The social determinants of mental health and disorder: evidence, prevention and recommendations
Affiliations.
- 1 Division of Psychiatry, University College London, London, UK.
- 2 City College, City University of New York, New York, NY, USA.
- 3 Graduate Center, City University of New York, New York, NY, USA.
- 4 School of Epidemiology and Public Health, University of Ottawa, Ottawa, ON, Canada.
- 5 Department of Psychiatry, University of Cambridge, Cambridge, UK.
- 6 Cambridgeshire & Peterborough NHS Foundation Trust, Cambridge, UK.
- 7 Medical Research Council Unit for Lifelong Health and Ageing, University College London, London, UK.
- 8 Centre for Longitudinal Studies, Social Research Institute, University College London, London, UK.
- 9 Camden and Islington NHS Foundation Trust, London, UK.
- 10 Department of Psychiatry, University of Oxford, Oxford, UK.
- 11 Institute for Mental Health, University of Birmingham, Birmingham, UK.
- PMID: 38214615
- PMCID: PMC10786006
- DOI: 10.1002/wps.21160
People exposed to more unfavourable social circumstances are more vulnerable to poor mental health over their life course, in ways that are often determined by structural factors which generate and perpetuate intergenerational cycles of disadvantage and poor health. Addressing these challenges is an imperative matter of social justice. In this paper we provide a roadmap to address the social determinants that cause mental ill health. Relying as far as possible on high-quality evidence, we first map out the literature that supports a causal link between social determinants and later mental health outcomes. Given the breadth of this topic, we focus on the most pervasive social determinants across the life course, and those that are common across major mental disorders. We draw primarily on the available evidence from the Global North, acknowledging that other global contexts will face both similar and unique sets of social determinants that will require equitable attention. Much of our evidence focuses on mental health in groups who are marginalized, and thus often exposed to a multitude of intersecting social risk factors. These groups include refugees, asylum seekers and displaced persons, as well as ethnoracial minoritized groups; lesbian, gay, bisexual, transgender and queer (LGBTQ+) groups; and those living in poverty. We then introduce a preventive framework for conceptualizing the link between social determinants and mental health and disorder, which can guide much needed primary prevention strategies capable of reducing inequalities and improving population mental health. Following this, we provide a review of the evidence concerning candidate preventive strategies to intervene on social determinants of mental health. These interventions fall broadly within the scope of universal, selected and indicated primary prevention strategies, but we also briefly review important secondary and tertiary strategies to promote recovery in those with existing mental disorders. Finally, we provide seven key recommendations, framed around social justice, which constitute a roadmap for action in research, policy and public health. Adoption of these recommendations would provide an opportunity to advance efforts to intervene on modifiable social determinants that affect population mental health.
Keywords: Mental health; marginalized groups; mental disorder; population mental health; prevention; social determinants; social justice; social risk factors.
© 2024 World Psychiatric Association.
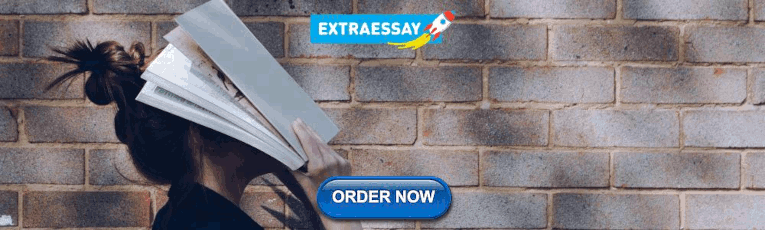
Grants and funding
- WT_/Wellcome Trust/United Kingdom
- MC_UU_00019/3/MRC_/Medical Research Council/United Kingdom
- Introduction
- Conclusions
- Article Information
Graphs show propensity score–weighted cumulative incidences, 3-year risk differences, and 3-year risk ratios of depression (A), substance use disorders (B), and anxiety disorders (C) among partners of stroke survivors (stroke-partner cohort), partners of individuals from the general population (GP-partner cohort), and partners of MI survivors (MI-partner cohort). Error bars denote 95% CIs.
Graphs show propensity score–weighted cumulative incidences, 3-year risk differences, and 3-year risk ratios of depression (A), substance use disorders (B), and anxiety disorders (C) among adult children of stroke survivors (stroke-offspring cohort), adult children of individuals from the general population (GP-offspring cohort), and adult children of MI survivors (MI-offspring cohort). Error bars denote 95% CIs.
eAppendix. Supplemental Methods
eTable 1. Overview of Studies Assessing Caregiving After Stroke and Various Psychological Aspects of Caregiver Burden
eTable 2. Codes and Definitions Used in This Study
eTable 3. Baseline Characteristics (N, %) of Partners of Stroke Patients, Partners of Individuals From the General Population, and Partners of Myocardial Infarction Patients Both Before and After Propensity Score Weighting
eTable 4. Numbers of Events and 3-Year Absolute Risks of Depression, Substance Use Disorders, Anxiety Disorders, Self-Harm or Suicide, and a Composite Outcome of Any Diagnosis of a Mental Health Condition
eTable 5. Associations Between Stroke in a Partner or Parent and Risk of Depression, Substance Use Disorders, Anxiety Disorders, Self-Harm or Suicide, and a Composite Outcome of Any Diagnosis of a Mental Health Condition, Stratified by Stroke Subtype
eTable 6. Associations Between Stroke in a Partner or Parent and Risk of Depression, Substance Use Disorders, Anxiety Disorders, Self-Harm or Suicide, and a Composite Outcome of Any Diagnosis of a Mental Health Condition, Stratified by Age Group
eTable 7. Associations Between Stroke in a Partner or Parent and Risk of Depression, Substance Use Disorders, Anxiety Disorders, Self-Harm or Suicide, and a Composite Outcome of Any Diagnosis of a Mental Health Condition, Stratified by Sex
eTable 8. Associations Between Stroke in a Partner or Parent and Risk of Depression, Substance Use Disorders, Anxiety Disorders, Self-Harm or Suicide, and a Composite Outcome of Any Diagnosis of a Mental Health Condition, Stratified by Number of Comorbidities
eTable 9. Associations Between Stroke in a Partner or Parent and Risk of Depression, Substance Use Disorders, Anxiety Disorders, Self-Harm or Suicide, and a Composite Outcome of Any Diagnosis of a Mental Health Condition, Stratified by Household Income
eTable 10. Associations Between Stroke in a Partner or Parent and Risk of Depression, Substance Use Disorders, Anxiety Disorders, Self-Harm or Suicide, and a Composite Outcome of Any Diagnosis of a Mental Health Condition, Stratified by Highest Achieved Education
eTable 11. Numbers of Events and 3-Year Absolute Risks of Depression (Defined From Either a Hospital-Based Diagnosis or ≥2 Prescriptions for an Antidepressant With Indication Code for Depression), Depression (Additionally Including Persistent Mood Disorders), and Nonmelanoma Skin Cancer
eTable 12. Associations Between Stroke in a Partner or Parent and Risk of Depression (Defined From Either a Hospital-Based Diagnosis or ≥2 Prescriptions for an Antidepressant With Indication Code for Depression), Depression (Additionally Including Persistent Mood Disorders), and Nonmelanoma Skin Cancer
eTable 13. Associations Between Stroke in a Partner or Parent and Risk of Depression, Substance Use Disorders, Anxiety Disorders, Self-Harm or Suicide, and a Composite Outcome of Any Diagnosis of a Mental Health Condition, When Performing a Complete-Case Analysis in Which Individuals With Missing Data on Household Income or Highest Achieved Education Were Excluded
eTable 14. Associations Between Stroke in a Partner or Parent and Risk of Depression, Substance Use Disorders, Anxiety Disorders, Self-Harm or Suicide, and a Composite Outcome of Any Diagnosis of a Mental Health Condition, When Performing an Analysis Setting the Index Date to the Stroke Admission Date Instead of the Discharge Date
eTable 15. Baseline Characteristics (N, %) of Adult Children of Stroke Patients, Adult Children of Individuals From the General Population, and Adult Children of Myocardial Infarction Patients Both Before and After Propensity Score Weighting
eFigure 1. Flowchart of Study Cohorts
eFigure 2. Directed Acyclic Graph Depicting Causal Assumptions in This Study
eFigure 3. Propensity Score Weighted Cumulative Incidences, 3-Year Risk Differences, and 3-Year Risk Ratios of Self-Harm or Suicide and Any Diagnosis of a Mental Health Condition Among Partners of Stroke Survivors (Stroke-Partner Cohort), Partners of Individuals From the General Population (GP-Partner Cohort), and Partners of Myocardial Infarction Survivors (MI-Partner Cohort)
eFigure 4. Propensity Score Weighted Cumulative Incidences, 3-Year Risk Differences, and 3-Year Risk Ratios of Self-Harm or Suicide and Any Diagnosis of a Mental Health Condition Among Adult Children of Stroke Survivors (Stroke-Offspring Cohort), Adult Children of Individuals From the General Population (GP-Offspring Cohort), and Adult Children of Myocardial Infarction Survivors (MI-Offspring Cohort)
eReferences
Data Sharing Statement
See More About
Sign up for emails based on your interests, select your interests.
Customize your JAMA Network experience by selecting one or more topics from the list below.
- Academic Medicine
- Acid Base, Electrolytes, Fluids
- Allergy and Clinical Immunology
- American Indian or Alaska Natives
- Anesthesiology
- Anticoagulation
- Art and Images in Psychiatry
- Artificial Intelligence
- Assisted Reproduction
- Bleeding and Transfusion
- Caring for the Critically Ill Patient
- Challenges in Clinical Electrocardiography
- Climate and Health
- Climate Change
- Clinical Challenge
- Clinical Decision Support
- Clinical Implications of Basic Neuroscience
- Clinical Pharmacy and Pharmacology
- Complementary and Alternative Medicine
- Consensus Statements
- Coronavirus (COVID-19)
- Critical Care Medicine
- Cultural Competency
- Dental Medicine
- Dermatology
- Diabetes and Endocrinology
- Diagnostic Test Interpretation
- Digital Health
- Drug Development
- Electronic Health Records
- Emergency Medicine
- End of Life, Hospice, Palliative Care
- Environmental Health
- Equity, Diversity, and Inclusion
- Facial Plastic Surgery
- Gastroenterology and Hepatology
- Genetics and Genomics
- Genomics and Precision Health
- Global Health
- Guide to Statistics and Methods
- Hair Disorders
- Health Care Delivery Models
- Health Care Economics, Insurance, Payment
- Health Care Quality
- Health Care Reform
- Health Care Safety
- Health Care Workforce
- Health Disparities
- Health Inequities
- Health Policy
- Health Systems Science
- History of Medicine
- Hypertension
- Images in Neurology
- Implementation Science
- Infectious Diseases
- Innovations in Health Care Delivery
- JAMA Infographic
- Law and Medicine
- Leading Change
- Less is More
- LGBTQIA Medicine
- Lifestyle Behaviors
- Medical Coding
- Medical Devices and Equipment
- Medical Education
- Medical Education and Training
- Medical Journals and Publishing
- Mobile Health and Telemedicine
- Narrative Medicine
- Neuroscience and Psychiatry
- Notable Notes
- Nutrition, Obesity, Exercise
- Obstetrics and Gynecology
- Occupational Health
- Ophthalmology
- Orthopedics
- Otolaryngology
- Pain Medicine
- Palliative Care
- Pathology and Laboratory Medicine
- Patient Care
- Patient Information
- Performance Improvement
- Performance Measures
- Perioperative Care and Consultation
- Pharmacoeconomics
- Pharmacoepidemiology
- Pharmacogenetics
- Pharmacy and Clinical Pharmacology
- Physical Medicine and Rehabilitation
- Physical Therapy
- Physician Leadership
- Population Health
- Primary Care
- Professional Well-being
- Professionalism
- Psychiatry and Behavioral Health
- Public Health
- Pulmonary Medicine
- Regulatory Agencies
- Reproductive Health
- Research, Methods, Statistics
- Resuscitation
- Rheumatology
- Risk Management
- Scientific Discovery and the Future of Medicine
- Sexual Health
- Shared Decision Making and Communication
- Sleep Medicine
- Sports Medicine
- Stem Cell Transplantation
- Substance Use and Addiction Medicine
- Surgical Innovation
- Surgical Pearls
- Teachable Moment
- Technology and Finance
- The Art of JAMA
- The Arts and Medicine
- The Rational Clinical Examination
- Tobacco and e-Cigarettes
- Translational Medicine
- Trauma and Injury
- Treatment Adherence
- Ultrasonography
- Users' Guide to the Medical Literature
- Vaccination
- Venous Thromboembolism
- Veterans Health
- Women's Health
- Workflow and Process
- Wound Care, Infection, Healing
Get the latest research based on your areas of interest.
Others also liked.
- Download PDF
- X Facebook More LinkedIn
Skajaa N , Farkas DK , Laugesen K, et al. Mental Health Conditions in Partners and Adult Children of Stroke Survivors. JAMA Netw Open. 2024;7(3):e243286. doi:10.1001/jamanetworkopen.2024.3286
Manage citations:
© 2024
- Permissions
Mental Health Conditions in Partners and Adult Children of Stroke Survivors
- 1 Department of Clinical Epidemiology, Department of Clinical Medicine, Aarhus University, Aarhus University Hospital, Aarhus, Denmark
- 2 Department of Epidemiology & Population Health, Stanford University, Stanford, California
- 3 Department of Neurology & Neurological Sciences, Stanford University, Stanford, California
- 4 Research Unit for Neurology, Odense University Hospital and University of Southern Denmark, Odense, Denmark
Question Are partners and adult children of stroke survivors at increased risk of depression, substance use disorders, anxiety disorders, and self-harm or suicide?
Findings In this cohort study or more than 1.9 million individuals, family members, particularly partners, of stroke survivors had moderately higher risks of several mental health conditions and self-harm or suicide compared with the Danish general population and, to a lesser extent, family members of myocardial infarction survivors.
Meaning These findings highlight the potential mental health consequences of stroke among family members and may possibly serve as a quantitative foundation for the development of future stroke rehabilitation services.
Importance Family caregiving after critical illness has been associated with several adverse health outcomes, including various aspects of mental health, but research focusing specifically on family members of stroke survivors is limited.
Objectives To examine the associations of stroke in a partner or parent with the risk of depression, substance use disorders, anxiety disorders, and self-harm or suicide.
Design, Setting, and Participants This nationwide, population-based cohort study used data from Danish nationwide administrative and clinical registries (2004-2021). Participants included partners and adult children of survivors of stroke. Data analysis was performed from March to December 2023.
Exposure Having a partner or parent who survived stroke.
Main Outcomes and Measures The Aalen-Johansen estimator was used to compute propensity score–weighted 3-year absolute risks, risk differences, and risk ratios for depression, substance use disorders, anxiety disorders, and self-harm or suicide among partners or children of survivors of stroke compared with partners or children of survivors of myocardial infarction (MI) and matched individuals from the general population.
Results The study included a total of 1 923 732 individuals: 70 917 partners of stroke survivors (median [IQR] age, 68 [59-76] years; 46 369 women [65%]), 70 664 partners of MI survivors (median [IQR] age, 65 [55-73] years; 51 849 women [73%]), 354 570 partners of individuals from the general population (median [IQR] age, 68 [59-76] years; 231 833 women [65%]), 207 386 adult children of stroke survivors (median [IQR] age, 45 [36-52] years; 99 382 women [48%]), 183 309 adult children of MI survivors (median [IQR] age, 42 [33-49] years; 88 078 women [48%]), and 1 036 886 adult children of individuals from the general population (median [IQR] age, 45 [36-52] years; 496 875 women [48%]). Baseline characteristics were well balanced across cohorts after propensity score weighting. Among partners of stroke survivors, the 3-year absolute risk was 1.0% for depression, 0.7% for substance use disorders, 0.3% for anxiety disorders, and 0.04% for self-harm or suicide. Risk ratio point estimates for the assessed outcomes ranged from 1.14 to 1.42 compared with the general population and from 1.04 to 1.09 compared with partners of MI survivors. The elevated risk of depression in partners of stroke survivors was more pronounced after severe or moderate stroke than after mild stroke. Among adult children of stroke survivors, the 3-year absolute risk was 0.6% for depression, 0.6% for substance use disorders, 0.2% for anxiety disorders, and 0.05% for self-harm or suicide. Both absolute risks and risk ratios for adult children of stroke survivors were smaller than those reported in the partner analyses.
Conclusions and Relevance In this cohort study of partners and adult children of stroke survivors, risks of several mental health conditions and self-harm or suicide were moderately higher compared with the general population and, to a lesser extent, partners and adult children of MI survivors. These findings highlight the potential consequences of stroke among family members, particularly partners, and its findings may possibly serve as a quantitative foundation for the development of future stroke rehabilitation services.
Stroke is common, disabling, and costly. 1 , 2 Survival after stroke has improved dramatically in recent decades in most geographic settings, including Denmark, 1 , 3 which has resulted in a surge in the absolute number of stroke survivors.
After surviving a stroke, patients often cope with persistent disability. Subsequent cardiovascular events and neurological and mental health complications are also common in these patients, 4 - 6 underscoring the challenges faced by patients and their families. Indeed, family members of stroke survivors may assume essential roles in the stroke recovery process, often acting as informal caregivers. 7 Caring for loved ones after illness can be grueling and may lead to chronic stress, fatigue, social isolation, and deleterious behavioral changes (eg, less exercise). 8 , 9 Other studies have found an association between caregiving and adverse health outcomes, most prominently psychological distress 10 (including suicidal ideation 11 ), cardiovascular disease, 9 , 12 and premature mortality. 13
Research focusing on mental health aspects of family members or caregivers specifically after stroke is limited. 14 - 22 Moreover, most earlier studies were small (<500 individuals) 14 - 18 , 20 , 21 and lacked longitudinal data based on a population-based study design with relevant comparison cohorts 14 - 22 (an overview of the existing literature is provided in eTable 1 in Supplement 1 ). To advance this body of research, the inclusion of relevant comparison cohorts remains essential. In this nationwide, population-based study, we (1) examine the associations of stroke in a partner or parent with depression, substance use disorders, anxiety disorders, self-harm or suicide, and a composite mental health condition outcome; (2) provide context to these risk estimates using general population (GP) (to fully understand the magnitude of effect estimates) and myocardial infarction (MI) (as an active comparator 23 , 24 ) comparison cohorts; and (3) explore the extent to which stroke severity, stroke subtype, age, sex, comorbidity, and socioeconomic position may moderate these associations.
This cohort study used data from Danish clinical and administrative registries with long-term, nationwide coverage. 25 Data sources (described in detail in the eAppendix in Supplement 1 ) were linked at the individual level using a unique personal identification number assigned to each resident. 26 The cumulative source population during the study period (2004-2021) was 6 157 156 individuals. All codes and definitions are listed in eTable 2 in Supplement 1 . We followed the Strengthening the Reporting of Observational Studies in Epidemiology ( STROBE ) reporting guidelines for cohort studies. Ethical approval and informed consent of study participants are not required for registry-based studies under Danish law. The study was approved by the Danish Data Protection Agency at Aarhus University.
In Denmark, health care is tax financed and, therefore, free of personal charge for all residents. Stroke management in Denmark is detailed in the eAppendix in Supplement 1 . In brief, specialized stroke rehabilitation is initiated during in-hospital care; after hospital discharge, rehabilitation is managed at the municipal level, and few data exist regarding its implementation and effectiveness. 27
We assembled 2 exposed cohorts: partners of stroke survivors (ie, the stroke-partner cohort) and adult children of stroke survivors (ie, the stroke-offspring cohort) (eFigure 1 in Supplement 1 ). From the Danish Stroke Registry, 28 we identified patients (aged ≥18 years) hospitalized with a first-time stroke (ischemic stroke or intracerebral hemorrhage) between May 1, 2004, and December 31, 2021, who were discharged from hospital alive. Using linked information in the Civil Registration System, 26 we identified stroke survivors’ partners and adult children. Partners were defined as follows: (1) 2 people who were married (including civil unions of same-sex couples), (2) 2 people who were cohabitating with a shared child, or (3) 2 cohabitating individuals of opposite sex, with an age difference less than 15 years and without a shared child. Both biological and adopted children were eligible for inclusion. We then assembled 2 unexposed comparison cohorts for each of the 2 exposed cohorts: partners and adult children of individuals from the GP without stroke or MI (GP-partner cohort and GP-offspring cohort) and partners and adult children of MI survivors (MI-partner cohort and MI-offspring cohort).
In all cohorts, we excluded individuals with a diagnosis of a mental health condition before the index date (defined as the partner’s or parent’s stroke discharge date for the stroke-partner and stroke-offspring cohorts, the matched partner’s or parent’s stroke discharge date for the GP-partner and GP-offspring cohorts, and the partner’s or parent’s MI discharge date for the MI-partner and MI-offspring cohorts) and those younger than 18 years. A full description of how cohorts were constructed is provided in the eAppendix in Supplement 1 . Follow-up for all cohort members began on the index date and continued for up to 3 years (data were available until the end of 2021) or until the occurrence of an outcome, death, or emigration, whichever occurred first.
We defined 4 outcomes with high clinical relevance and mechanistic plausibility 8 : (1) depression ( International Statistical Classification of Diseases and Related Health Problems, Tenth Revision [ ICD-10 ] codes F32-F33), (2) substance use disorders ( ICD-10 codes F10-F19), (3) anxiety disorders ( ICD-10 code F41), and (4) intentional self-harm or suicide (ie, suicide attempts or completions; ICD-10 codes X60-X84). As a fifth outcome, to facilitate a broad understanding of the overall mental health burden, we defined a composite outcome as the first occurrence of a hospital diagnosis of any mental health condition ( ICD-10 codes F00-F99 and G30). Self-harm or suicide was not included in the composite outcome definition. Mental health conditions were identified from hospital-based diagnoses in the Patient Registry 29 or the Psychiatric Central Research Database 30 ; self-harm or suicide was identified from hospital-based diagnoses or cause of death records in the Registry of Causes of Death 31 (see eTable 2 in Supplement 1 for precise definitions).
On the basis of the causal assumptions depicted in eFigure 2 in Supplement 1 , the following baseline characteristics, measured before the index date, were selected as potential confounders: demographics, year of index date, household income, highest achieved education, comorbidity, comedications, and health care utilization (see eAppendix and eTable 2 in Supplement 1 for definitions). In the stroke-partner and stroke-offspring cohorts only, we further obtained information on stroke severity at time of admission, as measured by the Scandinavian Stroke Scale (mild, 43-58; moderate, 26-42; or severe, 0-25) 32 and stroke subtype (ischemic stroke or intracerebral hemorrhage).
Data analysis was performed from March to December 2023. We used propensity score (PS) standardized morbidity ratio weighting to control for baseline imbalances between cohorts (eAppendix in Supplement 1 ). 33 This weighting approach reweighted the unexposed cohorts so that their covariate distribution resembled that in the exposed cohorts. Missing data on income (0.2%) and education (1.9%) were handled using a missing data indicator variable in the PS estimation. 34 We assessed covariate balance after weighting using standardized mean differences, with values less than 0.1 indicative of balance. 35
We used the Aalen-Johansen estimator, which accounts for death as a competing event, 36 to calculate weighted 3-year absolute risks, risk differences (RDs), and risk ratios (RRs) comparing the stroke-partner and stroke-offspring cohorts with the GP-partner and GP-offspring and MI-partner and MI-offspring cohorts. Corresponding 95% CIs were obtained through nonparametric bootstrapping using 200 resamples. 37
We stratified the analyses according to stroke severity, stroke subtype, age group, sex, number of baseline comorbidities, household income, and highest achieved education. PS weights were re-estimated within each examined stratum. 38
In sensitivity analyses, we (1) altered the depression definition also incorporating filled prescriptions, (2) altered the depression definition also incorporating persistent mood disorders ( ICD-10 codes F32-F34), (3) used nonmelanoma skin cancer as a negative control outcome, (4) performed a complete-case analysis, and (5) set the index date as the stroke admission date instead of the discharge date (eAppendix in Supplement 1 ). Statistical analysis was performed with SAS statistical software version 9.4 (SAS Institute), and data visualization was performed with RStudio software version 2023.06.01 (mounting R version 4.3.1; R Project for Statistical Computing).
A total of 1 923 732 individuals were included in the cohorts. The partner cohorts consisted of 70 917 partners of stroke survivors (91% ischemic strokes, 72% of mild severity; median [IQR] age, 68 [59-76] years; 46 369 women [65%]), 354 570 partners of individuals in the GP (median [IQR] age, 68 [59-76] years; 231 833 women [65%]), and 70 664 partners of MI survivors (median [IQR] age, 65 [55-73] years; 51 849 women [73%]) (eTable 3 in Supplement 1 ). Even before PS weighting, baseline characteristics were relatively well balanced across cohorts. The proportion with a high educational level (International Standard Classification of Education level 5-8) was 20% among partners of stroke survivors, 25% among partners in the GP, and 20% among partners of MI survivors. There were no clear differences across cohorts in health care utilization in the prior 3 years (eTable 3 in Supplement 1 ). After PS weighting, virtually all baseline characteristics had standardized mean differences less than 0.01. The RDs and RRs presented later in this article are PS weighted.
Among partners of stroke survivors, the 3-year absolute risk was 1.0% for depression, 0.7% for substance use disorders, 0.3% for anxiety disorders, 0.04% for self-harm or suicide, and 4.1% for the composite outcome of any diagnosis of a mental health condition ( Figure 1 and eTable 4 and eFigure 3 in Supplement 1 ). Compared with the GP-partner cohort, the RDs and RRs were 0.24% (95% CI, 0.16% to 0.32%) and 1.31 (95% CI, 1.19 to 1.41) for depression, 0.22% (95% CI, 0.15% to 0.28%) and 1.42 (95% CI, 1.29 to 1.55) for substance use disorders, 0.04% (95% CI, −0.01% to 0.08%) and 1.14 (95% CI, 0.95 to 1.33) for anxiety disorders, 0.01% (95% CI, −0.01% to 0.03%) and 1.25 (95% CI, 0.76 to 1.94) for self-harm or suicide, and 0.64% (95% CI, 0.48% to 0.82%) and 1.19 (95% CI, 1.14 to 1.24) for the composite outcome ( Table 1 , Figure 1 , and eFigure 3 in Supplement 1 ). Compared with the MI-partner cohort, the RDs and RRs were 0.08% (95% CI, −0.03% to 0.20%) and 1.08 (95% CI, 0.97 to 1.23) for depression, 0.06% (95% CI, −0.02% to 0.16%) and 1.09 (95% CI, 0.97 to 1.25) for substance use disorders, 0.01% (95% CI, −0.05% to 0.07%) and 1.04 (95% CI, 0.83 to 1.27) for anxiety disorders, 0.00% (95% CI, −0.02% to 0.02%) and 1.09 (95% CI, 0.60 to 2.04) for self-harm or suicide, and 0.15% (95% CI −0.04% to 0.40%) and 1.04 (95% CI, 0.99 to 1.11) for the composite outcome ( Table 1 , Figure 1 , and eFigure 3 in Supplement 1 ).
Stroke severity modified the association with depression ( Table 2 ). Compared with the GP-partner cohort, the RRs in the stroke-partner cohort were 1.26 (95% CI, 1.14-1.38) for mild stroke, 1.34 (95% CI, 1.11-1.61) for moderate stroke, and 1.46 (95% CI, 1.13-1.73) for severe stroke. Compared with the MI-partner cohort, the RRs in the stroke-partner cohort were 1.04 (95% CI, 0.90-1.15) for mild stroke, 1.12 (95% CI, 0.91-1.37) for moderate stroke, and 1.21 (95% CI, 0.95-1.49) for severe stroke. Additional subgroup results are presented in eTables 5 to 10 in Supplement 1 .
When depression was defined as either a hospital-based diagnosis or 2 or more prescriptions for an antidepressant with an indication code for depression, the absolute risks markedly increased in all cohorts (eTable 11 in Supplement 1 ). According to this definition, the RDs and RRs were 1.42% (95% CI, 1.18%-1.59%) and 1.19 (95% CI, 1.1601.21) compared with the GP-partner cohort and 0.41% (95% CI, 0.12%-0.66%) and 1.05 (95% CI, 1.01-1.08) compared with the MI-partner cohort (eTable 12 in Supplement 1 ). The inclusion of persistent mood disorders in the depression definition had no impact on results (eTables 11 and 12 in Supplement 1 ). When nonmelanoma skin cancer was used as a negative control outcome, the findings were virtually null (eTables 11 and 12 in Supplement 1 ). Finally, in a complete case analysis (eTable 13 in Supplement 1 ) and when the index date was set as the stroke admission date (eTable 14 in Supplement 1 ), the results were unchanged.
The offspring cohorts included 207 386 children of stroke survivors (91% ischemic strokes, 68% of mild severity; median [IQR] age, 45 [36-52] years; 99 382 women [48%]), 1 036 886 children of individuals in the GP (median [IQR] age, 45 [36-52] years; 496 875 women [48%]), and 183 309 children of MI survivors (median [IQR] age, 42 [33-49] years; 88 078 women [48%]) (eTable 15 in Supplement 1 ). As for partners, the baseline characteristics were well balanced even before PS weighting. As expected, the prevalence of comorbidities and drug use was lower in the offspring cohorts than in the partner cohorts (eTable 15 in Supplement 1 ). After PS weighting, virtually all baseline characteristics had standardized mean differences less than 0.01.
Except for self-harm or suicide, the absolute risks for the assessed outcomes were lower in the 3 offspring cohorts than in the 3 partner cohorts. Among children of stroke survivors, the 3-year absolute risk was 0.6% for depression, 0.6% for substance use disorders, 0.2% for anxiety disorders, 0.05% for self-harm or suicide, and 2.2% for the composite outcome ( Figure 2 and eTable 4 and eFigure 4 in Supplement 1 ). RDs and RRs were, in general, closer to the null in these analyses than those reported for the partner analyses ( Table 1 , Figure 2 , and eFigure 4 in Supplement 1 ). The RRs compared with the GP-offspring cohort were 1.07 (95% CI, 0.99-1.14) for depression, 1.11 (95% CI, 1.05-1.19) for substance use disorders, 1.10 (95% CI, 0.97-1.25) for anxiety disorders, 1.42 (95% CI, 1.11-1.84) for self-harm or suicide, and 1.09 (95% CI, 1.05-1.12) for the composite outcome. Compared with the MI-offspring cohort, RRs were virtually null for all outcomes, except for self-harm or suicide, for which the RR was 1.28 (95% CI, 0.91-1.77).
Stroke severity did not clearly modify the association with depression among adult children ( Table 2 ). Additional subgroup results are presented in eTables 5 to 10 in Supplement 1 .
The results of the sensitivity analyses were in line with those of the sensitivity analyses presented for the partner cohorts. See details in eTables 11 to 14 in Supplement 1 .
This cohort study examined the 3-year risk of depression, substance use disorders, anxiety disorders, and self-harm or suicide in more than 70 000 partners and more than 200 000 adult children of stroke survivors in Denmark. Our study had 4 main findings: (1) in partners of stroke survivors, RR point estimates for the assessed outcomes ranged from 1.14 to 1.42 compared with the GP and from 1.04 to 1.09 compared with partners of MI survivors; (2) the elevated risk of depression in partners of stroke survivors was more pronounced after severe or moderate stroke than after mild stroke; (3) relative risks found for adult children of stroke survivors were lower than those reported in the partner analyses; and (4) absolute risks were low for all outcomes (eg, 1% and 0.6% for depression in partners and adult children of stroke survivors, respectively). Consequently, despite observed relative risk increases, absolute RDs were marginal in all analyses.
Previous studies used self-administered and interview-based instruments (eg, the Caregiver Strain Index, the Relatives Stress Scale, and the Center for Epidemiologic Studies Depression Scale) to measure the extent of caregiver burden, as well as the presence of symptoms related to depression and anxiety. 14 - 22 A 2009 systematic review 19 (24 studies of 2619 caregivers of stroke survivors) found that the prevalence of caregiver burden was 25% to 54% across individual studies. A 2017 meta-analysis 22 (12 studies of 2059 caregivers of patients with stroke) reported a pooled prevalence of 40% for depressive symptoms and 21% for anxiety symptoms. That meta-analysis 22 further argued that the estimated pooled prevalence of depression among stroke caregivers is nearly 2-fold higher than the expected prevalence in the GP, citing an older study from 1992. 39
We believe our results are not directly comparable to those reported previously. Although the instruments used previously are useful in obtaining an assessment of the overall psychological distress associated with stroke caregiving, achieving high scores on such instruments does not in itself meet the criteria for diagnosing a mental health condition. In contrast, our assessment of outcomes relied primarily on diagnoses determined from hospital-based registries. 29 , 30 Because mental illness is often unrecognized and, if recognized, is treated in primary care settings with no hospital diagnosis given, registries tend to capture cases of severe mental illness, whereas mild cases are often missed. 40 For this reason, absolute risks observed in our study were almost certainly underestimated. Indeed, the inclusion of antidepressant use in the definition of depression clearly increased absolute risks; nonetheless, contrasts with either comparison remained largely intact.
Our study population comprised partners and adult children of stroke survivors, some of whom may have served as informal caregivers and others who may not have done so. In contrast, prior studies 14 - 22 focused exclusively on caregivers. In Denmark, postdischarge stroke rehabilitation is primarily managed by local municipalities. 27 It is possible this could lead to a comparatively lower caregiver burden for family members, in contrast to countries where patients and their families are often tasked with assuming a more extensive role in the rehabilitation process. Thus, on the basis of the assumption that a component of any true effect of stroke in a family member is mediated by the actual act of caregiving, our estimates may have been diluted toward the null (eFigure 2 in Supplement 1 ). In support of this possibility, RDs found for adult children, who, on average, may be less involved with informal caregiving than partners, were smaller.
Strokes identified in this study were generally of mild severity (ie, mild stroke comprised 72% of the strokes associated with the stroke-partner cohort and 68% of those associated with the stroke-offspring cohort). The rate of mild stroke has been increasing in Denmark. 3 This development could be explained by the early establishment of specialized stroke units and an effective prehospital response, 27 which may have lowered barriers to stroke workup. In contrast, the proportion of mild strokes (according to the National Institute of Health Stroke Scale) was 48% during 2012 to 2015 in the South London Stroke Register (United Kingdom) 41 and 58% during 1987 to 2009 in the Atherosclerosis Risk in Communities study (US). 42 Thus, stroke severity is likely to be milder on average in Denmark than in other countries, thereby potentially contributing to the modest overall RDs observed in this study. This possibility was supported by the finding that stroke severity appeared to modify the association between stroke in partners and the risk of depression.
Our study advances existing knowledge by including 2 separate comparison cohorts. As expected, associations were in general larger for the GP comparison than for the MI comparison. Although the effect of MI on long-term disability in survivors tends to be lower than that of stroke, 43 family members of MI survivors may also experience adverse psychological consequences. 44 As such, this comparison likely eliminated part of the causal pathway in addition to any potential unmeasured confounding (eFigure 2 in Supplement 1 ). Notwithstanding, this comparison cohort provided important context to risk estimates, suggesting that stroke and MI convey a roughly similar impact on the psychological consequences in family members.
Strengths of this study include its size; its high-quality data within a nationwide, population-based setting with complete long-term follow-up; and its use of comparison cohorts. Positive predictive values of stroke (90%-94%) 45 , 46 and MI (97%) 47 discharge diagnoses are high. Although hospital-based diagnoses of mental health conditions in the Danish registries have positive predictive values of acceptable standards, 30 , 48 , 49 nondifferential misclassification of outcomes may have been present. Motivated by the possibility of unmeasured confounding and diagnostic bias, we included an active comparator cohort, consisting of partners and adult children of MI survivors. Reassuringly, the null result for the negative control outcome (nonmelanoma skin cancer) and the minimal differences in baseline characteristics across cohorts both before and after weighting on a comprehensive set of possible confounders indicate that these sources of potential bias probably did not affect the study results; still, residual confounding is possible.
Although Denmark has a strong welfare and health care system, where stroke is well managed and stroke severity is relatively mild, no support system exists specifically for family members of stroke survivors, and the high relative risks for self-harm or suicide found in this study for both partners and adult children are concerning. It is possible that upgrading the current organization and services could lessen the burden on family members and lower risks of mental health conditions even more. In summary, this study highlights the potential consequences of stroke among family members, particularly partners, and its findings may possibly serve as a quantitative foundation for the development of future stroke rehabilitation services.
Accepted for Publication: January 29, 2024.
Published: March 14, 2024. doi:10.1001/jamanetworkopen.2024.3286
Open Access: This is an open access article distributed under the terms of the CC-BY License . © 2024 Skajaa N et al. JAMA Network Open .
Corresponding Author: Nils Skajaa, PhD, Department of Clinical Epidemiology, Department of Clinical Medicine, Aarhus University, Aarhus University Hospital, Olof Palmes Allé 43-45, DK, 8200 Aarhus N, Denmark ( [email protected] ).
Author Contributions: Drs Skajaa and Sørensen had full access to all of the data in the study and take responsibility for the integrity of the data and the accuracy of the data analysis.
Concept and design: Skajaa, Laugesen, Fuglsang, Plana-Ripoll, Gaist, Sørensen.
Acquisition, analysis, or interpretation of data: All authors.
Drafting of the manuscript: Skajaa, Henderson.
Critical review of the manuscript for important intellectual content: All authors.
Statistical analysis: Skajaa, Farkas.
Obtained funding: Skajaa.
Administrative, technical, or material support: Sørensen.
Supervision: Laugesen, Sørensen.
Conflict of Interest Disclosures: Dr Skajaa reported receiving personal fees from Pfizer outside the submitted work and research funding in the form of institutional research grants to and administered by Aarhus University outside the submitted work. Dr Henderson reported receiving research grants from the National Institutes of Health to their institution and grants from Health IQ Insurance outside the submitted work. Dr Gaist reported receiving personal fees from Pfizer and Bristol-Myers Squibb for speaker honoraria outside the submitted work. Dr Sørensen reported receiving research funding in the form of institutional research grants to and administered by Aarhus University outside the submitted work. No other disclosures were reported.
Funding/Support: This work was supported by a grant from The Lundbeck Foundation (grant R248-2017-521) and a grant from the Platform for Inequality Research at Aarhus University.
Role of the Funder/Sponsor: The funders had no role in the design and conduct of the study; collection, management, analysis, and interpretation of the data; preparation, review, or approval of the manuscript; and decision to submit the manuscript for publication.
Data Sharing Statement: See Supplement 2 .
- Register for email alerts with links to free full-text articles
- Access PDFs of free articles
- Manage your interests
- Save searches and receive search alerts

Official websites use .gov
A .gov website belongs to an official government organization in the United States.
Secure .gov websites use HTTPS
A lock ( ) or https:// means you've safely connected to the .gov website. Share sensitive information only on official, secure websites.
Adverse Childhood Experiences and Health Conditions and Risk Behaviors Among High School Students — Youth Risk Behavior Survey, United States, 2023
Supplements / October 10, 2024 / 73(4);39–49
Elizabeth A. Swedo, MD 1 ; Sanjana Pampati, PhD 2 ; Kayla N. Anderson, PhD 3 ; Evelyn Thorne, PhD 1 ; Izraelle I. McKinnon, PhD 2 ,4 ; Nancy D Brener, PhD 2 ; Joi Stinson, MPH 2 ; Jonetta J. Mpofu, PhD 2 ; Phyllis Holditch Niolon, PhD 1 ( View author affiliations )
Introduction
Limitations, future directions, acknowledgments.
- Article PDF
- Full Issue PDF
Adverse childhood experiences (ACEs) are preventable, potentially traumatic events occurring before age 18 years. Data on ACEs among adolescents in the United States have primarily been collected through parent report and have not included important violence-related ACEs, including physical, sexual, and emotional abuse. This report presents the first national prevalence of self-reported ACEs among U.S. high school students aged <18 years, estimates associations between ACEs and 16 health conditions and risk behaviors, and calculates population-attributable fractions of ACEs with these conditions and behaviors using cross-sectional, nationally representative 2023 Youth Risk Behavior Survey data. Exposures were lifetime prevalence of individual (emotional, physical, and sexual abuse; physical neglect; witnessed intimate partner violence; household substance use; household poor mental health; and incarcerated or detained parent or guardian) ACEs and cumulative ACEs count (zero, one, two or three, or four or more). Health conditions and risk behaviors included violence risk factors, substance use, sexual behaviors, weight and weight perceptions, mental health, and suicidal thoughts and behaviors. Bivariate analyses assessed associations between individual and cumulative ACEs and demographics. Adjusted prevalence ratios assessed associations between cumulative ACEs and health conditions and risk behaviors, accounting for demographics. Population-attributable fractions were calculated to determine the potential reduction in health conditions and risk behaviors associated with preventing ACEs. ACEs were common, with approximately three in four students (76.1%) experiencing one or more ACEs and approximately one in five students (18.5%) experiencing four or more ACEs. The most common ACEs were emotional abuse (61.5%), physical abuse (31.8%), and household poor mental health (28.4%). Students who identified as female; American Indian or Alaska Native; multiracial; or gay or lesbian, bisexual, questioning, or who describe their sexual identity in some other way experienced the highest number of ACEs. Population-attributable fractions associated with experiencing ACEs were highest for suicide attempts (89.4%), seriously considering attempting suicide (85.4%), and prescription opioid misuse (84.3%). ACEs are prevalent among students and contribute substantially to numerous health conditions and risk behaviors in adolescence. Policymakers and public health professionals can use these findings to understand the potential public health impact of ACEs prevention to reduce adolescent suicidal behaviors, substance use, sexual risk behaviors, and other negative health conditions and risk behaviors and to understand current effects of ACEs among U.S. high school students.

Adverse childhood experiences (ACEs) are preventable, potentially traumatic events occurring before age 18 years ( 1 ). For nearly three decades, considerable research, particularly among adults, has demonstrated the importance of ACEs’ contributions to negative health outcomes and diminished life opportunities ( 2 , 3 ). Retrospective surveys of adults have established that ACEs are prevalent: Approximately two thirds of U.S. adults have experienced at least one ACE and 17% of U.S. adults have experienced four or more ACEs ( 4 ). Despite the volume of research supporting strong associations between ACEs and negative outcomes in adulthood, relatively few studies have examined the role of ACEs in child and adolescent health ( 5 ). ACEs are associated with increased likelihood of numerous risk factors and health outcomes, including being in physical fights and carrying weapons ( 6 ); smoking, alcohol use, and illicit drug use in both adolescence and adulthood ( 2 ); early sexual initiation, teenage pregnancy, sexually transmitted infections, and multiple sexual partners ( 2 ); overweight or obesity ( 2 ); and various mental health conditions or symptoms, as well as suicide risk, across the lifespan ( 5 , 7 ).
Population-level estimates of ACEs among adolescents and their effects have largely been limited to parent-report or a small number of ACEs ( 8 ). Collecting and analyzing self-reported ACEs data among adolescents is valuable for several reasons, most notably the proximity of the event to the time of report and adolescents’ knowledge of their own experiences that might be unknown to or not disclosed by their parents ( 9 ). Retrospective reports of ACEs on surveys, such as the Behavioral Risk Factor Surveillance System (BRFSS), require adults to recall events that occurred decades ago, introducing the potential for significant recall bias ( 9 ). Collection of ACE data among adolescents decreases recall bias, improves the ability to track trends in ACEs over time, and permits quicker assessment of the impact of current prevention and mitigation efforts in disproportionately affected populations ( 9 ). Collection of self-reported ACEs data among adolescents, and not parent-proxy reporters, might improve accuracy of estimates: Adolescent-reported data potentially captures abuse- or neglect-related experiences that might have been perpetrated by the proxy reporters, and sensitive experiences not disclosed by adolescents to their parent.
This report presents the first lifetime national prevalence of self-reported individual and cumulative ACEs among U.S. high school students aged <18 years, associations between cumulative ACE exposure and negative health conditions and risk behaviors in adolescence, and population-attributable fractions related to ACEs for each condition and behavior. Policymakers and public health professionals can use this information to understand current prevalence of ACEs among U.S. high school students, and the proportion of negative health conditions and risk behaviors that could potentially be reduced or eliminated by implementing evidence-based strategies and approaches to prevent ACEs and mitigate their consequences.
Data Source
This report includes data from the 2023 Youth Risk Behavior Survey (YRBS) (N = 20,103), a cross-sectional, school-based survey conducted biennially since 1991. Each survey year, CDC collects data from a nationally representative sample of public and private school students in grades 9–12 in the 50 U.S. states and the District of Columbia. Additional information about YRBS sampling, data collection, response rates, and processing is available in the overview report of this supplement ( 8 ). Prevalence estimates for ACEs for the overall study population and by sex, race and ethnicity, grade, and sexual identity are available at https://nccd.cdc.gov/youthonline/App/Default.aspx . The full YRBS questionnaire, datasets, and documentation are available at https://www.cdc.gov/yrbs/index.html . Institutional review boards at CDC and ICF, the survey contractor, approved the protocol for YRBS. Data collection was conducted consistent with applicable Federal law and CDC policy.*
Information about question content and coding for all demographics, ACEs, and included health conditions and risk behaviors is presented ( Table 1 ). Students self-reported lifetime experiences of eight ACEs (emotional, physical, and sexual abuse; physical neglect; witnessing intimate partner violence; household substance use; household poor mental health; and parent or guardian incarcerated or detained). Questions align with and were adapted from the original ACEs included in the seminal CDC-Kaiser Permanente ACEs Study ( 3 ) and subsequently used for adult retrospective data collection from the BRFSS ( 4 ). Slight adaptations were made to ACEs questions to align with age of respondent (i.e., changes to the question stem from “before you were 18 years of age” to “during your life”), and to reduce the number of questions used to capture sexual abuse and household substance use. ACEs questions were cognitively tested with high school students to ensure fidelity to question intention and suitability for adolescent populations; cognitive testing results are available ( https://stacks.cdc.gov/view/cdc/150784 ). In addition to examination of the presence of individual ACEs, a cumulative ACEs count (cumulative ACEs) was calculated (zero, one, two or three, or four or more) following CDC guidelines for coding ACEs responses using YRBS data ( 10 ).
Students also self-reported on 16 measures across a spectrum of risk behaviors and health conditions. These included carrying a weapon at school, being in a physical fight, multiple forms of substance use (i.e., current electronic vapor product use, current alcohol use, current binge drinking, and current prescription opioid misuse), sexual behaviors (i.e., alcohol or drug use before last sexual intercourse, currently sexually active with multiple partners, and did not use a condom during last sexual intercourse), weight and perceived weight status, persistent feelings of sadness or hopelessness, and suicide risk (i.e., seriously considered attempting suicide or attempted suicide). Most questions referenced conditions or behaviors that took place during the past 12 months or past 30 days, increasing the chances that the condition or behavior took place after initial ACE exposure.
Demographic variables included sex (female or male) and race and ethnicity (American Indian or Alaska Native [AI/AN], Asian, Black or African American [Black], White, Hispanic or Latino [Hispanic], and multiracial [selected >1 racial category]). (Persons of Hispanic or Latino origin might be of any race but are categorized as Hispanic; all racial groups are non-Hispanic.) Prevalence estimates for Native Hawaiian or other Pacific Islander students had denominators <30 and were therefore considered statistically unreliable and were suppressed ( 8 ). Other demographic variables included age (≤14, 15, 16, and 17 years), and sexual identity (heterosexual, gay or lesbian, bisexual, questioning [I am not sure about my sexual identity/questioning], or describe identity in some other way [I describe my identity some other way]).
The analytic sample was restricted to those aged <18 years (n = 17,838) to ensure the adversity occurred during childhood. Weighted prevalence and 95% CIs for individual and cumulative ACEs count, overall and by each demographic, are presented. Demographic differences in the prevalence of individual ACEs and cumulative ACEs count were examined using pairwise t -test analyses. All prevalence estimates and measures of association used Taylor series linearization. Cumulative ACEs counts were only calculated for participants with complete data on at least five individual ACEs.
The weighted prevalence and 95% CI of each health condition and risk behavior by cumulative ACEs count are presented. Adjusted prevalence ratios (aPRs) were calculated using logistic regression with predicted marginals; models fit cumulative ACEs count as the independent variable and each risk behavior or health condition as the dependent variable, adjusting for sex, race and ethnicity, age, and sexual identity. Population-attributable fractions, adjusted for aforementioned model covariates, were calculated using Miettinen’s formula, aPRs, and weighted prevalence estimates of each health condition and risk behavior by each cumulative ACEs count level (Supplementary Table, https://stacks.cdc.gov/view/cdc/160323 ). These population-attributable fractions were used to ascertain the percentage reduction in the number of observed cases of each outcome that would be expected if ACEs exposure were incrementally reduced or eliminated in the study population ( 7 , 11 ). Findings were considered statistically significant if p<0.05. Prevalence ratios were considered statistically significant if 95% CI did not cross the value of 1.0. All analyses except estimates of population-attributable fractions were conducted in SAS-callable SUDAAN (version 11.0.3; RTI International) using sample weights to account for complex survey design.
The most common ACEs were emotional abuse (61.5%), physical abuse (31.8%), and household poor mental health (28.4%) ( Table 2 ). Experiences of specific ACEs varied by demographic characteristics. All ACEs, except physical neglect, were more common among female students compared with male students. Unique patterns were observed by race and ethnicity for individual ACEs. For example, AI/AN students had the highest prevalence of witnessed intimate partner violence (28.7%) and household substance use (34.2%) but one of the lowest prevalence estimates of physical abuse (35.7%). Asian students had the lowest prevalence of sexual abuse (4.3%), witnessed intimate partner violence (17.1%), household substance use (12.4%), household poor mental health (13.2%), and parent or guardian incarcerated or detained (4.6%), but had the highest prevalence of physical abuse (38.2%), along with Black students (38.2%). Prevalence differed by age for four out of eight ACEs; household substance use and household poor mental health were more commonly reported by students aged 17 years (26.4% and 30.3%, respectively) compared with students aged ≤14 years (21.3% and 24.7%, respectively). Students who identified as gay, lesbian, bisexual, or questioning (LGBQ+) had higher prevalence of all ACEs except physical neglect compared with heterosexual students. Heterosexual students (8.6%) experienced a higher prevalence of physical neglect than bisexual students (6.8%) and students who describe their identity in some other way (3.9%). Bisexual students experienced a higher prevalence of sexual abuse (19.6%) and household substance use (40.6%) compared with gay and lesbian students (10.0% and 30.1%, respectively). Students who describe their identity in some other way had higher prevalence of emotional abuse (85.0%), household substance use (43.0%), and household poor mental health (56.4%) compared with gay and lesbian students (77.0%, 30.1%, and 42.0%, respectively).
Three in four students (76.1%) experienced at least one ACE and nearly one in five (18.5%) experienced four or more ACEs ( Table 3 ). The prevalence of four or more ACEs was highest in female (23.9%), AI/AN (28.1%), multiracial (25.9%), and LGBQ+ students, particularly students who describe their identity in some other way (38.6%) and bisexual students (35.1%). AI/AN students had the highest prevalence of experiencing zero ACEs (30.9%).
As the number of ACEs increased for most conditions and behaviors, aPRs between cumulative ACEs count and health condition or risk behavior increased in magnitude, indicative of a dose-response relation ( Table 4 ). For 10 of 16 conditions and behaviors, those experiencing one ACE had significantly higher prevalence of each health condition or risk behavior than those experiencing zero ACEs. Students who experienced two or more ACEs had significantly higher prevalence of almost all conditions and behaviors compared with students with zero ACEs (excluding underweight and overweight or obesity for two or three ACEs and underweight for four or more ACEs). The strongest associations were observed between experiencing four or more ACEs and attempted suicide, seriously considered attempting suicide, and current prescription opioid misuse.
Population-attributable fractions due to ACEs (the potential reduction in each outcome if ACEs were reduced or eliminated in the study population) ranged greatly depending on the outcome and ACEs count ( Table 5 ). The largest potential reductions were estimated among students with four or more ACEs across all health conditions and risk behaviors (range of −6.7% [underweight] to 77.8% [attempted suicide]). The estimated overall potential percentage reductions in negative health conditions and risk behaviors associated with preventing all ACEs (one or more ACE) ranged from 4.2% for overweight or obesity to 89.4% for attempted suicide, with population-attributable fractions exceeding 50% for all conditions and behaviors except those related to weight. Substantial reductions associated with preventing all ACEs were estimated for seriously considered attempting suicide (85.4%), current prescription opioid misuse (84.3%), alcohol or drug use before last sexual intercourse (80.2%), current electronic vapor product use (73.2%), persistent feelings of sadness or hopelessness (65.6%), carrying a weapon at school (65.2%), current binge drinking (64.5%), and not using a condom during last sexual intercourse (64.0%).
This study is the first to present self-reported, nationally representative estimates of ACEs among U.S. high school students. ACEs were common among students, exceeding previous estimates from U.S. adults ( 4 ): Three in four students experienced one or more ACE and nearly one in five students experienced four or more ACEs. Approximately 60% of students experienced emotional abuse during their lifetime (61.5%) and approximately one in three (31.8%) experienced physical abuse. More than one in four students live in a household that has been affected by substance use (25.1%) or poor mental health (28.4%). Approximately one in five students has witnessed intimate partner violence (18.6%), and one in seven has had a parent or guardian incarcerated or detained (14.5%). Students who identified as female, AI/AN, multiracial, or LGBQ+ experienced the highest prevalence of ACEs. Results indicate that preventing and mitigating ACEs is critical to improving population-level adolescent behavioral health: Nearly 90% of suicidal behaviors, 84.3% of current prescription opioid misuse, and 65.6% of persistent feelings of sadness or hopelessness were associated with experiencing one or more ACE. Students with four or more ACEs had significantly increased prevalence ratios for 15 of 16 negative health conditions and risk behaviors compared with students with zero ACEs (e.g., aPR for attempted suicide = 12.42), demonstrating the marked association between cumulative ACEs and negative outcomes.
Results highlight the wide variety of individual student experiences across and within racial and ethnic groups. For example, although AI/AN students had the highest prevalence of four or more ACEs, they also had the highest prevalence of zero ACEs. Specific ACEs most prevalent among students differed by racial and ethnic identity, consistent with previous studies ( 12 ). For example, although Asian students had the lowest prevalence of four or more ACEs, they experienced comparatively high prevalence of emotional and physical abuse. Significantly fewer multiracial students experienced zero ACEs compared with all other racial and ethnic groups, except when compared to AI/AN students. Taken together, these findings indicate important racial and ethnic disparities in exposure to individual and cumulative ACEs. Variability of individual ACEs by racial and ethnic identity indicates that approaches designed to prevent or address the impact of ACEs might benefit from being tailored to specific cultural contexts, as opposed to a one-size-fits-all approach.
Although LGBQ+ students experienced a higher prevalence of ACEs than heterosexual students, bisexual students also experienced a disproportionate prevalence of certain ACEs compared with gay and lesbian students. These findings align with previous research, though the reason for the disproportionate prevalence of ACEs among bisexual students compared with gay and lesbian students remains unclear and needs to be further explored to guide prevention efforts ( 13 ). Students who described their sexual identity in some other way demonstrated the highest prevalence of four or more ACEs, significantly higher than heterosexual, gay and lesbian, and questioning students; these findings support the need to better understand this population of students and their prevention and intervention needs.
Female students had significantly higher prevalence of four or more ACEs than male students and had higher prevalence of all individual types of ACEs except for physical neglect. Although certain ACEs (e.g., physical and sexual abuse) might reflect directed violence based on their sex, other ACEs (e.g., household poor mental health) might instead reflect a greater awareness of household challenges among female students compared with male students.
Although it might be expected that older students would have experienced a greater number of ACEs than younger students, this study found only a modest increase between students aged ≤14 years and those aged 17 years. The only two ACEs that significantly increased between those aged ≤14 years and those aged 17 years were household substance use and household poor mental health, which more likely reflects greater awareness of household challenges among older adolescents than a true increase in prevalence. This supports the approach that preventing ACEs earlier in childhood helps to prevent negative impacts in adolescence and beyond.
Results from this study expand upon previous research indicating that preventing ACEs could result in sizable reductions in poor health conditions and risk behaviors among adult populations ( 7 ). However, this study is the first to document the critical, widespread contribution that preventing ACEs could have on reducing a wide spectrum of poor behavioral health conditions and risk behaviors among adolescents. With a high association of ACEs with attempted suicide (89.4%), with current prescription opioid misuse (84.3%), and with persistent feelings of sadness or hopelessness (65.6%), preventing ACEs could be a clear pathway to improving adolescent behavioral health amid an ongoing mental health crisis ( 5 ).
Students experiencing high cumulative (four or more) ACEs had significantly higher prevalence of overweight or obesity, consistent with previous studies linking ACEs with obesity ( 2 ). Such findings suggest the need to incorporate ACEs prevention and mitigation into interventions and policies that aim to address childhood obesity, such as incorporating social workers into family healthy weight programs to assist families in accessing benefit programs (e.g., Supplemental Nutrition Assistance Program or Women, Infants, and Children) that might alleviate financial stress and promote food security, and integration of Substance Abuse and Mental Health Services Administration’s guiding principles for trauma-informed care into behavioral interventions for weight ( 14 ).
Preventing ACEs is important because all children deserve to have safe, stable, nurturing relationships and environments in childhood so that they can flourish and thrive; experiencing abuse and neglect and witnessing violence disrupt that safety, stability, and nurturing. However, these findings suggest that preventing ACEs also is an important public health goal because many other health problems could be eliminated or reduced when ACEs are prevented ( 7 ). Preventing ACEs is possible and achievable, and CDC’s Preventing Adverse Childhood Experiences Resource for Action outlines six strategies for preventing ACEs and mitigating their negative consequences based on the best available evidence ( 1 ). This resource outlines approaches for advancing each strategy, with examples of evidence-based programs, policies, and practices for each approach. Strategies include increasing economic supports for families, promoting social norms that protect against ACEs, ensuring a strong start for children, teaching skills, and connecting youth to caring adults and activities. Tailored approaches to address the most prevalent ACEs experienced by U.S. high school students are needed. Examples include promoting of parenting skills and family relationship approaches to prevent emotional abuse, providing high-quality childcare to reduce risk for physical abuse and improve household mental health, and offering family-centered treatment for substance use disorders ( 1 ). Because more than three fourths of U.S. high school students have experienced ACEs, intervening to lessen long-term harms of ACEs is critical for improving public health. Policymakers and public health professionals in states and communities that implement evidence-based approaches to advance these strategies could prevent ACEs among children, and this study’s findings suggest that preventing ACEs might also translate into sizable reductions in suicidal behaviors, substance use, sexual risk behaviors, violence, and persistent feelings of sadness and hopelessness when these children reach adolescence.
General limitations for the YRBS are available in the overview report of this supplement ( 8 ). The findings in this report are subject to at least four additional limitations. First, although the eight ACEs categories measured on the YRBS are consistent with traditional measures of ACEs ( 3 ), these categories do not capture all potentially traumatic experiences in childhood or characteristics such as intensity or severity of the experience, which might have implications for health conditions and risk behaviors. This study dichotomized ACEs exposures; future studies could use available responses to explore the nuances of ACEs frequency on conditions and behaviors. Second, these data are cross-sectional. Whereas the ACEs questions measure lifetime occurrence and most conditions and behaviors of interest reflect current (past 30 days) or past-year experiences, for certain persons, ACEs might not have occurred before the outcome of interest. These effects, although strong and consistent, are correlative in nature and should not be interpreted as causal associations between ACEs and included risk behaviors and health conditions. Third, social determinants of health and other related risk factors that contribute to both ACEs and health conditions and risk behaviors could not be assessed, but these social and structural conditions shape the contexts in which adolescents live, play, and learn. Not analyzing these factors could lead to inaccurate estimations of the relations between ACEs and conditions and behaviors because the survey did not include underlying factors with strong influence on the context that shapes adolescents’ lives and communities. Finally, adolescents who have experienced multiple ACEs might be more likely to be engaged with the juvenile justice system, unstably housed, or to miss school frequently ( 15 ), all of which could affect their opportunity to participate in the school-based YRBS. As a result, estimates for ACEs might be underreported.
The findings in this report highlight the importance of preventing ACEs for adolescent well-being and as a prevention and reduction strategy for multiple other adolescent health and behavioral risks, such as suicide risk, substance use, sexual risk behaviors, and poor mental health. Ongoing monitoring of ACEs among adolescents is critical to understand trends in the prevalence of ACEs, inequities in the number of ACEs, and effects of ACEs prevention efforts over time. Future research in this area might examine how individual ACEs uniquely contribute to health conditions and risk behaviors, including across demographic groups, and identify protective factors and positive childhood experiences that might buffer against the negative effect of ACEs on adolescent health conditions and risk behaviors. In 2023, CDC’s National Center for Injury Prevention and Control partnered with the Division of Adolescent and School Health to obtain national level estimates of ACEs. Ongoing research and surveillance are warranted to support data-driven, evidence-based implementation of primary prevention approaches to prevent ACEs and to mitigate their harms. CDC supports a robust portfolio across the public health model to better monitor, understand, prevent, and respond to ACEs so that all children and families can thrive ( 1 ).
ACEs are common in U.S. high school students and have significant associations with negative health conditions and risk behaviors. Prevalence of individual ACEs differ by demographic characteristics, with the highest prevalence of ACEs disproportionately affecting female, AI/AN, multiracial, and LGBQ+ students. Preventing ACEs has considerable potential public health impact in adolescence and beyond, with substantial population-attributable fractions (>50%) for all violence, substance use, sexual, mental health, and suicide-related behaviors. Timely, adolescent-reported data on ACEs is needed to tailor prevention strategies to specific cultural contexts and populations. CDC’s Preventing Adverse Childhood Experiences Resource for Action provides evidence-based strategies and approaches to prevent ACEs and mitigate their consequences ( 1 ).
Maria Aslam, Division of Injury Prevention, National Center for Injury Prevention and Control, CDC; David Chyen, William A. Harris, Connie Lim, Cecily K. Mbaka, Zachary Myles, Lindsay Trujillo, National Center for Chronic Disease Prevention and Health Promotion, CDC.
Corresponding Author: Elizabeth A. Swedo, Division of Violence Prevention, National Center for Injury Prevention and Control, CDC. Telephone: 404-498-5277; Email: [email protected] .
1 Division of Violence Prevention, National Center for Injury Prevention and Control, CDC; 2 Division of Adolescent and School Health, National Center for Chronic Disease Prevention and Health Promotion, CDC; 3 Office of Strategy and Innovation, National Center for Injury Prevention and Control, CDC; 4 Epidemic Intelligence Service, CDC
Conflicts of Interest
All authors have completed and submitted the International Committee of Medical Journal Editors form for disclosure of potential conflicts of interest. Jonetta J. Mpofu reported being a board member of the American School Health Association. No other potential conflicts of interest were disclosed.
* 45 C.F.R. part 46.114; 21 C.F.R. part 56.114.
- CDC. Adverse childhood experiences (ACEs) prevention resource for action: a compilation of the best available evidence. Atlanta, GA: US Department of Health and Human Services, CDC; 2019. https://www.cdc.gov/violenceprevention/pdf/ACEs-Prevention-Resource_508.pdf
- Hughes K, Bellis MA, Hardcastle KA, et al. The effect of multiple adverse childhood experiences on health: a systematic review and meta-analysis. Lancet Public Health 2017;2:e356–66. https://doi.org/10.1016/S2468-2667(17)30118-4 PMID:29253477
- Felitti VJ, Anda RF, Nordenberg D, et al. Relationship of childhood abuse and household dysfunction to many of the leading causes of death in adults. The Adverse Childhood Experiences (ACE) Study. Am J Prev Med 1998;14:245–58. https://doi.org/10.1016/S0749-3797(98)00017-8 PMID:9635069
- Swedo EA, Aslam MV, Dahlberg LL, et al. Prevalence of adverse childhood experiences among U.S. adults—Behavioral Risk Factor Surveillance System, 2011–2020. MMWR Morb Mortal Wkly Rep 2023;72:707–15. https://doi.org/10.15585/mmwr.mm7226a2 PMID:37384554
- Anderson KN, Swedo EA, Trinh E, et al. Adverse childhood experiences during the COVID-19 pandemic and associations with poor mental health and suicidal behaviors among high school students—Adolescent Behaviors and Experiences Survey, United States, January–June 2021. MMWR Morb Mortal Wkly Rep 2022;71:1301–5. https://doi.org/10.1007/s10560-018-0532-x PMID:36227769
- Salo M, Appleton AA, Tracy M. Childhood adversity trajectories and violent behaviors in adolescence and early adulthood. J Interpers Violence 2022;37:NP13978–4007. https://doi.org/10.1177/08862605211006366 PMID:33858246
- Peterson C, Aslam MV, Niolon PH, et al. Economic burden of health conditions associated with adverse childhood experiences among U.S. adults. JAMA Netw Open 2023;6:e2346323. https://doi.org/10.1001/jamanetworkopen.2023.46323 PMID:38055277
- Brener ND, Mpofu JJ, Krause KH, et al. Overview and methods for the Youth Risk Behavior Surveillance System—United States, 2023. In: Youth Risk Behavior Surveillance—United States, 2023. MMWR Suppl 2024;73(No. Suppl-4):1−12.
- Anderson KN, Swedo EA, Clayton HB, Niolon PH, Shelby D, McDavid HK. Building infrastructure for surveillance of adverse and positive childhood experiences: integrated, multimethod approaches to generate data for prevention action. Am J Prev Med 2022;62(Suppl 1):S31–9. https://doi.org/10.1016/j.amepre.2021.11.017 PMID:35597581
- CDC. Guidance for analyzing 2021 ACEs & PCEs data: YRBS Youth Risk Behavior Survey. Atlanta, GA: US Department of Health and Human Services, CDC; 2023. https://www.cdc.gov/violenceprevention/pdf/YRBS-ACEs-PCEs-Analytic-Recommendations-CLEARED_508.pdf
- Miettinen OS. Proportion of disease caused or prevented by a given exposure, trait or intervention. Am J Epidemiol 1974;99:325–32. https://doi.org/10.1093/oxfordjournals.aje.a121617 PMID:4825599
- Mersky JP, Choi C, Plummer Lee C, Janczewski CE. Disparities in adverse childhood experiences by race/ethnicity, gender, and economic status: intersectional analysis of a nationally representative sample. Child Abuse Negl 2021;117:105066. https://doi.org/10.1016/j.chiabu.2021.105066 PMID:33845239
- Giano Z, Wheeler DL, Hubach RD. The frequencies and disparities of adverse childhood experiences in the U.S. BMC Public Health 2020;20:1327. https://doi.org/10.1186/s12889-020-09411-z PMID:32907569
- Substance Abuse and Mental Health Services Administration. A treatment improvement protocol: trauma-informed care in behavioral health services. Rockville, MD: US Department of Health and Human Services, Substance Abuse and Mental Health Services Administration; 2014. https://store.samhsa.gov/product/tip-57-trauma-informed-care-behavioral-health-services/sma14-4816
- Portwood SG, Lawler MJ, Roberts MC, editors. Handbook of adverse childhood experiences: a framework for collaborative health promotion. New York, NY: Springer; 2023.
Abbreviations: ACEs = adverse childhood experiences; BMI = body mass index. * Physical neglect is reverse coded and includes both “never” and “rarely” to align with previously published measures of neglect. https://www.cdc.gov/violenceprevention/aces/ace-brfss.html † Cumulative ACE count calculated only for participants with complete data on at least five individual ACEs. § BMI for each student was calculated from self-reported height and weight and then an obesity indicator and an overweight indicator were categorized based on sex- and age-specific reference data from the 2000 CDC Extended BMI-for-Age Growth Charts. https://www.cdc.gov/growthcharts/clinical_charts.htm
Abbreviation: IPV = intimate partner violence. * N = 17,838 respondents aged <18 years. The total number of students answering each question varied. Data might be missing because 1) the question did not appear in that student’s questionnaire, 2) the student did not answer the question, or 3) the response was set to missing because of an out-of-range response or logical inconsistency. Percentages in each category are calculated on the known data. † Male students significantly differed from female students, based on t -test analysis with Taylor series linearization (p<0.05). § Persons of Hispanic or Latino origin might be of any race but are categorized as Hispanic; all racial groups are non-Hispanic. ¶ American Indian or Alaska Native students significantly differed from Asian students, based on t -test analysis with Taylor series linearization (p<0.05). ** Asian students significantly differed from Black or African American students, based on t -test analysis with Taylor series linearization (p<0.05). †† Asian students significantly differed from Hispanic or Latino students, based on t -test analysis with Taylor series linearization (p<0.05). §§ Asian students significantly differed from White students, based on t -test analysis with Taylor series linearization (p<0.05). ¶¶ Asian students significantly differed from multiracial students, based on t -test analysis with Taylor series linearization (p<0.05). *** Black or African American students significantly differed from White students, based on t -test analysis with Taylor series linearization (p<0.05). ††† Black or African American students significantly differed from multiracial students, based on t -test analysis with Taylor series linearization (p<0.05). §§§ Black or African American students significantly differed from Hispanic or Latino students, based on t -test analysis with Taylor series linearization (p<0.05). ¶¶¶ Hispanic or Latino students significantly differed from White students (p<0.05). **** Hispanic or Latino students significantly differed from multiracial students (p<0.05). †††† Multiracial students significantly differed from White students (p<0.05). §§§§ Students aged ≤14 years significantly differed from students aged 16 years, based on t -test analysis with Taylor series linearization (p<0.05). ¶¶¶¶ Students aged ≤14 years significantly differed from students aged 17 years, based on t -test analysis with Taylor series linearization (p<0.05). ***** Students aged ≤14 years significantly differed from students aged 15 years, based on t -test analysis with Taylor series linearization (p<0.05). ††††† Students aged 15 years significantly differed from students aged 17 years, based on t -test analysis with Taylor series linearization (p<0.05). §§§§§ Heterosexual students significantly differed from gay or lesbian students, based on t -test analysis with Taylor series linearization (p<0.05). ¶¶¶¶¶ Heterosexual students significantly differed from bisexual students, based on t -test analysis with Taylor series linearization (p<0.05). ****** Heterosexual students significantly differed from students who describe their identity some other way, based on t -test analysis with Taylor series linearization (p<0.05). †††††† Heterosexual students significantly differed from students with who were questioning, based on t -test analysis with Taylor series linearization (p<0.05). §§§§§§ Gay or lesbian students significantly differed from students who describe their identity some other way, based on t -test analysis with Taylor series linearization (p<0.05). ¶¶¶¶¶¶ Gay or lesbian students significantly differed from bisexual students, based on t -test analysis with Taylor series linearization (p<0.05). ******* Gay or lesbian students significantly differed from questioning students, based on t -test analysis with Taylor series linearization (p<0.05). ††††††† Bisexual students significantly differed from questioning students, based on t -test analysis with Taylor series linearization (p<0.05). §§§§§§§ Bisexual students significantly differed from students who describe their identity some other way, based on t -test analysis with Taylor series linearization (p<0.05). ¶¶¶¶¶¶¶ Students who describe their identity in some other way significantly differed from students who were questioning, based on t -test with Taylor series linearization (p<0.05).
Abbreviation: ACEs = adverse childhood experiences. * Cumulative ACEs counts were only calculated for participants with complete data on at least five individual ACEs (N = 11,871). The total number of students answering each question varied. Data might be missing because 1) the question did not appear in that student’s questionnaire, 2) the student did not answer the question, or 3) the response was set to missing because of an out-of-range response or logical inconsistency. Percentages in each category are calculated on the known data. † Male students significantly differed from female students, based on t -test analysis with Taylor series linearization (p<0.05). § Persons of Hispanic or Latino origin might be of any race but are categorized as Hispanic; all racial groups are non-Hispanic. ¶ American Indian or Alaska Native students significantly differed from Asian students, based on t -test analysis with Taylor series linearization (p<0.05). ** Asian students significantly differed from multiracial students, based on t -test analysis with Taylor series linearization (p<0.05). †† Asian students significantly differed from Black or African American students, based on t -test analysis with Taylor series linearization (p<0.05). §§ Asian students significantly differed from White students, based on t -test analysis with Taylor series linearization (p<0.05). ¶¶ Asian students significantly differed from Hispanic or Latino students, based on t -test analysis with Taylor series linearization (p<0.05). *** Black or African American students significantly differed from multiracial students, based on t -test analysis with Taylor series linearization (p<0.05). ††† Black or African American students significantly differed from White students, based on t -test analysis with Taylor series linearization (p<0.05). §§§ Hispanic or Latino students significantly differed from multiracial students, based on t -test analysis with Taylor series linearization (p<0.05). ¶¶¶ Hispanic or Latino students significantly differed from White students, based on t -test analysis with Taylor series linearization (p<0.05). **** Multiracial students significantly differed from White students, based on t -test analysis with Taylor series linearization (p<0.05). †††† Students aged ≤14 years significantly differed from students aged 17 years, based on t -test analysis with Taylor series linearization (p<0.05). §§§§ Students aged 15 years significantly differed from students aged 17 years, based on t -test analysis with Taylor series linearization (p<0.05). ¶¶¶¶ Heterosexual students significantly differed from gay and lesbian students, based on t -test analysis with Taylor series linearization (p<0.05). ***** Heterosexual students significantly differed from bisexual students, based on t -test analysis with Taylor series linearization (p<0.05). ††††† Heterosexual students significantly differed from students who describe their identity some other way, based on t -test analysis with Taylor series linearization (p<0.05). §§§§§ Heterosexual students significantly differed from students with who were questioning, based on t -test analysis with Taylor series linearization (p<0.05). ¶¶¶¶¶ Gay or lesbian students significantly differed from bisexual students, based on t -test analysis with Taylor series linearization (p<0.05). ****** Gay or lesbian students significantly differed from students who describe their identity some other way, based on t -test analysis with Taylor series linearization (p<0.05). †††††† Bisexual students significantly differed from students who were questioning, based on t -test analysis with Taylor series linearization (p<0.05). §§§§§§ Students who were questioning significantly differed from students who described their identity some other way, based on t -test analysis with Taylor series linearization (p<0.05).
Abbreviations: ACEs = adverse childhood experiences; YRBS = Youth Risk Behavior Survey. * Cumulative ACEs counts were only calculated for participants with complete data on at least five individual ACEs (N = 11,871); the referent group includes those students with zero ACEs. The total number of students answering each question varied. Data might be missing because 1) the question did not appear in that student’s questionnaire, 2) the student did not answer the question, or 3) the response was set to missing because of an out-of-range response or logical inconsistency. † Because YRBS questionnaires differ by jurisdiction, students are not asked all national YRBS questions. Therefore, the total number of students included in each model varied. § Adjusted for sex, race and ethnicity, age, and sexual identity. ¶ Statistically significant; 95% CIs do not cross the null value of 1.0.
Abbreviation: ACEs = adverse childhood experiences. * Cumulative ACEs counts were only calculated for participants with complete data for at least five individual ACEs (N = 11,871); the referent group includes those students with zero ACEs. The total number of students answering each question varied. Data might be missing because 1) the question did not appear in that student’s questionnaire, 2) the student did not answer the question, or 3) the response was set to missing because of an out-of-range response or logical inconsistency. † Population-attributable fraction calculated using Miettinen’s formula and weighted after adjusting for sex, race and ethnicity, age, and sexual identity.
Suggested citation for this article: Swedo EA, Pampati S, Anderson KN, et al. Adverse Childhood Experiences and Health Conditions and Risk Behaviors Among High School Students — Youth Risk Behavior Survey, United States, 2023. MMWR Suppl 2024;73(Suppl-4):39–49. DOI: http://dx.doi.org/10.15585/mmwr.su7304a5 .
MMWR and Morbidity and Mortality Weekly Report are service marks of the U.S. Department of Health and Human Services. Use of trade names and commercial sources is for identification only and does not imply endorsement by the U.S. Department of Health and Human Services. References to non-CDC sites on the Internet are provided as a service to MMWR readers and do not constitute or imply endorsement of these organizations or their programs by CDC or the U.S. Department of Health and Human Services. CDC is not responsible for the content of pages found at these sites. URL addresses listed in MMWR were current as of the date of publication.
All HTML versions of MMWR articles are generated from final proofs through an automated process. This conversion might result in character translation or format errors in the HTML version. Users are referred to the electronic PDF version ( https://www.cdc.gov/mmwr ) and/or the original MMWR paper copy for printable versions of official text, figures, and tables.

Abstract – Mental health and inequity: A human rights approach to inequality, discrimination, and mental disability
Jonathan Kenneth Burns
Health and Human Rights 11/2
Published December 2009
Mental disability and mental health care have been neglected in the discourse around health, human rights, and equality. This is perplexing as mental disabilities are pervasive, affecting approximately 8% of the world’s population. Furthermore, the experience of persons with mental disability is one characterized by multiple interlinked levels of inequality and discrimination within society. Efforts directed toward achieving formal equality should not stand alone without similar efforts to achieve substantive equality for persons with mental disabilities. Structural factors such as poverty, inequality, homelessness, and discrimination contribute to risk for mental disability and impact negatively on the course and outcome of such disabilities. A human rights approach to mental disability means affirming the full personhood of those with mental disabilities by respecting their inherent dignity, their individual autonomy and independence, and their freedom to make their own choices. A rights-based approach requires us to examine and transform the language, terminology, and models of mental disability that have previously prevailed, especially within health discourse. Such an approach also requires us to examine the multiple ways in which inequality and discrimination characterize the lives of persons with mental disabilities and to formulate a response based on a human rights framework. In this article, I examine issues of terminology, models of understanding mental disability, and the implications of international treaties such as the United Nations Convention on the Rights of Persons with Disabilities for our response to the inequalities and discrimination that exist within society — both within and outside the health care system. Finally, while acknowledging that health care professionals have a role to play as advocates for equality, non-discrimination, and justice, I argue that it is persons with mental disabilities themselves who have the right to exercise agency in their own lives and who, consequently, should be at the center of advocacy movements and the setting of the advocacy agenda.
An official website of the United States government
Official websites use .gov A .gov website belongs to an official government organization in the United States.
Secure .gov websites use HTTPS A lock ( Lock Locked padlock icon ) or https:// means you've safely connected to the .gov website. Share sensitive information only on official, secure websites.
- Publications
- Account settings
- Advanced Search
- Journal List
Improved indoor lighting improved healthy aging at home – an intervention study in 77-year-old Norwegians
Helle k falkenberg, tor martin kvikstad, grethe eilertsen.
- Author information
- Article notes
- Copyright and License information
Correspondence: Helle K FalkenbergNational Centre for Optics, Vision and Eye Care, Faculty of Health and Social Sciences, University of South-Eastern Norway, Hasbergs vei 36, Kongsberg, 3616, Norway, Phone: Tel +473 100 8961Email [email protected]
Received 2018 Dec 18; Accepted 2019 Mar 29; Collection date 2019.
This work is published and licensed by Dove Medical Press Limited. The full terms of this license are available at https://www.dovepress.com/terms.php and incorporate the Creative Commons Attribution – Non Commercial (unported, v3.0) License ( http://creativecommons.org/licenses/by-nc/3.0/ ). By accessing the work you hereby accept the Terms. Non-commercial uses of the work are permitted without any further permission from Dove Medical Press Limited, provided the work is properly attributed. For permission for commercial use of this work, please see paragraphs 4.2 and 5 of our Terms ( https://www.dovepress.com/terms.php ).
Introduction: Healthy aging and good quality of life is important to allow older people to live at home. Lighting is a significant environmental attribute promoting visual, physical, and mental health. Due to normal visual age changes, older people need more light, but improving indoor lighting levels receives little attention.
Objective: To investigate the impact of improved home lighting on abilities to perform activities of daily living (ADLs) and quality of life in healthy older people.
Methods: Sixty healthy 77 years old living at home participated during the 4-month dark winter period. In the intervention group (IG, n=30), the living room lighting was optimized by providing lamps and a basic control system with three preset levels (normal, medium, low). Participants chose the light level and kept a diary. No change was implemented for the control group (CG, n=30). A questionnaire measured self-reported visual and general health and ability to perform ADL in regards to lighting before and after the intervention in both groups.
Results: In the IG, lighting levels significantly improved self-assessed lighting levels, abilities to perform ADLs, and read and write in the living room (all p <0.03). In the CG the only change was a deterioration in performing ADLs ( p <0.05). The difference in change was significant between the IG and CG (all p <0.02). “Normal” lighting was the preferred level and increased comfort and well-being. The IG also resumed visually demanding tasks, and acknowledged that avoiding these tasks were mainly due to poor lighting.
Conclusions: Good vision is essential in promoting healthy aging at home and require adequate lighting. This can easily be achieved using a basic light system. Adopting to higher lighting levels evolves quickly. Our results suggest that improved quality of light could improve quality of life, and lighting should be included as a factor promoting healthy aging at home.
Keywords: home environment, older people, aging, vision, health promotion, lighting intervention
Introduction
Increased life expectancy and improved living conditions in combination with an escalating older population with health care needs will lead to great challenges in Norway and internationally. 1 – 3 Arranging for people to remain living at home as long as possible may result in both improved quality of life, health and significant social economic effects. 4 Healthy aging is defined by the WHO as “the process of developing and maintaining the functional ability that enables wellbeing in older age.” 5 Functional ability is influenced by both environmental and individual capacities. Good vision is important to enable living at home and performing everyday tasks. Due to normal age-related vision loss, older people need correct glasses and more light to perform daily activities efficiently and safely. Reduced vision negatively effects general health and basic activities of daily living (ADLs, eg, dressing, transferring) and instrumental activities of daily living (IADLs, eg, reading 6 ). Poor vision is associated with an increased risk of trips and falls, often resulting in fractures and other injuries, 7 – 10 feelings of loneliness, depression, and anxiety. 9 , 11 – 15 Poor lighting exacerbates these problems, and older people commonly report difficulties reading in dim light and are only able to drive during the day. 16 – 19 Insufficient and poor lighting increase the risk of fall accidents among older people. 20 – 23 Lighting is thus a significant environmental factor promoting visual, physical, and mental health, 24 – 26 enabling successful aging at home. This was found more than 20 years ago, when Sörensen and Brunström showed that improved indoor lighting increased the quality of life in healthy older people living at home. 27 Since then, however, the need for more light to promote healthy aging at home, and to compensate for normal age-related vision loss, has received little attention in both the older population 28 – 32 among health care personnel, 19 and public health agencies. 4 The focus of lighting research has been on therapeutic effects of lighting in nursing homes and in clinical populations, such as those with dementia, depression, seasonal disorders, sleep disorders, and low vision. 33 – 38
The intervention described in this study is part of a larger study, where we previously have described the indoor home lighting levels, self-reported vision and general health, and ADLs in 114 healthy 75-year-old Norwegians. 31 Despite very low levels of indoor lighting, the participants were happy and healthy. We also found a large discrepancy between self-assessed health and recommended lighting levels, and low awareness of the effect of lighting on age-related vision loss or daily activities in the future. Consequently, the aims of the intervention were to investigate how improved lighting effected abilities to perform ADLs and health-related quality of life in healthy older persons living at home.
This intervention study forms Stages II–V of a study exploring how indoor lighting effects vision, health, ADL, and quality of life in healthy older persons living at home (see Figure 1 ).
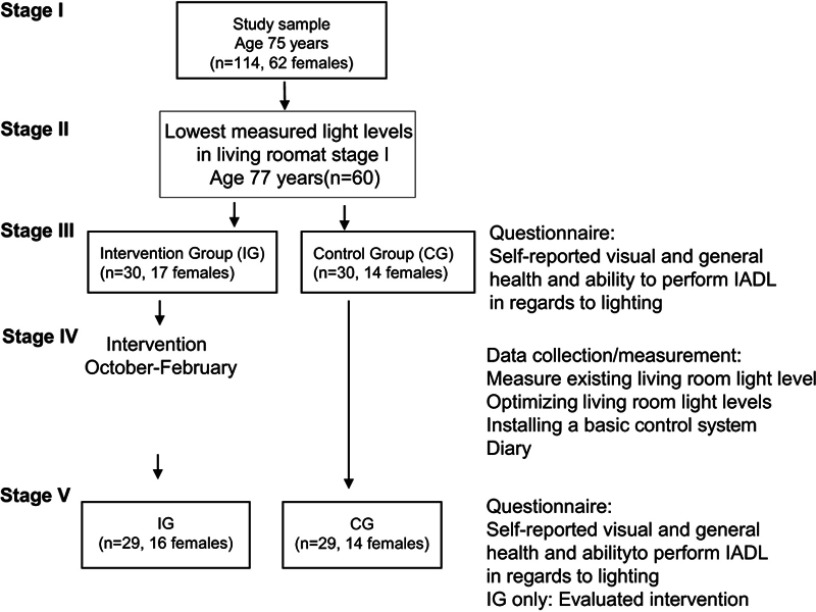
Flowchart describing the stages of the study, the intervention is described by Stages II–V. The baseline Stage I is described elsewhere. 31 .
The intervention took place in the living room in the private homes of healthy older persons living in the Southeast of Norway. The living room was chosen because this is where older people spend most of their waking hours, performing both hobbies, ADLs, IADLs, and social activities/interactions with friends.
The intervention was planned for two groups of 30 ( Figure 1 , Stage II) of the participants with the poorest living room lighting levels in Stage I. 31 The 77-year-old participants were stratified according to gender, housing, and co-habitancy (based on results from Stage I 31 ) to ensure equal allocation in the intervention group (IG) and the control group (CG), before being randomly assigned to one of the groups ( Figure 1 , Stage III). Exclusion criteria were recipient of any public health care, the presence of obvious cognitive impairment or palliation as documented in the medical journal or by clinical judgment by the health preventative nurse team (HPNT). Sociodemographic characteristics are presented in Table 1 .
Sociodemographic characteristics of the 77-year-old participants in the intervention and control group at Stage III and participants in the baseline study at Stage I
Notes: a Intervention group. b Control group. c Baseline data from the whole sample (Stage I).
Intervention (Stages III–V)
Figure 1 describes the 4-month intervention which occurred during the dark winter period (October–February) to avoid influence from natural daylight (Stages III–V). At the start and end of the intervention (Stages III and V) both the IG and CG self-reported visual and general health, and ability to perform IADLs in regards to lighting using a questionnaire. 31 In Stage IV, the lighting was optimized by 1) measuring light levels and supplementing existing lamps if necessary to achieve recommended living room lighting levels (200 lux) for older persons; 39 and 2) installing a wireless control system with three preset levels. During the intervention period, the IG kept a diary where they documented the amount of hours they used each of the three preset light levels.
Aspect on designing a successful home lighting intervention (Stage IV)
The intervention required evaluation of and physical change (adding lamps and control system) to the living room and private homes of older persons. To achieve a successful intervention, several aspects were important to consider. 39 , 40 The intrusion to the participant’s personal taste and interior had to be minimized, and both added lamps and the control system needed to be as inconspicuous as possible and not require permanent installations. Both lamps and the control system needed to be commercially available and universally designed. In collaboration with the lighting designers, the following criteria was required for all lamps: energy efficient, tolerate a 40 W light bulb, minimum level of glare, bulbs easy to replace, and reasonable cost and design. The participants (IG) chose from the preselected range of floor and table lamps. The control system was chosen upon fulfilling the following criteria: wireless, easy to use, programmable light levels, and reasonable cost and design. The control system connected up to seven lamps, and enabled three preset levels of lighting. In this intervention, we programmed three options: 200 lux (normal); 100 lux for watching TV (medium); and 50 lux for “candlelight dinner” (low). In addition, an “off” button turned off all the lamps connected to the control system. After a brief instruction, the participants found the control system easy to use.
Questionnaire (Stages III and V)
All participants reported sociodemographics, IADLs with respect to indoor lighting levels, symptoms of visual problems and general health-related quality of life (SF 36) in a questionnaire 31 with 55 questions including free comments. VASs were used to self-report on the ability to perform IADLs and visual health. VAS comprises a 100-mm horizontal line whose endpoints represent the positive and negative extremes pertaining to a particular statement, and the respondent marks the position that best represented their level of agreement with the statement. General health-related quality of life was assessed using SF-36 (Norwegian version 1.2 41 ) with 36 items that assess eight health scales (physical and social functioning, general and mental health, role physical and emotional, bodily pain, vitality, and health transition) over the past year. The physical health summary measure (PH) and mental-health summary (MH) measure are aggregated from the health scales. 42 The questionnaire took approximately 45 mins to complete, and all completed the questionnaire during a visit by the HPNT at Stages III and V. At the completion of the study (Stage V), the IG additionally assessed the lighting intervention answering eight questions, including a free text comment.
Light measurements (Stage IV)
The living room lighting optimization in the IG took place during the dark winter months (Stage IV). Lighting designers measured the lighting levels 80 cm above floor level in a 1×1-m 2 grid, according to international standard procedures. 31 , 43 The baseline study 31 showed that the average living room lighting level was just 35 lux, well below the recommended level of 200 lux. 39 If the recommended lighting level could not be achieved with existing lamps, extra lamps were added. Lamps were connected to the wireless control system. Participants were encouraged to use the “normal” level as this ensured the recommended 200 lux. The lighting designers also gave advice on good lighting conditions, ie, the position and direction of light, use of daylight, color and contrast, and to avoid glare. 39 , 44 After 1 week, participants were contacted to ensure that everything was working, and to answer any questions.
Data analysis
Continuously distributed location variables are presented as means with standard deviations. Because of very skewed distributions and small groups, nonparametric tests were used. Even if a few distributions indicated the use of Student’s t -test, we chose to use nonparametric test all over to avoid confusion. Differences between groups were tested using a Wilcoxon rank-sum test (Mann–Whitney test). For paired sample tests, Wilcoxon signed-rank test was used. All tests were two-tailed using the null hypothesis of equality. In some comparison of the two groups (IG and CG) involving counts, binominal tests were used. To compare more than two related samples, Friedmans’ test was used. The threshold for statistical significance level was set at 5% ( p <0.05). All computations were performed using the statistical package SPSS Statistics 25 (International Business Machines, USA).
After completing the questionnaire at Stage III, one participant in the IG withdrew from the study and one in the CG did not complete the final questionnaire. These two were excluded from the final analysis.
A typical living room measured about 30 m 2 . At the beginning of the intervention (Stage IV), the lighting designers added lamps to 26 living rooms to achieve the recommended lighting levels. The IG group reported significant larger improved ability to perform IADLs than the CG (mean difference between groups of 11.7 mm, p =0.003). For reading and writing in the living room a mean difference of 12.3 mm was reported with p =0.006 and at the dining table (mean difference between the groups of 4.8 mm, p =0.019). Table 2 shows the change in perceived indoor lighting environment, ability to perform IADLs and general health for the IG and CG between Stage III and Stage V. The IG group reported large and significant improvements in the self-assessed indoor lighting levels (mean improvement of 21.9 mm, p <0.001). They also reported an improved ability to perform IADLs (mean improvement of 8.8 mm, p =0.026), including reading and writing in the living room (mean improvement of 12.3 mm, p <0.001) or at the dining table (mean improvement of 3 mm, p =0.016). The only change in the CG was a significant deterioration in the ability to perform IADLs (mean deterioration of 2.9 mm, Wilcoxon, p <0.022). Further, the IG reported an improvement in 24 of the 28 items asking about the ability to perform ADLs. This was statistically better, compared to the CG ( p <0.001). Self-reported visual problems and health remained unchanged in both groups during the 4-month intervention (all p >0.05). The SF-36 mean PH scores for the IG and CG were 63.6 and 58, respectively, and 75.6 and 76.9 for mean MH scores. The SF-36 scores remained the same, and there was no difference within or between the two groups (all p >0.05).
Change in self-assessed VAS scores (mm) with regard to indoor lighting levels, IADLs and health between Stage V and Stage III
Notes: a Negative change indicates improvement, positive change indicates deterioration, b IG significantly worse at Stage III, c significantly better, d significantly worse.
All participants kept a diary documenting how the three preset light levels were used for each day. Figure 2 shows the total sum of hours used with the different light levels during the intervention period. As can be seen, the “normal” level is the preferred light level during the intervention period compared to the two lower light levels ( p <0.001). For all light levels the number of hours used peaked in the middle of the period, and tailors off at the start and end of the intervention. Further, there is a small drop around 75–85 days, coinciding with the Christmas and New Year holiday period.
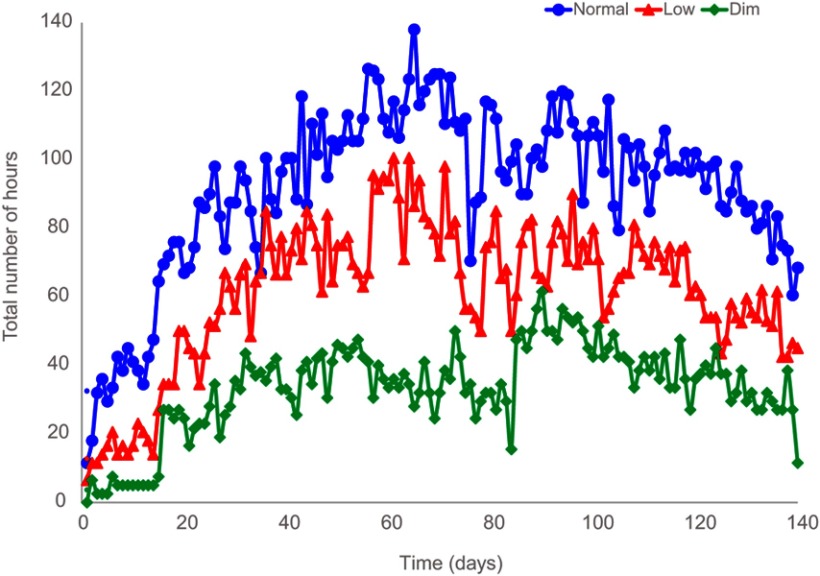
The amount of hours used per day (% hours, n=29) for the different preset levels during the intervention period.
With regards to the use of “normal” lighting, the proportion of persons who use this for a long time (5–8 hrs per day) is significantly increasing during the intervention period ( p <0.001). This is at the expense of the proportion who use it for shorter periods, which subsequently are decreasing.
After the intervention, 26 answered eight questions assessing the two intervention elements. Overall, their assessment showed that most were very satisfied with both the lighting and the control system. Twenty-two participants were very satisfied or satisfied with increased lighting levels, 21 were very satisfied or satisfied with the preset levels, and 23 reported to be very satisfied or satisfied with the control system. When asked to assess the importance of the different criteria applied to choose lamps and control system to include in the intervention, the ease of replacing bulbs, that lamps prevented glare and that the control system was easy to use was considered very important by 18, 19, and 19, respectively, and 4, 1, and 2 said it was important. The ability to use energy-saving bulbs and the cost of lamps or control system was considered very important by 12, 12, and 13, and not important by 5, 4, and 5, respectively. Comments from the free text were almost exclusively positive regarding the improved lighting. Several reported that they now realized that before the intervention, their lighting levels were poor leading to reduced visual ability. With the improved lighting, they were now able to resume some meaningful activities they had stopped performing due to poor lighting and vision. One commented that they used more light now, that the combination of being able to use “normal” lighting for reading, jigsaw puzzles and handicraft, while “cosy” lighting for watching TV and in social settings was very valuable. The importance of being able to vary the different light levels that were “just right” for different purposes was also commented, and further that the “normal” lighting improved their vision. Regarding the control system, comments were that the simple design of the preset levels was valuable, and that the wireless mode made it easy use. The participants found it very useful that lamps were controlled by one box. This made it easy to use more light because avoiding bending down, stretching or walking to reach individual light switches with a painful back or reduced mobility. Twenty-four reported they would recommend a similar system to friends. The two people who did not want to recommend the system to friends because this system could not dim the light (but had preset levels), and that the control system required extension cords to connect all the lamps to the system. After the intervention, 28 of the 29 participants wanted to keep the lamps and the control system.
This intervention investigated how improved lighting in the living room effected abilities to perform daily activities, perception of the home lighting environment, and health-related quality of life in healthy older persons living at home. The baseline study (Stage I) 31 revealed that healthy older persons overall were happy, but living in darkness, and unaware of any problems related to lighting and everyday tasks. However, this intervention showed that participants were even happier with the improved lighting levels.
The ability to perform meaningful activities (eg, IADLs) significantly improved with improved lighting in the IG. In contrast, the only change in the CG was a deterioration in the ability to perform IADLs. This is, as far as we know, the first time this has been shown in healthy older adults with normal vision. The difference can be explained by the knowledge that home lighting levels were very low before the start of the intervention, 31 similar to other studies, 27 , 32 , 45 and the significant improvement in lighting improved IADLs. These results are in line with previous studies in healthy older adults 27 and low vision. 38 The deterioration seen in the CG was not expected. One interpretation could be that the need for more lighting to compensate for normal age-related vision changes was absent in the CG, and further that they reported the ability to perform IADLs to be very easy at the start of the intervention. When ADLs become easier, one might infer that these activities are performed more often, enabling promotion of both cognitive and physical health. 3
Specifically the ability to read and write improved in the IG. Reading and writing are activities that are visually demanding and require more lighting with normal age changes. Most reading tasks benefit from specific task lighting, but this study also shows that improving the general lighting will benefit reading. Reading is considered an important and meaningful activity for most people, not only for pleasure, but also for accessing information and promoting independence and social communication. The ability to participate in meaningful conversations with others, and feeling a part of society reduces the risk of feeling of isolated. Further, the ability to read is a good measure of vision-related quality of life, 12 , 46 , 47 and reduced near vision is associated with reduction in depression and mental health. 15 , 48
The IG reported significant improvements in the home lighting environment after the intervention, and all but one wanted to keep the lighting system after the intervention ended. This indicates that lighting positively influences the perception of the home environment, and participants were happy with the intervention. That homes are perceived as a good place to be is even more important as older persons have to remain longer at home. Further, homes have to facilitate maintaining meaningful activities, and consequently our study confirms that lighting is a factor promoting several health aspects. This was supported by free comments, where some reported that ceasing performance of certain tasks was due to reduced vision because of poor lighting in the evening. Many older persons experience a reduction in social activities, and any factor contributing to improve this is important. 4 This emphasizes that lighting is an essential contributor to promote better health among older persons living at home, in line with other studies. 24 – 27 As such, we interpret our results to nuance and support the findings by Sörensen and Brunnström ; 27 that good lighting can promote health, even if we did not replicate this specifically. As our small sample consisted of healthy older adults and the intervention period was short, we did not expect a significant change in health.
The diary showed that the “normal” lighting was the preferred level during the whole period. This indicates that the “normal” lighting level quickly was adapted and perceived as helpful. Moreover, the diary showed a gradual increase in the use of more light during the darkest wintertime, before the overall use was reduced toward the end of the intervention period. The most plausible explanation for this is that the behavior is adapted to the seasonal variation in daylight during the intervention period. Interestingly, there is a marked dip for all three light levels, coinciding with the Christmas and New Year holiday period. We interpret this as an indication that participants were spending time outside their home, for example while visiting family. It further shows the compliance and diligence of the participants to log the use of light levels in their diary. Compliance is essential in order to change behavior and for a successful implementation. Compliance among older persons requires the intervention to be perceived as meaningful, simple, relatively easy to implement and improvements must be experienced relatively quickly. 49 – 51 With this in mind, our intervention seemed to fulfill criteria for a successful outcome, where the use of more light resulted in improved function and a positive perception of the home environment. The “normal” light was throughout the intervention the most used level. One explanation is described above that it quickly helped the ability to perform activities, and that it was perceived as pleasant. Another factor could be that instructing the participants that this was the recommended “normal” lighting levels, may have influenced the rapid adaptation. A simplistic interpretation from our intervention is that by informing people of what the lighting should be, and providing the system to achieve this, will make people change their behavior – as long as they find it useful. The fact that all but one wanted to keep the lamps and control system also indicates that this simple intervention were perceived as adding something positive to the homes and that the intervention has provided a permanent change to use higher lighting levels at home, in support of other studies. 27 , 38
Normal vision changes and increased prevalence of eye diseases with age means that older persons need more and better lighting. Lighting as an important factor in healthy aging at home was also founded by Haanes and colleagues. 29 They showed that small improvements, such as correct glasses, removal of earwax and use hearing aids, and increase the lighting levels had an encouraging effect on health in persons with a mean age of 88 years. However, their participants were too old and fragile to adapt fully to the advice given, and the authors concluded that these interventions should be applied earlier. Our study confirms that simple lighting improvements at home are wise to adapt already in the 70s to reduce the consequences of normal age-related vision impairments. Further, it suggests that lighting is important to promote better health and well-being in older persons living at home, in agreement with other studies. 20 , 23 , 52 We argue that better lighting also will reduce the risk of falls at home, as it is well documented that impaired vision increases the risk of falling. 24 – 26 As such, it is clearly necessary for health care providers to increase their knowledge and competency regarding indoor lighting. This includes the ability to assess whether the home lighting is universally designed, with lamps giving light without glare, lightbulbs being easy to change, and the ability to vary the level of lighting. In addition, new lightbulbs are energy efficient, and running costs are negligible. More information about what older people know about the effects of indoor lighting on vision, ADLs, and quality of life is also required.
Our study on healthy older persons adds to the knowledge that good lighting benefits daily activities including reading in persons with normal vision. 24 , 38 , 53 This confirms that lighting is an environmental characteristic positively influencing the quality of life, 27 , 38 and should be considered in promoting healthy aging.
Limitations
Strengths of the study include that the differences in sociodemographic variables between participants were small, and did not vary from the baseline study. In addition, the sample is representative of the Norwegian population born in 1934 54 with respect to the sociodemographic distribution, which we believe strengthens the potential generalizability of the study results. Another strength is the collaborative and multidisciplinary design and analysis by the multidisciplinary research group, which contribute to the validity of the results. Limitations include the confines of this basic intervention particularly when it comes to the diary entries, which might have caused a bias in the data. However, the diary logs showed that the participants were very thorough, and we believe any such biases to be small. Despite these limitations, we believe that this intervention study contributes important knowledge that may guide further research and clinical practice in this area.
The ability to perform meaningful activities is essential to maintain a good quality of life and facilitate healthy aging at home. This requires good vision and lighting. Our study showed that good lighting improved the ability to read and perform ADLs, and increased the home environment. Notably, improved lighting could easily be achieved with a basic low-cost control system. Our study confirms that lighting is an environmental characteristic influencing quality of life and should be considered in promoting healthy aging.
Acknowledgments
The authors want to acknowledge emeritus professor Gunnar Horgen, associate professor Ösp Egilsdottir RN, lighting designers Benedikte Nilsen Rauan, MultiConsult Oslo, Thomas Hummel ECT AS Drammen, Mari Sando ECT AS Drammen, and members of the health promotion nursing team in Drammen municipality, Norway for the support and assistance in carrying out the project.
This study was given financial support by the Norwegian State Housing Bank, Arena Health Innovation, Elko, Mulitconsult Oslo & ECT AS Drammen. Sponsors had no involvement in any of the stages from study design to submission of this paper for publication.
Abbreviation list
ADL, activities of daily living; IADL, instrumental activities of daily living; HPNT, health preventative nurse team; CG, control group; IG, intervention group; MH, mental health summary scores; PH, physical health summary scores.
Ethics approval and informed consent
All participated with written informed consent. The study was carried out in accordance with the Declaration of Helsinki, approved by the Regional Committee for Medical Research Ethics and the Ombudsman for Privacy in Research at the Norwegian Social Science Data Services.
Author contributions
Study design: HKF and GE contributed to the study design. All authors contributed to data analysis, drafting or revising the article, gave final approval of the version to be published, and agree to be accountable for all aspects of the work.
The authors reports no conflicts of interest in this work.
- 1. Christensen K, Doblhammer G, Rau R, Vaupel JW. Ageing populations: the challenges ahead. Lancet . 2009;374(9696):1196–1208. doi: 10.1016/S0140-6736(09)61460-4 [ DOI ] [ PMC free article ] [ PubMed ] [ Google Scholar ]
- 2. Rechel B, Grundy E, Robine JM, et al. Ageing in the European Union. Lancet . 2013;381(9874):1312–1322. doi: 10.1016/S0140-6736(12)62087-X [ DOI ] [ PubMed ] [ Google Scholar ]
- 3. WHO. Global Strategy and Action Plan on Ageing and Health . Geneva: World Health Organization; 2017. [ Google Scholar ]
- 4. WHO. WHO Housing and Health Guidelines . Geneva: World Health Organization; 2018. [ PubMed ] [ Google Scholar ]
- 5. WHO. World Report on Ageing and Health . World Health Organization; 2015.
- 6. Falkenberg HK, Rubin GS, Bex PJ. Acuity, crowding, reading and fixation stability. Vision Res . 2007;47(1):126–135. doi: 10.1016/j.visres.2006.09.014 [ DOI ] [ PubMed ] [ Google Scholar ]
- 7. Black A, Wood J. Vision and falls. Clin Exp Optom . 2005;88(4):212–222. [ DOI ] [ PubMed ] [ Google Scholar ]
- 8. White UE, Black AA, Wood JM, Delbaere K. Fear of falling in vision impairment. Optom Vis Sci . 2015;92(6):730–735. doi: 10.1097/OPX.0000000000000596 [ DOI ] [ PubMed ] [ Google Scholar ]
- 9. Freeman E, Muñoz B, Rubin G, West S. Visual field loss increases the risk of falls in older adults: the Salisbury eye evaluation. Invest Ophthalmol Vis Sci . 2007;48(10):4445–4450. doi: 10.1167/iovs.07-0326 [ DOI ] [ PubMed ] [ Google Scholar ]
- 10. Huang HC, Gau ML, Lin WC, George K. Assessing risk of falling in older adults. Public Health Nurs . 2003;20(5):399–411. [ DOI ] [ PubMed ] [ Google Scholar ]
- 11. Tsai S-Y, Cheng C-Y, Hsu W-M, Su T-P, Liu J-H, Chou P. Association between visual impairment and depression in the elderly. J Formos Med Assoc . 2003;102(2):86–90. [ PubMed ] [ Google Scholar ]
- 12. El-Gasim M, Munoz B, West SK, Scott AW. Associations between self-rated vision score, vision tests, and self-reported visual function in the salisbury eye evaluation study. Invest Ophthalmol Vis Sci . 2013;54(9):6439–6445. [ DOI ] [ PMC free article ] [ PubMed ] [ Google Scholar ]
- 13. Gall C, Brosel D, Sabel BA. Remaining visual field and preserved subjective visual functioning prevent mental distress in patients with visual field defects. Front Hum Neurosci . 2013;7:584. doi: 10.3389/fnhum.2013.00584 [ DOI ] [ PMC free article ] [ PubMed ] [ Google Scholar ]
- 14. Renaud J, Bedard E. Depression in the elderly with visual impairment and its association with quality of life. Clin Interv Aging . 2013;8:931–943. doi: 10.2147/CIA.S27717 [ DOI ] [ PMC free article ] [ PubMed ] [ Google Scholar ]
- 15. van der Aa HP, Comijs HC, Penninx BW, van Rens GH, van Nispen RM. Major depressive and anxiety disorders in visually impaired older adults. Invest Ophthalmol Vis Sci . 2015;56(2):849–854. doi: 10.1167/iovs.14-15848 [ DOI ] [ PubMed ] [ Google Scholar ]
- 16. Ball K, Owsley C, Stalvey B, Roenker DL, Sloane ME, Graves M. Driving avoidance and functional impairment in older drivers. Accid Anal Prev . 1998;30(3):313–322. [ DOI ] [ PubMed ] [ Google Scholar ]
- 17. Owsley C, McGwin G Jr. Vision and driving. Vision Res . 2010;50(23):2348–2361. doi: 10.1016/j.visres.2010.05.021 [ DOI ] [ PMC free article ] [ PubMed ] [ Google Scholar ]
- 18. Brabyn JA, Schneck ME, Lott LA, Haegerstrom-Portnoy G. Night driving self-restriction: vision function and gender differences. Optom Vis Sci . 2005;82(8):755–764. [ DOI ] [ PubMed ] [ Google Scholar ]
- 19. Houde SC, Huff MH. Age-related vision loss in older adults. A challenge for gerontological nurses. J Gerontol Nurs . 2003;29(4):25–33. [ DOI ] [ PubMed ] [ Google Scholar ]
- 20. Shi J, Zhou BY, Tao YK, et al. Incidence and associated factors for single and recurrent falls among the elderly in an urban community of Beijing. Biomed Environ Sci . 2014;27(12):939–949. doi: 10.3967/bes2014.134 [ DOI ] [ PubMed ] [ Google Scholar ]
- 21. Lopez-Soto PJ, Manfredini R, Smolensky MH, Rodriguez-Borrego MA. 24 hr pattern of falls in hospitalized and long-term care institutionalized elderly persons: a systematic review of the published literature. Chronobiol Int . 2015;32(4):548–556. doi: 10.3109/07420528.2014.987295 [ DOI ] [ PubMed ] [ Google Scholar ]
- 22. Crews JE, Chou CF, Stevens JA, Saaddine JB. Falls among persons aged >/=65 years with and without severe vision impairment - United States, 2014. Mmwr . 2016;65(17):433–437. doi: 10.15585/mmwr.mm6517a2 [ DOI ] [ PubMed ] [ Google Scholar ]
- 23. Brown MJ, Jacobs DE. Residential light and risk for depression and falls: results from the LARES study of eight European cities. Public Health Rep . 2011;126(Suppl 1):131–140. doi: 10.1177/00333549111260S117 [ DOI ] [ PMC free article ] [ PubMed ] [ Google Scholar ]
- 24. Stanley P, Yuanlong L. Inadequate light levels and their effect on falls and daily activities of community dwelling older adults: a review of literature. NZ J Occup Ther . 2012;59:39–42. [ Google Scholar ]
- 25. Boyce P. Lighting for the elderly. Technol Disabil . 2003;15(3):165–180. [ Google Scholar ]
- 26. Boyce PR. Human Factors in Lighting . London: Crc Press; 2014. [ Google Scholar ]
- 27. Sörensen S, Brunnström G. Quality of light and quality of life: an intervention study among older people. Light Res Technol . 1995;27(2):113–118. doi: 10.1177/14771535950270020501 [ DOI ] [ Google Scholar ]
- 28. Haanes GG, Kirkevold M, Hofoss D, Eilertsen G. Discrepancy between self-assessments and standardised tests of vision and hearing abilities in older people living at home: an ROC curve analysis. J Clin Nurs . 2015;24(23–24):3380–3388. doi: 10.1111/jocn.12967 [ DOI ] [ PubMed ] [ Google Scholar ]
- 29. Haanes GG, Kirkevold M, Hofoss D, Horgen G, Eilertsen G. An intervention designed to improve sensory impairments in the elderly and indoor lighting in their homes: an exploratory randomized controlled trial. J Multidiscip Healthc . 2015;8:11–20. doi: 10.2147/JMDH.S71718 [ DOI ] [ PMC free article ] [ PubMed ] [ Google Scholar ]
- 30. Hamel KA, Okita N, Higginson JS, Cavanagh PR. Foot clearance during stair descent: effects of age and illumination. Gait Posture . 2005;21(2):135–140. doi: 10.1016/j.gaitpost.2004.01.006 [ DOI ] [ PubMed ] [ Google Scholar ]
- 31. Eilertsen G, Horgen G, Kvikstad TM, Falkenberg HK. Happy living in darkness! indoor lighting in relation to activities of daily living, visual and general health in 75-year-olds living at home. J Hous Elderly . 2016;30(2):199–213. doi: 10.1080/02763893.2016.1162256 [ DOI ] [ Google Scholar ]
- 32. Hegde AL, Rhodes R. Assessment of lighting in independent living facilities and residents’ perceptions. J Appl Gerontol . 2009;29(3):381–390. [ Google Scholar ]
- 33. Campbell V, Crews J, Moriarty D, Zack M, Blackman D. Surveillance for sensory impairment, activity limitation, and health-related quality of life among older adults—United States, 1993–1997. MMWR CDC Surveill Summ . 1999;48(8):131–156. [ PubMed ] [ Google Scholar ]
- 34. Noell-Waggoner E. Lighting solutions for contemporary problems of older adults. J Psychosoc Nurs Ment Health Serv . 2004;42(7):14–20. [ DOI ] [ PubMed ] [ Google Scholar ]
- 35. Grandner MA, Kripke DF, Langer RD. Light exposure is related to social and emotional functioning and to quality of life in older women. Psychiatry Res . 2006;143(1):35–42. doi: 10.1016/j.psychres.2005.08.018 [ DOI ] [ PMC free article ] [ PubMed ] [ Google Scholar ]
- 36. De Lepeleire J, Bouwen A, De Coninck L, Buntinx F. Insufficient lighting in nursing homes. J Am Med Dir Assoc . 2007;8(5):314–317. doi: 10.1016/j.jamda.2007.01.003 [ DOI ] [ PubMed ] [ Google Scholar ]
- 37. Shikder S, Mourshed M, Price A. Therapeutic lighting design for the elderly: a review. Perspect Public Health . 2012;132(6):282–291. doi: 10.1177/1757913911422288 [ DOI ] [ PubMed ] [ Google Scholar ]
- 38. Brunnström G, Sörensen S, Alsterstad K, Sjöstrand J. Quality of light and quality of life–the effect of lighting adaptation among people with low vision. Ophthalmic Physiol Opt . 2004;24(4):274–280. doi: 10.1111/j.1475-1313.2004.00192.x [ DOI ] [ PubMed ] [ Google Scholar ]
- 39. Lyskultur. Belysning for eldre og svaksynte [Lighting Conditions for Elderly and Visually Impaired People] Vol. 11/97 Sandvika: Lyskultur; 1997. [ Google Scholar ]
- 40. Gitlin LN. Conducting research on home environments: lessons learned and new directions. Gerontologist . 2003;43(5):628–637. [ DOI ] [ PubMed ] [ Google Scholar ]
- 41. Loge JH, Kaasa S. Short form 36 (SF-36) health survey: normative data from the general Norwegian population. Scand J Soc Med . 1998;26(4):250–258. [ PubMed ] [ Google Scholar ]
- 42. Ware J, Snow KK, Kosinski M. SF-36 Health Survey: Manual and Interpretation Guide . Lincon, RI: Quality Metric Incorporated; 2002. [ Google Scholar ]
- 43. Bjørset -H-H. Lysteknikk: Lys og belysning [Lighting technology: Light and lighting] Oslo: Universitetsforlaget; 2000. [ Google Scholar ]
- 44. America IESoN. Lighting and the Visual Environment for Senior Living . New York: Illuminating Engineering Society; 2007. ANSI/IESNA RP-28-07 [ Google Scholar ]
- 45. Bakker R, Iofel Y, Lachs MS. Lighting levels in the Dwellings of homebound older adults. J Hous Elderly . 2004;18(2):17–27. doi: 10.1300/J081v18n02_03 [ DOI ] [ Google Scholar ]
- 46. Hazel CA, Petre KL, Armstrong RA, Benson MT, Frost NA. Visual function and subjective quality of life compared in subjects with acquired macular disease. Invest Ophthalmol Vis Sci . 2000;41(6):1309–1315. [ PubMed ] [ Google Scholar ]
- 47. Matthews K, Nazroo J, Whillans J. The consequences of self-reported vision change in later-life: evidence from the English longitudinal study of ageing. Public Health . 2017;142:7–14. doi: 10.1016/j.puhe.2016.09.034 [ DOI ] [ PubMed ] [ Google Scholar ]
- 48. Zhang X, Bullard K, Cotch M, et al. Association between depression and functional vision loss in persons 20 years of age or older in the united states, nhanes 2005–2008. JAMA Ophthalmol . 2013;131(5):573–581. doi: 10.1001/jamaophthalmol.2013.2597 [ DOI ] [ PMC free article ] [ PubMed ] [ Google Scholar ]
- 49. Dale B, Söderhamn U, Söderhamn O. Self-care ability among home-dwelling older people in rural areas in southern Norway. Scand J Caring Sci . 2012;26(1):113–122. doi: 10.1111/j.1471-6712.2011.00917.x [ DOI ] [ PubMed ] [ Google Scholar ]
- 50. Atreja A, Bellam N, Levy SR. Strategies to enhance patient adherence: making it simple. MedGenMed . 2005;7(1):4. [ PMC free article ] [ PubMed ] [ Google Scholar ]
- 51. Bunn F, Dickinson A, Barnett-Page E, McInnes E, Horton K. A systematic review of older people‘s perceptions of facilitators and barriers to participation in falls-prevention interventions. Ageing Soc . 2008;28(4):449–472. [ Google Scholar ]
- 52. Bhorade AM, Perlmutter MS, Wilson B, et al. Differences in vision between clinic and home and the effect of lighting in older adults with and without glaucoma. JAMA Ophthalmol . 2013;131(12):1554–1562. doi: 10.1001/jamaophthalmol.2013.4995 [ DOI ] [ PMC free article ] [ PubMed ] [ Google Scholar ]
- 53. Bowers AR, Meek C, Stewart N. Illumination and reading performance in age-related macular degeneration. Clin Exp Optom . 2001;84(3):139–147. [ DOI ] [ PubMed ] [ Google Scholar ]
- 54. Statistics Norway. Population Data. Statistics Norway. Available from: https://www.ssb.no/en/befolkning . 2018.
- View on publisher site
- PDF (502.2 KB)
- Collections
Similar articles
Cited by other articles, links to ncbi databases.
- Download .nbib .nbib
- Format: AMA APA MLA NLM
Add to Collections

An official website of the United States government
The .gov means it's official. Federal government websites often end in .gov or .mil. Before sharing sensitive information, make sure you're on a federal government site.
The site is secure. The https:// ensures that you are connecting to the official website and that any information you provide is encrypted and transmitted securely.
- Publications
- Account settings
- Browse Titles
NCBI Bookshelf. A service of the National Library of Medicine, National Institutes of Health.
Institute of Medicine (US) Committee on Prevention of Mental Disorders; Mrazek PJ, Haggerty RJ, editors. Reducing Risks for Mental Disorders: Frontiers for Preventive Intervention Research. Washington (DC): National Academies Press (US); 1994.

Reducing Risks for Mental Disorders: Frontiers for Preventive Intervention Research.
- Hardcopy Version at National Academies Press
H ardly a family in America has been untouched by mental illness. As many as one third of American adults will suffer a diagnosable mental disorder sometime in their life, and 20 percent have a mental disorder at any given time. Although research on the causes and treatment of mental disorders remains vitally important—and indeed major advances are leading to better lives for increasing numbers of people—much greater effort than ever before needs to be directed to prevention.
The Senate Appropriations Committee of the U.S. Congress believed that a strategic approach to the prevention of mental disorders was warranted. The Congress mandated the National Institute of Mental Health to enter into an agreement with the Institute of Medicine (IOM) to prepare an integrated report of current research with policy-oriented and detailed long-term recommendations for a prevention research agenda.
The specific tasks of the IOM committee, as negotiated with NIMH and the co-funding agencies, were as follows:
- Review the status of current research on the prevention of mental illness and problem behaviors and on the promotion of mental health throughout the life span. This should include an understanding of available research knowledge, research priorities, and research opportunities in the prevention research area.
- Review the existing federal presence in the prevention of mental disorders and the promotion of mental health, spanning the continuum from research to policy and services.
- Provide recommendations on federal policies and programs of research support leading to a prevention research agenda as well as on opportunities for maximization of involvement and improvement of coordination among federal agencies.
- Render a “capacity-building” plan for the development of personnel and resources necessary to ensure a cadre of prevention researchers.
- Provide an estimate of resources (funding, manpower, training opportunities) necessary to make effective scientific progress in prevention research.
- Prepare a final report that will encourage universities, colleges, hospitals, and federal agencies to foster prevention research.
The committee concludes that a critical mass of knowledge relevant to the prevention of mental disorders has accumulated and that opportunities now exist to effectively use this knowledge to launch a research agenda. Therefore the committee strongly recommends that an enhanced research agenda to prevent mental disorders be initiated and supported across all relevant federal agencies, including, but not limited to, the Departments of Health and Human Services, Education, Justice, Labor, Defense, and Housing and Urban Development, as well as state governments, universities, and private foundations. This agenda should facilitate development in three major areas:
- Building the infrastructure to coordinate research and service programs and to train and support new investigators.
- Expanding the knowledge base for preventive interventions.
- Conducting well-evaluated preventive interventions.
The three major areas to be developed are recommended in conjunction with use of the definitions of interventions for mental disorders and of prevention research developed in this report. The term prevention is reserved for only those interventions that occur before the initial onset of a disorder. These preventive interventions can be further classified into universal, selective, and indicated types, depending on the targeted population group. The term prevention research, as used in this report, refers only to preventive intervention research and is distinct from research that builds a broad scientific base for preventive interventions. This latter research is prevention-related, but it is not prevention research per se.
- BUILDING THE INFRASTRUCTURE
Preventive intervention research for mental disorders cannot thrive without providing for its infrastructure. Two areas are particularly important for moving ahead—coordination and research training.
- Coordination among federal agencies is needed for four reasons: (1) variation in the application of definitions has made it virtually impossible to assess the current activities and expenditures in preventive intervention research; (2) duplication of research activities and the lack of piggybacking of smaller projects onto larger ones contribute to waste of dollars and time, and, at the same time, gaps in research go undetected; (3) agencies conduct research or provide interventions for mental disorders (including addictions), educational disabilities, criminal behavior, and physical disorders as though these were separate conditions, whereas more often than not, coexisting disorders or problems occur; and (4) agencies have different strengths; for example, some are better at applying rigorous research methodologies to intervention programs, whereas others are better at reaching out into communities and forging alliances. Therefore the committee strongly recommends that a mechanism be created to coordinate research and services on prevention of mental disorders across the federal departments. One model for accomplishing this would be the establishment of a national scientific council on the prevention of mental disorders by Congress and/or the President. Such an overarching federal council could be operated out of the White House Office of Science and Technology Policy or another coordinating office within the Executive Office of the President.
- The committee also strongly recommends that Congress encourage the establishment of offices for prevention of mental disorders at the state level.
- Agencies must be required to identify their funded programs for the prevention of mental disorders, separately accounting for universal, selective, and indicated preventive interventions, using the definitions developed in this report.
- The National Institute of Mental Health (NIMH), the National Institute on Alcohol Abuse and Alcoholism (NIAAA), and the National Institute on Drug Abuse (NIDA) should consider including prevention researchers with broad mental health perspectives on their national advisory councils.
- Mental health reimbursement from existing health insurance should be provided for preventive interventions that have proved effective under rigorous research standards such as those described in this report.
- Dissemination activities should receive much higher priority than they have in the past.
- Congress and federal agencies should take steps immediately to develop and support the training of additional researchers who can develop new preventive intervention research trials as well as evaluate the effectiveness of current service projects.
- Research training should be focused on two groups—mid-career scientists and postdoctoral students.
- The number of institutional training programs focusing on preventive intervention research should be increased from 5 to 12 over the next five years, including one at every specialized prevention research center, known at NIMH as Preventive Intervention Research Centers (PIRCs), that is productive.
- Support for faculty within institutional training programs should be increased.
- A major effort should be made to encourage the prevention research training of minorities.
- The proposed national scientific council on the prevention of mental disorders should reevaluate the training needs for preventive intervention research after the first five years.
- EXPANDING THE KNOWLEDGE BASE
The committee believes that a viable research agenda for prevention of mental disorders rests on a firm stratum of health research in other fields. This knowledge base includes basic and applied research in the core sciences that is aimed at the causes and prevention of mental disorders. Included in this knowledge base are neurosciences, genetics, epidemiology, psychiatry, behavioral sciences (including developmental psychopathology), and risk research. It also includes evidence and lessons from other fields of research, such as prevention of physical illness and treatment of mental disorders. Therefore,
- Research to expand the knowledge base for preventive interventions should be continued.
- Support for research on potentially modifiable biological and psychosocial risk and protective factors for the onset of mental disorders should be increased. Priority should be given to research that illuminates the interaction of potentially modifiable biological and psychosocial risk and protective factors, rather than restricting the research to either biological or psychosocial factors alone.
- NIMH should support a series of prospective studies on well-defined general populations under the age of 18 to provide initial benchmark estimates of the prevalence and incidence of mental disorders and problem behaviors in this age group.
- A population laboratory should be established with the capacity for conducting longitudinal studies over the entire life span in order to generate understanding as to how risk factors and developmental transitions combine to influence the development of psychopathology.
- Whenever possible, research proposals relevant to the knowledge base for preventive interventions should explicitly state this connection, such as identification of potentially modifiable risk factors and possible avenues for preventive interventions.
- Treatment intervention research conducted under rigorous methodological standards that is directly relevant to preventive intervention research should continue to be supported—but not from the prevention research budget.
- Research should continue to be supported to determine which risk and protective factors are similar and which ones are different for treatment and prevention of a variety of mental disorders.
- Research should be supported to study the effects of social environments, such as families, peers, neighborhoods, and communities, on the individual and the effects of context on the onset of various mental disorders.
- Researchers working on relevant research in the core sciences should be encouraged to participate in activities such as forums and colloquia with preventive intervention researchers.
- A comprehensive, descriptive inventory of the activities in which the public engages to promote psychological well-being and mental health should be developed and supported.
- CONDUCTING WELL-EVALUATED INTERVENTIONS
The knowledge base for some mental disorders is now advanced enough that preventive intervention research programs, targeted at risk factors for these disorders, can rest on sound conceptual and empirical foundations. Therefore,
- Increased methodological rigor in all research trials, demonstration projects, and service program evaluations should be required.
- The concept of risk reduction, including the strengthening of protective factors, should be used as the best available theoretical model for guiding interventions to prevent the onset of mental disorders.
- Universal preventive interventions should continue to be supported in the areas of prenatal care, immunization, safety standards such as the use of seat belts and helmets, and control of the availability of alcohol.
- Research on selective and indicated interventions targeting high-risk groups and individuals should be given high priority.
- Priority should be given to preventive intervention research proposals that address well-validated clusters of biological and psychosocial risk and protective factors within a developmental life-span framework.
- Increased attention should be given to preventive intervention research that addresses the overlap between physical and mental illness.
- Research support should be developed in two waves over the next decade, initially focusing primarily on increasing research grant support for individual investigators and later on increasing support for preventive intervention research centers.
- Research on sequential preventive interventions aimed at multiple risks in infancy, early childhood, and elementary school age to prevent onset of multiple behavioral problems and mental disorders should be increased immediately and substantially.
- Research on preventive interventions aimed at major depressive disorder should be increased immediately and substantially.
- Research on preventive interventions aimed at alcohol abuse should be increased immediately.
- Support for pilot and confirmatory preventive intervention trials should be increased for conduct disorder.
- Research should be supported on alternative forms of intervention for the caregivers and family members of individuals with mental disorders, especially Alzheimer's disease and schizophrenia, to prevent the onset of stress-induced disorders among these caregivers.
- Over the next decade, as new specialized prevention research centers are initiated across the federal government, priority should be given to those that are sponsored through interagency agreement.
- Knowledge base research at the specialized prevention research centers should be supported by new research grants (RO1s) that do not use preventive intervention research dollars.
- Dissemination mechanisms, including publication in peer-reviewed journals, and knowledge exchange opportunities with other researchers and with representatives from the community should be mandated as part of the mission of each specialized prevention research center.
- The preventive intervention research cycle as described in this report should be used as a conceptual model for designing, conducting, and analyzing research programs.
- Increased attention to cultural diversity, ethical considerations, and benefit-cost and cost-effectiveness analyses should be an essential component of preventive intervention research.
- Community involvement should be increased to help identify disorders and problems that merit research and to support preventive intervention research programs.
There could be no wiser investment in our country than a commitment to foster the prevention of mental disorders and the promotion of mental health through rigorous research with the highest of methodological standards. Such a commitment would yield the potential for healthier lives for countless individuals and the general advancement of the nation's well-being. The committee recommends increased support for a research agenda, beginning with an increase of $50.5 million in fiscal year 1995. But even with the support of the federal government, the effort will not be easy. Overall, what is required is a national commitment to rigorous research and cooperation among federal, state, and local agencies, as well as universities, foundations, researchers, and communities.
- Cite this Page Institute of Medicine (US) Committee on Prevention of Mental Disorders; Mrazek PJ, Haggerty RJ, editors. Reducing Risks for Mental Disorders: Frontiers for Preventive Intervention Research. Washington (DC): National Academies Press (US); 1994. Abstract.
- PDF version of this title (7.8M)
In this Page
Recent activity.
- Abstract - Reducing Risks for Mental Disorders Abstract - Reducing Risks for Mental Disorders
Your browsing activity is empty.
Activity recording is turned off.
Turn recording back on
Connect with NLM
National Library of Medicine 8600 Rockville Pike Bethesda, MD 20894
Web Policies FOIA HHS Vulnerability Disclosure
Help Accessibility Careers
- - Google Chrome
Intended for healthcare professionals
- Access provided by Google Indexer
- My email alerts
- BMA member login
- Username * Password * Forgot your log in details? Need to activate BMA Member Log In Log in via OpenAthens Log in via your institution

Search form
- Advanced search
- Search responses
- Search blogs
- Ultra-processed food...
Ultra-processed food exposure and adverse health outcomes: umbrella review of epidemiological meta-analyses
Linked editorial.
Reasons to avoid ultra-processed foods
- Related content
- Peer review
- 1 Institute for Mental and Physical Health and Clinical Translation, Food & Mood Centre, School of Medicine, Deakin University, Barwon Health, Geelong, Victoria, Australia, 3220
- 2 Department of Epidemiology, Johns Hopkins Bloomberg School of Public Health, Baltimore, MD, USA
- 3 Welch Center for Prevention, Epidemiology, and Clinical Research, Johns Hopkins University, Baltimore, MD, USA
- 4 Chronic Disease and Ageing, School of Public Health and Preventive Medicine, Faculty of Medicine Nursing and Health Sciences, Monash University, Melbourne, Victoria, Australia
- 5 Sydney School of Public Health, Faculty of Medicine and Health, University of Sydney, Sydney, NSW, Australia
- 6 Institute for Physical Activity and Nutrition, School of Exercise and Nutrition Sciences, Deakin University, Geelong, Victoria, Australia
- 7 Université Sorbonne Paris Nord and Université Paris Cité, INSERM, INRAE, CNAM, Nutritional Epidemiology Research Team (EREN), Center of Research in Epidemiology and StatisticS (CRESS), F-93017 Bobigny, France
- 8 Centre for Adolescent Health, Murdoch Children’s Research Institute, Melbourne, Victoria, Australia
- 9 James Cook University, College of Public Health, Medical & Veterinary Sciences, Townsville, Queensland, Australia
- 10 School of Biotechnology, Dublin City University, Dublin, Ireland
- Correspondence to: M M Lane m.lane{at}deakin.edu.au (or @drmelissalane on Twitter/X)
- Accepted 19 January 2024
Objective To evaluate the existing meta-analytic evidence of associations between exposure to ultra-processed foods, as defined by the Nova food classification system, and adverse health outcomes.
Design Systematic umbrella review of existing meta-analyses.
Data sources MEDLINE, PsycINFO, Embase, and the Cochrane Database of Systematic Reviews, as well as manual searches of reference lists from 2009 to June 2023.
Eligibility criteria for selecting studies Systematic reviews and meta-analyses of cohort, case-control, and/or cross sectional study designs. To evaluate the credibility of evidence, pre-specified evidence classification criteria were applied, graded as convincing (“class I”), highly suggestive (“class II”), suggestive (“class III”), weak (“class IV”), or no evidence (“class V”). The quality of evidence was assessed using the GRADE (Grading of Recommendations, Assessment, Development, and Evaluations) framework, categorised as “high,” “moderate,” “low,” or “very low” quality.
Results The search identified 45 unique pooled analyses, including 13 dose-response associations and 32 non-dose-response associations (n=9 888 373). Overall, direct associations were found between exposure to ultra-processed foods and 32 (71%) health parameters spanning mortality, cancer, and mental, respiratory, cardiovascular, gastrointestinal, and metabolic health outcomes. Based on the pre-specified evidence classification criteria, convincing evidence (class I) supported direct associations between greater ultra-processed food exposure and higher risks of incident cardiovascular disease related mortality (risk ratio 1.50, 95% confidence interval 1.37 to 1.63; GRADE=very low) and type 2 diabetes (dose-response risk ratio 1.12, 1.11 to 1.13; moderate), as well as higher risks of prevalent anxiety outcomes (odds ratio 1.48, 1.37 to 1.59; low) and combined common mental disorder outcomes (odds ratio 1.53, 1.43 to 1.63; low). Highly suggestive (class II) evidence indicated that greater exposure to ultra-processed foods was directly associated with higher risks of incident all cause mortality (risk ratio 1.21, 1.15 to 1.27; low), heart disease related mortality (hazard ratio 1.66, 1.51 to 1.84; low), type 2 diabetes (odds ratio 1.40, 1.23 to 1.59; very low), and depressive outcomes (hazard ratio 1.22, 1.16 to 1.28; low), together with higher risks of prevalent adverse sleep related outcomes (odds ratio 1.41, 1.24 to 1.61; low), wheezing (risk ratio 1.40, 1.27 to 1.55; low), and obesity (odds ratio 1.55, 1.36 to 1.77; low). Of the remaining 34 pooled analyses, 21 were graded as suggestive or weak strength (class III-IV) and 13 were graded as no evidence (class V). Overall, using the GRADE framework, 22 pooled analyses were rated as low quality, with 19 rated as very low quality and four rated as moderate quality.
Conclusions Greater exposure to ultra-processed food was associated with a higher risk of adverse health outcomes, especially cardiometabolic, common mental disorder, and mortality outcomes. These findings provide a rationale to develop and evaluate the effectiveness of using population based and public health measures to target and reduce dietary exposure to ultra-processed foods for improved human health. They also inform and provide support for urgent mechanistic research.
Systematic review registration PROSPERO CRD42023412732.

- Download figure
- Open in new tab
- Download powerpoint
Introduction
Ultra-processed foods, as defined using the Nova food classification system, encompass a broad range of ready to eat products, including packaged snacks, carbonated soft drinks, instant noodles, and ready-made meals. 1 These products are characterised as industrial formulations primarily composed of chemically modified substances extracted from foods, along with additives to enhance taste, texture, appearance, and durability, with minimal to no inclusion of whole foods. 2 Analyses of worldwide ultra-processed food sales data and consumption patterns indicate a shift towards an increasingly ultra-processed global diet, 3 4 although considerable diversity exists within and between countries and regions. 5 6 Across high income countries, the share of dietary energy derived from ultra-processed foods ranges from 42% and 58% in Australia and the United States, respectively, to as low as 10% and 25% in Italy and South Korea. 5 6 In low and middle income countries such as Colombia and Mexico, for example, these figures range from 16% to 30% of total energy intake, respectively. 5 Notably, over recent decades, the availability and variety of ultra-processed products sold has substantially and rapidly increased in countries across diverse economic development levels, but especially in many highly populated low and middle income nations. 3
The shift from unprocessed and minimally processed foods to ultra-processed foods and their subsequent increasing contribution to global dietary patterns in recent years have been attributed to key drivers including behavioural mechanisms, food environments, and commercial influences on food choices. 7 8 9 10 11 These factors, combined with the specific features of ultra-processed foods, raise concerns about overall diet quality and the health of populations more broadly. For example, some characteristics of ultra-processed foods include alterations to food matrices and textures, potential contaminants from packaging material and processing, and the presence of food additives and other industrial ingredients, as well as nutrient poor profiles (for example, higher energy, salt, sugar, and saturated fat, with lower levels of dietary fibre, micronutrients, and vitamins). 6 12 Although mechanistic research is still in its infancy, emerging evidence suggests that such properties may pose synergistic or compounded consequences for chronic inflammatory diseases and may act through known or plausible physiological mechanisms including changes to the gut microbiome and increased inflammation. 12 13 14 15 16 Researchers, public health experts, and the general public have shown considerable interest in ultra-processed dietary patterns, foods, and their constituent parts given their potential role as modifiable risk factors for chronic diseases and mortality.
Although several meta-analyses have made efforts to consolidate the many individual original research articles that have investigated the associations between exposure to ultra-processed foods and the risk of adverse health outcomes in the past decade, 17 18 no comprehensive umbrella review has offered a broad overview and assessment of the existing meta-analytic evidence. Undertaking such a comprehensive review has the potential to enhance our understanding of these associations and provide valuable insights for better informing public health policies and strategies. This is particularly pertinent as the global debate continues regarding the need for public health measures to tackle exposure to ultra-processed foods in general populations. 19 20 To bridge this gap in evidence and contribute to the ongoing discussion on the role of ultra-processed food exposure in chronic diseases, we did an umbrella review to evaluate the evidence provided by meta-analyses of observational epidemiological studies exploring the associations between exposure to ultra-processed food and the risk of adverse health outcomes.
We conducted and reported this systematic umbrella review of meta-analyses (herein referred to as “meta-analysis studies”) in line with the Preferred Reporting Items for Systematic Reviews and Meta-Analyses (PRISMA) guidelines. 21
Inclusion criteria and searches
We found no existing pooled analyses of randomised controlled trials during the pilot phase of this review. Consequently, we refined our search approach and scope to focus on observational epidemiological studies. Thus, we outlined inclusion criteria in accordance with the population, exposure, comparisons, outcomes, and study design (PECOS) reporting structure. 22 Eligible meta-analysis studies comprised human populations across the life course, irrespective of health status (population). We also considered meta-analysis studies that examined associations of dietary intake of ultra-processed foods, as defined by the Nova food classification system (exposure), comparing dose-response (continuous exposure) and/or non-dose-response (categorical only or categorical and continuous exposure) associations of dietary intake of ultra-processed foods (comparison), with any adverse health endpoint (outcome). Included in our review were observational epidemiological study designs (for example, prospective cohort, case-control, and/or cross sectional) that pooled categorical or continuous outcome data by using meta-analysis (study design).
The lead author (MML) did a systematic search across MEDLINE, PsycINFO, Embase, and the Cochrane databases for studies spanning the period from 2009 to June 2023 (last update). The year 2009 aligns with the initial publication of the details and principles of the Nova food classification system, which introduced the concept of ultra-processed foods. 23 We applied no language limitations.
To identify relevant meta-analysis studies, we used key search terms and variations of text words related to ultra-processed food or Nova and meta-analysis study design: (“ultra-processed food” OR UPF OR “Nova food classification system”) AND (“meta-analysis” OR “systematic review”). The specific search strings for each database can be found in supplementary table A. We used Covidence systematic review software to do duplicate primary screening based on titles and abstracts (MML and EG) and duplicate secondary screening based on full text articles (MML and WM). We screened references cited within the eligible meta-analysis studies to identify any additional relevant meta-analysis studies (EG). Any disagreements between authors conducting eligibility screening were resolved through consensus. We included the most recent and/or largest meta-analysis study when multiple pooled analyses were available for the same adverse health outcome. This is consistent with the methods used in previous umbrella reviews. 24 25 26 In cases in which the most recent meta-analysis study examined non-dose-response and dose-response exposure to ultra-processed foods, we included both meta-analysed effect estimates.
Data extraction
We extracted characteristics of the original research articles included in the retained meta-analysis studies in duplicate by using a pre-piloted custom Microsoft Excel spreadsheet (MML, EG, SD, DNA, AJM, and SG). These data included details such as the outcome, spanning health domains such as mortality, cancer, and mental, respiratory, cardiovascular, gastrointestinal, and metabolic health outcomes. In addition, the data extraction encompassed details on the level of exposure comparison, distinguishing between dose-response (involving each additional serving per day or a 10% increment) and non-dose-response (encompassing categories such as high versus low, daily consumers versus not daily consumers, and frequent consumption versus no frequent consumption, as well as combinations of categories with continuous exposure including 1% or 10% increments). It also covered the total number of studies (original research articles), participants, and cases included in the pooled analysis. The extraction included effect estimates with 95% confidence intervals from both separate original research articles and those pooled from the meta-analysis studies, as well as the pooled effect size metric (hazard ratios, odds ratios, and risk ratios). Furthermore, we extracted details about the meta-analysis study, including the first author’s name, publication year, original research study design, and competing interests and funding disclosures of meta-analysis study authors. We prioritised pooled estimates with the largest number of prospective cohorts, given that prospective studies guarantee temporality in epidemiological associations and strongly limit reverse causality bias. 27 Additionally, we extracted pooled estimates for related health outcomes that were meta-analysed together and separately (for example, metabolic syndrome and its individual components including low high density lipoprotein cholesterol and hypertriglyceridaemia). If information was missing or unclear in the meta-analysis studies, we obtained the data from the original research articles or directly requested it from the corresponding author(s) of those meta-analysis studies. If discrepancies existed between the data reported in the original research articles and the meta-analyses, we prioritised extracting data from the original research article.
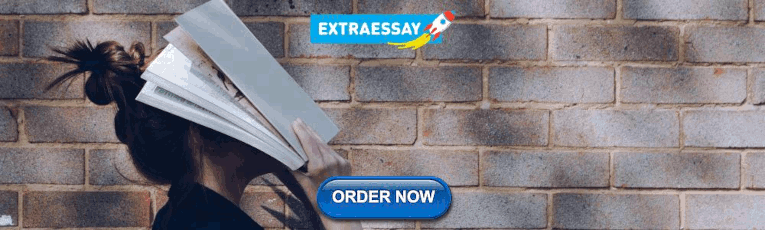
Data analysis
Operating according to previously published methods and guidance, 28 29 we used a random effects meta-analysis model to reanalyse the effect estimates for each outcome. As part of our main reanalysis, we took the following steps: entry of separate effect estimates and the total number of participants and cases from the original research articles; recalculation of the pooled effect estimates using the original metric used by the meta-analysis study authors (hazard ratio, odds ratio, and risk ratio with 95% confidence intervals); recalculation of the P value; and recalculation of the between study heterogeneity using the I 2 statistic. We also calculated 95% prediction intervals and assessed excess significance bias, small study effects, and the largest study significance (detailed below) as part of our reanalysis.
I 2 statistic
We used the I 2 statistic to assess the proportion of variability in a pooled analysis that was explained by between study heterogeneity, rather than by sampling error, and to reflect the extent to which 95% confidence intervals from the different original research articles overlapped with each other. 30 We considered a value of 50% to be moderate heterogeneity and a value of 75% or more to be high heterogeneity. 30
Prediction intervals
Unlike 95% confidence intervals, which give a range within which we can reasonably expect the true population parameter to fall based on our sample, 95% prediction intervals provide a range in which we can anticipate the value of an individual observation from future studies to fall. 31 In an umbrella review, if the 95% prediction intervals exclude the null, it indicates a statistically significant range of effect estimates. 31 Notably, outputs for tests of 95% prediction intervals, as well as the small study effects and excess significance bias (as described below), were available only for pooled analyses involving three or more original research articles (n=28).
Excess significance bias
We did a test for excess significance to determine whether the number of studies with nominally significant results (P<0.05) was higher than expected, based on statistical power. 32
Small study effects
We used Egger's regression asymmetry test to detect potential small study effects, whereby smaller studies sometimes show different, often larger, effect estimates than large studies. 33
Largest study significance
We assessed whether effect estimates from the largest original research article (that is, the study with the highest participant count) included in the pooled analyses had a P value below 0.05. This evaluation is expected to provide the most reliable and precise estimation considering the statistical power involved. 35 We evaluated the significance of the largest study across all 45 unique pooled analyses.
Visualisation
For visually comparative purposes, we developed forest plots whereby pooled effect estimates were harmonised to equivalent odds ratios with 95% confidence intervals by using methods presented in table 1 of Fusar-Poli et al (2018). 36 In this instance, an equivalent odds ratio >1 indicates higher odds, whereas an equivalent odds ratio <1 indicates lower odds, of an outcome.
Analysis software and code
We used the online version of the R statistical package called metaumbrella ( https://metaumbrella.org/app ) for data analyses. 37 The corresponding code repository is publicly accessible at GitHub ( https://github.com/cran/metaumbrella ). 37 Furthermore, the raw data are available at the Open Science Framework ( https://osf.io/8j2gt/ ), and a step-by-step analysis using metaumbrella is provided in supplementary table B.
Terminology
We used the terms “direct” and “inverse” to describe the direction of observed associations between ultra-processed food exposure and adverse health outcomes, with “direct” referring to a higher risk associated with greater exposure and “inverse” referring to a lower risk. We chose these terms over “positive” or “negative” associations to avoid ambiguous interpretations.
Credibility and quality assessment of evidence and methods
Credibility assessment of each pooled analysis using evidence classification criteria.
Using the data derived from our reanalyses, such as the P value, I 2 statistic, 95% prediction intervals, small study effects, excess significance bias, and largest study significance, we categorised each re-meta-analysed result of our umbrella review as convincing (“class I”), highly suggestive (“class II”), suggestive (“class III”), weak (“class IV”), or no evidence (“class V”) by following evidence classification criteria and previous umbrella reviews. 24 25 26 36 We determined these classifications on the basis of the criteria outlined in supplementary table C.
Quality assessment of each pooled analysis using GRADE
We used the GRADE (Grading of Recommendations, Assessment, Development, and Evaluation) system to evaluate the quality of evidence for each unique pooled analysis, and categorised them as either “high,” “moderate,” “low,” or “very low” (supplementary table D). 38 The GRADE approach initially considers all observational studies as evidence of low quality. 38 Of the eight criteria put forth in the GRADE method, five have the potential to diminish confidence in the accuracy of effect estimates, leading to downgrading: risk of bias, inconsistency of results across studies, indirectness of evidence, imprecision, and publication bias. 38 Additionally, three criteria are proposed to enhance confidence or upgrade it: a substantial effect size with no plausible confounders, a dose-response relation, and a conclusion that all plausible residual confounding would further support inferences regarding exposure effect. 38
Quality assessment of individual meta-analysis studies using AMSTAR 2
We used the AMSTAR 2 (A Measurement Tool to Assess Systematic Reviews – second edition) quality assessment tool to evaluate the quality of the included meta-analysis studies (supplementary table E). 39 This tool emphasises certain critical domains that could affect the reliability of a review. 39 The critical domains considered pertinent to our review included pre-specified review methods, the adequacy of the literature search, the rationale for excluding specific studies, the risk of bias in the included studies, the appropriateness of the meta-analytic methods, and the consideration of bias when interpreting the results (domains bolded in supplementary table E). 39 Following a recommendation from a recent review, 39 we used the AMSTAR 2 tool to do a qualitative assessment, considering the potential impact of a low rating for each item, particularly the critical domains outlined in supplementary table E. This meant that we did not quantify individual item ratings or combine them to create an overall score. 39
Patient and public involvement
The study and manuscript development did not involve patients or the public owing to the absence of funding for this research.
The systematic search identified 430 de-duplicated articles ( fig 1 ). After applying the eligibility criteria, we included 14 meta-analysis studies with 45 distinct pooled analyses. 17 18 40 41 42 43 44 45 46 47 48 49 50 51

Preferred Reporting Items for Systematic Reviews and Meta-Analyses (PRISMA) flowchart
Study characteristics
The range of adverse health outcomes reviewed across the 45 discrete pooled analyses included mortality, cancer, and mental, respiratory, cardiovascular, gastrointestinal, and metabolic health outcomes. All meta-analysis studies were published in the past three years, and none was funded by a company involved in the production of ultra-processed foods. The number of original research articles included in the pooled analyses was four on average and ranged from two to nine. The sum total number of participants included across the pooled analyses was 9 888 373 (ranging from 1113 17 to 962 593 48 ). Supplementary table F details the characteristics of the original research articles included in each of the pooled analyses, such as study design, population, and exposure measurement. Pooled analyses included estimates from original research articles that comprised either prospective cohorts (n=18), mixed study designs (n=15), or cross sectional designs (n=12). Most pooled analyses included adults as the main population, except for five, which included children and adolescents in examining mental health outcomes and respiratory conditions. 18 49 50 In 87% of pooled analyses, estimates of exposure to ultra-processed food were obtained from a combination of tools, including food frequency questionnaires, 24 hour dietary recalls, and dietary history, as reported in the meta-analysis studies. Six pooled analyses, pertaining to heart disease related mortality, 51 cancer related mortality, 51 respiratory conditions, 18 and non-alcohol fatty liver disease, 46 included estimates of exposure from food frequency questionnaires alone.
Each of the meta-analysis studies examined the non-dose-response associations between exposure to ultra-processed foods and adverse health outcomes. However, an additional analysis involving dose-response modelling of the ultra-processed food exposure variable was conducted in 13 pooled analyses across five meta-analysis studies. 40 42 43 47 51 The outcomes considered using this approach included all cause mortality and cardiovascular disease events, such as cardiovascular disease morbidity and mortality, associated with each increase in daily servings of ultra-processed food. 43 One meta-analysis study specifically pooled heart disease related deaths, such as ischaemic heart disease related mortality and cerebrovascular disease related mortality, with each 10% increase in total ultra-processed food exposure. 51 Additionally, associations for other outcomes, such as abdominal obesity, 42 overweight and obesity, 42 type 2 diabetes, 47 and breast, colorectal, and prostate cancers, 40 were modelled on the basis of each 10% increase in ultra-processed food exposure.
Results of syntheses
Figure 2 and figure 3 show the direction and sizes of effect estimates using equivalent odds ratios for both the non-dose-response and dose-response relations between exposure to ultra-processed foods and each adverse health outcome, respectively.

Forest plot of non-dose-response relations between greater exposure to ultra-processed foods and risk of adverse health outcomes, with credibility and GRADE (Grading of Recommendations, Assessment, Development, and Evaluation) quality assessments. Estimates are equivalent odds ratios, 36 with corresponding 95% confidence intervals (CIs). Cardiovascular disease events combined=morbidity+mortality; credibility=evidence classification criteria assessment; HDL=high density lipoprotein; k=number of original research articles. An interactive version of this graphic is available at https://public.flourish.studio/visualisation/16644020/

Forest plot of dose-response relations between greater exposure to ultra-processed foods and risk of adverse health outcomes, with credibility and GRADE (Grading of Recommendations, Assessment, Development, and Evaluation) quality assessments. Estimates are equivalent odds ratios, 36 with corresponding 95% confidence intervals (CIs). Cardiovascular disease events combined=morbidity+mortality; credibility=evidence classification criteria assessment; k=number of original research articles. An interactive version of this graphic is available at https://public.flourish.studio/visualisation/16645261/
On the basis of the random effects model, 32 (71%) distinct pooled analyses showed direct associations between greater ultra-processed food exposure and a higher risk of adverse health outcomes at the significance level of P≤0.05 (supplementary table G). Additionally, of these combined analyses, 11 (34%) showed continued statistical significance when a more stringent threshold was applied (P<1×10 −6 ) (data not shown). These included the incidence of all cause mortality, 43 cardiovascular disease related mortality, 43 heart disease related mortality, 51 type 2 diabetes (dose-response and non-dose-response), 47 and depressive outcomes, 50 as well as the prevalence of anxiety and combined common mental disorder outcomes, 50 adverse sleep related outcomes, 49 and wheezing. 18
We found evidence of moderate (I 2 =50-74.9%) to high (I 2 ≥75%) heterogeneity in 13 (29%) and eight (18%) of the 45 discrete pooled analyses, respectively (supplementary table G). The 95% prediction intervals were statistically significant for seven (25%) of the 28 pooled analyses with three or more original research articles (supplementary table G), including direct associations of greater ultra-processed food exposure with higher risks of all cause mortality, 43 cardiovascular disease related mortality, 43 common mental disorder outcomes, 50 Crohn’s disease, 48 obesity, 42 and type 2 diabetes (dose-response). 47 Additionally, we found evidence of excess significance bias in nine (32%) of the 28 pooled analyses with three or more original research articles listed in supplementary table G. This bias was evident in associations between higher ultra-processed food exposure and all cause mortality (dose-response and non-dose-response), 43 hypertension, 44 abdominal obesity, 42 metabolic syndrome, 45 non-alcoholic fatty liver disease, 46 obesity (dose-response and non-dose-response), 42 and type 2 diabetes. 47 Small study effects were evident in five (18%) of the 28 pooled analyses with three or more original research articles, as indicated in supplementary table G. We observed these effects in associations between higher ultra-processed food exposure and all cause mortality (dose-response and non-dose-response), 43 breast cancer, 40 metabolic syndrome, 45 and obesity (dose-response). 42
Effect estimates from the largest original research article were nominally statistically significant for 28 (62%) pooled analyses (supplementary table G) and pertained to associations of greater ultra-processed food exposure with higher risks of all cause mortality (dose-response and non-dose-response), 43 cardiovascular disease related mortality (dose-response and non-dose-response), 43 heart disease related mortality (dose-response and non-dose-response), 51 central nervous system tumours, 40 adverse sleep outcomes, 49 common mental disorder outcomes, 50 asthma, 18 wheezing, 18 cardiovascular disease events (dose-response and non-dose-response), 43 low high density lipoprotein concentrations, 17 abdominal obesity (dose-response and non-dose-response), 42 hyperglycaemia, 17 metabolic syndrome, 45 non-alcoholic fatty liver disease, 46 obesity and overweight (dose-response and non-dose-response), 42 and type 2 diabetes (dose-response and non-dose-response). 47
Credibility and GRADE quality assessments
Pooled effect estimates from nine dose-response and seven non-dose-response cohorts showed direct associations between greater exposure to ultra-processed foods and higher risks of incident all cause mortality (dose-response risk ratio 1.02, 95% confidence interval 1.01 to 1.03; credibility assessment class III; GRADE assessment moderate and non-dose-response risk ratio 1.21, 1.15 to 1.27; class II; low) 43 ( fig 4 ; supplementary tables D and G). Four dose-response and five non-dose-response cohorts informed the synthesis of associations between greater exposure to ultra-processed foods and higher risks of incident cardiovascular disease related mortality (dose-response risk ratio 1.05, 1.02 to 1.08; class IV; low and non-dose-response risk ratio 1.50, 1.37 to 1.63; class I; very low). 43 Effect estimates from two cohorts were pooled and showed limited evidence supporting direct associations between greater ultra-processed food exposure and a higher risk of incident cancer related mortality (hazard ratio 1.00, 0.81 to 1.24; class V; low). 51 We found further limited evidence for an association between greater ultra-processed food exposure and incident heart disease related mortality (dose-response hazard ratio 1.18, 0.95 to 1.47; class V; low and non-dose-response hazard ratio 1.66, 1.51 to 1.84; class II; low). 51

Credibility and GRADE (Grading of Recommendations, Assessment, Development, and Evaluation) ratings for associations between greater exposure to ultra-processed foods and risks of each adverse health outcome
Pooled analyses from seven cohort studies showed direct associations between greater exposure to ultra-processed foods and higher risks of incident cancer overall (hazard ratio 1.12, 1.06 to 1.19; class III; very low). 41 Synthesised analyses including mixed cohort and case-control study designs additionally showed direct associations with a risk of colorectal cancer (dose-response odds ratio 1.04, 1.01 to 1.07; class IV; low and non-dose-response odds ratio 1.23, 1.10 to 1.38; class III; very low). 40
We found limited evidence for pooled analyses, including mixed cohort and case-control study designs, of the association between greater ultra-processed food exposure and higher risks of breast cancer (dose-response odds ratio 1.03, 0.98 to 1.09; class V; low and non-dose-response odds ratio 1.15, 0.99 to 1.34; class V; very low), 40 central nervous system tumours (odds ratio 1.20, 0.87 to 1.65; class V; very low), chronic lymphocytic leukaemia (odds ratio 1.08, 0.80 to 1.45; class V; very low), pancreatic cancer (odds ratio 1.24, 0.85 to 1.79; class V; very low), and prostate cancer (dose-response odds ratio 0.99, 0.97 to 1.02; class V; moderate and non-dose-response odds ratio 1.02, 0.93 to 1.12; class V; low).
Mental health
Examining data from two to four cross sectional designs, we found evidence supporting direct associations between greater exposure to ultra-processed foods and a higher risk of the prevalence of adverse sleep related outcomes (odds ratio 1.41, 1.24 to 1.61; class II; low), 49 as well as anxiety outcomes (odds ratio 1.48, 1.37 to 1.59; class I; low). 50 We observed similar associations in separate assessments of prevalent combined common mental disorder outcomes across six cross sectional designs (odds ratio 1.53, 1.43 to 1.63; class I; low) 50 and incident depressive outcomes across two cohorts (odds ratio 1.22, 1.16 to 1.28; class II; low). 50
Respiratory health
Pooled analyses that included two cross sectional studies provided limited evidence of an association between greater exposure to ultra-processed foods and risks of prevalent asthma (risk ratio 1.20, 0.99 to 1.46; class V; very low) 18 and wheezing (risk ratio 1.40, 1.27 to 1.55; class II; low). 18
Cardiovascular health
Pooled analyses from six cohorts showed direct associations between greater ultra-processed food exposure and higher risks of incident cardiovascular disease events such as morbidity and mortality (dose-response risk ratio 1.04, 1.02 to 1.06; class III; low and non-dose-response risk ratio 1.35, 1.18 to 1.54; class III; very low), 43 as well as incident cardiovascular disease morbidity (dose-response risk ratio 1.04, 1.02 to 1.06; class III; low and non-dose-response risk ratio 1.20, 1.09 to 1.33; class III; low). 43 The higher risk of hypertension associated with greater ultra-processed food exposure was assessed using data from nine mixed cohorts and cross sectional study designs (odds ratio 1.23, 1.11 to 1.37; class III; very low). 44 We found weak to no evidence for associations between exposure to ultra-processed foods and hypertriglyceridaemia (odds ratio 0.95, 0.60 to 1.50; class V; very low) 17 and low high density lipoprotein concentrations (odds ratio 2.02, 1.27 to 3.21; class IV; very low). 17
Gastrointestinal health
We found weak or no evidence in pooled analyses incorporating data from four cohorts for associations between greater exposure to ultra-processed foods and higher risks of incident Crohn’s disease (hazard ratio 1.71, 1.37 to 2.14; class IV; low) 48 and ulcerative colitis (hazard ratio 1.17, 0.86 to 1.61; class V; very low). 48
Metabolic health
The risk of abdominal obesity was examined by synthesising effect estimates from mixed cohort and cross sectional study designs, which showed direct associations with greater ultra-processed food exposure (dose-response odds ratio 1.05, 1.02 to 1.07; class III; low and non-dose-response odds ratio 1.41, 1.18 to 1.68; class III; very low). 42 We found weak to no evidence for associations between exposure to ultra-processed foods and hyperglycaemia (odds ratio 1.10, 0.34 to 3.52; class V; very low), 17 metabolic syndrome (risk ratio 1.25, 1.09 to 1.42; class IV; very low), 45 non-alcoholic fatty liver disease (risk ratio 1.23, 1.03 to 1.46; class IV; very low), 46 and overweight and obesity (assessed together: dose-response odds ratio 1.03, 1.01 to 1.06; class IV; moderate and non-dose-response odds ratio 1.29, 1.05 to 1.58; class IV; low). 42 Effect estimates from four cross sectional studies informed pooled analyses of direct associations between greater ultra-processed food exposure and higher risk of the prevalence of overweight (dose-response odds ratio 1.06, 1.03 to 1.10; class III; low and non-dose-response odds ratio 1.36, 1.14 to 1.63; class III; very low). 42 Pooled analyses including seven cross sectional study designs further showed direct associations between greater ultra-processed food exposure and a higher prevalence of obesity (dose-response odds ratio 1.07, 1.03 to 1.11; class III; low and non-dose-response odds ratio 1.55, 1.36 to 1.77; class II; low). 42 The combined analysis of seven cohorts showed direct associations between greater exposure to ultra-processed foods and higher risk of incident type 2 diabetes (dose-response risk ratio 1.12, 1.11 to 1.13; class I; moderate and non-dose-response odds ratio 1.40, 1.23 to 1.59; class II; very low). 47
Quality assessment of individual meta-analysis studies using AMSTAR 2 tool
Although all of the authors of the meta-analysis studies used satisfactory literature search techniques (AMSTAR critical item 4) and accounted for the potential risk of bias in original research articles when interpreting and discussing their results (AMSTAR critical item 9), we considered the overall confidence in the results of seven meta-analysis studies to be low owing to lack of clarity as to whether the review methods were established before the conduct of the review (AMSTAR critical item 2) (supplementary table E). 17 40 41 42 44 45 51 Based on non-critical items, the confidence in the results of all meta-analysis studies was assessed as moderate. Notably, the most considerable limitations, for which all meta-analysis studies scored zero, were related to the review authors’ failure to provide an explanation for their selection of study designs for inclusion in the review (AMSTAR item 3) and their omission of information on funding sources for the studies included in the review (AMSTAR item 10). 39
Principal findings
Our umbrella review provides a comprehensive overview and evaluation of the evidence for associations between dietary exposure to ultra-processed foods and various adverse health outcomes. Our review included 45 distinct pooled analyses, encompassing a total population of 9 888 373 participants and spanning seven health parameters related to mortality, cancer, and mental, respiratory, cardiovascular, gastrointestinal, and metabolic health outcomes. Across the pooled analyses, greater exposure to ultra-processed foods, whether measured as higher versus lower consumption, additional servings per day, or a 10% increment, was consistently associated with a higher risk of adverse health outcomes (71% of outcomes).
Considering the evidence classification criteria assessments, we graded 9% of the pooled analyses as providing convincing evidence (class I), including those measuring risks of cardiovascular disease related mortality, common mental disorder outcomes, and type 2 diabetes (dose-response) ( fig 4 ). We graded 16% of pooled analyses (all non-dose-response) as providing highly suggestive evidence (class II), encompassing risks of all cause mortality, heart disease related mortality, adverse sleep related outcomes, wheezing, obesity, and type 2 diabetes. We graded approximately 29% of the pooled analyses as providing suggestive evidence (class III), covering a range of conditions from risks of abdominal obesity to overweight, with 18% graded as weak evidence (class IV), encompassing outcomes such as risks of colorectal cancer and overweight and obesity (evaluated together as single outcome). We graded the remaining 29% of pooled analyses as lacking evidence (class V), spanning conditions from asthma to ulcerative colitis. As previously noted, moderate to high levels of heterogeneity were observed across 45% of pooled analyses. Using GRADE assessments, which initially assign observational epidemiological studies as “low” quality evidence, 38 approximately 29% of the pooled analyses remained unchanged, indicating that no additional concerns were identified based on GRADE criteria, with a further 9% upgraded to a “moderate” rating owing to a dose-response gradient ( fig 4 ). Dose-response pooled analyses upgraded to “moderate” quality evidence related to all cause mortality, prostate cancer, overweight and obesity (assessed together), and type 2 diabetes. Associations were downgraded largely owing to inconsistencies or heterogeneity in the effect estimates found across the original research articles or owing to imprecision (that is, wide confidence intervals).
The heterogeneity and imprecision noted across several of the pooled analyses, as shown by both the evidence classification criteria and GRADE assessments, may be partly explained by the treatment of different effect estimates derived from original research articles (hazard ratios, odds ratios, and risk ratios) as approximately equivalent in various meta-analysis studies. 40 42 43 45 47 Such variations in scales may introduce heterogeneity and reduce precision in pooled estimates, even if the original research articles share conceptual similarities in exposures and outcomes. 52 Moreover, the synthesis of results based on three or fewer original research articles may contribute to heterogeneity and imprecision, 53 affecting outcomes assessed in our review such as certain cancers, asthma, and intermediate cardiometabolic risk factors. Although the pooled analyses relating to these outcomes were rated as having no or low quality evidence based on the evidence classification criteria and GRADE assessments, this does not necessarily negate the potential for an association, particularly as more data may become available in the future. Furthermore, considering the overall body of evidence, 93% of pooled analyses indicated point estimates in the same direction (greater than one) ( fig 2 and fig 3 ). The presence of 95% confidence intervals that included the null value in 24% of these pooled analyses signifies some uncertainty in the data, which may be partly due to insufficient sample size, particularly in analyses with a small number of original research articles and results showing wide confidence intervals. 54 This underscores the importance of conducting additional original research and subsequent meta-analyses in the respective disease areas.
Potential mechanisms of action
Understanding the aspects of ultra-processed dietary patterns that link them to poor health and early death requires more research. 12 55 The available evidence indicates that ultra-processed foods differ from unprocessed and minimally processed foods in several aspects, potentially explaining their plausible links with adverse health outcomes. These differences include poorer nutrient profiles, the displacement of non-ultra-processed foods from the diet, and alterations to the physical structure of consumables through intensive ultra-processing. More specifically, diets rich in ultra-processed foods are associated with markers of poor diet quality, with higher levels of added sugars, saturated fat, and sodium; higher energy density; and lower fibre, protein, and micronutrients. 6 56 Ultra-processed foods displace more nutritious foods in diets, such as fruits, vegetables, legumes, nuts, and seeds, 6 resulting in reduced intakes of beneficial bioactive compounds that are present in these foods, including polyphenols or phytoestrogens such as enterodiol. 57 58 Such nutrient-poor dietary profiles have been implicated in the prevalence and incidence of chronic diseases through various pathways, including inflammatory mechanisms. 13 14 16
The adverse health outcomes associated with ultra-processed foods may not be fully explained by their nutrient composition and energy density alone but also by physical and chemical properties associated with industrial processing methods, ingredients, and by-products. Firstly, alterations in the food matrix during intensive processing, also known as dietary reconstitution, may affect digestion, nutrient absorption, and feelings of satiety. 59 Secondly, emerging evidence in humans shows links between exposure to additives, including non-sugar sweeteners, emulsifiers, colorants, and nitrates/nitrites, and detrimental health outcomes. 60 61 62 63 64 65 A recent review of experimental research found that ultra-processed weight loss formulations composed of ostensibly balanced nutrient profiles but containing different additives, including non-sugar sweeteners, may have adverse effects on the gut microbiome—which is thought to play an important function in many of the diseases studied here—and related inflammation. 66 The World Health Organization recently warned against the ongoing use of sugar substitutes for weight control or non-communicable illnesses, 67 and, according to its new report, non-sugar sweeteners may also elevate the risk of cardiometabolic diseases and mortality. 67 In addition, citing “limited evidence” in humans, the International Agency for Research on Cancer recently classified the non-sugar sweetener aspartame as “possibly carcinogenic to humans” (group 2B). 68 A growing body of data shows instances of exposure to combinations of multiple additives, which may have potential “cocktail effects” with greater implications for human health than exposure to a single additive. 69 Thirdly, the intensive industrial processing of food may produce potentially harmful substances that have been linked to higher risks of chronic inflammatory diseases, including acrolein, acrylamide, advanced glycation end products, furans, heterocyclic amines, industrial trans-fatty acids, and polycyclic aromatic hydrocarbons. 12 70 Finally, ultra-processed foods can contain contaminants with health implications that migrate from packaging materials, such as bisphenols, microplastics, mineral oils, and phthalates. 12
Experimental evidence indicates a robust causal relation between ultra-processed diets and increased energy intake and weight gain (approximately 500 kcal (2000 kJ) per day and 0.9 kg during the ultra-processed diet). 71 Other experimental evidence has also shown that using the Nova food classification system for nutritional counselling and adjunctive to physical activity effectively prevents excessive weight gain in pregnant women with high body mass index. 72 The mechanisms contributing to the excess consumption effect of diets rich in ultra-processed foods seem to involve the nature of the energy source—specifically, whether it comes from solid foods or beverages. 71 Furthermore, the greater energy density, faster eating rate, and hyper-palatability attributed to ultra-processed foods are regarded as important factors influencing this effect. 73 The extensive marketing strategies used by ultra-processed food manufacturers, which involve visually captivating packaging with eye catching designs and health related assertions, have also been suggested as a potential contributing factor to excessive consumption. 74
Strengths and limitations of study and comparisons with other studies
Recognising the importance of establishing causality, we acknowledge that further randomised controlled trials are needed, particularly for outcomes for which strong meta-analytic epidemiological evidence exists, such as cardiometabolic disorder and common mental disorder outcomes. However, only short term trials testing the effect of ultra-processed food exposure on intermediate outcomes (such as alterations to body weight, insulin resistance, depressive and anxiety symptoms, gut microbiome, and inflammation) would be feasible. Setting up trials testing the effect of long term exposure to interventions with suspected deleterious properties (that is, diets rich in ultra-processed foods) on hard disease endpoints such as cardiovascular disease or cancer will not be possible, for obvious ethical reasons. In this context, our umbrella review of extant observational epidemiological research provides complementary insights and has implications for public health, especially in light of the current debate about tackling (or not) exposure to ultra-processed foods through public health measures. It stands as the first comprehensive synthesis of current evidence derived from meta-analyses of epidemiological studies, exploring the associations between dietary exposure to ultra-processed foods and various adverse health outcomes. We used rigorous systematic methods, including duplicate study selection and data extraction, alongside the evidence classification criteria and GRADE assessments, to evaluate the credibility and quality of the pooled analyses. An additional strength of our review is that we reviewed the competing interests and funding disclosures of the included meta-analysis studies, with none being funded by companies involved in the production of ultra-processed foods.
One limitation of umbrella reviews in general is their high level overview. As a result, we did not consider specific confounder or mediator adjustments and sensitivity analyses as part of our review, but these may be important factors, particularly in the context of ultra-processed foods. The consumption of ultra-processed foods is linked to a lower intake of unprocessed or minimally processed fruits, vegetables, legumes, and seafood. 6 This raises the question of whether the associations between exposure to ultra-processed foods and poorer health are due to an overall unhealthy dietary pattern. Although such analyses were beyond the scope of our review, which focused on evaluating overall associations between exposure to ultra-processed foods and adverse health outcomes, we note that a recent meta-analysis found that adjusting for diet quality or patterns does not change the consistent evidence for direct associations between greater ultra-processed food exposure and a higher risk of adverse health outcomes (as per inference criteria and sizes of effect estimates). 75 Furthermore, the inclusion of original research articles with different methods of assessing ultra-processed food intake, such as dietary history, food frequency questionnaires, food records, and 24 hour dietary recalls, introduces an inevitable measurement bias regardless of whether validated methods were applied. 76 Considering that observational epidemiological studies have inherent limitations is also important, with residual confounding being perhaps most pertinent. 77 However, the consistent findings across most pooled analyses in our review support the notion that residual confounding does not fully explain the observed associations.
Although our umbrella review provides a systematic synthesis of the role of ultra-processed dietary patterns in chronic disease outcomes, a related consideration is the possible heterogeneity of associations between subgroups and subcategories of ultra-processed foods and chronic disease outcomes. A meta-analysis by Chen and colleagues (2023), included in our review, established a clear link between overall consumption of ultra-processed foods and a higher risk of type 2 diabetes, consistently observed across multiple cohorts. 47 However, while certain subcategories of ultra-processed foods further showed higher risk, others were inversely associated, such as ultra-processed cereals, dark/wholegrain bread, packaged sweet and savoury snacks, fruit based products and yoghurt, and dairy based desserts. 47 These findings underscore the complexity of the relation between ultra-processed foods and adverse health. Nevertheless, although some subcategories of ultra-processed items may have better nutrient and ingredient profiles, the overall consumption of ultra-processed foods remains consistently associated with a higher risk of chronic diseases, as evidenced by our review. Some people have argued that understanding the differences within subcategories of ultra-processed foods may aid consumers in adopting a healthier dietary pattern compared with maximally reducing their consumption on the whole. 78 However, others propose that the focus should be on the overall quality of the diet, including all ultra-processed foods, and its link to higher disease risk, rather than specific subcategories or individual products. 79
When considering the above and examining subcategories of ultra-processed foods, composite interactions between various consumables within broader dietary patterns are unaccounted for. This limitation may partially account for differences in the strength of evidence observed in our review compared with another recent umbrella review focusing on dietary sugar consumption, including sugar sweetened beverages, a commonly consumed subcategory of ultra-processed foods. 25 That review found no convincing (class I) evidence for adverse health outcomes linked to dietary sugar or sugar sweetened beverage consumption. 25 In contrast, our umbrella review shows compelling evidence (class I) that supports direct associations between greater dietary exposure to ultra-processed foods and higher risks of adverse health outcomes spanning cardiometabolic diseases, common mental disorders, and mortality. These findings support recommendations to consider overall diet quality in nutritional epidemiology, 79 and they suggest that higher consumption of ultra-processed foods within broader dietary patterns may have synergistic or compounded consequences compared with lower intakes, as hypothesised elsewhere. 12 13 14 15
Policy implications
Organisations such as the American Heart Association have cautiously advised people to choose unprocessed and minimally processed foods over ultra-processed foods, noting the absence of a widely accepted definition for ultra-processed foods. 80 Although various food classification systems have been developed to classify foods on the basis of processing related criteria, 81 82 83 84 85 the most commonly used classification system worldwide is the Nova food classification system. 86 Furthermore, Nova has received recognition from authoritative reports by the Food and Agricultural Organisation of the United Nations and the Pan American Health Organization of the WHO. 87 88 89 90 91 A recent statement from the UK’s Scientific Advisory Committee on Nutrition (SACN) evaluated the Nova classification system among others and concluded that Nova is the only suitable classification for potential use in the country. 92 However, SACN expressed concerns about various key points. For example, it highlighted that the available studies applying the Nova system are primarily epidemiological in nature and may lack adequate consideration of confounding factors or covariates 92 . Criticisms of Nova as a classification system also exist, with concerns raised about its possible imprecision and inconsistency among evaluators. 93 94 95 96 In contrast, more recent assessments show acceptable construct validity and strong agreement among coders, 97 98 with the definitions and examples provided by the Nova system deemed adequate in classifying more than 70% of the food items reported in food frequency questionnaires from various cohorts from the US, 99 as well as more than 90% of the food items reported in 24 hour dietary recalls from participants in a national Brazilian dietary survey. 100 Recent efforts including best practice guidelines have further focused on improving the efficiency and transparency of the categorisation process for Nova food groups, which ultimately aim to enhance the accuracy of effect estimates. 101
Public health measures promoting a reduction or avoidance of ultra-processed products have already been implemented most comprehensively in Latin American countries. These strategies include octagonal front-of-pack warning labels, taxes on sugar sweetened beverages and ultra-processed foods, marketing restrictions, and bans in schools. 102 103 104 Since the introduction of the recommendation to avoid ultra-processed foods in the 2014 Dietary Guidelines for the Brazilian Population, 105 seven additional countries have adopted the term and similar recommendations. 106 Furthermore, similar strategies for paediatric development and prevention of liver disease have also been recommended by the UK’s First Steps Nutrition Trust and the European Association for the Study of the Liver-Lancet Commission, respectively. 107 108 We also note that WHO and the International Agency for Research on Cancer endorse public health strategies to limit the intake of components commonly present in ultra-processed foods, including high levels of added sugar and non-sugar sweeteners. 67 68 109 Importantly, sustained progress in implementing these strategies and the exploration of novel approaches mean that stakeholders need to be responsive and sensitive to factors that influence access to fresh produce and food choices, including the relatively greater time, effort, and (in some contexts) cost of preparing non-ultra-processed food. 95
Conclusions
This umbrella review reports a higher risk of adverse health outcomes associated with ultra-processed food exposure. The strongest available evidence pertained to direct associations between greater exposure to ultra-processed foods and higher risks of all cause mortality, cardiovascular disease related mortality, common mental disorder outcomes, overweight and obesity, and type 2 diabetes. Evidence for the associations of ultra-processed food exposure with asthma, gastrointestinal health, some cancers, and intermediate cardiometabolic risk factors remains limited and warrants further investigation. Coupled with existing population based strategies, we recommend urgent mechanistic research and the development and evaluation of comprehensive population based and public health strategies, including government led policy frameworks and dietary guidelines, aimed at targeting and reducing dietary exposure to ultra-processed foods for improved human health.
What is already known on this topic
Multiple meta-analyses have aimed to consolidate original epidemiological research investigating associations between ultra-processed food and adverse health outcomes
However, no comprehensive umbrella review has been conducted to provide a broad perspective and evaluate the meta-analytic evidence in this area
What this study adds
This umbrella review found consistent evidence of a higher risk of adverse health outcomes associated with greater ultra-processed food exposure
Convincing and highly suggestive evidence (classes I and II) related to early death and adverse cardiometabolic and mental health
These findings support urgent mechanistic research and public health actions that seek to target and minimise ultra-processed food consumption for improved population health
Ethics statements
Ethical approval.
Not required.
Data availability statement
To access additional data from this study, the code repository corresponding to the online version of the R statistical package, metaumbrella , can be found on GitHub at https://github.com/cran/metaumbrella . The raw data are available on the Open Science Framework at https://osf.io/8j2gt/ , and a step-by-step analysis using metaumbrella usage is provided in supplementary table B. For further assistance or inquiries, please contact the corresponding author at [email protected].
Contributors: MML was involved in conceptualisation, literature searching, data curation, project administration, resources, and writing the original draft of the manuscript. EG, SD, DNA, AJM, and SG were involved in conceptualisation and data curation. TS was involved in data visualisation. PB, ML, CMR, BS, MT, FNJ, and AO were involved in conceptualisation. WM was involved in conceptualisation, data curation, formal analysis, supervision, and data visualisation. All authors were involved in reviewing and editing the manuscript. MML is the guarantor. The corresponding author attests that all listed authors meet authorship criteria and that no others meeting the criteria have been omitted.
Funding: This work was not funded.
Competing interests: All authors have completed the ICMJE uniform disclosure form at www.icmje.org/coi_disclosure.pdf and declare: MML, EG, DNA, AJM, SG, FNJ, AO, and WM are affiliated with the Food & Mood Centre, Deakin University, which has received research funding support from Be Fit Food, Bega Dairy and Drinks, and the a2 Milk Company and philanthropic research funding support from the Waterloo Foundation, Wilson Foundation, the JTM Foundation, the Serp Hills Foundation, the Roberts Family Foundation, and the Fernwood Foundation; MML is secretary for the Melbourne Branch Committee of the Nutrition Society of Australia (unpaid) and has received travel funding support from the International Society for Nutritional Psychiatry Research, the Nutrition Society of Australia, the Australasian Society of Lifestyle Medicine, and the Gut Brain Congress and is an associate investigator for the MicroFit Study, an investigator-led randomised controlled trial exploring the effect of diets with varying levels of industrial processing on gut microbiome composition and partially funded by Be Fit Food (payment received by the Food and Mood Centre, Deakin University); AMJ is secretary for the International Society for Nutritional Psychiatry Research (unpaid) and an associate investigator for the MicroFit Study; SG is affiliated with Deakin University, which has received grant funding support from a National Health and Medical Research Council Synergy Grant (#GNT1182301) and Medical Research Future Fund Cardiovascular Health Mission (#MRF2022907), is affiliated with Monash University, which has received grant funding support from Medical Research Future Fund Consumer-led research (#MRF2022907), is secretary for the Australian Cardiovascular Health and Rehabilitation Association—Victoria and Tasmania—(unpaid), and has received travel funding support from the Institute for Mental and Physical Health and Clinical Translation and SOLVE-CHD (solving the long-standing evidence-practice gap associated with cardiac rehabilitation and secondary prevention of coronary heart disease); PB has received funding from an Australian Research Council Future Fellowship award (project number #FT220100690) and from Bloomberg Philanthropies; ML is affiliated with the Institute for Physical Activity and Nutrition, School of Exercise and Nutrition Sciences, Deakin University, which has received grant funding support from the Australian Research Council (#DP190101323), and has received royalties or license funding from Allen and Unwin (Public Health Nutrition: from Principles to Practice) and Routledge, Taylor and Francis Group (Healthy and Sustainable Food Systems) and consultation and remuneration funding support from WHO and Food Standards Australia New Zealand (in his role as a board member); CMR is affiliated with Johns Hopkins Bloomberg School of Public Health and Johns Hopkins University, which have received grant funding support from the National Heart, Lung, and Blood Institute, Bloomberg American Health Initiative, and the National Institute of Diabetes and Digestive and Kidney Diseases, was chair of the Data and Safety Monitoring Boards for the SUPER Trial: Effect of Dietary Sodium Reduction in Kidney Disease Patients with Albuminuria and the ADEPT Trial: A Clinical Trial of Low-Carbohydrate Dietary Pattern on Glycemic Outcomes, was on the Editorial Board of Diabetes Care (unpaid), was the immediate past chair for the Early Career Committee of the Council on Lifestyle and Cardiometabolic Health, American Heart Association (unpaid), and the Nutritional Epidemiology Research Interest Section of the American Society for Nutrition (unpaid), and has received funding support as an associate editor of Diabetes Care and editorial fellow of the Journal of the American Society of Nephrology ; FNJ has received fellowship funding support from the National Health and Medical Research Council (#1194982) and payment or honorariums for lectures, presentations, speakers bureaus, manuscript writing, or educational events from the Malaysian Society of Gastroenterology and Hepatology, JNPN Congress, American Nutrition Association, Personalised Nutrition Summit, and American Academy of Craniofacial Pain, is a Scientific Advisory Board member of Dauten Family Centre for Bipolar Treatment Innovation (unpaid) and Zoe Nutrition (unpaid), has written two books for commercial publication on the topic of nutritional psychiatry and gut health, and is the principal investigator for the MicroFit Study; AO has received fellowship funding support from the National Health and Medical Research Council (#2009295) and is affiliated with Deakin University, which has received grant funding support from the Medical Research Future Fund, Dasman Diabetes Institute, MTP Connect—Targeted Translation Research Accelerator Program, the National Health and Medical Research Council, Barwon Health, and the Waterloo Foundation, and has received funding support for academic editing and as a grant reviewer from SLACK Incorporated ( Psychiatric Annals ) and the National Health and Medical Research Council, respectively, and travel funding support from the International Society for Nutritional Psychiatry Research; WM is president of the International Society for Nutritional Psychiatry Research (unpaid), has received fellowship funding support from the National Health and Medical Research Council (#2008971) and Multiple Sclerosis Research Australia, consultation and remuneration funding support from Nutrition Research Australia and ParachuteBH, and travel funding support from the Nutrition Society of Australia, Mind Body Interface Symposium, and VitaFoods, and was the acting principal investigator and is an associate investigator for the MicroFit Study.
Transparency: The lead author (the manuscript’s guarantor) affirms that the manuscript is an honest, accurate, and transparent account of the study being reported; that no important aspects of the study have been omitted; and that any discrepancies from the study as planned (and, if relevant, registered) have been explained.
Dissemination to participants and related patient and public communities: We will share our review findings with academic, clinical, policy, and public audiences post-publication through various channels, including conferences as well as personal and institutional media hosted primarily by Deakin University and the Food and Mood Centre.
Provenance and peer review: Not commissioned; externally peer reviewed.
This is an Open Access article distributed in accordance with the Creative Commons Attribution Non Commercial (CC BY-NC 4.0) license, which permits others to distribute, remix, adapt, build upon this work non-commercially, and license their derivative works on different terms, provided the original work is properly cited and the use is non-commercial. See: http://creativecommons.org/licenses/by-nc/4.0/ .
- Monteiro CA ,
- Lawrence M ,
- Machado P ,
- Moubarac JC ,
- Del Bo’ C ,
- Martini D ,
- Bonaccio M ,
- Vitaglione P ,
- Swinburn BA ,
- Miranda L ,
- Aggarwal A ,
- Drewnowski A
- Luiten CM ,
- Steenhuis IHM ,
- Ni Mhurchu C ,
- Waterlander WE
- Kordahi MC ,
- Bonazzi E ,
- Deschasaux-Tanguy M ,
- Touvier M ,
- Chassaing B
- Martínez Leo EE ,
- Peñafiel AM ,
- Hernández Escalante VM ,
- Cabrera Araujo ZM
- Tristan Asensi M ,
- Napoletano A ,
- Pagliai G ,
- Madarena MP ,
- Iacoviello L ,
- Beattie S ,
- Monteiro CA
- McKenzie JE ,
- Bossuyt PM ,
- Morgan RL ,
- Thayer KA ,
- Schünemann HJ
- Veronese N ,
- Demurtas J ,
- Thompson T ,
- Belbasis L ,
- Evangelou E ,
- Ioannidis JP ,
- Borenstein M ,
- Hedges LV ,
- Higgins JP ,
- Rothstein HR
- Higgins JPT ,
- Altman DG ,
- the Cochrane Statistical Methods Group
- Thompson SG ,
- Spiegelhalter DJ
- Trikalinos TA
- Sterne JA ,
- Davey Smith G ,
- Schneider M ,
- Markozannes G ,
- Aretouli E ,
- Fusar-Poli P ,
- Gosling CJ ,
- Solanes A ,
- Reeves BC ,
- Isaksen IM ,
- Entezari MH ,
- Mohammadi H ,
- Henney AE ,
- Gillespie CS ,
- Cuthbertson DJ
- Khandpur N ,
- Desjardins C ,
- Mohammad D ,
- Delpino FM ,
- Figueiredo LM ,
- Flores TR ,
- Travica N ,
- Suksatan W ,
- Chandler J ,
- Higgins J ,
- Tobias DK ,
- Coletro HN ,
- Bressan J ,
- Martínez Steele E ,
- Chazelas E ,
- Valdés-Mas R ,
- Bettini S ,
- Boutet-Robinet E ,
- Cartier C ,
- Druesne-Pecollo N ,
- Chassaing B ,
- Compher C ,
- Bonhomme B ,
- Howland G ,
- ↵ World Health Organization Guidelines Review Committee Nutrition and Food Safety. Use of non-sugar sweeteners. 2023. https://www.who.int/publications/i/item/9789240073616 .
- Beland FA ,
- Lachenmeier DW ,
- Esseddik Y ,
- Buckley JP ,
- Ayuketah A ,
- Brychta R ,
- Sartorelli DS ,
- Crivellenti LC ,
- Baroni NF ,
- Fazzino TL ,
- Courville AB ,
- Moubarac J-C ,
- Dicken SJ ,
- Batterham RL
- Midthune D ,
- Freedman L ,
- Vadiveloo MK ,
- Scrinis G ,
- Lichtenstein AH ,
- Vadiveloo M ,
- Slimani N ,
- Deharveng G ,
- Southgate DA ,
- Eicher-Miller HA ,
- Fulgoni VL 3rd . ,
- Mendez MA ,
- Castro IR ,
- Louzada MLC ,
- Food Agriculture Organization (FAO) of the United Nations
- Food and Agriculture Organization of the United Nations (FAO)
- ↵ United Nations Children's Fund (UNICEF). Policy Brief: Sugar-Sweetened Beverage Taxation 2021. https://www.unicef.org/documents/advocacy-packages-food-environment-policies .
- ↵ FAO; IFAD; UNICEF; WFP; WHO. The State of Food Security and Nutrition in the World 2023. Urbanization, agrifood systems transformation and healthy diets across the rural–urban continuum. 2023. https://www.fao.org/documents/card/en?details=cc3017en .
- Moubarac JC
- ↵ Scientific Advisory Committee on Nutrition (SACN). SACN statement on processed foods and health. 2023. https://assets.publishing.service.gov.uk/media/64ac1fe7b504f7000ccdb89a/SACN-position-statement-Processed-Foods-and-Health.pdf .
- Gibney MJ ,
- Mullally D ,
- Braesco V ,
- Souchon I ,
- Sauvant P ,
- Ukwuani S ,
- Sommer EC ,
- Rossato S ,
- Drouin-Chartier J-P ,
- Louzada MLDC ,
- Silva KAAN ,
- Martinez-Steele E ,
- Popkin BM ,
- Barquera S ,
- Corvalan C ,
- Crosbie E ,
- Rincón-Gallardo Patiño S ,
- ↵ Ministry of Health of Brazil. Dietary guidelines for the Brazilian population. 2015. https://bvsms.saude.gov.br/bvs/publicacoes/dietary_guidelines_brazilian_population.pdf .
- Anastasiou K ,
- Ribeiro De Melo P ,
- Karlsen TH ,
- Zelber-Sagi S ,
- ↵ Childs R, Sibson V. Ultra-processed foods (UPF) in the diets of infants and young children in the UK: What they are, how they harm health, and what needs to be done to reduce intakes. 2023. https://www.unicef.org/documents/advocacy-packages-food-environment-policies .
- ↵ World Health Organization. Guideline: Sugars intake for adults and children. 2015 https://www.who.int/publications/i/item/9789241549028 .
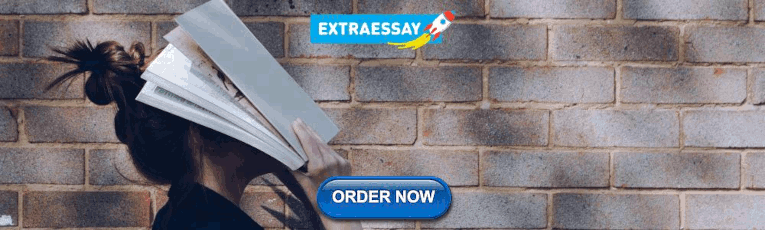
COMMENTS
Abstract. Background. The COVID-19 pandemic has profoundly influenced the world. In wave after wave, many countries suffered from the pandemic, which caused social instability, hindered global growth, and harmed mental health. Although research has been published on various mental health issues during the pandemic, some profound effects on ...
The abstract provides a concise summary of the key points discussed in the paper, highlighting the potential risks and benefits of social media use on mental health, the underlying mechanisms, and ...
[Show full abstract] and mental health service use is portrayed, and how characters respond to mental health scenes. Euphoria follows a group of high school students as they navigate adolescence ...
2. Conceptualising mental health and well-being. The terms "mental health" and "well-being" are often used together in the literature due to the connections that exist between the meaning of both terms, however their relationship is not frequently explored (Norwich et al., Citation 2022).Nowadays, two main conceptions explain the relationship between mental health and well-being, a one ...
Abstract. Mental health problems bring substantial individual, community and societal costs and the need for innovation to promote good mental health and to prevent and treat mental health problems has never been greater. ... Most exercises on mental health research priorities have mentioned childhood difficulties (Medical Research Council ...
7 NIHR Mental Health Policy Research Unit, Division of Psychiatry, UCL, London, UK. 8 McPin Foundation, London, UK. ... Psychiatry, University of Oxford, Oxford, UK. PMID: 33966543 DOI: 10.1080/09638237.2021.1898552 Abstract Mental health problems bring substantial individual, community and societal costs and the need for innovation to promote ...
Abstract and Figures. This manuscript summarizes areas of school mental health (SMH) research relevant to the interplay between students' academic and social-emotional outcomes. After ...
Abstract. The mental health of college students has been the source of research, projects, and public policies involving education, health, and psychology professionals. ... Despite several research interrelating mental health and university space, most were dedicated to data collection, using questionnaires, inventories, and scales ...
With an escalating global health burden of mental disorders (James et al., 2018),scientific communities today are facing urgent needs to (1) identify the biological underpinnings of mental disorders, biomarkers, and new treatment targets, and (2) identify strategies to scale up existing evidence-based treatments to address the massive dearth of mental health care, particularly in resource-poor ...
People exposed to more unfavourable social circumstances are more vulnerable to poor mental health over their life course, in ways that are often determined by structural factors which generate and perpetuate intergenerational cycles of disadvantage and poor health. ... 7 Medical Research Council Unit for Lifelong Health and Ageing, University ...
This article synthesizes 25 reviews published between 2019 and mid-2021 on how social media use affects adolescent well-being and mental health. It discusses the conceptualizations, interpretations, gaps, and directions for future research on this topic.
Abstract. Mental health outcomes have shown dramatic changes over the past half-century, yet these trends are still underexplored. ... I conclude with potential mechanisms and implications for future mental health research. Get full access to this article. View all access and purchase options for this article. Get Access.
Findings from existing research suggest that how people understand their own and others' mental health conditions affects their behaviour, in terms of how they may respond to others with a mental health condition, and also how they may seek and respond to treatment if they were experiencing a problem themselves (Dang et al., 2020; Goldney et al., 2005; Jorm, 2000; Jorm et al., 1997).
Abstract. Research suggests that multiple forms of relaxation training (e.g., progressive muscle relaxation, meditation, breathing exercises, visualization, and autogenics) can help individuals reduce stress, enhance relaxation states, and improve overall well-being. ... and positive mental health. Often these studies have examined specific ...
Research focusing on mental health aspects of family members or caregivers specifically after stroke is limited. 14-22 Moreover, most earlier studies were small (<500 individuals) 14-18,20,21 and lacked longitudinal data based on a population-based study design with relevant comparison cohorts 14-22 (an overview of the existing literature is ...
Adverse childhood experiences (ACEs) are potentially traumatic events before age 18 years that can affect health and risk behaviors. This report presents the first national prevalence of self-reported ACEs among high school students, including violence, substance use, sexual, and mental health-related ACEs.
This article examines the human rights issues of mental disability and mental health care, such as inequality, discrimination, and autonomy. It argues for a rights-based approach that respects the dignity and agency of persons with mental disabilities and challenges the prevailing models and language of mental disability.
[Show full abstract] the pandemic on mental health, identifying anxiety, depression, and stress as common challenges. It was observed that psychological resilience, influenced by factors such as ...
Abstract. Introduction: Healthy aging and good quality of life is important to allow older people to live at home. Lighting is a significant environmental attribute promoting visual, physical, and mental health. Due to normal visual age changes, older people need more light, but improving indoor lighting levels receives little attention.
Writing an Abstract Lesson An abstract is a summary of your research that can range anywhere from 150 words to 350 words. Your abstract should explain the research's topic and research question(s), the researchers' methodologies, and the overall findings. Often, in industry, an abstract holds importance because it is the first item a
Hardly a family in America has been untouched by mental illness. As many as one third of American adults will suffer a diagnosable mental disorder sometime in their life, and 20 percent have a mental disorder at any given time. Although research on the causes and treatment of mental disorders remains vitally important—and indeed major advances are leading to better lives for increasing ...
Conclusions Greater exposure to ultra-processed food was associated with a higher risk of adverse health outcomes, especially cardiometabolic, common mental disorder, and mortality outcomes. These findings provide a rationale to develop and evaluate the effectiveness of using population based and public health measures to target and reduce dietary exposure to ultra-processed foods for improved ...
Based on the desk research conducted, we have found children are vulnerable to mental health issues and more susceptible if coming from an urban poverty background. Available intervention systems ...
Conclusion Urban green spaces play a vital role in mitigating heat-related health risks, offering a potential strategy for urban planning to address climate change and enhance public health. Additional research is required to thoroughly comprehend the magnitude of urban greenery's impact on heat-related morbidity and mortality, as well as its interplay with other variables, including air ...
Writing an Abstract . Overview . An abstract is a summary of your research that can range anywhere from 150 words to 350 words. Your abstract should explain the research's topic and research question(s), the researchers' methodologies, and the overall findings. Often, in industry, an abstract holds importance because it is the first item ...
[Show full abstract] [CCEB]) and psychological conditions through the Brazilian version of the Problem Areas in Diabetes, associated with glycemic control, measured by glycated hemoglobin (HbA1c ...
Anthropological Quarterly 75.3 (2002) 557-574 Images of the World Trade Center site flooded the media in the aftermath of September 11, and have continued to do so in New York City.