
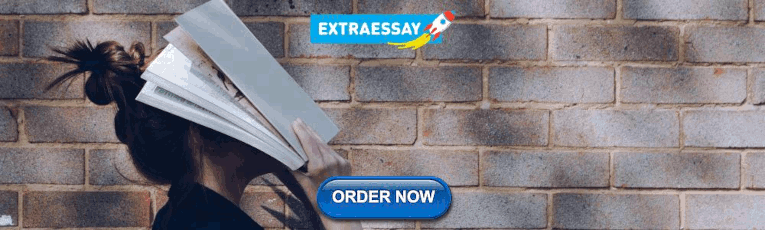
Quantitative Data Analysis 101
The lingo, methods and techniques, explained simply.
By: Derek Jansen (MBA) and Kerryn Warren (PhD) | December 2020
Quantitative data analysis is one of those things that often strikes fear in students. It’s totally understandable – quantitative analysis is a complex topic, full of daunting lingo , like medians, modes, correlation and regression. Suddenly we’re all wishing we’d paid a little more attention in math class…
The good news is that while quantitative data analysis is a mammoth topic, gaining a working understanding of the basics isn’t that hard , even for those of us who avoid numbers and math . In this post, we’ll break quantitative analysis down into simple , bite-sized chunks so you can approach your research with confidence.
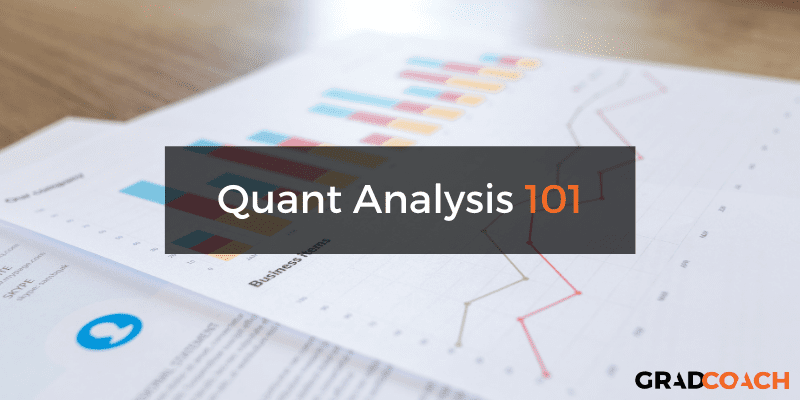
Overview: Quantitative Data Analysis 101
- What (exactly) is quantitative data analysis?
- When to use quantitative analysis
- How quantitative analysis works
The two “branches” of quantitative analysis
- Descriptive statistics 101
- Inferential statistics 101
- How to choose the right quantitative methods
- Recap & summary
What is quantitative data analysis?
Despite being a mouthful, quantitative data analysis simply means analysing data that is numbers-based – or data that can be easily “converted” into numbers without losing any meaning.
For example, category-based variables like gender, ethnicity, or native language could all be “converted” into numbers without losing meaning – for example, English could equal 1, French 2, etc.
This contrasts against qualitative data analysis, where the focus is on words, phrases and expressions that can’t be reduced to numbers. If you’re interested in learning about qualitative analysis, check out our post and video here .
What is quantitative analysis used for?
Quantitative analysis is generally used for three purposes.
- Firstly, it’s used to measure differences between groups . For example, the popularity of different clothing colours or brands.
- Secondly, it’s used to assess relationships between variables . For example, the relationship between weather temperature and voter turnout.
- And third, it’s used to test hypotheses in a scientifically rigorous way. For example, a hypothesis about the impact of a certain vaccine.
Again, this contrasts with qualitative analysis , which can be used to analyse people’s perceptions and feelings about an event or situation. In other words, things that can’t be reduced to numbers.
How does quantitative analysis work?
Well, since quantitative data analysis is all about analysing numbers , it’s no surprise that it involves statistics . Statistical analysis methods form the engine that powers quantitative analysis, and these methods can vary from pretty basic calculations (for example, averages and medians) to more sophisticated analyses (for example, correlations and regressions).
Sounds like gibberish? Don’t worry. We’ll explain all of that in this post. Importantly, you don’t need to be a statistician or math wiz to pull off a good quantitative analysis. We’ll break down all the technical mumbo jumbo in this post.
Need a helping hand?
As I mentioned, quantitative analysis is powered by statistical analysis methods . There are two main “branches” of statistical methods that are used – descriptive statistics and inferential statistics . In your research, you might only use descriptive statistics, or you might use a mix of both , depending on what you’re trying to figure out. In other words, depending on your research questions, aims and objectives . I’ll explain how to choose your methods later.
So, what are descriptive and inferential statistics?
Well, before I can explain that, we need to take a quick detour to explain some lingo. To understand the difference between these two branches of statistics, you need to understand two important words. These words are population and sample .
First up, population . In statistics, the population is the entire group of people (or animals or organisations or whatever) that you’re interested in researching. For example, if you were interested in researching Tesla owners in the US, then the population would be all Tesla owners in the US.
However, it’s extremely unlikely that you’re going to be able to interview or survey every single Tesla owner in the US. Realistically, you’ll likely only get access to a few hundred, or maybe a few thousand owners using an online survey. This smaller group of accessible people whose data you actually collect is called your sample .
So, to recap – the population is the entire group of people you’re interested in, and the sample is the subset of the population that you can actually get access to. In other words, the population is the full chocolate cake , whereas the sample is a slice of that cake.
So, why is this sample-population thing important?
Well, descriptive statistics focus on describing the sample , while inferential statistics aim to make predictions about the population, based on the findings within the sample. In other words, we use one group of statistical methods – descriptive statistics – to investigate the slice of cake, and another group of methods – inferential statistics – to draw conclusions about the entire cake. There I go with the cake analogy again…
With that out the way, let’s take a closer look at each of these branches in more detail.
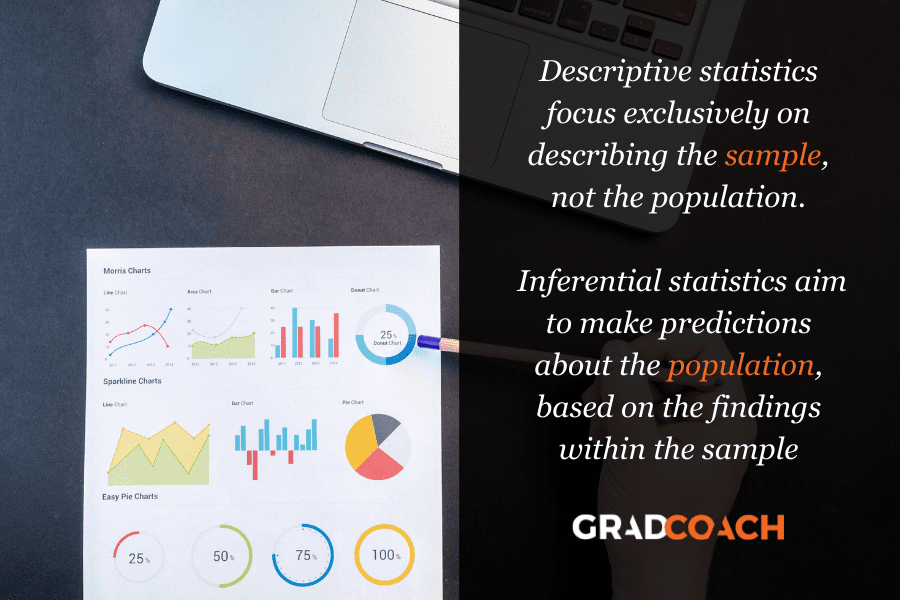
Branch 1: Descriptive Statistics
Descriptive statistics serve a simple but critically important role in your research – to describe your data set – hence the name. In other words, they help you understand the details of your sample . Unlike inferential statistics (which we’ll get to soon), descriptive statistics don’t aim to make inferences or predictions about the entire population – they’re purely interested in the details of your specific sample .
When you’re writing up your analysis, descriptive statistics are the first set of stats you’ll cover, before moving on to inferential statistics. But, that said, depending on your research objectives and research questions , they may be the only type of statistics you use. We’ll explore that a little later.
So, what kind of statistics are usually covered in this section?
Some common statistical tests used in this branch include the following:
- Mean – this is simply the mathematical average of a range of numbers.
- Median – this is the midpoint in a range of numbers when the numbers are arranged in numerical order. If the data set makes up an odd number, then the median is the number right in the middle of the set. If the data set makes up an even number, then the median is the midpoint between the two middle numbers.
- Mode – this is simply the most commonly occurring number in the data set.
- In cases where most of the numbers are quite close to the average, the standard deviation will be relatively low.
- Conversely, in cases where the numbers are scattered all over the place, the standard deviation will be relatively high.
- Skewness . As the name suggests, skewness indicates how symmetrical a range of numbers is. In other words, do they tend to cluster into a smooth bell curve shape in the middle of the graph, or do they skew to the left or right?
Feeling a bit confused? Let’s look at a practical example using a small data set.
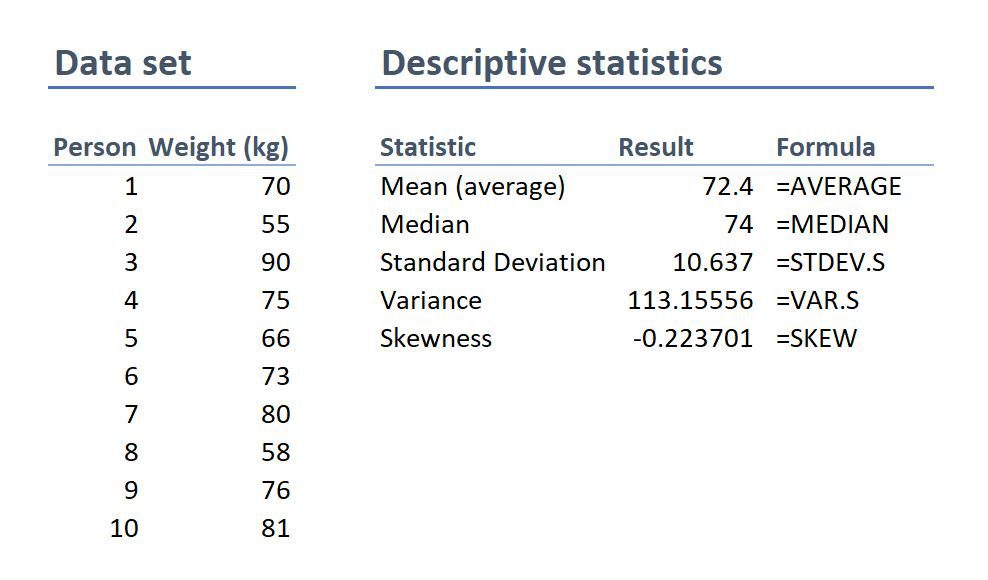
On the left-hand side is the data set. This details the bodyweight of a sample of 10 people. On the right-hand side, we have the descriptive statistics. Let’s take a look at each of them.
First, we can see that the mean weight is 72.4 kilograms. In other words, the average weight across the sample is 72.4 kilograms. Straightforward.
Next, we can see that the median is very similar to the mean (the average). This suggests that this data set has a reasonably symmetrical distribution (in other words, a relatively smooth, centred distribution of weights, clustered towards the centre).
In terms of the mode , there is no mode in this data set. This is because each number is present only once and so there cannot be a “most common number”. If there were two people who were both 65 kilograms, for example, then the mode would be 65.
Next up is the standard deviation . 10.6 indicates that there’s quite a wide spread of numbers. We can see this quite easily by looking at the numbers themselves, which range from 55 to 90, which is quite a stretch from the mean of 72.4.
And lastly, the skewness of -0.2 tells us that the data is very slightly negatively skewed. This makes sense since the mean and the median are slightly different.
As you can see, these descriptive statistics give us some useful insight into the data set. Of course, this is a very small data set (only 10 records), so we can’t read into these statistics too much. Also, keep in mind that this is not a list of all possible descriptive statistics – just the most common ones.
But why do all of these numbers matter?
While these descriptive statistics are all fairly basic, they’re important for a few reasons:
- Firstly, they help you get both a macro and micro-level view of your data. In other words, they help you understand both the big picture and the finer details.
- Secondly, they help you spot potential errors in the data – for example, if an average is way higher than you’d expect, or responses to a question are highly varied, this can act as a warning sign that you need to double-check the data.
- And lastly, these descriptive statistics help inform which inferential statistical techniques you can use, as those techniques depend on the skewness (in other words, the symmetry and normality) of the data.
Simply put, descriptive statistics are really important , even though the statistical techniques used are fairly basic. All too often at Grad Coach, we see students skimming over the descriptives in their eagerness to get to the more exciting inferential methods, and then landing up with some very flawed results.
Don’t be a sucker – give your descriptive statistics the love and attention they deserve!
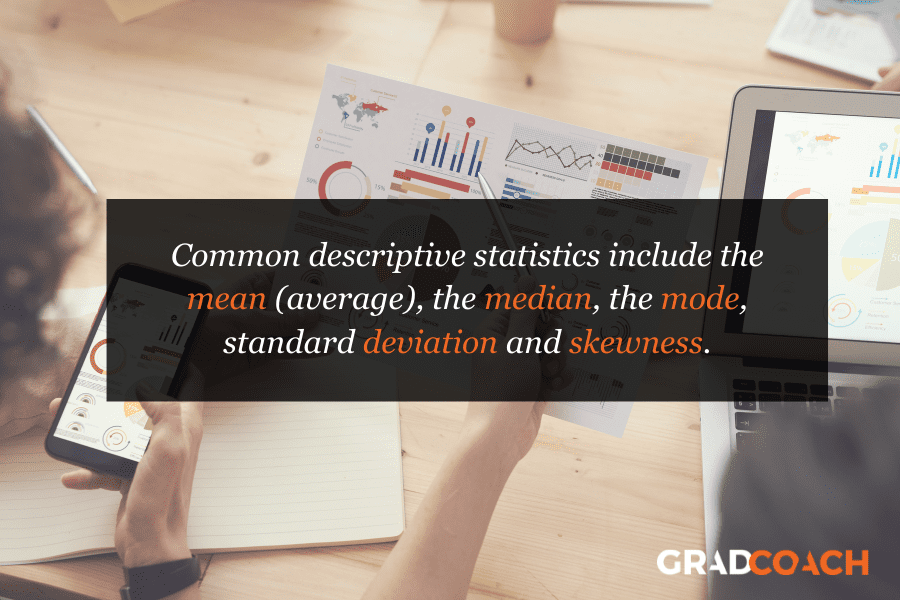
Branch 2: Inferential Statistics
As I mentioned, while descriptive statistics are all about the details of your specific data set – your sample – inferential statistics aim to make inferences about the population . In other words, you’ll use inferential statistics to make predictions about what you’d expect to find in the full population.
What kind of predictions, you ask? Well, there are two common types of predictions that researchers try to make using inferential stats:
- Firstly, predictions about differences between groups – for example, height differences between children grouped by their favourite meal or gender.
- And secondly, relationships between variables – for example, the relationship between body weight and the number of hours a week a person does yoga.
In other words, inferential statistics (when done correctly), allow you to connect the dots and make predictions about what you expect to see in the real world population, based on what you observe in your sample data. For this reason, inferential statistics are used for hypothesis testing – in other words, to test hypotheses that predict changes or differences.
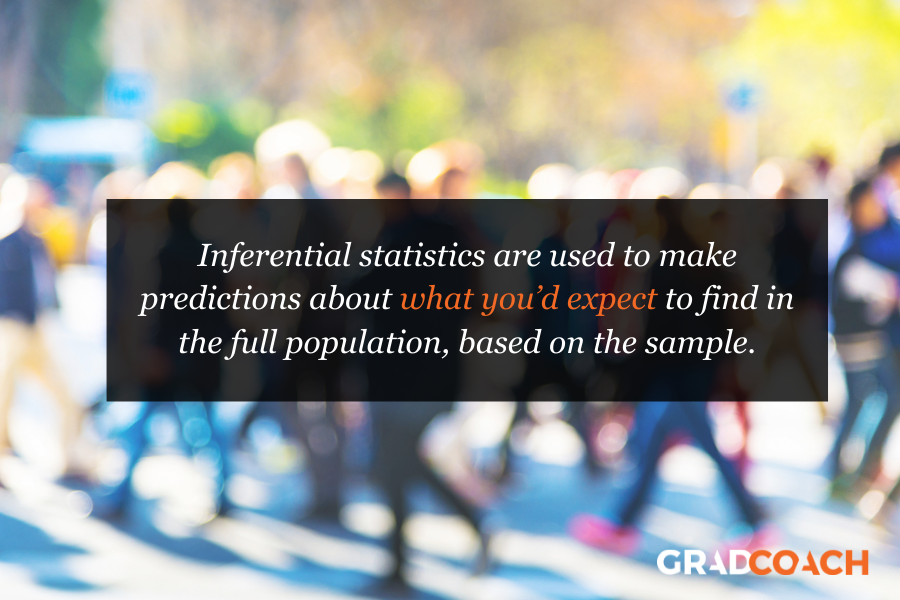
Of course, when you’re working with inferential statistics, the composition of your sample is really important. In other words, if your sample doesn’t accurately represent the population you’re researching, then your findings won’t necessarily be very useful.
For example, if your population of interest is a mix of 50% male and 50% female , but your sample is 80% male , you can’t make inferences about the population based on your sample, since it’s not representative. This area of statistics is called sampling, but we won’t go down that rabbit hole here (it’s a deep one!) – we’ll save that for another post .
What statistics are usually used in this branch?
There are many, many different statistical analysis methods within the inferential branch and it’d be impossible for us to discuss them all here. So we’ll just take a look at some of the most common inferential statistical methods so that you have a solid starting point.
First up are T-Tests . T-tests compare the means (the averages) of two groups of data to assess whether they’re statistically significantly different. In other words, do they have significantly different means, standard deviations and skewness.
This type of testing is very useful for understanding just how similar or different two groups of data are. For example, you might want to compare the mean blood pressure between two groups of people – one that has taken a new medication and one that hasn’t – to assess whether they are significantly different.
Kicking things up a level, we have ANOVA, which stands for “analysis of variance”. This test is similar to a T-test in that it compares the means of various groups, but ANOVA allows you to analyse multiple groups , not just two groups So it’s basically a t-test on steroids…
Next, we have correlation analysis . This type of analysis assesses the relationship between two variables. In other words, if one variable increases, does the other variable also increase, decrease or stay the same. For example, if the average temperature goes up, do average ice creams sales increase too? We’d expect some sort of relationship between these two variables intuitively , but correlation analysis allows us to measure that relationship scientifically .
Lastly, we have regression analysis – this is quite similar to correlation in that it assesses the relationship between variables, but it goes a step further to understand cause and effect between variables, not just whether they move together. In other words, does the one variable actually cause the other one to move, or do they just happen to move together naturally thanks to another force? Just because two variables correlate doesn’t necessarily mean that one causes the other.
Stats overload…
I hear you. To make this all a little more tangible, let’s take a look at an example of a correlation in action.
Here’s a scatter plot demonstrating the correlation (relationship) between weight and height. Intuitively, we’d expect there to be some relationship between these two variables, which is what we see in this scatter plot. In other words, the results tend to cluster together in a diagonal line from bottom left to top right.
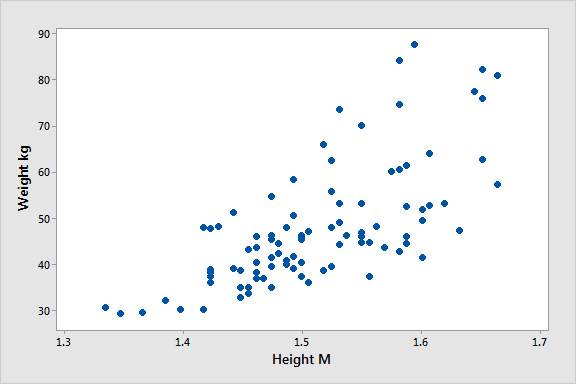
As I mentioned, these are are just a handful of inferential techniques – there are many, many more. Importantly, each statistical method has its own assumptions and limitations .
For example, some methods only work with normally distributed (parametric) data, while other methods are designed specifically for non-parametric data. And that’s exactly why descriptive statistics are so important – they’re the first step to knowing which inferential techniques you can and can’t use.
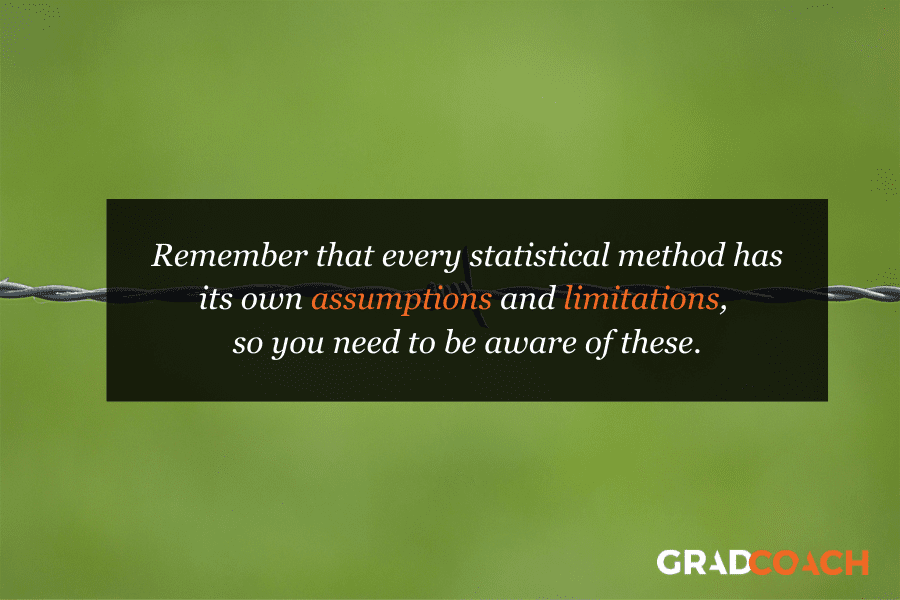
How to choose the right analysis method
To choose the right statistical methods, you need to think about two important factors :
- The type of quantitative data you have (specifically, level of measurement and the shape of the data). And,
- Your research questions and hypotheses
Let’s take a closer look at each of these.
Factor 1 – Data type
The first thing you need to consider is the type of data you’ve collected (or the type of data you will collect). By data types, I’m referring to the four levels of measurement – namely, nominal, ordinal, interval and ratio. If you’re not familiar with this lingo, check out the video below.
Why does this matter?
Well, because different statistical methods and techniques require different types of data. This is one of the “assumptions” I mentioned earlier – every method has its assumptions regarding the type of data.
For example, some techniques work with categorical data (for example, yes/no type questions, or gender or ethnicity), while others work with continuous numerical data (for example, age, weight or income) – and, of course, some work with multiple data types.
If you try to use a statistical method that doesn’t support the data type you have, your results will be largely meaningless . So, make sure that you have a clear understanding of what types of data you’ve collected (or will collect). Once you have this, you can then check which statistical methods would support your data types here .
If you haven’t collected your data yet, you can work in reverse and look at which statistical method would give you the most useful insights, and then design your data collection strategy to collect the correct data types.
Another important factor to consider is the shape of your data . Specifically, does it have a normal distribution (in other words, is it a bell-shaped curve, centred in the middle) or is it very skewed to the left or the right? Again, different statistical techniques work for different shapes of data – some are designed for symmetrical data while others are designed for skewed data.
This is another reminder of why descriptive statistics are so important – they tell you all about the shape of your data.
Factor 2: Your research questions
The next thing you need to consider is your specific research questions, as well as your hypotheses (if you have some). The nature of your research questions and research hypotheses will heavily influence which statistical methods and techniques you should use.
If you’re just interested in understanding the attributes of your sample (as opposed to the entire population), then descriptive statistics are probably all you need. For example, if you just want to assess the means (averages) and medians (centre points) of variables in a group of people.
On the other hand, if you aim to understand differences between groups or relationships between variables and to infer or predict outcomes in the population, then you’ll likely need both descriptive statistics and inferential statistics.
So, it’s really important to get very clear about your research aims and research questions, as well your hypotheses – before you start looking at which statistical techniques to use.
Never shoehorn a specific statistical technique into your research just because you like it or have some experience with it. Your choice of methods must align with all the factors we’ve covered here.
Time to recap…
You’re still with me? That’s impressive. We’ve covered a lot of ground here, so let’s recap on the key points:
- Quantitative data analysis is all about analysing number-based data (which includes categorical and numerical data) using various statistical techniques.
- The two main branches of statistics are descriptive statistics and inferential statistics . Descriptives describe your sample, whereas inferentials make predictions about what you’ll find in the population.
- Common descriptive statistical methods include mean (average), median , standard deviation and skewness .
- Common inferential statistical methods include t-tests , ANOVA , correlation and regression analysis.
- To choose the right statistical methods and techniques, you need to consider the type of data you’re working with , as well as your research questions and hypotheses.
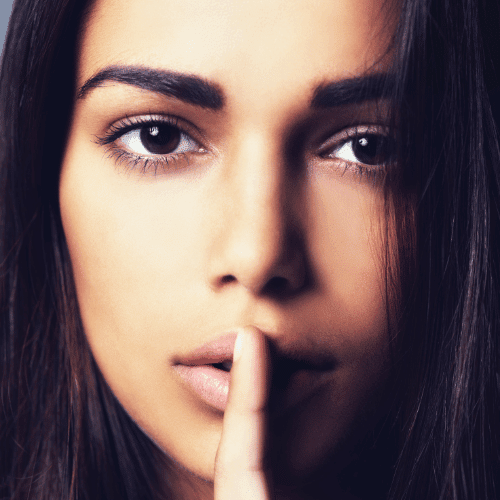
Psst... there’s more!
This post was based on one of our popular Research Bootcamps . If you're working on a research project, you'll definitely want to check this out ...
You Might Also Like:
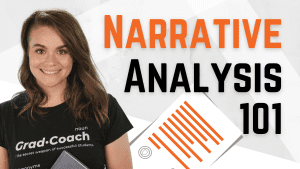
75 Comments
Hi, I have read your article. Such a brilliant post you have created.
Thank you for the feedback. Good luck with your quantitative analysis.
Thank you so much.
Thank you so much. I learnt much well. I love your summaries of the concepts. I had love you to explain how to input data using SPSS
Amazing and simple way of breaking down quantitative methods.
This is beautiful….especially for non-statisticians. I have skimmed through but I wish to read again. and please include me in other articles of the same nature when you do post. I am interested. I am sure, I could easily learn from you and get off the fear that I have had in the past. Thank you sincerely.
Send me every new information you might have.
i need every new information
Thank you for the blog. It is quite informative. Dr Peter Nemaenzhe PhD
It is wonderful. l’ve understood some of the concepts in a more compréhensive manner
Your article is so good! However, I am still a bit lost. I am doing a secondary research on Gun control in the US and increase in crime rates and I am not sure which analysis method I should use?
Based on the given learning points, this is inferential analysis, thus, use ‘t-tests, ANOVA, correlation and regression analysis’
Well explained notes. Am an MPH student and currently working on my thesis proposal, this has really helped me understand some of the things I didn’t know.
I like your page..helpful
wonderful i got my concept crystal clear. thankyou!!
This is really helpful , thank you
Thank you so much this helped
Wonderfully explained
thank u so much, it was so informative
THANKYOU, this was very informative and very helpful
This is great GRADACOACH I am not a statistician but I require more of this in my thesis
Include me in your posts.
This is so great and fully useful. I would like to thank you again and again.
Glad to read this article. I’ve read lot of articles but this article is clear on all concepts. Thanks for sharing.
Thank you so much. This is a very good foundation and intro into quantitative data analysis. Appreciate!
You have a very impressive, simple but concise explanation of data analysis for Quantitative Research here. This is a God-send link for me to appreciate research more. Thank you so much!
Avery good presentation followed by the write up. yes you simplified statistics to make sense even to a layman like me. Thank so much keep it up. The presenter did ell too. i would like more of this for Qualitative and exhaust more of the test example like the Anova.
This is a very helpful article, couldn’t have been clearer. Thank you.
Awesome and phenomenal information.Well done
The video with the accompanying article is super helpful to demystify this topic. Very well done. Thank you so much.
thank you so much, your presentation helped me a lot
I don’t know how should I express that ur article is saviour for me 🥺😍
It is well defined information and thanks for sharing. It helps me a lot in understanding the statistical data.
I gain a lot and thanks for sharing brilliant ideas, so wish to be linked on your email update.
Very helpful and clear .Thank you Gradcoach.
Thank for sharing this article, well organized and information presented are very clear.
VERY INTERESTING AND SUPPORTIVE TO NEW RESEARCHERS LIKE ME. AT LEAST SOME BASICS ABOUT QUANTITATIVE.
An outstanding, well explained and helpful article. This will help me so much with my data analysis for my research project. Thank you!
wow this has just simplified everything i was scared of how i am gonna analyse my data but thanks to you i will be able to do so
simple and constant direction to research. thanks
This is helpful
Great writing!! Comprehensive and very helpful.
Do you provide any assistance for other steps of research methodology like making research problem testing hypothesis report and thesis writing?
Thank you so much for such useful article!
Amazing article. So nicely explained. Wow
Very insightfull. Thanks
I am doing a quality improvement project to determine if the implementation of a protocol will change prescribing habits. Would this be a t-test?
The is a very helpful blog, however, I’m still not sure how to analyze my data collected. I’m doing a research on “Free Education at the University of Guyana”
tnx. fruitful blog!
So I am writing exams and would like to know how do establish which method of data analysis to use from the below research questions: I am a bit lost as to how I determine the data analysis method from the research questions.
Do female employees report higher job satisfaction than male employees with similar job descriptions across the South African telecommunications sector? – I though that maybe Chi Square could be used here. – Is there a gender difference in talented employees’ actual turnover decisions across the South African telecommunications sector? T-tests or Correlation in this one. – Is there a gender difference in the cost of actual turnover decisions across the South African telecommunications sector? T-tests or Correlation in this one. – What practical recommendations can be made to the management of South African telecommunications companies on leveraging gender to mitigate employee turnover decisions?
Your assistance will be appreciated if I could get a response as early as possible tomorrow
This was quite helpful. Thank you so much.
wow I got a lot from this article, thank you very much, keep it up
Thanks for yhe guidance. Can you send me this guidance on my email? To enable offline reading?
Thank you very much, this service is very helpful.
Every novice researcher needs to read this article as it puts things so clear and easy to follow. Its been very helpful.
Wonderful!!!! you explained everything in a way that anyone can learn. Thank you!!
I really enjoyed reading though this. Very easy to follow. Thank you
Many thanks for your useful lecture, I would be really appreciated if you could possibly share with me the PPT of presentation related to Data type?
Thank you very much for sharing, I got much from this article
This is a very informative write-up. Kindly include me in your latest posts.
Very interesting mostly for social scientists
Thank you so much, very helpfull
You’re welcome 🙂
woow, its great, its very informative and well understood because of your way of writing like teaching in front of me in simple languages.
I have been struggling to understand a lot of these concepts. Thank you for the informative piece which is written with outstanding clarity.
very informative article. Easy to understand
Beautiful read, much needed.
Always greet intro and summary. I learn so much from GradCoach
Quite informative. Simple and clear summary.
I thoroughly enjoyed reading your informative and inspiring piece. Your profound insights into this topic truly provide a better understanding of its complexity. I agree with the points you raised, especially when you delved into the specifics of the article. In my opinion, that aspect is often overlooked and deserves further attention.
Absolutely!!! Thank you
Thank you very much for this post. It made me to understand how to do my data analysis.
its nice work and excellent job ,you have made my work easier
Submit a Comment Cancel reply
Your email address will not be published. Required fields are marked *
Save my name, email, and website in this browser for the next time I comment.
- Print Friendly
Quantitative Data Analysis: A Comprehensive Guide
By: Ofem Eteng | Published: May 18, 2022
Related Articles
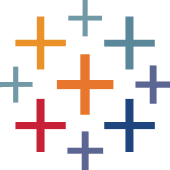
A healthcare giant successfully introduces the most effective drug dosage through rigorous statistical modeling, saving countless lives. A marketing team predicts consumer trends with uncanny accuracy, tailoring campaigns for maximum impact.
Table of Contents
These trends and dosages are not just any numbers but are a result of meticulous quantitative data analysis. Quantitative data analysis offers a robust framework for understanding complex phenomena, evaluating hypotheses, and predicting future outcomes.
In this blog, we’ll walk through the concept of quantitative data analysis, the steps required, its advantages, and the methods and techniques that are used in this analysis. Read on!
What is Quantitative Data Analysis?
Quantitative data analysis is a systematic process of examining, interpreting, and drawing meaningful conclusions from numerical data. It involves the application of statistical methods, mathematical models, and computational techniques to understand patterns, relationships, and trends within datasets.
Quantitative data analysis methods typically work with algorithms, mathematical analysis tools, and software to gain insights from the data, answering questions such as how many, how often, and how much. Data for quantitative data analysis is usually collected from close-ended surveys, questionnaires, polls, etc. The data can also be obtained from sales figures, email click-through rates, number of website visitors, and percentage revenue increase.
Quantitative Data Analysis vs Qualitative Data Analysis
When we talk about data, we directly think about the pattern, the relationship, and the connection between the datasets – analyzing the data in short. Therefore when it comes to data analysis, there are broadly two types – Quantitative Data Analysis and Qualitative Data Analysis.
Quantitative data analysis revolves around numerical data and statistics, which are suitable for functions that can be counted or measured. In contrast, qualitative data analysis includes description and subjective information – for things that can be observed but not measured.
Let us differentiate between Quantitative Data Analysis and Quantitative Data Analysis for a better understanding.
Data Preparation Steps for Quantitative Data Analysis
Quantitative data has to be gathered and cleaned before proceeding to the stage of analyzing it. Below are the steps to prepare a data before quantitative research analysis:
- Step 1: Data Collection
Before beginning the analysis process, you need data. Data can be collected through rigorous quantitative research, which includes methods such as interviews, focus groups, surveys, and questionnaires.
- Step 2: Data Cleaning
Once the data is collected, begin the data cleaning process by scanning through the entire data for duplicates, errors, and omissions. Keep a close eye for outliers (data points that are significantly different from the majority of the dataset) because they can skew your analysis results if they are not removed.
This data-cleaning process ensures data accuracy, consistency and relevancy before analysis.
- Step 3: Data Analysis and Interpretation
Now that you have collected and cleaned your data, it is now time to carry out the quantitative analysis. There are two methods of quantitative data analysis, which we will discuss in the next section.
However, if you have data from multiple sources, collecting and cleaning it can be a cumbersome task. This is where Hevo Data steps in. With Hevo, extracting, transforming, and loading data from source to destination becomes a seamless task, eliminating the need for manual coding. This not only saves valuable time but also enhances the overall efficiency of data analysis and visualization, empowering users to derive insights quickly and with precision
Hevo is the only real-time ELT No-code Data Pipeline platform that cost-effectively automates data pipelines that are flexible to your needs. With integration with 150+ Data Sources (40+ free sources), we help you not only export data from sources & load data to the destinations but also transform & enrich your data, & make it analysis-ready.
Start for free now!
Now that you are familiar with what quantitative data analysis is and how to prepare your data for analysis, the focus will shift to the purpose of this article, which is to describe the methods and techniques of quantitative data analysis.
Methods and Techniques of Quantitative Data Analysis
Quantitative data analysis employs two techniques to extract meaningful insights from datasets, broadly. The first method is descriptive statistics, which summarizes and portrays essential features of a dataset, such as mean, median, and standard deviation.
Inferential statistics, the second method, extrapolates insights and predictions from a sample dataset to make broader inferences about an entire population, such as hypothesis testing and regression analysis.
An in-depth explanation of both the methods is provided below:
- Descriptive Statistics
- Inferential Statistics
1) Descriptive Statistics
Descriptive statistics as the name implies is used to describe a dataset. It helps understand the details of your data by summarizing it and finding patterns from the specific data sample. They provide absolute numbers obtained from a sample but do not necessarily explain the rationale behind the numbers and are mostly used for analyzing single variables. The methods used in descriptive statistics include:
- Mean: This calculates the numerical average of a set of values.
- Median: This is used to get the midpoint of a set of values when the numbers are arranged in numerical order.
- Mode: This is used to find the most commonly occurring value in a dataset.
- Percentage: This is used to express how a value or group of respondents within the data relates to a larger group of respondents.
- Frequency: This indicates the number of times a value is found.
- Range: This shows the highest and lowest values in a dataset.
- Standard Deviation: This is used to indicate how dispersed a range of numbers is, meaning, it shows how close all the numbers are to the mean.
- Skewness: It indicates how symmetrical a range of numbers is, showing if they cluster into a smooth bell curve shape in the middle of the graph or if they skew towards the left or right.
2) Inferential Statistics
In quantitative analysis, the expectation is to turn raw numbers into meaningful insight using numerical values, and descriptive statistics is all about explaining details of a specific dataset using numbers, but it does not explain the motives behind the numbers; hence, a need for further analysis using inferential statistics.
Inferential statistics aim to make predictions or highlight possible outcomes from the analyzed data obtained from descriptive statistics. They are used to generalize results and make predictions between groups, show relationships that exist between multiple variables, and are used for hypothesis testing that predicts changes or differences.
There are various statistical analysis methods used within inferential statistics; a few are discussed below.
- Cross Tabulations: Cross tabulation or crosstab is used to show the relationship that exists between two variables and is often used to compare results by demographic groups. It uses a basic tabular form to draw inferences between different data sets and contains data that is mutually exclusive or has some connection with each other. Crosstabs help understand the nuances of a dataset and factors that may influence a data point.
- Regression Analysis: Regression analysis estimates the relationship between a set of variables. It shows the correlation between a dependent variable (the variable or outcome you want to measure or predict) and any number of independent variables (factors that may impact the dependent variable). Therefore, the purpose of the regression analysis is to estimate how one or more variables might affect a dependent variable to identify trends and patterns to make predictions and forecast possible future trends. There are many types of regression analysis, and the model you choose will be determined by the type of data you have for the dependent variable. The types of regression analysis include linear regression, non-linear regression, binary logistic regression, etc.
- Monte Carlo Simulation: Monte Carlo simulation, also known as the Monte Carlo method, is a computerized technique of generating models of possible outcomes and showing their probability distributions. It considers a range of possible outcomes and then tries to calculate how likely each outcome will occur. Data analysts use it to perform advanced risk analyses to help forecast future events and make decisions accordingly.
- Analysis of Variance (ANOVA): This is used to test the extent to which two or more groups differ from each other. It compares the mean of various groups and allows the analysis of multiple groups.
- Factor Analysis: A large number of variables can be reduced into a smaller number of factors using the factor analysis technique. It works on the principle that multiple separate observable variables correlate with each other because they are all associated with an underlying construct. It helps in reducing large datasets into smaller, more manageable samples.
- Cohort Analysis: Cohort analysis can be defined as a subset of behavioral analytics that operates from data taken from a given dataset. Rather than looking at all users as one unit, cohort analysis breaks down data into related groups for analysis, where these groups or cohorts usually have common characteristics or similarities within a defined period.
- MaxDiff Analysis: This is a quantitative data analysis method that is used to gauge customers’ preferences for purchase and what parameters rank higher than the others in the process.
- Cluster Analysis: Cluster analysis is a technique used to identify structures within a dataset. Cluster analysis aims to be able to sort different data points into groups that are internally similar and externally different; that is, data points within a cluster will look like each other and different from data points in other clusters.
- Time Series Analysis: This is a statistical analytic technique used to identify trends and cycles over time. It is simply the measurement of the same variables at different times, like weekly and monthly email sign-ups, to uncover trends, seasonality, and cyclic patterns. By doing this, the data analyst can forecast how variables of interest may fluctuate in the future.
- SWOT analysis: This is a quantitative data analysis method that assigns numerical values to indicate strengths, weaknesses, opportunities, and threats of an organization, product, or service to show a clearer picture of competition to foster better business strategies
How to Choose the Right Method for your Analysis?
Choosing between Descriptive Statistics or Inferential Statistics can be often confusing. You should consider the following factors before choosing the right method for your quantitative data analysis:
1. Type of Data
The first consideration in data analysis is understanding the type of data you have. Different statistical methods have specific requirements based on these data types, and using the wrong method can render results meaningless. The choice of statistical method should align with the nature and distribution of your data to ensure meaningful and accurate analysis.
2. Your Research Questions
When deciding on statistical methods, it’s crucial to align them with your specific research questions and hypotheses. The nature of your questions will influence whether descriptive statistics alone, which reveal sample attributes, are sufficient or if you need both descriptive and inferential statistics to understand group differences or relationships between variables and make population inferences.
Pros and Cons of Quantitative Data Analysis
1. Objectivity and Generalizability:
- Quantitative data analysis offers objective, numerical measurements, minimizing bias and personal interpretation.
- Results can often be generalized to larger populations, making them applicable to broader contexts.
Example: A study using quantitative data analysis to measure student test scores can objectively compare performance across different schools and demographics, leading to generalizable insights about educational strategies.
2. Precision and Efficiency:
- Statistical methods provide precise numerical results, allowing for accurate comparisons and prediction.
- Large datasets can be analyzed efficiently with the help of computer software, saving time and resources.
Example: A marketing team can use quantitative data analysis to precisely track click-through rates and conversion rates on different ad campaigns, quickly identifying the most effective strategies for maximizing customer engagement.
3. Identification of Patterns and Relationships:
- Statistical techniques reveal hidden patterns and relationships between variables that might not be apparent through observation alone.
- This can lead to new insights and understanding of complex phenomena.
Example: A medical researcher can use quantitative analysis to pinpoint correlations between lifestyle factors and disease risk, aiding in the development of prevention strategies.
1. Limited Scope:
- Quantitative analysis focuses on quantifiable aspects of a phenomenon , potentially overlooking important qualitative nuances, such as emotions, motivations, or cultural contexts.
Example: A survey measuring customer satisfaction with numerical ratings might miss key insights about the underlying reasons for their satisfaction or dissatisfaction, which could be better captured through open-ended feedback.
2. Oversimplification:
- Reducing complex phenomena to numerical data can lead to oversimplification and a loss of richness in understanding.
Example: Analyzing employee productivity solely through quantitative metrics like hours worked or tasks completed might not account for factors like creativity, collaboration, or problem-solving skills, which are crucial for overall performance.
3. Potential for Misinterpretation:
- Statistical results can be misinterpreted if not analyzed carefully and with appropriate expertise.
- The choice of statistical methods and assumptions can significantly influence results.
This blog discusses the steps, methods, and techniques of quantitative data analysis. It also gives insights into the methods of data collection, the type of data one should work with, and the pros and cons of such analysis.
Gain a better understanding of data analysis with these essential reads:
- Data Analysis and Modeling: 4 Critical Differences
- Exploratory Data Analysis Simplified 101
- 25 Best Data Analysis Tools in 2024
Carrying out successful data analysis requires prepping the data and making it analysis-ready. That is where Hevo steps in.
Want to give Hevo a try? Sign Up for a 14-day free trial and experience the feature-rich Hevo suite first hand. You may also have a look at the amazing Hevo price , which will assist you in selecting the best plan for your requirements.
Share your experience of understanding Quantitative Data Analysis in the comment section below! We would love to hear your thoughts.
Ofem is a freelance writer specializing in data-related topics, who has expertise in translating complex concepts. With a focus on data science, analytics, and emerging technologies.
No-code Data Pipeline for your Data Warehouse
- Data Analysis
- Data Warehouse
- Quantitative Data Analysis
Continue Reading
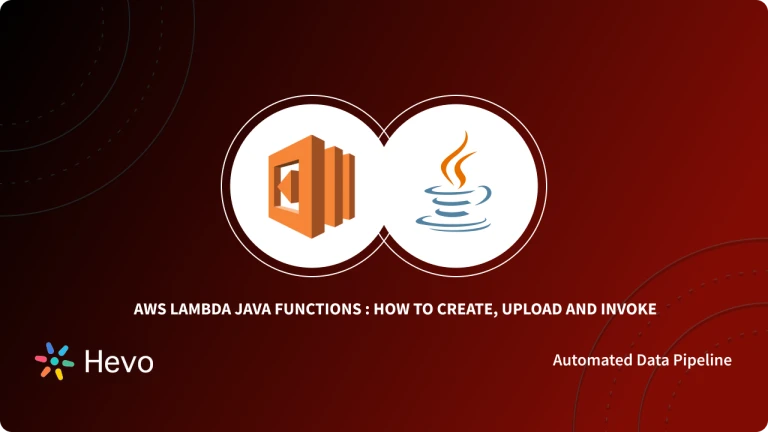
Skand Agrawal
Working With AWS Lambda Java Functions: 6 Easy Steps
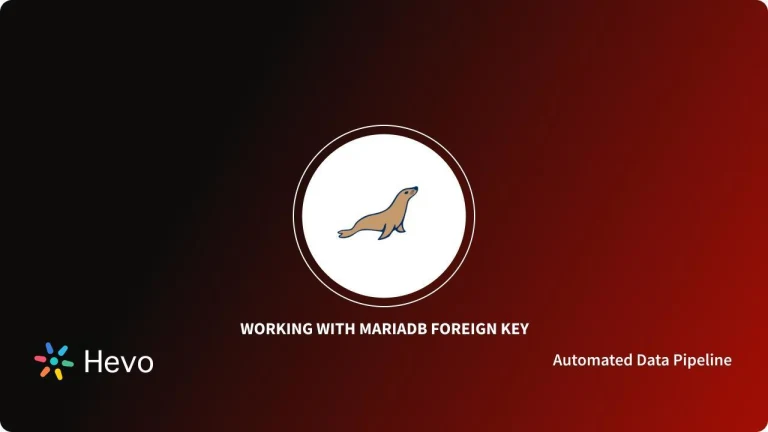
Harsh Varshney
MariaDB Foreign Key: A Comprehensive Guide 101
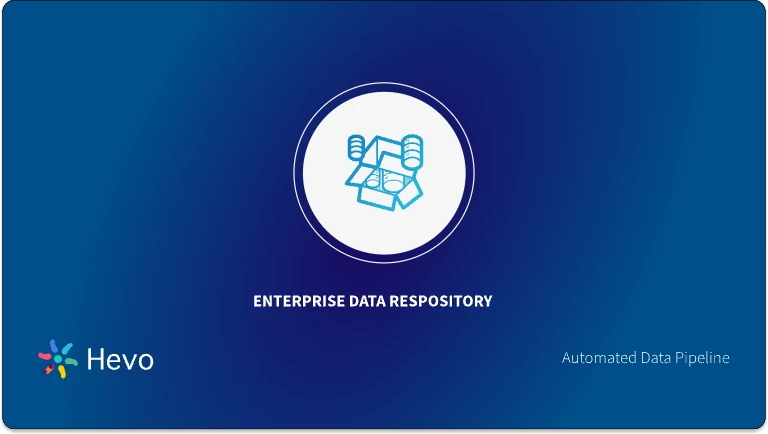
Manisha Sharma
Enterprise Data Repository: Types, Benefits, & Best Practices
I want to read this e-book.
Have a language expert improve your writing
Run a free plagiarism check in 10 minutes, automatically generate references for free.
- Knowledge Base
- Methodology
- What Is Quantitative Research? | Definition & Methods
What Is Quantitative Research? | Definition & Methods
Published on 4 April 2022 by Pritha Bhandari . Revised on 10 October 2022.
Quantitative research is the process of collecting and analysing numerical data. It can be used to find patterns and averages, make predictions, test causal relationships, and generalise results to wider populations.
Quantitative research is the opposite of qualitative research , which involves collecting and analysing non-numerical data (e.g. text, video, or audio).
Quantitative research is widely used in the natural and social sciences: biology, chemistry, psychology, economics, sociology, marketing, etc.
- What is the demographic makeup of Singapore in 2020?
- How has the average temperature changed globally over the last century?
- Does environmental pollution affect the prevalence of honey bees?
- Does working from home increase productivity for people with long commutes?
Table of contents
Quantitative research methods, quantitative data analysis, advantages of quantitative research, disadvantages of quantitative research, frequently asked questions about quantitative research.
You can use quantitative research methods for descriptive, correlational or experimental research.
- In descriptive research , you simply seek an overall summary of your study variables.
- In correlational research , you investigate relationships between your study variables.
- In experimental research , you systematically examine whether there is a cause-and-effect relationship between variables.
Correlational and experimental research can both be used to formally test hypotheses , or predictions, using statistics. The results may be generalised to broader populations based on the sampling method used.
To collect quantitative data, you will often need to use operational definitions that translate abstract concepts (e.g., mood) into observable and quantifiable measures (e.g., self-ratings of feelings and energy levels).
Prevent plagiarism, run a free check.
Once data is collected, you may need to process it before it can be analysed. For example, survey and test data may need to be transformed from words to numbers. Then, you can use statistical analysis to answer your research questions .
Descriptive statistics will give you a summary of your data and include measures of averages and variability. You can also use graphs, scatter plots and frequency tables to visualise your data and check for any trends or outliers.
Using inferential statistics , you can make predictions or generalisations based on your data. You can test your hypothesis or use your sample data to estimate the population parameter .
You can also assess the reliability and validity of your data collection methods to indicate how consistently and accurately your methods actually measured what you wanted them to.
Quantitative research is often used to standardise data collection and generalise findings . Strengths of this approach include:
- Replication
Repeating the study is possible because of standardised data collection protocols and tangible definitions of abstract concepts.
- Direct comparisons of results
The study can be reproduced in other cultural settings, times or with different groups of participants. Results can be compared statistically.
- Large samples
Data from large samples can be processed and analysed using reliable and consistent procedures through quantitative data analysis.
- Hypothesis testing
Using formalised and established hypothesis testing procedures means that you have to carefully consider and report your research variables, predictions, data collection and testing methods before coming to a conclusion.
Despite the benefits of quantitative research, it is sometimes inadequate in explaining complex research topics. Its limitations include:
- Superficiality
Using precise and restrictive operational definitions may inadequately represent complex concepts. For example, the concept of mood may be represented with just a number in quantitative research, but explained with elaboration in qualitative research.
- Narrow focus
Predetermined variables and measurement procedures can mean that you ignore other relevant observations.
- Structural bias
Despite standardised procedures, structural biases can still affect quantitative research. Missing data , imprecise measurements or inappropriate sampling methods are biases that can lead to the wrong conclusions.
- Lack of context
Quantitative research often uses unnatural settings like laboratories or fails to consider historical and cultural contexts that may affect data collection and results.
Quantitative research deals with numbers and statistics, while qualitative research deals with words and meanings.
Quantitative methods allow you to test a hypothesis by systematically collecting and analysing data, while qualitative methods allow you to explore ideas and experiences in depth.
In mixed methods research , you use both qualitative and quantitative data collection and analysis methods to answer your research question .
Data collection is the systematic process by which observations or measurements are gathered in research. It is used in many different contexts by academics, governments, businesses, and other organisations.
Operationalisation means turning abstract conceptual ideas into measurable observations.
For example, the concept of social anxiety isn’t directly observable, but it can be operationally defined in terms of self-rating scores, behavioural avoidance of crowded places, or physical anxiety symptoms in social situations.
Before collecting data , it’s important to consider how you will operationalise the variables that you want to measure.
Reliability and validity are both about how well a method measures something:
- Reliability refers to the consistency of a measure (whether the results can be reproduced under the same conditions).
- Validity refers to the accuracy of a measure (whether the results really do represent what they are supposed to measure).
If you are doing experimental research , you also have to consider the internal and external validity of your experiment.
Hypothesis testing is a formal procedure for investigating our ideas about the world using statistics. It is used by scientists to test specific predictions, called hypotheses , by calculating how likely it is that a pattern or relationship between variables could have arisen by chance.
Cite this Scribbr article
If you want to cite this source, you can copy and paste the citation or click the ‘Cite this Scribbr article’ button to automatically add the citation to our free Reference Generator.
Bhandari, P. (2022, October 10). What Is Quantitative Research? | Definition & Methods. Scribbr. Retrieved 14 May 2024, from https://www.scribbr.co.uk/research-methods/introduction-to-quantitative-research/
Is this article helpful?
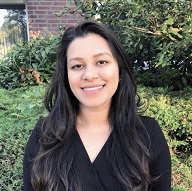
Pritha Bhandari
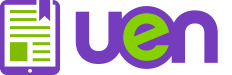
Part II: Data Analysis Methods in Quantitative Research
Data analysis methods in quantitative research.
We started this module with levels of measurement as a way to categorize our data. Data analysis is directed toward answering the original research question and achieving the study purpose (or aim). Now, we are going to delve into two main statistical analyses to describe our data and make inferences about our data:
Descriptive Statistics and Inferential Statistics.
Descriptive Statistics:
Before you panic, we will not be going into statistical analyses very deeply. We want to simply get a good overview of some of the types of general statistical analyses so that it makes some sense to us when we read results in published research articles.
Descriptive statistics summarize or describe the characteristics of a data set. This is a method of simply organizing and describing our data. Why? Because data that are not organized in some fashion are super difficult to interpret.
Let’s say our sample is golden retrievers (population “canines”). Our descriptive statistics tell us more about the same.
- 37% of our sample is male, 43% female
- The mean age is 4 years
- Mode is 6 years
- Median age is 5.5 years
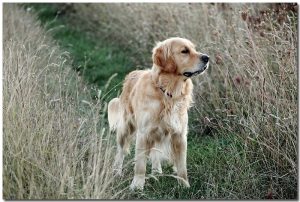
Let’s explore some of the types of descriptive statistics.
Frequency Distributions : A frequency distribution describes the number of observations for each possible value of a measured variable. The numbers are arranged from lowest to highest and features a count of how many times each value occurred.
For example, if 18 students have pet dogs, dog ownership has a frequency of 18.
We might see what other types of pets that students have. Maybe cats, fish, and hamsters. We find that 2 students have hamsters, 9 have fish, 1 has a cat.
You can see that it is very difficult to interpret the various pets into any meaningful interpretation, yes?
Now, let’s take those same pets and place them in a frequency distribution table.
As we can now see, this is much easier to interpret.
Let’s say that we want to know how many books our sample population of students have read in the last year. We collect our data and find this:
We can then take that table and plot it out on a frequency distribution graph. This makes it much easier to see how the numbers are disbursed. Easier on the eyes, yes?
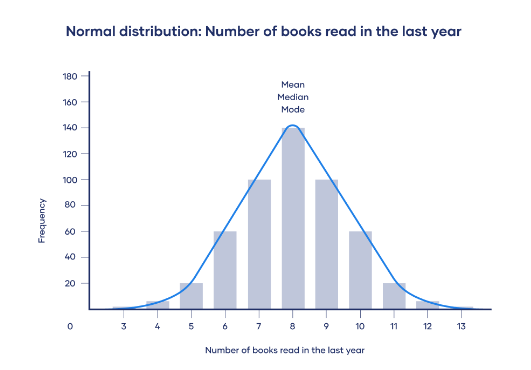
Here’s another example of symmetrical, positive skew, and negative skew:
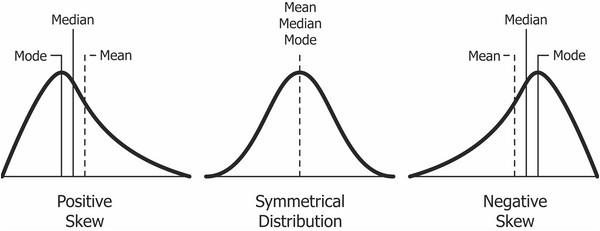
Correlation : Relationships between two research variables are called correlations . Remember, correlation is not cause-and-effect. Correlations simply measure the extent of relationship between two variables. To measure correlation in descriptive statistics, the statistical analysis called Pearson’s correlation coefficient I is often used. You do not need to know how to calculate this for this course. But, do remember that analysis test because you will often see this in published research articles. There really are no set guidelines on what measurement constitutes a “strong” or “weak” correlation, as it really depends on the variables being measured.
However, possible values for correlation coefficients range from -1.00 through .00 to +1.00. A value of +1 means that the two variables are positively correlated, as one variable goes up, the other goes up. A value of r = 0 means that the two variables are not linearly related.
Often, the data will be presented on a scatter plot. Here, we can view the data and there appears to be a straight line (linear) trend between height and weight. The association (or correlation) is positive. That means, that there is a weight increase with height. The Pearson correlation coefficient in this case was r = 0.56.
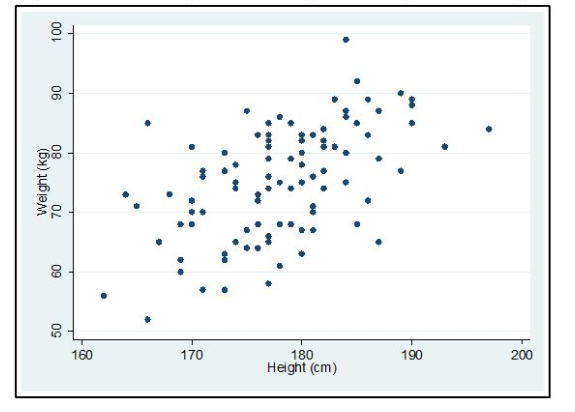
A type I error is made by rejecting a null hypothesis that is true. This means that there was no difference but the researcher concluded that the hypothesis was true.
A type II error is made by accepting that the null hypothesis is true when, in fact, it was false. Meaning there was actually a difference but the researcher did not think their hypothesis was supported.
Hypothesis Testing Procedures : In a general sense, the overall testing of a hypothesis has a systematic methodology. Remember, a hypothesis is an educated guess about the outcome. If we guess wrong, we might set up the tests incorrectly and might get results that are invalid. Sometimes, this is super difficult to get right. The main purpose of statistics is to test a hypothesis.
- Selecting a statistical test. Lots of factors go into this, including levels of measurement of the variables.
- Specifying the level of significance. Usually 0.05 is chosen.
- Computing a test statistic. Lots of software programs to help with this.
- Determining degrees of freedom ( df ). This refers to the number of observations free to vary about a parameter. Computing this is easy (but you don’t need to know how for this course).
- Comparing the test statistic to a theoretical value. Theoretical values exist for all test statistics, which is compared to the study statistics to help establish significance.
Some of the common inferential statistics you will see include:
Comparison tests: Comparison tests look for differences among group means. They can be used to test the effect of a categorical variable on the mean value of some other characteristic.
T-tests are used when comparing the means of precisely two groups (e.g., the average heights of men and women). ANOVA and MANOVA tests are used when comparing the means of more than two groups (e.g., the average heights of children, teenagers, and adults).
- t -tests (compares differences in two groups) – either paired t-test (example: What is the effect of two different test prep programs on the average exam scores for students from the same class?) or independent t-test (example: What is the difference in average exam scores for students from two different schools?)
- analysis of variance (ANOVA, which compares differences in three or more groups) (example: What is the difference in average pain levels among post-surgical patients given three different painkillers?) or MANOVA (compares differences in three or more groups, and 2 or more outcomes) (example: What is the effect of flower species on petal length, petal width, and stem length?)
Correlation tests: Correlation tests check whether variables are related without hypothesizing a cause-and-effect relationship.
- Pearson r (measures the strength and direction of the relationship between two variables) (example: How are latitude and temperature related?)
Nonparametric tests: Non-parametric tests don’t make as many assumptions about the data, and are useful when one or more of the common statistical assumptions are violated. However, the inferences they make aren’t as strong as with parametric tests.
- chi-squared ( X 2 ) test (measures differences in proportions). Chi-square tests are often used to test hypotheses. The chi-square statistic compares the size of any discrepancies between the expected results and the actual results, given the size of the sample and the number of variables in the relationship. For example, the results of tossing a fair coin meet these criteria. We can apply a chi-square test to determine which type of candy is most popular and make sure that our shelves are well stocked. Or maybe you’re a scientist studying the offspring of cats to determine the likelihood of certain genetic traits being passed to a litter of kittens.
Inferential Versus Descriptive Statistics Summary Table
Statistical Significance Versus Clinical Significance
Finally, when it comes to statistical significance in hypothesis testing, the normal probability value in nursing is <0.05. A p=value (probability) is a statistical measurement used to validate a hypothesis against measured data in the study. Meaning, it measures the likelihood that the results were actually observed due to the intervention, or if the results were just due by chance. The p-value, in measuring the probability of obtaining the observed results, assumes the null hypothesis is true.
The lower the p-value, the greater the statistical significance of the observed difference.
In the example earlier about our diabetic patients receiving online diet education, let’s say we had p = 0.05. Would that be a statistically significant result?
If you answered yes, you are correct!
What if our result was p = 0.8?
Not significant. Good job!
That’s pretty straightforward, right? Below 0.05, significant. Over 0.05 not significant.
Could we have significance clinically even if we do not have statistically significant results? Yes. Let’s explore this a bit.
Statistical hypothesis testing provides little information for interpretation purposes. It’s pretty mathematical and we can still get it wrong. Additionally, attaining statistical significance does not really state whether a finding is clinically meaningful. With a large enough sample, even a small very tiny relationship may be statistically significant. But, clinical significance is the practical importance of research. Meaning, we need to ask what the palpable effects may be on the lives of patients or healthcare decisions.
Remember, hypothesis testing cannot prove. It also cannot tell us much other than “yeah, it’s probably likely that there would be some change with this intervention”. Hypothesis testing tells us the likelihood that the outcome was due to an intervention or influence and not just by chance. Also, as nurses and clinicians, we are not concerned with a group of people – we are concerned at the individual, holistic level. The goal of evidence-based practice is to use best evidence for decisions about specific individual needs.
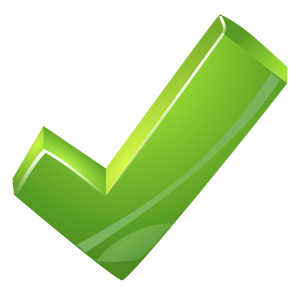
Additionally, begin your Discussion section. What are the implications to practice? Is there little evidence or a lot? Would you recommend additional studies? If so, what type of study would you recommend, and why?
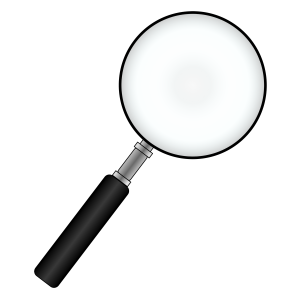
- Were all the important results discussed?
- Did the researchers discuss any study limitations and their possible effects on the credibility of the findings? In discussing limitations, were key threats to the study’s validity and possible biases reviewed? Did the interpretations take limitations into account?
- What types of evidence were offered in support of the interpretation, and was that evidence persuasive? Were results interpreted in light of findings from other studies?
- Did the researchers make any unjustifiable causal inferences? Were alternative explanations for the findings considered? Were the rationales for rejecting these alternatives convincing?
- Did the interpretation consider the precision of the results and/or the magnitude of effects?
- Did the researchers draw any unwarranted conclusions about the generalizability of the results?
- Did the researchers discuss the study’s implications for clinical practice or future nursing research? Did they make specific recommendations?
- If yes, are the stated implications appropriate, given the study’s limitations and the magnitude of the effects as well as evidence from other studies? Are there important implications that the report neglected to include?
- Did the researchers mention or assess clinical significance? Did they make a distinction between statistical and clinical significance?
- If clinical significance was examined, was it assessed in terms of group-level information (e.g., effect sizes) or individual-level results? How was clinical significance operationalized?
References & Attribution
“ Green check mark ” by rawpixel licensed CC0 .
“ Magnifying glass ” by rawpixel licensed CC0
“ Orange flame ” by rawpixel licensed CC0 .
Polit, D. & Beck, C. (2021). Lippincott CoursePoint Enhanced for Polit’s Essentials of Nursing Research (10th ed.). Wolters Kluwer Health
Vaid, N. K. (2019) Statistical performance measures. Medium. https://neeraj-kumar-vaid.medium.com/statistical-performance-measures-12bad66694b7
Evidence-Based Practice & Research Methodologies Copyright © by Tracy Fawns is licensed under a Creative Commons Attribution-NonCommercial-ShareAlike 4.0 International License , except where otherwise noted.
Share This Book
Learn / Guides / Quantitative data analysis guide
Back to guides
The ultimate guide to quantitative data analysis
Numbers help us make sense of the world. We collect quantitative data on our speed and distance as we drive, the number of hours we spend on our cell phones, and how much we save at the grocery store.
Our businesses run on numbers, too. We spend hours poring over key performance indicators (KPIs) like lead-to-client conversions, net profit margins, and bounce and churn rates.
But all of this quantitative data can feel overwhelming and confusing. Lists and spreadsheets of numbers don’t tell you much on their own—you have to conduct quantitative data analysis to understand them and make informed decisions.
Last updated
Reading time.
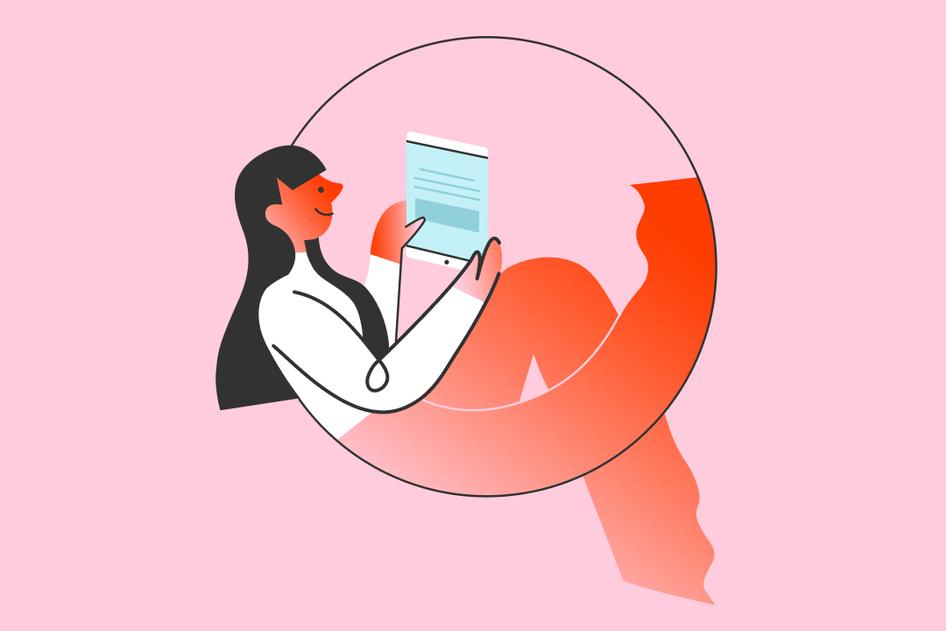
This guide explains what quantitative data analysis is and why it’s important, and gives you a four-step process to conduct a quantitative data analysis, so you know exactly what’s happening in your business and what your users need .
Collect quantitative customer data with Hotjar
Use Hotjar’s tools to gather the customer insights you need to make quantitative data analysis a breeze.
What is quantitative data analysis?
Quantitative data analysis is the process of analyzing and interpreting numerical data. It helps you make sense of information by identifying patterns, trends, and relationships between variables through mathematical calculations and statistical tests.
With quantitative data analysis, you turn spreadsheets of individual data points into meaningful insights to drive informed decisions. Columns of numbers from an experiment or survey transform into useful insights—like which marketing campaign asset your average customer prefers or which website factors are most closely connected to your bounce rate.
Without analytics, data is just noise. Analyzing data helps you make decisions which are informed and free from bias.
What quantitative data analysis is not
But as powerful as quantitative data analysis is, it’s not without its limitations. It only gives you the what, not the why . For example, it can tell you how many website visitors or conversions you have on an average day, but it can’t tell you why users visited your site or made a purchase.
For the why behind user behavior, you need qualitative data analysis , a process for making sense of qualitative research like open-ended survey responses, interview clips, or behavioral observations. By analyzing non-numerical data, you gain useful contextual insights to shape your strategy, product, and messaging.
Quantitative data analysis vs. qualitative data analysis
Let’s take an even deeper dive into the differences between quantitative data analysis and qualitative data analysis to explore what they do and when you need them.
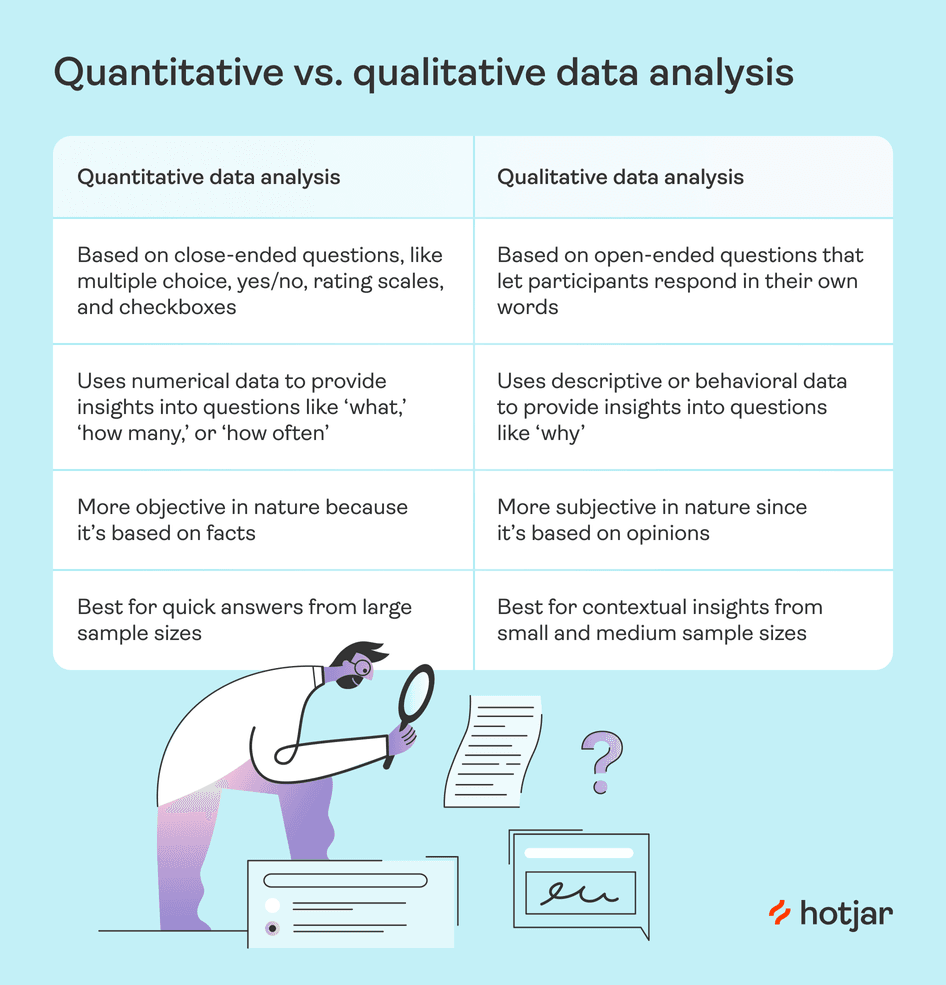
The bottom line: quantitative data analysis and qualitative data analysis are complementary processes. They work hand-in-hand to tell you what’s happening in your business and why.
💡 Pro tip: easily toggle between quantitative and qualitative data analysis with Hotjar Funnels .
The Funnels tool helps you visualize quantitative metrics like drop-off and conversion rates in your sales or conversion funnel to understand when and where users leave your website. You can break down your data even further to compare conversion performance by user segment.
Spot a potential issue? A single click takes you to relevant session recordings , where you see user behaviors like mouse movements, scrolls, and clicks. With this qualitative data to provide context, you'll better understand what you need to optimize to streamline the user experience (UX) and increase conversions .
Hotjar Funnels lets you quickly explore the story behind the quantitative data
4 benefits of quantitative data analysis
There’s a reason product, web design, and marketing teams take time to analyze metrics: the process pays off big time.
Four major benefits of quantitative data analysis include:
1. Make confident decisions
With quantitative data analysis, you know you’ve got data-driven insights to back up your decisions . For example, if you launch a concept testing survey to gauge user reactions to a new logo design, and 92% of users rate it ‘very good’—you'll feel certain when you give the designer the green light.
Since you’re relying less on intuition and more on facts, you reduce the risks of making the wrong decision. (You’ll also find it way easier to get buy-in from team members and stakeholders for your next proposed project. 🙌)
2. Reduce costs
By crunching the numbers, you can spot opportunities to reduce spend . For example, if an ad campaign has lower-than-average click-through rates , you might decide to cut your losses and invest your budget elsewhere.
Or, by analyzing ecommerce metrics , like website traffic by source, you may find you’re getting very little return on investment from a certain social media channel—and scale back spending in that area.
3. Personalize the user experience
Quantitative data analysis helps you map the customer journey , so you get a better sense of customers’ demographics, what page elements they interact with on your site, and where they drop off or convert .
These insights let you better personalize your website, product, or communication, so you can segment ads, emails, and website content for specific user personas or target groups.
4. Improve user satisfaction and delight
Quantitative data analysis lets you see where your website or product is doing well—and where it falls short for your users . For example, you might see stellar results from KPIs like time on page, but conversion rates for that page are low.
These quantitative insights encourage you to dive deeper into qualitative data to see why that’s happening—looking for moments of confusion or frustration on session recordings, for example—so you can make adjustments and optimize your conversions by improving customer satisfaction and delight.
💡Pro tip: use Net Promoter Score® (NPS) surveys to capture quantifiable customer satisfaction data that’s easy for you to analyze and interpret.
With an NPS tool like Hotjar, you can create an on-page survey to ask users how likely they are to recommend you to others on a scale from 0 to 10. (And for added context, you can ask follow-up questions about why customers selected the rating they did—rich qualitative data is always a bonus!)
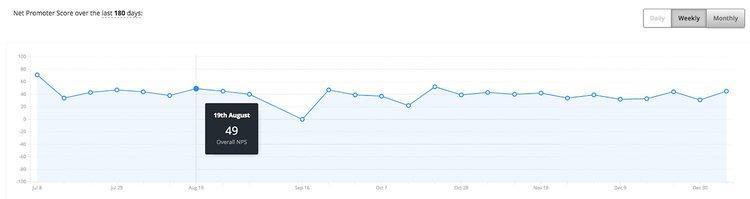
Hotjar graphs your quantitative NPS data to show changes over time
4 steps to effective quantitative data analysis
Quantitative data analysis sounds way more intimidating than it actually is. Here’s how to make sense of your company’s numbers in just four steps:
1. Collect data
Before you can actually start the analysis process, you need data to analyze. This involves conducting quantitative research and collecting numerical data from various sources, including:
Interviews or focus groups
Website analytics
Observations, from tools like heatmaps or session recordings
Questionnaires, like surveys or on-page feedback widgets
Just ensure the questions you ask in your surveys are close-ended questions—providing respondents with select choices to choose from instead of open-ended questions that allow for free responses.
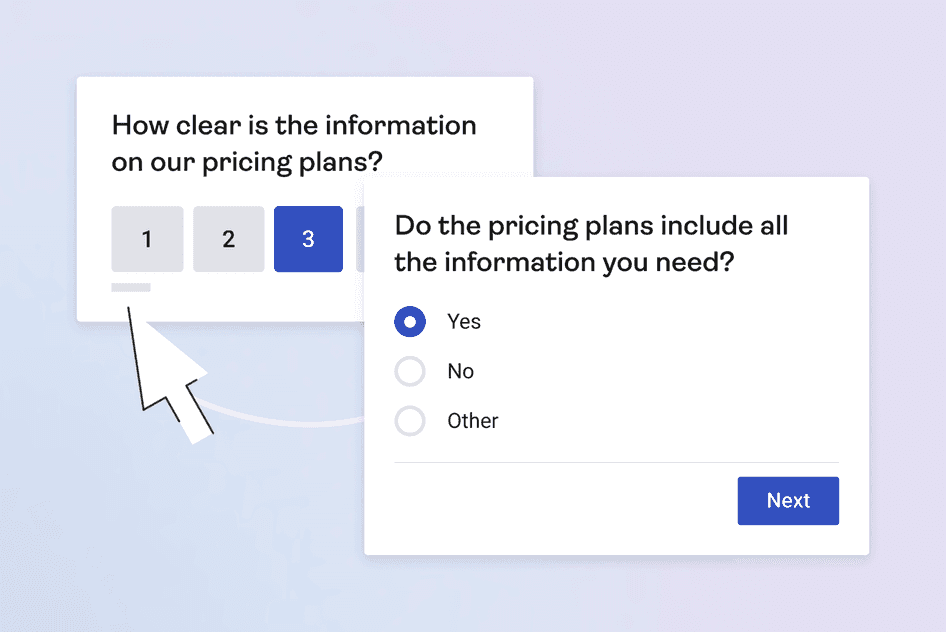
Hotjar’s pricing plans survey template provides close-ended questions
2. Clean data
Once you’ve collected your data, it’s time to clean it up. Look through your results to find errors, duplicates, and omissions. Keep an eye out for outliers, too. Outliers are data points that differ significantly from the rest of the set—and they can skew your results if you don’t remove them.
By taking the time to clean your data set, you ensure your data is accurate, consistent, and relevant before it’s time to analyze.
3. Analyze and interpret data
At this point, your data’s all cleaned up and ready for the main event. This step involves crunching the numbers to find patterns and trends via mathematical and statistical methods.
Two main branches of quantitative data analysis exist:
Descriptive analysis : methods to summarize or describe attributes of your data set. For example, you may calculate key stats like distribution and frequency, or mean, median, and mode.
Inferential analysis : methods that let you draw conclusions from statistics—like analyzing the relationship between variables or making predictions. These methods include t-tests, cross-tabulation, and factor analysis. (For more detailed explanations and how-tos, head to our guide on quantitative data analysis methods.)
Then, interpret your data to determine the best course of action. What does the data suggest you do ? For example, if your analysis shows a strong correlation between email open rate and time sent, you may explore optimal send times for each user segment.
4. Visualize and share data
Once you’ve analyzed and interpreted your data, create easy-to-read, engaging data visualizations—like charts, graphs, and tables—to present your results to team members and stakeholders. Data visualizations highlight similarities and differences between data sets and show the relationships between variables.
Software can do this part for you. For example, the Hotjar Dashboard shows all of your key metrics in one place—and automatically creates bar graphs to show how your top pages’ performance compares. And with just one click, you can navigate to the Trends tool to analyze product metrics for different segments on a single chart.
Hotjar Trends lets you compare metrics across segments
Discover rich user insights with quantitative data analysis
Conducting quantitative data analysis takes a little bit of time and know-how, but it’s much more manageable than you might think.
By choosing the right methods and following clear steps, you gain insights into product performance and customer experience —and you’ll be well on your way to making better decisions and creating more customer satisfaction and loyalty.
FAQs about quantitative data analysis
What is quantitative data analysis.
Quantitative data analysis is the process of making sense of numerical data through mathematical calculations and statistical tests. It helps you identify patterns, relationships, and trends to make better decisions.
How is quantitative data analysis different from qualitative data analysis?
Quantitative and qualitative data analysis are both essential processes for making sense of quantitative and qualitative research .
Quantitative data analysis helps you summarize and interpret numerical results from close-ended questions to understand what is happening. Qualitative data analysis helps you summarize and interpret non-numerical results, like opinions or behavior, to understand why the numbers look like they do.
If you want to make strong data-driven decisions, you need both.
What are some benefits of quantitative data analysis?
Quantitative data analysis turns numbers into rich insights. Some benefits of this process include:
Making more confident decisions
Identifying ways to cut costs
Personalizing the user experience
Improving customer satisfaction
What methods can I use to analyze quantitative data?
Quantitative data analysis has two branches: descriptive statistics and inferential statistics.
Descriptive statistics provide a snapshot of the data’s features by calculating measures like mean, median, and mode.
Inferential statistics , as the name implies, involves making inferences about what the data means. Dozens of methods exist for this branch of quantitative data analysis, but three commonly used techniques are:
Cross tabulation
Factor analysis

An official website of the United States government
The .gov means it’s official. Federal government websites often end in .gov or .mil. Before sharing sensitive information, make sure you’re on a federal government site.
The site is secure. The https:// ensures that you are connecting to the official website and that any information you provide is encrypted and transmitted securely.
- Publications
- Account settings
Preview improvements coming to the PMC website in October 2024. Learn More or Try it out now .
- Advanced Search
- Journal List
- J Korean Med Sci
- v.37(16); 2022 Apr 25

A Practical Guide to Writing Quantitative and Qualitative Research Questions and Hypotheses in Scholarly Articles
Edward barroga.
1 Department of General Education, Graduate School of Nursing Science, St. Luke’s International University, Tokyo, Japan.
Glafera Janet Matanguihan
2 Department of Biological Sciences, Messiah University, Mechanicsburg, PA, USA.
The development of research questions and the subsequent hypotheses are prerequisites to defining the main research purpose and specific objectives of a study. Consequently, these objectives determine the study design and research outcome. The development of research questions is a process based on knowledge of current trends, cutting-edge studies, and technological advances in the research field. Excellent research questions are focused and require a comprehensive literature search and in-depth understanding of the problem being investigated. Initially, research questions may be written as descriptive questions which could be developed into inferential questions. These questions must be specific and concise to provide a clear foundation for developing hypotheses. Hypotheses are more formal predictions about the research outcomes. These specify the possible results that may or may not be expected regarding the relationship between groups. Thus, research questions and hypotheses clarify the main purpose and specific objectives of the study, which in turn dictate the design of the study, its direction, and outcome. Studies developed from good research questions and hypotheses will have trustworthy outcomes with wide-ranging social and health implications.
INTRODUCTION
Scientific research is usually initiated by posing evidenced-based research questions which are then explicitly restated as hypotheses. 1 , 2 The hypotheses provide directions to guide the study, solutions, explanations, and expected results. 3 , 4 Both research questions and hypotheses are essentially formulated based on conventional theories and real-world processes, which allow the inception of novel studies and the ethical testing of ideas. 5 , 6
It is crucial to have knowledge of both quantitative and qualitative research 2 as both types of research involve writing research questions and hypotheses. 7 However, these crucial elements of research are sometimes overlooked; if not overlooked, then framed without the forethought and meticulous attention it needs. Planning and careful consideration are needed when developing quantitative or qualitative research, particularly when conceptualizing research questions and hypotheses. 4
There is a continuing need to support researchers in the creation of innovative research questions and hypotheses, as well as for journal articles that carefully review these elements. 1 When research questions and hypotheses are not carefully thought of, unethical studies and poor outcomes usually ensue. Carefully formulated research questions and hypotheses define well-founded objectives, which in turn determine the appropriate design, course, and outcome of the study. This article then aims to discuss in detail the various aspects of crafting research questions and hypotheses, with the goal of guiding researchers as they develop their own. Examples from the authors and peer-reviewed scientific articles in the healthcare field are provided to illustrate key points.
DEFINITIONS AND RELATIONSHIP OF RESEARCH QUESTIONS AND HYPOTHESES
A research question is what a study aims to answer after data analysis and interpretation. The answer is written in length in the discussion section of the paper. Thus, the research question gives a preview of the different parts and variables of the study meant to address the problem posed in the research question. 1 An excellent research question clarifies the research writing while facilitating understanding of the research topic, objective, scope, and limitations of the study. 5
On the other hand, a research hypothesis is an educated statement of an expected outcome. This statement is based on background research and current knowledge. 8 , 9 The research hypothesis makes a specific prediction about a new phenomenon 10 or a formal statement on the expected relationship between an independent variable and a dependent variable. 3 , 11 It provides a tentative answer to the research question to be tested or explored. 4
Hypotheses employ reasoning to predict a theory-based outcome. 10 These can also be developed from theories by focusing on components of theories that have not yet been observed. 10 The validity of hypotheses is often based on the testability of the prediction made in a reproducible experiment. 8
Conversely, hypotheses can also be rephrased as research questions. Several hypotheses based on existing theories and knowledge may be needed to answer a research question. Developing ethical research questions and hypotheses creates a research design that has logical relationships among variables. These relationships serve as a solid foundation for the conduct of the study. 4 , 11 Haphazardly constructed research questions can result in poorly formulated hypotheses and improper study designs, leading to unreliable results. Thus, the formulations of relevant research questions and verifiable hypotheses are crucial when beginning research. 12
CHARACTERISTICS OF GOOD RESEARCH QUESTIONS AND HYPOTHESES
Excellent research questions are specific and focused. These integrate collective data and observations to confirm or refute the subsequent hypotheses. Well-constructed hypotheses are based on previous reports and verify the research context. These are realistic, in-depth, sufficiently complex, and reproducible. More importantly, these hypotheses can be addressed and tested. 13
There are several characteristics of well-developed hypotheses. Good hypotheses are 1) empirically testable 7 , 10 , 11 , 13 ; 2) backed by preliminary evidence 9 ; 3) testable by ethical research 7 , 9 ; 4) based on original ideas 9 ; 5) have evidenced-based logical reasoning 10 ; and 6) can be predicted. 11 Good hypotheses can infer ethical and positive implications, indicating the presence of a relationship or effect relevant to the research theme. 7 , 11 These are initially developed from a general theory and branch into specific hypotheses by deductive reasoning. In the absence of a theory to base the hypotheses, inductive reasoning based on specific observations or findings form more general hypotheses. 10
TYPES OF RESEARCH QUESTIONS AND HYPOTHESES
Research questions and hypotheses are developed according to the type of research, which can be broadly classified into quantitative and qualitative research. We provide a summary of the types of research questions and hypotheses under quantitative and qualitative research categories in Table 1 .
Research questions in quantitative research
In quantitative research, research questions inquire about the relationships among variables being investigated and are usually framed at the start of the study. These are precise and typically linked to the subject population, dependent and independent variables, and research design. 1 Research questions may also attempt to describe the behavior of a population in relation to one or more variables, or describe the characteristics of variables to be measured ( descriptive research questions ). 1 , 5 , 14 These questions may also aim to discover differences between groups within the context of an outcome variable ( comparative research questions ), 1 , 5 , 14 or elucidate trends and interactions among variables ( relationship research questions ). 1 , 5 We provide examples of descriptive, comparative, and relationship research questions in quantitative research in Table 2 .
Hypotheses in quantitative research
In quantitative research, hypotheses predict the expected relationships among variables. 15 Relationships among variables that can be predicted include 1) between a single dependent variable and a single independent variable ( simple hypothesis ) or 2) between two or more independent and dependent variables ( complex hypothesis ). 4 , 11 Hypotheses may also specify the expected direction to be followed and imply an intellectual commitment to a particular outcome ( directional hypothesis ) 4 . On the other hand, hypotheses may not predict the exact direction and are used in the absence of a theory, or when findings contradict previous studies ( non-directional hypothesis ). 4 In addition, hypotheses can 1) define interdependency between variables ( associative hypothesis ), 4 2) propose an effect on the dependent variable from manipulation of the independent variable ( causal hypothesis ), 4 3) state a negative relationship between two variables ( null hypothesis ), 4 , 11 , 15 4) replace the working hypothesis if rejected ( alternative hypothesis ), 15 explain the relationship of phenomena to possibly generate a theory ( working hypothesis ), 11 5) involve quantifiable variables that can be tested statistically ( statistical hypothesis ), 11 6) or express a relationship whose interlinks can be verified logically ( logical hypothesis ). 11 We provide examples of simple, complex, directional, non-directional, associative, causal, null, alternative, working, statistical, and logical hypotheses in quantitative research, as well as the definition of quantitative hypothesis-testing research in Table 3 .
Research questions in qualitative research
Unlike research questions in quantitative research, research questions in qualitative research are usually continuously reviewed and reformulated. The central question and associated subquestions are stated more than the hypotheses. 15 The central question broadly explores a complex set of factors surrounding the central phenomenon, aiming to present the varied perspectives of participants. 15
There are varied goals for which qualitative research questions are developed. These questions can function in several ways, such as to 1) identify and describe existing conditions ( contextual research question s); 2) describe a phenomenon ( descriptive research questions ); 3) assess the effectiveness of existing methods, protocols, theories, or procedures ( evaluation research questions ); 4) examine a phenomenon or analyze the reasons or relationships between subjects or phenomena ( explanatory research questions ); or 5) focus on unknown aspects of a particular topic ( exploratory research questions ). 5 In addition, some qualitative research questions provide new ideas for the development of theories and actions ( generative research questions ) or advance specific ideologies of a position ( ideological research questions ). 1 Other qualitative research questions may build on a body of existing literature and become working guidelines ( ethnographic research questions ). Research questions may also be broadly stated without specific reference to the existing literature or a typology of questions ( phenomenological research questions ), may be directed towards generating a theory of some process ( grounded theory questions ), or may address a description of the case and the emerging themes ( qualitative case study questions ). 15 We provide examples of contextual, descriptive, evaluation, explanatory, exploratory, generative, ideological, ethnographic, phenomenological, grounded theory, and qualitative case study research questions in qualitative research in Table 4 , and the definition of qualitative hypothesis-generating research in Table 5 .
Qualitative studies usually pose at least one central research question and several subquestions starting with How or What . These research questions use exploratory verbs such as explore or describe . These also focus on one central phenomenon of interest, and may mention the participants and research site. 15
Hypotheses in qualitative research
Hypotheses in qualitative research are stated in the form of a clear statement concerning the problem to be investigated. Unlike in quantitative research where hypotheses are usually developed to be tested, qualitative research can lead to both hypothesis-testing and hypothesis-generating outcomes. 2 When studies require both quantitative and qualitative research questions, this suggests an integrative process between both research methods wherein a single mixed-methods research question can be developed. 1
FRAMEWORKS FOR DEVELOPING RESEARCH QUESTIONS AND HYPOTHESES
Research questions followed by hypotheses should be developed before the start of the study. 1 , 12 , 14 It is crucial to develop feasible research questions on a topic that is interesting to both the researcher and the scientific community. This can be achieved by a meticulous review of previous and current studies to establish a novel topic. Specific areas are subsequently focused on to generate ethical research questions. The relevance of the research questions is evaluated in terms of clarity of the resulting data, specificity of the methodology, objectivity of the outcome, depth of the research, and impact of the study. 1 , 5 These aspects constitute the FINER criteria (i.e., Feasible, Interesting, Novel, Ethical, and Relevant). 1 Clarity and effectiveness are achieved if research questions meet the FINER criteria. In addition to the FINER criteria, Ratan et al. described focus, complexity, novelty, feasibility, and measurability for evaluating the effectiveness of research questions. 14
The PICOT and PEO frameworks are also used when developing research questions. 1 The following elements are addressed in these frameworks, PICOT: P-population/patients/problem, I-intervention or indicator being studied, C-comparison group, O-outcome of interest, and T-timeframe of the study; PEO: P-population being studied, E-exposure to preexisting conditions, and O-outcome of interest. 1 Research questions are also considered good if these meet the “FINERMAPS” framework: Feasible, Interesting, Novel, Ethical, Relevant, Manageable, Appropriate, Potential value/publishable, and Systematic. 14
As we indicated earlier, research questions and hypotheses that are not carefully formulated result in unethical studies or poor outcomes. To illustrate this, we provide some examples of ambiguous research question and hypotheses that result in unclear and weak research objectives in quantitative research ( Table 6 ) 16 and qualitative research ( Table 7 ) 17 , and how to transform these ambiguous research question(s) and hypothesis(es) into clear and good statements.
a These statements were composed for comparison and illustrative purposes only.
b These statements are direct quotes from Higashihara and Horiuchi. 16
a This statement is a direct quote from Shimoda et al. 17
The other statements were composed for comparison and illustrative purposes only.
CONSTRUCTING RESEARCH QUESTIONS AND HYPOTHESES
To construct effective research questions and hypotheses, it is very important to 1) clarify the background and 2) identify the research problem at the outset of the research, within a specific timeframe. 9 Then, 3) review or conduct preliminary research to collect all available knowledge about the possible research questions by studying theories and previous studies. 18 Afterwards, 4) construct research questions to investigate the research problem. Identify variables to be accessed from the research questions 4 and make operational definitions of constructs from the research problem and questions. Thereafter, 5) construct specific deductive or inductive predictions in the form of hypotheses. 4 Finally, 6) state the study aims . This general flow for constructing effective research questions and hypotheses prior to conducting research is shown in Fig. 1 .

Research questions are used more frequently in qualitative research than objectives or hypotheses. 3 These questions seek to discover, understand, explore or describe experiences by asking “What” or “How.” The questions are open-ended to elicit a description rather than to relate variables or compare groups. The questions are continually reviewed, reformulated, and changed during the qualitative study. 3 Research questions are also used more frequently in survey projects than hypotheses in experiments in quantitative research to compare variables and their relationships.
Hypotheses are constructed based on the variables identified and as an if-then statement, following the template, ‘If a specific action is taken, then a certain outcome is expected.’ At this stage, some ideas regarding expectations from the research to be conducted must be drawn. 18 Then, the variables to be manipulated (independent) and influenced (dependent) are defined. 4 Thereafter, the hypothesis is stated and refined, and reproducible data tailored to the hypothesis are identified, collected, and analyzed. 4 The hypotheses must be testable and specific, 18 and should describe the variables and their relationships, the specific group being studied, and the predicted research outcome. 18 Hypotheses construction involves a testable proposition to be deduced from theory, and independent and dependent variables to be separated and measured separately. 3 Therefore, good hypotheses must be based on good research questions constructed at the start of a study or trial. 12
In summary, research questions are constructed after establishing the background of the study. Hypotheses are then developed based on the research questions. Thus, it is crucial to have excellent research questions to generate superior hypotheses. In turn, these would determine the research objectives and the design of the study, and ultimately, the outcome of the research. 12 Algorithms for building research questions and hypotheses are shown in Fig. 2 for quantitative research and in Fig. 3 for qualitative research.

EXAMPLES OF RESEARCH QUESTIONS FROM PUBLISHED ARTICLES
- EXAMPLE 1. Descriptive research question (quantitative research)
- - Presents research variables to be assessed (distinct phenotypes and subphenotypes)
- “BACKGROUND: Since COVID-19 was identified, its clinical and biological heterogeneity has been recognized. Identifying COVID-19 phenotypes might help guide basic, clinical, and translational research efforts.
- RESEARCH QUESTION: Does the clinical spectrum of patients with COVID-19 contain distinct phenotypes and subphenotypes? ” 19
- EXAMPLE 2. Relationship research question (quantitative research)
- - Shows interactions between dependent variable (static postural control) and independent variable (peripheral visual field loss)
- “Background: Integration of visual, vestibular, and proprioceptive sensations contributes to postural control. People with peripheral visual field loss have serious postural instability. However, the directional specificity of postural stability and sensory reweighting caused by gradual peripheral visual field loss remain unclear.
- Research question: What are the effects of peripheral visual field loss on static postural control ?” 20
- EXAMPLE 3. Comparative research question (quantitative research)
- - Clarifies the difference among groups with an outcome variable (patients enrolled in COMPERA with moderate PH or severe PH in COPD) and another group without the outcome variable (patients with idiopathic pulmonary arterial hypertension (IPAH))
- “BACKGROUND: Pulmonary hypertension (PH) in COPD is a poorly investigated clinical condition.
- RESEARCH QUESTION: Which factors determine the outcome of PH in COPD?
- STUDY DESIGN AND METHODS: We analyzed the characteristics and outcome of patients enrolled in the Comparative, Prospective Registry of Newly Initiated Therapies for Pulmonary Hypertension (COMPERA) with moderate or severe PH in COPD as defined during the 6th PH World Symposium who received medical therapy for PH and compared them with patients with idiopathic pulmonary arterial hypertension (IPAH) .” 21
- EXAMPLE 4. Exploratory research question (qualitative research)
- - Explores areas that have not been fully investigated (perspectives of families and children who receive care in clinic-based child obesity treatment) to have a deeper understanding of the research problem
- “Problem: Interventions for children with obesity lead to only modest improvements in BMI and long-term outcomes, and data are limited on the perspectives of families of children with obesity in clinic-based treatment. This scoping review seeks to answer the question: What is known about the perspectives of families and children who receive care in clinic-based child obesity treatment? This review aims to explore the scope of perspectives reported by families of children with obesity who have received individualized outpatient clinic-based obesity treatment.” 22
- EXAMPLE 5. Relationship research question (quantitative research)
- - Defines interactions between dependent variable (use of ankle strategies) and independent variable (changes in muscle tone)
- “Background: To maintain an upright standing posture against external disturbances, the human body mainly employs two types of postural control strategies: “ankle strategy” and “hip strategy.” While it has been reported that the magnitude of the disturbance alters the use of postural control strategies, it has not been elucidated how the level of muscle tone, one of the crucial parameters of bodily function, determines the use of each strategy. We have previously confirmed using forward dynamics simulations of human musculoskeletal models that an increased muscle tone promotes the use of ankle strategies. The objective of the present study was to experimentally evaluate a hypothesis: an increased muscle tone promotes the use of ankle strategies. Research question: Do changes in the muscle tone affect the use of ankle strategies ?” 23
EXAMPLES OF HYPOTHESES IN PUBLISHED ARTICLES
- EXAMPLE 1. Working hypothesis (quantitative research)
- - A hypothesis that is initially accepted for further research to produce a feasible theory
- “As fever may have benefit in shortening the duration of viral illness, it is plausible to hypothesize that the antipyretic efficacy of ibuprofen may be hindering the benefits of a fever response when taken during the early stages of COVID-19 illness .” 24
- “In conclusion, it is plausible to hypothesize that the antipyretic efficacy of ibuprofen may be hindering the benefits of a fever response . The difference in perceived safety of these agents in COVID-19 illness could be related to the more potent efficacy to reduce fever with ibuprofen compared to acetaminophen. Compelling data on the benefit of fever warrant further research and review to determine when to treat or withhold ibuprofen for early stage fever for COVID-19 and other related viral illnesses .” 24
- EXAMPLE 2. Exploratory hypothesis (qualitative research)
- - Explores particular areas deeper to clarify subjective experience and develop a formal hypothesis potentially testable in a future quantitative approach
- “We hypothesized that when thinking about a past experience of help-seeking, a self distancing prompt would cause increased help-seeking intentions and more favorable help-seeking outcome expectations .” 25
- “Conclusion
- Although a priori hypotheses were not supported, further research is warranted as results indicate the potential for using self-distancing approaches to increasing help-seeking among some people with depressive symptomatology.” 25
- EXAMPLE 3. Hypothesis-generating research to establish a framework for hypothesis testing (qualitative research)
- “We hypothesize that compassionate care is beneficial for patients (better outcomes), healthcare systems and payers (lower costs), and healthcare providers (lower burnout). ” 26
- Compassionomics is the branch of knowledge and scientific study of the effects of compassionate healthcare. Our main hypotheses are that compassionate healthcare is beneficial for (1) patients, by improving clinical outcomes, (2) healthcare systems and payers, by supporting financial sustainability, and (3) HCPs, by lowering burnout and promoting resilience and well-being. The purpose of this paper is to establish a scientific framework for testing the hypotheses above . If these hypotheses are confirmed through rigorous research, compassionomics will belong in the science of evidence-based medicine, with major implications for all healthcare domains.” 26
- EXAMPLE 4. Statistical hypothesis (quantitative research)
- - An assumption is made about the relationship among several population characteristics ( gender differences in sociodemographic and clinical characteristics of adults with ADHD ). Validity is tested by statistical experiment or analysis ( chi-square test, Students t-test, and logistic regression analysis)
- “Our research investigated gender differences in sociodemographic and clinical characteristics of adults with ADHD in a Japanese clinical sample. Due to unique Japanese cultural ideals and expectations of women's behavior that are in opposition to ADHD symptoms, we hypothesized that women with ADHD experience more difficulties and present more dysfunctions than men . We tested the following hypotheses: first, women with ADHD have more comorbidities than men with ADHD; second, women with ADHD experience more social hardships than men, such as having less full-time employment and being more likely to be divorced.” 27
- “Statistical Analysis
- ( text omitted ) Between-gender comparisons were made using the chi-squared test for categorical variables and Students t-test for continuous variables…( text omitted ). A logistic regression analysis was performed for employment status, marital status, and comorbidity to evaluate the independent effects of gender on these dependent variables.” 27
EXAMPLES OF HYPOTHESIS AS WRITTEN IN PUBLISHED ARTICLES IN RELATION TO OTHER PARTS
- EXAMPLE 1. Background, hypotheses, and aims are provided
- “Pregnant women need skilled care during pregnancy and childbirth, but that skilled care is often delayed in some countries …( text omitted ). The focused antenatal care (FANC) model of WHO recommends that nurses provide information or counseling to all pregnant women …( text omitted ). Job aids are visual support materials that provide the right kind of information using graphics and words in a simple and yet effective manner. When nurses are not highly trained or have many work details to attend to, these job aids can serve as a content reminder for the nurses and can be used for educating their patients (Jennings, Yebadokpo, Affo, & Agbogbe, 2010) ( text omitted ). Importantly, additional evidence is needed to confirm how job aids can further improve the quality of ANC counseling by health workers in maternal care …( text omitted )” 28
- “ This has led us to hypothesize that the quality of ANC counseling would be better if supported by job aids. Consequently, a better quality of ANC counseling is expected to produce higher levels of awareness concerning the danger signs of pregnancy and a more favorable impression of the caring behavior of nurses .” 28
- “This study aimed to examine the differences in the responses of pregnant women to a job aid-supported intervention during ANC visit in terms of 1) their understanding of the danger signs of pregnancy and 2) their impression of the caring behaviors of nurses to pregnant women in rural Tanzania.” 28
- EXAMPLE 2. Background, hypotheses, and aims are provided
- “We conducted a two-arm randomized controlled trial (RCT) to evaluate and compare changes in salivary cortisol and oxytocin levels of first-time pregnant women between experimental and control groups. The women in the experimental group touched and held an infant for 30 min (experimental intervention protocol), whereas those in the control group watched a DVD movie of an infant (control intervention protocol). The primary outcome was salivary cortisol level and the secondary outcome was salivary oxytocin level.” 29
- “ We hypothesize that at 30 min after touching and holding an infant, the salivary cortisol level will significantly decrease and the salivary oxytocin level will increase in the experimental group compared with the control group .” 29
- EXAMPLE 3. Background, aim, and hypothesis are provided
- “In countries where the maternal mortality ratio remains high, antenatal education to increase Birth Preparedness and Complication Readiness (BPCR) is considered one of the top priorities [1]. BPCR includes birth plans during the antenatal period, such as the birthplace, birth attendant, transportation, health facility for complications, expenses, and birth materials, as well as family coordination to achieve such birth plans. In Tanzania, although increasing, only about half of all pregnant women attend an antenatal clinic more than four times [4]. Moreover, the information provided during antenatal care (ANC) is insufficient. In the resource-poor settings, antenatal group education is a potential approach because of the limited time for individual counseling at antenatal clinics.” 30
- “This study aimed to evaluate an antenatal group education program among pregnant women and their families with respect to birth-preparedness and maternal and infant outcomes in rural villages of Tanzania.” 30
- “ The study hypothesis was if Tanzanian pregnant women and their families received a family-oriented antenatal group education, they would (1) have a higher level of BPCR, (2) attend antenatal clinic four or more times, (3) give birth in a health facility, (4) have less complications of women at birth, and (5) have less complications and deaths of infants than those who did not receive the education .” 30
Research questions and hypotheses are crucial components to any type of research, whether quantitative or qualitative. These questions should be developed at the very beginning of the study. Excellent research questions lead to superior hypotheses, which, like a compass, set the direction of research, and can often determine the successful conduct of the study. Many research studies have floundered because the development of research questions and subsequent hypotheses was not given the thought and meticulous attention needed. The development of research questions and hypotheses is an iterative process based on extensive knowledge of the literature and insightful grasp of the knowledge gap. Focused, concise, and specific research questions provide a strong foundation for constructing hypotheses which serve as formal predictions about the research outcomes. Research questions and hypotheses are crucial elements of research that should not be overlooked. They should be carefully thought of and constructed when planning research. This avoids unethical studies and poor outcomes by defining well-founded objectives that determine the design, course, and outcome of the study.
Disclosure: The authors have no potential conflicts of interest to disclose.
Author Contributions:
- Conceptualization: Barroga E, Matanguihan GJ.
- Methodology: Barroga E, Matanguihan GJ.
- Writing - original draft: Barroga E, Matanguihan GJ.
- Writing - review & editing: Barroga E, Matanguihan GJ.
- USC Libraries
- Research Guides
Organizing Your Social Sciences Research Paper
- Quantitative Methods
- Purpose of Guide
- Design Flaws to Avoid
- Independent and Dependent Variables
- Glossary of Research Terms
- Reading Research Effectively
- Narrowing a Topic Idea
- Broadening a Topic Idea
- Extending the Timeliness of a Topic Idea
- Academic Writing Style
- Applying Critical Thinking
- Choosing a Title
- Making an Outline
- Paragraph Development
- Research Process Video Series
- Executive Summary
- The C.A.R.S. Model
- Background Information
- The Research Problem/Question
- Theoretical Framework
- Citation Tracking
- Content Alert Services
- Evaluating Sources
- Primary Sources
- Secondary Sources
- Tiertiary Sources
- Scholarly vs. Popular Publications
- Qualitative Methods
- Insiderness
- Using Non-Textual Elements
- Limitations of the Study
- Common Grammar Mistakes
- Writing Concisely
- Avoiding Plagiarism
- Footnotes or Endnotes?
- Further Readings
- Generative AI and Writing
- USC Libraries Tutorials and Other Guides
- Bibliography
Quantitative methods emphasize objective measurements and the statistical, mathematical, or numerical analysis of data collected through polls, questionnaires, and surveys, or by manipulating pre-existing statistical data using computational techniques . Quantitative research focuses on gathering numerical data and generalizing it across groups of people or to explain a particular phenomenon.
Babbie, Earl R. The Practice of Social Research . 12th ed. Belmont, CA: Wadsworth Cengage, 2010; Muijs, Daniel. Doing Quantitative Research in Education with SPSS . 2nd edition. London: SAGE Publications, 2010.
Need Help Locating Statistics?
Resources for locating data and statistics can be found here:
Statistics & Data Research Guide
Characteristics of Quantitative Research
Your goal in conducting quantitative research study is to determine the relationship between one thing [an independent variable] and another [a dependent or outcome variable] within a population. Quantitative research designs are either descriptive [subjects usually measured once] or experimental [subjects measured before and after a treatment]. A descriptive study establishes only associations between variables; an experimental study establishes causality.
Quantitative research deals in numbers, logic, and an objective stance. Quantitative research focuses on numeric and unchanging data and detailed, convergent reasoning rather than divergent reasoning [i.e., the generation of a variety of ideas about a research problem in a spontaneous, free-flowing manner].
Its main characteristics are :
- The data is usually gathered using structured research instruments.
- The results are based on larger sample sizes that are representative of the population.
- The research study can usually be replicated or repeated, given its high reliability.
- Researcher has a clearly defined research question to which objective answers are sought.
- All aspects of the study are carefully designed before data is collected.
- Data are in the form of numbers and statistics, often arranged in tables, charts, figures, or other non-textual forms.
- Project can be used to generalize concepts more widely, predict future results, or investigate causal relationships.
- Researcher uses tools, such as questionnaires or computer software, to collect numerical data.
The overarching aim of a quantitative research study is to classify features, count them, and construct statistical models in an attempt to explain what is observed.
Things to keep in mind when reporting the results of a study using quantitative methods :
- Explain the data collected and their statistical treatment as well as all relevant results in relation to the research problem you are investigating. Interpretation of results is not appropriate in this section.
- Report unanticipated events that occurred during your data collection. Explain how the actual analysis differs from the planned analysis. Explain your handling of missing data and why any missing data does not undermine the validity of your analysis.
- Explain the techniques you used to "clean" your data set.
- Choose a minimally sufficient statistical procedure ; provide a rationale for its use and a reference for it. Specify any computer programs used.
- Describe the assumptions for each procedure and the steps you took to ensure that they were not violated.
- When using inferential statistics , provide the descriptive statistics, confidence intervals, and sample sizes for each variable as well as the value of the test statistic, its direction, the degrees of freedom, and the significance level [report the actual p value].
- Avoid inferring causality , particularly in nonrandomized designs or without further experimentation.
- Use tables to provide exact values ; use figures to convey global effects. Keep figures small in size; include graphic representations of confidence intervals whenever possible.
- Always tell the reader what to look for in tables and figures .
NOTE: When using pre-existing statistical data gathered and made available by anyone other than yourself [e.g., government agency], you still must report on the methods that were used to gather the data and describe any missing data that exists and, if there is any, provide a clear explanation why the missing data does not undermine the validity of your final analysis.
Babbie, Earl R. The Practice of Social Research . 12th ed. Belmont, CA: Wadsworth Cengage, 2010; Brians, Craig Leonard et al. Empirical Political Analysis: Quantitative and Qualitative Research Methods . 8th ed. Boston, MA: Longman, 2011; McNabb, David E. Research Methods in Public Administration and Nonprofit Management: Quantitative and Qualitative Approaches . 2nd ed. Armonk, NY: M.E. Sharpe, 2008; Quantitative Research Methods. Writing@CSU. Colorado State University; Singh, Kultar. Quantitative Social Research Methods . Los Angeles, CA: Sage, 2007.
Basic Research Design for Quantitative Studies
Before designing a quantitative research study, you must decide whether it will be descriptive or experimental because this will dictate how you gather, analyze, and interpret the results. A descriptive study is governed by the following rules: subjects are generally measured once; the intention is to only establish associations between variables; and, the study may include a sample population of hundreds or thousands of subjects to ensure that a valid estimate of a generalized relationship between variables has been obtained. An experimental design includes subjects measured before and after a particular treatment, the sample population may be very small and purposefully chosen, and it is intended to establish causality between variables. Introduction The introduction to a quantitative study is usually written in the present tense and from the third person point of view. It covers the following information:
- Identifies the research problem -- as with any academic study, you must state clearly and concisely the research problem being investigated.
- Reviews the literature -- review scholarship on the topic, synthesizing key themes and, if necessary, noting studies that have used similar methods of inquiry and analysis. Note where key gaps exist and how your study helps to fill these gaps or clarifies existing knowledge.
- Describes the theoretical framework -- provide an outline of the theory or hypothesis underpinning your study. If necessary, define unfamiliar or complex terms, concepts, or ideas and provide the appropriate background information to place the research problem in proper context [e.g., historical, cultural, economic, etc.].
Methodology The methods section of a quantitative study should describe how each objective of your study will be achieved. Be sure to provide enough detail to enable the reader can make an informed assessment of the methods being used to obtain results associated with the research problem. The methods section should be presented in the past tense.
- Study population and sampling -- where did the data come from; how robust is it; note where gaps exist or what was excluded. Note the procedures used for their selection;
- Data collection – describe the tools and methods used to collect information and identify the variables being measured; describe the methods used to obtain the data; and, note if the data was pre-existing [i.e., government data] or you gathered it yourself. If you gathered it yourself, describe what type of instrument you used and why. Note that no data set is perfect--describe any limitations in methods of gathering data.
- Data analysis -- describe the procedures for processing and analyzing the data. If appropriate, describe the specific instruments of analysis used to study each research objective, including mathematical techniques and the type of computer software used to manipulate the data.
Results The finding of your study should be written objectively and in a succinct and precise format. In quantitative studies, it is common to use graphs, tables, charts, and other non-textual elements to help the reader understand the data. Make sure that non-textual elements do not stand in isolation from the text but are being used to supplement the overall description of the results and to help clarify key points being made. Further information about how to effectively present data using charts and graphs can be found here .
- Statistical analysis -- how did you analyze the data? What were the key findings from the data? The findings should be present in a logical, sequential order. Describe but do not interpret these trends or negative results; save that for the discussion section. The results should be presented in the past tense.
Discussion Discussions should be analytic, logical, and comprehensive. The discussion should meld together your findings in relation to those identified in the literature review, and placed within the context of the theoretical framework underpinning the study. The discussion should be presented in the present tense.
- Interpretation of results -- reiterate the research problem being investigated and compare and contrast the findings with the research questions underlying the study. Did they affirm predicted outcomes or did the data refute it?
- Description of trends, comparison of groups, or relationships among variables -- describe any trends that emerged from your analysis and explain all unanticipated and statistical insignificant findings.
- Discussion of implications – what is the meaning of your results? Highlight key findings based on the overall results and note findings that you believe are important. How have the results helped fill gaps in understanding the research problem?
- Limitations -- describe any limitations or unavoidable bias in your study and, if necessary, note why these limitations did not inhibit effective interpretation of the results.
Conclusion End your study by to summarizing the topic and provide a final comment and assessment of the study.
- Summary of findings – synthesize the answers to your research questions. Do not report any statistical data here; just provide a narrative summary of the key findings and describe what was learned that you did not know before conducting the study.
- Recommendations – if appropriate to the aim of the assignment, tie key findings with policy recommendations or actions to be taken in practice.
- Future research – note the need for future research linked to your study’s limitations or to any remaining gaps in the literature that were not addressed in your study.
Black, Thomas R. Doing Quantitative Research in the Social Sciences: An Integrated Approach to Research Design, Measurement and Statistics . London: Sage, 1999; Gay,L. R. and Peter Airasain. Educational Research: Competencies for Analysis and Applications . 7th edition. Upper Saddle River, NJ: Merril Prentice Hall, 2003; Hector, Anestine. An Overview of Quantitative Research in Composition and TESOL . Department of English, Indiana University of Pennsylvania; Hopkins, Will G. “Quantitative Research Design.” Sportscience 4, 1 (2000); "A Strategy for Writing Up Research Results. The Structure, Format, Content, and Style of a Journal-Style Scientific Paper." Department of Biology. Bates College; Nenty, H. Johnson. "Writing a Quantitative Research Thesis." International Journal of Educational Science 1 (2009): 19-32; Ouyang, Ronghua (John). Basic Inquiry of Quantitative Research . Kennesaw State University.
Strengths of Using Quantitative Methods
Quantitative researchers try to recognize and isolate specific variables contained within the study framework, seek correlation, relationships and causality, and attempt to control the environment in which the data is collected to avoid the risk of variables, other than the one being studied, accounting for the relationships identified.
Among the specific strengths of using quantitative methods to study social science research problems:
- Allows for a broader study, involving a greater number of subjects, and enhancing the generalization of the results;
- Allows for greater objectivity and accuracy of results. Generally, quantitative methods are designed to provide summaries of data that support generalizations about the phenomenon under study. In order to accomplish this, quantitative research usually involves few variables and many cases, and employs prescribed procedures to ensure validity and reliability;
- Applying well established standards means that the research can be replicated, and then analyzed and compared with similar studies;
- You can summarize vast sources of information and make comparisons across categories and over time; and,
- Personal bias can be avoided by keeping a 'distance' from participating subjects and using accepted computational techniques .
Babbie, Earl R. The Practice of Social Research . 12th ed. Belmont, CA: Wadsworth Cengage, 2010; Brians, Craig Leonard et al. Empirical Political Analysis: Quantitative and Qualitative Research Methods . 8th ed. Boston, MA: Longman, 2011; McNabb, David E. Research Methods in Public Administration and Nonprofit Management: Quantitative and Qualitative Approaches . 2nd ed. Armonk, NY: M.E. Sharpe, 2008; Singh, Kultar. Quantitative Social Research Methods . Los Angeles, CA: Sage, 2007.
Limitations of Using Quantitative Methods
Quantitative methods presume to have an objective approach to studying research problems, where data is controlled and measured, to address the accumulation of facts, and to determine the causes of behavior. As a consequence, the results of quantitative research may be statistically significant but are often humanly insignificant.
Some specific limitations associated with using quantitative methods to study research problems in the social sciences include:
- Quantitative data is more efficient and able to test hypotheses, but may miss contextual detail;
- Uses a static and rigid approach and so employs an inflexible process of discovery;
- The development of standard questions by researchers can lead to "structural bias" and false representation, where the data actually reflects the view of the researcher instead of the participating subject;
- Results provide less detail on behavior, attitudes, and motivation;
- Researcher may collect a much narrower and sometimes superficial dataset;
- Results are limited as they provide numerical descriptions rather than detailed narrative and generally provide less elaborate accounts of human perception;
- The research is often carried out in an unnatural, artificial environment so that a level of control can be applied to the exercise. This level of control might not normally be in place in the real world thus yielding "laboratory results" as opposed to "real world results"; and,
- Preset answers will not necessarily reflect how people really feel about a subject and, in some cases, might just be the closest match to the preconceived hypothesis.
Research Tip
Finding Examples of How to Apply Different Types of Research Methods
SAGE publications is a major publisher of studies about how to design and conduct research in the social and behavioral sciences. Their SAGE Research Methods Online and Cases database includes contents from books, articles, encyclopedias, handbooks, and videos covering social science research design and methods including the complete Little Green Book Series of Quantitative Applications in the Social Sciences and the Little Blue Book Series of Qualitative Research techniques. The database also includes case studies outlining the research methods used in real research projects. This is an excellent source for finding definitions of key terms and descriptions of research design and practice, techniques of data gathering, analysis, and reporting, and information about theories of research [e.g., grounded theory]. The database covers both qualitative and quantitative research methods as well as mixed methods approaches to conducting research.
SAGE Research Methods Online and Cases
- << Previous: Qualitative Methods
- Next: Insiderness >>
- Last Updated: May 20, 2024 9:47 AM
- URL: https://libguides.usc.edu/writingguide
- Help & FAQ
Quantitative data analysis
Research output : Chapter in Book/Report/Conference proceeding › Chapter (Book) › Research › peer-review
This chapter provides a brief introduction to quantitative data analysis, explaining processes for the preparation of quantitative data and common techniques for data description and analysis. The context for the examples used in the chapter is a project management website, used by information technology (IT) students in the third year of their undergraduate degree. The description of data preparation includes data cleaning, as well as data transformation and integration. Statistics, used to investigate numerical data, include descriptive statistics for describing distributions and relationships between variables, and inferential statistics. The latter cover testing for differences between distributions using parametric and nonparametric tests (according to the type of data involved). Examples of different types of tests are provided, using the project management website dataset which is the focus of the chapter.
- Data preparation
- Descriptive statistics
- Graphical representation of data
- Inferential statistics
- Reporting of statistical tests
- Statistical analysis
Access to Document
- 10.1016/B978-0-08-102220-7.00018-2
Other files and links
- Link to publication in Scopus
T1 - Quantitative data analysis
AU - Sheard, Judithe
N2 - This chapter provides a brief introduction to quantitative data analysis, explaining processes for the preparation of quantitative data and common techniques for data description and analysis. The context for the examples used in the chapter is a project management website, used by information technology (IT) students in the third year of their undergraduate degree. The description of data preparation includes data cleaning, as well as data transformation and integration. Statistics, used to investigate numerical data, include descriptive statistics for describing distributions and relationships between variables, and inferential statistics. The latter cover testing for differences between distributions using parametric and nonparametric tests (according to the type of data involved). Examples of different types of tests are provided, using the project management website dataset which is the focus of the chapter.
AB - This chapter provides a brief introduction to quantitative data analysis, explaining processes for the preparation of quantitative data and common techniques for data description and analysis. The context for the examples used in the chapter is a project management website, used by information technology (IT) students in the third year of their undergraduate degree. The description of data preparation includes data cleaning, as well as data transformation and integration. Statistics, used to investigate numerical data, include descriptive statistics for describing distributions and relationships between variables, and inferential statistics. The latter cover testing for differences between distributions using parametric and nonparametric tests (according to the type of data involved). Examples of different types of tests are provided, using the project management website dataset which is the focus of the chapter.
KW - Data preparation
KW - Descriptive statistics
KW - Graphical representation of data
KW - Inferential statistics
KW - Reporting of statistical tests
KW - Statistical analysis
UR - http://www.scopus.com/inward/record.url?scp=85054205929&partnerID=8YFLogxK
U2 - 10.1016/B978-0-08-102220-7.00018-2
DO - 10.1016/B978-0-08-102220-7.00018-2
M3 - Chapter (Book)
SN - 9780081022207
BT - Research Methods
A2 - Williamson, Kirsty
A2 - Johanson, Graeme
PB - Elsevier
CY - Cambridge MA USA
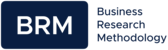
Quantitative Data Analysis
In quantitative data analysis you are expected to turn raw numbers into meaningful data through the application of rational and critical thinking. Quantitative data analysis may include the calculation of frequencies of variables and differences between variables. A quantitative approach is usually associated with finding evidence to either support or reject hypotheses you have formulated at the earlier stages of your research process .
The same figure within data set can be interpreted in many different ways; therefore it is important to apply fair and careful judgement.
For example, questionnaire findings of a research titled “A study into the impacts of informal management-employee communication on the levels of employee motivation: a case study of Agro Bravo Enterprise” may indicate that the majority 52% of respondents assess communication skills of their immediate supervisors as inadequate.
This specific piece of primary data findings needs to be critically analyzed and objectively interpreted through comparing it to other findings within the framework of the same research. For example, organizational culture of Agro Bravo Enterprise, leadership style, the levels of frequency of management-employee communications need to be taken into account during the data analysis.
Moreover, literature review findings conducted at the earlier stages of the research process need to be referred to in order to reflect the viewpoints of other authors regarding the causes of employee dissatisfaction with management communication. Also, secondary data needs to be integrated in data analysis in a logical and unbiased manner.
Let’s take another example. You are writing a dissertation exploring the impacts of foreign direct investment (FDI) on the levels of economic growth in Vietnam using correlation quantitative data analysis method . You have specified FDI and GDP as variables for your research and correlation tests produced correlation coefficient of 0.9.
In this case simply stating that there is a strong positive correlation between FDI and GDP would not suffice; you have to provide explanation about the manners in which the growth on the levels of FDI may contribute to the growth of GDP by referring to the findings of the literature review and applying your own critical and rational reasoning skills.
A set of analytical software can be used to assist with analysis of quantitative data. The following table illustrates the advantages and disadvantages of three popular quantitative data analysis software: Microsoft Excel, Microsoft Access and SPSS.
Advantages and disadvantages of popular quantitative analytical software
Quantitative data analysis with the application of statistical software consists of the following stages [1] :
- Preparing and checking the data. Input of data into computer.
- Selecting the most appropriate tables and diagrams to use according to your research objectives.
- Selecting the most appropriate statistics to describe your data.
- Selecting the most appropriate statistics to examine relationships and trends in your data.
It is important to note that while the application of various statistical software and programs are invaluable to avoid drawing charts by hand or undertake calculations manually, it is easy to use them incorrectly. In other words, quantitative data analysis is “a field where it is not at all difficult to carry out an analysis which is simply wrong, or inappropriate for your data or purposes. And the negative side of readily available specialist statistical software is that it becomes that much easier to generate elegantly presented rubbish” [2] .
Therefore, it is important for you to seek advice from your dissertation supervisor regarding statistical analyses in general and the choice and application of statistical software in particular.
My e-book, The Ultimate Guide to Writing a Dissertation in Business Studies: a step by step approach contains a detailed, yet simple explanation of quantitative data analysis methods . The e-book explains all stages of the research process starting from the selection of the research area to writing personal reflection. Important elements of dissertations such as research philosophy, research approach, research design, methods of data collection and data analysis are explained in simple words. John Dudovskiy
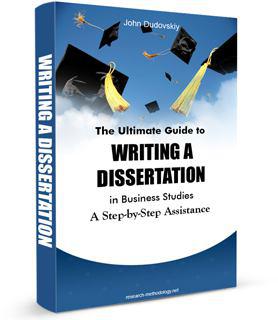
[1] Saunders, M., Lewis, P. & Thornhill, A. (2012) “Research Methods for Business Students” 6th edition, Pearson Education Limited.
[2] Robson, C. (2011) Real World Research: A Resource for Users of Social Research Methods in Applied Settings (3rd edn). Chichester: John Wiley.
Qualitative vs Quantitative Research Methods & Data Analysis
Saul Mcleod, PhD
Editor-in-Chief for Simply Psychology
BSc (Hons) Psychology, MRes, PhD, University of Manchester
Saul Mcleod, PhD., is a qualified psychology teacher with over 18 years of experience in further and higher education. He has been published in peer-reviewed journals, including the Journal of Clinical Psychology.
Learn about our Editorial Process
Olivia Guy-Evans, MSc
Associate Editor for Simply Psychology
BSc (Hons) Psychology, MSc Psychology of Education
Olivia Guy-Evans is a writer and associate editor for Simply Psychology. She has previously worked in healthcare and educational sectors.
On This Page:
What is the difference between quantitative and qualitative?
The main difference between quantitative and qualitative research is the type of data they collect and analyze.
Quantitative research collects numerical data and analyzes it using statistical methods. The aim is to produce objective, empirical data that can be measured and expressed in numerical terms. Quantitative research is often used to test hypotheses, identify patterns, and make predictions.
Qualitative research , on the other hand, collects non-numerical data such as words, images, and sounds. The focus is on exploring subjective experiences, opinions, and attitudes, often through observation and interviews.
Qualitative research aims to produce rich and detailed descriptions of the phenomenon being studied, and to uncover new insights and meanings.
Quantitative data is information about quantities, and therefore numbers, and qualitative data is descriptive, and regards phenomenon which can be observed but not measured, such as language.
What Is Qualitative Research?
Qualitative research is the process of collecting, analyzing, and interpreting non-numerical data, such as language. Qualitative research can be used to understand how an individual subjectively perceives and gives meaning to their social reality.
Qualitative data is non-numerical data, such as text, video, photographs, or audio recordings. This type of data can be collected using diary accounts or in-depth interviews and analyzed using grounded theory or thematic analysis.
Qualitative research is multimethod in focus, involving an interpretive, naturalistic approach to its subject matter. This means that qualitative researchers study things in their natural settings, attempting to make sense of, or interpret, phenomena in terms of the meanings people bring to them. Denzin and Lincoln (1994, p. 2)
Interest in qualitative data came about as the result of the dissatisfaction of some psychologists (e.g., Carl Rogers) with the scientific study of psychologists such as behaviorists (e.g., Skinner ).
Since psychologists study people, the traditional approach to science is not seen as an appropriate way of carrying out research since it fails to capture the totality of human experience and the essence of being human. Exploring participants’ experiences is known as a phenomenological approach (re: Humanism ).
Qualitative research is primarily concerned with meaning, subjectivity, and lived experience. The goal is to understand the quality and texture of people’s experiences, how they make sense of them, and the implications for their lives.
Qualitative research aims to understand the social reality of individuals, groups, and cultures as nearly as possible as participants feel or live it. Thus, people and groups are studied in their natural setting.
Some examples of qualitative research questions are provided, such as what an experience feels like, how people talk about something, how they make sense of an experience, and how events unfold for people.
Research following a qualitative approach is exploratory and seeks to explain ‘how’ and ‘why’ a particular phenomenon, or behavior, operates as it does in a particular context. It can be used to generate hypotheses and theories from the data.
Qualitative Methods
There are different types of qualitative research methods, including diary accounts, in-depth interviews , documents, focus groups , case study research , and ethnography.
The results of qualitative methods provide a deep understanding of how people perceive their social realities and in consequence, how they act within the social world.
The researcher has several methods for collecting empirical materials, ranging from the interview to direct observation, to the analysis of artifacts, documents, and cultural records, to the use of visual materials or personal experience. Denzin and Lincoln (1994, p. 14)
Here are some examples of qualitative data:
Interview transcripts : Verbatim records of what participants said during an interview or focus group. They allow researchers to identify common themes and patterns, and draw conclusions based on the data. Interview transcripts can also be useful in providing direct quotes and examples to support research findings.
Observations : The researcher typically takes detailed notes on what they observe, including any contextual information, nonverbal cues, or other relevant details. The resulting observational data can be analyzed to gain insights into social phenomena, such as human behavior, social interactions, and cultural practices.
Unstructured interviews : generate qualitative data through the use of open questions. This allows the respondent to talk in some depth, choosing their own words. This helps the researcher develop a real sense of a person’s understanding of a situation.
Diaries or journals : Written accounts of personal experiences or reflections.
Notice that qualitative data could be much more than just words or text. Photographs, videos, sound recordings, and so on, can be considered qualitative data. Visual data can be used to understand behaviors, environments, and social interactions.
Qualitative Data Analysis
Qualitative research is endlessly creative and interpretive. The researcher does not just leave the field with mountains of empirical data and then easily write up his or her findings.
Qualitative interpretations are constructed, and various techniques can be used to make sense of the data, such as content analysis, grounded theory (Glaser & Strauss, 1967), thematic analysis (Braun & Clarke, 2006), or discourse analysis.
For example, thematic analysis is a qualitative approach that involves identifying implicit or explicit ideas within the data. Themes will often emerge once the data has been coded .
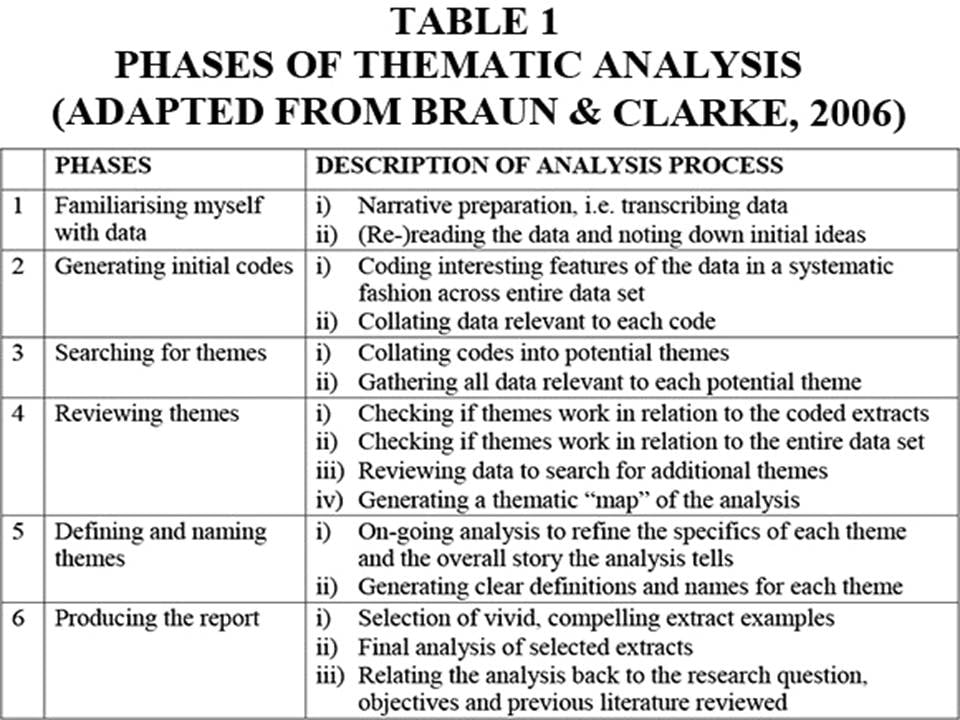
Key Features
- Events can be understood adequately only if they are seen in context. Therefore, a qualitative researcher immerses her/himself in the field, in natural surroundings. The contexts of inquiry are not contrived; they are natural. Nothing is predefined or taken for granted.
- Qualitative researchers want those who are studied to speak for themselves, to provide their perspectives in words and other actions. Therefore, qualitative research is an interactive process in which the persons studied teach the researcher about their lives.
- The qualitative researcher is an integral part of the data; without the active participation of the researcher, no data exists.
- The study’s design evolves during the research and can be adjusted or changed as it progresses. For the qualitative researcher, there is no single reality. It is subjective and exists only in reference to the observer.
- The theory is data-driven and emerges as part of the research process, evolving from the data as they are collected.
Limitations of Qualitative Research
- Because of the time and costs involved, qualitative designs do not generally draw samples from large-scale data sets.
- The problem of adequate validity or reliability is a major criticism. Because of the subjective nature of qualitative data and its origin in single contexts, it is difficult to apply conventional standards of reliability and validity. For example, because of the central role played by the researcher in the generation of data, it is not possible to replicate qualitative studies.
- Also, contexts, situations, events, conditions, and interactions cannot be replicated to any extent, nor can generalizations be made to a wider context than the one studied with confidence.
- The time required for data collection, analysis, and interpretation is lengthy. Analysis of qualitative data is difficult, and expert knowledge of an area is necessary to interpret qualitative data. Great care must be taken when doing so, for example, looking for mental illness symptoms.
Advantages of Qualitative Research
- Because of close researcher involvement, the researcher gains an insider’s view of the field. This allows the researcher to find issues that are often missed (such as subtleties and complexities) by the scientific, more positivistic inquiries.
- Qualitative descriptions can be important in suggesting possible relationships, causes, effects, and dynamic processes.
- Qualitative analysis allows for ambiguities/contradictions in the data, which reflect social reality (Denscombe, 2010).
- Qualitative research uses a descriptive, narrative style; this research might be of particular benefit to the practitioner as she or he could turn to qualitative reports to examine forms of knowledge that might otherwise be unavailable, thereby gaining new insight.
What Is Quantitative Research?
Quantitative research involves the process of objectively collecting and analyzing numerical data to describe, predict, or control variables of interest.
The goals of quantitative research are to test causal relationships between variables , make predictions, and generalize results to wider populations.
Quantitative researchers aim to establish general laws of behavior and phenomenon across different settings/contexts. Research is used to test a theory and ultimately support or reject it.
Quantitative Methods
Experiments typically yield quantitative data, as they are concerned with measuring things. However, other research methods, such as controlled observations and questionnaires , can produce both quantitative information.
For example, a rating scale or closed questions on a questionnaire would generate quantitative data as these produce either numerical data or data that can be put into categories (e.g., “yes,” “no” answers).
Experimental methods limit how research participants react to and express appropriate social behavior.
Findings are, therefore, likely to be context-bound and simply a reflection of the assumptions that the researcher brings to the investigation.
There are numerous examples of quantitative data in psychological research, including mental health. Here are a few examples:
Another example is the Experience in Close Relationships Scale (ECR), a self-report questionnaire widely used to assess adult attachment styles .
The ECR provides quantitative data that can be used to assess attachment styles and predict relationship outcomes.
Neuroimaging data : Neuroimaging techniques, such as MRI and fMRI, provide quantitative data on brain structure and function.
This data can be analyzed to identify brain regions involved in specific mental processes or disorders.
For example, the Beck Depression Inventory (BDI) is a clinician-administered questionnaire widely used to assess the severity of depressive symptoms in individuals.
The BDI consists of 21 questions, each scored on a scale of 0 to 3, with higher scores indicating more severe depressive symptoms.
Quantitative Data Analysis
Statistics help us turn quantitative data into useful information to help with decision-making. We can use statistics to summarize our data, describing patterns, relationships, and connections. Statistics can be descriptive or inferential.
Descriptive statistics help us to summarize our data. In contrast, inferential statistics are used to identify statistically significant differences between groups of data (such as intervention and control groups in a randomized control study).
- Quantitative researchers try to control extraneous variables by conducting their studies in the lab.
- The research aims for objectivity (i.e., without bias) and is separated from the data.
- The design of the study is determined before it begins.
- For the quantitative researcher, the reality is objective, exists separately from the researcher, and can be seen by anyone.
- Research is used to test a theory and ultimately support or reject it.
Limitations of Quantitative Research
- Context: Quantitative experiments do not take place in natural settings. In addition, they do not allow participants to explain their choices or the meaning of the questions they may have for those participants (Carr, 1994).
- Researcher expertise: Poor knowledge of the application of statistical analysis may negatively affect analysis and subsequent interpretation (Black, 1999).
- Variability of data quantity: Large sample sizes are needed for more accurate analysis. Small-scale quantitative studies may be less reliable because of the low quantity of data (Denscombe, 2010). This also affects the ability to generalize study findings to wider populations.
- Confirmation bias: The researcher might miss observing phenomena because of focus on theory or hypothesis testing rather than on the theory of hypothesis generation.
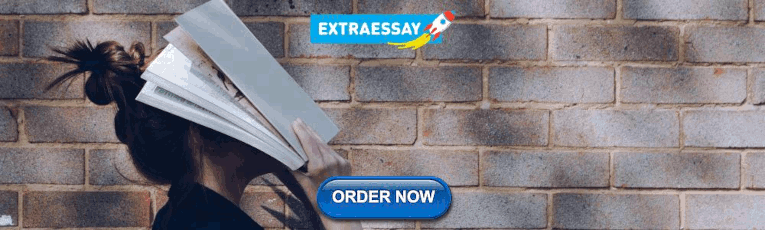
Advantages of Quantitative Research
- Scientific objectivity: Quantitative data can be interpreted with statistical analysis, and since statistics are based on the principles of mathematics, the quantitative approach is viewed as scientifically objective and rational (Carr, 1994; Denscombe, 2010).
- Useful for testing and validating already constructed theories.
- Rapid analysis: Sophisticated software removes much of the need for prolonged data analysis, especially with large volumes of data involved (Antonius, 2003).
- Replication: Quantitative data is based on measured values and can be checked by others because numerical data is less open to ambiguities of interpretation.
- Hypotheses can also be tested because of statistical analysis (Antonius, 2003).
Antonius, R. (2003). Interpreting quantitative data with SPSS . Sage.
Black, T. R. (1999). Doing quantitative research in the social sciences: An integrated approach to research design, measurement and statistics . Sage.
Braun, V. & Clarke, V. (2006). Using thematic analysis in psychology . Qualitative Research in Psychology , 3, 77–101.
Carr, L. T. (1994). The strengths and weaknesses of quantitative and qualitative research : what method for nursing? Journal of advanced nursing, 20(4) , 716-721.
Denscombe, M. (2010). The Good Research Guide: for small-scale social research. McGraw Hill.
Denzin, N., & Lincoln. Y. (1994). Handbook of Qualitative Research. Thousand Oaks, CA, US: Sage Publications Inc.
Glaser, B. G., Strauss, A. L., & Strutzel, E. (1968). The discovery of grounded theory; strategies for qualitative research. Nursing research, 17(4) , 364.
Minichiello, V. (1990). In-Depth Interviewing: Researching People. Longman Cheshire.
Punch, K. (1998). Introduction to Social Research: Quantitative and Qualitative Approaches. London: Sage
Further Information
- Designing qualitative research
- Methods of data collection and analysis
- Introduction to quantitative and qualitative research
- Checklists for improving rigour in qualitative research: a case of the tail wagging the dog?
- Qualitative research in health care: Analysing qualitative data
- Qualitative data analysis: the framework approach
- Using the framework method for the analysis of
- Qualitative data in multi-disciplinary health research
- Content Analysis
- Grounded Theory
- Thematic Analysis

Related Articles
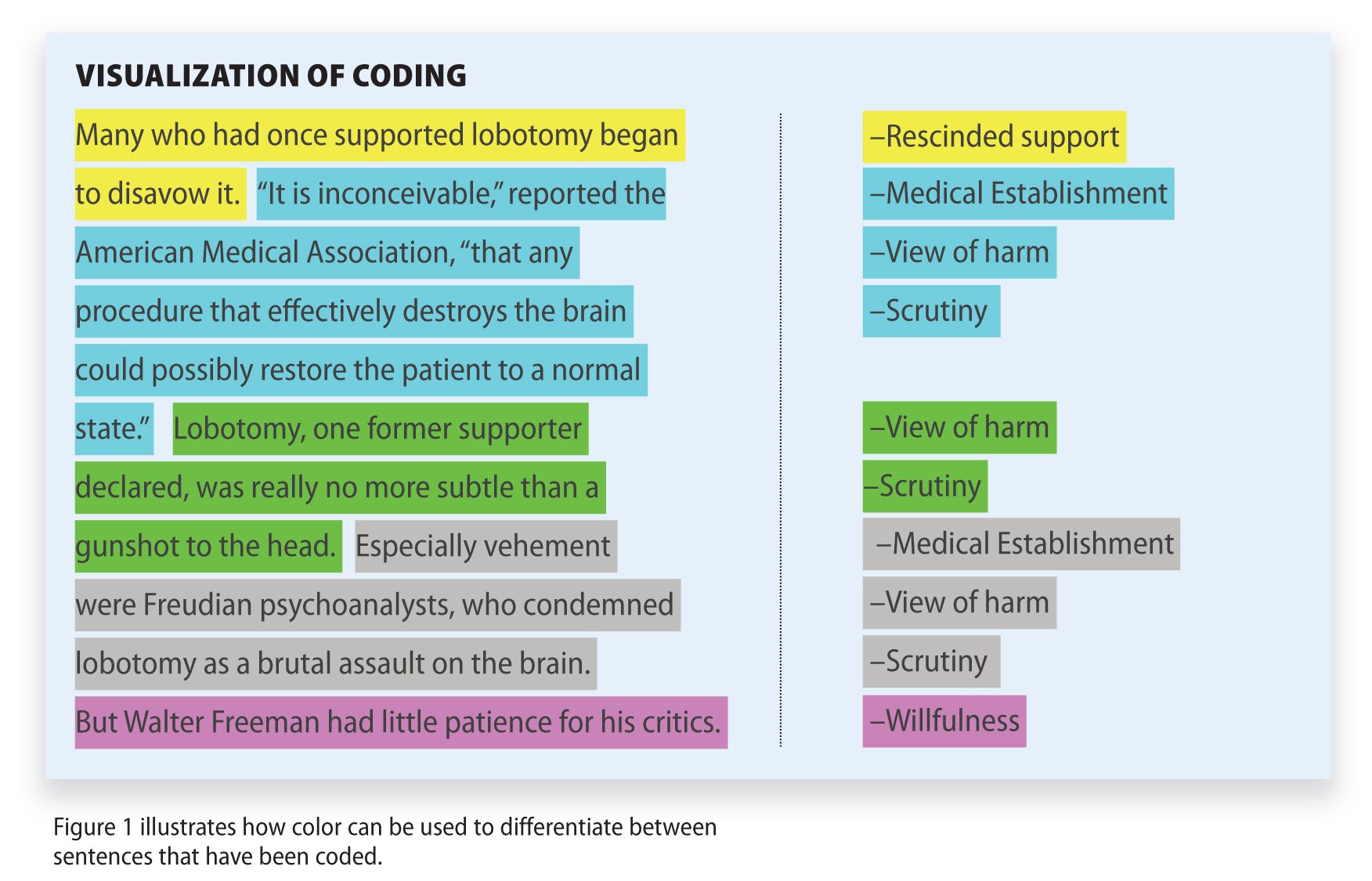
Research Methodology
Qualitative Data Coding
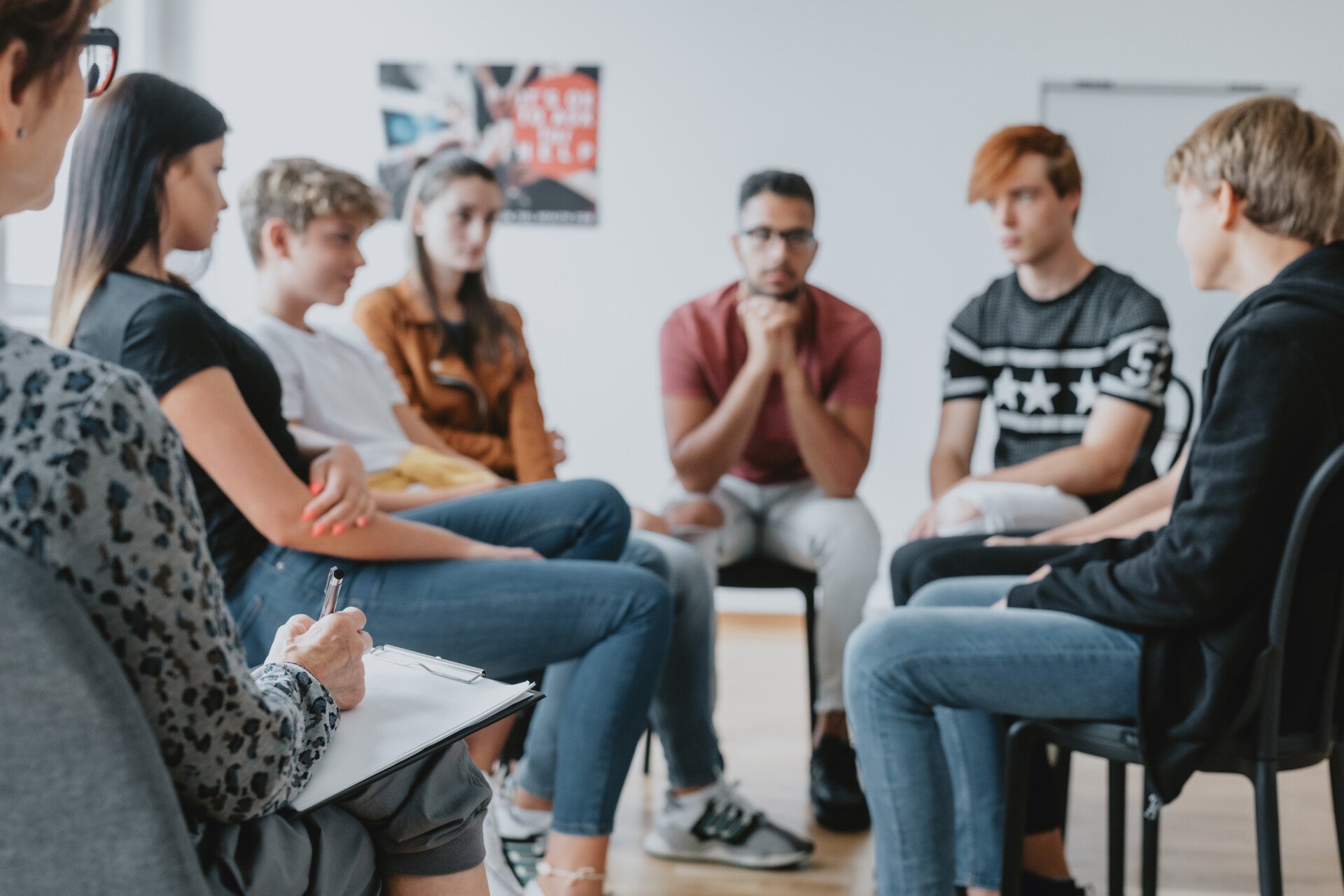
What Is a Focus Group?
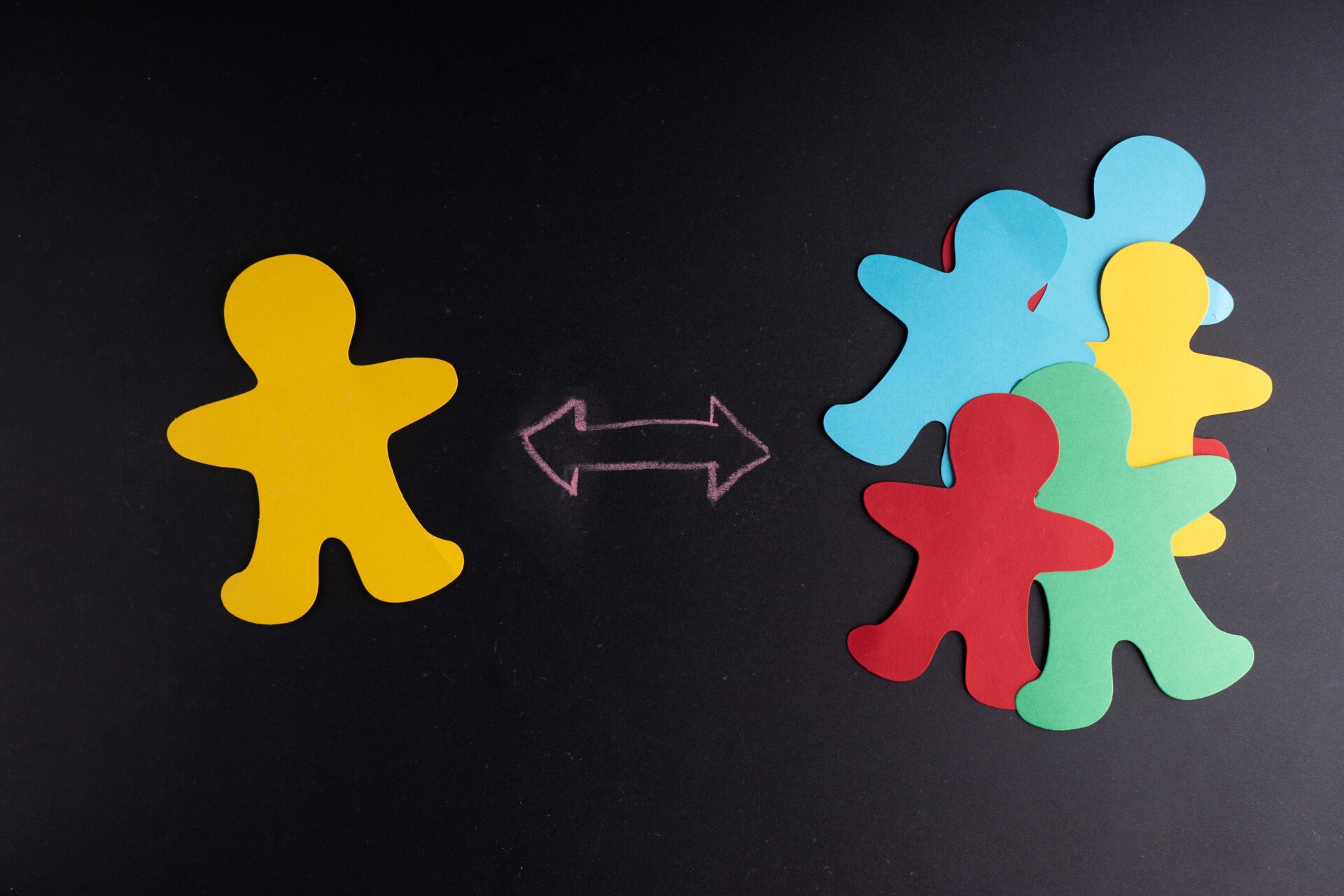
Cross-Cultural Research Methodology In Psychology
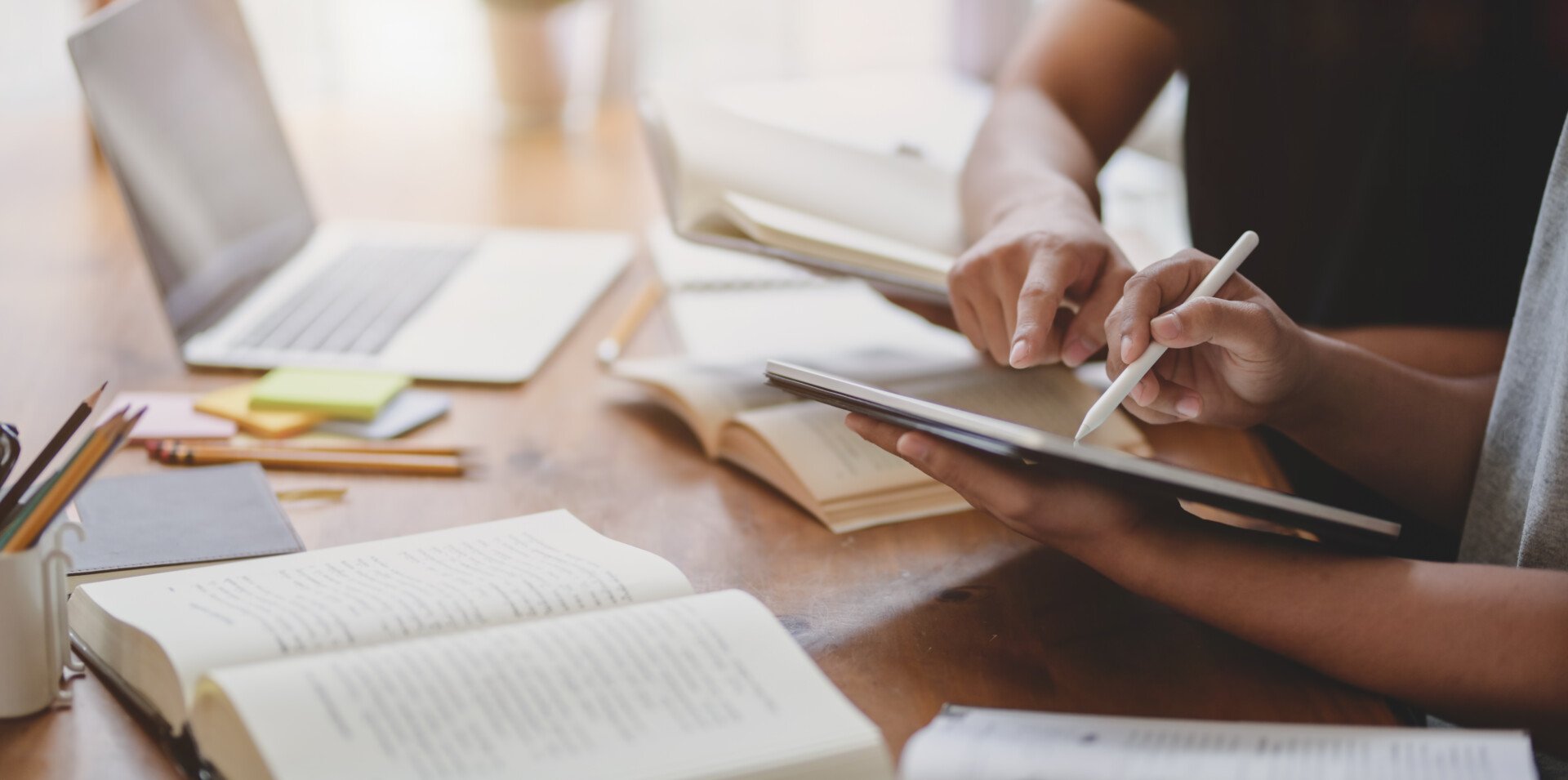
What Is Internal Validity In Research?
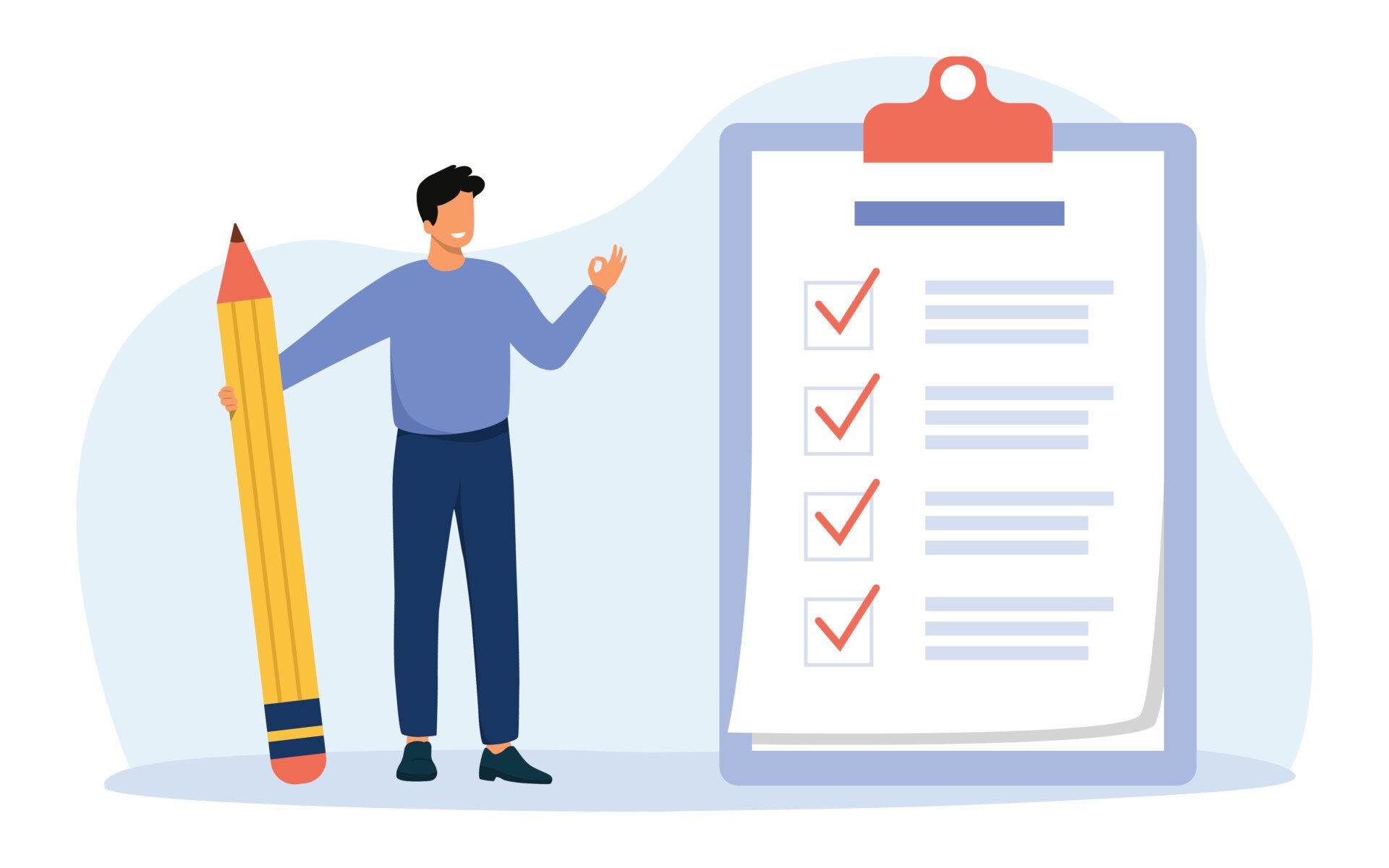
Research Methodology , Statistics
What Is Face Validity In Research? Importance & How To Measure
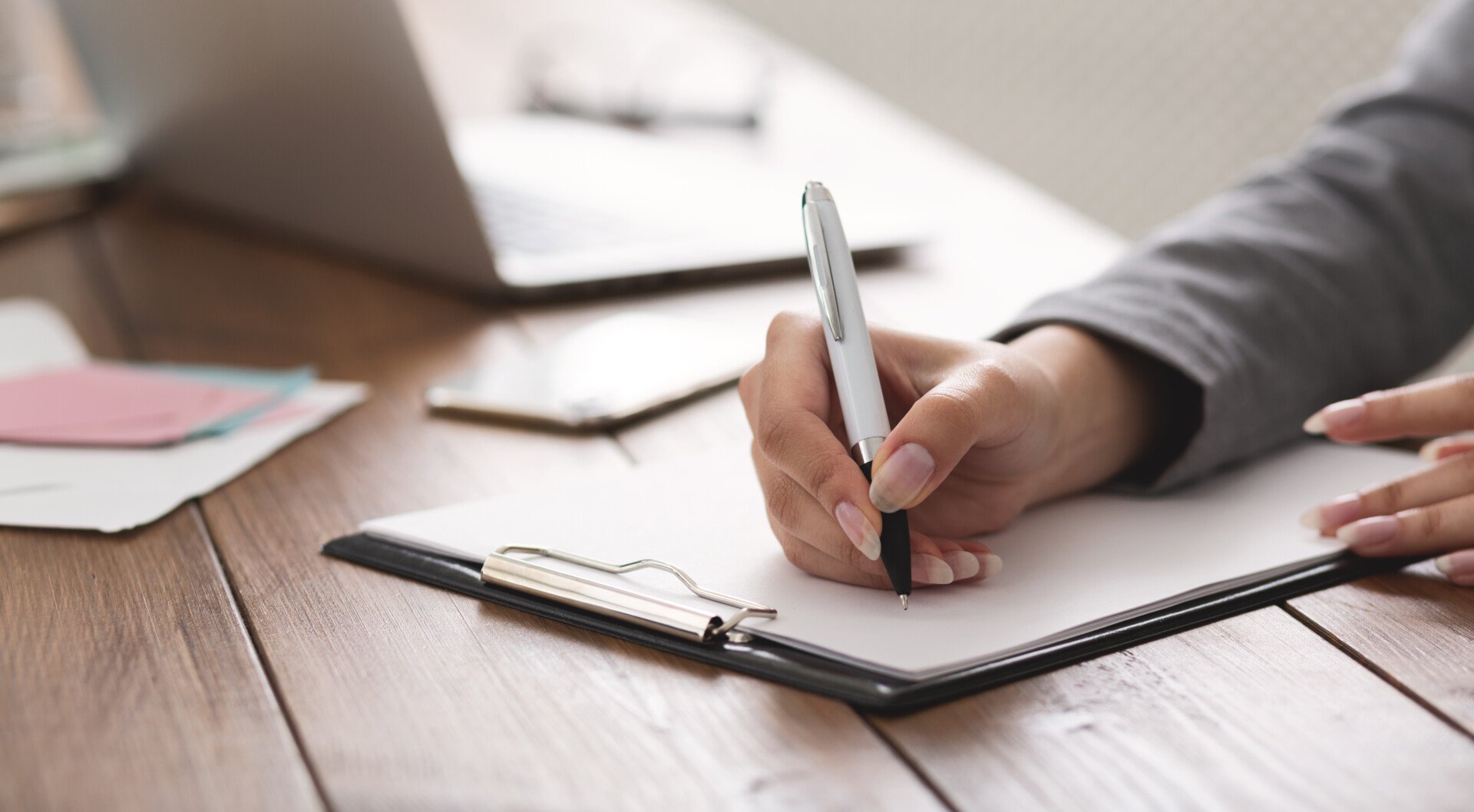
Criterion Validity: Definition & Examples
Quantitative research: Understanding the approaches and key elements
Quantitative research has many benefits and challenges but understanding how to properly conduct it can lead to a successful marketing research project.
Choosing the right quantitative approach
Editor’s note: Allison Von Borstel is the associate director of creative analytics at The Sound. This is an edited version of an article that originally appeared under the title “ Understanding Quantitative Research Approaches .”
What is quantitative research?
The systematic approaches that ground quantitative research involve hundreds or thousands of data points for one research project. The wonder of quantitative research is that each data point, or row in a spreadsheet, is a person and has a human story to tell.
Quantitative research aggregates voices and distills them into numbers that uncover trends, illuminates relationships and correlations that inform decision-making with solid evidence and clarity.
The benefits of quantitative approach es
Why choose a quantitative approach? Because you want a very clear story grounded in statistical rigor as a guide to making smart, data-backed decisions.
Quantitative approaches shine because they:
Involve a lot of people
Large sample sizes (think hundreds or thousands) enable researchers to generalize findings because the sample is representative of the total population.
They are grounded in statistical rigor
Allowing for precise measurement and analysis of data, providing statistically significant results that bolster confidence in research.
Reduce bias
Structured data collection and analysis methods enhance the reliability of findings.
Boost efficiency
Quantitative methods often follow a qualitative phase, allowing researchers to validate findings by reporting the perspective of hundreds of people in a fraction of the time.
Widen the analysis’ scope
The copious data collected in just a 20-minute (max) survey positions researchers to evaluate a broad spectrum of variables within the data. This thorough comprehension is instrumental when dealing with complex questions that require in-depth analysis.
Quantitative approaches have hurdles, which include:
Limited flexibility
Once a survey is fielded, or data is gathered, there’s no opportunity to ask a live follow-up question. While it is possible to follow-up with the same people for two surveys, the likelihood of sufficient responses is small.
Battling bots
One of the biggest concerns in data quality is making sure data represents people and not bots.
Missing body language cues
Numbers, words and even images lack the cues that a researcher could pick up on during an interview. Unlike in a qualitative focus group, where one might deduce that a person is uncertain of an answer, in quantitative research, a static response is what the researcher works with.
Understanding quantitative research methods
Quantitative approaches approach research from the same starting point as qualitative approaches – grounded in business objectives with a specific group of people to study.
Once research has kicked off, the business objective thoroughly explored and the approach selected, research follows a general outline:
Consider what data is needed
Think about what type of information needs to be gathered, with an approach in mind. While most quantitative research involves numbers, words and images also count.
- Numbers: Yes, the stereotypical rows of numbers in spreadsheets. Rows that capture people’s opinions and attitudes and are coded to numbers for comparative analytics. Numerical analysis is used for everything from descriptive statistics to regression/predictive analysis.
- Words: Text analysis employs a machine learning model to identify sentiment, emotion and meaning of text. Often used for sentiment analysis or content classification, it can be applied to single-word responses, elaborate open-ends, reviews or even social media posts.
- Images: Image analysis extracts meaningful information from images. A computer vision model that takes images as inputs and outputs numerical information (e.g., having a sample upload their favorite bag of chips and yielding the top three brands).
Design a survey
Create a survey to capture the data needed to address the objective. During this process, different pathways could be written to get a dynamic data set (capturing opinions that derive from various lived experiences). Survey logic is also written to provide a smooth UX experience for respondents.
Prepare the data
The quality of quantitative research rests heavily on the quality of data. After data is collected (typically by fielding a survey or collecting already-existing data, more on that in a bit), it’s time to clean the data.
Begin the analysis process
Now that you have a robust database (including numbers, words or images), it’s time to listen to the story that the data tells. Depending on the research approach used, advanced analytics come into play to tease out insights and nuances for the business objective.
Tell the story
Strip the quantitative jargon and convey the insights from the research. Just because it’s quantitative research does not mean the results have to be told in a monotone drone with a monochrome chart. Answer business objectives dynamically, knowing that research is grounded in statistically sound information.
The two options: Primary vs. secondary research
The two methods that encompass quantitative approaches are primary (collecting data oneself) and secondary (relying on already existing data).
Primary research is primarily used
Most research involves primary data collection – where the researcher collects data directly. The main approach in primary research is survey data collection.
The types of survey questions
Span various measurement scales (nominal, ordinal, interval and ratio) using a mix of question types (single and multi-choice, scales, matrix or open-ends).
Analysis methods
Custom surveys yield great data for a variety of methods in market analysis. Here are a couple favorites:
- Crosstabulation : Used to uncover insights that might not be obvious at first glance. This analysis organizes data into categories, revealing trends or patterns between variables.
- Sentiment analysis: Used to sift through text to gauge emotions, opinions and attitudes. This method helps understand perception, fine-tune strategies and effectively respond to feedback.
- Market sizing: Used to map out the dimensions of a market. By calculating the total potential demand for a product or service in a specific market, this method reveals the scope of opportunities needed to make informed decisions about investment and growth strategies.
- Conjoint analysis : Used to uncover what people value most in products or services. It breaks down features into bits and pieces and asks people to choose their ideal combo. By analyzing these preferences, this analysis reveals the hidden recipe for customer satisfaction.
- Job-To-Be-Done : Used to understand the underlying human motivations that drive people to act. People are multifaceted and experience a myriad of situations each day – meaning that a brand’s competition isn’t limited to in-category.
- Segmentation: Used to identify specific cohorts within a greater population. It groups people with similar characteristics, behaviors or needs together. This method helps tailor products or services to specific groups, boosting satisfaction and sales.
Statistical rigor
Regardless of method, a quantitative approach then enables researchers to draw inferences and make predictions based upon the confidence in the data (looking at confidence intervals, margin of error, etc.)
Let’s not forget secondary research
By accessing a wide range of existing information, this research can be a cost-effective way to gain insights or can supplement primary research findings.
Here are popular options:
Government sources
Government sources can be extremely in-depth, can range across multiple industries and markets and reflect millions of people. This type of data is often instrumental for longitudinal or cultural trends analysis.
Educational institutions
Research universities conduct in-depth studies on a variety of topics, often aggregating government data, nonprofit data and primary data.
Client data
This includes any research that was conducted for or by companies before the present research project. Whether it’s data gathered from customer reviews or prior quantitative work, these secondary resources can help extend findings and detect trends by connecting past data to future data.
Quantitative research enhances research projects
Quantitative research approaches are so much more than “how much” or “how many,” they reveal the why behind people’s actions, emotions and behaviors. By using standardized collection methods, like surveys, quant instills confidence and rigor in findings.
Canvs AI: Unlock critical insights from unstructured feedback Related Categories: Research Industry, Data Analysis, Quantitative Research Research Industry, Data Analysis, Quantitative Research, Artificial Intelligence / AI, Text Analytics
Segmentation in the pharma industry: How to create resilient strategies Related Categories: Research Industry, Sampling, Survey Research Research Industry, Sampling, Survey Research, Market Segmentation Studies, Segmentation Studies, Health Care (Healthcare), Health Care (Healthcare) Research, Patients , Questionnaire Analysis, Social Media Research
Leveraging AI to unlock qualitative research at scale Related Categories: Research Industry, Quantitative Research, Sampling, Hybrid Research (Qual/Quant) Research Industry, Quantitative Research, Sampling, Hybrid Research (Qual/Quant), Artificial Intelligence / AI, Qualitative Research, Attitude/Usage Studies, Consumer Research, Consumers, Data Visualization/Infographics
Situational choice experiments for marketing research Related Categories: Research Industry, Data Analysis, Survey Research Research Industry, Data Analysis, Survey Research, Conjoint Analysis/Trade-Off Analysis, Discrete Choice Modeling, Physicians, Software-Conjoint Analysis, Questionnaire Analysis
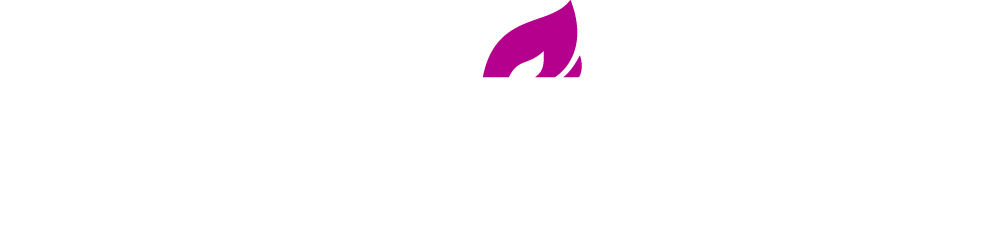
No products in the cart.
What is Qualitative Data Analysis Software (QDA Software)?
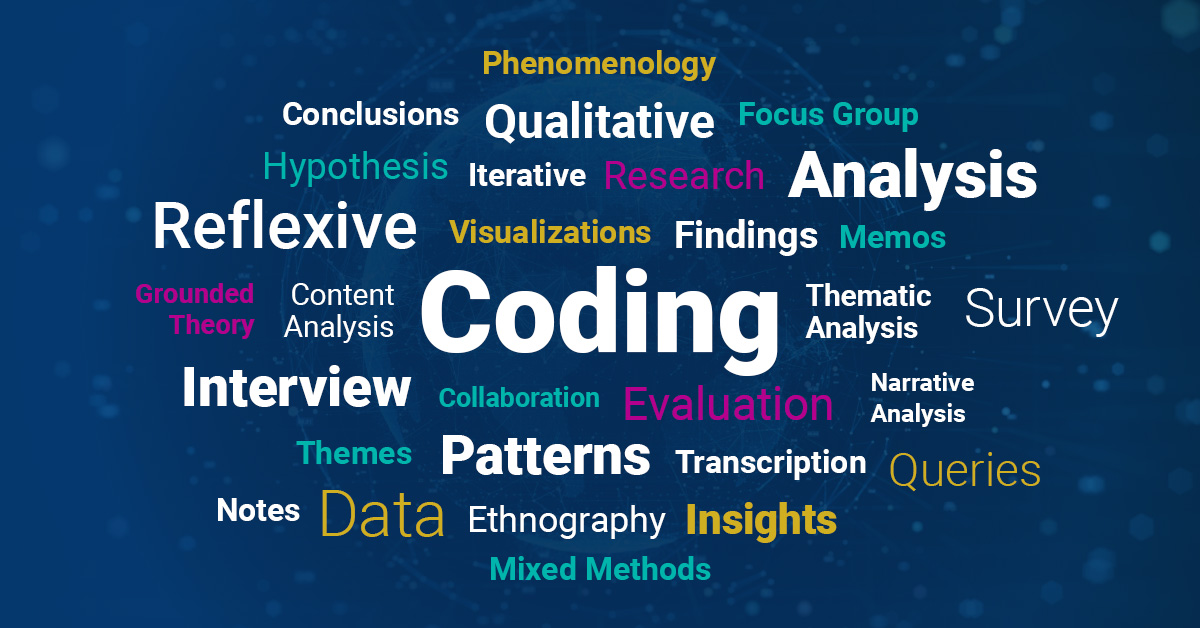
Qualitative Data Analysis Software (QDA software) allows researchers to organize, analyze and visualize their data, finding the patterns in qualitative data or unstructured data: interviews, surveys, field notes, videos, audio files, images, journal articles interviews, web content etc.
Quantitative vs. Qualitative Data Analysis
What is the difference between quantitative and qualitative data analysis. As the name implies, quantitative data analysis has to do with numbers. For example, any time you are doing statistical analysis, you are doing quantitative data analysis. Some examples of quantitative data analysis software are SPSS, STATA, SAS, and Lumivero’s own powerful statistics software, XLSTAT .
In contrast, qualitative analysis "helps you understand people’s perceptions and experiences by systematically coding and analyzing the data", as described in Qualitative vs Quantitative Research 101 . It tends to deal more with words than numbers. It can be useful when working with a lot of rich and deep data and when you aren’t trying to test something very specific. Some examples of qualitative data analysis software are MAXQDA, ATLAS.ti, Quirkos, and Lumivero’s NVivo, the leading tool for qualitative data analysis .
When would you use each one? Well, qualitative data analysis is often used for exploratory research or developing a theory, whereas quantitative is better if you want to test a hypothesis, find averages, and determine relationships between variables. With quantitative research you often want a large sample size to get relevant statistics. In contrast, qualitative research, because so much data in the form of text is involved, can have much smaller sample sizes and still yield valuable insights.
Of course, it’s not always so cut and dry, and many researchers end up taking a «mixed methods» approach, meaning that they combine both types of research. In this case they might use a combination of both types of software programs.
Learn how some qualitative researchers use QDA software for text analysis in the on-demand webinar Twenty-Five Qualitative Researchers Share How-To's for Data Analysis .

How is Qualitative Data Analysis Software Used for Research?
Qualitative Data Analysis Software works with any qualitative research methodology used by a researcher For example, software for qualitative data analysis can be used by a social scientist wanting to develop new concepts or theories may take a ‘grounded theory’ approach. Or a researcher looking for ways to improve health policy or program design might use ‘evaluation methods’. QDA software analysis tools don't favor a particular methodology — they're designed to facilitate common qualitative techniques no matter what method you use.
NVivo can help you to manage, explore and find patterns in your data and conduct thematic and sentiment analysis, but it cannot replace your analytical expertise.
Qualitative Research as an Iterative Process
Handling qualitative and mixed methods data is not usually a step-by-step process. Instead, it tends to be an iterative process where you explore, code, reflect, memo, code some more, query and so on. For example, this picture shows a path you might take to investigate an interesting theme using QDA software, like NVivo, to analyze data:
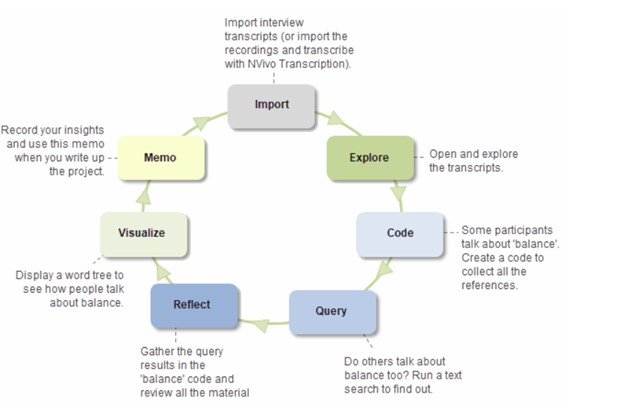
How Do I Choose the Best Approach for My Research Project with QDA Software?
Every research project is unique — the way you organize and analyze the material depends on your methodology, data and research design.
Here are some example scenarios for handling different types of research projects in QDA software — these are just suggestions to get you up and running.
A study with interviews exploring stakeholder perception of a community arts program
Your files consist of unstructured interview documents. You would set up a case for each interview participant, then code to codes and cases. You could then explore your data with simple queries or charts and use memos to record your discoveries.
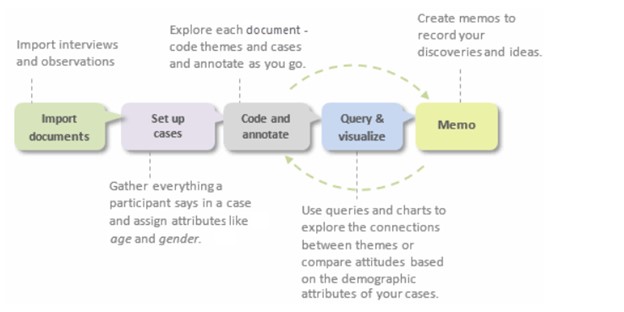
A study exploring community perceptions about climate change using autocoding with AI
Your files consist of structured, consistently formatted interviews (where each participant is asked the same set of questions). With AI, you could autocode the interviews and set up cases for each participant. Then code themes to query and visualize your data.
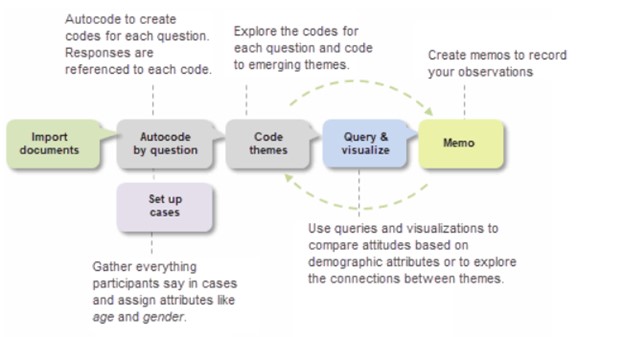
A literature review on adolescent depression
Your files consist of journal articles, books and web pages. You would classify your files before coding and querying them; and then you could critique each file in a memo. With Citavi integration in NVivo, you can import your Citavi references into NVivo.
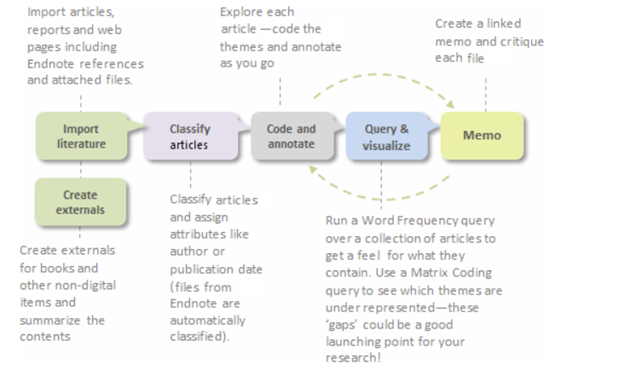
A social media study of the language used by members of an online community
Your files consist of Facebook data captured with NCapture. You would import it as a dataset ready to code and query. Use memos to record your insights.
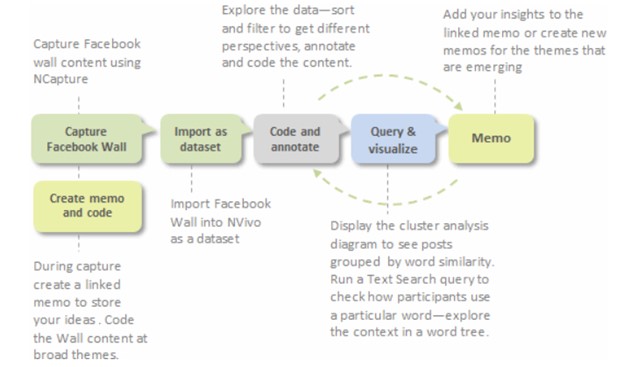
A quick analysis of a local government budget survey
Your file is a large dataset of survey responses. You would import it using the Survey Import Wizard, which prepares your data for analysis. As part of the import, choose to run automated insights with AI to identify and code to themes and sentiment so that you can quickly review results and report broad findings.
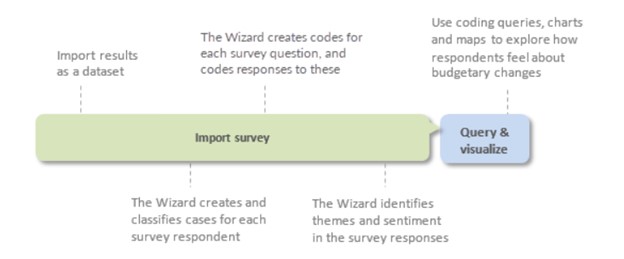
Ways to Get Started with Your Project with Qualitative Analysis Software
Since projects (and researchers) are unique there is no one 'best practice' approach to organizing and analyzing your data but there are some useful strategies to help you get up and running:
- Start now - don't wait until you have collected all the data. Import your research design, grant application or thesis proposal.
- Make a project journa l and state your research questions and record your goals. Why are you doing the project? What is it about? What do you expect to find and why?
- Make a mind map for your preliminary ideas. Show the relationships or patterns you expect to find in your data based on prior experience or preliminary reading.
- Import your interviews, field notes, focus groups —organize these files into folders for easy access.
- Set up an initial code structure based on your early reading and ideas—you could run a Word Frequency query over your data to tease out the common themes for creating your code structure.
- Set up cases for the people, places or other cases in your project.
- Explore your material and code themes as they emerge in your data mining —creating memos and describing your discoveries and interpretations.
- To protect your work, get in the habit of making regular back-ups.
QDA Analysis Tools Help You Work Toward Outcomes that are Robust and Transparent
Using QDA software to organize and analyze your data also increases the 'transparency' of your research outcomes—for example, you can:
- Demonstrate the evolution of your ideas in memos and maps.
- Document your early preconceptions and biases (in a memo or map) and demonstrate how these have been acknowledged and tested.
- Easily find illustrative quotes.
- Always return to the original context of your coded material.
- Save and revisit the queries and visualizations that helped you to arrive at your conclusions.
QDA software, like NVivo, can demonstrate the credibility of your findings in the following ways:
- If you used NVivo for your literature review, run a query or create a chart to demonstrate how your findings compare with the views of other authors.
- Was an issue or theme reported by more than one participant? Run a Matrix Coding query to see how many participants talked about a theme.
- Were multiple methods used to collect the data (interviews, observations, surveys)—and are the findings supported across these text data and video data files? Run a Matrix Coding query to see how often a theme is reported across all your files.

- If multiple researchers analyzed the material — were their findings consistent? Use coding stripes (or filter the contents in a code) to see how various team members have coded the material and run a Coding Comparison query to assess the level of agreement.
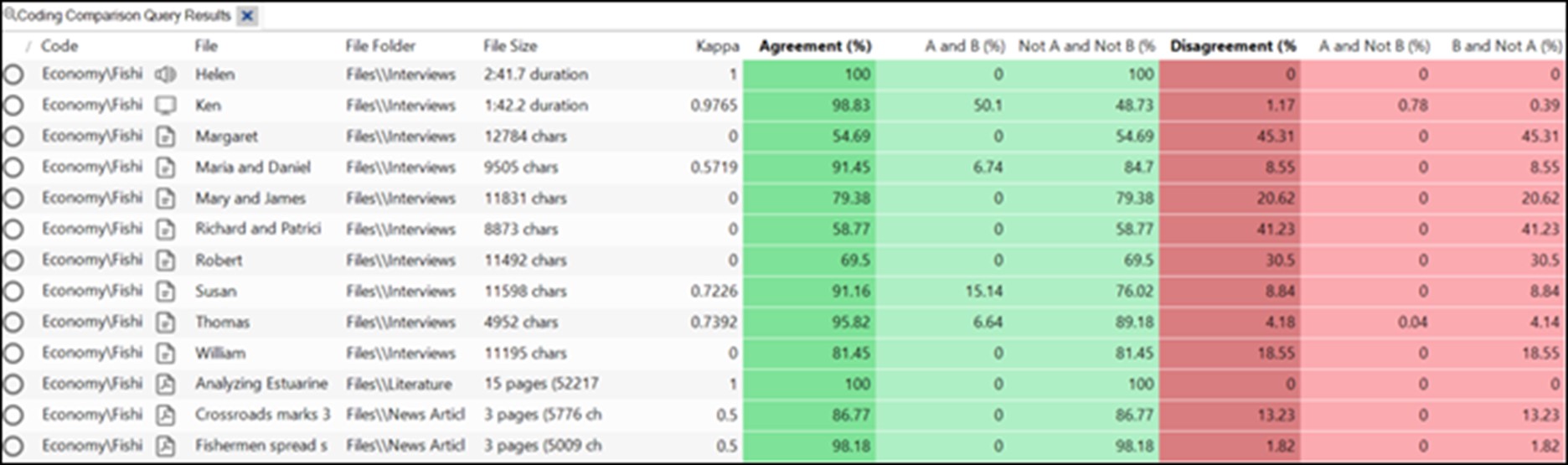
QDA Software Integrations
Many qualitative analysis software options have integration with other software to enhance your research process. NVivo integrates or can be used with the following software:
- NVivo Transcription to save you time and jump start your qualitative data analysis. Learn how in the on-demand webinar Transcription – Go Beyond the Words .
- Reference management software, like Lumivero’s Citavi, for reference management and writing. By combining Citavi and NVivo, you can create complicated searches for certain keywords, terms, and categories and make advanced search syntax, like wildcards, boolean operators, and regular expressions. This integration allows you to take your analyses beyond reference management by developing a central location to collect references and thoughts, analyze literature, and connect empirical data.
- Statistical software, like Lumivero’s XLSTAT , SPSS, or STATA to export your queries from NVivo to run statistical analysis
- Qualtrics, SurveyMonkey to import your survey results into NVivo to start analyzing.
Make Choosing QDA Software Easy — Try NVivo Today!
It's tough choosing QDA software! Test out NVivo, the most cited qualitative data analysis tool, by requesting a free 14-day trial of NVivo to start improving your qualitative and mixed methods research today.
Recent Articles
Thank you for visiting nature.com. You are using a browser version with limited support for CSS. To obtain the best experience, we recommend you use a more up to date browser (or turn off compatibility mode in Internet Explorer). In the meantime, to ensure continued support, we are displaying the site without styles and JavaScript.
- View all journals
- My Account Login
- Explore content
- About the journal
- Publish with us
- Sign up for alerts
- Systematic Review
- Open access
- Published: 17 May 2024
Risk factors and incidence of central venous access device-related thrombosis in hospitalized children: a systematic review and meta-analysis
- Maoling Fu 1 , 2 ,
- Quan Yuan 2 ,
- Qiaoyue Yang 1 , 2 ,
- Yaqi Yu 1 , 2 ,
- Wenshuai Song 1 , 2 ,
- Xiuli Qin 1 ,
- Ying Luo 1 ,
- Xiaoju Xiong 1 &
- Genzhen Yu 1
Pediatric Research ( 2024 ) Cite this article
66 Accesses
Metrics details
The risk factors for central venous access device-related thrombosis (CRT) in children are not fully understood. We used evidence-based medicine to find the risk factors for CRT by pooling current studies reporting risk factors of CRT, aiming to guide clinical diagnosis and treatment.
A systematic search of PubMed, Web of Science, Embase, Cochrane Library, Scopus, CNKI, Sinomed, and Wanfang databases was conducted. RevMan 5.4 was employed for data analysis.
The review included 47 studies evaluating 262,587 children with CVAD placement. Qualitative synthesis and quantitative meta-analysis identified D-dimer, location of insertion, type of catheter, number of lumens, catheter indwelling time, and central line-associated bloodstream infection as the most critical risk factors for CRT. Primarily due to observational design, the quality of evidence was regarded as low certainty for these risk factors according to the GRADE approach.
Because fewer high-quality studies are available, larger sample sizes and well-designed prospective studies are still needed to clarify the risk factors affecting CRT. In the future, developing pediatric-specific CRT risk assessment tools is important. Appropriate stratified preventive strategies for CRT according to risk assessment level will help improve clinical efficiency, avoid the occurrence of CRT, and alleviate unnecessary suffering of children.
This is the latest systematic review of risk factors and incidence of CRT in children.
A total of 47 studies involving 262,587 patients were included in our meta-analysis, according to which the pooled prevalence of CRT was 9.1%.
This study identified several of the most critical risk factors affecting CRT in children, including D-dimer, insertion location, type of catheter, number of lumens, catheter indwelling time, and central line-associated bloodstream infection (CLABSI).
Similar content being viewed by others
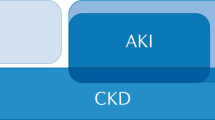
Acute kidney injury
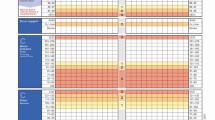
Using National Early Warning Score (NEWS) 2 to help manage medical emergencies in the dental practice
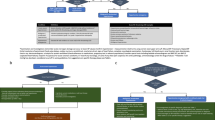
Management of hypertensive crisis: British and Irish Hypertension Society Position document
Introduction.
Central venous access device (CVAD) is an infusion device inserted through different parts to make the tip of the catheter to the vena cava. In the clinic, CVAD is mainly divided into the following four categories: tunneled central venous catheter (CVC), nontunneled CVC, peripherally inserted central catheter (PICC), and totally implantable venous access port (TIVAP). 1 Pediatric patients often require stable, multifunctional, and comfortable long-term vascular access due to factors such as poor puncture cooperation, small vessel diameter, poor peripheral venous visibility and tolerance, high water content in the body leading to easy dehydration, and easy changes in condition after diseases. 2 The application of CVAD can significantly reduce the frequency of venipuncture, relieve the stimulation of drugs on the venous blood vessels, alleviate the pain and fear of the children, improve their medication compliance, ensure the effectiveness of intravenous infusion, and improve the quality of disease treatment. 3 , 4 , 5 Therefore, CVAD is widely used in pediatric clinics and has become an indispensable aspect of complex medical care for children with severe and chronic diseases.
Although CVAD has become an important tool in the pediatric treatment and nursing process, there are also risks of complications related to it, including CVAD-related thrombosis (CRT), phlebitis, fluid and blood leakage at the puncture point, catheter displacement, catheter obstruction, central line-associated bloodstream infection (CLABSI) and so on. 6 , 7 Among these, CRT is one of the most common and serious complications. The prevalence of CRT in children varies significantly by country, age, disease, and medical institution, ranging from 2 to 81%, 4 , 8 , 9 , 10 while in Chinese children without prophylactic treatment ranges from 20 to 66%. 11 , 12 CRT has no obvious clinical symptoms in the early stage, but it may still cause serious side effects, not only increasing the patient pain and medical costs but also delaying treatment timing, affecting prognosis and quality of life, and in severe cases, may even lead to thromboembolism, endangering life. 13 , 14 , 15
Identifying risk factors and incidence of CRT facilitates clinical practitioners in the early identification of high-risk patients, designing specific preventive strategies, treatment regimens, and management plans, thereby effectively reducing the incidence of CRT in hospitalized children and alleviating unnecessary patient suffering. However, most current research on CRT involves only small-scale groups in isolated nursing units or specific disease types. To date, no up-to-date systematic review provides pooled estimates of the risk factors and prevalence of CRT in children. Therefore, this study had a dual purpose: 1. to explore potential risk factors for CRT in children and to determine a pooled level of CRT prevalence; and 2. to provide evidence-based recommendations to improve the recognition, control, and treatment of CRT in children, as well as better nursing management for CRT.
This review was conducted following the Preferred Reporting Items for Systematic Reviews and Meta-Analyses (PRISMA) guidelines. 16 The detailed research protocol can be accessed on the PROSPERO website (registration number: CRD42023421353).
Search strategy
Eight electronic databases were utilized to conduct a thorough literature search: PubMed, Web of Science, Embase, Cochrane Library, Scopus, China National Knowledge Infrastructure (CNKI), Sinomed, and Wanfang. The search in these databases was conducted from the earliest records available up to January 31st, 2024. The search strategy used a combination of Mesh terms and free words. The following Mesh terms and free words were mainly used: “child,” “children,” “adolescent,” “infant,” “pediatrics,” “central venous access device-related thrombosis,” “CRT,” “catheter-related thrombosis,” “catheter-related venous thrombosis,” “CVC-related thrombosis,” “risk factors,” “protective factors,” “predictors,” “causality,” “influencing factors”. The full search strategy for each database is available in the Supplementary Materials. In addition, we screened the reference lists of all included studies for relevant studies that met the criteria. Grey literature was searched as well. Some authors were contacted through email to gather more information or clarify any uncertainties.
Inclusion criteria
The study population was hospitalized children aged ≤18 years.
The primary research objective was to explore the risk factors for CRT.
The study results have at least one statistically significant predictor.
Case-control studies or cohort studies.
Published in English or Chinese.
Exclusion criteria
Catheter-related infection, catheter dysfunction, or other catheter complications as the primary outcome indicators.
Repeated published research.
Case reports, study designs, or clinical trials.
Reviews, editorials, letters, and conference abstracts.
In vitro or animal research.
Data were incomplete and could not be extracted.
Unable to find the original article.
Data extraction
Data from each eligible study were independently extracted by two reviewers using a pre-designed data collection form. Any disagreements were resolved by discussions among all authors. Data on the following characteristics were obtained from all included studies (see Supplementary Table S 1 for details):
Basic information: first author, country, year of publication, study duration, and study design.
Demographic characteristics: study population, sample size, number of CRT, and CRT rate.
Catheter-related features: catheter type, CRT type, and diagnostic method.
Potential risk factors for CRT: odds ratios (OR) or relative risks (RR) values and 95% confidence interval (CI) were extracted for each risk factor. If the study did not provide specific values, it was calculated by constructing a 2 × 2 contingency table.
Quality assessment
Two reviewers evaluated the quality of each study independently using the Risk of Bias Assessment for Nonrandomized Studies tool, 17 with any differences settled via group discussion. The tool assessed six domains of risk of bias: participant selection, confounding variables, exposure measurement, blinding of outcome assessment, incomplete outcome data, and selective outcome reporting. If all six domains were rated as low risk, the overall risk of bias for the study was low. The overall risk of bias was moderate if at least one domain was rated as unclear risk, and no domain was rated as high risk, and high if one or more domains were rated as high risk.
To ensure the accuracy of the assessment results, a third reviewer randomly selected five studies to check the data extraction and quality assessment.
Qualitative synthesis and quantitative meta-analysis
Qualitatively classify each risk factor as definite, likely, unclear, or not a risk factor based on the total number of studies with low and moderate bias risks and the proportion of studies demonstrating positive association (Box 1 in the supplementary material). If a risk factor was reported by more than two studies with low or moderate risk of bias, and the definition and reference range were sufficiently consistent, a quantitative meta-analysis was performed to estimate the combined OR.
Data were analyzed using Revman 5.4 software. In the meta-analysis of risk factors and CRT rate, the generic inverse variance method was applied, which only required effect estimate and standard error (SE). 18 The SE was obtained by inverse transforming the 95% CI applying the standard normal distribution. Heterogeneity tests were performed on the studies included in the Meta-analysis to examine for the combinability of the results of each independent study. P ≥ 0.05 and I-squared ( I 2 ) < 50% considered less heterogeneity between studies and therefore a fixed-effects model was chosen for the analysis, conversely, P < 0.05 or I 2 ≥ 50% considered greater heterogeneity, and a random-effects model was chosen.
Certainty of the evidence
The Grading of Recommendations Assessment, Development, and Evaluation (GRADE) method was used to assess the certainty of the evidence. In this method, observational studies were initially classified as low-quality evidence and then downgraded and upgraded according to five downgrading and three upgrading principles. The 5 downgrading factors included risk of bias, inconsistency, indirectness, imprecision, and publication bias, and the 3 upgrading factors included the magnitude of an effect, dose-response gradient, and effect of plausible residual confounding. Based on these considerations, the overall certainty of each piece of evidence was rated as one of four levels: high, moderate, low, or very low.
The initial search of the databases extracted a total of 4193 articles, of which 1656 were duplicates and removed. The titles and abstracts of the remaining 2537 articles were screened according to the inclusion criteria and 142 were selected for full-text search. After a rigorous eligibility review, 45 articles met the inclusion criteria. In addition, two articles were found to meet the eligibility criteria in a search of the reference lists of the selected articles and grey literature. In the end, a total of 47 articles were included in this review, of which 43 contributed to the qualitative synthesis and quantitative meta-analysis (Fig. 1 ).
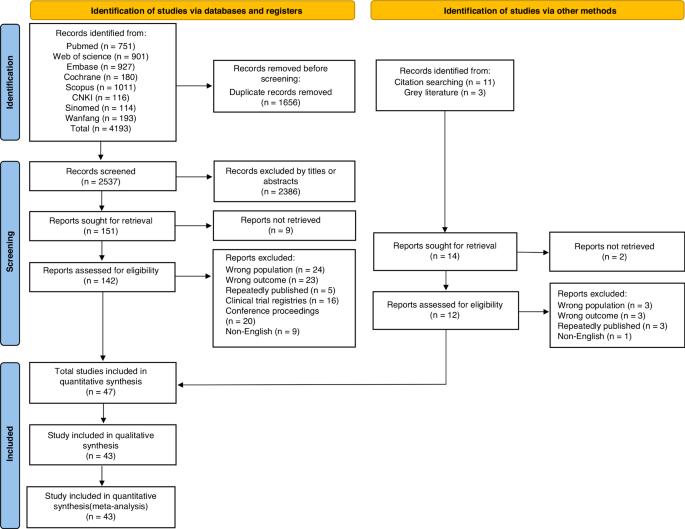
Demonstrate the screening and inclusion process for systematic literature search.
Of the 47 studies, 19 were prospective 4 , 13 , 19 , 20 , 21 , 22 , 23 , 24 , 25 , 26 , 27 , 28 , 29 , 30 , 31 , 32 , 33 , 34 , 35 and the rest were retrospective, 9 , 12 , 36 , 37 , 38 , 39 , 40 , 41 , 42 , 43 , 44 , 45 , 46 , 47 , 48 , 49 , 50 , 51 , 52 , 53 , 54 , 55 , 56 , 57 , 58 , 59 , 60 , 61 of which 10 were multicenter 4 , 9 , 13 , 21 , 23 , 26 , 27 , 28 , 49 , 59 and 37 were single-center. 12 , 19 , 20 , 22 , 24 , 25 , 29 , 30 , 31 , 32 , 33 , 34 , 35 , 36 , 37 , 38 , 39 , 40 , 41 , 42 , 43 , 44 , 45 , 46 , 47 , 48 , 50 , 51 , 52 , 53 , 54 , 55 , 56 , 57 , 58 , 60 , 61 The sample sizes ranged from 47 to 158,299, with the two largest being 71,782 13 and 158,299, 59 respectively. In addition, three studies constructed clinical prediction models. 22 , 28 , 47 Table 1 lists the summary characteristics of the included studies.
Study populations and CRT rates in included studies
These studies investigated a series of hospitalized children of different ages and departments, of which 12 studies with all hospitalized children as the study population, 12 studies with PICU hospitalized children as the study population, six studies with NICU hospitalized children as the study population, one study with all ICU hospitalized children as the study population, four studies with leukemia children as the study population, two studies with infants under 1-year-old as the study population, and the other ten studies with children with a specific disease as the study population.
The combined CRT rate was 9.1% (95% CI : 5.7–14.5%) with a high degree of heterogeneity ( I 2 = 100%). The combined CRT rate was 11.5% (95% CI : 5.7–23.1%; I 2 = 99%) in both male and female children. The frequency of CRT in PICU and NICU was available from 13 articles with 234,464 children and 7 articles with 6093 infants, which combined CRT rates were 10.7% (95% CI : 3.8–23.7%; I 2 = 100%), 2.9% (95% CI : 1.0–6.5%; I 2 = 96%), respectively. The combined CRT rate of children with leukemia was 13.0% (95% CI : 2.9–38.3%; I 2 = 98%) (Supplementary Material Figs. S 1 – 6 )
Quality of the CRT studies
The methodological quality of the included studies varied (Fig. 2 and Supplementary Material Fig. S 7 ). Nine studies had a low overall risk of bias, as all six domains were categorized as low risk. Four studies had a high overall risk of bias, three of which were associated with confounding variables and one to participant selection. The remaining 34 studies had a moderate overall risk of bias, with at least one of the six domains having an unclear risk.
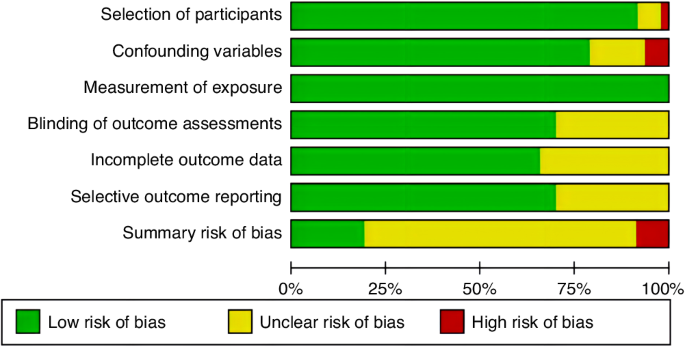
A summary presentation of the assessment results of risk of bias for the 47 studies.
Risk factors of CRT in included studies
The 47 included studies reported 61 statistically significant risk factors for CRT (Table 1 ). These factors were classified into three categories: patient-related risk factors (37.7%, 23/61); CVAD-related risk factors (34.4%, 21/61), and treatment-related risk factors (27.9%, 17/61).
Based on the qualitative synthesis, six variables were considered to be definite risk factors for CRT, including D-dimer, location of insertion, type of catheter, number of lumens, catheter indwelling time, and CLABSI. Eleven variables were considered likely associated with CRT, including gastrointestinal diseases, history of catheterization, thrombophilia, geographic location of line placement, catheter dysfunction, number of catheters, insertion length (cm), catheter to vein ratio, dialysis, hypertonic liquid, and cardiac catheterization. For 42 variables, the relationship with CRT was deemed unclear due to conflicting results from studies assessed as having low and moderate risk of bias, or because they were positively associated in only one study. Additionally, birth weight and gestational age were considered non-risk factors (Table 2 ).
Meta-analyses were implemented for risk factors that were reported by at least two low or moderate risk of bias studies with a consistent definition and reference range (Table 3 and Figs. 3 – 6 ).
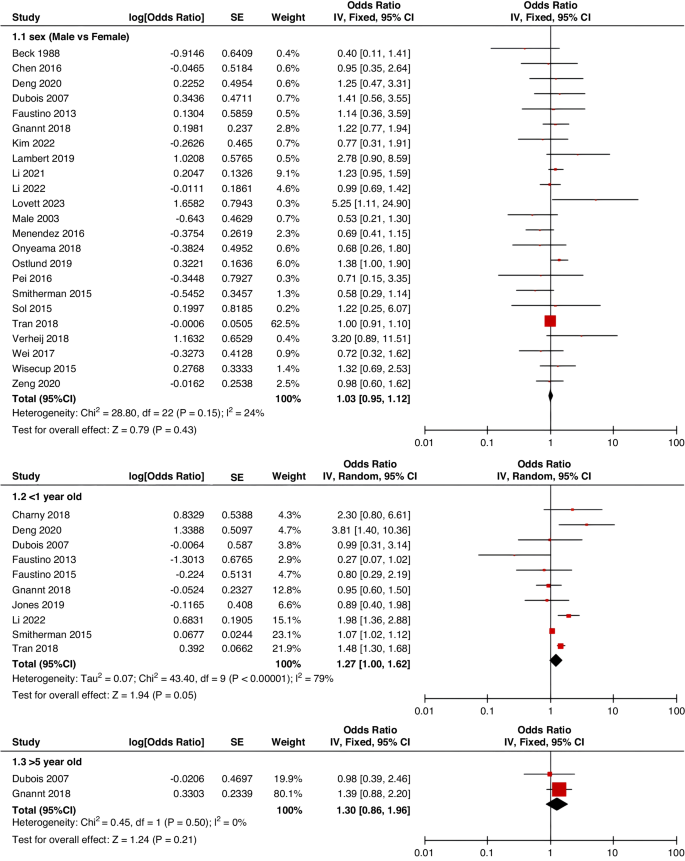
Forest plots of odds ratios (OR) that were included in the quantitative meta-analysis and the associated overall OR. For each OR, the size of the red square region is proportional to the corresponding study weight. Diamond shape intervals represent the overall OR. I 2 represents the fraction of variability among the individual OR that cannot be explained by sampling variability.
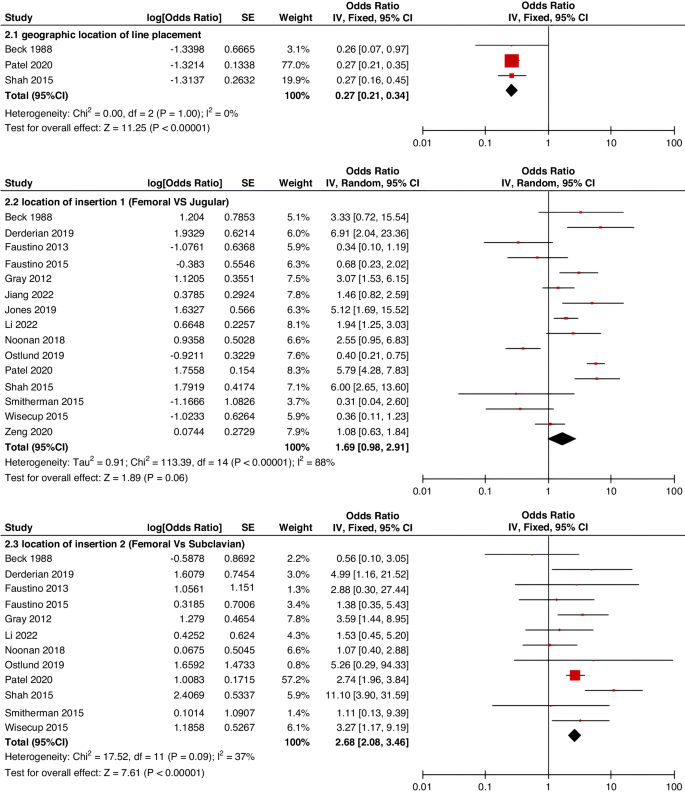
Forest plots of odds ratios (OR) that were included in the quantitative meta-analysis and the associated overall OR. For each OR, the size of the red square region is proportional to the corresponding study weight. Diamond shape intervals represent the overall OR. I 2 represents the fraction of variability among the individual OR that cannot be explained by sampling variability.
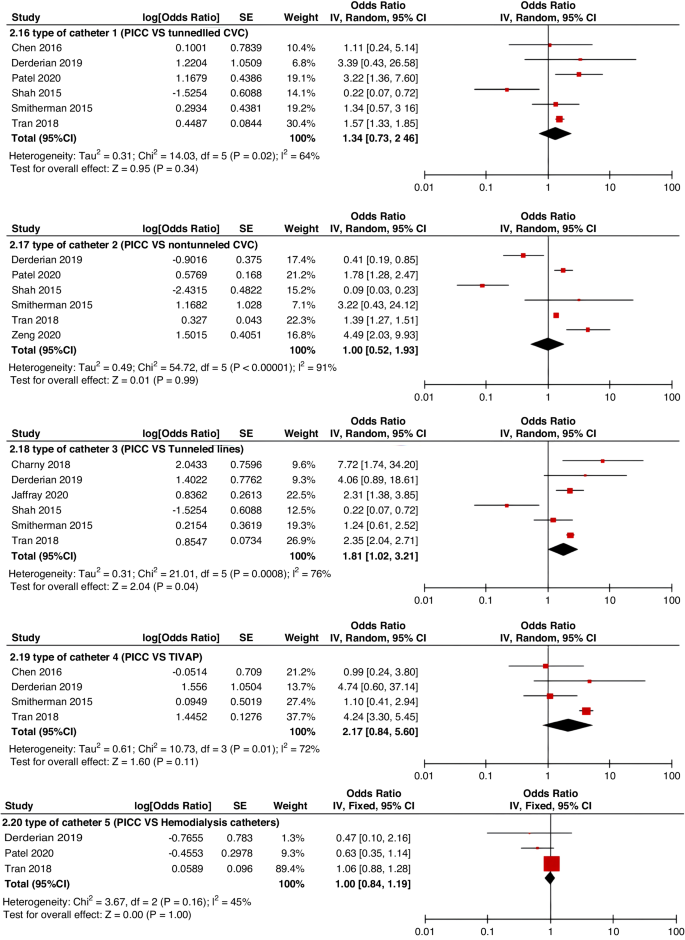
GRADE assessment of evidence
Supplementary Table S 2 shows GRADE assessments for the certainty of evidence. Due to the design of the observational studies, all evidence was initially rated as low certainty. Based on five downgrading and three upgrading principles, 17 pieces of evidence were still rated as low certainty, and the remaining 44 pieces of evidence were downgraded to very low certainty for serious inconsistency and imprecision.
Our study is the latest systematic review of risk factors and the incidence of CRT in hospitalized children. Based on 47 studies included in the current meta-analysis, which involved a total of 262,587 patients, the pooled prevalence of CRT is 9.1%. We conducted a qualitative synthesis analysis of 61 predictive factors and a quantitative meta-analysis of 38 factors, identifying six definite factors, 11 likely factors, and 42 unclear factors associated with CRT. Definite predictors included being of D-dimer, location of insertion, type of catheter, number of lumens, catheter indwelling time and CLABSI. The findings of our systematic review provide the latest comprehensive evidence summary that can inform the early identification of children at risk for CRT and the development of intervention measures to prevent and reduce CRT.
Implantable and temporary medical devices such as CVAD are exposed to blood for weeks to years depending on the type of CVAD in place. Since CVAD is an artificial surface and lacks an endothelial layer that inhibits platelet coagulation and adhesion, it is thought to potentially activate the contact pathways, ultimately leading to thrombosis. Assembly of artificial surface contact systems might be part of the host defense mechanism against foreign substances, but it can lead to kinin and thrombin generation, and complement activation. 62 This eventually promotes thrombosis and inflammation. The presence of CVAD is the most common risk factor for venous thromboembolism (VTE). CRT accounts for 10% of deep vein thrombosis (DVT) in adults and 50–80% in children. 10 , 55 , 63 The incidence of CRT in hospitalized children has increased significantly by 30–70% over the past 20 years, 64 , 65 which may cause serious medical complications besides increasing healthcare expenditures and length of stay.
We discover that a higher level of D-dimer is an independent risk factor for CRT in hospitalized children, consistent with the results of adult studies. 66 D-dimer is a soluble fibrin degradation product deriving from the plasmin-mediated degradation of cross-linked fibrin that is increased or positive in secondary hyperfibrinolysis, such as hypercoagulable states, disseminated intravascular coagulation, and thrombolytic therapy. 67 , 68 Increased D-dimer suggests an association with thrombotic disorders in the body of various origins and an increase in fibrinolytic activity. D-dimer has been extensively investigated for excluding the diagnosis of VTE and is used routinely for this indication. 67 , 69 Therefore, for early recognition and to reduce the incidence of CRT, D-dimer levels should be closely monitored before and after catheterization. However, the elevated D-dimer test results cannot fully explain the cause and location of CRT formation and must be analyzed in conjunction with clinical and other test results. Inherited thrombophilia, caused by genetic defects leading to a deficiency or abnormality in associated proteins, including protein C, protein S, antithrombin, the coagulation factor V Leiden mutation, and factor II mutation G20210A, 70 is considered a potential risk factor for CRT. The prevalence of thrombophilia varies widely among different populations, with a reported prevalence of 10% to 59% in pediatric VTE patients. 71 Children with gastrointestinal diseases like short bowel syndrome (SBS) and inflammatory bowel disease (IBD) have an increased risk of developing CRT during hospitalization. The precise mechanism behind this association is still uncertain according to current research. It may be attributed to the heightened inflammation levels during catheterization, particularly in patients with active IBD episodes or admissions during surgery, which leads to a period of increased inactivity. 55 This suggests that delaying placement during the most active period of inflammation may reduce the rate of thrombosis.
A narrative review pointed out that age is one of the most significant risk factors for VTE. In children, CRT shows a bimodal distribution, with the highest incidence rate in infancy and adolescence. 10 The higher incidence in infancy may be due in part to the smaller diameter of the vein, making insertion difficult and requiring multiple attempts. However, whether age is a risk factor for CRT is still highly controversial. The study by Chojnacka et al. did not find a statistically significant difference, 39 although a trend toward a similar bimodal distribution was found in the study population. Cancer, cardiovascular disease, sepsis, asphyxia, and neurological diseases are also considered unclear factors for CRT. Pediatric patients diagnosed with leukemia have multiple risk factors for VTE formation, such as the presence of hypercoagulable blast cells, the pro-thrombotic nature of the cancer itself, and treatment with steroids and L-asparaginase. Chen et al. 38 and Jaffray et al. 4 concluded that children with leukemia are more likely to develop CRT. Sepsis causes the coagulation mechanism to become fragile, which in turn activates the coagulation system and creates thrombosis. 72 However, a study by Onyeama et al. 52 showed that sepsis was significantly associated with a reduced incidence of CRT, and the exact mechanism is currently unknown.
The location of insertion and type of catheter are critical risk factors for CRT. The incidence of CRT is higher in femoral vein catheterizations compared to subclavian and jugular vein catheterizations in children, which is contrary to findings in adult patients. 73 The femoral location is a larger vessel and allows placement of a larger size catheter. Femoral CVAD is prioritized in urgent and emergency situations. In such cases, the patients tend to be more critically ill and often immobilized, further exacerbating the low-flow state. In addition, there may be vein compression and kinking beneath the inguinal ligament with leg movement, which may increase the risk of CRT. 27 PICC catheters provide a reliable medium to long-term route to intravenous therapy for children, but compared with other types of catheters, the risk of CRT is higher. We speculate that the long tunnel length and relatively large lumen size of the PICC, compared to the diameter of the vessel at the insertion site, may lead to increased blood flow obstruction. 52 Additionally, patients with PICC may be more likely to be diagnosed with symptomatic VTE than tunneled lines (TLs) because PICC is often placed in smaller vessels and journeys through the arm or leg causing limb pain and swelling, whereas TLs are located in the chest.
The risk of CRT increases with the number of lumens. A possible explanation for this finding is that multilumen catheters tend to have larger catheter sizes and thus occupy more area within the vessel lumen, leading to obstruction of normal blood flow within the veins. The relationship between CRT and CLABSI is bidirectional. Following catheter insertion, a fibrin sheath forms around the catheter. Microorganisms, especially staphylococcus aureus, easily adhere to the fibrin sheaths, and may lead to CLABSI. 74 Conversely, CLABSI can trigger inflammatory reactions, leading to further progression of thrombosis. CVAD duration is positively associated with the risk of CRT. Catheter placement may cause mechanical injury to the vein. As the indwelling duration increases, many damaged smooth muscle and endothelial cells become embedded within the fibrin, resulting in thrombus formation. In addition, prolonged indwelling increases the chance of platelet contact with the vessel lining, activating coagulation factors and thrombin, increasing the risk of thrombosis. 22 Therefore, nurses should perform routine maintenance of the catheter in children who require long-term CVAD indwelling. The duration of CVAD should be monitored, the necessity of its indwelling should be assessed daily, and the catheter should be removed as early as possible while ensuring treatment.
As obstruction of venous blood flow from the CVAD is considered an essential causative mechanism for the development of VTE, a high ratio between catheter size and vein diameter could be a risk factor for CRT. The 2012 international guidelines on pediatric CVC insertion recommend that the ratio between the catheter’s external diameter and the cannulated vein’s diameter should not exceed 0.33. 75 However, this suggestion is only based on expert opinions and currently lacks relevant clinical data support. Therefore, further research is still needed to verify it. Catheter dysfunction is mainly caused by small clots or fibrous sheaths wrapping around the tip of the catheter. Prolonged accumulation may lead to incomplete or complete blockage of blood vessels, becoming a gathering point for thrombosis. 74 Journeycake et al. observed that the risk of VTE was highest in pediatric cancer patients with multiple episodes of catheter dysfunction. 76 A study of pediatric brain tumor patients reported that VTE was more common in patients with catheter dysfunction. 77 Thus, these studies and the current data support the need to consider catheter dysfunction as a possible risk factor for CRT and to design further screening and intervention studies for early identification and prevention of catheter dysfunction.
The rationale for studying the relationship between the insertion side of CVAD and the risk of CRT is based on the anatomy of the upper body venous system. The left brachiocephalic vein is longer and courses more horizontally than the right side, thus entering the superior vena cava at a sharper angle. The right jugular vein is the most direct and shortest route for the CVAD to enter the heart. By contrast, the CVAD located in the left jugular vein has a greater distance to the heart and passes through 2 angles in the venous system, which may cause endothelial damage and increase the likelihood of blood flow obstruction and venous wall adhesion. 26 However, our meta-analysis did not find a statistically significant increase in the risk of CRT with left-sided placement compared to right-sided placement. The ideal location for the catheter tip is the junction of the superior vena cava and the right atrium. This location is preferred because of the higher blood flow rate, which may be protective against thrombosis. 43 Currently, the pediatric literature on the effect of optimal tip position on CRT is scarce and inconclusive. In addition, catheter tips do not always remain in that position after initial placement. Therefore, tip movement should be a significant concern in pediatric patients, especially active, growing, and requiring long-term catheter use.
Providing renal replacement therapy is a lifelong task for pediatric end-stage renal disease (ESRD) patients. Although successful transplantation can be achieved even in young patients, the lifespan of the graft is limited. Consequently, many transplant recipients may be put back on dialysis as part of their ESRD treatment. 78 CVC remains the main vascular access for hemodialysis in children. Long-term reliance on CVC is related to a high incidence of catheter dysfunction and failure. The frequent need for recurrent CVC placement in such patients leads to an elevated risk of central vein stenosis and CRT. Cardiac catheterization is also a possible risk factor for CRT. Appropriate anticoagulation is required during catheterization, without which the risk of thrombosis is up to 40%. However, the use of unfractionated heparin in pediatric patients is challenging because the coagulation system and heparin response are different from that of adults. 79 There’s a need for further research to determine if children are receiving adequate doses of heparin during cardiac catheterization to prevent thrombosis without increasing the risk of bleeding complications. The incidence of VTE in adult patients who are chronically bedridden and braked is 3.59 times higher than in patients with normal activity levels. 80 In critically ill or surgical children, mechanical ventilation is often performed in the early stages, requiring continuous use of multiple sedative or inotropic drugs to reduce cardiac load and protect pulmonary function. During sedation, the child is in a braked state, limb activity is reduced or even inactive, blood flow slows down, and blood stagnates in the veins, increasing the chance of platelet adhesion to the endothelium, which may increase the risk of CRT. Therefore, passive movements such as limb abduction, internal rotation, elbow flexion and elbow extension should be performed appropriately when the child’s condition permits.
Nutritional support is an important part of critical illness treatment, including enteral and parenteral nutrition (PN). CVAD is the supply channel for total parenteral nutrition (TPN), and some children may even need this method to provide calories for a long time. High glucose and calcium concentrations in PN are both possible triggers of CRT, and PN has been shown to upregulate the extrinsic coagulation cascade, especially with long-term use. 60 Diamanti et al. reported that the incidence rate of TPN complicated with CRT was 20%. 81 Mannitol or glycerol fructose are widely used as hypertonic drugs in clinical practice, which can increase plasma osmolality to dehydrate tissues after entering the body. At the same time, it may cause a cellular stress response, induce apoptosis, and can activate inflammatory cytokines and coagulation pathways to induce thrombosis. Jiang et al. 22 found vasoactive drugs to be a risk factor for CRT. The possible reason is that vasoactive drugs can cause strong vasoconstriction, endothelial function damage or impairment, and promote fibrinogen synthesis. However, this is contrary to the findings of Marquez et al. 28 and Faustino et al. 21 Therefore, larger prospective studies are still needed to assess this risk factor more precisely.
The strengths of this study include the systematic identification of all relevant studies of risk factors for CRT in hospitalized children and the classification of risk factors into three categories, patient-related risk factors, CVAD-related risk factors, and treatment-related risk factors, to offer a logical progression of the possible causes of CRT in children. However, several limitations of this systematic review should be stated. Firstly, as most of the studies originate from Western countries, extrapolating these results to Eastern populations is questionable. Second, significant heterogeneity was encountered in our analysis, potentially stemming from variations in regimen, duration, population enrolled, and center setting, among other factors. This diversity necessitates a cautious interpretation of the results. In addition, only a few high-quality studies with a low risk of bias, and many of the studies suffer from significant sources of bias. Furthermore, the effect in many occasions was assessed by very few studies. Therefore, the evidence to support it is low, which needs to be validated in future studies. Finally, risk factors for CRT could not be made causal assertions since the majority of studies were retrospective.
Conclusions
In conclusion, we have identified several critical factors that affect CRT, including D-dimer, location of insertion, type of catheter, number of lumens, catheter indwelling time, and CLABSI. Nevertheless, none of the included studies considered the impact of socio-demographic factors on CRT, such as parental education level, occupation, and family economic status. Therefore, larger sample sizes and well-designed prospective studies are still needed to clarify the predictors affecting CRT in the future. In addition, there is a lack of pediatric-specific CRT risk assessment tools, which need to be further developed and validated. Machine learning (ML), as a method for designing risk assessment models that help to efficiently explore and mine useful information, has been widely used in recent years to solve a variety of challenging medical problems. Likewise, the application of ML in CRT risk diagnosis may contribute to a more precise assessment. In clinical practice, it is necessary to take appropriate stratified preventive measures according to the level of CRT risk assessment of children, to improve the efficiency of clinical work, reduce the burden of clinical work, and minimize the occurrence of CRT under the premise of ensuring the safety of children.
Data availability
The datasets used and/or analyzed during the current study are available from the corresponding author upon reasonable request.
Yeow, M. et al. A systematic review and network meta-analysis of randomized controlled trials on choice of central venous access device for delivery of chemotherapy. J. Vasc. Surg. Venous Lymphat. Disord. 10 , 1184–91.e8 (2022).
Article PubMed Google Scholar
Cellini, M. et al. Guidelines of the Italian Association of Pediatric Hematology and Oncology for the management of the central venous access devices in pediatric patients with onco-hematological disease. J. Vasc. Access 23 , 3–17 (2022).
Ares, G. & Hunter, C. J. Central venous access in children: indications, devices, and risks. Curr. Opin. Pediatr. 29 , 340–346 (2017).
Jaffray, J. et al. Peripherally inserted central catheters lead to a high risk of venous thromboembolism in children. Blood 135 , 220–226 (2020).
Article CAS PubMed Google Scholar
Zhang, J. J. et al. Factors affecting mechanical complications of central venous access devices in children. Pediatr. Surg. Int. 38 , 1067–1073 (2022).
Article PubMed PubMed Central Google Scholar
Akhtar, N. & Lee, L. Utilization and Complications of Central Venous Access Devices in Oncology Patients. Curr. Oncol. 28 , 367–377 (2021).
Ullman, A. J., Marsh, N., Mihala, G., Cooke, M. & Rickard, C. M. Complications of Central Venous Access Devices: A Systematic Review. Pediatrics 136 , e1331–e1344 (2015).
Östlund, Å. et al. Erratum to ‘Incidence of and risk factors for venous thrombosis in children with percutaneous non-tunnelled central venous catheters’ (Br J Anaesth 2019; 123: 316-24). Br. J. Anaesth. 123 , 918 (2019).
McLaughlin, C. M. et al. Symptomatic catheter-associated thrombosis in pediatric trauma patients: Choose your access wisely. Surgery 166 , 1117–1121 (2019).
Citla Sridhar, D., Abou-Ismail, M. Y. & Ahuja, S. P. Central venous catheter-related thrombosis in children and adults. Thromb. Res. 187 , 103–112 (2020).
Zhou, X. et al. A retrospective analysis of risk factors associated with catheter-related thrombosis: a single-center study. Perfusion 35 , 806–813 (2020).
Li, S. et al. Risk factors for central venous catheter-related thrombosis in hospitalized children: a single-center a retrospective cohort study. Transl. Pediatr. 11 , 1840–1851 (2022).
Patel, N., Petersen, T. L., Simpson, P. M., Feng, M. & Hanson, S. J. Rates of Venous Thromboembolism and Central Line-Associated Bloodstream Infections Among Types of Central Venous Access Devices in Critically Ill Children. Crit. Care Med. 48 , 1340–1348 (2020).
Timsit, J. F. et al. A state of the art review on optimal practices to prevent, recognize, and manage complications associated with intravascular devices in the critically ill. Intensive Care Med. 44 , 742–759 (2018).
Ullman, A. J. et al. Pediatric central venous access devices: practice, performance, and costs. Pediatr. Res. 92 , 1381–1390 (2022).
Hutton, B. et al. The PRISMA extension statement for reporting of systematic reviews incorporating network meta-analyses of health care interventions: checklist and explanations. Ann. Intern Med. 162 , 777–784 (2015).
Kim, S. Y. et al. Testing a tool for assessing the risk of bias for nonrandomized studies showed moderate reliability and promising validity. J. Clin. Epidemiol. 66 , 408–414 (2013).
Borenstein, M., Hedges, L. V., Higgins, J. P. & Rothstein, H. R. A basic introduction to fixed-effect and random-effects models for meta-analysis. Res. Synth. Methods 1 , 97–111 (2010).
Beck, C., Dubois, J., Grignon, A., Lacroix, J. & David, M. Incidence and risk factors of catheter-related deep vein thrombosis in a pediatric intensive care unit: a prospective study. J. Pediatr. 133 , 237–241 (1998).
Dubois, J. et al. Incidence of deep vein thrombosis related to peripherally inserted central catheters in children and adolescents. Cmaj 177 , 1185–1190 (2007).
Faustino, E. V. et al. Incidence and acute complications of asymptomatic central venous catheter-related deep venous thrombosis in critically ill children. J. Pediatr. 162 , 387–391 (2013).
Jiang, W. et al. Construction and validation of a risk prediction model for central venous catheter-associated deep venous thromboses in children with congenital heart disease after surgery. Chin. J. Nurs. 57 , 2217–2224 (2022).
Google Scholar
Faustino, E. V. et al. Factor VIII May Predict Catheter-Related Thrombosis in Critically Ill Children: A Preliminary Study. Pediatr. Crit. Care Med. 16 , 497–504 (2015).
Jones, S., Butt, W., Monagle, P., Cain, T. & Newall, F. The natural history of asymptomatic central venous catheter-related thrombosis in critically ill children. Blood 133 , 857–866 (2019).
Kim, E. H. et al. Central venous catheter-related thrombosis in pediatric surgical patients: A prospective observational study. Paediatr. Anaesth. 32 , 563–571 (2022).
Male, C. et al. Central venous line-related thrombosis in children: association with central venous line location and insertion technique. Blood 101 , 4273–4278 (2003).
Male, C., Julian, J. A., Massicotte, P., Gent, M. & Mitchell, L. Significant association with location of central venous line placement and risk of venous thrombosis in children. Thromb. Haemost. 94 , 516–521 (2005).
Marquez, A., Shabanova, V. & Faustino, E. V. Prediction of Catheter-Associated Thrombosis in Critically Ill Children. Pediatr. Crit. Care Med. 17 , e521–e528 (2016).
Menéndez, J. J. et al. Incidence and risk factors of superficial and deep vein thrombosis associated with peripherally inserted central catheters in children. J. Thromb. Haemost. 14 , 2158–2168 (2016).
Rubio Longo, M. C. et al. Catheter-related deep vein thrombosis in newborn infants. Arch. Argent. Pediatr. 119 , 32–38 (2021).
PubMed Google Scholar
Östlund, Å. et al. Incidence of and risk factors for venous thrombosis in children with percutaneous non-tunnelled central venous catheters. Br. J. Anaesth. 123 , 316–324 (2019).
Sol, J. J. et al. Chronic Complications After Femoral Central Venous Catheter-related Thrombosis in Critically Ill Children. J. Pediatr. Hematol. Oncol. 37 , 462–467 (2015).
van Rooden, C. J. et al. Infectious complications of central venous catheters increase the risk of catheter-related thrombosis in hematology patients: a prospective study. J. Clin. Oncol. 23 , 2655–2660 (2005).
Zeng, X., Zhang, C. & Shi, Y. Analysis of risk factors for complicated catheter-related thrombosis in children. Chin. J. Emerg. Med. 29 , 719–723 (2020).
Wei, Y. et al. The incidence and risk factors of catheter-related-thrombosis during induction chemotherapy in acute lymphocytic leukemia children. Chin. J. Hematol. 38 , 313–317 (2017).
CAS Google Scholar
Deng, G. & Liao, Q. Analysis of risk factors for venous thrombosis after PICC placement in critically ill children. Int. I Nurs. 39 , 775–777 (2020).
Badheka, A. V. et al. Catheter related thrombosis in hospitalized infants: A neural network approach to predict risk factors. Thromb. Res. 200 , 34–40 (2021).
Chen, K. et al. Risk factors for central venous catheter-related thrombosis in children: a retrospective analysis. Blood Coagul. Fibrinol. 27 , 384–388 (2016).
Article Google Scholar
Chojnacka, K., Krasiński, Z., Wróblewska-Seniuk, K. & Mazela, J. Catheter-related venous thrombosis in NICU: A case-control retrospective study. J. Vasc. Access 23 , 88–93 (2022).
Derderian, S. C., Good, R., Vuille-Dit-Bille, R. N., Carpenter, T. & Bensard, D. D. Central venous lines in critically ill children: Thrombosis but not infection is site dependent. J. Pediatr. Surg. 54 , 1740–1743 (2019).
Diamond, C. E. et al. Catheter-Related Venous Thrombosis in Hospitalized Pediatric Patients with Inflammatory Bowel Disease: Incidence, Characteristics, and Role of Anticoagulant Thromboprophylaxis with Enoxaparin. J. Pediatr. 198 , 53–59 (2018).
Noailly Charny, P. A. et al. Increased Risk of Thrombosis Associated with Peripherally Inserted Central Catheters Compared with Conventional Central Venous Catheters in Children with Leukemia. J. Pediatr. 198 , 46–52 (2018).
Gnannt, R. et al. Increased risk of symptomatic upper-extremity venous thrombosis with multiple peripherally inserted central catheter insertions in pediatric patients. Pediatr. Radio. 48 , 1013–1020 (2018).
Gray, B. W. et al. Characterization of central venous catheter-associated deep venous thrombosis in infants. J. Pediatr. Surg. 47 , 1159–1166 (2012).
Haddad, H. et al. Routine surveillance ultrasound for the management of central venous catheters in neonates. J. Pediatr. 164 , 118–122 (2014).
Lambert, I., Tarima, S., Uhing, M. & Cohen, S. S. Risk Factors Linked to Central Catheter-Associated Thrombosis in Critically Ill Infants in the Neonatal Intensive Care Unit. Am. J. Perinatol. 36 , 291–295 (2019).
Li, H. et al. Prediction of central venous catheter-associated deep venous thrombosis in pediatric critical care settings. BMC Med. Inf. Decis. Mak. 21 , 332 (2021).
Article CAS Google Scholar
Lovett, M. E. et al. Catheter-associated deep vein thrombosis in children with severe traumatic brain injury: A single-center experience. Pediatr. Blood Cancer 70 , e30044 (2023).
MacLean, J. et al. Need for tissue plasminogen activator for central venous catheter dysfunction is significantly associated with thrombosis in pediatric cancer patients. Pediatr. Blood Cancer 65 , e27015 (2018).
Noonan, P. J., Hanson, S. J., Simpson, P. M., Dasgupta, M. & Petersen, T. L. Comparison of Complication Rates of Central Venous Catheters Versus Peripherally Inserted Central Venous Catheters in Pediatric Patients. Pediatr. Crit. Care Med. 19 , 1097–1105 (2018).
Pei, L. et al. Clinical characteristics and risk factors of symptomatic central venous catheter-related deep vein thrombosis in children. Chin. Pediatr. Emerg. Med. 23 , 450–454 (2016).
Onyeama, S. N. et al. Central Venous Catheter-associated Venous Thromboembolism in Children With Hematologic Malignancy. J. Pediatr. Hematol. Oncol. 40 , e519–e524 (2018).
Shah, S. H. et al. Clinical risk factors for central line-associated venous thrombosis in children. Front. Pediatr. 3 , 35 (2015).
Shin, H. S., Towbin, A. J., Zhang, B., Johnson, N. D. & Goldstein, S. L. Venous thrombosis and stenosis after peripherally inserted central catheter placement in children. Pediatr. Radio. 47 , 1670–1675 (2017).
Smitherman, A. B. et al. The incidence of catheter-associated venous thrombosis in noncritically ill children. Hosp. Pediatr. 5 , 59–66 (2015).
Steen, E. H. et al. Central Venous Catheter-Related Deep Vein Thrombosis in the Pediatric Cardiac Intensive Care Unit. J. Surg. Res 241 , 149–159 (2019).
Wang, J. & Ren, G. Peripherally inserted central catheter related venous thromboembolism in children with acute leukemia: a factorial analysis. Chin. J. Biomed. Eng. 27 , 288–293 (2021).
Dubbink-Verheij, G. H. et al. Femoral Vein Catheter is an Important Risk Factor for Catheter-related Thrombosis in (Near-)term Neonates. J. Pediatr. Hematol. Oncol. 40 , e64–e68 (2018).
Tran, M., Shein, S. L., Ji, X. & Ahuja, S. P. Identification of a “VTE-rich” population in pediatrics - Critically ill children with central venous catheters. Thromb. Res 161 , 73–77 (2018).
Wisecup, S., Eades, S. & Turiy, Y. Characterizing the Risk Factors Associated With Venous Thromboembolism in Pediatric Patients After Central Venous Line Placement. J. Pediatr. Pharm. Ther. 20 , 358–366 (2015).
Zhu, W., Zhang, H., Xing, Y. Clinical Characteristics of Venous Thrombosis Associated with Peripherally Inserted Central Venous Catheter in Premature Infants. Children 9 , https://doi.org/10.3390/children9081126 (2022).
Ekdahl, K. N. et al. Innate immunity activation on biomaterial surfaces: a mechanistic model and coping strategies. Adv. Drug Deliv. Rev. 63 , 1042–1050 (2011).
Article CAS PubMed PubMed Central Google Scholar
Takemoto, C. M. et al. Hospital-associated venous thromboembolism in children: incidence and clinical characteristics. J. Pediatr. 164 , 332–338 (2014).
Boulet, S. L. et al. Trends in venous thromboembolism-related hospitalizations, 1994-2009. Pediatrics 130 , e812–e820 (2012).
Raffini, L., Huang, Y. S., Witmer, C. & Feudtner, C. Dramatic increase in venous thromboembolism in children’s hospitals in the United States from 2001 to 2007. Pediatrics 124 , 1001–1008 (2009).
Lin, S., Zhu, N., YihanZhang, Du, L. & Zhang, S. Development and validation of a prediction model of catheter-related thrombosis in patients with cancer undergoing chemotherapy based on ultrasonography results and clinical information. J. Thromb. Thrombolysis 54 , 480–491 (2022).
Johnson, E. D., Schell, J. C. & Rodgers, G. M. The D-dimer assay. Am. J. Hematol. 94 , 833–839 (2019).
Favresse, J. et al. D-dimer: Preanalytical, analytical, postanalytical variables, and clinical applications. Crit. Rev. Clin. Lab Sci. 55 , 548–577 (2018).
Weitz, J. I., Fredenburgh, J. C. & Eikelboom, J. W. A Test in Context: D-Dimer. J. Am. Coll. Cardiol. 70 , 2411–2420 (2017).
Darlow, J. & Mould, H. Thrombophilia testing in the era of direct oral anticoagulants. Clin. Med. 21 , e487–e491 (2021).
Monagle, P. et al. American Society of Hematology 2018 Guidelines for management of venous thromboembolism: treatment of pediatric venous thromboembolism. Blood Adv. 2 , 3292–3316 (2018).
Meziani, F., Gando, S. & Vincent, J. L. Should all patients with sepsis receive anticoagulation? Yes. Intensive Care Med 43 , 452–454 (2017).
Saber, W. et al. Risk factors for catheter-related thrombosis (CRT) in cancer patients: a patient-level data (IPD) meta-analysis of clinical trials and prospective studies. J. Thromb. Haemost. 9 , 312–319 (2011).
Journeycake, J. M. & Buchanan, G. R. Thrombotic complications of central venous catheters in children. Curr. Opin. Hematol. 10 , 369–374 (2003).
Lamperti, M. et al. International evidence-based recommendations on ultrasound-guided vascular access. Intensive Care Med 38 , 1105–1117 (2012).
Journeycake, J. M. & Buchanan, G. R. Catheter-related deep venous thrombosis and other catheter complications in children with cancer. J. Clin. Oncol. 24 , 4575–4580 (2006).
Deitcher, S. R., Gajjar, A., Kun, L. & Heideman, R. L. Clinically evident venous thromboembolic events in children with brain tumors. J. Pediatr. 145 , 848–850 (2004).
Mandel-Shorer, N., Tzvi-Behr, S., Harvey, E. & Revel-Vilk, S. Central venous catheter-related venous thrombosis in children with end-stage renal disease undergoing hemodialysis. Thromb. Res 172 , 150–157 (2018).
Chen, D., Långström, S., Petäjä, J., Heikinheimo, M. & Pihkala, J. Thrombin formation and effect of unfractionated heparin during pediatric cardiac catheterization. Catheter Cardiovasc Inter. 81 , 1174–1179 (2013).
Reynolds, P. M. et al. Evaluation of Prophylactic Heparin Dosage Strategies and Risk Factors for Venous Thromboembolism in the Critically Ill Patient. Pharmacotherapy 39 , 232–241 (2019).
Diamanti, A. et al. Prevalence of life-threatening complications in pediatric patients affected by intestinal failure. Transpl. Proc. 39 , 1632–1633 (2007).
Download references
This study was supported by the Fundamental Research Funds for the Central Universities [grant numbers YCJJ20230244] and Tongji Hospital, Tongji Medical College, Huazhong University of Science and Technology Research Fund [grant numbers 2022C09].
Author information
Authors and affiliations.
Department of Nursing, Tongji Hospital, Tongji Medical College, Huazhong University of Science and Technology, Wuhan, China
Maoling Fu, Qiaoyue Yang, Yaqi Yu, Wenshuai Song, Xiuli Qin, Ying Luo, Xiaoju Xiong & Genzhen Yu
School of Nursing, Tongji Medical College, Huazhong University of Science and Technology, Wuhan, China
Maoling Fu, Quan Yuan, Qiaoyue Yang, Yaqi Yu & Wenshuai Song
You can also search for this author in PubMed Google Scholar
Contributions
GY and YL framed the review questions on the basis of input from MF and QY. YY and XQ conducted the literature search. MF, WS, and QY screened and evaluated the identified papers. GY and YY performed data extraction and analysis. MF, WS, XQ and QY prepared the initial manuscript with revisions and comments from GY, YL, and XX. All authors approved the final manuscript as submitted and agreed to be accountable for all aspects of the work.
Corresponding author
Correspondence to Genzhen Yu .
Ethics declarations
Competing interests.
The authors declare no competing interests.
Additional information
Publisher’s note Springer Nature remains neutral with regard to jurisdictional claims in published maps and institutional affiliations.
Supplementary information
Supplementary checklist, supplemental digital tables1, supplemental digital tables2, supplemental digital, rights and permissions.
Open Access This article is licensed under a Creative Commons Attribution 4.0 International License, which permits use, sharing, adaptation, distribution and reproduction in any medium or format, as long as you give appropriate credit to the original author(s) and the source, provide a link to the Creative Commons licence, and indicate if changes were made. The images or other third party material in this article are included in the article’s Creative Commons licence, unless indicated otherwise in a credit line to the material. If material is not included in the article’s Creative Commons licence and your intended use is not permitted by statutory regulation or exceeds the permitted use, you will need to obtain permission directly from the copyright holder. To view a copy of this licence, visit http://creativecommons.org/licenses/by/4.0/ .
Reprints and permissions
About this article
Cite this article.
Fu, M., Yuan, Q., Yang, Q. et al. Risk factors and incidence of central venous access device-related thrombosis in hospitalized children: a systematic review and meta-analysis. Pediatr Res (2024). https://doi.org/10.1038/s41390-024-03225-0
Download citation
Received : 06 October 2023
Revised : 18 March 2024
Accepted : 25 March 2024
Published : 17 May 2024
DOI : https://doi.org/10.1038/s41390-024-03225-0
Share this article
Anyone you share the following link with will be able to read this content:
Sorry, a shareable link is not currently available for this article.
Provided by the Springer Nature SharedIt content-sharing initiative
Quick links
- Explore articles by subject
- Guide to authors
- Editorial policies

- Python For Data Analysis
- Data Science
- Data Analysis with R
- Data Analysis with Python
- Data Visualization with Python
- Data Analysis Examples
- Math for Data Analysis
- Data Analysis Interview questions
- Artificial Intelligence
- Data Analysis Projects
- Machine Learning
- Deep Learning
- Computer Vision
- What is Data Analytics?
- What is Statistical Analysis in Data Science?
- What Is Spatial Analysis, and How Does It Work
- What is Data Analysis?
- What is Data Munging in Analysis?
- What is Geospatial Data Analysis?
- What is Exploratory Data Analysis ?
- Qualitative and Quantitative Data
- What are Descriptive Analytics?
- What is Prescriptive Analytics in Data Science?
- Qualitative Data
- Data Analysis Tutorial
- Why Data Visualization Matters in Data Analytics?
- What is Data Lineage?
- Data analysis using R
- What is Data Organization?
- What is a Data Science Platform?
What is Qualitative Data Analysis?
Understanding qualitative information analysis is important for researchers searching for to uncover nuanced insights from non-numerical statistics. By exploring qualitative statistics evaluation, you can still draw close its importance in studies, understand its methodologies, and determine while and the way to apply it successfully to extract meaningful insights from qualitative records.
The article goals to provide a complete manual to expertise qualitative records evaluation, masking its significance, methodologies, steps, advantages, disadvantages, and applications.
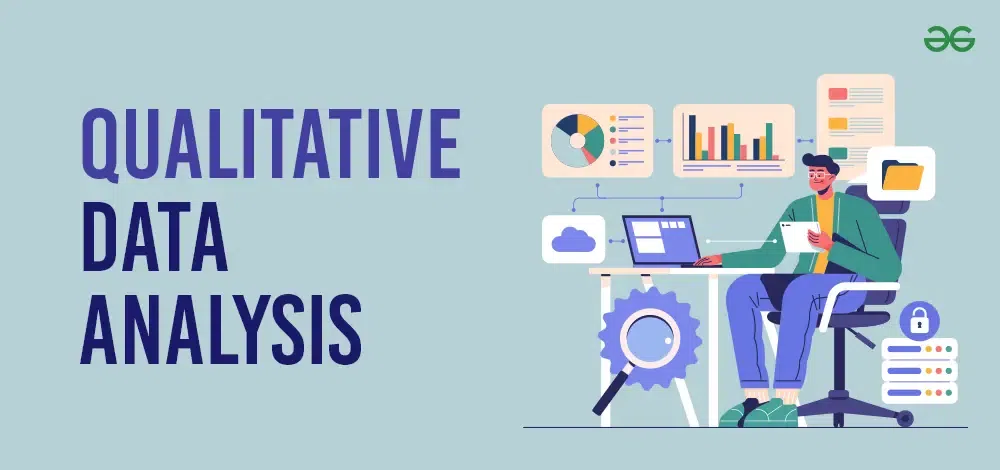
Table of Content
Understanding Qualitative Data Analysis
Importance of qualitative data analysis, steps to perform qualitative data analysis, 1. craft clear research questions, 2. gather rich customer insights, 3. organize and categorize data, 4. uncover themes and patterns : coding, 5. make hypotheses and validating, methodologies in qualitative data analysis, advantages of qualitative data analysis, disadvantages of qualitative data analysis, when qualitative data analysis is used, applications of qualitative data analysis.
Qualitative data analysis is the process of systematically examining and deciphering qualitative facts (such as textual content, pix, motion pictures, or observations) to discover patterns, themes, and meanings inside the statistics· Unlike quantitative statistics evaluation, which focuses on numerical measurements and statistical strategies, qualitative statistics analysis emphasizes know-how the context, nuances, and subjective views embedded inside the information.
Qualitative facts evaluation is crucial because it is going past the bloodless hard information and numbers to provide a richer expertise of why and the way things appear. Qualitative statistics analysis is important for numerous motives:
- Understanding Complexity and unveils the “Why” : Quantitative facts tells you “what” came about (e· g·, sales figures), however qualitative evaluation sheds light on the motives in the back of it (e·g·, consumer comments on product features).
- Contextual Insight : Numbers don’t exist in a vacuum. Qualitative information affords context to quantitative findings, making the bigger photo clearer· Imagine high customer churn – interviews would possibly monitor lacking functionalities or perplexing interfaces.
- Uncovers Emotions and Opinions: Qualitative records faucets into the human element· Surveys with open ended questions or awareness companies can display emotions, critiques, and motivations that can’t be captured by using numbers on my own.
- Informs Better Decisions: By understanding the “why” and the “how” at the back of customer behavior or employee sentiment, companies can make greater knowledgeable decisions about product improvement, advertising techniques, and internal techniques.
- Generates New Ideas : Qualitative analysis can spark clean thoughts and hypotheses· For example, via analyzing consumer interviews, commonplace subject matters may emerge that cause totally new product features.
- Complements Quantitative Data : While each facts sorts are precious, they paintings quality collectively· Imagine combining website site visitors records (quantitative) with person comments (qualitative) to apprehend user revel in on a particular webpage.
In essence, qualitative data evaluation bridges the gap among the what and the why, providing a nuanced know-how that empowers better choice making·
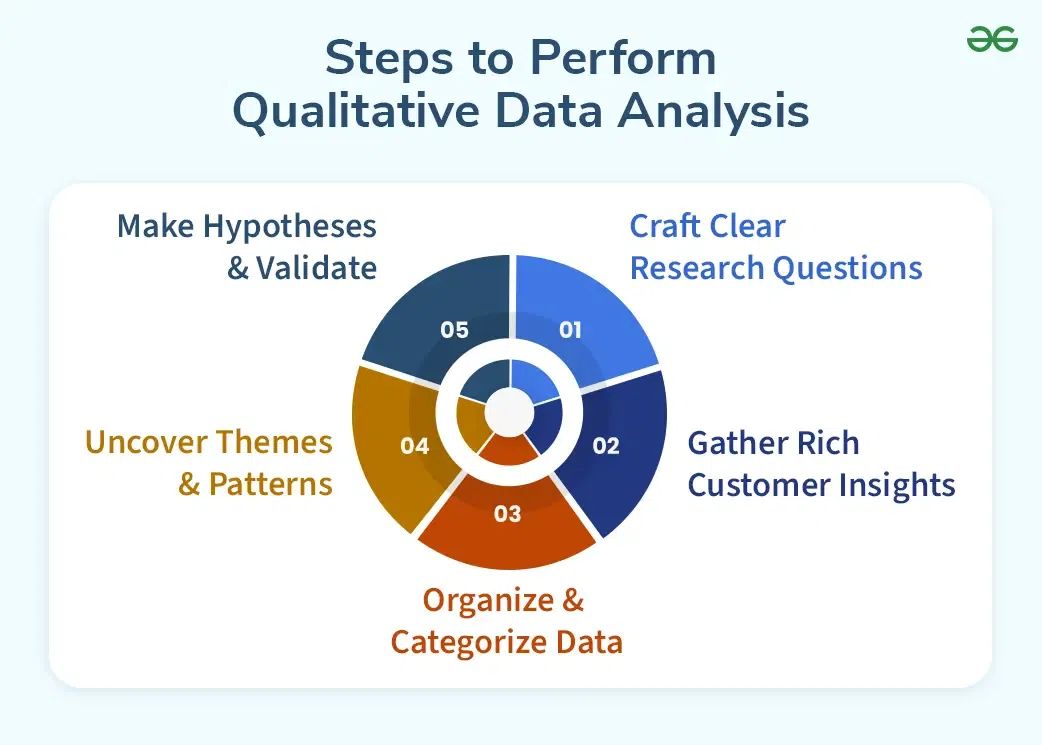
Qualitative data analysis process, follow the structure in below steps:
Qualitative information evaluation procedure, comply with the shape in underneath steps:
Before diving into evaluation, it is critical to outline clear and particular studies questions. These questions ought to articulate what you want to study from the data and manual your analysis towards actionable insights. For instance, asking “How do employees understand the organizational culture inside our agency?” helps makes a speciality of know-how personnel’ perceptions of the organizational subculture inside a selected business enterprise. By exploring employees’ perspectives, attitudes, and stories related to organizational tradition, researchers can find valuable insights into workplace dynamics, communication patterns, management patterns, and worker delight degrees.
There are numerous methods to acquire qualitative information, each offering specific insights into client perceptions and reviews.
- User Feedback: In-app surveys, app rankings, and social media feedback provide direct remarks from users approximately their studies with the products or services.
- In-Depth Interviews : One-on-one interviews allow for deeper exploration of particular topics and offer wealthy, special insights into individuals’ views and behaviors.
- Focus Groups : Facilitating group discussions allows the exploration of numerous viewpoints and permits individuals to construct upon every different’s ideas.
- Review Sites : Analyzing purchaser critiques on systems like Amazon, Yelp, or app shops can monitor not unusual pain points, pride levels, and areas for improvement.
- NPS Follow-Up Questions : Following up on Net Promoter Score (NPS) surveys with open-ended questions allows customers to elaborate on their rankings and provides qualitative context to quantitative ratings.
Efficient facts below is crucial for powerful analysis and interpretation.
- Centralize: Gather all qualitative statistics, along with recordings, notes, and transcripts, right into a valuable repository for smooth get admission to and control.
- Categorize through Research Question : Group facts primarily based at the specific studies questions they deal with. This organizational structure allows maintain consciousness in the course of analysis and guarantees that insights are aligned with the research objectives.
Coding is a scientific manner of assigning labels or categories to segments of qualitative statistics to uncover underlying issues and patterns.
- Theme Identification : Themes are overarching principles or ideas that emerge from the records· During coding, researchers perceive and label segments of statistics that relate to those themes, bearing in mind the identification of vital principles in the dataset.
- Pattern Detection : Patterns seek advice from relationships or connections between exceptional elements in the statistics. By reading coded segments, researchers can locate trends, repetitions, or cause-and-effect relationships, imparting deeper insights into patron perceptions and behaviors.
Based on the identified topics and styles, researchers can formulate hypotheses and draw conclusions about patron experiences and choices.
- Hypothesis Formulation: Hypotheses are tentative causes or predictions based on found styles within the information. Researchers formulate hypotheses to provide an explanation for why certain themes or styles emerge and make predictions approximately their effect on patron behavior.
- Validation : Researchers validate hypotheses by means of segmenting the facts based on one-of-a-kind standards (e.g., demographic elements, usage patterns) and analyzing variations or relationships inside the records. This procedure enables enhance the validity of findings and offers proof to assist conclusions drawn from qualitative evaluation.
There are five common methodologies utilized in Qualitative Data Analysis·
- Thematic Analysis : Thematic Analysis involves systematically figuring out and reading habitual subject matters or styles within qualitative statistics. Researchers begin with the aid of coding the facts, breaking it down into significant segments, and then categorizing these segments based on shared traits. Through iterative analysis, themes are advanced and refined, permitting researchers to benefit insight into the underlying phenomena being studied.
- Content Analysis: Content Analysis focuses on reading textual information to pick out and quantify particular styles or issues. Researchers code the statistics primarily based on predefined classes or subject matters, taking into consideration systematic agency and interpretation of the content. By analyzing how frequently positive themes occur and the way they’re represented inside the data, researchers can draw conclusions and insights relevant to their research objectives.
- Narrative Analysis: Narrative Analysis delves into the narrative or story within qualitative statistics, that specialize in its structure, content, and meaning. Researchers examine the narrative to understand its context and attitude, exploring how individuals assemble and speak their reports thru storytelling. By analyzing the nuances and intricacies of the narrative, researchers can find underlying issues and advantage a deeper know-how of the phenomena being studied.
- Grounded Theory : Grounded Theory is an iterative technique to growing and checking out theoretical frameworks primarily based on empirical facts. Researchers gather, code, and examine information without preconceived hypotheses, permitting theories to emerge from the information itself. Through constant assessment and theoretical sampling, researchers validate and refine theories, main to a deeper knowledge of the phenomenon under investigation.
- Phenomenological Analysis : Phenomenological Analysis objectives to discover and recognize the lived stories and views of people. Researchers analyze and interpret the meanings, essences, and systems of these reviews, figuring out not unusual topics and styles across individual debts. By immersing themselves in members’ subjective stories, researchers advantage perception into the underlying phenomena from the individuals’ perspectives, enriching our expertise of human behavior and phenomena.
- Richness and Depth: Qualitative records evaluation lets in researchers to discover complex phenomena intensive, shooting the richness and complexity of human stories, behaviors, and social processes.
- Flexibility : Qualitative techniques offer flexibility in statistics collection and evaluation, allowing researchers to conform their method based on emergent topics and evolving studies questions.
- Contextual Understanding: Qualitative evaluation presents perception into the context and meaning of information, helping researchers recognize the social, cultural, and historic elements that form human conduct and interactions.
- Subjective Perspectives : Qualitative methods allow researchers to explore subjective perspectives, beliefs, and reviews, offering a nuanced know-how of people’ mind, emotions, and motivations.
- Theory Generation : Qualitative information analysis can cause the generation of recent theories or hypotheses, as researchers uncover patterns, themes, and relationships in the records that might not were formerly recognized.
- Subjectivity: Qualitative records evaluation is inherently subjective, as interpretations can be stimulated with the aid of researchers’ biases, views, and preconceptions .
- Time-Intensive : Qualitative records analysis may be time-consuming, requiring giant data collection, transcription, coding, and interpretation.
- Generalizability: Findings from qualitative studies might not be effortlessly generalizable to larger populations, as the focus is often on know-how unique contexts and reviews in preference to making statistical inferences.
- Validity and Reliability : Ensuring the validity and reliability of qualitative findings may be difficult, as there are fewer standardized methods for assessing and establishing rigor in comparison to quantitative studies.
- Data Management : Managing and organizing qualitative information, together with transcripts, subject notes, and multimedia recordings, can be complicated and require careful documentation and garage.
- Exploratory Research: Qualitative records evaluation is nicely-suited for exploratory studies, wherein the aim is to generate hypotheses, theories, or insights into complex phenomena.
- Understanding Context : Qualitative techniques are precious for knowledge the context and which means of statistics, in particular in studies wherein social, cultural, or ancient factors are vital.
- Subjective Experiences : Qualitative evaluation is good for exploring subjective stories, beliefs, and views, providing a deeper knowledge of people’ mind, feelings, and behaviors.
- Complex Phenomena: Qualitative strategies are effective for studying complex phenomena that can not be effortlessly quantified or measured, allowing researchers to seize the richness and depth of human stories and interactions.
- Complementary to Quantitative Data: Qualitative information analysis can complement quantitative research by means of offering context, intensity, and insight into the meanings at the back of numerical statistics, enriching our knowledge of studies findings.
- Social Sciences: Qualitative information analysis is widely utilized in social sciences to apprehend human conduct, attitudes, and perceptions. Researchers employ qualitative methods to delve into the complexities of social interactions, cultural dynamics, and societal norms. By analyzing qualitative records which include interviews, observations, and textual resources, social scientists benefit insights into the elaborate nuances of human relationships, identity formation, and societal structures.
- Psychology : In psychology, qualitative data evaluation is instrumental in exploring and deciphering person reports, emotions, and motivations. Qualitative methods along with in-depth interviews, cognizance businesses, and narrative evaluation allow psychologists to delve deep into the subjective stories of individuals. This approach facilitates discover underlying meanings, beliefs, and emotions, dropping light on psychological processes, coping mechanisms, and personal narratives.
- Anthropology : Anthropologists use qualitative records evaluation to look at cultural practices, ideals, and social interactions inside various groups and societies. Through ethnographic research strategies such as player statement and interviews, anthropologists immerse themselves within the cultural contexts of different agencies. Qualitative analysis permits them to find the symbolic meanings, rituals, and social systems that form cultural identification and behavior.
- Qualitative Market Research : In the sphere of marketplace research, qualitative statistics analysis is vital for exploring consumer options, perceptions, and behaviors. Qualitative techniques which include consciousness groups, in-depth interviews, and ethnographic research permit marketplace researchers to gain a deeper understanding of customer motivations, choice-making methods, and logo perceptions· By analyzing qualitative facts, entrepreneurs can identify emerging developments, discover unmet wishes, and tell product development and advertising and marketing techniques.
- Healthcare: Qualitative statistics analysis plays a important function in healthcare studies via investigating patient experiences, delight, and healthcare practices. Researchers use qualitative techniques which includes interviews, observations, and patient narratives to explore the subjective reviews of people inside healthcare settings. Qualitative evaluation helps find affected person perspectives on healthcare services, treatment consequences, and pleasant of care, facilitating enhancements in patient-targeted care delivery and healthcare policy.
Qualitative data evaluation offers intensity, context, and know-how to investigate endeavors, enabling researchers to find wealthy insights and discover complicated phenomena via systematic examination of non-numerical information.
Please Login to comment...
Similar reads.
- Data Analysis
Improve your Coding Skills with Practice
What kind of Experience do you want to share?
A big data analysis of the adoption of quoting encouragement policy on Twitter during the 2020 U.S. presidential election
- Research Article
- Open access
- Published: 19 May 2024
Cite this article
You have full access to this open access article
- Amirhosein Bodaghi ORCID: orcid.org/0000-0002-9284-474X 1 &
- Jonathan J. H. Zhu 2
This research holds significance for the fields of social media and communication studies through its comprehensive evaluation of Twitter’s quoting encouragement policy enacted during the 2020 U.S. presidential election. In addressing a notable gap in the literature, this study introduces a framework that assesses both the quantitative and qualitative effects of specific platform-wide policy interventions, an aspect lacking in existing research. Employing a big data approach, the analysis includes 304 million tweets from a randomly sampled cohort of 86,334 users, using a systematic framework to examine pre-, within-, and post-intervals aligned with the policy timeline. Methodologically, SARIMAX models and linear regression are applied to the time series data on tweet types within each interval, offering an examination of temporal trends. Additionally, the study characterizes short-term and long-term adopters of the policy using text and sentiment analyses on quote tweets. Results show a significant retweeting decrease and modest quoting increase during the policy, followed by a swift retweeting resurgence and quoting decline post-policy. Users with fewer connections or higher activity levels adopt quoting more. Emerging quoters prefer shorter, positive quote texts. These findings hold implications for social media policymaking, providing evidence for refining existing policies and shaping effective interventions.
Avoid common mistakes on your manuscript.
Introduction
The introduction of the quote tweet feature by Twitter in April 2015 marked a significant development in the platform’s functionality. While a conventional retweet merely reproduces the original tweet, serving as a symbol of agreement and endorsement between users involved [ 1 ], the quote tweet feature allows users to include their own commentary when sharing a tweet. Consequently, this feature has given rise to various novel applications, including the expression of opinions, public replies, and content forwarding [ 2 ]. Notably, owing to the perennial significance of the US presidential elections [ 3 , 4 ], Twitter instituted a novel policy on October 9, 2020, advising users to abstain from mere retweeting and advocating instead for the utilization of quote tweets supplemented by individual perspectives. This policy remained in effect until December 16, 2020. Indeed, before the policy change, retweeting on Twitter was simple. With a single click, users could share a post with their followers. However, during the time policy was held, clicking the retweet button no longer automatically shared the post. Instead, Twitter prompted users to add their own thoughts or comments before sharing. This essentially created a “Quote Tweet.” This extra step was intended to encourage users to share more thoughtfully. Importantly, adding text to the quote tweet was optional. Users could still leave the comment section blank and share the post without any additional commentary. This option essentially replicated the old retweet functionality.
Significance of the research
This research holds significance in the realm of social media and communication studies, particularly in understanding the impact of policy interventions on user behavior. The significance can be delineated through various dimensions. First, the study provides a comprehensive evaluation of the effectiveness of Twitter’s quoting encouragement policy implemented during the 2020 U.S. presidential election. By employing a robust big data approach and sophisticated analytical methods, the research goes beyond anecdotal observations, offering a nuanced understanding of how such policies influence user engagement. This contribution is valuable for social media platforms seeking evidence-based insights into the outcomes of policy interventions, aiding in the refinement of existing policies and the formulation of new ones. Second, the findings offer actionable insights for social media policymakers and practitioners involved in the delicate task of shaping user behavior. Understanding the quantitative and qualitative effects of the policy shift allows for the optimization of future interventions, fostering more effective communication strategies on platforms like Twitter. Policymakers can leverage the identified user characteristics and behavioral patterns to tailor interventions that resonate with the diverse user base, thereby enhancing the impact of social media policies. Finally, the research enriches the theoretical landscape by applying the Motivation Crowding Theory, Theory of Planned Behavior (TPB), and Theory of Diffusion of Innovation (DOI) to the context of social media policy adoption. This interdisciplinary approach contributes to theoretical advancements, offering a framework that can be applied beyond the scope of this study. As theories from economics and psychology are employed to understand user behavior in the digital age, the research paves the way for cross-disciplinary collaborations and a more holistic comprehension of online interactions.
Research gap
Despite the existing body of literature on quoting behavior on Twitter, there is a conspicuous gap in addressing the unique policy implemented by Twitter from October 9 to December 16, 2020, encouraging users to quote instead of retweeting. Previous studies have explored the use of quotes in various contexts, such as political discourse and the spread of misinformation, but none have specifically examined the impact of a platform-wide policy shift promoting quoting behavior. In addition, while some studies have investigated user behaviors associated with quoting, retweeting, and other tweet types, there is a lack of a comprehensive framework that assesses the quantitative and qualitative effects of a specific policy intervention. The current study introduces a detailed evaluation framework, incorporating time series analysis, text analysis, and sentiment analysis, providing a nuanced understanding of the Twitter quoting encouragement policy’s impact on user engagement. Moreover, previous research has explored user characteristics in the context of social media engagement but has not specifically addressed how users' attributes may influence their response to a platform-wide policy change. The current study bridges this gap by investigating how factors like social network size and activity levels correlate with users’ adoption of the quoting encouragement policy. Finally, while some studies have assessed the immediate effects of policy interventions, there is a lack of research investigating the longitudinal impact after the withdrawal of such policies. The current study extends the temporal dimension by examining user behavior during the pre-, within-, and post-intervals, offering insights into the sustained effects and user adaptation following the cessation of the quoting encouragement policy. By addressing these research gaps, the current study seeks to provide a holistic examination of the quoting encouragement policy on Twitter, contributing valuable insights to the fields of social media studies, policy evaluation, and user behavior analysis.
Research objectives
This study aims to assess the effectiveness of the Twitter policy implemented from October 9 to December 16, 2020, which encouraged users to utilize the quote tweet feature instead of simple retweeting. Specifically, the research objectives are twofold: (1) to determine the adoption rate of this policy and evaluate its success, and (2) to identify user characteristics based on their reactions to this policy. The outcomes of this research contribute to both the evaluation of the Twitter policy and the development of more effective approaches to policymaking. Stier et al. [ 5 ] proposed a comprehensive framework comprising four phases for the policymaking process: agenda setting, policy formulation, policy implementation, and evaluation. According to this framework, the evaluation phase involves assessing the outcomes of the policy, considering the perspectives of stakeholders involved in the previous phases. In this context, the present research examines the Twitter quoting encouragement policy, which represents an intervention in the daily usage patterns of Twitter users, through both quantitative and qualitative analyses. The quantitative effects analysis, particularly the achievements observed, provide valuable insights for evaluating the efficacy of the quoting encouragement policy by Twitter. Additionally, the results obtained from the qualitative analyses facilitate policy implementation, which refers to the process of translating policies into practical action under the guidance of an authoritative body.
Quantitative effects
In this section, we present the hypotheses formulated to assess the quantitative effects of the Twitter quoting encouragement policy. The hypotheses are as follows:
H1: The intervention is expected to have a negative impact on users’ retweeting behavior. We hypothesize that the policy promoting the use of quote tweets instead of simple retweets will lead to a reduction in the frequency of retweeting among users.
H2: The intervention is unlikely to significantly affect other types of user behavior, such as posting original or reply tweets, as well as quotes. We anticipate that any observed changes in the rates of these tweet types would be of minimal magnitude and primarily influenced by factors unrelated to the intervention.
H3: The termination of the intervention is anticipated to have a positive effect on users' retweeting behavior. We hypothesize that the discontinuation of the policy encouraging quote tweets will result in an increase in users' retweeting activity.
H4: Similar to H2, the conclusion of the intervention is not expected to impact other tweet types (excluding quotes) in terms of posting behavior. This suggests the presence of a prevailing opinion inertia, where users tend to maintain their existing patterns and tendencies when posting original, reply, and non-quote tweets.
These hypotheses serve as a foundation for analyzing the quantitative effects of the Twitter quoting encouragement policy and investigating its influence on users’ tweet behaviors. Through rigorous analysis, we aim to shed light on the impact of the intervention and its implications for user engagement on the platform.
Qualitative effects
The qualitative effects can be examined from two distinct perspectives: User Characteristics and Text Characteristics. Moreover, the analysis encompasses three intervals, namely the Pre-Interval (prior to the policy implementation), Within Interval (during the policy implementation), and Post-Interval (after the policy withdrawal). The hypotheses for each perspective are as follows:
User characteristics
H5: Users with a larger social network (i.e., more friends) are expected to exhibit a lesser increase in their quoting rate during the Within Interval.
H6: Users who demonstrate a regular pattern of activity, characterized by a lower frequency of overall Twitter engagement (such as publishing at least one tweet type on more days), are more inclined to experience an elevation in their quoting rate during the Within Interval.
H7: Users who engage in a higher volume of retweeting activities during the Pre-Interval are more likely to observe an increase in their quoting rate during the Within Interval.
H8: The swifter users experience an increase in their quoting rate during the Within Interval, the sooner they are likely to discontinue quoting tweets upon entering the Post-Interval.
Text characteristics
H9: Short-term quoters tend to exhibit a comparatively smaller change in the length of their quote texts compared to long-term quoters. This is primarily due to the involuntary nature of the former, whereas the latter are more intentionally created.
H10: The sentiment of quote texts from short-term quoters is generally more likely to elicit a greater range of emotions compared to those from long-term quoters. This difference is attributable, at least in part, to the intervention's influence on short-term quoters.
H11: The quote texts of short-term quoters are generally more prone to receiving a higher number of retweets compared to those of long-term quoters. This can be attributed to factors such as longer text length, less deliberative content, and the presence of heightened emotional elements in the latter.
These hypotheses form the basis for analyzing the qualitative effects of the Twitter quoting encouragement policy, enabling a comprehensive understanding of user and text characteristics during different intervals. By examining these effects, we aim to shed light on the nuanced dynamics that underlie users’ quoting behavior and its implications on social interaction and engagement within the Twitter platform.
Theoretical framework
In alignment with the two main parts of this research, which examine the quantitative and qualitative effects of the recent Twitter policy, the theoretical framework is also divided into two contexts: one for quantitative analysis and the other for investigating qualitative effects. For the quantitative analyses, the motivation crowding theory has played a central role in shaping the corresponding hypotheses. This theory suggests that providing extrinsic incentives for specific behavior can sometimes undermine intrinsic motivation to engage in that behavior [ 6 ]. Although the motivation crowding theory originated in the realm of economics [ 7 ], this study aims to apply it to the adoption of policies within the context of Twitter. By treating the quoting encouragement policy as an incentive, this research seeks to quantify the impact of this incentive during its implementation and withdrawal. Hypotheses 1–4 have been formulated to guide these quantitative analyses and explore the potential influence of the undermining effect on the adoption rate after the policy withdrawal.
Regarding the qualitative analyses, the TPB and the DOI serve as foundational frameworks for developing hypotheses related to user and text characteristics. The TPB explains behavior based on individuals' beliefs through three key components: attitude, subjective norms, and perceived behavioral control, which collectively shape behavioral intentions. Drawing on the TPB, hypotheses 5–8 aim to characterize different users based on their behaviors and attitudes toward the new policy. The DOI provides a platform for distinguishing users based on the time of adoption. In line with this theory, hypotheses 9–11 have been formulated to address characteristics that facilitate early adoption based on the content of quote texts. Figure 1 illustrates the theoretical framework of this study, highlighting its key components.
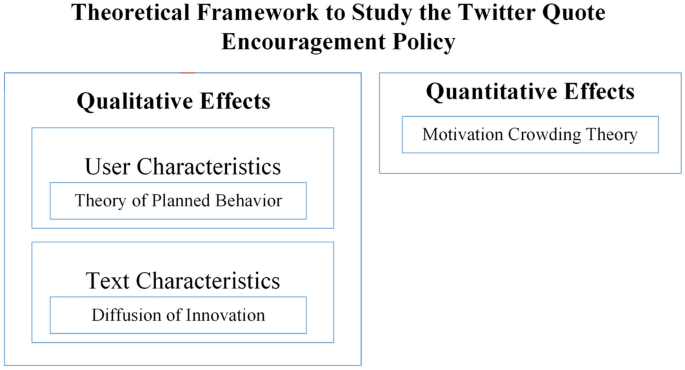
Uniqueness and generalizability
To the best of our knowledge, this research represents the first comprehensive study to investigate the impact of the quoting encouragement policy implemented by Twitter. In comparison to the limited existing studies that have examined Twitter policies in the past, this research distinguishes itself through both the scale of the dataset utilized and the breadth of the analyses conducted. These unique aspects contribute to the applicability of this study in two key areas: methodology and findings. In terms of methodology, the presented approach incorporates an interrupted time series analysis framework, coupled with text and sentiment analyses, to examine policy interventions on a large scale. This framework enables researchers to develop various approaches for analyzing interventions within the realm of social media and big data. With regards to the findings, the extraction of qualitative and quantitative patterns from such a vast dataset yields novel insights. Particularly noteworthy is the ability to juxtapose these macro and micro results, leading to a deeper understanding of the policy’s effects. The findings of this study hold potential value for practitioners and policymakers not only on Twitter but also on other global platforms like Instagram and YouTube. However, it is important to consider certain modifications, such as adapting the follower-to-following ratio, when applying these findings to undirected networks like Facebook, where mutual agreement is necessary for link creation. Moreover, the analysis of this policy, which was implemented during the presidential election, provides valuable insights into its potential impact on public attention. Public attention has recently been identified as a critical factor in the success of presidential candidates [ 8 ]. Therefore, understanding the effects of the quoting encouragement policy can contribute to a better understanding of the dynamics surrounding public attention during such critical periods. Indeed, the uniqueness of this research lies in its pioneering examination of the Twitter quoting encouragement policy, extensive dataset, and comprehensive analyses. These distinct features enhance the applicability of the research in terms of methodology and findings, with potential implications for other global platforms and the study of public attention in political contexts.
Literature review
Given the nature of this research, which focuses on a novel Twitter policy that promotes quoting instead of retweeting, the literature review examines three perspectives: (1) Quote, (2) Engagement, and (3) Hashtag Adoption. These perspectives encompass relevant aspects that align with the scope of this study.
Garimella et al. [ 2 ] conducted a study on the utilization of the newly introduced “quote RT” feature on Twitter, specifically examining its role in political discourse and the sharing of political opinions within the broader social network. Their findings indicated that users who were more socially connected and had a longer history on Twitter were more likely to employ quote RTs. Furthermore, they observed that quotes facilitated the dissemination of political discourse beyond its original source. In a different context, Jang et al. [ 9 ] employed the rate of quotes as a measure to identify and detect fake news on Twitter. Their research focused on leveraging quotes as a means of analyzing the spread of misinformation and distinguishing it from authentic news. Li et al. [ 10 ] tried to identify users with high dissemination capability under different topics. Additionally, Bodaghi et al. [ 11 ] investigated the characteristics of users involved in the propagation of fake news, considering quotes and their combined usage with other tweet types such as retweets and replies. Their analysis aimed to gain insights into the user behaviors associated with the dissemination of false information. South et al. [ 12 ] utilized the quoter model, which mimics the information generation process of social media accounts, to evaluate the reliability and resilience of information flow metrics within a news–network ecosystem. This study focused on assessing the validity of these metrics in capturing the dynamics between news outlets engaged in a similar information dissemination process. By reviewing these studies, we can identify their relevance to the understanding of quoting behavior and its implications within different contexts, such as political discourse and the spread of misinformation. However, it is important to note that these previous works primarily focused on the usage of quotes and their effects without specifically addressing the Twitter policy under investigation in this study.
The concept of engagement on social media platforms, particularly in relation to political communication and online interactions, has been extensively explored in previous studies. Boulianne et al. [ 13 ] conducted research on the engagement rate with candidates’ posts on social media and observed that attack posts tend to receive higher levels of engagement, while tagging is associated with a general trend of lower engagement. Lazarus et al. [ 14 ] focused on President Trump’s tweets and found that engagement levels vary depending on the substantive content of the tweet, with negatively toned tweets and tweets involving foreign policy receiving higher engagement compared to other types of tweets. Yue et al. [ 15 ] delved into how nonprofit executives in the U.S. engage with online audiences through various communication strategies and tactics. Ahmed et al. [ 16 ] examined Twitter political campaigns during the 2014 Indian general election. Bodaghi et al. [ 17 ] conducted a longitudinal analysis on Olympic gold medalists on Instagram, investigating their characteristics as well as the rate of engagement they receive from their followers. Hou et al. [ 18 ] studied the engagement differences between scholars and non-scholars on Twitter. Hoang et al. [ 19 ] aimed at predicting whether a post is going to be forwarded or not. Munoz et al. [ 20 ] proposed an index as a tool to measure engagement based on the tweet and follower approach.
The decision of an online social network user to join a discussion group is not solely influenced by the number of friends who are already members of the group. Backstrom et al. [ 21 ] discovered that factors such as the relationships between friends within the group and the level of activity in the group also play a significant role in the user’s decision. Hu et al. [ 22 ] performed an empirical study on Sina Weibo to understand the selectivity of retweeting behaviors. Moreover, Balestrucci et al. [ 23 ] studied how credulous users engage with social media content. Bodaghi et al. [ 24 ] explored the impact of dissenting opinions on the engagement rate during the process of information spreading on Twitter. Wells et al. [ 25 ] examined the interactions between candidate communications, social media, partisan media, and news media during the 2015–2016 American presidential primary elections. They found that social media activity, particularly in the form of retweets of candidate posts, significantly influenced news media coverage of specific candidates. Yang et al. [ 26 ] investigated the tweet features that trigger customer engagement and found a positive correlation between the rate of quoting and the number of positive quotes. Bodaghi et al. [ 27 ] studied the role of users’ position in Twitter graphs in their engagement with viral tweets. They demonstrated how different patterns of engagement can arise from various forms of graph structures, leading to the development of open-source software for characterizing spreaders [ 28 , 29 ].
Hashtag adoption
The adoption and usage of hashtags on Twitter have been investigated in several studies, shedding light on the factors influencing individual behavior and the role of social networks. Zhang et al. [ 30 ] explored the behavior of Twitter users in adopting hashtags and specifically focused on the effect of “structure diversity” on individual behavior. Their findings suggest that users' behavior in online social networks is not solely influenced by their friends but is also significantly affected by the number of groups to which these friends belong. Tian et al. [ 31 ] investigated the impact of preferred behaviors among a heterogeneous population on social propagation within multiplex-weighted networks. Their research shed light on the diverse adoption behaviors exhibited by individuals with varying personalities in real-world scenarios. Examining hashtag use on Twitter, Monster et al. [ 32 ] examined how social network size influences people's likelihood of adopting novel labels. They found that individuals who follow fewer users tend to use a larger number of unique hashtags to refer to events, indicating greater malleability and variability in hashtag use. Rathnayake [ 33 ] sought to conceptualize networking events from a platform-oriented view of media events, emphasizing the role of hashtags in bottom-up construction. Hashtags played a key role in this taxonomy, reflecting their significance in organizing and categorizing discussions around specific events. Furthermore, Bodaghi et al. [ 34 ] demonstrated that the size of a user's friend network also impacts broader aspects, such as their decision to participate in an information-spreading process. The characteristics and dynamics of an individual’s social network play a role in shaping their behavior and engagement with hashtags. These studies collectively contribute to our understanding of hashtag adoption and its relationship to social networks, providing insights into the factors that influence individuals’ decisions to adopt and use hashtags in online platforms like Twitter.
Method and analysis
Data collection.
For this study, a random sample of 86,334 users from the United States was selected. The data collection process involved crawling their tweets, specifically the last 3200 tweets if available, until October 2020. The crawling process continued for these users at seven additional time intervals until February 2021. This resulted in a total of eight waves of data, encompassing all the tweets from these 86,334 users starting from the 3200th tweet prior to the first crawling time in October 2020, up until their last tweet on February 2, 2021. The eight waves of crawled data were then merged into a final dataset, and any overlapping tweets were removed. The final dataset consists of a data frame containing 304,602,173 unique tweets from the 86,334 users. Each tweet in the dataset is associated with 23 features, resulting in a dataset size exceeding 31 GB. Additionally, another dataset was created by crawling the user characteristics of these 86,334 users, such as the number of followers, friends, and statuses. The dataset includes four types of tweets: Retweet, Quote, Reply, and Original. Each tweet in the dataset belongs to only one of these types (pure mode) or a combination of types (hybrid mode). The hybrid modes are represented in two forms: (1) a retweet of a quote and (2) a reply that contains a quote. To maintain consistency and focus on pure modes in the dataset, the former was considered solely as a retweet, and the latter was treated as a quote only. As a result, the approximate counts of the four tweet types (Retweet, Quote, Reply, and Original) in the dataset are 143 M, 23 M, 77 M, and 61 M, respectively. To ensure a more recent focus on activities, the analysis specifically considered data from October 9, 2019, onwards. This date, October 9, 2019, was chosen as it is one year prior to Twitter’s issuance of the quoting encouragement policy. By using this cutoff date, the analysis concentrates on the data relevant to the policy's implementation and subsequent effects.
Data exploration
This section explores three aspects of the data: (1) the average number of tweets per user in each tweet type, (2) the number of active users in each tweet type, and (3) the usage of hashtags. The analysis includes all 86,334 users in the dataset. The exploration is conducted across three intervals: (1) pre-interval (from October 9, 2019, to October 8, 2020), (2) within-interval (from October 9, 2020, to December 15, 2020), and (3) post-interval (from December 16, 2020, to February 2, 2021). The code used for these explorations is publicly available. Footnote 1 Figure 2 presents the results for the first two aspects. The plots on the left-hand side illustrate the average number of tweets published in each tweet type, namely Original, Quote, Reply, and Retweet. The plots on the right-hand side display the number of active users in each tweet type. Active users in a specific type on a given day are defined as users who have published at least one tweet in that type on that day.
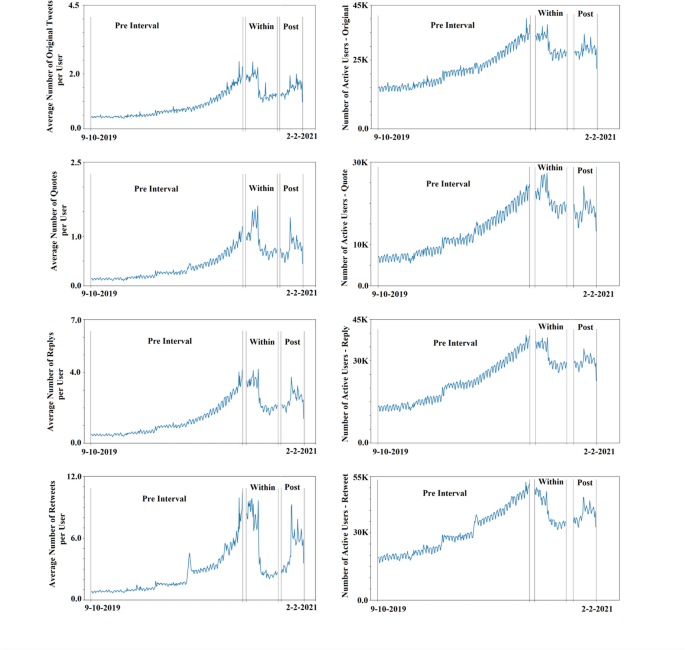
Daily rates of user activities during pre-, within-, and post-intervals
To analyze the usage of hashtags, the first step is to identify political hashtags. This involves extracting all the hashtags used in the dataset from September 1, 2020, to February 1, 2021, excluding the last day of the dataset (February 2, 2021) due to incomplete data collection. The following intervals are defined based on this period:
Pre-Interval: September 1, 2020, to October 8, 2020.
Within-Interval: October 9, 2020, to December 15, 2020.
Post-Interval: December 16, 2020, to February 1, 2021.
The extraction process yields a total of 1,126,587 hashtags. From this set, the 100 most frequently used hashtags are selected for further analysis. These selected hashtags are then reviewed and annotated by two referees, considering their political context. Through consensus between the referees, 32 hashtags out of the initial 100 are identified as political. The results of the usage analysis on these selected political hashtags are presented in Fig. 3 .
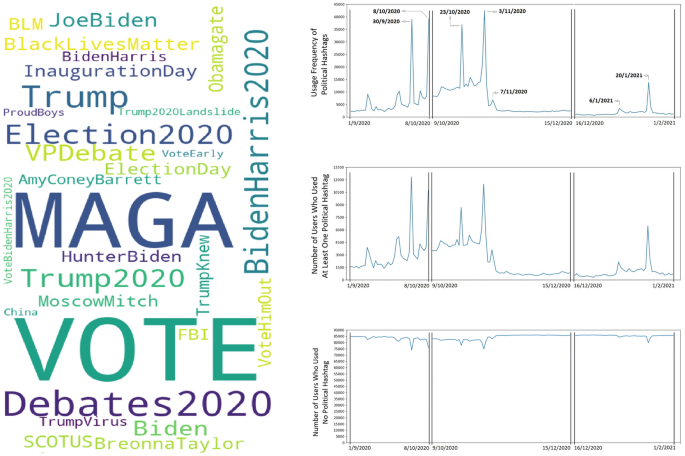
Usage of political hashtags. The left plot presents a word cloud depicting the 32 most frequently repeated political hashtags. The right plot displays the distribution of these political hashtags. The upper plot labels the significant dates associated with spikes in usage
Table 1 displays the key dates corresponding to the significant spikes observed in the plots depicted in Fig. 3 . These events directly influenced the patterns observed in the dataset.
Measurements of quantitative effects
To perform quantitative analysis, the data frame of each user was extracted by segregating all tweets associated with the same user ID. This process resulted in the creation of 86,334 individual data frames, each corresponding to a unique user. Subsequently, each user's data frame was divided into three distinct time intervals as follows:
Pre Interval [2019-10-09 to 2020-10-08]: This interval encompasses the year prior to the implementation of the new Twitter policy on 2020-10-09. Hence, the end of this interval is set as 2020-10-08.
Within Interval [2020-10-09 to 2020-12-15]: This interval spans from the policy’s inception on the first day, i.e., 2020-10-09, until its termination by Twitter on the last day, i.e., 2020-12-15.
Post Interval [2020-12-16 to 2021-02-02]: This interval commences on the day immediately following the removal of the policy, i.e., 2020-12-16, and continues until the last day on which a user published a tweet within the dataset. The dataset's coverage concludes on 2021-02-02, which represents the latest possible end date for this interval if a user had any tweet published on that date.
Impact analysis of the Twitter policy
The objective of this analysis is to assess the individual impact of the new Twitter policy, which promotes quoting instead of retweeting, on each user. Specifically, we aim to examine how the rate and quantity of published tweets per day have been altered following the implementation or removal of the new policy. Figure 4 illustrates the slopes and levels of a selected tweet type (quote) within each interval for a given user. Given the presence of four tweet types and three intervals, it is necessary to fit a total of 12 models for each user, corresponding to each tweet type within each interval.
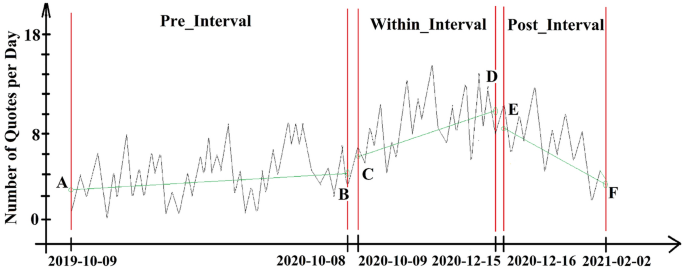
Slope and levels of number of quotes published by users during three intervals. This figure displays the slope and levels of the three intervals (pre-, within-, and post-intervals) for the number of quotes published by each user. The green lines depict the linear regression of the time series for each interval. The slope of pre-interval, within-interval, and post-interval corresponds to the slope of AB, CD, and EF lines, respectively. The start/end levels of pre-interval, within-interval, and post-interval are represented by A/B, C/D, and E/F, respectively
To analyze the impact of the new policy for each tweet type within a specific interval, we applied linear regression using the Ordinary Least Squares method (Eq. 1 ) in Python for users who had at least 7 data points with non-zero values.
where y is the number of tweets per day, x is the number of days, \(\alpha\) is the coefficient representing the slope, \(\varepsilon\) is the error, and \(\beta\) is the level. We then checked for the presence of autocorrelation in the residuals using the Durbin–Watson test (Eq. 2 ). If no autocorrelation was detected, we used linear regression to calculate the slopes and levels.
where d is the Durbin–Watson statistic, \({{\text{e}}}_{{\text{i}}}\) is the residual at observation i, n is the number of observations. The Durbin–Watson statistic ranges from 0 to 4. A value around 2 indicates no autocorrelation, while values significantly less than 2 suggest positive autocorrelation, and values significantly greater than 2 suggest negative autocorrelation. However, if autocorrelation was present, we employed linear regression with autoregressive errors (Eq. 3 ).
where \(\delta = {\widehat{{\varnothing }}}_{1}{\delta }_{i-1}+ {\widehat{{\varnothing }}}_{2}{\delta }_{i-2}+\dots + {\widehat{{\varnothing }}}_{p}{\delta }_{i-p}-{\widehat{\theta }}_{1}{e}_{i-1}- {\widehat{\theta }}_{2}{e}_{i-2}-\dots -{\widehat{\theta }}_{q}{e}_{i-q}+{\varepsilon }_{i}\)
In this equation, the errors are modelled using an ARIMA (p, d, q), where p and q represent the lags in the autoregressive (AR) and moving-average (MA) models, respectively, and d is the differencing value. We utilized the SARIMAX (p, d, q) model in Python to implement this regression, where the exogenous variable X (in Eq. 1 ) represents the number of days.
To determine the best values for the model's parameters, we conducted a grid search to generate a set of potential parameter combinations. We then evaluated the results for each combination based on the following criteria: (1) All achieved coefficients must be significant, (2) Akaike Information Criterion (AIC) based on Eq. ( 4 ) should be less than 5000, and (3) The Ljung–Box coefficient based on Eq. ( 5 ) should be significant (> 0.05).
where \(\mathcal{L}\) is the maximum log-likelihood of the model, k is the number of estimated parameters. A lower AIC value indicates a better estimation of the model orders.
where n is the sample size, \({\rho }_{k}\) is the sample autocorrelation at lag k. The test statistic follows a chi-squared distribution with degrees of freedom equal to the number of lags considered. The null hypothesis is that there is no autocorrelation up to the specified lag. A p-value greater than 0.05 suggests that there is no significant autocorrelation in the residuals, indicating an adequate fit. Finally, among the selected results, the model with the lowest sigma2 shown by Eq. ( 6 ), indicating less variance in the residuals, was chosen as the best-fit model.
where \({e}_{i}\) is the residual at observation i, \(\overline{e }\) is the mean of the residuals, and n is the number of observations. In the case of time series analysis, the residuals are the differences between the observed values and the values predicted by the ARIMA or SARIMAX model. The parameter values corresponding to this model were considered the optimal fit. The entire process for obtaining the slope and level findings is depicted in Fig. 5 , and the results are presented in Table 2 .
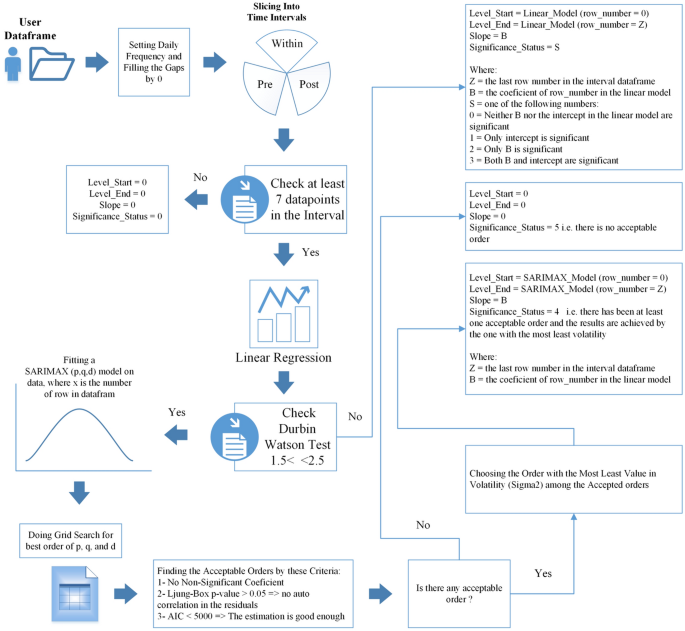
Flowchart illustrating the overall analysis procedure for slope and level assessments
Table 2 illustrates variations in the slope and level of tweeting between intervals. For instance, a level change of − 1.025 indicates a daily decrease of approximately 1.025 quotes from pre-interval to within-interval. Similarly, a slope change of 0.003 reflects an increase of around 0.003 quotes per day in the slope of quoting during the same transition. The table provides additional insights into slope and level changes for other tweet types across different intervals.
Analysis of qualitative effects
In this section, we aim to investigate the changes in user behavior towards the Twitter policy based on user characteristics such as the number of followers, number of friends, and number of statuses. To achieve this, we consider users whose obtained models are significant in both paired intervals (pre-within or within-post). We calculate the correlations between the values of these characteristics and the rate of change in the slope of each tweet type between the intervals. The results of this analysis are presented in Table 3 .
For instance based on Table 3 , investigating the relationships, a notable negative correlation of − 0.042 is observed between the number of friends a user has and the rate of slope change for quote publishing, specifically from the pre-interval to the within-interval. Additionally, a significant negative correlation of − 0.079 is evident between the number of quotes published in the post-interval and the number of retweets previously published in the within-interval. Further detailed explanations and implications are presented in the “ Results ” section.
The analysis of text characteristics focuses on examining the impact of the new policy on the length and sentiment of quote texts. Specifically, we are interested in understanding how the quote texts of two different user groups, namely “short-term quoters” and “long-term quoters,” have changed in terms of length and sentiment from the pre-interval to the within-interval. We define the two groups as follows:
Short-term Quoter: A user who did not engage in quoting during the pre-interval but started quoting in the within interval.
Long-term Quoter: A user who engaged in quoting during the pre-interval and continued to do so in the within interval. A quoter is defined as a user whose average number of quotes in all tweets exceeds a certain threshold.
For the analysis, we extract three characteristics from the quote text: (1) the number of characters (excluding spaces), (2) the sentiment score, and (3) the number of times the quote has been retweeted. We preprocess the text by performing tasks such as removing non-ASCII characters, emojis, mentions, and hashtags. To calculate the sentiment score, we utilize the sentiment analyzer from the Python NLTK package, which is based on VADER, Footnote 2 a lexicon, and rule-based sentiment analysis tool specifically designed for sentiments expressed in social media. The sentiment score calculated by VADER is a compound score that represents the overall sentiment of a text. The score is computed based on the valence (positivity or negativity) of individual words in the text (Eq. 7 ).
where \({S}_{compound}\) is the compound sentiment score, V is the valence score of word and is normalized to be between − 1 (most negative) and 1 (most positive), and I is the intensity of word. The weights are determined by the intensity of each word's sentiment. The rate of change in the average value of these characteristics from the pre to within intervals is then calculated for each user. Finally, we compute the average rates of change separately for short-term and long-term quoters, as presented in Table 4 .
As illustrated in Table 4 , 34,317 users in the dataset exhibited a quote-publishing rate exceeding 0.05 during the pre and within intervals, indicating more than 5 quotes per every 100 published tweets. These users observed a marginal increase (0.006) in the average sentiment of their tweets from pre-intervals to within-intervals. Conversely, 5900 users in the dataset, who had no quotes in the pre-interval but exceeded 0.01 of all their tweets as quotes during the pre and within intervals, experienced a decrease of 0.242 per day in their rate of retweeting from pre-intervals to within-intervals. Further detailed explanations and implications are presented in the “ Results ” section.
Impact of the Twitter policy
The findings of the impact analysis are presented in Table 2 , illustrating the changes in slopes for different tweet types. It is observed that the slope of each tweet type, except for quotes, decreased upon entering the within interval, while quotes experienced a slight increase (0.0031). Notably, prior to the implementation of the new policy, there was a substantial increase in the number of daily tweets across all types. Therefore, the decline in levels during the within intervals relative to the pre-intervals can be attributed to this initial surge in activity. Another significant result is the considerable decrease in the number of daily published quotes during the post-interval compared to the within-interval. Additionally, a significant decrease (− 2.785) and increase (11.587) are observed in the slope of retweets per day during the within and post intervals, respectively. These notable changes in both retweet and quote rates highlight the impact of Twitter's new policy. When examining these results from a broader perspective, two trends emerge: (1) during the transition from the pre-interval to the within interval, the slope of all tweet types, except for quotes, decreased, and (2) from the within-interval to the post-interval, the slope of all tweet types increased, except for quotes. These trends underscore the pronounced impact of the new policy implemented by Twitter. In conclusion, it can be inferred that the policy has achieved some progress. However, determining the true success of the policy requires considering Twitter's overarching goals within a broader context, encompassing both short-term and long-term consequences.
The correlations between user characteristics and slope changes in each tweet type during different intervals are presented in Table 3 . The results, particularly the correlations between slope changes in quoting and retweeting and other user characteristics, can be examined from three perspectives: the pre-within transition, within-post transition, and a comparison of pre-within to post-within.
Pre-within transitions
Regarding the pre-within transition, several noteworthy relationships can be observed. Firstly, there is an inverse relationship between the number of friends a user has and the slope change for the quote type. This suggests that users with a larger number of friends exhibit less improvement in their quoting rate during the within-interval (following the implementation of the Twitter policy). Similarly, the number of statuses published by users also demonstrates a negative correlation with the slope change for quotes. In other words, users who tend to publish a higher number of statuses show less inclination to increase their quoting rate during the within interval. Additionally, significant relationships emerge between the slope change in quoting during the pre-within interval and both retweet counts and the number of data points. This indicates that users who have engaged in more retweets are more likely to exhibit a propensity for quoting during the within-interval. Similar relationships can be observed between the slope change in quoting during the pre-within interval and other tweet types, suggesting that more active users are more influenced by the changes in the quoting behavior.
Within-post transitions
Analyzing the within-post transitions, several significant relationships can be observed. Firstly, the slope change in retweeting during the within-post interval exhibits a significant relationship with the number of quotes and original tweets during the within interval. This implies that users who have a higher number of quotes and original tweets in their activity would experience a greater increase in the retweeting rate after the policy cancellation (post-interval). However, the slope change in retweeting during the within-post interval does not show a significant relationship with the slope change in any other tweet type, except for an inverse relationship with original tweets. In other words, users who engage more in original tweets during the within-interval are likely to exhibit a lower increase in the rate of retweeting during the post-interval. Regarding the slope change in quoting during the within-post interval, a significant negative relationship is observed with the number of retweets during the within interval. This indicates that users who have a higher number of retweets during the within-interval are likely to experience a lower increase in the quoting rate during the post-interval. This relationship holds true for users who have quoted more during the within interval as well.
Pre-within to within-post comparison
Comparing the slope change in quoting and retweeting between the pre-within and within-post transitions, it can be observed that users who experienced an increase in their quoting or retweeting rate during the pre-within transition tend to exhibit a higher inclination to decrease it during the within-post transition. Additionally, a significant inverse relationship is evident between the slope change in quoting during the pre-within interval and the slope change in retweeting during the within-post interval. This implies that users who witnessed a greater increase in their quoting rate during the pre-within transition are likely to experience a larger decrease in their retweeting rate during the within-post transition.
The results of the text analysis, specifically length, sentiment, and the number of retweets, are presented in Table 4 . Examining the results reveals several key findings. Firstly, the quote texts of long-term quoters have undergone a reduction in length during the within interval compared to the pre-interval, across all threshold levels. However, for short-term quoters, this reduction in quote length only occurs at threshold levels equal to or above 0.05. Furthermore, among those whose quote texts have been shortened (at threshold levels of 0.05 and 0.075), short-term quoters experience a greater reduction in length compared to long-term quoters. Regarding sentiment analysis, the results indicate an overall increase in the sentiment score of quote texts from the pre-interval to the within interval. However, this increase is more pronounced for short-term quoters compared to long-term quoters.
Additionally, for both categories and across all threshold levels, the number of retweets received by quotes has decreased from the pre-interval to the within-interval. This decrease is particularly significant for long-term quoters, except at threshold level 0 for short-term quoters. This observation aligns with expectations since short-term quoters did not have any quotes during the pre-interval, resulting in their sentiment score being subtracted from the sentiment scores of quotes during the within interval. Notably, the decrease in the number of retweets is more substantial for long-term quoters, except at a threshold level of 0.075, where it is slightly higher for short-term quoters. In summary, by considering a threshold of 0.075 as an indicator, we can conclude that the Twitter policy has influenced quote texts in the following ways: (1) There is a greater reduction in the number of characters for short-term quoters compared to long-term quoters, and (2) The increase in sentiment score is more significant for short-term quoters relative to long-term quoters.
The findings pertaining to the hypotheses are outlined in Table 5 .
Quantitative findings
The quantitative analysis, based on hypotheses H1–4, reveals that the intervention has a negative impact on users’ retweeting behavior, while other tweet types remain relatively unaffected. However, the cessation of the intervention leads to an increase in the retweeting rate and a decrease in the quoting rate. When considering only the period when the policy was in effect, namely the within-interval, it can be concluded that the policy was partially successful. Despite a minor increase in the quoting rate, the significant decline in retweeting indicates a positive outcome. However, when examining the long-term effects after discontinuation of the policy, i.e., the post-interval, the policy can be regarded as a failure, as the retweeting rate experienced a dramatic increase while the quoting rate decreased substantially. Although Twitter did not enforce users to quote instead of retweeting nor provide any explicit promotion or reward for quoting, the quoting encouragement policy may have influenced users' perceptions and served as a virtual external incentive for initiating quoting behavior. This phenomenon can be explained by the adaptive nature of the brain in perceiving rewards based on recent levels and ranges of rewards, fictive outcomes, social comparisons, and other relevant factors [ 35 , 36 ]. The motivation crowding theory offers a framework for discussing this observation. When an extrinsic reward is removed, the level of intrinsic motivation diminishes compared to a scenario where no additional reward was initially provided [ 37 ]. In the case of Twitter's policy, users may have perceived the extrinsic incentive of adding a few extra characters to a retweet as rewarding and complied accordingly. However, once this external incentive was eliminated, the residual intrinsic motivation decreased below its initial level. This explains the subsequent decline in the quoting rate during the post-interval, accompanied by a surge in retweeting activity.
Qualitative findings
The qualitative analysis, focusing on hypotheses H5–11, reveals several noteworthy patterns. Users with a smaller number of friends and higher levels of overall tweet activity are more inclined to align with the policy and increase their quoting rate during the within interval. Furthermore, users who experienced an increase in their quoting rate during the within interval are more likely to decrease their quoting rate following the policy withdrawal in the post interval. Additionally, users who adopted quoting behavior as a result of the policy during the within interval demonstrated a tendency to publish quotes with shorter text length and more positive emotions. Two observed patterns can be explained respectively by the TPB and the DOI. The TPB posits that an individual’s behavioral intentions are influenced by three components, with subjective norms playing a significant role [ 38 ]. The impact of subjective norms is contingent upon the connections an individual has with others. Users with a smaller number of friends have fewer channels through which subjective norms can exert pressure. Consequently, these users are less influenced by societal norms that have not yet accommodated the new policy. Hence, users with fewer friends are more likely to be early adopters of the policy. Moreover, recent research [ 39 ] suggests that TPB, along with the theory of the Spiral of Silence, can potentially explain the avoidance of adoption, particularly when adoption involves expressing individual beliefs. Furthermore, the DOI provides insights into the adoption process, suggesting that adopters can be categorized into distinct groups based on the timing of their adoption [ 40 ]. Through this categorization, shared characteristics in terms of personality, socioeconomic status, and communication behaviors emerge. Early adopters, characterized by a greater tolerance for uncertainty and change, often exhibit higher levels of upward mobility within their social and socioeconomic contexts, as well as enhanced self-efficacy [ 41 ]. These characteristics are reflected in the more positive emotions expressed in their quote posts.
Implications
This study carries implications from both practical and theoretical perspectives. From a practical standpoint, the findings provide valuable guidance for practitioners in developing a multistage model that captures users’ behavior towards a new social media policy at an aggregate level. Such a model is crucial for designing efficient strategies aimed at expediting the adoption process among the majority of users. Leveraging the quantitative analysis method employed in this study, practitioners can first evaluate the impact of the policy, and then, using the qualitative analysis method, identify users who are more inclined to adopt or reject the policy based on their characteristics and text behavior. Gaining insights into user tendencies towards policy adoption or rejection in advance can inform a series of initiatives, including targeted user categorization to introduce or withhold the policy during its initial stages. An illustrative study by Xu et al. [ 42 ] explored public opinion on Twitter during Hurricane Irma across different stages, analyzing over 3.5 million tweets related to the disaster to discern distinct thematic patterns that emerged during each stage. Their findings assist practitioners in utilizing Twitter data to devise more effective strategies for crisis management. From a theoretical perspective, the findings contribute to the advancement of theories such as the TPB and the DOI in the realm of cyberspace. According to TPB, subjective norms play a significant role in shaping human behavior. This study revealed that users with a smaller number of friends are more inclined to accept the new policy. This suggests that users who have fewer connections are more likely to deviate from the prevailing norm in which the adoption of the new policy has not yet gained traction. Furthermore, the higher rates of positivity observed in the quote texts of short-term quoters, relative to their long-term counterparts, contribute to the extension of the Innovation Diffusion Theory regarding policy adoption and expand our understanding of the possible manifestations of early adopters' characteristics in the context of social media.
For a more nuanced understanding, it is noteworthy to explore the impact of events on user behavior. While events like debates can undeniably influence user activity levels, this impact is likely experienced across all types of users, such as quoters and retweeters. Our analysis, examining individual users across multiple time intervals that encompass these events, allows us to observe user-specific behavioral evolution. The extracted patterns thus represent dominant shifts in spreading behavior observed in the majority, irrespective of their original preference (retweeting or quoting). This observed consistency suggests that the policy's influence may extend beyond just event-driven fluctuations. The consistent shift in information-sharing behavior throughout the study period points towards the possible contribution of additional factors beyond isolated events.
Conclusion and future works
This research employed a big data approach to analyze the Twitter quoting encouragement policy, examining both its quantitative and qualitative effects. The research timeline was divided into three distinct intervals: pre, within, and post-intervals. Time series analysis was then utilized to identify changes in the rates of different tweet types across these intervals. Additionally, text and sentiment analysis, along with correlation methods, were applied to explore the relationships between user characteristics and their responses to the policy. The results revealed a short-term success followed by a long-term failure of the policy. Moreover, a set of user characteristics was identified, shedding light on their adherence to the policy and their quoting tendencies during the policy’s implementation. These findings have significant implications for the development and evaluation of new policies in the realm of social media, offering valuable insights for the design of more effective strategies.
The study of policy adoption on social media is still in its early stages, particularly in the realm of data analytics and behavioral research [ 43 ]. Future studies can build upon this research and explore additional factors and techniques to deepen our understanding. For example, the impact of aggregations, such as crowd emotional contagion, convergence behavior, and adaptive acceptance, can be modelled as exogenous factors in the analysis [ 44 , 45 ]. Additionally, incorporating new techniques for sentiment analysis, as highlighted in studies by Zhao et al. [ 46 ], and Erkantarci et al. [ 47 ], as well as semantic techniques [ 48 ], can further enhance computational analyses. Moreover, future research can consider factors related to the continuance of use [ 49 ] to examine the reasons behind policy rejection by users who initially adopted it. The inclusion of census data, search logs of users [ 50 ], user demographics [ 51 ], and the analysis of interconnections within a graph [ 52 ] would be valuable additions to the analysis. These additional data sources can provide a more comprehensive understanding of user behaviors and interactions. Furthermore, it is important to consider bot filtering techniques to ensure the accuracy and reliability of the findings. This step is particularly crucial for extending the research beyond Twitter and examining policy adoption in non-cyber spaces. By exploring these avenues of research, future studies can advance our knowledge of policy adoption on social media, providing valuable insights into user behaviors, motivations, and the effectiveness of policy interventions. Finally, this study’s data collection and storage methods share similarities with those employed in prior efforts [ 53 ]. However, there remains significant potential for innovation in this area.
Data availability
The datasets analyzed during the current study are available from the corresponding author on reasonable request.
Code availability
Codes are publicly available by this link: https://github.com/AmirhoseinBodaghi/TwitterPolicyProject .
https://github.com/AmirhoseinBodaghi/TwitterPolicyProject .
Valence Aware Dictionary and sEntiment Reasoner.
Weber, I., Garimella, V. R. K., & Batayneh, A. (2013). Secular vs. Islamist polarization in Egypt on twitter . ASONAM.
Book Google Scholar
Garimella, K., Weber, I., & Choudhury, M.D. (2016). Quote RTs on Twitter: Usage of the new feature for political discourse. WebSci’ 16 Germany.
Gallego, M., & Schofield, N. (2017). Modeling the effect of campaign advertising on US presidential elections when differences across states matter. Mathematical Social Sciences, 90 , 160–181.
Article Google Scholar
Jones, M. A., McCune, D., & Wilson, J. M. (2020). New quota-based apportionment methods: The allocation of delegates in the Republican Presidential Primary. Mathematical Social Sciences., 108 , 122–137.
Stier, S., Schünemann, W. J., & Steiger, S. (2018). Of activists and gatekeepers: Temporal and structural properties of policy networks on Twitter. New Media and Society, 20 (5), 1910–1930.
Frey, B. S., & Jegen, R. (2001). Motivation crowding theory. Journal of Economic Survey, 15 , 589–611.
Kreps, D. (1997). Intrinsic motivation and extrinsic incentives. American Economic Review, 87 , 359–364.
Google Scholar
Stiles, E. A., Swearingen, C. D., & Seiter, L. M. (2022). Life of the party: Social networks, public attention, and the importance of shocks in the presidential nomination process. Social Science Computer Review . https://doi.org/10.1177/08944393221074599
Jang, Y., Park, C. H., & Seo, Y. S. (2019). Fake news analysis modeling using quote retweet. Electronics, 8 (12), 1377.
Li, K., Zhu, H., Zhang, Y., & Wei, J. (2022). Dynamic evaluation method on dissemination capability of microblog users based on topic segmentation. Physica A: Statistical Mechanics and its Applications, 608 , 128264. https://doi.org/10.1016/j.physa.2022.128264
Bodaghi, A., & Oliveira, J. (2020). The characteristics of rumor spreaders on Twitter: A quantitative analysis on real data. Computer Communications, 160 , 674–687.
South, T., Smart, B., Roughan, M., & Mitchell, L. (2022). Information flow estimation: A study of news on Twitter. Online Social Networks and Media, 31 , 100231. https://doi.org/10.1016/j.osnem.2022.100231
Boulianne, S., & Larsson, A. O. (2021). Engagement with candidate posts on Twitter, Instagram, and Facebook during the 2019 election. New Media and Society, 1–22.
Lazarus, J., & Thornton, J. R. (2021). Bully pulpit? Twitter users’ engagement with president trump’s tweets. Social Science Computer Review., 39 (5), 961–980.
Yue, C. A., Qin, Y. S., Vielledent, M., Men, L. R., & Zhou, A. (2021). Leadership going social: How U.S. nonprofit executives engage publics on Twitter. Telematics and Informatics, 65 , 101710. https://doi.org/10.1016/j.tele.2021.101710
Ahmed, S., Jaidka, K., & Cho, J. (2021). The 2014 Indian elections on Twitter: A comparison of campaign strategies of political parties. Telematics and Informatics, 33 (4), 1071–1087.
Bodaghi, A., & Oliveira, J. (2022). A longitudinal analysis on Instagram characteristics of Olympic champions. Social Network Analysis and Mining, 12 , 3.
Hou, J., Wang, Y., Zhang, Y., & Wang, D. (2022). How do scholars and non-scholars participate in dataset dissemination on Twitter. Journal of Informetrics., 16 (1), 101223. https://doi.org/10.1016/j.joi.2021.101223
Hoang, T. B. N., & Mothe, J. (2018). Predicting information diffusion on Twitter—Analysis of predictive features. Journal of Computational Science, 28 , 257–264. https://doi.org/10.1016/j.jocs.2017.10.010
Munoz, M. M., Rojas-de-Gracia, M.-M., & Navas-Sarasola, C. (2022). Measuring engagement on Twitter using a composite index: An application to social media influencers. Journal of Informetrics, 16 (4), 101323. https://doi.org/10.1016/j.joi.2022.101323
Backstrom, L., Huttenlocher, D., Kleinberg, J., & Lan, X. (2006). Group formation in large social networks: membership, growth, and evolution. Proceedings of the 12th ACM SIGKDD international conference on Knowledge discovery and data mining (KDD ’06) (pp. 44–54). Association for Computing Machinery.
Chapter Google Scholar
Hu, J., Luo, Y., & Yu, J. (2018). An empirical study on selectiviey of retweeting behaviors under multiple exposures in social networks. Journal of Computational Science, 28 , 228–235. https://doi.org/10.1016/j.jocs.2017.11.004
Balestrucci, A., De Nicola, R., Petrocchi, M., & Trubiani, C. (2021). A behavioural analysis of credulous Twitter users. Online Social Networks and Media., 23 , 100133. https://doi.org/10.1016/j.osnem.2021.100133
Bodaghi, A., & Goliaei, S. (2018). A novel model for rumor spreading on social networks with considering the influence of dissenting opinions. Advances in Complex Systems, 21 , 1850011.
Wells, C., Shah, D., Lukito, J., Pelled, A., Pevehouse, J. C., & Yang, J. (2020). Trump, Twitter, and news media responsiveness: A media systems approach. New Media and Society, 22 (4), 659–682.
Yang, D., & Fujimura, S. (2019). What Will Influence customer's engagement the strategies and goals of tweet. IEEE international conference on industrial engineering and engineering management ( IEEM ), pp. 364–368.
Bodaghi, A., & Oliveira, J. (2022). The theater of fake news spreading, who plays which role? A study on real graphs of spreading on Twitter. Expert Systems with Applications, 189 , 116110.
Bodaghi, A., Oliveira, J., & Zhu, J. J. H. (2021). The fake news graph analyzer: An open-source software for characterizing spreaders in large diffusion graphs. Software Impacts. 100182.
Bodaghi, A., Oliveira, J., & Zhu, J. J. H. (2022). The Rumor Categorizer: An open-source software for analyzing rumor posts on Twitter. Software Impacts. 100232.
Zhang, A., Zheng, M., & Pang, B. (2018). Structural diversity effect on hashtag adoption in Twitter. Physica A: Statistical Mechanics and its Applications., 493 , 267–275.
Tian, Y., Tian, H., Cui, Y., Zhu, X., & Cui, Q. (2023). Influence of behavioral adoption preference based on heterogeneous population on multiple weighted networks. Applied Mathematics and Computation, 446 , 127880. https://doi.org/10.1016/j.amc.2023.127880
Monster, I., & Lev-Ari, S. (2018). The effect of social network size on hashtag adoption on Twitter. Cognitive Science, 42 (8), 3149–3158.
Rathnayake, C. (2021). Uptake, polymorphism, and the construction of networked events on Twitter. Telematics and Informatics, 57 , 101518.
Bodaghi, A., Goliaei, S., & Salehi, M. (2019). The number of followings as an influential factor in rumor spreading. Applied Mathematics and Computation, 357 , 167–184.
Seymour, B., & McClure, S. M. (2008). Anchors, scales and the relative coding of value in the brain. Current Opinion in Neurobiology, 18 , 173–178.
Murayama, K., Matsumoto, M., Izuma, K., & Matsumoto, K. (2010). Neural basis of the undermining effect of monetary reward on intrinsic motivation. Proceedings of the National Academy of Sciences of USA, 107 , 20911–20916.
Camerer, C. (2010). Removing financial incentives demotivates the brain. Proceedings of the National Academy of Sciences, 107 (49), 20849–20850.
Ajzen, I. (1991). The theory of planned behavior. Organizational Behavior and Human Decision Processes, 50 (2), 179–211.
Wu, T. Y., Xu, X., & Atkin, D. (2020). The alternatives to being silent: Exploring opinion expression avoidance strategies for discussing politics on Facebook. Internet Research, 30 (6), 1709–1729.
Everett, R. (2003). Diffusion of innovations (5th ed.). Simon and Schuster. ISBN 978-0-7432-5823-4.
Straub, E. T. (2009). Understanding technology adoption: Theory and future directions for informal learning. Review of Educational Research, 79 (2), 625–649.
Xu, Z., Lachlan, K., Ellis, L., & Rainear, A. M. (2020). Understanding public opinion in different disaster stages: A case study of Hurricane Irma. Internet Research, 30 (2), 695–709.
Motiwalla, L., Deokar, A. V., Sarnikar, S., & Dimoka, A. (2019). Leveraging data analytics for behavioral research. Information Systems Frontiers, 21 , 735–742.
Mirbabaie, M., Bunker, D., Stieglitz, S., & Deubel, A. (2020). Who sets the tone? Determining the impact of convergence behaviour archetypes in social media crisis communication. Information System Frontiers, 22 , 339–351. https://doi.org/10.1007/s10796-019-09917-x
Iannacci, F., Fearon, C., & Pole, K. (2021). From acceptance to adaptive acceptance of social media policy change: A set-theoretic analysis of B2B SMEs. Information Systems Frontiers, 23 , 663–680.
Zhao, X., & Wong, C. W. (2023). Automated measures of sentiment via transformer- and lexicon-based sentiment analysis (TLSA). Journal of Computational Social Science . https://doi.org/10.1007/s42001-023-00233-8
Erkantarci, B., & Bakal, G. (2023). An empirical study of sentiment analysis utilizing machine learning and deep learning algorithms. Journal of Computational Social Science . https://doi.org/10.1007/s42001-023-00236-5
Bodaghi, A., & Oliveira, J. (2024). A financial anomaly prediction approach using semantic space of news flow on twitter. Decision Analytics Journal, 10 , 100422. https://doi.org/10.1016/j.dajour.2024.100422
Franque, F. B., Oliveira, T., Tam, C., & Santini, F. O. (2020). A meta-analysis of the quantitative studies in continuance intention to use an information system. Internet Research, 31 (1), 123–158.
Feng, Y., & Shah, C. (2022). Unifying telescope and microscope: A multi-lens framework with open data for modeling emerging events. Information Processing and Management, 59 (2), 102811.
Brandt, J., Buckingham, K., Buntain, C., Anderson, W., Ray, S., Pool, J. R., & Ferrari, N. (2020). Identifying social media user demographics and topic diversity with computational social science: A case study of a major international policy forum. Journal of Computational Social Science, 3 , 167–188.
Antonakaki, D., Fragopoulou, P., & Ioannidis, S. (2021). A survey of Twitter research: Data model, graph structure, sentiment analysis and attacks. Expert Systems with Applications, 164 , 114006.
Bodaghi, A. (2019). Newly emerged rumors in Twitter. Zenodo. https://doi.org/10.5281/zenodo.2563864
Download references
Acknowledgements
The study was funded by City University of Hong Kong Centre for Communication Research (No. 9360120) and Hong Kong Institute of Data Science (No. 9360163). We would also like to express our sincere appreciation to Pastor David Senaratne and his team at Haggai Tourist Bungalow in Colombo, Sri Lanka, for their generous hospitality. Their support provided a conducive environment for the corresponding author to complete parts of this manuscript.
Author information
Authors and affiliations.
School of Computing, Ulster University, Belfast, Northern Ireland, UK
Amirhosein Bodaghi
Department of Media and Communication, City University of Hong Kong, Kowloon, Hong Kong
Jonathan J. H. Zhu
You can also search for this author in PubMed Google Scholar
Corresponding author
Correspondence to Amirhosein Bodaghi .
Ethics declarations
Conflict of interest.
On behalf of all authors, the corresponding author states that there is no conflict of interest.
Additional information
Publisher's note.
Springer Nature remains neutral with regard to jurisdictional claims in published maps and institutional affiliations.
Rights and permissions
Open Access This article is licensed under a Creative Commons Attribution 4.0 International License, which permits use, sharing, adaptation, distribution and reproduction in any medium or format, as long as you give appropriate credit to the original author(s) and the source, provide a link to the Creative Commons licence, and indicate if changes were made. The images or other third party material in this article are included in the article's Creative Commons licence, unless indicated otherwise in a credit line to the material. If material is not included in the article's Creative Commons licence and your intended use is not permitted by statutory regulation or exceeds the permitted use, you will need to obtain permission directly from the copyright holder. To view a copy of this licence, visit http://creativecommons.org/licenses/by/4.0/ .
Reprints and permissions
About this article
Bodaghi, A., Zhu, J.J.H. A big data analysis of the adoption of quoting encouragement policy on Twitter during the 2020 U.S. presidential election. J Comput Soc Sc (2024). https://doi.org/10.1007/s42001-024-00291-6
Download citation
Received : 06 January 2024
Accepted : 07 May 2024
Published : 19 May 2024
DOI : https://doi.org/10.1007/s42001-024-00291-6
Share this article
Anyone you share the following link with will be able to read this content:
Sorry, a shareable link is not currently available for this article.
Provided by the Springer Nature SharedIt content-sharing initiative
- Quote retweets
- Social media
- Time series analysis
- Text analysis
- Policy intervention
- Find a journal
- Publish with us
- Track your research
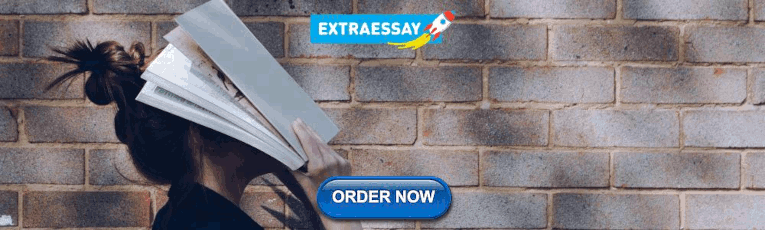
IMAGES
VIDEO
COMMENTS
The two "branches" of quantitative analysis. As I mentioned, quantitative analysis is powered by statistical analysis methods.There are two main "branches" of statistical methods that are used - descriptive statistics and inferential statistics.In your research, you might only use descriptive statistics, or you might use a mix of both, depending on what you're trying to figure out.
Quantitative data has to be gathered and cleaned before proceeding to the stage of analyzing it. Below are the steps to prepare a data before quantitative research analysis: Step 1: Data Collection. Before beginning the analysis process, you need data. Data can be collected through rigorous quantitative research, which includes methods such as ...
Revised on June 22, 2023. Quantitative research is the process of collecting and analyzing numerical data. It can be used to find patterns and averages, make predictions, test causal relationships, and generalize results to wider populations. Quantitative research is the opposite of qualitative research, which involves collecting and analyzing ...
Abstract. Quantitative data analysis serves as part of an essential process of evidence-making in health and social sciences. It is adopted for any types of research question and design whether it is descriptive, explanatory, or causal. However, compared with qualitative counterpart, quantitative data analysis has less flexibility.
It is important to know w hat kind of data you are planning to collect or analyse as this w ill. affect your analysis method. A 12 step approach to quantitative data analysis. Step 1: Start with ...
Quantitative method is the collection and analysis of numerical data to answer scientific research questions. Quantitative method is used to summarize, average, find patterns, make predictions, and test causal associations as well as generalizing results to wider populations.
8. Weight customer feedback. So far, the quantitative data analysis methods on this list have leveraged numeric data only. However, there are ways to turn qualitative data into quantifiable feedback and to mix and match data sources. For example, you might need to analyze user feedback from multiple surveys.
Revised on 10 October 2022. Quantitative research is the process of collecting and analysing numerical data. It can be used to find patterns and averages, make predictions, test causal relationships, and generalise results to wider populations. Quantitative research is the opposite of qualitative research, which involves collecting and ...
Data Analysis Methods in Quantitative Research. We started this module with levels of measurement as a way to categorize our data. Data analysis is directed toward answering the original research question and achieving the study purpose (or aim). Now, we are going to delve into two main statistical analyses to describe our data and make ...
Offers a guide through the essential steps required in quantitative data analysis; Helps in choosing the right method before starting the data collection process; Presents statistics without the math! Offers numerous examples from various diciplines in accounting and information systems; No need to invest in expensive and complex software packages
Here's how to make sense of your company's numbers in just four steps: 1. Collect data. Before you can actually start the analysis process, you need data to analyze. This involves conducting quantitative research and collecting numerical data from various sources, including: Interviews or focus groups.
Here is a basic guide for gathering quantitative data: Define the research question: The first step in gathering quantitative data is to clearly define the research question. This will help determine the type of data to be collected, the sample size, and the methods of data analysis.
Teaching quantitative data analysis is not teaching number crunching, but teaching a way of critical thinking for how to analyze the data. The goal of data analysis is to reveal the underlying patterns, trends, and relationships of a study's contextual situation. Learning data analysis is not learning how to use statistical tests to crunch ...
A research question is what a study aims to answer after data analysis and interpretation. The answer is written in length in the discussion section of the paper. ... In quantitative research, ... this suggests an integrative process between both research methods wherein a single mixed-methods research question can be developed.1 ...
Quantitative methods emphasize objective measurements and the statistical, mathematical, or numerical analysis of data collected through polls, questionnaires, and surveys, or by manipulating pre-existing statistical data using computational techniques.Quantitative research focuses on gathering numerical data and generalizing it across groups of people or to explain a particular phenomenon.
Abstract. This chapter provides a brief introduction to quantitative data analysis, explaining processes for the preparation of quantitative data and common techniques for data description and analysis. The context for the examples used in the chapter is a project management website, used by information technology (IT) students in the third ...
Replicable: Quantitative research aims to be replicable, meaning that other researchers should be able to conduct similar studies and obtain similar results using the same methods. Statistical analysis: Quantitative research involves using statistical tools and techniques to analyze the numerical data collected during the research process ...
Quantitative research is 'Explaining phenomena by collecting numerical data that are analysed using mathematically based methods (in particu-lar statistics)'. Let's go through this definition step by step. The first element is explaining phenomena. This is a key element of all research, be it quantitative or quali-tative.
Quantitative data analysis may include the calculation of frequencies of variables and differences between variables. A quantitative approach is usually associated with finding evidence to either support or reject hypotheses you have formulated at the earlier stages of your research process. The same figure within data set can be interpreted in ...
When collecting and analyzing data, quantitative research deals with numbers and statistics, while qualitative research deals with words and meanings. Both are important for gaining different kinds of knowledge. Quantitative research. Quantitative research is expressed in numbers and graphs. It is used to test or confirm theories and assumptions.
The main difference between quantitative and qualitative research is the type of data they collect and analyze. Quantitative research collects numerical data and analyzes it using statistical methods. The aim is to produce objective, empirical data that can be measured and expressed in numerical terms.
Quantitative research methods are concerned with the planning, design, and implementation of strategies to collect and analyze data. Descartes, the seventeenth-century philosopher, suggested that how the results are achieved is often more important than the results themselves, as the journey taken along the research path is a journey of discovery. . High-quality quantitative research is ...
Quantitative research enhances research projects. Quantitative research approaches are so much more than "how much" or "how many," they reveal the why behind people's actions, emotions and behaviors. By using standardized collection methods, like surveys, quant instills confidence and rigor in findings. Quantitative research has many ...
So, after analyzing the data the result will provide by qualitative and quantitative method of data results. Quantitative data analysis is mainly use numbers, graphs, charts, equations, statistics ...
The data for the Rasch analysis come from the large-scale research conducted in 2006-07, which investigated Croatian high school students' conceptual understanding of mechanics on a ...
The type of analysis you plan to conduct also dictates your choice of method. Qualitative data analysis is interpretive and seeks to understand the underlying reasons and motivations. In contrast ...
Published: Oct. 23, 2023. Qualitative Data Analysis Software (QDA software) allows researchers to organize, analyze and visualize their data, finding the patterns in qualitative data or unstructured data: interviews, surveys, field notes, videos, audio files, images, journal articles interviews, web content etc.
Qualitative synthesis and quantitative meta-analysis identified D-dimer, location of insertion, type of catheter, number of lumens, catheter indwelling time, and central line-associated ...
Understanding Qualitative Data Analysis. Qualitative data analysis is the process of systematically examining and deciphering qualitative facts (such as textual content, pix, motion pictures, or observations) to discover patterns, themes, and meanings inside the statistics· Unlike quantitative statistics evaluation, which focuses on numerical measurements and statistical strategies ...
This research holds significance for the fields of social media and communication studies through its comprehensive evaluation of Twitter's quoting encouragement policy enacted during the 2020 U.S. presidential election. In addressing a notable gap in the literature, this study introduces a framework that assesses both the quantitative and qualitative effects of specific platform-wide policy ...