- Privacy Policy

Home » Case Study – Methods, Examples and Guide
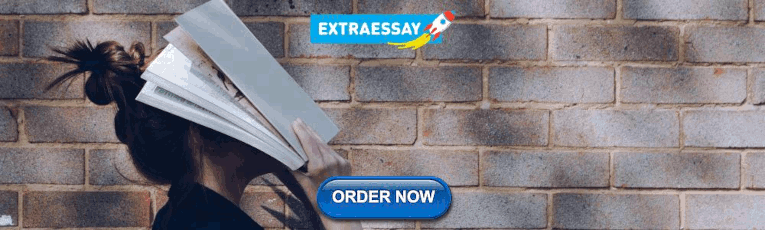
Case Study – Methods, Examples and Guide
Table of Contents

A case study is a research method that involves an in-depth examination and analysis of a particular phenomenon or case, such as an individual, organization, community, event, or situation.
It is a qualitative research approach that aims to provide a detailed and comprehensive understanding of the case being studied. Case studies typically involve multiple sources of data, including interviews, observations, documents, and artifacts, which are analyzed using various techniques, such as content analysis, thematic analysis, and grounded theory. The findings of a case study are often used to develop theories, inform policy or practice, or generate new research questions.
Types of Case Study
Types and Methods of Case Study are as follows:
Single-Case Study
A single-case study is an in-depth analysis of a single case. This type of case study is useful when the researcher wants to understand a specific phenomenon in detail.
For Example , A researcher might conduct a single-case study on a particular individual to understand their experiences with a particular health condition or a specific organization to explore their management practices. The researcher collects data from multiple sources, such as interviews, observations, and documents, and uses various techniques to analyze the data, such as content analysis or thematic analysis. The findings of a single-case study are often used to generate new research questions, develop theories, or inform policy or practice.
Multiple-Case Study
A multiple-case study involves the analysis of several cases that are similar in nature. This type of case study is useful when the researcher wants to identify similarities and differences between the cases.
For Example, a researcher might conduct a multiple-case study on several companies to explore the factors that contribute to their success or failure. The researcher collects data from each case, compares and contrasts the findings, and uses various techniques to analyze the data, such as comparative analysis or pattern-matching. The findings of a multiple-case study can be used to develop theories, inform policy or practice, or generate new research questions.
Exploratory Case Study
An exploratory case study is used to explore a new or understudied phenomenon. This type of case study is useful when the researcher wants to generate hypotheses or theories about the phenomenon.
For Example, a researcher might conduct an exploratory case study on a new technology to understand its potential impact on society. The researcher collects data from multiple sources, such as interviews, observations, and documents, and uses various techniques to analyze the data, such as grounded theory or content analysis. The findings of an exploratory case study can be used to generate new research questions, develop theories, or inform policy or practice.
Descriptive Case Study
A descriptive case study is used to describe a particular phenomenon in detail. This type of case study is useful when the researcher wants to provide a comprehensive account of the phenomenon.
For Example, a researcher might conduct a descriptive case study on a particular community to understand its social and economic characteristics. The researcher collects data from multiple sources, such as interviews, observations, and documents, and uses various techniques to analyze the data, such as content analysis or thematic analysis. The findings of a descriptive case study can be used to inform policy or practice or generate new research questions.
Instrumental Case Study
An instrumental case study is used to understand a particular phenomenon that is instrumental in achieving a particular goal. This type of case study is useful when the researcher wants to understand the role of the phenomenon in achieving the goal.
For Example, a researcher might conduct an instrumental case study on a particular policy to understand its impact on achieving a particular goal, such as reducing poverty. The researcher collects data from multiple sources, such as interviews, observations, and documents, and uses various techniques to analyze the data, such as content analysis or thematic analysis. The findings of an instrumental case study can be used to inform policy or practice or generate new research questions.
Case Study Data Collection Methods
Here are some common data collection methods for case studies:
Interviews involve asking questions to individuals who have knowledge or experience relevant to the case study. Interviews can be structured (where the same questions are asked to all participants) or unstructured (where the interviewer follows up on the responses with further questions). Interviews can be conducted in person, over the phone, or through video conferencing.
Observations
Observations involve watching and recording the behavior and activities of individuals or groups relevant to the case study. Observations can be participant (where the researcher actively participates in the activities) or non-participant (where the researcher observes from a distance). Observations can be recorded using notes, audio or video recordings, or photographs.
Documents can be used as a source of information for case studies. Documents can include reports, memos, emails, letters, and other written materials related to the case study. Documents can be collected from the case study participants or from public sources.
Surveys involve asking a set of questions to a sample of individuals relevant to the case study. Surveys can be administered in person, over the phone, through mail or email, or online. Surveys can be used to gather information on attitudes, opinions, or behaviors related to the case study.
Artifacts are physical objects relevant to the case study. Artifacts can include tools, equipment, products, or other objects that provide insights into the case study phenomenon.
How to conduct Case Study Research
Conducting a case study research involves several steps that need to be followed to ensure the quality and rigor of the study. Here are the steps to conduct case study research:
- Define the research questions: The first step in conducting a case study research is to define the research questions. The research questions should be specific, measurable, and relevant to the case study phenomenon under investigation.
- Select the case: The next step is to select the case or cases to be studied. The case should be relevant to the research questions and should provide rich and diverse data that can be used to answer the research questions.
- Collect data: Data can be collected using various methods, such as interviews, observations, documents, surveys, and artifacts. The data collection method should be selected based on the research questions and the nature of the case study phenomenon.
- Analyze the data: The data collected from the case study should be analyzed using various techniques, such as content analysis, thematic analysis, or grounded theory. The analysis should be guided by the research questions and should aim to provide insights and conclusions relevant to the research questions.
- Draw conclusions: The conclusions drawn from the case study should be based on the data analysis and should be relevant to the research questions. The conclusions should be supported by evidence and should be clearly stated.
- Validate the findings: The findings of the case study should be validated by reviewing the data and the analysis with participants or other experts in the field. This helps to ensure the validity and reliability of the findings.
- Write the report: The final step is to write the report of the case study research. The report should provide a clear description of the case study phenomenon, the research questions, the data collection methods, the data analysis, the findings, and the conclusions. The report should be written in a clear and concise manner and should follow the guidelines for academic writing.
Examples of Case Study
Here are some examples of case study research:
- The Hawthorne Studies : Conducted between 1924 and 1932, the Hawthorne Studies were a series of case studies conducted by Elton Mayo and his colleagues to examine the impact of work environment on employee productivity. The studies were conducted at the Hawthorne Works plant of the Western Electric Company in Chicago and included interviews, observations, and experiments.
- The Stanford Prison Experiment: Conducted in 1971, the Stanford Prison Experiment was a case study conducted by Philip Zimbardo to examine the psychological effects of power and authority. The study involved simulating a prison environment and assigning participants to the role of guards or prisoners. The study was controversial due to the ethical issues it raised.
- The Challenger Disaster: The Challenger Disaster was a case study conducted to examine the causes of the Space Shuttle Challenger explosion in 1986. The study included interviews, observations, and analysis of data to identify the technical, organizational, and cultural factors that contributed to the disaster.
- The Enron Scandal: The Enron Scandal was a case study conducted to examine the causes of the Enron Corporation’s bankruptcy in 2001. The study included interviews, analysis of financial data, and review of documents to identify the accounting practices, corporate culture, and ethical issues that led to the company’s downfall.
- The Fukushima Nuclear Disaster : The Fukushima Nuclear Disaster was a case study conducted to examine the causes of the nuclear accident that occurred at the Fukushima Daiichi Nuclear Power Plant in Japan in 2011. The study included interviews, analysis of data, and review of documents to identify the technical, organizational, and cultural factors that contributed to the disaster.
Application of Case Study
Case studies have a wide range of applications across various fields and industries. Here are some examples:
Business and Management
Case studies are widely used in business and management to examine real-life situations and develop problem-solving skills. Case studies can help students and professionals to develop a deep understanding of business concepts, theories, and best practices.
Case studies are used in healthcare to examine patient care, treatment options, and outcomes. Case studies can help healthcare professionals to develop critical thinking skills, diagnose complex medical conditions, and develop effective treatment plans.
Case studies are used in education to examine teaching and learning practices. Case studies can help educators to develop effective teaching strategies, evaluate student progress, and identify areas for improvement.
Social Sciences
Case studies are widely used in social sciences to examine human behavior, social phenomena, and cultural practices. Case studies can help researchers to develop theories, test hypotheses, and gain insights into complex social issues.
Law and Ethics
Case studies are used in law and ethics to examine legal and ethical dilemmas. Case studies can help lawyers, policymakers, and ethical professionals to develop critical thinking skills, analyze complex cases, and make informed decisions.
Purpose of Case Study
The purpose of a case study is to provide a detailed analysis of a specific phenomenon, issue, or problem in its real-life context. A case study is a qualitative research method that involves the in-depth exploration and analysis of a particular case, which can be an individual, group, organization, event, or community.
The primary purpose of a case study is to generate a comprehensive and nuanced understanding of the case, including its history, context, and dynamics. Case studies can help researchers to identify and examine the underlying factors, processes, and mechanisms that contribute to the case and its outcomes. This can help to develop a more accurate and detailed understanding of the case, which can inform future research, practice, or policy.
Case studies can also serve other purposes, including:
- Illustrating a theory or concept: Case studies can be used to illustrate and explain theoretical concepts and frameworks, providing concrete examples of how they can be applied in real-life situations.
- Developing hypotheses: Case studies can help to generate hypotheses about the causal relationships between different factors and outcomes, which can be tested through further research.
- Providing insight into complex issues: Case studies can provide insights into complex and multifaceted issues, which may be difficult to understand through other research methods.
- Informing practice or policy: Case studies can be used to inform practice or policy by identifying best practices, lessons learned, or areas for improvement.
Advantages of Case Study Research
There are several advantages of case study research, including:
- In-depth exploration: Case study research allows for a detailed exploration and analysis of a specific phenomenon, issue, or problem in its real-life context. This can provide a comprehensive understanding of the case and its dynamics, which may not be possible through other research methods.
- Rich data: Case study research can generate rich and detailed data, including qualitative data such as interviews, observations, and documents. This can provide a nuanced understanding of the case and its complexity.
- Holistic perspective: Case study research allows for a holistic perspective of the case, taking into account the various factors, processes, and mechanisms that contribute to the case and its outcomes. This can help to develop a more accurate and comprehensive understanding of the case.
- Theory development: Case study research can help to develop and refine theories and concepts by providing empirical evidence and concrete examples of how they can be applied in real-life situations.
- Practical application: Case study research can inform practice or policy by identifying best practices, lessons learned, or areas for improvement.
- Contextualization: Case study research takes into account the specific context in which the case is situated, which can help to understand how the case is influenced by the social, cultural, and historical factors of its environment.
Limitations of Case Study Research
There are several limitations of case study research, including:
- Limited generalizability : Case studies are typically focused on a single case or a small number of cases, which limits the generalizability of the findings. The unique characteristics of the case may not be applicable to other contexts or populations, which may limit the external validity of the research.
- Biased sampling: Case studies may rely on purposive or convenience sampling, which can introduce bias into the sample selection process. This may limit the representativeness of the sample and the generalizability of the findings.
- Subjectivity: Case studies rely on the interpretation of the researcher, which can introduce subjectivity into the analysis. The researcher’s own biases, assumptions, and perspectives may influence the findings, which may limit the objectivity of the research.
- Limited control: Case studies are typically conducted in naturalistic settings, which limits the control that the researcher has over the environment and the variables being studied. This may limit the ability to establish causal relationships between variables.
- Time-consuming: Case studies can be time-consuming to conduct, as they typically involve a detailed exploration and analysis of a specific case. This may limit the feasibility of conducting multiple case studies or conducting case studies in a timely manner.
- Resource-intensive: Case studies may require significant resources, including time, funding, and expertise. This may limit the ability of researchers to conduct case studies in resource-constrained settings.
About the author
Muhammad Hassan
Researcher, Academic Writer, Web developer
You may also like

Observational Research – Methods and Guide

Qualitative Research Methods

Transformative Design – Methods, Types, Guide

Quantitative Research – Methods, Types and...

Correlational Research – Methods, Types and...

Mixed Methods Research – Types & Analysis
Have a language expert improve your writing
Run a free plagiarism check in 10 minutes, generate accurate citations for free.
- Knowledge Base
Methodology
- What Is a Case Study? | Definition, Examples & Methods
What Is a Case Study? | Definition, Examples & Methods
Published on May 8, 2019 by Shona McCombes . Revised on November 20, 2023.
A case study is a detailed study of a specific subject, such as a person, group, place, event, organization, or phenomenon. Case studies are commonly used in social, educational, clinical, and business research.
A case study research design usually involves qualitative methods , but quantitative methods are sometimes also used. Case studies are good for describing , comparing, evaluating and understanding different aspects of a research problem .
Table of contents
When to do a case study, step 1: select a case, step 2: build a theoretical framework, step 3: collect your data, step 4: describe and analyze the case, other interesting articles.
A case study is an appropriate research design when you want to gain concrete, contextual, in-depth knowledge about a specific real-world subject. It allows you to explore the key characteristics, meanings, and implications of the case.
Case studies are often a good choice in a thesis or dissertation . They keep your project focused and manageable when you don’t have the time or resources to do large-scale research.
You might use just one complex case study where you explore a single subject in depth, or conduct multiple case studies to compare and illuminate different aspects of your research problem.
Research question | Case study |
---|---|
What are the ecological effects of wolf reintroduction? | Case study of wolf reintroduction in Yellowstone National Park |
How do populist politicians use narratives about history to gain support? | Case studies of Hungarian prime minister Viktor Orbán and US president Donald Trump |
How can teachers implement active learning strategies in mixed-level classrooms? | Case study of a local school that promotes active learning |
What are the main advantages and disadvantages of wind farms for rural communities? | Case studies of three rural wind farm development projects in different parts of the country |
How are viral marketing strategies changing the relationship between companies and consumers? | Case study of the iPhone X marketing campaign |
How do experiences of work in the gig economy differ by gender, race and age? | Case studies of Deliveroo and Uber drivers in London |
Receive feedback on language, structure, and formatting
Professional editors proofread and edit your paper by focusing on:
- Academic style
- Vague sentences
- Style consistency
See an example
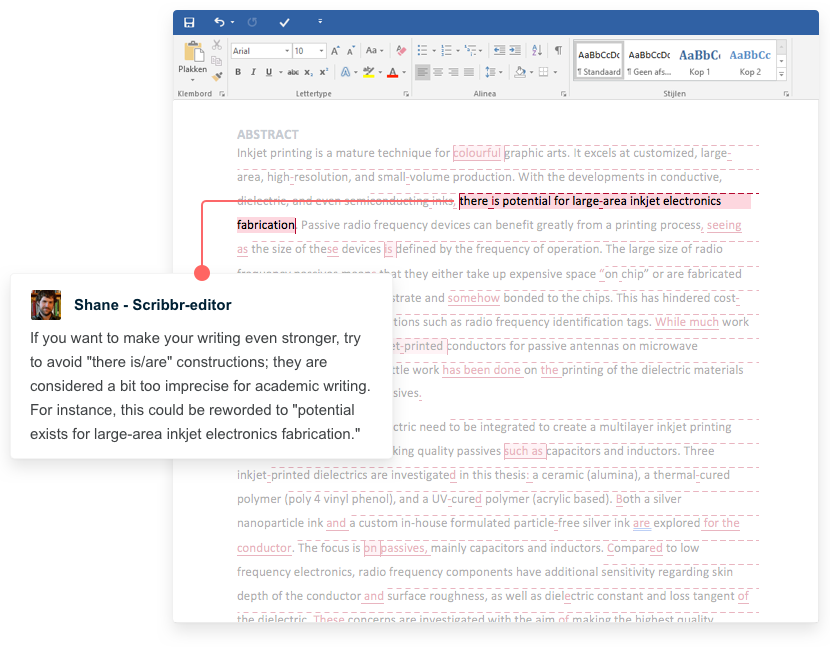
Once you have developed your problem statement and research questions , you should be ready to choose the specific case that you want to focus on. A good case study should have the potential to:
- Provide new or unexpected insights into the subject
- Challenge or complicate existing assumptions and theories
- Propose practical courses of action to resolve a problem
- Open up new directions for future research
TipIf your research is more practical in nature and aims to simultaneously investigate an issue as you solve it, consider conducting action research instead.
Unlike quantitative or experimental research , a strong case study does not require a random or representative sample. In fact, case studies often deliberately focus on unusual, neglected, or outlying cases which may shed new light on the research problem.
Example of an outlying case studyIn the 1960s the town of Roseto, Pennsylvania was discovered to have extremely low rates of heart disease compared to the US average. It became an important case study for understanding previously neglected causes of heart disease.
However, you can also choose a more common or representative case to exemplify a particular category, experience or phenomenon.
Example of a representative case studyIn the 1920s, two sociologists used Muncie, Indiana as a case study of a typical American city that supposedly exemplified the changing culture of the US at the time.
While case studies focus more on concrete details than general theories, they should usually have some connection with theory in the field. This way the case study is not just an isolated description, but is integrated into existing knowledge about the topic. It might aim to:
- Exemplify a theory by showing how it explains the case under investigation
- Expand on a theory by uncovering new concepts and ideas that need to be incorporated
- Challenge a theory by exploring an outlier case that doesn’t fit with established assumptions
To ensure that your analysis of the case has a solid academic grounding, you should conduct a literature review of sources related to the topic and develop a theoretical framework . This means identifying key concepts and theories to guide your analysis and interpretation.
There are many different research methods you can use to collect data on your subject. Case studies tend to focus on qualitative data using methods such as interviews , observations , and analysis of primary and secondary sources (e.g., newspaper articles, photographs, official records). Sometimes a case study will also collect quantitative data.
Example of a mixed methods case studyFor a case study of a wind farm development in a rural area, you could collect quantitative data on employment rates and business revenue, collect qualitative data on local people’s perceptions and experiences, and analyze local and national media coverage of the development.
The aim is to gain as thorough an understanding as possible of the case and its context.
Prevent plagiarism. Run a free check.
In writing up the case study, you need to bring together all the relevant aspects to give as complete a picture as possible of the subject.
How you report your findings depends on the type of research you are doing. Some case studies are structured like a standard scientific paper or thesis , with separate sections or chapters for the methods , results and discussion .
Others are written in a more narrative style, aiming to explore the case from various angles and analyze its meanings and implications (for example, by using textual analysis or discourse analysis ).
In all cases, though, make sure to give contextual details about the case, connect it back to the literature and theory, and discuss how it fits into wider patterns or debates.
If you want to know more about statistics , methodology , or research bias , make sure to check out some of our other articles with explanations and examples.
- Normal distribution
- Degrees of freedom
- Null hypothesis
- Discourse analysis
- Control groups
- Mixed methods research
- Non-probability sampling
- Quantitative research
- Ecological validity
Research bias
- Rosenthal effect
- Implicit bias
- Cognitive bias
- Selection bias
- Negativity bias
- Status quo bias
Cite this Scribbr article
If you want to cite this source, you can copy and paste the citation or click the “Cite this Scribbr article” button to automatically add the citation to our free Citation Generator.
McCombes, S. (2023, November 20). What Is a Case Study? | Definition, Examples & Methods. Scribbr. Retrieved August 9, 2024, from https://www.scribbr.com/methodology/case-study/
Is this article helpful?
Shona McCombes
Other students also liked, primary vs. secondary sources | difference & examples, what is a theoretical framework | guide to organizing, what is action research | definition & examples, get unlimited documents corrected.
✔ Free APA citation check included ✔ Unlimited document corrections ✔ Specialized in correcting academic texts

The Ultimate Guide to Qualitative Research - Part 1: The Basics
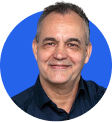
- Introduction and overview
- What is qualitative research?
- What is qualitative data?
- Examples of qualitative data
- Qualitative vs. quantitative research
- Mixed methods
- Qualitative research preparation
- Theoretical perspective
- Theoretical framework
- Literature reviews
Research question
- Conceptual framework
- Conceptual vs. theoretical framework
Data collection
- Qualitative research methods
- Focus groups
- Observational research
What is a case study?
Applications for case study research, what is a good case study, process of case study design, benefits and limitations of case studies.
- Ethnographical research
- Ethical considerations
- Confidentiality and privacy
- Power dynamics
- Reflexivity
Case studies
Case studies are essential to qualitative research , offering a lens through which researchers can investigate complex phenomena within their real-life contexts. This chapter explores the concept, purpose, applications, examples, and types of case studies and provides guidance on how to conduct case study research effectively.
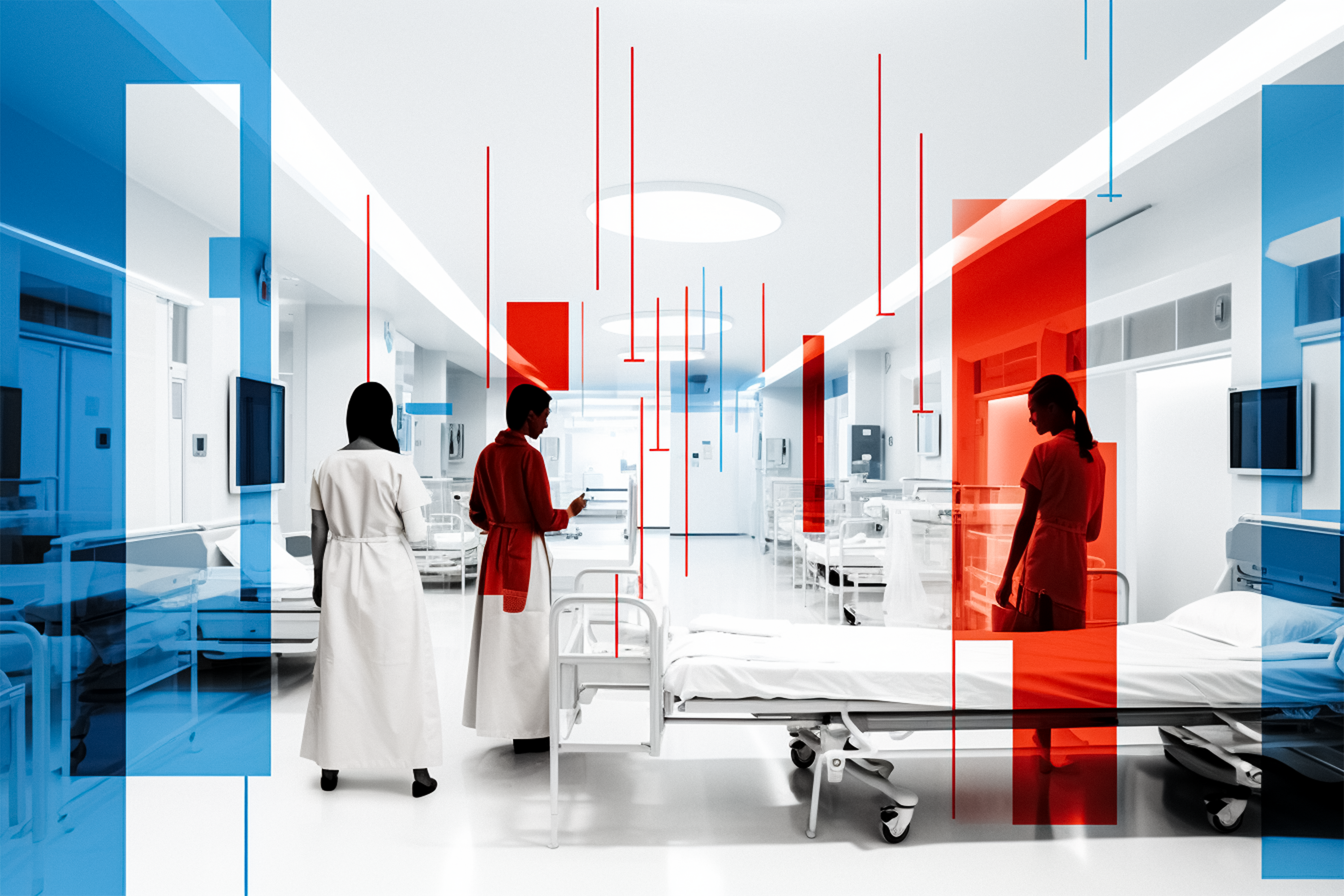
Whereas quantitative methods look at phenomena at scale, case study research looks at a concept or phenomenon in considerable detail. While analyzing a single case can help understand one perspective regarding the object of research inquiry, analyzing multiple cases can help obtain a more holistic sense of the topic or issue. Let's provide a basic definition of a case study, then explore its characteristics and role in the qualitative research process.
Definition of a case study
A case study in qualitative research is a strategy of inquiry that involves an in-depth investigation of a phenomenon within its real-world context. It provides researchers with the opportunity to acquire an in-depth understanding of intricate details that might not be as apparent or accessible through other methods of research. The specific case or cases being studied can be a single person, group, or organization – demarcating what constitutes a relevant case worth studying depends on the researcher and their research question .
Among qualitative research methods , a case study relies on multiple sources of evidence, such as documents, artifacts, interviews , or observations , to present a complete and nuanced understanding of the phenomenon under investigation. The objective is to illuminate the readers' understanding of the phenomenon beyond its abstract statistical or theoretical explanations.
Characteristics of case studies
Case studies typically possess a number of distinct characteristics that set them apart from other research methods. These characteristics include a focus on holistic description and explanation, flexibility in the design and data collection methods, reliance on multiple sources of evidence, and emphasis on the context in which the phenomenon occurs.
Furthermore, case studies can often involve a longitudinal examination of the case, meaning they study the case over a period of time. These characteristics allow case studies to yield comprehensive, in-depth, and richly contextualized insights about the phenomenon of interest.
The role of case studies in research
Case studies hold a unique position in the broader landscape of research methods aimed at theory development. They are instrumental when the primary research interest is to gain an intensive, detailed understanding of a phenomenon in its real-life context.
In addition, case studies can serve different purposes within research - they can be used for exploratory, descriptive, or explanatory purposes, depending on the research question and objectives. This flexibility and depth make case studies a valuable tool in the toolkit of qualitative researchers.
Remember, a well-conducted case study can offer a rich, insightful contribution to both academic and practical knowledge through theory development or theory verification, thus enhancing our understanding of complex phenomena in their real-world contexts.
What is the purpose of a case study?
Case study research aims for a more comprehensive understanding of phenomena, requiring various research methods to gather information for qualitative analysis . Ultimately, a case study can allow the researcher to gain insight into a particular object of inquiry and develop a theoretical framework relevant to the research inquiry.
Why use case studies in qualitative research?
Using case studies as a research strategy depends mainly on the nature of the research question and the researcher's access to the data.
Conducting case study research provides a level of detail and contextual richness that other research methods might not offer. They are beneficial when there's a need to understand complex social phenomena within their natural contexts.
The explanatory, exploratory, and descriptive roles of case studies
Case studies can take on various roles depending on the research objectives. They can be exploratory when the research aims to discover new phenomena or define new research questions; they are descriptive when the objective is to depict a phenomenon within its context in a detailed manner; and they can be explanatory if the goal is to understand specific relationships within the studied context. Thus, the versatility of case studies allows researchers to approach their topic from different angles, offering multiple ways to uncover and interpret the data .
The impact of case studies on knowledge development
Case studies play a significant role in knowledge development across various disciplines. Analysis of cases provides an avenue for researchers to explore phenomena within their context based on the collected data.
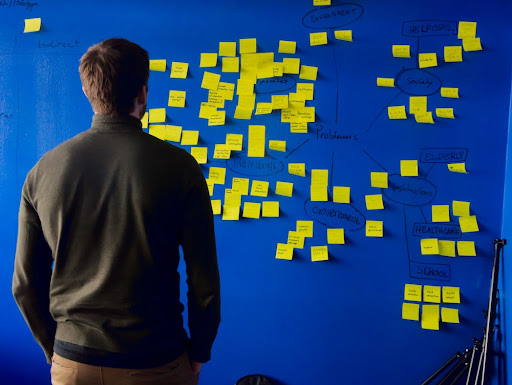
This can result in the production of rich, practical insights that can be instrumental in both theory-building and practice. Case studies allow researchers to delve into the intricacies and complexities of real-life situations, uncovering insights that might otherwise remain hidden.
Types of case studies
In qualitative research , a case study is not a one-size-fits-all approach. Depending on the nature of the research question and the specific objectives of the study, researchers might choose to use different types of case studies. These types differ in their focus, methodology, and the level of detail they provide about the phenomenon under investigation.
Understanding these types is crucial for selecting the most appropriate approach for your research project and effectively achieving your research goals. Let's briefly look at the main types of case studies.
Exploratory case studies
Exploratory case studies are typically conducted to develop a theory or framework around an understudied phenomenon. They can also serve as a precursor to a larger-scale research project. Exploratory case studies are useful when a researcher wants to identify the key issues or questions which can spur more extensive study or be used to develop propositions for further research. These case studies are characterized by flexibility, allowing researchers to explore various aspects of a phenomenon as they emerge, which can also form the foundation for subsequent studies.
Descriptive case studies
Descriptive case studies aim to provide a complete and accurate representation of a phenomenon or event within its context. These case studies are often based on an established theoretical framework, which guides how data is collected and analyzed. The researcher is concerned with describing the phenomenon in detail, as it occurs naturally, without trying to influence or manipulate it.
Explanatory case studies
Explanatory case studies are focused on explanation - they seek to clarify how or why certain phenomena occur. Often used in complex, real-life situations, they can be particularly valuable in clarifying causal relationships among concepts and understanding the interplay between different factors within a specific context.
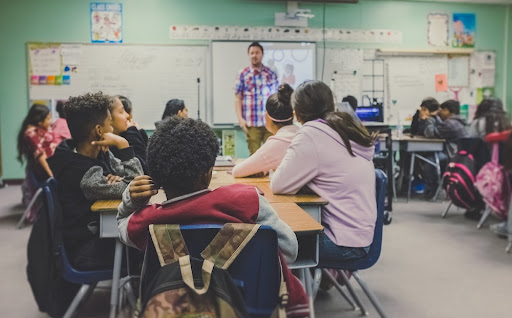
Intrinsic, instrumental, and collective case studies
These three categories of case studies focus on the nature and purpose of the study. An intrinsic case study is conducted when a researcher has an inherent interest in the case itself. Instrumental case studies are employed when the case is used to provide insight into a particular issue or phenomenon. A collective case study, on the other hand, involves studying multiple cases simultaneously to investigate some general phenomena.
Each type of case study serves a different purpose and has its own strengths and challenges. The selection of the type should be guided by the research question and objectives, as well as the context and constraints of the research.
The flexibility, depth, and contextual richness offered by case studies make this approach an excellent research method for various fields of study. They enable researchers to investigate real-world phenomena within their specific contexts, capturing nuances that other research methods might miss. Across numerous fields, case studies provide valuable insights into complex issues.
Critical information systems research
Case studies provide a detailed understanding of the role and impact of information systems in different contexts. They offer a platform to explore how information systems are designed, implemented, and used and how they interact with various social, economic, and political factors. Case studies in this field often focus on examining the intricate relationship between technology, organizational processes, and user behavior, helping to uncover insights that can inform better system design and implementation.
Health research
Health research is another field where case studies are highly valuable. They offer a way to explore patient experiences, healthcare delivery processes, and the impact of various interventions in a real-world context.
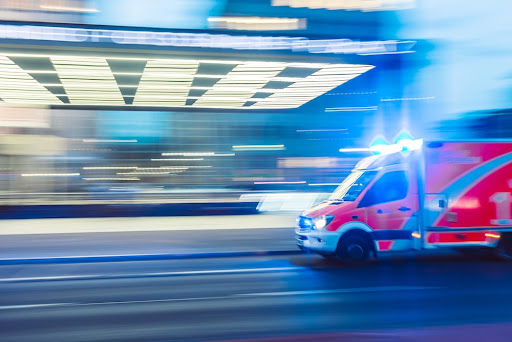
Case studies can provide a deep understanding of a patient's journey, giving insights into the intricacies of disease progression, treatment effects, and the psychosocial aspects of health and illness.
Asthma research studies
Specifically within medical research, studies on asthma often employ case studies to explore the individual and environmental factors that influence asthma development, management, and outcomes. A case study can provide rich, detailed data about individual patients' experiences, from the triggers and symptoms they experience to the effectiveness of various management strategies. This can be crucial for developing patient-centered asthma care approaches.
Other fields
Apart from the fields mentioned, case studies are also extensively used in business and management research, education research, and political sciences, among many others. They provide an opportunity to delve into the intricacies of real-world situations, allowing for a comprehensive understanding of various phenomena.
Case studies, with their depth and contextual focus, offer unique insights across these varied fields. They allow researchers to illuminate the complexities of real-life situations, contributing to both theory and practice.
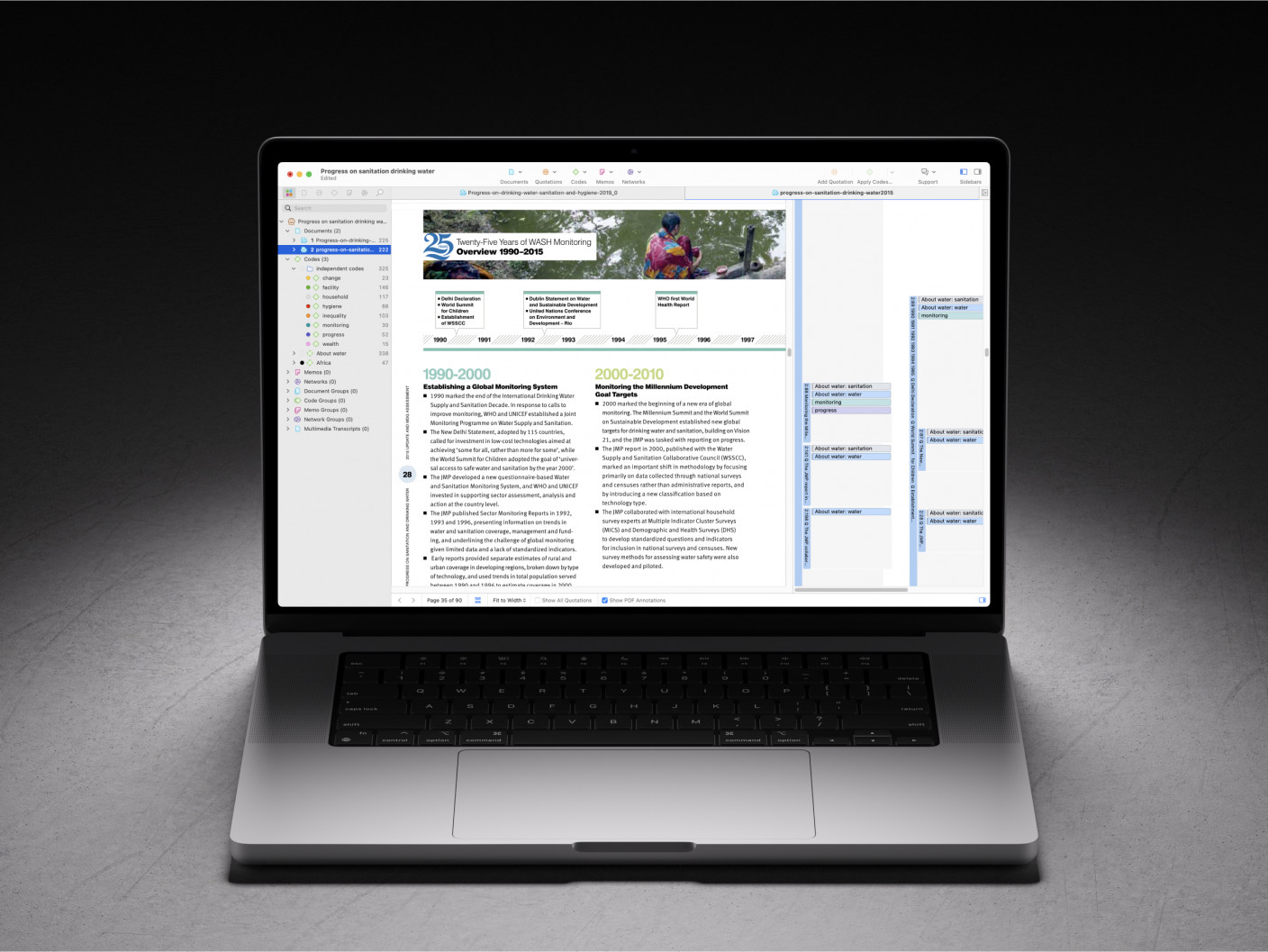
Whatever field you're in, ATLAS.ti puts your data to work for you
Download a free trial of ATLAS.ti to turn your data into insights.
Understanding the key elements of case study design is crucial for conducting rigorous and impactful case study research. A well-structured design guides the researcher through the process, ensuring that the study is methodologically sound and its findings are reliable and valid. The main elements of case study design include the research question , propositions, units of analysis, and the logic linking the data to the propositions.
The research question is the foundation of any research study. A good research question guides the direction of the study and informs the selection of the case, the methods of collecting data, and the analysis techniques. A well-formulated research question in case study research is typically clear, focused, and complex enough to merit further detailed examination of the relevant case(s).
Propositions
Propositions, though not necessary in every case study, provide a direction by stating what we might expect to find in the data collected. They guide how data is collected and analyzed by helping researchers focus on specific aspects of the case. They are particularly important in explanatory case studies, which seek to understand the relationships among concepts within the studied phenomenon.
Units of analysis
The unit of analysis refers to the case, or the main entity or entities that are being analyzed in the study. In case study research, the unit of analysis can be an individual, a group, an organization, a decision, an event, or even a time period. It's crucial to clearly define the unit of analysis, as it shapes the qualitative data analysis process by allowing the researcher to analyze a particular case and synthesize analysis across multiple case studies to draw conclusions.
Argumentation
This refers to the inferential model that allows researchers to draw conclusions from the data. The researcher needs to ensure that there is a clear link between the data, the propositions (if any), and the conclusions drawn. This argumentation is what enables the researcher to make valid and credible inferences about the phenomenon under study.
Understanding and carefully considering these elements in the design phase of a case study can significantly enhance the quality of the research. It can help ensure that the study is methodologically sound and its findings contribute meaningful insights about the case.
Ready to jumpstart your research with ATLAS.ti?
Conceptualize your research project with our intuitive data analysis interface. Download a free trial today.
Conducting a case study involves several steps, from defining the research question and selecting the case to collecting and analyzing data . This section outlines these key stages, providing a practical guide on how to conduct case study research.
Defining the research question
The first step in case study research is defining a clear, focused research question. This question should guide the entire research process, from case selection to analysis. It's crucial to ensure that the research question is suitable for a case study approach. Typically, such questions are exploratory or descriptive in nature and focus on understanding a phenomenon within its real-life context.
Selecting and defining the case
The selection of the case should be based on the research question and the objectives of the study. It involves choosing a unique example or a set of examples that provide rich, in-depth data about the phenomenon under investigation. After selecting the case, it's crucial to define it clearly, setting the boundaries of the case, including the time period and the specific context.
Previous research can help guide the case study design. When considering a case study, an example of a case could be taken from previous case study research and used to define cases in a new research inquiry. Considering recently published examples can help understand how to select and define cases effectively.
Developing a detailed case study protocol
A case study protocol outlines the procedures and general rules to be followed during the case study. This includes the data collection methods to be used, the sources of data, and the procedures for analysis. Having a detailed case study protocol ensures consistency and reliability in the study.
The protocol should also consider how to work with the people involved in the research context to grant the research team access to collecting data. As mentioned in previous sections of this guide, establishing rapport is an essential component of qualitative research as it shapes the overall potential for collecting and analyzing data.
Collecting data
Gathering data in case study research often involves multiple sources of evidence, including documents, archival records, interviews, observations, and physical artifacts. This allows for a comprehensive understanding of the case. The process for gathering data should be systematic and carefully documented to ensure the reliability and validity of the study.
Analyzing and interpreting data
The next step is analyzing the data. This involves organizing the data , categorizing it into themes or patterns , and interpreting these patterns to answer the research question. The analysis might also involve comparing the findings with prior research or theoretical propositions.
Writing the case study report
The final step is writing the case study report . This should provide a detailed description of the case, the data, the analysis process, and the findings. The report should be clear, organized, and carefully written to ensure that the reader can understand the case and the conclusions drawn from it.
Each of these steps is crucial in ensuring that the case study research is rigorous, reliable, and provides valuable insights about the case.
The type, depth, and quality of data in your study can significantly influence the validity and utility of the study. In case study research, data is usually collected from multiple sources to provide a comprehensive and nuanced understanding of the case. This section will outline the various methods of collecting data used in case study research and discuss considerations for ensuring the quality of the data.
Interviews are a common method of gathering data in case study research. They can provide rich, in-depth data about the perspectives, experiences, and interpretations of the individuals involved in the case. Interviews can be structured , semi-structured , or unstructured , depending on the research question and the degree of flexibility needed.
Observations
Observations involve the researcher observing the case in its natural setting, providing first-hand information about the case and its context. Observations can provide data that might not be revealed in interviews or documents, such as non-verbal cues or contextual information.
Documents and artifacts
Documents and archival records provide a valuable source of data in case study research. They can include reports, letters, memos, meeting minutes, email correspondence, and various public and private documents related to the case.
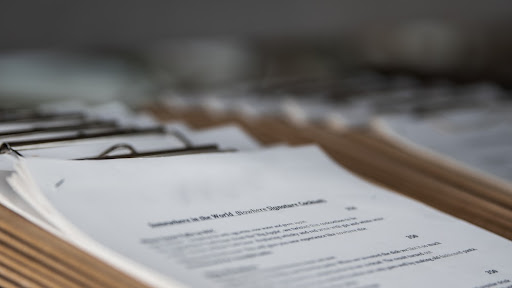
These records can provide historical context, corroborate evidence from other sources, and offer insights into the case that might not be apparent from interviews or observations.
Physical artifacts refer to any physical evidence related to the case, such as tools, products, or physical environments. These artifacts can provide tangible insights into the case, complementing the data gathered from other sources.
Ensuring the quality of data collection
Determining the quality of data in case study research requires careful planning and execution. It's crucial to ensure that the data is reliable, accurate, and relevant to the research question. This involves selecting appropriate methods of collecting data, properly training interviewers or observers, and systematically recording and storing the data. It also includes considering ethical issues related to collecting and handling data, such as obtaining informed consent and ensuring the privacy and confidentiality of the participants.
Data analysis
Analyzing case study research involves making sense of the rich, detailed data to answer the research question. This process can be challenging due to the volume and complexity of case study data. However, a systematic and rigorous approach to analysis can ensure that the findings are credible and meaningful. This section outlines the main steps and considerations in analyzing data in case study research.
Organizing the data
The first step in the analysis is organizing the data. This involves sorting the data into manageable sections, often according to the data source or the theme. This step can also involve transcribing interviews, digitizing physical artifacts, or organizing observational data.
Categorizing and coding the data
Once the data is organized, the next step is to categorize or code the data. This involves identifying common themes, patterns, or concepts in the data and assigning codes to relevant data segments. Coding can be done manually or with the help of software tools, and in either case, qualitative analysis software can greatly facilitate the entire coding process. Coding helps to reduce the data to a set of themes or categories that can be more easily analyzed.
Identifying patterns and themes
After coding the data, the researcher looks for patterns or themes in the coded data. This involves comparing and contrasting the codes and looking for relationships or patterns among them. The identified patterns and themes should help answer the research question.
Interpreting the data
Once patterns and themes have been identified, the next step is to interpret these findings. This involves explaining what the patterns or themes mean in the context of the research question and the case. This interpretation should be grounded in the data, but it can also involve drawing on theoretical concepts or prior research.
Verification of the data
The last step in the analysis is verification. This involves checking the accuracy and consistency of the analysis process and confirming that the findings are supported by the data. This can involve re-checking the original data, checking the consistency of codes, or seeking feedback from research participants or peers.
Like any research method , case study research has its strengths and limitations. Researchers must be aware of these, as they can influence the design, conduct, and interpretation of the study.
Understanding the strengths and limitations of case study research can also guide researchers in deciding whether this approach is suitable for their research question . This section outlines some of the key strengths and limitations of case study research.
Benefits include the following:
- Rich, detailed data: One of the main strengths of case study research is that it can generate rich, detailed data about the case. This can provide a deep understanding of the case and its context, which can be valuable in exploring complex phenomena.
- Flexibility: Case study research is flexible in terms of design , data collection , and analysis . A sufficient degree of flexibility allows the researcher to adapt the study according to the case and the emerging findings.
- Real-world context: Case study research involves studying the case in its real-world context, which can provide valuable insights into the interplay between the case and its context.
- Multiple sources of evidence: Case study research often involves collecting data from multiple sources , which can enhance the robustness and validity of the findings.
On the other hand, researchers should consider the following limitations:
- Generalizability: A common criticism of case study research is that its findings might not be generalizable to other cases due to the specificity and uniqueness of each case.
- Time and resource intensive: Case study research can be time and resource intensive due to the depth of the investigation and the amount of collected data.
- Complexity of analysis: The rich, detailed data generated in case study research can make analyzing the data challenging.
- Subjectivity: Given the nature of case study research, there may be a higher degree of subjectivity in interpreting the data , so researchers need to reflect on this and transparently convey to audiences how the research was conducted.
Being aware of these strengths and limitations can help researchers design and conduct case study research effectively and interpret and report the findings appropriately.
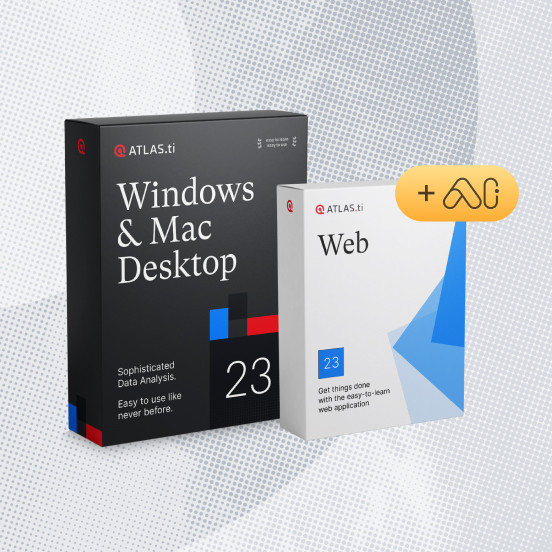
Ready to analyze your data with ATLAS.ti?
See how our intuitive software can draw key insights from your data with a free trial today.

Research Writing and Analysis
- NVivo Group and Study Sessions
- SPSS This link opens in a new window
- Statistical Analysis Group sessions
- Using Qualtrics
- Dissertation and Data Analysis Group Sessions
- Defense Schedule - Commons Calendar This link opens in a new window
- Research Process Flow Chart
- Research Alignment Chapter 1 This link opens in a new window
- Step 1: Seek Out Evidence
- Step 2: Explain
- Step 3: The Big Picture
- Step 4: Own It
- Step 5: Illustrate
- Annotated Bibliography
- Literature Review This link opens in a new window
- Systematic Reviews & Meta-Analyses
- How to Synthesize and Analyze
- Synthesis and Analysis Practice
- Synthesis and Analysis Group Sessions
- Problem Statement
- Purpose Statement
- Conceptual Framework
- Theoretical Framework
- Locating Theoretical and Conceptual Frameworks This link opens in a new window
- Quantitative Research Questions
- Qualitative Research Questions
- Trustworthiness of Qualitative Data
- Analysis and Coding Example- Qualitative Data
- Thematic Data Analysis in Qualitative Design
- Dissertation to Journal Article This link opens in a new window
- International Journal of Online Graduate Education (IJOGE) This link opens in a new window
- Journal of Research in Innovative Teaching & Learning (JRIT&L) This link opens in a new window
Writing a Case Study

What is a case study?

A Case study is:
- An in-depth research design that primarily uses a qualitative methodology but sometimes includes quantitative methodology.
- Used to examine an identifiable problem confirmed through research.
- Used to investigate an individual, group of people, organization, or event.
- Used to mostly answer "how" and "why" questions.
What are the different types of case studies?

Descriptive | This type of case study allows the researcher to: | How has the implementation and use of the instructional coaching intervention for elementary teachers impacted students’ attitudes toward reading? |
Explanatory | This type of case study allows the researcher to: | Why do differences exist when implementing the same online reading curriculum in three elementary classrooms? |
Exploratory | This type of case study allows the researcher to:
| What are potential barriers to student’s reading success when middle school teachers implement the Ready Reader curriculum online? |
Multiple Case Studies or Collective Case Study | This type of case study allows the researcher to: | How are individual school districts addressing student engagement in an online classroom? |
Intrinsic | This type of case study allows the researcher to: | How does a student’s familial background influence a teacher’s ability to provide meaningful instruction? |
Instrumental | This type of case study allows the researcher to: | How a rural school district’s integration of a reward system maximized student engagement? |
Note: These are the primary case studies. As you continue to research and learn
about case studies you will begin to find a robust list of different types.
Who are your case study participants?

|
This type of study is implemented to understand an individual by developing a detailed explanation of the individual’s lived experiences or perceptions.
|
| This type of study is implemented to explore a particular group of people’s perceptions. |
| This type of study is implemented to explore the perspectives of people who work for or had interaction with a specific organization or company. |
| This type of study is implemented to explore participant’s perceptions of an event. |
What is triangulation ?
Validity and credibility are an essential part of the case study. Therefore, the researcher should include triangulation to ensure trustworthiness while accurately reflecting what the researcher seeks to investigate.

How to write a Case Study?
When developing a case study, there are different ways you could present the information, but remember to include the five parts for your case study.

|
|
|
|
|

Was this resource helpful?
- << Previous: Thematic Data Analysis in Qualitative Design
- Next: Journal Article Reporting Standards (JARS) >>
- Last Updated: Jul 22, 2024 8:15 PM
- URL: https://resources.nu.edu/researchtools

Case Study Research Method in Psychology
Saul McLeod, PhD
Editor-in-Chief for Simply Psychology
BSc (Hons) Psychology, MRes, PhD, University of Manchester
Saul McLeod, PhD., is a qualified psychology teacher with over 18 years of experience in further and higher education. He has been published in peer-reviewed journals, including the Journal of Clinical Psychology.
Learn about our Editorial Process
Olivia Guy-Evans, MSc
Associate Editor for Simply Psychology
BSc (Hons) Psychology, MSc Psychology of Education
Olivia Guy-Evans is a writer and associate editor for Simply Psychology. She has previously worked in healthcare and educational sectors.
On This Page:
Case studies are in-depth investigations of a person, group, event, or community. Typically, data is gathered from various sources using several methods (e.g., observations & interviews).
The case study research method originated in clinical medicine (the case history, i.e., the patient’s personal history). In psychology, case studies are often confined to the study of a particular individual.
The information is mainly biographical and relates to events in the individual’s past (i.e., retrospective), as well as to significant events that are currently occurring in his or her everyday life.
The case study is not a research method, but researchers select methods of data collection and analysis that will generate material suitable for case studies.
Freud (1909a, 1909b) conducted very detailed investigations into the private lives of his patients in an attempt to both understand and help them overcome their illnesses.
This makes it clear that the case study is a method that should only be used by a psychologist, therapist, or psychiatrist, i.e., someone with a professional qualification.
There is an ethical issue of competence. Only someone qualified to diagnose and treat a person can conduct a formal case study relating to atypical (i.e., abnormal) behavior or atypical development.
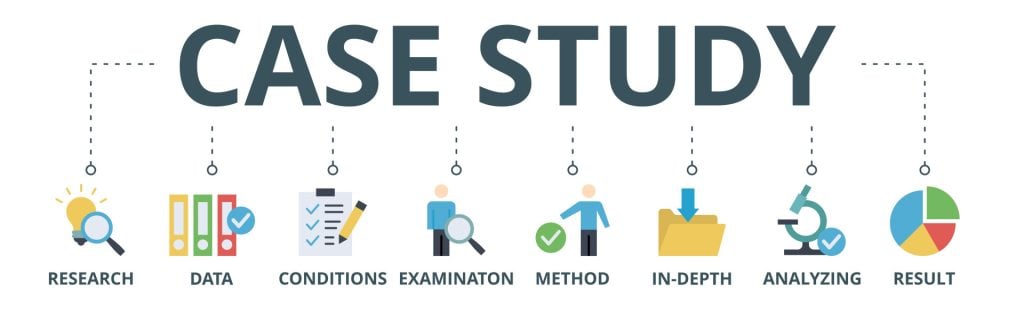
Famous Case Studies
- Anna O – One of the most famous case studies, documenting psychoanalyst Josef Breuer’s treatment of “Anna O” (real name Bertha Pappenheim) for hysteria in the late 1800s using early psychoanalytic theory.
- Little Hans – A child psychoanalysis case study published by Sigmund Freud in 1909 analyzing his five-year-old patient Herbert Graf’s house phobia as related to the Oedipus complex.
- Bruce/Brenda – Gender identity case of the boy (Bruce) whose botched circumcision led psychologist John Money to advise gender reassignment and raise him as a girl (Brenda) in the 1960s.
- Genie Wiley – Linguistics/psychological development case of the victim of extreme isolation abuse who was studied in 1970s California for effects of early language deprivation on acquiring speech later in life.
- Phineas Gage – One of the most famous neuropsychology case studies analyzes personality changes in railroad worker Phineas Gage after an 1848 brain injury involving a tamping iron piercing his skull.
Clinical Case Studies
- Studying the effectiveness of psychotherapy approaches with an individual patient
- Assessing and treating mental illnesses like depression, anxiety disorders, PTSD
- Neuropsychological cases investigating brain injuries or disorders
Child Psychology Case Studies
- Studying psychological development from birth through adolescence
- Cases of learning disabilities, autism spectrum disorders, ADHD
- Effects of trauma, abuse, deprivation on development
Types of Case Studies
- Explanatory case studies : Used to explore causation in order to find underlying principles. Helpful for doing qualitative analysis to explain presumed causal links.
- Exploratory case studies : Used to explore situations where an intervention being evaluated has no clear set of outcomes. It helps define questions and hypotheses for future research.
- Descriptive case studies : Describe an intervention or phenomenon and the real-life context in which it occurred. It is helpful for illustrating certain topics within an evaluation.
- Multiple-case studies : Used to explore differences between cases and replicate findings across cases. Helpful for comparing and contrasting specific cases.
- Intrinsic : Used to gain a better understanding of a particular case. Helpful for capturing the complexity of a single case.
- Collective : Used to explore a general phenomenon using multiple case studies. Helpful for jointly studying a group of cases in order to inquire into the phenomenon.
Where Do You Find Data for a Case Study?
There are several places to find data for a case study. The key is to gather data from multiple sources to get a complete picture of the case and corroborate facts or findings through triangulation of evidence. Most of this information is likely qualitative (i.e., verbal description rather than measurement), but the psychologist might also collect numerical data.
1. Primary sources
- Interviews – Interviewing key people related to the case to get their perspectives and insights. The interview is an extremely effective procedure for obtaining information about an individual, and it may be used to collect comments from the person’s friends, parents, employer, workmates, and others who have a good knowledge of the person, as well as to obtain facts from the person him or herself.
- Observations – Observing behaviors, interactions, processes, etc., related to the case as they unfold in real-time.
- Documents & Records – Reviewing private documents, diaries, public records, correspondence, meeting minutes, etc., relevant to the case.
2. Secondary sources
- News/Media – News coverage of events related to the case study.
- Academic articles – Journal articles, dissertations etc. that discuss the case.
- Government reports – Official data and records related to the case context.
- Books/films – Books, documentaries or films discussing the case.
3. Archival records
Searching historical archives, museum collections and databases to find relevant documents, visual/audio records related to the case history and context.
Public archives like newspapers, organizational records, photographic collections could all include potentially relevant pieces of information to shed light on attitudes, cultural perspectives, common practices and historical contexts related to psychology.
4. Organizational records
Organizational records offer the advantage of often having large datasets collected over time that can reveal or confirm psychological insights.
Of course, privacy and ethical concerns regarding confidential data must be navigated carefully.
However, with proper protocols, organizational records can provide invaluable context and empirical depth to qualitative case studies exploring the intersection of psychology and organizations.
- Organizational/industrial psychology research : Organizational records like employee surveys, turnover/retention data, policies, incident reports etc. may provide insight into topics like job satisfaction, workplace culture and dynamics, leadership issues, employee behaviors etc.
- Clinical psychology : Therapists/hospitals may grant access to anonymized medical records to study aspects like assessments, diagnoses, treatment plans etc. This could shed light on clinical practices.
- School psychology : Studies could utilize anonymized student records like test scores, grades, disciplinary issues, and counseling referrals to study child development, learning barriers, effectiveness of support programs, and more.
How do I Write a Case Study in Psychology?
Follow specified case study guidelines provided by a journal or your psychology tutor. General components of clinical case studies include: background, symptoms, assessments, diagnosis, treatment, and outcomes. Interpreting the information means the researcher decides what to include or leave out. A good case study should always clarify which information is the factual description and which is an inference or the researcher’s opinion.
1. Introduction
- Provide background on the case context and why it is of interest, presenting background information like demographics, relevant history, and presenting problem.
- Compare briefly to similar published cases if applicable. Clearly state the focus/importance of the case.
2. Case Presentation
- Describe the presenting problem in detail, including symptoms, duration,and impact on daily life.
- Include client demographics like age and gender, information about social relationships, and mental health history.
- Describe all physical, emotional, and/or sensory symptoms reported by the client.
- Use patient quotes to describe the initial complaint verbatim. Follow with full-sentence summaries of relevant history details gathered, including key components that led to a working diagnosis.
- Summarize clinical exam results, namely orthopedic/neurological tests, imaging, lab tests, etc. Note actual results rather than subjective conclusions. Provide images if clearly reproducible/anonymized.
- Clearly state the working diagnosis or clinical impression before transitioning to management.
3. Management and Outcome
- Indicate the total duration of care and number of treatments given over what timeframe. Use specific names/descriptions for any therapies/interventions applied.
- Present the results of the intervention,including any quantitative or qualitative data collected.
- For outcomes, utilize visual analog scales for pain, medication usage logs, etc., if possible. Include patient self-reports of improvement/worsening of symptoms. Note the reason for discharge/end of care.
4. Discussion
- Analyze the case, exploring contributing factors, limitations of the study, and connections to existing research.
- Analyze the effectiveness of the intervention,considering factors like participant adherence, limitations of the study, and potential alternative explanations for the results.
- Identify any questions raised in the case analysis and relate insights to established theories and current research if applicable. Avoid definitive claims about physiological explanations.
- Offer clinical implications, and suggest future research directions.
5. Additional Items
- Thank specific assistants for writing support only. No patient acknowledgments.
- References should directly support any key claims or quotes included.
- Use tables/figures/images only if substantially informative. Include permissions and legends/explanatory notes.
- Provides detailed (rich qualitative) information.
- Provides insight for further research.
- Permitting investigation of otherwise impractical (or unethical) situations.
Case studies allow a researcher to investigate a topic in far more detail than might be possible if they were trying to deal with a large number of research participants (nomothetic approach) with the aim of ‘averaging’.
Because of their in-depth, multi-sided approach, case studies often shed light on aspects of human thinking and behavior that would be unethical or impractical to study in other ways.
Research that only looks into the measurable aspects of human behavior is not likely to give us insights into the subjective dimension of experience, which is important to psychoanalytic and humanistic psychologists.
Case studies are often used in exploratory research. They can help us generate new ideas (that might be tested by other methods). They are an important way of illustrating theories and can help show how different aspects of a person’s life are related to each other.
The method is, therefore, important for psychologists who adopt a holistic point of view (i.e., humanistic psychologists ).
Limitations
- Lacking scientific rigor and providing little basis for generalization of results to the wider population.
- Researchers’ own subjective feelings may influence the case study (researcher bias).
- Difficult to replicate.
- Time-consuming and expensive.
- The volume of data, together with the time restrictions in place, impacted the depth of analysis that was possible within the available resources.
Because a case study deals with only one person/event/group, we can never be sure if the case study investigated is representative of the wider body of “similar” instances. This means the conclusions drawn from a particular case may not be transferable to other settings.
Because case studies are based on the analysis of qualitative (i.e., descriptive) data , a lot depends on the psychologist’s interpretation of the information she has acquired.
This means that there is a lot of scope for Anna O , and it could be that the subjective opinions of the psychologist intrude in the assessment of what the data means.
For example, Freud has been criticized for producing case studies in which the information was sometimes distorted to fit particular behavioral theories (e.g., Little Hans ).
This is also true of Money’s interpretation of the Bruce/Brenda case study (Diamond, 1997) when he ignored evidence that went against his theory.
Breuer, J., & Freud, S. (1895). Studies on hysteria . Standard Edition 2: London.
Curtiss, S. (1981). Genie: The case of a modern wild child .
Diamond, M., & Sigmundson, K. (1997). Sex Reassignment at Birth: Long-term Review and Clinical Implications. Archives of Pediatrics & Adolescent Medicine , 151(3), 298-304
Freud, S. (1909a). Analysis of a phobia of a five year old boy. In The Pelican Freud Library (1977), Vol 8, Case Histories 1, pages 169-306
Freud, S. (1909b). Bemerkungen über einen Fall von Zwangsneurose (Der “Rattenmann”). Jb. psychoanal. psychopathol. Forsch ., I, p. 357-421; GW, VII, p. 379-463; Notes upon a case of obsessional neurosis, SE , 10: 151-318.
Harlow J. M. (1848). Passage of an iron rod through the head. Boston Medical and Surgical Journal, 39 , 389–393.
Harlow, J. M. (1868). Recovery from the Passage of an Iron Bar through the Head . Publications of the Massachusetts Medical Society. 2 (3), 327-347.
Money, J., & Ehrhardt, A. A. (1972). Man & Woman, Boy & Girl : The Differentiation and Dimorphism of Gender Identity from Conception to Maturity. Baltimore, Maryland: Johns Hopkins University Press.
Money, J., & Tucker, P. (1975). Sexual signatures: On being a man or a woman.
Further Information
- Case Study Approach
- Case Study Method
- Enhancing the Quality of Case Studies in Health Services Research
- “We do things together” A case study of “couplehood” in dementia
- Using mixed methods for evaluating an integrative approach to cancer care: a case study


An official website of the United States government
The .gov means it’s official. Federal government websites often end in .gov or .mil. Before sharing sensitive information, make sure you’re on a federal government site.
The site is secure. The https:// ensures that you are connecting to the official website and that any information you provide is encrypted and transmitted securely.
- Publications
- Account settings
Preview improvements coming to the PMC website in October 2024. Learn More or Try it out now .
- Advanced Search
- Journal List
- Int J Qual Stud Health Well-being
Methodology or method? A critical review of qualitative case study reports
Despite on-going debate about credibility, and reported limitations in comparison to other approaches, case study is an increasingly popular approach among qualitative researchers. We critically analysed the methodological descriptions of published case studies. Three high-impact qualitative methods journals were searched to locate case studies published in the past 5 years; 34 were selected for analysis. Articles were categorized as health and health services ( n= 12), social sciences and anthropology ( n= 7), or methods ( n= 15) case studies. The articles were reviewed using an adapted version of established criteria to determine whether adequate methodological justification was present, and if study aims, methods, and reported findings were consistent with a qualitative case study approach. Findings were grouped into five themes outlining key methodological issues: case study methodology or method, case of something particular and case selection, contextually bound case study, researcher and case interactions and triangulation, and study design inconsistent with methodology reported. Improved reporting of case studies by qualitative researchers will advance the methodology for the benefit of researchers and practitioners.
Case study research is an increasingly popular approach among qualitative researchers (Thomas, 2011 ). Several prominent authors have contributed to methodological developments, which has increased the popularity of case study approaches across disciplines (Creswell, 2013b ; Denzin & Lincoln, 2011b ; Merriam, 2009 ; Ragin & Becker, 1992 ; Stake, 1995 ; Yin, 2009 ). Current qualitative case study approaches are shaped by paradigm, study design, and selection of methods, and, as a result, case studies in the published literature vary. Differences between published case studies can make it difficult for researchers to define and understand case study as a methodology.
Experienced qualitative researchers have identified case study research as a stand-alone qualitative approach (Denzin & Lincoln, 2011b ). Case study research has a level of flexibility that is not readily offered by other qualitative approaches such as grounded theory or phenomenology. Case studies are designed to suit the case and research question and published case studies demonstrate wide diversity in study design. There are two popular case study approaches in qualitative research. The first, proposed by Stake ( 1995 ) and Merriam ( 2009 ), is situated in a social constructivist paradigm, whereas the second, by Yin ( 2012 ), Flyvbjerg ( 2011 ), and Eisenhardt ( 1989 ), approaches case study from a post-positivist viewpoint. Scholarship from both schools of inquiry has contributed to the popularity of case study and development of theoretical frameworks and principles that characterize the methodology.
The diversity of case studies reported in the published literature, and on-going debates about credibility and the use of case study in qualitative research practice, suggests that differences in perspectives on case study methodology may prevent researchers from developing a mutual understanding of practice and rigour. In addition, discussion about case study limitations has led some authors to query whether case study is indeed a methodology (Luck, Jackson, & Usher, 2006 ; Meyer, 2001 ; Thomas, 2010 ; Tight, 2010 ). Methodological discussion of qualitative case study research is timely, and a review is required to analyse and understand how this methodology is applied in the qualitative research literature. The aims of this study were to review methodological descriptions of published qualitative case studies, to review how the case study methodological approach was applied, and to identify issues that need to be addressed by researchers, editors, and reviewers. An outline of the current definitions of case study and an overview of the issues proposed in the qualitative methodological literature are provided to set the scene for the review.
Definitions of qualitative case study research
Case study research is an investigation and analysis of a single or collective case, intended to capture the complexity of the object of study (Stake, 1995 ). Qualitative case study research, as described by Stake ( 1995 ), draws together “naturalistic, holistic, ethnographic, phenomenological, and biographic research methods” in a bricoleur design, or in his words, “a palette of methods” (Stake, 1995 , pp. xi–xii). Case study methodology maintains deep connections to core values and intentions and is “particularistic, descriptive and heuristic” (Merriam, 2009 , p. 46).
As a study design, case study is defined by interest in individual cases rather than the methods of inquiry used. The selection of methods is informed by researcher and case intuition and makes use of naturally occurring sources of knowledge, such as people or observations of interactions that occur in the physical space (Stake, 1998 ). Thomas ( 2011 ) suggested that “analytical eclecticism” is a defining factor (p. 512). Multiple data collection and analysis methods are adopted to further develop and understand the case, shaped by context and emergent data (Stake, 1995 ). This qualitative approach “explores a real-life, contemporary bounded system (a case ) or multiple bounded systems (cases) over time, through detailed, in-depth data collection involving multiple sources of information … and reports a case description and case themes ” (Creswell, 2013b , p. 97). Case study research has been defined by the unit of analysis, the process of study, and the outcome or end product, all essentially the case (Merriam, 2009 ).
The case is an object to be studied for an identified reason that is peculiar or particular. Classification of the case and case selection procedures informs development of the study design and clarifies the research question. Stake ( 1995 ) proposed three types of cases and study design frameworks. These include the intrinsic case, the instrumental case, and the collective instrumental case. The intrinsic case is used to understand the particulars of a single case, rather than what it represents. An instrumental case study provides insight on an issue or is used to refine theory. The case is selected to advance understanding of the object of interest. A collective refers to an instrumental case which is studied as multiple, nested cases, observed in unison, parallel, or sequential order. More than one case can be simultaneously studied; however, each case study is a concentrated, single inquiry, studied holistically in its own entirety (Stake, 1995 , 1998 ).
Researchers who use case study are urged to seek out what is common and what is particular about the case. This involves careful and in-depth consideration of the nature of the case, historical background, physical setting, and other institutional and political contextual factors (Stake, 1998 ). An interpretive or social constructivist approach to qualitative case study research supports a transactional method of inquiry, where the researcher has a personal interaction with the case. The case is developed in a relationship between the researcher and informants, and presented to engage the reader, inviting them to join in this interaction and in case discovery (Stake, 1995 ). A postpositivist approach to case study involves developing a clear case study protocol with careful consideration of validity and potential bias, which might involve an exploratory or pilot phase, and ensures that all elements of the case are measured and adequately described (Yin, 2009 , 2012 ).
Current methodological issues in qualitative case study research
The future of qualitative research will be influenced and constructed by the way research is conducted, and by what is reviewed and published in academic journals (Morse, 2011 ). If case study research is to further develop as a principal qualitative methodological approach, and make a valued contribution to the field of qualitative inquiry, issues related to methodological credibility must be considered. Researchers are required to demonstrate rigour through adequate descriptions of methodological foundations. Case studies published without sufficient detail for the reader to understand the study design, and without rationale for key methodological decisions, may lead to research being interpreted as lacking in quality or credibility (Hallberg, 2013 ; Morse, 2011 ).
There is a level of artistic license that is embraced by qualitative researchers and distinguishes practice, which nurtures creativity, innovation, and reflexivity (Denzin & Lincoln, 2011b ; Morse, 2009 ). Qualitative research is “inherently multimethod” (Denzin & Lincoln, 2011a , p. 5); however, with this creative freedom, it is important for researchers to provide adequate description for methodological justification (Meyer, 2001 ). This includes paradigm and theoretical perspectives that have influenced study design. Without adequate description, study design might not be understood by the reader, and can appear to be dishonest or inaccurate. Reviewers and readers might be confused by the inconsistent or inappropriate terms used to describe case study research approach and methods, and be distracted from important study findings (Sandelowski, 2000 ). This issue extends beyond case study research, and others have noted inconsistencies in reporting of methodology and method by qualitative researchers. Sandelowski ( 2000 , 2010 ) argued for accurate identification of qualitative description as a research approach. She recommended that the selected methodology should be harmonious with the study design, and be reflected in methods and analysis techniques. Similarly, Webb and Kevern ( 2000 ) uncovered inconsistencies in qualitative nursing research with focus group methods, recommending that methodological procedures must cite seminal authors and be applied with respect to the selected theoretical framework. Incorrect labelling using case study might stem from the flexibility in case study design and non-directional character relative to other approaches (Rosenberg & Yates, 2007 ). Methodological integrity is required in design of qualitative studies, including case study, to ensure study rigour and to enhance credibility of the field (Morse, 2011 ).
Case study has been unnecessarily devalued by comparisons with statistical methods (Eisenhardt, 1989 ; Flyvbjerg, 2006 , 2011 ; Jensen & Rodgers, 2001 ; Piekkari, Welch, & Paavilainen, 2009 ; Tight, 2010 ; Yin, 1999 ). It is reputed to be the “the weak sibling” in comparison to other, more rigorous, approaches (Yin, 2009 , p. xiii). Case study is not an inherently comparative approach to research. The objective is not statistical research, and the aim is not to produce outcomes that are generalizable to all populations (Thomas, 2011 ). Comparisons between case study and statistical research do little to advance this qualitative approach, and fail to recognize its inherent value, which can be better understood from the interpretive or social constructionist viewpoint of other authors (Merriam, 2009 ; Stake, 1995 ). Building on discussions relating to “fuzzy” (Bassey, 2001 ), or naturalistic generalizations (Stake, 1978 ), or transference of concepts and theories (Ayres, Kavanaugh, & Knafl, 2003 ; Morse et al., 2011 ) would have more relevance.
Case study research has been used as a catch-all design to justify or add weight to fundamental qualitative descriptive studies that do not fit with other traditional frameworks (Merriam, 2009 ). A case study has been a “convenient label for our research—when we ‘can't think of anything ‘better”—in an attempt to give it [qualitative methodology] some added respectability” (Tight, 2010 , p. 337). Qualitative case study research is a pliable approach (Merriam, 2009 ; Meyer, 2001 ; Stake, 1995 ), and has been likened to a “curious methodological limbo” (Gerring, 2004 , p. 341) or “paradigmatic bridge” (Luck et al., 2006 , p. 104), that is on the borderline between postpositivist and constructionist interpretations. This has resulted in inconsistency in application, which indicates that flexibility comes with limitations (Meyer, 2001 ), and the open nature of case study research might be off-putting to novice researchers (Thomas, 2011 ). The development of a well-(in)formed theoretical framework to guide a case study should improve consistency, rigour, and trust in studies published in qualitative research journals (Meyer, 2001 ).
Assessment of rigour
The purpose of this study was to analyse the methodological descriptions of case studies published in qualitative methods journals. To do this we needed to develop a suitable framework, which used existing, established criteria for appraising qualitative case study research rigour (Creswell, 2013b ; Merriam, 2009 ; Stake, 1995 ). A number of qualitative authors have developed concepts and criteria that are used to determine whether a study is rigorous (Denzin & Lincoln, 2011b ; Lincoln, 1995 ; Sandelowski & Barroso, 2002 ). The criteria proposed by Stake ( 1995 ) provide a framework for readers and reviewers to make judgements regarding case study quality, and identify key characteristics essential for good methodological rigour. Although each of the factors listed in Stake's criteria could enhance the quality of a qualitative research report, in Table I we present an adapted criteria used in this study, which integrates more recent work by Merriam ( 2009 ) and Creswell ( 2013b ). Stake's ( 1995 ) original criteria were separated into two categories. The first list of general criteria is “relevant for all qualitative research.” The second list, “high relevance to qualitative case study research,” was the criteria that we decided had higher relevance to case study research. This second list was the main criteria used to assess the methodological descriptions of the case studies reviewed. The complete table has been preserved so that the reader can determine how the original criteria were adapted.
Framework for assessing quality in qualitative case study research.
Checklist for assessing the quality of a case study report |
---|
Relevant for all qualitative research |
1. Is this report easy to read? |
2. Does it fit together, each sentence contributing to the whole? |
3. Does this report have a conceptual structure (i.e., themes or issues)? |
4. Are its issues developed in a series and scholarly way? |
5. Have quotations been used effectively? |
6. Has the writer made sound assertions, neither over- or under-interpreting? |
7. Are headings, figures, artefacts, appendices, indexes effectively used? |
8. Was it edited well, then again with a last minute polish? |
9. Were sufficient raw data presented? |
10. Is the nature of the intended audience apparent? |
11. Does it appear that individuals were put at risk? |
High relevance to qualitative case study research |
12. Is the case adequately defined? |
13. Is there a sense of story to the presentation? |
14. Is the reader provided some vicarious experience? |
15. Has adequate attention been paid to various contexts? |
16. Were data sources well-chosen and in sufficient number? |
17. Do observations and interpretations appear to have been triangulated? |
18. Is the role and point of view of the researcher nicely apparent? |
19. Is empathy shown for all sides? |
20. Are personal intentions examined? |
Added from Merriam ( ) |
21. Is the case study particular? |
22. Is the case study descriptive? |
23. Is the case study heuristic? |
Added from Creswell ( ) |
24. Was study design appropriate to methodology? |
Adapted from Stake ( 1995 , p. 131).
Study design
The critical review method described by Grant and Booth ( 2009 ) was used, which is appropriate for the assessment of research quality, and is used for literature analysis to inform research and practice. This type of review goes beyond the mapping and description of scoping or rapid reviews, to include “analysis and conceptual innovation” (Grant & Booth, 2009 , p. 93). A critical review is used to develop existing, or produce new, hypotheses or models. This is different to systematic reviews that answer clinical questions. It is used to evaluate existing research and competing ideas, to provide a “launch pad” for conceptual development and “subsequent testing” (Grant & Booth, 2009 , p. 93).
Qualitative methods journals were located by a search of the 2011 ISI Journal Citation Reports in Social Science, via the database Web of Knowledge (see m.webofknowledge.com). No “qualitative research methods” category existed in the citation reports; therefore, a search of all categories was performed using the term “qualitative.” In Table II , we present the qualitative methods journals located, ranked by impact factor. The highest ranked journals were selected for searching. We acknowledge that the impact factor ranking system might not be the best measure of journal quality (Cheek, Garnham, & Quan, 2006 ); however, this was the most appropriate and accessible method available.
International Journal of Qualitative Studies on Health and Well-being.
Journal title | 2011 impact factor | 5-year impact factor |
---|---|---|
2.188 | 2.432 | |
1.426 | N/A | |
0.839 | 1.850 | |
0.780 | N/A | |
0.612 | N/A |
Search strategy
In March 2013, searches of the journals, Qualitative Health Research , Qualitative Research , and Qualitative Inquiry were completed to retrieve studies with “case study” in the abstract field. The search was limited to the past 5 years (1 January 2008 to 1 March 2013). The objective was to locate published qualitative case studies suitable for assessment using the adapted criterion. Viewpoints, commentaries, and other article types were excluded from review. Title and abstracts of the 45 retrieved articles were read by the first author, who identified 34 empirical case studies for review. All authors reviewed the 34 studies to confirm selection and categorization. In Table III , we present the 34 case studies grouped by journal, and categorized by research topic, including health sciences, social sciences and anthropology, and methods research. There was a discrepancy in categorization of one article on pedagogy and a new teaching method published in Qualitative Inquiry (Jorrín-Abellán, Rubia-Avi, Anguita-Martínez, Gómez-Sánchez, & Martínez-Mones, 2008 ). Consensus was to allocate to the methods category.
Outcomes of search of qualitative methods journals.
Journal title | Date of search | Number of studies located | Number of full text studies extracted | Health sciences | Social sciences and anthropology | Methods |
---|---|---|---|---|---|---|
4 Mar 2013 | 18 | 16 | Barone ( ); Bronken et al. ( ); Colón-Emeric et al. ( ); Fourie and Theron ( ); Gallagher et al. ( ); Gillard et al. ( ); Hooghe et al. ( ); Jackson et al. ( ); Ledderer ( ); Mawn et al. ( ); Roscigno et al. ( ); Rytterström et al. ( ) | Nil | Austin, Park, and Goble ( ); Broyles, Rodriguez, Price, Bayliss, and Sevick ( ); De Haene et al. ( ); Fincham et al. ( ) | |
7 Mar 2013 | 11 | 7 | Nil | Adamson and Holloway ( ); Coltart and Henwood ( ) | Buckley and Waring ( ); Cunsolo Willox et al. ( ); Edwards and Weller ( ); Gratton and O'Donnell ( ); Sumsion ( ) | |
4 Mar 2013 | 16 | 11 | Nil | Buzzanell and D’Enbeau ( ); D'Enbeau et al. ( ); Nagar-Ron and Motzafi-Haller ( ); Snyder-Young ( ); Yeh ( ) | Ajodhia-Andrews and Berman ( ); Alexander et al. ( ); Jorrín-Abellán et al. ( ); Nairn and Panelli ( ); Nespor ( ); Wimpenny and Savin-Baden ( ) | |
Total | 45 | 34 | 12 | 7 | 15 |
In Table III , the number of studies located, and final numbers selected for review have been reported. Qualitative Health Research published the most empirical case studies ( n= 16). In the health category, there were 12 case studies of health conditions, health services, and health policy issues, all published in Qualitative Health Research . Seven case studies were categorized as social sciences and anthropology research, which combined case study with biography and ethnography methodologies. All three journals published case studies on methods research to illustrate a data collection or analysis technique, methodological procedure, or related issue.
The methodological descriptions of 34 case studies were critically reviewed using the adapted criteria. All articles reviewed contained a description of study methods; however, the length, amount of detail, and position of the description in the article varied. Few studies provided an accurate description and rationale for using a qualitative case study approach. In the 34 case studies reviewed, three described a theoretical framework informed by Stake ( 1995 ), two by Yin ( 2009 ), and three provided a mixed framework informed by various authors, which might have included both Yin and Stake. Few studies described their case study design, or included a rationale that explained why they excluded or added further procedures, and whether this was to enhance the study design, or to better suit the research question. In 26 of the studies no reference was provided to principal case study authors. From reviewing the description of methods, few authors provided a description or justification of case study methodology that demonstrated how their study was informed by the methodological literature that exists on this approach.
The methodological descriptions of each study were reviewed using the adapted criteria, and the following issues were identified: case study methodology or method; case of something particular and case selection; contextually bound case study; researcher and case interactions and triangulation; and, study design inconsistent with methodology. An outline of how the issues were developed from the critical review is provided, followed by a discussion of how these relate to the current methodological literature.
Case study methodology or method
A third of the case studies reviewed appeared to use a case report method, not case study methodology as described by principal authors (Creswell, 2013b ; Merriam, 2009 ; Stake, 1995 ; Yin, 2009 ). Case studies were identified as a case report because of missing methodological detail and by review of the study aims and purpose. These reports presented data for small samples of no more than three people, places or phenomenon. Four studies, or “case reports” were single cases selected retrospectively from larger studies (Bronken, Kirkevold, Martinsen, & Kvigne, 2012 ; Coltart & Henwood, 2012 ; Hooghe, Neimeyer, & Rober, 2012 ; Roscigno et al., 2012 ). Case reports were not a case of something, instead were a case demonstration or an example presented in a report. These reports presented outcomes, and reported on how the case could be generalized. Descriptions focussed on the phenomena, rather than the case itself, and did not appear to study the case in its entirety.
Case reports had minimal in-text references to case study methodology, and were informed by other qualitative traditions or secondary sources (Adamson & Holloway, 2012 ; Buzzanell & D'Enbeau, 2009 ; Nagar-Ron & Motzafi-Haller, 2011 ). This does not suggest that case study methodology cannot be multimethod, however, methodology should be consistent in design, be clearly described (Meyer, 2001 ; Stake, 1995 ), and maintain focus on the case (Creswell, 2013b ).
To demonstrate how case reports were identified, three examples are provided. The first, Yeh ( 2013 ) described their study as, “the examination of the emergence of vegetarianism in Victorian England serves as a case study to reveal the relationships between boundaries and entities” (p. 306). The findings were a historical case report, which resulted from an ethnographic study of vegetarianism. Cunsolo Willox, Harper, Edge, ‘My Word’: Storytelling and Digital Media Lab, and Rigolet Inuit Community Government (2013) used “a case study that illustrates the usage of digital storytelling within an Inuit community” (p. 130). This case study reported how digital storytelling can be used with indigenous communities as a participatory method to illuminate the benefits of this method for other studies. This “case study was conducted in the Inuit community” but did not include the Inuit community in case analysis (Cunsolo Willox et al., 2013 , p. 130). Bronken et al. ( 2012 ) provided a single case report to demonstrate issues observed in a larger clinical study of aphasia and stroke, without adequate case description or analysis.
Case study of something particular and case selection
Case selection is a precursor to case analysis, which needs to be presented as a convincing argument (Merriam, 2009 ). Descriptions of the case were often not adequate to ascertain why the case was selected, or whether it was a particular exemplar or outlier (Thomas, 2011 ). In a number of case studies in the health and social science categories, it was not explicit whether the case was of something particular, or peculiar to their discipline or field (Adamson & Holloway, 2012 ; Bronken et al., 2012 ; Colón-Emeric et al., 2010 ; Jackson, Botelho, Welch, Joseph, & Tennstedt, 2012 ; Mawn et al., 2010 ; Snyder-Young, 2011 ). There were exceptions in the methods category ( Table III ), where cases were selected by researchers to report on a new or innovative method. The cases emerged through heuristic study, and were reported to be particular, relative to the existing methods literature (Ajodhia-Andrews & Berman, 2009 ; Buckley & Waring, 2013 ; Cunsolo Willox et al., 2013 ; De Haene, Grietens, & Verschueren, 2010 ; Gratton & O'Donnell, 2011 ; Sumsion, 2013 ; Wimpenny & Savin-Baden, 2012 ).
Case selection processes were sometimes insufficient to understand why the case was selected from the global population of cases, or what study of this case would contribute to knowledge as compared with other possible cases (Adamson & Holloway, 2012 ; Bronken et al., 2012 ; Colón-Emeric et al., 2010 ; Jackson et al., 2012 ; Mawn et al., 2010 ). In two studies, local cases were selected (Barone, 2010 ; Fourie & Theron, 2012 ) because the researcher was familiar with and had access to the case. Possible limitations of a convenience sample were not acknowledged. Purposeful sampling was used to recruit participants within the case of one study, but not of the case itself (Gallagher et al., 2013 ). Random sampling was completed for case selection in two studies (Colón-Emeric et al., 2010 ; Jackson et al., 2012 ), which has limited meaning in interpretive qualitative research.
To demonstrate how researchers provided a good justification for the selection of case study approaches, four examples are provided. The first, cases of residential care homes, were selected because of reported occurrences of mistreatment, which included residents being locked in rooms at night (Rytterström, Unosson, & Arman, 2013 ). Roscigno et al. ( 2012 ) selected cases of parents who were admitted for early hospitalization in neonatal intensive care with a threatened preterm delivery before 26 weeks. Hooghe et al. ( 2012 ) used random sampling to select 20 couples that had experienced the death of a child; however, the case study was of one couple and a particular metaphor described only by them. The final example, Coltart and Henwood ( 2012 ), provided a detailed account of how they selected two cases from a sample of 46 fathers based on personal characteristics and beliefs. They described how the analysis of the two cases would contribute to their larger study on first time fathers and parenting.
Contextually bound case study
The limits or boundaries of the case are a defining factor of case study methodology (Merriam, 2009 ; Ragin & Becker, 1992 ; Stake, 1995 ; Yin, 2009 ). Adequate contextual description is required to understand the setting or context in which the case is revealed. In the health category, case studies were used to illustrate a clinical phenomenon or issue such as compliance and health behaviour (Colón-Emeric et al., 2010 ; D'Enbeau, Buzzanell, & Duckworth, 2010 ; Gallagher et al., 2013 ; Hooghe et al., 2012 ; Jackson et al., 2012 ; Roscigno et al., 2012 ). In these case studies, contextual boundaries, such as physical and institutional descriptions, were not sufficient to understand the case as a holistic system, for example, the general practitioner (GP) clinic in Gallagher et al. ( 2013 ), or the nursing home in Colón-Emeric et al. ( 2010 ). Similarly, in the social science and methods categories, attention was paid to some components of the case context, but not others, missing important information required to understand the case as a holistic system (Alexander, Moreira, & Kumar, 2012 ; Buzzanell & D'Enbeau, 2009 ; Nairn & Panelli, 2009 ; Wimpenny & Savin-Baden, 2012 ).
In two studies, vicarious experience or vignettes (Nairn & Panelli, 2009 ) and images (Jorrín-Abellán et al., 2008 ) were effective to support description of context, and might have been a useful addition for other case studies. Missing contextual boundaries suggests that the case might not be adequately defined. Additional information, such as the physical, institutional, political, and community context, would improve understanding of the case (Stake, 1998 ). In Boxes 1 and 2 , we present brief synopses of two studies that were reviewed, which demonstrated a well bounded case. In Box 1 , Ledderer ( 2011 ) used a qualitative case study design informed by Stake's tradition. In Box 2 , Gillard, Witt, and Watts ( 2011 ) were informed by Yin's tradition. By providing a brief outline of the case studies in Boxes 1 and 2 , we demonstrate how effective case boundaries can be constructed and reported, which may be of particular interest to prospective case study researchers.
Article synopsis of case study research using Stake's tradition
Ledderer ( 2011 ) used a qualitative case study research design, informed by modern ethnography. The study is bounded to 10 general practice clinics in Denmark, who had received federal funding to implement preventative care services based on a Motivational Interviewing intervention. The researcher question focussed on “why is it so difficult to create change in medical practice?” (Ledderer, 2011 , p. 27). The study context was adequately described, providing detail on the general practitioner (GP) clinics and relevant political and economic influences. Methodological decisions are described in first person narrative, providing insight on researcher perspectives and interaction with the case. Forty-four interviews were conducted, which focussed on how GPs conducted consultations, and the form, nature and content, rather than asking their opinion or experience (Ledderer, 2011 , p. 30). The duration and intensity of researcher immersion in the case enhanced depth of description and trustworthiness of study findings. Analysis was consistent with Stake's tradition, and the researcher provided examples of inquiry techniques used to challenge assumptions about emerging themes. Several other seminal qualitative works were cited. The themes and typology constructed are rich in narrative data and storytelling by clinic staff, demonstrating individual clinic experiences as well as shared meanings and understandings about changing from a biomedical to psychological approach to preventative health intervention. Conclusions make note of social and cultural meanings and lessons learned, which might not have been uncovered using a different methodology.
Article synopsis of case study research using Yin's tradition
Gillard et al. ( 2011 ) study of camps for adolescents living with HIV/AIDs provided a good example of Yin's interpretive case study approach. The context of the case is bounded by the three summer camps of which the researchers had prior professional involvement. A case study protocol was developed that used multiple methods to gather information at three data collection points coinciding with three youth camps (Teen Forum, Discover Camp, and Camp Strong). Gillard and colleagues followed Yin's ( 2009 ) principles, using a consistent data protocol that enhanced cross-case analysis. Data described the young people, the camp physical environment, camp schedule, objectives and outcomes, and the staff of three youth camps. The findings provided a detailed description of the context, with less detail of individual participants, including insight into researcher's interpretations and methodological decisions throughout the data collection and analysis process. Findings provided the reader with a sense of “being there,” and are discovered through constant comparison of the case with the research issues; the case is the unit of analysis. There is evidence of researcher immersion in the case, and Gillard reports spending significant time in the field in a naturalistic and integrated youth mentor role.
This case study is not intended to have a significant impact on broader health policy, although does have implications for health professionals working with adolescents. Study conclusions will inform future camps for young people with chronic disease, and practitioners are able to compare similarities between this case and their own practice (for knowledge translation). No limitations of this article were reported. Limitations related to publication of this case study were that it was 20 pages long and used three tables to provide sufficient description of the camp and program components, and relationships with the research issue.
Researcher and case interactions and triangulation
Researcher and case interactions and transactions are a defining feature of case study methodology (Stake, 1995 ). Narrative stories, vignettes, and thick description are used to provoke vicarious experience and a sense of being there with the researcher in their interaction with the case. Few of the case studies reviewed provided details of the researcher's relationship with the case, researcher–case interactions, and how these influenced the development of the case study (Buzzanell & D'Enbeau, 2009 ; D'Enbeau et al., 2010 ; Gallagher et al., 2013 ; Gillard et al., 2011 ; Ledderer, 2011 ; Nagar-Ron & Motzafi-Haller, 2011 ). The role and position of the researcher needed to be self-examined and understood by readers, to understand how this influenced interactions with participants, and to determine what triangulation is needed (Merriam, 2009 ; Stake, 1995 ).
Gillard et al. ( 2011 ) provided a good example of triangulation, comparing data sources in a table (p. 1513). Triangulation of sources was used to reveal as much depth as possible in the study by Nagar-Ron and Motzafi-Haller ( 2011 ), while also enhancing confirmation validity. There were several case studies that would have benefited from improved range and use of data sources, and descriptions of researcher–case interactions (Ajodhia-Andrews & Berman, 2009 ; Bronken et al., 2012 ; Fincham, Scourfield, & Langer, 2008 ; Fourie & Theron, 2012 ; Hooghe et al., 2012 ; Snyder-Young, 2011 ; Yeh, 2013 ).
Study design inconsistent with methodology
Good, rigorous case studies require a strong methodological justification (Meyer, 2001 ) and a logical and coherent argument that defines paradigm, methodological position, and selection of study methods (Denzin & Lincoln, 2011b ). Methodological justification was insufficient in several of the studies reviewed (Barone, 2010 ; Bronken et al., 2012 ; Hooghe et al., 2012 ; Mawn et al., 2010 ; Roscigno et al., 2012 ; Yeh, 2013 ). This was judged by the absence, or inadequate or inconsistent reference to case study methodology in-text.
In six studies, the methodological justification provided did not relate to case study. There were common issues identified. Secondary sources were used as primary methodological references indicating that study design might not have been theoretically sound (Colón-Emeric et al., 2010 ; Coltart & Henwood, 2012 ; Roscigno et al., 2012 ; Snyder-Young, 2011 ). Authors and sources cited in methodological descriptions were inconsistent with the actual study design and practices used (Fourie & Theron, 2012 ; Hooghe et al., 2012 ; Jorrín-Abellán et al., 2008 ; Mawn et al., 2010 ; Rytterström et al., 2013 ; Wimpenny & Savin-Baden, 2012 ). This occurred when researchers cited Stake or Yin, or both (Mawn et al., 2010 ; Rytterström et al., 2013 ), although did not follow their paradigmatic or methodological approach. In 26 studies there were no citations for a case study methodological approach.
The findings of this study have highlighted a number of issues for researchers. A considerable number of case studies reviewed were missing key elements that define qualitative case study methodology and the tradition cited. A significant number of studies did not provide a clear methodological description or justification relevant to case study. Case studies in health and social sciences did not provide sufficient information for the reader to understand case selection, and why this case was chosen above others. The context of the cases were not described in adequate detail to understand all relevant elements of the case context, which indicated that cases may have not been contextually bounded. There were inconsistencies between reported methodology, study design, and paradigmatic approach in case studies reviewed, which made it difficult to understand the study methodology and theoretical foundations. These issues have implications for methodological integrity and honesty when reporting study design, which are values of the qualitative research tradition and are ethical requirements (Wager & Kleinert, 2010a ). Poorly described methodological descriptions may lead the reader to misinterpret or discredit study findings, which limits the impact of the study, and, as a collective, hinders advancements in the broader qualitative research field.
The issues highlighted in our review build on current debates in the case study literature, and queries about the value of this methodology. Case study research can be situated within different paradigms or designed with an array of methods. In order to maintain the creativity and flexibility that is valued in this methodology, clearer descriptions of paradigm and theoretical position and methods should be provided so that study findings are not undervalued or discredited. Case study research is an interdisciplinary practice, which means that clear methodological descriptions might be more important for this approach than other methodologies that are predominantly driven by fewer disciplines (Creswell, 2013b ).
Authors frequently omit elements of methodologies and include others to strengthen study design, and we do not propose a rigid or purist ideology in this paper. On the contrary, we encourage new ideas about using case study, together with adequate reporting, which will advance the value and practice of case study. The implications of unclear methodological descriptions in the studies reviewed were that study design appeared to be inconsistent with reported methodology, and key elements required for making judgements of rigour were missing. It was not clear whether the deviations from methodological tradition were made by researchers to strengthen the study design, or because of misinterpretations. Morse ( 2011 ) recommended that innovations and deviations from practice are best made by experienced researchers, and that a novice might be unaware of the issues involved with making these changes. To perpetuate the tradition of case study research, applications in the published literature should have consistencies with traditional methodological constructions, and deviations should be described with a rationale that is inherent in study conduct and findings. Providing methodological descriptions that demonstrate a strong theoretical foundation and coherent study design will add credibility to the study, while ensuring the intrinsic meaning of case study is maintained.
The value of this review is that it contributes to discussion of whether case study is a methodology or method. We propose possible reasons why researchers might make this misinterpretation. Researchers may interchange the terms methods and methodology, and conduct research without adequate attention to epistemology and historical tradition (Carter & Little, 2007 ; Sandelowski, 2010 ). If the rich meaning that naming a qualitative methodology brings to the study is not recognized, a case study might appear to be inconsistent with the traditional approaches described by principal authors (Creswell, 2013a ; Merriam, 2009 ; Stake, 1995 ; Yin, 2009 ). If case studies are not methodologically and theoretically situated, then they might appear to be a case report.
Case reports are promoted by university and medical journals as a method of reporting on medical or scientific cases; guidelines for case reports are publicly available on websites ( http://www.hopkinsmedicine.org/institutional_review_board/guidelines_policies/guidelines/case_report.html ). The various case report guidelines provide a general criteria for case reports, which describes that this form of report does not meet the criteria of research, is used for retrospective analysis of up to three clinical cases, and is primarily illustrative and for educational purposes. Case reports can be published in academic journals, but do not require approval from a human research ethics committee. Traditionally, case reports describe a single case, to explain how and what occurred in a selected setting, for example, to illustrate a new phenomenon that has emerged from a larger study. A case report is not necessarily particular or the study of a case in its entirety, and the larger study would usually be guided by a different research methodology.
This description of a case report is similar to what was provided in some studies reviewed. This form of report lacks methodological grounding and qualities of research rigour. The case report has publication value in demonstrating an example and for dissemination of knowledge (Flanagan, 1999 ). However, case reports have different meaning and purpose to case study, which needs to be distinguished. Findings of our review suggest that the medical understanding of a case report has been confused with qualitative case study approaches.
In this review, a number of case studies did not have methodological descriptions that included key characteristics of case study listed in the adapted criteria, and several issues have been discussed. There have been calls for improvements in publication quality of qualitative research (Morse, 2011 ), and for improvements in peer review of submitted manuscripts (Carter & Little, 2007 ; Jasper, Vaismoradi, Bondas, & Turunen, 2013 ). The challenging nature of editor and reviewers responsibilities are acknowledged in the literature (Hames, 2013 ; Wager & Kleinert, 2010b ); however, review of case study methodology should be prioritized because of disputes on methodological value.
Authors using case study approaches are recommended to describe their theoretical framework and methods clearly, and to seek and follow specialist methodological advice when needed (Wager & Kleinert, 2010a ). Adequate page space for case study description would contribute to better publications (Gillard et al., 2011 ). Capitalizing on the ability to publish complementary resources should be considered.
Limitations of the review
There is a level of subjectivity involved in this type of review and this should be considered when interpreting study findings. Qualitative methods journals were selected because the aims and scope of these journals are to publish studies that contribute to methodological discussion and development of qualitative research. Generalist health and social science journals were excluded that might have contained good quality case studies. Journals in business or education were also excluded, although a review of case studies in international business journals has been published elsewhere (Piekkari et al., 2009 ).
The criteria used to assess the quality of the case studies were a set of qualitative indicators. A numerical or ranking system might have resulted in different results. Stake's ( 1995 ) criteria have been referenced elsewhere, and was deemed the best available (Creswell, 2013b ; Crowe et al., 2011 ). Not all qualitative studies are reported in a consistent way and some authors choose to report findings in a narrative form in comparison to a typical biomedical report style (Sandelowski & Barroso, 2002 ), if misinterpretations were made this may have affected the review.
Case study research is an increasingly popular approach among qualitative researchers, which provides methodological flexibility through the incorporation of different paradigmatic positions, study designs, and methods. However, whereas flexibility can be an advantage, a myriad of different interpretations has resulted in critics questioning the use of case study as a methodology. Using an adaptation of established criteria, we aimed to identify and assess the methodological descriptions of case studies in high impact, qualitative methods journals. Few articles were identified that applied qualitative case study approaches as described by experts in case study design. There were inconsistencies in methodology and study design, which indicated that researchers were confused whether case study was a methodology or a method. Commonly, there appeared to be confusion between case studies and case reports. Without clear understanding and application of the principles and key elements of case study methodology, there is a risk that the flexibility of the approach will result in haphazard reporting, and will limit its global application as a valuable, theoretically supported methodology that can be rigorously applied across disciplines and fields.
Conflict of interest and funding
The authors have not received any funding or benefits from industry or elsewhere to conduct this study.
- Adamson S, Holloway M. Negotiating sensitivities and grappling with intangibles: Experiences from a study of spirituality and funerals. Qualitative Research. 2012; 12 (6):735–752. doi: 10.1177/1468794112439008. [ CrossRef ] [ Google Scholar ]
- Ajodhia-Andrews A, Berman R. Exploring school life from the lens of a child who does not use speech to communicate. Qualitative Inquiry. 2009; 15 (5):931–951. doi: 10.1177/1077800408322789. [ CrossRef ] [ Google Scholar ]
- Alexander B. K, Moreira C, Kumar H. S. Resisting (resistance) stories: A tri-autoethnographic exploration of father narratives across shades of difference. Qualitative Inquiry. 2012; 18 (2):121–133. doi: 10.1177/1077800411429087. [ CrossRef ] [ Google Scholar ]
- Austin W, Park C, Goble E. From interdisciplinary to transdisciplinary research: A case study. Qualitative Health Research. 2008; 18 (4):557–564. doi: 10.1177/1049732307308514. [ PubMed ] [ CrossRef ] [ Google Scholar ]
- Ayres L, Kavanaugh K, Knafl K. A. Within-case and across-case approaches to qualitative data analysis. Qualitative Health Research. 2003; 13 (6):871–883. doi: 10.1177/1049732303013006008. [ PubMed ] [ CrossRef ] [ Google Scholar ]
- Barone T. L. Culturally sensitive care 1969–2000: The Indian Chicano Health Center. Qualitative Health Research. 2010; 20 (4):453–464. doi: 10.1177/1049732310361893. [ PubMed ] [ CrossRef ] [ Google Scholar ]
- Bassey M. A solution to the problem of generalisation in educational research: Fuzzy prediction. Oxford Review of Education. 2001; 27 (1):5–22. doi: 10.1080/03054980123773. [ CrossRef ] [ Google Scholar ]
- Bronken B. A, Kirkevold M, Martinsen R, Kvigne K. The aphasic storyteller: Coconstructing stories to promote psychosocial well-being after stroke. Qualitative Health Research. 2012; 22 (10):1303–1316. doi: 10.1177/1049732312450366. [ PubMed ] [ CrossRef ] [ Google Scholar ]
- Broyles L. M, Rodriguez K. L, Price P. A, Bayliss N. K, Sevick M. A. Overcoming barriers to the recruitment of nurses as participants in health care research. Qualitative Health Research. 2011; 21 (12):1705–1718. doi: 10.1177/1049732311417727. [ PubMed ] [ CrossRef ] [ Google Scholar ]
- Buckley C. A, Waring M. J. Using diagrams to support the research process: Examples from grounded theory. Qualitative Research. 2013; 13 (2):148–172. doi: 10.1177/1468794112472280. [ CrossRef ] [ Google Scholar ]
- Buzzanell P. M, D'Enbeau S. Stories of caregiving: Intersections of academic research and women's everyday experiences. Qualitative Inquiry. 2009; 15 (7):1199–1224. doi: 10.1177/1077800409338025. [ CrossRef ] [ Google Scholar ]
- Carter S. M, Little M. Justifying knowledge, justifying method, taking action: Epistemologies, methodologies, and methods in qualitative research. Qualitative Health Research. 2007; 17 (10):1316–1328. doi: 10.1177/1049732307306927. [ PubMed ] [ CrossRef ] [ Google Scholar ]
- Cheek J, Garnham B, Quan J. What's in a number? Issues in providing evidence of impact and quality of research(ers) Qualitative Health Research. 2006; 16 (3):423–435. doi: 10.1177/1049732305285701. [ PubMed ] [ CrossRef ] [ Google Scholar ]
- Colón-Emeric C. S, Plowman D, Bailey D, Corazzini K, Utley-Smith Q, Ammarell N, et al. Regulation and mindful resident care in nursing homes. Qualitative Health Research. 2010; 20 (9):1283–1294. doi: 10.1177/1049732310369337. [ PMC free article ] [ PubMed ] [ CrossRef ] [ Google Scholar ]
- Coltart C, Henwood K. On paternal subjectivity: A qualitative longitudinal and psychosocial case analysis of men's classed positions and transitions to first-time fatherhood. Qualitative Research. 2012; 12 (1):35–52. doi: 10.1177/1468794111426224. [ CrossRef ] [ Google Scholar ]
- Creswell J. W. Five qualitative approaches to inquiry. In: Creswell J. W, editor. Qualitative inquiry and research design: Choosing among five approaches. 3rd ed. Thousand Oaks, CA: Sage; 2013a. pp. 53–84. [ Google Scholar ]
- Creswell J. W. Qualitative inquiry and research design: Choosing among five approaches. 3rd ed. Thousand Oaks, CA: Sage; 2013b. [ Google Scholar ]
- Crowe S, Cresswell K, Robertson A, Huby G, Avery A, Sheikh A. The case study approach. BMC Medical Research Methodology. 2011; 11 (1):1–9. doi: 10.1186/1471-2288-11-100. [ PMC free article ] [ PubMed ] [ CrossRef ] [ Google Scholar ]
- Cunsolo Willox A, Harper S. L, Edge V. L, ‘My Word’: Storytelling and Digital Media Lab, & Rigolet Inuit Community Government Storytelling in a digital age: Digital storytelling as an emerging narrative method for preserving and promoting indigenous oral wisdom. Qualitative Research. 2013; 13 (2):127–147. doi: 10.1177/1468794112446105. [ CrossRef ] [ Google Scholar ]
- De Haene L, Grietens H, Verschueren K. Holding harm: Narrative methods in mental health research on refugee trauma. Qualitative Health Research. 2010; 20 (12):1664–1676. doi: 10.1177/1049732310376521. [ PubMed ] [ CrossRef ] [ Google Scholar ]
- D'Enbeau S, Buzzanell P. M, Duckworth J. Problematizing classed identities in fatherhood: Development of integrative case studies for analysis and praxis. Qualitative Inquiry. 2010; 16 (9):709–720. doi: 10.1177/1077800410374183. [ CrossRef ] [ Google Scholar ]
- Denzin N. K, Lincoln Y. S. Introduction: Disciplining the practice of qualitative research. In: Denzin N. K, Lincoln Y. S, editors. The SAGE handbook of qualitative research. 4th ed. Thousand Oaks, CA: Sage; 2011a. pp. 1–6. [ Google Scholar ]
- Denzin N. K, Lincoln Y. S, editors. The SAGE handbook of qualitative research. 4th ed. Thousand Oaks, CA: Sage; 2011b. [ Google Scholar ]
- Edwards R, Weller S. Shifting analytic ontology: Using I-poems in qualitative longitudinal research. Qualitative Research. 2012; 12 (2):202–217. doi: 10.1177/1468794111422040. [ CrossRef ] [ Google Scholar ]
- Eisenhardt K. M. Building theories from case study research. The Academy of Management Review. 1989; 14 (4):532–550. doi: 10.2307/258557. [ CrossRef ] [ Google Scholar ]
- Fincham B, Scourfield J, Langer S. The impact of working with disturbing secondary data: Reading suicide files in a coroner's office. Qualitative Health Research. 2008; 18 (6):853–862. doi: 10.1177/1049732307308945. [ PubMed ] [ CrossRef ] [ Google Scholar ]
- Flanagan J. Public participation in the design of educational programmes for cancer nurses: A case report. European Journal of Cancer Care. 1999; 8 (2):107–112. doi: 10.1046/j.1365-2354.1999.00141.x. [ PubMed ] [ CrossRef ] [ Google Scholar ]
- Flyvbjerg B. Five misunderstandings about case-study research. Qualitative Inquiry. 2006; 12 (2):219–245. doi: 10.1177/1077800405284.363. [ CrossRef ] [ Google Scholar ]
- Flyvbjerg B. Case study. In: Denzin N. K, Lincoln Y. S, editors. The SAGE handbook of qualitative research. 4th ed. Thousand Oaks, CA: Sage; 2011. pp. 301–316. [ Google Scholar ]
- Fourie C. L, Theron L. C. Resilience in the face of fragile X syndrome. Qualitative Health Research. 2012; 22 (10):1355–1368. doi: 10.1177/1049732312451871. [ PubMed ] [ CrossRef ] [ Google Scholar ]
- Gallagher N, MacFarlane A, Murphy A. W, Freeman G. K, Glynn L. G, Bradley C. P. Service users’ and caregivers’ perspectives on continuity of care in out-of-hours primary care. Qualitative Health Research. 2013; 23 (3):407–421. doi: 10.1177/1049732312470521. [ PubMed ] [ CrossRef ] [ Google Scholar ]
- Gerring J. What is a case study and what is it good for? American Political Science Review. 2004; 98 (2):341–354. doi: 10.1017/S0003055404001182. [ CrossRef ] [ Google Scholar ]
- Gillard A, Witt P. A, Watts C. E. Outcomes and processes at a camp for youth with HIV/AIDS. Qualitative Health Research. 2011; 21 (11):1508–1526. doi: 10.1177/1049732311413907. [ PubMed ] [ CrossRef ] [ Google Scholar ]
- Grant M, Booth A. A typology of reviews: An analysis of 14 review types and associated methodologies. Health Information and Libraries Journal. 2009; 26 :91–108. doi: 10.1111/j.1471-1842.2009.00848.x. [ PubMed ] [ CrossRef ] [ Google Scholar ]
- Gratton M.-F, O'Donnell S. Communication technologies for focus groups with remote communities: A case study of research with First Nations in Canada. Qualitative Research. 2011; 11 (2):159–175. doi: 10.1177/1468794110394068. [ CrossRef ] [ Google Scholar ]
- Hallberg L. Quality criteria and generalization of results from qualitative studies. International Journal of Qualitative Studies on Health and Wellbeing. 2013; 8 :1. doi: 10.3402/qhw.v8i0.20647. [ PMC free article ] [ PubMed ] [ CrossRef ] [ Google Scholar ]
- Hames I. Committee on Publication Ethics, 1. 2013, March. COPE Ethical guidelines for peer reviewers. Retrieved April 7, 2013, from http://publicationethics.org/resources/guidelines . [ Google Scholar ]
- Hooghe A, Neimeyer R. A, Rober P. “Cycling around an emotional core of sadness”: Emotion regulation in a couple after the loss of a child. Qualitative Health Research. 2012; 22 (9):1220–1231. doi: 10.1177/1049732312449209. [ PubMed ] [ CrossRef ] [ Google Scholar ]
- Jackson C. B, Botelho E. M, Welch L. C, Joseph J, Tennstedt S. L. Talking with others about stigmatized health conditions: Implications for managing symptoms. Qualitative Health Research. 2012; 22 (11):1468–1475. doi: 10.1177/1049732312450323. [ PMC free article ] [ PubMed ] [ CrossRef ] [ Google Scholar ]
- Jasper M, Vaismoradi M, Bondas T, Turunen H. Validity and reliability of the scientific review process in nursing journals—time for a rethink? Nursing Inquiry. 2013 doi: 10.1111/nin.12030. [ PubMed ] [ CrossRef ] [ Google Scholar ]
- Jensen J. L, Rodgers R. Cumulating the intellectual gold of case study research. Public Administration Review. 2001; 61 (2):235–246. doi: 10.1111/0033-3352.00025. [ CrossRef ] [ Google Scholar ]
- Jorrín-Abellán I. M, Rubia-Avi B, Anguita-Martínez R, Gómez-Sánchez E, Martínez-Mones A. Bouncing between the dark and bright sides: Can technology help qualitative research? Qualitative Inquiry. 2008; 14 (7):1187–1204. doi: 10.1177/1077800408318435. [ CrossRef ] [ Google Scholar ]
- Ledderer L. Understanding change in medical practice: The role of shared meaning in preventive treatment. Qualitative Health Research. 2011; 21 (1):27–40. doi: 10.1177/1049732310377451. [ PubMed ] [ CrossRef ] [ Google Scholar ]
- Lincoln Y. S. Emerging criteria for quality in qualitative and interpretive research. Qualitative Inquiry. 1995; 1 (3):275–289. doi: 10.1177/107780049500100301. [ CrossRef ] [ Google Scholar ]
- Luck L, Jackson D, Usher K. Case study: A bridge across the paradigms. Nursing Inquiry. 2006; 13 (2):103–109. doi: 10.1111/j.1440-1800.2006.00309.x. [ PubMed ] [ CrossRef ] [ Google Scholar ]
- Mawn B, Siqueira E, Koren A, Slatin C, Devereaux Melillo K, Pearce C, et al. Health disparities among health care workers. Qualitative Health Research. 2010; 20 (1):68–80. doi: 10.1177/1049732309355590. [ PMC free article ] [ PubMed ] [ CrossRef ] [ Google Scholar ]
- Merriam S. B. Qualitative research: A guide to design and implementation. 3rd ed. San Francisco, CA: Jossey-Bass; 2009. [ Google Scholar ]
- Meyer C. B. A case in case study methodology. Field Methods. 2001; 13 (4):329–352. doi: 10.1177/1525822x0101300402. [ CrossRef ] [ Google Scholar ]
- Morse J. M. Mixing qualitative methods. Qualitative Health Research. 2009; 19 (11):1523–1524. doi: 10.1177/1049732309349360. [ PubMed ] [ CrossRef ] [ Google Scholar ]
- Morse J. M. Molding qualitative health research. Qualitative Health Research. 2011; 21 (8):1019–1021. doi: 10.1177/1049732311404706. [ PubMed ] [ CrossRef ] [ Google Scholar ]
- Morse J. M, Dimitroff L. J, Harper R, Koontz A, Kumra S, Matthew-Maich N, et al. Considering the qualitative–quantitative language divide. Qualitative Health Research. 2011; 21 (9):1302–1303. doi: 10.1177/1049732310392386. [ PubMed ] [ CrossRef ] [ Google Scholar ]
- Nagar-Ron S, Motzafi-Haller P. “My life? There is not much to tell”: On voice, silence and agency in interviews with first-generation Mizrahi Jewish women immigrants to Israel. Qualitative Inquiry. 2011; 17 (7):653–663. doi: 10.1177/1077800411414007. [ CrossRef ] [ Google Scholar ]
- Nairn K, Panelli R. Using fiction to make meaning in research with young people in rural New Zealand. Qualitative Inquiry. 2009; 15 (1):96–112. doi: 10.1177/1077800408318314. [ CrossRef ] [ Google Scholar ]
- Nespor J. The afterlife of “teachers’ beliefs”: Qualitative methodology and the textline. Qualitative Inquiry. 2012; 18 (5):449–460. doi: 10.1177/1077800412439530. [ CrossRef ] [ Google Scholar ]
- Piekkari R, Welch C, Paavilainen E. The case study as disciplinary convention: Evidence from international business journals. Organizational Research Methods. 2009; 12 (3):567–589. doi: 10.1177/1094428108319905. [ CrossRef ] [ Google Scholar ]
- Ragin C. C, Becker H. S. What is a case?: Exploring the foundations of social inquiry. Cambridge: Cambridge University Press; 1992. [ Google Scholar ]
- Roscigno C. I, Savage T. A, Kavanaugh K, Moro T. T, Kilpatrick S. J, Strassner H. T, et al. Divergent views of hope influencing communications between parents and hospital providers. Qualitative Health Research. 2012; 22 (9):1232–1246. doi: 10.1177/1049732312449210. [ PMC free article ] [ PubMed ] [ CrossRef ] [ Google Scholar ]
- Rosenberg J. P, Yates P. M. Schematic representation of case study research designs. Journal of Advanced Nursing. 2007; 60 (4):447–452. doi: 10.1111/j.1365-2648.2007.04385.x. [ PubMed ] [ CrossRef ] [ Google Scholar ]
- Rytterström P, Unosson M, Arman M. Care culture as a meaning- making process: A study of a mistreatment investigation. Qualitative Health Research. 2013; 23 :1179–1187. doi: 10.1177/1049732312470760. [ PubMed ] [ CrossRef ] [ Google Scholar ]
- Sandelowski M. Whatever happened to qualitative description? Research in Nursing & Health. 2000; 23 (4):334–340. doi: 10.1002/1098-240X. [ PubMed ] [ CrossRef ] [ Google Scholar ]
- Sandelowski M. What's in a name? Qualitative description revisited. Research in Nursing & Health. 2010; 33 (1):77–84. doi: 10.1002/nur.20362. [ PubMed ] [ CrossRef ] [ Google Scholar ]
- Sandelowski M, Barroso J. Reading qualitative studies. International Journal of Qualitative Methods. 2002; 1 (1):74–108. [ Google Scholar ]
- Snyder-Young D. “Here to tell her story”: Analyzing the autoethnographic performances of others. Qualitative Inquiry. 2011; 17 (10):943–951. doi: 10.1177/1077800411425149. [ CrossRef ] [ Google Scholar ]
- Stake R. E. The case study method in social inquiry. Educational Researcher. 1978; 7 (2):5–8. [ Google Scholar ]
- Stake R. E. The art of case study research. Thousand Oaks, CA: Sage; 1995. [ Google Scholar ]
- Stake R. E. Case studies. In: Denzin N. K, Lincoln Y. S, editors. Strategies of qualitative inquiry. Thousand Oaks, CA: Sage; 1998. pp. 86–109. [ Google Scholar ]
- Sumsion J. Opening up possibilities through team research: Investigating infants’ experiences of early childhood education and care. Qualitative Research. 2013; 14 (2):149–165. doi: 10.1177/1468794112468471.. [ CrossRef ] [ Google Scholar ]
- Thomas G. Doing case study: Abduction not induction, phronesis not theory. Qualitative Inquiry. 2010; 16 (7):575–582. doi: 10.1177/1077800410372601. [ CrossRef ] [ Google Scholar ]
- Thomas G. A typology for the case study in social science following a review of definition, discourse, and structure. Qualitative Inquiry. 2011; 17 (6):511–521. doi: 10.1177/1077800411409884. [ CrossRef ] [ Google Scholar ]
- Tight M. The curious case of case study: A viewpoint. International Journal of Social Research Methodology. 2010; 13 (4):329–339. doi: 10.1080/13645570903187181. [ CrossRef ] [ Google Scholar ]
- Wager E, Kleinert S. Responsible research publication: International standards for authors. A position statement developed at the 2nd World Conference on Research Integrity, Singapore, July 22–24, 2010. In: Mayer T, Steneck N, editors. Promoting research integrity in a global environment. Singapore: Imperial College Press/World Scientific; 2010a. pp. 309–316. [ Google Scholar ]
- Wager E, Kleinert S. Responsible research publication: International standards for editors. A position statement developed at the 2nd World Conference on Research Integrity, Singapore, July 22–24, 2010. In: Mayer T, Steneck N, editors. Promoting research integrity in a global environment. Singapore: Imperial College Press/World Scientific; 2010b. pp. 317–328. [ Google Scholar ]
- Webb C, Kevern J. Focus groups as a research method: A critique of some aspects of their use in nursing research. Journal of Advanced Nursing. 2000; 33 (6):798–805. doi: 10.1046/j.1365-2648.2001.01720.x. [ PubMed ] [ CrossRef ] [ Google Scholar ]
- Wimpenny K, Savin-Baden M. Exploring and implementing participatory action synthesis. Qualitative Inquiry. 2012; 18 (8):689–698. doi: 10.1177/1077800412452854. [ CrossRef ] [ Google Scholar ]
- Yeh H.-Y. Boundaries, entities, and modern vegetarianism: Examining the emergence of the first vegetarian organization. Qualitative Inquiry. 2013; 19 (4):298–309. doi: 10.1177/1077800412471516. [ CrossRef ] [ Google Scholar ]
- Yin R. K. Enhancing the quality of case studies in health services research. Health Services Research. 1999; 34 (5 Pt 2):1209–1224. [ PMC free article ] [ PubMed ] [ Google Scholar ]
- Yin R. K. Case study research: Design and methods. 4th ed. Thousand Oaks, CA: Sage; 2009. [ Google Scholar ]
- Yin R. K. Applications of case study research. 3rd ed. Thousand Oaks, CA: Sage; 2012. [ Google Scholar ]
- Open access
- Published: 27 June 2011
The case study approach
- Sarah Crowe 1 ,
- Kathrin Cresswell 2 ,
- Ann Robertson 2 ,
- Guro Huby 3 ,
- Anthony Avery 1 &
- Aziz Sheikh 2
BMC Medical Research Methodology volume 11 , Article number: 100 ( 2011 ) Cite this article
793k Accesses
1098 Citations
43 Altmetric
Metrics details
The case study approach allows in-depth, multi-faceted explorations of complex issues in their real-life settings. The value of the case study approach is well recognised in the fields of business, law and policy, but somewhat less so in health services research. Based on our experiences of conducting several health-related case studies, we reflect on the different types of case study design, the specific research questions this approach can help answer, the data sources that tend to be used, and the particular advantages and disadvantages of employing this methodological approach. The paper concludes with key pointers to aid those designing and appraising proposals for conducting case study research, and a checklist to help readers assess the quality of case study reports.
Peer Review reports
Introduction
The case study approach is particularly useful to employ when there is a need to obtain an in-depth appreciation of an issue, event or phenomenon of interest, in its natural real-life context. Our aim in writing this piece is to provide insights into when to consider employing this approach and an overview of key methodological considerations in relation to the design, planning, analysis, interpretation and reporting of case studies.
The illustrative 'grand round', 'case report' and 'case series' have a long tradition in clinical practice and research. Presenting detailed critiques, typically of one or more patients, aims to provide insights into aspects of the clinical case and, in doing so, illustrate broader lessons that may be learnt. In research, the conceptually-related case study approach can be used, for example, to describe in detail a patient's episode of care, explore professional attitudes to and experiences of a new policy initiative or service development or more generally to 'investigate contemporary phenomena within its real-life context' [ 1 ]. Based on our experiences of conducting a range of case studies, we reflect on when to consider using this approach, discuss the key steps involved and illustrate, with examples, some of the practical challenges of attaining an in-depth understanding of a 'case' as an integrated whole. In keeping with previously published work, we acknowledge the importance of theory to underpin the design, selection, conduct and interpretation of case studies[ 2 ]. In so doing, we make passing reference to the different epistemological approaches used in case study research by key theoreticians and methodologists in this field of enquiry.
This paper is structured around the following main questions: What is a case study? What are case studies used for? How are case studies conducted? What are the potential pitfalls and how can these be avoided? We draw in particular on four of our own recently published examples of case studies (see Tables 1 , 2 , 3 and 4 ) and those of others to illustrate our discussion[ 3 – 7 ].
What is a case study?
A case study is a research approach that is used to generate an in-depth, multi-faceted understanding of a complex issue in its real-life context. It is an established research design that is used extensively in a wide variety of disciplines, particularly in the social sciences. A case study can be defined in a variety of ways (Table 5 ), the central tenet being the need to explore an event or phenomenon in depth and in its natural context. It is for this reason sometimes referred to as a "naturalistic" design; this is in contrast to an "experimental" design (such as a randomised controlled trial) in which the investigator seeks to exert control over and manipulate the variable(s) of interest.
Stake's work has been particularly influential in defining the case study approach to scientific enquiry. He has helpfully characterised three main types of case study: intrinsic , instrumental and collective [ 8 ]. An intrinsic case study is typically undertaken to learn about a unique phenomenon. The researcher should define the uniqueness of the phenomenon, which distinguishes it from all others. In contrast, the instrumental case study uses a particular case (some of which may be better than others) to gain a broader appreciation of an issue or phenomenon. The collective case study involves studying multiple cases simultaneously or sequentially in an attempt to generate a still broader appreciation of a particular issue.
These are however not necessarily mutually exclusive categories. In the first of our examples (Table 1 ), we undertook an intrinsic case study to investigate the issue of recruitment of minority ethnic people into the specific context of asthma research studies, but it developed into a instrumental case study through seeking to understand the issue of recruitment of these marginalised populations more generally, generating a number of the findings that are potentially transferable to other disease contexts[ 3 ]. In contrast, the other three examples (see Tables 2 , 3 and 4 ) employed collective case study designs to study the introduction of workforce reconfiguration in primary care, the implementation of electronic health records into hospitals, and to understand the ways in which healthcare students learn about patient safety considerations[ 4 – 6 ]. Although our study focusing on the introduction of General Practitioners with Specialist Interests (Table 2 ) was explicitly collective in design (four contrasting primary care organisations were studied), is was also instrumental in that this particular professional group was studied as an exemplar of the more general phenomenon of workforce redesign[ 4 ].
What are case studies used for?
According to Yin, case studies can be used to explain, describe or explore events or phenomena in the everyday contexts in which they occur[ 1 ]. These can, for example, help to understand and explain causal links and pathways resulting from a new policy initiative or service development (see Tables 2 and 3 , for example)[ 1 ]. In contrast to experimental designs, which seek to test a specific hypothesis through deliberately manipulating the environment (like, for example, in a randomised controlled trial giving a new drug to randomly selected individuals and then comparing outcomes with controls),[ 9 ] the case study approach lends itself well to capturing information on more explanatory ' how ', 'what' and ' why ' questions, such as ' how is the intervention being implemented and received on the ground?'. The case study approach can offer additional insights into what gaps exist in its delivery or why one implementation strategy might be chosen over another. This in turn can help develop or refine theory, as shown in our study of the teaching of patient safety in undergraduate curricula (Table 4 )[ 6 , 10 ]. Key questions to consider when selecting the most appropriate study design are whether it is desirable or indeed possible to undertake a formal experimental investigation in which individuals and/or organisations are allocated to an intervention or control arm? Or whether the wish is to obtain a more naturalistic understanding of an issue? The former is ideally studied using a controlled experimental design, whereas the latter is more appropriately studied using a case study design.
Case studies may be approached in different ways depending on the epistemological standpoint of the researcher, that is, whether they take a critical (questioning one's own and others' assumptions), interpretivist (trying to understand individual and shared social meanings) or positivist approach (orientating towards the criteria of natural sciences, such as focusing on generalisability considerations) (Table 6 ). Whilst such a schema can be conceptually helpful, it may be appropriate to draw on more than one approach in any case study, particularly in the context of conducting health services research. Doolin has, for example, noted that in the context of undertaking interpretative case studies, researchers can usefully draw on a critical, reflective perspective which seeks to take into account the wider social and political environment that has shaped the case[ 11 ].
How are case studies conducted?
Here, we focus on the main stages of research activity when planning and undertaking a case study; the crucial stages are: defining the case; selecting the case(s); collecting and analysing the data; interpreting data; and reporting the findings.
Defining the case
Carefully formulated research question(s), informed by the existing literature and a prior appreciation of the theoretical issues and setting(s), are all important in appropriately and succinctly defining the case[ 8 , 12 ]. Crucially, each case should have a pre-defined boundary which clarifies the nature and time period covered by the case study (i.e. its scope, beginning and end), the relevant social group, organisation or geographical area of interest to the investigator, the types of evidence to be collected, and the priorities for data collection and analysis (see Table 7 )[ 1 ]. A theory driven approach to defining the case may help generate knowledge that is potentially transferable to a range of clinical contexts and behaviours; using theory is also likely to result in a more informed appreciation of, for example, how and why interventions have succeeded or failed[ 13 ].
For example, in our evaluation of the introduction of electronic health records in English hospitals (Table 3 ), we defined our cases as the NHS Trusts that were receiving the new technology[ 5 ]. Our focus was on how the technology was being implemented. However, if the primary research interest had been on the social and organisational dimensions of implementation, we might have defined our case differently as a grouping of healthcare professionals (e.g. doctors and/or nurses). The precise beginning and end of the case may however prove difficult to define. Pursuing this same example, when does the process of implementation and adoption of an electronic health record system really begin or end? Such judgements will inevitably be influenced by a range of factors, including the research question, theory of interest, the scope and richness of the gathered data and the resources available to the research team.
Selecting the case(s)
The decision on how to select the case(s) to study is a very important one that merits some reflection. In an intrinsic case study, the case is selected on its own merits[ 8 ]. The case is selected not because it is representative of other cases, but because of its uniqueness, which is of genuine interest to the researchers. This was, for example, the case in our study of the recruitment of minority ethnic participants into asthma research (Table 1 ) as our earlier work had demonstrated the marginalisation of minority ethnic people with asthma, despite evidence of disproportionate asthma morbidity[ 14 , 15 ]. In another example of an intrinsic case study, Hellstrom et al.[ 16 ] studied an elderly married couple living with dementia to explore how dementia had impacted on their understanding of home, their everyday life and their relationships.
For an instrumental case study, selecting a "typical" case can work well[ 8 ]. In contrast to the intrinsic case study, the particular case which is chosen is of less importance than selecting a case that allows the researcher to investigate an issue or phenomenon. For example, in order to gain an understanding of doctors' responses to health policy initiatives, Som undertook an instrumental case study interviewing clinicians who had a range of responsibilities for clinical governance in one NHS acute hospital trust[ 17 ]. Sampling a "deviant" or "atypical" case may however prove even more informative, potentially enabling the researcher to identify causal processes, generate hypotheses and develop theory.
In collective or multiple case studies, a number of cases are carefully selected. This offers the advantage of allowing comparisons to be made across several cases and/or replication. Choosing a "typical" case may enable the findings to be generalised to theory (i.e. analytical generalisation) or to test theory by replicating the findings in a second or even a third case (i.e. replication logic)[ 1 ]. Yin suggests two or three literal replications (i.e. predicting similar results) if the theory is straightforward and five or more if the theory is more subtle. However, critics might argue that selecting 'cases' in this way is insufficiently reflexive and ill-suited to the complexities of contemporary healthcare organisations.
The selected case study site(s) should allow the research team access to the group of individuals, the organisation, the processes or whatever else constitutes the chosen unit of analysis for the study. Access is therefore a central consideration; the researcher needs to come to know the case study site(s) well and to work cooperatively with them. Selected cases need to be not only interesting but also hospitable to the inquiry [ 8 ] if they are to be informative and answer the research question(s). Case study sites may also be pre-selected for the researcher, with decisions being influenced by key stakeholders. For example, our selection of case study sites in the evaluation of the implementation and adoption of electronic health record systems (see Table 3 ) was heavily influenced by NHS Connecting for Health, the government agency that was responsible for overseeing the National Programme for Information Technology (NPfIT)[ 5 ]. This prominent stakeholder had already selected the NHS sites (through a competitive bidding process) to be early adopters of the electronic health record systems and had negotiated contracts that detailed the deployment timelines.
It is also important to consider in advance the likely burden and risks associated with participation for those who (or the site(s) which) comprise the case study. Of particular importance is the obligation for the researcher to think through the ethical implications of the study (e.g. the risk of inadvertently breaching anonymity or confidentiality) and to ensure that potential participants/participating sites are provided with sufficient information to make an informed choice about joining the study. The outcome of providing this information might be that the emotive burden associated with participation, or the organisational disruption associated with supporting the fieldwork, is considered so high that the individuals or sites decide against participation.
In our example of evaluating implementations of electronic health record systems, given the restricted number of early adopter sites available to us, we sought purposively to select a diverse range of implementation cases among those that were available[ 5 ]. We chose a mixture of teaching, non-teaching and Foundation Trust hospitals, and examples of each of the three electronic health record systems procured centrally by the NPfIT. At one recruited site, it quickly became apparent that access was problematic because of competing demands on that organisation. Recognising the importance of full access and co-operative working for generating rich data, the research team decided not to pursue work at that site and instead to focus on other recruited sites.
Collecting the data
In order to develop a thorough understanding of the case, the case study approach usually involves the collection of multiple sources of evidence, using a range of quantitative (e.g. questionnaires, audits and analysis of routinely collected healthcare data) and more commonly qualitative techniques (e.g. interviews, focus groups and observations). The use of multiple sources of data (data triangulation) has been advocated as a way of increasing the internal validity of a study (i.e. the extent to which the method is appropriate to answer the research question)[ 8 , 18 – 21 ]. An underlying assumption is that data collected in different ways should lead to similar conclusions, and approaching the same issue from different angles can help develop a holistic picture of the phenomenon (Table 2 )[ 4 ].
Brazier and colleagues used a mixed-methods case study approach to investigate the impact of a cancer care programme[ 22 ]. Here, quantitative measures were collected with questionnaires before, and five months after, the start of the intervention which did not yield any statistically significant results. Qualitative interviews with patients however helped provide an insight into potentially beneficial process-related aspects of the programme, such as greater, perceived patient involvement in care. The authors reported how this case study approach provided a number of contextual factors likely to influence the effectiveness of the intervention and which were not likely to have been obtained from quantitative methods alone.
In collective or multiple case studies, data collection needs to be flexible enough to allow a detailed description of each individual case to be developed (e.g. the nature of different cancer care programmes), before considering the emerging similarities and differences in cross-case comparisons (e.g. to explore why one programme is more effective than another). It is important that data sources from different cases are, where possible, broadly comparable for this purpose even though they may vary in nature and depth.
Analysing, interpreting and reporting case studies
Making sense and offering a coherent interpretation of the typically disparate sources of data (whether qualitative alone or together with quantitative) is far from straightforward. Repeated reviewing and sorting of the voluminous and detail-rich data are integral to the process of analysis. In collective case studies, it is helpful to analyse data relating to the individual component cases first, before making comparisons across cases. Attention needs to be paid to variations within each case and, where relevant, the relationship between different causes, effects and outcomes[ 23 ]. Data will need to be organised and coded to allow the key issues, both derived from the literature and emerging from the dataset, to be easily retrieved at a later stage. An initial coding frame can help capture these issues and can be applied systematically to the whole dataset with the aid of a qualitative data analysis software package.
The Framework approach is a practical approach, comprising of five stages (familiarisation; identifying a thematic framework; indexing; charting; mapping and interpretation) , to managing and analysing large datasets particularly if time is limited, as was the case in our study of recruitment of South Asians into asthma research (Table 1 )[ 3 , 24 ]. Theoretical frameworks may also play an important role in integrating different sources of data and examining emerging themes. For example, we drew on a socio-technical framework to help explain the connections between different elements - technology; people; and the organisational settings within which they worked - in our study of the introduction of electronic health record systems (Table 3 )[ 5 ]. Our study of patient safety in undergraduate curricula drew on an evaluation-based approach to design and analysis, which emphasised the importance of the academic, organisational and practice contexts through which students learn (Table 4 )[ 6 ].
Case study findings can have implications both for theory development and theory testing. They may establish, strengthen or weaken historical explanations of a case and, in certain circumstances, allow theoretical (as opposed to statistical) generalisation beyond the particular cases studied[ 12 ]. These theoretical lenses should not, however, constitute a strait-jacket and the cases should not be "forced to fit" the particular theoretical framework that is being employed.
When reporting findings, it is important to provide the reader with enough contextual information to understand the processes that were followed and how the conclusions were reached. In a collective case study, researchers may choose to present the findings from individual cases separately before amalgamating across cases. Care must be taken to ensure the anonymity of both case sites and individual participants (if agreed in advance) by allocating appropriate codes or withholding descriptors. In the example given in Table 3 , we decided against providing detailed information on the NHS sites and individual participants in order to avoid the risk of inadvertent disclosure of identities[ 5 , 25 ].
What are the potential pitfalls and how can these be avoided?
The case study approach is, as with all research, not without its limitations. When investigating the formal and informal ways undergraduate students learn about patient safety (Table 4 ), for example, we rapidly accumulated a large quantity of data. The volume of data, together with the time restrictions in place, impacted on the depth of analysis that was possible within the available resources. This highlights a more general point of the importance of avoiding the temptation to collect as much data as possible; adequate time also needs to be set aside for data analysis and interpretation of what are often highly complex datasets.
Case study research has sometimes been criticised for lacking scientific rigour and providing little basis for generalisation (i.e. producing findings that may be transferable to other settings)[ 1 ]. There are several ways to address these concerns, including: the use of theoretical sampling (i.e. drawing on a particular conceptual framework); respondent validation (i.e. participants checking emerging findings and the researcher's interpretation, and providing an opinion as to whether they feel these are accurate); and transparency throughout the research process (see Table 8 )[ 8 , 18 – 21 , 23 , 26 ]. Transparency can be achieved by describing in detail the steps involved in case selection, data collection, the reasons for the particular methods chosen, and the researcher's background and level of involvement (i.e. being explicit about how the researcher has influenced data collection and interpretation). Seeking potential, alternative explanations, and being explicit about how interpretations and conclusions were reached, help readers to judge the trustworthiness of the case study report. Stake provides a critique checklist for a case study report (Table 9 )[ 8 ].
Conclusions
The case study approach allows, amongst other things, critical events, interventions, policy developments and programme-based service reforms to be studied in detail in a real-life context. It should therefore be considered when an experimental design is either inappropriate to answer the research questions posed or impossible to undertake. Considering the frequency with which implementations of innovations are now taking place in healthcare settings and how well the case study approach lends itself to in-depth, complex health service research, we believe this approach should be more widely considered by researchers. Though inherently challenging, the research case study can, if carefully conceptualised and thoughtfully undertaken and reported, yield powerful insights into many important aspects of health and healthcare delivery.
Yin RK: Case study research, design and method. 2009, London: Sage Publications Ltd., 4
Google Scholar
Keen J, Packwood T: Qualitative research; case study evaluation. BMJ. 1995, 311: 444-446.
Article CAS PubMed PubMed Central Google Scholar
Sheikh A, Halani L, Bhopal R, Netuveli G, Partridge M, Car J, et al: Facilitating the Recruitment of Minority Ethnic People into Research: Qualitative Case Study of South Asians and Asthma. PLoS Med. 2009, 6 (10): 1-11.
Article Google Scholar
Pinnock H, Huby G, Powell A, Kielmann T, Price D, Williams S, et al: The process of planning, development and implementation of a General Practitioner with a Special Interest service in Primary Care Organisations in England and Wales: a comparative prospective case study. Report for the National Co-ordinating Centre for NHS Service Delivery and Organisation R&D (NCCSDO). 2008, [ http://www.sdo.nihr.ac.uk/files/project/99-final-report.pdf ]
Robertson A, Cresswell K, Takian A, Petrakaki D, Crowe S, Cornford T, et al: Prospective evaluation of the implementation and adoption of NHS Connecting for Health's national electronic health record in secondary care in England: interim findings. BMJ. 2010, 41: c4564-
Pearson P, Steven A, Howe A, Sheikh A, Ashcroft D, Smith P, the Patient Safety Education Study Group: Learning about patient safety: organisational context and culture in the education of healthcare professionals. J Health Serv Res Policy. 2010, 15: 4-10. 10.1258/jhsrp.2009.009052.
Article PubMed Google Scholar
van Harten WH, Casparie TF, Fisscher OA: The evaluation of the introduction of a quality management system: a process-oriented case study in a large rehabilitation hospital. Health Policy. 2002, 60 (1): 17-37. 10.1016/S0168-8510(01)00187-7.
Stake RE: The art of case study research. 1995, London: Sage Publications Ltd.
Sheikh A, Smeeth L, Ashcroft R: Randomised controlled trials in primary care: scope and application. Br J Gen Pract. 2002, 52 (482): 746-51.
PubMed PubMed Central Google Scholar
King G, Keohane R, Verba S: Designing Social Inquiry. 1996, Princeton: Princeton University Press
Doolin B: Information technology as disciplinary technology: being critical in interpretative research on information systems. Journal of Information Technology. 1998, 13: 301-311. 10.1057/jit.1998.8.
George AL, Bennett A: Case studies and theory development in the social sciences. 2005, Cambridge, MA: MIT Press
Eccles M, the Improved Clinical Effectiveness through Behavioural Research Group (ICEBeRG): Designing theoretically-informed implementation interventions. Implementation Science. 2006, 1: 1-8. 10.1186/1748-5908-1-1.
Article PubMed Central Google Scholar
Netuveli G, Hurwitz B, Levy M, Fletcher M, Barnes G, Durham SR, Sheikh A: Ethnic variations in UK asthma frequency, morbidity, and health-service use: a systematic review and meta-analysis. Lancet. 2005, 365 (9456): 312-7.
Sheikh A, Panesar SS, Lasserson T, Netuveli G: Recruitment of ethnic minorities to asthma studies. Thorax. 2004, 59 (7): 634-
CAS PubMed PubMed Central Google Scholar
Hellström I, Nolan M, Lundh U: 'We do things together': A case study of 'couplehood' in dementia. Dementia. 2005, 4: 7-22. 10.1177/1471301205049188.
Som CV: Nothing seems to have changed, nothing seems to be changing and perhaps nothing will change in the NHS: doctors' response to clinical governance. International Journal of Public Sector Management. 2005, 18: 463-477. 10.1108/09513550510608903.
Lincoln Y, Guba E: Naturalistic inquiry. 1985, Newbury Park: Sage Publications
Barbour RS: Checklists for improving rigour in qualitative research: a case of the tail wagging the dog?. BMJ. 2001, 322: 1115-1117. 10.1136/bmj.322.7294.1115.
Mays N, Pope C: Qualitative research in health care: Assessing quality in qualitative research. BMJ. 2000, 320: 50-52. 10.1136/bmj.320.7226.50.
Mason J: Qualitative researching. 2002, London: Sage
Brazier A, Cooke K, Moravan V: Using Mixed Methods for Evaluating an Integrative Approach to Cancer Care: A Case Study. Integr Cancer Ther. 2008, 7: 5-17. 10.1177/1534735407313395.
Miles MB, Huberman M: Qualitative data analysis: an expanded sourcebook. 1994, CA: Sage Publications Inc., 2
Pope C, Ziebland S, Mays N: Analysing qualitative data. Qualitative research in health care. BMJ. 2000, 320: 114-116. 10.1136/bmj.320.7227.114.
Cresswell KM, Worth A, Sheikh A: Actor-Network Theory and its role in understanding the implementation of information technology developments in healthcare. BMC Med Inform Decis Mak. 2010, 10 (1): 67-10.1186/1472-6947-10-67.
Article PubMed PubMed Central Google Scholar
Malterud K: Qualitative research: standards, challenges, and guidelines. Lancet. 2001, 358: 483-488. 10.1016/S0140-6736(01)05627-6.
Article CAS PubMed Google Scholar
Yin R: Case study research: design and methods. 1994, Thousand Oaks, CA: Sage Publishing, 2
Yin R: Enhancing the quality of case studies in health services research. Health Serv Res. 1999, 34: 1209-1224.
Green J, Thorogood N: Qualitative methods for health research. 2009, Los Angeles: Sage, 2
Howcroft D, Trauth E: Handbook of Critical Information Systems Research, Theory and Application. 2005, Cheltenham, UK: Northampton, MA, USA: Edward Elgar
Book Google Scholar
Blakie N: Approaches to Social Enquiry. 1993, Cambridge: Polity Press
Doolin B: Power and resistance in the implementation of a medical management information system. Info Systems J. 2004, 14: 343-362. 10.1111/j.1365-2575.2004.00176.x.
Bloomfield BP, Best A: Management consultants: systems development, power and the translation of problems. Sociological Review. 1992, 40: 533-560.
Shanks G, Parr A: Positivist, single case study research in information systems: A critical analysis. Proceedings of the European Conference on Information Systems. 2003, Naples
Pre-publication history
The pre-publication history for this paper can be accessed here: http://www.biomedcentral.com/1471-2288/11/100/prepub
Download references
Acknowledgements
We are grateful to the participants and colleagues who contributed to the individual case studies that we have drawn on. This work received no direct funding, but it has been informed by projects funded by Asthma UK, the NHS Service Delivery Organisation, NHS Connecting for Health Evaluation Programme, and Patient Safety Research Portfolio. We would also like to thank the expert reviewers for their insightful and constructive feedback. Our thanks are also due to Dr. Allison Worth who commented on an earlier draft of this manuscript.
Author information
Authors and affiliations.
Division of Primary Care, The University of Nottingham, Nottingham, UK
Sarah Crowe & Anthony Avery
Centre for Population Health Sciences, The University of Edinburgh, Edinburgh, UK
Kathrin Cresswell, Ann Robertson & Aziz Sheikh
School of Health in Social Science, The University of Edinburgh, Edinburgh, UK
You can also search for this author in PubMed Google Scholar
Corresponding author
Correspondence to Sarah Crowe .
Additional information
Competing interests.
The authors declare that they have no competing interests.
Authors' contributions
AS conceived this article. SC, KC and AR wrote this paper with GH, AA and AS all commenting on various drafts. SC and AS are guarantors.
Rights and permissions
This article is published under license to BioMed Central Ltd. This is an Open Access article distributed under the terms of the Creative Commons Attribution License ( http://creativecommons.org/licenses/by/2.0 ), which permits unrestricted use, distribution, and reproduction in any medium, provided the original work is properly cited.
Reprints and permissions
About this article
Cite this article.
Crowe, S., Cresswell, K., Robertson, A. et al. The case study approach. BMC Med Res Methodol 11 , 100 (2011). https://doi.org/10.1186/1471-2288-11-100
Download citation
Received : 29 November 2010
Accepted : 27 June 2011
Published : 27 June 2011
DOI : https://doi.org/10.1186/1471-2288-11-100
Share this article
Anyone you share the following link with will be able to read this content:
Sorry, a shareable link is not currently available for this article.
Provided by the Springer Nature SharedIt content-sharing initiative
- Case Study Approach
- Electronic Health Record System
- Case Study Design
- Case Study Site
- Case Study Report
BMC Medical Research Methodology
ISSN: 1471-2288
- General enquiries: [email protected]
- Bipolar Disorder
- Therapy Center
- When To See a Therapist
- Types of Therapy
- Best Online Therapy
- Best Couples Therapy
- Best Family Therapy
- Managing Stress
- Sleep and Dreaming
- Understanding Emotions
- Self-Improvement
- Healthy Relationships
- Student Resources
- Personality Types
- Sweepstakes
- Guided Meditations
- Verywell Mind Insights
- 2024 Verywell Mind 25
- Mental Health in the Classroom
- Editorial Process
- Meet Our Review Board
- Crisis Support
What Is a Case Study?
Weighing the pros and cons of this method of research
Verywell / Colleen Tighe
- Pros and Cons
What Types of Case Studies Are Out There?
Where do you find data for a case study, how do i write a psychology case study.
A case study is an in-depth study of one person, group, or event. In a case study, nearly every aspect of the subject's life and history is analyzed to seek patterns and causes of behavior. Case studies can be used in many different fields, including psychology, medicine, education, anthropology, political science, and social work.
The point of a case study is to learn as much as possible about an individual or group so that the information can be generalized to many others. Unfortunately, case studies tend to be highly subjective, and it is sometimes difficult to generalize results to a larger population.
While case studies focus on a single individual or group, they follow a format similar to other types of psychology writing. If you are writing a case study, we got you—here are some rules of APA format to reference.
At a Glance
A case study, or an in-depth study of a person, group, or event, can be a useful research tool when used wisely. In many cases, case studies are best used in situations where it would be difficult or impossible for you to conduct an experiment. They are helpful for looking at unique situations and allow researchers to gather a lot of˜ information about a specific individual or group of people. However, it's important to be cautious of any bias we draw from them as they are highly subjective.
What Are the Benefits and Limitations of Case Studies?
A case study can have its strengths and weaknesses. Researchers must consider these pros and cons before deciding if this type of study is appropriate for their needs.
One of the greatest advantages of a case study is that it allows researchers to investigate things that are often difficult or impossible to replicate in a lab. Some other benefits of a case study:
- Allows researchers to capture information on the 'how,' 'what,' and 'why,' of something that's implemented
- Gives researchers the chance to collect information on why one strategy might be chosen over another
- Permits researchers to develop hypotheses that can be explored in experimental research
On the other hand, a case study can have some drawbacks:
- It cannot necessarily be generalized to the larger population
- Cannot demonstrate cause and effect
- It may not be scientifically rigorous
- It can lead to bias
Researchers may choose to perform a case study if they want to explore a unique or recently discovered phenomenon. Through their insights, researchers develop additional ideas and study questions that might be explored in future studies.
It's important to remember that the insights from case studies cannot be used to determine cause-and-effect relationships between variables. However, case studies may be used to develop hypotheses that can then be addressed in experimental research.
Case Study Examples
There have been a number of notable case studies in the history of psychology. Much of Freud's work and theories were developed through individual case studies. Some great examples of case studies in psychology include:
- Anna O : Anna O. was a pseudonym of a woman named Bertha Pappenheim, a patient of a physician named Josef Breuer. While she was never a patient of Freud's, Freud and Breuer discussed her case extensively. The woman was experiencing symptoms of a condition that was then known as hysteria and found that talking about her problems helped relieve her symptoms. Her case played an important part in the development of talk therapy as an approach to mental health treatment.
- Phineas Gage : Phineas Gage was a railroad employee who experienced a terrible accident in which an explosion sent a metal rod through his skull, damaging important portions of his brain. Gage recovered from his accident but was left with serious changes in both personality and behavior.
- Genie : Genie was a young girl subjected to horrific abuse and isolation. The case study of Genie allowed researchers to study whether language learning was possible, even after missing critical periods for language development. Her case also served as an example of how scientific research may interfere with treatment and lead to further abuse of vulnerable individuals.
Such cases demonstrate how case research can be used to study things that researchers could not replicate in experimental settings. In Genie's case, her horrific abuse denied her the opportunity to learn a language at critical points in her development.
This is clearly not something researchers could ethically replicate, but conducting a case study on Genie allowed researchers to study phenomena that are otherwise impossible to reproduce.
There are a few different types of case studies that psychologists and other researchers might use:
- Collective case studies : These involve studying a group of individuals. Researchers might study a group of people in a certain setting or look at an entire community. For example, psychologists might explore how access to resources in a community has affected the collective mental well-being of those who live there.
- Descriptive case studies : These involve starting with a descriptive theory. The subjects are then observed, and the information gathered is compared to the pre-existing theory.
- Explanatory case studies : These are often used to do causal investigations. In other words, researchers are interested in looking at factors that may have caused certain things to occur.
- Exploratory case studies : These are sometimes used as a prelude to further, more in-depth research. This allows researchers to gather more information before developing their research questions and hypotheses .
- Instrumental case studies : These occur when the individual or group allows researchers to understand more than what is initially obvious to observers.
- Intrinsic case studies : This type of case study is when the researcher has a personal interest in the case. Jean Piaget's observations of his own children are good examples of how an intrinsic case study can contribute to the development of a psychological theory.
The three main case study types often used are intrinsic, instrumental, and collective. Intrinsic case studies are useful for learning about unique cases. Instrumental case studies help look at an individual to learn more about a broader issue. A collective case study can be useful for looking at several cases simultaneously.
The type of case study that psychology researchers use depends on the unique characteristics of the situation and the case itself.
There are a number of different sources and methods that researchers can use to gather information about an individual or group. Six major sources that have been identified by researchers are:
- Archival records : Census records, survey records, and name lists are examples of archival records.
- Direct observation : This strategy involves observing the subject, often in a natural setting . While an individual observer is sometimes used, it is more common to utilize a group of observers.
- Documents : Letters, newspaper articles, administrative records, etc., are the types of documents often used as sources.
- Interviews : Interviews are one of the most important methods for gathering information in case studies. An interview can involve structured survey questions or more open-ended questions.
- Participant observation : When the researcher serves as a participant in events and observes the actions and outcomes, it is called participant observation.
- Physical artifacts : Tools, objects, instruments, and other artifacts are often observed during a direct observation of the subject.
If you have been directed to write a case study for a psychology course, be sure to check with your instructor for any specific guidelines you need to follow. If you are writing your case study for a professional publication, check with the publisher for their specific guidelines for submitting a case study.
Here is a general outline of what should be included in a case study.
Section 1: A Case History
This section will have the following structure and content:
Background information : The first section of your paper will present your client's background. Include factors such as age, gender, work, health status, family mental health history, family and social relationships, drug and alcohol history, life difficulties, goals, and coping skills and weaknesses.
Description of the presenting problem : In the next section of your case study, you will describe the problem or symptoms that the client presented with.
Describe any physical, emotional, or sensory symptoms reported by the client. Thoughts, feelings, and perceptions related to the symptoms should also be noted. Any screening or diagnostic assessments that are used should also be described in detail and all scores reported.
Your diagnosis : Provide your diagnosis and give the appropriate Diagnostic and Statistical Manual code. Explain how you reached your diagnosis, how the client's symptoms fit the diagnostic criteria for the disorder(s), or any possible difficulties in reaching a diagnosis.
Section 2: Treatment Plan
This portion of the paper will address the chosen treatment for the condition. This might also include the theoretical basis for the chosen treatment or any other evidence that might exist to support why this approach was chosen.
- Cognitive behavioral approach : Explain how a cognitive behavioral therapist would approach treatment. Offer background information on cognitive behavioral therapy and describe the treatment sessions, client response, and outcome of this type of treatment. Make note of any difficulties or successes encountered by your client during treatment.
- Humanistic approach : Describe a humanistic approach that could be used to treat your client, such as client-centered therapy . Provide information on the type of treatment you chose, the client's reaction to the treatment, and the end result of this approach. Explain why the treatment was successful or unsuccessful.
- Psychoanalytic approach : Describe how a psychoanalytic therapist would view the client's problem. Provide some background on the psychoanalytic approach and cite relevant references. Explain how psychoanalytic therapy would be used to treat the client, how the client would respond to therapy, and the effectiveness of this treatment approach.
- Pharmacological approach : If treatment primarily involves the use of medications, explain which medications were used and why. Provide background on the effectiveness of these medications and how monotherapy may compare with an approach that combines medications with therapy or other treatments.
This section of a case study should also include information about the treatment goals, process, and outcomes.
When you are writing a case study, you should also include a section where you discuss the case study itself, including the strengths and limitiations of the study. You should note how the findings of your case study might support previous research.
In your discussion section, you should also describe some of the implications of your case study. What ideas or findings might require further exploration? How might researchers go about exploring some of these questions in additional studies?
Need More Tips?
Here are a few additional pointers to keep in mind when formatting your case study:
- Never refer to the subject of your case study as "the client." Instead, use their name or a pseudonym.
- Read examples of case studies to gain an idea about the style and format.
- Remember to use APA format when citing references .
Crowe S, Cresswell K, Robertson A, Huby G, Avery A, Sheikh A. The case study approach . BMC Med Res Methodol . 2011;11:100.
Crowe S, Cresswell K, Robertson A, Huby G, Avery A, Sheikh A. The case study approach . BMC Med Res Methodol . 2011 Jun 27;11:100. doi:10.1186/1471-2288-11-100
Gagnon, Yves-Chantal. The Case Study as Research Method: A Practical Handbook . Canada, Chicago Review Press Incorporated DBA Independent Pub Group, 2010.
Yin, Robert K. Case Study Research and Applications: Design and Methods . United States, SAGE Publications, 2017.
By Kendra Cherry, MSEd Kendra Cherry, MS, is a psychosocial rehabilitation specialist, psychology educator, and author of the "Everything Psychology Book."
- Affiliate Program

- UNITED STATES
- 台灣 (TAIWAN)
- TÜRKIYE (TURKEY)
- Academic Editing Services
- - Research Paper
- - Journal Manuscript
- - Dissertation
- - College & University Assignments
- Admissions Editing Services
- - Application Essay
- - Personal Statement
- - Recommendation Letter
- - Cover Letter
- - CV/Resume
- Business Editing Services
- - Business Documents
- - Report & Brochure
- - Website & Blog
- Writer Editing Services
- - Script & Screenplay
- Our Editors
- Client Reviews
- Editing & Proofreading Prices
- Wordvice Points
- Partner Discount
- Plagiarism Checker
- APA Citation Generator
- MLA Citation Generator
- Chicago Citation Generator
- Vancouver Citation Generator
- - APA Style
- - MLA Style
- - Chicago Style
- - Vancouver Style
- Writing & Editing Guide
- Academic Resources
- Admissions Resources
How to Write a Case Study | Examples & Methods
What is a case study?
A case study is a research approach that provides an in-depth examination of a particular phenomenon, event, organization, or individual. It involves analyzing and interpreting data to provide a comprehensive understanding of the subject under investigation.
Case studies can be used in various disciplines, including business, social sciences, medicine ( clinical case report ), engineering, and education. The aim of a case study is to provide an in-depth exploration of a specific subject, often with the goal of generating new insights into the phenomena being studied.
When to write a case study
Case studies are often written to present the findings of an empirical investigation or to illustrate a particular point or theory. They are useful when researchers want to gain an in-depth understanding of a specific phenomenon or when they are interested in exploring new areas of inquiry.
Case studies are also useful when the subject of the research is rare or when the research question is complex and requires an in-depth examination. A case study can be a good fit for a thesis or dissertation as well.
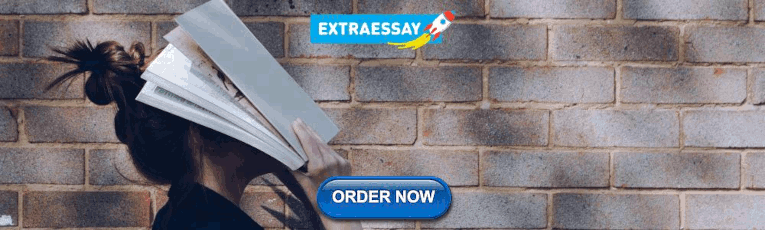
Case study examples
Below are some examples of case studies with their research questions:
How do small and medium-sized enterprises (SMEs) in developing countries manage risks? | Risk management practices in SMEs in Ghana |
What factors contribute to successful organizational change? | A case study of a successful organizational change at Company X |
How do teachers use technology to enhance student learning in the classroom? | The impact of technology integration on student learning in a primary school in the United States |
How do companies adapt to changing consumer preferences? | Coca-Cola’s strategy to address the declining demand for sugary drinks |
What are the effects of the COVID-19 pandemic on the hospitality industry? | The impact of COVID-19 on the hotel industry in Europe |
How do organizations use social media for branding and marketing? | The role of Instagram in fashion brand promotion |
How do businesses address ethical issues in their operations? | A case study of Nike’s supply chain labor practices |
These examples demonstrate the diversity of research questions and case studies that can be explored. From studying small businesses in Ghana to the ethical issues in supply chains, case studies can be used to explore a wide range of phenomena.
Outlying cases vs. representative cases
An outlying case stud y refers to a case that is unusual or deviates significantly from the norm. An example of an outlying case study could be a small, family-run bed and breakfast that was able to survive and even thrive during the COVID-19 pandemic, while other larger hotels struggled to stay afloat.
On the other hand, a representative case study refers to a case that is typical of the phenomenon being studied. An example of a representative case study could be a hotel chain that operates in multiple locations that faced significant challenges during the COVID-19 pandemic, such as reduced demand for hotel rooms, increased safety and health protocols, and supply chain disruptions. The hotel chain case could be representative of the broader hospitality industry during the pandemic, and thus provides an insight into the typical challenges that businesses in the industry faced.
Steps for Writing a Case Study
As with any academic paper, writing a case study requires careful preparation and research before a single word of the document is ever written. Follow these basic steps to ensure that you don’t miss any crucial details when composing your case study.
Step 1: Select a case to analyze
After you have developed your statement of the problem and research question , the first step in writing a case study is to select a case that is representative of the phenomenon being investigated or that provides an outlier. For example, if a researcher wants to explore the impact of COVID-19 on the hospitality industry, they could select a representative case, such as a hotel chain that operates in multiple locations, or an outlying case, such as a small bed and breakfast that was able to pivot their business model to survive during the pandemic. Selecting the appropriate case is critical in ensuring the research question is adequately explored.
Step 2: Create a theoretical framework
Theoretical frameworks are used to guide the analysis and interpretation of data in a case study. The framework should provide a clear explanation of the key concepts, variables, and relationships that are relevant to the research question. The theoretical framework can be drawn from existing literature, or the researcher can develop their own framework based on the data collected. The theoretical framework should be developed early in the research process to guide the data collection and analysis.
To give your case analysis a strong theoretical grounding, be sure to include a literature review of references and sources relating to your topic and develop a clear theoretical framework. Your case study does not simply stand on its own but interacts with other studies related to your topic. Your case study can do one of the following:
- Demonstrate a theory by showing how it explains the case being investigated
- Broaden a theory by identifying additional concepts and ideas that can be incorporated to strengthen it
- Confront a theory via an outlier case that does not conform to established conclusions or assumptions
Step 3: Collect data for your case study
Data collection can involve a variety of research methods , including interviews, surveys, observations, and document analyses, and it can include both primary and secondary sources . It is essential to ensure that the data collected is relevant to the research question and that it is collected in a systematic and ethical manner. Data collection methods should be chosen based on the research question and the availability of data. It is essential to plan data collection carefully to ensure that the data collected is of high quality
Step 4: Describe the case and analyze the details
The final step is to describe the case in detail and analyze the data collected. This involves identifying patterns and themes that emerge from the data and drawing conclusions that are relevant to the research question. It is essential to ensure that the analysis is supported by the data and that any limitations or alternative explanations are acknowledged.
The manner in which you report your findings depends on the type of research you are doing. Some case studies are structured like a standard academic paper, with separate sections or chapters for the methods section , results section , and discussion section , while others are structured more like a standalone literature review.
Regardless of the topic you choose to pursue, writing a case study requires a systematic and rigorous approach to data collection and analysis. By following the steps outlined above and using examples from existing literature, researchers can create a comprehensive and insightful case study that contributes to the understanding of a particular phenomenon.
Preparing Your Case Study for Publication
After completing the draft of your case study, be sure to revise and edit your work for any mistakes, including grammatical errors , punctuation errors , spelling mistakes, and awkward sentence structure . Ensure that your case study is well-structured and that your arguments are well-supported with language that follows the conventions of academic writing . To ensure your work is polished for style and free of errors, get English editing services from Wordvice, including our paper editing services and manuscript editing services . Let our academic subject experts enhance the style and flow of your academic work so you can submit your case study with confidence.
What is case study research?
Last updated
8 February 2023
Reviewed by
Cathy Heath
Short on time? Get an AI generated summary of this article instead
Suppose a company receives a spike in the number of customer complaints, or medical experts discover an outbreak of illness affecting children but are not quite sure of the reason. In both cases, carrying out a case study could be the best way to get answers.
Organization
Case studies can be carried out across different disciplines, including education, medicine, sociology, and business.
Most case studies employ qualitative methods, but quantitative methods can also be used. Researchers can then describe, compare, evaluate, and identify patterns or cause-and-effect relationships between the various variables under study. They can then use this knowledge to decide what action to take.
Another thing to note is that case studies are generally singular in their focus. This means they narrow focus to a particular area, making them highly subjective. You cannot always generalize the results of a case study and apply them to a larger population. However, they are valuable tools to illustrate a principle or develop a thesis.
Analyze case study research
Dovetail streamlines case study research to help you uncover and share actionable insights
- What are the different types of case study designs?
Researchers can choose from a variety of case study designs. The design they choose is dependent on what questions they need to answer, the context of the research environment, how much data they already have, and what resources are available.
Here are the common types of case study design:
Explanatory
An explanatory case study is an initial explanation of the how or why that is behind something. This design is commonly used when studying a real-life phenomenon or event. Once the organization understands the reasons behind a phenomenon, it can then make changes to enhance or eliminate the variables causing it.
Here is an example: How is co-teaching implemented in elementary schools? The title for a case study of this subject could be “Case Study of the Implementation of Co-Teaching in Elementary Schools.”
Descriptive
An illustrative or descriptive case study helps researchers shed light on an unfamiliar object or subject after a period of time. The case study provides an in-depth review of the issue at hand and adds real-world examples in the area the researcher wants the audience to understand.
The researcher makes no inferences or causal statements about the object or subject under review. This type of design is often used to understand cultural shifts.
Here is an example: How did people cope with the 2004 Indian Ocean Tsunami? This case study could be titled "A Case Study of the 2004 Indian Ocean Tsunami and its Effect on the Indonesian Population."
Exploratory
Exploratory research is also called a pilot case study. It is usually the first step within a larger research project, often relying on questionnaires and surveys . Researchers use exploratory research to help narrow down their focus, define parameters, draft a specific research question , and/or identify variables in a larger study. This research design usually covers a wider area than others, and focuses on the ‘what’ and ‘who’ of a topic.
Here is an example: How do nutrition and socialization in early childhood affect learning in children? The title of the exploratory study may be “Case Study of the Effects of Nutrition and Socialization on Learning in Early Childhood.”
An intrinsic case study is specifically designed to look at a unique and special phenomenon. At the start of the study, the researcher defines the phenomenon and the uniqueness that differentiates it from others.
In this case, researchers do not attempt to generalize, compare, or challenge the existing assumptions. Instead, they explore the unique variables to enhance understanding. Here is an example: “Case Study of Volcanic Lightning.”
This design can also be identified as a cumulative case study. It uses information from past studies or observations of groups of people in certain settings as the foundation of the new study. Given that it takes multiple areas into account, it allows for greater generalization than a single case study.
The researchers also get an in-depth look at a particular subject from different viewpoints. Here is an example: “Case Study of how PTSD affected Vietnam and Gulf War Veterans Differently Due to Advances in Military Technology.”
Critical instance
A critical case study incorporates both explanatory and intrinsic study designs. It does not have predetermined purposes beyond an investigation of the said subject. It can be used for a deeper explanation of the cause-and-effect relationship. It can also be used to question a common assumption or myth.
The findings can then be used further to generalize whether they would also apply in a different environment. Here is an example: “What Effect Does Prolonged Use of Social Media Have on the Mind of American Youth?”
Instrumental
Instrumental research attempts to achieve goals beyond understanding the object at hand. Researchers explore a larger subject through different, separate studies and use the findings to understand its relationship to another subject. This type of design also provides insight into an issue or helps refine a theory.
For example, you may want to determine if violent behavior in children predisposes them to crime later in life. The focus is on the relationship between children and violent behavior, and why certain children do become violent. Here is an example: “Violence Breeds Violence: Childhood Exposure and Participation in Adult Crime.”
Evaluation case study design is employed to research the effects of a program, policy, or intervention, and assess its effectiveness and impact on future decision-making.
For example, you might want to see whether children learn times tables quicker through an educational game on their iPad versus a more teacher-led intervention. Here is an example: “An Investigation of the Impact of an iPad Multiplication Game for Primary School Children.”
- When do you use case studies?
Case studies are ideal when you want to gain a contextual, concrete, or in-depth understanding of a particular subject. It helps you understand the characteristics, implications, and meanings of the subject.
They are also an excellent choice for those writing a thesis or dissertation, as they help keep the project focused on a particular area when resources or time may be too limited to cover a wider one. You may have to conduct several case studies to explore different aspects of the subject in question and understand the problem.
- What are the steps to follow when conducting a case study?
1. Select a case
Once you identify the problem at hand and come up with questions, identify the case you will focus on. The study can provide insights into the subject at hand, challenge existing assumptions, propose a course of action, and/or open up new areas for further research.
2. Create a theoretical framework
While you will be focusing on a specific detail, the case study design you choose should be linked to existing knowledge on the topic. This prevents it from becoming an isolated description and allows for enhancing the existing information.
It may expand the current theory by bringing up new ideas or concepts, challenge established assumptions, or exemplify a theory by exploring how it answers the problem at hand. A theoretical framework starts with a literature review of the sources relevant to the topic in focus. This helps in identifying key concepts to guide analysis and interpretation.
3. Collect the data
Case studies are frequently supplemented with qualitative data such as observations, interviews, and a review of both primary and secondary sources such as official records, news articles, and photographs. There may also be quantitative data —this data assists in understanding the case thoroughly.
4. Analyze your case
The results of the research depend on the research design. Most case studies are structured with chapters or topic headings for easy explanation and presentation. Others may be written as narratives to allow researchers to explore various angles of the topic and analyze its meanings and implications.
In all areas, always give a detailed contextual understanding of the case and connect it to the existing theory and literature before discussing how it fits into your problem area.
- What are some case study examples?
What are the best approaches for introducing our product into the Kenyan market?
How does the change in marketing strategy aid in increasing the sales volumes of product Y?
How can teachers enhance student participation in classrooms?
How does poverty affect literacy levels in children?
Case study topics
Case study of product marketing strategies in the Kenyan market
Case study of the effects of a marketing strategy change on product Y sales volumes
Case study of X school teachers that encourage active student participation in the classroom
Case study of the effects of poverty on literacy levels in children
Should you be using a customer insights hub?
Do you want to discover previous research faster?
Do you share your research findings with others?
Do you analyze research data?
Start for free today, add your research, and get to key insights faster
Editor’s picks
Last updated: 18 April 2023
Last updated: 27 February 2023
Last updated: 6 February 2023
Last updated: 5 February 2023
Last updated: 16 April 2023
Last updated: 9 March 2023
Last updated: 30 April 2024
Last updated: 12 December 2023
Last updated: 11 March 2024
Last updated: 4 July 2024
Last updated: 6 March 2024
Last updated: 5 March 2024
Last updated: 13 May 2024
Latest articles
Related topics, .css-je19u9{-webkit-align-items:flex-end;-webkit-box-align:flex-end;-ms-flex-align:flex-end;align-items:flex-end;display:-webkit-box;display:-webkit-flex;display:-ms-flexbox;display:flex;-webkit-flex-direction:row;-ms-flex-direction:row;flex-direction:row;-webkit-box-flex-wrap:wrap;-webkit-flex-wrap:wrap;-ms-flex-wrap:wrap;flex-wrap:wrap;-webkit-box-pack:center;-ms-flex-pack:center;-webkit-justify-content:center;justify-content:center;row-gap:0;text-align:center;max-width:671px;}@media (max-width: 1079px){.css-je19u9{max-width:400px;}.css-je19u9>span{white-space:pre;}}@media (max-width: 799px){.css-je19u9{max-width:400px;}.css-je19u9>span{white-space:pre;}} decide what to .css-1kiodld{max-height:56px;display:-webkit-box;display:-webkit-flex;display:-ms-flexbox;display:flex;-webkit-align-items:center;-webkit-box-align:center;-ms-flex-align:center;align-items:center;}@media (max-width: 1079px){.css-1kiodld{display:none;}} build next, decide what to build next, log in or sign up.
Get started for free
Have a language expert improve your writing
Run a free plagiarism check in 10 minutes, automatically generate references for free.
- Knowledge Base
- Methodology
- Case Study | Definition, Examples & Methods
Case Study | Definition, Examples & Methods
Published on 5 May 2022 by Shona McCombes . Revised on 30 January 2023.
A case study is a detailed study of a specific subject, such as a person, group, place, event, organisation, or phenomenon. Case studies are commonly used in social, educational, clinical, and business research.
A case study research design usually involves qualitative methods , but quantitative methods are sometimes also used. Case studies are good for describing , comparing, evaluating, and understanding different aspects of a research problem .
Table of contents
When to do a case study, step 1: select a case, step 2: build a theoretical framework, step 3: collect your data, step 4: describe and analyse the case.
A case study is an appropriate research design when you want to gain concrete, contextual, in-depth knowledge about a specific real-world subject. It allows you to explore the key characteristics, meanings, and implications of the case.
Case studies are often a good choice in a thesis or dissertation . They keep your project focused and manageable when you don’t have the time or resources to do large-scale research.
You might use just one complex case study where you explore a single subject in depth, or conduct multiple case studies to compare and illuminate different aspects of your research problem.
Research question | Case study |
---|---|
What are the ecological effects of wolf reintroduction? | Case study of wolf reintroduction in Yellowstone National Park in the US |
How do populist politicians use narratives about history to gain support? | Case studies of Hungarian prime minister Viktor Orbán and US president Donald Trump |
How can teachers implement active learning strategies in mixed-level classrooms? | Case study of a local school that promotes active learning |
What are the main advantages and disadvantages of wind farms for rural communities? | Case studies of three rural wind farm development projects in different parts of the country |
How are viral marketing strategies changing the relationship between companies and consumers? | Case study of the iPhone X marketing campaign |
How do experiences of work in the gig economy differ by gender, race, and age? | Case studies of Deliveroo and Uber drivers in London |
Prevent plagiarism, run a free check.
Once you have developed your problem statement and research questions , you should be ready to choose the specific case that you want to focus on. A good case study should have the potential to:
- Provide new or unexpected insights into the subject
- Challenge or complicate existing assumptions and theories
- Propose practical courses of action to resolve a problem
- Open up new directions for future research
Unlike quantitative or experimental research, a strong case study does not require a random or representative sample. In fact, case studies often deliberately focus on unusual, neglected, or outlying cases which may shed new light on the research problem.
If you find yourself aiming to simultaneously investigate and solve an issue, consider conducting action research . As its name suggests, action research conducts research and takes action at the same time, and is highly iterative and flexible.
However, you can also choose a more common or representative case to exemplify a particular category, experience, or phenomenon.
While case studies focus more on concrete details than general theories, they should usually have some connection with theory in the field. This way the case study is not just an isolated description, but is integrated into existing knowledge about the topic. It might aim to:
- Exemplify a theory by showing how it explains the case under investigation
- Expand on a theory by uncovering new concepts and ideas that need to be incorporated
- Challenge a theory by exploring an outlier case that doesn’t fit with established assumptions
To ensure that your analysis of the case has a solid academic grounding, you should conduct a literature review of sources related to the topic and develop a theoretical framework . This means identifying key concepts and theories to guide your analysis and interpretation.
There are many different research methods you can use to collect data on your subject. Case studies tend to focus on qualitative data using methods such as interviews, observations, and analysis of primary and secondary sources (e.g., newspaper articles, photographs, official records). Sometimes a case study will also collect quantitative data .
The aim is to gain as thorough an understanding as possible of the case and its context.
In writing up the case study, you need to bring together all the relevant aspects to give as complete a picture as possible of the subject.
How you report your findings depends on the type of research you are doing. Some case studies are structured like a standard scientific paper or thesis, with separate sections or chapters for the methods , results , and discussion .
Others are written in a more narrative style, aiming to explore the case from various angles and analyse its meanings and implications (for example, by using textual analysis or discourse analysis ).
In all cases, though, make sure to give contextual details about the case, connect it back to the literature and theory, and discuss how it fits into wider patterns or debates.
Cite this Scribbr article
If you want to cite this source, you can copy and paste the citation or click the ‘Cite this Scribbr article’ button to automatically add the citation to our free Reference Generator.
McCombes, S. (2023, January 30). Case Study | Definition, Examples & Methods. Scribbr. Retrieved 5 August 2024, from https://www.scribbr.co.uk/research-methods/case-studies/
Is this article helpful?
Shona McCombes
Other students also liked, correlational research | guide, design & examples, a quick guide to experimental design | 5 steps & examples, descriptive research design | definition, methods & examples.
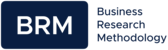
Case Studies
Case studies are a popular research method in business area. Case studies aim to analyze specific issues within the boundaries of a specific environment, situation or organization.
According to its design, case studies in business research can be divided into three categories: explanatory, descriptive and exploratory.
Explanatory case studies aim to answer ‘how’ or ’why’ questions with little control on behalf of researcher over occurrence of events. This type of case studies focus on phenomena within the contexts of real-life situations. Example: “An investigation into the reasons of the global financial and economic crisis of 2008 – 2010.”
Descriptive case studies aim to analyze the sequence of interpersonal events after a certain amount of time has passed. Studies in business research belonging to this category usually describe culture or sub-culture, and they attempt to discover the key phenomena. Example: “Impact of increasing levels of multiculturalism on marketing practices: A case study of McDonald’s Indonesia.”
Exploratory case studies aim to find answers to the questions of ‘what’ or ‘who’. Exploratory case study data collection method is often accompanied by additional data collection method(s) such as interviews, questionnaires, experiments etc. Example: “A study into differences of leadership practices between private and public sector organizations in Atlanta, USA.”
Advantages of case study method include data collection and analysis within the context of phenomenon, integration of qualitative and quantitative data in data analysis, and the ability to capture complexities of real-life situations so that the phenomenon can be studied in greater levels of depth. Case studies do have certain disadvantages that may include lack of rigor, challenges associated with data analysis and very little basis for generalizations of findings and conclusions.
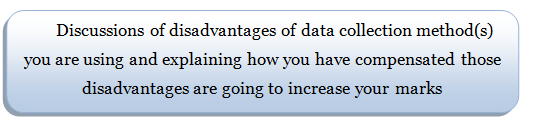
John Dudovskiy
- How to Order

Writing A Case Study
Case Study Examples

Brilliant Case Study Examples and Templates For Your Help
15 min read
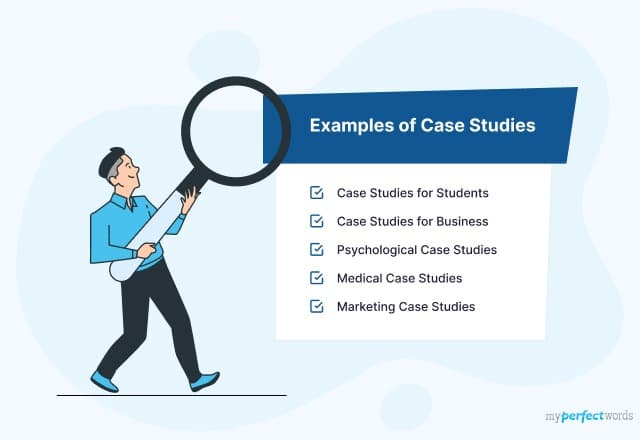
People also read
A Complete Case Study Writing Guide With Examples
Simple Case Study Format for Students to Follow
Understand the Types of Case Study Here
It’s no surprise that writing a case study is one of the most challenging academic tasks for students. You’re definitely not alone here!
Most people don't realize that there are specific guidelines to follow when writing a case study. If you don't know where to start, it's easy to get overwhelmed and give up before you even begin.
Don't worry! Let us help you out!
We've collected over 25 free case study examples with solutions just for you. These samples with solutions will help you win over your panel and score high marks on your case studies.
So, what are you waiting for? Let's dive in and learn the secrets to writing a successful case study.

- 1. An Overview of Case Studies
- 2. Case Study Examples for Students
- 3. Business Case Study Examples
- 4. Medical Case Study Examples
- 5. Psychology Case Study Examples
- 6. Sales Case Study Examples
- 7. Interview Case Study Examples
- 8. Marketing Case Study Examples
- 9. Tips to Write a Good Case Study
An Overview of Case Studies
A case study is a research method used to study a particular individual, group, or situation in depth. It involves analyzing and interpreting data from a variety of sources to gain insight into the subject being studied.
Case studies are often used in psychology, business, and education to explore complicated problems and find solutions. They usually have detailed descriptions of the subject, background info, and an analysis of the main issues.
The goal of a case study is to provide a comprehensive understanding of the subject. Typically, case studies can be divided into three parts, challenges, solutions, and results.
Here is a case study sample PDF so you can have a clearer understanding of what a case study actually is:
Case Study Sample PDF
How to Write a Case Study Examples
Learn how to write a case study with the help of our comprehensive case study guide.
Case Study Examples for Students
Quite often, students are asked to present case studies in their academic journeys. The reason instructors assign case studies is for students to sharpen their critical analysis skills, understand how companies make profits, etc.
Below are some case study examples in research, suitable for students:
|
Case Study Example in Software Engineering
Qualitative Research Case Study Sample
Software Quality Assurance Case Study
Social Work Case Study Example
Ethical Case Study
Case Study Example PDF
These examples can guide you on how to structure and format your own case studies.
Struggling with formatting your case study? Check this case study format guide and perfect your document’s structure today.
Business Case Study Examples
A business case study examines a business’s specific challenge or goal and how it should be solved. Business case studies usually focus on several details related to the initial challenge and proposed solution.
To help you out, here are some samples so you can create case studies that are related to businesses:
|
|
Here are some more business case study examples:
Business Case Studies PDF
Business Case Studies Example
Typically, a business case study discovers one of your customer's stories and how you solved a problem for them. It allows your prospects to see how your solutions address their needs.
Medical Case Study Examples
Medical case studies are an essential part of medical education. They help students to understand how to diagnose and treat patients.
Here are some medical case study examples to help you.
Medical Case Study Example
Nursing Case Study Example
Want to understand the various types of case studies? Check out our types of case study blog to select the perfect type.
Psychology Case Study Examples
Case studies are a great way of investigating individuals with psychological abnormalities. This is why it is a very common assignment in psychology courses.
By examining all the aspects of your subject’s life, you discover the possible causes of exhibiting such behavior.
For your help, here are some interesting psychology case study examples:
Psychology Case Study Example
Mental Health Case Study Example
Sales Case Study Examples
Case studies are important tools for sales teams’ performance improvement. By examining sales successes, teams can gain insights into effective strategies and create action plans to employ similar tactics.
By researching case studies of successful sales campaigns, sales teams can more accurately identify challenges and develop solutions.
Sales Case Study Example
Interview Case Study Examples
Interview case studies provide businesses with invaluable information. This data allows them to make informed decisions related to certain markets or subjects.
Interview Case Study Example
Marketing Case Study Examples
Marketing case studies are real-life stories that showcase how a business solves a problem. They typically discuss how a business achieves a goal using a specific marketing strategy or tactic.
They typically describe a challenge faced by a business, the solution implemented, and the results achieved.
This is a short sample marketing case study for you to get an idea of what an actual marketing case study looks like.
: ABC Solutions, a leading provider of tech products and services.
Engaging and informative content highlighting products and services. Incorporating real-world examples to showcase the impact of ABC Solutions. Utilizing analytics to refine content strategies. Aligning content with customer needs and pain points. Content marketing efforts led to a significant boost in brand visibility. Compelling narratives highlighting how products and services transformed businesses.
|
Here are some more popular marketing studies that show how companies use case studies as a means of marketing and promotion:
“Chevrolet Discover the Unexpected” by Carol H. Williams
This case study explores Chevrolet's “ DTU Journalism Fellows ” program. The case study uses the initials “DTU” to generate interest and encourage readers to learn more.
Multiple types of media, such as images and videos, are used to explain the challenges faced. The case study concludes with an overview of the achievements that were met.
Key points from the case study include:
- Using a well-known brand name in the title can create interest.
- Combining different media types, such as headings, images, and videos, can help engage readers and make the content more memorable.
- Providing a summary of the key achievements at the end of the case study can help readers better understand the project's impact.
“The Met” by Fantasy
“ The Met ” by Fantasy is a fictional redesign of the Metropolitan Museum of Art in New York City, created by the design studio Fantasy. The case study clearly and simply showcases the museum's website redesign.
The Met emphasizes the website’s features and interface by showcasing each section of the interface individually, allowing the readers to concentrate on the significant elements.
For those who prefer text, each feature includes an objective description. The case study also includes a “Contact Us” call-to-action at the bottom of the page, inviting visitors to contact the company.
Key points from this “The Met” include:
- Keeping the case study simple and clean can help readers focus on the most important aspects.
- Presenting the features and solutions with a visual showcase can be more effective than writing a lot of text.
- Including a clear call-to-action at the end of the case study can encourage visitors to contact the company for more information.
“Better Experiences for All” by Herman Miller
Herman Miller's minimalist approach to furniture design translates to their case study, “ Better Experiences for All ”, for a Dubai hospital. The page features a captivating video with closed-captioning and expandable text for accessibility.
The case study presents a wealth of information in a concise format, enabling users to grasp the complexities of the strategy with ease. It concludes with a client testimonial and a list of furniture items purchased from the brand.
Key points from the “Better Experiences” include:
- Make sure your case study is user-friendly by including accessibility features like closed captioning and expandable text.
- Include a list of products that were used in the project to guide potential customers.
“NetApp” by Evisort
Evisort's case study on “ NetApp ” stands out for its informative and compelling approach. The study begins with a client-centric overview of NetApp, strategically directing attention to the client rather than the company or team involved.
The case study incorporates client quotes and explores NetApp’s challenges during COVID-19. Evisort showcases its value as a client partner by showing how its services supported NetApp through difficult times.
- Provide an overview of the company in the client’s words, and put focus on the customer.
- Highlight how your services can help clients during challenging times.
- Make your case study accessible by providing it in various formats.
“Red Sox Season Campaign,” by CTP Boston
The “ Red Sox Season Campaign ” showcases a perfect blend of different media, such as video, text, and images. Upon visiting the page, the video plays automatically, there are videos of Red Sox players, their images, and print ads that can be enlarged with a click.
The page features an intuitive design and invites viewers to appreciate CTP's well-rounded campaign for Boston's beloved baseball team. There’s also a CTA that prompts viewers to learn how CTP can create a similar campaign for their brand.
Some key points to take away from the “Red Sox Season Campaign”:
- Including a variety of media such as video, images, and text can make your case study more engaging and compelling.
- Include a call-to-action at the end of your study that encourages viewers to take the next step towards becoming a customer or prospect.
“Airbnb + Zendesk” by Zendesk
The case study by Zendesk, titled “ Airbnb + Zendesk : Building a powerful solution together,” showcases a true partnership between Airbnb and Zendesk.
The article begins with an intriguing opening statement, “Halfway around the globe is a place to stay with your name on it. At least for a weekend,” and uses stunning images of beautiful Airbnb locations to captivate readers.
Instead of solely highlighting Zendesk's product, the case study is crafted to tell a good story and highlight Airbnb's service in detail. This strategy makes the case study more authentic and relatable.
Some key points to take away from this case study are:
- Use client's offerings' images rather than just screenshots of your own product or service.
- To begin the case study, it is recommended to include a distinct CTA. For instance, Zendesk presents two alternatives, namely to initiate a trial or seek a solution.
“Influencer Marketing” by Trend and WarbyParker
The case study "Influencer Marketing" by Trend and Warby Parker highlights the potential of influencer content marketing, even when working with a limited budget.
The “Wearing Warby” campaign involved influencers wearing Warby Parker glasses during their daily activities, providing a glimpse of the brand's products in use.
This strategy enhanced the brand's relatability with influencers' followers. While not detailing specific tactics, the case study effectively illustrates the impact of third-person case studies in showcasing campaign results.
Key points to take away from this case study are:
- Influencer marketing can be effective even with a limited budget.
- Showcasing products being used in everyday life can make a brand more approachable and relatable.
- Third-person case studies can be useful in highlighting the success of a campaign.
Marketing Case Study Template
Marketing Case Study Example
Now that you have read multiple case study examples, hop on to our tips.
Tips to Write a Good Case Study
Here are some note-worthy tips to craft a winning case study
- Define the purpose of the case study This will help you to focus on the most important aspects of the case. The case study objective helps to ensure that your finished product is concise and to the point.
- Choose a real-life example. One of the best ways to write a successful case study is to choose a real-life example. This will give your readers a chance to see how the concepts apply in a real-world setting.
- Keep it brief. This means that you should only include information that is directly relevant to your topic and avoid adding unnecessary details.
- Use strong evidence. To make your case study convincing, you will need to use strong evidence. This can include statistics, data from research studies, or quotes from experts in the field.
- Edit and proofread your work. Before you submit your case study, be sure to edit and proofread your work carefully. This will help to ensure that there are no errors and that your paper is clear and concise.
There you go!
We’re sure that now you have secrets to writing a great case study at your fingertips! This blog teaches the key guidelines of various case studies with samples. So grab your pen and start crafting a winning case study right away!
Having said that, we do understand that some of you might be having a hard time writing compelling case studies.
But worry not! Our expert case study writing service is here to take all your case-writing blues away!
With 100% thorough research guaranteed, our online essay service can craft an amazing case study within 24 hours!
So why delay? Let us help you shine in the eyes of your instructor!

Write Essay Within 60 Seconds!

Dr. Barbara is a highly experienced writer and author who holds a Ph.D. degree in public health from an Ivy League school. She has worked in the medical field for many years, conducting extensive research on various health topics. Her writing has been featured in several top-tier publications.

Paper Due? Why Suffer? That’s our Job!
Keep reading
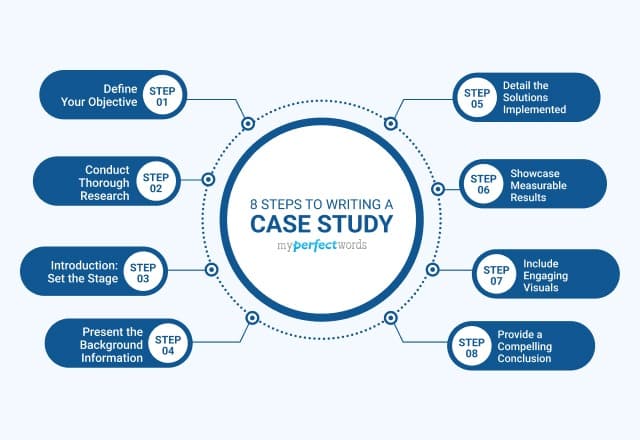

Investigating the Impact of Social Media Applications on Promoting EFL Learners' Oral Communication Skills: A Case Study of Saudi Universities
- Somia Ali Mohammed Idries Qassim University
- Mohammed AbdAlgane Qassim University
- Asjad Ahmed Saeed Balla Qassim University
- Awwad Othman Abdelaziz Ahmed Taif University
Social media platforms exert a substantial influence on the improvement of learners' spoken communication abilities. The objective of this study is to investigate the effects of incorporating social media platforms on enhancing the development of oral communication abilities among English as Foreign Language (EFL) learners enrolled in the English Department at Qassim University, Kingdom of Saudi Arabia (KSA). This study aims to examine the correlation between the utilization of social media applications and the enhancement of oral communication abilities among EFL learners, to determine the impact of social media on oral communication skills. The present study employed a descriptive-analytical methodology to explore the effects of utilizing social media applications on enhancing students' proficiency in oral communication abilities. To get adequate data for this study, a survey was conducted among a sample of 40 participants. The purpose of the questionnaire is to gather data regarding the learners' perspectives on their attitudes toward utilizing social media as a means of enhancing their oral communication abilities. The questionnaire comprises a total of ten items. The survey instrument employed in this study utilizes a close-ended question format, wherein participants are instructed to select the most suitable response option by marking it. The Likert Scale questionnaire was utilized to gather statistical data. The results of the study indicated that the utilization of social media platforms among EFL learners majoring in English at universities in Saudi Arabia yielded favorable results, leading to improvements in their spoken communication abilities.
Author Biographies
Somia ali mohammed idries, qassim university.
Department of English Language & Literature, College of Languages & Humanities
Mohammed AbdAlgane, Qassim University
Asjad ahmed saeed balla, qassim university, awwad othman abdelaziz ahmed, taif university.
Department of Foreign Languages, College of Arts
Abdalgane, M. (2022). The EFL Learning Process: An Examination of the Potential of Social Media. World J. Engl. Lang, 12, 69-75.
Aforo, A. A. (2014). Impact of social media on academic reading: A study at Kwame. Nkrumah University of Science and Technology, Kumasi, Ghana. Asian Journal of Humanities and Social Studies, 2(1), 92-99.
Ahmed, M. A. (2016). Using Facebook to develop grammar discussion and writing skills in English as a foreign language for university students. Sino-US English Teaching, 13(12), 932-952.
Albahiri, M. H., & Alhaj, A. A. M. (2020). Role of visual element in spoken English discourse: implications for YouTube technology in EFL classrooms. The Electronic Library. https://doi.org/10.1108/EL-07-2019-0172
Al Harbi, W. N. (2021). The Role of Social Media (YouTube and Snapchat) in Enhancing Saudi EFL Learners' Listening Comprehension Skills. https://doi.org/10.31235/osf.io/tpfxk
Ali, & Bin-Hady, W. (2019). A study of EFL students' attitudes, motivation and anxiety towards WhatsApp as a language learning tool. Arab World English Journal (AWEJ) Special Issue on CALL, (5).
Allam, M., Elyas, T., Bajnaid, A., & Rajab, H. (2017). Using Twitter as an ELT tool in the Saudi EFL context. International Journal of Linguistics, 9(5), 41-63. https://doi.org/10.5296/ijl.v9i5.11813
Almogheerah, A. (2021). Exploring the effect of using WhatsApp on Saudi female EFL students' idiom-learning. Arab World English Journal (AWEJ), 11. https://doi.org/10.2139/ssrn.3764287
Alshalan, K. (2019). Investigating EFL Saudi students’ vocabulary improvement in micro-blogging on Twitter at Imam University. International Journal of Linguistics, Literature and Translation, 2(2), 290245.
Alshammari, R., Parkes, M., & Adlington, R. (2017). Using WhatsApp in EFL instruction with Saudi Arabian university students. Arab World English Journal (AWEJ), 8. https://doi.org/10.24093/awej/vol8no4.5
Alsharidi, N. K. (2018). The use of Twitter amongst female Saudi EFL learners. International Journal of Applied Linguistics and English Literature, 7(4), 198-205. https://doi.org/10.7575/aiac.ijalel.v.7n.4p.198
Bensalem, E. (2018). The impact of WhatsApp on EFL students' vocabulary learning. Arab World English Journal (AWEJ) Volume, 9. Ahmed, M. A. (2016). Using Facebook to develop grammar discussion and writing skills in English as a foreign language for university students. Sino-US English Teaching, 13(12), 932-952. https://doi.org/10.5296/ijl.v9i5.11813
Boyd, D. M., & Ellison, N. B. (2007). Social network sites: Definition, history, and scholarship. Journal of Computer‐mediated Communication, 13(1), 210-230.
Bygate, M. (2002). Speaking. In R. Carter & D. Nunan (Eds.), The Cambridge Guide to Teaching English to Speakers of Other Languages (pp. 14–20). Cambridge: Cambridge University Press.
Eren, Ö. (2012). Students’ Attitudes towards Using Social Networking in Foreign [3]. Language Classes: A Facebook Example. International Journal of Business and Social Science, 288-294.
Ghoneim, N.M.M. and Abdelsalam, H.E. (2016). Using Voice Thread to develop EFL preservice teachers’ speaking skills. International Journal of English Language Teaching, 4(6), 13–31.
Hosseini, E. Z., Nasri, M., & Afghari, A. (2017). Looking beyond teachers’ classroom behavior: novice and experienced EFL teachers’ practice of pedagogical Knowledge to Improve Learners’ Motivational Strategies. Journal of Applied Linguistics and Language Research, 4(8), 183-200.
Khan, R. M. I., Radzuan, N. R. M., Farooqi, S. U. H., Shahbaz, M., & Khan, M. S. (2021). Learners' Perceptions on WhatsApp Integration as a Learning Tool to Develop EFL Vocabulary for Speaking Skill. International Journal of Language Education, 5(2), 1-14. https://doi.org/10.26858/ijole.v5i2.15787
Lau, R. Y. (2012). An empirical study of online social networking for enhancing counseling and related fields. International Journal of e-Education, e-Business, e-Management and e-Learning. https://doi.org/10.7763/IJEEEE.2012.V2.158
Manning, J. (2014). Social media, definition, and classes of social media. In book: Encyclopedia of Social Media and Politics (pp. 1158-1162). Sage Publications. Editors: Kerric Harvey.
Mao, J. (2014). Social media for learning: A mixed methods study on high school students' technology affordances and perspectives. Computers in Human Behavior, 33, 213-223. https://doi.org/10.1016/j.chb.2014.01.002
Marleni, Lusi. and Asilestari, Putri. (2018). The effect of using social media: WhatsApp toward the students’ speaking skill. Journal of English Language and Education, 3(2), 1-16.
Namaziandost, E., Abdi Saray, A., & Rahimi Esfahani, F. (2018). The effect of writing practice on improving speaking skill among pre-intermediate EFL learners. Theory and Practice in Language Studies, 8(1), 1690-1697.
Namaziandost, Ehsan. and Nasri, Mehdi. (2019). The impact of social media on EFL learners’ speaking skill: A survey study involving EFL teachers and students. Journal of Applied Linguistics and Language Research, 6(3), 199-215.
Nilayon, N., & Brahmakasikara, L. (2018). Using Social Network Sites for Language Learning and Video Conferencing Technology to Improve English Speaking Skills: A Case Study of Thai Undergraduate Students. LEARN Journal: Language Education and Acquisition Research Network, 11(1), 47-63.
Omar, H. (2015). The impact of using YouTube in EFL classroom on enhancing EFL students' content learning. Journal of College Teaching & Learning, 12(2), 121-126.
Rahmah, R.E. (2018). Sharing photographs on Instagram boosts students’ self-confidence in speaking English. Pedagogy: Journal of English Language Teaching, 6(2), 148–158. DOI: https://doi.org/10.32332/pedagogy.v6i2.1335 .
Schaffer, N. (2013). Maximize your social: A one-stop guide to building a social media strategy for marketing and business success. John Wiley & Sons.
Sevy-Biloon, J. and Chroman, T. (2019). Authentic use of technology to improve EFL communication and motivation through international language exchange video chat. Teaching English with Technology, 19(2), 44-58.
Top, E. (2012). Blogging as a social medium in undergraduate courses: Sense of community best predictor of perceived learning. The Internet and Higher Education, 15(1), 24-28. https://doi.org/10.1016/j.iheduc.2011.02.001 .
Zaitun, Z.; Hadi, M.S. and Indriani, E.D. (2021). Tik Tok as a media to enhancing the speaking skills of EFL students. Jurnal Studi Guru Dan Pembelajaran, 4(1), 89-94.
Copyright © 2015-2024 ACADEMY PUBLICATION — All Rights Reserved
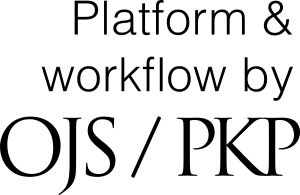
ORIGINAL RESEARCH article
Solid waste management service chain and sanitation safety: a case study of existing practice in addis ababa, ethiopia.
- Division of Water and Health, Ethiopian Institute of Water Resources, Addis Ababa University, Addis Ababa, Ethiopia
Background: Poor sanitation safety in municipal solid waste management can cause environmental and public health problems. This is the case in Ethiopia, where the sanitation safety standards are low in the municipal solid waste management operations. Therefore, the sanitation safety practices along the solid waste management service chains in Addis Ababa, Ethiopia are poorly understood, and this research will contribute new insights for the scientific community and can also inform policies and the current solid waste management operations in Addis Ababa.
Materials and Methods: This study evaluated the safety of sanitation practices in the solid waste management service chain using a community-based approach in Addis Ababa city from January to August 2023. We have interviewed 384 participants using a cluster-random sampling technique and collected data through direct observations and face-to-face interviews. The study employed descriptive statistics, factor analysis and multiple linear logistic regression to analyze the data.
Results: The findings of the study revealed significant variations in sanitation safety practices and risks among households during solid waste management. While 60% of households practiced solid waste segregation, only 15% of them followed safe segregation practices. The majority of households (85%) used unsafe segregation practices, such as mixing different types of waste and storing wet and dry waste together. Additionally, 85% of households used storage and transport containers that had leaks, potentially leading to contamination and infection. Furthermore, the study identified sanitation safety risks and practices at waste collection and transport sites. The risks included solid waste droppings during transport, inadequate vehicle cleaning and disinfection, lack of personal protective equipment (PPE) for workers, and uncovered waste collection vehicles, leading to environmental contamination. At transfer stations, the study found several risk factors, such as the lack of protection from animals and human activities, absence of shower facilities for workers, and inadequate storage facilities for PPE and tools. The transfer stations also lacked odor-neutralizing systems, proper waste handling practices, and physical fly barriers. Workers did not have the opportunity to shower after work, further increasing the risk. The sanitation safety practices and risks at solid waste treatment/disposal sites were also assessed. The study revealed medium risks associated with waste treatment/disposal operations, including working without PPE, handling contaminated containers and raw waste, and releasing airborne particulates that could be inhaled by workers or the nearby community. Factor analysis was conducted to categorize the variables related to sanitation safety practices. Six factors were identified, explaining approximately 60.6% of the overall variance. These factors represented different aspects of sanitation safety, including onsite waste handling practices, failure to maintain proper standards, risks related to unsafe waste storage, failure to properly store wastes at the household level, having safe storage practices, and unsafe waste segregation and storage. The study also examined the association between sanitation safety practices and sociodemographic factors using multiple linear regression analysis. Marital status, education, occupation, and income were found to be significant factors influencing sanitation safety practices during onsite waste handling. Income and marital status had the highest contribution, while occupation had the lowest contribution.
Conclusion and Recommendation: the research findings highlight the wide variation in sanitation safety practices and risks associated with solid waste management. The study emphasizes the need for improved waste management practices at the household level, waste collection and transport sites, transfer stations, and waste treatment/disposal sites. The identified risk factors should be addressed through targeted interventions, including public awareness campaigns, proper training of waste management workers, and the implementation of safety protocols and infrastructure improvements. Additionally, sociodemographic factors play a role in determining sanitation safety practices, emphasizing the importance of considering these factors when developing waste management strategies and interventions.
1 Introduction
The United Nations Environment Programme ( Wilson et al., 2015 ) global waste management outlook warns that the growing volume and complexity of garbage produced by the modern economy puts ecosystems and human health at risk. An estimated 11.2 billion tonnes of solid trash are collected annually worldwide, and around 5% of the global greenhouse gas emissions are caused by organic waste decomposition ( Ram et al., 2021 ). Solid waste management (SWM) is the process of collecting, treating, and disposing of solid materials that are discarded because they have served their purpose or are no longer useful. SWM can pose various environmental, health, and safety risks, such as pollution, disease transmission, fire, explosion, injury, and accidents ( Naidu et al., 2021 ).
How much people are exposed depends on many factors. It is important to consider how different solid waste management methods, ways of moving contaminants, and health effects are connected. People can get exposed by touching waste, breathing polluted air, or eating polluted food or water ( Alam et al., 2022 ).
Solid waste management has different activities along the service chain, which include generation, collection, transportation, treatment, reuse, recycling, and disposal. Risks are present at every step of the service chain, from the point of generation at homes to solid waste recycling and disposal ( Ike et al., 2018 ; Beka and Meng, 2021 ). Solid waste management workers can be affected by various health and sanitation safety risks, especially injuries, allergies, respiratory, gastrointestinal, and infectious diseases ( Cruvinel et al., 2019 ; Melaku and Tiruneh, 2020 ). For instance, according to a study among municipal solid waste workers in Egypt, poor personal hygiene, inadequate use of personal protective equipment, and failure to apply safety measures were associated with accidents and needle stick injuries in 46.5% and 32.7% of the study participants respectively ( Madian and Abd El-Wahed, 2018 ). A similar study also reported that 73.8% of the study participants had unsafe solid waste management practices which caused a high prevalence of gastrointestinal, respiratory, skin, and other infectious diseases ( Kasemy et al., 2021 ). Another similar assessment on occupational health and safety among scavengers in the Gaza Strip, Palestine, revealed that the occupational health and safety conditions of waste pickers are in a state of constant deterioration, primarily due to the informal nature of their work. These waste pickers are reportedly facing severe hardships, with the majority lacking access to potable water, adequate sanitation, and hygienic places to sleep and eat. Furthermore, none of the waste pickers have ever received occupational health and safety training, exacerbating their vulnerability and health risks ( Al-Khatib et al., 2020 ).
Improper disposal of household solid waste can cause environmental degradation and deterioration. When organic solids decompose, they produce odors, leachate, and other acids that can destroy plants, dissolve important soil minerals, and contaminate groundwater. This can lead to ecosystem disturbances by some organisms such as water hyacinth, which kills aquatic life and causes water-borne diseases such as cholera, diarrhea, dysentery, and typhoid ( Mandevere and Jerie, 2018 ; Rautela et al., 2021 ).
Waste generation in Addis Ababa is driven by rapid urbanization, population growth, and economic activities. The waste includes household, commercial, industrial, and healthcare waste, with a significant portion being organic waste ( Mekonnen et al., 2024 ). Waste collection in Addis Ababa faces several challenges, including inadequate coverage, irregular service, and insufficient infrastructure. Many areas, especially informal settlements, do not receive regular waste collection services. Collection is often done using outdated and insufficient equipment, leading to inefficiencies and environmental pollution. In recent years, efforts have been made to improve collection services through the involvement of private sector players and community-based organizations. These initiatives aim to enhance the reach and efficiency of waste collection services across the city ( Teshager Alemu, 2017 ). Transporting waste to disposal sites is another critical stage. The city’s waste transportation system is often hindered by traffic congestion, inadequate vehicles, and poor road conditions. This results in delays and increases the risk of waste being dumped illegally or improperly managed. The city has initiated waste-to-energy projects, such as the Reppie waste-to-energy facility, which aims to convert waste into electricity. However, these projects face challenges related to technology, maintenance, and operational sustainability ( Teshager Alemu, 2017 ). A significant portion of the waste generated in Addis Ababa is organic, making composting a viable treatment option. However, the city’s composting infrastructure is underdeveloped, and much of the organic waste ends up in landfills due to insufficient sorting at the source. Plastic and metal recycling facilities exist but are limited, affecting the overall efficiency of the waste management system ( Cheru, 2016 ).
The primary disposal site for Addis Ababa is the Repi landfill, also known as Koshe, which has been operational for several decades. Despite efforts to improve its management, the landfill remains a significant environmental and health concern. The city has explored waste-to-energy projects to reduce landfill dependency, but these initiatives are still in early stages ( Furgasa et al., 2023 ).
Municipal solid waste poses a risk to the environment and public health in Addis Ababa, as only a fraction of it is properly managed. Out of the daily waste generation, 65% is collected and disposed of, 5% is recycled, 5% is composted, and the rest 25% is left uncollected and dumped in unauthorized areas ( Gelan, 2021 ). In the city, inadequate household solid waste collection and disposal has led to significant waste piles in open temporary collection sites, building corridors and sewers. Until it is taken to the city’s disposal site, the collected garbage is kept at roadside and between community neighborhoods. Furthermore, the collected waste is entirely left outside for days, exposed to sun and rain, and different animals including street dogs, cattle and horse scatter the solid waste in the surrounding. The piles and scattered wastes produce an offensive odor, ruin the surrounding urban landscape, attract pests, and interfere with local people’s daily activities ( Mohammed and Elias, 2017 ). Meanwhile, uncollected waste is disposed informally, with a small percentage being burned and dumped in open areas, drainage canals, rivers and gorges, and on the street ( Gelan, 2021 ). The open-air burning and spontaneous combustion in dumping sites produce air pollution and unpleasant odors, which can travel several kilometers. These problems are exacerbated in areas where there is no solid waste collection at all such as in slum areas ( Mazhindu et al., 2010 ).
The improper management of the solid waste in the city has become a threat to the surface and groundwater sources. The solid waste management system has several problems, despite the gravity of the issue. For instance, a study conducted on occupational injuries and illness symptoms among Addis Ababa city solid waste collectors reported that only 43.6% of municipal solid waste collectors were using some form of personal protective equipment (PPE) while performing their duties. However, 22.5% of these PPE users stated that they did not use their PPE constantly while performing their duties, indicating their awareness gap. Another study on the occupational health conditions and contributing factors among municipal solid waste collectors reported that 71.1% of the study participants did not receive occupational safety training ( Melaku and Tiruneh, 2020 ). Approximately 74% of this study participants did not immediately manage their personal hygiene; 73.1% of municipal solid waste collectors have no access to PPE from their company and are forced to buy PPE for themselves ( Melaku et al., 2020 ).
The study conducted on groundwater pollution and public health risk analysis in the vicinity of Reppi solid waste dumping site also concluded that the solid waste disposal site significantly impacts groundwater pollution and public health ( Zedwie, 2007 ). A study carried out on the health risk assessment of heavy metals in exposed workers of municipal waste recycling facility in Iran showed that, waste recyclers, dismantlers and waste sorters have the highest exposure and public health risks to hazardous metals due to their occupational exposure who are working in the Municipal solid waste recycling sites ( Ghobakhloo et al., 2024 ).
Studies on sanitation safety measures, standards, and approaches to implementation practice along the whole solid waste management service chain are scarce. There is no community-based study on assessing the existing practice of sanitation safety along the solid waste management service chain in Addis Ababa, Ethiopia. The current safety practices, risks, and their impact on public health and the environment are not well understood. Studies that can help to propose corrective measures that can help to appropriately maintain the sanitation safety practices across the municipal solid waste management service chains are rare. Therefore, this study was designed to look how the sanitation safety standards are practiced/implemented along the solid waste management service chains in Addis Ababa and generate scientific evidence that can inform policies and the current solid waste management operations.
2 Materials and methods
2.1 description of study area and sampling sites.
The study area was Addis Ababa, the capital city of Ethiopia and the seat of both federal and regional governments. The city covers an area of 54 km 2 and has an altitude ranging from 2,000 m to 2,800 m. It is surrounded by the Oromia National Regional State and divided into 11 sub-cities and 116 Districts. The city has a population of approximately 6 million people and is experiencing rapid urbanization and infrastructure development. Addis Ababa hosts over 2,000 industries, such as potable water, cement, textile, beverage and alcohol, tobacco, leather, tannery, plastic, and food factories. The city is the country’s industrial, cultural, administrative, commercial, and modern hub, as well as one of the central hubs in Africa with many international organizations and institutions ( Spaliviero and Cheru, 2017 ). The African Union, United Nations Economic Commission for Africa, and more than a hundred embassies are in Addis Ababa. The city is regarded as Africa’s diplomatic capital and a symbol of humanitarian progress on the continent.
The study assessed the sanitation safety practices in 384 households (HHs) located in 23 districts of the ten sub-cities, which are represented by highlighted marks ( Figure 1 ), and field observations were conducted on the operations of four solid waste collection and transport operations, at four solid waste transfer stations and in one final disposal, and recycling center.
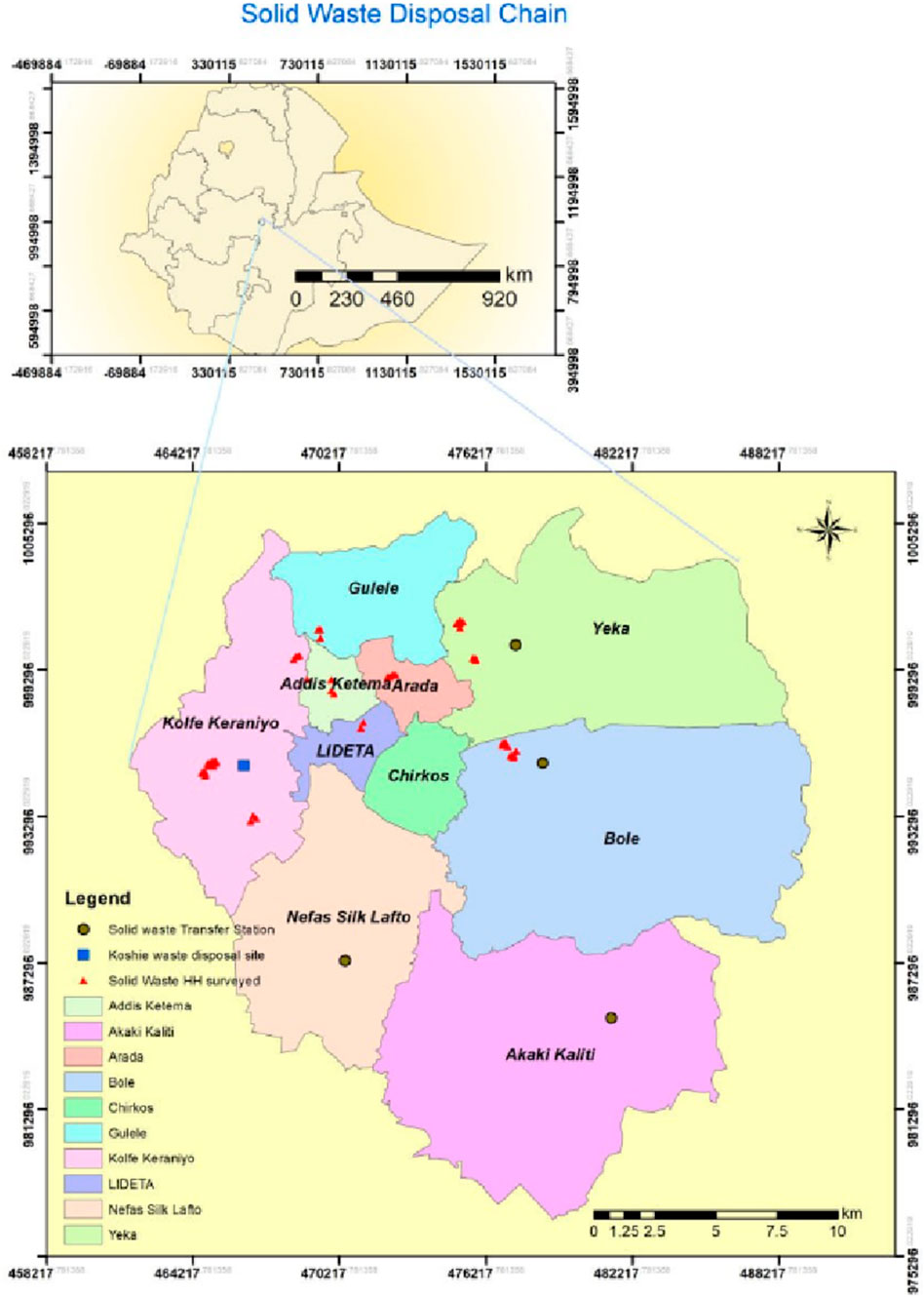
Figure 1 . Maps of study sites showing the solid waste management service chain.
2.2 Study design, and population selection
This study aimed to assess the sanitation safety of the solid waste management service chain in Addis Ababa city, Ethiopia, from January to August 2023. The study used a community-based cross-sectional design and collected both qualitative and quantitative data from various sources. The study population included community members who generated solid waste and solid waste management service providers who were involved in waste collection, transportation, treatment, and disposal. The study adapted tools from the World Health Organization (WHO) Water and Sanitation Safety planning manual ( Bartram, 2009 ; World Health Organization, 2015 ) and to measure the sanitation safety indicators along the service chain.
2.3 Sample size, sampling technique, and sampling procedure
2.3.1 sample size.
The sample size for the quantitative data was determined using the single population proportion formula. Given the parameters, the calculation was based on a 95% confidence level, represented by a Z value of 1.96, and a precision or margin of error set at 5%. In the absence of prior studies on sanitation safety along the solid waste management service chain in Addis Ababa, and lacking the time to conduct a pilot study, we assumed the proportion (P) to be 0.5. This assumption provides the most conservative estimate, ensuring the largest necessary sample size.
The formula ( Degu, 2005 ) used is as follows:
Where, n = the required sample size
p = the average proportion of in different settings.
Z = the critical value at 95% confidence level = 1.96.
d = precision (margin of error) = 5%.
To account for a potential non-response rate of 5%, the initial sample size of 384 was increased, resulting in a final sample size of 403 participants. This adjustment aims to mitigate the impact of non-participation and ensure sufficient data collection. Ultimately, data was collected from 385 participants, representing a 5% non-response rate.
The achieved sample size of 385 participants was designed to be representative of the broader population, based on several key factors. To enhance representativeness, a random sampling method was employed, ensuring that each member of the target population had an equal chance of being selected. This minimized selection bias and helped to achieve a sample that mirrors the population’s diversity. The sample covered various geographical areas within Addis Ababa and included diverse demographic segments such as different age groups, genders, socio-economic statuses, and educational backgrounds. This diversity helps in capturing a wide range of perspectives and behaviors related to sanitation safety.
While the calculated sample size included a 5% buffer for non-response, the final sample size of 385 falls slightly short of the intended 403. This slight shortfall is within acceptable limits and still allows for a reliable representation of the population. Efforts were made to follow up with non-respondents and encourage their participation to reduce non-response bias.
In the absence of previous studies specifically on sanitation safety along the solid waste management service chain in Addis Ababa, the use of a conservative proportion estimate ( p = 0.5) provided a robust and safe estimate for the required sample size. Careful design and implementation of the survey further enhanced representativeness. This included clear and unbiased questions, trained data collectors, and ensuring accessibility of the survey to all potential participants, including those with limited literacy or digital access.
The sample size of 385, despite falling slightly short of the intended 403, was calculated using rigorous statistical principles to ensure representativeness. Random sampling, demographic and geographical coverage, and efforts to minimize non-response bias were critical in achieving a representative sample. While the methodology provided a solid foundation, the actual representativeness also depended on the practical execution of the sampling and survey processes. By following these guidelines, the sample is designed to be a reliable representation of the population for the study on sanitation safety along the solid waste management service chain in Addis Ababa.
2.3.2 Sampling technique and sampling procedure
The sampling techniques used for the quantitative data included simple random sampling and cluster sampling. Figure 2 illustrates the household sampling procedures followed. For the qualitative data, purposive sampling was employed. The steps of the sampling procedure was as follows:
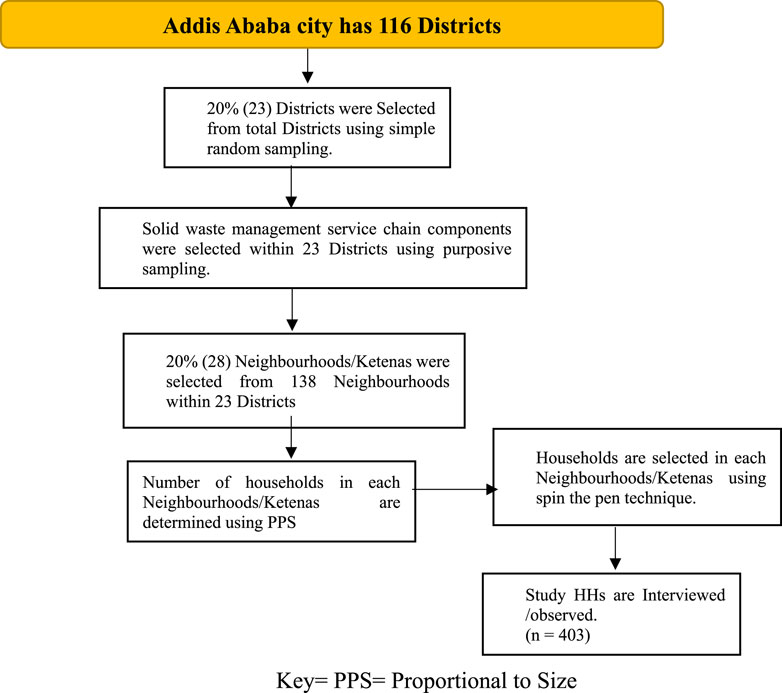
Figure 2 . Sampling technique and sampling procedure.
First, we identified the key actors involved in the solid waste management service chain in Addis Ababa, such as waste collectors, transporters, treatment plant operators, landfill workers, and municipal officials.
Second, we selected a representative sample of each actor group purposively based on their availability, willingness, and experience in the solid waste management service chain.
Third, we conducted in-depth interviews with the selected participants using a semi-structured interview guide.
2.4 Data collection methods and tools
The study utilized a cross-sectional research design to evaluate sanitation safety practices in solid waste management. The data collection process adhered to the methodology outlined in the WHO Sanitation Safety Planning Manual, Second Edition ( World Health Organization, 2015 ). A structured semi-quantitative risk assessment questionnaire was developed, taking into account the manual’s guidelines and tailored to the specific study context. The questionnaire encompassed multiple sections that addressed various facets of solid waste management, including household waste handling practices, waste collection and transport, transfer stations, and solid waste treatment/disposal sites.
To ensure the credibility and accuracy of the data, a pilot study was conducted with a small sample of participants. Study subjects and areas under the solid waste management service chain were selected from the total number of Addis Ababa city administration Districts using simple random and cluster sampling methods. The primary sampling units, Districts, were selected using simple random sampling techniques. Accordingly, 20% (23 out of 116) of the total Districts were selected for the household survey.
Following the selection of the primary sampling unit (Districts), secondary sampling unit (neighborhoods) were considered as clusters, assuming homogeneity among them concerning sanitation safety practices. Neighborhoods within the randomly selected Districts were included based on the Probability Proportion to Sampling from each sampled District. The sampling frame was constructed by obtaining a list of neighborhoods with their household size from the sampled Districts. Subsequently, neighborhoods were randomly selected from the 23 Districts, resulting in a total of 28 neighborhoods (20% of the 138 neighborhoods).
The number of households in each selected neighborhood was determined using the Probability Proportional to Size (PPS) method, where size is defined as the total number of households derived from the population size in the sampled neighborhoods. Finally, tertiary sampling units (households) were selected using the “spin the pen” technique to identify the starting point within a sampled neighborhood. Spinning a ballpoint pen at the center of the neighborhood helped the study team randomly choose a direction to follow. Once the starting household was identified, households who were beneficiaries of the solid waste service chain and residing in the sampled neighborhoods were interviewed/observed using a standardized questionnaire until the desired sample size per neighborhood was achieved.
Other components of the solid waste management service chain, including waste collection, transportation, treatment, and disposal sites and service providers, were purposively selected. Field observations were conducted on the operations of four solid waste collection and transport operations, four solid waste transfer stations, and one final disposal and recycling center. Key informant interviews were also conducted with responsible personnel at the solid waste collection and transport operations, solid waste transfer stations, and the final disposal and recycling center to obtain additional primary information on the practice of safe solid waste management operations. These personnel provided insights into the facilities and processes involved.
Data collectors, each holding a Bachelor of Science degree in Environmental Health, were carefully selected based on their expertise and experience. They underwent a comprehensive 2-day training program that included 1 day of theoretical training and 1 day of practical pretesting. The training covered the study’s objectives, ethical considerations, detailed instructions on administering the questionnaire, and techniques for accurate data recording.
The proficient data collectors conducted household surveys under the supportive supervision of field supervisors who possessed a Master of Science degree in Environmental Health. The field supervisors provided continuous guidance and quality control to ensure the reliability of the data collected. Additionally, four data collectors with a Master of Science degree in Environmental Health were assigned to collect qualitative data across the solid waste management service chain, conducting in-depth interviews and focus group discussions.
The questionnaires, initially designed in English, were translated into the local language, Amharic, to facilitate effective communication and ensure comprehension by the respondents. The translation process included back-translation to verify accuracy and cultural relevance. Based on feedback from the pilot study, the questionnaire was further refined to improve clarity and relevance.
Trained surveyors administered the finalized questionnaire to the selected households in face-to-face interviews, ensuring that all sections were thoroughly covered. The administration process included obtaining informed consent, explaining the purpose of the study, and ensuring the confidentiality of the responses. In addition to the survey, direct observations were conducted at waste collection and transport sites, transfer stations, and solid waste treatment/disposal sites. These observations aimed to evaluate sanitation safety practices and identify potential risks, providing a comprehensive understanding of the solid waste management system.
2.5 Data processing and analysis
The collected data underwent a series of steps, including data entry, cleaning, editing, and analysis, conducted by the principal investigators using SPSS version 26 (Statistical Package for the Social Sciences). These processes aimed to ensure the accuracy, consistency, and completeness of the data, enhancing the reliability of the analyzed results.
To categorize sanitation safety risk practices, the study followed the risk scoring system outlined in the WHO Sanitation Safety Planning Manual, Second Edition. Risk levels were classified as low risk, medium risk, high risk, and very high risk. Table 1 shows semi-quantitative risk assessment matrix we have used to analyse the sanitation safety practices along the solid waste management service chain:

Table 1 . Semi-quantitative risk assessment matrix for sanitation safety practices in solid waste management.
Diagnostic sanitary inspection questions were utilized to assign standard scores to each component of the safe solid waste management system, enabling the evaluation of risk levels associated with sanitation safety practices.
Descriptive statistics, such as frequency tables, percentages, means, and standard deviations, were employed to analyze most variables. These statistics provided a comprehensive overview of the data, allowing for a better understanding of the distribution and characteristics of the variables.
Additionally, factor analysis was conducted to assess the variability and identify common themes among observed, correlated variables related to sanitation safety practices. This analysis aimed to determine the relative importance of variables contributing to sanitation safety risks at the household level. The Kaiser-Meyer-Olkin (KMO) Measure of Sampling Adequacy value of 0.680 indicated that the manifest variables had enough in common to justify the use of factor analysis on the empirical data, supporting the validity of this technique. To enable linear regression analysis, transformations were applied to the originally categorical data, creating continuous data. Multiple linear regression was then conducted to estimate the relationship between sanitation safety practices and socio-demographic variables.
The data cleaning process ensured accuracy, consistency, and completeness of the data and variables, enhancing the reliability of the analyzed results.
3.1 Socio-demographic characteristics
A total of 384 individuals (95% participation rate) provided information on their gender, religion, education level, marital status, and income for the research. Table 2 presents the frequency (percentage) of these major socio-demographic characteristics of the study population.
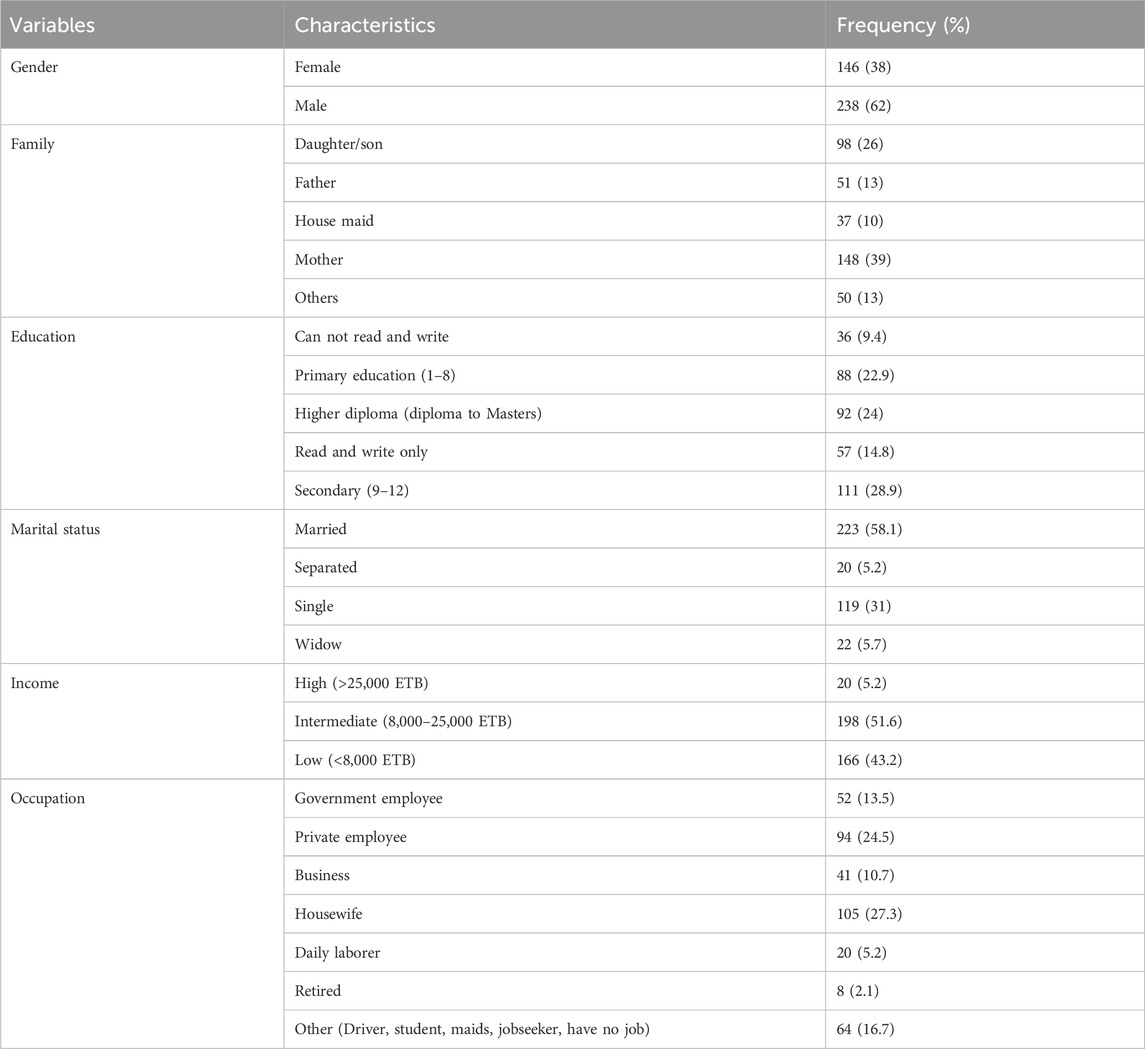
Table 2 . Socio-demographic characteristics of study participants.
3.2 Sanitation safety and risk assessment in solid waste management operations
3.2.1 sanitation safety and risk in household solid waste management.
The study aimed to assess sanitation safety practices and associated risks in household solid waste management within the study area. Sixteen indicators were used, categorized according to the WHO Sanitation Safety Planning Manual ( World Health Organization, 2015 ). These indicators were classified into four levels of sanitation safety risk: low (<6), intermediate (6–12), high (13–32), and very high (>32), with higher scores indicating higher risks.
Results revealed significant variations in sanitation safety practices and risks among households. Hazardous practices were observed, indicating significant risks to human health and the environment. For example, while 60% of households practiced solid waste segregation, only 15% implemented safe segregation practices, such as using separate bins, washing, and drying waste before storage, and using protective gloves and masks. The remaining 85% of households engaged in unsafe segregation practices, including mixing different waste types and storing wet and dry waste together without any protection. Additionally, 85% of households used leaky storage and transport containers, leading to potential contamination and infection.
Nevertheless, according to the risk level categorization by the World Health Organization (WHO), the findings indicated that a significant majority of households (88%) were classified as low risk. Conversely, 12% of households were categorized as having an intermediate risk level. Notably, no households were identified as having high or very high-risk scores.
Factor analysis was conducted to further examine sanitation safety practices and risks related to household solid waste management which helped to reduce the number of variables, categorize them into groups, and ascertain the significance of each variable in relation to the risks of sanitation safety at the household level ( Table 3 ). To determine the number of factors to retain, the eigenvalue-greater-than-one (1) retention criterion was utilized. Accordingly, six factors were retained, explaining approximately 60.6% of the overall variance. Conversely, the remaining ten factors were excluded as they collectively accounted for only about 39.9% of the total variance.
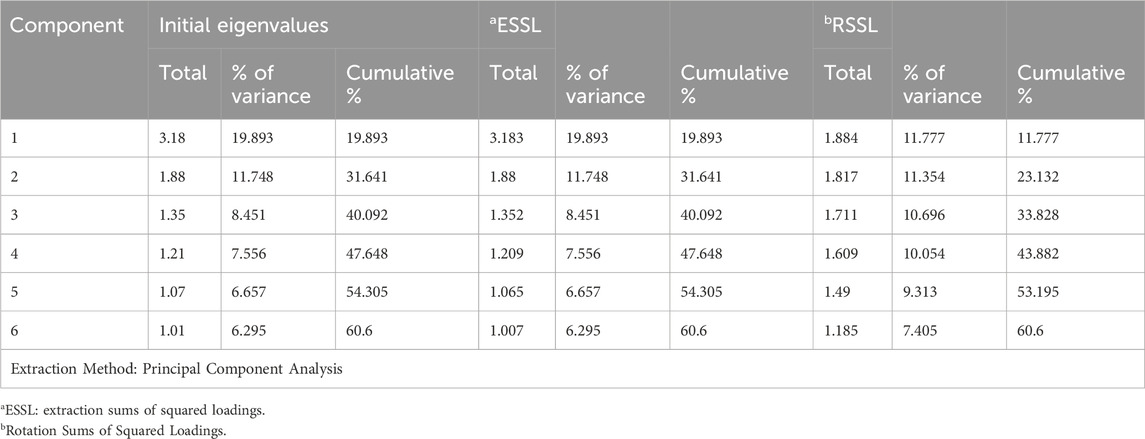
Table 3 . Factor analysis—total variance explained.
The varimax rotation with Kaiser normalization identified six factors from the indicator variables. The four variables that loaded high on Factor 1 were related to “sanitation safety practices during onsite waste handling.” These variables were access to a solid waste collection service, proper onsite solid waste storage, waste segregation at home, and handwashing after waste handling. Factor 2 represented “failure to maintain proper sanitation safety standards in waste storage and safe waste handling.” The three variables that loaded high on this factor were mixing hazardous wastes with other wastes, unclean waste container, and hand contamination due to lack of handwashing. Factor 3 depicted “risks related to unsafe management of waste storage at household level”. The three variables that loaded high on this factor were emission of airborne particulates from poor sealing of waste storage containers, exposure to sanitation safety risks during primary collection, and poor waste storage at household level. Factor 4 indicated “failure to properly store wastes at household level.” The four variables that loaded high on this factor were lack of access to handwashing facilities, presence of scavenging animals and rodents at waste storage container, presence of flies/bad smell in the storage container, and presence of accumulated refuse near to the houses (20 m). Factor 5 described “having safe storage at house”. The four variables that loaded high on this factor were airborne particulates from poor sealing of waste storage containers, presence of flies/bad smell in the storage container, accumulated refuse near household, and leak-free closed container for onsite storage. Factor 6 reflected “Unsafe waste segregation and storage.” The two variables that loaded high on this factor were safe waste segregation practice and waste scattering/splashing from waste storage container (especially solid waste, urine, faeces, tissue) and contaminates surfaces.
3.2.2 Sanitation safety practices and risk at the waste collection and transport sites
The study employed a health risk assessment matrix to evaluate sanitation safety practices and risks in solid waste collection and transport across four operation areas (Akaki kality, Bole, Yeka, and Nifas Silk-Lafto sites). Fourteen sanitation safety risks/practices were identified, including waste droppings during transport, inadequate vehicle cleaning and disinfection, lack of personal protective equipment (PPE) for workers, and uncovered waste collection vehicles, leading to environmental contamination. Observations at the collection and transport sites highlighted risks faced by workers, such as the inability to shower after work, handling different waste types, feeling stressed and disrespected, and wearing dirty and damaged PPE. These factors posed high threats to human health and the environment.
3.2.3 Sanitation safety and risk at transfer stations
The study assessed sanitation safety practices and risks at four transfer stations. Thirteen diagnostic indicators were collected, all of which (100%) were identified as risk factors for safe solid waste management at the transfer stations. Risk factors included lack of protection from animals, scavengers, and human activities, absence of shower facilities for workers, inadequate facilities for washing boots and tools, absence of separate storage facilities for workers’ clothing and PPE, lack of odor-neutralizing systems, failure to practice “first-in, first-out” waste handling, absence of physical fly barriers, and workers not showering after work. All these factors were observed as the highest risk factors related to ineffective sanitation safety practices during solid waste management at the transfer stations.
3.2.4 Sanitation safety and risk at solid waste treatment/disposal site
The study assessed sanitation safety practices and risks at Reppi/Koshe solid waste disposal and recycling site. The study utilized a sanitary safety inspection checklist adapted from WHO and other sources to assess sanitation safety practices and risks associated with solid waste disposal and reuse. Out of the eleven sanitary safety assessment questions, nine (82%) were identified as risk factors for safe solid waste management at the Solid Waste Treatment/Disposal Site which is classified as “medium risk” to workers, the nearby community, and the environment as per the WHO semi quantitative risk score levels due to waste treatment/disposal operations. Risk factors at the disposal site included working without personal protective clothing, handling contaminated containers and raw waste, splashing contaminated waste on operators, and releasing airborne particulates that could be inhaled by operators or the nearby community. Table 4 shows the summary of risk levels of sanitation safety practices along the solid waste management service chain evaluated by standard risk scores based sanitary inspection questions (SIQ).
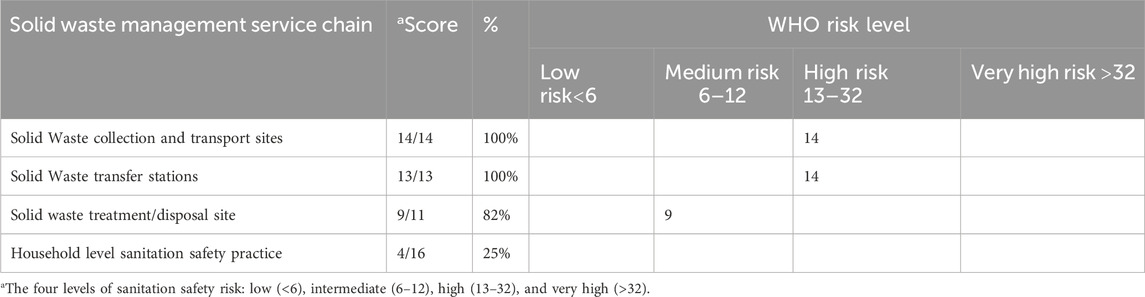
Table 4 . Summary of the risk levels of sanitation safety practices along the solid waste management service chain.
3.3 The association between sanitation safety practices and sociodemographic factors
Multiple linear regression was conducted to determine if the dependent variable shows a linear relationship with the independent variables (Socio demographic variables) ( Table 5 ). Correlation analysis was conducted to examine the strength of relationship between independent and outcome variables. It is observed that gender, marital status, education, occupation, and income are highly correlated. The multiple linear regression analysis shows that marital status, education, occupation, and income of the respondent are significant ( p < 0.05). More specifically, income and marital status have the highest contribution to applying sanitation safety practices during onsite waste handling; whereas occupation had the lowest contribution as indicated in a standardized beta coefficient column ( Table 5 ).
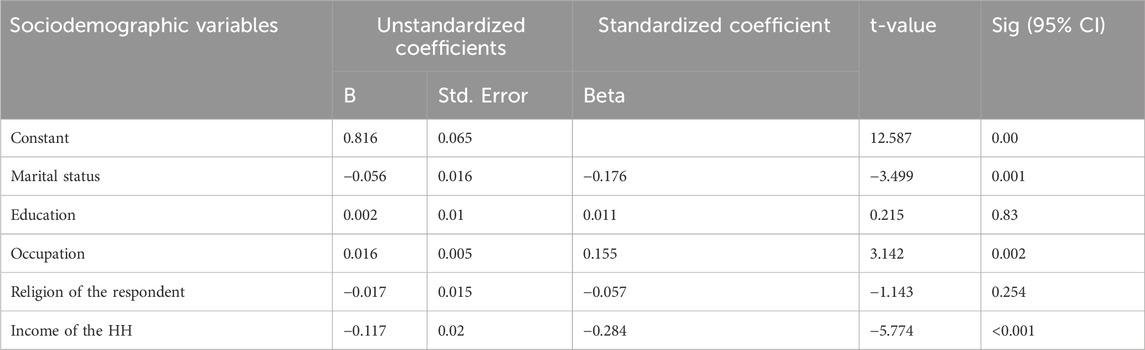
Table 5 . Multiple linear regression analysis of sanitation safety practices and sociodemographic characteristics of the households.
4 Discussion
The Our study’s results reflect a scenario where the majority of households exhibit intermediate risk in their waste management practices. This intermediate risk category suggests that while some waste management measures are in place, they are insufficient to mitigate potential adverse effects. Such practices include sporadic waste collection, improper disposal methods, and a lack of waste segregation, all of which contribute to increased risks of health and environmental degradation. This finding aligns with previous research conducted in developing countries, which similarly reports suboptimal waste management practices and the associated risks ( Srivastava et al., 2015 ; Mmereki et al., 2016 ; Serge Kubanza and Simatele, 2020 ).
For instance, studies in urban areas of developing countries frequently highlight challenges such as inadequate waste collection infrastructure, limited recycling facilities, and inefficient waste disposal practices ( Wilson and Velis, 2014 ). These deficiencies often result in health risks such as the spread of infectious diseases, including cholera and respiratory infections, and environmental problems such as soil and water contamination, as noted by several researchers ( Hoornweg and Bhada-Tata, 2012 ; Katiyar, 2016 ). The intermediate risk levels observed in our study reflect a similar pattern of inadequate waste management practices that have been documented globally.
The implications of these practices are profound. Poor waste management can lead to the accumulation of waste in public spaces, creating breeding grounds for vectors like mosquitoes and rodents, which can transmit diseases ( Akmal and Jamil, 2021 ). Furthermore, improper waste disposal can lead to the leaching of contaminants into groundwater and the emission of greenhouse gases from decomposing organic waste, both of which have long-term environmental consequences ( Kaza et al., 2018 ). The findings from this study are consistent with these observations, reinforcing the understanding that intermediate levels of risk in household waste management can have serious repercussions for public health and environmental sustainability.
Comparative analysis with similar research in developing countries reveals that our findings are part of a broader trend ( Wilson et al., 2012 ) which demonstrate that in many developing regions, the waste management systems are often inadequate due to infrastructural limitations, economic constraints, and insufficient regulatory frameworks. This study’s results contribute to a growing body of evidence indicating that without significant improvements in waste management practices, communities will continue to face health and environmental risks.
Socio-demographic factors, such as marital status, education, occupation, and income, were found to significantly influence sanitation safety practices. Married individuals tended to handle household waste more safely than single or divorced individuals. Income emerged as the most important factor for safe waste segregation and storage, as higher and middle-income households had better sanitation facilities and equipment. Education played a role in the safety of waste storage, as more educated individuals had greater awareness, knowledge, and access to information and technology for reducing sanitation risks. Occupation had the least impact on maintaining sanitation safety standards, with housewives, maids, and students being more exposed to unsafe waste handling practices than other professionals.
The findings align with a study conducted in the East Coast of Malaysia ( Fadhullah et al., 2022 ), which also identified income and marital status as significant influencers of sanitation safety practices. However, our study revealed a much lower percentage (15%) of households practicing safe waste segregation compared to the study in Malaysia. These differences may be attributed to socio-economic and cultural factors that influence waste management behaviors in different countries. Similarly, a study in Benin highlighted the influence of socio-demographic characteristics, including income, marital status, and education level, on adopting good hygiene and sanitation practices ( Sintondji et al., 2017 ). Our study is consistent with a study conducted in Bogotá, Colombia, which pointed out that low income and education levels impact households’ sanitation safety practices during solid waste management ( J Padilla and Trujillo, 2018 ).
Education emerged as a significant determinant contributing to household-level solid waste handling and transport. Better awareness of the risks associated with solid waste led to more careful and effective waste handling and transport practices. Households with higher education levels demonstrated greater awareness of the dangers of solid waste, as supported by evidence from various countries such as Malaysia ( Afroz, 2011 ; Al-Dailami et al., 2022 ) and Islamabad ( Anjum, 2013 ).
The findings from our study align with several other studies conducted globally. For instance, research in Benin highlighted the influence of socio-demographic characteristics, including income, marital status, and education level, on adopting good hygiene and sanitation practices ( Sintondji et al., 2017 ). The consistency of our findings with those from various regions underscores the universal impact of socio-demographic factors on waste management practices.
However, the disparities observed, such as the lower percentage of households practicing safe waste segregation in our study compared to Malaysia, suggest that socio-economic and cultural factors play a significant role in shaping waste management behaviors. These differences highlight the need for tailored interventions that consider the unique socio-demographic contexts of different communities.
Education emerged as a particularly significant determinant in enhancing household-level solid waste handling and transport. Households with higher education levels demonstrated a greater awareness of the risks associated with solid waste, leading to more careful and effective waste handling and transport practices. This finding is supported by evidence from multiple studies, which indicate that better-educated individuals are more likely to adopt safer sanitation practices due to their increased awareness and access to relevant information and technologies. The consistency of our findings with those from various regions underscores the universal impact of socio-demographic factors on waste management practices.
Education emerged as a particularly significant determinant in enhancing household-level solid waste handling and transport. Households with higher education levels demonstrated a greater awareness of the risks associated with solid waste, leading to more careful and effective waste handling and transport practices. This finding is supported by evidence from multiple studies, which indicate that better-educated individuals are more likely to adopt safer sanitation practices due to their increased awareness and access to relevant information and technologies ( Fadhullah et al., 2022 ; Habib, 2022 ).
The study also revealed unsafe and risky conditions during waste collection and transport operations, exposing workers to various risks. Inadequate access to personal protective equipment, sanitation facilities, and safe waste collection and transport equipment, along with low worker awareness of the risks associated with handling solid waste, contributed to these unsafe conditions. This finding is consistent with a study conducted in Alexandria, Egypt ( Abd El-Wahab et al., 2014 ) which identified municipal solid waste management as one of the most dangerous jobs, exposing households and workers to physical, biological, and chemical hazards and occupational-related morbidities.
At the transfer station, all thirteen diagnostic indicators were identified as risk factors, indicating that several factors negatively affect the service chain. The high risk scores for sanitation safety practices and risks at transfer stations reflect a poor solid waste management system. Inadequate facilities to prevent odors, waste scattering, waste scavengers, and protection for workers contribute to these risk factors. Studies have shown that inadequate and mismanaged waste transfer stations can have significant public health and environmental consequences ( Sarkhosh et al., 2017 ; Dixit et al., 2022 ). Similar findings have been reported in studies conducted in Addis Ababa ( Mohammed and Elias, 2017 ), North East of Tehran ( Daryabeigi Zand et al., 2019 ) and ( Nhubu et al., 2021 ) Harare, Zimbabwe which highlighted the associations between transfer stations near residential areas and adverse human health and environmental impacts, particularly regarding occupational health conditions.
Sanitation safety risk assessment during solid waste collection operations yielded a high-risk score of 14, indicating high risk levels ( Vimercati et al., 2016 ). Common risk factors along the sanitation service chain during collection and transport included waste dropping on the ground and scattering in the environment, leading to infections in humans and environmental contamination. The unhygienic condition of vehicles emerged as a major risk factor for worker and community contamination during solid waste collection and transport. Environmental impacts from collection and transport primarily arise from the operation of collection and transport vehicles ( Gupta et al., 2015 ), further emphasizing the risks posed to workers and the surrounding community. A study conducted in Ghana highlighted psychological stress and job satisfaction as significant factors ( Lissah et al., 2022 ; Tshivhase et al., 2022 ).
The study findings also revealed that 82% of sanitation safety standards were not followed during waste reuse/disposal operations, indicating significant risks associated with these practices. Workers and the nearby community are exposed to bad odors, direct contact with waste on the skin, handling contaminated containers and raw waste, and performing tasks without personal protective clothing. The reuse/disposal operations result in contaminated waste and leachate being splashed into the environment, posing serious risks to individuals. These findings are consistent with studies conducted in Darfur state, Sudan ( Adam et al., 2015 ), Freetown, Sierra ( Sankoh et al., 2013 ), Kolkata, India ( De and Debnath, 2016 ) and Umuahia, Nigeria ( Chibwe et al., 2021 ).
Overall, these findings emphasize the multifaceted nature of solid waste management issues, with socio-demographic factors, lack of adequate facilities, and unsafe practices contributing to significant health and environmental risks. Addressing these challenges requires a comprehensive approach, incorporating policy interventions, community education, and improved infrastructure to enhance sanitation safety practices and mitigate associated risks.
5 Conclusion
In conclusion, this study reveals significant deficiencies in sanitation safety practices throughout the entire solid waste management process, from households to waste collection and transport sites, transfer stations, and solid waste treatment/disposal sites. Hazardous practices were observed, posing risks to human health and the environment. Factors such as unsafe waste handling, inadequate storage, and improper waste segregation were identified as key contributors to these risks. To address these issues, it is recommended to implement targeted interventions. These include raising awareness among households about proper waste segregation and storage, enforcing regulations for regular cleaning and disinfection of waste collection vehicles, improving physical infrastructure at transfer stations, implementing proper waste handling practices at treatment/disposal sites, and establishing comprehensive policies and regulations alongside monitoring mechanisms. Tailoring interventions based on socio-demographic factors such as income, education, and marital status is essential to support vulnerable populations and improve waste management practices. Additionally, fostering international collaboration to exchange best practices adapted to local contexts is crucial. These proposed measures aim to enhance sanitation safety practices, mitigate health risks, and promote environmental sustainability. By addressing identified deficiencies through a coordinated approach, communities can establish safer and more effective solid waste management systems.
Data availability statement
The raw data supporting the conclusions of this article will be made available by the authors, without undue reservation.
Ethics statement
The study received ethical approval from the Ministry of Education National Research Ethics Review committee, in accordance with the Ethiopia National Research Ethics Review Guideline (Fifth Edition). Written informed consent was obtained from all participants who took part in the study, after explaining the purpose and significance of the research. Data collection proceeded only after obtaining fully informed verbal consent from the participants, and confidentiality measures were implemented to protect their privacy by excluding their names and personal identification information.
Author contributions
SS: Writing–original draft. SG: Writing–review and editing. AA: Writing–review and editing.
The author(s) declare that no financial support was received for the research, authorship, and/or publication of this article.
Acknowledgments
We would like to thank the experts from Addis Ababa Solid Waste Management Agency who helped us during the data collection process along the solid waste service chain.
Conflict of interest
The authors declare that the research was conducted in the absence of any commercial or financial relationships that could be construed as a potential conflict of interest.
Publisher’s note
All claims expressed in this article are solely those of the authors and do not necessarily represent those of their affiliated organizations, or those of the publisher, the editors and the reviewers. Any product that may be evaluated in this article, or claim that may be made by its manufacturer, is not guaranteed or endorsed by the publisher.
Supplementary material
The Supplementary Material for this article can be found online at: https://www.frontiersin.org/articles/10.3389/fenve.2024.1414669/full#supplementary-material
Abd El-Wahab, E. W., Eassa, S. M., Lotfi, S. E., El Masry, S. A., Shatat, H. Z., and Kotkat, A. M. (2014). Adverse health problems among municipality workers in Alexandria (Egypt). Int. J. Prev. Med. 5 (5), 545–556.
PubMed Abstract | Google Scholar
Adam, B., Elgader, A., and Abdelrhman, I. (2015). Health and environmental impacts due to final disposal of solid waste in Zalingy town-central Darfur State-Sudan. Int. J. Res. Granthaalayah 4 (11), 92–100.
Google Scholar
Afroz, R. (2011). Sustainable household waste management improvement in Dhaka city, Bangladesh. Int. J. Environ. Sustain. Dev. 10 (4), 433–448. doi:10.1504/ijesd.2011.047775
CrossRef Full Text | Google Scholar
Akmal, T., and Jamil, F. (2021). Assessing health damages from improper disposal of solid waste in metropolitan Islamabad–Rawalpindi, Pakistan. Sustainability 13 (5), 2717. doi:10.3390/su13052717
Alam, P., Sharholy, M., Khan, A. H., Ahmad, K., Alomayri, T., Radwan, N., et al. (2022). Energy generation and revenue potential from municipal solid waste using system dynamic approach. Chemosphere 299, 134351. doi:10.1016/j.chemosphere.2022.134351
PubMed Abstract | CrossRef Full Text | Google Scholar
Al-Dailami, A., Ahmad, I., Kamyab, H., Abdullah, N., Koji, I., Ashokkumar, V., et al. (2022). Sustainable solid waste management in Yemen: environmental, social aspects, and challenges. Biomass Convers. Biorefinery , 1–27. doi:10.1007/s13399-022-02871-w
Al-Khatib, I. A., Al-Sari, M. I., and Kontogianni, S. (2020). Assessment of occupational health and safety among scavengers in Gaza Strip, Palestine. J. Environ. public health 2020 (1), 3780431–3780439. doi:10.1155/2020/3780431
Anjum, R. (2013). Willingness to pay for solid waste management services: a case study of Islamabad . Islamabad, Pakistan: Pakistan Institute of Development Economics .
Bartram, J. (2009). Water safety plan manual: step-by-step risk management for drinking-water suppliers . Geneva, Switzerland: World Health Organization .
Beka, D. D., and Meng, X.-Z. (2021). Redesign solid waste collection and transference system for Addis Ababa (Ethiopia) based on the comparison with Shanghai, China. OALib 08 (5), 1–23. doi:10.4236/oalib.1107470
Cheru, M. (2016). Solid Waste Management in Addis Ababa: a new approach to improving the waste management system .
Chibwe, W., Mbewe, A., and Hazemba, A. N. (2021). The health effects of Chunga Dumpsite on surrounding communities in Lusaka, Zambia . medRxiv. 2021.12. 21.21268110.
Cruvinel, V. R. N., Marques, C. P., Cardoso, V., Novaes, MRCG, Araújo, W. N., Angulo-Tuesta, A., et al. (2019). Health conditions and occupational risks in a novel group: waste pickers in the largest open garbage dump in Latin America. BMC public health 19 (1), 581–615. doi:10.1186/s12889-019-6879-x
Daryabeigi Zand, A., Vaeziheir, A., and Hoveidi, H. (2019). Comparative evaluation of unmitigated options for solid waste transfer stations in North East of Tehran using rapid impact assessment matrix and Iranian Leopold matrix. Environ. Energy Econ. Res. 3 (3), 189–202.
De, S., and Debnath, B. J. P. E. S. (2016). Prevalence of health hazards associated with solid waste disposal-A case study of Kolkata, India , 35, 201–208.
Degu, G. (2005). Fasil tessema university of gondar .
Dixit, A., Singh, D., and Shukla, S. K. (2022). Changing scenario of municipal solid waste management in Kanpur city, India. J. Material Cycles Waste Manag. 24 (5), 1648–1662. doi:10.1007/s10163-022-01427-4
Fadhullah, W., Imran, N. I. N., Ismail, S. N. S., Jaafar, M. H., and Abdullah, H. (2022). Household solid waste management practices and perceptions among residents in the East Coast of Malaysia. BMC public health 22 (1), 1–20. doi:10.1186/s12889-021-12274-7
Furgasa, W., Hongbin, C., Mariye, M., Desalegne, D. G., Ararsa, F., and Abdela, S. (2023). Assessment of integrated solid waste management practices in Addis Ababa city: the case of akaki sub city, Ethiopia. IJSRP 13 (8), 1–24. doi:10.29322/ijsrp.13.08.2023.p14002
Gelan, E. (2021). Municipal solid waste management practices for achieving green architecture concepts in Addis Ababa, Ethiopia. Technologies 9 (3), 48. doi:10.3390/technologies9030048
Ghobakhloo, S., Mostafaii, G. R., Khoshakhlagh, A. H., Moda, H. M., and Gruszecka-Kosowska, A. (2024). Health risk assessment of heavy metals in exposed workers of municipal waste recycling facility in Iran. Chemosphere 346, 140627. doi:10.1016/j.chemosphere.2023.140627
Gupta, N., Yadav, K. K., and Kumar, V. (2015). A review on current status of municipal solid waste management in India. J. Environ. Sci. 37, 206–217. doi:10.1016/j.jes.2015.01.034
Habib, S. (2022). Impact of urbanization on sanitation management in Pakistan: the case of Islamabad capital territory. Ann. Hum. Soc. Sci. 3 (2), 495–508. doi:10.35484/ahss.2022(3-ii)47
Hoornweg, D., and Bhada-Tata, P. (2012). What a waste: a global review of solid waste management .
Ike, C., Ezeibe, C. C., Anijiofor, S. C., and Daud, N. N. N. (2018). Solid waste management in Nigeria: problems, prospects, and policies. J. Solid Waste Technol. Manag. 44 (2), 163–172. doi:10.5276/jswtm.2018.163
J Padilla, A., and Trujillo, J. C. (2018). Waste disposal and households’ heterogeneity. Identifying factors shaping attitudes towards source-separated recycling in Bogotá, Colombia. Waste Manag. 74, 16–33. doi:10.1016/j.wasman.2017.11.052
Kasemy, Z. A., Rohlman, D. S., and Abdel Latif, A. A. (2021). Health disorders among Egyptian municipal solid waste workers and assessment of their knowledge, attitude, and practice towards the hazardous exposure. Environ. Sci. Pollut. Res. 28, 30993–31002. doi:10.1007/s11356-021-12856-3
Katiyar, M. (2016). Solid waste management. RIET-IJSET: international journal of science. RIET-IJSET Int. J. Sci. Eng. Technol. 3 (2), 117–124. doi:10.5958/2395-3381.2016.00015.0
Kaza, S., Yao, L. C., Bhada-Tata, P., and Woerden, F. V. (2018). What a waste 2.0: a global snapshot of solid waste management to 2050 . Washington, DC, United States: World Bank Publications .
Lissah, S. Y., Ayanore, M. A., Krugu, J. K., Aberese-Ako, M., and Ruiter, R. A. C. (2022). “Our work, our health, No one’s concern”: domestic waste collectors’ perceptions of occupational safety and self-reported health issues in an urban town in Ghana. Int. J. Environ. Res. public health 19 (11), 6539. doi:10.3390/ijerph19116539
Madian, A. A. E.-A. M., and Abd El-Wahed, A. Y. (2018). Adverse health effects among solid waste collectors in Alexandria Governorate. Int. J. Occup. Health Public Health Nurs. 5 (2), 23–48.
Mandevere, B., and Jerie, S. (2018). Household solid waste management: how effective are the strategies used in Harare Zimbabwe. J Environ Waste Manag. Recycl. 2 (1). 16, 2018. 22 .
Mazhindu, E., Gumbo, T., and Gondo, T. (2010). Living with environmental health risks — the case of Addis Ababa. Ecohydrol. and Hydrobiology 10 (2-4), 281–286. doi:10.2478/v10104-011-0026-3
Mekonnen, T., Araya, M. M., Abeje, G., Chanie, A. A., Alemayehu, S., Yimam, Y., et al. (2024). “Evaluation of evolving waste management strategies in Addis Ababa city, Ethiopia: a life cycle assessment approach,” in EcoDesign for sustainable products, services and social systems II ( Springer ), 171–186.
Melaku, H. S., and Tiruneh, M. A. (2020). Occupational health conditions and associated factors among municipal solid waste collectors in Addis Ababa, Ethiopia. Risk Manag. Healthc. Policy 13, 2415–2423. doi:10.2147/rmhp.s276790
Melaku, H. S., Tiruneh, M. A. J. R. M., and Policy, H. (2020). Occupational health conditions and associated factors among municipal solid waste collectors in Addis Ababa, Ethiopia , 2415–2423.
Mmereki, D., Baldwin, A., and Li, B. (2016). A comparative analysis of solid waste management in developed, developing and lesser developed countries. Environ. Technol. Rev. 5 (1), 120–141. doi:10.1080/21622515.2016.1259357
Mohammed, A., and Elias, E. (2017) Domestic solid waste management and its environmental impacts in Addis Ababa city. Journal of Environment and Waste management. 4 (1), 194–203.
Naidu, R., Biswas, B., Willett, I. R., Cribb, J., Kumar Singh, B., Paul Nathanail, C., et al. (2021). Chemical pollution: a growing peril and potential catastrophic risk to humanity. Environ. Int. 156, 106616. doi:10.1016/j.envint.2021.106616
Nhubu, T., Murwira, T., Mugabe, J., Maposa, S., Dube, N., Chikukwa, P., et al. (2021). Assessment of the municipal solid waste transfer stations suitability in Harare . Zimbabwe .
Sintondji, R. O., Tossa, S. E. Y., Sogbohossou, N. O., Yabi, J. A., Adjahossou, R. A. D. C., Sinsin, B., et al. (2017). Socio-demographic characteristics of households as determinants of access to water, hygiene and sanitation in So-Ava, Benin. J. Environ. Sci. Public Health 1 (4), 253–267. doi:10.26502/jesph.96120023
Ram, C., Kumar, A., and Rani, P. (2021). Municipal solid waste management: a review of waste to energy (WtE) approaches. Bioresources 16 (2), 4275–4320. doi:10.15376/biores.16.2.ram
Rautela, R., Arya, S., Vishwakarma, S., Lee, J., Kim, K. H., and Kumar, S. (2021). E-waste management and its effects on the environment and human health. Sci. Total Environ. 773, 145623. doi:10.1016/j.scitotenv.2021.145623
Sankoh, F. P., Yan, X., and Tran, Q. (2013). Environmental and health impact of solid waste disposal in developing cities: a case study of granville brook dumpsite, Freetown, Sierra Leone. J. Environ. Prot. 2013.
Sarkhosh, M., Shamsipour, A., Yaghmaeian, K., Nabizadeh, R., Naddafi, K., and Mohseni, S. M. (2017). Dispersion modeling and health risk assessment of VOCs emissions from municipal solid waste transfer station in Tehran, Iran. J. Environ. Health Sci. Eng. 15, 4–7. doi:10.1186/s40201-017-0268-0
Serge Kubanza, N., and Simatele, M. D. (2020). Sustainable solid waste management in developing countries: a study of institutional strengthening for solid waste management in Johannesburg, South Africa. J. Environ. Plan. Manag. 63 (2), 175–188. doi:10.1080/09640568.2019.1576510
Spaliviero, M., and Cheru, F. (2017). The state of Addis Ababa 2017: the Addis Ababa we want. State Addis Ababa 2017 Addis Ababa we want .
Srivastava, V., Ismail, S. A., Singh, P., and Singh, R. P. (2015). Urban solid waste management in the developing world with emphasis on India: challenges and opportunities. Rev. Environ. Sci. Bio/Technology 14, 317–337. doi:10.1007/s11157-014-9352-4
Teshager Alemu, K. (2017). Formal and informal actors in Addis Ababa’s solid waste management system. IDS Bull. 48 (2). doi:10.19088/1968-2017.116
Tshivhase, S. E., Mashau, N. S., Ngobeni, T., and Ramathuba, D. U. (2022). Occupational health and safety hazards among solid waste handlers at a selected municipality South Africa. Health SA Gesondheid (Online) 27, 1–8. doi:10.4102/hsag.v27i0.1978
Vimercati, L., Baldassarre, A., Gatti, M., De Maria, L., Caputi, A., Dirodi, A., et al. (2016). Respiratory health in waste collection and disposal workers. Int. J. Environ. Res. public health 13 (7), 631. doi:10.3390/ijerph13070631
Wilson, D. C., and Velis, C. A. (2014). Cities and waste: current and emerging issues . London, England: SAGE Publications Sage UK , 797–799.
Wilson, D. C., Rodic, L., Scheinberg, A., Velis, C. A., and Alabaster, G. (2012). Comparative analysis of solid waste management in 20 cities. Waste Manag. and Res. J. a Sustain. Circular Econ. 30 (3), 237–254. doi:10.1177/0734242x12437569
Wilson, D. C., Rodic, L., Modak, P., Soos, R., Carpintero, A., Velis, K., et al. (2015). Global waste management outlook . Osaka, Japan: UNEP .
World Health Organization (2015). Sanitation safety planning: manual for safe use and disposal of wastewater greywater and Excreta . Geneva, Switzerland: World Health Organization .
Zedwie, T. (2007). Groundwater pollution and public health risk analysis in the vicinity of Reppi solid waste dumping site, Addis Ababa city, Ethiopia . Addis Ababa, Ethiopia: Citeseer .
Keywords: municipal waste, sanitation chain, sanitation safety, solid waste, waste collection, waste disposal
Citation: Sisay SF, Gari SR and Ambelu A (2024) Solid waste management service chain and sanitation safety: a case study of existing practice in Addis Ababa, Ethiopia. Front. Environ. Eng. 3:1414669. doi: 10.3389/fenve.2024.1414669
Received: 09 April 2024; Accepted: 29 July 2024; Published: 09 August 2024.
Reviewed by:
Copyright © 2024 Sisay, Gari and Ambelu. This is an open-access article distributed under the terms of the Creative Commons Attribution License (CC BY). The use, distribution or reproduction in other forums is permitted, provided the original author(s) and the copyright owner(s) are credited and that the original publication in this journal is cited, in accordance with accepted academic practice. No use, distribution or reproduction is permitted which does not comply with these terms.
*Correspondence: Shegaw Fentaye Sisay, [email protected]
Disclaimer: All claims expressed in this article are solely those of the authors and do not necessarily represent those of their affiliated organizations, or those of the publisher, the editors and the reviewers. Any product that may be evaluated in this article or claim that may be made by its manufacturer is not guaranteed or endorsed by the publisher.
Thank you for visiting nature.com. You are using a browser version with limited support for CSS. To obtain the best experience, we recommend you use a more up to date browser (or turn off compatibility mode in Internet Explorer). In the meantime, to ensure continued support, we are displaying the site without styles and JavaScript.
- View all journals
- Explore content
- About the journal
- Publish with us
- Sign up for alerts
- Open access
- Published: 08 August 2024
Precious2GPT: the combination of multiomics pretrained transformer and conditional diffusion for artificial multi-omics multi-species multi-tissue sample generation
- Denis Sidorenko ORCID: orcid.org/0009-0004-0571-5192 1 ,
- Stefan Pushkov 1 ,
- Akhmed Sakip 1 ,
- Geoffrey Ho Duen Leung 1 ,
- Sarah Wing Yan Lok 1 ,
- Anatoly Urban 1 ,
- Diana Zagirova 1 ,
- Alexander Veviorskiy 2 ,
- Nina Tihonova 2 ,
- Aleksandr Kalashnikov 2 ,
- Ekaterina Kozlova 1 ,
- Vladimir Naumov 1 ,
- Frank W. Pun ORCID: orcid.org/0000-0001-8801-6645 1 ,
- Alex Aliper 2 ,
- Feng Ren 3 &
- Alex Zhavoronkov ORCID: orcid.org/0000-0001-7067-8966 1 , 2 , 4
npj Aging volume 10 , Article number: 37 ( 2024 ) Cite this article
Metrics details
- Drug discovery
Synthetic data generation in omics mimics real-world biological data, providing alternatives for training and evaluation of genomic analysis tools, controlling differential expression, and exploring data architecture. We previously developed Precious1GPT, a multimodal transformer trained on transcriptomic and methylation data, along with metadata, for predicting biological age and identifying dual-purpose therapeutic targets potentially implicated in aging and age-associated diseases. In this study, we introduce Precious2GPT, a multimodal architecture that integrates Conditional Diffusion (CDiffusion) and decoder-only Multi-omics Pretrained Transformer (MoPT) models trained on gene expression and DNA methylation data. Precious2GPT excels in synthetic data generation, outperforming Conditional Generative Adversarial Networks (CGANs), CDiffusion, and MoPT. We demonstrate that Precious2GPT is capable of generating representative synthetic data that captures tissue- and age-specific information from real transcriptomics and methylomics data. Notably, Precious2GPT surpasses other models in age prediction accuracy using the generated data, and it can generate data beyond 120 years of age. Furthermore, we showcase the potential of using this model in identifying gene signatures and potential therapeutic targets in a colorectal cancer case study.
Similar content being viewed by others
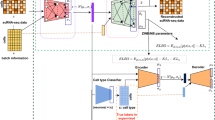
scDREAMER for atlas-level integration of single-cell datasets using deep generative model paired with adversarial classifier
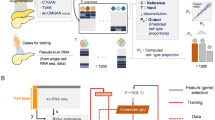
New generative methods for single-cell transcriptome data in bulk RNA sequence deconvolution
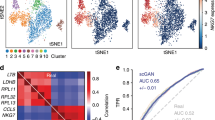
Construction of Precious2GPT
We propose a novel hybrid approach that combines the power of two generation models, CDiffusion and MoPT, to generate high-quality multi-omics methylation and expression data (Fig. 1 ). Our approach of constructing Precious2GPT (P2GPT) involves the following steps:
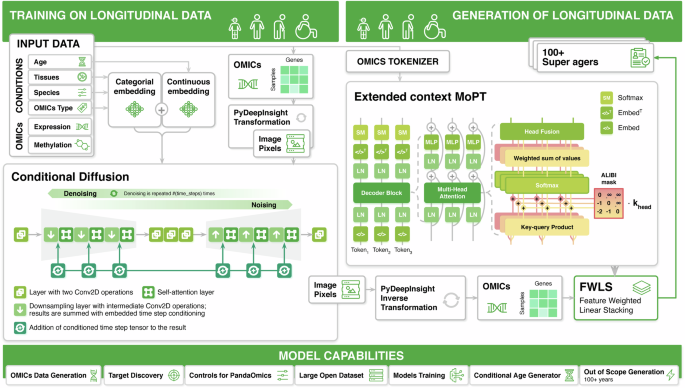
The top left section of the diagram delineates the diverse omics datasets (e.g., methylation and gene expression) collected under various conditions such as age, tissue type, species, and omics types. From this initial data representation, lines branch out to indicate two separate data processing streams feeding into the CDiffusion. One stream enters the Categorical Embedding, processing discrete data features and the other enters the Continuous Embedding, handling the age data. Adjacent to these embedding blocks, the PyDeepInsight Transformation highlights another preparatory step for the input data, which is processed in parallel to the embeddings and also fed into the CDiffusion. On the left side, CDiffusion is presented in detail to reflect its centrality in the data analysis pipeline. Beneath this architecture, an Inverse PyDeepInsight block reverts the transformed data back to its omics representation after processing through the CDiffusion model. The transformed outcomes are combined with results from the CDiffusion in the FWLS block. The top right section of the figure introduces the Omics Tokenizer, serving as the preliminary stage for the LLM generation. Below the tokenizer, a larger visual represents the architecture of the LLM model. Its output is directed back into the omics space to broaden interpretability and also channeled into the FWLS, where it is integrated with the CDiffusion generations. The bottom right of the illustration showcases the Model Capabilities block. This block emphasizes various practical applications of the developed framework, including omics data generation, assembly of large open datasets, facilitation of control mechanisms for PandaOmics, the model’s capacity for target discovery, out-of-domain extrapolations, and conditional age prediction.
Step 1: CDiffusion model generation
We employ the CDiffusion model to generate an initial dataset, which simulates gene expression levels based on the provided gene expression network. This network incorporates dependencies between genes, ensuring a biologically plausible gene expression pattern.
Step 2: MoPT model evaluation
Using the generated dataset from Step 1, we evaluate the quality of each gene’s generation using the MoPT model. The MoPT model calculates a quality score for each gene, reflecting the similarity between the synthetic data and real-world gene expression and DNA methylation profiles.
Step 3: Coefficient calculation
To create a balanced combination of the two models and reflect the proportion of quality contributed by each, we calculate coefficients based on the quality scores obtained from the MoPT model for each gene. These coefficients represent the relative importance of the CDiffusion and MoPT models in the final hybrid generation. To conduct the combination of our models, we employed the Feature Weighted Linear Stacking (FWLS 19 ) approach. FWLS is a technique that combines multiple models by assigning weights to each model based on their performance and using these weights to calculate a weighted average prediction. The FWLS formula is as follows:
Where \({y}_{{FWLS}}\) represents the final combined generation, \({y}_{{ij}}\) represents the generation of each individual model (MoPT and CDiffusion) for each gene, \({w}_{{ij}}\) represents the weight assigned to each model for each gene. In our study, we employed a linear regression approach to determine the optimal weights for model combination. The weight calculation formula using linear regression is as follows:
Where \(w\) represents the vector of weights, \(X\) represents the matrix of generations from individual models (MoPT and CDiffusion), \(y\) represents the actual target values for each subgroup of conditions (tissue, age, omics type, species). Once the weights are determined, they are used to calculate the final combined prediction by taking the weighted average of the individual predictions. This approach allows us to leverage the strengths of each model and minimize the impact of any individual model’s weaknesses.
P2GPT is capable of generating tissue-specific and accurate multi-omics data
We first tested 3 basic models including CGAN, CDiffusion, and MoPT, as well as the multi-omics versions of CDiffusion, MoPT, and their combination, P2GPT. As a simple model, CGAN was used to serve as the benchmark or baseline in the analysis to evaluate the performance of other models. All 6 models performed well in classifying tissues with both real (Table 1 ) and generated (Table 2 ) labels. Table 1 in columns 1 and 2 for the P2GPT and diffusion model presents the results with genes extrapolated from the landmark genes (obtained from the LINCS L1000 project) and the CGAN model uses all genes to generate. The comparison between all the 6 models tested using human expression, DNA methylation, and mice expression data showed that in the case of extremely underrepresented data such as the imbalance of tissue densities, which is common in practice, only the P2GPT model is capable of generating accurate omics data by combining the multi-omics models of CDiffusion and MoPT. We further visualized the distribution of data by UMAP dimensionality reduction which showed that using human expression data (Fig. 2A, B , Supplementary Fig. 1 ), the generated labels were highly concordant with the real labels, further suggesting that P2GPT was capable of synthesizing tissue-specific expression data accurately. On the other hand, real human DNA methylation data were not well clustered into tissue types (Fig. 2D , Supplementary Fig. 1 ). Despite this, they were also highly concordant with the real data in terms of similarity and distribution (Fig. 2C , Table 2 ). In mice expression data, P2GPT could also generate concordant and tissue-specific data, although less accurately than in human data (Fig. 2E, F , Table 2 ) (see method). Overall, this model has shown itself to work well, both for DNA methylation and expression, in the case of tissue-specific data generation.
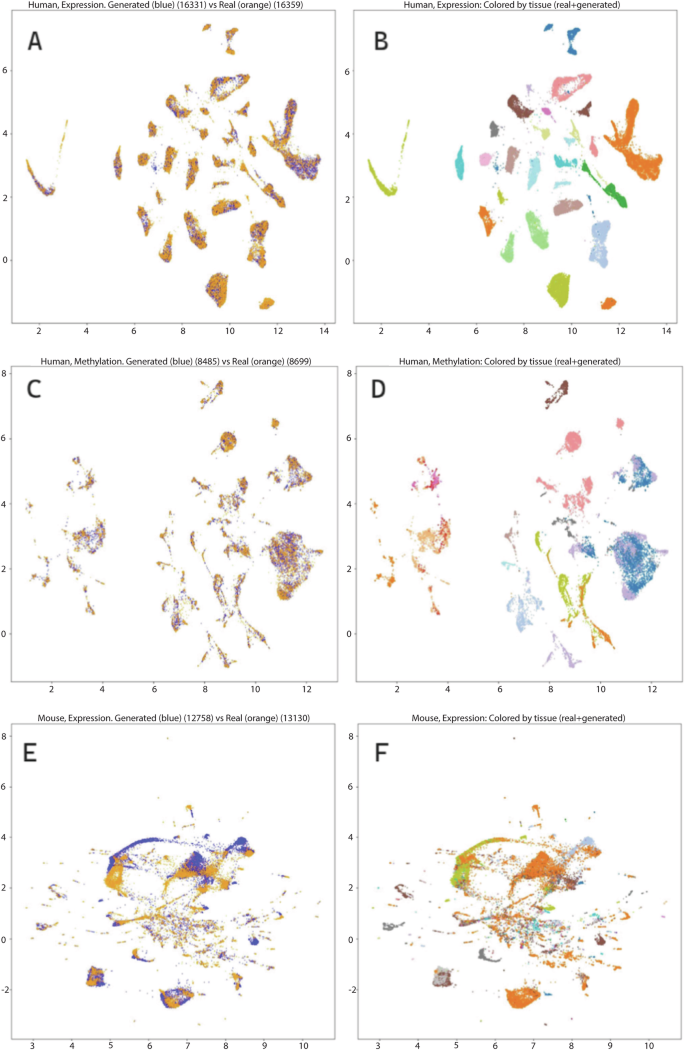
Each point represents an individual sample. A Human expression data colored by data type (orange, real; blue, generated). B Human expression data colored by tissue type. C Human methylation data colored by data type (real or generated). D Human methylation data colored by tissue type. E Mouse expression data colored by data type (real or generated). F Mouse expression data colored by tissue type.
P2GPT outperformed other models in age prediction using generated data
Next, we trained the CatBoost regression model using real data with age as the parameter and assessed the prediction performance of age in the generated data. Our results showed that P2GPT demonstrated the best performance, achieving the lowest mean absolute error (MAE) and highest R 2 score across all types of datasets tested when compared to other models (Tables 3 and 4 ).
It is important to highlight that the expression-based regressor error is notably substantial, especially in mice expression data. In contrast, the generative models with the age condition demonstrate a pronounced proficiency in terms of MAE and R 2 when applied to human DNA methylation data. This observation has guided subsequent experiments to also focus on DNA methylation with the age condition. A noteworthy characteristic is the enhanced quality achieved through the unique heterogeneity and the combination of these models which results in the optimal performance. Additionally, we have witnessed improvements not only in models that encompass multiple omics datasets but also in the results produced by their integrative combinations. Based on the figure with the results of the best regression on DNA methylation in Supplementary Fig. 2 , our model shows high quality in all tissues.
The effect of underrepresented data on P2GPT’s data synthesis
We then investigated how the representation of data affects the P2GPT’s ability to generate new synthetic data in each of the three data types (human expression, human DNA methylation, and mouse expression). The model was asked to generate 300 synthetic samples for each tissue with the additional condition of age from a uniform distribution of age values. We can see the model requires a relatively small amount of real data samples to start generating valid synthetic expression samples conditioned with specific age and tissue, while the error rate for the generation of DNA methylation samples is significantly higher (Fig. 3 ). We could not definitely state that the number of samples in a dataset is the main factor of successful generation of synthetic samples, but as we can see from trends on graphs it is one of the most important factors. Here, we defined correctly generated samples as those generated in strictly proper structure i.e. the order of genes and their generated omics numerical values were correct.
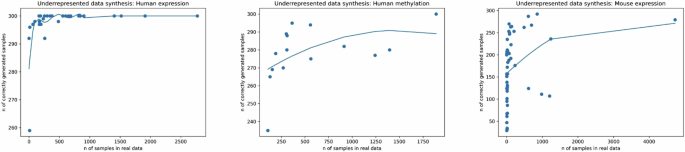
Each point is a specific tissue, while the x-axis shows the number of samples presented in the dataset, and the y-axis shows the number of correctly generated samples out of the expected 300 samples. Left: Human expression. Middle: Human methylation. Right: Mouse expression.
P2GPT is capable of generating highly accurate DNA methylation data across ages
Since our results from CatBoost regression analysis (Tables 3 and 4 ) indicated that DNA methylation data was the best data type for age prediction using the generated data, we used it to compare the similarity between real and generated data in terms of differential methylation. For each of the tissues, we compared the DNA methylation levels of samples of 80 ± 20 years old and 30 ± 20 years old in the real data, as well as those of 80 ± 20 years old (predicted) and 30 ± 20 years old (predicted) in the generated data. The significantly differentially methylated genes were then obtained from each of them to identify the number of intersections (Fig. 4 ). We then studied how much overlap there is between real and generated data on sets of differentially methylated genes (Table 5, MA ). We hypothesized that if the models captured a certain difference between groups, in this case, a 50-year difference, then we could generate the data for older people or mice. For certain tissues (e.g., leukocytes or liver), we observe that it is better to use a single model. However, in the case of the occipital lobe, blood, breast, and some other tissues, the P2GPT shows better performance. With buccal mucosa tissue, we have very little overlap, but this is because there are only 3 differentially methylated genes. Globally, based on statistics, we can say that our model P2GPT has become more stable between all tissues and there is no bias. In addition, we compared the DNA methylation levels in 80 years old samples between the real and generated data. We hypothesized that if the real and generated data shared high similarity, low counts of differentially methylated genes would be obtained. As shown by our results, for the majority of tissues analyzed, P2GPT demonstrated the lowest numbers of differentially methylated genes identified by the comparison between real and generated data (Table 5, DM ). This further suggests that our model can identify the difference in DNA methylation levels both between groups at different ages and preserve DNA methylation values with the same age group for real and generated data.
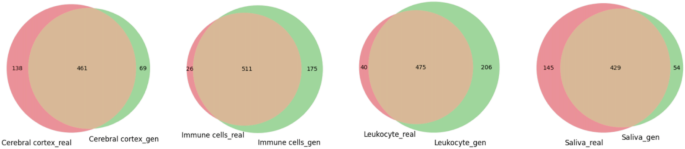
gen generated.
P2GPT can extrapolate age in generated data beyond the age range of the training dataset
Since MoPT could not be used to predict age in the generated data that was not present in the training real data, as an out-of-scope experiment, we used the diffusion model integrated in P2GPT to study its age prediction accuracy based on two conditions. The first condition involved the training exclusively on the DNA methylation data from individuals aged lower than 50 or 80 years old, while in the second condition, the model was trained using data encompassing all ages (ranging from 0 to 114 years old) in the real data. We show that the number of samples available per age group varies across tissues, with some tissues having underrepresented age groups (Fig. 5A ). Our results indicated that the quality of the model’s predictions was maintained particularly well in the crucial age component within PCA for specific tissues. Specifically, we obtained notable results for blood and examined the components that exhibited the highest correlation with age. We observed that the layer representing generated samples of older individuals was positioned right after the layer representing the 80–100 age group, regardless of the training condition (Fig. 5B, C ). Similarly, generated samples at older age were also positioned close to the real old samples in blood by setting the training threshold at 50 years old (Supplementary Fig. 3 ). This insightful finding confirms the model’s ability to generate age values that have not been encountered during training, indicating its capacity to extrapolate and generate realistic age predictions beyond the observed age range. Moreover, the results on tissues with the underrepresented 80–100 years old group (Supplementary Fig. 4A ) do not differ much between both training conditions. However, when it is well represented, the variance between samples is lower where the model was trained on all labels (Frontal Lobe in Supplementary Fig. 4B ). Similar results were also observed in the cerebral cortex (Supplementary Fig. 4C ), cerebellum (Supplementary Fig. 4D ) and buccal mucosa (Supplementary Fig. 4E ).
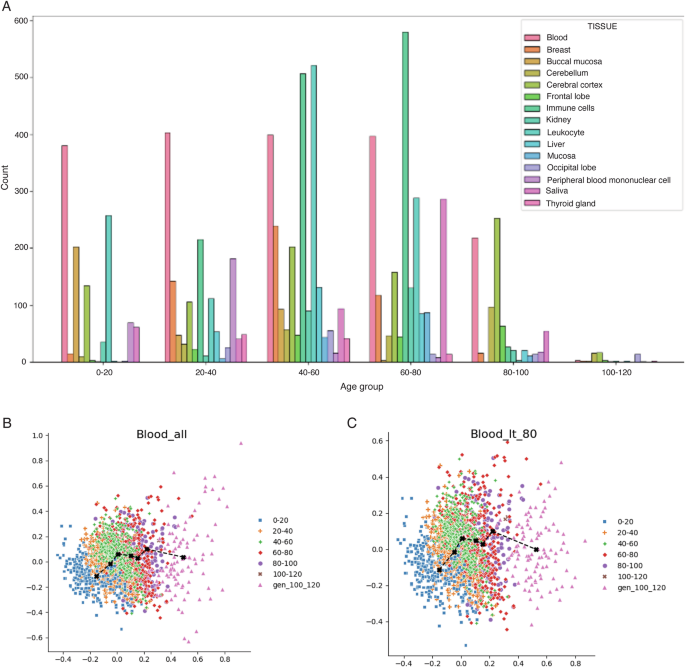
A Distribution of age groups in real methylation data for each tissue. B PCA for blood with real and generated data with different age bins for models trained on data with the whole age distribution. C PCA for blood with real and generated data with different age bins for models trained on data with age lower than 80. The black line in PCA is connected by cluster centroids of each age group from [0,20] to [100,120] for real data and [100,120] for generated blood data.
Applications of P2GPT-synthesized data in pathway enrichment analysis
To assess the applicability of P2GPT in biological data interpretation, we performed differential DNA methylation analysis between the 120 and 150 years old samples in each tissue generated by P2GPT, followed by pathway enrichment analysis on the differentially methylated genes based on the KEGG database to identify the pathways that were potentially related to aging. In blood, the enriched pathways for the differentially methylated genes were associated with immune function, organ development, and hormonal regulation (Supplementary Fig. 5 ). In the liver, pathways associated with cytokine receptor interactions and NOD-like receptor signaling were enriched, while pathways enriched in the older thyroid gland revealed a shift towards chronic inflammation and immune dysregulation as suggested by disrupted cytokine interactions, increased neutrophil extracellular trap formation, and alterations in hematopoietic cell lineage. Moreover, overrepresentation analysis showed that the differentially methylated genes were enriched in several hallmarks of aging across multiple tissues. In particular, inflammation was found to be enriched in 4 tissues (blood, liver, saliva, thyroid gland), while genomic instability was enriched in 2 tissues (liver, thyroid gland) and altered intercellular communication in 2 tissues (blood, thyroid gland). Here we took the conclusion of enriched pathways through all tissues in KEGG_2021_Human. Lists of pathways for selected tissues can be seen in Supplementary Table 1 . We also added a description of these pathways through GPT-4 based on the direction of methylation and importance in aging. It can be observed that most of the pathways are indeed related to aging. This suggests that our P2GPT model can generate old people data and find the most important biological signaling pathways of aging.
Case study experiment in colorectal carcinoma
To demonstrate the potential of in silico-generated gene expression data as control samples for actual colorectal cancer samples, we generated control samples for corresponding case samples of eight colorectal cancer (CRC) cell lines using our P2GPT model. Subsequently, eight case-control comparisons for the corresponding CRC cell lines were incorporated into a meta-analysis. CRC meta-analysis was constructed using a restricted subset of genes, referred to as the “landmark” genes, and another meta-analysis was created using a comprehensive subset of genes, termed “restored” by a CycleGAN model which is described in Supplementary materials. For each of the two meta-analyses, we extracted the common gene expression signatures across all eight cell lines which yield two lists of gene expression signatures. Each of them was subjected to Spearman’s correlation test with the gene expression signature obtained from the pre-calculated CRC meta-analysis on PandaOmics (Fig. 6 ). We observed that common gene expression signatures calculated on an extended number of genes (restored genes) exhibited greater similarity to the benchmark CRC signatures (r = 0.552) compared to signatures calculated on a limited number of genes (landmark genes, r = 0.497). Additionally, it was evident that the application of a gene expression significance threshold positively correlated with overall signature similarities. The combined landmark signature generated with the P2GPT model demonstrated strong similarity to the CRC benchmark signature (Fig. 6A ). However, its performance on the restored subset of genes was even better (Fig. 6B ). Consequently, the meta-analysis derived from comparisons created using the P2GPT on an extended number of genes was further employed for target identification analysis.
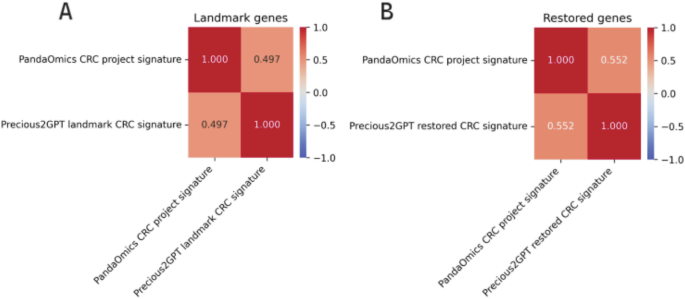
Spearman correlation coefficients between colon carcinoma signatures were calculated using only landmark genes ( A ) and all restored genes ( B ). The colon carcinoma signature (PandaOmics CRC project signature) was derived from the “expression analysis” section of manually curated colon carcinoma meta-analysis in PandaOmics and corresponded to the combined gene expression changes values for colon carcinoma. P2GPT CRC signature was collected from the corresponding meta-analysis in PandaOmics.
As previously, the manually curated CRC project available in PandaOmics served as a benchmark for target hypotheses. The Target ID results were compared between the CRC meta-analysis, containing case-control datasets that were obtained from patients, and the P2GPT CRC meta-analysis (refer to the previous section). This case study aimed to demonstrate that the in silico-generated control samples could be used as control samples for actual case samples. Therefore, to compare Target ID results, we only used omics scores for hypothesis ranking and included solely druggable target families 20 . The top 20 target hypotheses for both the benchmark and the P2GPT-restored CRC meta-analyses are depicted on a heatmap (Fig. 7 ). Using the Target ID approach the top genes that were highly scored in PandaOmics CRC meta-analysis and P2GPT-controls generated CRC meta-analysis were explored. By analyzing the overlapped genes, it was observed that both top 20 target hypotheses lists contain hits that are strongly associated with CRC pathology. For instance, AKT1, PTEN, and PIK3R1 are key modulators in the PI3K/AKT pathway while PLK1, CDK2, and MAPK14 are major drivers involved in cell cycle regulation. Being ranked top in both Target ID results, AKT has been extensively studied in disease pathogenesis 21 , 22 and is altered in CRC patients 23 . CDK2 is also highly scored in both meta-analyses. The CDK2 has been explored in the G1/S phase transition 24 and the CDK2 selective inhibitors have already been tested in CRC models 25 . Genes that were top scored only in P2GPT-controls generated CRC meta-analysis (PIK3CD, FYN, YES1, ATM, HRAS, TNFRSF1A, GSK3B, PLCG1, CSK, PIK3CA) are also related to pathogenesis. For example, PIK3CD was shown to be involved in AKT/GSK-3β/β-catenin signaling and could be considered as a potential target 26 , while mutations in PIK3CA were observed in 20% to 25% of CRC 27 and associated with shorter cancer-specific survival 28 . The results were supported by PandaOmics knowledge graph (Supplementary Fig. 6 ). Overall, our results suggested that our P2GPT model can be used to generate expression data that could be utilized in target discovery. We showed that gene expression changes between case and control (both real and generated) samples resulted in a similar disease-specific expression signature. At the same time, the Target ID approach applied for data from patients (colon carcinoma PandaOmics meta-analysis) and for P2GPT-controls generated colorectal cancer meta-analysis showed a strong overlap between well-known targets for colorectal carcinoma along with a new target hypothesis.
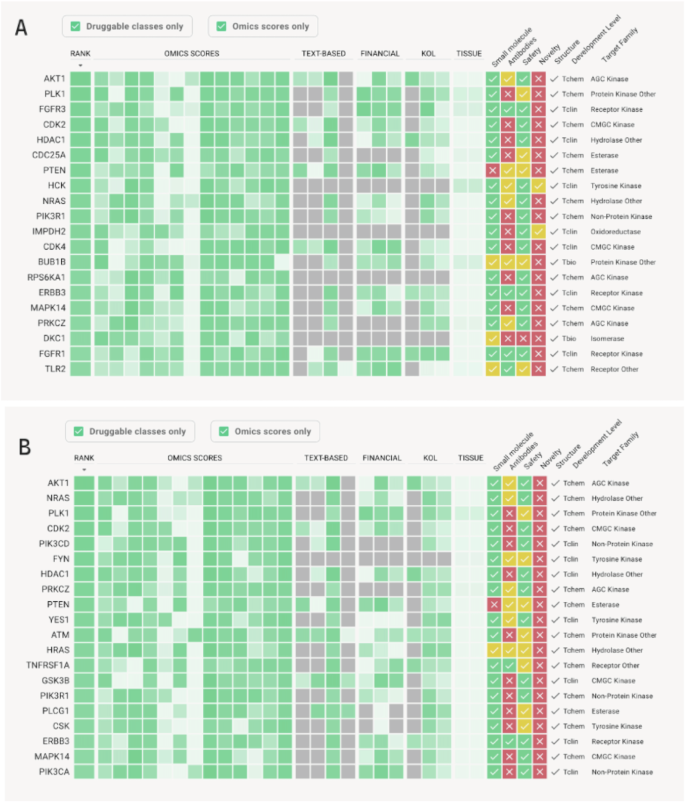
Results were derived from the in silico Target ID scoring approach for PandaOmics colorectal carcinoma meta-analysis ( A ) and P2GPT colon cancer meta-analysis ( B ). To validate our approach, only omics-based scores with the application of a druggability filter were taken into account and used for the composition of the scores for ranking.
Studies have identified several key biomarkers, proteins and pathways that play important roles in aging 29 , 30 . Despite this, the multifaceted process of aging still requires substantial understanding and unraveling of the complex biological data. To address this, the use of artificial intelligence (AI) in the field of aging research has been increasing recently. Indeed, deep learning-based approaches have been proposed to play vital roles in multiple areas facilitating aging research, such as predicting biological age 16 , 31 , 32 , developing biomarkers 15 , 33 , 34 , 35 , identifying therapeutic targets 15 , 20 , 36 , 37 , 38 , 39 , and generating novel compounds 34 , 40 , 41 . In fact, studies have also demonstrated the potential of applying AI models to identify targets implicated in aging and age-associated diseases, targeting established hallmarks of aging 20 , 30 , 37 , 42 .
In this study, we present a hybrid approach Precious2GPT (P2GPT) that combines the complementary strengths of the CDiffusion and MoPT models for generating high-quality multi-omics DNA methylation and expression data. Our approach reduces the limitations of individual models and leverages their strengths to enhance the generation process. This innovative approach has potential applications in various fields, including data analysis, algorithm development, and privacy preservation for multi-omics research. We demonstrate the effectiveness of our hybrid approach by comparing the quality of data generated using individual models of CGAN, CDiffusion, and MoPT, with the combined hybrid approach P2GPT. With the aid of tissue classification and age regression experiments, the performance of models was assessed in terms of their specificity to species and tissue types, as well as their capability to predict age based on learned patterns from real data.
Upon training the transformer-based model with this corpus, we demonstrated its high capability of generating new data conditioned on specific factors like age or tissue type. In our study, we encountered a primary challenge in generating tabular data from continuous gene expression and DNA methylation omics data. Previous works have attempted the conversion of table data to text before the application of the pretrained GPT-2 model 43 . Another approach addressed the complexity issue by using the GPT-2 architecture with a customized vocabulary to improve the efficiency during both training and inference of the model 44 . Hence, we devised an encoding scheme, wherein each gene and its corresponding omics value were represented as individual tokens. In essence, our approach treated the gene-omics data as pseudo-text, enabling us to utilize the transformer-based model, ultimately introducing the MoPT model. To evaluate the generated data with predictions of age and tissues for different data types and species, we highlight the potential of transformer architectures in bioinformatics tasks, which represents the first biomedical-specific adaptation of a language model for generating tabular data.
Synthetic data plays a crucial role in overcoming data insufficiency by providing synthetic controls that replicate the biological properties of real control samples, and enhance equity in differential expression analysis. The use of generated data also enables cost-effective testing of algorithms and pipelines in a virtual experimental platform, allowing researchers to mimic the effects of interventions under specific scenarios such as varying levels of noise and different degrees of differential expression 45 . Furthermore, the potential impact of alterations in genomic profiles can be predicted with synthetic gene knockdowns or knockins data 46 . Our P2GPT model demonstrated exceptional performance in classifying tissues based on synthetic data. The model’s accuracy is remarkable, with its predictions closely resembling those based on real biological datasets as evidenced by the high correlation coefficients in cross-validation studies and the model’s robustness when tested against known benchmarks. In the age regression analysis, P2GPT showcased its aptitude by accurately predicting the biological age of samples using synthetically generated DNA methylation patterns. The synthetic data, when compared against real-world epigenetic clocks, confirmed that P2GPT successfully captured the nuances of age-related changes, with a minimal margin of error. This reveals the potential for wide-ranging applications in biogerontology and personalized medicine.
Leveraging out-of-scope (OOS) experiments with the P2GPT model has revealed that across various tissues, aging is consistently associated with dysregulated immune function, chronic inflammation, and alteration in cell lineage and signaling pathways. Age-associated dysregulated immune function, accompanied by chronic inflammation (inflammaging), contributes to the process of immunosenescence observed in aged individuals 47 , 48 . The alteration in signaling pathways has been shown to trigger inflammaging and senescence across multiple tissues 30 . These biological processes markedly contribute to the increased disease burden observed in the elderly population and present potential targets for therapeutic intervention. The insights delivered by the P2GPT model’s OOS experiments underscore the value of advanced computational models in understanding the complex biological underpinnings of aging and spotlighting potential avenues to mitigate its detrimental effects on health. Our results showed that our model can be utilized to identify biologically relevant pathways and processes through synthetic data generation.
By combining MoPT and CDiffusion models using Feature Weighted Linear Stacking (FWLS), we aimed to improve the overall predictive performance and generalization ability. This approach integrates diverse perspectives and captures complementary information from each model, resulting in a more robust and accurate prediction. Applying FWLS during coefficient calculation allowed us to obtain more accurate predictions by incorporating individual model strengths. By considering model weights, we ensured that more accurate and reliable models had a higher impact on the final generation, mitigating biases or inaccuracies introduced by any single model and providing a more robust prediction. Our findings indicate that the coefficients derived from P2GPT allow a refined integration of the two models, leading to enhanced performance with improved generation quality. Despite the advancements achieved by integrating MoPT and CDiffusion models with FWLS, there are certain limitations in the current P2GPT model. Firstly, the complexity of the model poses a potential barrier to replication and broader application. The intricacies involved in managing and interpreting the combination of such models may limit their use by those without deep expertise in bioinformatics and access to substantial computational resources. Secondly, the current iteration of P2GPT processes primarily tabular data or bidimensional image data, and could not accommodate the analysis of graphical structures which represent complex biological interactions or pathways at this stage. Future extensions of the model that incorporate graph neural networks could enable the analysis of data represented in graph forms, such as protein-protein interaction networks or gene regulatory networks. Despite these limitations, the synergistic integration of MoPT and CDiffusion models through FWLS has successfully demonstrated an enhanced predictive capability.
Our findings underscore the versatility and effectiveness of transformer architectures in handling bioinformatics tasks. However, it is important to acknowledge that the success of our P2GPT is attributed to the generation of relatively large sequence lengths and the design of an effective encoding scheme. Future work can expand the application of our method in other bioinformatics tasks like survival analysis, cross-modality prediction, and generation of omics depending on the disease or drug, thereby broadening the usage of transformer architectures in the field. For instance, beyond aging research, P2GPT could facilitate the analysis of fundamental processes underlying tumor progression, resistance, and metastasis. Additionally, modeling the timing and administration methods of various therapy combinations could provide insights into how tumor cells develop resistance to drugs 49 , 50 , 51 . In addition, we envision further refining our hybrid approach by exploring additional generation models and incorporating various omics data types. Moreover, we believe that validation of synthetic data through downstream applications and benchmarking against real-world datasets would enhance the utility and robustness of synthetic multi-omics data. Lastly, we anticipate the future integration of P2GPT into clinical settings, enabling invaluable applications such as simulating tissue-specific biological data without invasive biopsies to predict treatment responses, predicting biological changes and disease progression trajectories, and incorporating various clinical parameters to enhance the accuracy for personalized disease monitoring and therapeutic strategies.
In summary, we developed Precious2GPT, a generative model capable of producing methylation and expression data, which are invaluable resources for aging research due to the scarcity of longitudinal biological data. Through multiple lines of evidence and validation, we demonstrated the significant potential of Precious2GPT in facilitating aging research. Future work addressing the aforementioned limitations would further strengthen the model’s applicability, accuracy, and comprehensiveness, providing a powerful tool for biological discovery and translational medical research.
Data sources
In this study, expression and methylation data were adopted across two species, human and mouse. Access to Genotype-Tissue Expression (GTEx) V8-protected data (phs000424) was authorized by the Data Access Committee of NCBI dbGAP. Human transcriptomic data 52 and sample attribute data were downloaded, constituting 12,453 samples. Complementary mouse transcriptomic data was sourced from ARHS4 database, V2.2 (12,541 samples). Mouse genes were mapped to their corresponding human orthologs with the use of Human Genome Organisation Gene Nomenclature Committee (HGNC) mappings 53 . Both GTEx and ARCHS4 RNA-seq data were procured in the form of raw gene counts. These datasets underwent log2 transformation, followed by quantile normalization applied to each tissue type separately within the expression datasets. After performing log2 transformation and quantile normalization, we preserved the target distribution to facilitate its application to novel samples. Human DNA methylation data was aggregated from the Illumina Infinium HumanMethylation450 BeadChip array datasets, retrieved from the China National Center for Bioinformation’s (CNCB) data repository (8,285 samples) 54 . Methylation beta values were mapped to genomic features based on the HumanMethylation450 v1.2 Manifest File. In detail, we intentionally focused our attention on the CpGs located exclusively within the TSS200 region, as these were interpreted as the most relevant to age prediction. The TSS200 region, defined as the area comprising 200 base pairs upstream of the transcription initiation site, is documented as crucial for gene regulation processes. Consequently, the beta values of the CpGs situated within a gene’s TSS200 were averaged for downstream analysis.
Preprocessing methods
For pictures construction, DeepInsight technique with the application of convolutional neural networks (CNNs) 55 , 56 and Kohonen’s self-organizing maps (SOMs) 57 , 58 was used to transform non-image data into image-like representations in CGAN and CDiffusion models. For the acceleration in training and inference processes of computationally heavy models, deep learning engaging CycleGAN 59 , 60 was employed to generate synthetic data in CDiffusion, MoPT and Precious2GPT models. In brief, generation methods work either with text or with pictures. We used DeepInsight to construct pictures for CGAN and CDiffusion models and in the CDiffusion part of Precious2GPT model. To compare individual genes in each pixel of images, SOM was used instead of the TSNE, UMAP and PCA algorithms. For each data set, we built a separate SOM of different dimensions to minimize space in the square image, and ach picture was colored by expression or methylation, along with the training set in different colors.
DeepInsight
CNNs was used to automatically extract features from spatially coherent pixels, detecting higher-order statistics and non-linear correlations, and to provide promising performance in learning complex patterns and relationships in the data. To improve the efficiency of CNNs, one-dimensional (1D) biological data was transformed into two-dimensional (2D) representations. DeepInsight is a methodology designed to transform non-image data into image-like representations, allowing convolutional neural networks (CNNs) to be applied more effectively. It serves as the basis for the DeepInsight-3D model, which extends this approach to multi-domain tabular datasets. The DeepInsight pipeline consists of the following steps (Supplementary Fig. 7 ):
Data normalization: The input data is normalized to ensure that all features have the same scale. This is typically achieved by applying min-max scaling, z-score normalization, or other suitable normalization techniques. Dimensionality reduction: The high-dimensional input data is transformed into a lower-dimensional representation. This can be done using dimensionality reduction techniques such as t-SNE, UMAP, or PCA. The resulting lower-dimensional data retains the most important information from the original data while reducing noise and computational complexity. Image generation: The lower-dimensional data is then converted into a 2D image-like representation. This is achieved by mapping each data point to a pixel in the image, with the pixel intensity representing the value of the corresponding feature. The resulting image preserves the spatial relationships between the data points, allowing CNNs to effectively capture local and global patterns in the data. Convolutional neural network (CNN) training: The generated images are used as input to a CNN, which is trained to perform a specific task, such as classification or regression. Recently developed techniques such as diffusion models can be used to effectively process such data Supplementary Fig. 7 . By transforming non-image data into image-like representations, DeepInsight-like models allow for the efficient application of image-oriented models to a wide range of data types, including biological data.
Kohonen’s self-organizing maps (SOMs) offer a promising alternative to PCA or UMAP for dimensionality reduction in the context of transforming non-image data into image-like representations. As an unsupervised learning algorithm, SOMs excel at converting high-dimensional data into lower-dimensional representations while preserving the topological structure of the input data. This ability to maintain the spatial relationships between data points makes SOMs particularly well-suited for generating images that can be fed into convolutional neural networks (CNNs). Unlike PCA, which focuses on linear relationships and maximizes variance, or UMAP, which aims to preserve both local and global structure, SOMs employ a competitive learning process that iteratively updates neuron weight vectors to better represent the input data. This results in a 2D grid of neurons that captures complex relationships between variables, potentially leading to more effective feature extraction and improved performance of the CNN. By incorporating Kohonen’s SOMs into the DeepInsight methodology, we can harness the unique advantages of this algorithm to enhance the analysis of non-image data using deep neural networks.
To speed up training and inference of heavy models (CDiffusion, MoPT and P2GPT), for we used extrapolation of all genes using the CycleGAN model during post-processing. In our heavy models, we trained them with different generations and then extrapolated the result using this model. In detail, our domain X consists of data for landmark 978 genes 59 and domain Y consists of desired output data for 11,278 genes, which are our intersections across several OMICS datasets and species types. The set of 978 genes serves as the starting point to generate synthetic output data for the 11,278 genes.
A CycleGAN comprises two generators (G & F) and two discriminators (Dx & Dy). Generator G transforms from domain X to Y (G: X → Y), while F does the vice versa, i.e., F: Y → X. Dx aims to distinguish between X and FX(Y), whereas Dy works on discriminating between Y and G(X). The training goes as follows: first, the generator G translates a sample data from domain X into a synthetic data of Domain Y. Subsequently, the generator F tries to regenerate the original sample from this synthetic data. The objective is to train the CycleGAN in learning the mapping such that the regenerated data closely matches the input data. This is referred to as forward-cycle consistency. A backward cycle consistency is simultaneously processed from Domain Y to X, and the whole cycle repeats continuously in learning. The network learns from the inconsistencies between the regenerated data and the original input data to increase the capabilities in generating synthetic data aligned with the target domain. Importantly, the discriminators Dx and Dy also participate in this training process, aiming to classify an instance from the actual dataset or a generated data by respective generators. As a result, CycleGAN has the ability to extrapolate the data from 978 genes to realistically simulate data for 11,278 genes even in cases where paired samples are lacking. Finally, we can say that this model greatly helped us in generating a large amount of data in a short time with minor losses in quality compared to the full set. In the production model we will of course eventually use the full data set, but for some experiments this is not necessary.
Generation methods
Mathematical formulation of conditional generation task.
In the context of conditionality, we aim to develop models that can generate data instances conditioned on multiple factors: tissue ( \(T\) ), age ( \(A\) ), species ( \(S\) ), and omics types ( \(D\) ). We represent the generated data as \(X\) , and the conditions as a tuple \(C=(T,A,S,D)\) . The conditional generation task is defined given a set of training data (D):
where \({X}_{i}\) represents the observed data instances and \({C}_{i}\) represents the corresponding conditions in order to learn a conditional generative model \(G\) that can sample data instances \(X\) conditioned on arbitrary conditions \(C\) .
The training objective of this model is to estimate the conditional probability distribution \(P({X|C})\) , such that
where \(G({X|C})\) represents the data generated by our model.
To evaluate the performance of Precious2GPT, Conditional Generative adversarial network (CGAN) was used as positive control in the validation experiments. Generative adversarial networks are more classical, easier to learn and faster in terms of speed of inference, which serve as a baseline for the other models. In some situations it has been observed that they can show themselves high and do not necessarily use complex patterns. In particular, if there is a generation task with one condition and we do not want to take into account the age condition. This generative model was trained to generate synthetic data using two networks, th generator \(G\) and the discriminator \(D\) . In CGAN, the generator \(G\) was trained to produce data samples that are indistinguishable from real data by a discriminator \({D}\) , whilst the generator took the conditions \(C\) as input and generates data \(\,X\) (Supplementary Fig. 8 ). In the context of multi-omics data integration, CGANs were employed to generate realistic images corresponding to expression or methylation data with additional conditions, tissue type, age, omics type, and species.
Diffusion models were employed to estimate the likelihood of generation data \({X}\) . The model was trained to sample data through a diffusion process conditioned on \(C\) , and the likelihood of data was maximized throughout the learning process. A PyTorch published on GitHub (available at https://github.com/tcapelle/Diffusion-Models-pytorch/tree/main ) 61 was implemented as the basis of the conditional diffusion (CDiffusion) model, and PyTorch’s embedding was applied to construct the conditionality on categorical features in this model.
A PyTorch implementation of the conditional diffusion model published on GitHub 61 (available at https://github.com/tcapelle/Diffusion-Models-pytorch/tree/main ) was the basis for the used CDiffusion model. In its basis, this implementation involves a U-Net block that has self-attention layers between the downsampling and upsampling layers. Standard diffusion models incorporate temporal information in a tensor (later referred to as the time step) that controls the noising/denoising process based on the current step through being embedded into every downsampling/upsampling layer of the U-Net block. This time step is initialized by ongoing positional encoding given the previous step’s tensor through a sinusoidal positional embedding (Supplementary Fig. 9 ).
The diffusion model is conditioned on categorical features of sets of genes by adding the conditions’ embeddings into the current time step. PyTorch’s nn.Embedding is used as a learnable embedding layer that stores embeddings mapping each class of a categorical condition (mapped to unique integers) into a tensor with the time step’s shape. However, such an embedding layer is not applicable for continuous features, such as age, so ages are fused in the time step by first undergoing a small network (three linear layers with ReLU activation functions) that transforms a single floating-point value (age) into the time step’s dimensions. Finally, the ages’ embeddings are similarly added to the time step. While the outlined approach works as is for single conditions, including multiple conditions leads to the optimizer tilting its attention towards the condition with higher embedded values. This is solved by normalizing all the conditions’ embeddings (categorical/continuous) before adding them to the time step.
To process large numbers of genes and omics values, memory efficient transformer architecture – MPT 14 was utilized in the construction of Precious2GPT. It incorporates a modified architecture inspired by GPT-2 6 , where the positional embeddings are replaced with a Linear basis matrix. This modification enhances extrapolation capabilities while requiring fewer GPU memory resources during model training. Following the retraining process, our model underwent biological adaptation to multi-omics data, ultimately presenting as the Multi-omics Pretrained Transformer (MoPT).
Model setup and training procedure
We prepared a tokenizer which consists of all possible genes from datasets, all 2-digit values for tokens referred to as age, tissues, species.
We utilized “mosaicml/mpt-7b” configuration from HuggingFace 14 . To properly set the number of parameters we considered Chinchilla scaling law 62 , which proposed that the number model parameters should be proportional to the number of tokens in training corpus in ratio of 1:20. For all three datasets we considered this law and got the next model sizes: 4.1, 1.7 and 1 million parameters for multi-omics dataset, expression and methylation respectively.
Learning curves for different datasets are represented in Supplementary Fig. 10 . For each dataset the evaluation set was 1000 samples uniformly distributed by all tissues.
MoPT generation procedure
To generate new omics samples we pass to the model desirable conditions on generation such as age, tissue, species and omics data type in form of plain string with spaces between conditions, e.g: “SPECIES Mouse dataset EXPRESSION TISSUE Brain MouseAGE84 ”. We utilized top-k together with top-p sampling, where k = 40, p = 0.9 and temperature = 0.8.
Validation experiments
Tissue classification.
To evaluate the quality of generated omics samples, the Logistic Regression model was used in the assessment for tissue classification tasks. The evaluation was based on the f1-score 63 , weighted by classes for both real and generated data, as the key metric for determining the reliability of the generated data. For each model, we built classification metrics twice. First, we generated synthetic samples in a 1:1 ratio with the original data, and the metrics were calculated on these samples, where we compared the real label with the one predicted by the classifier (Supplementary Fig. 11 ). We subsequently examined the performance of the classifier on uniformly generated labels from the total number of tissues to evaluate its effectiveness in handling unbalanced classes. In the case of multiple conditions, we additionally generated age between the minimum and maximum values present in our data, or other label types of dataset or species within each tissue.
In addition to the aforementioned metrics, UMAP 64 representations were used to visualize both synthesized and real tissue data in identifying disparities or similarities between the two distributions.
Age regression
To predict the age of generated data, a CatboostRegressor 65 model was applied solely based on gene omics values. The training dataset was composed of real samples paired with their respective age values as the target variable, while the synthesized samples were utilized as the testing data to generate predicted age values as the conditioning variable. The evaluation of performance by each model was presented as mean absolute error (MAE) 63 and R-squared (R 2 ) metrics.
Differential methylation analysis
To examine the sample homogeneity of real and generated data, we performed several statistical tests focusing on human methylation in multiple tissues. First, the nonparametric Mann–Whitney 66 statistical test was used where we fed methylation data from different age groups generated by the CDiffusion, MoPT and Precious2GPT models. To evaluate the ability of models in preserving the differentially methylated genes of distinct age groups, we identified differentially methylated genes between the samples obtained from 80 ± 20 vs 30 ± 20 years old individuals, in both real and generated data. We then calculated the rate of intersection by the number of intersected differentially methylated genes between two sets divided by the number of differentially methylated genes in the real data. Differential methylation analysis was also performed between the 80 ± 20 years old (real data) and 80 ± 20 years old (generated data) to assess the similarity between the real and generated data (Supplementary Fig. 12 ). To optimize statistical validity, multiple testing corrections of the Benjamini–Hochberg 67 hypotheses were used.
Out-of-scope experiment
To validate our Precious2GPT model for age prediction, we conducted several out-of-scope experiments involving the training of models with methylation data at different age thresholds.
Two models were trained for this purpose. One was trained with samples up to thresholds of 50 and 80 years old, and the other one was trained with the entire sample.
Using the model trained with distinct thresholds, we generated data for (threshold +20, threshold +40) years old, and compared the clusters created by the generated data with those of real data on PCA 68 representations.
We trained a model with available data from 100 samples per tissue for individuals aged between 120 and 150 years old, and generated the differentially methylation data for pathway analysis to predict aging-related alteration. The pathway analysis was conducted using the Python package gseapy 69 , with the KEGG-human database 70 and the 12 HALLMARKS lists serving as the enriched pathways for the differentially methylated genes.
Case study experiment
In the case study experiment focusing on colorectal cancer (CRC), we utilized Precious2GPT for CRC cell lines as synthetic controls, namely Caco2, Lovo, SW1417, NCI-716, RKO, HCT-8, SW480 and SK-CO-1, obtained from our internal laboratory, the Robotic Lab. We fed the gene expression data of the eight CRC cell lines as input to facilitate the generation of respective synthetic controls using the pre-trained Precious2GPT model. For the generated control samples, gene expression data was normalized and uploaded to our PandaOmics platform. Within the platform, individual comparisons (case vs. control) were established for each cell line. These eight comparisons were then incorporated into meta-analysis, and the results for CRC landmark genes (obtained from the LINCS L1000 project) were generated through TargetID panel and Knowledge graph. To evaluate the quality of control samples generated by Precious2GPT, we compared the AI-driven target prioritization results between the generated CRC data and the pre-calculated results using real data available on PandaOmics.
Data availability
Human transcriptomic data is available on the GTEx database. Mouse transcriptomic data is sourced from the ARCHS4 database. Human DNA methylation data is available from this study https://doi.org/10.3389/fgene.2021.810985 . PandaOmics is commercially available at https://pandaomics.com/ .
Huang, L. et al. Deep Learning Methods for Omics Data Imputation. Biology 12 , https://doi.org/10.3390/biology12101313 (2023).
Lee, M. Recent Advances in Generative Adversarial Networks for Gene Expression Data: A Comprehensive Review. Mathematics 11 , 3055 (2023).
Article Google Scholar
Killoran, N., Lee, L. J., Delong, A., Duvenaud, D. & Frey, B. J. Generating and designing DNA with deep generative models. arxiv , https://doi.org/10.48550/arXiv.1712.06148 (2017).
Lew, S., Solé-Casals, J., Caiafa, C. F. & Bau-Macià, J. A copula-based method for synthetic microarray data generation. In Barcelona Advances in Statistics , https://doi.org/10.13140/2.1.2281.9843 (2012).
Yang, L. et al. Diffusion Models: A Comprehensive Survey of Methods and Applications. ACM Comput. Surv. 56 , 1–39 (2023).
Wang, C., Li, M. & Smola, A. J. Language Models with Transformers. arxiv , https://doi.org/10.48550/arXiv.1904.09408 (2019).
Rigaill, G. et al. Synthetic data sets for the identification of key ingredients for RNA-seq differential analysis. Brief. Bioinform 19 , 65–76 (2018).
CAS PubMed Google Scholar
Mehrotra, S., Bronstein, R., Navarro-Gomez, D., Segrè, A.V. & Pierce, E. A. Evaluating Methods for Differential Gene Expression And Alternative Splicing Using Internal Synthetic Controls. bioRxiv , https://doi.org/10.1101/2020.08.05.238295 (2020).
Lui, J. C., Chen, W., Barnes, K. M. & Baron, J. Changes in gene expression associated with aging commonly originate during juvenile growth. Mech. Ageing Dev. 131 , 641–649 (2010).
Article CAS PubMed PubMed Central Google Scholar
Vinuela, A. et al. Age-dependent changes in mean and variance of gene expression across tissues in a twin cohort. Hum. Mol. Genet. 27 , 732–741 (2018).
Article CAS PubMed Google Scholar
Yusipov, I. et al. Age-related DNA methylation changes are sex-specific: a comprehensive assessment. Aging 12 , 24057–24080 (2020).
Urban, A. et al. Precious1GPT: multimodal transformer-based transfer learning for aging clock development and feature importance analysis for aging and age-related disease target discovery. Aging 15 , 4649–4666 (2023).
CAS PubMed PubMed Central Google Scholar
Xu, L., Skoularidou, M., Cuesta-Infante, A. & Veeramachaneni, K. Modeling Tabular data using Conditional GAN. arxiv , https://doi.org/10.48550/arXiv.1907.00503 (2019).
Team, T. M. N. Introducing MPT-7B: A New Standard for Open-Source, Commercially Usable LLMs (Databricks, 2023).
Mamoshina, P. et al. Machine Learning on Human Muscle Transcriptomic Data for Biomarker Discovery and Tissue-Specific Drug Target Identification. Front. Genet. 9 , 242 (2018).
Article PubMed PubMed Central Google Scholar
Galkin, F., Mamoshina, P., Kochetov, K., Sidorenko, D. & Zhavoronkov, A. DeepMAge: A Methylation Aging Clock Developed with Deep Learning. Aging Dis. 12 , 1252–1262, (2021).
Johnson, A. A., Shokhirev, M. N., Wyss-Coray, T. & Lehallier, B. Systematic review and analysis of human proteomics aging studies unveils a novel proteomic aging clock and identifies key processes that change with age. Ageing Res. Rev. 60 , 101070 (2020).
Hwangbo, N. et al. A Metabolomic Aging Clock Using Human Cerebrospinal Fluid. J. Gerontol. A Biol. Sci. Med. Sci. 77 , 744–754 (2022).
Sill, J., Takacs, G., Mackey, L. & Lin, D. Feature-Weighted Linear Stacking. arXiv , https://doi.org/10.48550/arXiv.0911.0460 (2009).
Pun, F. W. et al. Hallmarks of aging-based dual-purpose disease and age-associated targets predicted using PandaOmics AI-powered discovery engine. Aging 14 , 2475–2506 (2022).
Huang, H. et al. Targeting AKT with costunolide suppresses the growth of colorectal cancer cells and induces apoptosis in vitro and in vivo. J. Exp. Clin. Cancer Res. 40 , 114 (2021).
Hechtman, J. F. et al. AKT1 E17K in Colorectal Carcinoma Is Associated with BRAF V600E but Not MSI-H Status: A Clinicopathologic Comparison to PIK3CA Helical and Kinase Domain Mutants. Mol. Cancer Res. 13 , 1003–1008 (2015).
Roy, H. K. et al. AKT proto-oncogene overexpression is an early event during sporadic colon carcinogenesis. Carcinogenesis 23 , 201–205 (2002).
Horiuchi, D. et al. Chemical-genetic analysis of cyclin dependent kinase 2 function reveals an important role in cellular transformation by multiple oncogenic pathways. Proc. Natl Acad. Sci. USA 109 , E1019–E1027 (2012).
Lane, M. E. et al. A novel cdk2-selective inhibitor, SU9516, induces apoptosis in colon carcinoma cells. Cancer Res. 61 , 6170–6177 (2001).
Chen, J. S. et al. PIK3CD induces cell growth and invasion by activating AKT/GSK-3beta/beta-catenin signaling in colorectal cancer. Cancer Sci. 110 , 997–1011 (2019).
Voutsadakis, I. A. The Landscape of PIK3CA Mutations in Colorectal Cancer. Clin. Colorectal Cancer 20 , 201–215 (2021).
Article PubMed Google Scholar
Ogino, S. et al. PIK3CA mutation is associated with poor prognosis among patients with curatively resected colon cancer. J. Clin. Oncol. 27 , 1477–1484 (2009).
Moqri, M. et al. Biomarkers of aging for the identification and evaluation of longevity interventions. Cell 186 , 3758–3775 (2023).
Lopez-Otin, C., Blasco, M. A., Partridge, L., Serrano, M. & Kroemer, G. The hallmarks of aging. Cell 153 , 1194–1217 (2013).
Zhavoronkov, A., Bischof, E. & Lee, K. F. Artificial intelligence in longevity medicine. Nat. Aging 1 , 5–7 (2021).
Zhavoronkov, A., Kochetov, K., Diamandis, P. & Mitina, M. PsychoAge and SubjAge: development of deep markers of psychological and subjective age using artificial intelligence. Aging 12 , 23548–23577 (2020).
Zhavoronkov, A. & Mamoshina, P. Deep Aging Clocks: The Emergence of AI-Based Biomarkers of Aging and Longevity. Trends Pharm. Sci. 40 , 546–549 (2019).
Zhavoronkov, A. Artificial Intelligence for Drug Discovery, Biomarker Development, and Generation of Novel Chemistry. Mol. Pharm. 15 , 4311–4313 (2018).
Putin, E. et al. Deep biomarkers of human aging: Application of deep neural networks to biomarker development. Aging 8 , 1021–1033 (2016).
Zagirova, D. et al. Biomedical generative pre-trained based transformer language model for age-related disease target discovery. Aging 15 , 9293–9309 (2023).
Pun, F. W. et al. A comprehensive AI-driven analysis of large-scale omic datasets reveals novel dual-purpose targets for the treatment of cancer and aging. Aging Cell 22 , e14017 (2023).
Pun, F. W. et al. Identification of Therapeutic Targets for Amyotrophic Lateral Sclerosis Using PandaOmics - An AI-Enabled Biological Target Discovery Platform. Front. Aging Neurosci. 14 , 914017 (2022).
Pun, F. W., Ozerov, I. V. & Zhavoronkov, A. AI-powered therapeutic target discovery. Trends Pharm. Sci. 44 , 561–572 (2023).
Aliper, A. et al. In search for geroprotectors: in silico screening and in vitro validation of signalome-level mimetics of young healthy state. Aging 8 , 2127–2152 (2016).
Zeng, X. et al. Deep generative molecular design reshapes drug discovery. Cell Rep. Med. 3 , 100794 (2022).
Xie, C. et al. Amelioration of Alzheimer’s disease pathology by mitophagy inducers identified via machine learning and a cross-species workflow. Nat. Biomed. Eng. 6 , 76–93 (2022).
Borisov, V., Seßler, K., Leemann, T., Pawelczyk, M. & Kasneci, G. Language Models are Realistic Tabular Data Generators. arXiv , https://doi.org/10.48550/arXiv.2210.06280 (2022).
Solatorio, A.V. & Dupriez, O. REaLTabFormer: Generating Realistic Relational and Tabular Data using Transformers. arXiv , https://doi.org/10.48550/arXiv.2302.02041 (2023).
Fisch, K. M. et al. Omics Pipe: a community-based framework for reproducible multi-omics data analysis. Bioinformatics 31 , 1724–1728 (2015).
Mocellin, S. & Provenzano, M. RNA interference: learning gene knock-down from cell physiology. J. Transl. Med. 2 , 39 (2004).
Aiello, A. et al. Immunosenescence and Its Hallmarks: How to Oppose Aging Strategically? A Review of Potential Options for Therapeutic Intervention. Front. Immunol. 10 , 2247 (2019).
Ponnappan, S. & Ponnappan, U. Aging and immune function: molecular mechanisms to interventions. Antioxid. Redox Signal 14 , 1551–1585 (2011).
Blagosklonny, M. V. Selective protection of normal cells from chemotherapy, while killing drug-resistant cancer cells. Oncotarget 14 , 193–206 (2023).
Blagosklonny, M. V. Cancer prevention with rapamycin. Oncotarget 14 , 342–350 (2023).
Blagosklonny, M. V. My battle with cancer. Part 1. Oncoscience 11 , 1–14 (2024).
Consortium, G. The Genotype-Tissue Expression (GTEx) project. Nat. Genet. 45 , 580–585 (2013).
Seal, R. L. et al. Genenames.org: the HGNC resources in 2023. Nucleic Acids Res. 51 , D1003–D1009 (2023).
Xiong, Z., Li, M., Ma, Y., Li, R. & Bao, Y. GMQN: A Reference-Based Method for Correcting Batch Effects and Probe Bias in HumanMethylation BeadChip. Front. Genet. 12 , 810985 (2021).
Gao, Z., Tang, J., Xia, J., Zheng, C. H. & Wei, P. J. CNNGRN: A Convolutional Neural Network-Based Method for Gene Regulatory Network Inference From Bulk Time-Series Expression Data. IEEE/ACM Trans. Comput. Biol. Bioinform. 20 , 2853–2861 (2023).
Sharma, A., Vans, E., Shigemizu, D., Boroevich, K. A. & Tsunoda, T. DeepInsight: A methodology to transform a non-image data to an image for convolution neural network architecture. Sci. Rep. 9 , 11399 (2019).
Kohonen, T. The self-organizing map. Proc. IEEE 78 , 1464–1480 (1990).
Kohonen, T. Essentials of the self-organizing map. Neural Netw. 37 , 52–65 (2013).
Jeon, M. et al. Transforming L1000 profiles to RNA-seq-like profiles with deep learning. BMC Bioinforma. 23 , 374 (2022).
Article CAS Google Scholar
Zhu, J., Park, T., Isola, P. & Efros, A. A. Unpaired Image-to-Image Translation Using Cycle-Consistent Adversarial Networks. IEEE International Conference on Computer Vision (ICCV) , 2242-2251, (2017).
Capelle, T. Diffusion Models (GitHub, 2023).
Hoffmann, J. et al. Training Compute-Optimal Large Language Models. arXiv , https://doi.org/10.48550/arXiv.2203.15556 (2022).
Sasaki, Y. The truth of the F-measure . (Old Dominion University, 2007).
Mclnnes, L., Healy, J., Saul, N. & Großberger, L. UMAP: Uniform Manifold Approximation and Projection for Dimension Reduction. J. Open Source Softw. 3 , 861 (2018).
Dorogush, A.V., Ershov, V. & Gulin, A. CatBoost: gradient boosting with categorical features support. arxiv , https://doi.org/10.48550/arXiv.1810.11363 (2018).
Nachar, N. The Mann-Whitney U: A Test for Assessing Whether Two Independent Samples Come from the Same Distribution. Tutor. Quant. Methods Psychol. 4 , 13–20 (2008).
Tsybakov, A. B. Introduction to Nonparametric Estimation , 1st edn, (Springer, 2008).
Higgins-Chen, A. T. & Levine, M. E. Principal component analysis improves reliability of epigenetic aging biomarkers. Nat. Aging 2 , 578–579 (2022).
Fang, Z., Liu, X. & Peltz, G. GSEApy: a comprehensive package for performing gene set enrichment analysis in Python. Bioinformatics 39 , https://doi.org/10.1093/bioinformatics/btac757 (2023).
Kanehisa, M., Sato, Y., Kawashima, M., Furumichi, M. & Tanabe, M. KEGG as a reference resource for gene and protein annotation. Nucleic Acids Res. 44 , D457–462, (2016).
Download references
Acknowledgements
We thank Ms. Elizaveta Ekimova for her technical assistance with figure design. This study received no specific grant from any funding agency in the public, commercial, or not-for-profit sectors.
Author information
Authors and affiliations.
Insilico Medicine Hong Kong Ltd., Unit 310, 3/F, Building 8W Hong Kong Science and Technology Park, Hong Kong SAR, China
Denis Sidorenko, Stefan Pushkov, Akhmed Sakip, Geoffrey Ho Duen Leung, Sarah Wing Yan Lok, Anatoly Urban, Diana Zagirova, Ekaterina Kozlova, Vladimir Naumov, Frank W. Pun & Alex Zhavoronkov
Insilico Medicine AI Limited, Level 6, Unit 08, Block A, IRENA HQ Building, Masdar City, Abu Dhabi, UAE
Alexander Veviorskiy, Nina Tihonova, Aleksandr Kalashnikov, Alex Aliper & Alex Zhavoronkov
Insilico Medicine Shanghai Ltd., Suite 902, Tower C, Changtai Plaza, 2889 Jinke Road, Pudong, Shanghai, 201203, China
Buck Institute for Research on Aging, Novato, CA, 94945, USA
Alex Zhavoronkov
You can also search for this author in PubMed Google Scholar
Contributions
D.S. developed the main model, analyzed data, participated in result interpretation and project administration, and drafted the manuscript. S.P. developed LLM part of the model, A.S. developed the CDiffusion part of the model, S.P and A.S. analyzed data and participated in result interpretation. G.H.D.L. and S.W.Y.L. participated in result interpretation and revised the manuscript. A.U. calculated SOM, provided technical support, and reviewed the manuscript, D.Z. provided data collection and preprocessing, provided technical support, and reviewed the manuscript, A.V., N.T. provided a case study experiment, A.K. and E.K. provided technical support, and reviewed the manuscript. V.N., F.W.P., A.A. and F.R. provided scientific advice and reviewed the manuscript. A.Z. provided conceptualization, reviewed the manuscript, provided resources, and supervised the project. All authors read and approved the final manuscript.
Corresponding author
Correspondence to Alex Zhavoronkov .
Ethics declarations
Competing interests.
The authors are affiliated with Insilico Medicine, a commercial company developing and using generative artificial intelligence and other next-generation AI technologies and robotics for drug discovery, drug development, and aging research. Utilizing its generative AI platform and a range of deep aging clocks, Insilico Medicine has developed a portfolio of multiple therapeutic programs targeting fibrotic diseases, cancer, immunological diseases, and a range of age-related diseases.
Additional information
Publisher’s note Springer Nature remains neutral with regard to jurisdictional claims in published maps and institutional affiliations.
Supplementary information
Rights and permissions.
Open Access This article is licensed under a Creative Commons Attribution-NonCommercial-NoDerivatives 4.0 International License, which permits any non-commercial use, sharing, distribution and reproduction in any medium or format, as long as you give appropriate credit to the original author(s) and the source, provide a link to the Creative Commons licence, and indicate if you modified the licensed material. You do not have permission under this licence to share adapted material derived from this article or parts of it. The images or other third party material in this article are included in the article’s Creative Commons licence, unless indicated otherwise in a credit line to the material. If material is not included in the article’s Creative Commons licence and your intended use is not permitted by statutory regulation or exceeds the permitted use, you will need to obtain permission directly from the copyright holder. To view a copy of this licence, visit http://creativecommons.org/licenses/by-nc-nd/4.0/ .
Reprints and permissions
About this article
Cite this article.
Sidorenko, D., Pushkov, S., Sakip, A. et al. Precious2GPT: the combination of multiomics pretrained transformer and conditional diffusion for artificial multi-omics multi-species multi-tissue sample generation. npj Aging 10 , 37 (2024). https://doi.org/10.1038/s41514-024-00163-3
Download citation
Received : 19 March 2024
Accepted : 22 July 2024
Published : 08 August 2024
DOI : https://doi.org/10.1038/s41514-024-00163-3
Share this article
Anyone you share the following link with will be able to read this content:
Sorry, a shareable link is not currently available for this article.
Provided by the Springer Nature SharedIt content-sharing initiative
Quick links
- Explore articles by subject
- Guide to authors
- Editorial policies
Sign up for the Nature Briefing: Translational Research newsletter — top stories in biotechnology, drug discovery and pharma.

- Fact sheets
- Facts in pictures
- Publications
- Questions and answers
- Tools and toolkits
- Endometriosis
- Excessive heat
- Mental disorders
- Polycystic ovary syndrome
- All countries
- Eastern Mediterranean
- South-East Asia
- Western Pacific
- Data by country
- Country presence
- Country strengthening
- Country cooperation strategies
- News releases
- Feature stories
- Press conferences
- Commentaries
- Photo library
- Afghanistan
- Cholera
- Coronavirus disease (COVID-19)
- Greater Horn of Africa
- Israel and occupied Palestinian territory
- Disease Outbreak News
- Situation reports
- Weekly Epidemiological Record
- Surveillance
- Health emergency appeal
- International Health Regulations
- Independent Oversight and Advisory Committee
- Classifications
- Data collections
- Global Health Estimates
- Mortality Database
- Sustainable Development Goals
- Health Inequality Monitor
- Global Progress
- World Health Statistics
- Partnerships
- Committees and advisory groups
- Collaborating centres
- Technical teams
- Organizational structure
- Initiatives
- General Programme of Work
- WHO Academy
- Investment in WHO
- WHO Foundation
- External audit
- Financial statements
- Internal audit and investigations
- Programme Budget
- Results reports
- Governing bodies
- World Health Assembly
- Executive Board
- Member States Portal
Strengthening Vaccine Manufacturing: Announcing the Fifth Virtual cGMP Training Marathon - Institutionalizing Compliance and Continued Improvement
The World Health Organization's Local Production & Assistance Unit (LPA) , with support from key stakeholders, is pleased to announce the upcoming fifth “Virtual cGMP Training Marathon for Vaccine Manufacturing: Institutionalizing Compliance and Continued Improvement.” This annual event aims to bolster the capacities of Member States in producing quality-assured vaccines, ensuring equitable and timely access, and fortifying health security worldwide.
Access to quality-assured vaccines is a cornerstone of effective health systems and national immunization programs. However, many low- and middle-income countries (LMICs) face significant challenges in this area, often relying on imports that can be inconsistent. Strengthening local production capabilities is essential to overcoming these challenges.
Since 2020, the WHO LPA Unit has successfully conducted four Virtual cGMP Training Marathons, enhancing knowledge and preparedness among vaccine manufacturers globally. This year's marathon, themed “Institutionalizing Compliance and Continued Improvement,” will build on previous years’ successes by focusing on sustaining GMP compliance and fostering a culture of continuous quality improvement.
The 2024 training aims to support LMICs in strengthening their local vaccine manufacturing capabilities. By providing in-depth knowledge on current GMP standards, regulatory updates, and technological advancements, the training seeks to empower manufacturers and regulators to meet and exceed international quality benchmarks.
- Bio-Analytical Method Development and Validation for Biologicals
- Quality Risk Management Framework: A Proactive, Data-Driven Approach
- Process Validation of Biologicals: Current Regulatory Expectations
- Maintaining and Improving Process Performance: Monitoring and Trending
- Sterile Manufacturing: Navigating TRS 1044 Annex 2
- Technology Transfer Essentials for Bio-Pharmaceuticals
- Management of GXP Outsourced Activities: Challenges and Strategies
- Maintaining Compliant Critical Utilities from URS to PQ
- CAPA and RCA Investigation Management: Improving Effectiveness
- Recent cGMP Inspection Trends: Common Non-Compliances and Pitfalls
These sessions will be delivered virtually twice per week from September 10th to October 10th, 2024; 13:00 – 15:30 CEST, combining lectures, real-world examples, exercises, and case studies to ensure practical understanding and application of cGMP principles.
Participation
The training is open to professionals involved in vaccine, biological, and pharmaceutical manufacturing, particularly those from LMICs. Participants will have the opportunity to enhance their skills, adapt to regulatory changes, and implement effective quality management systems. Registration is required, and space is limited. Priority will be given to eligible participants from LMICs and those with active plans for WHO prequalification/EUL.
Register Now
To express your interest in participating, click here to register (Deadline: 6th September 2024). Selected participants will receive a confirmation email. For further inquiries, please contact the LPA Unit Secretariat at [email protected] .
- Open access
- Published: 08 August 2024
Drug repositioning based on residual attention network and free multiscale adversarial training
- Guanghui Li 1 ,
- Shuwen Li 1 ,
- Cheng Liang 2 ,
- Qiu Xiao 3 &
- Jiawei Luo 4
BMC Bioinformatics volume 25 , Article number: 261 ( 2024 ) Cite this article
Metrics details
Conducting traditional wet experiments to guide drug development is an expensive, time-consuming and risky process. Analyzing drug function and repositioning plays a key role in identifying new therapeutic potential of approved drugs and discovering therapeutic approaches for untreated diseases. Exploring drug-disease associations has far-reaching implications for identifying disease pathogenesis and treatment. However, reliable detection of drug-disease relationships via traditional methods is costly and slow. Therefore, investigations into computational methods for predicting drug-disease associations are currently needed.
This paper presents a novel drug-disease association prediction method, RAFGAE. First, RAFGAE integrates known associations between diseases and drugs into a bipartite network. Second, RAFGAE designs the Re_GAT framework, which includes multilayer graph attention networks (GATs) and two residual networks. The multilayer GATs are utilized for learning the node embeddings, which is achieved by aggregating information from multihop neighbors. The two residual networks are used to alleviate the deep network oversmoothing problem, and an attention mechanism is introduced to combine the node embeddings from different attention layers. Third, two graph autoencoders (GAEs) with collaborative training are constructed to simulate label propagation to predict potential associations. On this basis, free multiscale adversarial training (FMAT) is introduced. FMAT enhances node feature quality through small gradient adversarial perturbation iterations, improving the prediction performance. Finally, tenfold cross-validations on two benchmark datasets show that RAFGAE outperforms current methods. In addition, case studies have confirmed that RAFGAE can detect novel drug-disease associations.
Conclusions
The comprehensive experimental results validate the utility and accuracy of RAFGAE. We believe that this method may serve as an excellent predictor for identifying unobserved disease-drug associations.
Peer Review reports
Drugs play important roles in treating diseases and promoting the health of organisms [ 1 ]. However, traditional drug development is an extremely lengthy and expensive process [ 2 ]. Recent studies have estimated that the average development cost to approve a new drug is $2.6 billion and the average development time is 10 years [ 3 ]. Drug repositioning, which involves discovering new therapeutic outcomes for previously approved drugs, is considered an important alternative to traditional drug development [ 4 , 5 , 6 , 7 , 8 ]. This approach shortens drug development and research cycles to 7 years, reduces costs to $295 million, and is more reliable than novel drug development [ 9 ]. Therefore, using known drugs for new disease treatments is gaining popularity [ 10 , 11 ]. Traditional methods of discovering abnormal clinical manifestations through manual screening of clinical drug databases requires extensive experimentation. With the continuous accumulation of a wide variety of biological data, numerous computational methods based on data mining techniques have gained traction [ 12 ].
Matrix factorization aims to approximate the initial matrix by decomposing it into the product of two low-rank matrices, which are represented by hidden factor vectors in the k -dimension. The inner product of the drug and disease vectors represents the association between them. Previous studies have shown that matrix decomposition methods are effective computational methods for drug-disease association prediction [ 13 , 14 , 15 , 16 , 17 ]. For example, the similarity constrained matrix factorization method for the drug-disease association prediction (SCMFDD) method, proposed by Zhang et al., maps the associations between diseases and drugs into two low-ranking spaces and reveals the basic features. Then, drug similarity and disease similarity are introduced as increasing constraints [ 18 ]. Furthermore, Yang et al. proposed the multisimilarities bilinear matrix factorization (MSBMF) approach, which connects multiple disease and drug similarity matrices and extracts the effective latent features in the similarity matrix to infer associations between diseases and drugs [ 19 ]. In addition, Zhang et al. proposed a new drug repositioning method by using Bayesian inductive matrix completion (DRIMC), which uses the complement of Bayesian inductive matrices. This method integrates multiple similarities into a fused similarity matrix, where similarity information is described by similarity values between a drug or disease and its k -nearest neighbors. Finally, the disease-drug association is predicted via induction matrix completion [ 20 ].
Networks can represent the complex relationships among entities, and the methods used to construct biological networks can effectively utilize information from multiple biological entities to represent the degree of association between them [ 21 ]. The network-based method has produced good results in drug repositioning [ 22 , 23 , 24 ]. For instance, Zhao et al. first constructed a heterogeneous information network by combining drug-disease, protein-disease and drug-protein bioinformatics networks with disease and drug biology information. Then, the combined features of the nodes were learned from a biological and topological perspective via different representations. Moreover, random forest classifiers can be used to predict unknown associations [ 25 ]. Zhang et al. proposed a multiscale neighborhood topology learning method for drug repositioning (MTRD) to learn and integrate multiscale neighborhood topologies. This method involves the construction of different drug-disease heterogeneous networks to discover new drug-disease associations [ 26 ]. In addition, Luo et al. proposed a method named MBiRW that uses similarity matrices and known associations to construct heterogeneous networks and predicts unknown associations via the double random walk algorithm [ 27 ].
Although matrix factorization methods achieve good performance, they are weak in the interpretability of associations between diseases and drugs, whereas network methods are biased in representing higher-order networks. To solve these problems, several pioneering studies have focused on developing deep learning-based drug repositioning models [ 28 , 29 , 30 , 31 , 32 , 33 ]. For example, Zeng et al. first integrated multiple disease-drug biological networks and designed a multimodal deep autoencoder named deep learning-based drug repositioning (deepDR) for learning higher order neighborhood information of drug-disease associations [ 34 ]. Subsequently, Yu et al. constructed a graph convolutional network (GCN) architecture with attention mechanisms, i.e., the label-aware GCN (LAGCN). First, this method uses known drug-disease associations, disease-disease similarities and drug-drug similarities to construct heterogeneous networks and applies GCNs to the network. Next, the embeddings from multiple GCN layers are integrated via layer attention mechanisms. Finally, drug-disease pairs are scored on the basis of the integrated embeddings [ 35 ]. Feng et al. proposed Protein And Drug Molecule interaction prEdiction (PADME), a novel method to combine molecular GCNs for compound featurization with protein descriptors for drug-target interaction prediction [ 36 ]. Moreover, Meng et al. proposed a drug repositioning approach based on weighted bilinear neural collaborative filtering (DRWBNCF) on the basis of neighborhood interaction and collaborative filtering. Instead of using all neighbors, this method uses only the nearest neighbors, thus filtering out noise and yielding more precise results [ 37 ]. Recently, Gu et al. proposed a method named relations-enhanced drug-disease association (REDDA) for learning node features of heterogeneous networks and topological subnetworks. This method employs heterogeneous networks as the backbone and combines the backbone with three attention mechanisms [ 38 ]. Deep learning-based methods mainly construct heterogeneous networks by using supplementary information about diseases and drugs and learn the features of diseases and drugs by applying deep learning algorithms to these networks.
However, these deep learning-based approaches tend to have oversmoothing problems caused by the homogenization of node embeddings and are highly dependent on the input quality. In this paper, we present a novel method of drug repositioning named RAFGAE. This method combines residual networks, graph attention networks (GATs), graph autoencoders (GAEs) and adversarial training to predict unknown associations between diseases and drugs. First, we use disease semantic similarity, drug structural similarity and disease-drug associations to construct the initial input features. GATs are used to facilitate the learning of disease and drug embeddings in each layer and combine the embedding of different layers via attention mechanisms. Moreover, the initial residual and adaptive residual connections are adopted to alleviate the oversmoothing problem. Then, two GAEs are constructed on the basis of the disease space and drug space, and the information in these spaces can be integrated through synergistic training. Finally, the scores of the two GAEs are linearly combined by a balancing parameter to calculate the final prediction scores. On this basis, adversarial training is introduced to reduce invalid information and data noise, improving the input quality. The main contributions of RAFGAE can be summarized as follows:
RAFGAE is a complete deep learning approach that can effectively predict the associations between diseases and drugs.
RAFGAE designs the Re_GAT framework, which includes multilayer GATs and two residual networks. Multilayer GATs are utilized to learn the node embeddings by aggregating information from multihop neighbors, and two residual networks are used to alleviate the deep network oversmoothing problem. Then, an attention mechanism is introduced to combine the node embeddings of different attention layers.
RAFGAE performs adversarial training that may eliminate abnormal values, missing values and noise, increasing the input quality and prediction accuracy when extracting associations between diseases and drugs.
Our comprehensive experimental results demonstrate that the proposed RAFGAE method significantly outperforms five state-of-the-art methods on the benchmark dataset.
Results and discussion
Algorithm performance comparison.
To verify the performance of RAFGAE, we compare it with five recently proposed methods.
DRWBNCF [ 37 ], a method for drug repositioning on the basis of neighborhood interaction and collaborative filtering, uses only the nearest neighbors, rather than all neighbors, to filter out noisy information. A new weighted bilinear GCN encoder is then proposed.
LAGCN [ 35 ], a layer attention GCN method for drug repositioning, encodes a heterogeneous network combining known drug-disease associations, disease similarity and drug similarity information. To integrate all useful information, a layer attention mechanism is introduced into multiple GCN layers.
In bounded nuclear norm regularization (BNNR) [ 39 ], a heterogeneous network is constructed. This network combines known drug-disease associations, disease similarity and drug similarity information. The method tolerates noise by adding a regularization term to balance the rank properties and approximation error.
The neural inductive matrix completion with GCN (NIMCGCN) method [ 40 ], a method for the prediction of miRNA-disease associations) first employs GCN to learn the features of diseases and miRNAs from the disease and miRNA similarity networks. Then, neural induction matrix completion is applied for association matrix completion.
SCMFDD [ 18 ] (a similarity constraint matrix completion method for the prediction of drug-disease associations) projects known drug-disease association information into two low-rank spaces, revealing potential disease and drug embeddings, and then introduces drug featured-based and disease semantic similarities as constraints for drugs and diseases in the low-rank spaces.
The above methods also involve similarity-based graph neural network models. The parameters in these methods are set to either the optimal values via a grid search (for DRWBNCF, λ is selected from {0.1, 0.2, ..., 0.9}; for BNNR, α and β are chosen from {0.01, 0.1, 1, 10}; and for SCMFDD, k is selected from{5%, 10%, ..., 50%}) or the values recommended by the authors (for LAGCN, α = 4000, β =0.6, and γ = 0.4; and for NIMCGCN, α = 0.4, l = 3, and t = 2). Furthermore, to ensure a meaningful and relevant comparison, each of the comparison methods is initially evaluated via the same 10-fold cross-validation approach and on the same benchmarking sets as those for our proposed method, RAFGAE. This approach allows us to conduct a comprehensive and rigorous assessment of the performance of all the methods.
The area under the curve (AUC) values in Fig. 1 and Table 1 show a comparison of the model performance. On the F-dataset, RAFGAE achieves the highest AUC score of 0.9343, which is 7.28%, 4.50%, 3.13%, 4.31%, and 4.01% higher than those of SCMFDD, LAGCN, BNNR, NIMGCN, and DRWBNCF, respectively. Similarly, on the C-dataset, RAFGAE achieves the highest AUC score of 0.9346. By comparing the model proposed in this paper with other models, it is evident that introducing residual connections and adversarial training can enhance the predictive performance of our model. Overall, the above experiments show that RAFGAE is an excellent predictor of disease-drug relationships.
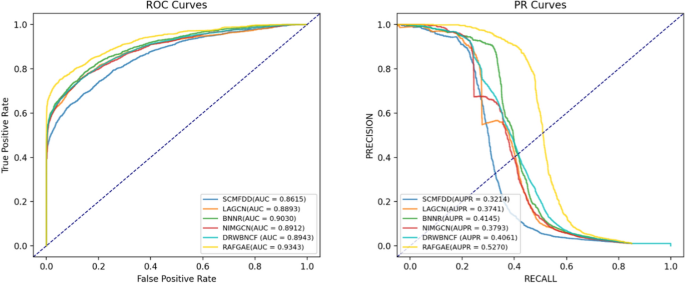
ROC curves and PR curves of RAFGAE and other models on the F-dataset
Ablation study
To quantitatively evaluate the importance of the two modules (the Re_GAT framework and the FMAT module) to RAFGAE, ablation experiments are conducted. The details of these variants of RAFGAE are listed below:
RAFGAE: The comprehensive RAFGAE framework consists of three main components: the Re_GAT framework, the FMAT module, and the GAE module.
GAE: The RAFGAE variant that includes only the GAE module.
FGAE: The RAFGAE variant that includes the FMAT and GAE modules but excludes the Re_GAT framework.
RAGAE: The RAFGAE variant that includes Re_GAT framework and the GAE module but excludes the FMAT module.
According to Fig. 2 and Table 2 , it is clear that RAFGAE achieved the highest AUC and area under the precision–recall (AUPR) curve values on both the F-dataset and the C-dataset. The RAGAE and FGAE results show the impacts of global neighborhood node information aggregation and adversarial feature enhancement on the RAFGAE performance, respectively. In addition, the GAE results demonstrate that combining the Re_GAT framework and the FMAT module can improve the predictive performance of the RAFGAE model. In comparing FGAE and RAGAE to GAE, the performance results imply that both the Re_GAT framework and the FMAT module can improve the model performance. The poor performance of GAE suggests that the use of multilayer attention networks to aggregate global information and the incorporation of residual architectures to address the potential oversmoothing problem can enhance the accuracy of drug-disease association prediction. Furthermore, the results indicate that the inclusion of the adversarial training module improves the input quality, thereby satisfying the requirements of deep neural networks for high-quality input features. These results demonstrate that the RAFGAE structure is reasonable.
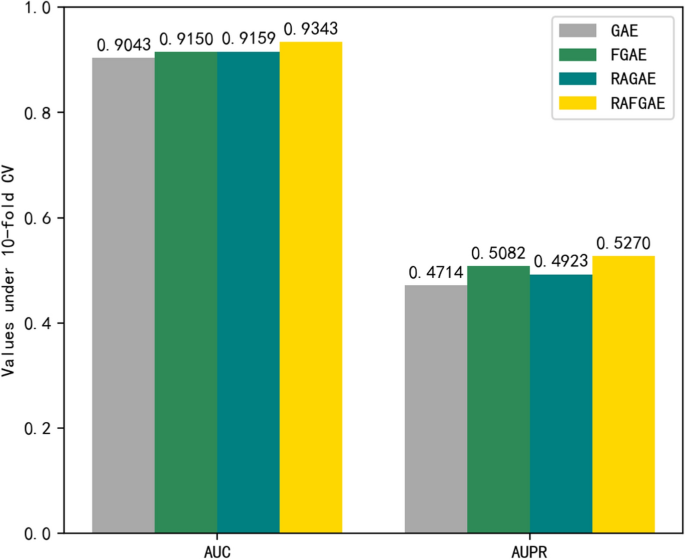
Results of RAFGAE and its variants in the ablation study on the F-dataset
Performance evaluation
To assess the effectiveness of RAFGAE in predicting known associations, tenfold cross validation (CV) is applied. In tenfold CV, the dataset is divided into ten folds. Nine folds are used as the training set, and the remaining fold is used to validate the performance of RAFGAE. This process is repeated 10 times, with each fold used as the testing fold once. Several important indicators are used to evaluate the performance of RAFGAE. The receiver operating characteristic (ROC) curve, which is based on the false-positive rate (FPR) and the true positive rate (TPR), is utilized. As the benchmark datasets used in this experiment are imbalanced, we also use the PR curve and calculate the area under the PR curve (AUPR) as two additional indicators. To further evaluate the overall performance of the prediction model from multiple perspectives, the F1 score and the Mathews correlation coefficient (MCC) are calculated.
The ROC and PR curves for the F-dataset are shown in Fig. 3 . RAFGAE achieves mean AUC and AUPR values of 0.9343 and 0.5270, respectively. The detailed results, including the F1-score and MCC, are presented in Table 3 . The results based on the C-dataset are shown in Table 4 . As shown in Tables 1 and 2 , the newly proposed RAFGAE model obtains good performance on the above two datasets, proving the effectiveness and robustness of this model.
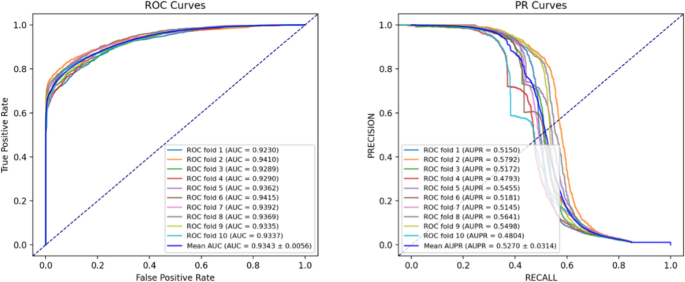
RAFGAE ROC and PR curves via tenfold CV on the F-dataset
Parameter adjustment
Since the hyperparameter settings can influence the performance of RAFGAE, we used tenfold CV on the F-dataset to analyze the impact of different parameter settings. In the Re_GAT framework, the weight α of the initial residual connection and the weight β of the adaptive residual connection can directly affect the result of feature fusion. To fully integrate adjacent node information and mitigate the oversmoothing problem, we adjust the α and β values within the following range: α ϵ {0.1 ~ 0.9} and β ϵ {0.1 ~ 0.9}. As shown in Fig. 4 , when α = 0.3 and β = 0.7, the AUC reaches its maximum value.
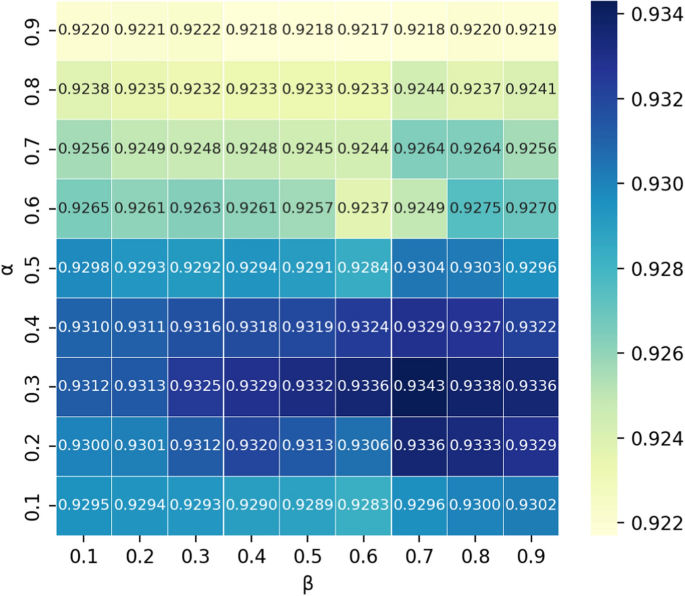
Effect of the α and β parameters on the AUC of RAFGAE
In addition, the features of diseases and drugs are extracted via GATs. The Re_GAT framework computes and aggregates different multilayer features via the GAT. We discuss the impact of GATs with different numbers of layers on association prediction. Figure 5 presents the results of the ROC curve analysis on the basis of tenfold CV.
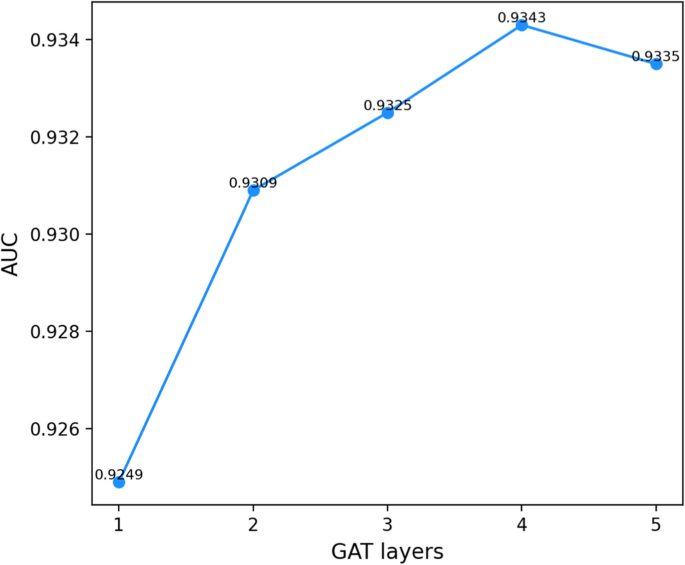
Effect of the number of GAT layers on the AUC of RAFGAE
To optimize the initial parameters, we use the Adam optimizer [ 41 ]. As in previous studies [ 42 , 43 ], we set the dropout and weight decay parameters to 0.5 and 10 –5 , respectively. We also evaluate the model performance by changing the dimensions of the GAE hidden layers. With the other parameters unchanged, the AUC value of RAFGAE generally increases as the embedding dimension of the GAE hidden layer increase and tends to stabilize when the dimension reaches 256. Finally, we set the embedding dimension of the hidden layer to 256. These results are shown in Fig. 6 .
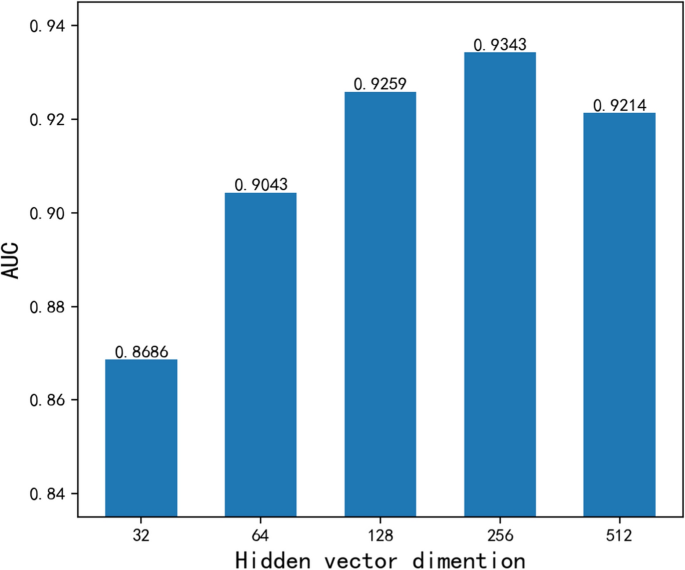
Effect of the hidden vector dimension on the AUC of RAFGAE
Case studies
To evaluate the practical ability of RAFGAE to predict unknown indications of approved drugs as well as new therapies for existing diseases, we train the RAFGAE model using all known associations as training data, and predict potential associations for known diseases or drugs. The predicted ranking of unknown indications of approved drugs and unknown therapies for existing diseases is validated on the public database, namely, the Comparative Toxicogenomics Database (CTD) [ 44 ].
To assess the ability of RAFGAE to discover new indications, we select two representative medicinal products. Table 5 shows the confirmation information for the top 10 candidate diseases and the known drug-disease associations. Among them, doxorubicin is a cytotoxic anthracycline antibiotic that is widely used to treat various cancers, including Kaposi sarcoma and metastatic cancer related to AIDS. Of the top 10 positive predictions, there were 7 tumor-related diseases that have been verified via reliable databases. Levodopa is a precursor of dopamine and is commonly used in the treatment of Parkinson's syndrome and Parkinson's syndrome-related disorders because of its ability to cross the blood–brain barrier. As shown in Table 5 , reliable sources have identified 7 of the top 10 associated diseases. This evidence suggests that RAFGAE can be trained on and can learn from existing biological information and can identify association markers that are not captured in the training set.
To validate the practical ability of RAFGAE to discover novel therapies, we select breast neoplasms and small-cell lung cancer as experimental cases. On the basis of the RAFGAE prediction results, the 10 drugs with the highest prediction scores are validated via the CTD. Table 6 shows similar results for the top 10 positive predictions. Breast neoplasms are among the most common malignancies in women and the leading cause of cancer-related disease in women. As shown in Table 6 , 9 of the top 10 drugs were verified via reliable sources. The high incidence rate and high mortality of small cell lung cancer worldwide make this complex tumor a difficult medical problem. In summary, 6 drugs have been confirmed by evidence from authoritative sources among the top 10 predicted drugs ranked by prediction score. In summary, case studies have shown that RAFGAE can identify the associations between diseases and drugs that are unknown in training datasets but that have been validated in real-world studies. Moreover, RAFGAE can make reliable predictions regarding unconfirmed potential associations between diseases and drugs. Therefore, RAFGAE has a noteworthy ability to uncover novel therapies/indications for existing diseases/drugs.
In this paper, a deep-learning methodology named RAFGAE is developed for elucidating drug-disease associations. The key innovation of RAFGAE is that it combines the Re_GAT framework and the FMAT algorithm, facilitating the learning of neighbor node information and enhancing the initial node features in the disease-drug bipartite network. Then, two GAEs with collaborative training are applied to integrate the disease and drug spaces for association prediction. Notably, unlike some previous predictors that consider only low-order neighbor information, the Re_GAT framework can account for both high-order and low-order neighbor information by using multilayer GATs. Moreover, residual networks are introduced to mitigate model data oversmoothing, enabling the full employment of graph structure information hidden in the bipartite network. To enhance the initial features of nodes and make the model more robust, the FMAT algorithm is employed. This algorithm adds gradient-based adversarial perturbation to the input characteristics. In addition, we construct two GAEs with collaborative training for label propagation, enabling the full integration of the drug and disease space information for association prediction and improving the robustness of the RAFGAE model.
With tenfold CV, the RAFGAE model achieves an AUC score of 0.9343, which is better than the AUC scores of five state-of-the-art predictors. Furthermore, the case study results show that RAFGAE can reposition several representative drugs for human diseases and can be applied as a reasonable and effective tool for predicting the relationships between diseases and drugs.
We propose a computational drug repurposing method. This method can effectively identify candidate drugs with potential for treating different diseases and has the potential to uncover new indications for approved drugs that were previously unexplored. RAFGAE can guide wet laboratory experiments, accelerating drug development, reducing costs, and expanding treatment options. The method combines multilayer neural networks with residual connections to capture global information and alleviate oversmoothing problems. We also employ adversarial perturbations to improve the input quality. This novel combination of techniques provides a new perspective for future research and can also serve as a valuable reference for similar studies, such as predicting the associations between ncRNAs and diseases, microbiome-disease associations, and screening ncRNA drug targets.
However, RAFGAE has certain limitations. In this study, the negative and positive samples of the benchmark dataset are unbalanced, and we use all the negative samples as negative samples for training the proposed model. However, these unknown samples considered negative samples may be potential correlations, which greatly impacts the prediction accuracy of the model. In the future, we will select negative samples to further improve the model accuracy. In terms of biological data, we simply apply the interaction network between drugs and diseases without establishing a more informative biological regulatory network, which may further improve performance. In future research, we will introduce other biological entities, such as proteins, pathways, and genes. In scenarios where drugs share the same or similar indications but lack structural similarity, the transmission of structural similarity information through a multilayer neural network can give rise to an "information leakage" problem, leading to a distorted view of the algorithm's performance in realistic drug repurposing settings. In our future research, we plan to address the problem of information leakage further by incorporating multiple drug similarities, such as target protein domain similarity, GO target protein annotation similarity, side effect similarity, and GIP similarity. This broader range of drug similarities can provide a more comprehensive features for drug repurposing. Similarly, incorporating disease similarities, such as disease ontology similarity, can help improve the accuracy and reliability of repositioning predictions by leveraging additional disease-related information.
Data preparation
We employ two benchmark datasets established by investigators. The first dataset is the F-dataset, which corresponds to Gottlieb's gold standard dataset [ 45 ]. The F-dataset contains 1933 known associations between diseases and drugs, including 313 diseases collected from the OMIM database [ 46 ] and 593 drugs obtained from the DrugBank database [ 47 ]. The second dataset is the C-dataset [ 24 ], which includes 2532 known associations between 409 diseases collected from the OMIM database and 663 drugs obtained from the DrugBank database. Table 7 summarizes the benchmark datasets in our proposal.
In this study, we calculated the drug structure similarity matrix X dr via the simplified molecular input line entry system (SMILES) chemical structure [ 48 ], which is represented as the Tanimoto index of chemical fingerprints of the drug pair via the Chemical Development Kit [ 49 ]. The disease semantic similarity matrix X di is computed from the semantic similarity of the disease phenotypes via information from the medical descriptions of the disease pairs [ 50 ].
After collecting the required data from different sources, we propose a prediction model with three individual modules to predict potential candidate diseases for drugs of interest. We first design the Re_GAT framework, which captures global structural information from a bipartite network. For the second module, we employ GAEs that use known associations between diseases and drugs to simulate label propagation to guide and predict unknown associations. On the basis of the above, we utilize the FMAT module for adversarial training to improve the input quality and increase the prediction accuracy. Figure 7 shows the overall workflow of RAFGAE.
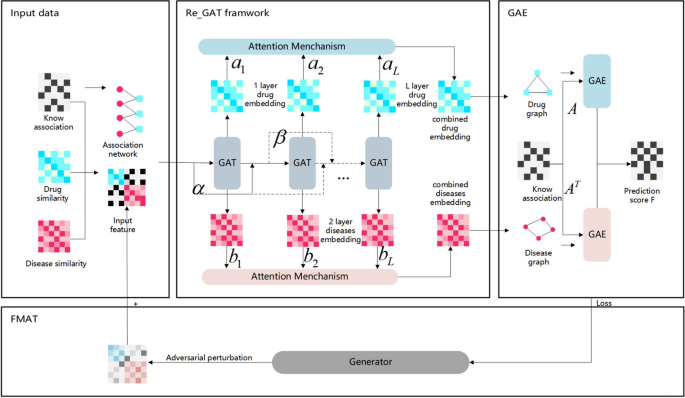
Flow chart of the RAFGAE calculation method
Re_GAT framework
Graph attention networks use a self-attention hidden layer to assign different attention scores to different neighbors, thus extracting the features of neighboring nodes more effectively.
The initial input to the Re_GAT framework can be described as follows:
where N represents the node count, F represents the dimension of the feature and h i ϵ R F represents the initial feature matrix of all the nodes. GATs calculate attention scores on the basis of the importance of neighbors and then aggregate neighbor features on the basis of the attention score.
The attention score is calculated as follows:
To adjust for the influence of different nodes, we use the softmax function for attention score normalization score:
By combining Formulas ( 3 ) and ( 4 ), the calculation formula for the attention score can be expressed as:
where a ij is the attention score, W is a learnable linear transformation matrix, a vector denotes the weight vector, σ () represents the LeakyReLU activation function, and ║ denotes the connection operation. After normalization, the following formula can be used to calculate the final output feature:
In this study, the drug-disease association matrix is given by matrix A , where the columns represent diseases and the rows represent drugs. The matrix A ( j , k ) = 1 if drug j is associated with disease k and 0 otherwise. Matrix A and its transposition matrix A T define the bipartite network G :
We create the initial input embedding H (0) as follows:
When combined with the bipartite network adjacency matrix G above, the graph attention network is defined as:
where H ( l ) represents the node embedding of the l -th layer, where l = 1, …, L , and GATs () represents a single attention layer, whereas the entire Re_GAT framework consists of multiple attention layers.
This study proposes a Re_GAT framework through two main strategies for forward propagation: (I) initial residual connection and adaptive residual connection; and (II) attention mechanism layer aggregation.
To facilitate the learning of feature information from higher-order neighbors, multiple attention layers are typically used, easily homogenizing the data and thus leading to oversmoothing problems. To alleviate the oversmoothing problem of deep CNNs, residual connections, also known as skip connections was first proposed for ResNet. Inspired by ResNet [ 51 ], recent studies have attempted to apply various residual connections to GATs to alleviate the oversmoothing problem. Several studies have shown that residual connections are necessary for deep GATs [ 52 ], not only to alleviate the oversmoothing problem, but also to give GATs a more stable gradient.
We sum the H ( l ) weights with H (0) and H ( l− 1) according to the scale coefficients α and β , respectively. We use the initial skip connection and the adaptive skip connection to mitigate the oversmoothing problem and accelerate the convergence of the GATs. The GAT formula of our model can be rewritten as:
where α and β are hyperparameters.
Inspired by LAGCN [ 35 ], the embedding of each layer captures structural information from different orders of the heterogeneous network. For instance, the initial layer obtains direct connection information, whereas the higher-order layers collect information about multihop neighbors through iterative update embedding. To fuse all useful information from multiple GAT layers, we use the attention mechanism. Since the Re_GAT framework calculates the embedding of different layers and the embeddings contain different information, we define the resulting GAT layer embedding as:
where Hdr l ϵ R Ndr × kl is the embedding of the drug in layer l and Hdi l ϵ R Ndi × kl is the embedding of the disease in layer l . We use attention mechanism layer aggregation to integrate multiple embedding matrices, and the final fused embedding matrix is as follows:
where, Hdr i and Hdi i are the l -layer embeddings of drugs and diseases, respectively, a i and b i are the attention factors that can be calculated via Formulas ( 2 ), ( 3 ) and ( 4 ), and L is the number of layers.
Constructing the feature similarity graph
A previous study showed that a similarity graph constructed using drug and disease features can be used to propagate labels [ 53 ]. We use the features C dr and C di to construct feature similarity graphs for diseases and drugs, respectively. These features are used for label propagation in the disease and drug spaces. The feature similarity graphs are constructed as follows. First, the Euclidean distance between nodes is calculated and ranked. Second, for each node i , its 10 nearest neighbors are selected. Finally, the adjacency matrix is defined as M , and the set of neighbors of node i is defined as N ( i ). The matrix M satisfies M ij = 1 when j belongs to N ( i ); otherwise, M ij = 0.
The self-loop adjacency matrix for the similarity graph S is constructed as follows:
where ⊙ is the Hadamard product. This method can be used to obtain both the drug similarity graph S dr and the disease similarity graph S di .
- Graph autoencoder
Previous studies have shown that the graph autoencoder may simulate label propagation by iteratively propagating label information on the graph [ 54 , 55 , 56 ]. The association matrix A can be considered initial label information. The initial label information and the similarity graph S calculated via the above method are input to the GAE. The encoder layer produces a hidden layer Z , whereas the decoder outputs the score F . The encoder of the GAE can be defined as:
where Φ denotes the weight matrix. Here, we use two GAEs to propagate label information on the drug and disease graphs. We can obtain the drug hidden layer Z dr and the disease hidden layer Z di , which are expressed as follows:
where S dr and S di denote the drug similarity graph and the disease similarity graph, respectively, and A denotes the association matrix.
The decoder of the GAE is applied to decode the hidden layer representation, which is defined as follows:
Therefore, the score matrices F dr and F di can be obtained by decoding Z dr and Z di , respectively.
Since F dr and F di are both low rank matrices [ 57 ], they need to satisfy the rank-sum inequality:
By performing a linear combination of F dr and F di , the final integrated score is obtained as follows:
where α ϵ (0,1) represents the balanced weight between the drug space and the disease space.
The GAE reconstruction error is the loss of cross-entropy between the final prediction and the true value:
As the information from the disease space and the drug space influences the predicted outcome, we use a cotraining approach to train the above two GAEs. The cotraining training loss L co is defined as:
The combined loss function can be rewritten as:
where L rdr and L rdi denote the reconstruction errors of the two GAEs in the drug space and the disease space, respectively.
Free multiscale adversarial training
In this section, we investigate how to effectively improve the input quality through data augmentation [ 58 ]. When neural networks are trained, the quality of the data is far more important than the quantity. By searching for and stamping out small perturbations that cause the classifier to fail, one may hope that adversarial training could benefit standard accuracy. Adversarial training is a well-studied method that increases the robustness and interpretability of neural networks. When the data distribution is sparse and discrete, the beneficial effect of adversarial perturbations on generalizability is prominent [ 59 ]. Inspired by this, we introduce free multiscale adversarial training (FMAT) to augment the node features [ 60 ].
Adversarial training first generates adversarial perturbations, which are then integrated into the training node features. Given a learning model f θ with parameters θ , we denote the perturbed feature as H adv = H + δ . Adversarial learning follows the min–max formulation:
where A represents the real value, D represents the data distribution, L represents the objective loss function, ε represents the perturbation budget, and ║║ p represents an l p -norm distance measure.
The saddle-point optimization problem can be solved via projected gradient descent (PGD), which implements inner maximization, and stochastic gradient descent (SGD), which implements outer minimization. The parameter δ is updated after each step:
where ∏ ║δ║≤ε is projected onto the ε -sphere under the l ∞ -norm . The initial layer of the Re_GAT framework can be rewritten as:
To effectively exploit the generalizability of adversarial perturbations and improve their diversity and quality, Chen et al. emphasized the importance of adapting to different types of data enhancements [ 61 ]. To achieve this, we introduce a 'free' training approach [ 62 ].
The calculation of δ is inefficient because the N -step update requires N forward and backward channels. This update runs N times completely forward and backward to obtain the worst perturbation δ N . However, the model weight θ is updated once to use only δ N . Model training is N times slower because of this process. In contrast, the 'free' training outputs the model weights θ on the same backward channel while calculating the δ gradient, allowing model weight updates to be calculated in parallel with perturbation updates.
'Free' training has the same robustness and accuracy as standard adversarial training does. However, the training costs are the same as those of clean training. The 'free' strategy accumulates a gradient of \(\nabla_{\theta } L\) in each iteration and updates the model weight θ through this gradient. During training process, the model runs the inner circle T times, each time calculating the gradient of θ t -1 and δ t by taking a step along the average gradient at H ( l ) + δ 0 , …, H ( l ) + δ T- 1 . Formally, the optimization step is
Availability of data and materials
We acquired the C-dataset of disease-drug associations, from the Comparative Toxicogenomics Database [ 44 ] ( http://ctdbase.org/ ). We screened the F-dataset of disease-drug interactions from the OMIM database [ 46 ] ( https://www.omim.org/ ) and DrugBank database [ 47 ] ( https://www.drugbank.ca/ ). These two datasets and the source code are available at: https://github.com/ghli16/RAFGAE .
Abbreviations
- Graph attention network
True positive rate
False-positive rate
Receiver operating characteristic
Area under ROC curve
Cross validation
Rifaioglu AS, Atas H, Martin MJ, Cetin-Atalay R, Atalay V, Doğan T. Recent applications of deep learning and machine intelligence on in silico drug discovery: methods, tools and databases. Brief Bioinform. 2019;20(5):1878–912.
Article CAS PubMed Google Scholar
Ashburn TT, Thor KB. Drug repositioning: identifying and developing new uses for existing drugs. Nat Rev Drug Discov. 2004;3(8):673–83.
Dickson M, Gagnon JP. Key factors in the rising cost of new drug discovery and development. Nat Rev Drug Discov. 2004;3(5):417–29.
Padhy BM, Gupta YK. Drug repositioning: re-investigating existing drugs for new therapeutic indications. J Postgrad Med. 2011;57(2):153.
Xue H, Li J, Xie H, Wang Y. Review of drug repositioning approaches and resources. Int J Biol Sci. 2018;14(10):1232.
Article CAS PubMed PubMed Central Google Scholar
Pushpakom S, Iorio F, Eyers PA, Escott KJ, Hopper S, Wells A, Doig A, Guilliams T, Latimer J, McNamee C, Norris A, Sanseau P, Cavalla C, Pirmohamed M. Drug repurposing: progress, challenges and recommendations. Nat Rev Drug Discov. 2019;18(1):41–58.
Baker NC, Ekins S, Williams AJ, Tropsha A. A bibliometric review of drug repurposing. Drug Discov Today. 2018;23(3):661–72.
Nosengo N. New tricks for old drugs. Nature. 2016;534(7607):314–6.
Article PubMed Google Scholar
Jarada TN, Rokne JG, Alhajj R. A review of computational drug repositioning: strategies, approaches, opportunities, challenges, and directions. J Cheminform. 2020;12(1):1–23.
Article Google Scholar
Mohamed K, Yazdanpanah N, Saghazadeh A, Rezaei N. Computational drug discovery and repurposing for the treatment of COVID-19: a systematic review. Bioorg Chem. 2021;106: 104490.
Fahimian G, Zahiri J, Arab SS, Sajedi RH. RepCOOL: computational drug repositioning via integrating heterogeneous biological networks. J Transl Med. 2020;18(1):1–10.
Traylor JI, Sheppard HE, Ravikumar V, Breshears J, Raza SM, Lin CY, Patel SR, DeMonte F. Computational drug repositioning identifies potentially active therapies for chordoma. Neurosurgery. 2021;88(2):428.
Bai L, Scott MK, Steinberg E, Kalesinskas L, Habtezion A, Shah NH, Khatri P. Computational drug repositioning of atorvastatin for ulcerative colitis. J Am Med Inform Assoc. 2021;28(11):2325–35.
Article PubMed PubMed Central Google Scholar
Dai W, Liu X, Gao Y, Chen L, Song J, Chen D, Gao K, Jiang YS, Yang YP, Chen JX, Lu P. Matrix factorization-based prediction of novel drug indications by integrating genomic space. Comput Math Methods Med. 2015;2015:275045.
Zhang W, Zou H, Luo L, Liu Q, Wu W, Xiao W. Predicting potential side effects of drugs by recommender methods and ensemble learning. Neurocomputing. 2016;173:979–87.
Huang F, Qiu Y, Li Q, Liu S, Ni F. Predicting drug-disease associations via multi-task learning based on collective matrix factorization. Front Bioeng Biotechnol. 2020;8:218.
Luo H, Li M, Wang S, Liu Q, Li Y, Wang J. Computational drug repositioning using low-rank matrix approximation and randomized algorithms. Bioinformatics. 2018;34(11):1904–12.
Zhang W, Yue X, Lin W, Wu W, Liu R, Huang F, Liu F. Predicting drug-disease associations by using similarity constrained matrix factorization. BMC Bioinform. 2018;19:1–12.
Yang M, Wu G, Zhao Q, Li Y, Wang J. Computational drug repositioning based on multi-similarities bilinear matrix factorization. Brief Bioinform. 2021;22(4):bbaa267.
Zhang W, Xu H, Li X, Gao Q, Wang L. DRIMC: an improved drug repositioning approach using Bayesian inductive matrix completion. Bioinformatics. 2020;36(9):2839–47.
Hu L, Zhang J, Pan X, Yan H, You ZH. HiSCF: leveraging higher-order structures for clustering analysis in biological networks. Bioinformatics. 2021;37(4):542–50.
Chu Y, Kaushik AC, Wang X, Wang W, Zhang Y, Shan X, Salahub DR, Xiong Y, Wei DQ. DTI-CDF: a cascade deep forest model towards the prediction of drug-target interactions based on hybrid features. Brief Bioinform. 2021;22(1):451–62.
Yang K, Zhao X, Waxman D, Zhao XM. Predicting drug-disease associations with heterogeneous network embedding. Chaos Interdiscip J Nonlinear Sci. 2019;29(12):123109.
Luo Y, Zhao X, Zhou J, Yang J, Zhang Y, Kuang W, Peng J, Chen L, Zeng J. A network integration approach for drug-target interaction prediction and computational drug repositioning from heterogeneous information. Nat Commun. 2017;8(1):573.
Zhao BW, Hu L, You ZH, Wang L, Su XR. HINGRL: predicting drug–disease associations with graph representation learning on heterogeneous information networks. Brief Bioinform. 2022;23(1):bbab515.
Zhang H, Cui H, Zhang T, Cao Y, Xuan P. Learning multi-scale heterogenous network topologies and various pairwise attributes for drug–disease association prediction. Brief Bioinform. 2022;23(2):bbac009.
Luo H, Wang J, Li M, Luo J, Peng X, Wu FX, Pan Y. Drug repositioning based on comprehensive similarity measures and bi-random walk algorithm. Bioinformatics. 2016;32(17):2664–71.
Cai L, Lu C, Xu J, Meng Y, Wang P, Fu X, Su Y. Drug repositioning based on the heterogeneous information fusion graph convolutional network. Brief Bioinform. 2021;22(6):bbab319.
Xuan P, Ye Y, Zhang T, Zhao L, Sun C. Convolutional neural network and bidirectional long short-term memory-based method for predicting drug–disease associations. Cells. 2019;8(7):705.
Liu H, Zhang W, Song Y, Deng L, Zhou S. HNet-DNN: inferring new drug–disease associations with deep neural network based on heterogeneous network features. J Chem Inf Model. 2020;60(4):2367–76.
Peng L, Tan J, Xiong W, Zhang L, Wang Z, Yuan R, Li Z, Chen X. Deciphering ligand–receptor-mediated intercellular communication based on ensemble deep learning and the joint scoring strategy from single-cell transcriptomic data. Comput Biol Med. 2023;2023: 107137.
Xuan P, Gao L, Sheng N, Zhang T, Nakaguchi T. Graph convolutional autoencoder and fully-connected autoencoder with attention mechanism based method for predicting drug-disease associations. IEEE J Biomed Health Inform. 2020;25(5):1793–804.
Coşkun M, Koyutürk M. Node similarity-based graph convolution for link prediction in biological networks. Bioinformatics. 2021;37(23):4501–8.
Zeng X, Zhu S, Liu X, Zhou Y, Nussinov R, Cheng F. deepDR: a network-based deep learning approach to in silico drug repositioning. Bioinformatics. 2019;35(24):5191–8.
Yu Z, Huang F, Zhao X, Xiao W, Zhang W. Predicting drug–disease associations through layer attention graph convolutional network. Brief Bioinform. 2021;22(4):bbaa243.
Feng Q, Dueva E, Cherkasov A, Ester M. PADME: a deep learning-based framework for drug–target interaction prediction. https://arxiv.org/abs/1807.09741 (2019).
Meng Y, Lu C, Jin M, Xu J, Zeng X, Yang J. A weighted bilinear neural collaborative filtering approach for drug repositioning. Brief Bioinform. 2022;23(2):bbab581.
Gu Y, Zheng S, Yin Q, Jiang R, Li J. REDDA: integrating multiple biological relations to heterogeneous graph neural network for drug-disease association prediction. Comput Biol Med. 2022;150: 106127.
Yang M, Luo H, Li Y, et al. Drug repositioning based on bounded nuclear norm regularization. Bioinformatics. 2019;35(14):i455–63.
Li J, Zhang S, Liu T, et al. Neural inductive matrix completion with graph convolutional networks for miRNA-disease association prediction. Bioinformatics. 2020;36(8):2538–46.
Kingma DP. A method for stochastic optimization. ArXiv Prepr. 2014.
Niu M, Zou Q, Wang C. GMNN2CD: identification of circRNA–disease associations based on variational inference and graph Markov neural networks. Bioinformatics. 2022;38(8):2246–53.
Shi Z, Zhang H, Jin C, Quan X, Yin Y. A representation learning model based on variational inference and graph autoencoder for predicting lncRNA-disease associations. BMC Bioinform. 2021;22(1):1–20.
Article CAS Google Scholar
Davis AP, Murphy CG, Johnson R, Lay JM, Lennon-Hopkins K, Saraceni-Richards C, Sciaky D, King BL, Rosenstein MC, Wiegers TC, Mattingly CJ. The comparative toxicogenomics database: update 2013. Nucleic Acids Res. 2013;41(D1):D1104–14.
Gottlieb A, Stein GY, Ruppin E, Sharan R. PREDICT: a method for inferring novel drug indications with application to personalized medicine. Mol Syst Biol. 2011;7(1):496.
Wishart DS, Knox C, Guo AC, Shrivastava S, Hassanali M, Stothard P, Chang Z, Woolsey J. DrugBank: a comprehensive resource for in silico drug discovery and exploration. Nucleic Acids Res. 2006;34(suppl_1):D668–72.
Hamosh A, Scott AF, Amberger JS, Bocchini CA, McKusick VA. Online Mendelian Inheritance in Man (OMIM), a knowledgebase of human genes and genetic disorders. Nucleic Acids Res. 2005;33(suppl_1):D514–7.
CAS PubMed Google Scholar
Vidal D, Thormann M, Pons M. LINGO, an efficient holographic text based method to calculate biophysical properties and intermolecular similarities. J Chem Inf Model. 2005;45(2):386–93.
Steinbeck C, Han Y, Kuhn S, Horlacher O, Luttmann E, Willighagen E. The Chemistry Development Kit (CDK): an open-source Java library for chemo-and bioinformatics. J Chem Inf Comput Sci. 2003;43(2):493–500.
Van Driel MA, Bruggeman J, Vriend G, Brunner HG, Leunissen JA. A text-mining analysis of the human phenome. Eur J Hum Genet. 2006;14(5):535–42.
Kaiming H, Shaoqing R, Jian S. Deep residual learning for image recognition. In Proceedings of the IEEE conference on computer vision and pattern recognition. 2016:770–778.
Sharma V, Dyreson C. Covid-19 screening using residual attention network an artificial intelligence approach. 2020 19th IEEE International Conference on Machine Learning and Applications (ICMLA). IEEE. 2020:1354–1361.
Belkin M, Niyogi P, Sindhwani V. Manifold regularization: A geometric framework for learning from labeled and unlabeled examples. J Mach Learn Res. 2006;7(11).
Kipf TN, Welling M. Variational graph auto-encoders. https://arxiv.org/abs/1611.07308 (2016).
Li G, Luo J, Xiao Q, Liang C, Ding P. Predicting microRNA-disease associations using label propagation based on linear neighborhood similarity. J Biomed Inform. 2018;82:169–77.
Wang F, Zhang C. Label propagation through linear neighborhoods. Proceedings of the 23rd international conference on Machine learning. 2006:985–992.
Bahdanau D, Cho K, Bengio Y. Neural machine translation by jointly learning to align and translate. https://arxiv.org/abs/1409.0473 (2014).
Krizhevsky A, Sutskever I, Hinton GE. Imagenet classification with deep convolutional neural networks. Commun ACM. 2017;60(6):84–90.
Gan Z, Chen YC, Li L, et al. Large-scale adversarial training for vision-and-language representation learning. Adv Neural Inf Process Syst. 2020;33:6616–28.
Google Scholar
Kong K, Li G, Ding M, Wu Z, Zhu C, Ghanem B, Taylor G, Goldstein T. Robust optimization as data augmentation for large-scale graphs. In Proceedings of the IEEE/CVF Conference on Computer Vision and Pattern Recognition. 2022:60–69.
Chen T, Kornblith S, Norouzi M, Hinton G. A simple framework for contrastive learning of visual representations. In International conference on machine learning. PMLR. 2020:1597–1607.
Shafahi A, Najibi M, Ghiasi MA, Xu Z, Dickerson J, Studer C, Davis LS, Taylor G, Goldstein T. Adversarial training for free!. Adv Neural Inf Process Syst. 2019;32.
Download references
Acknowledgements
Not applicable.
This work is supported by the National Natural Science Foundation of China (Grant Nos. 62362034, 61862025, 62372279, and 62002116), the Natural Science Foundation of Jiangxi Province (Grant Nos. 20232ACB202010, 20212BAB202009, 20181BAB211016), and the Natural Science Foundation of Shandong Province (Grant No. ZR2023MF119).
Author information
Authors and affiliations.
School of Information Engineering, East China Jiaotong University, Nanchang, China
Guanghui Li & Shuwen Li
School of Information Science and Engineering, Shandong Normal University, Jinan, China
Cheng Liang
College of Information Science and Engineering, Hunan Normal University, Changsha, China
College of Computer Science and Electronic Engineering, Hunan University, Changsha, China
You can also search for this author in PubMed Google Scholar
Contributions
GL and JL conceived and designed the study. GL and SL implemented the experiments and drafted the manuscript. CL and QX analyzed the results. All the authors have read and approved the final manuscript.
Corresponding authors
Correspondence to Guanghui Li or Jiawei Luo .
Ethics declarations
Ethics approval and consent to participate, consent for publication, competing interests.
The authors declare no competing interests.
Additional information
Publisher's note.
Springer Nature remains neutral with regard to jurisdictional claims in published maps and institutional affiliations.
Rights and permissions
Open Access This article is licensed under a Creative Commons Attribution-NonCommercial-NoDerivatives 4.0 International License, which permits any non-commercial use, sharing, distribution and reproduction in any medium or format, as long as you give appropriate credit to the original author(s) and the source, provide a link to the Creative Commons licence, and indicate if you modified the licensed material. You do not have permission under this licence to share adapted material derived from this article or parts of it. The images or other third party material in this article are included in the article’s Creative Commons licence, unless indicated otherwise in a credit line to the material. If material is not included in the article’s Creative Commons licence and your intended use is not permitted by statutory regulation or exceeds the permitted use, you will need to obtain permission directly from the copyright holder. To view a copy of this licence, visit http://creativecommons.org/licenses/by-nc-nd/4.0/ .
Reprints and permissions
About this article
Cite this article.
Li, G., Li, S., Liang, C. et al. Drug repositioning based on residual attention network and free multiscale adversarial training. BMC Bioinformatics 25 , 261 (2024). https://doi.org/10.1186/s12859-024-05893-5
Download citation
Received : 30 June 2023
Accepted : 06 August 2024
Published : 08 August 2024
DOI : https://doi.org/10.1186/s12859-024-05893-5
Share this article
Anyone you share the following link with will be able to read this content:
Sorry, a shareable link is not currently available for this article.
Provided by the Springer Nature SharedIt content-sharing initiative
- Residual network
- Adversarial training
- Drug-disease association
BMC Bioinformatics
ISSN: 1471-2105
- General enquiries: [email protected]
Information
- Author Services
Initiatives
You are accessing a machine-readable page. In order to be human-readable, please install an RSS reader.
All articles published by MDPI are made immediately available worldwide under an open access license. No special permission is required to reuse all or part of the article published by MDPI, including figures and tables. For articles published under an open access Creative Common CC BY license, any part of the article may be reused without permission provided that the original article is clearly cited. For more information, please refer to https://www.mdpi.com/openaccess .
Feature papers represent the most advanced research with significant potential for high impact in the field. A Feature Paper should be a substantial original Article that involves several techniques or approaches, provides an outlook for future research directions and describes possible research applications.
Feature papers are submitted upon individual invitation or recommendation by the scientific editors and must receive positive feedback from the reviewers.
Editor’s Choice articles are based on recommendations by the scientific editors of MDPI journals from around the world. Editors select a small number of articles recently published in the journal that they believe will be particularly interesting to readers, or important in the respective research area. The aim is to provide a snapshot of some of the most exciting work published in the various research areas of the journal.
Original Submission Date Received: .
- Active Journals
- Find a Journal
- Proceedings Series
- For Authors
- For Reviewers
- For Editors
- For Librarians
- For Publishers
- For Societies
- For Conference Organizers
- Open Access Policy
- Institutional Open Access Program
- Special Issues Guidelines
- Editorial Process
- Research and Publication Ethics
- Article Processing Charges
- Testimonials
- Preprints.org
- SciProfiles
- Encyclopedia
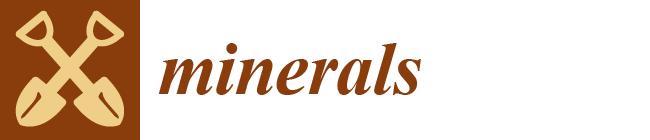
Article Menu

- Subscribe SciFeed
- Recommended Articles
- Google Scholar
- on Google Scholar
- Table of Contents
Find support for a specific problem in the support section of our website.
Please let us know what you think of our products and services.
Visit our dedicated information section to learn more about MDPI.
JSmol Viewer
Involvement of the northeastern margin of south china block in rodinia supercontinent evolution: a case study of neoproterozoic granitic gneiss in rizhao area, shandong province.
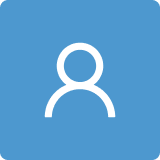
1. Introduction
2. geological setting and petrographic characteristics, 3. sampling and analytical methods, 3.1. whole-rock major- and trace- element analyses, 3.2. zircon u–pb, trace element, and lu–hf isotopic analyses, 4.1. whole-rock major- and trace-element concentrations, 4.2. zircon u–pb ages and trace element contents, 4.3. zircon lu-hf isotopes, 5. discussion, 5.1. genetic discrimination of zircon, 5.2. diagenetic age and characteristics of the magma source.
Click here to enlarge figure
Share and Cite
He, X.; Yang, Z.; Liu, K.; Zhu, W.; Zhan, H.; Yang, P.; Wei, T.; Wang, S.; Zhang, Y. Involvement of the Northeastern Margin of South China Block in Rodinia Supercontinent Evolution: A Case Study of Neoproterozoic Granitic Gneiss in Rizhao Area, Shandong Province. Minerals 2024 , 14 , 807. https://doi.org/10.3390/min14080807
He X, Yang Z, Liu K, Zhu W, Zhan H, Yang P, Wei T, Wang S, Zhang Y. Involvement of the Northeastern Margin of South China Block in Rodinia Supercontinent Evolution: A Case Study of Neoproterozoic Granitic Gneiss in Rizhao Area, Shandong Province. Minerals . 2024; 14(8):807. https://doi.org/10.3390/min14080807
He, Xiaolong, Zeyu Yang, Kai Liu, Wei Zhu, Honglei Zhan, Peng Yang, Tongzheng Wei, Shuxun Wang, and Yaoyao Zhang. 2024. "Involvement of the Northeastern Margin of South China Block in Rodinia Supercontinent Evolution: A Case Study of Neoproterozoic Granitic Gneiss in Rizhao Area, Shandong Province" Minerals 14, no. 8: 807. https://doi.org/10.3390/min14080807
Article Metrics
Article access statistics, supplementary material.
ZIP-Document (ZIP, 468 KiB)
Further Information
Mdpi initiatives, follow mdpi.
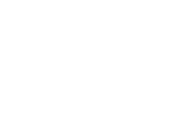
Subscribe to receive issue release notifications and newsletters from MDPI journals
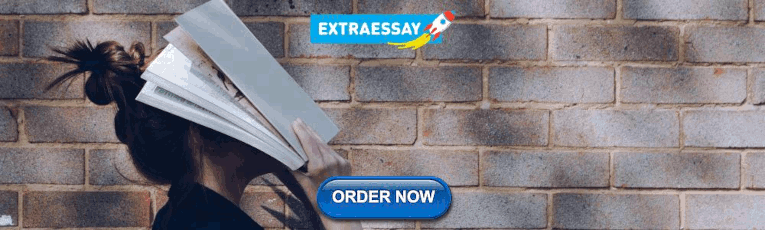
IMAGES
COMMENTS
Learn about case study methodology, a system of frameworks used to design and conduct research on one or more cases. Explore different types, purposes, and sources of case study research with examples from various disciplines.
Abstract A case study is one of the most commonly used methodologies of social research. This article attempts to look into the various dimensions of a case study research strategy, the different epistemological strands which determine the particular case study type and approach adopted in the field, discusses the factors which can enhance the effectiveness of a case study research, and the ...
Learn how to conduct a case study research with this guide that covers the definition, types, methods, steps, and examples. A case study is a qualitative method that involves an in-depth examination and analysis of a particular phenomenon or case.
Learn what a case study is, when to use it, and how to conduct one. Find out the steps, methods, and tips for writing a case study research design.
Qualitative case study methodology provides tools for researchers to study complex phenomena within their contexts. When the approach is applied correctly, it becomes a valuable method for health ...
Learn how to conduct case study research effectively with this guide. It covers the definition, characteristics, types, and examples of case studies in qualitative research.
Abstract Qualitative case study methodology enables researchers to conduct an in-depth exploration of intricate phenomena within some specific context. By keeping in mind research students, this article presents a systematic step-by-step guide to conduct a case study in the business discipline. Research students belonging to said discipline face issues in terms of clarity, selection, and ...
Learn how to conduct a case study using qualitative and quantitative methods. Find useful resources, tips, and examples for your research project.
Rather than discussing case study in general, a targeted step-by-step plan with real-time research examples to conduct a case study is given. Empirical material interpretation process.
Besides discussing case study design, data collection, and analysis, the refresher addresses several key features of case study research. First, an abbreviated definition of a "case study" will help identify the circumstances when you might choose to use the case study method instead of (or as a complement to) some other research method. Second, other features cover the choices you are ...
The case study research method originated in clinical medicine (the case history, i.e., the patient's personal history). In psychology, case studies are often confined to the study of a particular individual.
Abstract Qualitative case study methodology provides tools for researchers to study complex phenomena within their contexts. When the approach is applied correctly, it becomes a valuable method for health science research to develop theory, evaluate programs, and develop interventions. The purpose of this paper is to guide the novice researcher in identifying the key elements for designing and ...
Findings were grouped into five themes outlining key methodological issues: case study methodology or method, case of something particular and case selection, contextually bound case study, researcher and case interactions and triangulation, and study design inconsistent with methodology reported.
The case study approach allows in-depth, multi-faceted explorations of complex issues in their real-life settings. The value of the case study approach is well recognised in the fields of business, law and policy, but somewhat less so in health services research. Based on our experiences of conducting several health-related case studies, we reflect on the different types of case study design ...
A case study is an in-depth analysis of one individual or group. Learn more about how to write a case study, including tips and examples, and its importance in psychology.
Chapter 3: Method (Exploratory Case Study)Chapter 3: Method (Exploratory Case Study) This workbook Chapter workbook 3 of contains your is intended proposal: information to hel that y will u to help write you Ch to pt understand r 3 of your what proposal. should Each be included part of this in Issues to points consider to include regarding in ...
A case study is a research approach that provides an examination of a phenomenon, event, organization, or individual. Learn how to write a case study.
A case study deeply dives into a particular subject, such as a person, event, or group. Case studies are used in multiple areas of research. See examples of how to use case studies in your research.
A case study is a detailed study of a specific subject, such as a person, group, place, event, organisation, or phenomenon. Case studies are commonly used in social, educational, clinical, and business research. A case study research design usually involves qualitative methods, but quantitative methods are sometimes also used.
Case study methodology is a small -scale research. method which can readily be used by practitioner-. researchers from the TA community to test and develop. TA theory and to explore the processes ...
Case studies are a popular research method in business area. Case studies aim to analyze specific issues within the boundaries of a specific environment, situation or organization. According to its design, case studies in business research can be divided into three categories: explanatory, descriptive and exploratory.
Discover how to craft effective case studies with 28+ examples and guidelines. Learn from the best samples and templates for different subjects and topics.
The present study employed a descriptive-analytical methodology to explore the effects of utilizing social media applications on enhancing students' proficiency in oral communication abilities. To get adequate data for this study, a survey was conducted among a sample of 40 participants.
To ensure the credibility and accuracy of the data, a pilot study was conducted with a small sample of participants. Study subjects and areas under the solid waste management service chain were selected from the total number of Addis Ababa city administration Districts using simple random and cluster sampling methods.
A case study using colorectal cancer as an exemplary dataset was also conducted to highlight the potential and applicability of P2GPT in accurately generating simulated biological data for ...
Bio-Analytical Method Development and Validation for Biologicals; Quality Risk Management Framework: A Proactive, Data-Driven Approach ... 15:30 CEST, combining lectures, real-world examples, exercises, and case studies to ensure practical understanding and application of cGMP principles. Participation. The training is open to professionals ...
Finally, tenfold cross-validations on two benchmark datasets show that RAFGAE outperforms current methods. In addition, case studies have confirmed that RAFGAE can detect novel drug-disease associations. The comprehensive experimental results validate the utility and accuracy of RAFGAE. ... these unknown samples considered negative samples may ...
In this study, systematic petrology, geochemistry, isotopic chronology, and zircon Hf isotopic analyses were carried out on gneisses samples of biotite alkali feldspar granitic and biotite monzogranitic compositions in the Rizhao area.