Loading metrics
Open Access
Perspective
The Perspective section provides experts with a forum to comment on topical or controversial issues of broad interest.
See all article types »
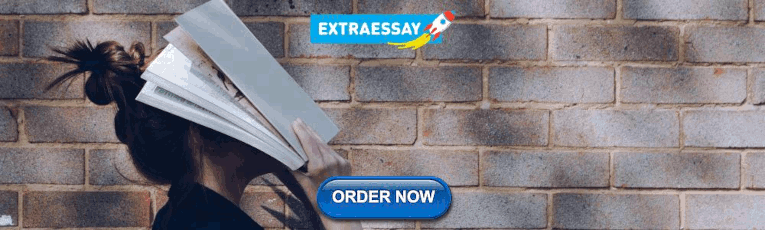
Behavioral ecology: New technology enables a more holistic view of complex animal behavior
* E-mail: [email protected]
Affiliation Department of Evolution and Ecology, University of California, Davis, California, United States of America

- Gail L. Patricelli
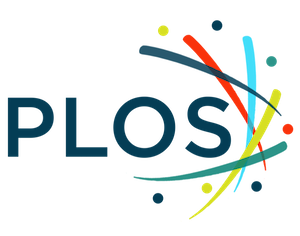
Published: August 24, 2023
- https://doi.org/10.1371/journal.pbio.3002264
- Reader Comments
As any animal observer will tell you, behavior is complex. A more holistic view of this complexity is emerging as technological advances enable the study of spatiotemporal variability and expand the focus from single components to behavioral systems.
Citation: Patricelli GL (2023) Behavioral ecology: New technology enables a more holistic view of complex animal behavior. PLoS Biol 21(8): e3002264. https://doi.org/10.1371/journal.pbio.3002264
Copyright: © 2023 Gail L. Patricelli. This is an open access article distributed under the terms of the Creative Commons Attribution License , which permits unrestricted use, distribution, and reproduction in any medium, provided the original author and source are credited.
Funding: The author received no specific funding for this work.
Competing interests: The author has declared that no competing interests exist.
This article is part of the PLOS Biology 20th Anniversary Collection.
Behavior is more than just a suite of traits; it is the crux where the inside of the organism meets and interacts with the external environment. On the inside of the organism, behavior emerges through an interaction of genetic, physiological, cognitive, and developmental processes, which can be affected, in turn, by that organism’s behavior and experience. Behavior is also how organisms respond to—and influence—the biotic and physical environment, which includes potential mates, rivals, offspring, group members, predators, prey, and pathogens—all with their own behaviors—interacting amidst changing seasons and climates. And behaviors may manifest at multiple scales, from individuals to swarms. For the past 20 years and going forward, many of the exciting frontiers in the study of animal behavior involve grappling with this complexity in a more holistic way, examining the causes and functions of variability in behavior over space and time, and scaling up from components to systems, examining interaction networks that function as a whole.
Many breakthroughs on key aspects of animal behavior (social behavior, mate choice, communication, predator–prey dynamics, foraging ecology, and migration) have been enabled by advances in technology that allow us to collect detailed and simultaneous data from many components of complex systems ( Box 1 ). These tools are made possible by increased computing power, rapid advances in machine learning, and the development of smaller, cheaper, and more powerful hardware. Such tools are pushing the study of behavior, like other fields of science, into the “big data” era.
Box 1. Technology allowing a more holistic view of animal behavior.
Advances in both hardware for collecting data and machine learning software to analyze those data are expanding the detail and the scale at which we can study behavior.
- Animal-borne telemetry tags, which collect or transmit data about movements and other measures, can be miniaturized to much less than a gram and provide precise locations with onboard GPS or data from sensors (e.g., accelerometers, physiological monitors, microphones, or light-level monitors). Tags may store or transmit data to other tags, land-based receiver arrays, or satellites (e.g., ICARUS or MOTUS). These tags reveal aspects of animal lives that were previously unobservable, helping to identify critical resources and habitats for protection (e.g., migration corridors and refueling sites), exposure and response to stressors (e.g., human activity, noise and light pollution), and cryptic behaviors (e.g., nocturnal movements, quiet communication, and visits to potential mates).
- Other key hardware includes synchronized microphone arrays to triangulate animal positions from the arrival time of their vocalizations [ 1 ], drones with imaging tools, terrestrial laser scanning (ground-based LiDAR) for detailed habitat measures, and Passive Integrated Transponders (PIT tags).
Machine learning
- Supervised machine learning, trained on human-annotated data sets, is automating tedious tasks and making detailed analysis of large datasets more feasible.
- Unsupervised machine learning can identify new patterns in movement tracks and other behavioral data, providing insights less limited by human biases and reducing (not eliminating) subjective decisions about which characteristics to measure.
- On videos, freely available software [ 2 ] uses machine learning to track position and orientation on multiple individuals, enabling the study of social networks and swarm dynamics. Machine learning can also be used for pose estimation by tracking the relative position of multiple body parts for biomechanical studies of behaviors (e.g., DEEPLabCut [ 3 ]).
- On audio recordings, machine learning is automating detection and identification of sounds from birds, bats, and other vocal animals, enabling acoustic monitoring over time, in remote locations, and at night [ 4 ] and increasing the feasibility of using synchronized microphone arrays to study vocal behavior and movements [ 1 ].
Spatiotemporal variability is a ubiquitous feature of animal behavior. By necessity, behaviors are often measured by choosing a few key characteristics that can be scored accurately and repeatably, often averaging multiple measures from consistent conditions. This allows behaviorists to examine, for example, the relationship between courtship rate and mating success, or dominance hierarchies in social groups. While important, there is increasing awareness that fascinating biology is being averaged away, such as differences among individuals in the ability to execute behaviors consistently or adapt to changing social and environmental situations, or variation among groups in the stability of social networks [ 5 – 7 ]. The past few decades have seen frameworks for understanding aspects of this behavioral variation, such as consistent individual differences (CIDs) and personality, behavioral reaction norms, and dynamic social network analyses, but the difficulty of collecting data has limited the scope of empirical work.
To capture and analyze variability itself, we need enough snapshots to make a movie, multiple measures of behaviors within and among individuals or groups, across time and context, so the patterns of change can be examined. New hardware and machine learning algorithms for tracking movements and recognizing patterns are opening exciting new opportunities for collecting such data [ 2 , 4 , 8 ].
For example, using GPS telemetry tags in the wild or overhead video in captive enclosures, it is increasingly feasible to study the causes and consequences of CIDs in behavior, such as activity level or aggressiveness, by tracking multiple individuals throughout development or among contexts. Patterns of behavioral variation can then be examined relative to genotype, epigenetics, experience, adult behavior (of the focal animal, their parents, and their offspring), and social group dynamics. In fish, for example, tracking has revealed that CIDs in behavior among clonal mollies raised in identical conditions are present from birth and strengthen over time [ 9 ] and that CIDs among sticklebacks in sociability and boldness can affect the movement and foraging performance of entire shoals [ 10 ].
Similar machine learning algorithms can track the position of body parts for pose estimation, automating frame-by frame analysis of biomechanics during courting, fighting, prey capture, locomotion, and other behaviors [ 3 , 8 ]. This can save time, expand the number of traits measured on focal or interacting individuals, and reduce subjectivity in analyses ( Box 1 ). These opportunities for high-resolution data collection will (I hope) inspire further development of theory in neglected areas, such as optimal tactics during courtship and other dynamic behavioral interactions [ 5 ].
New tracking tools are also helping us to scale up from spatiotemporal analyses of behavioral components to a systems-level view of the whole. The systems approach focuses on structure–function relationships, moving from cause-and-effect thinking to synergistic thinking, by emphasizing interactions, linkages, and integrated phenotypes [ 5 , 11 ].
For example, a hot topic of research for more than 20 years has been why sexual selection frequently favors complex courtship displays with components in different sensory modalities, combining songs, dances, colors, scents, and vibrations [ 5 , 6 , 11 ]. A recent comparative analysis of the famously complex and spectacular displays of 40 species of birds-of-paradise utilized video and audio recordings, as well as color patterns from museum skins, finding positive relationships instead of trade-offs between complexity in the acoustic, color, and behavioral display components [ 12 ]. The authors argued that integrated suites of traits evolve as a courtship phenotype, with functional overlap and interdependency providing robustness and promoting diversification. Further research is needed to determine whether similar patterns emerge in the complex courtship displays of other clades of birds, as well as clades of reptiles, amphibians, fishes, insects, and spiders. With machine learning tools for automated data collection, such broad comparative analyses are becoming possible with growing online databases, such as libraries of audio and video recordings and 3D scans of museum specimens. Ultimately, to understand the evolution of complex courtship phenotypes, as in birds-of-paradise, we must also understand how male display components interact to stimulate the females’ sensory, cognitive, and motivational systems to influence their mate choice. In other words, a holistic approach is also required to understand the aesthetic experiences and complex preferences of the females these courtship displays evolved to impress. This interface between behavioral ecology and neuroethology promises exciting discoveries about the evolution of some of nature’s most beautiful spectacles.
Systems-level analyses of multicomponent social groups have been similarly insightful. Tracking of large groups of birds is revealing surprisingly complex, multilevel social systems, from families, to cohesive groups of unrelated individuals, to fission–fusion dynamics among groups, to structured flocks of interacting species [ 13 ]. Tracking is also allowing the detailed examination of collective behaviors [ 10 , 14 ], exploring how behavioral rules followed by individuals scale into emergent properties of groups, such as swarming behavior of locusts. For example, by modelling how group size and spacing affect individuals’ views through the crowd, researchers are learning how geometry affects swarm dynamics and collective decisions.
Along with benefits of new technology, come challenges. To name a few, minimizing the impacts of our technology on animal bearers, finding meaningful biology in the output of black box algorithms, and not letting data volume and high statistical power substitute for thoughtful experimental design and biologically relevant effect sizes. Downloading data from satellites is no substitute for time in the field or lab learning about natural history and carefully observing behaviors, which is essential to inspire creativity and anchor us to the real world. At its best, new technology complements existing methods and helps to reveal hidden dimensions of behavior. Moving into the big data era, animal behavior, like other fields, can minimize pitfalls by increasing transparency, standardization, and sharing of data, algorithms, and statistical code.
As the pace of urbanization, habitat loss, climate change, and other human impacts increase, behavior will often be the first response, either allowing animals to adjust to change, or not. Behavioral changes are often the first signs scientists can measure as evidence of human impacts. Behavior is also what often inspires public fascination and concern about wildlife. Therefore, in addition to addressing basic questions about behavioral evolution, new technology and a more holistic view of animal behavior is key to understanding, predicting, and mitigating human impacts on wildlife. For example, behaviorists are revealing how noise and light pollution impact social behaviors, improving methods for population monitoring and restoration, and reducing human–wildlife conflict. The next 20 years will bring increased opportunity and increased necessity for animal behaviorists to engage actively with conservationists, policy makers, stakeholders, and the public to find solutions to these complex problems.
Acknowledgments
The author apologizes to the hundreds of authors and ideas in the field of animal behavior that there was insufficient space to credit here.
- View Article
- Google Scholar
- PubMed/NCBI
Thank you for visiting nature.com. You are using a browser version with limited support for CSS. To obtain the best experience, we recommend you use a more up to date browser (or turn off compatibility mode in Internet Explorer). In the meantime, to ensure continued support, we are displaying the site without styles and JavaScript.
- View all journals
- My Account Login
- Explore content
- About the journal
- Publish with us
- Sign up for alerts
- Open access
- Published: 20 October 2020
Deep learning-assisted comparative analysis of animal trajectories with DeepHL
- Takuya Maekawa ORCID: orcid.org/0000-0002-7227-580X 1 ,
- Kazuya Ohara 1 ,
- Yizhe Zhang 1 ,
- Matasaburo Fukutomi 2 ,
- Sakiko Matsumoto 3 ,
- Kentarou Matsumura 4 ,
- Hisashi Shidara ORCID: orcid.org/0000-0002-3992-9226 5 ,
- Shuhei J. Yamazaki 6 ,
- Ryusuke Fujisawa 7 ,
- Kaoru Ide 8 ,
- Naohisa Nagaya 9 ,
- Koji Yamazaki 10 ,
- Shinsuke Koike ORCID: orcid.org/0000-0002-2926-8196 11 ,
- Takahisa Miyatake ORCID: orcid.org/0000-0002-5476-0676 4 ,
- Koutarou D. Kimura ORCID: orcid.org/0000-0002-3359-1578 6 , 12 ,
- Hiroto Ogawa ORCID: orcid.org/0000-0002-4927-9714 5 ,
- Susumu Takahashi 8 &
- Ken Yoda 3
Nature Communications volume 11 , Article number: 5316 ( 2020 ) Cite this article
11k Accesses
32 Citations
19 Altmetric
Metrics details
- Animal migration
- Data mining
- Machine learning
A comparative analysis of animal behavior (e.g., male vs. female groups) has been widely used to elucidate behavior specific to one group since pre-Darwinian times. However, big data generated by new sensing technologies, e.g., GPS, makes it difficult for them to contrast group differences manually. This study introduces DeepHL, a deep learning-assisted platform for the comparative analysis of animal movement data, i.e., trajectories. This software uses a deep neural network based on an attention mechanism to automatically detect segments in trajectories that are characteristic of one group. It then highlights these segments in visualized trajectories, enabling biologists to focus on these segments, and helps them reveal the underlying meaning of the highlighted segments to facilitate formulating new hypotheses. We tested the platform on a variety of trajectories of worms, insects, mice, bears, and seabirds across a scale from millimeters to hundreds of kilometers, revealing new movement features of these animals.
Similar content being viewed by others
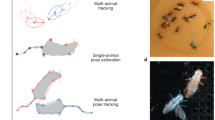
Quantifying behavior to understand the brain
Talmo D. Pereira, Joshua W. Shaevitz & Mala Murthy
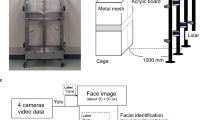
Development of a 3D tracking system for multiple marmosets under free-moving conditions
Terumi Yurimoto, Wakako Kumita, … Erika Sasaki
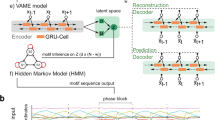
Identifying behavioral structure from deep variational embeddings of animal motion
Kevin Luxem, Petra Mocellin, … Pavol Bauer
Introduction
Recent advances in sensing technologies such as Global Positioning System (GPS) and computer vision provide “big behavioral data” of animals 1 , 2 , 3 , 4 , 5 . The challenge is how best to capitalize on such data to understand animal behavior, a challenge that has led to many significant cross-disciplinary research projects combining biology and information science 6 , 7 , 8 , 9 . One potentially powerful option involves deep learning artificial intelligence (AI). The recent rapid evolution of this has exceeded the capability of humans in a number of “intelligent” tasks requiring human creativity, including the game of Go 10 , 11 . Because big behavioral data require substantial effort for experts to analyze manually, and because the complexity of the data threatens to blur the capacity for insight, we believe that deep learning-oriented AI is an extremely promising tool to meet complex data challenges. Deep learning and classic machine learning have been used as support tools to quantify animal behavior (e.g., tracking 1 , 12 and behavior recognition 13 ) to reduce the effort involved in manual data labeling by biologists. In contrast, to take deep learning-assisted research one step further, this study leverages deep learning to assist high-level intelligent tasks associated with researchers requiring their insight, for example, the proposal of a hypothesis. Here, we showcase an example of this type of deep learning-assisted research, presenting a computational method that supports the comparative analysis of big behavioral data acquired by, and for, biologists.
Comparative methods have been used by biologists since pre-Darwinian times. Today, with the advent of animal-tracking technologies, comparative analysis, that is, comparison between two groups, for example, experimental vs. control groups and male vs. female groups, is one of the most fundamental approaches to animal behavior analysis. Regarding this, biologists have applied classic knowledge-driven approaches thus far, which are illustrated in the upper portion of Fig. 1 a. In this approach, the biologists typically visually compare huge amounts of time-series movement data, such as hundreds to thousands of trajectories, to identify the behavior that characterizes one group for elucidation, for example, sex-specific movement strategies, which requires substantial effort from the researchers. Then, based on the finding, the biologists design some statistical value computed from the behavioral data that well separates the two groups, which is called as a high-level feature in this study. After that, the biologists validate the finding using the computed high-level features with a statistical test (e.g., testing the significant difference in the high-level features between the male and female groups).
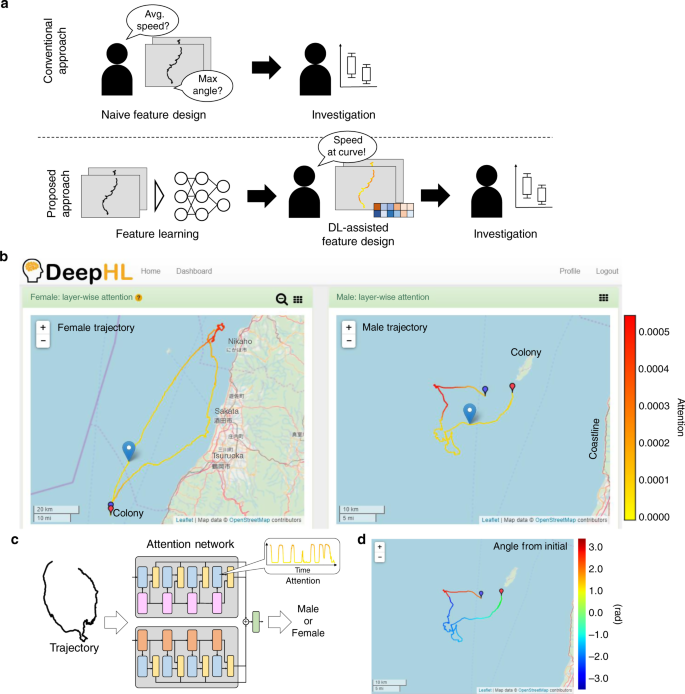
a Difference in research procedures between the conventional and proposed deep learning (DL)-assisted approaches. b Screenshot of the DeepHL web interface comparing the trajectories of a female (left) and male (right) streaked shearwater. Some characteristic segments of the trajectories are highlighted in red; these were detected by our neural network model trained to distinguish between the trajectories of male and female birds. A user can observe that there is something worth investigating in the highlighted segments. In this case, the female trajectory is highlighted when the female bird stays close to the coastline (see Supplementary Information , Application to the study of seabirds, for more detailed analysis). In contrast, the male trajectory is highlighted when the male bird travels away from the coastline. Note that the small blue and red pins on the maps indicate the starting and terminating points of the trajectories, respectively. The large blue pin on the map moves along the trajectory at a speed proportional to the actual movement speed (Supplementary Movie 1 ). c DeepHL extracts the segments in an input trajectory to which the neural network pays attention when classifying the trajectory by using a time series of the attention values. These segments reveal the importance of each data point. The input trajectory is colored by the time series of the attention values. The range of colors used to color the trajectories is shown on the right of b . d To facilitate a deeper understanding of the implications of the highlighted segments, DeepHL colors trajectories with the values of other sensor data or handcrafted features highly correlated with the attention values; the angle between the vertical axis ( y axis) and a line segment connecting the initial position and each point is used in this example. Points with large angles are focused as shown in the red segments in the male bird trajectory of b . Base map and data copyright OpenStreetMap contributors (License: www.openstreetmap.org/copyright ).
However, this approach possesses the potential risk of researchers overlooking an important high-level feature. This problem is obvious in the big data era. Although trajectory analysis based on classic machine learning has been studied, it still relies on features handcrafted by experienced researchers based on findings discovered by manually browsing a large amount of behavioral data or high-level features designed based on hypotheses formulated in advance, yielding a narrowly focused analysis.
In this study, we present a data-driven approach based on deep learning to support an analysis by biologists, as illustrated in the lower part of Fig. 1 a. Specifically, this study focuses on a comparative analysis, and a deep learning-based method is proposed to help identify the differences between the trajectory data of two groups. With this approach, to extract the high-level features from the trajectory data for a classification of the two groups, we leverage the feature learning capacity of deep learning, that is, learning of the high-level feature extraction processes performed within a deep neural network (DNN), which was originally conducted by experienced researchers. Although a DNN can extract high-level features objectively, unlike a classic approach, a DNN is regarded as a black box, making it difficult to interpret the meaning of the high-level features learned by the DNN, that is, to observe the group differences detected by the network. To address this problem, we developed DeepHL, a free, user-friendly, web-based software, in which an interpretable neural network with multi-scale layer-wise attention 14 is used to elucidate the characteristic segments in the trajectories to which the proposed DNN model focuses on in order to distinguish between the trajectories of the two groups (Fig. 1 b, c). Because this method informs researchers regarding “which parts of the trajectories they should focus on for further analysis,” researchers can save time and effort related to an otherwise manual analysis of huge numbers of trajectories to derive the characteristic segments. In addition, DeepHL finds handcrafted features prepared in advance that are highly correlated with the identified segments to help the researchers consider how best the segments can be explained. Thus, this method facilitates data-driven research for a comparative analysis by supporting knowledge discovery from the data. Figure 1 b shows example outputs of DeepHL when we compare trajectories from male seabirds to those from female seabirds. DeepHL automatically finds trajectory segments characteristic of each sex and then provides visualized trajectories highlighting the relevant segments to researchers. Based on the highlighted trajectories and highly correlated features, biologists develop a new hypothesis related to sex-related difference. Then, the biologists can design a high-level feature to validate the hypothesis.
In this study, we present our analysis on a variety of movement trajectories of worms, insects, and mice in laboratories, and animals in the wild, such as bears and seabirds using DeepHL. Behavioral data of these animals were provided by specialists who have been intensively studying the behavior of these animals by manual analysis and/or classic machine learning. We showed the ability of DeepHL to discover new biological insights that have not been found by the manual analysis or classic machine learning. We believe that DeepHL, a web-based open system, could be the first step to democratize AI for biologists who would otherwise have difficulty setting up computing environments for deep learning.
Here, we briefly introduce the pipeline of the proposed method: (i) DeepHL first trains our proposed network (hereafter called DeepHL-Net) on the trajectory data from two classes. (ii) The attention mechanism in DeepHL-Net then calculates the attention value of each data point in each trajectory for each layer in DeepHL-Net. (iii) Once the attention values are computed, some parts of the trajectories are highlighted by DeepHL using the attention output from a particular layer that is assumed to capture differences in the two classes. To help a user of DeepHL find such a layer (hereinafter, a “discriminator layer”) in DeepHL-Net, DeepHL calculates the score for each layer based on attention outputs from the layer. (iv) DeepHL also supports the user in explaining the meaning of the highlighted segments based on a list of handcrafted features from the trajectories prepared in advance by calculating the correlation between the attention values and each of the handcrafted features (Fig. 1 d).
Before describing our method in detail, herein we provide definitions of the features used in this study. Primitive features are basic features widely used in a locomotion analysis, that is, speed and relative angular speed. Handcrafted features are low-level features handcrafted by researchers, such as acceleration and distance from the initial position, and include the primitive features. High-level features are designed by researchers and characterize a group through a comparative analysis. A high-level feature is computed from the entire trajectory, such as the average movement speed and duration of stay at a feeding location. Although a DNN can also acquire high-level features or concepts, we found it difficult to comprehend these high-level features. A feature calculated in each layer in the DNN is simply called a feature. We explain our method in detail as follows.
Trajectory highlighting in DeepHL
We first explain the method of highlighting trajectories using deep learning. Our method assumes that there are two groups of animals with different properties, that is, class A and class B, and each trajectory belongs either to class A or B. We first convert the time series of the coordinates into time series of movement speed and relative angular speed (Fig. 2 a, b), which are widely used primitive features indicating movement velocity and orientation 15 , 16 , 17 , to achieve position and rotation-invariant trajectory analysis. (For animals that freely move on an agar plate, for example, their absolute coordinates are meaningless.) These features are then fed into DeepHL-Net. DeepHL allows a user to easily input other time series into DeepHL-Net, for example, original coordinates, other primitive features, and other sensor data.
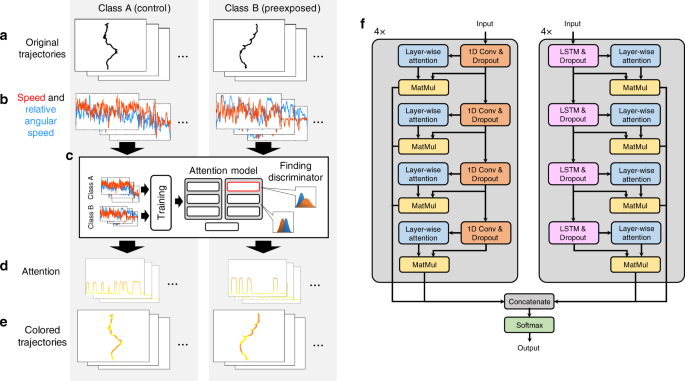
We assume that the trajectories of two classes are given: class A and class B in this example, which corresponds to worms without and with prior odor learning, respectively. a , b Trajectories, that is, a time series of two-dimensional coordinates, are converted into time series of speed and relative angular speed to achieve position- and rotation-invariant analysis. c DeepHL-Net is trained on the time series and then a discriminator layer is found using its attention values. d The discriminator layer outputs a time series of attention values when a trajectory is fed into the trained DeepHL-Net. The length of the time series is identical to that of the time series of speed and relative angular speed. e Each trajectory is colored with its corresponding attention values obtained by the layer. In our system, a large attention value is encoded as red and a small attention value is encoded as yellow, as shown in Fig. 1 b. f Proposed multi-scale layer-wise attention model (DeepHL-Net). The input and output of this model are the time-series primitive features and predicted class, respectively. The model consists of four stacks for the convolutional layers and four stacks for the LSTM layers to extract features at different levels of scale. Blocks labeled “1D Conv and Dropout” and “LSTM and Dropout” indicate the 1D convolutional layer and long short-term memory (LSTM) layer with dropout, respectively. The “Layer-wise attention” block calculates the attention of the outputs of a convolutional/LSTM layer using Eq. ( 1 ). The “MatMul” block multiplies the attention and the outputs of the layer to reflect the segments paid a high level of attention in the classification result. The “Softmax” block indicates the output softmax layer. For more details about the model, see “Methods” section.
DeepHL-Net is designed to classify a trajectory into either class A or B. We train DeepHL-Net on the extracted time series associated with their class labels (Fig. 2 c). Within DeepHL-Net, we identify a discriminator layer that detects characteristic segments, which is detailed later. Because DeepHL-Net is also designed to output the segments in a trajectory to which the discriminator layer pays attention, we color the trajectory using the attention information (Fig. 2 d, e). Figure 2 f shows the architecture of the proposed multi-scale layer-wise attention model (DeepHL-Net) comprising eight stacks of 1D convolutional or long short-term memory (LSTM) layers. Because different filter sizes are used in different convolutional stacks, these stacks are designed to extract features at different levels of scale. In addition, the 1D convolutional layers (orange-colored blocks in Fig. 2 f) extract short-term features. In contrast, the LSTM layers (pink-colored blocks in Fig. 2 f) tend to extract features reflecting long-term dependencies. Furthermore, more abstract features tend to be extracted in deeper layers in each stack. Therefore, the model is designed so that the layers extract features at different levels of temporal scale to classify trajectories. To elucidate which segments of the trajectories are considered to be important by each layer, we introduce an attention mechanism 14 into the model. As shown in Fig. 2 f, the outputs of each 1D convolutional/LSTM layer for an input trajectory are used to compute attention as follows:
Here, \({\bf{a}}\in {{\mathbb{R}}}^{1\times {l}_{\mathrm{MAX}}}\) , which shows the importance (i.e., attention) of each data point in the trajectory and is also used to color the trajectory, is an attention vector that has the same length as the trajectory, where l MAX is the maximum length of the input trajectories. Matrix \(Z\in {{\mathbb{R}}}^{{l}_{\mathrm{MAX}}\times N}\) is an output matrix of the 1D convolutional/LSTM layer, where N is the number of nodes in the convolutional/LSTM layer. Finally, \({W}_{\mathrm{a}}\in {{\mathbb{R}}}^{1\times N}\) and \({b}_{\mathrm{a}}\in {{\mathbb{R}}}^{1\times {l}_{\mathrm{MAX}}}\) are the weight matrix and bias, respectively. The softmax function ensures all the output values sum to 1, and the tanh function limits the output value of its input to a value between −1 and 1. Equation ( 1 ) is implemented as an artificial neuron in DeepHL-Net (“layer-wise attention”; aqua-colored blocks in Fig. 2 f). The attention is multiplied by the outputs of the 1D convolutional/LSTM layer to contrast the segments to which the layer pays attention (“MatMul”; khaki-colored blocks in Fig. 2 f). The multiplied outputs of all layers are concatenated and then used to output an estimate, that is, class A or B, in a densely connected output layer using the softmax function, that is, the final layer in DeepHL-Net (green-colored block in Fig. 2 f). As mentioned above, our model is designed to calculate attention information at different levels of scale (see “Methods” section for more details about the model).
Comparative analysis using DeepHL
A user of DeepHL discovers knowledge using a web page that displays highlighted trajectories (Fig. 1 b). We explain the usage of DeepHL through an analysis of the roundworm Caenorhabditis elegans , which is commonly used as a model animal in neuroscience to understand how learning modulates behavior 18 , 19 . Previous studies revealed that worms learn prior experience of the repulsive odor 2-nonanone in dopamine-dependent manner 20 , 21 : the worms preexposed to the odor migrate further away from the odor source more efficiently than naive worms do. Interestingly, the average speeds of the worms with or without odor learning are not significantly different, suggesting that the preexposed worms avoid the odor more efficiently. To comprehensively determine the high-level behavioral features characteristic of the repulsive odor learning, we compared the trajectories of naive worms (control class; 163 trajectories) to those of worms preexposed to the odor (preexposed class; 162 trajectories) using DeepHL. The positions of each worm’s centroid on a 9-cm agar plate were recorded at 1 Hz for 600 s (Fig. 3 a; see “Methods” and Supplementary Table 2 ). DeepHL-Net was trained on a multivariate time series of primitive features that DeepHL automatically extracts from the time series of trajectories (see “Methods,” Supplementary Information , Algorithm, and Supplementary Table 1 ). Here, the classification accuracy of the trained DeepHL-Net was 93.9% (see “Methods”), indicating that DeepHL-Net was properly trained. When the accuracy is low, for example, 50%, we can regard such a state as having no differences between the two classes or the training data having certain problems (e.g., an excessively small amount of data).
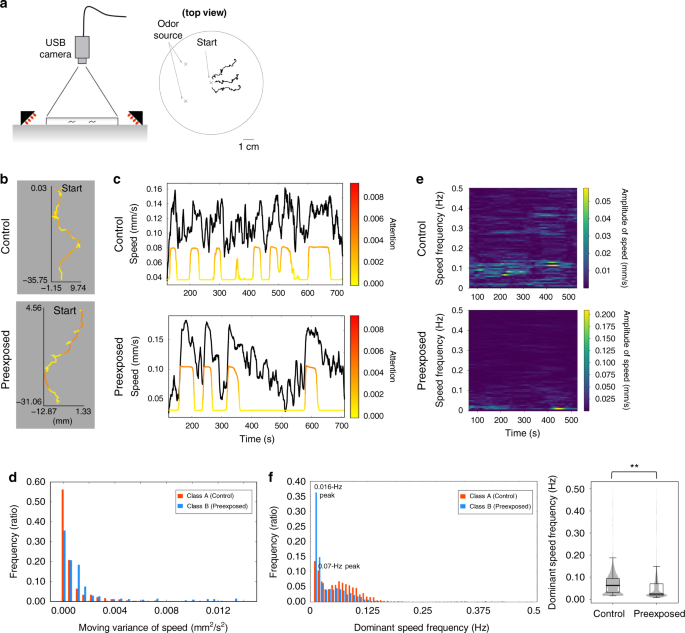
a The experimental setup (left) for monitoring the worm’s trajectory (right). b Example trajectories of worms colored by attentions of a discriminator layer. Segments of the trajectories corresponding to the run state of the worm are highlighted (in red). c Time series of the moving average of speed (black lines) associated with attention values (colored lines). The upper and lower graphs are obtained from the upper and lower trajectories shown in b , respectively. d Histograms showing the distributions of the moving variance of speed for each time slice within the highlighted trajectory segments. e Frequency analysis of the velocity of a preexposed or control worm. A 128-s-wide sliding window was shifted in 1-sample intervals and the amplitude of each frequency component was obtained from its fast Fourier transform (FFT). The upper and lower spectrograms were, respectively, obtained from the upper and lower trajectories shown in b . f Frequency analysis of the velocity of all the preexposed or control worms computed from entire trajectories. The histograms and box plot show the distributions of the dominant frequency of speed for each time slice. The dominant frequency is the one with the largest amplitude within each window. Significant difference in the dominant frequencies were observed by a generalized linear mixed model (GLMM) with Gaussian distributions ( t = −6.60; d.f. = 322.8; p = 1.68 × 10 −10 , effect size( r 2 ) = 0.232; ** p < 0.01; see “Methods”). The p value is two sided. The box plot shows the 25–75% quartile, with embedded bar representing the median; lower whiskers show Q1 − 1.5 × IQR (Q1: 25% quartile; IQR: interquartile range); upper whiskers show Q3 + 1.5 × IQR (Q3: 75% quartile). Control: n = 76, 784, preexposed: n = 75, 750.
In the following, we explain the process of knowledge discovery using the functions of DeepHL:
1. Screening layers: Because DeepHL-Net comprises several layers, DeepHL helps the user find discriminator layers by computing a score for each layer using the following criteria:
A discriminator layer should pay attention only to a portion of a trajectory. Technically speaking, an attention vector from the discriminator layer should have large values within limited segments. When the attention values are identical throughout the entire trajectory, the user cannot determine which part of the trajectory is characteristic of the class of interest.
It is desirable that the way attention is paid to the segments of trajectories belonging to one class by the layer is different from that for another class. Technically speaking, a distribution of attention values using the layer for one class should be different from that for another class. For example, when the layer exhibits large attention values to segments in trajectories belonging to only one class, the user can easily understand that these segments are characteristic of that class.
See “Methods” section for an equation to calculate the score. The DeepHL web interface provides a ranking of the layers based on the calculated scores, enabling the user to easily find high-scoring layers, which can provide an insightful highlight of the trajectory.
2. Showing colored trajectories: In this stage, the user compares trajectories colored by the identified discriminator layer. In the example of Fig. 3 b (colored by a discriminator layer with the highest score), only the relatively straight segments of the trajectories are highlighted in red. In contrast, the layer does not pay attention to segments representing more complex movement (yellow segments). The straight and complex movements reflect the two major behavioral states of the worms: “run” and “pirouette” 15 , 19 . DeepHL found that the run behavior of the preexposed class differs from that of the control class.
Note that, because the number of trajectories to be analyzed is large in many cases, DeepHL has a function for screening the trajectories when the user attempts to show highlighted trajectories by a discriminator layer. Especially when we deal with the trajectories of wild animals, not all trajectories include segments characteristic of a specific class. Therefore, DeepHL computes a score of each trajectory, enabling the user to focus mainly on, for example, trajectories with “female-like” segments. The score is calculated as V ( a ), where a is a time series of attention of the trajectory, to find trajectories with characteristic segments. When the variance value is small, this indicates that the layer does not pay attention to particular segments in the trajectory. In addition, the DeepHL web interface provides a classification result for each trajectory, permitting the user to ignore misclassified trajectories when the user browses trajectories.
3. Understanding meaning of highlights: DeepHL provides two functions to help the user understand the reason why a segment attracts attention using a discriminator layer. The first function provides the correlation between the time series of attention values and each of computed handcrafted features prepared in advance (or other sensor data). This reveals which handcrafted feature is related to the attention of the layer (Supplementary Table 1 ). The second function provides the difference in distributions of each handcrafted feature among the two classes within highlighted segments. This reveals which handcrafted feature has different distributions among the two classes within highlighted segments ( Supplementary Information , Algorithm).
Note that these handcrafted features are intended to help interpret the meaning of the attention of DeepHL-Net and that the handcrafted features do not always completely explain the meaning of the attention. As shown in the animal studies below, the biologists understand the meaning of such attention and then manually design interpretable high-level features, which are used in statistical tests, with the help of the functions.
In the worm example, the absolute correlation coefficient between the attention values of the layer and the moving average of the worm speed is the highest among all handcrafted features (Supplementary Table 3 ). Therefore, we then focus on the speed of the worms. Figure 3 c shows the moving averages of speed associated with the attention values. Here, we can employ the second function to reveal the difference in speed between preexposed and control worms within highlighted segments. Interestingly, the difference in distributions of speed itself between preexposed worms and control worms within highlighted segments is smaller than that of the moving variance of speed (0.22 vs. 0.25; see Supplementary Information , Algorithm for detailed description about difference computation). DeepHL also provides a graph of the distributions as shown in Fig. 3 d. The graph indicates that the changes in speed of preexposed worms are larger than those of control worms. As shown in the graph related to a control worm (Fig. 3 c, upper panel), we can see that, when attention values are high (colored line), the speed indicates tiny high-frequency changes (black line). In contrast, in one typical example of a preexposed worm (Fig. 3 c, lower panel), when attention values are high, the worm accelerates substantially and maintains a high speed, resulting in large low-frequency changes in speed as well as large moving variance of speed. DeepHL seems to detect the low-frequency changes in the speed of the preexposed worms as a characteristic behavior of the preexposed worms. Consistently, the lower frequency components of the speed of the preexposed worms are more dominant than those of the control worms (Fig. 3 e, f). These results suggest that, in worm odor avoidance behavior, two states for periodic changes in velocity—long-term changes with a peak at 2/128 Hz (i.e., 0.016 Hz; 64 s cycle) and short-term change that peak at 9/128 Hz (i.e., 0.07 Hz; 14.2 s cycle)—exist (Fig. 3 f), and that learning modulates the ratio between these two states to avoid odors efficiently. It is reasonable to speculate that maintaining a high speed (resulting in long-term speed changes) only during the run state contributes to efficient odor–source avoidance behavior. Note that these results were not predicted before this analysis because the average velocities of preexposed and control worms are essentially similar 20 , 22 . The biological significance of the worm and other animal analyses are described in the Supplementary Information.
Application to the study of mice
To test general applicability of DeepHL, we compared the behavioral patterns of normal and Parkinson’s disease (PD) mice freely moving in an open field (Fig. 4 a). Although the primary cause of PD is considered to be the loss of dopaminergic inputs to the striatum, the type of motor symptoms it induces remains unclear. Neurotoxic lesion animal models of PD have been utilized to elucidate the neuronal mechanisms underlying PD. However, in such models, the degree of dopaminergic cell loss can only be established post mortem. To estimate the degree of cell loss before death, several behavioral tests have been developed 23 , 24 . For instance, frequencies of ambulation, immobility, or fine movement epochs in open-field tests are evaluated. We compared normal mice to PD mice using DeepHL to discover a new high-level behavior feature. The classification accuracy for the mouse dataset is 74.7% (see “Methods” for further details). Figure 4 b shows typical examples of trajectories highlighted using a discriminator layer. Segments of the normal mouse trajectory that are far away from the initial position are highlighted in red (see also Fig. 4 d). In addition, DeepHL indicated that the attention values highly correlate with the straight-line distances from the initial position (highest; Supplementary Table 3 ). As shown in Fig. 4 c, when straight-line distances from the initial position exhibit high values, the attention values also increase. This result indicates that the behavior of visiting locations far away from the initial position is characteristic of normal mice.
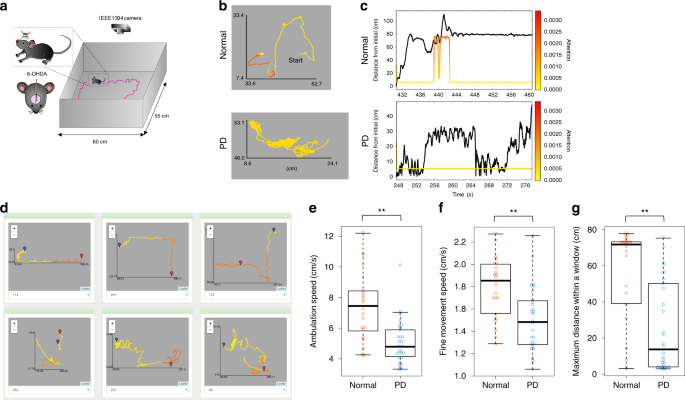
a Experimental apparatus and lesion protocol. b Example trajectories of mice colored by attention of the discriminator layer. The upper one is a trajectory of a normal mouse and the lower one is a trajectory of a 6-hydroxydopamine (OHDA) lesion mouse model of Parkinson’s disease (PD). The upper trajectory shows that when the mouse is far away from the initial position, the layer pays attention to the corresponding segments (red segments). c A time series of the straight-line distance from the initial position (black lines) associated with attention values (colored lines). d A screenshot of DeepHL for comparing multiple trajectories colored by the discriminator layer at a glance (normal mice). A blue balloon shows the initial position of each trajectory. e – g Average movement speed during ambulation periods, average movement speed during fine movement periods, and average maximum distance within a ±60-s window in a session of normal and PD mice for each entire trajectory (see “Methods”). Significant differences between normal and PD mice were observed for all three features (Wilcoxon rank-sum test, p = 3.486 × 10 −5 , p = 5.869 × 10 −4 , p = 2.666 × 10 −4 ; ** p < 0.01; n = 22 original 10-min trajectories from normal; n = 30 original 10-min trajectories from PD). The p values are two sided. The edges of the box plot correspond to 95% confidence intervals, the embedded bar represents the median, and whiskers show minimum and maximum values. Dots show values for individual sessions.
To investigate the usefulness of this finding in terms of PD mouse detection, we designed a new high-level movement feature based on it: the maximum straight-line distance within a ±60 s window. We compare its performance to the performances of existing high-level movement features, that is, ambulation and fine movement speeds (Fig. 4 e–g). We compute these three feature values for each entire trajectory and then evaluated the features using information gain 25 , which is used to evaluate classification features. A larger value of information gain indicates better classification performance. While the ambulation speed, fine movement speed, and maximum distance all exhibited statistical differences between the normal and PD groups, their information gains were 0.269, 0.184, and 0.287, respectively, indicating that the maximum distance is more useful for evaluating PD symptoms than conventional measures.
The results suggest that normal mice prefer exploring unvisited locations. This feature strongly relates to the straight-line distance from the initial position and differs from widely used existing high-level movement features based on speed. It is well known that rodents such as mice and rats spontaneously prefer to explore an environment, particularly in novel places. Thus, DeepHL may have revealed that the abnormal behavior of PD mice hinders such spontaneous behavioral traits.
Application to the study of red flour beetles
In addition to the PD and normal mice, DeepHL was used to detect dopamine-dependent differences in the trajectories of insects. Tonic immobility (TI), sometime called as “thanatosis” or “death-feigning,” is an antipredator behavior of many animals 26 , 27 . Miyatake et al. 28 performed a two-way artificial selection for the duration of TI, and established the strains with short (S strain) and long (L strain) duration of TI in the red flour beetle, Tribolium castaneum . Tribolium castaneum is an insect model species for which all the genomes are already known 29 . The S strain showed significantly higher levels of brain dopamine expression and a higher locomotor activity than those of the L strain 30 . In the present study, we analyzed 419 walking trails collected from S- and L-strain beetles on a treadmill using DeepHL (Fig. 5 a and Supplementary Table 2 ).
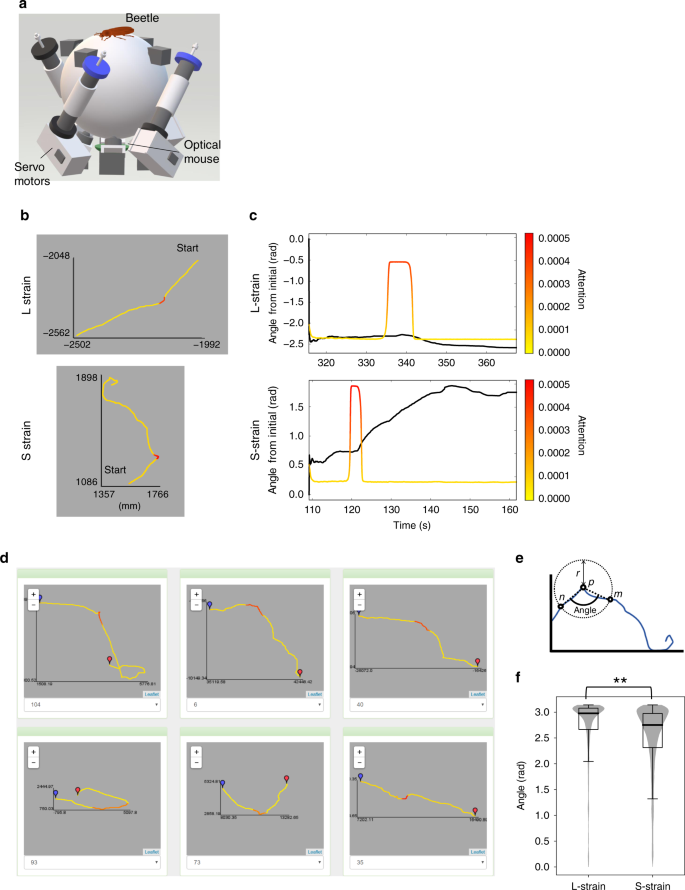
a Experimental apparatus (treadmill). b Example trajectories of the red flour beetles colored by the attention values of a discriminator layer. The upper one is a trajectory of the L-strain (long-strain) beetle and the lower one is a trajectory of the S-strain (short-strain) beetle. These trajectories show that segments corresponding to orientation change are highlighted. c Time series of the angle from the initial position (black lines) associated with attention values (colored lines). The upper and lower graphs are obtained from the upper and lower trajectories shown in b , respectively. The lower graph shows that the attention values have large positive values just before the angle increases. d Other trajectories belonging to the S-strain class colored by attention of the discriminator layer. e We assume a circle centered at each point ( p ) on a trajectory with radius r (100 mm) and obtain points n and m where the trajectory first crosses the circle before/after p . We then compute the angle between a line segment connecting p and n and one connecting p and m , showing the curvature around p . f Angles of the L and S strains. The box plot shows the 25–75% quartile, with embedded bar representing the median; lower whiskers show Q1 − 1.5 × IQR (Q1: 25% quartile; IQR: interquartile range); upper whiskers show the maximum values, that is, π , with the violin plots showing the distributions of data points. Significant difference between the L and S strains was observed using the two-sided ANOVA ( F = 12.57; d.f. = 1; p = 0.001; effect size ( η 2 ) = 0.09; ** p < 0.01; see “Methods”). # of data points for S strain is n = 185, 884; # of data points for L strain is n = 219, 497.
The classification accuracy for the beetle dataset is 84.5% (see “Methods” for further details). Figure 5 b shows typical examples of trajectories highlighted using a discriminator layer. The trajectories in Fig. 5 b appear to be highlighted when the beetles turn, which is the characteristic difference between the L and S strains detected by DeepHL. Consistently, the difference in distributions of the angle from the initial position between the S and L strains within highlighted segments is large (0.61). We can clearly see that the turn in the S-strain trajectories is sharp, and we found similar patterns in other trajectories. (See Fig. 5 d, generated by a function of DeepHL that allows the comparison of multiple trajectories at a glance.) Figure 5 c shows the angle from the initial position and attention values used for highlighting trajectories in Fig. 5 b, indicating increases in attention values just before increases in the angle for the S strain.
As shown in Fig. 5 e, we computed an angle of a trajectory segment for each point. Figure 5 f shows the distributions of the angles for the S and L strains (the number of data points for the S strain is 185,884 and the number of data points for the L strain is 219,497). We found that the angle for the S strain is significantly smaller than that for the L strain, indicating that the S-strain beetles walk with more angle changing. This finding related to angle change, which has not been discovered by prior studies 30 , may lead to new hypotheses concerning the survival strategy of the beetles. The L-strain beetles are known to perform death-feigning as an antipredator behavior. In contrast, the S-strain beetles are assumed to select a survival strategy of changing movement directions to escape from predators.
Application to the studies of crickets and animals in the wild
We also employed DeepHL to analyze context-dependent modulation of escape behavior in field crickets, Gryllus bimaculatus . Fukutomi et al. 31 , 32 revealed that an acoustic stimulus at high frequency (>10 kHz) preceding an air puff alters crickets’ moving direction in wind-elicited escape behavior, suggesting that the crickets recognize the high-frequency sound as the echolocation signal of bats and change their behaviors in the presence of predators. Here, we adopted DeepHL to compare two groups of escape movement: prestimulated and control (no sound). In this analysis, in addition to the speed and relative angular speed, we input additional sensor data measured using a treadmill, that is, a rotational speed of the body-axis computed from a body-axis angle measured using the treadmill, into DeepHL-Net. Figure 6 a shows typical trajectories colored by the attention values of a discriminator layer. DeepHL shows that the rotational speed of the body axis transiently elevated and peaked earlier in the prestimulated group ( Supplementary Information , Application to the study of crickets; Fig. 6 b), indicating that the sound preceding the air puff provoked the prompt rotational changes of the body axis.
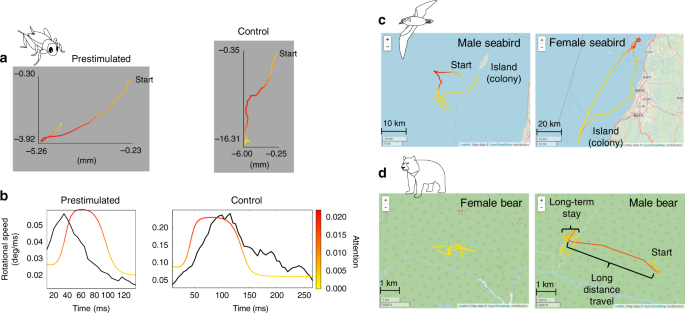
a Trajectories of the prestimulated and control crickets highlighted by DeepHL (see Supplementary Information , Application to the study of crickets, for more detail). b Time series of rotational speed (black lines) and attention value (colored lines) of the trajectories in a . A discriminator layer pays attention to the difference in the peaks of the rotational speed for the prestimulated and control classes. The high rotational speed was sustained in the control trajectory, which means that the crickets exhibited longer and larger turning movements in the control group. In contrast, the rotational speed transiently elevated and peaked earlier in the prestimulated group. c Trajectories of female and male seabirds highlighted by DeepHL. A discriminator layer pays attention to female trajectories when the locations of the female birds are close to the coastline (see Supplementary Information, Application to the study of seabirds). d Trajectories of female and male bears highlighted by DeepHL. The red segment is characteristic of the male bears, as detected by DeepHL. A discriminator layer pays attention to male trajectories when a male bear has traveled a long distance after/before staying in one place (see Supplementary Information, Application to the study of bears). Base map and data copyright OpenStreetMap contributors (License: www.openstreetmap.org/copyright ).
In addition, we applied DeepHL to the trajectories of wild animals. Figure 6 c shows GPS trajectories of female and male seabirds highlighted by a discriminator layer that pays attention to the migration direction of the birds from their colony. Our investigation revealed that the GPS measurements of the female seabirds are significantly closer to the coastline than those of the male seabirds. In this analysis, in addition to the speed and relative angular speed, we input the absolute coordinates (longitude and latitude) into DeepHL-Net because the absolute coordinates of the specific places such as colonies and feeding sites can affect the behavior of the seabirds. The longitude values were highly correlated with the attention values for the female seabirds. Because the coastline runs north–south, the distance between the coastline and a position is related to the longitude of the position. Therefore, this fact indicates that the behavior of the female seabirds is strongly affected by the distance from the coastline (see Supplementary Information, Application to the study of seabirds). As described above, because we can input an additional time series in addition to the speed and relative angular speed into DeepHL, we can see the effect of the time series on the animal behaviors. Figure 6 d shows the trajectories of female and male bears highlighted by a discriminator layer that pays attention to male trajectories when a male bear travels a long distance after/before it remains in one place. Our investigation revealed that the male bears combined long distance movements with short rests at many locations and the female bears remained in limited locations for a long time (see Supplementary Information, Application to the study of bears).
In the above analysis of the worms, mice, insects, seabirds, and bears, we could discover findings that were not revealed through a manual analysis or classic machine learning. Here, we can easily observe the differences between two groups from the highlighted trajectories of the seabirds and bears. Specifically, it is impossible to observe the relationships between the preferred locations of female seabirds and coastlines without visualization. For the mouse and beetle studies, we also observe the differences between the two groups from the highlighted trajectories. In contrast, it is difficult to find any differences between the two groups related to the worms and crickets by just browsing the highlighted trajectories. Therefore, leveraging both visualization functions and functions to help interpret the meanings of highlights is important to discover knowledge, which is facilitated by the DeepHL web interface (see also Supplementary Information, User guide to DeepHL).
In this study, we demonstrated that DeepHL is able to extract group differences in trajectories for a variety of taxa that operate across scales (for a quantitative evaluation of DeepHL using synthetic trajectory data, see Supplementary Information , Evaluation with synthetic data). This versatile trajectory analysis was possible because of the useful functions of DeepHL. Furthermore, we confirmed that DeepHL does not require a large number of trajectories to train DeepHL-Net (Supplementary Table 5 ).
Discovering high-level features hidden in temporal dynamics, for example, the frequencies of worm movement speeds and the sustained rotation speed of crickets found with the help of DeepHL, is difficult in classic machine learning without an algorithm specifically designed for each task using prior knowledge gained by manual analysis, which requires substantial effort. In fact, this finding related to the worms has not been discovered by prior study 17 mainly performed by specialists who have been intensively studying the behavior of worms based on a classic approach, which comprehensively extracts 333 handcrafted locomotion features, on the worm data that are also used in our study even though the finding of our study was obtained from the discriminator layer with the highest score. In addition, DeepHL was able to help in finding a prominent mouse movement feature related to exploration, which has not been a focus of prior studies and also obtained from a discriminator layer with the highest score. The discovered movement feature outperformed high-level features found in prior studies in terms of feature importance. This result is surprising because movement features of PD mice have been intensively studied by neuroscientists 23 , 24 . While the movement features of some animals such as seabirds and mice found with the help of DeepHL seem to be simple, the fact that these simple features have not been discovered after many years of research indicates the value of the findings given the difficulties of big behavioral data analysis based on classic approaches. Refer to Supplementary Information , Comparison with classic approaches, for analysis of the six animal species using classic approaches. Whereas the classification accuracy of a classic approach is not extremely different from that of DeepHL, as shown in Supplementary Information , Comparison with classic approaches, it was difficult to find fine-grained characteristics of animal behaviors by using the classic approach because it employs only high-level features prepared in advance extracted from a whole trajectory.
In this study, we find a discriminator layer that focuses on a part of trajectory. However, it is possible for many attention layers to focus on the full trajectory. In such a case, we can assume that the global features are important for classifying the trajectories. We believe that such global features can be easily identified through classic statistical techniques or manual analysis.
Trajectory data observed from wild animals can include different noises. For example, the trajectory data from bears are noisy because of the forest canopy, as shown in Fig. 6 d. When noises are included in the GPS measurements of both the male and female bears uniformly, we believe that DeepHL-Net can extract useful high-level features from the data. However, such noises can also degrade the classification performance. One possible solution to addressing this problem is to introduce a denoizing autoencoder 33 (e.g., reducing noises during the preprocessing). In addition, the seabird GPS data include few sudden large errors. We can remove such errors by thresholding calculated speed (see Supplementary Information , Application to the study of seabirds). Moreover, GPS signals can be lost for a moment. However, primitive features used in this study, that is, speed and relative angular speed, are robust against such missing measurements.
There are several deep learning studies related to our work. Endo et al. 34 visualize/generate typical trajectories for taxis using an autoencoder. In addition, several visualization tools for interpreting the behavior of LSTMs have been developed, although these mainly focus on natural language processing 35 , 36 . LSTMs have also been used to predict worm trajectories 37 , although these studies do not focus on comparative analysis. Recent deep learning studies have also employed attention mechanisms to visualize distinguishing features 38 , 39 . Attention mechanisms have also been actively studied in the computer vision field. Xu et al. 40 generated captions for an image by leveraging the attention of the input image to identify an important region in the image and generate each word. Zhang et al. 41 leveraged attention mechanisms to focus on foreground regions to alleviate distractions from the background for image-based salient object detection tasks. Park et al. 42 employed an attention mechanism to identify important regions in an image as well as generate textual descriptions using an LSTM for an image classification task. In the biology domain, Heras et al. 43 leveraged a deep attention network that predicts future turns of a zebrafish in a collective to identify surrounding zebrafish that affect the future turning of the focal zebrafish. Unlike in the above studies, in the present study, deep attention networks have been used to find distinguishing group-specific patterns in the trajectories.
Because the manual analysis of behavioral data is impractical for big behavioral data, we suggest that we are now on the cusp of changing the methods used for big data in biology research. We envision that DeepHL will transform the hitherto standard approach to comparative analysis from a hypothesis-driven approach, which relies on individual experience or manual analysis by researchers, to a data-driven approach. Owing to the useful functions proposed in this study, DeepHL enables researchers to easily extract insightful information hidden within a DNN that is trained on big data. Furthermore, because DeepHL is simply designed to find distinguishing trajectory segments between two groups, it can also be applied to a variety of comparative analyses, for example, old vs. young animals, free-ranging vs. captive animals, animals from different habitats, animals with different life-history stages, food storing vs. non-storing individuals/species, social vs. solitary individuals/species, and specialists vs. generalists. Although many functions of DeepHL are tailored to a trajectory analysis, DeepHL-Net can process any type of time-series data. As a part of a future study, we plan to apply our network to other time series such as sounds emitted by animals.
We believe that these animal behavior analyses contribute not only to biology research, but also to the sustainable development of our society and coexistence with wild animals by understanding animal behavior. In addition, livestock farming has many potential applications of our animal behavior analysis. For example, our method can be applied to identifying characteristic behaviors of disease animals, productive cows, and submissive cows in a social hierarchy. Moreover, because trajectory data are observed from any moving objects, DeepHL is capable of wide application. Specifically, we believe that DeepHL can also be applied to trajectory analyses for humans and automobiles, which can contribute to our society in various aspects: improvement of work efficiency (e.g., analyzing trajectories of workers in logistics centers), healthcare (e.g., comparing between patients and healthy subjects), and eco-safe driving.
DeepHL system architecture
The DeepHL system consists of three server computers. The first one is a web server that receives a trajectory data file from a user and provides analysis results to the user (Intel Xeon E5-2620 v4, 16 cores, 32 GB RAM, Ubuntu 14.04). The second one is a storage server that stores data files and analysis results. The third one is a GPU server that analyzes data provided by the user (Intel Xeon E5-2620 v4, 32 cores, 512 GB RAM, four NVIDIA Quadro P6000, Ubuntu 14.04). Supplementary Information , Algorithm, provides a complete description of the DeepHL method. DeepHL is accessible on the Internet through http://www-mmde.ist.osaka-u.ac.jp/maekawa/deephl/ . Supplementary Information , User guide to DeepHL, provides a user guide to DeepHL. In addition, Supplementary Information , Usage of Python-based Software, and Supplementary Software 1 present the Python code of DeepHL.
Preprocessing
An input trajectory is a series of timestamps and X / Y coordinates associated with a class label. To perform position- and rotation-independent analysis, we convert the series into time series of speed and relative angular speed and then standardize them ( Supplementary Information , Algorithm). Note that the absolute coordinates of wild animals, which can relate to the distance from a nest or feeding location, for example, are important in understanding behavior of the animals. Hence, DeepHL allows the original coordinates to be input to DeepHL-Net along with the speed and relative angular speed. In addition, other biological time-series sensor data measured by the user can be fed into DeepHL-Net when these time-series data are included in a data file uploaded by the user. For example, a time series of the heading direction of animals obtained from digital compasses can be useful for behavior understanding. Moreover, primitive features usually used in trajectory analysis can be easily fed into DeepHL-Net. DeepHL automatically computes the travel distance from the initial position, the straight-line distance from the initial position, and the angle from the initial position (Supplementary Table 1 ) as primitive features. Using the web interface of DeepHL, the user can easily select primitive features and other sensor data to be fed into DeepHL-Net ( Supplementary Information , User guide to DeepHL). See Supplementary Information , Effect of input features, for effects of input features on classification accuracy. Normally, the inputs of DeepHL-Net are two-dimensional time series, that is, speed and relative angular speed. When we input an additional time series (such as the original coordinates) into DeepHL-Net, the additional time series are added as additional dimensions of the inputs.
Multi-scale layer-wise attention model (DeepHL-Net)
Here, we explain DeepHL-Net shown in Fig. 2 f in detail. The input of the model is a time series of primitive features, that is, an l MAX × N f matrix, where l MAX is the maximum length of the input trajectories and N f is the dimensionality of the time series, that is, the number of the primitive features. Because the lengths of observed trajectories are not identical to each other in many cases, we fill in missing elements in the matrix with −1.0 and mask them when we train DeepHL-Net. In each 1D convolutional layer of the convolutional stacks, we extract features by convolving input features through the time dimension using a filter with a width (kernel size) of F t . We use different filter widths in the four convolutional stacks (3%, 6%, 9%, and 12% of l MAX ) to extract features at different levels of scale. We use a stride (step size) of one sample in terms of the time axis. We also use padding to allow the outputs of a layer to have the same length as the layer inputs. In addition, to reduce an overfitting, we employ a dropout, which is a simple regularization technique in which randomly selected neurons are dropped during training 44 . The dropout rate used in this study is 0.5.
In each LSTM layer of the LSTM stacks, we extract features considering the long-term dependencies of the input features. LSTM is a recurrent neural network architecture with memory cells, and it permits us to learn temporal relationships over a long time scale. LSTM learns long-term dependencies by employing memory cells that hold past information, updating the cell state using write, read, and reset operations with input, output, and forget gates (see Supplementary Information , Algorithm). In addition, we employ dropout to reduce overfitting. The attention information of each layer is computed by using Eq. ( 1 ), and then it is multiplied by the layer output. Here, the softmax and tanh functions in Eq. ( 1 ) are defined as follows:
Note that parameters in Eq. ( 1 ) for each layer, that is, W a and b a , as well as parameters in the convolutional and LSTM layers are estimated during the network training phase. Here, we introduced the tanh activation function into Eq. ( 1 ) to smooth out the output attention values. When an outlying large value is included in W a Z T + b a at time t , attention values other than time t become extremely small without using the tanh function. When we visualize a trajectory using such attention values, only a single data point is colored in red, making it difficult for a user to identify important segments.
Training and testing of DeepHL-Net
The DeepHL user can select the parameters of DeepHL-Net used in the analysis, that is, the number of convolutional/LSTM layers and the number of neurons in each layer (default: four layers with 16 neurons). Then, DeepHL-Net is trained on 80% of randomly selected trajectories to minimize the binary classification error of the training data, employing backpropagation based on Adam 45 ( Supplementary Information , Algorithm). (Note that each trajectory has a class label for binary classification.) Then, the trained DeepHL-Net is tested using the remaining 20% of trajectories to compute the classification accuracy, providing an indication of the degree of difference between the two classes.
Computing the score of each layer
To screen the layers in DeepHL-Net, we compute a score for each layer according to Eq. ( 4 )
Here, \({A}_{i,{C}_{\mathrm{A}}}\) is a set of attention vectors calculated from trajectories belonging to class A using the i th layer. In addition, \({A}_{i,{C}_{\mathrm{B}}}\) is a set of attention vectors calculated from trajectories belonging to class B using the i th layer. As mentioned in the main text, an attention vector from a discriminator layer should have large values within limited segments. Therefore, \({s}_{\mathrm{fc}}({A}_{i,{C}_{\mathrm{A}}},{A}_{i,{C}_{\mathrm{B}}})\) in Eq. ( 4 ) calculates the averaged variance of the attention values normalized by the average length of the trajectories, as described in Eq. ( 5 ). When the layer focuses on a part of a trajectory, the variance increases
Note that V ( ⋅ ) calculates the variance and l ( ⋅ ) calculates the average length of the trajectories. We take the square root of the average variance to derive the average standard deviation. Using \(l({A}_{i,{C}_{\mathrm{A}}}\cup {A}_{i,{C}_{\mathrm{B}}})\) , which calculates the average length of \({A}_{i,{C}_{\mathrm{A}}}\cup {A}_{i,{C}_{\mathrm{B}}}\) , we normalize the computed variance. Because the softmax function in Eq. ( 1 ) ensures that all values sum to 1, resulting in a larger variance for longer trajectories, we normalize the average variance using the average length.
In addition, as mentioned in the main text, the distribution of attention values by the layer for one class should be different from that for another class. Therefore, \({s}_{\mathrm{it}}({A}_{i,{C}_{\mathrm{A}}},{A}_{i,{C}_{\mathrm{B}}})\) calculates the difference between the distributions of the attention values of classes A and B as follows:
Here, h ( ⋅ ) calculates a normalized histogram of attention with 200 bins, and Intersect( ⋅ , ⋅ ) calculates the area overlap between two histograms, and is described as follows:
where H 1 ( i ) shows the normalized frequency of the i th bin of histogram H 1 . As described in Eq. ( 4 ), the final score is calculated as the sum of the two scores of \({s}_{\mathrm{fc}}({A}_{i,{C}_{\mathrm{A}}},{A}_{i,{C}_{\mathrm{B}}})\) and \({s}_{\mathrm{it}}({A}_{i,{C}_{\mathrm{A}}},{A}_{i,{C}_{\mathrm{B}}})\) .
Here, \({s}_{\mathrm{fc}}({A}_{i,{C}_{\mathrm{A}}},{A}_{i,{C}_{\mathrm{B}}})\) in Eq. ( 4 ) is used to find a layer that focuses only on a portion of a trajectory. Owing to the term, only a small important portion of trajectories is highlighted in many cases, as shown in Figs. 3 , 5 , and 6 , especially for the trajectories of beetles. However, substantial portions of several trajectories of the normal mice are highlighted, as shown in Fig. 4 d. Because the characteristics of the normal mouse trajectories are the distance from the initial position, the segments in the trajectories far from the initial position are highlighted.
Computing the correlation between attention values and handcrafted features
To help the user understand the meaning of the highlights, DeepHL automatically computes the Pearson correlation coefficients between the attention values of each layer and handcrafted features computed by DeepHL, as shown in Supplementary Table 1 . In addition, the correlation coefficients with sensor data and handcrafted features included in a trajectory data file are automatically computed. Computing the correlation with environmental sensor data can reveal the relationship between a behavior and environmental conditions. If a specific behavior is exhibited only when the temperature is high, for example, we can infer that the behavior relates to the high temperature condition. Furthermore, DeepHL automatically computes the moving average, moving variance, and derivative of each of the above features/sensor data, and then computes the correlation coefficients with the attention values, which are presented to the user (Supplementary Fig. 1 ).
Computing the difference between distributions of each handcrafted feature for the two classes within highlighted segments
To help the user understand the meaning of the highlights, DeepHL automatically computes the difference between distributions of each handcrafted feature for two classes within highlighted segments. The difference is computed as follows:
Here, \({F}_{j,{C}_{\mathrm{A}}}\) is a set of time series of the j th handcrafted feature calculated from trajectories belonging to class A. In addition, m ( ⋅ , ⋅ ) is a masking function that extracts feature values within highlighted segments. Because the softmax function in each attention layer ensures that all attention values in a sum of 1, we consider an attention value larger than c /(# time slices) as a potential attended value ( c = 1.2 in our implementation).
Data acquisition of worms
Data acquisition was performed according to Yamazoe-Umemoto et al. 22 . In brief, several worms were placed in the center of an agar plate in a 9-cm Petri dish, 30% 2-nonanone (v/v, EtOH) was spotted on the left side of the plate, which was covered by a lid and placed on the bench upside down. Then, the images of the plate were captured with a high-resolution USB camera for 12 min at 1 Hz. Because the worms do not exhibit odor avoidance behavior during the first 2 min because of the rapid increase in odor concentration 46 , the data for the following 10 min (i.e., 600 s) was used. From the images, individual worms were identified and the position of the centroid was recorded by an image processing software Move-tr/2D (v. 8.31; Library Inc., Japan). The number of recorded trajectories is 325 (Supplementary Table 2 ). The comparison was between the naive worms (control class) and the worms after preexposure to the odor (preexposed class).
DeepHL analysis of worms
A multivariate time series of movement speed, relative angular speed, distances from the initial position, and angle from the initial position extracted from the time series of trajectories was fed into DeepHL-Net, yielding a binary classification accuracy of 93.9%, where 20% of the data are used as test data. The discriminator layer used in this investigation has the highest score of all layers. As shown in Fig. 3 d, which was calculated from the moving variance of the speed within highlighted segments, we can state that the changes in the speed of preexposed worms is larger than those of control worms. Figure 3 e shows spectrograms of the speed calculated from entire trajectories (Fig. 3 c) with a 128-s wide sliding window shifted in 1-sample intervals. In addition, Fig. 3 f shows histograms of the dominant frequency of speed calculated from entire trajectories using the 128-s wide sliding window shifted in 1-sample intervals. These results also indicate the difference in the frequency of speed between the preexposed and control worms. Our investigation revealed that the dominant frequency of speed significantly differs between the preexposed and control worms using GLMM with Gaussian distributions ( t = −6.60; d.f. = 322.8; p = 1.68 × 10 −10 , effect size( r 2 ) = 0.232). The p value is two sided. Individual factors were treated as random effects. The number of data points for the control class is n = 76, 784 and that for the preexposed class is n = 75, 750. We used GLMM with Gaussian distributions because the objective variable has a continuous value and we used the lmerTest package (v. 2.0–36) of R (v. 3.4.3) for the analysis.
Data acquisition of mice
We collected 52 trajectories of normal mice and unilateral 6-hydroxydopamine (OHDA) lesion mouse models of PD while they freely moved for 10 min in an open field (60 × 55 cm 2 , wall height = 20 cm; normal: 22, PD: 30). The trajectories were detected by the animal’s head position, which was captured by an overhead digital video camera (60 fps). Two sets of small red and green light-emitting diodes were mounted above the animal’s head so that it could be located in each frame. Custom softwares based on Matlab (R2018b, Mathworks, MA, USA) and LabVIEW (Labview 2018, National Instruments, TX, USA) were used for tracking. We then created 30-s segments by splitting each trajectory because training a DNN requires a number of trajectories. We used 966 segments in total (normal: 374, PD: 592) collected from nine C57BL/6J mice (normal: 5, PD: 4). Note that we excluded 30-s segments that contain no movements of a mouse.
DeepHL analysis of mice
Movement speed, relative angular speed, travel distances, straight-line and travel distances from the initial position, and angle from the initial position were fed into our model. The accuracy for the binary classification of normal and 6-OHDA model mice was 74.7%, where 20% of the data are used as test data. The score of the discriminator layer was the highest of all LSTM layers and the sixth highest of all layers. Our investigation revealed that the behavior of visiting locations far away from the initial position can be characteristic of normal mice.
To evaluate PD symptoms from animal behaviors, previous studies have exclusively focused on the movement speed of animals in the open-field tests (frequency and bout duration of ambulation as well as immobility or fine movement) because typical symptoms in the animal model of PD are thought to be slowness of movement and a paucity of spontaneous movements. As shown in Fig. 4 e–g, we found significant differences in average movement speed during ambulation periods, average movement speed during fine movement periods, and average maximum distance within a ±60-s window in a session. These differences were derived from the findings of DeepHL using the two-sided Wilcoxon rank-sum test ( W = 544, p = 3.486 × 10 −5 , effect size (Cliff’s delta) = −0.648; W = 511, p = 5.869 × 10 −4 , effect size (Cliff’s delta) = −0.548; W = 521, p = 2.666 × 10 −4 , effect size (Cliff’s delta) = −0.579). The 95% confidence intervals are [1.222, 3.481], [0.139, 0.468], and [13.726, 43.175], respectively. We used the exactRankTests package (v. 0.8–29) of R (v. 3.2.3). Note that these behavioral features are extracted from original 10-min trajectories.
The maximum distance, which was derived from a finding of DeepHL, is more useful for evaluating the PD symptoms than conventional measures based on the movement speed. Note that the new feature is designed based on an insight drawn from an analysis by deep learning. These results suggest that DeepHL helps find a novel measure not directly linked to the movement speed, that is, a straight-line distance within a certain time window. When the aim of an animal is to visit all locations in an area, the travel distance over a short duration commonly becomes longer. Besides, it is well known that rodents, including mice and rats, spontaneously prefer to explore an environment, particularly in novel places. Thus, DeepHL may capture the fact that the abnormal behavior of the 6-OHDA lesion model of PD hinders such spontaneous behavioral traits of normal mice. Indeed, the 6-OHDA lesion mouse model appears to remain in the same place. Although this hypothesis should be verified based on the causality between behavioral traits and neural activity patterns underlying PD symptoms using neuronal recording together with its optogenetic manipulation in the basal ganglia and motor cortex 23 , it is beyond the scope of this study.
Behavioral features of mice
According to Kravitz et al. 23 , ambulation was defined as periods when the velocity of the animal’s center point averaged >2 cm/s for at least 0.5 s. Immobility was defined as continuous periods of time during which the average change of the trajectory was <1 cm for at least 1 s. Fine movement was defined as any movement that was not ambulation or immobility. Maximum travel distance within a ±60-s window was defined as the maximum straight-line distance between the center of the window and each point within the window. Note that each feature value is computed for each entire 10-min trajectory.
6-OHDA injection of mice
Under isoflurane anesthesia, 6-OHDA (4 mg/ml; Sigma) was injected through the implanted cannulae (AP −1.2 mm, ML 1.1 mm, DV 5.0 mm, 2 μl). Animals were allowed to recover for at least 1 week before post-lesion behavioral testing.
Histological verification of dopaminergic cell loss
After the mice were sacrificed by pentobarbital sodium overdose and perfused with formalin, their brains were frozen and cut coronally at 30 μl with a sliding microtome. For immunostaining, sections were divided into six interleaved sets. Immunohistochemistry was performed on the free-floating sections. Sections were pretreated with 3% hydrogen peroxide and incubated overnight with primary antibody mouse anti-tyrosine hydroxylase (1:1000; Millipore). As a secondary antibody, we used biotinylated donkey anti-mouse IgG (1:100; Jackson ImmunoResearch Inc.), followed by incubation with avidin–biotin–peroxidase complex solution (1:100; VECTASTAIN Elite ABC STANDARD KIT, Vector Laboratories). The immunoreactivities were visualized by 3-3′ diaminobenzidine tetrahydrochloride (Dojindo Laboratories). The degree of dopaminergic cell loss was estimated by dividing the number of cells manually counted across three sections of the SNc (most rostral, most caudal, and the intermediate between them) of the lesioned hemisphere from that of the non-lesioned hemisphere.
Data acquisition of beetles
In the present study, we analyzed 419 walking trails collected from S- and L-strain beetles freely moving on a treadmill 47 (tracking software: custom software based on OpenCV, v. 2.4.9) using DeepHL (Supplementary Table 2 ). The number of the S-strain (L-strain) beetles is 20, consisting of 10 males and 10 females. The sampling rate of the treadmill is ~14.3 Hz, and the average duration of the trajectories is 52 s.
DeepHL analysis of beetles
In addition to the movement speed and relative angular speed, the distances and angle from the initial position were fed into DeepHL-Net. The classification accuracy for the binary classification between S and L strains was 84.5%, where 20% of the data are used as test data. The score of the discriminator layer in Fig. 5 b was the third highest of all layers. Because the layer seems to focus on turns, we computed an angle of a trajectory segment for each point according to Fig. 5 e. Figure 5 f shows the average angles for the S and L strains (the number of data points for the S strain is 185,884 and the number of data points for the L strain is 219,497). We found that the angle for the S strain is significantly larger than that for the L strain, indicating that beetles of the S-strain beetles walk with more angle changing. Note that we used two-sided analysis of variance (ANOVA) ( F = 12.57; d.f. = 1; p = 0.001; effect size( η 2 ) = 0.09). Because multiple data points were computed from each individual beetle’s trajectory, we treated the individuals as a random factor. The 95% confidence interval is [0.05, 0.18]. We used JMP 12.2.0., SAS. This result could indicate the difference in strategies for survival between the S- and L-strain beetles. The L-strain beetles can survive because of their long duration of TI against predators. In contrast, the S-strain beetles attempt to escape from a predator by frequently changing their moving directions.
Previous studies have shown a significantly lower expression level of brain dopamine in the beetles derived from the L strain than those from the S strain 30 . Nishi et al. 48 showed that injection of caffeine, which activates dopamine, decreases the duration of immobility in the L strain of T. castaneum . These phenomena concerning dopamine show an analogy to PD, which alters walking patterns 49 . In many animals, dopamine expression level relates to movement patterns, and a specific trajectory segment pattern of the L strain might be similarly deeply affected. To test this new hypothesis, the relationship between dopamine expression and detailed analysis for walking ability, which should be done apart from the present study, should be examined in the future. In conclusion, the analysis using DeepHL revealed significantly different walking trajectories between beetles from the S and L strains using ANOVA: the S-strain beetles walk with more angle changes along the direction of travel compared to the L-strain beetles.
Ethics statement
The studies on streaked shearwaters, mice, and bears were approved by the Animal Experimental Committees of Nagoya University (streaked shearwaters), the Doshisha University Institutional Animal Care and Use Committees (mice), and the Institutional Animal Care and Use Committee of Tokyo University of Agriculture and Technology (bears), respectively. The research on streaked shearwaters was conducted with permits from the Ministry of the Environment, Japan. All experimental procedures used in the bear research followed the Guidelines Concerning Animal Experimentation of the Tokyo University of Agriculture and Technology and the Mammal Society of Japan. They specify no requirements for the treatment of insects in experiments. Details of animals used in this study are described in Supplementary Information , Animals.
Reporting summary
Further information on research design is available in the Nature Research Reporting Summary linked to this article.
Data availability
The dataset of the worms analyzed during the current study is available in the Dryad repository, https://doi.org/10.5061/dryad.37pvmcvf5 , and included in Supplementary Data 1 . The datasets of the mice, beetles, crickets, and seabirds analyzed during the current study are included in Supplementary Data 1 . The dataset of the bears are available from the corresponding author upon reasonable request because the release of the bear data can increase the likelihood of poaching and stir up the fear in residents. Source data are provided with this paper.
Code availability
The source code of DeepHL is distributed as Supplementary Software 1 . The most recent version of the software is available at https://doi.org/10.5281/zenodo.4023931 50 . The use of the software is exclusively limited to the purpose of undertaking academic, governmental, or not-for-profit research. The DeepHL web system is accessible on the Internet through http://www-mmde.ist.osaka-u.ac.jp/maekawa/deephl/ . We will keep the website operating and freely accessible for the foreseeable future.
Pérez-Escudero, A., Vicente-Page, J., Hinz, R. C., Arganda, S. & de Polavieja, G. G. idTracker: tracking individuals in a group by automatic identification of unmarked animals. Nat. Methods 11 , 743–748 (2014).
Article PubMed Google Scholar
Cagnacci, F., Boitani, L., Powell, R. A. & Boyce, M. S. Animal ecology meets GPS-based radiotelemetry : a perfect storm of opportunities and challenges. Philos. Trans. R. Soc. Ser. B 365 , 2157–2162 (2010).
Article Google Scholar
Block, B. A. et al. Tracking apex marine predator movements in a dynamic ocean. Nature 475 , 86–90 (2011).
Article CAS PubMed Google Scholar
Ropert-Coudert, Y. & Wilson, R. P. Trends and perspectives in animal-attached remote sensing. Front. Ecol. Environ. 3 , 437–444 (2005).
Gomez-Marin, A., Paton, J. J., Kampff, A. R., Costa, R. M. & Mainen, Z. F. Big behavioral data: psychology, ethology and the foundations of neuroscience. Nat. Neurosci. 17 , 1455–1462 (2014).
Zheng, Y. Trajectory data mining: an overview. ACM Trans. Intell. Syst. Technol. 6 , 1–41 (2015).
Wang, R. F. & Spelke, E. S. Human spatial representation: Insights from animals. Trends Cogn. Sci. 6 , 376–382 (2002).
Sugden, A. & Pennisi, E. When to go, where to stop. Science 313 , 775 (2006).
Article CAS Google Scholar
Trullier, O., Wiener, S. I., Berthoz, A. & Meyer, J. Biologically based artificial navigation systems: review and prospects. Prog. Neurobiol. 51 , 483–544 (1997).
Silver, D. et al. Mastering the game of Go with deep neural networks and tree search. Nature 529 , 484–489 (2016).
Article ADS CAS Google Scholar
Silver, D. et al. Mastering the game of Go without human knowledge. Nature 550 , 354–359 (2017).
Article ADS CAS PubMed Google Scholar
Mathis, A. et al. DeepLabCut: markerless pose estimation of user-defined body parts with deep learning. Nat. Neurosci. 21 , 1281–1289 (2018).
Kabra, M., Robie, A. A., Rivera-Alba, M., Branson, S. & Branson, K. JAABA: interactive machine learning for automatic annotation of animal behavior. Nat. Methods 10 , 64–67 (2013).
Bahdanau, D., Cho, K. & Bengio, Y. Neural machine translation by jointly learning to align and translate. Preprint at https://arxiv.org/abs/1409.0473 (2014).
Pierce-Shimomura, J. T., Morse, T. M. & Lockery, S. R. The fundamental role of pirouettes in Caenorhabditis elegans chemotaxis. J. Neurosci. 19 , 9557–9569 (1999).
Article CAS PubMed PubMed Central Google Scholar
Bennison, A. et al. Search and foraging behaviors from movement data: a comparison of methods. Ecol. Evol. 8 , 13–24 (2018).
Yamazaki, S. J. et al. STEFTR: A hybrid versatile method for state estimation and feature extraction from the trajectory of animal behavior. Front. Neurosci. 13 , 626 (2019).
Article PubMed PubMed Central Google Scholar
Bargmann, C. I. Chemosensation. in WormBook (ed. The C. elegans Research Community) (2006).
Lockery, S. R. The computational worm: spatial orientation and its neuronal basis in C. elegans . Curr. Opin. Neurobiol. 21 , 782–790 (2011).
Kimura, K. D., Fujita, K. & Katsura, I. Enhancement of odor avoidance regulated by dopamine signaling in Caenorhabditis elegans . J. Neurosci. 30 , 16365–16375 (2010).
Kimura, K. D. & Yamazaki, S. J. The data of ~160 worms during odor avoidance behavior before or after learning. Dryad https://doi.org/10.5061/dryad.37pvmcvf5 (2019).
Yamazoe-Umemoto, A., Fujita, K., Iino, Y., Iwasaki, Y. & Kimura, K. D. Modulation of different behavioral components by neuropeptide and dopamine signalings in non-associative odor learning of Caenorhabditis elegans . Neurosci. Res. 99 , 22–33 (2015).
Kravitz, A. V. et al. Regulation of parkinsonian motor behaviors by optogenetic control of basal ganglia circuitry. Nature 466 , 622–626 (2013).
Article ADS Google Scholar
Boix, J., Padel, T. & Paul, G. A partial lesion model of Parkinson’s disease in mice—characterization of a 6-OHDA-induced medial forebrain bundle lesion. Behav. Brain Res. 284 , 196–206 (2015).
Kullback, S. Information Theory and Statistics , Vol. 1 (Dover Publications, New York, 1959).
Rogers, S. M. & Simpson, S. J. Thanatosis. Curr. Biol. 24 , R1031–R1033 (2014).
Humphreys, R. K. & Ruxton, G. D. A review of thanatosis (death feigning) as an anti-predator behaviour. Behav. Ecol. Sociobiol. 72 , 22 (2018).
Miyatake, T. et al. Is death-feigning adaptive? Heritable variation in fitness difference of death-feigning behaviour. Proc. R. Soc. Ser. B 271 , 2293–2296 (2004).
Tribolium Genome Sequencing Consortium. The genome of the model beetle and pest Tribolium castaneum . Nature 452 , 949–955 (2008).
Miyatake, T. et al. Pleiotropic antipredator strategies, fleeing and feigning death, correlated with dopamine levels in Tribolium castaneum . Anim. Behav. 75 , 113–121 (2008).
Fukutomi, M. & Ogawa, H. Crickets alter wind-elicited escape strategies depending on acoustic context. Sci. Rep. 7 , 15158 (2017).
Article ADS PubMed PubMed Central Google Scholar
Fukutomi, M., Someya, M. & Ogawa, H. Auditory modulation of wind-elicited walking behavior in the cricket Gryllus bimaculatus . J. Exp. Biol. 218 , 3968–3977 (2015).
Vincent, P., Larochelle, H., Bengio, Y. & Manzagol, P.-A. Extracting and composing robust features with denoising autoencoders. In the 25th International Conference on Machine Learning , 1096–1103 (2008).
Endo, Y., Toda, H., Nishida, K. & Ikedo, J. Classifying spatial trajectories using representation learning. Int. J. Data Sci. Anal. 2, 107–117 (2016).
Karpathy, A., Johnson, J. & Fei-Fei, L. Visualizing and understanding recurrent networks. Preprint at https://arxiv.org/abs/1506.02078 (2015).
Strobelt, H., Gehrmann, S., Pfister, H. & Rush, A. M. LSTMVis: a tool for visual analysis of hidden state dynamics in recurrent neural networks. IEEE Trans. Vis. Comput. Graph. 24 , 667–676 (2018).
Li, K. et al. Recurrent neural networks with interpretable cells predict and classify worm behaviour. Preprint at bioRxiv 222208 (2017).
Lin, Z. et al. A structured self-attentive sentence embedding. Preprint at https://arxiv.org/abs/1703.03130 (2017).
Vaswani, A. et al. Attention is all you need. In Advances in Neural Information Processing Systems , 5998–6008 (2017).
Xu, K. et al. Show, attend and tell: neural image caption generation with visual attention. In International Conference on Machine Learning , 2048–2057 (2015).
Zhang, X., Wang, T., Qi, J., Lu, H. & Wang, G. Progressive attention guided recurrent network for salient object detection. In IEEE Conference on Computer Vision and Pattern Recognition , 714–722 (2018).
Park, D. H. et al. Multimodal explanations: justifying decisions and pointing to the evidence. In IEEE Conference on Computer Vision and Pattern Recognition , 8779–8788 (2018).
Heras, F. J., Romero-Ferrero, F., Hinz, R. C. & de Polavieja, G. G. Deep attention networks reveal the rules of collective motion in zebrafish. PLoS Comput. Biol. 15 , e1007354 (2019).
Srivastava, N., Hinton, G., Krizhevsky, A., Sutskever, I. & Salakhutdinov, R. Dropout: a simple way to prevent neural networks from overfitting. J. Mach. Learn. Res. 15 , 1929–1958 (2014).
MathSciNet MATH Google Scholar
Kingma, D. P. & Ba, J. L. Adam: a method for stochastic optimization. Preprint at https://arxiv.org/abs/1412.6980 . 1–15 (2014).
Tanimoto, Y. et al. Calcium dynamics regulating the timing of decision-making in C. elegans . eLife 6 , e21629 (2017).
Nagaya, N. et al. Anomalous diffusion on the servosphere: a potential tool for detecting inherent organismal movement patterns. PLoS ONE 12 , e0177480 (2017).
Nishi, Y., Sasaki, K. & Miyatake, T. Biogenic amines, caffeine and tonic immobility in Tribolium castaneum . J. Insect Physiol. 56 , 622–628 (2010).
Gelb, D. J., Oliver, E. & Gilman, S. Diagnostic criteria for Parkinson disease. Arch. Neurol. 56 , 33–39 (1999).
Maekawa, T. et al. Deep learning-assisted comparative analysis of animal trajectories with DeepHL. GitHub https://doi.org/10.5281/zenodo.4023931 (2020).
Download references
Acknowledgements
We thank Rory P. Wilson for comments on the manuscript. We are also grateful to Chinatsu Kozakai, Tomoya Abe, Masahiro Ogawa, and Maki Yamamoto for their field assistance. This work was supported by JSPS Kakenhi JP16H06539, JP16H06545, JP16H06544, JP16H06543, JP16H06541, JP17H05976, and JP17H05971.
Author information
Authors and affiliations.
Graduate School of Information Science and Technology, Osaka University, Osaka, Japan
Takuya Maekawa, Kazuya Ohara & Yizhe Zhang
Graduate School of Life Science, Hokkaido University, Hokkaido, Japan
Matasaburo Fukutomi
Graduate School of Environmental Studies, Nagoya University, Nagoya, Japan
Sakiko Matsumoto & Ken Yoda
Graduate School of Environmental and Life Science, Okayama University, Okayama, Japan
Kentarou Matsumura & Takahisa Miyatake
Department of Biological Sciences, Hokkaido University, Hokkaido, Japan
Hisashi Shidara & Hiroto Ogawa
Graduate School of Science, Osaka University, Osaka, Japan
Shuhei J. Yamazaki & Koutarou D. Kimura
Graduate School of Computer Science and Systems Engineering, Kyushu Institute of Technology, Iizuka, Japan
Ryusuke Fujisawa
Graduate School of Brain Science, Doshisha University, Kyotanabe, Japan
Kaoru Ide & Susumu Takahashi
Department of Intelligent Systems, Kyoto Sangyo University, Kyoto, Japan
Naohisa Nagaya
Department of Forest Science, Tokyo University of Agriculture, Tokyo, Japan
Koji Yamazaki
Graduate School of Agriculture, Tokyo University of Agriculture and Technology, Tokyo, Japan
Shinsuke Koike
Graduate School of Science, Nagoya City University, Nagoya, Japan
Koutarou D. Kimura
You can also search for this author in PubMed Google Scholar
Contributions
T.Maekawa conceived and directed the study, and performed method design, software implementation, data analysis, and manuscript writing. K.O. and Y.Z. performed method design, software implementation, and data analysis. K.D.K., S.J.Y., K.Yoda, S.T., H.O., H.S., M.F., T.Miyatake, K.M., and S.K. performed data collection, data analysis, and manuscript writing. S.M., R.F., N.N., K.Yamazaki, and K.I. performed data collection and developed data collection devices.
Corresponding author
Correspondence to Takuya Maekawa .
Ethics declarations
Competing interests.
The authors declare no competing interests.
Additional information
Peer review information Nature Communications thanks Gonzalo de Polavieja and the other, anonymous, reviewer(s) for their contribution to the peer review of this work. Peer reviewer reports are available.
Publisher’s note Springer Nature remains neutral with regard to jurisdictional claims in published maps and institutional affiliations.
Supplementary information
Supplementary information, peer review file, description of additional supplementary files, supplementary data 1, supplementary movie 1, supplementary software 1, reporting summary, source data, source data, rights and permissions.
Open Access This article is licensed under a Creative Commons Attribution 4.0 International License, which permits use, sharing, adaptation, distribution and reproduction in any medium or format, as long as you give appropriate credit to the original author(s) and the source, provide a link to the Creative Commons license, and indicate if changes were made. The images or other third party material in this article are included in the article’s Creative Commons license, unless indicated otherwise in a credit line to the material. If material is not included in the article’s Creative Commons license and your intended use is not permitted by statutory regulation or exceeds the permitted use, you will need to obtain permission directly from the copyright holder. To view a copy of this license, visit http://creativecommons.org/licenses/by/4.0/ .
Reprints and permissions
About this article
Cite this article.
Maekawa, T., Ohara, K., Zhang, Y. et al. Deep learning-assisted comparative analysis of animal trajectories with DeepHL. Nat Commun 11 , 5316 (2020). https://doi.org/10.1038/s41467-020-19105-0
Download citation
Received : 20 November 2019
Accepted : 25 September 2020
Published : 20 October 2020
DOI : https://doi.org/10.1038/s41467-020-19105-0
Share this article
Anyone you share the following link with will be able to read this content:
Sorry, a shareable link is not currently available for this article.
Provided by the Springer Nature SharedIt content-sharing initiative
This article is cited by
Ptds centertrack: pedestrian tracking in dense scenes with re-identification and feature enhancement.
- Jiazheng Wen
Machine Vision and Applications (2024)
Ponds as experimental arenas for studying animal movement: current research and future prospects
- Christer Brönmark
- Gustav Hellström
- Kaj Hulthén
Movement Ecology (2023)
Cross-species behavior analysis with attention-based domain-adversarial deep neural networks
- Takuya Maekawa
- Daiki Higashide
- Susumu Takahashi
Nature Communications (2021)
Unraveling hidden interactions in complex systems with deep learning
- Seungwoong Ha
- Hawoong Jeong
Scientific Reports (2021)
By submitting a comment you agree to abide by our Terms and Community Guidelines . If you find something abusive or that does not comply with our terms or guidelines please flag it as inappropriate.
Quick links
- Explore articles by subject
- Guide to authors
- Editorial policies
Sign up for the Nature Briefing newsletter — what matters in science, free to your inbox daily.

Animal Cognition
- Debbie M. Kelly
Latest articles
Perception of vocoded speech in domestic dogs.
- Amritha Mallikarjun
- Emily Shroads
- Rochelle S. Newman
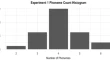
Figure-ground segmentation based on motion in the archerfish
- Svetlana Volotsky
- Ronen Segev
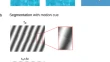
How to solve novel problems: the role of associative learning in problem-solving performance in wild great tits Parus major
- Laure Cauchard
- Pierre Bize
- Blandine Doligez
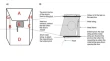
Social demonstration of colour preference improves the learning of associated demonstrated actions
- Na’ama Aljadeff
- Arnon Lotem
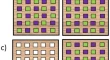
A preliminary analysis of the effect of individual differences on cognitive performance in young companion dogs
- Jordan G. Smith
- Sarah Krichbaum
- Jeffrey S. Katz
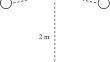
Journal updates
Morris animal foundation funding opportunity for equine behavior/welfare/cognition research.
Morris Animal Foundation is pleased to invest in equine behavior and welfare research, including cognitive research, through an annual call for research proposals .
Animal Cognition is now fully open access!
We are excited to announce that Animal Cognition has now become a fully open access (OA) journal as of January 2024. This means that we will only be publishing articles as Open Access meaning content will be and freely available to readers worldwide, enabling the widest possible dissemination and reuse.
In the News
Articles published in Animal Cognition are often featured in media outlets and blogs. Click here to see the most recent featured articles.
Follow Animal Cognition on Twitter
Stay up to date with the latest news: https://twitter.com/SpringerANCO
Journal information
- Biological Abstracts
- CAB Abstracts
- Current Contents/Agriculture, Biology & Environmental Sciences
- Google Scholar
- Japanese Science and Technology Agency (JST)
- Norwegian Register for Scientific Journals and Series
- OCLC WorldCat Discovery Service
- Science Citation Index Expanded (SCIE)
- TD Net Discovery Service
- UGC-CARE List (India)
- Zoological Record
Rights and permissions
Springer policies
© Springer-Verlag GmbH Germany, part of Springer Nature
- Find a journal
- Publish with us
- Track your research
- Reference Manager
- Simple TEXT file
People also looked at
Conceptual analysis article, critical problems for research in animal sheltering, a conceptual analysis.
- 1 Research Department, Austin Pets Alive!, Austin, TX, United States
- 2 Arkansas State University, Department of Political Science, Jonesboro, AR, United States
Animal shelter research has seen significant increases in participation over the past several decades from academic organizations, private organizations, public entities, and even corporations that aims to improve shelter programs, processes, operations, and outcomes for the various stakeholders/participants involved in a shelter system (animals, humans, the community, wildlife, and the environment). These efforts are scattered through a huge variety of different research areas that are challenging to define and scope for organizations seeking to start new lines of research inquiry. This work aims to enumerate some of the most critical outstanding problems for research in animal sheltering in a conceptual framework that is intended to help direct research conversations toward the research topics of highest impact (with the highest quality outcomes possible). To this end, we define seven (7) key areas for research: animal behavior, adoptions and special needs populations, medical conditions, disease transmission, community, ecology, and wellness (one health), operations, and public-private-academic-corporate collaboration. Within each of these areas, we review specific problems and highlight examples of successes in each area in the past several decades. We close with a discussion of some of the topics that were not detailed in this manuscript but, nonetheless, deserve some mention. Through this enumeration, we hope to spur conversation around innovative methodologies, technologies, and concepts in both research and practice in animal sheltering.
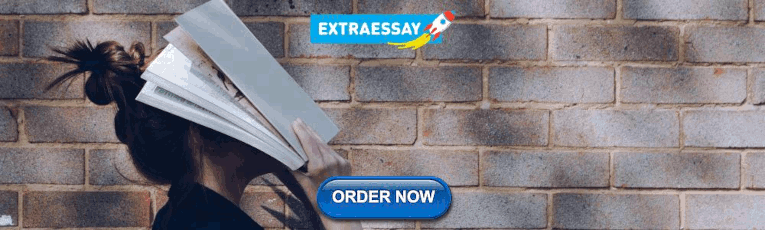
Introduction
Animal Sheltering in Western society, in some form, has existed since the mid-1800's (with the creation of both the Royal Society for the Prevention of Cruelty to Animals and the American Society for the Prevention of Cruelty to Animals in 1824 and 1866, respectively) and has been a constantly evolving field to both the benefit ( 1 , 2 ) and detriment ( 3 , 4 ), of its stakeholders: animals, pet owners, communities, and the organizations that tie these groups together. In the past several decades, a cultural shift has been occurring in which animal welfare ( 5 , 6 ) has received more attention, resources, and scrutiny than in the decades before. Success in sheltering is commonly measured by the Live Release Rate (hereafter LRR) that is obtained by dividing the total number of live animal outcomes (such as adoptions and transfers) by the total number of live animal intakes ( 7 ). Many cities have been able to increase their LRR and those of surrounding counties above 90 and even 95% ( 8 ), yet shelters still struggle with having adequate resources ( 9 ) and rural shelters may be more likely to struggle ( 10 ). The number of conceptual problems in sheltering is enormous, and as awareness of the needs of shelters continues to rise, more and more groups—academic, corporate, non-profit, and private—are looking for ways to contribute to the wider movement of animal welfare using their unique skills and talents. One difficulty for these potential partners is in understanding what the needs of shelters are and what high-value unsolved problems exist in the field.
Some of these are knowledge problems, others, implementation problems, and even more, systemic, cultural, and societal problems. Almost all require some manner of research to elucidate best practices and truths and differentiate them from traditions and myths. To aid interested parties in contributing to these areas of animal sheltering, we seek to enumerate and explain many of the critical problems for research in animal sheltering so that those organizations and interested parties might find a place to contribute. The key areas for future research were developed through a combination of both empirical and a priori traditions. The empirical approach used included input from animal sheltering professionals, including the responses of over 10 working groups representing more than 300 shelter professionals associated with Human Animal Support Services project to the question of what research needs were to advance animal sheltering. A priori observation and reflection of the researchers and reviews of the existing literature also helped to inform a lengthy list of research needs. These research needs were then thematically grouped in to the 7 key areas. Each of the areas was then evaluated on two factors: the degree of potential impact to animal sheltering and the difficulty in studying the problem.
Table 1 presents the 7 key areas identified. It also presents the impact potential for research in these areas by identifying the top-level impacts that advances in each topic area could have on the field of animal sheltering. While not intended to be all encompassing, this list captures the main topics generated by the authors and the consulted professionals.
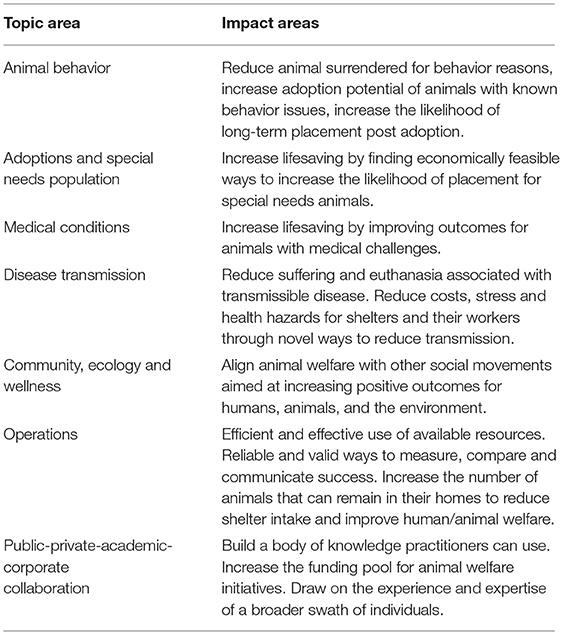
Table 1 . The 7 key problem areas identified in this work and their impact potential in the space of animal sheltering. While not intended to be all encompassing, this list captures the main topics generated by the authors and the consulted professionals.
Figure 1 provides an additional way of examining these topical areas. This figure provides examples of critical problems for research in animal sheltering and provides a way to compare and evaluate the areas in terms of the relative difficulty of studying the problems as well as the relative magnitude of the potential impact. In addition to evaluating these factors for the key research areas identified herein, this figures also shows a framework through which other researchers could evaluate the relative impacts and difficulty of other possible research topics.
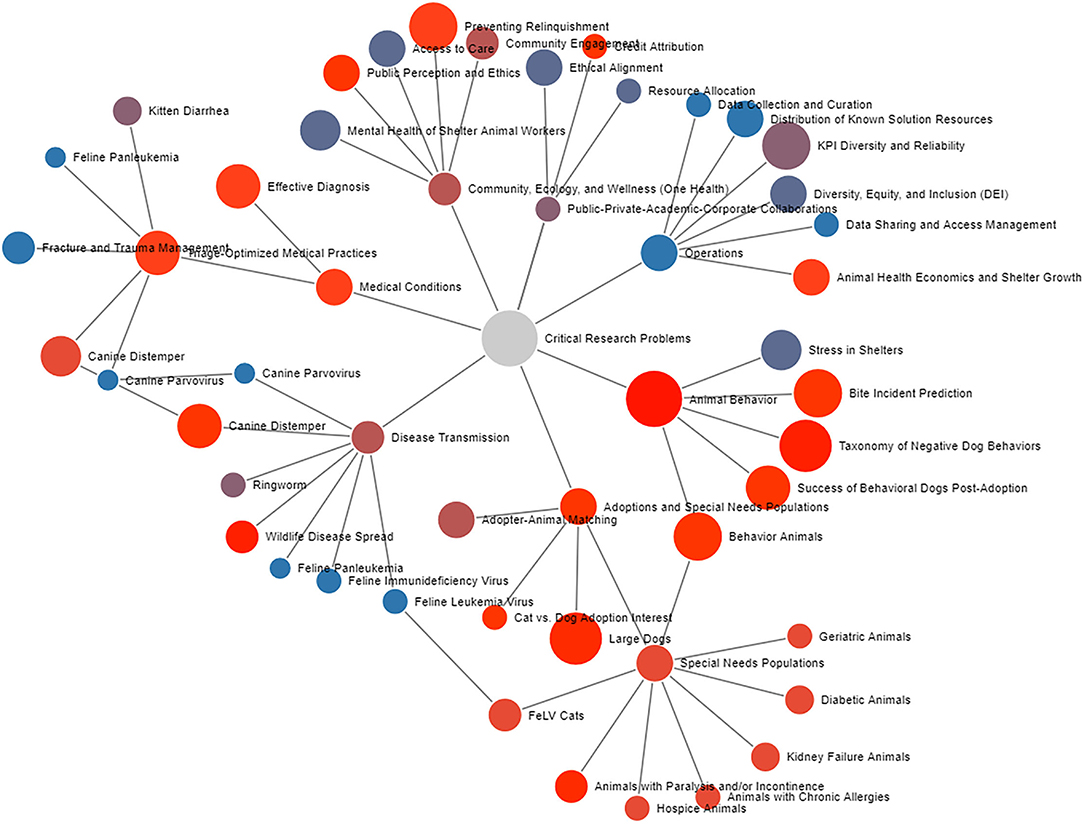
Figure 1 . A Force Directed Graph showing the various problems discussed in this article. The size of a node represents the relative impact a solution would have. The color represents the relative difficulty of studying the problem (with red being more difficult). Links relate the topics. An interactive version can be found here: https://codepen.io/kevroy314/pen/jONoXma . Click to isolate nodes and their neighbors. Drag to move around. Scroll to zoom.
Key Areas for Research
Animal behavior.
Animal behavior is one of the most challenging and complex topics in animal sheltering. Leaving aside controversies surrounding the ethics of adopting out animals with known behavior challenges or the ending of the life of an animal, whether for the protection of the public, retribution for an incident, quality of life, or any other justification related to behavioral issues, such as biting or inappropriate elimination, the practical need to better understand and modify animal behavior to improve the lives of animals and their caregivers to improve their chances of adoption and/or their probability of remaining in the home ( 11 ) is substantial. Here, we highlight 4 key areas in animal behavior that may have the biggest impact in a shelter setting and that may be underrepresented in the literature.
Efforts to form a typology of dog behaviors that may be problematic in the home, and, specifically, dog behavior that may be averse to a successful adoption and retention in a home ( 12 , 13 ) have been attempted in the past ( 14 – 16 ). Despite the interest in canine behavior in general rising sharply in the early 1990s and more recently ( 17 ), no consensus has been reached upon a singular behavioral classification and identification system that can be used to make decisions around best practices with dogs with histories of behavior problems or potential for behavior problems. Such a classification system should have the following properties [( 18 ) for a more detailed discussion of the difficulties surrounding some of these issues]:
• Objective measurability and reproducibility.
• Characterization of common temporal progressions.
• Understood correlations between related behaviors.
• Clinical relevance to predictability and intervention.
Somewhat recent attempts ( 18 , 19 ) at assessing the efficacy of behavioral evaluations have not been as promising as might be hoped given the 50+ year history of the field, and the impact of such a system, especially in establishing new interventions that can help these animals be successfully placed in homes, could be enormous given the extreme difficulty in achieving successful outcomes for dogs with behavior issues.
One key factor in negative animal behaviors, especially as pertaining to the adoptability of animals, is the stress they experience while in a shelter setting ( 20 – 22 ). Studies of animal stress date back to 1926 ( 23 ) with animals have often serving as a model for human stress ( 24 , 25 ). Practical tools are needed to assess the impact of shelter environmental improvements. Using Biomarkers to assess stress ( 26 ) across species ( 27 – 29 ) have shown significant success in recent years. Unfortunately, the practical measurement of such biomarkers in shelter settings remains unlikely due to resource and practical constraints. Non-invasive measures of stress are possible in many species [including thermographic ( 30 , 31 ), salivary ( 32 ), visual ( 33 ), and multimodal ( 34 ) systems], though their efficacy as an intervention target is unclear. A more thorough understanding of best practices around the reduction of stress for animals in shelters will allow for significant improvement in the quality of life of long-stay animals as well as the adoptability of animals that may show fewer behavioral issues once removed from a stressful environment, with some evidence showing changes in cortisol levels, a common biomarker for stress, with even a single night removed from the shelter environment in adult dogs ( 35 ).
A key element in the success of an animal with behavioral issues, post-adoption is not simply the cessation of negative behaviors, but also the match with an adopter who can maintain the environment necessary for permanent improvement in behavior as well as following up with those adopters to ensure continued success is achieved. Preparing adopters and proper matching is key given the frequency of post adoption behavioral issues among shelter animals ( 36 ). This problem comes down to two key sets of questions:
Given evidence suggesting choice of pet is often tied to factors like appearance more than behavioral considerations ( 37 ), how should shelters best match behavioral issues with potential adopters who can handle the maintenance surrounding those issues to reduce the chance of return ( 36 , 38 , 39 ) and adverse incidents such as bites or escape from a yard?
What risks (i.e., environment impact on biting) ( 40 ) exist in the home that might exacerbate issues surrounding behavior?
Finally, when it comes to animal behavior, especially canine behavior, one of the most critical incidents that can occur is a bite incident since these can result in serious injury to persons and potentially result in liability claims against the shelter ( 41 ). The previously mentioned issues all likely contribute to the probability of a bite incident occurring, but predictions of such events, even in aggregate across a city ( 42 ), are challenging at best. A successful bite prediction system would also pose ethical issues as individuals, shelters, and cities may choose to use such a system to decide which animal's lives should be preemptively ended, to avoid the potential risk and liability. It is critical, therefore, that the predictability of bite incidents increase at the same rate as our ability to reasonably intervene to prevent the incidents.
Adoptions and Special Needs Populations
The core problem with adoptions at shelters is always “how do we get as many animals out to good homes as quickly as possible?.” Of course, as with so many seemingly simple problems, the posing of the question in such a general manner means no obvious solutions present themselves. Properly reframing the question often begins to imply solutions. Preventing the surrender of animals to the shelter system is certainly a key component to assuring positive outcomes for animals and people alike. New programs, such as the Human Animal Support Services project, are focusing heavily on programs aimed at keeping animals out of the shelter altogether and in their original homes whenever possible. Further, this paradigm shift has the potential to profoundly impact positive outcomes for community cats, who may not be best served through adoption. Although adoption is not the only possible positive outcome for all animals that enter a shelter system, for many animals (and the humans who manage the systems of sheltering), it remains an important practical and ethical outcome. Here, we review 3 key areas in adoptions that remain complex and difficult despite extensive efforts in the sheltering community. For a more complete list of these challenge areas, see the American Pets Alive! Documentation on the topic (“American Pets Alive! Resources;” https://americanpetsalive.org/resources ) ( 43 ).
First and most critically, large dogs, often considered to be those weighing over approximately 35 pounds or 16 kilograms, consistently have more difficulty in being adopted ( 44 – 46 ). This can be due to factors such as the general public perception around larger breeds ( 47 , 48 ), city ordinances banning ownership of certain breeds ( 49 ), housing restrictions implemented at the facility level ( 50 ), or concerns around safety, behavior, and compatibility with other home residents ( 51 ). These issues are exacerbated by the difficulty in accurately identifying breed information in shelter animal populations ( 52 ). As a result of these complications around getting large breeds out of shelters, shelters often end up with a stagnant population of these animals that has less turnover than other, easier to adopt categories (puppies of any breed, for example). This can create a perception that the only populations present are these large breed animals. These factors result in many of these animals having long stays and, as mentioned in prior sections, increased stress and overall wellness difficulties that further worsen their adoptable potential. Moreover, animals in the shelter are less likely to behave the way they might otherwise in a home ( 53 ), further decreasing their chances for a positive outcome. A strategy around breaking this cycle and helping large dogs would alleviate significant amounts of trapped resources as site maintenance and housing can create substantial costs and reduce flexibility in serving other populations. The importance of providing an equal opportunity for these large breed dogs to stay in their home is one consideration beyond adoption in strategy design. For example, policies that disallow the use of size of a dog as criteria for access to housing (as discussed above) would help keep these animals out of the shelter system in the first place. Adequate access to resources to behavior training could be another community level intervention that could allow more of these animals to stay in their homes.
Other Special Populations
Beyond these major issues, there are numerous conditions of decreasing commonality that require increasingly complex adaptations of program and policy to accommodate. This article cannot enumerate all such conditions, but the following list, sorted roughly by difficulty, captures some of the most critical special needs populations that require specially trained homes to inhabit, making them more difficult to adopt out:
• Geriatric Animals.
• Animals with Chronic Allergies.
• Hospice Animals.
• Feline Leukemia Virus (FeLV) Cats.
• Kidney Failure Animals.
• Diabetic Animals.
• Behavior Animals.
• Animals with Paralysis and/or Incontinence.
Much of the care, maintenance, and treatment of these populations is well understood, but the problem of placing them in amenable homes is still a significant one. More research around interventions that can increase the likelihood of placement as well as the factors that impact the likelihood of special population placement may provide actionable insights [see ( 54 ) as an example in geriatric animals].
Finally, and significantly, a more thorough understanding of how to match adopters to animals ( 37 , 55 ), how to evaluate homes for safety and longevity of adoption outcomes ( 13 , 56 ), how to optimize placement of animals in homes ( 57 ), and what preferences exist when it comes to adoption practices around marketing, visitation, and engagement is desperately needed. This understanding will likely depend significantly on local cultural distinctions in populations ( 58 , 59 ) and is, therefore, difficult to examine systematically. More best practices around adoption matching and marketing would greatly simplify one of the most critical functions in animal shelters.
Unique Challenges of Cats
Another, potentially less obvious problem in sheltering is the difference in positive outcomes for cats vs. dogs. Best Friends, a national non-profit that provides the most comprehensive summary of annual shelter statistics reports that cats are still dying in shelters at a ratio of 2:1 when compared with dogs ( 60 ) despite approximately one-fourth of US households providing a home for cats ( 61 ). Many shelters consistently report difficulties in adopting out adult cats once they no longer have the appearance of a kitten ( 62 ). Further, shelter or municipal policies around the extermination of community cats ( 63 ) may also be a significant contributor to the numbers of cats not having successful outcomes in shelters.
Approaches to improving live outcomes for cats require shelters to explore ideas outside of the traditional intake to adoption framework. Some strategies that are specifically applicable to cats have been evaluated and shown to be effective such as trap-neuter-return and shelter-neuter-return, which could reduce the number of un-adoptable cats entering the shelter system ( 64 , 65 ), but more research into the social drivers and potential interventions for this issue are warranted. A development of the recognition of the ecology of community cats is an additional issue that is elaborated on in Section Operations.
Medical Conditions
In addition to its capacity as an adoption agency for unowned animals, animal shelters often perform a variety of medical services. These services depend on the location, resources, and risk tolerance each organization has, and it is often difficult for organizations to decide what to treat and what to not treat (whether euthanasia is then called for or not). One critical element of this that remains a challenge for all shelters is the effective, actionable diagnosis of disease [see, ( 66 )]. Many diseases have reliable tests (such as canine parvovirus) while others have a much more complicated history in the development of a reliable test [such as canine distemper, though many strongly claim RNA tests should be considered reliable; ( 67 – 69 )]. Cost is also a critical factor in shelter tests as even a relatively inexpensive (50 dollars) test in an outbreak scenario can be entirely impractical in a population of just a few dozen animals. Further research into low-cost testing is certainly needed for a wide variety of diseases.
Once the disease is identified, shelters often lack the resources for what would be considered “standard” care in a private practice. Some shelters opt to not offer reduced care and, instead, euthanize, while others choose to offer whatever care they can within their own ethical limitations of suffering and quality of life considerations. The need for significantly more research into evidence-based medical guidelines, and especially those that are specifically optimized for triage situations with limited resources and around medical conditions seen in shelters, is widely apparent. Some conditions, such as kitten diarrhea, may be somewhat understood in a general medical sense, but the treatments and time course do not scale appropriately for the model of a medium to large shelter.
Although many diseases could use additional scrutiny for the purposes outlined above, the following are of particular interest due to the costs, in either lives or resources, associated with typical treatment or management (T; indicates specific transmissible disease relevant to Section Disease Transmission):
• (T) Canine parvovirus ( 70 , 71 ).
• (T) Feline panleukemia ( 72 ).
• (T) Canine distemper ( 73 ).
• (T) Feline leukemia virus (FeLV) ( 74 ).
• (T) Feline immunodeficiency virus ( 75 – 78 ).
• Kitten diarrhea ( 79 ).
• Fracture and trauma management.
Disease Transmission
More so than the treatment of disease, the prevention of disease spread in the shelter environment is one of the most challenging, concretely measurable in the form of infection rates, yet ambiguous (difficult to diagnose in source) tasks a shelter may face. Shelters are examples of anthropogenic biological instability due to the housing of transient, displaced mixed-species of animals that may not have prior veterinary care or have been scavenging during times of homelessness ( 80 ). The disease transmission in shelters is further complicated by situation of overcrowding, poor levels of hygiene, and housing of multiple species which can add significant sources of stress for the animals and create a perfect environment for pathogen emergence and transmission ( 80 ). This transmission can quickly lead to a crisis in the shelter ( 81 ). Shelters that treat infectious disease like the canine parvovirus establish isolation areas in which only that disease is treated, but little is known about the ease with which these diseases spread under different quarantine practices.
Although there are many interesting diseases that are typically seen in shelters, some (such as those listed in Section Medical Conditions) are considered more impactful/deadly than others and, therefore, would make excellent targets for more detailed studies of disease spread.
While it is not officially recommended as a best practice ( 82 ), when shelters experience disease outbreaks, some may opt to depopulate, i.e., end the lives of their entire population, ( 83 ) rather than have it persist through many generations of animals flowing through the system. Better understanding of how to stem these outbreaks rapidly, efficiently, safely, in a resource-efficient manner, and given the constraints of a shelter environment (space, staffing, facility design, and the need to maintain normal operations) will allow shelters to avoid mass culling and take an approach that increases lifesaving with more confidence.
Community, Ecology, and Wellness (One Health)
Beyond the scope of the basic operations of a shelter in managing the conditions of individual animals and placing them in appropriate homes, shelters also serve a critical role in the community as providers of services that can enhance public perception and wellbeing ( 84 ). This collaboration requires an engaged community that recognizes the importance of animal welfare in the health and wellness of the larger, shared space. Best practices around establishing this type of engagement are not well identified in the existing body of knowledge. This is further confounded by variation in the distribution of resources and community attitudes in different geographic areas.
As animal shelters continue to evolve in response to societal shifts in attitudes toward animals, the focus of operations are changing from centering on adoptions to centering on the prevention of surrender of animals to the shelter in the first place [see ( 11 ) for a review]. This has already been discussed as it relates to community cats and behavior/health but there are many other human-centered reasons that animals are surrendered to shelters such as guardian health problems, housing insecurity, domestic violence, and many others ( 85 ). Our understanding of how human welfare intersects with animal welfare has the potential to have a dramatic impact on the way shelters operate in their communities. Some communities have hotlines, spay and neuter programs, and other medical/behavioral services that can potentially contribute to this issue, but the efficacy of such systems and the gaps they leave are not well understood. More significant study of the needs of local populations as they relate to shelter success is needed.
Local populations also differ in their perception and support of shelter policies, ethics, and the local system of laws that are intertwined with these efforts. No unified system of ethics is established in animal sheltering, and communities often do not understand the nuances of practices in shelters (especially regarding resource allocations and euthanasia practices). This makes galvanizing community support difficult, even in communities that have achieved remarkably high live release rates. Public perception, messaging, and ethical alignment will undoubtedly continue to be an ever-evolving socio-cultural landscape that is sorely in need of attention.
The mental health of volunteers, staff, and veterinarians ( 86 ) in animal shelters also requires much more attention than it often receives. Individuals that participate in euthanasia are reported to have higher work stress and lower job satisfaction than their counterparts ( 87 ). Suicide rates are significantly higher in the field of animal welfare than other high-stress fields ( 88 , 89 ), and more understanding and support is needing to help those working in these areas receive the help they need to continue to serve the community in a sustainable, healthy manner.
Access to Care
Access to veterinary care is emerging as a critical issue in animal welfare. Access to care is an aspect of the One Health approach to considering animal welfare due to the zoonotic potential of various diseases that can find reservoir in companion animals ( 90 ). In addition to being a risk to public health, lack of access to veterinary care can result in surrender of animals to shelters, stress to the caregiver/family ( 91 ) as well as stress to veterinarians who must counsel caregivers who cannot afford the recommended care ( 92 ). Shelters feel the impact of this as downstream recipients of animals when owners surrender due to an inability to access needed care. This can both drive surrender to shelters and result in a greater financial burden for shelters to meet medical needs that may be complicated by a historic lack of access to preventative or early intervention care. Further, shelters themselves compete in the market to employ veterinary professionals and support staff that may be further complicated by a shortage of veterinarians ( 93 , 94 ).
Access to care can be seen as a problem with multiple causes from cost to lack of transportation to the unequal distribution of veterinary resources across the landscape. Cost was identified as the most common barrier to accessing veterinary care in the Access to Veterinary Care Coalition report on this issue ( 91 ). In the past decade, costs for veterinary care have been outpacing increases in human health care ( 95 ). The average American spends 47% more on equivalent veterinary care today than a decade ago ( 96 ). The functional impact of this increasing cost is that fewer people are seeking care for their pets ( 97 ) resulting in what is considered the greatest current threat to companion animal welfare in the US ( 91 ). More research that identifies efficient, effective, and sustainable solutions to the cost of veterinary care will be key for animal shelters.
Key research questions in access to care can come down to three key areas:
Advances in areas like incremental care or spectrum of care, which are not equivalent but present different perspectives on the issue of cost-benefit analyses in treatment protocols, could reduce costs and prevent shelter surrenders but could also help shelters mitigate the increasing expense of medical treatment for animals in their care.
A deeper understanding of the number of animals surrendered for medical reasons, the types of these conditions and potential treatment routes pre-surrender would also add valuable knowledge to the animal sheltering and animal welfare communities.
Development of community-based solutions that focus on disease prevention when the cost is likely lower than when a disease process is more advanced. This includes the prevention of infectious disease transmission in the community and the development of effective education around other preventable conditions by pet guardians.
Ecology/Environment
The study of the ecology surrounding community cats has received significant attention over the past several years ( 63 , 64 , 98 – 102 ), and debates are likely to continue in this area to determine the most effective ways to ensure the health and safety of community cats and the organisms with which they interact. Additionally, ecological perspectives on the interaction between stray and roaming animals in general and the community are also of interest, but often only actively studied due to concerns over infectious disease spread such as the Rabies virus. Finally, the interaction of wildlife systems with domesticated animals may be of some interest both due to the spread of infectious disease and the more complex interactions these two animal groups may have with one another.
As animal welfare incorporates a One-health approach, further research that identifies strategies to reduce the environmental impact of shelter operations cannot be ignored. Effective ways of cleaning outdoor kennels without contributing to contaminate run-off, ecological disposal of animal waste and the evaluation of how large-scale animal transport can contribute to environmental degradation are just a few examples of the interaction of sheltering and the environment that are open for additional exploration.
In addition to the study of animal-centric, adoption-centric, and community-centric aspects of sheltering, the study of the operations that contribute to the ability of shelters to continually adapt, and advance is of critical importance if we are to have systems robust to disaster and capable of implementing our values and ethics on a global scale. Although blueprints do exist that can guide communities in setting up new shelters and enhancing existing shelters, significant problems remain in the space beyond the distribution of known solution resources. Here, we discuss 4 key operations problem areas with varying levels of complexity.
Data Problems
Shelters need to collect data to know how they are serving their animals, adopters, volunteers, staff, and community, and how to improve operations in all areas of the shelter. While the industry recognizes the need for quality data, significant barriers have been identified such as a lack of training and resources [( 103 ), additionally, see the Associate for Veterinary Informatics (AVI) for additional information on this topic; https://avinformatics.org/ ]. Solutions such as ShelterLuv, Chameleon, and PetPoint for database management go a long way to improving situations for shelters, but the ability to flexibly collect and curate all manner of useful data (including electronic medical records, location-based event history, and other meta-data about entities that comprise shelters) remains an open problem. It is also essential that the prioritization and understanding of the critical importance of data is shared by line staff as well as senior management. When line staff fail to understand the importance of complete data collection this action can be de-prioritized in fast paced shelter environment.
Beyond this, shelters need methods of protecting themselves in the sharing of data with the public, academic institutions, and each other. The public, which support shelters through taxes or donations, show widespread support, for example, for programs that reduce levels of shelter euthanasia shelters ( 104 ). The best practices around of performing data sharing and managing data access for shelters have yet to be established (though some progress has been made in recent months at the Municipal Shelter level). Over time, there have been attempts to create a single authoritative collection of sheltering data but to date, none have achieved high success. The current initiative that has achieved the most progress is Shelters Animals Count (SAC). SAC is a national database that relies on the voluntary participation by shelters and animal rescues to upload monthly sheltering summary statistics. Unfortunately, there is still relatively poor participation. For example, in 2020 there was participation by only 422 municipal shelters, 359 private shelters and 516 rescues ( 105 ). This can be contrasted with a 2014 estimate by the Humane Society of the United States of 3,500 municipal and non-profit shelters and over 10,000 rescue organizations ( 106 ). Despite the move toward increasing transparency in government, only a small handful of states and municipalities require reporting to their state and local governments, with even fewer providing enough clarity as to what should be reported for such reporting to be of use to the wider sheltering community. The result of this paucity and irregularity of data provides a significant challenge to researchers and policymakers in understanding what is happening across the nation regarding sheltering, though the contributions of states in which reporting is mandated effectively have provided a valuable starting point for these efforts.
KPI Problems
Once data is collected, linking that data down to trackable KPIs (Key Performance Indicators) that are useful to shelters in improving outcomes for animals is a challenge in and of itself. The standardization of KPIs and their strict definitions has suffered from some of the disagreement and difficulties surrounding data collection. The most marked attempt to create unified KPIs occurred in 2004 resulting in the Asilomar Accords. The Live Release Rate, and methods of fairly but consistently calculating it materialized as a critical outcome of the Accords ( 107 ). This measure has never been without controversy and is limited, in part, by the wide variance in the various ways in which animal shelters operate in their community and what their priority services are ( 108 ). As the operation of shelters have changed, with more innovative programs designed to prevent animals from ever entering the shelter system appearing, advancements in medical and behavioral interventions, and the geographically biased nature of animal population distributions ( 109 ), the use of a single KPI will likely remain a source of both conflict and difficulty for many shelters. A more diverse set of KPIs will allow for shelters to perform more nuanced comparisons of their successes and failures that will enable better sharing of solutions and resources. What this list of KPIs should entail remains an open problem [see ( 110 )].
Growth Problems
Finally, as some shelters begin to stabilize the animal welfare situation in their cities, adapting to the varying degrees and paces of growth in various organizations to ensure resources are being properly utilized to the benefit of animals and the community is a challenge, to say the least. The field of Health Economics in humans has a rich history ( 111 ), and a similar field in Animal Health Economics ( 112 , 113 ) will likely need to be expanded beyond its traditional focus on production animals so that organizations are not put in a position to blindly guess at the proper allocations or resources toward different intervention programs (such as a canine parvovirus treatment program, FeLV treatment program, behavior program, or kitten foster program).
One particularly challenging program area for shelters to understand in the context of growth, integration, and resource allocation is the management of foster programs. Foster programs have been fantastically successful as a method of expanding the effective capacity of shelters, increasing live outcomes ( 114 ), enhancing community engagement, increasing quality of life of animals in care ( 35 ), and providing special assistance for more difficult to adopt populations. However, a thorough understanding of how to best engage, utilize, and grow foster programs is lacking.
Diversity Equity and Inclusion
Researchers have evidenced that the oppression of non-human animals, disabled humans, and people of color are deeply interconnected ( 115 ). If animal shelters are to continue to function as key members of diverse communities it is essential that they pay increasing attention to issues of diversity, equity, and inclusion in their operations both internal and external. While the community of research in this space has assembled a basic understanding of some inequities that currently exist, many others have yet to be explored in a thorough way. For example, we know that African Americans are underrepresented in leadership positions ( 116 ). The homogeneity of animal shelters is not confined to the workforce alone. Two large survey-based studies found similar results in evaluating the demographics of animal welfare volunteers concluding that most volunteers were White females in the middle to upper middle class ( 117 , 118 ). Questions of why this lack of diversity persists and what successful strategies could be used to improve conditions would be of benefit as representation of communities within organizations that serve them allow those organizations to supply the appropriate services to maximize the community benefit and foster a highly participatory, engaged, fair, enthusiastic, and ethical social system.
Beyond direct engagement with shelters as volunteers or employees, there are fecund areas for research in the provisioning of shelter operations. As increases in public-private partnerships place more animal shelters in the business of providing animal control operations, the enforcement of ordinances becomes a key issue in balancing public demand for action and the ethics and priorities of animal welfare. A recently published commentary on the subject argues that there is inherent bias in the design and enforcement of public policy around animal welfare and urges a shift from enforcement to resource provision ( 119 ). Evaluating policies and enforcement and implementation of these policies and whether biases are leading to unequal burden are not well understood though it is difficult to not draw comparisons to the arena of policing and the long, complicated relationship between marginalized communities and law enforcement personnel. Additional challenges persist in understanding potential inequities between the surrender of animals and the adoption of animals and whether these differences enforce equity imbalances or are based on existing biases and structural inequities ( 120 ).
Public-Private-Academic-Corporate Collaborations
A less visible and virtually unstudied problem in animal sheltering is the ability for organizational entities of different types and with different incentives to collaborate to the benefit of animals, their owners, the community, and each other. The social network analysis of Reese and Ye ( 121 ) is a prime example of the complex collaborative relationships that can emerge between organizations to advance lifesaving in a community. Many questions in this space exist around the best ways for these organizations to interact (i.e., what roles are best served by what organizations, what incentives are best to ensure ethical treatment of all parties, and what restrictions should be put on various types of interactions). Legal restrictions around the use of shelter animals in research may be a barrier that exists to research collaborations between shelters and academic institutions. Dialogue, consensus, and potential legislative change may be needed between animal shelters, the veterinary community, and academia to address the negative consequences of legislation originally intended to protect animals from harm.
Public-private partnerships in other areas of medicine have become increasingly common and valuable ( 122 ), and corporate sponsorship of shelters has become increasingly common. Public-private shelter partnerships are also on the rise with some proposing this structure as the new standard in the field ( 123 ). Academic collaboration with animal shelters, where academic institutions take advantage of the wealth of available subjects and data in shelters, is still a relatively new concept. Though many potential pitfalls exist in these collaborations (including issues with credit attribution, resource allocation, and ethical alignment), the potential to accelerate the state of the art in animal sheltering via these collaborations is huge thanks to the varied strengths of each organizational type.
The seven key areas for research in animal sheltering outlined above are not the only areas that might be of interest to shelter practitioners and their partners. Some additional areas of interest were not mentioned specifically in this manuscript due to the well-researched nature of the topics, the lack of clear definition in the space, and/or their relative distance from the typical practices of an animal shelter. These areas, nonetheless, merit some mention due to their importance to the area of animal welfare research at large and potential intersection with some shelter practices (depending on specific shelter policy, philosophy, and operations).
A variety of interventions have been proposed that might address some of the problems mentioned in this manuscript. On the behavior side, playgroup services have been proposed that may aid in social development and lead to more positive behavioral outcomes for dogs ( 124 ). Moreover, foster programs that take advantage of these and other medical or behavioral services to accelerate positive outcomes for animals deserve significant attention ( 35 , 125 ). Foster programs can serve as an additional reservoir for animal populations, increase community engagement in the shelter system, and encourage positive outcomes for animals in the foster system through positive environmental enrichment in homes. In situations where foster homes are not available, additional environmental enrichment to achieve similar aims may be found through clever building and facility design at the shelter site ( 126 , 127 ). Finally, a variety of programmatic and procedural interventions around lost and found animals, self-service rehoming, and intake-to-placement optimization, and field services optimization that aim to prevent animals from entering the physical shelter facility can serve as systems optimizations that improve outcomes for all parties; though, more research is needed in these areas to examine their efficacy. Each of these intervention areas, and other innovations in sheltering, deserve significantly more attention than can be afforded in this outline, and future work should attempt to address them more directly.
In addition to a variety of community and ecology problems and interventions, ethical problems in the industry of animal sheltering are not specifically addressed in this work as these are not research topics per se , but more in the realm of philosophy. Future work should examine ethical questions surrounding the topics outlined in this manuscript and other sociological research questions related to the ethics of animal shelter practices.
In this work, we present a conceptual organization of topics for research in Animal Sheltering. These topics vary significantly in difficulty and impact but represent a large swath of needed scientific contributions in the literature. Many of these areas are being actively worked upon by various research institutions (i.e., significant work in animal diseases has occurred), but some have received little attention yet (i.e., operations research). Moreover, some of these areas are being examined, but due to resource and/or methodological constraints, progress is slow. By enumerating these problems, the community of researchers attempting to improve the function of shelters for animals, staff, volunteers, and the community can more carefully and wholistically consider the breadth of applicability of their ideas and investigations and hopefully, more productively contribute to the literature.
Author Contributions
The above document was drafted and originated by KH with review, added sections on community cats, DEI, and Access to Care and most edits provided by SN. Figures were generated by KH. Both authors contributed to the article and approved the submitted version.
Funding for publication was provided by the American Pets Alive! Organization, while funding for individual authors and the creation of the work herein was provided by their respective institutions or the authors themselves.
Conflict of Interest
The authors declare that the research was conducted in the absence of any commercial or financial relationships that could be construed as a potential conflict of interest.
Publisher's Note
All claims expressed in this article are solely those of the authors and do not necessarily represent those of their affiliated organizations, or those of the publisher, the editors and the reviewers. Any product that may be evaluated in this article, or claim that may be made by its manufacturer, is not guaranteed or endorsed by the publisher.
Acknowledgments
The authors would like to thank the Human Animal Support Services organization for their support and encouragement. Additionally, we would like to especially thank Dr. Nipuni Ratnayaka for her contributions to and review of some of the ideas in this document, Dr. Elizabeth Davis for her support in the development of these ideas, Rory Adams for his thoughtful comments around the topics in this document, Steve Porter for his commentary around shelter operations, Dr. Ellen Jefferson for her leadership and support of this work, and all of the staff, volunteers, and communities that continue to advance the state of the art in animal sheltering around the world. This work originally appeared in large part in a blog post from November of 2019. The original blog post can be found at: https://medium.com/@amparesearch/critical-problems-for-research-in-animal-sheltering-404395127b35 .
1. Bornstein D. How Smart Animal Shelters Aim for “Zero Kill” (2015). Availabe online at: https://opinionator.blogs.nytimes.com/2015/12/22/how-smart-animal-shelters-aim-for-zero-kill/ (accessed December 22, 2021).
2. Parlapiano A. Why Euthanasia Rates at Animal Shelters Have Plummeted. (2019). Available online at: https://www.nytimes.com/2019/09/03/upshot/why-euthanasia-rates-at-animal-shelters-have-plummeted.html (accessed September 3, 2021).
Google Scholar
3. Roberts SE, Jaremin B, Lloyd K. High-risk occupations for suicide. Psychol Med. (2013) 43:1231–40. doi: 10.1017/S0033291712002024
PubMed Abstract | CrossRef Full Text | Google Scholar
4. Auerbach K. The Transparent Shelter, Building Trust with Your Community. (2019). Available online at: https://www.maddiesfund.org/the-transparent-shelter-building-trust-with-your-community.htm?p=39694e56-a476-47af-eeac-28049e2f4b2e (accessed February 10, 2022).
5. Hewson CJ. What is animal welfare? common definitions and their practical consequences. Can Vet J . (2003) 44:496–9. Available online at: https://www.ncbi.nlm.nih.gov/pmc/articles/PMC340178/
PubMed Abstract | Google Scholar
6. Glassey S. Did Harvey Learn from katrina? initial observations of the response to companion animals during hurricane harvey. Animals. (2018) 8:47. doi: 10.3390/ani8040047
7. Weiss E, Patronek G, Slater M, Garrison L, Medicus K. Community partnering as a tool for improving live release rate in animal shelters in the United States. J Appl Anim Welf Sci. (2013) 16:221–38. doi: 10.1080/10888705.2013.803816
8. National Dashboard. Best Friends. (2021). Available online at: https://bestfriends.org/no-kill-2025/animal-shelter-statistics (accessed March 9, 2022).
9. Kresnye KC, Shih PC. We have a volunteer coordinator who is unfortunately a volunteer. In: Proceedings of the Fifth International Conference on Animal-Computer Interaction . New York, NY: ACM (2018). p. 1–6. doi: 10.1145/3295598.3295612
CrossRef Full Text | Google Scholar
10. Daly N. Why Animal Shelters Are Facing a New Crisis. National Geographic (2021). Available online at: https://www.nationalgeographic.com/animals/article/why-animal-shelters-are-facing-a-new-crisis . Published (accessed February 10, 2022).
11. Protopopova A, Gunter L. Adoption and relinquishment interventions at the animal shelter: a review. Anim Welf. (2017) 26:35–48. doi: 10.7120/09627286.26.1.035
12. Patronek GJ, Glickman LT, Beck AM, McCabe GP, Ecker C. Risk factors for relinquishment of cats to an animal shelter. J Am Vet Med Assoc . (1996) 209:582–8.
13. Salman MD, Hutchison J, Ruch-Gallie R, et al. Behavioral reasons for relinquishment of dogs and cats to 12 shelters. J Appl Anim Welf Sci. (2000) 3:93–106. doi: 10.1207/S15327604JAWS0302_2
14. Beaver BV. Clinical classification of canine aggression. Appl Anim Ethol. (1983) 10:35–43. doi: 10.1016/0304-3762(83)90110-4
15. Wright JC, Nesselrote MS. Classification of behavior problems in dogs: distributions of age, breed, sex and reproductive status. Appl Anim Behav Sci. (1987) 19:169–78. doi: 10.1016/0168-1591(87)90213-9
16. Landsberg GM, Hunthausen WL, Ackerman LJ. Behavior Problems of the Dog Cat3: Behavior Problems of the Dog Cat. (2012). Available online at: https://books.google.com/books?id=eYbVBMkYvSAC&pgis=1 (accessed March 9, 2022).
17. Dowling-Guyer S, Marder A, D'Arpino S. Behavioral traits detected in shelter dogs by a behavior evaluation. Appl Anim Behav Sci. (2011) 130:107–14. doi: 10.1016/j.applanim.2010.12.004
18. Patronek GJ, Bradley J. No better than flipping a coin: reconsidering canine behavior evaluations in animal shelters. J Vet Behav. (2016) 15:66–77. doi: 10.1016/j.jveb.2016.08.001
19. Mornement KM, Coleman GJ, Toukhsati S, Bennett PC. A review of behavioral assessment protocols used by australian animal shelters to determine the adoption suitability of dogs. J Appl Anim Welf Sci. (2010) 13:314–29. doi: 10.1080/10888705.2010.483856
20. McCobb EC, Patronek GJ, Marder A, Dinnage JD, Stone MS. Assessment of stress levels among cats in four animal shelters. J Am Vet Med Assoc. (2005) 226:548–55. doi: 10.2460/javma.2005.226.548
21. Gourkow N, Fraser D. The effect of housing and handling practices on the welfare, behaviour and selection of domestic cats (Felis sylvestris catus) by adopters in an animal shelter. Anim Welf. (2006) 15:371–7.
22. Taylor KD, Mills DS. The effect of the kennel environment on canine welfare: a critical review of experimental studies. Anim Welf . (2007) 16:435–47.
23. Cannon W. Physiological regulation of normal states: some tentative postulates concerning biological homeostatics in the scientific jubilee of charles richet. Science. (1926) 64:91–3. doi: 10.1126/science.64.1644.9
24. Campos AC, Fogaca M V, Aguiar DC, Guimaraes FS. Animal models of anxiety disorders and stress. Rev Bras Psiquiatr. (2013) 35:S101–11. doi: 10.1590/1516-4446-2013-1139
25. Patchev VK, Patchev AV. Experimental models of stress. Dialogues Clin Neurosci. (2006) 8:417–32. doi: 10.31887/DCNS.2006.8.4/vpatchev
26. Nater UM, Skoluda N, Strahler J. Biomarkers of stress in behavioural medicine. Curr Opin Psychiatry. (2013) 26:440–5. doi: 10.1097/YCO.0b013e328363b4ed
27. Campbell SA, Hughes HC, Griffin HE, Landi MS, Mallon FM. Some effects of limited exercise on purpose-bred beagles. Am J Vet Res . (1988) 49:1298–301.
28. Contarteze RVL, Manchado FDB, Gobatto CA, De Mello MAR. Stress biomarkers in rats submitted to swimming and treadmill running exercises. Comp Biochem Physiol Part A Mol Integr Physiol. (2008) 151:415–22. doi: 10.1016/j.cbpa.2007.03.005
29. Langgartner D, Füchsl AM, Kaiser LM, Meier T, Foertsch S, Buske C, et al. Biomarkers for classification and class prediction of stress in a murine model of chronic subordination stress. PLoS ONE. (2018) 13:e0202471. doi: 10.1371/journal.pone.0202471
30. Stewart M, Webster JR, Verkerk GA, Schaefer AL, Colyn JJ, Stafford KJ. Non-invasive measurement of stress in dairy cows using infrared thermography. Physiol Behav. (2007) 92:520–5. doi: 10.1016/j.physbeh.2007.04.034
31. Cannas S, Palestrini C, Canali E, Cozzi B, Ferri N, Heinzl E, et al. Thermography as a non-invasive measure of stress and fear of humans in sheep. Animals. (2018) 8:146. doi: 10.3390/ani8090146
32. Nemeth M, Pschernig E, Wallner B, Millesi E. Non-invasive cortisol measurements as indicators of physiological stress responses in guinea pigs. PeerJ. (2016) 4:e1590. doi: 10.7717/peerj.1590
33. Hong W, Kennedy A, Burgos-Artizzu XP, Zelikowsky M, Navonne SG, Perona P, et al. Automated measurement of mouse social behaviors using depth sensing, video tracking, and machine learning. Proc Natl Acad Sci. (2015) 112:E5351–60. doi: 10.1073/pnas.1515982112
34. Alberdi A, Aztiria A, Basarab A. Towards an automatic early stress recognition system for office environments based on multimodal measurements: a review. J Biomed Inform. (2016) 59:49–75. doi: 10.1016/j.jbi.2015.11.007
35. Gunter LM, Feuerbacher EN, Gilchrist RJ, Wynne CDL. Evaluating the effects of a temporary fostering program on shelter dog welfare. PeerJ. (2019) 7:e6620. doi: 10.7717/peerj.6620
36. Vitulová S, Voslárová E, Večerek V, Bedánová I. Behaviour of dogs adopted from an animal shelter. Acta Vet Brno. (2018) 87:155–63. doi: 10.2754/avb201887020155
37. Weiss E, Miller K, Mohan-Gibbons H, Vela C. Why did you choose this pet? : adopters and pet selection preferences in five animal shelters in the United States. Animals. (2012) 2:144–59. doi: 10.3390/ani2020144
38. Cafazzo S, Maragliano L, Bonanni R, Scholl F, Guarducci M, Scarcella R, et al. Behavioural and physiological indicators of shelter dogs' welfare: Reflections on the no-kill policy on free-ranging dogs in Italy revisited on the basis of 15years of implementation. Physiol Behav. (2014) 133:223–9. doi: 10.1016/j.physbeh.2014.05.046
39. Gates M, Zito S, Thomas J, Dale A. Post-adoption problem behaviours in adolescent and adult dogs rehomed through a New Zealand animal shelter. Animals. (2018) 8:93. doi: 10.3390/ani8060093
40. Messam LLM, Kass PH, Chomel BB, Hart LA. The human–canine environment: a risk factor for non-play bites? Vet J. (2008) 177:205–15. doi: 10.1016/j.tvjl.2007.08.020
41. Bernstein M. Preventing shelter liability with adoption contracts. Anim Law . (2016) 22:16. Available online at: https://link.gale.com/apps/doc/A530407550/AONE?u=googlescholar&sid=bookmark-AONE&xid=a3c322c7
42. Zapata J. Loose Dogs in Dallas: Strategic Recommendations to Improve Public Safety Animal Welfare in Dallas. Dallas, TX (2016). Available online at: https://dallascityhall.com/government/CouncilMeeting~Documents/loose-dogs-in-dallas-strategic-recommendations-to-improve-public-safety-and-animal-welfare_combined_083016.pdf
43. American Pets Alive! Resources. American Pets Alive! . (2022). Available online at: https://americanpetsalive.org/resources (accessed February 10, 2022).
44. DeLeeuw J. Animal Shelter Dogs: Factors Predicting Adoption Versus Euthanasia. (2008). Available online at: https://soar.wichita.edu/handle/10057/3647 (accessed March 9, 2022).
45. Diesel G, Pfeiffer DU, Brodbelt D. Factors affecting the success of rehoming dogs in the UK during 2005. Prev Vet Med. (2008) 84:228–41. doi: 10.1016/j.prevetmed.2007.12.004
46. Protopopova A, Gilmour AJ, Weiss RH, Shen JY, Wynne CDL. The effects of social training and other factors on adoption success of shelter dogs. Appl Anim Behav Sci. (2012) 142:61–8. doi: 10.1016/j.applanim.2012.09.009
47. Blecker D, Hiebert N, Kuhne F. Preliminary study of the impact of different dog features on humans in public. J Vet Behav. (2013) 8:170–4. doi: 10.1016/j.jveb.2012.06.005
48. Gunter LM, Barber RT, Wynne CDL. What's in a name? effect of breed perceptions & labeling on attractiveness, adoptions & length of stay for pit-bull-type dogs. PLoS ONE. (2016) 11:e0146857. doi: 10.1371/journal.pone.0146857
49. Nolen S. The dangerous dog debate: Breed bans are popular, but do they make the public safer? J Am Vet Med Assoc . (2017). Available online at: https://www.avma.org/javma-news/2017-11-15/dangerous-dog-debate
50. Graham TM, Rock MJ. The spillover effect of a flood on pets and their people: implications for rental housing. J Appl Anim Welf Sci. (2019) 22:229–39. doi: 10.1080/10888705.2018.1476863
51. Bir C, Widmar NO, Croney C. Public Perceptions of Dog Acquisitions: Sources, Rationales Expenditures. (2016). Available online at: https://www.purdue.edu/vet/CAWS/files/documents/20160602-public-perceptions-of-dog-acquisition.pdf (accessed March 9, 2022).
52. Gunter LM, Barber RT, Wynne CDL. A canine identity crisis: genetic breed heritage testing of shelter dogs. PLoS ONE. (2018) 13:e0202633. doi: 10.1371/journal.pone.0202633
53. Protopopova A. Effects of sheltering on physiology, immune function, behavior, and the welfare of dogs. Physiol Behav. (2016) 159:95–103. doi: 10.1016/j.physbeh.2016.03.020
54. Hawes S, Kerrigan J, Morris K. Factors informing outcomes for older cats and dogs in animal shelters. Anim an open access J from MDPI . (2018) 8:36. doi: 10.3390/ani8030036
55. Curb LA, Abramson CI, Grice JW, Kennison SM. The relationship between personality match and pet satisfaction among dog owners. Anthrozoos. (2013) 26:395–404. doi: 10.2752/175303713X13697429463673
56. Patronek GJ, Glickman LT, Beck AM, McCabe GP, Ecker C. Risk factors for relinquishment of dogs to an animal shelter. J Am Vet Med Assoc . (1996) 209:572–81.
57. O'Connor R, Coe JB, Niel L, Jones-Bitton A. Exploratory study of adopters' concerns prior to acquiring dogs or cats from animal shelters. Soc Anim. (2017) 25:362–83. doi: 10.1163/15685306-12341451
58. Brown S-E. Ethnic Variations in pet attachment among students at an American school of veterinary medicine. Soc Anim. (2002) 10:249–66. doi: 10.1163/156853002320770065
59. Herzog HA. Biology, culture, and the origins of pet-keeping. Anim Behav Cogn. (2014) 1:296. doi: 10.12966/abc.08.06.2014
60. Rayvid E, Patterson K. Best Friends Animal Society Releases Data Showing Most Significant Annual Decrease in Animals Killed in Shelters. bestfriends.org. (2021). Available online at: https://bestfriendsorg/about/media/best-friends-animal-society-releases-data-showing-most-significant-annual-decrease
61. AVMA. U.S. Pet Ownership Statistics. AVMAorg (2018). Available online at: https://wwwavmaorg/resources-tools/reports-statistics/us-pet-ownership-statistics
62. Zito S, Paterson M, Vankan D, Morton J, Bennett P, Phillips C. Determinants of cat choice and outcomes for adult cats and kittens adopted from an Australian animal shelter. Animals. (2015) 5:276–314. doi: 10.3390/ani5020276
63. Wolf PJ, Hamilton F. Managing free-roaming cats in U.S. cities: an object lesson in public policy and citizen action. J Urban Aff . (2020) 44:221–42. doi: 10.1080/07352166.2020.1742577
64. Levy JK, Isaza NM, Scott KC. Effect of high-impact targeted trap-neuter-return and adoption of community cats on cat intake to a shelter. Vet J. (2014) 201:269–74. doi: 10.1016/j.tvjl.2014.05.001
65. Johnson KL, Cicirelli J. Study of the effect on shelter cat intakes and euthanasia from a shelter neuter return project of 10,080 cats from March 2010 to June 2014. PeerJ. (2014) 2:e646. doi: 10.7717/peerj.646
66. Le Boedec K. A systematic review and meta-analysis of the association between Mycoplasma spp and upper and lower respiratory tract disease in cats. J Am Vet Med Assoc. (2017) 250:397–407. doi: 10.2460/javma.250.4.397
67. Frisk AL, König M, Moritz A, Baumgärtner W. Detection of canine distemper virus nucleoprotein RNA by reverse transcription-PCR using serum, whole blood, and cerebrospinal fluid from dogs with distemper. J Clin Microbiol. (1999) 37:3634–43. doi: 10.1128/JCM.37.11.3634-3643.1999
68. Wilkes RP, Tsai Y-L, Lee P-Y, Lee F-C, Chang H-FG, Wang H-TT. Rapid and sensitive detection of canine distemper virus by one-tube reverse transcription-insulated isothermal polymerase chain reaction. BMC Vet Res. (2014) 10:213. doi: 10.1186/s12917-014-0213-8
69. Costa VG da, Saivish MV, Rodrigues RL, Lima Silva RF de, Moreli ML, Krüger RH. Molecular and serological surveys of canine distemper virus: a meta-analysis of cross-sectional studies. PLoS ONE. (2019) 14:e0217594. doi: 10.1371/journal.pone.0217594
70. Horecka K, Porter S, Amirian ES, Jefferson E. A decade of treatment of canine parvovirus in an animal shelter : a retrospective study. Animals . (2020) 10:939. doi: 10.3390/ani10060939
71. Markovich JE, Stucker KM, Carr AH, Harbison CE, Scarlett JM, Parrish CR. Effects of canine parvovirus strain variations on diagnostic test results and clinical management of enteritis in dogs. J Am Vet Med Assoc. (2012) 241:66–72. doi: 10.2460/javma.241.1.66
72. Truyen U, Addie D, Belák S, Boucraut-Baralon C, Egberink H, Frymus T, et al. Feline panleukopenia: ABCD guidelines on prevention and management. J Feline Med Surg. (2009) 11:538–46. doi: 10.1016/j.jfms.2009.05.002
73. Martella V, Elia G, Buonavoglia C. Canine distemper virus. Vet Clin North Am Small Anim Pract. (2008) 38:787–97. doi: 10.1016/j.cvsm.2008.02.007
74. Little S, Bienzle D, Carioto L, Chisholm H, O'Brien E, Scherk M. Feline leukemia virus and feline immunodeficiency virus in Canada: recommendations for testing and management. Can Vet J . (2011) 52:849–55.
75. Hosie MJ, Addie D, Belák S, Boucraut-Baralon C, Egberink E, Frymus T, et al. Feline Immunodeficiency: ABCD guidelines on prevention and management. J Feline Med Surg. (2009) 11:575–84. doi: 10.1016/j.jfms.2009.05.006
76. Litster AL. Transmission of feline immunodeficiency virus (FIV) among cohabiting cats in two cat rescue shelters. Vet J. (2014) 201:184–8. doi: 10.1016/j.tvjl.2014.02.030
77. Beczkowski PM, Litster A, Lin TL, Mellor DJ, Willett BJ, Hosie MJ. Contrasting clinical outcomes in two cohorts of cats naturally infected with feline immunodeficiency virus (FIV). Vet Microbiol. (2015) 176:50–60. doi: 10.1016/j.vetmic.2014.12.023
78. Hartmann K. Clinical aspects of feline immunodeficiency and feline leukemia virus infection. Vet Immunol Immunopathol. (2011) 143:190–201. doi: 10.1016/j.vetimm.2011.06.003
79. Strong SJ, Gookin JL, Correa MT, Banks RE. Interventions and observations associated with survival of orphaned shelter kittens undergoing treatment for diarrhea. J Feline Med Surg. (2020) 22:292–8. doi: 10.1177/1098612X19840459
80. Pesavento PA, Murphy BG. Common and emerging infectious diseases in the animal shelter. Vet Pathol. (2014) 51:478–91. doi: 10.1177/0300985813511129
81. Priestnall SL, Mitchell JA, Walker CA, Erles K, Brownlie J. New and emerging pathogens in canine infectious respiratory disease. Vet Pathol. (2014) 51:492–504. doi: 10.1177/0300985813511130
82. Newbury S, Blinn MK, Bushby PA, et al. Guidelines for standards of care in animal shelters. Assoc Shelter Vet . (2010) 1–67. Available online at: https://www.sheltervet.org/assets/docs/shelter-standards-oct2011-wforward.pdf
83. Sabshin SJ, Levy JK, Tupler T, Tucker SJ, Greiner EC, Leutenegger CM. Enteropathogens identified in cats entering a florida animal shelter with normal feces or diarrhea. J Am Vet Med Assoc. (2012) 241:331–7. doi: 10.2460/javma.241.3.331
84. White SC, Jefferson E, Levy JK. Impact of publicly sponsored neutering programs on animal population dynamics at animal shelters: the new hampshire and austin experiences. J Appl Anim Welf Sci. (2010) 13:191–212. doi: 10.1080/10888700903579903
85. Lambert K, Coe J, Niel L, Dewey C, Sargeant JM. A systematic review and meta-analysis of the proportion of dogs surrendered for dog-related and owner-related reasons. Prev Vet Med. (2015) 118:148–60. doi: 10.1016/j.prevetmed.2014.11.002
86. Jones-Fairnie H, Ferroni P, Silburn S, Lawrence D. Suicide in Australian veterinarians. Aust Vet J. (2008) 86:114–6. doi: 10.1111/j.1751-0813.2008.00277.x
87. Scotney RL, McLaughlin D, Keates HL. A systematic review of the effects of euthanasia and occupational stress in personnel working with animals in animal shelters, veterinary clinics, and biomedical research facilities. J Am Vet Med Assoc. (2015) 247:1121–30. doi: 10.2460/javma.247.10.1121
88. Tomasi SE, Fechter-Leggett ED, Edwards NT, Reddish AD, Crosby AE, Nett RJ. Suicide among veterinarians in the United States from 1979 through 2015. J Am Vet Med Assoc. (2019) 254:104–12. doi: 10.2460/javma.254.1.104
89. Fink-Miller EL, Nestler LM. Suicide in physicians and veterinarians: risk factors and theories. Curr Opin Psychol. (2018) 22:23–6. doi: 10.1016/j.copsyc.2017.07.019
90. Day MJ, Breitschwerdt E, Cleaveland S, Karkare U, Khanna C, Kirpensteijn J, et al. Surveillance of zoonotic infectious disease transmitted by small companion animals. Emerg Infect Dis . (2012) 18:1–7. doi: 10.3201/eid1812.120664
91. Coalition A to VC. Access to Veterinary Care: Barriers, Current Practices, Public Policy. (2018). Available online at: https://pphe.utk.edu/wp-content/uploads/2020/09/avcc-report.pdf (accessed March 9, 2022).
92. Kipperman BS, Kass PH, Rishniw M. Factors that influence small animal veterinarians' opinions and actions regarding cost of care and effects of economic limitations on patient care and outcome and professional career satisfaction and burnout. J Am Vet Med Assoc. (2017) 250:785–94. doi: 10.2460/javma.250.7.785
93. Booth M, Rishniw M, Kogan LR. The shortage of veterinarians in emergency practice: a survey and analysis. J Vet Emerg Crit Care. (2021) 31:295–305. doi: 10.1111/vec.13039
94. Kreisler RE, Spindel ME, Rishniw M. Surveys of salary, benefits, and job responsibilities for veterinarians employed in the field of shelter medicine in the United States conducted in 2011 and 2018. Top Companion Anim Med. (2020) 39:100430. doi: 10.1016/j.tcam.2020.100430
95. Einav L, Finkelstein A, Gupta A. Is American pet health care (Also) uniquely inefficient? Am Econ Rev. (2017) 107:491–5. doi: 10.1257/aer.p20171087
96. Udell M. A new breed of health care. Mark Health Serv . (2014) 34:24–31.
97. Daneshvary N, Schwer RK. The nature of demand for companion pet health care. J Appl Bus Res. (2011) 9:24. doi: 10.19030/jabr.v9i4.5986
98. López-Jara MJ, Sacristán I, Farías AA, Maron-Perez F, Acuña F, Aguilar E, et al. Free-roaming domestic cats near conservation areas in Chile: spatial movements, human care and risks for wildlife. Perspect Ecol Conserv. (2021) 19:387–98. doi: 10.1016/j.pecon.2021.02.001
99. Loss SR, Will T, Marra PP. The impact of free-ranging domestic cats on wildlife of the United States. Nat Commun. (2013) 4:1396. doi: 10.1038/ncomms2380
100. Schmidt PM, Lopez RR, Collier BA. Survival, fecundity, and movements of free-roaming cats. J Wildl Manage. (2007) 71:915–9. doi: 10.2193/2006-066
101. Trouwborst A, McCormack PC, Martínez Camacho E. Domestic cats and their impacts on biodiversity: a blind spot in the application of nature conservation law. People Nat. (2020) 2:235–50. doi: 10.1002/pan3.10073
102. Gehrt SD, Wilson EC, Brown JL, Anchor C. Population ecology of free-roaming cats and interference competition by coyotes in urban parks. PLoS ONE. (2013) 8:e75718. doi: 10.1371/journal.pone.0075718
103. Vinic T, Dowling-Guyer S, Lindenmayer J, Lindsay A, Panofsky R, McCobb E. Survey of massachusetts animal shelter record-keeping practices in 2015. J Appl Anim Welf Sci. (2020) 23:385–401. doi: 10.1080/10888705.2019.1646135
104. Sinski JB, Gagné P. Give me shelter: the state of animal sheltering in Kentucky's county shelter system. Contemp Justice Rev. (2016) 19:250–66. doi: 10.1080/10282580.2016.1169706
105. Count SA. Shelter Animals Count—The National Database Project. Shelter Animals Count. Available online at: https://www.shelteranimalscount.org (accessed March 8, 2022).
106. States THS of the U. Pets By the Numbers Animal Sheltering. (2015). Available online at: https://humanepro.org/page/pets-by-the-numbers
107. Asilomar Accords (2004). Available online at: https://www.shelteranimalscount.org/docs/default-source/DataResources/2004aaccords5.pdf?sfvrsn=31c1ff76_0 (accessed March 8, 2022).
108. Ammons DN. Municipal Benchmarks: Assessing Local Performance and Establishing Community Standards. 3rd ed. Oxfordshire: Routledge (2014). doi: 10.4324/9781315702261
109. Rowan A, Kartal T. Dog population & dog sheltering trends in the United States of America. Animals. (2018) 8:68. doi: 10.3390/ani8050068
110. Pizano S. The Best Practice Playbook for Animal Shelters . Gainesville, FL: Team Shelter USA, LLC (2019).
111. Jakovljevic MM, Ogura S. Health economics at the crossroads of centuries – from the past to the future. Front Public Heal . (2016) 4:115. doi: 10.3389/fpubh.2016.00115
112. Dijkhuizen AA, Morris RS. Animal Health Economics: Principles and Applications. Sydney, NSW: University of Sydney (1997).
113. Rushton JA. Animal Health Economics: An Introduction. Oxfordshire: CABI (2015).
114. Patronek G, Crowe A. Factors associated with high live release for dogs at a large, open-admission, municipal shelter. Animals. (2018) 8:45. doi: 10.3390/ani8040045
115. Jenkins S, Montford KS, Taylor C. Disability and Animality: Crip Perspectives in Critical Animal Studies. Oxfordshire: Routledge (2020). doi: 10.4324/9781003014270
116. Brown S-E. The under-representation of African American employees in animal welfare organizations in the United States. Soc Anim. (2005) 13:153–62. doi: 10.1163/1568530054300217
117. Neumann SL. Animal welfare volunteers: who are they and why do they do what they do? Anthrozoos. (2010) 23:351–64. doi: 10.2752/175303710X12750451259372
118. Sisson DC. Control mutuality, social media, and organization-public relationships: a study of local animal welfare organizations' donors. Public Relat Rev. (2017) 43:179–89. doi: 10.1016/j.pubrev.2016.10.007
119. Hawes SM, Hupe T, Morris KN. Punishment to support: the need to align animal control enforcement with the human social justice movement. Animals. (2020) 10:1902. doi: 10.3390/ani10101902
120. Ly LH, Gordon E, Protopopova A. Inequitable flow of animals in and out of shelters: comparison of community-level vulnerability for owner-surrendered and subsequently adopted animals. Front Vet Sci . (2021) 8:389. doi: 10.3389/fvets.2021.784389
121. Reese LA, Ye M. Minding the Gap: networks of animal welfare service provision. Am Rev Public Adm. (2017) 47:503–19. doi: 10.1177/0275074015623377
122. Ballantyne A, Stewart C. Big data and public-private partnerships in healthcare and research. Asian Bioeth Rev. (2019) 11:315–26. doi: 10.1007/s41649-019-00100-7
123. Kim J. Social finance funding model for animal shelter programs: public–private partnerships using social impact bonds. Soc Anim. (2018) 26:259–76. doi: 10.1163/15685306-12341521
124. Flower S. The Effect of Play Group on the Behavior of Shelter Dogs. Hunt Coll City Univ New Yor (2016). Available online at: https://academicworks.cuny.edu/cgi/viewcontent.cgi?article=1122&context=hc_sas_etds (accessed March 9, 2022).
125. Mohan-Gibbons H, Weiss E, Garrison L, Allison M. Evaluation of a novel dog adoption program in two US Communities. PLoS ONE. (2014) 9:e91959. doi: 10.1371/journal.pone.0091959
126. Wagner D, Hurley K, Stavisky J. Shelter housing for cats: principles of design for health, welfare and rehoming. J Feline Med Surg. (2018) 20:635–42. doi: 10.1177/1098612X18781388
127. Coppola CL, Enns RM, Grandin T. Noise in the animal shelter environment: building design and the effects of daily noise exposure. J Appl Anim Welf Sci. (2006) 9:1–7. doi: 10.1207/s15327604jaws0901_1
Keywords: animal shelters, animal welfare, research problems, animal behavior, shelter adoption, disease transmission, one health
Citation: Horecka K and Neal S (2022) Critical Problems for Research in Animal Sheltering, a Conceptual Analysis. Front. Vet. Sci. 9:804154. doi: 10.3389/fvets.2022.804154
Received: 28 October 2021; Accepted: 22 February 2022; Published: 01 April 2022.
Reviewed by:
Copyright © 2022 Horecka and Neal. This is an open-access article distributed under the terms of the Creative Commons Attribution License (CC BY) . The use, distribution or reproduction in other forums is permitted, provided the original author(s) and the copyright owner(s) are credited and that the original publication in this journal is cited, in accordance with accepted academic practice. No use, distribution or reproduction is permitted which does not comply with these terms.
*Correspondence: Kevin Horecka, kevin.horecka@gmail.com
This article is part of the Research Topic
Reimagining Animal Sheltering: Support Services and Community-Driven Sheltering Methods
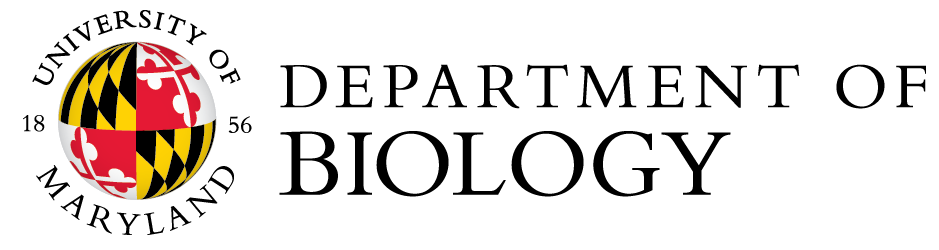
Global-scale Animal Ecology Reveals Behavioral Changes in Response to Climate Change
Using a new large-scale data archive of animal movement studies, an international team including University of Maryland biologists found that animals are responding in unexpected ways to climate change. The archive contains data from studies across the global Arctic and sub-Arctic, an enormous region that is experiencing some of the most dramatic effects of global warming, including animal declines.
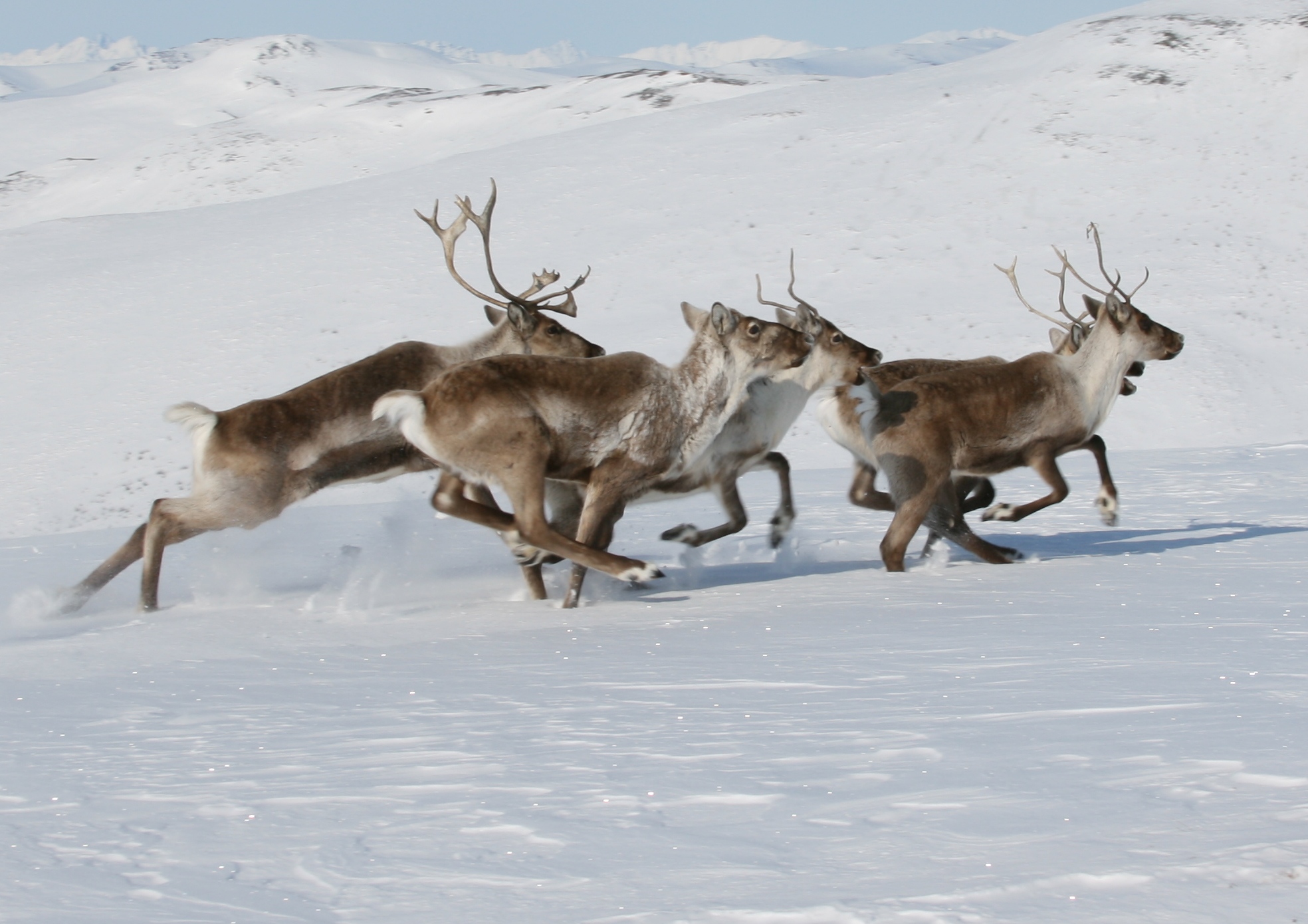
After developing the archive, the researchers used it to conduct three case studies that revealed surprising patterns and associations between climate change and the behavior of golden eagles, bears, caribou, moose and wolves. This work demonstrates both the feasibility and importance of studying animal ecology on extremely large scales. A research paper describing the archive and case studies appears in the November 6, 2020, issue of the journal Science .
“I’m really excited about how this work shows what you can learn from comparing data across populations on a very large scale,” said Elie Gurarie , an associate research scientist in UMD’s Department of Biology and a co-author of the paper. “I would say this is an early example of what we might call global animal movement ecology. We’re increasing our ability to monitor the pulse of animal populations across the Earth and ask big picture questions about what it means.”
Large-scale monitoring of things like sea-surface temperature and global forest cover have revealed important information about the response of Earth’s systems to climate change and human activity. But big-picture trends in animal behavior have been difficult to study, in part because animal ecology is not traditionally studied across landscapes that span entire regions of the globe, and also because the necessary data are collected by a variety of agencies and jurisdictions and are therefore neither standardized nor easily accessible.
To address these issues, Gurarie and his collaborators spent years building relationships with scientists from national, regional and First Nations governments and research groups throughout the Arctic to convey the benefits of sharing data in a global repository that they call the Arctic Animal Movement Archive (AAMA). Currently, the archive includes contributions from researchers from over 100 universities, government agencies and conservation groups across 17 countries.
The archive includes data from 201 terrestrial and marine animal tracking studies representing more than 8,000 animals between 1991 and the present. Using this data, Gurarie and members of his lab analyzed the movements of more than 900 female caribou from 2000 to 2017. They found that the iconic long-distance migrating caribou are giving birth earlier in the spring, roughly tracking rates of warming. But among the non-migratory mountain and lowland woodland caribou only the northern sub-populations are showing similar changes. While the drivers of these differences remain a mystery, understanding their behavior is critical to anticipating how they will respond as the Arctic continues to warm and many populations continue to decline.
“It’s tricky to predict how these trends might impact populations,” Gurarie said. “On the one hand, it can be better to give birth earlier, as it gives the calves more opportunity to grow during the summer season. On the other hand, giving birth too early may mean you literally don’t have time to reach the optimal calving grounds. The ability to look at biological processes, like birth, at such a large scale, across populations and subspecies and over millions of square kilometers, is unprecedented for a species in such a remote and harsh environment. These results reveal patterns that we would not have suspected, and point to further lines of inquiry about everything from caribou evolution to their ability to adapt to environmental changes moving forward."
Data analysis tools Gurarie developed to study caribou were also used for another case study led by his collaborators.
In an analysis comparing movements of more than 100 golden eagles from 1993 to 2017, Scott LaPoint, a researcher from Columbia University who is now at Black Rock Forest Consortium, found that immature birds migrating north in the spring arrived earlier following mild winters, while adult birds did not. The timing shift for young birds varied in response to a large-scale climate cycle called the Pacific Decadal Oscillation, which is being affected by climate change. Such age-related behavior changes could only be seen through decades of movement data and may have implications for breeding success.
A third study by Peter Mahoney of the University of Washington looked at the movement speeds of bears, caribou, moose and wolves from 1998 to 2019. His study showed that species respond differently to seasonal temperatures and winter snow conditions. Those differences could influence species interactions, food competition and predator-prey dynamics.
The scientists expect that other researchers will continue to mine the AAMA for answers to pressing questions about whether and how animals are responding to a changing Arctic. Meanwhile, the resource continues to expand as more data comes in from currently tracked animals and more researchers add their studies.
“This work has given us a baseline to understand the large-scale picture so we can get a sense of how animals and environments are really interacting across species and across space as the environment changes,” Gurarie said.
The researchers invite participation at https://www.movebank.org/cms/movebank-content/arctic-animal-movement-archive .
Additional authors of the paper from UMD are Emma Grier (B.S. '20, biological sciences; environmental sciences and policy) and Ophélie Couriot, a postdoctoral associate at the National Socio-Environmental Synthesis Center (SESYNC).
This work was supported by NASA ABoVE Animals on the Move (Award Nos. NNX15AU20A and NNX15AW71A). Gurarie’s work was also supported by the National Science Foundation (Award Nos. 1853465, 1915347). The content of this article does not necessarily reflect the views of these organizations .
The research paper , "Ecological insights from three decades of animal movement tracking across a changing Arctic," appears in the November 6, 2020 issue of the journal Science .
Writer: Kimbra Cutlip
Media Relations Contact: Abby Robinson, 301-405-5845, [email protected]
News Categories
- Undergraduates
- Graduate Students
Recent Posts
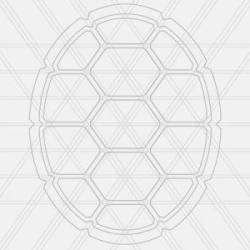
- Ecology, Evolution, and Behavior
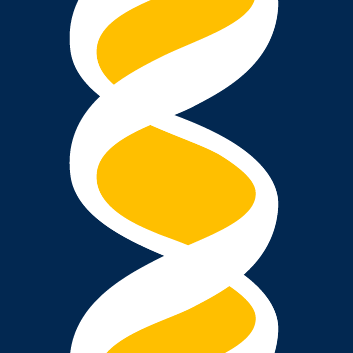
College of Biological Sciences
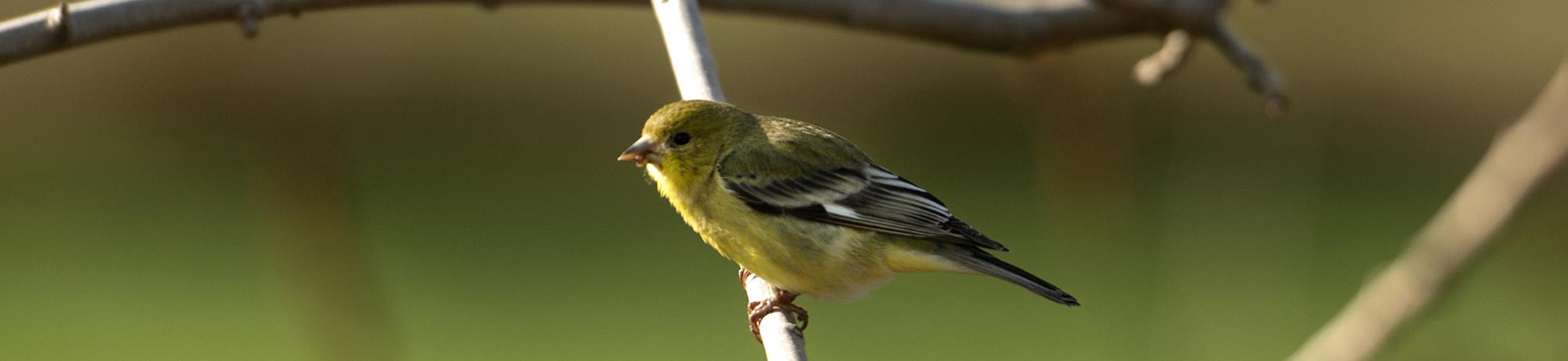
Department of Neurobiology, Physiology and Behavior
The Department of Neurobiology, Physiology and Behavior is a vibrant academic unit that investigates the fundamental processes shared by all animals. These include growth, reproduction, movement, response to stimuli, and maintenance of homeostasis. Faculty explore the highly integrated and precisely regulated physiological mechanisms underlying these vital functions. The department’s eponymous major offers students an exciting blend of hands-on labs, foundational courses in biological sciences, chemistry, mathematics, and physics and advanced topics like animal behavior, physiology of specific organ systems, developmental neurobiology, and endocrinology. Graduates pursue diverse career paths, including roles in medical professions, research and academia, and clinical settings.
Undergraduate Programs
Graduate programs, affiliated centers.
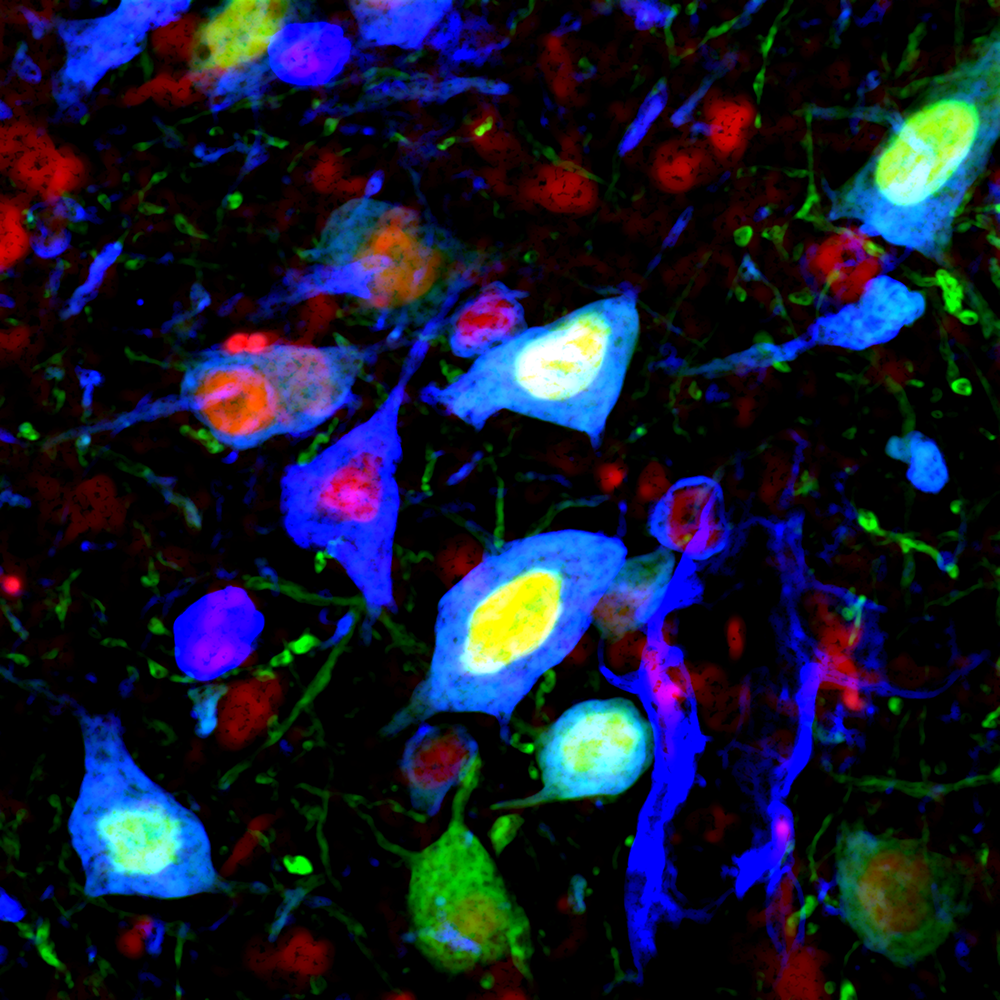
Center for Neuroscience
The Center for Neuroscience is dedicated to understanding all levels of brain development, function and dysfunction. These discoveries help provide the foundation for improvements in health and new therapies for disease.
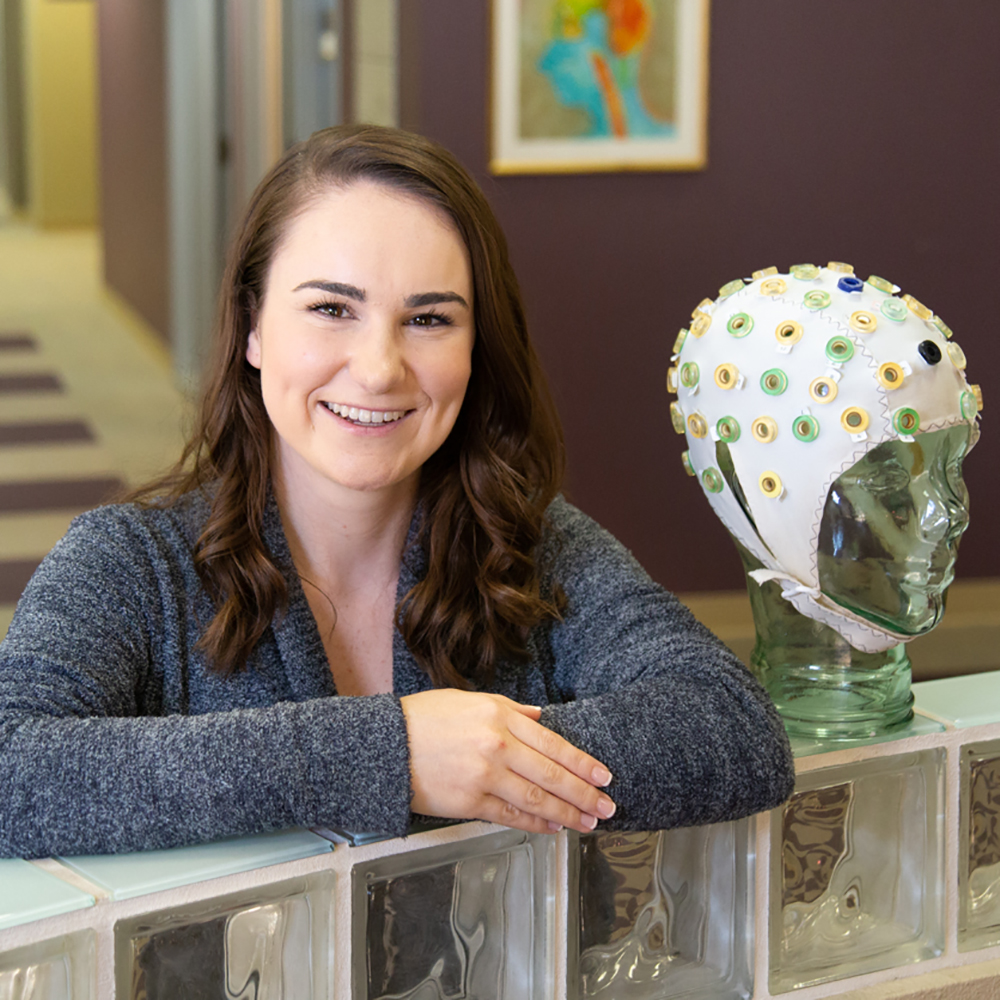
Center for Mind and Brain
The Center for Mind and Brain (CMB) is devoted to supporting the collaborative work of world-class scientists from a broad spectrum of disciplines encompassing divergent social, biological, computational and medical perspectives.
Academia.edu no longer supports Internet Explorer.
To browse Academia.edu and the wider internet faster and more securely, please take a few seconds to upgrade your browser .
- We're Hiring!
- Help Center
Animal Behavior
- Most Cited Papers
- Most Downloaded Papers
- Newest Papers
- Save to Library
- Last »
- Behavioral Ecology Follow Following
- Zoology Follow Following
- Animal Cognition Follow Following
- Animal Behaviour Follow Following
- Evolutionary Biology Follow Following
- Conservation Biology Follow Following
- Ecology Follow Following
- Animal Ecology Follow Following
- Primatology Follow Following
- Ethology Follow Following
Enter the email address you signed up with and we'll email you a reset link.
- Academia.edu Publishing
- We're Hiring!
- Help Center
- Find new research papers in:
- Health Sciences
- Earth Sciences
- Cognitive Science
- Mathematics
- Computer Science
- Academia ©2024
Smithsonian Voices
From the Smithsonian Museums
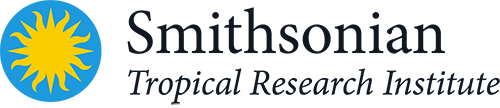
SMITHSONIAN TROPICAL RESEARCH INSTITUTE
Indigenous Marine Scientist Studies Fish Feeding Evolution in Panama
Through advanced isotopic analyses, Rodnyel Arosemena seeks to understand how fish in the Caribbean and the Pacific that had a common ancestor take advantage of the resources of their different environments today.
Leila Nilipour
:focal(1280x859:1281x860)/https://tf-cmsv2-smithsonianmag-media.s3.amazonaws.com/filer_public/17/d1/17d10c0e-9695-45d3-b086-caa210f0dfa6/2_a7s08978_rodnyel_arosemena_1.jpg)
Rodnyel Arosemena is of Dule (from Guna Yala) descent but grew up in the city. Unlike his parents, who grew up in the Guna Yala region, the Panamanian Caribbean Sea was not central to his childhood. His first memory of the beach is at the age of eight, but once he started college, his roots claimed him back: he is now a marine biologist. He is also one of the few people in the world trained in compound-specific stable isotope analysis of amino acids (CSIA-AA).
Arosemena was chosen to train in CSIA-AA after a year working with various marine labs at the Smithsonian Tropical Research Institute (STRI). This led him to spend two months at the University of Hong Kong, where STRI postdoctoral researcher Jon Cybulski taught him how to analyze fish muscle samples with the cutting-edge technique for a project on sister fish species across the isthmus of Panama.
The project led by STRI scientist Matthieu Leray aims to understand how the diet and resource utilization of fish sharing a common ancestor differs. These species, once connected but now geographically separated due to the formation of the isthmus, have adapted to distinct environmental conditions in the Caribbean and the Pacific.
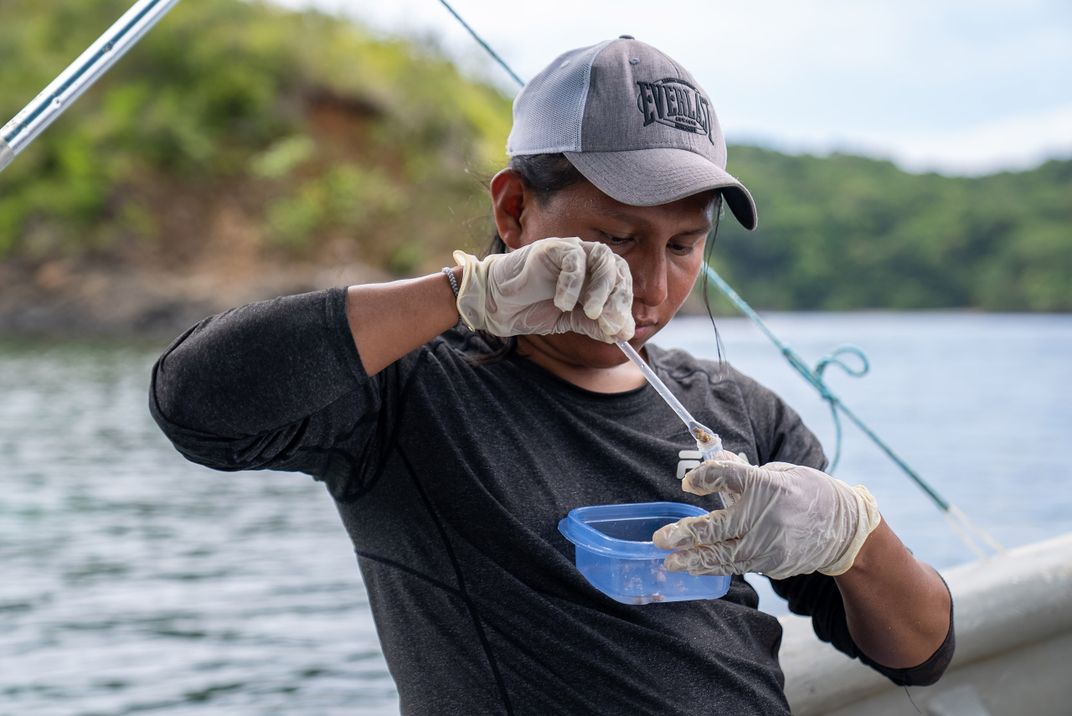
“Their dietary evolution can be evaluated by observing the animals’ eating habits, but the stable isotopes’ technique provides a more specific and detailed analyses,” Arosemena explains.
Most elements, such as carbon and nitrogen, possess multiple stable isotopes with slight differences in atomic masses. These variations cause them to behave differently biologically from one another, leading to varying ratios within an animal's body. In other words, analyzing their ratios may unveil what an animal has been eating during most of its life, shedding light on its food sources and environmental influences.
“Samples of fish muscles are used to examine the transfer of each amino acid during the feeding process, as well as how each organism takes advantage of nutrients and energy, providing a greater level of detail on resource use,” says Arosemena.
It is expected that, due to the elevated nutrient levels in the Pacific, there will likely be a wider range of dietary choices for the fish there compared to the Caribbean region.
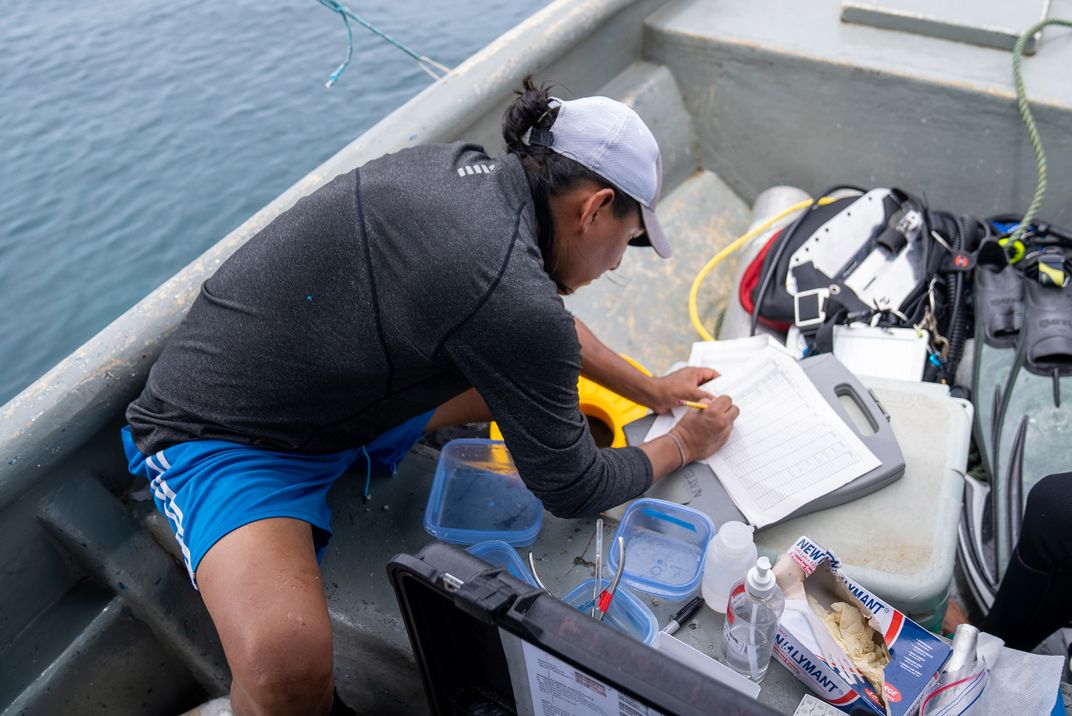
“The sister species separated by the isthmus of Panama are a unique system to understand how feeding strategies evolve in marine organisms in response to different environmental conditions,” said Leray. “This research is not only very important for understanding the mechanisms of adaptation and speciation in marine organisms, but it will also provide insights into how marine fishes may be able to cope with climate change related impacts, such as changes in prey availability or shifts in habitat suitability.”
For Arosemena, this and other experiences at the Smithsonian have allowed him to enrich the knowledge he had already acquired in college and have motivated him to continue advancing in his scientific career in marine biology.
“I am considering applying for a master's degree and continuing to dedicate myself to research,” Arosemena said. “All my work so far at STRI has been related to concepts such as evolution, resilience and adaptation and I believe that fish, because of their great diversity, would be an excellent starting point for my career.”
Headquartered in Panama, the Smithsonian Tropical Research Institute is a unit of the Smithsonian Institution in Washington, DC. The institute furthers the understanding of tropical biodiversity and its importance to human welfare, trains students to conduct research in the tropics and promotes conservation by increasing public awareness of the beauty and importance of tropical ecosystems.
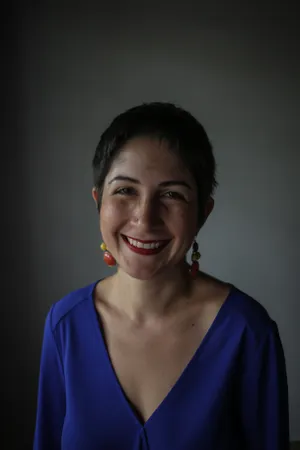
Leila Nilipour | READ MORE
Leila Nilipour is a bilingual storyteller based in Panama City.

Ask an Expert: Forever Chemicals, PFAS, and Environmental Health with Carmen Marsit
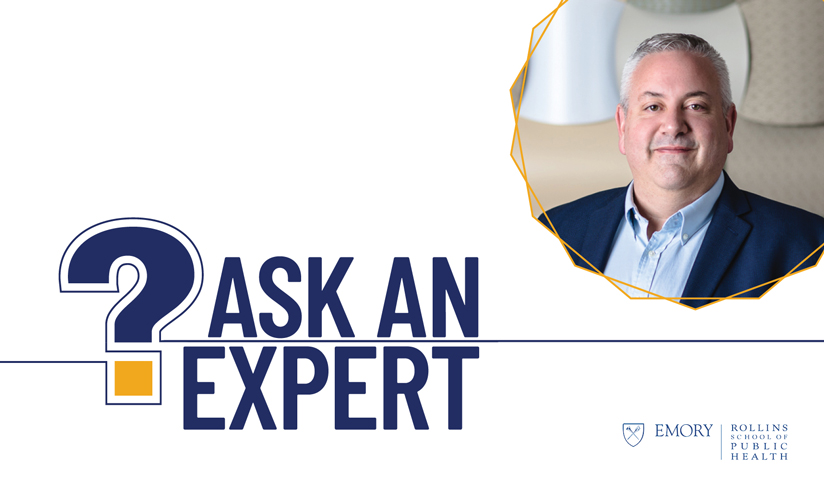
By Kelly Jordan
The terms “PFAS (or per- and polyfluoroalkyl substances)” and “forever chemicals” have popped up more frequently in news headlines as evidence about these substances and their harmful health effects continues to grow. Most recently, the U.S. Food and Drug Administration (FDA) made moves to end the use of grease-proofing in food packaging and the Environmental Protection Agency (EPA) launched a “ legally enforceable drinking water standard for PFAS .”
PFAS chemicals are human-made innovations developed in the 1950s to problem-solve major everyday issues, including everything from water-proofing and fire-proofing to non-stick and non-skid surface coatings. While revolutionary and effective in their use, researchers are now learning about the health associations and environmental consequences that can occur when the thousands of chemicals falling under the PFAS umbrella remain in the environment forever (hence, the nickname).
Carmen Marsit, PhD, Rollins Distinguished Professor of Environmental Health, shared insights on what you should know about this group of chemicals, their impacts on health, and the path ahead.
What do we know about PFAS exposures and their impacts on human health?
In the past five years, the literature has blown up with studies about these chemicals, with even low-dose exposures showing negative outcomes. These include reproductive and birth-related outcomes [including low-fertility, high blood pressure, and low birthweight], diabetes, metabolic disease, heart disease, cancer, and more.
How prevalent are PFAS chemicals?
These chemicals are in just about everything and the environment is polluted with them. So far, there has not been good regulation to protect people or the environment from this. For years, there were no regulations at all with people and companies throwing it in unregulated landfills where it was getting into the environment. I think that has led to a lot of the public outcry we’ve seen with demanding regulation.
READ RECENT NEWS COVERAGE ABOUT PFAS-RELATED RESEARCH BEING CONDUCTED IN ROME, GEORGIA
Are there stronger quantities of these chemicals in some countries over others?
Europe had concerns about this earlier and has already pushed some regulations around it. In the U.S, we're seeing this real push for it because a lot of the research is being done in the U.S. and is focused on this country’s populations. The reality is this is going to be everywhere. These chemicals are used in everything. That's actually a concern of mine even as we put policies in place here, is how much of this are we just going to push off to low- and middle-income countries [relating to manufacturing and waste disposal]?
Do forever chemicals also impact animal life or the environment?
It’s very likely that the same things we are seeing in people could be happening in animals too. Exposures could be affecting species out there and changing ecology. So if we see endocrine disruption occurrences, that same thing is happening out in the environment as well. This is also where the challenge comes in for food: Much of our food comes from these environments [fish swimming in contaminated streams, food grown in contaminated soil]. It’s difficult to avoid it.
What behavior changes have you personally made to limit your exposure?
It’s impossible to completely avoid exposure to forever chemicals because they really are in everything, including beauty products, food packaging, to-go containers, and plastics. Three changes we’ve made at home are moving from non-stick cookware to cast-iron, swapping out our plastic food containers for glass containers, and buying more fresh food so we can limit the amount of food packaging we are exposed to.
Looking ahead to the presidential election this November, what topics related to exposure science or climate change should be on people’s radars?
We need to be thinking about what the impacts of climate change are now and what they are going to continue to be, because they're going to continue to happen. Hopefully there are still opportunities for us to make some changes to reduce what could potentially happen. Extreme weather is going to happen [due to climate change]. Are we prepared as a country? That’s an important topic, the other one would be around these kinds of chemical regulations and how they can be improved.
What areas should the FDA, EPA, and other regulatory agencies focus on next?
We need better regulation around how these chemicals should be disposed. I also think looking more closely at consumer products is going to be really important. Grease shields are one piece of it, but they're in personal care products, they're in makeup, they're in other things people use every day on their bodies. So looking at where all of those pieces are and getting them out of those products is also going to be really important.
How do you stay motivated to do this type of research when the findings are often so negative?
A lot of what drives me is the hope that what we're doing will eventually lead to these types of policy changes that are finally starting to come down. One thing that's really frustrating is that so much research has to happen to prove these negative effects—which often takes several years— before we see those policy changes.
That's where some of the molecular-focused work several of us do within the HERCULES Exposome Research Center can be helpful. The idea there is, if we can understand at the molecular level what some of these chemicals do, can we move more quickly on proving that they're problematic instead of having to wait until we have cancer outcomes 30 years from now?
I’m also driven by the question, “How can we help?” Even if we get every needed regulation and these chemicals are no longer on the market, they will still exist in the environment forever. We have a whole generation of kids who have been exposed. Health inequities and issues related to access are at play. We have to find ways to protect people across the board. This keeps me motivated.
Associated Topics:
- Ask an Expert
- Environmental Health
- Climate and Health
- Community Health
- Emergency Preparedness
Related Links
- Rollins News
- In the Media
- Rollins Experts
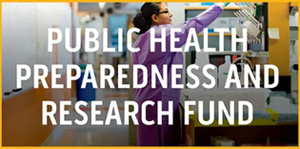

An official website of the United States government
The .gov means it’s official. Federal government websites often end in .gov or .mil. Before sharing sensitive information, make sure you’re on a federal government site.
The site is secure. The https:// ensures that you are connecting to the official website and that any information you provide is encrypted and transmitted securely.
- Publications
- Account settings
Preview improvements coming to the PMC website in October 2024. Learn More or Try it out now .
- Advanced Search
- Journal List
- Front Vet Sci
Critical Problems for Research in Animal Sheltering, a Conceptual Analysis
Kevin horecka.
1 Research Department, Austin Pets Alive!, Austin, TX, United States
2 Arkansas State University, Department of Political Science, Jonesboro, AR, United States
Animal shelter research has seen significant increases in participation over the past several decades from academic organizations, private organizations, public entities, and even corporations that aims to improve shelter programs, processes, operations, and outcomes for the various stakeholders/participants involved in a shelter system (animals, humans, the community, wildlife, and the environment). These efforts are scattered through a huge variety of different research areas that are challenging to define and scope for organizations seeking to start new lines of research inquiry. This work aims to enumerate some of the most critical outstanding problems for research in animal sheltering in a conceptual framework that is intended to help direct research conversations toward the research topics of highest impact (with the highest quality outcomes possible). To this end, we define seven (7) key areas for research: animal behavior, adoptions and special needs populations, medical conditions, disease transmission, community, ecology, and wellness (one health), operations, and public-private-academic-corporate collaboration. Within each of these areas, we review specific problems and highlight examples of successes in each area in the past several decades. We close with a discussion of some of the topics that were not detailed in this manuscript but, nonetheless, deserve some mention. Through this enumeration, we hope to spur conversation around innovative methodologies, technologies, and concepts in both research and practice in animal sheltering.
Introduction
Animal Sheltering in Western society, in some form, has existed since the mid-1800's (with the creation of both the Royal Society for the Prevention of Cruelty to Animals and the American Society for the Prevention of Cruelty to Animals in 1824 and 1866, respectively) and has been a constantly evolving field to both the benefit ( 1 , 2 ) and detriment ( 3 , 4 ), of its stakeholders: animals, pet owners, communities, and the organizations that tie these groups together. In the past several decades, a cultural shift has been occurring in which animal welfare ( 5 , 6 ) has received more attention, resources, and scrutiny than in the decades before. Success in sheltering is commonly measured by the Live Release Rate (hereafter LRR) that is obtained by dividing the total number of live animal outcomes (such as adoptions and transfers) by the total number of live animal intakes ( 7 ). Many cities have been able to increase their LRR and those of surrounding counties above 90 and even 95% ( 8 ), yet shelters still struggle with having adequate resources ( 9 ) and rural shelters may be more likely to struggle ( 10 ). The number of conceptual problems in sheltering is enormous, and as awareness of the needs of shelters continues to rise, more and more groups—academic, corporate, non-profit, and private—are looking for ways to contribute to the wider movement of animal welfare using their unique skills and talents. One difficulty for these potential partners is in understanding what the needs of shelters are and what high-value unsolved problems exist in the field.
Some of these are knowledge problems, others, implementation problems, and even more, systemic, cultural, and societal problems. Almost all require some manner of research to elucidate best practices and truths and differentiate them from traditions and myths. To aid interested parties in contributing to these areas of animal sheltering, we seek to enumerate and explain many of the critical problems for research in animal sheltering so that those organizations and interested parties might find a place to contribute. The key areas for future research were developed through a combination of both empirical and a priori traditions. The empirical approach used included input from animal sheltering professionals, including the responses of over 10 working groups representing more than 300 shelter professionals associated with Human Animal Support Services project to the question of what research needs were to advance animal sheltering. A priori observation and reflection of the researchers and reviews of the existing literature also helped to inform a lengthy list of research needs. These research needs were then thematically grouped in to the 7 key areas. Each of the areas was then evaluated on two factors: the degree of potential impact to animal sheltering and the difficulty in studying the problem.
Table 1 presents the 7 key areas identified. It also presents the impact potential for research in these areas by identifying the top-level impacts that advances in each topic area could have on the field of animal sheltering. While not intended to be all encompassing, this list captures the main topics generated by the authors and the consulted professionals.
The 7 key problem areas identified in this work and their impact potential in the space of animal sheltering. While not intended to be all encompassing, this list captures the main topics generated by the authors and the consulted professionals.
Figure 1 provides an additional way of examining these topical areas. This figure provides examples of critical problems for research in animal sheltering and provides a way to compare and evaluate the areas in terms of the relative difficulty of studying the problems as well as the relative magnitude of the potential impact. In addition to evaluating these factors for the key research areas identified herein, this figures also shows a framework through which other researchers could evaluate the relative impacts and difficulty of other possible research topics.

A Force Directed Graph showing the various problems discussed in this article. The size of a node represents the relative impact a solution would have. The color represents the relative difficulty of studying the problem (with red being more difficult). Links relate the topics. An interactive version can be found here: https://codepen.io/kevroy314/pen/jONoXma . Click to isolate nodes and their neighbors. Drag to move around. Scroll to zoom.
Key Areas for Research
Animal behavior.
Animal behavior is one of the most challenging and complex topics in animal sheltering. Leaving aside controversies surrounding the ethics of adopting out animals with known behavior challenges or the ending of the life of an animal, whether for the protection of the public, retribution for an incident, quality of life, or any other justification related to behavioral issues, such as biting or inappropriate elimination, the practical need to better understand and modify animal behavior to improve the lives of animals and their caregivers to improve their chances of adoption and/or their probability of remaining in the home ( 11 ) is substantial. Here, we highlight 4 key areas in animal behavior that may have the biggest impact in a shelter setting and that may be underrepresented in the literature.
Efforts to form a typology of dog behaviors that may be problematic in the home, and, specifically, dog behavior that may be averse to a successful adoption and retention in a home ( 12 , 13 ) have been attempted in the past ( 14 – 16 ). Despite the interest in canine behavior in general rising sharply in the early 1990s and more recently ( 17 ), no consensus has been reached upon a singular behavioral classification and identification system that can be used to make decisions around best practices with dogs with histories of behavior problems or potential for behavior problems. Such a classification system should have the following properties [( 18 ) for a more detailed discussion of the difficulties surrounding some of these issues]:
- Objective measurability and reproducibility.
- Characterization of common temporal progressions.
- Understood correlations between related behaviors.
- Clinical relevance to predictability and intervention.
Somewhat recent attempts ( 18 , 19 ) at assessing the efficacy of behavioral evaluations have not been as promising as might be hoped given the 50+ year history of the field, and the impact of such a system, especially in establishing new interventions that can help these animals be successfully placed in homes, could be enormous given the extreme difficulty in achieving successful outcomes for dogs with behavior issues.
One key factor in negative animal behaviors, especially as pertaining to the adoptability of animals, is the stress they experience while in a shelter setting ( 20 – 22 ). Studies of animal stress date back to 1926 ( 23 ) with animals have often serving as a model for human stress ( 24 , 25 ). Practical tools are needed to assess the impact of shelter environmental improvements. Using Biomarkers to assess stress ( 26 ) across species ( 27 – 29 ) have shown significant success in recent years. Unfortunately, the practical measurement of such biomarkers in shelter settings remains unlikely due to resource and practical constraints. Non-invasive measures of stress are possible in many species [including thermographic ( 30 , 31 ), salivary ( 32 ), visual ( 33 ), and multimodal ( 34 ) systems], though their efficacy as an intervention target is unclear. A more thorough understanding of best practices around the reduction of stress for animals in shelters will allow for significant improvement in the quality of life of long-stay animals as well as the adoptability of animals that may show fewer behavioral issues once removed from a stressful environment, with some evidence showing changes in cortisol levels, a common biomarker for stress, with even a single night removed from the shelter environment in adult dogs ( 35 ).
A key element in the success of an animal with behavioral issues, post-adoption is not simply the cessation of negative behaviors, but also the match with an adopter who can maintain the environment necessary for permanent improvement in behavior as well as following up with those adopters to ensure continued success is achieved. Preparing adopters and proper matching is key given the frequency of post adoption behavioral issues among shelter animals ( 36 ). This problem comes down to two key sets of questions:
Given evidence suggesting choice of pet is often tied to factors like appearance more than behavioral considerations ( 37 ), how should shelters best match behavioral issues with potential adopters who can handle the maintenance surrounding those issues to reduce the chance of return ( 36 , 38 , 39 ) and adverse incidents such as bites or escape from a yard?
What risks (i.e., environment impact on biting) ( 40 ) exist in the home that might exacerbate issues surrounding behavior?
Finally, when it comes to animal behavior, especially canine behavior, one of the most critical incidents that can occur is a bite incident since these can result in serious injury to persons and potentially result in liability claims against the shelter ( 41 ). The previously mentioned issues all likely contribute to the probability of a bite incident occurring, but predictions of such events, even in aggregate across a city ( 42 ), are challenging at best. A successful bite prediction system would also pose ethical issues as individuals, shelters, and cities may choose to use such a system to decide which animal's lives should be preemptively ended, to avoid the potential risk and liability. It is critical, therefore, that the predictability of bite incidents increase at the same rate as our ability to reasonably intervene to prevent the incidents.
Adoptions and Special Needs Populations
The core problem with adoptions at shelters is always “how do we get as many animals out to good homes as quickly as possible?.” Of course, as with so many seemingly simple problems, the posing of the question in such a general manner means no obvious solutions present themselves. Properly reframing the question often begins to imply solutions. Preventing the surrender of animals to the shelter system is certainly a key component to assuring positive outcomes for animals and people alike. New programs, such as the Human Animal Support Services project, are focusing heavily on programs aimed at keeping animals out of the shelter altogether and in their original homes whenever possible. Further, this paradigm shift has the potential to profoundly impact positive outcomes for community cats, who may not be best served through adoption. Although adoption is not the only possible positive outcome for all animals that enter a shelter system, for many animals (and the humans who manage the systems of sheltering), it remains an important practical and ethical outcome. Here, we review 3 key areas in adoptions that remain complex and difficult despite extensive efforts in the sheltering community. For a more complete list of these challenge areas, see the American Pets Alive! Documentation on the topic (“American Pets Alive! Resources;” https://americanpetsalive.org/resources ) ( 43 ).
First and most critically, large dogs, often considered to be those weighing over approximately 35 pounds or 16 kilograms, consistently have more difficulty in being adopted ( 44 – 46 ). This can be due to factors such as the general public perception around larger breeds ( 47 , 48 ), city ordinances banning ownership of certain breeds ( 49 ), housing restrictions implemented at the facility level ( 50 ), or concerns around safety, behavior, and compatibility with other home residents ( 51 ). These issues are exacerbated by the difficulty in accurately identifying breed information in shelter animal populations ( 52 ). As a result of these complications around getting large breeds out of shelters, shelters often end up with a stagnant population of these animals that has less turnover than other, easier to adopt categories (puppies of any breed, for example). This can create a perception that the only populations present are these large breed animals. These factors result in many of these animals having long stays and, as mentioned in prior sections, increased stress and overall wellness difficulties that further worsen their adoptable potential. Moreover, animals in the shelter are less likely to behave the way they might otherwise in a home ( 53 ), further decreasing their chances for a positive outcome. A strategy around breaking this cycle and helping large dogs would alleviate significant amounts of trapped resources as site maintenance and housing can create substantial costs and reduce flexibility in serving other populations. The importance of providing an equal opportunity for these large breed dogs to stay in their home is one consideration beyond adoption in strategy design. For example, policies that disallow the use of size of a dog as criteria for access to housing (as discussed above) would help keep these animals out of the shelter system in the first place. Adequate access to resources to behavior training could be another community level intervention that could allow more of these animals to stay in their homes.
Other Special Populations
Beyond these major issues, there are numerous conditions of decreasing commonality that require increasingly complex adaptations of program and policy to accommodate. This article cannot enumerate all such conditions, but the following list, sorted roughly by difficulty, captures some of the most critical special needs populations that require specially trained homes to inhabit, making them more difficult to adopt out:
- Geriatric Animals.
- Animals with Chronic Allergies.
- Hospice Animals.
- Feline Leukemia Virus (FeLV) Cats.
- Kidney Failure Animals.
- Diabetic Animals.
- Behavior Animals.
- Animals with Paralysis and/or Incontinence.
Much of the care, maintenance, and treatment of these populations is well understood, but the problem of placing them in amenable homes is still a significant one. More research around interventions that can increase the likelihood of placement as well as the factors that impact the likelihood of special population placement may provide actionable insights [see ( 54 ) as an example in geriatric animals].
Finally, and significantly, a more thorough understanding of how to match adopters to animals ( 37 , 55 ), how to evaluate homes for safety and longevity of adoption outcomes ( 13 , 56 ), how to optimize placement of animals in homes ( 57 ), and what preferences exist when it comes to adoption practices around marketing, visitation, and engagement is desperately needed. This understanding will likely depend significantly on local cultural distinctions in populations ( 58 , 59 ) and is, therefore, difficult to examine systematically. More best practices around adoption matching and marketing would greatly simplify one of the most critical functions in animal shelters.
Unique Challenges of Cats
Another, potentially less obvious problem in sheltering is the difference in positive outcomes for cats vs. dogs. Best Friends, a national non-profit that provides the most comprehensive summary of annual shelter statistics reports that cats are still dying in shelters at a ratio of 2:1 when compared with dogs ( 60 ) despite approximately one-fourth of US households providing a home for cats ( 61 ). Many shelters consistently report difficulties in adopting out adult cats once they no longer have the appearance of a kitten ( 62 ). Further, shelter or municipal policies around the extermination of community cats ( 63 ) may also be a significant contributor to the numbers of cats not having successful outcomes in shelters.
Approaches to improving live outcomes for cats require shelters to explore ideas outside of the traditional intake to adoption framework. Some strategies that are specifically applicable to cats have been evaluated and shown to be effective such as trap-neuter-return and shelter-neuter-return, which could reduce the number of un-adoptable cats entering the shelter system ( 64 , 65 ), but more research into the social drivers and potential interventions for this issue are warranted. A development of the recognition of the ecology of community cats is an additional issue that is elaborated on in Section Operations.
Medical Conditions
In addition to its capacity as an adoption agency for unowned animals, animal shelters often perform a variety of medical services. These services depend on the location, resources, and risk tolerance each organization has, and it is often difficult for organizations to decide what to treat and what to not treat (whether euthanasia is then called for or not). One critical element of this that remains a challenge for all shelters is the effective, actionable diagnosis of disease [see, ( 66 )]. Many diseases have reliable tests (such as canine parvovirus) while others have a much more complicated history in the development of a reliable test [such as canine distemper, though many strongly claim RNA tests should be considered reliable; ( 67 – 69 )]. Cost is also a critical factor in shelter tests as even a relatively inexpensive (50 dollars) test in an outbreak scenario can be entirely impractical in a population of just a few dozen animals. Further research into low-cost testing is certainly needed for a wide variety of diseases.
Once the disease is identified, shelters often lack the resources for what would be considered “standard” care in a private practice. Some shelters opt to not offer reduced care and, instead, euthanize, while others choose to offer whatever care they can within their own ethical limitations of suffering and quality of life considerations. The need for significantly more research into evidence-based medical guidelines, and especially those that are specifically optimized for triage situations with limited resources and around medical conditions seen in shelters, is widely apparent. Some conditions, such as kitten diarrhea, may be somewhat understood in a general medical sense, but the treatments and time course do not scale appropriately for the model of a medium to large shelter.
Although many diseases could use additional scrutiny for the purposes outlined above, the following are of particular interest due to the costs, in either lives or resources, associated with typical treatment or management (T; indicates specific transmissible disease relevant to Section Disease Transmission):
- (T) Canine parvovirus ( 70 , 71 ).
- (T) Feline panleukemia ( 72 ).
- (T) Canine distemper ( 73 ).
- (T) Feline leukemia virus (FeLV) ( 74 ).
- (T) Feline immunodeficiency virus ( 75 – 78 ).
- Kitten diarrhea ( 79 ).
- Fracture and trauma management.
Disease Transmission
More so than the treatment of disease, the prevention of disease spread in the shelter environment is one of the most challenging, concretely measurable in the form of infection rates, yet ambiguous (difficult to diagnose in source) tasks a shelter may face. Shelters are examples of anthropogenic biological instability due to the housing of transient, displaced mixed-species of animals that may not have prior veterinary care or have been scavenging during times of homelessness ( 80 ). The disease transmission in shelters is further complicated by situation of overcrowding, poor levels of hygiene, and housing of multiple species which can add significant sources of stress for the animals and create a perfect environment for pathogen emergence and transmission ( 80 ). This transmission can quickly lead to a crisis in the shelter ( 81 ). Shelters that treat infectious disease like the canine parvovirus establish isolation areas in which only that disease is treated, but little is known about the ease with which these diseases spread under different quarantine practices.
Although there are many interesting diseases that are typically seen in shelters, some (such as those listed in Section Medical Conditions) are considered more impactful/deadly than others and, therefore, would make excellent targets for more detailed studies of disease spread.
While it is not officially recommended as a best practice ( 82 ), when shelters experience disease outbreaks, some may opt to depopulate, i.e., end the lives of their entire population, ( 83 ) rather than have it persist through many generations of animals flowing through the system. Better understanding of how to stem these outbreaks rapidly, efficiently, safely, in a resource-efficient manner, and given the constraints of a shelter environment (space, staffing, facility design, and the need to maintain normal operations) will allow shelters to avoid mass culling and take an approach that increases lifesaving with more confidence.
Community, Ecology, and Wellness (One Health)
Beyond the scope of the basic operations of a shelter in managing the conditions of individual animals and placing them in appropriate homes, shelters also serve a critical role in the community as providers of services that can enhance public perception and wellbeing ( 84 ). This collaboration requires an engaged community that recognizes the importance of animal welfare in the health and wellness of the larger, shared space. Best practices around establishing this type of engagement are not well identified in the existing body of knowledge. This is further confounded by variation in the distribution of resources and community attitudes in different geographic areas.
As animal shelters continue to evolve in response to societal shifts in attitudes toward animals, the focus of operations are changing from centering on adoptions to centering on the prevention of surrender of animals to the shelter in the first place [see ( 11 ) for a review]. This has already been discussed as it relates to community cats and behavior/health but there are many other human-centered reasons that animals are surrendered to shelters such as guardian health problems, housing insecurity, domestic violence, and many others ( 85 ). Our understanding of how human welfare intersects with animal welfare has the potential to have a dramatic impact on the way shelters operate in their communities. Some communities have hotlines, spay and neuter programs, and other medical/behavioral services that can potentially contribute to this issue, but the efficacy of such systems and the gaps they leave are not well understood. More significant study of the needs of local populations as they relate to shelter success is needed.
Local populations also differ in their perception and support of shelter policies, ethics, and the local system of laws that are intertwined with these efforts. No unified system of ethics is established in animal sheltering, and communities often do not understand the nuances of practices in shelters (especially regarding resource allocations and euthanasia practices). This makes galvanizing community support difficult, even in communities that have achieved remarkably high live release rates. Public perception, messaging, and ethical alignment will undoubtedly continue to be an ever-evolving socio-cultural landscape that is sorely in need of attention.
The mental health of volunteers, staff, and veterinarians ( 86 ) in animal shelters also requires much more attention than it often receives. Individuals that participate in euthanasia are reported to have higher work stress and lower job satisfaction than their counterparts ( 87 ). Suicide rates are significantly higher in the field of animal welfare than other high-stress fields ( 88 , 89 ), and more understanding and support is needing to help those working in these areas receive the help they need to continue to serve the community in a sustainable, healthy manner.
Access to Care
Access to veterinary care is emerging as a critical issue in animal welfare. Access to care is an aspect of the One Health approach to considering animal welfare due to the zoonotic potential of various diseases that can find reservoir in companion animals ( 90 ). In addition to being a risk to public health, lack of access to veterinary care can result in surrender of animals to shelters, stress to the caregiver/family ( 91 ) as well as stress to veterinarians who must counsel caregivers who cannot afford the recommended care ( 92 ). Shelters feel the impact of this as downstream recipients of animals when owners surrender due to an inability to access needed care. This can both drive surrender to shelters and result in a greater financial burden for shelters to meet medical needs that may be complicated by a historic lack of access to preventative or early intervention care. Further, shelters themselves compete in the market to employ veterinary professionals and support staff that may be further complicated by a shortage of veterinarians ( 93 , 94 ).
Access to care can be seen as a problem with multiple causes from cost to lack of transportation to the unequal distribution of veterinary resources across the landscape. Cost was identified as the most common barrier to accessing veterinary care in the Access to Veterinary Care Coalition report on this issue ( 91 ). In the past decade, costs for veterinary care have been outpacing increases in human health care ( 95 ). The average American spends 47% more on equivalent veterinary care today than a decade ago ( 96 ). The functional impact of this increasing cost is that fewer people are seeking care for their pets ( 97 ) resulting in what is considered the greatest current threat to companion animal welfare in the US ( 91 ). More research that identifies efficient, effective, and sustainable solutions to the cost of veterinary care will be key for animal shelters.
Key research questions in access to care can come down to three key areas:
Advances in areas like incremental care or spectrum of care, which are not equivalent but present different perspectives on the issue of cost-benefit analyses in treatment protocols, could reduce costs and prevent shelter surrenders but could also help shelters mitigate the increasing expense of medical treatment for animals in their care.
A deeper understanding of the number of animals surrendered for medical reasons, the types of these conditions and potential treatment routes pre-surrender would also add valuable knowledge to the animal sheltering and animal welfare communities.
Development of community-based solutions that focus on disease prevention when the cost is likely lower than when a disease process is more advanced. This includes the prevention of infectious disease transmission in the community and the development of effective education around other preventable conditions by pet guardians.
Ecology/Environment
The study of the ecology surrounding community cats has received significant attention over the past several years ( 63 , 64 , 98 – 102 ), and debates are likely to continue in this area to determine the most effective ways to ensure the health and safety of community cats and the organisms with which they interact. Additionally, ecological perspectives on the interaction between stray and roaming animals in general and the community are also of interest, but often only actively studied due to concerns over infectious disease spread such as the Rabies virus. Finally, the interaction of wildlife systems with domesticated animals may be of some interest both due to the spread of infectious disease and the more complex interactions these two animal groups may have with one another.
As animal welfare incorporates a One-health approach, further research that identifies strategies to reduce the environmental impact of shelter operations cannot be ignored. Effective ways of cleaning outdoor kennels without contributing to contaminate run-off, ecological disposal of animal waste and the evaluation of how large-scale animal transport can contribute to environmental degradation are just a few examples of the interaction of sheltering and the environment that are open for additional exploration.
In addition to the study of animal-centric, adoption-centric, and community-centric aspects of sheltering, the study of the operations that contribute to the ability of shelters to continually adapt, and advance is of critical importance if we are to have systems robust to disaster and capable of implementing our values and ethics on a global scale. Although blueprints do exist that can guide communities in setting up new shelters and enhancing existing shelters, significant problems remain in the space beyond the distribution of known solution resources. Here, we discuss 4 key operations problem areas with varying levels of complexity.
Data Problems
Shelters need to collect data to know how they are serving their animals, adopters, volunteers, staff, and community, and how to improve operations in all areas of the shelter. While the industry recognizes the need for quality data, significant barriers have been identified such as a lack of training and resources [( 103 ), additionally, see the Associate for Veterinary Informatics (AVI) for additional information on this topic; https://avinformatics.org/ ]. Solutions such as ShelterLuv, Chameleon, and PetPoint for database management go a long way to improving situations for shelters, but the ability to flexibly collect and curate all manner of useful data (including electronic medical records, location-based event history, and other meta-data about entities that comprise shelters) remains an open problem. It is also essential that the prioritization and understanding of the critical importance of data is shared by line staff as well as senior management. When line staff fail to understand the importance of complete data collection this action can be de-prioritized in fast paced shelter environment.
Beyond this, shelters need methods of protecting themselves in the sharing of data with the public, academic institutions, and each other. The public, which support shelters through taxes or donations, show widespread support, for example, for programs that reduce levels of shelter euthanasia shelters ( 104 ). The best practices around of performing data sharing and managing data access for shelters have yet to be established (though some progress has been made in recent months at the Municipal Shelter level). Over time, there have been attempts to create a single authoritative collection of sheltering data but to date, none have achieved high success. The current initiative that has achieved the most progress is Shelters Animals Count (SAC). SAC is a national database that relies on the voluntary participation by shelters and animal rescues to upload monthly sheltering summary statistics. Unfortunately, there is still relatively poor participation. For example, in 2020 there was participation by only 422 municipal shelters, 359 private shelters and 516 rescues ( 105 ). This can be contrasted with a 2014 estimate by the Humane Society of the United States of 3,500 municipal and non-profit shelters and over 10,000 rescue organizations ( 106 ). Despite the move toward increasing transparency in government, only a small handful of states and municipalities require reporting to their state and local governments, with even fewer providing enough clarity as to what should be reported for such reporting to be of use to the wider sheltering community. The result of this paucity and irregularity of data provides a significant challenge to researchers and policymakers in understanding what is happening across the nation regarding sheltering, though the contributions of states in which reporting is mandated effectively have provided a valuable starting point for these efforts.
KPI Problems
Once data is collected, linking that data down to trackable KPIs (Key Performance Indicators) that are useful to shelters in improving outcomes for animals is a challenge in and of itself. The standardization of KPIs and their strict definitions has suffered from some of the disagreement and difficulties surrounding data collection. The most marked attempt to create unified KPIs occurred in 2004 resulting in the Asilomar Accords. The Live Release Rate, and methods of fairly but consistently calculating it materialized as a critical outcome of the Accords ( 107 ). This measure has never been without controversy and is limited, in part, by the wide variance in the various ways in which animal shelters operate in their community and what their priority services are ( 108 ). As the operation of shelters have changed, with more innovative programs designed to prevent animals from ever entering the shelter system appearing, advancements in medical and behavioral interventions, and the geographically biased nature of animal population distributions ( 109 ), the use of a single KPI will likely remain a source of both conflict and difficulty for many shelters. A more diverse set of KPIs will allow for shelters to perform more nuanced comparisons of their successes and failures that will enable better sharing of solutions and resources. What this list of KPIs should entail remains an open problem [see ( 110 )].
Growth Problems
Finally, as some shelters begin to stabilize the animal welfare situation in their cities, adapting to the varying degrees and paces of growth in various organizations to ensure resources are being properly utilized to the benefit of animals and the community is a challenge, to say the least. The field of Health Economics in humans has a rich history ( 111 ), and a similar field in Animal Health Economics ( 112 , 113 ) will likely need to be expanded beyond its traditional focus on production animals so that organizations are not put in a position to blindly guess at the proper allocations or resources toward different intervention programs (such as a canine parvovirus treatment program, FeLV treatment program, behavior program, or kitten foster program).
One particularly challenging program area for shelters to understand in the context of growth, integration, and resource allocation is the management of foster programs. Foster programs have been fantastically successful as a method of expanding the effective capacity of shelters, increasing live outcomes ( 114 ), enhancing community engagement, increasing quality of life of animals in care ( 35 ), and providing special assistance for more difficult to adopt populations. However, a thorough understanding of how to best engage, utilize, and grow foster programs is lacking.
Diversity Equity and Inclusion
Researchers have evidenced that the oppression of non-human animals, disabled humans, and people of color are deeply interconnected ( 115 ). If animal shelters are to continue to function as key members of diverse communities it is essential that they pay increasing attention to issues of diversity, equity, and inclusion in their operations both internal and external. While the community of research in this space has assembled a basic understanding of some inequities that currently exist, many others have yet to be explored in a thorough way. For example, we know that African Americans are underrepresented in leadership positions ( 116 ). The homogeneity of animal shelters is not confined to the workforce alone. Two large survey-based studies found similar results in evaluating the demographics of animal welfare volunteers concluding that most volunteers were White females in the middle to upper middle class ( 117 , 118 ). Questions of why this lack of diversity persists and what successful strategies could be used to improve conditions would be of benefit as representation of communities within organizations that serve them allow those organizations to supply the appropriate services to maximize the community benefit and foster a highly participatory, engaged, fair, enthusiastic, and ethical social system.
Beyond direct engagement with shelters as volunteers or employees, there are fecund areas for research in the provisioning of shelter operations. As increases in public-private partnerships place more animal shelters in the business of providing animal control operations, the enforcement of ordinances becomes a key issue in balancing public demand for action and the ethics and priorities of animal welfare. A recently published commentary on the subject argues that there is inherent bias in the design and enforcement of public policy around animal welfare and urges a shift from enforcement to resource provision ( 119 ). Evaluating policies and enforcement and implementation of these policies and whether biases are leading to unequal burden are not well understood though it is difficult to not draw comparisons to the arena of policing and the long, complicated relationship between marginalized communities and law enforcement personnel. Additional challenges persist in understanding potential inequities between the surrender of animals and the adoption of animals and whether these differences enforce equity imbalances or are based on existing biases and structural inequities ( 120 ).
Public-Private-Academic-Corporate Collaborations
A less visible and virtually unstudied problem in animal sheltering is the ability for organizational entities of different types and with different incentives to collaborate to the benefit of animals, their owners, the community, and each other. The social network analysis of Reese and Ye ( 121 ) is a prime example of the complex collaborative relationships that can emerge between organizations to advance lifesaving in a community. Many questions in this space exist around the best ways for these organizations to interact (i.e., what roles are best served by what organizations, what incentives are best to ensure ethical treatment of all parties, and what restrictions should be put on various types of interactions). Legal restrictions around the use of shelter animals in research may be a barrier that exists to research collaborations between shelters and academic institutions. Dialogue, consensus, and potential legislative change may be needed between animal shelters, the veterinary community, and academia to address the negative consequences of legislation originally intended to protect animals from harm.
Public-private partnerships in other areas of medicine have become increasingly common and valuable ( 122 ), and corporate sponsorship of shelters has become increasingly common. Public-private shelter partnerships are also on the rise with some proposing this structure as the new standard in the field ( 123 ). Academic collaboration with animal shelters, where academic institutions take advantage of the wealth of available subjects and data in shelters, is still a relatively new concept. Though many potential pitfalls exist in these collaborations (including issues with credit attribution, resource allocation, and ethical alignment), the potential to accelerate the state of the art in animal sheltering via these collaborations is huge thanks to the varied strengths of each organizational type.
The seven key areas for research in animal sheltering outlined above are not the only areas that might be of interest to shelter practitioners and their partners. Some additional areas of interest were not mentioned specifically in this manuscript due to the well-researched nature of the topics, the lack of clear definition in the space, and/or their relative distance from the typical practices of an animal shelter. These areas, nonetheless, merit some mention due to their importance to the area of animal welfare research at large and potential intersection with some shelter practices (depending on specific shelter policy, philosophy, and operations).
A variety of interventions have been proposed that might address some of the problems mentioned in this manuscript. On the behavior side, playgroup services have been proposed that may aid in social development and lead to more positive behavioral outcomes for dogs ( 124 ). Moreover, foster programs that take advantage of these and other medical or behavioral services to accelerate positive outcomes for animals deserve significant attention ( 35 , 125 ). Foster programs can serve as an additional reservoir for animal populations, increase community engagement in the shelter system, and encourage positive outcomes for animals in the foster system through positive environmental enrichment in homes. In situations where foster homes are not available, additional environmental enrichment to achieve similar aims may be found through clever building and facility design at the shelter site ( 126 , 127 ). Finally, a variety of programmatic and procedural interventions around lost and found animals, self-service rehoming, and intake-to-placement optimization, and field services optimization that aim to prevent animals from entering the physical shelter facility can serve as systems optimizations that improve outcomes for all parties; though, more research is needed in these areas to examine their efficacy. Each of these intervention areas, and other innovations in sheltering, deserve significantly more attention than can be afforded in this outline, and future work should attempt to address them more directly.
In addition to a variety of community and ecology problems and interventions, ethical problems in the industry of animal sheltering are not specifically addressed in this work as these are not research topics per se , but more in the realm of philosophy. Future work should examine ethical questions surrounding the topics outlined in this manuscript and other sociological research questions related to the ethics of animal shelter practices.
In this work, we present a conceptual organization of topics for research in Animal Sheltering. These topics vary significantly in difficulty and impact but represent a large swath of needed scientific contributions in the literature. Many of these areas are being actively worked upon by various research institutions (i.e., significant work in animal diseases has occurred), but some have received little attention yet (i.e., operations research). Moreover, some of these areas are being examined, but due to resource and/or methodological constraints, progress is slow. By enumerating these problems, the community of researchers attempting to improve the function of shelters for animals, staff, volunteers, and the community can more carefully and wholistically consider the breadth of applicability of their ideas and investigations and hopefully, more productively contribute to the literature.
Author Contributions
The above document was drafted and originated by KH with review, added sections on community cats, DEI, and Access to Care and most edits provided by SN. Figures were generated by KH. Both authors contributed to the article and approved the submitted version.
Funding for publication was provided by the American Pets Alive! Organization, while funding for individual authors and the creation of the work herein was provided by their respective institutions or the authors themselves.
Conflict of Interest
The authors declare that the research was conducted in the absence of any commercial or financial relationships that could be construed as a potential conflict of interest.
Publisher's Note
All claims expressed in this article are solely those of the authors and do not necessarily represent those of their affiliated organizations, or those of the publisher, the editors and the reviewers. Any product that may be evaluated in this article, or claim that may be made by its manufacturer, is not guaranteed or endorsed by the publisher.
Acknowledgments
The authors would like to thank the Human Animal Support Services organization for their support and encouragement. Additionally, we would like to especially thank Dr. Nipuni Ratnayaka for her contributions to and review of some of the ideas in this document, Dr. Elizabeth Davis for her support in the development of these ideas, Rory Adams for his thoughtful comments around the topics in this document, Steve Porter for his commentary around shelter operations, Dr. Ellen Jefferson for her leadership and support of this work, and all of the staff, volunteers, and communities that continue to advance the state of the art in animal sheltering around the world. This work originally appeared in large part in a blog post from November of 2019. The original blog post can be found at: https://medium.com/@amparesearch/critical-problems-for-research-in-animal-sheltering-404395127b35 .
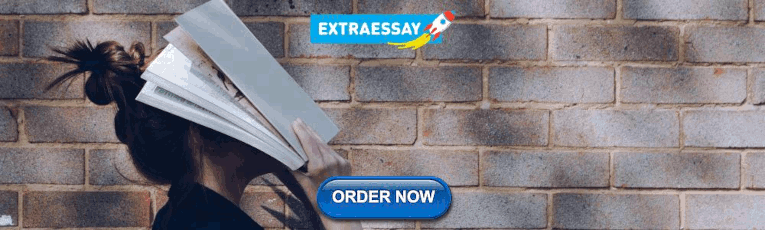
IMAGES
VIDEO
COMMENTS
Animal behaviour is the scientific study of the behaviour of animals. The discipline covers study under experimental conditions, behaviourism, or natural conditions, ethology. Latest Research and ...
Animal Behaviour is published for the Association for the Study of Animal Behaviour in collaboration with the Animal Behavior Society. First published in 1953, Animal Behaviour is a leading international publication and has wide appeal, containing critical reviews, original papers, and research articles on all aspects of animal behaviour. Book Reviews and Books Received sections are also included.
Moving into the big data era, animal behavior, like other fields, can minimize pitfalls by increasing transparency, standardization, and sharing of data, algorithms, and statistical code. As the pace of urbanization, habitat loss, climate change, and other human impacts increase, behavior will often be the first response, either allowing ...
A comparative analysis of animal behavior (e.g., male vs. female groups) has been widely used to elucidate behavior specific to one group since pre-Darwinian times. However, big data generated by ...
The Journal of Experimental Psychology: Animal Learning and Cognition ® publishes experimental and theoretical studies concerning all aspects of animal behavior processes. Studies of associative, nonassociative, cognitive, perceptual, and motivational processes are welcome. The journal emphasizes empirical reports but may include specialized reviews appropriate to the journal's content area.
This editorial initiative focusses on new insights, novel developments, current challenges, latest discoveries, recent advances, and future perspectives in the field of Animal Behavior and Welfare. The Research Topic solicited brief, forward-looking contributions from the editorial board members that describe the state of the art, outlining ...
The history of the application of operant learning to socially significant animal behavior is long and has resulted in tremendously effective technologies benefiting both animals and humans in many different practical settings (Alligood et al., 2017; Edwards & Poling, 2011; Mahoney et al., 2012).Over the past several decades, there has been a weakening of some of the connections between ...
Guidelines for the treatment of animals in behavioural research and teaching. ASAB Ethical Committee, ABS Animal Care Committee. Pages I-XI. View PDF. Previous vol/issue. Next vol/issue. Read the latest articles of Animal Behaviour at ScienceDirect.com, Elsevier's leading platform of peer-reviewed scholarly literature.
Animal Behavior - Science topic. Explore the latest full-text research PDFs, articles, conference papers, preprints and more on ANIMAL BEHAVIOR. Find methods information, sources, references or ...
Clinical animal behaviour is the management of problem animal behaviour, and emerged as a specific scientific discipline about 50 years ago [ 1 ]. As an academic discipline with a strong practical element, it is of interest to many members of the public, researchers and clinicians; many of whom are prepared to offer an opinion on best practice.
Research papers Animal Behaviour publishes original papers relating to all aspects of the behaviour of animals, including humans. Papers may be field, laboratory or theoretical studies. ... Statements on scientific integrity by the Association for the Study of Animal Behaviour and the Animal Behavior Society can be found at, respectively, ...
Animal Cognition is an interdisciplinary journal offering current research on all aspects of animal (and human) cognition in an evolutionary framework. Publishes original empirical and theoretical work, reviews, methods papers, short communications and correspondence. ... Morris Animal Foundation is pleased to invest in equine behavior and ...
Animal Behavior and Cognition, 4(4), 474-498. ... This paper represents a scientific review of what we know about cows in an attempt to place our understanding of them outside of the framework of their use as sources of food, clothing, work, and organ donors. The substantial and growing literature on the psychology of other animals indicating ...
The scientific study of animal behavior is also called ethology, a term used. first by the nineteenth-century French zoologist Isidore Geoffroy Saint Hilaire. but then used with its modern ...
Key Areas for Research Animal Behavior. Animal behavior is one of the most challenging and complex topics in animal sheltering. Leaving aside controversies surrounding the ethics of adopting out animals with known behavior challenges or the ending of the life of an animal, whether for the protection of the public, retribution for an incident, quality of life, or any other justification related ...
The ability to look at animal behavior across populations and subspecies over millions of square kilometers reveals unexpected patterns and enables researchers to ask big-picture questions about the impacts of climate change. Photo courtesy of NPS/Kyle Joly. ... A research paper describing the archive and case studies appears in the November 6, ...
1.1. The Positive Side of Sentience. Although today, the subjective experiences of animals receive considerably more attention than 50 or even 20 years ago, research is still focused on the negative experiences of animals [].Whilst this research has been fundamental in improving many practices involving animals, it has failed to take into account the importance of positive experiences and ...
The Department of Neurobiology, Physiology and Behavior is a vibrant academic unit that investigates the fundamental processes shared by all animals. These include growth, reproduction, movement, response to stimuli, and maintenance of homeostasis. Faculty explore the highly integrated and precisely regulated physiological mechanisms underlying these vital functions.
Transcranial low-level laser therapy enhances learning, memory, and neuroprogenitor cells after traumatic brain injury in mice. The use of transcranial low-level laser (light) therapy (tLLLT) to treat stroke and traumatic brain injury (TBI) is attracting increasing attention. We previously showed that LLLT using an 810-nm laser 4 h after ...
The behavior of living organisms is a visible manifestation of activity of the central nervous system. Thus, the study of behavior is a central feature of contemporary neuroscience research in animals. In some studies, the research emphasizes behavior itself, and the primary goal is to characterize behavior and its environmental determinants. In others, the behavior of an animal may be ...
The institute furthers the understanding of tropical biodiversity and its importance to human welfare, trains students to conduct research in the tropics and promotes conservation by increasing ...
Do forever chemicals also impact animal life or the environment? It's very likely that the same things we are seeing in people could be happening in animals too. Exposures could be affecting species out there and changing ecology. So if we see endocrine disruption occurrences, that same thing is happening out in the environment as well.
The goal of the research topic is to showcase those interdisciplinary studies that reverse the idea of bio-inspired robots by applying engineering methods (theory, simulation, or experiment) to study animal behaviour. After the peer review process, this topic accepted 5 articles, including 4 research articles and 1 perspective article.
Introduction. Animal Sheltering in Western society, in some form, has existed since the mid-1800's (with the creation of both the Royal Society for the Prevention of Cruelty to Animals and the American Society for the Prevention of Cruelty to Animals in 1824 and 1866, respectively) and has been a constantly evolving field to both the benefit (1, 2) and detriment (3, 4), of its stakeholders ...