Comparative Analysis of Learning Curve Models on Construction Productivity of Diaphragm Wall and Pile
G SaravanaPrabhu 1 and R Vidjeapriya 1
Published under licence by IOP Publishing Ltd IOP Conference Series: Materials Science and Engineering , Volume 1197 , International Conference on Advances in Civil Engineering (ICACE 2021) 25th-26th June 2021, Guntur, India Citation G SaravanaPrabhu and R Vidjeapriya 2021 IOP Conf. Ser.: Mater. Sci. Eng. 1197 012004 DOI 10.1088/1757-899X/1197/1/012004
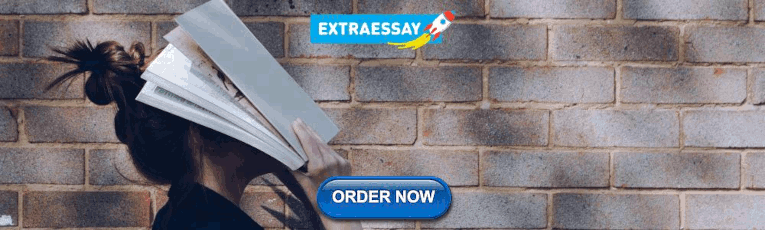
Article metrics
341 Total downloads
Share this article
Author e-mails.
Author affiliations
1 Division of Structural Engineering, Department of Civil Engineering, College of Engineering Guindy, Anna University Chennai, India
Buy this article in print
In the analysis of construction operations, Learning Curves (LC) are considered one of the most important factors that determines the on-site variation in the productivity, which is usually considered in the construction projects during the estimation and planning stage. This research attempts to assess the suitability of LC models for the analysis of the learning phenomenon using productivity data for fairly complicated construction operations concerning the Diaphragm Wall and Pile Construction process from large-scale construction projects. In this study, the role of different LC models (i.e., Wright or Straight Line, Quadratic, Cubic, Knecht or Combined Exponential Log-linear, Stanford B) is investigated by the comparison of their outcomes through the utilization of cumulative productivity data of the activities involved in the Diaphragm Wall and Pile Construction process. The two main research objectives are (i) the investigation of the model which is the best bit for the historical productivity data of the completed construction activities (ii) an endeavour is formed to work out which model predicts the future performance better. The best suited LC model is predicted based on the least deviation from the yielded results of each model with respect to the actual construction data. Analysis of the cumulative average productivity data predicted that the Knecht or Combined Exponential Log-linear Model best fits both the complete Diaphragm Wall and Pile Construction Process in both the cases of fitting Historical data and in predicting future performance.
Export citation and abstract BibTeX RIS
Content from this work may be used under the terms of the Creative Commons Attribution 3.0 licence . Any further distribution of this work must maintain attribution to the author(s) and the title of the work, journal citation and DOI.
The Infona portal uses cookies, i.e. strings of text saved by a browser on the user's device. The portal can access those files and use them to remember the user's data, such as their chosen settings (screen view, interface language, etc.), or their login data. By using the Infona portal the user accepts automatic saving and using this information for portal operation purposes. More information on the subject can be found in the Privacy Policy and Terms of Service. By closing this window the user confirms that they have read the information on cookie usage, and they accept the privacy policy and the way cookies are used by the portal. You can change the cookie settings in your browser.
- Login or register account
INFONA - science communication portal
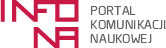
- advanced search
- conferences
- Collections
Learning curve models and applications: Literature review and research directions $("#expandableTitles").expandable();
- Contributors
Fields of science
- Bibliography
International Journal of Industrial Ergonomics > 2011 > 41 > 5 > 573-583
Identifiers
User assignment, assignment remove confirmation, you're going to remove this assignment. are you sure.
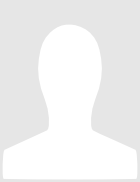
Michel Jose Anzanello
Flavio sanson fogliatto.
Learning curves Forgetting curves Production planning Task allocation
Additional information

- Read online
- Add to read later
- Add to collection
- Add to followed

Export to bibliography

- Terms of service
Accessibility options
- Report an error / abuse
Reporting an error / abuse
Sending the report failed.
Submitting the report failed. Please, try again. If the error persists, contact the administrator by writing to [email protected].
You can adjust the font size by pressing a combination of keys:
- CONTROL + + increase font size
- CONTROL + – decrease font
You can change the active elements on the page (buttons and links) by pressing a combination of keys:
- TAB go to the next element
- SHIFT + TAB go to the previous element
Recent advances in deep learning models: a systematic literature review
- Published: 25 April 2023
- Volume 82 , pages 44977–45060, ( 2023 )
Cite this article
- Ruchika Malhotra 1 &
- Priya Singh ORCID: orcid.org/0000-0001-7656-7108 1
1634 Accesses
6 Citations
Explore all metrics
In recent years, deep learning has evolved as a rapidly growing and stimulating field of machine learning and has redefined state-of-the-art performances in a variety of applications. There are multiple deep learning models that have distinct architectures and capabilities. Up to the present, a large number of novel variants of these baseline deep learning models is proposed to address the shortcomings of the existing baseline models. This paper provides a comprehensive review of one hundred seven novel variants of six baseline deep learning models viz. Convolutional Neural Network, Recurrent Neural Network, Long Short Term Memory, Generative Adversarial Network, Autoencoder and Transformer Neural Network. The current review thoroughly examines the novel variants of each of the six baseline models to identify the advancements adopted by them to address one or more limitations of the respective baseline model. It is achieved by critically reviewing the novel variants based on their improved approach. It further provides the merits and demerits of incorporating the advancements in novel variants compared to the baseline deep learning model. Additionally, it reports the domain, datasets and performance measures exploited by the novel variants to make an overall judgment in terms of the improvements. This is because the performance of the deep learning models are subject to the application domain, type of datasets and may also vary on different performance measures. The critical findings of the review would facilitate the researchers and practitioners with the most recent progressions and advancements in the baseline deep learning models and guide them in selecting an appropriate novel variant of the baseline to solve deep learning based tasks in a similar setting.
This is a preview of subscription content, log in via an institution to check access.
Access this article
Price includes VAT (Russian Federation)
Instant access to the full article PDF.
Rent this article via DeepDyve
Institutional subscriptions
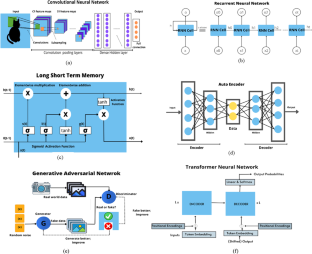
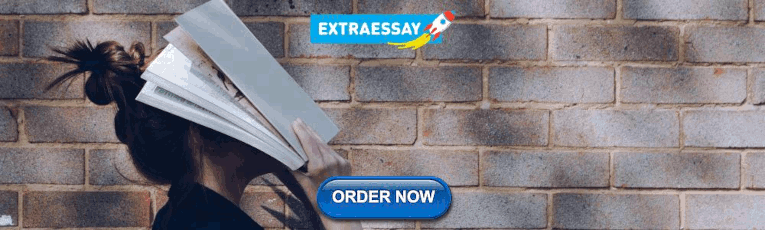
Similar content being viewed by others
- Deep Learning
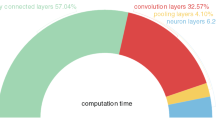
Various Frameworks and Libraries of Machine Learning and Deep Learning: A Survey
Zhaobin Wang, Ke Liu, … Yaonan Zhang
An Overview of Deep Learning
Data availability.
Data sharing is not applicable to this article as this is a review article. The detail of the selected primary studies is presented in Table 3 .
Abbreviations
Deep Leering
- Autoencoder
- Convolutional Neural Network
- Recurrent Neural Network
- Generative Adversarial Network
- Long Short-Term Memory
- Transformer Neural Network
Deep Learning Models
Systematic Literature Review
Novel Variant
Aberbour M, Mehrez H (1998) Architecture and design methodology of the RBF-DDA neural network. 1998 IEEE International Symposium on Circuits and Systems (ISCAS). 3:199–202. https://doi.org/10.1109/ISCAS.1998.703974
Ainslie J, Ontanon S, Alberti C, Cvicek V, Fisher Z, Pham P, Yang L (2020) ETC: Encoding long and structured inputs in transformers. arXiv preprint arXiv:2004.08483
Akhtar MM, Shatat RSA, Shatat ASA et al (2022) IoMT-based smart healthcare monitoring system using adaptive wavelet entropy deep feature fusion and improved RNN. Multimed Tools Appl. https://doi.org/10.1007/s11042-022-13934-5
Alexia JM (2018) The relativistic discriminator: a key element missing from standard GAN. International Conference on Learning Representations
Al-Sarem M, Boulila W, Al-Harby M, Qadir J, Alsaeedi A (2019) Deep learning-based rumor detection on microblogging platforms: A systematic review. IEEE Access 7:152788–152812. https://doi.org/10.1109/ACCESS.2019.2947855
Article Google Scholar
Alzubaidi L, Zhang J, Humaidi AJ et al (2021) Review of deep learning: concepts, CNN architectures, challenges, applications, future directions. J Big Data 8:53. https://doi.org/10.1186/s40537-021-00444-8
Amjady N (2001) Short-term hourly load forecasting using time-series modeling with peak load estimation capability. IEEE Trans Power Syst 16(4):798–805. https://doi.org/10.1109/59.962429
Arjovsky M, Chintala S, Bottou L (2017) Wasserstein generative adversarial networks. Int Conf Mach Learn 17:214–223
Google Scholar
Atassi A, Azami IEl, Sadiq A (2018) The new deep learning architecture based on GRU and word2vec. International Conference on Electronics, Control, Optimization and Computer Science (ICECOCS). https://doi.org/10.1109/ICECOCS.2018.8610611
Aygun RC, Yavuz AG (2017) Network anomaly detection with stochastically improved autoencoder based models. IEEE 4th International Conference on Cyber Security and Cloud Computing (CSCloud). IEEE, 2017. https://doi.org/10.1109/CSCloud.2017.39
Aziz MF, Mostafa SA, Mohd CF et al (2022) Integrating Elman recurrent neural network with particle swarm optimization algorithms for an improved hybrid training of multidisciplinary datasets. Expert Syst Appl 183(115441):0957–4174. https://doi.org/10.1016/j.eswa.2021.115441
Bhaskar, S, Thasleema TM. LSTM model for visual speech recognition through facial expressions. Multimed Tools Appl. https://doi.org/10.1007/s11042-022-12796-1
Biolchini L, Mian PG (2005) Systematic review in software engineering. System Engineering and Computer Science Department COPPE/UFRJ
Brock A, Jeff D, Karen S (2018) Large scale GAN training for high fidelity natural image synthesis. Large scale GAN training for high fidelity natural image synthesis
Buettner R, Bilo M, Bay N, Zubac T (2020) A systematic literature review of medical image analysis using deep learning. IEEE Symp Ind Electron Appl 1:4. https://doi.org/10.1109/ISIEA49364.2020.9188131
Cai C, Gou B, Khishe M et al (2023) Improved deep convolutional neural networks using chimp optimization algorithm for Covid19 diagnosis from the X-ray images. Expert Syst Appl 213:119206. https://doi.org/10.1016/j.eswa.2022.119206
Can Aygun R, Gokhan Yavuz A (2017) Network anomaly detection with stochastically improved autoencoder based models. 2017 IEEE 4th International Conference on Cyber Security and Cloud Computing (CSCloud). https://doi.org/10.1109/CSCloud.2017.39
Chen HF (2010) New approach to recursive identification for ARMAX systems. IEEE Trans Autom Control 55(4):868–879. https://doi.org/10.1109/TAC.2010.2041997
Article MathSciNet MATH Google Scholar
Chen G, Hu L, Zhang Q, Ren Z, Gao X, Cheng J (2020) ST-LSTM: Spatio-temporal graph based long short-term memory network for vehicle trajectory prediction. 2020 IEEE International Conference on Image Processing (ICIP). 608-612. https://doi.org/10.1109/ICIP40778.2020.9191332
Cheng Z, Wang S, Liu X, Zhu E (2021) Improved autoencoder for unsupervised anomaly detection. Int J Intell Syst 36(12):7103–7125. https://doi.org/10.1002/int.22582
Child R, Gray S, Radford A, Sutskever I (2019) Generating long sequences with sparse transformers. arXiv preprint arXiv:1904.10509
Choromanski K, Likhosherstov V, Dohan D, Song X, Gane A, Sarlos T, Weller A (2020) Rethinking attention with performers. International Conference on Learning Representations
Ciresan DC, Meier U, Gambardella LM, Schmidhuber J (2010) Deep big simple neural nets excel on hand-written digit recognition. Neural Comput 22:12. https://doi.org/10.1162/NECO_a_00052
Clauwaert J, Waegeman W (2022) Novel transformer networks for improved sequence labeling in genomics. IEEE/ACM Trans Comput Biol Bioinf 19(1):97–106. https://doi.org/10.1109/TCBB.2020.3035021
Connor JT, Martin RD, Atlas LE (1994) Recurrent neural networks and robust time series prediction. IEEE Trans Neural Networks 5(2):240–254. https://doi.org/10.1016/j.oceaneng.2020.107704
Cui J, Gao Q, Li D (2019) Improved long short-term memory network based short term load forecasting 2019 Chinese Automation Congress (CAC). 4428–4433. https://doi.org/10.1109/CAC48633.2019.8996379
Dai Z, Lai G, Yang Y, Le Q (2020) Funnel-transformer: Filtering out sequential redundancy for efficient language processing. Adv Neural Inf Process Syst 33:4271–4282
Das M, Pratama M, Ashfahani A, Samanta S (2019) FERNN: A fast and evolving recurrent neural network model for streaming data classification. International Joint Conference on Neural Networks (IJCNN), 1–8. https://doi.org/10.1109/IJCNN.2019.8851757
Dehuri S, Roy R, Cho SB, Ghosh A (2012) An improved swarm optimized functional link artificial neural network (ISO-FLANN) for classification. J Syst Softw 85(6):1333–1345. https://doi.org/10.1016/j.jss.2012.01.025
Denton E, Chintala S, Szlam A, Fergus R (2015) Deep generative image models using a Laplacian pyramid of adversarial networks. In: Proceedings of the 28th International Conference on Neural Information Processing Systems 1:1486–1494
Devlin J, Chang MW, Lee K, Toutanova K (2019) BERT: Pre-training of deep bidirectional transformers for language understanding. In: Proceedings of HLT-NAACL. Minneapolis, Minnesota. 4171–4186. https://doi.org/10.18653/v1/N19-1423
Ding Z, Liu XY, Yin M et al (2019) Tgan: Deep tensor generative adversarial nets for large image generation. International Conference on Learning Representations
Fang X, Zhang W, Guo Y, Wang J, Wang M, Li S (2022) A novel reinforced deep RNN–LSTM Algorithm: Energy management forecasting case study. IEEE Trans Industr Inf 18(8):5698–5704. https://doi.org/10.1109/TII.2021.3136562
Fetanat M, Stevens M, Jain P, Hayward C, Meijering E, Lovell NH (2022) Fully Elman Neural Network: A novel deep recurrent neural network optimized by an improved harris hawks algorithm for classification of pulmonary arterial Wedge Pressure. IEEE Trans Biomed Eng 69(5):1733–1744. https://doi.org/10.1109/TBME.2021.3129459
Gao F et al (2018) SD-CNN: A shallow-deep CNN for improved breast cancer diagnosis. Comput Med Imaging Graph 70:53–62
Gavrilescu R et al (2018) Faster R-CNN: an approach to real-time object detection. 2018 International Conference and Exposition on Electrical and Power Engineering (EPE). https://doi.org/10.1109/ICEPE.2018.8559776
Geng Z, Chen Z, Meng Q, Han Y (2022) Novel transformer based on gated convolutional neural network for dynamic soft sensor modeling of industrial processes. IEEE Trans Industr Inf 18(3):1521–1529. https://doi.org/10.1109/TII.2021.3086798
Gnanha AT, Cao W, Mao X, Wu S, Wong HS, Li Q (2022) The residual generator: An improved divergence minimization framework for GAN. Pattern Recognit 121:108222. https://doi.org/10.1016/j.patcog.2021.108222
Gong W, Chen H, Zhang Z, Zhang M, Wang R, Guan C, Wang Q (2019) A novel deep learning method for intelligent fault diagnosis of rotating machinery based on improved CNN-SVM and multichannel data fusion. Sensors 19(7):1693
Gu S, Feng Y (2019) Improving multi-head attention with capsule networks. In: Proceedings of NLPCC. 314–326. https://doi.org/10.1007/978-3-030-32233-5_25
Gu Q, Huang Z (2022) An improved convolutional neural network for wind turbine bearing fault diagnosis research method. In Proceedings of the 7th International Conference on Cyber Security and Information Engineering (ICCSIE '22). Association for Computing Machinery, New York, NY, USA, 725–729. https://doi.org/10.1145/3558819.3565179
Guan J, Pan C, Li S, Yu D (2019) Srdgan: learning the noise prior for super resolution with dual generative adversarial networks. International Conference on Learning Representations
Guo M, Zhang Y, Liu T (2019) Gaussian transformer: a lightweight approach for natural language inference. Proc AAAI Conf Artif Intell 33(1):6489–6496. https://doi.org/10.1609/aaai.v33i01.33016489
Guo Q, Qiu X, Xue X, Zhang Z (2019) Low-rank and locality constrained self-attention for sequence modeling. IEEE/ACM Trans Audio Speech Lang Process 27(12):2213–2222. https://doi.org/10.1109/TASLP.2019.2944078
Hah J, Lee W, Lee J, Par S (2018) Information-based boundary equilibrium generative adversarial networks with interpretable representation learning. Comput Intell Neurosci 2018:6465949. https://doi.org/10.1155/2018/6465949
Han L, Musunuri SH, Min MR, Gao R, Tian Y, Metaxas D (2022) AE-StyleGAN: Improved training of style-based autoencoders. IEEE/CVF Winter Conference on Applications of Computer Vision (WACV). 955–964. https://doi.org/10.1109/WACV51458.2022.00103
He W (2017) Load forecasting via deep neural networks. Procedia Comput Sci 122:308–314. https://doi.org/10.1016/j.procs.2017.11.374
He K, Zhang X, Ren S, Sun J (2016) Deep residual learning for image recognition. IEEE Conference on Computer Vision and Pattern Recognition (CVPR), 770–778. https://doi.org/10.1109/CVPR.2016.90
He Z, Shao H, Zhang X, Cheng J, Yang Y (2019) Improved deep transfer autoencoder for fault diagnosis of gearbox under variable working conditions with small training samples. IEEE Access 7:115368–115377. https://doi.org/10.1109/ACCESS.2019.2936243
He YL, Chen L, Gao Y, Ma JH, Xu Y, Zhu QX (2022) Novel double-layer bidirectional LSTM network with improved attention mechanism for predicting energy consumption. ISA Trans 127:350–360. https://doi.org/10.1016/j.isatra.2021.08.030
Heo YJ, Yeo WH, Kim BG (2022) DeepFake detection algorithm based on improved vision transformer. Appl Intell 53:7512–7527. https://doi.org/10.1007/s10489-022-03867-9
Hsu W, Zhang Y, Glass J (2016) A prioritized grid long short-term memory RNN for speech recognition. 2016 IEEE Spoken Language Technology Workshop (SLT), 467-473. https://doi.org/10.1109/SLT.2016.7846305
Hu L, Taylor G (2014) A novel hybrid technique for short-term electricity price forecasting in UK electricity markets. J Int Counc Electr Eng 4(2):114–120
Huang K, Wang X (2021) ADA-INCVAE: Improved data generation using variational autoencoder for imbalanced classification. Appl Intell 52:2838–28531. https://doi.org/10.1007/s10489-021-02566-1
Huang K, Wang X (2022) ADA-INCVAE: Improved data generation using variational autoencoder for imbalanced classification. Appl Intell 52:2838–2853. https://doi.org/10.1007/s10489-021-02566-1
Huang H, Li L, Ma H (2022) An improved cascade R-CNN-Based target detection algorithm for UAV Aerial Images, 7th International Conference on Image, Vision and Computing (ICIVC), 232–237. https://doi.org/10.1109/ICIVC55077.2022.9886321
Huang W, Gao X, Huang Y et al (2022) Improved convolutional neural network for laser welding defect prediction. Int J Precis Eng Manuf. https://doi.org/10.1007/s12541-022-00729-9
Isola P et al (2017) Image-to-image translation with conditional adversarial networks. Proceedings of the IEEE conference on computer vision and pattern recognition
Jabeen G, Rahim S, Afzal W et al (2022) Machine learning techniques for software vulnerability prediction: a comparative study. Appl Intell 52:17614–17635. https://doi.org/10.1007/s10489-022-03350-5
Joshy AA, Rajan R (2022) Automated dysarthria severity classification: A study on acoustic features and deep learning techniques. IEEE Trans Neural Syst Rehabil Eng 30:1147–1157. https://doi.org/10.1109/TNSRE.2022.3169814
Jung W et al (2019) Restructuring batch normalization to accelerate CNN training. Proc Mach Learn Syst 1:14–26
Karabayir I, Akbilgic O, Tas N (2021) A Novel Learning Algorithm to Optimize Deep Neural Networks: Evolved Gradient Direction Optimizer (EVGO). IEEE Trans Neural Netw Learn Syst 32(2):685–694. https://doi.org/10.1109/TNNLS.2020.2979121
Article MathSciNet Google Scholar
Karras T et al (2017) Progressive growing of gans for improved quality, stability, and variation. International Conference on Learning Representations
Karras T, Laine S, Aila T (2021) A Style-Based Generator Architecture for Generative Adversarial Networks. IEEE Trans Pattern Anal Mach Intell 42(12):4217–4228. https://doi.org/10.1109/TPAMI.2020.2970919
Khamparia A, Singh KM (2019) A systematic review on deep learning architectures and applications. Expert Systems 36:e12400. https://doi.org/10.1111/exsy.12400
Kisan K, Jatoth RK (2021) A new training scheme for neural network based non-linear channel equalizers in wireless communication system using Cuckoo Search Algorithm. AEU-Int J Electron Commun 138:153371. https://doi.org/10.1016/j.aeue.2020.153371
Kitchenham B, Brereton OP (2009) Systematic literature reviews in software engineering -a systematic literature review. Inf Softw Technol 51(1):7–17. https://doi.org/10.1016/j.infsof.2008.09.009
Krizhevsky A, Ilya S, Hinton GE (2012) Imagenet classification with deep convolutional neural networks. Adv Neural Inf Process Syst 60(6):84–90. https://doi.org/10.1145/3065386
Kumar A, Kumari P (2021) A pragmatic approach to face recognition using a novel deep learning algorithm. Inernational Conference on Advance Computing and Innovative Technologies in Engineering (ICACITE). 806–810. https://doi.org/10.1109/ICACITE51222.2021.9404697 .
Kumar N, Sukavanam N (2020) An improved CNN framework for detecting and tracking human body in unconstraint environment. Knowl Based Syst 193:105198. https://doi.org/10.1016/j.knosys.2019.105198
Kumar M, Mukherjee P, Verma K, Verma S, Rawat DB (2022) Improved deep convolutional neural network based malicious node detection and energy-efficient data transmission in wireless sensor networks. IEEE Trans Netw Sci Eng 9(5):3272–3281. https://doi.org/10.1109/TNSE.2021.3098011
Kuo PH, Huang CJ (2018) A high precision artificial neural networks model for short-term energy load forecasting. Energies 11(1):213. https://doi.org/10.3390/en11010213
Lata K, Dave M, Nishanth KN (2019) Image-to-Image translation using generative adversarial network. International conference on Electronics, Communication and Aerospace Technology (ICECA). 186–189. https://doi.org/10.1109/ICECA.2019.8822195
Ledig C et al (2017) Photo-realistic single image super-resolution using a generative adversarial network. IEEE Conference on Computer Vision and Pattern Recognition (CVPR), 2017, 105-114. https://doi.org/10.1109/CVPR.2017.19
Li X, Meng Y, Zhou M, Han Q, Wu F, Li J (2020) SAC: accelerating and structuring self-attention via sparse adaptive connection. In Proceedings of the 34th International Conference on Neural Information Processing Systems (NIPS'20). Curran Associates Inc., Red Hook, NY, USA. 426:16997–17008. https://doi.org/10.5555/3495724.3497150
Li Y, Zhou Z, Sun C, Chen X, Yan R (2022) Variational Attention-Based Interpretable Transformer Network for Rotary Machine Fault Diagnosis. In: IEEE Transactions on Neural Networks and Learning Systems. https://doi.org/10.1109/TNNLS.2022.3202234
Li J, Lu Y, Xu Z, Li S, Qian L (2022) MILP: A memory improved LSTM prediction algorithm for gradient transmission time in distributed deep learning. ICC 2022 - IEEE International Conference on Communications, 4462–4467
Li W, Chen J, Wang Z, Shen Z, Ma C and Cui X (2022). IFL-GAN: Improved federated learning generative adversarial network with maximum mean discrepancy model Aggregation. IEEE Trans Neural Netw Learn Syst. https://doi.org/10.1109/TNNLS.2022.3167482
Li C, Pan C, Chen F, Li J, Fu S, Zeng W (2022) A handwritten number recognition scheme based on improved convolutional neural network algorithm. Advances in artificial intelligence and security communications in computer and Information Science 1586. Springer. https://doi.org/10.1007/978-3-031-06767-9_33
Li Y, Xiao N, Ouyang W (2018) Improved boundary equilibrium generative adversarial networks. IEEE Access 6:11342–11348. https://doi.org/10.1109/ACCESS.2018.2804278
Li J, Yao P, Guo L, Zhang W (2019) Boosted transformer for image captioning. Appl Sci 9(16):3260. https://doi.org/10.3390/app9163260
Li Q, Zhao Y, Yu F (2020) A novel multichannel long short-term memory method with time series for soil temperature modeling. IEEE Access 8:182026–182043. https://doi.org/10.1109/ACCESS.2020.3028995
Li L, Kameoka H, Makino S (2022) FastMVAE2: On Improving and accelerating the fast variational autoencoder-based source separation algorithm for determined mixtures. IEEE/ACM Trans Audio Speech Lang Process 31:96–110. https://doi.org/10.1109/TASLP.2022.3214763
Li Y, Yang S, Zheng Y, Lu H (2022) Improved point-voxel region convolutional neural network: 3D object detectors for autonomous driving. IEEE Trans Intell Transp Syst 23(7):9311–9317. https://doi.org/10.1109/TITS.2021.3071790
Li X, Wei J, Jiao H (2022) Real-time tracking algorithm for aerial vehicles using improved convolutional neural network and transfer learning. IEEE Trans Intell Transp Syst 23(3):2296–2305. https://doi.org/10.1109/TITS.2021.3072872
Lin M, Qiang C, Shuicheng Y (2013) Network in network. Neural and Evolutionary Computing. https://doi.org/10.48550/arXiv.1312.4400
Lin T, Wang Y, Liu X, Qiu X (2022) A survey of transformers. AI Open 3:111–132. https://doi.org/10.1016/j.aiopen.2022.10.001
Liu B et al (2020) Traffic flow combination forecasting method based on improved LSTM and ARIMA. Int J Embedded Syst 12(1):22–30
Liu W, You J, Lee J (2021) HSIGAN: A Conditional hyperspectral image synthesis method with auxiliary classifier. IEEE J Sel Top Appl Earth Obs Remote Sens 14:330–3344. https://doi.org/10.1109/JSTARS.2021.3063911
Lodha I, Kolur L, Krishnan K, Dheenadayalan K, Sitaram D, Nandi S (2022) Cost-optimized video transfer using real-time super resolution convolutional neural networks. In: 5th Joint International Conference on Data Science & Management of Data (9th ACM IKDD CODS and 27th COMAD). Association for Computing Machinery, New York, NY, USA, 213–221. https://doi.org/10.1145/3493700.3493731
Lu S et al (2019) Psgan: A minimax game for personalized search with limited and noisy click data. Proceedings of the 42nd International ACM SIGIR Conference on Research and Development in Information Retrieval
Lu S, Dou Z, Jun X, Nie JY, Wen JR (2019) Psgan: A minimax game for personalized search with limited and noisy click data. Proceedings of the 42nd International ACM SIGIR Conference on Research and Development in Information Retrieval. 555–564. https://doi.org/10.1145/3331184.3331218
Luo X, Li J, Chen M, Yang X, Li X (2021) Ophthalmic Disease Detection via Deep Learning with a Novel Mixture Loss Function. IEEE J Biomed Health Inform 25(9):3332–3339. https://doi.org/10.1109/JBHI.2021.3083605
Lv W, Xiong J, Shi J et al (2021) A deep convolution generative adversarial networks based fuzzing framework for industry control protocols. J Intell Manuf 32:441–457. https://doi.org/10.1007/s10845-020-01584-z
Ma L, Jia X, Sun Q, Schiele B, Tuytelaars T, Van G (2017) Pose guided person image generation. Advances in neural information processing systems
Ma J et al (2013) Improved GaN-based LED grown on silicon (111) substrates using stress/dislocation-engineered interlayers. J Cryst Growth 370:265–268. https://doi.org/10.1016/j.jcrysgro.2012.10.028
Martín A, Camacho D (2022) Recent advances on effective and efficient deep learning-based solutions. Neural Comput & Applic 34:10205–10210. https://doi.org/10.1007/s00521-022-07344-9
Mashudi NA, Ahmad N, Noor NM (2022) LiWGAN: A Light Method to Improve the Performance of Generative Adversarial Network. IEEE Access 10:93155–93167. https://doi.org/10.1109/ACCESS.2022.3203065
McDowall TM, Ham FM (1997) Robust partial least-squares regression: A modular neural network approach. Appl Sci Artif Neural Netw 3077:344–355. https://doi.org/10.1117/12.271496
Article MATH Google Scholar
Mirza M, Osindero S (2014) Conditional generative adversarial nets. Computer Vision and Pattern Recognition
Mittal V, Gangodkar D, Pant B (2021) Deep Graph-Long Short-Term Memory: A Deep Learning Based Approach for Text Classification. Wireless Pers Commun 119:2287–2301. https://doi.org/10.1007/s11277-021-08331-4
Moon T, Choi H, Lee H, Song I (2015) Rnndrop: A novel dropout for rnns in asr. Automatic Speech Recognition and Understanding. 65–70, https://doi.org/10.1109/ASRU.2015.7404775
Mou L, Zhao P, Xie H, Chen H (2018) T-LSTM: A Long Short-Term Memory Neural Network Enhanced by Temporal Information for Traffic Flow Prediction. IEEE Access 7:98053–98060. https://doi.org/10.1109/ACCESS.2019.2929692
Nagabushanam P, George ST, Radha S (2020) EEG signal classification using LSTM and improved neural network algorithms. Soft Comput 24(13):9981–10003. https://doi.org/10.1007/s00500-019-04515-0
Neifar N, Mdhaffar A, Hamadou AB, Jmaiel M, and Freisleben B (2022) Disentangling temporal and amplitude variations in ECG synthesis using anchored GANs. In: Proceedings of the 37th ACM/SIGAPP Symposium on Applied Computing. https://doi.org/10.1145/3477314.3507300
Nguyen TT, Nguyen ND, Nahavandi S (2020) Deep Reinforcement Learning for Multiagent Systems: A Review of Challenges, Solutions, and Applications. IEEE Trans Cybern 50(9):3826–3839. https://doi.org/10.1109/TCYB.2020.2977374
Ni Q, Cao X (2022) MBGAN: An improved generative adversarial network with multi-head self-attention and bidirectional RNN for time series imputation. Eng Appl Artif Intell 115:105232. https://doi.org/10.1016/j.engappai.2022.105232
Odena A, Olah C, Shlens J (2017) Conditional image synthesis with auxiliary classifier gans. Int Conf Mach Learn 70(2642):2651
Ogundokun RO, Maskeliunas R, Misra S, Damaševičius R (2022). Improved CNN based on batch normalization and adam optimizer. In: Computational Science and Its Applications – ICCSA 2022 Workshops. ICCSA 2022. Lecture Notes in Computer Science, 13381. https://doi.org/10.1007/978-3-031-10548-7_43
Otter DW, Medina JR, Kalita JK (2021) A survey of the usages of deep learning for natural language processing. IEEE Trans Neural Netw Learn Syst 32(2):604–624. https://doi.org/10.1109/TNNLS.2020.2979670
Pal SK, Pramanik A, Maiti J et al (2021) Deep learning in multi-object detection and tracking: state of the art. Appl Intell 51:6400–6429. https://doi.org/10.1007/s10489-021-02293-7
Pandey A, Wang D (2022) Self-Attending RNN for speech enhancement to improve cross-corpus generalization. IEEE/ACM Trans Audio Speech Lang Process 30:1374–1385. https://doi.org/10.1109/TASLP.2022.3161143
Pandey A, Wang D (2022) Self-Attending RNN for speech enhancement to improve cross-corpus generalization. ACM Trans Audio Speech Lang Process 30:1374–1385. https://doi.org/10.1109/TASLP.2022.3161143
Playout C, Renaud D, Farida C (2018) A multitask learning architecture for simultaneous segmentation of bright and red lesions in fundus images. International Conference on Medical Image Computing and Computer-Assisted Intervention 11071. https://doi.org/10.1007/978-3-030-00934-2_12
Price SR, Steven RP, and Derek TA (2019) Introducing fuzzy layers for deep learning. IEEE International Conference on Fuzzy Systems (FUZZ-IEEE). https://doi.org/10.1109/FUZZ-IEEE.2019.8858790
Qing Y, Liu W, Feng L, Gao W (2021) Improved transformer net for hyperspectral image classification. Remote Sensing 13(11):2216. https://doi.org/10.3390/rs13112216
Qipeng G, Xipeng G, Pengfei L, Yunfan S, Xiangyang X, Zheng Z (2019) Star-transformer. In: Proceedings of HLT-NAACL. 1315–1325. https://doi.org/10.18653/v1/N19-1133
Qiu D, Cheng Y, Wang X (2022) Improved generative adversarial network for retinal image super-resolution. Comput Methods Programs Biomed 225(106995):10169–12607. https://doi.org/10.1016/j.cmpb.2022.106995
Rakotonirina NC and Rasoanaivo A (2020) ESRGAN+ : Further improving enhanced super-resolution generative adversarial network. IEEE International Conference on Acoustics, Speech and Signal Processing (ICASSP). 3637–3641. https://doi.org/10.1109/ICASSP40776.2020.9054071
Reis AFD, Medjahdi Y, Chang BS, Sublime J, Brante G, Bader CF (2022) Low Complexity LSTM-NN-Based Receiver for Vehicular Communications in the Presence of High-Power Amplifier Distortions. IEEE Access 10:121985–122000. https://doi.org/10.1109/ACCESS.2022.3223113
Roy A, Saffar M, Vaswani A, Grangier D (2021) Efficient content-based sparse attention with routing transformers. Trans Assoc Comput Linguist 9:53–68
Sermanet P, Eigen D, Zhang X, Mathieu M, Fergus R, LeCun Y (2013) Overfeat: Integrated recognition, localization and detection using convolutional networks. International Conference on Learning Representations
Serradilla O, Zugasti E, Rodriguez J et al. (2022) Deep learning models for predictive maintenance: a survey, comparison, challenges and prospects. Appl Intell. https://doi.org/10.1007/s10489-021-03004-y
She J, Gong S, Yang S, Yang H, Lu S (2022) Xigmoid: An approach to improve the gating mechanism of RNN. International Joint Conference on Neural Networks (IJCNN). 1–1. https://doi.org/10.1109/IJCNN55064.2022.9892346
Shi Y, Li Y, Fan J, Wang T, Yin T (2020) A novel network architecture of decision-making for self-driving vehicles based on long short-term memory and grasshopper optimization algorithm. IEEE Access 8:155429–155440. https://doi.org/10.1109/ACCESS.2020.3019048
Shi N, Chen Z, Chen L, Lee RST (2022) CNO-LSTM: A chaotic neural oscillatory long short-term memory model for text classification. IEEE Access 10:129564–129579. https://doi.org/10.1109/ACCESS.2022.3228600
Shrestha A, Mahmood A (2019) Review of deep learning algorithms and architectures. IEEE Access 7:53040–53065. https://doi.org/10.1109/ACCESS.2019.2912200
Simonyan K, Zisserman A (2015) Very deep convolutional networks for large-scale image recognition Very deep convolutional networks for large-scale image recognition. International Conference on Learning Representations
Singh SK, Yang R, Behjat A, Rai R, Chowdhury S, Matei I (2019) PI-LSTM: Physics-infused long short-term memory network. 18th IEEE International Conference On Machine Learning And Applications (ICMLA). 34–41. https://doi.org/10.1109/ICMLA.2019.00015
Singh R, Mary AB, Athisayamani S (2020) Banana leaf diseased image classification using novel HEAP Autoencoder (HAE) deep learning. Multimed Tools Appl 79:30601–30613. https://doi.org/10.1007/s11042-020-09521-1
Song J et al (2018) Multi-agent generative adversarial imitation learning. Advances in neural information processing systems 31. https://doi.org/10.5555/3305381.3305404
Song J et al (2018) Multi-agent generative adversarial imitation learning. Advances in neural information processing systems 31 (2018)
Song C, He Z, Yu Y, Zhang Z (2021) Low Resolution Face Recognition System Based on ESRGAN. 3rd International Conference on Applied Machine Learning (ICAML). 76–79. https://doi.org/10.1109/ICAML54311.2021.00024
Szegedy C, Liu W, Yangqing J et al (2015) Going deeper with convolutions. IEEE Conference on Computer Vision and Pattern Recognition (CVPR). 1–9. https://doi.org/10.1109/CVPR.2015.7298594
Tang X (2019) Large-scale computing systems workload prediction using parallel improved LSTM neural network. IEEE Access 7:40525–40533. https://doi.org/10.1109/ACCESS.2019.2905634
Tay Y, Bahri D, Yang L, Metzler D, Juan DC (2020). Sparse sinkhorn attention. International Conference on Machine Learning. 9438–9447. https://doi.org/10.5555/3524938.3525813
Tian C, Ma J, Zhang C, Zhan C (2018) A deep neural network model for short-term load forecast based on long short-term memory network and convolutional neural network. Energies 11:12. https://doi.org/10.3390/en11123493
Tripathi BK (2017) On the complex domain deep machine learning for face recognition. Appl Intell 47:382–396. https://doi.org/10.1007/s10489-017-0902-7
Uyar K, Taşdemir S, Ülker E, Ünlükal N, Solmaz M (2022) Improving efficiency in convolutional neural networks with 3D image filters. Biomed Signal Process Control 74(03563):1746–8094. https://doi.org/10.1016/j.bspc.2022.103563
Valliani AAA, Ranti D, Oermann EK (2019) Deep Learning and Neurology: A Systematic Review. Neurol Ther 8:351–365. https://doi.org/10.1007/s40120-019-00153-8
Venkatachalam K, Trojovský P, Pamucar D, Bacanin N, Simic V (2022) DWFH: An improved data-driven deep weather forecasting hybrid model using Transductive Long Short Term Memory (T-LSTM). Expert Syst Appl 213(119270):0957–4174. https://doi.org/10.1016/j.eswa.2022.119270
Vyas A, Katharopoulos A, Fleuret F (2020) Fast transformers with clustered attention. Adv Neural Inf Process Syst 33:21665–21674
Wang Z, Pan S (2021) An improved convolutional neural network based on noise layer. Knowledge Science, Engineering and Management . KSEM 2021. Lecture Notes in Computer Science. 12816. Springer, Cham. https://doi.org/10.1007/978-3-030-82147-0_6
Wang R, Zhang Y, Zhang J (2022) An efficient swin transformer-based method for underwater image enhancement. Multimed Tools Appl. https://doi.org/10.1007/s11042-022-14228-6
Wang H, Wu W, Su Y, Duan Y, Wang P (2019) Image super-resolution using a improved generative adversarial network. IEEE 9th International Conference on Electronics Information and Emergency Communication (ICEIEC). 312–315. https://doi.org/10.1109/ICEIEC.2019.8784610
Wang Z, Lin J, Wang Z (2017) Accelerating recurrent neural networks: A memory-efficient approach. IEEE Trans Very Large Scale Integr VLSI Syst 25(10):2763–2775. https://doi.org/10.1109/TVLSI.2017.2717950
Wang X, Yu K, Gu J et al (2018) Esrgan: Enhanced super-resolution generative adversarial networks. Proc European Conf Comput Vision (ECCV) 11133:63–79
Wang J, Zhang J, Wang X (2018) Bilateral LSTM: A two-dimensional long short-term memory model with multiply memory units for short-term cycle time forecasting in re-entrant manufacturing systems. IEEE Trans Ind Inf 14(2):748–758. https://doi.org/10.1109/TII.2017.2754641
Wang Y, Shen Y, Mao S, Chen X, Zou H (2019) LASSO and LSTM integrated temporal model for short-term solar intensity forecasting. IEEE Internet Things J 6(2):2933–2944. https://doi.org/10.1109/JIOT.2018.2877510
Wang Q, Peng RQ, Wang JQ, Li Z, Qu HB (2020) NEWLSTM: An optimized long short-term memory language model for sequence prediction. IEEE Access 8:65395–65401. https://doi.org/10.1109/ACCESS.2020.2985418
Wang J, Hou B, Ren B, Zhang Y, Yang M, Wang S, Jiao L (2022) Parameter selection of Touzi decomposition and a distribution improved autoencoder for PolSAR image classification. ISPRS J Photogramm Remote Sens 186:246–266. https://doi.org/10.1016/j.isprsjprs.2022.02.003
Wei L, Li J (2022) Short-term power load forecasting based on MA-LSTM. IEEE International Conference on Advances in Electrical Engineering and Computer Applications (AEECA). 1370–1374. https://doi.org/10.1109/AEECA55500.2022.9918996
Wei S, Zhang Y, Park SC (2021) A novel deep Autoencoder considering energy and label constraints for categorization. Expert Systems with Applications. 176:114936. https://doi.org/10.1016/j.eswa.2021.114936
Wu J, Liu J, Ma J et al (2020) Classification of power loads based on an improved denoising deconvolutional Autoencoder. Applied Soft Computing 87:105959. https://doi.org/10.1016/j.asoc.2019.105959
Chen Xi, Duan Y, Houthooft R et al (2016) Infogan: Interpretable representation learning by information maximizing generative adversarial nets. In: Proceedings of the 30th International Conference on Neural Information Processing Systems. 2180–2188
Xia L, Diao L, Jiang Z et al (2019) PAI-FCNN: FPGA based inference system for complex CNN models. 2019 IEEE 30th International Conference on Application-specific Systems, Architectures and Processors (ASAP). https://doi.org/10.1109/ASAP.2019.00-21
Xiong Y, Zeng Z, Chakraborty R, Tan M, Fung G, Li Y, Singh V (2021) Nyströmformer: A nyström-based algorithm for approximating self-attention. Proc AAAI Conf Artif Intell 35(16):14138–14148
Xu K, Shen X, Yao T, Tian X, Mei T (2018) Greedy layer-wise training of long short term memory networks. 2018 IEEE International Conference on Multimedia & Expo Workshops (ICMEW), 1–6. https://doi.org/10.1109/ICMEW.2018.8551584
Xu T, Zhang H, Li H et al (2017) Unpaired image-to-image translation using cycle-consistent adversarial networks. Proceedings of the IEEE international conference on computer vision. https://doi.org/10.1109/ICCV.2017.629
Xu X, Lu Y, Liu X et al (2020) Intelligent collision avoidance algorithms for USVs via deep reinforcement learning under COLREGs. Ocean Engineering 217:107704. https://doi.org/10.1016/j.oceaneng.2020.107704
Xu X, Xu H, Wang Y et al (2021) AENEA: A novel autoencoder-based network embedding algorithm. Peer-to-Peer Netw Appl 14:1829–1840. https://doi.org/10.1007/s12083-020-01043-9
Yang M, Xu S (2021) Orthogonal nonnegative matrix factorization using a novel deep autoencoder network. Knowledge-Based Systems 227:107236. https://doi.org/10.1016/j.knosys.2021.107236
Yang R, Xu M, Wang Z (2017) Decoder-side HEVC quality enhancement with scalable convolutional neural network. 2017 IEEE International Conference on Multimedia and Expo (ICME). 817-822. https://doi.org/10.1109/ICME.2017.8019299
Yang S, Yang J, Ge X, Wang X (2022) Medium and short-term prediction of power system load based on improved LSTM Algorithm. International Conference on Communications, Computing, Cybersecurity, and Informatics (CCCI). 1–7. https://doi.org/10.1109/CCCI55352.2022.9926584
Yang Y, Zheng K, Wu C, Yang Z (2019) Improving the classification effectiveness of intrusion detection by using improved conditional variational autoencoder and deep neural network. Sensors 19(11):2528. https://doi.org/10.3390/s19112528
Yang M, Nazir S, Xu Q et al (2020) Deep learning algorithms and multicriteria decision-making used in big data: A systematic literature review. Complexity 2020:1076–2787. https://doi.org/10.1155/2020/2836064
Yao L, Yazhuo G (2018) An improved LSTM structure for natural language processing. IEEE International Conference of Safety Produce Informatization (IICSPI). https://doi.org/10.1109/IICSPI.2018.8690387
Yin A, Zheng F, Tan J, Wang Y (2021) An improved variational autoencoder with reverse supervision for the obstacles recognition of UGVs. IEEE Sens J 21(10):11791–11798. https://doi.org/10.1109/JSEN.2020.3013668
Yuan X, He P, Zhu Q, Li X (2019) Adversarial examples: Attacks and defenses for deep learning. IEEE Trans Neural Netw Learn Syst 30(9):2805–2824. https://doi.org/10.1109/TNNLS.2018.2886017
Yuqian C, Song Y, Liu W, Zhang YJ et al (2021) CellTrack R-CNN: A novel end-to-end deep neural network for cell segmentation and tracking in microscopy images. 2021 IEEE 18th International Symposium on Biomedical Imaging. 779–782. https://doi.org/10.1109/ISBI48211.2021.9434057
Yuzhen L, and Salem FM (2017) Simplified gating in long short-term memory (lstm) recurrent neural networks. 2017 IEEE 60th International Midwest Symposium on Circuits and Systems (MWSCAS). 1601–1604. https://doi.org/10.1109/MWSCAS.2017.8053244
Zabalza J, Ren J, Zheng J et al (2016) Novel segmented stacked autoencoder for effective dimensionality reduction and feature extraction in hyperspectral imaging. Neurocomputing 185:1–10. https://doi.org/10.1016/j.neucom.2015.11.044
Zhang L, Suganthan PN (2017) Visual tracking with convolutional random vector functional link network. IEEE Trans Cybern 47:3243–3253. https://doi.org/10.1109/TCYB.2016.2588526
Zhang Z, Luo J, Liu J, Chen M, Zhang S, Zhu L (2022) DGGCNN: An improved generative grasping convolutional neural networks. 2022 Asia Conference on Advanced Robotics, Automation, and Control Engineering. 57–61. https://doi.org/10.1109/ARACE56528.2022.00019
Zhang Z, Luo J, Liu J et al (2022) DGGCNN: An improved generative grasping convolutional neural networks. 2022 Asia Conference on Advanced Robotics, Automation, and Control Engineering. 57–61. https://doi.org/10.1109/ARACE56528.2022.00019
Zhang H et al (2017) StackGAN: Text to photo-realistic image synthesis with stacked generative adversarial networks. IEEE Int Conf Comput Vision (ICCV) 2017:5908–5916. https://doi.org/10.1109/ICCV.2017.629
Zhang H, Xu T, Li H, Zhang S, Wang X, Huang X, Metaxas DN (2018) StackGAN++: Realistic image synthesis with stacked generative adversarial networks. IEEE Trans Pattern Anal Mach Intell 41(8):1947–1962. https://doi.org/10.1109/TPAMI.2018.2856256
Zhang Y, Cheng Y, Xu T, Wang G, Chen C, Yang T (2022) Fault prediction of railway turnout systems based on improved sparse autoencoder and gated recurrent unit network. IEEE Trans Intell Transp Syst 23(8):12711–12723. https://doi.org/10.1109/TITS.2021.3116966
Zhao J, Michael M, LeCun Y (2017) Energy-based generative adversarial network. 5th International Conference on Learning Representations
Zhao H, Zenget X, Zhang J et al (2011) Pipelined functional link artificial recurrent neural network with the decision feedback structure for nonlinear channel equalization. Inf Sci 181(17):3677–3692. https://doi.org/10.1016/j.ins.2011.04.033
Zhao R, Dong D, Wang Y et al (2022) Image-based crowd stability analysis using improved multi-column convolutional neural network. IEEE Trans Intell Transp Syst 23(6):5480–5489. https://doi.org/10.1109/TITS.2021.3054376
Zheng C et al (2019) A novel equivalent model of active distribution networks based on LSTM. IEEE Trans Neural Netw Learn Syst 30(9):2611–2624. https://doi.org/10.1109/TNNLS.2018.2885219
Zhong H, Wu J (2022) Image dehazing algorithm based on improved generative adversarial network. In: Proceedings of the 7th International Conference on Cyber Security and Information Engineering. 429–434. https://doi.org/10.1145/3558819.3565120
Zhou H, Zhang S, Peng J, Zhang S, Li J, Xiong H, Zhang W (2021) Informer: Beyond efficient transformer for long sequence time-series forecasting. Proc AAAI Conf Artif Intell 35(12):11106–11115
Download references
Author information
Authors and affiliations.
Department of Software Engineering, Delhi Technological University, Delhi, India
Ruchika Malhotra & Priya Singh
You can also search for this author in PubMed Google Scholar
Contributions
For the Systematic Literature Review-
1. Ruchika Malhotra proposed the idea for the article.
2. Primary Study selection was done by Priya Singh followed by a review by Dr. Ruchika Malhotra.
3. Data Extraction was done by Ruchika Malhotra and Priya Singh both separately, resolving differences where applicable at the time of merging.
4. Result Reporting was done by Priya Singh and reviewed by Ruchika Malhotra.
5. Proofreading and final review were done by Ruchika Malhotra and Priya Singh.
Corresponding author
Correspondence to Priya Singh .
Ethics declarations
Ethics approval and consent to participate.
Not applicable.
Consent for publication
The authors provide consent for publication.
Competing interests
The authors declare that they have no known competing financial interests or personal relationships that could have appeared to influence the work reported in this paper.
Conflict of interest
The authors certify that this manuscript has not been submitted to more than one journal for simultaneous consideration and it has not been published previously (partly or in full). The authors also certify that no funding has been received for the conduct of this study and the preparation of this manuscript. This article does not contain any studies with human participants or animals performed by any of the authors.
Additional information
Publisher's note.
Springer Nature remains neutral with regard to jurisdictional claims in published maps and institutional affiliations.
1.1 Quality assessment results
We provide the quality scores to 166 studies selected after Inclusion–Exclusion criteria according to 16 quality assessment questions stated in Table 2 . Table 10 reports the percentage of candidate studies that answered a given quality question as “Yes”, “Partly” or “No”.
Rights and permissions
Springer Nature or its licensor (e.g. a society or other partner) holds exclusive rights to this article under a publishing agreement with the author(s) or other rightsholder(s); author self-archiving of the accepted manuscript version of this article is solely governed by the terms of such publishing agreement and applicable law.
Reprints and permissions
About this article
Malhotra, R., Singh, P. Recent advances in deep learning models: a systematic literature review. Multimed Tools Appl 82 , 44977–45060 (2023). https://doi.org/10.1007/s11042-023-15295-z
Download citation
Received : 08 September 2022
Revised : 10 January 2023
Accepted : 06 April 2023
Published : 25 April 2023
Issue Date : December 2023
DOI : https://doi.org/10.1007/s11042-023-15295-z
Share this article
Anyone you share the following link with will be able to read this content:
Sorry, a shareable link is not currently available for this article.
Provided by the Springer Nature SharedIt content-sharing initiative
- Find a journal
- Publish with us
- Track your research
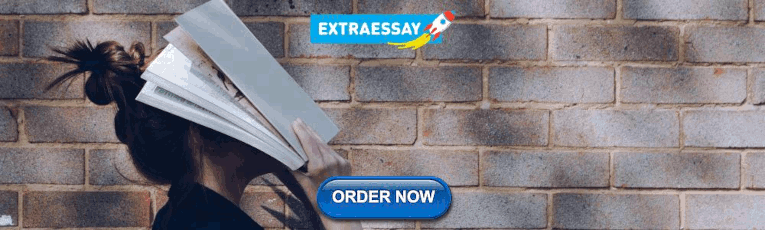
IMAGES
VIDEO
COMMENTS
This wide variety of applications justifies the relevance of LCs in industrial applications. This paper presents the state of the art in the literature on learning and forgetting curves, describing the existing models, their limitations, and reported applications. Directions for future research on the subject are eventually proposed.
This paper presents the state of the art in the literature on learning and forgetting curves, describing the existing models, their limitations, and reported applications. Directions for future ...
Log-linear and non-log-linear learning curve models for production research and cost estimation. The derivation of the mid-unit model is presented, a continuous form of the log-linear learning curve, which can accurately provide production cost estimates from either cumulative average costs or unit costs. Expand.
Learning Curves - Literature Review and Future Research Directions. Inside the Learning Curve: Opening Up the Black Box of the Learning Curve. The Learning-Quality Relationship. Forgetting Curves: A Review. Learning and Forgetting: A Conceptual Framework. The Organizational Learning Curve. The Learning Organization at the Steady State.
point onward, when we talk about the learning curve, this is what is meant. The preceding learning curve is defined for a single problem P. Sometimes we wish to study how a model performs over a range of problems or, more generally, a full distribution P over problems. The learning curve that considers such averaged performance is referred to ...
Theory and Models Learning Curves: The State of the Art and Research Directions, Flavio S. Anzanello Inside the Learning Curve: Opening the Black Box of the learning Curve, Michael A. Lapre Linking Quality to Learning - A Review, Mehmood Khan, Mohamad Y. Jaber, and Margaret Plaza Latent Growth Models for Operations Management Research: A Methodological Primer. Theory and Models Learning Curves ...
Learning curve models and applications: Literature review and research directions Michel Jose Anzanello*, Flavio Sanson Fogliatto1 Federal University of Rio Grande do Sul (UFRGS), Av. Osvaldo ...
Anzanello MJ, Fogliatto FS (2011) Learning curve models and applications: literature review and research directions. Int J Ind Ergon 41(5):573-583. Article Google Scholar Mosheiov G, Sidney JB (2003) Scheduling with general job-dependent learning curves. Eur J Oper Res 147(3):665-670
Written by international contributors, Learning Curves: Theory, Models, and Applications first draws a learning map that shows where learning is involved within organizations, then examines how it can be sustained, perfected, and accelerated. The book reviews empirical findings in the literature in terms of different sources for learning and ...
Further, despite the number of studies investigated, few explicitly described the LC model used to represent the impact of I4.0 technologies on learning. Our findings allowed the proposition of five research directions. Literature on both LC and I4.0 is still fragmented, poorly addressing their relationship.
This wide variety of applications justifies the relevance of LCs in industrial applications. This paper presents the state of the art in the literature on learning and forgetting curves, describing the existing models, their limitations, and reported applications. Directions for future research on the subject are eventually proposed.
Written by international contributors, Learning Curves: Theory, Models, and Applications first draws a learning map that shows where learning is involved within organizations, then examines how it can be sustained, perfected, and accelerated.The book reviews empirical findings in the literature in terms of different sources for learning and partial assessments of the steps that make up the ...
Learning curve models and applications: Literature review and research... Go to citation Crossref Google Scholar. ... Literature review . Constructing Qualitatively Derived Theory: Concept Construction and Concept Typologies ... Sage Research Methods Supercharging research opens in new tab;
Written by international contributors, Learning Curves: Theory, Models, and Applications first draws a learning map that shows where learning is involved within organizations, then examines how it can be sustained, perfected, and accelerated. The book reviews empirical findings in the literature in terms of different sources for learning and partia
[24] Anzanello M J and Fogliatto F S 2011 Learning curve models and applications: Literature review and research directions Int. J. Ind. Ergon. 41 573-83. Google Scholar [25] Jordan Srour F, Kiomjian D and Srour I M 2016 Learning curves in construction: A critical review and new model J. Constr. Eng. Manag. 142 6015004. Google Scholar
The operations management literature is replete with pieces of evidence and discussions on learning-by-doing, its theory, models, and applications (Glock et al., 2019). The learning curve (LC) is ...
Learning curves provide insight into the dependence of a learner's generalization performance on the training set size. This important tool can be used for model selection, to predict the effect of more training data, and to reduce the ...
AbstractThis paper brings forth from the literature a series of learning curve models and evaluates them through the lens of the construction industry. ... Anzanello, M. J., and Fogliatto, F. S. (2011). "Learning curve models and applications: Literature review and research directions." ... "The repetition effect in building and ...
This paper presents the state of the art in the literature on learning and forgetting curves, describing the existing models, their limitations, and reported applications. Directions for future research on the subject are eventually proposed.The Learning Curve (LC) models described here can be used in a wide variety of industrial applications ...
It maps this specific research area, synthesizes its research findings, highlights its key application areas and explores future research directions. This work achieves this goal by presenting the results of a systematic literature review on the applications of learning curves in production and operations management.
DOI: 10.1016/J.CIE.2018.10.030 Corpus ID: 70224169; Applications of learning curves in production and operations management: A systematic literature review @article{Glock2019ApplicationsOL, title={Applications of learning curves in production and operations management: A systematic literature review}, author={Christoph H. Glock and Eric H. Grosse and Mohamad Y. Jaber and Timothy L. Smunt ...
In recent years, deep learning has evolved as a rapidly growing and stimulating field of machine learning and has redefined state-of-the-art performances in a variety of applications. There are multiple deep learning models that have distinct architectures and capabilities. Up to the present, a large number of novel variants of these baseline deep learning models is proposed to address the ...
Learning Curve Defined Log-Linear Learning Curve Models Determining the Appropriate Learning Rate or Slope Estimating Requirements and Limitations and Caveats to Learning Curve Usage are explained. Foreword by John G. Carlson Preface Learning Curve Defined Log-Linear Learning Curve Models Determining the Appropriate Learning Rate or Slope Estimating Requirements Other Common Learning Curve ...