10.3 Glance at Genre: Thesis, Reasoning, and Evidence
Learning outcomes.
By the end of this section, you will be able to:
- Articulate and analyze key rhetorical concepts in presenting a position or an argument.
- Demonstrate awareness of context, audience, and purpose in a position argument.
- Identify the thesis and supporting evidence of a position argument.
- Distinguish different types of evidence used in a position argument.
The purpose of a position argument is to persuade readers to adopt a viewpoint. Writers of position arguments focus on a thesis that takes a stance on a debatable issue and support that thesis with reasoning and evidence. When writing persuasively, consider your audience and use the kinds of reasoning strategies and evidentiary appeals you believe will be convincing. In addition, use language with which your audience is most comfortable. In academic environments, academic language is generally most acceptable, although you may choose to challenge this notion for rhetorical purposes. Outside academic environments, tailor your language to connect best with your audience.
Reasoning is most effective when it is built on evidence that readers recognize as logical and practical. Suppose you want to persuade your audience that because of the insurrection at the U.S. Capitol on January 6, 2021, additional police should be hired to protect the building and the people who work there. You could include information about the number of police on duty that day, the number of people injured, and the amount of damage done. You then could explain how the number of police on duty was insufficient to protect the people and the Capitol.
Additionally, you identify and refute the counterclaims . An example of a counterclaim against hiring additional police officers might be that the cost is too high. Your response, then, might be that the cost could easily be shifted from another nationally funded source.
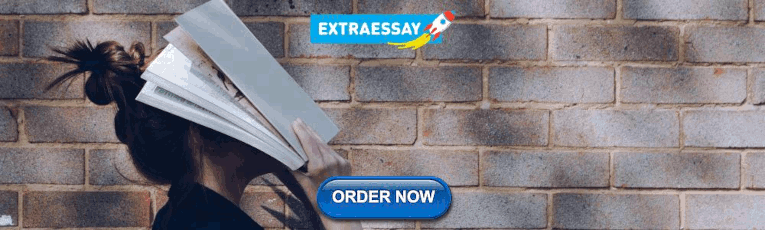
Characteristics of Position Arguments
The characteristics of a position argument include the following elements.
Ethos (Ethical Appeal)
You establish credibility by showing readers that your approach to the issue is fair and that you can be trusted. One way to demonstrate fairness and trustworthiness is to use neutral language that avoids name-calling. For instance, in your paper about hiring additional police to defend the Capitol, you would avoid taking political sides and would use neutral language when describing police, workers in the Capitol, and demonstrators.
To show trustworthiness, always follow these guidelines:
- Use only respected, reliable sources as evidence. Avoid sources that lean heavily to the political right or left or that are otherwise questionable as to accuracy. Reliable sources include scholarly, peer-reviewed articles and books; professional articles and books; and articles from magazines, newspapers, websites, and blogs. For more information about credible sources, see Research Process: Accessing and Recording Information and Annotated Bibliography: Gathering, Evaluating, and Documenting Sources.
- Present evidence from sources in the same context in which it was originally presented. Do not change the original author’s meaning or tone. Be especially careful of such changes when you paraphrase or summarize. See Spotlight on… Citation for more about paraphrasing and summarizing.
- Cite evidence to the proper sources. Use the citation style required by your instructor, usually MLA Documentation and Format or APA Documentation and Format Proper citations direct readers to more information about your sources and show you are not plagiarizing.
- Incorporate common ground between readers who support your position and those who do not. To do this, many authors use evidence pulled from patriotic or religious documents to create ethical appeal. For instance, regarding the activity that took place at the Capitol, both sides might find common ground in the First Amendment of the U.S. Constitution, which outlines rights of the people. The protestors might cite the section of the amendment that deals with freedom of assembly; those on the other side might point out that the amendment guarantees “the right of the people peaceably to assemble” and that the assembly was not peaceable.
Logos (Logical Appeal)
You appeal to your audience’s intelligence by showing that you understand the value of sound reasoning. To do this, state your position clearly and support it with rational arguments, critical thinking, and credible evidence. Also, avoid exaggerating or making claims you cannot support with reliable evidence. Many authors use facts and statistics to create logical appeal.
To appeal to logic, follow these guidelines:
- State your position clearly with easy-to-understand language. For example, to appeal to readers’ intelligence in your paper about hiring additional police to defend the Capitol, avoid using vocabulary that would feel unnatural. Instead of writing “The verbiage from the campaigners importuned the dispossession of their statesmen,” write “The protestors demanded the resignations of their congressional representatives.”
- Support your position with reasoning that is neither incomplete nor faulty. Sound reasoning is that which all can agree makes sense. For example, you would not contend that to be ready for future protests, the Capitol police force must be doubled from 2,000 to 4,000 because you cannot know the number needed at any time. However, you could argue that the Capitol police force and government leaders should study the January 6, 2021, riot to determine how many additional police are needed, should such an occasion arise again.
- Present your critical thinking through a well-constructed argument. By ordering your position argument in a manner that moves logically from one point to the next, you help guide readers through your thought process, which is reflected in the smooth flow of ideas that work together to support your thesis.
- Incorporate credible evidence from trusted and reliable academic, government, media, and professional sources. Using these sources shows readers that you recognize biased material and have excluded it from your paper.
Pathos (Emotional Appeal)
You appeal to your audience’s feelings—such as sympathy, anger, fear, insecurity, guilt, and conscience—to support your position.
For example, to appeal to your audience’s emotions in your paper about the need for more Capitol police, you might do the following:
- Help your readers understand feelings of fear. One way to appeal to this emotion is to quote from interviews with government workers and bystanders who were hiding behind locked doors and had no police protection.
- Use vivid description and concrete language to recreate images that showed lone officers overwhelmed by crowds of people and beaten.
- Use nonaggressive language to address the positions of readers who do not support your stance. For example, some readers may believe that the federal government spends too much money already and should not allocate more. By using language that is not inflammatory, you can show your empathy for others, and this may help you convince them to support your position.
Kairos (Timeliness)
The sense of timing—presenting your position at the right time—is critical in a position argument. The issue must be worthy of attention at the time it is presented for readers to feel a sense of urgency. For example, in an argumentative paper about the significance of the Black Lives Matter (BLM) movement, you could do the following:
- Point out the history of the BLM movement, which began in 2013 after the acquittal of the man accused of killing Trayvon Martin (1995–2012) in 2012.
- Note that today, most of the speeches delivered in BLM rallies held across the country reference the May 2020 murder of George Floyd (1973–2020).
- Emphasize that Floyd’s killing remains front and center in the minds of rally participants. In other words, the topic of Floyd’s death is timely, and related circumstances indicate a favorable time for action.
You can find further discussion about these appeals in Rhetorical Analysis: Interpreting the Art of Rhetoric .
These are key terms and characteristics of position arguments:
- Allusion : direct or implied reference to a person, place, work of literature, idea, event, or anything a writer expects readers to know about. Allusion is a frequently used literary device.
- Citation : reference to the source of information used in a writer’s research.
- Critical thinking : ability to identify and solve problems by gathering information about a topic and then analyzing and evaluating evidence to form a judgment.
- Counterclaim (dissenting opinion) : statement of what the other side might say in opposition to the stance the writer takes about an issue.
- Ethos : appeal to readers’ ethical sense; establishing authority and credibility.
- Evidence : facts and other information that prove or disprove the validity of something written or stated.
- Introduction : first part of a paper. In position arguments, the writer alerts readers to the issue or problem discussed and often presents the thesis at the end of the introduction.
- Kairos : appeal to timeliness of the subject matter.
- Logos : appeal to readers’ sense of logic, or reason.
- Pathos : appeal to readers’ emotions.
- Purpose : author’s reason for writing the paper. In a position argument, the purpose is to persuade readers to agree with the writer’s stance.
- Reasoning : logical and sensible explanation of a concept.
- Recursive : movement back and forth from one part of the writing process to another.
- Rhetorical appeals : methods of persuasion (ethos, logos, pathos, and kairos).
- Rhetorical question : questions intended to make a point rather than to get an answer. Rhetorical questions, which often have no answers or no obvious answers, appear frequently in argument writing as a way of capturing audience attention.
- Topic : subject of a paper. In this genre, the topic is a debatable issue.
- Thesis : declarative sentence (sometimes two) that states a writer’s position about the debatable issue, or topic, of the paper.
- Transitional words or phrases : words and phrases that help readers connect ideas from one sentence to another or from one paragraph to another. Transitions establish relationships among ideas.
As an Amazon Associate we earn from qualifying purchases.
This book may not be used in the training of large language models or otherwise be ingested into large language models or generative AI offerings without OpenStax's permission.
Want to cite, share, or modify this book? This book uses the Creative Commons Attribution License and you must attribute OpenStax.
Access for free at https://openstax.org/books/writing-guide/pages/1-unit-introduction
- Authors: Michelle Bachelor Robinson, Maria Jerskey, featuring Toby Fulwiler
- Publisher/website: OpenStax
- Book title: Writing Guide with Handbook
- Publication date: Dec 21, 2021
- Location: Houston, Texas
- Book URL: https://openstax.org/books/writing-guide/pages/1-unit-introduction
- Section URL: https://openstax.org/books/writing-guide/pages/10-3-glance-at-genre-thesis-reasoning-and-evidence
© Dec 19, 2023 OpenStax. Textbook content produced by OpenStax is licensed under a Creative Commons Attribution License . The OpenStax name, OpenStax logo, OpenStax book covers, OpenStax CNX name, and OpenStax CNX logo are not subject to the Creative Commons license and may not be reproduced without the prior and express written consent of Rice University.

What is a Genre?
Like the word research , the word genre also has many definitions. At its most basic level, genre is the French word for “type.” In the world of English for Academic Purposes, it refers to a communicative event that is widely recognized. In terms of research, some common genres include research articles, grant proposals, conference papers, posters, abstracts, and even job-related documents such as cover letters, research statements, etc. In this book, we are focused on the research article genre.
As genres have particular characteristics, one way of learning how to write better within a given genre is to explore the characteristics of it, which is one of our primary goals in this book. Before we explore the research article, however, it’s important to know about genre systems, which are interrelated text types that often work together to achieve a communicative goal.
Genre chains
The concept of genre chains was first discussed in Swales (2004) [1] , where he defined a “chain” as a genre that is an antecedent of another genre. When studying English for Academic Purposes, it is common to approach the learning academic writing, for example, by exploring genre chains because it helps us understand certain types of genre, like research writing, as it is systematized and chronologically organized in an order of sequences.
Genre ecologies
Genres are also sometimes conceptualized in terms of their ecologies, or interrelated and interacting genres Erickson (2000) [2] . In terms of the research article genre, it is helpful to envision the research write-up as only one piece of the communication that occurs between scholars. For example, lab reports, conference presentations and published conference proceedings, white papers, systematic reviews, and more are all part of ecologies that comprise a research communication genre.
- Swales, J. M. (2004). Research genres: Explorations and applications. Cambridge: Cambridge University Press. ↵
- Erickson, T. (2000). Making sense of computer-mediated communication (CMC): Conversations as genres, CMC systems as genre ecologies. In 33rd Hawaii International Conference on System Sciences , ed. R. H. Sprague, Jr. Maui: IEEE Computer Society Press. ↵
Preparing to Publish Copyright © 2023 by Sarah Huffman; Elena Cotos; and Kimberly Becker is licensed under a Creative Commons Attribution-NonCommercial-ShareAlike 4.0 International License , except where otherwise noted.
Purdue Online Writing Lab Purdue OWL® College of Liberal Arts
Where do I Begin?
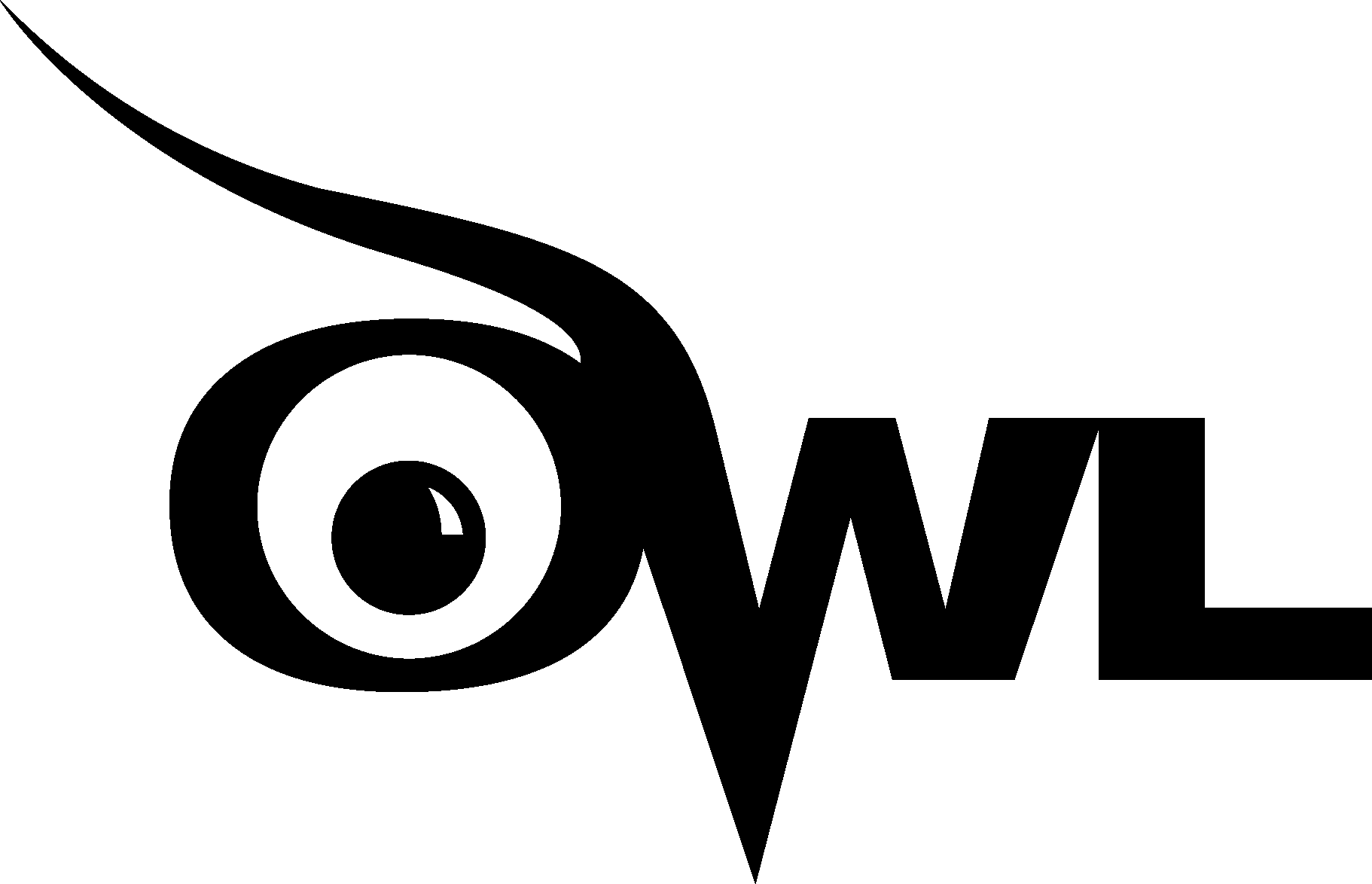
Welcome to the Purdue OWL
This page is brought to you by the OWL at Purdue University. When printing this page, you must include the entire legal notice.
Copyright ©1995-2018 by The Writing Lab & The OWL at Purdue and Purdue University. All rights reserved. This material may not be published, reproduced, broadcast, rewritten, or redistributed without permission. Use of this site constitutes acceptance of our terms and conditions of fair use.
This handout provides detailed information about how to write research papers including discussing research papers as a genre, choosing topics, and finding sources.
There is neither a template nor shortcut for writing a research paper; again, the process is, amongst other things, one of practice, experience, and organization, and begins with the student properly understanding the assignment at hand.
As many college students know, the writer may find himself composing three quite different research papers for three quite different courses all at the same time in a single semester. Each of these papers may have varying page lengths, guidelines, and expectations.
Therefore, in order for a student to become an experienced researcher and writer, she must not only pay particular attention to the genre, topic, and audience, but must also become skilled in researching, outlining, drafting, and revising.
For a discussion of where to begin one's research, see Research: Overview .
Outlining is an integral part of the process of writing. For a detailed discussion see Developing an Outline .
Drafting is one of the last stages in the process of writing a research paper. No drafting should take place without a research question or thesis statement; otherwise, the student will find himself writing without a purpose or direction. Think of the research question or thesis statement as a compass. The research the student has completed is a vast sea of information through which he must navigate; without a compass, the student will be tossed aimlessly about by the waves of sources. In the end, he might discover the Americas (though the journey will be much longer than needed), or—and what is more likely—he will sink.
For some helpful ideas concerning the initial stages of writing, see Starting the Writing Process .
Revising, Editing, Proofreading
Revising is the process consisting of:
- Major, sweeping, changes to the various drafts of a project
- An evaluation of word choice throughout the project
- The removal of paragraphs and sometimes, quite painfully, complete pages of text
- Rethinking the whole project and reworking it as needed
Editing is a process interested in the general appearance of a text, and includes the following:
- Analysis of the consistency of tone and voice throughout the project
- Correction of minor errors in mechanics and typography
- Evaluation of the logical flow of thought between paragraphs and major ideas
This process is best completed toward the final stages of the project, since much of what is written early on is bound to change anyway.
Proofreading is the final stage in the writing process, and consists of a detailed final reread in order to find any mistakes that may have been overlooked in the previous revisions.
For a discussion of proofreading, see Proofreading Your Writing .
- Getting Started
- Research Plan
- Paper Genre
- Choosing a Topic
- Finding Sources
- Evaluating Sources
- Taking Notes
Writing Guides

Chat with a Librarian
What is genre.
Genre defines your paper and how it will be presented. A genre can clue your audience in on what they can expect from your paper. It can also act as a guideline for your research and can help you when it is time to structure your paper.
Genre Guides
Below are some of the most common styles in academic writing. Click on the link for prewriting techniques and example outlines.
- Compare/Contrast Papers
- Persuasive & Argumentative Papers
- Writing as Inquiry Papers
- << Previous: Research Plan
- Next: Choosing a Topic >>
- Last Updated: Feb 13, 2024 9:45 AM
- URL: https://tstc.libguides.com/search
Background Texture Image Credit
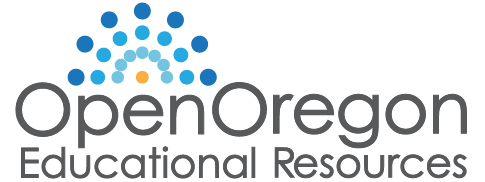
14.3 Methods for Studying Genres
The previous section outlined some key terms and definitions for the study of writing. This section builds on that by providing an overview of research tools that can be used to better understand writing-in-context. Some of these tools–like an interview–may seem more familiar to you than others (such as genre analysis). At the same time, an activity you probably engage in every day–observation–achieves importance when done in the context of research and analysis.
There is no one right way to “do” writing research. Choosing the right tools depends on what it is you hope to learn. As you study a genre, whether it is a resume, a report, a procedural, or a complaint letter, think creatively about which of the following methods might help you learn more about it.
Genre/Textual Analysis
If genres are the key object of study for writing researchers, then genre analysis is the key tool for studying those objects–for unlocking their meaning. While it is true that we can learn a great deal about genres by observing people using them and even asking their users about them (which I detail in the next section), there is often important meaning that goes unnoticed by the producers and users of a genre. This meaning is what writing researchers try to access by studying the genres themselves. To put it another way, genres often have embedded in them a kind of code or shorthand that can reveal important information about the context in which they are used. As someone learning to write in that context, such information can help you to advance in your writing skills more quickly.
So how do writing researchers do this analysis? In short, genre analysis involves picking apart and noting the various features of a particular text in order to figure out what they mean (i.e., why they are significant) for the people who use that genre. In that sense, writing researchers act as detectives, revealing clues in order to then piece them all together and generate a cohesive story about what those clues mean.
It probably will not surprise you to know that, once again, curiosity plays an important role in conducting genre analysis. While it can be tempting when looking at a text to think there is not much to say about it (this is especially true when you take something as everyday as your grocery list or the menu at a coffee shop), when we begin to ask questions, the complexity of a text (and the genre it represents) is pretty quickly revealed.
A precursor to genre analysis is what researchers call “document collection.” While it is true that the more samples of a genre you find the more reliable and extensive your analysis can and will be, analyzing even one text can reveal a great deal. So, if genre analysis sounds kind of overwhelming or challenging, start by looking at a single sample text rather than many.
The following questions can get you thinking about (and taking notes on) how you would describe a sample text, by focusing on its content , its form , and its presentation :
- Who and what is referenced in the document?
- What information is included in the document? How much?
- What is the rhetorical purpose of the document?
- How is information organized, from beginning to end? In other words, what appears where?
- What kinds of sentences are used (questions, statements, commands, etc.)?
- What do you notice about the kind of language that is used?
- How would you describe the tone of the writing?
- How does the text use rhetorical appeals (ethos, pathos, logos)?
- Are section headings used in the document?
- Does the document include text only, or text and images? What is the layout like?
- What font size and style is used?
- How would you describe the “look” of the document?
Figure 1 provides a sample police incident report and is followed by some notes you would be likely to make based on the suggested questions above. Alternate formats: Word version of incident report ; PDF version of incident report .
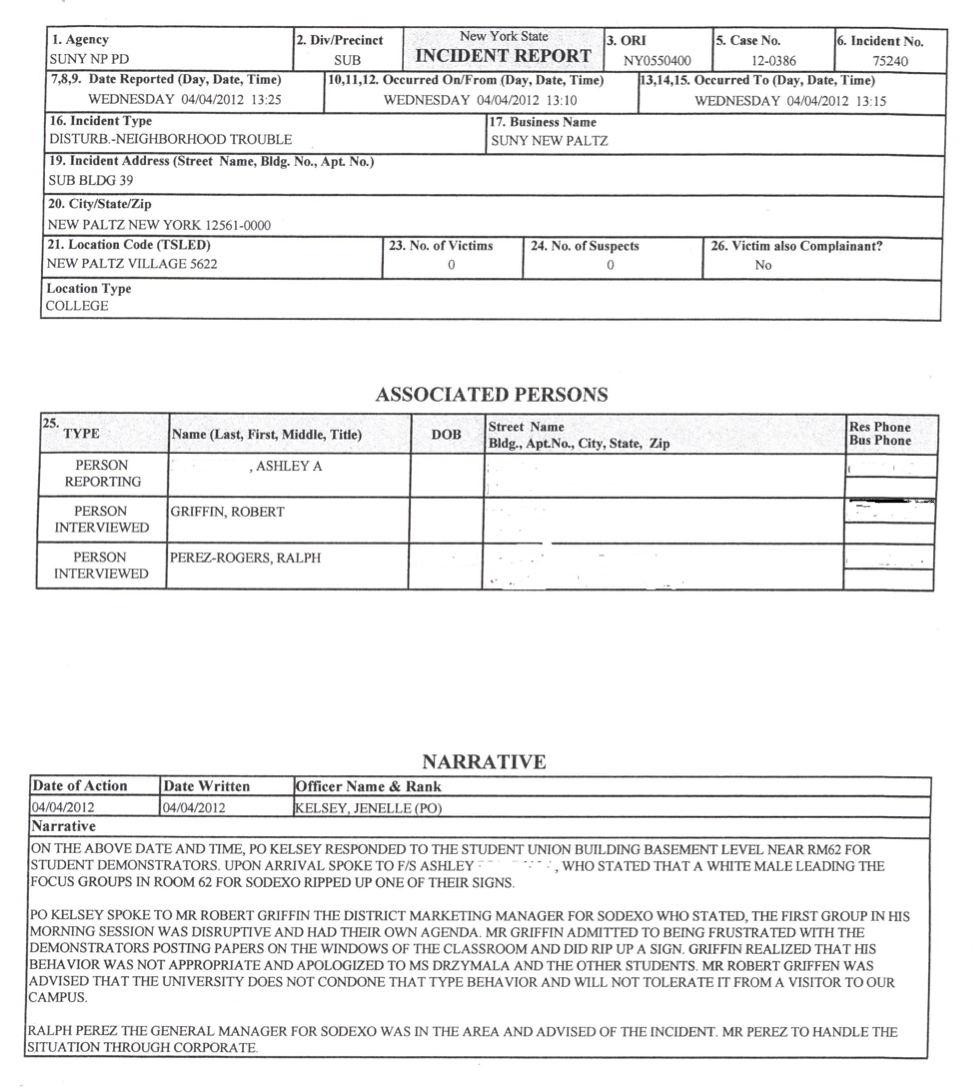
Case Study, Part One: Notes on the Incident Report
- When the incident occurred
- Where the incident occurred
- Who was involved
- How many were involved
- Who was interviewed
- What happened (narrative)
- Which precinct is responsible for investigating
- Type of incident, people involved (victims/suspects), and location
- Filing information (case and incident numbers)
- Three distinct sections
- Logistical info appears first, “associated persons” appears second, narrative of the event appears third
- Narrative uses simple declarative statements, typically with people occupying the place of subject
- Use of verbs is active but primarily neutral, with a focus on communication that transpired (“responded,” “spoke,” “admitted,” “realized,” “advised”), with two instances of the use of passive voice (“was advised”)
- Narrative is described with a neutral tone and feels formal
- Focus of narrative is on actions taken that
- Narrative is described in chronological order, beginning with officer responding to incident, moving through the incident, and providing information on follow-up
- Items used for referential or filing reasons are numbered
- Information is organized into boxes and tables
- Each section is clearly labeled
- Abbreviations are used throughout
- Report is typed
After you have answered these questions, it is time to start looking for patterns and connections that will help you draw conclusions about what these features mean. Doing so involves a kind of creative thinking that is best done by someone who has been involved in studying or practicing the profession under investigation. Generally speaking, it is best to give some consideration to how the features of a particular genre might be connected to goals, objectives, and values of a particular position, organization, and/or context, since it is that context that produced the need for that genre in the first place.
Case Study, Part Two: Analysis of the Incident Report
The content, form, and presentation of the police incident report form work together to present a verifiable , objective account. Used internally, the design of the form helps to create uniformity by directing the officer to include the information that is likely to prove most salient for police purposes and for easy retrieval should future incidents occur. This streamlined approach to documentation keeps the focus on material and factual evidence, which clearly relates to the fact that this is a document that may be used in a legal context.
Generally speaking, a document that pays little attention to design, but has a great deal of detailed content, might derive from a situation where people place heavy emphasis on the development of ideas but don’t necessarily need to act on those ideas; on the other hand, if a document makes heavy use of section headings in order to direct the reader more carefully, it might suggest a need for greater efficiency of time and/or a number of readers with different background knowledge. Of course, there are genres that will do both: include a great number of complex ideas, neatly organized into easily accessible sections. No matter what you find, there is an interpretation to be discovered and explained with evidence from the text itself. The connecting of evidence to interpretation/conclusion is genre analysis.
[ Genre Analysis Essay video without captions ; Genre Analysis Essay video with captions ]
Interviewing is something that happens informally all the time when we query colleagues or supervisors about how to write in a new genre. But a formal interview is a particular kind of research method that takes a bit of practice and can be quite difficult if you have never done it before. With a question we ask of a colleague, we usually have something very specific we want to know, but as a research method, interviews are usually used in order to answer a research question –and it is that distinction that you need to keep in mind.
A good research question, as you may have already learned in other college-level classes, does not have an easy answer. In fact, it usually does not have a single answer either; instead, it is a question that requires interpretation and that might be answered differently depending on who you ask. That said, it is answerable, meaning that given the right collection of evidence, you would be able to craft a response of some kind. In writing research, the interview is one way to collect just such evidence, since talking to someone about how, when, and why they use writing in their profession can provide all kinds of insight that you might miss if you were to analyze a text all by itself. Typically, these kinds of questions (of the “how,” “when,” and “why” variety) help writing researchers to understand the particular importance of writing to a specific profession, industry, organization, or even economy.
It is not uncommon for people in workplace settings not to realize just how much writing is a part of their everyday work practices. In the course of being asked questions, though, they often reveal the way that writing helps them accomplish their jobs successfully and make sure the company or organization runs effectively and achieves its goals. This is true whether you are interviewing a doctor, a firefighter, a restaurant manager, an electrician, a politician, a general contractor, or a computer specialist.
Here are some general advice and reminders for getting organized to conduct an interview:
- Practice good manners when scheduling the interview. This is an opportunity to practice being professional in your communication: everything you know about audience analysis should come into play as you request someone’s time and input.
- Be sure to practice your interview questions ahead of time. Questions that seem straightforward to you might not be clear to someone else; alternatively, they might clearly call for a different kind of answer than what you anticipated. The best way to know is to practice them on someone who is not your intended interviewee. Then, revise accordingly.
- Request permission to record the interview. You will be glad to have a record to return to if your interviewee says yes. Whether or not you record the interview, though, be sure to take notes in the interview (this is something you can and should practice in your practice interview as well). Recording devices can fail; writing during the interview can also help you to focus on what your interviewee is saying and to think of new, sometimes clarifying questions, as the interview proceeds.
Observation
Another powerful research tool is simply observing where the writing of a particular profession takes place. The values of a company or organization, the expectations they hold for their employees and various working conditions are often on display if you only look for them. For example:
- Is the workplace open to the public, or does it require secure entry?
- Do people work in offices or cubicles? Or maybe there is no individual work space at all?
- How many meeting rooms are there? How big are they?
- Are people milling around, or are they mostly on computers?
- How is the workplace decorated?
- What is the dress code?
The answers to these questions can lead to new insight regarding how genres are used and produced and help develop new questions for you to consider. Furthermore, observation also helps with imagining texts in use, which is so crucial to an effective analysis of your audience.
Genre Ecology Maps
A Genre Ecology Map, or GEM, is a visual representation of genres in action, interacting with one another. Let’s consider an earlier example: the job description. We could explain, using words, that the job description leads to job applications, which (often) lead to interviews and background checks, the hiring of an individual and all the associated paperwork, as well as training materials. But if we wanted to represent that visually, it would look something like Figure 2. Alternate formats: Word version of Job Application GEM ; PDF version of Job Application GEM .
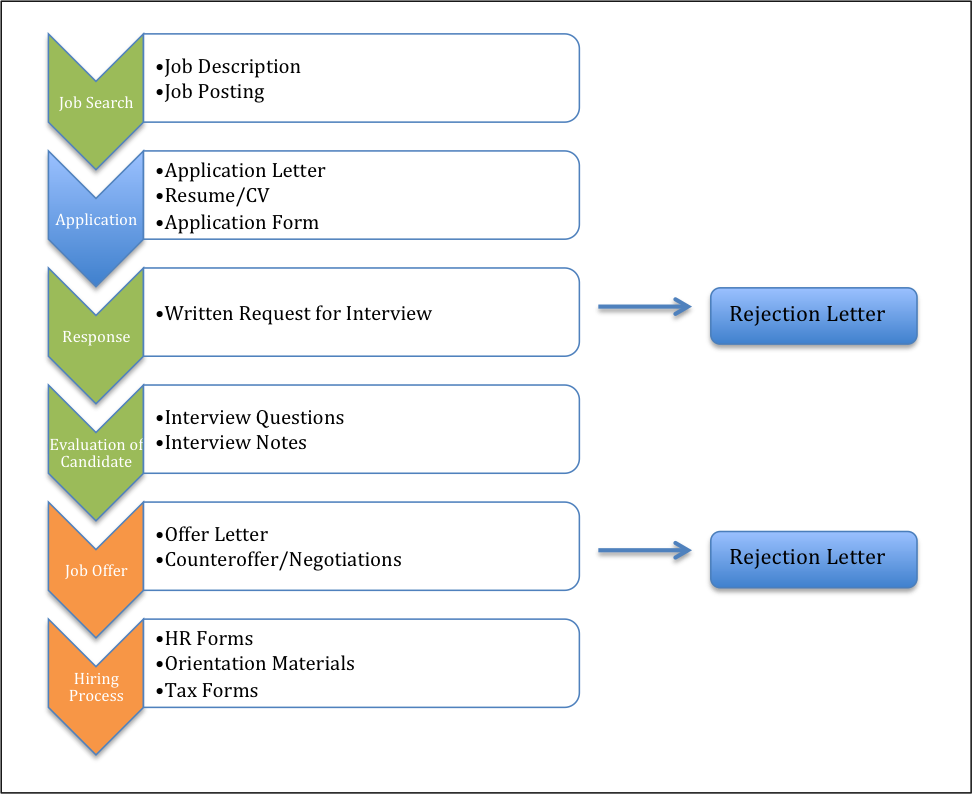
All of a sudden, with a visual illustration, we have a slightly different understanding of the complexity involved in the production and circulation of different kinds of writing. Figure 3 provides another example, one that captures the intersection of different writers, positions, and stakeholders (put another way: the intersection of different genre sets in the college classroom). Alternate formats: Word version of Classroom GEM ; PDF version of Classroom GEM .
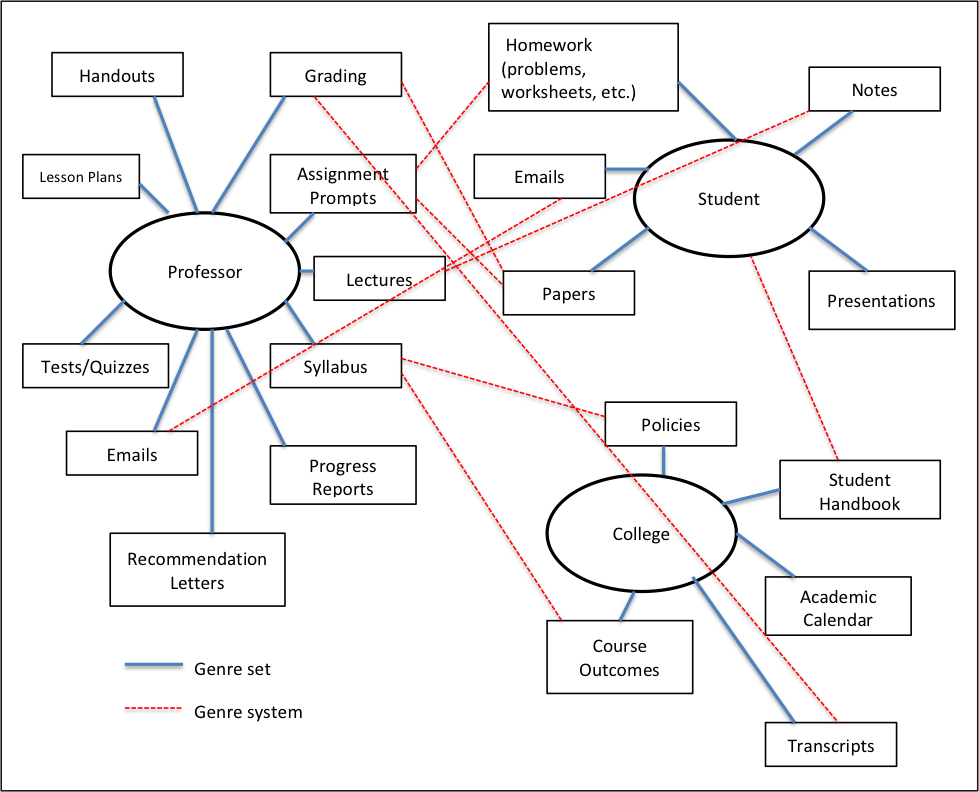
Particularly if you are a visual learner, maps like those above can help you to “see” genres in a way you might not otherwise and to reinforce what I have noted in sections above about how writing is not static but actually performs “actions” in various workplace settings.
CHAPTER ATTRIBUTION INFORMATION
This chapter was written by Allison Gross, Portland Community College, and is licensed CC-BY 4.0 .
Technical Writing Copyright © 2017 by Allison Gross, Annemarie Hamlin, Billy Merck, Chris Rubio, Jodi Naas, Megan Savage, and Michele DeSilva is licensed under a Creative Commons Attribution-NonCommercial-ShareAlike 4.0 International License , except where otherwise noted.
Share This Book
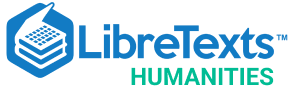
- school Campus Bookshelves
- menu_book Bookshelves
- perm_media Learning Objects
- login Login
- how_to_reg Request Instructor Account
- hub Instructor Commons
- Download Page (PDF)
- Download Full Book (PDF)
- Periodic Table
- Physics Constants
- Scientific Calculator
- Reference & Cite
- Tools expand_more
- Readability
selected template will load here
This action is not available.
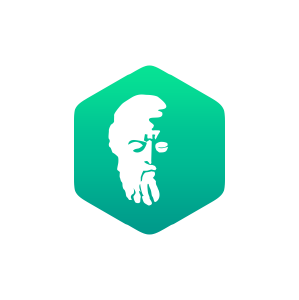
5.10: The Traditional Research Paper is Best
- Last updated
- Save as PDF
- Page ID 65300
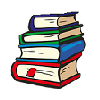
- Cheryl E. Ball & Drew M. Loewe ed.
- West Virginia University via Digital Publishing Institute and West Virginia University Libraries
Author: Alexandria Lockett, English, Spelman College
To understand the research paper and its contemporary significance, we must acknowledge how the Internet makes the process of research and of writing research much more complex. A vast majority of Internet users use the web and social media multiple times per day. Long gone are the days when one major function of the research paper was to bring students into contact with libraries. Today’s students need to also know how to navigate the Internet—a vast digital source of information whose system architecture affects the work of teaching and research.
Typically, a first-year college student’s research paper assignment might require 5–10 sources, whereas advanced students are probably asked to cite no more than 30 sources. These figures may stem from research concerns that emerged during an entirely different technological history. This number makes sense if we consider the physical labor involved in visiting the library, communicating with a librarian, finding the card catalog, writing down serial numbers, walking up several flights of stairs, locating the correct stack, browsing the stack, and using a step stool to reach the source in question—rinse and repeat. These spatio-temporal aspects of composing a research paper most likely affected source selection. For example, some textbook writers used to complain about how research papers often lacked primary sources and relied on questionable secondary materials despite physical libraries’ numerous resources.
The number of sources a paper should include remains an essential guideline that defines the research paper, which affects how students prioritize their efforts. Most college students will not have to worry about physically setting foot in a library building to meet the research paper’s quantitative source requirement. In fact, finding the number of sources is the easiest part for student writers, because a broad search will take less than one full second to retrieve millions and millions of sources on any given subject.
Of course, finding sources may be easy, but strategically incorporating them into an argument may seem impossible to today’s writers. How could any teacher reasonably expect a student to come up with a thesis when they are seconds away from an uncountable selection of sources and communities of knowledge? What incentive does any researcher have to make new ideas in the data deluge? When almost anything that can be conceived is searchable via the Internet, what is the researcher really responsible for? Verifying data? Deliberating about its significance? Informing their social media networks?
Unfortunately, the labor involved in researching and using the Internet for research tends to be ignored. Instructors may underestimate the nuances of popular databases and overestimate students’ frequent use of databases as competency. However, Internet research really is a lot of work. Researching “the research paper” via Google, Google News, and Google Scholar retrieves almost 19,000,000 results. Unaccompanied by quotes, the number of results exceeds one billion. Without awareness of the importance of Boolean logic, or operators that affect the scale of results, a researcher may find herself drowning in data. When plugged into proprietary databases available to most college and university students such as Proquest, JSTOR, ScienceDirect, and Academic Search Complete, the research paper displays several thousands of sources per database.
Consuming data dumps, whether by the dozens, hundreds, or thousands, would take decades to read, summarize, annotate, interpret, and analyze. These processes do not include the creative task of evaluating the patterns between data or learning more about the backgrounds, values, and beliefs of their authors—all of which were easy to take for granted when working with a limited number of print sources. Therefore, the 21st-century politics of research is defined by the problem of scope. There is simply too much information.
Although traditional research papers undoubtedly address the problem of how to evaluate and integrate sources, a contemporary first-year college writing student will probably be sensitive to her limitations as a single writer. What kind of original contribution can teachers reasonably expect the average high school or college student to create that they can’t instantly access via the Internet? It hardly seems appropriate, or fair, to ask any student, regardless of classification, to wade through oceanic swaths of online data for the purposes of making an original contribution, as a single author, to some public policy debate or academic discipline.
Moreover, there are few incentives to ethically conduct research when the paper is taught as a bureaucratic necessity of the high school or college experience. I could wax poetic about the joys of discovery and the wonderment of wandering aimlessly through scholarly work, but the research paper does not tend to encourage this openness. Students may believe that if they include a certain number of sources of a particular kind, and use the instructor’s preferred documentation style, that their research paper will be successful. Too often this simplistic approach is mistaken for laziness. But, most people cannot handle the chore of deciphering the data deluge. Plagiarism, then, becomes a major effect of the Internet’s causal effect on teaching and learning the research paper.
Thousands, if not millions, of students will use Google and Wikipedia as first steps towards plagiarizing work—plunging into an abyss of boredom or cultivating their curiosity about a subject. Their teachers will obediently, and sometimes zealously, police plagiarism with the assistance of Google’s robust search engines and Turnitin. Both the student and teacher will use social media to talk about their frustrations and joys in real-time. The student’s plagiarism will most certainly deserve a status update, some likes, and perhaps some comments. The teacher’s boring instruction and the difficulty of the assignment will end up discussed in text messages, and who knows which social media platforms or blogs. At worst, the student will complain about it to RateMyProfessor. com or in the teacher’s evaluations. These examples illustrate that the Internet and mobile technologies extend the reach of the research paper far beyond classrooms and institutions. In fact, Research 2.0 converges with offline human activity, extending its causal force across several media, very much affecting real life.
The Research Paper 2.0
The entire Internet user experience is embedded in knowledge economies, which impact how people learn. For example, Internet users’ attention is managed and directed by large private corporations like Apple, Microsoft, Facebook, Amazon, and their partnerships and affiliations with the handful of multinational
conglomerates that produce and own the media. The data collection practices and design of these companies’ websites direct users’ attention, which affects their research skills. The same Internet users will also participate in the development of revolutionary open-source, collaborative archives like Wikipedia, which models an unprecedented effort in collective intelligence.
By virtue of accessing and using the Internet, its users are researchers. As a landscape of big data, the Internet’s primary purpose is to facilitate research and its subsequent acts of storing, producing, and retrieving huge amounts of information (as it was when it was conceived at CERN). Unfortunately, the Internet’s global multidisciplinary, multi-sector, and multi-generational history and culture are largely unknown by most contemporary students—even though they interact with it every single day. Thus, the research paper in contemporary web settings should be designed to directly address any of the technological politics of blended learning and emerging technologies.
At best, research papers 2.0 will encourage students and instructors to reflect on how the Internet and its complex networked features mediate their research and writing process. Specifically, research 2.0 might include a much stronger emphasis on collaborative and professional writing. Students may organize online writing groups via Google+ or LinkedIn based on their topical interests to provide evidence of their ability to lead and contribute to a team. They might also contribute to crowdsourced, annotated bibliographies of paper mill websites to help the school’s integrity office, or participate in one of the Wikipedia edit-a-thons sponsored by Art + Feminism. Research 2.0—be it delivered through a paper, ePortfolio, Wikipedia, or Prezi—might include ethical evaluations of research scandals, the legality of citizen surveillance footage of police brutality, and a comparative analysis of big data websites like Data.gov or WikiLeaks.com. But not all of its topics need to be digitally themed, but it can and should use digital technologies and resources to refresh what the research paper can do in the 21st century.
One of research paper 2.0’s primary objectives should be bringing students into contact with research communities that synergize online experiences with offline social events. Towards this end, Wikipedia is an ideal space for (and subject of) research in 2.0 because it has been a subject associated with research writing conduct for over a decade. Most students’ experience with Wikipedia and academic writing is that its use is strictly forbidden.
When it is cited as a source in a research paper, teachers are annoyed or infuriated because they can’t understand why students don’t know better. Regardless of how much suspicion surrounds the veracity of Wikipedians’ knowledge, every Internet user consults this information resource. Furthermore, students and teachers would have a much different experience with Wikipedia, and research, if students understood the site from the perspective of its editors. Thus, the Wiki Education Foundation, an affiliate of the Wikimedia Foundation—the non-profit organization that runs Wikipedia among several other projects—has made strong attempts to connect Wikipedia to educational institutions through their Wikiedu.org platform.
Due to technological, and thus pedagogical limitations, the traditional research paper is incapable of translating the affordances of research writing to online environments. Therefore, research 2.0 should respond to the significance of human interaction with the Internet and the politics of big data. We live in a superabundance of learning spaces, and thus, infinite possibilities for research. However, few educational institutions and disciplines are cultivating the technical, scientific, and artistic competencies necessary for editing, navigating, and managing the Internet’s infinite retrieval mechanisms. When students are taught how to recognize that they have the power to diversify Internet content with high-quality research, the research paper 2.0 could play a major role in balancing the dynamics of knowledge production between traditional institutions and emerging media.
Further Reading
To learn more about how the purpose and genre of the American research paper has changed since the late 19th century, see John Scott Clark’s A Briefer Practical Rhetoric . Also important is Robert Morell Schmitz’s Preparing the Research Paper, A Handbook for Undergraduates. Additionally, Cecile Williams and Allan Stevenson’s A Research Manual and Florence Hilbish’s The Research Paper show that the research paper continued to be the central subject of writing manuals and textbooks throughout the mid-20th century.
For more information about the popularity of the research paper assignment, as well as teacher training in the genre, see James E. Ford and Dennis R. Perry’s Research Paper Instruction in the Undergraduate Writing Program , and Rethinking the Research Paper , written by Bruce Ballenger. Robert Davis and Mark Shadle’s Building a Mystery: Alternative Research Writing and the Academic Act of Seeking.
also discusses non-traditional approaches to research writing. Researchers Tere Vaden and Juha Suoranta have critically evaluated some of the ways in which educators ought to make sense of the politics of making information in Web 2.0 contexts in their book Wikiworld . In addition, for information on how researchers are measuring data and its volume, the following studies may be useful: “UC San Diego Experts Calculate How Much Information Americans Consume”; J.E. Short, R.E. Bohn, & C. Baru’s study, “How much information”; and Martin Hilbert’s “How to Measure ‘How Much Information?’ Theoretical, Methodological, and Statistical Challenges for the Social Sciences.”
big data, Boolean logic, data deluge, traditional research paper, web 2.0
Alexandria Lockett is an assistant professor of English at Spelman College. Her areas of applied expertise include technical and professional writing, teaching with technology, and writing program administration, with research interests in digital history, new media, surveillance, social movements, discourse theory, transdisciplinary communication, and cultural rhetoric. In the capacities of tutor, mentor, editor, career assistant, administrator, and instructor, she’s worked with diverse groups of college writers representing all classification levels including multilingual (ESL), first-generation, and students from underrepresented and diverse backgrounds at the University of Oklahoma and Pennsylvania State University. Her twitter handle is @MzJaneNova. Her public portfolio link is www.alexandrialockett.com
Our websites may use cookies to personalize and enhance your experience. By continuing without changing your cookie settings, you agree to this collection. For more information, please see our University Websites Privacy Notice .
Neag School of Education
Graduate Certificate in College Instruction
Exploring genres beyond the research paper.
By Sophie Buckner
Aw… the research essay. The staple in undergraduate writing. At best, the student research essay poses a provocative question and curates convincing and credible evidence to support the student writer’s own unique answer to that question. At its worst, the research essay is a frustrating document embodying the boredom and/or confusion of the student writer.
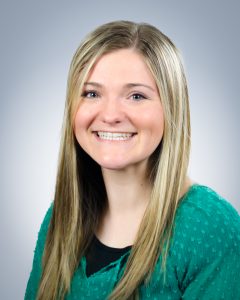
In my experience as an instructor in UConn’s First-Year Writing program, I have seen research papers all across this spectrum, but mostly, research paper assignments produce formulaic and unengaged writing. And despite the few excellent outliers, the task of grading such an assignment—which is generally the longest writing assignment in a class—is daunting to say the least.
Besides, as I think of the future writing of my students, I can’t help but wonder if these research essays will mean anything to them when they leave the university. Perhaps instructors could do their students (and themselves) a favor by assigning a variety of genres beyond the research paper.
While we focus so much of our attention on the traditional research paper, there are so many other forms of writing that are valuable and worth teaching our students. What about personal essays, blog posts, or op-eds? Or what about digital writing? Like podcasts or videos? All these types of writing have real audiences that will motivate students to analyze the context of their writing and see it as something more than a grade.
Additionally, many writing studies experts argue that when students make a personal connection to what they are writing about, they remember the material better and can better internalize the knowledge (Sommer and Saltz 2004; Bean 2011; Newkirk 2014). In his book Engaging Ideas , John C. Bean states:
As cognitive research has shown, to assimilate a new concept, learners must link it back to a structure of known material, determining how a new concept is both similar to and different from what the learner already knows. The more that unfamiliar material can be linked to the familiar ground of personal experience and already existing knowledge, the easier it is to learn. (151)
The formality of the traditional research essay generally bars “indulging” in personal reflection, but other genres can provide the personal connection to subject material that will allow students to fully understand and remember the material.
I assign what I call a non-traditional research essay. In this type of essay, students conduct research about their topic and then write small pieces in different genres. Students write poetry, short stories, mock news reports, fabricated journal entries, and so much more. Then they arrange their pieces together to create one whole. As students learn the conventions of different genres, this assignment also pushes them to think about how their ideas fit together, rather than just plugging them into a formula. This assignment in particular gets students excited about their research, and I’ve noticed that when students care about their material, the quality of their writing improves.
Another genre that I enjoy assigning is the op-ed. Op-eds are characteristically short, so students don’t feel overwhelmed. But students also learn to make their argument and back it up concisely. Another bonus of the op-ed genre is that students can have an audience for their writing besides the instructor. I have encouraged my students to send their writing to the local newspaper, and several have gotten their work published.
Although the traditional research essay may remain a staple in undergraduate education, it is not the only option. Sometimes, a different genre is more practical and will leave a longer-lasting impression. And it doesn’t hurt to have a little fun!
For ideas on how to incorporate alternative genres into your classroom, see:
- Engaging Ideas: The Professor’s Guide to Integrating Writing, Critical Thinking, and Active Learning in the Classroom by John C. Bean
- “Collage: Your Cheatin’ Art” by Peter Elbow
- Twenty-One Genres and How to Write Them by Brock Drethier
- Fearless Writing: Multigenre to Motivate and Inspire by Tom Romano
Additional Works Referenced
Bean, j. c. engaging ideas: the professor’s guide to integrating writing, critical thinking, and active learning in the classroom . (2 nd ed.) san francisco, ca: jossey-bass. 2011., newkirk, t. minds made for stories: how we really read and write informational and persuasive texts . portsmouth, nh: heinemann, 2014., sommers, n. and l. saltz. “the novice as expert: writing the freshman year.” college composition and communication , vol. 56, no 1, sep 2004, pp. 124-149. .
- Campus Library Info.
- ARC Homepage
- Library Resources
- Articles & Databases
- Books & Ebooks
Baker College Research Guides
- Research Guides
- General Education
COM 1010: Composition and Critical Thinking I
- Understanding Genre and Genre Analysis
- The Writing Process
- Essay Organization Help
- Understanding Memoir
- What is a Book Cover (Not an Infographic)?
- Understanding PowerPoint and Presentations
- Understanding Summary
- What is a Response?
- Structuring Sides of a Topic
- Locating Sources
- What is an Annotated Bibliography?
- What is a Peer Review?
- Understanding Images
- What is Literacy?
- What is an Autobiography?
- Shifting Genres
Understanding What is Meant by the Word "Genre"
What do we mean by genre? This means a type of writing, i.e., an essay, a poem, a recipe, an email, a tweet. These are all different types (or categories) of writing, and each one has its own format, type of words, tone, and so on. Analyzing a type of writing (or genre) is considered a genre analysis project. A genre analysis grants students the means to think critically about how a particular form of communication functions as well as a means to evaluate it.
Every genre (type of writing/writing style) has a set of conventions that allow that particular genre to be unique. These conventions include the following components:
- Tone: tone of voice, i.e. serious, humorous, scholarly, informal.
- Diction : word usage - formal or informal, i.e. “disoriented” (formal) versus “spaced out” (informal or colloquial).
- Content : what is being discussed/demonstrated in the piece? What information is included or needs to be included?
- Style / Format (the way it looks): long or short sentences? Bulleted list? Paragraphs? Short-hand? Abbreviations? Does punctuation and grammar matter? How detailed do you need to be? Single-spaced or double-spaced? Can pictures / should pictures be included? How long does it need to be / should be? What kind of organizational requirements are there?
- Expected Medium of Genre : where does the genre appear? Where is it created? i.e. can be it be online (digital) or does it need to be in print (computer paper, magazine, etc)? Where does this genre occur? i.e. flyers (mostly) occur in the hallways of our school, and letters of recommendation (mostly) occur in professors’ offices.
- Genre creates an expectation in the minds of its audience and may fail or succeed depending on if that expectation is met or not.
- Many genres have built-in audiences and corresponding publications that support them, such as magazines and websites.
- The goal of the piece that is written, i.e. a newspaper entry is meant to inform and/or persuade, and a movie script is meant to entertain.
- Basically, each genre has a specific task or a specific goal that it is created to attain.
- Understanding Genre
- Understanding the Rhetorical Situation
To understand genre, one has to first understand the rhetorical situation of the communication.
Below are some additional resources to assist you in this process:
- Reading and Writing for College
Genre Analysis
Genre analysis: A tool used to create genre awareness and understand the conventions of new writing situations and contexts. This a llows you to make effective communication choices and approach your audience and rhetorical situation appropriately
Basically, when we say "genre analysis," that is a fancy way of saying that we are going to look at similar pieces of communication - for example a handful of business memos - and determine the following:
- Tone: What was the overall tone of voice in the samples of that genre (piece of writing)?
- Diction : What was the overall type of writing in the three samples of that genre (piece of writing)? Formal or informal?
- Content : What types(s) of information is shared in those pieces of writing?
- Style / Format (the way it looks): Do the pieces of communication contain long or short sentences? Bulleted list? Paragraphs? Abbreviations? Does punctuation and grammar matter? How detailed do you need to be in that type of writing style? Single-spaced or double-spaced? Are pictures included? If so, why? How long does it need to be / should be? What kind of organizational requirements are there?
- Expected Medium of Genre : Where did the pieces appear? Were they online? Where? Were they in a printed, physical context? If so, what?
- Audience: What audience is this piece of writing trying to reach?
- Purpose : What is the goal of the piece of writing? What is its purpose? Example: the goal of the piece that is written, i.e. a newspaper entry is meant to inform and/or persuade, and a movie script is meant to entertain.
In other words, we are analyzing the genre to determine what are some commonalities of that piece of communication.
For additional help, see the following resource for Questions to Ask When Completing a Genre Analysis .
- << Previous: The Writing Process
- Next: Essay Organization Help >>
- Last Updated: Feb 23, 2024 2:08 PM
- URL: https://guides.baker.edu/com1010
- Search this Guide Search
Explore our publications and services.
University of michigan press.
Publishes award-winning books that advance humanities and social science fields, as well as English language teaching and regional resources.
Michigan Publishing Services
Assists the U-M community of faculty, staff, and students in achieving their publishing ambitions.
Deep Blue Repositories
Share and access research data, articles, chapters, dissertations and more produced by the U-M community.
A community-based, open source publishing platform that helps publishers present the full richness of their authors' research outputs in a durable, discoverable, accessible and flexible form. Developed by Michigan Publishing and University of Michigan Library.

- shopping_cart Cart
Browse Our Books
- See All Books
- Distributed Clients
Feature Selections
- New Releases
- Forthcoming
- Bestsellers
- Great Lakes
English Language Teaching
- Companion Websites
- Subject Index
- Resources for Teachers and Students
By Skill Area
- Academic Skills/EAP
- Teacher Training
For Authors
Prospective authors.
- Why Publish with Michigan?
- Open Access
- Our Publishing Program
- Submission Guidelines
Author's Guide
- Introduction
- Final Manuscript Preparation
- Production Process
- Marketing and Sales
- Guidelines for Indexing
For Instructors
- Exam Copies
- Desk Copies
For Librarians and Booksellers
- Our Ebook Collection
- Ordering Information for Booksellers
- Review Copies
Background and Contacts
- About the Press
- Customer Service
- Staff Directory
News and Information
- Conferences and Events
Policies and Requests
- Rights and Permissions
- Accessibility
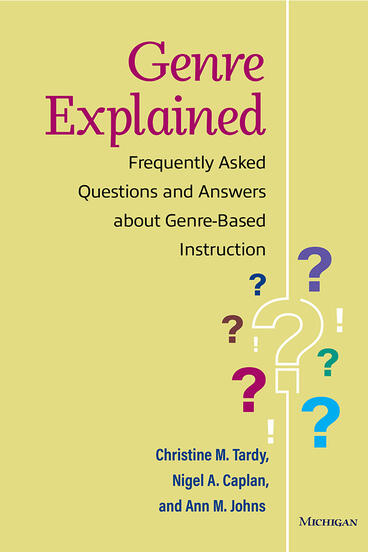
Genre Explained
Frequently asked questions and answers about genre-based instruction.
Genre Explained presents accessible, research-grounded answers to 40 questions that teachers frequently have about genre-based writing instruction
Table of contents
Table of Contents Foreword Introduction Part A: Understanding Genre-Based Instruction 1. What are genres? 2. What are the differences between genre and text? 3. What are some genres that students commonly encounter? 4. Is the 5PE a genre? 5. What are the differences between a genre and a mode? 6. What are the differences between a genre and a template? 7. What are the differences between genre and argument? 8. What is genre knowledge? Part B: Introducing Genre-Based Instruction 9. What is genre-based instruction? 10. What is genre analysis? 11. What are the roles of audience and context in genre-based instruction? 12. What is rhetorical moves analysis? 13. What does grammar mean in genre-based writing? 14. What is register? 15. How can grammar, vocabulary, and writing instruction be effectively integrated? 16. How can I teach coherence in genre-based instruction? 17. How can I teach cohesion in genre-based instruction? 18. How can I teach stance in a genre-based classroom? Part C: Designing a Genre-Based Course 19. What is the role of needs assessment in genre-based instruction? 20. What does a genre-based curriculum look like? 21. What does a genre-based unit look like? 22. How do I write a good assignment and prompt? 23. How do I teach students to analyze assignments for other classes? 24. How should I assess genre-based writing? 25. How do I write a genre-based rubric? 26. What is the role of written corrective feedback in genre-based writing? 27. What is the role of reflection in genre-based instruction? 28. How can I help students use their prior knowledge strategically in approaching a new genre? 29. How can I help students critique genres? Part D: Addressing Common Concerns 30. Is genre-based writing instruction only for advanced students? 31. Should I assign “essays” in genre-based instruction? 32. Should I assign “the research paper” in a genre-based curriculum? 33. How can students draw on their multilingual resources in genre-based instruction? 34. What do I do if I’m unfamiliar with the genres that students need to learn? 35. How do I find and use sample texts? 36. What role can emerging multimodal genres play in an academic writing class? Part E: Moving Forward with Genre-Based Instruction 37. How do I encourage colleagues to adopt genre-based instruction? 38. How do I talk about genre with faculty across the disciplines? 39. How do I explain genre to an administrator? 40. What do I read next?
Look Inside
Preview the Table of Contents
Description
The idea of teaching writing through genres—rather than, say, through prescriptive forms, templates, and rhetorical modes—is intuitively appealing. Yet many teachers have questions, and they are absolutely right to ask them: What are genres? What is genre-based instruction? What do students write if they don’t write essays? Isn’t it easier to teach and learn five-paragraph essays? What’s the role of language in genre teaching? And many more. These are all excellent questions and ones that new and experienced teachers alike have also struggled with. This book sets out to tackle some of the most common questions that teachers, teacher educators, and administrators may have when moving toward a genre-based teaching approach.
Christine M. Tardy is Professor of English Applied Linguistics at the University of Arizona. Nigel A. Caplan is an Associate Professor at the University of Delaware English Language Institute. Ann M. Johns is Professor Emerita of Linguistics and Writing Studies at San Diego State University.
"In short, I strongly believe that both seasoned and novice writing instructors, regardless of their previous familiarity with GBI theory, will consider this book a true gem due to its profound insights presented in the most accessible manner possible." — System - Wei Xu, University of Arizona
"This book offers reader-friendly, accessible answers to some of the most common questions writing teachers in higher education may have about genre and genre-based writing instruction. It does this without oversimplifying the complex nature of genre-based writing instruction. This book will be of use to educators at various stages of their career and with varying levels of familiarity with genre-based approaches. This is a book I know I will come back to regularly and will recommend to others often." — Journal of Second Language Writing - Angela Hakim, University of Arizona
"This volume offers a comprehensive and insightful exploration of the frequently asked questions and answers surrounding genre-based instruction (GBI). . . . Its comprehensive coverage, practical insights, and analysis of teaching materials make it an invaluable resource for educators seeking to implement effective genre-based instruction strategies." — English for Specific Purposes - Basim Alamri, English Language Institute, King Abdulaziz University, Saudi Arabia
"Genres are not set in stone; they always evolve to meet changing social needs. Genre Explained gives us the important insight that students are not just empowered by genre conventions; in fact, they can defy them to go beyond a prototypical representation of a genre and take actions appropriate for the 21st century." - TESOL Quarterly, Sachiko Yasuda, Kobe University
Help | Advanced Search
Computer Science > Computer Vision and Pattern Recognition
Title: mm1: methods, analysis & insights from multimodal llm pre-training.
Abstract: In this work, we discuss building performant Multimodal Large Language Models (MLLMs). In particular, we study the importance of various architecture components and data choices. Through careful and comprehensive ablations of the image encoder, the vision language connector, and various pre-training data choices, we identified several crucial design lessons. For example, we demonstrate that for large-scale multimodal pre-training using a careful mix of image-caption, interleaved image-text, and text-only data is crucial for achieving state-of-the-art (SOTA) few-shot results across multiple benchmarks, compared to other published pre-training results. Further, we show that the image encoder together with image resolution and the image token count has substantial impact, while the vision-language connector design is of comparatively negligible importance. By scaling up the presented recipe, we build MM1, a family of multimodal models up to 30B parameters, including both dense models and mixture-of-experts (MoE) variants, that are SOTA in pre-training metrics and achieve competitive performance after supervised fine-tuning on a range of established multimodal benchmarks. Thanks to large-scale pre-training, MM1 enjoys appealing properties such as enhanced in-context learning, and multi-image reasoning, enabling few-shot chain-of-thought prompting.
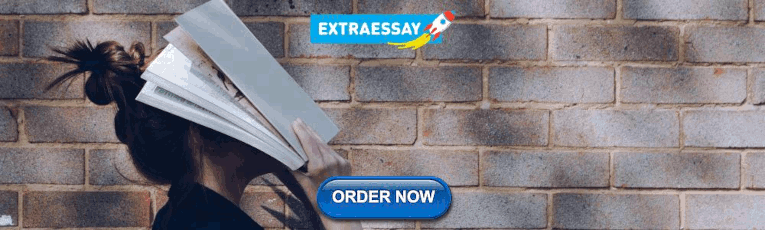
Submission history
Access paper:.
- Download PDF
- Other Formats
References & Citations
- Google Scholar
- Semantic Scholar
BibTeX formatted citation

Bibliographic and Citation Tools
Code, data and media associated with this article, recommenders and search tools.
- Institution
arXivLabs: experimental projects with community collaborators
arXivLabs is a framework that allows collaborators to develop and share new arXiv features directly on our website.
Both individuals and organizations that work with arXivLabs have embraced and accepted our values of openness, community, excellence, and user data privacy. arXiv is committed to these values and only works with partners that adhere to them.
Have an idea for a project that will add value for arXiv's community? Learn more about arXivLabs .
Thank you for visiting nature.com. You are using a browser version with limited support for CSS. To obtain the best experience, we recommend you use a more up to date browser (or turn off compatibility mode in Internet Explorer). In the meantime, to ensure continued support, we are displaying the site without styles and JavaScript.
- View all journals
- My Account Login
- Explore content
- About the journal
- Publish with us
- Sign up for alerts
- Open access
- Published: 26 March 2024
Predicting and improving complex beer flavor through machine learning
- Michiel Schreurs ORCID: orcid.org/0000-0002-9449-5619 1 , 2 , 3 na1 ,
- Supinya Piampongsant 1 , 2 , 3 na1 ,
- Miguel Roncoroni ORCID: orcid.org/0000-0001-7461-1427 1 , 2 , 3 na1 ,
- Lloyd Cool ORCID: orcid.org/0000-0001-9936-3124 1 , 2 , 3 , 4 ,
- Beatriz Herrera-Malaver ORCID: orcid.org/0000-0002-5096-9974 1 , 2 , 3 ,
- Christophe Vanderaa ORCID: orcid.org/0000-0001-7443-5427 4 ,
- Florian A. Theßeling 1 , 2 , 3 ,
- Łukasz Kreft ORCID: orcid.org/0000-0001-7620-4657 5 ,
- Alexander Botzki ORCID: orcid.org/0000-0001-6691-4233 5 ,
- Philippe Malcorps 6 ,
- Luk Daenen 6 ,
- Tom Wenseleers ORCID: orcid.org/0000-0002-1434-861X 4 &
- Kevin J. Verstrepen ORCID: orcid.org/0000-0002-3077-6219 1 , 2 , 3
Nature Communications volume 15 , Article number: 2368 ( 2024 ) Cite this article
7171 Accesses
681 Altmetric
Metrics details
- Chemical engineering
- Gas chromatography
- Machine learning
- Metabolomics
- Taste receptors
The perception and appreciation of food flavor depends on many interacting chemical compounds and external factors, and therefore proves challenging to understand and predict. Here, we combine extensive chemical and sensory analyses of 250 different beers to train machine learning models that allow predicting flavor and consumer appreciation. For each beer, we measure over 200 chemical properties, perform quantitative descriptive sensory analysis with a trained tasting panel and map data from over 180,000 consumer reviews to train 10 different machine learning models. The best-performing algorithm, Gradient Boosting, yields models that significantly outperform predictions based on conventional statistics and accurately predict complex food features and consumer appreciation from chemical profiles. Model dissection allows identifying specific and unexpected compounds as drivers of beer flavor and appreciation. Adding these compounds results in variants of commercial alcoholic and non-alcoholic beers with improved consumer appreciation. Together, our study reveals how big data and machine learning uncover complex links between food chemistry, flavor and consumer perception, and lays the foundation to develop novel, tailored foods with superior flavors.
Similar content being viewed by others
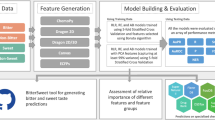
BitterSweet: Building machine learning models for predicting the bitter and sweet taste of small molecules
Rudraksh Tuwani, Somin Wadhwa & Ganesh Bagler
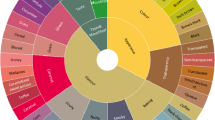
Sensory lexicon and aroma volatiles analysis of brewing malt
Xiaoxia Su, Miao Yu, … Tianyi Du
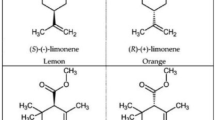
Predicting odor from molecular structure: a multi-label classification approach
Kushagra Saini & Venkatnarayan Ramanathan
Introduction
Predicting and understanding food perception and appreciation is one of the major challenges in food science. Accurate modeling of food flavor and appreciation could yield important opportunities for both producers and consumers, including quality control, product fingerprinting, counterfeit detection, spoilage detection, and the development of new products and product combinations (food pairing) 1 , 2 , 3 , 4 , 5 , 6 . Accurate models for flavor and consumer appreciation would contribute greatly to our scientific understanding of how humans perceive and appreciate flavor. Moreover, accurate predictive models would also facilitate and standardize existing food assessment methods and could supplement or replace assessments by trained and consumer tasting panels, which are variable, expensive and time-consuming 7 , 8 , 9 . Lastly, apart from providing objective, quantitative, accurate and contextual information that can help producers, models can also guide consumers in understanding their personal preferences 10 .
Despite the myriad of applications, predicting food flavor and appreciation from its chemical properties remains a largely elusive goal in sensory science, especially for complex food and beverages 11 , 12 . A key obstacle is the immense number of flavor-active chemicals underlying food flavor. Flavor compounds can vary widely in chemical structure and concentration, making them technically challenging and labor-intensive to quantify, even in the face of innovations in metabolomics, such as non-targeted metabolic fingerprinting 13 , 14 . Moreover, sensory analysis is perhaps even more complicated. Flavor perception is highly complex, resulting from hundreds of different molecules interacting at the physiochemical and sensorial level. Sensory perception is often non-linear, characterized by complex and concentration-dependent synergistic and antagonistic effects 15 , 16 , 17 , 18 , 19 , 20 , 21 that are further convoluted by the genetics, environment, culture and psychology of consumers 22 , 23 , 24 . Perceived flavor is therefore difficult to measure, with problems of sensitivity, accuracy, and reproducibility that can only be resolved by gathering sufficiently large datasets 25 . Trained tasting panels are considered the prime source of quality sensory data, but require meticulous training, are low throughput and high cost. Public databases containing consumer reviews of food products could provide a valuable alternative, especially for studying appreciation scores, which do not require formal training 25 . Public databases offer the advantage of amassing large amounts of data, increasing the statistical power to identify potential drivers of appreciation. However, public datasets suffer from biases, including a bias in the volunteers that contribute to the database, as well as confounding factors such as price, cult status and psychological conformity towards previous ratings of the product.
Classical multivariate statistics and machine learning methods have been used to predict flavor of specific compounds by, for example, linking structural properties of a compound to its potential biological activities or linking concentrations of specific compounds to sensory profiles 1 , 26 . Importantly, most previous studies focused on predicting organoleptic properties of single compounds (often based on their chemical structure) 27 , 28 , 29 , 30 , 31 , 32 , 33 , thus ignoring the fact that these compounds are present in a complex matrix in food or beverages and excluding complex interactions between compounds. Moreover, the classical statistics commonly used in sensory science 34 , 35 , 36 , 37 , 38 , 39 require a large sample size and sufficient variance amongst predictors to create accurate models. They are not fit for studying an extensive set of hundreds of interacting flavor compounds, since they are sensitive to outliers, have a high tendency to overfit and are less suited for non-linear and discontinuous relationships 40 .
In this study, we combine extensive chemical analyses and sensory data of a set of different commercial beers with machine learning approaches to develop models that predict taste, smell, mouthfeel and appreciation from compound concentrations. Beer is particularly suited to model the relationship between chemistry, flavor and appreciation. First, beer is a complex product, consisting of thousands of flavor compounds that partake in complex sensory interactions 41 , 42 , 43 . This chemical diversity arises from the raw materials (malt, yeast, hops, water and spices) and biochemical conversions during the brewing process (kilning, mashing, boiling, fermentation, maturation and aging) 44 , 45 . Second, the advent of the internet saw beer consumers embrace online review platforms, such as RateBeer (ZX Ventures, Anheuser-Busch InBev SA/NV) and BeerAdvocate (Next Glass, inc.). In this way, the beer community provides massive data sets of beer flavor and appreciation scores, creating extraordinarily large sensory databases to complement the analyses of our professional sensory panel. Specifically, we characterize over 200 chemical properties of 250 commercial beers, spread across 22 beer styles, and link these to the descriptive sensory profiling data of a 16-person in-house trained tasting panel and data acquired from over 180,000 public consumer reviews. These unique and extensive datasets enable us to train a suite of machine learning models to predict flavor and appreciation from a beer’s chemical profile. Dissection of the best-performing models allows us to pinpoint specific compounds as potential drivers of beer flavor and appreciation. Follow-up experiments confirm the importance of these compounds and ultimately allow us to significantly improve the flavor and appreciation of selected commercial beers. Together, our study represents a significant step towards understanding complex flavors and reinforces the value of machine learning to develop and refine complex foods. In this way, it represents a stepping stone for further computer-aided food engineering applications 46 .
To generate a comprehensive dataset on beer flavor, we selected 250 commercial Belgian beers across 22 different beer styles (Supplementary Fig. S1 ). Beers with ≤ 4.2% alcohol by volume (ABV) were classified as non-alcoholic and low-alcoholic. Blonds and Tripels constitute a significant portion of the dataset (12.4% and 11.2%, respectively) reflecting their presence on the Belgian beer market and the heterogeneity of beers within these styles. By contrast, lager beers are less diverse and dominated by a handful of brands. Rare styles such as Brut or Faro make up only a small fraction of the dataset (2% and 1%, respectively) because fewer of these beers are produced and because they are dominated by distinct characteristics in terms of flavor and chemical composition.
Extensive analysis identifies relationships between chemical compounds in beer
For each beer, we measured 226 different chemical properties, including common brewing parameters such as alcohol content, iso-alpha acids, pH, sugar concentration 47 , and over 200 flavor compounds (Methods, Supplementary Table S1 ). A large portion (37.2%) are terpenoids arising from hopping, responsible for herbal and fruity flavors 16 , 48 . A second major category are yeast metabolites, such as esters and alcohols, that result in fruity and solvent notes 48 , 49 , 50 . Other measured compounds are primarily derived from malt, or other microbes such as non- Saccharomyces yeasts and bacteria (‘wild flora’). Compounds that arise from spices or staling are labeled under ‘Others’. Five attributes (caloric value, total acids and total ester, hop aroma and sulfur compounds) are calculated from multiple individually measured compounds.
As a first step in identifying relationships between chemical properties, we determined correlations between the concentrations of the compounds (Fig. 1 , upper panel, Supplementary Data 1 and 2 , and Supplementary Fig. S2 . For the sake of clarity, only a subset of the measured compounds is shown in Fig. 1 ). Compounds of the same origin typically show a positive correlation, while absence of correlation hints at parameters varying independently. For example, the hop aroma compounds citronellol, and alpha-terpineol show moderate correlations with each other (Spearman’s rho=0.39 and 0.57), but not with the bittering hop component iso-alpha acids (Spearman’s rho=0.16 and −0.07). This illustrates how brewers can independently modify hop aroma and bitterness by selecting hop varieties and dosage time. If hops are added early in the boiling phase, chemical conversions increase bitterness while aromas evaporate, conversely, late addition of hops preserves aroma but limits bitterness 51 . Similarly, hop-derived iso-alpha acids show a strong anti-correlation with lactic acid and acetic acid, likely reflecting growth inhibition of lactic acid and acetic acid bacteria, or the consequent use of fewer hops in sour beer styles, such as West Flanders ales and Fruit beers, that rely on these bacteria for their distinct flavors 52 . Finally, yeast-derived esters (ethyl acetate, ethyl decanoate, ethyl hexanoate, ethyl octanoate) and alcohols (ethanol, isoamyl alcohol, isobutanol, and glycerol), correlate with Spearman coefficients above 0.5, suggesting that these secondary metabolites are correlated with the yeast genetic background and/or fermentation parameters and may be difficult to influence individually, although the choice of yeast strain may offer some control 53 .
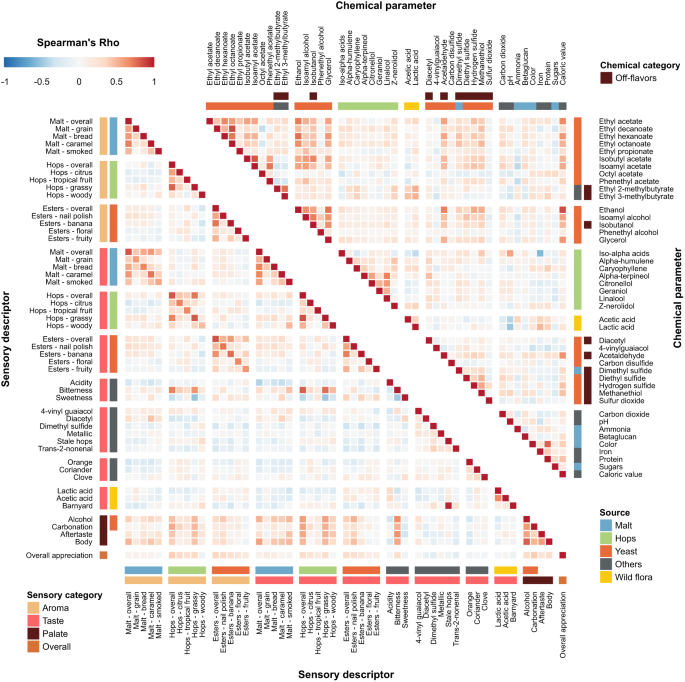
Spearman rank correlations are shown. Descriptors are grouped according to their origin (malt (blue), hops (green), yeast (red), wild flora (yellow), Others (black)), and sensory aspect (aroma, taste, palate, and overall appreciation). Please note that for the chemical compounds, for the sake of clarity, only a subset of the total number of measured compounds is shown, with an emphasis on the key compounds for each source. For more details, see the main text and Methods section. Chemical data can be found in Supplementary Data 1 , correlations between all chemical compounds are depicted in Supplementary Fig. S2 and correlation values can be found in Supplementary Data 2 . See Supplementary Data 4 for sensory panel assessments and Supplementary Data 5 for correlation values between all sensory descriptors.
Interestingly, different beer styles show distinct patterns for some flavor compounds (Supplementary Fig. S3 ). These observations agree with expectations for key beer styles, and serve as a control for our measurements. For instance, Stouts generally show high values for color (darker), while hoppy beers contain elevated levels of iso-alpha acids, compounds associated with bitter hop taste. Acetic and lactic acid are not prevalent in most beers, with notable exceptions such as Kriek, Lambic, Faro, West Flanders ales and Flanders Old Brown, which use acid-producing bacteria ( Lactobacillus and Pediococcus ) or unconventional yeast ( Brettanomyces ) 54 , 55 . Glycerol, ethanol and esters show similar distributions across all beer styles, reflecting their common origin as products of yeast metabolism during fermentation 45 , 53 . Finally, low/no-alcohol beers contain low concentrations of glycerol and esters. This is in line with the production process for most of the low/no-alcohol beers in our dataset, which are produced through limiting fermentation or by stripping away alcohol via evaporation or dialysis, with both methods having the unintended side-effect of reducing the amount of flavor compounds in the final beer 56 , 57 .
Besides expected associations, our data also reveals less trivial associations between beer styles and specific parameters. For example, geraniol and citronellol, two monoterpenoids responsible for citrus, floral and rose flavors and characteristic of Citra hops, are found in relatively high amounts in Christmas, Saison, and Brett/co-fermented beers, where they may originate from terpenoid-rich spices such as coriander seeds instead of hops 58 .
Tasting panel assessments reveal sensorial relationships in beer
To assess the sensory profile of each beer, a trained tasting panel evaluated each of the 250 beers for 50 sensory attributes, including different hop, malt and yeast flavors, off-flavors and spices. Panelists used a tasting sheet (Supplementary Data 3 ) to score the different attributes. Panel consistency was evaluated by repeating 12 samples across different sessions and performing ANOVA. In 95% of cases no significant difference was found across sessions ( p > 0.05), indicating good panel consistency (Supplementary Table S2 ).
Aroma and taste perception reported by the trained panel are often linked (Fig. 1 , bottom left panel and Supplementary Data 4 and 5 ), with high correlations between hops aroma and taste (Spearman’s rho=0.83). Bitter taste was found to correlate with hop aroma and taste in general (Spearman’s rho=0.80 and 0.69), and particularly with “grassy” noble hops (Spearman’s rho=0.75). Barnyard flavor, most often associated with sour beers, is identified together with stale hops (Spearman’s rho=0.97) that are used in these beers. Lactic and acetic acid, which often co-occur, are correlated (Spearman’s rho=0.66). Interestingly, sweetness and bitterness are anti-correlated (Spearman’s rho = −0.48), confirming the hypothesis that they mask each other 59 , 60 . Beer body is highly correlated with alcohol (Spearman’s rho = 0.79), and overall appreciation is found to correlate with multiple aspects that describe beer mouthfeel (alcohol, carbonation; Spearman’s rho= 0.32, 0.39), as well as with hop and ester aroma intensity (Spearman’s rho=0.39 and 0.35).
Similar to the chemical analyses, sensorial analyses confirmed typical features of specific beer styles (Supplementary Fig. S4 ). For example, sour beers (Faro, Flanders Old Brown, Fruit beer, Kriek, Lambic, West Flanders ale) were rated acidic, with flavors of both acetic and lactic acid. Hoppy beers were found to be bitter and showed hop-associated aromas like citrus and tropical fruit. Malt taste is most detected among scotch, stout/porters, and strong ales, while low/no-alcohol beers, which often have a reputation for being ‘worty’ (reminiscent of unfermented, sweet malt extract) appear in the middle. Unsurprisingly, hop aromas are most strongly detected among hoppy beers. Like its chemical counterpart (Supplementary Fig. S3 ), acidity shows a right-skewed distribution, with the most acidic beers being Krieks, Lambics, and West Flanders ales.
Tasting panel assessments of specific flavors correlate with chemical composition
We find that the concentrations of several chemical compounds strongly correlate with specific aroma or taste, as evaluated by the tasting panel (Fig. 2 , Supplementary Fig. S5 , Supplementary Data 6 ). In some cases, these correlations confirm expectations and serve as a useful control for data quality. For example, iso-alpha acids, the bittering compounds in hops, strongly correlate with bitterness (Spearman’s rho=0.68), while ethanol and glycerol correlate with tasters’ perceptions of alcohol and body, the mouthfeel sensation of fullness (Spearman’s rho=0.82/0.62 and 0.72/0.57 respectively) and darker color from roasted malts is a good indication of malt perception (Spearman’s rho=0.54).
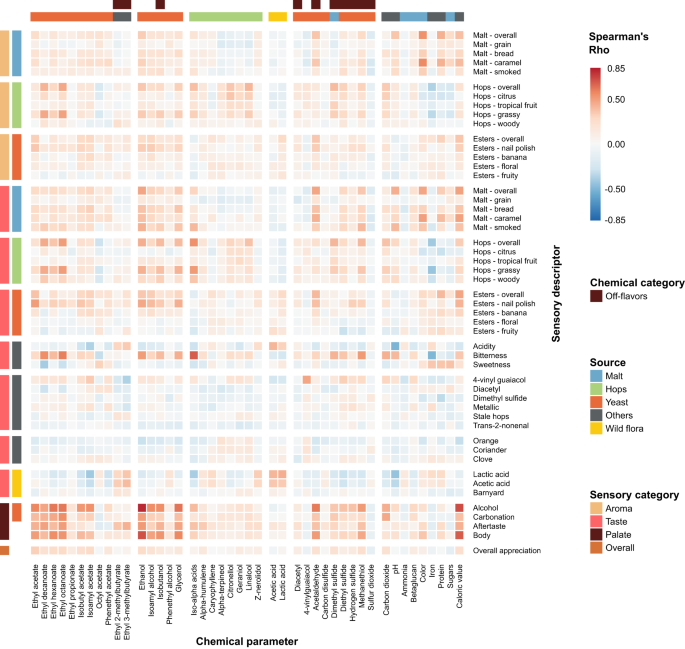
Heatmap colors indicate Spearman’s Rho. Axes are organized according to sensory categories (aroma, taste, mouthfeel, overall), chemical categories and chemical sources in beer (malt (blue), hops (green), yeast (red), wild flora (yellow), Others (black)). See Supplementary Data 6 for all correlation values.
Interestingly, for some relationships between chemical compounds and perceived flavor, correlations are weaker than expected. For example, the rose-smelling phenethyl acetate only weakly correlates with floral aroma. This hints at more complex relationships and interactions between compounds and suggests a need for a more complex model than simple correlations. Lastly, we uncovered unexpected correlations. For instance, the esters ethyl decanoate and ethyl octanoate appear to correlate slightly with hop perception and bitterness, possibly due to their fruity flavor. Iron is anti-correlated with hop aromas and bitterness, most likely because it is also anti-correlated with iso-alpha acids. This could be a sign of metal chelation of hop acids 61 , given that our analyses measure unbound hop acids and total iron content, or could result from the higher iron content in dark and Fruit beers, which typically have less hoppy and bitter flavors 62 .
Public consumer reviews complement expert panel data
To complement and expand the sensory data of our trained tasting panel, we collected 180,000 reviews of our 250 beers from the online consumer review platform RateBeer. This provided numerical scores for beer appearance, aroma, taste, palate, overall quality as well as the average overall score.
Public datasets are known to suffer from biases, such as price, cult status and psychological conformity towards previous ratings of a product. For example, prices correlate with appreciation scores for these online consumer reviews (rho=0.49, Supplementary Fig. S6 ), but not for our trained tasting panel (rho=0.19). This suggests that prices affect consumer appreciation, which has been reported in wine 63 , while blind tastings are unaffected. Moreover, we observe that some beer styles, like lagers and non-alcoholic beers, generally receive lower scores, reflecting that online reviewers are mostly beer aficionados with a preference for specialty beers over lager beers. In general, we find a modest correlation between our trained panel’s overall appreciation score and the online consumer appreciation scores (Fig. 3 , rho=0.29). Apart from the aforementioned biases in the online datasets, serving temperature, sample freshness and surroundings, which are all tightly controlled during the tasting panel sessions, can vary tremendously across online consumers and can further contribute to (among others, appreciation) differences between the two categories of tasters. Importantly, in contrast to the overall appreciation scores, for many sensory aspects the results from the professional panel correlated well with results obtained from RateBeer reviews. Correlations were highest for features that are relatively easy to recognize even for untrained tasters, like bitterness, sweetness, alcohol and malt aroma (Fig. 3 and below).
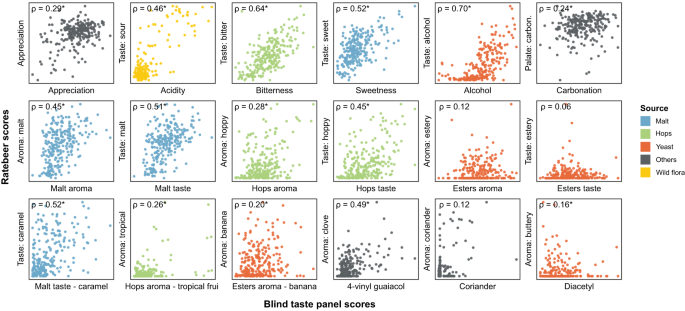
RateBeer text mining results can be found in Supplementary Data 7 . Rho values shown are Spearman correlation values, with asterisks indicating significant correlations ( p < 0.05, two-sided). All p values were smaller than 0.001, except for Esters aroma (0.0553), Esters taste (0.3275), Esters aroma—banana (0.0019), Coriander (0.0508) and Diacetyl (0.0134).
Besides collecting consumer appreciation from these online reviews, we developed automated text analysis tools to gather additional data from review texts (Supplementary Data 7 ). Processing review texts on the RateBeer database yielded comparable results to the scores given by the trained panel for many common sensory aspects, including acidity, bitterness, sweetness, alcohol, malt, and hop tastes (Fig. 3 ). This is in line with what would be expected, since these attributes require less training for accurate assessment and are less influenced by environmental factors such as temperature, serving glass and odors in the environment. Consumer reviews also correlate well with our trained panel for 4-vinyl guaiacol, a compound associated with a very characteristic aroma. By contrast, correlations for more specific aromas like ester, coriander or diacetyl are underrepresented in the online reviews, underscoring the importance of using a trained tasting panel and standardized tasting sheets with explicit factors to be scored for evaluating specific aspects of a beer. Taken together, our results suggest that public reviews are trustworthy for some, but not all, flavor features and can complement or substitute taste panel data for these sensory aspects.
Models can predict beer sensory profiles from chemical data
The rich datasets of chemical analyses, tasting panel assessments and public reviews gathered in the first part of this study provided us with a unique opportunity to develop predictive models that link chemical data to sensorial features. Given the complexity of beer flavor, basic statistical tools such as correlations or linear regression may not always be the most suitable for making accurate predictions. Instead, we applied different machine learning models that can model both simple linear and complex interactive relationships. Specifically, we constructed a set of regression models to predict (a) trained panel scores for beer flavor and quality and (b) public reviews’ appreciation scores from beer chemical profiles. We trained and tested 10 different models (Methods), 3 linear regression-based models (simple linear regression with first-order interactions (LR), lasso regression with first-order interactions (Lasso), partial least squares regressor (PLSR)), 5 decision tree models (AdaBoost regressor (ABR), extra trees (ET), gradient boosting regressor (GBR), random forest (RF) and XGBoost regressor (XGBR)), 1 support vector regression (SVR), and 1 artificial neural network (ANN) model.
To compare the performance of our machine learning models, the dataset was randomly split into a training and test set, stratified by beer style. After a model was trained on data in the training set, its performance was evaluated on its ability to predict the test dataset obtained from multi-output models (based on the coefficient of determination, see Methods). Additionally, individual-attribute models were ranked per descriptor and the average rank was calculated, as proposed by Korneva et al. 64 . Importantly, both ways of evaluating the models’ performance agreed in general. Performance of the different models varied (Table 1 ). It should be noted that all models perform better at predicting RateBeer results than results from our trained tasting panel. One reason could be that sensory data is inherently variable, and this variability is averaged out with the large number of public reviews from RateBeer. Additionally, all tree-based models perform better at predicting taste than aroma. Linear models (LR) performed particularly poorly, with negative R 2 values, due to severe overfitting (training set R 2 = 1). Overfitting is a common issue in linear models with many parameters and limited samples, especially with interaction terms further amplifying the number of parameters. L1 regularization (Lasso) successfully overcomes this overfitting, out-competing multiple tree-based models on the RateBeer dataset. Similarly, the dimensionality reduction of PLSR avoids overfitting and improves performance, to some extent. Still, tree-based models (ABR, ET, GBR, RF and XGBR) show the best performance, out-competing the linear models (LR, Lasso, PLSR) commonly used in sensory science 65 .
GBR models showed the best overall performance in predicting sensory responses from chemical information, with R 2 values up to 0.75 depending on the predicted sensory feature (Supplementary Table S4 ). The GBR models predict consumer appreciation (RateBeer) better than our trained panel’s appreciation (R 2 value of 0.67 compared to R 2 value of 0.09) (Supplementary Table S3 and Supplementary Table S4 ). ANN models showed intermediate performance, likely because neural networks typically perform best with larger datasets 66 . The SVR shows intermediate performance, mostly due to the weak predictions of specific attributes that lower the overall performance (Supplementary Table S4 ).
Model dissection identifies specific, unexpected compounds as drivers of consumer appreciation
Next, we leveraged our models to infer important contributors to sensory perception and consumer appreciation. Consumer preference is a crucial sensory aspects, because a product that shows low consumer appreciation scores often does not succeed commercially 25 . Additionally, the requirement for a large number of representative evaluators makes consumer trials one of the more costly and time-consuming aspects of product development. Hence, a model for predicting chemical drivers of overall appreciation would be a welcome addition to the available toolbox for food development and optimization.
Since GBR models on our RateBeer dataset showed the best overall performance, we focused on these models. Specifically, we used two approaches to identify important contributors. First, rankings of the most important predictors for each sensorial trait in the GBR models were obtained based on impurity-based feature importance (mean decrease in impurity). High-ranked parameters were hypothesized to be either the true causal chemical properties underlying the trait, to correlate with the actual causal properties, or to take part in sensory interactions affecting the trait 67 (Fig. 4A ). In a second approach, we used SHAP 68 to determine which parameters contributed most to the model for making predictions of consumer appreciation (Fig. 4B ). SHAP calculates parameter contributions to model predictions on a per-sample basis, which can be aggregated into an importance score.
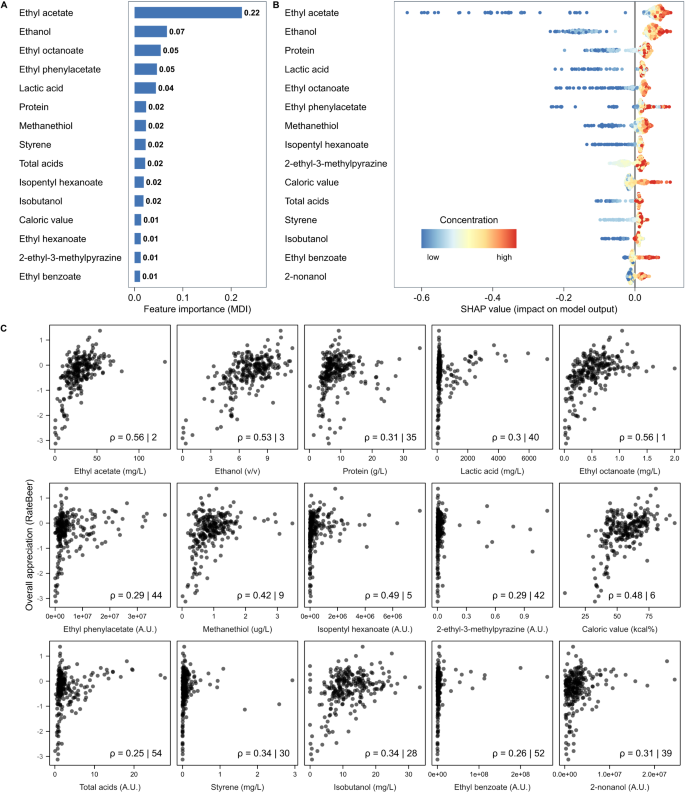
A The impurity-based feature importance (mean deviance in impurity, MDI) calculated from the Gradient Boosting Regression (GBR) model predicting RateBeer appreciation scores. The top 15 highest ranked chemical properties are shown. B SHAP summary plot for the top 15 parameters contributing to our GBR model. Each point on the graph represents a sample from our dataset. The color represents the concentration of that parameter, with bluer colors representing low values and redder colors representing higher values. Greater absolute values on the horizontal axis indicate a higher impact of the parameter on the prediction of the model. C Spearman correlations between the 15 most important chemical properties and consumer overall appreciation. Numbers indicate the Spearman Rho correlation coefficient, and the rank of this correlation compared to all other correlations. The top 15 important compounds were determined using SHAP (panel B).
Both approaches identified ethyl acetate as the most predictive parameter for beer appreciation (Fig. 4 ). Ethyl acetate is the most abundant ester in beer with a typical ‘fruity’, ‘solvent’ and ‘alcoholic’ flavor, but is often considered less important than other esters like isoamyl acetate. The second most important parameter identified by SHAP is ethanol, the most abundant beer compound after water. Apart from directly contributing to beer flavor and mouthfeel, ethanol drastically influences the physical properties of beer, dictating how easily volatile compounds escape the beer matrix to contribute to beer aroma 69 . Importantly, it should also be noted that the importance of ethanol for appreciation is likely inflated by the very low appreciation scores of non-alcoholic beers (Supplementary Fig. S4 ). Despite not often being considered a driver of beer appreciation, protein level also ranks highly in both approaches, possibly due to its effect on mouthfeel and body 70 . Lactic acid, which contributes to the tart taste of sour beers, is the fourth most important parameter identified by SHAP, possibly due to the generally high appreciation of sour beers in our dataset.
Interestingly, some of the most important predictive parameters for our model are not well-established as beer flavors or are even commonly regarded as being negative for beer quality. For example, our models identify methanethiol and ethyl phenyl acetate, an ester commonly linked to beer staling 71 , as a key factor contributing to beer appreciation. Although there is no doubt that high concentrations of these compounds are considered unpleasant, the positive effects of modest concentrations are not yet known 72 , 73 .
To compare our approach to conventional statistics, we evaluated how well the 15 most important SHAP-derived parameters correlate with consumer appreciation (Fig. 4C ). Interestingly, only 6 of the properties derived by SHAP rank amongst the top 15 most correlated parameters. For some chemical compounds, the correlations are so low that they would have likely been considered unimportant. For example, lactic acid, the fourth most important parameter, shows a bimodal distribution for appreciation, with sour beers forming a separate cluster, that is missed entirely by the Spearman correlation. Additionally, the correlation plots reveal outliers, emphasizing the need for robust analysis tools. Together, this highlights the need for alternative models, like the Gradient Boosting model, that better grasp the complexity of (beer) flavor.
Finally, to observe the relationships between these chemical properties and their predicted targets, partial dependence plots were constructed for the six most important predictors of consumer appreciation 74 , 75 , 76 (Supplementary Fig. S7 ). One-way partial dependence plots show how a change in concentration affects the predicted appreciation. These plots reveal an important limitation of our models: appreciation predictions remain constant at ever-increasing concentrations. This implies that once a threshold concentration is reached, further increasing the concentration does not affect appreciation. This is false, as it is well-documented that certain compounds become unpleasant at high concentrations, including ethyl acetate (‘nail polish’) 77 and methanethiol (‘sulfury’ and ‘rotten cabbage’) 78 . The inability of our models to grasp that flavor compounds have optimal levels, above which they become negative, is a consequence of working with commercial beer brands where (off-)flavors are rarely too high to negatively impact the product. The two-way partial dependence plots show how changing the concentration of two compounds influences predicted appreciation, visualizing their interactions (Supplementary Fig. S7 ). In our case, the top 5 parameters are dominated by additive or synergistic interactions, with high concentrations for both compounds resulting in the highest predicted appreciation.
To assess the robustness of our best-performing models and model predictions, we performed 100 iterations of the GBR, RF and ET models. In general, all iterations of the models yielded similar performance (Supplementary Fig. S8 ). Moreover, the main predictors (including the top predictors ethanol and ethyl acetate) remained virtually the same, especially for GBR and RF. For the iterations of the ET model, we did observe more variation in the top predictors, which is likely a consequence of the model’s inherent random architecture in combination with co-correlations between certain predictors. However, even in this case, several of the top predictors (ethanol and ethyl acetate) remain unchanged, although their rank in importance changes (Supplementary Fig. S8 ).
Next, we investigated if a combination of RateBeer and trained panel data into one consolidated dataset would lead to stronger models, under the hypothesis that such a model would suffer less from bias in the datasets. A GBR model was trained to predict appreciation on the combined dataset. This model underperformed compared to the RateBeer model, both in the native case and when including a dataset identifier (R 2 = 0.67, 0.26 and 0.42 respectively). For the latter, the dataset identifier is the most important feature (Supplementary Fig. S9 ), while most of the feature importance remains unchanged, with ethyl acetate and ethanol ranking highest, like in the original model trained only on RateBeer data. It seems that the large variation in the panel dataset introduces noise, weakening the models’ performances and reliability. In addition, it seems reasonable to assume that both datasets are fundamentally different, with the panel dataset obtained by blind tastings by a trained professional panel.
Lastly, we evaluated whether beer style identifiers would further enhance the model’s performance. A GBR model was trained with parameters that explicitly encoded the styles of the samples. This did not improve model performance (R2 = 0.66 with style information vs R2 = 0.67). The most important chemical features are consistent with the model trained without style information (eg. ethanol and ethyl acetate), and with the exception of the most preferred (strong ale) and least preferred (low/no-alcohol) styles, none of the styles were among the most important features (Supplementary Fig. S9 , Supplementary Table S5 and S6 ). This is likely due to a combination of style-specific chemical signatures, such as iso-alpha acids and lactic acid, that implicitly convey style information to the original models, as well as the low number of samples belonging to some styles, making it difficult for the model to learn style-specific patterns. Moreover, beer styles are not rigorously defined, with some styles overlapping in features and some beers being misattributed to a specific style, all of which leads to more noise in models that use style parameters.
Model validation
To test if our predictive models give insight into beer appreciation, we set up experiments aimed at improving existing commercial beers. We specifically selected overall appreciation as the trait to be examined because of its complexity and commercial relevance. Beer flavor comprises a complex bouquet rather than single aromas and tastes 53 . Hence, adding a single compound to the extent that a difference is noticeable may lead to an unbalanced, artificial flavor. Therefore, we evaluated the effect of combinations of compounds. Because Blond beers represent the most extensive style in our dataset, we selected a beer from this style as the starting material for these experiments (Beer 64 in Supplementary Data 1 ).
In the first set of experiments, we adjusted the concentrations of compounds that made up the most important predictors of overall appreciation (ethyl acetate, ethanol, lactic acid, ethyl phenyl acetate) together with correlated compounds (ethyl hexanoate, isoamyl acetate, glycerol), bringing them up to 95 th percentile ethanol-normalized concentrations (Methods) within the Blond group (‘Spiked’ concentration in Fig. 5A ). Compared to controls, the spiked beers were found to have significantly improved overall appreciation among trained panelists, with panelist noting increased intensity of ester flavors, sweetness, alcohol, and body fullness (Fig. 5B ). To disentangle the contribution of ethanol to these results, a second experiment was performed without the addition of ethanol. This resulted in a similar outcome, including increased perception of alcohol and overall appreciation.
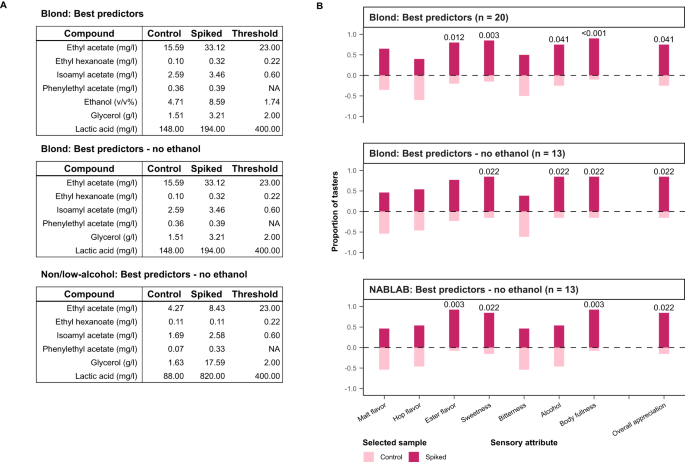
Adding the top chemical compounds, identified as best predictors of appreciation by our model, into poorly appreciated beers results in increased appreciation from our trained panel. Results of sensory tests between base beers and those spiked with compounds identified as the best predictors by the model. A Blond and Non/Low-alcohol (0.0% ABV) base beers were brought up to 95th-percentile ethanol-normalized concentrations within each style. B For each sensory attribute, tasters indicated the more intense sample and selected the sample they preferred. The numbers above the bars correspond to the p values that indicate significant changes in perceived flavor (two-sided binomial test: alpha 0.05, n = 20 or 13).
In a last experiment, we tested whether using the model’s predictions can boost the appreciation of a non-alcoholic beer (beer 223 in Supplementary Data 1 ). Again, the addition of a mixture of predicted compounds (omitting ethanol, in this case) resulted in a significant increase in appreciation, body, ester flavor and sweetness.
Predicting flavor and consumer appreciation from chemical composition is one of the ultimate goals of sensory science. A reliable, systematic and unbiased way to link chemical profiles to flavor and food appreciation would be a significant asset to the food and beverage industry. Such tools would substantially aid in quality control and recipe development, offer an efficient and cost-effective alternative to pilot studies and consumer trials and would ultimately allow food manufacturers to produce superior, tailor-made products that better meet the demands of specific consumer groups more efficiently.
A limited set of studies have previously tried, to varying degrees of success, to predict beer flavor and beer popularity based on (a limited set of) chemical compounds and flavors 79 , 80 . Current sensitive, high-throughput technologies allow measuring an unprecedented number of chemical compounds and properties in a large set of samples, yielding a dataset that can train models that help close the gaps between chemistry and flavor, even for a complex natural product like beer. To our knowledge, no previous research gathered data at this scale (250 samples, 226 chemical parameters, 50 sensory attributes and 5 consumer scores) to disentangle and validate the chemical aspects driving beer preference using various machine-learning techniques. We find that modern machine learning models outperform conventional statistical tools, such as correlations and linear models, and can successfully predict flavor appreciation from chemical composition. This could be attributed to the natural incorporation of interactions and non-linear or discontinuous effects in machine learning models, which are not easily grasped by the linear model architecture. While linear models and partial least squares regression represent the most widespread statistical approaches in sensory science, in part because they allow interpretation 65 , 81 , 82 , modern machine learning methods allow for building better predictive models while preserving the possibility to dissect and exploit the underlying patterns. Of the 10 different models we trained, tree-based models, such as our best performing GBR, showed the best overall performance in predicting sensory responses from chemical information, outcompeting artificial neural networks. This agrees with previous reports for models trained on tabular data 83 . Our results are in line with the findings of Colantonio et al. who also identified the gradient boosting architecture as performing best at predicting appreciation and flavor (of tomatoes and blueberries, in their specific study) 26 . Importantly, besides our larger experimental scale, we were able to directly confirm our models’ predictions in vivo.
Our study confirms that flavor compound concentration does not always correlate with perception, suggesting complex interactions that are often missed by more conventional statistics and simple models. Specifically, we find that tree-based algorithms may perform best in developing models that link complex food chemistry with aroma. Furthermore, we show that massive datasets of untrained consumer reviews provide a valuable source of data, that can complement or even replace trained tasting panels, especially for appreciation and basic flavors, such as sweetness and bitterness. This holds despite biases that are known to occur in such datasets, such as price or conformity bias. Moreover, GBR models predict taste better than aroma. This is likely because taste (e.g. bitterness) often directly relates to the corresponding chemical measurements (e.g., iso-alpha acids), whereas such a link is less clear for aromas, which often result from the interplay between multiple volatile compounds. We also find that our models are best at predicting acidity and alcohol, likely because there is a direct relation between the measured chemical compounds (acids and ethanol) and the corresponding perceived sensorial attribute (acidity and alcohol), and because even untrained consumers are generally able to recognize these flavors and aromas.
The predictions of our final models, trained on review data, hold even for blind tastings with small groups of trained tasters, as demonstrated by our ability to validate specific compounds as drivers of beer flavor and appreciation. Since adding a single compound to the extent of a noticeable difference may result in an unbalanced flavor profile, we specifically tested our identified key drivers as a combination of compounds. While this approach does not allow us to validate if a particular single compound would affect flavor and/or appreciation, our experiments do show that this combination of compounds increases consumer appreciation.
It is important to stress that, while it represents an important step forward, our approach still has several major limitations. A key weakness of the GBR model architecture is that amongst co-correlating variables, the largest main effect is consistently preferred for model building. As a result, co-correlating variables often have artificially low importance scores, both for impurity and SHAP-based methods, like we observed in the comparison to the more randomized Extra Trees models. This implies that chemicals identified as key drivers of a specific sensory feature by GBR might not be the true causative compounds, but rather co-correlate with the actual causative chemical. For example, the high importance of ethyl acetate could be (partially) attributed to the total ester content, ethanol or ethyl hexanoate (rho=0.77, rho=0.72 and rho=0.68), while ethyl phenylacetate could hide the importance of prenyl isobutyrate and ethyl benzoate (rho=0.77 and rho=0.76). Expanding our GBR model to include beer style as a parameter did not yield additional power or insight. This is likely due to style-specific chemical signatures, such as iso-alpha acids and lactic acid, that implicitly convey style information to the original model, as well as the smaller sample size per style, limiting the power to uncover style-specific patterns. This can be partly attributed to the curse of dimensionality, where the high number of parameters results in the models mainly incorporating single parameter effects, rather than complex interactions such as style-dependent effects 67 . A larger number of samples may overcome some of these limitations and offer more insight into style-specific effects. On the other hand, beer style is not a rigid scientific classification, and beers within one style often differ a lot, which further complicates the analysis of style as a model factor.
Our study is limited to beers from Belgian breweries. Although these beers cover a large portion of the beer styles available globally, some beer styles and consumer patterns may be missing, while other features might be overrepresented. For example, many Belgian ales exhibit yeast-driven flavor profiles, which is reflected in the chemical drivers of appreciation discovered by this study. In future work, expanding the scope to include diverse markets and beer styles could lead to the identification of even more drivers of appreciation and better models for special niche products that were not present in our beer set.
In addition to inherent limitations of GBR models, there are also some limitations associated with studying food aroma. Even if our chemical analyses measured most of the known aroma compounds, the total number of flavor compounds in complex foods like beer is still larger than the subset we were able to measure in this study. For example, hop-derived thiols, that influence flavor at very low concentrations, are notoriously difficult to measure in a high-throughput experiment. Moreover, consumer perception remains subjective and prone to biases that are difficult to avoid. It is also important to stress that the models are still immature and that more extensive datasets will be crucial for developing more complete models in the future. Besides more samples and parameters, our dataset does not include any demographic information about the tasters. Including such data could lead to better models that grasp external factors like age and culture. Another limitation is that our set of beers consists of high-quality end-products and lacks beers that are unfit for sale, which limits the current model in accurately predicting products that are appreciated very badly. Finally, while models could be readily applied in quality control, their use in sensory science and product development is restrained by their inability to discern causal relationships. Given that the models cannot distinguish compounds that genuinely drive consumer perception from those that merely correlate, validation experiments are essential to identify true causative compounds.
Despite the inherent limitations, dissection of our models enabled us to pinpoint specific molecules as potential drivers of beer aroma and consumer appreciation, including compounds that were unexpected and would not have been identified using standard approaches. Important drivers of beer appreciation uncovered by our models include protein levels, ethyl acetate, ethyl phenyl acetate and lactic acid. Currently, many brewers already use lactic acid to acidify their brewing water and ensure optimal pH for enzymatic activity during the mashing process. Our results suggest that adding lactic acid can also improve beer appreciation, although its individual effect remains to be tested. Interestingly, ethanol appears to be unnecessary to improve beer appreciation, both for blond beer and alcohol-free beer. Given the growing consumer interest in alcohol-free beer, with a predicted annual market growth of >7% 84 , it is relevant for brewers to know what compounds can further increase consumer appreciation of these beers. Hence, our model may readily provide avenues to further improve the flavor and consumer appreciation of both alcoholic and non-alcoholic beers, which is generally considered one of the key challenges for future beer production.
Whereas we see a direct implementation of our results for the development of superior alcohol-free beverages and other food products, our study can also serve as a stepping stone for the development of novel alcohol-containing beverages. We want to echo the growing body of scientific evidence for the negative effects of alcohol consumption, both on the individual level by the mutagenic, teratogenic and carcinogenic effects of ethanol 85 , 86 , as well as the burden on society caused by alcohol abuse and addiction. We encourage the use of our results for the production of healthier, tastier products, including novel and improved beverages with lower alcohol contents. Furthermore, we strongly discourage the use of these technologies to improve the appreciation or addictive properties of harmful substances.
The present work demonstrates that despite some important remaining hurdles, combining the latest developments in chemical analyses, sensory analysis and modern machine learning methods offers exciting avenues for food chemistry and engineering. Soon, these tools may provide solutions in quality control and recipe development, as well as new approaches to sensory science and flavor research.
Beer selection
250 commercial Belgian beers were selected to cover the broad diversity of beer styles and corresponding diversity in chemical composition and aroma. See Supplementary Fig. S1 .
Chemical dataset
Sample preparation.
Beers within their expiration date were purchased from commercial retailers. Samples were prepared in biological duplicates at room temperature, unless explicitly stated otherwise. Bottle pressure was measured with a manual pressure device (Steinfurth Mess-Systeme GmbH) and used to calculate CO 2 concentration. The beer was poured through two filter papers (Macherey-Nagel, 500713032 MN 713 ¼) to remove carbon dioxide and prevent spontaneous foaming. Samples were then prepared for measurements by targeted Headspace-Gas Chromatography-Flame Ionization Detector/Flame Photometric Detector (HS-GC-FID/FPD), Headspace-Solid Phase Microextraction-Gas Chromatography-Mass Spectrometry (HS-SPME-GC-MS), colorimetric analysis, enzymatic analysis, Near-Infrared (NIR) analysis, as described in the sections below. The mean values of biological duplicates are reported for each compound.
HS-GC-FID/FPD
HS-GC-FID/FPD (Shimadzu GC 2010 Plus) was used to measure higher alcohols, acetaldehyde, esters, 4-vinyl guaicol, and sulfur compounds. Each measurement comprised 5 ml of sample pipetted into a 20 ml glass vial containing 1.75 g NaCl (VWR, 27810.295). 100 µl of 2-heptanol (Sigma-Aldrich, H3003) (internal standard) solution in ethanol (Fisher Chemical, E/0650DF/C17) was added for a final concentration of 2.44 mg/L. Samples were flushed with nitrogen for 10 s, sealed with a silicone septum, stored at −80 °C and analyzed in batches of 20.
The GC was equipped with a DB-WAXetr column (length, 30 m; internal diameter, 0.32 mm; layer thickness, 0.50 µm; Agilent Technologies, Santa Clara, CA, USA) to the FID and an HP-5 column (length, 30 m; internal diameter, 0.25 mm; layer thickness, 0.25 µm; Agilent Technologies, Santa Clara, CA, USA) to the FPD. N 2 was used as the carrier gas. Samples were incubated for 20 min at 70 °C in the headspace autosampler (Flow rate, 35 cm/s; Injection volume, 1000 µL; Injection mode, split; Combi PAL autosampler, CTC analytics, Switzerland). The injector, FID and FPD temperatures were kept at 250 °C. The GC oven temperature was first held at 50 °C for 5 min and then allowed to rise to 80 °C at a rate of 5 °C/min, followed by a second ramp of 4 °C/min until 200 °C kept for 3 min and a final ramp of (4 °C/min) until 230 °C for 1 min. Results were analyzed with the GCSolution software version 2.4 (Shimadzu, Kyoto, Japan). The GC was calibrated with a 5% EtOH solution (VWR International) containing the volatiles under study (Supplementary Table S7 ).
HS-SPME-GC-MS
HS-SPME-GC-MS (Shimadzu GCMS-QP-2010 Ultra) was used to measure additional volatile compounds, mainly comprising terpenoids and esters. Samples were analyzed by HS-SPME using a triphase DVB/Carboxen/PDMS 50/30 μm SPME fiber (Supelco Co., Bellefonte, PA, USA) followed by gas chromatography (Thermo Fisher Scientific Trace 1300 series, USA) coupled to a mass spectrometer (Thermo Fisher Scientific ISQ series MS) equipped with a TriPlus RSH autosampler. 5 ml of degassed beer sample was placed in 20 ml vials containing 1.75 g NaCl (VWR, 27810.295). 5 µl internal standard mix was added, containing 2-heptanol (1 g/L) (Sigma-Aldrich, H3003), 4-fluorobenzaldehyde (1 g/L) (Sigma-Aldrich, 128376), 2,3-hexanedione (1 g/L) (Sigma-Aldrich, 144169) and guaiacol (1 g/L) (Sigma-Aldrich, W253200) in ethanol (Fisher Chemical, E/0650DF/C17). Each sample was incubated at 60 °C in the autosampler oven with constant agitation. After 5 min equilibration, the SPME fiber was exposed to the sample headspace for 30 min. The compounds trapped on the fiber were thermally desorbed in the injection port of the chromatograph by heating the fiber for 15 min at 270 °C.
The GC-MS was equipped with a low polarity RXi-5Sil MS column (length, 20 m; internal diameter, 0.18 mm; layer thickness, 0.18 µm; Restek, Bellefonte, PA, USA). Injection was performed in splitless mode at 320 °C, a split flow of 9 ml/min, a purge flow of 5 ml/min and an open valve time of 3 min. To obtain a pulsed injection, a programmed gas flow was used whereby the helium gas flow was set at 2.7 mL/min for 0.1 min, followed by a decrease in flow of 20 ml/min to the normal 0.9 mL/min. The temperature was first held at 30 °C for 3 min and then allowed to rise to 80 °C at a rate of 7 °C/min, followed by a second ramp of 2 °C/min till 125 °C and a final ramp of 8 °C/min with a final temperature of 270 °C.
Mass acquisition range was 33 to 550 amu at a scan rate of 5 scans/s. Electron impact ionization energy was 70 eV. The interface and ion source were kept at 275 °C and 250 °C, respectively. A mix of linear n-alkanes (from C7 to C40, Supelco Co.) was injected into the GC-MS under identical conditions to serve as external retention index markers. Identification and quantification of the compounds were performed using an in-house developed R script as described in Goelen et al. and Reher et al. 87 , 88 (for package information, see Supplementary Table S8 ). Briefly, chromatograms were analyzed using AMDIS (v2.71) 89 to separate overlapping peaks and obtain pure compound spectra. The NIST MS Search software (v2.0 g) in combination with the NIST2017, FFNSC3 and Adams4 libraries were used to manually identify the empirical spectra, taking into account the expected retention time. After background subtraction and correcting for retention time shifts between samples run on different days based on alkane ladders, compound elution profiles were extracted and integrated using a file with 284 target compounds of interest, which were either recovered in our identified AMDIS list of spectra or were known to occur in beer. Compound elution profiles were estimated for every peak in every chromatogram over a time-restricted window using weighted non-negative least square analysis after which peak areas were integrated 87 , 88 . Batch effect correction was performed by normalizing against the most stable internal standard compound, 4-fluorobenzaldehyde. Out of all 284 target compounds that were analyzed, 167 were visually judged to have reliable elution profiles and were used for final analysis.
Discrete photometric and enzymatic analysis
Discrete photometric and enzymatic analysis (Thermo Scientific TM Gallery TM Plus Beermaster Discrete Analyzer) was used to measure acetic acid, ammonia, beta-glucan, iso-alpha acids, color, sugars, glycerol, iron, pH, protein, and sulfite. 2 ml of sample volume was used for the analyses. Information regarding the reagents and standard solutions used for analyses and calibrations is included in Supplementary Table S7 and Supplementary Table S9 .
NIR analyses
NIR analysis (Anton Paar Alcolyzer Beer ME System) was used to measure ethanol. Measurements comprised 50 ml of sample, and a 10% EtOH solution was used for calibration.
Correlation calculations
Pairwise Spearman Rank correlations were calculated between all chemical properties.
Sensory dataset
Trained panel.
Our trained tasting panel consisted of volunteers who gave prior verbal informed consent. All compounds used for the validation experiment were of food-grade quality. The tasting sessions were approved by the Social and Societal Ethics Committee of the KU Leuven (G-2022-5677-R2(MAR)). All online reviewers agreed to the Terms and Conditions of the RateBeer website.
Sensory analysis was performed according to the American Society of Brewing Chemists (ASBC) Sensory Analysis Methods 90 . 30 volunteers were screened through a series of triangle tests. The sixteen most sensitive and consistent tasters were retained as taste panel members. The resulting panel was diverse in age [22–42, mean: 29], sex [56% male] and nationality [7 different countries]. The panel developed a consensus vocabulary to describe beer aroma, taste and mouthfeel. Panelists were trained to identify and score 50 different attributes, using a 7-point scale to rate attributes’ intensity. The scoring sheet is included as Supplementary Data 3 . Sensory assessments took place between 10–12 a.m. The beers were served in black-colored glasses. Per session, between 5 and 12 beers of the same style were tasted at 12 °C to 16 °C. Two reference beers were added to each set and indicated as ‘Reference 1 & 2’, allowing panel members to calibrate their ratings. Not all panelists were present at every tasting. Scores were scaled by standard deviation and mean-centered per taster. Values are represented as z-scores and clustered by Euclidean distance. Pairwise Spearman correlations were calculated between taste and aroma sensory attributes. Panel consistency was evaluated by repeating samples on different sessions and performing ANOVA to identify differences, using the ‘stats’ package (v4.2.2) in R (for package information, see Supplementary Table S8 ).
Online reviews from a public database
The ‘scrapy’ package in Python (v3.6) (for package information, see Supplementary Table S8 ). was used to collect 232,288 online reviews (mean=922, min=6, max=5343) from RateBeer, an online beer review database. Each review entry comprised 5 numerical scores (appearance, aroma, taste, palate and overall quality) and an optional review text. The total number of reviews per reviewer was collected separately. Numerical scores were scaled and centered per rater, and mean scores were calculated per beer.
For the review texts, the language was estimated using the packages ‘langdetect’ and ‘langid’ in Python. Reviews that were classified as English by both packages were kept. Reviewers with fewer than 100 entries overall were discarded. 181,025 reviews from >6000 reviewers from >40 countries remained. Text processing was done using the ‘nltk’ package in Python. Texts were corrected for slang and misspellings; proper nouns and rare words that are relevant to the beer context were specified and kept as-is (‘Chimay’,’Lambic’, etc.). A dictionary of semantically similar sensorial terms, for example ‘floral’ and ‘flower’, was created and collapsed together into one term. Words were stemmed and lemmatized to avoid identifying words such as ‘acid’ and ‘acidity’ as separate terms. Numbers and punctuation were removed.
Sentences from up to 50 randomly chosen reviews per beer were manually categorized according to the aspect of beer they describe (appearance, aroma, taste, palate, overall quality—not to be confused with the 5 numerical scores described above) or flagged as irrelevant if they contained no useful information. If a beer contained fewer than 50 reviews, all reviews were manually classified. This labeled data set was used to train a model that classified the rest of the sentences for all beers 91 . Sentences describing taste and aroma were extracted, and term frequency–inverse document frequency (TFIDF) was implemented to calculate enrichment scores for sensorial words per beer.
The sex of the tasting subject was not considered when building our sensory database. Instead, results from different panelists were averaged, both for our trained panel (56% male, 44% female) and the RateBeer reviews (70% male, 30% female for RateBeer as a whole).
Beer price collection and processing
Beer prices were collected from the following stores: Colruyt, Delhaize, Total Wine, BeerHawk, The Belgian Beer Shop, The Belgian Shop, and Beer of Belgium. Where applicable, prices were converted to Euros and normalized per liter. Spearman correlations were calculated between these prices and mean overall appreciation scores from RateBeer and the taste panel, respectively.
Pairwise Spearman Rank correlations were calculated between all sensory properties.
Machine learning models
Predictive modeling of sensory profiles from chemical data.
Regression models were constructed to predict (a) trained panel scores for beer flavors and quality from beer chemical profiles and (b) public reviews’ appreciation scores from beer chemical profiles. Z-scores were used to represent sensory attributes in both data sets. Chemical properties with log-normal distributions (Shapiro-Wilk test, p < 0.05 ) were log-transformed. Missing chemical measurements (0.1% of all data) were replaced with mean values per attribute. Observations from 250 beers were randomly separated into a training set (70%, 175 beers) and a test set (30%, 75 beers), stratified per beer style. Chemical measurements (p = 231) were normalized based on the training set average and standard deviation. In total, three linear regression-based models: linear regression with first-order interaction terms (LR), lasso regression with first-order interaction terms (Lasso) and partial least squares regression (PLSR); five decision tree models, Adaboost regressor (ABR), Extra Trees (ET), Gradient Boosting regressor (GBR), Random Forest (RF) and XGBoost regressor (XGBR); one support vector machine model (SVR) and one artificial neural network model (ANN) were trained. The models were implemented using the ‘scikit-learn’ package (v1.2.2) and ‘xgboost’ package (v1.7.3) in Python (v3.9.16). Models were trained, and hyperparameters optimized, using five-fold cross-validated grid search with the coefficient of determination (R 2 ) as the evaluation metric. The ANN (scikit-learn’s MLPRegressor) was optimized using Bayesian Tree-Structured Parzen Estimator optimization with the ‘Optuna’ Python package (v3.2.0). Individual models were trained per attribute, and a multi-output model was trained on all attributes simultaneously.
Model dissection
GBR was found to outperform other methods, resulting in models with the highest average R 2 values in both trained panel and public review data sets. Impurity-based rankings of the most important predictors for each predicted sensorial trait were obtained using the ‘scikit-learn’ package. To observe the relationships between these chemical properties and their predicted targets, partial dependence plots (PDP) were constructed for the six most important predictors of consumer appreciation 74 , 75 .
The ‘SHAP’ package in Python (v0.41.0) was implemented to provide an alternative ranking of predictor importance and to visualize the predictors’ effects as a function of their concentration 68 .
Validation of causal chemical properties
To validate the effects of the most important model features on predicted sensory attributes, beers were spiked with the chemical compounds identified by the models and descriptive sensory analyses were carried out according to the American Society of Brewing Chemists (ASBC) protocol 90 .
Compound spiking was done 30 min before tasting. Compounds were spiked into fresh beer bottles, that were immediately resealed and inverted three times. Fresh bottles of beer were opened for the same duration, resealed, and inverted thrice, to serve as controls. Pairs of spiked samples and controls were served simultaneously, chilled and in dark glasses as outlined in the Trained panel section above. Tasters were instructed to select the glass with the higher flavor intensity for each attribute (directional difference test 92 ) and to select the glass they prefer.
The final concentration after spiking was equal to the within-style average, after normalizing by ethanol concentration. This was done to ensure balanced flavor profiles in the final spiked beer. The same methods were applied to improve a non-alcoholic beer. Compounds were the following: ethyl acetate (Merck KGaA, W241415), ethyl hexanoate (Merck KGaA, W243906), isoamyl acetate (Merck KGaA, W205508), phenethyl acetate (Merck KGaA, W285706), ethanol (96%, Colruyt), glycerol (Merck KGaA, W252506), lactic acid (Merck KGaA, 261106).
Significant differences in preference or perceived intensity were determined by performing the two-sided binomial test on each attribute.
Reporting summary
Further information on research design is available in the Nature Portfolio Reporting Summary linked to this article.
Data availability
The data that support the findings of this work are available in the Supplementary Data files and have been deposited to Zenodo under accession code 10653704 93 . The RateBeer scores data are under restricted access, they are not publicly available as they are property of RateBeer (ZX Ventures, USA). Access can be obtained from the authors upon reasonable request and with permission of RateBeer (ZX Ventures, USA). Source data are provided with this paper.
Code availability
The code for training the machine learning models, analyzing the models, and generating the figures has been deposited to Zenodo under accession code 10653704 93 .
Tieman, D. et al. A chemical genetic roadmap to improved tomato flavor. Science 355 , 391–394 (2017).
Article ADS CAS PubMed Google Scholar
Plutowska, B. & Wardencki, W. Application of gas chromatography–olfactometry (GC–O) in analysis and quality assessment of alcoholic beverages – A review. Food Chem. 107 , 449–463 (2008).
Article CAS Google Scholar
Legin, A., Rudnitskaya, A., Seleznev, B. & Vlasov, Y. Electronic tongue for quality assessment of ethanol, vodka and eau-de-vie. Anal. Chim. Acta 534 , 129–135 (2005).
Loutfi, A., Coradeschi, S., Mani, G. K., Shankar, P. & Rayappan, J. B. B. Electronic noses for food quality: A review. J. Food Eng. 144 , 103–111 (2015).
Ahn, Y.-Y., Ahnert, S. E., Bagrow, J. P. & Barabási, A.-L. Flavor network and the principles of food pairing. Sci. Rep. 1 , 196 (2011).
Article CAS PubMed PubMed Central Google Scholar
Bartoshuk, L. M. & Klee, H. J. Better fruits and vegetables through sensory analysis. Curr. Biol. 23 , R374–R378 (2013).
Article CAS PubMed Google Scholar
Piggott, J. R. Design questions in sensory and consumer science. Food Qual. Prefer. 3293 , 217–220 (1995).
Article Google Scholar
Kermit, M. & Lengard, V. Assessing the performance of a sensory panel-panellist monitoring and tracking. J. Chemom. 19 , 154–161 (2005).
Cook, D. J., Hollowood, T. A., Linforth, R. S. T. & Taylor, A. J. Correlating instrumental measurements of texture and flavour release with human perception. Int. J. Food Sci. Technol. 40 , 631–641 (2005).
Chinchanachokchai, S., Thontirawong, P. & Chinchanachokchai, P. A tale of two recommender systems: The moderating role of consumer expertise on artificial intelligence based product recommendations. J. Retail. Consum. Serv. 61 , 1–12 (2021).
Ross, C. F. Sensory science at the human-machine interface. Trends Food Sci. Technol. 20 , 63–72 (2009).
Chambers, E. IV & Koppel, K. Associations of volatile compounds with sensory aroma and flavor: The complex nature of flavor. Molecules 18 , 4887–4905 (2013).
Pinu, F. R. Metabolomics—The new frontier in food safety and quality research. Food Res. Int. 72 , 80–81 (2015).
Danezis, G. P., Tsagkaris, A. S., Brusic, V. & Georgiou, C. A. Food authentication: state of the art and prospects. Curr. Opin. Food Sci. 10 , 22–31 (2016).
Shepherd, G. M. Smell images and the flavour system in the human brain. Nature 444 , 316–321 (2006).
Meilgaard, M. C. Prediction of flavor differences between beers from their chemical composition. J. Agric. Food Chem. 30 , 1009–1017 (1982).
Xu, L. et al. Widespread receptor-driven modulation in peripheral olfactory coding. Science 368 , eaaz5390 (2020).
Kupferschmidt, K. Following the flavor. Science 340 , 808–809 (2013).
Billesbølle, C. B. et al. Structural basis of odorant recognition by a human odorant receptor. Nature 615 , 742–749 (2023).
Article ADS PubMed PubMed Central Google Scholar
Smith, B. Perspective: Complexities of flavour. Nature 486 , S6–S6 (2012).
Pfister, P. et al. Odorant receptor inhibition is fundamental to odor encoding. Curr. Biol. 30 , 2574–2587 (2020).
Moskowitz, H. W., Kumaraiah, V., Sharma, K. N., Jacobs, H. L. & Sharma, S. D. Cross-cultural differences in simple taste preferences. Science 190 , 1217–1218 (1975).
Eriksson, N. et al. A genetic variant near olfactory receptor genes influences cilantro preference. Flavour 1 , 22 (2012).
Ferdenzi, C. et al. Variability of affective responses to odors: Culture, gender, and olfactory knowledge. Chem. Senses 38 , 175–186 (2013).
Article PubMed Google Scholar
Lawless, H. T. & Heymann, H. Sensory evaluation of food: Principles and practices. (Springer, New York, NY). https://doi.org/10.1007/978-1-4419-6488-5 (2010).
Colantonio, V. et al. Metabolomic selection for enhanced fruit flavor. Proc. Natl. Acad. Sci. 119 , e2115865119 (2022).
Fritz, F., Preissner, R. & Banerjee, P. VirtualTaste: a web server for the prediction of organoleptic properties of chemical compounds. Nucleic Acids Res 49 , W679–W684 (2021).
Tuwani, R., Wadhwa, S. & Bagler, G. BitterSweet: Building machine learning models for predicting the bitter and sweet taste of small molecules. Sci. Rep. 9 , 1–13 (2019).
Dagan-Wiener, A. et al. Bitter or not? BitterPredict, a tool for predicting taste from chemical structure. Sci. Rep. 7 , 1–13 (2017).
Pallante, L. et al. Toward a general and interpretable umami taste predictor using a multi-objective machine learning approach. Sci. Rep. 12 , 1–11 (2022).
Malavolta, M. et al. A survey on computational taste predictors. Eur. Food Res. Technol. 248 , 2215–2235 (2022).
Lee, B. K. et al. A principal odor map unifies diverse tasks in olfactory perception. Science 381 , 999–1006 (2023).
Mayhew, E. J. et al. Transport features predict if a molecule is odorous. Proc. Natl. Acad. Sci. 119 , e2116576119 (2022).
Niu, Y. et al. Sensory evaluation of the synergism among ester odorants in light aroma-type liquor by odor threshold, aroma intensity and flash GC electronic nose. Food Res. Int. 113 , 102–114 (2018).
Yu, P., Low, M. Y. & Zhou, W. Design of experiments and regression modelling in food flavour and sensory analysis: A review. Trends Food Sci. Technol. 71 , 202–215 (2018).
Oladokun, O. et al. The impact of hop bitter acid and polyphenol profiles on the perceived bitterness of beer. Food Chem. 205 , 212–220 (2016).
Linforth, R., Cabannes, M., Hewson, L., Yang, N. & Taylor, A. Effect of fat content on flavor delivery during consumption: An in vivo model. J. Agric. Food Chem. 58 , 6905–6911 (2010).
Guo, S., Na Jom, K. & Ge, Y. Influence of roasting condition on flavor profile of sunflower seeds: A flavoromics approach. Sci. Rep. 9 , 11295 (2019).
Ren, Q. et al. The changes of microbial community and flavor compound in the fermentation process of Chinese rice wine using Fagopyrum tataricum grain as feedstock. Sci. Rep. 9 , 3365 (2019).
Hastie, T., Friedman, J. & Tibshirani, R. The Elements of Statistical Learning. (Springer, New York, NY). https://doi.org/10.1007/978-0-387-21606-5 (2001).
Dietz, C., Cook, D., Huismann, M., Wilson, C. & Ford, R. The multisensory perception of hop essential oil: a review. J. Inst. Brew. 126 , 320–342 (2020).
CAS Google Scholar
Roncoroni, Miguel & Verstrepen, Kevin Joan. Belgian Beer: Tested and Tasted. (Lannoo, 2018).
Meilgaard, M. Flavor chemistry of beer: Part II: Flavor and threshold of 239 aroma volatiles. in (1975).
Bokulich, N. A. & Bamforth, C. W. The microbiology of malting and brewing. Microbiol. Mol. Biol. Rev. MMBR 77 , 157–172 (2013).
Dzialo, M. C., Park, R., Steensels, J., Lievens, B. & Verstrepen, K. J. Physiology, ecology and industrial applications of aroma formation in yeast. FEMS Microbiol. Rev. 41 , S95–S128 (2017).
Article PubMed PubMed Central Google Scholar
Datta, A. et al. Computer-aided food engineering. Nat. Food 3 , 894–904 (2022).
American Society of Brewing Chemists. Beer Methods. (American Society of Brewing Chemists, St. Paul, MN, U.S.A.).
Olaniran, A. O., Hiralal, L., Mokoena, M. P. & Pillay, B. Flavour-active volatile compounds in beer: production, regulation and control. J. Inst. Brew. 123 , 13–23 (2017).
Verstrepen, K. J. et al. Flavor-active esters: Adding fruitiness to beer. J. Biosci. Bioeng. 96 , 110–118 (2003).
Meilgaard, M. C. Flavour chemistry of beer. part I: flavour interaction between principal volatiles. Master Brew. Assoc. Am. Tech. Q 12 , 107–117 (1975).
Briggs, D. E., Boulton, C. A., Brookes, P. A. & Stevens, R. Brewing 227–254. (Woodhead Publishing). https://doi.org/10.1533/9781855739062.227 (2004).
Bossaert, S., Crauwels, S., De Rouck, G. & Lievens, B. The power of sour - A review: Old traditions, new opportunities. BrewingScience 72 , 78–88 (2019).
Google Scholar
Verstrepen, K. J. et al. Flavor active esters: Adding fruitiness to beer. J. Biosci. Bioeng. 96 , 110–118 (2003).
Snauwaert, I. et al. Microbial diversity and metabolite composition of Belgian red-brown acidic ales. Int. J. Food Microbiol. 221 , 1–11 (2016).
Spitaels, F. et al. The microbial diversity of traditional spontaneously fermented lambic beer. PLoS ONE 9 , e95384 (2014).
Blanco, C. A., Andrés-Iglesias, C. & Montero, O. Low-alcohol Beers: Flavor Compounds, Defects, and Improvement Strategies. Crit. Rev. Food Sci. Nutr. 56 , 1379–1388 (2016).
Jackowski, M. & Trusek, A. Non-Alcohol. beer Prod. – Overv. 20 , 32–38 (2018).
Takoi, K. et al. The contribution of geraniol metabolism to the citrus flavour of beer: Synergy of geraniol and β-citronellol under coexistence with excess linalool. J. Inst. Brew. 116 , 251–260 (2010).
Kroeze, J. H. & Bartoshuk, L. M. Bitterness suppression as revealed by split-tongue taste stimulation in humans. Physiol. Behav. 35 , 779–783 (1985).
Mennella, J. A. et al. A spoonful of sugar helps the medicine go down”: Bitter masking bysucrose among children and adults. Chem. Senses 40 , 17–25 (2015).
Wietstock, P., Kunz, T., Perreira, F. & Methner, F.-J. Metal chelation behavior of hop acids in buffered model systems. BrewingScience 69 , 56–63 (2016).
Sancho, D., Blanco, C. A., Caballero, I. & Pascual, A. Free iron in pale, dark and alcohol-free commercial lager beers. J. Sci. Food Agric. 91 , 1142–1147 (2011).
Rodrigues, H. & Parr, W. V. Contribution of cross-cultural studies to understanding wine appreciation: A review. Food Res. Int. 115 , 251–258 (2019).
Korneva, E. & Blockeel, H. Towards better evaluation of multi-target regression models. in ECML PKDD 2020 Workshops (eds. Koprinska, I. et al.) 353–362 (Springer International Publishing, Cham, 2020). https://doi.org/10.1007/978-3-030-65965-3_23 .
Gastón Ares. Mathematical and Statistical Methods in Food Science and Technology. (Wiley, 2013).
Grinsztajn, L., Oyallon, E. & Varoquaux, G. Why do tree-based models still outperform deep learning on tabular data? Preprint at http://arxiv.org/abs/2207.08815 (2022).
Gries, S. T. Statistics for Linguistics with R: A Practical Introduction. in Statistics for Linguistics with R (De Gruyter Mouton, 2021). https://doi.org/10.1515/9783110718256 .
Lundberg, S. M. et al. From local explanations to global understanding with explainable AI for trees. Nat. Mach. Intell. 2 , 56–67 (2020).
Ickes, C. M. & Cadwallader, K. R. Effects of ethanol on flavor perception in alcoholic beverages. Chemosens. Percept. 10 , 119–134 (2017).
Kato, M. et al. Influence of high molecular weight polypeptides on the mouthfeel of commercial beer. J. Inst. Brew. 127 , 27–40 (2021).
Wauters, R. et al. Novel Saccharomyces cerevisiae variants slow down the accumulation of staling aldehydes and improve beer shelf-life. Food Chem. 398 , 1–11 (2023).
Li, H., Jia, S. & Zhang, W. Rapid determination of low-level sulfur compounds in beer by headspace gas chromatography with a pulsed flame photometric detector. J. Am. Soc. Brew. Chem. 66 , 188–191 (2008).
Dercksen, A., Laurens, J., Torline, P., Axcell, B. C. & Rohwer, E. Quantitative analysis of volatile sulfur compounds in beer using a membrane extraction interface. J. Am. Soc. Brew. Chem. 54 , 228–233 (1996).
Molnar, C. Interpretable Machine Learning: A Guide for Making Black-Box Models Interpretable. (2020).
Zhao, Q. & Hastie, T. Causal interpretations of black-box models. J. Bus. Econ. Stat. Publ. Am. Stat. Assoc. 39 , 272–281 (2019).
Article MathSciNet Google Scholar
Hastie, T., Tibshirani, R. & Friedman, J. The Elements of Statistical Learning. (Springer, 2019).
Labrado, D. et al. Identification by NMR of key compounds present in beer distillates and residual phases after dealcoholization by vacuum distillation. J. Sci. Food Agric. 100 , 3971–3978 (2020).
Lusk, L. T., Kay, S. B., Porubcan, A. & Ryder, D. S. Key olfactory cues for beer oxidation. J. Am. Soc. Brew. Chem. 70 , 257–261 (2012).
Gonzalez Viejo, C., Torrico, D. D., Dunshea, F. R. & Fuentes, S. Development of artificial neural network models to assess beer acceptability based on sensory properties using a robotic pourer: A comparative model approach to achieve an artificial intelligence system. Beverages 5 , 33 (2019).
Gonzalez Viejo, C., Fuentes, S., Torrico, D. D., Godbole, A. & Dunshea, F. R. Chemical characterization of aromas in beer and their effect on consumers liking. Food Chem. 293 , 479–485 (2019).
Gilbert, J. L. et al. Identifying breeding priorities for blueberry flavor using biochemical, sensory, and genotype by environment analyses. PLOS ONE 10 , 1–21 (2015).
Goulet, C. et al. Role of an esterase in flavor volatile variation within the tomato clade. Proc. Natl. Acad. Sci. 109 , 19009–19014 (2012).
Article ADS CAS PubMed PubMed Central Google Scholar
Borisov, V. et al. Deep Neural Networks and Tabular Data: A Survey. IEEE Trans. Neural Netw. Learn. Syst. 1–21 https://doi.org/10.1109/TNNLS.2022.3229161 (2022).
Statista. Statista Consumer Market Outlook: Beer - Worldwide.
Seitz, H. K. & Stickel, F. Molecular mechanisms of alcoholmediated carcinogenesis. Nat. Rev. Cancer 7 , 599–612 (2007).
Voordeckers, K. et al. Ethanol exposure increases mutation rate through error-prone polymerases. Nat. Commun. 11 , 3664 (2020).
Goelen, T. et al. Bacterial phylogeny predicts volatile organic compound composition and olfactory response of an aphid parasitoid. Oikos 129 , 1415–1428 (2020).
Article ADS Google Scholar
Reher, T. et al. Evaluation of hop (Humulus lupulus) as a repellent for the management of Drosophila suzukii. Crop Prot. 124 , 104839 (2019).
Stein, S. E. An integrated method for spectrum extraction and compound identification from gas chromatography/mass spectrometry data. J. Am. Soc. Mass Spectrom. 10 , 770–781 (1999).
American Society of Brewing Chemists. Sensory Analysis Methods. (American Society of Brewing Chemists, St. Paul, MN, U.S.A., 1992).
McAuley, J., Leskovec, J. & Jurafsky, D. Learning Attitudes and Attributes from Multi-Aspect Reviews. Preprint at https://doi.org/10.48550/arXiv.1210.3926 (2012).
Meilgaard, M. C., Carr, B. T. & Carr, B. T. Sensory Evaluation Techniques. (CRC Press, Boca Raton). https://doi.org/10.1201/b16452 (2014).
Schreurs, M. et al. Data from: Predicting and improving complex beer flavor through machine learning. Zenodo https://doi.org/10.5281/zenodo.10653704 (2024).
Download references
Acknowledgements
We thank all lab members for their discussions and thank all tasting panel members for their contributions. Special thanks go out to Dr. Karin Voordeckers for her tremendous help in proofreading and improving the manuscript. M.S. was supported by a Baillet-Latour fellowship, L.C. acknowledges financial support from KU Leuven (C16/17/006), F.A.T. was supported by a PhD fellowship from FWO (1S08821N). Research in the lab of K.J.V. is supported by KU Leuven, FWO, VIB, VLAIO and the Brewing Science Serves Health Fund. Research in the lab of T.W. is supported by FWO (G.0A51.15) and KU Leuven (C16/17/006).
Author information
These authors contributed equally: Michiel Schreurs, Supinya Piampongsant, Miguel Roncoroni.
Authors and Affiliations
VIB—KU Leuven Center for Microbiology, Gaston Geenslaan 1, B-3001, Leuven, Belgium
Michiel Schreurs, Supinya Piampongsant, Miguel Roncoroni, Lloyd Cool, Beatriz Herrera-Malaver, Florian A. Theßeling & Kevin J. Verstrepen
CMPG Laboratory of Genetics and Genomics, KU Leuven, Gaston Geenslaan 1, B-3001, Leuven, Belgium
Leuven Institute for Beer Research (LIBR), Gaston Geenslaan 1, B-3001, Leuven, Belgium
Laboratory of Socioecology and Social Evolution, KU Leuven, Naamsestraat 59, B-3000, Leuven, Belgium
Lloyd Cool, Christophe Vanderaa & Tom Wenseleers
VIB Bioinformatics Core, VIB, Rijvisschestraat 120, B-9052, Ghent, Belgium
Łukasz Kreft & Alexander Botzki
AB InBev SA/NV, Brouwerijplein 1, B-3000, Leuven, Belgium
Philippe Malcorps & Luk Daenen
You can also search for this author in PubMed Google Scholar
Contributions
S.P., M.S. and K.J.V. conceived the experiments. S.P., M.S. and K.J.V. designed the experiments. S.P., M.S., M.R., B.H. and F.A.T. performed the experiments. S.P., M.S., L.C., C.V., L.K., A.B., P.M., L.D., T.W. and K.J.V. contributed analysis ideas. S.P., M.S., L.C., C.V., T.W. and K.J.V. analyzed the data. All authors contributed to writing the manuscript.
Corresponding author
Correspondence to Kevin J. Verstrepen .
Ethics declarations
Competing interests.
K.J.V. is affiliated with bar.on. The other authors declare no competing interests.
Peer review
Peer review information.
Nature Communications thanks Florian Bauer, Andrew John Macintosh and the other, anonymous, reviewer(s) for their contribution to the peer review of this work. A peer review file is available.
Additional information
Publisher’s note Springer Nature remains neutral with regard to jurisdictional claims in published maps and institutional affiliations.
Supplementary information
Supplementary information, peer review file, description of additional supplementary files, supplementary data 1, supplementary data 2, supplementary data 3, supplementary data 4, supplementary data 5, supplementary data 6, supplementary data 7, reporting summary, source data, source data, rights and permissions.
Open Access This article is licensed under a Creative Commons Attribution 4.0 International License, which permits use, sharing, adaptation, distribution and reproduction in any medium or format, as long as you give appropriate credit to the original author(s) and the source, provide a link to the Creative Commons licence, and indicate if changes were made. The images or other third party material in this article are included in the article’s Creative Commons licence, unless indicated otherwise in a credit line to the material. If material is not included in the article’s Creative Commons licence and your intended use is not permitted by statutory regulation or exceeds the permitted use, you will need to obtain permission directly from the copyright holder. To view a copy of this licence, visit http://creativecommons.org/licenses/by/4.0/ .
Reprints and permissions
About this article
Cite this article.
Schreurs, M., Piampongsant, S., Roncoroni, M. et al. Predicting and improving complex beer flavor through machine learning. Nat Commun 15 , 2368 (2024). https://doi.org/10.1038/s41467-024-46346-0
Download citation
Received : 30 October 2023
Accepted : 21 February 2024
Published : 26 March 2024
DOI : https://doi.org/10.1038/s41467-024-46346-0
Share this article
Anyone you share the following link with will be able to read this content:
Sorry, a shareable link is not currently available for this article.
Provided by the Springer Nature SharedIt content-sharing initiative
By submitting a comment you agree to abide by our Terms and Community Guidelines . If you find something abusive or that does not comply with our terms or guidelines please flag it as inappropriate.
Quick links
- Explore articles by subject
- Guide to authors
- Editorial policies
Sign up for the Nature Briefing: Translational Research newsletter — top stories in biotechnology, drug discovery and pharma.

- Share full article
Advertisement
Supported by
More Studies by Columbia Cancer Researchers Are Retracted
The studies, pulled because of copied data, illustrate the sluggishness of scientific publishers to address serious errors, experts said.

By Benjamin Mueller
Scientists in a prominent cancer lab at Columbia University have now had four studies retracted and a stern note added to a fifth accusing it of “severe abuse of the scientific publishing system,” the latest fallout from research misconduct allegations recently leveled against several leading cancer scientists.
A scientific sleuth in Britain last year uncovered discrepancies in data published by the Columbia lab, including the reuse of photos and other images across different papers. The New York Times reported last month that a medical journal in 2022 had quietly taken down a stomach cancer study by the researchers after an internal inquiry by the journal found ethics violations.
Despite that study’s removal, the researchers — Dr. Sam Yoon, chief of a cancer surgery division at Columbia University’s medical center, and Changhwan Yoon, a more junior biologist there — continued publishing studies with suspicious data. Since 2008, the two scientists have collaborated with other researchers on 26 articles that the sleuth, Sholto David, publicly flagged for misrepresenting experiments’ results.
One of those articles was retracted last month after The Times asked publishers about the allegations. In recent weeks, medical journals have retracted three additional studies, which described new strategies for treating cancers of the stomach, head and neck. Other labs had cited the articles in roughly 90 papers.
A major scientific publisher also appended a blunt note to the article that it had originally taken down without explanation in 2022. “This reuse (and in part, misrepresentation) of data without appropriate attribution represents a severe abuse of the scientific publishing system,” it said .
Still, those measures addressed only a small fraction of the lab’s suspect papers. Experts said the episode illustrated not only the extent of unreliable research by top labs, but also the tendency of scientific publishers to respond slowly, if at all, to significant problems once they are detected. As a result, other labs keep relying on questionable work as they pour federal research money into studies, allowing errors to accumulate in the scientific record.
“For every one paper that is retracted, there are probably 10 that should be,” said Dr. Ivan Oransky, co-founder of Retraction Watch, which keeps a database of 47,000-plus retracted studies. “Journals are not particularly interested in correcting the record.”
Columbia’s medical center declined to comment on allegations facing Dr. Yoon’s lab. It said the two scientists remained at Columbia and the hospital “is fully committed to upholding the highest standards of ethics and to rigorously maintaining the integrity of our research.”
The lab’s web page was recently taken offline. Columbia declined to say why. Neither Dr. Yoon nor Changhwan Yoon could be reached for comment. (They are not related.)
Memorial Sloan Kettering Cancer Center, where the scientists worked when much of the research was done, is investigating their work.
The Columbia scientists’ retractions come amid growing attention to the suspicious data that undergirds some medical research. Since late February, medical journals have retracted seven papers by scientists at Harvard’s Dana-Farber Cancer Institute . That followed investigations into data problems publicized by Dr. David , an independent molecular biologist who looks for irregularities in published images of cells, tumors and mice, sometimes with help from A.I. software.
The spate of misconduct allegations has drawn attention to the pressures on academic scientists — even those, like Dr. Yoon, who also work as doctors — to produce heaps of research.
Strong images of experiments’ results are often needed for those studies. Publishing them helps scientists win prestigious academic appointments and attract federal research grants that can pay dividends for themselves and their universities.
Dr. Yoon, a robotic surgery specialist noted for his treatment of stomach cancers, has helped bring in nearly $5 million in federal research money over his career.
The latest retractions from his lab included articles from 2020 and 2021 that Dr. David said contained glaring irregularities . Their results appeared to include identical images of tumor-stricken mice, despite those mice supposedly having been subjected to different experiments involving separate treatments and types of cancer cells.
The medical journal Cell Death & Disease retracted two of the latest studies, and Oncogene retracted the third. The journals found that the studies had also reused other images, like identical pictures of constellations of cancer cells.
The studies Dr. David flagged as containing image problems were largely overseen by the more senior Dr. Yoon. Changhwan Yoon, an associate research scientist who has worked alongside Dr. Yoon for a decade, was often a first author, which generally designates the scientist who ran the bulk of the experiments.
Kun Huang, a scientist in China who oversaw one of the recently retracted studies, a 2020 paper that did not include the more senior Dr. Yoon, attributed that study’s problematic sections to Changhwan Yoon. Dr. Huang, who made those comments this month on PubPeer, a website where scientists post about studies, did not respond to an email seeking comment.
But the more senior Dr. Yoon has long been made aware of problems in research he published alongside Changhwan Yoon: The two scientists were notified of the removal in January 2022 of their stomach cancer study that was found to have violated ethics guidelines.
Research misconduct is often pinned on the more junior researchers who conduct experiments. Other scientists, though, assign greater responsibility to the senior researchers who run labs and oversee studies, even as they juggle jobs as doctors or administrators.
“The research world’s coming to realize that with great power comes great responsibility and, in fact, you are responsible not just for what one of your direct reports in the lab has done, but for the environment you create,” Dr. Oransky said.
In their latest public retraction notices, medical journals said that they had lost faith in the results and conclusions. Imaging experts said some irregularities identified by Dr. David bore signs of deliberate manipulation, like flipped or rotated images, while others could have been sloppy copy-and-paste errors.
The little-noticed removal by a journal of the stomach cancer study in January 2022 highlighted some scientific publishers’ policy of not disclosing the reasons for withdrawing papers as long as they have not yet formally appeared in print. That study had appeared only online.
Roland Herzog, the editor of the journal Molecular Therapy, said that editors had drafted an explanation that they intended to publish at the time of the article’s removal. But Elsevier, the journal’s parent publisher, advised them that such a note was unnecessary, he said.
Only after the Times article last month did Elsevier agree to explain the article’s removal publicly with the stern note. In an editorial this week , the Molecular Therapy editors said that in the future, they would explain the removal of any articles that had been published only online.
But Elsevier said in a statement that it did not consider online articles “to be the final published articles of record.” As a result, company policy continues to advise that such articles be removed without an explanation when they are found to contain problems. The company said it allowed editors to provide additional information where needed.
Elsevier, which publishes nearly 3,000 journals and generates billions of dollars in annual revenue , has long been criticized for its opaque removals of online articles.
Articles by the Columbia scientists with data discrepancies that remain unaddressed were largely distributed by three major publishers: Elsevier, Springer Nature and the American Association for Cancer Research. Dr. David alerted many journals to the data discrepancies in October.
Each publisher said it was investigating the concerns. Springer Nature said investigations take time because they can involve consulting experts, waiting for author responses and analyzing raw data.
Dr. David has also raised concerns about studies published independently by scientists who collaborated with the Columbia researchers on some of their recently retracted papers. For example, Sandra Ryeom, an associate professor of surgical sciences at Columbia, published an article in 2003 while at Harvard that Dr. David said contained a duplicated image . As of 2021, she was married to the more senior Dr. Yoon, according to a mortgage document from that year.
A medical journal appended a formal notice to the article last week saying “appropriate editorial action will be taken” once data concerns had been resolved. Dr. Ryeom said in a statement that she was working with the paper’s senior author on “correcting the error.”
Columbia has sought to reinforce the importance of sound research practices. Hours after the Times article appeared last month, Dr. Michael Shelanski, the medical school’s senior vice dean for research, sent an email to faculty members titled “Research Fraud Accusations — How to Protect Yourself.” It warned that such allegations, whatever their merits, could take a toll on the university.
“In the months that it can take to investigate an allegation,” Dr. Shelanski wrote, “funding can be suspended, and donors can feel that their trust has been betrayed.”
Benjamin Mueller reports on health and medicine. He was previously a U.K. correspondent in London and a police reporter in New York. More about Benjamin Mueller
- Search for: Toggle Search
‘You Transformed the World,’ NVIDIA CEO Tells Researchers Behind Landmark AI Paper
Of GTC ’s 900+ sessions, the most wildly popular was a conversation hosted by NVIDIA founder and CEO Jensen Huang with seven of the authors of the legendary research paper that introduced the aptly named transformer — a neural network architecture that went on to change the deep learning landscape and enable today’s era of generative AI.
“Everything that we’re enjoying today can be traced back to that moment,” Huang said to a packed room with hundreds of attendees, who heard him speak with the authors of “ Attention Is All You Need .”
Sharing the stage for the first time, the research luminaries reflected on the factors that led to their original paper, which has been cited more than 100,000 times since it was first published and presented at the NeurIPS AI conference. They also discussed their latest projects and offered insights into future directions for the field of generative AI.
While they started as Google researchers, the collaborators are now spread across the industry, most as founders of their own AI companies.
“We have a whole industry that is grateful for the work that you guys did,” Huang said.
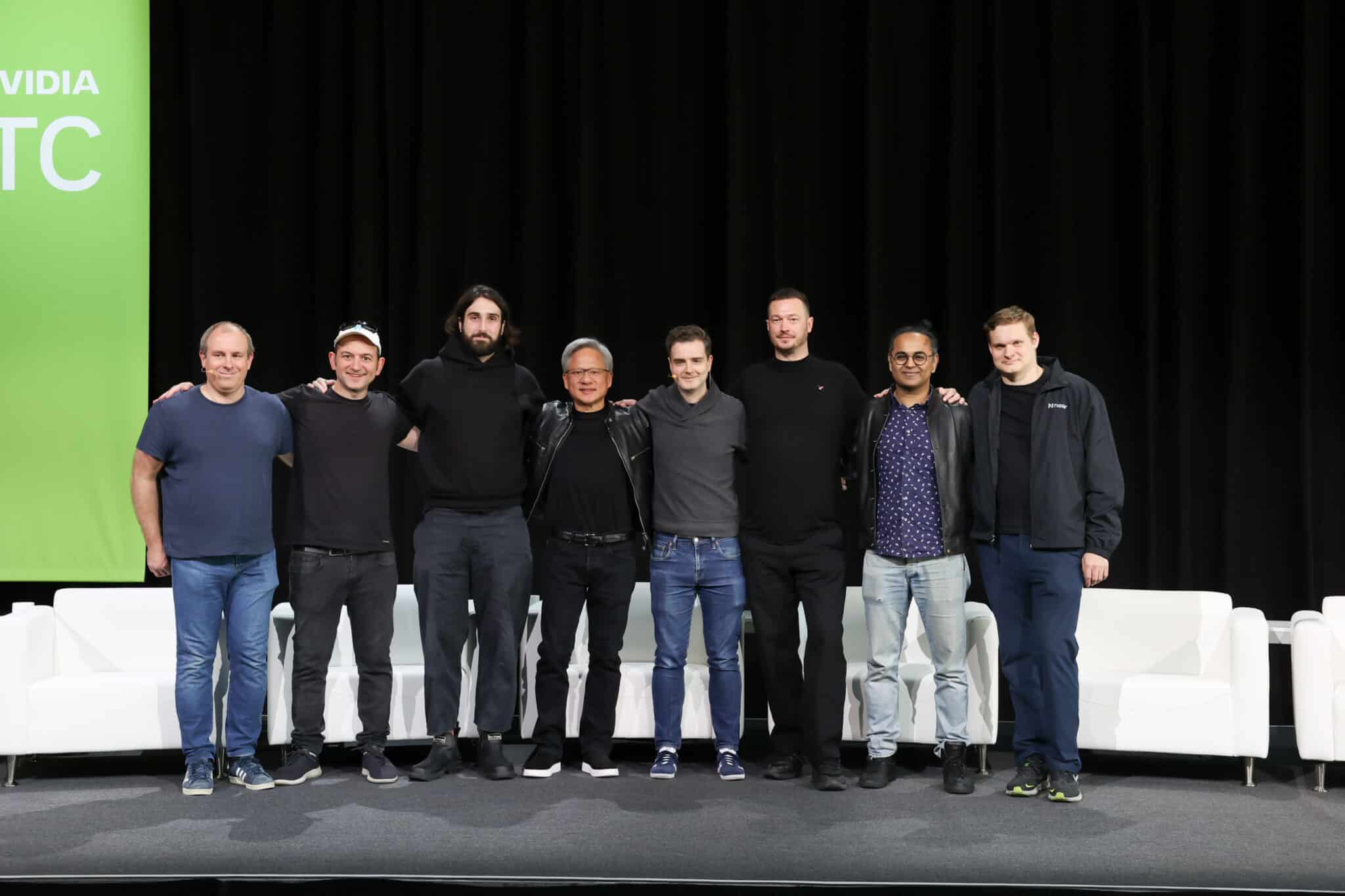
Origins of the Transformer Model
The research team initially sought to overcome the limitations of recurrent neural networks , or RNNs, which were then the state of the art for processing language data.
Noam Shazeer, cofounder and CEO of Character.AI, compared RNNs to the steam engine and transformers to the improved efficiency of internal combustion.
“We could have done the industrial revolution on the steam engine, but it would just have been a pain,” he said. “Things went way, way better with internal combustion.”
“Now we’re just waiting for the fusion,” quipped Illia Polosukhin, cofounder of blockchain company NEAR Protocol.
The paper’s title came from a realization that attention mechanisms — an element of neural networks that enable them to determine the relationship between different parts of input data — were the most critical component of their model’s performance.
“We had very recently started throwing bits of the model away, just to see how much worse it would get. And to our surprise it started getting better,” said Llion Jones, cofounder and chief technology officer at Sakana AI.
Having a name as general as “transformers” spoke to the team’s ambitions to build AI models that could process and transform every data type — including text, images, audio, tensors and biological data.
“That North Star, it was there on day zero, and so it’s been really exciting and gratifying to watch that come to fruition,” said Aidan Gomez, cofounder and CEO of Cohere. “We’re actually seeing it happen now.”
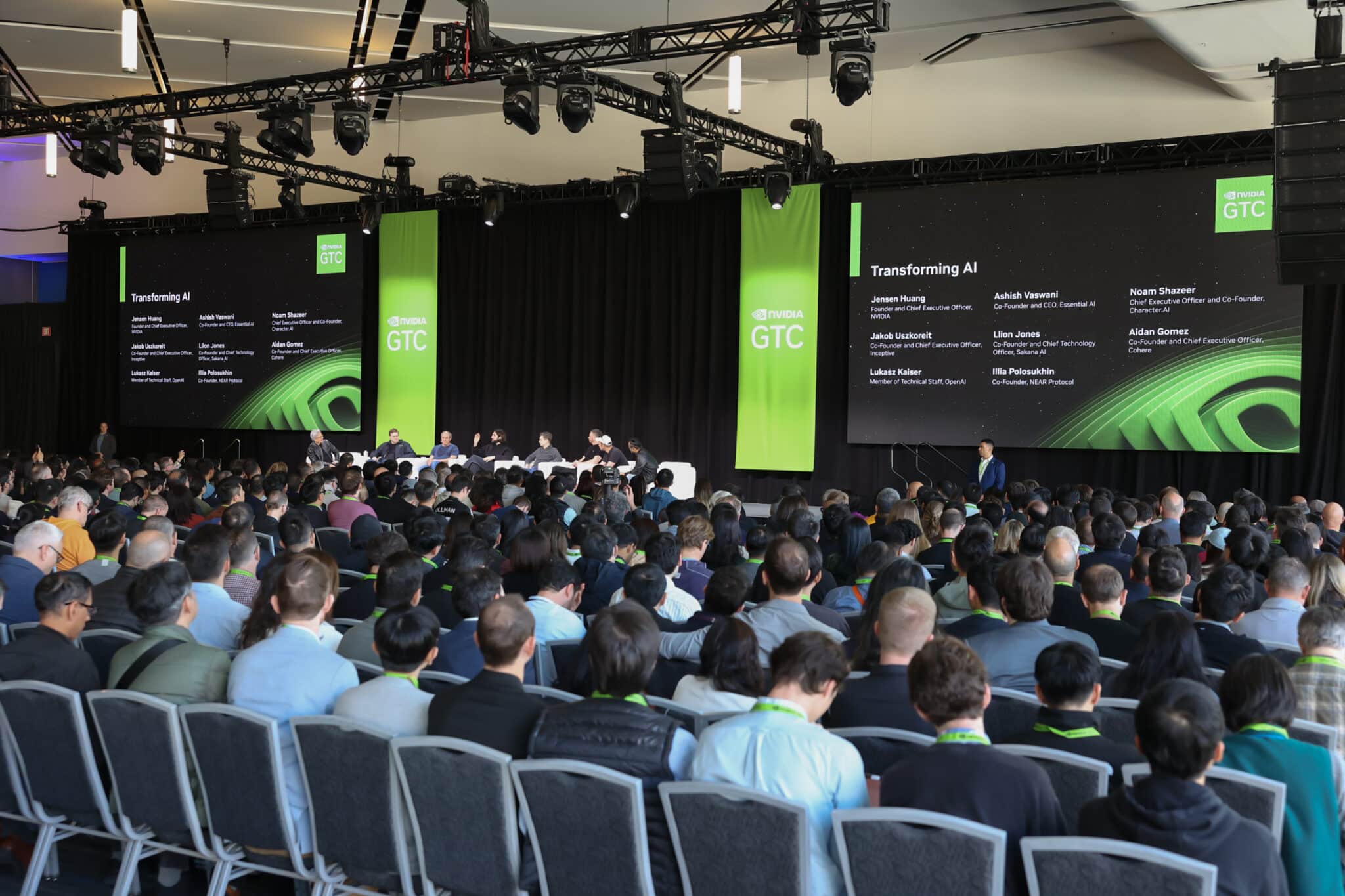
Envisioning the Road Ahead
Adaptive computation, where a model adjusts how much computing power is used based on the complexity of a given problem, is a key factor the researchers see improving in future AI models.
“It’s really about spending the right amount of effort and ultimately energy on a given problem,” said Jakob Uszkoreit, cofounder and CEO of biological software company Inceptive. “You don’t want to spend too much on a problem that’s easy or too little on a problem that’s hard.”
A math problem like two plus two, for example, shouldn’t be run through a trillion-parameter transformer model — it should run on a basic calculator, the group agreed.
They’re also looking forward to the next generation of AI models.
“I think the world needs something better than the transformer,” said Gomez. “I think all of us here hope it gets succeeded by something that will carry us to a new plateau of performance.”
“You don’t want to miss these next 10 years,” Huang said. “Unbelievable new capabilities will be invented.”
The conversation concluded with Huang presenting each researcher with a framed cover plate of the NVIDIA DGX-1 AI supercomputer, signed with the message, “You transformed the world.”
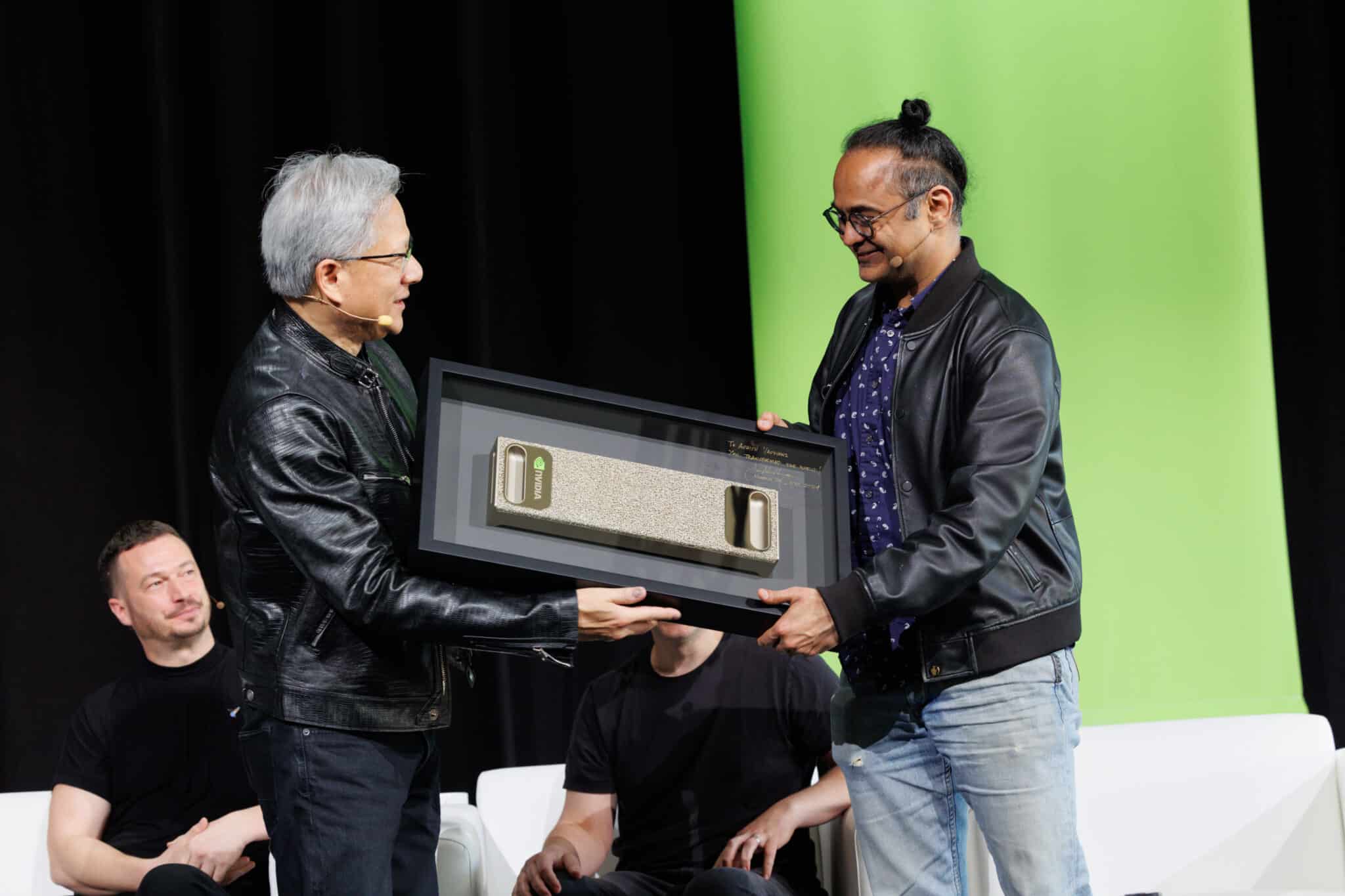
There’s still time to catch the session replay by registering for a virtual GTC pass — it’s free.
To discover the latest in generative AI, watch Huang’s GTC keynote address:
NVIDIA websites use cookies to deliver and improve the website experience. See our cookie policy for further details on how we use cookies and how to change your cookie settings.
Share on Mastodon
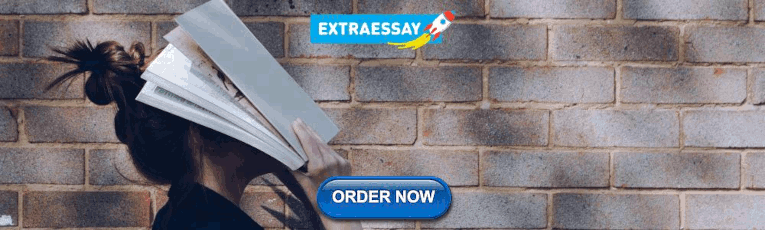
IMAGES
VIDEO
COMMENTS
Research: What it is. A research paper is the culmination and final product of an involved process of research, critical thinking, source evaluation, organization, and composition. It is, perhaps, helpful to think of the research paper as a living thing, which grows and changes as the student explores, interprets, and evaluates sources related ...
Identify key terms and characteristics of evidence-based research writing. Participate effectively in a continuing scholarly conversation by synthesizing research and discussing it with others. Identify and analyze genre conventions as shaped by purpose, culture, and expectation. Good writing satisfies audience expectations in genre, style, and ...
12.1 Introducing Research and Research Evidence; 12.2 Argumentative Research Trailblazer: Samin Nosrat; 12.3 Glance at Genre: ... Topic: subject of a paper. In this genre, the topic is a debatable issue. Thesis: declarative sentence (sometimes two) that states a writer's position about the debatable issue, ...
The thesis of the paper is supported by the evidence drawn from the research. In order to present an effective position or argument, the author must utilize clear writing, organization, and logic. Do not confuse this type of paper with a literature review, described in a later section. Webpage: Genre and the Research Paper (Purdue OWL, n.d.)
Like the word research, the word genre also has many definitions. At its most basic level, genre is the French word for "type.". In the world of English for Academic Purposes, it refers to a communicative event that is widely recognized. In terms of research, some common genres include research articles, grant proposals, conference papers ...
Research papers constitute their own genre and are qualitatively different from other forms of writing such as fiction, policy statements, personal journals, and news articles. ... Every research paper should have a title, an introduction with a thesis that relates to the literature, a body of argument that relates to the thesis, a conclusion ...
(1B) Student refers to a single, monolithic genre or structure for the research paper without recognition that different disciplines will have different genre requirements: ... Find a research paper that represents your best writing from (high school / last semester). If you have not done a research paper, please find a paper that is based on ...
Abstract. This book provides a rich and accessible account of genre studies by a world-renowned applied linguist. The hardback edition discusses today's research world, its various configurations ...
This handout provides detailed information about how to write research papers including discussing research papers as a genre, choosing topics, and finding sources. There is neither a template nor shortcut for writing a research paper; again, the process is, amongst other things, one of practice, experience, and organization, and begins with ...
What is genre? Genre defines your paper and how it will be presented. A genre can clue your audience in on what they can expect from your paper. It can also act as a guideline for your research and can help you when it is time to structure your paper.
Development" 287). Indeed, genre research forms a rich site for inter-disciplinarity, with Amy Devitt arguing, in her conclusion to Writing Genres, that further research on genre is needed, including cognitive studies, historical studies, and collaborative research between sociol-ogists and genre theorists (218). Joining this call, Bazerman ...
Multigenre: An Introduction. "A multigenre paper arises from research, experience, and imagination. It is not an uninterrupted, expository monolog nor a seamless narrative nor a collection of poems. A multigenre paper is composed of many genres and subgenres, each piece self-contained, making a point of its own, yet connected by theme or topic ...
14.3 Methods for Studying Genres. The previous section outlined some key terms and definitions for the study of writing. This section builds on that by providing an overview of research tools that can be used to better understand writing-in-context. Some of these tools-like an interview-may seem more familiar to you than others (such as ...
Further Reading. To learn more about how the purpose and genre of the American research paper has changed since the late 19th century, see John Scott Clark's A Briefer Practical Rhetoric.Also important is Robert Morell Schmitz's Preparing the Research Paper, A Handbook for Undergraduates.Additionally, Cecile Williams and Allan Stevenson's A Research Manual and Florence Hilbish's The ...
Exploring Genres Beyond the Research Paper. Aw… the research essay. The staple in undergraduate writing. At best, the student research essay poses a provocative question and curates convincing and credible evidence to support the student writer's own unique answer to that question. At its worst, the research essay is a frustrating document ...
The abstract of a research paper is a separate genre that arose as a result of a well- defined and generally accep ted communicative objective that most abstracts meet, independent o f the subject ...
Genre analysis: A tool used to create genre awareness and understand the conventions of new writing situations and contexts. This a llows you to make effective communication choices and approach your audience and rhetorical situation appropriately. Basically, when we say "genre analysis," that is a fancy way of saying that we are going to look at similar pieces of communication - for example a ...
The idea of teaching writing through genres—rather than, say, through prescriptive forms, templates, and rhetorical modes—is intuitively appealing. ... Genre Explained presents accessible, research-grounded answers to 40 questions that teachers frequently have about genre-based writing instruction. ... Should I assign "the research paper ...
medical research articles as a genre. John Skelton in "Analysis of the Structure of riginal O Research Papers: An Aid to riting W Original Papers for ublication" describes the difficulty of defining P structured writing in the field of medicine because of the lack of research that has been conducted on the genre (455).
The present paper reveals the usefulness of corpus-based analysis to discover the linguistic patterns selected and favoured by a specific genre. It analyzes the use of collocational frameworks, or discontinuous sequences of words, in a corpus of medical research papers and describes the intermediate words, or collocates, which fill these ...
Research proposals and reports submitted by novice writers may at times be rejected on grounds of their inability to demonstrate a need to carry out research in a suggested area. This genre-based investigation looked into how experienced writers use rhetorical steps and linguistic choices to establish research niches in the introductory ...
This paper reports a contrastive genre analysis of rhetorical structures of linguistic research articles written in English and Vietnamese. The quantitative method was employed with data from a corpus of 35 English and 35 Vietnamese research articles randomly selected from internationally and Vietnamese reputable journals of linguistics published in the five-year period from 2015 to 2019.
In scientific research and its application, scientific literature analysis is crucial as it allows researchers to build on the work of others. However, the fast growth of scientific knowledge has led to a massive increase in scholarly articles, making in-depth literature analysis increasingly challenging and time-consuming. The emergence of Large Language Models (LLMs) has offered a new way to ...
In this work, we discuss building performant Multimodal Large Language Models (MLLMs). In particular, we study the importance of various architecture components and data choices. Through careful and comprehensive ablations of the image encoder, the vision language connector, and various pre-training data choices, we identified several crucial design lessons. For example, we demonstrate that ...
The perception and appreciation of food flavor depends on many interacting chemical compounds and external factors, and therefore proves challenging to understand and predict. Here, we combine ...
"For every one paper that is retracted, there are probably 10 that should be," said Dr. Ivan Oransky, co-founder of Retraction Watch, which keeps a database of 47,000-plus retracted studies.
March 21, 2024 by Isha Salian. Of GTC 's 900+ sessions, the most wildly popular was a conversation hosted by NVIDIA founder and CEO Jensen Huang with seven of the authors of the legendary research paper that introduced the aptly named transformer — a neural network architecture that went on to change the deep learning landscape and enable ...
When junior Ava Franzoy was approached by School of Communication Studies Assistant Professor Jessica Frampton about conducting research, she had no idea that saying yes would eventually lead to the resulting paper, "An Investigation of Technology's Role in Coping with Infidelity," being published in the Qualitative Research Reports in Communication journal.