Null Hypothesis Definition and Examples
PM Images / Getty Images
- Chemical Laws
- Periodic Table
- Projects & Experiments
- Scientific Method
- Biochemistry
- Physical Chemistry
- Medical Chemistry
- Chemistry In Everyday Life
- Famous Chemists
- Activities for Kids
- Abbreviations & Acronyms
- Weather & Climate
- Ph.D., Biomedical Sciences, University of Tennessee at Knoxville
- B.A., Physics and Mathematics, Hastings College
In a scientific experiment, the null hypothesis is the proposition that there is no effect or no relationship between phenomena or populations. If the null hypothesis is true, any observed difference in phenomena or populations would be due to sampling error (random chance) or experimental error. The null hypothesis is useful because it can be tested and found to be false, which then implies that there is a relationship between the observed data. It may be easier to think of it as a nullifiable hypothesis or one that the researcher seeks to nullify. The null hypothesis is also known as the H 0, or no-difference hypothesis.
The alternate hypothesis, H A or H 1 , proposes that observations are influenced by a non-random factor. In an experiment, the alternate hypothesis suggests that the experimental or independent variable has an effect on the dependent variable .
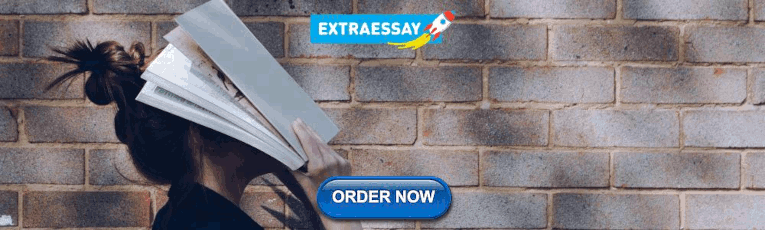
How to State a Null Hypothesis
There are two ways to state a null hypothesis. One is to state it as a declarative sentence, and the other is to present it as a mathematical statement.
For example, say a researcher suspects that exercise is correlated to weight loss, assuming diet remains unchanged. The average length of time to achieve a certain amount of weight loss is six weeks when a person works out five times a week. The researcher wants to test whether weight loss takes longer to occur if the number of workouts is reduced to three times a week.
The first step to writing the null hypothesis is to find the (alternate) hypothesis. In a word problem like this, you're looking for what you expect to be the outcome of the experiment. In this case, the hypothesis is "I expect weight loss to take longer than six weeks."
This can be written mathematically as: H 1 : μ > 6
In this example, μ is the average.
Now, the null hypothesis is what you expect if this hypothesis does not happen. In this case, if weight loss isn't achieved in greater than six weeks, then it must occur at a time equal to or less than six weeks. This can be written mathematically as:
H 0 : μ ≤ 6
The other way to state the null hypothesis is to make no assumption about the outcome of the experiment. In this case, the null hypothesis is simply that the treatment or change will have no effect on the outcome of the experiment. For this example, it would be that reducing the number of workouts would not affect the time needed to achieve weight loss:
H 0 : μ = 6
- Null Hypothesis Examples
"Hyperactivity is unrelated to eating sugar " is an example of a null hypothesis. If the hypothesis is tested and found to be false, using statistics, then a connection between hyperactivity and sugar ingestion may be indicated. A significance test is the most common statistical test used to establish confidence in a null hypothesis.
Another example of a null hypothesis is "Plant growth rate is unaffected by the presence of cadmium in the soil ." A researcher could test the hypothesis by measuring the growth rate of plants grown in a medium lacking cadmium, compared with the growth rate of plants grown in mediums containing different amounts of cadmium. Disproving the null hypothesis would set the groundwork for further research into the effects of different concentrations of the element in soil.
Why Test a Null Hypothesis?
You may be wondering why you would want to test a hypothesis just to find it false. Why not just test an alternate hypothesis and find it true? The short answer is that it is part of the scientific method. In science, propositions are not explicitly "proven." Rather, science uses math to determine the probability that a statement is true or false. It turns out it's much easier to disprove a hypothesis than to positively prove one. Also, while the null hypothesis may be simply stated, there's a good chance the alternate hypothesis is incorrect.
For example, if your null hypothesis is that plant growth is unaffected by duration of sunlight, you could state the alternate hypothesis in several different ways. Some of these statements might be incorrect. You could say plants are harmed by more than 12 hours of sunlight or that plants need at least three hours of sunlight, etc. There are clear exceptions to those alternate hypotheses, so if you test the wrong plants, you could reach the wrong conclusion. The null hypothesis is a general statement that can be used to develop an alternate hypothesis, which may or may not be correct.
- What Are Examples of a Hypothesis?
- What Is a Hypothesis? (Science)
- What 'Fail to Reject' Means in a Hypothesis Test
- What Are the Elements of a Good Hypothesis?
- Scientific Hypothesis Examples
- Null Hypothesis and Alternative Hypothesis
- What Is a Control Group?
- Understanding Simple vs Controlled Experiments
- Six Steps of the Scientific Method
- Scientific Method Vocabulary Terms
- Definition of a Hypothesis
- Type I and Type II Errors in Statistics
- How to Conduct a Hypothesis Test
- An Example of a Hypothesis Test
- Understanding Experimental Groups

An official website of the United States government
The .gov means it’s official. Federal government websites often end in .gov or .mil. Before sharing sensitive information, make sure you’re on a federal government site.
The site is secure. The https:// ensures that you are connecting to the official website and that any information you provide is encrypted and transmitted securely.
- Publications
- Account settings
Preview improvements coming to the PMC website in October 2024. Learn More or Try it out now .
- Advanced Search
- Journal List
- v.11(11); 2021 Jun
When are hypotheses useful in ecology and evolution?
Matthew g. betts.
1 Forest Biodiversity Research Network, Department of Forest Ecosystems and Society, Oregon State University, Corvallis OR, USA
Adam S. Hadley
David w. frey, sarah j. k. frey, dusty gannon, scott h. harris, urs g. kormann, kara leimberger, katie moriarty.
2 USDA Forest Service, Pacific Northwest Research Station, Corvallis OR, USA
Joseph M. Northrup
3 Wildlife Research and Monitoring Section, Ontario Ministry of Natural Resources and Forestry, Environmental and Life Sciences Graduate Program, Trent University, Peterborough ON, Canada
Josée S. Rousseau
Thomas d. stokely, jonathon j. valente, diego zárrate‐charry, associated data.
Data for the analysis of hypothesis use in ecology and evolution publications is available at https://figshare.com/articles/dataset/Betts_et_al_2021_When_are_hypotheses_useful_in_ecology_and_evolution_Ecology_and_Evolution/14110289 .
Research hypotheses have been a cornerstone of science since before Galileo. Many have argued that hypotheses (1) encourage discovery of mechanisms, and (2) reduce bias—both features that should increase transferability and reproducibility. However, we are entering a new era of big data and highly predictive models where some argue the hypothesis is outmoded. We hypothesized that hypothesis use has declined in ecology and evolution since the 1990s, given the substantial advancement of tools further facilitating descriptive, correlative research. Alternatively, hypothesis use may have become more frequent due to the strong recommendation by some journals and funding agencies that submissions have hypothesis statements. Using a detailed literature analysis ( N = 268 articles), we found prevalence of hypotheses in eco–evo research is very low (6.7%–26%) and static from 1990–2015, a pattern mirrored in an extensive literature search ( N = 302,558 articles). Our literature review also indicates that neither grant success nor citation rates were related to the inclusion of hypotheses, which may provide disincentive for hypothesis formulation. Here, we review common justifications for avoiding hypotheses and present new arguments based on benefits to the individual researcher. We argue that stating multiple alternative hypotheses increases research clarity and precision, and is more likely to address the mechanisms for observed patterns in nature. Although hypotheses are not always necessary, we expect their continued and increased use will help our fields move toward greater understanding, reproducibility, prediction, and effective conservation of nature.
We use a quantitative literature review to show that use of a priori hypotheses is still rare in the fields of ecology and evolution. We provide suggestions about the group and individual‐level benefits of hypothesis use.

1. INTRODUCTION
Why should ecologists have hypotheses? At the beginning of most science careers, there comes a time of “hypothesis angst” where students question the need for the hypothetico‐deductive approach their elders have deemed essential for good science. Why is it not sufficient to just have a research objective or question? Why can't we just collect observations and describe those in our research papers?
Research hypotheses are explanations for an observed phenomenon (Loehle, 1987 ; Wolff & Krebs, 2008 ) (see Box 1 ) and have been proposed as a central tool of science since Galileo and Francis Bacon in the mid‐1600s (Glass & Hall, 2008 ). Over the past century, there have been repeated calls for rigorous application of hypotheses in science, and arguments that hypothesis use is the cornerstone of the scientific method (Chamberlin, 1890 ; Popper, 1959 ; Romesburg, 1981 ). In a seminal paper in Science, Platt ( 1964 ) challenged all scientific fields to adopt and rigorously test multiple hypotheses (sensu Chamberlin, 1890 ), arguing that without such hypothesis tests, disciplines would be prone to “stamp collecting” (Landy, 1986 ). To constitute “strong inference,” Platt required the scientific method to be a three‐step process including (1) developing alternative hypotheses, (2) devising a set of “crucial” experiments to eliminate all but one hypothesis, and (3) performing the experiments (Elliott & Brook, 2007 ).
Definitions of hypotheses and associated terms
Hypothesis : An explanation for an observed phenomenon.
Research Hypothesis: A statement about a phenomenon that also includes the potential mechanism or cause of that phenomenon. Though a research hypothesis doesn't need to adhere to this strict framework it is often best described as the “if” in an “if‐then” statement. In other words, “if X is true” (where X is the mechanism or cause for an observed phenomenon) “then Y” (where Y is the outcome of a crucial test that supports the hypothesis). These can also be thought of as “ mechanistic hypotheses ” since they link with a causal mechanism. For example, trees grow slowly at high elevation because of nutrient limitation (hypothesis); if this is the case, fertilizing trees should result in more rapid growth (prediction).
Prediction: The potential outcome of a test that would support a hypothesis. Most researchers call the second part of the if‐then statement a “prediction”.
Multiple alternative hypotheses: Multiple plausible explanations for the same phenomenon.
Descriptive Hypothesis: Descriptive statements or predictions with the word “hypothesis” in front of them. Typically researchers state their guess about the results they expect and call this the “hypothesis” (e.g., “I hypothesize trees at higher elevation will grow slowly”).
Statistical Hypothesis : A predicted pattern in data that should occur if a research hypothesis is true.
Null Hypothesis : A concise statement expressing the concept of “no difference” between a sample and the population mean.
The commonly touted strengths of hypotheses are two‐fold. First, by adopting multiple plausible explanations for a phenomenon (hereafter “ multiple alternative hypotheses ”; Box 1 ), a researcher reduces the chance that they will become attached to a single possibility, thereby biasing research in favor of this outcome (Chamberlin, 1890 ); this “confirmation bias” is a well‐known human trait (Loehle, 1987 ; Rosen, 2016 ) and likely decreases reproducibility (Munafò et al., 2017 ). Second, various authors have argued that the a priori hypothesis framework forces one to think in advance about—and then test—various causes for patterns in nature (Wolff & Krebs, 2008 ), rather than simply examining the patterns themselves and coming up with explanations after the fact (so called “inductive research;” Romesburg, 1981 ). By understanding and testing mechanisms, science becomes more reliable and transferable (Ayres & Lombardero, 2017 ; Houlahan et al., 2017 ; Sutherland et al., 2013 ) (Figure 1 ). Importantly, both of these strengths should have strong, positive impacts on reproducibility of ecological and evolutionary studies (see Discussion).

Understanding mechanisms often increases model transferability. Panels (a and b) show snowshoe hares in winter and summer coloration, respectively. If a correlative (i.e., nonmechanistic) model for hare survival as a function of color was trained only on hares during the winter and then extrapolated into the summer months, it would perform poorly (white hares would die disproportionately under no‐snow conditions). On the other hand, a researcher testing mechanisms for hare survival would (ideally via experimentation) arrive at the conclusion that it is not the whiteness of hares, but rather blending with the background that confers survival (the “camouflage” hypothesis). Understanding mechanism results in model predictions being robust to novel conditions. Panel (c) Shows x and y geographic locations of training (blue filled circles) and testing (blue open circles) locations for a hypothetical correlative model. Even if the model performs well on these independent test data (predicting open to closed circles), there is no guarantee that it will predict well outside of the spatial bounds of the existing data (red circles). Nonstationarity (in this case caused by a nonlinear relationship between predictor and response variable; panel d) could result in correlative relationships shifting substantially if extrapolated to new times or places. However, mechanistic hypotheses aimed at understanding the underlying factors driving the distribution of this species would be more likely to elucidate this nonlinear relationship. In both of these examples, understanding drivers behind ecological patterns—via testing mechanistic hypotheses—is likely to enhance model transferability
However, we are entering a new era of ecological and evolutionary science that is characterized by massive datasets on genomes, species distributions, climate, land cover, and other remotely sensed information (e.g., bioacoustics, camera traps; Pettorelli et al., 2017 ). Exceptional computing power and new statistical and machine‐learning algorithms now enable thousands of statistical models to be run in minutes. Such datasets and methods allow for pattern recognition at unprecedented spatial scales and for huge numbers of taxa and processes. Indeed, there have been recent arguments in both the scientific literature and popular press to do away with the traditional scientific method and a priori hypotheses (Glass & Hall, 2008 ; Golub, 2010 ). These arguments go something along the lines of “if we can get predictions right most of the time, why do we need to know the cause?”
In this paper, we sought to understand if hypothesis use in ecology and evolution has shifted in response to these pressures on the discipline. We, therefore, hypothesized that hypothesis use has declined in ecology and evolution since the 1990s, given the substantial advancement of tools further facilitating descriptive, correlative research (e.g., Cutler et al., 2007 ; Elith et al., 2008 ). We predicted that this decline should be particularly evident in the applied conservation literature—where the emergence of machine‐learning models has resulted in an explosion of conservation‐oriented species distribution models (Elith et al., 2006 ). Our alternative hypothesis was that hypothesis use has become more frequent. The mechanism for such increases is that higher‐profile journals (e.g., Functional Ecology , Proceedings of the Royal Society of London Ser. B ) and competitive granting agencies (e.g., the U.S. National Science Foundation) now require or strongly encourage hypothesis statements.
As noted above, many have argued that hypotheses are useful and important for overall progress in science, because they facilitate the discovery of mechanisms, reduce bias, and increase reproducibility (Platt, 1964 ). However, for hypothesis use to be propagated among scientists, one would also expect hypotheses to confer benefits to the individual. We, therefore, tested whether hypothesis use was associated with individual‐level incentives relevant to academic success: publications, citations, and grants (Weinberg, 2010 ). If hypothesis use confers individual‐level advantages, then hypothesis‐based research should be (1) published in more highly ranked journals, (2) have higher citation rates, and (3) be supported by highly competitive funding sources.
Finally, we also present some common justifications for absence of hypotheses and suggest potential counterpoints researchers should consider prior to dismissing hypothesis use, including potential benefits to the individual researcher. We hope this communication provides practical recommendations for improving hypothesis use in ecology and evolution—particularly for new practitioners in the field (Box 2 ).
Recommendations for improving hypotheses use in ecology and evolution
Authors : Know that you are human and prone to confirmation bias and highly effective at false pattern recognition. Thus, inductive research and single working hypotheses should be rare in your research. Remember that if your work is to have a real “impact”, it needs to withstand multiple tests from other labs over the coming decades.
Editors and Reviewers : Reward research that is conducted using principles of sound scientific method. Be skeptical of research that smacks of data dredging, post hoc hypothesis development, and single hypotheses. If no hypotheses are stated in a paper and/or the paper is purely descriptive, ask whether the novelty of the system and question warrant this, or if the field would have been better served by a study with mechanistic hypotheses. If only single hypotheses are stated, ask whether appropriate precautions were taken for the researcher to avoid finding support for a pet idea (e.g., blinded experiments, randomized attribution of treatments, etc.). To paraphrase Platt ( 1964 ): beware of the person with only one method or one instrument, either experimental or theoretical.
Mentors : Encourage your advisees to think carefully about hypothesis use and teach them how to construct sound multiple, mechanistic hypotheses. Importantly, explain why hypotheses are important to the scientific method, the individual and group consequences of excluding them, and the rare instances where they may not be necessary.
Policymakers/media/educators/students/readers : Read scientific articles with skepticism; have a scrutinous eye out for single hypothesis studies and p‐hacking. Reward multi‐hypothesis, mechanistic, predictive science by giving it greater weight in policy decisions (Sutherland et al., 2013 ), more coverage in the media, greater leverage in education, and more citations in reports.
2.1. Literature analysis
To examine hypothesis use over time and test whether hypothesis presence was associated with research type (basic vs. applied), journal impact factor, citation rates, and grants, we sampled the ecology and evolution literature using a stratified random sample of ecology and evolution journals in existence before 1991. First, we randomly selected 19 journals across impact factor (IF) strata ranging from 0.5–10.0 in two bins (<3 IF and ≥3 IF; see Figure 3 for full journal list). We then added three multidisciplinary journals that regularly publish ecology and evolution articles ( Proceedings of the National Academy of Sciences, Science, and Nature ). From this sample of 22 journals, we randomly selected ecology and evolution articles within 5‐year strata beginning in 1991 (3 articles/journal per 5‐year bin) to ensure the full date range was evenly sampled. We removed articles in the following categories: editorials, corrections, reviews, opinions, and methods papers. In multidisciplinary journals, we examined only ecology, evolution, and conservation biology articles, as indicated by section headers in each journal. Once selected, articles were randomly distributed to the authors of the current paper (hereafter “reviewers:” MGB, ASH, DF, SF, DG, SH, HK, UK, KL, KM, JN, BP, JSR, TSS, JV, DZC) for detailed examination. On rare occasions, an article was not found, or reviewers were not able to complete their review. Ultimately, our final sample comprised 268 articles.

Frequency distributions showing proportion of various hypotheses types across ecology and evolution journals included in our detailed literature search. Hypothesis use varied greatly across publication outlets. We considered J. Applied Ecology, J. Wildlife Management, J. Soil, and Water Cons., Ecological Applications, Conservation Biology, and Biological Conservation to be applied journals; both applied and basic journals varied greatly in the prevalence of hypotheses
Reviewers were given a maximum of 10 min to find research hypothesis statements within the abstract or introduction of articles. We chose 10 min to simulate the amount of time that a journal editor pressed for time might spend evaluating the introductory material in an article. After this initial 10 min period, we determined: (1) whether or not an article contained at least one hypothesis, (2) whether hypotheses were mechanistic or not (i.e., the authors claimed to examine the mechanism for an observed phenomenon), (3) whether multiple alternative hypotheses were considered (sensu Chamberlin, 1890 ), and (4) whether hypotheses were “descriptive” (that is, they did not explore a mechanism but simply stated the expected direction of an effect; we define this as a “prediction” [Box 1 ]). It is important to note that to be identified as having hypotheses, articles did not need to contain the actual term “hypothesis” under our protocol; we also included articles using phrases such as “If X is true, we expected …” or “ we anticipated, ” both of which reflect a priori expectations from the data. We categorized each article as either basic (fundamental research without applications as a focus) or applied (clear management or conservation focus to article). Finally, we also examined all articles for funding sources and noted the presence of a national or international‐level competitive grant (e.g., National Science Foundation, European Union, Natural Sciences and Engineering Research Council). We assumed that published articles would have fidelity to the hypotheses stated in original grant proposals that funded the research, therefore, the acknowledgment of a successful grant is an indicator of financial reward for including hypotheses in initial proposals. Journal impact factors and individual article citation rates were gleaned directly from Web of Science. We reasoned that many researchers seek out journals with higher impact factors for the first submission of their manuscripts (Paine & Fox, 2018 ). Our assumption was that studies with more careful experimental design—including hypotheses—should be published where initially submitted, whereas those without may be eventually published, on average, in lower impact journals (Opthof et al., 2000 ). Ideally, we could have included articles that were rejected and never published in our analysis, but such articles are notoriously difficult to track (Thornton & Lee, 2000 ).
To support our detailed literature analysis, we also tested for temporal trends in hypothesis use within a broader sample of the ecology and evolution literature. For the same set of 22 journals in our detailed sample, we conducted a Web of Science search for articles containing “hypoth*” in the title or abstract. To calculate the proportion of articles with hypotheses (from 1990–2018), we divided the number of articles with hypotheses by the total number of articles ( N = 302,558). Because our search method does not include the main text of articles and excludes more subtle ways of stating hypotheses (e.g., “We expected…,” “We predicted…”), we acknowledge that the proportion of papers identified is likely to be an underestimate of the true proportions. Nevertheless, we do not expect that the degree of underestimation would change over time, so temporal trends in the proportion of papers containing hypotheses should be unbiased.
2.2. Statistical analysis
We used generalized linear mixed models (GLMMs) to test for change in the prevalance of various hypothesis types over time (descriptive, mechanistic, multiple, any hypothesis). Presence of a hypothesis was modeled as dichotomous (0,1) with binomial error structure, and “journal” was included as a random effect to account for potential lack of independence among articles published in the same outlet. The predictor variable (i.e., year) was scaled to enable convergence. Similarly, we tested for differences in hypothesis prevalence between basic and applied articles using GLMMs with “journal” as a random effect. Finally, we tested the hypothesis that hypothesis use might decline over time due to the emergence of machine‐learning in the applied conservation literature; specifically, we modeled “hypothesis presence” as a function of the statistical interaction between “year” and “basic versus applied” articles. We conducted this test for all hypothesis types. GLMMs were implemented in R (version 3.60) using the lme4 package (Bates et al., 2018 ). In three of our models, the “journal” random effect standard deviation was estimated to be zero or nearly zero (i.e., 10 –8 ). In such cases, the model with the random effect is exceptionally difficult to estimate, and the random effect standard deviation being estimated as approximately zero indicates the random effect was likely not needed.
We tested whether the presence of hypotheses influenced the likelihood of publication in a high‐impact journal using generalized linear models with a Gaussian error structure. We used the log of journal impact factor (+0.5) as the response variable to improve normality of model residuals. We tested the association between major competitive grants and the presence of a hypotheses using generalized linear models (logistic regression) with “hypothesis presence” (0,1) as a predictor and presence of a grant (0,1) as a response.
Finally, we tested whether hypotheses increase citation rates using linear mixed effects models (LMMs); presence of various hypotheses (0,1) were predictors in univariate models and average citations per year (log‐transformed) was the response. “Journal” was treated as a random effect, which assumes that articles within a particular journal are unlikely to be independent in their citation rates. LMMs were implemented in R using the lme4 package (Bates et al., 2015 ).
3.1. Trends in hypothesis use in ecology and evolution
In the ecology and evolution articles we examined in detail, the prevalence of multiple alternative hypotheses (6.7%) and mechanistic hypotheses (26%) was very low and showed no temporal trend (GLMM: multiple alternative: β ^ = 0.098 [95% CI: −0.383, 0.595], z = 0.40, p = 0.69, mechanistic: β ^ = 0.131 [95% CI: −0.149, 0.418], z = 0.92, p = 0.36, Figure 2a,b ). Descriptive hypothesis use was also low (8.5%), and although we observed a slight tendency to increase over time, 95% confidence intervals overlapped zero (GLMM: β ^ = 0.351 [95% CI: −0.088, 0.819], z = 1.53, p = 0.13, Figure 2c ). Although the proportion of papers containing no hypotheses appears to have declined (Figure 2d ), this effect was not statistically significant (GLMM: β ^ = −0.201 [95% CI: −0.483, 0.074], z = −1.41, p = 0.15). This overall pattern is consistent with a Web of Science search ( N = 302,558 articles) for the term “hypoth*” in titles or abstracts that shows essentially no trend over the same time period (Figure 2e,f ).

Trends in hypothesis use from 1991–2015 from a sample of the ecological and evolutionary literature ( N = 268, (a) multiple alternative hypotheses, (b) mechanistic hypotheses, (c) descriptive hypotheses [predictions], and (d) no hypotheses present). We detected no temporal trend in any of these variables. Lines reflect LOESS smoothing with 95% confidence intervals. Dots show raw data with darker colors indicating overlapping data points. The total number of publications in ecology and evolution in selected journals has increased (e), but use of the term “hypoth*” in the title or abstracts of these 302,558 articles has remained flat, and at very low prevalence (f)
Counter to our hypothesis, applied and basic articles did not show a statistically significant difference in the prevalence of either mechanistic (GLMM: β ^ = 0.054 [95% CI: −0.620, 0.728], z = 0.16, p = 0.875) or multiple alternative hypotheses (GLMM: β ^ = 0.517 [95% CI: −0.582, 1.80], z = 0.88, p = 0.375). Although both basic and applied ecology and evolution articles containing hypotheses were similarly rare overall, there was a tendency for applied ecology articles to show increasing prevalence of mechanistic hypothesis use over time, whereas basic ecology articles have remained relatively unchanged (Table S1 , Figure S1 ). However, there was substantial variation across both basic and applied journals in the prevalence of hypotheses (Figure 3 ).
3.2. Do hypotheses “pay?”
We found little evidence that presence of hypotheses increased paper citation rates. Papers with mechanistic (LMM: β ^ = −0.109 [95% CI: −0.329, 0.115], t = 0.042, p = 0.97, Figure 4a , middle panel) or multiple alternative hypotheses (LMM: β ^ = −0.008 [95% CI: −0.369, 0.391], t = 0.042, p = 0.96, Figure 4a , bottom panel) did not have higher average annual citation rates, nor did papers with at least one hypothesis type (LMM: β ^ = −0.024 [95% CI: −0.239, 0.194], t = 0.218, p = 0.83, Figure 4a , top panel).

Results of our detailed literature search showing the relationship between having a hypothesis (or not) and three commonly sought after scientific rewards (Average times a paper is cited/year, Journal impact factor, and the likelihood of having a major national competitive grant). We found no statistically significant relationships between having a hypothesis and citation rates or grants, but articles with hypotheses tended to be published in higher impact journals
On the other hand, journal articles containing mechanistic hypotheses tended to be published in higher impact journals (GLM: β ^ = 0.290 [95% CI: 0.083, 0.497], t = 2.74, p = 0.006) but only slightly so (Figure 4b , middle panel). Including multiple alternative hypotheses in papers did not have a statistically significant effect (GLM: = 0.339 [95% CI: −0.029, 0.707], t = 1.80, p = 0.072, Figure 4b , bottom panel).
Finally, we found no association between obtaining a competitive national or international grant and the presence of a hypothesis (logistic regression: mechanistic: β ^ = −0.090 [95% CI: −0.637, 0.453], z = −0.36, p =0 .745; multiple alternative: β ^ = 0.080 [95% CI: −0.891, 1.052], z = 0.49, p = 0.870; any hypothesis: β ^ = −0.005 [95% CI: −0.536, 0.525], z = −0.02, p = 0.986, Figure 4c ).
4. DISCUSSION
Overall, the prevalence of hypothesis use in the ecological and evolutionary literature is strikingly low and has been so for the past 25 years despite repeated calls to reverse this pattern (Elliott & Brook, 2007 ; Peters, 1991 ; Rosen, 2016 ; Sells et al., 2018 ). Why is this the case?
Clearly, hypotheses are not always necessary and a portion of the sampled articles may represent situations where hypotheses are truly not useful (see Box 3 : “When Are Hypotheses Not Useful?”). Some authors (Wolff & Krebs, 2008 ) overlook knowledge gathering and descriptive research as a crucial first step for making observations about natural phenomena—from which hypotheses can be formulated. This descriptive work is an important part of ecological science (Tewksbury et al., 2014 ), but may not benefit from strict use of hypotheses. Similarly, some efforts are simply designed to be predictive, such as auto‐recognition of species via machine learning (Briggs et al., 2012 ) or for prioritizing conservation efforts (Wilson et al., 2006 ), where the primary concern is correct identification and prediction rather than the biological or computational reasons for correct predictions (Box 3 ). However, it would be surprising if 75% of ecology since 1990 has been purely descriptive work from little‐known systems or purely predictive in nature. Indeed, the majority of the articles we observed did not fall into these categories.
When are hypotheses not useful?
Of course, there are a number of instances where hypotheses might not be useful or needed. It is important to recognize these instances to prevent the pendulum from swinging in a direction where without hypotheses, research ceases to be considered science (Wolff & Krebs, 2008 ). Below are several important types of ecological research where formulating hypotheses may not always be beneficial.
When the goal is prediction rather than understanding. Examples of this exception include species distribution models (Elith et al., 2008 ) where the question is not why species are distributed as they are, but simply where species are predicted to be. Such results can be useful in conservation planning (Guisan et al., 2013 ; see below). Another example lies in auto‐recognition of species (Briggs et al., 2012 ) where the primary concern is getting identification right rather than the biological or computational reasons for correct predictions. In such instances, complex algorithms can be very effective at uncovering patterns (e.g., deep learning). A caveat and critical component of such efforts is to ensure that such models are tested on independent data. Further, if model predictions are made beyond the spatial or temporal bounds of training or test data, extreme caution should be applied (see Figure 4 ).
When the goal is description rather than understanding. In many applications, the objective is to simply quantify a pattern in nature; for example, where on Earth is forest loss most rapid (Hansen et al., 2013 )? Further, sometimes so little is known about a system or species that formulating hypotheses is impossible and more description is necessary. In rare instances, an ecological system may be so poorly known and different to other systems that generating testable hypotheses would be extremely challenging. Darwin's observations while traveling on the Beagle are some of the best examples of such “hypothesis generating” science; these initial observations resulted in the formulation of one of the most extensively tested hypotheses in biology. However, such novelty should be uncommon in ecological and evolutionary research where theoretical and empirical precedent abounds (Sells et al., 2018 ). In the field of biogeography, there is the commonly held view that researchers should first observe and analyze patterns, and only then might explanations emerge (“pattern before process”); however, it has frequently been demonstrated that mechanistic hypotheses are useful even in disciplines where manipulative experiments are impossible (Crisp et al., 2011 ).
When the objective is a practical planning outcome such as reserve design. In many conservation planning efforts, the goal is not to uncover mechanisms, but rather simply to predict efficient methods or contexts for conserving species (Myers et al., 2000 ; Wilson et al., 2006 ). Perhaps this is the reason for such low prevalence of hypotheses in conservation journals (e.g., Conservation Biology).
Alternatively, researchers may not include hypotheses because they see little individual‐level incentive for their inclusion. Our results suggest that currently there are relatively few measurable benefits to individuals. Articles with mechanistic hypotheses do tend to be published in higher impact factor journals, which, for better or worse, is one of the key predictors in obtaining an academic job (van Dijk et al., 2014 ). However, few of the other typical academic metrics (i.e., citations or grant funding) appear to reward this behavior. Although hypotheses might be “useful” for overall progress in science (Platt, 1964 ), for their use to be propagated in the population of scientists, one would also expect them to provide benefits to the individuals conducting the science. Interestingly, the few existing papers on hypotheses (Loehle, 1987 ; Romesburg, 1981 ; Sells et al., 2018 ) tended to explain the advantages in terms of benefits to the group by offering arguments such as “because hypotheses help the field move forward more rapidly”.
Here we address some common justifications for hypotheses being unnecessary and show how one's first instinct to avoid hypotheses may be mistaken. We also present four reasons that use of hypotheses may be of individual self‐interest.
5. RESPONSES TO COMMON JUSTIFICATIONS FOR THE ABSENCE OF HYPOTHESES
During our collective mentoring at graduate and undergraduate levels, as well as examination of the literature, we have heard a number of common justifications for why hypotheses are not included. We must admit that many of us have, on occasion, rationalized absence of hypotheses in our own work using the same logic! We understand that clearly formulating and testing hypotheses can often be challenging, but propose that the justifications for avoiding hypotheses should be carefully considered.
- “ But I do have hypotheses ”. Simply using the word “hypothesis” does not a hypothesis make. A common pattern in the literature we reviewed was for researchers to state their guess about the results they expect and call this the “hypothesis” (e.g., “I hypothesize trees at higher elevation will grow slowly”). But these are usually predictions derived from an implicit theoretical model (Symes et al., 2015 ) or are simply descriptive statements with the word “hypothesis” in front of them (see Box 1 ). A research hypothesis must contain explanations for an observed phenomenon (Loehle, 1987 ; Wolff & Krebs, 2008 ). Such explanations are derived from existing or new theory (Symes et al., 2015 ). Making the link between the expected mechanism (hypothesis) and logical outcome if that mechanism were true (the prediction), is a key element of strong inference. Similarly, using “statistical hypotheses” and “null hypothesis testing” is not the same as developing mechanistic research hypotheses (Romesburg, 1981 ; Sells et al., 2018 ).
- “ Not enough is known about my system to formulate hypotheses ”. This is perhaps the most common defense against needing hypotheses (Golub, 2010 ). The argument goes that due to lack of previous research no mature theory has developed, so formal tests are impossible. Such arguments may have basis in some truly novel contexts (e.g., exploratory research on genomes) (Golub, 2010 ). But on close inspection, similar work has often been conducted in other geographic regions, systems, or with different taxa. If the response by a researcher is “but we really need to know if X pattern also applies in this region” (e.g., does succession influence bird diversity in forests of Western North America the same way as it does in Eastern forests), this is fine and it is certainly useful to accumulate descriptive studies globally for future synthetic work. However, continued efforts at description alone constitute missed opportunities for understanding the mechanisms behind a pattern (e.g., why does bird diversity decline when the forest canopy closes?). Often with a little planning, both the initial descriptive local interest question (e.g., “is it?”) and the broader interest question (i.e., “why?”) can both be tackled with minimal additional effort.
- “ What about Darwin? Many important discoveries have been made without hypotheses .” Several authors (and many students) have argued that many important and reliable patterns in nature have emerged outside of the hypothetico‐deductive (H‐D) method (Brush, 1974 ). For instance, Darwin's discovery of natural selection as a key force for evolution has been put forward as an example of how reliable ideas can emerge without the H‐D method (May, 1981 ; Milner, 2018 ). Examination of Darwin's notebooks has suggested that he did not propose explicit hypotheses and test them (Brush, 1974 ). However, Darwin himself wrote “all observation must be for or against some view if it is to be of any service!” (Ayala, 2009 ). In fact, Darwin actually put forward and empirically tested hypotheses in multiple fields, including geology, plant morphology and physiology, psychology, and evolution (Ayala, 2009 ). This debate suggests that, like Darwin, we should continue to value systematic observation and descriptive science (Tewksbury et al., 2014 ), but whenever possible, it should be with a view toward developing theory and testing hypotheses
The statement that “many important discoveries have been made without hypotheses” stems from a common misconception that somehow hypotheses spring fully formed into the mind, and that speculation, chance and induction play no role in the H‐D method. As noted by Loehle ( 1987 ; p. 402) “The H‐D method and strong inference, however, are valid no matter how theories are obtained. Dreams, crystal balls, or scribbled notebooks are all allowed. In fact, induction may be used to create empirical relations which then become candidates for hypothesis testing even though induction cannot be used to prove anything”. So, although induction has frequently been used to develop theory, it is an unreliable means to test theory (Popper, 1959 ). As is well‐known, Darwin's theory of natural selection was heavily debated in scientific circles at the time, and it is only through countless hypothesis tests that it remains the best explanation for evolution even today (Mayr, 2002 ).
- “ Ecology is too complex for hypotheses ”. In one of the most forcefully presented arguments for the H‐D method, Karl Popper ( 1959 ) argued that science should be done through a process of falsification; that is, multiple hypotheses should be constructed and the researcher's role is to successively eliminate these one at a time via experimentation until a single plausible hypothesis remains. This approach has caused some consternation among ecologists because the idea of single causes to phenomena doesn't match most of our experiences (Quinn & Dunham, 1983 ); rather, multiple interacting processes often overlap to drive observed patterns. For example, Robert Paine found that the distribution of a common seaweed was best explained by competition, physical disturbance, and dispersal ability (Paine, 1966 ).
It would be interesting if Popperian logic has inoculated ecology and evolution against the frequent application of hypotheses in research. Perhaps because the bar of falsification and testable mutually exclusive hypotheses is so high, many have opted to ignore the need for hypotheses altogether. If this is the case, our response is that in ecology and evolution we must not let Popperian perfection be the enemy of strong inference. With sufficient knowledge of a system, formal a priori hypotheses can be formulated that directly address the possibility of nonlinear relationships and interactions among variables. An example from conservation biology is the well‐explored hypothesis that the effects of habitat fragmentation should be greatest when habitat amount is low due to dispersal limitation (i.e., there should be a statistical interaction between fragmentation and habitat loss (Andrén, 1994 )).

Hypothesis generation is possible at all levels of organization, and does not need to get to the bottom of a causal hierarchy to be useful. As illustrated in this case study (after Betts et al., 2015 ), using published work by the authors, support for a hypothesis at one level often generates a subsequent question and hypotheses at the next. After each new finding we had to return to the white board and draw out new alternative hypotheses as we progressed further down the hierarchy. Supported hypotheses are shown in black and the alternative hypotheses that were eliminated are in grey. A single study is not expected to tackle an entire mechanistic hierarchy. In fact, we still have yet to uncover the physiological mechanisms involved in this phenomenon
- “ But my model predicts patterns well ”. An increasingly common justification for not presenting and testing research hypotheses seems to be the notion that if large datasets and complex modeling methods can predict outcomes effectively, what is the need for hypothesizing a mechanism (Glass & Hall, 2008 ; Golub, 2010 )? Indeed, some have argued that prediction is a gold standard in ecology and evolution (Houlahan et al., 2017 ). However, underlying such arguments is the critical assumption that the relationship between predictors (i.e., independent variables, 'x's) and responses ('y's) exhibit stationarity in time and space. Although this appears to be the case in cosmology (e.g., relativity is thought to apply wherever you are in the universe (Einstein, 1920 )), the assumption of stationarity has repeatedly been shown to be violated in ecological and evolutionary studies (Betts et al., 2006 ; Osborne et al., 2007 ; Thompson, 2005 ). Hence the well‐known maxim “correlation does not equal causation;” correlates of a phenomenon often shift, even if the underlying cause remains the same.
The advantage of understanding mechanism is that the relationship between cause and effect is less likely to shift in space and time than between the correlates of a phenomenon (Sells et al., 2018 ) (Figure 1 ). For instance, climate‐envelope models are still commonly used to predict future species distributions (Beale et al., 2008 ) despite the fact that links between correlates often fail (Gutiérrez et al., 2014 ) and climate per se may not be the direct driver of distributions. In an example from our own group, predictions that fit observed data well in the region where the model was built completely failed when predicted to a new region only 250 km away (Betts et al., 2006 ). Although it is true that mechanisms can also exhibit nonstationarity, at least in these instances logic can inform decisions about whether or not causal factors are likely to hold in a new place or time.
6. WHY SHOULD YOU HAVE HYPOTHESES? (A SELF‐INTERESTED PERSPECTIVE)
We have already described two arguments for hypothesis use, both of which should have positive influences on reproducibility and therefore progress in science: (1) multiple alternative hypotheses developed a priori prevent attachment to a single idea, and (2) hypotheses encourage exploration of mechanisms, which should increase the transferability of findings to new systems. Both these arguments have been made frequently in the eco‐evolutionary literature for decades (Elliott & Brook, 2007 ; Loehle, 1987 ; Rosen, 2016 ; Sells et al., 2018 ), but our results show that such arguments have been lost on the majority of researchers. One hypothesis recently proposed to explain why “poor methods persist [in science] despite perennial calls for improvements” is that such arguments have largely failed because they do not appeal to researcher self‐interest (Smaldino & McElreath, 2016 ). In periods of intense competition for grants and top‐tier publications, perhaps arguments that rely on altruism fall short. However, happily, there are at least four self‐interested reasons that students of ecological and evolutionary science should adopt the hypothetico‐deductive method.
- Clarity and Precision in Research
First, and most apparent during our review of the literature, hypotheses force clarity and precision in thinking. We often found it difficult to determine the core purpose of papers that lacked clear hypotheses. One of the key goals of scientific writing is to communicate ideas efficiently (Schimel, 2011 ). Increased clarity through use of hypotheses could potentially even explain the pattern for manuscripts using hypotheses getting published in higher impact journals. Editors are increasingly pressed for time and forced to reject the majority of papers submitted to higher impact outlets prior to detailed review (AAAS, 2018 ). “Unclear message” and “lack of clear hypotheses” are top reasons a paper ends up in the editor's reject pile (Eassom, 2018 ; Elsevier, 2015 ). If editors have to struggle as often as we did to determine the purpose of a paper, this does not bode well for future publication. Clearly, communication through succinctly stated hypotheses is likely to enhance publication success.
Hypotheses also provide crucial direction during study design. Nothing is more frustrating than realizing that your hard‐earned data cannot actually address the key study objectives or rule out alternative explanations. Developing clear hypotheses and, in particular, multiple alternative hypotheses ensures that you actually design your study in a way that can answer the key questions of interest.
- Personal Fulfillment
Second, science is more likely to be fulfilling and fun when the direction of research is clear, but perhaps more importantly, when questions are addressed with more than one plausible answer. Results are often disappointing or unfulfilling when the study starts out with a single biological hypothesis in mind (Symes et al., 2015 )—particularly if there is no support for this hypothesis. If multiple alternative hypotheses are well crafted, something interesting and rewarding will result regardless of the outcome. This results in a situation where researchers are much more likely to enjoy the process of science because the stress of wanting a particular end is removed. Subsequently, as Chamberlin ( 1890 ) proposed, “the dangers of parental affection for a favorite theory can be circumvented” which should reduce the risk of creeping bias. In our experience reviewing competitive grant proposals at the U.S. National Science Foundation, it is consistently the case that proposals testing several compelling hypotheses were more likely to be well received—presumably because reviewers are risk‐averse and understand that ultimately finding support for any of the outcomes will pay‐off. Why bet on just one horse when you can bet on them all?
- Intrinsic Value to Mechanism
Mechanism seems to have intrinsic value for humans—regardless of the practical application. Humans tend to be interested in acquiring understanding rather than just accumulating facts. As a species, we crave answers to the question “why.” Indeed, it is partly this desire for mechanism that is driving a recent perceived “crisis” in machine learning, with the entire field being referred to as “alchemy” (Hutson, 2018 ); algorithms continue to increase in performance, but the mechanisms for such improvements are often a mystery—even to the researchers themselves. “Because our model predicts well” is the unsatisfying scientific equivalent to a parent answering a child's “why?” with “because that's just the way it is.” This problem is beginning to spawn a new field in artificial intelligence “AI neuroscience” which attempts to get into the “black‐box” of machine‐learning algorithms to understand how and why they are predictive (Voosen, 2017 ).
Even in some of our most applied research, we find that managers and policymakers when confronted with a result (e.g., thinning trees to 70% of initial densities reduced bird diversity) want to know why (e.g., thinning eliminated nesting substrate for 4 species); If the answer to this question is not available, policy is much less likely to change (Sells et al., 2018 ). So, formulating mechanistic hypotheses will not only be more personally satisfying, but we expect it may also be more likely to result in real‐world changes.
- You Are More Likely To be Right
In a highly competitive era, it seems that in the quest for high publication rates and funding, researchers lose sight of the original aim of science: To discover a truth about nature that is transferable to other systems. In a recent poll conducted by Nature, more than 70% of researchers have tried and failed to reproduce another scientist's experiments (Baker, 2016 ). Ultimately, each researcher has a choice; put forward multiple explanations for a phenomenon on their own or risk “attachment” to a single hypothesis and run the risk of bias entering their work, rendering it irreproducible, and subsequently being found wrong by a future researcher. Imagine if Lamarck had not championed a single hypothesis for the mechanisms of evolution? Although Lamarck potentially had a vital impact as an early proponent of the idea that biological evolution occurred and proceeded in accordance with natural laws (Stafleu, 1971 ), unfortunately in the modern era he is largely remembered for his pet hypothesis. It may be a stretch to argue that he would have necessarily come up with natural selection, but if he had considered natural selection, the idea would have emerged 50 years earlier, substantially accelerating scientific progress and limiting his infamy as an early evolutionary biologist. An interesting contemporary example is provided by Prof. Amy Cuddy's research focused on “power posing” as a means to succeed. The work featured in one of the most viewed TED talks of all time but rather famously turned out to be irreproducible (Ranehill et al., 2015 ). When asked in a TED interview what she would do differently now, Prof. Cuddy noted that she would include a greater diversity of theory and multiple potential lines of evidence to “shed light on the psychological mechanisms” (Biello, 2017 ).
7. CONCLUSION
We acknowledge that formulating effective hypotheses can feel like a daunting hurdle for ecologists. However, we suggest that initial justifications for absence of hypotheses may often be unfounded. We argue that there are both selfish and altruistic reasons to include multiple alternative mechanistic hypotheses in your research: (1) testing multiple alternative hypotheses simultaneously makes for rapid and powerful progress which is to the benefit of all (Platt, 1964 ), (2) you lessen the chance that confirmation bias will result in you publishing an incorrect but provocative idea, (3) hypotheses provide clarity in design and writing, (4) research using hypotheses is more likely to be published in a high‐impact journal, and (5) you are able to provide satisfying answers to “why?” phenomena occur. However, few current academic metrics appear to reward use of hypotheses. Therefore, we propose that in order to promote hypothesis use we may need to provide additional incentives (Edwards & Roy, 2016 ; Smaldino & McElreath, 2016 ). We suggest editors reward research conducted using principles of sound scientific method and be skeptical of research that smacks of data dredging, post hoc hypothesis development, and single hypotheses. If no hypotheses are stated in a paper and/or the paper is purely descriptive, editors should ask whether the novelty of the system and question warrant this, or if the field would have been better served by a study with mechanistic hypotheses. Eleven of the top 20 ecology journals already indicate a desire for hypotheses in their instructions for authors—with some going as far as indicating “priority will be given” for manuscripts testing clearly stated hypotheses. Although hypotheses are not necessary in all instances, we expect that their continued and increased use will help our disciplines move toward greater understanding, higher reproducibility, better prediction, and more effective management and conservation of nature. We recommend authors, editors, and readers encourage their use (Box 2 ).
CONFLICT OF INTEREST
The authors have no conflicts of interests to declare.
AUTHOR CONTRIBUTIONS
Matthew G. Betts: Conceptualization (lead); data curation (lead); formal analysis (lead); funding acquisition (lead); investigation (lead); methodology (equal); project administration (lead); resources (lead); supervision (lead); visualization (lead); writing‐original draft (lead); writing‐review & editing (lead). Adam S. Hadley: Conceptualization (lead); data curation (lead); funding acquisition (equal); investigation (equal); methodology (lead); project administration (equal); resources (supporting); software (supporting); supervision (lead); validation (lead); visualization (lead); writing‐original draft (equal); writing‐review & editing (equal). David W. Frey: Conceptualization (supporting); data curation (supporting); formal analysis (supporting); funding acquisition (supporting); writing‐review & editing (supporting). Sarah J. K. Frey: Conceptualization (supporting); Investigation (equal); writing‐review & editing (equal). Dusty Gannon: Conceptualization (supporting); Investigation (equal); writing‐review & editing (equal). Scott H. Harris: Conceptualization (supporting); Investigation (equal); methodology (equal); writing‐review & editing (equal). Hankyu Kim: Conceptualization (supporting); Investigation (equal); Methodology (equal); writing‐review & editing (equal). Kara Leimberger: Conceptualization (supporting); Investigation (equal); Methodology (equal); writing‐review & editing (equal). Katie Moriarty: Conceptualization (supporting); Investigation (equal); methodology (equal); writing‐review & editing (equal). Joseph M. Northrup: Investigation (equal); methodology (equal); writing‐review & editing (equal). Ben Phalan: Investigation (equal); Methodology (equal); writing‐review & editing (equal). Josée S. Rousseau: Investigation (equal); Methodology (equal); writing‐review & editing (equal). Thomas D. Stokely: Investigation (equal); methodology (equal); writing‐review & editing (equal). Jonathon J. Valente: Investigation (equal); methodology (equal); writing‐review & editing (equal). Urs G. Kormann: Methodology (supporting); resources (equal); writing‐review & editing (supporting). Chris Wolf: Formal analysis (supporting); writing‐review & editing (supporting). Diego Zárrate‐Charry: Investigation (equal); Methodology (equal); writing‐review & editing (equal).
ETHICAL APPROVAL
The authors adhered to all standards for the ethical conduct of research.
Supporting information
Supplementary Material
ACKNOWLEDGMENTS
Funding from the National Science Foundation (NSF‐DEB‐1457837) to MGB and ASH supported this research. We thank Rob Fletcher, Craig Loehle and anonymous reviewers for thoughtful comments early versions of this manuscript, as well as Joe Nocera and his graduate student group at the University of New Brunswick for constructive comments on the penultimate version of the paper. The authors are also grateful for A. Dream for providing additional resources to enable the completion of this manuscript.
Betts MG, Hadley AS, Frey DW, et al. When are hypotheses useful in ecology and evolution? . Ecol Evol . 2021; 11 :5762–5776. 10.1002/ece3.7365 [ PMC free article ] [ PubMed ] [ CrossRef ] [ Google Scholar ]
Matthew G. Betts and Adam S. Hadley contributed equally to this manuscript.
DATA AVAILABILITY STATEMENT
- AAAS (2018). What percentage of submissions does Science accept? . AAAS Science Contributors. Retrieved from http://www.sciencemag.org/site/feature/contribinfo/faq/index.xhtml‐pct_faq [ Google Scholar ]
- Andrén, H. , & Andren, H. (1994). Effects of habitat fragmentation on birds and mammals in landscapes with different proportions of suitable habitat: A review . Oikos , 71 , 355–366. 10.2307/3545823 [ CrossRef ] [ Google Scholar ]
- Ayala, F. J. (2009). Darwin and the scientific method . Proceedings of the National Academy of Sciences , 106 , 10033–10039. 10.1073/pnas.0901404106 [ PMC free article ] [ PubMed ] [ CrossRef ] [ Google Scholar ]
- Ayres, M. P. , & Lombardero, M. J. (2017). Forest pests and their management in the Anthropocene . Canadian Journal of Forest Research , 48 , 292–301. 10.1139/cjfr-2017-0033 [ CrossRef ] [ Google Scholar ]
- Baker, M. (2016). 1,500 scientists lift the lid on reproducibility . Nature , 533 , 452–454. [ PubMed ] [ Google Scholar ]
- Bates, D. , Mächler, M. , Bolker, B. , & Walker, S. (2015). Fitting linear mixed‐effects models using lme4 . Journal of Statistical Software , 67 , 1–48. [ Google Scholar ]
- Bates, D. , Maechler, M. , & Bolker, B. (2018). ‘lme4’ Linear mixed‐effects models using S4 classes . R Core Team. Retrieved from https://cran.r‐project.org/web/packages/lme4/lme4.pdf [ Google Scholar ]
- Beale, C. M. , Lennon, J. J. , & Gimona, A. (2008). Opening the climate envelope reveals no macroscale associations with climate in European birds . Proceedings of the National Academy of Sciences , 105 , 14908–14912. 10.1073/pnas.0803506105 [ PMC free article ] [ PubMed ] [ CrossRef ] [ Google Scholar ]
- Betts, M. , Diamond, T. , Forbes, G. J. , Villard, M.‐A. , & Gunn, J. (2006). The importance of spatial autocorrelation, extent and resolution in predicting forest bird occurrence . Ecological Modeling , 191 , 197–224. [ Google Scholar ]
- Betts, M. G. , Hadley, A. S. , & Kress, J. (2015). Pollinator recognition in a keystone tropical plant . Proceedings of the National Academy of Sciences , 112 , 3433–3438. [ PMC free article ] [ PubMed ] [ Google Scholar ]
- Biello, D. (2017). Inside the debate about power posing: A Q & A with Amy Cuddy . Ideas.TED.com. Retrieved from https://ideas.ted.com/inside‐the‐debate‐about‐power‐posing‐a‐q‐a‐with‐amy‐cuddy/ [ Google Scholar ]
- Briggs, F. , Lakshminarayanan, B. , Neal, L. , Fern, X. Z. , Raich, R. , Hadley, S. J. K. , Hadley, A. S. , & Betts, M. G. (2012). Acoustic classification of multiple simultaneous bird species: A multi‐instance multi‐label approach . The Journal of the Acoustical Society of America , 131 , 4640–4650. 10.1121/1.4707424 [ PubMed ] [ CrossRef ] [ Google Scholar ]
- Brush, S. G. (1974). Should the history of science be rated X? Science , 183 , 1164–1172. [ PubMed ] [ Google Scholar ]
- Chamberlin, T. C. (1890). The method of multiple working hypotheses . Science , 15 , 92–96. [ PubMed ] [ Google Scholar ]
- Crisp, M. D. , Trewick, S. A. , & Cook, L. G. (2011). Hypothesis testing in biogeography . Trends in Ecology & Evolution , 26 , 66–72. 10.1016/j.tree.2010.11.005 [ PubMed ] [ CrossRef ] [ Google Scholar ]
- Cutler, D. R. , Edwards, T. C. , Beard, K. H. , Cutler, A. , Hess, K. T. , Gibson, J. , & Lawler, J. J. (2007). Random forests for classification in ecology . Ecology , 88 , 2783–2792. 10.1890/07-0539.1 [ PubMed ] [ CrossRef ] [ Google Scholar ]
- Eassom, H. (2018). 9 common reasons for rejection . Wiley: Discover the Future of Research. Retrieved from https://hub.wiley.com/community/exchanges/discover/blog/2018/2001/2031/2019‐common‐reasons‐for‐rejection [ Google Scholar ]
- Edwards, M. A. , & Roy, S. (2016). Academic research in the 21st century: Maintaining scientific integrity in a climate of perverse incentives and hypercompetition . Environmental Engineering Science , 34 , 51–61. 10.1089/ees.2016.0223 [ PMC free article ] [ PubMed ] [ CrossRef ] [ Google Scholar ]
- Egler, F. E. (1986). “Physics envy” in ecology . Bulletin of the Ecological Society of America , 67 , 233–235. [ Google Scholar ]
- Einstein, A. (1920). Relativity: The special and general theory (78 pp.). Henry Holt and Company. [ Google Scholar ]
- Elith, J. , Graham, C. H. , Anderson, R. P. , Dudík, M. , Ferrier, S. , Guisan, A. , Hijmans, R. J. , Huettmann, F. , Leathwick, J. R. , Lehmann, A. , Li, J. , Lohmann, L. G. , Loiselle, B. A. , Manion, G. , Moritz, C. , Nakamura, M. , Nakazawa, Y. , Overton, J. M. M. , Townsend Peterson, A. , … Zimmermann, N. E. (2006). Novel methods improve prediction of species' distributions from occurrence data . Ecography , 29 , 129–151. [ Google Scholar ]
- Elith, J. , Leathwick, J. R. , & Hastie, T. (2008). A working guide to boosted regression trees . Journal of Animal Ecology , 77 , 802–813. 10.1111/j.1365-2656.2008.01390.x [ PubMed ] [ CrossRef ] [ Google Scholar ]
- Elliott, L. P. , & Brook, B. W. (2007). Revisiting Chamberlin: Multiple working hypotheses for the 21st century . BioScience , 57 , 608–614. 10.1641/B570708 [ CrossRef ] [ Google Scholar ]
- Elsevier (2015). 5 ways you can ensure your manuscript avoids the desk reject pile . Elsevier Connect: https://www.elsevier.com/authors‐update/story/publishing‐tips/5‐ways‐you‐can‐ensure‐your‐manuscript‐avoids‐the‐desk‐reject‐pile [ Google Scholar ]
- Fahrig, L. (2003). Effects of habitat fragmentation on biodiversity . Annual Review of Ecology, Evolution, and Systematics , 34 , 487–515. [ Google Scholar ]
- Glass, D. J. , & Hall, N. (2008). A brief history of the hypothesis . Cell , 134 , 378–381. 10.1016/j.cell.2008.07.033 [ PubMed ] [ CrossRef ] [ Google Scholar ]
- Golub, T. (2010). Counterpoint: Data first . Nature , 464 , 679. 10.1038/464679a [ PubMed ] [ CrossRef ] [ Google Scholar ]
- Guisan, A. , Tingley, R. , Baumgartner, J. B. , Naujokaitis‐Lewis, I. , Sutcliffe, P. R. , Tulloch, A. I. T. , Regan, T. J. , Brotons, L. , McDonald‐Madden, E. , Mantyka‐Pringle, C. , Martin, T. G. , Rhodes, J. R. , Maggini, R. , Setterfield, S. A. , Elith, J. , Schwartz, M. W. , Wintle, B. A. , Broennimann, O. , Austin, M. , … Buckley, Y. M. (2013). Predicting species distributions for conservation decisions . Ecology Letters , 16 , 1424–1435. 10.1111/ele.12189 [ PMC free article ] [ PubMed ] [ CrossRef ] [ Google Scholar ]
- Hansen, M. C. , Potapov, P. V. , Moore, R. , Hancher, M. , Turubanova, S. A. , Tyukavina, A. , Thau, D. , Stehman, S. V. , Goetz, S. J. , Loveland, T. R. , Kommareddy, A. , Egorov, A. , Chini, L. , Justice, C. O. , & Townshend, J. R. G. (2013). High‐resolution global maps of 21st‐century forest cover change . Science , 342 , 850–853. 10.1126/science.1244693 [ PubMed ] [ CrossRef ] [ Google Scholar ]
- Houlahan, J. E. , McKinney, S. T. , Anderson, T. M. , & McGill, B. J. (2017). The priority of prediction in ecological understanding . Oikos , 126 , 1–7. [ Google Scholar ]
- Hutson, M. (2018). AI researchers allege that machine learning is alchemy . Science Posted in: Technology May 3, 2018. 10.1126/science.aau0577 [ CrossRef ]
- Illán, J. G. , Thomas, C. D. , Jones, J. A. , Wong, W.‐K. , Shirley, S. M. , & Betts, M. G. (2014). Precipitation and winter temperature predict long‐term range‐scale abundance changes in Western North American birds . Global Change Biology , 20 , 3351–3364. 10.1111/gcb.12642 [ PubMed ] [ CrossRef ] [ Google Scholar ]
- Lack, D. (1954). The natural regulation of animal numbers . Clarendon Press. [ Google Scholar ]
- Landy, F. (1986). Stamp collecting versus science ‐ Validation as hypothesis testing . American Psychologist , 41 , 1183–1192. [ Google Scholar ]
- Loehle, C. (1987). Hypothesis testing in ecology: Psychological aspects and the importance of theory maturation . The Quarterly Review of Biology , 62 , 397–409. [ PubMed ] [ Google Scholar ]
- May, R. M. (1981). The role of theory in ecology . American Zoologist , 21 , 903–910. [ Google Scholar ]
- Mayr, E. (2002). What evolution is . Basic Books. [ Google Scholar ]
- Milner, S. (2018). Newton didn't frame hypotheses. Why should we? Real Clear Science: Posted in: Physics Today April 25, 2018. 10.1063/PT.2016.2013.20180424a [ CrossRef ]
- Munafò, M. , Nosek, B. , Bishop, D. , Button, K. S. , Chambers, C. D. , du Sert, N. P. , Simonsohn, U. , Wagenmakers, E.‐J. , Ware, J. J. , & Ioannidis, J. P. A. (2017). A manifesto for reproducible science . Nature Human Behavior , 1 , 0021. [ PMC free article ] [ PubMed ] [ Google Scholar ]
- Myers, N. , Mittermeier, R. A. , Mittermeier, C. G. , da Fonseca, G. A. B. , & Kent, J. (2000). Biodiversity hotspots for conservation priorities . Nature , 403 , 853. [ PubMed ] [ Google Scholar ]
- O'Neill, R. V. , Johnson, A. R. , & King, A. W. (1989). A hierarchical framework for the analysis of scale . Landscape Ecology , 3 , 193–205. 10.1007/BF00131538 [ CrossRef ] [ Google Scholar ]
- Opthof, T. , Furstner, F. , van Geer, M. , & Coronel, R. (2000). Regrets or no regrets? No regrets! The fate of rejected manuscripts . Cardiovascular Research , 45 , 255–258. 10.1016/S0008-6363(99)00339-9 [ PubMed ] [ CrossRef ] [ Google Scholar ]
- Osborne Patrick, E. , Foody Giles, M. , & Suárez‐Seoane, S. (2007). Non‐stationarity and local approaches to modelling the distributions of wildlife . Diversity and Distributions , 13 , 313–323. 10.1111/j.1472-4642.2007.00344.x [ CrossRef ] [ Google Scholar ]
- Paine, C. E. T. , & Fox, C. W. (2018). The effectiveness of journals as arbiters of scientific impact . Ecology and Evolution , 8 , 9666–9685. [ PMC free article ] [ PubMed ] [ Google Scholar ]
- Paine, R. T. (1966). Food web complexity and species diversity . American Naturalist , 100 , 65–75. 10.1086/282400 [ CrossRef ] [ Google Scholar ]
- Peters, R. H. (1991). A critique for ecology . Nature. Cambridge University Press. [ Google Scholar ]
- Pettorelli, N. , Nagendra, H. , Rocchini, D. , Rowcliffe, M. , Williams, R. , Ahumada, J. , de Angelo, C. , Atzberger, C. , Boyd, D. , Buchanan, G. , Chauvenet, A. , Disney, M. , Duncan, C. , Fatoyinbo, T. , Fernandez, N. , Haklay, M. , He, K. , Horning, N. , Kelly, N. , … Wegmann, M. (2017). Remote sensing in ecology and conservation: Three years on . Remote Sensing in Ecology and Conservation , 3 , 53–56. [ Google Scholar ]
- Platt, J. R. (1964). Strong inference . Science , 146 , 347–353. [ PubMed ] [ Google Scholar ]
- Popper, K. (1959). The logic of scientific discovery . Basic Books. [ Google Scholar ]
- Quinn, J. F. , & Dunham, A. E. (1983). On hypothesis testing in ecology and evolution . The American Naturalist , 122 , 602–617. 10.1086/284161 [ CrossRef ] [ Google Scholar ]
- Ranehill, E. , Dreber, A. , Johannesson, M. , Leiberg, S. , Sul, S. , & Weber, R. A. (2015). Assessing the robustness of power posing: No effect on hormones and risk tolerance in a large sample of men and women . Psychological Science , 26 , 653–656. 10.1177/0956797614553946 [ PubMed ] [ CrossRef ] [ Google Scholar ]
- Romesburg, H. C. (1981). Wildlife science: Gaining reliable knowledge . The Journal of Wildlife Management , 45 , 293–313. 10.2307/3807913 [ CrossRef ] [ Google Scholar ]
- Rosen, J. (2016). Research protocols: A forest of hypotheses . Nature , 536 , 239–241. 10.1038/nj7615-239a [ PubMed ] [ CrossRef ] [ Google Scholar ]
- Schimel, J. (2011). Writing science: How to write papers that get cited and proposals that get funded . Oxford University Press. [ Google Scholar ]
- Sells, S. N. , Bassing, S. B. , Barker, K. J. , Forshee, S. C. , Keever, A. C. , Goerz, J. W. , & Mitchell, M. S. (2018). Increased scientific rigor will improve reliability of research and effectiveness of management . Journal of Wildlife Management , 82 , 485–494. 10.1002/jwmg.21413 [ CrossRef ] [ Google Scholar ]
- Smaldino, P. E. , & McElreath, R. (2016). The natural selection of bad science . Royal Society Open Science , 3 ( 9 ), 160384. 10.1098/rsos.160384 [ PMC free article ] [ PubMed ] [ CrossRef ] [ Google Scholar ]
- Stafleu, F. (1971). Lamarck: The birth of biology . Taxon , 20 , 397–442. 10.2307/1218244 [ PubMed ] [ CrossRef ] [ Google Scholar ]
- Sutherland, W. J. , Spiegelhalter, D. , & Burgman, M. A. (2013). Twenty tips for interpreting scientific claims . Nature , 503 , 335–337. [ PubMed ] [ Google Scholar ]
- Symes, L. B. , Serrell, N. , & Ayres, M. P. (2015). A pactical guide for mentoring scientific inquiry . The Bulletin of the Ecological Society of America , 96 , 352–367. [ Google Scholar ]
- Tewksbury, J. J. , Anderson, J. G. T. , Bakker, J. D. , Billo, T. J. , Dunwiddie, P. W. , Groom, M. J. , Hampton, S. E. , Herman, S. G. , Levey, D. J. , Machnicki, N. J. , del Rio, C. M. , Power, M. E. , Rowell, K. , Salomon, A. K. , Stacey, L. , Trombulak, S. C. , & Wheeler, T. A. (2014). Natural history's place in science and society . BioScience , 64 , 300–310. 10.1093/biosci/biu032 [ CrossRef ] [ Google Scholar ]
- Thompson, J. N. (2005). The geographic mosaic of coevolution . University of Chicago Press. [ Google Scholar ]
- Thornton, A. , & Lee, P. (2000). Publication bias in meta‐analysis: Its causes and consequences . Journal of Clinical Epidemiology , 53 , 207–216. 10.1016/S0895-4356(99)00161-4 [ PubMed ] [ CrossRef ] [ Google Scholar ]
- van Dijk, D. , Manor, O. , & Carey, L. B. (2014). Publication metrics and success on the academic job market . Current Biology , 24 , R516–R517. 10.1016/j.cub.2014.04.039 [ PubMed ] [ CrossRef ] [ Google Scholar ]
- Voosen, P. (2017). The AI detectives . Science , 357 , 22–27. 10.1126/science.357.6346.22 [ PubMed ] [ CrossRef ] [ Google Scholar ]
- Weinberg, R. (2010). Point: Hypotheses first . Nature , 464 , 678. 10.1038/464678a [ PubMed ] [ CrossRef ] [ Google Scholar ]
- Wilson, K. A. , McBride, M. F. , Bode, M. , & Possingham, H. P. (2006). Prioritizing global conservation efforts . Nature , 440 , 337. 10.1038/nature04366 [ PubMed ] [ CrossRef ] [ Google Scholar ]
- Wolff, J. O. , & Krebs, C. J. (2008). Hypothesis testing and the scientific method revisited . Acta Zoologica Sinica , 54 , 383–386. [ Google Scholar ]

- Science Notes Posts
- Contact Science Notes
- Todd Helmenstine Biography
- Anne Helmenstine Biography
- Free Printable Periodic Tables (PDF and PNG)
- Periodic Table Wallpapers
- Interactive Periodic Table
- Periodic Table Posters
- How to Grow Crystals
- Chemistry Projects
- Fire and Flames Projects
- Holiday Science
- Chemistry Problems With Answers
- Physics Problems
- Unit Conversion Example Problems
- Chemistry Worksheets
- Biology Worksheets
- Periodic Table Worksheets
- Physical Science Worksheets
- Science Lab Worksheets
- My Amazon Books
Null Hypothesis Examples

The null hypothesis (H 0 ) is the hypothesis that states there is no statistical difference between two sample sets. In other words, it assumes the independent variable does not have an effect on the dependent variable in a scientific experiment .
The null hypothesis is the most powerful type of hypothesis in the scientific method because it’s the easiest one to test with a high confidence level using statistics. If the null hypothesis is accepted, then it’s evidence any observed differences between two experiment groups are due to random chance. If the null hypothesis is rejected, then it’s strong evidence there is a true difference between test sets or that the independent variable affects the dependent variable.
- The null hypothesis is a nullifiable hypothesis. A researcher seeks to reject it because this result strongly indicates observed differences are real and not just due to chance.
- The null hypothesis may be accepted or rejected, but not proven. There is always a level of confidence in the outcome.
What Is the Null Hypothesis?
The null hypothesis is written as H 0 , which is read as H-zero, H-nought, or H-null. It is associated with another hypothesis, called the alternate or alternative hypothesis H A or H 1 . When the null hypothesis and alternate hypothesis are written mathematically, they cover all possible outcomes of an experiment.
An experimenter tests the null hypothesis with a statistical analysis called a significance test. The significance test determines the likelihood that the results of the test are not due to chance. Usually, a researcher uses a confidence level of 95% or 99% (p-value of 0.05 or 0.01). But, even if the confidence in the test is high, there is always a small chance the outcome is incorrect. This means you can’t prove a null hypothesis. It’s also a good reason why it’s important to repeat experiments.
Exact and Inexact Null Hypothesis
The most common type of null hypothesis assumes no difference between two samples or groups or no measurable effect of a treatment. This is the exact hypothesis . If you’re asked to state a null hypothesis for a science class, this is the one to write. It is the easiest type of hypothesis to test and is the only one accepted for certain types of analysis. Examples include:
There is no difference between two groups H 0 : μ 1 = μ 2 (where H 0 = the null hypothesis, μ 1 = the mean of population 1, and μ 2 = the mean of population 2)
Both groups have value of 100 (or any number or quality) H 0 : μ = 100
However, sometimes a researcher may test an inexact hypothesis . This type of hypothesis specifies ranges or intervals. Examples include:
Recovery time from a treatment is the same or worse than a placebo: H 0 : μ ≥ placebo time
There is a 5% or less difference between two groups: H 0 : 95 ≤ μ ≤ 105
An inexact hypothesis offers “directionality” about a phenomenon. For example, an exact hypothesis can indicate whether or not a treatment has an effect, while an inexact hypothesis can tell whether an effect is positive of negative. However, an inexact hypothesis may be harder to test and some scientists and statisticians disagree about whether it’s a true null hypothesis .
How to State the Null Hypothesis
To state the null hypothesis, first state what you expect the experiment to show. Then, rephrase the statement in a form that assumes there is no relationship between the variables or that a treatment has no effect.
Example: A researcher tests whether a new drug speeds recovery time from a certain disease. The average recovery time without treatment is 3 weeks.
- State the goal of the experiment: “I hope the average recovery time with the new drug will be less than 3 weeks.”
- Rephrase the hypothesis to assume the treatment has no effect: “If the drug doesn’t shorten recovery time, then the average time will be 3 weeks or longer.” Mathematically: H 0 : μ ≥ 3
This null hypothesis (inexact hypothesis) covers both the scenario in which the drug has no effect and the one in which the drugs makes the recovery time longer. The alternate hypothesis is that average recovery time will be less than three weeks:
H A : μ < 3
Of course, the researcher could test the no-effect hypothesis (exact null hypothesis): H 0 : μ = 3
The danger of testing this hypothesis is that rejecting it only implies the drug affected recovery time (not whether it made it better or worse). This is because the alternate hypothesis is:
H A : μ ≠ 3 (which includes μ <3 and μ >3)
Even though the no-effect null hypothesis yields less information, it’s used because it’s easier to test using statistics. Basically, testing whether something is unchanged/changed is easier than trying to quantify the nature of the change.
Remember, a researcher hopes to reject the null hypothesis because this supports the alternate hypothesis. Also, be sure the null and alternate hypothesis cover all outcomes. Finally, remember a simple true/false, equal/unequal, yes/no exact hypothesis is easier to test than a more complex inexact hypothesis.
- Adèr, H. J.; Mellenbergh, G. J. & Hand, D. J. (2007). Advising on Research Methods: A Consultant’s Companion . Huizen, The Netherlands: Johannes van Kessel Publishing. ISBN 978-90-79418-01-5 .
- Cox, D. R. (2006). Principles of Statistical Inference . Cambridge University Press. ISBN 978-0-521-68567-2 .
- Everitt, Brian (1998). The Cambridge Dictionary of Statistics . Cambridge, UK New York: Cambridge University Press. ISBN 978-0521593465.
- Weiss, Neil A. (1999). Introductory Statistics (5th ed.). ISBN 9780201598773.
Related Posts
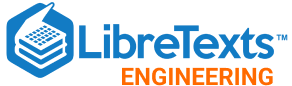
- school Campus Bookshelves
- menu_book Bookshelves
- perm_media Learning Objects
- login Login
- how_to_reg Request Instructor Account
- hub Instructor Commons
Margin Size
- Download Page (PDF)
- Download Full Book (PDF)
- Periodic Table
- Physics Constants
- Scientific Calculator
- Reference & Cite
- Tools expand_more
- Readability
selected template will load here
This action is not available.
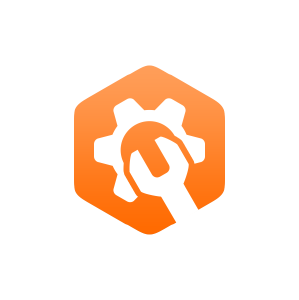
1.4: Environment and environmental science
- Last updated
- Save as PDF
- Page ID 12221
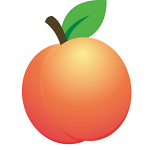
- Caralyn Zehnder, Kalina Manoylov, Samuel Mutiti, Christine Mutiti, Allison VandeVoort, & Donna Bennett
- Georgia College and State University via GALILEO Open Learning Materials
\( \newcommand{\vecs}[1]{\overset { \scriptstyle \rightharpoonup} {\mathbf{#1}} } \)
\( \newcommand{\vecd}[1]{\overset{-\!-\!\rightharpoonup}{\vphantom{a}\smash {#1}}} \)
\( \newcommand{\id}{\mathrm{id}}\) \( \newcommand{\Span}{\mathrm{span}}\)
( \newcommand{\kernel}{\mathrm{null}\,}\) \( \newcommand{\range}{\mathrm{range}\,}\)
\( \newcommand{\RealPart}{\mathrm{Re}}\) \( \newcommand{\ImaginaryPart}{\mathrm{Im}}\)
\( \newcommand{\Argument}{\mathrm{Arg}}\) \( \newcommand{\norm}[1]{\| #1 \|}\)
\( \newcommand{\inner}[2]{\langle #1, #2 \rangle}\)
\( \newcommand{\Span}{\mathrm{span}}\)
\( \newcommand{\id}{\mathrm{id}}\)
\( \newcommand{\kernel}{\mathrm{null}\,}\)
\( \newcommand{\range}{\mathrm{range}\,}\)
\( \newcommand{\RealPart}{\mathrm{Re}}\)
\( \newcommand{\ImaginaryPart}{\mathrm{Im}}\)
\( \newcommand{\Argument}{\mathrm{Arg}}\)
\( \newcommand{\norm}[1]{\| #1 \|}\)
\( \newcommand{\Span}{\mathrm{span}}\) \( \newcommand{\AA}{\unicode[.8,0]{x212B}}\)
\( \newcommand{\vectorA}[1]{\vec{#1}} % arrow\)
\( \newcommand{\vectorAt}[1]{\vec{\text{#1}}} % arrow\)
\( \newcommand{\vectorB}[1]{\overset { \scriptstyle \rightharpoonup} {\mathbf{#1}} } \)
\( \newcommand{\vectorC}[1]{\textbf{#1}} \)
\( \newcommand{\vectorD}[1]{\overrightarrow{#1}} \)
\( \newcommand{\vectorDt}[1]{\overrightarrow{\text{#1}}} \)
\( \newcommand{\vectE}[1]{\overset{-\!-\!\rightharpoonup}{\vphantom{a}\smash{\mathbf {#1}}}} \)
Viewed from space, Earth (Figure \(\PageIndex{1}\) ) offers no clues about the diversity of lifeforms that reside there. The first forms of life on Earth are thought to have been microorganisms that existed for billions of years in the ocean before plants and animals appeared. The mammals, birds, and plants so familiar to us are all relatively recent, originating 130 to 200 million years ago. Humans have inhabited this planet for only the last 2.5 million years, and only in the last 200,000 years have humans started looking like we do today. There are around 7.35 billion people today ( https://www.census.gov/popclock/ ).
.png?revision=1&size=bestfit&width=580&height=303)
The word environment describes living and nonliving surroundings relevant to organisms. It incorporates physical, chemical and biological factors and processes that determine the growth and survival of organisms, populations, and communities. All these components fit within the ecosystem concept as a way to organize all of the factors and processes that make up the environment. The ecosystem includes organisms and their environment within a specific area. Review the previous section for in-depth information regarding the Earth’s ecosystems. Today, human activities influence all of the Earth’s ecosystems.
Environmental science studies all aspects of the environment in an interdisciplinary way. This means that it requires the knowledge of various other subjects including biology, chemistry, physics, statistics, microbiology, biochemistry, geology, economics, law, sociology, etc. It is a relatively new field of study that has evolved from integrated use of many disciplines. Environmental engineering is one of the fastest growing and most complex disciplines of engineering. Environmental engineers solve problems and design systems using knowledge of environmental concepts and ecology, thereby providing solutions to various environmental problems. Environmentalism , in contrast, is a social movement through which citizens are involved in activism to further the protection of environmental landmarks and natural resources. This is not a field of science, but incorporates some aspects of environmental knowledge to advance conservation and sustainability efforts.
The Process of Science
Environmental science is a science, but what exactly is science? Science (from the Latin scientia, meaning “knowledge”) can be defined as all of the fields of study that attempt to comprehend the nature of the universe and all its parts. The scientific method is a method of research with defined steps that include experiments and careful observation. One of the most important aspects of this method is the testing of hypotheses by means of repeatable experiments. A hypothesis is a suggested explanation for an event, which can be tested. A theory is a tested and confirmed explanation for observations or phenomena that is supported by many repeated experiences and observations.
The scientific method
The scientific process typically starts with an observation (often a problem to be solved) that leads to a question. The scientific method consists of a series of well-defined steps. If a hypothesis is not supported by experimental data, a new hypothesis can be proposed. Let’s think about a simple problem that starts with an observation and apply the scientific method to solve the problem. One Monday morning, a student arrives in class and quickly discovers that the classroom is too warm. That is an observation that also describes a problem: the classroom is too warm. The student then asks a question: “Why is the classroom so warm?”
Proposing a Hypothesis
Recall that a hypothesis is a suggested explanation that can be tested. To solve a problem, several hypotheses may be proposed. For example, one hypothesis might be, “The classroom is warm because no one turned on the air conditioning.” But there could be other responses to the question, and therefore other hypotheses may be proposed. A second hypothesis might be, “The classroom is warm because there is a power failure, and so the air conditioning doesn’t work.” Once a hypothesis has been selected, the student can make a prediction. A prediction is similar to a hypothesis but it typically has the format “If . . . then . . . .” For example, the prediction for the first hypothesis might be, “If the student turns on the air conditioning, then the classroom will no longer be too warm.”
Testing a Hypothesis
A valid hypothesis must be testable. It should also be falsifiable, meaning that it can be disproven by experimental results. Importantly, science does not claim to “prove” anything because scientific understandings are always subject to modification with further information. This step — openness to disproving ideas — is what distinguishes sciences from non-sciences. The presence of the supernatural, for instance, is neither testable nor falsifiable.
To test a hypothesis, a researcher will conduct one or more experiments designed to eliminate, or disprove, the hypotheses. Each experiment will have one or more variables and one or more controls. A variable is any part of the experiment that can vary or change during the experiment. The independent variable is the variable that is manipulated throughout the course of the experiment. The dependent variable , or response variable is the variable by which we measure change in response to the independent variable. Ideally, all changes that we measure in the dependent variable are because of the manipulations we made to the independent variable. In most experiments, we will maintain one group that has had no experimental change made to it. This is the control group . It contains every feature of the experimental group except it is not given any manipulation. Therefore, if the results of the experimental group differ from the control group, the difference must be due to the hypothesized manipulation, rather than some outside factor. Look for the variables and controls in the examples that follow.
To test the hypothesis “The classroom is warm because no one turned on the air conditioning,” the student would find out if the air conditioning is on. If the air conditioning is turned on but does not work, there should be another reason, and this hypothesis should be rejected. To test the second hypothesis, the student could check if the lights in the classroom are functional. If so, there is no power failure and this hypothesis should be rejected. Each hypothesis should be tested by carrying out appropriate experiments. Be aware that rejecting one hypothesis does not determine whether or not the other hypotheses can be accepted; it simply eliminates one hypothesis that is not valid (Figure \(\PageIndex{2}\)). Using the scientific method, the hypotheses that are inconsistent with experimental data are rejected.
The scientific method may seem too rigid and structured. It is important to keep in mind that, although scientists often follow this sequence, there is flexibility. Sometimes an experiment leads to conclusions that favor a change in approach; often, an experiment brings entirely new scientific questions to the puzzle. Many times, science does not operate in a linear fashion; instead, scientists continually draw inferences and make generalizations, finding patterns as their research proceeds. Scientific reasoning is more complex than the scientific method alone suggests. Notice, too, that the scientific method can be applied to solving problems that aren’t necessarily scientific in nature.
.png?revision=1&size=bestfit&width=375&height=610)
Null Hypotheses in Ecology
Cite this chapter.
- Donald R. Strong Jr. 2
249 Accesses
8 Citations
Null hypotheses entertain the possibility that nothing has happened, that a process has not occurred, or that change has not been produced by a cause of interest. Null hypotheses are reference points against which alternatives should be contrasted. They are used not only in statistics but in all sciences. “This hypothesis…is… characteristic of all experimentation” (Fisher 1935). In physics for example, an important null hypothesis of the post-Newtonian era was that time is a variable which is independent of all other factors. Modern physics is based upon the alternative hypothesis that time can be a function of space and relative velocities. Another famous null hypothesis, that the speed of light is independent of its direction, inspired the Michelson-Morley experiments, which failed to disprove it. An example in chemistry is that there is no molecular property unique to life, that any synthesis by protoplasm can be repeated in the test tube. Modern biochemistry has failed to disprove this null hypothesis. But the term null hypothesis sounds odd in reference to much of physics and chemistry. It is not found in textbooks nor is it used frequently in conversation about these disciplines. Though all sciences use null hypotheses in principle, the ‘atomistic’ 1 sciences of physics and chemistry often use them implicitly. In atomistic sciences, fundamental units are simple and quite similar to one another, and effects of phenomena are commonly so distinct that the null state of no effect does not need special recognition.
This is a preview of subscription content, log in via an institution to check access.
Access this chapter
- Available as EPUB and PDF
- Read on any device
- Instant download
- Own it forever
- Compact, lightweight edition
- Dispatched in 3 to 5 business days
- Free shipping worldwide - see info
Tax calculation will be finalised at checkout
Purchases are for personal use only
Institutional subscriptions
Unable to display preview. Download preview PDF.
Abele, L. G. and K. Walters: 1979, ‘The stability-time hypothesis: reevaluation of the data’, Amer. Natur . 114 , 559.
Article Google Scholar
Abbot, I., Abbott, L. K., and P. R. Grant: 1977, ‘Comparative ecology of the Galapágos ground finches’, Ecol. Monog . 47 , 151–184.
Anderson, S.: 1974, ‘Patterns of faunal evolution’, The Quart. Rev. Biol . 49 , 311–332.
Auerbach, M. A.: 1979, ‘Some real communities are unstable’, Nature 279 , 821–822.
Beddington, J. R., Free, C. A., and J. H. Lawton: 1978, ‘Characteristics of successful natural enemies in models of biological control of insect pests’, Nature 273 , 513–519.
Bowman, R. I.: 1961, ‘Morphological variation and adaptation in the Galapágos finches’, Univ. Calif. Pub. Zool . 59 , 1–302
Google Scholar
Brown, W. H., and E. O. Wilson: 1956, ‘Character displacement’, Syst. Zool . 5 , 48–64.
Connell, J.: 1961, ‘The influence of interspecific competition and other factors on the distribution of the barnacle Chthamalus stellatusEcology 42 , 710–723.
Connor, E. F. and D. S. Simberloff: 1979, ‘The assembly of species communities: chance or competition? Ecology 60 , 1132–1141.
Cook, R. E.: 1969, ‘Variation in species density of North American birds’, Syst. Zool . 18 , 63–84.
Darwin, C.: 1859, The Origin of Species , Murray, London.
Dayton, P. K.: 1973, ‘Two cases of resource partitioning in an intertidal community: making the right predictions for the wrong reasons’, The American Naturalist 107 , 662–670.
Diamond, J. M.: 1975, ‘Assemble rules of species communities’, pp. 342–344 in M. Cody and J. Diamond (eds.), Ecology and Evolution of Communities , Harvard Univ. Press., Cambridge Mass.
Diamond, J. M.: 1978, ‘Niche-shifts and the rediscovery of interspecific competition’, American Scientist 66 , 322–331.
Elton, C. S.: 1946, Journ. Anim. Ecol . 15 , 54–68.
Emlen, J. M.: 1966, ‘The role of time and energy in food preference’, Amer. Natur . 107 , 580–584.
Fenchel, T.: 1975, ‘Character displacement and coexistence in mud snails’, Oecologia 20 , 19–32.
Fisher, R. A.: 1935, The Design of Experiments ii , Oliver and Boyd, London.
Gause, G. F.: 1934, The Struggle for Existence , Williams and Wilkins, Baltimore.
Grant, P. R.: 1966, ‘Ecological compatibility of bird species on islands’, Amer. Natur . 100 , 451–462.
Grant, P. R.: 1972, ‘Convergent and divergent character displacement’, Biol. Journ. Linn. Soc . 4 , 39–68.
Greenwood, J. J. D.: 1968, ‘Coexistence of avian congeners on islands’, Amer. Natur . 102 , 591–592.
Heatwole, H. and R. Levins: 1972, ‘The trophic structure, stability and faunal change during recolonization’, Ecology 53 , 531–534.
Heck, K. L.: 1976, ‘Some critical considerations of the theory of species packing’, Evol. Theory 1 , 247–258.
Heck, K. L., Van Belle, G., and D. Simberloff: 1975, ‘Explicit calculation of the rarefaction diversity measurement and the determination of sufficient sample size’, Ecology 56 , 1451–1461.
Huey, R. B., and E. R. Pianka: 1974, ‘Ecological character displacement in a lizard’, Amer. Zool . 14 , 1127–1136.
Hutchinson, G. E.: 1959, ‘Homage to Santa Rosalia, or why are there so many kinds of animals? Amer. Natur . 93 , 145–159.
Janzen, D. H.: 1966, ‘Coevolution of mutualism between ants and acacias in Central America’, Evolution 20 , 249–275.
Kuhn, T. S.: 1962, The Structure of Scientific Revolutions , 2nd ed., Univ. Chicago Press.
Lack, D.: 1947, The Galapágos Finches , Cambridge Univ. Press.
Lawlor, L. R.: 1978, ‘A comment on randomly constructed model ecosystems’, The American Naturalist 112 , 445–447.
Levin, S. A.: 1974, ‘Dispersion and population interactions’, The Amer. Natur . 108 , 207–228.
MacArthur, R. H. and E. O. Wilson: 1967, The theory of island biogeography , Princeton Univ. Press, Princeton, N.J.
MacFadyen, A.: 1975, ‘Some thoughts on the behaviour of ecologists’, Journ. Anim. Ecol ., 351–363.
May, R. M.: 1974, Stability and Complexity in Model Ecosystems , 2nd ed., Princeton Univ. Press. Princeton, N.J.
May, R. M.: 1978, ‘The evolution of ecological systems’, Scientific American 239 , 160–175.
Moreau, R. E.: 1948, ‘Ecological isolation in a rich tropical avifauna’, Journ. Anim. Ecol . 17 , 113–126.
Moreau, R. E.: 1966, The Bird Faunas of Africa and Its Islands , Academic Press, N.Y.
Murdoch, W. W.: 1966, ‘Community structure, population control, and competition — a critique’, The Amer. Natur . 100 , 219–226.
Paine, R. T.: 1966, ‘Food web complexity and species diversity’, Amer. Natur . 100 , 65–75.
Peters, R. H.: 1977, ‘The unpredictable problems of tropho-dynamics’, Env. Biol. Fish . 2 , 97–101.
Pimm, S. L., and J. H. Lawton: 1977, ‘Number of trophic levels in ecological communities’, Nature 268 , 329–331.
Popper, K. R.: 1968, The Logic of Scientific Discovery , Hutchinson, London.
Schoener, T. W.: 1965, ‘The evolution of bill size differences among sympatric congeneric species of birds’, Evolution 19 , 189–213.
Simberloff, D. S.: 1970, ‘The taxonomic diversity of island biotas’, Evolution 24 , 22–47.
Simberloff, D. S.: 1976, ‘Trophic structure determination and equilibrium in an arthropod community’, Ecology 57 , 395–398.
Stearns, S. C.: 1978, ‘Life–history tactics: a review of ideas’, T he Quarterly Review of Biology , 51 , 3–47.
Strong, D. R., Szyska, L. A., and D. S. Simberloff: 1979, Tests of community-wide character displacement against null hypotheses’, Evolution , in press.
Terborgh, J., Faaborg, K. and H. J. Brockmann: 1978, ‘Island colonization by lesser Antillean birds’, The Auk 95 , 59–72.
Williams, C. B.: 1947, ‘The generic relations of species in small ecological communities’, J. Anim. Ecol . 16 : 11–18.
Williams, C. B.: 1951, ‘Intra-generic competition as illustrated by Moreau’s records of East African birds’, Journ. Anim. Ecol . 20 , 246–253.
Williams, C. B.: 1964, Patterns in the Balance of Nature , Academic Press, Lond.
Wilson, E. O.: 1975, Sociobiology , Harvard.
Download references
Author information
Authors and affiliations.
Department of Biological Sciences, Florida State University, Tallahassee, USA
Donald R. Strong Jr.
You can also search for this author in PubMed Google Scholar
Editor information
Editors and affiliations.
Dept. of Philosophy, University of Helsinki, Finland
Esa Saarinen
Rights and permissions
Reprints and permissions
Copyright information
© 1982 D. Reidel Publishing Company, Dordrecht, Holland
About this chapter
Strong, D.R. (1982). Null Hypotheses in Ecology. In: Saarinen, E. (eds) Conceptual Issues in Ecology. Springer, Dordrecht. https://doi.org/10.1007/978-94-009-7796-9_10
Download citation
DOI : https://doi.org/10.1007/978-94-009-7796-9_10
Publisher Name : Springer, Dordrecht
Print ISBN : 978-90-277-1391-9
Online ISBN : 978-94-009-7796-9
eBook Packages : Springer Book Archive
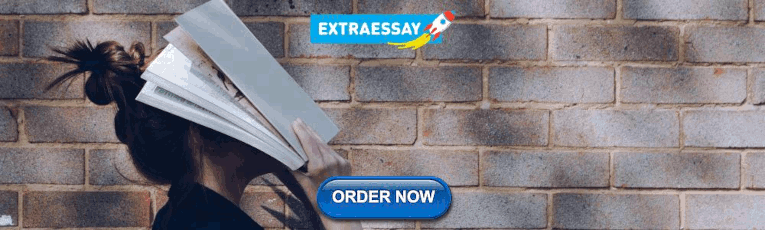
Share this chapter
Anyone you share the following link with will be able to read this content:
Sorry, a shareable link is not currently available for this article.
Provided by the Springer Nature SharedIt content-sharing initiative
- Publish with us
Policies and ethics
- Find a journal
- Track your research
- Skip to primary navigation
- Skip to main content
- Skip to footer
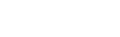
Understanding Science
How science REALLY works...
null hypothesis
Usually a statement asserting that there is no difference or no association between variables. The null hypothesis is a tool that makes it possible to use certain statistical tests to figure out if another hypothesis of interest is likely to be accurate or not.
Subscribe to our newsletter
- Understanding Science 101
- The science flowchart
- Science stories
- Grade-level teaching guides
- Teaching resource database
- Journaling tool
- Misconceptions
Best Practices in Science
The Null Hypothesis
Show Topics
Publications
- Journals and Blogs
The null hypothesis, as described by Anthony Greenwald in ‘Consequences of Prejudice Against the Null Hypothesis,’ is the hypothesis of no difference between treatment effects or of no association between variables. Unfortunately in academia, the ‘null’ is often associated with ‘insignificant,’ ‘no value,’ or ‘invalid.’ This association is due to the bias against papers that accept the null hypothesis by journals. This prejudice by journals to only accept papers that show ‘significant’ results (also known as rejecting this ‘null hypothesis’) puts added pressure on those working in academia, especially with their relevance and salaries often depend on publications. This pressure may also be correlated with increased scientific misconduct, which you can also read more about on this website by clicking here . If you would like to read publication, journal articles, and blogs about the null hypothesis, views on rejecting and accepting the null, and journal bias against the null hypothesis, please see the resources we have linked below.
Most scientific journals are prejudiced against papers that demonstrate support for null hypotheses and are unlikely to publish such papers and articles. This phenomenon leads to selective publishing of papers and ensures that the portion of articles that do get published is unrepresentative of the total research in the field.
Anderson, D. R., Burnham, K. P., & Thompson, W. L. (2000). Null hypothesis testing: problems, prevalence, and an alternative. The journal of wildlife management , 912-923.
Benjamini, Y., & Hochberg, Y. (1995). Controlling the false discovery rate: a practical and powerful approach to multiple testing. Journal of the royal statistical society . Series B (Methodological), 289-300.
Berger, J. O., & Sellke, T. (1987). Testing a point null hypothesis: The irreconcilability of p values and evidence. Journal of the American statistical Association , 82 (397), 112-122.
Blackwelder, W. C. (1982). “Proving the null hypothesis” in clinical trials. Controlled clinical trials , 3 (4), 345-353.
Dirnagl, U. (2010). Fighting publication bias: introducing the Negative Results section. Journal of cerebral blood flow and metabolism: official journal of the International Society of Cerebral Blood Flow and Metabolism , 30 (7), 1263.
Dickersin, K., Chan, S. S., Chalmersx, T. C., Sacks, H. S., & Smith, H. (1987). Publication bias and clinical trials. Controlled clinical trials , 8 (4), 343-353.
Efron, B. (2004). Large-scale simultaneous hypothesis testing: the choice of a null hypothesis. Journal of the American Statistical Association , 99 (465), 96-104.
Fanelli, D. (2010). Do pressures to publish increase scientists’ bias? An empirical support from US States Data. PloS one , 5 (4), e10271.
Fanelli, D. (2011). Negative results are disappearing from most disciplines and countries. Scientometrics , 90 (3), 891-904.
Greenwald, A. G. (1975). Consequences of Prejudice Against the Null Hypothesis. Psychological Bulletin , 82 (1).
Hubbard, R., & Armstrong, J. S. (1997). Publication bias against null results. Psychological Reports , 80 (1), 337-338.
I’ve Got Your Impact Factor Right Here (Science, February 24, 2012)
Johnson, R. T., & Dickersin, K. (2007). Publication bias against negative results from clinical trials: three of the seven deadly sins. Nature Clinical Practice Neurology , 3 (11), 590-591.
Keep negativity out of politics. We need more of it in journals (STAT, October 14, 2016)
Knight, J. (2003). Negative results: Null and void. Nature , 422 (6932), 554-555.
Koren, G., & Klein, N. (1991). Bias against negative studies in newspaper reports of medical research. Jama , 266 (13), 1824-1826.
Koren, G., Shear, H., Graham, K., & Einarson, T. (1989). Bias against the null hypothesis: the reproductive hazards of cocaine. The Lancet , 334 (8677), 1440-1442.
Krantz, D. (2012). The Null Hypothesis Testing Controversy in Psychology. Journal of American Statistical Association .
Lash, T. (2017). The Harm Done to Reproducibility by the Culture of Null Hypothesis Significance Testing. American Journal of Epidemiology .
Mahoney, M. J. (1977). Publication prejudices: An experimental study of confirmatory bias in the peer review system. Cognitive therapy and research , 1 (2), 161-175.
Matosin, N., Frank, E., Engel, M., Lum, J. S., & Newell, K. A. (2014). Negativity towards negative results: a discussion of the disconnect between scientific worth and scientific culture.
Nickerson, R. S. (2000). Null hypothesis significance testing: a review of an old and continuing controversy. Psychological methods , 5 (2), 241.
No result is worthless: the value of negative results in science (BioMed Central, October 10, 2012)
Negative Results: The Dark Matter of Research (American Journal Experts)
Neil Malhotra: Why No News Is Still Important News in Research (Stanford Graduate School of Business, October 27, 2014)
Null Hypothesis Definition and Example (Statistics How To, November 5, 2012)
Null Hypothesis Glossary Definition (Statlect Digital Textbook)
Opinion: Publish Negative Results (The Scientist, January 15, 2013)
Positives in negative results: when finding ‘nothing’ means something (The Conversation, September 24, 2014)
Rouder, J. N., Speckman, P. L., Sun, D., Morey, R. D., & Iverson, G. (2009). Bayesian t tests for accepting and rejecting the null hypothesis. Psychonomic bulletin & review , 16 (2), 225-237.
Unknown Unknowns: The War on Null and Negative Results (social science space, September 19, 2014)
Valuing Null and Negative Results in Scientific Publishing (Scholastica, November 4, 2015)
Vasilev, M. R. (2013). Negative results in European psychology journals. Europe’s Journal of Psychology , 9 (4), 717-730
Where have all the negative results gone? (bioethics.net, December 4, 2013)
Where to publish negative results (BitesizeBio, November 27, 2013)
Why it’s time to publish research “failures” (Elsevier, May 5, 2015)
Woolson, R. F., & Kleinman, J. C. (1989). Perspectives on statistical significance testing. Annual review of public health , 10 (1), 423-440.
Would you publish your negative results? If no, why? (ResearchGate, October 26, 2012)
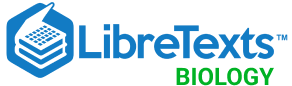
- school Campus Bookshelves
- menu_book Bookshelves
- perm_media Learning Objects
- login Login
- how_to_reg Request Instructor Account
- hub Instructor Commons
Margin Size
- Download Page (PDF)
- Download Full Book (PDF)
- Periodic Table
- Physics Constants
- Scientific Calculator
- Reference & Cite
- Tools expand_more
- Readability
selected template will load here
This action is not available.
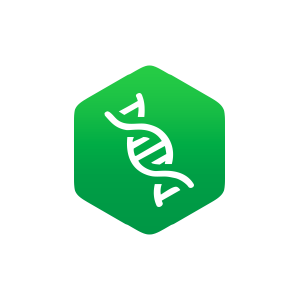
1.1: What is Environmental Science?
- Last updated
- Save as PDF
- Page ID 69344
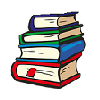
- Melissa Ha and Rachel Schleiger
- Yuba College & Butte College via ASCCC Open Educational Resources Initiative
\( \newcommand{\vecs}[1]{\overset { \scriptstyle \rightharpoonup} {\mathbf{#1}} } \)
\( \newcommand{\vecd}[1]{\overset{-\!-\!\rightharpoonup}{\vphantom{a}\smash {#1}}} \)
\( \newcommand{\id}{\mathrm{id}}\) \( \newcommand{\Span}{\mathrm{span}}\)
( \newcommand{\kernel}{\mathrm{null}\,}\) \( \newcommand{\range}{\mathrm{range}\,}\)
\( \newcommand{\RealPart}{\mathrm{Re}}\) \( \newcommand{\ImaginaryPart}{\mathrm{Im}}\)
\( \newcommand{\Argument}{\mathrm{Arg}}\) \( \newcommand{\norm}[1]{\| #1 \|}\)
\( \newcommand{\inner}[2]{\langle #1, #2 \rangle}\)
\( \newcommand{\Span}{\mathrm{span}}\)
\( \newcommand{\id}{\mathrm{id}}\)
\( \newcommand{\kernel}{\mathrm{null}\,}\)
\( \newcommand{\range}{\mathrm{range}\,}\)
\( \newcommand{\RealPart}{\mathrm{Re}}\)
\( \newcommand{\ImaginaryPart}{\mathrm{Im}}\)
\( \newcommand{\Argument}{\mathrm{Arg}}\)
\( \newcommand{\norm}[1]{\| #1 \|}\)
\( \newcommand{\Span}{\mathrm{span}}\) \( \newcommand{\AA}{\unicode[.8,0]{x212B}}\)
\( \newcommand{\vectorA}[1]{\vec{#1}} % arrow\)
\( \newcommand{\vectorAt}[1]{\vec{\text{#1}}} % arrow\)
\( \newcommand{\vectorB}[1]{\overset { \scriptstyle \rightharpoonup} {\mathbf{#1}} } \)
\( \newcommand{\vectorC}[1]{\textbf{#1}} \)
\( \newcommand{\vectorD}[1]{\overrightarrow{#1}} \)
\( \newcommand{\vectorDt}[1]{\overrightarrow{\text{#1}}} \)
\( \newcommand{\vectE}[1]{\overset{-\!-\!\rightharpoonup}{\vphantom{a}\smash{\mathbf {#1}}}} \)
What is Environmental Science?
Environmental science is the dynamic, interdisciplinary study of the interaction of living and non-living parts of the environment, with special focus on the impact of humans on the environment. The study of environmental science includes circumstances, objects, or conditions by which an organism or community is surrounded and the complex ways in which they interact.
Environmental Science is Interdisciplinary
Environmental science includes disciplines in the physical sciences (like geology, soil science, physical geography, chemistry, and atmospheric sciences), life sciences (like ecology, conservation biology, restoration biology, and population biology), social sciences (like human geography, economics, law, political science, and anthropology), and humanities (philosophy, ethics). As such, environmental scientists are a diverse bunch. However, they all come together for the focus of studying and identifying past, current, and future environmental issues while also exploring solutions to the environmental issues and also considering practical needs.
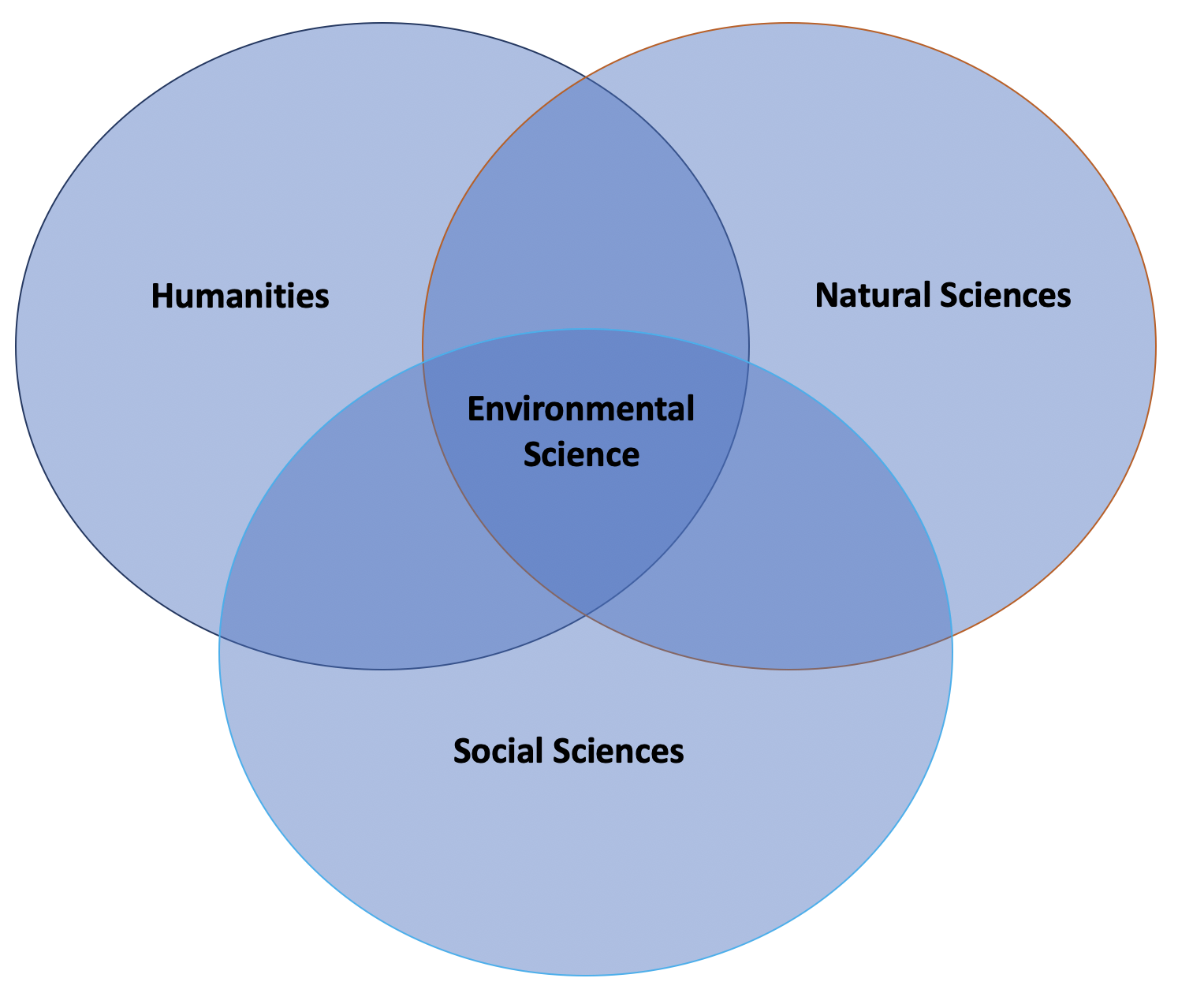
Why Study Environmental Science?
The need for equitable, ethical, and sustainable use of Earth’s resources by a global population that nears the carrying capacity of the planet requires us not only to understand how human behaviors affect the environment, but also the scientific principles that govern interactions between the living and non-living. Our future depends on our ability to understand and evaluate evidence-based arguments about the environmental consequences of human actions and technologies, and to make informed decisions based on those arguments.
From global climate change to habitat loss driven by human population growth and development, Earth is becoming a different planet—right before our eyes. The global scale and rate of environmental change are beyond anything in recorded human history. Our challenge is to acquire an improved understanding of Earth’s complex environmental systems; systems characterized by interactions within and among their natural and human components that link local to global and short-term to long-term phenomena, and individual behavior to collective action. The complexity of environmental challenges demands that we all participate in finding and implementing solutions leading to long-term environmental sustainability.
The Tragedy of the Commons
In his essay, The Tragedy of the Commons, Garrett Hardin (1968) looked at what happens when humans do not limit their actions by including the land as part of their ethic. The tragedy of the commons develops in the following way: Imagine a pasture open to all (the ‘commons’). It is to be expected that each herdsman will try to keep as many cattle as possible on the commons. As rational beings, each herdsman seeks to maximize their gain. Adding more cattle increases their profit, and they do not suffer any immediate negative consequence because the commons are shared by all. The rational herdsman concludes that the only sensible course is to add another animal to their herd, and then another, and so forth. However, this same conclusion is reached by each and every rational herdsman sharing the commons. Therein lies the tragedy: each person is locked into a system that compels them to increase their herd, without limit, in a world that is limited. Eventually this leads to the ruination of the commons. In a society that believes in the freedom of the commons, freedom brings ruin to all because each person acts selfishly.
Hardin went on to apply the situation to modern commons: overgrazing of public lands, overuse of public forests and parks, depletion of fish populations in the ocean, use of rivers as a common dumping ground for sewage, and fouling the air with pollution.
The “Tragedy of the Commons” is applicable to what is arguably the most consequential environmental problem: global climate change. The atmosphere is a commons into which countries are dumping carbon dioxide from the burning of fossil fuels. Although we know that the generation of greenhouse gases will have damaging effects upon the entire globe, we continue to burn fossil fuels. As a country, the immediate benefit from the continued use of fossil fuels is seen as a positive component (because of economic growth). All countries, however, will share the negative long-term effects.
Some Indicators of Global Environmental Stress
- Forests — Deforestation remains a main issue. 1 million hectares of forest were lost every year in the decade 1980-1990. The largest losses of forest area are taking place in the tropical moist deciduous forests, the zone best suited to human settlement and agriculture. Recent estimates suggest that nearly two-thirds of tropical deforestation is due to farmers clearing land for agriculture. There is increasing concern about the decline in forest quality associated with intensive use of forests and unregulated access.
- Soil — As much as 10% of the earth’s vegetated surface is now at least moderately degraded. Trends in soil quality and management of irrigated land raise serious questions about longer-term sustainability. It is estimated that about 20% of the world’s 250 million hectares of irrigated land are already degraded to the point where crop production is seriously reduced.
- Fresh water — Some 20% of the world’s population lacks access to safe water and 50% lacks access to safe sanitation. If current trends in water use persist, two-thirds of the world’s population could be living in countries experiencing moderate or high water stress by 2025.
- Marine fisheries — 25% of the world’s marine fisheries are being fished at their maximum level of productivity and 35% are overfished (yields are declining). In order to maintain current per capita consumption of fish, global fish harvests must be increased; much of the increase might come through aquaculture which is a known source of water pollution, wetland loss and mangrove swamp destruction.
- Biodiversity — Biodiversity is increasingly coming under threat from development, which destroys or degrades natural habitats, and from pollution from a variety of sources. The first comprehensive global assessment of biodiversity put the total number of species at close to 14 million and found that between 1% and 11% of the world’s species may be threatened by extinction every decade. Coastal ecosystems, which host a very large proportion of marine species, are at great risk with perhaps one-third of the world’s coasts at high potential risk of degradation and another 17% at moderate risk.
- Atmosphere — The Intergovernmental Panel on Climate Change has established that human activities are having a discernible influence on global climate. CO 2 emissions in most industrialized countries have risen during the past few years and countries generally failed to stabilize their greenhouse gas emissions at 1990 levels by 2000 as required by the Climate Change convention.
- Toxic chemicals — About 100,000 chemicals are now in commercial use and their potential impacts on human health and ecological function represent largely unknown risks. Persistent organic pollutants are now so widely distributed by air and ocean currents that they are found in the tissues of people and wildlife everywhere; they are of particular concern because of their high levels of toxicity and persistence in the environment.
- Hazardous wastes — Pollution from heavy metals, especially from their use in industry and mining, is also creating serious health consequences in many parts of the world. Incidents and accidents involving uncontrolled radioactive sources continue to increase, and particular risks are posed by the legacy of contaminated areas left from military activities involving nuclear materials.
- Waste — Domestic and industrial waste production continues to increase in both absolute and per capita terms, worldwide. In the developed world, per capita waste generation has increased threefold over the past 20 years; in developing countries, it is highly likely that waste generation will double during the next decade. The level of awareness regarding the health and environmental impacts of inadequate waste disposal remains rather poor; poor sanitation and waste management infrastructure is still one of the principal causes of death and disability for the urban poor.
Attributions
Modified by Melissa Ha and Rachel Schleiger from The Earth, Humans, & the Environment , Environment and Sustainability , and Environmental Ethics from Environmental Biology by Matthew R. Fisher (licensed under CC-BY )
Contributors and Attributions
- Essentials of Environmental Science by Kamala Doršner is licensed under CC BY 4.0. Modified from the original by Matthew R. Fisher.
- School Guide
- Mathematics
- Number System and Arithmetic
- Trigonometry
- Probability
- Mensuration
- Maths Formulas
- Integration Formulas
- Differentiation Formulas
- Trigonometry Formulas
- Algebra Formulas
- Mensuration Formula
- Statistics Formulas
- Trigonometric Table
Null Hypothesis
- Hypothesis Testing Formula
- SQLite IS NULL
- MySQL NOT NULL Constraint
- Real-life Applications of Hypothesis Testing
- Null in JavaScript
- SQL NOT NULL Constraint
- Null Cipher
- Hypothesis in Machine Learning
- Understanding Hypothesis Testing
- Difference between Null and Alternate Hypothesis
- Hypothesis Testing in R Programming
- SQL | NULL functions
- Kotlin Null Safety
- PHP | is_null() Function
- MySQL | NULLIF( ) Function
- Null-Coalescing Operator in C#
- Nullity of a Matrix
Null Hypothesis , often denoted as H 0, is a foundational concept in statistical hypothesis testing. It represents an assumption that no significant difference, effect, or relationship exists between variables within a population. It serves as a baseline assumption, positing no observed change or effect occurring. The null is t he truth or falsity of an idea in analysis.
In this article, we will discuss the null hypothesis in detail, along with some solved examples and questions on the null hypothesis.
Table of Content
What is Null Hypothesis?
Null hypothesis symbol, formula of null hypothesis, types of null hypothesis, null hypothesis examples, principle of null hypothesis, how do you find null hypothesis, null hypothesis in statistics, null hypothesis and alternative hypothesis, null hypothesis and alternative hypothesis examples, null hypothesis – practice problems.
Null Hypothesis in statistical analysis suggests the absence of statistical significance within a specific set of observed data. Hypothesis testing, using sample data, evaluates the validity of this hypothesis. Commonly denoted as H 0 or simply “null,” it plays an important role in quantitative analysis, examining theories related to markets, investment strategies, or economies to determine their validity.
Null Hypothesis Meaning
Null Hypothesis represents a default position, often suggesting no effect or difference, against which researchers compare their experimental results. The Null Hypothesis, often denoted as H 0 asserts a default assumption in statistical analysis. It posits no significant difference or effect, serving as a baseline for comparison in hypothesis testing.
The null Hypothesis is represented as H 0 , the Null Hypothesis symbolizes the absence of a measurable effect or difference in the variables under examination.
Certainly, a simple example would be asserting that the mean score of a group is equal to a specified value like stating that the average IQ of a population is 100.
The Null Hypothesis is typically formulated as a statement of equality or absence of a specific parameter in the population being studied. It provides a clear and testable prediction for comparison with the alternative hypothesis. The formulation of the Null Hypothesis typically follows a concise structure, stating the equality or absence of a specific parameter in the population.
Mean Comparison (Two-sample t-test)
H 0 : μ 1 = μ 2
This asserts that there is no significant difference between the means of two populations or groups.
Proportion Comparison
H 0 : p 1 − p 2 = 0
This suggests no significant difference in proportions between two populations or conditions.
Equality in Variance (F-test in ANOVA)
H 0 : σ 1 = σ 2
This states that there’s no significant difference in variances between groups or populations.
Independence (Chi-square Test of Independence):
H 0 : Variables are independent
This asserts that there’s no association or relationship between categorical variables.
Null Hypotheses vary including simple and composite forms, each tailored to the complexity of the research question. Understanding these types is pivotal for effective hypothesis testing.
Equality Null Hypothesis (Simple Null Hypothesis)
The Equality Null Hypothesis, also known as the Simple Null Hypothesis, is a fundamental concept in statistical hypothesis testing that assumes no difference, effect or relationship between groups, conditions or populations being compared.
Non-Inferiority Null Hypothesis
In some studies, the focus might be on demonstrating that a new treatment or method is not significantly worse than the standard or existing one.
Superiority Null Hypothesis
The concept of a superiority null hypothesis comes into play when a study aims to demonstrate that a new treatment, method, or intervention is significantly better than an existing or standard one.
Independence Null Hypothesis
In certain statistical tests, such as chi-square tests for independence, the null hypothesis assumes no association or independence between categorical variables.
Homogeneity Null Hypothesis
In tests like ANOVA (Analysis of Variance), the null hypothesis suggests that there’s no difference in population means across different groups.
- Medicine: Null Hypothesis: “No significant difference exists in blood pressure levels between patients given the experimental drug versus those given a placebo.”
- Education: Null Hypothesis: “There’s no significant variation in test scores between students using a new teaching method and those using traditional teaching.”
- Economics: Null Hypothesis: “There’s no significant change in consumer spending pre- and post-implementation of a new taxation policy.”
- Environmental Science: Null Hypothesis: “There’s no substantial difference in pollution levels before and after a water treatment plant’s establishment.”
The principle of the null hypothesis is a fundamental concept in statistical hypothesis testing. It involves making an assumption about the population parameter or the absence of an effect or relationship between variables.
In essence, the null hypothesis (H 0 ) proposes that there is no significant difference, effect, or relationship between variables. It serves as a starting point or a default assumption that there is no real change, no effect or no difference between groups or conditions.
The null hypothesis is usually formulated to be tested against an alternative hypothesis (H 1 or H [Tex]\alpha [/Tex] ) which suggests that there is an effect, difference or relationship present in the population.
Null Hypothesis Rejection
Rejecting the Null Hypothesis occurs when statistical evidence suggests a significant departure from the assumed baseline. It implies that there is enough evidence to support the alternative hypothesis, indicating a meaningful effect or difference. Null Hypothesis rejection occurs when statistical evidence suggests a deviation from the assumed baseline, prompting a reconsideration of the initial hypothesis.
Identifying the Null Hypothesis involves defining the status quotient, asserting no effect and formulating a statement suitable for statistical analysis.
When is Null Hypothesis Rejected?
The Null Hypothesis is rejected when statistical tests indicate a significant departure from the expected outcome, leading to the consideration of alternative hypotheses. It occurs when statistical evidence suggests a deviation from the assumed baseline, prompting a reconsideration of the initial hypothesis.
In statistical hypothesis testing, researchers begin by stating the null hypothesis, often based on theoretical considerations or previous research. The null hypothesis is then tested against an alternative hypothesis (Ha), which represents the researcher’s claim or the hypothesis they seek to support.
The process of hypothesis testing involves collecting sample data and using statistical methods to assess the likelihood of observing the data if the null hypothesis were true. This assessment is typically done by calculating a test statistic, which measures the difference between the observed data and what would be expected under the null hypothesis.
In the realm of hypothesis testing, the null hypothesis (H 0 ) and alternative hypothesis (H₁ or Ha) play critical roles. The null hypothesis generally assumes no difference, effect, or relationship between variables, suggesting that any observed change or effect is due to random chance. Its counterpart, the alternative hypothesis, asserts the presence of a significant difference, effect, or relationship between variables, challenging the null hypothesis. These hypotheses are formulated based on the research question and guide statistical analyses.
Difference Between Null Hypothesis and Alternative Hypothesis
The null hypothesis (H 0 ) serves as the baseline assumption in statistical testing, suggesting no significant effect, relationship, or difference within the data. It often proposes that any observed change or correlation is merely due to chance or random variation. Conversely, the alternative hypothesis (H 1 or Ha) contradicts the null hypothesis, positing the existence of a genuine effect, relationship or difference in the data. It represents the researcher’s intended focus, seeking to provide evidence against the null hypothesis and support for a specific outcome or theory. These hypotheses form the crux of hypothesis testing, guiding the assessment of data to draw conclusions about the population being studied.
Let’s envision a scenario where a researcher aims to examine the impact of a new medication on reducing blood pressure among patients. In this context:
Null Hypothesis (H 0 ): “The new medication does not produce a significant effect in reducing blood pressure levels among patients.”
Alternative Hypothesis (H 1 or Ha): “The new medication yields a significant effect in reducing blood pressure levels among patients.”
The null hypothesis implies that any observed alterations in blood pressure subsequent to the medication’s administration are a result of random fluctuations rather than a consequence of the medication itself. Conversely, the alternative hypothesis contends that the medication does indeed generate a meaningful alteration in blood pressure levels, distinct from what might naturally occur or by random chance.
People Also Read:
Mathematics Maths Formulas Probability and Statistics
Example 1: A researcher claims that the average time students spend on homework is 2 hours per night.
Null Hypothesis (H 0 ): The average time students spend on homework is equal to 2 hours per night. Data: A random sample of 30 students has an average homework time of 1.8 hours with a standard deviation of 0.5 hours. Test Statistic and Decision: Using a t-test, if the calculated t-statistic falls within the acceptance region, we fail to reject the null hypothesis. If it falls in the rejection region, we reject the null hypothesis. Conclusion: Based on the statistical analysis, we fail to reject the null hypothesis, suggesting that there is not enough evidence to dispute the claim of the average homework time being 2 hours per night.
Example 2: A company asserts that the error rate in its production process is less than 1%.
Null Hypothesis (H 0 ): The error rate in the production process is 1% or higher. Data: A sample of 500 products shows an error rate of 0.8%. Test Statistic and Decision: Using a z-test, if the calculated z-statistic falls within the acceptance region, we fail to reject the null hypothesis. If it falls in the rejection region, we reject the null hypothesis. Conclusion: The statistical analysis supports rejecting the null hypothesis, indicating that there is enough evidence to dispute the company’s claim of an error rate of 1% or higher.
Q1. A researcher claims that the average time spent by students on homework is less than 2 hours per day. Formulate the null hypothesis for this claim?
Q2. A manufacturing company states that their new machine produces widgets with a defect rate of less than 5%. Write the null hypothesis to test this claim?
Q3. An educational institute believes that their online course completion rate is at least 60%. Develop the null hypothesis to validate this assertion?
Q4. A restaurant claims that the waiting time for customers during peak hours is not more than 15 minutes. Formulate the null hypothesis for this claim?
Q5. A study suggests that the mean weight loss after following a specific diet plan for a month is more than 8 pounds. Construct the null hypothesis to evaluate this statement?
Summary – Null Hypothesis and Alternative Hypothesis
The null hypothesis (H 0 ) and alternative hypothesis (H a ) are fundamental concepts in statistical hypothesis testing. The null hypothesis represents the default assumption, stating that there is no significant effect, difference, or relationship between variables. It serves as the baseline against which the alternative hypothesis is tested. In contrast, the alternative hypothesis represents the researcher’s hypothesis or the claim to be tested, suggesting that there is a significant effect, difference, or relationship between variables. The relationship between the null and alternative hypotheses is such that they are complementary, and statistical tests are conducted to determine whether the evidence from the data is strong enough to reject the null hypothesis in favor of the alternative hypothesis. This decision is based on the strength of the evidence and the chosen level of significance. Ultimately, the choice between the null and alternative hypotheses depends on the specific research question and the direction of the effect being investigated.
FAQs on Null Hypothesis
What does null hypothesis stands for.
The null hypothesis, denoted as H 0 , is a fundamental concept in statistics used for hypothesis testing. It represents the statement that there is no effect or no difference, and it is the hypothesis that the researcher typically aims to provide evidence against.
How to Form a Null Hypothesis?
A null hypothesis is formed based on the assumption that there is no significant difference or effect between the groups being compared or no association between variables being tested. It often involves stating that there is no relationship, no change, or no effect in the population being studied.
When Do we reject the Null Hypothesis?
In statistical hypothesis testing, if the p-value (the probability of obtaining the observed results) is lower than the chosen significance level (commonly 0.05), we reject the null hypothesis. This suggests that the data provides enough evidence to refute the assumption made in the null hypothesis.
What is a Null Hypothesis in Research?
In research, the null hypothesis represents the default assumption or position that there is no significant difference or effect. Researchers often try to test this hypothesis by collecting data and performing statistical analyses to see if the observed results contradict the assumption.
What Are Alternative and Null Hypotheses?
The null hypothesis (H0) is the default assumption that there is no significant difference or effect. The alternative hypothesis (H1 or Ha) is the opposite, suggesting there is a significant difference, effect or relationship.
What Does it Mean to Reject the Null Hypothesis?
Rejecting the null hypothesis implies that there is enough evidence in the data to support the alternative hypothesis. In simpler terms, it suggests that there might be a significant difference, effect or relationship between the groups or variables being studied.
How to Find Null Hypothesis?
Formulating a null hypothesis often involves considering the research question and assuming that no difference or effect exists. It should be a statement that can be tested through data collection and statistical analysis, typically stating no relationship or no change between variables or groups.
How is Null Hypothesis denoted?
The null hypothesis is commonly symbolized as H 0 in statistical notation.
What is the Purpose of the Null hypothesis in Statistical Analysis?
The null hypothesis serves as a starting point for hypothesis testing, enabling researchers to assess if there’s enough evidence to reject it in favor of an alternative hypothesis.
What happens if we Reject the Null hypothesis?
Rejecting the null hypothesis implies that there is sufficient evidence to support an alternative hypothesis, suggesting a significant effect or relationship between variables.
What are Test for Null Hypothesis?
Various statistical tests, such as t-tests or chi-square tests, are employed to evaluate the validity of the Null Hypothesis in different scenarios.
Please Login to comment...
Similar reads.
- Geeks Premier League 2023
- Math-Concepts
- Geeks Premier League
- School Learning
Improve your Coding Skills with Practice
What kind of Experience do you want to share?
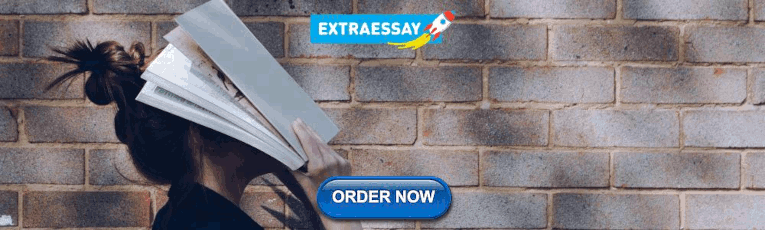
IMAGES
VIDEO
COMMENTS
Null Hypothesis Examples. "Hyperactivity is unrelated to eating sugar " is an example of a null hypothesis. If the hypothesis is tested and found to be false, using statistics, then a connection between hyperactivity and sugar ingestion may be indicated. A significance test is the most common statistical test used to establish confidence in a ...
Basic definitions. The null hypothesis and the alternative hypothesis are types of conjectures used in statistical tests to make statistical inferences, which are formal methods of reaching conclusions and separating scientific claims from statistical noise.. The statement being tested in a test of statistical significance is called the null hypothesis. . The test of significance is designed ...
Research hypotheses have been a cornerstone of science since before Galileo. Many have argued that hypotheses (1) encourage discovery of mechanisms, and (2) reduce bias—both features that should increase transferability and reproducibility. However, we are entering a new era of big data and highly predictive models where some argue the ...
A hypothesis, in scientific studies, is defined as a proposed explanation for an observed phenomena that can be subject to further testing. A well formulated hypothesis must do two things: be able ...
The null and alternative hypotheses offer competing answers to your research question. When the research question asks "Does the independent variable affect the dependent variable?": The null hypothesis ( H0) answers "No, there's no effect in the population.". The alternative hypothesis ( Ha) answers "Yes, there is an effect in the ...
The null hypothesis in statistics states that there is no difference between groups or no relationship between variables. It is one of two mutually exclusive hypotheses about a population in a hypothesis test. When your sample contains sufficient evidence, you can reject the null and conclude that the effect is statistically significant.
A null hypothesis is a specific testable investigation that denies something implied by the main hypothesis being studied. Unless null hypotheses are eliminated on the basis of contrary evidence, we cannot be confident of the main hypothesis. ... Environmental science is filled with many examples of uncertainty—in present values and future ...
Step 1: Figure out the hypothesis from the problem. The hypothesis is usually hidden in a word problem, and is sometimes a statement of what you expect to happen in the experiment. The hypothesis in the above question is "I expect the average recovery period to be greater than 8.2 weeks.". Step 2: Convert the hypothesis to math.
An example of the null hypothesis is that light color has no effect on plant growth. The null hypothesis (H 0) is the hypothesis that states there is no statistical difference between two sample sets. In other words, it assumes the independent variable does not have an effect on the dependent variable in a scientific experiment.
The empirical testing of formulated hypotheses is considered by some as the 'criterion of demarcation' that distinguishes science from other forms of knowledge (Ayala, 1994). An hypothesis is a "supposition made as a starting point for further investigation from known facts" (Pearsall & Trumble, 1996). Since an hypothesis is aimed at ...
The Process of Science. The scientific method. Proposing a Hypothesis; Testing a Hypothesis; Viewed from space, Earth (Figure \(\PageIndex{1}\)) offers no clues about the diversity of lifeforms that reside there.The first forms of life on Earth are thought to have been microorganisms that existed for billions of years in the ocean before plants and animals appeared.
In physics for example, an important null hypothesis of the post-Newtonian era was that time is a variable which is independent of all other factors. Modern physics is based upon the alternative hypothesis that time can be a function of space and relative velocities. Another famous null hypothesis, that the speed of light is independent of its ...
The null hypothesis is a tool that makes it possible to use certain statistical tests to figure out if another hypothesis of interest is likely to be accurate or not. Usually a statement asserting that there is no difference or no association between variables. The null hypothesis is a tool that makes it possible to use certain statistical ...
The null hypothesis, as described by Anthony Greenwald in 'Consequences of Prejudice Against the Null Hypothesis,' is the hypothesis of no difference between treatment effects or of no association between variables. Unfortunately in academia, the 'null' is often associated with 'insignificant,' 'no value,' or 'invalid.'.
Description. Applications of Hypothesis Testing for Environmental Science presents the theory and application of hypothesis testing in environmental science, allowing researchers to carry out suitable tests for decision-making on a variety of issues. This book works as a step-by-step resource to provide understanding of the concepts and ...
In statistics: Hypothesis testing. This assumption is called the null hypothesis and is denoted by H0. An alternative hypothesis (denoted Ha ), which is the opposite of what is stated in the null hypothesis, is then defined. The hypothesis-testing procedure involves using sample data to determine whether or not H0 can be rejected.
What is Environmental Science? Environmental science is the dynamic, interdisciplinary study of the interaction of living and non-living parts of the environment, with special focus on the impact of humans on the environment. The study of environmental science includes circumstances, objects, or conditions by which an organism or community is surrounded and the complex ways in which they interact.
The motivational and verificationist realities of normal science have fundamental implications for how the "null hypothesis" is interpreted, and whether or not it is "accepted." In a normal-science setting, multiple explanatory paradigms may offer competing accounts of demonstrated effects and differing predictions for what may be ...
Another famous null hypothesis, that the speed of light is independent of its direction, inspired the Michelson-Morley experiments, which failed to disprove it. ... Environmental Science. 2009; This essay grew out of an examination of one-tailed significance testing. ... A definition of vegetation science is given, spanning 6 levels of ...
Here, the null hypothesis of no linkage of biotic to environmental variables can be recreated by permuting the sample labels for one of the data matrices, and Fig. 1 shows a random permutation of the columns of the environmental matrix in relation to those of the biota. (It does not matter whether the columns of one or both matrices are ...
Hypothesis: An explanation for an observed phenomenon. Research Hypothesis: A statement about a phenomenon that also includes the potential mechanism or cause of that phenomenon. Though a research hypothesis doesn't need to adhere to this strict framework it is often best described as the "if" in an "if-then" statement.
Null hypothesis, often denoted as H0, is a foundational concept in statistical hypothesis testing. ... Environmental Science: Null Hypothesis: "There's no substantial difference in pollution levels before and after a water treatment plant's establishment. ... Alternative Hypothesis: Definition, Types and Examples
AHA Journals Null Hypothesis Collection. Publishing research with negative results—that is, null or inconclusive findings—is a critical but often overlooked task of biomedical journals. Without it, the scientific literature relies on highly selected pieces of evidence that viewed in isolation can distort a field.
I assume the authors are referring to a null hypothesis although a hypothesis was never truly stated and the purpose of the study. Page 10, line 294; "….towards the null since the patients with an early, large impact on urinary function …"