- Privacy Policy
Buy Me a Coffee

Home » Research Data – Types Methods and Examples
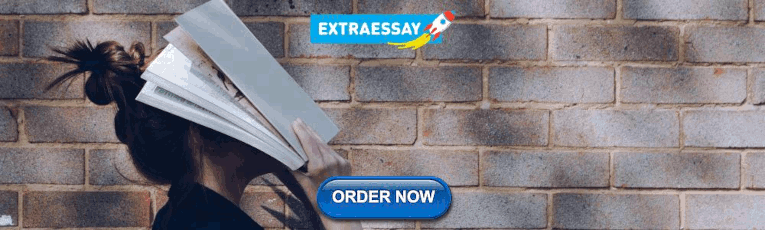
Research Data – Types Methods and Examples
Table of Contents

Research Data
Research data refers to any information or evidence gathered through systematic investigation or experimentation to support or refute a hypothesis or answer a research question.
It includes both primary and secondary data, and can be in various formats such as numerical, textual, audiovisual, or visual. Research data plays a critical role in scientific inquiry and is often subject to rigorous analysis, interpretation, and dissemination to advance knowledge and inform decision-making.
Types of Research Data
There are generally four types of research data:
Quantitative Data
This type of data involves the collection and analysis of numerical data. It is often gathered through surveys, experiments, or other types of structured data collection methods. Quantitative data can be analyzed using statistical techniques to identify patterns or relationships in the data.
Qualitative Data
This type of data is non-numerical and often involves the collection and analysis of words, images, or sounds. It is often gathered through methods such as interviews, focus groups, or observation. Qualitative data can be analyzed using techniques such as content analysis, thematic analysis, or discourse analysis.
Primary Data
This type of data is collected by the researcher directly from the source. It can include data gathered through surveys, experiments, interviews, or observation. Primary data is often used to answer specific research questions or to test hypotheses.
Secondary Data
This type of data is collected by someone other than the researcher. It can include data from sources such as government reports, academic journals, or industry publications. Secondary data is often used to supplement or support primary data or to provide context for a research project.
Research Data Formates
There are several formats in which research data can be collected and stored. Some common formats include:
- Text : This format includes any type of written data, such as interview transcripts, survey responses, or open-ended questionnaire answers.
- Numeric : This format includes any data that can be expressed as numerical values, such as measurements or counts.
- Audio : This format includes any recorded data in an audio form, such as interviews or focus group discussions.
- Video : This format includes any recorded data in a video form, such as observations of behavior or experimental procedures.
- Images : This format includes any visual data, such as photographs, drawings, or scans of documents.
- Mixed media: This format includes any combination of the above formats, such as a survey response that includes both text and numeric data, or an observation study that includes both video and audio recordings.
- Sensor Data: This format includes data collected from various sensors or devices, such as GPS, accelerometers, or heart rate monitors.
- Social Media Data: This format includes data collected from social media platforms, such as tweets, posts, or comments.
- Geographic Information System (GIS) Data: This format includes data with a spatial component, such as maps or satellite imagery.
- Machine-Readable Data : This format includes data that can be read and processed by machines, such as data in XML or JSON format.
- Metadata: This format includes data that describes other data, such as information about the source, format, or content of a dataset.
Data Collection Methods
Some common research data collection methods include:
- Surveys : Surveys involve asking participants to answer a series of questions about a particular topic. Surveys can be conducted online, over the phone, or in person.
- Interviews : Interviews involve asking participants a series of open-ended questions in order to gather detailed information about their experiences or perspectives. Interviews can be conducted in person, over the phone, or via video conferencing.
- Focus groups: Focus groups involve bringing together a small group of participants to discuss a particular topic or issue in depth. The group is typically led by a moderator who asks questions and encourages discussion among the participants.
- Observations : Observations involve watching and recording behaviors or events as they naturally occur. Observations can be conducted in person or through the use of video or audio recordings.
- Experiments : Experiments involve manipulating one or more variables in order to measure the effect on an outcome of interest. Experiments can be conducted in a laboratory or in the field.
- Case studies: Case studies involve conducting an in-depth analysis of a particular individual, group, or organization. Case studies typically involve gathering data from multiple sources, including interviews, observations, and document analysis.
- Secondary data analysis: Secondary data analysis involves analyzing existing data that was collected for another purpose. Examples of secondary data sources include government records, academic research studies, and market research reports.
Analysis Methods
Some common research data analysis methods include:
- Descriptive statistics: Descriptive statistics involve summarizing and describing the main features of a dataset, such as the mean, median, and standard deviation. Descriptive statistics are often used to provide an initial overview of the data.
- Inferential statistics: Inferential statistics involve using statistical techniques to draw conclusions about a population based on a sample of data. Inferential statistics are often used to test hypotheses and determine the statistical significance of relationships between variables.
- Content analysis : Content analysis involves analyzing the content of text, audio, or video data to identify patterns, themes, or other meaningful features. Content analysis is often used in qualitative research to analyze open-ended survey responses, interviews, or other types of text data.
- Discourse analysis: Discourse analysis involves analyzing the language used in text, audio, or video data to understand how meaning is constructed and communicated. Discourse analysis is often used in qualitative research to analyze interviews, focus group discussions, or other types of text data.
- Grounded theory : Grounded theory involves developing a theory or model based on an analysis of qualitative data. Grounded theory is often used in exploratory research to generate new insights and hypotheses.
- Network analysis: Network analysis involves analyzing the relationships between entities, such as individuals or organizations, in a network. Network analysis is often used in social network analysis to understand the structure and dynamics of social networks.
- Structural equation modeling: Structural equation modeling involves using statistical techniques to test complex models that include multiple variables and relationships. Structural equation modeling is often used in social science research to test theories about the relationships between variables.
Purpose of Research Data
Research data serves several important purposes, including:
- Supporting scientific discoveries : Research data provides the basis for scientific discoveries and innovations. Researchers use data to test hypotheses, develop new theories, and advance scientific knowledge in their field.
- Validating research findings: Research data provides the evidence necessary to validate research findings. By analyzing and interpreting data, researchers can determine the statistical significance of relationships between variables and draw conclusions about the research question.
- Informing policy decisions: Research data can be used to inform policy decisions by providing evidence about the effectiveness of different policies or interventions. Policymakers can use data to make informed decisions about how to allocate resources and address social or economic challenges.
- Promoting transparency and accountability: Research data promotes transparency and accountability by allowing other researchers to verify and replicate research findings. Data sharing also promotes transparency by allowing others to examine the methods used to collect and analyze data.
- Supporting education and training: Research data can be used to support education and training by providing examples of research methods, data analysis techniques, and research findings. Students and researchers can use data to learn new research skills and to develop their own research projects.
Applications of Research Data
Research data has numerous applications across various fields, including social sciences, natural sciences, engineering, and health sciences. The applications of research data can be broadly classified into the following categories:
- Academic research: Research data is widely used in academic research to test hypotheses, develop new theories, and advance scientific knowledge. Researchers use data to explore complex relationships between variables, identify patterns, and make predictions.
- Business and industry: Research data is used in business and industry to make informed decisions about product development, marketing, and customer engagement. Data analysis techniques such as market research, customer analytics, and financial analysis are widely used to gain insights and inform strategic decision-making.
- Healthcare: Research data is used in healthcare to improve patient outcomes, develop new treatments, and identify health risks. Researchers use data to analyze health trends, track disease outbreaks, and develop evidence-based treatment protocols.
- Education : Research data is used in education to improve teaching and learning outcomes. Data analysis techniques such as assessments, surveys, and evaluations are used to measure student progress, evaluate program effectiveness, and inform policy decisions.
- Government and public policy: Research data is used in government and public policy to inform decision-making and policy development. Data analysis techniques such as demographic analysis, cost-benefit analysis, and impact evaluation are widely used to evaluate policy effectiveness, identify social or economic challenges, and develop evidence-based policy solutions.
- Environmental management: Research data is used in environmental management to monitor environmental conditions, track changes, and identify emerging threats. Data analysis techniques such as spatial analysis, remote sensing, and modeling are used to map environmental features, monitor ecosystem health, and inform policy decisions.
Advantages of Research Data
Research data has numerous advantages, including:
- Empirical evidence: Research data provides empirical evidence that can be used to support or refute theories, test hypotheses, and inform decision-making. This evidence-based approach helps to ensure that decisions are based on objective, measurable data rather than subjective opinions or assumptions.
- Accuracy and reliability : Research data is typically collected using rigorous scientific methods and protocols, which helps to ensure its accuracy and reliability. Data can be validated and verified using statistical methods, which further enhances its credibility.
- Replicability: Research data can be replicated and validated by other researchers, which helps to promote transparency and accountability in research. By making data available for others to analyze and interpret, researchers can ensure that their findings are robust and reliable.
- Insights and discoveries : Research data can provide insights into complex relationships between variables, identify patterns and trends, and reveal new discoveries. These insights can lead to the development of new theories, treatments, and interventions that can improve outcomes in various fields.
- Informed decision-making: Research data can inform decision-making in a range of fields, including healthcare, business, education, and public policy. Data analysis techniques can be used to identify trends, evaluate the effectiveness of interventions, and inform policy decisions.
- Efficiency and cost-effectiveness: Research data can help to improve efficiency and cost-effectiveness by identifying areas where resources can be directed most effectively. By using data to identify the most promising approaches or interventions, researchers can optimize the use of resources and improve outcomes.
Limitations of Research Data
Research data has several limitations that researchers should be aware of, including:
- Bias and subjectivity: Research data can be influenced by biases and subjectivity, which can affect the accuracy and reliability of the data. Researchers must take steps to minimize bias and subjectivity in data collection and analysis.
- Incomplete data : Research data can be incomplete or missing, which can affect the validity of the findings. Researchers must ensure that data is complete and representative to ensure that their findings are reliable.
- Limited scope: Research data may be limited in scope, which can limit the generalizability of the findings. Researchers must carefully consider the scope of their research and ensure that their findings are applicable to the broader population.
- Data quality: Research data can be affected by issues such as measurement error, data entry errors, and missing data, which can affect the quality of the data. Researchers must ensure that data is collected and analyzed using rigorous methods to minimize these issues.
- Ethical concerns: Research data can raise ethical concerns, particularly when it involves human subjects. Researchers must ensure that their research complies with ethical standards and protects the rights and privacy of human subjects.
- Data security: Research data must be protected to prevent unauthorized access or use. Researchers must ensure that data is stored and transmitted securely to protect the confidentiality and integrity of the data.
About the author
Muhammad Hassan
Researcher, Academic Writer, Web developer
You may also like

Qualitative Data – Types, Methods and Examples

Secondary Data – Types, Methods and Examples

Information in Research – Types and Examples

Primary Data – Types, Methods and Examples

Quantitative Data – Types, Methods and Examples

Data Module #1: What is Research Data?
Defining research data.
- Qualitative vs. Quantitative
- Types of Research Data
- Data and Statistics
- Let's Review...
Data Module Quick Navigation
Data Modules Table of Contents
#1 - What is Research Data? #2 - Planning for Your Data Use #3 - Finding & Collecting Data #4 - Keeping Your Data Organized #5 - Intellectual Property & Ethics #6 - Storage, Backup, & Security #7 - Documentation
Library Resources

Module created by Aaron Albertson, Beth Hillemann, & Ron Joslin.

Many people think of data-driven research as something that primarily happens in the sciences. It is often thought of as involving a spreadsheet filled with numbers. Both of these beliefs are incorrect. Research data are collected and used in scholarship across all academic disciplines and, while it can consist of numbers in a spreadsheet, it also takes many different formats, including videos, images, artifacts, and diaries. Whether a psychologist collecting survey data to better understand human behavior, an artist using data to generate images and sounds, or an anthropologist using audio files to document observations about different cultures, scholarly research across all academic fields is increasingly data-driven.
In our Data Literacy Modules, we will demonstrate the ways in which research data are gathered and used across various academic disciplines by discussing it in a very broad sense. We define research data as: any information collected, stored, and processed to produce and validate original research results. Data might be used to prove or disprove a theory, bolster claims made in research, or to further the knowledge around a specific topic or problem.
Other Definitions of Research Data
There are many different definitions of research data available. Here are just a few examples of other definitions. We share these examples to illustrate there is not universal consensus on a definition, although many similarities are apparent.
- U.S. Office of Management & Budget
“research data, unlike other types of information, is collected, observed, or created, for purposes of analysis to produce original research results”
- University of Edinburgh
"...recorded factual material commonly accepted in the scientific community as necessary to validate research findings..."
- National Endowment for the Humanities
"...materials generated or collected during the course of conducting research..."
Research Data Formats
Research data takes many different forms. Data may be intangible as in measured numerical values found in a spreadsheet or an object as in physical research materials such samples of rocks, plants, or insects. Here are some examples of the formats that data can take:
- Next: Qualitative vs. Quantitative >>
- Last Updated: Feb 2, 2024 1:41 PM
- URL: https://libguides.macalester.edu/data1
When you choose to publish with PLOS, your research makes an impact. Make your work accessible to all, without restrictions, and accelerate scientific discovery with options like preprints and published peer review that make your work more Open.
- PLOS Biology
- PLOS Climate
- PLOS Complex Systems
- PLOS Computational Biology
- PLOS Digital Health
- PLOS Genetics
- PLOS Global Public Health
- PLOS Medicine
- PLOS Mental Health
- PLOS Neglected Tropical Diseases
- PLOS Pathogens
- PLOS Sustainability and Transformation
- PLOS Collections
Open Data is a strategy for incorporating research data into the permanent scientific record by releasing it under an Open Access license. Whether data is deposited in a purpose-built repository or published as Supporting Information alongside a research article, Open Data practices ensure that data remains accessible and discoverable. For verification, replication, reuse, and enhanced understanding of research.
Benefits of Open Data
Readers rely on raw scientific data to enhance their understanding of published research, for purposes of verification, replication and reanalysis, and to inform future investigations.
Ensure reproducibility Proactively sharing data ensures that your work remains reproducible over the long term.
Inspire trust Sharing data demonstrates rigor and signals to the community that the work has integrity.
Receive credit Making data public opens opportunities to get academic credit for collecting and curating data during the research process.
Make a contribution Access to data accelerates progress. According to the 2019 State of Open Data report, more than 70% of researchers use open datasets to inform their future research.
Preserve the scientific record Posting datasets in a repository or uploading them as Supporting Information prevents data loss.
Why do researchers choose to make their data public?
Watch the short video that explores the top benefits of data sharing, what types of research data you should share, and how you can get it ready to help ensure more impact for your research.
PLOS Open Data policy
Publishing in a PLOS journal carries with it a commitment to make the data underlying the conclusions in your research article publicly available upon publication.
Our data policy underscores the rigor of the research we publish, and gives readers a fuller understanding of each study.
Read more about Open Data
Data sharing has long been a hallmark of high-quality reproducible research. Now, Open Data is becoming...
For PLOS, increasing data-sharing rates—and especially increasing the amount of data shared in a repository—is a high priority.
Ensure that you’re publication-ready and ensure future reproducibility through good data management How you store your data matters. Even after…
Data repositories
All methods of data sharing data facilitate reproduction, improve trust in science, ensure appropriate credit, and prevent data loss. When you choose to deposit your data in a repository, those benefits are magnified and extended.
Data posted in a repository is…
…more discoverable.
Detailed metadata and bidirectional linking to and from related articles help to make data in public repositories easily findable.
…more reusable
Machine-readable data formatting allows research in a repository to be incorporated into future systematic reviews or meta analyses more easily.
…easier to cite
Repositories assign data its own unique DOI, distinct from that of related research articles, so datasets can accumulate citations in their own right, illustrating the importance and lasting relevance of the data itself.
…more likely to earn citations
A 2020 study of more than 500,000 published research articles found articles that link to data in a public repository were likely to have a 25% higher citation rate on average than articles where data is available on request or as Supporting Information.
Open Data is more discoverable and accessible than ever
Deposit your data in a repository and earn an accessible data icon.

You already know depositing research data in a repository yields benefits like improved reproducibility, discoverability, and more attention and citations for your research.
PLOS helps to magnify these benefits even further with our Accessible Data icon. When you link to select, popular data repositories, your article earns an eye-catching graphic with a link to the associated dataset, so it’s more visible to readers.
Participating data repositories include:
- Open Science Framework (OSF)
- Gene Expression Omnibus
- NCBI Bioproject
- NCBI Sequence Read Archive
- Demographic and Health Surveys
We aim to add more repositories to the list in future. Read more
The PLOS Open Science Toolbox
The future is open
The PLOS Open Science Toolbox is your source for sci-comm tips and best-practice. Learn practical strategies and hands-on tips to improve reproducibility, increase trust, and maximize the impact of your research through Open Science.
Sign up to have new issues delivered to your inbox every week.
Learn more about the benefits of Open Science. Open Science
- skip to Main Navigation
- skip to Main Content
- skip to Footer
- Accessibility feedback
- Data & Visualization and Research Support
- Data Management
Defining Research Data
One definition of research data is: "the recorded factual material commonly accepted in the scientific community as necessary to validate research findings." ( OMB Circular 110 ).
Research data covers a broad range of types of information (see examples below), and digital data can be structured and stored in a variety of file formats.
Note that properly managing data (and records) does not necessarily equate to sharing or publishing that data.
Examples of Research Data
Some examples of research data:
- Documents (text, Word), spreadsheets
- Laboratory notebooks, field notebooks, diaries
- Questionnaires, transcripts, codebooks
- Audiotapes, videotapes
- Photographs, films
- Protein or genetic sequences
- Test responses
- Slides, artifacts, specimens, samples
- Collection of digital objects acquired and generated during the process of research
- Database contents (video, audio, text, images)
- Models, algorithms, scripts
- Contents of an application (input, output, logfiles for analysis software, simulation software, schemas)
- Methodologies and workflows
- Standard operating procedures and protocols
Exclusions from Sharing
In addition to the other records to manage (below), some kinds of data may not be sharable due to the nature of the records themselves, or to ethical and privacy concerns. As defined by the OMB , this refers to:
- preliminary analyses,
- drafts of scientific papers,
- plans for future research,
- peer reviews, or
- communications with colleagues
Research data also do not include:
- Trade secrets, commercial information, materials necessary to be held confidential by a researcher until they are published, or similar information which is protected under law; and
- Personnel and medical information and similar information the disclosure of which would constitute a clearly unwarranted invasion of personal privacy, such as information that could be used to identify a particular person in a research study.
Some types of data, particularly software, may require special license to share. In those cases, contact the Office of Technology Transfer to review considerations for software generated in your research.
Other Records to Manage
Although they might not be addressed in an NSF data management plan, the following research records may also be important to manage during and beyond the life of a project.
- Correspondence (electronic mail and paper-based correspondence)
- Project files
- Grant applications
- Ethics applications
- Technical reports
- Research reports
- Signed consent forms
Adapted from Defining Research Data by the University of Oregon Libraries.
Opens in your default email client
URL copied to clipboard.
QR code for this page
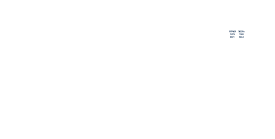
Popular pages
- COVID-19 Data Explorer
- CO₂ & Greenhouse Gas Emissions
- Child and Infant Mortality
- Artificial Intelligence
- Global Education
Research and data to make progress against the world’s largest problems.
4543 charts across 115 topics All free: open access and open source
Our Mission
What do we need to know to make the world a better place?
To make progress against the pressing problems the world faces, we need to be informed by the best research and data.
Our World in Data makes this knowledge accessible and understandable, to empower those working to build a better world.
We are a non-profit — all our work is free to use and open source. We believe the best research and data should be accessible to everyone. Consider supporting us if you find our work valuable.
Featured work
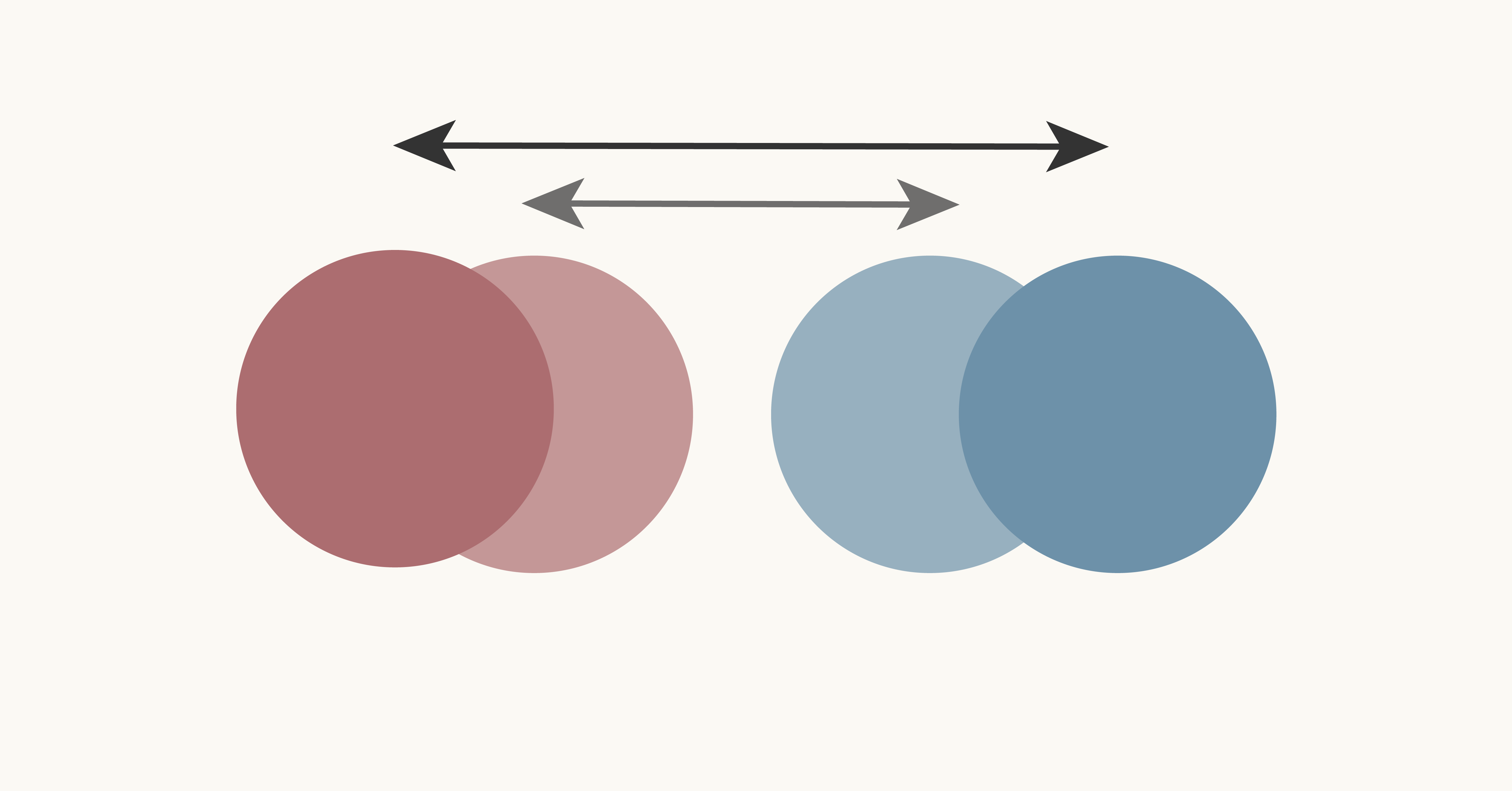
More people care about climate change than you think
The majority of people in every country support action on climate, but the public consistently underestimates this share.
Hannah Ritchie
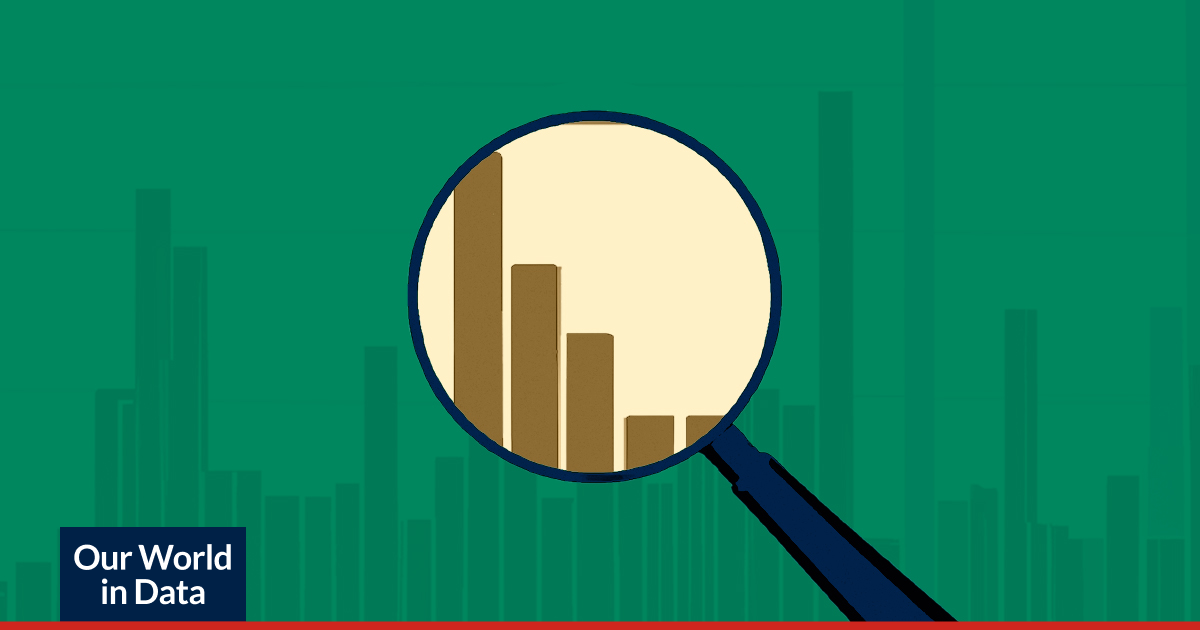
Browse our Data Insights
Bite-sized insights on how the world is changing, written by our team.
Our World in Data team
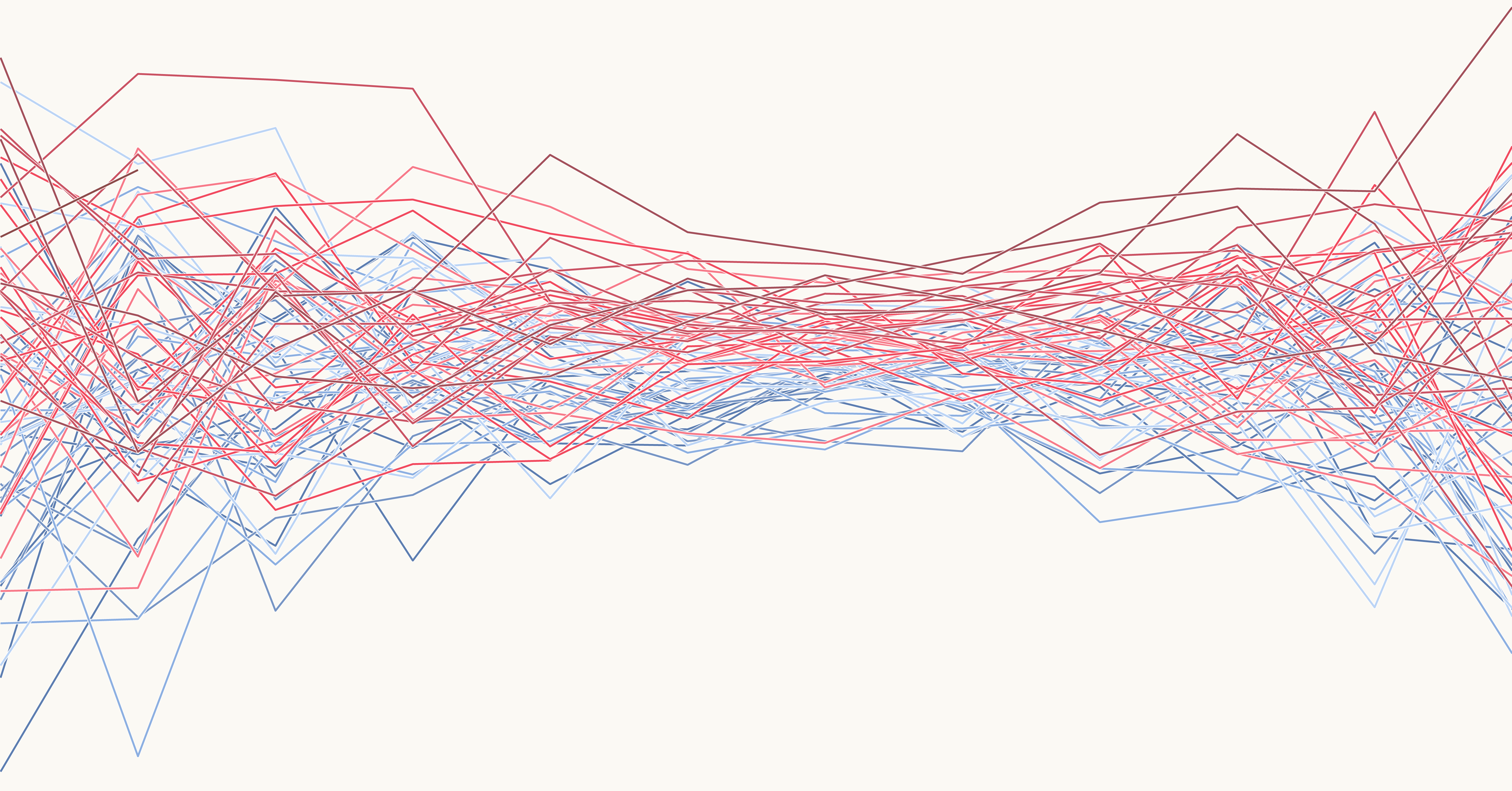
How much have temperatures risen in countries across the world?
Explore country-by-country data on monthly temperature anomalies.
Veronika Samborska
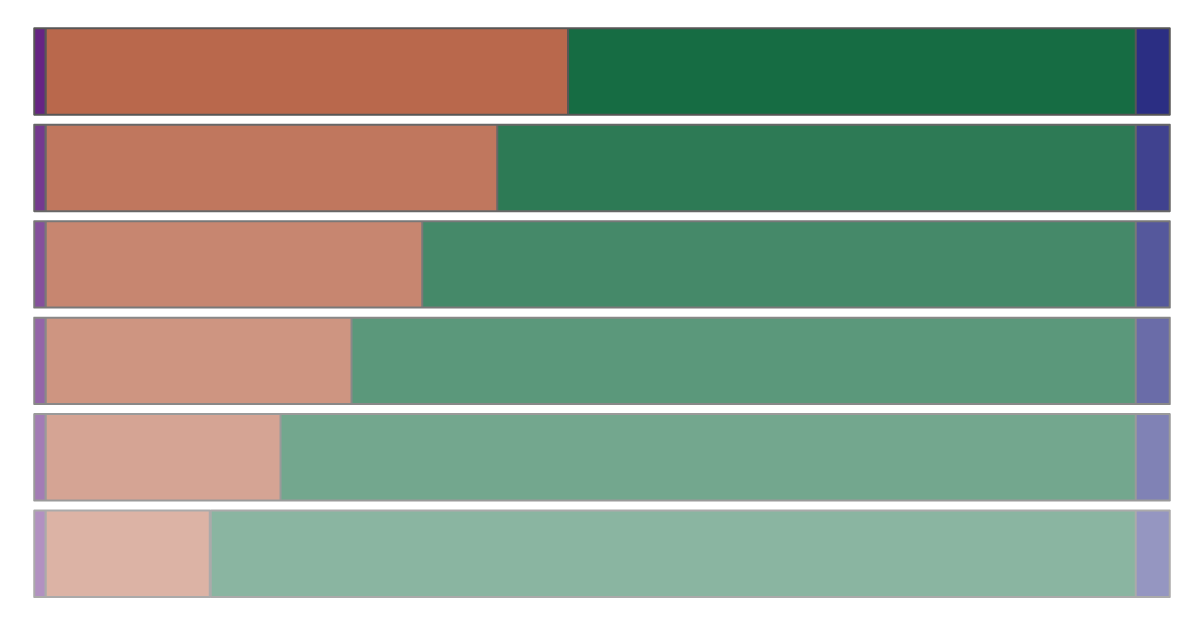
Why is improving agricultural productivity crucial to ending global hunger and protecting the world’s wildlife?
A future in which humanity produces more food on less land is not inevitable, but possible.

- War and Peace
How common are armed conflict and peace between and within countries? How is this changing over time? Explore research and data on war and peace.
Bastian Herre, Lucas Rodés-Guirao, Max Roser, Joe Hasell and Bobbie Macdonald
Latest Data Insights
Bite-sized insights on how the world is changing.
March 25, 2024
Richer countries often use much of their crops for animal feed and biofuels
March 22, 2024
Colonialism meant that for centuries, many territories and people were ruled from elsewhere
March 20, 2024
Plastic recycling rates are increasing, but slowly, in many regions
March 18, 2024
The modal age at death has been rising
Explore our data
Featured data from our collection of more than 4543 interactive charts.
Under-five mortality rate Long-run estimates combining data from UN & Gapminder
What share of children die before their fifth birthday.
What could be more tragic than the death of a young child? Child mortality, the death of children under the age of five, is still extremely common in our world today.
The historical data makes clear that it doesn’t have to be this way: it is possible for societies to protect their children and reduce child mortality to very low rates. For child mortality to reach low levels, many things have to go right at the same time: good healthcare , good nutrition , clean water and sanitation , maternal health , and high living standards . We can, therefore, think of child mortality as a proxy indicator of a country’s living conditions.
The chart shows our long-run data on child mortality, which allows you to see how child mortality has changed in countries around the world.
Share of population living in extreme poverty World Bank
What share of the population is living in extreme poverty.
The UN sets the “International Poverty Line” as a worldwide comparable definition for extreme poverty. Living in extreme poverty is currently defined as living on less than $2.15 per day. This indicator, published by the World Bank, has successfully drawn attention to the terrible depths of poverty of the poorest people in the world.
Two centuries ago, the majority of the world’s population was extremely poor. Back then, it was widely believed that widespread poverty was inevitable. This turned out to be wrong. Economic growth is possible and makes it possible for entire societies to leave the deep poverty of the past behind. Whether or not countries are leaving the worst poverty behind can be monitored by relying on this indicator.
Life expectancy at birth Long-run estimates collated from multiple sources by Our World in Data
How has people’s life expectancy changed over time.
Across the world, people are living longer. In 1900, the global average life expectancy of a newborn was 32 years. By 2021, this had more than doubled to 71 years.
Big improvements were achieved by countries around the world . The chart shows that life expectancy has more than doubled in every region of the world. This improvement is not only due to declining child mortality; life expectancy increased at all ages .
This visualization shows long-run estimates of life expectancy brought together by our team from several different data sources. It also shows that the COVID-19 pandemic led to reduced life expectancy worldwide.
Per capita CO₂ emissions Long-run estimates from the Global Carbon Budget
How have co₂ emissions per capita changed.
The main source of carbon dioxide (CO 2 ) emissions is the burning of fossil fuels. It is the primary greenhouse gas causing climate change .
Globally, CO 2 emissions have remained at just below 5 tonnes per person for over a decade. Between countries, however, there are large differences, and while emissions are rapidly increasing in some countries, they are rapidly falling in others.
The source for this CO 2 data is the Global Carbon Budget, a dataset we update yearly as soon as it is published. In addition to these production-based emissions, they publish consumption-based emissions for the last three decades, which can be viewed in our Greenhouse Gas Emissions Data Explorer .
GDP per capita Long-run estimates from the Maddison Project Database
How do average incomes compare between countries around the world.
GDP per capita is a very comprehensive measure of people’s average income . This indicator reveals how large the inequality between people in different countries is. In the poorest countries, people live on less than $1,000 per year, while in rich countries, the average income is more than 50 times higher.
The data shown is sourced from the Maddison Project Database. Drawing together the careful work of hundreds of economic historians, the particular value of this data lies in the historical coverage it provides. This data makes clear that the vast majority of people in all countries were poor in the past. It allows us to understand when and how the economic growth that made it possible to leave the deep poverty of the past behind was achieved.
Share of the population that is undernourished World Bank
What share of the population is suffering from hunger.
Hunger has been a severe problem for most of humanity throughout history. Growing enough food to feed one’s family was a constant struggle in daily life. Food shortages, malnutrition, and famines were common around the world.
The UN’s Food and Agriculture Organization publishes global data on undernourishment, defined as not consuming enough calories to maintain a normal, active, healthy life. These minimum requirements vary by a person’s sex, weight, height, and activity levels. This is considered in these national and global estimates.
The world has made much progress in reducing global hunger in recent decades. But we are still far away from an end to hunger, as this indicator shows. Tragically, nearly one in ten people still do not get enough food to eat and in recent years — especially during the pandemic — hunger levels have increased.
Literacy rate Long-run estimates collated from multiple sources by Our World in Data
When has literacy become a widespread skill.
Literacy is a foundational skill. Children need to learn to read so that they can read to learn. When we fail to teach this foundational skill, people have fewer opportunities to lead the rich and interesting lives that a good education offers.
The historical data shows that only a very small share of the population, a tiny elite, was able to read and write. Over the course of the last few generations, literacy levels increased, but it remains an important challenge for our time to provide this foundational skill to all.
At Our World in Data, we investigated the strengths and shortcomings of the available data on literacy. Based on this work, our team brought together the long-run data shown in the chart by combining several different sources, including the World Bank, the CIA Factbook, and a range of research publications.
Share of the population with access to electricity World Bank
Where do people lack access to even the most basic electricity supply.
Light at night makes it possible to get together after sunset; mobile phones allow us to stay in touch with those far away; the refrigeration of food reduces food waste; and household appliances free up time from household chores. Access to electricity improves people’s living conditions in many ways.
The World Bank data on the world map captures whether people have access to the most basic electricity supply — just enough to provide basic lighting and charge a phone or power a radio for 4 hours per day.
It shows that, especially in several African countries, a large share of the population lacks the benefits that basic electricity offers. No radio and no light at night.
Data explorers
Interactive visualization tools to explore a wide range of related indicators.
Data Explorer
Population & Demography
- Global Health
Subscribe to our newsletter
All our topics.
All our data, research, and writing — topic by topic.
Population and Demographic Change
- Population Change:
- Population Growth
- Age Structure
- Gender Ratio
- Births and Deaths:
- Life Expectancy
- Fertility Rate
- Geography of the World Population:
- Urbanization
- Health Risks:
- Lead Pollution
- Alcohol Consumption
- Opioids, Cocaine, Cannabis, and Other Illicit Drugs
- Air Pollution
- Outdoor Air Pollution
- Indoor Air Pollution
- Infectious Diseases:
- Coronavirus Pandemic (COVID-19)
- Mpox (monkeypox)
- Diarrheal Diseases
- Tuberculosis
- Health Institutions and Interventions:
- Vaccination
- Healthcare Spending
- Eradication of Diseases
- Life and Death:
- Causes of Death
- Mental Health
- Cardiovascular Diseases
- Burden of Disease
- Maternal Mortality
Energy and Environment
- Energy Systems:
- Access to Energy
- Fossil Fuels
- Renewable Energy
- Nuclear Energy
- Waste and Pollution:
- Plastic Pollution
- Climate and Air:
- CO₂ and Greenhouse Gas Emissions
- Climate Change
- Ozone Layer
- Clean Water and Sanitation
- Clean Water
- Water Use and Stress
- Environment and Ecosystems:
- Natural Disasters
- Biodiversity
- Environmental Impacts of Food Production
- Animal Welfare
- Forests and Deforestation
Food and Agriculture
- Hunger and Undernourishment
- Food Supply
- Food Prices
- Diet Compositions
- Human Height
- Micronutrient Deficiency
- Food Production:
- Agricultural Production
- Crop Yields
- Meat and Dairy Production
- Farm Size and Productivity
- Agricultural Inputs:
- Fertilizers
- Employment in Agriculture
Poverty and Economic Development
- Public Sector:
- State Capacity
- Government Spending
- Education Spending
- Military Personnel and Spending
- Poverty and Prosperity:
- Economic Inequality
- Economic Growth
- Economic Inequality by Gender
- Child Labor
- Working Hours
- Women’s Employment
- Global Connections:
- Trade and Globalization
Education and Knowledge
- Research and Development
Innovation and Technological Change
- Space Exploration and Satellites
- Technological Change
Living Conditions, Community, and Wellbeing
- Housing and Infrastructure:
- Light at Night
- Homelessness
- Relationships:
- Marriages and Divorces
- Loneliness and Social Connections
- Happiness and Wellbeing:
- Human Development Index (HDI)
- Happiness and Life Satisfaction
Human Rights and Democracy
- Human Rights
- Women’s Rights
- LGBT+ Rights
- Violence Against Children and Children’s Rights
Violence and War
- Nuclear Weapons
- Biological and Chemical Weapons
Our World in Data is free and accessible for everyone.
Help us do this work by making a donation.
Thank you for visiting nature.com. You are using a browser version with limited support for CSS. To obtain the best experience, we recommend you use a more up to date browser (or turn off compatibility mode in Internet Explorer). In the meantime, to ensure continued support, we are displaying the site without styles and JavaScript.
- View all journals
Research data articles from across Nature Portfolio
Research data comprises research observations or findings, such as facts, images, measurements, records and files in various formats, and can be stored in databases. Data publication and archiving is important for the reuse of research data and the reproducibility of scientific research.
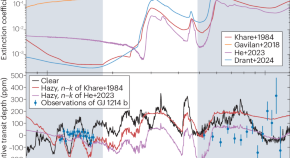
In an exoplanet atmosphere far, far away
JWST collects vast amounts of information about exoplanets light years away from Earth. Back home, the measured optical constants of laboratory aerosols are critically input parameters in models to interpret the observational results.
- Ella Sciamma-O’Brien
- Thomas Drant
- Nicholas Wogan
Related Subjects
Latest research and reviews.
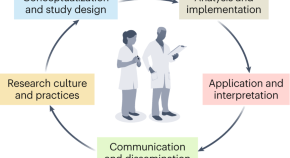
Recommendations for the responsible use and communication of race and ethnicity in neuroimaging research
This paper provides recommendations for researchers on responsibly conceptualizing, contextualizing and communicating issues related to race and ethnicity, including examples of important terms and frameworks.
- Carlos Cardenas-Iniguez
- Marybel Robledo Gonzalez
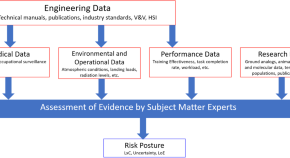
Levels of evidence for human system risk evaluation
- Jessica Ward
- Robert J. Reynolds
- Erik Antonsen
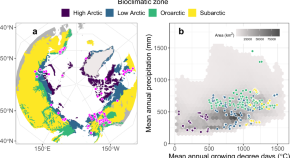
The Arctic Plant Aboveground Biomass Synthesis Dataset
- Logan T. Berner
- Kathleen M. Orndahl
- Scott J. Goetz
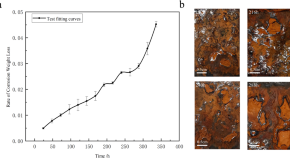
Corrosion behavior and cellular automata simulation of carbon steel in salt-spray environment
Turning alterations detected by mobile health technology in idiopathic rem sleep behavior disorder.
- Cinzia Zatti
- Andrea Pilotto
- Alessandro Padovani
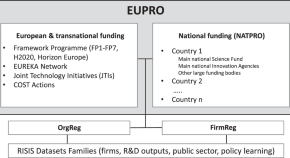
EUPRO - A reference database on project-based R&D collaboration networks
- Thomas Scherngell
- Michael Barber
- Xheneta Bilalli Shkodra
News and Comment
Understanding the provenance and quality of methods is essential for responsible reuse of fair data.
- Tracey L. Weissgerber
- Małgorzata Anna Gazda
- Malcolm R. Macleod
Cosmic recipe book updated
On the ethics of informed consent in genetic data collected before 1997.
- Martin Zieger
- Maria Eugenia D’Amato
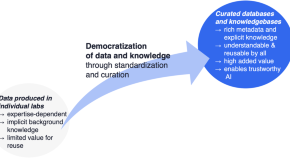
AI and the democratization of knowledge
The solution of the longstanding “protein folding problem” in 2021 showcased the transformative capabilities of AI in advancing the biomedical sciences. AI was characterized as successfully learning from protein structure data , which then spurred a more general call for AI-ready datasets to drive forward medical research. Here, we argue that it is the broad availability of knowledge , not just data, that is required to fuel further advances in AI in the scientific domain. This represents a quantum leap in a trend toward knowledge democratization that had already been developing in the biomedical sciences: knowledge is no longer primarily applied by specialists in a sub-field of biomedicine, but rather multidisciplinary teams, diverse biomedical research programs, and now machine learning. The development and application of explicit knowledge representations underpinning democratization is becoming a core scientific activity, and more investment in this activity is required if we are to achieve the promise of AI.
- Christophe Dessimoz
- Paul D. Thomas
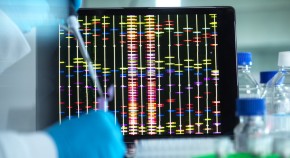
‘All of Us’ genetics chart stirs unease over controversial depiction of race
Debate over figure connecting genes, race and ethnicity reignites concerns among geneticists about how to represent human diversity.
Quick links
- Explore articles by subject
- Guide to authors
- Editorial policies

- Search Menu
- Browse content in Arts and Humanities
- Browse content in Archaeology
- Anglo-Saxon and Medieval Archaeology
- Archaeological Methodology and Techniques
- Archaeology by Region
- Archaeology of Religion
- Archaeology of Trade and Exchange
- Biblical Archaeology
- Contemporary and Public Archaeology
- Environmental Archaeology
- Historical Archaeology
- History and Theory of Archaeology
- Industrial Archaeology
- Landscape Archaeology
- Mortuary Archaeology
- Prehistoric Archaeology
- Underwater Archaeology
- Urban Archaeology
- Zooarchaeology
- Browse content in Architecture
- Architectural Structure and Design
- History of Architecture
- Residential and Domestic Buildings
- Theory of Architecture
- Browse content in Art
- Art Subjects and Themes
- History of Art
- Industrial and Commercial Art
- Theory of Art
- Biographical Studies
- Byzantine Studies
- Browse content in Classical Studies
- Classical History
- Classical Philosophy
- Classical Mythology
- Classical Literature
- Classical Reception
- Classical Art and Architecture
- Classical Oratory and Rhetoric
- Greek and Roman Epigraphy
- Greek and Roman Law
- Greek and Roman Papyrology
- Greek and Roman Archaeology
- Late Antiquity
- Religion in the Ancient World
- Digital Humanities
- Browse content in History
- Colonialism and Imperialism
- Diplomatic History
- Environmental History
- Genealogy, Heraldry, Names, and Honours
- Genocide and Ethnic Cleansing
- Historical Geography
- History by Period
- History of Emotions
- History of Agriculture
- History of Education
- History of Gender and Sexuality
- Industrial History
- Intellectual History
- International History
- Labour History
- Legal and Constitutional History
- Local and Family History
- Maritime History
- Military History
- National Liberation and Post-Colonialism
- Oral History
- Political History
- Public History
- Regional and National History
- Revolutions and Rebellions
- Slavery and Abolition of Slavery
- Social and Cultural History
- Theory, Methods, and Historiography
- Urban History
- World History
- Browse content in Language Teaching and Learning
- Language Learning (Specific Skills)
- Language Teaching Theory and Methods
- Browse content in Linguistics
- Applied Linguistics
- Cognitive Linguistics
- Computational Linguistics
- Forensic Linguistics
- Grammar, Syntax and Morphology
- Historical and Diachronic Linguistics
- History of English
- Language Acquisition
- Language Evolution
- Language Reference
- Language Variation
- Language Families
- Lexicography
- Linguistic Anthropology
- Linguistic Theories
- Linguistic Typology
- Phonetics and Phonology
- Psycholinguistics
- Sociolinguistics
- Translation and Interpretation
- Writing Systems
- Browse content in Literature
- Bibliography
- Children's Literature Studies
- Literary Studies (Asian)
- Literary Studies (European)
- Literary Studies (Eco-criticism)
- Literary Studies (Romanticism)
- Literary Studies (American)
- Literary Studies (Modernism)
- Literary Studies - World
- Literary Studies (1500 to 1800)
- Literary Studies (19th Century)
- Literary Studies (20th Century onwards)
- Literary Studies (African American Literature)
- Literary Studies (British and Irish)
- Literary Studies (Early and Medieval)
- Literary Studies (Fiction, Novelists, and Prose Writers)
- Literary Studies (Gender Studies)
- Literary Studies (Graphic Novels)
- Literary Studies (History of the Book)
- Literary Studies (Plays and Playwrights)
- Literary Studies (Poetry and Poets)
- Literary Studies (Postcolonial Literature)
- Literary Studies (Queer Studies)
- Literary Studies (Science Fiction)
- Literary Studies (Travel Literature)
- Literary Studies (War Literature)
- Literary Studies (Women's Writing)
- Literary Theory and Cultural Studies
- Mythology and Folklore
- Shakespeare Studies and Criticism
- Browse content in Media Studies
- Browse content in Music
- Applied Music
- Dance and Music
- Ethics in Music
- Ethnomusicology
- Gender and Sexuality in Music
- Medicine and Music
- Music Cultures
- Music and Religion
- Music and Media
- Music and Culture
- Music Education and Pedagogy
- Music Theory and Analysis
- Musical Scores, Lyrics, and Libretti
- Musical Structures, Styles, and Techniques
- Musicology and Music History
- Performance Practice and Studies
- Race and Ethnicity in Music
- Sound Studies
- Browse content in Performing Arts
- Browse content in Philosophy
- Aesthetics and Philosophy of Art
- Epistemology
- Feminist Philosophy
- History of Western Philosophy
- Metaphysics
- Moral Philosophy
- Non-Western Philosophy
- Philosophy of Science
- Philosophy of Language
- Philosophy of Mind
- Philosophy of Perception
- Philosophy of Action
- Philosophy of Law
- Philosophy of Religion
- Philosophy of Mathematics and Logic
- Practical Ethics
- Social and Political Philosophy
- Browse content in Religion
- Biblical Studies
- Christianity
- East Asian Religions
- History of Religion
- Judaism and Jewish Studies
- Qumran Studies
- Religion and Education
- Religion and Health
- Religion and Politics
- Religion and Science
- Religion and Law
- Religion and Art, Literature, and Music
- Religious Studies
- Browse content in Society and Culture
- Cookery, Food, and Drink
- Cultural Studies
- Customs and Traditions
- Ethical Issues and Debates
- Hobbies, Games, Arts and Crafts
- Lifestyle, Home, and Garden
- Natural world, Country Life, and Pets
- Popular Beliefs and Controversial Knowledge
- Sports and Outdoor Recreation
- Technology and Society
- Travel and Holiday
- Visual Culture
- Browse content in Law
- Arbitration
- Browse content in Company and Commercial Law
- Commercial Law
- Company Law
- Browse content in Comparative Law
- Systems of Law
- Competition Law
- Browse content in Constitutional and Administrative Law
- Government Powers
- Judicial Review
- Local Government Law
- Military and Defence Law
- Parliamentary and Legislative Practice
- Construction Law
- Contract Law
- Browse content in Criminal Law
- Criminal Procedure
- Criminal Evidence Law
- Sentencing and Punishment
- Employment and Labour Law
- Environment and Energy Law
- Browse content in Financial Law
- Banking Law
- Insolvency Law
- History of Law
- Human Rights and Immigration
- Intellectual Property Law
- Browse content in International Law
- Private International Law and Conflict of Laws
- Public International Law
- IT and Communications Law
- Jurisprudence and Philosophy of Law
- Law and Politics
- Law and Society
- Browse content in Legal System and Practice
- Courts and Procedure
- Legal Skills and Practice
- Primary Sources of Law
- Regulation of Legal Profession
- Medical and Healthcare Law
- Browse content in Policing
- Criminal Investigation and Detection
- Police and Security Services
- Police Procedure and Law
- Police Regional Planning
- Browse content in Property Law
- Personal Property Law
- Study and Revision
- Terrorism and National Security Law
- Browse content in Trusts Law
- Wills and Probate or Succession
- Browse content in Medicine and Health
- Browse content in Allied Health Professions
- Arts Therapies
- Clinical Science
- Dietetics and Nutrition
- Occupational Therapy
- Operating Department Practice
- Physiotherapy
- Radiography
- Speech and Language Therapy
- Browse content in Anaesthetics
- General Anaesthesia
- Neuroanaesthesia
- Browse content in Clinical Medicine
- Acute Medicine
- Cardiovascular Medicine
- Clinical Genetics
- Clinical Pharmacology and Therapeutics
- Dermatology
- Endocrinology and Diabetes
- Gastroenterology
- Genito-urinary Medicine
- Geriatric Medicine
- Infectious Diseases
- Medical Toxicology
- Medical Oncology
- Pain Medicine
- Palliative Medicine
- Rehabilitation Medicine
- Respiratory Medicine and Pulmonology
- Rheumatology
- Sleep Medicine
- Sports and Exercise Medicine
- Clinical Neuroscience
- Community Medical Services
- Critical Care
- Emergency Medicine
- Forensic Medicine
- Haematology
- History of Medicine
- Browse content in Medical Dentistry
- Oral and Maxillofacial Surgery
- Paediatric Dentistry
- Restorative Dentistry and Orthodontics
- Surgical Dentistry
- Browse content in Medical Skills
- Clinical Skills
- Communication Skills
- Nursing Skills
- Surgical Skills
- Medical Ethics
- Medical Statistics and Methodology
- Browse content in Neurology
- Clinical Neurophysiology
- Neuropathology
- Nursing Studies
- Browse content in Obstetrics and Gynaecology
- Gynaecology
- Occupational Medicine
- Ophthalmology
- Otolaryngology (ENT)
- Browse content in Paediatrics
- Neonatology
- Browse content in Pathology
- Chemical Pathology
- Clinical Cytogenetics and Molecular Genetics
- Histopathology
- Medical Microbiology and Virology
- Patient Education and Information
- Browse content in Pharmacology
- Psychopharmacology
- Browse content in Popular Health
- Caring for Others
- Complementary and Alternative Medicine
- Self-help and Personal Development
- Browse content in Preclinical Medicine
- Cell Biology
- Molecular Biology and Genetics
- Reproduction, Growth and Development
- Primary Care
- Professional Development in Medicine
- Browse content in Psychiatry
- Addiction Medicine
- Child and Adolescent Psychiatry
- Forensic Psychiatry
- Learning Disabilities
- Old Age Psychiatry
- Psychotherapy
- Browse content in Public Health and Epidemiology
- Epidemiology
- Public Health
- Browse content in Radiology
- Clinical Radiology
- Interventional Radiology
- Nuclear Medicine
- Radiation Oncology
- Reproductive Medicine
- Browse content in Surgery
- Cardiothoracic Surgery
- Gastro-intestinal and Colorectal Surgery
- General Surgery
- Neurosurgery
- Paediatric Surgery
- Peri-operative Care
- Plastic and Reconstructive Surgery
- Surgical Oncology
- Transplant Surgery
- Trauma and Orthopaedic Surgery
- Vascular Surgery
- Browse content in Science and Mathematics
- Browse content in Biological Sciences
- Aquatic Biology
- Biochemistry
- Bioinformatics and Computational Biology
- Developmental Biology
- Ecology and Conservation
- Evolutionary Biology
- Genetics and Genomics
- Microbiology
- Molecular and Cell Biology
- Natural History
- Plant Sciences and Forestry
- Research Methods in Life Sciences
- Structural Biology
- Systems Biology
- Zoology and Animal Sciences
- Browse content in Chemistry
- Analytical Chemistry
- Computational Chemistry
- Crystallography
- Environmental Chemistry
- Industrial Chemistry
- Inorganic Chemistry
- Materials Chemistry
- Medicinal Chemistry
- Mineralogy and Gems
- Organic Chemistry
- Physical Chemistry
- Polymer Chemistry
- Study and Communication Skills in Chemistry
- Theoretical Chemistry
- Browse content in Computer Science
- Artificial Intelligence
- Computer Architecture and Logic Design
- Game Studies
- Human-Computer Interaction
- Mathematical Theory of Computation
- Programming Languages
- Software Engineering
- Systems Analysis and Design
- Virtual Reality
- Browse content in Computing
- Business Applications
- Computer Security
- Computer Games
- Computer Networking and Communications
- Digital Lifestyle
- Graphical and Digital Media Applications
- Operating Systems
- Browse content in Earth Sciences and Geography
- Atmospheric Sciences
- Environmental Geography
- Geology and the Lithosphere
- Maps and Map-making
- Meteorology and Climatology
- Oceanography and Hydrology
- Palaeontology
- Physical Geography and Topography
- Regional Geography
- Soil Science
- Urban Geography
- Browse content in Engineering and Technology
- Agriculture and Farming
- Biological Engineering
- Civil Engineering, Surveying, and Building
- Electronics and Communications Engineering
- Energy Technology
- Engineering (General)
- Environmental Science, Engineering, and Technology
- History of Engineering and Technology
- Mechanical Engineering and Materials
- Technology of Industrial Chemistry
- Transport Technology and Trades
- Browse content in Environmental Science
- Applied Ecology (Environmental Science)
- Conservation of the Environment (Environmental Science)
- Environmental Sustainability
- Environmentalist Thought and Ideology (Environmental Science)
- Management of Land and Natural Resources (Environmental Science)
- Natural Disasters (Environmental Science)
- Nuclear Issues (Environmental Science)
- Pollution and Threats to the Environment (Environmental Science)
- Social Impact of Environmental Issues (Environmental Science)
- History of Science and Technology
- Browse content in Materials Science
- Ceramics and Glasses
- Composite Materials
- Metals, Alloying, and Corrosion
- Nanotechnology
- Browse content in Mathematics
- Applied Mathematics
- Biomathematics and Statistics
- History of Mathematics
- Mathematical Education
- Mathematical Finance
- Mathematical Analysis
- Numerical and Computational Mathematics
- Probability and Statistics
- Pure Mathematics
- Browse content in Neuroscience
- Cognition and Behavioural Neuroscience
- Development of the Nervous System
- Disorders of the Nervous System
- History of Neuroscience
- Invertebrate Neurobiology
- Molecular and Cellular Systems
- Neuroendocrinology and Autonomic Nervous System
- Neuroscientific Techniques
- Sensory and Motor Systems
- Browse content in Physics
- Astronomy and Astrophysics
- Atomic, Molecular, and Optical Physics
- Biological and Medical Physics
- Classical Mechanics
- Computational Physics
- Condensed Matter Physics
- Electromagnetism, Optics, and Acoustics
- History of Physics
- Mathematical and Statistical Physics
- Measurement Science
- Nuclear Physics
- Particles and Fields
- Plasma Physics
- Quantum Physics
- Relativity and Gravitation
- Semiconductor and Mesoscopic Physics
- Browse content in Psychology
- Affective Sciences
- Clinical Psychology
- Cognitive Psychology
- Cognitive Neuroscience
- Criminal and Forensic Psychology
- Developmental Psychology
- Educational Psychology
- Evolutionary Psychology
- Health Psychology
- History and Systems in Psychology
- Music Psychology
- Neuropsychology
- Organizational Psychology
- Psychological Assessment and Testing
- Psychology of Human-Technology Interaction
- Psychology Professional Development and Training
- Research Methods in Psychology
- Social Psychology
- Browse content in Social Sciences
- Browse content in Anthropology
- Anthropology of Religion
- Human Evolution
- Medical Anthropology
- Physical Anthropology
- Regional Anthropology
- Social and Cultural Anthropology
- Theory and Practice of Anthropology
- Browse content in Business and Management
- Business Strategy
- Business Ethics
- Business History
- Business and Government
- Business and Technology
- Business and the Environment
- Comparative Management
- Corporate Governance
- Corporate Social Responsibility
- Entrepreneurship
- Health Management
- Human Resource Management
- Industrial and Employment Relations
- Industry Studies
- Information and Communication Technologies
- International Business
- Knowledge Management
- Management and Management Techniques
- Operations Management
- Organizational Theory and Behaviour
- Pensions and Pension Management
- Public and Nonprofit Management
- Strategic Management
- Supply Chain Management
- Browse content in Criminology and Criminal Justice
- Criminal Justice
- Criminology
- Forms of Crime
- International and Comparative Criminology
- Youth Violence and Juvenile Justice
- Development Studies
- Browse content in Economics
- Agricultural, Environmental, and Natural Resource Economics
- Asian Economics
- Behavioural Finance
- Behavioural Economics and Neuroeconomics
- Econometrics and Mathematical Economics
- Economic Systems
- Economic History
- Economic Methodology
- Economic Development and Growth
- Financial Markets
- Financial Institutions and Services
- General Economics and Teaching
- Health, Education, and Welfare
- History of Economic Thought
- International Economics
- Labour and Demographic Economics
- Law and Economics
- Macroeconomics and Monetary Economics
- Microeconomics
- Public Economics
- Urban, Rural, and Regional Economics
- Welfare Economics
- Browse content in Education
- Adult Education and Continuous Learning
- Care and Counselling of Students
- Early Childhood and Elementary Education
- Educational Equipment and Technology
- Educational Strategies and Policy
- Higher and Further Education
- Organization and Management of Education
- Philosophy and Theory of Education
- Schools Studies
- Secondary Education
- Teaching of a Specific Subject
- Teaching of Specific Groups and Special Educational Needs
- Teaching Skills and Techniques
- Browse content in Environment
- Applied Ecology (Social Science)
- Climate Change
- Conservation of the Environment (Social Science)
- Environmentalist Thought and Ideology (Social Science)
- Natural Disasters (Environment)
- Social Impact of Environmental Issues (Social Science)
- Browse content in Human Geography
- Cultural Geography
- Economic Geography
- Political Geography
- Browse content in Interdisciplinary Studies
- Communication Studies
- Museums, Libraries, and Information Sciences
- Browse content in Politics
- African Politics
- Asian Politics
- Chinese Politics
- Comparative Politics
- Conflict Politics
- Elections and Electoral Studies
- Environmental Politics
- European Union
- Foreign Policy
- Gender and Politics
- Human Rights and Politics
- Indian Politics
- International Relations
- International Organization (Politics)
- International Political Economy
- Irish Politics
- Latin American Politics
- Middle Eastern Politics
- Political Methodology
- Political Communication
- Political Philosophy
- Political Sociology
- Political Behaviour
- Political Economy
- Political Institutions
- Political Theory
- Politics and Law
- Public Administration
- Public Policy
- Quantitative Political Methodology
- Regional Political Studies
- Russian Politics
- Security Studies
- State and Local Government
- UK Politics
- US Politics
- Browse content in Regional and Area Studies
- African Studies
- Asian Studies
- East Asian Studies
- Japanese Studies
- Latin American Studies
- Middle Eastern Studies
- Native American Studies
- Scottish Studies
- Browse content in Research and Information
- Research Methods
- Browse content in Social Work
- Addictions and Substance Misuse
- Adoption and Fostering
- Care of the Elderly
- Child and Adolescent Social Work
- Couple and Family Social Work
- Developmental and Physical Disabilities Social Work
- Direct Practice and Clinical Social Work
- Emergency Services
- Human Behaviour and the Social Environment
- International and Global Issues in Social Work
- Mental and Behavioural Health
- Social Justice and Human Rights
- Social Policy and Advocacy
- Social Work and Crime and Justice
- Social Work Macro Practice
- Social Work Practice Settings
- Social Work Research and Evidence-based Practice
- Welfare and Benefit Systems
- Browse content in Sociology
- Childhood Studies
- Community Development
- Comparative and Historical Sociology
- Economic Sociology
- Gender and Sexuality
- Gerontology and Ageing
- Health, Illness, and Medicine
- Marriage and the Family
- Migration Studies
- Occupations, Professions, and Work
- Organizations
- Population and Demography
- Race and Ethnicity
- Social Theory
- Social Movements and Social Change
- Social Research and Statistics
- Social Stratification, Inequality, and Mobility
- Sociology of Religion
- Sociology of Education
- Sport and Leisure
- Urban and Rural Studies
- Browse content in Warfare and Defence
- Defence Strategy, Planning, and Research
- Land Forces and Warfare
- Military Administration
- Military Life and Institutions
- Naval Forces and Warfare
- Other Warfare and Defence Issues
- Peace Studies and Conflict Resolution
- Weapons and Equipment
Research data
Research data for journals.
Oxford University Press (OUP) and the journals we publish enforce high standards of rigour in the materials we accept for publication. The availability of data, code and other materials underlying research publications offers greater transparency, better trust in the literature and better reproducibility. Reuse of data offers enormous potential for further scientific and economic development. Making available the data underlying research publications supports our educational and scholarly mission as a department of the University of Oxford.
OUP is a signatory to the Center for Open Science’s Transparency and Openness Promotion (TOP) Guidelines which served as a framework for our overall data policy approach. OUP also supports the Joint Declaration of Data Citation Principles and the recommendations of the FORCE11 Software Citation Implementation Group , as reflected in our data and software citation practices .
Data availability policies
Data availability statements, citing research data and software, choosing where to archive your data.
OUP encourages authors, where ethically feasible, to make the research data underlying their articles publicly available. Because the nature of research data varies widely between different disciplines, each individual journal has set a field-appropriate data availability policy based on one of the levels listed below. Please see the individual journal’s Instructions to Authors for further details on the data requirements and citation practices of that publication.
Level 1: The journal encourages all authors, where ethically possible, to publicly release all data underlying any published paper.
Level 2 : The journal encourages all authors, where ethically possible, to publicly release all data underlying any published paper. Authors must include a Data Availability Statement in their published article.
Level 3 : The journal requires all authors, where ethically possible, to publicly release all data underlying any published paper as a condition of publication. Authors must include a Data Availability Statement in their published article.
Level 4 : The journal requires all authors, where ethically possible, to publicly release all data underlying any published paper as a condition of publication. The data must undergo peer review along with the manuscript as part of the acceptance process. Authors must include a Data Availability Statement in their published article.
Data Availability Statements provide a standardised format to describe the availability of data underlying the research results of the article. The statement may refer to original data generated in the course of the study or to third-party data analysed in the article. The statement should describe and provide means of access, where applicable, by linking to the data or providing the required unique identifier.
Sample Data Availability Statements
Please see the Instructions to Authors of your chosen journal for their specific requirements in relation to Data Availability Statements.
OUP endorses the Joint Declaration of Data Citation Principles , and the recommendations of the FORCE11 Software Citation Implementation Group . Research data and software are legitimate, citable products of research for which citations should be given the same status as citations to publications.
In addition to references to data and software included in a data availability statement, when materials underlying the research article are available in an online source authors should include a full citation in their reference list. The citation should include a persistent link enabling readers to directly access the source, i.e. an online repository.
Citing data and software enables the authors to get credit for their contribution to the research article, and also increases the discoverability of the research data and software.
Data and software citations should include the following minimum information where available:
Author(s) / Creator(s)
Title / Software name
Publication venue (e.g repository or archive name)
Persistent identifier (e.g. DOI)
In addition, some journal reference styles may require the following information:
Version number or date of access
Bracketed description of material type (e.g. Dataset, Computer software)
*When citing software, if there is an article describing the software it is recommended to cite both the software and the article.
OUP encourages authors to share their data and other research materials to support the transparency and reproducibility of their research. Authors should consult the journal Instructions to Authors for recommendations/requirements on where to archive data. Many disciplines have specific requirements for depositing of data in recognised disciplinary repositories.
You may also refer to online resources such as FAIRsharing.org and re3data.org for lists of data repositories, including information on certification status and services offered.
Authors are highly encouraged to select a repository that issues DOIs as this helps to facilitate persistent linking to the dataset from the research article.
There are also a number of generalist data repositories that you may wish to consider when depositing your data:
Code Ocean (for data and code)
Dryad Digital Repository
Harvard Dataverse
Open Science Framework
A number of Oxford University Press journals sponsor the deposition of data by their authors in the Dryad Digital Repository:
Behavioral Ecology
Evolution Letters
Journal of Experimental Botany
Journal of the American Medical Informatics Association
Journal of Heredity
Ornithological Applications
Ornithology
Systematic Biology
Toxicological Sciences
For more information see the journal Instructions to Authors.
- About Oxford Academic
- Publish journals with us
- University press partners
- What we publish
- New features
- Open access
- Institutional account management
- Rights and permissions
- Get help with access
- Accessibility
- Advertising
- Media enquiries
- Oxford University Press
- Oxford Languages
- University of Oxford
Oxford University Press is a department of the University of Oxford. It furthers the University's objective of excellence in research, scholarship, and education by publishing worldwide
- Copyright © 2024 Oxford University Press
- Cookie settings
- Cookie policy
- Privacy policy
- Legal notice
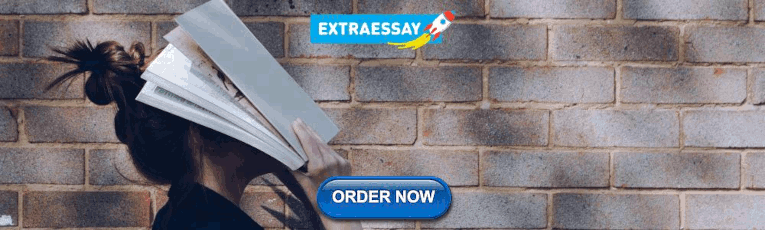
This Feature Is Available To Subscribers Only
Sign In or Create an Account
This PDF is available to Subscribers Only
For full access to this pdf, sign in to an existing account, or purchase an annual subscription.
- Skip to main content
- Skip to primary sidebar
- Skip to footer
- QuestionPro

- Solutions Industries Gaming Automotive Sports and events Education Government Travel & Hospitality Financial Services Healthcare Cannabis Technology Use Case NPS+ Communities Audience Contactless surveys Mobile LivePolls Member Experience GDPR Positive People Science 360 Feedback Surveys
- Resources Blog eBooks Survey Templates Case Studies Training Help center

Home Market Research
Data Analysis in Research: Types & Methods
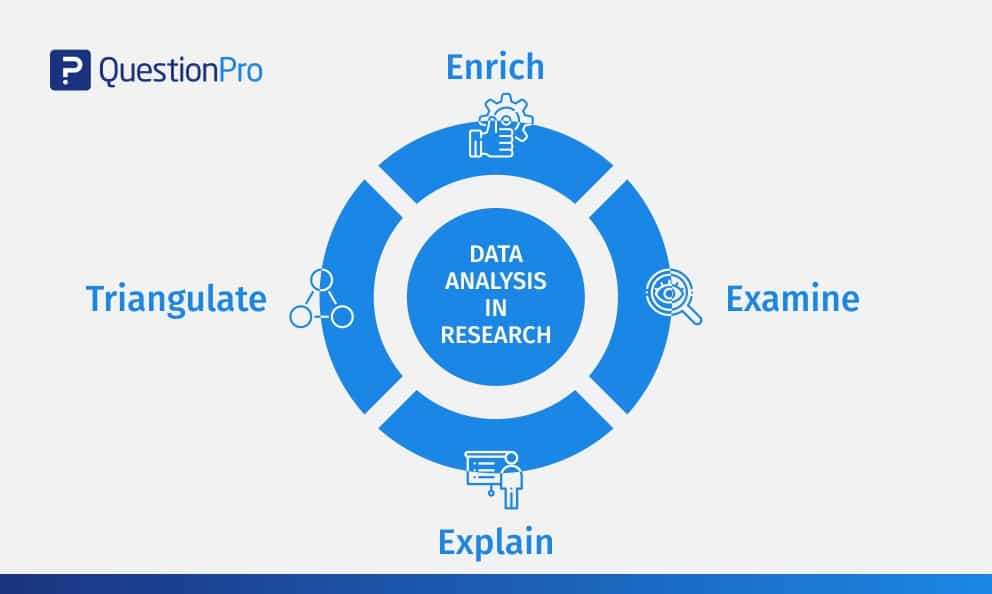
Content Index
Why analyze data in research?
Types of data in research, finding patterns in the qualitative data, methods used for data analysis in qualitative research, preparing data for analysis, methods used for data analysis in quantitative research, considerations in research data analysis, what is data analysis in research.
Definition of research in data analysis: According to LeCompte and Schensul, research data analysis is a process used by researchers to reduce data to a story and interpret it to derive insights. The data analysis process helps reduce a large chunk of data into smaller fragments, which makes sense.
Three essential things occur during the data analysis process — the first is data organization . Summarization and categorization together contribute to becoming the second known method used for data reduction. It helps find patterns and themes in the data for easy identification and linking. The third and last way is data analysis – researchers do it in both top-down and bottom-up fashion.
LEARN ABOUT: Research Process Steps
On the other hand, Marshall and Rossman describe data analysis as a messy, ambiguous, and time-consuming but creative and fascinating process through which a mass of collected data is brought to order, structure and meaning.
We can say that “the data analysis and data interpretation is a process representing the application of deductive and inductive logic to the research and data analysis.”
Researchers rely heavily on data as they have a story to tell or research problems to solve. It starts with a question, and data is nothing but an answer to that question. But, what if there is no question to ask? Well! It is possible to explore data even without a problem – we call it ‘Data Mining’, which often reveals some interesting patterns within the data that are worth exploring.
Irrelevant to the type of data researchers explore, their mission and audiences’ vision guide them to find the patterns to shape the story they want to tell. One of the essential things expected from researchers while analyzing data is to stay open and remain unbiased toward unexpected patterns, expressions, and results. Remember, sometimes, data analysis tells the most unforeseen yet exciting stories that were not expected when initiating data analysis. Therefore, rely on the data you have at hand and enjoy the journey of exploratory research.
Create a Free Account
Every kind of data has a rare quality of describing things after assigning a specific value to it. For analysis, you need to organize these values, processed and presented in a given context, to make it useful. Data can be in different forms; here are the primary data types.
- Qualitative data: When the data presented has words and descriptions, then we call it qualitative data . Although you can observe this data, it is subjective and harder to analyze data in research, especially for comparison. Example: Quality data represents everything describing taste, experience, texture, or an opinion that is considered quality data. This type of data is usually collected through focus groups, personal qualitative interviews , qualitative observation or using open-ended questions in surveys.
- Quantitative data: Any data expressed in numbers of numerical figures are called quantitative data . This type of data can be distinguished into categories, grouped, measured, calculated, or ranked. Example: questions such as age, rank, cost, length, weight, scores, etc. everything comes under this type of data. You can present such data in graphical format, charts, or apply statistical analysis methods to this data. The (Outcomes Measurement Systems) OMS questionnaires in surveys are a significant source of collecting numeric data.
- Categorical data: It is data presented in groups. However, an item included in the categorical data cannot belong to more than one group. Example: A person responding to a survey by telling his living style, marital status, smoking habit, or drinking habit comes under the categorical data. A chi-square test is a standard method used to analyze this data.
Learn More : Examples of Qualitative Data in Education
Data analysis in qualitative research
Data analysis and qualitative data research work a little differently from the numerical data as the quality data is made up of words, descriptions, images, objects, and sometimes symbols. Getting insight from such complicated information is a complicated process. Hence it is typically used for exploratory research and data analysis .
Although there are several ways to find patterns in the textual information, a word-based method is the most relied and widely used global technique for research and data analysis. Notably, the data analysis process in qualitative research is manual. Here the researchers usually read the available data and find repetitive or commonly used words.
For example, while studying data collected from African countries to understand the most pressing issues people face, researchers might find “food” and “hunger” are the most commonly used words and will highlight them for further analysis.
LEARN ABOUT: Level of Analysis
The keyword context is another widely used word-based technique. In this method, the researcher tries to understand the concept by analyzing the context in which the participants use a particular keyword.
For example , researchers conducting research and data analysis for studying the concept of ‘diabetes’ amongst respondents might analyze the context of when and how the respondent has used or referred to the word ‘diabetes.’
The scrutiny-based technique is also one of the highly recommended text analysis methods used to identify a quality data pattern. Compare and contrast is the widely used method under this technique to differentiate how a specific text is similar or different from each other.
For example: To find out the “importance of resident doctor in a company,” the collected data is divided into people who think it is necessary to hire a resident doctor and those who think it is unnecessary. Compare and contrast is the best method that can be used to analyze the polls having single-answer questions types .
Metaphors can be used to reduce the data pile and find patterns in it so that it becomes easier to connect data with theory.
Variable Partitioning is another technique used to split variables so that researchers can find more coherent descriptions and explanations from the enormous data.
LEARN ABOUT: Qualitative Research Questions and Questionnaires
There are several techniques to analyze the data in qualitative research, but here are some commonly used methods,
- Content Analysis: It is widely accepted and the most frequently employed technique for data analysis in research methodology. It can be used to analyze the documented information from text, images, and sometimes from the physical items. It depends on the research questions to predict when and where to use this method.
- Narrative Analysis: This method is used to analyze content gathered from various sources such as personal interviews, field observation, and surveys . The majority of times, stories, or opinions shared by people are focused on finding answers to the research questions.
- Discourse Analysis: Similar to narrative analysis, discourse analysis is used to analyze the interactions with people. Nevertheless, this particular method considers the social context under which or within which the communication between the researcher and respondent takes place. In addition to that, discourse analysis also focuses on the lifestyle and day-to-day environment while deriving any conclusion.
- Grounded Theory: When you want to explain why a particular phenomenon happened, then using grounded theory for analyzing quality data is the best resort. Grounded theory is applied to study data about the host of similar cases occurring in different settings. When researchers are using this method, they might alter explanations or produce new ones until they arrive at some conclusion.
LEARN ABOUT: 12 Best Tools for Researchers
Data analysis in quantitative research
The first stage in research and data analysis is to make it for the analysis so that the nominal data can be converted into something meaningful. Data preparation consists of the below phases.
Phase I: Data Validation
Data validation is done to understand if the collected data sample is per the pre-set standards, or it is a biased data sample again divided into four different stages
- Fraud: To ensure an actual human being records each response to the survey or the questionnaire
- Screening: To make sure each participant or respondent is selected or chosen in compliance with the research criteria
- Procedure: To ensure ethical standards were maintained while collecting the data sample
- Completeness: To ensure that the respondent has answered all the questions in an online survey. Else, the interviewer had asked all the questions devised in the questionnaire.
Phase II: Data Editing
More often, an extensive research data sample comes loaded with errors. Respondents sometimes fill in some fields incorrectly or sometimes skip them accidentally. Data editing is a process wherein the researchers have to confirm that the provided data is free of such errors. They need to conduct necessary checks and outlier checks to edit the raw edit and make it ready for analysis.
Phase III: Data Coding
Out of all three, this is the most critical phase of data preparation associated with grouping and assigning values to the survey responses . If a survey is completed with a 1000 sample size, the researcher will create an age bracket to distinguish the respondents based on their age. Thus, it becomes easier to analyze small data buckets rather than deal with the massive data pile.
LEARN ABOUT: Steps in Qualitative Research
After the data is prepared for analysis, researchers are open to using different research and data analysis methods to derive meaningful insights. For sure, statistical analysis plans are the most favored to analyze numerical data. In statistical analysis, distinguishing between categorical data and numerical data is essential, as categorical data involves distinct categories or labels, while numerical data consists of measurable quantities. The method is again classified into two groups. First, ‘Descriptive Statistics’ used to describe data. Second, ‘Inferential statistics’ that helps in comparing the data .
Descriptive statistics
This method is used to describe the basic features of versatile types of data in research. It presents the data in such a meaningful way that pattern in the data starts making sense. Nevertheless, the descriptive analysis does not go beyond making conclusions. The conclusions are again based on the hypothesis researchers have formulated so far. Here are a few major types of descriptive analysis methods.
Measures of Frequency
- Count, Percent, Frequency
- It is used to denote home often a particular event occurs.
- Researchers use it when they want to showcase how often a response is given.
Measures of Central Tendency
- Mean, Median, Mode
- The method is widely used to demonstrate distribution by various points.
- Researchers use this method when they want to showcase the most commonly or averagely indicated response.
Measures of Dispersion or Variation
- Range, Variance, Standard deviation
- Here the field equals high/low points.
- Variance standard deviation = difference between the observed score and mean
- It is used to identify the spread of scores by stating intervals.
- Researchers use this method to showcase data spread out. It helps them identify the depth until which the data is spread out that it directly affects the mean.
Measures of Position
- Percentile ranks, Quartile ranks
- It relies on standardized scores helping researchers to identify the relationship between different scores.
- It is often used when researchers want to compare scores with the average count.
For quantitative research use of descriptive analysis often give absolute numbers, but the in-depth analysis is never sufficient to demonstrate the rationale behind those numbers. Nevertheless, it is necessary to think of the best method for research and data analysis suiting your survey questionnaire and what story researchers want to tell. For example, the mean is the best way to demonstrate the students’ average scores in schools. It is better to rely on the descriptive statistics when the researchers intend to keep the research or outcome limited to the provided sample without generalizing it. For example, when you want to compare average voting done in two different cities, differential statistics are enough.
Descriptive analysis is also called a ‘univariate analysis’ since it is commonly used to analyze a single variable.
Inferential statistics
Inferential statistics are used to make predictions about a larger population after research and data analysis of the representing population’s collected sample. For example, you can ask some odd 100 audiences at a movie theater if they like the movie they are watching. Researchers then use inferential statistics on the collected sample to reason that about 80-90% of people like the movie.
Here are two significant areas of inferential statistics.
- Estimating parameters: It takes statistics from the sample research data and demonstrates something about the population parameter.
- Hypothesis test: I t’s about sampling research data to answer the survey research questions. For example, researchers might be interested to understand if the new shade of lipstick recently launched is good or not, or if the multivitamin capsules help children to perform better at games.
These are sophisticated analysis methods used to showcase the relationship between different variables instead of describing a single variable. It is often used when researchers want something beyond absolute numbers to understand the relationship between variables.
Here are some of the commonly used methods for data analysis in research.
- Correlation: When researchers are not conducting experimental research or quasi-experimental research wherein the researchers are interested to understand the relationship between two or more variables, they opt for correlational research methods.
- Cross-tabulation: Also called contingency tables, cross-tabulation is used to analyze the relationship between multiple variables. Suppose provided data has age and gender categories presented in rows and columns. A two-dimensional cross-tabulation helps for seamless data analysis and research by showing the number of males and females in each age category.
- Regression analysis: For understanding the strong relationship between two variables, researchers do not look beyond the primary and commonly used regression analysis method, which is also a type of predictive analysis used. In this method, you have an essential factor called the dependent variable. You also have multiple independent variables in regression analysis. You undertake efforts to find out the impact of independent variables on the dependent variable. The values of both independent and dependent variables are assumed as being ascertained in an error-free random manner.
- Frequency tables: The statistical procedure is used for testing the degree to which two or more vary or differ in an experiment. A considerable degree of variation means research findings were significant. In many contexts, ANOVA testing and variance analysis are similar.
- Analysis of variance: The statistical procedure is used for testing the degree to which two or more vary or differ in an experiment. A considerable degree of variation means research findings were significant. In many contexts, ANOVA testing and variance analysis are similar.
- Researchers must have the necessary research skills to analyze and manipulation the data , Getting trained to demonstrate a high standard of research practice. Ideally, researchers must possess more than a basic understanding of the rationale of selecting one statistical method over the other to obtain better data insights.
- Usually, research and data analytics projects differ by scientific discipline; therefore, getting statistical advice at the beginning of analysis helps design a survey questionnaire, select data collection methods, and choose samples.
LEARN ABOUT: Best Data Collection Tools
- The primary aim of data research and analysis is to derive ultimate insights that are unbiased. Any mistake in or keeping a biased mind to collect data, selecting an analysis method, or choosing audience sample il to draw a biased inference.
- Irrelevant to the sophistication used in research data and analysis is enough to rectify the poorly defined objective outcome measurements. It does not matter if the design is at fault or intentions are not clear, but lack of clarity might mislead readers, so avoid the practice.
- The motive behind data analysis in research is to present accurate and reliable data. As far as possible, avoid statistical errors, and find a way to deal with everyday challenges like outliers, missing data, data altering, data mining , or developing graphical representation.
LEARN MORE: Descriptive Research vs Correlational Research The sheer amount of data generated daily is frightening. Especially when data analysis has taken center stage. in 2018. In last year, the total data supply amounted to 2.8 trillion gigabytes. Hence, it is clear that the enterprises willing to survive in the hypercompetitive world must possess an excellent capability to analyze complex research data, derive actionable insights, and adapt to the new market needs.
LEARN ABOUT: Average Order Value
QuestionPro is an online survey platform that empowers organizations in data analysis and research and provides them a medium to collect data by creating appealing surveys.
MORE LIKE THIS
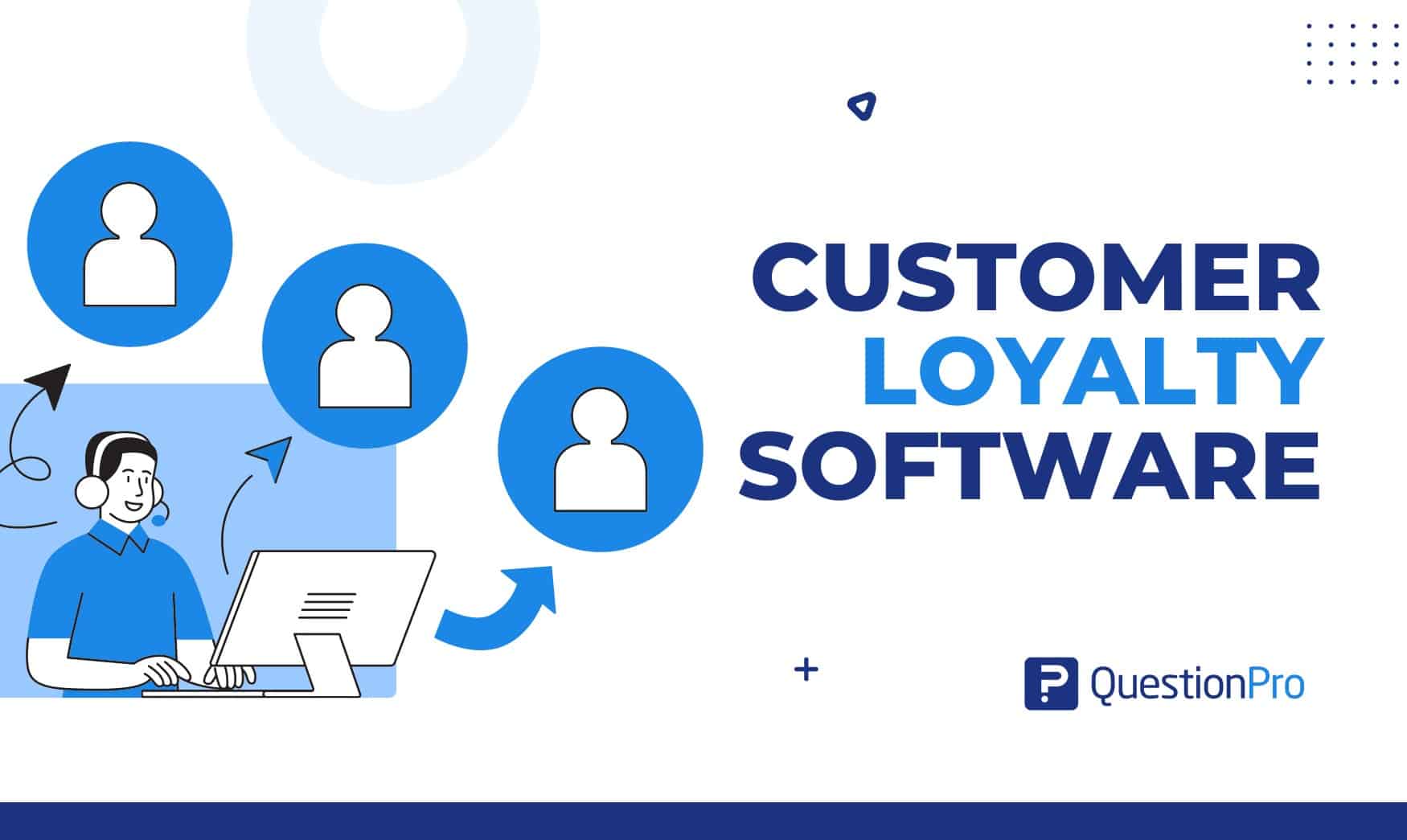
10 Top Customer Loyalty Software to Boost Your Business
Mar 25, 2024
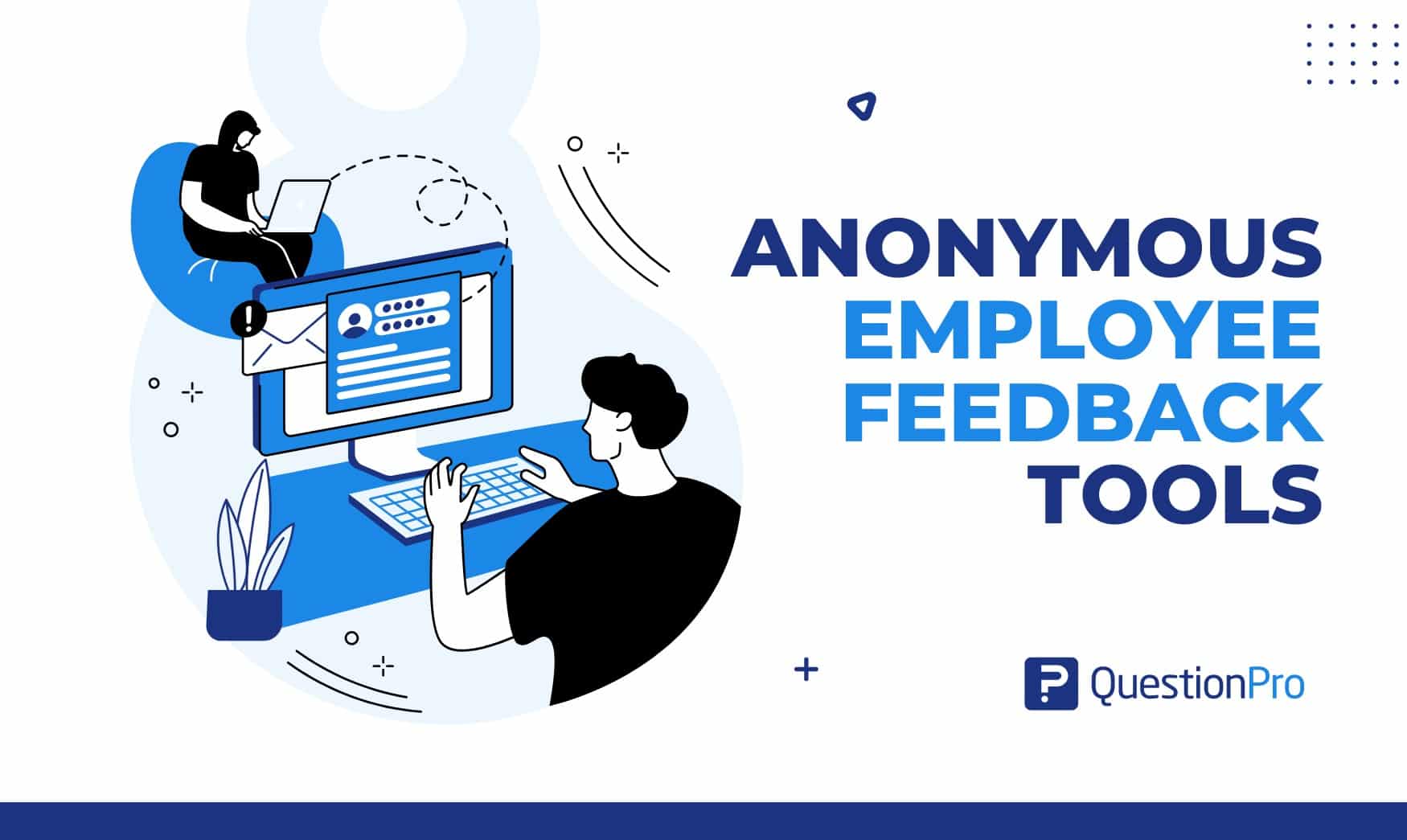
Top 13 Anonymous Employee Feedback Tools for 2024
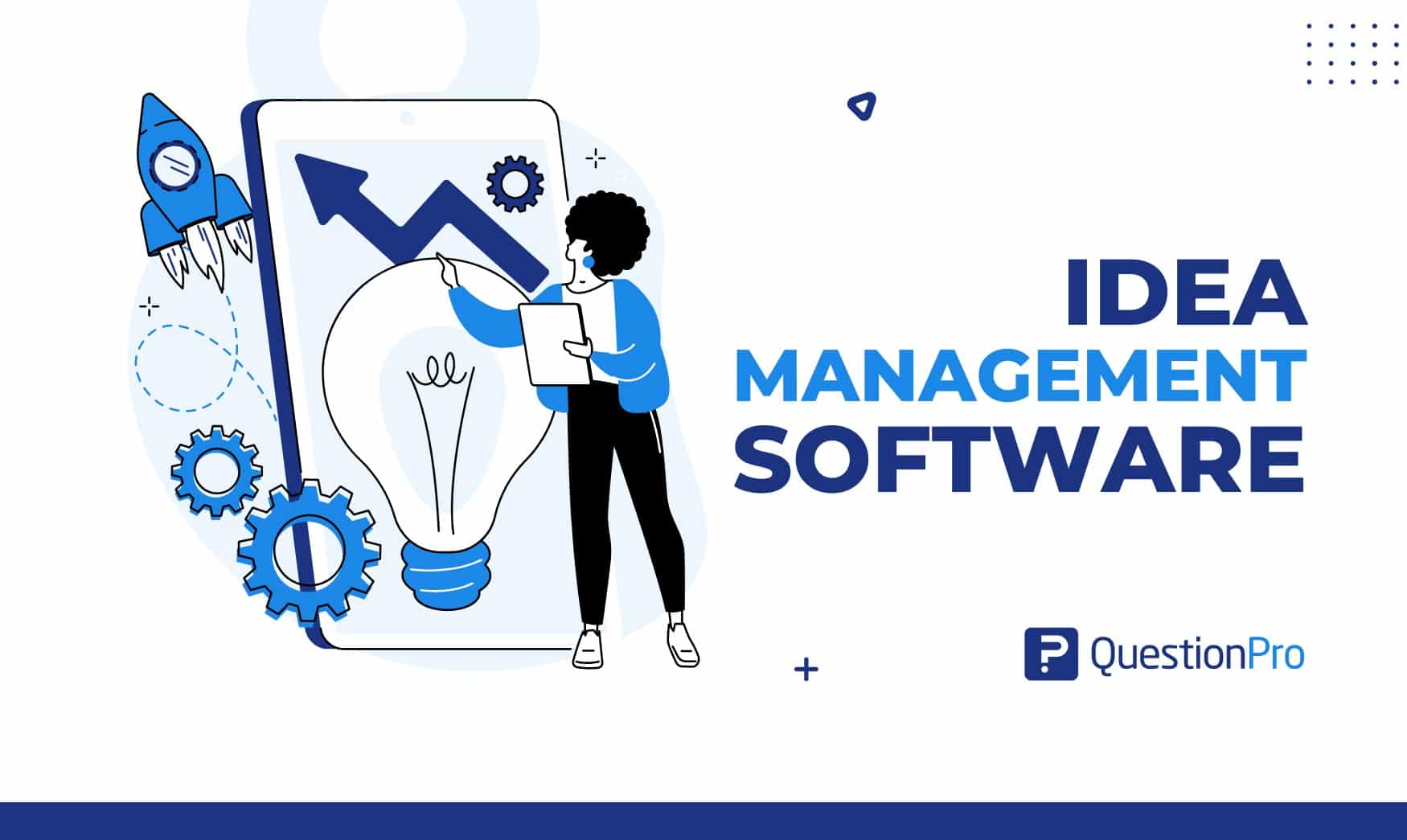
Unlocking Creativity With 10 Top Idea Management Software
Mar 23, 2024
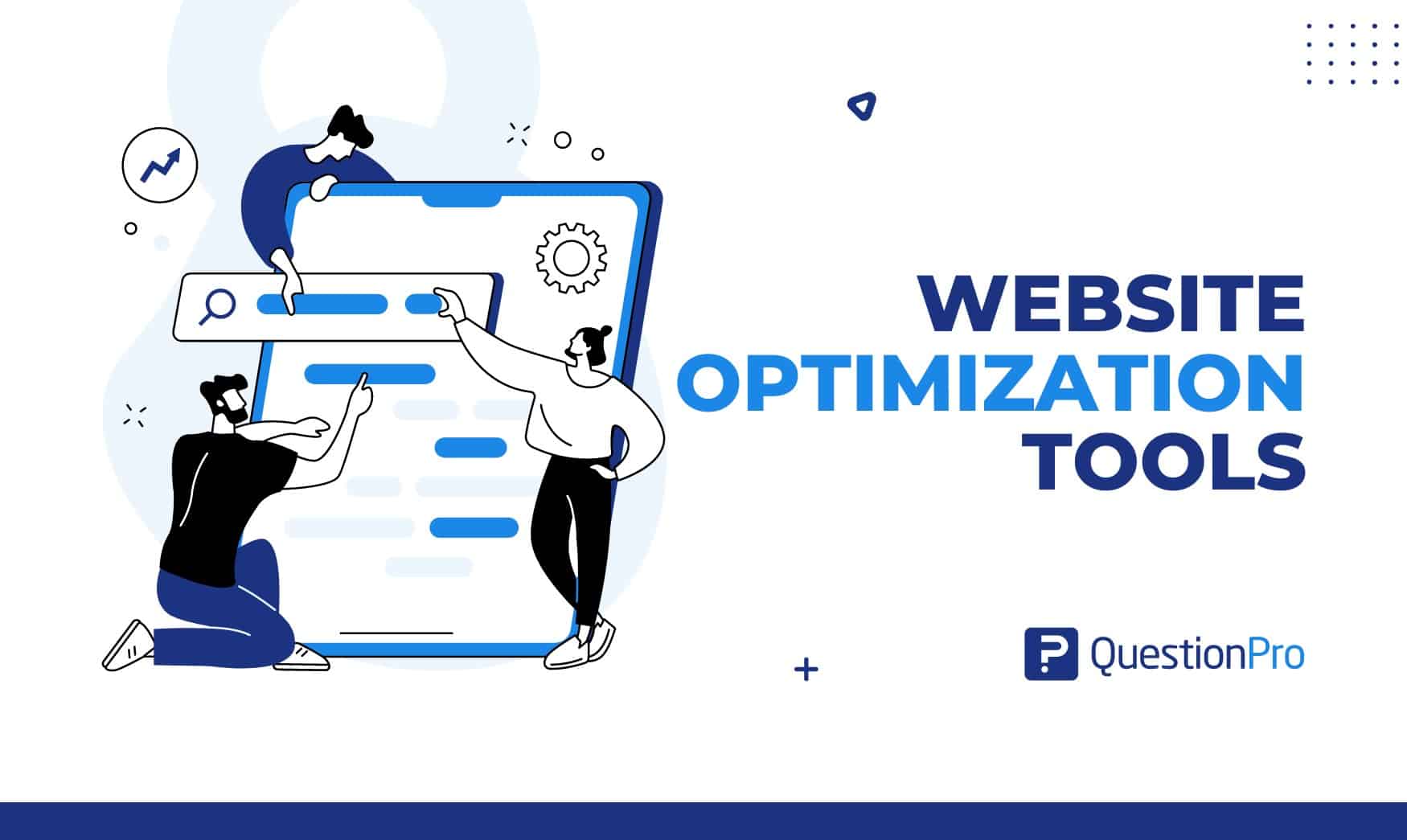
20 Best Website Optimization Tools to Improve Your Website
Mar 22, 2024
Other categories
- Academic Research
- Artificial Intelligence
- Assessments
- Brand Awareness
- Case Studies
- Communities
- Consumer Insights
- Customer effort score
- Customer Engagement
- Customer Experience
- Customer Loyalty
- Customer Research
- Customer Satisfaction
- Employee Benefits
- Employee Engagement
- Employee Retention
- Friday Five
- General Data Protection Regulation
- Insights Hub
- Life@QuestionPro
- Market Research
- Mobile diaries
- Mobile Surveys
- New Features
- Online Communities
- Question Types
- Questionnaire
- QuestionPro Products
- Release Notes
- Research Tools and Apps
- Revenue at Risk
- Survey Templates
- Training Tips
- Uncategorized
- Video Learning Series
- What’s Coming Up
- Workforce Intelligence
News alert: UC Berkeley has announced its next university librarian
Secondary menu
- Log in to your Library account
- Hours and Maps
- Connect from Off Campus
- UC Berkeley Home
Search form
Research methods--quantitative, qualitative, and more: overview.
- Quantitative Research
- Qualitative Research
- Data Science Methods (Machine Learning, AI, Big Data)
- Text Mining and Computational Text Analysis
- Evidence Synthesis/Systematic Reviews
- Get Data, Get Help!
About Research Methods
This guide provides an overview of research methods, how to choose and use them, and supports and resources at UC Berkeley.
As Patten and Newhart note in the book Understanding Research Methods , "Research methods are the building blocks of the scientific enterprise. They are the "how" for building systematic knowledge. The accumulation of knowledge through research is by its nature a collective endeavor. Each well-designed study provides evidence that may support, amend, refute, or deepen the understanding of existing knowledge...Decisions are important throughout the practice of research and are designed to help researchers collect evidence that includes the full spectrum of the phenomenon under study, to maintain logical rules, and to mitigate or account for possible sources of bias. In many ways, learning research methods is learning how to see and make these decisions."
The choice of methods varies by discipline, by the kind of phenomenon being studied and the data being used to study it, by the technology available, and more. This guide is an introduction, but if you don't see what you need here, always contact your subject librarian, and/or take a look to see if there's a library research guide that will answer your question.
Suggestions for changes and additions to this guide are welcome!
START HERE: SAGE Research Methods
Without question, the most comprehensive resource available from the library is SAGE Research Methods. HERE IS THE ONLINE GUIDE to this one-stop shopping collection, and some helpful links are below:
- SAGE Research Methods
- Little Green Books (Quantitative Methods)
- Little Blue Books (Qualitative Methods)
- Dictionaries and Encyclopedias
- Case studies of real research projects
- Sample datasets for hands-on practice
- Streaming video--see methods come to life
- Methodspace- -a community for researchers
- SAGE Research Methods Course Mapping
Library Data Services at UC Berkeley
Library Data Services Program and Digital Scholarship Services
The LDSP offers a variety of services and tools ! From this link, check out pages for each of the following topics: discovering data, managing data, collecting data, GIS data, text data mining, publishing data, digital scholarship, open science, and the Research Data Management Program.
Be sure also to check out the visual guide to where to seek assistance on campus with any research question you may have!
Library GIS Services
Other Data Services at Berkeley
D-Lab Supports Berkeley faculty, staff, and graduate students with research in data intensive social science, including a wide range of training and workshop offerings Dryad Dryad is a simple self-service tool for researchers to use in publishing their datasets. It provides tools for the effective publication of and access to research data. Geospatial Innovation Facility (GIF) Provides leadership and training across a broad array of integrated mapping technologies on campu Research Data Management A UC Berkeley guide and consulting service for research data management issues
General Research Methods Resources
Here are some general resources for assistance:
- Assistance from ICPSR (must create an account to access): Getting Help with Data , and Resources for Students
- Wiley Stats Ref for background information on statistics topics
- Survey Documentation and Analysis (SDA) . Program for easy web-based analysis of survey data.
Consultants
- D-Lab/Data Science Discovery Consultants Request help with your research project from peer consultants.
- Research data (RDM) consulting Meet with RDM consultants before designing the data security, storage, and sharing aspects of your qualitative project.
- Statistics Department Consulting Services A service in which advanced graduate students, under faculty supervision, are available to consult during specified hours in the Fall and Spring semesters.
Related Resourcex
- IRB / CPHS Qualitative research projects with human subjects often require that you go through an ethics review.
- OURS (Office of Undergraduate Research and Scholarships) OURS supports undergraduates who want to embark on research projects and assistantships. In particular, check out their "Getting Started in Research" workshops
- Sponsored Projects Sponsored projects works with researchers applying for major external grants.
- Next: Quantitative Research >>
- Last Updated: Apr 3, 2023 3:14 PM
- URL: https://guides.lib.berkeley.edu/researchmethods
- Skip to main content
- Keyboard shortcuts for audio player

- LISTEN & FOLLOW
- Apple Podcasts
- Google Podcasts
- Amazon Music
- Amazon Alexa
Your support helps make our show possible and unlocks access to our sponsor-free feed.
On eclipse day, hundreds of students will send up balloons for science

Geoff Brumfiel

Student volunteers prepare a balloon for a morning launch in Cumberland, Md. On April 8, eclipse day, hundreds of balloons will be launched into the path of the eclipse to study the atmosphere. Meredith Rizzo for NPR hide caption
Student volunteers prepare a balloon for a morning launch in Cumberland, Md. On April 8, eclipse day, hundreds of balloons will be launched into the path of the eclipse to study the atmosphere.
CUMBERLAND, Md. — It's a chilly March morning, and Mary Bowden is standing in the parking lot of a local community college.
Bowden is a professor of aerospace engineering at the University of Maryland. Nearby, her students are hustling around on a bright blue tarp, rolling out heavy cylinders of compressed gas and fiddling with boxes of electronics.
"This is our final, final dress rehearsal," Bowden says as she surveys the scene.
At the start of next month, a total solar eclipse will sweep across the continental United States. It will begin in Texas and move north through a dozen states before exiting the country through Maine and into Canada.

The eclipse gives astronomy clubs an opportunity to shine
On eclipse day — April 8 — dozens of student teams across the country will release hundreds of research balloons. The balloons will carry long, dangling strings of scientific instruments into the path of totality, the area on Earth's surface that will see the moon completely block the sun.
The effort, known as the Nationwide Eclipse Ballooning Project , is backed by NASA. It's an opportunity to make unique atmospheric measurements that can only be done during an eclipse, and a chance for students to learn skills they may someday use to launch satellites and astronauts into orbit. Bowden is guiding the University of Maryland team, which is made up of about 30 to 40 students.
"It's just a club," says Daniel Grammer, a junior who will be leading the team on eclipse day. "Everybody here volunteers to do it because they like to do it."
Floating laboratories
Deflated, the balloons look like giant party balloons. As they fill with helium, they begin to take shape — two white, upside-down teardrops bobbing gently in the spring air.

Saimah Siddiqui is a senior at the University of Maryland. She hopes her work on balloons will eventually lead to a career in mission control. Meredith Rizzo for NPR hide caption
Saimah Siddiqui is a senior at the University of Maryland. She hopes her work on balloons will eventually lead to a career in mission control.
Saimah Siddiqui is a senior and one of the "inflation leads" responsible for filling the balloons.
"Where are we at?" she asks as another student bends over the regulator of the helium tank. Siddiqui seems confident, and with good reason.
"I've done this so many times — this is probably my 30th launch or something," she says.
The scientific goal of this project is to study the atmosphere. As the eclipse shadow travels from south to north across the U.S., it briefly cools the air. Bowden says it's like dragging a swizzle stick through a cup of hot coffee.
"The eclipse itself is kind of stirring up the atmosphere as it traverses across the country," Bowden says. "What we're looking for is the signature, or the effect, of the movement of the shadow."

Balloons are a perfect way to train students: "It's a microcosm of a NASA launch, but cheap and fast — and you can do it again if you fail," says Mary Bowden. Meredith Rizzo for NPR hide caption
Balloons are a perfect way to train students: "It's a microcosm of a NASA launch, but cheap and fast — and you can do it again if you fail," says Mary Bowden.
The results will teach researchers more about how heat moves through the atmosphere. The data could be used to improve predictions of both weather and climate change.

Researchers watch and worry as balloons are blasted from the sky
When it comes to making these measurements, there's no better vehicle than a balloon . Unlike a rocket, balloons can drift gently in the eclipse zone for minutes or hours. And they travel at 75,000 to 80,000 feet in altitude, twice the altitude reached by a typical airliner.
Grammer says the view should be amazing.
"Hopefully we'll have livestream video from the balloon in flight," he says. "You'll see the shadow move across the Earth, and it'll be super cool to look at."
Runaway balloons
Things at the test launch appear to be going smoothly, but then, as Siddiqui begins to check her balloon to make sure it's got enough lifting power, it suddenly breaks loose and floats skyward.

Daniel Grammer, a junior, will be the flight director for the solar eclipse launch on April 8. "Everybody here volunteers to do it because they like to do it," he says. Meredith Rizzo for NPR hide caption
Daniel Grammer, a junior, will be the flight director for the solar eclipse launch on April 8. "Everybody here volunteers to do it because they like to do it," he says.
The whole team watches as one of their two balloons drifts slowly away.
Nobody panics. The students put their heads together to figure out what happened. It turns out they forgot to reset a device that is supposed to cut the string at the end of the flight. Normally, this allows the balloon to float away, and the scientific equipment to parachute back to the ground, where it can be recovered.
Instead, the device cut the balloon loose before the payload was even attached.
"I've never seen that happen!" Meredith Embrey says with a laugh. The junior is in charge of tying the scientific equipment to the balloon.
"The good thing is, we didn't lose the payload itself, and we always bring two spare balloons and double the amount of helium we need," Embrey says. "So we will start inflating and do another balloon."
It's a moment to learn from, and that's exactly the point.

Balloons are the perfect vehicle to study an eclipse. They fly higher than aircraft, and can stay in the eclipse zone longer than a sounding rocket. Meredith Rizzo for NPR hide caption
Balloons are the perfect vehicle to study an eclipse. They fly higher than aircraft, and can stay in the eclipse zone longer than a sounding rocket.
"The great thing about the program is that it's really both an education program and a research program," says Angela Des Jardins, a physicist at Montana State University and the principal investigator of the Nationwide Eclipse Ballooning Project.
Across the U.S., over 750 students making up 53 teams are participating in this project. The budding engineers are in charge of everything from scientific instruments to flight direction, weather forecasting, tracking stations, and more.

(Left) Jeremy Snyder, David Salako, and Rayne Wiser track the balloons from the ground. (Right) Launch Director Kruti Bhingradiya gives directions to the team. Meredith Rizzo for NPR hide caption
(Left) Jeremy Snyder, David Salako, and Rayne Wiser track the balloons from the ground. (Right) Launch Director Kruti Bhingradiya gives directions to the team.
"We're trying to give these students an opportunity outside the classroom," she says. The eclipse balloons are "a science project that gives them a little bit of a taste of what it would be like in the real world, working in an aerospace job."

Will you be celebrating the solar eclipse? NPR wants to hear from you
Balloons are a perfect prelude to launching rockets, says Bowden. "It's a microcosm of a NASA launch, but cheap and fast — and you can do it again if you fail."
Which is exactly what the team is doing now. With their string-cutting device fixed, they're racing to inflate another balloon. They've got to be quick because the wind is picking up. Siddiqui seems to love it — using engineering to solve problems on the fly, under pressure. She says she hopes to someday have a career launching rockets.
"Maybe like a flight controller/flight operator-type person for my full-time job," she muses as she watches the second balloon fill.
Meanwhile, Embrey and her fellow tie-on specialist Dan Gribok are doing final checks on the scientific instruments. They use red tape to close up the boxes that hold the cameras, measuring devices and transmitters.

Students ready their payloads ahead of the flight. The equipment includes cameras, tracking devices, and sensors to monitor conditions high above the Earth. Meredith Rizzo for NPR hide caption
Students ready their payloads ahead of the flight. The equipment includes cameras, tracking devices, and sensors to monitor conditions high above the Earth.
"Duct tape is an engineer's best friend, for sure," Grammer jokes as he looks on.
The radio crackles as the flight director, a spirited senior named Kruti Bhingradiya, calls out orders to the team.
"I hope you guys are ready, and if you're not, let me know right now," she says.
The payloads are tied to each balloon. Other students stand around, hands outstretched towards the floating spheres to make sure they don't bang into anything in the final moments before launch.
Bhingradiya calls for the launch area to be cleared of debris. Then she looks around. The team is ready.
"Three ... Two ... One ... Release!" she says.
And the students cheer as they watch their hard work drift off into the clouds.

A balloon floats skyward with scientific equipment in tow. On eclipse day dozens of teams will launch hundreds of balloons to study the atmosphere. Meredith Rizzo for NPR hide caption
A balloon floats skyward with scientific equipment in tow. On eclipse day dozens of teams will launch hundreds of balloons to study the atmosphere.
- eclipse science
- 2024 solar eclipse
- eclipse ballooning
- University of Maryland
- Share full article
Advertisement
Supported by
What the Data Says About Pandemic School Closures, Four Years Later
The more time students spent in remote instruction, the further they fell behind. And, experts say, extended closures did little to stop the spread of Covid.

By Sarah Mervosh , Claire Cain Miller and Francesca Paris
Four years ago this month, schools nationwide began to shut down, igniting one of the most polarizing and partisan debates of the pandemic.
Some schools, often in Republican-led states and rural areas, reopened by fall 2020. Others, typically in large cities and states led by Democrats, would not fully reopen for another year.
A variety of data — about children’s academic outcomes and about the spread of Covid-19 — has accumulated in the time since. Today, there is broad acknowledgment among many public health and education experts that extended school closures did not significantly stop the spread of Covid, while the academic harms for children have been large and long-lasting.
While poverty and other factors also played a role, remote learning was a key driver of academic declines during the pandemic, research shows — a finding that held true across income levels.
Source: Fahle, Kane, Patterson, Reardon, Staiger and Stuart, “ School District and Community Factors Associated With Learning Loss During the COVID-19 Pandemic .” Score changes are measured from 2019 to 2022. In-person means a district offered traditional in-person learning, even if not all students were in-person.
“There’s fairly good consensus that, in general, as a society, we probably kept kids out of school longer than we should have,” said Dr. Sean O’Leary, a pediatric infectious disease specialist who helped write guidance for the American Academy of Pediatrics, which recommended in June 2020 that schools reopen with safety measures in place.
There were no easy decisions at the time. Officials had to weigh the risks of an emerging virus against the academic and mental health consequences of closing schools. And even schools that reopened quickly, by the fall of 2020, have seen lasting effects.
But as experts plan for the next public health emergency, whatever it may be, a growing body of research shows that pandemic school closures came at a steep cost to students.
The longer schools were closed, the more students fell behind.
At the state level, more time spent in remote or hybrid instruction in the 2020-21 school year was associated with larger drops in test scores, according to a New York Times analysis of school closure data and results from the National Assessment of Educational Progress , an authoritative exam administered to a national sample of fourth- and eighth-grade students.
At the school district level, that finding also holds, according to an analysis of test scores from third through eighth grade in thousands of U.S. districts, led by researchers at Stanford and Harvard. In districts where students spent most of the 2020-21 school year learning remotely, they fell more than half a grade behind in math on average, while in districts that spent most of the year in person they lost just over a third of a grade.
( A separate study of nearly 10,000 schools found similar results.)
Such losses can be hard to overcome, without significant interventions. The most recent test scores, from spring 2023, show that students, overall, are not caught up from their pandemic losses , with larger gaps remaining among students that lost the most ground to begin with. Students in districts that were remote or hybrid the longest — at least 90 percent of the 2020-21 school year — still had almost double the ground to make up compared with students in districts that allowed students back for most of the year.
Some time in person was better than no time.
As districts shifted toward in-person learning as the year went on, students that were offered a hybrid schedule (a few hours or days a week in person, with the rest online) did better, on average, than those in places where school was fully remote, but worse than those in places that had school fully in person.
Students in hybrid or remote learning, 2020-21
80% of students
Some schools return online, as Covid-19 cases surge. Vaccinations start for high-priority groups.
Teachers are eligible for the Covid vaccine in more than half of states.
Most districts end the year in-person or hybrid.
Source: Burbio audit of more than 1,200 school districts representing 47 percent of U.S. K-12 enrollment. Note: Learning mode was defined based on the most in-person option available to students.
Income and family background also made a big difference.
A second factor associated with academic declines during the pandemic was a community’s poverty level. Comparing districts with similar remote learning policies, poorer districts had steeper losses.
But in-person learning still mattered: Looking at districts with similar poverty levels, remote learning was associated with greater declines.
A community’s poverty rate and the length of school closures had a “roughly equal” effect on student outcomes, said Sean F. Reardon, a professor of poverty and inequality in education at Stanford, who led a district-level analysis with Thomas J. Kane, an economist at Harvard.
Score changes are measured from 2019 to 2022. Poorest and richest are the top and bottom 20% of districts by percent of students on free/reduced lunch. Mostly in-person and mostly remote are districts that offered traditional in-person learning for more than 90 percent or less than 10 percent of the 2020-21 year.
But the combination — poverty and remote learning — was particularly harmful. For each week spent remote, students in poor districts experienced steeper losses in math than peers in richer districts.
That is notable, because poor districts were also more likely to stay remote for longer .
Some of the country’s largest poor districts are in Democratic-leaning cities that took a more cautious approach to the virus. Poor areas, and Black and Hispanic communities , also suffered higher Covid death rates, making many families and teachers in those districts hesitant to return.
“We wanted to survive,” said Sarah Carpenter, the executive director of Memphis Lift, a parent advocacy group in Memphis, where schools were closed until spring 2021 .
“But I also think, man, looking back, I wish our kids could have gone back to school much quicker,” she added, citing the academic effects.
Other things were also associated with worse student outcomes, including increased anxiety and depression among adults in children’s lives, and the overall restriction of social activity in a community, according to the Stanford and Harvard research .
Even short closures had long-term consequences for children.
While being in school was on average better for academic outcomes, it wasn’t a guarantee. Some districts that opened early, like those in Cherokee County, Ga., a suburb of Atlanta, and Hanover County, Va., lost significant learning and remain behind.
At the same time, many schools are seeing more anxiety and behavioral outbursts among students. And chronic absenteeism from school has surged across demographic groups .
These are signs, experts say, that even short-term closures, and the pandemic more broadly, had lasting effects on the culture of education.
“There was almost, in the Covid era, a sense of, ‘We give up, we’re just trying to keep body and soul together,’ and I think that was corrosive to the higher expectations of schools,” said Margaret Spellings, an education secretary under President George W. Bush who is now chief executive of the Bipartisan Policy Center.
Closing schools did not appear to significantly slow Covid’s spread.
Perhaps the biggest question that hung over school reopenings: Was it safe?
That was largely unknown in the spring of 2020, when schools first shut down. But several experts said that had changed by the fall of 2020, when there were initial signs that children were less likely to become seriously ill, and growing evidence from Europe and parts of the United States that opening schools, with safety measures, did not lead to significantly more transmission.
“Infectious disease leaders have generally agreed that school closures were not an important strategy in stemming the spread of Covid,” said Dr. Jeanne Noble, who directed the Covid response at the U.C.S.F. Parnassus emergency department.
Politically, though, there remains some disagreement about when, exactly, it was safe to reopen school.
Republican governors who pushed to open schools sooner have claimed credit for their approach, while Democrats and teachers’ unions have emphasized their commitment to safety and their investment in helping students recover.
“I do believe it was the right decision,” said Jerry T. Jordan, president of the Philadelphia Federation of Teachers, which resisted returning to school in person over concerns about the availability of vaccines and poor ventilation in school buildings. Philadelphia schools waited to partially reopen until the spring of 2021 , a decision Mr. Jordan believes saved lives.
“It doesn’t matter what is going on in the building and how much people are learning if people are getting the virus and running the potential of dying,” he said.
Pandemic school closures offer lessons for the future.
Though the next health crisis may have different particulars, with different risk calculations, the consequences of closing schools are now well established, experts say.
In the future, infectious disease experts said, they hoped decisions would be guided more by epidemiological data as it emerged, taking into account the trade-offs.
“Could we have used data to better guide our decision making? Yes,” said Dr. Uzma N. Hasan, division chief of pediatric infectious diseases at RWJBarnabas Health in Livingston, N.J. “Fear should not guide our decision making.”
Source: Fahle, Kane, Patterson, Reardon, Staiger and Stuart, “ School District and Community Factors Associated With Learning Loss During the Covid-19 Pandemic. ”
The study used estimates of learning loss from the Stanford Education Data Archive . For closure lengths, the study averaged district-level estimates of time spent in remote and hybrid learning compiled by the Covid-19 School Data Hub (C.S.D.H.) and American Enterprise Institute (A.E.I.) . The A.E.I. data defines remote status by whether there was an in-person or hybrid option, even if some students chose to remain virtual. In the C.S.D.H. data set, districts are defined as remote if “all or most” students were virtual.
An earlier version of this article misstated a job description of Dr. Jeanne Noble. She directed the Covid response at the U.C.S.F. Parnassus emergency department. She did not direct the Covid response for the University of California, San Francisco health system.
How we handle corrections
Sarah Mervosh covers education for The Times, focusing on K-12 schools. More about Sarah Mervosh
Claire Cain Miller writes about gender, families and the future of work for The Upshot. She joined The Times in 2008 and was part of a team that won a Pulitzer Prize in 2018 for public service for reporting on workplace sexual harassment issues. More about Claire Cain Miller
Francesca Paris is a Times reporter working with data and graphics for The Upshot. More about Francesca Paris
Read our research on: TikTok | Podcasts | Election 2024
Regions & Countries
What the data says about abortion in the u.s..
Pew Research Center has conducted many surveys about abortion over the years, providing a lens into Americans’ views on whether the procedure should be legal, among a host of other questions.
In a Center survey conducted nearly a year after the Supreme Court’s June 2022 decision that ended the constitutional right to abortion , 62% of U.S. adults said the practice should be legal in all or most cases, while 36% said it should be illegal in all or most cases. Another survey conducted a few months before the decision showed that relatively few Americans take an absolutist view on the issue .
Find answers to common questions about abortion in America, based on data from the Centers for Disease Control and Prevention (CDC) and the Guttmacher Institute, which have tracked these patterns for several decades:
How many abortions are there in the U.S. each year?
How has the number of abortions in the u.s. changed over time, what is the abortion rate among women in the u.s. how has it changed over time, what are the most common types of abortion, how many abortion providers are there in the u.s., and how has that number changed, what percentage of abortions are for women who live in a different state from the abortion provider, what are the demographics of women who have had abortions, when during pregnancy do most abortions occur, how often are there medical complications from abortion.
This compilation of data on abortion in the United States draws mainly from two sources: the Centers for Disease Control and Prevention (CDC) and the Guttmacher Institute, both of which have regularly compiled national abortion data for approximately half a century, and which collect their data in different ways.
The CDC data that is highlighted in this post comes from the agency’s “abortion surveillance” reports, which have been published annually since 1974 (and which have included data from 1969). Its figures from 1973 through 1996 include data from all 50 states, the District of Columbia and New York City – 52 “reporting areas” in all. Since 1997, the CDC’s totals have lacked data from some states (most notably California) for the years that those states did not report data to the agency. The four reporting areas that did not submit data to the CDC in 2021 – California, Maryland, New Hampshire and New Jersey – accounted for approximately 25% of all legal induced abortions in the U.S. in 2020, according to Guttmacher’s data. Most states, though, do have data in the reports, and the figures for the vast majority of them came from each state’s central health agency, while for some states, the figures came from hospitals and other medical facilities.
Discussion of CDC abortion data involving women’s state of residence, marital status, race, ethnicity, age, abortion history and the number of previous live births excludes the low share of abortions where that information was not supplied. Read the methodology for the CDC’s latest abortion surveillance report , which includes data from 2021, for more details. Previous reports can be found at stacks.cdc.gov by entering “abortion surveillance” into the search box.
For the numbers of deaths caused by induced abortions in 1963 and 1965, this analysis looks at reports by the then-U.S. Department of Health, Education and Welfare, a precursor to the Department of Health and Human Services. In computing those figures, we excluded abortions listed in the report under the categories “spontaneous or unspecified” or as “other.” (“Spontaneous abortion” is another way of referring to miscarriages.)
Guttmacher data in this post comes from national surveys of abortion providers that Guttmacher has conducted 19 times since 1973. Guttmacher compiles its figures after contacting every known provider of abortions – clinics, hospitals and physicians’ offices – in the country. It uses questionnaires and health department data, and it provides estimates for abortion providers that don’t respond to its inquiries. (In 2020, the last year for which it has released data on the number of abortions in the U.S., it used estimates for 12% of abortions.) For most of the 2000s, Guttmacher has conducted these national surveys every three years, each time getting abortion data for the prior two years. For each interim year, Guttmacher has calculated estimates based on trends from its own figures and from other data.
The latest full summary of Guttmacher data came in the institute’s report titled “Abortion Incidence and Service Availability in the United States, 2020.” It includes figures for 2020 and 2019 and estimates for 2018. The report includes a methods section.
In addition, this post uses data from StatPearls, an online health care resource, on complications from abortion.
An exact answer is hard to come by. The CDC and the Guttmacher Institute have each tried to measure this for around half a century, but they use different methods and publish different figures.
The last year for which the CDC reported a yearly national total for abortions is 2021. It found there were 625,978 abortions in the District of Columbia and the 46 states with available data that year, up from 597,355 in those states and D.C. in 2020. The corresponding figure for 2019 was 607,720.
The last year for which Guttmacher reported a yearly national total was 2020. It said there were 930,160 abortions that year in all 50 states and the District of Columbia, compared with 916,460 in 2019.
- How the CDC gets its data: It compiles figures that are voluntarily reported by states’ central health agencies, including separate figures for New York City and the District of Columbia. Its latest totals do not include figures from California, Maryland, New Hampshire or New Jersey, which did not report data to the CDC. ( Read the methodology from the latest CDC report .)
- How Guttmacher gets its data: It compiles its figures after contacting every known abortion provider – clinics, hospitals and physicians’ offices – in the country. It uses questionnaires and health department data, then provides estimates for abortion providers that don’t respond. Guttmacher’s figures are higher than the CDC’s in part because they include data (and in some instances, estimates) from all 50 states. ( Read the institute’s latest full report and methodology .)
While the Guttmacher Institute supports abortion rights, its empirical data on abortions in the U.S. has been widely cited by groups and publications across the political spectrum, including by a number of those that disagree with its positions .
These estimates from Guttmacher and the CDC are results of multiyear efforts to collect data on abortion across the U.S. Last year, Guttmacher also began publishing less precise estimates every few months , based on a much smaller sample of providers.
The figures reported by these organizations include only legal induced abortions conducted by clinics, hospitals or physicians’ offices, or those that make use of abortion pills dispensed from certified facilities such as clinics or physicians’ offices. They do not account for the use of abortion pills that were obtained outside of clinical settings .
(Back to top)
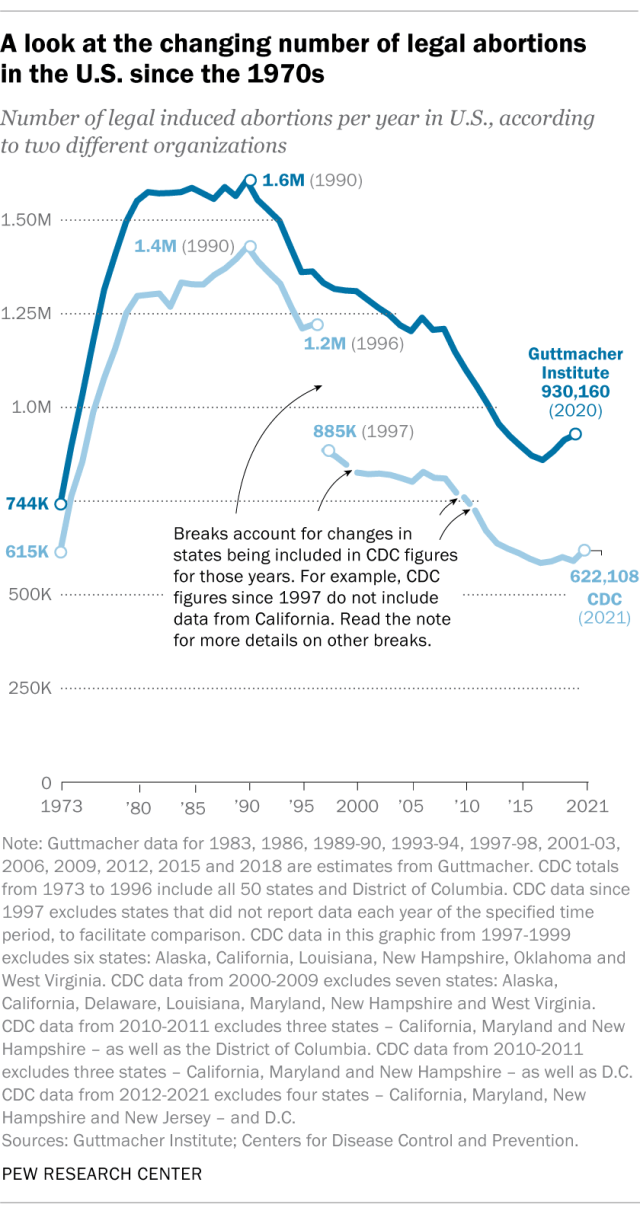
The annual number of U.S. abortions rose for years after Roe v. Wade legalized the procedure in 1973, reaching its highest levels around the late 1980s and early 1990s, according to both the CDC and Guttmacher. Since then, abortions have generally decreased at what a CDC analysis called “a slow yet steady pace.”
Guttmacher says the number of abortions occurring in the U.S. in 2020 was 40% lower than it was in 1991. According to the CDC, the number was 36% lower in 2021 than in 1991, looking just at the District of Columbia and the 46 states that reported both of those years.
(The corresponding line graph shows the long-term trend in the number of legal abortions reported by both organizations. To allow for consistent comparisons over time, the CDC figures in the chart have been adjusted to ensure that the same states are counted from one year to the next. Using that approach, the CDC figure for 2021 is 622,108 legal abortions.)
There have been occasional breaks in this long-term pattern of decline – during the middle of the first decade of the 2000s, and then again in the late 2010s. The CDC reported modest 1% and 2% increases in abortions in 2018 and 2019, and then, after a 2% decrease in 2020, a 5% increase in 2021. Guttmacher reported an 8% increase over the three-year period from 2017 to 2020.
As noted above, these figures do not include abortions that use pills obtained outside of clinical settings.
Guttmacher says that in 2020 there were 14.4 abortions in the U.S. per 1,000 women ages 15 to 44. Its data shows that the rate of abortions among women has generally been declining in the U.S. since 1981, when it reported there were 29.3 abortions per 1,000 women in that age range.
The CDC says that in 2021, there were 11.6 abortions in the U.S. per 1,000 women ages 15 to 44. (That figure excludes data from California, the District of Columbia, Maryland, New Hampshire and New Jersey.) Like Guttmacher’s data, the CDC’s figures also suggest a general decline in the abortion rate over time. In 1980, when the CDC reported on all 50 states and D.C., it said there were 25 abortions per 1,000 women ages 15 to 44.
That said, both Guttmacher and the CDC say there were slight increases in the rate of abortions during the late 2010s and early 2020s. Guttmacher says the abortion rate per 1,000 women ages 15 to 44 rose from 13.5 in 2017 to 14.4 in 2020. The CDC says it rose from 11.2 per 1,000 in 2017 to 11.4 in 2019, before falling back to 11.1 in 2020 and then rising again to 11.6 in 2021. (The CDC’s figures for those years exclude data from California, D.C., Maryland, New Hampshire and New Jersey.)
The CDC broadly divides abortions into two categories: surgical abortions and medication abortions, which involve pills. Since the Food and Drug Administration first approved abortion pills in 2000, their use has increased over time as a share of abortions nationally, according to both the CDC and Guttmacher.
The majority of abortions in the U.S. now involve pills, according to both the CDC and Guttmacher. The CDC says 56% of U.S. abortions in 2021 involved pills, up from 53% in 2020 and 44% in 2019. Its figures for 2021 include the District of Columbia and 44 states that provided this data; its figures for 2020 include D.C. and 44 states (though not all of the same states as in 2021), and its figures for 2019 include D.C. and 45 states.
Guttmacher, which measures this every three years, says 53% of U.S. abortions involved pills in 2020, up from 39% in 2017.
Two pills commonly used together for medication abortions are mifepristone, which, taken first, blocks hormones that support a pregnancy, and misoprostol, which then causes the uterus to empty. According to the FDA, medication abortions are safe until 10 weeks into pregnancy.
Surgical abortions conducted during the first trimester of pregnancy typically use a suction process, while the relatively few surgical abortions that occur during the second trimester of a pregnancy typically use a process called dilation and evacuation, according to the UCLA School of Medicine.
In 2020, there were 1,603 facilities in the U.S. that provided abortions, according to Guttmacher . This included 807 clinics, 530 hospitals and 266 physicians’ offices.
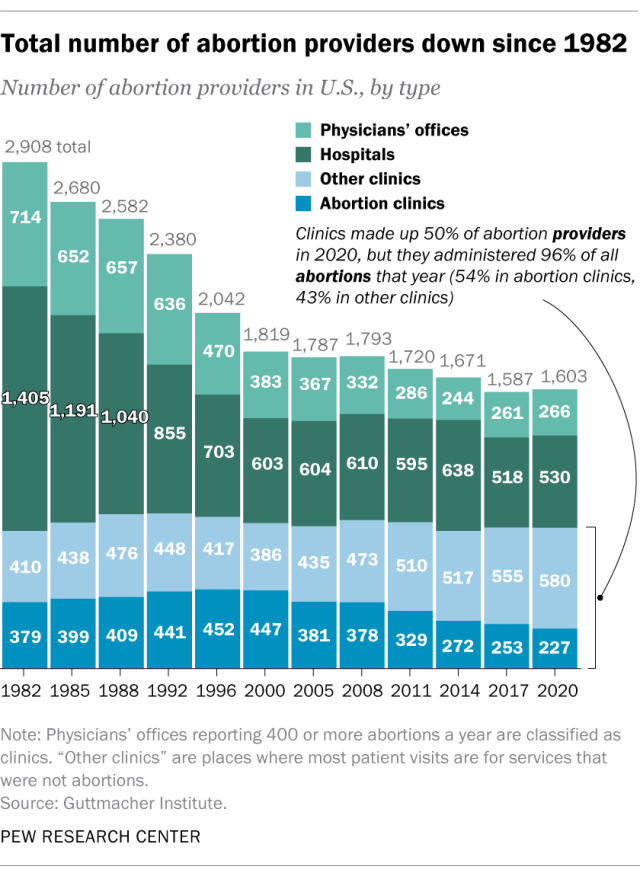
While clinics make up half of the facilities that provide abortions, they are the sites where the vast majority (96%) of abortions are administered, either through procedures or the distribution of pills, according to Guttmacher’s 2020 data. (This includes 54% of abortions that are administered at specialized abortion clinics and 43% at nonspecialized clinics.) Hospitals made up 33% of the facilities that provided abortions in 2020 but accounted for only 3% of abortions that year, while just 1% of abortions were conducted by physicians’ offices.
Looking just at clinics – that is, the total number of specialized abortion clinics and nonspecialized clinics in the U.S. – Guttmacher found the total virtually unchanged between 2017 (808 clinics) and 2020 (807 clinics). However, there were regional differences. In the Midwest, the number of clinics that provide abortions increased by 11% during those years, and in the West by 6%. The number of clinics decreased during those years by 9% in the Northeast and 3% in the South.
The total number of abortion providers has declined dramatically since the 1980s. In 1982, according to Guttmacher, there were 2,908 facilities providing abortions in the U.S., including 789 clinics, 1,405 hospitals and 714 physicians’ offices.
The CDC does not track the number of abortion providers.
In the District of Columbia and the 46 states that provided abortion and residency information to the CDC in 2021, 10.9% of all abortions were performed on women known to live outside the state where the abortion occurred – slightly higher than the percentage in 2020 (9.7%). That year, D.C. and 46 states (though not the same ones as in 2021) reported abortion and residency data. (The total number of abortions used in these calculations included figures for women with both known and unknown residential status.)
The share of reported abortions performed on women outside their state of residence was much higher before the 1973 Roe decision that stopped states from banning abortion. In 1972, 41% of all abortions in D.C. and the 20 states that provided this information to the CDC that year were performed on women outside their state of residence. In 1973, the corresponding figure was 21% in the District of Columbia and the 41 states that provided this information, and in 1974 it was 11% in D.C. and the 43 states that provided data.
In the District of Columbia and the 46 states that reported age data to the CDC in 2021, the majority of women who had abortions (57%) were in their 20s, while about three-in-ten (31%) were in their 30s. Teens ages 13 to 19 accounted for 8% of those who had abortions, while women ages 40 to 44 accounted for about 4%.
The vast majority of women who had abortions in 2021 were unmarried (87%), while married women accounted for 13%, according to the CDC , which had data on this from 37 states.
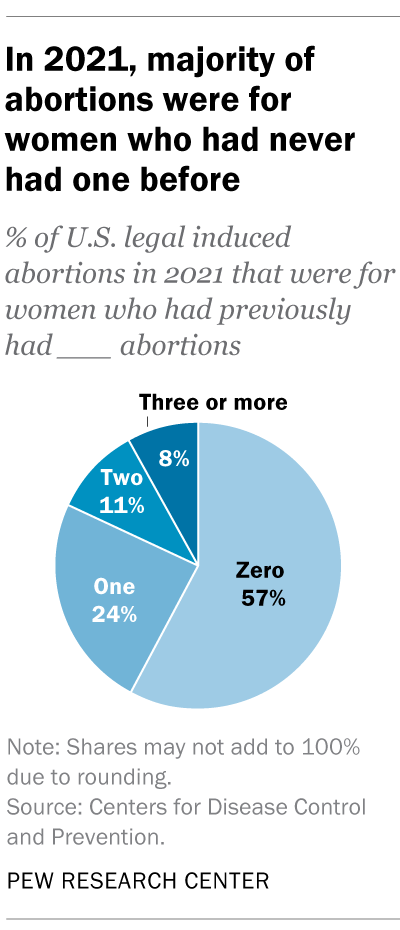
In the District of Columbia, New York City (but not the rest of New York) and the 31 states that reported racial and ethnic data on abortion to the CDC , 42% of all women who had abortions in 2021 were non-Hispanic Black, while 30% were non-Hispanic White, 22% were Hispanic and 6% were of other races.
Looking at abortion rates among those ages 15 to 44, there were 28.6 abortions per 1,000 non-Hispanic Black women in 2021; 12.3 abortions per 1,000 Hispanic women; 6.4 abortions per 1,000 non-Hispanic White women; and 9.2 abortions per 1,000 women of other races, the CDC reported from those same 31 states, D.C. and New York City.
For 57% of U.S. women who had induced abortions in 2021, it was the first time they had ever had one, according to the CDC. For nearly a quarter (24%), it was their second abortion. For 11% of women who had an abortion that year, it was their third, and for 8% it was their fourth or more. These CDC figures include data from 41 states and New York City, but not the rest of New York.
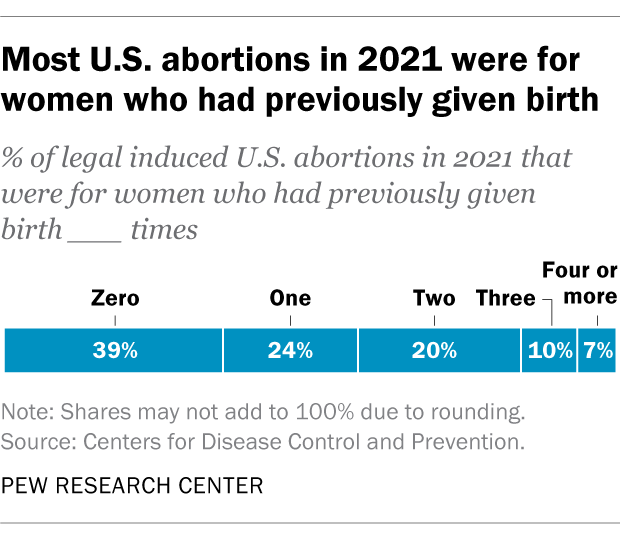
Nearly four-in-ten women who had abortions in 2021 (39%) had no previous live births at the time they had an abortion, according to the CDC . Almost a quarter (24%) of women who had abortions in 2021 had one previous live birth, 20% had two previous live births, 10% had three, and 7% had four or more previous live births. These CDC figures include data from 41 states and New York City, but not the rest of New York.
The vast majority of abortions occur during the first trimester of a pregnancy. In 2021, 93% of abortions occurred during the first trimester – that is, at or before 13 weeks of gestation, according to the CDC . An additional 6% occurred between 14 and 20 weeks of pregnancy, and about 1% were performed at 21 weeks or more of gestation. These CDC figures include data from 40 states and New York City, but not the rest of New York.
About 2% of all abortions in the U.S. involve some type of complication for the woman , according to an article in StatPearls, an online health care resource. “Most complications are considered minor such as pain, bleeding, infection and post-anesthesia complications,” according to the article.
The CDC calculates case-fatality rates for women from induced abortions – that is, how many women die from abortion-related complications, for every 100,000 legal abortions that occur in the U.S . The rate was lowest during the most recent period examined by the agency (2013 to 2020), when there were 0.45 deaths to women per 100,000 legal induced abortions. The case-fatality rate reported by the CDC was highest during the first period examined by the agency (1973 to 1977), when it was 2.09 deaths to women per 100,000 legal induced abortions. During the five-year periods in between, the figure ranged from 0.52 (from 1993 to 1997) to 0.78 (from 1978 to 1982).
The CDC calculates death rates by five-year and seven-year periods because of year-to-year fluctuation in the numbers and due to the relatively low number of women who die from legal induced abortions.
In 2020, the last year for which the CDC has information , six women in the U.S. died due to complications from induced abortions. Four women died in this way in 2019, two in 2018, and three in 2017. (These deaths all followed legal abortions.) Since 1990, the annual number of deaths among women due to legal induced abortion has ranged from two to 12.
The annual number of reported deaths from induced abortions (legal and illegal) tended to be higher in the 1980s, when it ranged from nine to 16, and from 1972 to 1979, when it ranged from 13 to 63. One driver of the decline was the drop in deaths from illegal abortions. There were 39 deaths from illegal abortions in 1972, the last full year before Roe v. Wade. The total fell to 19 in 1973 and to single digits or zero every year after that. (The number of deaths from legal abortions has also declined since then, though with some slight variation over time.)
The number of deaths from induced abortions was considerably higher in the 1960s than afterward. For instance, there were 119 deaths from induced abortions in 1963 and 99 in 1965 , according to reports by the then-U.S. Department of Health, Education and Welfare, a precursor to the Department of Health and Human Services. The CDC is a division of Health and Human Services.
Note: This is an update of a post originally published May 27, 2022, and first updated June 24, 2022.
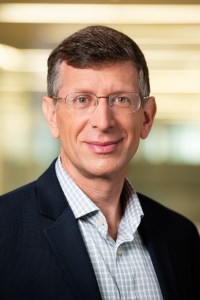
Sign up for our weekly newsletter
Fresh data delivered Saturday mornings
Key facts about the abortion debate in America
Public opinion on abortion, three-in-ten or more democrats and republicans don’t agree with their party on abortion, partisanship a bigger factor than geography in views of abortion access locally, do state laws on abortion reflect public opinion, most popular.
About Pew Research Center Pew Research Center is a nonpartisan fact tank that informs the public about the issues, attitudes and trends shaping the world. It conducts public opinion polling, demographic research, media content analysis and other empirical social science research. Pew Research Center does not take policy positions. It is a subsidiary of The Pew Charitable Trusts .
Have a language expert improve your writing
Run a free plagiarism check in 10 minutes, generate accurate citations for free.
- Knowledge Base
Methodology
- What Is Qualitative Research? | Methods & Examples
What Is Qualitative Research? | Methods & Examples
Published on June 19, 2020 by Pritha Bhandari . Revised on June 22, 2023.
Qualitative research involves collecting and analyzing non-numerical data (e.g., text, video, or audio) to understand concepts, opinions, or experiences. It can be used to gather in-depth insights into a problem or generate new ideas for research.
Qualitative research is the opposite of quantitative research , which involves collecting and analyzing numerical data for statistical analysis.
Qualitative research is commonly used in the humanities and social sciences, in subjects such as anthropology, sociology, education, health sciences, history, etc.
- How does social media shape body image in teenagers?
- How do children and adults interpret healthy eating in the UK?
- What factors influence employee retention in a large organization?
- How is anxiety experienced around the world?
- How can teachers integrate social issues into science curriculums?
Table of contents
Approaches to qualitative research, qualitative research methods, qualitative data analysis, advantages of qualitative research, disadvantages of qualitative research, other interesting articles, frequently asked questions about qualitative research.
Qualitative research is used to understand how people experience the world. While there are many approaches to qualitative research, they tend to be flexible and focus on retaining rich meaning when interpreting data.
Common approaches include grounded theory, ethnography , action research , phenomenological research, and narrative research. They share some similarities, but emphasize different aims and perspectives.
Note that qualitative research is at risk for certain research biases including the Hawthorne effect , observer bias , recall bias , and social desirability bias . While not always totally avoidable, awareness of potential biases as you collect and analyze your data can prevent them from impacting your work too much.
Prevent plagiarism. Run a free check.
Each of the research approaches involve using one or more data collection methods . These are some of the most common qualitative methods:
- Observations: recording what you have seen, heard, or encountered in detailed field notes.
- Interviews: personally asking people questions in one-on-one conversations.
- Focus groups: asking questions and generating discussion among a group of people.
- Surveys : distributing questionnaires with open-ended questions.
- Secondary research: collecting existing data in the form of texts, images, audio or video recordings, etc.
- You take field notes with observations and reflect on your own experiences of the company culture.
- You distribute open-ended surveys to employees across all the company’s offices by email to find out if the culture varies across locations.
- You conduct in-depth interviews with employees in your office to learn about their experiences and perspectives in greater detail.
Qualitative researchers often consider themselves “instruments” in research because all observations, interpretations and analyses are filtered through their own personal lens.
For this reason, when writing up your methodology for qualitative research, it’s important to reflect on your approach and to thoroughly explain the choices you made in collecting and analyzing the data.
Qualitative data can take the form of texts, photos, videos and audio. For example, you might be working with interview transcripts, survey responses, fieldnotes, or recordings from natural settings.
Most types of qualitative data analysis share the same five steps:
- Prepare and organize your data. This may mean transcribing interviews or typing up fieldnotes.
- Review and explore your data. Examine the data for patterns or repeated ideas that emerge.
- Develop a data coding system. Based on your initial ideas, establish a set of codes that you can apply to categorize your data.
- Assign codes to the data. For example, in qualitative survey analysis, this may mean going through each participant’s responses and tagging them with codes in a spreadsheet. As you go through your data, you can create new codes to add to your system if necessary.
- Identify recurring themes. Link codes together into cohesive, overarching themes.
There are several specific approaches to analyzing qualitative data. Although these methods share similar processes, they emphasize different concepts.
Qualitative research often tries to preserve the voice and perspective of participants and can be adjusted as new research questions arise. Qualitative research is good for:
- Flexibility
The data collection and analysis process can be adapted as new ideas or patterns emerge. They are not rigidly decided beforehand.
- Natural settings
Data collection occurs in real-world contexts or in naturalistic ways.
- Meaningful insights
Detailed descriptions of people’s experiences, feelings and perceptions can be used in designing, testing or improving systems or products.
- Generation of new ideas
Open-ended responses mean that researchers can uncover novel problems or opportunities that they wouldn’t have thought of otherwise.
Researchers must consider practical and theoretical limitations in analyzing and interpreting their data. Qualitative research suffers from:
- Unreliability
The real-world setting often makes qualitative research unreliable because of uncontrolled factors that affect the data.
- Subjectivity
Due to the researcher’s primary role in analyzing and interpreting data, qualitative research cannot be replicated . The researcher decides what is important and what is irrelevant in data analysis, so interpretations of the same data can vary greatly.
- Limited generalizability
Small samples are often used to gather detailed data about specific contexts. Despite rigorous analysis procedures, it is difficult to draw generalizable conclusions because the data may be biased and unrepresentative of the wider population .
- Labor-intensive
Although software can be used to manage and record large amounts of text, data analysis often has to be checked or performed manually.
If you want to know more about statistics , methodology , or research bias , make sure to check out some of our other articles with explanations and examples.
- Chi square goodness of fit test
- Degrees of freedom
- Null hypothesis
- Discourse analysis
- Control groups
- Mixed methods research
- Non-probability sampling
- Quantitative research
- Inclusion and exclusion criteria
Research bias
- Rosenthal effect
- Implicit bias
- Cognitive bias
- Selection bias
- Negativity bias
- Status quo bias
Quantitative research deals with numbers and statistics, while qualitative research deals with words and meanings.
Quantitative methods allow you to systematically measure variables and test hypotheses . Qualitative methods allow you to explore concepts and experiences in more detail.
There are five common approaches to qualitative research :
- Grounded theory involves collecting data in order to develop new theories.
- Ethnography involves immersing yourself in a group or organization to understand its culture.
- Narrative research involves interpreting stories to understand how people make sense of their experiences and perceptions.
- Phenomenological research involves investigating phenomena through people’s lived experiences.
- Action research links theory and practice in several cycles to drive innovative changes.
Data collection is the systematic process by which observations or measurements are gathered in research. It is used in many different contexts by academics, governments, businesses, and other organizations.
There are various approaches to qualitative data analysis , but they all share five steps in common:
- Prepare and organize your data.
- Review and explore your data.
- Develop a data coding system.
- Assign codes to the data.
- Identify recurring themes.
The specifics of each step depend on the focus of the analysis. Some common approaches include textual analysis , thematic analysis , and discourse analysis .
Cite this Scribbr article
If you want to cite this source, you can copy and paste the citation or click the “Cite this Scribbr article” button to automatically add the citation to our free Citation Generator.
Bhandari, P. (2023, June 22). What Is Qualitative Research? | Methods & Examples. Scribbr. Retrieved March 25, 2024, from https://www.scribbr.com/methodology/qualitative-research/
Is this article helpful?
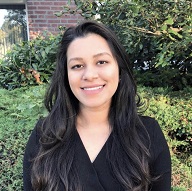
Pritha Bhandari
Other students also liked, qualitative vs. quantitative research | differences, examples & methods, how to do thematic analysis | step-by-step guide & examples, "i thought ai proofreading was useless but..".
I've been using Scribbr for years now and I know it's a service that won't disappoint. It does a good job spotting mistakes”
Mobile Menu Overlay
The White House 1600 Pennsylvania Ave NW Washington, DC 20500
FACT SHEET: President Biden Issues Executive Order and Announces New Actions to Advance Women’s Health Research and Innovation
In his State of the Union address, President Biden laid out his vision for transforming women’s health research and improving women’s lives all across America. The President called on Congress to make a bold, transformative investment of $12 billion in new funding for women’s health research. This investment would be used to create a Fund for Women’s Health Research at the National Institutes of Health (NIH) to advance a cutting-edge, interdisciplinary research agenda and to establish a new nationwide network of research centers of excellence and innovation in women’s health—which would serve as a national gold standard for women’s health research across the lifespan.
It is long past time to ensure women get the answers they need when it comes to their health—from cardiovascular disease to autoimmune diseases to menopause-related conditions. To pioneer the next generation of discoveries, the President and the First Lady launched the first-ever White House Initiative on Women’s Health Research , which aims to fundamentally change how we approach and fund women’s health research in the United States.
Today, President Biden is signing a new Executive Order that will direct the most comprehensive set of executive actions ever taken to expand and improve research on women’s health. These directives will ensure women’s health is integrated and prioritized across the federal research portfolio and budget, and will galvanize new research on a wide range of topics, including women’s midlife health.
The President and First Lady are also announcing more than twenty new actions and commitments by federal agencies, including through the U.S. Department of Health and Human Services (HHS), the Department of Defense (DoD), the Department of Veterans Affairs (VA), and the National Science Foundation (NSF). This includes the launch of a new NIH-wide effort that will direct key investments of $200 million in Fiscal Year 2025 to fund new, interdisciplinary women’s health research—a first step towards the transformative central Fund on Women’s Health that the President has called on Congress to invest in. These actions also build on the First Lady’s announcement last month of the Advanced Research Projects Agency for Health (ARPA-H) Sprint for Women’s Health , which committed $100 million towards transformative research and development in women’s health.
Today, the President is issuing an Executive Order that will:
- Integrate Women’s Health Across the Federal Research Portfolio . The Executive Order directs the Initiative’s constituent agencies to develop and strengthen research and data standards on women’s health across all relevant research and funding opportunities, with the goal of helping ensure that the Administration is better leveraging every dollar of federal funding for health research to improve women’s health. These actions will build on the NIH’s current policy to ensure that research it funds considers women’s health in the development of study design and in data collection and analysis. Agencies will take action to ensure women’s health is being considered at every step in the research process—from the applications that prospective grantees submit to the way that they report on grant implementation.
- Prioritize Investments in Women’s Health Research . The Executive Order directs the Initiative’s constituent agencies to prioritize funding for women’s health research and encourage innovation in women’s health, including through ARPA-H and multi-agency initiatives such as the Small Business Innovation Research Program and the Small Business Technology Transfer Program. These entities are dedicated to high-impact research and innovation, including through the support of early-stage small businesses and entrepreneurs engaged in research and innovation. The Executive Order further directs HHS and NSF to study ways to leverage artificial intelligence to advance women’s health research. These additional investments—across a wide range of agencies—will support innovation and open new doors to breakthroughs in women’s health.
- Galvanize New Research on Women’s Midlife Health . To narrow research gaps on diseases and conditions associated with women’s midlife health or that are more likely to occur after menopause, such as rheumatoid arthritis, heart attack, and osteoporosis, the President is directing HHS to: expand data collection efforts related to women’s midlife health; launch a comprehensive research agenda that will guide future investments in menopause-related research; identify ways to improve management of menopause-related issues and the clinical care that women receive; and develop new resources to help women better understand their options for menopause-related symptoms prevention and treatment. The Executive Order also directs the DoD and VA to study and take steps to improve the treatment of, and research related to, menopause for Service women and women veterans.
- Assess Unmet Needs to Support Women’s Health Research . The Executive Order directs the Office of Management and Budget and the Gender Policy Council to lead a robust effort to assess gaps in federal funding for women’s health research and identify changes—whether statutory, regulatory, or budgetary—that are needed to maximally support the broad scope of women’s health research across the federal government. Agencies will also be required to report annually on their investments in women’s health research, as well as progress towards their efforts to improve women’s health.
Today, agencies are also announcing new actions they are taking to promote women’s health research , as part of their ongoing efforts through the White House Initiative on Women’s Health Research. Agencies are announcing actions to:
Prioritize and Increase Investments in Women’s Health Research
- Launch an NIH-Cross Cutting Effort to Transform Women’s Health Throughout the Lifespan. NIH is launching an NIH-wide effort to close gaps in women’s health research across the lifespan. This effort—which will initially be supported by $200 million from NIH beginning in FY 2025—will allow NIH to catalyze interdisciplinary research, particularly on issues that cut across the traditional mandates of the institutes and centers at NIH. It will also allow NIH to launch ambitious, multi-faceted research projects such as research on the impact of perimenopause and menopause on heart health, brain health and bone health. In addition, the President’s FY25 Budget Request would double current funding for the NIH Office of Research on Women’s Health to support new and existing initiatives that emphasize women’s health research.
This coordinated, NIH-wide effort will be co-chaired by the NIH Office of the Director, the Office of Research on Women’s Health, and the institute directors from the National Institute on Aging; the National Heart, Lung, and Blood Institute; the National Institute on Drug Abuse; the Eunice Kennedy Shriver National Institute of Child Health and Human Development; the National Institute on Arthritis, Musculoskeletal and Skin Diseases.
- Invest in Research on a Wide Range of Women’s Health Issues. The bipartisan Congressionally Directed Medical Research Program (CDMRP), led out of DoD, funds research on women’s health encompassing a range of diseases and conditions that affect women uniquely, disproportionately, or differently from men. While the programs and topic areas directed by Congress differ each year, CDMRP has consistently funded research to advance women’s health since its creation in 1993. In Fiscal Year 2022, DoD implemented nearly $490 million in CDMRP investments towards women’s health research projects ranging from breast and ovarian cancer to lupus to orthotics and prosthetics in women. In Fiscal Year 2023, DoD anticipates implementing approximately $500 million in CDMRP funding for women’s health research, including in endometriosis, rheumatoid arthritis, and chronic fatigue.
- Call for New Proposals on Emerging Women’s Health Issues . Today, NSF is calling for new research and education proposals to advance discoveries and innovations related to women’s health. To promote multidisciplinary solutions to women’s health disparities, NSF invites applications that would improve women’s health through a wide range of disciplines—from computational research to engineering biomechanics. This is the first time that NSF has broadly called for novel and transformative research that is focused entirely on women’s health topics, and proposals will be considered on an ongoing basis.
- Increase Research on How Environmental Factors Affect Women’s Health. The Environmental Protection Agency (EPA) is updating its grant solicitations and contracts to ensure that applicants prioritize, as appropriate, the consideration of women’s exposures and health outcomes. These changes will help ensure that women’s health is better accounted for across EPA’s research portfolio and increase our knowledge of women’s environmental health—from endocrine disruption to toxic exposure.
- Create a Dedicated, One-Stop Shop for NIH Funding Opportunities on Women’s Health. Researchers are often unaware of existing opportunities to apply for federal funding. To help close this gap, NIH is issuing a new Notice of Special Interest that identifies current, open funding opportunities related to women’s health research across a wide range of health conditions and all Institutes, Centers, and Offices. The NIH Office of Research on Women’s Health will build on this new Notice by creating a dedicated one-stop shop on open funding opportunities related to women’s health research. This will make it easier for researchers and institutions to find and apply for funding—instead of having to search across each of NIH’s 27 institutes for funding opportunities.
Foster Innovation and Discovery in Women’s Health
- Accelerate Transformative Research and Development in Women’s Health. ARPA-H’s Sprint for Women’s Health launched in February 2024 commits $100 million to transformative research and development in women’s health. ARPA-H is soliciting ideas for novel groundbreaking research and development to address women’s health, as well as opportunities to accelerate and scale tools, products, and platforms with the potential for commercialization to improve women’s health outcomes.
- Support Private Sector Innovation Through Additional Federal Investments in Women’s Health Research. The NIH’s competitive Small Business Innovation Research Program and the Small Business Technology Transfer Program is committing to further increasing—by 50 percent—its investments in supporting innovators and early-stage small businesses engaged in research and development on women’s health. These programs will solicit new proposals on promising women’s health innovation and make evidence-based investments that bridge the gap between performance of basic science and commercialization of resulting innovations. This commitment for additional funds builds on the investments the Administration has already made to increase innovation in women’s health through small businesses, including by increasing investments by sevenfold between Fiscal Year 2021 and Fiscal Year 2023.
- Advance Initiatives to Protect and Promote the Health of Women. The Food and Drug Administration (FDA) seeks to advance efforts to help address gaps in research and availability of products for diseases and conditions that primarily impact women, or for which scientific considerations may be different for women, and is committed to research and regulatory initiatives that facilitate the development of safe and effective medical products for women. FDA also plans to issue guidance for industry that relates to the inclusion of women in clinical trials and conduct outreach to stakeholders to discuss opportunities to advance women’s health across the lifespan. And FDA’s Office of Women’s Health will update FDA’s framework for women’s health research and seek to fund research with an emphasis on bridging gaps in knowledge on important women’s health topics, including sex differences and conditions that uniquely or disproportionately impact women.
- Use Biomarkers to Improve the Health of Women Through Early Detection and Treatment of Conditions, such as Endometriosis. NIH will launch a new initiative dedicated to research on biomarker discovery and validation to help improve our ability to prevent, diagnose, and treat conditions that affect women uniquely, including endometriosis. This NIH initiative will accelerate our ability to identify new pathways for diagnosis and treatment by encouraging multi-sector collaboration and synergistic research that will speed the transfer of knowledge from bench to bedside.
- Leverage Engineering Research to Improve Women’s Health . The NSF Engineering Research Visioning Alliance (ERVA) is convening national experts to identify high-impact research opportunities in engineering that can improve women’s health. ERVA’s Transforming Women’s Health Outcomes Through Engineering visioning event will be held in June 2024, and will bring together experts from across engineering—including those in microfluidics, computational modeling, artificial intelligence/imaging, and diagnostic technologies and devices—to evaluate the landscape for new applications in women’s health. Following this event, ERVA will issue a report and roadmap on critical areas where engineering research can impact women’s health across the lifespan.
- Drive Engineering Innovations in Women’s Health Discovery . NSF awardees at Texas A&M University will hold a conference in summer 2024 to collectively identify challenges and opportunities in improving women’s health through engineering. Biomedical engineers and scientists will explore and identify how various types of engineering tools, including biomechanics and immuno-engineering, can be applied to women’s health and spark promising new research directions.
Expand and Leverage Data Collection and Analysis Related to Women’s Health
- Help Standardize Data to Support Research on Women’s Health. NIH is launching an effort to identify and develop new common data elements related to women’s health that will help researchers share and combine datasets, promote interoperability, and improve the accuracy of datasets when it comes to women’s health. NIH will initiate this process by convening data and scientific experts across the federal government to solicit feedback on the need to develop new NIH-endorsed common data elements—which are widely used in both research and clinical settings. By advancing new tools to capture more data about women’s health, NIH will give researchers and clinicians the tools they need to enable more meaningful data collection, analysis, and reporting and comprehensively improve our knowledge of women’s health.
- Reflect Women’s Health Needs in National Coverage Determinations. The Centers for Medicare & Medicaid Services (CMS) will strengthen its review process, including through Coverage with Evidence Development guidance, to ensure that new medical services and technologies work well in women, as applicable, before being covered nationally through the Medicare program. This will help ensure that Medicare funds are used for treatments with a sufficient evidence base to show that they actually work in women, who make up more than half of the Medicare population.
- Leverage Data and Quality Measures to Advance Women’s Health Research. The Centers for Disease Control and Prevention (CDC) and the Health Resources and Services Administration (HRSA) are building on existing datasets to improve the collection, analysis, and reporting of information on women’s health. The CDC is expanding the collection of key quality measures across a woman’s lifespan, including to understand the link between pregnancy and post-partum hypertension and heart disease, and plans to release the Million Hearts Hypertension in Pregnancy Change Package. This resource will feature a menu of evidence-informed strategies by which clinicians can change care processes. Each strategy includes tested tools and resources to support related clinical quality improvement. HRSA is modernizing its Uniform Data System in ways that will improve the ability to assess how women are being served through HRSA-funded health centers. By improving the ability to analyze data on key clinical quality measures, CDC and HRSA can help close gaps in women’s health care access and identify new opportunities for high-impact research.
Strengthen Coordination, Infrastructure, and Training to Support Women’s Health Research
- Launch New Joint Collaborative to Improve Women’s Health Research for Service Members and Veterans. DoD and VA are launching a new Women’s Health Research collaborative to explore opportunities that further promote joint efforts to advance women’s health research and improve evidence-based care for Service members and veterans. The collaborative will increase coordination with the goal of helping improve care across the lifespan for women in the military and women veterans. The Departments will further advance research on key women’s health issues and develop a roadmap to close pressing research gaps, including those specifically affecting Service women and women veterans.
- Coordinate Research to Advance the Health of Women in the Military. DoD will invest $10 million, contingent on available funds, in the Military Women’s Health Research Partnership. This Partnership is led by the Uniformed Services University and advances and coordinates women’s health research across the Department. The Partnership is supporting research in a wide range of health issues affecting women in the military, including cancers, mental and behavioral health, and the unique health care needs of Active Duty Service Women. In addition, the Uniformed Services University established a dedicated Director of Military Women’s Health Research Program, a role that is responsible for identifying research gaps, fostering collaboration, and coordinating and aligning a unified approach to address the evolving needs of Active Duty Service Women.
- Support EPA-Wide Research and Dissemination of Data on Women’s Health. EPA is establishing a Women’s Health Community of Practice to coordinate research and data dissemination. EPA also plans to direct the Board of Scientific Counselors to identify ways to advance EPA’s research with specific consideration of the intersection of environmental factors and women’s health, including maternal health.
- Expand Fellowship Training in Women’s Health Research. CDC, in collaboration with the CDC Foundation and American Board of Obstetrics and Gynecology, is expanding training in women’s health research and public health surveillance to OBGYNs, nurses and advanced practice nurses. Through fellowships and public health experiences with CDC, these clinicians will gain public health research skills to improve the health of women and children exposed to or affected by infectious diseases, mental health and substance use disorders. CDC will invite early career clinicians to train in public health and policy to become future leaders in women’s health research.
Improve Women’s Health Across the Lifespan
- Create a Comprehensive Research Agenda on Menopause. To help women get the answers they need about menopause, NIH will launch its first-ever Pathways to Prevention series on menopause and the treatment of menopausal symptoms. Pathways to Prevention is an independent, evidence-based process to synthesize the current state of the evidence, identify gaps in existing research, and develop a roadmap that can be used to help guide the field forward. The report, once completed, will help guide innovation and investments in menopause-related research and care across the federal government and research community.
- Improve Primary Care and Preventive Services for Women . The Agency for Healthcare Research and Quality (AHRQ) will issue a Notice of Intent to publish a funding opportunity announcement for research to advance the science of primary care, which will include a focus on women’s health. Through this funding opportunity, AHRQ will build evidence about key elements of primary care that influence patient outcomes and advance health equity—focusing on women of color—such as care coordination, continuity of care, comprehensiveness of care, person-centered care, and trust. The results from the funding opportunity will shed light on vital targets for improvements in the delivery of primary healthcare across a woman’s lifespan, including women’s health preventive services, prevention and management of multiple chronic diseases, perinatal care, transition from pediatric to adult care, sexual and reproductive health, and care of older adults.
- Promote the Health of American Indian and Alaska Native Women. The Indian Health Service is launching a series of engagements, including focus groups, to better understand tribal beliefs related to menopause in American Indian and Alaska Native Women. This series will inform new opportunities to expand culturally informed patient care and research as well as the development of new resources and educational materials.
- Connect Research to Real-World Outcomes to Improve Women’s Mental and Behavioral Health. The Substance Abuse and Mental Health Services Administration (SAMHSA) is supporting a range of health care providers to address the unique needs of women with or at risk for mental health and substance use disorders. Building on its current efforts to provide technical assistance through various initiatives , SAMHSA intends, contingent on available funds, to launch a new comprehensive Women’s Behavioral Health Technical Assistance Center. This center will identify and improve the implementation of best practices in women’s behavioral health across the life span; identify and fill critical gaps in knowledge of and resources for women’s behavioral health; and provide learning opportunities, training, and technical assistance for healthcare providers.
- Support Research on Maternal Health Outcomes. USDA will fund research to help recognize early warning signs of maternal morbidity and mortality in recipients of Special Supplemental Nutrition Program for Women, Infants, and Children (WIC), and anticipates awarding up to $5 million in Fiscal Year 2023 to support maternal health research through WIC. In addition, research being conducted through the Agricultural Research Service’s Human Nutrition Research Centers is focusing on women’s health across the lifespan, including the nutritional needs of pregnant and breastfeeding women and older adults.
Stay Connected
We'll be in touch with the latest information on how President Biden and his administration are working for the American people, as well as ways you can get involved and help our country build back better.
Opt in to send and receive text messages from President Biden.

An official website of the United States government
The .gov means it’s official. Federal government websites often end in .gov or .mil. Before sharing sensitive information, make sure you’re on a federal government site.
The site is secure. The https:// ensures that you are connecting to the official website and that any information you provide is encrypted and transmitted securely.
- Publications
- Account settings
Preview improvements coming to the PMC website in October 2024. Learn More or Try it out now .
- Advanced Search
- Journal List
- Neurol Res Pract

How to use and assess qualitative research methods
Loraine busetto.
1 Department of Neurology, Heidelberg University Hospital, Im Neuenheimer Feld 400, 69120 Heidelberg, Germany
Wolfgang Wick
2 Clinical Cooperation Unit Neuro-Oncology, German Cancer Research Center, Heidelberg, Germany
Christoph Gumbinger
Associated data.
Not applicable.
This paper aims to provide an overview of the use and assessment of qualitative research methods in the health sciences. Qualitative research can be defined as the study of the nature of phenomena and is especially appropriate for answering questions of why something is (not) observed, assessing complex multi-component interventions, and focussing on intervention improvement. The most common methods of data collection are document study, (non-) participant observations, semi-structured interviews and focus groups. For data analysis, field-notes and audio-recordings are transcribed into protocols and transcripts, and coded using qualitative data management software. Criteria such as checklists, reflexivity, sampling strategies, piloting, co-coding, member-checking and stakeholder involvement can be used to enhance and assess the quality of the research conducted. Using qualitative in addition to quantitative designs will equip us with better tools to address a greater range of research problems, and to fill in blind spots in current neurological research and practice.
The aim of this paper is to provide an overview of qualitative research methods, including hands-on information on how they can be used, reported and assessed. This article is intended for beginning qualitative researchers in the health sciences as well as experienced quantitative researchers who wish to broaden their understanding of qualitative research.
What is qualitative research?
Qualitative research is defined as “the study of the nature of phenomena”, including “their quality, different manifestations, the context in which they appear or the perspectives from which they can be perceived” , but excluding “their range, frequency and place in an objectively determined chain of cause and effect” [ 1 ]. This formal definition can be complemented with a more pragmatic rule of thumb: qualitative research generally includes data in form of words rather than numbers [ 2 ].
Why conduct qualitative research?
Because some research questions cannot be answered using (only) quantitative methods. For example, one Australian study addressed the issue of why patients from Aboriginal communities often present late or not at all to specialist services offered by tertiary care hospitals. Using qualitative interviews with patients and staff, it found one of the most significant access barriers to be transportation problems, including some towns and communities simply not having a bus service to the hospital [ 3 ]. A quantitative study could have measured the number of patients over time or even looked at possible explanatory factors – but only those previously known or suspected to be of relevance. To discover reasons for observed patterns, especially the invisible or surprising ones, qualitative designs are needed.
While qualitative research is common in other fields, it is still relatively underrepresented in health services research. The latter field is more traditionally rooted in the evidence-based-medicine paradigm, as seen in " research that involves testing the effectiveness of various strategies to achieve changes in clinical practice, preferably applying randomised controlled trial study designs (...) " [ 4 ]. This focus on quantitative research and specifically randomised controlled trials (RCT) is visible in the idea of a hierarchy of research evidence which assumes that some research designs are objectively better than others, and that choosing a "lesser" design is only acceptable when the better ones are not practically or ethically feasible [ 5 , 6 ]. Others, however, argue that an objective hierarchy does not exist, and that, instead, the research design and methods should be chosen to fit the specific research question at hand – "questions before methods" [ 2 , 7 – 9 ]. This means that even when an RCT is possible, some research problems require a different design that is better suited to addressing them. Arguing in JAMA, Berwick uses the example of rapid response teams in hospitals, which he describes as " a complex, multicomponent intervention – essentially a process of social change" susceptible to a range of different context factors including leadership or organisation history. According to him, "[in] such complex terrain, the RCT is an impoverished way to learn. Critics who use it as a truth standard in this context are incorrect" [ 8 ] . Instead of limiting oneself to RCTs, Berwick recommends embracing a wider range of methods , including qualitative ones, which for "these specific applications, (...) are not compromises in learning how to improve; they are superior" [ 8 ].
Research problems that can be approached particularly well using qualitative methods include assessing complex multi-component interventions or systems (of change), addressing questions beyond “what works”, towards “what works for whom when, how and why”, and focussing on intervention improvement rather than accreditation [ 7 , 9 – 12 ]. Using qualitative methods can also help shed light on the “softer” side of medical treatment. For example, while quantitative trials can measure the costs and benefits of neuro-oncological treatment in terms of survival rates or adverse effects, qualitative research can help provide a better understanding of patient or caregiver stress, visibility of illness or out-of-pocket expenses.
How to conduct qualitative research?
Given that qualitative research is characterised by flexibility, openness and responsivity to context, the steps of data collection and analysis are not as separate and consecutive as they tend to be in quantitative research [ 13 , 14 ]. As Fossey puts it : “sampling, data collection, analysis and interpretation are related to each other in a cyclical (iterative) manner, rather than following one after another in a stepwise approach” [ 15 ]. The researcher can make educated decisions with regard to the choice of method, how they are implemented, and to which and how many units they are applied [ 13 ]. As shown in Fig. 1 , this can involve several back-and-forth steps between data collection and analysis where new insights and experiences can lead to adaption and expansion of the original plan. Some insights may also necessitate a revision of the research question and/or the research design as a whole. The process ends when saturation is achieved, i.e. when no relevant new information can be found (see also below: sampling and saturation). For reasons of transparency, it is essential for all decisions as well as the underlying reasoning to be well-documented.

Iterative research process
While it is not always explicitly addressed, qualitative methods reflect a different underlying research paradigm than quantitative research (e.g. constructivism or interpretivism as opposed to positivism). The choice of methods can be based on the respective underlying substantive theory or theoretical framework used by the researcher [ 2 ].
Data collection
The methods of qualitative data collection most commonly used in health research are document study, observations, semi-structured interviews and focus groups [ 1 , 14 , 16 , 17 ].
Document study
Document study (also called document analysis) refers to the review by the researcher of written materials [ 14 ]. These can include personal and non-personal documents such as archives, annual reports, guidelines, policy documents, diaries or letters.
Observations
Observations are particularly useful to gain insights into a certain setting and actual behaviour – as opposed to reported behaviour or opinions [ 13 ]. Qualitative observations can be either participant or non-participant in nature. In participant observations, the observer is part of the observed setting, for example a nurse working in an intensive care unit [ 18 ]. In non-participant observations, the observer is “on the outside looking in”, i.e. present in but not part of the situation, trying not to influence the setting by their presence. Observations can be planned (e.g. for 3 h during the day or night shift) or ad hoc (e.g. as soon as a stroke patient arrives at the emergency room). During the observation, the observer takes notes on everything or certain pre-determined parts of what is happening around them, for example focusing on physician-patient interactions or communication between different professional groups. Written notes can be taken during or after the observations, depending on feasibility (which is usually lower during participant observations) and acceptability (e.g. when the observer is perceived to be judging the observed). Afterwards, these field notes are transcribed into observation protocols. If more than one observer was involved, field notes are taken independently, but notes can be consolidated into one protocol after discussions. Advantages of conducting observations include minimising the distance between the researcher and the researched, the potential discovery of topics that the researcher did not realise were relevant and gaining deeper insights into the real-world dimensions of the research problem at hand [ 18 ].
Semi-structured interviews
Hijmans & Kuyper describe qualitative interviews as “an exchange with an informal character, a conversation with a goal” [ 19 ]. Interviews are used to gain insights into a person’s subjective experiences, opinions and motivations – as opposed to facts or behaviours [ 13 ]. Interviews can be distinguished by the degree to which they are structured (i.e. a questionnaire), open (e.g. free conversation or autobiographical interviews) or semi-structured [ 2 , 13 ]. Semi-structured interviews are characterized by open-ended questions and the use of an interview guide (or topic guide/list) in which the broad areas of interest, sometimes including sub-questions, are defined [ 19 ]. The pre-defined topics in the interview guide can be derived from the literature, previous research or a preliminary method of data collection, e.g. document study or observations. The topic list is usually adapted and improved at the start of the data collection process as the interviewer learns more about the field [ 20 ]. Across interviews the focus on the different (blocks of) questions may differ and some questions may be skipped altogether (e.g. if the interviewee is not able or willing to answer the questions or for concerns about the total length of the interview) [ 20 ]. Qualitative interviews are usually not conducted in written format as it impedes on the interactive component of the method [ 20 ]. In comparison to written surveys, qualitative interviews have the advantage of being interactive and allowing for unexpected topics to emerge and to be taken up by the researcher. This can also help overcome a provider or researcher-centred bias often found in written surveys, which by nature, can only measure what is already known or expected to be of relevance to the researcher. Interviews can be audio- or video-taped; but sometimes it is only feasible or acceptable for the interviewer to take written notes [ 14 , 16 , 20 ].
Focus groups
Focus groups are group interviews to explore participants’ expertise and experiences, including explorations of how and why people behave in certain ways [ 1 ]. Focus groups usually consist of 6–8 people and are led by an experienced moderator following a topic guide or “script” [ 21 ]. They can involve an observer who takes note of the non-verbal aspects of the situation, possibly using an observation guide [ 21 ]. Depending on researchers’ and participants’ preferences, the discussions can be audio- or video-taped and transcribed afterwards [ 21 ]. Focus groups are useful for bringing together homogeneous (to a lesser extent heterogeneous) groups of participants with relevant expertise and experience on a given topic on which they can share detailed information [ 21 ]. Focus groups are a relatively easy, fast and inexpensive method to gain access to information on interactions in a given group, i.e. “the sharing and comparing” among participants [ 21 ]. Disadvantages include less control over the process and a lesser extent to which each individual may participate. Moreover, focus group moderators need experience, as do those tasked with the analysis of the resulting data. Focus groups can be less appropriate for discussing sensitive topics that participants might be reluctant to disclose in a group setting [ 13 ]. Moreover, attention must be paid to the emergence of “groupthink” as well as possible power dynamics within the group, e.g. when patients are awed or intimidated by health professionals.
Choosing the “right” method
As explained above, the school of thought underlying qualitative research assumes no objective hierarchy of evidence and methods. This means that each choice of single or combined methods has to be based on the research question that needs to be answered and a critical assessment with regard to whether or to what extent the chosen method can accomplish this – i.e. the “fit” between question and method [ 14 ]. It is necessary for these decisions to be documented when they are being made, and to be critically discussed when reporting methods and results.
Let us assume that our research aim is to examine the (clinical) processes around acute endovascular treatment (EVT), from the patient’s arrival at the emergency room to recanalization, with the aim to identify possible causes for delay and/or other causes for sub-optimal treatment outcome. As a first step, we could conduct a document study of the relevant standard operating procedures (SOPs) for this phase of care – are they up-to-date and in line with current guidelines? Do they contain any mistakes, irregularities or uncertainties that could cause delays or other problems? Regardless of the answers to these questions, the results have to be interpreted based on what they are: a written outline of what care processes in this hospital should look like. If we want to know what they actually look like in practice, we can conduct observations of the processes described in the SOPs. These results can (and should) be analysed in themselves, but also in comparison to the results of the document analysis, especially as regards relevant discrepancies. Do the SOPs outline specific tests for which no equipment can be observed or tasks to be performed by specialized nurses who are not present during the observation? It might also be possible that the written SOP is outdated, but the actual care provided is in line with current best practice. In order to find out why these discrepancies exist, it can be useful to conduct interviews. Are the physicians simply not aware of the SOPs (because their existence is limited to the hospital’s intranet) or do they actively disagree with them or does the infrastructure make it impossible to provide the care as described? Another rationale for adding interviews is that some situations (or all of their possible variations for different patient groups or the day, night or weekend shift) cannot practically or ethically be observed. In this case, it is possible to ask those involved to report on their actions – being aware that this is not the same as the actual observation. A senior physician’s or hospital manager’s description of certain situations might differ from a nurse’s or junior physician’s one, maybe because they intentionally misrepresent facts or maybe because different aspects of the process are visible or important to them. In some cases, it can also be relevant to consider to whom the interviewee is disclosing this information – someone they trust, someone they are otherwise not connected to, or someone they suspect or are aware of being in a potentially “dangerous” power relationship to them. Lastly, a focus group could be conducted with representatives of the relevant professional groups to explore how and why exactly they provide care around EVT. The discussion might reveal discrepancies (between SOPs and actual care or between different physicians) and motivations to the researchers as well as to the focus group members that they might not have been aware of themselves. For the focus group to deliver relevant information, attention has to be paid to its composition and conduct, for example, to make sure that all participants feel safe to disclose sensitive or potentially problematic information or that the discussion is not dominated by (senior) physicians only. The resulting combination of data collection methods is shown in Fig. 2 .

Possible combination of data collection methods
Attributions for icons: “Book” by Serhii Smirnov, “Interview” by Adrien Coquet, FR, “Magnifying Glass” by anggun, ID, “Business communication” by Vectors Market; all from the Noun Project
The combination of multiple data source as described for this example can be referred to as “triangulation”, in which multiple measurements are carried out from different angles to achieve a more comprehensive understanding of the phenomenon under study [ 22 , 23 ].
Data analysis
To analyse the data collected through observations, interviews and focus groups these need to be transcribed into protocols and transcripts (see Fig. 3 ). Interviews and focus groups can be transcribed verbatim , with or without annotations for behaviour (e.g. laughing, crying, pausing) and with or without phonetic transcription of dialects and filler words, depending on what is expected or known to be relevant for the analysis. In the next step, the protocols and transcripts are coded , that is, marked (or tagged, labelled) with one or more short descriptors of the content of a sentence or paragraph [ 2 , 15 , 23 ]. Jansen describes coding as “connecting the raw data with “theoretical” terms” [ 20 ]. In a more practical sense, coding makes raw data sortable. This makes it possible to extract and examine all segments describing, say, a tele-neurology consultation from multiple data sources (e.g. SOPs, emergency room observations, staff and patient interview). In a process of synthesis and abstraction, the codes are then grouped, summarised and/or categorised [ 15 , 20 ]. The end product of the coding or analysis process is a descriptive theory of the behavioural pattern under investigation [ 20 ]. The coding process is performed using qualitative data management software, the most common ones being InVivo, MaxQDA and Atlas.ti. It should be noted that these are data management tools which support the analysis performed by the researcher(s) [ 14 ].

From data collection to data analysis
Attributions for icons: see Fig. Fig.2, 2 , also “Speech to text” by Trevor Dsouza, “Field Notes” by Mike O’Brien, US, “Voice Record” by ProSymbols, US, “Inspection” by Made, AU, and “Cloud” by Graphic Tigers; all from the Noun Project
How to report qualitative research?
Protocols of qualitative research can be published separately and in advance of the study results. However, the aim is not the same as in RCT protocols, i.e. to pre-define and set in stone the research questions and primary or secondary endpoints. Rather, it is a way to describe the research methods in detail, which might not be possible in the results paper given journals’ word limits. Qualitative research papers are usually longer than their quantitative counterparts to allow for deep understanding and so-called “thick description”. In the methods section, the focus is on transparency of the methods used, including why, how and by whom they were implemented in the specific study setting, so as to enable a discussion of whether and how this may have influenced data collection, analysis and interpretation. The results section usually starts with a paragraph outlining the main findings, followed by more detailed descriptions of, for example, the commonalities, discrepancies or exceptions per category [ 20 ]. Here it is important to support main findings by relevant quotations, which may add information, context, emphasis or real-life examples [ 20 , 23 ]. It is subject to debate in the field whether it is relevant to state the exact number or percentage of respondents supporting a certain statement (e.g. “Five interviewees expressed negative feelings towards XYZ”) [ 21 ].
How to combine qualitative with quantitative research?
Qualitative methods can be combined with other methods in multi- or mixed methods designs, which “[employ] two or more different methods [ …] within the same study or research program rather than confining the research to one single method” [ 24 ]. Reasons for combining methods can be diverse, including triangulation for corroboration of findings, complementarity for illustration and clarification of results, expansion to extend the breadth and range of the study, explanation of (unexpected) results generated with one method with the help of another, or offsetting the weakness of one method with the strength of another [ 1 , 17 , 24 – 26 ]. The resulting designs can be classified according to when, why and how the different quantitative and/or qualitative data strands are combined. The three most common types of mixed method designs are the convergent parallel design , the explanatory sequential design and the exploratory sequential design. The designs with examples are shown in Fig. 4 .

Three common mixed methods designs
In the convergent parallel design, a qualitative study is conducted in parallel to and independently of a quantitative study, and the results of both studies are compared and combined at the stage of interpretation of results. Using the above example of EVT provision, this could entail setting up a quantitative EVT registry to measure process times and patient outcomes in parallel to conducting the qualitative research outlined above, and then comparing results. Amongst other things, this would make it possible to assess whether interview respondents’ subjective impressions of patients receiving good care match modified Rankin Scores at follow-up, or whether observed delays in care provision are exceptions or the rule when compared to door-to-needle times as documented in the registry. In the explanatory sequential design, a quantitative study is carried out first, followed by a qualitative study to help explain the results from the quantitative study. This would be an appropriate design if the registry alone had revealed relevant delays in door-to-needle times and the qualitative study would be used to understand where and why these occurred, and how they could be improved. In the exploratory design, the qualitative study is carried out first and its results help informing and building the quantitative study in the next step [ 26 ]. If the qualitative study around EVT provision had shown a high level of dissatisfaction among the staff members involved, a quantitative questionnaire investigating staff satisfaction could be set up in the next step, informed by the qualitative study on which topics dissatisfaction had been expressed. Amongst other things, the questionnaire design would make it possible to widen the reach of the research to more respondents from different (types of) hospitals, regions, countries or settings, and to conduct sub-group analyses for different professional groups.
How to assess qualitative research?
A variety of assessment criteria and lists have been developed for qualitative research, ranging in their focus and comprehensiveness [ 14 , 17 , 27 ]. However, none of these has been elevated to the “gold standard” in the field. In the following, we therefore focus on a set of commonly used assessment criteria that, from a practical standpoint, a researcher can look for when assessing a qualitative research report or paper.
Assessors should check the authors’ use of and adherence to the relevant reporting checklists (e.g. Standards for Reporting Qualitative Research (SRQR)) to make sure all items that are relevant for this type of research are addressed [ 23 , 28 ]. Discussions of quantitative measures in addition to or instead of these qualitative measures can be a sign of lower quality of the research (paper). Providing and adhering to a checklist for qualitative research contributes to an important quality criterion for qualitative research, namely transparency [ 15 , 17 , 23 ].
Reflexivity
While methodological transparency and complete reporting is relevant for all types of research, some additional criteria must be taken into account for qualitative research. This includes what is called reflexivity, i.e. sensitivity to the relationship between the researcher and the researched, including how contact was established and maintained, or the background and experience of the researcher(s) involved in data collection and analysis. Depending on the research question and population to be researched this can be limited to professional experience, but it may also include gender, age or ethnicity [ 17 , 27 ]. These details are relevant because in qualitative research, as opposed to quantitative research, the researcher as a person cannot be isolated from the research process [ 23 ]. It may influence the conversation when an interviewed patient speaks to an interviewer who is a physician, or when an interviewee is asked to discuss a gynaecological procedure with a male interviewer, and therefore the reader must be made aware of these details [ 19 ].
Sampling and saturation
The aim of qualitative sampling is for all variants of the objects of observation that are deemed relevant for the study to be present in the sample “ to see the issue and its meanings from as many angles as possible” [ 1 , 16 , 19 , 20 , 27 ] , and to ensure “information-richness [ 15 ]. An iterative sampling approach is advised, in which data collection (e.g. five interviews) is followed by data analysis, followed by more data collection to find variants that are lacking in the current sample. This process continues until no new (relevant) information can be found and further sampling becomes redundant – which is called saturation [ 1 , 15 ] . In other words: qualitative data collection finds its end point not a priori , but when the research team determines that saturation has been reached [ 29 , 30 ].
This is also the reason why most qualitative studies use deliberate instead of random sampling strategies. This is generally referred to as “ purposive sampling” , in which researchers pre-define which types of participants or cases they need to include so as to cover all variations that are expected to be of relevance, based on the literature, previous experience or theory (i.e. theoretical sampling) [ 14 , 20 ]. Other types of purposive sampling include (but are not limited to) maximum variation sampling, critical case sampling or extreme or deviant case sampling [ 2 ]. In the above EVT example, a purposive sample could include all relevant professional groups and/or all relevant stakeholders (patients, relatives) and/or all relevant times of observation (day, night and weekend shift).
Assessors of qualitative research should check whether the considerations underlying the sampling strategy were sound and whether or how researchers tried to adapt and improve their strategies in stepwise or cyclical approaches between data collection and analysis to achieve saturation [ 14 ].
Good qualitative research is iterative in nature, i.e. it goes back and forth between data collection and analysis, revising and improving the approach where necessary. One example of this are pilot interviews, where different aspects of the interview (especially the interview guide, but also, for example, the site of the interview or whether the interview can be audio-recorded) are tested with a small number of respondents, evaluated and revised [ 19 ]. In doing so, the interviewer learns which wording or types of questions work best, or which is the best length of an interview with patients who have trouble concentrating for an extended time. Of course, the same reasoning applies to observations or focus groups which can also be piloted.
Ideally, coding should be performed by at least two researchers, especially at the beginning of the coding process when a common approach must be defined, including the establishment of a useful coding list (or tree), and when a common meaning of individual codes must be established [ 23 ]. An initial sub-set or all transcripts can be coded independently by the coders and then compared and consolidated after regular discussions in the research team. This is to make sure that codes are applied consistently to the research data.
Member checking
Member checking, also called respondent validation , refers to the practice of checking back with study respondents to see if the research is in line with their views [ 14 , 27 ]. This can happen after data collection or analysis or when first results are available [ 23 ]. For example, interviewees can be provided with (summaries of) their transcripts and asked whether they believe this to be a complete representation of their views or whether they would like to clarify or elaborate on their responses [ 17 ]. Respondents’ feedback on these issues then becomes part of the data collection and analysis [ 27 ].
Stakeholder involvement
In those niches where qualitative approaches have been able to evolve and grow, a new trend has seen the inclusion of patients and their representatives not only as study participants (i.e. “members”, see above) but as consultants to and active participants in the broader research process [ 31 – 33 ]. The underlying assumption is that patients and other stakeholders hold unique perspectives and experiences that add value beyond their own single story, making the research more relevant and beneficial to researchers, study participants and (future) patients alike [ 34 , 35 ]. Using the example of patients on or nearing dialysis, a recent scoping review found that 80% of clinical research did not address the top 10 research priorities identified by patients and caregivers [ 32 , 36 ]. In this sense, the involvement of the relevant stakeholders, especially patients and relatives, is increasingly being seen as a quality indicator in and of itself.
How not to assess qualitative research
The above overview does not include certain items that are routine in assessments of quantitative research. What follows is a non-exhaustive, non-representative, experience-based list of the quantitative criteria often applied to the assessment of qualitative research, as well as an explanation of the limited usefulness of these endeavours.
Protocol adherence
Given the openness and flexibility of qualitative research, it should not be assessed by how well it adheres to pre-determined and fixed strategies – in other words: its rigidity. Instead, the assessor should look for signs of adaptation and refinement based on lessons learned from earlier steps in the research process.
Sample size
For the reasons explained above, qualitative research does not require specific sample sizes, nor does it require that the sample size be determined a priori [ 1 , 14 , 27 , 37 – 39 ]. Sample size can only be a useful quality indicator when related to the research purpose, the chosen methodology and the composition of the sample, i.e. who was included and why.
Randomisation
While some authors argue that randomisation can be used in qualitative research, this is not commonly the case, as neither its feasibility nor its necessity or usefulness has been convincingly established for qualitative research [ 13 , 27 ]. Relevant disadvantages include the negative impact of a too large sample size as well as the possibility (or probability) of selecting “ quiet, uncooperative or inarticulate individuals ” [ 17 ]. Qualitative studies do not use control groups, either.
Interrater reliability, variability and other “objectivity checks”
The concept of “interrater reliability” is sometimes used in qualitative research to assess to which extent the coding approach overlaps between the two co-coders. However, it is not clear what this measure tells us about the quality of the analysis [ 23 ]. This means that these scores can be included in qualitative research reports, preferably with some additional information on what the score means for the analysis, but it is not a requirement. Relatedly, it is not relevant for the quality or “objectivity” of qualitative research to separate those who recruited the study participants and collected and analysed the data. Experiences even show that it might be better to have the same person or team perform all of these tasks [ 20 ]. First, when researchers introduce themselves during recruitment this can enhance trust when the interview takes place days or weeks later with the same researcher. Second, when the audio-recording is transcribed for analysis, the researcher conducting the interviews will usually remember the interviewee and the specific interview situation during data analysis. This might be helpful in providing additional context information for interpretation of data, e.g. on whether something might have been meant as a joke [ 18 ].
Not being quantitative research
Being qualitative research instead of quantitative research should not be used as an assessment criterion if it is used irrespectively of the research problem at hand. Similarly, qualitative research should not be required to be combined with quantitative research per se – unless mixed methods research is judged as inherently better than single-method research. In this case, the same criterion should be applied for quantitative studies without a qualitative component.
The main take-away points of this paper are summarised in Table Table1. 1 . We aimed to show that, if conducted well, qualitative research can answer specific research questions that cannot to be adequately answered using (only) quantitative designs. Seeing qualitative and quantitative methods as equal will help us become more aware and critical of the “fit” between the research problem and our chosen methods: I can conduct an RCT to determine the reasons for transportation delays of acute stroke patients – but should I? It also provides us with a greater range of tools to tackle a greater range of research problems more appropriately and successfully, filling in the blind spots on one half of the methodological spectrum to better address the whole complexity of neurological research and practice.
Take-away-points
Acknowledgements
Abbreviations, authors’ contributions.
LB drafted the manuscript; WW and CG revised the manuscript; all authors approved the final versions.
no external funding.
Guidelines on the responsible use of generative AI in research developed by the European Research Area Forum
The Commission, together with the European Research Area countries and stakeholders, has put forward a set of guidelines to support the European research community in their responsible use of generative artificial intelligence (AI).
With the rapid spread of the use of this technology in all domains including in science, these recommendations address key opportunities and challenges. Building on the principles of research integrity, they offer guidance to researchers, research organisations, and research funders to ensure a coherent approach across Europe. The principles framing the new guidelines are based on existing frameworks such as the European Code of Conduct for Research Integrity and the guidelines on trustworthy AI .
AI is transforming research, making scientific work more efficient and accelerating discovery. While generative AI tools offer speed and convenience in producing text, images and code, researchers must also be mindful of the technology’s limitations, including plagiarism, revealing sensitive information, or inherent biases in the models.
Margrethe Vestager, Executive Vice-President said:
We are committed to innovation of AI and innovation with AI. And we will do our best to build a thriving AI ecosystem in Europe. With these guidelines, we encourage the research community to use generative AI to help supercharge European science and its applications to the benefit of society and for all of us.
Iliana Ivanova, Commissioner for Innovation, Research, Culture, Education and Youth said:
Generative AI can hugely boost research, but its use demands transparency and responsibility. These guidelines aim to uphold scientific integrity and preserve public trust in science amidst rapid technological advancements. I call on the scientific community to join us in turning these guidelines into the reference for European research.
Key takeaways from the guidelines include:
- Researchers refrain from using generative AI tools in sensitive activities such as peer reviews or evaluations and use generative AI respecting privacy, confidentiality, and intellectual property rights.
- Research organisations should facilitate the responsible use of generative AI and actively monitor how these tools are developed and used within their organisations.
- Funding organisations should support applicants in using generative AI transparently
As generative AI is constantly evolving, these guidelines will be updated with regular feedback from the scientific community and stakeholders.
Share your views
The widespread uptake of generative AI has triggered numerous institutional responses. While the EU is taking the global lead with its AI Act regulating AI products, many academic institutions and organisations across Europe have been developing guidelines on the use generative AI. The goal of the ERA Forum representatives, including Member States, Horizon Europe associated countries, and other research and innovation stakeholders, was to develop a guidance that could bring consistency across countries and research organisations.
More information
Artificial Intelligence in Science
Press contact:
EC Spokesperson for Research, Science and Innovation
Share this page
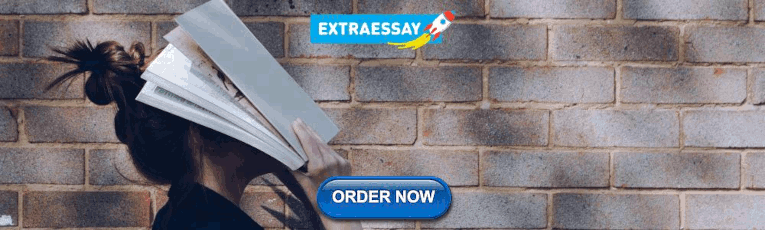
IMAGES
VIDEO
COMMENTS
Data collection is a systematic process of gathering observations or measurements. Whether you are performing research for business, governmental or academic purposes, data collection allows you to gain first-hand knowledge and original insights into your research problem. While methods and aims may differ between fields, the overall process of ...
Research data refers to any information or evidence gathered through systematic investigation or experimentation to support or refute a hypothesis or answer a research question. It includes both primary and secondary data, and can be in various formats such as numerical, textual, audiovisual, or visual. Research data plays a critical role in ...
Research data are collected and used in scholarship across all academic disciplines and, while it can consist of numbers in a spreadsheet, it also takes many different formats, including videos, images, artifacts, and diaries. Whether a psychologist collecting survey data to better understand human behavior, an artist using data to generate ...
Open Data is a strategy for incorporating research data into the permanent scientific record by releasing it under an Open Access license. Whether data is deposited in a purpose-built repository or published as Supporting Information alongside a research article, Open Data practices ensure that data remains accessible and discoverable. For ...
Research methods are specific procedures for collecting and analyzing data. Developing your research methods is an integral part of your research design. When planning your methods, there are two key decisions you will make. First, decide how you will collect data. Your methods depend on what type of data you need to answer your research question:
A research design is a strategy for answering your research question using empirical data. Creating a research design means making decisions about: Your overall research objectives and approach. Whether you'll rely on primary research or secondary research. Your sampling methods or criteria for selecting subjects. Your data collection methods.
Defining Research Data. One definition of research data is: "the recorded factual material commonly accepted in the scientific community as necessary to validate research findings." ( OMB Circular 110 ). Research data covers a broad range of types of information (see examples below), and digital data can be structured and stored in a variety of ...
At Our World in Data, we investigated the strengths and shortcomings of the available data on literacy. Based on this work, our team brought together the long-run data shown in the chart by combining several different sources, including the World Bank, the CIA Factbook, and a range of research publications. Explore and learn more about this data.
Research data comprises research observations or findings, such as facts, images, measurements, records and files in various formats, and can be stored in databases. Data publication and archiving ...
At Elsevier we believe there are 10 aspects of highly effective data and which can function as a roadmap for the development of better data management processes and systems throughout the data lifecycle. These are detailed below: Stored: The first step in the hierarchy of research data needs is that data that have been acquired need to be ...
Citing data and software enables the authors to get credit for their contribution to the research article, and also increases the discoverability of the research data and software. Data and software citations should include the following minimum information where available: Author (s) / Creator (s) Date. Title / Software name.
Introduction. Over the past decades, science has experienced rapid growth in the volume of data available for research—from a relative paucity of data in many areas to what has been recently described as a data deluge [1].Data volumes have increased exponentially across all fields of science and human endeavour, including data from sky, earth, and ocean observatories; social media such as ...
data analysis, the process of systematically collecting, cleaning, transforming, describing, modeling, and interpreting data, generally employing statistical techniques. Data analysis is an important part of both scientific research and business, where demand has grown in recent years for data-driven decision making.
Definition of research in data analysis: According to LeCompte and Schensul, research data analysis is a process used by researchers to reduce data to a story and interpret it to derive insights. The data analysis process helps reduce a large chunk of data into smaller fragments, which makes sense. Three essential things occur during the data ...
Library Data Services Program and Digital Scholarship Services. The LDSP offers a variety of services and tools! From this link, check out pages for each of the following topics: discovering data, managing data, collecting data, GIS data, text data mining, publishing data, digital scholarship, open science, and the Research Data Management Program.
Doing qualitative research is not easy and may require a complete rethink of how research is conducted, particularly for researchers who are more familiar with quantitative approaches. There are many ways of conducting qualitative research, and this paper has covered some of the practical issues regarding data collection, analysis, and management.
Abstract. Statistical methods involved in carrying out a study include planning, designing, collecting data, analysing, drawing meaningful interpretation and reporting of the research findings. The statistical analysis gives meaning to the meaningless numbers, thereby breathing life into a lifeless data. The results and inferences are precise ...
The following principles underpin Elsevier's research data policy: Research data should be made available free of charge to all researchers wherever possible and with minimal reuse restrictions. Researchers should remain in control of how and when their research data is accessed and used, and should be recognized and valued for the ...
AHRQ's database on Social Determinants of Health (SDOH) was created under a project funded by the Patient Centered Outcomes Research (PCOR) Trust Fund. The purpose of this project is to create easy to use, easily linkable SDOH-focused data to use in PCOR research, inform approaches to address ...
The data could be used to improve predictions of both weather and climate change. ... "The great thing about the program is that it's really both an education program and a research program," says ...
However, themes in qualitative research largely emerge during data analysis, which is where our focus remains. Process of Theme Development. For QCSR, the grounded theory process has been used most often. This is primarily due to the in-depth nature of the study and the variety of data sources that are collected for QCSR. Hence, we shall be ...
The A.E.I. data defines remote status by whether there was an in-person or hybrid option, even if some students chose to remain virtual. In the C.S.D.H. data set, districts are defined as remote ...
The CDC data that is highlighted in this post comes from the agency's "abortion surveillance" reports, which have been published annually since 1974 (and which have included data from 1969). Its figures from 1973 through 1996 include data from all 50 states, the District of Columbia and New York City - 52 "reporting areas" in all.
Qualitative research involves collecting and analyzing non-numerical data (e.g., text, video, or audio) to understand concepts, opinions, or experiences. It can be used to gather in-depth insights into a problem or generate new ideas for research. Qualitative research is the opposite of quantitative research, which involves collecting and ...
Support EPA-Wide Research and Dissemination of Data on Women's Health. EPA is establishing a Women's Health Community of Practice to coordinate research and data dissemination. EPA also plans ...
The Potential Of Clinical Data To Support Research. Most clinical data—about 80%—is unstructured, taking the form of doctors' notes, imaging scans, pathology reports and other forms that ...
How to conduct qualitative research? Given that qualitative research is characterised by flexibility, openness and responsivity to context, the steps of data collection and analysis are not as separate and consecutive as they tend to be in quantitative research [13, 14].As Fossey puts it: "sampling, data collection, analysis and interpretation are related to each other in a cyclical ...
The Commission, together with the European Research Area countries and stakeholders, has put forward a set of guidelines to support the European research community in their responsible use of generative artificial intelligence (AI).. With the rapid spread of the use of this technology in all domains including in science, these recommendations address key opportunities and challenges.
Identifying upstream factors associated with firearm recovery in crimes can inform violence prevention efforts, making communities safer." — Sonia L. Robinson, research data analyst, UC Davis Violence Prevention Research Program The study noted some similarities to what researchers had discovered earlier.
A technique developed by Penn State scientists could improve forecasts — including where the most powerful winds will occur during weather events like the 2020 Midwest Derecho, one of the most costly storms in modern U.S. history.