Study Design 101: Meta-Analysis
- Case Report
- Case Control Study
- Cohort Study
- Randomized Controlled Trial
- Practice Guideline
- Systematic Review
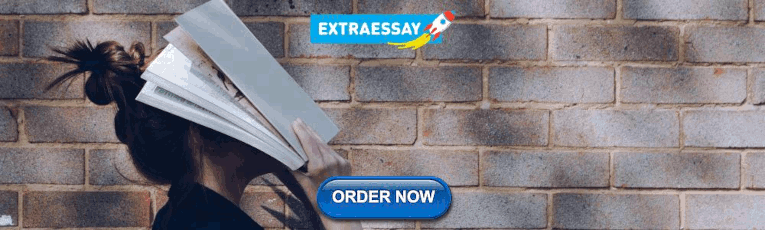
Meta-Analysis
- Helpful Formulas
- Finding Specific Study Types
A subset of systematic reviews; a method for systematically combining pertinent qualitative and quantitative study data from several selected studies to develop a single conclusion that has greater statistical power. This conclusion is statistically stronger than the analysis of any single study, due to increased numbers of subjects, greater diversity among subjects, or accumulated effects and results.
Meta-analysis would be used for the following purposes:
- To establish statistical significance with studies that have conflicting results
- To develop a more correct estimate of effect magnitude
- To provide a more complex analysis of harms, safety data, and benefits
- To examine subgroups with individual numbers that are not statistically significant
If the individual studies utilized randomized controlled trials (RCT), combining several selected RCT results would be the highest-level of evidence on the evidence hierarchy, followed by systematic reviews, which analyze all available studies on a topic.
- Greater statistical power
- Confirmatory data analysis
- Greater ability to extrapolate to general population affected
- Considered an evidence-based resource
Disadvantages
- Difficult and time consuming to identify appropriate studies
- Not all studies provide adequate data for inclusion and analysis
- Requires advanced statistical techniques
- Heterogeneity of study populations
Design pitfalls to look out for
The studies pooled for review should be similar in type (i.e. all randomized controlled trials).
Are the studies being reviewed all the same type of study or are they a mixture of different types?
The analysis should include published and unpublished results to avoid publication bias.
Does the meta-analysis include any appropriate relevant studies that may have had negative outcomes?
Fictitious Example
Do individuals who wear sunscreen have fewer cases of melanoma than those who do not wear sunscreen? A MEDLINE search was conducted using the terms melanoma, sunscreening agents, and zinc oxide, resulting in 8 randomized controlled studies, each with between 100 and 120 subjects. All of the studies showed a positive effect between wearing sunscreen and reducing the likelihood of melanoma. The subjects from all eight studies (total: 860 subjects) were pooled and statistically analyzed to determine the effect of the relationship between wearing sunscreen and melanoma. This meta-analysis showed a 50% reduction in melanoma diagnosis among sunscreen-wearers.
Real-life Examples
Goyal, A., Elminawy, M., Kerezoudis, P., Lu, V., Yolcu, Y., Alvi, M., & Bydon, M. (2019). Impact of obesity on outcomes following lumbar spine surgery: A systematic review and meta-analysis. Clinical Neurology and Neurosurgery, 177 , 27-36. https://doi.org/10.1016/j.clineuro.2018.12.012
This meta-analysis was interested in determining whether obesity affects the outcome of spinal surgery. Some previous studies have shown higher perioperative morbidity in patients with obesity while other studies have not shown this effect. This study looked at surgical outcomes including "blood loss, operative time, length of stay, complication and reoperation rates and functional outcomes" between patients with and without obesity. A meta-analysis of 32 studies (23,415 patients) was conducted. There were no significant differences for patients undergoing minimally invasive surgery, but patients with obesity who had open surgery had experienced higher blood loss and longer operative times (not clinically meaningful) as well as higher complication and reoperation rates. Further research is needed to explore this issue in patients with morbid obesity.
Nakamura, A., van Der Waerden, J., Melchior, M., Bolze, C., El-Khoury, F., & Pryor, L. (2019). Physical activity during pregnancy and postpartum depression: Systematic review and meta-analysis. Journal of Affective Disorders, 246 , 29-41. https://doi.org/10.1016/j.jad.2018.12.009
This meta-analysis explored whether physical activity during pregnancy prevents postpartum depression. Seventeen studies were included (93,676 women) and analysis showed a "significant reduction in postpartum depression scores in women who were physically active during their pregnancies when compared with inactive women." Possible limitations or moderators of this effect include intensity and frequency of physical activity, type of physical activity, and timepoint in pregnancy (e.g. trimester).
Related Terms
A document often written by a panel that provides a comprehensive review of all relevant studies on a particular clinical or health-related topic/question.
Publication Bias
A phenomenon in which studies with positive results have a better chance of being published, are published earlier, and are published in journals with higher impact factors. Therefore, conclusions based exclusively on published studies can be misleading.
Now test yourself!
1. A Meta-Analysis pools together the sample populations from different studies, such as Randomized Controlled Trials, into one statistical analysis and treats them as one large sample population with one conclusion.
a) True b) False
2. One potential design pitfall of Meta-Analyses that is important to pay attention to is:
a) Whether it is evidence-based. b) If the authors combined studies with conflicting results. c) If the authors appropriately combined studies so they did not compare apples and oranges. d) If the authors used only quantitative data.
Evidence Pyramid - Navigation
- Meta- Analysis
- Case Reports
- << Previous: Systematic Review
- Next: Helpful Formulas >>

- Last Updated: Sep 25, 2023 10:59 AM
- URL: https://guides.himmelfarb.gwu.edu/studydesign101

- Himmelfarb Intranet
- Privacy Notice
- Terms of Use
- GW is committed to digital accessibility. If you experience a barrier that affects your ability to access content on this page, let us know via the Accessibility Feedback Form .
- Himmelfarb Health Sciences Library
- 2300 Eye St., NW, Washington, DC 20037
- Phone: (202) 994-2850
- [email protected]
- https://himmelfarb.gwu.edu
Study designs: Part 8 - Meta-analysis (I)
Affiliations.
- 1 Tata Memorial Centre, Homi Bhabha National Institute, Mumbai, Maharashtra, India.
- 2 Director, Jawaharlal Institute of Postgraduate Medical Education and Research, Puducherry, India.
- PMID: 33489837
- PMCID: PMC7819365
- DOI: 10.4103/picr.PICR_283_20
A systematic review is a form of secondary research that answers a clearly formulated research question using systematic and defined methods to identify, collect, appraise, and summarize all the primary research evidence on that topic. In this article, we look at meta-analysis - the statistical technique of combining the results of studies included in a systematic review.
Keywords: Research design; meta-analysis; review; systematic.
Copyright: © 2020 Perspectives in Clinical Research.
- Meta-Analysis/Meta-Synthesis
Meta Analysis
Meta-analysis is a set of statistical techniques for synthesizing data across studies. It is a statistical method for combining the findings from quantitative studies. It evaluates, synthesizes, and summarizes results. It may be conducted independently or as a specialized subset of a systematic review. A systematic review attempts to collate empirical evidence that fits predefined eligibility criteria to answer a specific research question. Meta-analysis is a quantitative, formal, epidemiological study design used to systematically assess the results of previous research to derive conclusions about that body of research (Haidrich, 2010). Rigorously conducted meta-analyses are useful tools in evidence-based medicine . Outcomes from a meta-analysis may include a more precise estimate of the effect of a treatment or risk factor for disease or other outcomes. Not all systematic reviews include meta-analysis , but all meta-analyses are found in systematic reviews (Haidrich, 2010).
A Meta analysis is appropriate when a group of studies report quantitative results rather than qualitative findings or theory, if they examine the same or similar constructs or relationships, if they are derived from similar research designs and report the simple relationships between two variables rather than relationships that have been adjusted for the effect of additional variables (siddaway, et al., 2019).
Haidich A. B. (2010). Meta-analysis in medical research. Hippokratia , 14 (Suppl 1), 29–37.
Siddaway, A. P., Wood, A. M., & Hedges, L. V. (2019). How to do a systematic review: A best practice guide for conducting and reporting narrative reviews, meta-analyses, and meta-syntheses. Annual Review of Psychology, 70 , 747–770.
Meta Synthesis
A meta synthesis is the systematic review and integration of findings from qualitative studies (Lachal et al., 2017). Reviews of qualitative information can be conducted and reported using the same replicable, rigorous, and transparent methodology and presentation. A meta-synthesis can be used when a review aims to integrate qualitative research. A meta-synthesis attempts to synthesize qualitative studies on a topic to identify key themes, concepts, or theories that provide novel or more powerful explanations for the phenomenon under review (Siddaway et al., 2019).
Lachal, J., Revah-Levy, A., Orri, M., & Moro, M. R. (2017). Metasynthesis: An original method to synthesize qualitative literature in psychiatry. Frontiers in Psychiatry, 8 , 269 .
Siddaway, A. P., Wood, A. M., & Hedges, L. V. (2019). How to do a systematic review: A best practice guide for conducting and reporting narrative reviews, meta-analyses, and meta-syntheses. Annual Review of Psychology, 70 , 747–770 .
- << Previous: Rapid Review
- Next: Selecting a Review Type >>
- Types of Questions
- Key Features and Limitations
- Is a Systematic Review Right for Your Research?
- Integrative Review
- Scoping Review
- Rapid Review
- Selecting a Review Type
- Reducing Bias
- Guidelines for Student Researchers
- Training Resources
- Register Your Protocol
- Handbooks & Manuals
- Reporting Guidelines
- PRESS 2015 Guidelines
- Search Strategies
- Selected Databases
- Grey Literature
- Handsearching
- Citation Searching
- Study Types & Terminology
- Quantitative vs. Qualitative Research
- Critical Appraisal of Studies
- Broad Functionality Programs & Tools
- Search Strategy Tools
- Deduplication Tools
- CItation Screening
- Critical Appraisal Tools
- Quality Assessment/Risk of Bias Tools
- Data Collection/Extraction
- Meta Analysis Tools
- Books on Systematic Reviews
- Finding Systematic Review Articles in the Databases
- Systematic Review Journals
- More Resources
- Evidence-Based Practice Research in Nursing
- Citation Management Programs
- Last Updated: Mar 20, 2024 2:16 PM
- URL: https://libguides.adelphi.edu/Systematic_Reviews
- How it works
Meta-Analysis – Guide with Definition, Steps & Examples
Published by Owen Ingram at April 26th, 2023 , Revised On April 26, 2023
“A meta-analysis is a formal, epidemiological, quantitative study design that uses statistical methods to generalise the findings of the selected independent studies. “
Meta-analysis and systematic review are the two most authentic strategies in research. When researchers start looking for the best available evidence concerning their research work, they are advised to begin from the top of the evidence pyramid. The evidence available in the form of meta-analysis or systematic reviews addressing important questions is significant in academics because it informs decision-making.
What is Meta-Analysis
Meta-analysis estimates the absolute effect of individual independent research studies by systematically synthesising or merging the results. Meta-analysis isn’t only about achieving a wider population by combining several smaller studies. It involves systematic methods to evaluate the inconsistencies in participants, variability (also known as heterogeneity), and findings to check how sensitive their findings are to the selected systematic review protocol.
When Should you Conduct a Meta-Analysis?
Meta-analysis has become a widely-used research method in medical sciences and other fields of work for several reasons. The technique involves summarising the results of independent systematic review studies.
The Cochrane Handbook explains that “an important step in a systematic review is the thoughtful consideration of whether it is appropriate to combine the numerical results of all, or perhaps some, of the studies. Such a meta-analysis yields an overall statistic (together with its confidence interval) that summarizes the effectiveness of an experimental intervention compared with a comparator intervention” (section 10.2).
A researcher or a practitioner should choose meta-analysis when the following outcomes are desirable.
For generating new hypotheses or ending controversies resulting from different research studies. Quantifying and evaluating the variable results and identifying the extent of conflict in literature through meta-analysis is possible.
To find research gaps left unfilled and address questions not posed by individual studies. Primary research studies involve specific types of participants and interventions. A review of these studies with variable characteristics and methodologies can allow the researcher to gauge the consistency of findings across a wider range of participants and interventions. With the help of meta-analysis, the reasons for differences in the effect can also be explored.
To provide convincing evidence. Estimating the effects with a larger sample size and interventions can provide convincing evidence. Many academic studies are based on a very small dataset, so the estimated intervention effects in isolation are not fully reliable.
Elements of a Meta-Analysis
Deeks et al. (2019), Haidilch (2010), and Grant & Booth (2009) explored the characteristics, strengths, and weaknesses of conducting the meta-analysis. They are briefly explained below.
Characteristics:
- A systematic review must be completed before conducting the meta-analysis because it provides a summary of the findings of the individual studies synthesised.
- You can only conduct a meta-analysis by synthesising studies in a systematic review.
- The studies selected for statistical analysis for the purpose of meta-analysis should be similar in terms of comparison, intervention, and population.
Strengths:
- A meta-analysis takes place after the systematic review. The end product is a comprehensive quantitative analysis that is complicated but reliable.
- It gives more value and weightage to existing studies that do not hold practical value on their own.
- Policy-makers and academicians cannot base their decisions on individual research studies. Meta-analysis provides them with a complex and solid analysis of evidence to make informed decisions.
Criticisms:
- The meta-analysis uses studies exploring similar topics. Finding similar studies for the meta-analysis can be challenging.
- When and if biases in the individual studies or those related to reporting and specific research methodologies are involved, the meta-analysis results could be misleading.
Steps of Conducting the Meta-Analysis
The process of conducting the meta-analysis has remained a topic of debate among researchers and scientists. However, the following 5-step process is widely accepted.
Step 1: Research Question
The first step in conducting clinical research involves identifying a research question and proposing a hypothesis . The potential clinical significance of the research question is then explained, and the study design and analytical plan are justified.
Step 2: Systematic Review
The purpose of a systematic review (SR) is to address a research question by identifying all relevant studies that meet the required quality standards for inclusion. While established journals typically serve as the primary source for identified studies, it is important to also consider unpublished data to avoid publication bias or the exclusion of studies with negative results.
While some meta-analyses may limit their focus to randomized controlled trials (RCTs) for the sake of obtaining the highest quality evidence, other experimental and quasi-experimental studies may be included if they meet the specific inclusion/exclusion criteria established for the review.
Step 3: Data Extraction
After selecting studies for the meta-analysis, researchers extract summary data or outcomes, as well as sample sizes and measures of data variability for both intervention and control groups. The choice of outcome measures depends on the research question and the type of study, and may include numerical or categorical measures.
For instance, numerical means may be used to report differences in scores on a questionnaire or changes in a measurement, such as blood pressure. In contrast, risk measures like odds ratios (OR) or relative risks (RR) are typically used to report differences in the probability of belonging to one category or another, such as vaginal birth versus cesarean birth.
Step 4: Standardisation and Weighting Studies
After gathering all the required data, the fourth step involves computing suitable summary measures from each study for further examination. These measures are typically referred to as Effect Sizes and indicate the difference in average scores between the control and intervention groups. For instance, it could be the variation in blood pressure changes between study participants who used drug X and those who used a placebo.
Since the units of measurement often differ across the included studies, standardization is necessary to create comparable effect size estimates. Standardization is accomplished by determining, for each study, the average score for the intervention group, subtracting the average score for the control group, and dividing the result by the relevant measure of variability in that dataset.
In some cases, the results of certain studies must carry more significance than others. Larger studies, as measured by their sample sizes, are deemed to produce more precise estimates of effect size than smaller studies. Additionally, studies with less variability in data, such as smaller standard deviation or narrower confidence intervals, are typically regarded as higher quality in study design. A weighting statistic that aims to incorporate both of these factors, known as inverse variance, is commonly employed.
Step 5: Absolute Effect Estimation
The ultimate step in conducting a meta-analysis is to choose and utilize an appropriate model for comparing Effect Sizes among diverse studies. Two popular models for this purpose are the Fixed Effects and Random Effects models. The Fixed Effects model relies on the premise that each study is evaluating a common treatment effect, implying that all studies would have estimated the same Effect Size if sample variability were equal across all studies.
Conversely, the Random Effects model posits that the true treatment effects in individual studies may vary from each other, and endeavors to consider this additional source of interstudy variation in Effect Sizes. The existence and magnitude of this latter variability is usually evaluated within the meta-analysis through a test for ‘heterogeneity.’
Forest Plot
The results of a meta-analysis are often visually presented using a “Forest Plot”. This type of plot displays, for each study, included in the analysis, a horizontal line that indicates the standardized Effect Size estimate and 95% confidence interval for the risk ratio used. Figure A provides an example of a hypothetical Forest Plot in which drug X reduces the risk of death in all three studies.
However, the first study was larger than the other two, and as a result, the estimates for the smaller studies were not statistically significant. This is indicated by the lines emanating from their boxes, including the value of 1. The size of the boxes represents the relative weights assigned to each study by the meta-analysis. The combined estimate of the drug’s effect, represented by the diamond, provides a more precise estimate of the drug’s effect, with the diamond indicating both the combined risk ratio estimate and the 95% confidence interval limits.
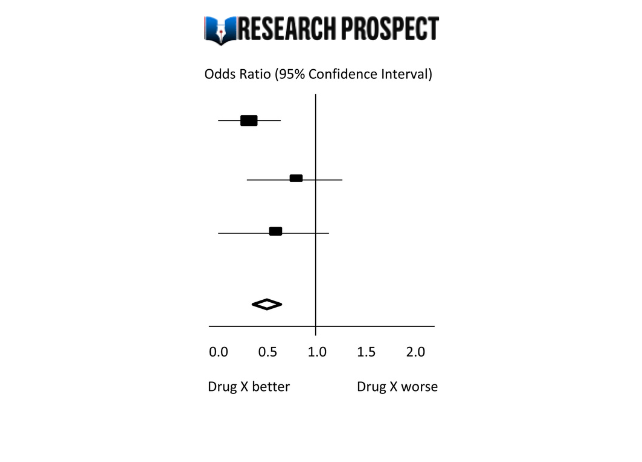
Figure-A: Hypothetical Forest Plot
Relevance to Practice and Research
Evidence Based Nursing commentaries often include recently published systematic reviews and meta-analyses, as they can provide new insights and strengthen recommendations for effective healthcare practices. Additionally, they can identify gaps or limitations in current evidence and guide future research directions.
The quality of the data available for synthesis is a critical factor in the strength of conclusions drawn from meta-analyses, and this is influenced by the quality of individual studies and the systematic review itself. However, meta-analysis cannot overcome issues related to underpowered or poorly designed studies.
Therefore, clinicians may still encounter situations where the evidence is weak or uncertain, and where higher-quality research is required to improve clinical decision-making. While such findings can be frustrating, they remain important for informing practice and highlighting the need for further research to fill gaps in the evidence base.
Methods and Assumptions in Meta-Analysis
Ensuring the credibility of findings is imperative in all types of research, including meta-analyses. To validate the outcomes of a meta-analysis, the researcher must confirm that the research techniques used were accurate in measuring the intended variables. Typically, researchers establish the validity of a meta-analysis by testing the outcomes for homogeneity or the degree of similarity between the results of the combined studies.
Homogeneity is preferred in meta-analyses as it allows the data to be combined without needing adjustments to suit the study’s requirements. To determine homogeneity, researchers assess heterogeneity, the opposite of homogeneity. Two widely used statistical methods for evaluating heterogeneity in research results are Cochran’s-Q and I-Square, also known as I-2 Index.
Difference Between Meta-Analysis and Systematic Reviews
Meta-analysis and systematic reviews are both research methods used to synthesise evidence from multiple studies on a particular topic. However, there are some key differences between the two.
Systematic reviews involve a comprehensive and structured approach to identifying, selecting, and critically appraising all available evidence relevant to a specific research question. This process involves searching multiple databases, screening the identified studies for relevance and quality, and summarizing the findings in a narrative report.
Meta-analysis, on the other hand, involves using statistical methods to combine and analyze the data from multiple studies, with the aim of producing a quantitative summary of the overall effect size. Meta-analysis requires the studies to be similar enough in terms of their design, methodology, and outcome measures to allow for meaningful comparison and analysis.
Therefore, systematic reviews are broader in scope and summarize the findings of all studies on a topic, while meta-analyses are more focused on producing a quantitative estimate of the effect size of an intervention across multiple studies that meet certain criteria. In some cases, a systematic review may be conducted without a meta-analysis if the studies are too diverse or the quality of the data is not sufficient to allow for statistical pooling.
Software Packages For Meta-Analysis
Meta-analysis can be done through software packages, including free and paid options. One of the most commonly used software packages for meta-analysis is RevMan by the Cochrane Collaboration.
Assessing the Quality of Meta-Analysis
Assessing the quality of a meta-analysis involves evaluating the methods used to conduct the analysis and the quality of the studies included. Here are some key factors to consider:
- Study selection: The studies included in the meta-analysis should be relevant to the research question and meet predetermined criteria for quality.
- Search strategy: The search strategy should be comprehensive and transparent, including databases and search terms used to identify relevant studies.
- Study quality assessment: The quality of included studies should be assessed using appropriate tools, and this assessment should be reported in the meta-analysis.
- Data extraction: The data extraction process should be systematic and clearly reported, including any discrepancies that arose.
- Analysis methods: The meta-analysis should use appropriate statistical methods to combine the results of the included studies, and these methods should be transparently reported.
- Publication bias: The potential for publication bias should be assessed and reported in the meta-analysis, including any efforts to identify and include unpublished studies.
- Interpretation of results: The results should be interpreted in the context of the study limitations and the overall quality of the evidence.
- Sensitivity analysis: Sensitivity analysis should be conducted to evaluate the impact of study quality, inclusion criteria, and other factors on the overall results.
Overall, a high-quality meta-analysis should be transparent in its methods and clearly report the included studies’ limitations and the evidence’s overall quality.
Hire an Expert Writer
Orders completed by our expert writers are
- Formally drafted in an academic style
- Free Amendments and 100% Plagiarism Free – or your money back!
- 100% Confidential and Timely Delivery!
- Free anti-plagiarism report
- Appreciated by thousands of clients. Check client reviews
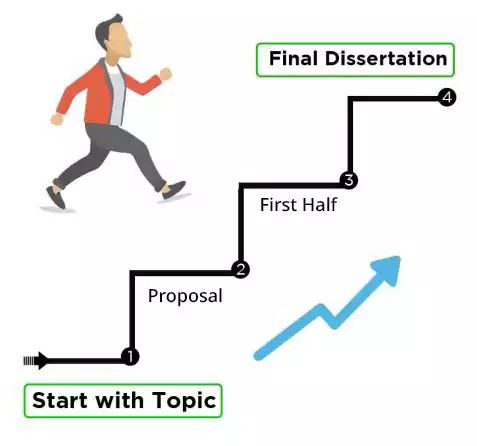
Examples of Meta-Analysis
- STANLEY T.D. et JARRELL S.B. (1989), « Meta-regression analysis : a quantitative method of literature surveys », Journal of Economics Surveys, vol. 3, n°2, pp. 161-170.
- DATTA D.K., PINCHES G.E. et NARAYANAN V.K. (1992), « Factors influencing wealth creation from mergers and acquisitions : a meta-analysis », Strategic Management Journal, Vol. 13, pp. 67-84.
- GLASS G. (1983), « Synthesising empirical research : Meta-analysis » in S.A. Ward and L.J. Reed (Eds), Knowledge structure and use : Implications for synthesis and interpretation, Philadelphia : Temple University Press.
- WOLF F.M. (1986), Meta-analysis : Quantitative methods for research synthesis, Sage University Paper n°59.
- HUNTER J.E., SCHMIDT F.L. et JACKSON G.B. (1982), « Meta-analysis : cumulating research findings across studies », Beverly Hills, CA : Sage.
Frequently Asked Questions
What is a meta-analysis in research.
Meta-analysis is a statistical method used to combine results from multiple studies on a specific topic. By pooling data from various sources, meta-analysis can provide a more precise estimate of the effect size of a treatment or intervention and identify areas for future research.
Why is meta-analysis important?
Meta-analysis is important because it combines and summarizes results from multiple studies to provide a more precise and reliable estimate of the effect of a treatment or intervention. This helps clinicians and policymakers make evidence-based decisions and identify areas for further research.
What is an example of a meta-analysis?
A meta-analysis of studies evaluating physical exercise’s effect on depression in adults is an example. Researchers gathered data from 49 studies involving a total of 2669 participants. The studies used different types of exercise and measures of depression, which made it difficult to compare the results.
Through meta-analysis, the researchers calculated an overall effect size and determined that exercise was associated with a statistically significant reduction in depression symptoms. The study also identified that moderate-intensity aerobic exercise, performed three to five times per week, was the most effective. The meta-analysis provided a more comprehensive understanding of the impact of exercise on depression than any single study could provide.
What is the definition of meta-analysis in clinical research?
Meta-analysis in clinical research is a statistical technique that combines data from multiple independent studies on a particular topic to generate a summary or “meta” estimate of the effect of a particular intervention or exposure.
This type of analysis allows researchers to synthesise the results of multiple studies, potentially increasing the statistical power and providing more precise estimates of treatment effects. Meta-analyses are commonly used in clinical research to evaluate the effectiveness and safety of medical interventions and to inform clinical practice guidelines.
Is meta-analysis qualitative or quantitative?
Meta-analysis is a quantitative method used to combine and analyze data from multiple studies. It involves the statistical synthesis of results from individual studies to obtain a pooled estimate of the effect size of a particular intervention or treatment. Therefore, meta-analysis is considered a quantitative approach to research synthesis.
You May Also Like
A variable is a characteristic that can change and have more than one value, such as age, height, and weight. But what are the different types of variables?
Sampling methods are used to to draw valid conclusions about a large community, organization or group of people, but they are based on evidence and reasoning.
Action research for my dissertation?, A brief overview of action research as a responsive, action-oriented, participative and reflective research technique.
USEFUL LINKS
LEARNING RESOURCES
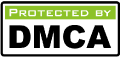
COMPANY DETAILS
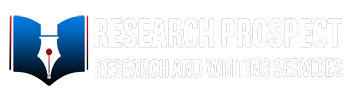
- How It Works
Loading metrics
Open Access
Research Matters
Research Matters articles provide a forum for scientists to communicate why basic research in their field matters.
See all article types »
Meta-research: Why research on research matters
* E-mail: [email protected]
Affiliations Meta-Research Innovation Center at Stanford (METRICS), Stanford University, Stanford, California, United States of America, Department of Medicine, Department of Health Research and Policy, and Department of Biomedical Data Science, Stanford University School of Medicine, Stanford, California, United States of America, Department of Statistics, Stanford University School of Humanities and Sciences, Stanford, California, United States of America

- John P. A. Ioannidis
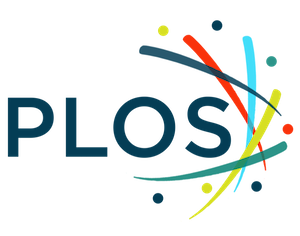
Published: March 13, 2018
- https://doi.org/10.1371/journal.pbio.2005468
- Reader Comments
Meta-research is the study of research itself: its methods, reporting, reproducibility, evaluation, and incentives. Given that science is the key driver of human progress, improving the efficiency of scientific investigation and yielding more credible and more useful research results can translate to major benefits. The research enterprise grows very fast. Both new opportunities for knowledge and innovation and new threats to validity and scientific integrity emerge. Old biases abound, and new ones continuously appear as novel disciplines emerge with different standards and challenges. Meta-research uses an interdisciplinary approach to study, promote, and defend robust science. Major disruptions are likely to happen in the way we pursue scientific investigation, and it is important to ensure that these disruptions are evidence based.
Citation: Ioannidis JPA (2018) Meta-research: Why research on research matters. PLoS Biol 16(3): e2005468. https://doi.org/10.1371/journal.pbio.2005468
Copyright: © 2018 Ioannidis John P. A.. This is an open access article distributed under the terms of the Creative Commons Attribution License , which permits unrestricted use, distribution, and reproduction in any medium, provided the original author and source are credited.
Funding: Laura and John Arnold Foundation. The Meta-Research Innovation Center at Stanford (METRICS) has been funded by the Laura and John Arnold Foundation. The work of John Ioannidis is funded by an unrestricted gift from Sue and Bob O’Donnell. The funder had no role in study design, data collection and analysis, decision to publish, or preparation of the manuscript.
Competing interests: The authors have declared that no competing interests exist.
Abbreviations: NIH, National Institutes of Health; R&D, Research and Development; STEM, Science, Technology, Engineering, and Math
Provenance: Commissioned; not externally peer reviewed
Science, like all human endeavors, is prone to biases. Yet science can assess its own methods, reporting, reproducibility, evaluation, and incentives [ 1 ]. A relatively new discipline, called meta-research, covers a wide range of theoretical, observational, and experimental investigations designed to study research itself and its practices. The objective is to understand and improve how we perform, communicate, verify, evaluate, and reward research [ 1 ].
Before elaborating on a discipline that studies biases, I should disclose some of my own. First, all scientists are meta-researchers to some extent, though most usually work on focused subject matter disciplines. And though the advice of my early lab mentors—“focus, focus, focus”—still rings in my ears, the piles on my desk and the files in my computers can be notoriously unfocused. I don’t have attention-deficit disorder, but plain unconstrained curiosity. What attracted me to science was its vastness and diversity. In my early training years, I enjoyed roaming in libraries in Athens and Boston, discovering scientific journals with fancy names, encountering intriguing articles, drifting from my initial search. Without yet realizing it, I was interested primarily in research itself apparently, much as others were interested primarily in Caenorhabditis elegans , volcanic eruptions, or automata.
Science and its literature is a marvelous maze of data, arguments, biases, errors, and the greatest achievements of humans. What can be more rewarding to study scientifically? Thirty years later, I still feel like a researcher-in-training—actually, in early training—barely scratching the surface. However, much has changed. Thirty years ago, articles had to be handpicked like flowers one by one from their journal shelves and photocopied one page at a time. Now, one can text mine a million articles overnight. Good research, however, still takes time and focus. Take, for example, a recent project I worked on with my friend David Chavalarias. We text mined 12,821,790 abstracts and 843,884 full-text articles. We initially joked that it would take two days max. Eventually, it took four years of work with innumerable iterations, meticulous corrections, and repeated downloads.
My other personal bias is a heightened interest in methods rather than results. Result narratives are supposedly always exciting. I find them unbearably boring. Conversely, methods typically are missing in action, left unsung, or hidden in small print. Many researchers hope to clarify how to do experiments chatting in corridors or conferences. Study design and analysis are still mostly taught (if at all) in statistics-lite courses. Most of us have mastered how to write papers through reading other (mostly poorly reported) papers. We freely volunteer peer review but lack formal training on how to do it. In many fields, issues surrounding reproducibility were dormant until recently.
Science remains the key driver of human progress, yet we have little evidence on how to best fund science and incentivize high-quality work. We do know that leaving research practices to serendipity, biasing influences, methodological illiteracy, and statistical innumeracy is inefficient. Science needs science to avoid wasted effort and optimize resources. Amateur approaches face the current gigantic magnitudes of the research endeavor. Google Scholar currently includes about 180,000,000 documents, accruing approximately 4,000,000 new papers annually [ 2 ]. Along this universe of visible (published) matter, dark matter abounds; probably most observations and data analyses remain unpublished. Ulrich’s directory includes more than 40,000 refereed academic journals, and this is probably an underestimate [ 3 ]. Thousands of journals follow predatory practices or have uncertain value. The Science, Technology, Engineering, and Math (STEM) publishing business market size ($28 billion) roughly equals the National Institutes of Health (NIH) budget. Webometrics lists 26,368 research-producing universities [ 4 ], and many other entities generate research. Probably 100,000 biomedical conferences happen annually [ 5 ]. Global Research and Development (R&D) investment recently exceeded $2 trillion per year. Industry has the lion’s share, while public funding is limited for basic research and it is even more sparse for evidence-based evaluation research. Financial conflicts may shape research agendas, results, and interpretations [ 6 ]. Consider that the $1 trillion tobacco industry still runs “research” on its products despite killing millions of people who use them as directed. Big Pharma, another behemoth of similar financial magnitude, but which probably saves lives (albeit often at high cost), has to sponsor most research on its own products. Understanding who should do what and how in research needs better study.
Science is no longer the occupation of few intellectual dilettanti. Millions (co)author scientific papers. Even more people participate in research. Currently, health record databases engulf hundreds of millions of individuals. Social media databases generate the possibility of using data on billions—active monthly Facebook users, for example, exceeded 2 billion by July 2017.
Currently, generated research data are massive but also fragmented and often nontransparent. Full data sharing and preregistration of protocols are still uncommon in most fields [ 7 ]. We need to understand whether results and inferences are correct, modestly biased, or plain wrong. Comparing patterns of data and biases across the vast number of available studies, one can help answer this important question [ 8 ]. We have mapped 235 biases in biomedical research alone [ 9 ]. With increasing research complexity, multifarious choices emerge on how to design studies and analyze data. With 20 binary choices, 2 20 = 1,048,576 different ways exist to analyze the same data. Therefore, almost any result is possible, unless we safeguard methods and analysis standards. Surveys show that questionable research practices are used by most scientists: not fraud (which is rare) but “cutting corners” to achieve more interesting-looking results [ 10 ]. Understanding the boundaries between bias and creative exploration is important. Efforts to reproduce high-profile studies have shown high rates of nonreproducibility [ 11 ] and most scientists agree that a reproducibility crisis exists [ 12 ]. Meta-analyses—efforts to combine all data on a given question—become increasingly popular but face their own problems and biases [ 13 ].
How should a scientist best train, work, collaborate, and contribute to scientific and broader communities? Researchers spend most of their time on grants [ 14 ] and administrative chores of unclear utility. Journal peer review takes another 64 million hours annually for biomedical papers alone [ 15 ]. Justifiably, we all despise bureaucracy and obstructions. Poor research practices make things worse.
Thousands of new scientific fields emerge, merge, split, and evolve [ 16 ]. Different disciplines may differ in research standards and challenges ( Box 1 ). Meta-research can help us disseminate efficient research practices and abandon wasteful ones. Publication and peer review models, scientific education, funding, and academic reward systems need to adapt successfully to a rapidly changing world. Some predict [ 17 ] that even researchers may disappear within decades, replaced by artificial intelligence. While this sounds extreme, several aspects of current “business as usual” in research will face disruption. Even 1% improvement in the yield and translation of useful discoveries effected through better research practices reflects value equivalent of many Nobel or Breakthrough prizes.
Box 1. Features of research practices, opportunities, and threats that vary across fields.
- ◦ Type of mix of research (basic, applied translational, evaluation, implementation)
- ◦ Types of study designs commonly used or misused
- ◦ Types of experimental/measurement tools commonly used or misused
- ◦ Types of statistical methods commonly used or misused
- ◦ Types of common biases encountered and whether they are easy to fix or not
- ◦ Extent of use of methods to prevent or correct for biases
- ◦ Prevalence of different types of questionable/detrimental research practices
- ◦ Distribution of effect sizes observed
- ◦ Typical heterogeneity of results across studies
- ◦ Proportion of results that are true, exaggerated, or entirely false
- ◦ Reputational impact for bias or wrong, refuted results
- ◦ Proportion of studies and analyses that are published
- ◦ Number and types of available publication venues
- ◦ Implementation of prepublication peer review (e.g., preprints)
- ◦ Implementation of postpublication peer review
- ◦ Extent from adoption of various research reporting standards
- ◦ Commonly accepted authorship and contributorship norms
- ◦ Extent of adoption of team science and consortia
- ◦ Type of training for scientists in the field
- ◦ Extent of methodological and statistical literacy/numeracy
- ◦ Extent and enforcement of preregistration of protocols
- ◦ Extent of use of replication studies
- ◦ Extent of use of exact replication versus corroboration or triangulation
- ◦ Extent of sharing of primary raw data and/or processed data
- ◦ Extent of sharing of software and code
- ◦ Extent and types of evidence synthesis used
- ◦ Main funders (government, industry, other) and types of studies that they fund
- ◦ Project-based versus person-based funding
- ◦ Mix and interplay of institutions performing research (university, industry, other)
- ◦ Types of metrics and criteria used for assessing researchers and institutions
- ◦ Typical conflicts of interest operating in the field
- ◦ Completeness of disclosure of conflicts of interest
- ◦ Extent and fidelity of dissemination of research findings to the general public
- ◦ Extent of public misperceptions about the field
- ◦ Threats from antiscience advocates attacking the field
Meta-research is interdisciplinary. For example, it benefits from better tools and methods in statistics and informatics. Complex issues of behavior change converge on modeling, psychology, sociology, and behavioral economics. Newly introduced, sophisticated measurement tools and techniques in various disciplines introduce new, peculiar errors and biases; their understanding requires combining expertise in biology, bioengineering, and data sciences. Properly communicating science and its value requires combining expertise in multiple fields and has become increasingly critical nowadays, when mistrust of science runs high and multiple interests hold a stake in influencing research results. Some interests set out to manipulate science and cause damage when their intentional bias pollutes the scientific record (e.g., tobacco companies or climate change deniers). Meta-research may be our best chance to defend science, gain public support for research, and counter antiscience movements. It may help provide a correcting mechanism closer to real time than the self-correcting scientific process that otherwise may take much longer.
Moreover, bird’s-eye metaviews of science are not separate and detached from focused field-specific research. In my experience, inspiration for new projects has often come from mistakes, shortcomings, or difficulties that I encountered while doing field-specific research. It is sometimes difficult to convey a message that something is wrong. However, it is paradoxically easier when the message says that thousands or millions of papers are doing something wrong rather than arousing personal animosity for a single failed paper. It is also easier when the constructive critique comes from within a field, recognized as necessary improvement rather than intrusion. Learning by collaborating with researchers in diverse disciplines and trying to understand the daily challenges in a specific field can be a highly rewarding experience for a meta-researcher. We need scientific curiosity but also intellectual humility and commitment to improve our efforts.
- View Article
- PubMed/NCBI
- Google Scholar
- 4. Webometrics. List of universities (as of January 2017). [Cited 21 January 2018]. Available from: http://www.webometrics.info/en/node/54 .
- USC Libraries
- Research Guides
Organizing Your Social Sciences Research Paper
- Types of Research Designs
- Purpose of Guide
- Design Flaws to Avoid
- Independent and Dependent Variables
- Glossary of Research Terms
- Reading Research Effectively
- Narrowing a Topic Idea
- Broadening a Topic Idea
- Extending the Timeliness of a Topic Idea
- Academic Writing Style
- Choosing a Title
- Making an Outline
- Paragraph Development
- Research Process Video Series
- Executive Summary
- The C.A.R.S. Model
- Background Information
- The Research Problem/Question
- Theoretical Framework
- Citation Tracking
- Content Alert Services
- Evaluating Sources
- Primary Sources
- Secondary Sources
- Tiertiary Sources
- Scholarly vs. Popular Publications
- Qualitative Methods
- Quantitative Methods
- Insiderness
- Using Non-Textual Elements
- Limitations of the Study
- Common Grammar Mistakes
- Writing Concisely
- Avoiding Plagiarism
- Footnotes or Endnotes?
- Further Readings
- Generative AI and Writing
- USC Libraries Tutorials and Other Guides
- Bibliography
Introduction
Before beginning your paper, you need to decide how you plan to design the study .
The research design refers to the overall strategy and analytical approach that you have chosen in order to integrate, in a coherent and logical way, the different components of the study, thus ensuring that the research problem will be thoroughly investigated. It constitutes the blueprint for the collection, measurement, and interpretation of information and data. Note that the research problem determines the type of design you choose, not the other way around!
De Vaus, D. A. Research Design in Social Research . London: SAGE, 2001; Trochim, William M.K. Research Methods Knowledge Base. 2006.
General Structure and Writing Style
The function of a research design is to ensure that the evidence obtained enables you to effectively address the research problem logically and as unambiguously as possible . In social sciences research, obtaining information relevant to the research problem generally entails specifying the type of evidence needed to test the underlying assumptions of a theory, to evaluate a program, or to accurately describe and assess meaning related to an observable phenomenon.
With this in mind, a common mistake made by researchers is that they begin their investigations before they have thought critically about what information is required to address the research problem. Without attending to these design issues beforehand, the overall research problem will not be adequately addressed and any conclusions drawn will run the risk of being weak and unconvincing. As a consequence, the overall validity of the study will be undermined.
The length and complexity of describing the research design in your paper can vary considerably, but any well-developed description will achieve the following :
- Identify the research problem clearly and justify its selection, particularly in relation to any valid alternative designs that could have been used,
- Review and synthesize previously published literature associated with the research problem,
- Clearly and explicitly specify hypotheses [i.e., research questions] central to the problem,
- Effectively describe the information and/or data which will be necessary for an adequate testing of the hypotheses and explain how such information and/or data will be obtained, and
- Describe the methods of analysis to be applied to the data in determining whether or not the hypotheses are true or false.
The research design is usually incorporated into the introduction of your paper . You can obtain an overall sense of what to do by reviewing studies that have utilized the same research design [e.g., using a case study approach]. This can help you develop an outline to follow for your own paper.
NOTE : Use the SAGE Research Methods Online and Cases and the SAGE Research Methods Videos databases to search for scholarly resources on how to apply specific research designs and methods . The Research Methods Online database contains links to more than 175,000 pages of SAGE publisher's book, journal, and reference content on quantitative, qualitative, and mixed research methodologies. Also included is a collection of case studies of social research projects that can be used to help you better understand abstract or complex methodological concepts. The Research Methods Videos database contains hours of tutorials, interviews, video case studies, and mini-documentaries covering the entire research process.
Creswell, John W. and J. David Creswell. Research Design: Qualitative, Quantitative, and Mixed Methods Approaches . 5th edition. Thousand Oaks, CA: Sage, 2018; De Vaus, D. A. Research Design in Social Research . London: SAGE, 2001; Gorard, Stephen. Research Design: Creating Robust Approaches for the Social Sciences . Thousand Oaks, CA: Sage, 2013; Leedy, Paul D. and Jeanne Ellis Ormrod. Practical Research: Planning and Design . Tenth edition. Boston, MA: Pearson, 2013; Vogt, W. Paul, Dianna C. Gardner, and Lynne M. Haeffele. When to Use What Research Design . New York: Guilford, 2012.
Action Research Design
Definition and Purpose
The essentials of action research design follow a characteristic cycle whereby initially an exploratory stance is adopted, where an understanding of a problem is developed and plans are made for some form of interventionary strategy. Then the intervention is carried out [the "action" in action research] during which time, pertinent observations are collected in various forms. The new interventional strategies are carried out, and this cyclic process repeats, continuing until a sufficient understanding of [or a valid implementation solution for] the problem is achieved. The protocol is iterative or cyclical in nature and is intended to foster deeper understanding of a given situation, starting with conceptualizing and particularizing the problem and moving through several interventions and evaluations.
What do these studies tell you ?
- This is a collaborative and adaptive research design that lends itself to use in work or community situations.
- Design focuses on pragmatic and solution-driven research outcomes rather than testing theories.
- When practitioners use action research, it has the potential to increase the amount they learn consciously from their experience; the action research cycle can be regarded as a learning cycle.
- Action research studies often have direct and obvious relevance to improving practice and advocating for change.
- There are no hidden controls or preemption of direction by the researcher.
What these studies don't tell you ?
- It is harder to do than conducting conventional research because the researcher takes on responsibilities of advocating for change as well as for researching the topic.
- Action research is much harder to write up because it is less likely that you can use a standard format to report your findings effectively [i.e., data is often in the form of stories or observation].
- Personal over-involvement of the researcher may bias research results.
- The cyclic nature of action research to achieve its twin outcomes of action [e.g. change] and research [e.g. understanding] is time-consuming and complex to conduct.
- Advocating for change usually requires buy-in from study participants.
Coghlan, David and Mary Brydon-Miller. The Sage Encyclopedia of Action Research . Thousand Oaks, CA: Sage, 2014; Efron, Sara Efrat and Ruth Ravid. Action Research in Education: A Practical Guide . New York: Guilford, 2013; Gall, Meredith. Educational Research: An Introduction . Chapter 18, Action Research. 8th ed. Boston, MA: Pearson/Allyn and Bacon, 2007; Gorard, Stephen. Research Design: Creating Robust Approaches for the Social Sciences . Thousand Oaks, CA: Sage, 2013; Kemmis, Stephen and Robin McTaggart. “Participatory Action Research.” In Handbook of Qualitative Research . Norman Denzin and Yvonna S. Lincoln, eds. 2nd ed. (Thousand Oaks, CA: SAGE, 2000), pp. 567-605; McNiff, Jean. Writing and Doing Action Research . London: Sage, 2014; Reason, Peter and Hilary Bradbury. Handbook of Action Research: Participative Inquiry and Practice . Thousand Oaks, CA: SAGE, 2001.
Case Study Design
A case study is an in-depth study of a particular research problem rather than a sweeping statistical survey or comprehensive comparative inquiry. It is often used to narrow down a very broad field of research into one or a few easily researchable examples. The case study research design is also useful for testing whether a specific theory and model actually applies to phenomena in the real world. It is a useful design when not much is known about an issue or phenomenon.
- Approach excels at bringing us to an understanding of a complex issue through detailed contextual analysis of a limited number of events or conditions and their relationships.
- A researcher using a case study design can apply a variety of methodologies and rely on a variety of sources to investigate a research problem.
- Design can extend experience or add strength to what is already known through previous research.
- Social scientists, in particular, make wide use of this research design to examine contemporary real-life situations and provide the basis for the application of concepts and theories and the extension of methodologies.
- The design can provide detailed descriptions of specific and rare cases.
- A single or small number of cases offers little basis for establishing reliability or to generalize the findings to a wider population of people, places, or things.
- Intense exposure to the study of a case may bias a researcher's interpretation of the findings.
- Design does not facilitate assessment of cause and effect relationships.
- Vital information may be missing, making the case hard to interpret.
- The case may not be representative or typical of the larger problem being investigated.
- If the criteria for selecting a case is because it represents a very unusual or unique phenomenon or problem for study, then your interpretation of the findings can only apply to that particular case.
Case Studies. Writing@CSU. Colorado State University; Anastas, Jeane W. Research Design for Social Work and the Human Services . Chapter 4, Flexible Methods: Case Study Design. 2nd ed. New York: Columbia University Press, 1999; Gerring, John. “What Is a Case Study and What Is It Good for?” American Political Science Review 98 (May 2004): 341-354; Greenhalgh, Trisha, editor. Case Study Evaluation: Past, Present and Future Challenges . Bingley, UK: Emerald Group Publishing, 2015; Mills, Albert J. , Gabrielle Durepos, and Eiden Wiebe, editors. Encyclopedia of Case Study Research . Thousand Oaks, CA: SAGE Publications, 2010; Stake, Robert E. The Art of Case Study Research . Thousand Oaks, CA: SAGE, 1995; Yin, Robert K. Case Study Research: Design and Theory . Applied Social Research Methods Series, no. 5. 3rd ed. Thousand Oaks, CA: SAGE, 2003.
Causal Design
Causality studies may be thought of as understanding a phenomenon in terms of conditional statements in the form, “If X, then Y.” This type of research is used to measure what impact a specific change will have on existing norms and assumptions. Most social scientists seek causal explanations that reflect tests of hypotheses. Causal effect (nomothetic perspective) occurs when variation in one phenomenon, an independent variable, leads to or results, on average, in variation in another phenomenon, the dependent variable.
Conditions necessary for determining causality:
- Empirical association -- a valid conclusion is based on finding an association between the independent variable and the dependent variable.
- Appropriate time order -- to conclude that causation was involved, one must see that cases were exposed to variation in the independent variable before variation in the dependent variable.
- Nonspuriousness -- a relationship between two variables that is not due to variation in a third variable.
- Causality research designs assist researchers in understanding why the world works the way it does through the process of proving a causal link between variables and by the process of eliminating other possibilities.
- Replication is possible.
- There is greater confidence the study has internal validity due to the systematic subject selection and equity of groups being compared.
- Not all relationships are causal! The possibility always exists that, by sheer coincidence, two unrelated events appear to be related [e.g., Punxatawney Phil could accurately predict the duration of Winter for five consecutive years but, the fact remains, he's just a big, furry rodent].
- Conclusions about causal relationships are difficult to determine due to a variety of extraneous and confounding variables that exist in a social environment. This means causality can only be inferred, never proven.
- If two variables are correlated, the cause must come before the effect. However, even though two variables might be causally related, it can sometimes be difficult to determine which variable comes first and, therefore, to establish which variable is the actual cause and which is the actual effect.
Beach, Derek and Rasmus Brun Pedersen. Causal Case Study Methods: Foundations and Guidelines for Comparing, Matching, and Tracing . Ann Arbor, MI: University of Michigan Press, 2016; Bachman, Ronet. The Practice of Research in Criminology and Criminal Justice . Chapter 5, Causation and Research Designs. 3rd ed. Thousand Oaks, CA: Pine Forge Press, 2007; Brewer, Ernest W. and Jennifer Kubn. “Causal-Comparative Design.” In Encyclopedia of Research Design . Neil J. Salkind, editor. (Thousand Oaks, CA: Sage, 2010), pp. 125-132; Causal Research Design: Experimentation. Anonymous SlideShare Presentation; Gall, Meredith. Educational Research: An Introduction . Chapter 11, Nonexperimental Research: Correlational Designs. 8th ed. Boston, MA: Pearson/Allyn and Bacon, 2007; Trochim, William M.K. Research Methods Knowledge Base. 2006.
Cohort Design
Often used in the medical sciences, but also found in the applied social sciences, a cohort study generally refers to a study conducted over a period of time involving members of a population which the subject or representative member comes from, and who are united by some commonality or similarity. Using a quantitative framework, a cohort study makes note of statistical occurrence within a specialized subgroup, united by same or similar characteristics that are relevant to the research problem being investigated, rather than studying statistical occurrence within the general population. Using a qualitative framework, cohort studies generally gather data using methods of observation. Cohorts can be either "open" or "closed."
- Open Cohort Studies [dynamic populations, such as the population of Los Angeles] involve a population that is defined just by the state of being a part of the study in question (and being monitored for the outcome). Date of entry and exit from the study is individually defined, therefore, the size of the study population is not constant. In open cohort studies, researchers can only calculate rate based data, such as, incidence rates and variants thereof.
- Closed Cohort Studies [static populations, such as patients entered into a clinical trial] involve participants who enter into the study at one defining point in time and where it is presumed that no new participants can enter the cohort. Given this, the number of study participants remains constant (or can only decrease).
- The use of cohorts is often mandatory because a randomized control study may be unethical. For example, you cannot deliberately expose people to asbestos, you can only study its effects on those who have already been exposed. Research that measures risk factors often relies upon cohort designs.
- Because cohort studies measure potential causes before the outcome has occurred, they can demonstrate that these “causes” preceded the outcome, thereby avoiding the debate as to which is the cause and which is the effect.
- Cohort analysis is highly flexible and can provide insight into effects over time and related to a variety of different types of changes [e.g., social, cultural, political, economic, etc.].
- Either original data or secondary data can be used in this design.
- In cases where a comparative analysis of two cohorts is made [e.g., studying the effects of one group exposed to asbestos and one that has not], a researcher cannot control for all other factors that might differ between the two groups. These factors are known as confounding variables.
- Cohort studies can end up taking a long time to complete if the researcher must wait for the conditions of interest to develop within the group. This also increases the chance that key variables change during the course of the study, potentially impacting the validity of the findings.
- Due to the lack of randominization in the cohort design, its external validity is lower than that of study designs where the researcher randomly assigns participants.
Healy P, Devane D. “Methodological Considerations in Cohort Study Designs.” Nurse Researcher 18 (2011): 32-36; Glenn, Norval D, editor. Cohort Analysis . 2nd edition. Thousand Oaks, CA: Sage, 2005; Levin, Kate Ann. Study Design IV: Cohort Studies. Evidence-Based Dentistry 7 (2003): 51–52; Payne, Geoff. “Cohort Study.” In The SAGE Dictionary of Social Research Methods . Victor Jupp, editor. (Thousand Oaks, CA: Sage, 2006), pp. 31-33; Study Design 101. Himmelfarb Health Sciences Library. George Washington University, November 2011; Cohort Study. Wikipedia.
Cross-Sectional Design
Cross-sectional research designs have three distinctive features: no time dimension; a reliance on existing differences rather than change following intervention; and, groups are selected based on existing differences rather than random allocation. The cross-sectional design can only measure differences between or from among a variety of people, subjects, or phenomena rather than a process of change. As such, researchers using this design can only employ a relatively passive approach to making causal inferences based on findings.
- Cross-sectional studies provide a clear 'snapshot' of the outcome and the characteristics associated with it, at a specific point in time.
- Unlike an experimental design, where there is an active intervention by the researcher to produce and measure change or to create differences, cross-sectional designs focus on studying and drawing inferences from existing differences between people, subjects, or phenomena.
- Entails collecting data at and concerning one point in time. While longitudinal studies involve taking multiple measures over an extended period of time, cross-sectional research is focused on finding relationships between variables at one moment in time.
- Groups identified for study are purposely selected based upon existing differences in the sample rather than seeking random sampling.
- Cross-section studies are capable of using data from a large number of subjects and, unlike observational studies, is not geographically bound.
- Can estimate prevalence of an outcome of interest because the sample is usually taken from the whole population.
- Because cross-sectional designs generally use survey techniques to gather data, they are relatively inexpensive and take up little time to conduct.
- Finding people, subjects, or phenomena to study that are very similar except in one specific variable can be difficult.
- Results are static and time bound and, therefore, give no indication of a sequence of events or reveal historical or temporal contexts.
- Studies cannot be utilized to establish cause and effect relationships.
- This design only provides a snapshot of analysis so there is always the possibility that a study could have differing results if another time-frame had been chosen.
- There is no follow up to the findings.
Bethlehem, Jelke. "7: Cross-sectional Research." In Research Methodology in the Social, Behavioural and Life Sciences . Herman J Adèr and Gideon J Mellenbergh, editors. (London, England: Sage, 1999), pp. 110-43; Bourque, Linda B. “Cross-Sectional Design.” In The SAGE Encyclopedia of Social Science Research Methods . Michael S. Lewis-Beck, Alan Bryman, and Tim Futing Liao. (Thousand Oaks, CA: 2004), pp. 230-231; Hall, John. “Cross-Sectional Survey Design.” In Encyclopedia of Survey Research Methods . Paul J. Lavrakas, ed. (Thousand Oaks, CA: Sage, 2008), pp. 173-174; Helen Barratt, Maria Kirwan. Cross-Sectional Studies: Design Application, Strengths and Weaknesses of Cross-Sectional Studies. Healthknowledge, 2009. Cross-Sectional Study. Wikipedia.
Descriptive Design
Descriptive research designs help provide answers to the questions of who, what, when, where, and how associated with a particular research problem; a descriptive study cannot conclusively ascertain answers to why. Descriptive research is used to obtain information concerning the current status of the phenomena and to describe "what exists" with respect to variables or conditions in a situation.
- The subject is being observed in a completely natural and unchanged natural environment. True experiments, whilst giving analyzable data, often adversely influence the normal behavior of the subject [a.k.a., the Heisenberg effect whereby measurements of certain systems cannot be made without affecting the systems].
- Descriptive research is often used as a pre-cursor to more quantitative research designs with the general overview giving some valuable pointers as to what variables are worth testing quantitatively.
- If the limitations are understood, they can be a useful tool in developing a more focused study.
- Descriptive studies can yield rich data that lead to important recommendations in practice.
- Appoach collects a large amount of data for detailed analysis.
- The results from a descriptive research cannot be used to discover a definitive answer or to disprove a hypothesis.
- Because descriptive designs often utilize observational methods [as opposed to quantitative methods], the results cannot be replicated.
- The descriptive function of research is heavily dependent on instrumentation for measurement and observation.
Anastas, Jeane W. Research Design for Social Work and the Human Services . Chapter 5, Flexible Methods: Descriptive Research. 2nd ed. New York: Columbia University Press, 1999; Given, Lisa M. "Descriptive Research." In Encyclopedia of Measurement and Statistics . Neil J. Salkind and Kristin Rasmussen, editors. (Thousand Oaks, CA: Sage, 2007), pp. 251-254; McNabb, Connie. Descriptive Research Methodologies. Powerpoint Presentation; Shuttleworth, Martyn. Descriptive Research Design, September 26, 2008; Erickson, G. Scott. "Descriptive Research Design." In New Methods of Market Research and Analysis . (Northampton, MA: Edward Elgar Publishing, 2017), pp. 51-77; Sahin, Sagufta, and Jayanta Mete. "A Brief Study on Descriptive Research: Its Nature and Application in Social Science." International Journal of Research and Analysis in Humanities 1 (2021): 11; K. Swatzell and P. Jennings. “Descriptive Research: The Nuts and Bolts.” Journal of the American Academy of Physician Assistants 20 (2007), pp. 55-56; Kane, E. Doing Your Own Research: Basic Descriptive Research in the Social Sciences and Humanities . London: Marion Boyars, 1985.
Experimental Design
A blueprint of the procedure that enables the researcher to maintain control over all factors that may affect the result of an experiment. In doing this, the researcher attempts to determine or predict what may occur. Experimental research is often used where there is time priority in a causal relationship (cause precedes effect), there is consistency in a causal relationship (a cause will always lead to the same effect), and the magnitude of the correlation is great. The classic experimental design specifies an experimental group and a control group. The independent variable is administered to the experimental group and not to the control group, and both groups are measured on the same dependent variable. Subsequent experimental designs have used more groups and more measurements over longer periods. True experiments must have control, randomization, and manipulation.
- Experimental research allows the researcher to control the situation. In so doing, it allows researchers to answer the question, “What causes something to occur?”
- Permits the researcher to identify cause and effect relationships between variables and to distinguish placebo effects from treatment effects.
- Experimental research designs support the ability to limit alternative explanations and to infer direct causal relationships in the study.
- Approach provides the highest level of evidence for single studies.
- The design is artificial, and results may not generalize well to the real world.
- The artificial settings of experiments may alter the behaviors or responses of participants.
- Experimental designs can be costly if special equipment or facilities are needed.
- Some research problems cannot be studied using an experiment because of ethical or technical reasons.
- Difficult to apply ethnographic and other qualitative methods to experimentally designed studies.
Anastas, Jeane W. Research Design for Social Work and the Human Services . Chapter 7, Flexible Methods: Experimental Research. 2nd ed. New York: Columbia University Press, 1999; Chapter 2: Research Design, Experimental Designs. School of Psychology, University of New England, 2000; Chow, Siu L. "Experimental Design." In Encyclopedia of Research Design . Neil J. Salkind, editor. (Thousand Oaks, CA: Sage, 2010), pp. 448-453; "Experimental Design." In Social Research Methods . Nicholas Walliman, editor. (London, England: Sage, 2006), pp, 101-110; Experimental Research. Research Methods by Dummies. Department of Psychology. California State University, Fresno, 2006; Kirk, Roger E. Experimental Design: Procedures for the Behavioral Sciences . 4th edition. Thousand Oaks, CA: Sage, 2013; Trochim, William M.K. Experimental Design. Research Methods Knowledge Base. 2006; Rasool, Shafqat. Experimental Research. Slideshare presentation.
Exploratory Design
An exploratory design is conducted about a research problem when there are few or no earlier studies to refer to or rely upon to predict an outcome . The focus is on gaining insights and familiarity for later investigation or undertaken when research problems are in a preliminary stage of investigation. Exploratory designs are often used to establish an understanding of how best to proceed in studying an issue or what methodology would effectively apply to gathering information about the issue.
The goals of exploratory research are intended to produce the following possible insights:
- Familiarity with basic details, settings, and concerns.
- Well grounded picture of the situation being developed.
- Generation of new ideas and assumptions.
- Development of tentative theories or hypotheses.
- Determination about whether a study is feasible in the future.
- Issues get refined for more systematic investigation and formulation of new research questions.
- Direction for future research and techniques get developed.
- Design is a useful approach for gaining background information on a particular topic.
- Exploratory research is flexible and can address research questions of all types (what, why, how).
- Provides an opportunity to define new terms and clarify existing concepts.
- Exploratory research is often used to generate formal hypotheses and develop more precise research problems.
- In the policy arena or applied to practice, exploratory studies help establish research priorities and where resources should be allocated.
- Exploratory research generally utilizes small sample sizes and, thus, findings are typically not generalizable to the population at large.
- The exploratory nature of the research inhibits an ability to make definitive conclusions about the findings. They provide insight but not definitive conclusions.
- The research process underpinning exploratory studies is flexible but often unstructured, leading to only tentative results that have limited value to decision-makers.
- Design lacks rigorous standards applied to methods of data gathering and analysis because one of the areas for exploration could be to determine what method or methodologies could best fit the research problem.
Cuthill, Michael. “Exploratory Research: Citizen Participation, Local Government, and Sustainable Development in Australia.” Sustainable Development 10 (2002): 79-89; Streb, Christoph K. "Exploratory Case Study." In Encyclopedia of Case Study Research . Albert J. Mills, Gabrielle Durepos and Eiden Wiebe, editors. (Thousand Oaks, CA: Sage, 2010), pp. 372-374; Taylor, P. J., G. Catalano, and D.R.F. Walker. “Exploratory Analysis of the World City Network.” Urban Studies 39 (December 2002): 2377-2394; Exploratory Research. Wikipedia.
Field Research Design
Sometimes referred to as ethnography or participant observation, designs around field research encompass a variety of interpretative procedures [e.g., observation and interviews] rooted in qualitative approaches to studying people individually or in groups while inhabiting their natural environment as opposed to using survey instruments or other forms of impersonal methods of data gathering. Information acquired from observational research takes the form of “ field notes ” that involves documenting what the researcher actually sees and hears while in the field. Findings do not consist of conclusive statements derived from numbers and statistics because field research involves analysis of words and observations of behavior. Conclusions, therefore, are developed from an interpretation of findings that reveal overriding themes, concepts, and ideas. More information can be found HERE .
- Field research is often necessary to fill gaps in understanding the research problem applied to local conditions or to specific groups of people that cannot be ascertained from existing data.
- The research helps contextualize already known information about a research problem, thereby facilitating ways to assess the origins, scope, and scale of a problem and to gage the causes, consequences, and means to resolve an issue based on deliberate interaction with people in their natural inhabited spaces.
- Enables the researcher to corroborate or confirm data by gathering additional information that supports or refutes findings reported in prior studies of the topic.
- Because the researcher in embedded in the field, they are better able to make observations or ask questions that reflect the specific cultural context of the setting being investigated.
- Observing the local reality offers the opportunity to gain new perspectives or obtain unique data that challenges existing theoretical propositions or long-standing assumptions found in the literature.
What these studies don't tell you
- A field research study requires extensive time and resources to carry out the multiple steps involved with preparing for the gathering of information, including for example, examining background information about the study site, obtaining permission to access the study site, and building trust and rapport with subjects.
- Requires a commitment to staying engaged in the field to ensure that you can adequately document events and behaviors as they unfold.
- The unpredictable nature of fieldwork means that researchers can never fully control the process of data gathering. They must maintain a flexible approach to studying the setting because events and circumstances can change quickly or unexpectedly.
- Findings can be difficult to interpret and verify without access to documents and other source materials that help to enhance the credibility of information obtained from the field [i.e., the act of triangulating the data].
- Linking the research problem to the selection of study participants inhabiting their natural environment is critical. However, this specificity limits the ability to generalize findings to different situations or in other contexts or to infer courses of action applied to other settings or groups of people.
- The reporting of findings must take into account how the researcher themselves may have inadvertently affected respondents and their behaviors.
Historical Design
The purpose of a historical research design is to collect, verify, and synthesize evidence from the past to establish facts that defend or refute a hypothesis. It uses secondary sources and a variety of primary documentary evidence, such as, diaries, official records, reports, archives, and non-textual information [maps, pictures, audio and visual recordings]. The limitation is that the sources must be both authentic and valid.
- The historical research design is unobtrusive; the act of research does not affect the results of the study.
- The historical approach is well suited for trend analysis.
- Historical records can add important contextual background required to more fully understand and interpret a research problem.
- There is often no possibility of researcher-subject interaction that could affect the findings.
- Historical sources can be used over and over to study different research problems or to replicate a previous study.
- The ability to fulfill the aims of your research are directly related to the amount and quality of documentation available to understand the research problem.
- Since historical research relies on data from the past, there is no way to manipulate it to control for contemporary contexts.
- Interpreting historical sources can be very time consuming.
- The sources of historical materials must be archived consistently to ensure access. This may especially challenging for digital or online-only sources.
- Original authors bring their own perspectives and biases to the interpretation of past events and these biases are more difficult to ascertain in historical resources.
- Due to the lack of control over external variables, historical research is very weak with regard to the demands of internal validity.
- It is rare that the entirety of historical documentation needed to fully address a research problem is available for interpretation, therefore, gaps need to be acknowledged.
Howell, Martha C. and Walter Prevenier. From Reliable Sources: An Introduction to Historical Methods . Ithaca, NY: Cornell University Press, 2001; Lundy, Karen Saucier. "Historical Research." In The Sage Encyclopedia of Qualitative Research Methods . Lisa M. Given, editor. (Thousand Oaks, CA: Sage, 2008), pp. 396-400; Marius, Richard. and Melvin E. Page. A Short Guide to Writing about History . 9th edition. Boston, MA: Pearson, 2015; Savitt, Ronald. “Historical Research in Marketing.” Journal of Marketing 44 (Autumn, 1980): 52-58; Gall, Meredith. Educational Research: An Introduction . Chapter 16, Historical Research. 8th ed. Boston, MA: Pearson/Allyn and Bacon, 2007.
Longitudinal Design
A longitudinal study follows the same sample over time and makes repeated observations. For example, with longitudinal surveys, the same group of people is interviewed at regular intervals, enabling researchers to track changes over time and to relate them to variables that might explain why the changes occur. Longitudinal research designs describe patterns of change and help establish the direction and magnitude of causal relationships. Measurements are taken on each variable over two or more distinct time periods. This allows the researcher to measure change in variables over time. It is a type of observational study sometimes referred to as a panel study.
- Longitudinal data facilitate the analysis of the duration of a particular phenomenon.
- Enables survey researchers to get close to the kinds of causal explanations usually attainable only with experiments.
- The design permits the measurement of differences or change in a variable from one period to another [i.e., the description of patterns of change over time].
- Longitudinal studies facilitate the prediction of future outcomes based upon earlier factors.
- The data collection method may change over time.
- Maintaining the integrity of the original sample can be difficult over an extended period of time.
- It can be difficult to show more than one variable at a time.
- This design often needs qualitative research data to explain fluctuations in the results.
- A longitudinal research design assumes present trends will continue unchanged.
- It can take a long period of time to gather results.
- There is a need to have a large sample size and accurate sampling to reach representativness.
Anastas, Jeane W. Research Design for Social Work and the Human Services . Chapter 6, Flexible Methods: Relational and Longitudinal Research. 2nd ed. New York: Columbia University Press, 1999; Forgues, Bernard, and Isabelle Vandangeon-Derumez. "Longitudinal Analyses." In Doing Management Research . Raymond-Alain Thiétart and Samantha Wauchope, editors. (London, England: Sage, 2001), pp. 332-351; Kalaian, Sema A. and Rafa M. Kasim. "Longitudinal Studies." In Encyclopedia of Survey Research Methods . Paul J. Lavrakas, ed. (Thousand Oaks, CA: Sage, 2008), pp. 440-441; Menard, Scott, editor. Longitudinal Research . Thousand Oaks, CA: Sage, 2002; Ployhart, Robert E. and Robert J. Vandenberg. "Longitudinal Research: The Theory, Design, and Analysis of Change.” Journal of Management 36 (January 2010): 94-120; Longitudinal Study. Wikipedia.
Meta-Analysis Design
Meta-analysis is an analytical methodology designed to systematically evaluate and summarize the results from a number of individual studies, thereby, increasing the overall sample size and the ability of the researcher to study effects of interest. The purpose is to not simply summarize existing knowledge, but to develop a new understanding of a research problem using synoptic reasoning. The main objectives of meta-analysis include analyzing differences in the results among studies and increasing the precision by which effects are estimated. A well-designed meta-analysis depends upon strict adherence to the criteria used for selecting studies and the availability of information in each study to properly analyze their findings. Lack of information can severely limit the type of analyzes and conclusions that can be reached. In addition, the more dissimilarity there is in the results among individual studies [heterogeneity], the more difficult it is to justify interpretations that govern a valid synopsis of results. A meta-analysis needs to fulfill the following requirements to ensure the validity of your findings:
- Clearly defined description of objectives, including precise definitions of the variables and outcomes that are being evaluated;
- A well-reasoned and well-documented justification for identification and selection of the studies;
- Assessment and explicit acknowledgment of any researcher bias in the identification and selection of those studies;
- Description and evaluation of the degree of heterogeneity among the sample size of studies reviewed; and,
- Justification of the techniques used to evaluate the studies.
- Can be an effective strategy for determining gaps in the literature.
- Provides a means of reviewing research published about a particular topic over an extended period of time and from a variety of sources.
- Is useful in clarifying what policy or programmatic actions can be justified on the basis of analyzing research results from multiple studies.
- Provides a method for overcoming small sample sizes in individual studies that previously may have had little relationship to each other.
- Can be used to generate new hypotheses or highlight research problems for future studies.
- Small violations in defining the criteria used for content analysis can lead to difficult to interpret and/or meaningless findings.
- A large sample size can yield reliable, but not necessarily valid, results.
- A lack of uniformity regarding, for example, the type of literature reviewed, how methods are applied, and how findings are measured within the sample of studies you are analyzing, can make the process of synthesis difficult to perform.
- Depending on the sample size, the process of reviewing and synthesizing multiple studies can be very time consuming.
Beck, Lewis W. "The Synoptic Method." The Journal of Philosophy 36 (1939): 337-345; Cooper, Harris, Larry V. Hedges, and Jeffrey C. Valentine, eds. The Handbook of Research Synthesis and Meta-Analysis . 2nd edition. New York: Russell Sage Foundation, 2009; Guzzo, Richard A., Susan E. Jackson and Raymond A. Katzell. “Meta-Analysis Analysis.” In Research in Organizational Behavior , Volume 9. (Greenwich, CT: JAI Press, 1987), pp 407-442; Lipsey, Mark W. and David B. Wilson. Practical Meta-Analysis . Thousand Oaks, CA: Sage Publications, 2001; Study Design 101. Meta-Analysis. The Himmelfarb Health Sciences Library, George Washington University; Timulak, Ladislav. “Qualitative Meta-Analysis.” In The SAGE Handbook of Qualitative Data Analysis . Uwe Flick, editor. (Los Angeles, CA: Sage, 2013), pp. 481-495; Walker, Esteban, Adrian V. Hernandez, and Micheal W. Kattan. "Meta-Analysis: It's Strengths and Limitations." Cleveland Clinic Journal of Medicine 75 (June 2008): 431-439.
Mixed-Method Design
- Narrative and non-textual information can add meaning to numeric data, while numeric data can add precision to narrative and non-textual information.
- Can utilize existing data while at the same time generating and testing a grounded theory approach to describe and explain the phenomenon under study.
- A broader, more complex research problem can be investigated because the researcher is not constrained by using only one method.
- The strengths of one method can be used to overcome the inherent weaknesses of another method.
- Can provide stronger, more robust evidence to support a conclusion or set of recommendations.
- May generate new knowledge new insights or uncover hidden insights, patterns, or relationships that a single methodological approach might not reveal.
- Produces more complete knowledge and understanding of the research problem that can be used to increase the generalizability of findings applied to theory or practice.
- A researcher must be proficient in understanding how to apply multiple methods to investigating a research problem as well as be proficient in optimizing how to design a study that coherently melds them together.
- Can increase the likelihood of conflicting results or ambiguous findings that inhibit drawing a valid conclusion or setting forth a recommended course of action [e.g., sample interview responses do not support existing statistical data].
- Because the research design can be very complex, reporting the findings requires a well-organized narrative, clear writing style, and precise word choice.
- Design invites collaboration among experts. However, merging different investigative approaches and writing styles requires more attention to the overall research process than studies conducted using only one methodological paradigm.
- Concurrent merging of quantitative and qualitative research requires greater attention to having adequate sample sizes, using comparable samples, and applying a consistent unit of analysis. For sequential designs where one phase of qualitative research builds on the quantitative phase or vice versa, decisions about what results from the first phase to use in the next phase, the choice of samples and estimating reasonable sample sizes for both phases, and the interpretation of results from both phases can be difficult.
- Due to multiple forms of data being collected and analyzed, this design requires extensive time and resources to carry out the multiple steps involved in data gathering and interpretation.
Burch, Patricia and Carolyn J. Heinrich. Mixed Methods for Policy Research and Program Evaluation . Thousand Oaks, CA: Sage, 2016; Creswell, John w. et al. Best Practices for Mixed Methods Research in the Health Sciences . Bethesda, MD: Office of Behavioral and Social Sciences Research, National Institutes of Health, 2010Creswell, John W. Research Design: Qualitative, Quantitative, and Mixed Methods Approaches . 4th edition. Thousand Oaks, CA: Sage Publications, 2014; Domínguez, Silvia, editor. Mixed Methods Social Networks Research . Cambridge, UK: Cambridge University Press, 2014; Hesse-Biber, Sharlene Nagy. Mixed Methods Research: Merging Theory with Practice . New York: Guilford Press, 2010; Niglas, Katrin. “How the Novice Researcher Can Make Sense of Mixed Methods Designs.” International Journal of Multiple Research Approaches 3 (2009): 34-46; Onwuegbuzie, Anthony J. and Nancy L. Leech. “Linking Research Questions to Mixed Methods Data Analysis Procedures.” The Qualitative Report 11 (September 2006): 474-498; Tashakorri, Abbas and John W. Creswell. “The New Era of Mixed Methods.” Journal of Mixed Methods Research 1 (January 2007): 3-7; Zhanga, Wanqing. “Mixed Methods Application in Health Intervention Research: A Multiple Case Study.” International Journal of Multiple Research Approaches 8 (2014): 24-35 .
Observational Design
This type of research design draws a conclusion by comparing subjects against a control group, in cases where the researcher has no control over the experiment. There are two general types of observational designs. In direct observations, people know that you are watching them. Unobtrusive measures involve any method for studying behavior where individuals do not know they are being observed. An observational study allows a useful insight into a phenomenon and avoids the ethical and practical difficulties of setting up a large and cumbersome research project.
- Observational studies are usually flexible and do not necessarily need to be structured around a hypothesis about what you expect to observe [data is emergent rather than pre-existing].
- The researcher is able to collect in-depth information about a particular behavior.
- Can reveal interrelationships among multifaceted dimensions of group interactions.
- You can generalize your results to real life situations.
- Observational research is useful for discovering what variables may be important before applying other methods like experiments.
- Observation research designs account for the complexity of group behaviors.
- Reliability of data is low because seeing behaviors occur over and over again may be a time consuming task and are difficult to replicate.
- In observational research, findings may only reflect a unique sample population and, thus, cannot be generalized to other groups.
- There can be problems with bias as the researcher may only "see what they want to see."
- There is no possibility to determine "cause and effect" relationships since nothing is manipulated.
- Sources or subjects may not all be equally credible.
- Any group that is knowingly studied is altered to some degree by the presence of the researcher, therefore, potentially skewing any data collected.
Atkinson, Paul and Martyn Hammersley. “Ethnography and Participant Observation.” In Handbook of Qualitative Research . Norman K. Denzin and Yvonna S. Lincoln, eds. (Thousand Oaks, CA: Sage, 1994), pp. 248-261; Observational Research. Research Methods by Dummies. Department of Psychology. California State University, Fresno, 2006; Patton Michael Quinn. Qualitiative Research and Evaluation Methods . Chapter 6, Fieldwork Strategies and Observational Methods. 3rd ed. Thousand Oaks, CA: Sage, 2002; Payne, Geoff and Judy Payne. "Observation." In Key Concepts in Social Research . The SAGE Key Concepts series. (London, England: Sage, 2004), pp. 158-162; Rosenbaum, Paul R. Design of Observational Studies . New York: Springer, 2010;Williams, J. Patrick. "Nonparticipant Observation." In The Sage Encyclopedia of Qualitative Research Methods . Lisa M. Given, editor.(Thousand Oaks, CA: Sage, 2008), pp. 562-563.
Philosophical Design
Understood more as an broad approach to examining a research problem than a methodological design, philosophical analysis and argumentation is intended to challenge deeply embedded, often intractable, assumptions underpinning an area of study. This approach uses the tools of argumentation derived from philosophical traditions, concepts, models, and theories to critically explore and challenge, for example, the relevance of logic and evidence in academic debates, to analyze arguments about fundamental issues, or to discuss the root of existing discourse about a research problem. These overarching tools of analysis can be framed in three ways:
- Ontology -- the study that describes the nature of reality; for example, what is real and what is not, what is fundamental and what is derivative?
- Epistemology -- the study that explores the nature of knowledge; for example, by what means does knowledge and understanding depend upon and how can we be certain of what we know?
- Axiology -- the study of values; for example, what values does an individual or group hold and why? How are values related to interest, desire, will, experience, and means-to-end? And, what is the difference between a matter of fact and a matter of value?
- Can provide a basis for applying ethical decision-making to practice.
- Functions as a means of gaining greater self-understanding and self-knowledge about the purposes of research.
- Brings clarity to general guiding practices and principles of an individual or group.
- Philosophy informs methodology.
- Refine concepts and theories that are invoked in relatively unreflective modes of thought and discourse.
- Beyond methodology, philosophy also informs critical thinking about epistemology and the structure of reality (metaphysics).
- Offers clarity and definition to the practical and theoretical uses of terms, concepts, and ideas.
- Limited application to specific research problems [answering the "So What?" question in social science research].
- Analysis can be abstract, argumentative, and limited in its practical application to real-life issues.
- While a philosophical analysis may render problematic that which was once simple or taken-for-granted, the writing can be dense and subject to unnecessary jargon, overstatement, and/or excessive quotation and documentation.
- There are limitations in the use of metaphor as a vehicle of philosophical analysis.
- There can be analytical difficulties in moving from philosophy to advocacy and between abstract thought and application to the phenomenal world.
Burton, Dawn. "Part I, Philosophy of the Social Sciences." In Research Training for Social Scientists . (London, England: Sage, 2000), pp. 1-5; Chapter 4, Research Methodology and Design. Unisa Institutional Repository (UnisaIR), University of South Africa; Jarvie, Ian C., and Jesús Zamora-Bonilla, editors. The SAGE Handbook of the Philosophy of Social Sciences . London: Sage, 2011; Labaree, Robert V. and Ross Scimeca. “The Philosophical Problem of Truth in Librarianship.” The Library Quarterly 78 (January 2008): 43-70; Maykut, Pamela S. Beginning Qualitative Research: A Philosophic and Practical Guide . Washington, DC: Falmer Press, 1994; McLaughlin, Hugh. "The Philosophy of Social Research." In Understanding Social Work Research . 2nd edition. (London: SAGE Publications Ltd., 2012), pp. 24-47; Stanford Encyclopedia of Philosophy . Metaphysics Research Lab, CSLI, Stanford University, 2013.
Sequential Design
- The researcher has a limitless option when it comes to sample size and the sampling schedule.
- Due to the repetitive nature of this research design, minor changes and adjustments can be done during the initial parts of the study to correct and hone the research method.
- This is a useful design for exploratory studies.
- There is very little effort on the part of the researcher when performing this technique. It is generally not expensive, time consuming, or workforce intensive.
- Because the study is conducted serially, the results of one sample are known before the next sample is taken and analyzed. This provides opportunities for continuous improvement of sampling and methods of analysis.
- The sampling method is not representative of the entire population. The only possibility of approaching representativeness is when the researcher chooses to use a very large sample size significant enough to represent a significant portion of the entire population. In this case, moving on to study a second or more specific sample can be difficult.
- The design cannot be used to create conclusions and interpretations that pertain to an entire population because the sampling technique is not randomized. Generalizability from findings is, therefore, limited.
- Difficult to account for and interpret variation from one sample to another over time, particularly when using qualitative methods of data collection.
Betensky, Rebecca. Harvard University, Course Lecture Note slides; Bovaird, James A. and Kevin A. Kupzyk. "Sequential Design." In Encyclopedia of Research Design . Neil J. Salkind, editor. (Thousand Oaks, CA: Sage, 2010), pp. 1347-1352; Cresswell, John W. Et al. “Advanced Mixed-Methods Research Designs.” In Handbook of Mixed Methods in Social and Behavioral Research . Abbas Tashakkori and Charles Teddle, eds. (Thousand Oaks, CA: Sage, 2003), pp. 209-240; Henry, Gary T. "Sequential Sampling." In The SAGE Encyclopedia of Social Science Research Methods . Michael S. Lewis-Beck, Alan Bryman and Tim Futing Liao, editors. (Thousand Oaks, CA: Sage, 2004), pp. 1027-1028; Nataliya V. Ivankova. “Using Mixed-Methods Sequential Explanatory Design: From Theory to Practice.” Field Methods 18 (February 2006): 3-20; Bovaird, James A. and Kevin A. Kupzyk. “Sequential Design.” In Encyclopedia of Research Design . Neil J. Salkind, ed. Thousand Oaks, CA: Sage, 2010; Sequential Analysis. Wikipedia.
Systematic Review
- A systematic review synthesizes the findings of multiple studies related to each other by incorporating strategies of analysis and interpretation intended to reduce biases and random errors.
- The application of critical exploration, evaluation, and synthesis methods separates insignificant, unsound, or redundant research from the most salient and relevant studies worthy of reflection.
- They can be use to identify, justify, and refine hypotheses, recognize and avoid hidden problems in prior studies, and explain data inconsistencies and conflicts in data.
- Systematic reviews can be used to help policy makers formulate evidence-based guidelines and regulations.
- The use of strict, explicit, and pre-determined methods of synthesis, when applied appropriately, provide reliable estimates about the effects of interventions, evaluations, and effects related to the overarching research problem investigated by each study under review.
- Systematic reviews illuminate where knowledge or thorough understanding of a research problem is lacking and, therefore, can then be used to guide future research.
- The accepted inclusion of unpublished studies [i.e., grey literature] ensures the broadest possible way to analyze and interpret research on a topic.
- Results of the synthesis can be generalized and the findings extrapolated into the general population with more validity than most other types of studies .
- Systematic reviews do not create new knowledge per se; they are a method for synthesizing existing studies about a research problem in order to gain new insights and determine gaps in the literature.
- The way researchers have carried out their investigations [e.g., the period of time covered, number of participants, sources of data analyzed, etc.] can make it difficult to effectively synthesize studies.
- The inclusion of unpublished studies can introduce bias into the review because they may not have undergone a rigorous peer-review process prior to publication. Examples may include conference presentations or proceedings, publications from government agencies, white papers, working papers, and internal documents from organizations, and doctoral dissertations and Master's theses.
Denyer, David and David Tranfield. "Producing a Systematic Review." In The Sage Handbook of Organizational Research Methods . David A. Buchanan and Alan Bryman, editors. ( Thousand Oaks, CA: Sage Publications, 2009), pp. 671-689; Foster, Margaret J. and Sarah T. Jewell, editors. Assembling the Pieces of a Systematic Review: A Guide for Librarians . Lanham, MD: Rowman and Littlefield, 2017; Gough, David, Sandy Oliver, James Thomas, editors. Introduction to Systematic Reviews . 2nd edition. Los Angeles, CA: Sage Publications, 2017; Gopalakrishnan, S. and P. Ganeshkumar. “Systematic Reviews and Meta-analysis: Understanding the Best Evidence in Primary Healthcare.” Journal of Family Medicine and Primary Care 2 (2013): 9-14; Gough, David, James Thomas, and Sandy Oliver. "Clarifying Differences between Review Designs and Methods." Systematic Reviews 1 (2012): 1-9; Khan, Khalid S., Regina Kunz, Jos Kleijnen, and Gerd Antes. “Five Steps to Conducting a Systematic Review.” Journal of the Royal Society of Medicine 96 (2003): 118-121; Mulrow, C. D. “Systematic Reviews: Rationale for Systematic Reviews.” BMJ 309:597 (September 1994); O'Dwyer, Linda C., and Q. Eileen Wafford. "Addressing Challenges with Systematic Review Teams through Effective Communication: A Case Report." Journal of the Medical Library Association 109 (October 2021): 643-647; Okoli, Chitu, and Kira Schabram. "A Guide to Conducting a Systematic Literature Review of Information Systems Research." Sprouts: Working Papers on Information Systems 10 (2010); Siddaway, Andy P., Alex M. Wood, and Larry V. Hedges. "How to Do a Systematic Review: A Best Practice Guide for Conducting and Reporting Narrative Reviews, Meta-analyses, and Meta-syntheses." Annual Review of Psychology 70 (2019): 747-770; Torgerson, Carole J. “Publication Bias: The Achilles’ Heel of Systematic Reviews?” British Journal of Educational Studies 54 (March 2006): 89-102; Torgerson, Carole. Systematic Reviews . New York: Continuum, 2003.
- << Previous: Purpose of Guide
- Next: Design Flaws to Avoid >>
- Last Updated: Apr 1, 2024 9:56 AM
- URL: https://libguides.usc.edu/writingguide
Evidence-Based Practice
- Introduction to EBP
- PICO(T) for Clinical Questions
- Qualitative Questions This link opens in a new window
- 3. Appraise
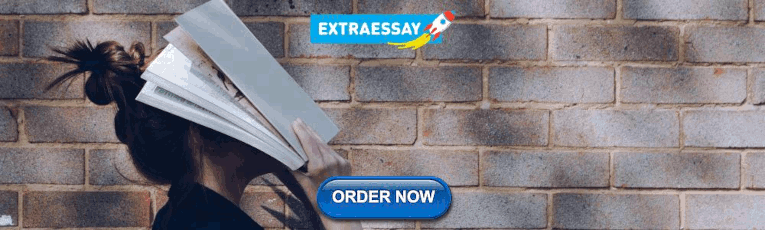
Suggested Research Designs
Study design glossary.
- Resources about EBP
Systematic Reviews and Meta-Analyses are generally considered to be the highest quality of evidence. But the type of study you need may differ based on what type of research question you are trying to answer. You'll want to identify which are the most valid research designs that can "prove" the research question with the understanding that there may not be much research done on that research question.
Table source: OHSU's Evidence-Based Practice Toolkit for Nursing

"Evidence Pyramid" is a product of Tufts University and is licensed under BY-NC-SA license 4.0
Systematic Review A summary of the clinical literature. A systematic review is a critical assessment and evaluation of all research studies that address a particular clinical issue. The researchers use an organized method of locating, assembling, and evaluating a body of literature on a particular topic using a set of specific criteria. A systematic review typically includes a description of the findings of the collection of research studies. (AHRQ Glossary of Terms)
Meta-Analysis A work consisting of studies using a quantitative method of combining the results of independent studies (usually drawn from the published literature) and synthesizing summaries and conclusions which may be used to evaluate therapeutic effectiveness, plan new studies, etc. It is often an overview of clinical trials. It is usually called a meta-analysis by the author or sponsoring body and should be differentiated from reviews of literature. (PubMed)
Randomized Controlled Trial A controlled clinical trial that randomly (by chance) assigns participants to two or more groups. There are various methods to randomize study participants to their groups. (AHRQ Glossary of Terms)
Controlled Clinical Trial A type of clinical trial comparing the effectiveness of one medication or treatment with the effectiveness of another medication or treatment. In many controlled trials, the other treatment is a placebo (inactive substance) and is considered the "control." (AHRQ Glossary of Terms)
Cohort Study A clinical research study in which people who presently have a certain condition or receive a particular treatment are followed over time and compared with another group of people who are not affected by the condition. (AHRQ Glossary of Terms)
Case Control Study The observational epidemiologic study of persons with the disease (or other outcome variable) of interest and a suitable control (comparison, reference) group of persons without the disease. The relationship of an attribute to the disease is examined by comparing the diseased and nondiseased with regard to how frequently the attribute is present or, if quantitative, the levels of the attribute, in each of the groups. (OCEBM Table of Evidence Glossary)
Case Series A group or series of case reports involving patients who were given similar treatment. Reports of case series usually contain detailed information about the individual patients. This includes demographic information (for example, age, gender, ethnic origin) and information on diagnosis, treatment, response to treatment, and follow-up after treatment. (OCEBM Table of Evidence Glossary)
Case Study An investigation of a single subject or a single unit, which could be a small number of individuals who seem to be representative of a larger group or very different from it. (Dictionary of Nursing Theory and Research, Fourth Edition)
Editorial Work consisting of a statement of the opinions, beliefs, and policy of the editor or publisher of a journal, usually on current matters of medical or scientific significance to the medical community or society at large. The editorials published by editors of journals representing the official organ of a society or organization are generally substantive. (PubMed)
Opinion A belief or conclusion held with confidence but not substantiated by positive knowledge or proof. (The Free Dictionary)
Animal Research A laboratory experiment using animals to study the development and progression of diseases. Animal studies also test how safe and effective new treatments are before they are tested in people.(NCI Dictionary of Cancer Terms)
In Vitro Research In the laboratory (outside the body). The opposite of in vivo (in the body). (NCI Dictionary of Cancer Terms)
- << Previous: 5. Assess
- Next: Resources about EBP >>
- Last Updated: Mar 13, 2024 11:48 AM
- URL: https://researchguides.gonzaga.edu/EBP
FDA: New Guidance for Non-interventional Studies of Drug Safety and Effectiveness
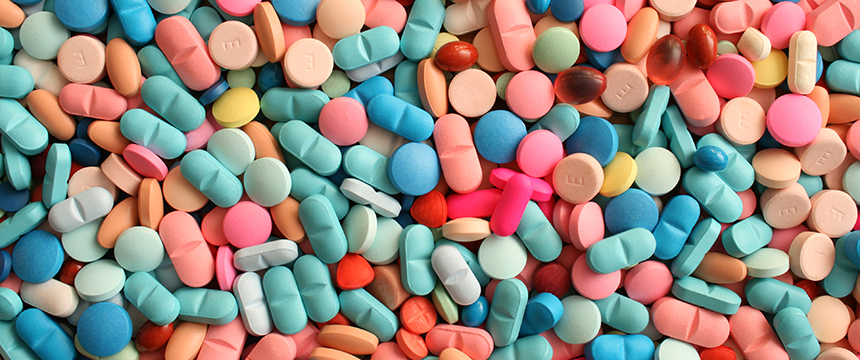
The U.S. Food and Drug Administration (FDA) has recently issued guidance for sponsors and investigators interested in submitting a non-interventional study, commonly known as an observational study, to contribute evidence of a drug’s safety and effectiveness. These drug studies aim to distinguish the drug’s effects from other factors like disease progression, placebo responses, or observer bias. Non-interventional studies, such as those using electronic health record data in cohort designs, may yield inaccurate conclusions due to confounding factors like noncomparable treatment groups or bias leading researchers to choosing specific types of participants thereby missing data. Addressing these issues is essential for obtaining accurate data and results. FDA’s guidance assists sponsors in recognizing and mitigating these challenges before finalizing study protocols and statistical analysis plans (SAP).
Understanding Non-Interventional Studies: Background
Non-interventional studies are invaluable for gaining insights into the actual safety and effectiveness of drugs in real-world settings. These studies involve patients receiving approved drugs as part of routine medical care, without specific interventions being dictated by a protocol.
There are various types of non-interventional study designs, including observational cohort studies, case-control studies, and self-controlled studies. These designs enable researchers to observe outcomes of interest without disrupting the natural course of treatment.
The reliability and relevance of real-world data (RWD) are crucial for the success of non-interventional studies. RWD further encompasses a wide range of information on patient health status and healthcare delivery from diverse sources. In these studies, the quality and consistency of RWD from different sources are pivotal for ensuring accurate conclusions are drawn.
Overview of Research Guidance
FDA has provided guidance to researchers regarding the:
- Proposed approach;
- Elements of a study design;
- Selection of data sources; and
- Analytic approach to reviewing data.
Summary of the Proposed Approach
Sponsors should first finalize their protocol by outlining the detailed plan for conducting the study. This protocol should encompass the study’s roadmap, covering aspects such as the research question, hypothesis, rationale for study design choice, selection of data sources, results of preliminary studies, approach to causal inference, and ethical considerations. Engaging with the FDA early is important for assuring alignment with expectations.
Study Design
Crafting a robust study design is the cornerstone of any successful research endeavor. The study design elements of the proposed research should include a schema outlining the overall study design and a causal diagram specifying the theorized causal relationship. Additionally, critical components such as the source population, eligibility criteria, conceptual and operational definitions for key variables, relevant covariates, index date determination, and start and end of follow-up periods should be concisely described in the protocol.
Data Sources
Selecting appropriate data sources is key for the success of a non-interventional study. The description of the proposed data source(s) should include details on how the data were originally collected and the rationale for choosing the data source(s). The description of the sources should also address the relevance to the drug-outcome association, information on confounding factors, data reliability, common data models used, timing and completeness of key data elements, appropriateness of coding based on operational definitions, alignment with the target patient population, and quality assurance activities. Additionally, plans for data quality assurance and potential links to other data sources should be outlined.
Analytic Approach
Once the study protocol is established, the focus shifts to the analytic approach. Sponsors must craft a detailed SAP that aligns with study objectives. This includes specifying the statistical approach for evaluating treatment effects, handling intercurrent events and censoring rules, and accounting for potential confounding factors, including assessment of unmeasured confounding factors.
The SAP also considers potential overadjustment of intermediate variables on the causal pathway, outlines approaches for subgroup analyses, addresses unequal detection of outcomes across compared groups, evaluates the possibility of reverse causality, describes methods for handling missing or misclassified data, and outlines strategies for managing multiplicity, such as analyzing multiple exposures or outcomes.
Non-interventional studies play a vital role in the evaluation of drug safety and effectiveness. FDA’s guidance offers recommendations to sponsors considering the submission of non-interventional studies to the FDA to support the demonstration of a drug’s safety and effectiveness in connection with RWD. The development of this draft guidance reflects the increasing interest among stakeholders in leveraging non-interventional studies to provide evidence of a drug’s safety and efficacy. By carefully considering the outlined recommendations at each stage of the research process, researchers can enhance the quality and reliability of their study outcomes.
Foley is here to help you address the short- and long-term impacts in the wake of regulatory changes. We have the resources to help you navigate these and other important legal considerations related to business operations and industry-specific issues. Please reach out to the authors, your Foley relationship partner, our Health Care & Life Science Sector , or to our Health Care Practice Group with any questions.
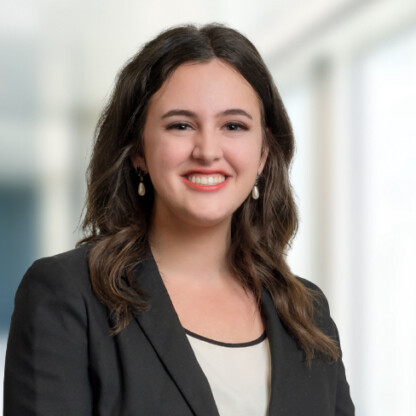
Jordan H. Smiley
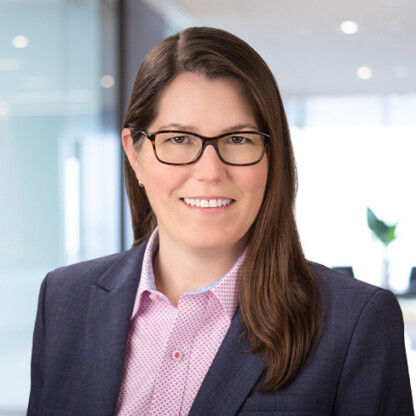
Kyle Y. Faget
Related insights, health-related social needs: three trends in leveraging community partnerships, hhs updates pixels and trackers guidance for hipaa regulated entities, clinical research: fda issues draft guidance on informed consent.
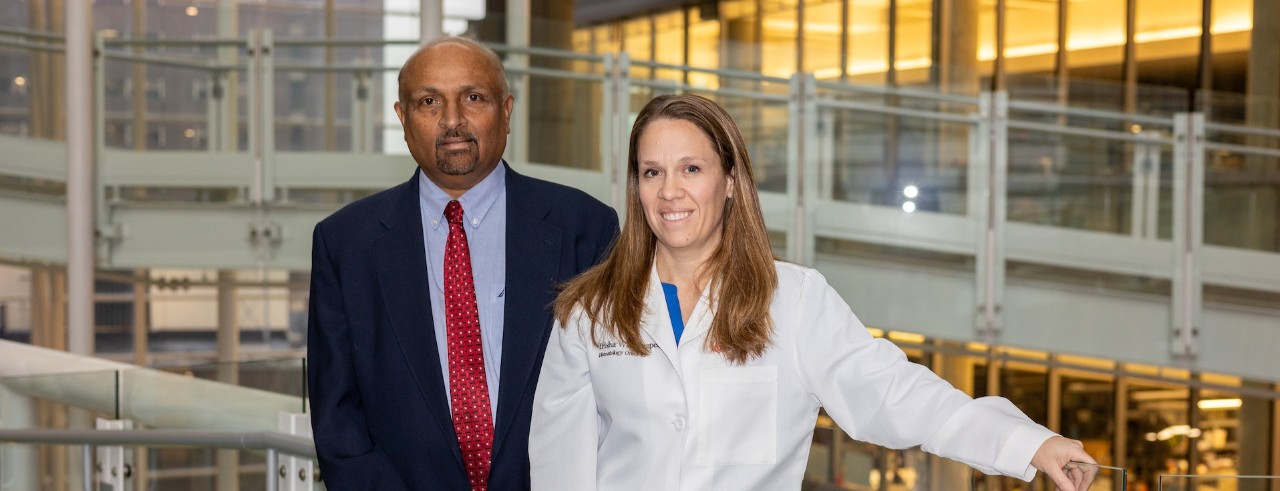
Collaborative University of Cincinnati Cancer Center team opens Phase 2 brain tumor trial
Falk catalyst award funds next phase of research seeking new treatments for glioblastomas.

A multidisciplinary team of University of Cincinnati Cancer Center researchers have opened a Phase 2 clinical trial to test a new combination treatment for glioblastomas (GBM), the most deadly form of brain tumors.
The team, led by UC’s Pankaj Desai, PhD, and Trisha Wise-Draper, MD, PhD, has been awarded a Catalyst Research Award from the Dr. Ralph and Marian Falk Medical Research Trust to move the trial forward.
Study background
Difficult to diagnose at early stages, GBMs are aggressive brain tumors that become symptomatic once the tumor is substantial. Current treatments include immediate surgery to safely remove as much tumor as possible, radiation and chemotherapy, but the tumor often recurs or becomes resistant to treatments. The average patient survives no more than 15 months after diagnosis.
Drug-based treatments for GBMs face an additional challenge known as the blood-brain barrier, which only allows certain compounds into the brain based on their physical and chemical properties.
The research team is focused on the use of a drug called letrozole that has been used for more than 20 years as a treatment for breast cancer. The drug targets an enzyme called aromatase that is present in the breast cancer cells and helps the cells grow.
Early research in Desai’s lab found that aromatase was present in brain tumor cells, making letrozole a potential new treatment for GBMs.
The team is testing the drug letrozole as a treatment for glioblastoma, a deadly and aggressive brain tumor. Photo/National Cancer Institute.
Phase 0/1 trial results
To bring letrozole from Desai’s lab to patients’ bedsides, he collaborated with Wise-Draper and neuro-oncologists and neurosurgeons at UC’s Brain Tumor Center to launch a Phase 0/1 clinical trial.
“In the academic setting, we are very good at doing molecular research that enhances our understanding of the mechanism of disease and preclinical characterization of efficacy, safety and other aspects of drug development research,” said Desai, professor and chair of the Pharmaceutical Sciences Division and director of the drug development graduate program in UC’s James L. Winkle College of Pharmacy. “But you can’t translate this into a clinical trial without a Phase 1 clinical trial expert like Dr. Wise-Draper and the experts at the Brain Tumor Center.”
The researchers published the results of the Phase 0/1 trial March 26 in Clinical Cancer Research , a journal of the American Association for Cancer Research.
Pankaj Desai, PhD. Photo/Andrew Higley/UC Marketing + Brand.
“Letrozole was safe up to the highest dose, and there were no safety concerns in the Phase 0/1 trial,” said Wise-Draper, section head of Medical Oncology and professor in the Division of Hematology/Oncology in UC’s College of Medicine. “The biggest conclusion is that it was safe and that we could reach what we felt was going to be the effective dose based on Dr. Desai’s preclinical work.”
The research team collected tumor tissues from patients enrolled in the Phase 0/1 trial and found that letrozole was crossing the blood-brain barrier when they analyzed the samples in Desai’s lab.
“We can categorically show that in humans the drug actually crosses and reaches the brain tumor at concentrations that we believe are likely to be most efficacious,” Desai said.
Phase 2 trial design
Trisha Wise-Draper, MD, PhD. Photo/Nyla Sauter/University of Cincinnati Cancer Center.
Since GBMs are aggressive and complicated tumors, Desai said most likely new effective treatments will be combinations of drugs instead of one single drug.
In the Phase 2 trial, patients will be given letrozole in combination with a chemotherapy drug called temozolomide that is already approved as a GBM treatment. Desai said preclinical research in his lab and input from Brain Tumor Center collaborators, including neuro-oncologist and former UC faculty member Soma Sengupta, suggested this combination treatment could be more effective than letrozole alone.
A total of 19 patients with recurrent GBM who are no longer eligible for additional surgery will be enrolled in the first stage of the trial. The results from this trial will guide the design of future larger Phase 2 trials.
The team estimates it will complete enrollment within two years, and two patients have already been enrolled.
Collaboration and funding support
Wise-Draper and Desai have worked together on various research projects for nearly 15 years and said this project would not be moving forward without the varied expertise each team member brings.
“I think collaboration with multidisciplinary teams is critical to be able to have the expertise and all the components you need, including biostatistics, pharmacokinetics, clinical, basic science and neuro-oncology expertise,” Wise-Draper said. “The future of all science is team science. No one really can do everything on their own anymore because we’re all too specialized.”
“Only academic centers with integrated scientific and clinical expertise are able to move their molecules from the research bench to clinical trials,” Desai added. “It takes a lot of persistence, ups and downs, highs and lows of funding, but we have been supported by a very strong team of people. It’s a journey that has taken a while and a lot of hard work by a number of people, and we’re in a very exciting stage.”
Early-stage support for the preclinical and clinical trial studies was provided by the UC Brain Tumor Center, where investigators from UC’s colleges of Medicine, Pharmacy, Engineering and Applied Science and Cincinnati Children’s Hospital collaborate on brain tumor research.
UC’s Brain Tumor Center provided direct support for the completion of the Phase 0/1 trial and some of the correlative mechanistic studies that will continue during the Phase 2 trials using funds raised in the annual Walk Ahead for a Brain Tumor Discoveries fundraiser.
The Falk Catalyst Award provides up to $350,000 in seed funding to support translational research projects, which the researchers said was crucial in opening the new trial.
“Oftentimes the funding is somewhat limited for initial clinical trial development compared to many other more early-stage studies that you can do,” Desai said. “So that gap is filled by foundations like the Falk Medical Research Trust, and that really is very helpful and plays a critical role in accelerating clinical development.”
“It would not be possible if we didn’t have the funding to be able to bring this combination into patients that desperately need new treatment options,” Wise-Draper said.
As the clinical trial progresses, the team is also collaborating to find other drugs to combine with letrozole to treat GBMs, funded by a $1.19 million National Institutes of Health/National Institute of Neurological Disorders and Stroke grant . The team is already preparing a proposal for larger confirmatory Phase 2 studies and expanding the opportunities for cutting-edge brain tumor clinical trials in Cincinnati.
Desai said the ongoing research includes additional collaboration from experts including David Plas, PhD, Biplab DasGupta, PhD, and Tim Phoenix, PhD (molecular/cancer biology); Gary Gudelsky, PhD (neuro-pharmacology) Rekha Chaudhary, MD, and Lalanthica Yogendran, MD (neuro-oncology); Mario Medvedovic, PhD (bioinformatics and genomics); and Shesh Rai, PhD (biostatistics). Many graduate students, postdoctoral fellows and the clinical trials support staff also provide essential support for the project.
Impact Lives Here
The University of Cincinnati is leading public urban universities into a new era of innovation and impact. Our faculty, staff and students are saving lives, changing outcomes and bending the future in our city's direction. Next Lives Here.
For more information on the trial, please call 513-584-7698 or email [email protected] .
The team also recently published research in the International Journal of Molecular Sciences reviewing approaches to overcome treatment resistance to temozolomide to treat GBMs.
Featured photo at top of Drs. Desai and Wise-Draper. Photo/Nyla Sauter/University of Cincinnati Cancer Center.
- Clinical Research
- Faculty Staff
- College of Pharmacy
- College of Medicine
- UC Gardner Neuroscience Institute
- Academic Health Center
- Neurology & Rehabilitation Medicine
- UC Cancer Institute
Related Stories
March 26, 2024
A multidisciplinary team of University of Cincinnati Cancer Center researchers have opened a Phase 2 clinical trial to test a new combination treatment for glioblastomas, the most deadly form of brain tumors.
Learning more about how cancer affects stroke risk
October 16, 2023
A collaborative team led by University of Cincinnati, University of North Carolina and Duke University researchers is studying how specific cancers and treatments affect patients' risk of stroke.
A potential new treatment for brain tumors
September 23, 2022
The University of Cincinnati's Pankaj Desai, PhD, has received a $1.19 million grant from the National Institutes of Health/National Institute of Neurological Disorders and Stroke to continue research into the use of a drug called letrozole to treat glioblastomas, the most deadly form of brain tumors.
The association of vasomotor symptoms with fracture risk and bone mineral density in postmenopausal women: a systematic review and meta-analysis of observational studies
- Open access
- Published: 02 April 2024
Cite this article
You have full access to this open access article
- Panagiotis Anagnostis ORCID: orcid.org/0000-0002-8949-4312 1 ,
- Konstantinos Lallas 2 ,
- Anna Pappa 1 ,
- Georgios Avgeris 1 ,
- Kristina Beta 1 ,
- Dimitrios Damakis 1 ,
- Eirini Fountoukidou 1 ,
- Maria Zidrou 1 ,
- Irene Lambrinoudaki 3 &
- Dimitrios G. Goulis 1
Background/Aims
Vasomotor symptoms (VMS) adversely affect postmenopausal quality of life. However, their association with bone health has not been elucidated. This study aimed to systematically review and meta-analyze the evidence regarding the association of VMS with fracture risk and bone mineral density (BMD) in peri- and postmenopausal women.
A literature search was conducted in PubMed, Scopus and Cochrane databases until 31 August 2023. Fracture, low BMD (osteoporosis/osteopenia) and mean change in lumbar spine (LS) and femoral neck (FN) BMD were assessed. The results are presented as odds ratio (OR) and mean difference (MD), respectively, with a 95% confidence interval (95% CI). The I 2 index quantified heterogeneity.
Twenty studies were included in the qualitative and 12 in the quantitative analysis ( n =49,659). No difference in fractures between women with and without VMS was found ( n =5, OR 1.04, 95% CI 0.93–1.16, I 2 16%). However, VMS were associated with low BMD ( n =5, OR 1.54, 95% CI 1.42–1.67, I 2 0%). This difference was evident for LS (MD -0.019 g/cm 2 , 95% CI -0.03 to -0.008, I 2 85.2%), but not for FN BMD (MD -0.010 g/cm 2 , 95% CI -0.021 to 0.001, I 2 78.2%). These results were independent of VMS severity, age and study design. When the analysis was confined to studies that excluded menopausal hormone therapy use, the association with BMD remained significant.
Conclusions
The presence of VMS is associated with low BMD in postmenopausal women, although it does not seem to increase fracture risk.
Avoid common mistakes on your manuscript.
Introduction
Vasomotor symptoms (VMS), mainly hot flushes and night sweats, are quite common in women entering menopause and adversely affect their quality of life [ 1 , 2 ]. An abrupt increase in VMS occurs during the first two years before the final menstrual period (FMP) (i.e., during the late perimenopausal phase) in 60-80% of women, presenting a peak one year after the FMP. VMS persist in 50% of cases during the first four years after the FMP [ 1 , 2 ]. Interestingly, 10% of postmenopausal women may experience VMS even 12 years after their FMP [ 1 ]. The prevalence of VMS varies across different geographic regions and is estimated at 22-63% in Asia, 36-50% in North America and 45-74% in Europe [ 3 ].
VMS reflect the fluctuations in the hormonal milieu during the transition to menopause [ 1 , 2 ]. Except for their impact on quality of life, severe VMS have been associated with increased prevalence of cardiovascular risk factors, such as type 2 diabetes mellitus (T2DM), smoking and obesity [ 4 ], predisposing to increased risk of cardiovascular disease (CVD), especially in women younger than 60 years [ 5 ]. Furthermore, the peak VMS prevalence coincides with accelerated bone loss during the menopausal transition [ 6 ]. Therefore, the severity of VMS has been proposed as a marker of climacteric bone loss [ 6 ] . The first report of a potentially adverse effect of VMS on peri- and postmenopausal bone health was made in 1994 by Lee et al. , who showed that the prevalence of osteoporosis in postmenopausal women who recalled severe and persistent VMS was higher than those who had no VMS [ 7 ]. After that, several studies of different designs had contradictory results regarding the impact of VMS both on fracture risk and low BMD in peri- and postmenopausal women [ 8 , 9 , 10 , 11 , 12 , 13 ].
The primary aim of this study was to systematically review and meta-analyze the available evidence regarding the association between VMS and the risk of fractures or low BMD (osteopenia/osteoporosis) in peri- and postmenopausal women. The secondary endpoint was to investigate the difference in BMD between women with and without VMS.
Materials and Methods
Guidelines followed.
The present study followed the MOOSE (Meta-analyses Of Observational Studies in Epidemiology) guidelines [ 14 ] and has been registered in the International Prospective Register of Systematic Reviews (PROSPERO) system (CRD42023387161).
Search strategy
Six reviewers (G.A, K.B, D.D, M.Z, A.P, E.F.) conducted a literature search in the electronic databases MEDLINE (PubMed), Scopus and Cochrane (CENTRAL) until 31 August 2023. A representative example of search strategy in MEDLINE was : (“hot flush*”[tiab] OR “hot flash*”[tiab] OR “night sweat*”[tiab] OR “vasomotor symptom*”[tiab] OR “menopausal symptom*”[tiab] OR “postmenopausal symptom*”[tiab] OR “post-menopausal symptom*”[tiab] OR climacteric[tiab]) AND (fracture[MeSH] OR fracture*[tiab] OR “bone density”[MeSH] OR “bone mineral density”[tiab] OR BMD[tiab] OR “bone mass”[tiab]). Two senior authors resolved discrepancies. Furthermore, conference abstracts, grey literature (using Opengrey.eu) and reference sections of the included studies were screened manually. E-mails were sent to the authors of the eligible studies to obtain missing data.
Study selection
The following PICO (Population, Intervention, Comparison and Outcome) elements were set as inclusion criteria: (i) Population: peri- or postmenopausal women, (ii) Intervention: VMS (hot flushes and/or night sweats), (iii) Comparison: peri- or postmenopausal women without VMS, (iv) Outcome: fractures (vertebral, non-vertebral, hip), low BMD (defined as osteopenia or osteoporosis) and mean difference in BMD between groups. Observational studies (cohort, cross-sectional and case-control) and randomized-controlled trials were included in the analysis. Exclusion criteria were: (i) studies that involved solely premenopausal women, (ii) studies that did not provide information on fracture incidence or BMD, (iii) non-English papers and (iv) animal studies.
Menopause was defined as the absence of menstruation for ≥12 months (natural menopause) or the period of time starting immediately after bilateral oophorectomy (surgical menopause). Women were classified as “premenopausal” if they had no change in menstrual regularity during the past year, or as “perimenopausal”, if they reported menstruation during the past three months, but with decreased irregularity (early perimenopausal) or no menses during the past 3-11 months (late perimenopausal).
Data extraction
Two independent researchers extracted data from eligible studies that met the predefined criteria. A standardized form was used to record: (i) first author, (ii) year of publication, (iii) country in which the study was conducted, (iv) study design, (v) total sample size, (vi) mean participants’ age, (vii) number of patients with and without VMS, (viii) type and severity of VMS, (ix) number of patients with fractures (hip, vertebral, non-vertebral), (x) number of patients with low BMD, (xi) BMD at lumbar spine (LS), femoral neck (FN) and total hip (TH) BMD in VMS and non-VMS groups.
Risk of bias and study quality assessment
The Newcastle-Ottawa scale (NOS) was used to evaluate the quality of the selected studies. This scale consists of the following domains: (i) selection of participants, with a maximum rating of four stars, (ii) comparability of study groups, with a maximum of two stars, and (iii) assessment of outcome or exposure, with the highest rating reaching three stars. According to NOS, a study is characterized as “good quality” if it gets 3-4 stars in the selection domain, 1-2 stars in the comparability domain, and 2-3 stars in the outcome/exposure domain. “Fair quality” is considered when the study gets two, 1-2 and 2-3 stars in the selection, comparability and outcome/exposure domain, respectively. Finally, a study is characterized as “poor quality” in the case of 0-1, 0 and 0-1 stars in the selection, comparability and outcome/exposure domain, respectively [ 15 ].
Statistical analysis
The dichotomous data results regarding the association between VMS status and the risk of fracture or low BMD were expressed as odds ratio (OR), with 95% confidence interval (CI), whereas the difference in BMD between women with and without VMS was expressed as mean difference (MD) with 95% CI. Heterogeneity among studies was quantified by the I 2 index, with values 30% being considered as “low”, 30- 60% as “moderate” and >60% as “high heterogeneity” [ 16 ]. The fixed effect model was used for low heterogeneity, whereas the random effects model was used for cases with moderate or high heterogeneity.
Subgroup and sensitivity analysis was implicated to eliminate possible sources of heterogeneity. Also, in order to investigate the effect of possible modifiers (i.e., baseline patients’ characteristics, such as age) on the outcome, meta-regression analysis (based on the random effects model) was conducted. The risk of publication bias was examined with the Harbord-Egger’s test [ 16 ]. All statistical tests were two-sided, with a p- value <0.05 considered significant. All analyses were conducted using the Review Manager ( RevMan 5.4.1 software) computer program (Copenhagen: The Nordic Cochrane Centre, The Cochrane Collaboration, September 2020).
Descriptive analysis
The initial search provided 1,959 results after excluding duplicates, 96 of which were reviewed for full-text eligibility. Of those, 76 articles were excluded with reasons (Supplementary Table 1). Finally, 20 studies were selected for qualitative and 12 for quantitative analysis [ 7 , 8 , 9 , 10 , 12 , 13 , 17 , 18 , 19 , 20 , 21 , 22 ] (eight studies [ 11 , 23 , 24 , 25 , 26 , 27 , 28 , 29 ] were excluded from the meta-analysis due to a lack of extractable data for comparisons). Notably, the study by Nudy et al. [ 11 ], and Hippisley-Cox et al. [ 29 ], were excluded since they did not provide the exact number of patients with VMS, either as hot flushes or night sweats. A flow chart diagram is illustrated in Fig. 1 .
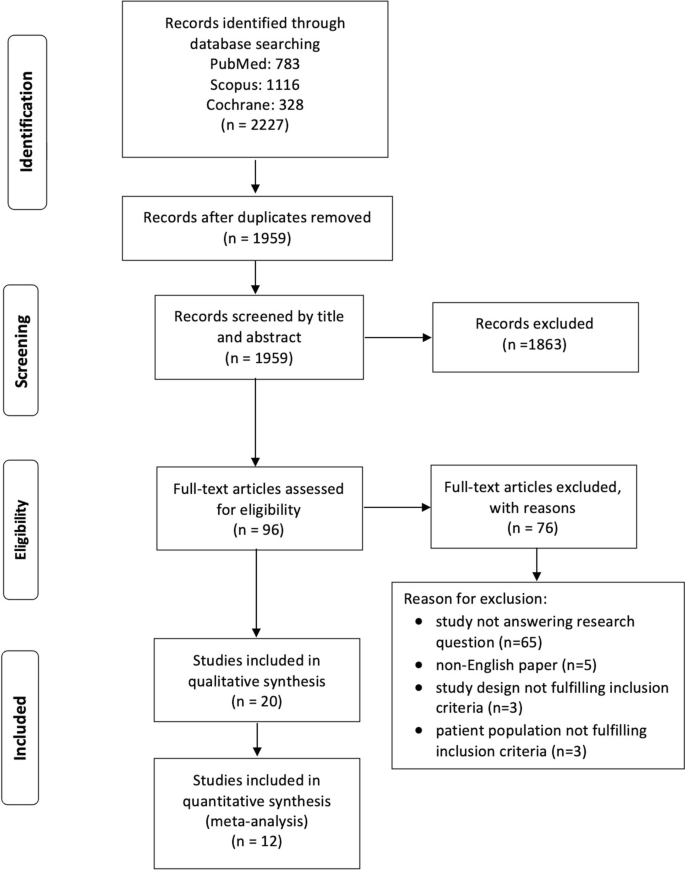
Flow chart diagram
Five [ 7 , 8 , 12 , 19 , 22 ] studies investigated the association between VMS and fracture risk, whereas five [ 7 , 10 , 12 , 13 , 21 ] addressed the occurrence of low BMD (osteopenia or osteoporosis). Furthermore, eight [ 8 , 9 , 12 , 17 , 18 , 19 , 20 , 22 ], seven [ 8 , 9 , 12 , 18 , 19 , 20 , 22 ] and four [ 9 , 18 , 20 , 22 ] studies provided data regarding the difference in LS, FN, TH BMD, respectively, between patients with and without VMS. Overall, 49,659 patients were included in the analysis.
VMS and risk of fracture
The five studies [ 7 , 8 , 12 , 19 , 22 ] assessing the association between VMS and fractures, found no increased risk in women with VMS compared with those without VMS (OR 1.04, 95% 0.93–1.16, I 2 16%) (Fig. 2 ). Regarding the type of fracture, three studies provided data on vertebral fractures showing again no association with VMS (OR 0.95, 95% CI 0.77–1.19, I 2 0%) [ 7 , 8 , 19 ]. Only the study by Crandall et al ., published in 2015 [ 8 ], provided data on hip fractures, showing increased risk in women with moderate/severe VMS (compared with no VMS) [hazard ratio (HR) 1.78, 95% CI 1.20–2.64].
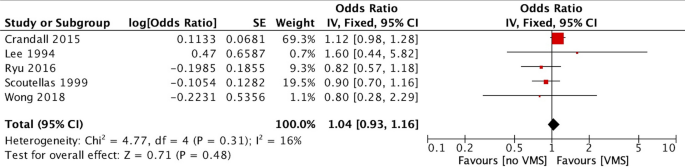
Forest plot of the fracture risk between women with and without vasomotor symptoms (VMS)
VMS and risk of low BMD (osteopenia or osteoporosis)
Since only three studies provided data for the diagnosis of osteoporosis and two studies for osteopenia, these were combined into one category termed “low BMD” [ 7 , 10 , 12 , 13 , 21 ]. The presence of VMS was associated with an increased risk of low BMD compared with the absence of VMS (OR 1.55, 95% CI 1.43–1.68, I 2 0%) (Fig. 3 ).
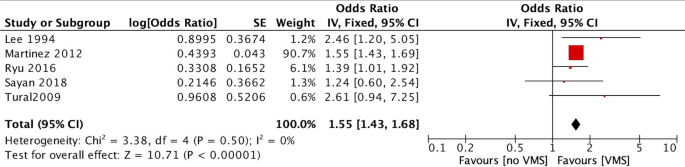
Forest plot of the low BMD risk (osteopenia or osteoporosis) between women with and without vasomotor symptoms (VMS)
VMS and mean difference in BMD
Regarding LS BMD, patients with VMS had lower values compared with those without VMS ( n =8, MD -0.019 g/cm 2 , 95% CI -0.03 to -0.008, I 2 85.2%) [ 8 , 9 , 12 , 17 , 18 , 19 , 20 , 22 ]. In contrast, there was no difference in FN ( n =7) [ 8 , 9 , 12 , 18 , 19 , 20 , 22 ] or TH BMD ( n =4) [ 9 , 18 , 20 , 22 ] between women with and without VMS [MD -0.010 g/cm 2 (95% CI -0.021 to 0.001, I 2 78.2%) and -0.040 g/cm 2 (95% CI -0.0 to 0.008, I 2 94.2%, respectively)]. The difference in LS and FN BMD between women with and without VMS is presented in Fig. 4 .
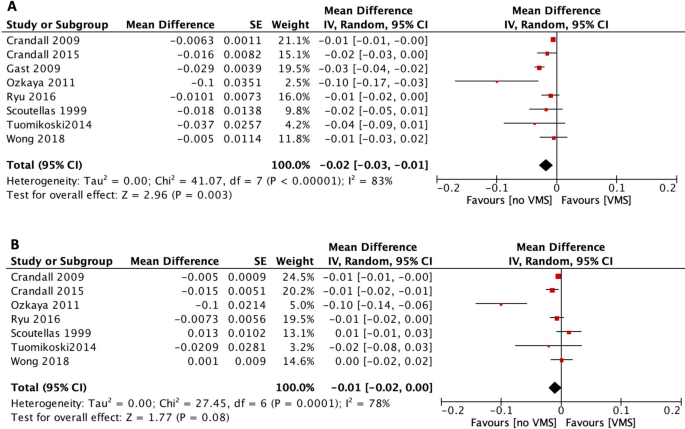
Forest plot of the difference in A ) lumbar spine (LS) and B ) femoral neck (FN) BMD between women with and without vasomotor symptoms (VMS)
Subgroup analysis
The effect of vms severity on fracture risk and bmd.
Three studies [ 7 , 8 , 12 ] were eligible for comparing moderate/severe VMS and no VMS, while two studies [ 8 , 12 ] provided data on mild VMS. No association with the risk of fracture was found when the analysis was confined to these subgroups [OR 1.08 (95% CI 0.79–1.46, I 2 40%) and 1.08 (95% CI 0.60–1.94, I 2 64%) for moderate/severe and mild VMS, respectively, compared with no VMS]. However, both moderate/severe ( n =4) [ 7 , 10 , 12 , 13 ] and mild VMS ( n =2) [ 10 , 12 ] were associated with an increased risk of low BMD compared with no VMS [OR 1.79 (95% CI 1.63–1.96, I 2 0%) and 1.28 (95% CI 1.15–1.41, I 2 0%), respectively].
Moreover, women with moderate/severe VMS demonstrated lower LS ( n =4, MD -0.025 g/cm 2 , 95% CI -0.042 to -0.008, I 2 72.9%) [ 8 , 12 , 17 , 20 ] and FN BMD ( n =3, MD -0.011 g/cm 2 , 95% CI -0.02 to -0.004, I 2 0%), respectively) than those without VMS [ 8 , 12 , 20 ]. Mild VMS were also associated with lower BMD in LS, but not FN, compared with no VMS. There were no available data regarding the effect of VMS severity on TH BMD.
The effect of study design on the risk of fracture and BMD
Regarding fracture risk, there was still no association with VMS, when the analysis was limited to cohort studies ( n =2, OR 1.11, 95% CI 0.98–1.28) [ 8 , 22 ] or case-control studies ( n =2, OR 0.86, 95% CI 0.61–1.22) [ 7 , 12 ]. Furthermore, when data from case-control studies [ 7 , 12 , 13 ] were analyzed separately, the risk of low BMD still was increased in women with a history of VMS (OR 1.39, 95% CI 1.06–1.82) similarly to cross-sectional studies (OR 1.56, 95% CI 1.43–1.69) [ 10 , 21 ]. Regarding BMD, cohort and cross-sectional studies showed similar differences for LS and FN between groups.
The effect of age on fracture risk and BMD
Only one study provided sufficient data for different age groups regarding the fracture risk [ 8 ], showing an increased risk of hip fracture in patients with VMS only for the older age group (70-79 years old; HR 1.93, 95% CI 1.11–3.53). No such risk was demonstrated for vertebral and non-vertebral fractures [ 8 ].
Notably, meta-regression analysis showed that age was not a predictor neither for fracture risk ( p =0.11) nor for low BMD ( p =0.707). Age also did not affect MD in LS ( p =0.807) and FN BMD ( p =0.498) between groups.
Sensitivity analysis
A sensitivity analysis was performed according to study quality and MHT use. Classification of studies according to NOS is presented in Supplementary Table 2. After excluding two fair-quality studies [ 12 , 18 ], the risk estimation for fractures, low BMD and LS or FN BMD remained unaltered.
Concerning MHT use, there were four studies [ 13 , 17 , 19 , 22 ] in which MHT was used by a variable proportion of patients (although MHT was included in the multivariable-adjusted models). However, the association between VMS and low BMD and MD in LS BMD between groups remained significant after restricting the analysis to studies where MHT was an exclusion criterion, but presence of VMS and fracture risk remained not significant (OR 1.12, 95% CI 0.98–1.28).
Publication bias
Publication bias was not evident regarding the risk of fracture ( p =0.546) or low BMD ( p =0.435), and TH BMD (p>0.20). However, Egger’s test showed significance for MD in LS ( p =0.09) and FN BMD ( p =0.06), possibly due to the effect of small sample size on study outcomes (Supplementary Figure 1).
This study shows that the presence of VMS in peri- and postmenopausal women is associated with a higher degree of bone loss, since these women are more prone to osteopenia or osteoporosis than those without VMS. This effect is independent of age, VMS severity, study quality and MHT use, although moderate/severe VMS affect LS and FN BMD to a greater extent than mild VMS. However, no association between VMS and fracture risk was found.
This meta-analysis is the first considering the effect of VMS on bone health in postmenopausal women. Recent studies have underscored the impact of the severity of VMS on the cardiovascular system, including risk factors, such as diabetes, smoking, obesity [ 4 ], and CVD events, especially in women younger than 60 years [ 5 ]. Therefore, the presence and severity of VMS appear as key players in the accelerated bone loss in peri- and postmenopausal women, necessitating the administration of MHT. Another clinical implication is that women who experience moderate/severe VMS constitute a distinct postmenopausal population at higher risk of osteoporosis and, potentially, fractures and should be screened by dual-energy X-ray absorptiometry (DXA) earlier than the generally recommended threshold of 65 years [ 30 ].
Estrogens are essential for acquiring and maintaining peak bone mass by acting at the osteoclast and osteoblast levels [ 31 ]. In particular, estrogen receptors α upregulate the expression of Wnt co-receptor lipoprotein receptor-related protein (LRP)-5, which is essential for osteoblast differentiation. They also inhibit osteoblast apoptosis by decreasing the production of Fas ligand (FasL) [ 31 ]. At the osteoclast level, they prevent osteoclast differentiation and proliferation indirectly through the osteoprotegerin (OPG)/receptor activator of NF-κB (RANK)/RANK ligand (RANKL) axis [ 31 ]. They also induce osteoclast apoptosis (through the FasL) and inhibit osteocyte apoptosis by increasing the expression of semaphorin 3A [ 31 ]. During the menopausal transition (i.e., from the first year before and three years after the FMP), a 9-10% bone loss occurs [ 32 ]. This fact is of clinical relevance in women with early menopause (<45 years), a category that is predisposed to increased fracture risk [ 33 ].
The exact pathogenetic mechanisms linking VMS with bone loss are not fully understood. Most [ 34 , 35 ], but not all studies [ 36 ], have shown that the decline in estradiol (E 2 ) concentrations is associated with VMS. However, the individual’s sensitivity to the fluctuations rather than the absolute decrease in E 2 concentrations seem to account for the association of VMS with accelerated bone loss [ 17 ]. Furthermore, increased sympathetic activity has been reported in women with VMS [ 37 ], which fosters bone loss through increased bone resorption and decreased bone formation. Indeed, β 2 - and α-adrenergic receptors have been identified in human osteoblasts [ 38 , 39 ]. Cortisol concentrations also increase after hot flushes, contributing to bone loss [ 40 ]. Another potential mechanism could be endothelial dysfunction [ 41 ] and increased concentrations of inflammatory markers, such as interleukin-8 and tumor necrosis factor α; these molecules have been identified in the presence of VMS and may impair bone quality [ 42 ]. Finally, VMS are associated with increased oxidative stress, which inhibits the Wnt pathway and, subsequently, bone formation [ 43 ].
Some limitations should be acknowledged, such as the heterogeneity regarding the definition of VMS and study design, and the increased risk of recall or selection bias. However, the effect of these limitations on the present study’s validity was mitigated by applying meta-regression, subgroup and sensitivity analysis, which did not significantly modify the study’s outcomes (except for the effect of MHT on fracture risk). The heterogeneity in the DXA device and the number of vertebrae assessed constitutes another limitation. Finally, no subgroup analysis for peri- and postmenopausal women could be performed by available data.
In conclusion, this study shows that the history of VMS (hot flushes and/or night sweats), especially in their moderate/severe form, is associated with greater bone loss, compared with no VMS, in peri- and postmenopausal women. This association involves both LS and FN BMD, potentially predisposing the woman to an increased risk of fracture, although this was not proven by the present study. This aspect of postmenopausal health, in combination with CVD, should be considered in future studies, designating the potentially beneficial role of MHT in this population.
Politi MC, Schleinitz MD, Col NF (2008) Revisiting the duration of vasomotor symptoms of menopause: a meta-analysis. J Gen Intern Med 23:1507–1513
Article PubMed PubMed Central Google Scholar
Williams RE, Kalilani L, DiBenedetti DB, Zhou X, Granger AL, Fehnel SE, Levine KB, Jordan J, Clark RV (2008) Frequency and severity of vasomotor symptoms among peri- and postmenopausal women in the United States. Climacteric 11:32–43
Article CAS PubMed Google Scholar
Palacios S, Henderson VW, Siseles N, Tan D, Villaseca P (2010) Age of menopause and impact of climacteric symptoms by geographical region. Climacteric 13:419–428
Armeni E, Kopanos S, Verykouki E, et al. (2023) The severity of menopausal symptoms is associated with diabetes, and cardiometabolic risk factors in middle-aged women. Minerva Endocrinol (Torino)
Armeni A, Anagnostis P, Armeni E, Mili N, Goulis D, Lambrinoudaki I (2023) Vasomotor symptoms and risk of cardiovascular disease in peri- and postmenopausal women: A systematic review and meta-analysis. Maturitas 171:13–20
Article PubMed Google Scholar
Sowers MR, Jannausch M, McConnell D, Little R, Greendale GA, Finkelstein JS, Neer RM, Johnston J, Ettinger B (2006) Hormone predictors of bone mineral density changes during the menopausal transition. J Clin Endocrinol Metab 91:1261–1267
Lee SJ, Kanis JA (1994) An association between osteoporosis and premenstrual symptoms and postmenopausal symptoms. Bone Miner 24:127–134
Crandall CJ, Aragaki A, Cauley JA et al (2015) Associations of menopausal vasomotor symptoms with fracture incidence. J Clin Endocrinol Metab 100:524–534
Crandall CJ, Zheng Y, Crawford SL, Thurston RC, Gold EB, Johnston JM, Greendale GA (2009) Presence of vasomotor symptoms is associated with lower bone mineral density: a longitudinal analysis. Menopause 16:239–246
Martinez Perez JA, Palacios S, Chavida F, Perez M (2013) Severity of menopausal symptoms and cardiovascular and osteoporosis risk factors. Climacteric 16:226–234
Nudy M, Jiang X, Aragaki AK et al (2020) The severity of vasomotor symptoms and number of menopausal symptoms in postmenopausal women and select clinical health outcomes in the Women’s Health Initiative Calcium and Vitamin D randomized clinical trial. Menopause 27:1265–1273
Ryu KJ, Park HT, Kim YJ, Yi KW, Shin JH, Hur JY, Kim T (2016) Vasomotor symptoms and osteoporosis in Korean postmenopausal women. Maturitas 87:27–32
Sayan S, Pekin T, Yildizhan B (2018) Relationship between vasomotor symptoms and metabolic syndrome in postmenopausal women. J Int Med Res 46:4157–4166
Stroup DF, Berlin JA, Morton SC, Olkin I, Williamson GD, Rennie D, Moher D, Becker BJ, Sipe TA, Thacker SB (2000) Meta-analysis of observational studies in epidemiology: a proposal for reporting. Meta-analysis Of Observational Studies in Epidemiology (MOOSE) group. JAMA 283:2008–2012
Wells GA SB, O’Connell D, Peterson J, Welch V, Tugwell P (2000) The Newcastle-Ottawa Scale (NOS) for assessing the quality of non-randomised studies in meta-analyses. In: 3rd symposium on systematic reviews: beyond the basics. http://www.ohri.ca/programs/clinical_epidemiology/oxford.asp
Higgins JPT, Thomas J, Chandler J, Cumpston M, Li T, Page MJ, Welch VAe (2023) Cochrane Handbook for Systematic Reviews of Interventions version 6.4 (updated August 2023). Cochrane
Gast GC, Grobbee DE, Pop VJ, Keyzer JJ, Wijnands-van Gent CJ, Samsioe GN, Nilsson PM, van der Schouw YT (2009) Vasomotor symptoms are associated with a lower bone mineral density. Menopause 16:231–238
Ozkaya E, Cakir E, Kara F, Okuyan E, Cakir C, Ustun G, Kucukozkan T (2011) Impact of hot flashes and night sweats on carotid intima-media thickness and bone mineral density among postmenopausal women. Int J Gynaecol Obstet 113:235–238
Scoutellas V, O’Neill TW, Lunt M, Reeve J, Silman AJ (1999) Does the presence of postmenopausal symptoms influence susceptibility to vertebral deformity? European Vertebral Osteoporosis Study (EVOS) Group. Maturitas 32:179–187
Tuomikoski P, Ylikorkala O, Mikkola TS (2015) Postmenopausal hot flushes and bone mineral density: a longitudinal study. Acta Obstet Gynecol Scand 94:198–203
Tural A, Yoldemir T, Erenus M (2009) Assessment of bone mineral density should be considered earlier in perimenopausal women with vasomotor symptoms. Int J Gynaecol Obstet 107:114–116
Wong EMM, Tomlinson G, Pinto MM, Berger C, Cheung AM, Prior JC (2018) Women's Mid-Life Night Sweats and 2-Year Bone Mineral Density Changes: A Prospective, Observational Population-Based Investigation from the Canadian Multicentre Osteoporosis Study (CaMos). Int J Environ Res Public Health 15:
Grainge MJ, Coupland CA, Cliffe SJ, Chilvers CE, Hosking DJ (2001) Reproductive, menstrual and menopausal factors: which are associated with bone mineral density in early postmenopausal women? Osteoporos Int 12:777–787
Jaber RM, Khalil IA, Almohtasib YS, Hamdan M, AlRyalat SA (2022) Association of menopausal symptom severity and osteoporotic fractures: a case-control study. J Women Aging 34:93–100
Naessen T, Persson I, Ljunghall S, Bergstrom R (1992) Women with climacteric symptoms: a target group for prevention of rapid bone loss and osteoporosis. Osteoporos Int 2:225–231
Ahn S, Song R (2009) Bone mineral density and perceived menopausal symptoms: factors influencing low back pain in postmenopausal women. J Adv Nurs 65:1228–1236
Coronado PJ, Monroy M, Fasero M, Sanchez-Borrego R, Palacios S, Rejas J, Ruiz MA, AcgftsopvotC Short-Form (2021) Population-based norms for the Cervantes-SF short-form questionnaire assessing health-related quality of life in menopause. Maturitas 146:34–41
Crandall CJ, Larson J, Cauley JA, Schousboe JT, LaCroix AZ, Robbins JA, Watts NB, Ensrud KE (2019) Do Additional Clinical Risk Factors Improve the Performance of Fracture Risk Assessment Tool (FRAX) Among Postmenopausal Women? Findings From the Women’s Health Initiative Observational Study and Clinical Trials. JBMR Plus 3:e10239
Hippisley-Cox J, Coupland C (2009) Predicting risk of osteoporotic fracture in men and women in England and Wales: prospective derivation and validation of QFractureScores. BMJ 339:b4229
Shuhart CR, Yeap SS, Anderson PA, Jankowski LG, Lewiecki EM, Morse LR, Rosen HN, Weber DR, Zemel BS, Shepherd JA (2019) Executive Summary of the 2019 ISCD Position Development Conference on Monitoring Treatment, DXA Cross-calibration and Least Significant Change, Spinal Cord Injury, Peri-prosthetic and Orthopedic Bone Health, Transgender Medicine, and Pediatrics. J Clin Densitom 22:453-471
Anagnostis P, Bosdou JK, Vaitsi K, Goulis DG, Lambrinoudaki I (2021) Estrogen and bones after menopause: a reappraisal of data and future perspectives. Hormones (Athens) 20:13–21
Greendale GA, Sowers M, Han W, Huang MH, Finkelstein JS, Crandall CJ, Lee JS, Karlamangla AS (2012) Bone mineral density loss in relation to the final menstrual period in a multiethnic cohort: results from the Study of Women’s Health Across the Nation (SWAN). J Bone Miner Res 27:111–118
Anagnostis P, Siolos P, Gkekas NK et al (2019) Association between age at menopause and fracture risk: a systematic review and meta-analysis. Endocrine 63:213–224
Guthrie JR, Dennerstein L, Taffe JR, Lehert P, Burger HG (2005) Hot flushes during the menopause transition: a longitudinal study in Australian-born women. Menopause 12:460–467
Overlie I, Moen MH, Holte A, Finset A (2002) Androgens and estrogens in relation to hot flushes during the menopausal transition. Maturitas 41:69–77
Randolph JF Jr, Sowers M, Bondarenko I, Gold EB, Greendale GA, Bromberger JT, Brockwell SE, Matthews KA (2005) The relationship of longitudinal change in reproductive hormones and vasomotor symptoms during the menopausal transition. J Clin Endocrinol Metab 90:6106–6112
Mashchak CA, Kletzky OA, Artal R, Mishell DR Jr (1984) The relation of physiological changes to subjective symptoms in postmenopausal women with and without hot flushes. Maturitas 6:301–308
Kondo H, Nifuji A, Takeda S, Ezura Y, Rittling SR, Denhardt DT, Nakashima K, Karsenty G, Noda M (2005) Unloading induces osteoblastic cell suppression and osteoclastic cell activation to lead to bone loss via sympathetic nervous system. J Biol Chem 280:30192–30200
Togari A (2002) Adrenergic regulation of bone metabolism: possible involvement of sympathetic innervation of osteoblastic and osteoclastic cells. Microsc Res Tech 58:77–84
Cignarelli M, Cicinelli E, Corso M, Cospite MR, Garruti G, Tafaro E, Giorgino R, Schonauer S (1989) Biophysical and endocrine-metabolic changes during menopausal hot flashes: increase in plasma free fatty acid and norepinephrine levels. Gynecol Obstet Invest 27:34–37
Hildreth KL, Ozemek C, Kohrt WM, Blatchford PJ, Moreau KL (2018) Vascular dysfunction across the stages of the menopausal transition is associated with menopausal symptoms and quality of life. Menopause 25:1011–1019
Huang WY, Hsin IL, Chen DR, Chang CC, Kor CT, Chen TY, Wu HM (2017) Circulating interleukin-8 and tumor necrosis factor-alpha are associated with hot flashes in healthy postmenopausal women. PLoS One 12:e0184011
Manolagas SC, Almeida M (2007) Gone with the Wnts: beta-catenin, T-cell factor, forkhead box O, and oxidative stress in age-dependent diseases of bone, lipid, and glucose metabolism. Mol Endocrinol 21:2605–2614
Download references
Open access funding provided by HEAL-Link Greece.
Author information
Authors and affiliations.
1st Department of Obstetrics and Gynecology, Medical School, Unit of Reproductive Endocrinology, Aristotle University of Thessaloniki, Thessaloniki, Greece
Panagiotis Anagnostis, Anna Pappa, Georgios Avgeris, Kristina Beta, Dimitrios Damakis, Eirini Fountoukidou, Maria Zidrou & Dimitrios G. Goulis
Department of Medical Oncology, School of Medicine, Aristotle University of Thessaloniki, Thessaloniki, Greece
Konstantinos Lallas
2nd Department of Obstetrics and Gynecology, Medical School, National and Kapodistrian University of Athens, Athens, Greece
Irene Lambrinoudaki
You can also search for this author in PubMed Google Scholar
Corresponding author
Correspondence to Panagiotis Anagnostis .
Ethics declarations
Competing interest.
Panagiotis Anagnostis, Konstantinos Lallas, Anna Pappa, Georgios Avgeris, Kristina Beta, Dimitrios Damakis, Eirini Fountoukidou, Maria Zidrou, Irene Lambrinoudaki and Dimitrios G. Goulis declare that they have no conflict of interest.
Additional information
Publisher's note.
Springer Nature remains neutral with regard to jurisdictional claims in published maps and institutional affiliations.
Supplementary Information
Below is the link to the electronic supplementary material.
Supplementary file1 (DOCX 21 KB)
Supplementary file2 (docx 15 kb), 198_2024_7075_moesm3_esm.tiff.
Supplementary file3 Funnel plots assessing publication bias for each outcome and p-value from Egger’s test: a) risk of fracture ( p =0.425); b) low BMD ( p =0.470); c) LS BMD ( p =0.06); d) FN BMD ( p =0.11); e) TH BMD, p =0.08 (TIFF 29512 KB)
Rights and permissions
Open Access This article is licensed under a Creative Commons Attribution-NonCommercial 4.0 International License, which permits any non-commercial use, sharing, adaptation, distribution and reproduction in any medium or format, as long as you give appropriate credit to the original author(s) and the source, provide a link to the Creative Commons licence, and indicate if changes were made. The images or other third party material in this article are included in the article's Creative Commons licence, unless indicated otherwise in a credit line to the material. If material is not included in the article's Creative Commons licence and your intended use is not permitted by statutory regulation or exceeds the permitted use, you will need to obtain permission directly from the copyright holder. To view a copy of this licence, visit http://creativecommons.org/licenses/by-nc/4.0/ .
Reprints and permissions
About this article
Anagnostis, P., Lallas, K., Pappa, A. et al. The association of vasomotor symptoms with fracture risk and bone mineral density in postmenopausal women: a systematic review and meta-analysis of observational studies. Osteoporos Int (2024). https://doi.org/10.1007/s00198-024-07075-8
Download citation
Received : 07 December 2023
Accepted : 21 March 2024
Published : 02 April 2024
DOI : https://doi.org/10.1007/s00198-024-07075-8
Share this article
Anyone you share the following link with will be able to read this content:
Sorry, a shareable link is not currently available for this article.
Provided by the Springer Nature SharedIt content-sharing initiative
- Osteoporosis
- hot flushes
- Night sweats
- Vasomotor symptoms
- Postmenopausal women
- Find a journal
- Publish with us
- Track your research
We've detected unusual activity from your computer network
To continue, please click the box below to let us know you're not a robot.
Why did this happen?
Please make sure your browser supports JavaScript and cookies and that you are not blocking them from loading. For more information you can review our Terms of Service and Cookie Policy .
For inquiries related to this message please contact our support team and provide the reference ID below.

An official website of the United States government
The .gov means it’s official. Federal government websites often end in .gov or .mil. Before sharing sensitive information, make sure you’re on a federal government site.
The site is secure. The https:// ensures that you are connecting to the official website and that any information you provide is encrypted and transmitted securely.
- Publications
- Account settings
Preview improvements coming to the PMC website in October 2024. Learn More or Try it out now .
- Advanced Search
- Journal List
- Hippokratia
- v.14(Suppl 1); 2010 Dec
Meta-analysis in medical research
The objectives of this paper are to provide an introduction to meta-analysis and to discuss the rationale for this type of research and other general considerations. Methods used to produce a rigorous meta-analysis are highlighted and some aspects of presentation and interpretation of meta-analysis are discussed.
Meta-analysis is a quantitative, formal, epidemiological study design used to systematically assess previous research studies to derive conclusions about that body of research. Outcomes from a meta-analysis may include a more precise estimate of the effect of treatment or risk factor for disease, or other outcomes, than any individual study contributing to the pooled analysis. The examination of variability or heterogeneity in study results is also a critical outcome. The benefits of meta-analysis include a consolidated and quantitative review of a large, and often complex, sometimes apparently conflicting, body of literature. The specification of the outcome and hypotheses that are tested is critical to the conduct of meta-analyses, as is a sensitive literature search. A failure to identify the majority of existing studies can lead to erroneous conclusions; however, there are methods of examining data to identify the potential for studies to be missing; for example, by the use of funnel plots. Rigorously conducted meta-analyses are useful tools in evidence-based medicine. The need to integrate findings from many studies ensures that meta-analytic research is desirable and the large body of research now generated makes the conduct of this research feasible.
Important medical questions are typically studied more than once, often by different research teams in different locations. In many instances, the results of these multiple small studies of an issue are diverse and conflicting, which makes the clinical decision-making difficult. The need to arrive at decisions affecting clinical practise fostered the momentum toward "evidence-based medicine" 1 – 2 . Evidence-based medicine may be defined as the systematic, quantitative, preferentially experimental approach to obtaining and using medical information. Therefore, meta-analysis, a statistical procedure that integrates the results of several independent studies, plays a central role in evidence-based medicine. In fact, in the hierarchy of evidence ( Figure 1 ), where clinical evidence is ranked according to the strength of the freedom from various biases that beset medical research, meta-analyses are in the top. In contrast, animal research, laboratory studies, case series and case reports have little clinical value as proof, hence being in the bottom.

Meta-analysis did not begin to appear regularly in the medical literature until the late 1970s but since then a plethora of meta-analyses have emerged and the growth is exponential over time ( Figure 2 ) 3 . Moreover, it has been shown that meta-analyses are the most frequently cited form of clinical research 4 . The merits and perils of the somewhat mysterious procedure of meta-analysis, however, continue to be debated in the medical community 5 – 8 . The objectives of this paper are to introduce meta-analysis and to discuss the rationale for this type of research and other general considerations.

Meta-Analysis and Systematic Review
Glass first defined meta-analysis in the social science literature as "The statistical analysis of a large collection of analysis results from individual studies for the purpose of integrating the findings" 9 . Meta-analysis is a quantitative, formal, epidemiological study design used to systematically assess the results of previous research to derive conclusions about that body of research. Typically, but not necessarily, the study is based on randomized, controlled clinical trials. Outcomes from a meta-analysis may include a more precise estimate of the effect of treatment or risk factor for disease, or other outcomes, than any individual study contributing to the pooled analysis. Identifying sources of variation in responses; that is, examining heterogeneity of a group of studies, and generalizability of responses can lead to more effective treatments or modifications of management. Examination of heterogeneity is perhaps the most important task in meta-analysis. The Cochrane collaboration has been a long-standing, rigorous, and innovative leader in developing methods in the field 10 . Major contributions include the development of protocols that provide structure for literature search methods, and new and extended analytic and diagnostic methods for evaluating the output of meta-analyses. Use of the methods outlined in the handbook should provide a consistent approach to the conduct of meta-analysis. Moreover, a useful guide to improve reporting of systematic reviews and meta-analyses is the PRISMA (Preferred Reporting Items for Systematic reviews and Meta-analyses) statement that replaced the QUOROM (QUality Of Reporting of Meta-analyses) statement 11 – 13 .
Meta-analyses are a subset of systematic review. A systematic review attempts to collate empirical evidence that fits prespecified eligibility criteria to answer a specific research question. The key characteristics of a systematic review are a clearly stated set of objectives with predefined eligibility criteria for studies; an explicit, reproducible methodology; a systematic search that attempts to identify all studies that meet the eligibility criteria; an assessment of the validity of the findings of the included studies (e.g., through the assessment of risk of bias); and a systematic presentation and synthesis of the attributes and findings from the studies used. Systematic methods are used to minimize bias, thus providing more reliable findings from which conclusions can be drawn and decisions made than traditional review methods 14 , 15 . Systematic reviews need not contain a meta-analysisthere are times when it is not appropriate or possible; however, many systematic reviews contain meta-analyses 16 .
The inclusion of observational medical studies in meta-analyses led to considerable debate over the validity of meta-analytical approaches, as there was necessarily a concern that the observational studies were likely to be subject to unidentified sources of confounding and risk modification 17 . Pooling such findings may not lead to more certain outcomes. Moreover, an empirical study showed that in meta-analyses were both randomized and non-randomized was included, nonrandomized studies tended to show larger treatment effects 18 .
Meta-analyses are conducted to assess the strength of evidence present on a disease and treatment. One aim is to determine whether an effect exists; another aim is to determine whether the effect is positive or negative and, ideally, to obtain a single summary estimate of the effect. The results of a meta-analysis can improve precision of estimates of effect, answer questions not posed by the individual studies, settle controversies arising from apparently conflicting studies, and generate new hypotheses. In particular, the examination of heterogeneity is vital to the development of new hypotheses.
Individual or Aggregated Data
The majority of meta-analyses are based on a series of studies to produce a point estimate of an effect and measures of the precision of that estimate. However, methods have been developed for the meta-analyses to be conducted on data obtained from original trials 19 , 20 . This approach may be considered the "gold standard" in metaanalysis because it offers advantages over analyses using aggregated data, including a greater ability to validate the quality of data and to conduct appropriate statistical analysis. Further, it is easier to explore differences in effect across subgroups within the study population than with aggregated data. The use of standardized individual-level information may help to avoid the problems encountered in meta-analyses of prognostic factors 21 , 22 . It is the best way to obtain a more global picture of the natural history and predictors of risk for major outcomes, such as in scleroderma 23 – 26 .This approach relies on cooperation between researchers who conducted the relevant studies. Researchers who are aware of the potential to contribute or conduct these studies will provide and obtain additional benefits by careful maintenance of original databases and making these available for future studies.
Literature Search
A sound meta-analysis is characterized by a thorough and disciplined literature search. A clear definition of hypotheses to be investigated provides the framework for such an investigation. According to the PRISMA statement, an explicit statement of questions being addressed with reference to participants, interventions, comparisons, outcomes and study design (PICOS) should be provided 11 , 12 . It is important to obtain all relevant studies, because loss of studies can lead to bias in the study. Typically, published papers and abstracts are identified by a computerized literature search of electronic databases that can include PubMed ( www.ncbi.nlm.nih.gov./entrez/query.fcgi ), ScienceDirect ( www.sciencedirect.com ), Scirus ( www.scirus.com/srsapp ), ISI Web of Knowledge ( http://www.isiwebofknowledge.com ), Google Scholar ( http://scholar.google.com ) and CENTRAL (Cochrane Central Register of Controlled Trials, http://www.mrw.interscience.wiley.com/cochrane/cochrane_clcentral_articles_fs.htm ). PRISMA statement recommends that a full electronic search strategy for at least one major database to be presented 12 . Database searches should be augmented with hand searches of library resources for relevant papers, books, abstracts, and conference proceedings. Crosschecking of references, citations in review papers, and communication with scientists who have been working in the relevant field are important methods used to provide a comprehensive search. Communication with pharmaceutical companies manufacturing and distributing test products can be appropriate for studies examining the use of pharmaceutical interventions.
It is not feasible to find absolutely every relevant study on a subject. Some or even many studies may not be published, and those that are might not be indexed in computer-searchable databases. Useful sources for unpublished trials are the clinical trials registers, such as the National Library of Medicine's ClinicalTrials.gov Website. The reviews should attempt to be sensitive; that is, find as many studies as possible, to minimize bias and be efficient. It may be appropriate to frame a hypothesis that considers the time over which a study is conducted or to target a particular subpopulation. The decision whether to include unpublished studies is difficult. Although language of publication can provide a difficulty, it is important to overcome this difficulty, provided that the populations studied are relevant to the hypothesis being tested.
Inclusion or Exclusion Criteria and Potential for Bias
Studies are chosen for meta-analysis based on inclusion criteria. If there is more than one hypothesis to be tested, separate selection criteria should be defined for each hypothesis. Inclusion criteria are ideally defined at the stage of initial development of the study protocol. The rationale for the criteria for study selection used should be clearly stated.
One important potential source of bias in meta-analysis is the loss of trials and subjects. Ideally, all randomized subjects in all studies satisfy all of the trial selection criteria, comply with all the trial procedures, and provide complete data. Under these conditions, an "intention-totreat" analysis is straightforward to implement; that is, statistical analysis is conducted on all subjects that are enrolled in a study rather than those that complete all stages of study considered desirable. Some empirical studies had shown that certain methodological characteristics, such as poor concealment of treatment allocation or no blinding in studies exaggerate treatment effects 27 . Therefore, it is important to critically appraise the quality of studies in order to assess the risk of bias.
The study design, including details of the method of randomization of subjects to treatment groups, criteria for eligibility in the study, blinding, method of assessing the outcome, and handling of protocol deviations are important features defining study quality. When studies are excluded from a meta-analysis, reasons for exclusion should be provided for each excluded study. Usually, more than one assessor decides independently which studies to include or exclude, together with a well-defined checklist and a procedure that is followed when the assessors disagree. Two people familiar with the study topic perform the quality assessment for each study, independently. This is followed by a consensus meeting to discuss the studies excluded or included. Practically, the blinding of reviewers from details of a study such as authorship and journal source is difficult.
Before assessing study quality, a quality assessment protocol and data forms should be developed. The goal of this process is to reduce the risk of bias in the estimate of effect. Quality scores that summarize multiple components into a single number exist but are misleading and unhelpful 28 . Rather, investigators should use individual components of quality assessment and describe trials that do not meet the specified quality standards and probably assess the effect on the overall results by excluding them, as part of the sensitivity analyses.
Further, not all studies are completed, because of protocol failure, treatment failure, or other factors. Nonetheless, missing subjects and studies can provide important evidence. It is desirable to obtain data from all relevant randomized trials, so that the most appropriate analysis can be undertaken. Previous studies have discussed the significance of missing trials to the interpretation of intervention studies in medicine 29 , 30 . Journal editors and reviewers need to be aware of the existing bias toward publishing positive findings and ensure that papers that publish negative or even failed trials be published, as long as these meet the quality guidelines for publication.
There are occasions when authors of the selected papers have chosen different outcome criteria for their main analysis. In practice, it may be necessary to revise the inclusion criteria for a meta-analysis after reviewing all of the studies found through the search strategy. Variation in studies reflects the type of study design used, type and application of experimental and control therapies, whether or not the study was published, and, if published, subjected to peer review, and the definition used for the outcome of interest. There are no standardized criteria for inclusion of studies in meta-analysis. Universal criteria are not appropriate, however, because meta-analysis can be applied to a broad spectrum of topics. Published data in journal papers should also be cross-checked with conference papers to avoid repetition in presented data.
Clearly, unpublished studies are not found by searching the literature. It is possible that published studies are systemically different from unpublished studies; for example, positive trial findings may be more likely to be published. Therefore, a meta-analysis based on literature search results alone may lead to publication bias.
Efforts to minimize this potential bias include working from the references in published studies, searching computerized databases of unpublished material, and investigating other sources of information including conference proceedings, graduate dissertations and clinical trial registers.
Statistical analysis
The most common measures of effect used for dichotomous data are the risk ratio (also called relative risk) and the odds ratio. The dominant method used for continuous data are standardized mean difference (SMD) estimation. Methods used in meta-analysis for post hoc analysis of findings are relatively specific to meta-analysis and include heterogeneity analysis, sensitivity analysis, and evaluation of publication bias.
All methods used should allow for the weighting of studies. The concept of weighting reflects the value of the evidence of any particular study. Usually, studies are weighted according to the inverse of their variance 31 . It is important to recognize that smaller studies, therefore, usually contribute less to the estimates of overall effect. However, well-conducted studies with tight control of measurement variation and sources of confounding contribute more to estimates of overall effect than a study of identical size less well conducted.
One of the foremost decisions to be made when conducting a meta-analysis is whether to use a fixed-effects or a random-effects model. A fixed-effects model is based on the assumption that the sole source of variation in observed outcomes is that occurring within the study; that is, the effect expected from each study is the same. Consequently, it is assumed that the models are homogeneous; there are no differences in the underlying study population, no differences in subject selection criteria, and treatments are applied the same way 32 . Fixed-effect methods used for dichotomous data include most often the Mantel-Haenzel method 33 and the Peto method 34 (only for odds ratios).
Random-effects models have an underlying assumption that a distribution of effects exists, resulting in heterogeneity among study results, known as τ2. Consequently, as software has improved, random-effects models that require greater computing power have become more frequently conducted. This is desirable because the strong assumption that the effect of interest is the same in all studies is frequently untenable. Moreover, the fixed effects model is not appropriate when statistical heterogeneity (τ2) is present in the results of studies in the meta-analysis. In the random-effects model, studies are weighted with the inverse of their variance and the heterogeneity parameter. Therefore, it is usually a more conservative approach with wider confidence intervals than the fixed-effects model where the studies are weighted only with the inverse of their variance. The most commonly used random-effects method is the DerSimonian and Laird method 35 . Furthermore, it is suggested that comparing the fixed-effects and random-effect models developed as this process can yield insights to the data 36 .
Heterogeneity
Arguably, the greatest benefit of conducting metaanalysis is to examine sources of heterogeneity, if present, among studies. If heterogeneity is present, the summary measure must be interpreted with caution 37 . When heterogeneity is present, one should question whether and how to generalize the results. Understanding sources of heterogeneity will lead to more effective targeting of prevention and treatment strategies and will result in new research topics being identified. Part of the strategy in conducting a meta-analysis is to identify factors that may be significant determinants of subpopulation analysis or covariates that may be appropriate to explore in all studies.
To understand the nature of variability in studies, it is important to distinguish between different sources of heterogeneity. Variability in the participants, interventions, and outcomes studied has been described as clinical diversity, and variability in study design and risk of bias has been described as methodological diversity 10 . Variability in the intervention effects being evaluated among the different studies is known as statistical heterogeneity and is a consequence of clinical or methodological diversity, or both, among the studies. Statistical heterogeneity manifests itself in the observed intervention effects varying by more than the differences expected among studies that would be attributable to random error alone. Usually, in the literature, statistical heterogeneity is simply referred to as heterogeneity.
Clinical variation will cause heterogeneity if the intervention effect is modified by the factors that vary across studies; most obviously, the specific interventions or participant characteristics that are often reflected in different levels of risk in the control group when the outcome is dichotomous. In other words, the true intervention effect will differ for different studies. Differences between studies in terms of methods used, such as use of blinding or differences between studies in the definition or measurement of outcomes, may lead to differences in observed effects. Significant statistical heterogeneity arising from differences in methods used or differences in outcome assessments suggests that the studies are not all estimating the same effect, but does not necessarily suggest that the true intervention effect varies. In particular, heterogeneity associated solely with methodological diversity indicates that studies suffer from different degrees of bias. Empirical evidence suggests that some aspects of design can affect the result of clinical trials, although this may not always be the case.
The scope of a meta-analysis will largely determine the extent to which studies included in a review are diverse. Meta-analysis should be conducted when a group of studies is sufficiently homogeneous in terms of subjects involved, interventions, and outcomes to provide a meaningful summary. However, it is often appropriate to take a broader perspective in a meta-analysis than in a single clinical trial. Combining studies that differ substantially in design and other factors can yield a meaningless summary result, but the evaluation of reasons for the heterogeneity among studies can be very insightful. It may be argued that these studies are of intrinsic interest on their own, even though it is not appropriate to produce a single summary estimate of effect.
Variation among k trials is usually assessed using Cochran's Q statistic, a chi-squared (χ 2 ) test of heterogeneity with k-1 degrees of freedom. This test has relatively poor power to detect heterogeneity among small numbers of trials; consequently, an α-level of 0.10 is used to test hypotheses 38 , 39 .
Heterogeneity of results among trials is better quantified using the inconsistency index I 2 , which describes the percentage of total variation across studies 40 . Uncertainty intervals for I 2 (dependent on Q and k) are calculated using the method described by Higgins and Thompson 41 . Negative values of I 2 are put equal to zero, consequently I 2 lies between 0 and 100%. A value >75% may be considered substantial heterogeneity 41 . This statistic is less influenced by the number of trials compared with other methods used to estimate the heterogeneity and provides a logical and readily interpretable metric but it still can be unstable when only a few studies are combined 42 .
Given that there are several potential sources of heterogeneity in the data, several steps should be considered in the investigation of the causes. Although random-effects models are appropriate, it may be still very desirable to examine the data to identify sources of heterogeneity and to take steps to produce models that have a lower level of heterogeneity, if appropriate. Further, if the studies examined are highly heterogeneous, it may be not appropriate to present an overall summary estimate, even when random effects models are used. As Petiti notes 43 , statistical analysis alone will not make contradictory studies agree; critically, however, one should use common sense in decision-making. Despite heterogeneity in responses, if all studies had a positive point direction and the pooled confidence interval did not include zero, it would not be logical to conclude that there was not a positive effect, provided that sufficient studies and subject numbers were present. The appropriateness of the point estimate of the effect is much more in question.
Some of the ways to investigate the reasons for heterogeneity; are subgroup analysis and meta-regression. The subgroup analysis approach, a variation on those described above, groups categories of subjects (e.g., by age, sex) to compare effect sizes. The meta-regression approach uses regression analysis to determine the influence of selected variables (the independent variables) on the effect size (the dependent variable). In a meta-regresregression, studies are regarded as if they were individual patients, but their effects are properly weighted to account for their different variances 44 .
Sensitivity analyses have also been used to examine the effects of studies identified as being aberrant concerning conduct or result, or being highly influential in the analysis. Recently, another method has been proposed that reduces the weight of studies that are outliers in meta-analyses 45 . All of these methods for examining heterogeneity have merit, and the variety of methods available reflects the importance of this activity.
Presentation of results
A useful graph, presented in the PRISMA statement 11 , is the four-phase flow diagram ( Figure 3 ).

This flow-diagram depicts the flow of information through the different phases of a systematic review or meta-analysis. It maps out the number of records identified, included and excluded, and the reasons for exclusions. The results of meta-analyses are often presented in a forest plot, where each study is shown with its effect size and the corresponding 95% confidence interval ( Figure 4 ).

The pooled effect and 95% confidence interval is shown in the bottom in the same line with "Overall". In the right panel of Figure 4 , the cumulative meta-analysis is graphically displayed, where data are entered successively, typically in the order of their chronological appearance 46 , 47 . Such cumulative meta-analysis can retrospectively identify the point in time when a treatment effect first reached conventional levels of significance. Cumulative meta-analysis is a compelling way to examine trends in the evolution of the summary-effect size, and to assess the impact of a specific study on the overall conclusions 46 . The figure shows that many studies were performed long after cumulative meta-analysis would have shown a significant beneficial effect of antibiotic prophylaxis in colon surgery.
Biases in meta-analysis
Although the intent of a meta-analysis is to find and assess all studies meeting the inclusion criteria, it is not always possible to obtain these. A critical concern is the papers that may have been missed. There is good reason to be concerned about this potential loss because studies with significant, positive results (positive studies) are more likely to be published and, in the case of interventions with a commercial value, to be promoted, than studies with non-significant or "negative" results (negative studies). Studies that produce a positive result, especially large studies, are more likely to have been published and, conversely, there has been a reluctance to publish small studies that have non-significant results. Further, publication bias is not solely the responsibility of editorial policy as there is reluctance among researchers to publish results that were either uninteresting or are not randomized 48 . There are, however, problems with simply including all studies that have failed to meet peer-review standards. All methods of retrospectively dealing with bias in studies are imperfect.
It is important to examine the results of each meta-analysis for evidence of publication bias. An estimation of likely size of the publication bias in the review and an approach to dealing with the bias is inherent to the conduct of many meta-analyses. Several methods have been developed to provide an assessment of publication bias; the most commonly used is the funnel plot. The funnel plot provides a graphical evaluation of the potential for bias and was developed by Light and Pillemer 49 and discussed in detail by Egger and colleagues 50 , 51 . A funnel plot is a scatterplot of treatment effect against a measure of study size. If publication bias is not present, the plot is expected to have a symmetric inverted funnel shape, as shown in Figure 5A .

In a study in which there is no publication bias, larger studies (i.e., have lower standard error) tend to cluster closely to the point estimate. As studies become less precise, such as in smaller trials (i.e., have a higher standard error), the results of the studies can be expected to be more variable and are scattered to both sides of the more precise larger studies. Figure 5A shows that the smaller, less precise studies are, indeed, scattered to both sides of the point estimate of effect and that these seem to be symmetrical, as an inverted funnel-plot, showing no evidence of publication bias. In contrast to Figure 5A , Figure 5B shows evidence of publication bias. There is evidence of the possibility that studies using smaller numbers of subjects and showing an decrease in effect size (lower odds ratio) were not published.
Asymmetry of funnel plots is not solely attributable to publication bias, but may also result from clinical heterogeneity among studies. Sources of clinical heterogeneity include differences in control or exposure of subjects to confounders or effect modifiers, or methodological heterogeneity between studies; for example, a failure to conceal treatment allocation. There are several statistical tests for detecting funnel plot asymmetry; for example, Eggers linear regression test 50 , and Begg's rank correlation test 52 but these do not have considerable power and are rarely used. However, the funnel plot is not without problems. If high precision studies really are different than low precision studies with respect to effect size (e.g., due different populations examined) a funnel plot may give a wrong impression of publication bias 53 . The appearance of the funnel plot plot can change quite dramatically depending on the scale on the y-axis - whether it is the inverse square error or the trial size 54 .
Other types of biases in meta-analysis include the time lag bias, selective reporting bias and the language bias. The time lag bias arises from the published studies, when those with striking results are published earlier than those with non-significant findings 55 . Moreover, it has been shown that positive studies with high early accrual of patients are published sooner than negative trials with low early accrual 56 . However, missing studies, either due to publication bias or time-lag bias may increasingly be identified from trials registries.
The selective reporting bias exists when published articles have incomplete or inadequate reporting. Empirical studies have shown that this bias is widespread and of considerable importance when published studies were compared with their study protocols 29 , 30 . Furthermore, recent evidence suggests that selective reporting might be an issue in safety outcomes and the reporting of harms in clinical trials is still suboptimal 57 . Therefore, it might not be possible to use quantitative objective evidence for harms in performing meta-analyses and making therapeutic decisions.
Excluding clinical trials reported in languages other than English from meta-analyses may introduce the language bias and reduce the precision of combined estimates of treatment effects. Trials with statistically significant results have been shown to be published in English 58 . In contrast, a later more extensive investigation showed that trials published in languages other than English tend to be of lower quality and produce more favourable treatment effects than trials published in English and concluded that excluding non-English language trials has generally only modest effects on summary treatment effect estimates but the effect is difficult to predict for individual meta-analyses 59 .
Evolution of meta-analyses
The classical meta-analysis compares two treatments while network meta-analysis (or multiple treatment metaanalysis) can provide estimates of treatment efficacy of multiple treatment regimens, even when direct comparisons are unavailable by indirect comparisons 60 . An example of a network analysis would be the following. An initial trial compares drug A to drug B. A different trial studying the same patient population compares drug B to drug C. Assume that drug A is found to be superior to drug B in the first trial. Assume drug B is found to be equivalent to drug C in a second trial. Network analysis then, allows one to potentially say statistically that drug A is also superior to drug C for this particular patient population. (Since drug A is better than drug B, and drug B is equivalent to drug C, then drug A is also better to drug C even though it was not directly tested against drug C.)
Meta-analysis can also be used to summarize the performance of diagnostic and prognostic tests. However, studies that evaluate the accuracy of tests have a unique design requiring different criteria to appropriately assess the quality of studies and the potential for bias. Additionally, each study reports a pair of related summary statistics (for example, sensitivity and specificity) rather than a single statistic (such as a risk ratio) and hence requires different statistical methods to pool the results of the studies 61 . Various techniques to summarize results from diagnostic and prognostic test results have been proposed 62 – 64 . Furthermore, there are many methodologies for advanced meta-analysis that have been developed to address specific concerns, such as multivariate meta-analysis 65 – 67 , and special types of meta-analysis in genetics 68 but will not be discussed here.
Meta-analysis is no longer a novelty in medicine. Numerous meta-analyses have been conducted for the same medical topic by different researchers. Recently, there is a trend to combine the results of different meta-analyses, known as a meta-epidemiological study, to assess the risk of bias 79 , 70 .
Conclusions
The traditional basis of medical practice has been changed by the use of randomized, blinded, multicenter clinical trials and meta-analysis, leading to the widely used term "evidence-based medicine". Leaders in initiating this change have been the Cochrane Collaboration who have produced guidelines for conducting systematic reviews and meta-analyses 10 and recently the PRISMA statement, a helpful resource to improve reporting of systematic reviews and meta-analyses has been released 11 . Moreover, standards by which to conduct and report meta-analyses of observational studies have been published to improve the quality of reporting 71 .
Meta-analysis of randomized clinical trials is not an infallible tool, however, and several examples exist of meta-analyses which were later contradicted by single large randomized controlled trials, and of meta-analyses addressing the same issue which have reached opposite conclusions 72 . A recent example, was the controversy between a meta-analysis of 42 studies 73 and the subsequent publication of the large-scale trial (RECORD trial) that did not support the cardiovascular risk of rosiglitazone 74 . However, the reason for this controversy was explained by the numerous methodological flaws found both in the meta-analysis and the large clinical trial 75 .
No single study, whether meta-analytic or not, will provide the definitive understanding of responses to treatment, diagnostic tests, or risk factors influencing disease. Despite this limitation, meta-analytic approaches have demonstrable benefits in addressing the limitations of study size, can include diverse populations, provide the opportunity to evaluate new hypotheses, and are more valuable than any single study contributing to the analysis. The conduct of the studies is critical to the value of a meta-analysis and the methods used need to be as rigorous as any other study conducted.
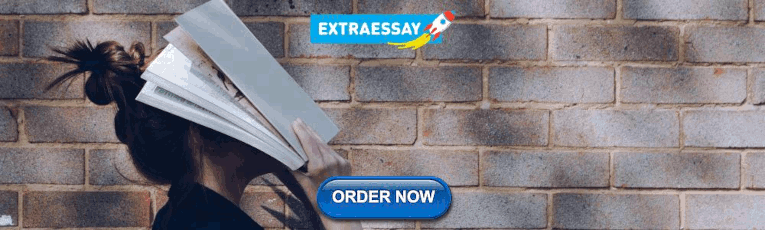
IMAGES
VIDEO
COMMENTS
2.1 Step 1: defining the research question. The first step in conducting a meta-analysis, as with any other empirical study, is the definition of the research question. Most importantly, the research question determines the realm of constructs to be considered or the type of interventions whose effects shall be analyzed.
This study looked at surgical outcomes including "blood loss, operative time, length of stay, complication and reoperation rates and functional outcomes" between patients with and without obesity. A meta-analysis of 32 studies (23,415 patients) was conducted. There were no significant differences for patients undergoing minimally invasive ...
A systematic review collects all possible studies related to a given topic and design, and reviews and analyzes their results [ 1 ]. During the systematic review process, the quality of studies is evaluated, and a statistical meta-analysis of the study results is conducted on the basis of their quality. A meta-analysis is a valid, objective ...
Keywords: Research design, review, systematic, meta-analysis In a previous article in this series, we looked at systematic reviews - their methods, uses, and limitations.[ 1 ] Systematic reviews are frequently followed by the use of a mathematical or statistical technique to pool the data from individual studies included in the review, to ...
The goal of this study is to present a brief theoretical foundation, computational resources and workflow outline along with a working example for performing systematic or rapid reviews of basic research followed by meta-analysis. Conventional meta-analytic techniques are extended to accommodate methods and practices found in basic research.
Next, researchers must decide which studies to include in the meta-analysis. Some guidelines for literature reviews recommend limiting the sample to studies published in renowned academic journals to ensure the quality of ndings (e.g., Kraus et al. 2020). For meta-analysis, however, Steel et al. (2021) advocate for the inclusion of
Study designs: Part 8 - Meta-analysis (I) : Perspectives in Clinical Research ... The term "heterogeneity" refers to variability between the results of different studies on a research question. On any question, results of the available studies are unlikely to be identical, and a certain amount of variation between these is expected just by ...
Partitioning heterogeneity: In this approach, the overall heterogeneity between studies is calculated as the weighted sum of the squared deviations from the fixed effects estimate. The studies are then divided (partitioned) according to the variable of interest, and meta-analysis is performed within each group.
ABSTRACT. Meta-study is a method for analysing the content and the process of knowledge production in a body of qualitative research. Conducting a meta-study involves four steps: (1) meta-data-analysis which involves the study of empirical findings; (2) meta-method which examines the epistemological soundness and rigour of methods; (3) meta-theory which examines the structures, assumptions ...
A systematic review is a form of secondary research that answers a clearly formulated research question using systematic and defined methods to identify, collect, appraise, and summarize all the primary research evidence on that topic. ... Study designs: Part 8 - Meta-analysis (I) Perspect Clin Res. 2020 Oct-Dec;11(4):178-181. doi: 10.4103/picr ...
Meta-analysis is a quantitative, formal, epidemiological study design used to systematically assess the results of previous research to derive conclusions about that body of research (Haidrich, 2010). Rigorously conducted meta-analyses are useful tools in evidence-based medicine. Outcomes from a meta-analysis may include a more precise estimate ...
Definition. "A meta-analysis is a formal, epidemiological, quantitative study design that uses statistical methods to generalise the findings of the selected independent studies. Meta-analysis and systematic review are the two most authentic strategies in research. When researchers start looking for the best available evidence concerning ...
In addition to having a lower probability of discovering true effects (Button et al., 2013), study design/test combinations that cannot reliably detect a wide range of effects also have a lower probability that statistically significant results represent true effects (Ioannidis, 2005).In addition, such study design/test combinations tend to be associated with questionable research practices ...
In this series on research study designs, we have so far looked at different types of primary research designs which attempt to answer a specific question. ... Study Design Meta-analysis. Methods ...
Meta-Analysis With Complex Research Designs: Dealing With Dependence From Multiple Measures and Multiple Group Comparisons. Nancy Scammacca, ... A case study using effect sizes from a recent meta-analysis of reading interventions is presented to compare the results obtained from different approaches to dealing with dependence. Overall, mean ...
Meta-research is the study of research itself: its methods, reporting, reproducibility, evaluation, and incentives. Given that science is the key driver of human progress, improving the efficiency of scientific investigation and yielding more credible and more useful research results can translate to major benefits. The research enterprise grows very fast. Both new opportunities for knowledge ...
In this segment, we discuss systematic review, which is a study design used to summarize the results of several primary research studies. Systematic reviews often also use meta-analysis, which is a statistical tool to mathematically collate the results of various research studies to obtain a pooled estimate of treatment effect; this will be ...
The case study research design is also useful for testing whether a specific theory and model actually applies to phenomena in the real world. It is a useful design when not much is known about an issue or phenomenon. ... The Handbook of Research Synthesis and Meta-Analysis. 2nd edition. New York: Russell Sage Foundation, 2009; Guzzo, Richard A ...
Meta-Analysis. A work consisting of studies using a quantitative method of combining the results of independent studies (usually drawn from the published literature) and synthesizing summaries and conclusions which may be used to evaluate therapeutic effectiveness, plan new studies, etc. It is often an overview of clinical trials.
Although the studies included in a meta‐analysis have the same research hypothesis, there is still the potential for several areas of heterogeneity. 86 Heterogeneity may exist in various parts of the studies' design and conduct, including participant selection, interventions/exposures or outcomes studied, data collection, data analyses and ...
Meta-study is a method for analysing the content and the process. of knowledge production in a body of qualitative research. Conducting a meta-study involves four steps: (1) meta-data-. analysis ...
The design of a study defines the study type (descriptive, correlational, semi-experimental, experimental, review, meta-analytic) and sub-type (e.g., descriptive-longitudinal case study), research ...
Meta-analysis would be used for the following purposes: To establish statistical significance with studies that have conflicting results. To develop a more correct estimate of effect magnitude. To provide a more complex analysis of harms, safety data, and benefits. To examine subgroups with individual numbers that are not statistically significant.
This protocol should encompass the study's roadmap, covering aspects such as the research question, hypothesis, rationale for study design choice, selection of data sources, results of preliminary studies, approach to causal inference, and ethical considerations. Engaging with the FDA early is important for assuring alignment with expectations.
To bring letrozole from Desai's lab to patients' bedsides, he collaborated with Wise-Draper and neuro-oncologists and neurosurgeons at UC's Brain Tumor Center to launch a Phase 0/1 clinical trial. "In the academic setting, we are very good at doing molecular research that enhances our understanding of the mechanism of disease and preclinical characterization of efficacy, safety and ...
Background/Aims Vasomotor symptoms (VMS) adversely affect postmenopausal quality of life. However, their association with bone health has not been elucidated. This study aimed to systematically review and meta-analyze the evidence regarding the association of VMS with fracture risk and bone mineral density (BMD) in peri- and postmenopausal women. Methods A literature search was conducted in ...
Digging into the latest research, scrutinizing complex mathematical formulas and parsing tens of thousands of data points, he discovered what he says are flaws that skewed the results.
Meta-Analysis and Systematic Review. Glass first defined meta-analysis in the social science literature as "The statistical analysis of a large collection of analysis results from individual studies for the purpose of integrating the findings" 9.Meta-analysis is a quantitative, formal, epidemiological study design used to systematically assess the results of previous research to derive ...
The study of grassroots design—applying structured, creative processes to the usability or aesthetics of a product without input from professional design consultancies—remains under investigated. ... Findings from the research will inform questions to be investigated in the recently collected 2022 Annual Business Survey (ABS) that for the ...