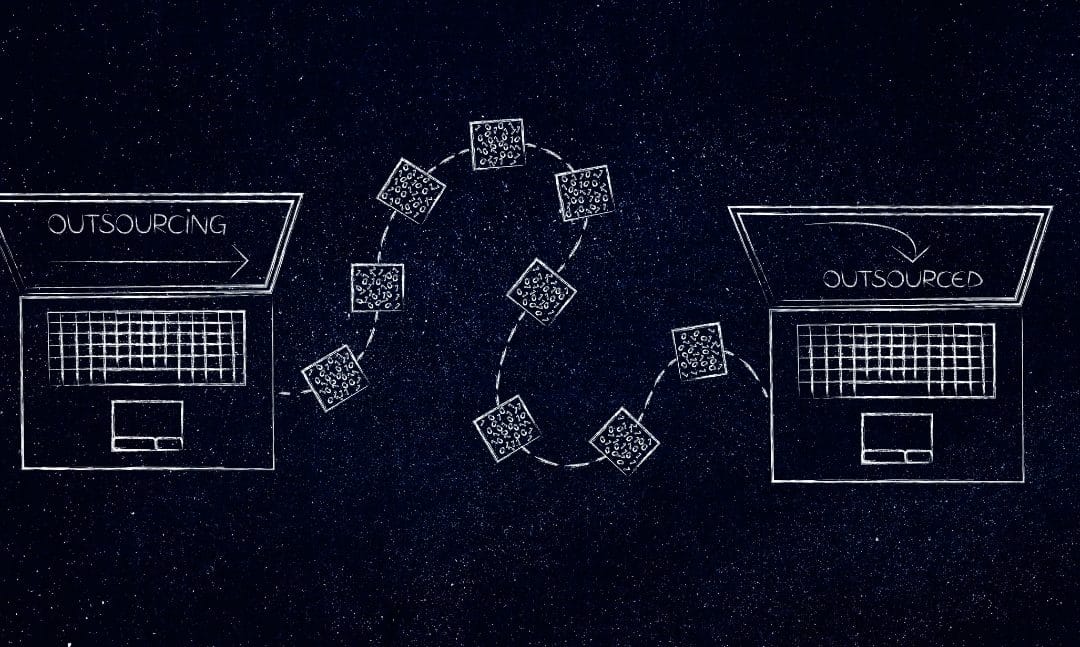
Data Analytics Outsourcing: Pros and Cons Explained
In today's data-driven world, businesses are constantly swimming in a sea of information, seeking the...
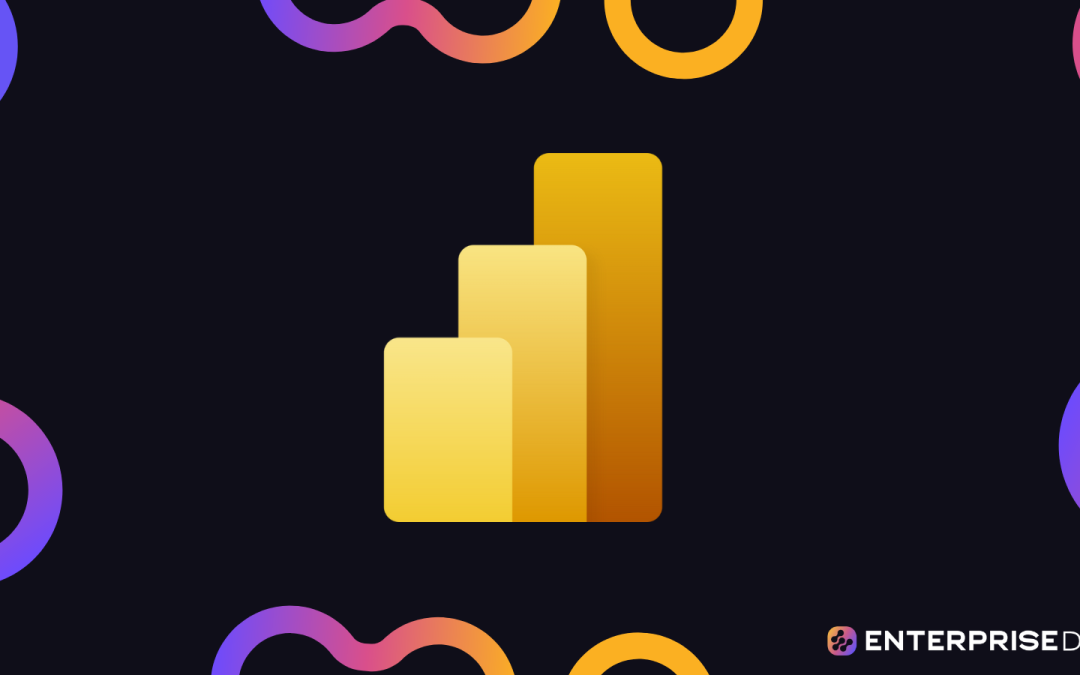
What Does a Data Analyst Do on a Daily Basis?
In the digital age, data plays a significant role in helping organizations make informed decisions and...
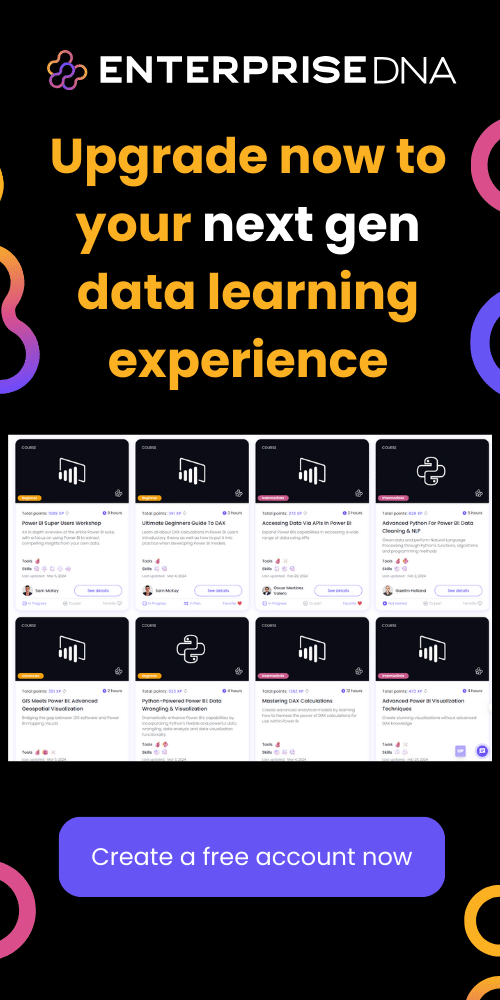
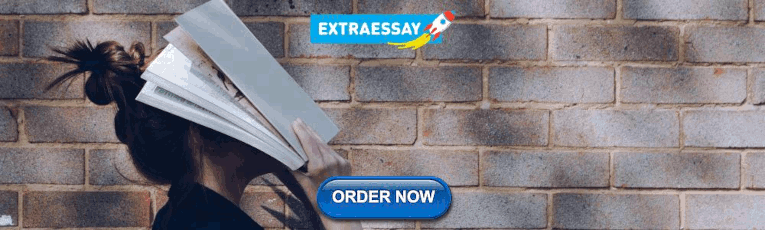
IMAGES
VIDEO
COMMENTS
First, we'll see the default analyses that Excel makes automatically. Excel shows the most commonly used analyzes by default. Steps: Click any data from the dataset. Next, click as follows: Home > Analyze Data. Soon after, you will get an Analyze Data field on the right side of your Excel window.
Data Analysis Using Excel Case Study. Data analysis is an essential skill in today's business world. As organizations deal with increasing amounts of data, it becomes crucial for professionals to make sense of this information and derive useful insights. Excel is a powerful and versatile tool that can assist in analyzing and presenting data ...
Mastering Excel for Data Analysis: - Comprehensive project or case study integrating various Excel skills. Since Excel is another essential skill for data analysts, I have decided to teach each ...
Select Analysis ToolPak from Add-ins and click on the Go. 5. Select the checkbox for the Analysis ToolPak and click OK. To access Analysis ToolPak Click on the Data tab and then from the Analysis group click On Data Analysis. The Analysis ToolPak adds the following functionality to Excel: · Anova: Single Factor.
This Excel Case Study is perfect for those who want to put their knowledge about Data Analysis in Excel into practice with selected, real-life scenarios. This makes the case study a great follow-up to BIDA's Fundamentals of Data Analysis in Excel. Led by Joseph Yeates. Level 3. 100% online and self-paced.
Once you grasp the basics of Excel PivotTables, you're ready to see how this powerful data analysis tool can add value in real-world situations. In this course— the final installment in a two ...
Simply select a cell in a data range > select the Analyze Data button on the Home tab. Analyze Data in Excel will analyze your data, and return interesting visuals about it in a task pane. If you're interested in more specific information, you can enter a question in the query box at the top of the pane, and press Enter.
The first course: Excel Fundamentals for Data Analysis, covers data preparation and cleaning but also teaches some of the prerequisites for this course like tables and named ranges as well as text, lookup and logical functions. To get the most out of this course we would recommend you do the first course or have experience with these topics.
Analyze business data using descriptive and inferential statistics in Excel. Construct and interpret statistical models to make informed business decisions. Implement regression analysis in Excel to predict future trends and make data-driven decisions. Utilize data analytics techniques to analyze large datasets and extract meaningful insights.
May 5, 2022. --. 5. Introduction ; so i found this interesting data set on kaggle * and decided to run complete analysis on it using only excel. This easy to follow analysis can serve as a guide ...
Even today Excel is widely used for its powerful data analysis capabilities. In this article, I will walk you through some of the very useful Excel methods to analyse data and prepare a visually appealing dashboard to present to end users. For this section, I have used the Air quality data shared in Kaggle. Here is a snapshot of the data set,
Review practical case studies that showcase how this powerful data analysis tool is used in the real world. Once you grasp the basics of Excel PivotTables, you're ready to see how this powerful data analysis tool can add value in real-world situations. In this courseâ the final installment in a two-part seriesâ instructor Chris Dutton dives ...
If you wanna make your career in Data Science domain, then check our course now. We provide hands-on practical learning experience to our learners with 1-1 L...
A Supply Chain Analysis Project using Microsoft Excel and Microsoft Power BI. Supply chain analytics plays a crucial role in driving data-driven decision-making across industries, including manufacturing, retail, healthcare, and logistics. In this report, I presented an analysis of a Fashion and Beauty startup's supply chain data ...
Share your videos with friends, family, and the world
The Data Analysis in Excel Certificate Program at a Glance. CFI's Data Analysis in Excel (DAE) Certificate Program positions you to master the world of data analysis using Microsoft Excel. Save hours by automating and streamlining data collection, transformation, and analysis. The DAE Certificate program is designed to ropel your career ...
In this Excel case study, you'll investigate a dataset from an example telecom company, Databel, and analyze their churn rates. Understand why customers churn Analyzing churn doesn't just mean knowing the churn rate: it's also about figuring out why customers are churning at the rate they are and how to reduce churn.
May 9, 2022. My last post ; Case Study — Data Analysis using excel*, was so well received;It did 100% beyond my expectations in terms of views and reviews. This post is simply my own review of ...
In this case study, I analyze historical data from a Chicago based bike-share company in order to identify trends in how their customers use bikes differently. The main tools I use are spreadsheets, SQL and Tableau. Here are the highlights: Tableau Dashboard: Cyclistic Bikeshare in Chicago. Slides: Where Rubber Meets Road in Converting Casual ...
A data analytics case study comprises essential elements that structure the analytical journey: Problem Context: A case study begins with a defined problem or question. It provides the context for the data analysis, setting the stage for exploration and investigation.. Data Collection and Sources: It involves gathering relevant data from various sources, ensuring data accuracy, completeness ...
10 top Excel functions in data analysis. While Excel has hundreds of built-in functions, you might gravitate to a few common ones more often than others. Some top Excel functions that can help you efficiently clean and manipulate your data include the following. Read more: How to Earn a Microsoft Excel Certification (+ Why You Should) 1. EXACT
Case Study: Product Sales Performance Analysis Using Excel. My journey to becoming a Data Scientist through the Women Techsters Fellowship Class of 2023, organized by Tech4Dev in Partnership with ...
If the issue persists, it's likely a problem on our side. Unexpected token < in JSON at position 4. keyboard_arrow_up. content_copy. SyntaxError: Unexpected token < in JSON at position 4. Refresh. Explore and run machine learning code with Kaggle Notebooks | Using data from FitBit Fitness Tracker Data.