- DOI: 10.1080/0969594X.2023.2182737
- Corpus ID: 257242352
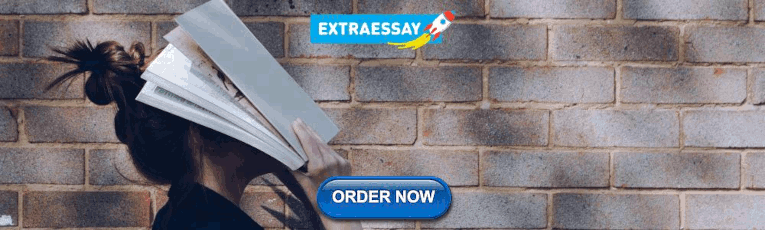
Data literacy assessments: a systematic literature review
- Yingqi Cui , Fu Chen , +2 authors M. Cutumisu
- Published in Assessment in Education… 2 January 2023
- Education, Computer Science
- Assessment in Education: Principles, Policy & Practice
13 Citations
Development and validation of a data literacy assessment scale, a strategic approach to information literacy: data literacy. a systematic review, how professional learning networks can support teachers’ data literacy: in conversation with experts, datalivr: transformation of data literacy education through virtual reality with chatgpt-powered enhancements, health data visualization literacy skills of young adults with down syndrome and the barriers to inference-making, the connections between citizenship education and mathematics education, the effectiveness of virtual laboratory integrated blacksmith ethnophysics in enhancing data literacy and graphic representation skills, feedback practices and transparency in data analysis.
- Highly Influenced
Pedagogical framework for cultivating children's data agency and creative abilities in the age of AI
Assessing information, media and data literacy in academic libraries: approaches and challenges in the research literature on the topic, 68 references, data literacy training and use for educational professionals, exploring the challenge of developing student teacher data literacy, incorporating data literacy into information literacy programs: core competencies and contents, data literacy assessment instrument for preparing 21 cs literacy: preliminary study.
- Highly Influential
A classroom data literacy intervention for pre-service teachers
The effects of a data use intervention on educators’ satisfaction and data literacy, centering complexity in ‘educators’ data literacy’ to support future practices in faculty development: a systematic review of the literature, a continuum of data literacy for teaching, data literacy: in search of a name and identity, a systemic view of implementing data literacy in educator preparation, related papers.
Showing 1 through 3 of 0 Related Papers
Dimensions of teachers’ data literacy: A systematic review of literature from 1990 to 2021
- Open access
- Published: 06 May 2024
- Volume 36 , pages 145–200, ( 2024 )
Cite this article
You have full access to this open access article
- Jihyun Lee ORCID: orcid.org/0000-0001-5896-0686 1 ,
- Dennis Alonzo 1 ,
- Kim Beswick 1 ,
- Jan Michael Vincent Abril 1 ,
- Adrian W. Chew 1 &
- Cherry Zin Oo 2
3000 Accesses
2 Citations
2 Altmetric
Explore all metrics
The current study presents a systematic review of teachers’ data literacy, arising from a synthesis of 83 empirical studies published between 1990 to 2021. Our review identified 95 distinct indicators across five dimensions: (a) knowledge about data, (b) skills in using data, (c) dispositions towards data use, (d) data application for various purposes, and (e) data-related behaviors. Our findings indicate that teachers' data literacy goes beyond addressing the needs of supporting student learning and includes elements such as teacher reflection, collaboration, communication, and participation in professional development. Considering these findings, future policies should acknowledge the significance of teacher dispositions and behaviors in relation to data, recognizing that they are as important as knowledge and skills acquisition. Additionally, prioritizing the provision of system-level support to foster teacher collaboration within in-school professional development programs may prove useful in enhancing teachers’ data literacy.
Similar content being viewed by others
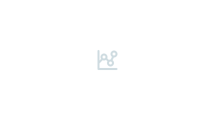
How Teachers Use Data: Description and Differences Across PreK Through Third Grade
Teachers’ data literacy for learning analytics: a central predictor for digital data use in upper secondary schools, teacher capacity for and beliefs about data-driven decision making: a literature review of international research.
Avoid common mistakes on your manuscript.
1 Introduction
In recent years, there has been a growing recognition of the importance of teachers’ data literacy for educational policy, research, and practice. This trend was ignited in 2009 when Arne Duncan, the former Secretary of Education of the United States, advocated evidence-driven practices in schools to enhance student performance (Mandinach & Gummer, 2016 ). Since then, there has been an increasing expectation for teachers to engage in data-informed practices to guide teaching and decision-making in schools. Following this trend, educational researchers have also increasingly directed their attention towards offering conceptual and theoretical foundations for teachers’ data literacy.
Various organizations and researchers have provided the definitions of teachers’ data literacy. For example, drawing on the opinions of diverse stakeholder groups, Data Quality Campaign ( 2014 ) defined teachers’ data literacy as teachers’ capabilities to “continuously, effectively, and ethically access, interpret, act on, and communicate multiple types of data from state, local, classroom, and other sources to improve outcomes for students in a manner appropriate to educators' professional roles and responsibilities” (p. 1). Kippers et al. ( 2018 ) defined teachers’ data literacy as “educators’ ability to set a purpose, collect, analyze, and interpret data and take instructional action” (p. 21). Similarly, teachers’ data literacy has been defined as “one’s ability, or the broad knowledge and skills, needed to engage in data use or implement a data use inquiry process (Abrams et al., 2021 , p. 100,868).
The data literacy for teachers (DLFT) framework proposed by Mandinach and Gummer defined teachers’ data literacy as “… the ability to transform information into actionable instructional knowledge and practices by collecting, analyzing, and interpreting all types of data to help determine instructional steps” (Gummer & Mandinach, 2015 , p. 2). In recent years, much of the research efforts to provide a theoretical framework on teachers’ data literacy has been led by Mandinach and Gummer (Gummer & Mandinach, 2015 ; Mandinach & Gummer, 2012 , 2013a , 2016 ; Mandinach et al., 2015 ). As far as we can ascertain, their work presents the most comprehensive framework of teachers’ data literacy in the current literature. The primary sources of Mandinach and Gummer’s DLFT framework were their previous works, Mandinach and Gummer ( 2012 ) and Mandinach et al. ( 2015 ). Their DLFT framework was developed as the results of the analysis of the teacher licensure documents across the US states (Mandinach et al., 2015 ) and the text analysis of the perspectives and definitions provided by 55 researchers and professional development providers during a braining storming at the conference held in 2012 (cf. Mandinach & Gummer, 2012 ). There are five components in the framework: (a) identifying problems and framing questions, (b) using data, (c) transforming data into information, (d) transforming information into decisions, and (e) evaluating outcomes. Their framework aimed to identify “the specific knowledge, skills, and dispositions teachers need to use data effectively and responsibly” (Mandinach & Gummer, 2016 , p. 366). However, a potential sixth dimension, “dispositions, habits of mind, or factors that influence data use” (Mandinach & Gummer, 2016 , p. 372) was mentioned but not included in the framework.
2 The present study
In the present study, we conducted a systematic review of the empirical studies on teachers’ data literacy and data use published in academic journals between 1990 and 2021. Our primary purpose was to enhance the conceptual clarity of teachers’ data literacy by providing its updated definition, indicators, and dimensions.
We argue that there are several reasons to justify the need for this systematic review. Firstly, we update, complement, and compare our review outcomes and the DLFT framework in Mandinach and Gummer ( 2016 ). A systematic review of research studies on teachers’ data use was conducted by Mandinach and Gummer ( 2013b ), but the study selection was limited to years between 2001 and 2009. Therefore, one of the aims of the present study is to compare our systematic review outcomes against the dimensions and specific indicators identified in the DLFT framework (Mandinach & Gummer, 2016 ). The present literature search spans a period from 1990 to 2021. We have set 1990 as the lower-boundary year because “during the 1990s, a new hypothesis – that the quality of teaching would provide a high-leverage policy target – began to gain currency” (Darling-Hammond et al., 2003 , p. 5).
Secondly, it appears that much work on teachers’ data literacy, including that of Mandinach and Gummer, has tended to focus on teachers’ data use in relation to teaching (e.g., Beck et al., 2020 ; Datnow et al., 2012 ) and instructional improvement (e.g., Datnow et al., 2021 ; Kerr et al., 2006 ; Wachen et al., 2018 ) or in relation to student academic performance (e.g., Poortman & Schildkamp, 2016 ; Staman et al., 2017 ). However, we argue that classroom teachers’ tasks and responsibilities go beyond teaching itself and include many other tasks such as advising/counselling, organising excursions, and administrative work (e.g., Albiladi et al., 2020 ; Kallemeyn, 2014 ). Our review, therefore, examines how teachers’ data use practices may be manifested across a range of teacher responsibilities beyond teaching and teaching-related tasks.
Thirdly, there has been a relative lack of attention to teachers’ personal dispositions in data literacy research. Dispositions refer to a person's inherent tendencies, attitudes, approaches, and inclinations towards ways of thinking, behaving, and believing (Lee & Stankov, 2018 ; Mischel & Shoda, 1995 ). According to Katz ( 1993 ), a disposition can be defined as “a tendency to exhibit frequently, consciously, and voluntarily a pattern of behavior that is directed to a broad goal” (p. 2). In the context of education, disposition refers to the attitudes, beliefs, and values that influence a teacher’s actions, decision-making, and interactions with various stakeholders including students, colleagues, and school leaders (Darling-Hammond et al., 2003 ). While teachers’ dispositions were mentioned in Mandinach and Gummer ( 2016 ), dispositions were not included in their DLFT framework. Teacher educators have long emphasized that accomplished teachers need to possess extensive knowledge, skills, and a range of dispositions to support the learning of all students in the classroom, engage in on-going professional development, and continuously strive to enhance their own learning throughout their careers (Darling-Hammond et al., 2003 ; Sykes, 1999 ). Therefore, we aim to identify a range of teachers’ dispositions in relation to data literacy and data use in the school contexts.
Fourthly, we argue that teachers’ data literacy may be more important in the current context of the rapidly evolving data and digital landscape influenced by the technical advancements in artificial intelligence. Teachers may encounter significant challenges in comprehending and addressing a wide array of issues, both anticipated and unforeseen, as well as observed and unobserved situations, stemming from various artificial intelligence tools and automated machines. In this sense, comprehending the nature, types, and functions of data is crucial for teachers. Without such understanding, the educational community and teaching workforce may soon find themselves in an increasingly worrisome situation when it comes to evaluating data and information.
Finally, we argue that there is a need to update conceptual clarity regarding teachers’ data literacy in the current literature. Several systematic review studies have focused on features in professional development interventions (PDIs) aimed at improving teachers’ data use in schools (e.g., Ansyari et al., 2020 ; 2022 ; Espin et al., 2021 ), emphasizing the need to understand data literacy as a continuum spanning from pre-service to in-service teachers and from novice to veteran educators (Beck & Nunnaley, 2021 ). Other systematic review studies have given substantial attention to data-based decision-making (DBDM) in the schools (e.g., Espin et al., 2021 ; Filderman et al., 2018 ; Gesel et al., 2021 ; Hoogland et al., 2016 ). For example, Hoogland et al. ( 2016 ) investigated the prerequisites for data-based decision-making (DBDM) in the classroom, highlighting nine themes that influence DBDM, such as collaboration, leadership, culture, time, and resources. These systematic reviews are highly relevant to the current review, as the PDIs, understanding the continuum, or data-based decision-making would require a clear and updated understanding of what teachers’ data literacy should be. We hope that the current study’s definition, indicators, and dimensions of teachers’ data literacy may be useful in conjunction with other systematic review studies on teachers’ data use and factors influencing teachers’ data use.
3.1 Data sources and selection of the studies
Our strategies for literature search were based on the guidelines of the Preferred Reporting Items for Systematic Reviews and Meta-Analyses (PRISMA), a framework for reporting and synthesising literature review (Moher et al., 2009 ). In accordance with PRISMA suggestions, we followed the four steps in locating and reviewing the relevant studies. First, we conducted initial searches to identify relevant studies, using three databases: Scopus, ProQuest, and Web of Science. Keywords in our search were teacher, school, data, data use, data literacy, evidence-based, and decision-making (see Table 1 for the detailed search strategy syntax). This initial search, using the combination of the identified keywords, yielded 2,414 journal articles (see Fig. 1 ). After removing duplicates, 1,976 articles remained.
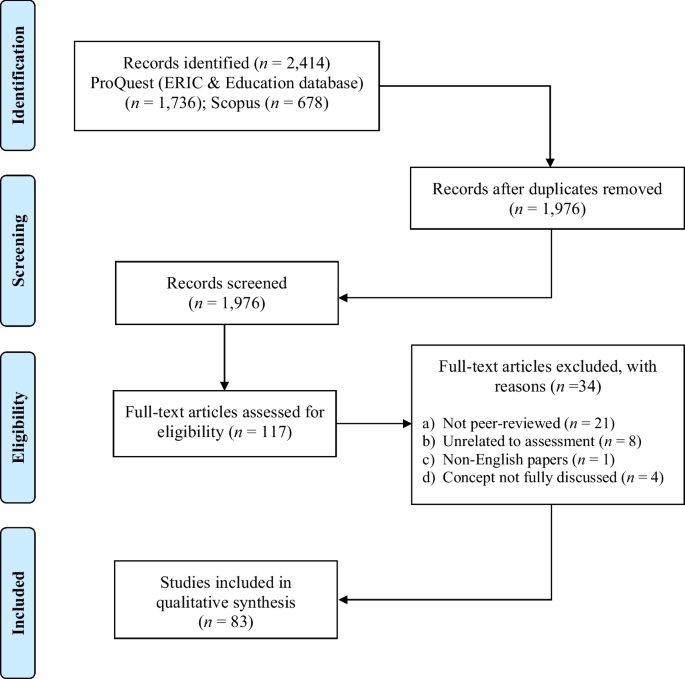
Study selection flow using PRISMA guidelines
Secondly, we set and applied the inclusion criteria to screen the studies. The inclusion criteria were: (a) topics relating to the key words, (b) school context of primary or secondary school settings (i.e., excluding studies focusing on university, vocational education, and adult learning), (c) the full text written in English (excluding studies if the full text is presented in another language or if only the abstract was presented in English), (d) peer-reviewed empirical studies (across quantitative, qualitative, and mixed-methods) published in academic journals (excluding book chapters, conference papers, thesis) to ensure the inclusion of the published work that has undergone peer-review process, and finally, (e) published studies from 1990 onwards. The titles and abstracts of the studies were reviewed to assess their eligibility based on the inclusion criteria. As a result of applying these criteria, 117 articles were selected for the next step, full-text review.
Thirdly, we evaluated the eligibility of the full-text versions of the published studies. This full-text review resulted in a further exclusion of 34 studies as they were found to not meet all the inclusion criteria. We also examined whether the studies included data literacy or data-driven decision-making. Following these assessments, we identified 83 articles that met all the inclusion criteria.
Finally, we reviewed, coded, and analyzed the final set of the selected studies. The analysis approaches are described below.
3.2 Approach to analysis
We employed a thematic synthesis methodology, following the framework outlined by Thomas & Harden ( 2008 ). The coding and analysis process consisted of three main stages: (a) conducting a line-by-line reading and coding of the text, (b) identifying specific descriptive codes, and (c) generating analytical themes by grouping conceptually inter-related descriptive codes. The final analytic process was, therefore, categorizing and naming related descriptive codes to produce analytical themes. During the development of the analytic themes, we utilized an inductive approach, organizing conceptually interconnected codes into broader themes.
The first author developed the descriptive and analytical themes, which were then reviewed by another two authors. To ensure coding rigor and consistency, three authors independently coded the same two articles, and then compared the coding to address any inconsistencies and reach a consensus. This process was repeated in four iterations. Once the three authors who were involved in the initial coding reached the consensus, the remaining authors double-checked the final outputs of the thematic analysis (i.e., codes, and themes). We have labelled descriptive codes as ‘indicators’ of teachers' data literacy, while the broader groups of descriptive codes, referred to as analytic themes, represent ‘dimensions’ of teachers’ data literacy.
4.1 Characteristics of the reviewed studies
The main purpose of the present study was to examine the conceptualization of teachers’ data literacy from 83 peer-reviewed empirical studies. Table 2 presents the studies included in our systematic review, along with the summary of the study characteristics such as country, school-level, study focus (i.e., main constructs), study purposes/objectives, research method, data collection tools, and sample size. Figure 2 presents the number of the reviewed studies by publication year. We found that since 2015, there has been an increase in the number of published empirical studies on teachers' data literacy.
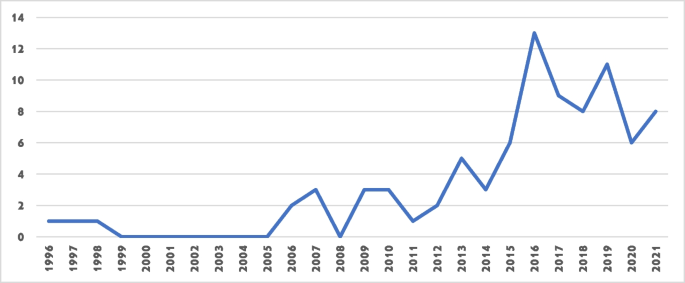
Number of the reviewed studies by publication year
Out of 83 studies, 50 were conducted in the United States. Thirteen studies were from Netherlands, four from Belgium, three from Australia, two from each of Canada and the United Kingdom, and one study for each of the following ten countries: China, Denmark, Germany, Indonesia, Ireland, Kenya, Korea, Norway, South Africa, and Sweden. Therefore, more than half of the studies (i.e., 58 studies, 70%) were conducted in the English-speaking countries. In terms of school-settings, studies were mostly conducted in primary school settings or in combination with high school: 36 studies in primary school settings, 16 in secondary school settings, and 30 studies were in both primary and secondary school settings. The most common design was qualitative ( n = 35 studies), followed by mixed methods ( n = 30) and quantitative ( n = 18). Multiple sources of data collection (e.g., interview and survey) were used in 22 studies. The most commonly used data collection tool was interview ( n = 55), which was followed by surveys ( n = 37) and observation ( n = 25). A smaller set of studies used focus group discussion ( n = 18) and document analysis ( n = 19). A few studies used students’ standardised assessment data ( n = 4), field notes ( n = 4), and teacher performance on data literacy test ( n = 4).
We also reviewed the study topics and found that there are seven foci among the reviewed studies: (a) factors influencing teachers’ data use ( n = 29), (b) specific practices in teachers’ data use ( n = 27), (c) teachers’ data use to enhance teaching practices ( n = 25), (d) teachers’ data use for various purposes ( n = 24), (e) approaches to improve teachers’ data literacy ( n = 22), (f) approaches to improve teachers’ assessment literacy ( n = 19), and (g) teachers’ data use to improve student learning outcomes ( n = 19).
4.2 Dimensions and indicators of teaches’ data literacy
Our thematic analysis identified 95 descriptive codes (see Table 3 ). Careful review of the identified descriptive codes suggested that they can be viewed as indicators of teachers’ knowledge, attitudes, behaviors, and dispositions in data use. These indicators were further organized into inter-related concepts, which formed analytic themes; we refer to these as ‘dimensions’ (see Table 3 ). There were five broad dimensions that emerged from the indicators: knowledge about data (Dimension 1), skills in using data (Dimension 2), dispositions towards data use (Dimension 3), data application for various purposes (Dimension 4), and data-related behaviors (Dimension 5).
It is necessary to point out that Dimension 1 pertains to understanding the nature of data itself, focusing on knowledge about data. On the other hand, Dimension 2 revolves around data-related skills in the actual use of data , encompassing a spectrum of sequences including data generation, processing, and production. These two dimensions, i.e., knowledge and skills, are highly interconnected and complement each other. Proficiency in data-use skills (Dimension 2) may not be developed without a solid understanding of how data can be utilised, for instance, in teaching practices or school improvement in data use (Dimension 1). Conversely, teachers' understanding of how data can enhance teaching practices (Dimension 1) can guide them in determining specific approaches to analysing particular datasets (Dimension 2). While we acknowledge the complementary nature of knowledge and skills, it is important to note that certain aspects of knowledge and skills may not completely overlap. For instance, a teacher who understands the process of creating state-level assessment data may not necessarily possess the technical expertise required to analyze state-level data, taking into account measurement errors. Therefore, we maintain knowledge and skills as two distinct dimensions to highlight both as the core components of teachers’ data literacy.
Within each of the five broad dimensions, we also uncovered sub-themes to illuminate the constituent elements of those dimensions. Under Dimension 1, four sub-themes emerged: “knowledge about data”, knowledge about data for “teaching practices”, understanding “data culture in the school”, and understanding the use of “external assessment”. Dimension 2 featured sub-themes highlighting the sequential stages of data utilization: “data generation & collection”, “data analysis”, “data interpretation”, “data integration”, “evaluation”, and “reporting”. Within Dimension 3, we identified dispositions towards data use, encompassing sub-themes such as confidence, values/beliefs, trust/respect, and anxiety. Dimension 4 revealed various purposes of data applications, categorized into three sub-themes: “teaching,” “student learning,” and “school improvement.” Lastly, Dimension 5 delineated teachers’ behaviors related to data into two sub-themes: “communication & discussion” and “participation & engagement.”
In the following passages we provide detailed descriptions of the indicators and their associated dimensions. Figure 3 presents a visual a summary of these indicators and dimensions.
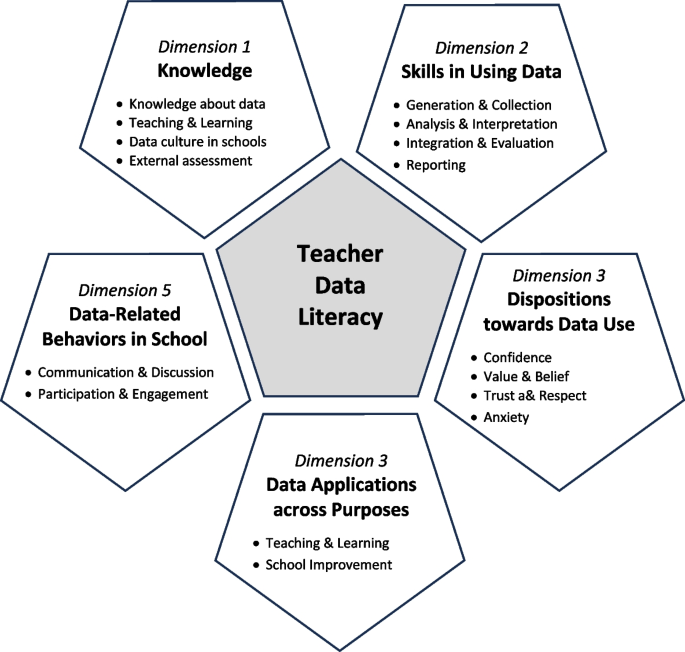
A summary of the dimensions and indicators of teachers’ data literacy
4.2.1 Dimension 1. Knowledge about data
The first dimension of teachers’ data literacy pertains to teachers’ knowledge about data . Many studies recognized the importance of data-related knowledge to be utilized in the schools (e.g., Jacobs et al., 2009 ; Omoso et al., 2019 ; Schildkamp et al., 2017 ). Our review revealed four major ways that teachers' data-related knowledge can be manifested. Firstly, teachers’ knowledge about data involves their understanding of the necessary steps in data analysis procedures (Ebbeler et al., 2016 ; Snodgrass Rangel et al., 2016 ; Vanlommel et al., 2021 ; Wardrip & Herman, 2018 ) and understanding of different data types to be used for varying purposes (Abdusyakur & Poortman, 2019 ; Beck et al., 2020 ; Howley et al., 2013 ; Reeves et al., 2016 ).
Secondly, teachers’ knowledge about data involves their capability to relate the insights gleaned from data to inform their teaching practices (Abrams et al., 2016 ; Jimerson et al., 2016 ). Specifically, data-literate teachers leverage student assessment data to evaluate learning progress (Abrams et al., 2016 ; Jimerson, 2014 ; Jimerson & Wayman, 2015 ; Jimerson et al., 2016 ; Snodgrass Rangel et al., 2016 ), to tailor classroom instruction based on data insights (Mokhtari et al., 2009 ; Poortman & Schildkamp, 2016 ; Staman et al., 2017 ; van der Scheer & Visscher, 2018 ), and to ensure alignment between instructional approaches and appropriate assessment methods (Howley et al., 2013 ; Marsh & Farrell, 2015 ; van der Scheer & Visscher, 2018 ).
Thirdly, teachers’ data literacy extends to understanding of the school culture surrounding data utilization (e.g., Andersen, 2020 ; Schildkamp, 2019 ; Wachen et al., 2018 ). This encompasses recognizing the conditions that may facilitate or hinder teachers’ data use (Abdusyakur & Poortman, 2019 ; Anderson et al., 2010 ; Keuning et al., 2017 ) and navigating various challenges associated with using assessment data in the school (Datnow et al., 2012 ; Ford, 2018 ; Kanjee & Moloi, 2014 ; Thomas & Huffman, 2011 ).
Lastly, teachers’ knowledge about data includes understanding of externally administered assessment data and data system, such as state-level assessment policies related to data use (Copp, 2017 ; Hardy, 2019 ; Reed, 2015 ) and understanding the broader state-level contexts that impact data utilization within the school (Datnow et al., 2013 ; Dunn et al., 2013a ; Ford, 2018 ; Omoso et al., 2019 ; Powell et al., 2021 ). Teachers may need to have thorough knowledge of educational government policies to ensure alignment between state-level curriculum initiatives and school-level assessment policies (Anderson et al., 2010 ; Copp, 2017 ; Gelderblom et al., 2016 ; Hardy, 2015 ).
In summary, existing literature highlights that data-literate teachers would have a comprehensive understanding of a diverse range of data sources and purposes, regularly reviewing and evaluating student outcomes from various channels. Consequently, if teachers face excessive pressure to meet accountability measures and improve standardized testing results, it could potentially hinder their overall development and growth in a broad spectrum of data-related knowledge.
4.2.2 Dimension 2. Skills in using data
Skills in using data is the second key dimension in teachers’ data literacy. There were a wide range of specific data-skills mentioned in the literature, spanning from data generation and collection (Farley-Ripple et al., 2019 ; Jimerson & Wayman, 2015 ) to data analysis (Farley-Ripple et al., 2019 ; Jimerson & Wayman, 2015 ; Marsh et al., 2010 ), data interpretation and integration (Jimerson & Wayman, 2015 ; Marsh et al., 2010 ), evaluation (Andersen, 2020 ; Dunn et al., 2013b ; Thomas & Huffman, 2011 ), and report writing (Farley-Ripple et al., 2019 ; Jimerson & Wayman, 2015 ). These indicators (see Table 3 ) emphasize that teachers’ data literacy requires proficiency across the entire sequence, across different stages of data generation, processing, and production.
Teachers’ skills in data use also involve selecting specific data types appropriate for different purposes (Anderson et al., 2010 ; Jimerson et al., 2016 ; Kanjee & Moloi, 2014 ), analysing multiple sources of data on student learning outcomes (Datnow et al., 2012 ; Vanlommel et al., 2021 ; von der Embse et al., 2021 ), and integrating multiple data sources to arrive at a holistic assessment of student progress (Brunner et al., 2005 ; Farley-Ripple et al., 2019 ; Ford, 2018 ; Jacobs et al., 2009 ; Mausethagen et al., 2018 ). For example, teachers may need to apply different data analytic approaches when evaluating student outcomes based on school-based versus externally administered standardized assessments (Copp, 2017 ; Curry et al., 2016 ; Omoso et al., 2019 ; Wardrip & Herman, 2018 ; Zeuch et al., 2017 ). Data-literate teachers may also plan data analysis for targeted purposes, such as analyzing students’ social-emotional outcomes (Abrams et al., 2021 ; Jimerson et al., 2021 ; von der Embse et al., 2021 ; Wardrip & Herman, 2018 ), identifying individual students’ learning needs, making recommendations for curriculum revisions, or evaluating pedagogical approaches (Dunn et al., 2013a ; Snodgrass Rangel et al., 2016 ; Wolff et al., 2019 ; Young, 2006 ).
In summary, this “skills” dimension highlights the importance of teachers possessing a diverse array of competencies to leverage data effectively. The literature reviewed identified various aspects of teachers’ data use, spanning the spectrum from data collection and generation to analysis, interpretation, integration across multiple sources, evaluation, and reporting.
4.2.3 Dimension 3. Dispositions towards data use
While somewhat overlooked in data literacy literature, teachers’ disposition is a crucial component of their data literacy. Our review identified four major types of such dispositions in the context of teachers’ data literacy (see Table 3 ). Firstly, studies have underscored that teachers’ confidence in using data may be necessary when making data-driven school-level decisions, for example, to design intervention programs (Andersen, 2020 ; Keuning et al., 2017 ; Staman et al., 2017 ; Thompson, 2012 ), or to develop strategic plans for school improvement (Dunn et al., 2013b ; Poortman & Schildkamp, 2016 ). Researchers also claimed that teachers may need to feel confident in many steps of data processes, across accessing, analyzing, interpreting, evaluating, and discussing data within the school environment (Abrams et al., 2021 ; Dunn et al., 2013a ; von der Embse et al., 2021 ).
The second disposition pertains to teachers valuing and believing in the importance of data use in schools. Data-literate teachers would recognize the usefulness of data in informing school improvement and enhancing student performance (Howley et al., 2013 ; Poortman & Schildkamp, 2016 ; Prenger & Schildkamp, 2018 ). They would also place value on collaboration among colleagues and actively seek institutional support for effective data use (Kallemeyn, 2014 ; Marsh & Farrell, 2015 ; Nicholson et al., 2017 ; Poortman & Schildkamp, 2016 ). Furthermore, they would appreciate the pivotal role of school leaders in supporting and promoting teachers’ data use within the school (Albiladi et al., 2020 ; Curry et al., 2016 ; Joo, 2020 ; Young, 2006 ).
A third type of teacher disposition that our review identified is trust in and respect towards colleagues and school leaders . Teachers often work collaboratively in the school environment when they learn about and utilise school-level data. In this sense, teacher collaboration and sustaining trusting relationships are fundamental in fostering a school culture that appreciates data-driven decision-making, as well as for encouraging teachers to further develop their own data knowledge and skills (Abrams et al., 2021 ; Andersen, 2020 ; Keuning et al., 2017 ). Mutual trust and respect among teachers can allow them to have open and honest conversations about their experiences and share any concerns arising from data use in the school context (Andersen, 2020 ; Datnow et al., 2013 ; Ford, 2018 ; Wachen et al., 2018 ).
Lastly, data anxiety may play a role when teachers use or are expected to use data in the school (Abrams et al., 2021 ; Dunn et al., 2013b ; Reeves et al., 2016 ). Teachers may experience data anxiety when they are expected to effectively analyze student assessment outcomes (Dunn et al., 2013b ; Powell et al., 2021 ), when they are introduced to new data management systems in the school, when they feel pressured to quickly grasp the school’s data management system (Andersen, 2020 ; Dunn et al., 2013a ), or when they are tasked with developing specific strategies to assess and enhance student learning outcomes (Dunn et al., 2013a , b ; Jimerson et al., 2019 ). These types of teacher responsibilities demand proficient data skills and knowledge, which not all teachers may possess, and thus, anxiety may hinder their ability to further develop their data literacy.
In summary, teacher dispositions towards data use can impact their effective utilization of data or impede the capacity to further develop their own data literacy. Our review also illuminated that it is not just individual teachers’ confidence or anxiety towards data use, but also the social dynamics within the school environment, including teacher collaboration, trust and respect, and relationships with the school management team, that can influence teachers’ data literacy. Therefore, fostering a collaborative climate within the school community and creating more opportunities for data use may strengthen a data-driven culture within the school.
4.2.4 Dimension 4. Data applications for various purposes
Our review suggests that teachers' data literacy can be manifested in their use of data for multiple purposes, primarily in three areas: (a) to enhance teaching practices (e.g., Datnow et al., 2012 , 2021 ; Farrell, 2015 ; Gelderblom et al., 2016 ; Wachen et al., 2018 ), (b) to support student learning (e.g., Joo, 2020 ; Lockton et al., 2020 ; Staman et al., 2017 ; Vanlommel et al., 2021 ; van der Scheer & Visscher, 2018 ), and (c) to make plans and strategies for school improvement (e.g., Abdusyakur & Poortman, 2019 ; Jimerson et al., 2021 ; Kallemeyn, 2014 ).
With respect to teaching enhancement purposes, teachers use data to inform their lesson plans (Ford, 2018 ; Gelderblom et al., 2016 ; Snodgrass Rangel et al., 2016 ; Reeves et al., 2016 ), set lesson objectives (Kallemeyn, 2014 ; Snodgrass Rangel et al., 2016 ; Reeves et al., 2016 ), develop differentiated instructions (Beck et al., 2020 ; Datnow et al., 2012 ; Farley-Ripple et al., 2019 ), and provide feedback to students (Gelderblom et al., 2016 ; Andersen, 2020 ; Jimerson et al., 2019 ; Marsh & Farrell, 2015 ). Furthermore, teachers use data to reflect on their own teaching practices (Datnow et al., 2021 ; Ford, 2018 ; Jimerson et al., 2019 ; Snodgrass Rangel et al., 2016 ) and evaluate the impact of using data on teaching and learning outcomes (Gelderblom et al., 2016 ; Marsh & Farrell, 2015 ).
In relation to supporting student learning, teachers use data to recognize individual students’ learning needs (Curry et al., 2016 ; Gelderblom et al., 2016 ), guide students to learning new or challenging concepts (Abrams et al., 2021 ; Keuning et al., 2017 ; Marsh et al., 2010 ; Reeves et al., 2016 ), set learning goals (Abdusyakur & Poortman, 2019 ; Curry et al., 2016 ), and monitor learning progress (Curry et al., 2016 ; Gelderblom et al., 2016 ; Marsh et al., 2010 ).
In terms of guiding school improvement strategies, teachers use data to develop school-based intervention programs (Abdusyakur & Poortman, 2019 ; Jimerson et al., 2021 ; Kallemeyn, 2014 ; Thompson, 2012 ), make decisions about school directions (Huffman & Kalnin, 2003 ; Prenger & Schildkamp, 2018 ; Schildkamp, 2019 ), and evaluate school performance for meeting the accountability requirements (Hardy, 2015 ; Jacobs et al., 2009 ; Jimerson & Wayman, 2015 ; Marsh et al., 2010 ; Omoso et al., 2019 ; Snodgrass Rangel et al., 2019 ).
In summary, the literature indicates that data-literate teachers use data for multiple purposes and consider it essential in fulfilling their various roles and responsibilities within the school. Teachers’ data use for supporting student learning tends to focus primarily on helping students achieve better learning outcomes; in contrast, teachers’ data use for teaching enhancement includes a broader range of data processes and practices.
4.2.5 Dimension 5. Data-related behavior
The fifth and final dimension we identified pertains to teachers' data-related behaviors within and outside the school context. Within this dimension, there appear to be two distinctive sets of teacher behaviors: (a) teachers’ data use to enhance communication and discussion with various stakeholders such as colleagues (Datnow et al., 2013 ; Van Gasse et al., 2017 ), school leaders (Jimerson, 2014 ; Marsh & Farrell, 2015 ; Nicholson et al., 2017 ), and parents (Jimerson & Wayman, 2015 ; Jimerson et al., 2019 ); and (b) teachers’ participation in and engagement with learning about data use (Schildkamp et al., 2019 ; Wardrip & Herman, 2018 ) and data culture in schools (Datnow et al., 2021 ; Keuning et al., 2016 ). These behaviors were found to be integral aspects of teachers' data literacy. Teacher engagement with data is manifested in multiple ways, such as involvement in team-based approaches to data utilization (Michaud, 2016 ; Schildkamp et al., 2017 ; Wardrip & Herman, 2018 ; Young, 2006 ), active participation in creating a school culture of data use (Abrams et al., 2021 ; Albiladi et al., 2020 ), evaluation of the organizational culture and conditions pertaining to data use (Andersen, 2020 ; Datnow et al., 2021 ; Lockton et al., 2020 ), and participation in professional development opportunities focused on data literacy (Ebbeler et al., 2016 ; O’Brien et al., 2022 ; Schildkamp et al., 2017 ).
In summary, this dimension highlights that teachers’ data literacy includes various forms of their active engagement and behavior to enhance the effective use and understanding of data. Our findings also indicate that teacher communication and discussions regarding data primarily focus on student assessment data with various stakeholder groups including colleagues, school leaders, and parents.
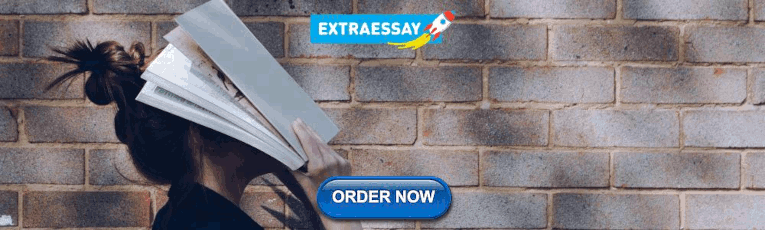
5 Discussion
The present study reviews 83 empirical studies on teachers' data literacy published in peer-reviewed journals from 1990 to 2021, and we identified 95 specific indicators categorized across five dimensions: (a) knowledge about data , (b) skills in using data , (c) dispositions towards data use , (d) data applications for various purposes , and (e) data-related behaviors in the school . Our review of the identified indicators of this study has led to the following definition of teachers’ data literacy:
A set of knowledge, skills, and dispositions that empower teachers to utilize data for various purposes, including generating, collecting, analyzing, interpreting, integrating, evaluating, reporting, and communicating, aimed at enhancing teaching, supporting student learning, engaging in school improvement, and fostering self-reflection. Teachers’ data literacy also involves the appreciation for working together with colleagues and school leaders to (a) assess organizational conditions for data use, (b) foster a supportive school culture, and (c) engage in ongoing learning to optimize the effective utilization of data.
Our analysis also revealed several noteworthy findings that are presented in the following sections.
5.1 Teachers’ data literacy and assessment literacy
There have been concerns expressed by scholars about conceptual fuzziness in teachers’ data literacy and assessment literacy (cf. Brookhart, 2011 ; Ebbeler et al., 2016 ; Mandinach, 2014 ; Mandinach & Gummer, 2016 ). Indeed, student assessment data are the most salient form of data in the school (Mandinach & Schildkamp, 2021 ). The research trend of recognising the importance of teachers’ data literacy is often based on the premise that teachers’ data literacy would enhance teaching and ultimately improve student outcomes (cf. Ebbeler et al., 2016 ; Mandinach & Gummer, 2016 ; Poortman & Schildkamp, 2016 ; Thompson, 2012 ; Van Gasse et al., 2018 ; Zhao et al., 2016 ). Furthermore, the systemic pressure on schools to meet accountability requirements has also impacted their endeavors to utilize, assess, and demonstrate school performance based on student assessment data in recent years (Abdusyakur & Poortman, 2019 ; Farrell, 2015 ; Schildkamp et al., 2017 ; Weiss, 2012 ). In these contexts, it is not surprising that educational practitioners would think about student assessment data when they are expected to improve their data skills.
In this light, we have tallied the teacher data literacy indicators that directly relate to student assessment or about students’ learning outcomes . In Table 3 , the symbol “⁑” is used for the indicators related to student assessment, and “ξ” is used for the indicators related to students’ learning outcomes. We found that there were only 19 out of 95 indicators that directly related to student assessment (e.g., knowledge about different purposes of assessment, understanding the alignment between instruction and assessment, understanding state-level assessment policies on data use). Similarly, there were only 13 out of 95 indicators that directly related to students’ learning outcomes (e.g., identifying evidence of student learning outcomes, understanding student learning outcomes using multiple sources).
Our review demonstrates that teachers regularly interact with a diverse array of data and undertake various tasks closely associated with its utilization. Therefore, teachers' data literacy encompasses more than just its use in student assessment and learning outcomes; it extends to understanding students’ social-emotional learning and higher-order thinking skills, assessing school conditions for data use, reflecting on teaching practices, and communicating with colleagues. Consequently, limiting the perspective of teachers’ data literacy solely to assessment literacy may impede their full utilization and appreciation of data applications essential to their multifaceted work in supporting and enhancing student and school outcomes.
5.2 Teachers’ data literacy and data-related dispositions
We found that one of the key aspects of teachers’ data literacy is teachers’ dispositions towards data use. As noted by Mandinach and Gummer ( 2012 , 2016 ), this aspect of teacher characteristics has not received as much research attention as data knowledge or data skills. It is perhaps due to ‘literacy’ being traditionally linked to knowledge and skills (Shavelson et al., 2005 ; also see Mandinach & Gummer, 2012 ) or due to the research trend of unpacking teachers’ needs and pedagogical approaches in specific subject/learning domains (Sykes, 1999 ; see Mandinach & Gummer, 2016 ). However, our review suggests that teacher dispositions towards data use are required in virtually all aspects of data use and data analyses processes. We also found that the most important data-related teacher disposition was confidence . The data literacy literature recognized the importance of teacher confidence, with respect to accessing, collecting, analysing, integrating, evaluating, discussing, and making decisions, suggesting that for teachers to be data literate, confidence may be required in every step of data use. There has been extensive research that has demonstrated a strong link between confidence and learning motivation, indicating that individuals tend to gravitate towards domains in which they feel comfortable and confident (e.g., Lee & Durksen, 2018 ; Lee & Stankov, 2018 ; Stankov & Lee, 2008 ). Our review findings contribute to this existing body of research, emphasizing the importance of confidence in teachers’ data utilization. This underscores the necessity for policies and professional development initiatives aimed at enhancing teachers’ data use to also prioritize strategies for building teachers’ confidence in this area.
Our findings also indicate that teachers’ data literacy is associated with their trust in colleagues and school leaders, as well as their respect for the leadership team's role in leading data use and school improvement (Andersen, 2020 ; Ford, 2018 ; Wachen et al., 2018 ). This suggests that for teachers to be effective data users, they need to feel empowered to voice concerns and express frustrations with colleagues (Andersen, 2020 ; Ford, 2018 ; Wachen et al., 2018 ), seek help when necessary (Wardrip & Herman, 2018 ; Young, 2006 ), and collaboratively develop strategies for effective collaboration within the school (Datnow et al., 2013 ; Huffman & Kalnin, 2003 ; Michaud, 2016 ; Van Gasse et al., 2021 ).
Many teacher tasks are deeply intertwined with human relationships (Lee, 2021 ) and often completed through collaborative efforts (Li et al., 2022 ). Therefore, school leaders and policymakers may recognize that fostering teachers’ data literacy may necessitate cultivating open, honest, and trusting school environments conducive to collaboration. Notably, the social aspect of data literacy was not prominently evident in dimensions related to teachers' knowledge and skills, which suggests that teachers may enhance their knowledge and skills independently from others in the school environment. However, fostering teacher dispositions, such as active engagement in effective data use within the school, appears to be influenced by collaborative relationships with colleagues, as well as the supportive roles of school leaders.
5.3 Teachers’ data literacy and data-related behaviors
Our review showed that teachers’ data literacy goes beyond the knowledge, skills, and dispositions that are required to effectively use data; it also involves a range of behaviors that enhance their ways of using and learning about data. Within this dimension, we noted two sub-categories, communication/discussion and participation/engagement. Therefore, one core aspect of teacher behaviors related to data was found to be communicating with various stakeholders such as colleagues, parents, and school leaders to discuss instructional approaches (e.g., Datnow et al., 2013 ; Militello et al., 2013 ; van der Scheer & Visscher, 2018 ) and assessment results (e.g., Curry et al., 2016 ; Howley et al., 2013 ). The other aspect—participation and engagement—underscores the importance of teacher involvement in team-based learning regarding data use (e.g., Andersen, 2020 ; Young, 2006 ), active engagement in establishing conducive school conditions and fostering a culture of data use within the school community (e.g., Datnow et al., 2021 ; Keuning et al., 2016 ), and proactive participation in professional development to enhance knowledge and skills (e.g., Ebbeler et al., 2016 ; van der Scheer & Visscher, 2018 ). Existing studies on data literacy have not given substantial attention to the importance of teachers' behaviors related to data. However, we argue that teachers’ behaviors related to data deserve recognition as a distinct category within the concept of teachers’ data literacy.
Dimension 4 (about teachers’ disposition) and Dimension 5 (about teachers’ behaviors) would be correlated. For example, teachers who are confident in data use may be more inclined to lead the discussions with other colleagues about data use in the school, and they may pursue additional learning opportunities to become an effective leader in school data use. Trust and respect within the school communities mentioned above would also influence how teachers behave in order to collectively enhance data literacy within the school. Studies (e.g., Ebbeler et al., 2016 ; van der Scheer & Visscher, 2018 ) have highlighted teacher participation in professional development, but there has been a relative lack of research attention to examine the collaborative nature of teacher engagement and learning within the professional settings. With the rapid evolution of educational tools and applications driven by learning analytics and artificial intelligence, the influx of data generated in this new era poses a significant challenge for teachers and school leaders. Accordingly, teacher collaboration in learning and addressing data-related challenges in schools will increasingly become a paramount concern, more so than ever before. In this regard, future policies concerning data use may prioritize the expansion of teacher collaboration and mutual learning as essential components of in-school professional development activities.
5.4 Reflections on Mandinach and Gummer’s ( 2016 ) DLFT framework
We have compared the indicators and dimensions arising from the present study and those in Mandinach and Gummer's ( 2016 ) “data literacy for teachers” (DLFT) framework. For this purpose, the conceptually similar indicators of Mandinach and Gummer ( 2016 ) are included in Table 3 alongside the corresponding indicators identified in this study. As can be seen in Table 3 , some indicators were identified in both studies, but there were also notable differences between the two sets of indicators.
Firstly, it appears that there were more fine-grained indicators across the five dimensions arising from the present study, compared to those included in Mandinach and Gummer’s ( 2016 ) DLFT framework. For instance, our study identified the importance of teacher knowledge about externally administered assessments and associated policies to guide teacher use of data, which were not a part of Mandinach and Gummer’s ( 2016 ) DLFT framework. Overall, 95 indicators of the present study, compared to 59 indicators within Mandinach and Gummer’s ( 2016 ) DLFT framework, indicates the level of details incorporated in our framework.
Secondly, perhaps the most important discrepancy is articulated in our Dimension 3 “Dispositions towards Data Use”. We have identified 25 specific indicators under this dimension, which were clustered into confidence, values/belief, trust/respect, and anxiety. These four constructs were identified as the most prominently featured psychological dispositions when teachers deal with data in the school. In Mandinach and Gummer ( 2016 ), “Dispositions, habits of mind, or factors that include data use” is mentioned, but they “chose not to include them in the conceptual framework… [due to the nature of] these positions as general to effective teaching, rather than specific to data use. They are likely to influence data literacy but are seen as more general habits of mind of good teaching” (p. 372). As such, their framework did not include dispositions as integral part of teachers’ data literacy. We argue that teacher dispositions are an essential component of teachers’ data literacy. Perhaps this discrepancy may have arisen from the views that the teacher dispositions identified in Mandinach and Gummer ( 2016 ) are general teacher qualities – such as “belief that all students can learn”, “belief in data/think critically” and “belief that improvement in education requires a continuous inquiry cycle” (p. 372). On the other hand, teachers’ dispositions in our framework were all specific to data use – such as “confidence in integrating data from multiple sources”, “confidence in discussing data with colleagues”, “trust in principals’ leadership in data use”, “trust in open and honest discussions about data use with colleagues”, and “anxiety in using data to make decision”.
On a related point, and thirdly, our framework has two separate dimensions, one focusing on individuals’ psychological dispositions under “Dimension 3: Dispositions towards Data Use”, and the other centered on behaviors “Dimension 5: Data-Related Behavior”. Most of the indicators under the behavioral dimensions were found to be social interactions, communication, discussion, participation, and engagement, as mentioned above. In Mandinach and Gummer ( 2016 ), psychological dispositions (such as belief) and behavioral tendencies (such as ethical use of data, collaboration, and communication skills) were grouped into one dimension of “Dispositions, habits of mind, or factors that include data use”. Considering these, it appears that there was less emphasis on the dispositions and behavioral tendencies in Mandinach and Gummer ( 2016 ).
On the other hand, Mandinach and Gummer ( 2016 ) offered a fine-grained description of skill-related indicators within their DLFT framework. For example, our indicator of “selecting data appropriate for different purposes” was described with more granularity in the DLFT framework: “understand what data are appropriate”, “use qualitative and quantitative data”, “prioritize data”, and “understand specificity of data to question/problem”. Likewise, our indicator of “describing data patterns” was further divided into “understand data properties”, “access patterns and trends”, “drill down into data” and “examine data” in the DLFT framework. Additionally, two indicators within the Mandinach and Gummer’ ( 2016 ) framework—“understand student privacy” and “ethics use of data, including the protection of privacy and confidentiality of data”—did not fit into any of the indicators or dimensions of the present study. This is because we were unable to locate empirical studies that directly examined ethical data management and data use among teachers. Therefore, data ethics issues, which we believe to be an important aspect of teachers’ data literacy, were omitted from our framework.
Finally, we also note the differences between the broad dimensions proposed by Mandinach and Gummer's ( 2016 ) DLFT framework and our framework. The DLFT framework consisted of: (a) identifying problems and framing questions, (b) using data, (c) transforming data into information, (d) transforming information into decisions, and (e) evaluating outcomes. These five dimensions are primarily about data skills, which was just one dimension of our framework. Furthermore, their indicator descriptions suggest heavy emphasis on data use to inform teaching and learning. In contrast, our dimensions and indicators illustrate the broader purposes and contexts of teachers' data use, highlighting the significance of fostering teacher dispositions and data-related behaviors through effective leadership and a collaborative school environment. In particular, the detailed descriptors for each of the indicators under Dimensions 3, 4, and 5 of the present study are the strengths of our framework, as they illustrate a wide range of varied and specific purposes and data-related dispositions and behaviors related to teachers’ data literacy; these descriptions are relatively sparse in Mandinach and Gummer ( 2016 ).
5.5 Limitations of the present study and future research directions
We acknowledge several limitations of the present study. First, our review focused on empirical studies published in journal articles, and omitted government documents, books, and book chapters and publications by professional organizations. Second, we did not differentiate the studies based on in-service teachers vs. pre-service teachers. Future studies may look into potential differences between these two groups and suggest policy directions and strategies for teacher preparation. Third, teachers may possess discipline-unique capabilities and inclinations, and thus it may be worthwhile to identify teacher characteristics across different disciplines (e.g., Science vs. English) and examine the influences of discipline contexts on teachers’ data use and data literacy. Fourth, exploring teachers’ data literacy required for students at different levels of schooling (e.g., early childhood, primary, and secondary) and for students with diverse needs (e.g., learning difficulties, dyslexia) may provide further insights into the specific expectations within the daily tasks and responsibilities of teachers. Fifth, most of the reviewed studies were conducted in Western or English-speaking countries, and thus our findings may have limited relevance to teacher data literacy in different world regions. Future studies may investigate cross-country characteristics in teachers’ data literacy. Sixth, our research also reveals that current studies of teachers’ data literacy have not explored the possible connections between technological advancements, particularly in AI-based systems, and teachers’ data literacy. This suggests a need to investigate the link between teachers’ data literacy and their proficiency in understanding emerging technologies such as AI-based systems. It is anticipated that discussions on data ethics will emerge as a crucial aspect of teachers’ data literacy in the era of artificial intelligence (AI). Finally, our review did not include, and thus future reviews may examine, system-level contextual factors (e.g., digital technology infrastructure, schools’ socio-economic standing) and their influences on teacher practices in data use.
6 Conclusion
Our review of 83 empirical studies published between 1990 and 2021 produced 95 specific indicators of teachers’ data literacy. The indicators were further categorised into five dimensions: (a) knowledge about data , (b) skills in using data , (c) dispositions towards data use , (d) data applications for various purposes , and (e) data-related behaviors . Our findings suggest that teachers' data literacy encompasses more than just knowledge and skills; it also includes a wide range of dispositions and behaviors. Additionally, teacher data literacy extends beyond assessing student learning outcomes and meeting accountability requirements and includes teachers’ reflection and engagement in professional development.
Data availability
Data sharing not applicable to this article as no datasets were generated or analysed during the current study.
*indicates the studies under the systematic review of this investigation
*Abdusyakur, I., & Poortman, C. L. (2019). Study on data use in Indonesian primary schools. Journal of Professional Capital and Community, 4 (3), 198-215. https://doi.org/10.1108/JPCC-11-2018-0029
*Abrams, L., Varier, D., & Jackson, L. (2016). Unpacking instructional alignment: The influence of teachers’ use of assessment data on instruction. Perspectives in Education, 34 (4), 15–28. https://doi.org/10.18820/2519593X/pie.v34i4.2
*Abrams, L. M., Varier, D., & Mehdi, T. (2021). The intersection of school context and teachers’ data use practice: Implications for an integrated approach to capacity building. Studies in Educational Evaluation , 69 , 100868. https://doi.org/10.1016/j.stueduc.2020.100868
*Albiladi, W. S., Lasater, K., & Bengtson, E. (2020). Data use among principals and teachers: divergent paths or common ground? Implications for the leadership preparation programs . Journal of School Administration Research and Development, 5 (2), 63–76.
*Andersen, I. G. (2020). What went wrong? Examining teachers’ data use and instructional decision making through a bottom-up data intervention in Denmark. International Journal of Educational Research, 102 , 101585. https://doi.org/10.1016/j.ijer.2020.101585
*Anderson, S., Leithwood, K., & Strauss, T. (2010). Leading data use in schools: Organizational conditions and practices at the school and district levels. Leadership and Policy in Schools , 9 (3), 292–327. https://doi.org/10.1080/15700761003731492
Ansyari, M. F., Groot, W., & De Witte, K. (2020). Tracking the process of data use professional development interventions for instructional improvement: A systematic literature review. Educational Research Review, 31 , 100362.
Article Google Scholar
Ansyari, M. F., Groot, W., & De Witte, K. (2022). A systematic review and meta-analysis of data use professional development interventions. Journal of Professional Capital and Community, 7 (3), 256–289.
*Beck, J. S., Morgan, J. J., Brown, N., Whitesides, H., & Riddle, D. R. (2020). “Asking, Learning, Seeking Out”: An exploration of data literacy for teaching. The Educational Forum , 84 (2), 150–165. https://doi.org/10.1080/00131725.2020.1674438
Beck, J. S., & Nunnaley, D. (2021). A continuum of data literacy for teaching. Studies in Educational Evaluation, 69 , 100871.
*Bianco, S. D. (2010). Improving student outcomes: data-driven instruction and fidelity of implementation in a response to intervention (RTI) model. Teaching Exceptional Children Plus , 6 (5).
Brookhart, S. M. (2011). Educational assessment knowledge and skills for teachers. Educational Measurement: Issues and Practice, 30 (1), 3–12.
*Brunner, C., Fasca, C., Heinze, J., Honey, M., Light, D., Mardinach, E., & Wexler, D. (2005). Linking data and learning: The grow network study. Journal of Education for Students Placed at Risk (JESPAR) , 10 (3), 241–267. https://doi.org/10.1207/s15327671espr1003_2
*Chen, L.-L. (2019). Enhancing teaching with effective data mining protocols. Journal of Educational Technology Systems , 47 (4), 500–512. https://doi.org/10.1177/0047239519835846
*Copp, D. T. (2016). The impact of teacher attitudes and beliefs about large-scale assessment on the use of provincial data for instructional change. Education Policy Analysis Archives , 24 , 109. https://doi.org/10.14507/epaa.24.2522
*Copp, D. T. (2017). Policy incentives in Canadian large-scale assessment: How policy levers influence teacher decisions about instructional change. Education Policy Analysis Archives , 25 , 115. https://doi.org/10.14507/epaa.25.3299
*Curry, K. A., Mwavita, M., Holter, A., & Harris, E. (2016). Getting assessment right at the classroom level: using formative assessment for decision making. Educational Assessment, Evaluation and Accountability, 28 (1), 89–104. https://doi.org/10.1007/s11092-015-9226-5
Darling-Hammond, L., Hightower, A. M., Husbands, J., LaFors, J. R., Young, V. M. & Christopher, C. (2003) Building instructional quality: ‘inside-out’ and ‘outside-in’ perspectives on San Diego’s school reform (Seattle, WA, Center for the Study of Teaching and Policy, University of Washington).
Data Quality Campaign. (2014). Teacher data literacy: It's about time.
*Datnow, A., Park, V., & Kennedy-Lewis, B. (2012). High school teachers' use of data to inform instruction. Journal of Education for Students Placed at Risk (JESPAR), 17 (4), 247–265. https://doi.org/10.1080/10824669.2012.718944
*Datnow, A., Park, V., & Kennedy‐Lewis, B. (2013). Affordances and constraints in the context of teacher collaboration for the purpose of data use. Journal of Educational Administration , 51 (3), 341–362. https://doi.org/10.1108/09578231311311500
*Datnow, A., Lockton, M., & Weddle, H. (2021). Capacity building to bridge data use and instructional improvement through evidence on student thinking. Studies in Educational Evaluation , 69 , 100869. https://doi.org/10.1016/j.stueduc.2020.100869
*Dunn, K. E., Airola, D. T., Lo, W.-J., & Garrison, M. (2013a). Becoming data driven: The influence of teachers’ sense of efficacy on concerns related to data-driven decision making. The Journal of Experimental Education , 81 (2), 222–241. https://doi.org/10.1080/00220973.2012.699899
*Dunn, K. E., Airola, D. T., Lo, W.-J., & Garrison, M. (2013b). What teachers think about what they can do with data: Development and validation of the data driven decision-making efficacy and anxiety inventory. Contemporary Educational Psychology, 38 (1), 87-98. https://doi.org/10.1016/j.cedpsych.2012.11.002
*Ebbeler, J., Poortman, C. L., Schildkamp, K., & Pieters, J. M. (2016). Effects of a data use intervention on educators’ use of knowledge and skills. Studies in Educational Evaluation, 48 , 19-31. https://doi.org/10.1016/j.stueduc.2015.11.002
*Ebbeler, J., Poortman, C.L., Schildkamp, K. & Pieters, J. M. (2017). The effects of a data use intervention on educators’ satisfaction and data literacy. Educational Assessment, Evaluation and Accountability, 29 , 83–105. https://doi.org/10.1007/s11092-016-9251z
Espin, C. A., van den Bosch, R. M., van der Liende, M., Rippe, R. C., Beutick, M., Langa, A., & Mol, S. E. (2021). A systematic review of CBM professional development materials: Are teachers receiving sufficient instruction in data-based decision-making? Journal of Learning Disabilities, 54 (4), 256–268.
*Farley-Ripple, E., & Buttram, J. (2015). The development of capacity for data use: The role of teacher networks in an elementary school. Teachers College Record, 117 (4), 1–34.
*Farley-Ripple, E. N., Jennings, A. S., & Buttram, J. (2019). Toward a framework for classifying teachers’ use of assessment data. AERA Open, 5 (4). https://doi.org/10.1177/2332858419883571 .
*Farrell, C. C. (2015). Designing school systems to encourage data use and instructional improvement: A comparison of school districts and charter management organizations. Educational Administration Quarterly, 51 (3), 438–471. https://doi.org/10.1177/0013161x14539806
Filderman, M. J., Toste, J. R., Didion, L. A., Peng, P., & Clemens, N. H. (2018). Data-based decision making in reading interventions: A synthesis and meta-analysis of the effects for struggling readers. The Journal of Special Education, 52 (3), 174–187.
*Ford, T. G. (2018). Pointing teachers in the wrong direction: Understanding Louisiana elementary teachers’ use of compass high-stakes teacher evaluation data. Educational Assessment, Evaluation and Accountability , 30 (3), 251–283. https://doi.org/10.1007/s11092-018-9280-x
*Gelderblom, G., Schildkamp, K., Pieters, J., & Ehren, M. (2016). Data-based decision making for instructional improvement in primary education. International Journal of Educational Research , 80 , 1–14. https://doi.org/10.1016/j.ijer.2016.07.004
Gesel, S. A., LeJeune, L. M., Chow, J. C., Sinclair, A. C., & Lemons, C. J. (2021). A meta-analysis of the impact of professional development on teachers’ knowledge, skill, and self-efficacy in data-based decision-making. Journal of Learning Disabilities, 54 (4), 269–283.
Gummer, E., & Mandinach, E. B. (2015). Building a conceptual framework for data literacy. Teachers College Record, 117 (4), 1–22. https://www.tcrecord.org
*Hardy, I. (2015). Data, numbers and accountability: The complexity, nature and effects of data use in schools. British Journal of Educational Studies , 63 (4), 467–486. https://doi.org/10.1080/00071005.2015.1066489
*Hardy, I. (2019). Governing teachers’ work and learning through data: Australian insights. Cambridge Journal of Education , 49 (4), 501–517. https://doi.org/10.1080/0305764X.2018.1557594
*Hodges, C. A. (1996). How valid and useful are alternative assessments for decision‐making in primary grade classrooms? Reading Research and Instruction , 36 (2), 157–173. https://doi.org/10.1080/19388079709558235
Hoogland, I., Schildkamp, K., Van der Kleij, F., Heitink, M., Kippers, W., Veldkamp, B., & Dijkstra, A. M. (2016). Prerequisites for data-based decision making in the classroom: Research evidence and practical illustrations. Teaching and Teacher Education, 60 , 377–386.
*Howley, M. D., Howley, A., Henning, J. E., Gilla, M. B., & Weade, G. (2013). Intersecting domains of assessment knowledge: School typologies based on interviews with secondary teachers. Educational Assessment , 18 (1), 26–48. https://doi.org/10.1080/10627197.2013.761527
*Huffman, D., & Kalnin, J. (2003). Collaborative inquiry to make data-based decisions in schools. Teaching and Teacher Education , 19 (6), 569–580. https://doi.org/10.1016/S0742-051X(03)00054-4
*Jacobs, J., Gregory, A., Hoppey, D., & Yendol-Hoppey, D. (2009). Data literacy: Understanding teachers’ data use in a context of accountability and response to intervention. Action in Teacher Education , 31 (3), 41–55. https://doi.org/10.1080/01626620.2009.10463527
*Jimerson, J. B., & Wayman, J. (2015). Professional learning for using data: Examining teacher needs and supports. Teachers College Record, 117 (4), 1–36.
*Jimerson, J. B., Cho, V., & Wayman, J. C. (2016). Student-involved data use: Teacher practices and considerations for professional learning. Teaching and Teacher Education , 60 , 413–424. https://doi.org/10.1016/j.tate.2016.07.008
*Jimerson, J. B., Cho, V., Scroggins, K. A., Balial, R., & Robinson, R. R. (2019). How and why teachers engage students with data. Educational Studies, 45 (6), 667–691. https://doi.org/10.1080/03055698.2018.1509781
*Jimerson, J. B., Garry, V., Poortman, C. L., & Schildkamp, K. (2021). Implementation of a collaborative data use model in a United States context. Studies in Educational Evaluation , 69 , 100866. https://doi.org/10.1016/j.stueduc.2020.100866
*Jimerson, J. B. (2014). Thinking about data: Exploring the development of mental models for “data use” among teachers and school leaders. Studies in Educational Evaluation, 42 , 5–14. https://doi.org/10.1016/j.stueduc.2013.10.010
*Joo, Y. H. (2020). Promoting sustainable data-based decision-making in the Korean educational information disclosure system. Sustainability , 12 (17), 6762. https://doi.org/10.3390/su12176762
*Kallemeyn, L. M. (2014). School-level organisational routines for learning: supporting data use. Journal of Educational Administration, 52 (4), 529–548. https://doi.org/10.1108/JEA-02-2013-0025
*Kanjee, A., & Moloi, Q. (2014). South African teachers’ use of national assessment data. South African Journal of Childhood Education , 4 (2), 24. https://doi.org/10.4102/sajce.v4i2.206
Katz, L. (1993). Dispositions as Educational Goals. ERIC Digest #211.
*Kerr, K. A., Marsh, J. A., Ikemoto, G. S., Darilek, H., & Barney, H. (2006). Strategies to promote data use for instructional improvement: Actions, outcomes, and lessons from three urban districts. American Journal of Education , 112 (4), 496–520. https://doi.org/10.1086/505057
*Keuning, T., Geel, M. V., Visscher, A., Fox, J.-P., & Moolenaar, N. M. (2016). The transformation of schools’ social networks during a data-based decision making reform. Teachers College Record, 118 (9), 1–33. https://doi.org/10.1177/016146811611800908
*Keuning, T., Van Geel, M., & Visscher, A. (2017). Why a data-based decision-making intervention works in some schools and not in others. Learning Disabilities Research & Practice, 32 (1), 32-45. https://doi.org/10.1111/ldrp.12124
*Kippers, W. B., Poortman, C. L., Schildkamp, K., & Visscher, A. J. (2018). Data literacy: What do educators learn and struggle with during a data use intervention? Studies in Educational Evaluation, 56 , 21–31 . https://doi.org/10.1016/j.stueduc.2017.11.001
Lee, J. (2021). Teacher–student relationships and academic achievement in Confucian educational countries/systems from PISA 2012 perspectives. Educational Psychology, 41 (6), 764–785.
Lee, J., & Durksen, T. (2018). Dimensions of academic interest among undergraduate students: Passion, confidence, aspiration, and self-expression. Educational Psychology, 38 (2), 120–138. https://doi.org/10.1080/01443410.2017.1342770
Lee, J., & Stankov, L. (2018). Non-cognitive predictors of academic achievement: Evidence from TIMSS and PISA. Learning and Individual Differences, 65 , 50–64.
Li, L., Zhu, H., & Li, H. (2022). School leadership enhances secondary students’ achievement in rural China through teacher commitment and collaborative culture. Frontiers in Psychology, 13 , 894688.
*Lockton, M., Weddle, H., & Datnow, A. (2020). When data don’t drive: Teacher agency in data use efforts in low-performing schools. School Effectiveness and School Improvement , 31 (2), 243–265. https://doi.org/10.1080/09243453.2019.1647442
Mandinach, E. B. (2012). A perfect time for data use: Using data-driven decision making to inform practice. Educational Psychologist, 47 (2), 71–85. https://doi.org/10.1080/00461520.2012.667064
Mandinach, E. B., & Gummer, E. S. (2013a). Defining data literacy: A report on a convening of experts. Journal of Educational Research and Policy Studies, 13 (2), 6–28.
Google Scholar
Mandinach, E. B., & Gummer, E. S. (2013b). A systemic view of implementing data literacy in educator preparation. Educational Researcher, 42 (1), 30–37. https://doi.org/10.3102/0013189x12459803
Mandinach, E. B., & Gummer, E. S. (2016). What does it mean for teachers to be data literate: Laying out the skills, knowledge, and dispositions. Teaching and Teacher Education, 60 , 366–376. https://doi.org/10.1016/j.tate.2016.07.011
Mandinach, E. B., & Schildkamp, K. (2021). Misconceptions about data-based decision making in education: An exploration of the literature. Studies in Educational Evaluation, 69 , 100842. https://doi.org/10.1016/j.stueduc.2020.100842
Mandinach, E. B., Parton, B. M., Gummer, E. S., & Anderson, R. B. (2015). Responsible data use as a skill in educators’ data literacy. Phi Delta Kappan, 96 (5), 25e28.
Mandinach, E. B. (2014). What's the difference between assessment literacy and data literacy?. In Presentation made at the data quality Campaign webinar. Retrieved from http://dataqualitycampaign.org/files/events/Data%20literacy%20event%20slides.pdf
*Marsh, J. A., & Farrell, C. C. (2015). How leaders can support teachers with data-driven decision making: A framework for understanding capacity building. Educational Management Administration & Leadership , 43 (2), 269–289. https://doi.org/10.1177/1741143214537229
*Marsh, J. A., Sloan McCombs, J., & Martorell, F. (2010). How instructional coaches support data-driven decision making: Policy implementation and effects in Florida middle schools. Educational Policy , 24 (6), 872–907. https://doi.org/10.1177/0895904809341467
*Mausethagen, S., Prøitz, T., & Skedsmo, G. (2018). Teachers’ use of knowledge sources in ‘result meetings’: Thin data and thick data use. Teachers and Teaching , 24 (1), 37–49. https://doi.org/10.1080/13540602.2017.1379986
*McDonald, J.P. (2019). Toward more effective data use in teaching. Phi Delta Kappan , 100 (6), 50–54. https://doi.org/10.1177/0031721719834029
*Michaud, R. (2016). The nature of teacher learning in collaborative data teams. The Qualitative Report, 21 (3), 529–545. Retrieved from http://nsuworks.nova.edu/tqr/vol21/iss3/7
*Militello, M., Bass, L., Jackson, K., & Wang, Y. (2013). How data are used and misused in schools: Perceptions from teachers and principals. Education Sciences , 3 (2), 98–120. https://doi.org/10.3390/educsci3020098
*Mills, M., Mockler, N., Stacey, M., & Taylor, B. (2021). Teachers’ orientations to educational research and data in England and Australia: Implications for teacher professionalism. Teaching Education, 32 (1), 77–98. https://doi.org/10.1080/10476210.2020.1843617
Mischel, W., & Shoda, Y. (1995). A cognitive-affective system theory of personality: Reconceptualizing situations, dispositions, dynamics, and invariance in personality structure. Psychological Review, 102 (2), 246–268.
Moher, D., Liberati, A., Tetzlaff, J., & Altman, D. G. (2009). Preferred reporting items for systematic reviews and meta-analyses: The PRISMA statement. BMJ, 339 , b2535. https://doi.org/10.1136/bmj.b2535
*Mokhtari, K., Thoma, J., & Edwards, P. (2009). How one elementary school uses data to help raise students’ reading achievement. The Reading Teacher , 63 (4), 334–337. https://www.jstor.org/stable/30249385
*Nicholson, J., Capitelli, S., Richert, A. E., Wilson, C., & Bove, C. (2017). Teacher leaders building foundations for data-informed teacher learning in one urban elementary school. The New Educator , 13 (2), 170–189. https://doi.org/10.1080/1547688X.2016.1140856
*O’Brien, S., McNamara, G., O’Hara, J., & Brown, M. (2022). Learning by doing: Evaluating the key features of a professional development intervention for teachers in data-use, as part of whole school self-evaluation process. Professional Development in Education , 48 (2), 273–297. https://doi.org/10.1080/19415257.2020.1720778
*Omoso, E., Schildkamp, K., & Pieters, J. (2019). Data use in Kenyan secondary schools. Journal of Professional Capital and Community , 4 (3), 216–231. https://doi.org/10.1108/JPCC-11-2018-0027
*Park, V., & Datnow, A. (2009). Co-constructing distributed leadership: District and school connections in data-driven decision-making. School Leadership & Management , 29 (5), 477–494. https://doi.org/10.1080/13632430903162541
*Poortman, C. L., & Schildkamp, K. (2016). Solving student achievement problems with a data use intervention for teachers. Teaching and Teacher Education, 60 , 425-433. https://doi.org/10.1016/j.tate.2016.06.010
*Powell, S. R., Lembke, E. S., Ketterlin-Geller, L. R., Petscher, Y., Hwang, J., Bos, S. E., Cox, T., Hirt, S., Mason, E. N., Pruitt-Britton, T., Thomas, E., & Hopkins, S. (2021). Data-based individualisation in mathematics to support middle school teachers and their students with mathematics learning difficulty. Studies in Educational Evaluation, 69 , 100897. https://doi.org/10.1016/j.stueduc.2020.100897
*Prenger, R., & Schildkamp, K. (2018). Data-based decision making for teacher and student learning: A psychological perspective on the role of the teacher. Educational Psychology , 38 (6), 734–752. https://doi.org/10.1080/01443410.2018.1426834
*Reed, D. K. (2015). Middle level teachers’ perceptions of interim reading assessments: An exploratory study of data-based decision making. RMLE Online , 38 (6), 1–13. https://doi.org/10.1080/19404476.2015.11462119
*Reeves, T. D., Summers, K. H., & Grove, E. (2016). Examining the landscape of teacher learning for data use: The case of Illinois. Cogent Education , 3 (1), 1211476. https://doi.org/10.1080/2331186X.2016.1211476
*Reeves, T. D. (2017). School level and other differences in Illinois teachers’ use of data to inform instruction. Mid-Western Educational Researcher , 29 (4), 332–354.
Schildkamp, K. (2019). Data-based decision-making for school improvement: Research insights and gaps. Educational Research, 61 (3), 257–273. https://doi.org/10.1080/00131881.2019.1625716
*Schildkamp, K., Poortman, C., Luyten, H., & Ebbeler, J. (2017). Factors promoting and hindering data-based decision making in schools . School Effectiveness and School Improvement, 28 (2), 242–258. https://doi.org/10.1080/09243453.2016.1256901
*Schildkamp, K., Smit, M., & Blossing, U. (2019). Professional development in the use of data: From data to knowledge in data teams. Scandinavian Journal of Educational Research , 63 (3), 393–411. https://doi.org/10.1080/00313831.2017.1376350
Shavelson, R. J., Ruiz-Primo, M. A., & Wiley, E. W. (2005). Windows into the mind. Higher Education, 49 (4), 413–430.
*Snodgrass Rangel, V., Monroy, C., & Bell, E. (2016). Science teachers’ data use practices: A descriptive analysis. Education Policy Analysis Archives , 24 , 86. https://doi.org/10.14507/epaa.24.2348
*Snodgrass Rangel, V. S., Bell, E., & Monroy, C. (2019). Teachers’ sensemaking and data use implementation in science classrooms. Education and Urban Society, 51 (4), 526–554. https://doi.org/10.1177/0013124517727053
*Staman, L., Timmermans, A. C., & Visscher, A. J. (2017). Effects of a data-based decision making intervention on student achievement. Studies in Educational Evaluation, 55 , 58–67. https://doi.org/10.1016/j.stueduc.2017.07.002
Stankov, L., & Lee, J. (2008). Confidence and cognitive test performance. Journal of Educational Psychology, 100 (4), 961–976. https://doi.org/10.1037/a0012546
Sykes, G. (1999). Teacher and student learning: Strengthening their connection. In L. Darling-Hammond & G. Sykes (Eds.), Teaching as the learning profession: Handbook of policy and practice (pp. 151–179). Jossey-Bass.
Thomas, J., & Harden, A. (2008). Methods for the thematic synthesis of qualitative research in systematic reviews. BMC Medical Research Methodology, 8 , 45. https://doi.org/10.1186/1471-2288-8-45
*Thomas, K., & Huffman, D. (2011). Navigating the challenges of helping teachers use data to inform educational decisions. Administrative Issues Journal , 1 (2). https://doi.org/10.5929/2011.1.2.7
*Thompson, C. J. (2012). Improving the use of data in early reading intervention programs in northwest Florida. Journal of Educational Research and Practice, 2 (1), 1-14.
*van Der Scheer, E. A., & Visscher, A. J. (2018). Effects of a data-based decision-making intervention for teachers on students’ mathematical achievement. Journal of Teacher Education , 69 (3), 307–320. https://doi.org/10.1177/0022487117704170
*Van Gasse, R., Vanlommel, K., Vanhoof, J., & Van Petegem, P. (2017). The impact of collaboration on teachers’ individual data use. School Effectiveness and School Improvement , 28 (3), 489–504. https://doi.org/10.1080/09243453.2017.1321555
*Van Gasse, R., Vanhoof, J., & Van Petegem, P. (2018). Instrumental, conceptual and symbolic effects of data use: The impact of collaboration and expectations. Educational Studies, 44 (5), 521–534. https://doi.org/10.1080/03055698.2017.1382328
*Van Gasse, R., Goffin, E., Vanhoof, J., & Van Petegem, P. (2021). For squad-members only! Why some teachers are more popular to interact with than others in data use. Studies in Educational Evaluation , 69 , 100881. https://doi.org/10.1016/j.stueduc.2020.100881
*Vanlommel, K., & Schildkamp, K. (2019). How do teachers make sense of data in the context of high-stakes decision making? American Educational Research Journal, 56 (3), 792–821. https://doi.org/10.3102/0002831218803891
*Vanlommel, K., Vanhoof, J., & Van Petegem, P. (2016). Data use by teachers: The impact of motivation, decision-making style, supportive relationships and reflective capacity. Educational Studies , 42 (1), 36–53. https://doi.org/10.1080/03055698.2016.1148582
*Vanlommel, K., Van Gasse, R., Vanhoof, J., & Van Petegem, P. (2021). Sorting pupils into their next educational track: How strongly do teachers rely on data-based or intuitive processes when they make the transition decision? Studies in Educational Evaluation , 69 , 100865. https://doi.org/10.1016/j.stueduc.2020.100865
*von der Embse, N. P., Kilgus, S. P., Eklund, K., Zahn, M., Peet, C., & Durango, S. (2021). Promoting effective decision making: Training educators to collect and use social–emotional skill assessment data to inform tier 2 interventions. School Psychology Review, 51 (5), 574-588. https://doi.org/10.1080/2372966X.2020.1827680
*Wachen, J., Harrison, C., & Cohen-Vogel, L. (2018). Data use as instructional reform: exploring educators’ reports of classroom practice. Leadership and Policy in Schools , 17 (2), 296–325. https://doi.org/10.1080/15700763.2016.1278244
*Wardrip, P. S., & Herman, P. (2018). ‘We’re keeping on top of the students’: Making sense of test data with more informal data in a grade-level instructional team. Teacher Development , 22 (1), 31–50. https://doi.org/10.1080/13664530.2017.1308428
Weiss, J. (2012). Data for improvement, data for accountability. Teachers College Record, 114 (11), 110307.
*Wolff, A., Wermelinger, M., & Petre, M. (2019). Exploring design principles for data literacy activities to support children’s inquiries from complex data. International Journal of Human-Computer Studies , 129 , 41–54. https://doi.org/10.1016/j.ijhcs.2019.03.006
*Young, V. M. (2006). Teachers’ use of data: Loose coupling, agenda setting, and team norms. American Journal of Education , 112 (4), 521–548. https://doi.org/10.1086/505058
*Zeuch, N., Förster, N., & Souvignier, E. (2017). Assessing teachers’ competencies to read and interpret graphs from learning progress assessment: Results from tests and interviews. Learning Disabilities Research & Practice , 32 (1), 61–70. https://doi.org/10.1111/ldrp.12126
*Zhao, X., Van den Heuvel-Panhuizen, M., & Veldhuis, M. (2016). Teachers’ use of classroom assessment techniques in primary mathematics education—An explorative study with six Chinese teachers. International Journal of STEM Education, 3 (1), 19. https://doi.org/10.1186/s40594-016-0051-2
Download references
Acknowledgements
This work was supported by the Faculty of Arts, Design & Architecture and the School of Education at UNSW, Sydney.
Open Access funding enabled and organized by CAUL and its Member Institutions.
Author information
Authors and affiliations.
School of Education, University of New South Wales, Sydney, NSW, Australia
Jihyun Lee, Dennis Alonzo, Kim Beswick, Jan Michael Vincent Abril & Adrian W. Chew
Yangon University of Education, Yangon, Myanmar
Cherry Zin Oo
You can also search for this author in PubMed Google Scholar
Contributions
Jihyun Lee: Conceptualization; Data curation; Formal analysis; Funding acquisition; Investigation; Methodology; Supervision; Validation; Visualization; Roles/Writing—original draft; and Writing—review & editing
Dennis Alonzo: Conceptualization; Formal analysis; Funding acquisition; Investigation; Methodology; Project administration; Resources
Kim Beswick: Conceptualization; Funding acquisition; Investigation; Writing—review & editing
Jan Abril: Data curation; Validation
Adrian W. Chew: Data curation; Visualization
Cherry Zin O: Data curation; Formal analysis
Corresponding author
Correspondence to Jihyun Lee .
Ethics declarations
Conflict of interest statement.
There is no conflict of interest.
Additional information
Publisher's note.
Springer Nature remains neutral with regard to jurisdictional claims in published maps and institutional affiliations.
Data Management Statement
The manuscript has no data attached, as it is a systematic review.
Rights and permissions
Open Access This article is licensed under a Creative Commons Attribution 4.0 International License, which permits use, sharing, adaptation, distribution and reproduction in any medium or format, as long as you give appropriate credit to the original author(s) and the source, provide a link to the Creative Commons licence, and indicate if changes were made. The images or other third party material in this article are included in the article's Creative Commons licence, unless indicated otherwise in a credit line to the material. If material is not included in the article's Creative Commons licence and your intended use is not permitted by statutory regulation or exceeds the permitted use, you will need to obtain permission directly from the copyright holder. To view a copy of this licence, visit http://creativecommons.org/licenses/by/4.0/ .
Reprints and permissions
About this article
Lee, J., Alonzo, D., Beswick, K. et al. Dimensions of teachers’ data literacy: A systematic review of literature from 1990 to 2021. Educ Asse Eval Acc 36 , 145–200 (2024). https://doi.org/10.1007/s11092-024-09435-8
Download citation
Received : 06 November 2023
Accepted : 27 March 2024
Published : 06 May 2024
Issue Date : May 2024
DOI : https://doi.org/10.1007/s11092-024-09435-8
Share this article
Anyone you share the following link with will be able to read this content:
Sorry, a shareable link is not currently available for this article.
Provided by the Springer Nature SharedIt content-sharing initiative
- Data literacy
- Find a journal
- Publish with us
- Track your research

Data literacy assessments: a systematic literature review
Chat with paper, feedback practices and transparency in data analysis, open data work for empowered deliberative democracy: findings from a living lab study, development and validation of a data literacy assessment scale, a strategic approach to information literacy: data literacy. a systematic review, research data literacy, what is data literacy, got game a choice-based learning assessment of data literacy and visualization skills, optimizing data visualization for reproductive, maternal, newborn, child health, and nutrition (rmnch&n) policymaking: data visualization preferences and interpretation capacity among decision-makers in tanzania, data literacy and research data management in two top universities in poland. raising awareness, trending questions (2).
The current state of research on data literacy is still emerging in education, with a need for high-quality assessment tools to monitor progress and support teaching and learning.
The paper provides a systematic review of available data literacy assessments, including information on educational levels, data literacy definitions and competencies, assessment types and item formats, and reliability and validity evidence.
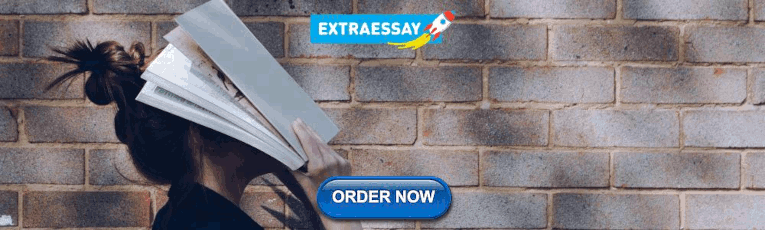
IMAGES
VIDEO
COMMENTS
The results can help researchers and practitioners better understand the current state of data literacy assessments in terms of issues related to 1) educational levels and audiences; 2) data literacy definitions and competencies; 3) assessment types and item formats; and 4) reliability and validity evidence. The results from the present review ...
This paper outlines a systematic literature review that investigated gaps in the current framing of data and faculty development, and explores how these gaps prevent the formulation of potential pathways and principles for fostering educators’ data literacy.
With the exponential increase in the volume of data available in the 21st century, data literacy skills have become vitally important in work places and everyday life. This paper provides a systematic review of available data literacy assessments targeted at different audiences and educational levels.
The results can help researchers and practitioners better understand the current state of data lit-eracy assessments in terms of issues related to 1) educational levels and audiences; 2)...
Our review identified 95 distinct indicators across five dimensions: (a) knowledge about data, (b) skills in using data, (c) dispositions towards data use, (d) data application for various purposes, and (e) data-related behaviors.
The paper provides a systematic review of available data literacy assessments, including information on educational levels, data literacy definitions and competencies, assessment types and item formats, and reliability and validity evidence.
This article explores issues around the growing need for data-driven decision making in programs in schools of education. The issues are complex and the actors needed to bring about change are multiple. A systems perspective to explore course and programmatic implementation is presented.
The data literacy scale developed in this study has respectable reliability and construct validity, supported by a concept analysis of data literacy, a comparative analysis of data literacy competency frameworks, an expert panel review, exploratory factor analysis, and confirmatory factor analysis.
The results can help researchers and practitioners better understand the current state of data literacy assessments in terms of issues related to 1) educational levels and audiences; 2) data literacy definitions and competencies; 3) assessment types and item formats; and 4) reliability and validity evidence.
The review of 71 articles revealed that data literacy education predominantly relies on existing learn-ing theories and teaching strategies, lacks empirical studies, and lacks agreement about what competencies constitute data literacy.