Techniques of Knowledge Representation
Artificial Intelligence (AI) is concerned with developing computer programs that can perform tasks that require human intelligence. One of the essential aspects of AI is knowledge representation, which involves capturing and storing human knowledge in a way that machines can understand and use. Knowledge representation is crucial in AI because it helps machines reason, make decisions, and solve problems like humans.
In this article, we will explore the different knowledge representation techniques in AI, including logical representation, semantic network representation, frame representation, and production rules. We will also discuss the different types of knowledge that need to be represented in AI, the cycle of knowledge representation in AI and the relationship between knowledge and intelligence.
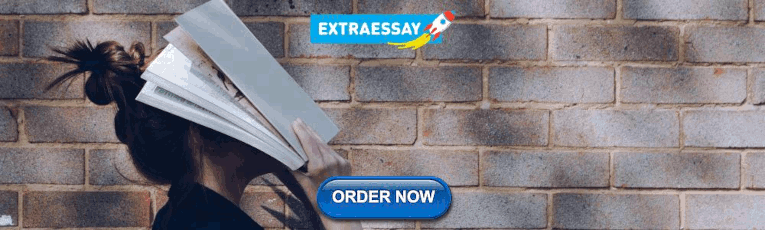
Introduction
AI aims to replicate human intelligence in machines to perform complex tasks, including perception, reasoning, decision-making, and problem-solving. However, achieving this goal requires machines to have access to human knowledge and use it to perform these tasks. Knowledge representation, which refers to the techniques of capturing and storing human knowledge in a way that machines can understand and use, is a critical component of AI. The main challenge in knowledge representation is finding a way to represent knowledge that is understandable by machines and can be used for reasoning and problem-solving.
To address this challenge, various techniques of knowledge representation in AI have been developed, such as rule-based systems, semantic networks, frames, ontologies, and logic-based representations. These techniques provide a structured way to represent knowledge and enable machines to reason about it and perform complex tasks.
What is Knowledge Representation?
Knowledge representation is the process of representing information in a structured form that is easily understood by both humans and machines. It is a fundamental task in artificial intelligence (AI) and computer science that involves organizing knowledge into a form that can be used for reasoning, problem-solving, and decision-making.
The goal of knowledge representation is to make explicit the relationships between concepts, ideas, and objects in a way that can be used to make inferences and draw conclusions. To achieve this, various knowledge representation techniques can be used, such as logical representation, semantic network representation, frame representation, and production rules.
In practical terms, with the help of techniques of knowledge representation AI is used to build intelligent systems that can understand natural language, recognize patterns, learn from data, and make predictions. For example, a knowledge representation system might be used to build a chatbot that can answer questions about a particular topic or a recommendation engine that can suggest products based on a user's preferences.
The Different Kinds of Knowledge that Need to be Represented in AI
The knowledge that needs to be represented in AI can be classified into several categories, including objects, events, performance, facts, meta-knowledge, and knowledge-base.
Objects refer to things in the world that have physical properties and can be observed, touched, or manipulated. Examples of objects include cars, buildings, and people. Object-oriented programming is an example of a technique that uses objects to represent knowledge in AI.
Events refer to actions or occurrences that take place in the world. Examples of events include driving a car, cooking food, or attending a concert. Event-based systems use events to represent knowledge in AI.
Performance
Performance refers to the behavior of agents or systems that perform a task. It includes the goals and objectives of the task and the criteria used to evaluate performance. Performance-based systems use performance to represent knowledge in AI.
Facts refer to propositions that are either true or false. They are statements that can be verified using evidence or logical deduction. Examples of facts include "the sky is blue," "the earth revolves around the sun," and "water boils at 100 degrees Celsius." Knowledge-based systems use facts to represent knowledge in AI.
Meta-Knowledge
Meta-knowledge refers to knowledge about knowledge. It includes information about the structure and organization of knowledge, the sources of knowledge, and the reliability and validity of knowledge. Meta-knowledge is essential in AI because it helps machines reason about the quality and validity of the knowledge they are using.
Knowledge-Base
A knowledge base is a collection of knowledge that is organized and stored in a way that machines can access and use it. It includes facts, rules, procedures, and other knowledge relevant to a particular domain. Knowledge-based systems use a knowledge base to represent knowledge in AI.
Techniques of Knowledge Representation in AI
There are several knowledge representation techniques in AI, including logical representation, semantic network representation, frame representation, and production rules. Each of these techniques has its syntax and semantics, advantages, and disadvantages.
Logical Representation
Logical Representation is a fundamental method of communicating knowledge to machines through a well-defined syntax with precise rules. This syntax should be unambiguous and able to handle prepositions, making it an ideal way to represent facts. There are two types of logical representation: Propositional Logic and First-order Logic.
Propositional Logic , also known as propositional calculus or statement logic, is a formal system of logic that deals with the relationships between propositions, which are statements that are either true or false. Propositional logic is based on the Boolean system, which means that propositions are evaluated as either true or false. In propositional logic, propositions are combined using logical connectives such as "and", "or", and "not", and the resulting compound propositions can also be evaluated as true or false based on the truth values of their component propositions.
First-order logic (FOL) , also known as first-order predicate calculus (FOPC) or first-order logic with identity, is an extension of propositional logic that allows for the representation of more complex relationships between objects. In FOL, propositions are constructed using predicates, which are statements that describe properties or relations between objects, and quantifiers, which specify the scope of the variables in the proposition.
FOL allows for more precise and flexible reasoning about the relationships between objects and is widely used in mathematics, computer science, and philosophy.
In logic, we use symbols and operators to represent concepts like truth, negation, conjunction, disjunction, implication, quantification, and identity. There are different types of logical representation like propositional logic, first-order logic, and higher-order logic.
The semantics of logical representation involves assigning meaning to these symbols and formulas. This is done by defining a set of axioms and rules for manipulating these symbols.
There are several advantages to using logical representation, such as its ability to facilitate logical reasoning and serve as the foundation for programming languages. However, there are also some limitations and challenges associated with this method. One disadvantage is that logical representations can be restrictive and difficult to work with. Additionally, this approach may not be very intuitive, and the process of inference may not always be efficient.
- It is Monday.
- The Sun rises from the North (False proposition)
- 3+3= 8(False proposition)
- 7 is a prime number.
Semantic Network Representation
A semantic network is a graphical representation of knowledge, where nodes represent concepts or objects, and links represent relationships between them. The syntax of a semantic network consists of nodes and links, and the semantics involve defining the meaning of each node and link.
One of the main advantages of semantic networks is that they can be easily visualized, making them more intuitive to understand than logical representations. Additionally, they can categorize objects and link them together.
However, there are some drawbacks associated with this representation method. For instance, semantic networks can be computationally expensive at runtime, as traversing the entire network tree may be necessary to answer certain questions. Furthermore, modeling the vastness of human-like memory is not practical. Semantic networks also lack quantifier equivalents such as "for all" or "for some", and do not have standard definitions for link names. Additionally, they are not inherently intelligent and depend on the creator of the system.
Example: The following are a few statements that must be represented with nodes and arcs:
- Jerry is a cat.
- Jerry is a mammal
- Jerry is owned by Priya.
- Jerry is brown-colored.
- All Mammals are animals.
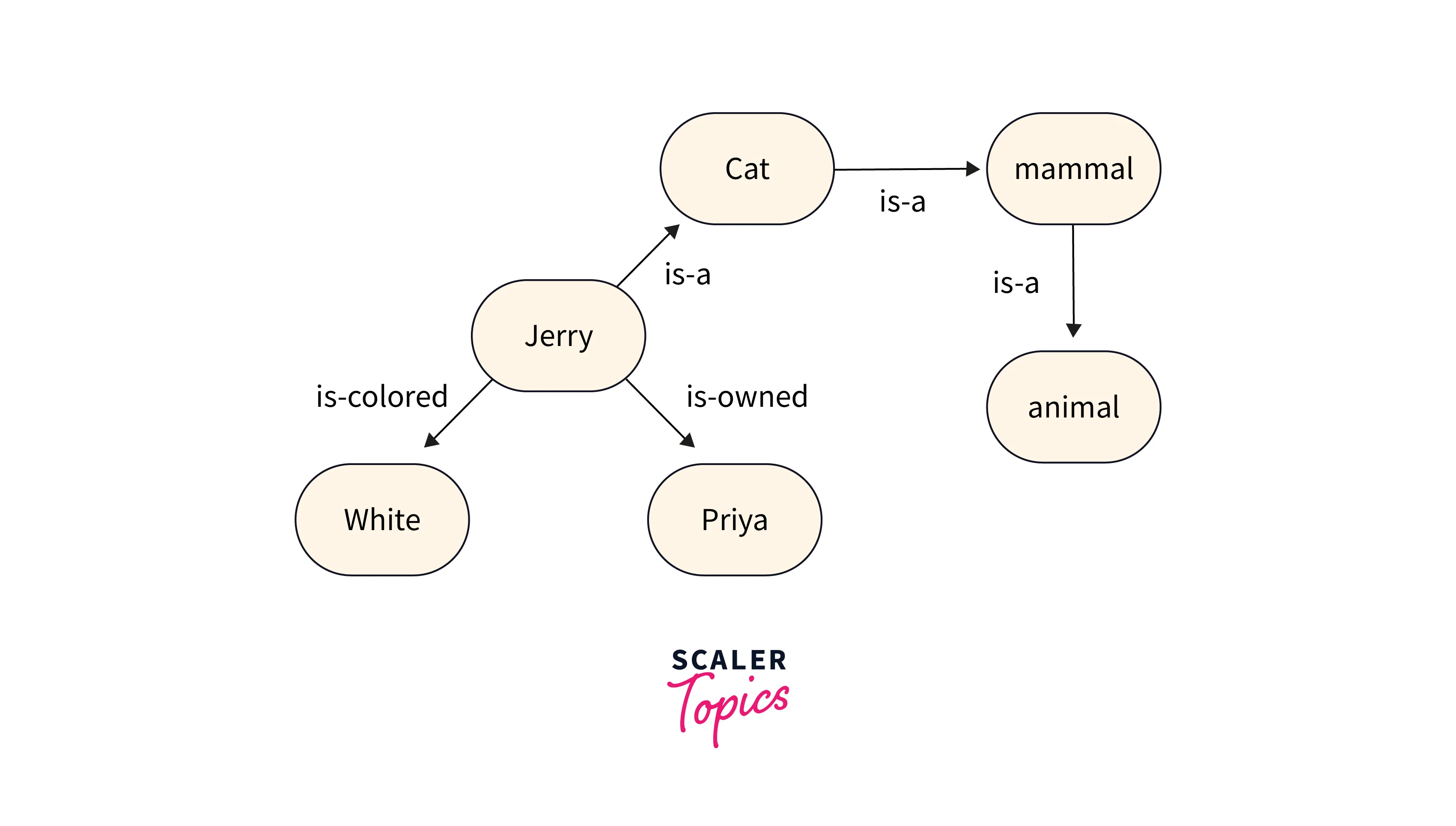
Frame Representation
Frame representation is a technique for organizing knowledge in a hierarchical structure. A frame is a structured record that describes an entity in the world by using a collection of attributes and their corresponding values. In artificial intelligence, frames serve as a data structure that divides knowledge into substructures by representing typical situations.
The syntax of a frame consists of attributes and values, and the semantics involve defining the meaning of each attribute and value. The frame representation method offers several advantages in the field of artificial intelligence. One of its key strengths is its ability to simplify programming by grouping related data. It is also a highly flexible approach utilized in various AI applications. Moreover, the visual nature of frame representation makes it easy to comprehend.
However, there are also some limitations associated with frame representation. For instance, the inference mechanism in frame systems can be challenging to process, and the approach is not always the most efficient. Additionally, the generalized nature of frame representation means that it may not always be the best fit for more specific or complex scenarios.
Example: Consider the example of a book frame.
Production Rules
Production rules are a set of IF-THEN statements that represent knowledge. The IF part of a rule is a condition, and the THEN part is an action to be taken if the condition is met. Production rules can be used to represent a wide range of knowledge, including facts, procedures, and heuristics.
The production rules system is composed of three key components:
- The set of production rules
- The working memory
- The recognize-act-cycle
The syntax of a production rule consists of IF-THEN statements, and the semantics involve defining the meaning of the conditions and actions. One advantage of this system is that production rules can be expressed in natural language, which makes them easier to understand and modify. Additionally, the modularity of the production rules system allows for easy removal or modification of individual rules.
However, there are also some drawbacks to the production rules system. They do not possess any learning capabilities and cannot store the result of a problem for future use. Furthermore, they can become complex and difficult to maintain as the number of rules increases.
- IF (at auto-rickshaw stop AND rickshaw arrives) THEN action (get into the rickshaw)
- IF (in the rickshaw AND paid AND empty seat) THEN action (sit down).
- IF (in rickshaw AND unpaid) THEN action (pay charges).
- IF (rickshaw arrives at destination) THEN action (get down from the rickshaw).
Cycle of Knowledge Representation in AI
The cycle of knowledge representation in AI consists of five stages: perception, learning, knowledge representation, reasoning, planning, and execution.
Perception is the process of acquiring information from the environment through sensors. This information is then processed and interpreted to generate knowledge.
Learning is the process of acquiring new knowledge from experience. This can be achieved through supervised learning, unsupervised learning, or reinforcement learning.
Knowledge Representation & Reasoning
Knowledge representation and reasoning is the stage where acquired knowledge is transformed into a form that can be processed by machines. This involves choosing an appropriate KR technique and representing knowledge using that technique. Reasoning involves using the knowledge represented to draw inferences and make decisions.
Planning is the stage where the system uses the acquired knowledge and reasoning to generate a sequence of actions to achieve a particular goal. This involves selecting the most appropriate actions to achieve the goal while taking into account any constraints or limitations.
Execution is the final stage where the system performs the planned actions. The success of the execution depends on the accuracy and completeness of the knowledge representation, reasoning, and planning.
What is the Relation between Knowledge & Intelligence?
Knowledge and intelligence are closely related concepts, but they are not the same thing. Knowledge is information that is acquired through experience or education. Intelligence is the ability to learn, reason, and solve problems. Knowledge is necessary for intelligence, but it is not sufficient.
Intelligence involves the ability to use the techniques of knowledge representation flexibly and adaptively. This requires not only acquiring knowledge but also being able to reason with it, apply it to new situations, and use it to solve problems. The ability to learn from experience and adapt to new situations is a key aspect of intelligence.
For instance, techniques of knowledge representation in AI can help AI systems learn and reason about language, enabling them to communicate effectively with humans. They can also be used to represent knowledge about a particular domain, such as medicine or finance, allowing AI systems to reason about complex problems in those domains.
However, techniques of knowledge representation in AI alone are not sufficient to create truly intelligent AI systems. Intelligence also involves the ability to learn from experience and adapt to new situations, which requires the use of machine learning algorithms and other advanced AI techniques.
- Knowledge representation is a critical component of AI that enables machines to reason about the world in a way that is similar to how humans reason.
- Various knowledge representation techniques in AIsuch as logical representation, semantic network representation, frame representation, and production rules can be used to represent knowledge.
- The cycle of knowledge representation in AI involves perception, learning, knowledge representation, reasoning, planning, and execution, all of which rely on the use of techniques of knowledge representation.
- The relationship between knowledge and intelligence is that knowledge is necessary for intelligence, but intelligence requires more than just knowledge.
- Overall, advances in techniques of knowledge representation in AI and AI algorithms have the potential to revolutionize many fields, from medicine and finance to transportation and education.
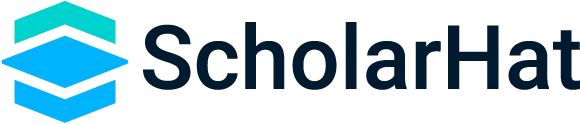
01 Beginner
- Introduction to Artificial Intelligence
- Agents in AI
- Environment in AI
02 Intermediate
- Search Algorithms in AI - Types of Search Algorithms in AI & Techniques
Knowledge Representation in AI - Types, Issues, & Techniques
03 advanced.
- Expert System in AI
- What is Environment in AI - Types of Environment in AI ( Full Guide )
- Natural Language Processing in AI
- Computer Vision in AI
- Ethics & Future of AI
04 Training Programs
- Data Structures and Algorithms Training
Artificial Intelligence Certification Course
- Data Science with Python Certification Course
- Artificialintelligence
- Knowledge Representation ..
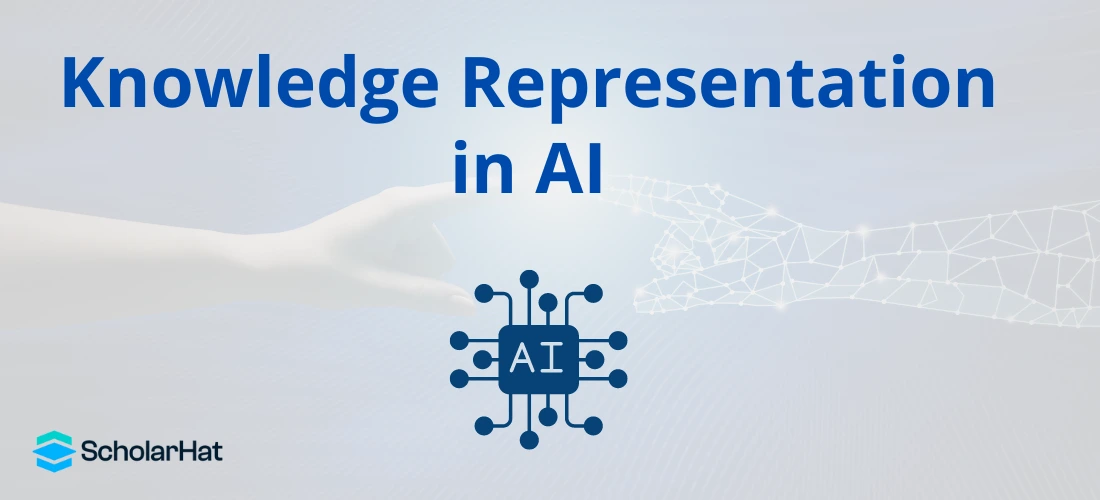
Knowledge Representation in AI - Overview
Artificial intelligence (AI) is based on the core idea of knowledge representation, which tries to capture and organize knowledge in a meaningful and structured fashion. It entails archiving data and making it available to AI systems so they may learn, reason, and make decisions based on knowledge. In this thorough book, Learn Artificial Intelligence , we will delve into the complexities of knowledge representation in AI, looking at its different forms, the knowledge cycle, approaches, strategies, benefits, drawbacks, practical applications, difficulties, and future directions.
What is Knowledge Representation in AI?
The process of encoding information in a way that an AI system can comprehend and use is known as knowledge representation in AI. It entails converting information and ideas from the real world into a form that computers can use, analyze, and make conclusions from. AI systems may imitate human cognitive functions including problem-solving, decision-making, and language comprehension thanks to this representation.
Types of Knowledge in AI
Knowledge in AI can be divided into various types of knowledge in AI, each of which serves a particular function in the process of knowledge representation as a whole.
1.) Declarative Knowledge
Declarative knowledge is the representation of information, facts, and claims about the outside world. Without outlining the method of knowledge acquisition or application, it concentrates on what is true or incorrect. Declarative knowledge is frequently modeled using logic-based formalisms and serves as the basis for other types of knowledge.
2.) Procedural Knowledge
Imperative knowledge, usually referred to as procedural knowledge, specifies how to carry out tasks or actions. It contains detailed guidelines, techniques, and rules that assist AI systems in carrying out particular tasks. AI systems need procedural knowledge to effectively complete complicated tasks and address issues.
3.) Meta Knowledge
Also known as knowledge about knowledge, meta-knowledge is the study of the composition, arrangement, and characteristics of knowledge. It aids AI systems in managing and making sense of their own information, allowing them to adjust, learn, and develop over time.
The Knowledge Cycle in AI
The continual process of obtaining, representing, reasoning, & updating knowledge within an AI system is referred to as the "knowledge cycle" in AI.
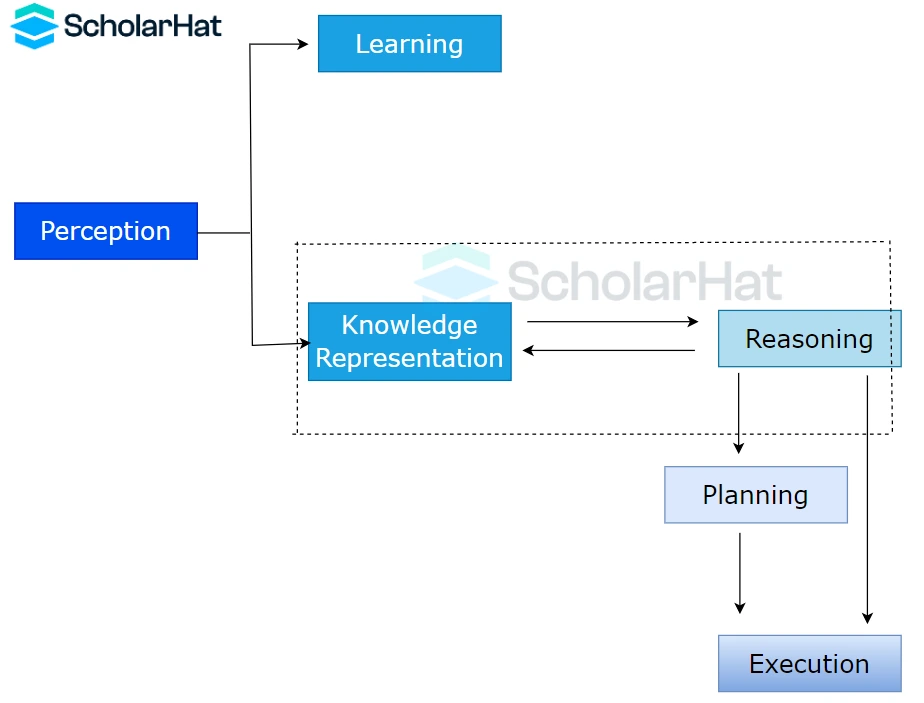
The following stages make up this process:
- Knowledge Acquisition: Information is gathered from a variety of sources, including databases, documents, experts, and even other AI systems. The goal of this stage is to gather pertinent knowledge and convert it into an appropriate representation format.
- Knowledge Representation: The key stage of the knowledge cycle is knowledge representation, where acquired information is organized and encoded in a language that AI systems can comprehend and use. The effectiveness and efficiency of knowledge processing are greatly influenced by the representation approach chosen.
- Knowledge Reasoning: During this phase, AI systems use the knowledge that has been encoded to carry out reasoning tasks like inference, deduction, or induction. AI systems can reason to create new knowledge from existing knowledge and to make defensible decisions based on the information at hand.
- Knowledge Update: The knowledge representation has to be updated when new information becomes available or as outdated knowledge is amended or rendered invalid. This phase guarantees that AI systems are current and flexible enough to respond to changing conditions.
Approaches to Knowledge Representation in AI
AI uses a variety of Approaches to knowledge representation, each with unique advantages and disadvantages. Several of the frequently used Approaches to knowledge representation include:
- Logic-Based Methods: Knowledge is represented using formal logic in logic-based techniques, such as propositional logic, first-order logic, or fuzzy logic. These methods enable exact and rigorous representation, allowing AI systems to carry out logical inference and reasoning.
- Semantic Networks: Semantic networks are ways to describe knowledge by connecting labeled edges (relationships) to nodes (concepts). With this method, knowledge graphs may be navigated and explored by AI systems and emphasize the connections between concepts.
- Frames or scripts: Frames and scripts capture the characteristics, traits, and behaviors of things or circumstances and convey knowledge as organized frames or templates. This method promotes reasoning based on common scenarios and makes it easier to convey complex knowledge.
- Ontologies: Ontologies define concepts, relationships, and limitations within a certain domain to offer a formal representation of knowledge. They give AI systems the ability to reason and infer using domain-specific information, improving accuracy and understanding of the context.
Techniques of Knowledge Representation in AI
There are other techniques of Knowledge Representation in addition to the different approaches.
- Rule-Based Systems: Using a set of rules, or production rules, rule-based systems express knowledge. AI systems may make judgments based on certain conditions thanks to these rules, which are made up of conditions and actions.
- Semantic Web Technologies: Semantic web technologies like the Resource Description Framework (RDF) and the Web Ontology Language (OWL) offer standardized formats for expressing and transferring knowledge on the web. These tools make it possible for AI systems to access and incorporate data from many sources.
- Neural Networks: Through the training process, neural networks, in particular deep learning models, can learn and implicitly represent knowledge. These models are excellent at identifying intricate patterns and connections in unstructured data, including speech, texts, and photographs.
- Statistical Models: Using probabilistic linkages and statistical inference, statistical models, such as Bayesian networks or Markov models, express knowledge. These models give AI systems the ability to reason and decide in the face of uncertainty.
Advantages and Limitations of Different Techniques of Knowledge Representation
Each method of knowledge representation has unique benefits and drawbacks that make it suitable for various AI applications . Several of the strategies discussed above have the following advantages and limitations:
Logic-Based Methods
- Formal and accurate representation.
- Facilitates deduction and logical reasoning.
Limitations
- Difficulty dealing with uncertainty and insufficient data.
- limited scalability for huge knowledge sets.
Semantic networks
- Emphasises linkages and interactions between concepts.
- Allows for flexible knowledge graph traversal.
- Difficulties in modeling sophisticated knowledge structures.
- Absence of formal semantics and reasoning abilities.
Scripts and frames
- Records specific characteristics and actions of things or circumstances.
- Backs up logic with common scenarios.
- Demands the explicit description of all potential circumstances.
- Minimal generalization outside of established frames or scripts.
- Supports reasoning & inference based on domain constraints.
- Offers a formal representation of knowledge relevant to a domain.
- Ontology construction requires topic specialists.
- Maintaining and updating them as knowledge expands presents difficulties.
Real-World Applications of Knowledge Representation in AI
AI's knowledge representation is used in a variety of fields and industries.
Here are a few significant real-world examples:
- Expert Systems: To simulate human competence and offer wise decision support, expert systems in AI make use of knowledge representation techniques. They are commonly utilized in industries like engineering, finance, and medicine where expertise in a certain topic is essential.
- Natural Language Processing: The ability of AI systems to comprehend, decipher, and produce human language is made possible by knowledge representation, which is a key component of NLP applications. Applications for NLP include sentiment analysis, language translation, chatbots, and virtual assistants.
- Robotics and autonomous systems: Knowledge representation helps with perception, planning, & decision-making in these systems. It enables robots to comprehend their surroundings, gain knowledge from their past, and efficiently communicate with people.
- Recommender Systems: Recommender systems employ knowledge representation to model item features, comprehend user preferences, and generate tailored recommendations. They are widely utilized in content recommendation engines, music and video streaming platforms, and e-commerce.
Challenges and Future Directions in Knowledge Representation in AI
Several obstacles and potential directions still exist in knowledge representation, despite the substantial progress made in this area. Among the principal difficulties are:
- Scalability: Scalability becomes a significant difficulty as knowledge's volume and complexity rise. Large knowledge bases must be efficiently represented and processed using sophisticated methods and distributed computing concepts.
- Information that is Uncertain or Incomplete: AI systems frequently work with information that is uncertain or incomplete. A major research area is improving knowledge representation approaches to manage uncertainty and reason with inadequate data.
- Knowledge Fusion and Integration: Combining and integrating knowledge from various sources and modalities is a difficult task. The goal of future research is to create methods that make it possible for heterogeneous knowledge to be seamlessly integrated for better AI performance.
- Explainability & Interpretability: AI systems should be able to justify their decisions with explanations. Building trust, assuring ethical AI, and satisfying legal standards all depend on the development of clear and understandable knowledge representation approaches.
Best Practices of Knowledge Representation in AI
AI knowledge representation entails gathering and arranging data for computational use. Top practices consist of:
- Expressivity: Use a representation language that fully expresses the constraints, relationships, supporting concepts, and domain knowledge.
- Formality: Offer well-defined syntax and semantics for defensible inference and automated reasoning.
- Ontology: Create an ontology to specify domain concepts, entities, and relationships, creating consensus.
- Modularity: In order to facilitate maintenance, reuse, and scalability, break complex knowledge down into modules.
- Granularity: Represent knowledge at the proper level of detail to facilitate sound deliberation and judgment.
- Probabilistic reasoning and uncertainty: Bayesian networks and fuzzy logic are two tools for dealing with uncertainty.
- Logic and inference: Based on the knowledge type and problem domain, choose the best logical processes.
- Scalability: Create the system with the ability to efficiently manage vast knowledge bases, facilitating quick retrieval and inference.
- Integration with Learning: Include learning algorithms as well as fresh information gleaned from data.
- Evaluation and iteration: Based on input and performance indicators, continuously assess and improve the representation.
Resources for further learning and practice
Online tutorials and courses: Explore Additional Learning and Practice Resources with us at https://www.scholarhat.com/training/artificial-intelligence-certification-training . There are numerous online tutorials and courses available that specifically focus on Artificial Intelligence.
Artificial intelligence's foundation is knowledge representation, which enables AI systems to comprehend, reason, and arrive at defensible decisions. We examined all facets of knowledge representation in AI in this thorough guide, from its definition to its types, methodologies, strategies, benefits, drawbacks, applications, difficulties, and future directions. Unlocking the potential of knowledge representation will be essential for creating intelligent systems that can solve difficult issues and supplement human abilities as AI develops.
About Author
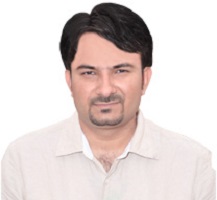
- 22+ Video Courses
- 750+ Hands-On Labs
- 300+ Quick Notes
- 55+ Skill Tests
- 45+ Interview Q&A Courses
- 10+ Real-world Projects
- Career Coaching Sessions
- Email Support
We use cookies to make interactions with our websites and services easy and meaningful. Please read our Privacy Policy for more details.
Laboratory for Intelligent Probabilistic Systems
Princeton University Department of Computer Science
What is representation learning?
Roger Grosse · February 25, 2013
In my last post , I argued that a major distinction in machine learning is between predictive learning and representation learning. Now I'll take a stab at summarizing what representation learning is about. Or, at least, what I think of as the first principal component of representation learning.
A good starting point is the notion of representation from David Marr's classic book, Vision: a Computational Investigation [1, p. 20-24]. In Marr's view, a representation is a formal system which "makes explicit certain entities and types of information," and which can be operated on by an algorithm in order to achieve some information processing goal (such as obtaining a depth image from two stereo views). Representations differ in terms of what information they make explicit and in terms of what algorithms they support. He gives the example of Arabic and Roman numerals (which Shamim also mentioned): the fact that operations can be applied to particular columns of Arabic numerals in meaningful ways allows for simple and efficient algorithms for addition and multiplication.
I'm sure a lot of others have proposed similar views, but being from MIT, I'm supposed to attribute everything to Marr.
I'm not going to say much about the first half of the definition, about making information explicit. While Marr tends to focus on clean representations where elements of the representation directly correspond to meaningful things in the world, in machine learning we're happy to work with messier representations. Since we've built up a powerful toolbox of statistical algorithms, it suffices to work with representations which merely correlate enough with properties of the world to be useful.
What I want to focus on is the second part, the notion that representations are meant to be operated on by algorithms. An algorithm is a systematic way of repeatedly applying mathematical operations to a representation in order to achieve some computational goal. Asking what algorithms a representation supports, therefore, is a matter of asking what mathematical operations can be meaningfully applied to it.
Consider some of the most widely used unsupervised learning techniques and the sorts of representations they produce:
- Clustering maps data points to a discrete set (say, the integers) where the only meaningful operation is equality.
- Nonlinear dimensionality reduction algorithms (e.g. multidimensional scaling, Isomap) map data points to a low-dimensional space where Euclidean distance is meaningful.
- Linear dimensionality reduction algorithms like PCA and factor analysis map data points to a low-dimensional space where Euclidean distance, linear combination, and dot products are all meaningful.
This list doesn't tell the whole story: there are a lot of variants on each of these categories, and there are more nuances in terms of precisely which operations are meaningful. For instance, in some versions of MDS, only the rank order of the Euclidean distances is meaningful. Still, I think it gives a useful coarse description of each of these categories.
There are also a wide variety of representation learning algorithms built around mathematical operations other than the ones given above:
- Greater/less than. TrueSkill is a model of people's performance in multiplayer games which represents each player with a scalar, and whoever has a larger value is expected to win. [2]
- $L_p$ distance . There is a variant of MDS which uses general $L_p$ distance rather than Euclidean distance. [3]
- Hamming distance. Semantic hashing is a technique in image retrieval which tries to represent images in terms of binary representations where the Hamming distance reflects the semantic dissimilarity between the images. The idea is that we can use the binary representation as a "hash key" and find semantically similar images by flipping random bits and finding the corresponding hash table entries. [4]
- Projection onto an axis. We might want the individual coordinates of a representation to represent something meaningful. As Shepard discusses, MDS with L1 distance often results in such a representation. Another MDS-based technique he describes is INDSCAL, which tries to model multiple subjects' similarity judgments with MDS in a single coordinate system, but where the individual axes can be rescaled for each subject. [3]
- Matrix multiplication. Paccanaro and Hinton [5] proposed a model called linear relational embedding, where entities are represented by vectors and relations between them are represented by matrices. Matrix multiplication corresponds to function composition: if we compose "father of" and "father of," we get the relation "paternal grandfather of."
Roger Shepard's classic 1980 paper, " Multidimensional scaling, tree fitting, and clustering ," [3] highlights a variety of different representation learning methods, and I took a lot of my examples from it. The paper is written from a cognitive science perspective, where the algorithms are used to model human similarity judgments and reaction time data, with the goal of understanding what our internal mental representations might be like. But the same kinds of models are widely used today, albeit in a more modern form, in statistics and machine learning.
One of the most exciting threads of representation learning in recent years has been learning feature representations which could be fed into standard machine learning (usually supervised learning) algorithms. Depending on the intended learning algorithm, the representation has to support some set of operations. For instance,
- For nonparametric regression/classification methods like nearest neighbors or an SVM with an RBF kernel, we want Euclidean distance to be meaningful.
- For linear classifiers, we want dot products to be meaningful.
- For decision trees or L1 regularization, we want projection onto an axis to be meaningful.
For instance, PCA is often used as a preprocessing step in linear regression or kernel SVMs, because it produces a low-dimensional representation in which Euclidean distance and dot products are likely to be more semantically meaningful than the same operations in the original space. However, it would probably not be a good preprocessing step for decision trees or L1 regularization, since the axes themselves (other than possibly the first few) don't have any special meaning. The issues have become somewhat blurred with the advent of deep learning, since we can argue endlessly about which parts of the representation are semantically meaningful. Still, I think these issues at least implicitly guide the research in the area.
The question of which operations and algorithms are supported is, of course, only one aspect of the representation learning problem. We also want representations which where both the mapping and the inverse mapping can be computed efficiently, which can be learned in a data efficient way, and so on. But I still find this a useful way to think about the relationships between different representation learning algorithms.
[1] David Marr. Vision: a Computational Investigation . W. H. Freeman and company, 1982.
[2] Ralf Herbrich, Tom Minka, and Thore Graepel, TrueSkill(TM): A Bayesian Skill Rating System , in Advances in Neural Information Processing Systems 20 , MIT Press, January 2007
[3] Roger Shepard. "Multidimensional scaling, tree-fitting, and clustering." Science, 1980.
[4] Ruslan Salakhutdinov and Geoffrey Hinton. "Semantic Hashing." SIGIR Workshop on Information Retrieval and Applications of Graphical Models, 2007.
[5] Alberto Paccanaro and Geoffrey Hinton. "Learning distributed representations of concepts using linear relational embedding." KDE 2000.
Share: Twitter , Facebook
Knowledge Representation in AI: The Foundation of Intelligent Systems

Knowledge representation plays a crucial role in the field of artificial intelligence (AI), serving as the bedrock for the development of intelligent systems. By allowing AI systems to organize and process information in a way that imitates human cognition, knowledge representation bridges the gap between raw data and meaningful insights. This article explores the various techniques and approaches used in AI knowledge representation , highlighting its importance and applications in different domains.
Key Takeaways:
- AI knowledge representation is essential for organizing and processing information in a way that mimics human cognition.
- Techniques like semantic networks , frames , and ontologies enable AI systems to capture and reason with hierarchical, associative, and formal relationships.
- Knowledge representation is crucial for AI reasoning, decision-making, and providing valuable recommendations in various domains.
- Challenges in knowledge representation include ambiguity and vagueness in natural language and handling complex and scalable data.
- Applications of knowledge representation span expert systems , natural language processing , and robotics , enhancing capabilities in decision-making, language understanding, and physical interaction.
Understanding Knowledge Representation

Knowledge representation is a fundamental process in artificial intelligence (AI) that enables AI systems to comprehend and manipulate information. By structuring and organizing data in a way that mirrors human understanding, knowledge representation facilitates the reasoning and decision-making capabilities of AI systems. Various techniques, such as semantic networks , frames , and ontologies , are employed to capture the hierarchical, associative, and formal relationships between concepts, enhancing the AI system’s ability to generate intelligent responses.
One common technique used in knowledge representation is semantic networks . These networks utilize interconnected nodes and edges to represent concepts and the relationships between them. This approach allows for the capture of hierarchical knowledge structures, enabling AI systems to understand the context and associations between different concepts. Frames , on the other hand, involve organizing knowledge into predefined categories known as frames, which contain attributes, properties, and relationships. This technique allows for the structured representation of knowledge, making it easier for AI systems to process and reason with information.
“Knowledge representation enables AI systems to capture the essence of human cognition, allowing them to mimic our thought processes and generate intelligent responses.” – AI Expert
Ontologies provide a formal structure for knowledge representation and are often used in domains where precise classification and categorization are required. These hierarchical representations allow for efficient retrieval and inference by AI systems, enabling them to navigate complex knowledge domains effectively. By employing these techniques, AI systems can understand and interpret information in a manner similar to human cognition, facilitating problem-solving, decision-making, and generating valuable insights.
Table: Comparison of Knowledge Representation Techniques
The choice of knowledge representation technique depends on the specific requirements and characteristics of the AI application. Each technique has its strengths and limitations, and the selection should be based on the nature of the knowledge and the intended use of the AI system.
Importance of Knowledge Representation in AI
Knowledge representation plays a crucial role in the field of artificial intelligence (AI) by enabling reasoning and decision-making. AI systems need a structured format to process and understand information, allowing them to derive valuable insights and make informed choices. By representing knowledge in a way that mirrors human cognition, AI systems can effectively solve complex problems and provide relevant recommendations in various domains.
AI reasoning and decision-making heavily rely on the structured representation of knowledge. Without a well-organized framework, AI systems would struggle to comprehend and analyze vast amounts of information. Knowledge representation allows AI systems to capture the hierarchical, associative, and formal relationships among concepts, providing a foundation for intelligent reasoning.
Additionally, knowledge representation empowers AI systems to handle uncertainty and ambiguity inherent in human language and real-world data. By encapsulating knowledge in structured formats such as semantic networks, frames, and ontologies, AI systems can better understand context, disambiguate meaning, and make accurate interpretations. This enhanced comprehension enables AI systems to generate intelligent responses and contribute to effective decision-making processes.
The importance of knowledge representation in AI cannot be overstated. It forms the basis for AI systems to reason, learn, and make informed decisions. By representing knowledge in a structured and meaningful way, AI systems can bridge the gap between raw data and valuable insights, leading to advancements in various domains and contributing to the development of more intelligent systems.
Techniques of Knowledge Representation
In the field of artificial intelligence (AI), several techniques are commonly used for knowledge representation. These techniques play a vital role in enabling AI systems to understand and process information effectively. Some of the prominent techniques are semantic networks, frames, and ontologies.
1. Semantic Networks:
Semantic networks are graphical representations that depict the relationships between different concepts. In a semantic network, nodes represent individual concepts, while edges illustrate the connections or associations between these concepts. This technique allows AI systems to capture hierarchical and associative knowledge, enabling them to understand complex relationships and make informed decisions. Semantic networks provide a structured framework for organizing information, making it easier for AI systems to retrieve relevant data and draw meaningful insights.
Frames involve the organization of knowledge into predefined categories known as frames. Each frame consists of attributes, properties, and relationships that define a particular concept or object. By using frames, AI systems can represent knowledge in a structured manner, allowing for efficient reasoning and decision-making. Frames provide a way to capture important characteristics and associations related to specific concepts, enhancing the AI system’s understanding and ability to generate intelligent responses.
3. Ontologies:
Ontologies provide a formal and explicit representation of knowledge. They establish a hierarchy of concepts and their relationships, similar to a taxonomy. Ontologies not only define the concepts but also specify the properties, attributes, and constraints associated with each concept. This technique enables AI systems to reason and infer based on the defined rules and relationships within the ontology. Ontologies offer a systematic approach to knowledge representation, facilitating efficient knowledge acquisition, storage, and retrieval.
These techniques of knowledge representation, namely semantic networks, frames, and ontologies, provide AI systems with the necessary tools to organize, understand, and reason with information effectively. Each technique has its unique advantages and applications, allowing AI systems to tackle complex problems and generate intelligent responses. The choice of technique depends on the specific requirements and characteristics of the AI system and the domain in which it operates.
Challenges in Knowledge Representation
Knowledge representation in AI faces various challenges that impact its effectiveness and efficiency. Two major challenges in knowledge representation are Ambiguity and Vagueness , and Scalability and Complexity .
Ambiguity and Vagueness
Ambiguity refers to the presence of multiple interpretations or meanings for a given piece of information. In natural language, words and phrases may have different meanings depending on the context, making it challenging to represent knowledge accurately. Vagueness, on the other hand, refers to the lack of clarity or precision in defining concepts or boundaries.
The representation of ambiguous and vague information requires AI systems to handle uncertainty and make probabilistic inferences. Techniques such as fuzzy logic and probabilistic reasoning can be used to manage ambiguity and vagueness , allowing AI systems to make informed decisions even when dealing with imprecise or uncertain knowledge.
Scalability and Complexity
As knowledge bases grow in size and complexity, maintaining a coherent and efficient representation becomes a significant challenge. The scalability challenge arises due to the need to handle vast amounts of data and ensure quick and accurate retrieval. AI systems must efficiently organize and index knowledge for efficient search and retrieval, which becomes increasingly difficult as the volume of data increases.
Furthermore, the complexity of knowledge representation stems from the need to capture diverse and intricate relationships between concepts and entities. Representing highly interconnected knowledge requires sophisticated techniques such as ontologies and semantic networks to maintain the integrity and richness of the information.
By addressing these challenges, AI researchers and developers can enhance the quality and effectiveness of knowledge representation, thereby improving the overall performance of AI systems in reasoning, decision-making, and problem-solving tasks.
Applications of Knowledge Representation

Knowledge representation plays a vital role in various domains, including expert systems , natural language processing , and robotics . These applications leverage the power of knowledge representation techniques to enhance AI capabilities and enable intelligent interactions with humans and the physical world.
Expert Systems
In the field of expert systems , knowledge representation is instrumental in replicating the decision-making abilities of human experts. By utilizing techniques such as semantic networks, frames, and ontologies, AI systems can capture and organize domain-specific knowledge. This structured representation allows expert systems to provide valuable advice, recommendations, and solutions in various industries such as healthcare, finance, and engineering.
Natural Language Processing
Natural language processing (NLP) involves the understanding and generation of human language by AI systems. Knowledge representation plays a crucial role in NLP, enabling machines to comprehend the context of a conversation, identify relationships between words and phrases, and generate coherent responses. By representing knowledge in a structured format, AI systems can analyze and interpret language more effectively, leading to improved communication and interaction with humans.
Knowledge representation is essential in the field of robotics as it enables machines to navigate and interact with the physical world. By representing knowledge about the environment, objects, and actions, AI systems can understand their surroundings and make informed decisions. This allows robots to perform tasks efficiently, ensure safe movements, and adapt to dynamic situations. Knowledge representation empowers robots to learn from previous experiences and apply that knowledge in real-time, enhancing their overall functionality and autonomy.
As AI continues to advance, knowledge representation will play an even more significant role in shaping intelligent systems. Its applications in expert systems, natural language processing, and robotics demonstrate the wide-reaching impact of structured knowledge on enhancing AI capabilities. By refining knowledge representation techniques , AI researchers and practitioners are working towards developing more intelligent, adaptable, and autonomous systems that can revolutionize various industries and improve the lives of individuals worldwide.
The Future of Knowledge Representation
As artificial intelligence (AI) continues to advance and transform various industries, the field of knowledge representation is also evolving to keep pace with the growing complexity of data. Advancements in knowledge representation are crucial for AI systems to effectively handle dynamic and unstructured data, enabling them to make more intelligent decisions and generate meaningful insights.
One of the key areas of focus for future advancements in knowledge representation is the handling of dynamic data. Traditional knowledge representation techniques are often based on static knowledge bases, which can limit the ability of AI systems to adapt and learn from real-time data. By developing techniques that can handle dynamic data, AI systems will be able to continuously update and refine their knowledge, leading to more accurate and up-to-date decision-making.
Another important aspect of future knowledge representation is the handling of unstructured data. Unstructured data, such as text, images, and videos, poses a significant challenge for AI systems, as it lacks a predefined format and structure. Advancements in natural language processing and computer vision are enabling AI systems to better understand and interpret unstructured data, allowing for more effective knowledge representation and analysis.
In summary, the future of knowledge representation in AI holds immense potential for advancements in handling dynamic and unstructured data. By developing techniques that can adapt to real-time data and effectively interpret unstructured information, AI systems will be able to achieve greater levels of understanding and cognition. These advancements will pave the way for more intelligent AI systems that can make informed decisions, provide valuable insights, and revolutionize various industries.
Knowledge representation forms the very essence of AI intelligence . With a structured framework to organize and process information, AI systems gain the ability to think, learn, and reason – mirroring human cognition. The techniques and approaches employed in knowledge representation enable AI systems to decipher intricate data, solve complex problems, and make informed decisions.
As technology continues to advance, optimizing knowledge representation techniques will unlock new frontiers for AI systems to achieve unprecedented levels of understanding and cognition. These advancements empower AI to handle dynamic and unstructured data, paving the way for even smarter systems.
The future of knowledge representation holds immense potential, propelling AI intelligence to greater heights. By continuously refining these techniques, AI systems will transcend their current capabilities, bridging the gap between raw data and meaningful insights. This progress will steer AI towards enhanced cognitive abilities and pave the path for a future where intelligent systems become an indispensable part of our lives.
What is knowledge representation in AI?
Knowledge representation is the process of structuring and organizing information in a format that AI systems can comprehend and manipulate.
Why is knowledge representation important in AI?
Knowledge representation is crucial in AI because it enables reasoning, decision-making, and the generation of meaningful insights from data.
What are the techniques used in knowledge representation?
The techniques used in knowledge representation include semantic networks, frames, and ontologies.
What challenges does knowledge representation face?
Knowledge representation faces challenges related to ambiguity and vagueness in natural language, as well as scalability and complexity as knowledge bases grow in size.
Where is knowledge representation applied?
Knowledge representation finds applications in expert systems, natural language processing, and robotics, among other domains.
How is knowledge representation advancing in the future?
Efforts are being made to develop more sophisticated approaches to handle dynamic and unstructured data, allowing for even smarter AI systems.

The Quest for Transparency in NLP Systems: Understanding the Black Box

Ensuring Fairness in Language Models: Overcoming Bias and Inequality

Protecting User Privacy: Essential Strategies in NLP Applications

Navigating the Ethical Landscape of AI and NLP: Challenges and Solutions

Unveiling Bias in NLP Algorithms: A Path Towards Fairer AI

The Future is Now: Real-time NLP Analysis in Big Data Environments
- Free Onboarding
- Privacy Policy
- Terms and Conditions
- Affiliate Program 💵
- Why Libraria AI?
- Documentation
- API Playground
- Ecommerce AI
- Preprocessing Content with AI
- Libraria AI Internal Chat
- Chatbase vs Libraria AI
- AI Preprocessing
- Images in your AI Chatbot
- Chat History
- Hispanoamérica
- Work at ArchDaily
- Terms of Use
- Privacy Policy
- Cookie Policy
From Representation to Reality: 19 Projects that Rethink Representation Techniques
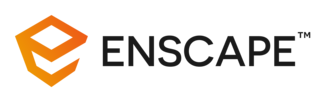
- Written by Agustina Coulleri
- Published on April 04, 2022
Graphics, even before language and writing, were the first means of communication and significance for humanity. Drawing is the act of replacing reality with representation, that is, replacing objects with images encoded in each of the graphic representation systems.
In architecture, graphics stimulate the imagination and are the basis of project thinking since they do not only constitute our code of communication but configure our ability to express ourselves in disciplinary terms. In fact, at first, the drawing is constructed in the mind of the architect, before it looks for support from any type of instrument.
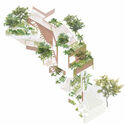
In this way, the drawing, in all its forms, guarantees learning and better management of the architectural space. As Rafael Moneo said: "Visual education gives a certain discipline in the eyes and in the hand and both come from the learning of drawing. Drawing helps you explain and is a way to help you get a judgment of the scenes, to see the corporeity of the scenes [...] Visual education gives the drawing."
The following collection of drawings shows the changes and similarities between the author’s graphic and the finished work. Some are generative drawings, process drawings, and others are post-project drawings; making up a collection of graphic explorations of great inspiration for us.
Vegan House / Block Architects
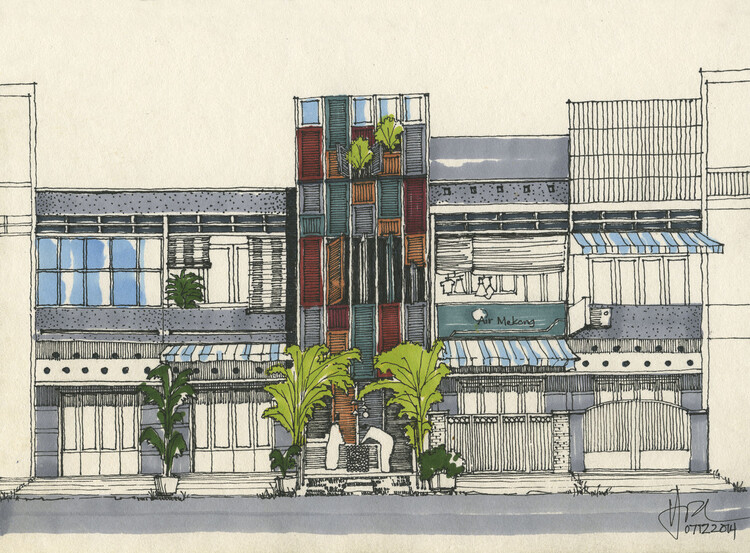
House in Melgaço / Nuno Brandão Costa
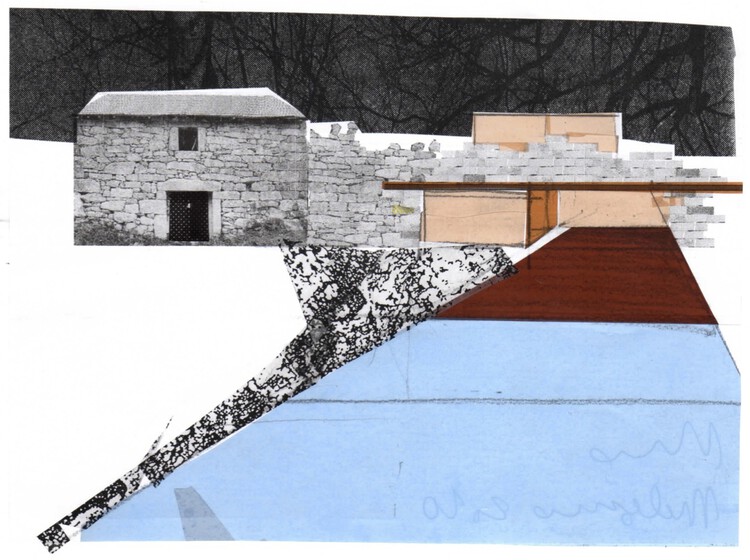
INES Innovation Center / Pezo von Ellrichshausen
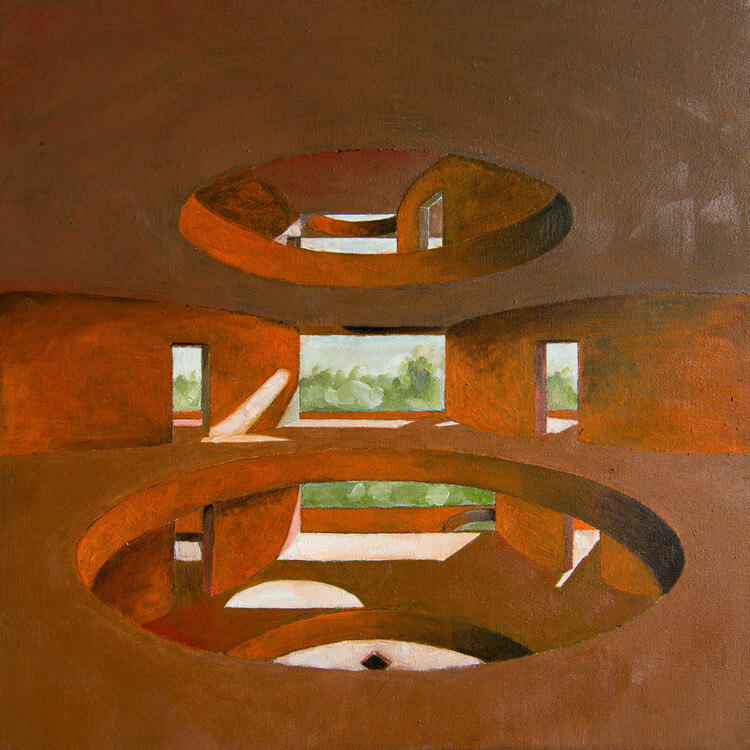
Innovation Studio / Sir Peter Cook
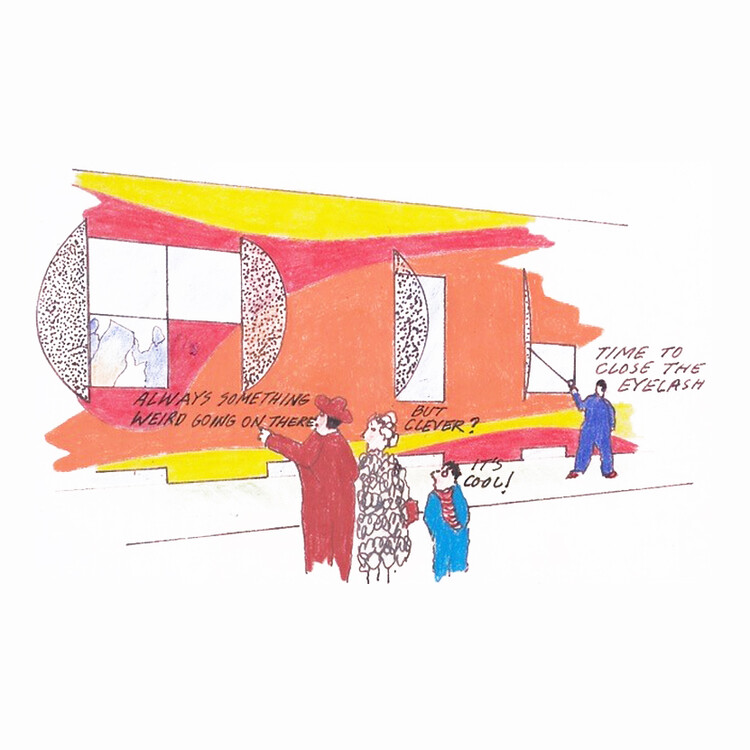
Infinity Bench / Azócar Catrón Arquitectos
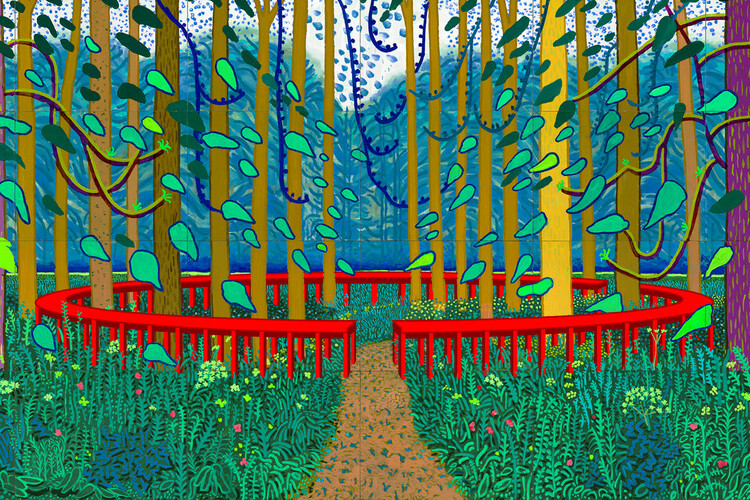
Le Monde Group Headquarters / Snøhetta
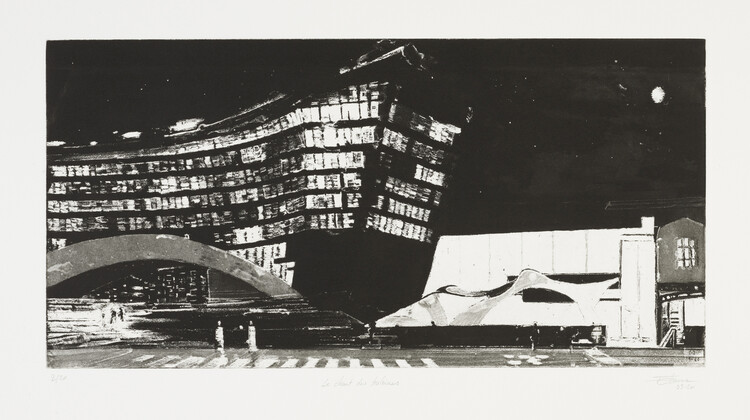
Mies Missing Materiality Installation / Anna & Eugeni Bach
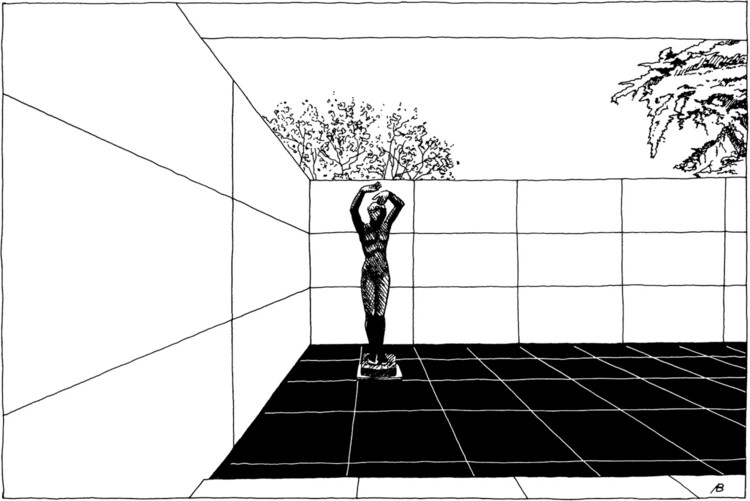
Peppertree Villa / Luigi Rosselli
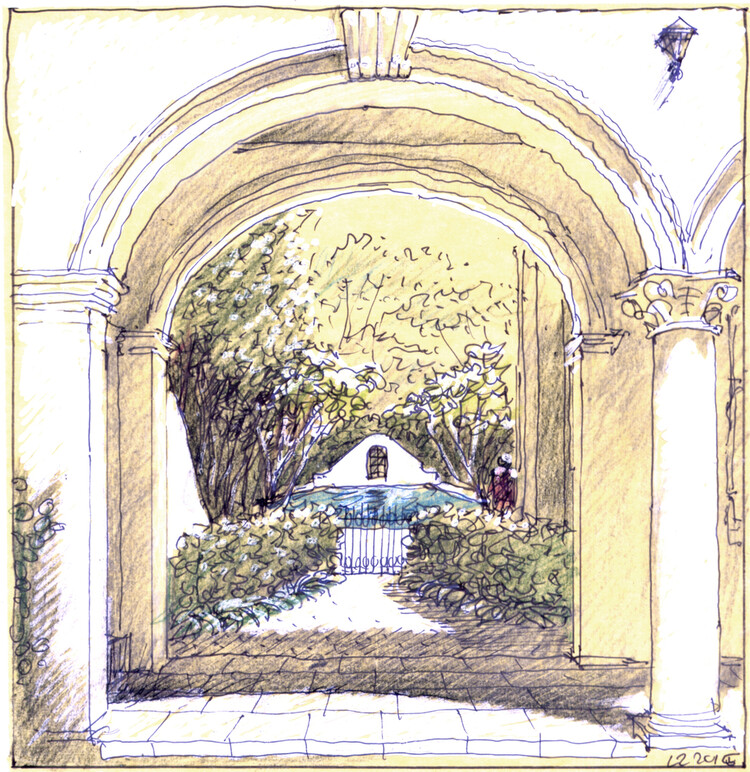
Collective Housing 110 Rooms / MAIO
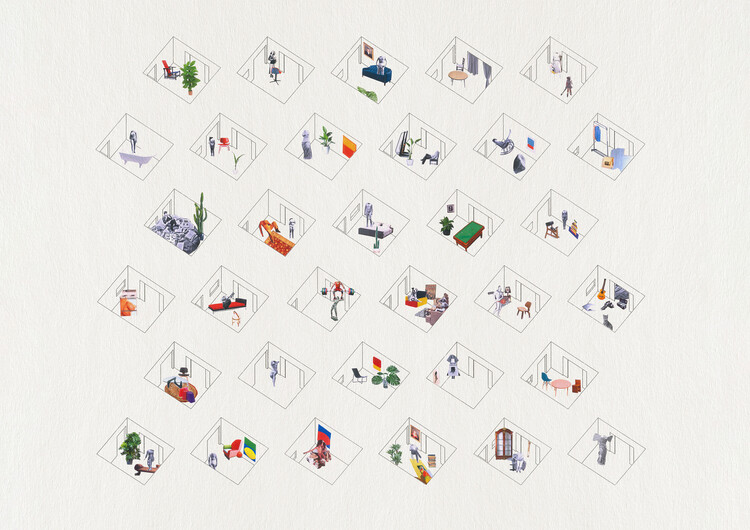
Tower House / Albor Arquitectos
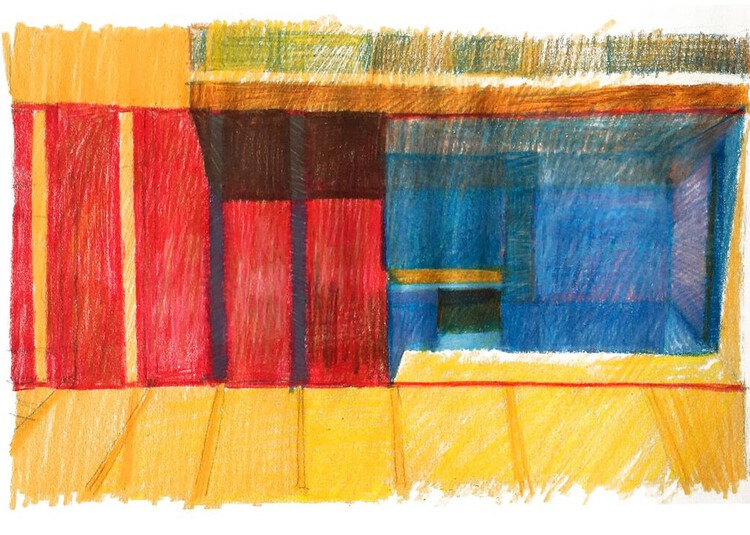
Meloso Restaurant / t-unoauno + arqaz arquitectura
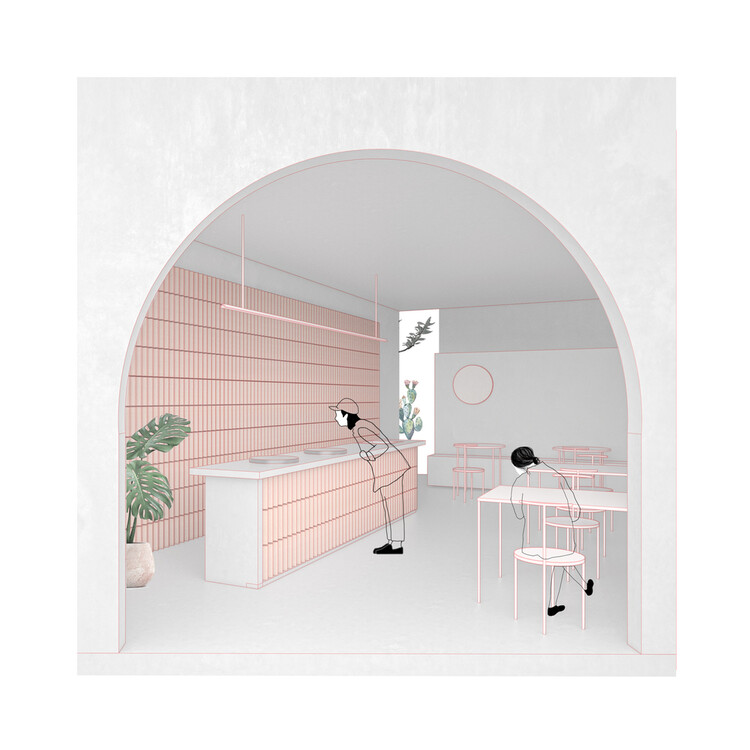
BT House / Studio Guilherme Torres
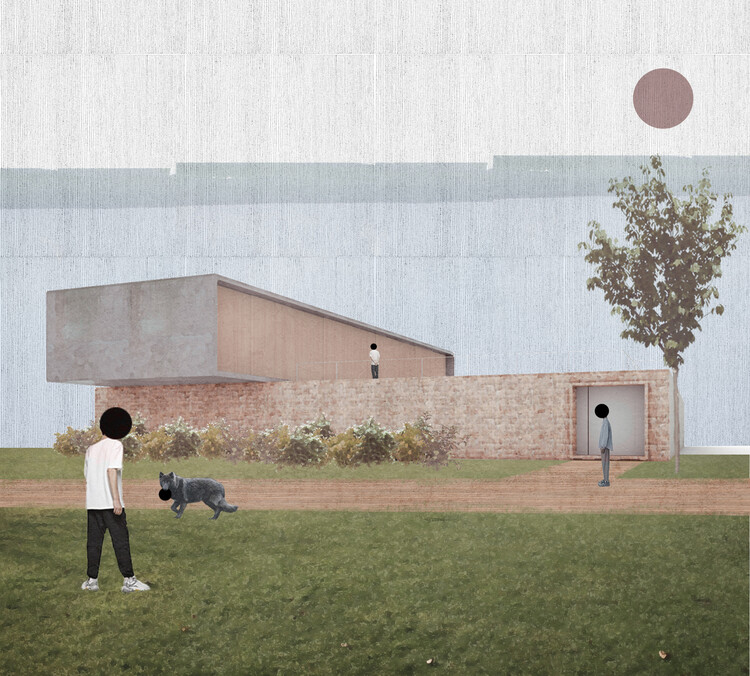
Types of Spaces Installation at CONCÉNTRICO Festival / Palma + HANGHAR
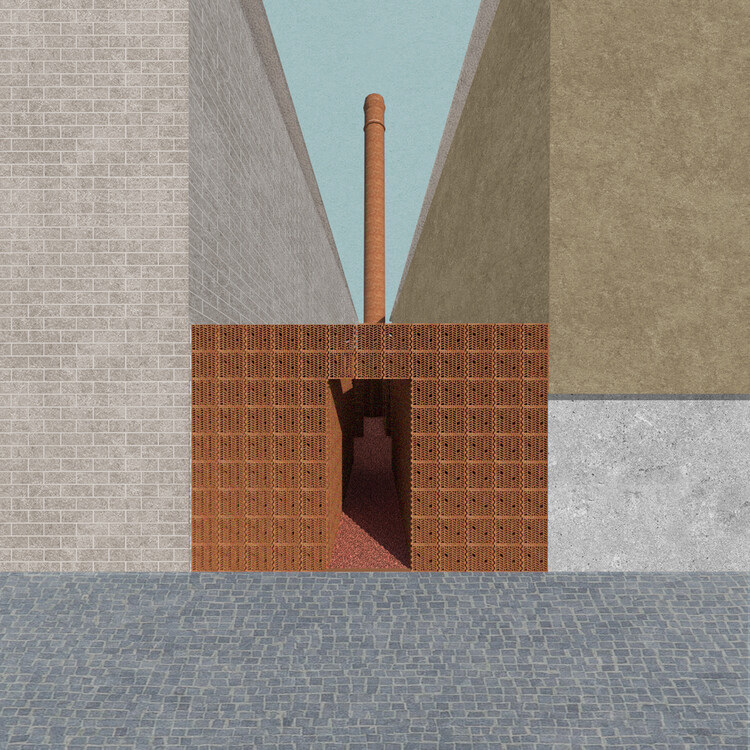
Miraflores House / Gerardo Caballero Maite Fernandez Arquitectos
.jpg?1647015994)
This is my Square Intervention / Conjuntos Empáticos
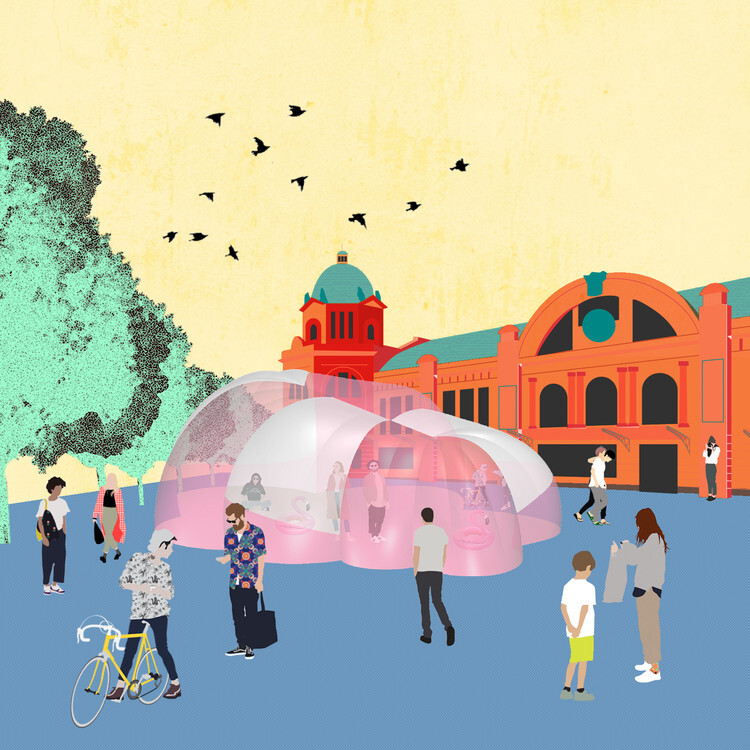
Ha House / VTN Architects
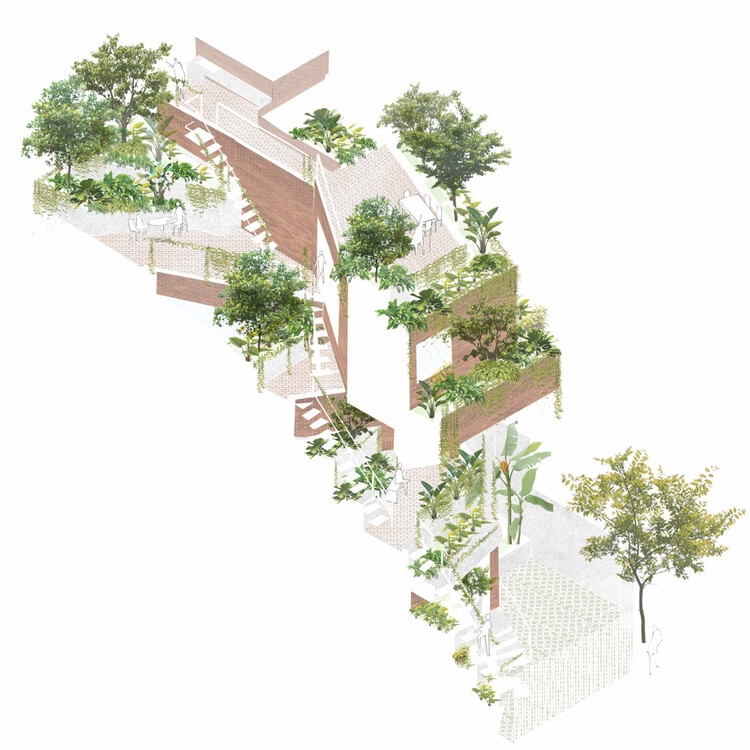
Run Run Run Intervention / Andrés Jaque / Office for Political Innovation
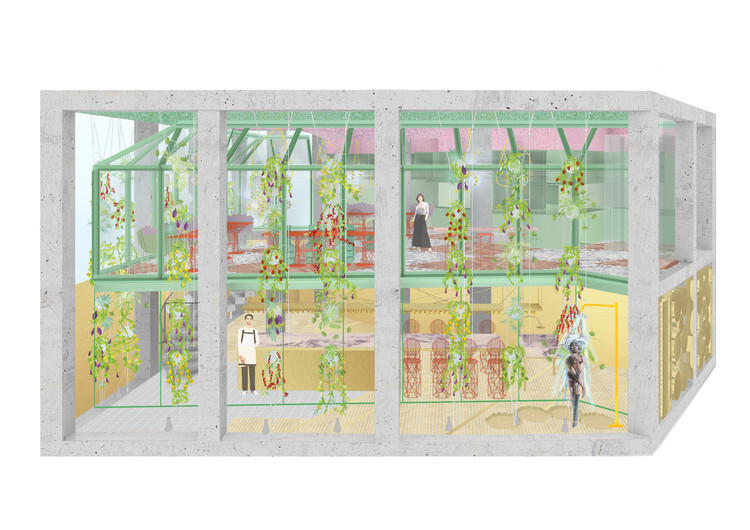
Bus House / OOIIO Architecture
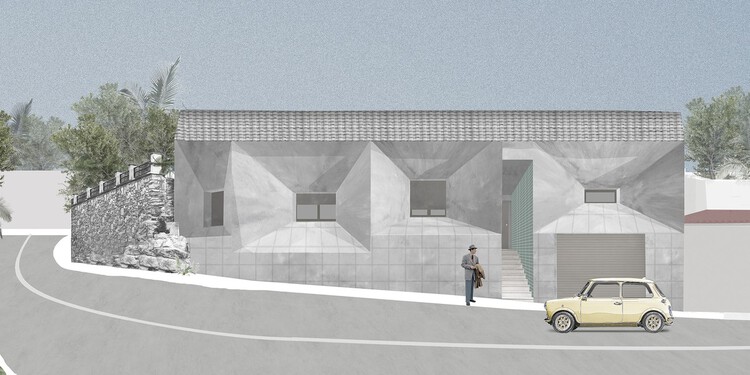
Tea Room / Natura Futura Arquitectura
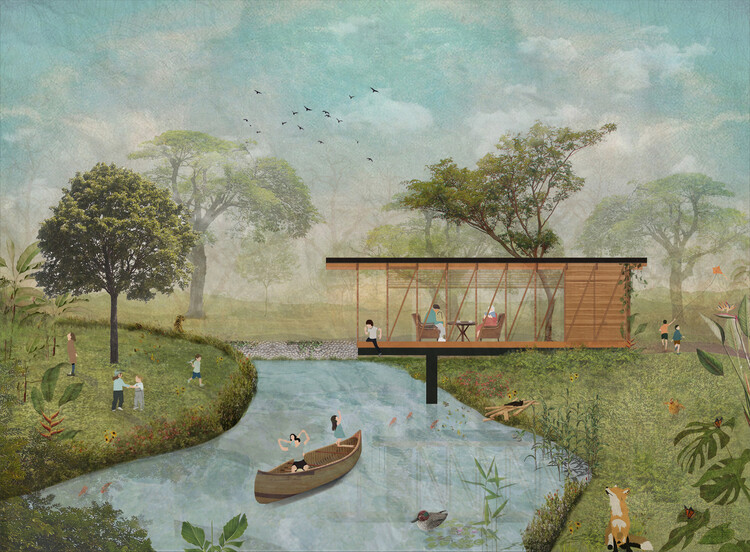
This article is part of the ArchDaily Topics: The Future of Architectural Visualizations , proudly presented by Enscape , the most intuitive real-time rendering and virtual reality plugin for Revit, SketchUp, Rhino, Archicad, and Vectorworks. Enscape plugs directly into your modeling software, giving you an integrated visualization and design workflow. Every month we explore a topic in-depth through articles, interviews, news, and projects. Learn more about our ArchDaily topics . As always, at ArchDaily we welcome the contributions of our readers; if you want to submit an article or project, contact us .
Image gallery
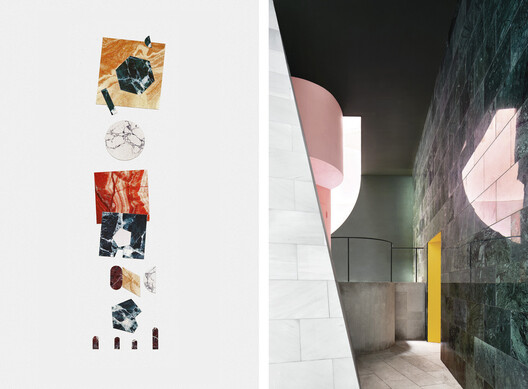
- Sustainability
想阅读文章的中文版本吗?
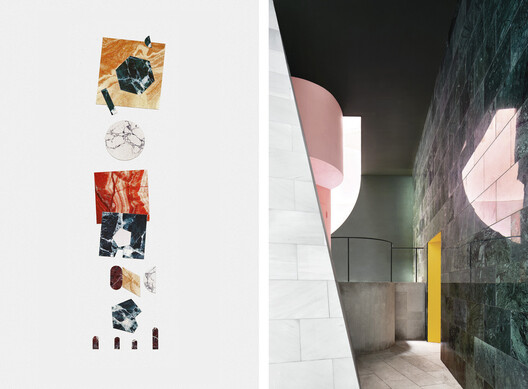
由表现到真实:19 个重新思考建筑图纸表现手法的项目
You've started following your first account, did you know.
You'll now receive updates based on what you follow! Personalize your stream and start following your favorite authors, offices and users.
Subscribe to the PwC Newsletter
Join the community, add a new evaluation result row, representation learning.
3722 papers with code • 5 benchmarks • 9 datasets
Representation Learning is a process in machine learning where algorithms extract meaningful patterns from raw data to create representations that are easier to understand and process. These representations can be designed for interpretability, reveal hidden features, or be used for transfer learning. They are valuable across many fundamental machine learning tasks like image classification and retrieval .
Deep neural networks can be considered representation learning models that typically encode information which is projected into a different subspace. These representations are then usually passed on to a linear classifier to, for instance, train a classifier.
Representation learning can be divided into:
- Supervised representation learning : learning representations on task A using annotated data and used to solve task B
- Unsupervised representation learning : learning representations on a task in an unsupervised way (label-free data). These are then used to address downstream tasks and reducing the need for annotated data when learning news tasks. Powerful models like GPT and BERT leverage unsupervised representation learning to tackle language tasks.
More recently, self-supervised learning (SSL) is one of the main drivers behind unsupervised representation learning in fields like computer vision and NLP.
Here are some additional readings to go deeper on the task:
- Representation Learning: A Review and New Perspectives - Bengio et al. (2012)
- A Few Words on Representation Learning - Thalles Silva
( Image credit: Visualizing and Understanding Convolutional Networks )

Benchmarks Add a Result

Most implemented papers
Unsupervised representation learning with deep convolutional generative adversarial networks.
In recent years, supervised learning with convolutional networks (CNNs) has seen huge adoption in computer vision applications.
Neural Discrete Representation Learning
Learning useful representations without supervision remains a key challenge in machine learning.
Momentum Contrast for Unsupervised Visual Representation Learning

This enables building a large and consistent dictionary on-the-fly that facilitates contrastive unsupervised learning.
Deep High-Resolution Representation Learning for Visual Recognition
High-resolution representations are essential for position-sensitive vision problems, such as human pose estimation, semantic segmentation, and object detection.
Deep High-Resolution Representation Learning for Human Pose Estimation
We start from a high-resolution subnetwork as the first stage, gradually add high-to-low resolution subnetworks one by one to form more stages, and connect the mutli-resolution subnetworks in parallel.
High-Resolution Representations for Labeling Pixels and Regions
The proposed approach achieves superior results to existing single-model networks on COCO object detection.
InfoGAN: Interpretable Representation Learning by Information Maximizing Generative Adversarial Nets
This paper describes InfoGAN, an information-theoretic extension to the Generative Adversarial Network that is able to learn disentangled representations in a completely unsupervised manner.
Improved Baselines with Momentum Contrastive Learning
Contrastive unsupervised learning has recently shown encouraging progress, e. g., in Momentum Contrast (MoCo) and SimCLR.
Domain-Adversarial Training of Neural Networks

Our approach is directly inspired by the theory on domain adaptation suggesting that, for effective domain transfer to be achieved, predictions must be made based on features that cannot discriminate between the training (source) and test (target) domains.
Bootstrap your own latent: A new approach to self-supervised Learning
From an augmented view of an image, we train the online network to predict the target network representation of the same image under a different augmented view.
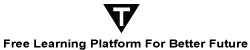
Artificial Intelligence
Control System
- Interview Q
Intelligent Agent
Problem-solving, adversarial search, knowledge represent, uncertain knowledge r., subsets of ai, artificial intelligence mcq, related tutorials.
Let's suppose we are taking an entity, Peter. Peter is an engineer as a profession, and his age is 25, he lives in city London, and the country is England. So following is the frame representation for this:
Advantages of frame representation:
- The frame knowledge representation makes the programming easier by grouping the related data.
- The frame representation is comparably flexible and used by many applications in AI.
- It is very easy to add slots for new attribute and relations.
- It is easy to include default data and to search for missing values.
- Frame representation is easy to understand and visualize.
Disadvantages of frame representation:
- In frame system inference mechanism is not be easily processed.
- Inference mechanism cannot be smoothly proceeded by frame representation.
- Frame representation has a much generalized approach.
4. Production Rules
Production rules system consist of ( condition, action ) pairs which mean, "If condition then action". It has mainly three parts:
- The set of production rules
- Working Memory
- The recognize-act-cycle
In production rules agent checks for the condition and if the condition exists then production rule fires and corresponding action is carried out. The condition part of the rule determines which rule may be applied to a problem. And the action part carries out the associated problem-solving steps. This complete process is called a recognize-act cycle.
The working memory contains the description of the current state of problems-solving and rule can write knowledge to the working memory. This knowledge match and may fire other rules.
If there is a new situation (state) generates, then multiple production rules will be fired together, this is called conflict set. In this situation, the agent needs to select a rule from these sets, and it is called a conflict resolution.
- IF (at bus stop AND bus arrives) THEN action (get into the bus)
- IF (on the bus AND paid AND empty seat) THEN action (sit down).
- IF (on bus AND unpaid) THEN action (pay charges).
- IF (bus arrives at destination) THEN action (get down from the bus).
Advantages of Production rule:
- The production rules are expressed in natural language.
- The production rules are highly modular, so we can easily remove, add or modify an individual rule.
Disadvantages of Production rule:
- Production rule system does not exhibit any learning capabilities, as it does not store the result of the problem for the future uses.
- During the execution of the program, many rules may be active hence rule-based production systems are inefficient.

- Send your Feedback to [email protected]
Help Others, Please Share

Learn Latest Tutorials

Transact-SQL

Reinforcement Learning

R Programming

React Native

Python Design Patterns

Python Pillow

Python Turtle

Preparation

Verbal Ability

Interview Questions

Company Questions
Trending Technologies

Cloud Computing

Data Science

Machine Learning

B.Tech / MCA

Data Structures

Operating System

Computer Network

Compiler Design

Computer Organization

Discrete Mathematics

Ethical Hacking

Computer Graphics

Software Engineering

Web Technology

Cyber Security

C Programming

Data Mining

Data Warehouse

An introduction to representation learning
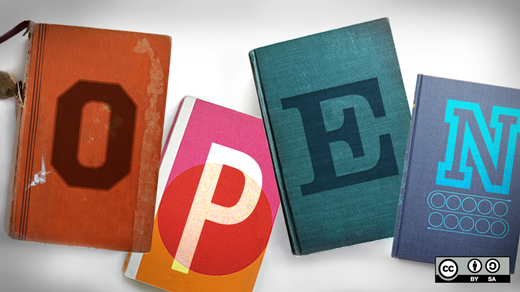
Opensource.com
Although many companies today possess massive amounts of data, the vast majority of that data is often unstructured and unlabeled. In fact, the amount of data that is appropriately labeled for a specific business need is typically quite small (possibly even zero), and acquiring new labels is usually a slow, expensive endeavor. As a result, algorithms that can extract features from unlabeled data to improve the performance of data-limited tasks are quite valuable.
Most machine learning practitioners are first exposed to feature extraction techniques through unsupervised learning . In unsupervised learning, an algorithm attempts to discover the latent features that describe a data set's "structure" under certain (either explicit or implicit) assumptions. For example, low-rank singular value decomposition (of which principal component analysis is a specific example ) factors a data matrix into three reduced rank matrices that minimize the squared error of the reconstructed data matrix.
Representation learning
Although traditional unsupervised learning techniques will always be staples of machine learning pipelines, representation learning has emerged as an alternative approach to feature extraction with the continued success of deep learning. In representation learning, features are extracted from unlabeled data by training a neural network on a secondary, supervised learning task.
Due to its popularity, word2vec has become the de facto "Hello, world!" application of representation learning. When applying deep learning to natural language processing (NLP) tasks, the model must simultaneously learn several language concepts:
- the meanings of words
- how words are combined to form concepts (i.e., syntax)
- how concepts relate to the task at hand
For example, you may have heard of the neural network that generates color names . Essentially, the model was trained to generate names for colors from RGB values using paint swatch data. While the concept was really neat, the results were fairly underwhelming; the model seemed to produce nonsensical color names and randomly pair names with colors. The task, as attempted, was simply too difficult for the model to learn, given the paucity of data.
Word2vec makes NLP problems like these easier to solve by providing the learning algorithm with pre-trained word embeddings, effectively removing the word meaning subtask from training. The word2vec model was inspired by the distributional hypothesis , which suggests words found in similar contexts often have similar meanings. Specifically, the model is trained to predict a central word given its surrounding words in a window of a certain size. For example, for the sentence "Natural language processing can be difficult" and a window size of three, the input/target combinations for the neural network would be:
["natural", "processing"] → "language" ["language", "can"] → "processing" ["processing", "be"] → "can" ["can", "difficult"] → "be"
When trained with enough data, the word embeddings tend to capture word meanings quite well, and are even able to perform analogies, e.g., the vector for "Paris" minus the vector for "France" plus the vector for "Italy" is very close to the vector for "Rome." Indeed, by incorporating word2vec vectors into the color names model, I achieved much more compelling results .
Customer2vec
Red Hat , like many business-to-business (B2B) companies, is often faced with data challenges that are distinct from those faced by business-to-consumer (B2C) companies. Typically, B2B companies handle orders of magnitude fewer "customers" than their B2C counterparts. Further, feedback cycles are generally much longer for B2B companies due to the nature of multi-year contracts. However, like B2C companies, many B2B companies possess mountains of behavioral data. Representation learning algorithms give B2B companies like Red Hat the ability to better optimize business strategies with limited historical context by extracting meaningful information from unlabeled data.
In many ways, web activity data resembles the data found in NLP tasks. There are terms (= specific URLs), sentences (= days of activity), and documents (= individual customers). With that being the case, web activity data is a perfect candidate for doc2vec . Doc2vec is a generalization of word2vec that, in addition to considering context words, considers the specific document when predicting a target word. This architecture allows the algorithm to learn meaningful representations of documents, which, in this instance, correspond to customers.
As an example, Red Hat data can be seen in Figure 1, where each line represents a different customer, each number represents a distinct URL, and the special [START] and [STOP] tokens denote the start and end of a day of activity. Once the data is in this format, training the model is as simple as two lines of code with gensim (Figure 2). These customer representations can then be used to form better segments for sales campaigns and product recommendations.
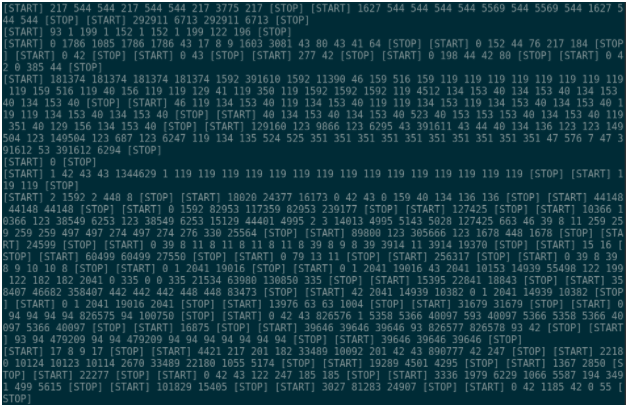
opensource.com
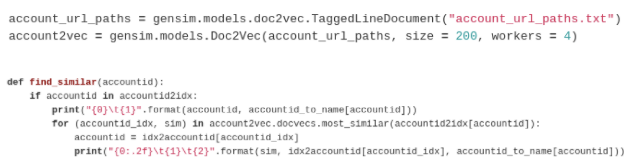
But representation learning models can be even more flexible than word2vec and doc2vec. For example, I've found that predicting at-bat outcomes for Major League Baseball batter/pitcher pairs can generate highly intuitive player embeddings.
Duplicate detection
Red Hat is also exploring the applicability of representation learning for detecting duplicate support content. Here, "duplicate" doesn't mean "exact copy," rather it indicates content that is conceptually redundant. Duplicate content can cause issues with information retrieval and introduce challenges when identifying support trends, so being able to effectively detect and remove duplicate content is important.
One strategy for duplicate detection is to look for similar latent semantic analysis (LSA) vectors, but there are assumptions and design elements in LSA that limit its effectiveness. Specifically, the model
- ignores word ordering
- implicitly assumes a Gaussian distribution on the term values by minimizing the squared error on the reconstructed matrix
- assumes that the term values are generated from a linear combination of the latent document and term vectors
Neural networks relax these assumptions, which makes them a good candidate for learning semantic representations of documents.
But the question remains of how to use a neural network for duplicate detection without any labeled data. To do so, we adapted the Deep Semantic Similarity Model (DSSM) developed by Microsoft Research for the task ( a Keras implementation of the model can be found on my GitHub ). The original motivation for the DSSM was to improve the relevance of search results by mapping documents and queries into a latent semantic space and use the cosine similarity of these vectors as a proxy for relevance. Essentially, the model compresses the documents and queries down to their essential concepts. To adapt the model for duplicate detection, we simply used document titles in place of queries and trained an otherwise nearly identical architecture (though we did use internally trained word2vec embeddings instead of letter-gram representations for words). The semantic document vectors were then used to find conceptually similar content.
Learn more about representation learning
This barely scratches the surface of representation learning, which is an active area of machine learning research (along with the closely related field of transfer learning ). For an extensive, technical introduction to representation learning, I highly recommend the "Representation Learning" chapter in Goodfellow, Bengio, and Courville's new Deep Learning textbook. For more information on word2vec, I recommend checking out this nice introduction by the folks over at DeepLearning4J .
Article republished with permission from MLConf.com . Use promo code "Redhat18" to save 18% off registration for the 2017 Machine Learning Conference in Atlanta, which will be held September 15.
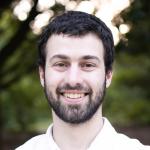
Comments are closed.
Related content.
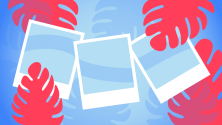
Find the perfect open source tool
Project management, business intelligence, reporting, and more. Check these popular projects.
Architectural Representation I
Architectural Representation I: Origins + Originality
Architectural representation is an ideology—a source of ideas and visionary theorizing that has a set of origins and qualities. As such, it’s prudent to study the origins of conventional techniques of architectural representation to be informed about their intentions and the specific contexts that conditioned their development.
Representation is not a conclusive index of an architecture already designed and completed, in the past tense. Rather, representation is integral to the design process and the production of architecture—it is present and future tense: an active participant in exploring and making. It occurs in multiple instances and forms along a project’s evolutionary path. Though not deterministic of the architecture, representation techniques selected to visualize ideas influence the evolution and outcome of the work.
The course initiates with an analysis of conventional representation techniques and their intentions. Using this knowledge as a platform, the class pivots to consider representational riffs emerging in response to the contemporary context—those that explore the limits of our “origin arsenal” and question what each offers for the present. Possible paradigms of architectural spaces generated from representation (rather than the other way around) will be presented and discussed.
“Architectural Representation I: Origins + Originality” will involve readings, lectures, and discussions framing the backstory on conventional techniques as well as contemporary critical stances in relation to these techniques. Students will be required to complete weekly representation exercises in relation to each course topic by experimenting with new representations of their design work being produced in parallel courses. These design exercises will be presented to and discussed by the class.
The final project will involve isolating a representation from concurrent studio work and critically evaluating the architectural possibilities that extend from its close reading and revision. The final project will require articulation of the goals of the original representation technique and the specific aims toward originality in the tweaking of this technique, as suited to the design project.
- Business Essentials
- Leadership & Management
- Credential of Leadership, Impact, and Management in Business (CLIMB)
- Entrepreneurship & Innovation
- Digital Transformation
- Finance & Accounting
- Business in Society
- For Organizations
- Support Portal
- Media Coverage
- Founding Donors
- Leadership Team
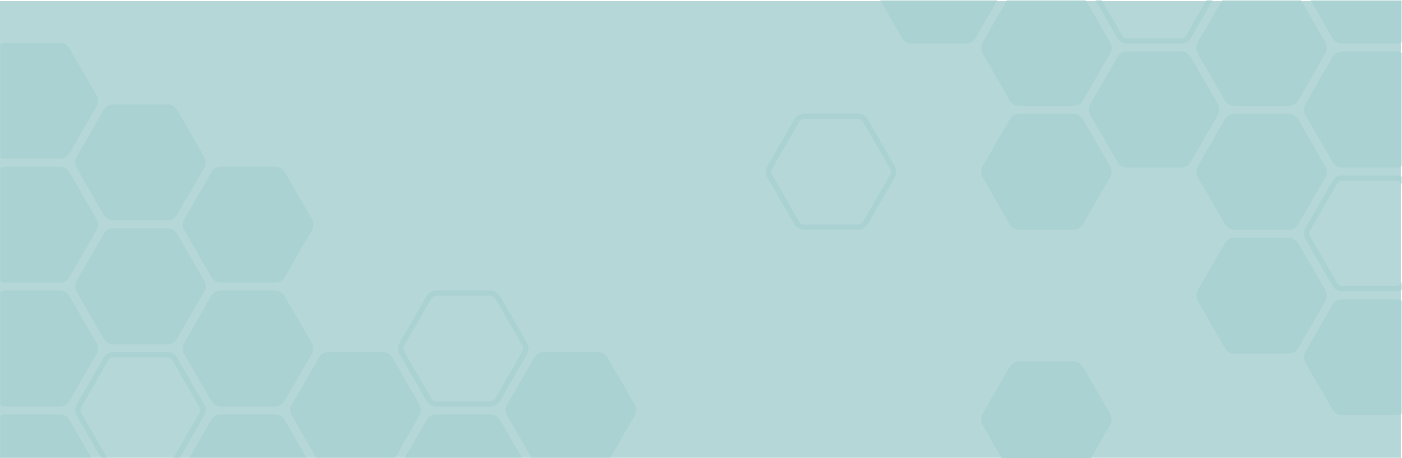
- Harvard Business School →
- HBS Online →
- Business Insights →
Business Insights
Harvard Business School Online's Business Insights Blog provides the career insights you need to achieve your goals and gain confidence in your business skills.
- Career Development
- Communication
- Decision-Making
- Earning Your MBA
- Negotiation
- News & Events
- Productivity
- Staff Spotlight
- Student Profiles
- Work-Life Balance
- AI Essentials for Business
- Alternative Investments
- Business Analytics
- Business Strategy
- Business and Climate Change
- Design Thinking and Innovation
- Digital Marketing Strategy
- Disruptive Strategy
- Economics for Managers
- Entrepreneurship Essentials
- Financial Accounting
- Global Business
- Launching Tech Ventures
- Leadership Principles
- Leadership, Ethics, and Corporate Accountability
- Leading with Finance
- Management Essentials
- Negotiation Mastery
- Organizational Leadership
- Power and Influence for Positive Impact
- Strategy Execution
- Sustainable Business Strategy
- Sustainable Investing
- Winning with Digital Platforms
17 Data Visualization Techniques All Professionals Should Know
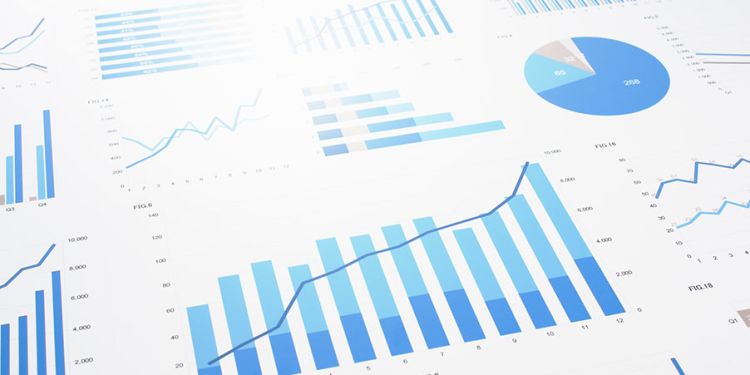
- 17 Sep 2019
There’s a growing demand for business analytics and data expertise in the workforce. But you don’t need to be a professional analyst to benefit from data-related skills.
Becoming skilled at common data visualization techniques can help you reap the rewards of data-driven decision-making , including increased confidence and potential cost savings. Learning how to effectively visualize data could be the first step toward using data analytics and data science to your advantage to add value to your organization.
Several data visualization techniques can help you become more effective in your role. Here are 17 essential data visualization techniques all professionals should know, as well as tips to help you effectively present your data.
Access your free e-book today.
What Is Data Visualization?
Data visualization is the process of creating graphical representations of information. This process helps the presenter communicate data in a way that’s easy for the viewer to interpret and draw conclusions.
There are many different techniques and tools you can leverage to visualize data, so you want to know which ones to use and when. Here are some of the most important data visualization techniques all professionals should know.
Data Visualization Techniques
The type of data visualization technique you leverage will vary based on the type of data you’re working with, in addition to the story you’re telling with your data .
Here are some important data visualization techniques to know:
- Gantt Chart
- Box and Whisker Plot
- Waterfall Chart
- Scatter Plot
- Pictogram Chart
- Highlight Table
- Bullet Graph
- Choropleth Map
- Network Diagram
- Correlation Matrices
1. Pie Chart

Pie charts are one of the most common and basic data visualization techniques, used across a wide range of applications. Pie charts are ideal for illustrating proportions, or part-to-whole comparisons.
Because pie charts are relatively simple and easy to read, they’re best suited for audiences who might be unfamiliar with the information or are only interested in the key takeaways. For viewers who require a more thorough explanation of the data, pie charts fall short in their ability to display complex information.
2. Bar Chart

The classic bar chart , or bar graph, is another common and easy-to-use method of data visualization. In this type of visualization, one axis of the chart shows the categories being compared, and the other, a measured value. The length of the bar indicates how each group measures according to the value.
One drawback is that labeling and clarity can become problematic when there are too many categories included. Like pie charts, they can also be too simple for more complex data sets.
3. Histogram

Unlike bar charts, histograms illustrate the distribution of data over a continuous interval or defined period. These visualizations are helpful in identifying where values are concentrated, as well as where there are gaps or unusual values.
Histograms are especially useful for showing the frequency of a particular occurrence. For instance, if you’d like to show how many clicks your website received each day over the last week, you can use a histogram. From this visualization, you can quickly determine which days your website saw the greatest and fewest number of clicks.
4. Gantt Chart

Gantt charts are particularly common in project management, as they’re useful in illustrating a project timeline or progression of tasks. In this type of chart, tasks to be performed are listed on the vertical axis and time intervals on the horizontal axis. Horizontal bars in the body of the chart represent the duration of each activity.
Utilizing Gantt charts to display timelines can be incredibly helpful, and enable team members to keep track of every aspect of a project. Even if you’re not a project management professional, familiarizing yourself with Gantt charts can help you stay organized.
5. Heat Map
A heat map is a type of visualization used to show differences in data through variations in color. These charts use color to communicate values in a way that makes it easy for the viewer to quickly identify trends. Having a clear legend is necessary in order for a user to successfully read and interpret a heatmap.
There are many possible applications of heat maps. For example, if you want to analyze which time of day a retail store makes the most sales, you can use a heat map that shows the day of the week on the vertical axis and time of day on the horizontal axis. Then, by shading in the matrix with colors that correspond to the number of sales at each time of day, you can identify trends in the data that allow you to determine the exact times your store experiences the most sales.
6. A Box and Whisker Plot

A box and whisker plot , or box plot, provides a visual summary of data through its quartiles. First, a box is drawn from the first quartile to the third of the data set. A line within the box represents the median. “Whiskers,” or lines, are then drawn extending from the box to the minimum (lower extreme) and maximum (upper extreme). Outliers are represented by individual points that are in-line with the whiskers.
This type of chart is helpful in quickly identifying whether or not the data is symmetrical or skewed, as well as providing a visual summary of the data set that can be easily interpreted.
7. Waterfall Chart

A waterfall chart is a visual representation that illustrates how a value changes as it’s influenced by different factors, such as time. The main goal of this chart is to show the viewer how a value has grown or declined over a defined period. For example, waterfall charts are popular for showing spending or earnings over time.
8. Area Chart

An area chart , or area graph, is a variation on a basic line graph in which the area underneath the line is shaded to represent the total value of each data point. When several data series must be compared on the same graph, stacked area charts are used.
This method of data visualization is useful for showing changes in one or more quantities over time, as well as showing how each quantity combines to make up the whole. Stacked area charts are effective in showing part-to-whole comparisons.
9. Scatter Plot

Another technique commonly used to display data is a scatter plot . A scatter plot displays data for two variables as represented by points plotted against the horizontal and vertical axis. This type of data visualization is useful in illustrating the relationships that exist between variables and can be used to identify trends or correlations in data.
Scatter plots are most effective for fairly large data sets, since it’s often easier to identify trends when there are more data points present. Additionally, the closer the data points are grouped together, the stronger the correlation or trend tends to be.
10. Pictogram Chart
Pictogram charts , or pictograph charts, are particularly useful for presenting simple data in a more visual and engaging way. These charts use icons to visualize data, with each icon representing a different value or category. For example, data about time might be represented by icons of clocks or watches. Each icon can correspond to either a single unit or a set number of units (for example, each icon represents 100 units).
In addition to making the data more engaging, pictogram charts are helpful in situations where language or cultural differences might be a barrier to the audience’s understanding of the data.
11. Timeline

Timelines are the most effective way to visualize a sequence of events in chronological order. They’re typically linear, with key events outlined along the axis. Timelines are used to communicate time-related information and display historical data.
Timelines allow you to highlight the most important events that occurred, or need to occur in the future, and make it easy for the viewer to identify any patterns appearing within the selected time period. While timelines are often relatively simple linear visualizations, they can be made more visually appealing by adding images, colors, fonts, and decorative shapes.
12. Highlight Table

A highlight table is a more engaging alternative to traditional tables. By highlighting cells in the table with color, you can make it easier for viewers to quickly spot trends and patterns in the data. These visualizations are useful for comparing categorical data.
Depending on the data visualization tool you’re using, you may be able to add conditional formatting rules to the table that automatically color cells that meet specified conditions. For instance, when using a highlight table to visualize a company’s sales data, you may color cells red if the sales data is below the goal, or green if sales were above the goal. Unlike a heat map, the colors in a highlight table are discrete and represent a single meaning or value.
13. Bullet Graph

A bullet graph is a variation of a bar graph that can act as an alternative to dashboard gauges to represent performance data. The main use for a bullet graph is to inform the viewer of how a business is performing in comparison to benchmarks that are in place for key business metrics.
In a bullet graph, the darker horizontal bar in the middle of the chart represents the actual value, while the vertical line represents a comparative value, or target. If the horizontal bar passes the vertical line, the target for that metric has been surpassed. Additionally, the segmented colored sections behind the horizontal bar represent range scores, such as “poor,” “fair,” or “good.”
14. Choropleth Maps

A choropleth map uses color, shading, and other patterns to visualize numerical values across geographic regions. These visualizations use a progression of color (or shading) on a spectrum to distinguish high values from low.
Choropleth maps allow viewers to see how a variable changes from one region to the next. A potential downside to this type of visualization is that the exact numerical values aren’t easily accessible because the colors represent a range of values. Some data visualization tools, however, allow you to add interactivity to your map so the exact values are accessible.
15. Word Cloud

A word cloud , or tag cloud, is a visual representation of text data in which the size of the word is proportional to its frequency. The more often a specific word appears in a dataset, the larger it appears in the visualization. In addition to size, words often appear bolder or follow a specific color scheme depending on their frequency.
Word clouds are often used on websites and blogs to identify significant keywords and compare differences in textual data between two sources. They are also useful when analyzing qualitative datasets, such as the specific words consumers used to describe a product.
16. Network Diagram

Network diagrams are a type of data visualization that represent relationships between qualitative data points. These visualizations are composed of nodes and links, also called edges. Nodes are singular data points that are connected to other nodes through edges, which show the relationship between multiple nodes.
There are many use cases for network diagrams, including depicting social networks, highlighting the relationships between employees at an organization, or visualizing product sales across geographic regions.
17. Correlation Matrix

A correlation matrix is a table that shows correlation coefficients between variables. Each cell represents the relationship between two variables, and a color scale is used to communicate whether the variables are correlated and to what extent.
Correlation matrices are useful to summarize and find patterns in large data sets. In business, a correlation matrix might be used to analyze how different data points about a specific product might be related, such as price, advertising spend, launch date, etc.
Other Data Visualization Options
While the examples listed above are some of the most commonly used techniques, there are many other ways you can visualize data to become a more effective communicator. Some other data visualization options include:
- Bubble clouds
- Circle views
- Dendrograms
- Dot distribution maps
- Open-high-low-close charts
- Polar areas
- Radial trees
- Ring Charts
- Sankey diagram
- Span charts
- Streamgraphs
- Wedge stack graphs
- Violin plots

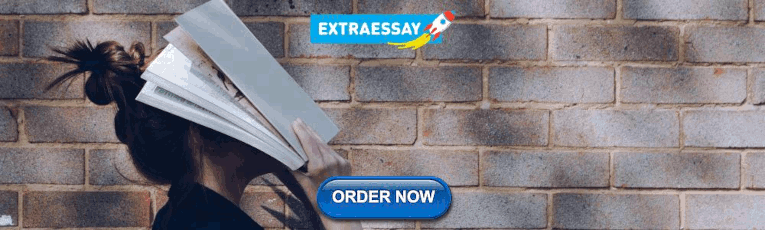
Tips For Creating Effective Visualizations
Creating effective data visualizations requires more than just knowing how to choose the best technique for your needs. There are several considerations you should take into account to maximize your effectiveness when it comes to presenting data.
Related : What to Keep in Mind When Creating Data Visualizations in Excel
One of the most important steps is to evaluate your audience. For example, if you’re presenting financial data to a team that works in an unrelated department, you’ll want to choose a fairly simple illustration. On the other hand, if you’re presenting financial data to a team of finance experts, it’s likely you can safely include more complex information.
Another helpful tip is to avoid unnecessary distractions. Although visual elements like animation can be a great way to add interest, they can also distract from the key points the illustration is trying to convey and hinder the viewer’s ability to quickly understand the information.
Finally, be mindful of the colors you utilize, as well as your overall design. While it’s important that your graphs or charts are visually appealing, there are more practical reasons you might choose one color palette over another. For instance, using low contrast colors can make it difficult for your audience to discern differences between data points. Using colors that are too bold, however, can make the illustration overwhelming or distracting for the viewer.
Related : Bad Data Visualization: 5 Examples of Misleading Data
Visuals to Interpret and Share Information
No matter your role or title within an organization, data visualization is a skill that’s important for all professionals. Being able to effectively present complex data through easy-to-understand visual representations is invaluable when it comes to communicating information with members both inside and outside your business.
There’s no shortage in how data visualization can be applied in the real world. Data is playing an increasingly important role in the marketplace today, and data literacy is the first step in understanding how analytics can be used in business.
Are you interested in improving your analytical skills? Learn more about Business Analytics , our eight-week online course that can help you use data to generate insights and tackle business decisions.
This post was updated on January 20, 2022. It was originally published on September 17, 2019.

About the Author

- Conferences
- Last updated March 18, 2024
- In Developers Corner , Top AI Tools
Representation Learning – Complete Guide for Beginners
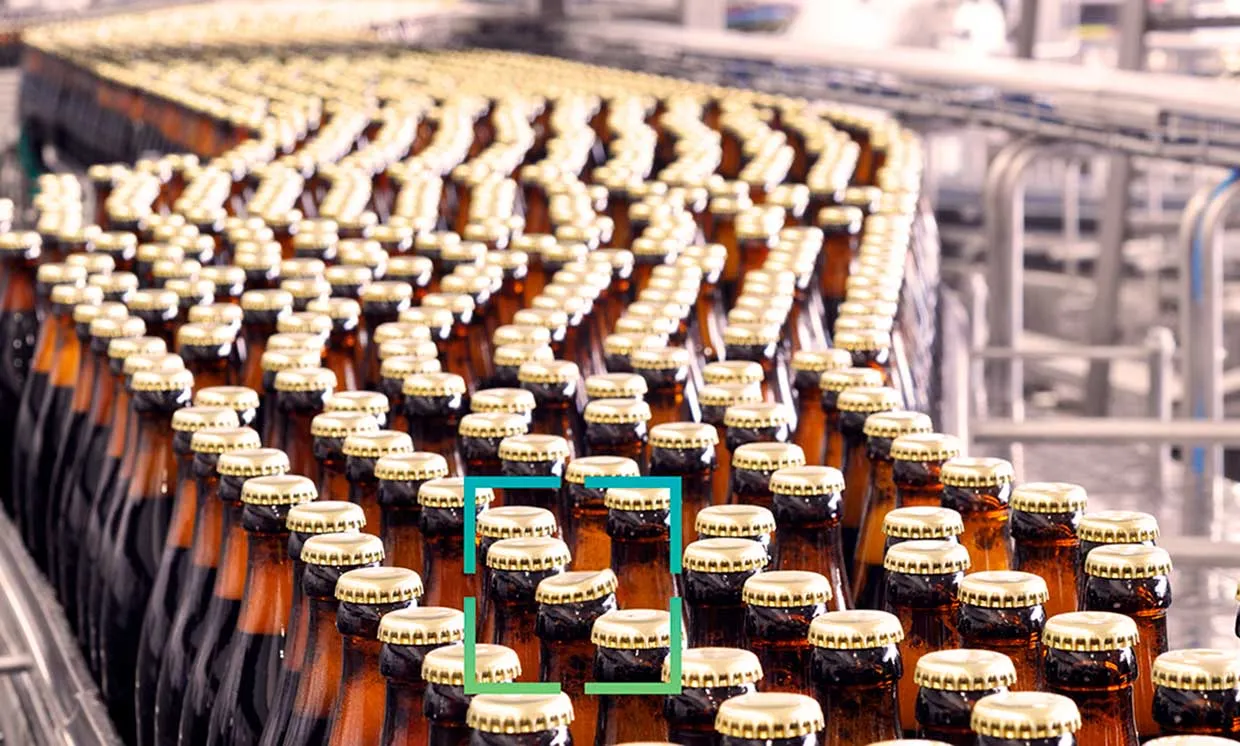
- Published on November 4, 2021
- by Vijaysinh Lendave
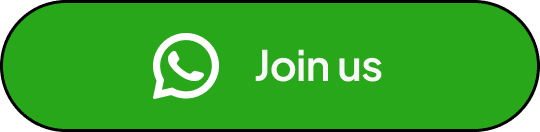
Representation learning is a very important aspect of machine learning which automatically discovers the feature patterns in the data. When the machine is provided with the data, it learns the representation itself without any human intervention. The goal of representation learning is to train machine learning algorithms to learn useful representations, such as those that are interpretable, incorporate latent features, or can be used for transfer learning. In this article, we will discuss the concept of representation learning along with its need and different approaches. The major points to be covered in this article are listed below.
Table of contents
Need of representation learning, what is representation learning, what is supervised learning, what is unsupervised learning, supervised dictionary learning, multi-layer perceptron, neural networks.
- K-Means Clustering
Local Linear Embedding
- Unsupervised Dictionary Mining
Deep Architectures Methods
Restricted boltzmann machine (rbms), autoencoders, final words.
Let’s start the discussion by understanding what is the actual need for representation learning.
Assume you’re developing a machine-learning algorithm to predict dog breeds based on pictures. Because image data provides all of the answers, the engineer must rely heavily on it when developing the algorithm. Each observation or feature in the data describes the qualities of the dogs. The machine learning system that predicts the outcome must comprehend how each attribute interacts with other outcomes such as Pug, Golden Retriever, and so on.
As a result, if there is any noise or irregularity in the input, the result can be drastically different, which is a risk with most machine learning algorithms. The majority of machine learning algorithms have only a basic understanding of the data. So in such cases, the solution is to provide a more abstract representation of data. It’s impossible to tell which features should be extracted for many tasks. This is where the concept of representation learning takes shape.
Representation learning is a class of machine learning approaches that allow a system to discover the representations required for feature detection or classification from raw data. The requirement for manual feature engineering is reduced by allowing a machine to learn the features and apply them to a given activity.
In representation learning, data is sent into the machine, and it learns the representation on its own. It is a way of determining a data representation of the features, the distance function, and the similarity function that determines how the predictive model will perform. Representation learning works by reducing high-dimensional data to low-dimensional data, making it easier to discover patterns and anomalies while also providing a better understanding of the data’s overall behaviour.
Basically, Machine learning tasks such as classification frequently demand input that is mathematically and computationally convenient to process, which motivates representation learning. Real-world data, such as photos, video, and sensor data, has resisted attempts to define certain qualities algorithmically. An approach is to examine the data for such traits or representations rather than depending on explicit techniques.
Methods of Representation Learning
We must employ representation learning to ensure that the model provides invariant and untangled outcomes in order to increase its accuracy and performance. In this section, we’ll look at how representation learning can improve the model’s performance in three different learning frameworks: supervised learning, unsupervised learning.
This is referred to as supervised learning when the ML or DL model maps the input X to the output Y. The computer tries to correct itself by comparing model output to ground truth, and the learning process optimizes the mapping from input to output. This process is repeated until the optimization function reaches global minima.
Even when the optimization function reaches the global minima, new data does not always perform well, resulting in overfitting. While supervised learning does not necessitate a significant amount of data to learn the mapping from input to output, it does necessitate the learned features. The prediction accuracy can improve by up to 17 percent when the learned attributes are incorporated into the supervised learning algorithm.
Using labelled input data, features are learned in supervised feature learning. Supervised neural networks, multilayer perceptrons, and (supervised) dictionary learning are some examples.
Unsupervised learning is a sort of machine learning in which the labels are ignored in favour of the observation itself. Unsupervised learning isn’t used for classification or regression; instead, it’s used to uncover underlying patterns, cluster data, denoise it, detect outliers, and decompose data, among other things.
When working with data x, we must be very careful about whatever features z we use to ensure that the patterns produced are accurate. It has been observed that having more data does not always imply having better representations. We must be careful to develop a model that is both flexible and expressive so that the extracted features can convey critical information.
Unsupervised feature learning learns features from unlabeled input data by following the methods such as Dictionary learning, independent component analysis, autoencoders, matrix factorization, and various forms of clustering are among examples.
In the next section, we will see more about these methods and workflow, how they learn the representation in detail.
Supervised Methods
Dictionary learning creates a set of representative elements (dictionary) from the input data, allowing each data point to be represented as a weighted sum of the representative elements. By minimizing the average representation error (across the input data) and applying L1 regularization to the weights, the dictionary items and weights may be obtained i.e., the representation of each data point has only a few nonzero weights.
For optimizing dictionary elements, supervised dictionary learning takes advantage of both the structure underlying the input data and the labels. The supervised dictionary learning technique uses dictionary learning to solve classification issues by optimizing dictionary elements, data point weights, and classifier parameters based on the input data.
A minimization problem is formulated, with the objective function consisting of the classification error, the representation error, an L1 regularization on the representing weights for each data point (to enable sparse data representation), and an L2 regularization on the parameters of the classification algorithm.
The perceptron is the most basic neural unit, consisting of a succession of inputs and weights that are compared to the ground truth. A multi-layer perceptron, or MLP, is a feed-forward neural network made up of layers of perceptron units. MLP is made up of three-node layers: an input, a hidden layer, and an output layer. MLP is commonly referred to as the vanilla neural network because it is a very basic artificial neural network.
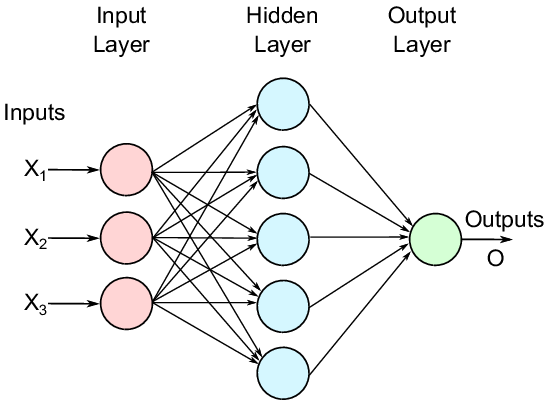
This notion serves as a foundation for hidden variables and representation learning. Our goal in this theorem is to determine the variables or required weights that can represent the underlying distribution of the entire data so that when we plug those variables or required weights into unknown data, we receive results that are almost identical to the original data. In a word, artificial neural networks (ANN) assist us in extracting meaningful patterns from a dataset.
Neural networks are a class of learning algorithms that employ a “network” of interconnected nodes in various layers. It’s based on the animal nervous system, with nodes resembling neurons and edges resembling synapses. The network establishes computational rules for passing input data from the network’s input layer to the network’s output layer, and each edge has an associated weight.
The relationship between the input and output layers, which is parameterized by the weights, is described by a network function associated with a neural network. Various learning tasks can be achieved by minimizing a cost function over the network function (w) with correctly defined network functions.
Unsupervised Methods
Learning Representation from unlabeled data is referred to as unsupervised feature learning. Unsupervised Representation learning frequently seeks to uncover low-dimensional features that encapsulate some structure beneath the high-dimensional input data.
K-Means Clustering
K-means clustering is a vector quantization approach. An n-vector set is divided into k clusters (i.e. subsets) via K-means clustering, with each vector belonging to the cluster with the closest mean. Despite the use of inferior greedy techniques, the problem is computationally NP-hard.
K-means clustering divides an unlabeled collection of inputs into k groups before obtaining centroids-based features. These characteristics can be honed in a variety of ways. The simplest method is to add k binary features to each sample, with each feature j having a value of one of the k-means learned jth centroid is closest to the sample under consideration. Cluster distances can be used as features after being processed with a radial basis function.
LLE is a nonlinear learning strategy for constructing low-dimensional neighbour-preserving representations from high-dimensional (unlabeled) input. LLE’s main goal is to reconstruct high-dimensional data using lower-dimensional points while keeping some geometric elements of the original data set’s neighbours.
There are two major steps in LLE. The first step is “neighbour-preserving,” in which each input data point Xi is reconstructed as a weighted sum of K nearest neighbour data points, with the optimal weights determined by minimizing the average squared reconstruction error (i.e., the difference between an input point and its reconstruction) while keeping the weights associated with each point equal to one.
The second stage involves “dimension reduction,” which entails searching for vectors in a lower-dimensional space that reduce the representation error while still using the optimal weights from the previous step.
The weights are optimized given fixed data in the first stage, which can be solved as a least-squares problem. Lower-dimensional points are optimized with fixed weights in the second phase, which can be solved using sparse eigenvalue decomposition.
Unsupervised Dictionary Mining
For optimizing dictionary elements, unsupervised dictionary learning does not use data labels and instead relies on the structure underlying the data. Sparse coding, which seeks to learn basic functions (dictionary elements) for data representation from unlabeled input data, is an example of unsupervised dictionary learning.
When the number of vocabulary items exceeds the dimension of the input data, sparse coding can be used to learn overcomplete dictionaries. K-SVD is an algorithm for learning a dictionary of elements that allows for sparse representation.
Deep learning architectures for feature learning are inspired by the hierarchical architecture of the biological brain system, which stacks numerous layers of learning nodes. The premise of distributed representation is typically used to construct these architectures: observable data is generated by the interactions of many diverse components at several levels.
In multilayer learning frameworks, RBMs (restricted Boltzmann machines) are widely used as building blocks. An RBM is a bipartite undirected network having a set of binary hidden variables, visible variables, and edges connecting the hidden and visible nodes. It’s a variant of the more general Boltzmann machines, with the added constraint of no intra-node connections. In an RBM, each edge has a weight assigned to it. The connections and weights define an energy function that can be used to generate a combined distribution of visible and hidden nodes.
For unsupervised representation learning, an RBM can be thought of as a single-layer design. The visible variables, in particular, relate to the input data, whereas the hidden variables correspond to the feature detectors. Hinton’s contrastive divergence (CD) approach can be used to train the weights by maximizing the probability of visible variables.
Deep network representations have been found to be insensitive to complex noise or data conflicts. This can be linked to the architecture to some extent. The employment of convolutional layers and max-pooling, for example, can be proven to produce transformation insensitivity.
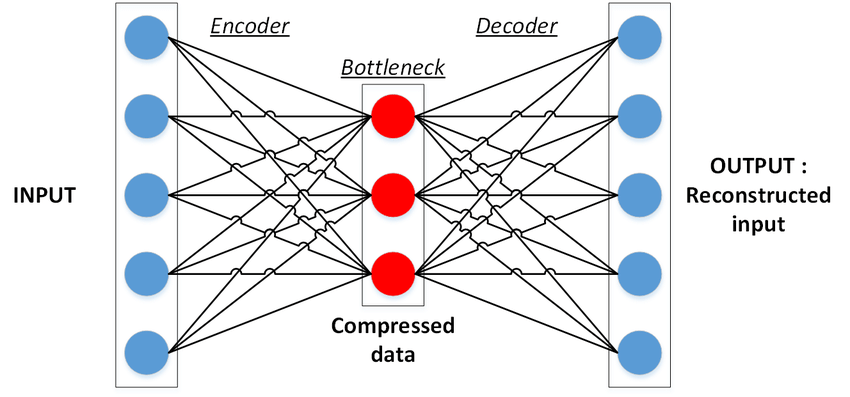
Autoencoders are therefore neural networks that may be taught to do representation learning. Autoencoders seek to duplicate their input to their output using an encoder and a decoder. Autoencoders are typically trained via recirculation, a learning process that compares the activation of the input network to the activation of the reconstructed input.
Unlike typical learning tasks like classification, which has the end goal of reducing misclassifications, representation learning is an intermediate goal of machine learning making it difficult to articulate a straight and obvious training target. In this post, we understood how to overcome such difficulties from scratch. From the starting, we have seen what was the actual need for this method and understood different methodologies in supervised, unsupervised, and some deep learning frameworks.
Access all our open Survey & Awards Nomination forms in one place
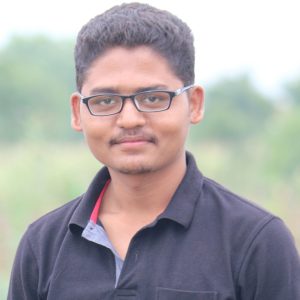
Vijaysinh Lendave

7 Leading Data Science and AI Institutes in India
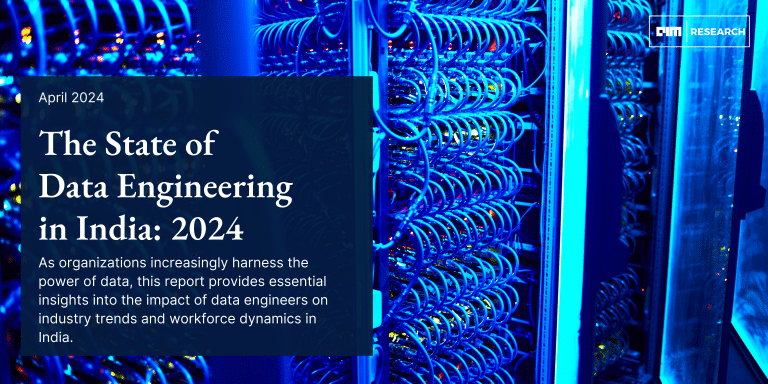
The State of Data Engineering in India: 2024
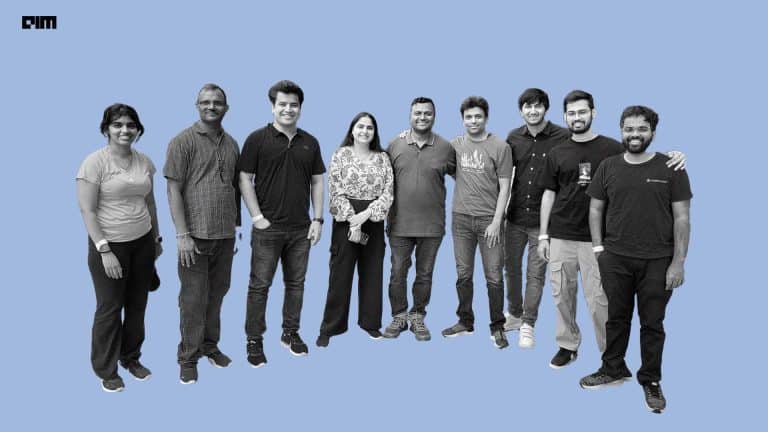
Data Science Hiring Process at Confluent
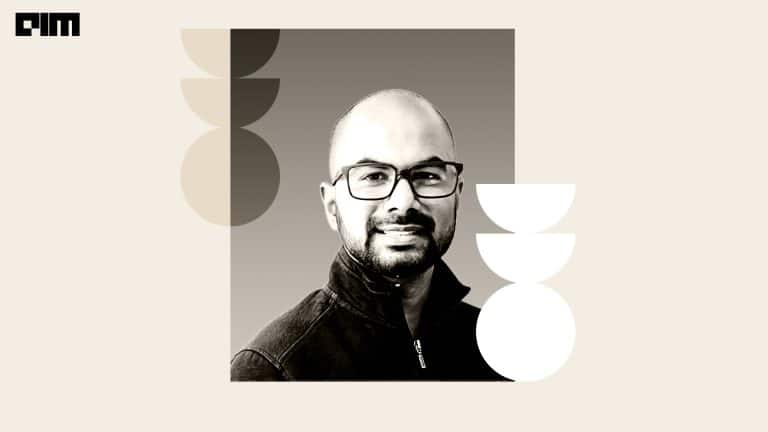
Supercharge Your Data Science Career: Strategies for Solid Foundation
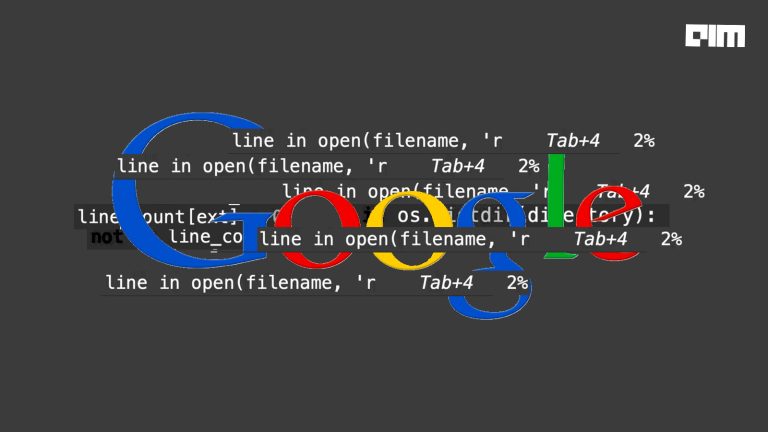
Google Research Introduce PERL, a New Method to Improve RLHF
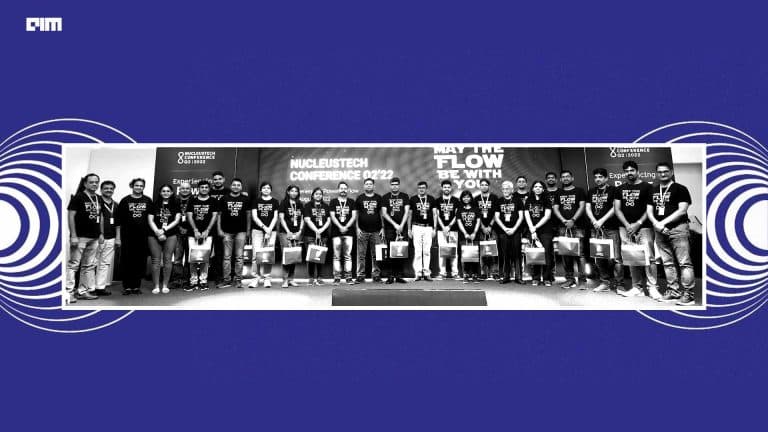
Data Science Hiring Process at Nucleus Software
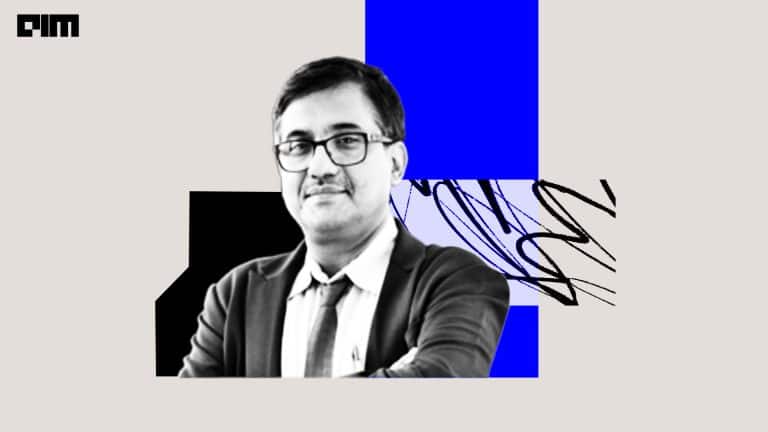
[Exclusive] Pushpak Bhattacharyya on Understanding Complex Human Emotions in LLMs

Data Science Hiring Process at Verizon
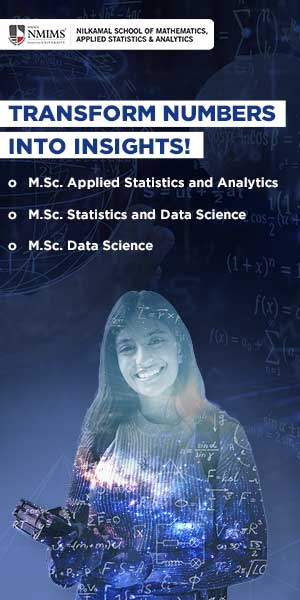
CORPORATE TRAINING PROGRAMS ON GENERATIVE AI
Generative ai skilling for enterprises, our customized corporate training program on generative ai provides a unique opportunity to empower, retain, and advance your talent., upcoming large format conference, data engineering summit 2024, may 30 and 31, 2024 | 📍 bangalore, india, download the easiest way to stay informed.

‘Pronoun Illness’ is Ola’s Problem, Not India’s ‘Rich Culture’
“Better to send this illness back to where it came from. Our culture has always had respect for all. No need for new pronouns.”
The World Needs Something Better Than the Transformer
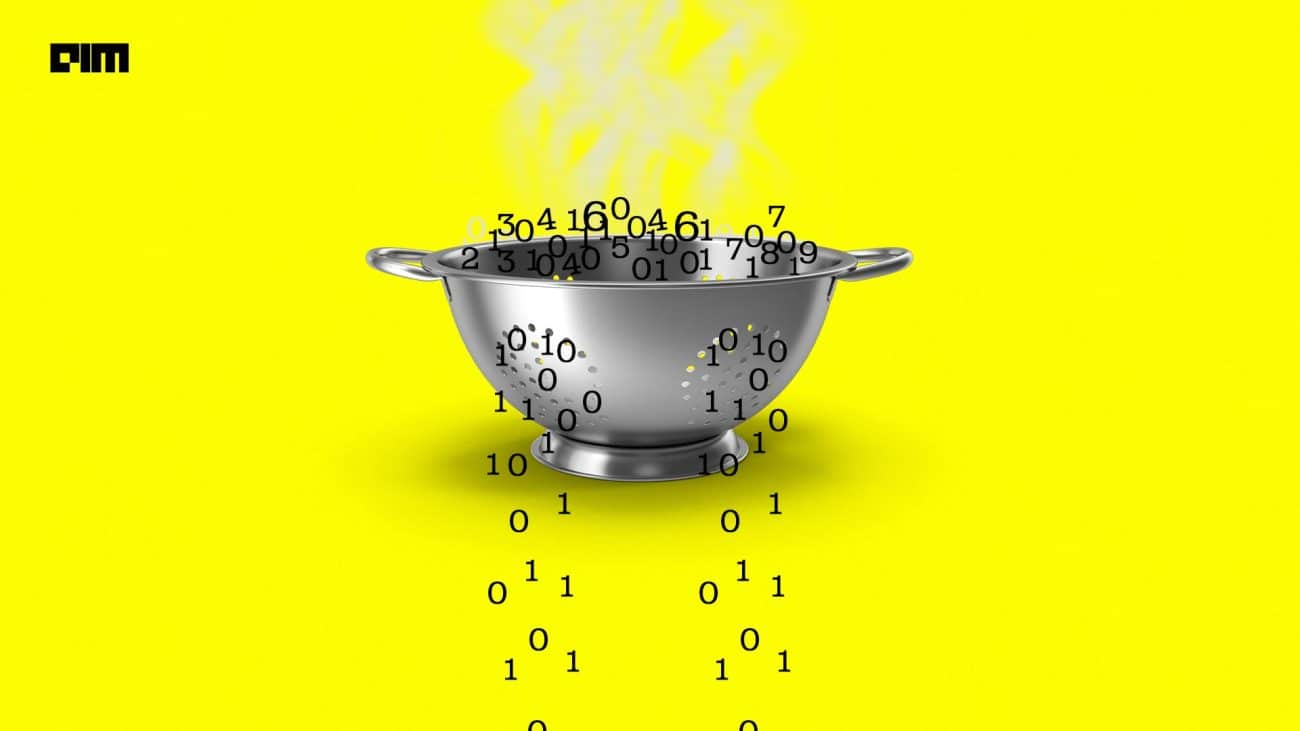
Why India has Less than 2000 AI Senior Engineers?
Top editorial picks, isomorphic labs has the potential to build multi-$100 bn business, tredence appoints munjay singh as chief operating officer, gnani.ai unveils india’s first voice-first slm for vernacular languages, “google is dancing to its own music,” says sundar pichai, subscribe to the belamy: our weekly newsletter, biggest ai stories, delivered to your inbox every week., also in news.
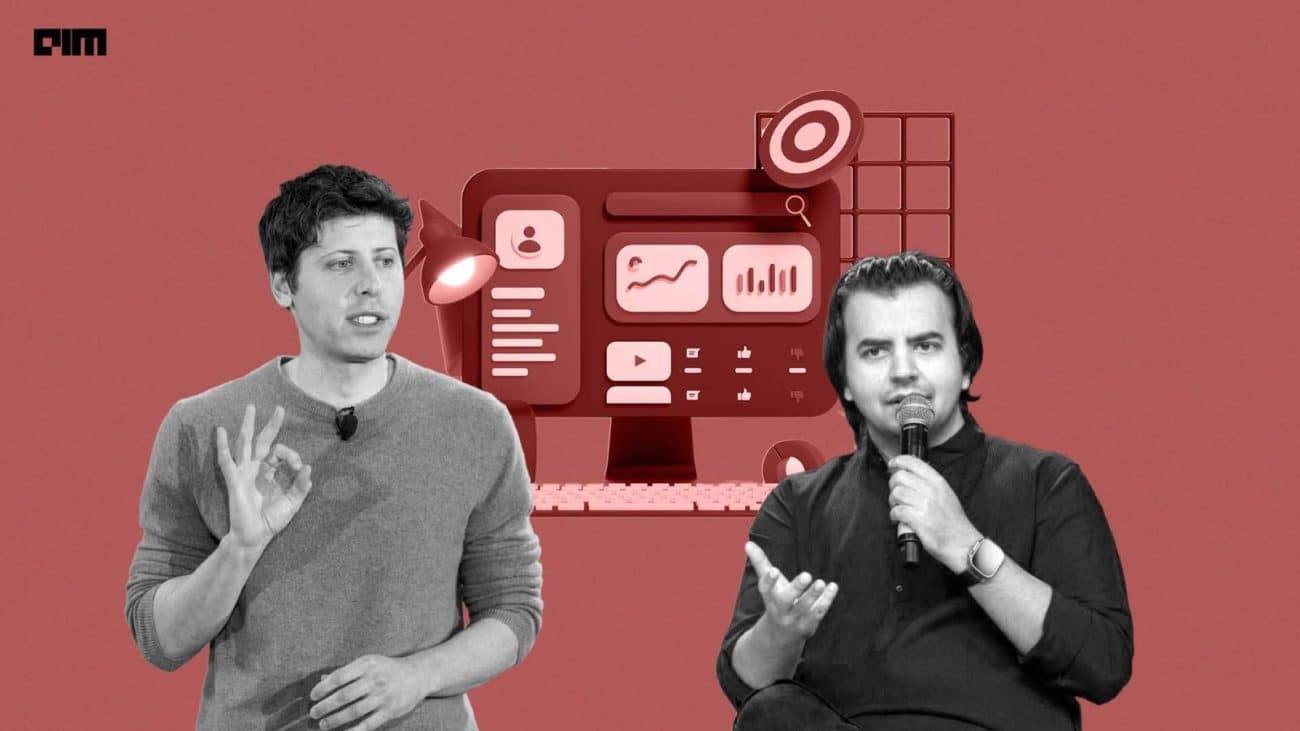
OpenAI Introducing Media Manager Tool in India Could Hurt Ola Krutrim’s Ego
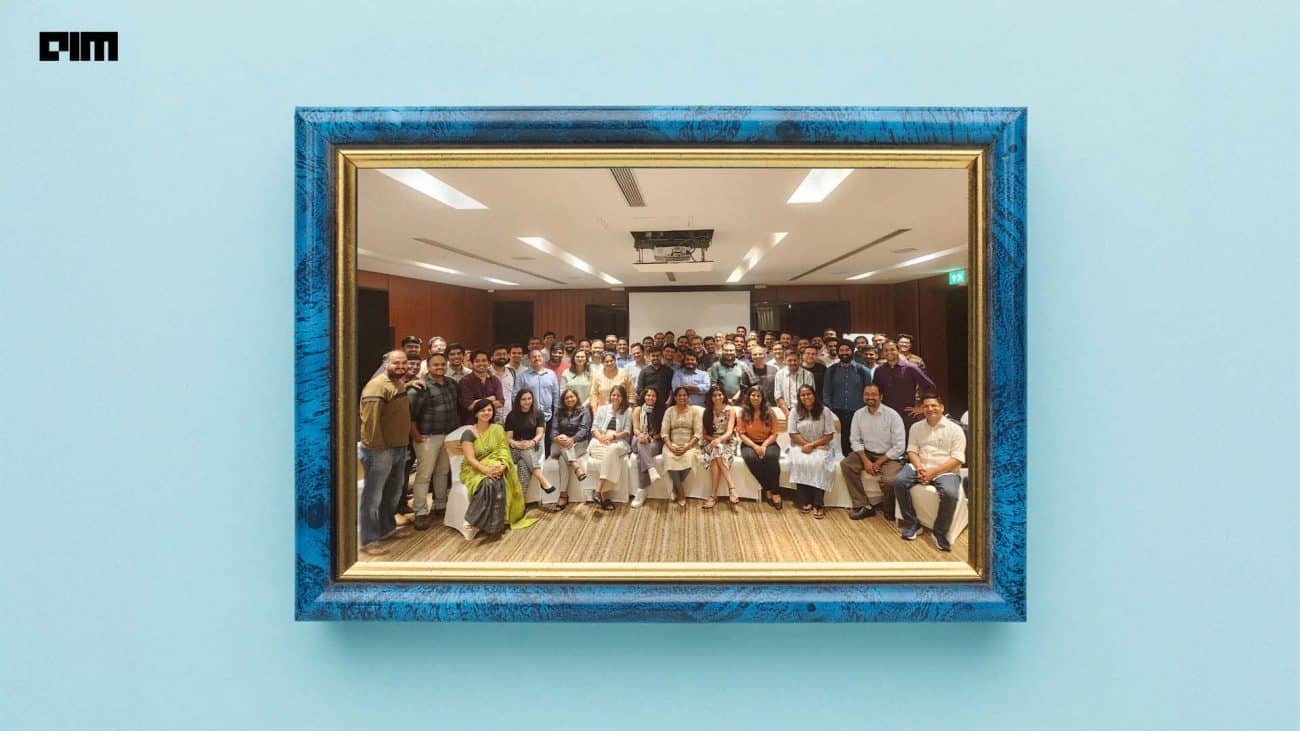
Data Science Hiring Process at Razorpay
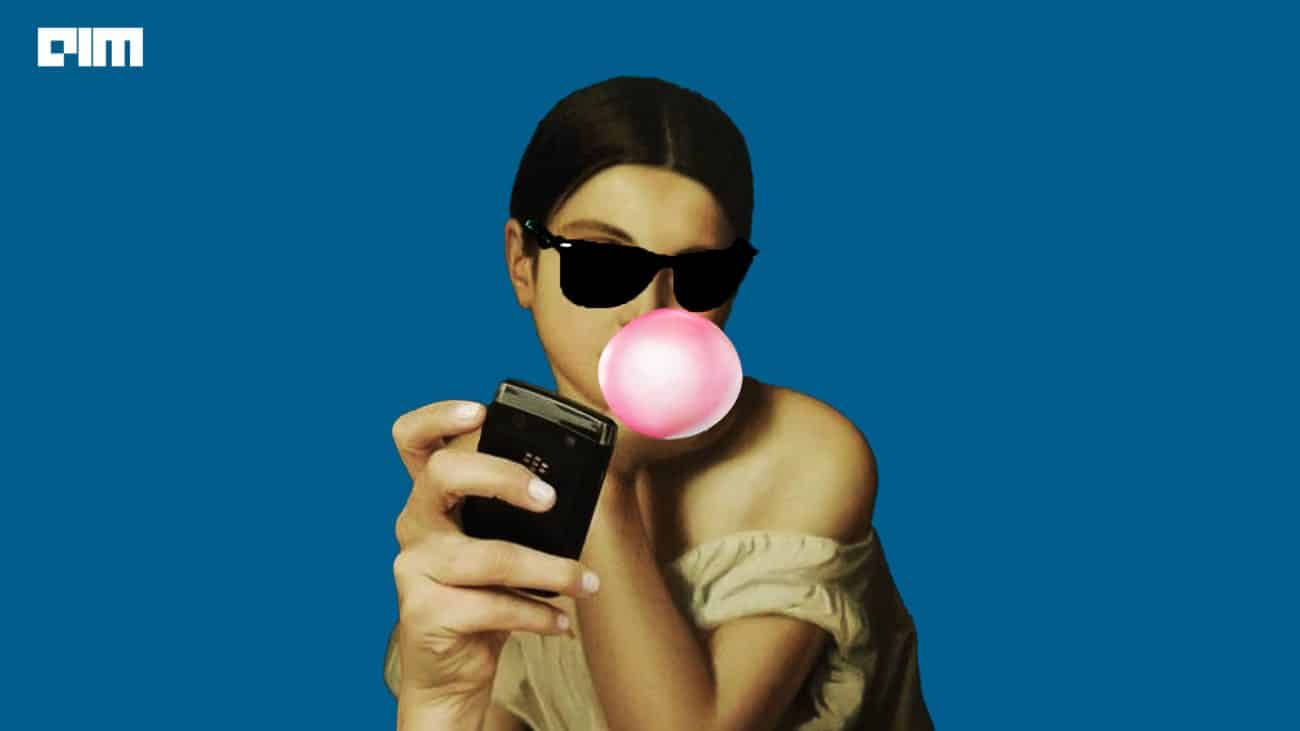
Is GenAI Spoiling Gen Z?
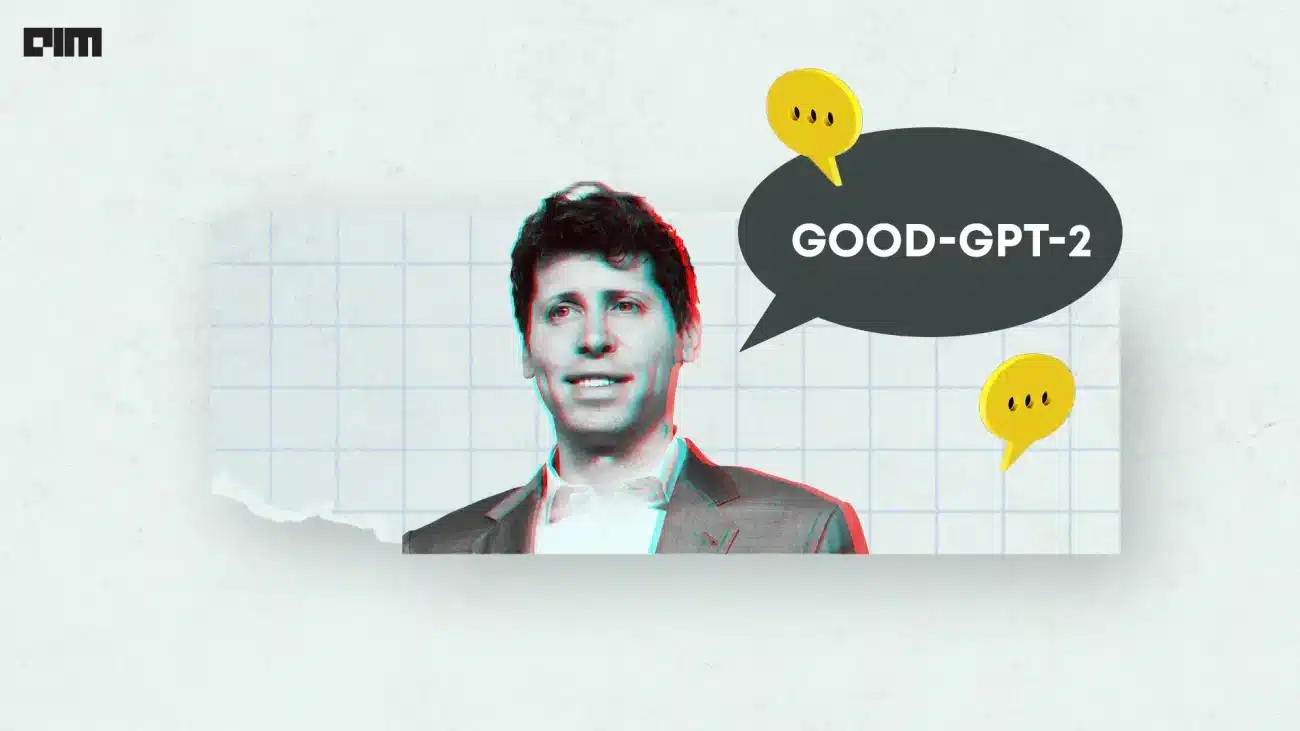
good-gpt-2-chatbot Gone Rogue
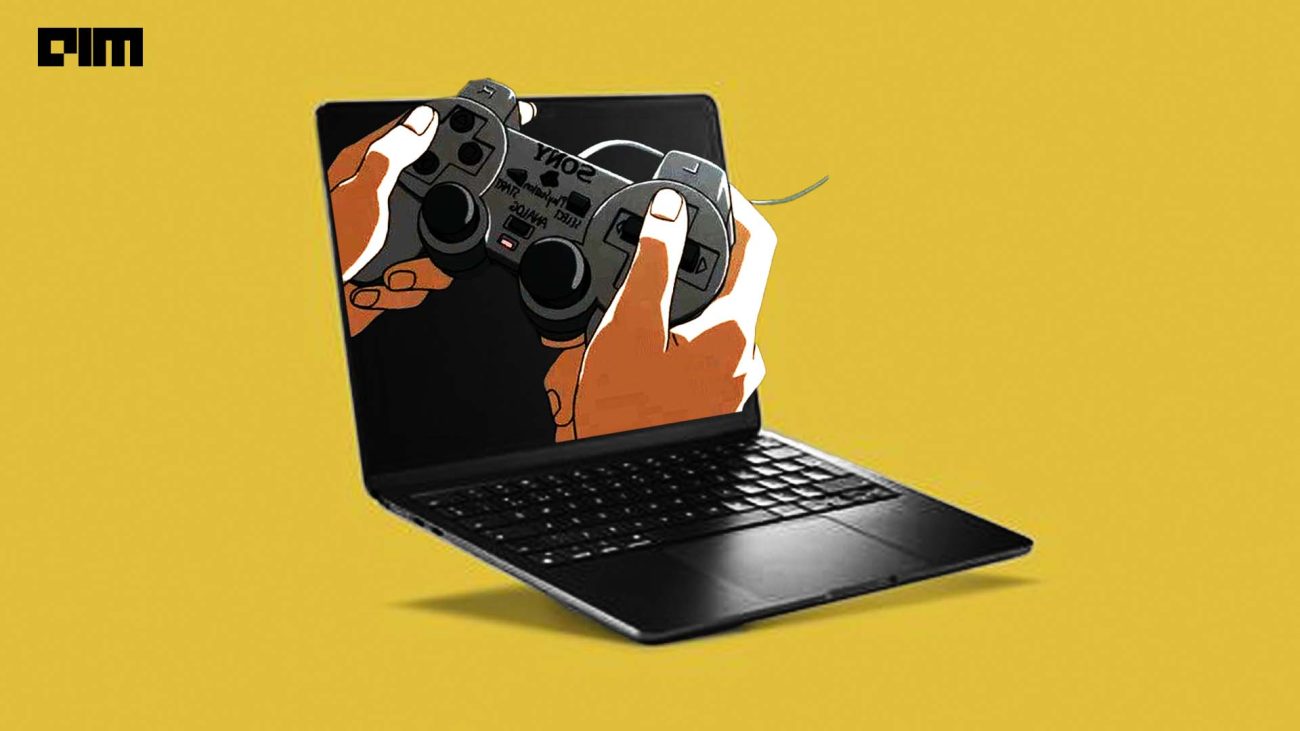
The Rise of AI-Powered Gaming Laptops
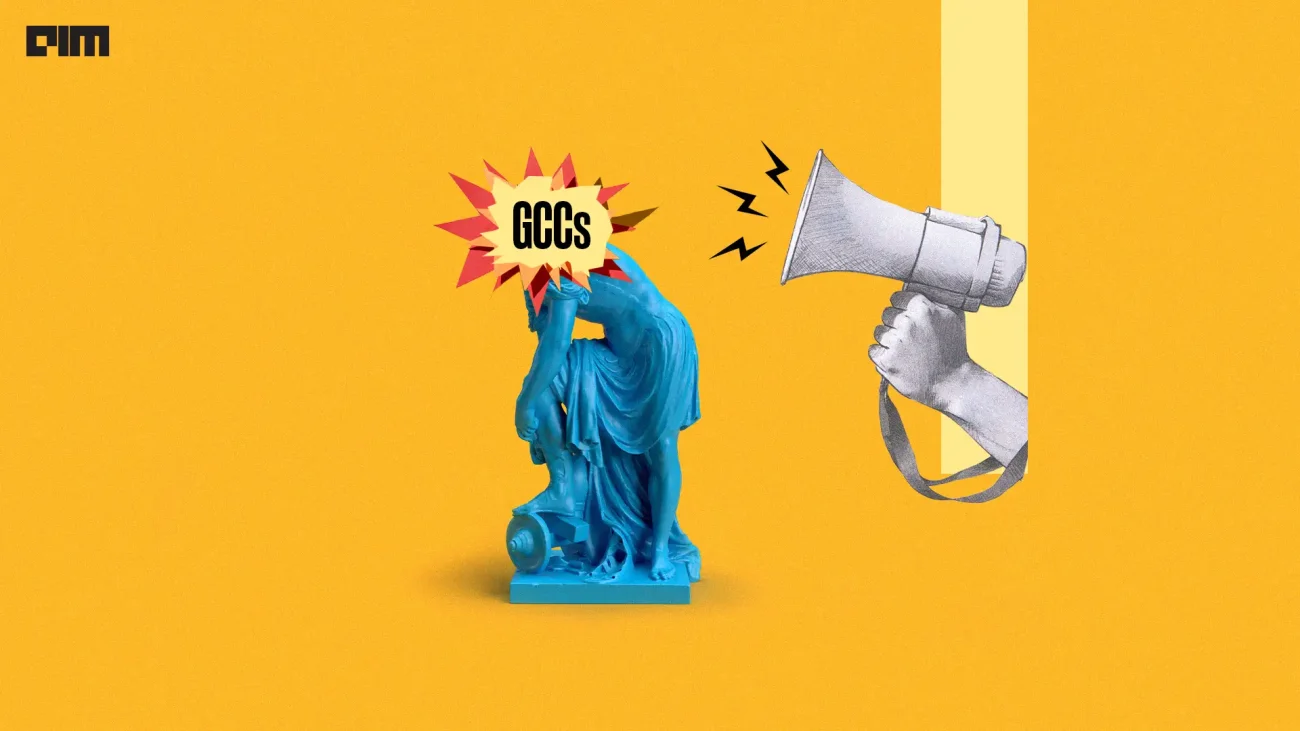
GCCs in India Are Facing a Branding Problem
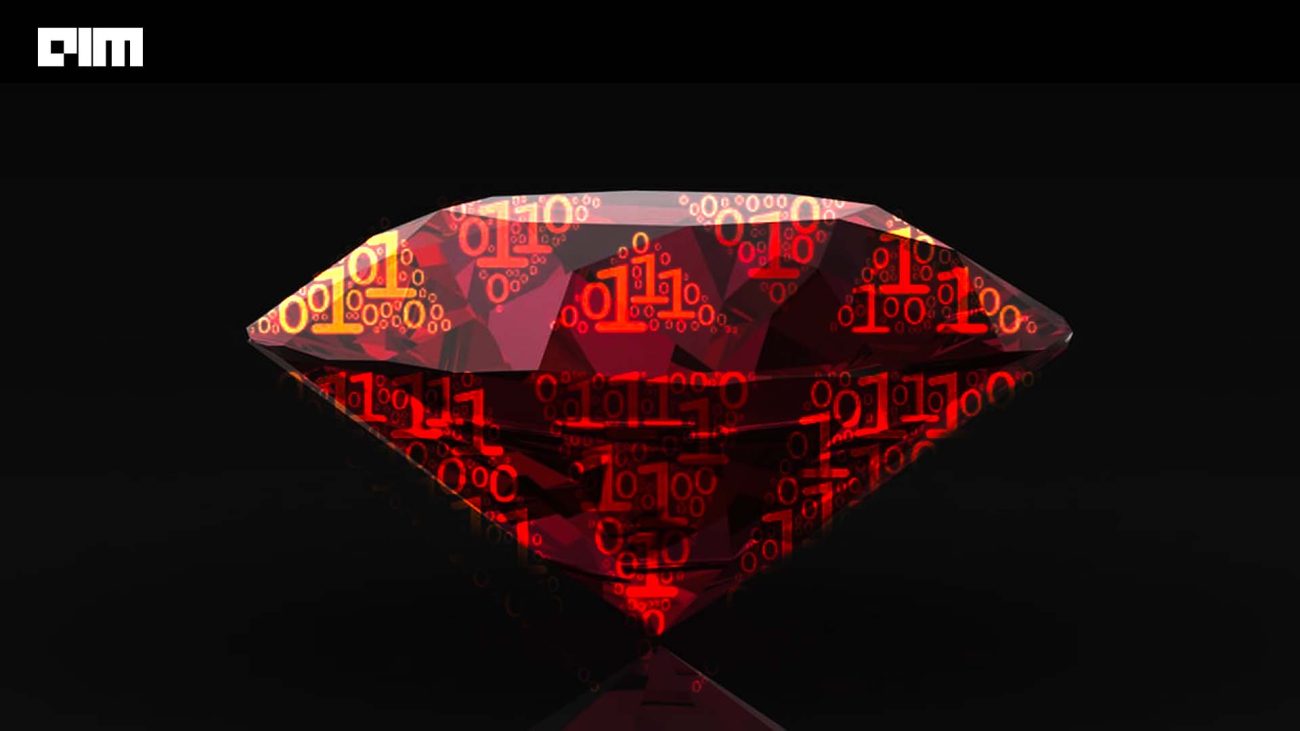
Can Ruby Survive as the ‘Human-First’ Programming Language?
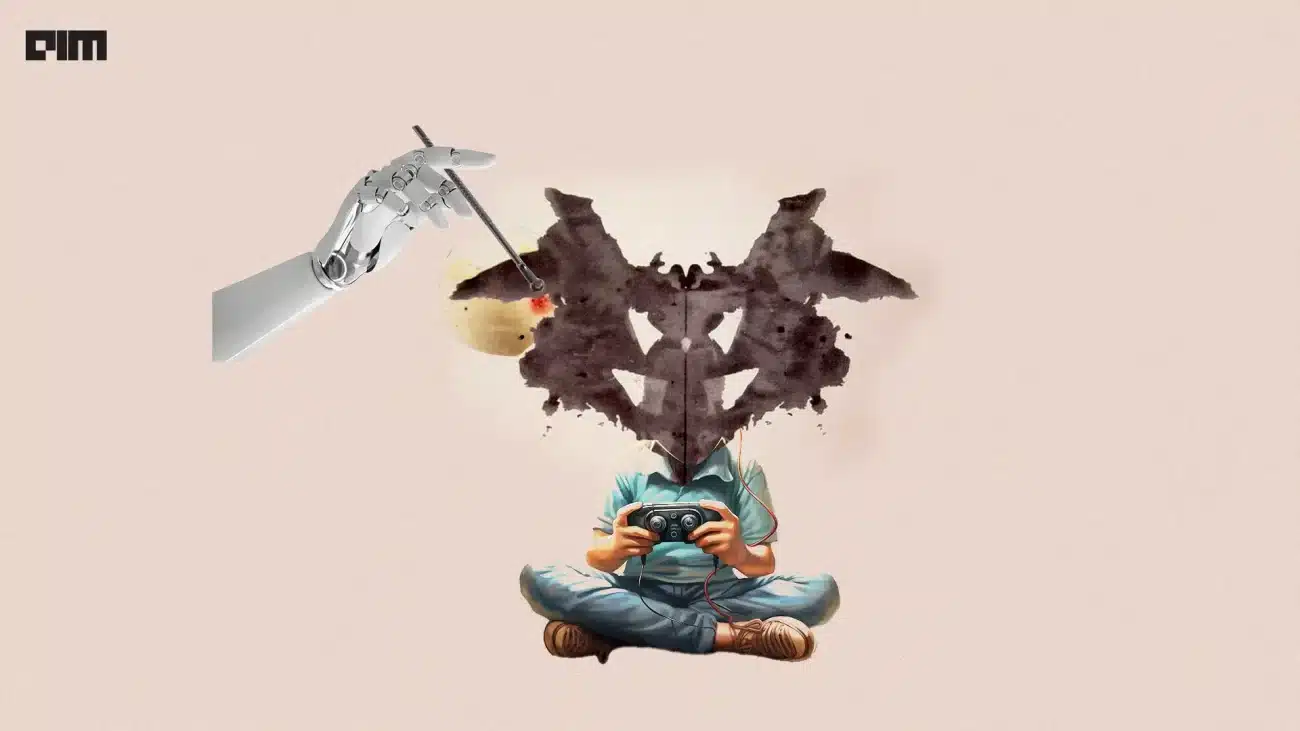
AGI is a Rorschach Test for People to Project Their Technology Anxieties
Ai courses & careers.

Gyan AI Unveils Smaller-Scale Maths LLM, Paramanu-Ganita, Outperforming LLama, Falcon
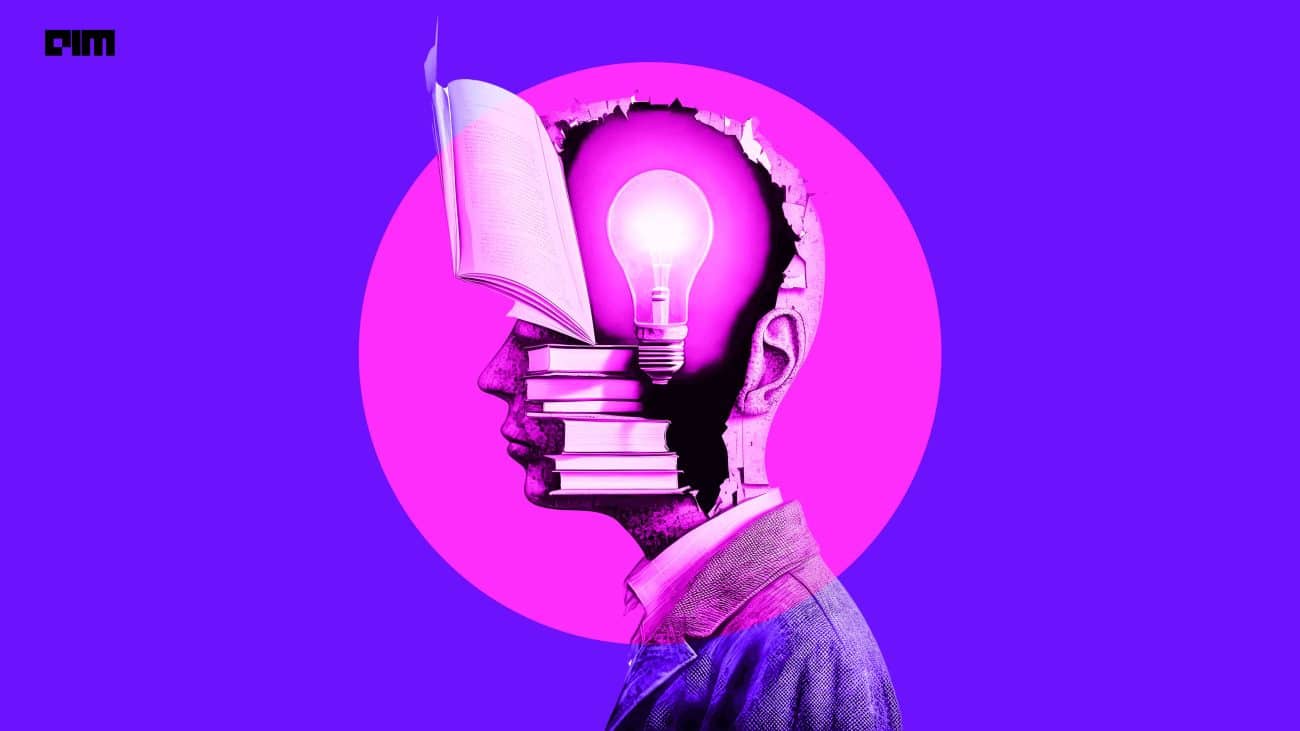
10 Free Online AI Courses to Learn from the Best
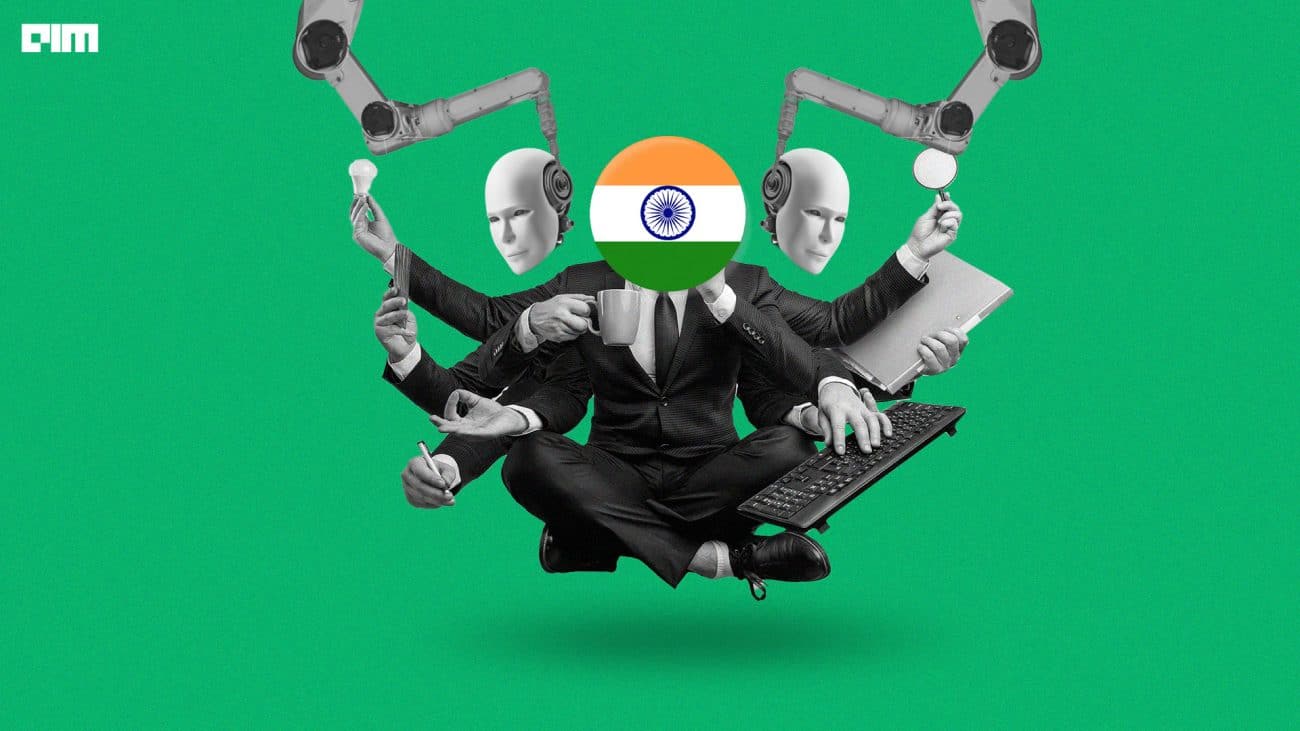
India is a Goldmine for AI Talent
Industry insights.
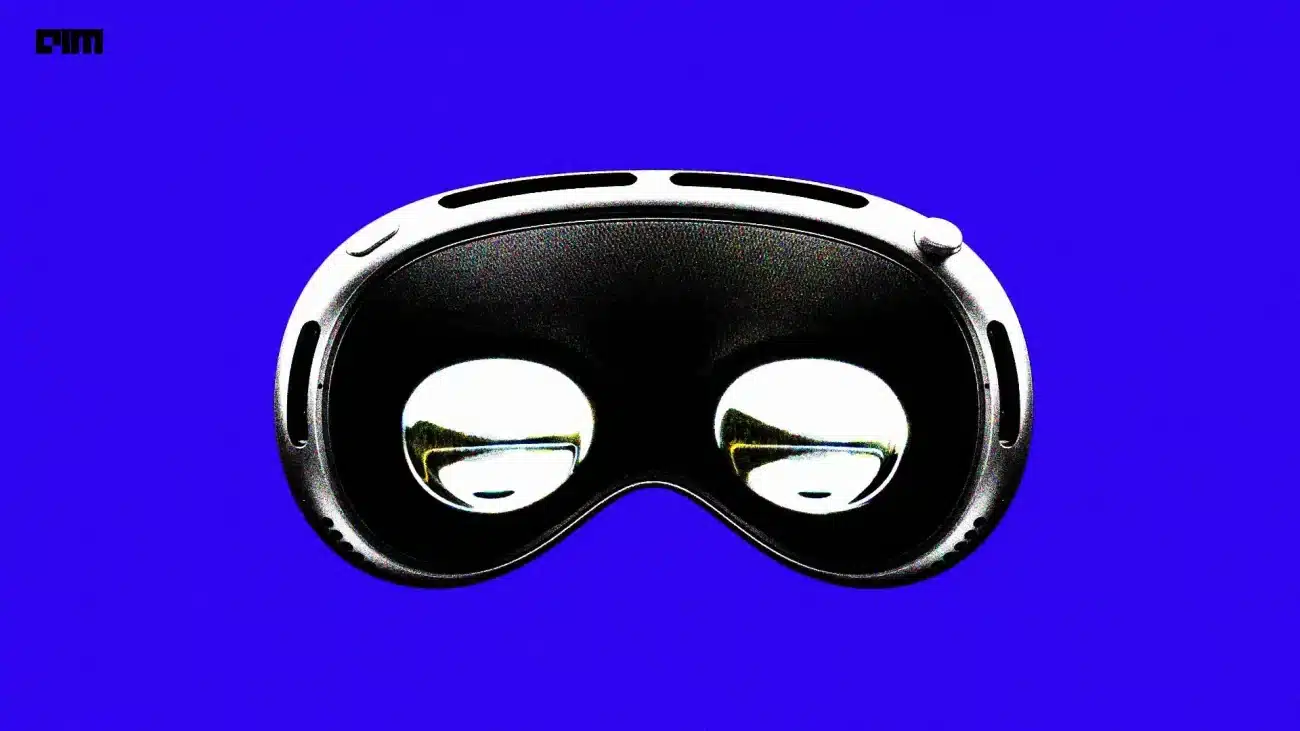
Doctors in India Use Apple Vision Pro to Perform 30+ Surgeries

Google DeepMind Unveils AlphaFold 3, Achieves 50% Better Prediction Accuracy
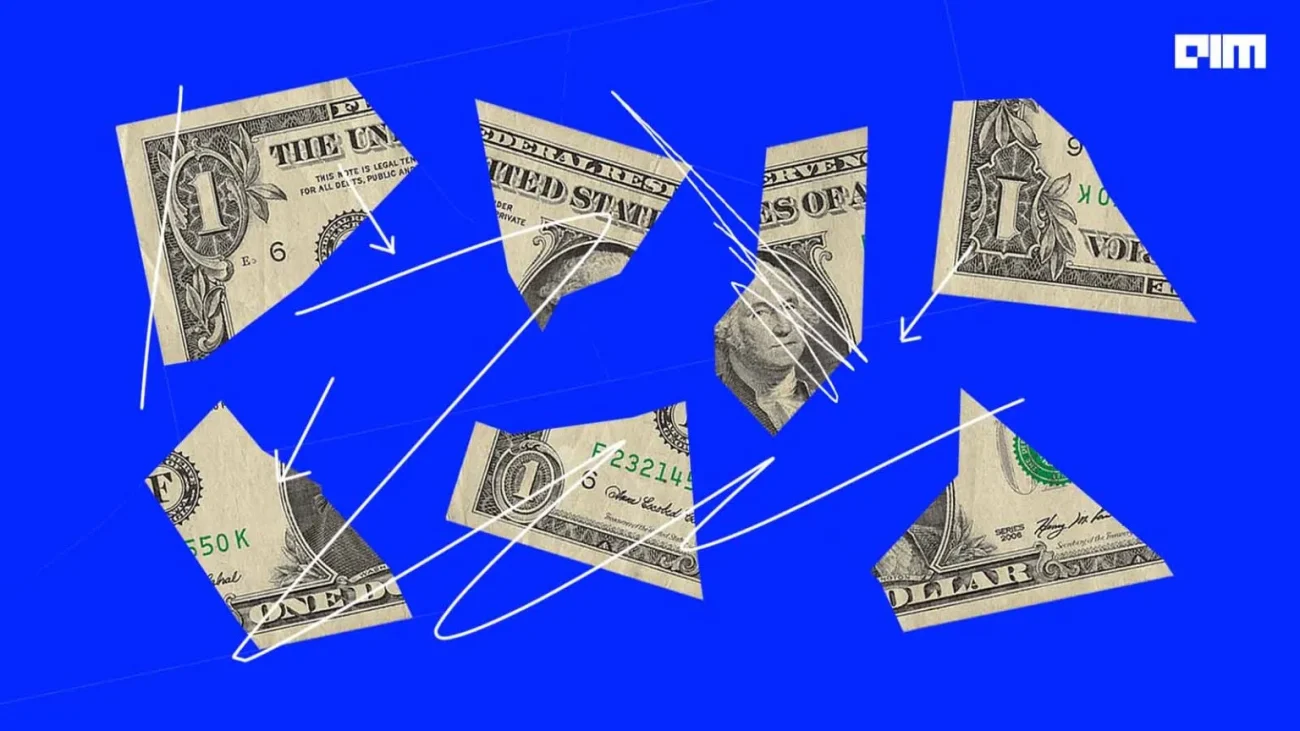
Setu and Sarvam AI Unveils Sesame, India’s First Domain Specific LLM for BFSI Sector
Check our industry research reports.
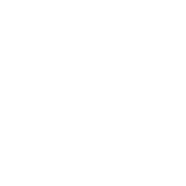
AI Forum for India
Our discord community for ai ecosystem, in collaboration with nvidia. .
Interview with Vivek Raghvan Co-founder Sarvam AI
"> "> Flagship Events
Rising 2024 | de&i in tech summit, april 4 and 5, 2024 | 📍 hilton convention center, manyata tech park, bangalore, machinecon gcc summit 2024, june 28 2024 | 📍bangalore, india, machinecon usa 2024, 26 july 2024 | 583 park avenue, new york, cypher india 2024, september 25-27, 2024 | 📍bangalore, india, cypher usa 2024, nov 21-22 2024 | 📍santa clara convention center, california, usa, genai corner.
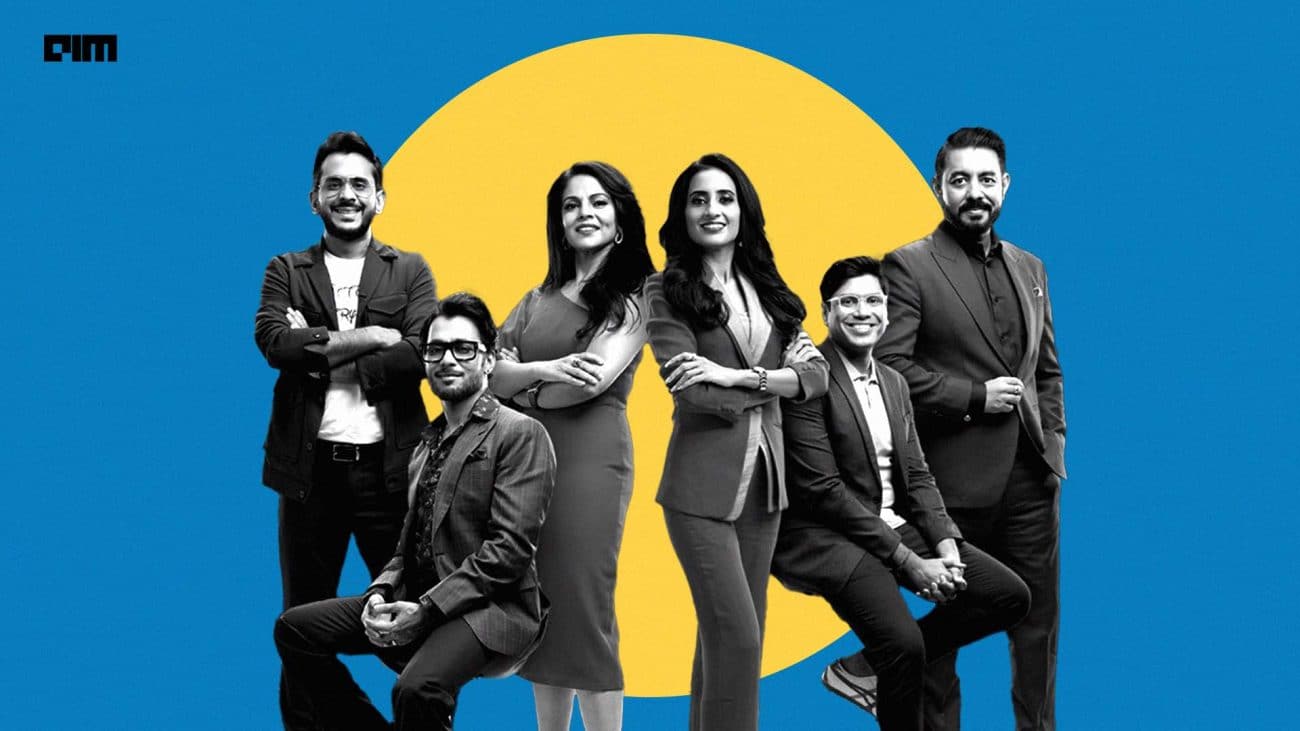
7 AI Startups that Featured on Shark Tank India Season 3

Top 9 Semiconductor GCCs in India
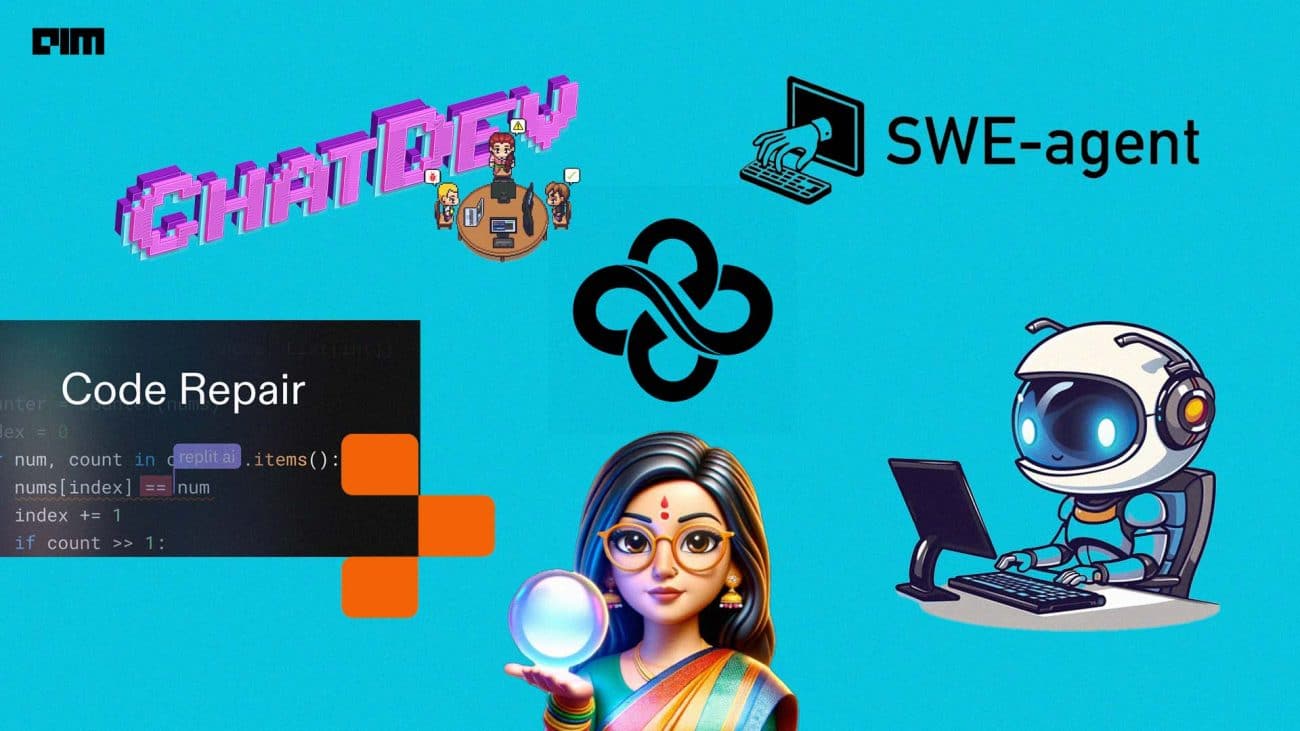
Top 6 Devin Alternatives to Automate Your Coding Tasks
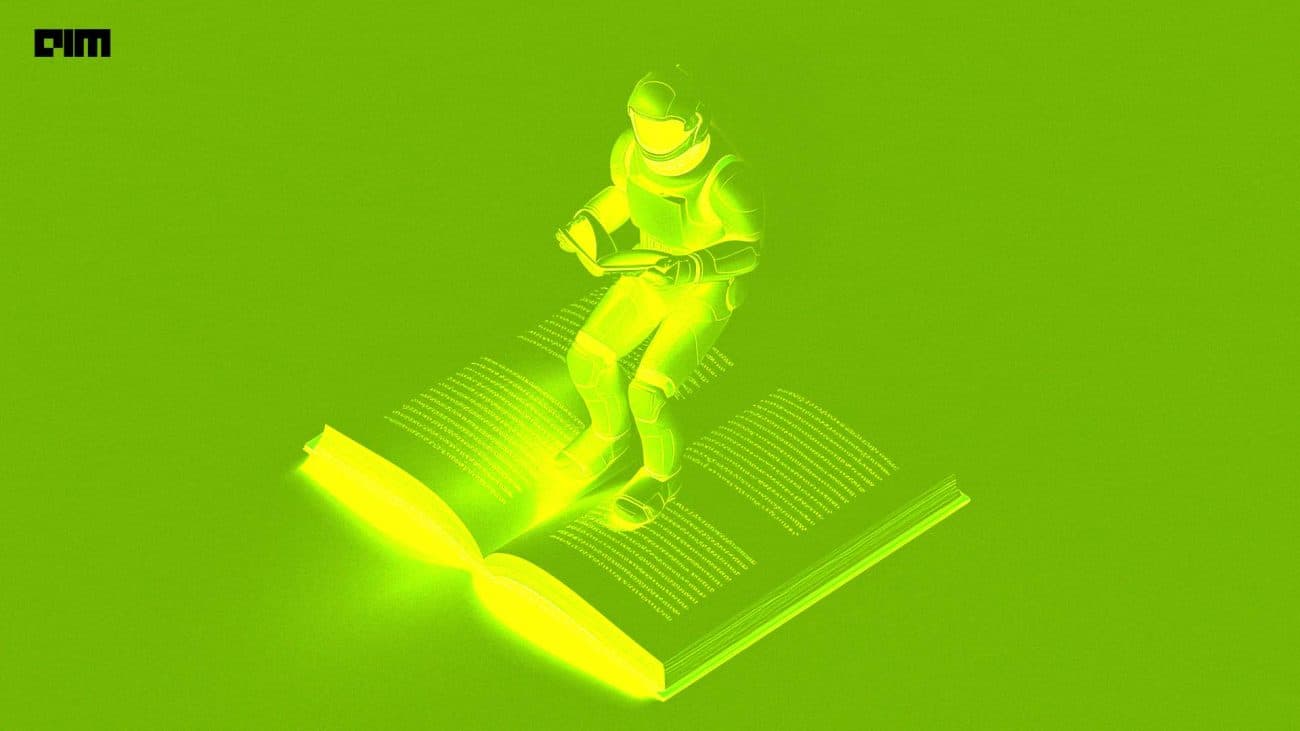
10 Free AI Courses by NVIDIA

Top 6 AI/ML Hackathons to Participate in 2024
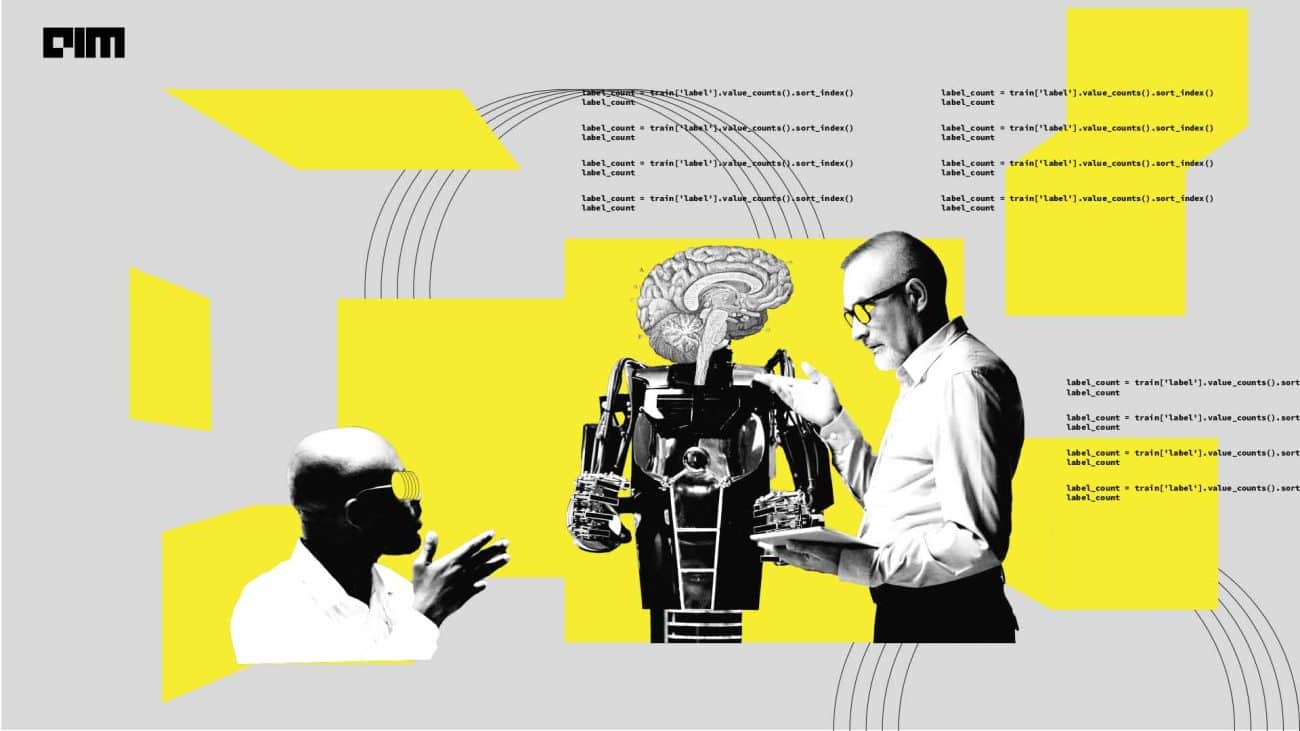
What’s Devin Up to?

10 Underrated Women in AI to Watchout For
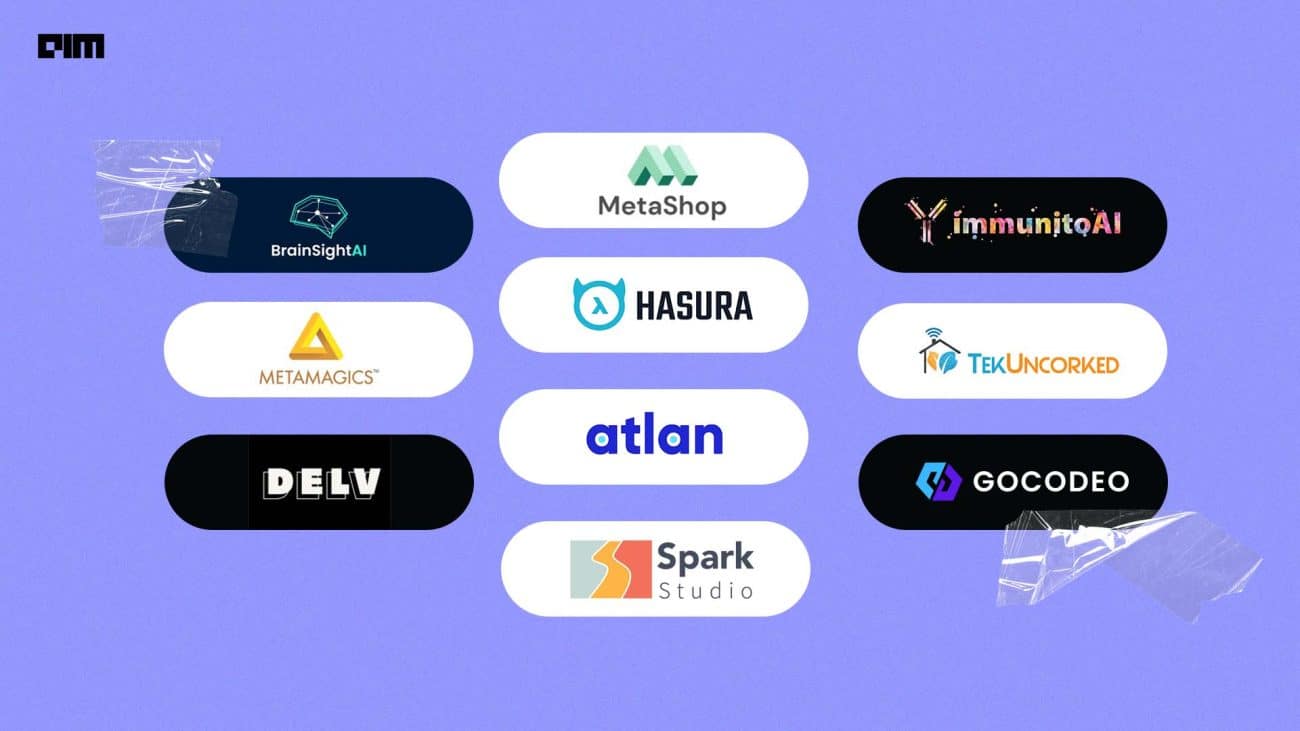
10 AI Startups Run by Incredible Women Entrepreneurs
Data dialogues.
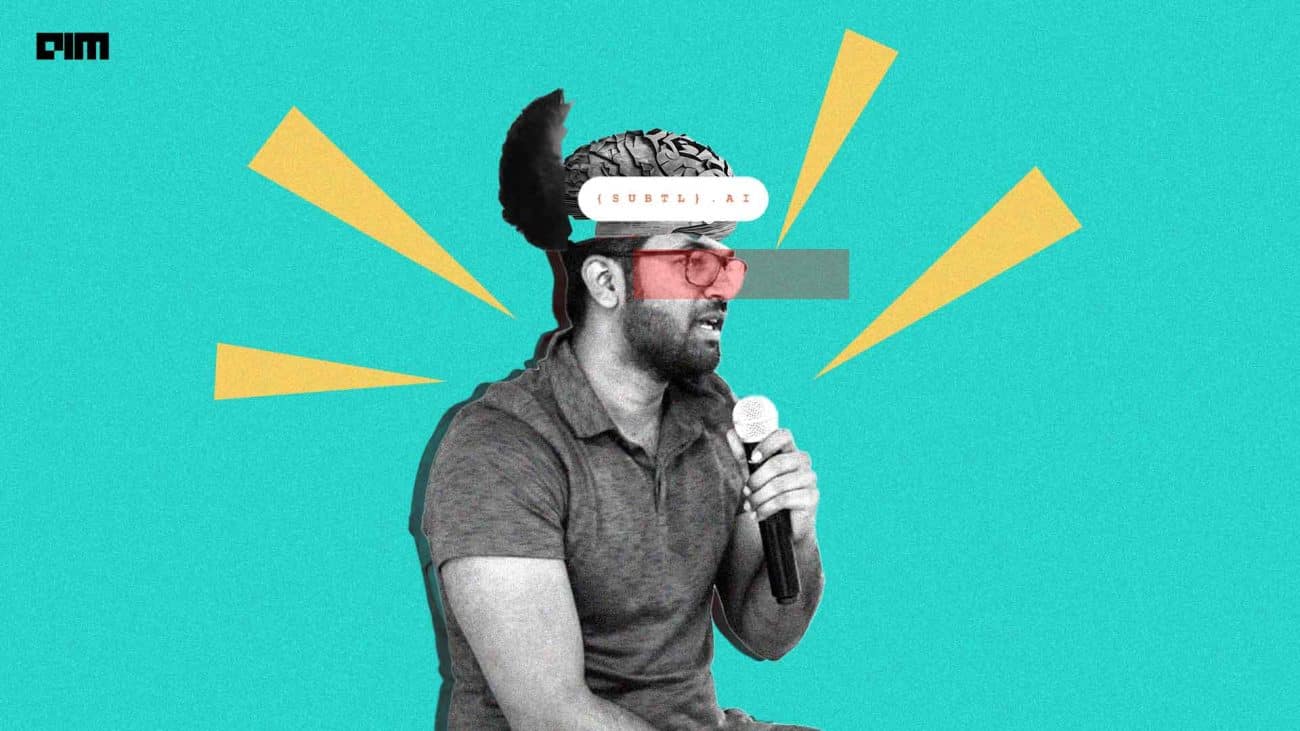
Meet the Indian AI Startup Building a Private Perplexity for Enterprise
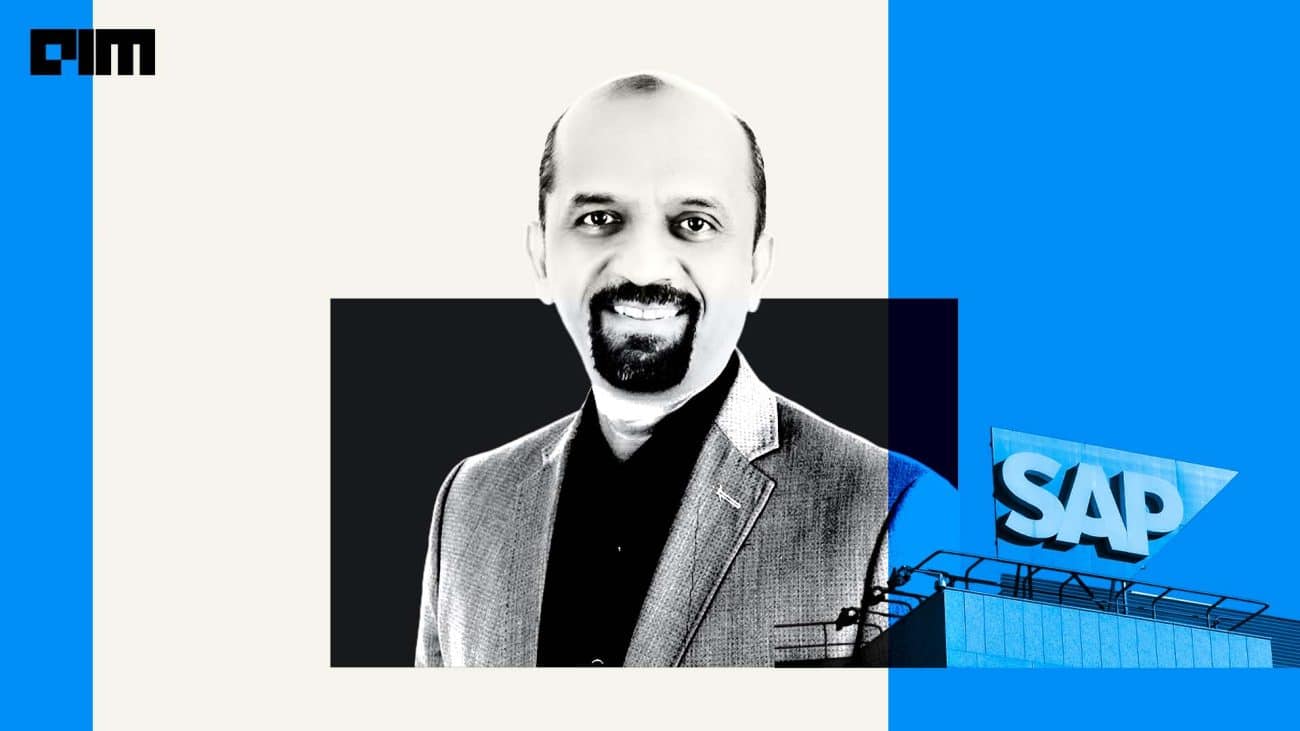
SAP Has Over 15,000 Customers in India
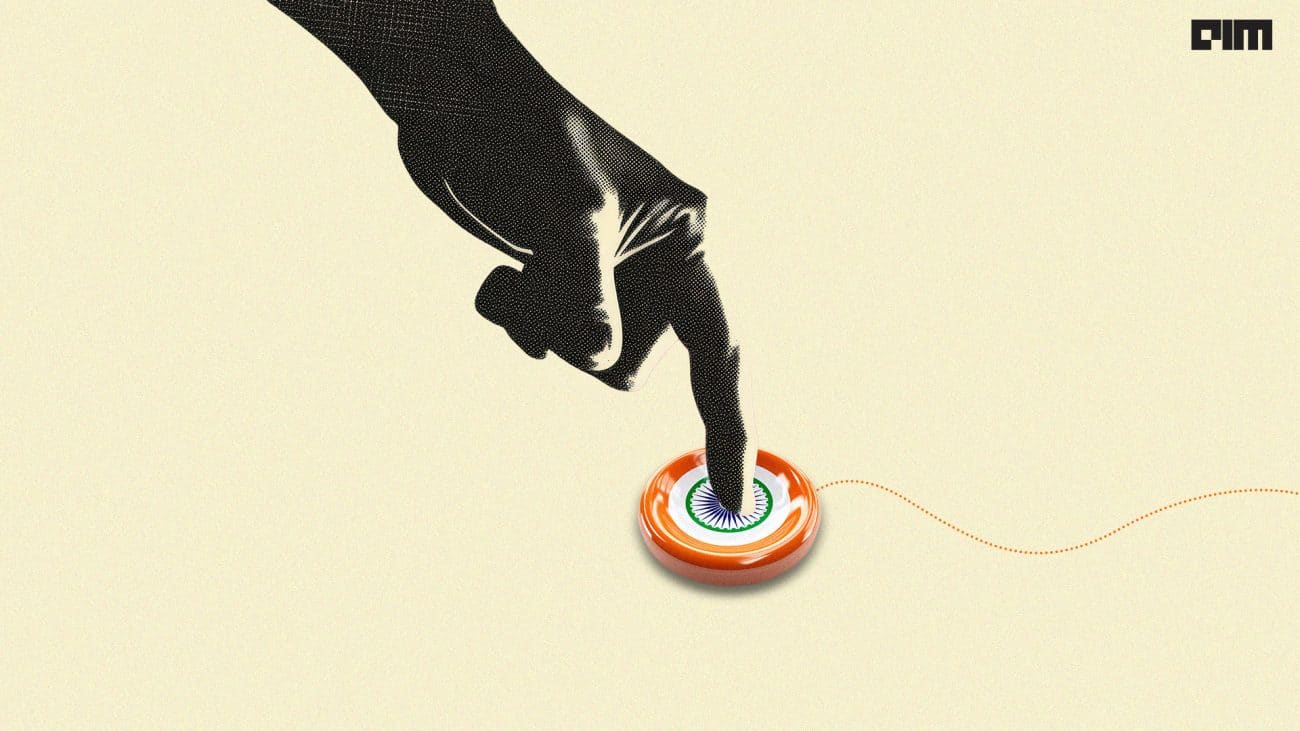
Soket AI Labs Becomes the First Indian Startup to Build Solutions Towards Ethical AGI
Zerodha cto warns companies to not look at ai as a solution chasing a problem.
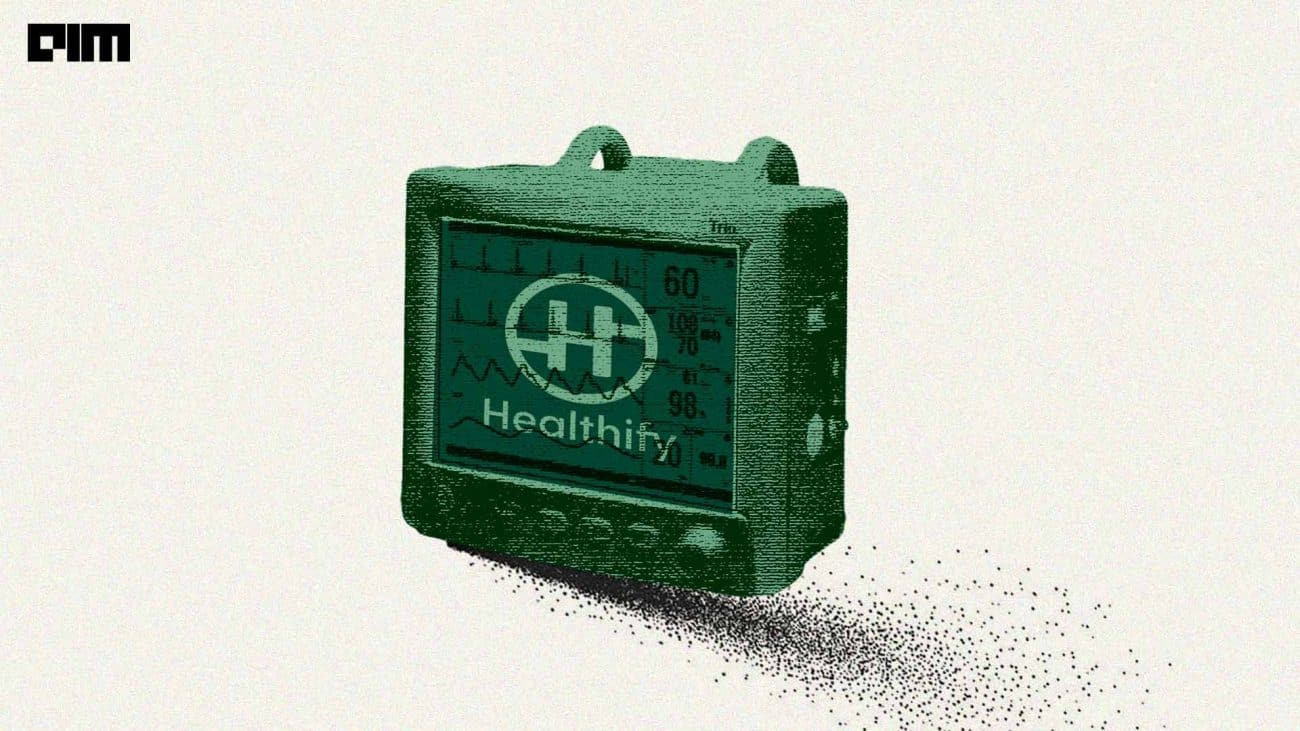
Healthify Uses OpenAI’s GPTs to Help Indians Make Better Health Choices
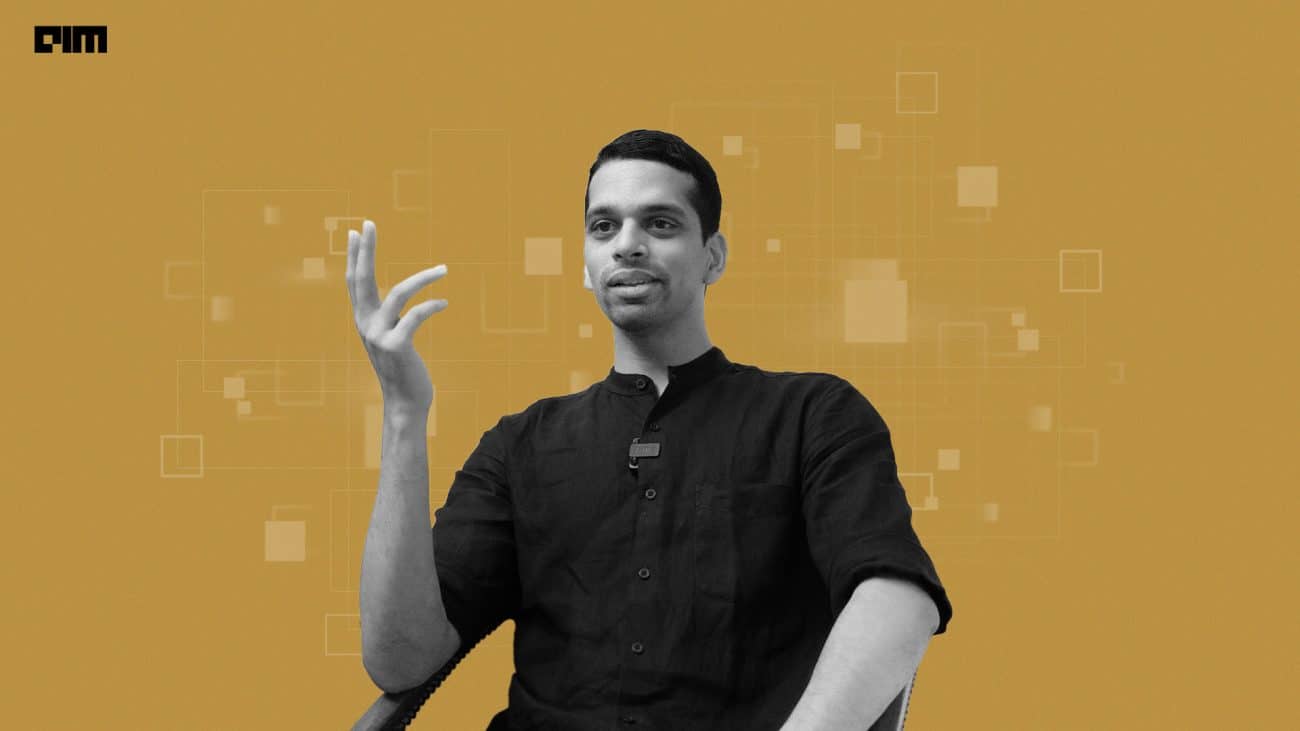
Zerodha CTO Says He Stopped Googling Technical Stuff Over the Past Year
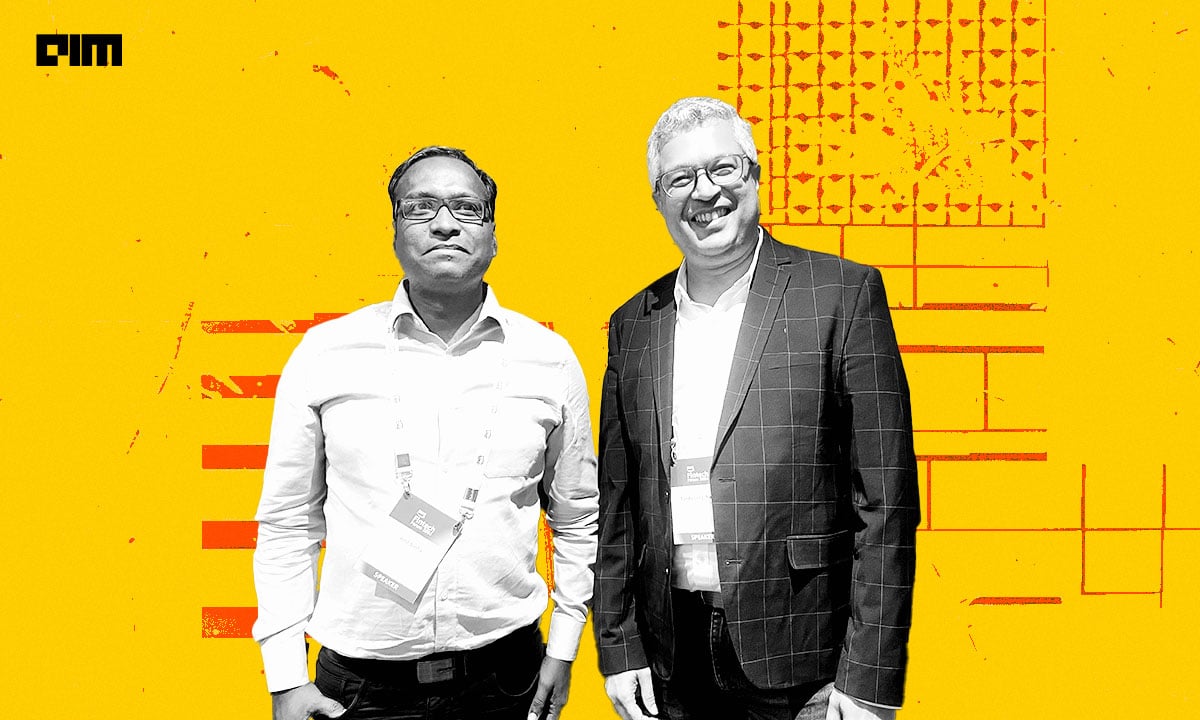
Fibe Leverages Amazon Bedrock to Increase Customer Support Efficiency by 30%
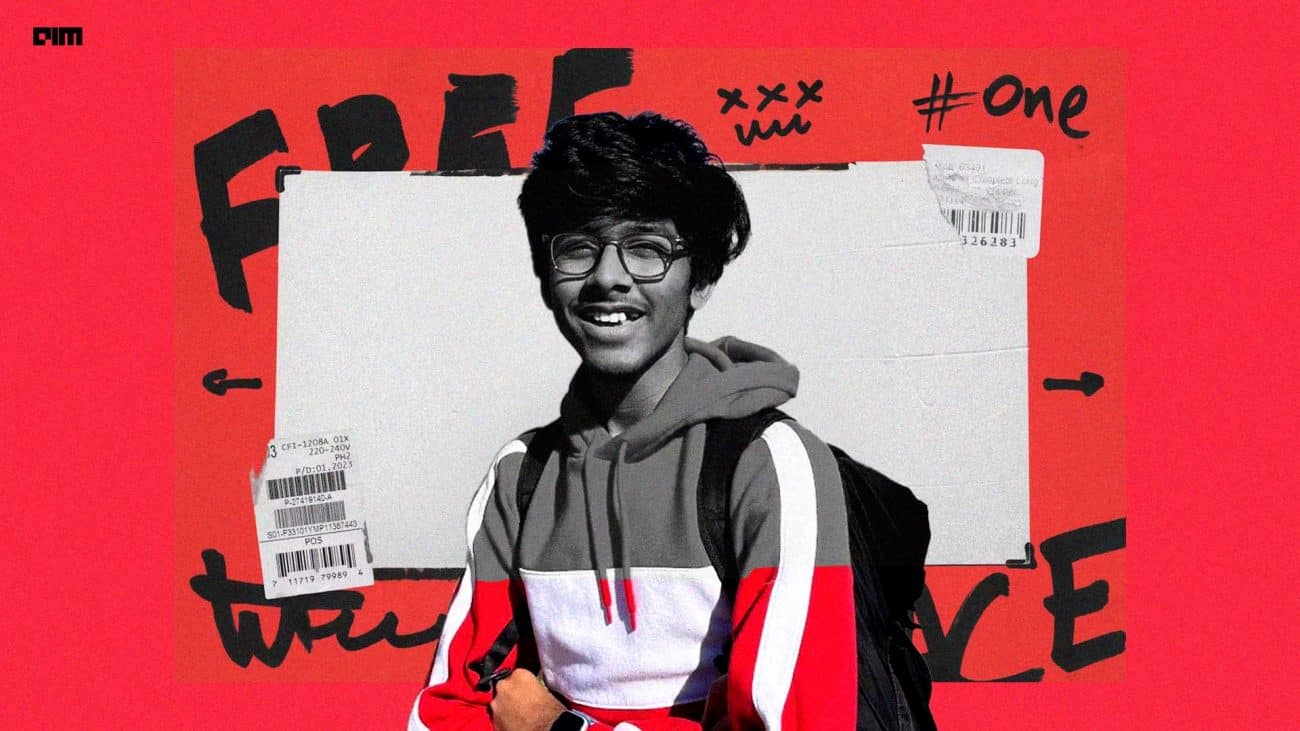
This 18-Year-Old Programmer is Creating an Open Source Alternative to Redis
Future talks.

As GitHub Begins Technical Preview of Copilot Workspace, an Engineer Answers How it Differs from Devin

T-Hub Supported MATH is Launching AI Career Finder to Create AI Jobs

Quora’s Poe Eats Google’s Lunch

Zoho Collaborates with Intel to Optimise & Accelerate Video AI Workloads

Rakuten Certified as Best Firm for Data Scientists for the 2nd Time

This Indian Logistics Company Developed an LLM to Enhance Last-Mile Delivery
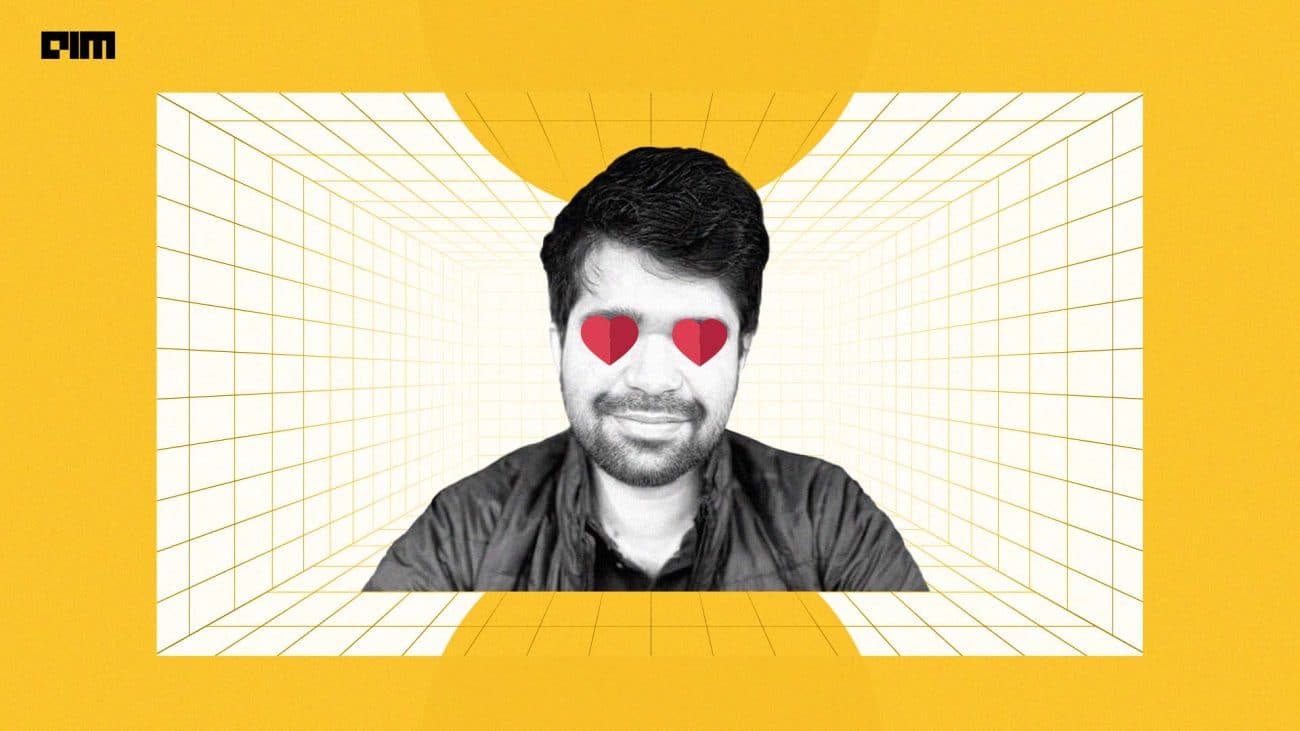
Perplexity AI Reviews with Pro Access
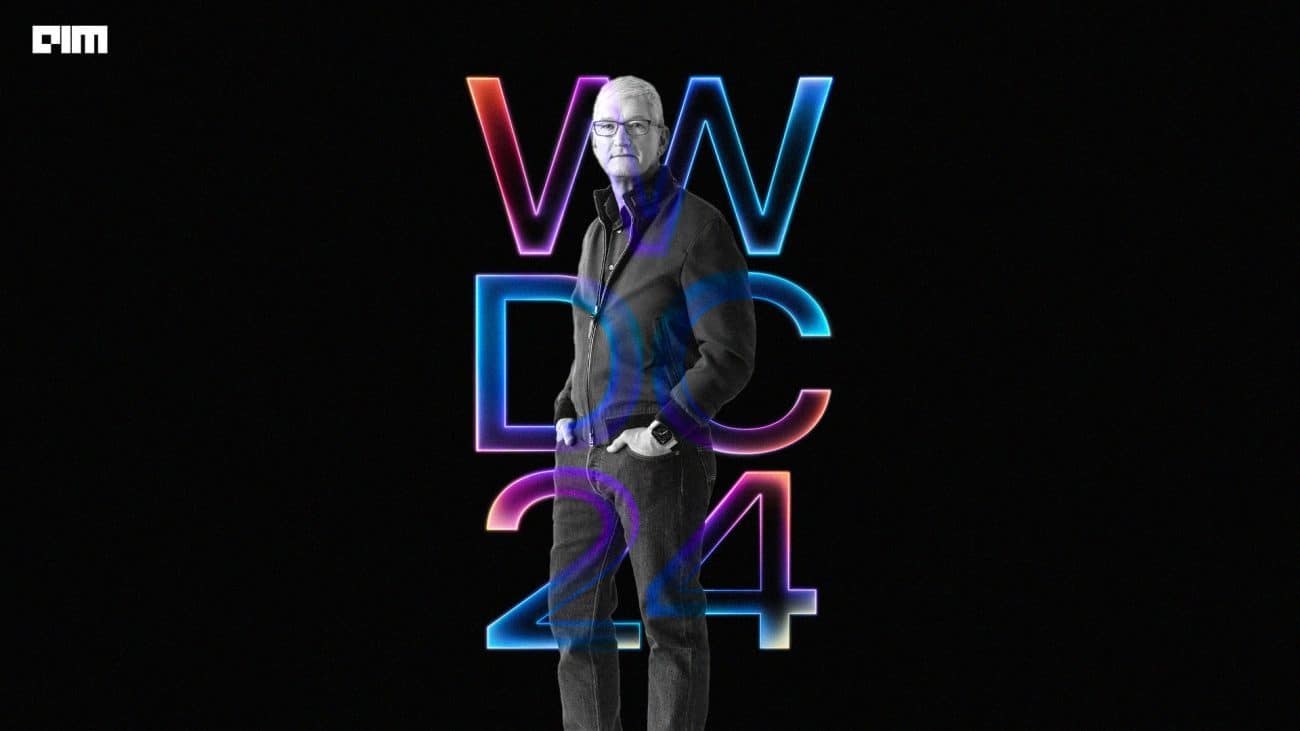
What to Expect at the ‘Absolutely Incredible’ Apple WWDC 2024
Developer’s corner.
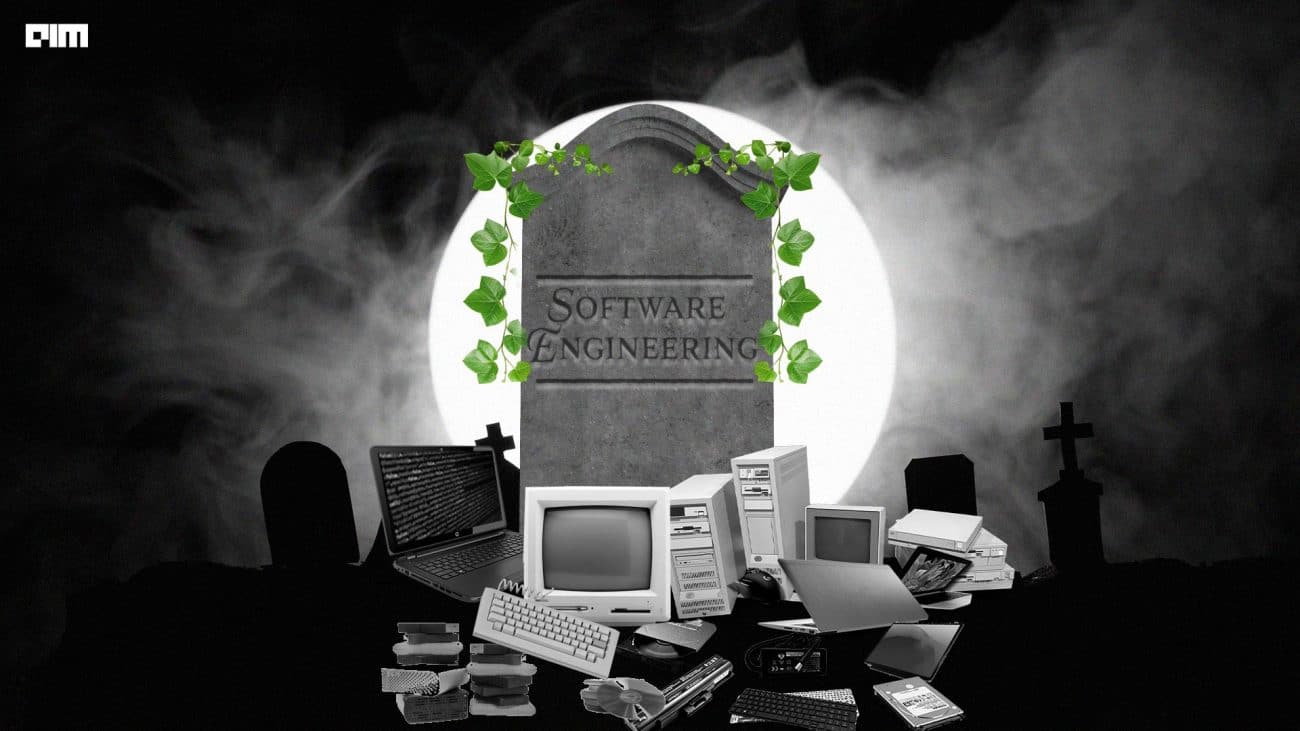
Software Engineering Jobs are Dying

Open-Source MS-DOS 4.0 Inspires Aspiring Developers to Embrace Retro Revolution
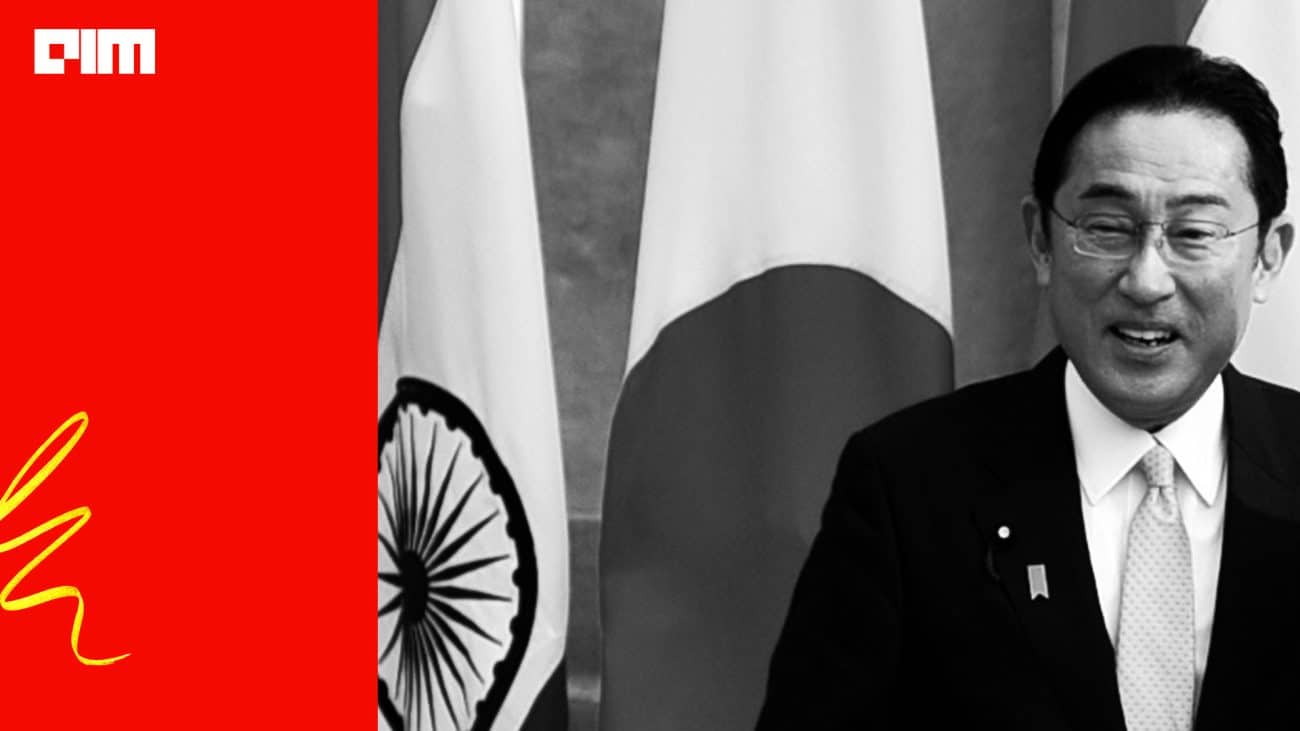
Japan is the Next Big Hub for Indian Tech Talent
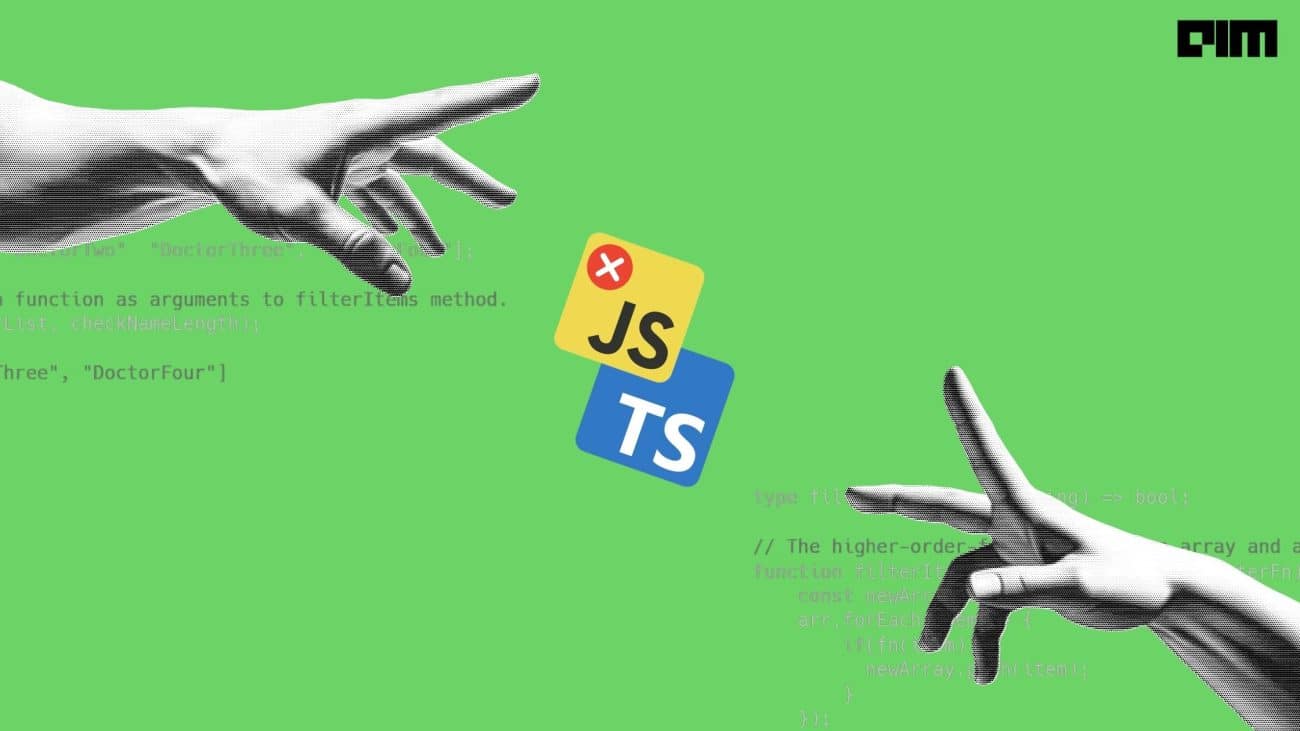
Will TypeScript Wipe Out JavaScript?
In case you missed it.

Meta Forces Developers Cite ‘Llama 3’ in their AI Development

Why Developers Hate Jira
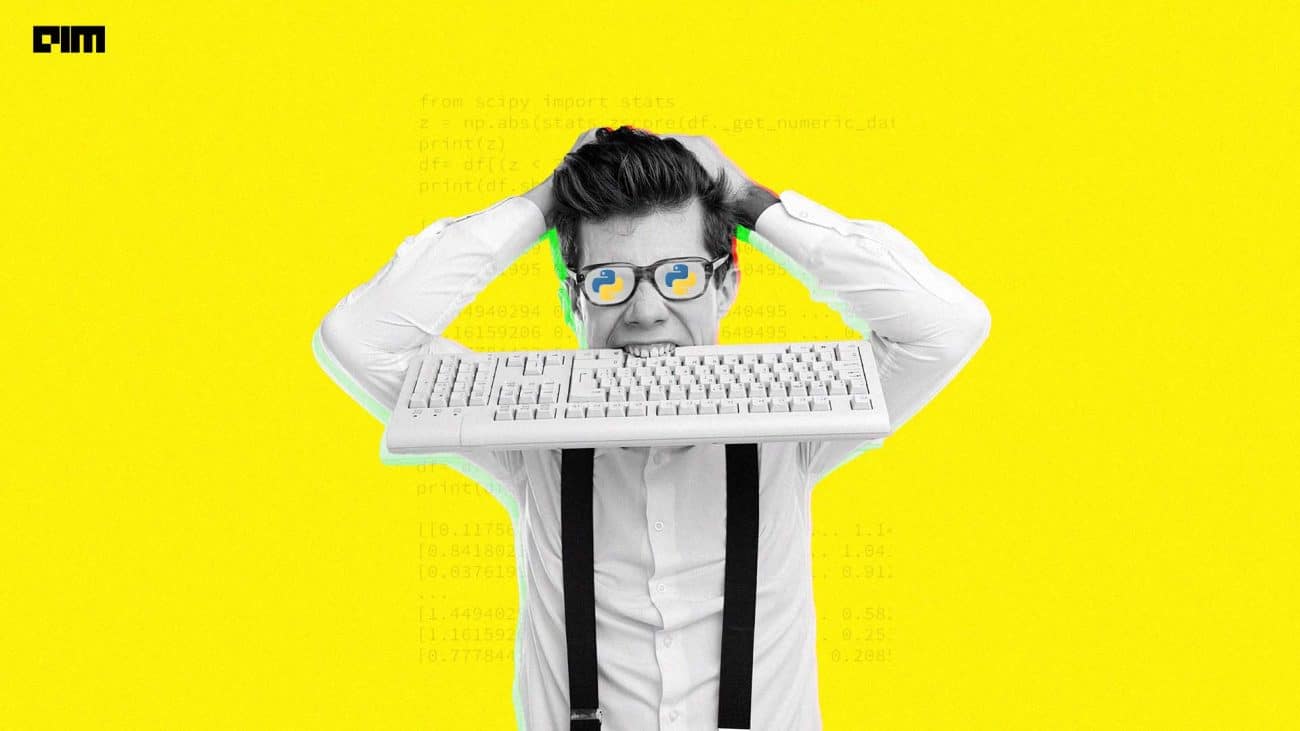
Which is the Most Frustrating Programming Language?
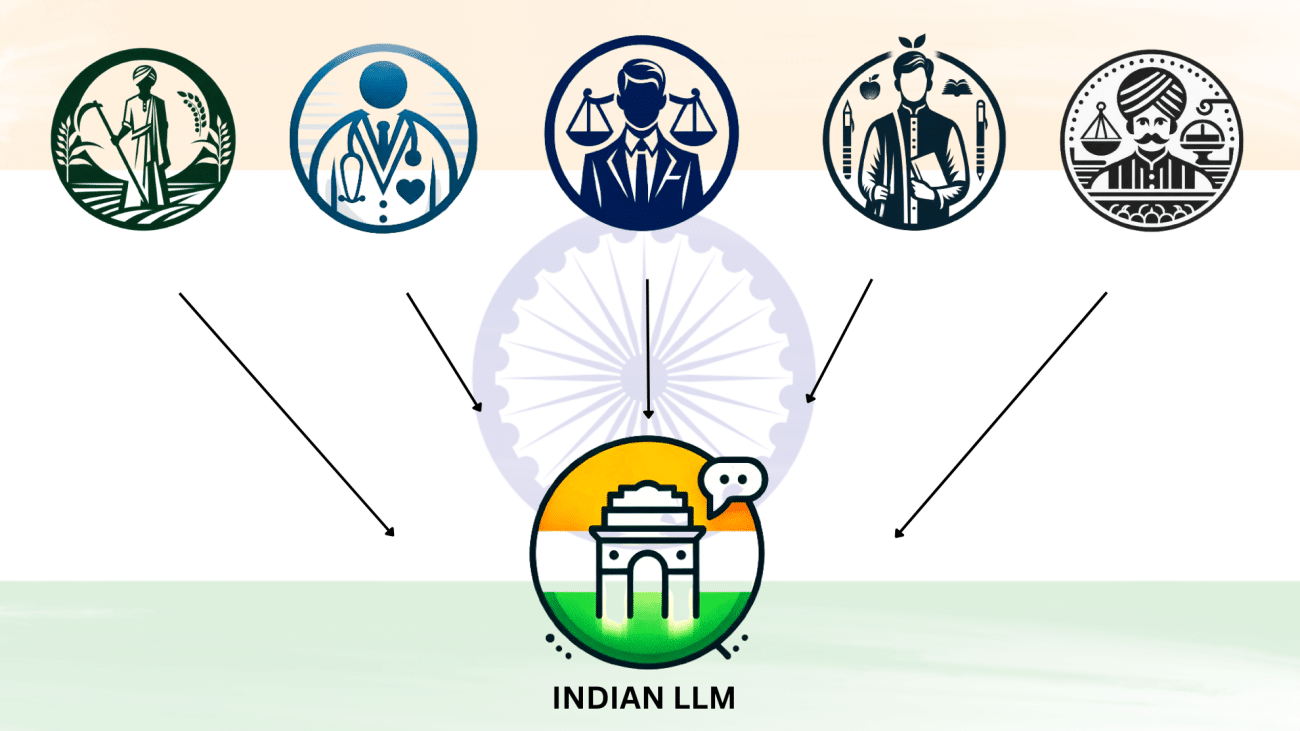
AI4Bharat Rolls Out IndicLLMSuite for Building LLMs in Indian Languages
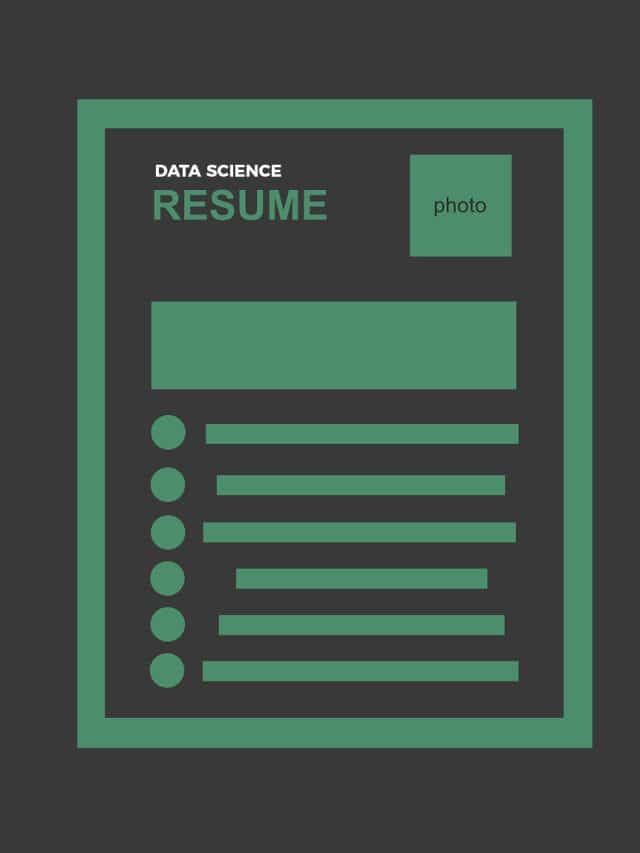
8 Best AI Resume Builders for Freshers in 2024
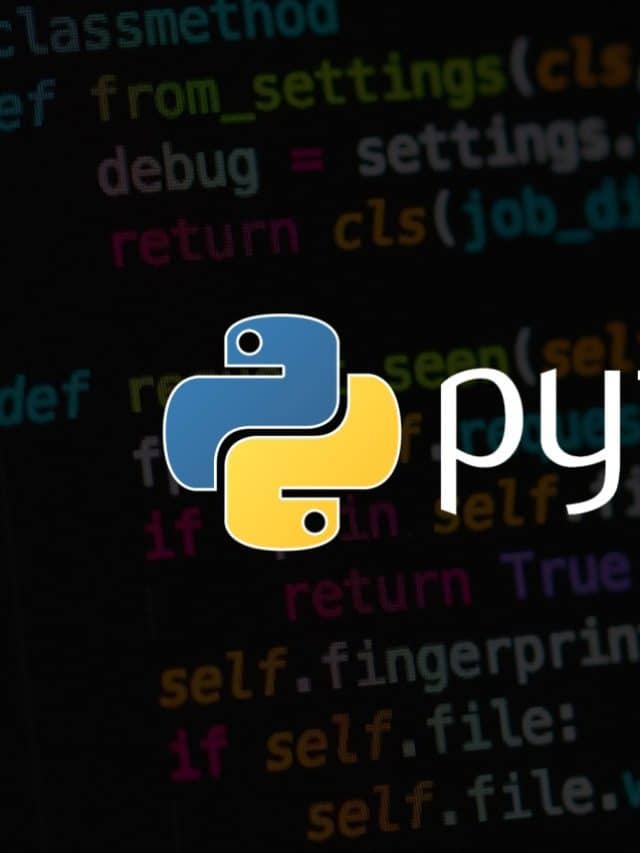
8 Best AI Libraries for Python Developers
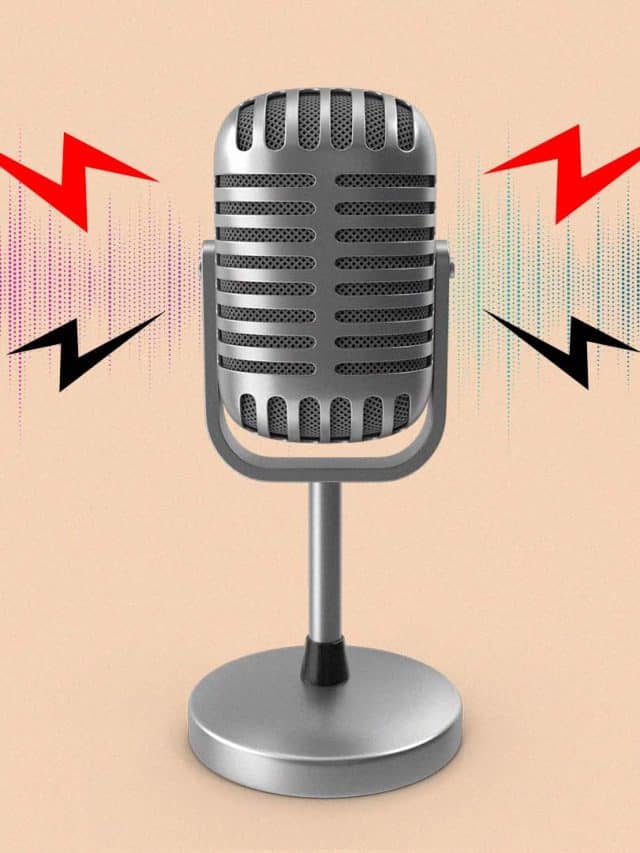
8 Best AI Voice Generators Available for Free to Use in 2024
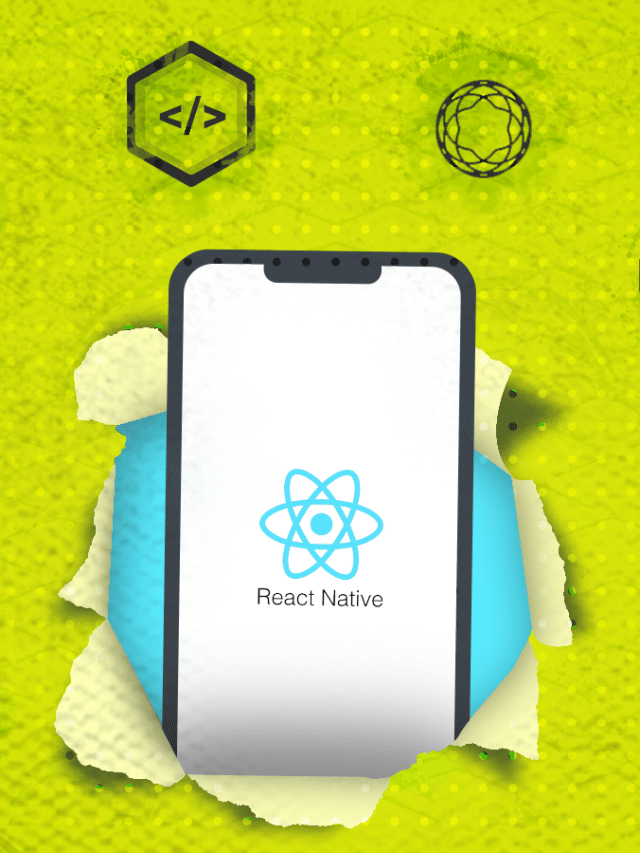
10 Best React Project Ideas For Beginners
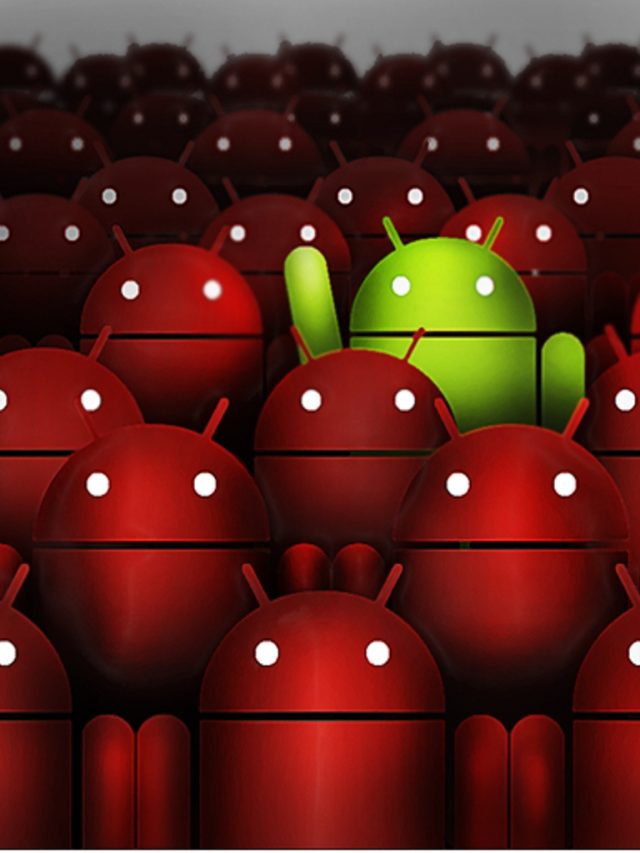
8 Best AI Image Generator Apps Free for Android Users in 2024

9 Best AI Tools for Excel and Google Spread Sheet Automation
Also in trends.
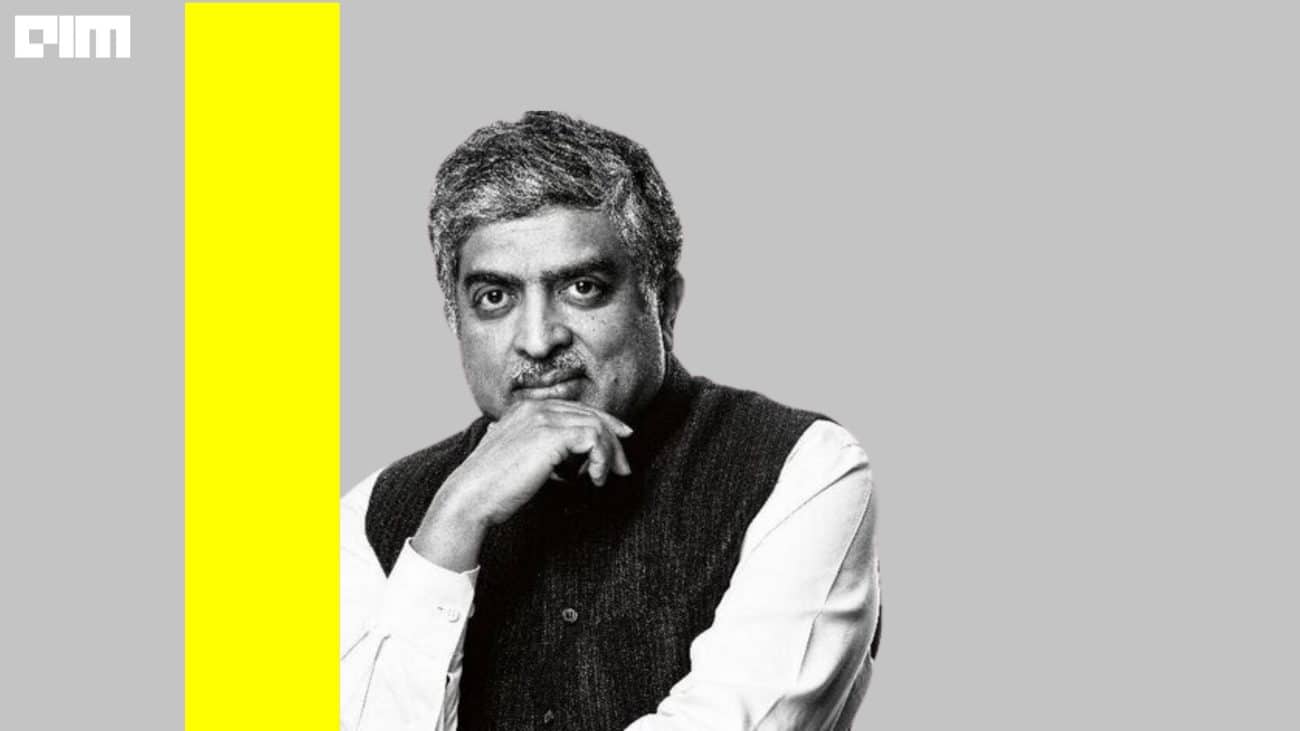
‘Winners in AI will be those who meet customers where they are,’ says Nandan Nilekani
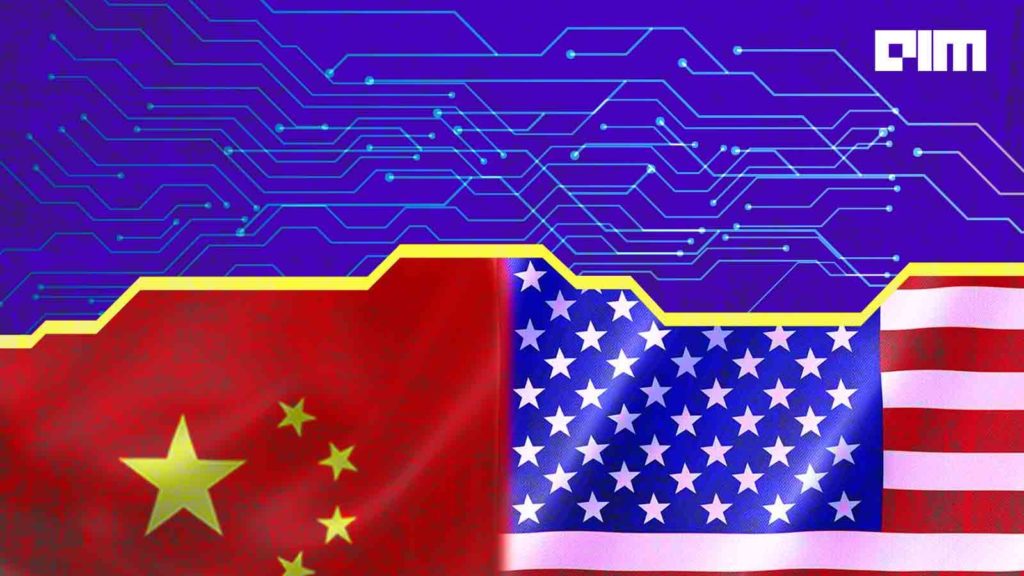
US is Two to Three Years Ahead of China in AI
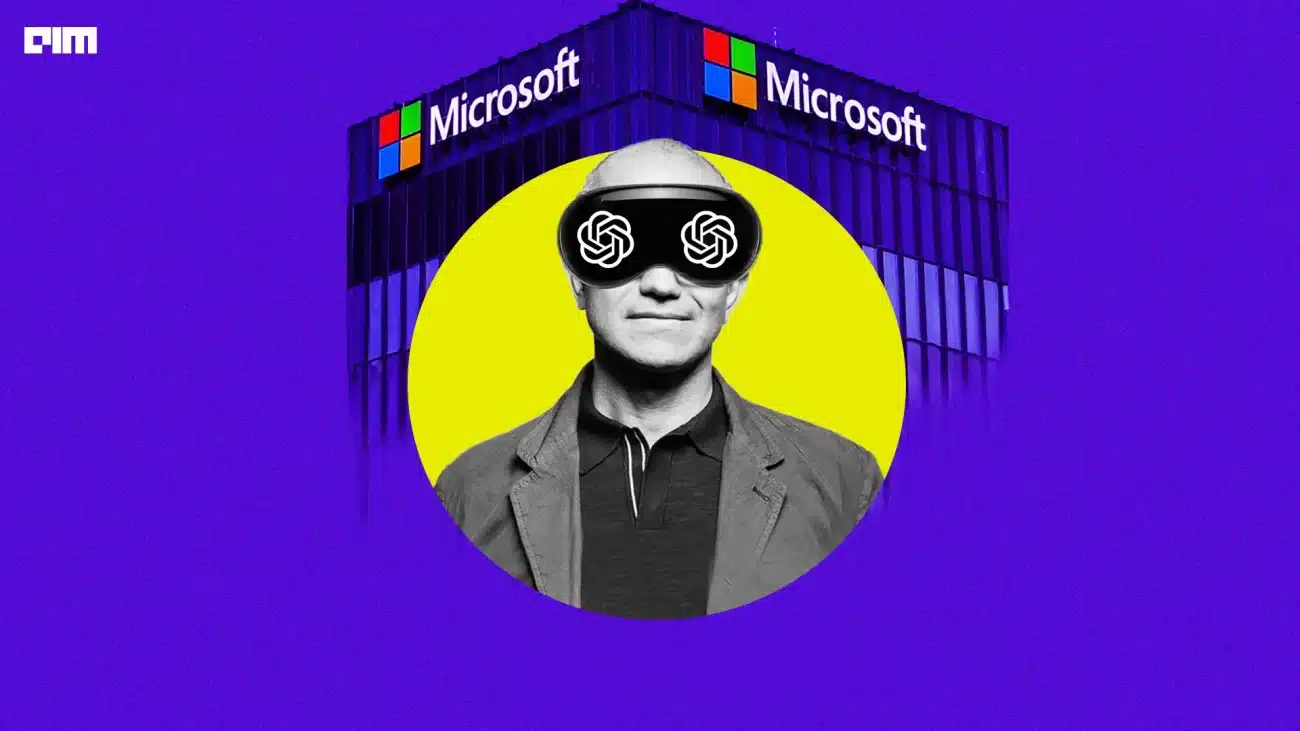
Microsoft and OpenAI Announce $2 Million for Societal Resilience Fund
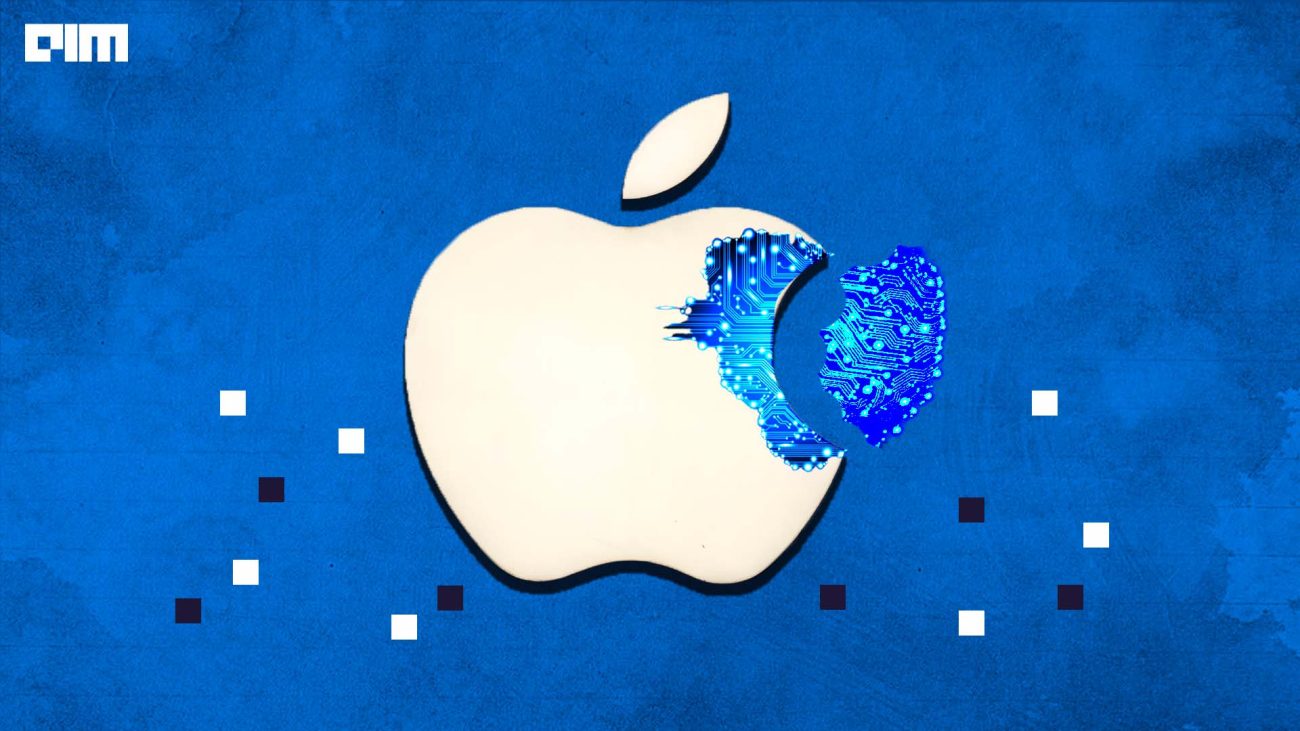
iPad Pro with M4 Chip Enables Seamless AI Tasks

Infosys & ServiceNow Boost Collaboration for Gen AI-Powered Solutions
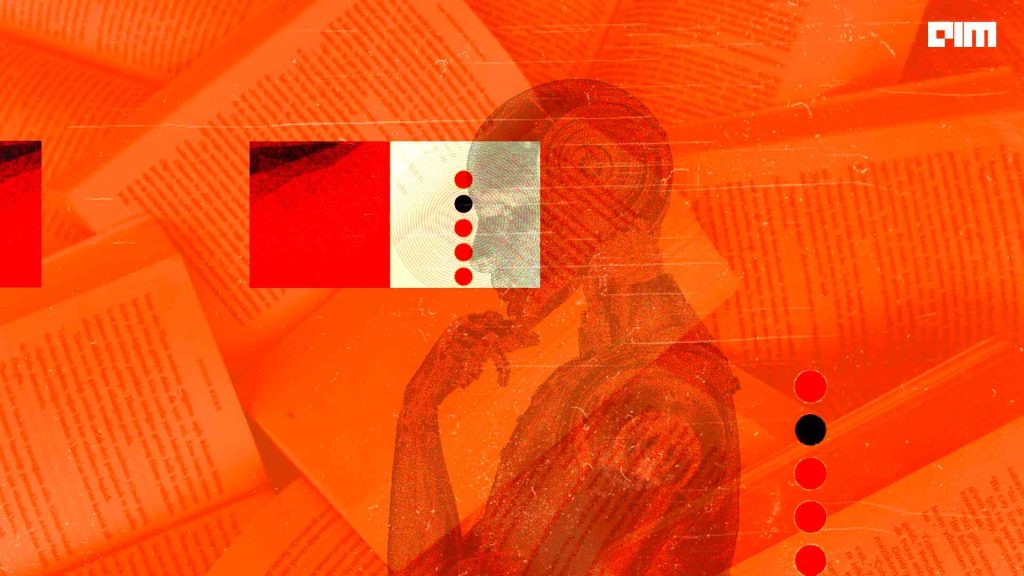
The Inventor of LSTM Unveils New Architecture for LLMs to Replace Transformers
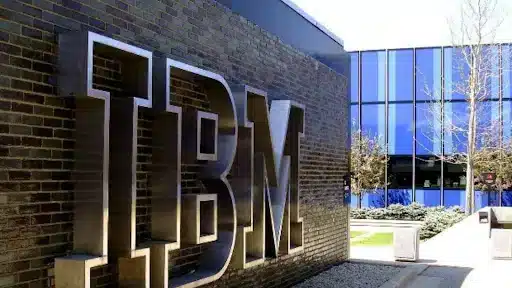
LTIMindtree, IBM Setup Watsonx Center of Excellence for GenAI in India
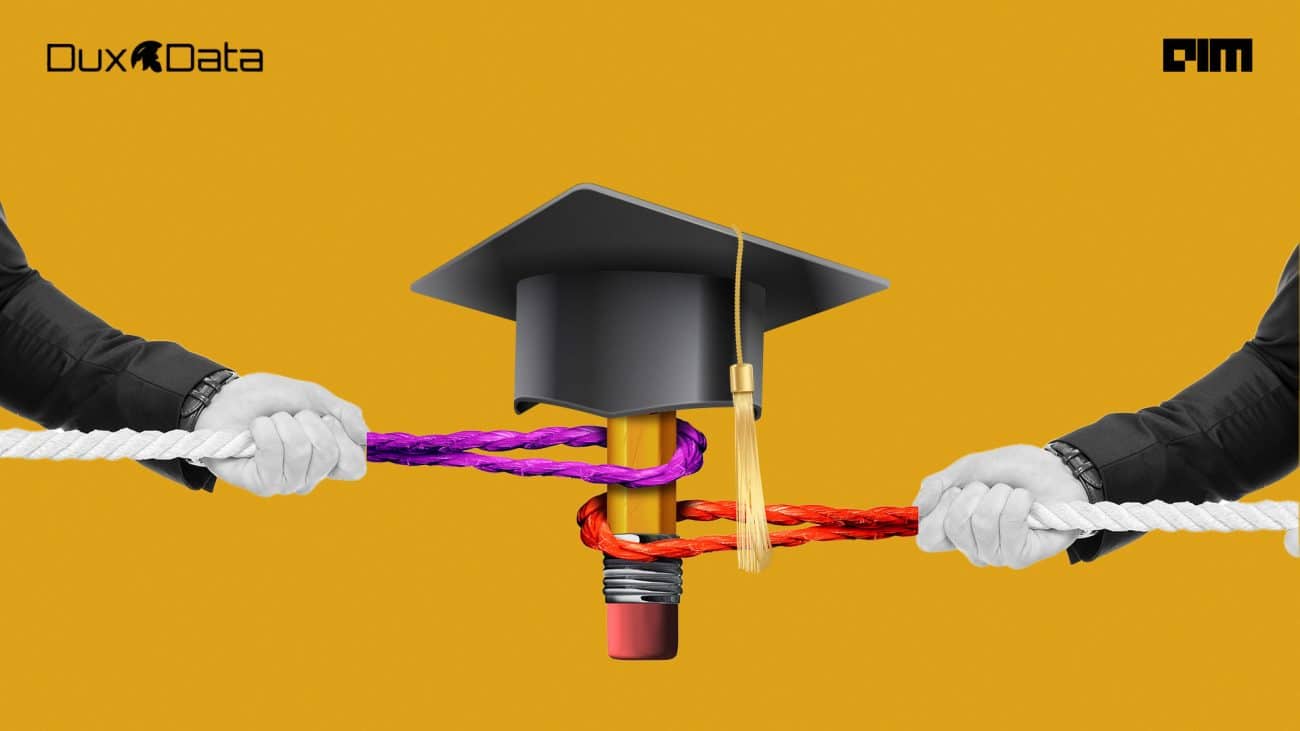
Leading the Way: DuxData’s Data Leadership & Strategy Course Prepares Data Pros for the AI Era
World's biggest media & analyst firm specializing in ai, advertise with us, aim publishes every day, and we believe in quality over quantity, honesty over spin. we offer a wide variety of branding and targeting options to make it easy for you to propagate your brand., branded content, aim brand solutions, a marketing division within aim, specializes in creating diverse content such as documentaries, public artworks, podcasts, videos, articles, and more to effectively tell compelling stories., corporate upskilling, adasci corporate training program on generative ai provides a unique opportunity to empower, retain and advance your talent, with machinehack you can not only find qualified developers with hiring challenges but can also engage the developer community and your internal workforce by hosting hackathons., talent assessment, conduct customized online assessments on our powerful cloud-based platform, secured with best-in-class proctoring, research & advisory, aim research produces a series of annual reports on ai & data science covering every aspect of the industry. request customised reports & aim surveys for a study on topics of your interest., conferences & events, immerse yourself in ai and business conferences tailored to your role, designed to elevate your performance and empower you to accomplish your organization’s vital objectives., aim launches the 3rd edition of data engineering summit. may 30-31, bengaluru.
Join the forefront of data innovation at the Data Engineering Summit 2024, where industry leaders redefine technology’s future.
© Analytics India Magazine Pvt Ltd & AIM Media House LLC 2024
- Terms of use
- Privacy Policy
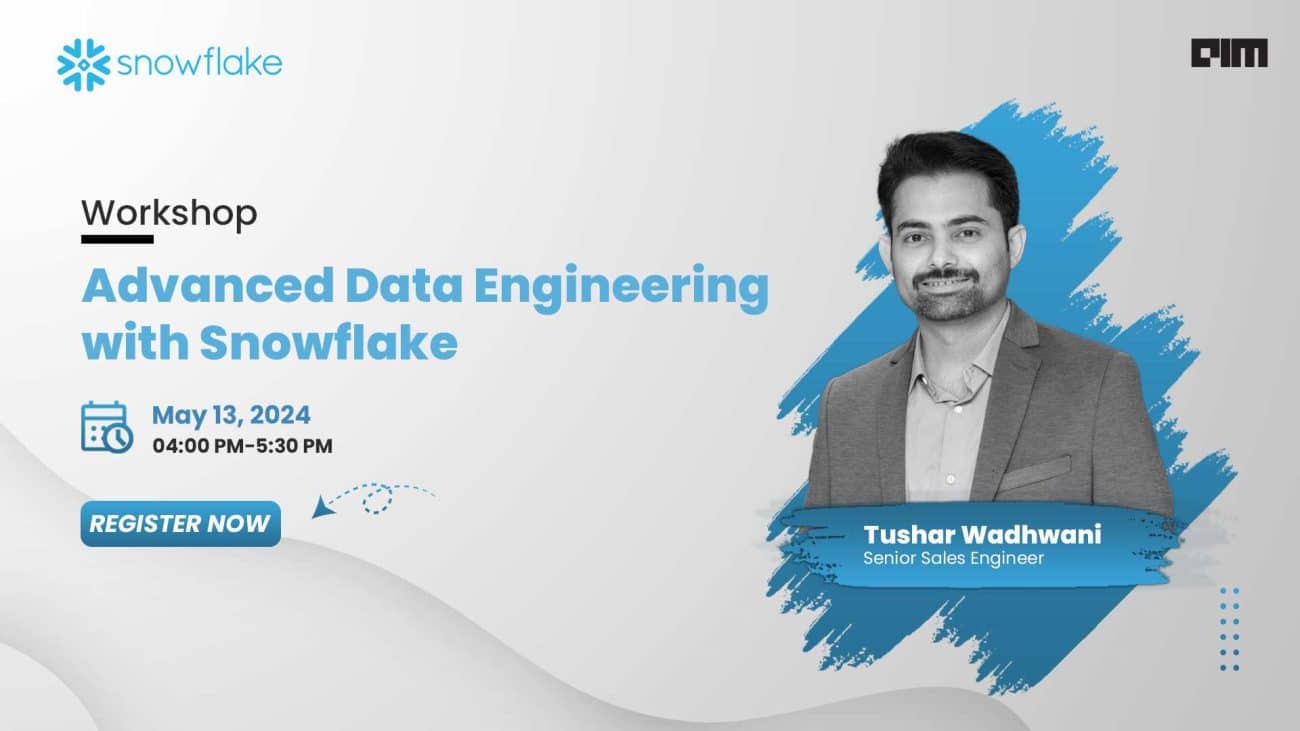
All Courses
- Interview Questions
- Free Courses
- Career Guide
- PGP in Data Science and Business Analytics
- PG Program in Data Science and Business Analytics Classroom
- PGP in Data Science and Engineering (Data Science Specialization)
- PGP in Data Science and Engineering (Bootcamp)
- PGP in Data Science & Engineering (Data Engineering Specialization)
- Master of Data Science (Global) – Deakin University
- MIT Data Science and Machine Learning Course Online
- Master’s (MS) in Data Science Online Degree Programme
- MTech in Data Science & Machine Learning by PES University
- Data Analytics Essentials by UT Austin
- Data Science & Business Analytics Program by McCombs School of Business
- MTech In Big Data Analytics by SRM
- M.Tech in Data Engineering Specialization by SRM University
- M.Tech in Big Data Analytics by SRM University
- PG in AI & Machine Learning Course
- Weekend Classroom PG Program For AI & ML
- AI for Leaders & Managers (PG Certificate Course)
- Artificial Intelligence Course for School Students
- IIIT Delhi: PG Diploma in Artificial Intelligence
- Machine Learning PG Program
- MIT No-Code AI and Machine Learning Course
- Study Abroad: Masters Programs
- MS in Information Science: Machine Learning From University of Arizon
- SRM M Tech in AI and ML for Working Professionals Program
- UT Austin Artificial Intelligence (AI) for Leaders & Managers
- UT Austin Artificial Intelligence and Machine Learning Program Online
- MS in Machine Learning
- IIT Roorkee Full Stack Developer Course
- IIT Madras Blockchain Course (Online Software Engineering)
- IIIT Hyderabad Software Engg for Data Science Course (Comprehensive)
- IIIT Hyderabad Software Engg for Data Science Course (Accelerated)
- IIT Bombay UX Design Course – Online PG Certificate Program
- Online MCA Degree Course by JAIN (Deemed-to-be University)
- Cybersecurity PG Course
- Online Post Graduate Executive Management Program
- Product Management Course Online in India
- NUS Future Leadership Program for Business Managers and Leaders
- PES Executive MBA Degree Program for Working Professionals
- Online BBA Degree Course by JAIN (Deemed-to-be University)
- MBA in Digital Marketing or Data Science by JAIN (Deemed-to-be University)
- Master of Business Administration- Shiva Nadar University
- Post Graduate Diploma in Management (Online) by Great Lakes
- Online MBA Programs
- Cloud Computing PG Program by Great Lakes
- University Programs
- Stanford Design Thinking Course Online
- Design Thinking : From Insights to Viability
- PGP In Strategic Digital Marketing
- Post Graduate Diploma in Management
- Master of Business Administration Degree Program
- MS in Business Analytics in USA
- MS in Machine Learning in USA
- Study MBA in Germany at FOM University
- M.Sc in Big Data & Business Analytics in Germany
- Study MBA in USA at Walsh College
- MS Data Analytics
- MS Artificial Intelligence and Machine Learning
- MS in Data Analytics
- Master of Business Administration (MBA)
- MS in Information Science: Machine Learning
- MS in Machine Learning Online
- MIT Data Science Program
- AI For Leaders Course
- Data Science and Business Analytics Course
- Cyber Security Course
- PG Program Online Artificial Intelligence Machine Learning
- PG Program Online Cloud Computing Course
- Data Analytics Essentials Online Course
- MIT Programa Ciencia De Dados Machine Learning
- MIT Programa Ciencia De Datos Aprendizaje Automatico
- Program PG Ciencia Datos Analitica Empresarial Curso Online
- Mit Programa Ciencia De Datos Aprendizaje Automatico
- Online Data Science Business Analytics Course
- Online Ai Machine Learning Course
- Online Full Stack Software Development Course
- Online Cloud Computing Course
- Cybersecurity Course Online
- Online Data Analytics Essentials Course
- Ai for Business Leaders Course
- Mit Data Science Program
- No Code Artificial Intelligence Machine Learning Program
- MS Information Science Machine Learning University Arizona
- Wharton Online Advanced Digital Marketing Program
- Representation of Knowledge
- Categories of knowledge
- Types of Knowledge Representation
- Requirements of knowledge Representation
- Components of knowledge Representation system
What is Knowledge Representation in AI? | Techniques used in Knowledge Representation?

In the field of AI, there are many complex tasks required to evaluate either in the field of Machine learning or deep learning. It is indeed necessary to automate a knowledge processing system in such system. Knowledge representation is one such process which depends on the logical situation and enable a strategy to take a decision in acquiring knowledge. There are many types and levels of knowledge acquired by human in daily life but machines find difficult to interpret all types of knowledge. For such conditions, knowledge representation is used.
In knowledge representation algorithms, AI agents tend to think and they contribute in taking decisions. With the aid of such complex thinking, they are capable to solve the complex problems indulged in real world scenarios that are hard and time consuming for a human being to interpret.
Learn all about AI and its application .
In AI systems, knowledge is represented in the following manner.
Events: Events are the occurrence of things in the real world. Anything which happens in real time are considered as the events. It is an important element as it is the initial thing to be considered in knowledge representation.
Objects are nothing but the facts that are actually true. Such facts can be habitual or a universal truth such as ‘The Sun rises in the East’, ‘Dogs are faithful’ or any facts which holds true in any events.
- Meta-Knowledge: It is those knowledges which are already been acquired either by human brain or machine.
- Knowledge base : It is the core component of the agents acquiring knowledge.
- Performance: It describes about how good the knowledge is acquired and it can be applied to machines.
Knowledge can be categorized into two major types:
- Tacit knowledge
- Explicit knowledge
Tacit knowledge is the knowledge which exists within a human being. It does correspond to informal or implicit type of knowledge. It is quite difficult to articulate formally and is also difficult to communicate and share.
Explicit knowledge is the knowledge which exists outside a human being. It corresponds to formal type of knowledge. It is easier to articulate compared to tacit knowledge and is easier to share, store or even process.
Declarative Knowledge
Procedural knowledge, meta knowledge, heuristic knowledge, structural knowledge.
Below is the all types of Knowledge Representation with Examples
It is the segment of knowledge which stores factual information in a memory and it seem to be static in nature. These can be things or events or processes and the domain of such knowledge find the relation between events or things.
This knowledge is less general compared to declarative knowledge and is also known called imperative knowledge. It can have the potential to declare the accomplishment of a particular thing. It is generally used by modern mobile robots where they can be planned to attack into a building or perform navigation in a room. If we consider declarative knowledge implanted into a modern robot, they it will be assigned just a map instead of detailed plan of attack into a building.
In the field of AI, the knowledge of pre-defined knowledge is known as meta knowledge. A study of planning, tagging and learning are some of the examples of meta knowledge. This model tends to change with time and utilize a different specification. A knowledge engineer may utilize different forms of meta-knowledge given below:
Accuracy, Applicability, Assessment, Consistency, Completeness, Disambiguation, Justification, Life Span, Purpose, Source, Reliability.
This knowledge is also known as Shallow knowledge and it follows the principle of thumb rule. It is very efficient in reasoning process as it solves the problems based on the records of past problems or the problems which are compiled by experts. It provides knowledge based on the experiences it gathered during the past problems.
Know about the new developments in AI ethics .
This is the most basic knowledge used and applied in problem solving. It tries to find out a relationship between concepts and objects.
Let us describe a relationship of the knowledge along with a flowchart.

As we can see, declarative knowledge is represented as describing one and procedural knowledge is represented as doing one. Now one more inference is, declarative knowledge is termed as explicit while procedural knowledge is termed as tacit. If the knowledge can be articulated, it is a declarative knowledge and if cannot be articulated, it is known as procedural knowledge.
Learn what the top AI startups in India are working on.
Knowledge representation often provides information about those things which occur very common and make a pattern. Such patterned description is known as schemas. There are various types of schema which are
- Frames – They contain information of all the attributes present in a given object. As for example, the description of a girl includes hair, facial pattern, eyes, etc. are considered as the frames. They represent knowledge of concepts and objects.
- Scripts- They are often used to explain series of events which follow a sequence. For example, a hotel scene. They represent knowledge of events.
- Stereotypes – They used to describe the characteristics present in a people.
- Rule models- In a production system, they describe features which are shared commonly among a set of rules.
A knowledge representation has the following requirements
- It should have the adequacy or fulfillment to represent all types of knowledge present in the domain. It is also known as representational adequacy.
- It should be capable enough to manipulate the representational structure in order to derive new structures which also should be corresponding to the new knowledge extracted from the old. It is also referred as inferential adequacy.
- It should be able to indulge the additional information into the knowledge structure which can be further used to focus on inference mechanisms in the best possible direction. It is sometimes known as inferential efficiency.
- It should acquire new knowledge with the help of automatic methods rather than relying on human source. This process is known as acquisitional efficiency.
The knowledge representation function contains the following components.
- KR and Reasoning
- Planning and Execution
Perception helps in extracting the information and can be helpful in telling us the status of AI system. It can detect any irregularity in the system and make us ready to decide whether an AI system has the potentiality of damage or not.
Learning component captures the data which are already sensed by the perception component. Learning component tries to enable the computer to learn just like human instead of always programming it. This component solely tries to focus on how to self-improve the AI system.
KR and reasoning are used in AI to acquire knowledge in the smartest way. It focuses on the behavior of an AI agent and make sure that it more or less behaves like human. It is used to formalize the knowledge in the knowledge base.
Planning and execution try to find the optimal solution of the current state and tries to understand the impact of the same. Now it tries to seek out the solution that the final state holds and then it will try to terminate the entire process with a solution here itself.
The below diagram shows how the process of knowledge representation works.

Different approaches are used by knowledge representation system. Those are
- Simple relational knowledge
This knowledge is used to store data systematically and in the form of columns. The only thing to know is they contain relation with each other and they very little chances to make an inference which can be later used in inference engines.
The above table can give answers to
- Who plays in rock style?
- Who plays trumpet in rock style?
2. Inheritable knowledge
This type of knowledge can be passed on other agents without having a need of learning again. If an AI agent learns something from a human, then it can pass it to other agents and they can inherit the same without learning again.
This type of knowledge is generally obtained from associated objects and tries to prescribe a new structure which extracts all or selective attributes from existing objects. This type of knowledge is indulged in the design hierarchies which is found in physical, functional and process domains. So the parent attributes try to inherit the knowledge within the hierarchy to prescribe to the child elements.
3 . Inferential knowledge
It defines the knowledge as a formal logic condition and has a strict rule. The knowledge is extracted from objects by studying the relation between them. If we take a word to make an inference, it will difficult except we take a phrase to get more meaningful insights of that same word. In linguistic, this approach is known as semantics. The new information extracted from the existing information does not require gathering of data from the source but they analyze the existing information in order to generate new knowledge.
4. Procedural knowledge
This knowledge tends to represent control information which uses the knowledge keeps embedded in the knowledge itself. This approach can easily represent heuristic or domain specific knowledge. They are represented as small programs of how to proceed and perform specific things. They may include inferential efficiency but they do not have inferential adequacy or acquisitional efficiency.
Knowledge representation theory is suitable when intelligent behavior solely depends on explicitly represented knowledge. Knowledge representation is not capable to solve anything by itself if a system fails to reason what it has represented explicitly in the mist effective way. Knowledge representation is a study of the information we can extract in a computationally dependable way or investigating the area within the theories of KR hypothesis. If a theory consumes classical first order logic assumptions, then knowledge representation is the basis of this investigation or else it is recommended to explore other theories.
To know more about AI and its various concepts, take a program which covers the subject extensively. Great Learning ’s PGP- AIML offers you mentored learning along with industry insights. Visit our website to learn more.

Top Free Courses

What is Artificial Intelligence in 2024? Types, Trends, and Future of it?

What is Ridge Regression?

Top 20 Generative AI Applications/ Use Cases Across Industries

What is Time Complexity And Why Is It Essential?

Advantages and Disadvantages of Artificial Intelligence

Generative Artificial Intelligence Implications for Industry Experts
Leave a comment cancel reply.
Your email address will not be published. Required fields are marked *
Save my name, email, and website in this browser for the next time I comment.

Table of contents
What Is Knowledge Representation?
Cite this chapter.
- Nick Cercone 3 &
- Gordon McCalla 4
Part of the book series: Symbolic Computation ((1064))
110 Accesses
3 Citations
In this chapter, we overview eight major approaches to knowledge representation: logical representations, semantic networks, procedural representations, logic programming formalisms, frame-based representations, production system architectures, and knowledge representation languages. The fundamentals of each approach are described, and then elaborated upon through illustrative examples chosen from actual systems which employ the approach. Where appropriate, comparisons among the various schemes are drawn. The chapter concludes with a set of general principles which have grown out of the different approaches.
This is a preview of subscription content, log in via an institution to check access.
Access this chapter
- Available as PDF
- Read on any device
- Instant download
- Own it forever
- Compact, lightweight edition
- Dispatched in 3 to 5 business days
- Free shipping worldwide - see info
- Durable hardcover edition
Tax calculation will be finalised at checkout
Purchases are for personal use only
Institutional subscriptions
Unable to display preview. Download preview PDF.
Author information
Authors and affiliations.
Laboratory for Computer and Communications Research, School of Computing Science, Simon Fraser University, Burnaby, British Columbia, Canada, V5A 1S6
Nick Cercone
Department of Computational Science, University of Saskatchewan, Saskatoon, Saskatchewan, Canada, S7N 0W0
Gordon McCalla
You can also search for this author in PubMed Google Scholar
Editor information
Editors and affiliations.
Computing Science Department, Simon Fraser University, V5A 1S6, Burnaby, British Columbia, Canada
Department of Computational Science, University of Saskatchewan, S7N 0W0, Saskatoon, Saskatchewan, Canada
Rights and permissions
Reprints and permissions
Copyright information
© 1987 Springer-Verlag New York Inc.
About this chapter
Cercone, N., McCalla, G. (1987). What Is Knowledge Representation?. In: Cercone, N., McCalla, G. (eds) The Knowledge Frontier. Symbolic Computation. Springer, New York, NY. https://doi.org/10.1007/978-1-4612-4792-0_1
Download citation
DOI : https://doi.org/10.1007/978-1-4612-4792-0_1
Publisher Name : Springer, New York, NY
Print ISBN : 978-1-4612-9158-9
Online ISBN : 978-1-4612-4792-0
eBook Packages : Springer Book Archive
Share this chapter
Anyone you share the following link with will be able to read this content:
Sorry, a shareable link is not currently available for this article.
Provided by the Springer Nature SharedIt content-sharing initiative
- Publish with us
Policies and ethics
- Find a journal
- Track your research
Data Science Introduction
- What Is Data Science? A Beginner's Guide To Data Science
- Data Science Tutorial – Learn Data Science from Scratch!
- What are the Best Books for Data Science?
- Top 15 Hot Artificial Intelligence Technologies
- Top 8 Data Science Tools Everyone Should Know
- Top 10 Data Analytics Tools You Need To Know In 2024
- 5 Data Science Projects – Data Science Projects For Practice
- Top 10 Data Science Applications with Real Life Examples in 2024
- Who is a Data Scientist?
- SQL For Data Science: One stop Solution for Beginners
Statistical Inference
- All You Need To Know About Statistics And Probability
- A Complete Guide To Math And Statistics For Data Science
- Introduction To Markov Chains With Examples – Markov Chains With Python
What is Fuzzy Logic in AI and What are its Applications?
- How To Implement Bayesian Networks In Python? – Bayesian Networks Explained With Examples
- All You Need To Know About Principal Component Analysis (PCA)
- Python for Data Science – How to Implement Python Libraries
Machine Learning
What is machine learning machine learning for beginners.
- Which is the Best Book for Machine Learning?
- Mathematics for Machine Learning: All You Need to Know
- Top 10 Machine Learning Frameworks You Need to Know
- Predicting the Outbreak of COVID-19 Pandemic using Machine Learning
Introduction To Machine Learning: All You Need To Know About Machine Learning
- Machine Learning Tutorial for Beginners
Top 10 Applications of Machine Learning in Daily Life
- Machine Learning Algorithms
How To Implement Find-S Algorithm In Machine Learning?
- What is Cross-Validation in Machine Learning and how to implement it?
- All You Need To Know About The Breadth First Search Algorithm
Supervised Learning
- What is Supervised Learning and its different types?
- Linear Regression Algorithm from Scratch
How To Implement Linear Regression for Machine Learning?
- Introduction to Classification Algorithms
How To Implement Classification In Machine Learning?
- Naive Bayes Classifier: Learning Naive Bayes with Python
- A Comprehensive Guide To Naive Bayes In R
- A Complete Guide On Decision Tree Algorithm
- Decision Tree: How To Create A Perfect Decision Tree?
- What is Overfitting In Machine Learning And How To Avoid It?
- How To Use Regularization in Machine Learning?
Unsupervised Learning
- What is Unsupervised Learning and How does it Work?
- K-means Clustering Algorithm: Know How It Works
- KNN Algorithm: A Practical Implementation Of KNN Algorithm In R
- Implementing K-means Clustering on the Crime Dataset
- K-Nearest Neighbors Algorithm Using Python
- Apriori Algorithm : Know How to Find Frequent Itemsets
- What Are GANs? How and why you should use them!
Q Learning: All you need to know about Reinforcement Learning
Miscellaneous.
- Data Science vs Machine Learning - What's The Difference?
- AI vs Machine Learning vs Deep Learning
- Data Analyst vs Data Engineer vs Data Scientist: Salary, Skills, Responsibilities
- Data Science vs Big Data vs Data Analytics
Career Opportunities
- Data Science Career Opportunities: Your Guide To Unlocking Top Data Scientist Jobs
- Data Scientist Skills – What Does It Take To Become A Data Scientist?
- 10 Skills To Master For Becoming A Data Scientist
- Data Scientist Resume Sample – How To Build An Impressive Data Scientist Resume
- Data Scientist Salary – How Much Does A Data Scientist Earn?
- Machine Learning Engineer vs Data Scientist : Career Comparision
- How To Become A Machine Learning Engineer? – Learning Path
Interview Questions
- Top Machine Learning Interview Questions You Must Prepare In 2024
- Top Data Science Interview Questions For Budding Data Scientists In 2024
- 120+ Data Science Interview Questions And Answers for 2024
Artificial Intelligence
What is knowledge representation in ai techniques you need to know.
Human beings are good at understanding, reasoning and interpreting knowledge. And using this knowledge, they are able to perform various actions in the real world. But how do machines perform the same? In this article, we will learn about Knowledge Representation in AI and how it helps the machines perform reasoning and interpretation using Artificial Intelligence in the following sequence:
What is Knowledge Representation?
Different types of knowledge.
- Cycle of Knowledge Representation
- What is the relation between Knowledge & Intelligence?
- Techniques of Knowledge Representation
Representation Requirements
- Approaches to Knowledge Representation with Example
Knowledge Representation in AI describes the representation of knowledge. Basically, it is a study of how the beliefs, intentions , and judgments of an intelligent agent can be expressed suitably for automated reasoning. One of the primary purposes of Knowledge Representation includes modeling intelligent behavior for an agent.
Knowledge Representation and Reasoning ( KR, KRR ) represents information from the real world for a computer to understand and then utilize this knowledge to solve complex real-life problems like communicating with human beings in natural language. Knowledge representation in AI is not just about storing data in a database, it allows a machine to learn from that knowledge and behave intelligently like a human being.
The different kinds of knowledge that need to be represented in AI include:
- Performance
- Meta-Knowledge
- Knowledge-base
Now that you know about Knowledge representation in AI, let’s move on and know about the different types of Knowledge.
There are 5 types of Knowledge such as:
Declarative Knowledge – It includes concepts, facts, and objects and expressed in a declarative sentence.
Structural Knowledge – It is a basic problem-solving knowledge that describes the relationship between concepts and objects.
Procedural Knowledge – This is responsible for knowing how to do something and includes rules, strategies, procedures, etc.
Meta Knowledge – Meta Knowledge defines knowledge about other types of Knowledge.
Heuristic Knowledge – This represents some expert knowledge in the field or subject.
These are the important types of Knowledge Representation in AI. Now, let’s have a look at the cycle of knowledge representation and how it works.
Cycle of Knowledge Representation in AI
Artificial Intelligent Systems usually consist of various components to display their intelligent behavior. Some of these components include:
- Knowledge Representation & Reasoning
Here is an example to show the different components of the system and how it works:
The above diagram shows the interaction of an AI system with the real world and the components involved in showing intelligence.
- The Perception component retrieves data or information from the environment. with the help of this component, you can retrieve data from the environment, find out the source of noises and check if the AI was damaged by anything. Also, it defines how to respond when any sense has been detected.
- Then, there is the Learning Component that learns from the captured data by the perception component. The goal is to build computers that can be taught instead of programming them. Learning focuses on the process of self-improvement. In order to learn new things, the system requires knowledge acquisition, inference, acquisition of heuristics, faster searches, etc.
- The main component in the cycle is Knowledge Representation and Reasoning which shows the human-like intelligence in the machines. Knowledge representation is all about understanding intelligence. Instead of trying to understand or build brains from the bottom up, its goal is to understand and build intelligent behavior from the top-down and focus on what an agent needs to know in order to behave intelligently. Also, it defines how automated reasoning procedures can make this knowledge available as needed.
- The Planning and Execution components depend on the analysis of knowledge representation and reasoning. Here, planning includes giving an initial state, finding their preconditions and effects, and a sequence of actions to achieve a state in which a particular goal holds. Now once the planning is completed, the final stage is the execution of the entire process.
So, these are the different components of the cycle of Knowledge Representation in AI. Now, let’s understand the relationship between knowledge and intelligence.
Top 10 Trending Technologies to Learn in 2024 | Edureka
What is the relation between knowledge & intelligence.
In the real world, knowledge plays a vital role in intelligence as well as creating artificial intelligence . It demonstrates the intelligent behavior in AI agents or systems . It is possible for an agent or system to act accurately on some input only when it has the knowledge or experience about the input.
Let’s take an example to understand the relationship:
In this example, there is one decision-maker whose actions are justified by sensing the environment and using knowledge. But, if we remove the knowledge part here, it will not be able to display any intelligent behavior.
Now that you know the relationship between knowledge and intelligence, let’s move on to the techniques of Knowledge Representation in AI.
Techniques of Knowledge Representation in AI
There are four techniques of representing knowledge such as:
Now, let’s discuss these techniques in detail.
Logical Representation
Logical representation is a language with some definite rules which deal with propositions and has no ambiguity in representation. It represents a conclusion based on various conditions and lays down some important communication rules . Also, it consists of precisely defined syntax and semantics which supports the sound inference. Each sentence can be translated into logics using syntax and semantics.
Advantages:
- Logical representation helps to perform logical reasoning.
- This representation is the basis for the programming languages.
Disadvantages:
- Logical representations have some restrictions and are challenging to work with.
- This technique may not be very natural, and inference may not be very efficient.
Semantic Network Representation
Semantic networks work as an alternative of predicate logic for knowledge representation. In Semantic networks, you can represent your knowledge in the form of graphical networks. This network consists of nodes representing objects and arcs which describe the relationship between those objects. Also, it categorizes the object in different forms and links those objects.
This representation consist of two types of relations:
- IS-A relation (Inheritance)
- Kind-of-relation
- Semantic networks are a natural representation of knowledge.
- Also, it conveys meaning in a transparent manner.
- These networks are simple and easy to understand.
- Semantic networks take more computational time at runtime.
- Also, these are inadequate as they do not have any equivalent quantifiers.
- These networks are not intelligent and depend on the creator of the system.
Frame Representation
A frame is a record like structure that consists of a collection of attributes and values to describe an entity in the world. These are the AI data structure that divides knowledge into substructures by representing stereotypes situations. Basically, it consists of a collection of slots and slot values of any type and size. Slots have names and values which are called facets.
- It makes the programming easier by grouping the related data.
- Frame representation is easy to understand and visualize.
- It is very easy to add slots for new attributes and relations.
- Also, it is easy to include default data and search for missing values.
- In frame system inference, the mechanism cannot be easily processed.
- The inference mechanism cannot be smoothly proceeded by frame representation.
- It has a very generalized approach.
Production Rules
In production rules, agent checks for the condition and if the condition exists then production rule fires and corresponding action is carried out. The condition part of the rule determines which rule may be applied to a problem. Whereas, the action part carries out the associated problem-solving steps. This complete process is called a recognize-act cycle.
The production rules system consists of three main parts:
- The set of production rules
- Working Memory
- The recognize-act-cycle
The production rules are expressed in natural language.
The production rules are highly modular and can be easily removed or modified.
It does not exhibit any learning capabilities and does not store the result of the problem for future uses.
During the execution of the program, many rules may be active. Thus, rule-based production systems are inefficient.
So, these were the important techniques for Knowledge Representation in AI. Now, let’s have a look at the requirements for these representations.
A good knowledge representation system must have properties such as:
Representational Accuracy: It should represent all kinds of required knowledge.
Inferential Adequacy : It should be able to manipulate the representational structures to produce new knowledge corresponding to the existing structure.
Inferential Efficiency : The ability to direct the inferential knowledge mechanism into the most productive directions by storing appropriate guides.
Acquisitional efficiency : The ability to acquire new knowledge easily using automatic methods.
Now, let’s have a look at some of the approaches to Knowledge Representation in AI along with different examples.
Approaches to Knowledge Representation in AI
There are different approaches to knowledge representation such as:
1. Simple Relational Knowledge
It is the simplest way of storing facts which uses the relational method. Here, all the facts about a set of the object are set out systematically in columns. Also, this approach of knowledge representation is famous in database systems where the relationship between different entities is represented. Thus, there is little opportunity for inference.
This is an example of representing simple relational knowledge.
2. Inheritable Knowledge
In the inheritable knowledge approach, all data must be stored into a hierarchy of classes and should be arranged in a generalized form or a hierarchal manner. Also, this approach contains inheritable knowledge which shows a relation between instance and class, and it is called instance relation. In this approach, objects and values are represented in Boxed nodes.
3. Inferential Knowledge
The inferential knowledge approach represents knowledge in the form of formal logic . Thus, it can be used to derive more facts. Also, it guarantees correctness.
Statement 1 : John is a cricketer.
Statement 2 : All cricketers are athletes.
Then it can be represented as;
Cricketer(John) ∀x = Cricketer (x) ———-> Athelete (x)s
These were some of the approaches to knowledge representation in AI along with examples. With this, we have come to the end of our article. I hope you understood what is Knowledge Representation in AI and its different types.
Learn more about this trending OpenAI chatbot concept and implementation join the ChatGPT training .
Also, check out the AI and Deep Learning with TensorFlow Course is curated by industry professionals as per the industry requirements & demands. Thus, You will master the concepts such as SoftMax function, Autoencoder Neural Networks, Restricted Boltzmann Machine (RBM) and work with libraries like Keras & TFLearn. The course has been specially curated by industry experts with real-time case studies.
Got a question for us? Please mention it in the comments section of “Knowledge Representation in AI” and we will get back to you.
Recommended videos for you
Introduction to mahout, recommended blogs for you, most frequently asked artificial intelligence interview questions in 2024, fuzzy k-means clustering in mahout, best laptop for machine learning in 2024, best generative ai learning path in 2024, understanding different types of artificial intelligence with examples, introduction to clustering in mahout, supervised learning in apache mahout, artificial intelligence pros and cons: everything you need to know ai, a step by step guide to install tensorflow, deep learning : perceptron learning algorithm, artificial intelligence algorithms: all you need to know, what is em algorithm in machine learning, join the discussion cancel reply, trending courses in artificial intelligence, human-computer interaction (hci) for ai syste ....
- 2k Enrolled Learners
- Weekend/Weekday
Prompt Engineering Course
- 3k Enrolled Learners
ChatGPT Complete Course: Beginners to Advance ...
- 13k Enrolled Learners
Artificial Intelligence Certification Course
- 15k Enrolled Learners
Generative AI in Business: University of Camb ...
- 0k Enrolled Learners
Reinforcement Learning
Graphical models certification training, introduction to generative ai, artificial intelligence (ai) course for begin ....
- 4k Enrolled Learners
MLOps Certification Course Online
- 6k Enrolled Learners
Browse Categories
Subscribe to our newsletter, and get personalized recommendations..
Already have an account? Sign in .
20,00,000 learners love us! Get personalised resources in your inbox.
At least 1 upper-case and 1 lower-case letter
Minimum 8 characters and Maximum 50 characters
We have recieved your contact details.
You will recieve an email from us shortly.
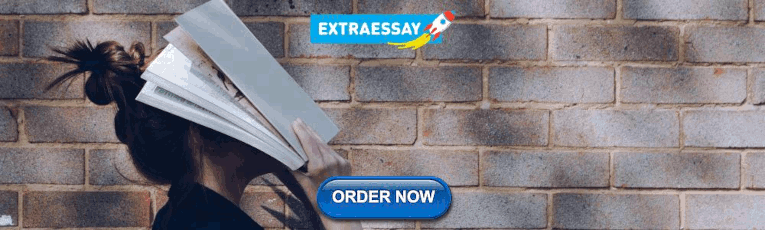
IMAGES
VIDEO
COMMENTS
Role 1: A Knowledge Representation Is a Surrogate. Any intelligent entity that wants to reason about its world encounters an important, inescapable fact: Reasoning is a process that goes on internally, but most things it wants to reason about exist only externally. A pro-gram (or person) engaged in planning the assembly of a bicycle, for ...
Knowledge representation, which refers to the techniques of capturing and storing human knowledge in a way that machines can understand and use, is a critical component of AI. The main challenge in knowledge representation is finding a way to represent knowledge that is understandable by machines and can be used for reasoning and problem-solving.
Knowledge representation and reasoning (KRR, KR&R, KR²) is the field of artificial intelligence (AI) dedicated to representing information about the world in a form that a computer system can use to solve complex tasks such as diagnosing a medical condition or having a dialog in a natural language.Knowledge representation incorporates findings from psychology about how humans solve problems ...
A script is a structured representation describing a stereotyped sequence of events in a particular context. Scripts are used to organize events in knowledge bases Scripts are very related to the idea of frames Philipp Koehn Artificial Intelligence: Knowledge Representation 7 March 2024
Techniques of Knowledge Representation in AI There are other techniques of Knowledge Representation in addition to the different approaches. Rule-Based Systems: Using a set of rules, or production rules, rule-based systems express knowledge. AI systems may make judgments based on certain conditions thanks to these rules, which are made up of ...
Asking what algorithms a representation supports, therefore, is a matter of asking what mathematical operations can be meaningfully applied to it. Consider some of the most widely used unsupervised learning techniques and the sorts of representations they produce: Clustering maps data points to a discrete set (say, the integers) where the only ...
There are four major techniques out there that represent the knowledge in AI: Logical representation; Semantic networks; Production rules; Frame representation; Logical Representation. Logical representation is the basic form of knowledge representation to the machines where a defined syntax with basic rules is used.
Techniques of Knowledge Representation. In the field of artificial intelligence (AI), several techniques are commonly used for knowledge representation. These techniques play a vital role in enabling AI systems to understand and process information effectively. Some of the prominent techniques are semantic networks, frames, and ontologies. 1.
Zhiyuan Liu, Yankai Lin, Maosong Sun. Provides a comprehensive overview of the representation learning techniques for natural language processing. Presents a self-contained reference resource with a rich blend of theory, algorithms and applications. Covers from word embedding to pre-trained language models. This book is open access, which means ...
In this chapter, we overview eight major approaches to knowledge representation: logical representations, semantic networks, procedural representations, logic programming formalisms, frame-based representations, production system architectures, and knowledge representation languages. The fundamentals of each approach are described, and then ...
Graphics, even before language and writing, were the first means of communication and significance for humanity. Drawing is the act of replacing reality with representation, that is, replacing ...
Representation Learning is a process in machine learning where algorithms extract meaningful patterns from raw data to create representations that are easier to understand and process. These representations can be designed for interpretability, reveal hidden features, or be used for transfer learning. They are valuable across many fundamental machine learning tasks like image classification ...
Techniques of knowledge representation. There are mainly four ways of knowledge representation which are given as follows: Logical Representation. Semantic Network Representation. Frame Representation. Production Rules. 1. Logical Representation. Logical representation is a language with some concrete rules which deals with propositions and has ...
Once the knowledge representation is completed, another important feature of AI system is the reasoning. However, although knowledge representation and reasoning are two separate entities, they are tightly coupled with each other for effective results. Analysis of reasoning and knowledge representation leads to planning and education.
Representation learning. Although traditional unsupervised learning techniques will always be staples of machine learning pipelines, representation learning has emerged as an alternative approach to feature extraction with the continued success of deep learning. In representation learning, features are extracted from unlabeled data by training ...
Architectural Representation I: Origins + Originality. Architectural representation is an ideology—a source of ideas and visionary theorizing that has a set of origins and qualities. As such, it's prudent to study the origins of conventional techniques of architectural representation to be informed about their intentions and the specific ...
It is argued that keeping in mind all five of these roles that a representation plays provides a usefully broad perspective that sheds light on some longstanding disputes and can invigorate both research and practice in the field. Although knowledge representation is one of the central and, in some ways, most familiar concepts in AI, the most fundamental question about it -- What is it? -- has ...
Here are some important data visualization techniques to know: 1. Pie Chart. Pie charts are one of the most common and basic data visualization techniques, used across a wide range of applications. Pie charts are ideal for illustrating proportions, or part-to-whole comparisons.
Representation learning is a class of machine learning approaches that allow a system to discover the representations required for feature detection or classification from raw data. The requirement for manual feature engineering is reduced by allowing a machine to learn the features and apply them to a given activity.
Knowledge representation is one such process which depends on the logical situation and enable a strategy to take a decision in acquiring knowledge. There are many types and levels of knowledge acquired by human in daily life but machines find difficult to interpret all types of knowledge. For such conditions, knowledge representation is used.
representation is provided in (Brachman and Smith. 1980). while summaries for the nonspecialist are given in (Barr and Feigenbaum. 1981) and (Brachman and Levesque. 1985). This paper is intended to provide some background to knowledge representation research by mapping out the basic approaches to
Knowledge Representation in AI describes the representation of knowledge. Basically, it is a study of how the beliefs, intentions, and judgments of an intelligent agent can be expressed suitably for automated reasoning. One of the primary purposes of Knowledge Representation includes modeling intelligent behavior for an agent.
the use of lexical and syntactic features to the use of advanced embedding techniques, especially in the last two years. Using advanced embedding representations has proved its e ectiveness in most RE tasks (such as requirement analysis, extracting requirements from reviews and forums, and semantic-level quality tasks).
• Use unsupervised learning techniques for unsupervised learning: including clustering and anomaly detection. • Build recommender systems with a collaborative filtering approach and a content-based deep learning method. • Build a deep reinforcement learning model. The Machine Learning Specialization is a foundational online program ...
In biomedicine, the critical task is to decode Drug-Drug Interactions (DDIs) from complex biomedical texts. The scientific community employs Knowledge Graph Embedding (KGE) methods, enhanced with advanced neural network technologies, including capsule networks. However, existing methodologies primarily focus on the structural details of individual entities or relations within Biomedical ...