- How it works
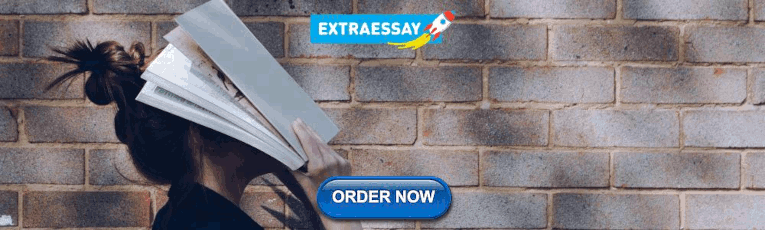
"Christmas Offer"
Terms & conditions.
As the Christmas season is upon us, we find ourselves reflecting on the past year and those who we have helped to shape their future. It’s been quite a year for us all! The end of the year brings no greater joy than the opportunity to express to you Christmas greetings and good wishes.
At this special time of year, Research Prospect brings joyful discount of 10% on all its services. May your Christmas and New Year be filled with joy.
We are looking back with appreciation for your loyalty and looking forward to moving into the New Year together.
"Claim this offer"
In unfamiliar and hard times, we have stuck by you. This Christmas, Research Prospect brings you all the joy with exciting discount of 10% on all its services.
Offer valid till 5-1-2024
We love being your partner in success. We know you have been working hard lately, take a break this holiday season to spend time with your loved ones while we make sure you succeed in your academics
Discount code: RP0996Y
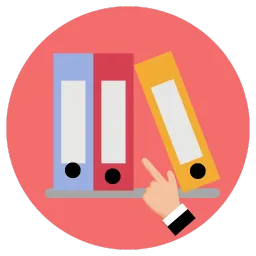
Hypothesis Testing – A Complete Guide with Examples
Published by Alvin Nicolas at August 14th, 2021 , Revised On October 26, 2023
In statistics, hypothesis testing is a critical tool. It allows us to make informed decisions about populations based on sample data. Whether you are a researcher trying to prove a scientific point, a marketer analysing A/B test results, or a manufacturer ensuring quality control, hypothesis testing plays a pivotal role. This guide aims to introduce you to the concept and walk you through real-world examples.
What is a Hypothesis and a Hypothesis Testing?
A hypothesis is considered a belief or assumption that has to be accepted, rejected, proved or disproved. In contrast, a research hypothesis is a research question for a researcher that has to be proven correct or incorrect through investigation.
What is Hypothesis Testing?
Hypothesis testing is a scientific method used for making a decision and drawing conclusions by using a statistical approach. It is used to suggest new ideas by testing theories to know whether or not the sample data supports research. A research hypothesis is a predictive statement that has to be tested using scientific methods that join an independent variable to a dependent variable.
Example: The academic performance of student A is better than student B
Characteristics of the Hypothesis to be Tested
A hypothesis should be:
- Clear and precise
- Capable of being tested
- Able to relate to a variable
- Stated in simple terms
- Consistent with known facts
- Limited in scope and specific
- Tested in a limited timeframe
- Explain the facts in detail
What is a Null Hypothesis and Alternative Hypothesis?
A null hypothesis is a hypothesis when there is no significant relationship between the dependent and the participants’ independent variables .
In simple words, it’s a hypothesis that has been put forth but hasn’t been proved as yet. A researcher aims to disprove the theory. The abbreviation “Ho” is used to denote a null hypothesis.
If you want to compare two methods and assume that both methods are equally good, this assumption is considered the null hypothesis.
Example: In an automobile trial, you feel that the new vehicle’s mileage is similar to the previous model of the car, on average. You can write it as: Ho: there is no difference between the mileage of both vehicles. If your findings don’t support your hypothesis and you get opposite results, this outcome will be considered an alternative hypothesis.
If you assume that one method is better than another method, then it’s considered an alternative hypothesis. The alternative hypothesis is the theory that a researcher seeks to prove and is typically denoted by H1 or HA.
If you support a null hypothesis, it means you’re not supporting the alternative hypothesis. Similarly, if you reject a null hypothesis, it means you are recommending the alternative hypothesis.
Example: In an automobile trial, you feel that the new vehicle’s mileage is better than the previous model of the vehicle. You can write it as; Ha: the two vehicles have different mileage. On average/ the fuel consumption of the new vehicle model is better than the previous model.
If a null hypothesis is rejected during the hypothesis test, even if it’s true, then it is considered as a type-I error. On the other hand, if you don’t dismiss a hypothesis, even if it’s false because you could not identify its falseness, it’s considered a type-II error.
Hire an Expert Researcher
Orders completed by our expert writers are
- Formally drafted in academic style
- 100% Plagiarism free & 100% Confidential
- Never resold
- Include unlimited free revisions
- Completed to match exact client requirements
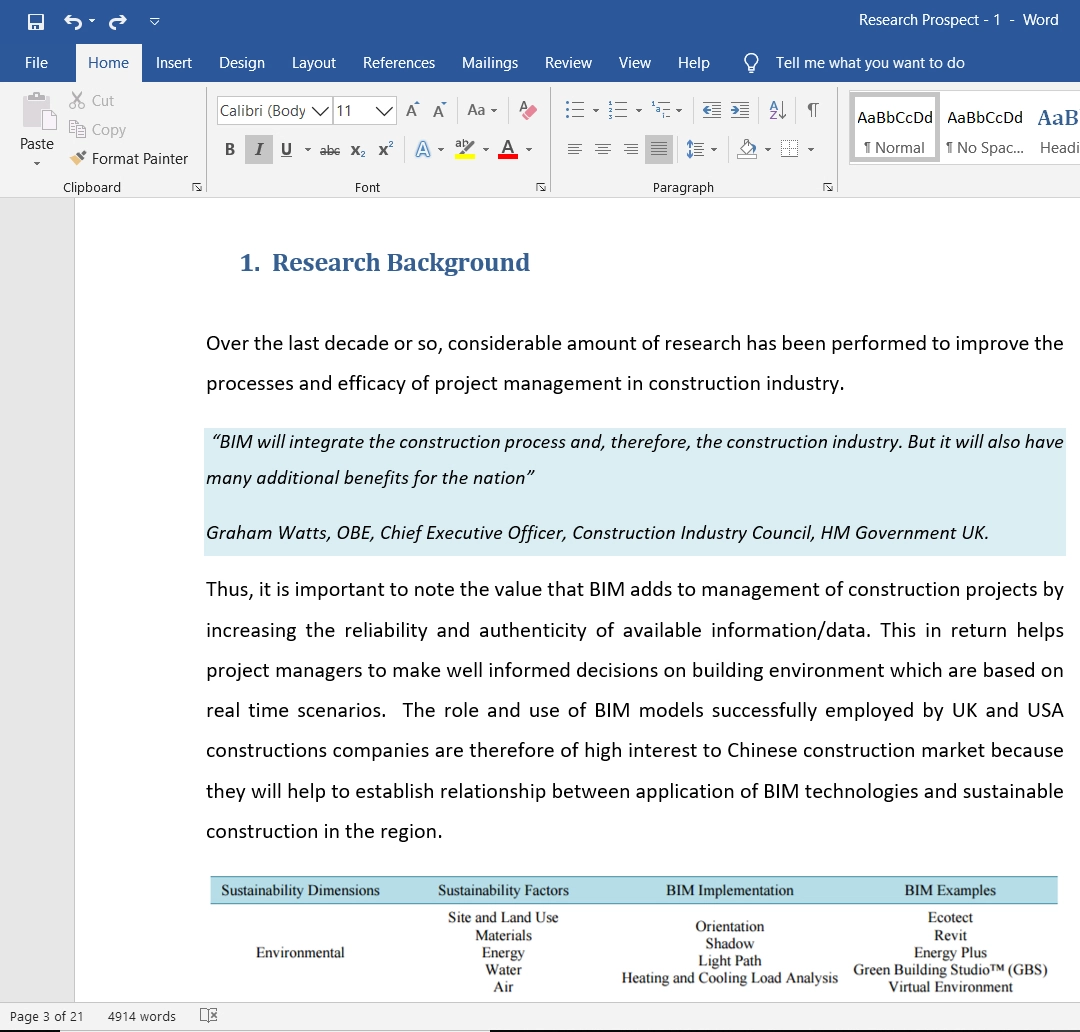
How to Conduct Hypothesis Testing?
Here is a step-by-step guide on how to conduct hypothesis testing.
Step 1: State the Null and Alternative Hypothesis
Once you develop a research hypothesis, it’s important to state it is as a Null hypothesis (Ho) and an Alternative hypothesis (Ha) to test it statistically.
A null hypothesis is a preferred choice as it provides the opportunity to test the theory. In contrast, you can accept the alternative hypothesis when the null hypothesis has been rejected.
Example: You want to identify a relationship between obesity of men and women and the modern living style. You develop a hypothesis that women, on average, gain weight quickly compared to men. Then you write it as: Ho: Women, on average, don’t gain weight quickly compared to men. Ha: Women, on average, gain weight quickly compared to men.
Step 2: Data Collection
Hypothesis testing follows the statistical method, and statistics are all about data. It’s challenging to gather complete information about a specific population you want to study. You need to gather the data obtained through a large number of samples from a specific population.
Example: Suppose you want to test the difference in the rate of obesity between men and women. You should include an equal number of men and women in your sample. Then investigate various aspects such as their lifestyle, eating patterns and profession, and any other variables that may influence average weight. You should also determine your study’s scope, whether it applies to a specific group of population or worldwide population. You can use available information from various places, countries, and regions.
Step 3: Select Appropriate Statistical Test
There are many types of statistical tests , but we discuss the most two common types below, such as One-sided and two-sided tests.
Note: Your choice of the type of test depends on the purpose of your study
One-sided Test
In the one-sided test, the values of rejecting a null hypothesis are located in one tail of the probability distribution. The set of values is less or higher than the critical value of the test. It is also called a one-tailed test of significance.
Example: If you want to test that all mangoes in a basket are ripe. You can write it as: Ho: All mangoes in the basket, on average, are ripe. If you find all ripe mangoes in the basket, the null hypothesis you developed will be true.
Two-sided Test
In the two-sided test, the values of rejecting a null hypothesis are located on both tails of the probability distribution. The set of values is less or higher than the first critical value of the test and higher than the second critical value test. It is also called a two-tailed test of significance.
Example: Nothing can be explicitly said whether all mangoes are ripe in the basket. If you reject the null hypothesis (Ho: All mangoes in the basket, on average, are ripe), then it means all mangoes in the basket are not likely to be ripe. A few mangoes could be raw as well.
Get statistical analysis help at an affordable price
- An expert statistician will complete your work
- Rigorous quality checks
- Confidentiality and reliability
- Any statistical software of your choice
- Free Plagiarism Report

Step 4: Select the Level of Significance
When you reject a null hypothesis, even if it’s true during a statistical hypothesis, it is considered the significance level . It is the probability of a type one error. The significance should be as minimum as possible to avoid the type-I error, which is considered severe and should be avoided.
If the significance level is minimum, then it prevents the researchers from false claims.
The significance level is denoted by P, and it has given the value of 0.05 (P=0.05)
If the P-Value is less than 0.05, then the difference will be significant. If the P-value is higher than 0.05, then the difference is non-significant.
Example: Suppose you apply a one-sided test to test whether women gain weight quickly compared to men. You get to know about the average weight between men and women and the factors promoting weight gain.
Step 5: Find out Whether the Null Hypothesis is Rejected or Supported
After conducting a statistical test, you should identify whether your null hypothesis is rejected or accepted based on the test results. It would help if you observed the P-value for this.
Example: If you find the P-value of your test is less than 0.5/5%, then you need to reject your null hypothesis (Ho: Women, on average, don’t gain weight quickly compared to men). On the other hand, if a null hypothesis is rejected, then it means the alternative hypothesis might be true (Ha: Women, on average, gain weight quickly compared to men. If you find your test’s P-value is above 0.5/5%, then it means your null hypothesis is true.
Step 6: Present the Outcomes of your Study
The final step is to present the outcomes of your study . You need to ensure whether you have met the objectives of your research or not.
In the discussion section and conclusion , you can present your findings by using supporting evidence and conclude whether your null hypothesis was rejected or supported.
In the result section, you can summarise your study’s outcomes, including the average difference and P-value of the two groups.
If we talk about the findings, our study your results will be as follows:
Example: In the study of identifying whether women gain weight quickly compared to men, we found the P-value is less than 0.5. Hence, we can reject the null hypothesis (Ho: Women, on average, don’t gain weight quickly than men) and conclude that women may likely gain weight quickly than men.
Did you know in your academic paper you should not mention whether you have accepted or rejected the null hypothesis?
Always remember that you either conclude to reject Ho in favor of Haor do not reject Ho . It would help if you never rejected Ha or even accept Ha .
Suppose your null hypothesis is rejected in the hypothesis testing. If you conclude reject Ho in favor of Haor do not reject Ho, then it doesn’t mean that the null hypothesis is true. It only means that there is a lack of evidence against Ho in favour of Ha. If your null hypothesis is not true, then the alternative hypothesis is likely to be true.
Example: We found that the P-value is less than 0.5. Hence, we can conclude reject Ho in favour of Ha (Ho: Women, on average, don’t gain weight quickly than men) reject Ho in favour of Ha. However, rejected in favour of Ha means (Ha: women may likely to gain weight quickly than men)
Frequently Asked Questions
What are the 3 types of hypothesis test.
The 3 types of hypothesis tests are:
- One-Sample Test : Compare sample data to a known population value.
- Two-Sample Test : Compare means between two sample groups.
- ANOVA : Analyze variance among multiple groups to determine significant differences.
What is a hypothesis?
A hypothesis is a proposed explanation or prediction about a phenomenon, often based on observations. It serves as a starting point for research or experimentation, providing a testable statement that can either be supported or refuted through data and analysis. In essence, it’s an educated guess that drives scientific inquiry.
What are null hypothesis?
A null hypothesis (often denoted as H0) suggests that there is no effect or difference in a study or experiment. It represents a default position or status quo. Statistical tests evaluate data to determine if there’s enough evidence to reject this null hypothesis.
What is the probability value?
The probability value, or p-value, is a measure used in statistics to determine the significance of an observed effect. It indicates the probability of obtaining the observed results, or more extreme, if the null hypothesis were true. A small p-value (typically <0.05) suggests evidence against the null hypothesis, warranting its rejection.
What is p value?
The p-value is a fundamental concept in statistical hypothesis testing. It represents the probability of observing a test statistic as extreme, or more so, than the one calculated from sample data, assuming the null hypothesis is true. A low p-value suggests evidence against the null, possibly justifying its rejection.
What is a t test?
A t-test is a statistical test used to compare the means of two groups. It determines if observed differences between the groups are statistically significant or if they likely occurred by chance. Commonly applied in research, there are different t-tests, including independent, paired, and one-sample, tailored to various data scenarios.
When to reject null hypothesis?
Reject the null hypothesis when the test statistic falls into a predefined rejection region or when the p-value is less than the chosen significance level (commonly 0.05). This suggests that the observed data is unlikely under the null hypothesis, indicating evidence for the alternative hypothesis. Always consider the study’s context.
You May Also Like
A confounding variable can potentially affect both the suspected cause and the suspected effect. Here is all you need to know about accounting for confounding variables in research.
Experimental research refers to the experiments conducted in the laboratory or under observation in controlled conditions. Here is all you need to know about experimental research.
Ethnography is a type of research where a researcher observes the people in their natural environment. Here is all you need to know about ethnography.
As Featured On
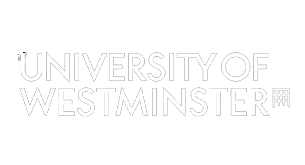
USEFUL LINKS
LEARNING RESOURCES
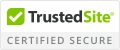
COMPANY DETAILS
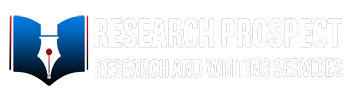
Splash Sol LLC

- How It Works
Module 9: Hypothesis Testing With One Sample
Null and alternative hypotheses, learning outcomes.
- Describe hypothesis testing in general and in practice
The actual test begins by considering two hypotheses . They are called the null hypothesis and the alternative hypothesis . These hypotheses contain opposing viewpoints.
H 0 : The null hypothesis: It is a statement about the population that either is believed to be true or is used to put forth an argument unless it can be shown to be incorrect beyond a reasonable doubt.
H a : The alternative hypothesis : It is a claim about the population that is contradictory to H 0 and what we conclude when we reject H 0 .
Since the null and alternative hypotheses are contradictory, you must examine evidence to decide if you have enough evidence to reject the null hypothesis or not. The evidence is in the form of sample data.
After you have determined which hypothesis the sample supports, you make adecision. There are two options for a decision . They are “reject H 0 ” if the sample information favors the alternative hypothesis or “do not reject H 0 ” or “decline to reject H 0 ” if the sample information is insufficient to reject the null hypothesis.
Mathematical Symbols Used in H 0 and H a :
H 0 always has a symbol with an equal in it. H a never has a symbol with an equal in it. The choice of symbol depends on the wording of the hypothesis test. However, be aware that many researchers (including one of the co-authors in research work) use = in the null hypothesis, even with > or < as the symbol in the alternative hypothesis. This practice is acceptable because we only make the decision to reject or not reject the null hypothesis.
H 0 : No more than 30% of the registered voters in Santa Clara County voted in the primary election. p ≤ 30
H a : More than 30% of the registered voters in Santa Clara County voted in the primary election. p > 30
A medical trial is conducted to test whether or not a new medicine reduces cholesterol by 25%. State the null and alternative hypotheses.
H 0 : The drug reduces cholesterol by 25%. p = 0.25
H a : The drug does not reduce cholesterol by 25%. p ≠ 0.25
We want to test whether the mean GPA of students in American colleges is different from 2.0 (out of 4.0). The null and alternative hypotheses are:
H 0 : μ = 2.0
H a : μ ≠ 2.0
We want to test whether the mean height of eighth graders is 66 inches. State the null and alternative hypotheses. Fill in the correct symbol (=, ≠, ≥, <, ≤, >) for the null and alternative hypotheses. H 0 : μ __ 66 H a : μ __ 66
- H 0 : μ = 66
- H a : μ ≠ 66
We want to test if college students take less than five years to graduate from college, on the average. The null and alternative hypotheses are:
H 0 : μ ≥ 5
H a : μ < 5
We want to test if it takes fewer than 45 minutes to teach a lesson plan. State the null and alternative hypotheses. Fill in the correct symbol ( =, ≠, ≥, <, ≤, >) for the null and alternative hypotheses. H 0 : μ __ 45 H a : μ __ 45
- H 0 : μ ≥ 45
- H a : μ < 45
In an issue of U.S. News and World Report , an article on school standards stated that about half of all students in France, Germany, and Israel take advanced placement exams and a third pass. The same article stated that 6.6% of U.S. students take advanced placement exams and 4.4% pass. Test if the percentage of U.S. students who take advanced placement exams is more than 6.6%. State the null and alternative hypotheses.
H 0 : p ≤ 0.066
H a : p > 0.066
On a state driver’s test, about 40% pass the test on the first try. We want to test if more than 40% pass on the first try. Fill in the correct symbol (=, ≠, ≥, <, ≤, >) for the null and alternative hypotheses. H 0 : p __ 0.40 H a : p __ 0.40
- H 0 : p = 0.40
- H a : p > 0.40
Concept Review
In a hypothesis test , sample data is evaluated in order to arrive at a decision about some type of claim. If certain conditions about the sample are satisfied, then the claim can be evaluated for a population. In a hypothesis test, we: Evaluate the null hypothesis , typically denoted with H 0 . The null is not rejected unless the hypothesis test shows otherwise. The null statement must always contain some form of equality (=, ≤ or ≥) Always write the alternative hypothesis , typically denoted with H a or H 1 , using less than, greater than, or not equals symbols, i.e., (≠, >, or <). If we reject the null hypothesis, then we can assume there is enough evidence to support the alternative hypothesis. Never state that a claim is proven true or false. Keep in mind the underlying fact that hypothesis testing is based on probability laws; therefore, we can talk only in terms of non-absolute certainties.
Formula Review
H 0 and H a are contradictory.
- OpenStax, Statistics, Null and Alternative Hypotheses. Provided by : OpenStax. Located at : http://cnx.org/contents/[email protected]:58/Introductory_Statistics . License : CC BY: Attribution
- Introductory Statistics . Authored by : Barbara Illowski, Susan Dean. Provided by : Open Stax. Located at : http://cnx.org/contents/[email protected] . License : CC BY: Attribution . License Terms : Download for free at http://cnx.org/contents/[email protected]
- Simple hypothesis testing | Probability and Statistics | Khan Academy. Authored by : Khan Academy. Located at : https://youtu.be/5D1gV37bKXY . License : All Rights Reserved . License Terms : Standard YouTube License
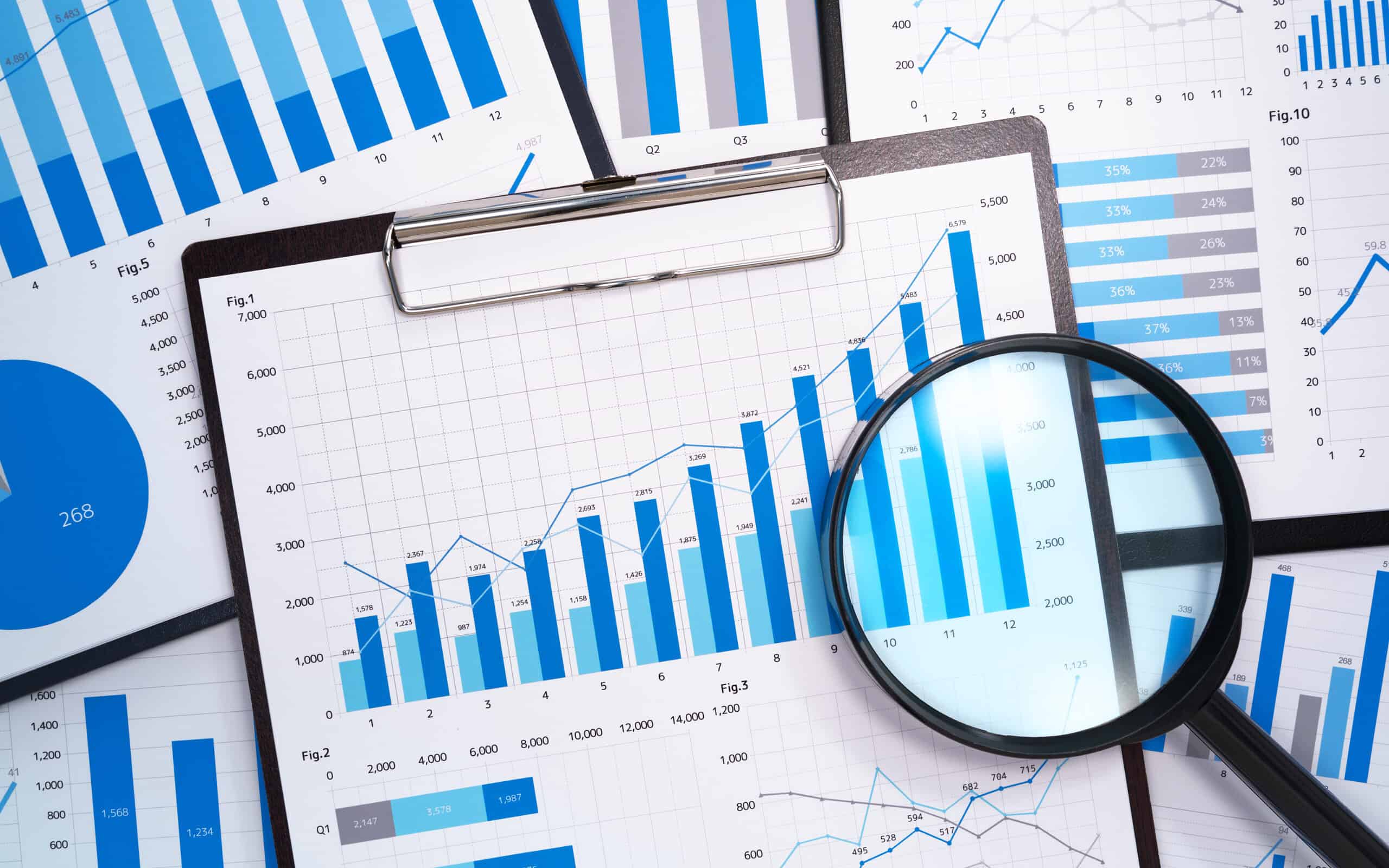
Formulating the Alternate Hypothesis: Guidelines and Examples
Updated: July 5, 2023 by Ken Feldman
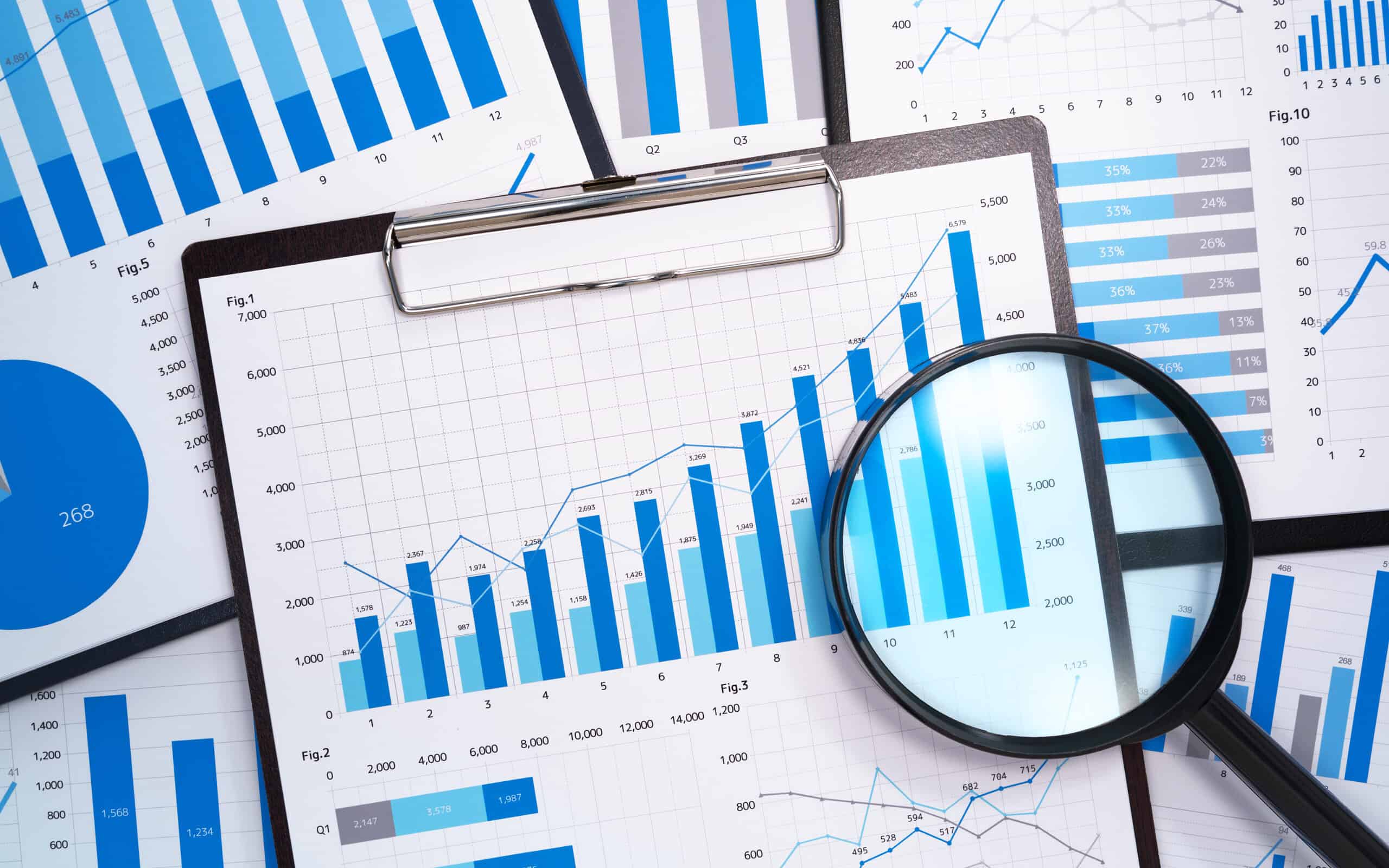
Hypothesis testing is a branch of statistics in which, using data from a sample, an inference is made about a population parameter or a population probability distribution .
First, a hypothesis statement and assumption is made about the population parameter or probability distribution. This initial statement is called the Null Hypothesis and is denoted by H o.
An alternative or alternate hypothesis (denoted Ha ) is then stated, which will be the opposite of the Null Hypothesis.
The hypothesis testing process and analysis involves using sample data to determine whether or not you can be statistically confident that you can reject or fail to reject the H o. If the H o is rejected, the statistical conclusion is that the alternative or alternate hypothesis Ha is true.
Overview: What is the Alternate Hypothesis (Ha)?
Hypothesis testing applies to all forms of statistical inquiry. For example, it can be used to determine whether there are differences between population parameters or an understanding about slopes of regression lines or equality of probability distributions.
In all cases, the first thing you do is state the Null Hypothesis. The word “null” in the context of hypothesis testing means “nothing” or “zero.”
If we wanted to test whether there was a difference in two population means based on the calculations from two samples, we would state the Null Hypothesis in the form of:
Ho: mu1 = mu2 or mu1 – mu2 = 0
In other words, there is no difference, or the difference is zero. Note that the notation is in the form of a population parameter, not a sample statistic.
The analysis of the Null Hypothesis is designed to test the Null, which will determine whether the Null should be rejected so that the Alternate Hypothesis is defaulted to and assumed to be true, or not to reject the Null so it is assumed to be the true condition.
A classic example is when you get the results back from your doctor after taking a blood test. The Null is written to state that there is no infection. Remember, the Null is always in the form of “nothingness.” The alternate hypothesis is that you have an infection. Once the test is done, the lab will determine whether the Null can be rejected or not. If the test shows an infection, the Null will be rejected, and the Alternate will be assumed to be true. If the test shows no infection, we cannot reject the Null.
While the Null can only be written in one form — “equal to” or “no difference” — the Alternate can be written for three conditions. For example, a marketing director wants to improve sales. She designs and launches a new social media campaign, collecting sample data for sales activity prior to the new campaign. After six months, sample sales data was collected to determine whether the campaign was successful. Hypothesis testing was used to statistically confirm whether the campaign was successful or not. The Null Hypothesis was written as: Ho: muBefore = muAfter, where the claim was that the population average sales before the campaign is the same as the population average sales after the campaign. In other words, the campaign had no effect on sales.
The Alternate Hypothesis can now be written in one of three forms:
- muBefore does not equal muAfter : The average of the population average sales before is not equal to the population average sales after, but you don’t know if it was more or less. You would have to look at the actual numbers to understand this result.
- muBefore less than muAfter : In this form, if you reject the Null, your conclusion will be that the sales after the campaign are greater than before the campaign. Put another way, the campaign increased average sales.
- muBefore greater than muAfter : Again, if you reject the Null, the alternate says that average sales before were greater than after the campaign. The campaign seems to have been a failure.
3 benefits of the Alternate Hypothesis
The stating and testing of the Null and the default to the Alternate hypothesis is the foundation of hypothesis testing. By doing so, you set the parameters for your statistical inference.
1. There can be a statistical assurance of determining differences between population parameters
Just looking at the mathematical difference between the means of two samples and making a decision is woefully inadequate. By statistically testing the Null hypothesis, you will have more confidence in any inferences you want to make about populations based on your samples. If you reject the Null, you’ll know which Alternate is most appropriate.
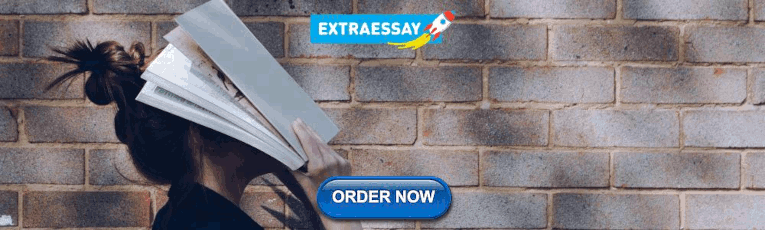
2. Statistically based estimation of the probability of a population distribution
Many statistical tests require assumptions of specific distributions. Many of these tests assume that the population follows the normal distribution . If it doesn’t, the test may be invalid. Rejecting the Null will establish whether the estimated distribution fulfills any test assumption or not.
3. You can assess the strength of your conclusions as to what to do with the Alternate hypothesis
Hypothesis testing calculations will provide some relative strength to your decisions as to whether you reject or fail to reject the Null hypothesis and, therefore, the Alternate.
Why is the Alternate Hypothesis important to understand?
It is the interpretation of the statistics relative to the Null and Alternate hypotheses that is important.
Properly write the Alternate hypothesis to capture what you are seeking to prove
The Alternate can take the form of “not equal,” “less than,” or “greater than.”
Frame your statement and select an appropriate alpha risk
You don’t want to place too big of a hurdle (or burde)n on your decision-making relative to action on the Null hypothesis by selecting an alpha value that is too high or too low. The Alternate can really reflect the true condition of the population, so failing to reject the Null too often can mask the truth.
Knowing there are decision errors when deciding how to respond to the Null Hypothesis
Since your decision relative to rejecting or not rejecting the Null impacts the Alternate Hypothesis, it’s important to understand how that decision works.
An industry example of using the Alternate Hypothesis
The director of manufacturing at a medium-size window manufacturer recently had an older machine retrofitted to increase run speed of the equipment. The supplier, after doing the retrofit, claimed that the machine was now running significantly faster. He showed a comparison of the sample average run speed before the retrofit and the sample average run speed after the retrofit. Looking at the two averages, it appeared that the supplier was correct and that the retrofit did indeed increase the speed.
However, having had some training in Lean Six Sigma, the director asked his local Black Belt for some help in doing a hypothesis test on the data to see if there was truly a statistically significant improvement of at least 100 rpm. The Null hypothesis was written as:
mu1-mu2= -100 rpms
That is the Before speed minus the After speed equaled -100 rpms. This was slightly different than the format he was used to seeing, where the Null would be reflective of “no difference” rather than a value of interest.
The Alternate hypothesis was written to reflect an increase of at least 100 rpms. That form was written as:
mu1 – mu2 greater -100
That would mean the After speed was more than 100 rpms faster than the Before speed. Fortunately, the results indicated that the Null hypothesis should be rejected and that in actuality, the difference was greater than the 100 rpms he wanted.
3 best practices when thinking about the Alternate Hypothesis
Using hypothesis testing to help make better data-driven decisions requires that you properly address the Null and Alternate Hypotheses.
1. Always use the proper nomenclature when stating the Alternate Hypothesis
The Alternate should be in the form of “not equal to,” “greater than,” “less than,” or “equal to some value of interest.”
2. Be sure that the Alternate statement reflects what you want to learn about the process characteristics
The writing of the Alternate Hypothesis can vary, so be sure you understand exactly what condition you are testing against.
3. Pick a reasonable alpha risk so you are not always failing to reject the Null Hypothesis
Being too cautious will lead you to not reject the Null enough so you will never learn anything about your population data.
Frequently Asked Questions (FAQ) about the Alternate Hypothesis
What form should the Alternate Hypothesis be written in?
The Alternate Hypothesis should be in the form of “not equal to,” “greater than,” “less than,” or “equal to some value of interest.”
When do you default to the Alternate Hypothesis?
If your analysis of the sample data suggests that you should reject the Null Hypothesis, you will default to the statement of the Alternate Hypothesis.
Should I be disappointed if I default to the Alternate Hypothesis?
Usually no. Typically, you want the actions you took to have had some impact or effect. Rejecting the Null and defaulting to the Alternate signals that something did, in fact, happen — and that might be a good thing.
Using an Alternate Hypothesis (Ha)
The Alternate Hypothesis is the default should you reject the Null. It is an indication that something has happened that is significant. Often, that is what you want to see if you’re comparing a before and after situation.
While the form of the Null Hypothesis is usually written in a single format, the format of the Alternate can be written a number of different ways. This provides more sensitivity to your interpretation of the population data and will therefore provide a richer insight for decision-making.
Join 65,000 Black Belts and Register For The Industry Leading ISIXSIGMA Newsletter Today
About the author.
Ken Feldman
Follow Me On:
- En español – ExME
- Em português – EME
Hypothesis Testing
Posted on 1st August 2014 by Ashline Amilcar
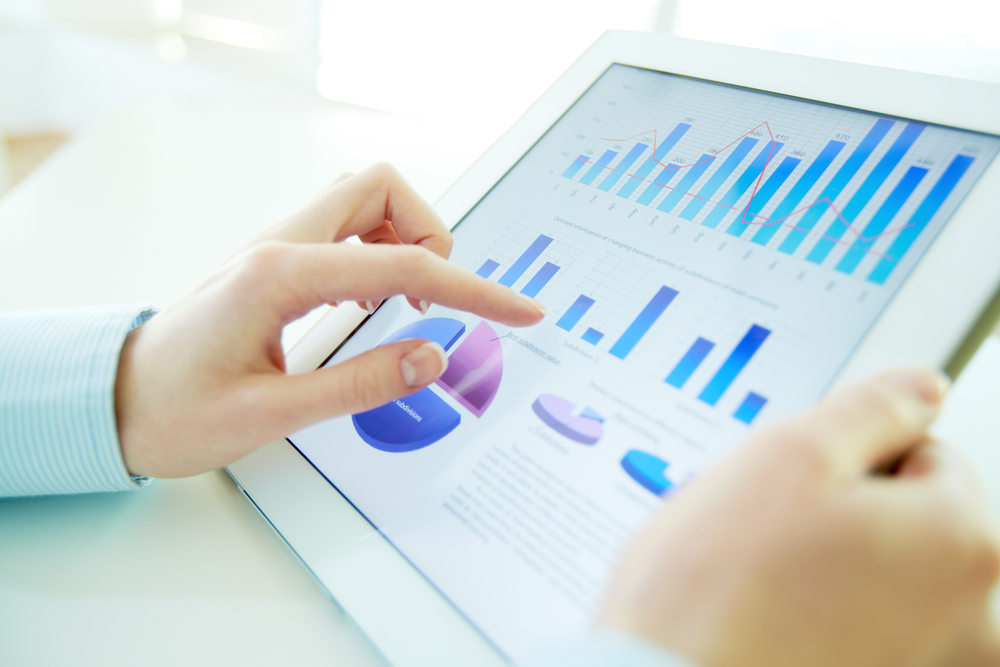
Most of the time in a research project, it is not economically feasible to work with an entire population . It is preferable to select an adequate sample and statistically infer characteristics of the population. One of the most used methods to make statistical inference is the hypothesis testing: a hypothesis testing requires establishing a null hypothesis (Ho) that is assumed to be true and an alternative hypothesis (Ha) that contradicts the null. The alternative hypothesis represents what we would expect if the null hypothesis were false.
- Hypothesis: “Value of the systolic blood pressure(SBP) of the population is 120mmHg on average”.
- Ho: “The mean of systolic blood pressure of the population is 120mmHg” (Ho: SBP=120mmHg)
- *Ha: The mean of systolic blood pressure of the population is not 120mmHg (Ha: SBP ≠120mmg)
*Notice when we state that, in the alternative hypothesis, the SBP is different from 120 mmHg we also mean that it can be greater than 120 mmHg or lesser.
In the example above after performing the experiment, suppose that the result of the mean of the SBP is greater than 120mmHg, what is the probability that we obtain this result because we selected by chance only participants with high SBP values? In other words we can´t automatically conclude from the sample that the population´s SBP is 120mmHg on average just because we have reached this conclusion in the sample; not until we test the hypothesis that the average in the sample is the same as the average in the population.
When performing a hypothesis test to be able to make inference, we assume that Ho is true. If we conclude wrongly that it is false and reject it, we make an error ( type I error ). The significance level (α) is the probability that this type I error has occurred. The maximum type I error commonly allowed is 5%; 1% significance level and 0.1% are also used when a high grade of certainty is needed.
Most statistical tests calculates a p-value to be compared with an established significance level . P-value reflects, assuming that Ho is true , how well data in the sample are consistent with the Ho. Small p-values provide high evidence against the Ho and indicate that it is very unlikely that the obtained result from the sample is due to chance. If p-value is smaller than the established significance level, the null hypothesis is rejected and we accept the alternative. The result of the research is usually called statistically significant for a p-value <0.05.
Suppose that the p-value is <0.001 for the above example, If we repeat the experiment several times, the probability that we observe SBP= 120mmHg more times is very low, Ho is rejected in this case and Ha becomes a better option for conclusion.
Ashline Amilcar
Leave a reply cancel reply.
Your email address will not be published. Required fields are marked *
Save my name, email, and website in this browser for the next time I comment.
No Comments on Hypothesis Testing
Hi Ashline, In this statement “The result of the research is usually called statistically significant for a p-value >0.05.” do you mean <0.05 ? Thanks, this is a nice summary for understanding tests of hypothesis in journal articles.
Hi Judy. I´m glad that the post helped you, thanks for comment. You are absolutely right, the correct sentence is “The result of the research is usually called statistically significant for a p-value <0.05". I apologize for the error, I will get it corrected soon.
Subscribe to our newsletter
You will receive our monthly newsletter and free access to Trip Premium.
Related Articles
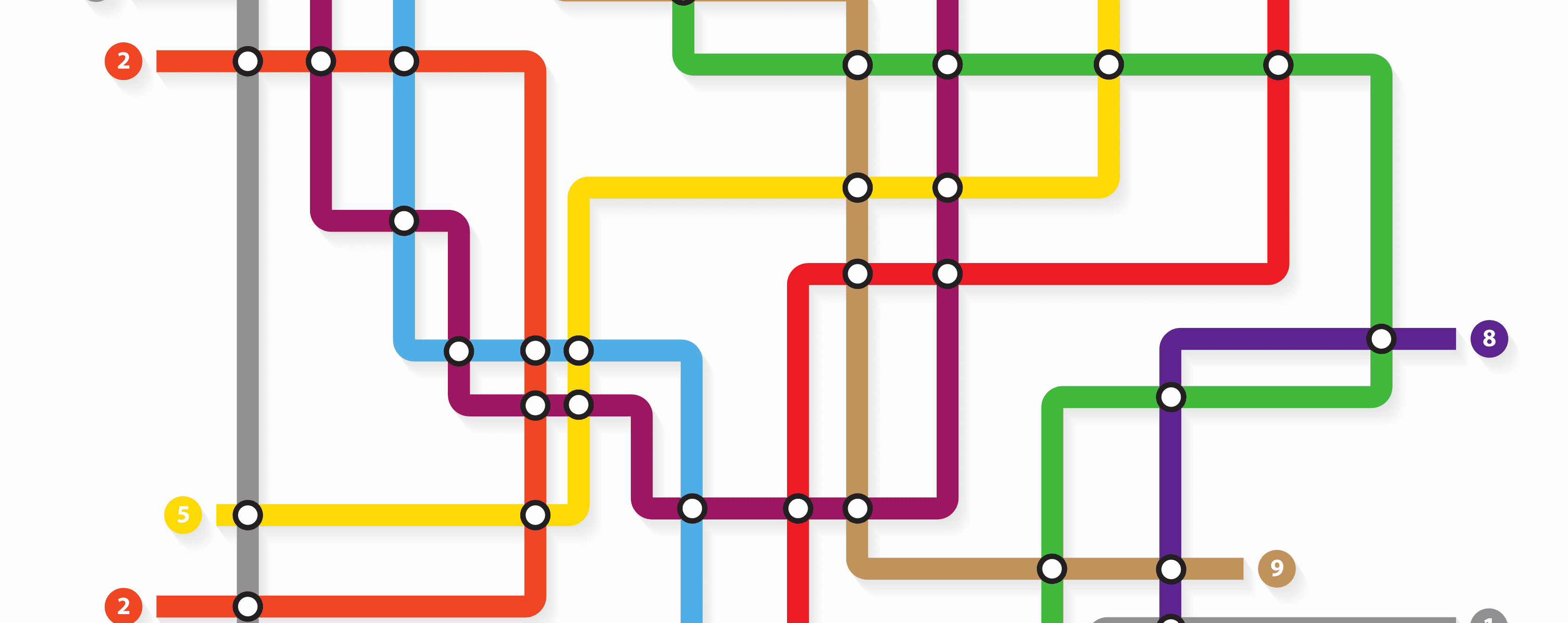
Making sense of medical statistics: a bite sized visual guide
This blog is a review of a newly published book, which has the overall aim of giving health professionals a ‘working understanding’ of medical statistics.
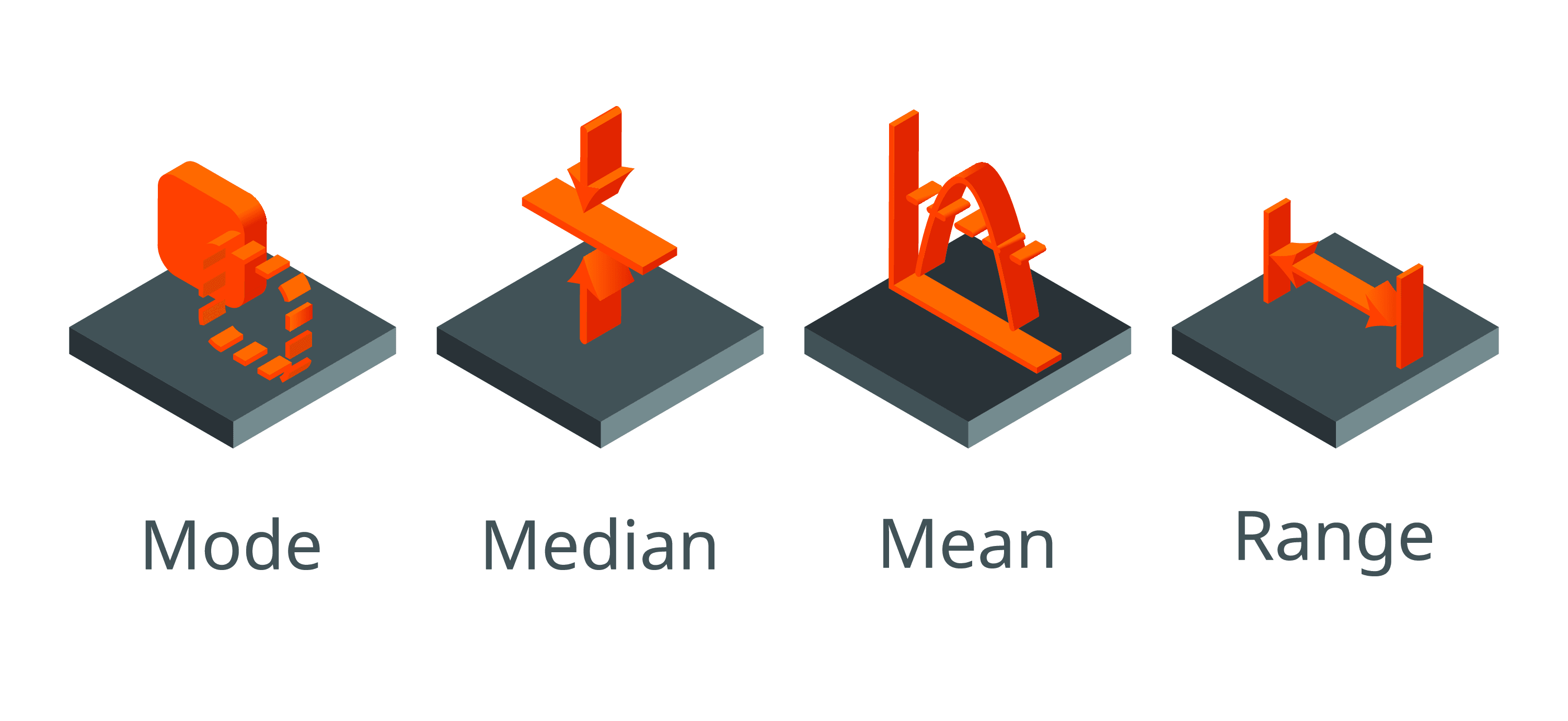
A Brief Introduction to Statistical Averages
This tutorial provides an introduction to statistical averages (mean, median and mode) for beginners to the topic.
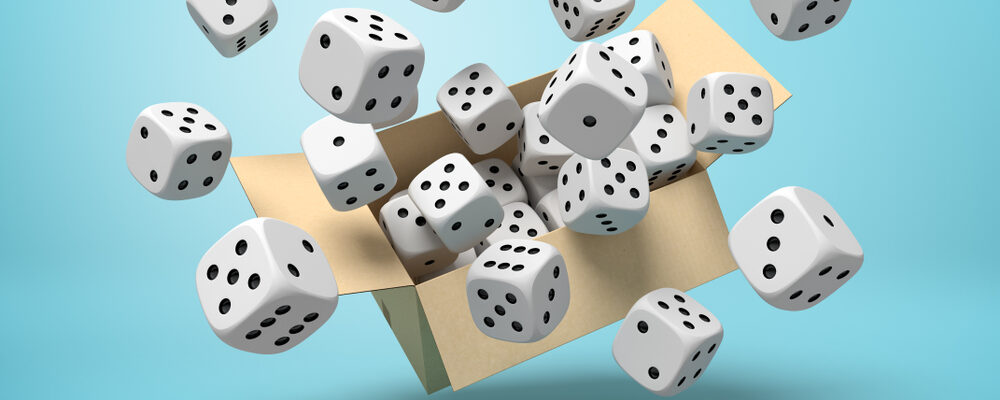
An Introduction to Probability
A tutorial for understanding and calculating probability. We go back to basics for beginners or for those just wanting a refresher.
Learn Math and Stats with Dr. G
A shortcut is the longest distance between two points.
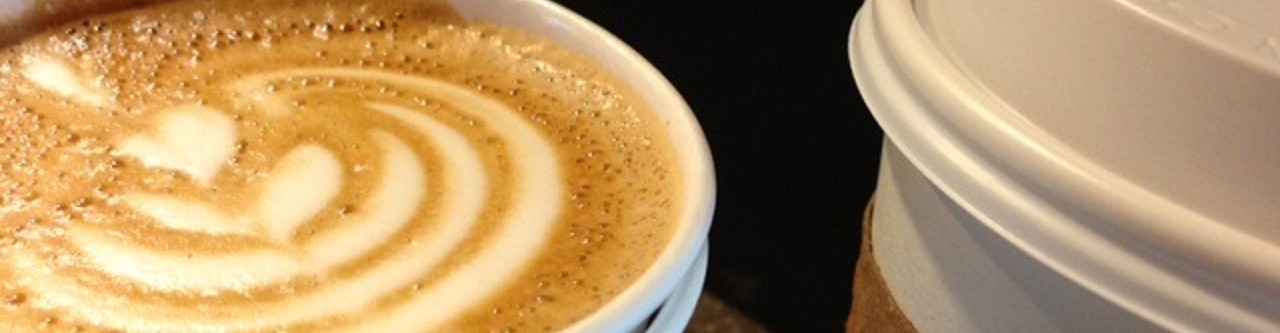
One Way ANOVA By Hand
ANOVA Testing Example
Excel Example for this ANOVA
See a HOW TO Video of this Example
A research study compared the ounces of coffee consumed daily between three groups. Group1 was Italians, Group 2 French, and Group 3 American. Determine if there is a significant difference among the groups using a 5% level (alpha is .05).
Group1: Italian
Group 2: French
Group 3: American
The Results of this study are in the following table:
Note that here in this example:
“n” is the sample size for each group
“M” is the “sample mean” for each group
“s^2” is the sample variance for each group
N = n1 + n2 + n3 = 70+ 70+70 = 210
NOTE that “N” is the combined sample size for all three groups.
Step 1: The null and alternative hypothesis Ho and Ha
Ho (the null) will represent that all the groups are statistically the same.
Ho: mean group 1 = mean group 2 = mean group 3
The Ha (alternative or research) hypothesis will represent that at least one of the groups is statistically significantly different.
Ha: mean group 1 ≠ mean group 2 ≠ mean group 3
Step 2: Determine the “degrees of freedom” also called df for each group and for the combination of groups:
The df BETWEEN is calculated by subtracting 1 from the number of groups you have. We have three groups here.
df BETWEEN = 3 – 1 = 2 (Used as the numerator or top df)
Next, the df WITHIN is calculated by first determining the individual df for each group and then adding them together:
df group1 = 70 – 1 = 69
df group 2 = 70 – 1 = 69
df group 3 = 70 – 1 = 69
df WITHIN = 69 + 69 + 69 = 207 (Used as the denominator or bottom df)
Step 3: Use the F-Table or a technology to get the “cut-off” values for this F-test ANOVA. Remember that all hypothesis tests have cut-off values that you use to determine if your F-test result is in the rejection region or not.
NOTE: The F-Table cannot contain all possible values. So most F Tables contain some values and you will choose the closet value to your df numbers.
IMPORTANT: Because of this, different textbooks or examples will have slightly different cut-off values for their F-tests. In this case, I am using the table below and my cut-off value is 3.07. However, a similar example might have a cut-off of 3.09 for example.
To get a more exact cut-off, use Excel to run the ANOVA. Excel will generate the p values for you.
Excel Example of ANOVA
Therefore, our cut-off value for the F-test is 3.07 here.
Step 4: Run the F-test to determine the F values. Then compare the F test value results to the cut-off values.
Running an F-test by hand has a few steps.
First Step:
Calculate the grand mean (GM) = (4.0 + 3.7 + 3.4) / 3 = 3.7
This grand mean is the sum of all of your individual means, divided by the total number of your groups. We have three groups. So we add the three group means together and divide by 3.
Second Step
Calculate the variance of the means. NOTE that 3.7 is the grand mean. Note that 4.0, 3.7, and 3.4 are individual group means. Note that “2” is the df BETWEEN.
s^2M = ( [ 4.0 – 3.7 ]^2 + [3.7 – 3.7 ]^2 + [3.4 – 3.7]^2 ) / dfBETWEEN
= ( [ 4.0 – 3.7 ]^2 + [3.7 – 3.7 ]^2 + [3.4 – 3.7]^2 ) / 2
= ( [ .3 ]^2 + [0 ]^2 + [-.3]^2 ) / 2
= ( .09 + 0 + .09 ) / 2
What did we do here?
The s^M is computed by subtracting the grand mean in this problem of 3.7 that we calculated in the first step from each individual group mean. Then, we squared each difference. Then, we added those results. Then, we divided by the dfBETWEEN which is 2 in this example.
Do not let formulas scare you.
Next, we can calculate the variance between the groups or s^2BETWEEN
s^2 BETWEEN = s^2M*n = .09 * 70 = 6.3
Remember, the s^2M was just calculated in the second step. The “n” is the size of each group sample. If your groups are different sizes, use the average size.
Fourth Step
Now we will calculate the WITHIN variance called s^2WITHIN
s^2WITHIN = (4.4 + 5.2 + 6.1)/3 = 5.233
Where did these numbers come from?
Here, these are the three individual variances of each group. See the table at the start of the example. You will see that Group 1 has a variance (s^2) of 4.4 and group 2 has a variance or 5.2, and group 3 has a variance of 6.1. To get the average of the three group variances, we add them together and divide by 3. This is what we did here in the fourth step.
Now we are ready to calculate F because we have calculated all the parts that we need for F.
F = s^2BETWEEN / s^2WITHIN
F = 6.3/5.233 = 1.20
The s^2 BETWEEN is what we calculated in the third step above and the s^2WITHIN is what we calculated in the fourth step above.
The F test is the between variance divided by the within variance.
Our F-test result here is 1.20
Step 5: Determine the final result and conclusion for your ANOVA F-test
The F-test is 1.20.
The cut-off value is 3.07
We can reject Ho only when our test value (1.20 in this case) is LARGER THAN our cut-off value (3.07 in this case).
However, in our example here, the F-test < cut-off
1.20 < 3.07
Therefore we CANNOT reject Ho
In conclusion, there is NOT a significant difference between our three groups.
We can say there is not enough evidence to conclude that people from the three groups of Italian, French, and American drink a sig diff amount of coffee per day. Therefore, statistically, we say that the amount of coffee consumed by these groups is NOT sig diff.
NOTE: These results are made up and are pretend. This is not a real test, nor a real conclusion. This is pretend and only for example purposes.
What is a statistical test?
A statistical test is a way to evaluate the evidence the data provides against a hypothesis. This hypothesis is called the null hypothesis and is often referred to as H0 . Under H0, data are generated by random processes. In other words, the controlled processes (the experimental manipulations for example) do not affect the data. Usually, H0 is a statement of equality (equality between averages or between variances or between a correlation coefficient and zero, for example).
H0 is usually opposed to a hypothesis called the alternative hypothesis , referred to as H1 or Ha . Most of the time, the alternative hypothesis is the one the user would like to demonstrate. It involves a statement of difference (difference between averages for example).
If the data does not provide enough evidence against H0, H0 is not rejected. If instead, the data shows strong evidence against H0, H0 is rejected and Ha is considered as true with a quantified (low) risk of being wrong. A statistical test allows to reject / not to reject the H0 hypothesis. Let’s have a look at an example ! Suppose you're comparing two varieties of apples and you're wondering whether the average size of apples from variety 1 differs from the average size of apples from variety 2. Here's how we would write down the null and alternative hypotheses:
H0: average size of apple from variety 1 = average size of apple from variety 2.
Ha: average size of apple from variety 1 ≠ average size of apple from variety 2.

Other examples of null hypotheses versus alternative challenging hypotheses
H0: the insulin rate of the group of patients receiving a placebo is equal to the insulin rate of patients receiving a medication.
Ha: the insulin rate of the group of patients receiving a placebo is different from the insulin rate of patients receiving a medication.
H0: the presence of attribute A does not affect consumer preference toward this product.
Ha: the presence of attribute A affects consumer preference toward this product.
H0: there is no trend in this time series.
Ha: there is a trend in this time series.
H0: Corn fields submitted to fertilizers A, B, C or D produce equivalent yields.
Ha: at least one fertilizer induces a difference in corn yield.
How to interpret the output of a statistical test: the significance level alpha and the p-value
When setting up a study, a risk threshold above which H0 should not be rejected must be specified. This threshold is referred to as the significance level alpha and should lay between 0 and 1. Low alpha’s are more conservative. The choice of alpha should depend on how dangerous it is to reject H0 while it is true. For example, in a study aiming at demonstrating the benefits of a medical treatment, alpha should be low. On the other hand, when screening the effects of many attributes on the appreciation of a product, alpha’s could be more moderate. Very often, alpha is set at 0.05 or 0.01 or 0.001.
The statistical test produces a number called p-value (that is also bounded between 0 and 1). The p-value is the probability of obtaining the data or more extreme data under the null hypothesis.
More practically, the p-value should be compared to alpha:
If p-value < alpha , we reject H0 and accept Ha with a risk proportional to p-value of being wrong.
If p-value > alpha , we do not reject H0, but this does not necessarily imply that we should accept it. It either means that H0 is true, or that H0 is false but our experiment and statistical test were not “strong” enough to lead to a p-value lower than alpha.
What is statistical power and in what case can we accept H0?
Statistically speaking, the ability of an experiment/a test to lead to a rejection of the null hypothesis is called statistical power . The power of an experiment increases with alpha, with the precision of the measurements and with the number of repetitions. Power also changes according to the type of statistical tests being used (see the last section of this tutorial). Power may be computed before or after an experiment. It equals 1 minus the risk of being wrong when accepting H0 (also called risk beta). So the higher the power, the lower the What is the difference between a parametric and a nonparametric test? risk of being wrong when accepting H0 (when p-value > alpha, of course).
In summary, if p > alpha AND if statistical power is high enough (usually higher than 0.95), then we may accept H0 with a risk proportional to (1 – Power) of being wrong.
What are the kinds of statistical tests?
A statistical test can be:
Parametric or nonparametric
two-tailed or one-tailed
Paired or independant samples
How do I know what statistical test to use?
Here is a grid which will help you choose an appropriate test according to your question.[
How can I run a statistical test in XLSTAT?
Was this article useful?
Similar articles
- Free Case Studies and White Papers
- How to interpret goodness of fit statistics in regression analysis?
- Webinar XLSTAT: Sensory data analysis - Part 1 - Evaluating differences between products
- Which statistical model should you choose?
- Comparison of Supervised Machine Learning Algorithms
- Which clustering method should you choose?
Expert Software for Better Insights, Research, and Outcomes
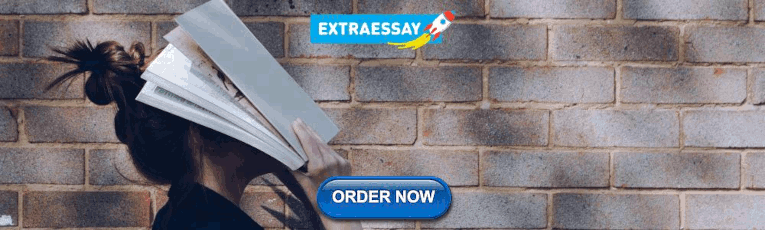
IMAGES
VIDEO
COMMENTS
Learn how to write null and alternative hypotheses for different statistical tests. The null hypothesis claims there's no effect in the population, while the alternative hypothesis claims there is an effect.
There are 5 main steps in hypothesis testing: State your research hypothesis as a null hypothesis and alternate hypothesis (H o) and (H a or H 1). Collect data in a way designed to test the hypothesis. Perform an appropriate statistical test. Decide whether to reject or fail to reject your null hypothesis. Present the findings in your results ...
H 0 (Null Hypothesis): Population parameter =, ≤, ≥ some value. H A (Alternative Hypothesis): Population parameter <, >, ≠ some value. Note that the null hypothesis always contains the equal sign. We interpret the hypotheses as follows: Null hypothesis: The sample data provides no evidence to support some claim being made by an individual.
Learn how to conduct hypothesis testing, a scientific method to make decisions and draw conclusions based on sample data. See examples of null and alternative hypotheses, one-sided and two-sided tests, and significance levels.
Learn how to formulate and test null and alternative hypotheses in statistics. See examples of different types of hypotheses and symbols, and how to evaluate sample data to make a decision.
Learn how to formulate and test hypotheses about population parameters using statistical methods. See examples of null and alternative hypotheses, type I and type II errors, and test statistics.
The actual test begins by considering two hypotheses.They are called the null hypothesis and the alternative hypothesis.These hypotheses contain opposing viewpoints. \(H_0\): The null hypothesis: It is a statement of no difference between the variables—they are not related. This can often be considered the status quo and as a result if you cannot accept the null it requires some action.
Hypothesis Testing Step 1: State the Hypotheses. In all three examples, our aim is to decide between two opposing points of view, Claim 1 and Claim 2. In hypothesis testing, Claim 1 is called the null hypothesis (denoted " Ho "), and Claim 2 plays the role of the alternative hypothesis (denoted " Ha ").
The alternative hypothesis, Ha, usually represents what we want to check or what we suspect is really going on. 5 Step 1: Stating the hypotheses Ho and Ha. The null hypothesis has the form: H0: µ = µ 0 (where µ 0 is a specific number). The alternative hypothesis takes one of the following three forms (depending on the context): Ha : µ < µ ...
Research hypotheses should be clear and specific, yet also succinct. A hypothesis should also be testable. If we state a hypothesis that is impossible to test, it forecloses any further investigation. To the contrary, a hypothesis should be what directs and demands investigation. In addition, a hypothesis should be directional, when possible.
1. Always use the proper nomenclature when stating the Alternate Hypothesis. The Alternate should be in the form of "not equal to," "greater than," "less than," or "equal to some value of interest.". 2. Be sure that the Alternate statement reflects what you want to learn about the process characteristics.
HA (Alternative Hypothesis): μ ≠ 10 inches. This is an example of a two-tailed hypothesis test because the alternative hypothesis contains the not equal "≠" sign. The botanist believes that the new fertilizer will influence plant growth, but doesn't specify whether it will cause average growth to increase or decrease.
One of the most used methods to make statistical inference is the hypothesis testing: a hypothesis testing requires establishing a null hypothesis (Ho) that is assumed to be true and an alternative hypothesis (Ha) that contradicts the null. The alternative hypothesis represents what we would expect if the null hypothesis were false. Example:
The null hypothesis would be H0: µ ≥ 70 inches and the alternative hypothesis would be Ha: µ < 70 inches. A two-tailed hypothesis involves making an "equal to" or "not equal to" statement. For example, suppose we assume the mean height of a male in the U.S. is equal to 70 inches. The null hypothesis would be H0: µ = 70 inches and ...
There is a paired data (also called correlated data) t-test that compares two samples from data that is related (like pretest score and post test score). t -test = (sample mean - population mean)/ [stddev/sqrt (n)] The sample mean "x" is 4.6 oz. The "mean" is the population mean of 4 oz. The sample std dev is .22 oz. n = 25.
The general procedure for testing the null hypothesis is as follows: Suppose you perform a statistical test of the null hypothesis with α = .05 and obtain a p-value of p = .04, thereby rejecting the null hypothesis. This does not mean there is a 4% probability of the null hypothesis being true, i.e. P(H0) =.04.
Step 1: The null and alternative hypothesis Ho and Ha. Ho (the null) will represent that all the groups are statistically the same. Ho: mean group 1 = mean group 2 = mean group 3 . The Ha (alternative or research) hypothesis will represent that at least one of the groups is statistically significantly different.
Hypothesis tests and condence intervals The 95% condence interval for is the set of values, 0, such that the null hypothesis H 0: = 0 would not be rejected (by a two-sided test with = 5%). The 95% CI for is the set of plausible values of . If a value of is plausible, then as a null hypothesis, it would not be rejected.
H0 is usually opposed to a hypothesis called the alternative hypothesis, referred to as H1 or Ha. Most of the time, the alternative hypothesis is the one the user would like to demonstrate. It involves a statement of difference (difference between averages for example). If the data does not provide enough evidence against H0, H0 is not rejected.
H0 H 0 : p>= 0.3 p>= 0.3; Ha H a : p <0.3 p <0.3. With the rationale that H0 must include the equality, which in this case is greater or equal to 30%. Her solution than failed to reject the null hypothesis and concluded that the researcher's claim is therefore correct. To me, this seems like assuming the claim to be true and giving it the ...