Guide to Writing the Results and Discussion Sections of a Scientific Article
A quality research paper has both the qualities of in-depth research and good writing ( Bordage, 2001 ). In addition, a research paper must be clear, concise, and effective when presenting the information in an organized structure with a logical manner ( Sandercock, 2013 ).
In this article, we will take a closer look at the results and discussion section. Composing each of these carefully with sufficient data and well-constructed arguments can help improve your paper overall.
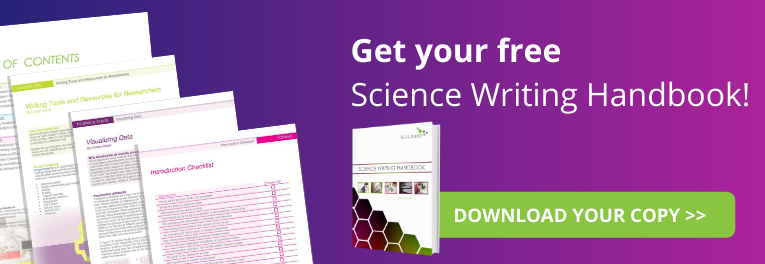
The results section of your research paper contains a description about the main findings of your research, whereas the discussion section interprets the results for readers and provides the significance of the findings. The discussion should not repeat the results.
Let’s dive in a little deeper about how to properly, and clearly organize each part.
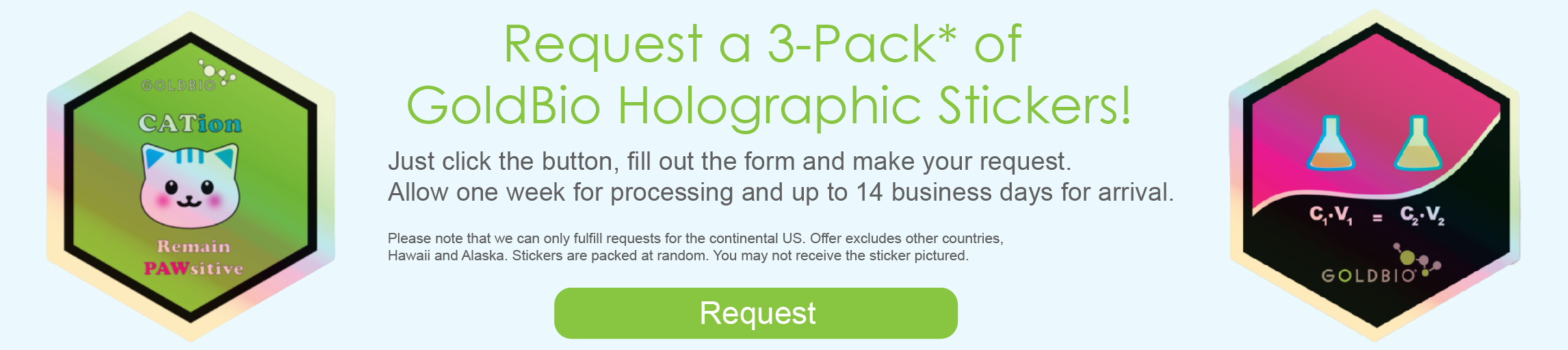
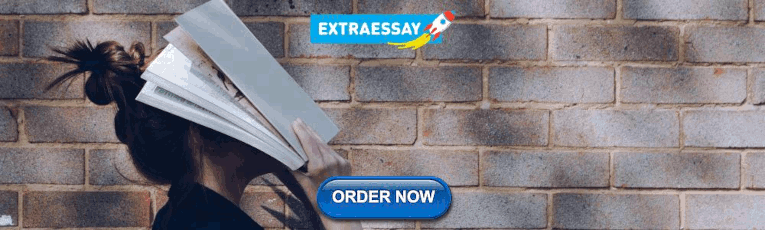
How to Organize the Results Section
Since your results follow your methods, you’ll want to provide information about what you discovered from the methods you used, such as your research data. In other words, what were the outcomes of the methods you used?
You may also include information about the measurement of your data, variables, treatments, and statistical analyses.
To start, organize your research data based on how important those are in relation to your research questions. This section should focus on showing major results that support or reject your research hypothesis. Include your least important data as supplemental materials when submitting to the journal.
The next step is to prioritize your research data based on importance – focusing heavily on the information that directly relates to your research questions using the subheadings.
The organization of the subheadings for the results section usually mirrors the methods section. It should follow a logical and chronological order.
Subheading organization
Subheadings within your results section are primarily going to detail major findings within each important experiment. And the first paragraph of your results section should be dedicated to your main findings (findings that answer your overall research question and lead to your conclusion) (Hofmann, 2013).
In the book “Writing in the Biological Sciences,” author Angelika Hofmann recommends you structure your results subsection paragraphs as follows:
- Experimental purpose
- Interpretation
Each subheading may contain a combination of ( Bahadoran, 2019 ; Hofmann, 2013, pg. 62-63):
- Text: to explain about the research data
- Figures: to display the research data and to show trends or relationships, for examples using graphs or gel pictures.
- Tables: to represent a large data and exact value
Decide on the best way to present your data — in the form of text, figures or tables (Hofmann, 2013).
Data or Results?
Sometimes we get confused about how to differentiate between data and results . Data are information (facts or numbers) that you collected from your research ( Bahadoran, 2019 ).
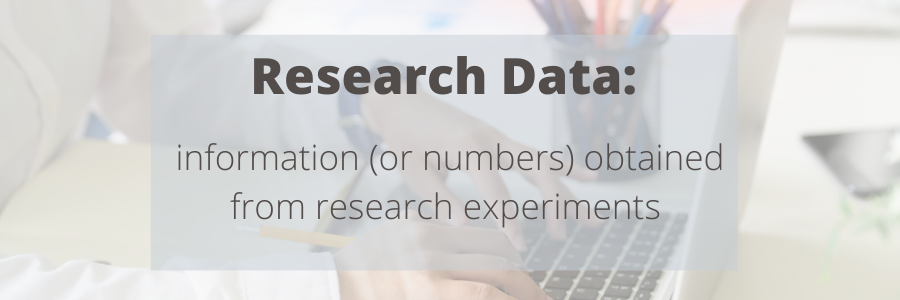
Whereas, results are the texts presenting the meaning of your research data ( Bahadoran, 2019 ).
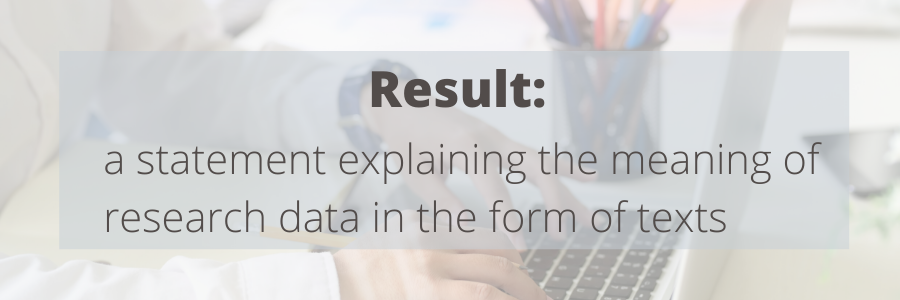
One mistake that some authors often make is to use text to direct the reader to find a specific table or figure without further explanation. This can confuse readers when they interpret data completely different from what the authors had in mind. So, you should briefly explain your data to make your information clear for the readers.
Common Elements in Figures and Tables
Figures and tables present information about your research data visually. The use of these visual elements is necessary so readers can summarize, compare, and interpret large data at a glance. You can use graphs or figures to compare groups or patterns. Whereas, tables are ideal to present large quantities of data and exact values.
Several components are needed to create your figures and tables. These elements are important to sort your data based on groups (or treatments). It will be easier for the readers to see the similarities and differences among the groups.
When presenting your research data in the form of figures and tables, organize your data based on the steps of the research leading you into a conclusion.
Common elements of the figures (Bahadoran, 2019):
- Figure number
- Figure title
- Figure legend (for example a brief title, experimental/statistical information, or definition of symbols).
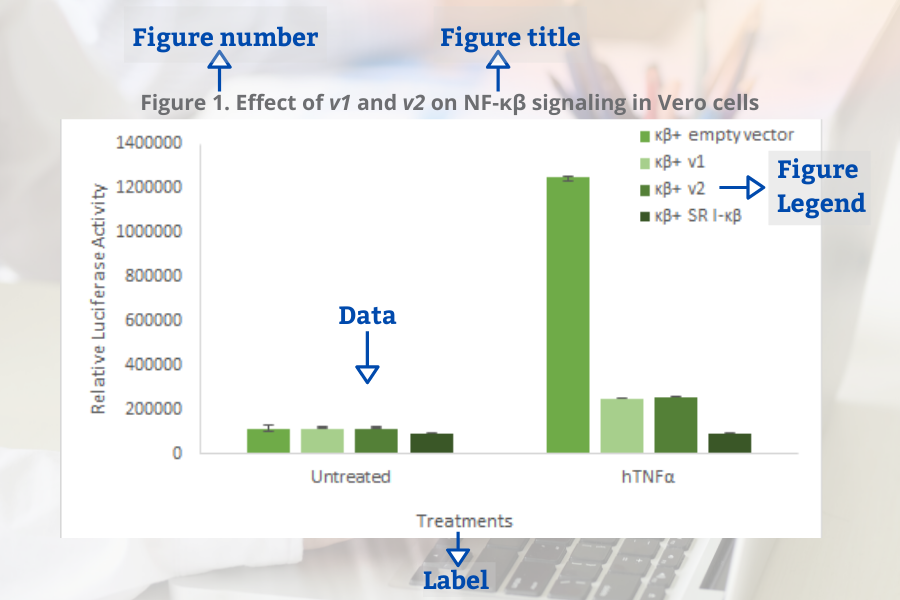
Tables in the result section may contain several elements (Bahadoran, 2019):
- Table number
- Table title
- Row headings (for example groups)
- Column headings
- Row subheadings (for example categories or groups)
- Column subheadings (for example categories or variables)
- Footnotes (for example statistical analyses)
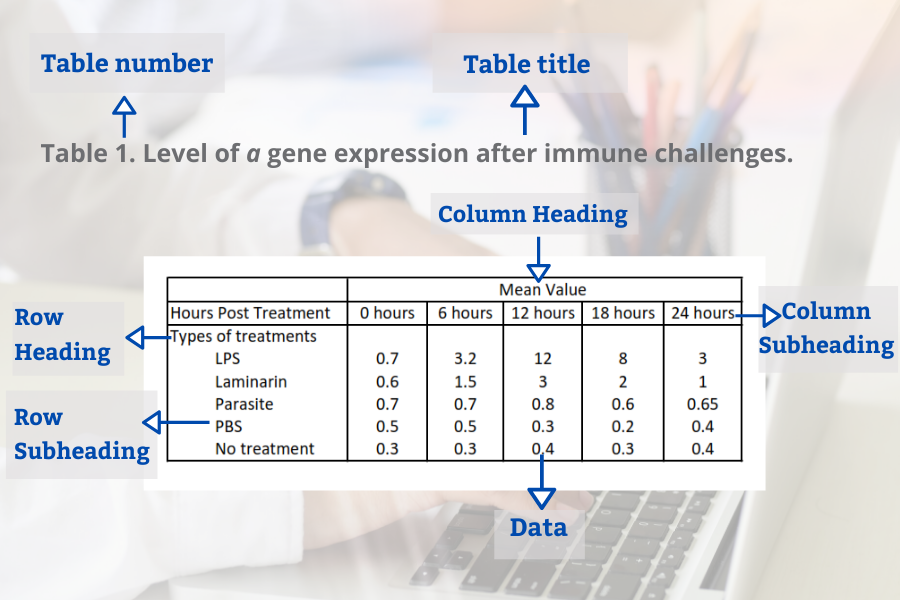
Tips to Write the Results Section
- Direct the reader to the research data and explain the meaning of the data.
- Avoid using a repetitive sentence structure to explain a new set of data.
- Write and highlight important findings in your results.
- Use the same order as the subheadings of the methods section.
- Match the results with the research questions from the introduction. Your results should answer your research questions.
- Be sure to mention the figures and tables in the body of your text.
- Make sure there is no mismatch between the table number or the figure number in text and in figure/tables.
- Only present data that support the significance of your study. You can provide additional data in tables and figures as supplementary material.
How to Organize the Discussion Section
It’s not enough to use figures and tables in your results section to convince your readers about the importance of your findings. You need to support your results section by providing more explanation in the discussion section about what you found.
In the discussion section, based on your findings, you defend the answers to your research questions and create arguments to support your conclusions.
Below is a list of questions to guide you when organizing the structure of your discussion section ( Viera et al ., 2018 ):
- What experiments did you conduct and what were the results?
- What do the results mean?
- What were the important results from your study?
- How did the results answer your research questions?
- Did your results support your hypothesis or reject your hypothesis?
- What are the variables or factors that might affect your results?
- What were the strengths and limitations of your study?
- What other published works support your findings?
- What other published works contradict your findings?
- What possible factors might cause your findings different from other findings?
- What is the significance of your research?
- What are new research questions to explore based on your findings?
Organizing the Discussion Section
The structure of the discussion section may be different from one paper to another, but it commonly has a beginning, middle-, and end- to the section.
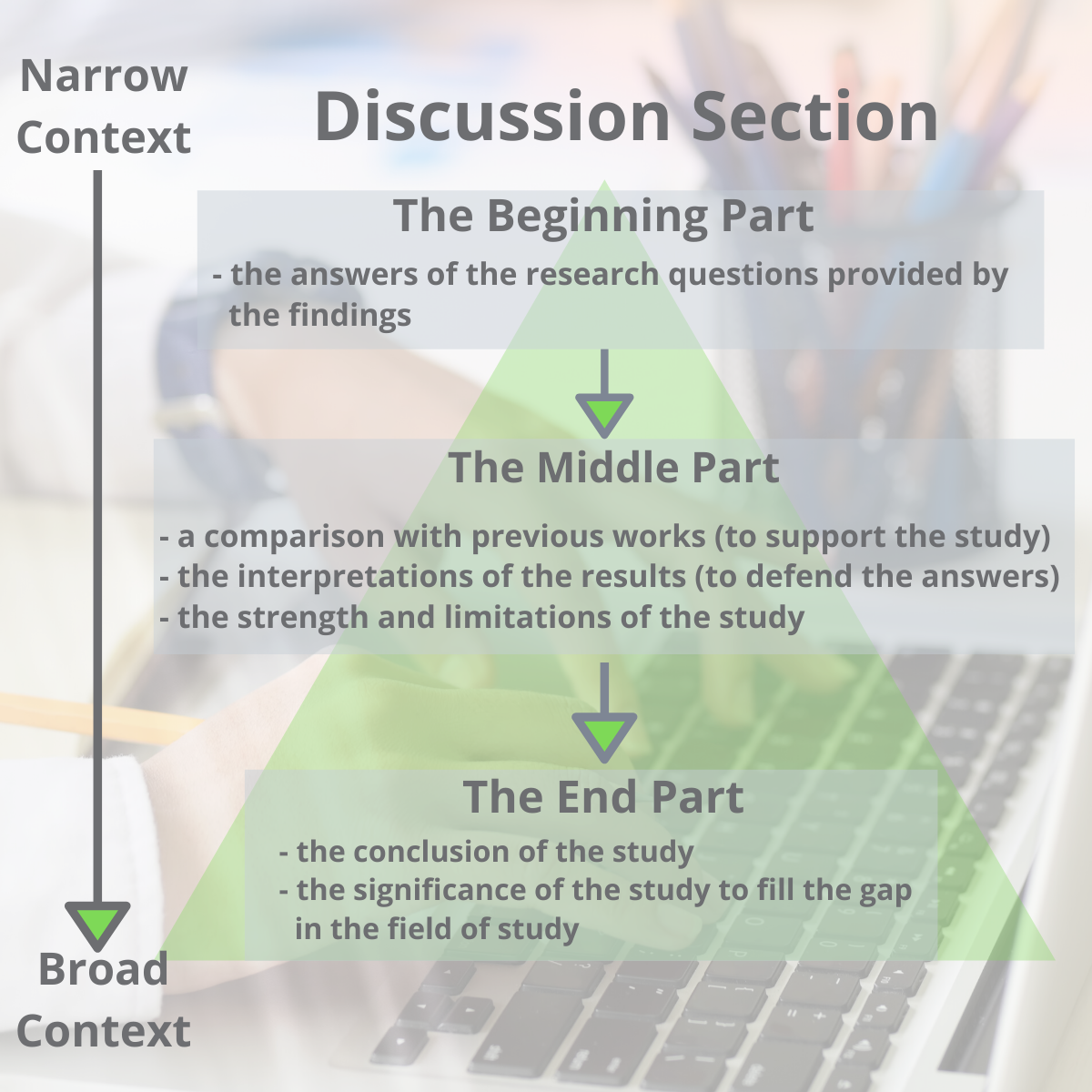
One way to organize the structure of the discussion section is by dividing it into three parts (Ghasemi, 2019):
- The beginning: The first sentence of the first paragraph should state the importance and the new findings of your research. The first paragraph may also include answers to your research questions mentioned in your introduction section.
- The middle: The middle should contain the interpretations of the results to defend your answers, the strength of the study, the limitations of the study, and an update literature review that validates your findings.
- The end: The end concludes the study and the significance of your research.
Another possible way to organize the discussion section was proposed by Michael Docherty in British Medical Journal: is by using this structure ( Docherty, 1999 ):
- Discussion of important findings
- Comparison of your results with other published works
- Include the strengths and limitations of the study
- Conclusion and possible implications of your study, including the significance of your study – address why and how is it meaningful
- Future research questions based on your findings
Finally, a last option is structuring your discussion this way (Hofmann, 2013, pg. 104):
- First Paragraph: Provide an interpretation based on your key findings. Then support your interpretation with evidence.
- Secondary results
- Limitations
- Unexpected findings
- Comparisons to previous publications
- Last Paragraph: The last paragraph should provide a summarization (conclusion) along with detailing the significance, implications and potential next steps.
Remember, at the heart of the discussion section is presenting an interpretation of your major findings.
Tips to Write the Discussion Section
- Highlight the significance of your findings
- Mention how the study will fill a gap in knowledge.
- Indicate the implication of your research.
- Avoid generalizing, misinterpreting your results, drawing a conclusion with no supportive findings from your results.
Aggarwal, R., & Sahni, P. (2018). The Results Section. In Reporting and Publishing Research in the Biomedical Sciences (pp. 21-38): Springer.
Bahadoran, Z., Mirmiran, P., Zadeh-Vakili, A., Hosseinpanah, F., & Ghasemi, A. (2019). The principles of biomedical scientific writing: Results. International journal of endocrinology and metabolism, 17(2).
Bordage, G. (2001). Reasons reviewers reject and accept manuscripts: the strengths and weaknesses in medical education reports. Academic medicine, 76(9), 889-896.
Cals, J. W., & Kotz, D. (2013). Effective writing and publishing scientific papers, part VI: discussion. Journal of clinical epidemiology, 66(10), 1064.
Docherty, M., & Smith, R. (1999). The case for structuring the discussion of scientific papers: Much the same as that for structuring abstracts. In: British Medical Journal Publishing Group.
Faber, J. (2017). Writing scientific manuscripts: most common mistakes. Dental press journal of orthodontics, 22(5), 113-117.
Fletcher, R. H., & Fletcher, S. W. (2018). The discussion section. In Reporting and Publishing Research in the Biomedical Sciences (pp. 39-48): Springer.
Ghasemi, A., Bahadoran, Z., Mirmiran, P., Hosseinpanah, F., Shiva, N., & Zadeh-Vakili, A. (2019). The Principles of Biomedical Scientific Writing: Discussion. International journal of endocrinology and metabolism, 17(3).
Hofmann, A. H. (2013). Writing in the biological sciences: a comprehensive resource for scientific communication . New York: Oxford University Press.
Kotz, D., & Cals, J. W. (2013). Effective writing and publishing scientific papers, part V: results. Journal of clinical epidemiology, 66(9), 945.
Mack, C. (2014). How to Write a Good Scientific Paper: Structure and Organization. Journal of Micro/ Nanolithography, MEMS, and MOEMS, 13. doi:10.1117/1.JMM.13.4.040101
Moore, A. (2016). What's in a Discussion section? Exploiting 2‐dimensionality in the online world…. Bioessays, 38(12), 1185-1185.
Peat, J., Elliott, E., Baur, L., & Keena, V. (2013). Scientific writing: easy when you know how: John Wiley & Sons.
Sandercock, P. M. L. (2012). How to write and publish a scientific article. Canadian Society of Forensic Science Journal, 45(1), 1-5.
Teo, E. K. (2016). Effective Medical Writing: The Write Way to Get Published. Singapore Medical Journal, 57(9), 523-523. doi:10.11622/smedj.2016156
Van Way III, C. W. (2007). Writing a scientific paper. Nutrition in Clinical Practice, 22(6), 636-640.
Vieira, R. F., Lima, R. C. d., & Mizubuti, E. S. G. (2019). How to write the discussion section of a scientific article. Acta Scientiarum. Agronomy, 41.
Related Articles
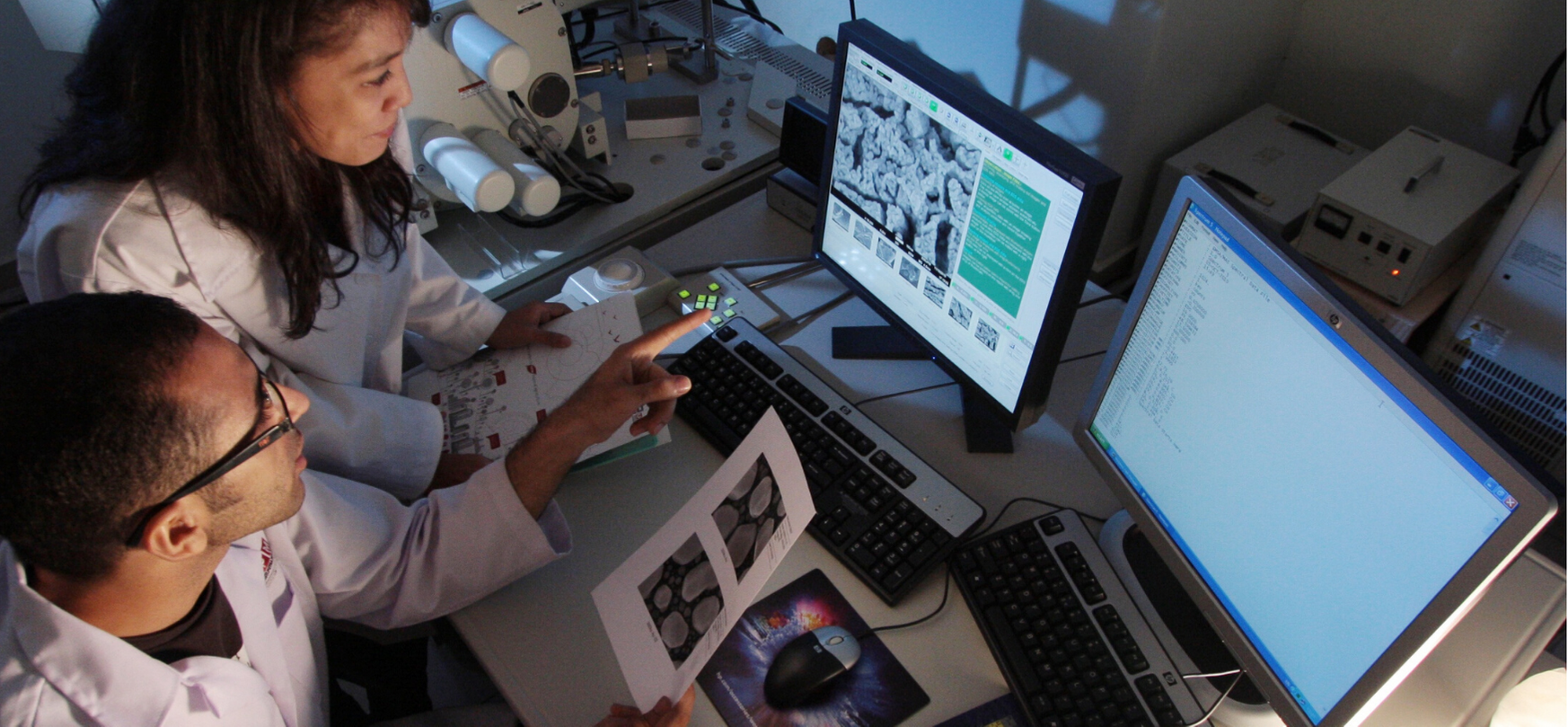
A quality research paper has both the qualities of in-depth research and good writing (Bordage, 200...
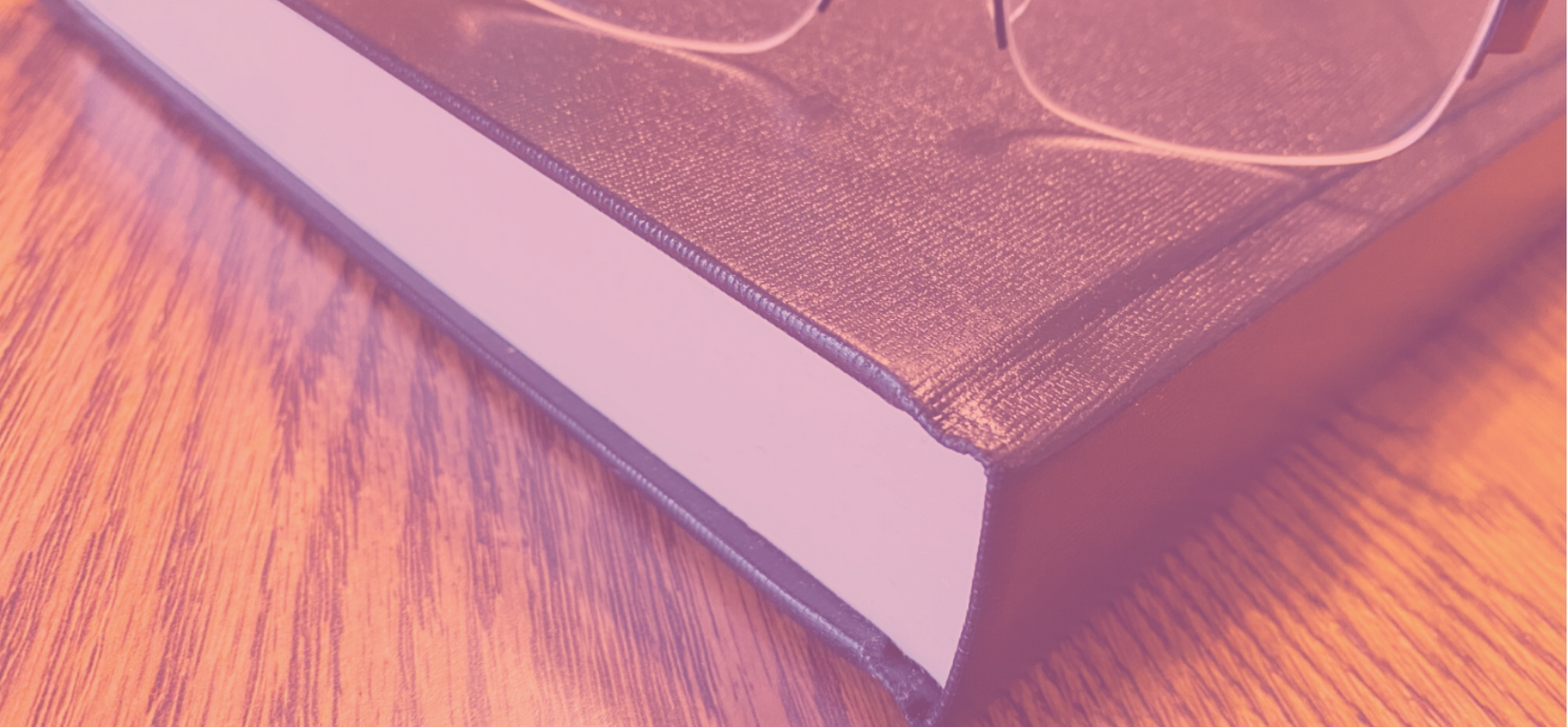
How to Survive and Complete a Thesis or a Dissertation
Writing a thesis or a dissertation can be a challenging process for many graduate students. There ar...
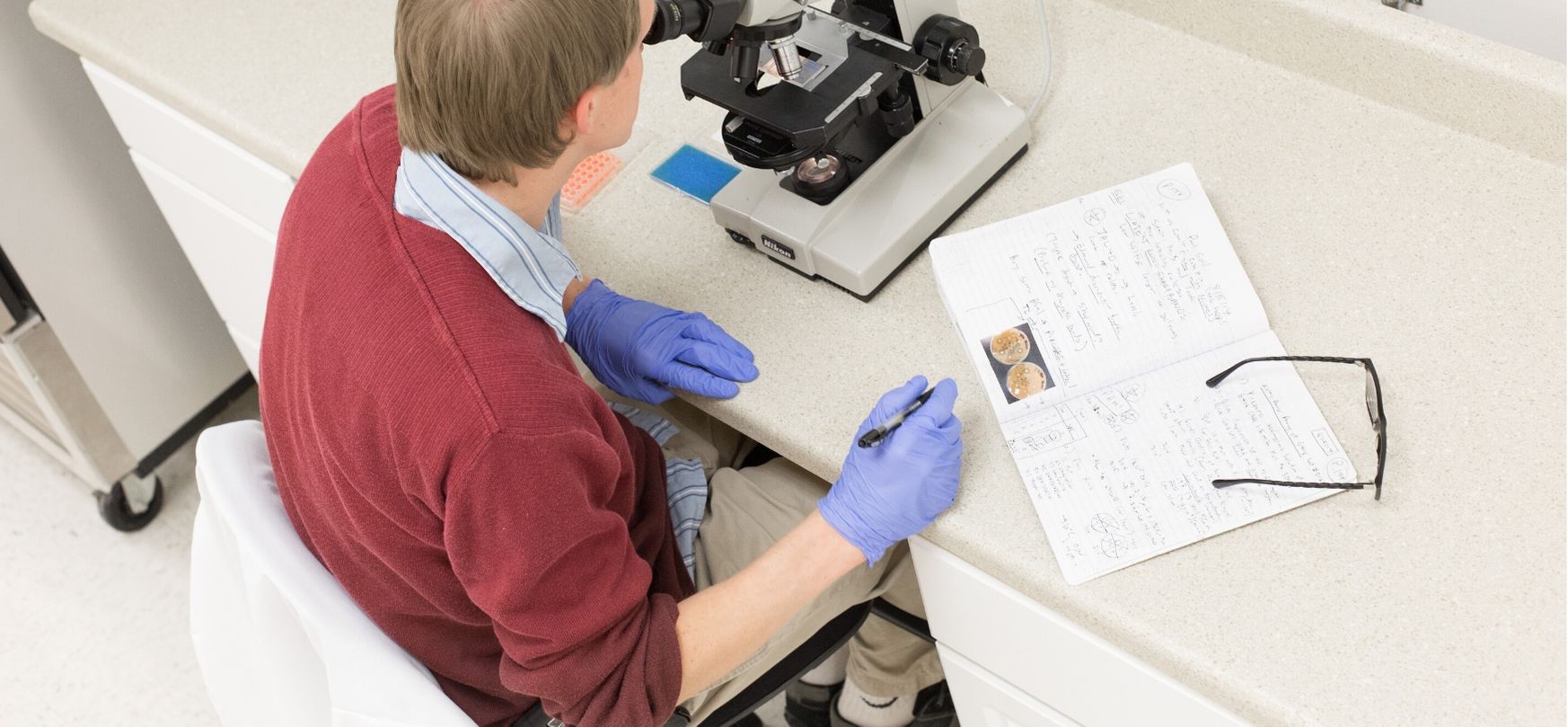
15 Laboratory Notebook Tips to Help with your Research Manuscript
Your lab notebook is a foundation to your research manuscript. It serves almost as a rudimentary dra...
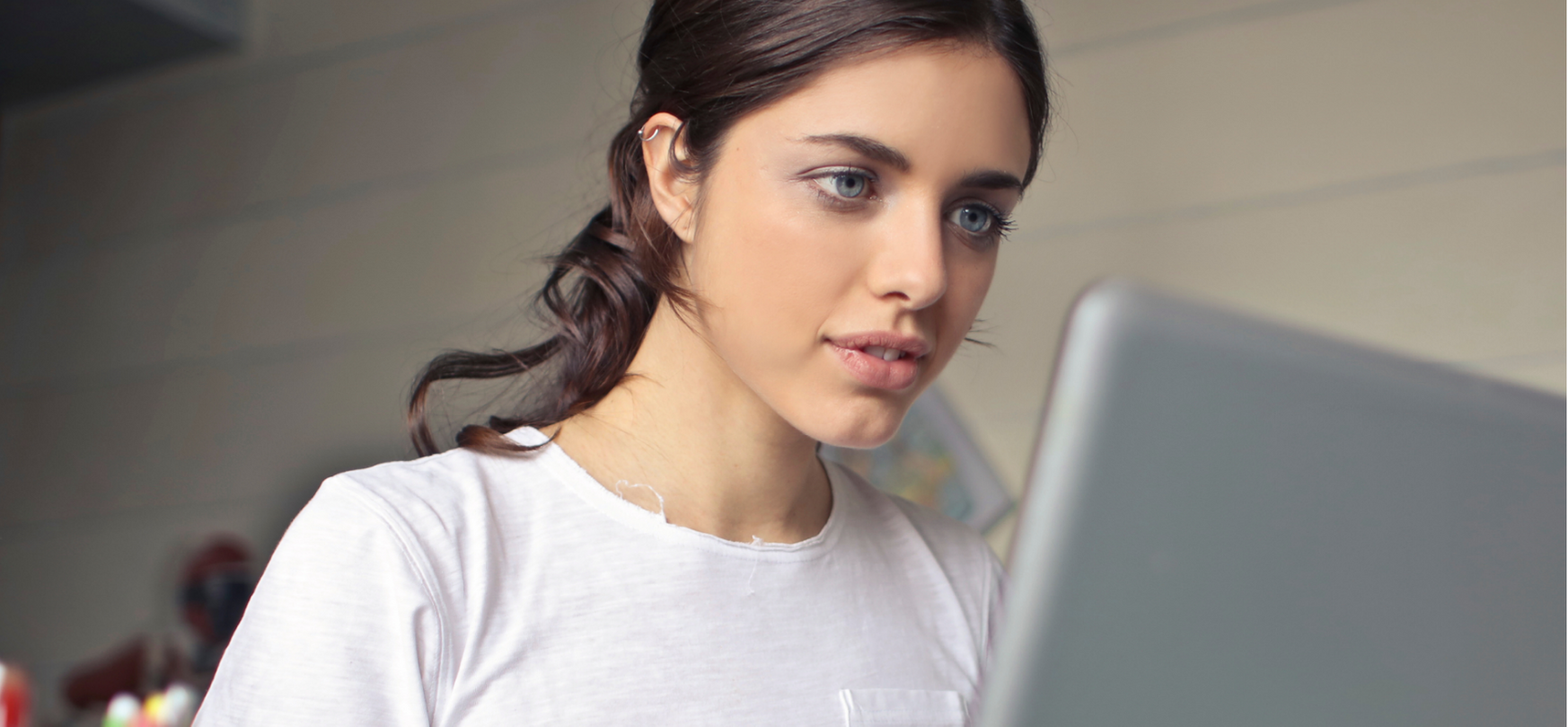
What is a Good Way to Read a Scientific Paper?
Have you wished you could read a scientific article like reading a newspaper article? More often, it...
Join our list to receive promos and articles.
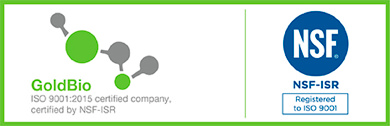
- Competent Cells
- Lab Startup
- Z')" data-type="collection" title="Products A->Z" target="_self" href="/collection/products-a-to-z">Products A->Z
- GoldBio Resources
- GoldBio Sales Team
- GoldBio Distributors
- Duchefa Direct
- Sign up for Promos
- Terms & Conditions
- ISO Certification
- Agarose Resins
- Antibiotics & Selection
- Biochemical Reagents
- Bioluminescence
- Buffers & Reagents
- Cell Culture
- Cloning & Induction
- Competent Cells and Transformation
- Detergents & Membrane Agents
- DNA Amplification
- Enzymes, Inhibitors & Substrates
- Growth Factors and Cytokines
- Lab Tools & Accessories
- Plant Research and Reagents
- Protein Research & Analysis
- Protein Expression & Purification
- Reducing Agents
- How it works
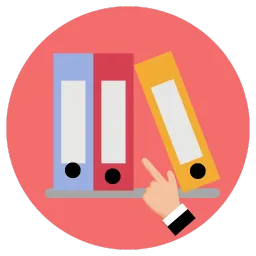
How to Write a Dissertation Discussion Chapter – A Quick Guide with Examples
Published by Alvin Nicolas at August 12th, 2021 , Revised On July 12, 2024
Dissertation discussion is the chapter where you explore the relevance, significance, and meanings of your findings – allowing you to showcase your talents in describing and analyzing the results of your study.
Here, you will be expected to demonstrate how your research findings answer the research questions established or test the hypothesis .
The arguments you assert in the dissertation analysis and discussions chapter lay the foundations of your conclusion . It is critically important to discuss the results in a precise manner.
To help you understand how to write a dissertation discussion chapter, here is the list of the main elements of this section so you stay on the right track when writing:
- Summary: Start by providing a summary of your key research findings
- Interpretations: What is the significance of your findings?
- Implications: Why are your findings important to academic and scientific communities, and what purpose would they serve?
- Limitations: When and where will your results have no implications?
- Future Recommendations : Advice for other researchers and scientists who explore the topic further in future.
The dissertation discussion chapter should be carefully drafted to ensure that the results mentioned in your research align with your research question, aims, and objectives.
Considering the importance of this chapter for all students working on their dissertations, we have comprehensive guidelines on how to write a dissertation discussion chapter.
The discussion and conclusion chapters often overlap. Depending on your university, you may be asked to group these two sections in one chapter – Discussion and Conclusion.
In some cases, the results and discussion are put together under the Results and Discussion chapter. Here are some dissertation examples of working out the best structure for your dissertation.
Alternatively, you can look for the required dissertation structure in your handbook or consult your supervisor.
Steps of How to Write Dissertation Discussion Chapter
1. provide a summary of your findings.
Start your discussion by summarising the key findings of your research questions. Avoid repeating the information you have already stated in the previous chapters.
You will be expected to clearly express your interpretation of results to answer the research questions established initially in one or two paragraphs.
Here are some examples of how to present the summary of your findings ;
- “The data suggests that”,
- “The results confirm that”,
- “The analysis indicates that”,
- “The research shows a relationship between”, etc.
2. Interpretations of Results
Your audience will expect you to provide meanings of the results, although they might seem obvious to you. The results and their interpretations should be linked to the research questions so the reader can understand the value your research has added to the literature.
There are many ways of interpreting the data, but your chosen approach to interpreting the data will depend on the type of research involved . Some of the most common strategies employed include;
- Describing how and why you ended up with unexpected findings and explaining their importance in detail
- Relating your findings with previous studies conducted
- Explaining your position with logical arguments when/if any alternative explanations are suggested
- An in-depth discussion around whether or not the findings answered your research questions and successfully tested the hypothesis
Examples of how you can start your interpretation in the Discussion chapter are –
- “Findings of this study contradict those of Allen et al. (2014) that”,
- “Contrary to the hypothesized association,” “Confirming the hypothesis…”,
- “The findings confirm that A is….. even though Allen et al. (2014) and Michael (2012) suggested B was …..”
3. Implications of your Study
What practical and theoretical implications will your study have for other researchers and the scientific community as a whole?
It is vital to relate your results to the knowledge in the existing literature so the readers can establish how your research will contribute to the existing data.
When thinking of the possible consequences of your findings, you should ask yourself these;
- Are your findings in line with previous studies? What contribution did your research make to them?
- Why are your results entirely different from other studies on the same topic?
- Did your findings approve or contradict existing knowledge?
- What are the practical implications of your study?
Remember that as the researcher, you should aim to let your readers know why your study will contribute to the existing literature. Possible ways of starting this particular section are;
- “The findings show that A….. whereas Lee (2017) and John (2013) suggested that B”, “The results of this study completely contradict the claims made in theories”,
- “These results are not in line with the theoretical perspectives”,
- “The statistical analysis provides a new understanding of the relationship between A and B”,
- “Future studies should take into consideration the findings of this study because”
“Improve your work’s language, structure, style, and overall quality. Get help from our experienced dissertation editors to improve the quality of your paper to First Class Standard. Click here to learn more about our Dissertation, Editing, and Improvement Services .
Looking for dissertation help?
Researchprospect to the rescue, then.
We have expert writers on our team who are skilled at helping students with dissertations across various disciplines. Guaranteeing 100% satisfaction!
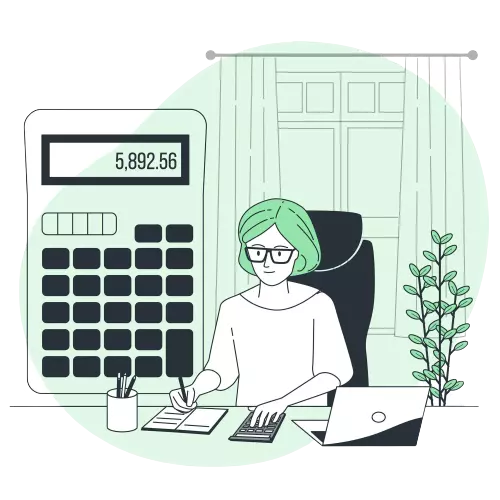
4. Recognise the Limitations of your Research
Almost every academic research has some limitations. Acknowledging them will only add to your credibility as a scientific researcher.
In addition to the possible human errors, it’s important to take into account other factors that might have influenced the results of your study, including but not limited to unexpected research obstacles, specific methodological choices , and the overall research design.
Avoid mentioning any limitations that may not be relevant to your research aim, but clearly state the limitations that may have affected your results.
For example, if you used a sample size that included a tiny population, you may not generalise your results.
Similarly, obstacles faced in collecting data from the participants can influence the findings of your study. Make a note of all such research limitations , but explain to the reader why your results are still authentic.
- The small sample size limited the generalisability of the results.
- The authenticity of the findings may have been influenced by….
- The obstacles in collecting data resulted in…
- It is beyond the framework of this research…
5. Provide Recommendations for Future Research
The limitations of your research work directly result in future recommendations . However, it should be noted that your recommendations for future research work should include the areas that your own work could not report so other researchers can build on them.
Sometimes the recommendations are a part of the conclusion chapter . Some examples;
- More research is needed to be performed….
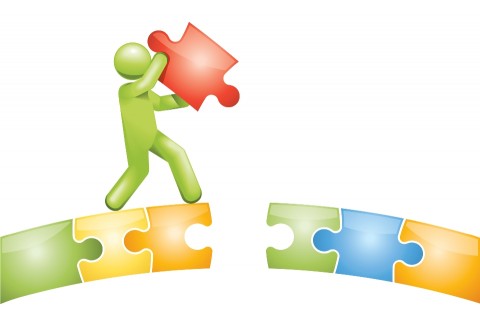
The Purpose of Dissertation Discussion Chapter
Remember that the discussion section of a dissertation is the heart of your research because a) it will indicate your stance on the topic of research, and b) it answers the research questions initially established in the Introduction chapter .
Every piece of information you present here will add value to the existing literature within your field of study. How you structured your findings in the preceding chapter will help you determine the best structure for your dissertation discussion section.
For example, it might be logical to structure your analysis/discussions by theme if you chose the pattern in your findings section.
But generally, discussion based on research questions is the more widely used structure in academia because this pattern clearly indicates how you have addressed the aim of your research.
Most UK universities require the supervisor or committee members to comment on the extent to which each research question has been answered. You will be doing them a great favour if you structure your discussion so that each research question is laid out separately.
Irrespective of whether you are writing an essay, dissertation, or chapter of a dissertation , all pieces of writing should start with an introduction .
Once your readers have read through your study results, you might want to highlight the contents of the subsequent discussion as an introduction paragraph (summary of your results – as explained above).
Likewise, the discussion chapter is expected to end with a concluding paragraph – allowing you the opportunity to summarise your interpretations.
The dissertation analysis & discussion chapter is usually very long, so it will make sense to emphasise the critical points in a concluding paragraph so the reader can grasp the essential information. This will also help to make sure the reader understands your analysis.
Also Read: Research Discussion Of Findings
Useful Tips
Presentation of graphs, tables, and figures.
In the 1990s and early 2000s, students spent days creating graphs and charts for their statistical analysis work . Thanks to technology, you can produce even more accurate graphs and figures today in a shorter period.
Using Microsoft Word, STATA, SPSS, Microsoft Excel and other statistical analysis software, we can now draw beautiful-looking figures, tables , and graphs with just a few clicks and make them appear in our document at the desired place. But there are downsides to being too dependent on technology.
Many students make the common mistake of using colours to represent variables when really they have to print their dissertation paper final copy in black and white.
Any colours on graphs and figures will eventually be viewed in the grayscale presentation. Recognizing different shades of grey on the same chart or graph can sometimes be a little confusing.
For example, green and purple appear as pretty much the same shade of grey on a line chat, meaning your chart will become unreadable to the marker.
Another trap you may fall into is the unintentional stuffing of the dissertation chapter with graphs and figures. Even though it is essential to show numbers and statistics, you don’t want to overwhelm your readers with too many.
It may not be necessary to have a graph/table under each sub-heading. Only you can best judge whether or not you need to have a graph/table under a particular sub-heading as the writer.
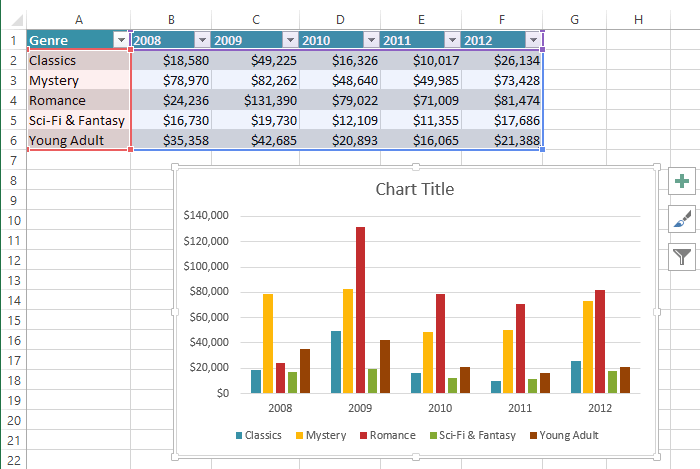
Relating to Previous Chapters
As a student, it can be challenging to develop your own analysis and discussion of results. One of the excellent discussion chapter requirements is to showcase your ability to relate previous research to your research results.
Avoid repeating the same information over and over. Many students fall into this trap which negatively affects the mark of their overall dissertation paper .
Concise and to-the-point information will help you effectively convey your point to the readers.
Although you must demonstrate how your findings relate to previous research, it is equally important to ensure you are not simply rewriting what has already been said in the introduction and literature review chapters.
The best strategy is to use examples from previous sections to postulate an argument.
Hyperlinks are recommended to take the reader from one section to another. This is especially important for submitting electronic documents as .word or .pdf files. Hyperlinking is tedious and time-consuming, so you should allow for this in your dissertation timeline to avoid rushing in the closing stages.
Also read: How to Write the Abstract for the Dissertation.
Using Subsections and Subheadings
You might want to reflect on the structure of the discussion in your organizstion of the dissertation discussion chapter, and for that, you will need to create sub-sections.
It is essential to keep subsections to the point and as short as possible. Use a layer of subheadings if possible.
For example
Subsection 4.1 of Chapter 4- Discussion can be further divided into sections 4.1.1 and 4.2.2. After three numerical layers (4.1.1, 4.2.2, and 4.2.3), any subheadings need not appear in the contents table.
The titles of all subsections will appear on your table of contents so choose the wordings carefully. A title too long or too short might confuse the reader. A one or two-word subheading will not give the reader enough information to understand the section.
Likewise, using a research question or long sentences in the subheading is not recommended. It might help to examine how other researchers and writers create these subheadings.
Critical Thinking
Your critical thinking skills are the crux of your dissertation discussion chapter. You will do yourself a great disservice if you fail to put the critical thinking element into the equation.
After all, this exercise aims to showcase clarity in your thoughts and arguments. Markers of the dissertation give more importance to the analysis and discussion chapter. But you could be marked negatively if this particular chapter lacks critical thinking.
Many students struggle to distinguish between fundamental descriptive analysis and critical thinking with their opinions on the research topic.
Critical thinking is a skill developed over time, and it might be daunting for you to come to terms with the idea of critical thinking and its use in your analysis. But even if you are no expert, you must try your best.

“Still unsure about how to write a dissertation discussion chapter ? Why not take advantage of our UK-based dissertation writing service ? Your dissertation is essential to your degree, so you cannot risk failing it.
With our custom writing service , you are guaranteed to have all your dissertation paper elements put into the right place. Our expert academics can help you with your full dissertation paper or a part of it. Click here to learn more about our dissertation services.
Duplication of Content
Another critical error students make reaffirming the point the graph/chart was supposed to make. Writing out the same information as presented in the graph defeats the whole purpose of having them in the first place.
You will be expected to form your opinions and arguments based on the findings (as presented by the graphs), so keep an eye on this mistake. Finally, avoid simply inserting a graph without any explanation whatsoever.
It should be noted that there is no correct or incorrect number of charts/figures one can use in the dissertation findings and discussion chapter. A balance must be struck.
Avoid Over Interpretation
This is a major no-no when writing a dissertation discussion. Do not make an argument that isn’t backed by your collected data.
The results and interpretations that cannot be supported should not be mentioned. Your research will be deemed unauthentic and will also be questioned by your supervisor if you do so. Results should be interpreted without any bias.
How to Write the Findings of a Dissertation.
Do not Speculate
Speculation in the discussion chapter of your dissertation is discouraged. Your dissertation’s discussion is based on your collected data and how it relates to your research questions. Thus, speculating here will undoubtedly undermine your research’s credibility.
Also, try not to generalise your findings. If your research is based on a specific population, do not state that the same findings might apply in every case. As indicated previously, it is essential to acknowledge the limitations of your research.
On the other hand, if you think your discussion needs to address other populations as well, start your sentence like this ‘We speculate that..’ or ‘It is speculated that..’ This will keep you from getting into any trouble.
What are the elements of the Dissertation Discussion?
The list of the main elements of the discussion chapter are:
- Implications : Why are your findings important to academic and scientific communities, and what purpose would they serve?
- Future Recommendations: Advice for other researchers and scientists who explore the topic further in future.
What are the steps of writing a Dissertation Discussion Chapter?
- Write a summary of the findings
- Provide a summary of your findings
- Interpretations of Results
- Recognise the Limitations of your research
- Provide Recommendations for Future Research.
Can we use graphs and charts in the Dissertation Discussion Chapter?
Yes, using graphs to aid your statistical results and enhance presentation is essential, but do not overwhelm it with a lot of graphs in multiple colours.
You May Also Like
Not sure how to write dissertation title page? All dissertations must have a dissertation title page where necessary information should be clearly presented
Anyone who supports you in your research should be acknowledged in dissertation acknowledgments. Learn more on how to write dissertation acknowledgements.
Have you failed dissertation, assignment, exam or coursework? Don’t panic because you are not alone. Get help from our professional UK qualified writers!
USEFUL LINKS
LEARNING RESOURCES
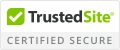
COMPANY DETAILS
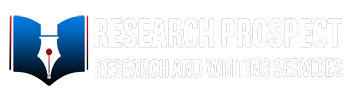
- How It Works

- LEARNING SKILLS
- Writing a Dissertation or Thesis
- Results and Discussion
Search SkillsYouNeed:
Learning Skills:
- A - Z List of Learning Skills
- What is Learning?
- Learning Approaches
- Learning Styles
- 8 Types of Learning Styles
- Understanding Your Preferences to Aid Learning
- Lifelong Learning
- Decisions to Make Before Applying to University
- Top Tips for Surviving Student Life
- Living Online: Education and Learning
- 8 Ways to Embrace Technology-Based Learning Approaches
- Critical Thinking Skills
- Critical Thinking and Fake News
- Understanding and Addressing Conspiracy Theories
- Critical Analysis
- Study Skills
- Exam Skills
- How to Write a Research Proposal
- Ethical Issues in Research
- Dissertation: The Introduction
- Researching and Writing a Literature Review
- Writing your Methodology
- Dissertation: Results and Discussion
- Dissertation: Conclusions and Extras
Writing Your Dissertation or Thesis eBook

Part of the Skills You Need Guide for Students .
- Research Methods
- Teaching, Coaching, Mentoring and Counselling
- Employability Skills for Graduates
Subscribe to our FREE newsletter and start improving your life in just 5 minutes a day.
You'll get our 5 free 'One Minute Life Skills' and our weekly newsletter.
We'll never share your email address and you can unsubscribe at any time.
Writing your Dissertation: Results and Discussion
When writing a dissertation or thesis, the results and discussion sections can be both the most interesting as well as the most challenging sections to write.
You may choose to write these sections separately, or combine them into a single chapter, depending on your university’s guidelines and your own preferences.
There are advantages to both approaches.
Writing the results and discussion as separate sections allows you to focus first on what results you obtained and set out clearly what happened in your experiments and/or investigations without worrying about their implications.This can focus your mind on what the results actually show and help you to sort them in your head.
However, many people find it easier to combine the results with their implications as the two are closely connected.
Check your university’s requirements carefully before combining the results and discussions sections as some specify that they must be kept separate.
Results Section
The Results section should set out your key experimental results, including any statistical analysis and whether or not the results of these are significant.
You should cover any literature supporting your interpretation of significance. It does not have to include everything you did, particularly for a doctorate dissertation. However, for an undergraduate or master's thesis, you will probably find that you need to include most of your work.
You should write your results section in the past tense: you are describing what you have done in the past.
Every result included MUST have a method set out in the methods section. Check back to make sure that you have included all the relevant methods.
Conversely, every method should also have some results given so, if you choose to exclude certain experiments from the results, make sure that you remove mention of the method as well.
If you are unsure whether to include certain results, go back to your research questions and decide whether the results are relevant to them. It doesn’t matter whether they are supportive or not, it’s about relevance. If they are relevant, you should include them.
Having decided what to include, next decide what order to use. You could choose chronological, which should follow the methods, or in order from most to least important in the answering of your research questions, or by research question and/or hypothesis.
You also need to consider how best to present your results: tables, figures, graphs, or text. Try to use a variety of different methods of presentation, and consider your reader: 20 pages of dense tables are hard to understand, as are five pages of graphs, but a single table and well-chosen graph that illustrate your overall findings will make things much clearer.
Make sure that each table and figure has a number and a title. Number tables and figures in separate lists, but consecutively by the order in which you mention them in the text. If you have more than about two or three, it’s often helpful to provide lists of tables and figures alongside the table of contents at the start of your dissertation.
Summarise your results in the text, drawing on the figures and tables to illustrate your points.
The text and figures should be complementary, not repeat the same information. You should refer to every table or figure in the text. Any that you don’t feel the need to refer to can safely be moved to an appendix, or even removed.
Make sure that you including information about the size and direction of any changes, including percentage change if appropriate. Statistical tests should include details of p values or confidence intervals and limits.
While you don’t need to include all your primary evidence in this section, you should as a matter of good practice make it available in an appendix, to which you should refer at the relevant point.
For example:
Details of all the interview participants can be found in Appendix A, with transcripts of each interview in Appendix B.
You will, almost inevitably, find that you need to include some slight discussion of your results during this section. This discussion should evaluate the quality of the results and their reliability, but not stray too far into discussion of how far your results support your hypothesis and/or answer your research questions, as that is for the discussion section.
See our pages: Analysing Qualitative Data and Simple Statistical Analysis for more information on analysing your results.
Discussion Section
This section has four purposes, it should:
- Interpret and explain your results
- Answer your research question
- Justify your approach
- Critically evaluate your study
The discussion section therefore needs to review your findings in the context of the literature and the existing knowledge about the subject.
You also need to demonstrate that you understand the limitations of your research and the implications of your findings for policy and practice. This section should be written in the present tense.
The Discussion section needs to follow from your results and relate back to your literature review . Make sure that everything you discuss is covered in the results section.
Some universities require a separate section on recommendations for policy and practice and/or for future research, while others allow you to include this in your discussion, so check the guidelines carefully.
Starting the Task
Most people are likely to write this section best by preparing an outline, setting out the broad thrust of the argument, and how your results support it.
You may find techniques like mind mapping are helpful in making a first outline; check out our page: Creative Thinking for some ideas about how to think through your ideas. You should start by referring back to your research questions, discuss your results, then set them into the context of the literature, and then into broader theory.
This is likely to be one of the longest sections of your dissertation, and it’s a good idea to break it down into chunks with sub-headings to help your reader to navigate through the detail.
Fleshing Out the Detail
Once you have your outline in front of you, you can start to map out how your results fit into the outline.
This will help you to see whether your results are over-focused in one area, which is why writing up your research as you go along can be a helpful process. For each theme or area, you should discuss how the results help to answer your research question, and whether the results are consistent with your expectations and the literature.
The Importance of Understanding Differences
If your results are controversial and/or unexpected, you should set them fully in context and explain why you think that you obtained them.
Your explanations may include issues such as a non-representative sample for convenience purposes, a response rate skewed towards those with a particular experience, or your own involvement as a participant for sociological research.
You do not need to be apologetic about these, because you made a choice about them, which you should have justified in the methodology section. However, you do need to evaluate your own results against others’ findings, especially if they are different. A full understanding of the limitations of your research is part of a good discussion section.
At this stage, you may want to revisit your literature review, unless you submitted it as a separate submission earlier, and revise it to draw out those studies which have proven more relevant.
Conclude by summarising the implications of your findings in brief, and explain why they are important for researchers and in practice, and provide some suggestions for further work.
You may also wish to make some recommendations for practice. As before, this may be a separate section, or included in your discussion.
The results and discussion, including conclusion and recommendations, are probably the most substantial sections of your dissertation. Once completed, you can begin to relax slightly: you are on to the last stages of writing!
Continue to: Dissertation: Conclusion and Extras Writing your Methodology
See also: Writing a Literature Review Writing a Research Proposal Academic Referencing What Is the Importance of Using a Plagiarism Checker to Check Your Thesis?
How Do I Write the Discussion Chapter?
Reflecting on and Comparing Your Data, Recognising the Strengths and Limitations
- First Online: 19 October 2023
Cite this chapter
- Sue Reeves ORCID: orcid.org/0000-0002-3017-0559 3 &
- Bartek Buczkowski ORCID: orcid.org/0000-0002-4146-3664 4
760 Accesses
The Discussion chapter brings an opportunity to write an academic argument that contains a detailed critical evaluation and analysis of your research findings. This chapter addresses the purpose and critical nature of the discussion, contains a guide to selecting key results to discuss, and details how best to structure the discussion with subsections and paragraphs. We also present a list of points to do and avoid when writing the discussion together with a Discussion chapter checklist.
This is a preview of subscription content, log in via an institution to check access.
Access this chapter
Subscribe and save.
- Get 10 units per month
- Download Article/Chapter or eBook
- 1 Unit = 1 Article or 1 Chapter
- Cancel anytime
- Available as EPUB and PDF
- Read on any device
- Instant download
- Own it forever
- Compact, lightweight edition
- Dispatched in 3 to 5 business days
- Free shipping worldwide - see info
Tax calculation will be finalised at checkout
Purchases are for personal use only
Institutional subscriptions
Braun V, Clarke V (2013) Successful qualitative research: a practical guide for beginners. SAGE Publications, London
Google Scholar
McGregor SLT (2018) Understanding and evaluating research: a critical guide. SAGE Publications, Los Angeles, CA
Book Google Scholar
PLOS (2023) Author resources. How to write discussions and conclusions. Accessed Mar 3, 2023, from https://plos.org/resource/how-to-write-conclusions/ . Accessed 3 Mar 2023
Further Reading
Cottrell S (2017) Critical thinking skills: effective analysis, argument and reflection, 3rd edn. Palgrave, London
Download references
Author information
Authors and affiliations.
University of Roehampton, London, UK
Manchester Metropolitan University, Manchester, UK
Bartek Buczkowski
You can also search for this author in PubMed Google Scholar
Rights and permissions
Reprints and permissions
Copyright information
© 2023 The Author(s), under exclusive license to Springer Nature Switzerland AG
About this chapter
Reeves, S., Buczkowski, B. (2023). How Do I Write the Discussion Chapter?. In: Mastering Your Dissertation. Springer, Cham. https://doi.org/10.1007/978-3-031-41911-9_9
Download citation
DOI : https://doi.org/10.1007/978-3-031-41911-9_9
Published : 19 October 2023
Publisher Name : Springer, Cham
Print ISBN : 978-3-031-41910-2
Online ISBN : 978-3-031-41911-9
eBook Packages : Biomedical and Life Sciences Biomedical and Life Sciences (R0)
Share this chapter
Anyone you share the following link with will be able to read this content:
Sorry, a shareable link is not currently available for this article.
Provided by the Springer Nature SharedIt content-sharing initiative
- Publish with us
Policies and ethics
- Find a journal
- Track your research
Have a language expert improve your writing
Run a free plagiarism check in 10 minutes, automatically generate references for free.
- Knowledge Base
- Dissertation
- How to Write a Discussion Section | Tips & Examples
How to Write a Discussion Section | Tips & Examples
Published on 21 August 2022 by Shona McCombes . Revised on 25 October 2022.
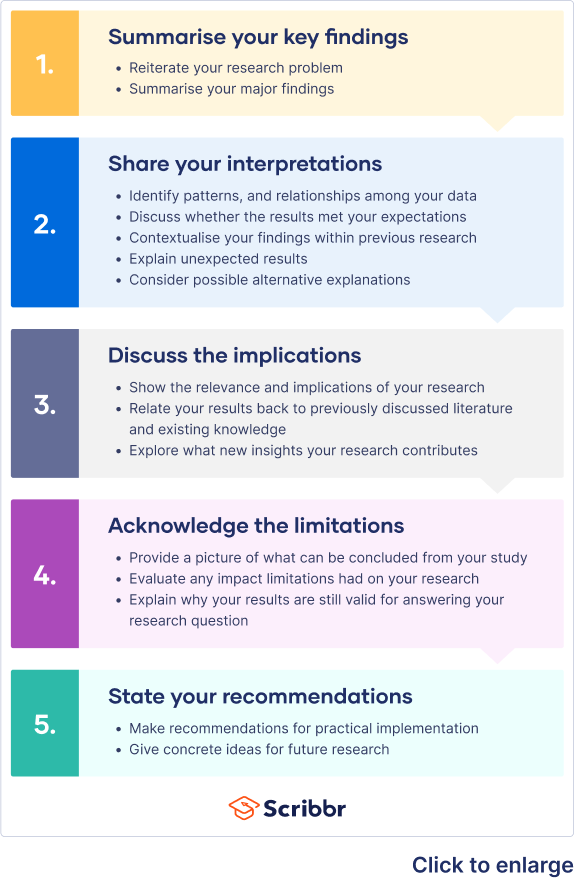
The discussion section is where you delve into the meaning, importance, and relevance of your results .
It should focus on explaining and evaluating what you found, showing how it relates to your literature review , and making an argument in support of your overall conclusion . It should not be a second results section .
There are different ways to write this section, but you can focus your writing around these key elements:
- Summary: A brief recap of your key results
- Interpretations: What do your results mean?
- Implications: Why do your results matter?
- Limitations: What can’t your results tell us?
- Recommendations: Avenues for further studies or analyses
Instantly correct all language mistakes in your text
Be assured that you'll submit flawless writing. Upload your document to correct all your mistakes.
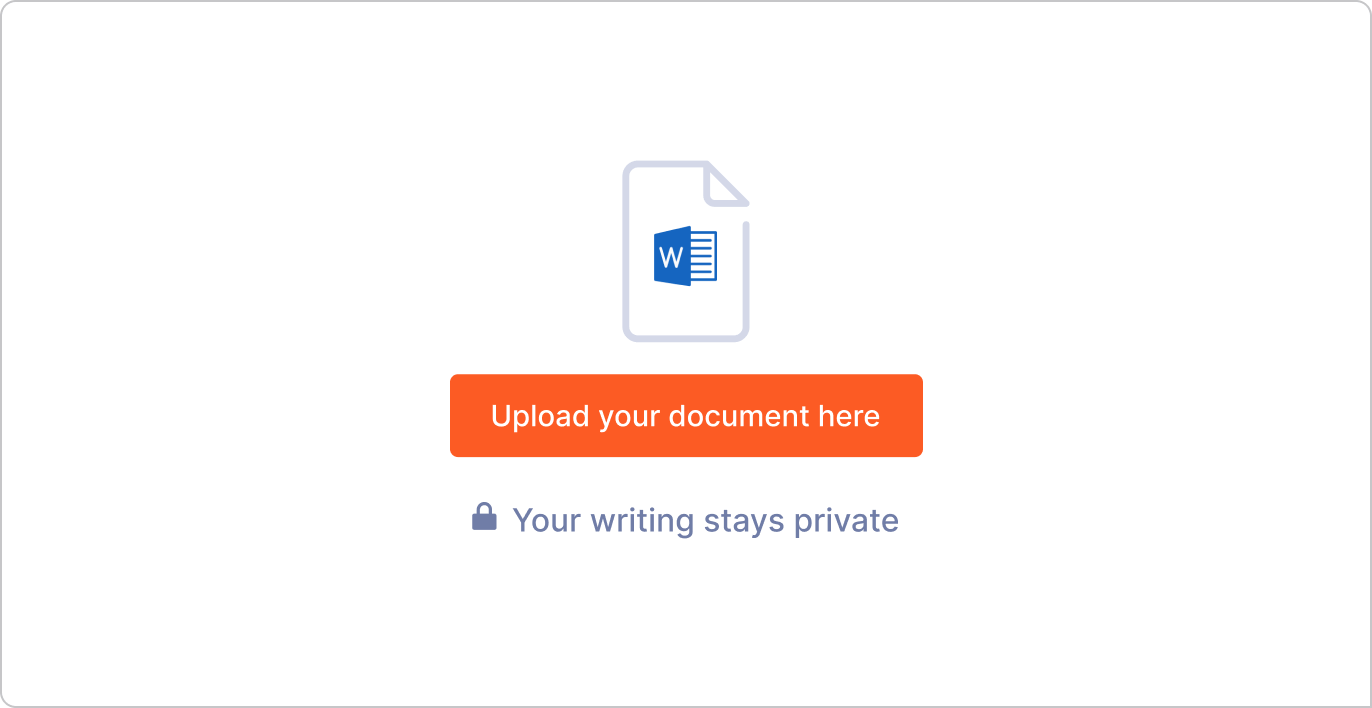
Table of contents
What not to include in your discussion section, step 1: summarise your key findings, step 2: give your interpretations, step 3: discuss the implications, step 4: acknowledge the limitations, step 5: share your recommendations, discussion section example.
There are a few common mistakes to avoid when writing the discussion section of your paper.
- Don’t introduce new results: You should only discuss the data that you have already reported in your results section .
- Don’t make inflated claims: Avoid overinterpretation and speculation that isn’t directly supported by your data.
- Don’t undermine your research: The discussion of limitations should aim to strengthen your credibility, not emphasise weaknesses or failures.
The only proofreading tool specialized in correcting academic writing
The academic proofreading tool has been trained on 1000s of academic texts and by native English editors. Making it the most accurate and reliable proofreading tool for students.
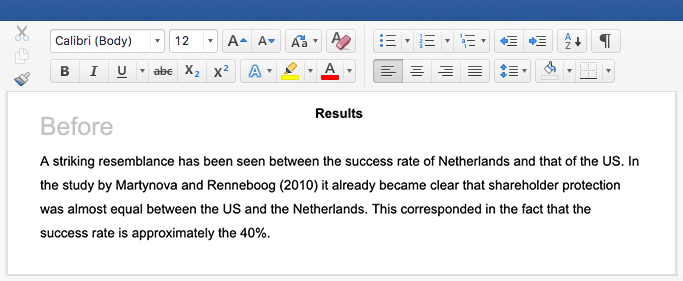
Correct my document today
Start this section by reiterating your research problem and concisely summarising your major findings. Don’t just repeat all the data you have already reported – aim for a clear statement of the overall result that directly answers your main research question . This should be no more than one paragraph.
Many students struggle with the differences between a discussion section and a results section . The crux of the matter is that your results sections should present your results, and your discussion section should subjectively evaluate them. Try not to blend elements of these two sections, in order to keep your paper sharp.
- The results indicate that …
- The study demonstrates a correlation between …
- This analysis supports the theory that …
- The data suggest that …
The meaning of your results may seem obvious to you, but it’s important to spell out their significance for your reader, showing exactly how they answer your research question.
The form of your interpretations will depend on the type of research, but some typical approaches to interpreting the data include:
- Identifying correlations , patterns, and relationships among the data
- Discussing whether the results met your expectations or supported your hypotheses
- Contextualising your findings within previous research and theory
- Explaining unexpected results and evaluating their significance
- Considering possible alternative explanations and making an argument for your position
You can organise your discussion around key themes, hypotheses, or research questions, following the same structure as your results section. Alternatively, you can also begin by highlighting the most significant or unexpected results.
- In line with the hypothesis …
- Contrary to the hypothesised association …
- The results contradict the claims of Smith (2007) that …
- The results might suggest that x . However, based on the findings of similar studies, a more plausible explanation is x .
As well as giving your own interpretations, make sure to relate your results back to the scholarly work that you surveyed in the literature review . The discussion should show how your findings fit with existing knowledge, what new insights they contribute, and what consequences they have for theory or practice.
Ask yourself these questions:
- Do your results support or challenge existing theories? If they support existing theories, what new information do they contribute? If they challenge existing theories, why do you think that is?
- Are there any practical implications?
Your overall aim is to show the reader exactly what your research has contributed, and why they should care.
- These results build on existing evidence of …
- The results do not fit with the theory that …
- The experiment provides a new insight into the relationship between …
- These results should be taken into account when considering how to …
- The data contribute a clearer understanding of …
- While previous research has focused on x , these results demonstrate that y .
Prevent plagiarism, run a free check.
Even the best research has its limitations. Acknowledging these is important to demonstrate your credibility. Limitations aren’t about listing your errors, but about providing an accurate picture of what can and cannot be concluded from your study.
Limitations might be due to your overall research design, specific methodological choices , or unanticipated obstacles that emerged during your research process.
Here are a few common possibilities:
- If your sample size was small or limited to a specific group of people, explain how generalisability is limited.
- If you encountered problems when gathering or analysing data, explain how these influenced the results.
- If there are potential confounding variables that you were unable to control, acknowledge the effect these may have had.
After noting the limitations, you can reiterate why the results are nonetheless valid for the purpose of answering your research question.
- The generalisability of the results is limited by …
- The reliability of these data is impacted by …
- Due to the lack of data on x , the results cannot confirm …
- The methodological choices were constrained by …
- It is beyond the scope of this study to …
Based on the discussion of your results, you can make recommendations for practical implementation or further research. Sometimes, the recommendations are saved for the conclusion .
Suggestions for further research can lead directly from the limitations. Don’t just state that more studies should be done – give concrete ideas for how future work can build on areas that your own research was unable to address.
- Further research is needed to establish …
- Future studies should take into account …
- Avenues for future research include …
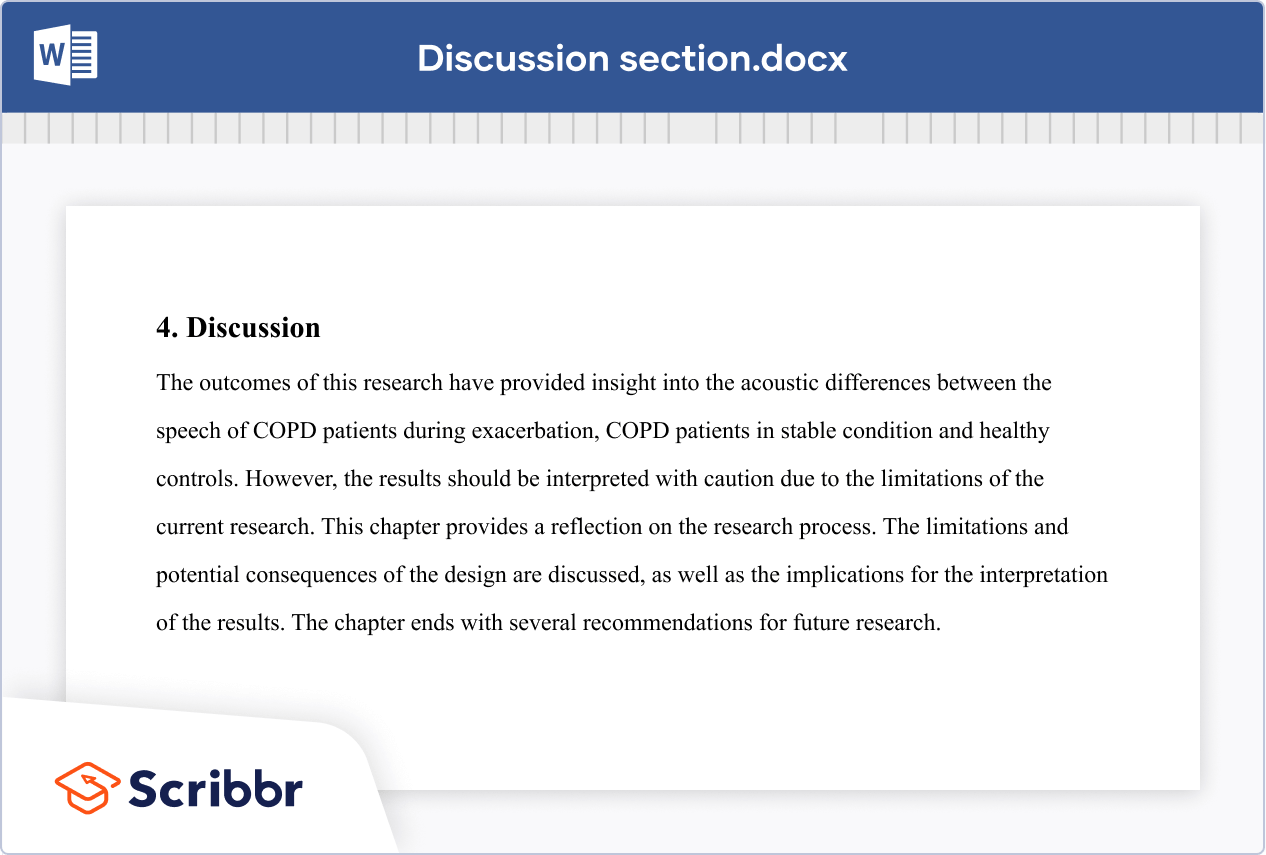
Cite this Scribbr article
If you want to cite this source, you can copy and paste the citation or click the ‘Cite this Scribbr article’ button to automatically add the citation to our free Reference Generator.
McCombes, S. (2022, October 25). How to Write a Discussion Section | Tips & Examples. Scribbr. Retrieved 3 September 2024, from https://www.scribbr.co.uk/thesis-dissertation/discussion/
Is this article helpful?
Shona McCombes
Other students also liked, how to write a results section | tips & examples, research paper appendix | example & templates, how to write a thesis or dissertation introduction.
- USC Libraries
- Research Guides
Organizing Your Social Sciences Research Paper
- 8. The Discussion
- Purpose of Guide
- Design Flaws to Avoid
- Independent and Dependent Variables
- Glossary of Research Terms
- Reading Research Effectively
- Narrowing a Topic Idea
- Broadening a Topic Idea
- Extending the Timeliness of a Topic Idea
- Academic Writing Style
- Applying Critical Thinking
- Choosing a Title
- Making an Outline
- Paragraph Development
- Research Process Video Series
- Executive Summary
- The C.A.R.S. Model
- Background Information
- The Research Problem/Question
- Theoretical Framework
- Citation Tracking
- Content Alert Services
- Evaluating Sources
- Primary Sources
- Secondary Sources
- Tiertiary Sources
- Scholarly vs. Popular Publications
- Qualitative Methods
- Quantitative Methods
- Insiderness
- Using Non-Textual Elements
- Limitations of the Study
- Common Grammar Mistakes
- Writing Concisely
- Avoiding Plagiarism
- Footnotes or Endnotes?
- Further Readings
- Generative AI and Writing
- USC Libraries Tutorials and Other Guides
- Bibliography
The purpose of the discussion section is to interpret and describe the significance of your findings in relation to what was already known about the research problem being investigated and to explain any new understanding or insights that emerged as a result of your research. The discussion will always connect to the introduction by way of the research questions or hypotheses you posed and the literature you reviewed, but the discussion does not simply repeat or rearrange the first parts of your paper; the discussion clearly explains how your study advanced the reader's understanding of the research problem from where you left them at the end of your review of prior research.
Annesley, Thomas M. “The Discussion Section: Your Closing Argument.” Clinical Chemistry 56 (November 2010): 1671-1674; Peacock, Matthew. “Communicative Moves in the Discussion Section of Research Articles.” System 30 (December 2002): 479-497.
Importance of a Good Discussion
The discussion section is often considered the most important part of your research paper because it:
- Most effectively demonstrates your ability as a researcher to think critically about an issue, to develop creative solutions to problems based upon a logical synthesis of the findings, and to formulate a deeper, more profound understanding of the research problem under investigation;
- Presents the underlying meaning of your research, notes possible implications in other areas of study, and explores possible improvements that can be made in order to further develop the concerns of your research;
- Highlights the importance of your study and how it can contribute to understanding the research problem within the field of study;
- Presents how the findings from your study revealed and helped fill gaps in the literature that had not been previously exposed or adequately described; and,
- Engages the reader in thinking critically about issues based on an evidence-based interpretation of findings; it is not governed strictly by objective reporting of information.
Annesley Thomas M. “The Discussion Section: Your Closing Argument.” Clinical Chemistry 56 (November 2010): 1671-1674; Bitchener, John and Helen Basturkmen. “Perceptions of the Difficulties of Postgraduate L2 Thesis Students Writing the Discussion Section.” Journal of English for Academic Purposes 5 (January 2006): 4-18; Kretchmer, Paul. Fourteen Steps to Writing an Effective Discussion Section. San Francisco Edit, 2003-2008.
Structure and Writing Style
I. General Rules
These are the general rules you should adopt when composing your discussion of the results :
- Do not be verbose or repetitive; be concise and make your points clearly
- Avoid the use of jargon or undefined technical language
- Follow a logical stream of thought; in general, interpret and discuss the significance of your findings in the same sequence you described them in your results section [a notable exception is to begin by highlighting an unexpected result or a finding that can grab the reader's attention]
- Use the present verb tense, especially for established facts; however, refer to specific works or prior studies in the past tense
- If needed, use subheadings to help organize your discussion or to categorize your interpretations into themes
II. The Content
The content of the discussion section of your paper most often includes :
- Explanation of results : Comment on whether or not the results were expected for each set of findings; go into greater depth to explain findings that were unexpected or especially profound. If appropriate, note any unusual or unanticipated patterns or trends that emerged from your results and explain their meaning in relation to the research problem.
- References to previous research : Either compare your results with the findings from other studies or use the studies to support a claim. This can include re-visiting key sources already cited in your literature review section, or, save them to cite later in the discussion section if they are more important to compare with your results instead of being a part of the general literature review of prior research used to provide context and background information. Note that you can make this decision to highlight specific studies after you have begun writing the discussion section.
- Deduction : A claim for how the results can be applied more generally. For example, describing lessons learned, proposing recommendations that can help improve a situation, or highlighting best practices.
- Hypothesis : A more general claim or possible conclusion arising from the results [which may be proved or disproved in subsequent research]. This can be framed as new research questions that emerged as a consequence of your analysis.
III. Organization and Structure
Keep the following sequential points in mind as you organize and write the discussion section of your paper:
- Think of your discussion as an inverted pyramid. Organize the discussion from the general to the specific, linking your findings to the literature, then to theory, then to practice [if appropriate].
- Use the same key terms, narrative style, and verb tense [present] that you used when describing the research problem in your introduction.
- Begin by briefly re-stating the research problem you were investigating and answer all of the research questions underpinning the problem that you posed in the introduction.
- Describe the patterns, principles, and relationships shown by each major findings and place them in proper perspective. The sequence of this information is important; first state the answer, then the relevant results, then cite the work of others. If appropriate, refer the reader to a figure or table to help enhance the interpretation of the data [either within the text or as an appendix].
- Regardless of where it's mentioned, a good discussion section includes analysis of any unexpected findings. This part of the discussion should begin with a description of the unanticipated finding, followed by a brief interpretation as to why you believe it appeared and, if necessary, its possible significance in relation to the overall study. If more than one unexpected finding emerged during the study, describe each of them in the order they appeared as you gathered or analyzed the data. As noted, the exception to discussing findings in the same order you described them in the results section would be to begin by highlighting the implications of a particularly unexpected or significant finding that emerged from the study, followed by a discussion of the remaining findings.
- Before concluding the discussion, identify potential limitations and weaknesses if you do not plan to do so in the conclusion of the paper. Comment on their relative importance in relation to your overall interpretation of the results and, if necessary, note how they may affect the validity of your findings. Avoid using an apologetic tone; however, be honest and self-critical [e.g., in retrospect, had you included a particular question in a survey instrument, additional data could have been revealed].
- The discussion section should end with a concise summary of the principal implications of the findings regardless of their significance. Give a brief explanation about why you believe the findings and conclusions of your study are important and how they support broader knowledge or understanding of the research problem. This can be followed by any recommendations for further research. However, do not offer recommendations which could have been easily addressed within the study. This would demonstrate to the reader that you have inadequately examined and interpreted the data.
IV. Overall Objectives
The objectives of your discussion section should include the following: I. Reiterate the Research Problem/State the Major Findings
Briefly reiterate the research problem or problems you are investigating and the methods you used to investigate them, then move quickly to describe the major findings of the study. You should write a direct, declarative, and succinct proclamation of the study results, usually in one paragraph.
II. Explain the Meaning of the Findings and Why They are Important
No one has thought as long and hard about your study as you have. Systematically explain the underlying meaning of your findings and state why you believe they are significant. After reading the discussion section, you want the reader to think critically about the results and why they are important. You don’t want to force the reader to go through the paper multiple times to figure out what it all means. If applicable, begin this part of the section by repeating what you consider to be your most significant or unanticipated finding first, then systematically review each finding. Otherwise, follow the general order you reported the findings presented in the results section.
III. Relate the Findings to Similar Studies
No study in the social sciences is so novel or possesses such a restricted focus that it has absolutely no relation to previously published research. The discussion section should relate your results to those found in other studies, particularly if questions raised from prior studies served as the motivation for your research. This is important because comparing and contrasting the findings of other studies helps to support the overall importance of your results and it highlights how and in what ways your study differs from other research about the topic. Note that any significant or unanticipated finding is often because there was no prior research to indicate the finding could occur. If there is prior research to indicate this, you need to explain why it was significant or unanticipated. IV. Consider Alternative Explanations of the Findings
It is important to remember that the purpose of research in the social sciences is to discover and not to prove . When writing the discussion section, you should carefully consider all possible explanations for the study results, rather than just those that fit your hypothesis or prior assumptions and biases. This is especially important when describing the discovery of significant or unanticipated findings.
V. Acknowledge the Study’s Limitations
It is far better for you to identify and acknowledge your study’s limitations than to have them pointed out by your professor! Note any unanswered questions or issues your study could not address and describe the generalizability of your results to other situations. If a limitation is applicable to the method chosen to gather information, then describe in detail the problems you encountered and why. VI. Make Suggestions for Further Research
You may choose to conclude the discussion section by making suggestions for further research [as opposed to offering suggestions in the conclusion of your paper]. Although your study can offer important insights about the research problem, this is where you can address other questions related to the problem that remain unanswered or highlight hidden issues that were revealed as a result of conducting your research. You should frame your suggestions by linking the need for further research to the limitations of your study [e.g., in future studies, the survey instrument should include more questions that ask..."] or linking to critical issues revealed from the data that were not considered initially in your research.
NOTE: Besides the literature review section, the preponderance of references to sources is usually found in the discussion section . A few historical references may be helpful for perspective, but most of the references should be relatively recent and included to aid in the interpretation of your results, to support the significance of a finding, and/or to place a finding within a particular context. If a study that you cited does not support your findings, don't ignore it--clearly explain why your research findings differ from theirs.
V. Problems to Avoid
- Do not waste time restating your results . Should you need to remind the reader of a finding to be discussed, use "bridge sentences" that relate the result to the interpretation. An example would be: “In the case of determining available housing to single women with children in rural areas of Texas, the findings suggest that access to good schools is important...," then move on to further explaining this finding and its implications.
- As noted, recommendations for further research can be included in either the discussion or conclusion of your paper, but do not repeat your recommendations in the both sections. Think about the overall narrative flow of your paper to determine where best to locate this information. However, if your findings raise a lot of new questions or issues, consider including suggestions for further research in the discussion section.
- Do not introduce new results in the discussion section. Be wary of mistaking the reiteration of a specific finding for an interpretation because it may confuse the reader. The description of findings [results section] and the interpretation of their significance [discussion section] should be distinct parts of your paper. If you choose to combine the results section and the discussion section into a single narrative, you must be clear in how you report the information discovered and your own interpretation of each finding. This approach is not recommended if you lack experience writing college-level research papers.
- Use of the first person pronoun is generally acceptable. Using first person singular pronouns can help emphasize a point or illustrate a contrasting finding. However, keep in mind that too much use of the first person can actually distract the reader from the main points [i.e., I know you're telling me this--just tell me!].
Analyzing vs. Summarizing. Department of English Writing Guide. George Mason University; Discussion. The Structure, Format, Content, and Style of a Journal-Style Scientific Paper. Department of Biology. Bates College; Hess, Dean R. "How to Write an Effective Discussion." Respiratory Care 49 (October 2004); Kretchmer, Paul. Fourteen Steps to Writing to Writing an Effective Discussion Section. San Francisco Edit, 2003-2008; The Lab Report. University College Writing Centre. University of Toronto; Sauaia, A. et al. "The Anatomy of an Article: The Discussion Section: "How Does the Article I Read Today Change What I Will Recommend to my Patients Tomorrow?” The Journal of Trauma and Acute Care Surgery 74 (June 2013): 1599-1602; Research Limitations & Future Research . Lund Research Ltd., 2012; Summary: Using it Wisely. The Writing Center. University of North Carolina; Schafer, Mickey S. Writing the Discussion. Writing in Psychology course syllabus. University of Florida; Yellin, Linda L. A Sociology Writer's Guide . Boston, MA: Allyn and Bacon, 2009.
Writing Tip
Don’t Over-Interpret the Results!
Interpretation is a subjective exercise. As such, you should always approach the selection and interpretation of your findings introspectively and to think critically about the possibility of judgmental biases unintentionally entering into discussions about the significance of your work. With this in mind, be careful that you do not read more into the findings than can be supported by the evidence you have gathered. Remember that the data are the data: nothing more, nothing less.
MacCoun, Robert J. "Biases in the Interpretation and Use of Research Results." Annual Review of Psychology 49 (February 1998): 259-287; Ward, Paulet al, editors. The Oxford Handbook of Expertise . Oxford, UK: Oxford University Press, 2018.
Another Writing Tip
Don't Write Two Results Sections!
One of the most common mistakes that you can make when discussing the results of your study is to present a superficial interpretation of the findings that more or less re-states the results section of your paper. Obviously, you must refer to your results when discussing them, but focus on the interpretation of those results and their significance in relation to the research problem, not the data itself.
Azar, Beth. "Discussing Your Findings." American Psychological Association gradPSYCH Magazine (January 2006).
Yet Another Writing Tip
Avoid Unwarranted Speculation!
The discussion section should remain focused on the findings of your study. For example, if the purpose of your research was to measure the impact of foreign aid on increasing access to education among disadvantaged children in Bangladesh, it would not be appropriate to speculate about how your findings might apply to populations in other countries without drawing from existing studies to support your claim or if analysis of other countries was not a part of your original research design. If you feel compelled to speculate, do so in the form of describing possible implications or explaining possible impacts. Be certain that you clearly identify your comments as speculation or as a suggestion for where further research is needed. Sometimes your professor will encourage you to expand your discussion of the results in this way, while others don’t care what your opinion is beyond your effort to interpret the data in relation to the research problem.
- << Previous: Using Non-Textual Elements
- Next: Limitations of the Study >>
- Last Updated: Sep 4, 2024 9:40 AM
- URL: https://libguides.usc.edu/writingguide

- Dissertation & Thesis Guides
- Basics of Dissertation & Thesis Writing
- How to Write a Dissertation Discussion Chapter: Guide & Examples
- Speech Topics
- Basics of Essay Writing
- Essay Topics
- Other Essays
- Main Academic Essays
- Research Paper Topics
- Basics of Research Paper Writing
- Miscellaneous
- Chicago/ Turabian
- Data & Statistics
- Methodology
- Admission Writing Tips
- Admission Advice
- Other Guides
- Student Life
- Studying Tips
- Understanding Plagiarism
- Academic Writing Tips

- Essay Guides
- Research Paper Guides
- Formatting Guides
- Basics of Research Process
- Admission Guides
How to Write a Dissertation Discussion Chapter: Guide & Examples

Table of contents

Use our free Readability checker
Dissertation discussion section is a chapter that interprets the results obtained from research and offers an in-depth analysis of findings. In this section, students need to analyze the outcomes, evaluate their significance, and compare them to previous research. The discussion section may also explore the limitations of the study and suggest further research perspectives.
If you are stuck with your thesis or dissertation discussion chapter, you are in the right place to complete this section successfully. This article will outline our best solutions and methods on how to write the discussion of a dissertation or thesis. We also will share advanced dissertation discussion examples to help you finalize your PhD work. Feel like academic writing gives you hassles? Remember that you can always rely on academic experts qualified in your field to get professional dissertation help online .
What Is a Dissertation Discussion?
First and foremost, students need to have a clear understanding of what dissertation discussion is. This is not the same as your results section , where you share data from your research. You are going deeper into the explanation of the existing data in your thesis or dissertation discussion section. In other words, you illustrate practical implications of your research and how the data can be used, researched further, or limited. What will make your discussion section of a dissertation excellent:
- clear structure
- practical implication
- elaboration on future work on this topic.
This section should go after research methodology and before the dissertation conclusion . It should be directly relevant to questions posed in your introduction. The biggest mistake you can make is to rewrite your result chapter with other words and add some limitations and recommendation paragraphs. However, this is an entirely different type of writing you need to complete.
Purpose of a Dissertation Discussion Chapter
A dissertation discussion section is critical to explaining students’ findings and the application of data to real-life cases. As we mentioned before, this section will often be read right after the dissertation methods . It evaluates and elaborates on findings and helps to understand the importance of your performed thesis research. A dissertation discussion opens a new perspective on further research on the same field or topic. It also outlines critical data to consider in subsequent studies. In a nutshell, this is the section where you explain your work to a broad audience.
Structure of a Dissertation Discussion Section
Let’s start your writing journey of this research part with a clear delineation of what it should include and then briefly discuss each component. Here are some basic things you need to consider for an excellent discussion chapter of dissertation :
- Brief summary It does not mean copying an introduction section. However, the first few paragraphs will make an overview of your findings and topic.
- Interpretations This is a critical component of your work — elaborate on your results and explain possible ways of using them.
- Implication Research work is not just 100+ pages of text. Students should explain and illustrate how it could be used for solving practical problems.
- Constraints This is where you outline your limitations. For instance, your research was done only on students, and it may have different results with elderly people.
- Recommendations You can also define possible ways of future research on the exact topic when writing a discussion for your thesis or dissertation. Tell readers, for example, that it would be helpful to run similar research in other specific circumstances.
How to Write a Dissertation Discussion Chapter?
One of the most commonly asked questions for our experts is how to write the discussion section of a dissertation or thesis. We understand why it can be complicated to get a clear answer. Students often think that this section is similar to the result chapter and just retells it in other words. But it is not so. Let’s go through all steps to writing a discussion in a dissertation, and share our best examples from academic papers.
1. Remind Your Research Questions & Objectives
Writing the discussion chapter of a dissertation is not a big deal if you understand its aim and each component in a text structure. First of all, you need to evaluate how your results help to answer research questions you defined in the beginning. It is not about repeating the result, you did it in previous paragraphs. However, dissertation or thesis discussion should underline how your findings help to answer the research problem. Start writing from a brief intro by recalling research questions or hypotheses . Then, show how your results answer them or support a hypothesis in your work.
2. Sum Up Key Findings
Next part of your discussion for dissertation is to provide a short summary of previous data. But do not respite the same summary paragraphs from results or introduction of a dissertation . Here researchers should be more thoughtful and go deeper into the work’s aims. Try to explain in a few sentences what you get from running research. For instance, starters usually write the statement that “our data proves that…” or “survey results illustrate a clear correlation between a and b that is critical for proving our working hypothesis…”. A discussion chapter of your dissertation is not just a fixation on results but a more profound summary connected to research goals and purpose. Here is an example: Summary of Findings Example
According to the data, implementing the co-orientation theory was successful and can be used for the same circumstances in the future. As we found, most participants agreed with the importance of those theses on the five fundamental reforms. It means that the results identified a successful government work in choosing the messages to communicate about examined reforms. At the same time, the situation is not so favorable with implementing the principles of two-way symmetrical communications. According to the results, people did not feel that the government had a mutual, open, and equal dialogue with the public about the reforms.
3. Interpret the Results
The most critical part of a discussion section is to explain and enact the results you’ve got. It is the most significant part of any text. Students should be clear about what to include in these paragraphs. Here is some advice to make this elaboration structured:
- Identify correlations or patterns in the data for dissertation discussion.
- Underline how results can answer research questions or prove your hypothesis.
- Emphasize how your findings are connected to the previous topic studies.
- Point out essential statements you can use in future research.
- Evaluate the significance of your results and any unexpected data you have.
- What others can learn from your research and how this work contributes to the field.
- Consider any possible additional or unique explanation of your findings.
- Go deeper with options of how results can be applied in practice.
Writing a dissertation discussion chapter can be tough, but here is a great sample to learn from. Example of Interpretations in Disssertation Discussion
Our study underlines the importance of future research on using TikTok for political communication. As discussed above, TikTok is the most commonly used social media platform for many young voters. This means that political discussion will also move to this platform. Our research and typology of political communication content can be used in the future planning of effective political campaigns. For example, we can assume that “play videos” have enormous potential to facilitate complicated topics and provide specific agenda settings. We also identified additional affordances of TikTok used for political communication, such as built-in video editors, playlists for specific topics, a green screen for news explainers, and duets for reflection on news and discussion. It means that these features make TikTok suitable for efficient political communications.
4. Discuss How Your Findings Relate to the Literature
Here we came to the implications of your findings for the dissertation discussion. In other words, this is a few sentences on how your work is connected to other studies on the same research topic or what literature gap you are going to fill with the data and research you launched. Remember to mention how your study address the limitations you have discovered while writing a literature review . First, outline how your hypothesis relates to theories or previous works in the field. Maybe, you challenged some theories or tried to define your own. Be specific in this section. Second, define a practical implementation of your work. Maybe, it can support recommendations or change legislation. Discussion chapter of a thesis is a place where you explain your work, make it valuable, and incorporate additional meaning for some specific data. Example of Implications in Disssertation Discussion
As we pointed out in the literature review, there are few works on using TikTok affordances for political communications, and this topic can be expanded in the future. Government institutions have already understood the importance of this platform for efficient communication with younger audiences, and we will see more political projects on TikTok. That is why expanding research on using TikTok for political communication will be enormous in the following years. Our work is one of the first research on the role of emerging media in war communication and can be used as a practical guide for government's strategic planning in times of emergencies.
5. Mention Possible Limitations
It is pretty tricky to conduct research without limitations. You will always have some, which does not mean that your work is not good. When you write a discussion chapter in a thesis or dissertation, focus on what may influence your results and how changing independent variables can affect your data collection methods and final outcomes. Here are some points to consider when you structure your dissertation discussion limitation part:
- If results can change in case you change the reference group?
- What will happen with data if it changes circumstances?
- What could influence results?
Critical thinking and analysis can help you to outline possible limitations. It can be the age of the reference group, change of questionnaire in a survey, or specific use of data extraction equipment. Be transparent about what could affect your results. Example of Complications
Although this study has provided critical first insights into the effects of multimodal disinformation and rebuttals, there are some limitations. First and most importantly, the effects of multimodal disinformation and rebuttals partially depend on the topic of the message. Although fact-checkers reduce credibility of disinformation in both settings, and attitudinal congruence plays a consistent role in conditioning responses to multimodal disinformation, visuals do not have the same impact on affecting the credibility of news on school shootings and refugees.
6. Provide Recommendations for Further Research
Writing a dissertation discussion also makes a connection to possible future research. So, other scientists may complete that. While elaborating on possible implementations of your study, you may also estimate future approaches in topic research. Here are some points to consider while your discussion in thesis writing:
- Outline questions related to your topic that you did not answer in defined study or did not outline as research questions. There are other possible gaps to research.
- Suggest future research based on limitations. For example, if you define surveyed people’s age as a limitation, recommend running another survey for older or younger recipients.
Example of Recommendations
As we mentioned before, our study has some limitations, as the research was conducted based on data from United State citizens. However, for a better understanding of government communication practices, it would be productive to implement the same research in other countries. Some cultural differences can influence the communication strategies the government uses in times of emergency. Another possible way to examine this topic is to conduct research using a specific period of time. For future studies, it will be beneficial to expand the number of survey recipients.
7. Conclude Your Thesis/ Dissertation Discussion
You are almost done, the last step is to provide a brief summary of a section. It is not the same as a conclusion for whole research. However, you need to briefly outline key points from the dissertation discussion. To finalize writing the discussion section of a dissertation, go through the text and check if there is no unimportant information. Do not overload the text with relevant data you did not present in the result section. Be specific in your summary paragraphs. It is a holistic view of everything you pointed out. Provide a few sentences to systemize all you outlined in the text. Example of a Concluding Summary in a Dissertation Discussion Section
To summarize, Airbnb has expertise in communicating CSR and CSA campaigns. We defined their communication strategy about the program for Ukrainian refugees as quite successful. They applied all the principles of CSR communication best practices, used dialogic theory to engage with the public on social media, and created clear messaging on applying for the program. Airbnb examples of CSR communication can be used by other businesses to create a communication strategy for unplanned CSR campaigns. Moreover, it can be further researched how Airbnb's CSR campaign influenced the organizational reputation in the future.
Dissertation Discussion Example
If we need to share one piece of practical advice, it would be to use thesis or dissertation discussion examples when writing your own copy. StudyCrumb provides the best samples from real students' work to help you understand the stylistic and possible structure of this part. It does not mean you need to copy and paste them into your work. However, you can use a dissertation discussion example for inspiration and brainstorming ideas for breaking writing blocks. Here’s a doctoral thesis discussion chapter example.

Dissertation Discussion Writing Tips
Before reading this blog, you should already know how to write a thesis discussion. However, we would share some essential tips you need to have in mind while working on the document.
- Be consistent Your dissertation discussion chapter is a part of bigger research, and it should be in line with your whole work.
- Understand your reader You are writing an academic text that will be analyzed by professionals and experts in the same field. Be sure that you are not trying to simplify your discussion.
- Be logical Do not jump into a new line of discussion if you did not delineate it as a research question at the beginning.
- Be clear Do not include any data that was not presented in the result section.
- Consider word choice Use such terms as “our data indicate…” or “our data suggests…” instead of “the data proves.”
- Use proper format Follow the formatting rules specified by a specific paper style (e.g., APA style format , MLA format , or Chicago format ) or provided by your instructor.
Bottom Line on Writing a Dissertation Discussion Chapter
At this stage, it should not be a question for you on how to write a discussion chapter in a PhD thesis or dissertation. Let’s make it clear. It is not a result section but still a place to elaborate on data and go deeper with explanations. Dissertation discussion section includes some intro, result interpretations, limitations, and recommendations for future research. Our team encourages you to use examples before starting your own piece of writing. It will help you to realize the purpose and structure of this chapter and inspire better texts! If you have other questions regarding the PhD writing process, check our blog for more insights. From detailed instruction on how to write a dissertation or guide on formatting a dissertation appendix , we’ve got you covered.

Order dissertation discussion from our proficient writers. They will take a significant burden off of you. Instead, they will carry out high-level academic work in a short time.
FAQ About Dissertation Discussion Chapter
1. where does a discussion section go in a dissertation.
Dissertation discussion section is used to go right after the result chapter. The logic is simple — you share your data and then go to the elaboration and explanation of it. Check the sample thesis we provide to students for details on structure.
2. How long should a dissertation discussion chapter be?
It is not a surprise that dissertation discussion chapter is extremely significant for the research. Here you will go into the details of your study and interpret results to prove or not your hypothesis. It should take almost 25% of your work.
3. What tense should I use in a dissertation discussion?
Thesis or dissertation discussion used to have some rules on using tenses. You need to use the present tense when referring to established facts and use the past tense when referring to previous studies. And check your text before submission to ensure that you did not miss something.
4. What not to include in a dissertation discussion section?
The answer is easy. Discussion section of a dissertation should not include any new findings or describe some unsupported claims. Also, do not try to feel all possible gaps with one research. It may be better to outline your ideas for future studies in recommendations.

Joe Eckel is an expert on Dissertations writing. He makes sure that each student gets precious insights on composing A-grade academic writing.
You may also like

Frequently asked questions
What’s the difference between results and discussion.
The results chapter or section simply and objectively reports what you found, without speculating on why you found these results. The discussion interprets the meaning of the results, puts them in context, and explains why they matter.
In qualitative research , results and discussion are sometimes combined. But in quantitative research , it’s considered important to separate the objective results from your interpretation of them.
Frequently asked questions: Dissertation
Dissertation word counts vary widely across different fields, institutions, and levels of education:
- An undergraduate dissertation is typically 8,000–15,000 words
- A master’s dissertation is typically 12,000–50,000 words
- A PhD thesis is typically book-length: 70,000–100,000 words
However, none of these are strict guidelines – your word count may be lower or higher than the numbers stated here. Always check the guidelines provided by your university to determine how long your own dissertation should be.
A dissertation prospectus or proposal describes what or who you plan to research for your dissertation. It delves into why, when, where, and how you will do your research, as well as helps you choose a type of research to pursue. You should also determine whether you plan to pursue qualitative or quantitative methods and what your research design will look like.
It should outline all of the decisions you have taken about your project, from your dissertation topic to your hypotheses and research objectives , ready to be approved by your supervisor or committee.
Note that some departments require a defense component, where you present your prospectus to your committee orally.
A thesis is typically written by students finishing up a bachelor’s or Master’s degree. Some educational institutions, particularly in the liberal arts, have mandatory theses, but they are often not mandatory to graduate from bachelor’s degrees. It is more common for a thesis to be a graduation requirement from a Master’s degree.
Even if not mandatory, you may want to consider writing a thesis if you:
- Plan to attend graduate school soon
- Have a particular topic you’d like to study more in-depth
- Are considering a career in research
- Would like a capstone experience to tie up your academic experience
The conclusion of your thesis or dissertation should include the following:
- A restatement of your research question
- A summary of your key arguments and/or results
- A short discussion of the implications of your research
The conclusion of your thesis or dissertation shouldn’t take up more than 5–7% of your overall word count.
For a stronger dissertation conclusion , avoid including:
- Important evidence or analysis that wasn’t mentioned in the discussion section and results section
- Generic concluding phrases (e.g. “In conclusion …”)
- Weak statements that undermine your argument (e.g., “There are good points on both sides of this issue.”)
Your conclusion should leave the reader with a strong, decisive impression of your work.
While it may be tempting to present new arguments or evidence in your thesis or disseration conclusion , especially if you have a particularly striking argument you’d like to finish your analysis with, you shouldn’t. Theses and dissertations follow a more formal structure than this.
All your findings and arguments should be presented in the body of the text (more specifically in the discussion section and results section .) The conclusion is meant to summarize and reflect on the evidence and arguments you have already presented, not introduce new ones.
A theoretical framework can sometimes be integrated into a literature review chapter , but it can also be included as its own chapter or section in your dissertation . As a rule of thumb, if your research involves dealing with a lot of complex theories, it’s a good idea to include a separate theoretical framework chapter.
A literature review and a theoretical framework are not the same thing and cannot be used interchangeably. While a theoretical framework describes the theoretical underpinnings of your work, a literature review critically evaluates existing research relating to your topic. You’ll likely need both in your dissertation .
While a theoretical framework describes the theoretical underpinnings of your work based on existing research, a conceptual framework allows you to draw your own conclusions, mapping out the variables you may use in your study and the interplay between them.
A thesis or dissertation outline is one of the most critical first steps in your writing process. It helps you to lay out and organize your ideas and can provide you with a roadmap for deciding what kind of research you’d like to undertake.
Generally, an outline contains information on the different sections included in your thesis or dissertation , such as:
- Your anticipated title
- Your abstract
- Your chapters (sometimes subdivided into further topics like literature review , research methods , avenues for future research, etc.)
When you mention different chapters within your text, it’s considered best to use Roman numerals for most citation styles. However, the most important thing here is to remain consistent whenever using numbers in your dissertation .
In most styles, the title page is used purely to provide information and doesn’t include any images. Ask your supervisor if you are allowed to include an image on the title page before doing so. If you do decide to include one, make sure to check whether you need permission from the creator of the image.
Include a note directly beneath the image acknowledging where it comes from, beginning with the word “ Note .” (italicized and followed by a period). Include a citation and copyright attribution . Don’t title, number, or label the image as a figure , since it doesn’t appear in your main text.
Definitional terms often fall into the category of common knowledge , meaning that they don’t necessarily have to be cited. This guidance can apply to your thesis or dissertation glossary as well.
However, if you’d prefer to cite your sources , you can follow guidance for citing dictionary entries in MLA or APA style for your glossary.
A glossary is a collection of words pertaining to a specific topic. In your thesis or dissertation, it’s a list of all terms you used that may not immediately be obvious to your reader. In contrast, an index is a list of the contents of your work organized by page number.
The title page of your thesis or dissertation goes first, before all other content or lists that you may choose to include.
The title page of your thesis or dissertation should include your name, department, institution, degree program, and submission date.
Glossaries are not mandatory, but if you use a lot of technical or field-specific terms, it may improve readability to add one to your thesis or dissertation. Your educational institution may also require them, so be sure to check their specific guidelines.
A glossary or “glossary of terms” is a collection of words pertaining to a specific topic. In your thesis or dissertation, it’s a list of all terms you used that may not immediately be obvious to your reader. Your glossary only needs to include terms that your reader may not be familiar with, and is intended to enhance their understanding of your work.
A glossary is a collection of words pertaining to a specific topic. In your thesis or dissertation, it’s a list of all terms you used that may not immediately be obvious to your reader. In contrast, dictionaries are more general collections of words.
An abbreviation is a shortened version of an existing word, such as Dr. for Doctor. In contrast, an acronym uses the first letter of each word to create a wholly new word, such as UNESCO (an acronym for the United Nations Educational, Scientific and Cultural Organization).
As a rule of thumb, write the explanation in full the first time you use an acronym or abbreviation. You can then proceed with the shortened version. However, if the abbreviation is very common (like PC, USA, or DNA), then you can use the abbreviated version from the get-go.
Be sure to add each abbreviation in your list of abbreviations !
If you only used a few abbreviations in your thesis or dissertation , you don’t necessarily need to include a list of abbreviations .
If your abbreviations are numerous, or if you think they won’t be known to your audience, it’s never a bad idea to add one. They can also improve readability, minimizing confusion about abbreviations unfamiliar to your reader.
A list of abbreviations is a list of all the abbreviations that you used in your thesis or dissertation. It should appear at the beginning of your document, with items in alphabetical order, just after your table of contents .
Your list of tables and figures should go directly after your table of contents in your thesis or dissertation.
Lists of figures and tables are often not required, and aren’t particularly common. They specifically aren’t required for APA-Style, though you should be careful to follow their other guidelines for figures and tables .
If you have many figures and tables in your thesis or dissertation, include one may help you stay organized. Your educational institution may require them, so be sure to check their guidelines.
A list of figures and tables compiles all of the figures and tables that you used in your thesis or dissertation and displays them with the page number where they can be found.
The table of contents in a thesis or dissertation always goes between your abstract and your introduction .
You may acknowledge God in your dissertation acknowledgements , but be sure to follow academic convention by also thanking the members of academia, as well as family, colleagues, and friends who helped you.
A literature review is a survey of credible sources on a topic, often used in dissertations , theses, and research papers . Literature reviews give an overview of knowledge on a subject, helping you identify relevant theories and methods, as well as gaps in existing research. Literature reviews are set up similarly to other academic texts , with an introduction , a main body, and a conclusion .
An annotated bibliography is a list of source references that has a short description (called an annotation ) for each of the sources. It is often assigned as part of the research process for a paper .
In a thesis or dissertation, the discussion is an in-depth exploration of the results, going into detail about the meaning of your findings and citing relevant sources to put them in context.
The conclusion is more shorter and more general: it concisely answers your main research question and makes recommendations based on your overall findings.
In the discussion , you explore the meaning and relevance of your research results , explaining how they fit with existing research and theory. Discuss:
- Your interpretations : what do the results tell us?
- The implications : why do the results matter?
- The limitation s : what can’t the results tell us?
Results are usually written in the past tense , because they are describing the outcome of completed actions.
The results chapter of a thesis or dissertation presents your research results concisely and objectively.
In quantitative research , for each question or hypothesis , state:
- The type of analysis used
- Relevant results in the form of descriptive and inferential statistics
- Whether or not the alternative hypothesis was supported
In qualitative research , for each question or theme, describe:
- Recurring patterns
- Significant or representative individual responses
- Relevant quotations from the data
Don’t interpret or speculate in the results chapter.
To automatically insert a table of contents in Microsoft Word, follow these steps:
- Apply heading styles throughout the document.
- In the references section in the ribbon, locate the Table of Contents group.
- Click the arrow next to the Table of Contents icon and select Custom Table of Contents.
- Select which levels of headings you would like to include in the table of contents.
Make sure to update your table of contents if you move text or change headings. To update, simply right click and select Update Field.
All level 1 and 2 headings should be included in your table of contents . That means the titles of your chapters and the main sections within them.
The contents should also include all appendices and the lists of tables and figures, if applicable, as well as your reference list .
Do not include the acknowledgements or abstract in the table of contents.
The abstract appears on its own page in the thesis or dissertation , after the title page and acknowledgements but before the table of contents .
An abstract for a thesis or dissertation is usually around 200–300 words. There’s often a strict word limit, so make sure to check your university’s requirements.
In a thesis or dissertation, the acknowledgements should usually be no longer than one page. There is no minimum length.
The acknowledgements are generally included at the very beginning of your thesis , directly after the title page and before the abstract .
Yes, it’s important to thank your supervisor(s) in the acknowledgements section of your thesis or dissertation .
Even if you feel your supervisor did not contribute greatly to the final product, you must acknowledge them, if only for a very brief thank you. If you do not include your supervisor, it may be seen as a snub.
In the acknowledgements of your thesis or dissertation, you should first thank those who helped you academically or professionally, such as your supervisor, funders, and other academics.
Then you can include personal thanks to friends, family members, or anyone else who supported you during the process.
Ask our team
Want to contact us directly? No problem. We are always here for you.
- Email [email protected]
- Start live chat
- Call +1 (510) 822-8066
- WhatsApp +31 20 261 6040
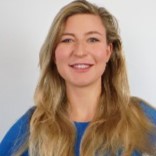
Our team helps students graduate by offering:
- A world-class citation generator
- Plagiarism Checker software powered by Turnitin
- Innovative Citation Checker software
- Professional proofreading services
- Over 300 helpful articles about academic writing, citing sources, plagiarism, and more
Scribbr specializes in editing study-related documents . We proofread:
- PhD dissertations
- Research proposals
- Personal statements
- Admission essays
- Motivation letters
- Reflection papers
- Journal articles
- Capstone projects
Scribbr’s Plagiarism Checker is powered by elements of Turnitin’s Similarity Checker , namely the plagiarism detection software and the Internet Archive and Premium Scholarly Publications content databases .
The add-on AI detector is powered by Scribbr’s proprietary software.
The Scribbr Citation Generator is developed using the open-source Citation Style Language (CSL) project and Frank Bennett’s citeproc-js . It’s the same technology used by dozens of other popular citation tools, including Mendeley and Zotero.
You can find all the citation styles and locales used in the Scribbr Citation Generator in our publicly accessible repository on Github .

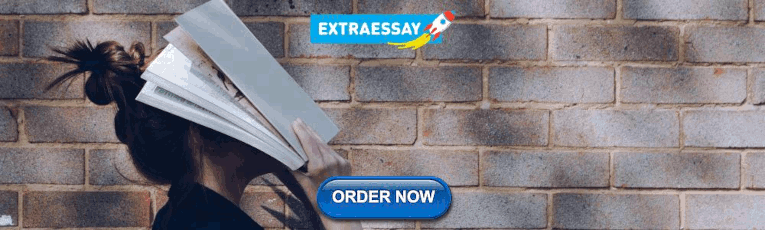
Dissertation Planner - Writing the Results and Discussion: Results and discussion
- Learning from lectures
- Managing your time
- Effective reading
- Evaluating Information
- Critical thinking
- Presentation skills
- Studying online
- Writing home
- Maths and Statistics Support
- Problem solving
- Maths skills by discipline
- Introduction to research skills
- Primary research
- Research methods
- Managing data
- Research ethics
- Searching and Generative AI
- Citing and referencing
- Searching the literature
- What is academic responsibility?
- Referencing software
- Conduct Officer/Panel
- Intellectual property and copyright
- Digital skills home
What’s the difference between the results and discussion of a dissertation? What's the best way to structure them?
- Read our guide on Writing the results and discussion for answers to these questions and more.
- You can also watch the Writing the Dissertation: the Results and Discussion webinar , which covers the overarching purpose of both chapters/sections in the context of the dissertation, defines the relationship between the results and discussion, and establishes a structural template for the discussion.
Look at our Writi ng critically guide for tips on constructing an argument and some of the common mistakes to avoid when interpreting your results.
If you need to interpret, visually present, or otherwise process data in these chapters, our how-to guides and videos on mathematics and statistics might prove useful.
- Last Updated: Aug 14, 2024 11:36 AM
- URL: https://library.soton.ac.uk/sash/dissertation-planner/results-and-discussion

Library Guides
Dissertations 5: findings, analysis and discussion: home.
- Results/Findings
Alternative Structures
The time has come to show and discuss the findings of your research. How to structure this part of your dissertation?
Dissertations can have different structures, as you can see in the dissertation structure guide.
Dissertations organised by sections
Many dissertations are organised by sections. In this case, we suggest three options. Note that, if within your course you have been instructed to use a specific structure, you should do that. Also note that sometimes there is considerable freedom on the structure, so you can come up with other structures too.
A) More common for scientific dissertations and quantitative methods:
- Results chapter
- Discussion chapter
Example:
- Introduction
- Literature review
- Methodology
- (Recommendations)
if you write a scientific dissertation, or anyway using quantitative methods, you will have some objective results that you will present in the Results chapter. You will then interpret the results in the Discussion chapter.
B) More common for qualitative methods
- Analysis chapter. This can have more descriptive/thematic subheadings.
- Discussion chapter. This can have more descriptive/thematic subheadings.
- Case study of Company X (fashion brand) environmental strategies
- Successful elements
- Lessons learnt
- Criticisms of Company X environmental strategies
- Possible alternatives
C) More common for qualitative methods
- Analysis and discussion chapter. This can have more descriptive/thematic titles.
- Case study of Company X (fashion brand) environmental strategies
If your dissertation uses qualitative methods, it is harder to identify and report objective data. Instead, it may be more productive and meaningful to present the findings in the same sections where you also analyse, and possibly discuss, them. You will probably have different sections dealing with different themes. The different themes can be subheadings of the Analysis and Discussion (together or separate) chapter(s).
Thematic dissertations
If the structure of your dissertation is thematic , you will have several chapters analysing and discussing the issues raised by your research. The chapters will have descriptive/thematic titles.
- Background on the conflict in Yemen (2004-present day)
- Classification of the conflict in international law
- International law violations
- Options for enforcement of international law
- Next: Results/Findings >>
- Last Updated: Aug 4, 2023 2:17 PM
- URL: https://libguides.westminster.ac.uk/c.php?g=696975
CONNECT WITH US
- +44 (0) 207 391 9032
Recent Posts
- What Is an Internship? Everything You Should Know
- How Long Should a Thesis Statement Be?
- How to Write a Character Analysis Essay
- Best Colours for Your PowerPoint Presentation: How to Choose
- How to Write a Nursing Essay
- Top 5 Essential Skills You Should Build As An International Student
- How Professional Editing Services Can Take Your Writing to the Next Level
- How to Write an Effective Essay Outline
- How to Write a Law Essay: A Comprehensive Guide with Examples
- What Are the Limitations of ChatGPT?
- Academic News
- Custom Essays
- Dissertation Writing
- Essay Marking
- Essay Writing
- Essay Writing Companies
- Model Essays
- Model Exam Answers
- Oxbridge Essays Updates
- PhD Writing
- Significant Academics
- Student News
- Study Skills
- University Applications
- University Essays
- University Life
- Writing Tips
Dissertation findings and discussion sections
(Last updated: 2 March 2020)
Since 2006, Oxbridge Essays has been the UK’s leading paid essay-writing and dissertation service
We have helped 10,000s of undergraduate, Masters and PhD students to maximise their grades in essays, dissertations, model-exam answers, applications and other materials. If you would like a free chat about your project with one of our UK staff, then please just reach out on one of the methods below.
Granted that at some point in the discussion you are going to have to link back to this previous research. But you still have the opportunity to demonstrate how you have met that coveted gap in the research and generally made a useful contribution to knowledge.
There are many ways to write up both your findings and discussion. In shorter dissertations, it might make sense to have both of these comprise one section. In longer pieces of work, these chapters are usually separate.
Information contained in this section will highlight the finer details of writing up your findings and discussion sections. We will use the model of Description – Analysis – Synthesis , which are typically the three components readers expect to see in these two sections.
Preparing to write
We also assume that you have used some sort of software program to help you with the organisation of your findings. If you have not completed this process, you must do so before beginning to write. If not, your findings chapter may end up a confusing and unorganised mess of random information. If you need help in this area, make sure to seek it out before beginning to put your findings down on paper.
One of the main issues that students tend to encounter when writing up their findings is the amount of data to include. By the end of the research process, you've probably collected very large amounts of data . Not all of this can possibly appear in your dissertation without completely overwhelming the reader. As a result, you need to be able to make smart decisions about what to include and what to leave out.
One of the easiest ways to approach this task is to create an outline. In approaching the outline, it is in your best interest to focus on two key points. Firstly, you need to focus on answering your research questions. Secondly, you must include any particularly interesting findings that have cropped up as you completed your research.
An outline will give you the structure you need, and should make the whole process of presenting your findings easier. We realise that it is going to be a difficult process to pick and choose pieces of data to include. But you must be diligent in the work that you cut out. A findings chapter that is long and confusing is going to put the reader off reading the rest of your work.
Introducing your findings
It can be up to 40% of the total word count within your dissertation writing . This is a huge chunk of information, so it's essential that it is clearly organised and that the reader knows what is supposed to be happening. One of the ways you can achieve this is through a logical and organised introduction.
There are four main components that your introduction should include:
Reminding the reader of what you set out to do
A brief description of how you intend approaching the write up of the results
Placing the research in context
Letting the reader know where they can find the research instruments (i.e. the Appendix)
With a findings chapter, there should be no suspense for the reader. You need to tell them what they need to know right from the beginning. This way, they'll have a clear idea about what is still to come. A good introduction will start by telling the reader where you have come from in the research process and what the outcome was (in a couple of paragraphs or less).
You need to highlight the structure of the chapter (as you generally will do with all chapters) and where the reader might find any further information (e.g. in the appendices).
Organisation of data
This is really going to depend on the type of project you have created .
For example, if you have completed a qualitative research project, you might have identified some key themes within the software program you used to organise your data. In this case, highlighting these themes in your findings chapter may be the most appropriate way to proceed. Not only are you using information that you have already documented, you are telling a story in each of your sections (which can be useful in qualitative research).
But what if you undertook a more quantitative type study? You might be better off structuring your findings chapter in relation to your research questions or your hypotheses. This assumes, of course, that you have more than one research question or hypothesis. Otherwise you would end up just having one really long section.
This brings us to our next student mistake – trying to do too much within one section.
Subheadings are ultimately going to be your friend throughout your dissertation writing . Not only do they organise your information into logical pieces, they give the reader guidelines for where your research might be going. This is also a break for the reader. Looking at pages and pages of text without any breaks can be daunting and overwhelming for a reader. You don't want to overwhelm someone who is going to mark your work and who is responsible for your success (or failure).
When writing your introduction, be clear, organised and methodical. Tell the reader what they need to know and try to organise the information in a way that makes the most sense to you and your project. If in doubt, discuss this with your supervisor before you start writing.
Presentation of qualitative data
If you have conducted things like interviews or observations, you are likely to have transcripts that encompass pages and pages of work.
Putting this all together cohesively within one chapter can be particularly challenging. This is true for two reasons. First, it is always difficult to determine what you are going to cut and/or include. Secondly, unlike quantitative data, it can often be difficult to represent qualitative data through figures and tables, so condensing the information into a visual representation is simply not possible. As a writer, it is important to address both these challenges.
When considering how to present your qualitative data, it may be helpful to begin with the initial outline you have created (and the one described above). Within each of your subsections, you are going to have themes or headings that represent impactful talking points that you want to focus on.
Once you have these headings, it might be helpful to go back to your data and highlight specific lines that can/might be used as examples in your writing. If you have used multiple different instruments to collect data (e.g. interviews and observations), you are going to want to ensure that you are using both examples within each section (if possible). This is so that you can demonstrate to more well-rounded perspective of the points you are trying to make. Once you have identified some key examples for each section, you might still have to do some further cutting/editing.
Once you have your examples firmly selected for each subsection, you want to ensure that you are including enough information. This way, the reader will understand the context and circumstances around what you are trying to ‘prove’. You must set up the examples you have chosen in a clear and coherent way.
Students often make the mistake of including quotations without any other information. It is important that you embed your quotes/examples within your own thoughts. Usually this means writing about the example both before and after. So you might say something like, “One of the main topics that my participants highlighted was the need for more teachers in elementary schools. This was a focal point for 7 of my 12 participants, and examples of their responses included: [insert example] by participant 3 and [insert example] by participant 9. The reoccurring focus by participants on the need for more teachers demonstrates [insert critical thought here]. By embedding your examples in the context, you are essentially highlighting to the reader what you want them to remember.
Aside from determining what to include, the presentation of such data is also essential. Participants, when speaking in an interview might not do so in a linear way. Instead they might jump from one thought to another and might go off topic here and there.
It is your job to present the reader with information on your theme/heading without including all the extra information. So the quotes need to be paired down to incorporate enough information for the reader to be able to understand, while removing the excess.
Finding this balance can be challenging. You have likely worked with the data for a long time and so it might make sense to you. Try to see your writing through the eyes of someone else, which should help you write more clearly.
Presentation of quantitative data
Something to consider first with numeric data is that presentation style depends what department you are submitting to. In the hard sciences, there is likely an expectation of heavy numeric input and corresponding statistics to accompany the findings. In the arts and humanities, however, such a detailed analysis might not be as common. Therefore as you write out your quantitative findings, take your audience into consideration.
Just like with the qualitative data, you must ensure that your data is appropriately organised. Again, you've likely used a software program to run your statistical analysis, and you have an outline and subheadings where you can focus your findings. There are many software programs available and it is important that you have used one that is most relevant to your field of study.
For some, Microsoft Excel may be sufficient for basic analysis. Others may rely on SPSS, Stata, R, or any of the other programs available through your institution or online. Whatever program you have used, make sure that you document what you have done and the variables that have affected your analysis.
One common mistake found in student writing is the presentation of the statistical analysis. During your analysis of the data, you are likely to have run multiple different analyses from regressions to correlations. Often, we see students presenting multiple different statistical analyses without any real understanding of what the tests mean.
Presentation of quantitative data is more than just about numbers and tables. You must explain your findings and justify why you have run/presented the tests that you have. You could also explain how they relate to the research question. However, depending on how you have organised your work, this might end up in the discussion section.
Students who are not confident with statistical analysis often have a tendency to revert back to their secondary school mathematics skills. They commonly document the mean, median, and mode for all of their results. Now, these three outcomes can be important. But having a good understanding of why you are proceeding with this strategy of analysis is going to be essential in a primarily quantitative study.
That noted, there are different expectations for an undergraduate dissertation and a PhD thesis, so knowing what these expectations are can be really helpful before you begin.
Presentation of graphs, tables, and figures
The first is the use of colour and/or variables. Depending on the presentation of your dissertation, you may be required to print out a final copy for the marker(s). In many cases, this final copy must be printed in black and white. This means that any figures or graphs that you create must be readable in a black and white (or greyscale) format.
This can be challenging because there are only so many distinct shades of grey. In a pie chart, you might show one section as purple and the other as green. Yet when printed, both the purple and the green translate to approximately the same shade of grey, making your graph suddenly unreadable.
Another common error is overwhelming the reader with graphs and tables. Let's think about your outline and subheadings. If you're including a table under each subheadings, it needs to be relevant to the information that is being discussed in that chapter. There is no correct or incorrect number of graphs that should exist within the section, but you should use your judgement about what looks appropriate.
The final mistake we see is the duplication of writing (or absence of writing) when presenting a graph. Some students will present their findings in a graph or table and then write out this information again below the graph. This defeats the entire purpose of using the graph in the first place. So avoid this at all times.
Conversely, other students sometimes include a graph or figure but nothing else. Doing this denies the reader of context or purpose of said graph or figure. At some point, a balance needs to be struck where the reader has the information they require to really understand the point being made within the section.
Analysis and synthesis in a discussion
The purpose of a discussion chapter.
The structure of your discussion chapter is really going to depend on what you are trying to do and how you have structured your findings. If you chose to structure your findings by theme, it might make sense to continue this into the analysis chapter.
Other people might structure it according to the research questions. This clearly indicates to the reader how you have addressed your study. Marking a dissertation usually requires the marker to comment on the extent to which the research questions have been addressed. So by structuring a dissertation that lays out each research question for the marker, you are making their job easier. Needless to say, this a great thing.
Like any other chapter in your thesis, an introduction is an essential component of your discussion. By this point, the reader has gone through your findings and is now looking for your interpretation. Therefore, at the end of your discussion introduction you should highlight the content that each of the subsections will cover.
A conclusion to your discussion section (or a chapter summary) is also going to be beneficial. The length of the analysis chapter is usually quite long, so a wrap up of the key points at the end can help the reader digest your work. It can also help ensure that the reader actually understands the points you are trying to highlight within your project.
Critical thinking
Without any critical thinking, you are really doing yourself a disservice. It will affect the mark that you obtain on your overall dissertation. This is why the analysis chapter is usually weighted quite heavily on the marking rubric.
We tell students about critical thinking and the importance of it on a daily basis. And yet, there does seem to be a general confusion about what critical thinking entails, i.e. what constitutes critical thinking versus what is a simple description.
Critical thinking asks you to provide your own opinion on your topic, which can be daunting at first. For much of your academic career, you've likely been asked to use research to justify a position that has already been set. Unlike critical thinking, this requires you to use other people’s ideas. But even if you're new to it, try and get to grips with what critical thinking entails and use it in your work.
Creating sub-sections
Subheadings need to be informative but not too long. It is possible to layer your subheadings, so you might have a Chapter 2, a Section 2.1 and then a 2.1.1 and 2.2.2. Usually anything after 3 numerical points does not get a number and would not appear in your table of contents.
When creating titles for your subheadings, consider how they are going to look in the table of contents. They need to fit on one line, ideally, so putting your research question as the subheading might end up being too long. Conversely, one- or two-word subheadings usually doesn't give enough information about the purpose of the section.
Finding this balance is important. But remember you can always edit your subheadings retrospectively.
Linking to previous chapters
Ideally, you will be able to concisely and effectively link your research to what has been researched previously. But this can be a challenge. You don't want to repeat what has been said in your literature review or the findings . But you need to pull examples from both of these sections in order to make the points that you need to.
So, how do you tackle this?
One way is by referring the reader back to previous chapters, sections, or subsections. This process can generally be done at the end. You can put in a place holder until you know how your sections will be numbered. For example you might write: “In Section XYZ, the theme of … was discussed. Findings from this study indicate…. (see Section XYZ for details)”. While ‘XYZ’ is obviously not going to be the same section, by using the same abbreviation, you can then search ‘XYZ’ after you have completed writing and replace each term with the appropriate number. This also makes the proofreading process easier.
If you are submitting an electronic version of this document, you may also consider hyperlinks to take the reader to the different sections. But be aware that this can be considerably more work, so you should allow for this in your timescale if it's something you wish to implement.
Let's outline the main takeaway points:
It is essential that you keep in mind the ‘describe, analyse, synthesise’ model.
The findings chapter is essentially the describe part. You need to ensure that you have clearly identified data that relates to your research questions, hypotheses, or themes of your study.
For the ‘describe’ component, you are not looking to support your work with other research, but rather to present your contribution. It is also important to consider your data in the ‘describe’ section. If you have qualitative data, ensure that you have edited the quotes and examples to a reasonable length. Pick quotes that accurately represent your theme. Try not to focus solely on one or two participants (if possible). Ensure that you are demonstrating links between multiple instruments, if you used them.
If you are using quantitative data, be careful about how many statistical tests you run. Make sure you can justify why you chose one particular test over another. When presenting graphs, use a colour scheme that's appropriate for the reader when printing in black and white. Ensure that graphs and tables are appropriately explained, but that the information provided is not duplicated.
From the ‘describe’ element, you move into the 'analysis' and 'synthesis'. These parts usually appear in the discussion and ask you to employ your critical thinking skills to demonstrate how your research fits into the bigger picture. It is often the case that your analysis holds the most weight in the marking scheme. So you should spend considerable time ensuring this section is appropriate. It needs to demonstrate how you have attempted to answer your research questions.
Finally, create an outline before you begin. While this might seem tedious at first, filling in the sections with the appropriate information will mean that you are not writing things over and over again. It'll also make sure you do not go wildly off topic. It is always beneficial to have a second set of eyes assess your work for any errors or omissions. Many students choose to contact professional editors to help with this as they hold the relevant expertise to guide you on the correct path to creating a perfect discussion section that is ready for submission.
In terms of presentation, both the findings and discussion chapters will benefit from a clear and logical introduction and chapter summary. Remember that both of these chapters are meant to inform. You are leading the reader on a journey, so make sure they stay on the path and arrive at the final destination with you!
Writing your dissertation methodology
10 tips on writing a dissertation literature review, dissertation introduction, conclusion and abstract.
- dissertation chapters
- dissertation discussion
- dissertation findings
- dissertation help
- dissertation writing service
- study skills
- writing tips
Writing Services
- Essay Plans
- Critical Reviews
- Literature Reviews
- Presentations
- Dissertation Title Creation
- Dissertation Proposals
- Dissertation Chapters
- PhD Proposals
- Journal Publication
- CV Writing Service
- Business Proofreading Services
Editing Services
- Proofreading Service
- Editing Service
- Academic Editing Service
Additional Services
- Marking Services
- Consultation Calls
- Personal Statements
- Tutoring Services
Our Company
- Frequently Asked Questions
- Become a Writer
Terms & Policies
- Fair Use Policy
- Policy for Students in England
- Privacy Policy
- Terms & Conditions
- [email protected]
- Contact Form
Payment Methods
Cryptocurrency payments.

Free Download
Discussion Chapter Template
The fastest (and smartest) way to craft a strong discussion section for your dissertation, thesis or research project.
Available in Google Doc, Word & PDF format 4.9 star rating, 5000 + downloads
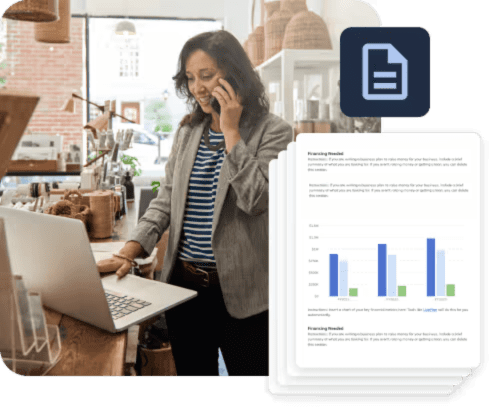
Step-by-step instructions
Tried & tested academic format
Fill-in-the-blanks simplicity
Pro tips, tricks and resources
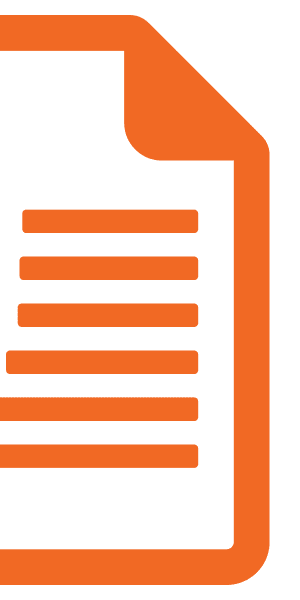
What It Covers
This template covers all the core components required in the discussion chapter (or section) of a typical dissertation or thesis, including:
- The opening/ overview section
- Overview of key findings
- Interpretation of the findings
- Concluding summary
The purpose of each section is explained in plain language, followed by an overview of the key elements that you need to cover. The template also includes practical examples to help you understand exactly what’s required, along with links to additional free resources (articles, videos, etc.) to help you along your research journey.
The cleanly formatted Google Doc can be downloaded as a fully editable MS Word Document (DOCX format), so you can use it as-is or convert it to LaTeX.
download your copy
100% Free to use. Instant access.
I agree to receive the free template and other useful resources.
Download Now (Instant Access)

FAQs: Thesis Discussion Template
Faq: thesis discussion template, what types of dissertations/theses can this template be used for.
The discussion chapter template follows the standard format for academic research projects, which means it will be suitable for the majority of dissertations, theses and research projects (especially those within the sciences).
Keep in mind that the exact requirements for the discussion chapter/section will vary between universities and degree programs. For example, your university may require that the discussion chapter and conclusion chapter are merged into one, or that the results and discussion are covered together (this is often the case with qualitative research). So, be sure to double-check your university’s requirements before you finalise your structure.
Is this template for an undergrad, Master or PhD-level thesis?
This template can be used for a dissertation, thesis or research project at any level of study. Doctoral-level projects typically require the discussion chapter to be more extensive/comprehensive, but the structure will typically remain the same. Again, be sure to check your university’s requirements and norms in terms of document structure.
How long should the discussion chapter be?
This can vary a fair deal, depending on the level of study (undergrad, Master or Doctoral), the field of research, as well as your university’s specific requirements. Therefore, it’s best to check with your university or review past dissertations from your program to get an accurate estimate.
Can I share this template with my friends/colleagues?
Yes, you’re welcome to share this template in its original format (no editing allowed). If you want to post about it on your blog or social media, please reference this page as your source.
What format is the template (DOC, PDF, PPT, etc.)?
The dissertation discussion chapter template is provided as a Google Doc. You can download it in MS Word format or make a copy to your Google Drive. You’re also welcome to convert it to whatever format works best for you, such as LaTeX or PDF.
Do you have templates for the other chapters?
Yes, we do. We are constantly developing our collection of free resources to help students complete their dissertations and theses. You can view all of our template resources here .
Can Grad Coach help me with my discussion/analysis?
Yes, we can provide coaching-based assistance with your discussion chapter (or any other chapter). If you’re interested, get in touch to discuss our private coaching services .
Additional Resources
If you’re working on a dissertation or thesis, you’ll also want to check these out…
1-On-1 Private Coaching
Research Bootcamps
The Grad Coach YouTube Channel
The Grad Coach Podcast
Training videos | Faqs

Academic Phrases for Writing Results & Discussion Sections of a Research Paper
Overview | Abstract | Introduction | Literature Review | Materials & Methods | Results & Discussion | Conclusion & Future Work | Acknowledgements & Appendix
The results and discussion sections are one of the challenging sections to write. It is important to plan this section carefully as it may contain a large amount of scientific data that needs to be presented in a clear and concise fashion. The purpose of a Results section is to present the key results of your research. Results and discussions can either be combined into one section or organized as separate sections depending on the requirements of the journal to which you are submitting your research paper. Use subsections and subheadings to improve readability and clarity. Number all tables and figures with descriptive titles. Present your results as figures and tables and point the reader to relevant items while discussing the results. This section should highlight significant or interesting findings along with P values for statistical tests. Be sure to include negative results and highlight potential limitations of the paper. You will be criticised by the reviewers if you don’t discuss the shortcomings of your research. This often makes up for a great discussion section, so do not be afraid to highlight them.
The results and discussion section of your research paper should include the following:
- Comparison with prior studies
- Limitations of your work
- Casual arguments
- Speculations
- Deductive arguments
1. Findings
From the short review above, key findings emerge: __ We describe the results of __, which show __ This suggests that __ We showed that __ Our findings on __ at least hint that __ This is an important finding in the understanding of the __ The present study confirmed the findings about __ Another promising finding was that __ Our results demonstrated that __ This result highlights that little is known about the __ A further novel finding is that __ Together, the present findings confirm __ The implications of these findings are discussed in __ The results demonstrate two things. First, __. Second, __ The results of the experiment found clear support for the __ This analysis found evidence for __ Planned comparisons revealed that __ Our results casts a new light on __ This section summarises the findings and contributions made. It performs well, giving good results. This gives clearly better results than __ The results confirm that this a good choice for __ From the results, it is clear that __ In this section, we will illustrate some experimental results. This delivers significantly better results due to __ The result now provides evidence to __ It leads to good results, even if the improvement is negligible. This yields increasingly good results on data. The result of this analysis is then compared with the __ The applicability of these new results are then tested on __ This is important to correctly interpret the results. The results are substantially better than __ The results lead to similar conclusion where __ Superior results are seen for __ From these results it is clear that __ Extensive results carried out show that this method improves __ We obtain good results with this simple method. However, even better results are achieved when using our algorithm. It is worth discussing these interesting facts revealed by the results of __ Overall, our method was the one that obtained the most robust results. Slightly superior results are achieved with our algorithm. The result is equal to or better than a result that is currently accepted.
2. Comparison with prior studies
The results demonstrated in this chapter match state of the art methods. Here we compare the results of the proposed method with those of the traditional methods. These results go beyond previous reports, showing that __ In line with previous studies __ This result ties well with previous studies wherein __ Contrary to the findings of __ we did not find __ They have demonstrated that __ Others have shown that __ improves __ By comparing the results from __, we hope to determine __ However, in line with the ideas of __, it can be concluded that __ When comparing our results to those of older studies, it must be pointed out that __ We have verified that using __ produces similar results Overall these findings are in accordance with findings reported by __ Even though we did not replicate the previously reported __, our results suggest that __ A similar conclusion was reached by __ However, when comparing our results to those of older studies, it must be pointed out __ This is consistent with what has been found in previous __ A similar pattern of results was obtained in __ The findings are directly in line with previous findings These basic findings are consistent with research showing that __ Other results were broadly in line with __
3. Limitations of your work
Because of the lack of __ we decided to not investigate __ One concern about the findings of __ was that __ Because of this potential limitation, we treat __ The limitations of the present studies naturally include __ Regarding the limitations of __, it could be argued that __ Another limitation of this __ This limitation is apparent in many __ Another limitation in __ involves the issue of __ The main limitation is the lack of __ One limitation is found in this case. One limitation of these methods however is that they __ It presents some limitations such as __ Although widely accepted, it suffers from some limitations due to __ An apparent limitation of the method is __ There are several limitations to this approach. One limitation of our implementation is that it is __ A major source of limitation is due to __ The approach utilised suffers from the limitation that __ The limitations are becoming clear __ It suffers from the same limitations associated with a __
4. Casual arguments
A popular explanation of __ is that __ It is by now generally accepted that __ A popular explanation is that __ As it is not generally agreed that __ These are very small and difficult to observe. It is important to highlight the fact that __ It is notable that __ An important question associated with __ is __ This did not impair the __ This is important because there is __ This implies that __ is associated with __ This is indicative for lack of __ This will not be biased by __ There were also some important differences in __ It is interesting to note that, __ It is unlikely that __ This may alter or improve aspects of __ In contrast, this makes it possible to __ This is particularly important when investigating __ This has been used to successfully account for __ This introduces a possible confound in __ This was included to verify that __
5. Speculations
However, we acknowledge that there are considerable discussions among researchers as to __ We speculate that this might be due to __ There are reasons to doubt this explanation of __ It remains unclear to which degree __ are attributed to __ However, __ does seem to improve __ This does seem to depend on __ It is important to note, that the present evidence relies on __ The results show that __ does not seem to impact the __ However, the extent to which it is possible to __ is unknown Alternatively, it could simply mean that __ It is difficult to explain such results within the context of __ It is unclear whether this is a suitable for __ This appears to be a case of __ From this standpoint, __ can be considered as __ To date, __remain unknown Under certain assumptions, this can be construed as __ Because of this potential limitation, we treat __ In addition, several questions remain unanswered. At this stage of understanding, we believe__ Therefore, it remains unclear whether __ This may explain why __
6. Deductive arguments
A difference between these __ can only be attributable to __ Nonetheless, we believe that it is well justified to __ This may raise concerns about __ which can be addressed by __ As discussed, this is due to the fact that __ Results demonstrate that this is not necessarily true. These findings support the notion that __ is not influenced by __ This may be the reason why we did not find __ In order to test whether this is equivalent across __, we __ Therefore, __ can be considered to be equivalent for __
Similar Posts
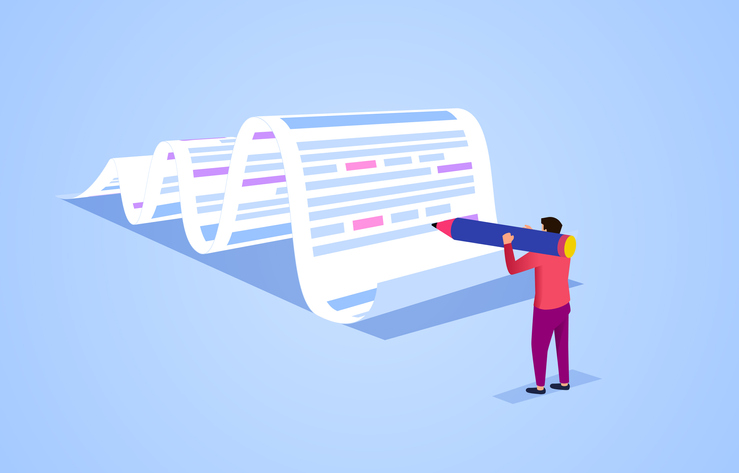
Academic Phrases for Writing Conclusion Section of a Research Paper
In this blog, we discuss phrases related to conclusion section such as summary of results and future work.
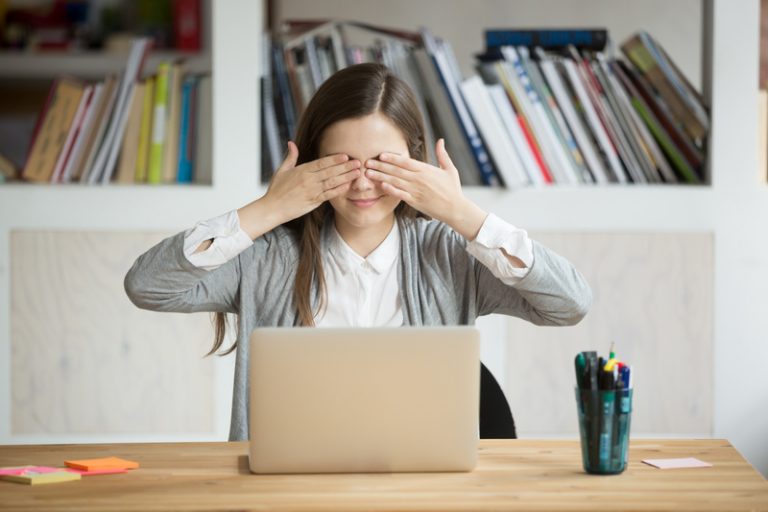
How to Write a Research Paper? A Beginners Guide with Useful Academic Phrases
This blog explains how to write a research paper and provides writing ideas in the form of academic phrases.

Academic Phrases for Writing Literature Review Section of a Research Paper
In this blog, we discuss phrases related to literature review such as summary of previous literature, research gap and research questions.
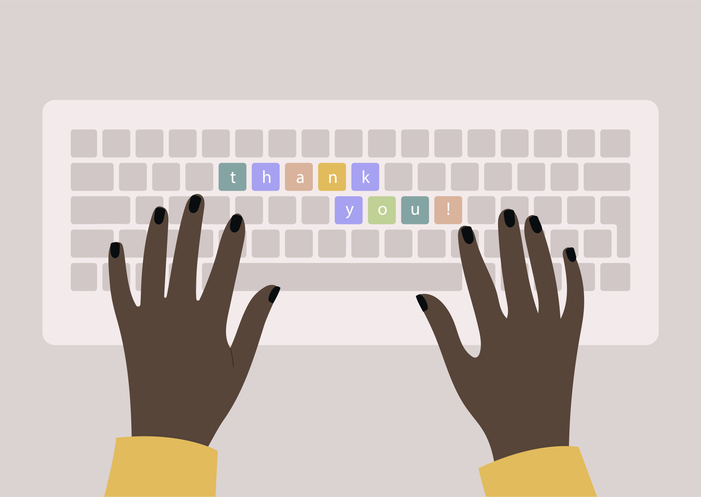
Academic Phrases for Writing Acknowledgements & Appendix Sections of a Research Paper
In this blog, we discuss phrases related to thanking colleagues, acknowledging funders and writing the appendix section.
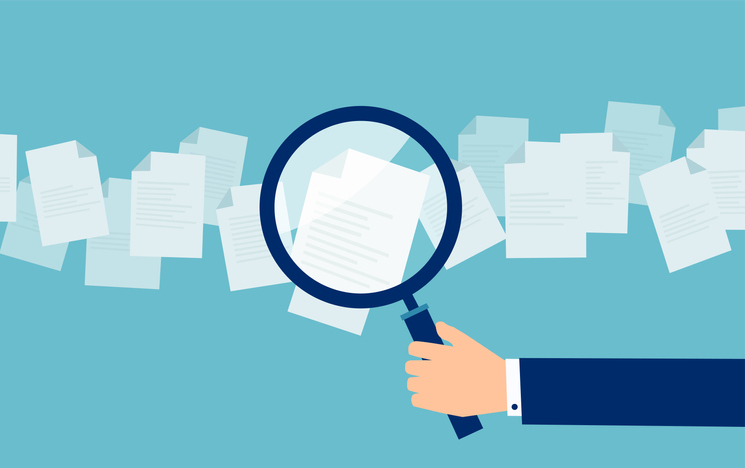
Academic Phrases for Writing Abstract Section of a Research Paper
In this blog, we discuss phrases related to the abstract section. An abstract is a self-contained and short synopsis that describes a larger work.

Academic Writing Resources – Academic PhraseBank | Academic Vocabulary & Word Lists
In this blog, we review various academic writing resources such as academic phrasebank, academic wordlists, academic vocabulary training sites.
32 Comments
Awesome vocab given, I am really thankful. keep it up!
Why didn’t I find this earlier? Thank you very much! Bless your soul!
thank you!! very useful!!!
Thank you, thank you thank you!!
I’m currently writing up my PhD thesis and as a non-native English speaker, I find this site extremely useful. Thanks for making it!
Very ve4y resourceful..well done Sam
Plesse add me to your mailing list Email: [email protected]
Hi, would like to clarify if that is “casual” or “causal”? Thanks!
Hi there, it should read “causal.”
Thanx for this. so helpful!
Very helpful. Thanks
thank you so much
- Pingback: Scholarly Paraphrasing Tool and Essay Rewriter for Rewording Academic Papers - Ref-N-Write: Scientific Research Paper Writing Software Tool - Improve Academic English Writing Skills
thankyouuuuuu
thank you very much
wow thanks for the help!!
Quite interesting! Thanks a lot!
This is ammmaazzinggg, too bad im in my last year of university this is very handy!!!
Extremely Useful. Thank-you so much.
This is an excellent collection of phrases for effective writing
Thank you so much, it has been helpful.
I found it extremely important!!!
It is a precise, brief and important guides;
It is a very important which gives a guide;
It is a very important guiding explanation for writing result and discussion;
It is a very important guiding academic phrases for writing;
thank you so much.I was in need of this.
- Pingback: Research Paper Structure – Main Sections and Parts of a Research Paper
Thank you so much!!! They are so helpful!
thank its very important.
This is timely, I needed it. Thanks
This is very helpful. Thanks.
You saved my Bachoelor thesis! Huge thanks
Leave a Reply Cancel reply
Your email address will not be published. Required fields are marked *
Save my name, email, and website in this browser for the next time I comment.
- 91 Share Facebook
- 68 Share Twitter
- 53 Share LinkedIn
- 0.1K Share Email
Thank you for visiting nature.com. You are using a browser version with limited support for CSS. To obtain the best experience, we recommend you use a more up to date browser (or turn off compatibility mode in Internet Explorer). In the meantime, to ensure continued support, we are displaying the site without styles and JavaScript.
- View all journals
- Explore content
- About the journal
- Publish with us
- Sign up for alerts
- Open access
- Published: 30 August 2024
A deep generative modeling architecture for designing lattice-constrained perovskite materials
- Ericsson Tetteh Chenebuah ORCID: orcid.org/0000-0003-2878-3484 1 , 2 ,
- Michel Nganbe 1 &
- Alain Beaudelaire Tchagang 1 , 2
npj Computational Materials volume 10 , Article number: 198 ( 2024 ) Cite this article
Metrics details
- Computational methods
- Materials for energy and catalysis
In modern materials discovery, materials are now efficiently screened using machine learning (ML) techniques with target-specific properties for meeting various engineering applications. However, a major challenge that persists with deep generative ML approach is the issue related to lattice reconstruction at the decoding phase, leading to the generation of materials with low symmetry, unfeasible atomic coordination, and triclinic behavioral properties in the crystal lattice. To address this concern, the present research makes a contribution by proposing a Lattice-Constrained Materials Generative Model (LCMGM) for designing new and polymorphic perovskite materials with crystal conformities that are consistent with predefined geometrical and thermodynamic stability constraints at the encoding phase. A comparison with baseline models such as Physics Guided Crystal Generative Model (PGCGM) and Fourier-Transformed Crystal Property (FTCP), confirms the potential of the LCMGM for improved training stability, better chemical learning effect and higher geometrical conformity. The new materials emerging from this research are Density Functional Theory (DFT) validated and openly made available in the Mendeley data repository: https://doi.org/10.17632/m262xxpgn2.1 .
Similar content being viewed by others
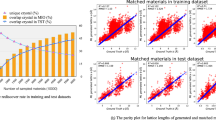
Physics guided deep learning for generative design of crystal materials with symmetry constraints
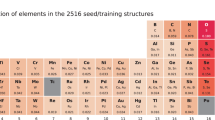
Data-driven discovery of 2D materials by deep generative models
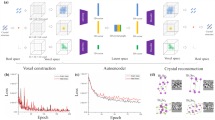
Constrained crystals deep convolutional generative adversarial network for the inverse design of crystal structures
Introduction.
The discovery of novel materials with phenomenal target properties is a major driving force in advancing technological innovation. Recent advances have shown that deep generative machine learning techniques are better alternatives over traditional first-principles and/or Edisonian-based synthetic chemistries for novel materials discovery. Famous for their speed, reliability, and low cost, Deep Generative Models (DGM) can intelligently identify hidden patterns and correlations in a training dataset by solving an inverse design scheme. Given a predefined target property of interest, the inverse design scheme seeks to find the corresponding unknown material, which is represented in the form of constitutive atoms and unit cell geometry 1 . Examples of two common DGM techniques with proven applications in materials discovery include Variational Autoencoders (VAE) 2 and Generative Adversarial Networks (GAN) 3 . VAEs are based on Bayes theorem and operate by transforming data into a smooth and continuous Gaussian latent space where vectorial or semantic sampling exploration can be smartly conducted for new materials generation. On the other hand, GANs are fundamentally different and involve adversarial interests between two interconnected models, which are usually in the form of a generative model and a critic/discriminative model. By competing against each other, the generative model learns the underlying variability within the training data distribution, and hence, recommends new material candidates that are unique but share common characteristics with the training data examples. In several works of literature, the practicality of VAE and GAN have been demonstrated for screening stable and synthesizable materials 4 , 5 , 6 . For instance, the iMatGen is considered as the pioneering study that first demonstrated the applicability of VAE in the computational prediction of new material compositions. Using a three-dimensional grid of image-based representation, the iMatGen was able to screen over 20,000 vanadium-oxide materials, which were all embedded within a target-learnable latent space of a VAE model 4 . Likewise, a Fourier-Transformed Crystal Property (FTCP) representation was developed from a VAE model and was used to discover general inorganic crystals that are characterized by their high chemical stability. The FTCP strongly relies on a target-structured latent space, in addition to local perturbative sampling operations for promoting higher exploitation (i.e. chemical validity) at the expense of exploration (i.e. chemical variety) 5 . In the Evolutionary Variational Autoencoder model for Perovskite Discovery (EVAPD), a genetic algorithm was hybridized with a semi-supervised VAE (SS-VAE) model, and was shown to generate host perovskite candidates for photovoltaic and/or optoelectronic applications 6 . A common trait with the aforementioned VAE studies is in the conceptualization of a hyper-dimensionally reduced latent space of the original data, which can be organized in a semi-supervisory manner for target-specific search operations. Furthermore, considering the contributions made by GAN models, a CubicGAN was designed for screening new material prototypes of the cubic crystal system using implicit chemical rules from space group and elemental embedding 7 . Bismuth-selenide materials were inversely designed using a constrained crystals deep convolutional generative adversarial network (CCDCGAN) architecture, which was optimized on the formation energy stability parameter 8 . In a different study, the MatGAN was designed using an improvised Wasserstein GAN (WGAN) 9 and was demonstrated to generate charge-neutral and electronegativity-balanced materials even though no chemical rules were directly enforced in the modeling architecture 10 . In the Physics Guided Crystal Generative Model (PGCGM), a semi-supervisory GAN was proposed for predicting diverse and stable inorganic materials based on customized oriented losses on the structural symmetry 11 . These studies and many more illustrate the strong capability of DGM techniques for screening novel materials with promising opportunities for further improvements in future design architectures and sampling strategies.
Although DGMs have contributed significantly in the field of novel materials discovery, they also face several challenges and issues that limit their modeling capabilities. For instance, a general concern with VAE models is in their repercussive decoding performance for input feature embedding that are not in discrete or one-hot encoded forms, such as lattice parameters and atomic coordination. This deficiency is evident in VAE-designed FTCP and EVAPD, as both models report unavoidable lattice reconstruction errors at the decoding phase, translating into the screening of new materials that are characterized by their high asymmetrisation (i.e. low symmetry), unfeasible atomic coordination, and triclinic behavioral properties. Materials with such high level of lattice asymmetrisation are structurally complex, anisotropic, and difficult to index in powder diffraction experiments 12 . A possible solution to this challenge could be by thoroughly discretizing all feature embedding to minimize modeling training errors, ultimately reflecting in improved decoding accuracy. However, such approach could be detrimental to the model’s assimilation, as unit cell information that is variably distributed is bound to be under-categorized and/or poorly described if represented in discrete forms. Another interesting solution could be by extending the descriptor design to incorporate space group affine transformative features for constraining the lattice. Such method was proposed by the PGCGM 11 and was found to achieve great success in the generation of high precision crystals with fractional coordination and lattice parameters that are physically constrained. However, the PGCGM may potentially be prone to training instabilities related to posterior and mode collapse 13 , 14 , which is a consequence of its singular reliance on the auxiliary conditional GAN.
To address the identified challenges, the current study proposes a Lattice-Constrained Materials Generative Model (LCMGM) by leveraging on the technical strengths of both a semi-supervisory VAE (SS-VAE) model 15 and an auxiliary GAN (A-GAN) model 16 , 17 . The modeling architecture is comprised of three phases for efficiently designing materials with crystal lattice conformities that are consistent with predefined symmetrical constraints at the encoding phase. The first modeling phase incorporates the SS-VAE model for discretely encoding the training data into a latent space that is effectively organized on primitive Bravais crystal systems and stability criterion in the formation energy. The second phase hybridizes the A-GAN model for exploring the chemical combinatorial design space as it explicitly learns the geometrical constraints (in terms of lattice and motifs) of the encoded SS-VAE features. By concentrating specifically on encoded representations, the A-GAN operates similarly to an explorative sampling approach, but with greater training stability due to the simplified representation of data points for learning geometrical behaviors. Moreover, the current study focusses on the generation of novel perovskites due to their interesting chemical diversity, appealing properties and multifunctionality for many engineering applications. Ideally, the ternary/single \(\,{{ABX}}_{3}\) perovskite can be geometrically described as a perfect \({Pm}\bar{3}{m}\) cubic crystal structure with the \(B\) -site cation situated at the center of a six-fold \(\,{{BX}}_{6}\) octahedron, whereas the \({A}\) -site cation occupies a twelve-fold cavity, as coordinated by \({X}\) -site anions within a three-dimensional polyhedral setting 18 . The double \({A}_{2}B{B}^{{\prime} }{X}_{6}\) perovskite can be seen as a higher derivative of the \(\,{{ABX}}_{3}\) , and is formed due to cationic displacements and/or local ionic-site sharing at the \(B\) -site of a perovskite crystal structure 19 , 20 , 21 . Statistically, the design space afforded by perovskite multi-stoichiometries is indeed very large, as random chemical elements across the periodic table are allowed to occupy distinctive ionic sites within the perovskite crystal lattice. For instance, roughly permuting the 94 naturally occurring chemical elements while mindful of the possibilities of charge imbalances from Jahn-Teller electronic instabilities 21 and ionic-swapping antiperovskite forms 22 , could potentially result into \({{\mathbb{C}}}_{3}^{94}=\mathrm{134,044}\,\) and \(\,{{\mathbb{C}}}_{4}^{94}=\mathrm{3,049,501}\,\) unique inorganic compounds that adopt the \(\,{{ABX}}_{3}\,\) and \(\,{A}_{2}B{B}^{{\prime} }{X}_{6}\,\) perovskite stoichiometries, respectively. Besides, this rough estimation does not additionally consider more complex perovskite forms such as the 2D Ruddlesden-Popper structures 23 , 24 , 25 , complex stoichiometrical derivatives 26 , hybrid organic-inorganic compositions 27 , 28 , and polymorphic variants 29 . With the number of unknown perovskite compounds far exceeding known compounds, the developed LCMGM design can be seen as a pragmatic tool for accelerating the search of unknown perovskite compositions. In the current study, the LCMGM is demonstrated for designing unique and polymorphic chemistries, as well as stable and charge-balanced inorganic perovskites that adopt the ternary \({{ABX}}_{3}\) and the quaternary \({A}_{2}B{B}^{{\prime} }{X}_{6}\) stoichiometries. The perovskite discovery process is conditioned to conform to a specific crystal system of interest, as predefined by induced geometrical constraints at the encoding phase. In the third modeling phase, the new materials are pre-optimized using Bayesian optimization 30 and are further validated via first-principles Density Functional Theory (DFT) calculations 31 , 32 .
Data preprocessing
The present research uses data from the Open Quantum Materials Database (OQMD) ( v1.0 ) 33 and Materials Project (MP) ( v2021.05.13 ) 34 . Both databases are queried to retrieve crystal structures that generally adopt the \({{ABX}}_{3}\,\) and \(\,{A}_{2}B{B}^{{\prime} }{X}_{6}\,\) perovskite stoichiometries. For modeling lattice constraints, conventional cells are preferred over primitive cells, as the former provide higher symmetrical representation for better alignment with experimental physical data (e.g. XRD data). This is essential for comprehensively learning the periodic behavior of the crystal structure 12 . For all samples, the conventional unit cells are processed using the SpacegroupAnalyzer of the pymatgen.symmetry.analyzer module 35 , 36 . The processed dataset contains only Bravais crystal systems with significant sample sizes for training. They include cubic, monoclinic, orthorhombic, tetragonal and trigonal systems. For hexagonal and triclinic systems that are not considered in this study, the LCMGM can equally be used to simulate their lattice properties provided sufficient training data are used in the training process. Overall, the data extraction and cleaning process resulted in 63,016 samples from the OQMD and 6,540 samples from MP. The datasets are not mixed due to the non-negligible differences at which both databases perform simulation for obtaining total energies using first-principles calculations 37 . Instead, a transfer learning approach is adopted by training and validating the model with the more numerous OQMD samples, and then using the transferable knowledge to learn the distribution of the higher-fidelity MP test set for evaluating the performance of the model and generating new compounds. More details on data curation and statistics, as well as the contribution of each considered crystal system and their respective stoichiometries, are provided in Methods.
Invertible mesh-grid descriptor design
For generative modeling, materials descriptors are expected to be two-way in form and application 1 , 4 , 5 , 6 , 7 , 8 , 10 , 11 . The two-way analogy refers to a descriptor’s capability in accurately predicting interested targets (i.e. forward design), while simultaneously being recoverable/invertible after passing through a machine learning pipeline (i.e. inverse design). In the present study, a user-interpretable perovskite descriptor is designed that projects input feature embedding into a discretized mesh-grid array of image pixels. Such mesh-grid concept was previously implemented in the iMatGen study for screening binary vanadium-oxide materials 4 but not for perovskites to the best of our knowledge. Hence, suitable modifications are made for adopting the descriptor concept to \(\,{{ABX}}_{3}\,\) and \(\,{A}_{2}B{B}^{{\prime} }{X}_{6}\,\) perovskite stoichiometries, while at the same time including diffraction fingerprints for adequately modeling the periodic behavior of a reciprocal crystal lattice 5 , 38 , 39 . The mesh-grid design consists of three feature arrays that are all one-hot encoded, namely: label mesh, property mesh and X-Ray Diffraction (XRD) mesh. The label mesh serves the purpose of identification by accommodating the atomic numbers of distinct atoms in the conventional unit cell, the type of stoichiometry, and the number of atoms. The property mesh on the other hand contains the discretized thermochemistry features of distinct atoms in the respective cell, and assists in the forward modeling of interested target properties 40 , 41 , 42 . Finally, the XRD mesh fingerprints computes diffraction patterns by taking into account the Fourier-transformed atomic scattering factor and the Lorentz polarization factor in the reciprocal space of a crystal lattice. The XRD mesh is crucial in modeling the lattice constraints, as it provides relevant information related to the Bravais crystal systems. The patterns are determined using the XRDCalculator of the pymatgen.analysis.diffraction module 36 , 43 . As a result, each mesh-grid feature is projected onto a 32 × 32 two-dimensional array, and assembling all meshes together produces a 32 × 32 × 3 invertible RGB image, which serves as input into the SS-VAE model. As an example, Fig. 1A illustrates a ball-and-stick chemical model of La 2 VReO 6 double perovskite belonging to the \({Fm}\bar{3}{m}\) cubic system 44 . The corresponding XRD pattern and mesh-grid feature projections are depicted in Fig. 1B , C , respectively. The fully-enmeshed representation in RGB image format is illustrated in Fig. 1D revealing the displacement of pixels across overlaid meshes. Further details about the mesh-grid descriptor design are provided in the Supplemental Information .
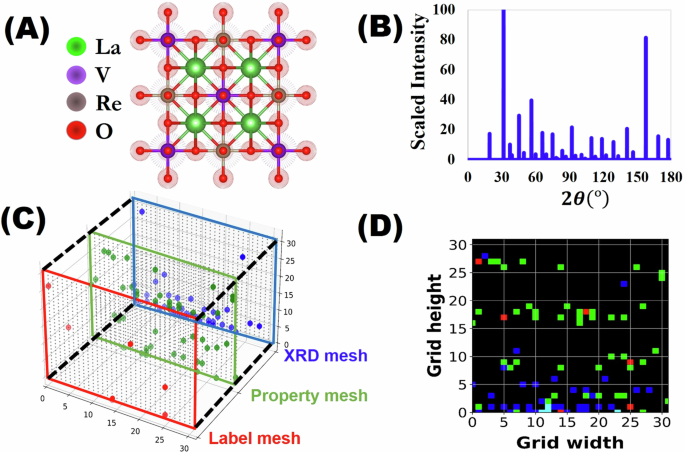
A Chemical model of La 2 VReO 6 double perovskite; ( B ) Computed XRD pattern; ( C ) Mesh-grid projections of input features embedding; ( D ) Fully-enmeshed RGB image format.
Simulation phases in the Lattice-Constrained Materials Generative Model (LCMGM)
As illustrated in Fig. 2 , designing new perovskites with the LCMGM can be broken down into three phases, namely: semi-supervised variational autoencoder (SS-VAE) training phase; auxiliary generative adversarial network (A-GAN) training phase; and geometrical optimization phase. The SS-VAE 15 is a variant of the traditional VAE 2 that integrates a supervised learning algorithm for organizing the latent space. The SS-VAE learns the structure-to-property relationship of the perovskite design space by projecting fully-enmeshed perovskites \(\,\left\{{x}_{i}\right\}\subseteq X\in {\left\{\mathrm{0,1}\right\}}^{R}\) onto a smooth and continuous Gaussian latent space \(\,\left\{{\vec{z}}_{i}\right\}\subseteq \vec{Z}\in {{\mathbb{R}}}^{Q}\) , whereby \(Q\,\ll\, {R}\) denotes dimensionality reduction or feature extraction. By coupling dual Multi-Layer Perceptron (MLP) networks for regressively predicting the formation energy target ( \({E}_{f}\) ) and multi-classifying crystal systems ( \({C}_{s}\) ), regions of interest in the latent space can be visibly identified for screening target materials. Statistically, the interested region can be described as the specific location in hyperdimension where the probability of finding highly stable perovskite data points, which are of the intentional crystal system, is relatively highest. Highly stable perovskites are defined as compounds with formation energy at \(\,{E}_{f}\) ≤ −1.5 eV/atom, as motivated by the FTCP study 5 for designing highly stable photovoltaic materials. It shall be noted that chemical compounds with negative formation energy, which means below a threshold value of zero ( \({E}_{f}\) < 0 eV) are generally considered energetically favorable or stable. By applying a negative, more stringent formation energy threshold, the current study ensures that the model captures only highly stable candidates in the generative inverse design. The dimensionally reduced data points ( \(\vec{z}\) ) within the interested region are extracted and fed to the A-GAN for further analysis. The present research prefers variational autoencoders over simple autoencoders for dimensional reduction in order to take advantage of the KL divergence loss term 45 for regularizing the latent space. This ensures that the latent space distribution is theoretically meaningful and continuous, as the data points are well clustered and organized. Moreover, the A-GAN 16 , 17 is a variant of the traditional GAN model 3 that incorporates an auxiliary predictive model at the discriminator’s branch for regularizing the generator’s assimilation rate. In the present research, the A-GAN is used to generate synthetic latent space vectors (i.e. \(\,{\vec{z}}^{* }\Rightarrow {{\mathbb{R}}}^{Q}\) ) that are of close similarity with their extracted ( \(\vec{z}\) ) SS-VAE counterparts. In addition, the auxiliary model simulates the geometrical constraints by predicting the lattice features (i.e. \({L}_{p}\) and \(\,{\vec{R}}_{\left(x,y,z\right)}\) ) of the original \(\vec{z}\) representations. Therefore, in addition to generating \(\,{\vec{z}}^{* }\) , the A-GAN implicitly learns their corresponding lattice constraints from randomly sampled forms by mapping \(\,{\vec{z}}^{* }\) to their synthetic lattice parameters (i.e. \(\,{L}_{p}^{* }=[a,b,{c;}\,\alpha ,\beta ,\gamma]\in {{\mathbb{R}}}^{\left(2\times 3\right)}\) ) and three-dimensional atomic coordination ( \({\vec{R}}_{\left(x,y,z\right)}^{* }\in {{\mathbb{R}}}^{\left(40\times 3\right)}\) ) as unknown target properties. Besides, an underlining advantage with the present modeling approach is in the autonomous learning of the geometrical features by the discriminator without the need of providing direct side information as inputs. That is to say, unlike the typical conditional-GAN architecture that directly inputs priori knowledge in the form of images (in this case encoded vectors) and labels (lattice features) into the discriminator 46 , the present A-GAN only supplies images as input. The discriminator therefore serves the purpose of assessing the authenticity of the image, as well as predicting their corresponding labels. In the final phase of learning, the synthetic latent space vectors ( \({\vec{z}}^{* }\) ) are decoded to produce de novo chemical compositions \(\,\left\{{x}_{i}^{* }\right\}\subseteq {X}^{* }\in {\left\{\mathrm{0,1}\right\}}^{R}\) that adopt the \({{ABX}}_{3}\) and \({A}_{2}B{B}^{{\prime} }{X}_{6}\) perovskite stoichiometries. The decoded perovskites are thus accompanied by their learnt lattice and coordination features, and are screened primarily based on two criteria: (1) charge neutrality as it relates to common and uncommon oxidation states from the revised Shannon ionic radii data 47 ; and (2) thermodynamic/energetic stability. In general, charge-balanced compounds are proven to be more stable due to reduced electronic or Coulombic interaction of constitutive ions at the atomic level 48 . In order not to omit any potentially intriguing compound, the predictive threshold for selecting stable compounds is relaxed to include all screened candidates with negative formation energy values. In the final modeling phase, all screened chemical compositions ( \({X}^{* }\) ) and their corresponding lattice features ( \({L}_{p}^{* }\,\) and \(\,{\vec{R}}_{\left(x,y,z\right)}^{* }\) ) are passed through two geometrical optimization stages to ascertain their chemical and geometrical validity. The first stage involves Bayesian Optimization (BO) for performing pre-Density Functional Theory (DFT) relaxation. In principle, BOs are powerful active learning techniques that can be used to find the global solution of an unknown objective function via the approximation of surrogate Gaussian Process models 30 . They have been utilized in the field of computational materials informatics for solving several optimization challenges 49 , 50 . In the present research, the BO algorithm time- and cost-efficiently determines the optimized lattice configuration (i.e. pre-relaxed state) that best captures the lowest DFT-predicted total energy ( \({E}_{{tot}}\) ) of the screened candidates. As for the second optimization stage, actual DFT validation is performed on the BO pre-relaxed candidates using the Quantum Espresso (QE) software package 51 . QE implements thorough DFT stress relaxation on all angles and axes of the variable-cell geometry for all examined perovskite candidates. Perovskite candidates that successfully converge upon DFT calculations are selected for property determination. Further context and mathematical descriptions of the distinctive training phases of the LCMGM are provided in Methods .

Modeling architecture of the Lattice-Constrained Materials Generative Model (LCMGM) comprising three design phases: semi-supervised variational autoencoder (SS-VAE) training phase; auxiliary generative adversarial network (A-GAN) training phase; and geometrical optimization phase in the Bayesian Optimization (BO) and Density Functional Theory (DFT).
Simulating the forward design for modeling interested target properties
For evaluating the structure-to-property relationship of the mesh-grid descriptor design, the forward design is simulated by mapping \({X}\) perovskites in the training dataset to their deterministic target properties \(y\) (i.e. \(\,f\left(X\right)\,\mapsto y\) ). The target properties of interest include the formation energy ( \({E}_{f}\) ) and crystallographic attributes that define the geometrical lattice constraints. The geometrical lattice constraints are characterized by their crystal system ( \({C}_{s}\) ), fractional atomic coordination ( \({\vec{R}}_{\left(x,y,z\right)}\) ), lattice edges ( \({L}_{P(a,b,c)}\) ), and inter-axial angles ( \({L}_{P(\alpha ,\beta ,\gamma )}\) ). For each target, the forward design is implemented using similar two-dimensional convolutional neural networks (Conv2D) with final output layers and training losses that are pertinent to the respective target objective. For instance, predicting regressive/continuous targets in \(\,{E}_{f}\) , \(\,{\vec{R}}_{\left(x,y,z\right)}\) , \(\,{L}_{P(a,b,c)}\,\) and \(\,{L}_{P(\alpha ,\beta ,\gamma )}\) utilizes standardized metrics losses in the mean absolute error (MAE) and coefficient of determination (R 2 ) for monitoring the modeling performance. For multi-classifying \(\,{C}_{s}\) , F1-accuracy and One-vs-Rest (OvR) Receiver Operating Characteristics (ROC) are rather used. Table 1 reports the average predictive performance on interested target properties from a five-fold cross-validation experiment. As evaluated on validation and test sets, it can be observed that the model effectively learns \(\,{C}_{{s}}\) for OQMD validation dataset and MP testing dataset. On average, the overall F1-accuracies in the multi-classification of \(\,{C}_{{s}}\,\) are determined at 95.20% and 92.98% while Area Under Curve (AUC) from ROC investigations are estimated at 99.40% and 97.82% for validation set and test set, respectively. The good modeling of the \(\,{C}_{{s}}\) target is due to the periodic effect of the XRD mesh, as the model proficiently assimilates peak patterns for distinguishing different crystal systems. Moreover, it should be noted that in the multi-classification of \(\,{C}_{{s}}\) , overrepresented crystal systems in the training dataset are downsized to ensure equal number of training examples from each class are used in the classification experiment. The downsizing measure mitigates the biased adjudication of the classification model to the majority class. In the subsequent inverse design modeling however, all training examples in the dataset are used, in order to promote a more explorative learning of new perovskite chemistries. Noticeable differences in modeling accuracies across validation and test sets can be observed for regressive target variables. For instance, average MAE scores on \({E}_{f}\,\) are reported at 0.0764 eV/atom and 0.1932 eV/atom for validation and test sets, respectively. The observed disparity in MAE is expected, given the differences in sensitive parametric choices for determining DFT total energies across both databases. In a past study moreover, the uncertainty in high-throughput simulations among interoperable crystal databases was discussed, thereby establishing the need for thorough standardization, as it relates to DFT parameterization 37 . The present research still takes advantage of the transfer learning approach given that the main objective in the simulation of target properties is towards evaluating the structure-to-property relationship of the invertible meh-grid descriptor for regularizing the latent space. Besides, the parity scatter plots from the regressive fittings of actual versus predicted results for validation and test sets demonstrate the effective capability of the invertible mesh-grid descriptor for modeling the targets. As illustrated in Fig. 3A, B , the average overall R 2 values for predicting \({E}_{f}\,\) are estimated at 96.66% and 91.50% while for predicting \(\,{L}_{P(a,b,c)}\) , the reported scores are at 94.49% and 86.27%, for validation and test sets, respectively. Given that \(\,{E}_{f}\,\) is thermodynamically obtained by accounting for the energy difference between constituents and compound 33 , 52 , the simulative effect of the Conv2D model in predicting \(\,{E}_{f}\,\) is suggested to rely on the atomic-label and thermochemistry property meshes of the invertible descriptor design. On further inspecting stoichiometric-specific results for most target properties, lower marginal errors are generally observed for \(\,{A}_{2}B{B}^{{\prime} }{X}_{6}\,\) compounds compared to their \(\,{{ABX}}_{3}\,\) counterparts. As previously established in past studies 6 , 41 , thermodynamic properties belonging to the double perovskites are better predicted due to the contributing effect of more constitutive chemical elements for developing the thermochemical representations.
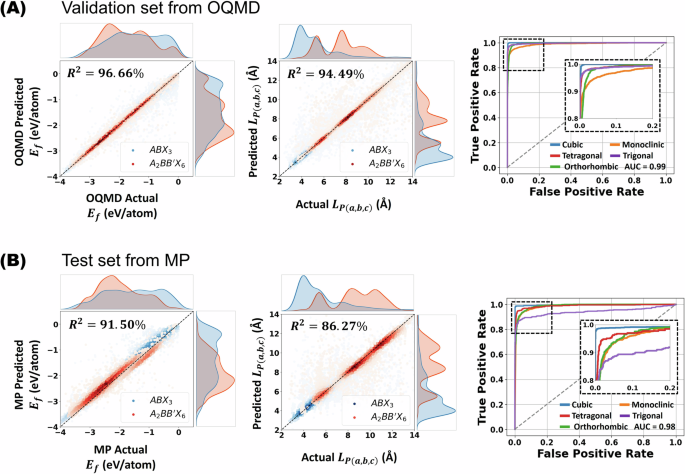
A OQMD validation set and ( B ) MP test set.
Simulating the inverse design towards novel perovskite discovery
To exemplify the target-learning effect of the SS-VAE model, Fig. 4 graphs latent space projections of OQMD training and MP testing data points for real and t-distributed stochastic neighbor embedding (t-SNE) principal components 53 . The real space projections are the identified two-dimensional planes (i.e. \([{z}_{x},{z}_{y}]\in \vec{z}\) ) that best capture the clustering of interested data points from a stochastic training process. The interested cluster region is the learnable chemical compositional space for extracting targeted-test data points that satisfy both stability and crystal system type. For assessing the effect of sample size, the experiment is conducted with increasing number of training samples. As illustrated in Fig. 4 , the clustering of stable data points for all crystal systems are seen to improve with increasing number of training data. For the case where the maximum number of training data samples are used (i.e. ~63,000 samples), the latent space can be further observed with more specific clusters that are based on stoichiometrical types, despite not being provided with such direct information for this specific task via the target-learning MLP networks. For extracting test-data points, the real vectorial space is used instead of the t-SNE principal component analysis (PCA) as data-projection via PCA is irreversible from loss of information. It is important to mention that entirely new data points are not directly sampled from the latent space because reconstructing such new data points from the SS-VAE domain leads to distorted structural features in decoding errors from predicting their geometrical properties. As such, clustered regions of interest in the real space are exploited for extracting only test data points. For instance, strictly limiting the search to known perovskite data points around the stable-cubic \(\,{A}_{2}B{B}^{{\prime} }{X}_{6}\,\) region in Fig. 4 provides roughly 1,072 test data points with 78% of them satisfying both stable and cubic design conditions. Hence, choosing to strictly exploit only the interested region is bound to promote higher validity in crystal system type at the expense of chemical diversity due to the restricted proportion of targeted data points. The current study prefers such economical approach in order to ensure that the crystal design conditions are effectively maintained, while relying on the A-GAN for further chemical and geometrical analysis. As such, the known test data points emerging from the targeted region, along with their known lattice features, are subjected to the A-GAN modeling phase for assimilating lattice features to their corresponding chemical representations. Figure 5 illustrates the learning evolution in generator and discriminator performances, as it relates to the training of extracted stable-cubic data points by the A-GAN model. As the number of training epochs increases, the divergence in log-losses between real and fake data generation is observed to decrease, as can be seen in Fig. 5A , as the discriminator is seemingly unable to distinguish between real and fake latent space representations. Likewise, with increasing number of training epoch, the RMSE losses are observed to decrease in Fig. 5B , which is due to the improved assimilation of the A-GAN model in learning the geometrical lattice constraints of the latent space representations. As a result, the deep learning of latent space vectors and their corresponding constraints enables the A-GAN to produce new chemical representations that are essentially different from their real space counterparts while being confined to the extracted data distribution within the interested cluster region. To illustrate the better chemical learning effect of the A-GAN over traditional VAE’s latent space sampling techniques by interpolation, Fig. 6 compares the diversity in chemical elements across the periodic table for over 40,000 newly generated stable-cubic perovskites that are prevalent from Spherical Linear Interpolation (SLERP) sampling operations 54 and the A-GAN explorative processes. From the investigation, candidates generated by the A-GAN are seen to be much chemically diverse, and as a result, preferably suited for finding unknown compositions. The synthetic representations are post-processed in a decoder, which reconstructs the encrypted chemical representations into their corresponding mesh-grid representations. In the Supplemental Information, an overview of the LCMGM architectural design and hyperparameters, as well as some selected prototypical structures for querying the A-GAN model are provided in details.
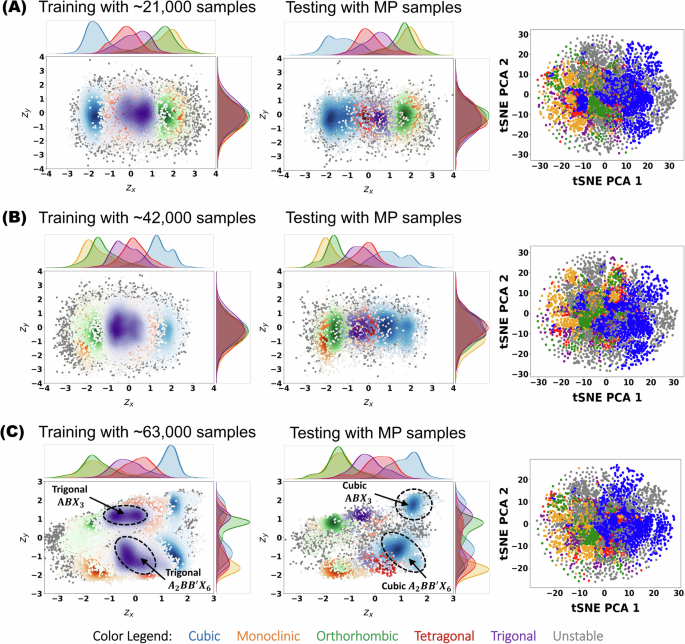
A Training with ~21,000 (one-third) OQMD samples; ( B ) Training with ~42,000 (two-thirds) OQMD samples; ( C ) Training with ~63,000 (full) OQMD samples.
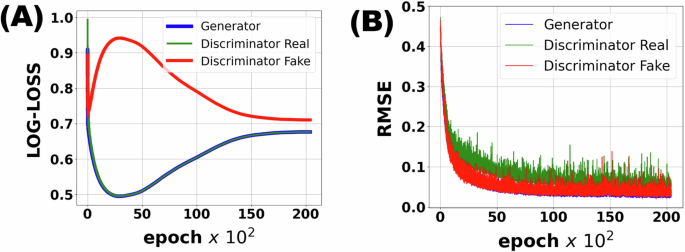
A Generator and discriminator log-losses for learning the latent space data distribution; ( B ) Evolution in RMSE accuracy for generative and discriminative (real and fake/synthetic) models with respect to the assimilation of the geometrical lattice constraints.
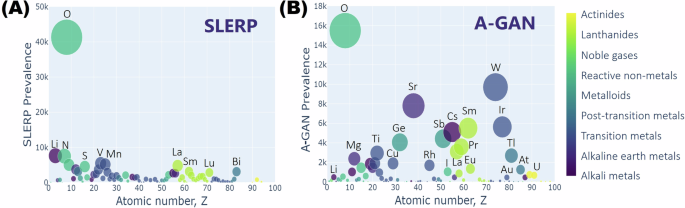
A Spherical Linear Interpolation (SLERP) sampling technique and ( B ) Auxiliary Generative Adversarial Network (A-GAN).
Geometrical optimization of newly generated perovskites
The BO algorithm is used to preliminarily find the most preferred crystal lattice parameters that best minimize the total DFT-computed energy ( \({E}_{{tot}}\) ), while ensuring that constitutive atoms and crystal system conformities remain unchanged. Because the BO perturbs input features in its search, the label mesh of the mesh-grid descriptor design is modified to directly accommodate geometrical attributes in the lattice parameters and atomic coordination. Figure 7A displays regressive fitting from the forward design mapping of perovskites in the MP dataset to their corresponding \(\,{E}_{{tot}}\,\) targets. The MAE and R 2 values for predicting the standard \({E}_{{tot}}\,\) are estimated at 2.309 eV and 99.14%, respectively. Considering the normalization of \(\,{E}_{{tot}}\,\) with respect to the total number of atoms in the unit cell (i.e. eV/atom), the prediction errors are updated at 0.184 eV/atom and 96.05% in the same manner. The present research preferably uses the normalized \(\,{E}_{{tot}}\) for simulating the objective function because the label-mesh of the invertible mesh-grid descriptor encodes distinctive atoms per site and not the entirety of atoms in the unit cell. Figure 7B demonstrates the convergence of the BO algorithm in the process of finding the minimized \(\,{E}_{{tot}}\,\) for a newly designed monoclinic candidate by the LCMGM. In finding the optimized geometry, the function values are seen to exhaust the search space with achieved convergence by the 70 th iterative mark, which corresponds to the lowest possible \(\,{E}_{{tot}}\,\) solution for the altered geometry. To further illustrate the pre-relaxation importance of the BO algorithm, Table 2 compares the close matching in optimized lattice parameters \(a\) , \({b}\) , \({c}\,\) and \(\,\beta\) for over 100 newly generated candidates that were subjected to the BO algorithm. For cases where BO was implemented, lattice parameters \(a\) , \({c}\,\) and \(\,\beta\) were found to be consistently closer to their final DFT relaxed forms. Their calculated average absolute differences were seen to improve by about 2.43%, 10.98%, and 14.99%, respectively. However, an inconsistency challenge is observed with respect to the relaxation of the \(b\) edge vectors, as their generated forms without BO were closer to the final DFT relaxed forms for crystal systems that are neither cubic nor monoclinic. The reason for such discrepancy is an ongoing study, and is investigated across various perspectives that revolve around the used acquisition function, local minim possibilities, in addition to DFT’s mechanism in altering the unit cell configurations in its process of relaxing the stresses applied on each atom 31 , 32 , 51 .
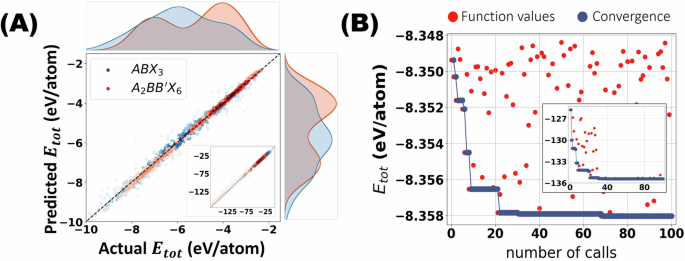
A Regression fitting in the prediction of the normalized \(\,{E}_{{tot}}\,\) (eV/atom); B BO convergence per iteration for finding the optimized geometry that best minimizes the functional value. For both ( A , B ), the figure insets demonstrate the same application with respect to the standard \({E}_{{tot}}\,\) (eV).
Structural match rate analysis of rediscovered perovskite chemistries by the LCMGM
To examine the model’s precision for rediscovering known perovskite structures as exact duplicates, over 12,000 structures were randomly generated by the LCMGM for each crystal system, resulting in approximately 75,000 structures for evaluation. Upon verifying the occurrence of the generated structures, it was found that about 32% were recognized chemical compositions present in either the OQMD training or MP testing sets. For compositions present in the MP test set, their structures were further checked to determine geometrical similarity by taking into account the invariances arising from crystal symmetry and coordination. Using the StructureMatcher submodule from Pymatgen 36 , two identical crystal structures successfully matched by satisfying ltol = 0.2 , stol = 0.3 , and angle_tol = 5.0 matching criteria. The results from the investigation are reported in terms of match rate and Root Mean Squared (RMS) displacements between paired sites of two identical structures. The evaluation is further compared with the results presented in the PGCGM 11 baseline study, as it relates to RMS investigations for generated structures found in the training and testing sets 33 , 34 , 55 . In addition, comparisons are drawn with FTCP 5 and CubicGAN 7 models, based on generated crystals that are matched with the Bayesian Optimization with Symmetry Relaxation (BOWSR) 49 versions. As presented in Table 3 , crystals generated by the LCMGM were seen to marginally outperform the PGCGM with lower average results in RMS values. For the FTCP model, a relatively lower match rate at 0.8% can be seen, which is suggested to be a consequence of the model’s inability to generate symmetrically constrained crystals, as the majority of the materials produced by the FTCP are triclinic. Figure 8 displays the distribution of RMS values with respect to crystal system types and provides an assessment of the LCMGM’s ability to match structures of known crystal systems. The assessment reveals that cubic and tetragonal structures are better discovered with lower RMS values, whereas trigonal systems correspond to higher RMS values. This suggests that the model is better capable at producing more realistic cubic and tetragonal structures in comparison to other crystal systems.
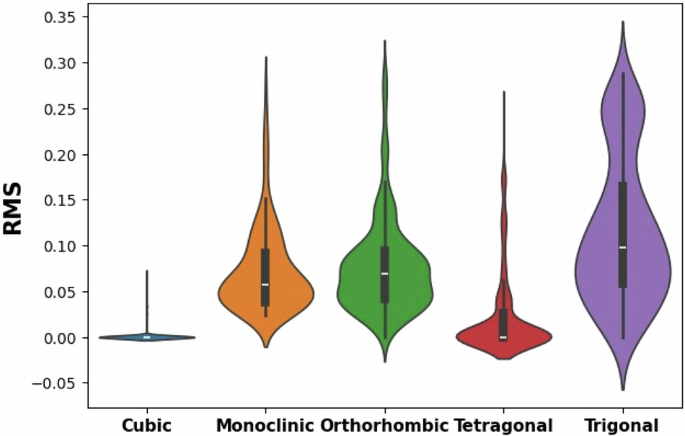
The lower Root Mean Squared (RMS) displacement values for cubic and tetragonal systems indicates the model's strong ability to realistically discover materials in both systems.
DFT validation and property determination
Emerging from the DFT validation phase, the current study presents 124 new inorganic materials consisting of 65 \({{ABX}}_{3}\,\) and 59 \(\,{A}_{2}B{B}^{{\prime} }{X}_{6}\,\) chemical compositions that were designed using the LCMGM. Their DFT computed total energies and forces were confirmed to converge upon spin-polarized calculations on the variable-cell geometry of the relaxed crystal structure. In addition, the materials were checked to ensure they satisfied chemical uniqueness, stability and charge neutrality requirements. The designed materials include 23 cubic, 18 monoclinic, 24 orthorhombic, 25 tetragonal and 34 trigonal structures. Using the Pymatgen SpacegroupAnalyzer.get_lattice_type module 36 that is interfaced with the spglib software 56 , the crystal system types of the new materials were found to be consistent with predefined lattice constraints at the encoding design phase. On surveying through standard materials databases such as Materials Project (MP) 34 , Open Quantum Materials Database (OQMD) 33 and Novel Materials Discovery (NOMAD) 57 , 72 of the new materials are identified as newly discovered perovskite chemistries. Considering the anionic occupation at the \(X\) -site of a standard perovskite stoichiometry, about 45% of the new materials are perovskite-oxides with the remainder being primarily halides, sulfides, hydrides, nitrides, and selenides. For assessing thermodynamic stability and functionality, the new materials are further subjected to property determination via DFT and machine learning techniques. To estimate thermodynamic stability based on energy above convex hull ( \({E}_{{hull}}\) ), the standard pymatgen.analysis.phase_diagram 58 , 59 was used, as it relates to comparing the stability of a material against its system. According to the investigation, about 40% of the new materials satisfy synthesis specification below 0.08 eV/atom, as suggested in a study on sulfides and oxides 60 . Table 4 reports the top-three stable candidates for each stoichiometry, as it relates to their crystal system type. For these compounds, the relative energies ( \({E}_{{rel}}\) ) 27 , 33 , 52 with respect to their atomic and solid constituents are computed using DFT. An examination of dynamic stability and statistical thermodynamics is presented in a case study involving three materials that are characterized by their low \({E}_{{hull}}\) values. The selected materials include Cs 2 LiScF 6 (cubic- \({Fm}\bar{3}m\) ), Rb 2 LiMnF 6 (cubic- \({Fm}\bar{3}m\) ), and RbVCl 3 (tetragonal- \(P4/{mmm}\) ). For these materials, the phonon dispersion relations and density of states (DOS) are analyzed by calculating the forces exerted on non-equilibria supercell configurations, based on the finite displacement method (FDM) 61 . As illustrated in Fig. 9 , the dispersion curves of these materials along high symmetry lines are shown with no imaginary frequencies, thereby indicating their dynamic stabilities, and agreeing with previous investigations from the \({E}_{{hull}}\,\) analysis. The phonon calculations are performed using the phonopy open-source software package 62 . In the Supplemental Information , a full breakdown of all new materials is provided, in addition to property determination, as it relates to lattice features and thermodynamic stability. Equally provided in Methods are further explanations for supporting the DFT calculations.
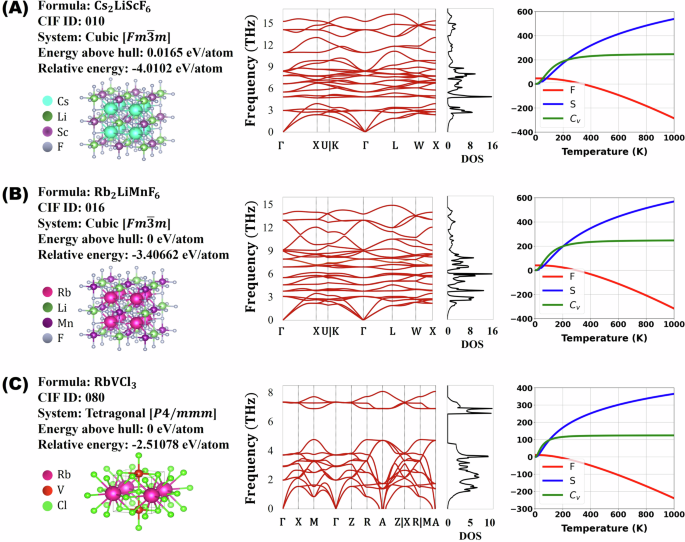
A Cs 2 LiScF 6 , ( B ) Rb 2 LiMnF 6 , and ( C ) RbVCl 3 , as it relates to DFT-computed phonon band structures, phonon density of states (DOS) and thermal properties. The thermal properties in F , S and C v correspond to Helmholtz Free Energy (kJ/mol), Entropy (J/K.mol), and Specific Heat Capacity at constant volume (J/K.mol), respectively.
A case study on the properties of perovskite duplicates in polymorphic forms
Among the new materials introduced in this research, 52 were found to be existing perovskite chemistries or compositions with the majority identifiable in the OQMD training dataset as polymorphs, meaning they have different crystal systems or space group number. These existing material compositions were investigated to assess the LCMGM capability for generating crystals that are potentially more stable or with different functional properties than their polymorphic counterparts. Considering preference based on stability, it was found that 14 of the presented materials have formation energies lower than the most stable polymorph that is associated with their compositional system. Examples are the RbMgCl 3 63 and RbVCl 3 64 crystals, both previously characterized as hexagonal- \(P{6}_{3}/{mmc}\) , but rediscovered by the LCMGM in marginally favorable states as orthorhombic- \({Amm}2\) and tetragonal- \(P4/{mmm}\) , respectively. The phonon band structure and thermal properties for the tetragonal- \(P4/{mmm}\) RbVCl 3 crystal are illustrated in Fig. 9C . Moreover, upon evaluating the model’s functionality for screening polymorphic variations with contrasting electrical properties, it was found that 16 of the presented materials exhibited bandgap properties that were considerably different from their most stable known forms. A case study is presented for the photoluminescence-tuning CsZnBr 3 perovskite 65 , which was generated by the LCMGM in cubic- \({Pm}\bar{3}m\) form, but is found in the OQMD database (ID: 1985788) as triclinic- \(P\bar{1}\) in its most stable state. To verify the properties of both crystal versions, DFT calculations based on recommended GGA-PBEsol Precision v1.3.0 functionals from the Standard Solid-State Pseudopotentials (SSSP) library 66 was used for further relaxing their structures. The DFT parameters for simulating both crystal structures were consistent in terms of pseudopotentials, convergence criteria for energy and force, as well as mesh size. A report on their computed XRD patterns, dynamic stabilities and electrical behaviors are displayed in Fig. 10 . From the investigation, it can be observed that the phonon dispersion curves of the LCMGM generated cubic crystal is ideally stable due to a clear absence of phonon bands in the imaginary frequency domain. For the OQMD triclinic polymorph on the other hand, the crystal also exhibits good stability, although it can be seen with phonon bands at the gamma point slightly extending into the imaginary frequency domain. Furthermore, considering the dramatic change in electrical behavior due to the nature of their different structures, the generated cubic crystal is displayed using electronic band structures with a lower bandgap at \({E}_{g}\,\) = 0.29 eV, which potentially makes the material more suitable for a variety of low-energy optical applications. In contrast, the triclinic polymorph is a large bandgap crystal with \({E}_{g}\,\) = 3.584 eV, and as such, may be more suitable for high-energy absorption engineering applications.

For each material, lattice features, computed XRD, TEM images, phonon band structures, electronic band structures, and density of states (DOS) are shown.
Application in photovoltaics and optoelectronics
Although the present research primary focused the LCMGM for designing perovskites with stability and geometrical conformity, some of the newly designed perovskites were identified to exhibit semiconducting energy bandgaps for potential serviceability in a variety of optical and electronic applications. They include solar cells, quantum dots, light-emitting diodes (LEDs), photodetectors, sensors, and many more. Specifically, using DFT calculations with GGA-PBE pseudopotentials, the electrical behaviors of the 124 newly designed materials were examined by evaluating their energy bandgaps ( \({E}_{g}\) ) and band structures. From the analysis, about 80% were calculated to exhibit metallic properties with \({E}_{g}\) ≅ 0 eV due to their overlapping valence and conduction bands across the fermi energy level. Considering the other non-metallic compositions as characterized by their finite bandgaps, further investigations are performed to identify special semiconducting candidates with promising potential as host materials in photovoltaics and/or optoelectronic applications. The special candidates are thoroughly examined on denser k -points grid mesh using GGA-PBEsol Precision v1.3.0 functionals from the SSSP library 66 . The six new materials and their corresponding lattice, electrical and stability properties are reported in Table 5 . Their bandgaps are seen to equivalently align with photons near the infrared to ultraviolet region (NIR-VIS-UV) of the electromagnetic spectrum for absorbing and emitting light. Among them, four stand out for single junction solar cell applications due to their bandgaps falling within the Shockley-Quessier limits for optimized Power Conversion Efficiencies (PCE) in excess of 30% 67 , 68 . They include Sr 2 CWO 6 (cubic- \({Fm}\bar{3}m\) ), TaAlO 3 (monoclinic- \(P{2}_{1}/m\) ), RbZnH 3 (orthorhombic- \({Amm}2\) ), and Rb 2 AuLuF 6 (trigonal- \(P\bar{3}m1\) ) with bandgaps within the 1.3–1.6 eV range. For these materials, the electronic band structures, projected density of states (P-DOS), computed XRD patterns and monocrystalline Transmission Electron Microscopic (TEM) images are illustrated in Fig. 11 . The band-structures are visualized along high symmetry lines in the irreducible Brillouin zone of the crystal lattice 35 , 69 and are seen to be indirect, due to their valence band maximum (VBM) and conduction band minimum (CBM) occurring at different points in the k -momentum space.
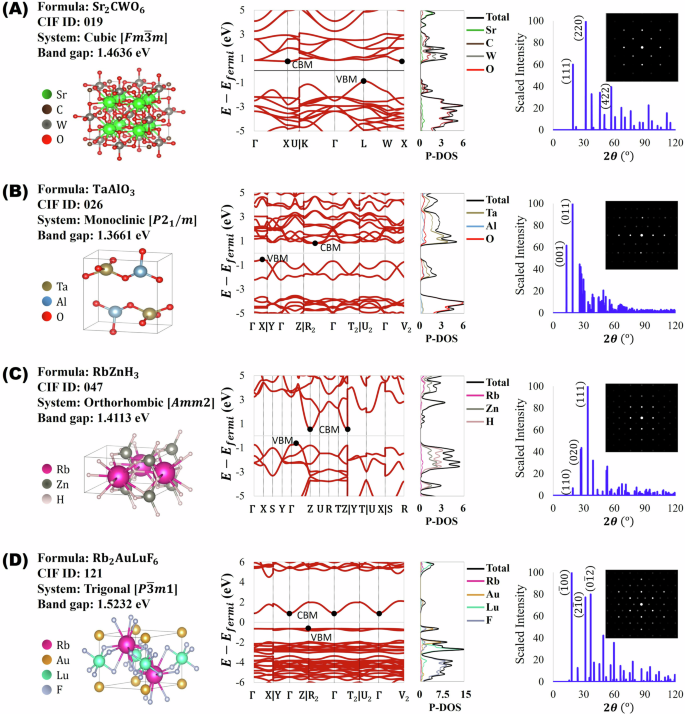
A Sr 2 CWO 6 cubic- \({Fm}\bar{3}m\) ; B TaAlO 3 monoclinic- \(P{2}_{1}/m\) ; C RbZnH 3 orthorhombic- \({Amm}2\) ; D Rb 2 AuLuF 6 trigonal- \({P}\bar{3}m1\) . For each material, the electrical band structures, projected density of states (P-DOS), computed X-ray Diffraction (XRD) patterns, and Transmission Electron Microscope (TEM) monocrystalline images, are illustrated. VBM and CBM are valence band maximum and conduction band minimum, respectively. TEM beam direction is towards the \(\left(001\right)\,\) crystal plane.
Experimental impact and potential improvements of the LCMGM model
Given the unlimited chemical combinatorial search space afforded by perovskite stoichiometries, the LCMGM can be seen as a pragmatic tool for rapidly designing novel perovskites that are potentially stable and synthesizable. Unlike the FTCP 5 and our previous EVAPD 6 and Target-Learning Variational Autoencoder (TL-VAE) 70 models that optimize for stability based on a strict formation energy perspective, the LCMGM includes the extra element of geometrical conformity for addressing the challenge of lattice asymmetrisation (i.e. low degree of symmetry), which is persistent with the aforementioned models in specific, and Deep Generative Models (DGM) in general. The concept of hybridizing the SS-VAE with the A-GAN critically assists in improving the modeling performance due to the forced learning of the geometrical constraints 71 , 72 . Simultaneously, the hybridization approach eliminates the concern of lattice reconstruction at the decoding phase, thereby addressing the concern of generating materials that are majorly of the triclinic crystal system. Comparing the overall modeling complexity of the LCMGM with respect to the Physics Guided Crystal Generative Model (PGCGM) 11 further elucidates on the issue of better training stability. Unlike the PGCGM that supplies high-dimensional input features such as affine matrix into the generator phase, the LCMGM’s generator has the advantage of analyzing low-dimensional features from the extraction of data points in the SS-VAE’s latent space. Furthermore, comparing the descriptor designs between LCMGM and FTCP further demonstrates the improved periodic modeling effect of the LCMGM, as it pertains to capturing essential features in the reciprocal crystal lattice. The FTCP projects features into the reciprocal space by using a robust Fourier transforming approach. In contrast, the LCMGM mesh-grid descriptor concept resorts to discretized XRD patterns with seven times lesser pixel attributes. Besides, by examining the correlation of the decoded XRD patterns to the crystal system conditions that are set at the design phase, the reliability and consistency of the LCMGM can be checked for constrained lattice learning. For over 70,000 newly generated crystals, Table 6 reports on the average MSE scores in pixel attributes for decoded XRD meshes with respect to their actual diffraction forms, as well as the novelty in new perovskite chemistries learnt by the LCMGM. From the report, it can be observed that new crystals generated as cubic and tetragonal structures slightly edge their other crystal system peers in terms of design consistency with an average MSE score of 0.078 for both systems. As an example, Fig. 12 depicts decoded, actual, and overlaid XRD meshes for a newly generated MnPtO 3 monoclinic crystal structure. Using a trained model that accurately recognizes XRD patterns, the decoded mesh of the case-studied crystal was predicted to be monoclinic, thereby agreeing with the geometrical lattice constraints that was set at the design phase for screening monoclinic crystals. In overlaying the decoded and actual calculated meshes, the MSE discrepancy in pixel attributes was estimated at 0.0527 MSE with low peaks ranging from 10 to 25 on the grid width axis for both images, corresponding to 60° to 150° in fingerprinted 2θ diffraction angle.
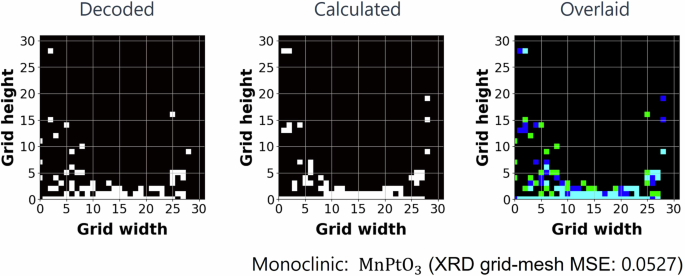
In both decoded and calculated images, low peaks can be observed from 10 to 25 on the grid width axis, corresponding to 60° to 150° in fingerprinted 2θ diffraction angle.
As demonstrated in the present research, the LCMGM is specially optimized for designing new and polymorphic perovskites that are stable while geometrically conforming to a particular crystal system of interest. This is primarily due to the incorporation of the supervisory learning arms for simulating deterministic perovskite’s target properties, as it relates to the formation energy, primitive Bravais crystal systems, and lattice constraints. As such, to capture other target properties that are of interest to materials scientists and engineers, the LCMGM will require substantial remodeling in order to reliably simulate the target property of interest prior to novel materials generation. In future studies, targets such as the energy bandgap, ionic diffusivity, and critical temperature will be considered for developing robust DGM frameworks, which can serve in multifunctional engineering applications. Moreover, the LCMGM could equally be extended to more complex perovskite stoichiometries and/or material classes. The challenge, however, with undertaking such expansion is in the accommodation of crucial thermochemistry or relational properties for modeling complex material forms with respect to the label and property arrays of the mesh-grid descriptor. As such, the expansion process will necessitate adjustments in the mesh-grid features, potentially leading to an increase in pixel contribution and/or computational cost for DGM image analysis. Furthermore, the LCMGM was demonstrated for five out of seven crystal systems due to the relative availability of training data. Hence, to account for a more generalized modeling architecture that considers all crystal symmetrisation possibilities, the training process will essentially require significant high-fidelity data contribution for marginalized primitive and non-primitive crystal systems. Moreover, suitable modifications are currently being considered in future studies to broaden the LCMGM to space-group specifications and not just crystal systems, as demonstrated in the PGCGM study 11 . Finally, the Bayesian Optimization (BO) algorithm for pre-DFT relaxation is implemented at the third phase of learning for preliminary geometrical optimization. For a more comprehensive approach, the BO may be directly infused in the first or second phase of learning for creating an adaptive machine learning framework. This also is an area of future studies.
Dataset curation and statistics
For downloading training and testing data, QMPYRester and MPRester APIs are imported using the Python programming language for OQMD 33 and MP 34 , respectively. Both databases are queried with anonymous ABC3 and ABC2D6 generic/formula filters for extracting \({{ABX}}_{3}\) and \({A}_{2}B{B}^{{\prime} }{X}_{6}\) stoichiometries, respectively. So far, querying the OQMD ( v1.0 ) and legacy version ( v2021.05.13 ) of MP produces 72,747 (35,325 \({{ABX}}_{3}\) and 37,422 \(\,{A}_{2}B{B}^{{\prime} }{X}_{6}\) ) and 8,238 (4,358 \({{ABX}}_{3}\) and 3,880 \(\,{A}_{2}B{B}^{{\prime} }{X}_{6}\) ) samples, respectively. For consistency, both datasets are screened to include only energetically favorable entries, as defined by their formation energies \({E}_{f}\) ≤ 0 eV/atom. Moreover, the crude data provided by OQMD and MP are normally in their primitive standard unit cells. Hence, by taking advantage of symmetry operations, the Pymatgen SpacegroupAnalyzer.get_conventional_standard_structure 36 is used to transform the primitive CIF structures into conventional standard forms. The conventional structures are further scrutinized to select only entries with constitutive atoms no more than forty in the unit cell ( \({n}_{{atoms}}\,\) ≤ 40). Limiting to forty atoms is due to the relatively small number of data samples beyond this value. Besides, working with larger supercells excessively increases the cost of computation for constrained lattice learning and subsequent DFT validation. Furthermore, the training dataset is crosschecked to exclude structures with Bravais crystal systems that are apparently underrepresented, which in the present study case were the pure hexagonal and triclinic systems. Overall, the data cleaning exercise resulted into 63,016 samples from the OQMD, consisting of 29,881 \(\,{{ABX}}_{3}\,\) and 33,135 \(\,{A}_{2}B{B}^{{\prime} }{X}_{6}\,\) structures. Likewise, preprocessed data from MP resulted into 6,540 samples, consisting of 3,283 \(\,{{ABX}}_{3}\,\) and 3257 \(\,{A}_{2}B{B}^{{\prime} }{X}_{6}\) structures. Figure 13 graphs the relative contribution in processed OQMD and MP data entries for selected cubic, orthorhombic, monoclinic, trigonal, and tetragonal crystal systems, as it relates to the considered stoichiometries. It can be observed that cubic crystal systems have a significantly higher data availability due to their extensively studied nature. They constitute about 40% and 54% of all data entries, for OQMD and MP, respectively. To better understand the targeted data region for performing generative operations, Kernel Density Estimate (KDE) curves are displayed for relating the range of formation energy values with respect to the selected crystal systems. Approximately 62% of OQMD samples and 69% of MP samples are found to meet predefined stability criteria based on formation energy \({E}_{f}\) ≤ −1.5 eV/atom for organizing the SS-VAE’s latent space. For inspecting stability based on the energy above hull parameter, 6% of OQMD samples and 24% of MP samples are found to be at the convex hull \({E}_{{hull}}\) = 0 eV/atom, with cubic and monoclinic crystal systems constituting the majority of highly stable and potentially synthesizable structures.
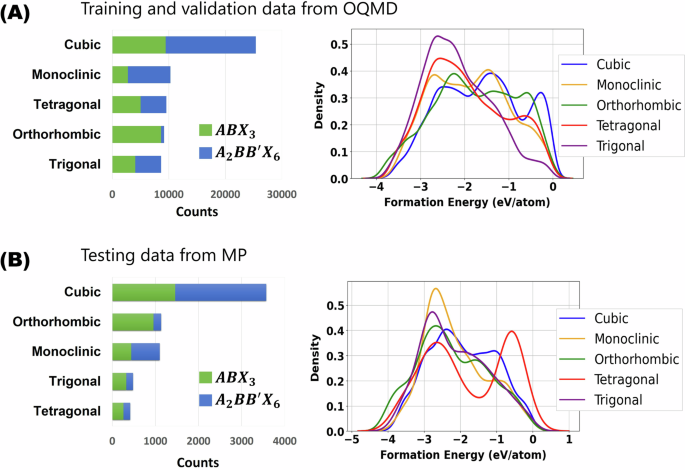
A Training and validation data from OQMD; ( B ) Generative testing data from MP.
LCMGM training phases and related equations
The LCMGM can be broken down into three modeling phases, namely: semi-supervised variational autoencoder (SS-VAE) training phase; auxiliary generative adversarial network (A-GAN) training phase; and geometrical optimization phase. In the Supplemental Material , a full breakdown of neural network architecture and hyperparameter tunings are provided.
Phase 1: Semi-supervised variational autoencoder (SS-VAE) training
Given the training perovskite data samples, as represented in their fully enmeshed forms (i.e. \(\,\left\{{x}_{i}\right\}\subseteq X\in {\left\{\mathrm{0,1}\right\}}^{R}\) ), the SS-VAE model projects \({e}\left(X\right)\,\) onto a smooth and continuous Gaussian latent space (i.e. \(\,\left\{{\vec{z}}_{i}\right\}\subseteq \vec{Z}\in {{\mathbb{R}}}^{Q}\) ) using a recognition network model. In this context, \({R}\) is the input image dimension (i.e. \(32\times 32\times 3\) ) and \(Q=\,\) 256 is the latent space dimension. Based on Bayes theorem, the SS-VAE model learns the probability distribution \({q}_{\phi }\left(\vec{z}{|x}\right)\) at the encoding phase and approximates the true posterior distribution \({p}_{\theta }\left(\vec{z}{|x}\right)\) at the decoding phase. The learning process is optimized using the Kullback-Leibler (KL) loss metric 45 , which aims at minimizing the measurable distance between both probability distributions for regularizing the latent space. In addition, the SS-VAE optimization process includes reconstructive losses, by ensuring that the inputs \({X}\) remain in close resemblance with their decoded/output forms \(\,{X}^{* }=d\left(\vec{z}\right)\) , where \(d\left(.\right)\,\) is the decoder function. By coupling dual Multi-Layer Perceptron (MLP) supervisory networks, the latent space is optimized for regressively predicting the formation energy target ( \({E}_{f}\) ) and classifying primitive crystal systems ( \({C}_{s}\) ). The latent space vectors \(\vec{z}\) are therefore mapped to their corresponding targets, i.e. \({f}_{1}\left(\vec{z}\right)\longmapsto {E}_{f}\) and \(\,{f}_{2}\left(\vec{z}\right)\longmapsto {C}_{s}\) , where \({f}_{1}\,\) and \({f}_{2}\,\) are feed forward functions for modeling \({E}_{f}\) and \({C}_{s}\) , respectively. The overall objective functions comprise supervisory and unsupervisory training losses, as mathematically described using the Equations -1 and -2 2 , 15 :
For \(i=\mathrm{1,2},\ldots ,{n}\) data entries, Equation-1 describes the loss/objective functions of the conventional VAE model (i.e. \(\,{{\mathscr{L}}}_{{vae}}\left(\phi ,\theta \right)\) ), as it relates to KL and reconstruction. \(\phi\) and \(\,\theta\) are learning parameters corresponding to recognition and generative models, respectively. Equation-2 relates the overall objective functions (i.e. \(\,{{\mathscr{L}}}_{{vae}}^{* }\) ) by the effected MLP networks. In the present research, the training losses for regression and multi-classification are the Mean Squared Error (MSE) and categorical cross-entropy, respectively.
Phase 2: Auxiliary generative adversarial network (A-GAN) training
The A-GAN model serves the primary purpose of generating synthetic latent space vectors \(\,{\vec{z}}^{* }\Rightarrow {{\mathbb{R}}}^{256}\) that are in close similarities with their extracted \(\vec{z}\) counterparts from the SS-VAE model. Given a random noise variable \({\vec{n}}^{* }\Rightarrow {{\mathbb{R}}}^{100}\) that is provided as input into the generator, the value/loss function \(V\left(G,D\right)\) of a traditional GAN can be described as in Equation-3 3 :
\({G}\) and \({D}\) are differentiable functions represented by generator and discriminator neural networks, respectively. \({\mathbb{E}}\) is the expected log-likelihood for maximizing \({D}\) and minimizing \(G\) with respect to the prior distribution on the input noise variable, \({p}_{{\vec{n}}^{* }}\left({\vec{n}}^{* }\right)\) . In the current study, Equation-3 is adapted to include a regressive loss function for minimizing the error in learning geometrical features. Moreover, the A-GAN’s generator is architectured to operate in a manner similar to a query unit, taking as input both the concatenated seed noise vector \({\vec{n}}^{* }\) and randomly sampled geometries in terms of lattice parameters \({L}_{p}^{* }=\left[a,b,{c;}\,\alpha ,\beta ,\gamma \right]\in {{\mathbb{R}}}^{\left(2\times 3\right)}\) and three-dimensional atomic coordinates \(\,{\vec{R}}_{\left(x,y,z\right)}^{* }\in {{\mathbb{R}}}^{\left(40\times 3\right)}\) . Hence, in addition to generating \(\,{\vec{z}}^{* }\) , the A-GAN implicitly learns the lattice constraints of the real space \(\vec{z}\) representations by predicting their known lattice parameters and coordination as unknown targets. By doing so, the inferred knowledge gained from the prediction process gives meaning (i.e. regularize) to the randomly sampled \({L}_{p}^{* }\,\) and \({\vec{R}}_{\left(x,y,z\right)}^{* }\) via a continuous learning sequence that is optimized/updated by backpropagation. As a result, querying the fully trained A-GAN model to produce de novo chemical representations will require as input a conformational geometry that is of interest to the user, as defined by \({L}_{p}^{* }\) and \(\,{\vec{R}}_{\left(x,y,z\right)}^{* }\) . Besides, in querying new structures, prototypes could as well be used to further narrow the search to highly feasible candidates. This study utilized such an approach by choosing prototypical structures recognized in the Inorganic Crystal Structure Database (ICSD) 55 . A list of such structures is provided in the Supplemental Information .
Phase 3: Geometrical Bayesian Optimization (BO)
Given a generated perovskite unit cell by the LCMGM, as characterized by their constitutive atoms and lattice geometry/parameters, the goal of the BO algorithm is to minimize the unknown/costly objective function while ensuring that predefined lattice constraints on the crystal system type are maintained. In this study, the objective function is the normalized total DFT energy \(\,{E}_{{tot}}\) (eV/atom). Mathematically, optimizing for lattice parameter \({L}_{{P|opt}}^{* }\) can be expressed using Equation-4 30 , 49 :
In this study, the BO approximates the defined objective function using cheaper surrogate models in Gaussian Processes (GP) 73 . In conducting explorative and exploitative GP operations, the expected improvement is used as the acquisition function and is regulated by necessary geometrical constraints that characterize the respective crystal system. For instance, geometrically optimizing a monoclinic perovskite candidate is conditional on four variabilities in search space dimensionality, given that monoclinic cells generally have three unequal lattice edge vectors and one non-orthogonal inter-axial angle (i.e. \(\,\beta \ne 90{\rm{^\circ }}\) ). As a result, Table 7 outlines the search space dimensionalities for simulating the BO algorithm, as it relates to the considered crystal systems. The definitions for lattice parameters are based on the Crystallographic Information Files (CIF) that are standardized in the Materials Project 34 database. For example, trigonal cells, sometimes referred to as rhombohedral cells, may in special instances be categorized in the hexagonal crystal class, and as such, share similar symmetrical operations 74 . Hence, the current study equally applies intuitive crystallographic knowledge in further narrowing the search space, as it relates to the geometrical boundary conditions from the preprocessed training and test data distribution.
Density Functional Theory (DFT) simulation
DFT is used to chemically and geometrically validate the new materials emerging from the LCMGM inverse design pipeline. In this study, DFT computations were enabled by using the open-sourced Quantum Espresso (QE) v7.0 software package 51 . For all newly generated perovskite candidates, QE performs plane-wave spin-polarized DFT relaxations on the variable-cell geometry of the conventional unit cell. The pseudopotential class for approximating Coulombic interactions in the nucleus of an atom is the Perdew-Burke-Ernzerhof (PBE) 75 —Projector Augmented Wave (PAW) functional 76 of the Generalized Gradient Approximation (GGA). The Brillouin zone of the reciprocal crystal lattice is sampled using a fine k -points grid mesh size at 0.2 Å −1 , as recommended by the Materials Cloud 77 . Self-consistent field (SCF) electronic convergence is achieved by setting energy accuracy, pressure and force at 1.0e-7 Rydberg, 0.5 kbar and 1.0e-3 Rydberg/Bohr, respectively. The energy cut-off threshold for charge density (ρ) is set no less than nine times the corresponding value for wave function (ψ) from the chemical element pseudopotential’s condition. To ensure a smooth integration of electron occupation across the fermi energy level, Gaussian-smearing with low broadening (0.01 Rydberg) is used. The Broyden-Fletcher-Goldfarb-Shanno (BFGS) iterative algorithm 78 is applied for ionic and cell optimizations. Moreover, for performing detailed property determination and evaluating band structures, the PBEsol Precision v1.3.0 pseudopotentials from the Standard Solid-State Pseudopotentials (SSSP) library 66 is used on denser k -points grids corresponding to 0.1 Å −1 . In addition, DFT is strictly used for calculating the relative energies \({E}_{{rel}}\) presented in Table 4 by accounting for the total energy difference between bulk crystalline forms and summed constitutive atomic forms, at the same level of theory with the bulk crystalline calculations. Mathematically, \({E}_{{rel}}\) can be expressed as in Equation-5:
\({E}_{{tot}}\) is the normalized DFT-computed total energy of the bulk system. \({E}_{i}\) as a function of \({x}\) is the DFT-computed total energy for \(i=\mathrm{1,2,3},\ldots ,{x}\) isolated atoms or molecules belonging to the bulk system, at the same level of theory as the bulk system calculation 27 , 33 , 52 . For computing \(\,{E}_{i}\) , the isolated atoms and diatomic molecules, such as hydrides, nitrides, oxides and halides, are placed at the center of the bulk crystal lattice associated with the relaxed perovskite structure. The bonding distance between similar atoms of a diatomic molecule is obtained from the standard NIST reference for Computational Chemistry Comparison and Benchmark DataBase (CCCBDB). The energy above hull \(\,{E}_{{hull}}\) parameter is estimated using the standard pymatgen.analysis.phase_diagram analyzer (i.e. get_e_above_hull ) 58 , 59 that considers related material’s systems from the Materials Project (MP) database, which were originally determined using the Vienna Ab Initio Simulation Package (VASP) 79 . Because the present research uses freely distributed QE and not VASP for performing DFT, a model is built for learning the distribution in total DFT energies for compounds in the MP dataset (i.e. Fig. 7A ). The actual DFT values of the presented materials are compared to their predicted values, and are thus used to estimate \(\,{E}_{{hull}}\) . Figure 14 further displays the relationship among energy values for the new materials, as it relates to DFT calculated total energy by QE in Rydberg (Ry) versus predicted total energy in electron-volts (eV), as well as \({E}_{{hull}}\) versus formation energy \(\,{E}_{f}\) estimations. To validate the technicality of our approach for calibrating stability, phonon calculations are performed for assessing dynamic stability of some selected candidates with low \({E}_{{hull}}\) values. Their phonon band structures are displayed without imaginary frequencies in Fig. 9 that indicates good stability.

A DFT-computed total energy, \({E}_{{tot}}\) (Ry) versus model-predicted \({E}_{{tot}}\) (eV); ( B ) energy above convex hull \({E}_{{hull}}\) versus formation energy \(\,{E}_{f}\) , based on phase diagram analysis.
Data availability
The Crystallographic Information Files (CIF) and Quantum Espresso (QE) geometrical optimization output files of the newly designed materials can be found in the Mendeley data repository at https://doi.org/10.17632/m262xxpgn2.1 .
Code availability
The code used to produce the results in this paper is available at https://github.com/chenebuah/LCMGM .
Fuhr, A. S. & Sumpter, B. G. Deep generative models for materials discovery and machine learning-accelerated innovation. Front. Mater. 9 , 865270 (2022).
Article Google Scholar
Kingma, D. P. & Welling, N. Auto-encoding variational bayes. arXiv https://doi.org/10.48550/arXiv.1312.6114 (2013).
Goodfellow, I. J. et al. Generative Adversarial Networks. arXiv https://doi.org/10.48550/arXiv.1406.2661 (2014).
Noh, J. et al. Inverse design of solid-state materials via a continuous representation. Matter 1 , 1370–1384 (2019).
Ren, Z. et al. An invertible crystallographic representation for general inverse design of inorganic crystals with targeted properties. Matter 5 , 314–335 (2022).
Article CAS Google Scholar
Chenebuah, E. T., Nganbe, M. & Tchagang, A. B. An evolutionary variational autoencoder for perovskite discovery. Front. Mater. 10 , 1233961 (2023).
Zhao, Y. et al. High-throughput discovery of novel cubic crystal materials using deep generative neural networks. Adv. Sci. 8 , 2100566 (2021).
Long, T. et al. Constrained crystals deep convolutional generative adversarial network for the inverse design of crystal structures. npj Comput. Mater. 7 , 66 (2021).
Arjovsky, M., Chintala, S., Bottou, L. & Wasserstein, G. A. N. arXiv preprint arXiv:1701.07875v3 https://doi.org/10.48550/arXiv.1701.07875 (2017).
Dan, Y. et al. Generative adversarial networks (GAN) based efficient sampling of chemical composition space for inverse design of inorganic materials. npj Comput. Mater. 6 , 84 (2020).
Zhao, Y. et al. Physics guided deep learning for generative design of crystal materials with symmetry constraints. npj Comput. Mater. 9 , 38, https://doi.org/10.1038/s41524-023-00987-9 (2023).
Pandey, A., Dalal, S., Dutta, S. & Dixit, A. Structural characterization of polycrystalline thin films by X-ray diffraction techniques. J. Mater. Sci.: Mater. Electron 32 , 1341–1368, https://doi.org/10.1007/s10854-020-04998-w (2021).
Thanh-Tung, H. & Tran, T. On catastrophic forgetting and mode collapse in generative adversarial networks. arXiv https://doi.org/10.48550/arXiv.1807.04015 (2018).
Lucas, J., Tucker, G., Grosse, R. B. & Norouzi, M. Understanding Posterior Collapse in Generative Latent Variable Models. https://paperswithcode.com/paper/understanding-posterior-collapse-in (2019).
Kingma, D. P., Rezende, D. J., Mohamed, S. & Welling, M. Semi-supervised learning with deep generative models. arXiv https://doi.org/10.48550/arXiv.1406.5298 (2014).
Odena, A., Olah, C., & Shlens, J. Conditional image synthesis with auxiliary classifier GANs. arXiv https://doi.org/10.48550/arXiv.1610.09585 (2016).
Odena, A. Semi-supervised learning with generative adversarial networks. arXiv https://doi.org/10.48550/arXiv.1606.01583 (2016).
Johnsson, M. & Lemmens, P. Crystallography and chemistry of perovskites. arXiv https://doi.org/10.48550/arXiv.cond-mat/0506606 (2005).
Woodward, P. M. Octahedral tilting in perovskites. I. Geometrical considerations. Acta Cryst. B53 , 32–43 (1997).
Woodward, P. M. Octahedral tilting in perovskites. II. Structure stabilizing forces. Acta Cryst. B53 , 44–66 (1997).
Lufaso, M. W. & Woodward, P. M. Jahn–teller distortions, cation ordering and octahedral tilting in perovskites. Acta Cryst. B60 , 10–20 (2004).
Wang, Y. et al. Antiperovskites with exceptional functionalities. Adv. Mater. 32 , 1905007 (2020).
Ruddlesden, S. N. & Popper, P. The compound Sr 3 Ti 2 O 7 and its structure. Acta Cryst 11 , 54–55 (1958).
Ruddlesden, S. N. & Popper, P. New compounds of the K 2 NiF 4 type. Acta Cryst 10 , 538–539 (1957).
Lu, J. et al. Origin and physical effects of edge states in two-dimensional ruddlesden-popper perovskites. iScience 25 , 104420 (2022).
Article CAS PubMed PubMed Central Google Scholar
Knapp, M. C. & Woodward, P. M. A-site cation ordering in AA′BB′O 6 perovskites. J. Solid State Chem. 179 , 1076–1085 (2006).
Kim, C., Huan, T., Krishnan, S. & Ramprasad, R. A hybrid organic-inorganic perovskite dataset. Sci. Data 4 , 170057 (2017).
Article PubMed PubMed Central Google Scholar
Lee, J.-W. et al. Dynamic structural property of organic-inorganic metal halide perovskite. iScience 24 , 101959 (2021).
Article CAS PubMed Google Scholar
Zhao, X.-G., Dalpian, G. M., Wang, A. & Zunger, A. Polymorphous nature of cubic halide perovskites. Phys. Rev. B 101 , 155137 (2020).
Snoek, J., Larochelle, H. & Adams, R. P. Practical Bayesian optimization of machine learning algorithms. arXiv https://doi.org/10.48550/arXiv.1206.2944 (2012).
Cohen, A. J., Mori- Sánchez, P. & Yang, W. Challenges for density functional theory. Chem. Rev. 112 , 289–320 (2012).
Jain, A. et al. A high-throughput infrastructure for density functional theory calculations. Comput. Mater. Sci. 50 , 2295–2310 (2011).
Kirklin, S. et al. The open quantum materials database (OQMD): assessing the accuracy of DFT formation energies. npj Comput. Mater. 1 , 15010 (2015).
Jain, A. et al. Commentary: the materials project: a materials genome approach to accelerating materials innovation. Apl. Mater. 1 , 011002 (2013).
Setyawan, W. & Curtarolo, S. High-throughput electronic band structure calculations: challenges and tools. Comput. Mater. Sci. 49 , 299–312 (2010).
Ong, S. P. et al. Python materials genomics (pymatgen): a robust, open-source python library for materials analysis. Comput. Mater. Sci. 68 , 314–319 (2013).
Hedge, V.I., et al. Quantifying uncertainty in high-throughput density functional theory: a comparison of AFLOW, materials project, and OQMD. arXiv https://doi.org/10.48550/arXiv.2007.01988 (2022).
Simon, S. H. The Oxford Solid State Basics, Vol. 290 (Oxford Univ Pr, 2013).
Chenebuah, E. T., Nganbe, M. & Tchagang, A. B. A fourier-transformed feature engineering design for predicting ternary perovskite properties by coupling a two-dimensional convolutional neural network with a support vector machine (Conv2D-SVM). Mater. Res. Express 10 , 026301, https://doi.org/10.1088/2053-1591/acb683 (2023).
Xie, T. & Grossman, J. C. Crystal graph convolutional neural networks for an accurate and Interpretable prediction of material properties. Phys. Rev. Lett. 120 , 145301, https://doi.org/10.1103/physrevlett.120.145301 (2018).
Chenebuah, E. T., Nganbe, M. & Tchagang, A. B. Comparative analysis of machine learning approaches on the prediction of the electronic properties of perovskites: a case study of ABX 3 and A 2 BB′X 6 . Mater. Today Commun. 27 , 102462, https://doi.org/10.1016/j.mtcomm.2021.102462 (2021).
Li, C. et al. A progressive learning method for predicting the band gap of ABO 3 perovskites using an instrumental variable. J. Mater. Chem. C 8 , 3127–3136, https://doi.org/10.1039/C9TC06632B (2020).
De Graef, M., & McHenry, M. E. Structure of Materials: An Introduction to Crystallography, Diffraction and Symmetry . 2nd edn, Vol. 268 (Cambridge University Press, 2012).
Chen, S. H., Xiao, Z. R., Liu, Y. P., Lee, P. H. & Wang, Y. K. First-principle calculation on nearly half-metallic antiferromagnetic behavior of double perovskites La 2 VReO 6 . J. Magn. Magn. Mater. 323 , 175–178, https://doi.org/10.1016/j.jmmm.2010.08.059 (2011).
Kullback, S. & Leibler, R. A. On information and sufficiency. JSTOR 22 , 79–86, https://doi.org/10.1214/aoms/1177729694 (1951).
Mirza, M. & Osindero, S. Conditional generative adversarial nets. arXiv https://doi.org/10.48550/arXiv.1411.1784 (2014).
Shannon, R. D. Revised effective ionic radii and systematic studies of interatomic distances in halides and chalcogenides. Acta Cryst. A32 , 751–767, https://doi.org/10.1107/S0567739476001551 (1976).
Galembeck, F. & Burgo, T. A. L. in Chemical Electrostatics https://doi.org/10.1007/978-3-319-52374-3_2 (Springer, Cham, 2017).
Zuo, Y. et al. Accelerating materials discovery with Bayesian optimization and graph deep learning. Mater. Today 51 , 126–135 (2021).
Packwood, D. Bayesian Optimization for Materials Science 1st edn, Vol. 2365–6344 (Springer Singapore. (2017).
Giannozzi, P. et al. Quantum espresso: a modular and open-source software project for quantum simulations of materials. J. Phys. Condens. Matter 21 , 395502 (2009).
Article PubMed Google Scholar
Emery, A. & Wolverton, C. High-throughput DFT calculations of formation energy, stability and oxygen vacancy formation energy of ABO 3 perovskites. Sci. Data 4 , 170153 (2017).
van der Maaten, L. & Hinton, G. Visualizing data using t-SNE. JMLR 9 , 2579–2605 (2008).
Google Scholar
Shoemake, K. Animating rotation with quaternion curves. SIGGRAPH Comput. Graph 19 , 245–254, https://doi.org/10.1145/325165.325242 (1985).
Belsky, A., Hellenbrandt, M., Karen, V. L. & Luksch, P. New developments in the inorganic crystal structure database (ICSD): accessibility in support of materials research and design. Acta Cryst. B58 , 364–369 (2002).
Togo, A. & Tanaka I. Spglib: a software library for crystal symmetry search. arXiv https://doi.org/10.48550/arXiv.1808.01590 (2018).
Draxl, C. & Scheffler, M. Nomad: the fair concept for big data-driven materials science. MRS Bull 43 , 676–682 (2018).
Ong, S. P., Wang, L., Kang, B. & Ceder, G. Li-Fe-P-O 2 phase diagram from first principles calculations. Chem. Mater. 20 , 1798–1807 (2008).
Ong, S. P., Jain, A., Hautier, G., Kang, B. & Ceder, G. Thermal stabilities of delithiated olivine MPO 4 (M=Fe, Mn) cathodes investigated using first principles calculations. Electrochem. Comm. 12 , 427–430 (2010).
Singh, A. K., Montoya, J. H., Gregoire, J. M. & Persson, K. A. Robust and synthesizable photocatalysts for CO 2 reduction: a data-driven materials discovery. Nat. Commun. 10 , 443 (2019).
Kresse, G., Furthmüller, J. & Hafner, J. Ab initio force constant approach to phonon dispersion relations of diamond and graphite. EPL 32 , 729 (1995).
Togo, A. First-principles phonon calculations with phonopy and phono3py. J. Phys. Soc. Jpn. 92 , 012001 (2023).
Holm, J. L., Holm, B. J., Rinnan, B. & Grønvold, F. The enthalpies of fusion and the heat capacities of K 2 MgCl 4 , Rb 2 MgCl 4 , Cs 2 MgCl 4 , KMgCl 3 , RbMgCl 3 , and CsMgCl 3 . J. Chem. Thermodyn. 5 , 97–106 (1973).
Niel, M., Cros, C., Pouchard, M. & Chaminade, J.-P. Contribution a l'étude des propriétés magnétiques des phases AVX 3 (A = Rb, Cs; X = Cl, Br, I). J. Solid State Chem. 20 , 1–8 (1976).
Cui, K. et al. Intense blue emission from one-pot synthesized quaternary CsZn x Pb 1-x Br 3 perovskite quantum dots. Opt. Mater. 136 , 113441 (2023).
Prandini, G., Marrazzo, A., Castelli, I. E., Mounet, N. & Marzari, N. Precision and efficiency in solid-state pseudopotential calculations. npj Comput. Mater. 4 , 72 (2018).
Shockley, W. & Queisser, H. J. Detailed balance limit of efficiency of p‐n junction solar cells. J. Appl. Phys. 32 , 510–519 (1961).
Rühle, S. Tabulated values of the Shockley–Queisser limit for single junction solar cells. Sol. Energy 130 , 139–147 (2016).
Hinuma, Y., Pizzi, G., Kumagai, Y., Oba, F. & Tanaka, I. Band structure diagram paths based on crystallography. Comp. Mat. Sci. 128 , 140, https://doi.org/10.1016/j.commatsci.2016.10.015 (2017).
Chenebuah, E. T., Nganbe, M. & Tchagang, A. Target-learning the latent space of a variational autoencoder model for the inverse design of stable perovskites. In: Proc. Canadian Conference on Artificial Intelligence . (2023).
Szegedy, C. et al. Going deeper with convolutions. arXiv https://doi.org/10.48550/arXiv.1409.4842 (2014).
Sutskever, I., Vinyals, O. & Le, Q. V. Sequence to sequence learning with neural networks. arXiv https://doi.org/10.48550/arXiv.1409.3215 (2014).
Rasmussen, C. E. & Williams, C. K. I. Gaussian Processes for Machine Learning . (MIT Press, 2005).
Hahn, T. (Ed.). International Tables for Crystallography 5th edn (Springer Dordrecht, 2005).
Perdew, J. P., Burke, K. & Ernzerhof, M. Generalized gradient approximation made simple. Phys. Rev. Lett. 77 , 3865–3868 (1996).
Blöchl, P. E. Projector augmented-wave method. Phys. Rev. B 50 , 17953–17979 (1994).
Talirz, L. et al. Materials cloud, a platform for open computational science. Sci. Data 7 , 1–12 (2020).
Fletcher, R. & Reeves, C. M. Function minimization by conjugate gradients. Comput. J. 7 , 149–154 (1964).
Kresse, G. & Furthmüller, J. Efficiency of ab-initio total energy calculations for metals and semiconductors using a plane-wave basis set. Comput. Mater. Sci. 6 , 15–50 (1996).
Download references
Acknowledgements
This research was supported by the National Research Council of Canada (NRC) through its Artificial Intelligence for Design Program led by the Digital Technologies Research Centre.
Author information
Authors and affiliations.
Department of Mechanical Engineering, University of Ottawa, 161 Louis-Pasteur, Ottawa, ON, K1N 6N5, Canada
Ericsson Tetteh Chenebuah, Michel Nganbe & Alain Beaudelaire Tchagang
Digital Technologies Research Center, National Research Council of Canada, 1200 Montréal Road, Ottawa, ON, K1A 0R6, Canada
Ericsson Tetteh Chenebuah & Alain Beaudelaire Tchagang
You can also search for this author in PubMed Google Scholar
Contributions
Conceptualization, E.T.C.; Methodology, E.T.C. and A.B.T.; Software, E.T.C.; Investigation, E.T.C.; Data Curation: E.T.C.; Writing—Original Draft, E.T.C.; Writing - Review and Editing, E.T.C., M.N., and A.B.T; Funding Acquisition and Supervision, M.N. and A.B.T.
Corresponding author
Correspondence to Ericsson Tetteh Chenebuah .
Ethics declarations
Competing interests.
All authors declare no competing interests.
Additional information
Publisher’s note Springer Nature remains neutral with regard to jurisdictional claims in published maps and institutional affiliations.
Supplementary information
Supplemental information, rights and permissions.
Open Access This article is licensed under a Creative Commons Attribution 4.0 International License, which permits use, sharing, adaptation, distribution and reproduction in any medium or format, as long as you give appropriate credit to the original author(s) and the source, provide a link to the Creative Commons licence, and indicate if changes were made. The images or other third party material in this article are included in the article’s Creative Commons licence, unless indicated otherwise in a credit line to the material. If material is not included in the article’s Creative Commons licence and your intended use is not permitted by statutory regulation or exceeds the permitted use, you will need to obtain permission directly from the copyright holder. To view a copy of this licence, visit http://creativecommons.org/licenses/by/4.0/ .
Reprints and permissions
About this article
Cite this article.
Chenebuah, E.T., Nganbe, M. & Tchagang, A.B. A deep generative modeling architecture for designing lattice-constrained perovskite materials. npj Comput Mater 10 , 198 (2024). https://doi.org/10.1038/s41524-024-01381-9
Download citation
Received : 12 January 2024
Accepted : 07 August 2024
Published : 30 August 2024
DOI : https://doi.org/10.1038/s41524-024-01381-9
Share this article
Anyone you share the following link with will be able to read this content:
Sorry, a shareable link is not currently available for this article.
Provided by the Springer Nature SharedIt content-sharing initiative
Quick links
- Explore articles by subject
- Guide to authors
- Editorial policies
Sign up for the Nature Briefing newsletter — what matters in science, free to your inbox daily.

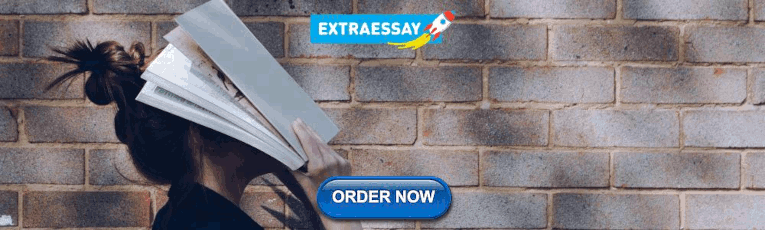
IMAGES
VIDEO
COMMENTS
The discussion section is where you delve into the meaning, importance, and relevance of your results.. It should focus on explaining and evaluating what you found, showing how it relates to your literature review and paper or dissertation topic, and making an argument in support of your overall conclusion.It should not be a second results section.. There are different ways to write this ...
Tips to Write the Results Section. Direct the reader to the research data and explain the meaning of the data. Avoid using a repetitive sentence structure to explain a new set of data. Write and highlight important findings in your results. Use the same order as the subheadings of the methods section.
The discussion chapter is where you interpret and explain your results within your thesis or dissertation. This contrasts with the results chapter, where you merely present and describe the analysis findings (whether qualitative or quantitative). In the discussion chapter, you elaborate on and evaluate your research findings, and discuss the ...
Here are some examples of how to present the summary of your findings; "The data suggests that", "The results confirm that", "The analysis indicates that", "The research shows a relationship between", etc. 2. Interpretations of Results. Your audience will expect you to provide meanings of the results, although they might seem ...
The results chapter (also referred to as the findings or analysis chapter) is one of the most important chapters of your dissertation or thesis because it shows the reader what you've found in terms of the quantitative data you've collected. It presents the data using a clear text narrative, supported by tables, graphs and charts.
When writing a dissertation or thesis, the results and discussion sections can be both the most interesting as well as the most challenging sections to write. You may choose to write these sections separately, or combine them into a single chapter, depending on your university's guidelines and your own preferences.
Guide contents. As part of the Writing the Dissertation series, this guide covers the most common conventions of the results and discussion chapters, giving you the necessary knowledge, tips and guidance needed to impress your markers! The sections are organised as follows: The Difference - Breaks down the distinctions between the results and discussion chapters.
Begin with a clear statement of the principal findings. This will reinforce the main take-away for the reader and set up the rest of the discussion. Explain why the outcomes of your study are important to the reader. Discuss the implications of your findings realistically based on previous literature, highlighting both the strengths and ...
Here are a few best practices: Your results should always be written in the past tense. While the length of this section depends on how much data you collected and analysed, it should be written as concisely as possible. Only include results that are directly relevant to answering your research questions.
The results chapter in a dissertation or thesis (or any formal academic research piece) is where you objectively and neutrally present the findings of your qualitative analysis (or analyses if you used multiple qualitative analysis methods). This chapter can sometimes be combined with the discussion chapter (where you interpret the data and ...
The best option to provide scaffolding to your discussion chapter is by following the sequence of the previous two chapters in your dissertation—the Methods and Results. This repeated sequence demonstrates your awareness of your reader, and you are making your dissertation or thesis easy to follow.
The results section is where you report the main findings of your research. Watch this video to learn how to report your results concisely and objectively in...
Table of contents. What not to include in your discussion section. Step 1: Summarise your key findings. Step 2: Give your interpretations. Step 3: Discuss the implications. Step 4: Acknowledge the limitations. Step 5: Share your recommendations. Discussion section example.
II. The Content. The content of the discussion section of your paper most often includes:. Explanation of results: Comment on whether or not the results were expected for each set of findings; go into greater depth to explain findings that were unexpected or especially profound.If appropriate, note any unusual or unanticipated patterns or trends that emerged from your results and explain their ...
Tips for Writing the Discussion Section. Start with the big picture - WHY is your study important? o Think of yourself as telling the story of how your findings answer the question you posed and why your findings matter, how your field's status quo or understanding is changed by your results. o Clearly signal that you are answering the ...
A discussion chapter of your dissertation is not just a fixation on results but a more profound summary connected to research goals and purpose. Here is an example: Summary of Findings Example According to the data, implementing the co-orientation theory was successful and can be used for the same circumstances in the future.
In a thesis or dissertation, the discussion is an in-depth exploration of the results, going into detail about the meaning of your findings and citing relevant sources to put them in context. The conclusion is more shorter and more general: it concisely answers your main research question and makes recommendations based on your overall findings.
Step 1: Craft a brief introduction section. As with all chapters in your dissertation or thesis, the conclusions chapter needs to start with a brief introduction. In this introductory section, you'll want to tell the reader what they can expect to find in the chapter, and in what order.
Read our guide on Writing the results and discussion for answers to these questions and more. You can also watch the Writing the Dissertation: the Results and Discussion webinar , which covers the overarching purpose of both chapters/sections in the context of the dissertation, defines the relationship between the results and discussion, and ...
if you write a scientific dissertation, or anyway using quantitative methods, you will have some objective results that you will present in the Results chapter. You will then interpret the results in the Discussion chapter. B) More common for qualitative methods. - Analysis chapter. This can have more descriptive/thematic subheadings.
Since 2006, Oxbridge Essays has been the UK's leading paid essay-writing and dissertation service. We have helped 10,000s of undergraduate, Masters and PhD students to maximise their grades in essays, dissertations, model-exam answers, applications and other materials. If you would like a free chat about your project with one of our UK staff ...
This template covers all the core components required in the discussion chapter (or section) of a typical dissertation or thesis, including: The purpose of each section is explained in plain language, followed by an overview of the key elements that you need to cover. The template also includes practical examples to help you understand exactly ...
In this blog, we discuss phrases related to results and discussion sections such as findings, limitations, arguments, and comparison to previous studies. The results and discussion sections are one of the challenging sections to write. It is important to plan this section carefully as it may contain a large amount of scientific data that needs to be presented in a clear and concise fashion.
The discovery of novel materials with phenomenal target properties is a major driving force in advancing technological innovation. Recent advances have shown that deep generative machine learning ...