- Skip to main content
- Skip to primary sidebar
- Skip to footer
- QuestionPro

- Solutions Industries Gaming Automotive Sports and events Education Government Travel & Hospitality Financial Services Healthcare Cannabis Technology Use Case NPS+ Communities Audience Contactless surveys Mobile LivePolls Member Experience GDPR Positive People Science 360 Feedback Surveys
- Resources Blog eBooks Survey Templates Case Studies Training Help center

Home Market Research
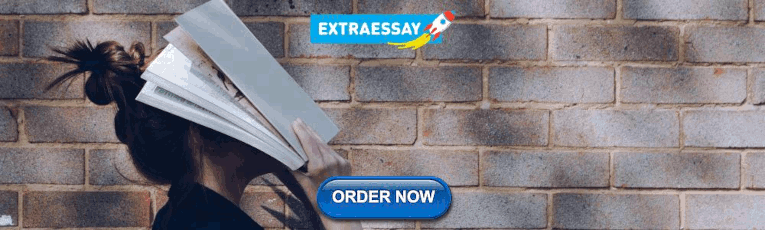
Research Hypothesis: What It Is, Types + How to Develop?
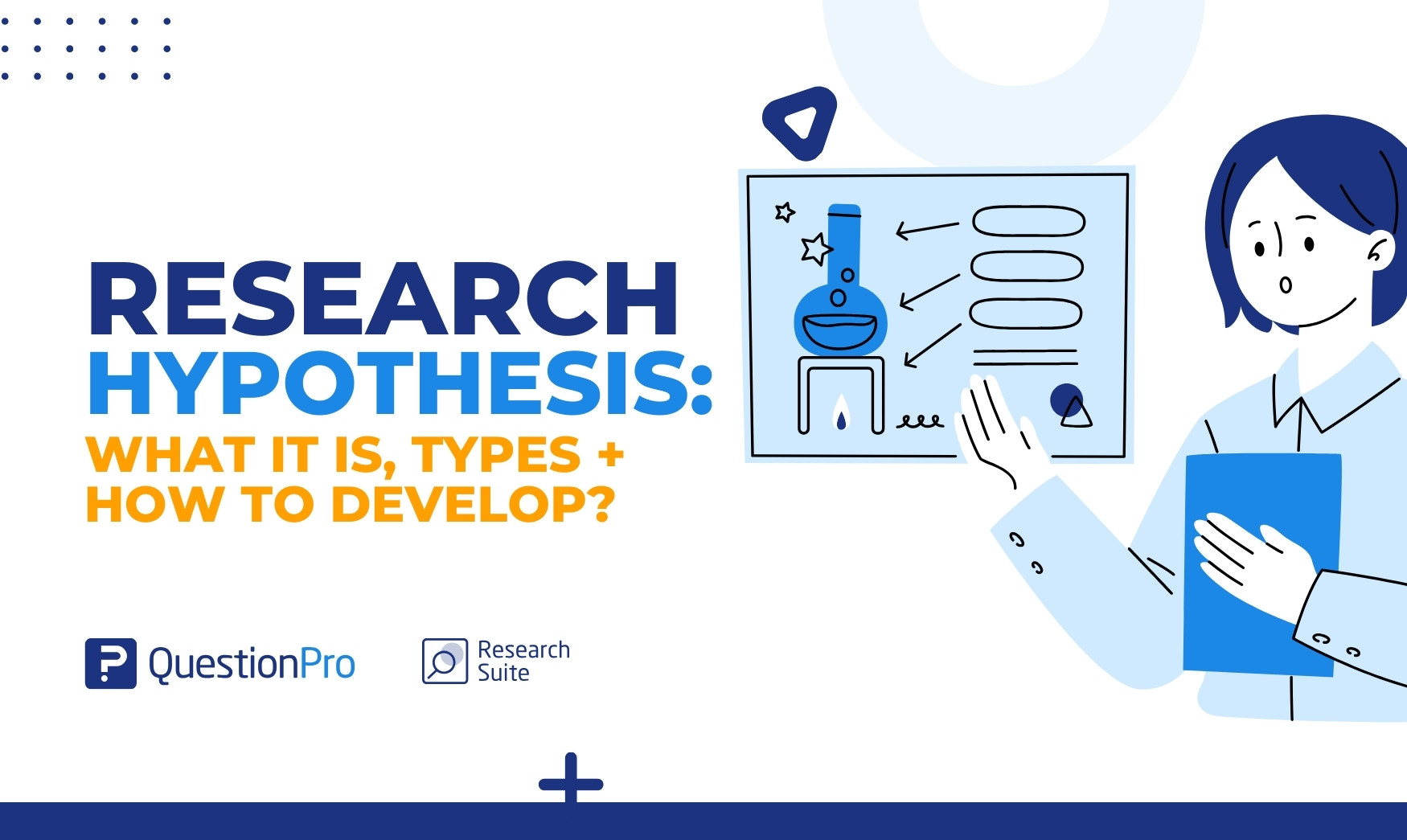
A research study starts with a question. Researchers worldwide ask questions and create research hypotheses. The effectiveness of research relies on developing a good research hypothesis. Examples of research hypotheses can guide researchers in writing effective ones.
In this blog, we’ll learn what a research hypothesis is, why it’s important in research, and the different types used in science. We’ll also guide you through creating your research hypothesis and discussing ways to test and evaluate it.
What is a Research Hypothesis?
A hypothesis is like a guess or idea that you suggest to check if it’s true. A research hypothesis is a statement that brings up a question and predicts what might happen.
It’s really important in the scientific method and is used in experiments to figure things out. Essentially, it’s an educated guess about how things are connected in the research.
A research hypothesis usually includes pointing out the independent variable (the thing they’re changing or studying) and the dependent variable (the result they’re measuring or watching). It helps plan how to gather and analyze data to see if there’s evidence to support or deny the expected connection between these variables.
Importance of Hypothesis in Research
Hypotheses are really important in research. They help design studies, allow for practical testing, and add to our scientific knowledge. Their main role is to organize research projects, making them purposeful, focused, and valuable to the scientific community. Let’s look at some key reasons why they matter:
- A research hypothesis helps test theories.
A hypothesis plays a pivotal role in the scientific method by providing a basis for testing existing theories. For example, a hypothesis might test the predictive power of a psychological theory on human behavior.
- It serves as a great platform for investigation activities.
It serves as a launching pad for investigation activities, which offers researchers a clear starting point. A research hypothesis can explore the relationship between exercise and stress reduction.
- Hypothesis guides the research work or study.
A well-formulated hypothesis guides the entire research process. It ensures that the study remains focused and purposeful. For instance, a hypothesis about the impact of social media on interpersonal relationships provides clear guidance for a study.
- Hypothesis sometimes suggests theories.
In some cases, a hypothesis can suggest new theories or modifications to existing ones. For example, a hypothesis testing the effectiveness of a new drug might prompt a reconsideration of current medical theories.
- It helps in knowing the data needs.
A hypothesis clarifies the data requirements for a study, ensuring that researchers collect the necessary information—a hypothesis guiding the collection of demographic data to analyze the influence of age on a particular phenomenon.
- The hypothesis explains social phenomena.
Hypotheses are instrumental in explaining complex social phenomena. For instance, a hypothesis might explore the relationship between economic factors and crime rates in a given community.
- Hypothesis provides a relationship between phenomena for empirical Testing.
Hypotheses establish clear relationships between phenomena, paving the way for empirical testing. An example could be a hypothesis exploring the correlation between sleep patterns and academic performance.
- It helps in knowing the most suitable analysis technique.
A hypothesis guides researchers in selecting the most appropriate analysis techniques for their data. For example, a hypothesis focusing on the effectiveness of a teaching method may lead to the choice of statistical analyses best suited for educational research.
Characteristics of a Good Research Hypothesis
A hypothesis is a specific idea that you can test in a study. It often comes from looking at past research and theories. A good hypothesis usually starts with a research question that you can explore through background research. For it to be effective, consider these key characteristics:
- Clear and Focused Language: A good hypothesis uses clear and focused language to avoid confusion and ensure everyone understands it.
- Related to the Research Topic: The hypothesis should directly relate to the research topic, acting as a bridge between the specific question and the broader study.
- Testable: An effective hypothesis can be tested, meaning its prediction can be checked with real data to support or challenge the proposed relationship.
- Potential for Exploration: A good hypothesis often comes from a research question that invites further exploration. Doing background research helps find gaps and potential areas to investigate.
- Includes Variables: The hypothesis should clearly state both the independent and dependent variables, specifying the factors being studied and the expected outcomes.
- Ethical Considerations: Check if variables can be manipulated without breaking ethical standards. It’s crucial to maintain ethical research practices.
- Predicts Outcomes: The hypothesis should predict the expected relationship and outcome, acting as a roadmap for the study and guiding data collection and analysis.
- Simple and Concise: A good hypothesis avoids unnecessary complexity and is simple and concise, expressing the essence of the proposed relationship clearly.
- Clear and Assumption-Free: The hypothesis should be clear and free from assumptions about the reader’s prior knowledge, ensuring universal understanding.
- Observable and Testable Results: A strong hypothesis implies research that produces observable and testable results, making sure the study’s outcomes can be effectively measured and analyzed.
When you use these characteristics as a checklist, it can help you create a good research hypothesis. It’ll guide improving and strengthening the hypothesis, identifying any weaknesses, and making necessary changes. Crafting a hypothesis with these features helps you conduct a thorough and insightful research study.
Types of Research Hypotheses
The research hypothesis comes in various types, each serving a specific purpose in guiding the scientific investigation. Knowing the differences will make it easier for you to create your own hypothesis. Here’s an overview of the common types:
01. Null Hypothesis
The null hypothesis states that there is no connection between two considered variables or that two groups are unrelated. As discussed earlier, a hypothesis is an unproven assumption lacking sufficient supporting data. It serves as the statement researchers aim to disprove. It is testable, verifiable, and can be rejected.
For example, if you’re studying the relationship between Project A and Project B, assuming both projects are of equal standard is your null hypothesis. It needs to be specific for your study.
02. Alternative Hypothesis
The alternative hypothesis is basically another option to the null hypothesis. It involves looking for a significant change or alternative that could lead you to reject the null hypothesis. It’s a different idea compared to the null hypothesis.
When you create a null hypothesis, you’re making an educated guess about whether something is true or if there’s a connection between that thing and another variable. If the null view suggests something is correct, the alternative hypothesis says it’s incorrect.
For instance, if your null hypothesis is “I’m going to be $1000 richer,” the alternative hypothesis would be “I’m not going to get $1000 or be richer.”
03. Directional Hypothesis
The directional hypothesis predicts the direction of the relationship between independent and dependent variables. They specify whether the effect will be positive or negative.
If you increase your study hours, you will experience a positive association with your exam scores. This hypothesis suggests that as you increase the independent variable (study hours), there will also be an increase in the dependent variable (exam scores).
04. Non-directional Hypothesis
The non-directional hypothesis predicts the existence of a relationship between variables but does not specify the direction of the effect. It suggests that there will be a significant difference or relationship, but it does not predict the nature of that difference.
For example, you will find no notable difference in test scores between students who receive the educational intervention and those who do not. However, once you compare the test scores of the two groups, you will notice an important difference.
05. Simple Hypothesis
A simple hypothesis predicts a relationship between one dependent variable and one independent variable without specifying the nature of that relationship. It’s simple and usually used when we don’t know much about how the two things are connected.
For example, if you adopt effective study habits, you will achieve higher exam scores than those with poor study habits.
06. Complex Hypothesis
A complex hypothesis is an idea that specifies a relationship between multiple independent and dependent variables. It is a more detailed idea than a simple hypothesis.
While a simple view suggests a straightforward cause-and-effect relationship between two things, a complex hypothesis involves many factors and how they’re connected to each other.
For example, when you increase your study time, you tend to achieve higher exam scores. The connection between your study time and exam performance is affected by various factors, including the quality of your sleep, your motivation levels, and the effectiveness of your study techniques.
If you sleep well, stay highly motivated, and use effective study strategies, you may observe a more robust positive correlation between the time you spend studying and your exam scores, unlike those who may lack these factors.
07. Associative Hypothesis
An associative hypothesis proposes a connection between two things without saying that one causes the other. Basically, it suggests that when one thing changes, the other changes too, but it doesn’t claim that one thing is causing the change in the other.
For example, you will likely notice higher exam scores when you increase your study time. You can recognize an association between your study time and exam scores in this scenario.
Your hypothesis acknowledges a relationship between the two variables—your study time and exam scores—without asserting that increased study time directly causes higher exam scores. You need to consider that other factors, like motivation or learning style, could affect the observed association.
08. Causal Hypothesis
A causal hypothesis proposes a cause-and-effect relationship between two variables. It suggests that changes in one variable directly cause changes in another variable.
For example, when you increase your study time, you experience higher exam scores. This hypothesis suggests a direct cause-and-effect relationship, indicating that the more time you spend studying, the higher your exam scores. It assumes that changes in your study time directly influence changes in your exam performance.
09. Empirical Hypothesis
An empirical hypothesis is a statement based on things we can see and measure. It comes from direct observation or experiments and can be tested with real-world evidence. If an experiment proves a theory, it supports the idea and shows it’s not just a guess. This makes the statement more reliable than a wild guess.
For example, if you increase the dosage of a certain medication, you might observe a quicker recovery time for patients. Imagine you’re in charge of a clinical trial. In this trial, patients are given varying dosages of the medication, and you measure and compare their recovery times. This allows you to directly see the effects of different dosages on how fast patients recover.
This way, you can create a research hypothesis: “Increasing the dosage of a certain medication will lead to a faster recovery time for patients.”
10. Statistical Hypothesis
A statistical hypothesis is a statement or assumption about a population parameter that is the subject of an investigation. It serves as the basis for statistical analysis and testing. It is often tested using statistical methods to draw inferences about the larger population.
In a hypothesis test, statistical evidence is collected to either reject the null hypothesis in favor of the alternative hypothesis or fail to reject the null hypothesis due to insufficient evidence.
For example, let’s say you’re testing a new medicine. Your hypothesis could be that the medicine doesn’t really help patients get better. So, you collect data and use statistics to see if your guess is right or if the medicine actually makes a difference.
If the data strongly shows that the medicine does help, you say your guess was wrong, and the medicine does make a difference. But if the proof isn’t strong enough, you can stick with your original guess because you didn’t get enough evidence to change your mind.
How to Develop a Research Hypotheses?
Step 1: identify your research problem or topic..
Define the area of interest or the problem you want to investigate. Make sure it’s clear and well-defined.
Start by asking a question about your chosen topic. Consider the limitations of your research and create a straightforward problem related to your topic. Once you’ve done that, you can develop and test a hypothesis with evidence.
Step 2: Conduct a literature review
Review existing literature related to your research problem. This will help you understand the current state of knowledge in the field, identify gaps, and build a foundation for your hypothesis. Consider the following questions:
- What existing research has been conducted on your chosen topic?
- Are there any gaps or unanswered questions in the current literature?
- How will the existing literature contribute to the foundation of your research?
Step 3: Formulate your research question
Based on your literature review, create a specific and concise research question that addresses your identified problem. Your research question should be clear, focused, and relevant to your field of study.
Step 4: Identify variables
Determine the key variables involved in your research question. Variables are the factors or phenomena that you will study and manipulate to test your hypothesis.
- Independent Variable: The variable you manipulate or control.
- Dependent Variable: The variable you measure to observe the effect of the independent variable.
Step 5: State the Null hypothesis
The null hypothesis is a statement that there is no significant difference or effect. It serves as a baseline for comparison with the alternative hypothesis.
Step 6: Select appropriate methods for testing the hypothesis
Choose research methods that align with your study objectives, such as experiments, surveys, or observational studies. The selected methods enable you to test your research hypothesis effectively.
Creating a research hypothesis usually takes more than one try. Expect to make changes as you collect data. It’s normal to test and say no to a few hypotheses before you find the right answer to your research question.
Testing and Evaluating Hypotheses
Testing hypotheses is a really important part of research. It’s like the practical side of things. Here, real-world evidence will help you determine how different things are connected. Let’s explore the main steps in hypothesis testing:
- State your research hypothesis.
Before testing, clearly articulate your research hypothesis. This involves framing both a null hypothesis, suggesting no significant effect or relationship, and an alternative hypothesis, proposing the expected outcome.
- Collect data strategically.
Plan how you will gather information in a way that fits your study. Make sure your data collection method matches the things you’re studying.
Whether through surveys, observations, or experiments, this step demands precision and adherence to the established methodology. The quality of data collected directly influences the credibility of study outcomes.
- Perform an appropriate statistical test.
Choose a statistical test that aligns with the nature of your data and the hypotheses being tested. Whether it’s a t-test, chi-square test, ANOVA, or regression analysis, selecting the right statistical tool is paramount for accurate and reliable results.
- Decide if your idea was right or wrong.
Following the statistical analysis, evaluate the results in the context of your null hypothesis. You need to decide if you should reject your null hypothesis or not.
- Share what you found.
When discussing what you found in your research, be clear and organized. Say whether your idea was supported or not, and talk about what your results mean. Also, mention any limits to your study and suggest ideas for future research.
The Role of QuestionPro to Develop a Good Research Hypothesis
QuestionPro is a survey and research platform that provides tools for creating, distributing, and analyzing surveys. It plays a crucial role in the research process, especially when you’re in the initial stages of hypothesis development. Here’s how QuestionPro can help you to develop a good research hypothesis:
- Survey design and data collection: You can use the platform to create targeted questions that help you gather relevant data.
- Exploratory research: Through surveys and feedback mechanisms on QuestionPro, you can conduct exploratory research to understand the landscape of a particular subject.
- Literature review and background research: QuestionPro surveys can collect sample population opinions, experiences, and preferences. This data and a thorough literature evaluation can help you generate a well-grounded hypothesis by improving your research knowledge.
- Identifying variables: Using targeted survey questions, you can identify relevant variables related to their research topic.
- Testing assumptions: You can use surveys to informally test certain assumptions or hypotheses before formalizing a research hypothesis.
- Data analysis tools: QuestionPro provides tools for analyzing survey data. You can use these tools to identify the collected data’s patterns, correlations, or trends.
- Refining your hypotheses: As you collect data through QuestionPro, you can adjust your hypotheses based on the real-world responses you receive.
A research hypothesis is like a guide for researchers in science. It’s a well-thought-out idea that has been thoroughly tested. This idea is crucial as researchers can explore different fields, such as medicine, social sciences, and natural sciences. The research hypothesis links theories to real-world evidence and gives researchers a clear path to explore and make discoveries.
QuestionPro Research Suite is a helpful tool for researchers. It makes creating surveys, collecting data, and analyzing information easily. It supports all kinds of research, from exploring new ideas to forming hypotheses. With a focus on using data, it helps researchers do their best work.
Are you interested in learning more about QuestionPro Research Suite? Take advantage of QuestionPro’s free trial to get an initial look at its capabilities and realize the full potential of your research efforts.
LEARN MORE FREE TRIAL
MORE LIKE THIS
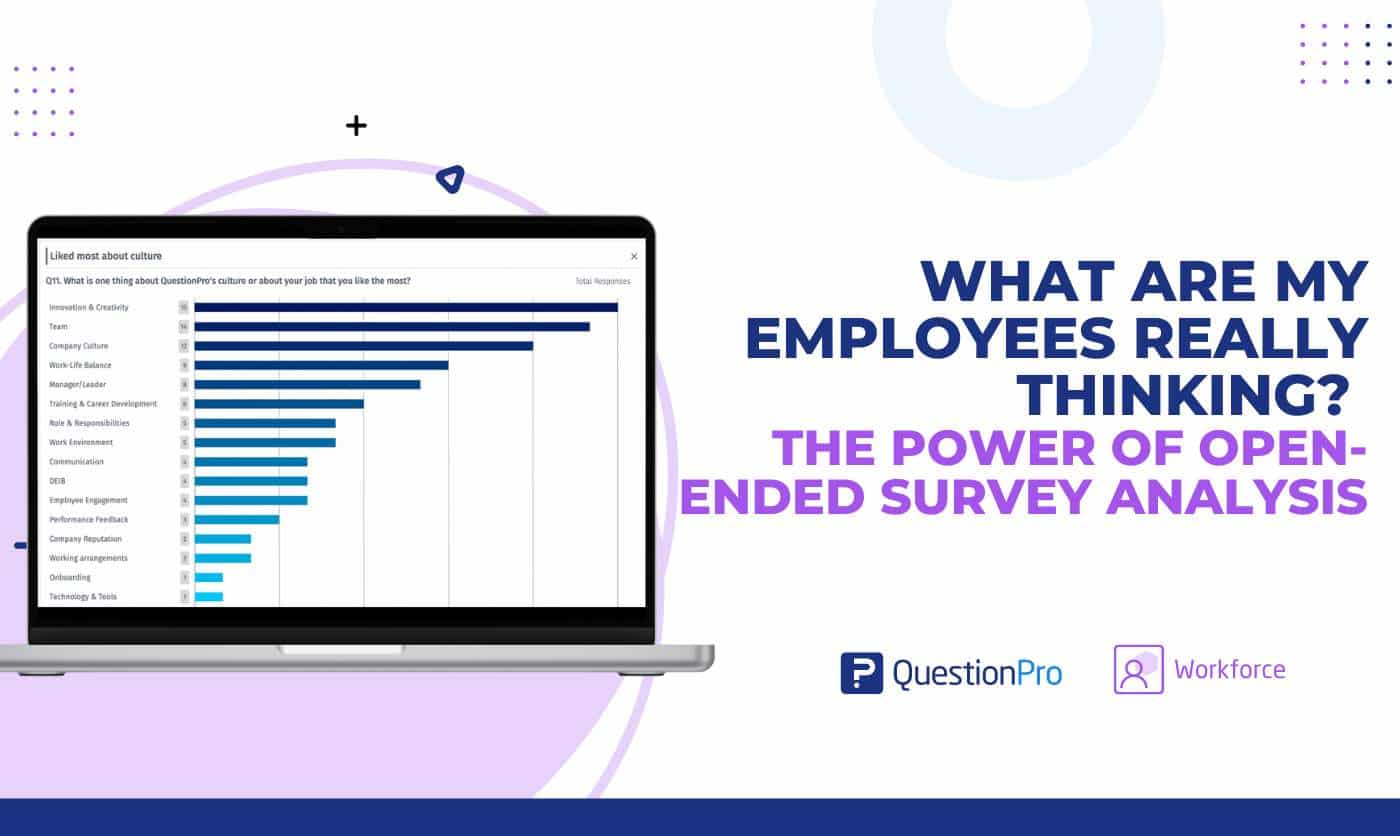
What Are My Employees Really Thinking? The Power of Open-ended Survey Analysis
May 24, 2024
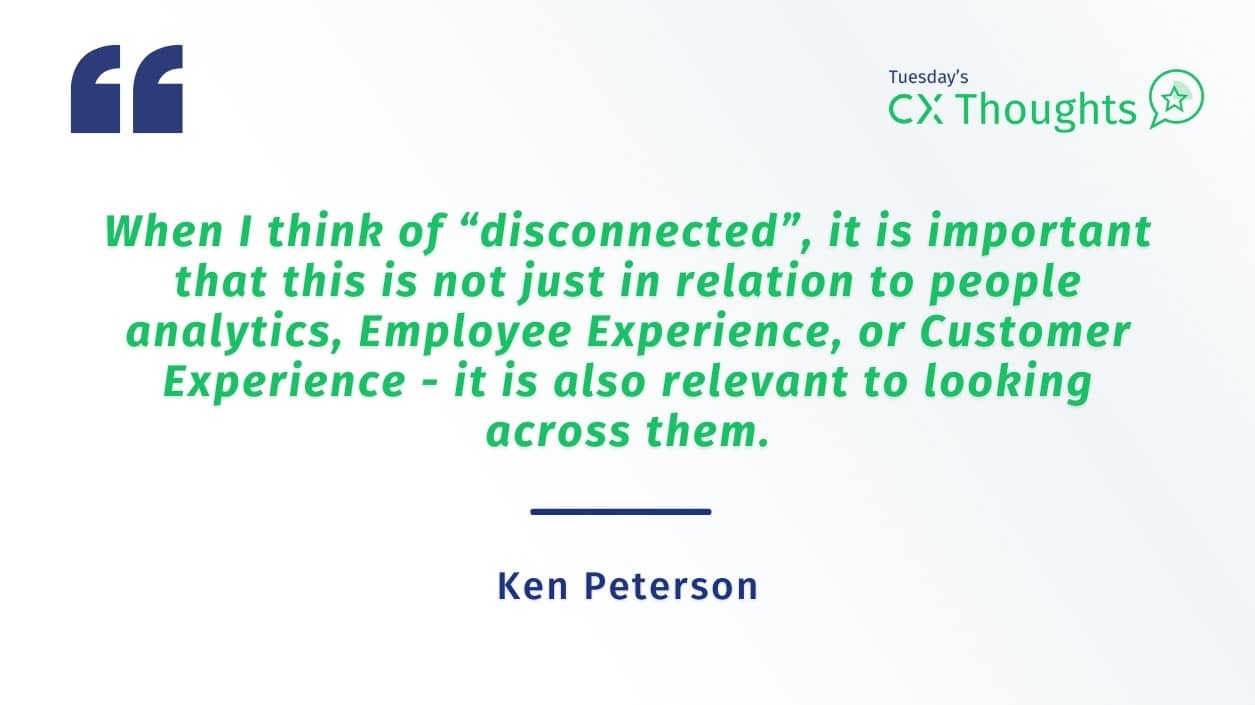
I Am Disconnected – Tuesday CX Thoughts
May 21, 2024
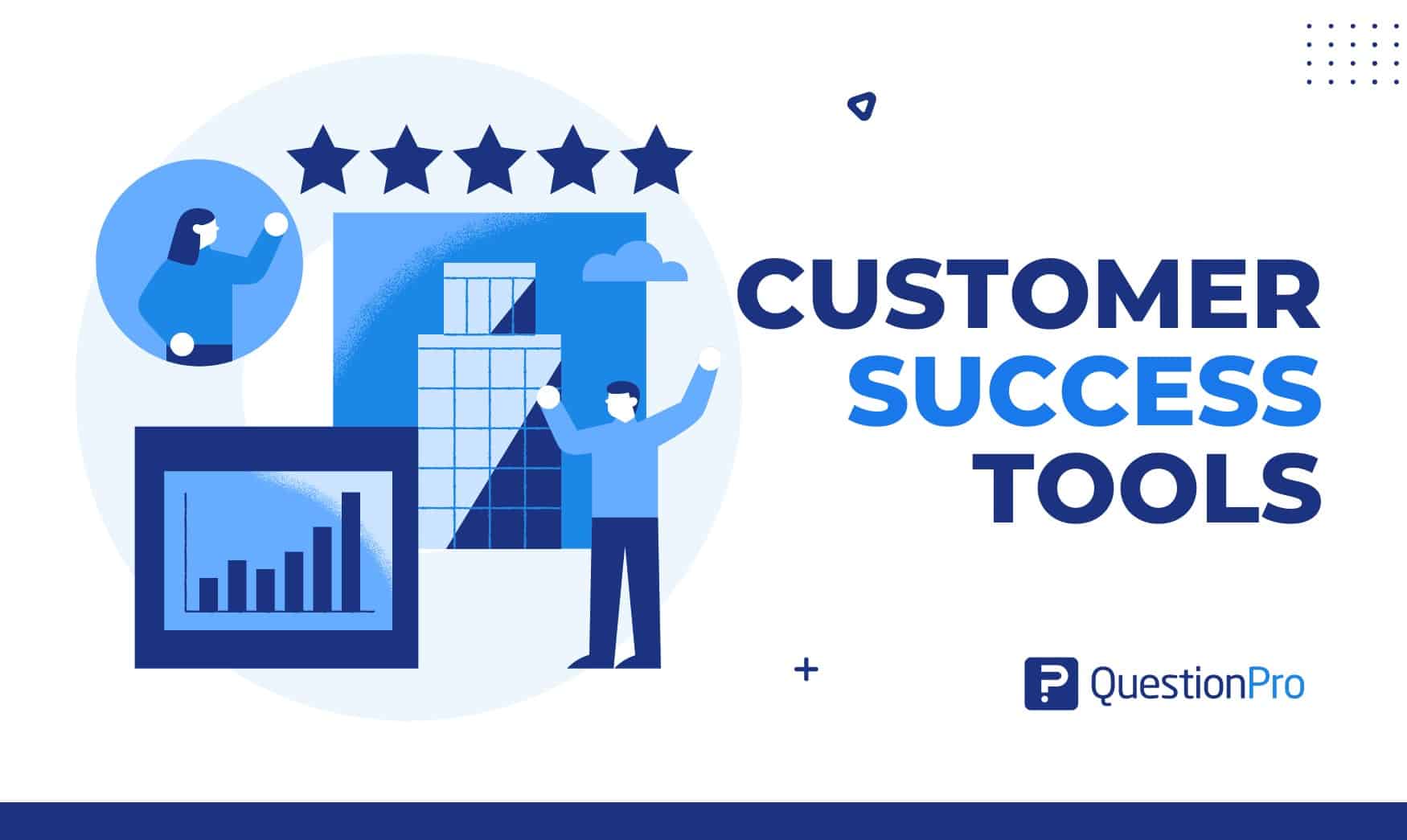
20 Best Customer Success Tools of 2024
May 20, 2024
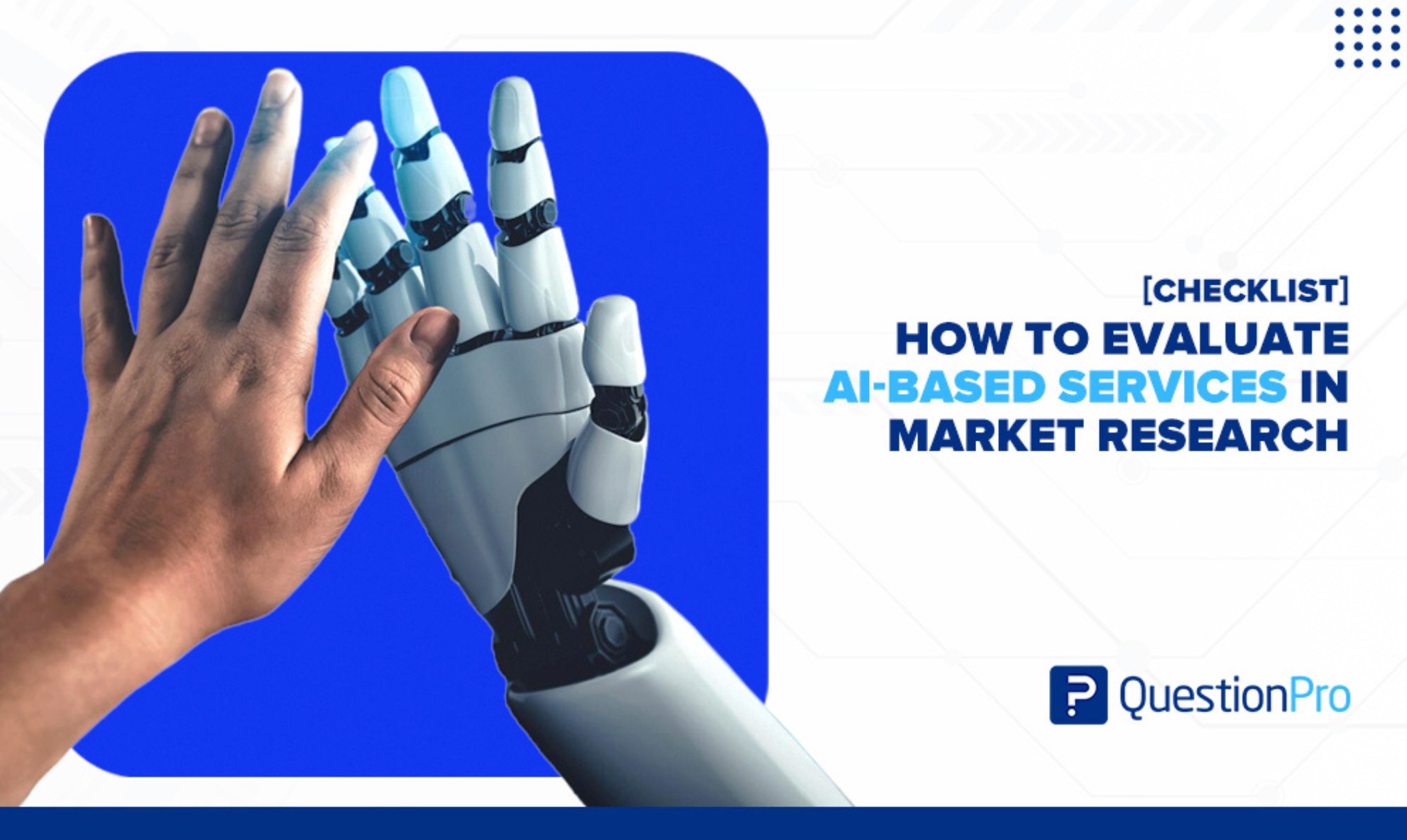
AI-Based Services Buying Guide for Market Research (based on ESOMAR’s 20 Questions)
Other categories.
- Academic Research
- Artificial Intelligence
- Assessments
- Brand Awareness
- Case Studies
- Communities
- Consumer Insights
- Customer effort score
- Customer Engagement
- Customer Experience
- Customer Loyalty
- Customer Research
- Customer Satisfaction
- Employee Benefits
- Employee Engagement
- Employee Retention
- Friday Five
- General Data Protection Regulation
- Insights Hub
- Life@QuestionPro
- Market Research
- Mobile diaries
- Mobile Surveys
- New Features
- Online Communities
- Question Types
- Questionnaire
- QuestionPro Products
- Release Notes
- Research Tools and Apps
- Revenue at Risk
- Survey Templates
- Training Tips
- Uncategorized
- Video Learning Series
- What’s Coming Up
- Workforce Intelligence
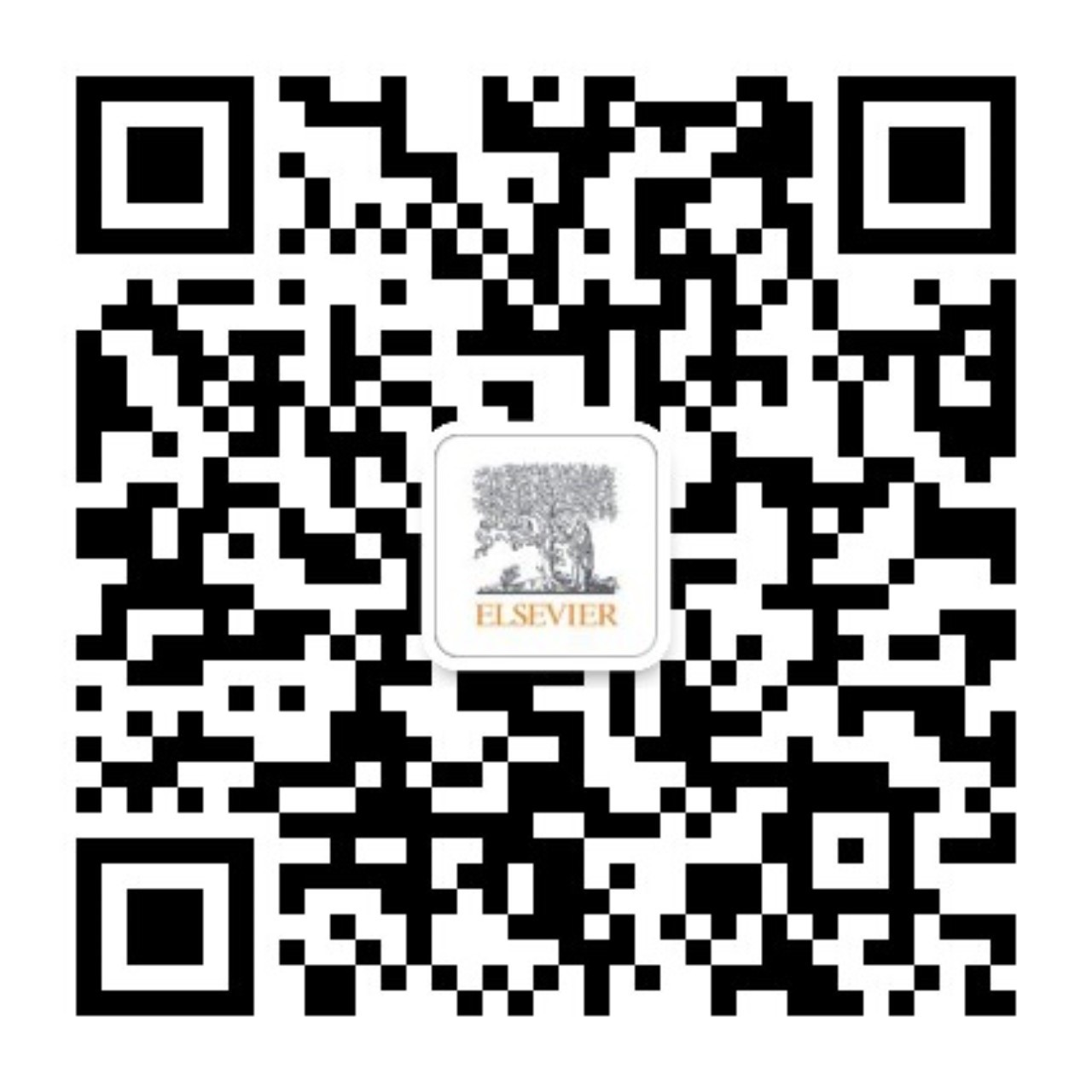
- Manuscript Preparation
What is and How to Write a Good Hypothesis in Research?
- 4 minute read
- 317.8K views
Table of Contents
One of the most important aspects of conducting research is constructing a strong hypothesis. But what makes a hypothesis in research effective? In this article, we’ll look at the difference between a hypothesis and a research question, as well as the elements of a good hypothesis in research. We’ll also include some examples of effective hypotheses, and what pitfalls to avoid.
What is a Hypothesis in Research?
Simply put, a hypothesis is a research question that also includes the predicted or expected result of the research. Without a hypothesis, there can be no basis for a scientific or research experiment. As such, it is critical that you carefully construct your hypothesis by being deliberate and thorough, even before you set pen to paper. Unless your hypothesis is clearly and carefully constructed, any flaw can have an adverse, and even grave, effect on the quality of your experiment and its subsequent results.
Research Question vs Hypothesis
It’s easy to confuse research questions with hypotheses, and vice versa. While they’re both critical to the Scientific Method, they have very specific differences. Primarily, a research question, just like a hypothesis, is focused and concise. But a hypothesis includes a prediction based on the proposed research, and is designed to forecast the relationship of and between two (or more) variables. Research questions are open-ended, and invite debate and discussion, while hypotheses are closed, e.g. “The relationship between A and B will be C.”
A hypothesis is generally used if your research topic is fairly well established, and you are relatively certain about the relationship between the variables that will be presented in your research. Since a hypothesis is ideally suited for experimental studies, it will, by its very existence, affect the design of your experiment. The research question is typically used for new topics that have not yet been researched extensively. Here, the relationship between different variables is less known. There is no prediction made, but there may be variables explored. The research question can be casual in nature, simply trying to understand if a relationship even exists, descriptive or comparative.
How to Write Hypothesis in Research
Writing an effective hypothesis starts before you even begin to type. Like any task, preparation is key, so you start first by conducting research yourself, and reading all you can about the topic that you plan to research. From there, you’ll gain the knowledge you need to understand where your focus within the topic will lie.
Remember that a hypothesis is a prediction of the relationship that exists between two or more variables. Your job is to write a hypothesis, and design the research, to “prove” whether or not your prediction is correct. A common pitfall is to use judgments that are subjective and inappropriate for the construction of a hypothesis. It’s important to keep the focus and language of your hypothesis objective.
An effective hypothesis in research is clearly and concisely written, and any terms or definitions clarified and defined. Specific language must also be used to avoid any generalities or assumptions.
Use the following points as a checklist to evaluate the effectiveness of your research hypothesis:
- Predicts the relationship and outcome
- Simple and concise – avoid wordiness
- Clear with no ambiguity or assumptions about the readers’ knowledge
- Observable and testable results
- Relevant and specific to the research question or problem
Research Hypothesis Example
Perhaps the best way to evaluate whether or not your hypothesis is effective is to compare it to those of your colleagues in the field. There is no need to reinvent the wheel when it comes to writing a powerful research hypothesis. As you’re reading and preparing your hypothesis, you’ll also read other hypotheses. These can help guide you on what works, and what doesn’t, when it comes to writing a strong research hypothesis.
Here are a few generic examples to get you started.
Eating an apple each day, after the age of 60, will result in a reduction of frequency of physician visits.
Budget airlines are more likely to receive more customer complaints. A budget airline is defined as an airline that offers lower fares and fewer amenities than a traditional full-service airline. (Note that the term “budget airline” is included in the hypothesis.
Workplaces that offer flexible working hours report higher levels of employee job satisfaction than workplaces with fixed hours.
Each of the above examples are specific, observable and measurable, and the statement of prediction can be verified or shown to be false by utilizing standard experimental practices. It should be noted, however, that often your hypothesis will change as your research progresses.
Language Editing Plus
Elsevier’s Language Editing Plus service can help ensure that your research hypothesis is well-designed, and articulates your research and conclusions. Our most comprehensive editing package, you can count on a thorough language review by native-English speakers who are PhDs or PhD candidates. We’ll check for effective logic and flow of your manuscript, as well as document formatting for your chosen journal, reference checks, and much more.
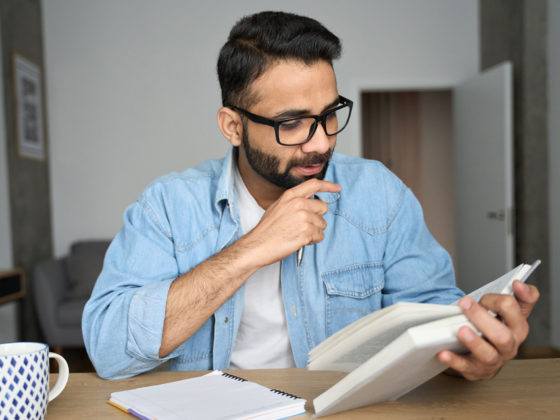
- Research Process
Systematic Literature Review or Literature Review?
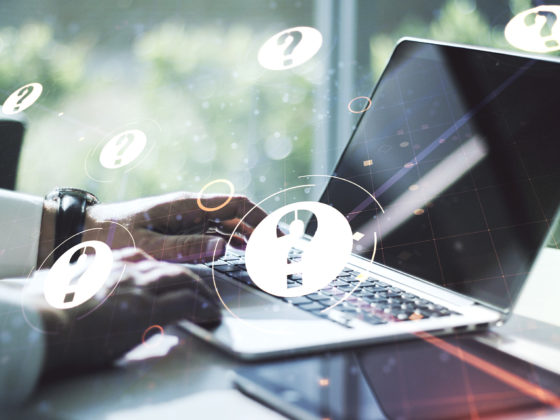
What is a Problem Statement? [with examples]
You may also like.
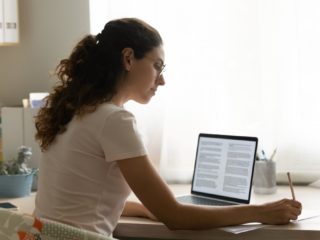
Make Hook, Line, and Sinker: The Art of Crafting Engaging Introductions
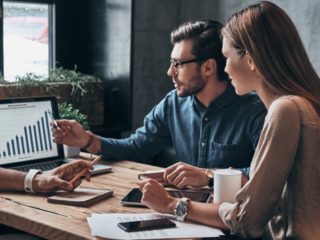
Can Describing Study Limitations Improve the Quality of Your Paper?
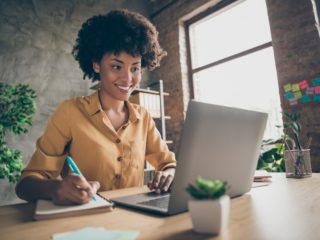
A Guide to Crafting Shorter, Impactful Sentences in Academic Writing
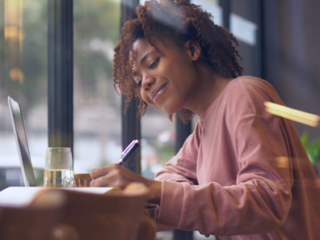
6 Steps to Write an Excellent Discussion in Your Manuscript
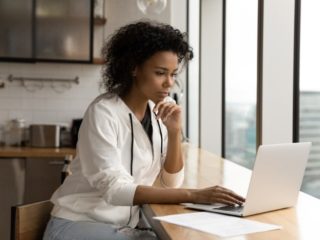
How to Write Clear and Crisp Civil Engineering Papers? Here are 5 Key Tips to Consider
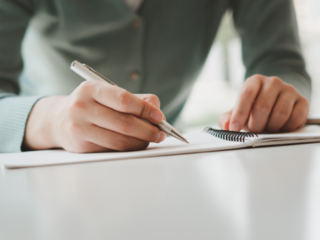
The Clear Path to An Impactful Paper: ②
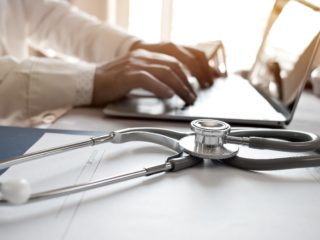
The Essentials of Writing to Communicate Research in Medicine
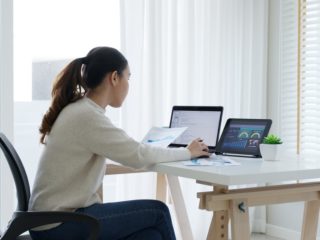
Changing Lines: Sentence Patterns in Academic Writing
Input your search keywords and press Enter.
- Bipolar Disorder
- Therapy Center
- When To See a Therapist
- Types of Therapy
- Best Online Therapy
- Best Couples Therapy
- Best Family Therapy
- Managing Stress
- Sleep and Dreaming
- Understanding Emotions
- Self-Improvement
- Healthy Relationships
- Student Resources
- Personality Types
- Guided Meditations
- Verywell Mind Insights
- 2024 Verywell Mind 25
- Mental Health in the Classroom
- Editorial Process
- Meet Our Review Board
- Crisis Support
How to Write a Great Hypothesis
Hypothesis Definition, Format, Examples, and Tips
Kendra Cherry, MS, is a psychosocial rehabilitation specialist, psychology educator, and author of the "Everything Psychology Book."
:max_bytes(150000):strip_icc():format(webp)/IMG_9791-89504ab694d54b66bbd72cb84ffb860e.jpg)
Amy Morin, LCSW, is a psychotherapist and international bestselling author. Her books, including "13 Things Mentally Strong People Don't Do," have been translated into more than 40 languages. Her TEDx talk, "The Secret of Becoming Mentally Strong," is one of the most viewed talks of all time.
:max_bytes(150000):strip_icc():format(webp)/VW-MIND-Amy-2b338105f1ee493f94d7e333e410fa76.jpg)
Verywell / Alex Dos Diaz
- The Scientific Method
Hypothesis Format
Falsifiability of a hypothesis.
- Operationalization
Hypothesis Types
Hypotheses examples.
- Collecting Data
A hypothesis is a tentative statement about the relationship between two or more variables. It is a specific, testable prediction about what you expect to happen in a study. It is a preliminary answer to your question that helps guide the research process.
Consider a study designed to examine the relationship between sleep deprivation and test performance. The hypothesis might be: "This study is designed to assess the hypothesis that sleep-deprived people will perform worse on a test than individuals who are not sleep-deprived."
At a Glance
A hypothesis is crucial to scientific research because it offers a clear direction for what the researchers are looking to find. This allows them to design experiments to test their predictions and add to our scientific knowledge about the world. This article explores how a hypothesis is used in psychology research, how to write a good hypothesis, and the different types of hypotheses you might use.
The Hypothesis in the Scientific Method
In the scientific method , whether it involves research in psychology, biology, or some other area, a hypothesis represents what the researchers think will happen in an experiment. The scientific method involves the following steps:
- Forming a question
- Performing background research
- Creating a hypothesis
- Designing an experiment
- Collecting data
- Analyzing the results
- Drawing conclusions
- Communicating the results
The hypothesis is a prediction, but it involves more than a guess. Most of the time, the hypothesis begins with a question which is then explored through background research. At this point, researchers then begin to develop a testable hypothesis.
Unless you are creating an exploratory study, your hypothesis should always explain what you expect to happen.
In a study exploring the effects of a particular drug, the hypothesis might be that researchers expect the drug to have some type of effect on the symptoms of a specific illness. In psychology, the hypothesis might focus on how a certain aspect of the environment might influence a particular behavior.
Remember, a hypothesis does not have to be correct. While the hypothesis predicts what the researchers expect to see, the goal of the research is to determine whether this guess is right or wrong. When conducting an experiment, researchers might explore numerous factors to determine which ones might contribute to the ultimate outcome.
In many cases, researchers may find that the results of an experiment do not support the original hypothesis. When writing up these results, the researchers might suggest other options that should be explored in future studies.
In many cases, researchers might draw a hypothesis from a specific theory or build on previous research. For example, prior research has shown that stress can impact the immune system. So a researcher might hypothesize: "People with high-stress levels will be more likely to contract a common cold after being exposed to the virus than people who have low-stress levels."
In other instances, researchers might look at commonly held beliefs or folk wisdom. "Birds of a feather flock together" is one example of folk adage that a psychologist might try to investigate. The researcher might pose a specific hypothesis that "People tend to select romantic partners who are similar to them in interests and educational level."
Elements of a Good Hypothesis
So how do you write a good hypothesis? When trying to come up with a hypothesis for your research or experiments, ask yourself the following questions:
- Is your hypothesis based on your research on a topic?
- Can your hypothesis be tested?
- Does your hypothesis include independent and dependent variables?
Before you come up with a specific hypothesis, spend some time doing background research. Once you have completed a literature review, start thinking about potential questions you still have. Pay attention to the discussion section in the journal articles you read . Many authors will suggest questions that still need to be explored.
How to Formulate a Good Hypothesis
To form a hypothesis, you should take these steps:
- Collect as many observations about a topic or problem as you can.
- Evaluate these observations and look for possible causes of the problem.
- Create a list of possible explanations that you might want to explore.
- After you have developed some possible hypotheses, think of ways that you could confirm or disprove each hypothesis through experimentation. This is known as falsifiability.
In the scientific method , falsifiability is an important part of any valid hypothesis. In order to test a claim scientifically, it must be possible that the claim could be proven false.
Students sometimes confuse the idea of falsifiability with the idea that it means that something is false, which is not the case. What falsifiability means is that if something was false, then it is possible to demonstrate that it is false.
One of the hallmarks of pseudoscience is that it makes claims that cannot be refuted or proven false.
The Importance of Operational Definitions
A variable is a factor or element that can be changed and manipulated in ways that are observable and measurable. However, the researcher must also define how the variable will be manipulated and measured in the study.
Operational definitions are specific definitions for all relevant factors in a study. This process helps make vague or ambiguous concepts detailed and measurable.
For example, a researcher might operationally define the variable " test anxiety " as the results of a self-report measure of anxiety experienced during an exam. A "study habits" variable might be defined by the amount of studying that actually occurs as measured by time.
These precise descriptions are important because many things can be measured in various ways. Clearly defining these variables and how they are measured helps ensure that other researchers can replicate your results.
Replicability
One of the basic principles of any type of scientific research is that the results must be replicable.
Replication means repeating an experiment in the same way to produce the same results. By clearly detailing the specifics of how the variables were measured and manipulated, other researchers can better understand the results and repeat the study if needed.
Some variables are more difficult than others to define. For example, how would you operationally define a variable such as aggression ? For obvious ethical reasons, researchers cannot create a situation in which a person behaves aggressively toward others.
To measure this variable, the researcher must devise a measurement that assesses aggressive behavior without harming others. The researcher might utilize a simulated task to measure aggressiveness in this situation.
Hypothesis Checklist
- Does your hypothesis focus on something that you can actually test?
- Does your hypothesis include both an independent and dependent variable?
- Can you manipulate the variables?
- Can your hypothesis be tested without violating ethical standards?
The hypothesis you use will depend on what you are investigating and hoping to find. Some of the main types of hypotheses that you might use include:
- Simple hypothesis : This type of hypothesis suggests there is a relationship between one independent variable and one dependent variable.
- Complex hypothesis : This type suggests a relationship between three or more variables, such as two independent and dependent variables.
- Null hypothesis : This hypothesis suggests no relationship exists between two or more variables.
- Alternative hypothesis : This hypothesis states the opposite of the null hypothesis.
- Statistical hypothesis : This hypothesis uses statistical analysis to evaluate a representative population sample and then generalizes the findings to the larger group.
- Logical hypothesis : This hypothesis assumes a relationship between variables without collecting data or evidence.
A hypothesis often follows a basic format of "If {this happens} then {this will happen}." One way to structure your hypothesis is to describe what will happen to the dependent variable if you change the independent variable .
The basic format might be: "If {these changes are made to a certain independent variable}, then we will observe {a change in a specific dependent variable}."
A few examples of simple hypotheses:
- "Students who eat breakfast will perform better on a math exam than students who do not eat breakfast."
- "Students who experience test anxiety before an English exam will get lower scores than students who do not experience test anxiety."
- "Motorists who talk on the phone while driving will be more likely to make errors on a driving course than those who do not talk on the phone."
- "Children who receive a new reading intervention will have higher reading scores than students who do not receive the intervention."
Examples of a complex hypothesis include:
- "People with high-sugar diets and sedentary activity levels are more likely to develop depression."
- "Younger people who are regularly exposed to green, outdoor areas have better subjective well-being than older adults who have limited exposure to green spaces."
Examples of a null hypothesis include:
- "There is no difference in anxiety levels between people who take St. John's wort supplements and those who do not."
- "There is no difference in scores on a memory recall task between children and adults."
- "There is no difference in aggression levels between children who play first-person shooter games and those who do not."
Examples of an alternative hypothesis:
- "People who take St. John's wort supplements will have less anxiety than those who do not."
- "Adults will perform better on a memory task than children."
- "Children who play first-person shooter games will show higher levels of aggression than children who do not."
Collecting Data on Your Hypothesis
Once a researcher has formed a testable hypothesis, the next step is to select a research design and start collecting data. The research method depends largely on exactly what they are studying. There are two basic types of research methods: descriptive research and experimental research.
Descriptive Research Methods
Descriptive research such as case studies , naturalistic observations , and surveys are often used when conducting an experiment is difficult or impossible. These methods are best used to describe different aspects of a behavior or psychological phenomenon.
Once a researcher has collected data using descriptive methods, a correlational study can examine how the variables are related. This research method might be used to investigate a hypothesis that is difficult to test experimentally.
Experimental Research Methods
Experimental methods are used to demonstrate causal relationships between variables. In an experiment, the researcher systematically manipulates a variable of interest (known as the independent variable) and measures the effect on another variable (known as the dependent variable).
Unlike correlational studies, which can only be used to determine if there is a relationship between two variables, experimental methods can be used to determine the actual nature of the relationship—whether changes in one variable actually cause another to change.
The hypothesis is a critical part of any scientific exploration. It represents what researchers expect to find in a study or experiment. In situations where the hypothesis is unsupported by the research, the research still has value. Such research helps us better understand how different aspects of the natural world relate to one another. It also helps us develop new hypotheses that can then be tested in the future.
Thompson WH, Skau S. On the scope of scientific hypotheses . R Soc Open Sci . 2023;10(8):230607. doi:10.1098/rsos.230607
Taran S, Adhikari NKJ, Fan E. Falsifiability in medicine: what clinicians can learn from Karl Popper [published correction appears in Intensive Care Med. 2021 Jun 17;:]. Intensive Care Med . 2021;47(9):1054-1056. doi:10.1007/s00134-021-06432-z
Eyler AA. Research Methods for Public Health . 1st ed. Springer Publishing Company; 2020. doi:10.1891/9780826182067.0004
Nosek BA, Errington TM. What is replication ? PLoS Biol . 2020;18(3):e3000691. doi:10.1371/journal.pbio.3000691
Aggarwal R, Ranganathan P. Study designs: Part 2 - Descriptive studies . Perspect Clin Res . 2019;10(1):34-36. doi:10.4103/picr.PICR_154_18
Nevid J. Psychology: Concepts and Applications. Wadworth, 2013.
By Kendra Cherry, MSEd Kendra Cherry, MS, is a psychosocial rehabilitation specialist, psychology educator, and author of the "Everything Psychology Book."
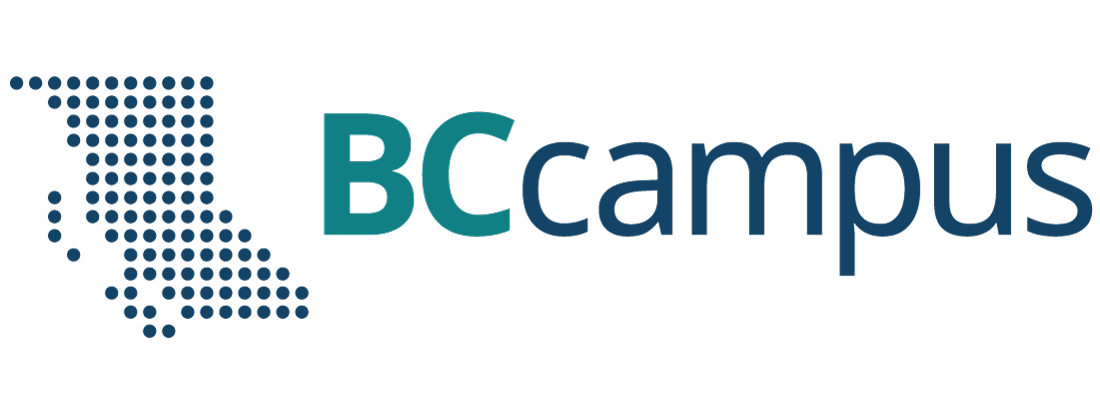
Want to create or adapt books like this? Learn more about how Pressbooks supports open publishing practices.
Chapter 3: Developing a Research Question
3.4 Hypotheses
When researchers do not have predictions about what they will find, they conduct research to answer a question or questions with an open-minded desire to know about a topic, or to help develop hypotheses for later testing. In other situations, the purpose of research is to test a specific hypothesis or hypotheses. A hypothesis is a statement, sometimes but not always causal, describing a researcher’s expectations regarding anticipated finding. Often hypotheses are written to describe the expected relationship between two variables (though this is not a requirement). To develop a hypothesis, one needs to understand the differences between independent and dependent variables and between units of observation and units of analysis. Hypotheses are typically drawn from theories and usually describe how an independent variable is expected to affect some dependent variable or variables. Researchers following a deductive approach to their research will hypothesize about what they expect to find based on the theory or theories that frame their study. If the theory accurately reflects the phenomenon it is designed to explain, then the researcher’s hypotheses about what would be observed in the real world should bear out.
Sometimes researchers will hypothesize that a relationship will take a specific direction. As a result, an increase or decrease in one area might be said to cause an increase or decrease in another. For example, you might choose to study the relationship between age and legalization of marijuana. Perhaps you have done some reading in your spare time, or in another course you have taken. Based on the theories you have read, you hypothesize that “age is negatively related to support for marijuana legalization.” What have you just hypothesized? You have hypothesized that as people get older, the likelihood of their support for marijuana legalization decreases. Thus, as age moves in one direction (up), support for marijuana legalization moves in another direction (down). If writing hypotheses feels tricky, it is sometimes helpful to draw them out and depict each of the two hypotheses we have just discussed.
Note that you will almost never hear researchers say that they have proven their hypotheses. A statement that bold implies that a relationship has been shown to exist with absolute certainty and there is no chance that there are conditions under which the hypothesis would not bear out. Instead, researchers tend to say that their hypotheses have been supported (or not). This more cautious way of discussing findings allows for the possibility that new evidence or new ways of examining a relationship will be discovered. Researchers may also discuss a null hypothesis, one that predicts no relationship between the variables being studied. If a researcher rejects the null hypothesis, he or she is saying that the variables in question are somehow related to one another.
Quantitative and qualitative researchers tend to take different approaches when it comes to hypotheses. In quantitative research, the goal often is to empirically test hypotheses generated from theory. With a qualitative approach, on the other hand, a researcher may begin with some vague expectations about what he or she will find, but the aim is not to test one’s expectations against some empirical observations. Instead, theory development or construction is the goal. Qualitative researchers may develop theories from which hypotheses can be drawn and quantitative researchers may then test those hypotheses. Both types of research are crucial to understanding our social world, and both play an important role in the matter of hypothesis development and testing. In the following section, we will look at qualitative and quantitative approaches to research, as well as mixed methods.
Text attributions This chapter has been adapted from Chapter 5.2 in Principles of Sociological Inquiry , which was adapted by the Saylor Academy without attribution to the original authors or publisher, as requested by the licensor, and is licensed under a CC BY-NC-SA 3.0 License .
Research Methods for the Social Sciences: An Introduction Copyright © 2020 by Valerie Sheppard is licensed under a Creative Commons Attribution-NonCommercial-ShareAlike 4.0 International License , except where otherwise noted.
Share This Book

What Is A Research (Scientific) Hypothesis? A plain-language explainer + examples
By: Derek Jansen (MBA) | Reviewed By: Dr Eunice Rautenbach | June 2020
If you’re new to the world of research, or it’s your first time writing a dissertation or thesis, you’re probably noticing that the words “research hypothesis” and “scientific hypothesis” are used quite a bit, and you’re wondering what they mean in a research context .
“Hypothesis” is one of those words that people use loosely, thinking they understand what it means. However, it has a very specific meaning within academic research. So, it’s important to understand the exact meaning before you start hypothesizing.
Research Hypothesis 101
- What is a hypothesis ?
- What is a research hypothesis (scientific hypothesis)?
- Requirements for a research hypothesis
- Definition of a research hypothesis
- The null hypothesis
What is a hypothesis?
Let’s start with the general definition of a hypothesis (not a research hypothesis or scientific hypothesis), according to the Cambridge Dictionary:
Hypothesis: an idea or explanation for something that is based on known facts but has not yet been proved.
In other words, it’s a statement that provides an explanation for why or how something works, based on facts (or some reasonable assumptions), but that has not yet been specifically tested . For example, a hypothesis might look something like this:
Hypothesis: sleep impacts academic performance.
This statement predicts that academic performance will be influenced by the amount and/or quality of sleep a student engages in – sounds reasonable, right? It’s based on reasonable assumptions , underpinned by what we currently know about sleep and health (from the existing literature). So, loosely speaking, we could call it a hypothesis, at least by the dictionary definition.
But that’s not good enough…
Unfortunately, that’s not quite sophisticated enough to describe a research hypothesis (also sometimes called a scientific hypothesis), and it wouldn’t be acceptable in a dissertation, thesis or research paper . In the world of academic research, a statement needs a few more criteria to constitute a true research hypothesis .
What is a research hypothesis?
A research hypothesis (also called a scientific hypothesis) is a statement about the expected outcome of a study (for example, a dissertation or thesis). To constitute a quality hypothesis, the statement needs to have three attributes – specificity , clarity and testability .
Let’s take a look at these more closely.
Need a helping hand?
Hypothesis Essential #1: Specificity & Clarity
A good research hypothesis needs to be extremely clear and articulate about both what’ s being assessed (who or what variables are involved ) and the expected outcome (for example, a difference between groups, a relationship between variables, etc.).
Let’s stick with our sleepy students example and look at how this statement could be more specific and clear.
Hypothesis: Students who sleep at least 8 hours per night will, on average, achieve higher grades in standardised tests than students who sleep less than 8 hours a night.
As you can see, the statement is very specific as it identifies the variables involved (sleep hours and test grades), the parties involved (two groups of students), as well as the predicted relationship type (a positive relationship). There’s no ambiguity or uncertainty about who or what is involved in the statement, and the expected outcome is clear.
Contrast that to the original hypothesis we looked at – “Sleep impacts academic performance” – and you can see the difference. “Sleep” and “academic performance” are both comparatively vague , and there’s no indication of what the expected relationship direction is (more sleep or less sleep). As you can see, specificity and clarity are key.
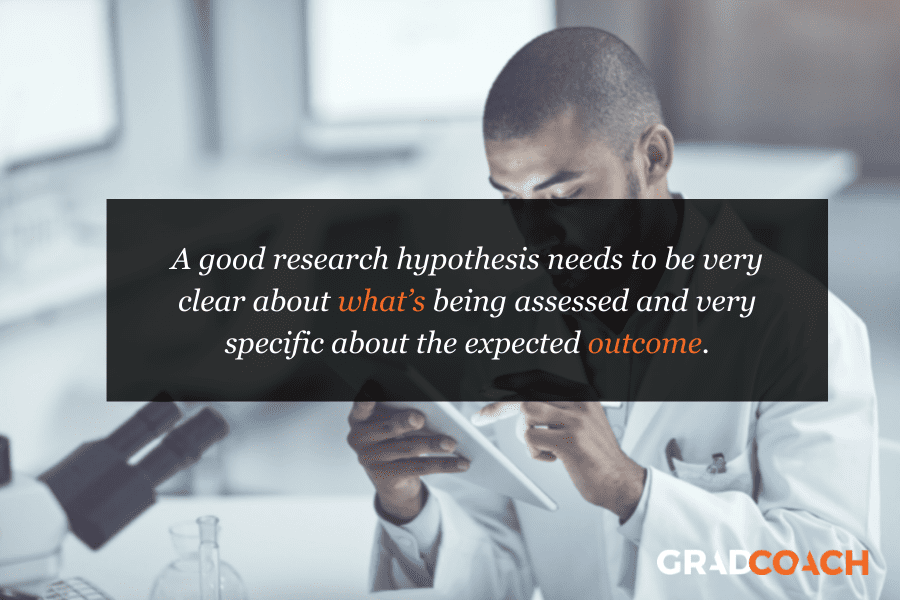
Hypothesis Essential #2: Testability (Provability)
A statement must be testable to qualify as a research hypothesis. In other words, there needs to be a way to prove (or disprove) the statement. If it’s not testable, it’s not a hypothesis – simple as that.
For example, consider the hypothesis we mentioned earlier:
Hypothesis: Students who sleep at least 8 hours per night will, on average, achieve higher grades in standardised tests than students who sleep less than 8 hours a night.
We could test this statement by undertaking a quantitative study involving two groups of students, one that gets 8 or more hours of sleep per night for a fixed period, and one that gets less. We could then compare the standardised test results for both groups to see if there’s a statistically significant difference.
Again, if you compare this to the original hypothesis we looked at – “Sleep impacts academic performance” – you can see that it would be quite difficult to test that statement, primarily because it isn’t specific enough. How much sleep? By who? What type of academic performance?
So, remember the mantra – if you can’t test it, it’s not a hypothesis 🙂
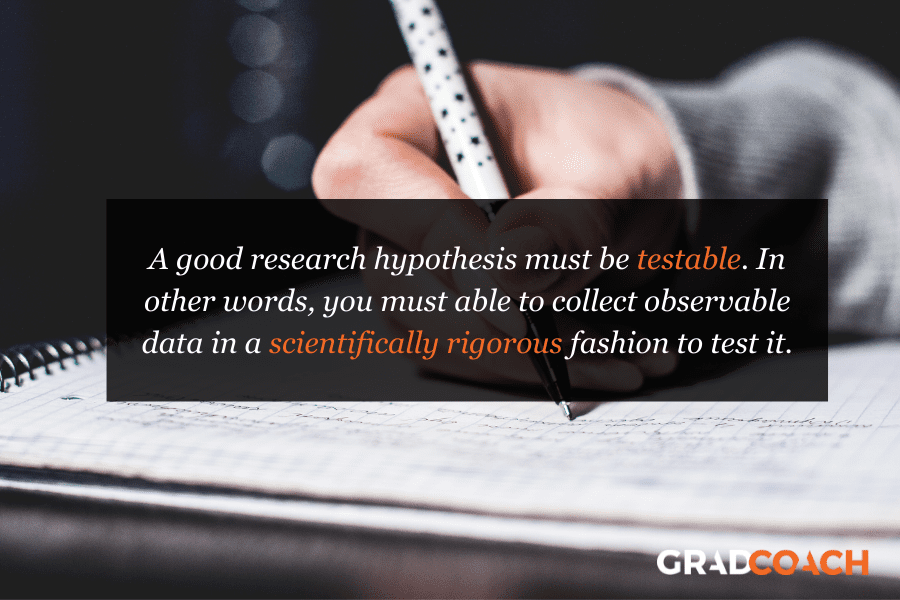
Defining A Research Hypothesis
You’re still with us? Great! Let’s recap and pin down a clear definition of a hypothesis.
A research hypothesis (or scientific hypothesis) is a statement about an expected relationship between variables, or explanation of an occurrence, that is clear, specific and testable.
So, when you write up hypotheses for your dissertation or thesis, make sure that they meet all these criteria. If you do, you’ll not only have rock-solid hypotheses but you’ll also ensure a clear focus for your entire research project.
What about the null hypothesis?
You may have also heard the terms null hypothesis , alternative hypothesis, or H-zero thrown around. At a simple level, the null hypothesis is the counter-proposal to the original hypothesis.
For example, if the hypothesis predicts that there is a relationship between two variables (for example, sleep and academic performance), the null hypothesis would predict that there is no relationship between those variables.
At a more technical level, the null hypothesis proposes that no statistical significance exists in a set of given observations and that any differences are due to chance alone.
And there you have it – hypotheses in a nutshell.
If you have any questions, be sure to leave a comment below and we’ll do our best to help you. If you need hands-on help developing and testing your hypotheses, consider our private coaching service , where we hold your hand through the research journey.
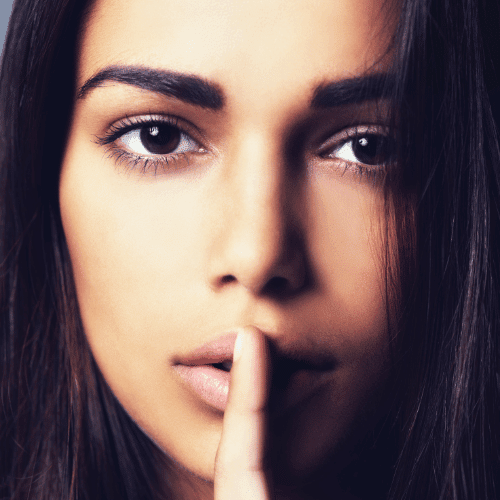
Psst... there’s more!
This post was based on one of our popular Research Bootcamps . If you're working on a research project, you'll definitely want to check this out ...
You Might Also Like:
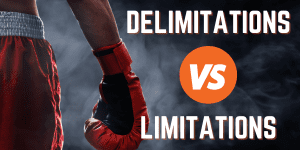
16 Comments
Very useful information. I benefit more from getting more information in this regard.
Very great insight,educative and informative. Please give meet deep critics on many research data of public international Law like human rights, environment, natural resources, law of the sea etc
In a book I read a distinction is made between null, research, and alternative hypothesis. As far as I understand, alternative and research hypotheses are the same. Can you please elaborate? Best Afshin
This is a self explanatory, easy going site. I will recommend this to my friends and colleagues.
Very good definition. How can I cite your definition in my thesis? Thank you. Is nul hypothesis compulsory in a research?
It’s a counter-proposal to be proven as a rejection
Please what is the difference between alternate hypothesis and research hypothesis?
It is a very good explanation. However, it limits hypotheses to statistically tasteable ideas. What about for qualitative researches or other researches that involve quantitative data that don’t need statistical tests?
In qualitative research, one typically uses propositions, not hypotheses.
could you please elaborate it more
I’ve benefited greatly from these notes, thank you.
This is very helpful
well articulated ideas are presented here, thank you for being reliable sources of information
Excellent. Thanks for being clear and sound about the research methodology and hypothesis (quantitative research)
I have only a simple question regarding the null hypothesis. – Is the null hypothesis (Ho) known as the reversible hypothesis of the alternative hypothesis (H1? – How to test it in academic research?
this is very important note help me much more
Trackbacks/Pingbacks
- What Is Research Methodology? Simple Definition (With Examples) - Grad Coach - […] Contrasted to this, a quantitative methodology is typically used when the research aims and objectives are confirmatory in nature. For example,…
Submit a Comment Cancel reply
Your email address will not be published. Required fields are marked *
Save my name, email, and website in this browser for the next time I comment.
- Print Friendly
Public Health Notes
Your partner for better health, hypothesis in research: definition, types and importance .
April 21, 2020 Kusum Wagle Epidemiology 0
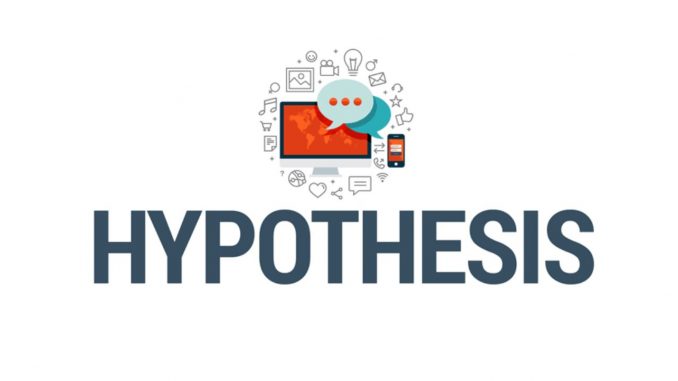
Table of Contents
What is Hypothesis?
- Hypothesis is a logical prediction of certain occurrences without the support of empirical confirmation or evidence.
- In scientific terms, it is a tentative theory or testable statement about the relationship between two or more variables i.e. independent and dependent variable.
Different Types of Hypothesis:
1. Simple Hypothesis:
- A Simple hypothesis is also known as composite hypothesis.
- In simple hypothesis all parameters of the distribution are specified.
- It predicts relationship between two variables i.e. the dependent and the independent variable
2. Complex Hypothesis:
- A Complex hypothesis examines relationship between two or more independent variables and two or more dependent variables.
3. Working or Research Hypothesis:
- A research hypothesis is a specific, clear prediction about the possible outcome of a scientific research study based on specific factors of the population.
4. Null Hypothesis:
- A null hypothesis is a general statement which states no relationship between two variables or two phenomena. It is usually denoted by H 0 .
5. Alternative Hypothesis:
- An alternative hypothesis is a statement which states some statistical significance between two phenomena. It is usually denoted by H 1 or H A .
6. Logical Hypothesis:
- A logical hypothesis is a planned explanation holding limited evidence.
7. Statistical Hypothesis:
- A statistical hypothesis, sometimes called confirmatory data analysis, is an assumption about a population parameter.
Although there are different types of hypothesis, the most commonly and used hypothesis are Null hypothesis and alternate hypothesis . So, what is the difference between null hypothesis and alternate hypothesis? Let’s have a look:
Major Differences Between Null Hypothesis and Alternative Hypothesis:
Importance of hypothesis:.
- It ensures the entire research methodologies are scientific and valid.
- It helps to assume the probability of research failure and progress.
- It helps to provide link to the underlying theory and specific research question.
- It helps in data analysis and measure the validity and reliability of the research.
- It provides a basis or evidence to prove the validity of the research.
- It helps to describe research study in concrete terms rather than theoretical terms.
Characteristics of Good Hypothesis:
- Should be simple.
- Should be specific.
- Should be stated in advance.
References and For More Information:
https://ocw.jhsph.edu/courses/StatisticalReasoning1/PDFs/2009/BiostatisticsLecture4.pdf
https://keydifferences.com/difference-between-type-i-and-type-ii-errors.html
https://www.khanacademy.org/math/ap-statistics/tests-significance-ap/error-probabilities-power/a/consequences-errors-significance
https://stattrek.com/hypothesis-test/hypothesis-testing.aspx
http://davidmlane.com/hyperstat/A2917.html
https://study.com/academy/lesson/what-is-a-hypothesis-definition-lesson-quiz.html
https://keydifferences.com/difference-between-null-and-alternative-hypothesis.html
https://blog.minitab.com/blog/adventures-in-statistics-2/understanding-hypothesis-tests-why-we-need-to-use-hypothesis-tests-in-statistics
- Characteristics of Good Hypothesis
- complex hypothesis
- example of alternative hypothesis
- example of null hypothesis
- how is null hypothesis different to alternative hypothesis
- Importance of Hypothesis
- null hypothesis vs alternate hypothesis
- simple hypothesis
- Types of Hypotheses
- what is alternate hypothesis
- what is alternative hypothesis
- what is hypothesis?
- what is logical hypothesis
- what is null hypothesis
- what is research hypothesis
- what is statistical hypothesis
- why is hypothesis necessary
Copyright © 2024 | WordPress Theme by MH Themes

- Table of Contents
- Random Entry
- Chronological
- Editorial Information
- About the SEP
- Editorial Board
- How to Cite the SEP
- Special Characters
- Advanced Tools
- Support the SEP
- PDFs for SEP Friends
- Make a Donation
- SEPIA for Libraries
- Entry Contents
Bibliography
Academic tools.
- Friends PDF Preview
- Author and Citation Info
- Back to Top
Normative Theories of Rational Choice: Expected Utility
We must often make decisions under conditions of uncertainty. Pursuing a degree in biology may lead to lucrative employment, or to unemployment and crushing debt. A doctor’s appointment may result in the early detection and treatment of a disease, or it may be a waste of money. Expected utility theory is an account of how to choose rationally when you are not sure which outcome will result from your acts. Its basic slogan is: choose the act with the highest expected utility.
This article discusses expected utility theory as a normative theory—that is, a theory of how people should make decisions. In classical economics, expected utility theory is often used as a descriptive theory—that is, a theory of how people do make decisions—or as a predictive theory—that is, a theory that, while it may not accurately model the psychological mechanisms of decision-making, correctly predicts people’s choices. Expected utility theory makes faulty predictions about people’s decisions in many real-life choice situations (see Kahneman & Tversky 1982); however, this does not settle whether people should make decisions on the basis of expected utility considerations.
The expected utility of an act is a weighted average of the utilities of each of its possible outcomes, where the utility of an outcome measures the extent to which that outcome is preferred, or preferable, to the alternatives. The utility of each outcome is weighted according to the probability that the act will lead to that outcome. Section 1 fleshes out this basic definition of expected utility in more rigorous terms, and discusses its relationship to choice. Section 2 discusses two types of arguments for expected utility theory: representation theorems, and long-run statistical arguments. Section 3 considers objections to expected utility theory; section 4 discusses its applications in philosophy of religion, economics, ethics, and epistemology.
1.1 Conditional Probabilities
1.2 outcome utilities, 2.1 long-run arguments, 2.2 representation theorems, 3.1 maximizing expected utility is impossible, 3.2 maximizing expected utility is irrational, 4.1 economics and public policy, 4.3 epistemology, other internet resources, related entries, 1. defining expected utility.
The concept of expected utility is best illustrated by example. Suppose I am planning a long walk, and need to decide whether to bring my umbrella. I would rather not tote the umbrella on a sunny day, but I would rather face rain with the umbrella than without it. There are two acts available to me: taking my umbrella, and leaving it at home. Which of these acts should I choose?
This informal problem description can be recast, slightly more formally, in terms of three sorts of entities. First, there are outcomes —objects of non-instrumental preferences. In the example, we might distinguish three outcomes: either I end up dry and unencumbered; I end up dry and encumbered by an unwieldy umbrella; or I end up wet. Second, there are states —things outside the decision-maker’s control which influence the outcome of the decision. In the example, there are two states: either it is raining, or it is not. Finally, there are acts —objects of the decision-maker’s instrumental preferences, and in some sense, things that she can do. In the example, there are two acts: I may either bring the umbrella; or leave it at home. Expected utility theory provides a way of ranking the acts according to how choiceworthy they are: the higher the expected utility, the better it is to choose the act. (It is therefore best to choose the act with the highest expected utility—or one of them, in the event that several acts are tied.)
Following general convention, I will make the following assumptions about the relationships between acts, states, and outcomes.
- States, acts, and outcomes are propositions, i.e., sets of possibilities. There is a maximal set of possibilities, \(\Omega\), of which each state, act, or outcome is a subset.
- The set of acts, the set of states, and the set of outcomes are all partitions on \(\Omega\). In other words, acts and states are individuated so that every possibility in \(\Omega\) is one where exactly one state obtains, the agent performs exactly one act, and exactly one outcome ensues.
- Acts and states are logically independent, so that no state rules out the performance of any act.
- I will assume for the moment that, given a state of the world, each act has exactly one possible outcome. (Section 1.1 briefly discusses how one might weaken this assumption.)
So the example of the umbrella can be depicted in the following matrix, where each column corresponds to a state of the world; each row corresponds to an act; and each entry corresponds to the outcome that results when the act is performed in the state of the world.
Having set up the basic framework, I can now rigorously define expected utility. The expected utility of an act \(A\) (for instance, taking my umbrella) depends on two features of the problem:
- The value of each outcome, measured by a real number called a utility .
- The probability of each outcome conditional on \(A\).
Given these three pieces of information, \(A\)’s expected utility is defined as:
where \(O\) is is the set of outcomes, \(P_{A}(o)\) is the probability of outcome \(o\) conditional on \(A\), and \(U(o)\) is the utility of \(o\).
The next two subsections will unpack the conditional probability function \(P_A\) and the utility function \(U\).
The term \(P_{A}(o)\) represents the probability of \(o\) given \(A\)—roughly, how likely it is that outcome \(o\) will occur, on the supposition that the agent chooses act \(A\). (For the axioms of probability, see the entry on interpretations of probability .) To understand what this means, we must answer two questions. First, which interpretation of probability is appropriate? And second, what does it mean to assign a probability on the supposition that the agent chooses act \(A\)?
Expected utility theorists often interpret probability as measuring individual degree of belief, so that a proposition \(E\) is likely (for an agent) to the extent that that agent is confident of \(E\) (see, for instance, Ramsey 1926, Savage 1972, Jeffrey 1983). But nothing in the formalism of expected utility theory forces this interpretation on us. We could instead interpret probabilities as objective chances (as in von Neumann and Morgenstern 1944), or as the degrees of belief that are warranted by the evidence, if we thought these were a better guide to rational action. (See the entry on interpretations of probability for discussion of these and other options.)
What is it to have a probability on the supposition that the agent chooses \(A\)? Here, there are two basic types of answer, corresponding to evidential decision theory and causal decision theory.
According to evidential decision theory, endorsed by Jeffrey (1983), the relevant suppositional probability \(P_{A}(o)\) is the conditional probability \(P(o \mid A)\), defined as the ratio of two unconditional probabilities: \(P(A \amp o) / P(A)\).
Against Jeffrey’s definition of expected utility, Spohn (1977) and Levi (1991) object that a decision-maker should not assign probabilities to the very acts under deliberation: when freely deciding whether to perform an act \(A\), you shouldn’t take into account your beliefs about whether you will perform \(A\). If Spohn and Levi are right, then Jeffrey’s ratio is undefined (since its denominator is undefined).
Nozick (1969) raises another objection: Jeffrey’s definition gives strange results in the Newcomb Problem . A predictor hands you a closed box, containing either $0 or $1 million, and offers you an open box, containing an additional $1,000. You can either refuse the open box (“one-box”) or take the open box (“two-box”). But there’s a catch: the predictor has predicted your choice beforehand, and all her predictions are 90% accurate. In other words, the probability that you one-box, given that she predicts you one-box, is 90%, and the probability that you two-box, given that she predicts you two-box, is 90%. Finally, the contents of the closed box depend on the prediction: if the predictor thought you would two-box, she put nothing in the closed box, while if she thought you would one-box, she put $1 million in the closed box. The matrix for your decision looks like this:
Two-boxing dominates one-boxing: in every state, two-boxing yields a better outcome. Yet on Jeffrey’s definition of conditional probability, one-boxing has a higher expected utility than two-boxing. There is a high conditional probability of finding $1 million is in the closed box, given that you one-box, so one-boxing has a high expected utility. Likewise, there is a high conditional probability of finding nothing in the closed box, given that you two-box, so two-boxing has a low expected utility.
Causal decision theory is an alternative proposal that gets around these problems. It does not require (but still permits) acts to have probabilities, and it recommends two-boxing in the Newcomb problem.
Causal decision theory comes in many varieties, but I’ll consider a representative version proposed by Savage (1972), which calculates \(P_{A}(o)\) by summing the probabilities of states that, when combined with the act \(A\), lead to the outcome \(o\). Let \(f_{A,s}(o)\) be a of outcomes, which maps \(o\) to 1 if \(o\) results from performing \(A\) in state s , maps \(o\) to 0 otherwise. Then
On Savage’s proposal, two-boxing comes out with a higher expected utility than one-boxing. This result holds no matter which probabilities you assign to the states prior to your decision. Let \(x\) be the probability you assign to the state that the closed box contains $1 million. According to Savage, the expected utilities of one-boxing and two-boxing, respectively, are:
As long as the larger monetary amounts are assigned strictly larger utilities, the second sum (the utility of two-boxing) is guaranteed to be larger than the first (the utility of one-boxing).
Savage assumes that each act and state are enough to uniquely determine an outcome. But there are cases where this assumption breaks down. Suppose you offer to sell me the following gamble: you will toss a coin; if the coin lands heads, I win $100; and if the coin lands tails, I lose $100. But I refuse the gamble, and the coin is never tossed. There is no outcome that would have resulted, had the coin been tossed—I might have won $100, and I might have lost $100.
We can generalze Savage’s proposal by letting \(f_{A,s}\) be a probability function that maps outcomes to real numbers in the \([0, 1]\) interval. Lewis (1981), Skyrms (1980), and Sobel (1994) equate \(f_{A,s}\) with the objective chance that \(o\) would be the outcome if state \(s\) obtained and the agent chose action \(A\).
In some cases—most famously the Newcomb problem—the Jeffrey definition and the Savage definition of expected utility come apart. But whenever the following two conditions are satisfied, they agree.
- Acts are probabilistically independent of states. In formal terms, for all acts \(A\) and states \(s\), \[ P(s) = P(s \mid A) = \frac{P(s \amp A)}{P(A)}. \] (This is the condition that is violated in the Newcomb problem.)
- For all outcomes \(o\), acts \(A\), and states \(s\), \(f_{A,s}(o)\) is equal to the conditional probability of \(o\) given \(A\) and \(s\); in formal terms, \[ f_{A,s}(o) = P(o \mid A \amp s) = \frac{P(o \amp A \amp s)}{P(A \amp s)}.\] (The need for this condition arises when acts and states fail to uniquely determine an outcome; see Lewis 1981.)
The term \(U(o)\) represents the utility of the outcome \(o\)—roughly, how valuable \(o\) is. Formally, \(U\) is a function that assigns a real number to each of the outcomes. (The units associated with \(U\) are typically called utiles , so that if \(U(o) = 2\), we say that \(o\) is worth 2 utiles.) The greater the utility, the more valuable the outcome.
What kind of value is measured in utiles? Utiles are typically not taken to be units of currency, like dollars, pounds, or yen. Bernoulli (1738) argued that money and other goods have diminishing marginal utility: as an agent gets richer, every successive dollar (or gold watch, or apple) is less valuable to her than the last. He gives the following example: It makes rational sense for a rich man, but not for a pauper, to pay 9,000 ducats in exchange for a lottery ticket that yields a 50% chance at 20,000 ducats and a 50% chance at nothing. Since the lottery gives the two men the same chance at each monetary prize, the prizes must have different values depending on whether the player is poor or rich.
Classic utilitarians such as Bentham (1789), Mill (1861), and Sidgwick (1907) interpreted utility as a measure of pleasure or happiness. For these authors, to say \(A\) has greater utility than \(B\) (for an agent or a group of agents) is to say that \(A\) results in more pleasure or happiness than \(B\) (for that agent or group of agents).
One objection to this interpretation of utility is that there may not be a single good (or indeed any good) which rationality requires us to seek. But if we understand “utility” broadly enough to include all potentially desirable ends—pleasure, knowledge, friendship, health and so on—it’s not clear that there is a unique correct way to make the tradeoffs between different goods so that each outcome receives a utility. There may be no good answer to the question of whether the life of an ascetic monk contains more or less good than the life of a happy libertine—but assigning utilities to these options forces us to compare them.
Contemporary decision theorists typically interpret utility as a measure of preference, so that to say that \(A\) has greater utility than \(B\) (for an agent) is simply to say that the agent prefers \(A\) to \(B\). It is crucial to this approach that preferences hold not just between outcomes (such as amounts of pleasure, or combinations of pleasure and knowledge), but also between uncertain prospects (such as a lottery that pays $1 million dollars if a particular coin lands heads, and results in an hour of painful electric shocks if the coin lands tails). Section 2 of this article addresses the formal relationship between preference and choice in detail.
Expected utility theory does not require that preferences be selfish or self-interested. Someone can prefer giving money to charity over spending the money on lavish dinners, or prefer sacrificing his own life over allowing his child to die. Sen (1977) suggests that each person’s psychology is best represented using three rankings: one representing the person’s narrow self-interest, a second representing the person’s self-interest construed more broadly to account for feelings of sympathy (e.g., suffering when watching another person suffer), and a third representing the person’s commitments, which may require her to act against her self-interest broadly construed.
Broome (1991, Ch. 6) interprets utilities as measuring comparisons of objective betterness and worseness, rather than personal preferences: to say that \(A\) has a greater utility than \(B\) is to say that \(A\) is objectively better than \(B\), or that a rational person would prefer \(A\) to \(B\). Just as there is nothing in the formalism of probability theory that requires us to use subjective rather than objective probabilities, so there is nothing in the formalism of expected utility theory that requires us to use subjective rather than objective values.
Those who interpret utilities in terms of personal preference face a special challenge: the so-called problem of interpersonal utility comparisons . When making decisions about how to distribute shared resources, we often want to know if our acts would make Alice better off than Bob—and if so, how much better off. But if utility is a measure of individual preference, there is no clear, meaningful way of making these comparisons. Alice’s utilities are constituted by Alice’s preferences, Bob’s utilities are constituted by Bob’s preferences, and there are no preferences spanning Alice and Bob. We can’t assume that Alice’s utility 10 is equivalent to Bob’s utility 10, any more than we can assume that getting an A grade in differential equations is equivalent to getting an A grade in basket weaving.
Now is a good time to consider which features of the utility function carry meaningful information. Comparisons are informative: if \(U(o_1) \gt U(o_2)\) (for a person), then \(o_1\) is better than (or preferred to) \(o_2\). But it is not only comparisons that are informative—the utility function must carry other information, if expected utility theory is to give meaningful results.
To see why, consider the umbrella example again. This time, I’ve filled in a probability for each state, and a utility for each outcome.
The expected utility of taking the umbrella is
while the expected utility of leaving the umbrella is
Since \(EU(\take) \gt EU(\leave)\), expected utility theory tells me that taking the umbrella is better than leaving it.
But now, suppose we change the utilities of the outcomes: instead of using \(U\), we use \(U'\).
The new expected utility of taking the umbrella is
while the new expected utility of leaving the umbrella is
Since \(EU'(\take) \lt EU'(\leave)\), expected utility theory tells me that leaving the umbrella is better than taking it.
The utility functions \(U\) and \(U'\) rank the outcomes in exactly the same way: free, dry is best; encumbered, dry ranks in the middle; and wet is worst. Yet expected utility theory gives different advice in the two versions of the problem. So there must be some substantive difference between preferences appropriately described by \(U\), and preferences appropriately described by \(U'\). Otherwise, expected utility theory is fickle, and liable to change its advice when fed different descriptions of the same problem.
When do two utility functions represent the same basic state of affairs? Measurement theory answers the question by characterizing the allowable transformations of a utility function—ways of changing it that leave all of its meaningful features intact. If we characterize the allowable transformations of a utility function, we have thereby specified which of its features are meaningful.
Defenders of expected utility theory typically require that utility be measured by a linear scale , where the allowable transformations are all and only the positive linear transformations, i.e., functions \(f\) of the form
for real numbers \(x \gt 0\) and \(y\).
Positive linear transformations of outcome utilities will never affect the verdicts of expected utility theory: if \(A\) has greater expected utility than \(B\) where utility is measured by function \(U\), then \(A\) will also have greater expected utility than \(B\) where utility is measured by any positive linear transformation of \(U\).
2. Arguments for Expected Utility Theory
Why choose acts that maximize expected utility? One possible answer is that expected utility theory is rational bedrock—that means-end rationality essentially involves maximizing expected utility. For those who find this answer unsatisfying, however, there are two further sources of justification. First, there are long-run arguments, which rely on evidence that expected-utility maximization is a profitable policy in the long term. Second, there are arguments based on representation theorems, which suggest that certain rational constraints on preference entail that all rational agents maximize expected utility.
One reason for maximizing expected utility is that it makes for good policy in the long run. Feller (1968) gives a version of this argument. He relies on two mathematical facts about probabilities: the strong and weak laws of large numbers . Both these facts concern sequences of independent, identically distributed trials—the sort of setup that results from repeatedly betting the same way on a sequence of roulette spins or craps games. Both the weak and strong laws of large numbers say, roughly, that over the long run, the average amount of utility gained per trial is overwhelmingly likely to be close to the expected value of an individual trial.
The weak law of large numbers states that where each trial has an expected value of \(\mu\), for any arbitrarily small real numbers \(\epsilon \gt 0\) and \(\delta \gt 0\), there is some finite number of trials \(n\), such that for all \(m\) greater than or equal to \(n\), with probability at least \(1-\delta\), the gambler’s average gains for the first \(m\) trials will fall within \(\epsilon\) of \(\mu\). In other words, in a long run of similar gamble, the average gain per trial is highly likely to become arbitrarily close to the gamble’s expected value within a finite amount of time. So in the finite long run, the average value associated with a gamble is overwhelmingly likely to be close to its expected value.
The strong law of large numbers states that where each trial has an expected value of \(\mu\), with probability 1, for any arbitrarily small real number \(\epsilon \gt 0\),as the number of trials increases, the gambler’s average winnings per trial will fall within \(\epsilon\) of \(\mu\). In other words, as the number of repetitions of a gamble approaches infinity, the average gain per trial will become arbitrarily close to the gamble’s expected value with probability 1. So in the long run, the average value associated with a gamble is virtually certain to equal its expected value.
There are several objections to these long run arguments. First, many decisions cannot be repeated over indefinitely many similar trials. Decisions about which career to pursue, whom to marry, and where to live, for instance, are made at best a small finite number of times. Furthermore, where these decisions are made more than once, different trials involve different possible outcomes, with different probabilities. It is not clear why long-run considerations about repeated gambles should bear on these single-case choices.
Second, the argument relies on two independence assumptions, one or both of which may fail. One assumption holds that the probabilities of the different trials are independent. This is true of casino gambles, but not true of other choices where we wish to use decision theory—e.g., choices about medical treatment. My remaining sick after one course of antibiotics makes it more likely I will remain sick after the next course, since it increases the chance that antibiotic-resistant bacteria will spread through my body. The argument also requires that the utilities of different trials be independent, so that winning a prize on one trial makes the same contribution to the decision-maker’s overall utility no matter what she wins on other trials. But this assumption is violated in many real-world cases. Due to the diminishing marginal utility of money, winning $10 million on ten games of roulette is not worth ten times as much as winning $1 million on one game of roulette.
A third problem is that the strong and weak laws of large numbers are modally weak. Neither law entails that if a gamble were repeated indefinitely (under the appropriate assumptions), the average utility gain per trial would be close to the game’s expected utility. They establish only that the average utility gain per trial would with high probability be close to the game’s expected utility. But high probability—even probability 1—is not certainty. (Standard probability theory rejects Cournot’s Principle , which says events with low or zero probability will not happen. But see Shafer (2005) for a defense of Cournot’s Principle.) For any sequence of independent, identically distributed trials, it is possible for the average utility payoff per trial to diverge arbitrarily far from the expected utility of an individual trial.
A second type of argument for expected utility theory relies on so-called representation theorems. We follow Zynda’s (2000) formulation of this argument—slightly modified to reflect the role of utilities as well as probabilities. The argument has three premises:
The Rationality Condition. The axioms of expected utility theory are the axioms of rational preference.
Representability. If a person’s preferences obey the axioms of expected utility theory, then she can be represented as having degrees of belief that obey the laws of the probability calculus [and a utility function such that she prefers acts with higher expected utility].
The Reality Condition. If a person can be represented as having degrees of belief that obey the probability calculus [and a utility function such that she prefers acts with higher expected utility], then the person really has degrees of belief that obey the laws of the probability calculus [and really does prefer acts with higher expected utility].
These premises entail the following conclusion.
If a person [fails to prefer acts with higher expected utility], then that person violates at least one of the axioms of rational preference.
If the premises are true, the argument shows that there is something wrong with people whose preferences are at odds with expected utility theory—they violate the axioms of rational preference. Let us consider each of the premises in greater detail, beginning with the key premise, Representability.
A probability function and a utility function together represent a set of preferences just in case the following formula holds for all values of \(A\) and \(B\) in the domain of the preference relation
Mathematical proofs of Representability are called representation theorems . Section 2.1 surveys three of the most influential representation theorems, each of which relies on a different set of axioms.
No matter which set of axioms we use, the Rationality Condition is controversial. In some cases, preferences that seem rationally permissible—perhaps even rationally required—violate the axioms of expected utility theory. Section 3 discusses such cases in detail.
The Reality Condition is also controversial. Hampton (1994), Zynda (2000), and Meacham and Weisberg (2011) all point out that to be representable using a probability and utility function is not to have a probability and utility function. After all, an agent who can be represented as an expected utility maximizer with degrees of belief that obey the probability calculus, can also be represented as someone who fails to maximize expected utility with degrees of belief that violate the probability calculus. Why think the expected utility representation is the right one?
There are several options. Perhaps the defender of representation theorems can stipulate that what it is to have particular degrees of belief and utilities is just to have the corresponding preferences. The main challenge for defenders of this response is to explain why representations in terms of expected utility are explanatorily useful, and why they are better than alternative representations. Or perhaps probabilities and utilities are a good cleaned-up theoretical substitutes for our folk notions of belief and desire—precise scientific substitutes for our folk concepts. Meacham and Weisberg challenge this response, arguing that probabilities and utilities are poor stand-ins for our folk notions. A third possibility, suggested by Zynda, is that facts about degrees of belief are made true independently of the agent’s preferences, and provide a principled way to restrict the range of acceptable representations. The challenge for defenders of this type of response is to specify what these additional facts are.
I now turn to consider three influential representation theorems. These representation theorems differ from each other in three of philosophically significant ways.
First, different representation theorems disagree about the objects of preference and utility. Are they repeatable? Must they be wholly within the agent’s control
Second, representation theorems differ in their treatment of probability. They disagree about which entities have probabilities, and about whether the same objects can have both probabilities and utilities.
Third, while every representation theorem proves that for a suitable preference ordering, there exist a probability and utility function representing the preference ordering, they differ how unique this probability and utility function are. In other words, they differ as to which transformations of the probability and utility functions are allowable.
2.2.1 Ramsey
The idea of a representation theorem for expected utility dates back to Ramsey (1926). (His sketch of a representation theorem is subsequently filled in by Bradley (2004) and Elliott (2017).) Ramsey assumes that preferences are defined over a domain of gambles, which yield one prize on the condition that a proposition \(P\) is true, and a different prize on the condition that \(P\) is false. (Examples of gambles: you receive a onesie if you’re having a baby and a bottle of scotch otherwise; you receive twenty dollars if Bojack wins the Kentucky Derby and lose a dollar otherwise.)
Ramsey calls a proposition ethically neutral when “two possible worlds differing only in regard to [its truth] are always of equal value”. For an ethically neutral proposition, probability 1/2 can be defined in terms of preference: such a proposition has probability 1/2 just in case you are indifferent as to which side of it you bet on. (So if Bojack wins the Kentucky Derby is an ethically neutral proposition, it has probability 1/2 just in case you are indifferent between winning twenty dollars if it’s true and losing a dollar otherwise, and winning twenty dollars if it’s false and losing a dollar otherwise.)
By positing an ethically neutral proposition with probability 1/2, together with a rich space of prizes, Ramsey defines numerical utilities for prizes. (The rough idea is that if you are indifferent between receiving a middling prize \(m\) for certain, and a gamble that yields a better prize \(b\) if the ethically neutral proposition is true and a worse prize \(w\) if it is falls, then the utility of \(m\) is halfway between the utilities of \(b\) and \(w\).) Using these numerical utilities, he then exploits the definition of expected utility to define probabilities for all other propositions.
The rough idea is to exploit the richness of the space of prizes, which ensures that for any gamble \(g\) that yields better prize \(b\) if \(E\) is true and worse prize \(w\) if \(E\) is false, the agent is indifferent between \(g\) and some middling prize \(m\). This means that \(EU(g) = EU(m)\). Using some algebra, plus the fact that \(EU(g) = P(E)U(b) + (1-P(E))U(w)\), Ramsey shows that
2.2.2 Von Neumann and Morgenstern
Von Neumann and Morgenstern (1944) claim that preferences are defined over a domain of lotteries . Some of these lotteries are constant , and yield a single prize with certainty. (Prizes might include a banana, a million dollars, a million dollars’ worth of debt, death, or a new car.) Lotteries can also have other lotteries as prizes, so that one can have a lottery with a 40% chance of yielding a banana, and a 60% chance of yielding a 50-50 gamble between a million dollars and death.) The domain of lotteries is closed under a mixing operation, so that if \(L\) and \(L'\) are lotteries and \(x\) is a real number in the \([0, 1]\) interval, then there is a lottery \(x L + (1-x) L'\) that yields \(L\) with probability \(x\) and \(L'\) with probability \(1-x\). They show that every preference relation obeying certain axioms can be represented by the probabilities used to define the lotteries, together with a utility function which is unique up to positive linear transformation.
2.2.3 Savage
Instead of taking probabilities for granted, as von Neumann and Morgenstern do, Savage (1972) defines them in terms of preferences over acts. Savage posits three separate domains. Probability attaches to events , which we can think of as disjunctions of states, while utility and intrinsic preference attach to outcomes . Expected utility and non-intrinsic preference attach to acts .
For Savage, acts, states, and outcomes must satisfy certain constraints. Acts must be wholly under the agent’s control (so publishing my paper in Mind is not an act, since it depends partly on the editor’s decision, which I do not control). Outcomes must have the same utility regardless of which state obtains (so "I win a fancy car" is not an outcome, since the utility of the fancy car will be greater in states where the person I most want to impress wishes I had a fancy car, and less in states where I lose my driver’s license). No state can rule out the performance of any act, and an act and a state together must determine an outcome with certainty. For each outcome \(o\), there is a constant act which yields \(o\) in every state. (Thus, if world peace is an outcome, there is an act that results in world peace, no matter what the state of the world.) Finally, he assumes for any two acts \(A\) and \(B\) and any event \(E\), there is a mixed act \(A_E \amp B_{\sim E}\) that yields the same outcome as \(A\) if \(E\) is true, and the same outcome as \(B\) otherwise. (Thus, if world peace and the end of the world are both outcomes, then there is a mixed act that results in world peace if a certain coin lands heads, and the end of the world otherwise.)
Savage postulates a preference relation over acts, and gives axioms governing that preference relation. He then defines subjective probabilities, or degrees of belief, in terms of preferences. The key move is to define an “at least as likely as” relation between events; I paraphrase here.
Suppose \(A\) and \(B\) are constant acts such that \(A\) is preferred to \(B\). Then \(E\) is at least as likely as \(F\) just in case the agent either prefers \(A_E \amp B_{\sim E}\) (the act that yields \(A\) if \(E\) obtains, and \(B\) otherwise) to \(A_F \amp B_{\sim F}\) (the act that yields \(A\) if \(F\) obtains, and \(B\) otherwise), or else is indifferent between \(A_E \amp B_{\sim E}\) and \(A_F \amp B_{\sim F}\).
The thought behind the definition is that the agent considers \(E\) at least as likely as \(F\) just in case she would not rather bet on \(F\) than on \(E\)).
Savage then gives axioms constraining rational preference, and shows that any set of preferences satisfying those axioms yields an “at least as likely” relation that can be uniquely represented by a probability function. In other words, there is one and only one probability function \(P\) such that for all \(E\) and \(F\), \(P(E) \ge P(F)\) if and only if \(E\) is at least as likely as \(F\). Every preference relation obeying Savage’s axioms is represented by this probability function \(P\), together with a utility function which is unique up to positive linear transformation.
Savage’s representation theorem gives strong results: starting with a preference ordering alone, we can find a single probability function, and a narrow class of utility functions, which represent that preference ordering. The downside, however, is that Savage has to build implausibly strong assumptions about the domain of acts.
Luce and Suppes (1965) point out that Savage’s constant acts are implausible. (Recall that constant acts yield the same outcome and the same amount of value in every state.) Take some very good outcome—total bliss for everyone. Is there really a constant act that has this outcome in every possible state, including states where the human race is wiped out by a meteor? Savage’s reliance on a rich space of mixed acts is also problematic. Savage has had to assume that any two outcomes and any event, there is a mixed act that yields the first outcome if the event occurs, and the second outcome otherwise? Is there really an act that yields total bliss if everyone is killed by an antibiotic-resistant plague, and total misery otherwise? Luce and Krantz (1971) suggest ways of reformulating Savage’s representation theorem that weaken these assumptions, but Joyce (1999) argues that even on the weakened assumptions, the domain of acts remains implausibly rich.
2.2.4 Bolker and Jeffrey
Bolker (1966) proves a general representation theorem about mathematical expectations, which Jeffrey (1983) uses as the basis for a philosophical account of expected utility theory. Bolker’s theorem assumes a single domain of propositions, which are objects of preference, utility, and probability alike. Thus, the proposition that it will rain today has a utility, as well as a probability. Jeffrey interprets this utility as the proposition’s news value —a measure of how happy or disappointed I would be to learn that the proposition was true. By convention, he sets the value of the necessary proposition at 0—the necessary proposition is no news at all! Likewise, the proposition that I take my umbrella to work, which is an act, has a probability as well as a utility. Jeffrey interprets this to mean that I have degrees of belief about what I will do.
Bolker gives axioms constraining preference, and shows that any preferences satisfying his axioms can be represented by a probability measure \(P\) and a utility measure \(U\). However, Bolker’s axioms do not ensure that \(P\) is unique, or that \(U\) is unique up to positive linear transformation. Nor do they allow us to define comparative probability in terms of preference. Instead, where \(P\) and \(U\) jointly represent a preference ordering, Bolker shows that the pair \(\langle P, U \rangle\) is unique up to a fractional linear transformation.
In technical terms, where \(U\) is a utility function normalized so that \(U(\Omega) = 0\), \(inf\) is the greatest lower bound of the values assigned by \(U\), \(sup\) is the least upper bound of the values assigned by by \(U\), and \(\lambda\) is a parameter falling between \(-1/inf\) and \(-1/sup\), the fractional linear transformation \(\langle P_{\lambda}, U_{\lambda} \rangle\) of \(\langle P, U \rangle\) corresponding to \(\lambda\) is given by:
Notice that fractional linear transformations of a probability-utility pair can disagree with the original pair about which propositions are likelier than which others.
Joyce (1999) shows that with additional resources, Bolker’s theorem can be modified to pin down a unique \(P\), and a \(U\) that is unique up to positive linear transformation. We need only supplement the preference ordering with a primitive “more likely than” relation, governed by its own set of axioms, and linked to belief by several additional axioms. Joyce modifies Bolker’s result to show that given these additional axioms, the “more likely than” relation is represented by a unique \(P\), and the preference ordering is represented by \(P\) together with a utility function that is unique up to positive linear transformation.
2.2.5 Summary
Together, these four representation theorems above can be summed up in the following table.
Notice that the order of construction differs between theorems: Ramsey constructs a representation of probability using utility, while von Neumann and Morgenstern begin with probabilities and construct a representation of utility. Thus, although the arrows represent a mathematical relationship of representation, they cannot represent a metaphysical relationship of grounding. The Reality Condition needs to be justified independently of any representation theorem.
Suitably structured ordinal probabilities (the relations picked out by “at least as likely as”, “more likely than”, and “equally likely”) stand in one-to-one correspondence with the cardinal probability functions. Finally, the grey line from preferences to ordinal probabilities indicates that every probability function satisfying Savage’s axioms is represented by a unique cardinal probability—but this result does not hold for Jeffrey’s axioms.
Notice that it is often possible to follow the arrows in circles—from preference to ordinal probability, from ordinal probability to cardinal probability, from cardinal probability and preference to expected utility, and from expected utility back to preference. Thus, although the arrows represent a mathematical relationship of representation, they do not represent a metaphysical relationship of grounding. This fact drives home the importance of independently justifying the Reality Condition—representation theorems cannot justify expected utility theory without additional assumptions.
3. Objections to Expected Utility Theory
Ought implies can, but is it humanly possible to maximize expected utility? March and Simon (1958) point out that in order to compute expected utilities, an agent needs a dauntingly complex understanding of the available acts, the possible outcomes, and the values of those outcomes, and that choosing the best act is much more demanding than choosing an act that is merely good enough. Similar points appear in Lindblom (1959), Feldman (2006), and Smith (2010).
McGee (1991) argues that maximizing expected utility is not mathematically possible even for an ideal computer with limitless memory. In order to maximize expected utility, we would have to accept any bet we were offered on the truths of arithmetic, and reject any bet we were offered on false sentences in the language of arithmetic. But arithmetic is undecidable, so no Turing machine can determine whether a given arithmetical sentence is true or false.
One response to these difficulties is the bounded rationality approach, which aims to replace expected utility theory with some more tractable rules. Another is to argue that the demands of expected utility theory are more tractable than they appear (Burch-Brown 2014; see also Greaves 2016), or that the relevant “ought implies can” principle is false (Srinivasan 2015).
A variety of authors have given examples in which expected utility theory seems to give the wrong prescriptions. Sections 3.2.1 and 3.2.2 discuss examples where rationality seems to permit preferences inconsistent with expected utility theory. These examples suggest that maximizing expected utility is not necessary for rationality. Section 3.2.3 discusses examples where expected utility theory permits preferences that seem irrational. These examples suggest that maximizing expected utility is not sufficient for rationality. Section 3.2.4 discusses an example where expected utility theory requires preferences that seem rationally forbidden—a challenge to both the necessity and the sufficiency of expected utility for rationality.
3.2.1 Counterexamples Involving Transitivity and Completeness
Expected utility theory implies that the structure of preferences mirrors the structure of the greater-than relation between real numbers. Thus, according to expected utility theory, preferences must be transitive : If \(A\) is preferred to \(B\) (so that \(U(A) \gt U(B)\)), and \(B\) is preferred to \(C\) (so that \(U(B) \gt U(C)\)), then \(A\) must be preferred to \(C\) (since it must be that \(U(A) \gt U(C)\)). Likewise, preferences must be complete : for any two options, either one must be preferred to the other, or the agent must be indifferent between them (since of their two utilities, either one must be greater or the two must be equal). But there are cases where rationality seems to permit (or perhaps even require) failures of transitivity and failures of completeness.
An example of preferences that are not transitive, but nonetheless seem rationally permissible, is Quinn’s puzzle of the self-torturer (1990). The self-torturer is hooked up to a machine with a dial with settings labeled 0 to 1,000, where setting 0 does nothing, and each successive setting delivers a slightly more powerful electric shock. Setting 0 is painless, while setting 1,000 causes excruciating agony, but the difference between any two adjacent settings is so small as to be imperceptible. The dial is fitted with a ratchet, so that it can be turned up but never down. Suppose that at each setting, the self-torturer is offered $10,000 to move up to the next, so that for tolerating setting \(n\), he receives a payoff of \(n {\cdot} {$10,000}\). It is permissible for the self-torturer to prefer setting \(n+1\) to setting \(n\) for each \(n\) between 0 and 999 (since the difference in pain is imperceptible, while the difference in monetary payoffs is significant), but not to prefer setting 1,000 to setting 0 (since the pain of setting 1,000 may be so unbearable that no amount of money will make up for it.
It also seems rationally permissible to have incomplete preferences. For some pairs of actions, an agent may have no considered view about which she prefers. Consider Jane, an electrician who has never given much thought to becoming a professional singer or a professional astronaut. (Perhaps both of these options are infeasible, or perhaps she considers both of them much worse than her steady job as an electrician). It is false that Jane prefers becoming a singer to becoming an astronaut, and it is false that she prefers becoming an astronaut to becoming a singer. But it is also false that she is indifferent between becoming a singer and becoming an astronaut. She prefers becoming a singer and receiving a $100 bonus to becoming a singer, and if she were indifferent between becoming a singer and becoming an astronaut, she would be rationally compelled to prefer being a singer and receiving a $100 bonus to becoming an astronaut.
There is one key difference between the two examples considered above. Jane’s preferences can be extended , by adding new preferences without removing any of the ones she has, in a way that lets us represent her as an expected utility maximizer. On the other hand, there is no way of extended the self-torturer’s preferences so that he can be represented as an expected utility maximizer. Some of his preferences would have to be altered. One popular response to incomplete preferences is to claim that, while rational preferences need not satisfy the axioms of a given representation theorem (see section 2.2), it must be possible to extend them so that they satisfy the axioms. From this weaker requirement on preferences—that they be extendible to a preference ordering that satisfies the relevant axioms—one can prove the existence halves of the relevant representation theorems. However, one can no longer establish that each preference ordering has a representation which is unique up to allowable transformations.
No such response is available in the case of the self-torturer, whose preferences cannot be extended to satisfy the axioms of expected utility theory. See the entry on preferences for a more extended discussion of the self-torturer case.
3.2.2 Counterexamples Involving Independence
Allais (1953) and Ellsberg (1961) propose examples of preferences that cannot be represented by an expected utility function, but that nonetheless seem rational. Both examples involve violations of Savage’s Independence axiom:
Independence . Suppose that \(A\) and \(A^*\) are two acts that produce the same outcomes in the event that \(E\) is false. Then, for any act \(B\), one must have \(A\) is preferred to \(A^*\) if and only if \(A_E \amp B_{\sim E}\) is preferred to \(A^*_E \amp B_{\sim E}\) The agent is indifferent between \(A\) and \(A^*\) if and only if she is indifferent between \(A_E \amp B_{\sim E}\) and \(A^*_E \amp B_{\sim E}\)
In other words, if two acts have the same consequences whenever \(E\) is false, then the agent’s preferences between those two acts should depend only on their consequences when \(E\) is true. On Savage’s definition of expected utility, expected utility theory entails Independence. And on Jeffrey’s definition, expected utility theory entails Independence in the presence of the assumption that the states are probabilistically independent of the acts.
The first counterexample, the Allais Paradox, involves two separate decision problems in which a ticket with a number between 1 and 100 is drawn at random. In the first problem, the agent must choose between these two lotteries:
- Lottery \(A\)
- • $100 million with certainty
- Lottery \(B\)
- • $500 million if one of tickets 1–10 is drawn
- • $100 million if one of tickets 12–100 is drawn
- • Nothing if ticket 11 is drawn
In the second decision problem, the agent must choose between these two lotteries:
- Lottery \(C\)
- • $100 million if one of tickets 1–11 is drawn
- • Nothing otherwise
- Lottery \(D\)
It seems reasonable to prefer \(A\) (which offers a sure $100 million) to \(B\) (where the added 10% chance at $500 million is more than offset by the risk of getting nothing). It also seems reasonable to prefer \(D\) (an 10% chance at a $500 million prize) to \(C\) (a slightly larger 11% chance at a much smaller $100 million prize). But together, these preferences (call them the Allais preferences ) violate Independence. Lotteries \(A\) and \(C\) yield the same $100 million prize for tickets 12–100. They can be converted into lotteries \(B\) and \(D\) by replacing this $100 million prize with $0.
Because they violate Independence, the Allais preferences are incompatible with expected utility theory. This incompatibility does not require any assumptions about the relative utilities of the $0, the $100 million, and the $500 million. Where $500 million has utility \(x\), $100 million has utility \(y\), and $0 has utility \(z\), the expected utilities of the lotteries are as follows.
It is easy to see that the condition under which \(EU(A) \gt EU(B)\) is exactly the same as the condition under which \(EU(C) \gt EU(D)\): both inequalities obtain just in case \(0.11y \gt 0.10x + 0.01z\)
The Ellsberg Paradox also involves two decision problems that generate a violation of the sure-thing principle. In each of them, a ball is drawn from an urn containing 30 red balls, and 60 balls that are either white or yellow in unknown proportions. In the first decision problem, the agent must choose between the following lotteries:
- Lottery \(R\)
- • Win $100 if a red ball is drawn
- • Lose $100 otherwise
- Lottery \(W\)
- • Win $100 if a white ball is drawn
In the second decision problem, the agent must choose between the following lotteries:
- Lottery \(RY\)
- • Win $100 if a red or yellow ball is drawn
- Lottery \(WY\)
- • Win $100 if a white or yellow ball is drawn
It seems reasonable to prefer \(R\) to \(W\), but at the same time prefer \(WY\) to \(RY\). (Call this combination of preferences the Ellsberg preferences .) Like the Allais preferences, the Ellsberg preferences violate Independence. Lotteries \(W\) and \(R\) yield a $100 loss if a yellow ball is drawn; they can be converted to lotteries \(RY\) and \(WY\) simply by replacing this $100 loss with a sure $100 gain.
Because they violate independence, the Ellsberg preferences are incompatible with expected utility theory. Again, this incompatibility does not require any assumptions about the relative utilities of winning $100 and losing $100. Nor do we need any assumptions about where between 0 and 1/3 the probability of drawing a yellow ball falls. Where winning $100 has utility \(w\) and losing $100 has utility \(l\),
It is easy to see that the condition in which \(EU(R) \gt EU(W)\) is exactly the same as the condition under which \(EU(RY) \gt EU(WY)\): both inequalities obtain just in case \(1/3\,w + P(W)l \gt 1/3\,l + P(W)w\).
There are three notable responses to the Allais and Ellsberg paradoxes. First, one might follow Savage (101 ff) and Raiffa (1968, 80–86), and defend expected utility theory on the grounds that the Allais and Ellsberg preferences are irrational.
Second, one might follow Buchak (2013) and claim that that the Allais and Ellsberg preferences are rationally permissible, so that expected utility theory fails as a normative theory of rationality. Buchak develops an a more permissive theory of rationality, with an extra parameter representing the decision-maker’s attitude toward risk. This risk parameter interacts with the utilities of outcomes and their conditional probabilities on acts to determine the values of acts. One setting of the risk parameter yields expected utility theory as a special case, but other, “risk-averse” settings rationalise the Allais preferences.
Third, one might follow Loomes and Sugden (1986), Weirich (1986), and Pope (1995) and argue that the outcomes in the Allais and Ellsberg paradoxes can be re-described to accommodate the Allais and Ellsberg preferences. The alleged conflict between the Allais and Ellsberg preferences on the one hand, and expected utility theory on the other, was based on the assumption that a given sum of money has the same utility no matter how it is obtained. Some authors challenge this assumption. Loomes and Sugden suggest that in addition to monetary amounts, the outcomes of the gambles include feelings of disappointment (or elation) at getting less (or more) than expected. Pope distinguishes “post-outcome” feelings of elation or disappointment from “pre-outcome” feelings of excitement, fear, boredom, or safety, and points out that both may affect outcome utilities. Weirich suggests that the value of a monetary sum depends partly on the risks that went into obtaining it, irrespective of the gambler’s feelings, so that (for instance) $100 million as the result of a sure bet is more than $100 million from a gamble that might have paid nothing.
Broome (1991, Ch. 5) raises a worry about this re-description solution. Any preferences can be justified by re-describing the space of outcomes, thus rendering the axioms of expected utility theory devoid of content. Broome rebuts this objection by suggesting an additional constraint on preference: if \(A\) is preferred to \(B\), then \(A\) and \(B\) must differ in some way that justifies preferring one to the other. An expected utility theorist can then count the Allais and Ellsberg preferences as rational if, and only if, there is a non-monetary difference that justifies placing outcomes of equal monetary value at different spots in one’s preference ordering.
3.2.3 Counterexamples Involving Probability 0 Events
Above, we’ve seen purported examples of rational preferences that violate expected utility theory. There are also purported examples of irrational preferences that satisfy expected utility theory.
On a typical understanding of expected utility theory, when two acts are tied for having the highest expected utility, agents are required to be indifferent between them. Skyrms (1980, p. 74) points out that this view lets us derive strange conclusions about events with probability 0. For instance, suppose you are about to throw a point-sized dart at a round dartboard. Classical probability theory countenances situations in which the dart has probability 0 of hitting any particular point. You offer me the following lousy deal: if the dart hits the board at its exact center, then you will charge me $100; otherwise, no money will change hands. My decision problem can be captured with the following matrix:
Expected utility theory says that it is permissible for me to accept the deal—accepting has expected utility of 0. (This is so on both the Jeffrey definition and the Savage definition, if we assume that how the dart lands is probabilistically independent of how you bet.) But common sense says it is not permissible for me to accept the deal. Refusing weakly dominates accepting: it yields a better outcome in some states, and a worse outcome in no state.
Skyrms suggests augmenting the laws of classical probability with an extra requirement that only impossibilities are assigned probability 0. Easwaran (2014) argues that we should instead reject the view that expected utility theory commands indifference between acts with equal expected utility. Instead, expected utility theory is not a complete theory of rationality: when two acts have the same expected utility, it does not tell us which to prefer. We can use non-expected-utility considerations like weak dominance as tiebreakers.
3.2.4 Counterexamples Involving Unbounded Utility
A utility function \(U\) is bounded above if there is a limit to how good things can be according to \(U\), or more formally, if there is some least natural number \(sup\) such that for every \(A\) in \(U\)’s domain, \(U(A) \le sup\). Likewise, \(U\) is bounded below if there is a limit to how bad things can be according to \(U\), or more formally, if there is some greatest natural number \(inf\) such that for every \(A\) in \(U\)’s domain, \(U(A) \ge inf\). Expected utility theory can run into trouble when utility functions are unbounded above, below, or both.
One problematic example is the St. Petersburg game, originally published by Bernoulli. Suppose that a coin is tossed until it lands tails for the first time. If it lands tails on the first toss, you win $2; if it lands tails on the second toss, you win $4; if it lands tails on the third toss, you win $8, and if it lands tails on the \(n\)th toss, you win $\(2^n\). Assuming each dollar is worth one utile, the expected value of the St Petersburg game is
It turns out that this sum diverges; the St Petersburg game has infinite expected utility. Thus, according to expected utility theory, you should prefer the opportunity to play the St Petersburg game to any finite sum of money, no matter how large. Furthermore, since an infinite expected utility multiplied by any nonzero chance is still infinite, anything that has a positive probability of yielding the St Petersburg game has infinite expected utility. Thus, according to expected utility theory, you should prefer any chance at playing the St Petersburg game, however slim, to any finite sum of money, however large.
Nover and Hájek (2004) argue that in addition to the St. Petersburg game, which has infinite expected utility, there are other infinitary games whose expected utilities are undefined, even though rationality mandates certain preferences among them.
One response to these problematic infinitary games is to argue that the decision problems themselves are ill-posed (Jeffrey (1983, 154); another is to adopt a modified version of expected utility theory that agrees with its verdicts in the ordinary case, but yields intuitively reasonable verdicts about the infinitary games (Thalos and Richardson 2013) (Fine 2008) (Colyvan 2006, 2008) (Easwaran 2008).
4. Applications
In the 1940s and 50s, expected utility theory gained currency in the US for its potential to provide a mechanism that would explain the behavior of macro-economic variables. As it became apparent that expected utility theory did not accurately predict the behaviors of real people, its proponents instead advanced the view that it might serve instead as a theory of how rational people should respond to uncertainty (see Herfeld 2017).
Expected utility theory has a variety of applications in public policy. In welfare economics, Harsanyi (1953) reasons from expected utility theory to the claim that the most socially just arrangement is the one that maximizes total welfare distributed across a society society. The theory of expected utility also has more direct applications. Howard (1980) introduces the concept of a micromort , or a one-in-a-million chance of death, and uses expected utility calculations to gauge which mortality risks are acceptable. In health policy, quality-adjusted life years, or QALYs, are measures of the expected utilities of different health interventions used to guide health policy (see Weinstein et al 2009). McAskill (2015) uses expected utility theory to address the central question of effective altruism : “How can I do the most good?” (Utilties in these applications are most naturally interpreted as measuring something like happiness or wellbeing, rather than subjective preference satisfaction for an individual agent.)
Another area where expected utility theory finds applications is in insurance sales. Like casinos, insurance companies take on calculated risks with the aim of long-term financial gain, and must take into account the chance of going broke in the short run.
Utilitarians, along with their descendants contemporary consequentialists, hold that the rightness or wrongness of an act is determined by the moral goodness or badness of its consequences. Some consequentialists, such as (Railton 1984), interpret this to mean that we ought to do whatever will in fact have the best consequences. But it is difficult—perhaps impossible—to know the long-term consequences of our acts (Lenman 2000, Howard-Snyder 2007). In light of this observation, Jackson (1991) argues that the right act is the one with the greatest expected moral value, not the one that will in fact yield the best consequences.
As Jackson notes, the expected moral value of an act depends on which probability function we work with. Jackson argues that, while every probability function is associated with an “ought”, the “ought” that matters most to action is the one associated with the decision-maker’s degrees of belief at the time of action. Other authors claim priority for other “oughts”: Mason (2013) favors the probability function that is most reasonable for the agent to adopt in response to her evidence, given her epistemic limitations, while Oddie and Menzies (1992) favor the objective chance function as a measure of objective rightness. (They appeal to a more complicated probability function to define a notion of “subjective rightness” for decisionmakers who are ignorant of the objective chances.)
Still others (Smart 1973, Timmons 2002) argue that even if that we ought to do whatever will have the best consequences, expected utility theory can play the role of a decision procedure when we are uncertain what consequences our acts will have. Feldman (2006) objects that expected utility calculations are horribly impractical. In most real life decisions, the steps required to compute expected utilities are beyond our ken: listing the possible outcomes of our acts, assigning each outcome a utility and a conditional probability given each act, and performing the arithmetic necessary to expected utility calculations.
The expected-utility-maximizing version of consequentialism is not strictly speaking a theory of rational choice. It is a theory of moral choice, but whether rationality requires us to do what is morally best is up for debate.
Expected utility theory can be used to address practical questions in epistemology. One such question is when to accept a hypothesis. In typical cases, the evidence is logically compatible with multiple hypotheses, including hypotheses to which it lends little inductive support. Furthermore, scientists do not typically accept only those hypotheses that are most probable given their data. When is a hypothesis likely enough to deserve acceptance?
Bayesians, such as Maher (1993), suggest that this decision be made on expected utility grounds. Whether to accept a hypothesis is a decision problem, with acceptance and rejection as acts. It can be captured by the following decision matrix:
On Savage’s definition, the expected utility of accepting the hypothesis is determined by the probability of the hypothesis, together with the utilities of each of the four outcomes. (We can expect Jeffrey’s definition to agree with Savage’s on the plausible assumption that, given the evidence in our possession, the hypothesis is probabilistically independent of whether we accept or reject it.) Here, the utilities can be understood as purely epistemic values, since it is epistemically valuable to believe interesting truths, and to reject falsehoods.
Critics of the Bayesian approach, such as Mayo (1996), object that scientific hypotheses cannot sensibly be given probabilities. Mayo argues that in order to assign a useful probability to an event, we need statistical evidence about the frequencies of similar events. But scientific hypotheses are either true once and for all, or false once and for all—there is no population of worlds like ours from which we can meaningfully draw statistics. Nor can we use subjective probabilities for scientific purposes, since this would be unacceptably arbitrary. Therefore, the expected utilities of acceptance and rejection are undefined, and we ought to use the methods of traditional statistics, which rely on comparing the probabilities of our evidence conditional on each of the hypotheses.
Expected utility theory also provides guidance about when to gather evidence. Good (1967) argues on expected utility grounds that it is always rational to gather evidence before acting, provided that evidence is free of cost. The act with the highest expected utility after the extra evidence is in will always be always at least as good as the act with the highest expected utility beforehand.
In epistemic decision theory , expected utilities are used to assess belief states as rational or irrational. If we think of belief formation as a mental act, facts about the contents of the agent’s beliefs as events, and closeness to truth as a desirable feature of outcomes, then we can use expected utility theory to evaluate degrees of belief in terms of their expected closeness to truth. The entry on epistemic utility arguments for probabilism includes an overview of expected utility arguments for a variety of epistemic norms, including conditionalization and the Principal Principle.
Kaplan (1968), argues that expected utility considerations can be used to fix a standard of proof in legal trials. A jury deciding whether to acquit or convict faces the following decision problem:
Kaplan shows that \(EU(convict) > EU(acquit)\) whenever
Qualitatively, this means that the standard of proof increases as the disutility of convicting an innocent person \((U(\mathrm{true~conviction})-U(\mathrm{false~acquittal}))\) increases, or as the disutility of acquitting a guilty person \((U(\mathrm{true~acquittal})-U(\mathrm{false~conviction}))\) decreases.
Critics of this decision-theoretic approach, such as Laudan (2006), argue that it’s difficult or impossible to bridge the gap between the evidence admissible in court, and the real probability of the defendant’s guilt. The probability guilt depends on three factors: the distribution of apparent guilt among the genuinely guilty, the distribution of apparent guilt among the genuinely innocent, and the ratio of genuinely guilty to genuinely innocent defendants who go to trial (see Bell 1987). Obstacles to calculating any of these factors will block the inference from a judge or jury’s perception of apparent guilt to a true probability of guilt.
- Allais M., 1953, “Le Comportement de l’Homme Rationnel devant le Risque: Critique des Postulats et Axiomes de l’École Americaine”, Econometrica , 21: 503–546.
- Bell, R., 1987, “Decision Theory and Due Process: A Critique of the Supreme Court’s Lawmaking for Burdens of Proof”, Journal of Criminal Law and Criminology , 78: 557-585.
- Bentham, J., 1961. An Introduction to the Principles of Morals and Legislation, Garden City: Doubleday. Originally published in 1789.
- Bernoulli, D., 1738, “Specimen theoriae novae de mensura sortis”, Commentarii Academiae Scientiarum Imperialis Petropolitanae 5. Translated by Louise Somer and reprinted as “Exposition of a New Theory on the Measurement of Risk” 1954, Econometrica , 22: 23–36.
- Bolker, E., 1966, “Functions Resembling Quotients of Measures”, Transactions of the American Mathematical Society , 2: 292–312.
- Bradley, R., 2004, “Ramsey’s representation theorem”, Dialectica , 58: 483–497.
- Broome, J., 1991, Weighing Goods: Equality, Uncertainty and Time , Oxford: Blackwell, doi:10.1002/9781119451266
- Burch-Brown, J.M., 2014, “Clues for Consequentialists”, Utilitas , 26: 105-119.
- Buchak, L., 2013, Risk and Rationality , Oxford: Oxford University Press.
- Colyvan, M., 2006, “No Expectations”, Mind , 116: 695–702.
- Colyvan, M., 2008, “Relative Expectation Theory”, Journal of Philosophy , 105: 37–44.
- Easwaran, K., 2014, “Regularity and Hyperreal Credences”, The Philosophical Review , 123: 1–41.
- Easwaran, K., 2008, “Strong and Weak Expectations”, Mind , 117: 633–641.
- Elliott, E., 2017, “Ramsey without Ethical Neutrality: A New Representation Theorem”, Mind , 126: 1-51.
- Ellsberg, D., 1961, “Risk, Ambiguity, and the Savage Axioms”, Quarterly Journal of Economics , 75: 643–669.
- Feldman, F. 2006, “Actual utility, the objection from impracticality, and the move to expected utility”, Philosophical Studies , 129 : 49–79.
- Fine, T., 2008, “Evaluating the Pasadena, Altadena, and St Petersburg Gambles”, Mind , 117: 613–632.
- Good, I.J., 1967, “On the Principle of Total Evidence”, The British Journal for the Philosophy of Science , 17: 319–321
- Greaves, H. 2016, “Cluelessness”, Proceedings of the Aristotelian Society , 116: 311-339.
- Hampton, J., “The Failure of Expected-Utility Theory as a Theory of Reason”, Economics and Philosophy , 10: 195–242.
- Harsanyi, J.C., 1953, “Cardinal utility in welfare economics and in the theory of risk-taking”, Journal of Political Economy , 61: 434–435.
- Herfeld, C., “From Theories of Human Behavior to Rules of Rational Choice: Tracing a Normative Turn at the Cowles Commission, 1943-1954”, History of Political Economy , 50: 1-48.
- Howard, R.A., 1980, “On Making Life and Death Decisions”, in R.C. Schwing and W.A. Albers, Societal Risk Assessment: How Safe is Safe Enough? , New York: Plenum Press.
- Howard-Snyder, F., 1997, “The Rejection of Objective Consequentialism”, Utilitas , 9: 241–248.
- Jackson, F., 1991, “Decision-theoretic consequentialism and the nearest and dearest objection”, Ethics , 101: 461–482.
- Jeffrey, R., 1983, The Logic of Decision , 2 nd edition, Chicago: University of Chicago Press.
- Jevons, W.S., 1866, “A General Mathematical Theory of Political Economy”, Journal of the Royal Statistical Society , 29: 282–287.
- Joyce, J., 1999, The Foundations of Causal Decision Theory , Cambridge: Cambridge University Press.
- Kahneman, D. & Tversky A., Judgment Under Uncertainty: Heuristics and Biases , New York: Cambridge University Press.
- Kaplan, J., 1968, “Decision Theory and the Factfinding Process”, Stanford Law Review , 20: 1065-1092.
- Kolmogorov, A. N., 1933, Grundbegriffe der Wahrscheinlichkeitrechnung, Ergebnisse Der Mathematik ; translated as Foundations of Probability , New York: Chelsea Publishing Company, 1950.
- Laudan, L., 2006, Truth, Error, and Criminal Law , Cambridge: Cambridge University Press.
- Lenman, J., 2000. “Consequentialism and cluelessness”, Philosophy and Public Affairs , 29(4): 342–370.
- Lewis, D., 1981, “Causal Decision Theory”, Australasian Journal of Philosophy , 59: 5–30.
- Levi, I., 1991, “Consequentialism and Sequential Choice”, in M. Bacharach and S. Hurley (eds.), Foundations of Decision Theory , Oxford: Basil Blackwell Ltd, 92–12.
- Lindblom, C.E., 1959, “The Science of ‘Muddling Through’”, Public Administration Review , 19: 79–88.
- Loomes, G. And Sugden, R., 1986, “Disappointment and Dynamic Consistency in Choice Under Uncertainty”, The Review of Economic Studies , 53(2): 271–282.
- Maher, P., 1993, Betting on Theories , Cambridge: Cambridge University Press.
- March, J.G. and Simon, H., 1958, Organizations , New York: Wiley.
- Mason, E., 2013, “Objectivism and Prospectivism About Rightness”, Journal of Ethics and Social Philosophy , 7: 1–21.
- Mayo, D., 1996, Error and the Growth of Experimental Knowledge , Chicago: University of Chicago Press.
- McAskill, W., 2015, Doing Good Better , New York: Gotham Books.
- McGee, V., 1991, “We Turing Machines Aren’t Expected-Utility Maximizers (Even Ideally)”, Philosophical Studies , 64: 115-123.
- Meacham, C. and Weisberg, J., 2011, “Representation Theorems and the Foundations of Decision Theory”, Australasian Journal of Philosophy , 89: 641–663.
- Menger, K., 1871, Grundsätze der Volkswirtschaftslehre , translated by James Dingwall and Bert F. Hoselitz as Principles of Economics , New York: New York University Press, 1976; reprinted online , Ludwig von Mises Institute, 2007.
- Mill, J. S., 1861. Utilitarianism. Edited with an introduction by Roger Crisp. New York: Oxford University Press, 1998.
- von Neumann, J., and Morgenstern, O., 1944, Theory of Games and Economic Behavior , Princeton: Princeton University Press.
- Nover, H. & Hájek, A., 2004, “Vexing expectations”, Mind , 113: 237–249.
- Nozick, R., 1969, “Newcomb’s Problem and Two Principles of Choice,” in Nicholas Rescher (ed.), Essays in Honor of Carl G. Hempel , Dordrecht: Reidel, 114–115.
- Oliver, A., 2003, “A quantitative and qualitative test of the Allais paradox using health outcomes”, Journal of Economic Psychology , 24: 35–48.
- Pope, R., 1995, “Towards a More Precise Decision Framework: A Separation of the Negative Utility of Chance from Diminishing Marginal Utility and the Preference for Safety”, Theory and Decision , 39: 241–265.
- Raiffa, H., 1968, Decision analysis: Introductory lectures on choices under uncertainty , Reading, MA: Addison-Wesley.
- Ramsey, F. P., 1926, “Truth and Probability”, in Foundations of Mathematics and other Essays, R. B. Braithwaite (ed.), London: Kegan, Paul, Trench, Trubner, & Co., 1931, 156–198; reprinted in Studies in Subjective Probability , H. E. Kyburg, Jr. and H. E. Smokler (eds.), 2nd edition, New York: R. E. Krieger Publishing Company, 1980, 23–52; reprinted in Philosophical Papers , D. H. Mellor (ed.), Cambridge: Cambridge University Press, 1990.
- Savage, L.J., 1972, The Foundations of Statistics , 2 nd edition, New York: Dover Publications, Inc.
- Sen, A., 1977, “Rational Fools: A Critique of the Behavioral Foundations of Economic Theory”, Philosophy and Public Affairs , 6: 317–344.
- Shafer, G., 2007, “From Cournot’s principle to market efficiency”, in Augustin Cournot: Modelling Economics , Jean-Philippe Touffut (ed.), Cheltenham: Edward Elgar, 55–95.
- Sidgwick, H., 1907. The Methods of Ethics, Seventh Edition. London: Macmillan; first edition, 1874.
- Simon, H., 1956, “A Behavioral Model of Rational Choice”, The Quarterly Journal of Economics , 69: 99–118.
- Skyrms, B., 1980. Causal Necessity: A Pragmatic Investigation of the Necessity of Laws , New Haven, CT: Yale University Press.
- Smith, H.M., “Subjective Rightness”, Social and Political Philosophy , 27: 64-110.
- Sobel, J.H., 1994, Taking Chances: Essays on Rational Choice , Cambridge: Cambridge University Press.
- Spohn, W., 1977, “Where Luce and Krantz do really generalize Savage’s decision model”, Erkenntnis , 11: 113–134.
- Srinivasan, A., 2015, “Normativity Without Cartesian Privilege”, Noûs , 25: 273-299.
- Suppes, P., 2002, Representation and Invariance of Scientific Structures , Stanford: CSLI Publications.
- Thalos, M. and Richardson, O., 2013, “Capitalization in the St. Petersburg game: Why statistical distributions matter”, Politics, Philosophy & Economics , 13: 292-313.
- Weinstein, M.C., Torrence, G., and McGuire, A., 2009 “QALYs: the basics”, Value in Health , 12: S5–S9.
- Weirich, P., 1986, “Expected Utility and Risk”, British Journal for the Philosophy of Science , 37: 419–442.
- Zynda, L., 2000, “Representation Theorems and Realism about Degrees of Belief”, Philosophy of Science , 67: 45–69.
How to cite this entry . Preview the PDF version of this entry at the Friends of the SEP Society . Look up topics and thinkers related to this entry at the Internet Philosophy Ontology Project (InPhO). Enhanced bibliography for this entry at PhilPapers , with links to its database.
- Decisions, Games, and Rational Choice , materials for a course taught in Spring 2008 by Robert Stalnaker, MIT OpenCourseWare.
- Microeconomic Theory III , materials for a course taught in Spring 2010 by Muhamet Yildiz, MIT OpenCourseWare.
- Choice Under Uncertainty , class lecture notes by Jonathan Levin.
- Expected Utility Theory , by Philippe Mongin, entry for The Handbook of Economic Methodology.
- The Origins of Expected Utility Theory , essay by Yvan Lengwiler.
decision theory | decision theory: causal | Pascal’s wager | preferences | probability, interpretations of | Ramsey, Frank: and intergenerational welfare economics | rational choice, normative: rivals to expected utility | risk
Copyright © 2023 by R. A. Briggs < formal . epistemology @ gmail . com >
- Accessibility
Support SEP
Mirror sites.
View this site from another server:
- Info about mirror sites
The Stanford Encyclopedia of Philosophy is copyright © 2023 by The Metaphysics Research Lab , Department of Philosophy, Stanford University
Library of Congress Catalog Data: ISSN 1095-5054
- Search Menu
Sign in through your institution
- Browse content in Arts and Humanities
- Browse content in Archaeology
- Anglo-Saxon and Medieval Archaeology
- Archaeological Methodology and Techniques
- Archaeology by Region
- Archaeology of Religion
- Archaeology of Trade and Exchange
- Biblical Archaeology
- Contemporary and Public Archaeology
- Environmental Archaeology
- Historical Archaeology
- History and Theory of Archaeology
- Industrial Archaeology
- Landscape Archaeology
- Mortuary Archaeology
- Prehistoric Archaeology
- Underwater Archaeology
- Urban Archaeology
- Zooarchaeology
- Browse content in Architecture
- Architectural Structure and Design
- History of Architecture
- Residential and Domestic Buildings
- Theory of Architecture
- Browse content in Art
- Art Subjects and Themes
- History of Art
- Industrial and Commercial Art
- Theory of Art
- Biographical Studies
- Byzantine Studies
- Browse content in Classical Studies
- Classical History
- Classical Philosophy
- Classical Mythology
- Classical Literature
- Classical Reception
- Classical Art and Architecture
- Classical Oratory and Rhetoric
- Greek and Roman Papyrology
- Greek and Roman Epigraphy
- Greek and Roman Law
- Greek and Roman Archaeology
- Late Antiquity
- Religion in the Ancient World
- Digital Humanities
- Browse content in History
- Colonialism and Imperialism
- Diplomatic History
- Environmental History
- Genealogy, Heraldry, Names, and Honours
- Genocide and Ethnic Cleansing
- Historical Geography
- History by Period
- History of Emotions
- History of Agriculture
- History of Education
- History of Gender and Sexuality
- Industrial History
- Intellectual History
- International History
- Labour History
- Legal and Constitutional History
- Local and Family History
- Maritime History
- Military History
- National Liberation and Post-Colonialism
- Oral History
- Political History
- Public History
- Regional and National History
- Revolutions and Rebellions
- Slavery and Abolition of Slavery
- Social and Cultural History
- Theory, Methods, and Historiography
- Urban History
- World History
- Browse content in Language Teaching and Learning
- Language Learning (Specific Skills)
- Language Teaching Theory and Methods
- Browse content in Linguistics
- Applied Linguistics
- Cognitive Linguistics
- Computational Linguistics
- Forensic Linguistics
- Grammar, Syntax and Morphology
- Historical and Diachronic Linguistics
- History of English
- Language Evolution
- Language Reference
- Language Acquisition
- Language Variation
- Language Families
- Lexicography
- Linguistic Anthropology
- Linguistic Theories
- Linguistic Typology
- Phonetics and Phonology
- Psycholinguistics
- Sociolinguistics
- Translation and Interpretation
- Writing Systems
- Browse content in Literature
- Bibliography
- Children's Literature Studies
- Literary Studies (Romanticism)
- Literary Studies (American)
- Literary Studies (Asian)
- Literary Studies (European)
- Literary Studies (Eco-criticism)
- Literary Studies (Modernism)
- Literary Studies - World
- Literary Studies (1500 to 1800)
- Literary Studies (19th Century)
- Literary Studies (20th Century onwards)
- Literary Studies (African American Literature)
- Literary Studies (British and Irish)
- Literary Studies (Early and Medieval)
- Literary Studies (Fiction, Novelists, and Prose Writers)
- Literary Studies (Gender Studies)
- Literary Studies (Graphic Novels)
- Literary Studies (History of the Book)
- Literary Studies (Plays and Playwrights)
- Literary Studies (Poetry and Poets)
- Literary Studies (Postcolonial Literature)
- Literary Studies (Queer Studies)
- Literary Studies (Science Fiction)
- Literary Studies (Travel Literature)
- Literary Studies (War Literature)
- Literary Studies (Women's Writing)
- Literary Theory and Cultural Studies
- Mythology and Folklore
- Shakespeare Studies and Criticism
- Browse content in Media Studies
- Browse content in Music
- Applied Music
- Dance and Music
- Ethics in Music
- Ethnomusicology
- Gender and Sexuality in Music
- Medicine and Music
- Music Cultures
- Music and Media
- Music and Religion
- Music and Culture
- Music Education and Pedagogy
- Music Theory and Analysis
- Musical Scores, Lyrics, and Libretti
- Musical Structures, Styles, and Techniques
- Musicology and Music History
- Performance Practice and Studies
- Race and Ethnicity in Music
- Sound Studies
- Browse content in Performing Arts
- Browse content in Philosophy
- Aesthetics and Philosophy of Art
- Epistemology
- Feminist Philosophy
- History of Western Philosophy
- Metaphysics
- Moral Philosophy
- Non-Western Philosophy
- Philosophy of Language
- Philosophy of Mind
- Philosophy of Perception
- Philosophy of Science
- Philosophy of Action
- Philosophy of Law
- Philosophy of Religion
- Philosophy of Mathematics and Logic
- Practical Ethics
- Social and Political Philosophy
- Browse content in Religion
- Biblical Studies
- Christianity
- East Asian Religions
- History of Religion
- Judaism and Jewish Studies
- Qumran Studies
- Religion and Education
- Religion and Health
- Religion and Politics
- Religion and Science
- Religion and Law
- Religion and Art, Literature, and Music
- Religious Studies
- Browse content in Society and Culture
- Cookery, Food, and Drink
- Cultural Studies
- Customs and Traditions
- Ethical Issues and Debates
- Hobbies, Games, Arts and Crafts
- Natural world, Country Life, and Pets
- Popular Beliefs and Controversial Knowledge
- Sports and Outdoor Recreation
- Technology and Society
- Travel and Holiday
- Visual Culture
- Browse content in Law
- Arbitration
- Browse content in Company and Commercial Law
- Commercial Law
- Company Law
- Browse content in Comparative Law
- Systems of Law
- Competition Law
- Browse content in Constitutional and Administrative Law
- Government Powers
- Judicial Review
- Local Government Law
- Military and Defence Law
- Parliamentary and Legislative Practice
- Construction Law
- Contract Law
- Browse content in Criminal Law
- Criminal Procedure
- Criminal Evidence Law
- Sentencing and Punishment
- Employment and Labour Law
- Environment and Energy Law
- Browse content in Financial Law
- Banking Law
- Insolvency Law
- History of Law
- Human Rights and Immigration
- Intellectual Property Law
- Browse content in International Law
- Private International Law and Conflict of Laws
- Public International Law
- IT and Communications Law
- Jurisprudence and Philosophy of Law
- Law and Politics
- Law and Society
- Browse content in Legal System and Practice
- Courts and Procedure
- Legal Skills and Practice
- Primary Sources of Law
- Regulation of Legal Profession
- Medical and Healthcare Law
- Browse content in Policing
- Criminal Investigation and Detection
- Police and Security Services
- Police Procedure and Law
- Police Regional Planning
- Browse content in Property Law
- Personal Property Law
- Study and Revision
- Terrorism and National Security Law
- Browse content in Trusts Law
- Wills and Probate or Succession
- Browse content in Medicine and Health
- Browse content in Allied Health Professions
- Arts Therapies
- Clinical Science
- Dietetics and Nutrition
- Occupational Therapy
- Operating Department Practice
- Physiotherapy
- Radiography
- Speech and Language Therapy
- Browse content in Anaesthetics
- General Anaesthesia
- Neuroanaesthesia
- Clinical Neuroscience
- Browse content in Clinical Medicine
- Acute Medicine
- Cardiovascular Medicine
- Clinical Genetics
- Clinical Pharmacology and Therapeutics
- Dermatology
- Endocrinology and Diabetes
- Gastroenterology
- Genito-urinary Medicine
- Geriatric Medicine
- Infectious Diseases
- Medical Toxicology
- Medical Oncology
- Pain Medicine
- Palliative Medicine
- Rehabilitation Medicine
- Respiratory Medicine and Pulmonology
- Rheumatology
- Sleep Medicine
- Sports and Exercise Medicine
- Community Medical Services
- Critical Care
- Emergency Medicine
- Forensic Medicine
- Haematology
- History of Medicine
- Browse content in Medical Skills
- Clinical Skills
- Communication Skills
- Nursing Skills
- Surgical Skills
- Browse content in Medical Dentistry
- Oral and Maxillofacial Surgery
- Paediatric Dentistry
- Restorative Dentistry and Orthodontics
- Surgical Dentistry
- Medical Ethics
- Medical Statistics and Methodology
- Browse content in Neurology
- Clinical Neurophysiology
- Neuropathology
- Nursing Studies
- Browse content in Obstetrics and Gynaecology
- Gynaecology
- Occupational Medicine
- Ophthalmology
- Otolaryngology (ENT)
- Browse content in Paediatrics
- Neonatology
- Browse content in Pathology
- Chemical Pathology
- Clinical Cytogenetics and Molecular Genetics
- Histopathology
- Medical Microbiology and Virology
- Patient Education and Information
- Browse content in Pharmacology
- Psychopharmacology
- Browse content in Popular Health
- Caring for Others
- Complementary and Alternative Medicine
- Self-help and Personal Development
- Browse content in Preclinical Medicine
- Cell Biology
- Molecular Biology and Genetics
- Reproduction, Growth and Development
- Primary Care
- Professional Development in Medicine
- Browse content in Psychiatry
- Addiction Medicine
- Child and Adolescent Psychiatry
- Forensic Psychiatry
- Learning Disabilities
- Old Age Psychiatry
- Psychotherapy
- Browse content in Public Health and Epidemiology
- Epidemiology
- Public Health
- Browse content in Radiology
- Clinical Radiology
- Interventional Radiology
- Nuclear Medicine
- Radiation Oncology
- Reproductive Medicine
- Browse content in Surgery
- Cardiothoracic Surgery
- Gastro-intestinal and Colorectal Surgery
- General Surgery
- Neurosurgery
- Paediatric Surgery
- Peri-operative Care
- Plastic and Reconstructive Surgery
- Surgical Oncology
- Transplant Surgery
- Trauma and Orthopaedic Surgery
- Vascular Surgery
- Browse content in Science and Mathematics
- Browse content in Biological Sciences
- Aquatic Biology
- Biochemistry
- Bioinformatics and Computational Biology
- Developmental Biology
- Ecology and Conservation
- Evolutionary Biology
- Genetics and Genomics
- Microbiology
- Molecular and Cell Biology
- Natural History
- Plant Sciences and Forestry
- Research Methods in Life Sciences
- Structural Biology
- Systems Biology
- Zoology and Animal Sciences
- Browse content in Chemistry
- Analytical Chemistry
- Computational Chemistry
- Crystallography
- Environmental Chemistry
- Industrial Chemistry
- Inorganic Chemistry
- Materials Chemistry
- Medicinal Chemistry
- Mineralogy and Gems
- Organic Chemistry
- Physical Chemistry
- Polymer Chemistry
- Study and Communication Skills in Chemistry
- Theoretical Chemistry
- Browse content in Computer Science
- Artificial Intelligence
- Computer Architecture and Logic Design
- Game Studies
- Human-Computer Interaction
- Mathematical Theory of Computation
- Programming Languages
- Software Engineering
- Systems Analysis and Design
- Virtual Reality
- Browse content in Computing
- Business Applications
- Computer Security
- Computer Games
- Computer Networking and Communications
- Digital Lifestyle
- Graphical and Digital Media Applications
- Operating Systems
- Browse content in Earth Sciences and Geography
- Atmospheric Sciences
- Environmental Geography
- Geology and the Lithosphere
- Maps and Map-making
- Meteorology and Climatology
- Oceanography and Hydrology
- Palaeontology
- Physical Geography and Topography
- Regional Geography
- Soil Science
- Urban Geography
- Browse content in Engineering and Technology
- Agriculture and Farming
- Biological Engineering
- Civil Engineering, Surveying, and Building
- Electronics and Communications Engineering
- Energy Technology
- Engineering (General)
- Environmental Science, Engineering, and Technology
- History of Engineering and Technology
- Mechanical Engineering and Materials
- Technology of Industrial Chemistry
- Transport Technology and Trades
- Browse content in Environmental Science
- Applied Ecology (Environmental Science)
- Conservation of the Environment (Environmental Science)
- Environmental Sustainability
- Environmentalist Thought and Ideology (Environmental Science)
- Management of Land and Natural Resources (Environmental Science)
- Natural Disasters (Environmental Science)
- Nuclear Issues (Environmental Science)
- Pollution and Threats to the Environment (Environmental Science)
- Social Impact of Environmental Issues (Environmental Science)
- History of Science and Technology
- Browse content in Materials Science
- Ceramics and Glasses
- Composite Materials
- Metals, Alloying, and Corrosion
- Nanotechnology
- Browse content in Mathematics
- Applied Mathematics
- Biomathematics and Statistics
- History of Mathematics
- Mathematical Education
- Mathematical Finance
- Mathematical Analysis
- Numerical and Computational Mathematics
- Probability and Statistics
- Pure Mathematics
- Browse content in Neuroscience
- Cognition and Behavioural Neuroscience
- Development of the Nervous System
- Disorders of the Nervous System
- History of Neuroscience
- Invertebrate Neurobiology
- Molecular and Cellular Systems
- Neuroendocrinology and Autonomic Nervous System
- Neuroscientific Techniques
- Sensory and Motor Systems
- Browse content in Physics
- Astronomy and Astrophysics
- Atomic, Molecular, and Optical Physics
- Biological and Medical Physics
- Classical Mechanics
- Computational Physics
- Condensed Matter Physics
- Electromagnetism, Optics, and Acoustics
- History of Physics
- Mathematical and Statistical Physics
- Measurement Science
- Nuclear Physics
- Particles and Fields
- Plasma Physics
- Quantum Physics
- Relativity and Gravitation
- Semiconductor and Mesoscopic Physics
- Browse content in Psychology
- Affective Sciences
- Clinical Psychology
- Cognitive Psychology
- Cognitive Neuroscience
- Criminal and Forensic Psychology
- Developmental Psychology
- Educational Psychology
- Evolutionary Psychology
- Health Psychology
- History and Systems in Psychology
- Music Psychology
- Neuropsychology
- Organizational Psychology
- Psychological Assessment and Testing
- Psychology of Human-Technology Interaction
- Psychology Professional Development and Training
- Research Methods in Psychology
- Social Psychology
- Browse content in Social Sciences
- Browse content in Anthropology
- Anthropology of Religion
- Human Evolution
- Medical Anthropology
- Physical Anthropology
- Regional Anthropology
- Social and Cultural Anthropology
- Theory and Practice of Anthropology
- Browse content in Business and Management
- Business Ethics
- Business Strategy
- Business History
- Business and Technology
- Business and Government
- Business and the Environment
- Comparative Management
- Corporate Governance
- Corporate Social Responsibility
- Entrepreneurship
- Health Management
- Human Resource Management
- Industrial and Employment Relations
- Industry Studies
- Information and Communication Technologies
- International Business
- Knowledge Management
- Management and Management Techniques
- Operations Management
- Organizational Theory and Behaviour
- Pensions and Pension Management
- Public and Nonprofit Management
- Strategic Management
- Supply Chain Management
- Browse content in Criminology and Criminal Justice
- Criminal Justice
- Criminology
- Forms of Crime
- International and Comparative Criminology
- Youth Violence and Juvenile Justice
- Development Studies
- Browse content in Economics
- Agricultural, Environmental, and Natural Resource Economics
- Asian Economics
- Behavioural Finance
- Behavioural Economics and Neuroeconomics
- Econometrics and Mathematical Economics
- Economic History
- Economic Systems
- Economic Methodology
- Economic Development and Growth
- Financial Markets
- Financial Institutions and Services
- General Economics and Teaching
- Health, Education, and Welfare
- History of Economic Thought
- International Economics
- Labour and Demographic Economics
- Law and Economics
- Macroeconomics and Monetary Economics
- Microeconomics
- Public Economics
- Urban, Rural, and Regional Economics
- Welfare Economics
- Browse content in Education
- Adult Education and Continuous Learning
- Care and Counselling of Students
- Early Childhood and Elementary Education
- Educational Equipment and Technology
- Educational Strategies and Policy
- Higher and Further Education
- Organization and Management of Education
- Philosophy and Theory of Education
- Schools Studies
- Secondary Education
- Teaching of a Specific Subject
- Teaching of Specific Groups and Special Educational Needs
- Teaching Skills and Techniques
- Browse content in Environment
- Applied Ecology (Social Science)
- Climate Change
- Conservation of the Environment (Social Science)
- Environmentalist Thought and Ideology (Social Science)
- Natural Disasters (Environment)
- Social Impact of Environmental Issues (Social Science)
- Browse content in Human Geography
- Cultural Geography
- Economic Geography
- Political Geography
- Browse content in Interdisciplinary Studies
- Communication Studies
- Museums, Libraries, and Information Sciences
- Browse content in Politics
- African Politics
- Asian Politics
- Chinese Politics
- Comparative Politics
- Conflict Politics
- Elections and Electoral Studies
- Environmental Politics
- European Union
- Foreign Policy
- Gender and Politics
- Human Rights and Politics
- Indian Politics
- International Relations
- International Organization (Politics)
- International Political Economy
- Irish Politics
- Latin American Politics
- Middle Eastern Politics
- Political Behaviour
- Political Economy
- Political Institutions
- Political Methodology
- Political Communication
- Political Philosophy
- Political Sociology
- Political Theory
- Politics and Law
- Politics of Development
- Public Policy
- Public Administration
- Quantitative Political Methodology
- Regional Political Studies
- Russian Politics
- Security Studies
- State and Local Government
- UK Politics
- US Politics
- Browse content in Regional and Area Studies
- African Studies
- Asian Studies
- East Asian Studies
- Japanese Studies
- Latin American Studies
- Middle Eastern Studies
- Native American Studies
- Scottish Studies
- Browse content in Research and Information
- Research Methods
- Browse content in Social Work
- Addictions and Substance Misuse
- Adoption and Fostering
- Care of the Elderly
- Child and Adolescent Social Work
- Couple and Family Social Work
- Direct Practice and Clinical Social Work
- Emergency Services
- Human Behaviour and the Social Environment
- International and Global Issues in Social Work
- Mental and Behavioural Health
- Social Justice and Human Rights
- Social Policy and Advocacy
- Social Work and Crime and Justice
- Social Work Macro Practice
- Social Work Practice Settings
- Social Work Research and Evidence-based Practice
- Welfare and Benefit Systems
- Browse content in Sociology
- Childhood Studies
- Community Development
- Comparative and Historical Sociology
- Economic Sociology
- Gender and Sexuality
- Gerontology and Ageing
- Health, Illness, and Medicine
- Marriage and the Family
- Migration Studies
- Occupations, Professions, and Work
- Organizations
- Population and Demography
- Race and Ethnicity
- Social Theory
- Social Movements and Social Change
- Social Research and Statistics
- Social Stratification, Inequality, and Mobility
- Sociology of Religion
- Sociology of Education
- Sport and Leisure
- Urban and Rural Studies
- Browse content in Warfare and Defence
- Defence Strategy, Planning, and Research
- Land Forces and Warfare
- Military Administration
- Military Life and Institutions
- Naval Forces and Warfare
- Other Warfare and Defence Issues
- Peace Studies and Conflict Resolution
- Weapons and Equipment
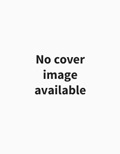
- < Previous chapter
- Next chapter >
II.1 Utility Hypothesis
- Published: November 1987
- Cite Icon Cite
- Permissions Icon Permissions
This is the first of six chapters in Part II about demand and utility cost, a typical area for what is understood as choice theory. It discusses utility hypothesis and the theory of value. Its five sections are: needs of measurement (of utility); common practice and (William) Fleetwood; parallels in theory (as applied to utility construction); revealed preference (as applied to demand functions); and the classical case (of the utility function).
Signed in as
Institutional accounts.
- GoogleCrawler [DO NOT DELETE]
- Google Scholar Indexing
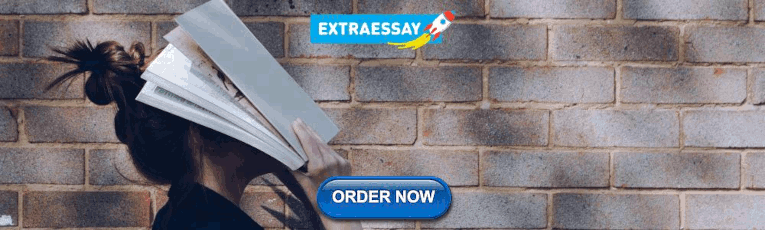
Personal account
- Sign in with email/username & password
- Get email alerts
- Save searches
- Purchase content
- Activate your purchase/trial code
- Add your ORCID iD
Institutional access
Sign in with a library card.
- Sign in with username/password
- Recommend to your librarian
- Institutional account management
- Get help with access
Access to content on Oxford Academic is often provided through institutional subscriptions and purchases. If you are a member of an institution with an active account, you may be able to access content in one of the following ways:
IP based access
Typically, access is provided across an institutional network to a range of IP addresses. This authentication occurs automatically, and it is not possible to sign out of an IP authenticated account.
Choose this option to get remote access when outside your institution. Shibboleth/Open Athens technology is used to provide single sign-on between your institution’s website and Oxford Academic.
- Click Sign in through your institution.
- Select your institution from the list provided, which will take you to your institution's website to sign in.
- When on the institution site, please use the credentials provided by your institution. Do not use an Oxford Academic personal account.
- Following successful sign in, you will be returned to Oxford Academic.
If your institution is not listed or you cannot sign in to your institution’s website, please contact your librarian or administrator.
Enter your library card number to sign in. If you cannot sign in, please contact your librarian.
Society Members
Society member access to a journal is achieved in one of the following ways:
Sign in through society site
Many societies offer single sign-on between the society website and Oxford Academic. If you see ‘Sign in through society site’ in the sign in pane within a journal:
- Click Sign in through society site.
- When on the society site, please use the credentials provided by that society. Do not use an Oxford Academic personal account.
If you do not have a society account or have forgotten your username or password, please contact your society.
Sign in using a personal account
Some societies use Oxford Academic personal accounts to provide access to their members. See below.
A personal account can be used to get email alerts, save searches, purchase content, and activate subscriptions.
Some societies use Oxford Academic personal accounts to provide access to their members.
Viewing your signed in accounts
Click the account icon in the top right to:
- View your signed in personal account and access account management features.
- View the institutional accounts that are providing access.
Signed in but can't access content
Oxford Academic is home to a wide variety of products. The institutional subscription may not cover the content that you are trying to access. If you believe you should have access to that content, please contact your librarian.
For librarians and administrators, your personal account also provides access to institutional account management. Here you will find options to view and activate subscriptions, manage institutional settings and access options, access usage statistics, and more.
Our books are available by subscription or purchase to libraries and institutions.
- About Oxford Academic
- Publish journals with us
- University press partners
- What we publish
- New features
- Open access
- Rights and permissions
- Accessibility
- Advertising
- Media enquiries
- Oxford University Press
- Oxford Languages
- University of Oxford
Oxford University Press is a department of the University of Oxford. It furthers the University's objective of excellence in research, scholarship, and education by publishing worldwide
- Copyright © 2024 Oxford University Press
- Cookie settings
- Cookie policy
- Privacy policy
- Legal notice
This Feature Is Available To Subscribers Only
Sign In or Create an Account
This PDF is available to Subscribers Only
For full access to this pdf, sign in to an existing account, or purchase an annual subscription.

An official website of the United States government
The .gov means it’s official. Federal government websites often end in .gov or .mil. Before sharing sensitive information, make sure you’re on a federal government site.
The site is secure. The https:// ensures that you are connecting to the official website and that any information you provide is encrypted and transmitted securely.
- Publications
- Account settings
Preview improvements coming to the PMC website in October 2024. Learn More or Try it out now .
- Advanced Search
- Journal List
- R Soc Open Sci
- v.10(8); 2023 Aug
- PMC10465209
On the scope of scientific hypotheses
William hedley thompson.
1 Department of Applied Information Technology, University of Gothenburg, Gothenburg, Sweden
2 Institute of Neuroscience and Physiology, Sahlgrenska Academy, University of Gothenburg, Gothenburg, Sweden
3 Department of Pedagogical, Curricular and Professional Studies, Faculty of Education, University of Gothenburg, Gothenburg, Sweden
4 Department of Clinical Neuroscience, Karolinska Institutet, Stockholm, Sweden
Associated Data
This article has no additional data.
Hypotheses are frequently the starting point when undertaking the empirical portion of the scientific process. They state something that the scientific process will attempt to evaluate, corroborate, verify or falsify. Their purpose is to guide the types of data we collect, analyses we conduct, and inferences we would like to make. Over the last decade, metascience has advocated for hypotheses being in preregistrations or registered reports, but how to formulate these hypotheses has received less attention. Here, we argue that hypotheses can vary in specificity along at least three independent dimensions: the relationship, the variables, and the pipeline. Together, these dimensions form the scope of the hypothesis. We demonstrate how narrowing the scope of a hypothesis in any of these three ways reduces the hypothesis space and that this reduction is a type of novelty. Finally, we discuss how this formulation of hypotheses can guide researchers to formulate the appropriate scope for their hypotheses and should aim for neither too broad nor too narrow a scope. This framework can guide hypothesis-makers when formulating their hypotheses by helping clarify what is being tested, chaining results to previous known findings, and demarcating what is explicitly tested in the hypothesis.
1. Introduction
Hypotheses are an important part of the scientific process. However, surprisingly little attention is given to hypothesis-making compared to other skills in the scientist's skillset within current discussions aimed at improving scientific practice. Perhaps this lack of emphasis is because the formulation of the hypothesis is often considered less relevant, as it is ultimately the scientific process that will eventually decide the veracity of the hypothesis. However, there are more hypotheses than scientific studies as selection occurs at various stages: from funder selection and researcher's interests. So which hypotheses are worthwhile to pursue? Which hypotheses are the most effective or pragmatic for extending or enhancing our collective knowledge? We consider the answer to these questions by discussing how broad or narrow a hypothesis can or should be (i.e. its scope).
We begin by considering that the two statements below are both hypotheses and vary in scope:
- H 1 : For every 1 mg decrease of x , y will increase by, on average, 2.5 points.
- H 2 : Changes in x 1 or x 2 correlate with y levels in some way.
Clearly, the specificity of the two hypotheses is very different. H 1 states a precise relationship between two variables ( x and y ), while H 2 specifies a vaguer relationship and does not specify which variables will show the relationship. However, they are both still hypotheses about how x and y relate to each other. This claim of various degrees of the broadness of hypotheses is, in and of itself, not novel. In Epistemetrics, Rescher [ 1 ], while drawing upon the physicist Duhem's work, develops what he calls Duhem's Law. This law considers a trade-off between certainty or precision in statements about physics when evaluating them. Duhem's Law states that narrower hypotheses, such as H 1 above, are more precise but less likely to be evaluated as true than broader ones, such as H 2 above. Similarly, Popper, when discussing theories, describes the reverse relationship between content and probability of a theory being true, i.e. with increased content, there is a decrease in probability and vice versa [ 2 ]. Here we will argue that it is important that both H 1 and H 2 are still valid scientific hypotheses, and their appropriateness depends on certain scientific questions.
The question of hypothesis scope is relevant since there are multiple recent prescriptions to improve science, ranging from topics about preregistrations [ 3 ], registered reports [ 4 ], open science [ 5 ], standardization [ 6 ], generalizability [ 7 ], multiverse analyses [ 8 ], dataset reuse [ 9 ] and general questionable research practices [ 10 ]. Within each of these issues, there are arguments to demarcate between confirmatory and exploratory research or normative prescriptions about how science should be done (e.g. science is ‘bad’ or ‘worse’ if code/data are not open). Despite all these discussions and improvements, much can still be done to improve hypothesis-making. A recent evaluation of preregistered studies in psychology found that over half excluded the preregistered hypotheses [ 11 ]. Further, evaluations of hypotheses in ecology showed that most hypotheses are not explicitly stated [ 12 , 13 ]. Other research has shown that obfuscated hypotheses are more prevalent in retracted research [ 14 ]. There have been recommendations for simpler hypotheses in psychology to avoid misinterpretations and misspecifications [ 15 ]. Finally, several evaluations of preregistration practices have found that a significant proportion of articles do not abide by their stated hypothesis or add additional hypotheses [ 11 , 16 – 18 ]. In sum, while multiple efforts exist to improve scientific practice, our hypothesis-making could improve.
One of our intentions is to provide hypothesis-makers with tools to assist them when making hypotheses. We consider this useful and timely as, with preregistrations becoming more frequent, the hypothesis-making process is now open and explicit . However, preregistrations are difficult to write [ 19 ], and preregistered articles can change or omit hypotheses [ 11 ] or they are vague and certain degrees of freedom hard to control for [ 16 – 18 ]. One suggestion has been to do less confirmatory research [ 7 , 20 ]. While we agree that all research does not need to be confirmatory, we also believe that not all preregistrations of confirmatory work must test narrow hypotheses. We think there is a possible point of confusion that the specificity in preregistrations, where researcher degrees of freedom should be stated, necessitates the requirement that the hypothesis be narrow. Our belief that this confusion is occurring is supported by the study Akker et al . [ 11 ] where they found that 18% of published psychology studies changed their preregistered hypothesis (e.g. its direction), and 60% of studies selectively reported hypotheses in some way. It is along these lines that we feel the framework below can be useful to help formulate appropriate hypotheses to mitigate these identified issues.
We consider this article to be a discussion of the researcher's different choices when formulating hypotheses and to help link hypotheses over time. Here we aim to deconstruct what aspects there are in the hypothesis about their specificity. Throughout this article, we intend to be neutral to many different philosophies of science relating to the scientific method (i.e. how one determines the veracity of a hypothesis). Our idea of neutrality here is that whether a researcher adheres to falsification, verification, pragmatism, or some other philosophy of science, then this framework can be used when formulating hypotheses. 1
The framework this article advocates for is that there are (at least) three dimensions that hypotheses vary along regarding their narrowness and broadness: the selection of relationships, variables, and pipelines. We believe this discussion is fruitful for the current debate regarding normative practices as some positions make, sometimes implicit, commitments about which set of hypotheses the scientific community ought to consider good or permissible. We proceed by outlining a working definition of ‘scientific hypothesis' and then discuss how it relates to theory. Then, we justify how hypotheses can vary along the three dimensions. Using this framework, we then discuss the scopes in relation to appropriate hypothesis-making and an argument about what constitutes a scientifically novel hypothesis. We end the article with practical advice for researchers who wish to use this framework.
2. The scientific hypothesis
In this section, we will describe a functional and descriptive role regarding how scientists use hypotheses. Jeong & Kwon [ 21 ] investigated and summarized the different uses the concept of ‘hypothesis’ had in philosophical and scientific texts. They identified five meanings: assumption, tentative explanation, tentative cause, tentative law, and prediction. Jeong & Kwon [ 21 ] further found that researchers in science and philosophy used all the different definitions of hypotheses, although there was some variance in frequency between fields. Here we see, descriptively , that the way researchers use the word ‘hypothesis’ is diverse and has a wide range in specificity and function. However, whichever meaning a hypothesis has, it aims to be true, adequate, accurate or useful in some way.
Not all hypotheses are ‘scientific hypotheses'. For example, consider the detective trying to solve a crime and hypothesizing about the perpetrator. Such a hypothesis still aims to be true and is a tentative explanation but differs from the scientific hypothesis. The difference is that the researcher, unlike the detective, evaluates the hypothesis with the scientific method and submits the work for evaluation by the scientific community. Thus a scientific hypothesis entails a commitment to evaluate the statement with the scientific process . 2 Additionally, other types of hypotheses can exist. As discussed in more detail below, scientific theories generate not only scientific hypotheses but also contain auxiliary hypotheses. The latter refers to additional assumptions considered to be true and not explicitly evaluated. 3
Next, the scientific hypothesis is generally made antecedent to the evaluation. This does not necessitate that the event (e.g. in archaeology) or the data collection (e.g. with open data reuse) must be collected before the hypothesis is made, but that the evaluation of the hypothesis cannot happen before its formulation. This claim state does deny the utility of exploratory hypothesis testing of post hoc hypotheses (see [ 25 ]). However, previous results and exploration can generate new hypotheses (e.g. via abduction [ 22 , 26 – 28 ], which is the process of creating hypotheses from evidence), which is an important part of science [ 29 – 32 ], but crucially, while these hypotheses are important and can be the conclusion of exploratory work, they have yet to be evaluated (by whichever method of choice). Hence, they still conform to the antecedency requirement. A further way to justify the antecedency is seen in the practice of formulating a post hoc hypothesis, and considering it to have been evaluated is seen as a questionable research practice (known as ‘hypotheses after results are known’ or HARKing [ 33 ]). 4
While there is a varying range of specificity, is the hypothesis a critical part of all scientific work, or is it reserved for some subset of investigations? There are different opinions regarding this. Glass and Hall, for example, argue that the term only refers to falsifiable research, and model-based research uses verification [ 36 ]. However, this opinion does not appear to be the consensus. Osimo and Rumiati argue that any model based on or using data is never wholly free from hypotheses, as hypotheses can, even implicitly, infiltrate the data collection [ 37 ]. For our definition, we will consider hypotheses that can be involved in different forms of scientific evaluation (i.e. not just falsification), but we do not exclude the possibility of hypothesis-free scientific work.
Finally, there is a debate about whether theories or hypotheses should be linguistic or formal [ 38 – 40 ]. Neither side in this debate argues that verbal or formal hypotheses are not possible, but instead, they discuss normative practices. Thus, for our definition, both linguistic and formal hypotheses are considered viable.
Considering the above discussion, let us summarize the scientific process and the scientific hypothesis: a hypothesis guides what type of data are sampled and what analysis will be done. With the new observations, evidence is analysed or quantified in some way (often using inferential statistics) to judge the hypothesis's truth value, utility, credibility, or likelihood. The following working definition captures the above:
- Scientific hypothesis : an implicit or explicit statement that can be verbal or formal. The hypothesis makes a statement about some natural phenomena (via an assumption, explanation, cause, law or prediction). The scientific hypothesis is made antecedent to performing a scientific process where there is a commitment to evaluate it.
For simplicity, we will only use the term ‘hypothesis’ for ‘scientific hypothesis' to refer to the above definition for the rest of the article except when it is necessary to distinguish between other types of hypotheses. Finally, this definition could further be restrained in multiple ways (e.g. only explicit hypotheses are allowed, or assumptions are never hypotheses). However, if the definition is more (or less) restrictive, it has little implication for the argument below.
3. The hypothesis, theory and auxiliary assumptions
While we have a definition of the scientific hypothesis, we have yet to link it with how it relates to scientific theory, where there is frequently some interconnection (i.e. a hypothesis tests a scientific theory). Generally, for this paper, we believe our argument applies regardless of how scientific theory is defined. Further, some research lacks theory, sometimes called convenience or atheoretical studies [ 41 ]. Here a hypothesis can be made without a wider theory—and our framework fits here too. However, since many consider hypotheses to be defined or deducible from scientific theory, there is an important connection between the two. Therefore, we will briefly clarify how hypotheses relate to common formulations of scientific theory.
A scientific theory is generally a set of axioms or statements about some objects, properties and their relations relating to some phenomena. Hypotheses can often be deduced from the theory. Additionally, a theory has boundary conditions. The boundary conditions specify the domain of the theory stating under what conditions it applies (e.g. all things with a central neural system, humans, women, university teachers) [ 42 ]. Boundary conditions of a theory will consequently limit all hypotheses deduced from the theory. For example, with a boundary condition ‘applies to all humans’, then the subsequent hypotheses deduced from the theory are limited to being about humans. While this limitation of the hypothesis by the theory's boundary condition exists, all the considerations about a hypothesis scope detailed below still apply within the boundary conditions. Finally, it is also possible (depending on the definition of scientific theory) for a hypothesis to test the same theory under different boundary conditions. 5
The final consideration relating scientific theory to scientific hypotheses is auxiliary hypotheses. These hypotheses are theories or assumptions that are considered true simultaneously with the theory. Most philosophies of science from Popper's background knowledge [ 24 ], Kuhn's paradigms during normal science [ 44 ], and Laktos' protective belt [ 45 ] all have their own versions of this auxiliary or background information that is required for the hypothesis to test the theory. For example, Meelh [ 46 ] auxiliary theories/assumptions are needed to go from theoretical terms to empirical terms (e.g. neural activity can be inferred from blood oxygenation in fMRI research or reaction time to an indicator of cognition) and auxiliary theories about instruments (e.g. the experimental apparatus works as intended) and more (see also Other approaches to categorizing hypotheses below). As noted in the previous section, there is a difference between these auxiliary hypotheses, regardless of their definition, and the scientific hypothesis defined above. Recall that our definition of the scientific hypothesis included a commitment to evaluate it. There are no such commitments with auxiliary hypotheses, but rather they are assumed to be correct to test the theory adequately. This distinction proves to be important as auxiliary hypotheses are still part of testing a theory but are separate from the hypothesis to be evaluated (discussed in more detail below).
4. The scope of hypotheses
In the scientific hypothesis section, we defined the hypothesis and discussed how it relates back to the theory. In this section, we want to defend two claims about hypotheses:
- (A1) Hypotheses can have different scopes . Some hypotheses are narrower in their formulation, and some are broader.
- (A2) The scope of hypotheses can vary along three dimensions relating to relationship selection , variable selection , and pipeline selection .
A1 may seem obvious, but it is important to establish what is meant by narrower and broader scope. When a hypothesis is very narrow, it is specific. For example, it might be specific about the type of relationship between some variables. In figure 1 , we make four different statements regarding the relationship between x and y . The narrowest hypothesis here states ‘there is a positive linear relationship with a magnitude of 0.5 between x and y ’ ( figure 1 a ), and the broadest hypothesis states ‘there is a relationship between x and y ’ ( figure 1 d ). Note that many other hypotheses are possible that are not included in this example (such as there being no relationship).

Examples of narrow and broad hypotheses between x and y . Circles indicate a set of possible relationships with varying slopes that can pivot or bend.
We see that the narrowest of these hypotheses claims a type of relationship (linear), a direction of the relationship (positive) and a magnitude of the relationship (0.5). As the hypothesis becomes broader, the specific magnitude disappears ( figure 1 b ), the relationship has additional options than just being linear ( figure 1 c ), and finally, the direction of the relationship disappears. Crucially, all the examples in figure 1 can meet the above definition of scientific hypotheses. They are all statements that can be evaluated with the same scientific method. There is a difference between these statements, though— they differ in the scope of the hypothesis . Here we have justified A1.
Within this framework, when we discuss whether a hypothesis is narrower or broader in scope, this is a relation between two hypotheses where one is a subset of the other. This means that if H 1 is narrower than H 2 , and if H 1 is true, then H 2 is also true. This can be seen in figure 1 a–d . Suppose figure 1 a , the narrowest of all the hypotheses, is true. In that case, all the other broader statements are also true (i.e. a linear correlation of 0.5 necessarily entails that there is also a positive linear correlation, a linear correlation, and some relationship). While this property may appear trivial, it entails that it is only possible to directly compare the hypothesis scope between two hypotheses (i.e. their broadness or narrowness) where one is the subset of the other. 6
4.1. Sets, disjunctions and conjunctions of elements
The above restraint defines the scope as relations between sets. This property helps formalize the framework of this article. Below, when we discuss the different dimensions that can impact the scope, these become represented as a set. Each set contains elements. Each element is a permissible situation that allows the hypothesis to be accepted. We denote elements as lower case with italics (e.g. e 1 , e 2 , e 3 ) and sets as bold upper case (e.g. S ). Each of the three different dimensions discussed below will be formalized as sets, while the total number of elements specifies their scope.
Let us reconsider the above restraint about comparing hypotheses as narrower or broader. This can be formally shown if:
- e 1 , e 2 , e 3 are elements of S 1 ; and
- e 1 and e 2 are elements of S 2 ,
then S 2 is narrower than S 1 .
Each element represents specific propositions that, if corroborated, would support the hypothesis. Returning to figure 1 a , b , the following statements apply to both:
- ‘There is a positive linear relationship between x and y with a slope of 0.5’.
Whereas the following two apply to figure 1 b but not figure 1 a :
- ‘There is a positive linear relationship between x and y with a slope of 0.4’ ( figure 1 b ).
- ‘There is a positive linear relationship between x and y with a slope of 0.3’ ( figure 1 b ).
Figure 1 b allows for a considerably larger number of permissible situations (which is obvious as it allows for any positive linear relationship). When formulating the hypothesis in figure 1 b , we do not need to specify every single one of these permissible relationships. We can simply specify all possible positive slopes, which entails the set of permissible elements it includes.
That broader hypotheses have more elements in their sets entails some important properties. When we say S contains the elements e 1 , e 2 , and e 3 , the hypothesis is corroborated if e 1 or e 2 or e 3 is the case. This means that the set requires only one of the elements to be corroborated for the hypothesis to be considered correct (i.e. the positive linear relationship needs to be 0.3 or 0.4 or 0.5). Contrastingly, we will later see cases when conjunctions of elements occur (i.e. both e 1 and e 2 are the case). When a conjunction occurs, in this formulation, the conjunction itself becomes an element in the set (i.e. ‘ e 1 and e 2 ’ is a single element). Figure 2 illustrates how ‘ e 1 and e 2 ’ is narrower than ‘ e 1 ’, and ‘ e 1 ’ is narrower than ‘ e 1 or e 2 ’. 7 This property relating to the conjunction being narrower than individual elements is explained in more detail in the pipeline selection section below.

Scope as sets. Left : four different sets (grey, red, blue and purple) showing different elements which they contain. Right : a list of each colour explaining which set is a subset of the other (thereby being ‘narrower’).
4.2. Relationship selection
We move to A2, which is to show the different dimensions that a hypothesis scope can vary along. We have already seen an example of the first dimension of a hypothesis in figure 1 , the relationship selection . Let R denote the set of all possible configurations of relationships that are permissible for the hypothesis to be considered true. For example, in the narrowest formulation above, there was one allowed relationship for the hypothesis to be true. Consequently, the size of R (denoted | R |) is one. As discussed above, in the second narrowest formulation ( figure 1 b ), R has more possible relationships where it can still be considered true:
- r 1 = ‘a positive linear relationship of 0.1’
- r 2 = ‘a positive linear relationship of 0.2’
- r 3 = ‘a positive linear relationship of 0.3’.
Additionally, even broader hypotheses will be compatible with more types of relationships. In figure 1 c , d , nonlinear and negative relationships are also possible relationships included in R . For this broader statement to be affirmed, more elements are possible to be true. Thus if | R | is greater (i.e. contains more possible configurations for the hypothesis to be true), then the hypothesis is broader. Thus, the scope of relating to the relationship selection is specified by | R |. Finally, if |R H1 | > |R H2 | , then H 1 is broader than H 2 regarding the relationship selection.
Figure 1 is an example of the relationship narrowing. That the relationship became linear is only an example and does not necessitate a linear relationship or that this scope refers only to correlations. An alternative example of a relationship scope is a broad hypothesis where there is no knowledge about the distribution of some data. In such situations, one may assume a uniform relationship or a Cauchy distribution centred at zero. Over time the specific distribution can be hypothesized. Thereafter, the various parameters of the distribution can be hypothesized. At each step, the hypothesis of the distribution gets further specified to narrower formulations where a smaller set of possible relationships are included (see [ 47 , 48 ] for a more in-depth discussion about how specific priors relate to more narrow tests). Finally, while figure 1 was used to illustrate the point of increasingly narrow relationship hypotheses, it is more likely to expect the narrowest relationship, within fields such as psychology, to have considerable uncertainty and be formulated with confidence or credible intervals (i.e. we will rarely reach point estimates).
4.3. Variable selection
We have demonstrated that relationship selection can affect the scope of a hypothesis. Additionally, at least two other dimensions can affect the scope of a hypothesis: variable selection and pipeline selection . The variable selection in figure 1 was a single bivariate relationship (e.g. x 's relationship with y ). However, it is not always the case that we know which variables will be involved. For example, in neuroimaging, we can be confident that one or more brain regions will be processing some information following a stimulus. Still, we might not be sure which brain region(s) this will be. Consequently, our hypothesis becomes broader because we have selected more variables. The relationship selection may be identical for each chosen variable, but the variable selection becomes broader. We can consider the following three hypotheses to be increasing in their scope:
- H 1 : x relates to y with relationship R .
- H 2 : x 1 or x 2 relates to y with relationship R .
- H 3 : x 1 or x 2 or x 3 relates to y with relationship R .
For H 1 –H 3 above, we assume that R is the same. Further, we assume that there is no interaction between these variables.
In the above examples, we have multiple x ( x 1 , x 2 , x 3 , … , x n ). Again, we can symbolize the variable selection as a non-empty set XY , containing either a single variable or many variables. Our motivation for designating it XY is that the variable selection can include multiple possibilities for both the independent variable ( x ) and the dependent variable ( y ). Like with relationship selection, we can quantify the broadness between two hypotheses with the size of the set XY . Consequently, | XY | denotes the total scope concerning variable selection. Thus, in the examples above | XY H1 | < | XY H2 | < | XY H3 |. Like with relationship selection, hypotheses that vary in | XY | still meet the definition of a hypothesis. 8
An obvious concern for many is that a broader XY is much easier to evaluate as correct. Generally, when | XY 1 | > | XY 2 |, there is a greater chance of spurious correlations when evaluating XY 1 . This concern is an issue relating to the evaluation of hypotheses (e.g. applying statistics to the evaluation), which will require additional assumptions relating to how to evaluate the hypotheses. Strategies to deal with this apply some correction or penalization for multiple statistical testing [ 49 ] or partial pooling and regularizing priors [ 50 , 51 ]. These strategies aim to evaluate a broader variable selection ( x 1 or x 2 ) on equal or similar terms to a narrow variable selection ( x 1 ).
4.4. Pipeline selection
Scientific studies require decisions about how to perform the analysis. This scope considers transformations applied to the raw data ( XY raw ) to achieve some derivative ( XY ). These decisions can also involve selection procedures that drop observations deemed unreliable, standardizing, correcting confounding variables, or different philosophies. We can call the array of decisions and transformations used as the pipeline . A hypothesis varies in the number of pipelines:
- H 1 : XY has a relationship(s) R with pipeline p 1 .
- H 2 : XY has a relationship(s) R with pipeline p 1 or pipeline p 2 .
- H 3 : XY has a relationship(s) R with pipeline p 1 or pipeline p 2 , or pipeline p 3 .
Importantly, the pipeline here considers decisions regarding how the hypothesis shapes the data collection and transformation. We do not consider this to include decisions made regarding the assumptions relating to the statistical inference as those relate to operationalizing the evaluation of the hypothesis and not part of the hypothesis being evaluated (these assumptions are like auxiliary hypotheses, which are assumed to be true but not explicitly evaluated).
Like with variable selection ( XY ) and relationship selection ( R ), we can see that pipelines impact the scope of hypotheses. Again, we can symbolize the pipeline selection with a set P . As previously, | P | will denote the dimension of the pipeline selection. In the case of pipeline selection, we are testing the same variables, looking for the same relationship, but processing the variables or relationships with different pipelines to evaluate the relationship. Consequently, | P H1 | < | P H2 | < | P H3 |.
These issues regarding pipelines have received attention as the ‘garden of forking paths' [ 52 ]. Here, there are calls for researchers to ensure that their entire pipeline has been specified. Additionally, recent work has highlighted the diversity of results based on multiple analytical pipelines [ 53 , 54 ]. These results are often considered a concern, leading to calls that results should be pipeline resistant.
The wish for pipeline-resistant methods entails that hypotheses, in their narrowest form, are possible for all pipelines. Consequently, a narrower formulation will entail that this should not impact the hypothesis regardless of which pipeline is chosen. Thus the conjunction of pipelines is narrower than single pipelines. Consider the following three scenarios:
- H 3 : XY has a relationship(s) R with pipeline p 1 and pipeline p 2 .
In this instance, since H 1 is always true if H 3 is true, thus H 3 is a narrower formulation than H 1 . Consequently, | P H3 | < | P H1 | < | P H2 |. Decreasing the scope of the pipeline dimension also entails the increase in conjunction of pipelines (i.e. creating pipeline-resistant methods) rather than just the reduction of disjunctional statements.
4.5. Combining the dimensions
In summary, we then have three different dimensions that independently affect the scope of the hypothesis. We have demonstrated the following general claim regarding hypotheses:
- The variables XY have a relationship R with pipeline P .
And that the broadness and narrowness of a hypothesis depend on how large the three sets XY , R and P are. With this formulation, we can conclude that hypotheses have a scope that can be determined with a 3-tuple argument of (| R |, | XY |, | P |).
While hypotheses can be formulated along these three dimensions and generally aim to be reduced, it does not entail that these dimensions behave identically. For example, the relationship dimensions aim to reduce the number of elements as far as possible (e.g. to an interval). Contrastingly, for both variables and pipeline, the narrower hypothesis can reduce to single variables/pipelines or become narrower still and become conjunctions where all variables/pipelines need to corroborate the hypothesis (i.e. regardless of which method one follows, the hypothesis is correct).
5. Additional possible dimensions
No commitment is being made about the exhaustive nature of there only being three dimensions that specify the hypothesis scope. Other dimensions may exist that specify the scope of a hypothesis. For example, one might consider the pipeline dimension as two different dimensions. The first would consider the experimental pipeline dimension regarding all variables relating to the experimental setup to collect data, and the latter would be the analytical pipeline dimension regarding the data analysis of any given data snapshot. Another possible dimension is adding the number of situations or contexts under which the hypothesis is valid. For example, any restraint such as ‘in a vacuum’, ‘under the speed of light’, or ‘in healthy human adults' could be considered an additional dimension of the hypothesis. There is no objection to whether these should be additional dimensions of the hypothesis. However, as stated above, these usually follow from the boundary conditions of the theory.
6. Specifying the scope versus assumptions
We envision that this framework can help hypothesis-makers formulate hypotheses (in research plans, registered reports, preregistrations etc.). Further, using this framework while formulating hypotheses can help distinguish between auxiliary hypotheses and parts of the scientific hypothesis being tested. When writing preregistrations, it can frequently occur that some step in the method has two alternatives (e.g. a preprocessing step), and there is not yet reason to choose one over the other, and the researcher needs to make a decision. These following scenarios are possible:
- 1. Narrow pipeline scope . The researcher evaluates the hypothesis with both pipeline variables (i.e. H holds for both p 1 and p 2 where p 1 and p 2 can be substituted with each other in the pipeline).
- 2. Broad pipeline scope. The researcher evaluates the hypothesis with both pipeline variables, and only one needs to be correct (i.e. H holds for either p 1 or p 2 where p 1 and p 2 can be substituted with each other in the pipeline). The result of this experiment may help motivate choosing either p 1 or p 2 in future studies.
- 3. Auxiliary hypothesis. Based on some reason (e.g. convention), the researcher assumes p 1 and evaluates H assuming p 1 is true.
Here we see that the same pipeline step can be part of either the auxiliary hypotheses or the pipeline scope. This distinction is important because if (3) is chosen, the decision becomes an assumption that is not explicitly tested by the hypothesis. Consequently, a researcher confident in the hypothesis may state that the auxiliary hypothesis p 1 was incorrect, and they should retest their hypothesis using different assumptions. In the cases where this decision is part of the pipeline scope, the hypothesis is intertwined with this decision, removing the eventual wiggle-room to reject auxiliary hypotheses that were assumed. Furthermore, starting with broader pipeline hypotheses that gradually narrow down can lead to a more well-motivated protocol for approaching the problem. Thus, this framework can help researchers while writing their hypotheses in, for example, preregistrations because they can consider when they are committing to a decision, assuming it, or when they should perhaps test a broader hypothesis with multiple possible options (discussed in more detail in §11 below).
7. The reduction of scope in hypothesis space
Having established that different scopes of a hypothesis are possible, we now consider how the hypotheses change over time. In this section, we consider how the scope of the hypothesis develops ideally within science.
Consider a new research question. A large number of hypotheses are possible. Let us call this set of all possible hypotheses the hypothesis space . Hypotheses formulated within this space can be narrower or broader based on the dimensions discussed previously ( figure 3 ).

Example of hypothesis space. The hypothesis scope is expressed as cuboids in three dimensions (relationship ( R ), variable ( XY ), pipeline ( P )). The hypothesis space is the entire possible space within the three dimensions. Three hypotheses are shown in the hypothesis space (H 1 , H 2 , H 3 ). H 2 and H 3 are subsets of H 1 .
After the evaluation of the hypothesis with the scientific process, the hypothesis will be accepted or rejected. 9 The evaluation could be done through falsification or via verification, depending on the philosophy of science commitments. Thereafter, other narrower formulations of the hypothesis can be formulated by reducing the relationship, variable or pipeline scope. If a narrower hypothesis is accepted, more specific details about the subject matter are known, or a theory has been refined in greater detail. A narrower hypothesis will entail a more specific relationship, variable or pipeline detailed in the hypothesis. Consequently, hypotheses linked to each other in this way will become narrower over time along one or more dimensions. Importantly, considering that the conjunction of elements is narrower than single elements for pipelines and variables, this process of narrower hypotheses will lead to more general hypotheses (i.e. they have to be applied in all conditions and yield less flexibility when they do not apply). 10
Considering that the scopes of hypotheses were defined as sets above, some properties can be deduced from this framework about how narrower hypotheses relate to broader hypotheses. Let us consider three hypotheses (H 1 , H 2 , and H 3 ; figure 3 ). H 2 and H 3 are non-overlapping subsets of H 1 . Thus H 2 and H 3 are both narrower in scope than H 1 . Thus the following is correct:
- P1: If H 1 is false, then H 2 is false, and H 2 does not need to be evaluated.
- P2: If H 2 is true, then the broader H 1 is true, and H 1 does not need to be evaluated.
- P3: If H 1 is true and H 2 is false, some other hypothesis H 3 of similar scope to H 2 is possible.
For example, suppose H 1 is ‘there is a relationship between x and y ’, H 2 is ‘there is a positive relationship between x and y ’, and H 3 is ‘a negative relationship between x and y ’. In that case, it becomes apparent how each of these follows. 11 Logically, many deductions from set theory are possible but will not be explored here. Instead, we will discuss two additional consequences of hypothesis scopes: scientific novelty and applications for the researcher who formulates a hypothesis.
P1–P3 have been formulated as hypotheses being true or false. In practice, hypotheses are likely evaluated probabilistically (e.g. ‘H 1 is likely’ or ‘there is evidence in support of H 1 ’). In these cases, P1–P3 can be rephrased to account for this by substituting true/false with statements relating to evidence. For example, P2 could read: ‘If there is evidence in support of H 2 , then there is evidence in support of H 1 , and H 1 does not need to be evaluated’.
8. Scientific novelty as the reduction of scope
Novelty is a key concept that repeatedly occurs in multiple aspects of the scientific enterprise, from funding to publishing [ 55 ]. Generally, scientific progress establishes novel results based on some new hypothesis. Consequently, the new hypothesis for the novel results must be narrower than previously established knowledge (i.e. the size of the scopes is reduced). Otherwise, the result is trivial and already known (see P2 above). Thus, scientific work is novel if the scientific process produces a result based on hypotheses with either a smaller | R |, | XY |, or | P | compared to previous work.
This framework of dimensions of the scope of a hypothesis helps to demarcate when a hypothesis and the subsequent result are novel. If previous studies have established evidence for R 1 (e.g. there is a positive relationship between x and y ), a hypothesis will be novel if and only if it is narrower than R 1 . Thus, if R 2 is narrower in scope than R 1 (i.e. | R 2 | < | R 1 |), R 2 is a novel hypothesis.
Consider the following example. Study 1 hypothesizes, ‘There is a positive relationship between x and y ’. It identifies a linear relationship of 0.6. Next, Study 2 hypothesizes, ‘There is a specific linear relationship between x and y that is 0.6’. Study 2 also identifies the relationship of 0.6. Since this was a narrower hypothesis, Study 2 is novel despite the same result. Frequently, researchers claim that they are the first to demonstrate a relationship. Being the first to demonstrate a relationship is not the final measure of novelty. Having a narrower hypothesis than previous researchers is a sign of novelty as it further reduces the hypothesis space.
Finally, it should be noted that novelty is not the only objective of scientific work. Other attributes, such as improving the certainty of a current hypothesis (e.g. through replications), should not be overlooked. Additional scientific explanations and improved theories are other aspects. Additionally, this definition of novelty relating to hypothesis scope does not exclude other types of novelty (e.g. new theories or paradigms).
9. How broad should a hypothesis be?
Given the previous section, it is elusive to conclude that the hypothesis should be as narrow as possible as it entails maximal knowledge gain and scientific novelty when formulating hypotheses. Indeed, many who advocate for daring or risky tests seem to hold this opinion. For example, Meehl [ 46 ] argues that we should evaluate theories based on point (or interval) prediction, which would be compatible with very narrow versions of relationships. We do not necessarily think that this is the most fruitful approach. In this section, we argue that hypotheses should aim to be narrower than current knowledge , but too narrow may be problematic .
Let us consider the idea of confirmatory analyses. These studies will frequently keep the previous hypothesis scopes regarding P and XY but aim to become more specific regarding R (i.e. using the same method and the same variables to detect a more specific relationship). A very daring or narrow hypothesis is to minimize R to include the fewest possible relationships. However, it becomes apparent that simply pursuing specificness or daringness is insufficient for selecting relevant hypotheses. Consider a hypothetical scenario where a researcher believes virtual reality use leads people to overestimate the amount of exercise they have done. If unaware of previous studies on this project, an apt hypothesis is perhaps ‘increased virtual reality usage correlates with a less accuracy of reported exercise performed’ (i.e. R is broad). However, a more specific and more daring hypothesis would be to specify the relationship further. Thus, despite not knowing if there is a relationship at all, a more daring hypothesis could be: ‘for every 1 h of virtual reality usage, there will be, on average, a 0.5% decrease in the accuracy of reported exercise performed’ (i.e. R is narrow). We believe it would be better to establish the broader hypothesis in any scenario here for the first experiment. Otherwise, if we fail to confirm the more specific formulation, we could reformulate another equally narrow relative to the broader hypothesis. This process of tweaking a daring hypothesis could be pursued ad infinitum . Such a situation will neither quickly identify the true hypothesis nor effectively use limited research resources.
By first discounting a broader hypothesis that there is no relationship, it will automatically discard all more specific formulations of that relationship in the hypothesis space. Returning to figure 3 , it will be better to establish H 1 before attempting H 2 or H 3 to ensure the correct area in the hypothesis space is being investigated. To provide an analogy: when looking for a needle among hay, first identify which farm it is at, then which barn, then which haystack, then which part of the haystack it is at before we start picking up individual pieces of hay. Thus, it is preferable for both pragmatic and cost-of-resource reasons to formulate sufficiently broad hypotheses to navigate the hypothesis space effectively.
Conversely, formulating too broad a relationship scope in a hypothesis when we already have evidence for narrower scope would be superfluous research (unless the evidence has been called into question by, for example, not being replicated). If multiple studies have supported the hypothesis ‘there is a 20-fold decrease in mortality after taking some medication M’, it would be unnecessary to ask, ‘Does M have any effect?’.
Our conclusion is that the appropriate scope of a hypothesis, and its three dimensions, follow a Goldilocks-like principle where too broad is superfluous and not novel, while too narrow is unnecessary or wasteful. Considering the scope of one's hypothesis and how it relates to previous hypotheses' scopes ensures one is asking appropriate questions.
Finally, there has been a recent trend in psychology that hypotheses should be formal [ 38 , 56 – 60 ]. Formal theories are precise since they are mathematical formulations entailing that their interpretations are clear (non-ambiguous) compared to linguistic theories. However, this literature on formal theories often refers to ‘precise predictions’ and ‘risky testing’ while frequently referencing Meehl, who advocates for narrow hypotheses (e.g. [ 38 , 56 , 59 ]). While perhaps not intended by any of the proponents, one interpretation of some of these positions is that hypotheses derived from formal theories will be narrow hypotheses (i.e. the quality of being ‘precise’ can mean narrow hypotheses with risky tests and non-ambiguous interpretations simultaneously). However, the benefit from the clarity (non-ambiguity) that formal theories/hypotheses bring also applies to broad formal hypotheses as well. They can include explicit but formalized versions of uncertain relationships, multiple possible pipelines, and large sets of variables. For example, a broad formal hypothesis can contain a hyperparameter that controls which distribution the data fit (broad relationship scope), or a variable could represent a set of formalized explicit pipelines (broad pipeline scope) that will be tested. In each of these instances, it is possible to formalize non-ambiguous broad hypotheses from broad formal theories that do not yet have any justification for being overly narrow. In sum, our argumentation here stating that hypotheses should not be too narrow is not an argument against formal theories but rather that hypotheses (derived from formal theories) do not necessarily have to be narrow.
10. Other approaches to categorizing hypotheses
The framework we present here is a way of categorizing hypotheses into (at least) three dimensions regarding the hypothesis scope, which we believe is accessible to researchers and help link scientific work over time while also trying to remain neutral with regard to a specific philosophy of science. Our proposal does not aim to be antagonistic or necessarily contradict other categorizing schemes—but we believe that our framework provides benefits.
One recent categorization scheme is the Theoretical (T), Auxiliary (A), Statistical (S) and Inferential (I) assumption model (together becoming the TASI model) [ 61 , 62 ]. Briefly, this model considers theory to generate theoretical hypotheses. To translate from theoretical unobservable terms (e.g. personality, anxiety, mass), auxiliary assumptions are needed to generate an empirical hypothesis. Statistical assumptions are often needed to test the empirical hypothesis (e.g. what is the distribution, is it skewed or not) [ 61 , 62 ]. Finally, additional inferential assumptions are needed to generalize to a larger population (e.g. was there a random and independent sampling from defined populations). The TASI model is insightful and helpful in highlighting the distance between a theory and the observation that would corroborate/contradict it. Part of its utility is to bring auxiliary hypotheses into the foreground, to improve comparisons between studies and improve theory-based interventions [ 63 , 64 ].
We do agree with the importance of being aware of or stating the auxiliary hypotheses, but there are some differences between the frameworks. First, the number of auxiliary assumptions in TASI can be several hundred [ 62 ], whereas our framework will consider some of them as part of the pipeline dimension. Consider the following four assumptions: ‘the inter-stimulus interval is between 2000 ms and 3000 ms', ‘the data will be z-transformed’, ‘subjects will perform correctly’, and ‘the measurements were valid’. According to the TASI model, all these will be classified similarly as auxiliary assumptions. Contrarily, within our framework, it is possible to consider the first two as part of the pipeline dimension and the latter two as auxiliary assumptions, and consequently, the first two become integrated as part of the hypothesis being tested and the latter two auxiliary assumptions. A second difference between the frameworks relates to non-theoretical studies (convenience, applied or atheoretical). Our framework allows for the possibility that the hypothesis space generated by theoretical and convenience studies can interact and inform each other within the same framework . Contrarily, in TASI, the theory assumptions no longer apply, and a different type of hypothesis model is needed; these assumptions must be replaced by another group of assumptions (where ‘substantive application assumptions' replace the T and the A, becoming SSI) [ 61 ]. Finally, part of our rationale for our framework is to be able to link and track hypotheses and hypothesis development together over time, so our classification scheme has different utility.
Another approach which has some similar utility to this framework is theory construction methodology (TCM) [ 57 ]. The similarity here is that TCM aims to be a practical guide to improve theory-making in psychology. It is an iterative process which relates theory, phenomena and data. Here hypotheses are not an explicit part of the model. However, what is designated as ‘proto theory’ could be considered a hypothesis in our framework as they are a product of abduction, shaping the theory space. Alternatively, what is deduced to evaluate the theory can also be considered a hypothesis. We consider both possible and that our framework can integrate with these two steps, especially since TCM does not have clear guidelines for how to do each step.
11. From theory to practice: implementing this framework
We believe that many practising researchers can relate to many aspects of this framework. But, how can a researcher translate the above theoretical framework to their work? The utility of this framework lies in bringing these three scopes of a hypothesis together and explaining how each can be reduced. We believe researchers can use this framework to describe their current practices more clearly. Here we discuss how it can be helpful for researchers when formulating, planning, preregistering, and discussing the evaluation of their scientific hypotheses. These practical implications are brief, and future work can expand on the connection between the full interaction between hypothesis space and scope. Furthermore, both authors have the most experience in cognitive neuroscience, and some of the practical implications may revolve around this type of research and may not apply equally to other fields.
11.1. Helping to form hypotheses
Abduction, according to Peirce, is a hypothesis-making exercise [ 22 , 26 – 28 ]. Given some observations, a general testable explanation of the phenomena is formed. However, when making the hypothesis, this statement will have a scope (either explicitly or implicitly). Using our framework, the scope can become explicit. The hypothesis-maker can start with ‘The variables XY have a relationship R with pipeline P ’ as a scaffold to form the hypothesis. From here, the hypothesis-maker can ‘fill in the blanks’, explicitly adding each of the scopes. Thus, when making a hypothesis via abduction and using our framework, the hypothesis will have an explicit scope when it is made. By doing this, there is less chance that a formulated hypothesis is unclear, ambiguous, and needs amending at a later stage.
11.2. Assisting to clearly state hypotheses
A hypothesis is not just formulated but also communicated. Hypotheses are stated in funding applications, preregistrations, registered reports, and academic articles. Further, preregistered hypotheses are often omitted or changed in the final article [ 11 ], and hypotheses are not always explicitly stated in articles [ 12 ]. How can this framework help to make better hypotheses? Similar to the previous point, filling in the details of ‘The variables XY have a relationship R with pipeline P ’ is an explicit way to communicate the hypothesis. Thinking about each of these dimensions should entail an appropriate explicit scope and, hopefully, less variation between preregistered and reported hypotheses. The hypothesis does not need to be a single sentence, and details of XY and P will often be developed in the methods section of the text. However, using this template as a starting point can help ensure the hypothesis is stated, and the scope of all three dimensions has been communicated.
11.3. Helping to promote explicit and broad hypotheses instead of vague hypotheses
There is an important distinction between vague hypotheses and broad hypotheses, and this framework can help demarcate between them. A vague statement would be: ‘We will quantify depression in patients after treatment’. Here there is uncertainty relating to how the researcher will go about doing the experiment (i.e. how will depression be quantified?). However, a broad statement can be uncertain, but the uncertainty is part of the hypothesis: ‘Two different mood scales (S 1 or S 2 ) will be given to patients and test if only one (or both) changed after treatment’. This latter statement is transparently saying ‘S 1 or S 2 ’ is part of a broad hypothesis—the uncertainty is whether the two different scales are quantifying the same construct. We keep this uncertainty within the broad hypothesis, which will get evaluated, whereas a vague hypothesis has uncertainty as part of the interpretation of the hypothesis. This framework can be used when formulating hypotheses to help be broad (where needed) but not vague.
11.4. Which hypothesis should be chosen?
When considering the appropriate scope above, we argued for a Goldilocks-like principle of determining the hypothesis that is not too broad or too narrow. However, when writing, for example, a preregistration, how does one identify this sweet spot? There is no easy or definite universal answer to this question. However, one possible way is first to identify the XY , R , and P of previous hypotheses. From here, identify what a non-trivial step is to improve our knowledge of the research area. So, for example, could you be more specific about the exact nature of the relationship between the variables? Does the pipeline correspond to today's scientific standards, or were some suboptimal decisions made? Is there another population that you think the previous result also applies to? Do you think that maybe a more specific construct or subpopulation might explain the previous result? Could slightly different constructs (perhaps easier to quantify) be used to obtain a similar relationship? Are there even more constructs to which this relationship should apply simultaneously? Are you certain of the direction of the relationship? Answering affirmatively to any of these questions will likely make a hypothesis narrower and connect to previous research while being clear and explicit. Moreover, depending on the research question, answering any of these may be sufficiently narrow to be a non-trivial innovation. However, there are many other ways to make a hypothesis narrower than these guiding questions.
11.5. The confirmatory–exploratory continuum
Research is often dichotomized into confirmatory (testing a hypothesis) or exploratory (without a priori hypotheses). With this framework, researchers can consider how their research acts on some hypothesis space. Confirmatory and exploratory work has been defined in terms of how each interacts with the researcher's degrees of freedom (where confirmatory aims to reduce while exploratory utilizes them [ 30 ]). Both broad confirmatory and narrow exploratory research are possible using this definition and possible within this framework. How research interacts with the hypothesis space helps demarcate it. For example, if a hypothesis reduces the scope, it becomes more confirmatory, and trying to understand data given the current scope would be more exploratory work. This further could help demarcate when exploration is useful. Future theoretical work can detail how different types of research impact the hypothesis space in more detail.
11.6. Understanding when multiverse analyses are needed
Researchers writing a preregistration may face many degrees of freedom they have to choose from, and different researchers may motivate different choices. If, when writing such a preregistration, there appears to be little evidential support for certain degrees of freedom over others, the researcher is left with the option to either make more auxiliary assumptions or identify when an investigation into the pipeline scope is necessary by conducting a multiverse analysis that tests the impact of the different degrees of freedom on the result (see [ 8 ]). Thus, when applying this framework to explicitly state what pipeline variables are part of the hypothesis or an auxiliary assumption, the researcher can identify when it might be appropriate to conduct a multiverse analysis because they are having difficulty formulating hypotheses.
11.7. Describing novelty
Academic journals and research funders often ask for novelty, but the term ‘novelty’ can be vague and open to various interpretations [ 55 ]. This framework can be used to help justify the novelty of research. For example, consider a scenario where a previous study has established a psychological construct (e.g. well-being) that correlates with a certain outcome measure (e.g. long-term positive health outcomes). This framework can be used to explicitly justify novelty by (i) providing a more precise understanding of the relationship (e.g. linear or linear–plateau) or (ii) identifying more specific variables related to well-being or health outcomes. Stating how some research is novel is clearer than merely stating that the work is novel. This practice might even help journals and funders identify what type of novelty they would like to reward. In sum, this framework can help identify and articulate how research is novel.
11.8. Help to identify when standardization of pipelines is beneficial or problematic to a field
Many consider standardization in a field to be important for ensuring the comparability of results. Standardization of methods and tools entails that the pipeline P is identical (or at least very similar) across studies. However, in such cases, the standardized pipeline becomes an auxiliary assumption representing all possible pipelines. Therefore, while standardized pipelines have their benefits, this assumption becomes broader without validating (e.g. via multiverse analysis) which pipelines a standardized P represents. In summary, because this framework helps distinguish between auxiliary assumptions and explicit parts of the hypothesis and identifies when a multiverse analysis is needed, it can help determine when standardizations of pipelines are representative (narrower hypotheses) or assumptive (broader hypotheses).
12. Conclusion
Here, we have argued that the scope of a hypothesis is made up of three dimensions: the relationship ( R ), variable ( XY ) and pipeline ( P ) selection. Along each of these dimensions, the scope can vary. Different types of scientific enterprises will often have hypotheses that vary the size of the scopes. We have argued that this focus on the scope of the hypothesis along these dimensions helps the hypothesis-maker formulate their hypotheses for preregistrations while also helping demarcate auxiliary hypotheses (assumed to be true) from the hypothesis (those being evaluated during the scientific process).
Hypotheses are an essential part of the scientific process. Considering what type of hypothesis is sufficient or relevant is an essential job of the researcher that we think has been overlooked. We hope this work promotes an understanding of what a hypothesis is and how its formulation and reduction in scope is an integral part of scientific progress. We hope it also helps clarify how broad hypotheses need not be vague or inappropriate.
Finally, we applied this idea of scopes to scientific progress and considered how to formulate an appropriate hypothesis. We have also listed several ways researchers can practically implement this framework today. However, there are other practicalities of this framework that future work should explore. For example, it could be used to differentiate and demarcate different scientific contributions (e.g. confirmatory studies, exploration studies, validation studies) with how their hypotheses interact with the different dimensions of the hypothesis space. Further, linking hypotheses over time within this framework can be a foundation for open hypothesis-making by promoting explicit links to previous work and detailing the reduction of the hypothesis space. This framework helps quantify the contribution to the hypothesis space of different studies and helps clarify what aspects of hypotheses can be relevant at different times.
Acknowledgements
We thank Filip Gedin, Kristoffer Sundberg, Jens Fust, and James Steele for valuable feedback on earlier versions of this article. We also thank Mark Rubin and an unnamed reviewer for valuable comments that have improved the article.
1 While this is our intention, we cannot claim that every theory has been accommodated.
2 Similar requirements of science being able to evaluate the hypothesis can be found in pragmatism [ 22 ], logical positivism [ 23 ] and falsification [ 24 ].
3 Although when making inferences about a failed evaluation of a scientific hypothesis it is possible, due to underdetermination, to reject the auxiliary hypothesis instead of rejecting the hypothesis. However, that rejection occurs at a later inference stage. The evaluation using the scientific method aims to test the scientific hypothesis, not the auxiliary assumptions.
4 Although some have argued that this practice is not as problematic or questionable (see [ 34 , 35 ]).
5 Alternatively, theories sometimes expand their boundary conditions. A theory that was previously about ‘humans' can be used with a more inclusive boundary condition. Thus it is possible for the hypothesis-maker to use a theory about humans (decision making) and expand it to fruit flies or plants (see [ 43 ]).
6 A similarity exists here with Popper, where he uses set theory in a similar way to compare theories (not hypotheses). Popper also discusses how theories with overlapping sets but neither is a subset are also comparable (see [ 24 , §§32–34]). We do not exclude this possibility but can require additional assumptions.
7 When this could be unclear, we place the element within quotation marks.
8 Here, we have assumed that there is no interaction between these variables in variable selection. If an interaction between x 1 and x 2 is hypothesized, this should be viewed as a different variable compared to ‘ x 1 or x 2 ’. The motivation behind this is because the hypothesis ‘ x 1 or x 2 ’ is not a superset of the interaction (i.e. ‘ x 1 or x 2 ’ is not necessarily true when the interaction is true). The interaction should, in this case, be considered a third variable (e.g. I( x 1 , x 2 )) and the hypothesis ‘ x 1 or x 2 or I( x 1 , x 2 )’ is broader than ‘ x 1 or x 2 ’.
9 Or possibly ambiguous or inconclusive.
10 This formulation of scope is compatible with different frameworks from the philosophy of science. For example, by narrowing the scope would in a Popperian terminology mean prohibiting more basic statements (thus a narrower hypothesis has a higher degree of falsifiability). The reduction of scope in the relational dimension would in Popperian terminology mean increase in precision (e.g. a circle is more precise than an ellipse since circles are a subset of possible ellipses), whereas reduction in variable selection and pipeline dimension would mean increase universality (e.g. ‘all heavenly bodies' is more universal than just ‘planets') [ 24 ]. For Meehl the reduction of the relationship dimension would amount to decreasing the relative tolerance of a theory to the Spielraum [ 46 ] .
11 If there is no relationship between x and y , we do not need to test if there is a positive relationship. If we know there is a positive relationship between x and y , we do not need to test if there is a relationship. If we know there is a relationship but there is not a positive relationship, then it is possible that they have a negative relationship.
Data accessibility
Declaration of ai use.
We have not used AI-assisted technologies in creating this article.
Authors' contributions
W.H.T.: conceptualization, investigation, writing—original draft, writing—review and editing; S.S.: investigation, writing—original draft, writing—review and editing.
Both authors gave final approval for publication and agreed to be held accountable for the work performed therein.
Conflict of interest declaration
We declare we have no competing interests.
We received no funding for this study.
Experiments on Decisions under Risk: The Expected Utility Hypothesis
- © 1980
- Paul J. H. Schoemaker 0
Graduate School of Business, Center for Decision Research, University of Chicago, USA
You can also search for this author in PubMed Google Scholar
687 Accesses
76 Citations
3 Altmetric
This is a preview of subscription content, log in via an institution to check access.
Access this book
- Available as PDF
- Read on any device
- Instant download
- Own it forever
- Compact, lightweight edition
- Dispatched in 3 to 5 business days
- Free shipping worldwide - see info
Tax calculation will be finalised at checkout
Other ways to access
Licence this eBook for your library
Institutional subscriptions
About this book
Similar content being viewed by others.
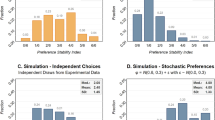
The risk elicitation puzzle revisited: Across-methods (in)consistency?
Precis of risk and rationality.
Expected Utility Hypothesis
- European Union (EU)
- information
- probability
- utility theory
Table of contents (8 chapters)
Front matter, introduction.
Paul J. H. Schoemaker
Expected Utility Theory
Alternative descriptive models, a positivistic test of eu theory, an experimental study of insurance decisons, statistical knowledge and gambling decisions, risk taking and problem context in the domain of losses, back matter, authors and affiliations, bibliographic information.
Book Title : Experiments on Decisions under Risk: The Expected Utility Hypothesis
Authors : Paul J. H. Schoemaker
DOI : https://doi.org/10.1007/978-94-017-5040-0
Publisher : Springer Dordrecht
eBook Packages : Springer Book Archive
Copyright Information : Springer Science+Business Media Dordrecht 1980
Softcover ISBN : 978-94-017-5042-4 Published: 23 August 2014
eBook ISBN : 978-94-017-5040-0 Published: 11 November 2013
Edition Number : 1
Number of Pages : XXIII, 211
Topics : Operations Research/Decision Theory
- Publish with us
Policies and ethics
- Find a journal
- Track your research
- Scientific Methods
What is Hypothesis?
We have heard of many hypotheses which have led to great inventions in science. Assumptions that are made on the basis of some evidence are known as hypotheses. In this article, let us learn in detail about the hypothesis and the type of hypothesis with examples.
A hypothesis is an assumption that is made based on some evidence. This is the initial point of any investigation that translates the research questions into predictions. It includes components like variables, population and the relation between the variables. A research hypothesis is a hypothesis that is used to test the relationship between two or more variables.
Characteristics of Hypothesis
Following are the characteristics of the hypothesis:
- The hypothesis should be clear and precise to consider it to be reliable.
- If the hypothesis is a relational hypothesis, then it should be stating the relationship between variables.
- The hypothesis must be specific and should have scope for conducting more tests.
- The way of explanation of the hypothesis must be very simple and it should also be understood that the simplicity of the hypothesis is not related to its significance.
Sources of Hypothesis
Following are the sources of hypothesis:
- The resemblance between the phenomenon.
- Observations from past studies, present-day experiences and from the competitors.
- Scientific theories.
- General patterns that influence the thinking process of people.
Types of Hypothesis
There are six forms of hypothesis and they are:
- Simple hypothesis
- Complex hypothesis
- Directional hypothesis
- Non-directional hypothesis
- Null hypothesis
- Associative and casual hypothesis
Simple Hypothesis
It shows a relationship between one dependent variable and a single independent variable. For example – If you eat more vegetables, you will lose weight faster. Here, eating more vegetables is an independent variable, while losing weight is the dependent variable.
Complex Hypothesis
It shows the relationship between two or more dependent variables and two or more independent variables. Eating more vegetables and fruits leads to weight loss, glowing skin, and reduces the risk of many diseases such as heart disease.
Directional Hypothesis
It shows how a researcher is intellectual and committed to a particular outcome. The relationship between the variables can also predict its nature. For example- children aged four years eating proper food over a five-year period are having higher IQ levels than children not having a proper meal. This shows the effect and direction of the effect.
Non-directional Hypothesis
It is used when there is no theory involved. It is a statement that a relationship exists between two variables, without predicting the exact nature (direction) of the relationship.
Null Hypothesis
It provides a statement which is contrary to the hypothesis. It’s a negative statement, and there is no relationship between independent and dependent variables. The symbol is denoted by “H O ”.
Associative and Causal Hypothesis
Associative hypothesis occurs when there is a change in one variable resulting in a change in the other variable. Whereas, the causal hypothesis proposes a cause and effect interaction between two or more variables.
Examples of Hypothesis
Following are the examples of hypotheses based on their types:
- Consumption of sugary drinks every day leads to obesity is an example of a simple hypothesis.
- All lilies have the same number of petals is an example of a null hypothesis.
- If a person gets 7 hours of sleep, then he will feel less fatigue than if he sleeps less. It is an example of a directional hypothesis.
Functions of Hypothesis
Following are the functions performed by the hypothesis:
- Hypothesis helps in making an observation and experiments possible.
- It becomes the start point for the investigation.
- Hypothesis helps in verifying the observations.
- It helps in directing the inquiries in the right direction.
How will Hypothesis help in the Scientific Method?
Researchers use hypotheses to put down their thoughts directing how the experiment would take place. Following are the steps that are involved in the scientific method:
- Formation of question
- Doing background research
- Creation of hypothesis
- Designing an experiment
- Collection of data
- Result analysis
- Summarizing the experiment
- Communicating the results
Frequently Asked Questions – FAQs
What is hypothesis.
A hypothesis is an assumption made based on some evidence.
Give an example of simple hypothesis?
What are the types of hypothesis.
Types of hypothesis are:
- Associative and Casual hypothesis
State true or false: Hypothesis is the initial point of any investigation that translates the research questions into a prediction.
Define complex hypothesis..
A complex hypothesis shows the relationship between two or more dependent variables and two or more independent variables.
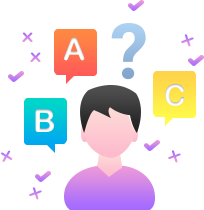
Put your understanding of this concept to test by answering a few MCQs. Click ‘Start Quiz’ to begin!
Select the correct answer and click on the “Finish” button Check your score and answers at the end of the quiz
Visit BYJU’S for all Physics related queries and study materials
Your result is as below
Request OTP on Voice Call
Leave a Comment Cancel reply
Your Mobile number and Email id will not be published. Required fields are marked *
Post My Comment

Register with BYJU'S & Download Free PDFs
Register with byju's & watch live videos.
Why is immunotheraphy not being used as a tool in the war against Alzheimer's Disease?
3-minute read.
Editor's note: Alexander Roberts is a longtime contributor to lohud.com, The Journal News and the USA TODAY Network. This is the first of a regular, monthly series of columns titled The Roberts Report.
Are drug companies delaying a potential cure for Alzheimer’s Disease?
The most powerful weapon against disease is not a drug. It is the body’s own immune system that constantly senses and neutralizes a huge variety of external and internal threats, from pathogens to abnormal cells. Dr. James Allison, together with Dr. Tasuku Honjo, received the 2018 Nobel Prize in Medicine for demonstrating that boosting the body’s systemic immune system could fight cancer. Their research launched an explosion of life-saving immunotherapies that can treat formerly incurable cancers.
Curiously, the immunotherapy revolution has bypassed age-related neurodegenerative diseases like Alzheimer’s. Critics blame two factors: the stubborn myth that the blood- brain barrier prevents the immune system from operating in the brain, and a 25-year preoccupation--some would say obsession--with an Alzheimer’s treatment that has yielded minimal results.
Challenging the immune system’s lack of brain access
The myth of the immune system’s lack of access to the brain was debunked 25 years ago by a professor of neuroimmunology and her team at the Weizmann Institute of Science in Israel. Professor Michal Schwartz felt it made no sense that the blood’s cell repair system would be off limits to one of its most critical organs. She successfully challenged the myth with seminal discoveries that immune cells are guardians of the brain, needed for life-long brain maintenance and repair. Schwartz is recognized as one of the world’s foremost neuroimmunologists, receiving the Israel Prize, that nation’s highest honor for life sciences, and was listed last year in Forbes among the top 50 most influential women in science and technology.
How it works
After establishing the immune system operates in the brain, Schwartz set out to prove that the breakthrough drugs that jumpstart the body’s immune system to fight cancer could be modified to fight Alzheimer’s and other dementias.
When the immune system is overwhelmed or exhausted, switches in the blood called “checkpoints” that turn immune response on and off become stuck on “off.” The antibodies used by oncologists are directed to the checkpoints. They disable the checkpoint inhibitor proteins to get the T cells working again. By 2016, in experiments on mice that modeled Alzheimer’s Disease, Schwartz’s team used modified checkpoint antibodies to rejuvenate the immune system in the brain. They were able to arrest, and even reverse cognitive decline. The benefit did not depend on whether the Alzheimer’s was early or late stage. Mice ravaged by Alzheimer’s showed improved cognition and could navigate mazes from their youth.
Galvanized by those achievements, the Israeli scientist embarked on developing and testing an immune checkpoint inhibitor therapy for Alzheimer’s. She again encountered a roadblock.
The amyloid hypothesis
Schwartz began testing her understanding of the brain’s immune system amid ongoing attempts by numerous companies to develop a treatment that targets the accumulated Beta-amyloid and Beta-amyloid plaques associated with the brains of Alzheimer patients. It’s called the Amyloid Hypothesis that Beta-amyloid deposits cause Alzheimer’s. Beta-amyloid is a sticky protein known to interfere in the work of synapses and neurons. Despite disappointing results and over 20 anti-amyloid drugs that failed in clinical trials, the hypothesis persists. A 2019 article by Sharon Begley in the magazine STAT noted:
“The most influential researchers have long believed so dogmatically in one theory of Alzheimer’s that they systematically thwarted alternative approaches. Several scientists described those who controlled the Alzheimer’s agenda as a ‘cabal’.”
The FDA approves an anti-amyloid drug
Begley, an award-winning science writer whose resume included science columnist at the Wall Street Journal and science editor at Newsweek unfortunately did not live to see the excitement followed by controversy that erupted over the release of Aduhelm (aducanumab) by Biogen, the first drug shown to slow the disease by removing amyloid plaques.
That drug’s approval in June 2021 occurred over the objections of an advisory council of 15 senior FDA officials who cited weak clinical evidence of effectiveness and serious side effects. In a statement at the time, a former Biogen senior medical director who designed the late-stage clinical trials, Dr. Vissia Viglietta said, “This approval shouldn’t have happened. It defeats everything I believe in scientifically and it lowers the rigor of regulatory bodies.”
Biogen announced in January of this year it was discontinuing the drug.
Like Aduhelm, the only other approved drug for Alzheimer’s, Leqembi (lecanemab), must be taken very early by patients with mild cognitive impairment or mild dementia, when they can still function independently. Also based on removing amyloid plaques, Leqembi won’t improve cognition or halt progression of the disease, much less cure it. The drug slowed progression about 4 ½ months during the 18-month clinical trial compared to placebo. After the initial disease stages, Leqembi provides no benefit compared to placebo.
In March, the FDA was set to approve a third anti-amyloid drug, this one developed by Ely Lilly called donanemab. It had slightly better results in clinical trials, but its March approval was unexpectedly delayed by the FDA, pending an independent advisory committee review.
A new market for the Amyloid Hypothesis
Despite the modest results of their anti-amyloid medications, which all have serious side effects, such as brain swelling and in rare cases death, the drug companies are doubling down on the Amyloid Hypothesis. Clinical trials at Eli Lilly will purportedly suggest that donanemab should be taken by all non-cognitively impaired individuals with evidence of amyloid plaques. This despite studies indicating 30%-50% of elderly people (mean age of 85) have such plaques and will never suffer symptoms.
Research on immune checkpoint inhibitors for Alzheimer’s
With help from the Weizmann Institute, Schwartz founded ImmunoBrain Checkpoint , a clinical-stage biopharmaceutical company testing a new antibody specific to immune checkpoints called Aboo2. Tailored to treat Alzheimer’s disease, it blocks a checkpoint protein called PD-L1. According to a company spokesman, “Ab002 is the ONLY therapeutic agent in development for AD with preclinical data suggesting simultaneous therapeutic effects on Amyloid pathology, Tau pathology [another toxic protein associated with Alzheimer’s] and neuroinflammation.”
ImmunoBrain Checkpoint is now conducting a Phase 1 clinical trial with Alzheimer’s patients in the U.K., Europe and Israel. Based on a preclinical study, Schwartz says Aboo2 has a significantly lower potential for side effects compared to antibodies used in cancer therapies.
The company’s approach recently attracted significant support with a $5 million grant from the National Institute on Aging, a division of the National Institutes of Health, and $1 million from the Alzheimer’s Association.
According to Alzheimer’s Association media director Niles Frantz, “Providing a $1 million research grant clearly demonstrates that we believe the area of research shows promise. Many of the research projects funded by the Alzheimer's Association generate significant new and compelling data, which enables the researcher(s) to secure additional funding to extend the time and/or expand the scope of their work.”
With the cost of bringing a drug to market at $1 billion, companies like ImmunoBrain need a major pharmaceutical firm to back it, which would require a willingness to consider alternatives to the Amyloid Hypothesis.
More from Alexander Roberts: The anxiety epidemic: A manufactured crisis
The human cost of Alzheimer’s Disease
Christa Daniello has worked with Alzheimer’s patients for over 25 years at The Osborn Senior Living in Rye, New York, where she is a vice president, and said the cost of the disease is significant.
“You never forget it when the wife and children of a 65-year-old Harvard graduate stand before you crying, with a husband and father who no longer recognizes them. It’s heart-breaking and it’s time for the drug companies to try a different approach.”
Alexander Roberts is a former New York City television news reporter and founder and CEO emeritus of the nonprofit Community Housing Innovations, based in White Plains, New York.
Research Assistant
How to apply.
A cover letter is required for consideration for this position and should be attached as the first page of your resume. The cover letter should address your specific interest in the position and outline skills and experience that directly relate to this position.
The Department of Psychiatry is applicants for a full-time Research Assistant to EEG studies for Dr. Soo-Eun Chang at the Rachel Upjohn Building in Ann Arbor. The position involves supporting multiple ongoing research studies which use EEG and behavioral data collection to investigate the neurophysiological bases of childhood brain development and speech disorders such as stuttering. The ability to work on short-term projects as requested is also required.
Why Join Michigan Medicine?
Michigan Medicine is one of the largest health care complexes in the world and has been the site of many groundbreaking medical and technological advancements since the opening of the U-M Medical School in 1850. Michigan Medicine is comprised of over 30,000 employees and our vision is to attract, inspire, and develop outstanding people in medicine, sciences, and healthcare to become one of the world’s most distinguished academic health systems. In some way, great or small, every person here helps to advance this world-class institution. Work at Michigan Medicine and become a victor for the greater good.
What Benefits can you Look Forward to?
- Excellent medical, dental and vision coverage effective on your very first day
- 2:1 Match on retirement savings
Responsibilities*
In this role you will oversee all aspects of day-to-day study activities including recruitment, screening, enrollment, consenting, scheduling visits, and EEG visits, assisting with other data collection, interfacing with parents and families, providing payment to families upon completion of the study, maintaining all study documents, and other tasks as needed.
Required Qualifications*
To be considered for this position, you must have:
- a Bachelor's Degree in Psychology, Neuroscience, Communication Sciences or a related field, or an equivalent combination of education and experience.
- at least one year of relevant experience.
- outstanding organizational skills.
- meticulous attention to detail.
- excellent verbal and written communication skills.
- availability to work occasional evenings and weekends.
Desired Qualifications*
Other qualifications that would help prepare you for this role include:
- previous work experience as a research assistant on studies involving children and their families.
- knowledge of and interest in speech, language, and/or neurodevelopmental disorders.
Work Schedule
Weekdays. Some late afternoon (e.g. 3 to 8 PM) and weekend shifts may be required to support study objectives.
Work Locations
Rachel Upjohn Building, 4250 Plymouth Road, Ann Arbor, MI.
Additional Information
The Department of Psychiatry is firmly committed to advancing inclusion, diversity, equity, accessibility, and belonging. These values are core to our mission, and we strive to create a culture where each team member feels respected, valued, and safe. We strongly support recruiting and cultivating a diverse workforce as a reflection of our commitment to serve the diverse people of the State of Michigan, and the world.
Background Screening
Michigan Medicine conducts background screening and pre-employment drug testing on job candidates upon acceptance of a contingent job offer and may use a third-party administrator to conduct background screenings. Background screenings are performed in compliance with the Fair Credit Report Act. Pre-employment drug testing applies to all selected candidates, including new or additional faculty and staff appointments, as well as transfers from other U-M campuses.
In addition to the screenings indicated above under Michigan law, a criminal history check including fingerprinting is required as a condition of transfer or employment for this position.
Application Deadline
Job openings are posted for a minimum of seven calendar days. The review and selection process may begin as early as the eighth day after posting. This opening may be removed from posting boards and filled anytime after the minimum posting period has ended.
The University of Michigan participates with the federal EVerify system. Individuals hired into positions that are funded by a federal contract with the FAR EVerify clause must have their identity and work eligibility confirmed by the EVerify system. This position is identified as a position that may include the EVerify requirement.
U-M EEO/AA Statement
The University of Michigan is an equal opportunity/affirmative action employer.
Supporting Texas Power
Wednesday, May 22, 2024 • Brian Lopez : contact
A group of University of Texas at Arlington researchers has received a federal grant to find ways to increase the reliability and resilience of the Texas power grid, provide relief from power transmission congestion, reduce customer bills and facilitate the production of Texas clean energy.
UTA is partnering with the Pacific Northwest National Laboratory (PNNL) on this grant and will provide support for the ongoing Texas Aggregated Distributed Energy Resources (ADER) Pilot Project.
“We are very excited to have received this grant,” said Yichen Zhang, principal investigator and assistant professor in the Department of Electrical Engineering. “This project has the possibility to support the Texas power grid and benefit the people of Texas.”
This work will be supported by a $1.6 million Biden-Harris Administration grant, with the goal of improving regional and state wholesale electricity markets.
The team will primarily look at the viability of adopting ADER into wholesale electricity markets, specifically in the Electric Reliability Council of Texas (ERCOT). ADER is the aggregation of behind-the-meter energy devices and capabilities that can be called upon to accomplish an action, such as reducing energy consumption or providing more energy.
This includes looking to develop Emerge-ADER: Energy Market Evaluation and Resource Planning for Grid Evolution with Aggregated Distributed Energy Resources, a holistic bottom-up ADER planning platform.
“Distributed energy resources are a key component of the transition to a more secure, clean and resilient energy future. Leveraging their flexibility, diversity and distributed nature, the ADERs enable their participation in the wholesale energy markets. It also enhances market efficiency, reliability and sustainability,” said Wei-Jen Lee, interim chair of the Department of Electrical Engineering.
“The capabilities of distributed energy resources to support the larger grid have been sitting dormant for a long time, and I’m glad to see the right partners coming together to bridge the gap and make this possible,” said Chris Boyer, associate professor in the Resource and Energy Engineering Program.
Peter Crouch, dean of the College of Engineering, said as the Dallas-Fort Worth Metroplex continues to grow at a rapid pace, the strain on the electric grid will only increase.
“This research is an important step in understanding how to ensure that Texans will have access to power during extreme weather events, preventing loss of revenue and lives,” Crouch said.
Addressing the needs of the industry is key to the project. The team will closely coordinate with the Texas ADER task force to support its ongoing pilot project. The Texas ADER Task Force consists of utilities, utility commissions, independent system operators, aggregator providers, retail electric providers and more.
Feng Pan, senior researcher at PNNL, said they are thrilled to be part of this unique collaboration between leading institutions in academia, national laboratories, industry and state agencies aimed at advancing ADER.
“PNNL brings to the table its cutting-edge electricity market simulation framework, including the HIPPO simulation framework, coupled with extensive experience in optimization modeling and high-performance computing. These resources will be instrumental in supporting the ADER research initiative and the ongoing ADER Pilot Project in Texas.”
“The project objectives are highly aligned with our task force,” added Jason Ryan, executive vice president for regulatory services and government affairs at CenterPoint Energy and the ADER task force chair. “We look forward to the success of this project in partnership with the Emerge-ADER team and the Department of Energy Grid Deployment Office as significant for future deployment of ADERs in Texas.”
AI is poised to drive 160% increase in data center power demand
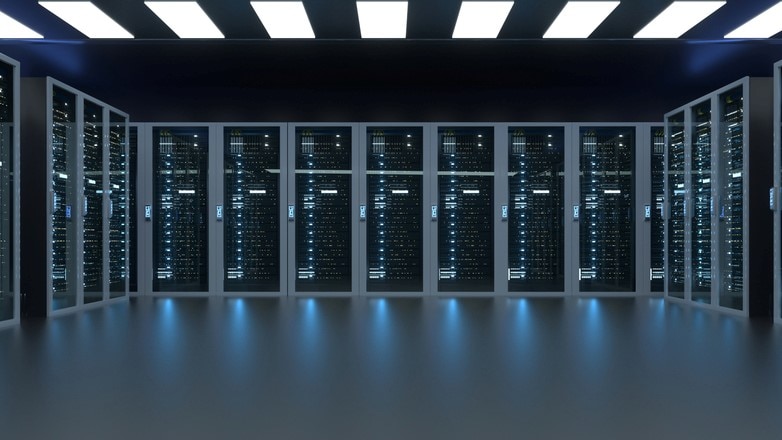
On average, a ChatGPT query needs nearly 10 times as much electricity to process as a Google search. In that difference lies a coming sea change in how the US, Europe, and the world at large will consume power — and how much that will cost.
For years, data centers displayed a remarkably stable appetite for power, even as their workloads mounted. Now, as the pace of efficiency gains in electricity use slows and the AI revolution gathers steam, Goldman Sachs Research estimates that data center power demand will grow 160% by 2030.
At present, data centers worldwide consume 1-2% of overall power, but this percentage will likely rise to 3-4% by the end of the decade. In the US and Europe, this increased demand will help drive the kind of electricity growth that hasn’t been seen in a generation. Along the way, the carbon dioxide emissions of data centers may more than double between 2022 and 2030.
How much power do data centers consume?
In a series of three reports, Goldman Sachs Research analysts lay out the US, European, and global implications of this spike in electricity demand. It isn’t that our demand for data has been meager in the recent past. In fact, data center workloads nearly tripled between 2015 and 2019. Through that period, though, data centers’ demand for power remained flattish, at about 200 terawatt-hours per year. In part, this was because data centers kept growing more efficient in how they used the power they drew, according to the Goldman Sachs Research reports, led by Carly Davenport, Alberto Gandolfi, and Brian Singer.
But since 2020, the efficiency gains appear to have dwindled, and the power consumed by data centers has risen. Some AI innovations will boost computing speed faster than they ramp up their electricity use, but the widening use of AI will still imply an increase in the technology’s consumption of power. A single ChatGPT query requires 2.9 watt-hours of electricity, compared with 0.3 watt-hours for a Google search, according to the International Energy Agency. Goldman Sachs Research estimates the overall increase in data center power consumption from AI to be on the order of 200 terawatt-hours per year between 2023 and 2030. By 2028, our analysts expect AI to represent about 19% of data center power demand.
In tandem, the expected rise of data center carbon dioxide emissions will represent a “social cost” of $125-140 billion (at present value), our analysts believe. “Conversations with technology companies indicate continued confidence in driving down energy intensity but less confidence in meeting absolute emissions forecasts on account of rising demand,” they write. They expect substantial investments by tech firms to underwrite new renewables and commercialize emerging nuclear generation capabilities. And AI may also provide benefits by accelerating innovation — for example, in health care, agriculture, education, or in emissions-reducing energy efficiencies.
US electricity demand is set to surge
Over the last decade, US power demand growth has been roughly zero, even though the population and its economic activity have increased. Efficiencies have helped; one example is the LED light, which drives lower power use. But that is set to change. Between 2022 and 2030, the demand for power will rise roughly 2.4%, Goldman Sachs Research estimates — and around 0.9 percent points of that figure will be tied to data centers.
That kind of spike in power demand hasn’t been seen in the US since the early years of this century. It will be stoked partly by electrification and industrial reshoring, but also by AI . Data centers will use 8% of US power by 2030, compared with 3% in 2022.
US utilities will need to invest around $50 billion in new generation capacity just to support data centers alone. In addition, our analysts expect incremental data center power consumption in the US will drive around 3.3 billion cubic feet per day of new natural gas demand by 2030, which will require new pipeline capacity to be built.
Europe needs $1 trillion-plus to prepare its power grid for AI
Over the past 15 years, Europe's power demand has been severely hit by a sequence of shocks: the global financial crisis, the covid pandemic, and the energy crisis triggered by the war in Ukraine. But it has also suffered due to a slower-than-expected pick up in electrification and the ongoing de-industrialization of the European economy. As a result, since a 2008 peak, electricity demand has cumulatively declined by nearly 10%.
Going forward, between 2023 and 2033, thanks to both the expansion of data centers and an acceleration of electrification, Europe’s power demand could grow by 40% and perhaps even 50%, according to Goldman Sachs Research. At the moment, around 15% of the world’s data centers are located in Europe. By 2030, the power needs of these data centers will match the current total consumption of Portugal, Greece, and the Netherlands combined.
Data center power demand will rise in two kinds of European countries, our analysts write. The first sort is those with cheap and abundant power from nuclear, hydro, wind, or solar sources, such as the Nordic nations, Spain and France. The second kind will include countries with large financial services and tech companies, which offer tax breaks or other incentives to attract data centers. The latter category includes Germany, the UK, and Ireland.
Europe has the oldest power grid in the world, so keeping new data centers electrified will require more investment. Our analysts expect nearly €800 billion ($861 billion) in spending on transmission and distribution over the coming decade, as well as nearly €850 billion in investment on solar, onshore wind, and offshore wind energy.
This article is being provided for educational purposes only. The information contained in this article does not constitute a recommendation from any Goldman Sachs entity to the recipient, and Goldman Sachs is not providing any financial, economic, legal, investment, accounting, or tax advice through this article or to its recipient. Neither Goldman Sachs nor any of its affiliates makes any representation or warranty, express or implied, as to the accuracy or completeness of the statements or any information contained in this article and any liability therefore (including in respect of direct, indirect, or consequential loss or damage) is expressly disclaimed.
Explore More Insights
Sign up for briefings, a newsletter from goldman sachs about trends shaping markets, industries and the global economy..
Thank you for subscribing to BRIEFINGS: a newsletter from Goldman Sachs about trends shaping markets, industries and the global economy.
Some error occurred. Please refresh the page and try again.
Invalid input parameters. Please refresh the page and try again.
Connect With Us
Rating Action Commentary
Fitch Affirms Gainesville Regional Utilities, FL's Revs at 'A+'; Outlook Stable
Wed 22 May, 2024 - 12:59 PM ET
Fitch Ratings - New York - 22 May 2024: Fitch Ratings has affirmed the ratings on the following bonds issued by the city of Gainesville, FL on behalf of Gainesville Regional Utilities (GRU):
--Approximately $1.8 billion in outstanding utility system revenue bonds at 'A+'.
Fitch has assessed the system's standalone credit profile (SCP) at 'a+'. The SCP represents the credit profile of the utility on a standalone basis, irrespective of its relationship with and the credit quality of the city of Gainesville, FL (Issuer Default Rating [IDR] 'AA'/Stable).
The Rating Outlook is Stable.
- Gainesville (FL) /Utility System Revenues/1 LT
VIEW ADDITIONAL RATING DETAILS
The 'A+' bonds rating and SCP reflect GRU's strong financial profile in the context of its very strong revenue defensibility and strong operating risk profile. The combined utility system's very strong revenue defensibility is supported by its monopolistic position as an essential services provider to a service area with favorable demand characteristics, coupled with autonomous rate-setting ability and relatively affordable rates.
The system's strong operating risk profile reflects a stable and low operating cost burden generated by a diverse, mainly owned resource base and a capital spending pattern that is commensurate with the needs of system assets.
GRU's financial profile primarily reflects the utility's leverage ratio, calculated as net adjusted debt to adjusted funds available for debt service (FADS), which has historically exceeded 10x and increased to 11.9x in FY 2023, following a $150 million debt issuance to fund capital projects through 2025. Although leverage was elevated in FY 2023, Fitch believes the utility' financial plan, which includes base rate increases for the electric and wastewater businesses through 2027 and a significant reduction of the general fund transfer payment beginning in 2024, will result in near-term improvement in the utility's financial metrics.
Based on Fitch's scenario analysis, leverage is expected to decline below 10x in both the base case and rating case by FY 2024 and trend toward 9x thereafter. The current ratings and Stable Outlook remain tied to expectations of lower future leverage, which is contingent on GRU's ability to execute its current financial plan, including approved reductions to the utility's general fund transfer .
The rating also incorporates Fitch's expectation that the utility's transition in governance structure from city commission oversight to that of a five-member independent board should not preclude repayment of existing debt & contractual obligations, materially change the operational practices of the utility nor prevent the utility from executing its previously-established financial plan. The five-member independent board was appointed on May 16th, 2024.
The bonds are secured by a first lien on the net revenues of GRU, which includes the combined electric, gas, water, wastewater and telecom systems (collectively, the system).
KEY RATING DRIVERS
Revenue Defensibility - 'aa'
Very Strong Revenue Defensibility
GRU's revenue defensibility assessment reflects the very strong revenue framework through the provision of monopolistic services to a growing service area, a strong local economy and independent ability to adjust rates. Customer growth trends have been solid and the city's unemployment rate remains below the national average.
The service territory extends into the county with roughly 40% of the customer base residing outside the city of Gainesville's limits. The customer base is well diversified and exhibits no concentration. The strong local economy Is anchored by the University of Florida, but the university does not receive electric service from GRU.
Residential electric rates remain well above the state average, but electric charges are affordable especially when compared to the broader service territory's somewhat higher median household income (MHI). Rates will rise over the next several years, which could pressure affordability over time. Retail rates for the other utility services are competitive.
Operating Risk - 'a'
Low Cost Burden, Diverse Resources
The utility's strong operating risk assessment reflects the electric system's low operating cost, which was 12.7 cents/KWh in FY 2023. Historically, GRU's operating cost burden has ranged between 12.5 cents/kWh and 13.5 cents/kWh, demonstrating relative stability of costs amid recent increases in fuel costs and natural gas prices in 2021 and 2022. GRU's diverse power supply portfolio includes owned resources of natural gas, diesel, coal, and biomass plants.
The utility continues to review its resource portfolio and will publish an integrated resource plan (IRP) later in 2024. The utility is actively reducing emissions by investing in its owned resources through modernization, gasification, and retrofits of diesel and coal units. GRU further maintains adequate water supply, and treatment capacity remains sufficient at both the water and wastewater facilities.
While the IRP will be published later this year, GRU's current estimated capital needs of $570 million through 2028 are manageable and will be funded with a combination of remaining 2023 bond proceeds, excess cash flows, and future debt issuance.
Financial Profile - 'a'
Strong Financial Profile; High Leverage to Decline
GRU's financial profile is assessed as strong despite currently high leverage at 11.9x in fiscal 2023. Additionally, the strong financial profile also incorporates Fitch's view that combined utility systems have additional leverage headroom relative to electric-only service-providers. Coverage of full obligations remains in excess of 1x at 1.48x, and liquidity adequate at 193 days cash on hand. When factoring GRU's lines of credit, the liquidity cushion rises to 325 days.
Leverage increased from 9.5x in 2022 to 11.9x primarily due to $150 million in debt issuance to fund capital needs. Despite the elevated leverage metric in 2023, Fitch anticipates that leverage will decline below 10x based on improved financial performance and limited future debt issuances. GRU's forecast includes electric & wastewater base rate increases, that are subject to board approval, through 2027.
Based on future rate increases, coupled with significantly reduced general fund transfers beginning in 2024, the utility expects to generate excess cash flow that will primarily be used to accelerate debt repayment. In Fitch's base and rating case scenarios, leverage is expected to remain below 10x through 2028.
Asymmetric Additional Risk Considerations
There are no additional asymmetric risks affecting the rating.
RATING SENSITIVITIES
Factors that could, individually or collectively, lead to negative rating action/downgrade.
--Failure to reduce leverage to near or below 10.0x on a sustained basis in Fitch's rating case scenario;
--Ineffective collaboration between GRU and the new board resulting in a failure to implement planned rate increases, and reductions in the general fund transfer, and/or deviation from existing financial plan or policies that weakens financial performance
--Sustained increase in the operating cost burden to above 16 cents / kWh that leads to a lower operating risk profile assessment.
Factors that Could, Individually or Collectively, Lead to Positive Rating Action/Upgrade
--Further deleveraging that leads to a leverage ratio consistently below 8.0x in Fitch's rating case scenario.
GRU provides retail electric, gas, water, wastewater and telecommunications services to approximately 281,000 total customers across the five utility systems. GRU's vertically integrated electric utility is the largest system, accounting for over two-thirds of total system revenues. The water (8% of total revenues), wastewater (11%) and gas systems serve territories similar to (and overlapping) the electric system. Each utility is self-supporting and exhibits no customer concentration.
Fitch considers GRU to be a related entity to the city of Gainesville for rating purposes as GRU is a utility enterprise fund of the city and makes annual transfer payments to the city's general fund. The city is the issuer of GRU's bonds, but the credit quality of the city does not currently constrain GRU's ratings. However, as a result of being a related entity, GRU's ratings could become constrained by a material decline in the general credit quality of the city.
GRU was previously governed by the Gainesville City Commission, but following the passage of CS/HB 1645, a five-member independent board known as the Gainesville Regional Utilities Authority (GRUA) was established. The GRUA board was most recently appointed on May 16th 2024 and will assume the previous responsibilities of the commission including approving rates for GRU, collaborating on the budgeting process.
Sources of Information
In addition to the sources of information identified in Fitch's applicable criteria specified below, this action was informed by information from Lumesis.
REFERENCES FOR SUBSTANTIALLY MATERIAL SOURCE CITED AS KEY DRIVER OF RATING
The principal sources of information used in the analysis are described in the Applicable Criteria.
ESG Considerations
The highest level of ESG credit relevance is a score of '3', unless otherwise disclosed in this section. A score of '3' means ESG issues are credit-neutral or have only a minimal credit impact on the entity, either due to their nature or the way in which they are being managed by the entity. Fitch's ESG Relevance Scores are not inputs in the rating process; they are an observation on the relevance and materiality of ESG factors in the rating decision. For more information on Fitch's ESG Relevance Scores, visit https://www.fitchratings.com/topics/esg/products#esg-relevance-scores .
Additional information is available on www.fitchratings.com
PARTICIPATION STATUS
The rated entity (and/or its agents) or, in the case of structured finance, one or more of the transaction parties participated in the rating process except that the following issuer(s), if any, did not participate in the rating process, or provide additional information, beyond the issuer’s available public disclosure.
APPLICABLE CRITERIA
- U.S. Public Sector, Revenue-Supported Entities Rating Criteria (pub. 12 Jan 2024) (including rating assumption sensitivity)
- U.S. Public Power Rating Criteria (pub. 08 Mar 2024) (including rating assumption sensitivity)
APPLICABLE MODELS
Numbers in parentheses accompanying applicable model(s) contain hyperlinks to criteria providing description of model(s).
- FAST Econometric API - Fitch Analytical Stress Test Model, v3.0.0 ( 1 )
ADDITIONAL DISCLOSURES
- Dodd-Frank Rating Information Disclosure Form
- Solicitation Status
- Endorsement Policy
ENDORSEMENT STATUS
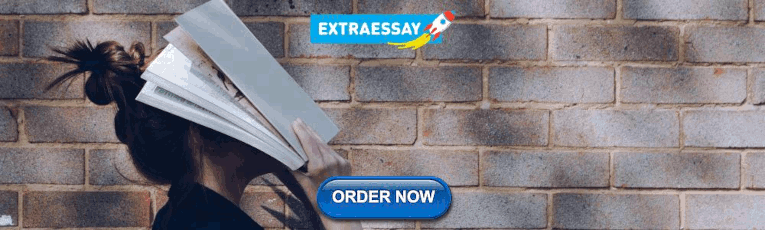
IMAGES
VIDEO
COMMENTS
Simple hypothesis. A simple hypothesis is a statement made to reflect the relation between exactly two variables. One independent and one dependent. Consider the example, "Smoking is a prominent cause of lung cancer." The dependent variable, lung cancer, is dependent on the independent variable, smoking. 4.
What they need at the start of their research is to formulate a scientific hypothesis that revisits conventional theories, real-world processes, and related evidence to propose new studies and test ideas in an ethical way.3 Such a hypothesis can be of most benefit if published in an ethical journal with wide visibility and exposure to relevant ...
A research hypothesis helps test theories. A hypothesis plays a pivotal role in the scientific method by providing a basis for testing existing theories. For example, a hypothesis might test the predictive power of a psychological theory on human behavior. It serves as a great platform for investigation activities.
An effective hypothesis in research is clearly and concisely written, and any terms or definitions clarified and defined. Specific language must also be used to avoid any generalities or assumptions. Use the following points as a checklist to evaluate the effectiveness of your research hypothesis: Predicts the relationship and outcome.
A hypothesis is a tentative statement about the relationship between two or more variables. It is a specific, testable prediction about what you expect to happen in a study. It is a preliminary answer to your question that helps guide the research process. Consider a study designed to examine the relationship between sleep deprivation and test ...
Developing a hypothesis (with example) Step 1. Ask a question. Writing a hypothesis begins with a research question that you want to answer. The question should be focused, specific, and researchable within the constraints of your project. Example: Research question.
3.4 Hypotheses. When researchers do not have predictions about what they will find, they conduct research to answer a question or questions with an open-minded desire to know about a topic, or to help develop hypotheses for later testing. In other situations, the purpose of research is to test a specific hypothesis or hypotheses.
A hypothesis (from the Greek, foundation) is a logical construct, interposed between a problem and its solution, which represents a proposed answer to a research question. It gives direction to the investigator's thinking about the problem and, therefore, facilitates a solution. Unlike facts and assumptions (presumed true and, therefore, not ...
Formulating Hypotheses for Different Study Designs. Generating a testable working hypothesis is the first step towards conducting original research. Such research may prove or disprove the proposed hypothesis. Case reports, case series, online surveys and other observational studies, clinical trials, and narrative reviews help to generate ...
This statement is based on background research and current knowledge.8,9 The research hypothesis makes a specific prediction about a new phenomenon10 or a formal statement on the expected relationship between an independent variable and a dependent variable.3,11 It provides a tentative answer to the research question to be tested or explored.4.
A research hypothesis (also called a scientific hypothesis) is a statement about the expected outcome of a study (for example, a dissertation or thesis). To constitute a quality hypothesis, the statement needs to have three attributes - specificity, clarity and testability. Let's take a look at these more closely.
A research hypothesis is a prediction of the outcome of a study. The prediction may be based on an educated guess or a formal . theory. Example 1 is a hypothesis for a nonexperimental study.
3.6 utility hypothesis A research hypothesis is a statement of expectation or prediction that will be tested by research. Before formulating your research hypothesis, read about the topic of interest to you. From your reading, which may include articles, books and/or cases, you should gain sufficient
A research hypothesis is a specification of a testable prediction about what a researcher expects as the outcome of the study. It comprises certain aspects such as the population, variables, and the relationship between the variables. It states the specific role of the position of individual elements through empirical verification.
rela onship between variables. When formula ng a hypothesis deduc ve. reasoning is u lized as it aims in tes ng a theory or rela onships. Finally, hypothesis helps in discussion of ndings and ...
2. Complex Hypothesis: A Complex hypothesis examines relationship between two or more independent variables and two or more dependent variables. 3. Working or Research Hypothesis: A research hypothesis is a specific, clear prediction about the possible outcome of a scientific research study based on specific factors of the population. 4.
Two-boxing dominates one-boxing: in every state, two-boxing yields a better outcome. Yet on Jeffrey's definition of conditional probability, one-boxing has a higher expected utility than two-boxing. There is a high conditional probability of finding $1 million is in the closed box, given that you one-box, so one-boxing has a high expected utility.
Transferability Defined. Transferability is a process of abstraction used to apply information drawn from specific persons, settings, and eras to others that have not been directly studied. It is often linked with generalization, a similar process that is much more widely discussed in the social science literature.
Expected utility theory (EUT) is an axiomatic theory of choice under risk that has held a central role in economic theory since the 1940s. The hypothesis is that, under certain assumptions, an individual's preferences towards lotteries can be represented as a linear function of the utility of each option multiplied by the probabilities of each option.
This is the first of six chapters in Part II about demand and utility cost, a typical area for what is understood as choice theory. It discusses utility hypothesis and the theory of value. Its five sections are: needs of measurement (of utility); common practice and (William) Fleetwood; parallels in theory (as applied to utility construction ...
The utility of this framework lies in bringing these three scopes of a hypothesis together and explaining how each can be reduced. We believe researchers can use this framework to describe their current practices more clearly. ... Future theoretical work can detail how different types of research impact the hypothesis space in more detail. 11.6 ...
The expected utility hypothesis has come under scrutiny in recent years from a number of different quarters. This book brings together these many studies and relates them to the large body of literature on individual de cision making under risk. Although this paradigm may be appropriate for describing behavior under many conditions of ...
Functions of Hypothesis. Following are the functions performed by the hypothesis: Hypothesis helps in making an observation and experiments possible. It becomes the start point for the investigation. Hypothesis helps in verifying the observations. It helps in directing the inquiries in the right direction.
"The fact there's been so little funded research in the cancer etiology of Asian Americans continues to perpetuate the sense that the cancer burden in these populations is very low," said Scarlett Lin Gomez, PhD, MPH, co-leader of the Cancer Control Program at the Helen Diller Family Comprehensive Cancer Center, a professor of ...
Feature papers represent the most advanced research with significant potential for high impact in the field. A Feature Paper should be a substantial original Article that involves several techniques or approaches, provides an outlook for future research directions and describes possible research applications. ... A Study on an IoT-Based SCADA ...
The amyloid hypothesis. ... Many of the research projects funded by the Alzheimer's Association generate significant new and compelling data, which enables the researcher(s) to secure additional ...
Summary. The Department of Psychiatry is applicants for a full-time Research Assistant to EEG studies for Dr. Soo-Eun Chang at the Rachel Upjohn Building in Ann Arbor. The position involves supporting multiple ongoing research studies which use EEG and behavioral data collection to investigate the neurophysiological bases of childhood brain ...
Wednesday, May 22, 2024 • Brian Lopez : contact. A group of University of Texas at Arlington researchers has received a federal grant to find ways to increase the reliability and resilience of the Texas power grid, provide relief from power transmission congestion, reduce customer bills and facilitate the production of Texas clean energy.
Goldman Sachs Research estimates the overall increase in data center power consumption from AI to be on the order of 200 terawatt-hours per year between 2023 and 2030. By 2028, our analysts expect AI to represent about 19% of data center power demand. In tandem, the expected rise of data center carbon dioxide emissions will represent a ...
The utility's strong operating risk assessment reflects the electric system's low operating cost, which was 12.7 cents/KWh in FY 2023. Historically, GRU's operating cost burden has ranged between 12.5 cents/kWh and 13.5 cents/kWh, demonstrating relative stability of costs amid recent increases in fuel costs and natural gas prices in 2021 and 2022.