- Skip to main content
- Skip to primary sidebar
- Skip to footer
- QuestionPro

- Solutions Industries Gaming Automotive Sports and events Education Government Travel & Hospitality Financial Services Healthcare Cannabis Technology Use Case NPS+ Communities Audience Contactless surveys Mobile LivePolls Member Experience GDPR Positive People Science 360 Feedback Surveys
- Resources Blog eBooks Survey Templates Case Studies Training Help center

Home Market Research
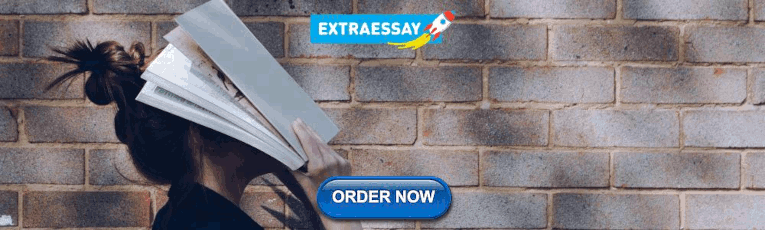
Data Analysis in Research: Types & Methods
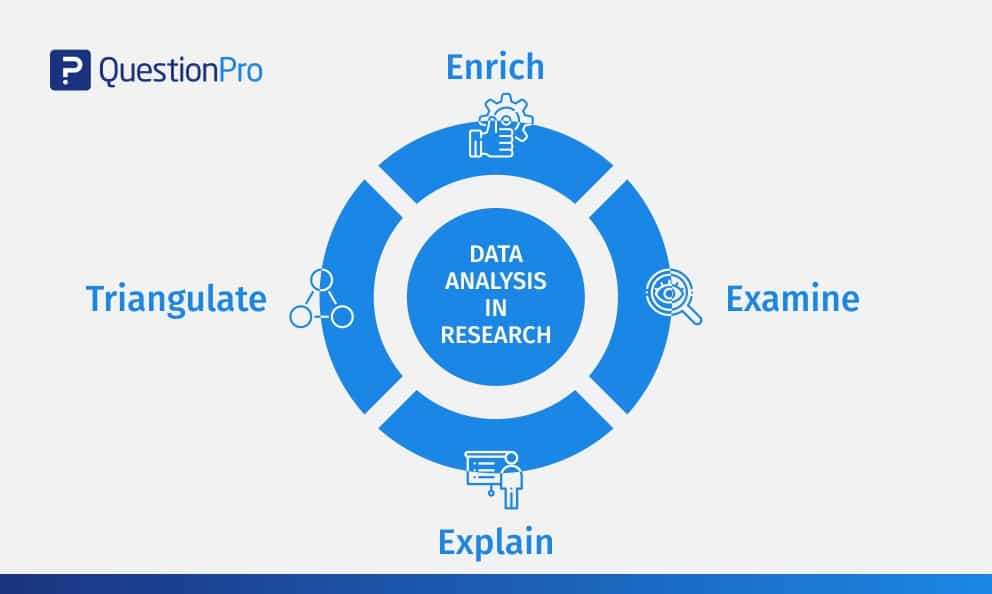
Content Index
Why analyze data in research?
Types of data in research, finding patterns in the qualitative data, methods used for data analysis in qualitative research, preparing data for analysis, methods used for data analysis in quantitative research, considerations in research data analysis, what is data analysis in research.
Definition of research in data analysis: According to LeCompte and Schensul, research data analysis is a process used by researchers to reduce data to a story and interpret it to derive insights. The data analysis process helps reduce a large chunk of data into smaller fragments, which makes sense.
Three essential things occur during the data analysis process — the first is data organization . Summarization and categorization together contribute to becoming the second known method used for data reduction. It helps find patterns and themes in the data for easy identification and linking. The third and last way is data analysis – researchers do it in both top-down and bottom-up fashion.
LEARN ABOUT: Research Process Steps
On the other hand, Marshall and Rossman describe data analysis as a messy, ambiguous, and time-consuming but creative and fascinating process through which a mass of collected data is brought to order, structure and meaning.
We can say that “the data analysis and data interpretation is a process representing the application of deductive and inductive logic to the research and data analysis.”
Researchers rely heavily on data as they have a story to tell or research problems to solve. It starts with a question, and data is nothing but an answer to that question. But, what if there is no question to ask? Well! It is possible to explore data even without a problem – we call it ‘Data Mining’, which often reveals some interesting patterns within the data that are worth exploring.
Irrelevant to the type of data researchers explore, their mission and audiences’ vision guide them to find the patterns to shape the story they want to tell. One of the essential things expected from researchers while analyzing data is to stay open and remain unbiased toward unexpected patterns, expressions, and results. Remember, sometimes, data analysis tells the most unforeseen yet exciting stories that were not expected when initiating data analysis. Therefore, rely on the data you have at hand and enjoy the journey of exploratory research.
Create a Free Account
Every kind of data has a rare quality of describing things after assigning a specific value to it. For analysis, you need to organize these values, processed and presented in a given context, to make it useful. Data can be in different forms; here are the primary data types.
- Qualitative data: When the data presented has words and descriptions, then we call it qualitative data . Although you can observe this data, it is subjective and harder to analyze data in research, especially for comparison. Example: Quality data represents everything describing taste, experience, texture, or an opinion that is considered quality data. This type of data is usually collected through focus groups, personal qualitative interviews , qualitative observation or using open-ended questions in surveys.
- Quantitative data: Any data expressed in numbers of numerical figures are called quantitative data . This type of data can be distinguished into categories, grouped, measured, calculated, or ranked. Example: questions such as age, rank, cost, length, weight, scores, etc. everything comes under this type of data. You can present such data in graphical format, charts, or apply statistical analysis methods to this data. The (Outcomes Measurement Systems) OMS questionnaires in surveys are a significant source of collecting numeric data.
- Categorical data: It is data presented in groups. However, an item included in the categorical data cannot belong to more than one group. Example: A person responding to a survey by telling his living style, marital status, smoking habit, or drinking habit comes under the categorical data. A chi-square test is a standard method used to analyze this data.
Learn More : Examples of Qualitative Data in Education
Data analysis in qualitative research
Data analysis and qualitative data research work a little differently from the numerical data as the quality data is made up of words, descriptions, images, objects, and sometimes symbols. Getting insight from such complicated information is a complicated process. Hence it is typically used for exploratory research and data analysis .
Although there are several ways to find patterns in the textual information, a word-based method is the most relied and widely used global technique for research and data analysis. Notably, the data analysis process in qualitative research is manual. Here the researchers usually read the available data and find repetitive or commonly used words.
For example, while studying data collected from African countries to understand the most pressing issues people face, researchers might find “food” and “hunger” are the most commonly used words and will highlight them for further analysis.
LEARN ABOUT: Level of Analysis
The keyword context is another widely used word-based technique. In this method, the researcher tries to understand the concept by analyzing the context in which the participants use a particular keyword.
For example , researchers conducting research and data analysis for studying the concept of ‘diabetes’ amongst respondents might analyze the context of when and how the respondent has used or referred to the word ‘diabetes.’
The scrutiny-based technique is also one of the highly recommended text analysis methods used to identify a quality data pattern. Compare and contrast is the widely used method under this technique to differentiate how a specific text is similar or different from each other.
For example: To find out the “importance of resident doctor in a company,” the collected data is divided into people who think it is necessary to hire a resident doctor and those who think it is unnecessary. Compare and contrast is the best method that can be used to analyze the polls having single-answer questions types .
Metaphors can be used to reduce the data pile and find patterns in it so that it becomes easier to connect data with theory.
Variable Partitioning is another technique used to split variables so that researchers can find more coherent descriptions and explanations from the enormous data.
LEARN ABOUT: Qualitative Research Questions and Questionnaires
There are several techniques to analyze the data in qualitative research, but here are some commonly used methods,
- Content Analysis: It is widely accepted and the most frequently employed technique for data analysis in research methodology. It can be used to analyze the documented information from text, images, and sometimes from the physical items. It depends on the research questions to predict when and where to use this method.
- Narrative Analysis: This method is used to analyze content gathered from various sources such as personal interviews, field observation, and surveys . The majority of times, stories, or opinions shared by people are focused on finding answers to the research questions.
- Discourse Analysis: Similar to narrative analysis, discourse analysis is used to analyze the interactions with people. Nevertheless, this particular method considers the social context under which or within which the communication between the researcher and respondent takes place. In addition to that, discourse analysis also focuses on the lifestyle and day-to-day environment while deriving any conclusion.
- Grounded Theory: When you want to explain why a particular phenomenon happened, then using grounded theory for analyzing quality data is the best resort. Grounded theory is applied to study data about the host of similar cases occurring in different settings. When researchers are using this method, they might alter explanations or produce new ones until they arrive at some conclusion.
LEARN ABOUT: 12 Best Tools for Researchers
Data analysis in quantitative research
The first stage in research and data analysis is to make it for the analysis so that the nominal data can be converted into something meaningful. Data preparation consists of the below phases.
Phase I: Data Validation
Data validation is done to understand if the collected data sample is per the pre-set standards, or it is a biased data sample again divided into four different stages
- Fraud: To ensure an actual human being records each response to the survey or the questionnaire
- Screening: To make sure each participant or respondent is selected or chosen in compliance with the research criteria
- Procedure: To ensure ethical standards were maintained while collecting the data sample
- Completeness: To ensure that the respondent has answered all the questions in an online survey. Else, the interviewer had asked all the questions devised in the questionnaire.
Phase II: Data Editing
More often, an extensive research data sample comes loaded with errors. Respondents sometimes fill in some fields incorrectly or sometimes skip them accidentally. Data editing is a process wherein the researchers have to confirm that the provided data is free of such errors. They need to conduct necessary checks and outlier checks to edit the raw edit and make it ready for analysis.
Phase III: Data Coding
Out of all three, this is the most critical phase of data preparation associated with grouping and assigning values to the survey responses . If a survey is completed with a 1000 sample size, the researcher will create an age bracket to distinguish the respondents based on their age. Thus, it becomes easier to analyze small data buckets rather than deal with the massive data pile.
LEARN ABOUT: Steps in Qualitative Research
After the data is prepared for analysis, researchers are open to using different research and data analysis methods to derive meaningful insights. For sure, statistical analysis plans are the most favored to analyze numerical data. In statistical analysis, distinguishing between categorical data and numerical data is essential, as categorical data involves distinct categories or labels, while numerical data consists of measurable quantities. The method is again classified into two groups. First, ‘Descriptive Statistics’ used to describe data. Second, ‘Inferential statistics’ that helps in comparing the data .
Descriptive statistics
This method is used to describe the basic features of versatile types of data in research. It presents the data in such a meaningful way that pattern in the data starts making sense. Nevertheless, the descriptive analysis does not go beyond making conclusions. The conclusions are again based on the hypothesis researchers have formulated so far. Here are a few major types of descriptive analysis methods.
Measures of Frequency
- Count, Percent, Frequency
- It is used to denote home often a particular event occurs.
- Researchers use it when they want to showcase how often a response is given.
Measures of Central Tendency
- Mean, Median, Mode
- The method is widely used to demonstrate distribution by various points.
- Researchers use this method when they want to showcase the most commonly or averagely indicated response.
Measures of Dispersion or Variation
- Range, Variance, Standard deviation
- Here the field equals high/low points.
- Variance standard deviation = difference between the observed score and mean
- It is used to identify the spread of scores by stating intervals.
- Researchers use this method to showcase data spread out. It helps them identify the depth until which the data is spread out that it directly affects the mean.
Measures of Position
- Percentile ranks, Quartile ranks
- It relies on standardized scores helping researchers to identify the relationship between different scores.
- It is often used when researchers want to compare scores with the average count.
For quantitative research use of descriptive analysis often give absolute numbers, but the in-depth analysis is never sufficient to demonstrate the rationale behind those numbers. Nevertheless, it is necessary to think of the best method for research and data analysis suiting your survey questionnaire and what story researchers want to tell. For example, the mean is the best way to demonstrate the students’ average scores in schools. It is better to rely on the descriptive statistics when the researchers intend to keep the research or outcome limited to the provided sample without generalizing it. For example, when you want to compare average voting done in two different cities, differential statistics are enough.
Descriptive analysis is also called a ‘univariate analysis’ since it is commonly used to analyze a single variable.
Inferential statistics
Inferential statistics are used to make predictions about a larger population after research and data analysis of the representing population’s collected sample. For example, you can ask some odd 100 audiences at a movie theater if they like the movie they are watching. Researchers then use inferential statistics on the collected sample to reason that about 80-90% of people like the movie.
Here are two significant areas of inferential statistics.
- Estimating parameters: It takes statistics from the sample research data and demonstrates something about the population parameter.
- Hypothesis test: I t’s about sampling research data to answer the survey research questions. For example, researchers might be interested to understand if the new shade of lipstick recently launched is good or not, or if the multivitamin capsules help children to perform better at games.
These are sophisticated analysis methods used to showcase the relationship between different variables instead of describing a single variable. It is often used when researchers want something beyond absolute numbers to understand the relationship between variables.
Here are some of the commonly used methods for data analysis in research.
- Correlation: When researchers are not conducting experimental research or quasi-experimental research wherein the researchers are interested to understand the relationship between two or more variables, they opt for correlational research methods.
- Cross-tabulation: Also called contingency tables, cross-tabulation is used to analyze the relationship between multiple variables. Suppose provided data has age and gender categories presented in rows and columns. A two-dimensional cross-tabulation helps for seamless data analysis and research by showing the number of males and females in each age category.
- Regression analysis: For understanding the strong relationship between two variables, researchers do not look beyond the primary and commonly used regression analysis method, which is also a type of predictive analysis used. In this method, you have an essential factor called the dependent variable. You also have multiple independent variables in regression analysis. You undertake efforts to find out the impact of independent variables on the dependent variable. The values of both independent and dependent variables are assumed as being ascertained in an error-free random manner.
- Frequency tables: The statistical procedure is used for testing the degree to which two or more vary or differ in an experiment. A considerable degree of variation means research findings were significant. In many contexts, ANOVA testing and variance analysis are similar.
- Analysis of variance: The statistical procedure is used for testing the degree to which two or more vary or differ in an experiment. A considerable degree of variation means research findings were significant. In many contexts, ANOVA testing and variance analysis are similar.
- Researchers must have the necessary research skills to analyze and manipulation the data , Getting trained to demonstrate a high standard of research practice. Ideally, researchers must possess more than a basic understanding of the rationale of selecting one statistical method over the other to obtain better data insights.
- Usually, research and data analytics projects differ by scientific discipline; therefore, getting statistical advice at the beginning of analysis helps design a survey questionnaire, select data collection methods, and choose samples.
LEARN ABOUT: Best Data Collection Tools
- The primary aim of data research and analysis is to derive ultimate insights that are unbiased. Any mistake in or keeping a biased mind to collect data, selecting an analysis method, or choosing audience sample il to draw a biased inference.
- Irrelevant to the sophistication used in research data and analysis is enough to rectify the poorly defined objective outcome measurements. It does not matter if the design is at fault or intentions are not clear, but lack of clarity might mislead readers, so avoid the practice.
- The motive behind data analysis in research is to present accurate and reliable data. As far as possible, avoid statistical errors, and find a way to deal with everyday challenges like outliers, missing data, data altering, data mining , or developing graphical representation.
LEARN MORE: Descriptive Research vs Correlational Research The sheer amount of data generated daily is frightening. Especially when data analysis has taken center stage. in 2018. In last year, the total data supply amounted to 2.8 trillion gigabytes. Hence, it is clear that the enterprises willing to survive in the hypercompetitive world must possess an excellent capability to analyze complex research data, derive actionable insights, and adapt to the new market needs.
LEARN ABOUT: Average Order Value
QuestionPro is an online survey platform that empowers organizations in data analysis and research and provides them a medium to collect data by creating appealing surveys.
MORE LIKE THIS
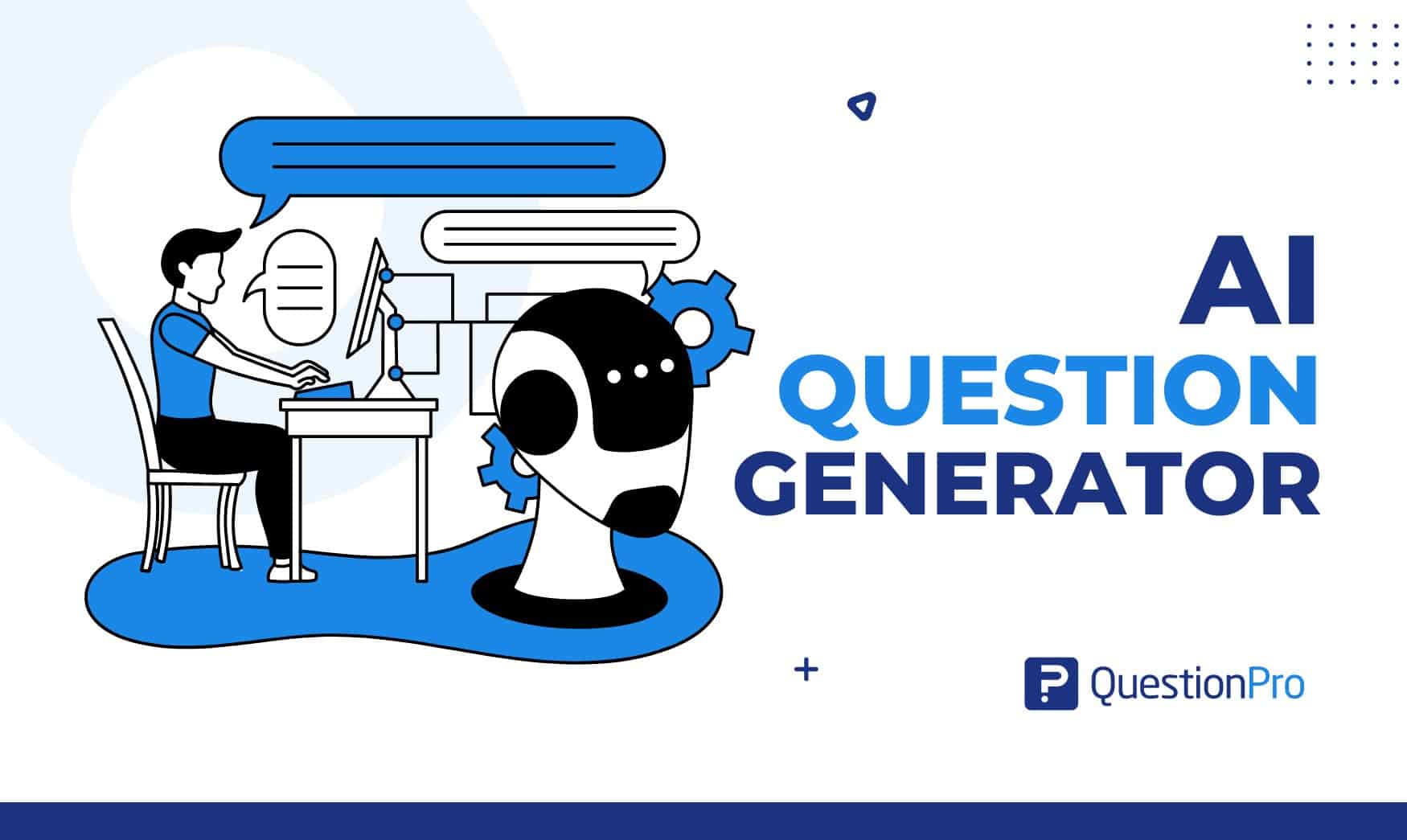
AI Question Generator: Create Easy + Accurate Tests and Surveys
Apr 6, 2024
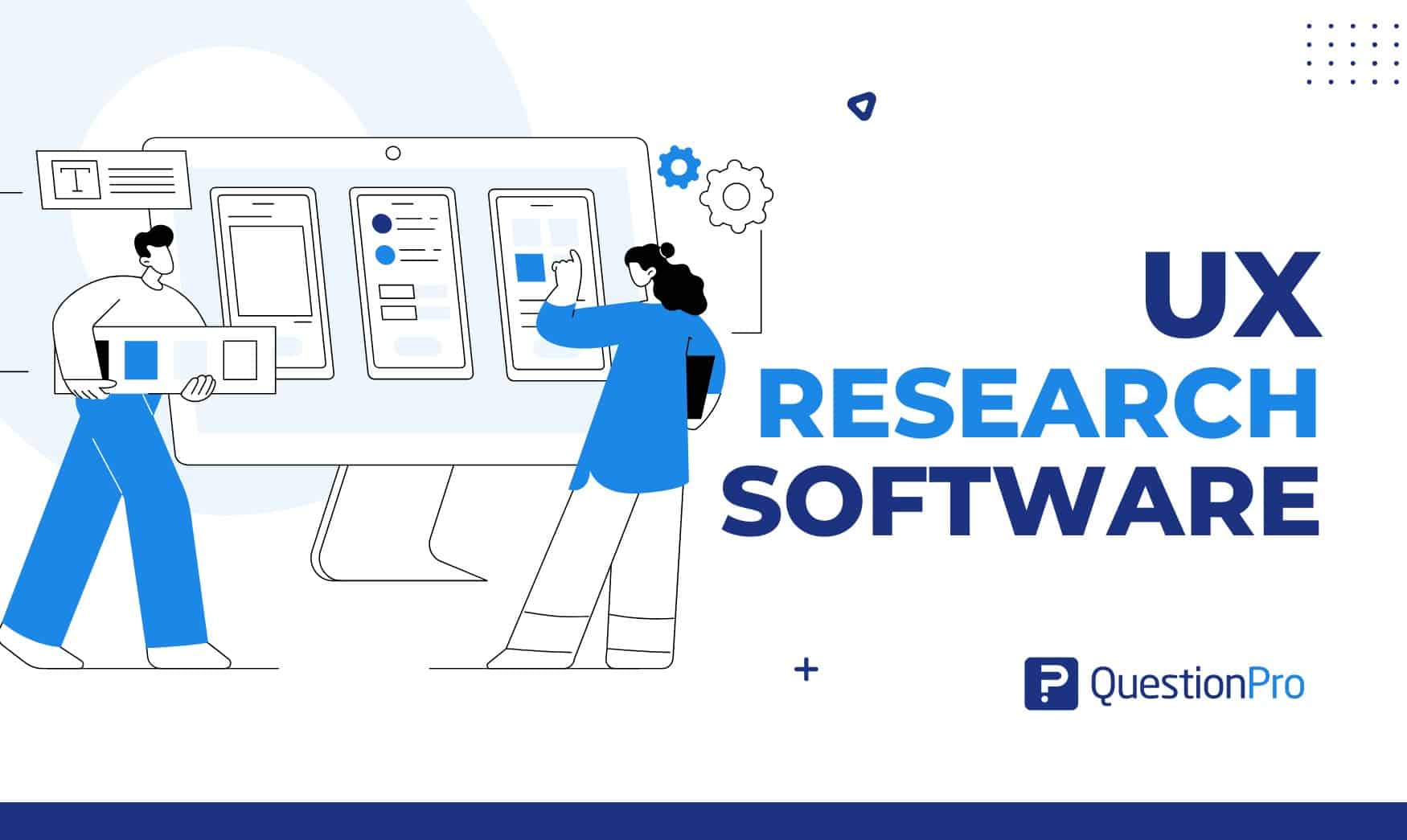
Top 17 UX Research Software for UX Design in 2024
Apr 5, 2024
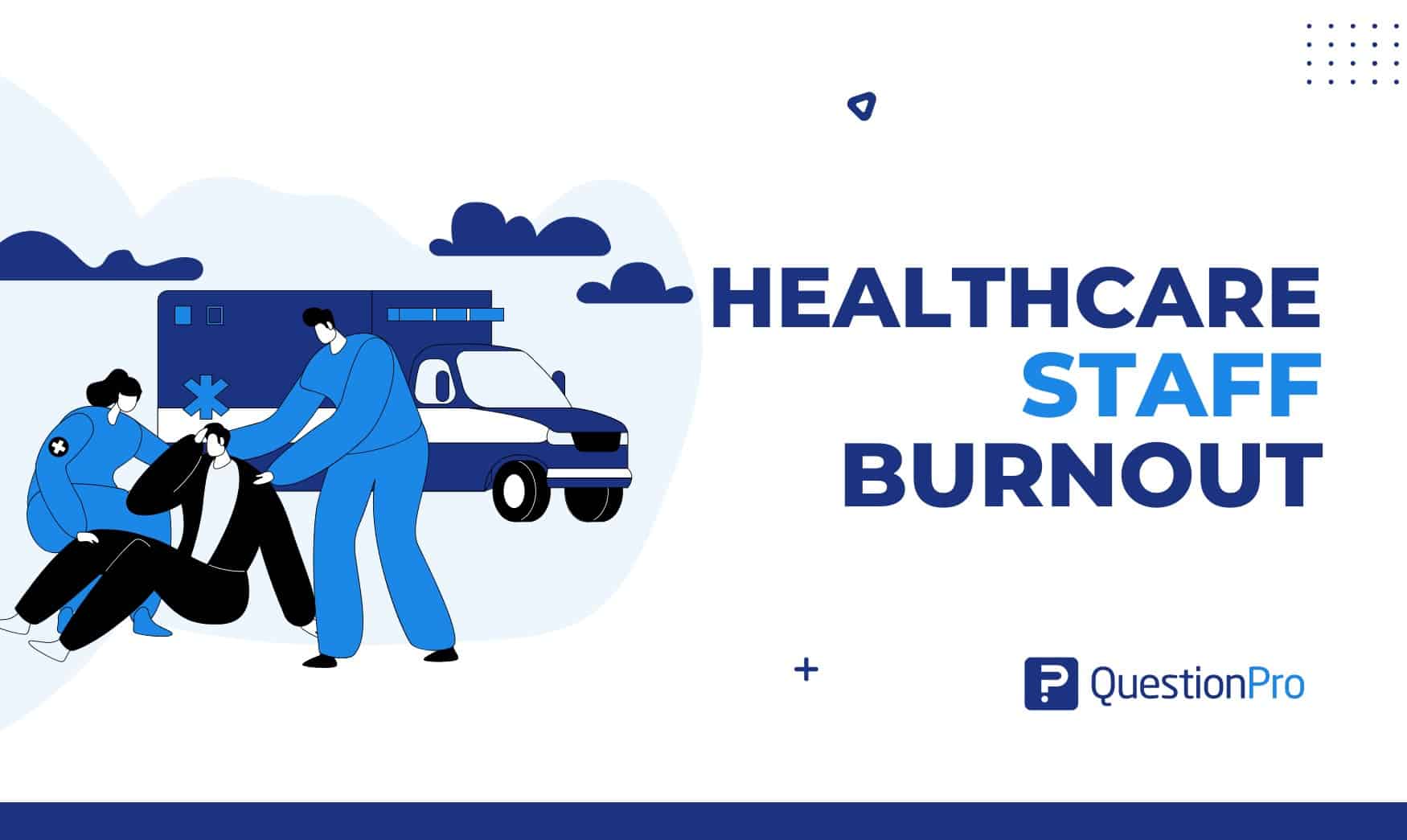
Healthcare Staff Burnout: What it Is + How To Manage It
Apr 4, 2024
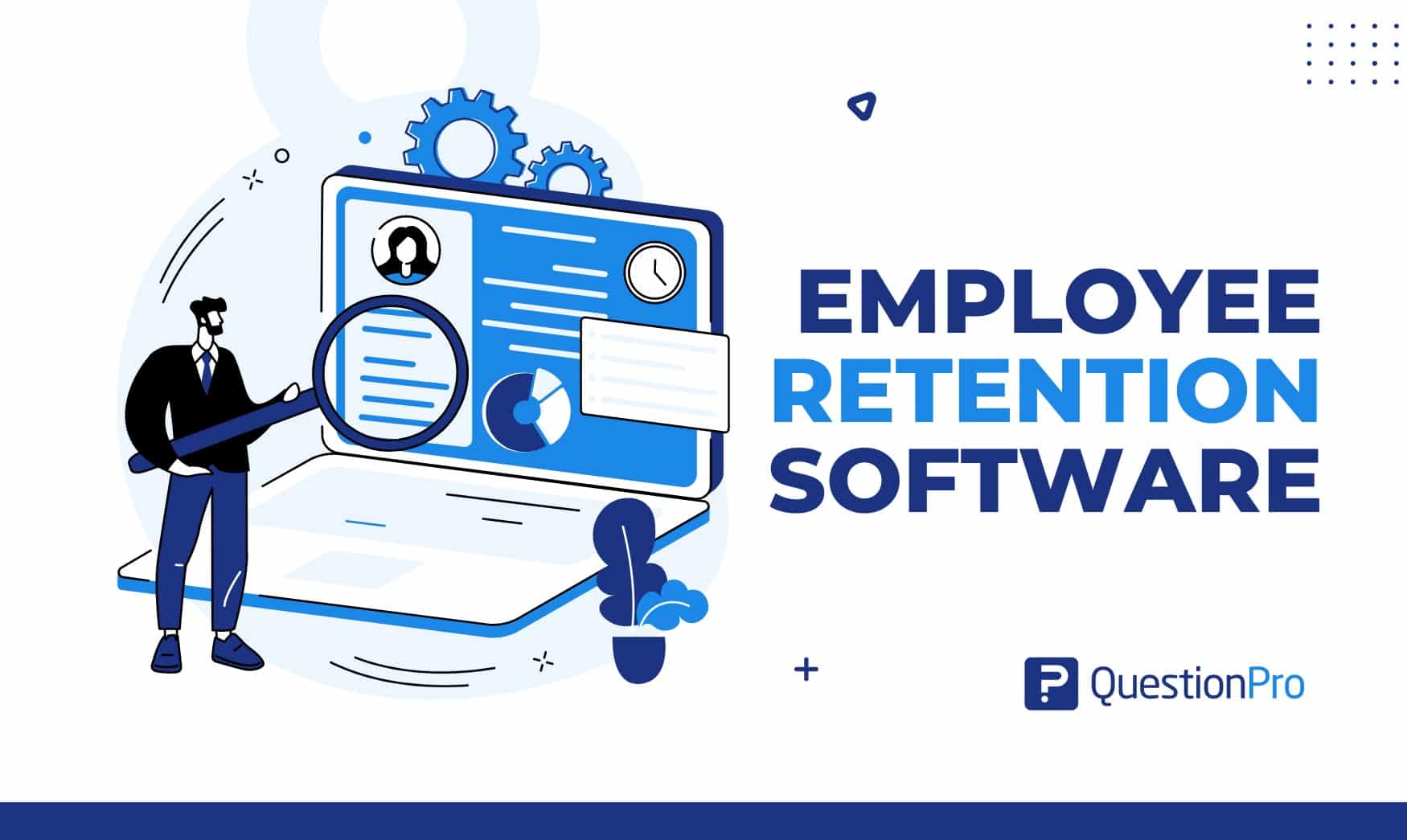
Top 15 Employee Retention Software in 2024
Other categories.
- Academic Research
- Artificial Intelligence
- Assessments
- Brand Awareness
- Case Studies
- Communities
- Consumer Insights
- Customer effort score
- Customer Engagement
- Customer Experience
- Customer Loyalty
- Customer Research
- Customer Satisfaction
- Employee Benefits
- Employee Engagement
- Employee Retention
- Friday Five
- General Data Protection Regulation
- Insights Hub
- Life@QuestionPro
- Market Research
- Mobile diaries
- Mobile Surveys
- New Features
- Online Communities
- Question Types
- Questionnaire
- QuestionPro Products
- Release Notes
- Research Tools and Apps
- Revenue at Risk
- Survey Templates
- Training Tips
- Uncategorized
- Video Learning Series
- What’s Coming Up
- Workforce Intelligence
Your Modern Business Guide To Data Analysis Methods And Techniques

Table of Contents
1) What Is Data Analysis?
2) Why Is Data Analysis Important?
3) What Is The Data Analysis Process?
4) Types Of Data Analysis Methods
5) Top Data Analysis Techniques To Apply
6) Quality Criteria For Data Analysis
7) Data Analysis Limitations & Barriers
8) Data Analysis Skills
9) Data Analysis In The Big Data Environment
In our data-rich age, understanding how to analyze and extract true meaning from our business’s digital insights is one of the primary drivers of success.
Despite the colossal volume of data we create every day, a mere 0.5% is actually analyzed and used for data discovery , improvement, and intelligence. While that may not seem like much, considering the amount of digital information we have at our fingertips, half a percent still accounts for a vast amount of data.
With so much data and so little time, knowing how to collect, curate, organize, and make sense of all of this potentially business-boosting information can be a minefield – but online data analysis is the solution.
In science, data analysis uses a more complex approach with advanced techniques to explore and experiment with data. On the other hand, in a business context, data is used to make data-driven decisions that will enable the company to improve its overall performance. In this post, we will cover the analysis of data from an organizational point of view while still going through the scientific and statistical foundations that are fundamental to understanding the basics of data analysis.
To put all of that into perspective, we will answer a host of important analytical questions, explore analytical methods and techniques, while demonstrating how to perform analysis in the real world with a 17-step blueprint for success.
What Is Data Analysis?
Data analysis is the process of collecting, modeling, and analyzing data using various statistical and logical methods and techniques. Businesses rely on analytics processes and tools to extract insights that support strategic and operational decision-making.
All these various methods are largely based on two core areas: quantitative and qualitative research.
To explain the key differences between qualitative and quantitative research, here’s a video for your viewing pleasure:
Gaining a better understanding of different techniques and methods in quantitative research as well as qualitative insights will give your analyzing efforts a more clearly defined direction, so it’s worth taking the time to allow this particular knowledge to sink in. Additionally, you will be able to create a comprehensive analytical report that will skyrocket your analysis.
Apart from qualitative and quantitative categories, there are also other types of data that you should be aware of before dividing into complex data analysis processes. These categories include:
- Big data: Refers to massive data sets that need to be analyzed using advanced software to reveal patterns and trends. It is considered to be one of the best analytical assets as it provides larger volumes of data at a faster rate.
- Metadata: Putting it simply, metadata is data that provides insights about other data. It summarizes key information about specific data that makes it easier to find and reuse for later purposes.
- Real time data: As its name suggests, real time data is presented as soon as it is acquired. From an organizational perspective, this is the most valuable data as it can help you make important decisions based on the latest developments. Our guide on real time analytics will tell you more about the topic.
- Machine data: This is more complex data that is generated solely by a machine such as phones, computers, or even websites and embedded systems, without previous human interaction.
Why Is Data Analysis Important?
Before we go into detail about the categories of analysis along with its methods and techniques, you must understand the potential that analyzing data can bring to your organization.
- Informed decision-making : From a management perspective, you can benefit from analyzing your data as it helps you make decisions based on facts and not simple intuition. For instance, you can understand where to invest your capital, detect growth opportunities, predict your income, or tackle uncommon situations before they become problems. Through this, you can extract relevant insights from all areas in your organization, and with the help of dashboard software , present the data in a professional and interactive way to different stakeholders.
- Reduce costs : Another great benefit is to reduce costs. With the help of advanced technologies such as predictive analytics, businesses can spot improvement opportunities, trends, and patterns in their data and plan their strategies accordingly. In time, this will help you save money and resources on implementing the wrong strategies. And not just that, by predicting different scenarios such as sales and demand you can also anticipate production and supply.
- Target customers better : Customers are arguably the most crucial element in any business. By using analytics to get a 360° vision of all aspects related to your customers, you can understand which channels they use to communicate with you, their demographics, interests, habits, purchasing behaviors, and more. In the long run, it will drive success to your marketing strategies, allow you to identify new potential customers, and avoid wasting resources on targeting the wrong people or sending the wrong message. You can also track customer satisfaction by analyzing your client’s reviews or your customer service department’s performance.
What Is The Data Analysis Process?

When we talk about analyzing data there is an order to follow in order to extract the needed conclusions. The analysis process consists of 5 key stages. We will cover each of them more in detail later in the post, but to start providing the needed context to understand what is coming next, here is a rundown of the 5 essential steps of data analysis.
- Identify: Before you get your hands dirty with data, you first need to identify why you need it in the first place. The identification is the stage in which you establish the questions you will need to answer. For example, what is the customer's perception of our brand? Or what type of packaging is more engaging to our potential customers? Once the questions are outlined you are ready for the next step.
- Collect: As its name suggests, this is the stage where you start collecting the needed data. Here, you define which sources of data you will use and how you will use them. The collection of data can come in different forms such as internal or external sources, surveys, interviews, questionnaires, and focus groups, among others. An important note here is that the way you collect the data will be different in a quantitative and qualitative scenario.
- Clean: Once you have the necessary data it is time to clean it and leave it ready for analysis. Not all the data you collect will be useful, when collecting big amounts of data in different formats it is very likely that you will find yourself with duplicate or badly formatted data. To avoid this, before you start working with your data you need to make sure to erase any white spaces, duplicate records, or formatting errors. This way you avoid hurting your analysis with bad-quality data.
- Analyze : With the help of various techniques such as statistical analysis, regressions, neural networks, text analysis, and more, you can start analyzing and manipulating your data to extract relevant conclusions. At this stage, you find trends, correlations, variations, and patterns that can help you answer the questions you first thought of in the identify stage. Various technologies in the market assist researchers and average users with the management of their data. Some of them include business intelligence and visualization software, predictive analytics, and data mining, among others.
- Interpret: Last but not least you have one of the most important steps: it is time to interpret your results. This stage is where the researcher comes up with courses of action based on the findings. For example, here you would understand if your clients prefer packaging that is red or green, plastic or paper, etc. Additionally, at this stage, you can also find some limitations and work on them.
Now that you have a basic understanding of the key data analysis steps, let’s look at the top 17 essential methods.
17 Essential Types Of Data Analysis Methods
Before diving into the 17 essential types of methods, it is important that we go over really fast through the main analysis categories. Starting with the category of descriptive up to prescriptive analysis, the complexity and effort of data evaluation increases, but also the added value for the company.
a) Descriptive analysis - What happened.
The descriptive analysis method is the starting point for any analytic reflection, and it aims to answer the question of what happened? It does this by ordering, manipulating, and interpreting raw data from various sources to turn it into valuable insights for your organization.
Performing descriptive analysis is essential, as it enables us to present our insights in a meaningful way. Although it is relevant to mention that this analysis on its own will not allow you to predict future outcomes or tell you the answer to questions like why something happened, it will leave your data organized and ready to conduct further investigations.
b) Exploratory analysis - How to explore data relationships.
As its name suggests, the main aim of the exploratory analysis is to explore. Prior to it, there is still no notion of the relationship between the data and the variables. Once the data is investigated, exploratory analysis helps you to find connections and generate hypotheses and solutions for specific problems. A typical area of application for it is data mining.
c) Diagnostic analysis - Why it happened.
Diagnostic data analytics empowers analysts and executives by helping them gain a firm contextual understanding of why something happened. If you know why something happened as well as how it happened, you will be able to pinpoint the exact ways of tackling the issue or challenge.
Designed to provide direct and actionable answers to specific questions, this is one of the world’s most important methods in research, among its other key organizational functions such as retail analytics , e.g.
c) Predictive analysis - What will happen.
The predictive method allows you to look into the future to answer the question: what will happen? In order to do this, it uses the results of the previously mentioned descriptive, exploratory, and diagnostic analysis, in addition to machine learning (ML) and artificial intelligence (AI). Through this, you can uncover future trends, potential problems or inefficiencies, connections, and casualties in your data.
With predictive analysis, you can unfold and develop initiatives that will not only enhance your various operational processes but also help you gain an all-important edge over the competition. If you understand why a trend, pattern, or event happened through data, you will be able to develop an informed projection of how things may unfold in particular areas of the business.
e) Prescriptive analysis - How will it happen.
Another of the most effective types of analysis methods in research. Prescriptive data techniques cross over from predictive analysis in the way that it revolves around using patterns or trends to develop responsive, practical business strategies.
By drilling down into prescriptive analysis, you will play an active role in the data consumption process by taking well-arranged sets of visual data and using it as a powerful fix to emerging issues in a number of key areas, including marketing, sales, customer experience, HR, fulfillment, finance, logistics analytics , and others.

As mentioned at the beginning of the post, data analysis methods can be divided into two big categories: quantitative and qualitative. Each of these categories holds a powerful analytical value that changes depending on the scenario and type of data you are working with. Below, we will discuss 17 methods that are divided into qualitative and quantitative approaches.
Without further ado, here are the 17 essential types of data analysis methods with some use cases in the business world:
A. Quantitative Methods
To put it simply, quantitative analysis refers to all methods that use numerical data or data that can be turned into numbers (e.g. category variables like gender, age, etc.) to extract valuable insights. It is used to extract valuable conclusions about relationships, differences, and test hypotheses. Below we discuss some of the key quantitative methods.
1. Cluster analysis
The action of grouping a set of data elements in a way that said elements are more similar (in a particular sense) to each other than to those in other groups – hence the term ‘cluster.’ Since there is no target variable when clustering, the method is often used to find hidden patterns in the data. The approach is also used to provide additional context to a trend or dataset.
Let's look at it from an organizational perspective. In a perfect world, marketers would be able to analyze each customer separately and give them the best-personalized service, but let's face it, with a large customer base, it is timely impossible to do that. That's where clustering comes in. By grouping customers into clusters based on demographics, purchasing behaviors, monetary value, or any other factor that might be relevant for your company, you will be able to immediately optimize your efforts and give your customers the best experience based on their needs.
2. Cohort analysis
This type of data analysis approach uses historical data to examine and compare a determined segment of users' behavior, which can then be grouped with others with similar characteristics. By using this methodology, it's possible to gain a wealth of insight into consumer needs or a firm understanding of a broader target group.
Cohort analysis can be really useful for performing analysis in marketing as it will allow you to understand the impact of your campaigns on specific groups of customers. To exemplify, imagine you send an email campaign encouraging customers to sign up for your site. For this, you create two versions of the campaign with different designs, CTAs, and ad content. Later on, you can use cohort analysis to track the performance of the campaign for a longer period of time and understand which type of content is driving your customers to sign up, repurchase, or engage in other ways.
A useful tool to start performing cohort analysis method is Google Analytics. You can learn more about the benefits and limitations of using cohorts in GA in this useful guide . In the bottom image, you see an example of how you visualize a cohort in this tool. The segments (devices traffic) are divided into date cohorts (usage of devices) and then analyzed week by week to extract insights into performance.
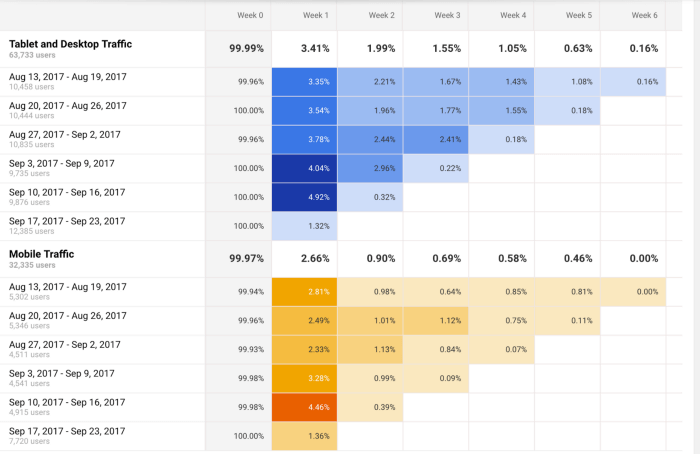
3. Regression analysis
Regression uses historical data to understand how a dependent variable's value is affected when one (linear regression) or more independent variables (multiple regression) change or stay the same. By understanding each variable's relationship and how it developed in the past, you can anticipate possible outcomes and make better decisions in the future.
Let's bring it down with an example. Imagine you did a regression analysis of your sales in 2019 and discovered that variables like product quality, store design, customer service, marketing campaigns, and sales channels affected the overall result. Now you want to use regression to analyze which of these variables changed or if any new ones appeared during 2020. For example, you couldn’t sell as much in your physical store due to COVID lockdowns. Therefore, your sales could’ve either dropped in general or increased in your online channels. Through this, you can understand which independent variables affected the overall performance of your dependent variable, annual sales.
If you want to go deeper into this type of analysis, check out this article and learn more about how you can benefit from regression.
4. Neural networks
The neural network forms the basis for the intelligent algorithms of machine learning. It is a form of analytics that attempts, with minimal intervention, to understand how the human brain would generate insights and predict values. Neural networks learn from each and every data transaction, meaning that they evolve and advance over time.
A typical area of application for neural networks is predictive analytics. There are BI reporting tools that have this feature implemented within them, such as the Predictive Analytics Tool from datapine. This tool enables users to quickly and easily generate all kinds of predictions. All you have to do is select the data to be processed based on your KPIs, and the software automatically calculates forecasts based on historical and current data. Thanks to its user-friendly interface, anyone in your organization can manage it; there’s no need to be an advanced scientist.
Here is an example of how you can use the predictive analysis tool from datapine:

**click to enlarge**
5. Factor analysis
The factor analysis also called “dimension reduction” is a type of data analysis used to describe variability among observed, correlated variables in terms of a potentially lower number of unobserved variables called factors. The aim here is to uncover independent latent variables, an ideal method for streamlining specific segments.
A good way to understand this data analysis method is a customer evaluation of a product. The initial assessment is based on different variables like color, shape, wearability, current trends, materials, comfort, the place where they bought the product, and frequency of usage. Like this, the list can be endless, depending on what you want to track. In this case, factor analysis comes into the picture by summarizing all of these variables into homogenous groups, for example, by grouping the variables color, materials, quality, and trends into a brother latent variable of design.
If you want to start analyzing data using factor analysis we recommend you take a look at this practical guide from UCLA.
6. Data mining
A method of data analysis that is the umbrella term for engineering metrics and insights for additional value, direction, and context. By using exploratory statistical evaluation, data mining aims to identify dependencies, relations, patterns, and trends to generate advanced knowledge. When considering how to analyze data, adopting a data mining mindset is essential to success - as such, it’s an area that is worth exploring in greater detail.
An excellent use case of data mining is datapine intelligent data alerts . With the help of artificial intelligence and machine learning, they provide automated signals based on particular commands or occurrences within a dataset. For example, if you’re monitoring supply chain KPIs , you could set an intelligent alarm to trigger when invalid or low-quality data appears. By doing so, you will be able to drill down deep into the issue and fix it swiftly and effectively.
In the following picture, you can see how the intelligent alarms from datapine work. By setting up ranges on daily orders, sessions, and revenues, the alarms will notify you if the goal was not completed or if it exceeded expectations.
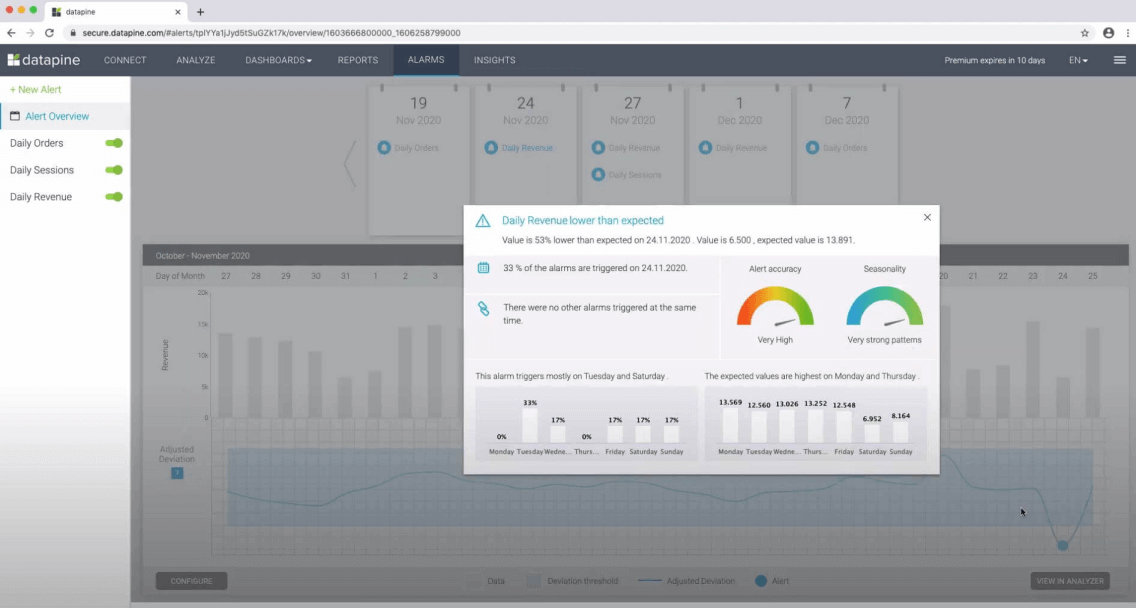
7. Time series analysis
As its name suggests, time series analysis is used to analyze a set of data points collected over a specified period of time. Although analysts use this method to monitor the data points in a specific interval of time rather than just monitoring them intermittently, the time series analysis is not uniquely used for the purpose of collecting data over time. Instead, it allows researchers to understand if variables changed during the duration of the study, how the different variables are dependent, and how did it reach the end result.
In a business context, this method is used to understand the causes of different trends and patterns to extract valuable insights. Another way of using this method is with the help of time series forecasting. Powered by predictive technologies, businesses can analyze various data sets over a period of time and forecast different future events.
A great use case to put time series analysis into perspective is seasonality effects on sales. By using time series forecasting to analyze sales data of a specific product over time, you can understand if sales rise over a specific period of time (e.g. swimwear during summertime, or candy during Halloween). These insights allow you to predict demand and prepare production accordingly.
8. Decision Trees
The decision tree analysis aims to act as a support tool to make smart and strategic decisions. By visually displaying potential outcomes, consequences, and costs in a tree-like model, researchers and company users can easily evaluate all factors involved and choose the best course of action. Decision trees are helpful to analyze quantitative data and they allow for an improved decision-making process by helping you spot improvement opportunities, reduce costs, and enhance operational efficiency and production.
But how does a decision tree actually works? This method works like a flowchart that starts with the main decision that you need to make and branches out based on the different outcomes and consequences of each decision. Each outcome will outline its own consequences, costs, and gains and, at the end of the analysis, you can compare each of them and make the smartest decision.
Businesses can use them to understand which project is more cost-effective and will bring more earnings in the long run. For example, imagine you need to decide if you want to update your software app or build a new app entirely. Here you would compare the total costs, the time needed to be invested, potential revenue, and any other factor that might affect your decision. In the end, you would be able to see which of these two options is more realistic and attainable for your company or research.
9. Conjoint analysis
Last but not least, we have the conjoint analysis. This approach is usually used in surveys to understand how individuals value different attributes of a product or service and it is one of the most effective methods to extract consumer preferences. When it comes to purchasing, some clients might be more price-focused, others more features-focused, and others might have a sustainable focus. Whatever your customer's preferences are, you can find them with conjoint analysis. Through this, companies can define pricing strategies, packaging options, subscription packages, and more.
A great example of conjoint analysis is in marketing and sales. For instance, a cupcake brand might use conjoint analysis and find that its clients prefer gluten-free options and cupcakes with healthier toppings over super sugary ones. Thus, the cupcake brand can turn these insights into advertisements and promotions to increase sales of this particular type of product. And not just that, conjoint analysis can also help businesses segment their customers based on their interests. This allows them to send different messaging that will bring value to each of the segments.
10. Correspondence Analysis
Also known as reciprocal averaging, correspondence analysis is a method used to analyze the relationship between categorical variables presented within a contingency table. A contingency table is a table that displays two (simple correspondence analysis) or more (multiple correspondence analysis) categorical variables across rows and columns that show the distribution of the data, which is usually answers to a survey or questionnaire on a specific topic.
This method starts by calculating an “expected value” which is done by multiplying row and column averages and dividing it by the overall original value of the specific table cell. The “expected value” is then subtracted from the original value resulting in a “residual number” which is what allows you to extract conclusions about relationships and distribution. The results of this analysis are later displayed using a map that represents the relationship between the different values. The closest two values are in the map, the bigger the relationship. Let’s put it into perspective with an example.
Imagine you are carrying out a market research analysis about outdoor clothing brands and how they are perceived by the public. For this analysis, you ask a group of people to match each brand with a certain attribute which can be durability, innovation, quality materials, etc. When calculating the residual numbers, you can see that brand A has a positive residual for innovation but a negative one for durability. This means that brand A is not positioned as a durable brand in the market, something that competitors could take advantage of.
11. Multidimensional Scaling (MDS)
MDS is a method used to observe the similarities or disparities between objects which can be colors, brands, people, geographical coordinates, and more. The objects are plotted using an “MDS map” that positions similar objects together and disparate ones far apart. The (dis) similarities between objects are represented using one or more dimensions that can be observed using a numerical scale. For example, if you want to know how people feel about the COVID-19 vaccine, you can use 1 for “don’t believe in the vaccine at all” and 10 for “firmly believe in the vaccine” and a scale of 2 to 9 for in between responses. When analyzing an MDS map the only thing that matters is the distance between the objects, the orientation of the dimensions is arbitrary and has no meaning at all.
Multidimensional scaling is a valuable technique for market research, especially when it comes to evaluating product or brand positioning. For instance, if a cupcake brand wants to know how they are positioned compared to competitors, it can define 2-3 dimensions such as taste, ingredients, shopping experience, or more, and do a multidimensional scaling analysis to find improvement opportunities as well as areas in which competitors are currently leading.
Another business example is in procurement when deciding on different suppliers. Decision makers can generate an MDS map to see how the different prices, delivery times, technical services, and more of the different suppliers differ and pick the one that suits their needs the best.
A final example proposed by a research paper on "An Improved Study of Multilevel Semantic Network Visualization for Analyzing Sentiment Word of Movie Review Data". Researchers picked a two-dimensional MDS map to display the distances and relationships between different sentiments in movie reviews. They used 36 sentiment words and distributed them based on their emotional distance as we can see in the image below where the words "outraged" and "sweet" are on opposite sides of the map, marking the distance between the two emotions very clearly.

Aside from being a valuable technique to analyze dissimilarities, MDS also serves as a dimension-reduction technique for large dimensional data.
B. Qualitative Methods
Qualitative data analysis methods are defined as the observation of non-numerical data that is gathered and produced using methods of observation such as interviews, focus groups, questionnaires, and more. As opposed to quantitative methods, qualitative data is more subjective and highly valuable in analyzing customer retention and product development.
12. Text analysis
Text analysis, also known in the industry as text mining, works by taking large sets of textual data and arranging them in a way that makes it easier to manage. By working through this cleansing process in stringent detail, you will be able to extract the data that is truly relevant to your organization and use it to develop actionable insights that will propel you forward.
Modern software accelerate the application of text analytics. Thanks to the combination of machine learning and intelligent algorithms, you can perform advanced analytical processes such as sentiment analysis. This technique allows you to understand the intentions and emotions of a text, for example, if it's positive, negative, or neutral, and then give it a score depending on certain factors and categories that are relevant to your brand. Sentiment analysis is often used to monitor brand and product reputation and to understand how successful your customer experience is. To learn more about the topic check out this insightful article .
By analyzing data from various word-based sources, including product reviews, articles, social media communications, and survey responses, you will gain invaluable insights into your audience, as well as their needs, preferences, and pain points. This will allow you to create campaigns, services, and communications that meet your prospects’ needs on a personal level, growing your audience while boosting customer retention. There are various other “sub-methods” that are an extension of text analysis. Each of them serves a more specific purpose and we will look at them in detail next.
13. Content Analysis
This is a straightforward and very popular method that examines the presence and frequency of certain words, concepts, and subjects in different content formats such as text, image, audio, or video. For example, the number of times the name of a celebrity is mentioned on social media or online tabloids. It does this by coding text data that is later categorized and tabulated in a way that can provide valuable insights, making it the perfect mix of quantitative and qualitative analysis.
There are two types of content analysis. The first one is the conceptual analysis which focuses on explicit data, for instance, the number of times a concept or word is mentioned in a piece of content. The second one is relational analysis, which focuses on the relationship between different concepts or words and how they are connected within a specific context.
Content analysis is often used by marketers to measure brand reputation and customer behavior. For example, by analyzing customer reviews. It can also be used to analyze customer interviews and find directions for new product development. It is also important to note, that in order to extract the maximum potential out of this analysis method, it is necessary to have a clearly defined research question.
14. Thematic Analysis
Very similar to content analysis, thematic analysis also helps in identifying and interpreting patterns in qualitative data with the main difference being that the first one can also be applied to quantitative analysis. The thematic method analyzes large pieces of text data such as focus group transcripts or interviews and groups them into themes or categories that come up frequently within the text. It is a great method when trying to figure out peoples view’s and opinions about a certain topic. For example, if you are a brand that cares about sustainability, you can do a survey of your customers to analyze their views and opinions about sustainability and how they apply it to their lives. You can also analyze customer service calls transcripts to find common issues and improve your service.
Thematic analysis is a very subjective technique that relies on the researcher’s judgment. Therefore, to avoid biases, it has 6 steps that include familiarization, coding, generating themes, reviewing themes, defining and naming themes, and writing up. It is also important to note that, because it is a flexible approach, the data can be interpreted in multiple ways and it can be hard to select what data is more important to emphasize.
15. Narrative Analysis
A bit more complex in nature than the two previous ones, narrative analysis is used to explore the meaning behind the stories that people tell and most importantly, how they tell them. By looking into the words that people use to describe a situation you can extract valuable conclusions about their perspective on a specific topic. Common sources for narrative data include autobiographies, family stories, opinion pieces, and testimonials, among others.
From a business perspective, narrative analysis can be useful to analyze customer behaviors and feelings towards a specific product, service, feature, or others. It provides unique and deep insights that can be extremely valuable. However, it has some drawbacks.
The biggest weakness of this method is that the sample sizes are usually very small due to the complexity and time-consuming nature of the collection of narrative data. Plus, the way a subject tells a story will be significantly influenced by his or her specific experiences, making it very hard to replicate in a subsequent study.
16. Discourse Analysis
Discourse analysis is used to understand the meaning behind any type of written, verbal, or symbolic discourse based on its political, social, or cultural context. It mixes the analysis of languages and situations together. This means that the way the content is constructed and the meaning behind it is significantly influenced by the culture and society it takes place in. For example, if you are analyzing political speeches you need to consider different context elements such as the politician's background, the current political context of the country, the audience to which the speech is directed, and so on.
From a business point of view, discourse analysis is a great market research tool. It allows marketers to understand how the norms and ideas of the specific market work and how their customers relate to those ideas. It can be very useful to build a brand mission or develop a unique tone of voice.
17. Grounded Theory Analysis
Traditionally, researchers decide on a method and hypothesis and start to collect the data to prove that hypothesis. The grounded theory is the only method that doesn’t require an initial research question or hypothesis as its value lies in the generation of new theories. With the grounded theory method, you can go into the analysis process with an open mind and explore the data to generate new theories through tests and revisions. In fact, it is not necessary to collect the data and then start to analyze it. Researchers usually start to find valuable insights as they are gathering the data.
All of these elements make grounded theory a very valuable method as theories are fully backed by data instead of initial assumptions. It is a great technique to analyze poorly researched topics or find the causes behind specific company outcomes. For example, product managers and marketers might use the grounded theory to find the causes of high levels of customer churn and look into customer surveys and reviews to develop new theories about the causes.
How To Analyze Data? Top 17 Data Analysis Techniques To Apply

Now that we’ve answered the questions “what is data analysis’”, why is it important, and covered the different data analysis types, it’s time to dig deeper into how to perform your analysis by working through these 17 essential techniques.
1. Collaborate your needs
Before you begin analyzing or drilling down into any techniques, it’s crucial to sit down collaboratively with all key stakeholders within your organization, decide on your primary campaign or strategic goals, and gain a fundamental understanding of the types of insights that will best benefit your progress or provide you with the level of vision you need to evolve your organization.
2. Establish your questions
Once you’ve outlined your core objectives, you should consider which questions will need answering to help you achieve your mission. This is one of the most important techniques as it will shape the very foundations of your success.
To help you ask the right things and ensure your data works for you, you have to ask the right data analysis questions .
3. Data democratization
After giving your data analytics methodology some real direction, and knowing which questions need answering to extract optimum value from the information available to your organization, you should continue with democratization.
Data democratization is an action that aims to connect data from various sources efficiently and quickly so that anyone in your organization can access it at any given moment. You can extract data in text, images, videos, numbers, or any other format. And then perform cross-database analysis to achieve more advanced insights to share with the rest of the company interactively.
Once you have decided on your most valuable sources, you need to take all of this into a structured format to start collecting your insights. For this purpose, datapine offers an easy all-in-one data connectors feature to integrate all your internal and external sources and manage them at your will. Additionally, datapine’s end-to-end solution automatically updates your data, allowing you to save time and focus on performing the right analysis to grow your company.
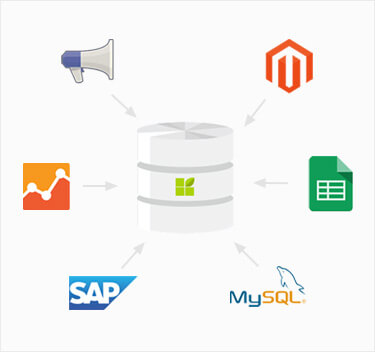
4. Think of governance
When collecting data in a business or research context you always need to think about security and privacy. With data breaches becoming a topic of concern for businesses, the need to protect your client's or subject’s sensitive information becomes critical.
To ensure that all this is taken care of, you need to think of a data governance strategy. According to Gartner , this concept refers to “ the specification of decision rights and an accountability framework to ensure the appropriate behavior in the valuation, creation, consumption, and control of data and analytics .” In simpler words, data governance is a collection of processes, roles, and policies, that ensure the efficient use of data while still achieving the main company goals. It ensures that clear roles are in place for who can access the information and how they can access it. In time, this not only ensures that sensitive information is protected but also allows for an efficient analysis as a whole.
5. Clean your data
After harvesting from so many sources you will be left with a vast amount of information that can be overwhelming to deal with. At the same time, you can be faced with incorrect data that can be misleading to your analysis. The smartest thing you can do to avoid dealing with this in the future is to clean the data. This is fundamental before visualizing it, as it will ensure that the insights you extract from it are correct.
There are many things that you need to look for in the cleaning process. The most important one is to eliminate any duplicate observations; this usually appears when using multiple internal and external sources of information. You can also add any missing codes, fix empty fields, and eliminate incorrectly formatted data.
Another usual form of cleaning is done with text data. As we mentioned earlier, most companies today analyze customer reviews, social media comments, questionnaires, and several other text inputs. In order for algorithms to detect patterns, text data needs to be revised to avoid invalid characters or any syntax or spelling errors.
Most importantly, the aim of cleaning is to prevent you from arriving at false conclusions that can damage your company in the long run. By using clean data, you will also help BI solutions to interact better with your information and create better reports for your organization.
6. Set your KPIs
Once you’ve set your sources, cleaned your data, and established clear-cut questions you want your insights to answer, you need to set a host of key performance indicators (KPIs) that will help you track, measure, and shape your progress in a number of key areas.
KPIs are critical to both qualitative and quantitative analysis research. This is one of the primary methods of data analysis you certainly shouldn’t overlook.
To help you set the best possible KPIs for your initiatives and activities, here is an example of a relevant logistics KPI : transportation-related costs. If you want to see more go explore our collection of key performance indicator examples .

7. Omit useless data
Having bestowed your data analysis tools and techniques with true purpose and defined your mission, you should explore the raw data you’ve collected from all sources and use your KPIs as a reference for chopping out any information you deem to be useless.
Trimming the informational fat is one of the most crucial methods of analysis as it will allow you to focus your analytical efforts and squeeze every drop of value from the remaining ‘lean’ information.
Any stats, facts, figures, or metrics that don’t align with your business goals or fit with your KPI management strategies should be eliminated from the equation.
8. Build a data management roadmap
While, at this point, this particular step is optional (you will have already gained a wealth of insight and formed a fairly sound strategy by now), creating a data governance roadmap will help your data analysis methods and techniques become successful on a more sustainable basis. These roadmaps, if developed properly, are also built so they can be tweaked and scaled over time.
Invest ample time in developing a roadmap that will help you store, manage, and handle your data internally, and you will make your analysis techniques all the more fluid and functional – one of the most powerful types of data analysis methods available today.
9. Integrate technology
There are many ways to analyze data, but one of the most vital aspects of analytical success in a business context is integrating the right decision support software and technology.
Robust analysis platforms will not only allow you to pull critical data from your most valuable sources while working with dynamic KPIs that will offer you actionable insights; it will also present them in a digestible, visual, interactive format from one central, live dashboard . A data methodology you can count on.
By integrating the right technology within your data analysis methodology, you’ll avoid fragmenting your insights, saving you time and effort while allowing you to enjoy the maximum value from your business’s most valuable insights.
For a look at the power of software for the purpose of analysis and to enhance your methods of analyzing, glance over our selection of dashboard examples .
10. Answer your questions
By considering each of the above efforts, working with the right technology, and fostering a cohesive internal culture where everyone buys into the different ways to analyze data as well as the power of digital intelligence, you will swiftly start to answer your most burning business questions. Arguably, the best way to make your data concepts accessible across the organization is through data visualization.
11. Visualize your data
Online data visualization is a powerful tool as it lets you tell a story with your metrics, allowing users across the organization to extract meaningful insights that aid business evolution – and it covers all the different ways to analyze data.
The purpose of analyzing is to make your entire organization more informed and intelligent, and with the right platform or dashboard, this is simpler than you think, as demonstrated by our marketing dashboard .

This visual, dynamic, and interactive online dashboard is a data analysis example designed to give Chief Marketing Officers (CMO) an overview of relevant metrics to help them understand if they achieved their monthly goals.
In detail, this example generated with a modern dashboard creator displays interactive charts for monthly revenues, costs, net income, and net income per customer; all of them are compared with the previous month so that you can understand how the data fluctuated. In addition, it shows a detailed summary of the number of users, customers, SQLs, and MQLs per month to visualize the whole picture and extract relevant insights or trends for your marketing reports .
The CMO dashboard is perfect for c-level management as it can help them monitor the strategic outcome of their marketing efforts and make data-driven decisions that can benefit the company exponentially.
12. Be careful with the interpretation
We already dedicated an entire post to data interpretation as it is a fundamental part of the process of data analysis. It gives meaning to the analytical information and aims to drive a concise conclusion from the analysis results. Since most of the time companies are dealing with data from many different sources, the interpretation stage needs to be done carefully and properly in order to avoid misinterpretations.
To help you through the process, here we list three common practices that you need to avoid at all costs when looking at your data:
- Correlation vs. causation: The human brain is formatted to find patterns. This behavior leads to one of the most common mistakes when performing interpretation: confusing correlation with causation. Although these two aspects can exist simultaneously, it is not correct to assume that because two things happened together, one provoked the other. A piece of advice to avoid falling into this mistake is never to trust just intuition, trust the data. If there is no objective evidence of causation, then always stick to correlation.
- Confirmation bias: This phenomenon describes the tendency to select and interpret only the data necessary to prove one hypothesis, often ignoring the elements that might disprove it. Even if it's not done on purpose, confirmation bias can represent a real problem, as excluding relevant information can lead to false conclusions and, therefore, bad business decisions. To avoid it, always try to disprove your hypothesis instead of proving it, share your analysis with other team members, and avoid drawing any conclusions before the entire analytical project is finalized.
- Statistical significance: To put it in short words, statistical significance helps analysts understand if a result is actually accurate or if it happened because of a sampling error or pure chance. The level of statistical significance needed might depend on the sample size and the industry being analyzed. In any case, ignoring the significance of a result when it might influence decision-making can be a huge mistake.
13. Build a narrative
Now, we’re going to look at how you can bring all of these elements together in a way that will benefit your business - starting with a little something called data storytelling.
The human brain responds incredibly well to strong stories or narratives. Once you’ve cleansed, shaped, and visualized your most invaluable data using various BI dashboard tools , you should strive to tell a story - one with a clear-cut beginning, middle, and end.
By doing so, you will make your analytical efforts more accessible, digestible, and universal, empowering more people within your organization to use your discoveries to their actionable advantage.
14. Consider autonomous technology
Autonomous technologies, such as artificial intelligence (AI) and machine learning (ML), play a significant role in the advancement of understanding how to analyze data more effectively.
Gartner predicts that by the end of this year, 80% of emerging technologies will be developed with AI foundations. This is a testament to the ever-growing power and value of autonomous technologies.
At the moment, these technologies are revolutionizing the analysis industry. Some examples that we mentioned earlier are neural networks, intelligent alarms, and sentiment analysis.
15. Share the load
If you work with the right tools and dashboards, you will be able to present your metrics in a digestible, value-driven format, allowing almost everyone in the organization to connect with and use relevant data to their advantage.
Modern dashboards consolidate data from various sources, providing access to a wealth of insights in one centralized location, no matter if you need to monitor recruitment metrics or generate reports that need to be sent across numerous departments. Moreover, these cutting-edge tools offer access to dashboards from a multitude of devices, meaning that everyone within the business can connect with practical insights remotely - and share the load.
Once everyone is able to work with a data-driven mindset, you will catalyze the success of your business in ways you never thought possible. And when it comes to knowing how to analyze data, this kind of collaborative approach is essential.
16. Data analysis tools
In order to perform high-quality analysis of data, it is fundamental to use tools and software that will ensure the best results. Here we leave you a small summary of four fundamental categories of data analysis tools for your organization.
- Business Intelligence: BI tools allow you to process significant amounts of data from several sources in any format. Through this, you can not only analyze and monitor your data to extract relevant insights but also create interactive reports and dashboards to visualize your KPIs and use them for your company's good. datapine is an amazing online BI software that is focused on delivering powerful online analysis features that are accessible to beginner and advanced users. Like this, it offers a full-service solution that includes cutting-edge analysis of data, KPIs visualization, live dashboards, reporting, and artificial intelligence technologies to predict trends and minimize risk.
- Statistical analysis: These tools are usually designed for scientists, statisticians, market researchers, and mathematicians, as they allow them to perform complex statistical analyses with methods like regression analysis, predictive analysis, and statistical modeling. A good tool to perform this type of analysis is R-Studio as it offers a powerful data modeling and hypothesis testing feature that can cover both academic and general data analysis. This tool is one of the favorite ones in the industry, due to its capability for data cleaning, data reduction, and performing advanced analysis with several statistical methods. Another relevant tool to mention is SPSS from IBM. The software offers advanced statistical analysis for users of all skill levels. Thanks to a vast library of machine learning algorithms, text analysis, and a hypothesis testing approach it can help your company find relevant insights to drive better decisions. SPSS also works as a cloud service that enables you to run it anywhere.
- SQL Consoles: SQL is a programming language often used to handle structured data in relational databases. Tools like these are popular among data scientists as they are extremely effective in unlocking these databases' value. Undoubtedly, one of the most used SQL software in the market is MySQL Workbench . This tool offers several features such as a visual tool for database modeling and monitoring, complete SQL optimization, administration tools, and visual performance dashboards to keep track of KPIs.
- Data Visualization: These tools are used to represent your data through charts, graphs, and maps that allow you to find patterns and trends in the data. datapine's already mentioned BI platform also offers a wealth of powerful online data visualization tools with several benefits. Some of them include: delivering compelling data-driven presentations to share with your entire company, the ability to see your data online with any device wherever you are, an interactive dashboard design feature that enables you to showcase your results in an interactive and understandable way, and to perform online self-service reports that can be used simultaneously with several other people to enhance team productivity.
17. Refine your process constantly
Last is a step that might seem obvious to some people, but it can be easily ignored if you think you are done. Once you have extracted the needed results, you should always take a retrospective look at your project and think about what you can improve. As you saw throughout this long list of techniques, data analysis is a complex process that requires constant refinement. For this reason, you should always go one step further and keep improving.
Quality Criteria For Data Analysis
So far we’ve covered a list of methods and techniques that should help you perform efficient data analysis. But how do you measure the quality and validity of your results? This is done with the help of some science quality criteria. Here we will go into a more theoretical area that is critical to understanding the fundamentals of statistical analysis in science. However, you should also be aware of these steps in a business context, as they will allow you to assess the quality of your results in the correct way. Let’s dig in.
- Internal validity: The results of a survey are internally valid if they measure what they are supposed to measure and thus provide credible results. In other words , internal validity measures the trustworthiness of the results and how they can be affected by factors such as the research design, operational definitions, how the variables are measured, and more. For instance, imagine you are doing an interview to ask people if they brush their teeth two times a day. While most of them will answer yes, you can still notice that their answers correspond to what is socially acceptable, which is to brush your teeth at least twice a day. In this case, you can’t be 100% sure if respondents actually brush their teeth twice a day or if they just say that they do, therefore, the internal validity of this interview is very low.
- External validity: Essentially, external validity refers to the extent to which the results of your research can be applied to a broader context. It basically aims to prove that the findings of a study can be applied in the real world. If the research can be applied to other settings, individuals, and times, then the external validity is high.
- Reliability : If your research is reliable, it means that it can be reproduced. If your measurement were repeated under the same conditions, it would produce similar results. This means that your measuring instrument consistently produces reliable results. For example, imagine a doctor building a symptoms questionnaire to detect a specific disease in a patient. Then, various other doctors use this questionnaire but end up diagnosing the same patient with a different condition. This means the questionnaire is not reliable in detecting the initial disease. Another important note here is that in order for your research to be reliable, it also needs to be objective. If the results of a study are the same, independent of who assesses them or interprets them, the study can be considered reliable. Let’s see the objectivity criteria in more detail now.
- Objectivity: In data science, objectivity means that the researcher needs to stay fully objective when it comes to its analysis. The results of a study need to be affected by objective criteria and not by the beliefs, personality, or values of the researcher. Objectivity needs to be ensured when you are gathering the data, for example, when interviewing individuals, the questions need to be asked in a way that doesn't influence the results. Paired with this, objectivity also needs to be thought of when interpreting the data. If different researchers reach the same conclusions, then the study is objective. For this last point, you can set predefined criteria to interpret the results to ensure all researchers follow the same steps.
The discussed quality criteria cover mostly potential influences in a quantitative context. Analysis in qualitative research has by default additional subjective influences that must be controlled in a different way. Therefore, there are other quality criteria for this kind of research such as credibility, transferability, dependability, and confirmability. You can see each of them more in detail on this resource .
Data Analysis Limitations & Barriers
Analyzing data is not an easy task. As you’ve seen throughout this post, there are many steps and techniques that you need to apply in order to extract useful information from your research. While a well-performed analysis can bring various benefits to your organization it doesn't come without limitations. In this section, we will discuss some of the main barriers you might encounter when conducting an analysis. Let’s see them more in detail.
- Lack of clear goals: No matter how good your data or analysis might be if you don’t have clear goals or a hypothesis the process might be worthless. While we mentioned some methods that don’t require a predefined hypothesis, it is always better to enter the analytical process with some clear guidelines of what you are expecting to get out of it, especially in a business context in which data is utilized to support important strategic decisions.
- Objectivity: Arguably one of the biggest barriers when it comes to data analysis in research is to stay objective. When trying to prove a hypothesis, researchers might find themselves, intentionally or unintentionally, directing the results toward an outcome that they want. To avoid this, always question your assumptions and avoid confusing facts with opinions. You can also show your findings to a research partner or external person to confirm that your results are objective.
- Data representation: A fundamental part of the analytical procedure is the way you represent your data. You can use various graphs and charts to represent your findings, but not all of them will work for all purposes. Choosing the wrong visual can not only damage your analysis but can mislead your audience, therefore, it is important to understand when to use each type of data depending on your analytical goals. Our complete guide on the types of graphs and charts lists 20 different visuals with examples of when to use them.
- Flawed correlation : Misleading statistics can significantly damage your research. We’ve already pointed out a few interpretation issues previously in the post, but it is an important barrier that we can't avoid addressing here as well. Flawed correlations occur when two variables appear related to each other but they are not. Confusing correlations with causation can lead to a wrong interpretation of results which can lead to building wrong strategies and loss of resources, therefore, it is very important to identify the different interpretation mistakes and avoid them.
- Sample size: A very common barrier to a reliable and efficient analysis process is the sample size. In order for the results to be trustworthy, the sample size should be representative of what you are analyzing. For example, imagine you have a company of 1000 employees and you ask the question “do you like working here?” to 50 employees of which 49 say yes, which means 95%. Now, imagine you ask the same question to the 1000 employees and 950 say yes, which also means 95%. Saying that 95% of employees like working in the company when the sample size was only 50 is not a representative or trustworthy conclusion. The significance of the results is way more accurate when surveying a bigger sample size.
- Privacy concerns: In some cases, data collection can be subjected to privacy regulations. Businesses gather all kinds of information from their customers from purchasing behaviors to addresses and phone numbers. If this falls into the wrong hands due to a breach, it can affect the security and confidentiality of your clients. To avoid this issue, you need to collect only the data that is needed for your research and, if you are using sensitive facts, make it anonymous so customers are protected. The misuse of customer data can severely damage a business's reputation, so it is important to keep an eye on privacy.
- Lack of communication between teams : When it comes to performing data analysis on a business level, it is very likely that each department and team will have different goals and strategies. However, they are all working for the same common goal of helping the business run smoothly and keep growing. When teams are not connected and communicating with each other, it can directly affect the way general strategies are built. To avoid these issues, tools such as data dashboards enable teams to stay connected through data in a visually appealing way.
- Innumeracy : Businesses are working with data more and more every day. While there are many BI tools available to perform effective analysis, data literacy is still a constant barrier. Not all employees know how to apply analysis techniques or extract insights from them. To prevent this from happening, you can implement different training opportunities that will prepare every relevant user to deal with data.
Key Data Analysis Skills
As you've learned throughout this lengthy guide, analyzing data is a complex task that requires a lot of knowledge and skills. That said, thanks to the rise of self-service tools the process is way more accessible and agile than it once was. Regardless, there are still some key skills that are valuable to have when working with data, we list the most important ones below.
- Critical and statistical thinking: To successfully analyze data you need to be creative and think out of the box. Yes, that might sound like a weird statement considering that data is often tight to facts. However, a great level of critical thinking is required to uncover connections, come up with a valuable hypothesis, and extract conclusions that go a step further from the surface. This, of course, needs to be complemented by statistical thinking and an understanding of numbers.
- Data cleaning: Anyone who has ever worked with data before will tell you that the cleaning and preparation process accounts for 80% of a data analyst's work, therefore, the skill is fundamental. But not just that, not cleaning the data adequately can also significantly damage the analysis which can lead to poor decision-making in a business scenario. While there are multiple tools that automate the cleaning process and eliminate the possibility of human error, it is still a valuable skill to dominate.
- Data visualization: Visuals make the information easier to understand and analyze, not only for professional users but especially for non-technical ones. Having the necessary skills to not only choose the right chart type but know when to apply it correctly is key. This also means being able to design visually compelling charts that make the data exploration process more efficient.
- SQL: The Structured Query Language or SQL is a programming language used to communicate with databases. It is fundamental knowledge as it enables you to update, manipulate, and organize data from relational databases which are the most common databases used by companies. It is fairly easy to learn and one of the most valuable skills when it comes to data analysis.
- Communication skills: This is a skill that is especially valuable in a business environment. Being able to clearly communicate analytical outcomes to colleagues is incredibly important, especially when the information you are trying to convey is complex for non-technical people. This applies to in-person communication as well as written format, for example, when generating a dashboard or report. While this might be considered a “soft” skill compared to the other ones we mentioned, it should not be ignored as you most likely will need to share analytical findings with others no matter the context.
Data Analysis In The Big Data Environment
Big data is invaluable to today’s businesses, and by using different methods for data analysis, it’s possible to view your data in a way that can help you turn insight into positive action.
To inspire your efforts and put the importance of big data into context, here are some insights that you should know:
- By 2026 the industry of big data is expected to be worth approximately $273.4 billion.
- 94% of enterprises say that analyzing data is important for their growth and digital transformation.
- Companies that exploit the full potential of their data can increase their operating margins by 60% .
- We already told you the benefits of Artificial Intelligence through this article. This industry's financial impact is expected to grow up to $40 billion by 2025.
Data analysis concepts may come in many forms, but fundamentally, any solid methodology will help to make your business more streamlined, cohesive, insightful, and successful than ever before.
Key Takeaways From Data Analysis
As we reach the end of our data analysis journey, we leave a small summary of the main methods and techniques to perform excellent analysis and grow your business.
17 Essential Types of Data Analysis Methods:
- Cluster analysis
- Cohort analysis
- Regression analysis
- Factor analysis
- Neural Networks
- Data Mining
- Text analysis
- Time series analysis
- Decision trees
- Conjoint analysis
- Correspondence Analysis
- Multidimensional Scaling
- Content analysis
- Thematic analysis
- Narrative analysis
- Grounded theory analysis
- Discourse analysis
Top 17 Data Analysis Techniques:
- Collaborate your needs
- Establish your questions
- Data democratization
- Think of data governance
- Clean your data
- Set your KPIs
- Omit useless data
- Build a data management roadmap
- Integrate technology
- Answer your questions
- Visualize your data
- Interpretation of data
- Consider autonomous technology
- Build a narrative
- Share the load
- Data Analysis tools
- Refine your process constantly
We’ve pondered the data analysis definition and drilled down into the practical applications of data-centric analytics, and one thing is clear: by taking measures to arrange your data and making your metrics work for you, it’s possible to transform raw information into action - the kind of that will push your business to the next level.
Yes, good data analytics techniques result in enhanced business intelligence (BI). To help you understand this notion in more detail, read our exploration of business intelligence reporting .
And, if you’re ready to perform your own analysis, drill down into your facts and figures while interacting with your data on astonishing visuals, you can try our software for a free, 14-day trial .
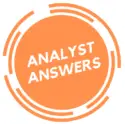
Data & Finance for Work & Life

Data Analysis: Types, Methods & Techniques (a Complete List)
( Updated Version )
While the term sounds intimidating, “data analysis” is nothing more than making sense of information in a table. It consists of filtering, sorting, grouping, and manipulating data tables with basic algebra and statistics.
In fact, you don’t need experience to understand the basics. You have already worked with data extensively in your life, and “analysis” is nothing more than a fancy word for good sense and basic logic.
Over time, people have intuitively categorized the best logical practices for treating data. These categories are what we call today types , methods , and techniques .
This article provides a comprehensive list of types, methods, and techniques, and explains the difference between them.
For a practical intro to data analysis (including types, methods, & techniques), check out our Intro to Data Analysis eBook for free.
Descriptive, Diagnostic, Predictive, & Prescriptive Analysis
If you Google “types of data analysis,” the first few results will explore descriptive , diagnostic , predictive , and prescriptive analysis. Why? Because these names are easy to understand and are used a lot in “the real world.”
Descriptive analysis is an informational method, diagnostic analysis explains “why” a phenomenon occurs, predictive analysis seeks to forecast the result of an action, and prescriptive analysis identifies solutions to a specific problem.
That said, these are only four branches of a larger analytical tree.
Good data analysts know how to position these four types within other analytical methods and tactics, allowing them to leverage strengths and weaknesses in each to uproot the most valuable insights.
Let’s explore the full analytical tree to understand how to appropriately assess and apply these four traditional types.
Tree diagram of Data Analysis Types, Methods, and Techniques
Here’s a picture to visualize the structure and hierarchy of data analysis types, methods, and techniques.
If it’s too small you can view the picture in a new tab . Open it to follow along!

Note: basic descriptive statistics such as mean , median , and mode , as well as standard deviation , are not shown because most people are already familiar with them. In the diagram, they would fall under the “descriptive” analysis type.
Tree Diagram Explained
The highest-level classification of data analysis is quantitative vs qualitative . Quantitative implies numbers while qualitative implies information other than numbers.
Quantitative data analysis then splits into mathematical analysis and artificial intelligence (AI) analysis . Mathematical types then branch into descriptive , diagnostic , predictive , and prescriptive .
Methods falling under mathematical analysis include clustering , classification , forecasting , and optimization . Qualitative data analysis methods include content analysis , narrative analysis , discourse analysis , framework analysis , and/or grounded theory .
Moreover, mathematical techniques include regression , Nïave Bayes , Simple Exponential Smoothing , cohorts , factors , linear discriminants , and more, whereas techniques falling under the AI type include artificial neural networks , decision trees , evolutionary programming , and fuzzy logic . Techniques under qualitative analysis include text analysis , coding , idea pattern analysis , and word frequency .
It’s a lot to remember! Don’t worry, once you understand the relationship and motive behind all these terms, it’ll be like riding a bike.
We’ll move down the list from top to bottom and I encourage you to open the tree diagram above in a new tab so you can follow along .
But first, let’s just address the elephant in the room: what’s the difference between methods and techniques anyway?
Difference between methods and techniques
Though often used interchangeably, methods ands techniques are not the same. By definition, methods are the process by which techniques are applied, and techniques are the practical application of those methods.
For example, consider driving. Methods include staying in your lane, stopping at a red light, and parking in a spot. Techniques include turning the steering wheel, braking, and pushing the gas pedal.
Data sets: observations and fields
It’s important to understand the basic structure of data tables to comprehend the rest of the article. A data set consists of one far-left column containing observations, then a series of columns containing the fields (aka “traits” or “characteristics”) that describe each observations. For example, imagine we want a data table for fruit. It might look like this:
Now let’s turn to types, methods, and techniques. Each heading below consists of a description, relative importance, the nature of data it explores, and the motivation for using it.
Quantitative Analysis
- It accounts for more than 50% of all data analysis and is by far the most widespread and well-known type of data analysis.
- As you have seen, it holds descriptive, diagnostic, predictive, and prescriptive methods, which in turn hold some of the most important techniques available today, such as clustering and forecasting.
- It can be broken down into mathematical and AI analysis.
- Importance : Very high . Quantitative analysis is a must for anyone interesting in becoming or improving as a data analyst.
- Nature of Data: data treated under quantitative analysis is, quite simply, quantitative. It encompasses all numeric data.
- Motive: to extract insights. (Note: we’re at the top of the pyramid, this gets more insightful as we move down.)
Qualitative Analysis
- It accounts for less than 30% of all data analysis and is common in social sciences .
- It can refer to the simple recognition of qualitative elements, which is not analytic in any way, but most often refers to methods that assign numeric values to non-numeric data for analysis.
- Because of this, some argue that it’s ultimately a quantitative type.
- Importance: Medium. In general, knowing qualitative data analysis is not common or even necessary for corporate roles. However, for researchers working in social sciences, its importance is very high .
- Nature of Data: data treated under qualitative analysis is non-numeric. However, as part of the analysis, analysts turn non-numeric data into numbers, at which point many argue it is no longer qualitative analysis.
- Motive: to extract insights. (This will be more important as we move down the pyramid.)
Mathematical Analysis
- Description: mathematical data analysis is a subtype of qualitative data analysis that designates methods and techniques based on statistics, algebra, and logical reasoning to extract insights. It stands in opposition to artificial intelligence analysis.
- Importance: Very High. The most widespread methods and techniques fall under mathematical analysis. In fact, it’s so common that many people use “quantitative” and “mathematical” analysis interchangeably.
- Nature of Data: numeric. By definition, all data under mathematical analysis are numbers.
- Motive: to extract measurable insights that can be used to act upon.
Artificial Intelligence & Machine Learning Analysis
- Description: artificial intelligence and machine learning analyses designate techniques based on the titular skills. They are not traditionally mathematical, but they are quantitative since they use numbers. Applications of AI & ML analysis techniques are developing, but they’re not yet mainstream enough to show promise across the field.
- Importance: Medium . As of today (September 2020), you don’t need to be fluent in AI & ML data analysis to be a great analyst. BUT, if it’s a field that interests you, learn it. Many believe that in 10 year’s time its importance will be very high .
- Nature of Data: numeric.
- Motive: to create calculations that build on themselves in order and extract insights without direct input from a human.
Descriptive Analysis
- Description: descriptive analysis is a subtype of mathematical data analysis that uses methods and techniques to provide information about the size, dispersion, groupings, and behavior of data sets. This may sounds complicated, but just think about mean, median, and mode: all three are types of descriptive analysis. They provide information about the data set. We’ll look at specific techniques below.
- Importance: Very high. Descriptive analysis is among the most commonly used data analyses in both corporations and research today.
- Nature of Data: the nature of data under descriptive statistics is sets. A set is simply a collection of numbers that behaves in predictable ways. Data reflects real life, and there are patterns everywhere to be found. Descriptive analysis describes those patterns.
- Motive: the motive behind descriptive analysis is to understand how numbers in a set group together, how far apart they are from each other, and how often they occur. As with most statistical analysis, the more data points there are, the easier it is to describe the set.
Diagnostic Analysis
- Description: diagnostic analysis answers the question “why did it happen?” It is an advanced type of mathematical data analysis that manipulates multiple techniques, but does not own any single one. Analysts engage in diagnostic analysis when they try to explain why.
- Importance: Very high. Diagnostics are probably the most important type of data analysis for people who don’t do analysis because they’re valuable to anyone who’s curious. They’re most common in corporations, as managers often only want to know the “why.”
- Nature of Data : data under diagnostic analysis are data sets. These sets in themselves are not enough under diagnostic analysis. Instead, the analyst must know what’s behind the numbers in order to explain “why.” That’s what makes diagnostics so challenging yet so valuable.
- Motive: the motive behind diagnostics is to diagnose — to understand why.
Predictive Analysis
- Description: predictive analysis uses past data to project future data. It’s very often one of the first kinds of analysis new researchers and corporate analysts use because it is intuitive. It is a subtype of the mathematical type of data analysis, and its three notable techniques are regression, moving average, and exponential smoothing.
- Importance: Very high. Predictive analysis is critical for any data analyst working in a corporate environment. Companies always want to know what the future will hold — especially for their revenue.
- Nature of Data: Because past and future imply time, predictive data always includes an element of time. Whether it’s minutes, hours, days, months, or years, we call this time series data . In fact, this data is so important that I’ll mention it twice so you don’t forget: predictive analysis uses time series data .
- Motive: the motive for investigating time series data with predictive analysis is to predict the future in the most analytical way possible.
Prescriptive Analysis
- Description: prescriptive analysis is a subtype of mathematical analysis that answers the question “what will happen if we do X?” It’s largely underestimated in the data analysis world because it requires diagnostic and descriptive analyses to be done before it even starts. More than simple predictive analysis, prescriptive analysis builds entire data models to show how a simple change could impact the ensemble.
- Importance: High. Prescriptive analysis is most common under the finance function in many companies. Financial analysts use it to build a financial model of the financial statements that show how that data will change given alternative inputs.
- Nature of Data: the nature of data in prescriptive analysis is data sets. These data sets contain patterns that respond differently to various inputs. Data that is useful for prescriptive analysis contains correlations between different variables. It’s through these correlations that we establish patterns and prescribe action on this basis. This analysis cannot be performed on data that exists in a vacuum — it must be viewed on the backdrop of the tangibles behind it.
- Motive: the motive for prescriptive analysis is to establish, with an acceptable degree of certainty, what results we can expect given a certain action. As you might expect, this necessitates that the analyst or researcher be aware of the world behind the data, not just the data itself.
Clustering Method
- Description: the clustering method groups data points together based on their relativeness closeness to further explore and treat them based on these groupings. There are two ways to group clusters: intuitively and statistically (or K-means).
- Importance: Very high. Though most corporate roles group clusters intuitively based on management criteria, a solid understanding of how to group them mathematically is an excellent descriptive and diagnostic approach to allow for prescriptive analysis thereafter.
- Nature of Data : the nature of data useful for clustering is sets with 1 or more data fields. While most people are used to looking at only two dimensions (x and y), clustering becomes more accurate the more fields there are.
- Motive: the motive for clustering is to understand how data sets group and to explore them further based on those groups.
- Here’s an example set:

Classification Method
- Description: the classification method aims to separate and group data points based on common characteristics . This can be done intuitively or statistically.
- Importance: High. While simple on the surface, classification can become quite complex. It’s very valuable in corporate and research environments, but can feel like its not worth the work. A good analyst can execute it quickly to deliver results.
- Nature of Data: the nature of data useful for classification is data sets. As we will see, it can be used on qualitative data as well as quantitative. This method requires knowledge of the substance behind the data, not just the numbers themselves.
- Motive: the motive for classification is group data not based on mathematical relationships (which would be clustering), but by predetermined outputs. This is why it’s less useful for diagnostic analysis, and more useful for prescriptive analysis.
Forecasting Method
- Description: the forecasting method uses time past series data to forecast the future.
- Importance: Very high. Forecasting falls under predictive analysis and is arguably the most common and most important method in the corporate world. It is less useful in research, which prefers to understand the known rather than speculate about the future.
- Nature of Data: data useful for forecasting is time series data, which, as we’ve noted, always includes a variable of time.
- Motive: the motive for the forecasting method is the same as that of prescriptive analysis: the confidently estimate future values.
Optimization Method
- Description: the optimization method maximized or minimizes values in a set given a set of criteria. It is arguably most common in prescriptive analysis. In mathematical terms, it is maximizing or minimizing a function given certain constraints.
- Importance: Very high. The idea of optimization applies to more analysis types than any other method. In fact, some argue that it is the fundamental driver behind data analysis. You would use it everywhere in research and in a corporation.
- Nature of Data: the nature of optimizable data is a data set of at least two points.
- Motive: the motive behind optimization is to achieve the best result possible given certain conditions.
Content Analysis Method
- Description: content analysis is a method of qualitative analysis that quantifies textual data to track themes across a document. It’s most common in academic fields and in social sciences, where written content is the subject of inquiry.
- Importance: High. In a corporate setting, content analysis as such is less common. If anything Nïave Bayes (a technique we’ll look at below) is the closest corporations come to text. However, it is of the utmost importance for researchers. If you’re a researcher, check out this article on content analysis .
- Nature of Data: data useful for content analysis is textual data.
- Motive: the motive behind content analysis is to understand themes expressed in a large text
Narrative Analysis Method
- Description: narrative analysis is a method of qualitative analysis that quantifies stories to trace themes in them. It’s differs from content analysis because it focuses on stories rather than research documents, and the techniques used are slightly different from those in content analysis (very nuances and outside the scope of this article).
- Importance: Low. Unless you are highly specialized in working with stories, narrative analysis rare.
- Nature of Data: the nature of the data useful for the narrative analysis method is narrative text.
- Motive: the motive for narrative analysis is to uncover hidden patterns in narrative text.
Discourse Analysis Method
- Description: the discourse analysis method falls under qualitative analysis and uses thematic coding to trace patterns in real-life discourse. That said, real-life discourse is oral, so it must first be transcribed into text.
- Importance: Low. Unless you are focused on understand real-world idea sharing in a research setting, this kind of analysis is less common than the others on this list.
- Nature of Data: the nature of data useful in discourse analysis is first audio files, then transcriptions of those audio files.
- Motive: the motive behind discourse analysis is to trace patterns of real-world discussions. (As a spooky sidenote, have you ever felt like your phone microphone was listening to you and making reading suggestions? If it was, the method was discourse analysis.)
Framework Analysis Method
- Description: the framework analysis method falls under qualitative analysis and uses similar thematic coding techniques to content analysis. However, where content analysis aims to discover themes, framework analysis starts with a framework and only considers elements that fall in its purview.
- Importance: Low. As with the other textual analysis methods, framework analysis is less common in corporate settings. Even in the world of research, only some use it. Strangely, it’s very common for legislative and political research.
- Nature of Data: the nature of data useful for framework analysis is textual.
- Motive: the motive behind framework analysis is to understand what themes and parts of a text match your search criteria.
Grounded Theory Method
- Description: the grounded theory method falls under qualitative analysis and uses thematic coding to build theories around those themes.
- Importance: Low. Like other qualitative analysis techniques, grounded theory is less common in the corporate world. Even among researchers, you would be hard pressed to find many using it. Though powerful, it’s simply too rare to spend time learning.
- Nature of Data: the nature of data useful in the grounded theory method is textual.
- Motive: the motive of grounded theory method is to establish a series of theories based on themes uncovered from a text.
Clustering Technique: K-Means
- Description: k-means is a clustering technique in which data points are grouped in clusters that have the closest means. Though not considered AI or ML, it inherently requires the use of supervised learning to reevaluate clusters as data points are added. Clustering techniques can be used in diagnostic, descriptive, & prescriptive data analyses.
- Importance: Very important. If you only take 3 things from this article, k-means clustering should be part of it. It is useful in any situation where n observations have multiple characteristics and we want to put them in groups.
- Nature of Data: the nature of data is at least one characteristic per observation, but the more the merrier.
- Motive: the motive for clustering techniques such as k-means is to group observations together and either understand or react to them.
Regression Technique
- Description: simple and multivariable regressions use either one independent variable or combination of multiple independent variables to calculate a correlation to a single dependent variable using constants. Regressions are almost synonymous with correlation today.
- Importance: Very high. Along with clustering, if you only take 3 things from this article, regression techniques should be part of it. They’re everywhere in corporate and research fields alike.
- Nature of Data: the nature of data used is regressions is data sets with “n” number of observations and as many variables as are reasonable. It’s important, however, to distinguish between time series data and regression data. You cannot use regressions or time series data without accounting for time. The easier way is to use techniques under the forecasting method.
- Motive: The motive behind regression techniques is to understand correlations between independent variable(s) and a dependent one.
Nïave Bayes Technique
- Description: Nïave Bayes is a classification technique that uses simple probability to classify items based previous classifications. In plain English, the formula would be “the chance that thing with trait x belongs to class c depends on (=) the overall chance of trait x belonging to class c, multiplied by the overall chance of class c, divided by the overall chance of getting trait x.” As a formula, it’s P(c|x) = P(x|c) * P(c) / P(x).
- Importance: High. Nïave Bayes is a very common, simplistic classification techniques because it’s effective with large data sets and it can be applied to any instant in which there is a class. Google, for example, might use it to group webpages into groups for certain search engine queries.
- Nature of Data: the nature of data for Nïave Bayes is at least one class and at least two traits in a data set.
- Motive: the motive behind Nïave Bayes is to classify observations based on previous data. It’s thus considered part of predictive analysis.
Cohorts Technique
- Description: cohorts technique is a type of clustering method used in behavioral sciences to separate users by common traits. As with clustering, it can be done intuitively or mathematically, the latter of which would simply be k-means.
- Importance: Very high. With regard to resembles k-means, the cohort technique is more of a high-level counterpart. In fact, most people are familiar with it as a part of Google Analytics. It’s most common in marketing departments in corporations, rather than in research.
- Nature of Data: the nature of cohort data is data sets in which users are the observation and other fields are used as defining traits for each cohort.
- Motive: the motive for cohort analysis techniques is to group similar users and analyze how you retain them and how the churn.
Factor Technique
- Description: the factor analysis technique is a way of grouping many traits into a single factor to expedite analysis. For example, factors can be used as traits for Nïave Bayes classifications instead of more general fields.
- Importance: High. While not commonly employed in corporations, factor analysis is hugely valuable. Good data analysts use it to simplify their projects and communicate them more clearly.
- Nature of Data: the nature of data useful in factor analysis techniques is data sets with a large number of fields on its observations.
- Motive: the motive for using factor analysis techniques is to reduce the number of fields in order to more quickly analyze and communicate findings.
Linear Discriminants Technique
- Description: linear discriminant analysis techniques are similar to regressions in that they use one or more independent variable to determine a dependent variable; however, the linear discriminant technique falls under a classifier method since it uses traits as independent variables and class as a dependent variable. In this way, it becomes a classifying method AND a predictive method.
- Importance: High. Though the analyst world speaks of and uses linear discriminants less commonly, it’s a highly valuable technique to keep in mind as you progress in data analysis.
- Nature of Data: the nature of data useful for the linear discriminant technique is data sets with many fields.
- Motive: the motive for using linear discriminants is to classify observations that would be otherwise too complex for simple techniques like Nïave Bayes.
Exponential Smoothing Technique
- Description: exponential smoothing is a technique falling under the forecasting method that uses a smoothing factor on prior data in order to predict future values. It can be linear or adjusted for seasonality. The basic principle behind exponential smoothing is to use a percent weight (value between 0 and 1 called alpha) on more recent values in a series and a smaller percent weight on less recent values. The formula is f(x) = current period value * alpha + previous period value * 1-alpha.
- Importance: High. Most analysts still use the moving average technique (covered next) for forecasting, though it is less efficient than exponential moving, because it’s easy to understand. However, good analysts will have exponential smoothing techniques in their pocket to increase the value of their forecasts.
- Nature of Data: the nature of data useful for exponential smoothing is time series data . Time series data has time as part of its fields .
- Motive: the motive for exponential smoothing is to forecast future values with a smoothing variable.
Moving Average Technique
- Description: the moving average technique falls under the forecasting method and uses an average of recent values to predict future ones. For example, to predict rainfall in April, you would take the average of rainfall from January to March. It’s simple, yet highly effective.
- Importance: Very high. While I’m personally not a huge fan of moving averages due to their simplistic nature and lack of consideration for seasonality, they’re the most common forecasting technique and therefore very important.
- Nature of Data: the nature of data useful for moving averages is time series data .
- Motive: the motive for moving averages is to predict future values is a simple, easy-to-communicate way.
Neural Networks Technique
- Description: neural networks are a highly complex artificial intelligence technique that replicate a human’s neural analysis through a series of hyper-rapid computations and comparisons that evolve in real time. This technique is so complex that an analyst must use computer programs to perform it.
- Importance: Medium. While the potential for neural networks is theoretically unlimited, it’s still little understood and therefore uncommon. You do not need to know it by any means in order to be a data analyst.
- Nature of Data: the nature of data useful for neural networks is data sets of astronomical size, meaning with 100s of 1000s of fields and the same number of row at a minimum .
- Motive: the motive for neural networks is to understand wildly complex phenomenon and data to thereafter act on it.
Decision Tree Technique
- Description: the decision tree technique uses artificial intelligence algorithms to rapidly calculate possible decision pathways and their outcomes on a real-time basis. It’s so complex that computer programs are needed to perform it.
- Importance: Medium. As with neural networks, decision trees with AI are too little understood and are therefore uncommon in corporate and research settings alike.
- Nature of Data: the nature of data useful for the decision tree technique is hierarchical data sets that show multiple optional fields for each preceding field.
- Motive: the motive for decision tree techniques is to compute the optimal choices to make in order to achieve a desired result.
Evolutionary Programming Technique
- Description: the evolutionary programming technique uses a series of neural networks, sees how well each one fits a desired outcome, and selects only the best to test and retest. It’s called evolutionary because is resembles the process of natural selection by weeding out weaker options.
- Importance: Medium. As with the other AI techniques, evolutionary programming just isn’t well-understood enough to be usable in many cases. It’s complexity also makes it hard to explain in corporate settings and difficult to defend in research settings.
- Nature of Data: the nature of data in evolutionary programming is data sets of neural networks, or data sets of data sets.
- Motive: the motive for using evolutionary programming is similar to decision trees: understanding the best possible option from complex data.
- Video example :
Fuzzy Logic Technique
- Description: fuzzy logic is a type of computing based on “approximate truths” rather than simple truths such as “true” and “false.” It is essentially two tiers of classification. For example, to say whether “Apples are good,” you need to first classify that “Good is x, y, z.” Only then can you say apples are good. Another way to see it helping a computer see truth like humans do: “definitely true, probably true, maybe true, probably false, definitely false.”
- Importance: Medium. Like the other AI techniques, fuzzy logic is uncommon in both research and corporate settings, which means it’s less important in today’s world.
- Nature of Data: the nature of fuzzy logic data is huge data tables that include other huge data tables with a hierarchy including multiple subfields for each preceding field.
- Motive: the motive of fuzzy logic to replicate human truth valuations in a computer is to model human decisions based on past data. The obvious possible application is marketing.
Text Analysis Technique
- Description: text analysis techniques fall under the qualitative data analysis type and use text to extract insights.
- Importance: Medium. Text analysis techniques, like all the qualitative analysis type, are most valuable for researchers.
- Nature of Data: the nature of data useful in text analysis is words.
- Motive: the motive for text analysis is to trace themes in a text across sets of very long documents, such as books.
Coding Technique
- Description: the coding technique is used in textual analysis to turn ideas into uniform phrases and analyze the number of times and the ways in which those ideas appear. For this reason, some consider it a quantitative technique as well. You can learn more about coding and the other qualitative techniques here .
- Importance: Very high. If you’re a researcher working in social sciences, coding is THE analysis techniques, and for good reason. It’s a great way to add rigor to analysis. That said, it’s less common in corporate settings.
- Nature of Data: the nature of data useful for coding is long text documents.
- Motive: the motive for coding is to make tracing ideas on paper more than an exercise of the mind by quantifying it and understanding is through descriptive methods.
Idea Pattern Technique
- Description: the idea pattern analysis technique fits into coding as the second step of the process. Once themes and ideas are coded, simple descriptive analysis tests may be run. Some people even cluster the ideas!
- Importance: Very high. If you’re a researcher, idea pattern analysis is as important as the coding itself.
- Nature of Data: the nature of data useful for idea pattern analysis is already coded themes.
- Motive: the motive for the idea pattern technique is to trace ideas in otherwise unmanageably-large documents.
Word Frequency Technique
- Description: word frequency is a qualitative technique that stands in opposition to coding and uses an inductive approach to locate specific words in a document in order to understand its relevance. Word frequency is essentially the descriptive analysis of qualitative data because it uses stats like mean, median, and mode to gather insights.
- Importance: High. As with the other qualitative approaches, word frequency is very important in social science research, but less so in corporate settings.
- Nature of Data: the nature of data useful for word frequency is long, informative documents.
- Motive: the motive for word frequency is to locate target words to determine the relevance of a document in question.
Types of data analysis in research
Types of data analysis in research methodology include every item discussed in this article. As a list, they are:
- Quantitative
- Qualitative
- Mathematical
- Machine Learning and AI
- Descriptive
- Prescriptive
- Classification
- Forecasting
- Optimization
- Grounded theory
- Artificial Neural Networks
- Decision Trees
- Evolutionary Programming
- Fuzzy Logic
- Text analysis
- Idea Pattern Analysis
- Word Frequency Analysis
- Nïave Bayes
- Exponential smoothing
- Moving average
- Linear discriminant
Types of data analysis in qualitative research
As a list, the types of data analysis in qualitative research are the following methods:
Types of data analysis in quantitative research
As a list, the types of data analysis in quantitative research are:
Data analysis methods
As a list, data analysis methods are:
- Content (qualitative)
- Narrative (qualitative)
- Discourse (qualitative)
- Framework (qualitative)
- Grounded theory (qualitative)
Quantitative data analysis methods
As a list, quantitative data analysis methods are:
Tabular View of Data Analysis Types, Methods, and Techniques
About the author.
Noah is the founder & Editor-in-Chief at AnalystAnswers. He is a transatlantic professional and entrepreneur with 5+ years of corporate finance and data analytics experience, as well as 3+ years in consumer financial products and business software. He started AnalystAnswers to provide aspiring professionals with accessible explanations of otherwise dense finance and data concepts. Noah believes everyone can benefit from an analytical mindset in growing digital world. When he's not busy at work, Noah likes to explore new European cities, exercise, and spend time with friends and family.
File available immediately.
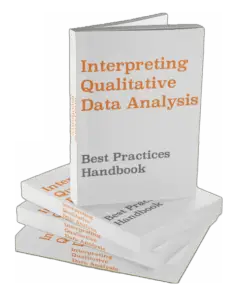
Notice: JavaScript is required for this content.
- University Libraries
- Research Guides
- Topic Guides
- Research Methods Guide
- Data Analysis
Research Methods Guide: Data Analysis
- Introduction
- Research Design & Method
- Survey Research
- Interview Research
- Resources & Consultation
Tools for Analyzing Survey Data
- R (open source)
- Stata
- DataCracker (free up to 100 responses per survey)
- SurveyMonkey (free up to 100 responses per survey)
Tools for Analyzing Interview Data
- AQUAD (open source)
- NVivo
Data Analysis and Presentation Techniques that Apply to both Survey and Interview Research
- Create a documentation of the data and the process of data collection.
- Analyze the data rather than just describing it - use it to tell a story that focuses on answering the research question.
- Use charts or tables to help the reader understand the data and then highlight the most interesting findings.
- Don’t get bogged down in the detail - tell the reader about the main themes as they relate to the research question, rather than reporting everything that survey respondents or interviewees said.
- State that ‘most people said …’ or ‘few people felt …’ rather than giving the number of people who said a particular thing.
- Use brief quotes where these illustrate a particular point really well.
- Respect confidentiality - you could attribute a quote to 'a faculty member', ‘a student’, or 'a customer' rather than ‘Dr. Nicholls.'
Survey Data Analysis
- If you used an online survey, the software will automatically collate the data – you will just need to download the data, for example as a spreadsheet.
- If you used a paper questionnaire, you will need to manually transfer the responses from the questionnaires into a spreadsheet. Put each question number as a column heading, and use one row for each person’s answers. Then assign each possible answer a number or ‘code’.
- When all the data is present and correct, calculate how many people selected each response.
- Once you have calculated how many people selected each response, you can set up tables and/or graph to display the data. This could take the form of a table or chart.
- In addition to descriptive statistics that characterize findings from your survey, you can use statistical and analytical reporting techniques if needed.
Interview Data Analysis
- Data Reduction and Organization: Try not to feel overwhelmed by quantity of information that has been collected from interviews- a one-hour interview can generate 20 to 25 pages of single-spaced text. Once you start organizing your fieldwork notes around themes, you can easily identify which part of your data to be used for further analysis.
- What were the main issues or themes that struck you in this contact / interviewee?"
- Was there anything else that struck you as salient, interesting, illuminating or important in this contact / interviewee?
- What information did you get (or failed to get) on each of the target questions you had for this contact / interviewee?
- Connection of the data: You can connect data around themes and concepts - then you can show how one concept may influence another.
- Examination of Relationships: Examining relationships is the centerpiece of the analytic process, because it allows you to move from simple description of the people and settings to explanations of why things happened as they did with those people in that setting.
- << Previous: Interview Research
- Next: Resources & Consultation >>
- Last Updated: Aug 21, 2023 10:42 AM

What Is Research Methodology? A Plain-Language Explanation & Definition (With Examples)
By Derek Jansen (MBA) and Kerryn Warren (PhD) | June 2020 (Last updated April 2023)
If you’re new to formal academic research, it’s quite likely that you’re feeling a little overwhelmed by all the technical lingo that gets thrown around. And who could blame you – “research methodology”, “research methods”, “sampling strategies”… it all seems never-ending!
In this post, we’ll demystify the landscape with plain-language explanations and loads of examples (including easy-to-follow videos), so that you can approach your dissertation, thesis or research project with confidence. Let’s get started.
Research Methodology 101
- What exactly research methodology means
- What qualitative , quantitative and mixed methods are
- What sampling strategy is
- What data collection methods are
- What data analysis methods are
- How to choose your research methodology
- Example of a research methodology
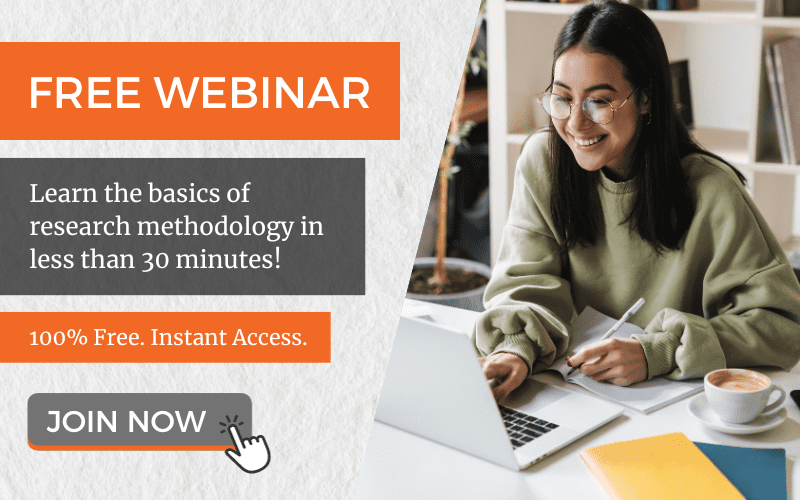
What is research methodology?
Research methodology simply refers to the practical “how” of a research study. More specifically, it’s about how a researcher systematically designs a study to ensure valid and reliable results that address the research aims, objectives and research questions . Specifically, how the researcher went about deciding:
- What type of data to collect (e.g., qualitative or quantitative data )
- Who to collect it from (i.e., the sampling strategy )
- How to collect it (i.e., the data collection method )
- How to analyse it (i.e., the data analysis methods )
Within any formal piece of academic research (be it a dissertation, thesis or journal article), you’ll find a research methodology chapter or section which covers the aspects mentioned above. Importantly, a good methodology chapter explains not just what methodological choices were made, but also explains why they were made. In other words, the methodology chapter should justify the design choices, by showing that the chosen methods and techniques are the best fit for the research aims, objectives and research questions.
So, it’s the same as research design?
Not quite. As we mentioned, research methodology refers to the collection of practical decisions regarding what data you’ll collect, from who, how you’ll collect it and how you’ll analyse it. Research design, on the other hand, is more about the overall strategy you’ll adopt in your study. For example, whether you’ll use an experimental design in which you manipulate one variable while controlling others. You can learn more about research design and the various design types here .
Need a helping hand?
What are qualitative, quantitative and mixed-methods?
Qualitative, quantitative and mixed-methods are different types of methodological approaches, distinguished by their focus on words , numbers or both . This is a bit of an oversimplification, but its a good starting point for understanding.
Let’s take a closer look.
Qualitative research refers to research which focuses on collecting and analysing words (written or spoken) and textual or visual data, whereas quantitative research focuses on measurement and testing using numerical data . Qualitative analysis can also focus on other “softer” data points, such as body language or visual elements.
It’s quite common for a qualitative methodology to be used when the research aims and research questions are exploratory in nature. For example, a qualitative methodology might be used to understand peoples’ perceptions about an event that took place, or a political candidate running for president.
Contrasted to this, a quantitative methodology is typically used when the research aims and research questions are confirmatory in nature. For example, a quantitative methodology might be used to measure the relationship between two variables (e.g. personality type and likelihood to commit a crime) or to test a set of hypotheses .
As you’ve probably guessed, the mixed-method methodology attempts to combine the best of both qualitative and quantitative methodologies to integrate perspectives and create a rich picture. If you’d like to learn more about these three methodological approaches, be sure to watch our explainer video below.
What is sampling strategy?
Simply put, sampling is about deciding who (or where) you’re going to collect your data from . Why does this matter? Well, generally it’s not possible to collect data from every single person in your group of interest (this is called the “population”), so you’ll need to engage a smaller portion of that group that’s accessible and manageable (this is called the “sample”).
How you go about selecting the sample (i.e., your sampling strategy) will have a major impact on your study. There are many different sampling methods you can choose from, but the two overarching categories are probability sampling and non-probability sampling .
Probability sampling involves using a completely random sample from the group of people you’re interested in. This is comparable to throwing the names all potential participants into a hat, shaking it up, and picking out the “winners”. By using a completely random sample, you’ll minimise the risk of selection bias and the results of your study will be more generalisable to the entire population.
Non-probability sampling , on the other hand, doesn’t use a random sample . For example, it might involve using a convenience sample, which means you’d only interview or survey people that you have access to (perhaps your friends, family or work colleagues), rather than a truly random sample. With non-probability sampling, the results are typically not generalisable .
To learn more about sampling methods, be sure to check out the video below.
What are data collection methods?
As the name suggests, data collection methods simply refers to the way in which you go about collecting the data for your study. Some of the most common data collection methods include:
- Interviews (which can be unstructured, semi-structured or structured)
- Focus groups and group interviews
- Surveys (online or physical surveys)
- Observations (watching and recording activities)
- Biophysical measurements (e.g., blood pressure, heart rate, etc.)
- Documents and records (e.g., financial reports, court records, etc.)
The choice of which data collection method to use depends on your overall research aims and research questions , as well as practicalities and resource constraints. For example, if your research is exploratory in nature, qualitative methods such as interviews and focus groups would likely be a good fit. Conversely, if your research aims to measure specific variables or test hypotheses, large-scale surveys that produce large volumes of numerical data would likely be a better fit.
What are data analysis methods?
Data analysis methods refer to the methods and techniques that you’ll use to make sense of your data. These can be grouped according to whether the research is qualitative (words-based) or quantitative (numbers-based).
Popular data analysis methods in qualitative research include:
- Qualitative content analysis
- Thematic analysis
- Discourse analysis
- Narrative analysis
- Interpretative phenomenological analysis (IPA)
- Visual analysis (of photographs, videos, art, etc.)
Qualitative data analysis all begins with data coding , after which an analysis method is applied. In some cases, more than one analysis method is used, depending on the research aims and research questions . In the video below, we explore some common qualitative analysis methods, along with practical examples.
Moving on to the quantitative side of things, popular data analysis methods in this type of research include:
- Descriptive statistics (e.g. means, medians, modes )
- Inferential statistics (e.g. correlation, regression, structural equation modelling)
Again, the choice of which data collection method to use depends on your overall research aims and objectives , as well as practicalities and resource constraints. In the video below, we explain some core concepts central to quantitative analysis.
How do I choose a research methodology?
As you’ve probably picked up by now, your research aims and objectives have a major influence on the research methodology . So, the starting point for developing your research methodology is to take a step back and look at the big picture of your research, before you make methodology decisions. The first question you need to ask yourself is whether your research is exploratory or confirmatory in nature.
If your research aims and objectives are primarily exploratory in nature, your research will likely be qualitative and therefore you might consider qualitative data collection methods (e.g. interviews) and analysis methods (e.g. qualitative content analysis).
Conversely, if your research aims and objective are looking to measure or test something (i.e. they’re confirmatory), then your research will quite likely be quantitative in nature, and you might consider quantitative data collection methods (e.g. surveys) and analyses (e.g. statistical analysis).
Designing your research and working out your methodology is a large topic, which we cover extensively on the blog . For now, however, the key takeaway is that you should always start with your research aims, objectives and research questions (the golden thread). Every methodological choice you make needs align with those three components.
Example of a research methodology chapter
In the video below, we provide a detailed walkthrough of a research methodology from an actual dissertation, as well as an overview of our free methodology template .
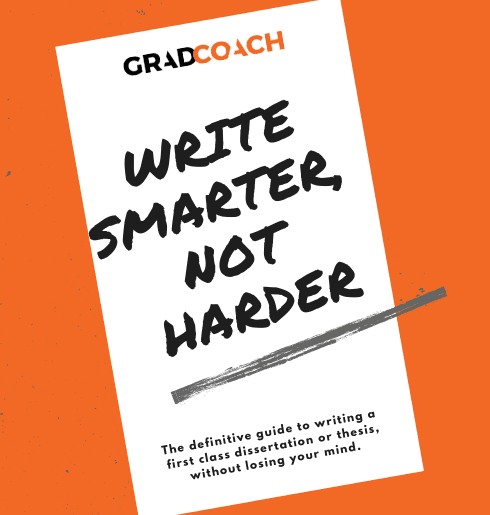
Psst… there’s more (for free)
This post is part of our dissertation mini-course, which covers everything you need to get started with your dissertation, thesis or research project.
You Might Also Like:
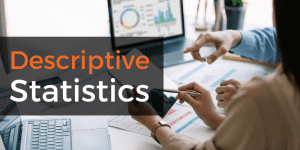
198 Comments
Thank you for this simple yet comprehensive and easy to digest presentation. God Bless!
You’re most welcome, Leo. Best of luck with your research!
I found it very useful. many thanks
This is really directional. A make-easy research knowledge.
Thank you for this, I think will help my research proposal
Thanks for good interpretation,well understood.
Good morning sorry I want to the search topic
Thank u more
Thank you, your explanation is simple and very helpful.
Very educative a.nd exciting platform. A bigger thank you and I’ll like to always be with you
That’s the best analysis
So simple yet so insightful. Thank you.
This really easy to read as it is self-explanatory. Very much appreciated…
Thanks for this. It’s so helpful and explicit. For those elements highlighted in orange, they were good sources of referrals for concepts I didn’t understand. A million thanks for this.
Good morning, I have been reading your research lessons through out a period of times. They are important, impressive and clear. Want to subscribe and be and be active with you.
Thankyou So much Sir Derek…
Good morning thanks so much for the on line lectures am a student of university of Makeni.select a research topic and deliberate on it so that we’ll continue to understand more.sorry that’s a suggestion.
Beautiful presentation. I love it.
please provide a research mehodology example for zoology
It’s very educative and well explained
Thanks for the concise and informative data.
This is really good for students to be safe and well understand that research is all about
Thank you so much Derek sir🖤🙏🤗
Very simple and reliable
This is really helpful. Thanks alot. God bless you.
very useful, Thank you very much..
thanks a lot its really useful
in a nutshell..thank you!
Thanks for updating my understanding on this aspect of my Thesis writing.
thank you so much my through this video am competently going to do a good job my thesis
Very simple but yet insightful Thank you
This has been an eye opening experience. Thank you grad coach team.
Very useful message for research scholars
Really very helpful thank you
yes you are right and i’m left
Research methodology with a simplest way i have never seen before this article.
wow thank u so much
Good morning thanks so much for the on line lectures am a student of university of Makeni.select a research topic and deliberate on is so that we will continue to understand more.sorry that’s a suggestion.
Very precise and informative.
Thanks for simplifying these terms for us, really appreciate it.
Thanks this has really helped me. It is very easy to understand.
I found the notes and the presentation assisting and opening my understanding on research methodology
Good presentation
Im so glad you clarified my misconceptions. Im now ready to fry my onions. Thank you so much. God bless
Thank you a lot.
thanks for the easy way of learning and desirable presentation.
Thanks a lot. I am inspired
Well written
I am writing a APA Format paper . I using questionnaire with 120 STDs teacher for my participant. Can you write me mthology for this research. Send it through email sent. Just need a sample as an example please. My topic is ” impacts of overcrowding on students learning
Thanks for your comment.
We can’t write your methodology for you. If you’re looking for samples, you should be able to find some sample methodologies on Google. Alternatively, you can download some previous dissertations from a dissertation directory and have a look at the methodology chapters therein.
All the best with your research.
Thank you so much for this!! God Bless
Thank you. Explicit explanation
Thank you, Derek and Kerryn, for making this simple to understand. I’m currently at the inception stage of my research.
Thnks a lot , this was very usefull on my assignment
excellent explanation
I’m currently working on my master’s thesis, thanks for this! I’m certain that I will use Qualitative methodology.
Thanks a lot for this concise piece, it was quite relieving and helpful. God bless you BIG…
I am currently doing my dissertation proposal and I am sure that I will do quantitative research. Thank you very much it was extremely helpful.
Very interesting and informative yet I would like to know about examples of Research Questions as well, if possible.
I’m about to submit a research presentation, I have come to understand from your simplification on understanding research methodology. My research will be mixed methodology, qualitative as well as quantitative. So aim and objective of mixed method would be both exploratory and confirmatory. Thanks you very much for your guidance.
OMG thanks for that, you’re a life saver. You covered all the points I needed. Thank you so much ❤️ ❤️ ❤️
Thank you immensely for this simple, easy to comprehend explanation of data collection methods. I have been stuck here for months 😩. Glad I found your piece. Super insightful.
I’m going to write synopsis which will be quantitative research method and I don’t know how to frame my topic, can I kindly get some ideas..
Thanks for this, I was really struggling.
This was really informative I was struggling but this helped me.
Thanks a lot for this information, simple and straightforward. I’m a last year student from the University of South Africa UNISA South Africa.
its very much informative and understandable. I have enlightened.
An interesting nice exploration of a topic.
Thank you. Accurate and simple🥰
This article was really helpful, it helped me understanding the basic concepts of the topic Research Methodology. The examples were very clear, and easy to understand. I would like to visit this website again. Thank you so much for such a great explanation of the subject.
Thanks dude
Thank you Doctor Derek for this wonderful piece, please help to provide your details for reference purpose. God bless.
Many compliments to you
Great work , thank you very much for the simple explanation
Thank you. I had to give a presentation on this topic. I have looked everywhere on the internet but this is the best and simple explanation.
thank you, its very informative.
Well explained. Now I know my research methodology will be qualitative and exploratory. Thank you so much, keep up the good work
Well explained, thank you very much.
This is good explanation, I have understood the different methods of research. Thanks a lot.
Great work…very well explanation
Thanks Derek. Kerryn was just fantastic!
Great to hear that, Hyacinth. Best of luck with your research!
Its a good templates very attractive and important to PhD students and lectuter
Thanks for the feedback, Matobela. Good luck with your research methodology.
Thank you. This is really helpful.
You’re very welcome, Elie. Good luck with your research methodology.
Well explained thanks
This is a very helpful site especially for young researchers at college. It provides sufficient information to guide students and equip them with the necessary foundation to ask any other questions aimed at deepening their understanding.
Thanks for the kind words, Edward. Good luck with your research!
Thank you. I have learned a lot.
Great to hear that, Ngwisa. Good luck with your research methodology!
Thank you for keeping your presentation simples and short and covering key information for research methodology. My key takeaway: Start with defining your research objective the other will depend on the aims of your research question.
My name is Zanele I would like to be assisted with my research , and the topic is shortage of nursing staff globally want are the causes , effects on health, patients and community and also globally
Thanks for making it simple and clear. It greatly helped in understanding research methodology. Regards.
This is well simplified and straight to the point
Thank you Dr
I was given an assignment to research 2 publications and describe their research methodology? I don’t know how to start this task can someone help me?
Sure. You’re welcome to book an initial consultation with one of our Research Coaches to discuss how we can assist – https://gradcoach.com/book/new/ .
Thanks a lot I am relieved of a heavy burden.keep up with the good work
I’m very much grateful Dr Derek. I’m planning to pursue one of the careers that really needs one to be very much eager to know. There’s a lot of research to do and everything, but since I’ve gotten this information I will use it to the best of my potential.
Thank you so much, words are not enough to explain how helpful this session has been for me!
Thanks this has thought me alot.
Very concise and helpful. Thanks a lot
Thank Derek. This is very helpful. Your step by step explanation has made it easier for me to understand different concepts. Now i can get on with my research.
I wish i had come across this sooner. So simple but yet insightful
really nice explanation thank you so much
I’m so grateful finding this site, it’s really helpful…….every term well explained and provide accurate understanding especially to student going into an in-depth research for the very first time, even though my lecturer already explained this topic to the class, I think I got the clear and efficient explanation here, much thanks to the author.
It is very helpful material
I would like to be assisted with my research topic : Literature Review and research methodologies. My topic is : what is the relationship between unemployment and economic growth?
Its really nice and good for us.
THANKS SO MUCH FOR EXPLANATION, ITS VERY CLEAR TO ME WHAT I WILL BE DOING FROM NOW .GREAT READS.
Short but sweet.Thank you
Informative article. Thanks for your detailed information.
I’m currently working on my Ph.D. thesis. Thanks a lot, Derek and Kerryn, Well-organized sequences, facilitate the readers’ following.
great article for someone who does not have any background can even understand
I am a bit confused about research design and methodology. Are they the same? If not, what are the differences and how are they related?
Thanks in advance.
concise and informative.
Thank you very much
How can we site this article is Harvard style?
Very well written piece that afforded better understanding of the concept. Thank you!
Am a new researcher trying to learn how best to write a research proposal. I find your article spot on and want to download the free template but finding difficulties. Can u kindly send it to my email, the free download entitled, “Free Download: Research Proposal Template (with Examples)”.
Thank too much
Thank you very much for your comprehensive explanation about research methodology so I like to thank you again for giving us such great things.
Good very well explained.Thanks for sharing it.
Thank u sir, it is really a good guideline.
so helpful thank you very much.
Thanks for the video it was very explanatory and detailed, easy to comprehend and follow up. please, keep it up the good work
It was very helpful, a well-written document with precise information.
how do i reference this?
MLA Jansen, Derek, and Kerryn Warren. “What (Exactly) Is Research Methodology?” Grad Coach, June 2021, gradcoach.com/what-is-research-methodology/.
APA Jansen, D., & Warren, K. (2021, June). What (Exactly) Is Research Methodology? Grad Coach. https://gradcoach.com/what-is-research-methodology/
Your explanation is easily understood. Thank you
Very help article. Now I can go my methodology chapter in my thesis with ease
I feel guided ,Thank you
This simplification is very helpful. It is simple but very educative, thanks ever so much
The write up is informative and educative. It is an academic intellectual representation that every good researcher can find useful. Thanks
Wow, this is wonderful long live.
Nice initiative
thank you the video was helpful to me.
Thank you very much for your simple and clear explanations I’m really satisfied by the way you did it By now, I think I can realize a very good article by following your fastidious indications May God bless you
Thanks very much, it was very concise and informational for a beginner like me to gain an insight into what i am about to undertake. I really appreciate.
very informative sir, it is amazing to understand the meaning of question hidden behind that, and simple language is used other than legislature to understand easily. stay happy.
This one is really amazing. All content in your youtube channel is a very helpful guide for doing research. Thanks, GradCoach.
research methodologies
Please send me more information concerning dissertation research.
Nice piece of knowledge shared….. #Thump_UP
This is amazing, it has said it all. Thanks to Gradcoach
This is wonderful,very elaborate and clear.I hope to reach out for your assistance in my research very soon.
This is the answer I am searching about…
realy thanks a lot
Thank you very much for this awesome, to the point and inclusive article.
Thank you very much I need validity and reliability explanation I have exams
Thank you for a well explained piece. This will help me going forward.
Very simple and well detailed Many thanks
This is so very simple yet so very effective and comprehensive. An Excellent piece of work.
I wish I saw this earlier on! Great insights for a beginner(researcher) like me. Thanks a mil!
Thank you very much, for such a simplified, clear and practical step by step both for academic students and general research work. Holistic, effective to use and easy to read step by step. One can easily apply the steps in practical terms and produce a quality document/up-to standard
Thanks for simplifying these terms for us, really appreciated.
Thanks for a great work. well understood .
This was very helpful. It was simple but profound and very easy to understand. Thank you so much!
Great and amazing research guidelines. Best site for learning research
hello sir/ma’am, i didn’t find yet that what type of research methodology i am using. because i am writing my report on CSR and collect all my data from websites and articles so which type of methodology i should write in dissertation report. please help me. i am from India.
how does this really work?
perfect content, thanks a lot
As a researcher, I commend you for the detailed and simplified information on the topic in question. I would like to remain in touch for the sharing of research ideas on other topics. Thank you
Impressive. Thank you, Grad Coach 😍
Thank you Grad Coach for this piece of information. I have at least learned about the different types of research methodologies.
Very useful content with easy way
Thank you very much for the presentation. I am an MPH student with the Adventist University of Africa. I have successfully completed my theory and starting on my research this July. My topic is “Factors associated with Dental Caries in (one District) in Botswana. I need help on how to go about this quantitative research
I am so grateful to run across something that was sooo helpful. I have been on my doctorate journey for quite some time. Your breakdown on methodology helped me to refresh my intent. Thank you.
thanks so much for this good lecture. student from university of science and technology, Wudil. Kano Nigeria.
It’s profound easy to understand I appreciate
Thanks a lot for sharing superb information in a detailed but concise manner. It was really helpful and helped a lot in getting into my own research methodology.
Comment * thanks very much
This was sooo helpful for me thank you so much i didn’t even know what i had to write thank you!
You’re most welcome 🙂
Simple and good. Very much helpful. Thank you so much.
This is very good work. I have benefited.
Thank you so much for sharing
This is powerful thank you so much guys
I am nkasa lizwi doing my research proposal on honors with the university of Walter Sisulu Komani I m on part 3 now can you assist me.my topic is: transitional challenges faced by educators in intermediate phase in the Alfred Nzo District.
Appreciate the presentation. Very useful step-by-step guidelines to follow.
I appreciate sir
wow! This is super insightful for me. Thank you!
Indeed this material is very helpful! Kudos writers/authors.
I want to say thank you very much, I got a lot of info and knowledge. Be blessed.
I want present a seminar paper on Optimisation of Deep learning-based models on vulnerability detection in digital transactions.
Need assistance
Dear Sir, I want to be assisted on my research on Sanitation and Water management in emergencies areas.
I am deeply grateful for the knowledge gained. I will be getting in touch shortly as I want to be assisted in my ongoing research.
The information shared is informative, crisp and clear. Kudos Team! And thanks a lot!
hello i want to study
Hello!! Grad coach teams. I am extremely happy in your tutorial or consultation. i am really benefited all material and briefing. Thank you very much for your generous helps. Please keep it up. If you add in your briefing, references for further reading, it will be very nice.
All I have to say is, thank u gyz.
Good, l thanks
thank you, it is very useful
Trackbacks/Pingbacks
- What Is A Literature Review (In A Dissertation Or Thesis) - Grad Coach - […] the literature review is to inform the choice of methodology for your own research. As we’ve discussed on the Grad Coach blog,…
- Free Download: Research Proposal Template (With Examples) - Grad Coach - […] Research design (methodology) […]
- Dissertation vs Thesis: What's the difference? - Grad Coach - […] and thesis writing on a daily basis – everything from how to find a good research topic to which…
Submit a Comment Cancel reply
Your email address will not be published. Required fields are marked *
Save my name, email, and website in this browser for the next time I comment.
- Print Friendly
Have a language expert improve your writing
Run a free plagiarism check in 10 minutes, generate accurate citations for free.
- Knowledge Base
Methodology
- What Is a Research Design | Types, Guide & Examples
What Is a Research Design | Types, Guide & Examples
Published on June 7, 2021 by Shona McCombes . Revised on November 20, 2023 by Pritha Bhandari.
A research design is a strategy for answering your research question using empirical data. Creating a research design means making decisions about:
- Your overall research objectives and approach
- Whether you’ll rely on primary research or secondary research
- Your sampling methods or criteria for selecting subjects
- Your data collection methods
- The procedures you’ll follow to collect data
- Your data analysis methods
A well-planned research design helps ensure that your methods match your research objectives and that you use the right kind of analysis for your data.
Table of contents
Step 1: consider your aims and approach, step 2: choose a type of research design, step 3: identify your population and sampling method, step 4: choose your data collection methods, step 5: plan your data collection procedures, step 6: decide on your data analysis strategies, other interesting articles, frequently asked questions about research design.
- Introduction
Before you can start designing your research, you should already have a clear idea of the research question you want to investigate.
There are many different ways you could go about answering this question. Your research design choices should be driven by your aims and priorities—start by thinking carefully about what you want to achieve.
The first choice you need to make is whether you’ll take a qualitative or quantitative approach.
Qualitative research designs tend to be more flexible and inductive , allowing you to adjust your approach based on what you find throughout the research process.
Quantitative research designs tend to be more fixed and deductive , with variables and hypotheses clearly defined in advance of data collection.
It’s also possible to use a mixed-methods design that integrates aspects of both approaches. By combining qualitative and quantitative insights, you can gain a more complete picture of the problem you’re studying and strengthen the credibility of your conclusions.
Practical and ethical considerations when designing research
As well as scientific considerations, you need to think practically when designing your research. If your research involves people or animals, you also need to consider research ethics .
- How much time do you have to collect data and write up the research?
- Will you be able to gain access to the data you need (e.g., by travelling to a specific location or contacting specific people)?
- Do you have the necessary research skills (e.g., statistical analysis or interview techniques)?
- Will you need ethical approval ?
At each stage of the research design process, make sure that your choices are practically feasible.
Receive feedback on language, structure, and formatting
Professional editors proofread and edit your paper by focusing on:
- Academic style
- Vague sentences
- Style consistency
See an example
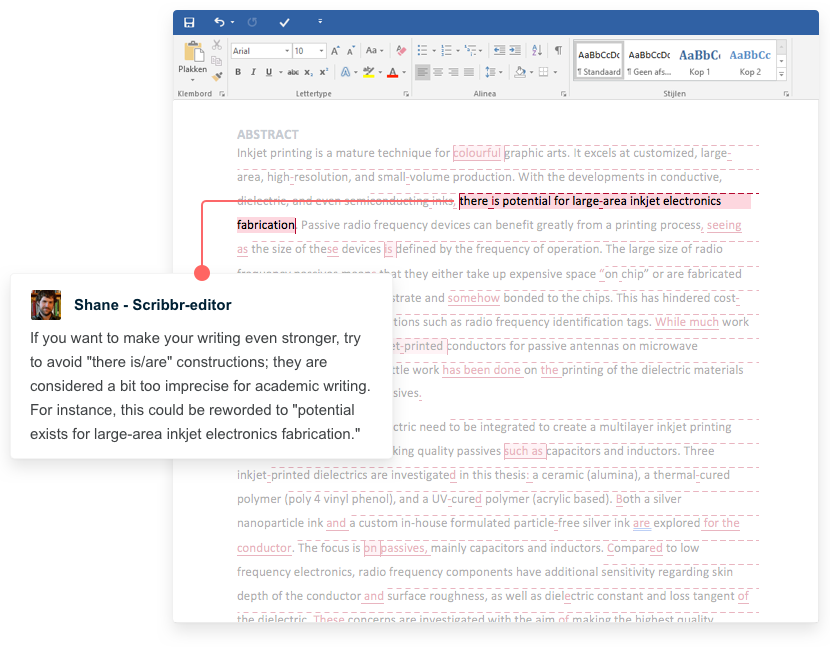
Within both qualitative and quantitative approaches, there are several types of research design to choose from. Each type provides a framework for the overall shape of your research.
Types of quantitative research designs
Quantitative designs can be split into four main types.
- Experimental and quasi-experimental designs allow you to test cause-and-effect relationships
- Descriptive and correlational designs allow you to measure variables and describe relationships between them.
With descriptive and correlational designs, you can get a clear picture of characteristics, trends and relationships as they exist in the real world. However, you can’t draw conclusions about cause and effect (because correlation doesn’t imply causation ).
Experiments are the strongest way to test cause-and-effect relationships without the risk of other variables influencing the results. However, their controlled conditions may not always reflect how things work in the real world. They’re often also more difficult and expensive to implement.
Types of qualitative research designs
Qualitative designs are less strictly defined. This approach is about gaining a rich, detailed understanding of a specific context or phenomenon, and you can often be more creative and flexible in designing your research.
The table below shows some common types of qualitative design. They often have similar approaches in terms of data collection, but focus on different aspects when analyzing the data.
Your research design should clearly define who or what your research will focus on, and how you’ll go about choosing your participants or subjects.
In research, a population is the entire group that you want to draw conclusions about, while a sample is the smaller group of individuals you’ll actually collect data from.
Defining the population
A population can be made up of anything you want to study—plants, animals, organizations, texts, countries, etc. In the social sciences, it most often refers to a group of people.
For example, will you focus on people from a specific demographic, region or background? Are you interested in people with a certain job or medical condition, or users of a particular product?
The more precisely you define your population, the easier it will be to gather a representative sample.
- Sampling methods
Even with a narrowly defined population, it’s rarely possible to collect data from every individual. Instead, you’ll collect data from a sample.
To select a sample, there are two main approaches: probability sampling and non-probability sampling . The sampling method you use affects how confidently you can generalize your results to the population as a whole.
Probability sampling is the most statistically valid option, but it’s often difficult to achieve unless you’re dealing with a very small and accessible population.
For practical reasons, many studies use non-probability sampling, but it’s important to be aware of the limitations and carefully consider potential biases. You should always make an effort to gather a sample that’s as representative as possible of the population.
Case selection in qualitative research
In some types of qualitative designs, sampling may not be relevant.
For example, in an ethnography or a case study , your aim is to deeply understand a specific context, not to generalize to a population. Instead of sampling, you may simply aim to collect as much data as possible about the context you are studying.
In these types of design, you still have to carefully consider your choice of case or community. You should have a clear rationale for why this particular case is suitable for answering your research question .
For example, you might choose a case study that reveals an unusual or neglected aspect of your research problem, or you might choose several very similar or very different cases in order to compare them.
Data collection methods are ways of directly measuring variables and gathering information. They allow you to gain first-hand knowledge and original insights into your research problem.
You can choose just one data collection method, or use several methods in the same study.
Survey methods
Surveys allow you to collect data about opinions, behaviors, experiences, and characteristics by asking people directly. There are two main survey methods to choose from: questionnaires and interviews .
Observation methods
Observational studies allow you to collect data unobtrusively, observing characteristics, behaviors or social interactions without relying on self-reporting.
Observations may be conducted in real time, taking notes as you observe, or you might make audiovisual recordings for later analysis. They can be qualitative or quantitative.
Other methods of data collection
There are many other ways you might collect data depending on your field and topic.
If you’re not sure which methods will work best for your research design, try reading some papers in your field to see what kinds of data collection methods they used.
Secondary data
If you don’t have the time or resources to collect data from the population you’re interested in, you can also choose to use secondary data that other researchers already collected—for example, datasets from government surveys or previous studies on your topic.
With this raw data, you can do your own analysis to answer new research questions that weren’t addressed by the original study.
Using secondary data can expand the scope of your research, as you may be able to access much larger and more varied samples than you could collect yourself.
However, it also means you don’t have any control over which variables to measure or how to measure them, so the conclusions you can draw may be limited.
Here's why students love Scribbr's proofreading services
Discover proofreading & editing
As well as deciding on your methods, you need to plan exactly how you’ll use these methods to collect data that’s consistent, accurate, and unbiased.
Planning systematic procedures is especially important in quantitative research, where you need to precisely define your variables and ensure your measurements are high in reliability and validity.
Operationalization
Some variables, like height or age, are easily measured. But often you’ll be dealing with more abstract concepts, like satisfaction, anxiety, or competence. Operationalization means turning these fuzzy ideas into measurable indicators.
If you’re using observations , which events or actions will you count?
If you’re using surveys , which questions will you ask and what range of responses will be offered?
You may also choose to use or adapt existing materials designed to measure the concept you’re interested in—for example, questionnaires or inventories whose reliability and validity has already been established.
Reliability and validity
Reliability means your results can be consistently reproduced, while validity means that you’re actually measuring the concept you’re interested in.
For valid and reliable results, your measurement materials should be thoroughly researched and carefully designed. Plan your procedures to make sure you carry out the same steps in the same way for each participant.
If you’re developing a new questionnaire or other instrument to measure a specific concept, running a pilot study allows you to check its validity and reliability in advance.
Sampling procedures
As well as choosing an appropriate sampling method , you need a concrete plan for how you’ll actually contact and recruit your selected sample.
That means making decisions about things like:
- How many participants do you need for an adequate sample size?
- What inclusion and exclusion criteria will you use to identify eligible participants?
- How will you contact your sample—by mail, online, by phone, or in person?
If you’re using a probability sampling method , it’s important that everyone who is randomly selected actually participates in the study. How will you ensure a high response rate?
If you’re using a non-probability method , how will you avoid research bias and ensure a representative sample?
Data management
It’s also important to create a data management plan for organizing and storing your data.
Will you need to transcribe interviews or perform data entry for observations? You should anonymize and safeguard any sensitive data, and make sure it’s backed up regularly.
Keeping your data well-organized will save time when it comes to analyzing it. It can also help other researchers validate and add to your findings (high replicability ).
On its own, raw data can’t answer your research question. The last step of designing your research is planning how you’ll analyze the data.
Quantitative data analysis
In quantitative research, you’ll most likely use some form of statistical analysis . With statistics, you can summarize your sample data, make estimates, and test hypotheses.
Using descriptive statistics , you can summarize your sample data in terms of:
- The distribution of the data (e.g., the frequency of each score on a test)
- The central tendency of the data (e.g., the mean to describe the average score)
- The variability of the data (e.g., the standard deviation to describe how spread out the scores are)
The specific calculations you can do depend on the level of measurement of your variables.
Using inferential statistics , you can:
- Make estimates about the population based on your sample data.
- Test hypotheses about a relationship between variables.
Regression and correlation tests look for associations between two or more variables, while comparison tests (such as t tests and ANOVAs ) look for differences in the outcomes of different groups.
Your choice of statistical test depends on various aspects of your research design, including the types of variables you’re dealing with and the distribution of your data.
Qualitative data analysis
In qualitative research, your data will usually be very dense with information and ideas. Instead of summing it up in numbers, you’ll need to comb through the data in detail, interpret its meanings, identify patterns, and extract the parts that are most relevant to your research question.
Two of the most common approaches to doing this are thematic analysis and discourse analysis .
There are many other ways of analyzing qualitative data depending on the aims of your research. To get a sense of potential approaches, try reading some qualitative research papers in your field.
If you want to know more about the research process , methodology , research bias , or statistics , make sure to check out some of our other articles with explanations and examples.
- Simple random sampling
- Stratified sampling
- Cluster sampling
- Likert scales
- Reproducibility
Statistics
- Null hypothesis
- Statistical power
- Probability distribution
- Effect size
- Poisson distribution
Research bias
- Optimism bias
- Cognitive bias
- Implicit bias
- Hawthorne effect
- Anchoring bias
- Explicit bias
A research design is a strategy for answering your research question . It defines your overall approach and determines how you will collect and analyze data.
A well-planned research design helps ensure that your methods match your research aims, that you collect high-quality data, and that you use the right kind of analysis to answer your questions, utilizing credible sources . This allows you to draw valid , trustworthy conclusions.
Quantitative research designs can be divided into two main categories:
- Correlational and descriptive designs are used to investigate characteristics, averages, trends, and associations between variables.
- Experimental and quasi-experimental designs are used to test causal relationships .
Qualitative research designs tend to be more flexible. Common types of qualitative design include case study , ethnography , and grounded theory designs.
The priorities of a research design can vary depending on the field, but you usually have to specify:
- Your research questions and/or hypotheses
- Your overall approach (e.g., qualitative or quantitative )
- The type of design you’re using (e.g., a survey , experiment , or case study )
- Your data collection methods (e.g., questionnaires , observations)
- Your data collection procedures (e.g., operationalization , timing and data management)
- Your data analysis methods (e.g., statistical tests or thematic analysis )
A sample is a subset of individuals from a larger population . Sampling means selecting the group that you will actually collect data from in your research. For example, if you are researching the opinions of students in your university, you could survey a sample of 100 students.
In statistics, sampling allows you to test a hypothesis about the characteristics of a population.
Operationalization means turning abstract conceptual ideas into measurable observations.
For example, the concept of social anxiety isn’t directly observable, but it can be operationally defined in terms of self-rating scores, behavioral avoidance of crowded places, or physical anxiety symptoms in social situations.
Before collecting data , it’s important to consider how you will operationalize the variables that you want to measure.
A research project is an academic, scientific, or professional undertaking to answer a research question . Research projects can take many forms, such as qualitative or quantitative , descriptive , longitudinal , experimental , or correlational . What kind of research approach you choose will depend on your topic.
Cite this Scribbr article
If you want to cite this source, you can copy and paste the citation or click the “Cite this Scribbr article” button to automatically add the citation to our free Citation Generator.
McCombes, S. (2023, November 20). What Is a Research Design | Types, Guide & Examples. Scribbr. Retrieved April 5, 2024, from https://www.scribbr.com/methodology/research-design/
Is this article helpful?
Shona McCombes
Other students also liked, guide to experimental design | overview, steps, & examples, how to write a research proposal | examples & templates, ethical considerations in research | types & examples, "i thought ai proofreading was useless but..".
I've been using Scribbr for years now and I know it's a service that won't disappoint. It does a good job spotting mistakes”
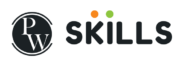
Data Analysis Techniques in Research – Methods, Tools & Examples
By Varun Saharawat | January 22, 2024
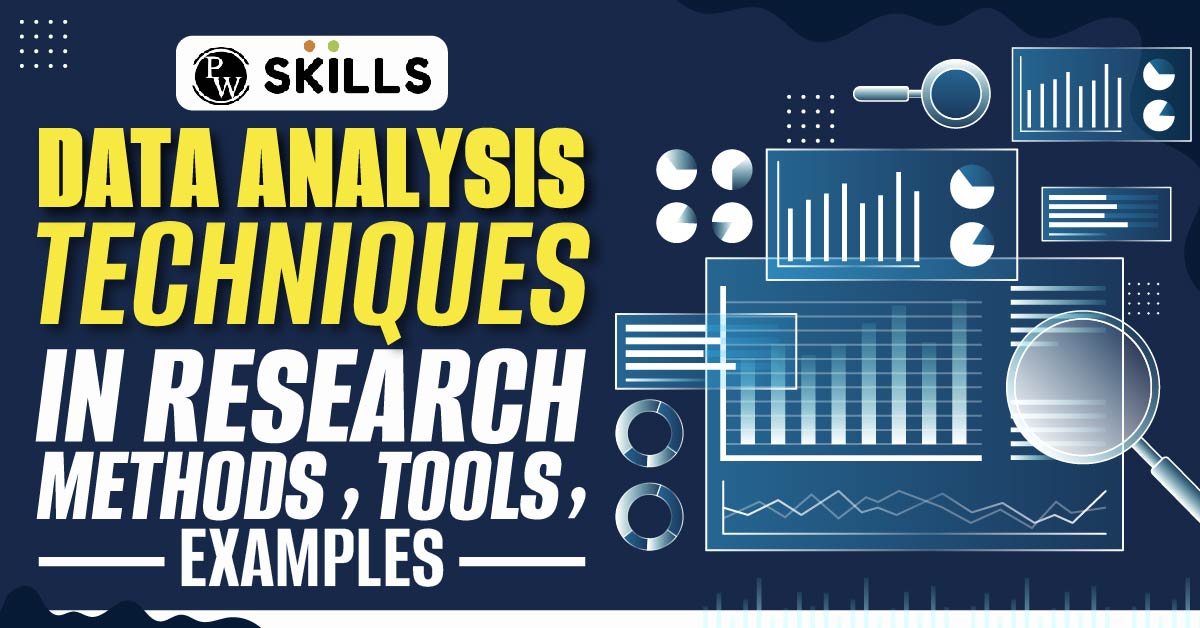
Data analysis techniques in research are essential because they allow researchers to derive meaningful insights from data sets to support their hypotheses or research objectives.
Data Analysis Techniques in Research : While various groups, institutions, and professionals may have diverse approaches to data analysis, a universal definition captures its essence. Data analysis involves refining, transforming, and interpreting raw data to derive actionable insights that guide informed decision-making for businesses.
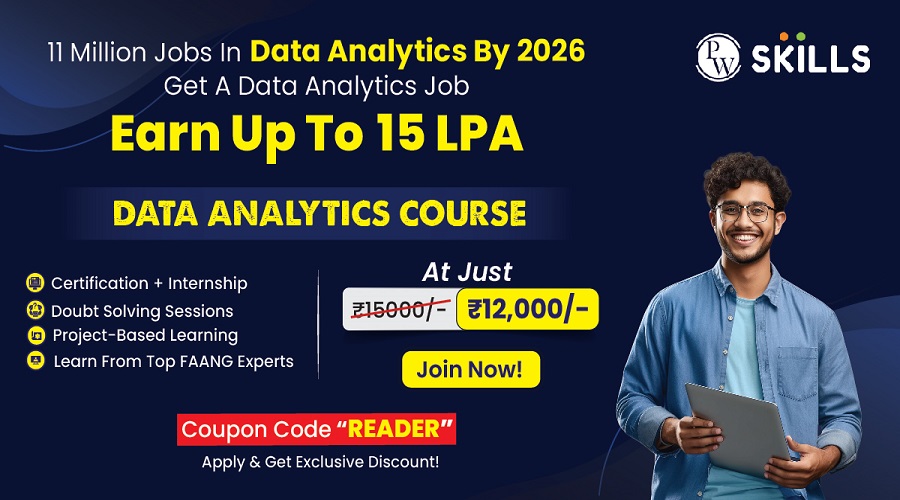
A straightforward illustration of data analysis emerges when we make everyday decisions, basing our choices on past experiences or predictions of potential outcomes.
If you want to learn more about this topic and acquire valuable skills that will set you apart in today’s data-driven world, we highly recommend enrolling in the Data Analytics Course by Physics Wallah . And as a special offer for our readers, use the coupon code “READER” to get a discount on this course.
Table of Contents
What is Data Analysis?
Data analysis is the systematic process of inspecting, cleaning, transforming, and interpreting data with the objective of discovering valuable insights and drawing meaningful conclusions. This process involves several steps:
- Inspecting : Initial examination of data to understand its structure, quality, and completeness.
- Cleaning : Removing errors, inconsistencies, or irrelevant information to ensure accurate analysis.
- Transforming : Converting data into a format suitable for analysis, such as normalization or aggregation.
- Interpreting : Analyzing the transformed data to identify patterns, trends, and relationships.
Types of Data Analysis Techniques in Research
Data analysis techniques in research are categorized into qualitative and quantitative methods, each with its specific approaches and tools. These techniques are instrumental in extracting meaningful insights, patterns, and relationships from data to support informed decision-making, validate hypotheses, and derive actionable recommendations. Below is an in-depth exploration of the various types of data analysis techniques commonly employed in research:
1) Qualitative Analysis:
Definition: Qualitative analysis focuses on understanding non-numerical data, such as opinions, concepts, or experiences, to derive insights into human behavior, attitudes, and perceptions.
- Content Analysis: Examines textual data, such as interview transcripts, articles, or open-ended survey responses, to identify themes, patterns, or trends.
- Narrative Analysis: Analyzes personal stories or narratives to understand individuals’ experiences, emotions, or perspectives.
- Ethnographic Studies: Involves observing and analyzing cultural practices, behaviors, and norms within specific communities or settings.
2) Quantitative Analysis:
Quantitative analysis emphasizes numerical data and employs statistical methods to explore relationships, patterns, and trends. It encompasses several approaches:
Descriptive Analysis:
- Frequency Distribution: Represents the number of occurrences of distinct values within a dataset.
- Central Tendency: Measures such as mean, median, and mode provide insights into the central values of a dataset.
- Dispersion: Techniques like variance and standard deviation indicate the spread or variability of data.
Diagnostic Analysis:
- Regression Analysis: Assesses the relationship between dependent and independent variables, enabling prediction or understanding causality.
- ANOVA (Analysis of Variance): Examines differences between groups to identify significant variations or effects.
Predictive Analysis:
- Time Series Forecasting: Uses historical data points to predict future trends or outcomes.
- Machine Learning Algorithms: Techniques like decision trees, random forests, and neural networks predict outcomes based on patterns in data.
Prescriptive Analysis:
- Optimization Models: Utilizes linear programming, integer programming, or other optimization techniques to identify the best solutions or strategies.
- Simulation: Mimics real-world scenarios to evaluate various strategies or decisions and determine optimal outcomes.
Specific Techniques:
- Monte Carlo Simulation: Models probabilistic outcomes to assess risk and uncertainty.
- Factor Analysis: Reduces the dimensionality of data by identifying underlying factors or components.
- Cohort Analysis: Studies specific groups or cohorts over time to understand trends, behaviors, or patterns within these groups.
- Cluster Analysis: Classifies objects or individuals into homogeneous groups or clusters based on similarities or attributes.
- Sentiment Analysis: Uses natural language processing and machine learning techniques to determine sentiment, emotions, or opinions from textual data.
Also Read: AI and Predictive Analytics: Examples, Tools, Uses, Ai Vs Predictive Analytics
Data Analysis Techniques in Research Examples
To provide a clearer understanding of how data analysis techniques are applied in research, let’s consider a hypothetical research study focused on evaluating the impact of online learning platforms on students’ academic performance.
Research Objective:
Determine if students using online learning platforms achieve higher academic performance compared to those relying solely on traditional classroom instruction.
Data Collection:
- Quantitative Data: Academic scores (grades) of students using online platforms and those using traditional classroom methods.
- Qualitative Data: Feedback from students regarding their learning experiences, challenges faced, and preferences.
Data Analysis Techniques Applied:
1) Descriptive Analysis:
- Calculate the mean, median, and mode of academic scores for both groups.
- Create frequency distributions to represent the distribution of grades in each group.
2) Diagnostic Analysis:
- Conduct an Analysis of Variance (ANOVA) to determine if there’s a statistically significant difference in academic scores between the two groups.
- Perform Regression Analysis to assess the relationship between the time spent on online platforms and academic performance.
3) Predictive Analysis:
- Utilize Time Series Forecasting to predict future academic performance trends based on historical data.
- Implement Machine Learning algorithms to develop a predictive model that identifies factors contributing to academic success on online platforms.
4) Prescriptive Analysis:
- Apply Optimization Models to identify the optimal combination of online learning resources (e.g., video lectures, interactive quizzes) that maximize academic performance.
- Use Simulation Techniques to evaluate different scenarios, such as varying student engagement levels with online resources, to determine the most effective strategies for improving learning outcomes.
5) Specific Techniques:
- Conduct Factor Analysis on qualitative feedback to identify common themes or factors influencing students’ perceptions and experiences with online learning.
- Perform Cluster Analysis to segment students based on their engagement levels, preferences, or academic outcomes, enabling targeted interventions or personalized learning strategies.
- Apply Sentiment Analysis on textual feedback to categorize students’ sentiments as positive, negative, or neutral regarding online learning experiences.
By applying a combination of qualitative and quantitative data analysis techniques, this research example aims to provide comprehensive insights into the effectiveness of online learning platforms.
Also Read: Learning Path to Become a Data Analyst in 2024
Data Analysis Techniques in Quantitative Research
Quantitative research involves collecting numerical data to examine relationships, test hypotheses, and make predictions. Various data analysis techniques are employed to interpret and draw conclusions from quantitative data. Here are some key data analysis techniques commonly used in quantitative research:
1) Descriptive Statistics:
- Description: Descriptive statistics are used to summarize and describe the main aspects of a dataset, such as central tendency (mean, median, mode), variability (range, variance, standard deviation), and distribution (skewness, kurtosis).
- Applications: Summarizing data, identifying patterns, and providing initial insights into the dataset.
2) Inferential Statistics:
- Description: Inferential statistics involve making predictions or inferences about a population based on a sample of data. This technique includes hypothesis testing, confidence intervals, t-tests, chi-square tests, analysis of variance (ANOVA), regression analysis, and correlation analysis.
- Applications: Testing hypotheses, making predictions, and generalizing findings from a sample to a larger population.
3) Regression Analysis:
- Description: Regression analysis is a statistical technique used to model and examine the relationship between a dependent variable and one or more independent variables. Linear regression, multiple regression, logistic regression, and nonlinear regression are common types of regression analysis .
- Applications: Predicting outcomes, identifying relationships between variables, and understanding the impact of independent variables on the dependent variable.
4) Correlation Analysis:
- Description: Correlation analysis is used to measure and assess the strength and direction of the relationship between two or more variables. The Pearson correlation coefficient, Spearman rank correlation coefficient, and Kendall’s tau are commonly used measures of correlation.
- Applications: Identifying associations between variables and assessing the degree and nature of the relationship.
5) Factor Analysis:
- Description: Factor analysis is a multivariate statistical technique used to identify and analyze underlying relationships or factors among a set of observed variables. It helps in reducing the dimensionality of data and identifying latent variables or constructs.
- Applications: Identifying underlying factors or constructs, simplifying data structures, and understanding the underlying relationships among variables.
6) Time Series Analysis:
- Description: Time series analysis involves analyzing data collected or recorded over a specific period at regular intervals to identify patterns, trends, and seasonality. Techniques such as moving averages, exponential smoothing, autoregressive integrated moving average (ARIMA), and Fourier analysis are used.
- Applications: Forecasting future trends, analyzing seasonal patterns, and understanding time-dependent relationships in data.
7) ANOVA (Analysis of Variance):
- Description: Analysis of variance (ANOVA) is a statistical technique used to analyze and compare the means of two or more groups or treatments to determine if they are statistically different from each other. One-way ANOVA, two-way ANOVA, and MANOVA (Multivariate Analysis of Variance) are common types of ANOVA.
- Applications: Comparing group means, testing hypotheses, and determining the effects of categorical independent variables on a continuous dependent variable.
8) Chi-Square Tests:
- Description: Chi-square tests are non-parametric statistical tests used to assess the association between categorical variables in a contingency table. The Chi-square test of independence, goodness-of-fit test, and test of homogeneity are common chi-square tests.
- Applications: Testing relationships between categorical variables, assessing goodness-of-fit, and evaluating independence.
These quantitative data analysis techniques provide researchers with valuable tools and methods to analyze, interpret, and derive meaningful insights from numerical data. The selection of a specific technique often depends on the research objectives, the nature of the data, and the underlying assumptions of the statistical methods being used.
Also Read: Analysis vs. Analytics: How Are They Different?
Data Analysis Methods
Data analysis methods refer to the techniques and procedures used to analyze, interpret, and draw conclusions from data. These methods are essential for transforming raw data into meaningful insights, facilitating decision-making processes, and driving strategies across various fields. Here are some common data analysis methods:
- Description: Descriptive statistics summarize and organize data to provide a clear and concise overview of the dataset. Measures such as mean, median, mode, range, variance, and standard deviation are commonly used.
- Description: Inferential statistics involve making predictions or inferences about a population based on a sample of data. Techniques such as hypothesis testing, confidence intervals, and regression analysis are used.
3) Exploratory Data Analysis (EDA):
- Description: EDA techniques involve visually exploring and analyzing data to discover patterns, relationships, anomalies, and insights. Methods such as scatter plots, histograms, box plots, and correlation matrices are utilized.
- Applications: Identifying trends, patterns, outliers, and relationships within the dataset.
4) Predictive Analytics:
- Description: Predictive analytics use statistical algorithms and machine learning techniques to analyze historical data and make predictions about future events or outcomes. Techniques such as regression analysis, time series forecasting, and machine learning algorithms (e.g., decision trees, random forests, neural networks) are employed.
- Applications: Forecasting future trends, predicting outcomes, and identifying potential risks or opportunities.
5) Prescriptive Analytics:
- Description: Prescriptive analytics involve analyzing data to recommend actions or strategies that optimize specific objectives or outcomes. Optimization techniques, simulation models, and decision-making algorithms are utilized.
- Applications: Recommending optimal strategies, decision-making support, and resource allocation.
6) Qualitative Data Analysis:
- Description: Qualitative data analysis involves analyzing non-numerical data, such as text, images, videos, or audio, to identify themes, patterns, and insights. Methods such as content analysis, thematic analysis, and narrative analysis are used.
- Applications: Understanding human behavior, attitudes, perceptions, and experiences.
7) Big Data Analytics:
- Description: Big data analytics methods are designed to analyze large volumes of structured and unstructured data to extract valuable insights. Technologies such as Hadoop, Spark, and NoSQL databases are used to process and analyze big data.
- Applications: Analyzing large datasets, identifying trends, patterns, and insights from big data sources.
8) Text Analytics:
- Description: Text analytics methods involve analyzing textual data, such as customer reviews, social media posts, emails, and documents, to extract meaningful information and insights. Techniques such as sentiment analysis, text mining, and natural language processing (NLP) are used.
- Applications: Analyzing customer feedback, monitoring brand reputation, and extracting insights from textual data sources.
These data analysis methods are instrumental in transforming data into actionable insights, informing decision-making processes, and driving organizational success across various sectors, including business, healthcare, finance, marketing, and research. The selection of a specific method often depends on the nature of the data, the research objectives, and the analytical requirements of the project or organization.
Also Read: Quantitative Data Analysis: Types, Analysis & Examples
Data Analysis Tools
Data analysis tools are essential instruments that facilitate the process of examining, cleaning, transforming, and modeling data to uncover useful information, make informed decisions, and drive strategies. Here are some prominent data analysis tools widely used across various industries:
1) Microsoft Excel:
- Description: A spreadsheet software that offers basic to advanced data analysis features, including pivot tables, data visualization tools, and statistical functions.
- Applications: Data cleaning, basic statistical analysis, visualization, and reporting.
2) R Programming Language:
- Description: An open-source programming language specifically designed for statistical computing and data visualization.
- Applications: Advanced statistical analysis, data manipulation, visualization, and machine learning.
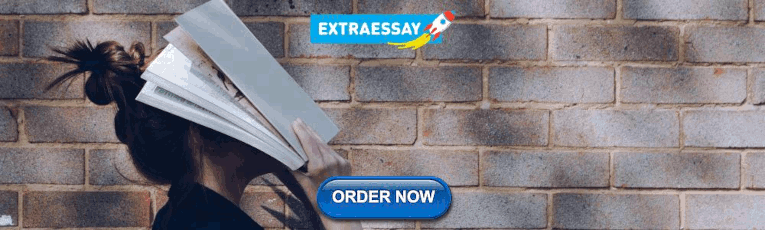
3) Python (with Libraries like Pandas, NumPy, Matplotlib, and Seaborn):
- Description: A versatile programming language with libraries that support data manipulation, analysis, and visualization.
- Applications: Data cleaning, statistical analysis, machine learning, and data visualization.
4) SPSS (Statistical Package for the Social Sciences):
- Description: A comprehensive statistical software suite used for data analysis, data mining, and predictive analytics.
- Applications: Descriptive statistics, hypothesis testing, regression analysis, and advanced analytics.
5) SAS (Statistical Analysis System):
- Description: A software suite used for advanced analytics, multivariate analysis, and predictive modeling.
- Applications: Data management, statistical analysis, predictive modeling, and business intelligence.
6) Tableau:
- Description: A data visualization tool that allows users to create interactive and shareable dashboards and reports.
- Applications: Data visualization , business intelligence , and interactive dashboard creation.
7) Power BI:
- Description: A business analytics tool developed by Microsoft that provides interactive visualizations and business intelligence capabilities.
- Applications: Data visualization, business intelligence, reporting, and dashboard creation.
8) SQL (Structured Query Language) Databases (e.g., MySQL, PostgreSQL, Microsoft SQL Server):
- Description: Database management systems that support data storage, retrieval, and manipulation using SQL queries.
- Applications: Data retrieval, data cleaning, data transformation, and database management.
9) Apache Spark:
- Description: A fast and general-purpose distributed computing system designed for big data processing and analytics.
- Applications: Big data processing, machine learning, data streaming, and real-time analytics.
10) IBM SPSS Modeler:
- Description: A data mining software application used for building predictive models and conducting advanced analytics.
- Applications: Predictive modeling, data mining, statistical analysis, and decision optimization.
These tools serve various purposes and cater to different data analysis needs, from basic statistical analysis and data visualization to advanced analytics, machine learning, and big data processing. The choice of a specific tool often depends on the nature of the data, the complexity of the analysis, and the specific requirements of the project or organization.
Also Read: How to Analyze Survey Data: Methods & Examples
Importance of Data Analysis in Research
The importance of data analysis in research cannot be overstated; it serves as the backbone of any scientific investigation or study. Here are several key reasons why data analysis is crucial in the research process:
- Data analysis helps ensure that the results obtained are valid and reliable. By systematically examining the data, researchers can identify any inconsistencies or anomalies that may affect the credibility of the findings.
- Effective data analysis provides researchers with the necessary information to make informed decisions. By interpreting the collected data, researchers can draw conclusions, make predictions, or formulate recommendations based on evidence rather than intuition or guesswork.
- Data analysis allows researchers to identify patterns, trends, and relationships within the data. This can lead to a deeper understanding of the research topic, enabling researchers to uncover insights that may not be immediately apparent.
- In empirical research, data analysis plays a critical role in testing hypotheses. Researchers collect data to either support or refute their hypotheses, and data analysis provides the tools and techniques to evaluate these hypotheses rigorously.
- Transparent and well-executed data analysis enhances the credibility of research findings. By clearly documenting the data analysis methods and procedures, researchers allow others to replicate the study, thereby contributing to the reproducibility of research findings.
- In fields such as business or healthcare, data analysis helps organizations allocate resources more efficiently. By analyzing data on consumer behavior, market trends, or patient outcomes, organizations can make strategic decisions about resource allocation, budgeting, and planning.
- In public policy and social sciences, data analysis is instrumental in developing and evaluating policies and interventions. By analyzing data on social, economic, or environmental factors, policymakers can assess the effectiveness of existing policies and inform the development of new ones.
- Data analysis allows for continuous improvement in research methods and practices. By analyzing past research projects, identifying areas for improvement, and implementing changes based on data-driven insights, researchers can refine their approaches and enhance the quality of future research endeavors.
However, it is important to remember that mastering these techniques requires practice and continuous learning. That’s why we highly recommend the Data Analytics Course by Physics Wallah . Not only does it cover all the fundamentals of data analysis, but it also provides hands-on experience with various tools such as Excel, Python, and Tableau. Plus, if you use the “ READER ” coupon code at checkout, you can get a special discount on the course.
For Latest Tech Related Information, Join Our Official Free Telegram Group : PW Skills Telegram Group
Data Analysis Techniques in Research FAQs
What are the 5 techniques for data analysis.
The five techniques for data analysis include: Descriptive Analysis Diagnostic Analysis Predictive Analysis Prescriptive Analysis Qualitative Analysis
What are techniques of data analysis in research?
Techniques of data analysis in research encompass both qualitative and quantitative methods. These techniques involve processes like summarizing raw data, investigating causes of events, forecasting future outcomes, offering recommendations based on predictions, and examining non-numerical data to understand concepts or experiences.
What are the 3 methods of data analysis?
The three primary methods of data analysis are: Qualitative Analysis Quantitative Analysis Mixed-Methods Analysis
What are the four types of data analysis techniques?
The four types of data analysis techniques are: Descriptive Analysis Diagnostic Analysis Predictive Analysis Prescriptive Analysis
- The 11 Best Analytical Tools For Data Analysis in 2024
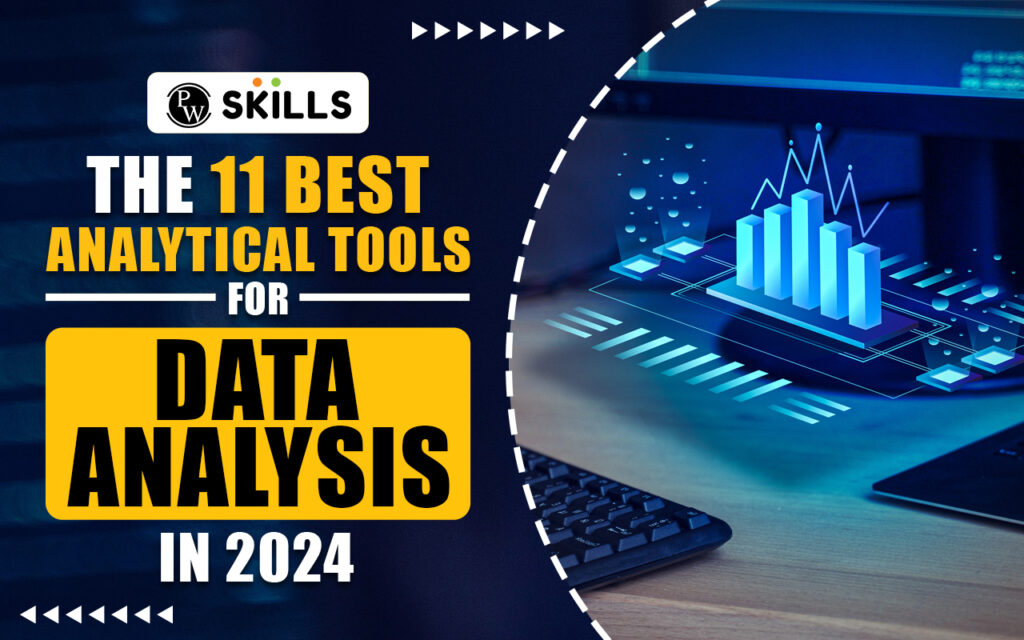
Data Analytical tools help to extract important insights from raw and unstructured data. Read this article to get a list…
- 10 Most Popular Big Data Analytics Tools
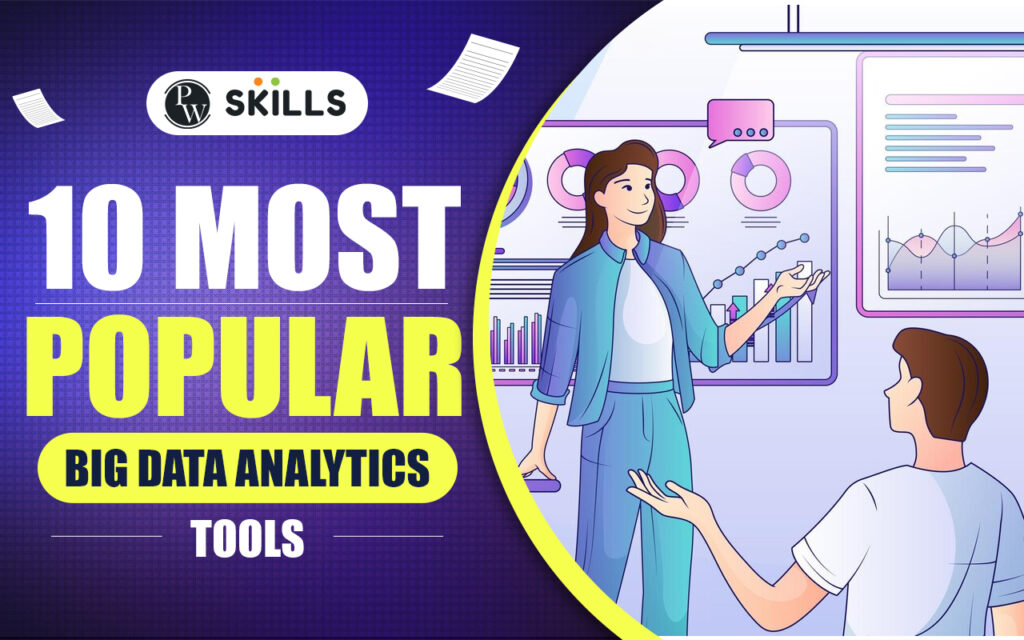
The world of big data analytics tools is diverse, with each tool offering a unique set of skills. Choose your…
- Top 20 Big Data Tools Used By Professionals
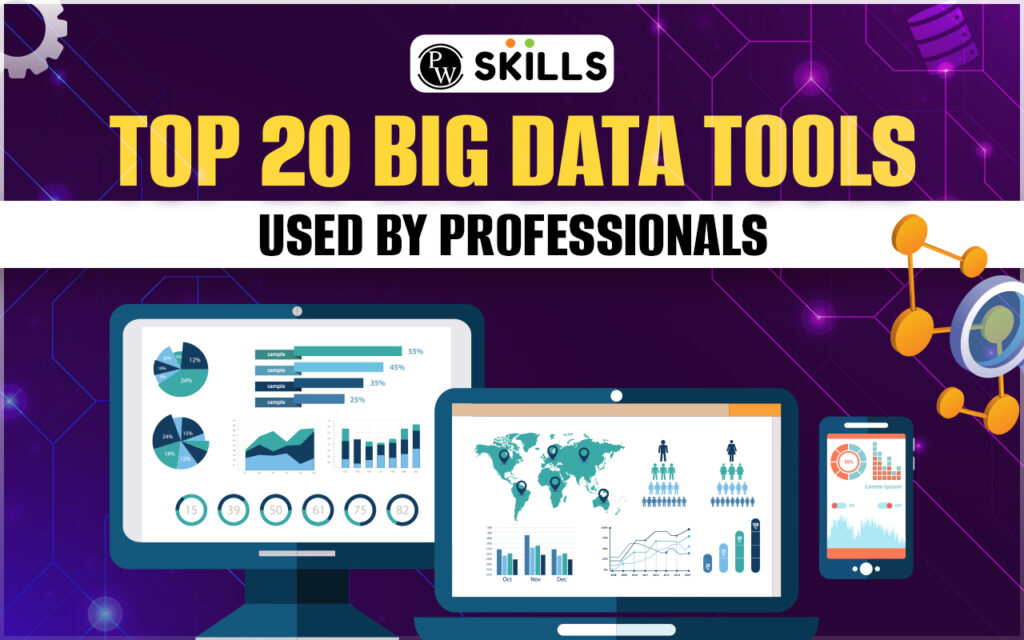
There are plenty of big data tools available online for free. However, some of the handpicked big data tools used…
Related Articles
- Top Best Big Data Analytics Classes 2024
- Best Courses For Data Analytics: Top 10 Courses For Your Career in Trend
- Big Data and Analytics – Definition, Benefits, and More
- Big Data Defined: Examples and Benefits
- Best 5 Unique Strategies to Use Artificial Intelligence Data Analytics
- Best BI Tool: Top 15 Business Intelligence Tools (BI Tools)
- Applications of Big Data
- Resources Home 🏠
- Try SciSpace Copilot
- Search research papers
- Add Copilot Extension
- Try AI Detector
- Try Paraphraser
- Try Citation Generator
- April Papers
- June Papers
- July Papers

A Comprehensive Guide to Methodology in Research
Table of Contents
Research methodology plays a crucial role in any study or investigation. It provides the framework for collecting, analyzing, and interpreting data, ensuring that the research is reliable, valid, and credible. Understanding the importance of research methodology is essential for conducting rigorous and meaningful research.
In this article, we'll explore the various aspects of research methodology, from its types to best practices, ensuring you have the knowledge needed to conduct impactful research.
What is Research Methodology?
Research methodology refers to the system of procedures, techniques, and tools used to carry out a research study. It encompasses the overall approach, including the research design, data collection methods, data analysis techniques, and the interpretation of findings.
Research methodology plays a crucial role in the field of research, as it sets the foundation for any study. It provides researchers with a structured framework to ensure that their investigations are conducted in a systematic and organized manner. By following a well-defined methodology, researchers can ensure that their findings are reliable, valid, and meaningful.
When defining research methodology, one of the first steps is to identify the research problem. This involves clearly understanding the issue or topic that the study aims to address. By defining the research problem, researchers can narrow down their focus and determine the specific objectives they want to achieve through their study.
How to Define Research Methodology
Once the research problem is identified, researchers move on to defining the research questions. These questions serve as a guide for the study, helping researchers to gather relevant information and analyze it effectively. The research questions should be clear, concise, and aligned with the overall goals of the study.
After defining the research questions, researchers need to determine how data will be collected and analyzed. This involves selecting appropriate data collection methods, such as surveys, interviews, observations, or experiments. The choice of data collection methods depends on various factors, including the nature of the research problem, the target population, and the available resources.
Once the data is collected, researchers need to analyze it using appropriate data analysis techniques. This may involve statistical analysis, qualitative analysis, or a combination of both, depending on the nature of the data and the research questions. The analysis of data helps researchers to draw meaningful conclusions and make informed decisions based on their findings.
Role of Methodology in Research
Methodology plays a crucial role in research, as it ensures that the study is conducted in a systematic and organized manner. It provides a clear roadmap for researchers to follow, ensuring that the research objectives are met effectively. By following a well-defined methodology, researchers can minimize bias, errors, and inconsistencies in their study, thus enhancing the reliability and validity of their findings.
In addition to providing a structured approach, research methodology also helps in establishing the reliability and validity of the study. Reliability refers to the consistency and stability of the research findings, while validity refers to the accuracy and truthfulness of the findings. By using appropriate research methods and techniques, researchers can ensure that their study produces reliable and valid results, which can be used to make informed decisions and contribute to the existing body of knowledge.
Steps in Choosing the Right Research Methodology
Choosing the appropriate research methodology for your study is a critical step in ensuring the success of your research. Let's explore some steps to help you select the right research methodology:
Identifying the Research Problem
The first step in choosing the right research methodology is to clearly identify and define the research problem. Understanding the research problem will help you determine which methodology will best address your research questions and objectives.
Identifying the research problem involves a thorough examination of the existing literature in your field of study. This step allows you to gain a comprehensive understanding of the current state of knowledge and identify any gaps that your research can fill. By identifying the research problem, you can ensure that your study contributes to the existing body of knowledge and addresses a significant research gap.
Once you have identified the research problem, you need to consider the scope of your study. Are you focusing on a specific population, geographic area, or time frame? Understanding the scope of your research will help you determine the appropriate research methodology to use.
Reviewing Previous Research
Before finalizing the research methodology, it is essential to review previous research conducted in the field. This will allow you to identify gaps, determine the most effective methodologies used in similar studies, and build upon existing knowledge.
Reviewing previous research involves conducting a systematic review of relevant literature. This process includes searching for and analyzing published studies, articles, and reports that are related to your research topic. By reviewing previous research, you can gain insights into the strengths and limitations of different methodologies and make informed decisions about which approach to adopt.
During the review process, it is important to critically evaluate the quality and reliability of the existing research. Consider factors such as the sample size, research design, data collection methods, and statistical analysis techniques used in previous studies. This evaluation will help you determine the most appropriate research methodology for your own study.
Formulating Research Questions
Once the research problem is identified, formulate specific and relevant research questions. These questions will guide your methodology selection process by helping you determine what type of data you need to collect and how to analyze it.
Formulating research questions involves breaking down the research problem into smaller, more manageable components. These questions should be clear, concise, and measurable. They should also align with the objectives of your study and provide a framework for data collection and analysis.
When formulating research questions, consider the different types of data that can be collected, such as qualitative or quantitative data. Depending on the nature of your research questions, you may need to employ different data collection methods, such as interviews, surveys, observations, or experiments. By carefully formulating research questions, you can ensure that your chosen methodology will enable you to collect the necessary data to answer your research questions effectively.
Implementing the Research Methodology
After choosing the appropriate research methodology, it is time to implement it. This stage involves collecting data using various techniques and analyzing the gathered information. Let's explore two crucial aspects of implementing the research methodology:
Data Collection Techniques
Data collection techniques depend on the chosen research methodology. They can include surveys, interviews, observations, experiments, or document analysis. Selecting the most suitable data collection techniques will ensure accurate and relevant data for your study.
Data Analysis Methods
Data analysis is a critical part of the research process. It involves interpreting and making sense of the collected data to draw meaningful conclusions. Depending on the research methodology, data analysis methods can include statistical analysis, content analysis, thematic analysis, or grounded theory.
Ensuring the Validity and Reliability of Your Research
In order to ensure the validity and reliability of your research findings, it is important to address these two key aspects:
Understanding Validity in Research
Validity refers to the accuracy and soundness of a research study. It is crucial to ensure that the research methods used effectively measure what they intend to measure. Researchers can enhance validity by using proper sampling techniques, carefully designing research instruments, and ensuring accurate data collection.
Ensuring Reliability in Your Study
Reliability refers to the consistency and stability of the research results. It is important to ensure that the research methods and instruments used yield consistent and reproducible results. Researchers can enhance reliability by using standardized procedures, ensuring inter-rater reliability, and conducting pilot studies.
A comprehensive understanding of research methodology is essential for conducting high-quality research. By selecting the right research methodology, researchers can ensure that their studies are rigorous, reliable, and valid. It is crucial to follow the steps in choosing the appropriate methodology, implement the chosen methodology effectively, and address validity and reliability concerns throughout the research process. By doing so, researchers can contribute valuable insights and advances in their respective fields.
You might also like

AI for Meta Analysis — A Comprehensive Guide
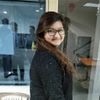
Cybersecurity in Higher Education: Safeguarding Students and Faculty Data

How To Write An Argumentative Essay
- Privacy Policy
Buy Me a Coffee

Home » Research Methodology – Types, Examples and writing Guide
Research Methodology – Types, Examples and writing Guide
Table of Contents

Research Methodology
Definition:
Research Methodology refers to the systematic and scientific approach used to conduct research, investigate problems, and gather data and information for a specific purpose. It involves the techniques and procedures used to identify, collect , analyze , and interpret data to answer research questions or solve research problems . Moreover, They are philosophical and theoretical frameworks that guide the research process.
Structure of Research Methodology
Research methodology formats can vary depending on the specific requirements of the research project, but the following is a basic example of a structure for a research methodology section:
I. Introduction
- Provide an overview of the research problem and the need for a research methodology section
- Outline the main research questions and objectives
II. Research Design
- Explain the research design chosen and why it is appropriate for the research question(s) and objectives
- Discuss any alternative research designs considered and why they were not chosen
- Describe the research setting and participants (if applicable)
III. Data Collection Methods
- Describe the methods used to collect data (e.g., surveys, interviews, observations)
- Explain how the data collection methods were chosen and why they are appropriate for the research question(s) and objectives
- Detail any procedures or instruments used for data collection
IV. Data Analysis Methods
- Describe the methods used to analyze the data (e.g., statistical analysis, content analysis )
- Explain how the data analysis methods were chosen and why they are appropriate for the research question(s) and objectives
- Detail any procedures or software used for data analysis
V. Ethical Considerations
- Discuss any ethical issues that may arise from the research and how they were addressed
- Explain how informed consent was obtained (if applicable)
- Detail any measures taken to ensure confidentiality and anonymity
VI. Limitations
- Identify any potential limitations of the research methodology and how they may impact the results and conclusions
VII. Conclusion
- Summarize the key aspects of the research methodology section
- Explain how the research methodology addresses the research question(s) and objectives
Research Methodology Types
Types of Research Methodology are as follows:
Quantitative Research Methodology
This is a research methodology that involves the collection and analysis of numerical data using statistical methods. This type of research is often used to study cause-and-effect relationships and to make predictions.
Qualitative Research Methodology
This is a research methodology that involves the collection and analysis of non-numerical data such as words, images, and observations. This type of research is often used to explore complex phenomena, to gain an in-depth understanding of a particular topic, and to generate hypotheses.
Mixed-Methods Research Methodology
This is a research methodology that combines elements of both quantitative and qualitative research. This approach can be particularly useful for studies that aim to explore complex phenomena and to provide a more comprehensive understanding of a particular topic.
Case Study Research Methodology
This is a research methodology that involves in-depth examination of a single case or a small number of cases. Case studies are often used in psychology, sociology, and anthropology to gain a detailed understanding of a particular individual or group.
Action Research Methodology
This is a research methodology that involves a collaborative process between researchers and practitioners to identify and solve real-world problems. Action research is often used in education, healthcare, and social work.
Experimental Research Methodology
This is a research methodology that involves the manipulation of one or more independent variables to observe their effects on a dependent variable. Experimental research is often used to study cause-and-effect relationships and to make predictions.
Survey Research Methodology
This is a research methodology that involves the collection of data from a sample of individuals using questionnaires or interviews. Survey research is often used to study attitudes, opinions, and behaviors.
Grounded Theory Research Methodology
This is a research methodology that involves the development of theories based on the data collected during the research process. Grounded theory is often used in sociology and anthropology to generate theories about social phenomena.
Research Methodology Example
An Example of Research Methodology could be the following:
Research Methodology for Investigating the Effectiveness of Cognitive Behavioral Therapy in Reducing Symptoms of Depression in Adults
Introduction:
The aim of this research is to investigate the effectiveness of cognitive-behavioral therapy (CBT) in reducing symptoms of depression in adults. To achieve this objective, a randomized controlled trial (RCT) will be conducted using a mixed-methods approach.
Research Design:
The study will follow a pre-test and post-test design with two groups: an experimental group receiving CBT and a control group receiving no intervention. The study will also include a qualitative component, in which semi-structured interviews will be conducted with a subset of participants to explore their experiences of receiving CBT.
Participants:
Participants will be recruited from community mental health clinics in the local area. The sample will consist of 100 adults aged 18-65 years old who meet the diagnostic criteria for major depressive disorder. Participants will be randomly assigned to either the experimental group or the control group.
Intervention :
The experimental group will receive 12 weekly sessions of CBT, each lasting 60 minutes. The intervention will be delivered by licensed mental health professionals who have been trained in CBT. The control group will receive no intervention during the study period.
Data Collection:
Quantitative data will be collected through the use of standardized measures such as the Beck Depression Inventory-II (BDI-II) and the Generalized Anxiety Disorder-7 (GAD-7). Data will be collected at baseline, immediately after the intervention, and at a 3-month follow-up. Qualitative data will be collected through semi-structured interviews with a subset of participants from the experimental group. The interviews will be conducted at the end of the intervention period, and will explore participants’ experiences of receiving CBT.
Data Analysis:
Quantitative data will be analyzed using descriptive statistics, t-tests, and mixed-model analyses of variance (ANOVA) to assess the effectiveness of the intervention. Qualitative data will be analyzed using thematic analysis to identify common themes and patterns in participants’ experiences of receiving CBT.
Ethical Considerations:
This study will comply with ethical guidelines for research involving human subjects. Participants will provide informed consent before participating in the study, and their privacy and confidentiality will be protected throughout the study. Any adverse events or reactions will be reported and managed appropriately.
Data Management:
All data collected will be kept confidential and stored securely using password-protected databases. Identifying information will be removed from qualitative data transcripts to ensure participants’ anonymity.
Limitations:
One potential limitation of this study is that it only focuses on one type of psychotherapy, CBT, and may not generalize to other types of therapy or interventions. Another limitation is that the study will only include participants from community mental health clinics, which may not be representative of the general population.
Conclusion:
This research aims to investigate the effectiveness of CBT in reducing symptoms of depression in adults. By using a randomized controlled trial and a mixed-methods approach, the study will provide valuable insights into the mechanisms underlying the relationship between CBT and depression. The results of this study will have important implications for the development of effective treatments for depression in clinical settings.
How to Write Research Methodology
Writing a research methodology involves explaining the methods and techniques you used to conduct research, collect data, and analyze results. It’s an essential section of any research paper or thesis, as it helps readers understand the validity and reliability of your findings. Here are the steps to write a research methodology:
- Start by explaining your research question: Begin the methodology section by restating your research question and explaining why it’s important. This helps readers understand the purpose of your research and the rationale behind your methods.
- Describe your research design: Explain the overall approach you used to conduct research. This could be a qualitative or quantitative research design, experimental or non-experimental, case study or survey, etc. Discuss the advantages and limitations of the chosen design.
- Discuss your sample: Describe the participants or subjects you included in your study. Include details such as their demographics, sampling method, sample size, and any exclusion criteria used.
- Describe your data collection methods : Explain how you collected data from your participants. This could include surveys, interviews, observations, questionnaires, or experiments. Include details on how you obtained informed consent, how you administered the tools, and how you minimized the risk of bias.
- Explain your data analysis techniques: Describe the methods you used to analyze the data you collected. This could include statistical analysis, content analysis, thematic analysis, or discourse analysis. Explain how you dealt with missing data, outliers, and any other issues that arose during the analysis.
- Discuss the validity and reliability of your research : Explain how you ensured the validity and reliability of your study. This could include measures such as triangulation, member checking, peer review, or inter-coder reliability.
- Acknowledge any limitations of your research: Discuss any limitations of your study, including any potential threats to validity or generalizability. This helps readers understand the scope of your findings and how they might apply to other contexts.
- Provide a summary: End the methodology section by summarizing the methods and techniques you used to conduct your research. This provides a clear overview of your research methodology and helps readers understand the process you followed to arrive at your findings.
When to Write Research Methodology
Research methodology is typically written after the research proposal has been approved and before the actual research is conducted. It should be written prior to data collection and analysis, as it provides a clear roadmap for the research project.
The research methodology is an important section of any research paper or thesis, as it describes the methods and procedures that will be used to conduct the research. It should include details about the research design, data collection methods, data analysis techniques, and any ethical considerations.
The methodology should be written in a clear and concise manner, and it should be based on established research practices and standards. It is important to provide enough detail so that the reader can understand how the research was conducted and evaluate the validity of the results.
Applications of Research Methodology
Here are some of the applications of research methodology:
- To identify the research problem: Research methodology is used to identify the research problem, which is the first step in conducting any research.
- To design the research: Research methodology helps in designing the research by selecting the appropriate research method, research design, and sampling technique.
- To collect data: Research methodology provides a systematic approach to collect data from primary and secondary sources.
- To analyze data: Research methodology helps in analyzing the collected data using various statistical and non-statistical techniques.
- To test hypotheses: Research methodology provides a framework for testing hypotheses and drawing conclusions based on the analysis of data.
- To generalize findings: Research methodology helps in generalizing the findings of the research to the target population.
- To develop theories : Research methodology is used to develop new theories and modify existing theories based on the findings of the research.
- To evaluate programs and policies : Research methodology is used to evaluate the effectiveness of programs and policies by collecting data and analyzing it.
- To improve decision-making: Research methodology helps in making informed decisions by providing reliable and valid data.
Purpose of Research Methodology
Research methodology serves several important purposes, including:
- To guide the research process: Research methodology provides a systematic framework for conducting research. It helps researchers to plan their research, define their research questions, and select appropriate methods and techniques for collecting and analyzing data.
- To ensure research quality: Research methodology helps researchers to ensure that their research is rigorous, reliable, and valid. It provides guidelines for minimizing bias and error in data collection and analysis, and for ensuring that research findings are accurate and trustworthy.
- To replicate research: Research methodology provides a clear and detailed account of the research process, making it possible for other researchers to replicate the study and verify its findings.
- To advance knowledge: Research methodology enables researchers to generate new knowledge and to contribute to the body of knowledge in their field. It provides a means for testing hypotheses, exploring new ideas, and discovering new insights.
- To inform decision-making: Research methodology provides evidence-based information that can inform policy and decision-making in a variety of fields, including medicine, public health, education, and business.
Advantages of Research Methodology
Research methodology has several advantages that make it a valuable tool for conducting research in various fields. Here are some of the key advantages of research methodology:
- Systematic and structured approach : Research methodology provides a systematic and structured approach to conducting research, which ensures that the research is conducted in a rigorous and comprehensive manner.
- Objectivity : Research methodology aims to ensure objectivity in the research process, which means that the research findings are based on evidence and not influenced by personal bias or subjective opinions.
- Replicability : Research methodology ensures that research can be replicated by other researchers, which is essential for validating research findings and ensuring their accuracy.
- Reliability : Research methodology aims to ensure that the research findings are reliable, which means that they are consistent and can be depended upon.
- Validity : Research methodology ensures that the research findings are valid, which means that they accurately reflect the research question or hypothesis being tested.
- Efficiency : Research methodology provides a structured and efficient way of conducting research, which helps to save time and resources.
- Flexibility : Research methodology allows researchers to choose the most appropriate research methods and techniques based on the research question, data availability, and other relevant factors.
- Scope for innovation: Research methodology provides scope for innovation and creativity in designing research studies and developing new research techniques.
Research Methodology Vs Research Methods
About the author.
Muhammad Hassan
Researcher, Academic Writer, Web developer
You may also like

How to Cite Research Paper – All Formats and...

Data Collection – Methods Types and Examples

Delimitations in Research – Types, Examples and...

Research Paper Format – Types, Examples and...

Research Process – Steps, Examples and Tips

Research Design – Types, Methods and Examples
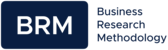
Basic statistical tools in research and data analysis
Zulfiqar ali.
Department of Anaesthesiology, Division of Neuroanaesthesiology, Sheri Kashmir Institute of Medical Sciences, Soura, Srinagar, Jammu and Kashmir, India
S Bala Bhaskar
1 Department of Anaesthesiology and Critical Care, Vijayanagar Institute of Medical Sciences, Bellary, Karnataka, India
Statistical methods involved in carrying out a study include planning, designing, collecting data, analysing, drawing meaningful interpretation and reporting of the research findings. The statistical analysis gives meaning to the meaningless numbers, thereby breathing life into a lifeless data. The results and inferences are precise only if proper statistical tests are used. This article will try to acquaint the reader with the basic research tools that are utilised while conducting various studies. The article covers a brief outline of the variables, an understanding of quantitative and qualitative variables and the measures of central tendency. An idea of the sample size estimation, power analysis and the statistical errors is given. Finally, there is a summary of parametric and non-parametric tests used for data analysis.
INTRODUCTION
Statistics is a branch of science that deals with the collection, organisation, analysis of data and drawing of inferences from the samples to the whole population.[ 1 ] This requires a proper design of the study, an appropriate selection of the study sample and choice of a suitable statistical test. An adequate knowledge of statistics is necessary for proper designing of an epidemiological study or a clinical trial. Improper statistical methods may result in erroneous conclusions which may lead to unethical practice.[ 2 ]
Variable is a characteristic that varies from one individual member of population to another individual.[ 3 ] Variables such as height and weight are measured by some type of scale, convey quantitative information and are called as quantitative variables. Sex and eye colour give qualitative information and are called as qualitative variables[ 3 ] [ Figure 1 ].

Classification of variables
Quantitative variables
Quantitative or numerical data are subdivided into discrete and continuous measurements. Discrete numerical data are recorded as a whole number such as 0, 1, 2, 3,… (integer), whereas continuous data can assume any value. Observations that can be counted constitute the discrete data and observations that can be measured constitute the continuous data. Examples of discrete data are number of episodes of respiratory arrests or the number of re-intubations in an intensive care unit. Similarly, examples of continuous data are the serial serum glucose levels, partial pressure of oxygen in arterial blood and the oesophageal temperature.
A hierarchical scale of increasing precision can be used for observing and recording the data which is based on categorical, ordinal, interval and ratio scales [ Figure 1 ].
Categorical or nominal variables are unordered. The data are merely classified into categories and cannot be arranged in any particular order. If only two categories exist (as in gender male and female), it is called as a dichotomous (or binary) data. The various causes of re-intubation in an intensive care unit due to upper airway obstruction, impaired clearance of secretions, hypoxemia, hypercapnia, pulmonary oedema and neurological impairment are examples of categorical variables.
Ordinal variables have a clear ordering between the variables. However, the ordered data may not have equal intervals. Examples are the American Society of Anesthesiologists status or Richmond agitation-sedation scale.
Interval variables are similar to an ordinal variable, except that the intervals between the values of the interval variable are equally spaced. A good example of an interval scale is the Fahrenheit degree scale used to measure temperature. With the Fahrenheit scale, the difference between 70° and 75° is equal to the difference between 80° and 85°: The units of measurement are equal throughout the full range of the scale.
Ratio scales are similar to interval scales, in that equal differences between scale values have equal quantitative meaning. However, ratio scales also have a true zero point, which gives them an additional property. For example, the system of centimetres is an example of a ratio scale. There is a true zero point and the value of 0 cm means a complete absence of length. The thyromental distance of 6 cm in an adult may be twice that of a child in whom it may be 3 cm.
STATISTICS: DESCRIPTIVE AND INFERENTIAL STATISTICS
Descriptive statistics[ 4 ] try to describe the relationship between variables in a sample or population. Descriptive statistics provide a summary of data in the form of mean, median and mode. Inferential statistics[ 4 ] use a random sample of data taken from a population to describe and make inferences about the whole population. It is valuable when it is not possible to examine each member of an entire population. The examples if descriptive and inferential statistics are illustrated in Table 1 .
Example of descriptive and inferential statistics

Descriptive statistics
The extent to which the observations cluster around a central location is described by the central tendency and the spread towards the extremes is described by the degree of dispersion.
Measures of central tendency
The measures of central tendency are mean, median and mode.[ 6 ] Mean (or the arithmetic average) is the sum of all the scores divided by the number of scores. Mean may be influenced profoundly by the extreme variables. For example, the average stay of organophosphorus poisoning patients in ICU may be influenced by a single patient who stays in ICU for around 5 months because of septicaemia. The extreme values are called outliers. The formula for the mean is

where x = each observation and n = number of observations. Median[ 6 ] is defined as the middle of a distribution in a ranked data (with half of the variables in the sample above and half below the median value) while mode is the most frequently occurring variable in a distribution. Range defines the spread, or variability, of a sample.[ 7 ] It is described by the minimum and maximum values of the variables. If we rank the data and after ranking, group the observations into percentiles, we can get better information of the pattern of spread of the variables. In percentiles, we rank the observations into 100 equal parts. We can then describe 25%, 50%, 75% or any other percentile amount. The median is the 50 th percentile. The interquartile range will be the observations in the middle 50% of the observations about the median (25 th -75 th percentile). Variance[ 7 ] is a measure of how spread out is the distribution. It gives an indication of how close an individual observation clusters about the mean value. The variance of a population is defined by the following formula:

where σ 2 is the population variance, X is the population mean, X i is the i th element from the population and N is the number of elements in the population. The variance of a sample is defined by slightly different formula:

where s 2 is the sample variance, x is the sample mean, x i is the i th element from the sample and n is the number of elements in the sample. The formula for the variance of a population has the value ‘ n ’ as the denominator. The expression ‘ n −1’ is known as the degrees of freedom and is one less than the number of parameters. Each observation is free to vary, except the last one which must be a defined value. The variance is measured in squared units. To make the interpretation of the data simple and to retain the basic unit of observation, the square root of variance is used. The square root of the variance is the standard deviation (SD).[ 8 ] The SD of a population is defined by the following formula:

where σ is the population SD, X is the population mean, X i is the i th element from the population and N is the number of elements in the population. The SD of a sample is defined by slightly different formula:

where s is the sample SD, x is the sample mean, x i is the i th element from the sample and n is the number of elements in the sample. An example for calculation of variation and SD is illustrated in Table 2 .
Example of mean, variance, standard deviation

Normal distribution or Gaussian distribution
Most of the biological variables usually cluster around a central value, with symmetrical positive and negative deviations about this point.[ 1 ] The standard normal distribution curve is a symmetrical bell-shaped. In a normal distribution curve, about 68% of the scores are within 1 SD of the mean. Around 95% of the scores are within 2 SDs of the mean and 99% within 3 SDs of the mean [ Figure 2 ].

Normal distribution curve
Skewed distribution
It is a distribution with an asymmetry of the variables about its mean. In a negatively skewed distribution [ Figure 3 ], the mass of the distribution is concentrated on the right of Figure 1 . In a positively skewed distribution [ Figure 3 ], the mass of the distribution is concentrated on the left of the figure leading to a longer right tail.

Curves showing negatively skewed and positively skewed distribution
Inferential statistics
In inferential statistics, data are analysed from a sample to make inferences in the larger collection of the population. The purpose is to answer or test the hypotheses. A hypothesis (plural hypotheses) is a proposed explanation for a phenomenon. Hypothesis tests are thus procedures for making rational decisions about the reality of observed effects.
Probability is the measure of the likelihood that an event will occur. Probability is quantified as a number between 0 and 1 (where 0 indicates impossibility and 1 indicates certainty).
In inferential statistics, the term ‘null hypothesis’ ( H 0 ‘ H-naught ,’ ‘ H-null ’) denotes that there is no relationship (difference) between the population variables in question.[ 9 ]
Alternative hypothesis ( H 1 and H a ) denotes that a statement between the variables is expected to be true.[ 9 ]
The P value (or the calculated probability) is the probability of the event occurring by chance if the null hypothesis is true. The P value is a numerical between 0 and 1 and is interpreted by researchers in deciding whether to reject or retain the null hypothesis [ Table 3 ].
P values with interpretation

If P value is less than the arbitrarily chosen value (known as α or the significance level), the null hypothesis (H0) is rejected [ Table 4 ]. However, if null hypotheses (H0) is incorrectly rejected, this is known as a Type I error.[ 11 ] Further details regarding alpha error, beta error and sample size calculation and factors influencing them are dealt with in another section of this issue by Das S et al .[ 12 ]
Illustration for null hypothesis

PARAMETRIC AND NON-PARAMETRIC TESTS
Numerical data (quantitative variables) that are normally distributed are analysed with parametric tests.[ 13 ]
Two most basic prerequisites for parametric statistical analysis are:
- The assumption of normality which specifies that the means of the sample group are normally distributed
- The assumption of equal variance which specifies that the variances of the samples and of their corresponding population are equal.
However, if the distribution of the sample is skewed towards one side or the distribution is unknown due to the small sample size, non-parametric[ 14 ] statistical techniques are used. Non-parametric tests are used to analyse ordinal and categorical data.
Parametric tests
The parametric tests assume that the data are on a quantitative (numerical) scale, with a normal distribution of the underlying population. The samples have the same variance (homogeneity of variances). The samples are randomly drawn from the population, and the observations within a group are independent of each other. The commonly used parametric tests are the Student's t -test, analysis of variance (ANOVA) and repeated measures ANOVA.
Student's t -test
Student's t -test is used to test the null hypothesis that there is no difference between the means of the two groups. It is used in three circumstances:

where X = sample mean, u = population mean and SE = standard error of mean

where X 1 − X 2 is the difference between the means of the two groups and SE denotes the standard error of the difference.
- To test if the population means estimated by two dependent samples differ significantly (the paired t -test). A usual setting for paired t -test is when measurements are made on the same subjects before and after a treatment.
The formula for paired t -test is:

where d is the mean difference and SE denotes the standard error of this difference.
The group variances can be compared using the F -test. The F -test is the ratio of variances (var l/var 2). If F differs significantly from 1.0, then it is concluded that the group variances differ significantly.
Analysis of variance
The Student's t -test cannot be used for comparison of three or more groups. The purpose of ANOVA is to test if there is any significant difference between the means of two or more groups.
In ANOVA, we study two variances – (a) between-group variability and (b) within-group variability. The within-group variability (error variance) is the variation that cannot be accounted for in the study design. It is based on random differences present in our samples.
However, the between-group (or effect variance) is the result of our treatment. These two estimates of variances are compared using the F-test.
A simplified formula for the F statistic is:

where MS b is the mean squares between the groups and MS w is the mean squares within groups.
Repeated measures analysis of variance
As with ANOVA, repeated measures ANOVA analyses the equality of means of three or more groups. However, a repeated measure ANOVA is used when all variables of a sample are measured under different conditions or at different points in time.
As the variables are measured from a sample at different points of time, the measurement of the dependent variable is repeated. Using a standard ANOVA in this case is not appropriate because it fails to model the correlation between the repeated measures: The data violate the ANOVA assumption of independence. Hence, in the measurement of repeated dependent variables, repeated measures ANOVA should be used.
Non-parametric tests
When the assumptions of normality are not met, and the sample means are not normally, distributed parametric tests can lead to erroneous results. Non-parametric tests (distribution-free test) are used in such situation as they do not require the normality assumption.[ 15 ] Non-parametric tests may fail to detect a significant difference when compared with a parametric test. That is, they usually have less power.
As is done for the parametric tests, the test statistic is compared with known values for the sampling distribution of that statistic and the null hypothesis is accepted or rejected. The types of non-parametric analysis techniques and the corresponding parametric analysis techniques are delineated in Table 5 .
Analogue of parametric and non-parametric tests

Median test for one sample: The sign test and Wilcoxon's signed rank test
The sign test and Wilcoxon's signed rank test are used for median tests of one sample. These tests examine whether one instance of sample data is greater or smaller than the median reference value.
This test examines the hypothesis about the median θ0 of a population. It tests the null hypothesis H0 = θ0. When the observed value (Xi) is greater than the reference value (θ0), it is marked as+. If the observed value is smaller than the reference value, it is marked as − sign. If the observed value is equal to the reference value (θ0), it is eliminated from the sample.
If the null hypothesis is true, there will be an equal number of + signs and − signs.
The sign test ignores the actual values of the data and only uses + or − signs. Therefore, it is useful when it is difficult to measure the values.
Wilcoxon's signed rank test
There is a major limitation of sign test as we lose the quantitative information of the given data and merely use the + or – signs. Wilcoxon's signed rank test not only examines the observed values in comparison with θ0 but also takes into consideration the relative sizes, adding more statistical power to the test. As in the sign test, if there is an observed value that is equal to the reference value θ0, this observed value is eliminated from the sample.
Wilcoxon's rank sum test ranks all data points in order, calculates the rank sum of each sample and compares the difference in the rank sums.
Mann-Whitney test
It is used to test the null hypothesis that two samples have the same median or, alternatively, whether observations in one sample tend to be larger than observations in the other.
Mann–Whitney test compares all data (xi) belonging to the X group and all data (yi) belonging to the Y group and calculates the probability of xi being greater than yi: P (xi > yi). The null hypothesis states that P (xi > yi) = P (xi < yi) =1/2 while the alternative hypothesis states that P (xi > yi) ≠1/2.
Kolmogorov-Smirnov test
The two-sample Kolmogorov-Smirnov (KS) test was designed as a generic method to test whether two random samples are drawn from the same distribution. The null hypothesis of the KS test is that both distributions are identical. The statistic of the KS test is a distance between the two empirical distributions, computed as the maximum absolute difference between their cumulative curves.
Kruskal-Wallis test
The Kruskal–Wallis test is a non-parametric test to analyse the variance.[ 14 ] It analyses if there is any difference in the median values of three or more independent samples. The data values are ranked in an increasing order, and the rank sums calculated followed by calculation of the test statistic.
Jonckheere test
In contrast to Kruskal–Wallis test, in Jonckheere test, there is an a priori ordering that gives it a more statistical power than the Kruskal–Wallis test.[ 14 ]
Friedman test
The Friedman test is a non-parametric test for testing the difference between several related samples. The Friedman test is an alternative for repeated measures ANOVAs which is used when the same parameter has been measured under different conditions on the same subjects.[ 13 ]
Tests to analyse the categorical data
Chi-square test, Fischer's exact test and McNemar's test are used to analyse the categorical or nominal variables. The Chi-square test compares the frequencies and tests whether the observed data differ significantly from that of the expected data if there were no differences between groups (i.e., the null hypothesis). It is calculated by the sum of the squared difference between observed ( O ) and the expected ( E ) data (or the deviation, d ) divided by the expected data by the following formula:

A Yates correction factor is used when the sample size is small. Fischer's exact test is used to determine if there are non-random associations between two categorical variables. It does not assume random sampling, and instead of referring a calculated statistic to a sampling distribution, it calculates an exact probability. McNemar's test is used for paired nominal data. It is applied to 2 × 2 table with paired-dependent samples. It is used to determine whether the row and column frequencies are equal (that is, whether there is ‘marginal homogeneity’). The null hypothesis is that the paired proportions are equal. The Mantel-Haenszel Chi-square test is a multivariate test as it analyses multiple grouping variables. It stratifies according to the nominated confounding variables and identifies any that affects the primary outcome variable. If the outcome variable is dichotomous, then logistic regression is used.
SOFTWARES AVAILABLE FOR STATISTICS, SAMPLE SIZE CALCULATION AND POWER ANALYSIS
Numerous statistical software systems are available currently. The commonly used software systems are Statistical Package for the Social Sciences (SPSS – manufactured by IBM corporation), Statistical Analysis System ((SAS – developed by SAS Institute North Carolina, United States of America), R (designed by Ross Ihaka and Robert Gentleman from R core team), Minitab (developed by Minitab Inc), Stata (developed by StataCorp) and the MS Excel (developed by Microsoft).
There are a number of web resources which are related to statistical power analyses. A few are:
- StatPages.net – provides links to a number of online power calculators
- G-Power – provides a downloadable power analysis program that runs under DOS
- Power analysis for ANOVA designs an interactive site that calculates power or sample size needed to attain a given power for one effect in a factorial ANOVA design
- SPSS makes a program called SamplePower. It gives an output of a complete report on the computer screen which can be cut and paste into another document.
It is important that a researcher knows the concepts of the basic statistical methods used for conduct of a research study. This will help to conduct an appropriately well-designed study leading to valid and reliable results. Inappropriate use of statistical techniques may lead to faulty conclusions, inducing errors and undermining the significance of the article. Bad statistics may lead to bad research, and bad research may lead to unethical practice. Hence, an adequate knowledge of statistics and the appropriate use of statistical tests are important. An appropriate knowledge about the basic statistical methods will go a long way in improving the research designs and producing quality medical research which can be utilised for formulating the evidence-based guidelines.
Financial support and sponsorship
Conflicts of interest.
There are no conflicts of interest.
Introducing the Data and Research Methods track
This summer, HKS announced the introduction of a new Data and Research Methods track—a Science, Technology, Engineering, and Mathematics (STEM)-designated pathway—for MPP , MPA , and MC/MPA students. (The MPA/ID Program is already STEM-designated.)
Students who pursue the Data and Research Methods track will build quantitative analysis and research methodology skills by successfully completing at least 16 credits from a list of qualifying courses.
As a prospective student, you may have questions about what this new track means for your potential academic future at HKS. Read on for answers to some frequently asked questions about this new offering.
Do I need to apply for the Data and Research Methods track when applying?
No, you do not need to take any action at the point of application. You will have an opportunity to declare interest in this track once you have been admitted to and enroll at HKS.
What are the requirements to pursue the Data and Research Methods track?
To complete the Data and Research Methods track, you:
- Must be an MPP, MPA, or MC/MPA student
- Enroll in and fulfill all MPP, MPA, or MC/MPA Program degree requirements
- Complete at least 16 course credits from a list of qualifying courses
- Must complete at least 4 credits from Group A of the qualifying courses (quantitative analysis) and at least 4 credits from Group B (research methods)
- Earn a minimum grade of B- or better in each qualifying course
What courses qualify for the Data and Research Methods track?
The qualifying courses are subject to change based on the course catalogue each year, but here are some examples from the 2023-2024 academic year.
Group A (Quantitative Analysis)
- Introduction to Budgeting and Financial Management
- Machine Learning & Big Data Analytics
- Data and Information Visualization
- Politics and Policies: What Can Data Tell Us?
- Energy and Environmental Economics and Policy
- City Politics Field Lab: Political Representation and Accountability
Group B (Research Methods)
- Thinking Analytically in an Uncertain World
- Emerging Tech: Security, Strategy, and Risk
- Mixed Methods Analytics for Leaders & Policymakers
- Confronting Climate Change: A Foundation in Science, Technology and Policy
- Technology and Public Interest: From Democracy to Technocracy and Back
- Science of Behavior Change

Get to know HKS: Admissions & Financial Aid Coordinator Gareth Jones
Master of Science in Threat and Response Management
Introduction to statistics and research methods bootcamp.
The Statistics and Research Methods Bootcamp will provide a foundation for the use of statistics in data analysis and offer students an introduction to quantitative and qualitative methods in support of program coursework and the completion of a Capstone project.
Topics covered include: formulating a research question, identifying data sources, engaging in a literature review, acquiring IRB approval, understanding statistics in context, developing research projects, and analyzing and interpreting quantitative and qualitative data.
- Python for Data Science
- Statistics for Data Science
- Decision-Making and Risk Management
Thank you for visiting nature.com. You are using a browser version with limited support for CSS. To obtain the best experience, we recommend you use a more up to date browser (or turn off compatibility mode in Internet Explorer). In the meantime, to ensure continued support, we are displaying the site without styles and JavaScript.
- View all journals
- My Account Login
- Explore content
- About the journal
- Publish with us
- Sign up for alerts
- Open access
- Published: 26 March 2024
Predicting and improving complex beer flavor through machine learning
- Michiel Schreurs ORCID: orcid.org/0000-0002-9449-5619 1 , 2 , 3 na1 ,
- Supinya Piampongsant 1 , 2 , 3 na1 ,
- Miguel Roncoroni ORCID: orcid.org/0000-0001-7461-1427 1 , 2 , 3 na1 ,
- Lloyd Cool ORCID: orcid.org/0000-0001-9936-3124 1 , 2 , 3 , 4 ,
- Beatriz Herrera-Malaver ORCID: orcid.org/0000-0002-5096-9974 1 , 2 , 3 ,
- Christophe Vanderaa ORCID: orcid.org/0000-0001-7443-5427 4 ,
- Florian A. Theßeling 1 , 2 , 3 ,
- Łukasz Kreft ORCID: orcid.org/0000-0001-7620-4657 5 ,
- Alexander Botzki ORCID: orcid.org/0000-0001-6691-4233 5 ,
- Philippe Malcorps 6 ,
- Luk Daenen 6 ,
- Tom Wenseleers ORCID: orcid.org/0000-0002-1434-861X 4 &
- Kevin J. Verstrepen ORCID: orcid.org/0000-0002-3077-6219 1 , 2 , 3
Nature Communications volume 15 , Article number: 2368 ( 2024 ) Cite this article
50k Accesses
850 Altmetric
Metrics details
- Chemical engineering
- Gas chromatography
- Machine learning
- Metabolomics
- Taste receptors
The perception and appreciation of food flavor depends on many interacting chemical compounds and external factors, and therefore proves challenging to understand and predict. Here, we combine extensive chemical and sensory analyses of 250 different beers to train machine learning models that allow predicting flavor and consumer appreciation. For each beer, we measure over 200 chemical properties, perform quantitative descriptive sensory analysis with a trained tasting panel and map data from over 180,000 consumer reviews to train 10 different machine learning models. The best-performing algorithm, Gradient Boosting, yields models that significantly outperform predictions based on conventional statistics and accurately predict complex food features and consumer appreciation from chemical profiles. Model dissection allows identifying specific and unexpected compounds as drivers of beer flavor and appreciation. Adding these compounds results in variants of commercial alcoholic and non-alcoholic beers with improved consumer appreciation. Together, our study reveals how big data and machine learning uncover complex links between food chemistry, flavor and consumer perception, and lays the foundation to develop novel, tailored foods with superior flavors.
Similar content being viewed by others
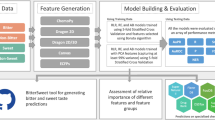
BitterSweet: Building machine learning models for predicting the bitter and sweet taste of small molecules
Rudraksh Tuwani, Somin Wadhwa & Ganesh Bagler
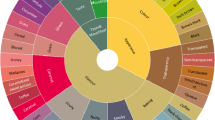
Sensory lexicon and aroma volatiles analysis of brewing malt
Xiaoxia Su, Miao Yu, … Tianyi Du
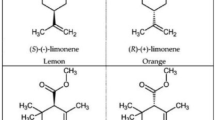
Predicting odor from molecular structure: a multi-label classification approach
Kushagra Saini & Venkatnarayan Ramanathan
Introduction
Predicting and understanding food perception and appreciation is one of the major challenges in food science. Accurate modeling of food flavor and appreciation could yield important opportunities for both producers and consumers, including quality control, product fingerprinting, counterfeit detection, spoilage detection, and the development of new products and product combinations (food pairing) 1 , 2 , 3 , 4 , 5 , 6 . Accurate models for flavor and consumer appreciation would contribute greatly to our scientific understanding of how humans perceive and appreciate flavor. Moreover, accurate predictive models would also facilitate and standardize existing food assessment methods and could supplement or replace assessments by trained and consumer tasting panels, which are variable, expensive and time-consuming 7 , 8 , 9 . Lastly, apart from providing objective, quantitative, accurate and contextual information that can help producers, models can also guide consumers in understanding their personal preferences 10 .
Despite the myriad of applications, predicting food flavor and appreciation from its chemical properties remains a largely elusive goal in sensory science, especially for complex food and beverages 11 , 12 . A key obstacle is the immense number of flavor-active chemicals underlying food flavor. Flavor compounds can vary widely in chemical structure and concentration, making them technically challenging and labor-intensive to quantify, even in the face of innovations in metabolomics, such as non-targeted metabolic fingerprinting 13 , 14 . Moreover, sensory analysis is perhaps even more complicated. Flavor perception is highly complex, resulting from hundreds of different molecules interacting at the physiochemical and sensorial level. Sensory perception is often non-linear, characterized by complex and concentration-dependent synergistic and antagonistic effects 15 , 16 , 17 , 18 , 19 , 20 , 21 that are further convoluted by the genetics, environment, culture and psychology of consumers 22 , 23 , 24 . Perceived flavor is therefore difficult to measure, with problems of sensitivity, accuracy, and reproducibility that can only be resolved by gathering sufficiently large datasets 25 . Trained tasting panels are considered the prime source of quality sensory data, but require meticulous training, are low throughput and high cost. Public databases containing consumer reviews of food products could provide a valuable alternative, especially for studying appreciation scores, which do not require formal training 25 . Public databases offer the advantage of amassing large amounts of data, increasing the statistical power to identify potential drivers of appreciation. However, public datasets suffer from biases, including a bias in the volunteers that contribute to the database, as well as confounding factors such as price, cult status and psychological conformity towards previous ratings of the product.
Classical multivariate statistics and machine learning methods have been used to predict flavor of specific compounds by, for example, linking structural properties of a compound to its potential biological activities or linking concentrations of specific compounds to sensory profiles 1 , 26 . Importantly, most previous studies focused on predicting organoleptic properties of single compounds (often based on their chemical structure) 27 , 28 , 29 , 30 , 31 , 32 , 33 , thus ignoring the fact that these compounds are present in a complex matrix in food or beverages and excluding complex interactions between compounds. Moreover, the classical statistics commonly used in sensory science 34 , 35 , 36 , 37 , 38 , 39 require a large sample size and sufficient variance amongst predictors to create accurate models. They are not fit for studying an extensive set of hundreds of interacting flavor compounds, since they are sensitive to outliers, have a high tendency to overfit and are less suited for non-linear and discontinuous relationships 40 .
In this study, we combine extensive chemical analyses and sensory data of a set of different commercial beers with machine learning approaches to develop models that predict taste, smell, mouthfeel and appreciation from compound concentrations. Beer is particularly suited to model the relationship between chemistry, flavor and appreciation. First, beer is a complex product, consisting of thousands of flavor compounds that partake in complex sensory interactions 41 , 42 , 43 . This chemical diversity arises from the raw materials (malt, yeast, hops, water and spices) and biochemical conversions during the brewing process (kilning, mashing, boiling, fermentation, maturation and aging) 44 , 45 . Second, the advent of the internet saw beer consumers embrace online review platforms, such as RateBeer (ZX Ventures, Anheuser-Busch InBev SA/NV) and BeerAdvocate (Next Glass, inc.). In this way, the beer community provides massive data sets of beer flavor and appreciation scores, creating extraordinarily large sensory databases to complement the analyses of our professional sensory panel. Specifically, we characterize over 200 chemical properties of 250 commercial beers, spread across 22 beer styles, and link these to the descriptive sensory profiling data of a 16-person in-house trained tasting panel and data acquired from over 180,000 public consumer reviews. These unique and extensive datasets enable us to train a suite of machine learning models to predict flavor and appreciation from a beer’s chemical profile. Dissection of the best-performing models allows us to pinpoint specific compounds as potential drivers of beer flavor and appreciation. Follow-up experiments confirm the importance of these compounds and ultimately allow us to significantly improve the flavor and appreciation of selected commercial beers. Together, our study represents a significant step towards understanding complex flavors and reinforces the value of machine learning to develop and refine complex foods. In this way, it represents a stepping stone for further computer-aided food engineering applications 46 .
To generate a comprehensive dataset on beer flavor, we selected 250 commercial Belgian beers across 22 different beer styles (Supplementary Fig. S1 ). Beers with ≤ 4.2% alcohol by volume (ABV) were classified as non-alcoholic and low-alcoholic. Blonds and Tripels constitute a significant portion of the dataset (12.4% and 11.2%, respectively) reflecting their presence on the Belgian beer market and the heterogeneity of beers within these styles. By contrast, lager beers are less diverse and dominated by a handful of brands. Rare styles such as Brut or Faro make up only a small fraction of the dataset (2% and 1%, respectively) because fewer of these beers are produced and because they are dominated by distinct characteristics in terms of flavor and chemical composition.
Extensive analysis identifies relationships between chemical compounds in beer
For each beer, we measured 226 different chemical properties, including common brewing parameters such as alcohol content, iso-alpha acids, pH, sugar concentration 47 , and over 200 flavor compounds (Methods, Supplementary Table S1 ). A large portion (37.2%) are terpenoids arising from hopping, responsible for herbal and fruity flavors 16 , 48 . A second major category are yeast metabolites, such as esters and alcohols, that result in fruity and solvent notes 48 , 49 , 50 . Other measured compounds are primarily derived from malt, or other microbes such as non- Saccharomyces yeasts and bacteria (‘wild flora’). Compounds that arise from spices or staling are labeled under ‘Others’. Five attributes (caloric value, total acids and total ester, hop aroma and sulfur compounds) are calculated from multiple individually measured compounds.
As a first step in identifying relationships between chemical properties, we determined correlations between the concentrations of the compounds (Fig. 1 , upper panel, Supplementary Data 1 and 2 , and Supplementary Fig. S2 . For the sake of clarity, only a subset of the measured compounds is shown in Fig. 1 ). Compounds of the same origin typically show a positive correlation, while absence of correlation hints at parameters varying independently. For example, the hop aroma compounds citronellol, and alpha-terpineol show moderate correlations with each other (Spearman’s rho=0.39 and 0.57), but not with the bittering hop component iso-alpha acids (Spearman’s rho=0.16 and −0.07). This illustrates how brewers can independently modify hop aroma and bitterness by selecting hop varieties and dosage time. If hops are added early in the boiling phase, chemical conversions increase bitterness while aromas evaporate, conversely, late addition of hops preserves aroma but limits bitterness 51 . Similarly, hop-derived iso-alpha acids show a strong anti-correlation with lactic acid and acetic acid, likely reflecting growth inhibition of lactic acid and acetic acid bacteria, or the consequent use of fewer hops in sour beer styles, such as West Flanders ales and Fruit beers, that rely on these bacteria for their distinct flavors 52 . Finally, yeast-derived esters (ethyl acetate, ethyl decanoate, ethyl hexanoate, ethyl octanoate) and alcohols (ethanol, isoamyl alcohol, isobutanol, and glycerol), correlate with Spearman coefficients above 0.5, suggesting that these secondary metabolites are correlated with the yeast genetic background and/or fermentation parameters and may be difficult to influence individually, although the choice of yeast strain may offer some control 53 .
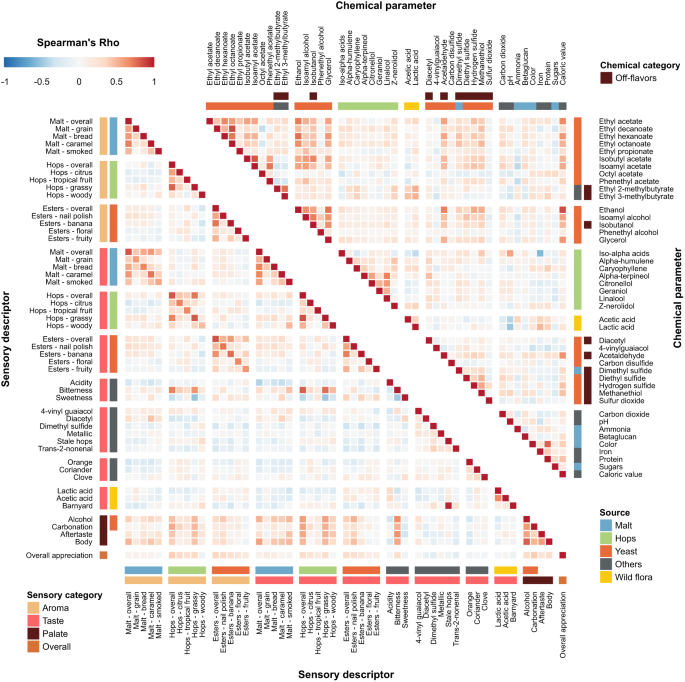
Spearman rank correlations are shown. Descriptors are grouped according to their origin (malt (blue), hops (green), yeast (red), wild flora (yellow), Others (black)), and sensory aspect (aroma, taste, palate, and overall appreciation). Please note that for the chemical compounds, for the sake of clarity, only a subset of the total number of measured compounds is shown, with an emphasis on the key compounds for each source. For more details, see the main text and Methods section. Chemical data can be found in Supplementary Data 1 , correlations between all chemical compounds are depicted in Supplementary Fig. S2 and correlation values can be found in Supplementary Data 2 . See Supplementary Data 4 for sensory panel assessments and Supplementary Data 5 for correlation values between all sensory descriptors.
Interestingly, different beer styles show distinct patterns for some flavor compounds (Supplementary Fig. S3 ). These observations agree with expectations for key beer styles, and serve as a control for our measurements. For instance, Stouts generally show high values for color (darker), while hoppy beers contain elevated levels of iso-alpha acids, compounds associated with bitter hop taste. Acetic and lactic acid are not prevalent in most beers, with notable exceptions such as Kriek, Lambic, Faro, West Flanders ales and Flanders Old Brown, which use acid-producing bacteria ( Lactobacillus and Pediococcus ) or unconventional yeast ( Brettanomyces ) 54 , 55 . Glycerol, ethanol and esters show similar distributions across all beer styles, reflecting their common origin as products of yeast metabolism during fermentation 45 , 53 . Finally, low/no-alcohol beers contain low concentrations of glycerol and esters. This is in line with the production process for most of the low/no-alcohol beers in our dataset, which are produced through limiting fermentation or by stripping away alcohol via evaporation or dialysis, with both methods having the unintended side-effect of reducing the amount of flavor compounds in the final beer 56 , 57 .
Besides expected associations, our data also reveals less trivial associations between beer styles and specific parameters. For example, geraniol and citronellol, two monoterpenoids responsible for citrus, floral and rose flavors and characteristic of Citra hops, are found in relatively high amounts in Christmas, Saison, and Brett/co-fermented beers, where they may originate from terpenoid-rich spices such as coriander seeds instead of hops 58 .
Tasting panel assessments reveal sensorial relationships in beer
To assess the sensory profile of each beer, a trained tasting panel evaluated each of the 250 beers for 50 sensory attributes, including different hop, malt and yeast flavors, off-flavors and spices. Panelists used a tasting sheet (Supplementary Data 3 ) to score the different attributes. Panel consistency was evaluated by repeating 12 samples across different sessions and performing ANOVA. In 95% of cases no significant difference was found across sessions ( p > 0.05), indicating good panel consistency (Supplementary Table S2 ).
Aroma and taste perception reported by the trained panel are often linked (Fig. 1 , bottom left panel and Supplementary Data 4 and 5 ), with high correlations between hops aroma and taste (Spearman’s rho=0.83). Bitter taste was found to correlate with hop aroma and taste in general (Spearman’s rho=0.80 and 0.69), and particularly with “grassy” noble hops (Spearman’s rho=0.75). Barnyard flavor, most often associated with sour beers, is identified together with stale hops (Spearman’s rho=0.97) that are used in these beers. Lactic and acetic acid, which often co-occur, are correlated (Spearman’s rho=0.66). Interestingly, sweetness and bitterness are anti-correlated (Spearman’s rho = −0.48), confirming the hypothesis that they mask each other 59 , 60 . Beer body is highly correlated with alcohol (Spearman’s rho = 0.79), and overall appreciation is found to correlate with multiple aspects that describe beer mouthfeel (alcohol, carbonation; Spearman’s rho= 0.32, 0.39), as well as with hop and ester aroma intensity (Spearman’s rho=0.39 and 0.35).
Similar to the chemical analyses, sensorial analyses confirmed typical features of specific beer styles (Supplementary Fig. S4 ). For example, sour beers (Faro, Flanders Old Brown, Fruit beer, Kriek, Lambic, West Flanders ale) were rated acidic, with flavors of both acetic and lactic acid. Hoppy beers were found to be bitter and showed hop-associated aromas like citrus and tropical fruit. Malt taste is most detected among scotch, stout/porters, and strong ales, while low/no-alcohol beers, which often have a reputation for being ‘worty’ (reminiscent of unfermented, sweet malt extract) appear in the middle. Unsurprisingly, hop aromas are most strongly detected among hoppy beers. Like its chemical counterpart (Supplementary Fig. S3 ), acidity shows a right-skewed distribution, with the most acidic beers being Krieks, Lambics, and West Flanders ales.
Tasting panel assessments of specific flavors correlate with chemical composition
We find that the concentrations of several chemical compounds strongly correlate with specific aroma or taste, as evaluated by the tasting panel (Fig. 2 , Supplementary Fig. S5 , Supplementary Data 6 ). In some cases, these correlations confirm expectations and serve as a useful control for data quality. For example, iso-alpha acids, the bittering compounds in hops, strongly correlate with bitterness (Spearman’s rho=0.68), while ethanol and glycerol correlate with tasters’ perceptions of alcohol and body, the mouthfeel sensation of fullness (Spearman’s rho=0.82/0.62 and 0.72/0.57 respectively) and darker color from roasted malts is a good indication of malt perception (Spearman’s rho=0.54).
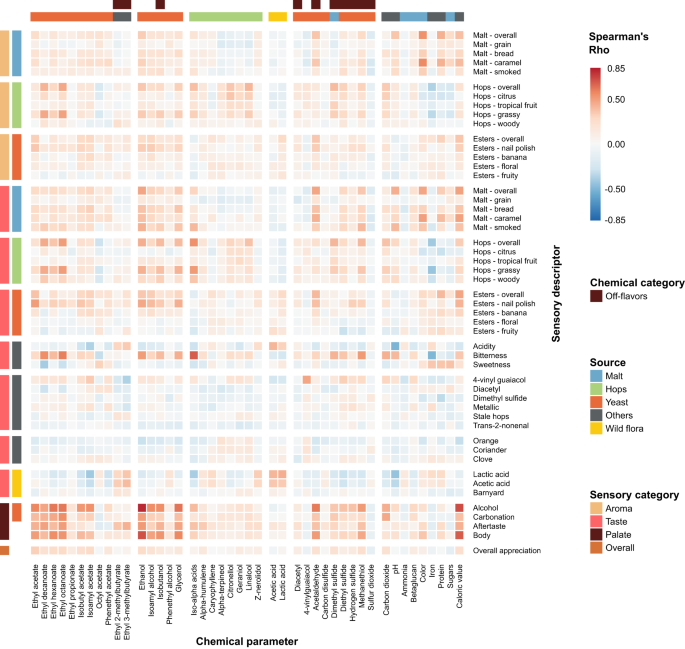
Heatmap colors indicate Spearman’s Rho. Axes are organized according to sensory categories (aroma, taste, mouthfeel, overall), chemical categories and chemical sources in beer (malt (blue), hops (green), yeast (red), wild flora (yellow), Others (black)). See Supplementary Data 6 for all correlation values.
Interestingly, for some relationships between chemical compounds and perceived flavor, correlations are weaker than expected. For example, the rose-smelling phenethyl acetate only weakly correlates with floral aroma. This hints at more complex relationships and interactions between compounds and suggests a need for a more complex model than simple correlations. Lastly, we uncovered unexpected correlations. For instance, the esters ethyl decanoate and ethyl octanoate appear to correlate slightly with hop perception and bitterness, possibly due to their fruity flavor. Iron is anti-correlated with hop aromas and bitterness, most likely because it is also anti-correlated with iso-alpha acids. This could be a sign of metal chelation of hop acids 61 , given that our analyses measure unbound hop acids and total iron content, or could result from the higher iron content in dark and Fruit beers, which typically have less hoppy and bitter flavors 62 .
Public consumer reviews complement expert panel data
To complement and expand the sensory data of our trained tasting panel, we collected 180,000 reviews of our 250 beers from the online consumer review platform RateBeer. This provided numerical scores for beer appearance, aroma, taste, palate, overall quality as well as the average overall score.
Public datasets are known to suffer from biases, such as price, cult status and psychological conformity towards previous ratings of a product. For example, prices correlate with appreciation scores for these online consumer reviews (rho=0.49, Supplementary Fig. S6 ), but not for our trained tasting panel (rho=0.19). This suggests that prices affect consumer appreciation, which has been reported in wine 63 , while blind tastings are unaffected. Moreover, we observe that some beer styles, like lagers and non-alcoholic beers, generally receive lower scores, reflecting that online reviewers are mostly beer aficionados with a preference for specialty beers over lager beers. In general, we find a modest correlation between our trained panel’s overall appreciation score and the online consumer appreciation scores (Fig. 3 , rho=0.29). Apart from the aforementioned biases in the online datasets, serving temperature, sample freshness and surroundings, which are all tightly controlled during the tasting panel sessions, can vary tremendously across online consumers and can further contribute to (among others, appreciation) differences between the two categories of tasters. Importantly, in contrast to the overall appreciation scores, for many sensory aspects the results from the professional panel correlated well with results obtained from RateBeer reviews. Correlations were highest for features that are relatively easy to recognize even for untrained tasters, like bitterness, sweetness, alcohol and malt aroma (Fig. 3 and below).
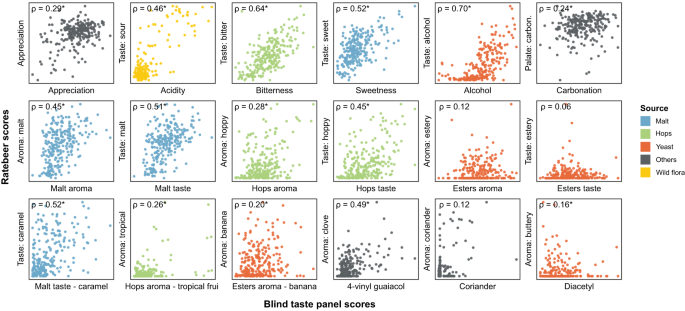
RateBeer text mining results can be found in Supplementary Data 7 . Rho values shown are Spearman correlation values, with asterisks indicating significant correlations ( p < 0.05, two-sided). All p values were smaller than 0.001, except for Esters aroma (0.0553), Esters taste (0.3275), Esters aroma—banana (0.0019), Coriander (0.0508) and Diacetyl (0.0134).
Besides collecting consumer appreciation from these online reviews, we developed automated text analysis tools to gather additional data from review texts (Supplementary Data 7 ). Processing review texts on the RateBeer database yielded comparable results to the scores given by the trained panel for many common sensory aspects, including acidity, bitterness, sweetness, alcohol, malt, and hop tastes (Fig. 3 ). This is in line with what would be expected, since these attributes require less training for accurate assessment and are less influenced by environmental factors such as temperature, serving glass and odors in the environment. Consumer reviews also correlate well with our trained panel for 4-vinyl guaiacol, a compound associated with a very characteristic aroma. By contrast, correlations for more specific aromas like ester, coriander or diacetyl are underrepresented in the online reviews, underscoring the importance of using a trained tasting panel and standardized tasting sheets with explicit factors to be scored for evaluating specific aspects of a beer. Taken together, our results suggest that public reviews are trustworthy for some, but not all, flavor features and can complement or substitute taste panel data for these sensory aspects.
Models can predict beer sensory profiles from chemical data
The rich datasets of chemical analyses, tasting panel assessments and public reviews gathered in the first part of this study provided us with a unique opportunity to develop predictive models that link chemical data to sensorial features. Given the complexity of beer flavor, basic statistical tools such as correlations or linear regression may not always be the most suitable for making accurate predictions. Instead, we applied different machine learning models that can model both simple linear and complex interactive relationships. Specifically, we constructed a set of regression models to predict (a) trained panel scores for beer flavor and quality and (b) public reviews’ appreciation scores from beer chemical profiles. We trained and tested 10 different models (Methods), 3 linear regression-based models (simple linear regression with first-order interactions (LR), lasso regression with first-order interactions (Lasso), partial least squares regressor (PLSR)), 5 decision tree models (AdaBoost regressor (ABR), extra trees (ET), gradient boosting regressor (GBR), random forest (RF) and XGBoost regressor (XGBR)), 1 support vector regression (SVR), and 1 artificial neural network (ANN) model.
To compare the performance of our machine learning models, the dataset was randomly split into a training and test set, stratified by beer style. After a model was trained on data in the training set, its performance was evaluated on its ability to predict the test dataset obtained from multi-output models (based on the coefficient of determination, see Methods). Additionally, individual-attribute models were ranked per descriptor and the average rank was calculated, as proposed by Korneva et al. 64 . Importantly, both ways of evaluating the models’ performance agreed in general. Performance of the different models varied (Table 1 ). It should be noted that all models perform better at predicting RateBeer results than results from our trained tasting panel. One reason could be that sensory data is inherently variable, and this variability is averaged out with the large number of public reviews from RateBeer. Additionally, all tree-based models perform better at predicting taste than aroma. Linear models (LR) performed particularly poorly, with negative R 2 values, due to severe overfitting (training set R 2 = 1). Overfitting is a common issue in linear models with many parameters and limited samples, especially with interaction terms further amplifying the number of parameters. L1 regularization (Lasso) successfully overcomes this overfitting, out-competing multiple tree-based models on the RateBeer dataset. Similarly, the dimensionality reduction of PLSR avoids overfitting and improves performance, to some extent. Still, tree-based models (ABR, ET, GBR, RF and XGBR) show the best performance, out-competing the linear models (LR, Lasso, PLSR) commonly used in sensory science 65 .
GBR models showed the best overall performance in predicting sensory responses from chemical information, with R 2 values up to 0.75 depending on the predicted sensory feature (Supplementary Table S4 ). The GBR models predict consumer appreciation (RateBeer) better than our trained panel’s appreciation (R 2 value of 0.67 compared to R 2 value of 0.09) (Supplementary Table S3 and Supplementary Table S4 ). ANN models showed intermediate performance, likely because neural networks typically perform best with larger datasets 66 . The SVR shows intermediate performance, mostly due to the weak predictions of specific attributes that lower the overall performance (Supplementary Table S4 ).
Model dissection identifies specific, unexpected compounds as drivers of consumer appreciation
Next, we leveraged our models to infer important contributors to sensory perception and consumer appreciation. Consumer preference is a crucial sensory aspects, because a product that shows low consumer appreciation scores often does not succeed commercially 25 . Additionally, the requirement for a large number of representative evaluators makes consumer trials one of the more costly and time-consuming aspects of product development. Hence, a model for predicting chemical drivers of overall appreciation would be a welcome addition to the available toolbox for food development and optimization.
Since GBR models on our RateBeer dataset showed the best overall performance, we focused on these models. Specifically, we used two approaches to identify important contributors. First, rankings of the most important predictors for each sensorial trait in the GBR models were obtained based on impurity-based feature importance (mean decrease in impurity). High-ranked parameters were hypothesized to be either the true causal chemical properties underlying the trait, to correlate with the actual causal properties, or to take part in sensory interactions affecting the trait 67 (Fig. 4A ). In a second approach, we used SHAP 68 to determine which parameters contributed most to the model for making predictions of consumer appreciation (Fig. 4B ). SHAP calculates parameter contributions to model predictions on a per-sample basis, which can be aggregated into an importance score.
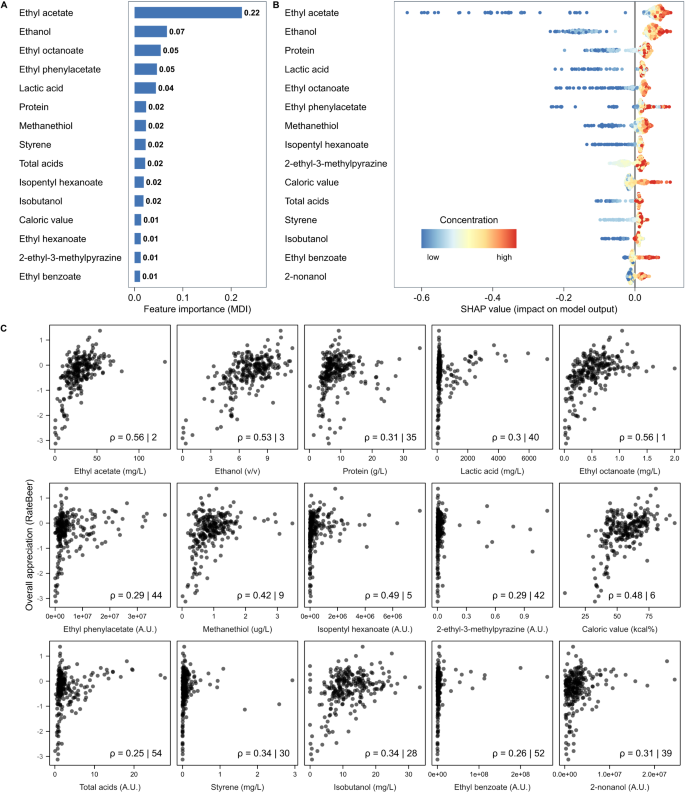
A The impurity-based feature importance (mean deviance in impurity, MDI) calculated from the Gradient Boosting Regression (GBR) model predicting RateBeer appreciation scores. The top 15 highest ranked chemical properties are shown. B SHAP summary plot for the top 15 parameters contributing to our GBR model. Each point on the graph represents a sample from our dataset. The color represents the concentration of that parameter, with bluer colors representing low values and redder colors representing higher values. Greater absolute values on the horizontal axis indicate a higher impact of the parameter on the prediction of the model. C Spearman correlations between the 15 most important chemical properties and consumer overall appreciation. Numbers indicate the Spearman Rho correlation coefficient, and the rank of this correlation compared to all other correlations. The top 15 important compounds were determined using SHAP (panel B).
Both approaches identified ethyl acetate as the most predictive parameter for beer appreciation (Fig. 4 ). Ethyl acetate is the most abundant ester in beer with a typical ‘fruity’, ‘solvent’ and ‘alcoholic’ flavor, but is often considered less important than other esters like isoamyl acetate. The second most important parameter identified by SHAP is ethanol, the most abundant beer compound after water. Apart from directly contributing to beer flavor and mouthfeel, ethanol drastically influences the physical properties of beer, dictating how easily volatile compounds escape the beer matrix to contribute to beer aroma 69 . Importantly, it should also be noted that the importance of ethanol for appreciation is likely inflated by the very low appreciation scores of non-alcoholic beers (Supplementary Fig. S4 ). Despite not often being considered a driver of beer appreciation, protein level also ranks highly in both approaches, possibly due to its effect on mouthfeel and body 70 . Lactic acid, which contributes to the tart taste of sour beers, is the fourth most important parameter identified by SHAP, possibly due to the generally high appreciation of sour beers in our dataset.
Interestingly, some of the most important predictive parameters for our model are not well-established as beer flavors or are even commonly regarded as being negative for beer quality. For example, our models identify methanethiol and ethyl phenyl acetate, an ester commonly linked to beer staling 71 , as a key factor contributing to beer appreciation. Although there is no doubt that high concentrations of these compounds are considered unpleasant, the positive effects of modest concentrations are not yet known 72 , 73 .
To compare our approach to conventional statistics, we evaluated how well the 15 most important SHAP-derived parameters correlate with consumer appreciation (Fig. 4C ). Interestingly, only 6 of the properties derived by SHAP rank amongst the top 15 most correlated parameters. For some chemical compounds, the correlations are so low that they would have likely been considered unimportant. For example, lactic acid, the fourth most important parameter, shows a bimodal distribution for appreciation, with sour beers forming a separate cluster, that is missed entirely by the Spearman correlation. Additionally, the correlation plots reveal outliers, emphasizing the need for robust analysis tools. Together, this highlights the need for alternative models, like the Gradient Boosting model, that better grasp the complexity of (beer) flavor.
Finally, to observe the relationships between these chemical properties and their predicted targets, partial dependence plots were constructed for the six most important predictors of consumer appreciation 74 , 75 , 76 (Supplementary Fig. S7 ). One-way partial dependence plots show how a change in concentration affects the predicted appreciation. These plots reveal an important limitation of our models: appreciation predictions remain constant at ever-increasing concentrations. This implies that once a threshold concentration is reached, further increasing the concentration does not affect appreciation. This is false, as it is well-documented that certain compounds become unpleasant at high concentrations, including ethyl acetate (‘nail polish’) 77 and methanethiol (‘sulfury’ and ‘rotten cabbage’) 78 . The inability of our models to grasp that flavor compounds have optimal levels, above which they become negative, is a consequence of working with commercial beer brands where (off-)flavors are rarely too high to negatively impact the product. The two-way partial dependence plots show how changing the concentration of two compounds influences predicted appreciation, visualizing their interactions (Supplementary Fig. S7 ). In our case, the top 5 parameters are dominated by additive or synergistic interactions, with high concentrations for both compounds resulting in the highest predicted appreciation.
To assess the robustness of our best-performing models and model predictions, we performed 100 iterations of the GBR, RF and ET models. In general, all iterations of the models yielded similar performance (Supplementary Fig. S8 ). Moreover, the main predictors (including the top predictors ethanol and ethyl acetate) remained virtually the same, especially for GBR and RF. For the iterations of the ET model, we did observe more variation in the top predictors, which is likely a consequence of the model’s inherent random architecture in combination with co-correlations between certain predictors. However, even in this case, several of the top predictors (ethanol and ethyl acetate) remain unchanged, although their rank in importance changes (Supplementary Fig. S8 ).
Next, we investigated if a combination of RateBeer and trained panel data into one consolidated dataset would lead to stronger models, under the hypothesis that such a model would suffer less from bias in the datasets. A GBR model was trained to predict appreciation on the combined dataset. This model underperformed compared to the RateBeer model, both in the native case and when including a dataset identifier (R 2 = 0.67, 0.26 and 0.42 respectively). For the latter, the dataset identifier is the most important feature (Supplementary Fig. S9 ), while most of the feature importance remains unchanged, with ethyl acetate and ethanol ranking highest, like in the original model trained only on RateBeer data. It seems that the large variation in the panel dataset introduces noise, weakening the models’ performances and reliability. In addition, it seems reasonable to assume that both datasets are fundamentally different, with the panel dataset obtained by blind tastings by a trained professional panel.
Lastly, we evaluated whether beer style identifiers would further enhance the model’s performance. A GBR model was trained with parameters that explicitly encoded the styles of the samples. This did not improve model performance (R2 = 0.66 with style information vs R2 = 0.67). The most important chemical features are consistent with the model trained without style information (eg. ethanol and ethyl acetate), and with the exception of the most preferred (strong ale) and least preferred (low/no-alcohol) styles, none of the styles were among the most important features (Supplementary Fig. S9 , Supplementary Table S5 and S6 ). This is likely due to a combination of style-specific chemical signatures, such as iso-alpha acids and lactic acid, that implicitly convey style information to the original models, as well as the low number of samples belonging to some styles, making it difficult for the model to learn style-specific patterns. Moreover, beer styles are not rigorously defined, with some styles overlapping in features and some beers being misattributed to a specific style, all of which leads to more noise in models that use style parameters.
Model validation
To test if our predictive models give insight into beer appreciation, we set up experiments aimed at improving existing commercial beers. We specifically selected overall appreciation as the trait to be examined because of its complexity and commercial relevance. Beer flavor comprises a complex bouquet rather than single aromas and tastes 53 . Hence, adding a single compound to the extent that a difference is noticeable may lead to an unbalanced, artificial flavor. Therefore, we evaluated the effect of combinations of compounds. Because Blond beers represent the most extensive style in our dataset, we selected a beer from this style as the starting material for these experiments (Beer 64 in Supplementary Data 1 ).
In the first set of experiments, we adjusted the concentrations of compounds that made up the most important predictors of overall appreciation (ethyl acetate, ethanol, lactic acid, ethyl phenyl acetate) together with correlated compounds (ethyl hexanoate, isoamyl acetate, glycerol), bringing them up to 95 th percentile ethanol-normalized concentrations (Methods) within the Blond group (‘Spiked’ concentration in Fig. 5A ). Compared to controls, the spiked beers were found to have significantly improved overall appreciation among trained panelists, with panelist noting increased intensity of ester flavors, sweetness, alcohol, and body fullness (Fig. 5B ). To disentangle the contribution of ethanol to these results, a second experiment was performed without the addition of ethanol. This resulted in a similar outcome, including increased perception of alcohol and overall appreciation.
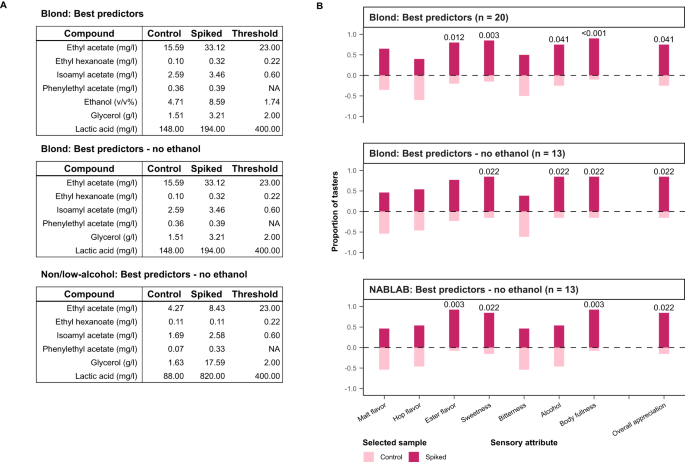
Adding the top chemical compounds, identified as best predictors of appreciation by our model, into poorly appreciated beers results in increased appreciation from our trained panel. Results of sensory tests between base beers and those spiked with compounds identified as the best predictors by the model. A Blond and Non/Low-alcohol (0.0% ABV) base beers were brought up to 95th-percentile ethanol-normalized concentrations within each style. B For each sensory attribute, tasters indicated the more intense sample and selected the sample they preferred. The numbers above the bars correspond to the p values that indicate significant changes in perceived flavor (two-sided binomial test: alpha 0.05, n = 20 or 13).
In a last experiment, we tested whether using the model’s predictions can boost the appreciation of a non-alcoholic beer (beer 223 in Supplementary Data 1 ). Again, the addition of a mixture of predicted compounds (omitting ethanol, in this case) resulted in a significant increase in appreciation, body, ester flavor and sweetness.
Predicting flavor and consumer appreciation from chemical composition is one of the ultimate goals of sensory science. A reliable, systematic and unbiased way to link chemical profiles to flavor and food appreciation would be a significant asset to the food and beverage industry. Such tools would substantially aid in quality control and recipe development, offer an efficient and cost-effective alternative to pilot studies and consumer trials and would ultimately allow food manufacturers to produce superior, tailor-made products that better meet the demands of specific consumer groups more efficiently.
A limited set of studies have previously tried, to varying degrees of success, to predict beer flavor and beer popularity based on (a limited set of) chemical compounds and flavors 79 , 80 . Current sensitive, high-throughput technologies allow measuring an unprecedented number of chemical compounds and properties in a large set of samples, yielding a dataset that can train models that help close the gaps between chemistry and flavor, even for a complex natural product like beer. To our knowledge, no previous research gathered data at this scale (250 samples, 226 chemical parameters, 50 sensory attributes and 5 consumer scores) to disentangle and validate the chemical aspects driving beer preference using various machine-learning techniques. We find that modern machine learning models outperform conventional statistical tools, such as correlations and linear models, and can successfully predict flavor appreciation from chemical composition. This could be attributed to the natural incorporation of interactions and non-linear or discontinuous effects in machine learning models, which are not easily grasped by the linear model architecture. While linear models and partial least squares regression represent the most widespread statistical approaches in sensory science, in part because they allow interpretation 65 , 81 , 82 , modern machine learning methods allow for building better predictive models while preserving the possibility to dissect and exploit the underlying patterns. Of the 10 different models we trained, tree-based models, such as our best performing GBR, showed the best overall performance in predicting sensory responses from chemical information, outcompeting artificial neural networks. This agrees with previous reports for models trained on tabular data 83 . Our results are in line with the findings of Colantonio et al. who also identified the gradient boosting architecture as performing best at predicting appreciation and flavor (of tomatoes and blueberries, in their specific study) 26 . Importantly, besides our larger experimental scale, we were able to directly confirm our models’ predictions in vivo.
Our study confirms that flavor compound concentration does not always correlate with perception, suggesting complex interactions that are often missed by more conventional statistics and simple models. Specifically, we find that tree-based algorithms may perform best in developing models that link complex food chemistry with aroma. Furthermore, we show that massive datasets of untrained consumer reviews provide a valuable source of data, that can complement or even replace trained tasting panels, especially for appreciation and basic flavors, such as sweetness and bitterness. This holds despite biases that are known to occur in such datasets, such as price or conformity bias. Moreover, GBR models predict taste better than aroma. This is likely because taste (e.g. bitterness) often directly relates to the corresponding chemical measurements (e.g., iso-alpha acids), whereas such a link is less clear for aromas, which often result from the interplay between multiple volatile compounds. We also find that our models are best at predicting acidity and alcohol, likely because there is a direct relation between the measured chemical compounds (acids and ethanol) and the corresponding perceived sensorial attribute (acidity and alcohol), and because even untrained consumers are generally able to recognize these flavors and aromas.
The predictions of our final models, trained on review data, hold even for blind tastings with small groups of trained tasters, as demonstrated by our ability to validate specific compounds as drivers of beer flavor and appreciation. Since adding a single compound to the extent of a noticeable difference may result in an unbalanced flavor profile, we specifically tested our identified key drivers as a combination of compounds. While this approach does not allow us to validate if a particular single compound would affect flavor and/or appreciation, our experiments do show that this combination of compounds increases consumer appreciation.
It is important to stress that, while it represents an important step forward, our approach still has several major limitations. A key weakness of the GBR model architecture is that amongst co-correlating variables, the largest main effect is consistently preferred for model building. As a result, co-correlating variables often have artificially low importance scores, both for impurity and SHAP-based methods, like we observed in the comparison to the more randomized Extra Trees models. This implies that chemicals identified as key drivers of a specific sensory feature by GBR might not be the true causative compounds, but rather co-correlate with the actual causative chemical. For example, the high importance of ethyl acetate could be (partially) attributed to the total ester content, ethanol or ethyl hexanoate (rho=0.77, rho=0.72 and rho=0.68), while ethyl phenylacetate could hide the importance of prenyl isobutyrate and ethyl benzoate (rho=0.77 and rho=0.76). Expanding our GBR model to include beer style as a parameter did not yield additional power or insight. This is likely due to style-specific chemical signatures, such as iso-alpha acids and lactic acid, that implicitly convey style information to the original model, as well as the smaller sample size per style, limiting the power to uncover style-specific patterns. This can be partly attributed to the curse of dimensionality, where the high number of parameters results in the models mainly incorporating single parameter effects, rather than complex interactions such as style-dependent effects 67 . A larger number of samples may overcome some of these limitations and offer more insight into style-specific effects. On the other hand, beer style is not a rigid scientific classification, and beers within one style often differ a lot, which further complicates the analysis of style as a model factor.
Our study is limited to beers from Belgian breweries. Although these beers cover a large portion of the beer styles available globally, some beer styles and consumer patterns may be missing, while other features might be overrepresented. For example, many Belgian ales exhibit yeast-driven flavor profiles, which is reflected in the chemical drivers of appreciation discovered by this study. In future work, expanding the scope to include diverse markets and beer styles could lead to the identification of even more drivers of appreciation and better models for special niche products that were not present in our beer set.
In addition to inherent limitations of GBR models, there are also some limitations associated with studying food aroma. Even if our chemical analyses measured most of the known aroma compounds, the total number of flavor compounds in complex foods like beer is still larger than the subset we were able to measure in this study. For example, hop-derived thiols, that influence flavor at very low concentrations, are notoriously difficult to measure in a high-throughput experiment. Moreover, consumer perception remains subjective and prone to biases that are difficult to avoid. It is also important to stress that the models are still immature and that more extensive datasets will be crucial for developing more complete models in the future. Besides more samples and parameters, our dataset does not include any demographic information about the tasters. Including such data could lead to better models that grasp external factors like age and culture. Another limitation is that our set of beers consists of high-quality end-products and lacks beers that are unfit for sale, which limits the current model in accurately predicting products that are appreciated very badly. Finally, while models could be readily applied in quality control, their use in sensory science and product development is restrained by their inability to discern causal relationships. Given that the models cannot distinguish compounds that genuinely drive consumer perception from those that merely correlate, validation experiments are essential to identify true causative compounds.
Despite the inherent limitations, dissection of our models enabled us to pinpoint specific molecules as potential drivers of beer aroma and consumer appreciation, including compounds that were unexpected and would not have been identified using standard approaches. Important drivers of beer appreciation uncovered by our models include protein levels, ethyl acetate, ethyl phenyl acetate and lactic acid. Currently, many brewers already use lactic acid to acidify their brewing water and ensure optimal pH for enzymatic activity during the mashing process. Our results suggest that adding lactic acid can also improve beer appreciation, although its individual effect remains to be tested. Interestingly, ethanol appears to be unnecessary to improve beer appreciation, both for blond beer and alcohol-free beer. Given the growing consumer interest in alcohol-free beer, with a predicted annual market growth of >7% 84 , it is relevant for brewers to know what compounds can further increase consumer appreciation of these beers. Hence, our model may readily provide avenues to further improve the flavor and consumer appreciation of both alcoholic and non-alcoholic beers, which is generally considered one of the key challenges for future beer production.
Whereas we see a direct implementation of our results for the development of superior alcohol-free beverages and other food products, our study can also serve as a stepping stone for the development of novel alcohol-containing beverages. We want to echo the growing body of scientific evidence for the negative effects of alcohol consumption, both on the individual level by the mutagenic, teratogenic and carcinogenic effects of ethanol 85 , 86 , as well as the burden on society caused by alcohol abuse and addiction. We encourage the use of our results for the production of healthier, tastier products, including novel and improved beverages with lower alcohol contents. Furthermore, we strongly discourage the use of these technologies to improve the appreciation or addictive properties of harmful substances.
The present work demonstrates that despite some important remaining hurdles, combining the latest developments in chemical analyses, sensory analysis and modern machine learning methods offers exciting avenues for food chemistry and engineering. Soon, these tools may provide solutions in quality control and recipe development, as well as new approaches to sensory science and flavor research.
Beer selection
250 commercial Belgian beers were selected to cover the broad diversity of beer styles and corresponding diversity in chemical composition and aroma. See Supplementary Fig. S1 .
Chemical dataset
Sample preparation.
Beers within their expiration date were purchased from commercial retailers. Samples were prepared in biological duplicates at room temperature, unless explicitly stated otherwise. Bottle pressure was measured with a manual pressure device (Steinfurth Mess-Systeme GmbH) and used to calculate CO 2 concentration. The beer was poured through two filter papers (Macherey-Nagel, 500713032 MN 713 ¼) to remove carbon dioxide and prevent spontaneous foaming. Samples were then prepared for measurements by targeted Headspace-Gas Chromatography-Flame Ionization Detector/Flame Photometric Detector (HS-GC-FID/FPD), Headspace-Solid Phase Microextraction-Gas Chromatography-Mass Spectrometry (HS-SPME-GC-MS), colorimetric analysis, enzymatic analysis, Near-Infrared (NIR) analysis, as described in the sections below. The mean values of biological duplicates are reported for each compound.
HS-GC-FID/FPD
HS-GC-FID/FPD (Shimadzu GC 2010 Plus) was used to measure higher alcohols, acetaldehyde, esters, 4-vinyl guaicol, and sulfur compounds. Each measurement comprised 5 ml of sample pipetted into a 20 ml glass vial containing 1.75 g NaCl (VWR, 27810.295). 100 µl of 2-heptanol (Sigma-Aldrich, H3003) (internal standard) solution in ethanol (Fisher Chemical, E/0650DF/C17) was added for a final concentration of 2.44 mg/L. Samples were flushed with nitrogen for 10 s, sealed with a silicone septum, stored at −80 °C and analyzed in batches of 20.
The GC was equipped with a DB-WAXetr column (length, 30 m; internal diameter, 0.32 mm; layer thickness, 0.50 µm; Agilent Technologies, Santa Clara, CA, USA) to the FID and an HP-5 column (length, 30 m; internal diameter, 0.25 mm; layer thickness, 0.25 µm; Agilent Technologies, Santa Clara, CA, USA) to the FPD. N 2 was used as the carrier gas. Samples were incubated for 20 min at 70 °C in the headspace autosampler (Flow rate, 35 cm/s; Injection volume, 1000 µL; Injection mode, split; Combi PAL autosampler, CTC analytics, Switzerland). The injector, FID and FPD temperatures were kept at 250 °C. The GC oven temperature was first held at 50 °C for 5 min and then allowed to rise to 80 °C at a rate of 5 °C/min, followed by a second ramp of 4 °C/min until 200 °C kept for 3 min and a final ramp of (4 °C/min) until 230 °C for 1 min. Results were analyzed with the GCSolution software version 2.4 (Shimadzu, Kyoto, Japan). The GC was calibrated with a 5% EtOH solution (VWR International) containing the volatiles under study (Supplementary Table S7 ).
HS-SPME-GC-MS
HS-SPME-GC-MS (Shimadzu GCMS-QP-2010 Ultra) was used to measure additional volatile compounds, mainly comprising terpenoids and esters. Samples were analyzed by HS-SPME using a triphase DVB/Carboxen/PDMS 50/30 μm SPME fiber (Supelco Co., Bellefonte, PA, USA) followed by gas chromatography (Thermo Fisher Scientific Trace 1300 series, USA) coupled to a mass spectrometer (Thermo Fisher Scientific ISQ series MS) equipped with a TriPlus RSH autosampler. 5 ml of degassed beer sample was placed in 20 ml vials containing 1.75 g NaCl (VWR, 27810.295). 5 µl internal standard mix was added, containing 2-heptanol (1 g/L) (Sigma-Aldrich, H3003), 4-fluorobenzaldehyde (1 g/L) (Sigma-Aldrich, 128376), 2,3-hexanedione (1 g/L) (Sigma-Aldrich, 144169) and guaiacol (1 g/L) (Sigma-Aldrich, W253200) in ethanol (Fisher Chemical, E/0650DF/C17). Each sample was incubated at 60 °C in the autosampler oven with constant agitation. After 5 min equilibration, the SPME fiber was exposed to the sample headspace for 30 min. The compounds trapped on the fiber were thermally desorbed in the injection port of the chromatograph by heating the fiber for 15 min at 270 °C.
The GC-MS was equipped with a low polarity RXi-5Sil MS column (length, 20 m; internal diameter, 0.18 mm; layer thickness, 0.18 µm; Restek, Bellefonte, PA, USA). Injection was performed in splitless mode at 320 °C, a split flow of 9 ml/min, a purge flow of 5 ml/min and an open valve time of 3 min. To obtain a pulsed injection, a programmed gas flow was used whereby the helium gas flow was set at 2.7 mL/min for 0.1 min, followed by a decrease in flow of 20 ml/min to the normal 0.9 mL/min. The temperature was first held at 30 °C for 3 min and then allowed to rise to 80 °C at a rate of 7 °C/min, followed by a second ramp of 2 °C/min till 125 °C and a final ramp of 8 °C/min with a final temperature of 270 °C.
Mass acquisition range was 33 to 550 amu at a scan rate of 5 scans/s. Electron impact ionization energy was 70 eV. The interface and ion source were kept at 275 °C and 250 °C, respectively. A mix of linear n-alkanes (from C7 to C40, Supelco Co.) was injected into the GC-MS under identical conditions to serve as external retention index markers. Identification and quantification of the compounds were performed using an in-house developed R script as described in Goelen et al. and Reher et al. 87 , 88 (for package information, see Supplementary Table S8 ). Briefly, chromatograms were analyzed using AMDIS (v2.71) 89 to separate overlapping peaks and obtain pure compound spectra. The NIST MS Search software (v2.0 g) in combination with the NIST2017, FFNSC3 and Adams4 libraries were used to manually identify the empirical spectra, taking into account the expected retention time. After background subtraction and correcting for retention time shifts between samples run on different days based on alkane ladders, compound elution profiles were extracted and integrated using a file with 284 target compounds of interest, which were either recovered in our identified AMDIS list of spectra or were known to occur in beer. Compound elution profiles were estimated for every peak in every chromatogram over a time-restricted window using weighted non-negative least square analysis after which peak areas were integrated 87 , 88 . Batch effect correction was performed by normalizing against the most stable internal standard compound, 4-fluorobenzaldehyde. Out of all 284 target compounds that were analyzed, 167 were visually judged to have reliable elution profiles and were used for final analysis.
Discrete photometric and enzymatic analysis
Discrete photometric and enzymatic analysis (Thermo Scientific TM Gallery TM Plus Beermaster Discrete Analyzer) was used to measure acetic acid, ammonia, beta-glucan, iso-alpha acids, color, sugars, glycerol, iron, pH, protein, and sulfite. 2 ml of sample volume was used for the analyses. Information regarding the reagents and standard solutions used for analyses and calibrations is included in Supplementary Table S7 and Supplementary Table S9 .
NIR analyses
NIR analysis (Anton Paar Alcolyzer Beer ME System) was used to measure ethanol. Measurements comprised 50 ml of sample, and a 10% EtOH solution was used for calibration.
Correlation calculations
Pairwise Spearman Rank correlations were calculated between all chemical properties.
Sensory dataset
Trained panel.
Our trained tasting panel consisted of volunteers who gave prior verbal informed consent. All compounds used for the validation experiment were of food-grade quality. The tasting sessions were approved by the Social and Societal Ethics Committee of the KU Leuven (G-2022-5677-R2(MAR)). All online reviewers agreed to the Terms and Conditions of the RateBeer website.
Sensory analysis was performed according to the American Society of Brewing Chemists (ASBC) Sensory Analysis Methods 90 . 30 volunteers were screened through a series of triangle tests. The sixteen most sensitive and consistent tasters were retained as taste panel members. The resulting panel was diverse in age [22–42, mean: 29], sex [56% male] and nationality [7 different countries]. The panel developed a consensus vocabulary to describe beer aroma, taste and mouthfeel. Panelists were trained to identify and score 50 different attributes, using a 7-point scale to rate attributes’ intensity. The scoring sheet is included as Supplementary Data 3 . Sensory assessments took place between 10–12 a.m. The beers were served in black-colored glasses. Per session, between 5 and 12 beers of the same style were tasted at 12 °C to 16 °C. Two reference beers were added to each set and indicated as ‘Reference 1 & 2’, allowing panel members to calibrate their ratings. Not all panelists were present at every tasting. Scores were scaled by standard deviation and mean-centered per taster. Values are represented as z-scores and clustered by Euclidean distance. Pairwise Spearman correlations were calculated between taste and aroma sensory attributes. Panel consistency was evaluated by repeating samples on different sessions and performing ANOVA to identify differences, using the ‘stats’ package (v4.2.2) in R (for package information, see Supplementary Table S8 ).
Online reviews from a public database
The ‘scrapy’ package in Python (v3.6) (for package information, see Supplementary Table S8 ). was used to collect 232,288 online reviews (mean=922, min=6, max=5343) from RateBeer, an online beer review database. Each review entry comprised 5 numerical scores (appearance, aroma, taste, palate and overall quality) and an optional review text. The total number of reviews per reviewer was collected separately. Numerical scores were scaled and centered per rater, and mean scores were calculated per beer.
For the review texts, the language was estimated using the packages ‘langdetect’ and ‘langid’ in Python. Reviews that were classified as English by both packages were kept. Reviewers with fewer than 100 entries overall were discarded. 181,025 reviews from >6000 reviewers from >40 countries remained. Text processing was done using the ‘nltk’ package in Python. Texts were corrected for slang and misspellings; proper nouns and rare words that are relevant to the beer context were specified and kept as-is (‘Chimay’,’Lambic’, etc.). A dictionary of semantically similar sensorial terms, for example ‘floral’ and ‘flower’, was created and collapsed together into one term. Words were stemmed and lemmatized to avoid identifying words such as ‘acid’ and ‘acidity’ as separate terms. Numbers and punctuation were removed.
Sentences from up to 50 randomly chosen reviews per beer were manually categorized according to the aspect of beer they describe (appearance, aroma, taste, palate, overall quality—not to be confused with the 5 numerical scores described above) or flagged as irrelevant if they contained no useful information. If a beer contained fewer than 50 reviews, all reviews were manually classified. This labeled data set was used to train a model that classified the rest of the sentences for all beers 91 . Sentences describing taste and aroma were extracted, and term frequency–inverse document frequency (TFIDF) was implemented to calculate enrichment scores for sensorial words per beer.
The sex of the tasting subject was not considered when building our sensory database. Instead, results from different panelists were averaged, both for our trained panel (56% male, 44% female) and the RateBeer reviews (70% male, 30% female for RateBeer as a whole).
Beer price collection and processing
Beer prices were collected from the following stores: Colruyt, Delhaize, Total Wine, BeerHawk, The Belgian Beer Shop, The Belgian Shop, and Beer of Belgium. Where applicable, prices were converted to Euros and normalized per liter. Spearman correlations were calculated between these prices and mean overall appreciation scores from RateBeer and the taste panel, respectively.
Pairwise Spearman Rank correlations were calculated between all sensory properties.
Machine learning models
Predictive modeling of sensory profiles from chemical data.
Regression models were constructed to predict (a) trained panel scores for beer flavors and quality from beer chemical profiles and (b) public reviews’ appreciation scores from beer chemical profiles. Z-scores were used to represent sensory attributes in both data sets. Chemical properties with log-normal distributions (Shapiro-Wilk test, p < 0.05 ) were log-transformed. Missing chemical measurements (0.1% of all data) were replaced with mean values per attribute. Observations from 250 beers were randomly separated into a training set (70%, 175 beers) and a test set (30%, 75 beers), stratified per beer style. Chemical measurements (p = 231) were normalized based on the training set average and standard deviation. In total, three linear regression-based models: linear regression with first-order interaction terms (LR), lasso regression with first-order interaction terms (Lasso) and partial least squares regression (PLSR); five decision tree models, Adaboost regressor (ABR), Extra Trees (ET), Gradient Boosting regressor (GBR), Random Forest (RF) and XGBoost regressor (XGBR); one support vector machine model (SVR) and one artificial neural network model (ANN) were trained. The models were implemented using the ‘scikit-learn’ package (v1.2.2) and ‘xgboost’ package (v1.7.3) in Python (v3.9.16). Models were trained, and hyperparameters optimized, using five-fold cross-validated grid search with the coefficient of determination (R 2 ) as the evaluation metric. The ANN (scikit-learn’s MLPRegressor) was optimized using Bayesian Tree-Structured Parzen Estimator optimization with the ‘Optuna’ Python package (v3.2.0). Individual models were trained per attribute, and a multi-output model was trained on all attributes simultaneously.
Model dissection
GBR was found to outperform other methods, resulting in models with the highest average R 2 values in both trained panel and public review data sets. Impurity-based rankings of the most important predictors for each predicted sensorial trait were obtained using the ‘scikit-learn’ package. To observe the relationships between these chemical properties and their predicted targets, partial dependence plots (PDP) were constructed for the six most important predictors of consumer appreciation 74 , 75 .
The ‘SHAP’ package in Python (v0.41.0) was implemented to provide an alternative ranking of predictor importance and to visualize the predictors’ effects as a function of their concentration 68 .
Validation of causal chemical properties
To validate the effects of the most important model features on predicted sensory attributes, beers were spiked with the chemical compounds identified by the models and descriptive sensory analyses were carried out according to the American Society of Brewing Chemists (ASBC) protocol 90 .
Compound spiking was done 30 min before tasting. Compounds were spiked into fresh beer bottles, that were immediately resealed and inverted three times. Fresh bottles of beer were opened for the same duration, resealed, and inverted thrice, to serve as controls. Pairs of spiked samples and controls were served simultaneously, chilled and in dark glasses as outlined in the Trained panel section above. Tasters were instructed to select the glass with the higher flavor intensity for each attribute (directional difference test 92 ) and to select the glass they prefer.
The final concentration after spiking was equal to the within-style average, after normalizing by ethanol concentration. This was done to ensure balanced flavor profiles in the final spiked beer. The same methods were applied to improve a non-alcoholic beer. Compounds were the following: ethyl acetate (Merck KGaA, W241415), ethyl hexanoate (Merck KGaA, W243906), isoamyl acetate (Merck KGaA, W205508), phenethyl acetate (Merck KGaA, W285706), ethanol (96%, Colruyt), glycerol (Merck KGaA, W252506), lactic acid (Merck KGaA, 261106).
Significant differences in preference or perceived intensity were determined by performing the two-sided binomial test on each attribute.
Reporting summary
Further information on research design is available in the Nature Portfolio Reporting Summary linked to this article.
Data availability
The data that support the findings of this work are available in the Supplementary Data files and have been deposited to Zenodo under accession code 10653704 93 . The RateBeer scores data are under restricted access, they are not publicly available as they are property of RateBeer (ZX Ventures, USA). Access can be obtained from the authors upon reasonable request and with permission of RateBeer (ZX Ventures, USA). Source data are provided with this paper.
Code availability
The code for training the machine learning models, analyzing the models, and generating the figures has been deposited to Zenodo under accession code 10653704 93 .
Tieman, D. et al. A chemical genetic roadmap to improved tomato flavor. Science 355 , 391–394 (2017).
Article ADS CAS PubMed Google Scholar
Plutowska, B. & Wardencki, W. Application of gas chromatography–olfactometry (GC–O) in analysis and quality assessment of alcoholic beverages – A review. Food Chem. 107 , 449–463 (2008).
Article CAS Google Scholar
Legin, A., Rudnitskaya, A., Seleznev, B. & Vlasov, Y. Electronic tongue for quality assessment of ethanol, vodka and eau-de-vie. Anal. Chim. Acta 534 , 129–135 (2005).
Loutfi, A., Coradeschi, S., Mani, G. K., Shankar, P. & Rayappan, J. B. B. Electronic noses for food quality: A review. J. Food Eng. 144 , 103–111 (2015).
Ahn, Y.-Y., Ahnert, S. E., Bagrow, J. P. & Barabási, A.-L. Flavor network and the principles of food pairing. Sci. Rep. 1 , 196 (2011).
Article CAS PubMed PubMed Central Google Scholar
Bartoshuk, L. M. & Klee, H. J. Better fruits and vegetables through sensory analysis. Curr. Biol. 23 , R374–R378 (2013).
Article CAS PubMed Google Scholar
Piggott, J. R. Design questions in sensory and consumer science. Food Qual. Prefer. 3293 , 217–220 (1995).
Article Google Scholar
Kermit, M. & Lengard, V. Assessing the performance of a sensory panel-panellist monitoring and tracking. J. Chemom. 19 , 154–161 (2005).
Cook, D. J., Hollowood, T. A., Linforth, R. S. T. & Taylor, A. J. Correlating instrumental measurements of texture and flavour release with human perception. Int. J. Food Sci. Technol. 40 , 631–641 (2005).
Chinchanachokchai, S., Thontirawong, P. & Chinchanachokchai, P. A tale of two recommender systems: The moderating role of consumer expertise on artificial intelligence based product recommendations. J. Retail. Consum. Serv. 61 , 1–12 (2021).
Ross, C. F. Sensory science at the human-machine interface. Trends Food Sci. Technol. 20 , 63–72 (2009).
Chambers, E. IV & Koppel, K. Associations of volatile compounds with sensory aroma and flavor: The complex nature of flavor. Molecules 18 , 4887–4905 (2013).
Pinu, F. R. Metabolomics—The new frontier in food safety and quality research. Food Res. Int. 72 , 80–81 (2015).
Danezis, G. P., Tsagkaris, A. S., Brusic, V. & Georgiou, C. A. Food authentication: state of the art and prospects. Curr. Opin. Food Sci. 10 , 22–31 (2016).
Shepherd, G. M. Smell images and the flavour system in the human brain. Nature 444 , 316–321 (2006).
Meilgaard, M. C. Prediction of flavor differences between beers from their chemical composition. J. Agric. Food Chem. 30 , 1009–1017 (1982).
Xu, L. et al. Widespread receptor-driven modulation in peripheral olfactory coding. Science 368 , eaaz5390 (2020).
Kupferschmidt, K. Following the flavor. Science 340 , 808–809 (2013).
Billesbølle, C. B. et al. Structural basis of odorant recognition by a human odorant receptor. Nature 615 , 742–749 (2023).
Article ADS PubMed PubMed Central Google Scholar
Smith, B. Perspective: Complexities of flavour. Nature 486 , S6–S6 (2012).
Pfister, P. et al. Odorant receptor inhibition is fundamental to odor encoding. Curr. Biol. 30 , 2574–2587 (2020).
Moskowitz, H. W., Kumaraiah, V., Sharma, K. N., Jacobs, H. L. & Sharma, S. D. Cross-cultural differences in simple taste preferences. Science 190 , 1217–1218 (1975).
Eriksson, N. et al. A genetic variant near olfactory receptor genes influences cilantro preference. Flavour 1 , 22 (2012).
Ferdenzi, C. et al. Variability of affective responses to odors: Culture, gender, and olfactory knowledge. Chem. Senses 38 , 175–186 (2013).
Article PubMed Google Scholar
Lawless, H. T. & Heymann, H. Sensory evaluation of food: Principles and practices. (Springer, New York, NY). https://doi.org/10.1007/978-1-4419-6488-5 (2010).
Colantonio, V. et al. Metabolomic selection for enhanced fruit flavor. Proc. Natl. Acad. Sci. 119 , e2115865119 (2022).
Fritz, F., Preissner, R. & Banerjee, P. VirtualTaste: a web server for the prediction of organoleptic properties of chemical compounds. Nucleic Acids Res 49 , W679–W684 (2021).
Tuwani, R., Wadhwa, S. & Bagler, G. BitterSweet: Building machine learning models for predicting the bitter and sweet taste of small molecules. Sci. Rep. 9 , 1–13 (2019).
Dagan-Wiener, A. et al. Bitter or not? BitterPredict, a tool for predicting taste from chemical structure. Sci. Rep. 7 , 1–13 (2017).
Pallante, L. et al. Toward a general and interpretable umami taste predictor using a multi-objective machine learning approach. Sci. Rep. 12 , 1–11 (2022).
Malavolta, M. et al. A survey on computational taste predictors. Eur. Food Res. Technol. 248 , 2215–2235 (2022).
Lee, B. K. et al. A principal odor map unifies diverse tasks in olfactory perception. Science 381 , 999–1006 (2023).
Mayhew, E. J. et al. Transport features predict if a molecule is odorous. Proc. Natl. Acad. Sci. 119 , e2116576119 (2022).
Niu, Y. et al. Sensory evaluation of the synergism among ester odorants in light aroma-type liquor by odor threshold, aroma intensity and flash GC electronic nose. Food Res. Int. 113 , 102–114 (2018).
Yu, P., Low, M. Y. & Zhou, W. Design of experiments and regression modelling in food flavour and sensory analysis: A review. Trends Food Sci. Technol. 71 , 202–215 (2018).
Oladokun, O. et al. The impact of hop bitter acid and polyphenol profiles on the perceived bitterness of beer. Food Chem. 205 , 212–220 (2016).
Linforth, R., Cabannes, M., Hewson, L., Yang, N. & Taylor, A. Effect of fat content on flavor delivery during consumption: An in vivo model. J. Agric. Food Chem. 58 , 6905–6911 (2010).
Guo, S., Na Jom, K. & Ge, Y. Influence of roasting condition on flavor profile of sunflower seeds: A flavoromics approach. Sci. Rep. 9 , 11295 (2019).
Ren, Q. et al. The changes of microbial community and flavor compound in the fermentation process of Chinese rice wine using Fagopyrum tataricum grain as feedstock. Sci. Rep. 9 , 3365 (2019).
Hastie, T., Friedman, J. & Tibshirani, R. The Elements of Statistical Learning. (Springer, New York, NY). https://doi.org/10.1007/978-0-387-21606-5 (2001).
Dietz, C., Cook, D., Huismann, M., Wilson, C. & Ford, R. The multisensory perception of hop essential oil: a review. J. Inst. Brew. 126 , 320–342 (2020).
CAS Google Scholar
Roncoroni, Miguel & Verstrepen, Kevin Joan. Belgian Beer: Tested and Tasted. (Lannoo, 2018).
Meilgaard, M. Flavor chemistry of beer: Part II: Flavor and threshold of 239 aroma volatiles. in (1975).
Bokulich, N. A. & Bamforth, C. W. The microbiology of malting and brewing. Microbiol. Mol. Biol. Rev. MMBR 77 , 157–172 (2013).
Dzialo, M. C., Park, R., Steensels, J., Lievens, B. & Verstrepen, K. J. Physiology, ecology and industrial applications of aroma formation in yeast. FEMS Microbiol. Rev. 41 , S95–S128 (2017).
Article PubMed PubMed Central Google Scholar
Datta, A. et al. Computer-aided food engineering. Nat. Food 3 , 894–904 (2022).
American Society of Brewing Chemists. Beer Methods. (American Society of Brewing Chemists, St. Paul, MN, U.S.A.).
Olaniran, A. O., Hiralal, L., Mokoena, M. P. & Pillay, B. Flavour-active volatile compounds in beer: production, regulation and control. J. Inst. Brew. 123 , 13–23 (2017).
Verstrepen, K. J. et al. Flavor-active esters: Adding fruitiness to beer. J. Biosci. Bioeng. 96 , 110–118 (2003).
Meilgaard, M. C. Flavour chemistry of beer. part I: flavour interaction between principal volatiles. Master Brew. Assoc. Am. Tech. Q 12 , 107–117 (1975).
Briggs, D. E., Boulton, C. A., Brookes, P. A. & Stevens, R. Brewing 227–254. (Woodhead Publishing). https://doi.org/10.1533/9781855739062.227 (2004).
Bossaert, S., Crauwels, S., De Rouck, G. & Lievens, B. The power of sour - A review: Old traditions, new opportunities. BrewingScience 72 , 78–88 (2019).
Google Scholar
Verstrepen, K. J. et al. Flavor active esters: Adding fruitiness to beer. J. Biosci. Bioeng. 96 , 110–118 (2003).
Snauwaert, I. et al. Microbial diversity and metabolite composition of Belgian red-brown acidic ales. Int. J. Food Microbiol. 221 , 1–11 (2016).
Spitaels, F. et al. The microbial diversity of traditional spontaneously fermented lambic beer. PLoS ONE 9 , e95384 (2014).
Blanco, C. A., Andrés-Iglesias, C. & Montero, O. Low-alcohol Beers: Flavor Compounds, Defects, and Improvement Strategies. Crit. Rev. Food Sci. Nutr. 56 , 1379–1388 (2016).
Jackowski, M. & Trusek, A. Non-Alcohol. beer Prod. – Overv. 20 , 32–38 (2018).
Takoi, K. et al. The contribution of geraniol metabolism to the citrus flavour of beer: Synergy of geraniol and β-citronellol under coexistence with excess linalool. J. Inst. Brew. 116 , 251–260 (2010).
Kroeze, J. H. & Bartoshuk, L. M. Bitterness suppression as revealed by split-tongue taste stimulation in humans. Physiol. Behav. 35 , 779–783 (1985).
Mennella, J. A. et al. A spoonful of sugar helps the medicine go down”: Bitter masking bysucrose among children and adults. Chem. Senses 40 , 17–25 (2015).
Wietstock, P., Kunz, T., Perreira, F. & Methner, F.-J. Metal chelation behavior of hop acids in buffered model systems. BrewingScience 69 , 56–63 (2016).
Sancho, D., Blanco, C. A., Caballero, I. & Pascual, A. Free iron in pale, dark and alcohol-free commercial lager beers. J. Sci. Food Agric. 91 , 1142–1147 (2011).
Rodrigues, H. & Parr, W. V. Contribution of cross-cultural studies to understanding wine appreciation: A review. Food Res. Int. 115 , 251–258 (2019).
Korneva, E. & Blockeel, H. Towards better evaluation of multi-target regression models. in ECML PKDD 2020 Workshops (eds. Koprinska, I. et al.) 353–362 (Springer International Publishing, Cham, 2020). https://doi.org/10.1007/978-3-030-65965-3_23 .
Gastón Ares. Mathematical and Statistical Methods in Food Science and Technology. (Wiley, 2013).
Grinsztajn, L., Oyallon, E. & Varoquaux, G. Why do tree-based models still outperform deep learning on tabular data? Preprint at http://arxiv.org/abs/2207.08815 (2022).
Gries, S. T. Statistics for Linguistics with R: A Practical Introduction. in Statistics for Linguistics with R (De Gruyter Mouton, 2021). https://doi.org/10.1515/9783110718256 .
Lundberg, S. M. et al. From local explanations to global understanding with explainable AI for trees. Nat. Mach. Intell. 2 , 56–67 (2020).
Ickes, C. M. & Cadwallader, K. R. Effects of ethanol on flavor perception in alcoholic beverages. Chemosens. Percept. 10 , 119–134 (2017).
Kato, M. et al. Influence of high molecular weight polypeptides on the mouthfeel of commercial beer. J. Inst. Brew. 127 , 27–40 (2021).
Wauters, R. et al. Novel Saccharomyces cerevisiae variants slow down the accumulation of staling aldehydes and improve beer shelf-life. Food Chem. 398 , 1–11 (2023).
Li, H., Jia, S. & Zhang, W. Rapid determination of low-level sulfur compounds in beer by headspace gas chromatography with a pulsed flame photometric detector. J. Am. Soc. Brew. Chem. 66 , 188–191 (2008).
Dercksen, A., Laurens, J., Torline, P., Axcell, B. C. & Rohwer, E. Quantitative analysis of volatile sulfur compounds in beer using a membrane extraction interface. J. Am. Soc. Brew. Chem. 54 , 228–233 (1996).
Molnar, C. Interpretable Machine Learning: A Guide for Making Black-Box Models Interpretable. (2020).
Zhao, Q. & Hastie, T. Causal interpretations of black-box models. J. Bus. Econ. Stat. Publ. Am. Stat. Assoc. 39 , 272–281 (2019).
Article MathSciNet Google Scholar
Hastie, T., Tibshirani, R. & Friedman, J. The Elements of Statistical Learning. (Springer, 2019).
Labrado, D. et al. Identification by NMR of key compounds present in beer distillates and residual phases after dealcoholization by vacuum distillation. J. Sci. Food Agric. 100 , 3971–3978 (2020).
Lusk, L. T., Kay, S. B., Porubcan, A. & Ryder, D. S. Key olfactory cues for beer oxidation. J. Am. Soc. Brew. Chem. 70 , 257–261 (2012).
Gonzalez Viejo, C., Torrico, D. D., Dunshea, F. R. & Fuentes, S. Development of artificial neural network models to assess beer acceptability based on sensory properties using a robotic pourer: A comparative model approach to achieve an artificial intelligence system. Beverages 5 , 33 (2019).
Gonzalez Viejo, C., Fuentes, S., Torrico, D. D., Godbole, A. & Dunshea, F. R. Chemical characterization of aromas in beer and their effect on consumers liking. Food Chem. 293 , 479–485 (2019).
Gilbert, J. L. et al. Identifying breeding priorities for blueberry flavor using biochemical, sensory, and genotype by environment analyses. PLOS ONE 10 , 1–21 (2015).
Goulet, C. et al. Role of an esterase in flavor volatile variation within the tomato clade. Proc. Natl. Acad. Sci. 109 , 19009–19014 (2012).
Article ADS CAS PubMed PubMed Central Google Scholar
Borisov, V. et al. Deep Neural Networks and Tabular Data: A Survey. IEEE Trans. Neural Netw. Learn. Syst. 1–21 https://doi.org/10.1109/TNNLS.2022.3229161 (2022).
Statista. Statista Consumer Market Outlook: Beer - Worldwide.
Seitz, H. K. & Stickel, F. Molecular mechanisms of alcoholmediated carcinogenesis. Nat. Rev. Cancer 7 , 599–612 (2007).
Voordeckers, K. et al. Ethanol exposure increases mutation rate through error-prone polymerases. Nat. Commun. 11 , 3664 (2020).
Goelen, T. et al. Bacterial phylogeny predicts volatile organic compound composition and olfactory response of an aphid parasitoid. Oikos 129 , 1415–1428 (2020).
Article ADS Google Scholar
Reher, T. et al. Evaluation of hop (Humulus lupulus) as a repellent for the management of Drosophila suzukii. Crop Prot. 124 , 104839 (2019).
Stein, S. E. An integrated method for spectrum extraction and compound identification from gas chromatography/mass spectrometry data. J. Am. Soc. Mass Spectrom. 10 , 770–781 (1999).
American Society of Brewing Chemists. Sensory Analysis Methods. (American Society of Brewing Chemists, St. Paul, MN, U.S.A., 1992).
McAuley, J., Leskovec, J. & Jurafsky, D. Learning Attitudes and Attributes from Multi-Aspect Reviews. Preprint at https://doi.org/10.48550/arXiv.1210.3926 (2012).
Meilgaard, M. C., Carr, B. T. & Carr, B. T. Sensory Evaluation Techniques. (CRC Press, Boca Raton). https://doi.org/10.1201/b16452 (2014).
Schreurs, M. et al. Data from: Predicting and improving complex beer flavor through machine learning. Zenodo https://doi.org/10.5281/zenodo.10653704 (2024).
Download references
Acknowledgements
We thank all lab members for their discussions and thank all tasting panel members for their contributions. Special thanks go out to Dr. Karin Voordeckers for her tremendous help in proofreading and improving the manuscript. M.S. was supported by a Baillet-Latour fellowship, L.C. acknowledges financial support from KU Leuven (C16/17/006), F.A.T. was supported by a PhD fellowship from FWO (1S08821N). Research in the lab of K.J.V. is supported by KU Leuven, FWO, VIB, VLAIO and the Brewing Science Serves Health Fund. Research in the lab of T.W. is supported by FWO (G.0A51.15) and KU Leuven (C16/17/006).
Author information
These authors contributed equally: Michiel Schreurs, Supinya Piampongsant, Miguel Roncoroni.
Authors and Affiliations
VIB—KU Leuven Center for Microbiology, Gaston Geenslaan 1, B-3001, Leuven, Belgium
Michiel Schreurs, Supinya Piampongsant, Miguel Roncoroni, Lloyd Cool, Beatriz Herrera-Malaver, Florian A. Theßeling & Kevin J. Verstrepen
CMPG Laboratory of Genetics and Genomics, KU Leuven, Gaston Geenslaan 1, B-3001, Leuven, Belgium
Leuven Institute for Beer Research (LIBR), Gaston Geenslaan 1, B-3001, Leuven, Belgium
Laboratory of Socioecology and Social Evolution, KU Leuven, Naamsestraat 59, B-3000, Leuven, Belgium
Lloyd Cool, Christophe Vanderaa & Tom Wenseleers
VIB Bioinformatics Core, VIB, Rijvisschestraat 120, B-9052, Ghent, Belgium
Łukasz Kreft & Alexander Botzki
AB InBev SA/NV, Brouwerijplein 1, B-3000, Leuven, Belgium
Philippe Malcorps & Luk Daenen
You can also search for this author in PubMed Google Scholar
Contributions
S.P., M.S. and K.J.V. conceived the experiments. S.P., M.S. and K.J.V. designed the experiments. S.P., M.S., M.R., B.H. and F.A.T. performed the experiments. S.P., M.S., L.C., C.V., L.K., A.B., P.M., L.D., T.W. and K.J.V. contributed analysis ideas. S.P., M.S., L.C., C.V., T.W. and K.J.V. analyzed the data. All authors contributed to writing the manuscript.
Corresponding author
Correspondence to Kevin J. Verstrepen .
Ethics declarations
Competing interests.
K.J.V. is affiliated with bar.on. The other authors declare no competing interests.
Peer review
Peer review information.
Nature Communications thanks Florian Bauer, Andrew John Macintosh and the other, anonymous, reviewer(s) for their contribution to the peer review of this work. A peer review file is available.
Additional information
Publisher’s note Springer Nature remains neutral with regard to jurisdictional claims in published maps and institutional affiliations.
Supplementary information
Supplementary information, peer review file, description of additional supplementary files, supplementary data 1, supplementary data 2, supplementary data 3, supplementary data 4, supplementary data 5, supplementary data 6, supplementary data 7, reporting summary, source data, source data, rights and permissions.
Open Access This article is licensed under a Creative Commons Attribution 4.0 International License, which permits use, sharing, adaptation, distribution and reproduction in any medium or format, as long as you give appropriate credit to the original author(s) and the source, provide a link to the Creative Commons licence, and indicate if changes were made. The images or other third party material in this article are included in the article’s Creative Commons licence, unless indicated otherwise in a credit line to the material. If material is not included in the article’s Creative Commons licence and your intended use is not permitted by statutory regulation or exceeds the permitted use, you will need to obtain permission directly from the copyright holder. To view a copy of this licence, visit http://creativecommons.org/licenses/by/4.0/ .
Reprints and permissions
About this article
Cite this article.
Schreurs, M., Piampongsant, S., Roncoroni, M. et al. Predicting and improving complex beer flavor through machine learning. Nat Commun 15 , 2368 (2024). https://doi.org/10.1038/s41467-024-46346-0
Download citation
Received : 30 October 2023
Accepted : 21 February 2024
Published : 26 March 2024
DOI : https://doi.org/10.1038/s41467-024-46346-0
Share this article
Anyone you share the following link with will be able to read this content:
Sorry, a shareable link is not currently available for this article.
Provided by the Springer Nature SharedIt content-sharing initiative
By submitting a comment you agree to abide by our Terms and Community Guidelines . If you find something abusive or that does not comply with our terms or guidelines please flag it as inappropriate.
Quick links
- Explore articles by subject
- Guide to authors
- Editorial policies
Sign up for the Nature Briefing: Translational Research newsletter — top stories in biotechnology, drug discovery and pharma.

- Original article
- Open access
- Published: 24 November 2021
Reducing maritime accidents in ships by tackling human error: a bibliometric review and research agenda
- Carine Dominguez-Péry ORCID: orcid.org/0000-0002-4288-6810 1 ,
- Lakshmi Narasimha Raju Vuddaraju 1 ,
- Isabelle Corbett-Etchevers 1 &
- Rana Tassabehji 2
Journal of Shipping and Trade volume 6 , Article number: 20 ( 2021 ) Cite this article
17k Accesses
15 Citations
1 Altmetric
Metrics details
Over the past decade the number of maritime transportation accidents has fallen. However, as shipping vessels continue to increase in size, one single incident, such as the oil spills from ‘super’ tankers, can have catastrophic and long-term consequences for marine ecosystems, the environment and local economies. Maritime transport accidents are complex and caused by a combination of events or processes that might ultimately result in the loss of human and marine life, and irreversible ecological, environmental and economic damage. Many studies point to direct or indirect human error as a major cause of maritime accidents, which raises many unanswered questions about the best way to prevent catastrophic human error in maritime contexts. This paper takes a first step towards addressing some of these questions by improving our understanding of upstream maritime accidents from an organisation science perspective—an area of research that is currently underdeveloped. This will provide new and relevant insights by both clarifying how ships can be described in terms of organisations and by considering them in a whole ecosystem and industry. A bibliometric review of extant literature of the causes of maritime accidents related to human error was conducted, and the findings revealed three main root causes of human and organisational error, namely, human resources and management, socio-technical Information Systems and Information Technologies, and individual/cognition-related errors. As a result of the bibliometric review, this paper identifies the gaps and limitations in the literature and proposes a research agenda to enhance our current understanding of the role of human error in maritime accidents. This research agenda proposes new organisational theory perspectives—including considering ships as organisations; types of organisations (highly reliable organisations or self-organised); complex systems and socio-technical systems theories for digitalised ships; the role of power; and developing dynamic safety capabilities for learning ships. By adopting different theoretical perspectives and adapting research methods from social and human sciences, scholars can advance human error in maritime transportation, which can ultimately contribute to addressing human errors and improving maritime transport safety for the wider benefit of the environment and societies ecologies and economies.
Introduction
The global shipping industry is responsible for transporting as much as 90% of world trade (SSR 2021 ). Over the past decade, improved ship design, technology, regulation and risk management systems have contributed to a 70% drop in reported shipping losses (SSR 2021 ). However, while the frequency of maritime accidents may be in decline, one single incident can have catastrophic and long-term consequences for marine ecosystems, the environment and local economies (Roberts et al. 2002 ). This is exacerbated further by the fact that maritime transportation vessels are increasing in size and the amounts of cargo on-board with them. For instance, in September 2019, Brazil’s north-eastern state of Bahia declared an emergency after an oil spill from the tanker Bouboulina contaminated kilometres of coastal beaches. In August 2020, Mauritius also declared a state of environmental emergency after the MV Wakashio ran aground at Pointe d’Esny, spilling oil into an area renowned as a sanctuary for rare wildlife. These types of accidents attract the attention of the media and heighten the concerns of people around the world, as images of the damage to marine wildlife and the environment are graphically visible.
Despite the ostensible fall in total reported losses, the number of accidents Footnote 1 , especially those related to passenger/car carrier vessels and ro-ros has increased, as has the number of reported casualties (SSR 2021 ). Therefore, this study's starting point was to understand further why maritime accidents with such wide-ranging consequences continue to occur.
Maritime transport accidents are complex (Guven-Koçak 2015 ) and caused by a combination of events or processes (Soares and Teixeira 2001 ) involving various actors that ultimately lead to disastrous consequences including loss of human and marine life and irreparable ecological, environmental and economic damage (Harrald et al. 1998 ). Apart from uncontrollable acts of God defined as ‘an extreme interruption with a natural cause (e.g. earthquake, storm, etc.)’ (Kristiansen 2005 :14), the literature consistently highlights human error (HE) as one of the main contributing factors in more than 85% of cases of maritime accidents (Acejo et al. 2018 ; Galieriková, 2019 ). Furthermore, experts estimate that 30–50% of oil spills are caused directly or indirectly by HE (Michel and Fingas 2016 ). Despite this, there is a surprising dearth of research in the management literature investigating HE in the maritime context (Berkowitz et al. 2019 ). This leads us to question the role of humans in the maritime transport ecosystem and ask: ‘ What is the current state-of-the-art research regarding human error as the main cause of maritime transportation accidents? How have researchers considered and framed human error? What research agenda is recommended to integrate the “human” further to avoid human error from an organisation science perspective, including team, organisational and collaborative networks/ecosystems?
This paper aims to address these questions by improving our understanding of maritime accidents and prevention from an organisational perspective, which is currently underdeveloped in organisation science. In order to achieve these objectives, a bibliometric review is conducted. The bibliometric review (BR) is a quantitative approach that uses co-citation analysis to visualise the literature in the field (van Oorschot et al. 2018 ). This reduces the reviewers’ subjectivity and bias and will generate a more systematic and encompassing picture of HE research in the field of maritime transportation.
The paper is organised as follows. The first part lays out the general context of the maritime transportation industry, the main causes of vessel accidents and the role of HE in maritime accidents. Then the five-step bibliometric review method adopted for this study is described. The findings are collated, analysed and discussed to provide a deeper understanding of what currently constitutes HE. Finally, a research agenda to investigate maritime accidents and HE from a socio-organisational perspective to prevent future accidents is proposed.
Accidents in maritime shipping
The maritime transportation industry’s distinct maritime culture is characterised by its global nature, working conditions, autonomy and complexity (Güven-Koçak 2015 ). The global nature of the shipping industry means worldwide competition is driving ship-owners to seek ever-increasing cost-efficiencies (Lützhöft et al. 2011 ). Maritime shipping is heavily influenced by the global economic, trade and environmental trends and were significantly impacted by the economic downturn in 2020 resulting from the COVID-19 pandemic. According to UNCTAD ( 2020 ), the total world fleet consists of 98,140 commercial ships over 100 gross tons (GT). Of these, the number of gas carriers, oil tankers, bulk carriers and container ships grew most rapidly over the year to 2020. Despite the advances in technology, processes, procedures, training and regulations, a total of 193 vessels exceeding 100 GT were lost over the 3 years from 2017, mainly through sinking (62%), grounding (15%), fire/explosion (10%), machinery damage/failure (6%) (SSR 2021 : 14). The type of cargo and size of vessel have a big impact on the extent and consequences of an accident at sea. Crude oil alone accounted for around 17–20% of total seaborne goods loaded between 2010 and 2019, and the amount of crude oil transported annually averages around 1,800 million metric tons (UNCTAD STAT 2019 ). In addition to the type of cargo, the increasing size of vessels can impact safety, effective fire prevention and salvage in the event of an accident (SSR 2021 ), highlighted so vividly by the recent case of the Ever Given ‘wedged’ in the Suez Canal (Guardian 2021 ).
Over the past 50 years, the size and capacity of vessels have increased by 1,500%, with the largest container ships now being as big as the largest oil tanker and bigger than the largest cruise ships (UNCTAD 2020 ). According to the ITOPF ( 2019 ), between 2010 and 2018, 91% of all oil spills resulted from 10 incidents, an increase from the previous decade where 75% of oil spills resulted from 10 incidents. Indeed, many studies identify collision/allision as a major cause of oil spill accidents in over half of the cases, most occurring while the vessels are underway or in open water (Eliopoulou and Papanikolaou 2007 ; Uğurlu et al. 2015). The catastrophic and often long-term human, economic and ecological consequences of accidents involving large vessels carrying increased volumes of highly toxic pollutants can be felt globally (ITOPF 2019 ; Chen et al. 2018 ). The focus of this study is to investigate human error (HE) in all types of maritime transportation, with a view to better understanding these errors in order to prevent future devastating accidents.
In addition to increasing the size of vessels, another very common way ship-owners reduce their fixed costs is by hiring multinational crews from developing countries or reducing the number of crew members on-board (Lützhöft et al. 2011 ). This often leads to de-prioritising employee training (Güven-Koçak 2015 ) and increased communication and comprehension problems between the multi-lingual and multi-cultural crew, who cannot effectively communicate with and understand one another. Crew members also inevitably transfer their cultural perspectives, stereotypes, and racial prejudices, leading to cultural tensions and strained relationships. These tensions are further exacerbated by long working hours, a noisy environment, a sense of isolation and loneliness, poor and often shared living conditions with little privacy, and the impossibility of getting away to enjoy free time alone (Güven-Koçak 2015 ). Living and working under such conditions for long periods can affect crew morale and raise stress levels, ultimately leading to fatigue, loss of concentration and focus, lower productivity (Alderton et al. 2004 ) and ultimately accidents.
Human error (HE) as the central cause of accidents
The complexity and lack of standardisation in maritime accident reporting often mean it is difficult and time-consuming to uncover detailed causal factors (Grech et al. 2002 ). Despite this, HE has been identified as one of the primary factors in over 75% of maritime accidents (Acejo et al. 2018 ; Celik and Cebi 2008 ). In an analysis of 177 maritime accident reports, Grech et al. ( 2002 ) found one aspect of HE – lack of situation awareness—to be a severe problem in the maritime domain. Specifically, ‘ shortcomings of the cognitive psychology paradigm of perception, cognition and projection of future events’ ( ibid. p.2), where HE resulted from a failure to anticipate future actions, a failure to correctly perceive information, a failure to correctly integrate or comprehend information and/or the system. In the context of advancing on-board digital systems, these human failings are particularly concerning as they suggest that as the crew become over-reliant on new technologies, the problems of situational awareness will grow considerably and have more of a negative impact on safety.
How have researchers considered and framed HE?
Having reviewed the literature, what is apparent is the different ways in which the concept of ‘human error’ is defined. ‘Human errors’ are the consequences focusing on individual actions leading to errors resulting from intentional actions (Reason 1990 ), a deviation from the performance of an action (Leveson 2011 ), a slip (Norman 1981 ) or a human disturbance that leads to an accident (Rasmussen 2000 ). For some, HE also includes organisational factors (Reason 1997 ; Dekker 2006 ). A selection of these definitions is summarised in Table 1 .
The definition of HE has evolved from being seen as a slip (Norman 1980 ) to a more complex interaction between people, tools and tasks in an organisational environment (Dekker 2002 ). How HE is defined is mainly dependent on the perspective of the discipline evaluating it. For instance, from the engineering discipline, HE is considered a set of causes that need to be tackled to avoid accidents. However, from the perspective of human factors and ergonomics (HFE), HE is more complex and includes aspects of organisational factors and has no systematic solutions to solve the causes. However, the terms are generally ill-defined with little distinction between them and are often used interchangeably.
In reviewing human factors that contribute to organisational accidents in shipping, Hetherington et al. ( 2006 ) developed a framework highlighting three areas common to accidents that can potentially improve shipping safety if moderated. These are (1) personnel issues (fatigue, stress, health, situation awareness, teamwork, decision-making, communication) which were immediate causes (2) organisational and management issues (safety culture) which were underlying causes, and (3) design issues (automation). As with all such studies, there are acknowledged limitations. In this case, there are only 20 studies, many of which lack measures of the impact of specific human behaviours on accidents. This, however, does not invalidate this study but rather highlights the need for more robust research in this complex area.
Researchers have used techniques such as Human Factor Analysis and Classification System (HFACS) and Fuzzy Analytical Hierarchy Process (FAHP) to further investigate causal links and weightings of HE in shipping accidents. For instance, operator failure due to lack of skills, misperception or error of judgement (Celik and Cebi 2008 ), fatigue and miscommunication more recently (Ung 2019 ; Yıldırım et al. 2017). These studies concluded that HE was one of the leading causes of shipping accidents. While these studies offer high level and general insights into the role of shipping, they do not sufficiently explain the role of HE in shipping accidents from an organisational or ecosystem perspective.
By applying a bibliometric review approach, this paper explores the literature in more depth to understand the themes related to the causes of maritime accidents and, more specifically, the aspects attributed to HE.
Methodology
A bibliometric review (BR) methodology was selected for this paper. It is a systematic approach consistent with the paper’s objective of presenting the state-of-the-art of published research on the causes of human error in maritime transportation accidents. Bibliometric reviews mobilise quantitative rather than qualitative techniques, reducing researcher subjectivity and bias, and are increasingly being used by scholars to map the development and structure of a scientific field (Zupic and Cater, 2015 ). They can combine co-citation analysis and bibliographic coupling to map the network of publications and arrive at distinct clusters of thematically related publications (van Oorschot et al. 2018 : 2). Bibliometric reviews also include other complementary analyses such as co-occurrence of keywords (where two or more keywords appear together in a document), co-word analysis (words that occur more frequently together with titles and abstracts) and co-citation of authors (Munim et al. 2020 ). The bibliometric review in this study followed the workflow process proposed by Zupic and Cater ( 2015 :5) summarised in Fig. 1 .
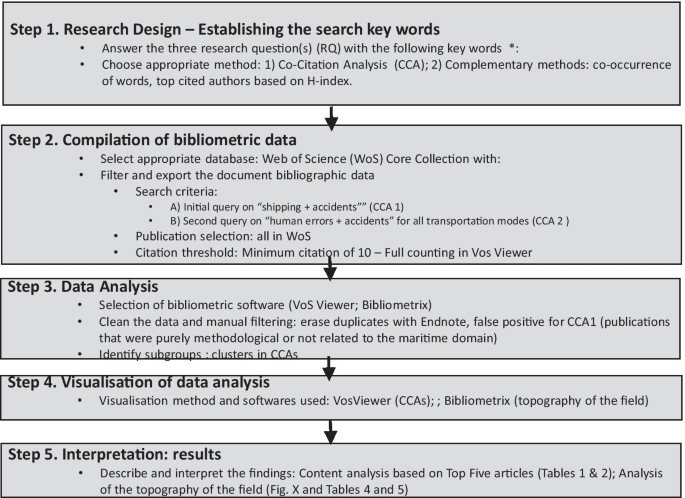
Bibliometric review workflow (adapted from Zupic and Cater ( 2015 :5))
Research design (Step 1) The initial broad review of the maritime transportation literature highlighted the important issues of ‘human error’ in maritime ship accidents. Therefore, the research question to direct this study, established at the outset of the paper, is, ‘ what is the current state-of-the-art of the research regarding HE as the main cause in maritime transportation accidents?’ In order to have a complete view of how this human dimension is handled in the field of maritime transportation, the appropriate methods selected were, (1) co-citation analysis to visualise the seminal publications related to these keywords (CCA); (2) the co-occurrence of words to complete the structuring of main topics to provide a topography of the field; and (3) top-cited authors based on the h-index analysis in order to further analyse the most recently developed topics and concepts.
Compilation of bibliometric data (Step 2) The Web of Science (WoS), which contains over 33,000 journals, including books, conference proceedings, data sets and patents dating back to 1900, was used as the core database for this bibliometric search. The WOS content is curated by experts and provides the data for Journal Impact Factor scores. The metadata and citation data are considered high quality and reliable (Haraldstad and Christophersen 2015 ) and, in line with other studies, is considered most appropriate for bibliometric reviews (Zupic and Cater 2015 ).
The initial search using the keywords “Shipping + Accidents” resulted in 1661 publications and was the basis for Stage 1 of the co-citation analysis. Several false positives were encountered. This is where ostensibly relevant articles that had keywords matching the search terms, on close reading, were found not to be related to the maritime domain and so were excluded. However, the seminal books that were most cited were included in the dataset. Articles that were purely about research methodologies with no relation to the maritime context were also excluded; for instance Yang et al. ( 2013 ) focuses only on fuzzy logic techniques and Saaty ( 1980 ) focuses only on the Analytic Hierarchy Process (AHP). This resulted in a total of 191 publications. The second search using the keywords “Accidents + Human Error” resulted in 2019 publications and was the basis for Stage 2 of the co-citation analysis (CCA). After filtering the data, this resulted in 225 articles.
For each search, a citation threshold was set at ten, which means that only documents that obtained at least ten local citations were included in the network. Furthermore, the entire counting method was used to select the articles for the CCAs. Any co-authored documents are counted and where ‘a link between [two authors] has a strength of 2 [this] indicates that both authors have co-authored two documents’ (Van Eck and Waltman 2013 , p.32).
Data analysis and visualisation (Step 3 and 4) To provide a complete bibliometric analysis, we used VosViewer software for the co-citation analysis (CCA) and Bibliometrix software for the bibliometric citation analysis (Munim et al. 2020 ) to identify the most influential articles, journals, authors and institutions. VosViewer software was used to generate a Co-Citation Analysis (CCA) of cited articles that were co-cited at least 10 times. Regarding CCAs, an overview of the major publications classified in clusters corresponding to seminal themes of interest-based on the dataset collated using the keywords “Shipping + Accidents” (CCA1) and “Accidents and Human Errors” (CCA2) was presented. These are further considered in the discussion section. Bibliometrix software provides a topography of the field with co-occurrence of keywords, a co-word analysis (Figs. 4 , 5 ). Finally, the top 20 authors resulting from the keywords are presented in Table 4 following Munim et al. ( 2020 ).
Interpretation (Step 5) At this stage, the researchers evaluated the top five papers of each cluster to interpret their content and were labelled according to the keywords (see Tables 2 , 3 ). The analysis of the CCA was supplemented with a topography of the field (analysis of top 20 authors for “Accidents + shipping” and “Accidents + Human error”) and discussed in the following section.
Discussion of findings
Co-citation analysis (cca 1): understanding shipping accidents.
The initial query using the keywords “Shipping + Accidents” was grouped into four clusters illustrated in Fig. 2 . Two clusters (A and C) focus on human error, whereas the other two (B and D) refer to engineering or other causes.
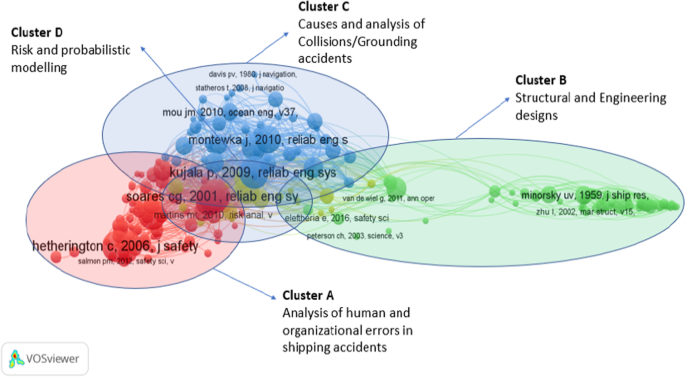
CCA clusters based on keywords “Shipping + Accidents”
Figure 2 presents the four main clusters identified in Stage 1 of the bibliometric review with CCA based on shipping accidents and illustrates the clusters with the most weight within the overall map based on the total articles per cluster and the average number of citations per article as summarised in Table 2 . Cluster A and C in Fig. 2 focus on finding and/or explaining the causes (with methods such as Root Cause Analysis) of HE. Cluster B deals with technical, engineering and other structural design issues, while Cluster D is related to risk and probabilistic modelling with mathematical models. As Clusters B and D were not related to HE, they are excluded from the analysis below.
Cluster A is labelled “ Analysis of Human and organisational errors in shipping accidents ”. It gathers 73 of the most cited co-cited references. Most research papers in this cluster describe and/or analyse the human error. In Table 6 (in the “ Appendix ”), the main themes were classified into three categories: Managerial and Human Resources, Socio-technical use and Individual and Cognitive approaches to explain, predict and/or prevent maritime shipping accidents. Cluster A contains the most significant proportion of references and overlaps extensively with Cluster C (Collision/Grounding accidents).
Cluster C is labelled “Collisions/Grounding accidents” . This cluster has 47 cited references and has extensive connections with Cluster A, which incorporates human and organisational errors as the leading causes of groundings and collisions. However, in Cluster D, HE is considered one of many other mathematical variables in risk models and algorithms; nevertheless, it overlooks the different dimensions that constitute HE (such as fatigue, organisation choices etc.).
This initial search confirms that HE is the central concern related to shipping accidents, highlighted in more than 63% of articles in Clusters A and C. To examine further the results of clusters A and C, all the articles were reviewed by the researchers concentrating on their titles and abstracts. These were classified into three main topics related to HE, namely (1) managerial and human resources, (2) socio-technical use, and (3) individual errors analysed with a cognitive approach. These categories are used to evaluate the literature identified in each of the respective clusters (A and C) and are summarised in Tables 6 and 7 in the “ Appendix ”.
Co-citation analysis (CCA2): understanding the role of “human error” in maritime accidents
In the second stage of the CCA process, another query using the keywords “Accidents + Human error” was conducted to refine our understanding of human error. A total of 225 articles resulted and were grouped into 5 clusters described in Table 3 and illustrated in Fig. 3 .
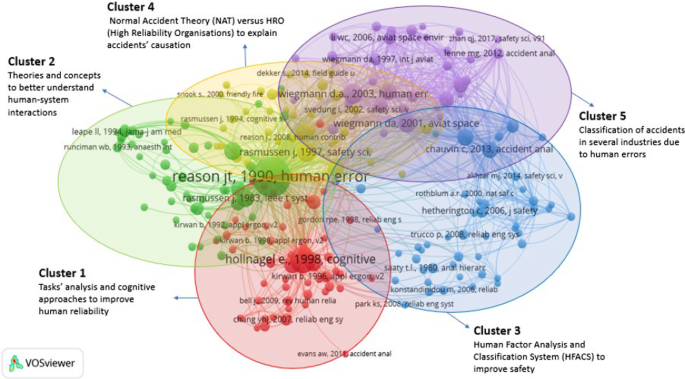
CCA clusters based on keywords “Accidents + Human error”
Cluster 1 focuses on an individual unit of analysis looking into tasks and cognitive reactions. Cluster 2 proposes the main theories around man–machine interactions (particularly Information Technologies and Systems) with the work of Reason ( 1990 ) linking all the other clusters. Cluster 4 adopts a more structural unit of analysis based on ship structures and illustrates the theoretical debate between Normal Accident Theory (NAT) and High-Reliability Organisations (HRO). Cluster 3 is centred in the Human Factor Analysis and Classification System (HFACS) related to safety, and Cluster 5 centres on identifying contributing factors and classifying accidents in several industries.
Cluster 1 was labelled Task analysis and cognitive approaches to improving human reliability . It gathers 51 co-cited references. Most papers propose methods or models to assess the risk of accidents to better predict them (Hollnagel 1998 ; Swain and Guttmann 1983 ; Shorrock and Kirwan 2002 ). Most research approaches adopt a cognitive understanding of HE (Hollnagel 1998 ; Shorrock and Kirwan 2002 ; Chang and Mosleh 2007 ). Kirwan ( 1994 ) focuses on tasks performed by humans as they interact with systems or technologies and the related risks. The top ten articles of this cluster are oriented toward improving human reliability.
Cluster 2 was labelled Theories and concepts to better understand human-system interactions . This has 51 co-cited references that are primarily dominated by the work of Reason, who proposed the theoretical integration of several previously independent literatures (Reason 1990 ). He further proposed two ways of modelling HE: using a person or a systems approach (Reason 2000 ). Other articles focus on socio-technical use, such as Rasmussen ( 1983 ), who develops theoretical backgrounds related to introducing information technology, digital computers and knowledge. Endsley ( 1995 ) discusses several methods to measure situation awareness, and Norman ( 1981 ) suggests a theory of action to avoid action slips.
Cluster 3 was labelled Human Factor Analysis and Classification System (HFACS) to improve safety. Cluster 3 gathers 48 co-cited references. Most papers investigate human error using the HFACS method to analyse multiple accidents (Celik and Cebi 2008 ; Chauvin et al. 2013 ; Chen et al. 2013 ). Chen et al. ( 2013 ) develop an HFACS dedicated to Maritime Accidents. Hetherington et al. ( 2006 ) raise the issue of aggregating the causal factors of HE within the maritime context, while Trucco et al. ( 2008 ) propose an innovative approach to integrate the human and organisational factors into risk analysis.
Cluster 4 was labelled Explaining accident causes using two theoretical approaches . Cluster 4 gathers 41 co-cited references. This cluster illustrates the theoretical debate between Normal Accident Theory (NAT) and High-Reliability Organisations (HRO) to explain the causes of accidents.
Cluster 5 was labelled Classification of accidents in several industries due to human error . Cluster 5 gathers 34 co-cited references with several classifications of accidents due to HE. Shappell and Wiegmann ( 1997 ) propose a taxonomy of unsafe operations. Reinach and Viale ( 2006 ) investigate six accidents, highlighting 36 probable contributing factors. Based on an analysis of 508 mining accidents, Patterson and Shappell ( 2010 ) classify main causations between operator error and system deficiencies
Overall, Fig. 3 identifies relevant literature tackling managerial and human resources issues. Clusters 3 and 5 adopt quantitative methods and provide statistics and factor weightings to describe the cause of accidents. Cluster 1 represents individual and cognitive issues with HFACS as the main method. Socio-technical issues are addressed in Clusters 2 and 4 but mainly with theoretical approaches coming from psychology, cognitive sciences and ergonomics.
Both CCA1 and CCA2 are complementary. Figure 2 of CCA1 (‘Shipping + Accidents’) provides the whole landscape of seminal publications (including papers and books) related to accidents in maritime transportation. Two clusters of CCA2 are more related to understanding human error in accidents (A and C). To go further, Fig. 3 with CCA2 ‘Accidents + Human error’ provides the seminal books and papers related to the analysis of what human error is, their causes and recommendations to cope with them, whatever the types of transportation. CCA1 is focused on maritime transportation, whereas CCA2 includes all types of transportation modes that tackle the HE question.
There is minimal overlap between CCA1 and CCA2. There are only two authors that belong both to CCA1 and CCA2. One is Reason for his seminal book on HE that is both co-cited in maritime and other transportation fields to study accidents. The other is Hetherington et al. ( 2006 ), who is one of the main cited papers to study HE in maritime.
This review highlights the limited understanding of HE and the lack of depth that would fully explain HE and on-board group behaviours, both from human resources and socio-technical perspectives.
Topography of the research field
To further develop the bibliometric review and compliment the co-citation analysis, the following section presents a topography of the field, following Munim et al.’s ( 2020 ) approach, which further maps the structure of the research themes related to the research keywords.
Topography of ‘shipping and accidents’ research
There has been a growing trend in the number of articles’ citations related to ‘Shipping and Accidents’, particularly over the last decade, illustrated in Fig. 4 . This suggests the growing interest and importance of this topic.
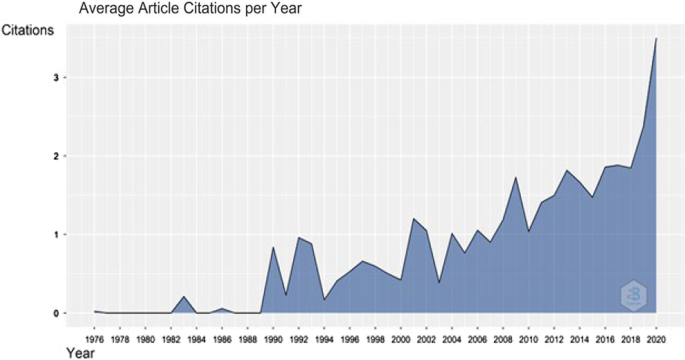
Average citations per year for the keywords “Shipping + Accidents”
To understand this trend in more depth, centrality and density measures of the main topics are calculated and presented visually in Fig. 5 . Centrality (Callon centrality) measures the strength of association between the keywords in one cluster with another cluster. Density (Callon density) measures the aggregate strength of the relationships between the keywords in the same cluster (Cobo et al., 2011 ).
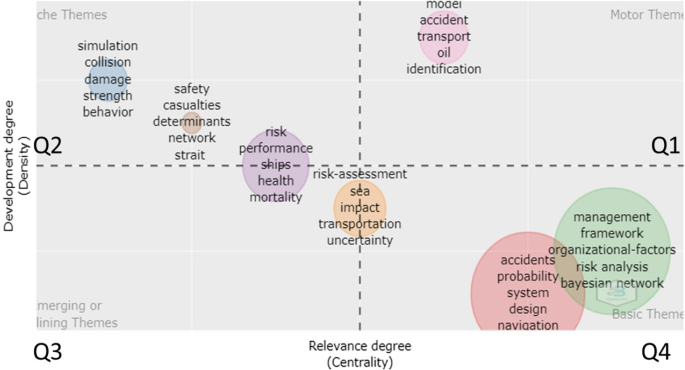
Thematic map with co-occurrence of keywords for ‘Shipping + Accidents’
Based on keyword co-occurrence centrality, the themes in quadrant Q1 (top right) called motor themes are topics that act as a bridge between other topics. The keywords in quadrant Q2 (top left) indicate highly developed or niche themes. The keywords in quadrant Q3 (bottom left) display emerging topics in a particular field. Finally, the keywords in quadrant Q4 (bottom right) indicate basic and transversal themes currently under development.
This thematic map shows that the most well developed and highly researched themes are related to models of accidents related to transportation, specifically in the context of oil spills and using identification systems such as AIS. In addition, the basic topics that are underdeveloped are related to frameworks, organisational factors, risk analysis and Bayesian networks, followed by probability of accidents related to design engineering.
The themes in the top right Q1 are fundamental to structure the research field. The keywords in the theme (model, accident, transport, oil, identification) are related to accident modelling, oil transportation and identification of risks. Q1 has strong connections with the keywords (sea, impact, transportation, uncertainty) (between Q2 and Q3). The cluster in Q1 is also connected with the themes of Q3 and Q4 regarding quantitative analysis of accidents and behavioural factors. The keywords in Q2 (simulation, damage, collision, strength) are research fields related to collision simulations and strength behaviour simulations of maritime structures. The other cluster (safety, casualties, determinants, network) has specialised themes; it is pretty isolated with strong internal ties but weak relations with other themes. The themes in Q4 (accident, probability, system, design, navigation) are related to a quantitative analysis of accidents, human error quantification and decision making (consistent with clusters B and D of CCA1). The other themes in Q4 (management, framework, organizational factors, risk analysis, Bayesian networks) are related to human and organizational factors related to the maritime industry and risk analysis using Bayesian networks (consistent with cluster C of CCA1). These themes have strong external ties with all other clusters (Fig. 6 ).
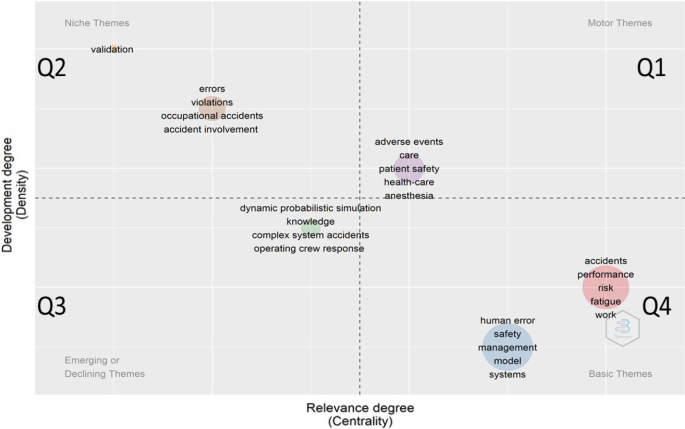
Thematic map with co-occurrence of keywords for “Human error + Accidents”
Topography of ‘human error + accidents’ research
In order to understand shipping accidents in more depth, the topography of the research related to the keywords ‘human error + accidents’ was also developed. While there were studies related to human error in other fields (such as medicine), only those related to the maritime sector are commented upon in this section.
The keywords in the Q4 are basic themes still in development with many external links but not necessarily strong with all the other clusters of Fig. 6 . On one side, the cluster related to (Accidents, performance, risk, fatigue, work) corresponds to the themes developed by the top 20 authors in marine technology and reliability engineering (See Table 4 ). On the other hand, the other cluster with keywords (human error, safety, management, models) is related to the themes developed by the top 20 authors in human factors and ergonomics (See Table 5 ). The clusters on the Q2 (errors, violations, occupational accidents, accidental involvement) are specialised and isolated themes.
As a conclusion, we can see that the keywords related to the understanding of human error with organisational insights (human error, safety, management systems, organisational factors, accidents, Bayesian network, performance, risks, fatigue and work) are promising fields of research as shown in Figs. 5 and 6 . Having highlighted the most interesting keywords and their co-occurrences, we further develop the literature by looking at the top-cited papers of the top 20 authors.
Focused literature review: shipping + accidents
To review the literature for both keywords “Shipping + Accidents” and then “Human error + Accidents”, the most cited papers of the top 20 authors highlighted by the Bibliometrix software (Table 4 ) were selected and analysed. Firstly, papers published before 2015 that were cited at least 40 times were selected; second, from 2015 to date (2021), the papers cited 15 or more times were included as they would highlight important and emerging topics. For the keywords “Shipping + Accidents”, this led to a comprehensive database of 222 articles. Table 4 below shows the top 20 authors according to their h-index provided by Bibliometrix.
All papers most cited and more recently published enter into one of the cluster labels of CCA1. Below we only focus on papers related to clusters A and C as they are related to HE in shipping accidents. Papers related to Cluster A split into two categories: first, some papers focus on the scope of HE from an individual cognitive approach with several methods: second, other papers adopt a monograph or historical approach to highlight human factors.
Firstly, Celik and Cebi ( 2008 ) develop a Human Factor Analysis and Classification System (HFACS) for HE in shipping accidents to improve group decision-making. In this model, the organisational influences are described as “big categories” (resource management, organisational climate and organisational processes). Supervision causes are described as inadequate or inappropriate. Finally, “communication, coordination and planning factors” are categorised as “personnel factors” and considered group-related activities. These models provide useful categories but do not fully describe how organisations act in a dynamic context.
Secondly, Graziano et al. ( 2016 ) propose a classification of HE taxonomy based on collision and grounding reports with four main categories: task errors, cognitive domain, technical equipment and performance. Interestingly, internal and external communication errors are highlighted as one key task; external communication includes communication between pilots, other vessels, tugs, VTS and on-shore. The main novelty of this paper is the description of the leading technical equipment which mediates HE, the most frequent being radars, followed by VHF and paper charts. All in all, we can infer from these categories of errors that they occur in situations where internal teams and/or external groups and stakeholders are involved.
Thirdly, Wu et al. ( 2017 ) propose a cognitive reliability and error analysis with evidential-based reasoning with original variables such as linguistic issues and incomplete information on-board. Akyuz and Celik ( 2014 ) similarly provide an HFCAS model combined with cognitive maps and highlight, in all categories of the model, the lack of knowledge or training as the major causes of accidents. They recommend studying ships in team contexts (including better diversity management on-board) and training them to adapt according to unexpected circumstances. In this paper, the recommendations are drawn on the necessity to adopt continuous learning, whatever the categories of HE.
Regarding monograph or historical approaches, Islam et al. ( 2017 ) develop a monograph for HE in operations maintenance useful for chief engineers and captains. Interestingly, the major causes of accidents come from deficiencies in knowledge (lack of experience) or insufficient training followed by seafarers' fatigue. Hansen developed several historical analyses of death on-board that enlarged and refined the human factors currently considered in studies. For instance, in their analysis, which covers the period between 1986 and 1993, Hansen and Pedersen ( 1996 ) concluded that the maritime workplace is a high risk where half of the deaths are due to the workplace and the lifestyle of seafarers. Hansen and Jensen ( 1998 ) undertook a unique study on the risks related to female seafarers and showed that major risks are due to their lifestyle (notably the consumption of alcohol and tobacco) and the fact that they “adopt the traditional male jobs at sea”. Roberts and Hansen ( 2002 ) highlighted several factors that concern both individuals (notably the age of the vessel as being one of the most important ones), several factors related to the working conditions (such as change of ship due to lost employment, daily routine duties, lifestyle) and the use of space on board (walking from one place to another, falling in docks when hazardous access and working practices are adopted). In a nutshell, most results of this cluster are oriented toward results aiming at facilitating decision-making but mostly at the level of individuals.
Complementary papers related to Cluster C are characterised by a diversity of research methods such as Bayesian networks (Hänninen and Kujala 2012 ), identification of events and processes of risks (Montewka et al. 2014b ), what-if analysis, association rules (Weng and Li 2019 ), scenario-event tree (Chai et al. 2017 ), binary logistic regressions (Weng and Yang 2015 ) and accident reports (Wróbel et al. 2017 ). They also sometimes develop research on specific ships such as ROPAX, cruise ships or tankers. Finally, they also propose tools or methods that improve safety: for instance, a ship collision alert system (Goerlandt et al. 2015 ) or a method for detecting possible near-miss ship collisions (Zhang et al. 2016 ).
This cluster provides interesting categorisations of human and organisational factors but always in “big categories” regarding the organisation of ships that remain static (except for Aps et al. 2015 ) and still mainly focused on “individuals” as units of analysis and not groups or networks. For instance, Hänninen and Kujala ( 2012 ) highlight the changing course in an encounter situation, the officer of the watch, the situation assessment, danger detection, personal conditions and other distractions (maintenance routines, fatigue, bridge view) as the main causes of accidents. Hänninen and Kujala ( 2014 ) integrate a new and interesting variable—the role of port state control in accidents—broadening the scope of study from the ship to her wider network. Regarding the automation and digitalisation of ships, Wróbel et al. ( 2017 ) provide one of the few analyses of the evolution of accidents with unmanned ships, arguing that if the number of navigational accidents falls, other types of accidents, such as fire on board, will increase with potentially worse consequences.
All in all, these pieces of research provide an interesting categorisation of the causes of HE. However, they all remain static pictures without providing a dynamic analysis, which would be a good basis for adaptive decision-making in specific contexts and building learning recommendations. Most studies still focus on individuals as units of analysis; few consider groups, and even fewer include the whole network of the ship. Research that includes “organisational factors” does not describe their workplaces nor the working conditions and routines on-board. Few studies recommend the necessity of a dynamic learning culture on-board offering ships the possibility to continuously adapt to the unexpected. This paper contends that these approaches will provide an in-depth understanding of the causes of accidents on ships, moving from a “technical structure” described through static categories to a real organisation with human beings on-board, able to adapt accordingly to their specific contexts. Finally, even though the digitalisation of ships is a reality, very few studies consider the use of technical tools as a cause of potential accidents.
Focused literature review: “human error + accidents”
The search keywords used led to papers related predominantly to transportation modes in aviation or rail. However, all papers related to maritime transportation that were cited 10 or more times were all included (see Table 5 ). The papers were reviewed to ensure a complete understanding of the content and themes within them. This led to a complete database of 241 articles.
The close analysis of the top 20 authors revealed three main academic disciplines that are currently structuring the field grouped as follows: (1) Human Factors and Ergonomics (HFE) on one side and (2) Marine Technology and Transportation Engineering (MTTE) and Reliability Engineering (RE) on the other side. HFE constitutes 11 top-cited authors Footnote 2 and publishes topics inspired from clusters 1, 2, 3 and 4 in CCA 1; (3) MTTE and RE consist of six authors Footnote 3 who publish on topics related to those in Clusters 3 and 5. The research of these authors is in the context of different modes of transportation (including maritime, rail, road, aviation) or other industries (health, mining, nuclear). Some authors are specialised in specific transportation modes—for instance, Shappell and Wiegmann in aviation, Mosleh in nuclear and Akyuz and Celik in maritime.
Our analysis highlights two main contributions of HFE:
Frameworks or models based on complex systems and sociotechnical systems theories (such as ACCIMAP, Human Factor Analysis and Classification System (HFACS), Systems Theoretic Accident Model and Processes (STAMP), Causal Analysis based on STAMP (CAST), Critical Path Analysis EAST, Functional Resonance Analysis Method (FRAM) to better assess risks based on taxonomies of human errors. Jenkins et al. ( 2017 ) and Hulme et al. ( 2019 ) propose a good synthesis and comparisons of them.
A diversity of industries and transportation modes can benefit or complement others (Banks et al. 2019 ; Grant et al. 2018 ; Hulme et al. 2019 ). There is, for instance, a historic move in the literature from research in the aviation industry that started to study the concept of situation awareness that is then applied to the maritime context. Indeed, Grant et al. ( 2018 ) recently proposed a generic accident causation model that could fit several industries using ‘systems thinking’.
There remain gaps and limitations in the HFE literature. For instance, the term HE does not sit easily with sociotechnical systems theories and concepts on which all these frameworks and models are based (Stanton et al. 2016 ), and specific phenomena such as the effects of communication and compounded information on performance are still under researched. Another limitation is the difficulty to model the different flows of information between separate teams (Jenkins et al. 2010 ). Furthermore, except for Harvey and Stanton ( 2014 ), there is still very little research focusing on the cognition of systems and large and distributed networks as units of analysis. An exception is Salmon et al. ( 2015 ) who study situation awareness at the level of systems. They present ten challenges for improving the understanding of interactions between social, technical and organisations, integrating the openness in systems, developing an understanding of what happens across boundaries (notably communication and coordination), culture, responsibility (with external pressure) and finally emerging behaviours (being more adaptive) and the ability to cope with changes. All these are still relevant and remain potentially fruitful areas for future research.
In the area of MTTE and RE overall, researchers tend to quantify HE in order to avoid researcher subjectivity using a range of methods such as fuzzy process on HFCAS (Celik and Cebi 2008 ), methods to set up the probabilities of human errors with the Error Producing Conditions (EPC) (Akyuz and Celik 2016 ) or weights related to causes (Akyuz et al. 2017 ) or the development of human error indexes (Khan et al. 2006 ). These methods are sometimes complemented by qualitative approaches such as the Why-because graphs of Chen et al. ( 2013 ). Furthermore, research in this field examines accidents in fine-grain looking at the specificities of different types of accidents, such as grounding (Akyuz and Celik 2015 ), fire (Akyuz et al., 2018 ), explosions (Baalisampang et al., 2018 ), offshore (Khan et al. 2016; Ren et al. 2008; Islam et al. 2017 ), and also different types of ships (Akyuz et al., 2017 ). To a lesser extent, there is also some research into the interactions between human and information systems (Mokhtari 2007 ).
However, similar to HFE, there are also gaps and limitations in the MTTE and RE literature that can provide an opportunity for future research. For example, much of the literature in this field, that highlighted that most current causes of HE relate to collective actions, is based on the modelling and analysis of cognitive and individual units of analysis (for instance, Akyuz and Celik 2014 ), which are mostly related to stress, fatigue, health except for Fan et al. ( 2018 ); Fan et al. ( 2018 ) mention the emotions of seafarers. Moreover, while Baalisampang et al. ( 2018 ) extended these individual factors to include elements such as knowledge, competencies, expectations, goals and attention, combined with workplaces factors (site and equipment design, work environment) and managerial factors (organisation of work, job design and information transfer), these are still not fully developed. Furthermore, when reviewing accident reports (for instance, Baalisampang et al. 2018 ), researchers do not address the lack of standardisation of these reports (Celik and Cebi 2008 ), which is a considerable limitation and an area for future work. Finally, as ships are becoming increasingly more automated, there are still very few studies investigating the on-board use of information systems and technologies and their interactions with the shore to improve communication and coordination.
All in all, this previous work has built a solid foundation for analysing HE to better prevent accidents. In the research agenda below, we propose how organisation and management sciences can bring new insights to advance human error research in maritime transportation.
Research agenda: propositions for studying human error in maritime accidents
Having evaluated the findings from the bibliometric review, it was clear that accidents are mainly explained from an engineering perspective. Human errors remain under-explored from organisational and network perspectives. In this section, five propositions for theoretically framing future research approaches are presented. Each of these theoretical management approaches can help improve our understanding of HE in the context of maritime accidents.
Ships as organisations: a novel perspective
The findings from this study revealed that the literature on maritime accidents has not fully conceptualised ships as organisations. Neither has it considered how these organisations behave according to the different temporalities in navigation. So, apart from individual and cognitive-based approaches, how can ships be conceptualised as organisations? Here, the conceptualisation of ships as temporary organisations generally follows navigational routines but, in cases of imminent accidents, develop crisis navigation routines.
From this perspective, merchant ships can be considered as organisations that go from point A to point B in order to deliver products. They are characterised by an organised (collective) course of action ‘aimed at evoking a non-routine process and/or completing a non-routine product’ (Packendorff 1995 ). Routines are defined as “repetitive, recognizable patterns of interdependent actions, carried out by multiple actors” (Feldman and Pentland 2003 ). The temporary time frame of the navigating crew is particularly relevant when considering safety management on-board. This is similar to project-based organisations characterised by a once-in-a-lifetime task with a predetermined delivery date, subject to performance goals and consisting of several complex and/or interdependent activities (Packendorff 1995 ).
Indeed, the analogy of merchant ships and temporary organisations is helpful to distinguish two types of temporalities: regular navigation and the period before an accident. When there are no accidents, the ship’s organisation and the environment are stable most of the time. The objectives of the ship are clear (to go from point A to point B), and actors behave according to a highly centralised and rational organisation that follows relatively standardised and shared routines (Degani and Wiener 1993 ), which we call ‘regular routine navigation’. This is empirically similar to formal quality management systems. However, during the period just before the accident (which can be short depending on the context), the crew and its network (notably for remote-controlled ships) try to make sense of the situation and adapt to it. Adopting a routine lens to study how routines cease or are transformed during an accident could be an interesting perspective yet not explored.
The transition between ‘regular routine navigation’ and ‘crisis routine navigation’ depends on the type of accident and can range from a few minutes to hours or days. During this transition time, which we term ‘crisis routine navigation’, actors on-board are aware of the imminence of the accident; behaviours on-board change due to uncertainty. As a result, there is an increase in stress (Sheridan 2008 ) that may lead to phenomena such as “out-of-the-loop” performance. This is characterised by actors’ failure to observe parameter changes and intervene when necessary, an over-reliance and absolute trust in information technology artefacts, a loss of situation awareness and finally, deterioration of an actor’s manual skills (Kaber and Endsley 1997 ). In such circumstances, both social cooperation modes and decision-making are affected. In the case of disaster management, resilience is critical. This is the system’s ability to anticipate and respond to anomalous circumstances to maintain safe functioning and recover and return to a stable equilibrium (Sheridan 2008 ; Normandin and Therrien 2016 ). Further research is needed to study ships as organisations that also include the specificities of their culture.
In the literature, as highlighted in Fig. 3 , the leading theory related to ships seen as organisations is the debate between High Reliable Organisations (HRO) and Normal Accident Theory (NAT). This controversy questions two domains, which raises new research questions: firstly, are there alternative theoretical models that can describe ships in practice? Second, with all the technologies and potential resources available today to secure ships, is it still relevant to consider the assumptions of NAT as reliable?
Ships: High Reliable Organisations (HRO) or self-organisations embedded in ecosystems?
Arguably, ships can be characterised as HRO and are perceived as one of the most highly centralised and rational types of transportation modes. Like the airline industry, maritime navigation has adopted standardised routines such as Cockpit Resource Management (CRM) implemented to provide checklist procedures that need to be accomplished by coordinated actions and communications between the captain and the other pilot(s) in a flight (Degani and Wiener 1993 ). According to the ‘high-reliability theory’, extremely safe operations are possible, even with extremely hazardous technologies, if appropriate organisational design and management techniques are followed (Sagan 1993 ).
However, accidents still do happen in HRO. Normal accident theory (NAT) presents a much more pessimistic prediction – specifically that ‘serious accidents with complex high technology systems are inevitable’ (Sagan 1993 :13). This empirical observation presents new research questions, such as, is the NAT still relevant today? Should we extend HRO theory to propose new concepts that would better describe ships as they function in real conditions? Could another way to manage resources and trade-off decisions concerning investments on ships avoid accidents? Has the maritime industry learnt from the aviation industry (International Air Transport Association congress of 1975) that it is machines that have to be adapted to human-beings and not the reverse (Clostermann 2017 :20)?
By applying Normal Accident Theory, ships can be considered to be an assemblage of components that are self-organised. From this perspective, we propose that ethnographic studies can better describe and shed light on working conditions on ships in real-life settings. From a theoretical perspective, we suggest exploring new concepts to study ships, notably in the case of imminent accidents. For instance, applying the concept of self-organisation of different maritime agents/stakeholders coordinating ports, ships and operations (Caschili and Medda 2012 ; Watson et al. 2021 ). More broadly, as ships are being increasingly managed remotely, this implies that their whole ecosystem and interactions with other stakeholders need to be considered in any future research. This includes the near network of shipping (incorporating the ship owner, insurances, port state control, VTS) and in a larger ecosystem representing the choices of the whole industry (flag ship, meta-organisations, countries that develop their marine policy).
Even though ships can be characterised as HRO, the proposition here is that their real organisational mode may be closer to self-organisation depending on the temporality of the accident. This is in direct opposition to the HRO view. The response to any accident is organisationally hierarchical and procedures officially documented according to quality management linked to the International Maritime Organisation (IMO) (Ismael 2011 ).
Digitalisation of ships and management of information systems
Many maritime vessels already use a range of information technologies (IT) and information systems (IS) with a host of different navigational equipment and sensors to assist them to navigate safely and efficiently, including Electronic Chart Display Information System (ECDIS) as a modern replacement for paper-based navigational charts, the Automatic Identification System (AIS) and radar (Radio Detection and Ranging) help improve situational awareness of other vessels and obstacles (Harti-Mokhtari et al., 2007). Furthermore, as Artificial Intelligence (AI) and machine learning develop at a pace, more vessels are using autonomous and semi-autonomous technologies that are monitored remotely from shore-based facilities requiring highly reliable and efficient communication channels (Hogg and Ghosh 2016 ).
These new technologies and other integrated bridge equipment mean that crew on-board ships increasingly rely on them. “Unlike in static situations where human–machine systems have complete control, in dynamic situations like navigation, changes occur rapidly giving only partial control to the operator” (Hoc 2000 : 835). This creates socio-technical systems that incorporate complex interactions between humans, machines and other environmental aspects (Baxter and Sommerville 2011 ). In this context, three main settings are particularly impacted by the socio-technical use of IT/IS, where human error can occur. Namely, IT/IS implementation, IT/IS use in navigation practice and IT/IS-based decision-making. For instance, the improper consideration of human–computer-interaction in the design of the technologies, the often ad-hoc way in which new and emerging technologies are implemented, and inadequate user training can all lead to inevitable human error (Lützhöft et al. 2011 ).
Similarly, the objectives of improving navigation safety are inextricably linked to a set of daily decisions taken by several interdependent actors on-board. This process is increasingly dependent on the diffusion and integration of data, information and knowledge between humans and technological devices in order to make decisions and take appropriate actions. Poor systems interfaces and improper allocation of functions to human and computer controllers can result in misinterpretation and misunderstanding of data and information being displayed, which leads to poor decision-making, degraded performance and ultimately accidents (Kaber and Endsley 1997 ).
Although ship systems are becoming increasingly well-equipped, technologically advanced and more reliable (Rothblum 2002 ), maritime accidents still happen. No technology is used in isolation, but rather the maritime system incorporates people, the environment (socio-technical and natural), and the organisation. In order to better understand the complexities, issues and problems, and how to avoid the repetition of accidents, all the different IT/IS technologies on-board a vessel must be considered holistically as part of the complex maritime ecosystem (Güven-Koçak, 2015 ; Watson et al. 2021 ). This digital transformation in the industry driven by new technologies such as AI and big data generates new operational challenges and risks such as cyber-attacks for the maritime sector that need further investigation (Munim et al. 2020 ).
One theoretical lens suggested continuing to develop complex systems and sociotechnical systems theories. Ships can be considered complex systems through this theoretical lens, both internally as an organisation and concerning their environment (Sovacool, 2008 ). These are large, tightly coupled systems (Perrow 1984 ) where socio-technical interdependencies (Thompson 1967 ) are high due to their complexity. Internally, a ship is a complex system involving a collection of crew members and the range of instruments and computer networks that support them. None of the crew possesses the complete plan or vision to navigate the ship. However, collectively they use information from the crew in conjunction with instrument observations and procedures to keep the vessel on the course (Ismael 2011 ). The more complicated the interdependence of systems and subsystems, the higher they become prone to failure due to their complexity, speed of interaction, tight coupling and limitations of their human operators and their designers (Sovacool 2008 ; Lützhöft et al. 2011 : 285). Consequently, from this perspective, ship-related maritime accidents can be characterised by a high level of complexity due to the interrelations of multiple and combined causes and the variability of contexts.
Orlikowski’s ( 1992 ) structuration theory, where technology is embedded with structure, can also offer insights into how human agents carry out their routines and the intervention that changes the relationship between human agents and organisational structure (Barley 1986 ) in the maritime context. Since technology is not always used by knowledgeable agents, this theoretical lens can explain how agents use these new technologies in their daily routines, and how they enact new structures or “technology-in-practice” (Orlikowski, 2000 ) to better understand human error.
De Vries ( 2017 ) is one of the few researchers in the maritime domain that showed how navigation safety of seagoing vessels can be improved through the socio-technical interaction of humans, technology, organisations and the environment drawing on Hollnagel et al.’s ( 2014 ) Functional Resonance Analysis Method (FRAM). Building on this work, De Vries and Bligård ( 2019 ) further demonstrated the benefits of applying a socio-technical systems perspective to influence navigation assistance assessment and design. Furthermore, they showed how discussions with stakeholders such as users, designers, managers, and regulators contributed to safe operations in the maritime context. However, these studies are few, and by applying a socio-technical perspective to the design of on-board systems to ensure they are compatible with and adapted to the human operator to improve performance (Brett et al. 2011 ) is a fruitful area of research for understanding and ultimately reducing human error in maritime transportation accidents.
As a consequence of these fast-paced technological developments, further research is needed on the interaction of ships within their broad and complex maritime ecosystems. These include but are not limited to the maritime environment, navigation and technologies, and the international organisations that frame, govern and regulate today’s shipping industry. This idea of improvement relies on developing standards in an industry that is more and more digitalised and interconnected (Watson et al. 2021 ). By improving our understanding of the maritime industry's emerging needs, which is partly considered self-organisations within an ecosystem, and partly tightly coupled with other systems, future accidents can be reduced.
Power Lens: a missing link
Organisations of all types, including ships and their ecosystems, are fundamentally underpinned by power relationships and issues. However, there is limited literature on this topic in the maritime context. At the level of the ship, a unique aspect of maritime culture is absolute autonomy and a strong power culture where the captain, known as “master under God”, is in full charge. While at sea, the captain has full authority over the ship, her occupants, and operations and is responsible for all safety issues (Güven-Koçak 2015 ), including final decisions and the responsibility related to accidents such as grounding. The captain and officers can exercise their judgement to make necessary decisions, such as changing routes, arrival ports or schedules.
With increasing links between the sea and the shore, communications between the ship-owner, who manages the ships from the shore, and the captain who stays on-board, may sometimes not be very effective. For example, in the Torrey Canyon oil tanker wrecked off the coast of Cornwall, this was initially attributed to several human errors. However, a more detailed examination identified management decisions ‘that put pressure on the captain’ and ‘equipment design issues’ related to activation of the autopilot mode (Harvey et al. 2013 ) as contributing factors to the disaster. Despite this, the literature hardly mentions in any depth communications issues between the vessels at sea and the shore and the pressure from the shore, in some cases due to trade-offs between security and profit that the captain and its crew experience. The few papers that deal with this issue mention “external pressure” as a factor without providing any details.
At the level of the ecosystem of ships, having multiple actors in this domain makes it difficult to legally assign responsibilities in the case of an accident. Empirical data suggests that diverging political interests stall proper investigation and prevention of similar accidents. Thus, the appearance of a mysterious oil spill on the north-east coast of Brazil in September 2019 is most probably linked to crude oil from Venezuela that was carried by the Greek-flagged ship Bouboulina (BBC 2019 ). There is strong evidence that the company, the captain and the vessel’s crew failed to communicate to authorities about the oil spill/release of the crude oil in the Atlantic Ocean.
The broad literature on power is diverse and complex, and its ramifications for the study of organisations have remained largely unexplored (Haugaard and Clegg 2012 ), especially in the maritime transportation sector. Indeed, power concerns the ways that social relationships shape capabilities, decisions and changes within organisations. Organisational power is bounded by the capacity of the decision-makers to gather and analyse complex data, which are often multi-dimensional and constrained by prior experiences, learning and knowledge (Haugaard and Clegg 2012 ). As such, the sources of power—reward, sanction, expertise, reference value and legitimacy—can also trigger conflict, especially when there is a divergence of objectives and strategies for achieving those objectives (Fulconis and Lissillour, 2021 ).
Of the few studies that examine power, Lissillour and Bonet Fernandez ( 2020 ) adopt a Bourdieusian perspective to understand the balance of power in the governance of the global maritime chain. They highlight the conflict of interest between the different global maritime stakeholders. In the context of human error and accidents, the maritime transportation stakeholders – which includes vessel owners, ship captains, classification authorities, insurers, customers and many others – often have differing and competing priorities between safety and economic interests. Often their strategies for managing these most effectively also diverge, leading to tensions and conflicts and ultimately trickle down to operational and human errors resulting in catastrophic accidents. Research should be developed to further understand the interactions among all the stakeholders at the level of the network of actors cooperating in the case of accidents, including the meta-organisations in this wider network (Berkowitz and Dumez 2016 ) acting to regulate the industry and sustain the oceans.
In the context of maritime transportation, there are several meta-organisations (Berkowitz and Dumez 2016 ) operating to regulate the industry with significant consequences on the collective actions of ships in their daily activities. More research is needed to build on Harvey et al. ( 2013 )'s work to further develop and mobilise the concept of meta-organisations. Other theoretical backgrounds, such as neo-institutionalism (DiMaggio and Powell, 1983 ), can shed light on potential isomorphism behaviours at the industry level. This can then be applied to the maritime context to explore how to better cope with accidents, reduce their often catastrophic consequences, and ultimately reduce them.
Since organisations are neither rational nor natural, the theories of power can translate practice to theory and highlight the phenomena of changing organisational practises (Haugaard and Clegg 2012 ). Thus, future studies could use the lens of power theories with human error in the maritime accident context at the centre of the analysis to better understand communication and coordination issues and the stakes and conflicts of interest of the power relationships between the different actors.
Developing dynamic safety capabilities for learning ships
In addition to more collaborative relationships, each ship and related stakeholders should develop their capacities to learn from the past to reduce future accidents. In this area, we propose to develop the concept of dynamic safety capability within the literature on learning organisations. Several streams of research have explored how organisations can learn from rare events such as crises or accidents. Developing alertness to weak cues in the environment is the first step for developing intelligence. Attentional triangulation (Rerup, 2009 ) combines three forms of attention – stability, coherence and vividness- for anticipating and preventing unexpected events. Previous studies have tended to base their analysis on the concept of situation awareness, mainly focusing on individuals (Hetherington et al. 2006 ). Very few studies have mobilised situation awareness through teams and systems (Stanton et al. 2015 ). Thus, dynamic capabilities can provide an interesting perspective for encompassing the previous concepts concerned with issues of adaptation and growth.
Different kinds of dynamic capabilities have already been identified in the literature. A dynamic safety capability is an organisation’s capacity to “generate, reconfigure, and adapt organisational routines to sustain high levels of safety performance in organisations characterised by change and uncertainty” (Griffin et al. 2016 : 249). Dynamic safety capability relies on three processes of organisational learning. Experience is first accumulated through tacit learning from ongoing action and events. Then the tacit learning is articulated and shared through collective discussions and processes of sense-making. Finally, knowledge is formalised into regulatory procedures (Griffin et al. 2016 ). Since crises remain rare events, the authors suggest using the simulation of high-risk environments and their potential consequences to allowing participants to engage in sense-making and focus on team communication and coordination processes. This literature provides rich insights into the importance of developing the ability to share knowledge and learn. However, most of the disaster cases investigated by Griffin et al. ( 2016 ) dealt with stable organisations.
Further research could focus on the mechanisms, processes and related skills for developing a safety capability aboard extreme cases such as tankers. In such temporary organisations, a salient issue is the ability to share knowledge among highly dispersed teams in terms of role tasks. In addition, these teams that frequently change, have to manage the continuity of routines through periods of transitions. These organisations, partly similar to SMEs, have to develop a certain level of absorptive capacity (Benhayoun et al. 2020 ) to identify and capture the external information that comes from the ecosystem to support on-board decision-making. The temporality of ships, which partly prevents routines for learning from rare events, questions how they can become learning organisations. This raises new research questions such as: How could we reconcile ships being both temporary and learning organisations? What is the subculture that would allow ships to move from a culture of adjustment (Baumler et al. 2020 ) to become learning organisations?
Under the umbrella term of “human error”, the literature presents many different explanations for accidents, including flaws in structural and engineering designs, cognitive limits and organisational choices. Can all these causes be considered to be “human” errors? In principle, at some point, the causes of all accidents can be related to the “human”, but in providing such a vague catch-all term, the real issues fail to be identified and addressed. This paper suggests that research from the disciplines of human and social sciences, particularly organisation studies, can provide new and relevant insights by clarifying how ships can be described in terms of organisations and by considering them in a whole ecosystem and industry.
The main contributions of this paper are twofold. First, four thematic clusters were identified through a bibliometric review of the causes of maritime accidents related to human error. Among them, the analysis of human and organisational errors showed that the three main causes are related to human resources and management, socio-technical IT/IS, and individual and cognitive errors. A second search on “human error” highlighted five clusters that confirm these three main root causes and provide several references for each of them. Second, the paper provides a critical analysis of the papers published by the top 20 authors cited both for shipping accidents and human error. Finally, several theoretical concepts and propositions for future researchers and practitioners to help tackle the causes of human error in the context of maritime accidents were suggested.
The implications of this study are several. First, the proposed agenda for future researchers can advance the field of human error in the maritime transport context by providing different theoretical perspectives and adapting research methods from social and human sciences. Second, this study highlights the gap in our current understanding of the role of human error in maritime accidents, which can feed into curricula for the education and training of maritime cadets, seafarers and other personnel. Finally, by understanding these gaps, maritime organisations and stakeholders can implement policies that will embed human factors more specifically with the ultimate objective of improving safety in maritime transportation.
Availability of data and materials
All data generated and analysed during this study are available through Web of Science and are included in this published article (please see references).
In line with academic literature (Rothblum 2002 :1) this paper refers to accidents and distinguishes between accidents and incidents depending on the severity of damage. Insurance company reports (SSR 2021 ) refer to any damage no matter the severity (including sinking of a vessel) as an “incident”.
(N.A. Stanton; G.H. Walker; P.H. Seong; S.A. Shappell; D.A. Wiegmann; S.W.A. Dekker; W. Jung; M.G. Lenne; S. Nazir, P. Waterson and J. Kim).
(E. Akyuz; J. Wang; M. Celik; F. Khan; Mosleh; R. Abassi).
Acejo I, Sampson H, Turgo N, Ellis N, Tang L (2018) The causes of maritime accidents in the period 2002–2016, Seafarers International Research Centre (SIRC), Cardiff University, United Kingdom. Availalbe from http://orca.cf.ac.uk/117481/1/Sampson_The%20causes%20of%20maritime%20accidents%20in%20the%20period%202002-2016.pdf
Akyuz E, Celik M (2014) Utilisation of cognitive map in modelling human error in marine accident analysis and prevention. Saf Sci 70:19–28
Article Google Scholar
Akyuz E, Celik M (2015) Application of CREAM human reliability model to cargo loading process of LPG tankers. J Loss Prev Process Ind 34:39–48. https://doi.org/10.1016/j.jlp.2015.01.019
Akyuz E, Celik E (2016) A modified human reliability analysis for cargo operation in single point mooring (SPM) off-shore units. Appl Ocean Res 58:11–20. https://doi.org/10.1016/j.apor.2016.03.012
Akyuz E, Celik E, Celik M (2017) A practical application of human reliability assessment for operating procedures of the emergency fire pump at ship. Ships Offshore Struct 13(2):208–216. https://doi.org/10.1080/17445302.2017.1354658
Akyuz E, Celik M, Akgun I, Cicek K (2018) Prediction of human error probabilities in a critical marine engineering operation on-board chemical tanker ship: the case of ship bunkering. Saf Sci 110:102–109. https://doi.org/10.1016/j.ssci.2018.08.002
Alderton T, Bloor M, Kahveci E, Lane T, Sampson H, Zhao M, Wu B (2004) The global seafarer: living and working conditions in a globalized industry. International Labour Organization, Geneva
Google Scholar
Aps R, Fetissov M, Goerlandt F, Helferich J, Kopti M, Kujala P (2015) Towards STAMP based dynamic safety management of eco-socio-technical maritime transport system. Procedia Eng 128:64–73
Baalisampang T, Abbassi R, Garaniya V, Khan F, Dadashzadeh M (2018) Review and analysis of fire and explosion accidents in maritime transportation. Ocean Eng 158:350–366. https://doi.org/10.1016/j.oceaneng.2018.04.022
Banda OA, Goerlandt F, Montewka J, Kujala P (2015) A risk analysis of winter navigation in Finnish sea areas. Accid Anal Prev 79:100–116. https://doi.org/10.1016/j.aap.2015.03.024
Banks VA, Stanton NA, Plant KL (2019) Who is responsible for automated driving? A macro-level insight into automated driving in the United Kingdom using the Risk Management Framework and Social Network Analysis. Appl Ergonom 81:102904
Barley SR (1986) Technology as an occasion for structuring: evidence from observations of CT scanners and the social order of radiology departments. Adm Sci Q 31:78–108
Baumler R, De Klerk Y, Manuel ME, Carballo L (2020) A culture of adjustment – evaluating the implementation of the current maritime regulatory framework on rest and work hours. World Maritime University, Malmo
Book Google Scholar
Baxter G, Sommerville I (2011) Socio-technical systems: from design methods to systems engineering. Interact Comput 23(1):4–17
BBC (2019) ‘Brazil oil spill: where has it come from?’ (BBC News Online 1st November, 2019). https://www.bbc.com/news/world-latin-america-50223106
Benhayoun L, Le Dain MA, Dominguez-Péry C, Lyons AC (2020) SMEs embedded in collaborative innovation networks: how to measure their absorptive capacity? Technol Forecast Soc Change 159:120–196
Berkowitz H, Dumez H (2016) The concept of meta-organization: issues for management studies. Eur Manag Rev 13(2):149–156
Berkowitz H, Prideaux M, Lelong S, Frey F (2019) The urgency of sustainable ocean studies in management. M@n@gement 22(2):297–315
Brett BE, Rothblum AM, Lyle WA, Durgavich J, Sargent MG, Downer KF (2011) Predicting total system performance: the benefit of integrating human performance models. Proc Hum Fact Ergon Soc Annu Meet 55(1):2020–2024. https://doi.org/10.1177/1071181311551421
Caschili S, Medda FR (2012) A review of the maritime container shipping industry as a complex adaptive system. Interdiscip Descr Complex Syst INDECS 10(1):1–15
Celik M, Cebi S (2008) Analytical HFACS for investigating human errors in shipping accidents. Accid Anal Prev 41(1):66–75
Chai T, Weng J, De-qi X (2017) Development of a quantitative risk assessment model for ship collisions in fairways. Saf Sci 91:71–83
Chang YHJ, Mosleh A (2007) Cognitive modeling and dynamic probabilistic simulation of operating crew response to complex system accidents: part 1: overview of the IDAC model. Reliab Eng Syst Saf 92(8):997–1013. https://doi.org/10.1016/j.ress.2006.05.014
Chauvin C, Lardjane S, Morel G, Clostermann J-P, Langard B (2013) Human and organisational factors in maritime accidents: analysis of collisions at sea using the HFACS. Accid Anal Prev 59:26–37. https://doi.org/10.1016/j.aap.2013.05.006
Chen S-T, Wall A, Davies P, Yang Z, Wang J, Chou Y-H (2013) A Human and Organisational Factors (HOFs) analysis method for marine casualties using HFACS-Maritime Accidents (HFACS-MA). Saf Sci 60:105–114. https://doi.org/10.1016/j.ssci.2013.06.009
Chen J, Zhang W, Li S, Zhang F, Zhu Y, Huang X (2018) Identifying critical factors of oil spill in the tanker shipping industry worldwide. J Clean Prod 180:1–10. https://doi.org/10.1016/j.jclepro.2017.12.238
Clostermann J-P (2017) La conduite du navire marchand. Facteurs humains dans une activité à risques. InfoMer, Marines éditions. 3ème edition.
Cobo MJ, López-Herrera AG, Herrera-Viedma E, Herrera F (2011) Science mapping software tools: review, analysis, and cooperative study among tools. J Am Soc Inf Sci Technol 62(7):1382–1402
de Vries L (2017) Work as done? Understanding the practice of socio-technical work in the maritime domain. J Cogn Eng Decis Mak 11(3):270–295
de Vries L, Bligård LO (2019) Visualising safety: the potential for using socio-technical systems models in prospective safety assessment and design. Saf Sci 111:80–93
Degani A, Wiener EL (1993) Cockpit checklists: concepts, design, and use. Hum Factors 35(2):345–359
Dekker SW (2002) Reconstructing human contributions to accidents: the new view on error and performance. J Saf Res 33(3):371–385
Dekker S (2006) The field guide to understanding human error. Ashgate Publishing, Ltd., Farnham
DiMaggio PJ, Powell WW (1983) The iron cage revisited: institutional isomorphism and collective rationality in organizational fields. Am Sociol Rev 48:147–160
ElBardissi AW, Wiegmann DA, Dearani JA, Daly RC, Sundt TM (2007) Application of the human factors analysis and classification system methodology to the cardiovascular surgery operating room. Ann Thorac Surg 83(4):1412–1419. https://doi.org/10.1016/j.athoracsur.2006.11.002
Eliopoulou E, Papanikolaou A (2007) Casualty analysis of large tankers. J Mar Sci Technol 12(4):240–250. https://doi.org/10.1007/s00773-007-0255-8
Endsley MR (1995) Measurement of situation awareness in dynamic systems. Hum Factors J Hum Factors Ergon Soc 37(1):65–84. https://doi.org/10.1518/001872095779049499
Fan S, Zhang J, Blanco-Davis E, Yang Z, Wang J, Yan X (2018) Effects of seafarers’ emotion on human performance using bridge simulation. Ocean Eng 170:111–119
Feldman MS, Pentland BT (2003) Reconceptualizing organizational routines as a source of flexibility and change. Adm Sci Q 48(1):94–118
Fowler TG, Sørgård E (2000) Modeling ship transportation risk. Risk Anal 20(2):225–244. https://doi.org/10.1111/0272-4332.202022
Fulconis F, Lissillour R (2021) Toward a behavioral approach of international shipping: a study of the inter-organisational dynamics of maritime safety. J Shipping Trade 6(1):1–23
Galieriková A (2019) The human factor and maritime safety. Transp Res Procedia 40:1319–1326
Goerlandt F, Kujala P (2011) Traffic simulation based ship collision probability modeling. Reliab Eng Syst Saf 96(1):91–107
Goerlandt F, Montewka J (2015a) Maritime transportation risk analysis: review and analysis in light of some foundational issues. Reliab Eng Syst Saf 138:115–134. https://doi.org/10.1016/j.ress.2015.01.025
Goerlandt F, Montewka J (2015b) A framework for risk analysis of maritime transportation systems: a case study for oil spill from tankers in a ship–ship collision. Saf Sci 76:42–66. https://doi.org/10.1016/j.ssci.2015.02.009
Goerlandt F, Ståhlberg K, Kujala P (2012) Influence of impact scenario models on collision risk analysis. Ocean Eng 47:74–87. https://doi.org/10.1016/j.oceaneng.2012.03.006
Goerlandt F, Montewka J, Kuzmin V, Kujala P (2015) A risk-informed ship collision alert system: framework and application. Saf Sci 77:182–204
Grant E, Salmon PM, Stevens NJ, Goode N, Read GJ (2018) Back to the future: What do accident causation models tell us about accident prediction? Safety Sci 104:99–109
Graziano A, Teixeira AP, Soares CG (2016) Classification of human errors in grounding and collision accidents using the TRACEr taxonomy. Saf Sci 86:245–257
Grech MR, Horberry T, Smith A (2002) Human error in maritime operations: analyses of accident reports using the Leximancer tool. In: Proceedings of the human factors and ergonomics society annual meeting, vol 46(19). Sage Publications, Los Angeles, pp 1718–1721
Griffin MA, Cordery J, Soo C (2016) Dynamic safety capability: how organizations proactively change core safety systems. Organ Psychol Rev 6(3):248–272
Guardian (2021) 'Ever Given, the ship that blocked the Suez Canal, to be released after settlement agreed’ Reuters Online Mon 5 Jul 2021 00.10 BST https://www.theguardian.com/world/2021/jul/05/ever-given-ship-that-blocked-the-suez-canal-to-be-released-after-settlement-agreed
Güven-Koçak S (2015) Maritime informatics framework and literature survey-ecosystem perspective. In: Twenty-first American conference on information systems, Puerto Rico
Hänninen M, Kujala P (2012) Influences of variables on ship collision probability in a Bayesian belief network model. Reliab Eng Syst Saf 102:27–40
Hänninen M, Kujala P (2014) Bayesian network modeling of Port State Control inspection findings and ship accident involvement. Expert Syst Appl 41(4):1632–1646
Hansen HL, Jensen J (1998) Female seafarers adopt the high risk lifestyle of male seafarers. Occup Environ Med 55(1):49–51
Hansen HL, Pedersen G (1996) Influence of occupational accidents and deaths related to lifestyle on mortality among merchant seafarers. Int J Epidemiol 25(6):1237–1243
Haraldstad AMB, Christophersen E (2015) Literature searches and reference management. In: Laake P, Breien Benestad H, Reino B (eds) Research in medical and biological sciences. (Second edition), Academic Press, pp 125–165.
Harrald JR, Mazzuchi TA, Spahn J, Van Dorp R, Merrick J, Shrestha S, Grabowski M (1998) Using system simulation to model the impact of human error in a maritime system. Saf Sci 30(1):235–247. https://doi.org/10.1016/S0925-7535(98)00048-4
Harvey C, Stanton N, Zheng P (2013) Safety at sea: human factors aboard ship The Ergonomist, Issue 517, July, 2013. http://archived.ciehf.org/safety-at-sea-human-factors-aboard-ship/
Harvey C, Stanton NA (2014) Safety in system-of-systems: ten key challenges. Saf Sci 70:358–366
Haugaard M, Clegg SR (eds) (2012) Power and politics. Sage Publications
Hetherington C, Flin R, Mearns K (2006) Safety in shipping: the human element. J Safety Res 37(4):401–411. https://doi.org/10.1016/j.jsr.2006.04.007
Hoc JM (2000) From human–machine interaction to human–machine cooperation. Ergonomics 43(7):833–843
Hogg T, Ghosh S (2016) Autonomous merchant vessels: examination of factors that impact the effective implementation of unmanned ships. Aust J Marit Ocean Aff 8(3):206–222
Hollnagel E (1998) Cognitive reliability and error analysis method (CREAM). Elsevier , Amsterdam
Hollnagel E (2016) Barriers and accident prevention. Routledge , Milton Park
Hollnagel E, Alm H, Axelsson B, Ros A, Shamoun S, Cook R (2014) A FRAM (Functional Resonance Analysis Method) analysis of labour-and-delivery: locating risk in a complex system. International Forum on Quality and Safety in healthcare, Paris, France
Hulme A, Stanton NA, Walker GH, Waterson P, Salmon PM (2019) What do applications of systems thinking accident analysis methods tell us about accident causation? A systematic review of applications between 1990 and 2018. Saf Sci 117:164–183
Islam R, Yu H, Abbassi R, Garaniya V, Khan F (2017) Development of a monograph for human error likelihood assessment in marine operations. Saf Sci 91:33–39
Ismael JT (2011) Self-organization and self-governance. Philos Soc Sci 41(3):327–351
ITOPF (2019) Oil tanker spill statistics published. https://www.itopf.org/news-events/news/2019-oil-tanker-spill-statistics-published/ . Retrieved August 4, 2020
Jenkins DP, Salmon PM, Stanton NA, Walker GH (2010) A systemic approach to accident analysis: a case study of the Stockwell shooting. Ergonomics 53(1):1–17
Jenkins D, Salmon PS, Walker GH (2017) Event analysis of systemic team-work. Modelling command and control. CRC Press, Boca Raton, pp 49–118
Kaber DB, Endsley MR (1997) Out-of-the-loop performance problems and the use of intermediate levels of automation for improved control system functioning and safety. Process Saf Prog 16(3):editor126–131
Kaber DB, Endsley MR (1997) Out-of-the-loop performance problems and the use of intermediate levels of automation for improved control system functioning and safety. Process Saf Prog 16(3):126–131
Khan FI, Amyotte PR, DiMattia DG (2006) HEPI: A new tool for human error probability calculation for offshore operation. Saf Sci 44(4):313–334
Khan B, Khan F, Veitch B, Yang M (2018) An operational risk analysis tool to analyze marine transportation in Arctic waters. Reliab Eng Syst Saf 169:485–502. https://doi.org/10.1016/j.ress.2017.09.014
Kirwan B (1994) A guide to practical human reliability assessment. CRC Press , Boca Raton
Kristiansen S (2005) Maritime transportation: safety management and risk analysis, 1st edn. Routledge, Milton Park . https://doi.org/10.4324/978080473369
Kujala P, Hanninen M, Arola T, Ylitalo J (2009) Analysis of the marine traffic safety in the Gulf of Finland. Reliab Eng Syst Saf 94(8):1349–1357
Kum S, Sahin B (2015) A root cause analysis for Arctic Marine accidents from 1993 to 2011. Saf Sci 74:206–220. https://doi.org/10.1016/j.ssci.2014.12.010
Lenné MG, Salmon PM, Liu CC, Trotter M (2012) A systems approach to accident causation in mining: an application of the HFACS method. Accid Anal Prev 48:111–117. https://doi.org/10.1016/j.aap.2011.05.026
Leveson NG (2011) Applying systems thinking to analyze and learn from events. Saf Sci 49(1):55–64. https://doi.org/10.1016/j.ssci.2009.12.021
Li S, Meng Q, Qu X (2012) An overview of maritime waterway quantitative risk assessment models. Risk Anal 32(3):496–512. https://doi.org/10.1111/j.1539-6924.2011.01697.x
Lissillour R, Bonet Fernandez D (2020) The balance of power in the governance of the global maritime safety: the role of classification societies from a habitus perspective. Supply Chain Forum Int J. https://doi.org/10.1080/16258312.2020.1824533
Lützhöft M, Grech MR, Porathe T (2011) Information environment, fatigue, and culture in the maritime domain. Rev Hum Factors Ergon 7(1):280–322
Michel J, Fingas M (2016) Oil spills: causes, consequences, prevention and countermeasures. In: Fossil fuels: current status and future directions, pp 159–201
Minorsky UV (1959) An analysis of ship collisions with reference to nuclear power plants. J Ship Res 3(2):1–4
Mokhtari AH (2007) Impact of automatic identification system (AIS) on safety of marine navigation. Liverpool John Moores University, Liverpool
Montewka J, Hinz T, Kujala P, Matusiak J (2010) Probability modelling of vessel collisions. Reliab Eng Syst Saf 95(5):573–589
Montewka J, Ehlers S, Goerlandt F, Hinz T, Tabri K, Kujala P (2014a) A framework for risk assessment for maritime transportation systems: a case study for open sea collisions involving RoPax vessels. Reliab Eng Syst Saf 124(13):142–157
Montewka J, Goerlandt F, Kujala P (2014b) On a systematic perspective on risk for formal safety assessment (FSA). Reliab Eng Syst Saf 127:77–85
Munim ZH, Dushenko M, Jimenez VJ, Shakil MH, Imset M (2020) Big data and artificial intelligence in the maritime industry: a bibliometric review and future research directions. Marit Policy Manag 47(5):577–597
Norman DA (1980) Twelve issues for cognitive science. Cogn Sci 4(1):1–32
Norman DA (1981) Categorization of action slips. Psychol Rev 88(1):1–15. https://doi.org/10.1037//0033-295X.88.1.1
Normandin JM, Therrien MC (2016) Resilience factors reconciled with complexity: the dynamics of order and disorder. J Conting Crisis Manag 24(2):107–118
Orlikowski WJ (1992) The duality of technology: rethinking the concept of technology in organizations. Organ Sci 3(3):398–427
Orlikowski WJ (2000) Using technology and constituting structures: a practice lens for studying technology in organizations. Organ Sci 11(4):404–428
Packendorff J (1995) Inquiring into the temporary organization: new directions for project management research. Scand J Manag 11(4):319–333
Patterson JM, Shappell SA (2010) Operator error and system deficiencies: analysis of 508 mining incidents and accidents from Queensland, Australia using HFACS. Accid Anal Prev 42(4):1379–1385. https://doi.org/10.1016/j.aap.2010.02.018
Pedersen PT (2010) Review and application of ship collision and grounding analysis procedures. Mar Struct 23:241–262. https://doi.org/10.1016/j.marstruc.2010.05.001
Perrow C (1984) Normal Accidents: living with High-Risk Technologies. Basic Books, New York
Perrow C (1999) Normal accidents: living with high-risk technologies. Princeton University Press, Princeton
Rasmussen J (1983) Skills, rules, and knowledge; signals, signs, and symbols, and other distinctions in human performance models. IEEE Trans Syst Man Cybern SMC 13(3):257–266. https://doi.org/10.1109/TSMC.1983.6313160
Rasmussen J (1997) Risk management in a dynamic society: a modelling problem. Saf Sci 27(2–3):183–213. https://doi.org/10.1016/S0925-7535(97)00052-0
Rasmussen J (2000) Human factors in a dynamic information society: where are we heading? Ergonomics 43(7):869–879
Reason J (1990) Human error. Cambridge University Press , Cambridge
Reason J (1997) Managing the risks of organizational accidents. Routledge , Milton Park
Reason J (2000) Human error: models and management. BMJ 320(7237):768–770. https://doi.org/10.1136/bmj.320.7237.768
Reinach S, Viale A (2006) Application of a human error framework to conduct train accident/incident investigations. Accid Anal Prev 38(2):396–406. https://doi.org/10.1016/j.aap.2005.10.013
Rerup C (2009) Attentional triangulation: learning from unexpected rare crises. Organ Sci 20(5):876–893
Roberts SE, Hansen HL (2002) An analysis of the causes of mortality among seafarers in the British merchant fleet (1986–1995) and recommendations for their reduction. Occup Med 52(4):195–202
Roberts CM, McClean CJ, Veron JEN, Hawkins JP, Allen GR, McAllister DE, Mittermeier CG, Schueler FW, Spalding M, Wells F, Vynne C, Werner TB (2002) Marine biodiversity hotspots and conservation priorities for tropical reefs. Science 295(5558):1280–1284. https://doi.org/10.1126/science.1067728
Rothblum AM (2002) Keys to successful incident inquiry. In: Human factors in incident investigation and analysis, 2nd international workshop on human factors in offshore operations (HFW2002), Houston, TX
Saaty T (1980) The analytic hierarchy process (AHP) for decision making. In Kobe, Japan, pp 1–69
Sagan S (1993) The limits of safety: organizations, accidents, and nuclear weapons. Princeton University Press, Princeton
Salmon PM, Walker GH, Stanton NA (2015) Pilot error versus sociotechnical systems failure: a distributed situation awareness analysis of Air France 447. Theor Issues Ergon Sci 17(1):64–79. https://doi.org/10.1080/1463922x.2015.1106618
Shappell SA, Wiegmann DA (1997) A human error approach to accident investigation: the taxonomy of unsafe operations. Int J Aviat Psychol 7(4):269–291. https://doi.org/10.1207/s15327108ijap0704_2
Sheridan TB (2008) Risk, human error, and system resilience: fundamental ideas. Hum Factors 50(3):418–426
Shorrock ST, Kirwan B (2002) Development and application of a human error identification tool for air traffic control. Appl Ergon 33(4):319–336. https://doi.org/10.1016/S0003-6870(02)00010-8
Simonsen BC (1997) Mechanics of ship grounding. Department of Naval Architecture and Offshore Engineering, Milton Park, p 260
Soares CG, Teixeira AP (2001) Risk assessment in maritime transportation. Reliab Eng Syst Saf 74(3):299–309
Sovacool BK (2008) The costs of failure: a preliminary assessment of major energy accidents, 1907–2007. Energy Policy 36(5):1802–1820
SSR (2021) Safety and shipping review 2021—allianz global corporate & specialty (AGCS). https://www.agcs.allianz.com/news-and-insights/reports/shipping-safety.html
Stanton NA, Salmon PM, Walker GH (2015) Let the reader decide: a paradigm shift for situation awareness in sociotechnical systems. J Cogn Eng Decis Mak 9(1):44–50
Stanton NA, Plant KL, Roberts AP, Harvey C, Thomas TG (2016) Extending helicopter operations to meet future integrated transportation needs. Appl Ergon 53:364–373
Swain AD, Guttmann HE (1983) Handbook of human-reliability analysis with emphasis on nuclear power plant applications. Final report (NUREG/CR-1278; SAND-80–0200). Sandia National Labs., Albuquerque, NM (USA). Doi: https://doi.org/10.2172/5752058
Terndrup Pedersen P, Zhang S (1998) On Impact mechanics in ship collisions. Mar Struct 11(10):429–449. https://doi.org/10.1016/S0951-8339(99)00002-7
Thompson JD (1967) Organizations. Action: Social Science Bases of Administrative
Trucco P, Cagno E, Ruggeri F, Grande O (2008) A Bayesian Belief Network modelling of organisational factors in risk analysis: a case study in maritime transportation. Reliab Eng Syst Saf 93(6):845–856. https://doi.org/10.1016/j.ress.2007.03.035
Uğurlu Ö, Köse E, Yıldırım U, Yüksekyıldız E (2015a) Marine accident analysis for collision and grounding in oil tanker using FTA method. Marit Policy Manag 42(2):163–185. https://doi.org/10.1080/03088839.2013.856524
UNCTAD (2020) Review of Maritime Transport 2000. United Nations, Geneva
UNCTAD STAT (2019) World seaborne trade by types of cargo and by group of economies, annual. https://unctadstat.unctad.org/wds/TableViewer/tableView.aspx?ReportId=32363
Ung ST (2019) Evaluation of human error contribution to oil tanker collision using fault tree analysis and modified fuzzy Bayesian Network based CREAM. Ocean Eng 179:159–172
Van Eck NJ, Waltman L (2013) Vosviewer manual. Leiden: Univeristeit Leiden 1(1):1–53
van Oorschot JAWH, Hofman E, Halman JIM (2018) A bibliometric review of the innovation adoption literature. Technol Forecast Soc Change 134(2018):1–21
Wang G, Chen Y, Zhang H, Peng H (2002) Longitudinal strength of ships with accidental damages. Mar Struct 15(2):119–138. https://doi.org/10.1016/S0951-8339(01)00018-1
Watson R, Haraldson S, Lind M, Rygh T, Singh S, Voorspuij J, Ward R (2021) Foundations of maritime informatics. The World of Shipping. In: An international conference on maritime affairs, Portugal, January, 16
Weng J, Li G (2019) Exploring shipping accident contributory factors using association rules. J Transp Saf Secur 11(1):36–57
Weng J, Yang D (2015) Investigation of shipping accident injury severity and mortality. Accid Anal Prev 76:92–101
Woods DD, Johannesen LJ, Cook RI, Sarter NB (1994) Behind human error: cognitive systems, computers and hindsight. University of Dayton Research Institute, Dayton
Wróbel K, Montewka J, Kujala P (2017) Towards the assessment of potential impact of unmanned vessels on maritime transportation safety. Reliab Eng Syst Saf 165:155–169
Wu B, Yan X, Wang Y, Zhang D, Guedes Soares C (2017) Three-stage decision-making model under restricted conditions for emergency response to ships not under control. Risk Anal 37(12):2455–2474
Yang ZL, Bonsall S, Wall A, Wang J, Usman M (2013) A modified CREAM to human reliability quantification in marine engineering. Ocean Eng 58:293–303
Zhang W, Goerlandt F, Kujala P, Wang Y (2016) An advanced method for detecting possible near miss ship collisions from AIS data. Ocean Eng 124:141–156
Zupic I, Cater T (2015) Bibliometric methods in management and organization. Organ Res Methods 18(3):429–472
Download references
Acknowledgements
The authors of this paper wish to thank the Master 2 ARAMIS group 2019–2020, at the University of Grenoble-Alpes, for their exploratory thesis on the prevention of recurrence of oil spills.
This research has been developed owing to the IDEX-IRS funding of the University of Grenoble-Alpes (OCEAN project). This work is supported by the French National Research Agency in the framework of the "Investissements d’avenir” program (ANR-15-IDEX-02).
Author information
Authors and affiliations.
Grenoble INP*, CERAG, Univ. Grenoble Alpes, 38000, Grenoble, France
Carine Dominguez-Péry, Lakshmi Narasimha Raju Vuddaraju & Isabelle Corbett-Etchevers
Visiting Fellow at the University of Bath School of Management, Bath, UK
Rana Tassabehji
You can also search for this author in PubMed Google Scholar
Contributions
The order of the authors reflects their level of contribution to the paper. All authors read and approved the final manuscript.
Corresponding author
Correspondence to Carine Dominguez-Péry .
Ethics declarations
Competing interests.
The authors declare that they have no competing interests.
Additional information
Publisher's note.
Springer Nature remains neutral with regard to jurisdictional claims in published maps and institutional affiliations.
Rights and permissions
Open Access This article is licensed under a Creative Commons Attribution 4.0 International License, which permits use, sharing, adaptation, distribution and reproduction in any medium or format, as long as you give appropriate credit to the original author(s) and the source, provide a link to the Creative Commons licence, and indicate if changes were made. The images or other third party material in this article are included in the article's Creative Commons licence, unless indicated otherwise in a credit line to the material. If material is not included in the article's Creative Commons licence and your intended use is not permitted by statutory regulation or exceeds the permitted use, you will need to obtain permission directly from the copyright holder. To view a copy of this licence, visit http://creativecommons.org/licenses/by/4.0/ .
Reprints and permissions
About this article
Cite this article.
Dominguez-Péry, C., Vuddaraju, L.N.R., Corbett-Etchevers, I. et al. Reducing maritime accidents in ships by tackling human error: a bibliometric review and research agenda. J. shipp. trd. 6 , 20 (2021). https://doi.org/10.1186/s41072-021-00098-y
Download citation
Received : 22 March 2021
Accepted : 13 October 2021
Published : 24 November 2021
DOI : https://doi.org/10.1186/s41072-021-00098-y
Share this article
Anyone you share the following link with will be able to read this content:
Sorry, a shareable link is not currently available for this article.
Provided by the Springer Nature SharedIt content-sharing initiative
- Ship accident
- Human error
- Socio-technical use of information technologies
- Organisation
- Bibliometric review
National Center for Science and Engineering Statistics
- 2022 - 2023
- 2021 - 2022
- 2020 - 2021
- All previous cycle years
The Survey of Federal Funds for Research and Development is an annual census of federal agencies that conduct research and development (R&D) programs and the primary source of information about U.S. federal funding for R&D.
Survey Info
- tag for use when URL is provided --> Methodology
- tag for use when URL is provided --> Data
- tag for use when URL is provided --> Analysis
The Survey of Federal Funds for Research and Development (R&D) is the primary source of information about federal funding for R&D in the United States. The survey is an annual census completed by the federal agencies that conduct R&D programs. Actual data are collected for the fiscal year just completed; estimates are obtained for the current fiscal year.
Areas of Interest
- Government Funding for Science and Engineering
- Research and Development
Survey Administration
Synectics for Management Decisions, Inc. (Synectics) performed the data collection for volume 72 (FYs 2022–23) under contract to the National Center for Science and Engineering Statistics.
Survey Details
- Survey Description (PDF 127 KB)
- Data Tables (PDF 4.8 MB)
Featured Survey Analysis
Federal R&D Obligations Increased 0.4% in FY 2022; Estimated to Decline in FY 2023
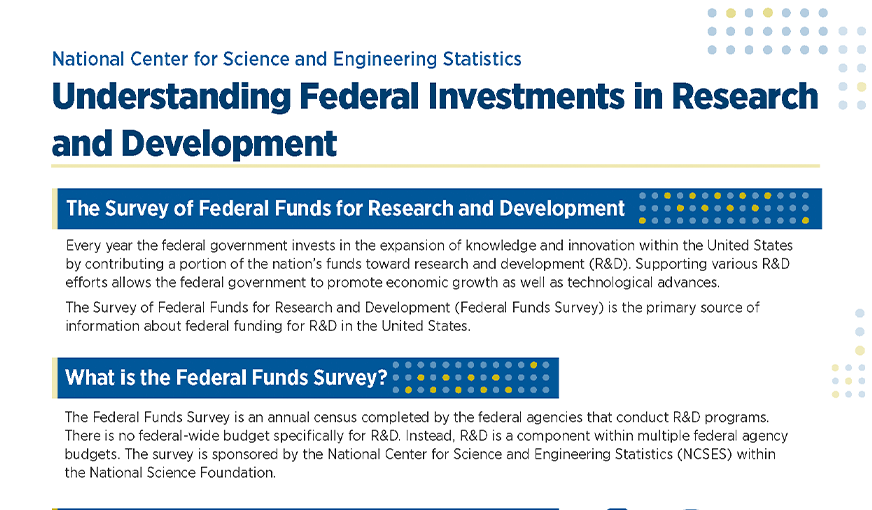
Survey of Federal Funds for R&D Overview
Methodology, survey description, survey overview (fys 2022–23 survey cycle; volume 72).
The annual Survey of Federal Funds for Research and Development (Federal Funds for R&D) is the primary source of information about federal funding for R&D in the United States. The results of the survey are also used in the federal government’s calculation of U.S. gross domestic product at the national and state level, used for policy analysis, and used for budget purposes for the Federal Laboratory Consortium for Technology Transfer, the Small Business Innovation Research, and the Small Business Technology Transfer. The survey is sponsored by the National Center for Science and Engineering Statistics (NCSES) within the National Science Foundation (NSF).
Data collection authority
The information is solicited under the authority of the National Science Foundation Act of 1950, as amended, and the America COMPETES Reauthorization Act of 2010.
Major changes to recent survey cycle
Key survey information, initial survey year, reference period.
FYs 2022–23.
Response unit
Federal agencies.
Sample or census
Population size.
The population consists of the 32 federal agencies that conduct R&D programs, excluding the Central Intelligence Agency (CIA).
Sample size
Not applicable; the survey is a census of all federal agencies that conduct R&D programs, excluding the CIA.
Key variables
Key variables of interest are listed below.
The survey provides data on federal obligations by the following key variables:
- Federal agency
- Field of R&D (formerly field of science and engineering)
- Geographic location (within the United States and by foreign country or economy)
- Performer (type of organization doing the work)
- R&D plant (facilities and major equipment)
- Type of R&D (research, development, test, and evaluation [RDT&E] for Department of Defense [DOD] agencies)
- Basic research
- Applied research
- Development, also known as experimental development
The survey provides data on federal outlays by the following key variables:
- R&D (RDT&E for DOD agencies)
R&D plant
Note that the variables “R&D,” “type of R&D,” and “R&D plant” in this survey use definitions comparable to those used by the Office of Management and Budget Circular A-11 , Section 84 (Schedule C).
Survey Design
Target population.
The population consists of the federal agencies that conduct R&D programs, excluding the CIA. For the FYs 2022–23 cycle, a total of 32 federal agencies (14 federal departments and 18 independent agencies) reported R&D data.
Sampling frame
The survey is a census of all federal agencies that conduct R&D programs, excluding the CIA. The agencies are identified from information in the president’s budget submitted to Congress. The Analytical Perspectives volume and the “Detailed Budget Estimates by Agency” section of the appendix to the president’s budget identify agencies that receive funding for R&D.
Sample design
Not applicable.
Data Collection and Processing
Data collection.
Synectics for Management Decisions, Inc. (Synectics) performed the data collection for volume 72 (FYs 2022–23) under contract to NCSES. Agencies were initially contacted by e-mail to verify the contact information of each agency-level survey respondent. A Web-based data collection system is used for the survey. Multiple subdivisions of some federal departments were permitted to submit information to create a complete accounting of the departments’ R&D funding activities.
Data collection for Federal Funds for R&D began in May 2023 and continued into September 2023.
Data processing
A Web-based data collection system is used to collect and manage data for the survey. This Web-based system was designed to help improve survey reporting and reduce data collection and processing costs by offering respondents direct online reporting and editing.
All data collection efforts, data imports, and trend checking are accomplished using the Web-based data collection system. The Web-based data collection system has a component that allows survey respondents to enter their data online; it also has a component that allows the contractor to monitor support requests, data entry, and data issues.
Estimation techniques
Published totals are created by summing respondent data, there are no survey weights or other adjustments.
Survey Quality Measures
Sampling error, coverage error.
Given the existence of a complete list of all eligible agencies, there is no known coverage error. The CIA is purposely excluded.
Nonresponse error
There is no unit nonresponse. To increase item response, agencies are encouraged to estimate when actual data are unavailable. The survey instrument allows respondents to enter data or skip data fields. There are several possible sources of nonresponse error by respondents, including inadvertently skipping data fields or skipping data fields when data are unavailable.
Measurement error
Some measurement problems are known to exist in the Federal Funds of R&D data. Some agencies cannot report the full costs of R&D, the final performer of R&D, or R&D plant data.
For example, DOD does not include headquarters’ costs of planning and administering R&D programs, which are estimated at a fraction of 1% of its total cost. DOD has stated that identification of amounts at this level is impracticable.
The National Institutes of Health (NIH) in the Department of Health and Human Services currently has many of its awards in its financial system without any field of R&D code. Therefore, NIH uses an alternate source to estimate its research dollars by field of R&D. NIH uses scientific class codes (based upon history of grant, content of the title, and the name of the awarding institute or center) as an approximation for field of R&D.
The National Aeronautics and Space Administration (NASA) does not include any field of R&D codes in its financial database. Consequently, NASA must estimate what percentage of the agency’s research dollars are allocated into the fields of R&D.
Also, agencies are required to report the ultimate performer of R&D. However, through past workshops, NCSES has learned that some agencies do not always track their R&D dollars to the ultimate performer of R&D. This leads to some degree of misclassification of performers of R&D, but NCSES has not determined the extent of the errors in performer misclassification by the reporting agencies.
R&D plant data are underreported to some extent because of the difficulty some agencies, particularly DOD and NASA, encounter in identifying and reporting these data. DOD’s respondents report obligations for R&D plant funded under the agency’s appropriation for construction, but they are able to identify only a small portion of the R&D plant support that is within R&D contracts funded from DOD’s appropriation for RDT&E. Similarly, NASA respondents cannot separately identify the portions of industrial R&D contracts that apply to R&D plant because these data are subsumed in the R&D data covering industrial performance. NASA R&D plant data for other performing sectors are reported separately.
Data Availability and Comparability
Data availability.
Annual data are available for FYs 1951–2023.
Data comparability
Until the release of volume 71 (FYs 2021–22) the information included in this survey had been unchanged since volume 23 (FYs 1973–75), when federal obligations for research to universities and colleges by agency and detailed field of science and engineering were added to the survey. Other variables (such as type of R&D and type of performer) are available from the early 1950s on. The volume 71 survey revisions maintained the four main R&D crosscuts (i.e., type of R&D, field of R&D [previously referred to as field of science and engineering], type of performer, and geographic area) collected previously. However, there were revisions within these crosscuts to ensure consistency with other NCSES surveys. These include revisions to the fields of R&D and the type of performer categories (see Technical Notes, table A-3 for a crosswalk of the fields of science and engineering to the fields of R&D). In addition, new variables were added, such as field of R&D for experimental development (whereas before, the survey participants had only reported fields of R&D [formerly fields of science] for basic research and applied research). Grants and contracts for extramural R&D performers and obligations to University Affiliated Research Centers were also added in volume 71.
Every time new data are released, there may be changes to past years’ data because agencies sometimes update older information or reclassify responses for prior years as additional budget data become available. For trend comparisons, use the historical data from only the most recent publication, which incorporates changes agencies have made in prior year data to reflect program reclassifications or other corrections. Do not use data published earlier.
Data Products
Publications.
NCSES publishes data from this survey annually in tables and analytic reports available at Federal Funds for R&D Survey page and in the Science and Engineering State Profiles .
Electronic access
Access to the data for major data elements are available in NCSES’s interactive data tool at https://ncsesdata.nsf.gov/ .
Technical Notes
Survey overview, data collection and processing methods, data comparability (changes), definitions.
Purpose. The annual Survey of Federal Funds for Research and Development (Federal Funds for R&D) is the primary source of information about federal funding for R&D in the United States. The results of the survey are also used in the federal government’s calculation of U.S. gross domestic product at the national and state level, for policy analysis, and for budget purposes for the Federal Laboratory Consortium for Technology Transfer, the Small Business Innovation Research, and the Small Business Technology Transfer. In addition, as of volume 71, the Survey of Federal Science and Engineering Support to Universities, Colleges, and Nonprofit Institutions (Federal S&E Support Survey) was integrated into this survey as a module, making Federal Funds for R&D the comprehensive data source on federal science and engineering (S&E) funding to individual academic and nonprofit institutions.
Data collection authority. The information is solicited under the authority of the National Science Foundation Act of 1950, as amended, and the America COMPETES Reauthorization Act of 2010.
Survey contractor. Synectics for Management Decisions, Inc. (Synectics).
Survey sponsor. The National Center for Science and Engineering Statistics (NCSES) within the National Science Foundation (NSF).
Frequency . Annual.
Initial survey year . 1951.
Reference period . FYs 2022–23.
Response unit. Federal agencies.
Sample or census. Census.
Population size. For the FYs 2022–23 cycle, a total of 32 federal agencies reported R&D data. (See section “ Survey Design ” for details.)
Sample size. Not applicable; the survey is a census of all federal agencies that conduct R&D programs, excluding the Central Intelligence Agency (CIA).
Target population. The population consists of the federal agencies that conduct R&D programs, excluding the CIA. For the FYs 2022–23 cycle, a total of 32 federal agencies (14 federal departments and 18 independent agencies) reported R&D data.
Sampling f rame. The survey is a census of all federal agencies that conduct R&D programs, excluding the CIA. The agencies are identified from information in the president’s budget submitted to Congress. The Analytical Perspectives volume and the “Detailed Budget Estimates by Agency” section of the appendix to the president’s budget identify agencies that receive funding for R&D.
Sample design. Not applicable.
Data collection. Data for FYs 2022–23 (volume 72) were collected by Synectics under contract to NCSES (for a full list of fiscal years canvassed by survey volume reference, see Table A-4 ). Data collection began with an e-mail to each agency to verify the name, phone number, and e-mail address of each agency-level survey respondent. A Web-based data collection system is used for the survey. Because multiple subdivisions of some federal departments completed the survey, there were 72 agency-level respondents: 6 federal departments that reported for themselves, 48 agencies within another 8 federal departments, and 18 independent agencies. However, lower offices could also be authorized to enter data: in Federal Funds for R&D nomenclature, agency-level offices could authorize program offices, program offices could authorize field offices, and field offices could authorize branch offices. When these suboffices are included, there were 725 total respondents: 72 agencies, 95 program offices, 178 field offices, and 380 branch offices.
Since volume 66, each survey cycle collects information for 2 federal government fiscal years: the fiscal year just completed (FY 2022—i.e., 1 October 2021 through 30 September 2022) and the current fiscal year during the start of the survey collection period (i.e., FY 2023). FY 2022 data are completed transactions. FY 2023 data are estimates of congressional appropriation actions and apportionment and reprogramming decisions.
Data collection began on 10 May 2023, and the requested due date for data submissions was 5 August 2023. Data collection was extended until all surveyed agencies provided complete and final survey data in September 2023.
Mode. Federal Funds for R&D uses a Web-based data collection system. The Web-based system consists of a data collection component that allows survey respondents to enter their data online and a monitoring component that allows the data collection contractor to monitor support requests, data entry, and data issues. The Web-based system’s two components are password protected so that only authorized respondents and staff can access them. However, some agencies submit their data in alternative formats such as Excel files, which are later imported into the Web-based system. All edit and trend checks are accomplished through the Web-based system. Final submission occurs through the Web-based system after all edit failures and trend checks have been resolved.
Response rate. The unit response rate is 100%.
Data checking . Data errors in Federal Funds for R&D are flagged automatically by the Web-based data collection system: respondents cannot submit their final data to NCSES until all required fields have been completed without errors. Once data are submitted, specially written SAS programs are run to check each agency’s submission to identify possible discrepancies, to ensure data from all suboffices are included correctly, and to check that there were no inadvertent shifts in reporting from one year to the next. As always, respondents are contacted to resolve potential reporting errors that cannot be reconciled by the narratives. Explanations of questionable data are noted by the survey respondents for NCSES review.
Imputation . None.
Weighting. None.
Variance estimation. Not applicable.
Sampling error. Not applicable.
Coverage error. Given the existence of a complete list of all eligible agencies, there is no known coverage error. The CIA is purposely excluded.
Nonresponse error. There is no unit nonresponse. To increase item response, agencies are encouraged to estimate when actual data are unavailable. The survey instrument allows respondents to enter data or skip data fields; however, blank fields are not accepted for survey submission, and respondents must either populate the fields with data or with $0 if the question is not applicable. There are several possible sources of nonresponse error by respondents, including inadvertently skipping data fields, skipping data fields when data are unavailable, or entering $0 when specific data are unavailable.
Measurement error . Some measurement problems are known to exist in the Federal Funds of R&D data. Some agencies cannot report the full costs of R&D, the final performer of R&D, or R&D plant data.
For example, the Department of Defense (DOD) does not include headquarters’ costs of planning and administering R&D programs, which are estimated at a fraction of 1% of its total cost. DOD has stated that identification of amounts at this level is impracticable.
The National Institutes of Health (NIH) in the Department of Health and Human Services (HHS) currently has many of its awards in its financial system without any field of R&D code. Therefore, NIH uses an alternate source to estimate its research dollars by field of R&D. NIH uses scientific class codes (based upon history of grant, content of the title, and the name of the awarding institute or center) as an approximation for field of R&D.
Agencies are asked to report the ultimate performer of R&D. However, through past workshops, NCSES has learned that some agencies do not always track their R&D dollars to the ultimate performer of R&D. In the case of transfers to other federal agencies, the originating agency often does not have information on the final disposition of funding made by the receiving agency. Therefore, intragovernmental transfers, which are classified as federal intramural funding, may have some degree of extramural performance. This leads to some degree of misclassification of performers of R&D, but NCSES has not determined the extent of the errors in performer misclassification by the reporting agencies.
Differences in agency and NCSES classification of some performers will also lead to some degree of measurement error. For example, although many university research foundations are legally organized as nonprofit organizations and may be classified as such within a reporting agency’s own system of record, NCSES classifies these as component units of higher education. These classification differences may contribute to differences in findings by the Federal Funds for R&D and the Federal S&E Support Survey in federal agency obligations to both higher education and nonprofit institutions.
R&D plant data are underreported to some extent because of the difficulty some agencies, particularly DOD and NASA, encounter in identifying and reporting these data. DOD’s respondents report obligations for R&D plant that are funded under the agency’s appropriation for construction, but they are able to identify only a small portion of the R&D plant support that is within R&D contracts funded from DOD’s appropriation for research, development, testing, and evaluation (RDT&E). Similarly, NASA respondents cannot separately identify the portions of industrial R&D contracts that apply to R&D plant because these data are subsumed in the R&D data covering industrial performance. NASA R&D plant data for other performing sectors are reported separately.
Data revisions. When completing the current year’s survey, agencies naturally revise their estimates for the last year of the previous report—in this case, FY 2022. Sometimes, survey submissions also reflect reappraisals and revisions in classification of various aspects of agencies’ R&D programs; in those instances, NCSES requests that agencies provide revised prior year data to maintain consistency and comparability with the most recent R&D concepts.
For trend comparisons, use the historical data from only the most recent publication, which incorporates changes agencies have made in prior year data to reflect program reclassifications or other corrections. Do not use data published earlier.
Changes in survey coverage and population. This cycle (volume 72, FYs 2022–23), one department, the Department of Homeland Security (DHS), became the agency respondent instead of continuing to delegate that role to its bureaus; one agency was added as a respondent—the Department of Agriculture’s (USDA’s) Natural Resources Conservation Service; one agency, the Department of Transportation’s Maritime Administration, resumed reporting; and two agencies, the Department of Treasury’s Internal Revenue Service (IRS) and the independent agency the Federal Communications Commission, ceased to report.
Changes in questionnaire .
- No changes were made to the questionnaire for volume 72.
- The survey was redesigned for volume 71 (FYs 2021–22). The Federal S&E Support Survey was integrated as the final two questions in the Federal Funds for R&D questionnaire. (NCSES will continue to publish these data separately at https://ncses.nsf.gov/surveys/federal-support-survey/ .)
- Four other new questions were added to the standard and DOD versions of the questionnaire; the questions covered, for the fiscal year just completed (FY 2021), R&D deobligations (Standard and DOD Question 4), nonfederal R&D obligations by type of agreement (Standard Question 10 and DOD Question 11), R&D obligations provided to other federal agencies (Standard Question 11 and DOD Question 12), and R&D and R&D plant obligations to university affiliated research centers (Standard Question 17 and DOD Question 19). One new question added solely to the DOD questionnaire (DOD Question 6) was about obligations for Small Business Innovation Research and Small Business Technology Transfer for the fiscal year just completed and the current fiscal year at the time of collection (i.e., FYs 2021 and 2022). Many of the other survey questions were reorganized and revised.
- For volume 71, some changes were made within the questions for consistency with other NCSES surveys. Among the performer categories, federally funded R&D centers (FFRDCs), which in previous volumes were included among the extramural performers, became one of the intramural performers. Other changes include retitling of certain performer categories, where “industry” was changed to “businesses” and “universities and colleges” was changed to “higher education.”
- For volume 71, “field of R&D” was used instead of the former “field of science and engineering.” The survey started collecting field of R&D information for experimental development obligations; previously, field of R&D information was collected only for research obligations.
- For volume 71, federal obligations for research performed at higher education institutions, by detailed field of R&D was asked of all agencies. Previously these data had only been collected from the Departments of Agriculture, Defense, Energy, HHS, and Homeland Security; NASA; and NSF.
- For volume 71, geographic distribution of R&D obligations was asked of all agencies. Previously, these data had only been collected from the Departments of Agriculture, Commerce, Defense, Energy, HHS, Homeland Security; NASA; and NSF. Agencies are asked to provide the principal location (state or outlying area) of the work performed by the primary contractor, grantee, or intramural organization; assign the obligations to the location of the headquarters of the U.S. primary contractor, grantee, or intramural organization; or, for DOD agencies, list the funds as undistributed for classified funds.
- For volume 71, collection of data on funding type (stimulus and non-stimulus) was limited to Question 5 on type of R&D.
- For volume 71, grants and contracts for extramural R&D performers and obligations to University Affiliated Research Centers were added.
- For volume 70 (FYs 2020–21), agencies were requested to report COVID-19 pandemic-related R&D from the agency’s initial appropriations, as well as from any stimulus funds received from the Coronavirus Aid, Relief, and Economic Security (CARES) Act, plus any other pandemic-related supplemental appropriations. Two tables in the questionnaire were modified to collect the stimulus and non-stimulus amounts separately (tables 1 and 2), and seven tables in the questionnaire (tables 6.1, 6.2, 7.1, 11.1, 11.2, 12.1, and 13.1) were added for respondents to specify stimulus and non-stimulus funding by various categories. The data on stimulus funding is reported in volume 70’s data table 132. The Biomedical Advanced Research and Development Authority accounted for 66% of all COVID-19 R&D in FY 2020; these obligations primarily include transfers to the other agencies to help facilitate execution of contractual awards under Operation Warp Speed.
- For volume 70 (FYs 2020–21), the optional narrative tables that ask for comparisons of the R&D obligations reported in Federal Funds for R&D with corresponding amounts in the Federal S&E Support Survey (standard questionnaire only) were renumbered from tables 6B and 6C to tables 6A and 6B.
- In volumes 68 (FYs 2018–19) and 69 (FYs 2019–20), table 6A, which collected information on federal intramural R&D obligations, was deactivated, and agencies were instructed not to complete it.
- For volumes 66 (FYs 2016–17) and 67 (FYs 2017–18), table 6A (formerly table VI.A) was included, but it was modified so that it no longer collected laboratory names.
- Starting with volume 66 (FYs 2016–17), the survey collects 2 federal government fiscal years—actual data for the fiscal year just completed and estimates for the current fiscal year. Previously, the survey also collected projected obligations for the next fiscal year based on the president’s budget request to Congress. For volume 66, data were collected for only 2 fiscal years due to the delayed FY 2018 budget formulation process. However, after consultation with data users, NCSES determined that the projections were not as useful as the budget authority data presented in the budget request.
- In volume 66, the survey table numbering was changed from Roman numerals I–XI and, for selected agencies, the letters A–E, to Arabic numerals 1–16. The order of tables remained the same.
- In the volume 66 DOD-version of the questionnaire, the definition of major systems development was changed to represent DOD Budget Activities 4 through 6 instead of Budget Activities 4 through 7, and questions relating to funding for Operational Systems Development (Budget Activity 7) were added to the instrument. The survey’s narrative tables 6 and 11 were removed from the DOD-version of the questionnaire.
- For volume 65 (FYs 2015–17), the survey reintroduced table VI.A to collect information on federal intramural R&D obligations, including the names and addresses of all federal laboratories that received federal intramural R&D obligations. The table was included in both the standard and DOD questionnaires.
- For volume 62 (FYs 2012–14), the survey added table VI.A to the standard questionnaire for that volume only to collect information on FY 2012 federal intramural R&D obligations, including the names and addresses of all federal laboratories that received federal intramural R&D obligations.
- In volumes 59 (FYs 2009–11) and 60 (FYs 2010–12), questions relating to funding from the American Recovery and Reinvestment Act of 2009 (ARRA) were added to the data collection instruments. The survey collected separate outlays and obligations for ARRA and non-ARRA sources of funding, by performer and geography for FYs 2009 and 2010.
- Starting with volume 59 (FYs 2009–11), federal funding data were requested in actual dollars (instead of rounded in thousands, as was done through volume 58).
Changes in reporting procedures or classification.
- FY 2022. During the volume 72 cycle (FYs 2022–23), NASA revised its FY 2021 data by field of R&D and performer categories based on improved classification procedures developed during the volume 72 reporting period.
- FY 2021. During the volume 71 cycle (FYs 2021–22), NCSES decided to remove “U.S.” from names like “U.S. Space Force” to conform with other surveys. For Federal Funds for R&D, this change will first appear in the detailed statistical tables.
- FY 2020. For volume 70 (FYs 2020 and 2021), data include obligations from supplemental COVID-19 pandemic-related appropriations (e.g., CARES Act) plus any other pandemic-related supplemental appropriations.
- FY 2020. The Department of Energy’s (DOE’s) Naval Reactor Program reclassified some of its R&D obligations from industry-administered FFRDCs to the industry sector.
- FY 2020. The Department of the Air Force (AF) and the DOE’s Energy Efficiency and Renewable Energy (EERE) partially revised their FY 2019 data. AF revised its operational system development classified program numbers for businesses excluding business or industry-administered FFRDCs, and EERE revised its outlay numbers.
- FY 2019. For volume 69 (FYs 2019–20), FY 2020 preliminary data do not include obligations from supplemental COVID-19 pandemic-related appropriations (e.g., CARES Act).
- FY 2019. The Biomedical Advanced Research and Development Authority began reporting. For volume 69 (FYs 2019–20), it could not submit any geographical data, so its data were reported as undistributed on the state tables.
- FY 2019. The U.S. Agency for Global Media (formerly the Broadcasting Board of Governors), which did not report data between FY 2008 and FY 2018, resumed reporting.
- FY 2018. The HHS Centers for Medicare and Medicaid (CMS) funding was reported by the CMS Office of Financial Management at an agency-wide level instead of by the CMS Center for Medicare and Medicaid Innovation and its R&D group, the Office of Research, Development, and Information, which used to report at a component level.
- FY 2018. The Department of State added the Global Health Programs R&D funding.
- FY 2018. The Department of Veterans Affairs added funds for the Medical Services support to the existing R&D funding to fully report the total cost of intramural R&D. Although the Medical Services do not directly fund specific R&D activities, they host intramural research programs that were not previously reported.
- FY 2018. DHS’s Countering Weapons of Mass Destruction (CWMD) Office was established on 7 December 2017. CWMD consolidated primarily the Domestic Nuclear Detection Office (DNDO) and a majority of the Office of Health Affairs, as well as other DHS elements. Prior to FY 2018, data reported for the CWMD would have been under the DNDO.
- FY 2018. DOE revised its FYs 2016 and 2017 data after discovering its Office of Fossil Energy reported “in thousands” instead of actual dollars for volumes 66 (FYs 2016–17) and 67 (FYs 2017–18).
- FY 2018. USDA’s Economic Research Service (ERS) partially revised its FYs 2009 and 2010 data during the volume 61 (FYs 2011–13) cycle. NCSES discovered a discrepancy that was corrected during the volume 68 cycle, completing the revision.
- FY 2018. DHS’s Transportation Security Administration, which did not report data between FY 2010 and FY 2017, resumed reporting for volume 68 (FYs 2018–19).
- FY 2018. DHS’s U.S. Secret Service, which did not report data between FY 2009 and FY 2017, resumed reporting for volume 68 (FYs 2018–19).
- FY 2018. NCSES discovered that in some past volumes, the obligations reported for basic research in certain foreign countries were greater than the corresponding obligations reported for R&D; the following data were corrected as a result: DOD and Chemical and Biological Defense FY 2003 data, defense agencies and activities FY 2003 and FY 2011 data, AF FY 2009 data, and Department of the Navy FY 2005, FY 2011, and FY 2013 data; DOE and Office of Science FY 2009 data; HHS and Centers for Disease Control and Prevention (CDC) FY 2008 and FY 2017 data; and NSF FY 2001 data. NCSES also discovered that some obligations reported for academic performers were greater than the corresponding obligations reported for total performers, and DOD and AF FY 2009 data, DOE and Fossil Energy FY 1999 data, and NASA FY 2008 data were corrected. Finally, NCSES discovered a problem with FY 2017 HHS CDC personnel costs data, which were then also corrected.
- FY 2017. The Department of the Treasury’s IRS performed a detailed evaluation and assessment of its programs and determined that none of its functions can be defined as R&D activity as defined in Office of Management and Budget (OMB) Circular A-11. The review included discussions with program owners and relevant contractors who perform work on behalf of the IRS. The IRS also provided a negative response to the OMB data call on R&D under Circular A-11 for the same reference period (FYs 2017–18). Despite no longer having any R&D obligations, the IRS still sponsors an FFRDC, the Center for Enterprise Modernization.
- FY 2017. NASA estimated that the revised OMB definition for "experimental development" reduced its reported R&D total by about $2.7 billion in FY 2017 and $2.9 billion in FY 2018 from what would have been reported under the previous definition prior to volume 66 (FYs 2016–17).
- FY 2017. The Patient-Centered Outcomes Research Trust Fund (PCORTF) was established by Congress through the Patient Protection and Affordable Care Act of 2010, signed by the president on 23 March 2010. PCORTF began reporting for volume 67 (FYs 2017–18), but it also submitted data for FYs 2011–16.
- FY 2017. The Tennessee Valley Authority, which did not report data between FY 1999 and FY 2016, resumed reporting for volume 67 (FYs 2017–18).
- FY 2017. The U.S. Postal Service, which did not report data between FY 1999 and FY 2016, resumed reporting for volume 67 (FYs 2017–18) and submitted data for FYs 2015–16.
- FY 2017. During the volume 67 (FYs 2017–18) data collection, DHS’s Science and Technology Directorate revised its FY 2016 data.
- FY 2016. The Administrative Office of the U.S. Courts began reporting as of volume 66 (FYs 2016–17).
- Beginning with FY 2016, the totals reported for development obligations and outlays represent a refinement to this category by more narrowly defining it to be “experimental development.” Most notably, totals for development do not include the DOD Budget Activity 7 (Operational System Development) obligations and outlays. Those funds, previously included in DOD’s development totals, support the development efforts to upgrade systems that have been fielded or have received approval for full rate production and anticipate production funding in the current or subsequent fiscal year. Therefore, the data are not directly comparable with totals reported in previous years.
- Prior to the volume 66 launch, the definitions of basic research, applied research, experimental development, R&D, and R&D plant were revised to match the definitions used by OMB in the July 2016 version of Circular A-11, Section 84 (Schedule C).
- FYs 2016–17. Before the volume 66 survey cycle, NSF updated the list of foreign performers in Federal Funds R&D to match the list of countries and territories in the Department of State’s Bureau of Intelligence and Research fact sheet of Independent States in the World and fact sheet of Dependencies and Areas of Special Sovereignty. Country lists in volume 66 data tables and later may differ from those in previous reports.
- FY 2015. The HHS Administration for Community Living (ACL) began reporting in FY 2015, replacing the Administration on Aging, which was transferred to ACL when ACL was established on 18 April 2012. Several programs that serve older adults and people with disabilities were transferred from other agencies to ACL, including a number of programs from the Department of Education due to the 2014 Workforce Innovation and Opportunities Act.
- FY 2015. The Department of the Interior’s Bureau of Land Management and U.S. Fish and Wildlife Service, which did not report data between FY 1999 and FY 2014, resumed reporting.
- In January 2014, all Research and Innovative Technology Administration programs were transferred into the Office of the Assistant Secretary for Research and Technology in the Office of the Secretary of Transportation.
- FY 2014. DHS’s Domestic Nuclear Detection Office began reporting for FY 2014.
- FY 2014. The Department of State data for FY 2014 were excluded due to their poor quality.
- FY 2013. NASA revamped its reporting process so that the data for FY 2012 forward are not directly comparable with totals reported in previous years.
- FY 2012. NASA began reporting International Space Station (ISS) obligations as research rather than R&D plant.
- Starting with volume 62 (FYs 2012–14), an “undistributed” category was added to the geographic location tables for DOD obligations for which the location of performance is not reported. It includes DOD obligations for industry R&D that were included in individual state totals prior to FY 2012 and DOD obligations for other performers that were not reported prior to FY 2011. This change was applied retroactively to FY 2011 data.
- Starting with volume 61 (FYs 2011–13), DOD subagencies other than the Defense Advanced Research Projects Agency were reported as an aggregate total under other defense agencies to enable complete reporting of DOD R&D (both unclassified and classified). Consequently, DOD began reporting additional classified R&D not previously reported by its subagencies.
- FY 2011. USDA’s ERS partially revised its data for FYs 2009 and 2010 during the volume 61 (FYs 2011–13) cycle.
- FY 2010. NASA resumed reporting ISS obligations as R&D plant.
- FYs 2000–09. Beginning in FY 2000, AF did not report Budget Activity 6.7 Operational Systems Development data because the agency misunderstood the reporting requirements. During the volume 57 data collection cycle, AF edited prior year data for FYs 2000–07 to include Budget Activity 6.7 Operational Systems Development data. These data revisions were derived from FY 2007 distribution percentages that were then applied backward to revise data for FYs 2000–06.
- FYs 2006–07. NASA’s R&D obligations decreased by $1 billion. Of this amount, $850 million was accounted for by obligations for operational projects that NASA excluded in FY 2007 but reported in FY 2006. The remainder was from an overall decrease in obligations between FYs 2006 and 2007.
- FY 2006. NASA reclassified funding for the following items as operational costs: Space Operations, the Hubble Space Telescope, the Stratospheric Observatory for Infrared Astronomy, and the James Webb Space Telescope. This funding was previously reported as R&D plant.
- FYs 2005–07. Before the volume 55 survey cycle, NSF updated the list of foreign performers in Federal Funds R&D to match the list of countries and territories in the Department of State’s Bureau of Intelligence and Research fact sheet of Independent States in the World and fact sheet of Dependencies and Areas of Special Sovereignty. Area and country lists in volume 55 data tables and later may differ from those in previous reports.
- FYs 2004–06. NASA implemented a full-cost budget approach, which includes all of the direct and indirect costs for procurement, personnel, travel, and other infrastructure-related expenses relative to a particular program and project. NASA’s data for FY 2004 and later years may not be directly comparable with its data for FY 2003 and earlier years.
- FY 2004. NIH revised its financial database; beginning with FY 2004, NIH records no longer contain information on the field of S&E. Data for FY 2004 and later years are not directly comparable with data for FY 2003 and earlier years.
- Data for FYs 2003–06 from the Substance Abuse and Mental Health Services Administration (SAMHSA) are estimates based on SAMHSA's obligations by program activity budget and previously reported funding for development.
- FY 2003. SAMHSA reclassified some of its funding categories as non-R&D that had been considered to be R&D in prior years.
- On 25 November 2002, the president signed the Homeland Security Act of 2002, establishing DHS. DHS includes the R&D activities previously reported by the Federal Emergency Management Agency, the Science and Technology Directorate, the Transportation Security Administration, the U.S. Coast Guard, and the U.S. Secret Service.
- FY 2000. NASA reclassified the ISS as a physical asset, reclassified ISS Research as equipment, and transferred funding for the program from R&D to R&D plant.
- FY 2000. NIH reclassified as research the activities that it had previously classified as development. NIH data for FY 2000 forward reflect this change. For more information on the classification changes at NASA and NIH, refer to Classification Revisions Reduce Reported Federal Development Obligations (InfoBrief NSF 02-309), February 2002, available at https://www.nsf.gov/statistics/nsf02309 .
- FYs 1996–98. The lines on the survey instrument for the special foreign currency program and for detailed field of S&E were eliminated beginning with the volume 46 survey cycle. Two tables depicting data on foreign performers by region, country, and agency that were removed before publication of volume 43 were reinstated with volume 46.
- FYs 1994–96. During the volume 44 survey cycle, the Director for Defense Research and Engineering (DDR&E) at DOD requested that NSF further clarify the true character of DOD’s R&D program, particularly as it compares with other federal agencies, by adding more detail to development obligations reported by DOD respondents. Specifically, DOD requested that NSF allow DOD agencies to report development obligations in two separate categories: advanced technology development and major systems development. An excerpt from a letter written by Robert V. Tuohy, Chief, Program Analysis and Integration at DDR&E, to John E. Jankowski, Program Director, Research and Development Statistics Program, Division of Science Resources Statistics, NSF, explains the reasoning behind the DDR&E request: “The DOD’s R&D program is divided into two major pieces, Science and Technology (S&T) and Major Systems Development. The other federal agencies’ entire R&D programs are equivalent in nature to DOD’s S&T program, with the exception of the Department of Energy and possibly NASA. Comparing those other agency programs to DOD’s program, including the development of weapons systems such as F-22 Fighter and the New Attack Submarine, is misleading.”
- FYs 1990–92. Since volume 40, DOD has reported research obligations and development obligations separately. Tables reporting obligations for research, by state and performer, and obligations for development, by state and performer, were specifically created for DOD. Circumstances specific to DOD are (1) DOD funds the preponderance of federal development and (2) DOD development funded at institutions of higher education is typically performed at university-affiliated nonacademic laboratories, which are separate from universities’ academic departments, where university research is typically performed.
Agency and subdivision. An agency is an organization of the federal government whose principal executive officer reports to the president. The Library of Congress and the Administrative Office of the U.S. Courts are also included in the survey, even though the chief officer of the Library of Congress reports to Congress and the U.S. Courts are part of the judicial branch. Subdivision refers to any organizational unit of a reporting agency, such as a bureau, division, office, or service.
Development . See R&D and R&D plant.
Fields of R&D (formerly fields of science and engineering ) . A list of the 41 fields of R&D reported on can be found on the survey questionnaire. In the data tables, the fields are grouped into 9 major areas: computer and information sciences; geosciences, atmospheric sciences, and ocean sciences; life sciences; mathematics and statistics; physical sciences; psychology; social sciences; engineering; and other fields. Table A-3 provides a crosswalk of the fields of science and engineering used in volume 70 and earlier surveys to the revised fields of R&D collected under volume 71.
Federal obligations for research performed at higher education institutions , by detailed field of R&D . As of volume 71, all respondents were required to report these obligations. Previously, this information was reported by seven agencies (the Departments of Agriculture, Defense, Energy, Health and Human Services, and Homeland Security; NASA; and NSF).
Geographic distribution of R&D obligations. As of volume 71, all respondents were required to respond to this portion of the survey. Previously, the 11 largest R&D funding agencies responded to this portion (the Departments of Agriculture, Commerce, Defense, Energy, Health and Human Services, Homeland Security, the Interior, and Transportation; the Environmental Protection Agency; NASA; and NSF). Respondents are asked to provide the principal location (state or outlying area) of the work performed by the primary contractor, grantee, or intramural organization, assign the obligations to the location of the headquarters of the U.S. primary contractor, grantee, or intramural organization, or list the funds as undistributed.
Obligations and outlays. Obligations represent the amounts for orders placed, contracts awarded, services received, and similar transactions during a given period, regardless of when funds were appropriated and when future payment of money is required. Outlays represent the amounts for checks issued and cash payments made during a given period, regardless of when funds were appropriated.
Performer. A group or organization carrying out an operational function or an extramural organization or a person receiving support or providing services under a contract or grant.
- Intramural performers are agencies of the federal government, including federal employees who work on R&D both onsite and offsite and, as of volume 71, FFRDCs.
- Federal. The work of agencies of the federal government is carried out directly by agency personnel. Obligations reported under this category are for activities performed or to be performed by the reporting agency itself or are for funds that the agency transfers to another federal agency for performance of R&D (intragovernmental transfers). Although the receiving agency may obligate these funds to extramural performers (businesses, universities and colleges, other nonprofit institutions, FFRDCs, nonfederal government, and foreign) they are reported as part of the federal sector by the originating agency. Federal activities cover not only actual intramural R&D performance but also the costs associated with administration of intramural R&D programs and extramural R&D procurements by federal personnel. Intramural activities also include the costs of supplies and off-the-shelf equipment (equipment that has gone beyond the development or prototype stage) procured for use in intramural R&D. For example, an operational launch vehicle purchased from an extramural source by NASA and used for intramural performance of R&D is reported as a part of the cost of intramural R&D.
- Federally funded research and development centers (FFRDCs) —R&D-performing organizations that are exclusively or substantially financed by the federal government and are supported by the federal government either to meet a particular R&D objective or in some instances to provide major facilities at universities for research and associated training purposes. Each center is administered by an industrial firm, a university, or another nonprofit institution (see https://www.nsf.gov/statistics/ffrdclist/ for the Master Government List of FFRDCs maintained by NSF).
- Extramural performers are organizations outside the federal sector that perform R&D with federal funds under contract, grant, or cooperative agreement. Only costs associated with actual R&D performance are reported. Types of extramural performers:
- Businesses (previously “ Industry or i ndustr ial firms ”) —Organizations that may legally distribute net earnings to individuals or to other organizations.
- Higher education institutions (previously “ Universities and colleges ”) —Institutions of higher education in the United States that engage primarily in providing resident or accredited instruction for a not less than a 2-year program above the secondary school level that is acceptable for full credit toward a bachelor’s degree or that provide not less than a 1-year program of training above the secondary school level that prepares students for gainful employment in a recognized occupation. Included are colleges of liberal arts; schools of arts and sciences; professional schools, as in engineering and medicine, including affiliated hospitals and associated research institutes; and agricultural experiment stations. Other examples of universities and colleges include community colleges, 4-year colleges, universities, and freestanding professional schools (medical schools, law schools, etc.).
- Other nonprofit institutions —Private organizations other than educational institutions whose net earnings do not benefit either private stockholders or individuals and other private organizations organized for the exclusive purpose of turning over their entire net earnings to such nonprofit organizations. Examples of nonprofit institutions include foundations, trade associations, charities, and research organizations.
- State and local governments —State and local government agencies, excluding state or local universities and colleges, agricultural experiment stations, medical schools, and affiliated hospitals. (Federal R&D funds obligated directly to such state and local institutions are excluded in this category. However, they are included under the universities and colleges category in this report.) R&D activities under the state and local governments category are performed either by the state or local agencies themselves or by other organizations under grants or contracts from such agencies. Regardless of the ultimate performer, federal R&D funds directed to state and local governments are reported only under this sector.
- Non-U.S. performers (previously “Foreign performers”) —Other nations’ citizens, organizations, universities and colleges, governments, as well as international organizations located outside the United States, that perform R&D. In most cases, foreigners performing R&D in the United States are not reported here. Excluded from this category are U.S. agencies, U.S. organizations, or U.S. citizens performing R&D abroad for the federal government. Examples of foreign performers include the North Atlantic Treaty Organization, the United Nations Educational, Scientific, and Cultural Organization, and the World Health Organization. An exception in the past was made in the case of U.S. citizens performing R&D abroad under special foreign-currency funds; these activities were included under the foreign performers category but have not been collected since the mid-1990s.
- Private individuals —When an R&D grant or contract is awarded directly to a private individual, obligations incurred are placed under the category businesses.
R &D and R&D plant. Amounts for R&D and R&D plant include all direct, incidental, or related costs resulting from, or necessary to, performance of R&D and costs of R&D plant as defined below, regardless of whether R&D is performed by a federal agency (intramurally) or by private individuals and organizations under grant or contract (extramurally). R&D excludes routine product testing, quality control, mapping and surveys, collection of general-purpose statistics, experimental production, and the training of scientific personnel.
- Research is defined as systematic study directed toward fuller scientific knowledge or understanding of the subject studied. Research is classified as either basic or applied, according to the objectives of the sponsoring agency.
- Basic research is defined as experimental or theoretical work undertaken primarily to acquire new knowledge of the underlying foundations of phenomena and observable facts. Basic research may include activities with broad or general applications in mind, such as the study of how plant genomes change, but should exclude research directed toward a specific application or requirement, such as the optimization of the genome of a specific crop species.
- Applied research is defined as original investigation undertaken in order to acquire new knowledge. Applied research is, however, directed primarily toward a specific practical aim or objective.
- Development , also known as experimental development, is defined as creative and systematic work, drawing on knowledge gained from research and practical experience, which is directed at producing new products or processes or improving existing products or processes. Like research, experimental development will result in gaining additional knowledge.
For reporting experimental development activities, the following are included:
The production of materials, devices, and systems or methods, including the design, construction, and testing of experimental prototypes.
Technology demonstrations, in cases where a system or component is being demonstrated at scale for the first time, and it is realistic to expect additional refinements to the design (feedback R&D) following the demonstration. However, not all activities that are identified as “technology demonstrations” are R&D.
However, experimental development excludes the following:
User demonstrations where the cost and benefits of a system are being validated for a specific use case. This includes low-rate initial production activities.
Pre-production development, which is defined as non-experimental work on a product or system before it goes into full production, including activities such as tooling and development of production facilities.
To better differentiate between the part of the federal R&D budget that supports science and key enabling technologies (including technologies for military and nondefense applications) and the part that primarily supports testing and evaluation (mostly of defense-related systems), NSF collects development dollars from DOD in two categories: advanced technology development and major systems development.
DOD uses RDT&E Budget Activities 1–7 to classify data into the survey categories. Within DOD’s research categories, basic research is classified as Budget Activity 1, and applied research is classified as Budget Activity 2. Within DOD’s development categories, advanced technology development is classified as Budget Activity 3. Starting in volume 66, major systems development is classified as Budget Activities 4–6 instead of Budget Activities 4–7 and includes advanced component development and prototypes, system development and demonstration, and RDT&E management support; data on Budget Activity 7, operational systems development, is collected separately. (Note: As a historical artifact from previous DOD budget authority terminology, funds for Budget Activity categories 1 through 7 are sometimes referred to as 6.1 through 6.7 monies.)
- Demonstration includes amounts for activities that are part of R&D (i.e., that are intended to prove or to test whether a technology or method does in fact work). Demonstrations intended primarily to make information available about new technologies or methods are excluded.
- R&D plant is defined as spending on both R&D facilities and major equipment as defined in OMB Circular A-11 Section 84 (Schedule C) and includes physical assets, such as land, structures, equipment, and intellectual property (e.g., software or applications) that have an estimated useful life of 2 years or more. Reporting for R&D plant includes the purchase, construction, manufacture, rehabilitation, or major improvement of physical assets regardless of whether the assets are owned or operated by the federal government, states, municipalities, or private individuals. The cost of the asset includes both its purchase price and all other costs incurred to bring it to a form and location suitable for use.
- For reporting construction of R&D facilities and major moveable R&D equipment, include the following:
Construction of facilities that are necessary for the execution of an R&D program. This may include land, major fixed equipment, and supporting infrastructure such as a sewer line, or housing at a remote location. Many laboratory buildings will include a mixture of R&D facilities and office space. The fraction of the building that is considered to be used for R&D may be calculated based on the percentage of square footage that is used for R&D.
Acquisition, design, or production of major movable equipment, such as mass spectrometers, research vessels, DNA sequencers, and other movable major instrumentation for use in R&D activities.
Programs of $1 million or more that are devoted to the purchase or construction of R&D major equipment.
Exclude the following:
Construction of other non-R&D facilities.
Minor equipment purchases, such as personal computers, standard microscopes, and simple spectrometers (report these costs under total R&D, not R&D Plant).
Obligations for foreign R&D plant are limited to federal funds for facilities that are located abroad and used in support of foreign R&D.
Technical Tables
Questionnaires, view archived questionnaires, key data tables.
Recommended data tables
Research, development, and R&D plant
Research and experimental development, research obligations, geographic distribution of obligations, data tables, research, development, test, and evaluation (rdt&e), intramural obligations for research and experimental development and r&d plant, basic research obligations, applied research obligations, experimental development obligations, obligations to university affiliated research centers: fy 2022, research obligations to higher education performers, basic research obligations to higher education performers, applied research obligations to higher education performers, experimental development obligations to higher education performers, foreign performer obligations, by region, country or economy, and agency, geographic distribution of department of defense rdt&e obligations, outlays, by agency, obligations, by agency, obligations, by performer: fys 1967–2023, obligations, by detailed field of science and engineering, obligations, by state or location, general notes.
These tables present the results of volume 72 (FYs 2022–23) of the Survey of Federal Funds for Research and Development. This annual census, completed by the federal agencies that conduct research and development (R&D) programs, is the primary source of information about federal funding for R&D in the United States. Actual data are collected for the fiscal year just completed; estimates are obtained for the current fiscal year.
Acknowledgments and Suggested Citation
Acknowledgments, suggested citation.
Christopher V. Pece of the National Center for Science and Engineering Statistics (NCSES) developed and coordinated this report under the guidance of Amber Levanon Seligson, NCSES Program Director, and the leadership of Emilda B. Rivers, NCSES Director; Christina Freyman NCSES Deputy Director; and John Finamore, NCSES Chief Statistician. Gary Anderson and Jock Black (NCSES) reviewed the report.
Under contract to NCSES, Synectics for Management Decisions, Inc. conducted the survey and prepared the statistics for this report. Synectics staff members who made significant contributions include LaVonda Scott, Elizabeth Walter, Suresh Kaja, Peter Ahn, and John Millen.
NCSES thanks the federal agency staff that provided information for this report.
National Center for Science and Engineering Statistics (NCSES). 2024. Federal Funds for Research and Development: Fiscal Years 202 2 –2 3 . NSF 24-321. Alexandria, VA: National Science Foundation. Available at https://ncses.nsf.gov/surveys/federal-funds-research-development/2022-2023#data
Featured Analysis
Definitions of research and development, related content, related collections, survey contact.
For additional information about this survey or the methodology, contact
Get e-mail updates from NCSES
NCSES is an official statistical agency. Subscribe below to receive our latest news and announcements.
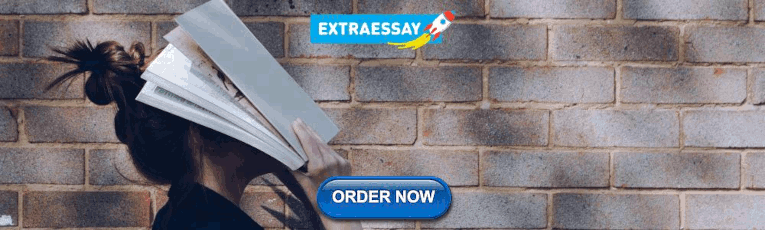
IMAGES
VIDEO
COMMENTS
Methods used for data analysis in qualitative research. There are several techniques to analyze the data in qualitative research, but here are some commonly used methods, Content Analysis: It is widely accepted and the most frequently employed technique for data analysis in research methodology. It can be used to analyze the documented ...
A method of data analysis that is the umbrella term for engineering metrics and insights for additional value, direction, and context. By using exploratory statistical evaluation, data mining aims to identify dependencies, relations, patterns, and trends to generate advanced knowledge.
Data Analysis. Definition: Data analysis refers to the process of inspecting, cleaning, transforming, and modeling data with the goal of discovering useful information, drawing conclusions, and supporting decision-making. It involves applying various statistical and computational techniques to interpret and derive insights from large datasets.
data analysis, the process of systematically collecting, cleaning, transforming, describing, modeling, and interpreting data, generally employing statistical techniques. Data analysis is an important part of both scientific research and business, where demand has grown in recent years for data-driven decision making.Data analysis techniques are used to gain useful insights from datasets, which ...
Description: Quantitative data analysis is a high-level branch of data analysis that designates methods and techniques concerned with numbers instead of words. It accounts for more than 50% of all data analysis and is by far the most widespread and well-known type of data analysis.
Below we present a step-by-step guide for analysing data for two different types of research questions. The data analysis methods described here are based on basic content analysis as described by Elo and Kyngäs 4 and Graneheim and Lundman, 5 and the integrative review as described by Whittemore and Knafl, 6 but modified to be applicable to ...
Research methods are specific procedures for collecting and analyzing data. Developing your research methods is an integral part of your research design. When planning your methods, there are two key decisions you will make. First, decide how you will collect data. Your methods depend on what type of data you need to answer your research question:
Data Analysis and Presentation Techniques that Apply to both Survey and Interview Research. Create a documentation of the data and the process of data collection. Analyze the data rather than just describing it - use it to tell a story that focuses on answering the research question. Use charts or tables to help the reader understand the data ...
Your research methodology discusses and explains the data collection and analysis methods you used in your research. A key part of your thesis, dissertation, or research paper, the methodology chapter explains what you did and how you did it, allowing readers to evaluate the reliability and validity of your research and your dissertation topic.
Written by Coursera Staff • Updated on Apr 1, 2024. Data analysis is the practice of working with data to glean useful information, which can then be used to make informed decisions. "It is a capital mistake to theorize before one has data. Insensibly one begins to twist facts to suit theories, instead of theories to suit facts," Sherlock ...
Qualitative data analysis all begins with data coding, after which an analysis method is applied. In some cases, more than one analysis method is used, depending on the research aims and research questions. In the video below, we explore some common qualitative analysis methods, along with practical examples.
For many researchers unfamiliar with qualitative research, determining how to conduct qualitative analyses is often quite challenging. Part of this challenge is due to the seemingly limitless approaches that a qualitative researcher might leverage, as well as simply learning to think like a qualitative researcher when analyzing data. From framework analysis (Ritchie & Spencer, 1994) to content ...
A research design is a strategy for answering your research question using empirical data. Creating a research design means making decisions about: Your overall research objectives and approach. Whether you'll rely on primary research or secondary research. Your sampling methods or criteria for selecting subjects. Your data collection methods.
Data analysis techniques in research are essential because they allow researchers to derive meaningful insights from data sets to support their hypotheses or research objectives.. Data Analysis Techniques in Research: While various groups, institutions, and professionals may have diverse approaches to data analysis, a universal definition captures its essence.
Research methodology refers to the system of procedures, techniques, and tools used to carry out a research study. It encompasses the overall approach, including the research design, data collection methods, data analysis techniques, and the interpretation of findings. Research methodology plays a crucial role in the field of research, as it ...
Qualitative Research Methodology. This is a research methodology that involves the collection and analysis of non-numerical data such as words, images, and observations. This type of research is often used to explore complex phenomena, to gain an in-depth understanding of a particular topic, and to generate hypotheses.
Data analysis in research is the systematic process of investigating facts and figures to make conclusions about a specific question or topic; there are two major types of data analysis methods in ...
Data analysis is simply the process of converting the gathered data to meanin gf ul information. Different techniques such as modeling to reach trends, relatio nships, and therefore conclusions to ...
How to conduct qualitative research? Given that qualitative research is characterised by flexibility, openness and responsivity to context, the steps of data collection and analysis are not as separate and consecutive as they tend to be in quantitative research [13, 14].As Fossey puts it: "sampling, data collection, analysis and interpretation are related to each other in a cyclical ...
There are differences between qualitative data analysis and quantitative data analysis. In qualitative researches using interviews, focus groups, experiments etc. data analysis is going to involve identifying common patterns within the responses and critically analyzing them in order to achieve research aims and objectives. Data analysis for ...
Research Methodology and Data Analysis Second Edition. Zainudin Awang. UiTM Press, 2012 - Education - 334 pages. This book provides proper direction in doing research especially towards the understanding of research objectives, and research hypotheses. The book also guides in research methodology such as the methods of designing a questionnaire ...
Statistical methods involved in carrying out a study include planning, designing, collecting data, analysing, drawing meaningful interpretation and reporting of the research findings. The statistical analysis gives meaning to the meaningless numbers, thereby breathing life into a lifeless data. The results and inferences are precise only if ...
Students who pursue the Data and Research Methods track will build quantitative analysis and research methodology skills by successfully completing at least 16 credits from a list of qualifying courses. As a prospective student, you may have questions about what this new track means for your potential academic future at HKS.
The Statistics and Research Methods Bootcamp will provide a foundation for the use of statistics in data analysis and offer students an introduction to quantitative and qualitative methods in support of program coursework and the completion of a Capstone project.
Data is an important resource for assisting companies in making informed decisions, but you need a comprehensive data strategy to manage it effectively. A data strategy is like a blueprint for how your company gathers, maintains, and interprets data and how your company will approach other important aspects of data like governance and security.
Applied Research is a multidisciplinary journal that connects fundamental and applied research across the physical, natural, and life sciences, and engineering. Abstract This paper presents a simple method to transform two-dimensional data sets into a format that can be easily processed into sound files.
For each beer, we measure over 200 chemical properties, perform quantitative descriptive sensory analysis with a trained tasting panel and map data from over 180,000 consumer reviews to train 10 ...
Complementary papers related to Cluster C are characterised by a diversity of research methods such as Bayesian networks (Hänninen and Kujala 2012), identification of events and processes of risks (Montewka et al. 2014b), what-if analysis, association rules (Weng and Li 2019), scenario-event tree (Chai et al. 2017), binary logistic regressions ...
Data collection. Data for FYs 2022-23 (volume 72) were collected by Synectics under contract to NCSES (for a full list of fiscal years canvassed by survey volume reference, see Table A-4). Data collection began with an e-mail to each agency to verify the name, phone number, and e-mail address of each agency-level survey respondent.