
- SUGGESTED TOPICS
- The Magazine
- Newsletters
- Managing Yourself
- Managing Teams
- Work-life Balance
- The Big Idea
- Data & Visuals
- Reading Lists
- Case Selections
- HBR Learning
- Topic Feeds
- Account Settings
- Email Preferences
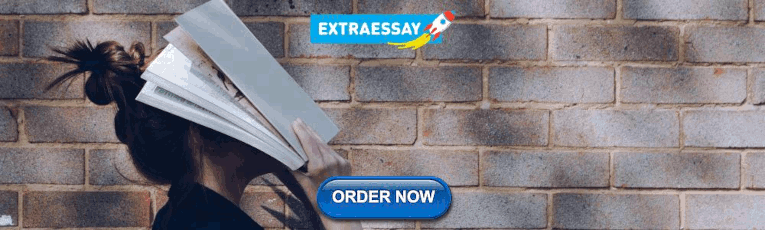
A Leader’s Framework for Decision Making
- David J. Snowden
- Mary E. Boone

Wise executives tailor their approach to fit the complexity of the circumstances they face.
Reprint: R0711C
Many executives are surprised when previously successful leadership approaches fail in new situations, but different contexts call for different kinds of responses. Before addressing a situation, leaders need to recognize which context governs it—and tailor their actions accordingly.
Snowden and Boone have formed a new perspective on leadership and decision making that’s based on complexity science. The result is the Cynefin framework, which helps executives sort issues into five contexts:
Simple contexts are characterized by stability and cause-and-effect relationships that are clear to everyone. Often, the right answer is self-evident. In this realm of “known knowns,” leaders must first assess the facts of a situation—that is, “sense” it—then categorize and respond to it.
Complicated contexts may contain multiple right answers, and though there is a clear relationship between cause and effect, not everyone can see it. This is the realm of “known unknowns.” Here, leaders must sense, analyze, and respond.
In a complex context, right answers can’t be ferreted out at all; rather, instructive patterns emerge if the leader conducts experiments that can safely fail. This is the realm of “unknown unknowns,” where much of contemporary business operates. Leaders in this context need to probe first, then sense, and then respond.
In a chaotic context, searching for right answers is pointless. The relationships between cause and effect are impossible to determine because they shift constantly and no manageable patterns exist. This is the realm of unknowables (the events of September 11, 2001, fall into this category). In this domain, a leader must first act to establish order, sense where stability is present, and then work to transform the situation from chaos to complexity.
The fifth context, disorder , applies when it is unclear which of the other four contexts is predominant. The way out is to break the situation into its constituent parts and assign each to one of the other four realms. Leaders can then make decisions and intervene in contextually appropriate ways.
In January 1993, a gunman murdered seven people in a fast-food restaurant in Palatine, a suburb of Chicago. In his dual roles as an administrative executive and spokesperson for the police department, Deputy Chief Walter Gasior suddenly had to cope with several different situations at once. He had to deal with the grieving families and a frightened community, help direct the operations of an extremely busy police department, and take questions from the media, which inundated the town with reporters and film crews. “There would literally be four people coming at me with logistics and media issues all at once,” he recalls. “And in the midst of all this, we still had a department that had to keep running on a routine basis.”

- DS David J. Snowden ( [email protected] ) is the founder and chief scientific officer of Cognitive Edge, an international research network. He is based primarily in Lockeridge, England.
- MB Mary E. Boone ( [email protected] ) is the president of Boone Associates, a consulting firm in Essex, Connecticut, and the author of numerous books and articles, including Managing Interactively (McGraw-Hill, 2001).
Partner Center

How it works
For Business
Join Mind Tools
Article • 10 min read
The Cynefin Framework
Using the most appropriate problem-solving process.
By the Mind Tools Content Team
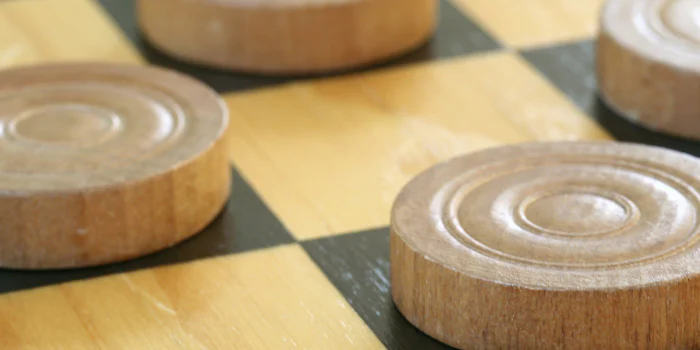
The most effective leaders understand that problem solving is not a "one-size-fits-all" process. They know that their actions depend on the situation, and they make better decisions by adapting their approach to changing circumstances.
But how do you know which approach you should use in a particular situation? And how can you avoid making the wrong decision?
In this article we'll look at the Cynefin framework, a tool that helps you make better decisions by assessing the situation you find yourself in.
About the Tool
Cynefin, pronounced "ku-nev-in," is a Welsh word that translates as "place" or "habitat." However, it can also be used to describe the elements of our situation and personal history that influence our thoughts and decisions in ways we don't understand.
Scholar David J. Snowden used the word to describe a framework he developed in 1999, based on concepts from knowledge management and organizational strategy. Along with his colleague Mary Boone, he published the framework in the November 2007 issue of the Harvard Business Review .
The Cynefin framework (Figure 1 below) is a problem-solving tool that helps you put situations into five "domains" defined by cause-and-effect relationships. This helps you assess your situation more accurately and respond appropriately.
Figure 1: The Cynefin Framework
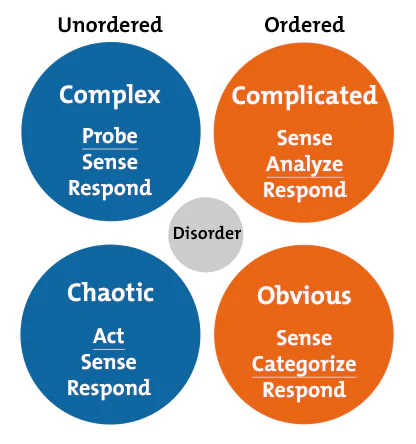
Based on the Cynefin framework diagram by David Snowden, see http://cognitive-edge.com . Reproduced with permission.
The "obvious" domain was originally called "simple," but this was updated in 2014.
You can use the Cynefin framework in a variety of situations to categorize a problem or decision and respond accordingly. For example, it is useful in product development, marketing and organizational strategy. It can also help you make better decisions in a crisis or emergency.
It helps you avoid using the same management style or decision-making approach in all situations – a mistake that can be costly to your team or organization– by encouraging you to be flexible and adaptable when making decisions, and to adjust your management style to fit your circumstances.
The Five Domains
Let's look at each of the five domains in greater detail.
Obvious Contexts – "The Domain of Best Practice"
In "obvious" contexts, your options are clear and cause-and-effect relationships are apparent to everyone involved.
Here, there are often explicit steps in place that dictate the next stage of the process. For example, problems encountered at help desks or call centers are often predictable, and there are processes in place to handle most of them.
Snowden argues that you need to "Sense – Categorize – Respond" to obvious decisions. Put simply, you should assess the situation, categorize its type, and then base your response on best practice. There is often one established "correct" answer, based on an existing process or procedure.
However, there is a danger that obvious contexts may be oversimplified. This often happens when leaders, or an entire organization, experience success and then become complacent. To avoid this, make sure that there are clear communication channels in place, so that team members can report any situations that don't fit with any established category.
Another challenge is that leaders may not be receptive to new ideas because of past experiences and success. For example, some people might automatically assume that previous solutions will work again. To overcome this, stay open to new ideas and be willing to pursue innovative suggestions.
Complicated Contexts – "The Domain of Experts"
"Complicated" problems might have several "correct" solutions. Here, there is a clear relationship between cause and effect, but it may not be visible to everyone, because the problem is... complicated. For example, you might see several symptoms of a problem but not know how to fix it.
The decision-making approach here is to "Sense – Analyze – Respond." In other words, you need to assess the situation, analyze what is known (often with the help of experts), and decide on the best response, using good practice.
Leaders may rely too heavily on experts in complicated situations, while dismissing or overlooking creative solutions from other people. To overcome this, assemble a team of people from a wide variety of backgrounds (including rebels and dissenters), and use tools such as Crawford's Slip Writing Method to ensure that everyone's views are heard.
Complex Contexts – "The Domain of Emergence"
It might be impossible to identify one "correct" solution, or spot cause-and-effect relationships, in "complex" situations. According to Snowden and Boone, many business situations fall into this category.
Complex contexts are often unpredictable, and the best approach here is to "Probe – Sense – Respond." Rather than trying to control the situation or insisting on a plan of action, it's often best to be patient, look for patterns, and encourage a solution to emerge.
It can be helpful to conduct business experiments in these situations, and accept failure as part of the learning process. Make sure that you have processes in place to guide your team's thinking – even a simple set of rules can lead to better solutions than no guidance at all.
Communication is essential here, too. Gather a diverse group of people to come up with innovative, creative solutions to complex problems. Use brainstorming tools such as Random Input or Provocation to generate new ideas, and encourage your team to debate the possibilities.
Complicated and complex situations are similar in some ways, and it can be challenging to tell which of them you're experiencing. However, if you need to make a decision based on incomplete data, for example, you're likely to be in a complex situation.
Chaotic Contexts – "The Domain of Rapid Response"
In "chaotic" situations, no relationship between cause and effect exists, so your primary goal is to establish order and stability. Crisis and emergency scenarios often fall into this domain.
The decision-making approach here is to "Act – Sense – Respond." You need to act decisively to address the most pressing issues, sense where there is stability and where there isn't, and then respond to move the situation from chaos to complexity.
To navigate chaotic situations successfully, conduct a Risk Analysis to identify possible risks, prioritize them with a Risk Impact/Probability Chart , and make sure that you have a comprehensive crisis plan in place. It's impossible to prepare for every situation, but planning for identifiable risks is often helpful.
Reliable information is critical in uncertain and chaotic situations, so make sure you know how to communicate in a crisis .
It can be extremely difficult to identify when you're in a "disorder" situation. Here, it isn't clear which of the other four domains is dominant, and people generally rely on decision-making techniques that are known and comfortable. Your primary goal in this situation is to gather more information , so that you can move into a known domain and then take the appropriate action.
José and his team recently rolled out an innovative new e-reader. However, it has developed an issue, and no one can agree on what's causing it. Dissatisfied customers are returning the product and the company's reputation has taken a hit. José is managing a number of issues. He has to help his team uncover the cause of the problem so it can be fixed, he's working with marketing to compensate customers, and he's answering questions from the media about the e-reader's issue.
He uses the Cynefin framework to gain a better understanding of the situation, and he categorizes it as "complicated," which means he needs to take a Sense – Analyze – Respond approach.
So, he brings in experts from research and development, IT and manufacturing to help him diagnose the problem. Working closely with his team, these experts list the quality concerns and then focus on each one individually to find the root cause of the problem.
After several days of analysis, everyone agrees that the problem is caused by dry solder joints. Working together, the consultants and José's team come up with a clear plan to address this and ensure that no more faulty e-readers are shipped.
The Cynefin framework was developed by David J. Snowden in 1999. It aims to help leaders understand that every situation is different and requires a unique approach to decision making.
The framework outlines five situational domains that are defined by cause-and-effect relationships. They are:
- Complicated.
Each of these domains has a specific decision-making approach that helps you make better sense of the situation, and choose the most appropriate way forward.
Apply This to Your Life
Practice using the Cynefin framework the next time you have an important decision to make at work. Aim to identify the domain you're in correctly, and use the appropriate decision-making approach to process information and move forward.
Snowden, D. and Boone, M. (2007). 'A Leader’s Framework for Decision Making,' Harvard Business Review , November 2007. (Available here .)
You've accessed 1 of your 2 free resources.
Get unlimited access
Discover more content
The iron triangle of project management.
Balancing Your Budget, Scope and Schedule
Gap Analysis
Reaching Your Ideal Future State
Add comment
Comments (0)
Be the first to comment!
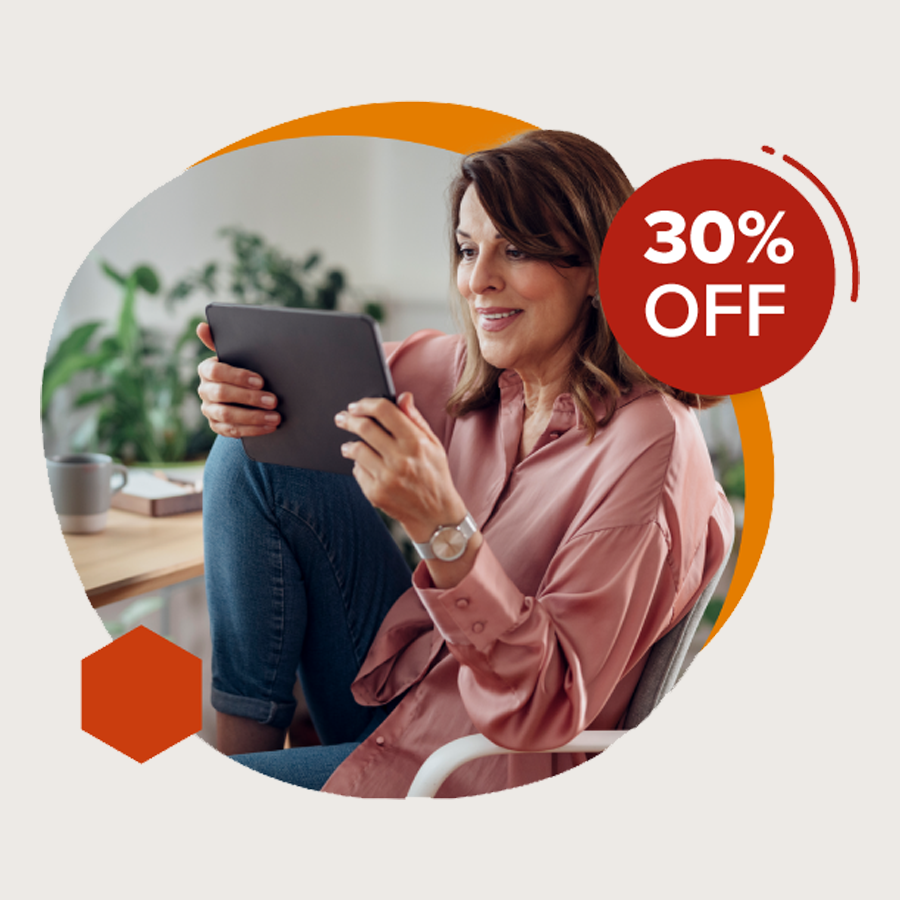
Gain essential management and leadership skills
Busy schedule? No problem. Learn anytime, anywhere.
Subscribe to unlimited access to meticulously researched, evidence-based resources.
Join today and take advantage of our 30% offer, available until May 31st .
Sign-up to our newsletter
Subscribing to the Mind Tools newsletter will keep you up-to-date with our latest updates and newest resources.
Subscribe now
Business Skills
Personal Development
Leadership and Management
Member Extras
Most Popular
Latest Updates
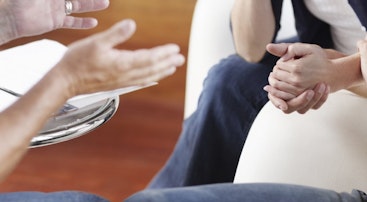
Winning Body Language
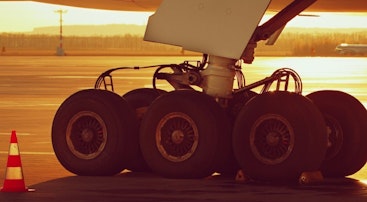
Business Stripped Bare
Mind Tools Store
About Mind Tools Content
Discover something new today
Nine ways to get the best from x (twitter).
Growing Your Business Quickly and Safely on Social Media
Managing Your Emotions at Work
Controlling Your Feelings... Before They Control You
How Emotionally Intelligent Are You?
Boosting Your People Skills
Self-Assessment
What's Your Leadership Style?
Learn About the Strengths and Weaknesses of the Way You Like to Lead
Recommended for you
An overview of knowledge management.
Knowledge Management and the Challenges in Promoting Effective Knowledge Sharing
Business Operations and Process Management
Strategy Tools
Customer Service
Business Ethics and Values
Handling Information and Data
Project Management
Knowledge Management
Self-Development and Goal Setting
Time Management
Presentation Skills
Learning Skills
Career Skills
Communication Skills
Negotiation, Persuasion and Influence
Working With Others
Difficult Conversations
Creativity Tools
Self-Management
Work-Life Balance
Stress Management and Wellbeing
Coaching and Mentoring
Change Management
Team Management
Managing Conflict
Delegation and Empowerment
Performance Management
Leadership Skills
Developing Your Team
Talent Management
Problem Solving
Decision Making
Member Podcast
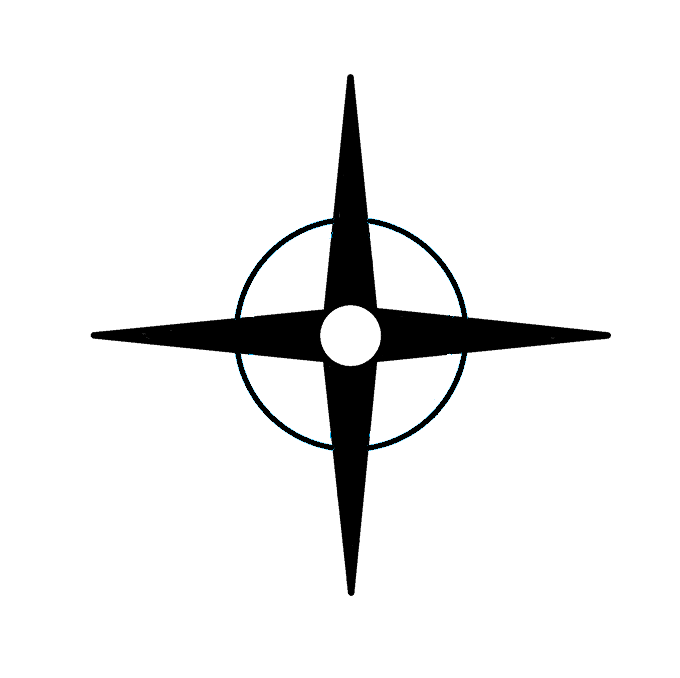
The Cynefin Framework for Decision Making
Discover a model that helps leaders categorize problems and apply appropriate decision-making approaches.
Essential Insights
- The Cynefin Framework categorizes situations into five domains to guide decision-making based on complexity.
- It emphasizes understanding the nature of the problem before selecting an appropriate approach.
- This tool helps leaders avoid placing a complex issue into a simple framework, which can lead to ineffective solutions.
"The Cynefin framework distinguishes between systems that are ordered, unordered, complex, or chaotic." - Dave Snowden
Introduction
In the dynamic landscape of leadership, decisions play a pivotal role in shaping the trajectory of organizations. One framework that has garnered significant attention in recent years for its ability to navigate the complexities of decision-making is the Cynefin Framework. The Cynefin Framework, developed by Dave Snowden, offers a unique perspective on decision-making by categorizing situations into five domains: Simple, Complicated, Complex, Chaotic, and Disorder. Each domain requires a distinct approach, providing leaders with a structured way to analyze and respond to challenges effectively. Understanding the Cynefin Framework is essential for leaders as it equips them with the tools to make informed decisions in various contexts. By recognizing the nature of a situation and applying the appropriate strategies outlined in the framework, leaders can navigate uncertainty with confidence and clarity. Leaders must consider the nuances of each domain within the Cynefin Framework and tailor their decision-making processes accordingly. While some situations may call for ordered and structured responses, others may require adaptive and emergent approaches. Being able to discern between these domains is crucial for effective leadership in today's ever-evolving business landscape. As we delve into the intricacies of the Cynefin Framework for Decision Making, it is evident that this topic holds immense significance for leaders seeking to enhance their decision-making capabilities. By embracing the principles of the framework and integrating them into their leadership practices, individuals can navigate complexity, drive innovation, and steer their organizations towards success.
Cynefin Framework Defined
The Cynefin Framework is a conceptual tool used in the field of leadership and decision-making to help individuals understand the nature of a given situation and make appropriate choices. Developed by Dave Snowden, the framework categorizes situations into five domains: Simple, Complicated, Complex, Chaotic, and Disorder. By placing a particular scenario into one of these domains, leaders can better determine the most suitable approach for problem-solving and decision-making. The Cynefin Framework emphasizes the importance of recognizing the unique characteristics of each domain and adapting one's leadership style accordingly to effectively navigate diverse challenges.
Importance of Cynefin Framework
The Cynefin Framework is crucial for leaders as it provides a way to categorize complex systems and situations into distinct domains - simple, complicated, complex, and chaotic. By understanding which domain a particular issue falls into, leaders can tailor their approach and decision-making strategies accordingly. This framework helps leaders avoid a one-size-fits-all approach, enabling them to adapt, navigate uncertainty, and make more effective choices based on the nature of the problem at hand. Embracing the Cynefin Framework empowers leaders to tackle challenges with a more nuanced and context-specific perspective, ultimately leading to better outcomes.
Cynefin Framework
The Cynefin Framework for Decision Making is a valuable tool for leaders to navigate complex situations effectively. Developed by Dave Snowden, this framework categorizes situations into five domains: Simple, Complicated, Complex, Chaotic, and Disorder. Each domain requires different approaches to decision making in order to achieve successful outcomes. In the Simple domain, situations are clear and cause-and-effect relationships are easily identifiable. Leaders can apply best practices and standard operating procedures to address issues in this domain. The Complicated domain involves multiple factors and requires expert knowledge to analyze and solve problems. Leaders in this domain need to consult with specialists and conduct in-depth analysis to make informed decisions. The Complex domain is characterized by uncertainty and unpredictability, making it challenging for leaders to determine cause-and-effect relationships. In this domain, leaders must adopt a probe-sense-respond approach by running small experiments, observing patterns, and adjusting their strategies accordingly. The Chaotic domain is marked by extreme volatility and requires immediate action to stabilize the situation. Leaders must act decisively to contain chaos and restore order. Lastly, the Disorder domain represents a state of confusion where the nature of the problem is unclear. Leaders need to first assess the situation and then categorize it into one of the four domains of the Cynefin Framework. Once the domain is identified, leaders can apply the appropriate decision-making methods to address the issue effectively and lead their teams toward success.
Application Ideas
The Cynefin Framework provides leaders with a valuable tool to analyze and categorize the complexity of situations they encounter. This framework outlines five domains: Simple, Complicated, Complex, Chaotic, and Disorder. To apply the Cynefin Framework effectively, leaders must first recognize the domain in which their current situation falls. In the Simple domain, characterized by known cause-and-effect relationships, leaders can apply best practices and standard operating procedures to find solutions. Making decisions based on past experiences and established rules is key in this domain. In the Complicated domain, where cause and effect relationships are not immediately clear, leaders should consult with experts and analyze data to develop a proper course of action. It is crucial to deeply investigate the issue at hand and gather all pertinent information to make an informed decision. Leaders should focus on understanding the underlying factors contributing to the problem and leveraging expertise to navigate through the complexity. When facing situations in the Complex domain, where cause and effect relationships are only understood in hindsight, leaders should foster creativity and experimentation. Embracing diverse perspectives and encouraging collaboration within teams can lead to innovative solutions in this domain. Leaders should be open to adaptability, continuously experimenting, and learning from failures to navigate complexity effectively. In the Chaotic domain, characterized by high unpredictability and turbulence, leaders must act swiftly and decisively to restore order. Implementing rapid response mechanisms, containing the immediate crisis, and gradually transitioning to a more stable state are vital in this domain. Leaders should focus on crisis management, restoring order, and creating a sense of direction amidst chaos to move towards a more manageable situation.
Reflection Questions
- How can you identify and classify the different types of problems within the Cynefin Framework in your current leadership challenges?
- What strategies can you use to effectively navigate the complex domain of the Cynefin Framework while making decisions?
- How can you ensure that you are not simplifying problems that actually belong in the complex or chaotic domains of the Cynefin Framework?
- What steps can you take to encourage innovative solutions when facing problems that fall within the complex domain?
- How can you leverage the Cynefin Framework to improve team collaboration and decision-making processes?
- What are some potential pitfalls to avoid when applying the Cynefin Framework in your leadership role?
- How can you use the Cynefin Framework to better understand the dynamics of uncertainty and ambiguity in your organization?
- What can you do to continuously assess and reassess the situational context of your leadership challenges through the lens of the Cynefin Framework?
Related Topics
- Adaptive Leadership
- An approach that focuses on navigating complex challenges by empowering teams to experiment and learn from their experiences.
- Sensemaking
- The process of interpreting and making sense of information in uncertain and complex situations to guide decision-making.
- An acronym for volatility, uncertainty, complexity, and ambiguity, describing the unpredictable nature of today's business environment.
- Complex Systems Thinking
- An approach that analyzes systems as a whole, emphasizing the interactions and relationships between components to understand complex phenomena.
Recommended Reading
Shop Leadership on Amazon
- Cynefin - Weaving Sense-Making into the Fabric of Our World by Dave Snowden
- Navigating Complexity by Jean Boulton, Peter Allen, and Cliff Bowman
- Complexity: A Guided Tour by Melanie Mitchell
FAQs About Cynefin Framework
What is the cynefin framework.
The Cynefin Framework is a sense-making model developed by Dave Snowden to help leaders understand the complexity of different situations. It categorizes problems into five domains: Simple, Complicated, Complex, Chaotic, and Disorder. By recognizing the domain a situation falls into, leaders can determine the appropriate approach to address it effectively. The framework assists in decision-making by offering insights into the nature of the challenge at hand and guiding leaders on the best course of action.
What are the main domains in the Cynefin Framework?
In the Cynefin Framework, there are five main domains: Simple, Complicated, Complex, Chaos, and Disorder. Each domain represents a different context in which leaders may find themselves operating. The Simple domain is characterized by clear cause-and-effect relationships, the Complicated domain involves experts and known solutions, the Complex domain is where solutions emerge through experimentation and adaptation, Chaos represents a turbulent environment requiring immediate action, and Disorder indicates uncertainty about which domain is in play. Understanding these domains can help leaders navigate different situations effectively.
What are the key principles of the Cynefin Framework?
In the Cynefin Framework, there are four key domains that represent different types of systems: Simple, Complicated, Complex, and Chaotic. Each domain has its principles that guide decision-making. In the Simple domain, best practices and clear cause-and-effect relationships apply. In the Complicated domain, expertise and analysis are crucial in determining the right course of action. The Complex domain involves emergent practices, where solutions are discovered through experimentation and adaptation. Lastly, the Chaotic domain requires immediate action to stabilize the situation before transitioning to one of the other domains. Understanding these principles helps leaders navigate various situations effectively.
How can the Cynefin Framework help leaders make better decisions?
The Cynefin Framework is a sense-making model that helps leaders understand the complexity of a situation and make appropriate decisions based on that understanding. By categorizing problems into five domains – Simple, Complicated, Complex, Chaotic, and Disorder – leaders can tailor their approaches to each domain. In the Simple domain, where the relationship between cause and effect is clear, leaders can apply best practices. In the Complicated domain, where causal relationships may require analysis, experts are often needed. In the Complex domain, where outcomes are emergent and unpredictable, experimentation and probes are crucial. In the Chaotic domain, where urgent action is required, leaders must stabilize the situation before moving forward. By using the Cynefin Framework, leaders can navigate uncertainty and make more informed decisions.
Teach About Cynefin Framework
Here are some ideas for teaching Cynefin Framework to your team, club, group, etc.
Case Studies Analysis
- Provide case studies involving real-life scenarios or experiences your team is currently working through or may likely face in the future.
- Divide participants into groups to analyze the cases, identify key communication challenges, and propose effective strategies for executive communication.
- Encourage discussion on the potential impact of the skills and application ideas discussed in the case study.
- Learn more about case studies
- Below is an example case study about Cynefin Framework. Consider creating your own case studies for situations your team is currently facing or is likely to encounter in the future.
Case Study: Applying the Cynefin Framework in a Team Decision-Making Process Imagine that you are leading a project team that is tasked with developing a new product for your company. As the project progresses, various challenges arise, and the team is struggling to make decisions effectively. By introducing the Cynefin Framework to the team during a training session, you guide them to categorize the project's issues into simple, complicated, complex, and chaotic domains. Through this exercise, the team gains a better understanding of the nature of each problem they face and can apply appropriate strategies to address them. The team starts to use the framework regularly in their decision-making processes, leading to improved outcomes and a more cohesive approach to tackling challenges.
Guest Speaker Sessions
- Invite experienced members of your team or subject matter experts to share insights, best practices, and real-world examples of Cynefin Framework.
- Organize Q&A sessions where participants can engage directly with the guest speakers to gain valuable perspectives and advice.
- Encourage participants to reflect on how they can apply the insights gained to their current situations.
Book Club Discussion
- Select a book for your team to review. A few recommended books about Cynefin Framework are listed above. Solicit book ideas from your team members.
- Communicate the reading schedule, meeting date, time, and location well in advance. Consider setting a pace that is manageable for all members to encourage thorough reading and reflection.
- Prepare a list of open-ended questions that prompt analysis, personal reflection, and connections to current situations and challenges. These questions should serve as a guide rather than a strict agenda. Invite participants to share discussion questions.
- During the discussion, encourage contributions from all members while being mindful of potentially dominating voices. Use facilitation techniques such as directing questions to quieter members or breaking into smaller groups if the club is large.
Lead a Group Discussion About Cynefin Framework
- Clearly define the goals of the discussion you want to have with your team. Are you aiming to explore new ideas, solve a problem, make a decision, or share knowledge? Understanding the purpose will shape the direction of the discussion.
- Establish the scope of the topic to keep the discussion focused and prevent it from veering off into tangential areas. It's important to communicate these boundaries to participants ahead of time.
- A list of potential questions about Cynefin Framework are listed above in the "Reflection Questions" section.
- Conclude the discussion by summarizing the key points, insights gained, and any decisions made. If applicable, outline any action items or follow-up tasks that emerged from the discussion. Assign responsibilities and deadlines to ensure accountability.
Affiliate Disclaimer
Some of the links on this website may be affiliate links. This means that, at no additional cost to you, we may earn a commission if you click through and make a purchase. Your support through these affiliate links helps sustain and improve the quality of the content we provide.
Subscribe to Leader Navigation
- 1.1 In journals
- 1.2 Blogs, company web sites, reports, gray literature and the like
- 2 Wiki members
This section is for links to published case studies and articles
In journals
- Households’ aspirations for rural development through agriculture Mausch et al., in SAGE JOURNALS, use of SenseMaker®
- Implementation of a SenseMaker® research project among Syrian refugees in Lebanon Bakhache et al., in Global Health Action (2017), use of SenseMaker®
- SenseMaker® as a monitoring and evaluation tool to provide new insights on gender-based violence programs and services in Lebanon Bartels SA et al., in Evaluation and Program Planning (2019), use of SenseMaker®
- Luke Metelerkamp, Scott Drimie & Reinette Biggs (2019) We’re ready, the system’s not – youth perspectives on agricultural careers in South Africa , Agrekon, 58:2, 154-179, DOI: 10.1080/03031853.2018.1564680
- Omoding, J., Walters, G., Andama, E., Carvalho, S., Colomer, J., Cracco, M., ... & Schelle, C. (2020). Analysing and Applying Stakeholder Perceptions to Improve Protected Area Governance in Ugandan Conservation Landscapes . Land, 9(6), 207.
- Van der Merwe, Liza & Biggs, Reinette & Preiser, Rika. (2020). Sensemaking as an approach for resilience assessment in an Essential Service Organization . Environment Systems and Decisions. 40. 84-106. 10.1007/s10669-019-09743-1.
- Walters, Gretchen et al.(2021) “COVID-19, Indigenous peoples, local communities and natural resource governance.” .
- Fraulin, G., Lee, S., Lusamba, S. et al. “It was with my consent since he was providing me with money”: a mixed methods study of adolescent perspectives on peacekeeper-perpetrated sexual exploitation and abuse in the Democratic Republic of Congo. Confl Health 15, 80 (2021). https://doi.org/10.1186/s13031-021-00414-0
- Illuminating and mitigating the evolving impacts of COVID-19 on ethnocultural communities: a participatory action mixed-methods study Denise Campbell-Scherer, Yvonne Chiu, Nicole Naadu Ofosu et al., in CMAJ (2021), use of SenseMaker®
- Group singing as a resource for the development of a healthy public David Camlin et al., in NATURE, use of SenseMaker®
- Mausch, K., Harris, D., Dilley, L. et al. (2021). Not All About Farming: Understanding Aspirations Can Challenge Assumptions About Rural Development . Eur J Dev Res 33: 861-884. https://doi.org/10.1057/s41287-021-00398-w
- Groot, B., de Kock, L., Liu, Y., Dedding, C., Schrijver, J., Teunissen, T., van Hartingsveldt, M., Menderink, J., Lengams, Y., Lindenberg, J., & Abma, T. (2021). The Value of Active Arts Engagement on Health and Well-Being of Older Adults: A Nation-Wide Participatory Study . International journal of environmental research and public health, 18(15), 8222. https://doi.org/10.3390/ijerph18158222
- Johan Enqvist, Gina Ziervogel, Luke Metelerkamp, John van Breda, Ntombikayise Dondi, Thabo Lusithi, Apiwe Mdunyelwa, Zinzi Mgwigwi, Mpumelelo Mhlalisi, Siya Myeza, Gciniwe Nomela, Ann October, Welekazi Rangana & Maggie Yalabi (2022) Informality and water justice: community perspectives on water issues in Cape Town’s low-income neighbourhoods , International Journal of Water Resources Development, 38:1, 108-129, DOI: 10.1080/07900627.2020.1841605
- Ziervogel, G., Enqvist, J., Metelerkamp, L., & van Breda, J. (2022). Supporting transformative climate adaptation: community-level capacity building and knowledge co-creation in South Africa. Climate Policy, 22(5), 607-622. https://www.tandfonline.com/doi/pdf/10.1080/14693062.2020.1863180
- Dohmen MDW, van den Eijnde C, Thielman CLE, Lindenberg J, Huijg JM, Abma TA (2022). Good Care during COVID-19: A Narrative Approach to Care Home Staff's Experiences of the Pandemic . Int J Environ Res Public Health. 19(4):2106. doi: 10.3390/ijerph19042106. Use of SenseMaker
- Informal and Incidental Learning in the Clinical Learning Environment: Learning Through Complexity and Uncertainty During COVID-19 Papanagnou, Dimitrios MD, MPH1; Watkins, Karen E. PhD2; Lundgren, Henriette PhD3; Alcid, Grace A. MA4; Ziring, Deborah MD5; Marsick, Victoria J. PhD6. Informal and Incidental Learning in the Clinical Learning Environment: Learning Through Complexity and Uncertainty During COVID-19. Academic Medicine: August 2022 - Volume 97 - Issue 8 - p 1137-1143 doi: 10.1097/ACM.0000000000004717 Use of the Cynefin Framework
- Christine Wamsler, Gustav Osberg, Anna Panagiotou, Beth Smith, Peter Stanbridge, Walter Osika & Luis Mundaca (2022) Meaning-making in a context of climate change: supporting agency and political engagement , Climate Policy, DOI: 10.1080/14693062.2022.2121254 Use of SenseMaker®
- Joshi, D.; Panagiotou, A.; Bisht, M.; Udalagama, U.; Schindler, A (2023) A. Digital Ethnography? Our Experiences in the Use of SenseMaker for Understanding Gendered Climate Vulnerabilities amongst Marginalized Agrarian Communities , Sustainability, 15, 7196. https://doi.org/10.3390/su15097196 Use of SenseMaker®
- Cunningham C, Vosloo M, Wallis LA (2023) Interprofessional sense-making in the emergency department: A SenseMaker study , PLoS One 18(3). doi: 10.1371/journal.pone.0282307. PMID: 36893158 Use of SenseMaker®
- Gambir, K., Matsika, A.B., Panagiotou, A. et al. (2024) Associations between child marriage and food insecurity in Zimbabwe: a participatory mixed methods study , BMC Public Health 24, 13 (2024). https://doi.org/10.1186/s12889-023-17408-7 Use of SenseMaker®
- Schlaile, M., Hector, V., Peters, L., Bäuerle, L., Smith, B., Hilt, A. & Graupe, S. (2024) Innovation Amidst Turmoil: A SenseMaker Study of Managerial Responses to the COVID‑19 Crisis in Germany. , Journal of Innovation Economics & Management, 43, 285-318. https://doi.org/10.3917/jie.pr1.0154 Use of SenseMaker®
- Osberg, G., M. Islar, and C. Wamsler. (2024) Toward a post-carbon society: supporting agency for collaborative climate action. , Ecology and Society 29(1):16. https://doi.org/10.5751/ES-14619-290116 Use of SenseMaker®
Blogs, company web sites, reports, gray literature and the like
- Using the SenseMaker® site to illustrate how to format
- Die Politik der kleinen Schritte: Sensemaking und kollektive Veränderung unter komplexen Bedingungen . Von Anne Caspari (LiFT)
- The Future of Higher Education in Moldova: a look into students' perceptions . (2020)
- Related: Sensemaker: Combining Stories, Numbers and Data Analytics to Uncover Hidden Dimensions of Relationships . A method note on the application of the project above.
- Using sensemaker to measure, learn and communicate about smallholder farmer inclusion . Leuven: VECO. Online. http://betterevaluation . org/resources/example/sensemaker_veco. Deprez, S., Huyghe, C., & Van Gool Maldonado, C. (2012)
- A Student Sense of Belonging At Georgetown: First Generation Student Undergraduate Experiences . Georgetown University: The Hub for Equity and Innovation in Higher Education. Morrison, Molly, Young, Toddchelle, Elmendorf, Heidi. (2020)
- SenseMaker: the lived experience of nursing in Northern Ireland during a pandemic 2020/2021 . Northern Ireland: Royal College of Nursing. (2021)
- PwC’s Data and Analytics Survey 2016
- Building Back Better: Making Sense of Drought and Resilience in Afghanistan , by UNDP (2020)
- Making sense of a water crisis documentary, by the Western Cape Water Caucus and researchers from University of Cape Town’s African Climate and Development Initiative (2021)
- Citizen Engagement & Democratic Innovation Case Studies including Measuring the Mountain, Valley Stories, Malmö Libraries, My Malmö, The People's Platform, Citizens Take Over Europe, Extinction Rebellion UK, and Live learning Welsh Storybook, with more coming soon.
- PONTE Media Futures fund and PONTE Media Futures Results
- Covid-19, Indigenous Peoples, Local communities and natural resource governance . A study from the Institute of Geography and Sustainability of the University of Lausanne (2022)
- How is life: micro-narratives on the impact of the Ukraine crisis in the Republic of Moldova . UNDP Moldova partnering with The Cynefin Company to explore the experiences of both Ukrainians refugees in Moldova, and the local Moldovan citizens. (2022)
- Evaluation of the School Meals Programme . A report from RSM Ireland evaluating the impact and directions of the School Meals Programme. (2023)
- Enlightened Gardens: Innovation Beyond the Obvious . Using SenseMaker® to understand consumers' attitudes towards urban gardening and lighting. (2011)
Wiki members
Anyone should feel free to write a case study, please do so as a separate article here and link
- longer description (using case template to illustrate formatting)
Navigation menu

Want to create or adapt books like this? Learn more about how Pressbooks supports open publishing practices.
6 The Cynefin Framework: Framing Decisions and Understanding Context
The Cynefin Framework
Framing Decisions and Understanding Context: The Cynefin Framework
An impactful project requires skills in logic, analysis and evaluation and applying those skills to persuasive reasoning and decision-making. This is based on critical, agile , and creative thinking.
This combination of application and learning will help you feel more confident as you develop good strategies of logic and reasoning you can use in your work and use these for strategic purposes. It will also help you to be confidence in the insights and recommendations you make to your sponsors and your agency or department leaders.
It is essential to understand the nature of the problems, challenges and opportunities that exist in your context and which influence and shape the decisions to be made.
David Snowden and the Cynefin Framework he has developed will help you in this regard. David Snowden of The Cognitive Edge has spent many years developing the concept of The Cynefin Framework which is a problem and decision-framing approach. You may have explored this concept in your other units. This complexity-framing model is one of the key thinking models that we use at QUT. If you have used it already keep going as each time you approach a new issue or problem with The Cynefin Framework, it is likely that you will learn something new.
Understanding an industry’s context and its competitive environment is a highly complex task. Decisions must be made with less than perfect knowledge, and often include highly dynamic variables, especially when technological disruption is likely to upend long-held assumptions. David Snowden proposes a decision framework for senior strategists and marketers.
David Snowden notes that when we are confronted with contexts and situations when we need to decide and act, “ … we will interpret the situation according to our personal preference for action” (Snowden, 2010).
To address this, The Cynefin Framework “gives us a very easy way of deciding how to work but it [also] gives us a divergent [way of thinking] – we sometimes call this requisite applicability. It basically says dependent on which space you’re in you should think differently, should analyze differently, rather the one-size-fits-all which has been a tradition of management theory” (Snowden, 2010).
To begin, watch the following video.
https://mediahub.qut.edu.au/media/t/0_pzhvy38l
http://<iframe id=”kmsembed-0_pzhvy38l” width=”400″ height=”285″ src=”https://mediahub.qut.edu.au/embed/secure/iframe/entryId/0_pzhvy38l/uiConfId/23452303″ class=”kmsembed” allowfullscreen webkitallowfullscreen mozAllowFullScreen allow=”autoplay *; fullscreen *; encrypted-media *” referrerPolicy=”no-referrer-when-downgrade” sandbox=”allow-forms allow-same-origin allow-scripts allow-top-navigation allow-pointer-lock allow-popups allow-modals allow-orientation-lock allow-popups-to-escape-sandbox allow-presentation allow-top-navigation-by-user-activation” frameborder=”0″ title=”Kaltura Player”></iframe>
Activity: How to use the Cynefin Framework
Consider where in the decision framework the set of decisions you need to make, should fit. Consider the likely mindset of the organisations that would potentially be making these long term strategic decisions. Ponder this for a moment.
As you watch the video, listen carefully for the nuances about each space, and be careful about the ‘cliff space’ between Simple and Chaotic. Also please have the reflection questions below in mind as you watch.
Consider your observations and reactions in the context of your current, and perhaps, past situations of decisions making, actions and outcomes. The image below summarises the emergent practices for each quadrant.
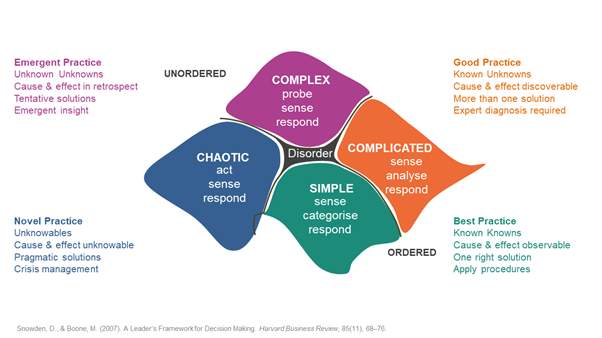
- Consider your observations and reactions in the context of your current, and perhaps, past situations of decisions making, actions and outcomes.
- How does the framework add to your understanding of how you, your teams and your organisation overall see and make decisions?
- How might you use this complexity decision framework in your own work? In your HIP thinking or as you approach the opportunity or challenge?
- Think about your own view of the world. What is your default approach to issues and decision situations?
- Think about how you might share with your immediate team your insights and preferences. How might you deal with these in your work context? What impact will your preferences and those of others, have on the outcomes?
David Snowden suggests that by addressing issues from the place of complexity thinking, we not only have a way to work but the Cynefin Framework also gives us a divergent way of thinking. This is based on the idea that the more input into our thinking and decisions models, the more possibilities and opportunities we can explore.
To sum up, Snowden says The Cynefin Framework “gives us a very easy way of deciding how to work but it (also) gives us a divergent (way of thinking) – we sometimes call this requisite applicability. It basically says dependent on which space you’re in, you should think differently, should analyze differently, rather than one-size-fits-all which has been a tradition of management theory”.2
Here is a final word that might help you put this lesson in context and get us ready for the next lesson. The famous American General Stanley McChrystal3 discusses complicated and complex in detail. He represents the differences in this way:
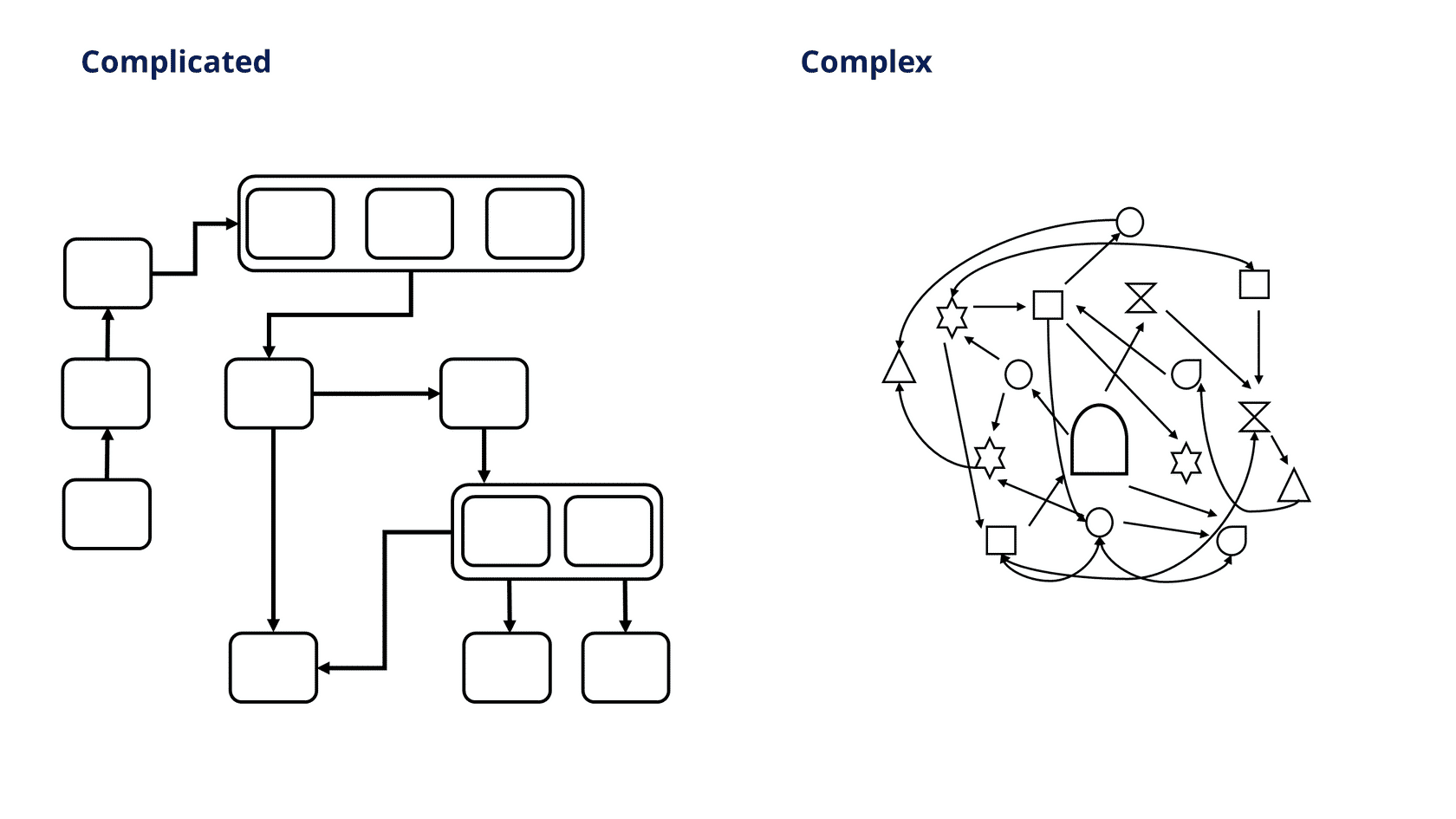
Adapted from: McChrystal, S. C., Tantum; Silverman, David; Fussel, Chris. (2015). Team of Teams: New Rules of Engagement for a Complex World. Portfolio Penguin.
He says that complexity occurs when the number of interactions between components increases dramatically. His wrap-up statement is that the world has become in many ways less predictable despite technology and data-gathering and mining advances.
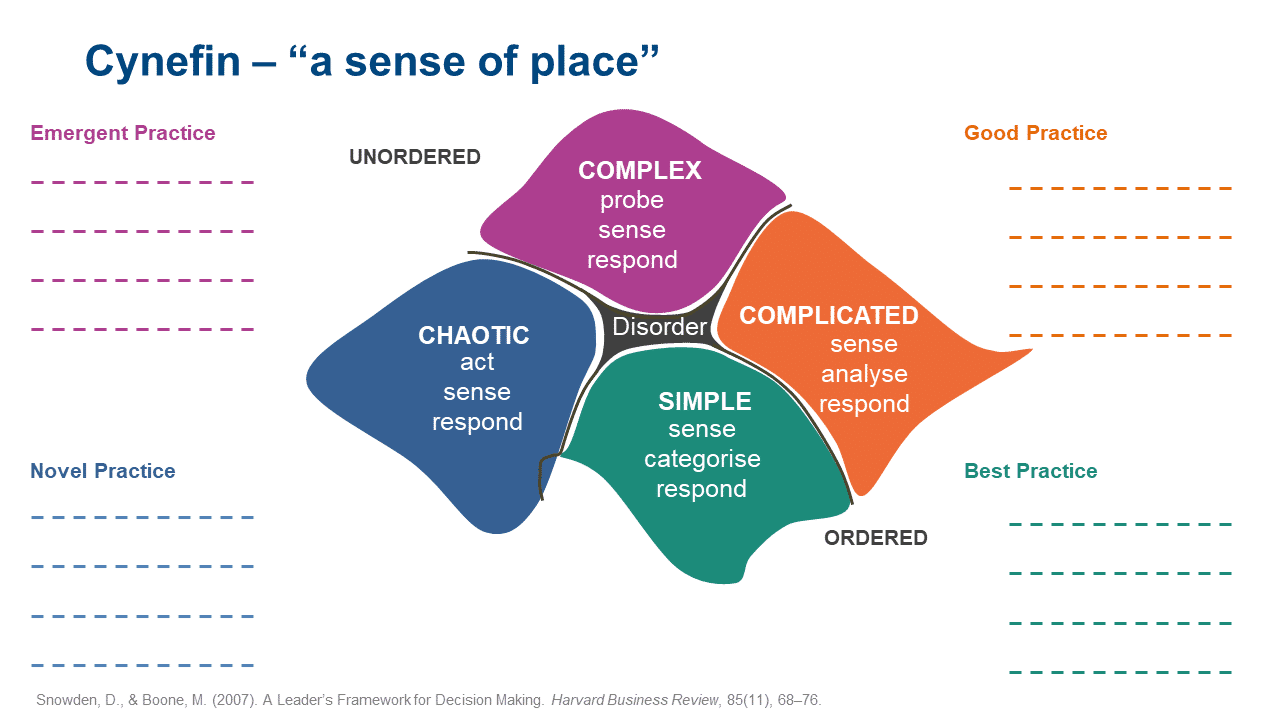
Decision Framework Template: Cynefin template ppt 2
- Snowden, D. J., & Boone, M. E. (2007). A leader’s framework for decision making. Harvard business review, 85(11), 68.
- McChrystal, S. C., Tantum; Silverman, David; Fussel, Chris. (2015). Team of Teams: New Rules of Engagement for a Complex World. Portfolio Penguin.
The Context: Internal and External Copyright © by Antony Peloso. All Rights Reserved.
Share This Book
HYPOTHESIS AND THEORY article
Cynefin as reference framework to facilitate insight and decision-making in complex contexts of biomedical research.
- 1 German Center for Neurodegenerative Diseases (DZNE) Dresden, Dresden, Germany
- 2 Center for Regenerative Therapies Dresden (CRTD), Technische Universität Dresden, Dresden, Germany
The Cynefin scheme is a concept of knowledge management, originally devised to support decision making in management, but more generally applicable to situations, in which complexity challenges the quality of insight, prediction, and decision. Despite the fact that life itself, and especially the brain and its diseases, are complex to the extent that complexity could be considered their cardinal feature, complex problems in biomedicine are often treated as if they were actually not more than the complicated sum of solvable sub-problems. Because of the emergent properties of complex contexts this is not correct. With a set of clear criteria Cynefin helps to set apart complex problems from “simple/obvious,” “complicated,” “chaotic,” and “disordered” contexts in order to avoid misinterpreting the relevant causality structures. The distinction comes with the insight, which specific kind of knowledge is possible in each of these categories and what are the consequences for resulting decisions and actions. From student's theses over the publication and grant writing process to research politics, misinterpretation of complexity can have problematic or even dangerous consequences, especially in clinical contexts. Conceptualization of problems within a straightforward reference language like Cynefin improves clarity and stringency within projects and facilitates communication and decision-making about them.
Introduction
We regard many, if not most important biological and medical questions as complex, because they do not have single straightforward solutions. Life itself is complex and is more than the sum of its part: simple, direct causalities are only found within defined functional modules, such as chemical reactions and physical interactions (and even there can be challenged). Insight on larger-scale questions about “life” cannot be fully reconstructed bottom-up only from such modules. In large networks, probabilistic relationships and highly indirect influences blur mechanistic insight. Complex problems and questions in the life sciences have “ emerging properties ” that preclude that there are single true answers to questions of causalities. Cancer, neurodegeneration, consciousness, etc., cannot be comprehensively understood simply by adding up insights from partial aspects. They require a more holistic perspective, which, however, might ultimately be impossible to gain. While the large questions of life are the most obvious manifestations of a problem with complexity, the issue actually permeates biomedical research at every level.
In the clinical context this is much more obvious than in basic research. If an average 75 year-old patient accumulates five or more medical diagnoses, which all might lead to a more or less specific treatment, the resulting matrix of past, present, and future interactions at the level of the development of the disease, the co-developing (intrinsic) strategies of coping with the problems and the, in most cases: pharmacological, (extrinsic) treatments amount to a high-dimensional complexity of causalities and interferences that cannot be grasped–especially not intuitively in the emergency room. Even if these networks could be modeled theoretically, this will be of limited value in the acute situation. Medicine for older people is, even more than for younger subjects, a medicine of complexity and so is the underlying biology. The reason that many, especially chronic, diseases have not yet been conquered lies in exactly their complexity and our imperfect grip on this complexity.
The problem is, however, neither limited to aged subjects or humans, nor to medicine. Biomedical research as the science of life is interested in causes and mechanisms underlying complex quantitative processes. Phenotypes tend to be gradual and are often found in a normal distribution. The strengths of reductionism generally turn into a risk, when the key to the sought solution lies in the emergent properties, which hide in the observable and measurable phenotypic appearance of complex traits, behaviors, diseases, etc., Most human traits, including the susceptibility to disease, are highly polygenic and to a variable degree subject to environmental and behavioral influences. The co-development of physiological traits with pathology results in extreme multi-dimensional complexity, challenging our ability to understand appropriately and act accordingly. In principle, the problem is thus relevant to any combination of physiological (and pathological) traits and across scales. This most elusive aspect of the picture might be the most critical one, especially for example, if it comes to translation from models to the clinic. Controlling for complexity must not result in ignoring it. The interpretation of the results might fail, if the full context is not appreciated, even though we might not be able to fully comprehend it and factor it in. So the first step is to be clearer in language and conceptualization.
While the diagnosis of complexity has thus become ubiquitous, concrete systems approaches in biology and medicine are attempts to extract usable insight from this complexity in order to guide novel approaches to gain insight on one side for treatment and for prevention on the other. With “systems biology,” “systems genetics,” “systems medicine” etc., sub-disciplines have evolved that specifically and by definition address the complexity of life, but the very same challenges permeate everyday research and medicine much more than is often realized. Quantitative biology calls for a particular mindset that is not always appreciated outside the seemingly fenced-off “systems” fields. In reality, however, essentially all biology is “systems,” because all biology deals with life and essentially all of biology has become quantitative. A better way to integrate “systems” ideas into normal biology and medicine is thus necessary.
In addition, outside the inner, concrete medical or clinical context the desire for insight into complex biological causalities is interwoven with a variable degree of translational intentions and aims, adding new layers of interdependencies and influences. Across the highly diverse spectrum of stakeholders from academic science and education over industrial research and development with its economic perspective, to societal and political interest groups, including patients, there is a wide range of perceptions of how our answers to complex questions and problems in biomedicine should look. Interpretation and decisions are not pure. The construct of science in our society is “multirational,” which means that overall aims, strategies, approaches, etc., do not follow one single rationale, but might be ill-aligned or conflicting. The perspective of a zebrafish or mouse researcher on “Alzheimer” is different from a clinician or a care professional, who nominally acts in the same field.
In this situation, it is an additional problem that biology as a whole has been relatively theory-adverse in coming to terms with what, in such situation, might constitute “mechanisms” and how we can interpret them. There are of course activities toward this end [for example in the work by Lindley Darden ( Craver and Darden, 2013 )], but appreciation has not generally caught on that a constant reflection on implicit assumptions and standards as well as the fundamental properties of the subjects are required in order to be successful.
It is also important to realize that scientific culture matters. Focus on the inner circle of biological reasoning has possibly prevented us from realizing that with “Cynefin” a relatively straightforward tool has been around for almost two decades which might help to change this situation and which we should now discover for the life sciences.
The Cynefin Framework
The Cynefin framework has been a influential concepts in management but largely unknown in the natural sciences and medicine. Cynefin was first published in 2000 by Snowden (2000) . A later paper in the Harvard Business Review from 2007 provided a first generalization as strategy model irradiating well beyond the original management contexts ( Snowden and Boone, 2007 ). Cynefin ( kuh-ne-vin ) is the welsh word for habitat or rootedness and was used by Snowden as metaphor for a conceptual framework for “time and place” to make decisions in complex situations. Complexity is certainly no privilege to business and economy, however, and the arguments that Snowden and others developed generalize easily to other fields. It has, for example, been famously used to describe (and make sense of) the decision making during the G. W. Bush presidency ( ONeill, 2004 ). An application to later administrations might be similarly enlightening.
There have also been some applications to medicine ( Mark, 2006 ; Sturmberg and Martin, 2008 ; Van Beurden et al., 2013 ) but by and large, Cynefin has not yet had a measurable impact on life sciences. This might have cultural reasons and be partly due to the emphasis on decision making that Snowden's original text had, but the tools from Cynefin to make sense of complexity appear to be highly useful for current biology and medicine as well.
The Cynefin framework allows contextualization of situations that require a decision and response by providing a reference language (Figure 1 ). The key assumption is that such situations fall into one of five categories, which call for substantially different but definable conclusions and mode of actions, and that awareness of these principal differences allows more structured insight and better-informed decisions.
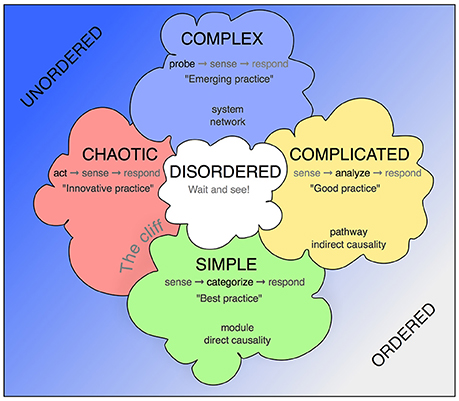
Figure 1 . The Cynefin scheme. This version is one of the many renderings of the Cynefin framework highlighting and explaining the five core contexts. Unlike some other replications, this version uses the arrangement from Snowden's original publication from 2007, in which the square stands on one corner, so that the “simple/obvious” category is found at the base and the “complex” category at the top. More commonly found is an arrangement with “chaotic” and “simple” next to each other at the bottom and “complex” and “complicated” as a top row. The version here has the advantage of highlighting the complex contents and assigning the simple contexts in their modularity a role at the basis. In some fields, quintessential concepts from biomedicine have been added.
Simple or Obvious Contexts
These are the domain of clear-cut causality, in which B follows from A. Dealing with simple or obvious contexts requires to describe (“sense”) the situation, assign it to pre-established categories and act according to the defined procedure for that category. The resulting action can adhere to fixed routines, called “best practice” or standard operating procedures. Simple context are the procedural backbone of more complex contexts. In the language of systems biology they relate to the modules, self-contained processes and functional units, such as protein-protein interactions, basic chemical reactions, etc.
In research, simple contexts comprise only what is known already: the “known knowns.” But what is known does not raise questions anymore and without questions no science exists. Simple contexts are thus only the building blocks for addressing the larger questions. Standard operating procedures can invariably apply only to methods that are used, not the entire experiment. Mistaking questions as simple and thus applying only the SOPs will almost invariably miss the point.
A single mutation, for example, does not generate a simple context. The knockout of any gene has reverberations throughout the genome that go far beyond the immediate function of that gene. Relatedly, the same mutation has different consequences on different genetic backgrounds ( Sittig et al., 2016 ). Even classical Mendelian genetics is not simple or obvious, and monogenic does not mean mono-causal.
Complicated Contexts
Contexts, in which causalities can at least in theory (or retrospect) be known but are non-linear and difficult to untangle are called “complicated.” In this category the causalities are potentially discoverable and this is why at this level the first research questions can appear. We here deal with “unknown knowns.” which means that the questions can be addressed by not venturing too far from what is established, often in a way of extrapolation or combination, sideways application or, to some degree, generalization.
Complicated contexts have no single, obvious best solution and thus are the domain of experts (and consortia). Hence not best but only “good practice.” is possible. In research complicated contexts are common, but they are not necessarily the most important and interesting contexts, because they ultimately do not leave the comfort zone in terms of the openness of the possible question. Complicated problems can certainly be very complicated and pose large organizational and other challenges. Many cohort studies, for example, would initially fall into this category of massive undertakings, that while addressing complex questions, by design are “only” complicated. The distinction and its consequences are critical. Only once the data starts becoming available the efforts to untangle complexity within it can be started. This level has a different structure than the organization of the data acquisition. The genome project is an example of such project, in which the highly complicated endeavor led to a excessively complex data set.
If seen from the biological fact not the technological achievement, one would say that, conversely to common perception, complex situations might require approximations by means of complicated tools, whose inherent limitations have to be recognized and factored into the interpretation.
Boyle, Li and Pritchard have argued that for complex traits, the classical description as “polygenic” is not fully appropriate because the underlying causal networks extend to essentially the entire genome with minimal effect sizes from most genes, which in their entirety nevertheless explain most of the variance. They have coined the term “omnigenic” for this relationship ( Boyle et al., 2017 ).
In addition, much of the more classical exploratory research (expeditions, field studies, etc.,) will be found in this category, but, again, not necessarily their results and the ensuing next questions. A consequential mistake would arise from the failure to realize that more than complicated research this is needed to address complexity.
Complex Contexts
Although it is only one of the five contexts described by the Cynefin framework, complexity actually lies at the heart of the idea. The entire framework has been designed in order to identify this critical category correctly and allow decisions (actions) that are appropriate to the specific demands of these contexts, also in relation to the others. The complex contexts are those of the “known unknowns,” in which neither “best” nor “good” practice can be used, but “emergent practice” is needed. This description alone already suggests, why for the research context this category is fundamental.
As stated above, “complex” and “complicated” is not the same. In complex contexts we face a situation, in which key aspects are non-observable, latent or emergent. Here, (as well as the chaotic situation described next) action has to precede proper apprehension, in complex contexts in the form of probing the context. The complex situation is such that it has to be probed through (experimental) perturbations in order to reveal enough of the underlying causality structure to allow proper diagnosis and consequently insight and decision. That action is required before insight is possible is, fundamentally different from simple/obvious or complicated contexts, where a certain level understanding can or even must precede action. Higher-order biological functions or diseases like neurodegeneration or cancer obviously have these complex properties but the feature pervades seemingly much simpler contexts. Reducing complex processes of life, for example, to the addition of single-gene effects and accepting descriptive changes in individual signaling pathways (e.g., through KEGG) as sufficient approximation to complexity and as convincing “mechanism” might be deceiving and result in false explanations and simplifications ( van Swinderen, 2005 ). The same is true, if we blindly assume that causalities uniformly act across scales, from molecules to behavioral and social. And finally, the same macroscopic outcome can be achieved through multiple, different mechanisms ( Marder, 2011 ).
Figure 2 shows how the Cynefin framework can be applied to the central relationship between allele frequency and effect size in complex, polygenic traits ( Manolio et al., 2009 ). It becomes obvious that different positions within the relationship depicted by that graph require very different approaches, decisions and actions. In some sense, the various fields of the graph are not comparable. It is not simple or even only complicated to close the gap between the calculated heritability of a trait and the total effect size of the known genetic variants (“missing heritability”).
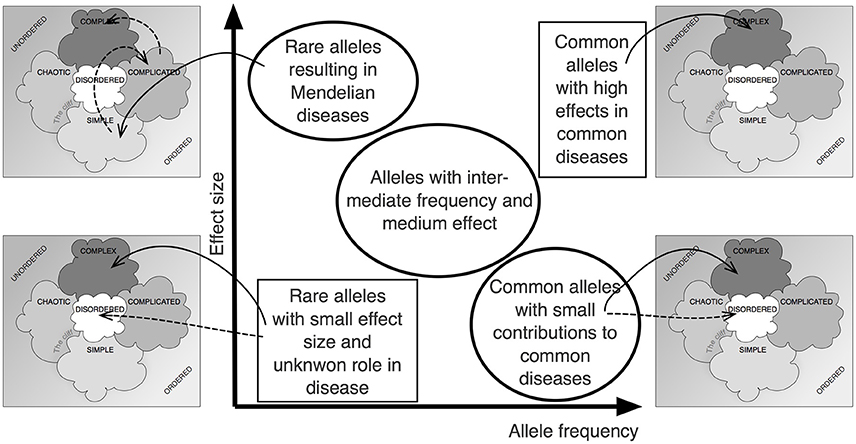
Figure 2 . The Cynefin scheme in complex genetics. Plotting effect size over allele frequency has been an important approach to visualize the complex situation underlying the noted “missing heritability,” the difference between overall heritability and the additive effect of identifiable gene loci ( Manolio et al., 2009 ). Applying the Cynefin scheme to this pattern highlights the fact that contexts differ vastly across that scheme and that, thus, different consequences must arise. The statement that the situation is “complex” becomes enriched by details.
Chaotic Contexts
Biological systems can be chaotic for various reasons: massive acute or late-stage disease, such as cancer are examples of chaotic breakdown of formerly orderly systems, but false security in dealing with biomedical phenomena are another, more subtle source of chaotic biomedical systems.
In the first cases, the reasons for chaos are immanent, in the seconds extrinsic or technical.
Many biological systems are said to be “poised at equilibrium,” which is often compared to a tight-rope walk and means that they border to chaos and fall ( Mora and Bialek, 2011 ). Biological systems tend to push the extremes, and the resulting equilibria are consequently fragile. If they break down, the picture does not allow conclusions about the previous, orderly state and about the rules that governed it. Correctly diagnosing chaos and distinguishing it from complexity is crucial for understanding biological systems. A single-gene knockout might have reverberating consequences for large genetic networks, so that despite the defined molecular lesion the consequences become unintelligible. Despite being seemingly “simple” no assessable cause-consequence relationship remains detectable at the systems level. The mutation's effects dissolve in a flurry of loosely interacting changes across the system, often manifesting itself in high variance. But in many experimental settings, chaotic conditions might actually be hidden.
Complexity has to be distinguished from chaos because both require different actions. In chaotic systems, like in complex contexts, action is required, but in chaos this action first consists of crisis intervention to steer the system into calmer waters. This intervention goes beyond probing and, importantly, is not targeting insight and decision making but is an emergency measure for stabilization.
Practical medicine might run much greater danger than pure research to face chaotic situations that result in conditions which are much closer to the leadership issues that Snowden described in his original publication. An acute ischemic stroke, for example, calls for lysis treatment within the shortest possible time; all further diagnosis and therapy must wait.
Nevertheless, there have been only few direct applications of the Cynefin framework to Medicine. Pubmed as the main publication data base in this area currently (September 2017) lists a mere 10 reports with the keyword, mostly related to public health issues, not research or clinical practice. And none to decision making in medical emergencies.
In research, chaotically failing experiments might have to be abandoned with less dire consequences than the death of a patient but structurally, the problem is similar.
Chaotic contexts require “innovative practice” and cannot be dealt with the common toolbox. Note that the intervention might or must nevertheless follow simple protocols and that even the next steps might by necessity apply merely complicated routines, failing to address the underlying complexity. According to the logic of Cynefin, it thus becomes evident, in which respect such solutions are limited. The reference framework has the purpose to point to such critical limitations (as well as to the opportunities).
Disordered Contexts
A key insight by Snowden and colleagues has been that many contexts cannot be categorized immediately and require a “wait and see” approach in order to appreciate into which of the other four causality categories they would fall. Especially complex contexts have a propensity to immanent change and development.
In the scientific context the “disordered” category is particularly interesting, because it includes many white spots on the map of knowledge. Being alert, sensitive and patient to recognize windows of opportunities in contexts that are not yet ready for mainstream creates opportunities. Applying Cynefin helps identifying contexts, which are worth further observation and careful probing. This does not implicate that the frontiers of knowledge necessarily have to present themselves as “disordered,” but only that “disordered” might be an indicator of novelty. The category will also include ballast, observations at the limits of technical resolution or plainly erroneous material (in which “wait and see” will ultimately not be fruitful).
In complex genetics, for example, common polymorphisms with small effect sizes test the limits of resolution and require distinction from mere stochasticity and noise in the system, before any incorporation into complex models of polygenic causality is possible.
The boundary between “simple” and “chaotic” contexts, in schematic drawings of the Cynefin framework often rendered as cliff, is dangerous, because a categorization as “simple” or “obvious” might lead to false security and premature conclusions. Because a straightforward causality is mistakenly assumed, the conclusions and resulting actions are inappropriate, leading to chaos. The context changes from seemingly simple to chaotic. In medicine this might relate to missing the chance for the appropriate surgical intervention because under an incorrect causality model symptoms have been treated too long with inadequate alternative medicine. In fundamental research similar constellations might underlie much of the irreproducibility crisis or whenever overly simplistic models of disease lead to the identification of pharmacological targets that fail to live up to the expectations in following clinical applications. A key insight from the Cynefin framework is that there is often no easy way back from the resulting chaotic conditions to simpler contexts.
There is a tendency in research (as in management as one learns) to move all phenomena to the complicated and simple fields, rather than to complexity, where, in fact, most issues will be and remain at home. Incorrectly identifying a context as complex, when it is only complicated, has little damaging consequence (except for the “impact” of the resulting paper), whereas missing the complexity of a context and mislabeling it as only complicated (or even simple) might have dire consequences. It is better to err on the more demanding side and re-categorize downwards than to “fall from the cliff.” The worst scenario would be the failure to recognize that this fall has happened.
In a small departure from the original Cynefin design one might with reference to biomedical research be inclined to extend the cliff also a distance into the complicated contexts.
Complex Biomedical Problems have Aspects that are Complicated or Even Simple/obvious
While most biomedical questions are complex, most biomedical problems have parts that are complicated or simple. This implies that to a certain extent, an action according to these categories will be appropriate. In Parkinson's disease (PD), treatment with dopamine precursor levodopa (L-dopa) usually is the initial therapy of choice and for basic diagnostics guidelines or SOPs can be developed at this level ( Berardelli et al., 2013 ; Martí and Tolosa, 2013 ). At a later stage of the disease, to rely only on these SOPs will miserably fail and an extensive individual fine-tuning will become necessary. In systems biology, such basic, relatively self-contained units are called “modules” and although the concepts are not entirely congruent, to think of identifiable simple or moderately complicated relationships within complex contexts as modules will often be helpful and informative. But the disease (or other scientific problem) is more than the sum of the modules and any module points outside of itself. From a practical point of view, to break down complexity into manageable units is tempting and even necessary. But this approach might lead to the implicit belief that by working off the list of modules, the larger question can be answered. However, no additive bottom-up strategy can be successful in answering complex questions. This lies in the particular emergent properties of complex contexts. What is needed is a framework to make best use of modules to capture the complexity of their context. In research, individual classical experiments are such modules. But many publications fall short of highlighting (to say nothing of explaining) the gap between the sum of the experiments and their results and the greater answer that is actually sought. Raising awareness of the general properties of complex contexts, for example within a framework, such as Cynefin, can improve judgment and decision, put insight into perspective, facilitate realistic concrete translation and reduce the risk that complexity is simply ignored.
Decision Making in Research
In contrast to management, where Cynefin came from, biomedical research usually does not overtly emphasize decision making. This is different in the purely clinical setting, but a potentially damaging misconception lies in the implicit idea that in most other biomedical contexts decision making would not be a key issue. But this is not correct. While there are parts of medicine that are closer to management of the kind represented by “ Harvard Business Review ” than research (for example administering a hospital or managing a large clinical study), science itself is in fact crucially dependent on decision making and a particular kind of management as well. But this is rarely made explicit and is often left to intuition or neglected as soft factor with marginal impact. But research is action, organization, communication, and leadership as much as it is creativity, knowledge, persistence and luck. There is also a “market” for research results, spanning out between grant writing, collaborating, publishing and lobbying that sets the stage, on which researchers from their Ph.D. level onwards have to act. There is no such thing as pure science, and science does not naturally self-evolve. Science is led and managed, and whether we like it or not, science has consistently to be “sold” at every level. This is no digression from the elysian, innocent conditions in an ideal past but a fundamental property, because science itself is a complex human endeavor.
Every experiment requires decisions during every single phase. When experiments (and the questions they attempt to answer) are simple, this is no issue. The staged “experiment” that the physics teacher performs to demonstrate F = m × a is predictable down to the boredom of the pupils. Such experiments are replicative and “best practice,” not set up to push the borders of knowledge in general, but only in the audience. The experiments, as any application of “best practice,” are not used to inform larger decisions.
If a diabetic patient routinely measures blood glucose to decide on the required dose of insulin in the next injection, the underlying causality context is “simple.” Nevertheless, the same context is complex from a scientific perspective, resulting in different options and resulting decision-making processes. The practical level differs profoundly from the scientific level. And despite standard operating procedures of insulin measurement and injections schemes, this approach might fail, because the underlying complex reality cannot be captured with this approach.
As most real and novel experimental contexts are complicated or complex, various levels of management and decision making are necessary to initiate the research, perform it, interpret it, communicate it and thereby make it successful. This starts, of course, with the implicit and explicit decisions on the right questions.
In complex research contexts with their emergent properties causality cannot be fully predicted a priori but only approximated to guide the experimental process. The stringency of the hypotheses varies. Iterative processes with computational modeling and experimental verification lie at the heart of any “systems” approach as the accepted answer to biological and medical complexity. Initial questions here tend to be extremely open, so that they do not qualify as “hypothesis” anymore, although this might be a semantic issue and the decision lie in the eyes of the beholder. As a consequence, the answers deduced from the results of such research might be more concrete than the question that has originally been asked, invariably leading to a retrograde, reconstruction of a “storyline” that satisfies our desire for simplicity and a narrative structure. From a communication point of view this might be justifiable and even necessary, but it would require a wider appreciation that such reduced stories are punchlines of a generally complex world in order to avoid larger scale misunderstandings and inflated hopes for translation. Reductionism, simplification, narrative structures, etc., will always be necessary but need to be seen in the correct context of the real complexity (or chaos or disorderedness).
The Cynefin framework is one way of sensitizing at least the researchers, grant agencies, journalists and politicians (as the mainly involved managers) to the the complexity in biomedical research and its consequences to insight and decision making.
“Case manager” are an established solution to addressing (and reducing) clinical complexity in cancer, dementia and neurodegeneration and arrive at executable best solutions ( Huston, 2002 ). The Cynefin scheme provides a straightforward framework to convey the necessity and benefits of such solutions to all stakeholders. Multi-disciplinary case management in science is still rare.
Case Study: Parkinson's Disease
Parkinson's disease (PD) is caused by the degeneration of dopaminergic neurons in the substantia nigra of the midbrain resulting in a characteristic pattern of motoric symptoms. This is a “simple,” monocausal description of the disease that is widely found in publications and is about the level of knowledge even of many health professionals. The obvious treatment option according to this description has been the extrinsic replacement of dopamine in the form of L-dopa, which indeed has the power of relieving the symptoms. But generally not quite. Some symptoms respond better than others, the effect might fluctuate over time and it wears off ( Salat and Tolosa, 2013 ). There is also a massive inter-individual variation in the response. Treatment with L-dopa is initially the approach of choice in most patients, but over time inherent limits become apparent.
But there is a large variation in the clinical picture and the genetic causes anyway ( Riess et al., 2000 ; Mullin and Schapira, 2015 ). The classical triade of tremor, rigor, and akinesia is found to a variable degree, there are non-motor symptoms, including dementia, the disease has a long clinically silent latency period, during which conditions, such as certain sleep disturbances might show up as first indicators and there are mixed forms with other types of neurodegeneration ( Berardelli et al., 2013 ; Martí and Tolosa, 2013 ). Such situation obviously qualifies as “complicated” at the very least. Treatment requires expert knowledge in weighing symptoms and progression against treatment options. There is generally no single correct solution that fits all situations. Therapy belongs, at least temporally, into the hands of specialists, who can collect that information. We are at the upper-right quadrant of the Cynefin scheme.
But the disease is actually complex and while treating it as “complicated” is better than simplifying it, still has serious limitations. The genetics of PD reveal that the sporadic forms of the disease are polygenic with low effect sizes for individual loci ( Riess et al., 2000 ; Mullin and Schapira, 2015 ). Most of these do not show any overt relationship to the dopaminergic system. The main pathogenic mechanism relates to the accumulation of alpha-synuclein, but mutations related to the alpha-synuclein gene account for only a very small subset of the disease ( Lashuel et al., 2013 ). Polymorphisms in the Lrkk2 gene shows a high association with sporadic PD, but the effects are highly variable and no single mechanism has as yet emerged, how Lrkk2 might be causally involved in PD. It seems that no such single, unique relationship exists ( Esteves et al., 2014 ).
In addition, environmental factors obviously play an important role, ranging from toxins, especially pesticides, to lifestyle risk and resilience factors ( Checkoway and Nelson, 1999 ). The relative contribution and interaction of all of these is not really known and no comprehensive model of the disease exists. By all standards, PD qualifies as “complex” with all the consequences for insight and decision making. Sticking with a “simple” disease concept is prone to failure, with all the consequences of “falling over the cliff.” Concretely, the patient might be overwhelmed by side-effects, might not be treated for the full range of symptoms, and critical differential diagnoses are missed. Appreciating that the situation is complicated, in contrast, still misses the critical point that there are aspects which cannot be known in complex contexts and, most importantly, that finding solutions are approximations.
Organizational Consequences
The original Cynefin framework emphasizes the social and cultural context. The approach has been designed to elucidate the relationship between individual, experience and context. The generalization to a tool of knowledge management allows the application to science and research at the level of contents, as outlined in this article, not just organization. But there are of course opportunities for combinations of the different domains, insight and knowledge on one side and decision-making and organization on the other. In fact they are closely related and will constantly inform and influence each other.
Some life-scientists might at first tend to find Cynefin rather common-sensical, but what they criticize in the concept might actually be an advantage. First in the sense of Ockham's razor, that the more simple description might actually the better one, but also by facilitating acceptance on the long run. Snowden characterized his tool as one whose outcome could be sketched on a napkin.
The point is not to invent a new layer of complexity that has to be dealt with in addition to the complexity that prevails anyway but to develop a culture in biomedical research that appreciates and accepts complexity and provides a reference framework and common language.
Many scientists experience the current organization of science and research as increasingly inadequate. Research in the life sciences is becoming more and more differentiated and specialized and takes place under an increasing numbers of preconditions that we are usually not aware of. These conditions includes opportunities and constraints and require additional efforts to decipher their impact and act accordingly.
Conclusions
The reproducibility crisis, the conceptual and practical challenges of multi-omics and big data, the discussions around personalized medicine, the heritability gap in genome wide association studies, the failure of clinical trials for cancer and neurodegeneration after successful animal experiments and the tremendous difficulties in defining criteria for satisfying “mechanistic” explanations of a biological observation are all examples of struggles with complexity in biomedicine. Living organism are complex, because they are adaptive, dynamic, self-organizing, developing, learning, etc., and have emergent properties, but in most scientific projects, from design to publication, and from Ph.D. theses over project reviews to institutional evaluations and research politics, consequences of complexity on results, insight and decisions are not considered systematically. The Cynefin scheme, originally published as tool for decision-making in management has developed into a broadly applicable approach for knowledge management, but remains to be discovered also by biologists and clinical researchers to conceptually and practically deal with complexity. Cynefin is no substitute for concrete scientific solutions to the consequences of complexity, but provides a common reference language and conceptual framework to talk about complexity and draw the appropriate conclusions for insight, decisions and actions. For biomedical research in general, Cynefin helps to capture the critical consequences of complexity at the place, where they arise, and prevents their sequestration into the specialist domains of “systems” biomedicine.
Author Contributions
The author confirms being the sole contributor of this work and approved it for publication.
Conflict of Interest Statement
The author declares that the research was conducted in the absence of any commercial or financial relationships that could be construed as a potential conflict of interest.
Acknowledgments
I would like to thank the Helmholtz Academy and Dr. Oliver Mack (osb Vienna) for first exposing me to Cynefin, the participants of the 2017 Systems Genetics workshop (funded by Bundesministerium für Bildung und Forschung, BMBF) in Frauenchiemsee, Germany, for insightful discussion, and in particular Rob Williams for specific comments on the manuscript and for alerting me to a number of important references.
Berardelli, A., Wenning, G. K., Antonini, A., Berg, D., Bloem, B. R., Bonifati, V., et al. (2013). EFNS/MDS-ES/ENS [corrected] recommendations for the diagnosis of Parkinson's disease. Eur. J. Neurol. 20, 16–34. doi: 10.1111/ene.12022
PubMed Abstract | CrossRef Full Text | Google Scholar
Boyle, E. A., Li, Y. I., and Pritchard, J. K. (2017). An expanded view of complex traits: from polygenic to omnigenic. Cell 169, 1177–1186. doi: 10.1016/j.cell.2017.05.038
Checkoway, H., and Nelson, L. M. (1999). Epidemiologic approaches to the study of Parkinson's disease etiology. Epidemiology 10, 327–336. doi: 10.1097/00001648-199905000-00023
Craver, C. F., and Darden, L. (2013). In Search of Mechanisms: Discoveries Across the Life Sciences . Chicago, IL: University of Chicago Press.
Google Scholar
Esteves, A. R., Swerdlow, R. H., and Cardoso, S. M. (2014). LRRK2, a puzzling protein: insights into Parkinson's disease pathogenesis. Exp. Neurol. 261, 206–216. doi: 10.1016/j.expneurol.2014.05.025
Huston, C. J. (2002). The role of the case manager in a disease management program. Lippincotts. Case Manage. 7, 221–227. doi: 10.1097/00129234-200211000-00003
Lashuel, H. A., Overk, C. R., Oueslati, A., and Masliah, E. (2013). The many faces of α-synuclein: from structure and toxicity to therapeutic target. Nat. Rev. Neurosci. 14, 38–48. doi: 10.1038/nrn3406
Manolio, T. A., Collins, F. S., Cox, N. J., Goldstein, D. B., Hindorff, L. A., Hunter, D. J., et al. (2009). Finding the missing heritability of complex diseases. Nature 461, 747–753. doi: 10.1038/nature08494
Marder, E. (2011). Variability, compensation, and modulation in neurons and circuits. Proc. Natl. Acad. Sci. U.S.A. 108, 15542–15548. doi: 10.1073/pnas.1010674108
Mark, A. L. (2006). Notes from a small Island: researching organisational behaviour in healthcare from a UK perspective. J. Organ. Behav. 27, 851–867. doi: 10.1002/job.414
CrossRef Full Text | Google Scholar
Martí, M. J., and Tolosa, E. (2013). Parkinson disease: new guidelines for diagnosis of Parkinson disease. Nat. Rev. Neurol. 9, 190–191. doi: 10.1038/nrneurol.2013.47
Mora, T., and Bialek, W. (2011). Are biological systems poised at criticality? J. Stat. Phys. 144, 268–302. doi: 10.1007/s10955-011-0229-4
Mullin, S., and Schapira, A. (2015). The genetics of Parkinson's disease. Br. Med. Bull. 114, 39–52. doi: 10.1093/bmb/ldv022
ONeill, L.-J. (2004). Faith and decision-making in the Bush presidency: the God elephant in the middle of America's living-room. Emerg. Complex. Organ. 6, 149–156.
Riess, O., Kuhn, W., and Krüger, R. (2000). Genetic influence on the development of Parkinson's disease. J. Neurol . 247 (Suppl. 2), II69–II74. doi: 10.1007/PL00007764
Salat, D., and Tolosa, E. (2013). Levodopa in the treatment of Parkinson's disease: current status and new developments. J. Parkinsons. Dis. 3, 255–269. doi: 10.3233/JPD-130186
Sittig, L. J., Carbonetto, P., Engel, K. A., Krauss, K. S., Barrios-Camacho, C. M., and Palmer, A. A. (2016). Genetic background limits generalizability of genotype-phenotype relationships. Neuron 91, 1–8. doi: 10.1016/j.neuron.2016.08.013
Snowden, D. (2000). “Cynefin: a sense of time and space, the social ecology of knowledge management,” in Knowledge Horizons: The Present and the Promise of Knowledge Management , eds C. Despres and D. Chauvel (Boston: Butterworth-Heinemann), 344.
Snowden, D. J., and Boone, M. E. (2007). A leader's framework for decision making. Harv. Bus. Rev . 85, 68–76.
PubMed Abstract | Google Scholar
Sturmberg, J. P., and Martin, C. M. (2008). Knowing–in medicine. J. Eval. Clin. Pract. 14, 767–770. doi: 10.1111/j.1365-2753.2008.01011.x
Van Beurden, E. K., Kia, A. M., Zask, A., Dietrich, U., and Rose, L. (2013). Making sense in a complex landscape: how the cynefin framework from complex adaptive systems theory can inform health promotion practice. Health Promot. Int. 28, 73–83. doi: 10.1093/heapro/dar089
van Swinderen, B. (2005). Flexibility in a gene network affecting a simple behavior in Drosophila melanogaster . Genetics 169, 2151–2163. doi: 10.1534/genetics.104.032631
Keywords: complexity, decision making, neurodegeneration, management, systems biology, systems medicine
Citation: Kempermann G (2017) Cynefin as Reference Framework to Facilitate Insight and Decision-Making in Complex Contexts of Biomedical Research. Front. Neurosci . 11:634. doi: 10.3389/fnins.2017.00634
Received: 24 August 2017; Accepted: 31 October 2017; Published: 14 November 2017.
Reviewed by:
Copyright © 2017 Kempermann. This is an open-access article distributed under the terms of the Creative Commons Attribution License (CC BY) . The use, distribution or reproduction in other forums is permitted, provided the original author(s) or licensor are credited and that the original publication in this journal is cited, in accordance with accepted academic practice. No use, distribution or reproduction is permitted which does not comply with these terms.
*Correspondence: Gerd Kempermann, [email protected] ; [email protected]
Disclaimer: All claims expressed in this article are solely those of the authors and do not necessarily represent those of their affiliated organizations, or those of the publisher, the editors and the reviewers. Any product that may be evaluated in this article or claim that may be made by its manufacturer is not guaranteed or endorsed by the publisher.

Victor Leung
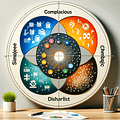
Understanding the Cynefin Framework - Navigating Complexity in Decision-Making

In today’s fast-paced and intricately connected world, decision-making has become an increasingly complex task. The Cynefin Framework, developed by Dave Snowden in 1999, offers a practical approach to understanding and addressing this complexity. This blog post delves into the fundamentals of the Cynefin Framework and explores how it can be effectively used in various organizational contexts.
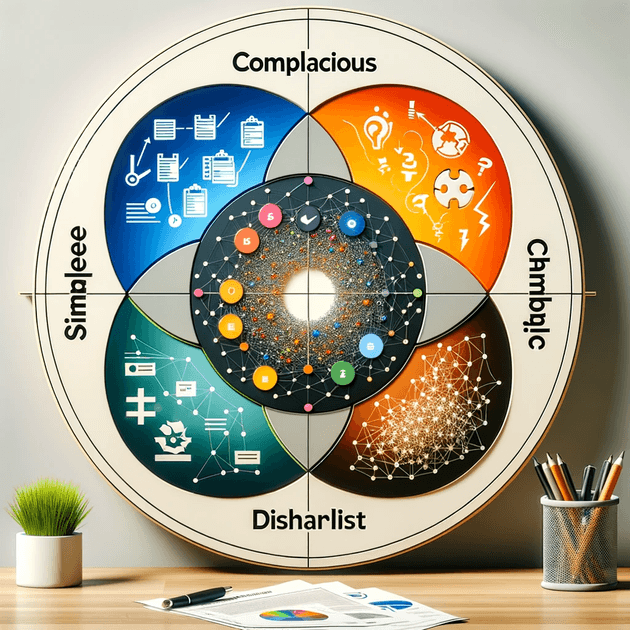
What is the Cynefin Framework?
The Cynefin Framework (pronounced "kuh-nev-in") is a conceptual tool designed to help leaders, managers, and decision-makers navigate the complexity of real-world situations. It offers a typology of contexts that guides users in identifying the nature of the challenges they face and selecting appropriate strategies for action.
The Five Domains of Cynefin
The framework categorizes problems and situations into five domains:
Simple/Obvious: These are situations where cause and effect are clear, and the right answer is evident. Best practice is applied here.
Complicated: In this domain, cause and effect are not immediately apparent but can be discerned through analysis or expertise. Good practice is often used in these scenarios.
Complex: Here, cause and effect are only visible in retrospect. These situations require probing, sensing, and responding. Emergent practice is necessary in this domain.
Chaotic: In chaotic contexts, there is no clear relationship between cause and effect. Rapid, decisive action is needed to establish order and prevent damage.
Disorder: This is the central domain, representing confusion or situations where it is unclear which of the other four domains applies. Identifying the correct domain is the first step in moving forward.
Applying the Cynefin Framework
1. problem identification:.
Simple/Obvious: Identify the problem, categorize it, and apply a known solution.
Complicated: Engage experts to analyze and diagnose, then apply existing methodologies or create new ones.
Complex: Experiment and learn by trial and error. Engage in a pattern of probing, sensing, and responding.
Chaotic: Act immediately to re-establish order, then understand the situation and respond accordingly.
2. Leadership and Management Implications:
In Simple contexts, traditional command-and-control approaches can work well.
Complicated contexts benefit from a more analytical and expert-driven approach.
Complex situations require a more adaptive leadership style, promoting innovation and creativity.
In Chaotic scenarios, rapid decision-making and clear directives are crucial.
3. Organizational Learning and Adaptation:
Organizations can use the Cynefin Framework to adapt their strategies, structures, and processes based on the nature of their challenges.
It encourages a shift from a one-size-fits-all approach to a more nuanced understanding of different situations.
The Cynefin Framework is a powerful tool for understanding and responding to the complexities of modern decision-making. By categorizing problems into distinct domains, it helps leaders choose appropriate strategies and actions. In a world where one misstep can lead to significant consequences, having a structured approach to complexity is invaluable. Whether you are leading a small team or a large corporation, the Cynefin Framework can provide clarity and direction in a complex and uncertain world.
By embracing the principles of the Cynefin Framework, organizations can improve their decision-making processes, adapt to changing circumstances, and navigate the complexities of their environments more effectively.

Ready for more?
You are using an outdated browser. Please upgrade your browser to improve your experience.
- Keynote speeches
Dealing with complexity in the Never Normal
My conversation with Dave Snowden, Founder and Chief Scientific Officer at the Cynefin Company
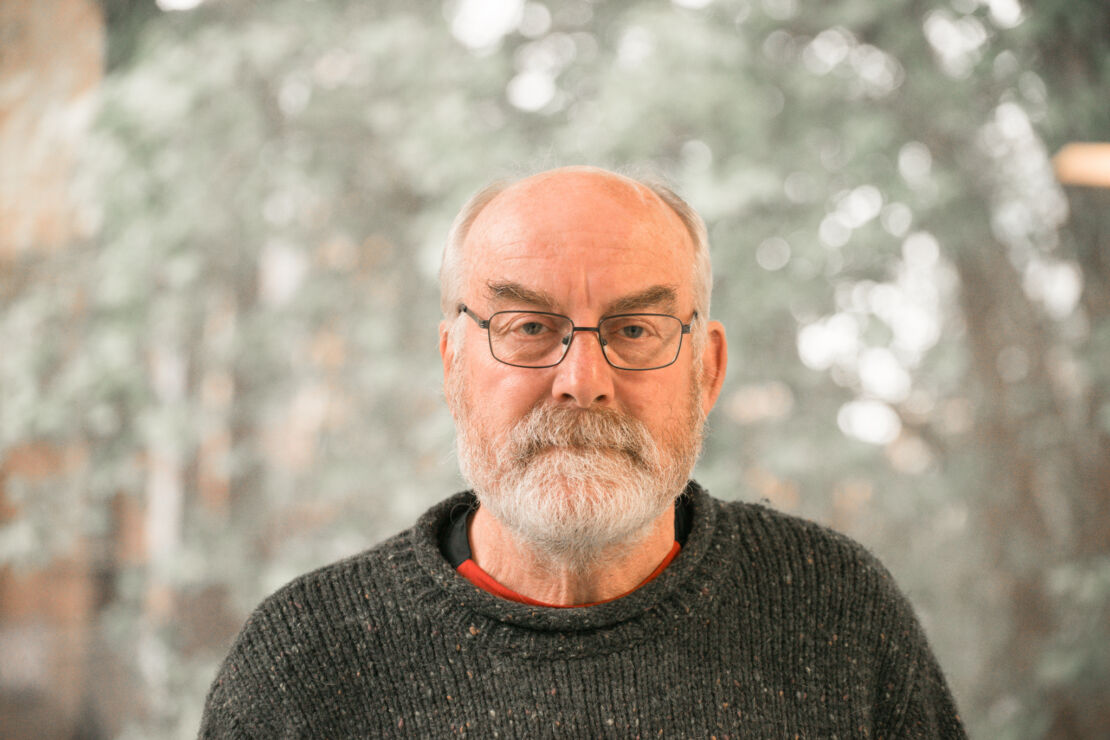
Ever since I came across the Cynefin sensemaking framework , I’ve been deeply intrigued with it, as well as with its maker Dave Snowden , Founder and Chief Scientific Officer at the Cynefin Company. Anything that can help us better understand the complexity of life and all things organizational, is pretty essential in these challenging times and so I really wanted to share Dave’s vision and approach in this Never Normal newsletter.
The Cynefin Framework
In our current period of high uncertainty and complexity, leaders can sometimes find it difficult to set a course of action. And that makes the Cynefin framework extra relevant, as it has been specifically developed to help them understand their challenges and to make decisions depending on their context. If a situation is clear, for instance, they’ll need to react very differently than when their context is chaotic and they have very little information about it.
It sounds deceivingly obvious, but still too many leaders disregard their context and that can have far reaching implications. Some of the benefits of the framework are that no one wastes energy in overthinking what is actually pretty routine on the one hand, but also that we never try to fit the complex into standard solutions on the other.
The Cynefin framework distinguishes 5 different domains: the clear, the complicated, the complex, the chaotic and, in the middle - where you don’t yet understand your context - the confused. Each of these domains, then, offers different types of constraints and different preferred reactions. For instance, while leaders in a chaotic context must first act, then sense, and then respond to a situation, those in a complicated context must sense, analyze, and respond, in that order.
Since there’s no better way to let you discover the framework than by listening to Dave himself, here is a video of him doing so:
20 years in the making
The wonderful part about Dave’s framework is that it took him no less than 20 years to develop it into its current form, with many different iterations since the first edition (and many probably to come). He was originally inspired by his late friend Max Boisot ’s I-Space framework (visual below) and from there started to build a whole theory of knowledge management which eventually evolved into a two by two matrix. The fact that he worked on it for so long and still keeps working on it, is probably where its power and success lies. And why his seminal article ” Complex Acts of Knowing – Paradox and Descriptive Self Awareness ”, evolved to become the ninth most cited paper in knowledge management.
A science-based approach
Dave has always been deeply suspicious of management gurus and their oversimplification of all things organizational. He condemns how a lot of these experts tend to gather 10 or 15 successful cases and then derive practice from that, confusing correlation with causation: “especially when things become highly uncertain, case studies are the last thing you need to rely on because they're what people say happened in the past”.
Instead, he uses natural science as an enabling constraint, because “complexity in human systems needs to be a transdisciplinary study, which has to bring in anthropology, cognitive neuroscience, material engagement theory, constructive theory, theoretical physics etc.”
We proved that human beings can create order.
One of the most important things Dave managed to accomplish with his framework is to contradict the currently over-popular idea that everything is complex. “With Cynefin, we proved that human beings can create order. And that there's nothing wrong with that. Processes are very valuable. But they also only work for linear, structured, ordered systems. They do not in a complex context.”
Dave’s company was actually one of the few to thrive during COVID-19 times, because the pandemic truly made people understand the importance of dealing with complexity in intelligent ways. It’s also why he was able to develop a field guide for the EU to help decision makers navigate times of crisis using the Cynefin framework as a compass. And we do see leaders everywhere waking up to the fact that trying to understand the successes of other companies (which operated in very different contexts anyway) will not help them make decisions in chaotic and complex situations. They ought to be looking into methods and tools that were developed on the basis of natural science on human cognition and on systems.
Complex methods & micro-change
It was interesting to learn how the Cynefin Company handles complex situations with methods that are in themselves also complex. For instance, rather than having one systems analyst tackling a complex IT problem, they work with “entangled trios”: for instance, a young programmer working together with a systems architect as well as a user. And it’s never just the one trio, it would always be a group of different trios at the same time.
And that was a returning theme in our conversation: many little steps at the same time. The same went for a big residential care home project in the Netherlands where they organized continuous capture of self-signified micro-narratives in order to detect weak signals of abuse.
So, first, what is a “self-signified micro-narrative”? It is a short personal story that respondents offer as an answer to a question from researchers which they (the respondents) can then categorize within a pre-existing frame. These micro-narratives are then clustered into a map of common narratives which can offer insights into the specific attitudes, perceptions, or motivations concerning the question being analyzed. The method allows for an examination of dominant behaviors, emerging trends and weak signals.
As a concrete example, the Cynefin Company used that same micro-narrative method in South Wales, where children's ethnographers gathered stories from a depressed industrial area which were then compilated into a narrative landscape. The minister then looked at the latter and told them “I need more stories like these and fewer stories like those”.
This offered a unique theory of change because no one talks about idealized qualities here. Instead it’s a really pragmatic method centered on “more like this, fewer like that”. After that, transgenerational pairs - teenagers together with people from their grandparents’ generation - were told to come up with ideas for change, to create “more stories like this, fewer stories like that”. And if the ideas were plausible, they were put in a trio with somebody from local or national government who could then help make the idea work. The result was a whole portfolio of micro projects rather than the traditional grand strategy overdesign promising wonders in three years’ time.
And there was that red thread again, of cleverly combining small steps rather than putting all of your hope into one grand strategy: “Complexity is all about making micro changes in the present and micro nudging the system so that its evolutionary potential can be realized.”
Though suspicious of overly simplified methods and scenarios, Dave is at the same time also pretty level-headed about complexity: “My experience is that most people at C-level understand complexity intuitively because they live it. They haven't got to be at board level without understanding it.”
That intuition is why they for instance respond so well to “dark constraints” which is a reference to dark matter: “you can see the evidence of a constraint, but you don't know what the constraint is”. The reason why Dave’s team introduced that term into their lexicon was to give language to things that most C-level people “feel” rather than “know”, so that they can at least discuss it. “Consultants want everything always to be certain, but it can be really useful to talk about uncertainties, about emergence in a clear language.”
Distributed leadership
Individuality has always been an important feature of our North Atlantic (Dave does not want to use ‘Western’ because there isn’t an East and a West according to him) leadership models. “Social atomists believe that the individual is the primary unit of analysis. And so they train their leaders as individuals. This individuality, for instance reflected in Protestantism, in fact lies at the root of capitalism and the justification of individual selfishness in every other domain.”
“Societies in Latin America, Europe, Asia, or the Celtic fringe of Europe and Southern Europe, on the other hand, are communitarian. In their view, individuals only have meaning within the context of the societies in which they were born and grew up. The science is now backing that up. We're social creatures, not individual creatures. Multiple paths influence what you are and you are only partially aware of those.”
Dave believes that the concept of collectivist ontologies is fundamental. This is where we’ll all be evolving in the future, because it’s the most adapted to our reality. He loves to tell leaders in Singapore (where he had some projects running) that they should send their elite scholars to universities in Latin America and Africa, not in the States, because that's the future.
Leadership becomes a set of interactions and processes.
That is why Dave and his team are also working on distributing leadership into combinations of three roles in order to allow decisions to be made at a lot quicker. They believe that the approach takes about 60 to 70% of the bureaucracy out of a system. Leadership is no longer a matter of an individual leading, here, but it becomes a set of interactions and processes. This approach may sound strange at first, but we all have seen great leaders in big organizations surround themselves with people who compliment their weaknesses. Dave’s approach is merely a formalization of that into a process. And there is a lot of room to play, here, as well. One of the three roles, for example, could be an avatar, or even a committee rather than a person.
Distributed leadership does not only make the decision process faster, it also makes it better: “Human beings actually did not evolve to make decisions as individuals. We did evolve to make decisions in extended family groups and clans and tribes. That’s why, as clans we're really good at decision making. As individuals, we're really bad.”
There is a natural limit to this distributed leadership, of course. “Three people from very different backgrounds can have a quick conversation and make a decision. If you go above five, they organize into their tribes and it becomes very difficult to get a decision. Three is the optimal number. And the theoretical maximum is five, which is actually the average size of the adult members of a family group going back for thousands of years.”
Leadership emerges
Dave also likes to warn people against talking about leadership competences and desired behavior because they have no causal capability. He does not believe that “leader X is good at Y because of his competences Z and A”. Good leadership and competences emerge, according to him. It's the context of work which produces them. “That means that you need to set up processes so that people get similar experiences and similar encounters over time that will allow them to develop in different ways. And distributing leadership into roles is a very efficient method for that, which he and his team already facilitated in many healthcare and government organizations.
Taylor wasn’t that bad
We also talked quite a while about scientific management, which a lot of people get wrong, according to Dave: “People just forget how bad it was before Frederick Taylor came along. He actually humanized the workplace. His view is not perfect by today’s standards, obviously, but you need to compare with what went before. He introduced lifetime employment and proper apprenticeships. He talked about trust and responsibility without bureaucratic control.”
We are switching back to an ecological metaphor for management, which is much closer to how complexity works.
“But in the eighties and nineties, management theory transitioned to systems thinking, particularly to systems dynamics and cybernetics. There was a switch from a military framework under Taylor into an engineering metaphor. They have a bad reputation today, but military frameworks are actually quite flexible. And that engineering metaphor gets encapsulated in business process re-engineering at the hard end Senge's learning organization at the soft end. And all of a sudden engineers are trying to define what the system should be and trying to define targets in advance to handle uncertainty. Whereas up to that time people managed things. They sometimes made long-term plans, but not that frequently. The budget cycle wasn't as dramatic as it is now. But today, the interesting part is that we seem to be switching back to an ecological metaphor, which is actually much closer to how complexity works.”
What I perhaps loved most about our conversation was the uncovering of all the nuances of complexity. Not everything is complex, even if that increasingly popular narrative would have us believe that. We can create order, depending on the situation. And other situations can perhaps not be ‘solved’ in ways that we would like them to, but we can certainly manage them, if we understand how to respond.
I think that this realization is highly relevant in times when too many people seem to be retreating in some sort of paralysis, even some kind of planned helplessness when it comes to tackling some of the huge challenges that await us. And I think that the Cynefin framework and Dave’s many methods (you can find some of them open sourced on the Cynefin wiki here) are a really useful place to start.
The Phoenix and the Unicorn
Order this book in ....
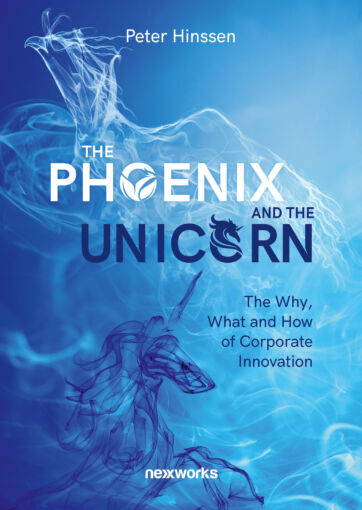
You may also like ...
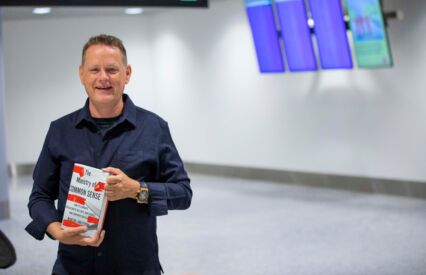
A holistic approach for the Never Normal
The Never Normal
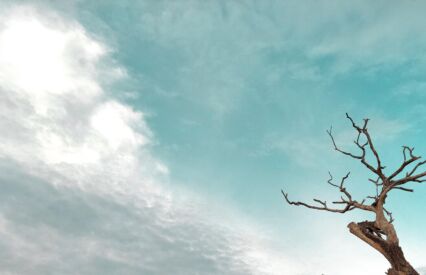
Resilience is just as much about governance as it is about culture
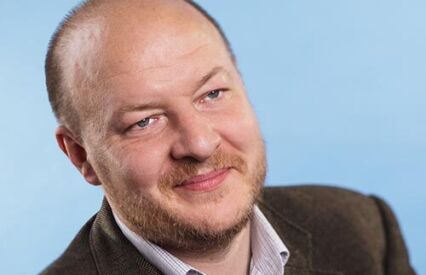
Mapping the future
© Peter Hinssen Privacy Policy Cookie Policy
Explore by Resource
- Policy & Doctrine
- Knowledge Check
Explore Collections
Browse by subject, explore by discipline.
- Public Affairs
- Visual Information
- Broadcasting
The Cynefin Framework Sense-Making Guide
As the information environment gets larger with more players becoming involved, problems are becoming increasingly more complex. It is important for leaders to think through problems and situations to discover new solutions and make innovative plans.
The Cynefin framework is a sense-making tool used to help leaders think through a problem or situation to find a solution. It encourages decisions to be made based on circumstances and addresses the uncertainty of complex projects and systems. It breaks down problems to fit into four domains: Obvious, Complicated, Complex and Chaotic. In between these domains, you have "disorder," or "confusion." Disorder is used when you don't know where you are or when you are between two domains. The first step out of disorder is to gather more information to move into a defined domain. This framework improves sense-making and the likelihood of operation planning success by providing direction for a leader to find the best solution.
This framework complements the traditional communication strategy and research approaches such as the Military Decision Making Process (MDMP) and the Joint Planning Process (JPP), as a tool for problem framing before problem-solving. In addition, Cynefin recognizes the causal differences that exist between different types of systems in complex social environments. Communication professionals must think differently about different problems. There is no one-size-fits-all approach and the actions taken depend on which domain a problem is in.
Explore to learn more about the four domains, the leadership role and the potential dangers while problem-solving in each domain.
Cynefin Framework
Complicated.
- is easily predictable
- is a repeating pattern
- has clear cause and effect
- has a single, obvious answer
- has known-knowns (awareness and understanding of all parts of the problem)
WHEN THE PROBLEM IS OBVIOUS
Just as the solution to walking in the rain is using an umbrella, an obvious solution to a problem requires you to:
- Use "best-practice" solutions
- Communicate in clear and direct ways
- Avoid over complicating the problem
- Ensure that proper processes are in place
- Delegate roles and duties
- Use fact-based management (acting in a straight-forward manner based on knowledge of the ordered world)
DANGER SIGNALS
- Complacency and comfort
- Desire to over or underestimate complexity of the problem
- Inflexible or outdated thinking
- No challenge of received wisdom
- Over-reliance on best practice if context shifts
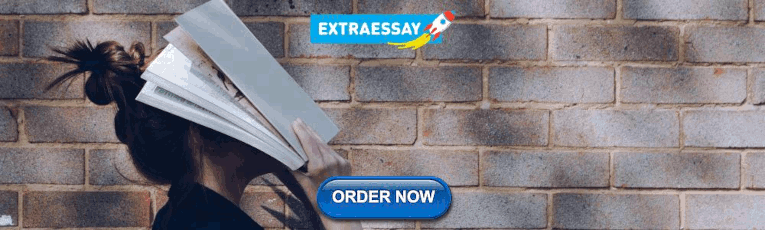
RESPONSE TO DANGER SIGNALS
- Create communication channels to spark ideas and challenge orthodoxy
- Stay connected without micromanaging
- Avoid assuming things are simple or chaotic; analyze the problem and evaluate appropriately
- Recognize both the value and limitations of best practice
- needs expert knowledge to assess the problem
- has a cause-and-effect relationship that is discoverable but not apparent
- has more than one possible "correct" answer
- has known unknowns (you know the questions but don't know the answers yet)
WHEN THE PROBLEM IS COMPLICATED
Just as a hurricane requires you to watch the news to know how and when to evacuate, a complicated problem requires you to:
- Coordinate a panel of experts
- Use "good-practice" solutions
- Listen to conflicting advice
- Determine a course of action
- Execute the plan
- Experts overconfident in their own solutions or in the efficacy of past solutions
- Over-analyzation a.k.a. "Analysis Paralysis"
- Viewpoints of non-experts excluded
- Encourage external and internal stakeholders to challenge expert opinions to combat outdated or inflexible thinking
- Use experiments and games to force people to think outside of the familiar
- is in flux and unpredictable
- has multiple, competing ideas for cause and effect
- requires creative and innovative approaches
- has solutions that are emergent and instructive
- needs deep analysis of the context to ideate solutions
- has unknown-unknowns (unaware of what questions to even ask)
WHEN THE PROBLEM IS COMPLEX
Just as the best way to avoid a tornado's path is uncertain and unpredictable, a complex problem's solution requires you to:
- Develop and experiment to gather more knowledge and understanding of how the problem operates in the environment
- Use "emergent-practice" solutions
- Use methods that can help generate ideas: open up discussion, set barriers, stimulate attractors, encourage dissent and diversity, manage starting conditions and monitor emergency
- Execute and evaluate the results of each experiment to begin determining next steps
- Use pattern-based leadership (responsive and reactive leadership in a disordered world)
- Temptation to fall back into habitual command-and-control mode
- Temptation to look for facts rather than allowing patterns to emerge
- Desire for accelerated resolution of problems or exploitation of opportunities
- Be patient and allow time for reflection
- Use approaches that encourage interaction and communication so patterns can emerge and ideas can flow
- has HIGH turbulence (no control over the situation, high tension)
- has no clear cause-and-effect relationships so there is no point in looking for right answers
- is unknowable (nothing about the problem or answer is known)
- requires many decisions to make and no time to think through logically
WHEN THE PROBLEM IS CHAOTIC
Just as the appropriate response to snow in the desert would be inconceivable and unknown, a chaotic problem requires you to:
- Prioritize containment until the long-term solution can be determined
- Take immediate action to reestablish order (command-and-control)
- Use "novel-practice" solutions
- Provide clear and direct communication
- Take action to move or remediate your problem to be "complex"
- Applying a command-and-control approach longer than needed
- "Cult of the leader"
- Missed opportunity for innovation
- Set up mechanisms, such as parallel teams, to take advantage of opportunities afforded by a chaotic environment
- Encourage advisers to challenge your point of view once the crisis has abated
Discover More You May Like
Dinfos live: pa & vi office management techniques, standard operating procedure (sop) template, how to create your own sop document, visual information and video production plan, get the 411 on information disorder, safeguarding your social media accounts.
- The Real Option Resource
The IT Risk Manager
… based on practice rather than theory.
Given When Then – A Cynefin Case Study
Dan and I created the “Given When Then” pattern on August 23rd 2004, or rather, that was the day we realised we needed the “Given” part . On November 30th, I wrote a series of blogs explaining the format that Dan and I had created in its more familiar form. JBehave II , JBehave III , JBehave IV and JBehave and Postmodernism .
This experience report is best explored by considering it through the lens of the Cynefin framework. Although I read the Cynefin whitepaper before this time, I did not understand it until fairly recently.

Neither Dan or I deliberately set out to create the “Given When Then” framework. We were both working on other projects. Dan was working on BDD where he was trying to change the language of TDD using NLP to make it easier for people to learn TDD. I was trying to work out an analysis approach that would work with Extreme Programming. Dan had replaced TDD’s assert with “Should” and was having more success explaining TDD to people. A week or two before, we had traveled back from the Agile Development Conference together and we had realised that “should” was the language of specification.
On the day that Dan and I first came up with “Given”, our goal was for Dan to explain BDD with Mock Objects and Patterns to me as I was going on sales visits to clients and talking about something I had never actually done. As an amusing aside, several years later Liz Keogh told me it took her six months to remove the visitor pattern from JBehave v1.
The key point is that Dan and I were working on oblique problems. Dan was trying to create a better way to teach TDD and I was trying to learn TDD. We were not deliberately trying to create a pattern to allow non technical people to effectively communicate with Agile developers.
Activated Individuals
Dan and I did not create the “GIVEN” word. We tripped over it. It was literally a movie moment when I said something and Dan and I looked at each other realising it was something useful.
The night before I had been to see a friend researching a PhD in Historiography. Historiography is the study of Post-Modernism as applied to how history is understood and taught. Historiography shows us that the way History is taught, understood and interpreted is more a function of the context than the events themselves.
When Dan showed me the code for TDD with Mocks, my head was full of “Context” (thanks to my friend Rob) which meant I recognised mock objects as context. When I said “Mocks provide the context” Dan and I both realised it was significant as our goals had activated us to the importance of the statement.
The key point which Dave Snowden makes in his talks is that you need individuals in a heightened state of awareness to spot something important* You need experts to spot something subtle and significant.
Recipe Books and Chefs
Both Dan and I were “Chefs” in Dave Snowden’s language. I had a decade of experience of Business Analysis and was used to coming up with new approaches when needed. Dan had several lifetimes worth of experience as a developer, and in particular coaching developers.
As Chefs we recognised that “context” was a new ingredient that was missing from the meal shared between Agile developers and non-developers trying to communicate to them.
This was only possible because we had both served our apprenticeships, and had an understanding of how to combine ingredients that we knew the “GIVEN” was a new ingredient**.
BDD was designed for developers to more easily learn TDD. Dan and I adapted it to communicate between Non technical people and developers.
Actually, the “Given When Then” format is an exaptation of TDD. TDD has the steps Setup – Execute – Assert. Dan and I exapted the TDD form into a specification format that non developers could use to communicate more effectively to developers.
Multiple Safe to Fail Experiments.
I went off to develop Feature Injection while Dan developed JBehave and promoted BDD. Rather than focus all his attention on one BDD solution, Dan promoted and supported many open source communities as they developed tools. From JBehave, through rSpec, to Cucumber and SpecFlow, Dan has supported them.
So Dan engaged in Multiple Safe to Fail experiments in his search to realise a BDD tool that worked.
Thanks to Cynefin, I now have a better understanding of what happened when we discovered the Given When Then format. As a result in the future, I will have a better understanding the context I need to create in order for innovation to occur.
- GIVEN I use Cynefin to understand the world
- WHEN I look at situations going on around me
- THEN I’ll find new meaning
* I’ve watched three of Dave’s keynotes to find the point where he says this but could not find it. My wording may be wrong.
**A while later I realised that GWT was a subset of the use case. Unfortunately the Use Case is so bloated as a tool that it is not focused on specifying behaviour. The Use Case has become the jack of all trades and master of none.
Share this:
About theitriskmanager.
3 responses to “ Given When Then – A Cynefin Case Study ”

Recently I’ve picked up on the suggestion that ‘Multiple Safe to Fail Experiments’ benefit from containing mutually exclusive hypothesis, either refuting or the constraining the existence of one another. Clearly Dan’s support of BDD tooling community does not fulfil the pattern to this extent, it’s interesting to consider what he could have done that would of. Although an evolutionary rather than parallel path, I feel it’s Liz Keogh who’s been most vocal in (re-)examining BDD from a Cynefin perspective.
As well as the tool approach, other people implemented GWT with other tools, or manually. Most famously Steve Freeman on the Sierra project.
I’m not sure I follow the comment on Liz. She is examining BDD through the lens of Cynefin. This post attempts to understand the creation of the GWT pattern using Cynefin. Could you expand on the point you are making?
“The key point which Dave Snowden makes in his talks is that you need individuals in a heightened state of awareness to spot something important* You need experts to spot something subtle and significant.”
thank you so much. this is for me what is the real value of a coach.
We (I) as a coach spot the “subtle and significant” things the teams I coach trip over.
Leave a comment Cancel reply
- The Others…
- The Real Option Resource
- February 2024
- December 2023
- October 2022
- February 2022
- January 2022
- December 2021
- November 2021
- October 2021
- August 2021
- February 2019
- January 2019
- December 2018
- September 2018
- August 2018
- January 2018
- November 2017
- October 2017
- September 2017
- August 2017
- February 2017
- January 2017
- December 2016
- November 2016
- August 2016
- November 2015
- October 2015
- August 2015
- February 2015
- January 2015
- December 2014
- September 2014
- August 2014
- February 2014
- December 2013
- November 2013
- September 2013
- August 2013
- December 2012
- November 2012
- February 2012
- January 2012
- December 2011
- November 2011
- October 2011
- September 2011
- August 2011
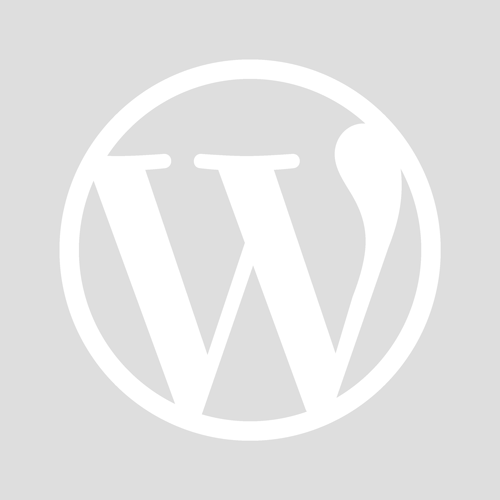
- Already have a WordPress.com account? Log in now.
- Subscribe Subscribed
- Copy shortlink
- Report this content
- View post in Reader
- Manage subscriptions
- Collapse this bar

An official website of the United States government
The .gov means it’s official. Federal government websites often end in .gov or .mil. Before sharing sensitive information, make sure you’re on a federal government site.
The site is secure. The https:// ensures that you are connecting to the official website and that any information you provide is encrypted and transmitted securely.
- Publications
- Account settings
Preview improvements coming to the PMC website in October 2024. Learn More or Try it out now .
- Advanced Search
- Journal List
- Front Neurosci
Cynefin as Reference Framework to Facilitate Insight and Decision-Making in Complex Contexts of Biomedical Research
Gerd kempermann.
1 German Center for Neurodegenerative Diseases (DZNE) Dresden, Dresden, Germany
2 Center for Regenerative Therapies Dresden (CRTD), Technische Universität Dresden, Dresden, Germany
The Cynefin scheme is a concept of knowledge management, originally devised to support decision making in management, but more generally applicable to situations, in which complexity challenges the quality of insight, prediction, and decision. Despite the fact that life itself, and especially the brain and its diseases, are complex to the extent that complexity could be considered their cardinal feature, complex problems in biomedicine are often treated as if they were actually not more than the complicated sum of solvable sub-problems. Because of the emergent properties of complex contexts this is not correct. With a set of clear criteria Cynefin helps to set apart complex problems from “simple/obvious,” “complicated,” “chaotic,” and “disordered” contexts in order to avoid misinterpreting the relevant causality structures. The distinction comes with the insight, which specific kind of knowledge is possible in each of these categories and what are the consequences for resulting decisions and actions. From student's theses over the publication and grant writing process to research politics, misinterpretation of complexity can have problematic or even dangerous consequences, especially in clinical contexts. Conceptualization of problems within a straightforward reference language like Cynefin improves clarity and stringency within projects and facilitates communication and decision-making about them.
Introduction
We regard many, if not most important biological and medical questions as complex, because they do not have single straightforward solutions. Life itself is complex and is more than the sum of its part: simple, direct causalities are only found within defined functional modules, such as chemical reactions and physical interactions (and even there can be challenged). Insight on larger-scale questions about “life” cannot be fully reconstructed bottom-up only from such modules. In large networks, probabilistic relationships and highly indirect influences blur mechanistic insight. Complex problems and questions in the life sciences have “ emerging properties ” that preclude that there are single true answers to questions of causalities. Cancer, neurodegeneration, consciousness, etc., cannot be comprehensively understood simply by adding up insights from partial aspects. They require a more holistic perspective, which, however, might ultimately be impossible to gain. While the large questions of life are the most obvious manifestations of a problem with complexity, the issue actually permeates biomedical research at every level.
In the clinical context this is much more obvious than in basic research. If an average 75 year-old patient accumulates five or more medical diagnoses, which all might lead to a more or less specific treatment, the resulting matrix of past, present, and future interactions at the level of the development of the disease, the co-developing (intrinsic) strategies of coping with the problems and the, in most cases: pharmacological, (extrinsic) treatments amount to a high-dimensional complexity of causalities and interferences that cannot be grasped–especially not intuitively in the emergency room. Even if these networks could be modeled theoretically, this will be of limited value in the acute situation. Medicine for older people is, even more than for younger subjects, a medicine of complexity and so is the underlying biology. The reason that many, especially chronic, diseases have not yet been conquered lies in exactly their complexity and our imperfect grip on this complexity.
The problem is, however, neither limited to aged subjects or humans, nor to medicine. Biomedical research as the science of life is interested in causes and mechanisms underlying complex quantitative processes. Phenotypes tend to be gradual and are often found in a normal distribution. The strengths of reductionism generally turn into a risk, when the key to the sought solution lies in the emergent properties, which hide in the observable and measurable phenotypic appearance of complex traits, behaviors, diseases, etc., Most human traits, including the susceptibility to disease, are highly polygenic and to a variable degree subject to environmental and behavioral influences. The co-development of physiological traits with pathology results in extreme multi-dimensional complexity, challenging our ability to understand appropriately and act accordingly. In principle, the problem is thus relevant to any combination of physiological (and pathological) traits and across scales. This most elusive aspect of the picture might be the most critical one, especially for example, if it comes to translation from models to the clinic. Controlling for complexity must not result in ignoring it. The interpretation of the results might fail, if the full context is not appreciated, even though we might not be able to fully comprehend it and factor it in. So the first step is to be clearer in language and conceptualization.
While the diagnosis of complexity has thus become ubiquitous, concrete systems approaches in biology and medicine are attempts to extract usable insight from this complexity in order to guide novel approaches to gain insight on one side for treatment and for prevention on the other. With “systems biology,” “systems genetics,” “systems medicine” etc., sub-disciplines have evolved that specifically and by definition address the complexity of life, but the very same challenges permeate everyday research and medicine much more than is often realized. Quantitative biology calls for a particular mindset that is not always appreciated outside the seemingly fenced-off “systems” fields. In reality, however, essentially all biology is “systems,” because all biology deals with life and essentially all of biology has become quantitative. A better way to integrate “systems” ideas into normal biology and medicine is thus necessary.
In addition, outside the inner, concrete medical or clinical context the desire for insight into complex biological causalities is interwoven with a variable degree of translational intentions and aims, adding new layers of interdependencies and influences. Across the highly diverse spectrum of stakeholders from academic science and education over industrial research and development with its economic perspective, to societal and political interest groups, including patients, there is a wide range of perceptions of how our answers to complex questions and problems in biomedicine should look. Interpretation and decisions are not pure. The construct of science in our society is “multirational,” which means that overall aims, strategies, approaches, etc., do not follow one single rationale, but might be ill-aligned or conflicting. The perspective of a zebrafish or mouse researcher on “Alzheimer” is different from a clinician or a care professional, who nominally acts in the same field.
In this situation, it is an additional problem that biology as a whole has been relatively theory-adverse in coming to terms with what, in such situation, might constitute “mechanisms” and how we can interpret them. There are of course activities toward this end [for example in the work by Lindley Darden (Craver and Darden, 2013 )], but appreciation has not generally caught on that a constant reflection on implicit assumptions and standards as well as the fundamental properties of the subjects are required in order to be successful.
It is also important to realize that scientific culture matters. Focus on the inner circle of biological reasoning has possibly prevented us from realizing that with “Cynefin” a relatively straightforward tool has been around for almost two decades which might help to change this situation and which we should now discover for the life sciences.
The Cynefin framework
The Cynefin framework has been a influential concepts in management but largely unknown in the natural sciences and medicine. Cynefin was first published in 2000 by Snowden ( 2000 ). A later paper in the Harvard Business Review from 2007 provided a first generalization as strategy model irradiating well beyond the original management contexts (Snowden and Boone, 2007 ). Cynefin ( kuh-ne-vin ) is the welsh word for habitat or rootedness and was used by Snowden as metaphor for a conceptual framework for “time and place” to make decisions in complex situations. Complexity is certainly no privilege to business and economy, however, and the arguments that Snowden and others developed generalize easily to other fields. It has, for example, been famously used to describe (and make sense of) the decision making during the G. W. Bush presidency (ONeill, 2004 ). An application to later administrations might be similarly enlightening.
There have also been some applications to medicine (Mark, 2006 ; Sturmberg and Martin, 2008 ; Van Beurden et al., 2013 ) but by and large, Cynefin has not yet had a measurable impact on life sciences. This might have cultural reasons and be partly due to the emphasis on decision making that Snowden's original text had, but the tools from Cynefin to make sense of complexity appear to be highly useful for current biology and medicine as well.
The Cynefin framework allows contextualization of situations that require a decision and response by providing a reference language (Figure (Figure1). 1 ). The key assumption is that such situations fall into one of five categories, which call for substantially different but definable conclusions and mode of actions, and that awareness of these principal differences allows more structured insight and better-informed decisions.

The Cynefin scheme. This version is one of the many renderings of the Cynefin framework highlighting and explaining the five core contexts. Unlike some other replications, this version uses the arrangement from Snowden's original publication from 2007, in which the square stands on one corner, so that the “simple/obvious” category is found at the base and the “complex” category at the top. More commonly found is an arrangement with “chaotic” and “simple” next to each other at the bottom and “complex” and “complicated” as a top row. The version here has the advantage of highlighting the complex contents and assigning the simple contexts in their modularity a role at the basis. In some fields, quintessential concepts from biomedicine have been added.
Simple or obvious contexts
These are the domain of clear-cut causality, in which B follows from A. Dealing with simple or obvious contexts requires to describe (“sense”) the situation, assign it to pre-established categories and act according to the defined procedure for that category. The resulting action can adhere to fixed routines, called “best practice” or standard operating procedures. Simple context are the procedural backbone of more complex contexts. In the language of systems biology they relate to the modules, self-contained processes and functional units, such as protein-protein interactions, basic chemical reactions, etc.
In research, simple contexts comprise only what is known already: the “known knowns.” But what is known does not raise questions anymore and without questions no science exists. Simple contexts are thus only the building blocks for addressing the larger questions. Standard operating procedures can invariably apply only to methods that are used, not the entire experiment. Mistaking questions as simple and thus applying only the SOPs will almost invariably miss the point.
A single mutation, for example, does not generate a simple context. The knockout of any gene has reverberations throughout the genome that go far beyond the immediate function of that gene. Relatedly, the same mutation has different consequences on different genetic backgrounds (Sittig et al., 2016 ). Even classical Mendelian genetics is not simple or obvious, and monogenic does not mean mono-causal.
Complicated contexts
Contexts, in which causalities can at least in theory (or retrospect) be known but are non-linear and difficult to untangle are called “complicated.” In this category the causalities are potentially discoverable and this is why at this level the first research questions can appear. We here deal with “unknown knowns.” which means that the questions can be addressed by not venturing too far from what is established, often in a way of extrapolation or combination, sideways application or, to some degree, generalization.
Complicated contexts have no single, obvious best solution and thus are the domain of experts (and consortia). Hence not best but only “good practice.” is possible. In research complicated contexts are common, but they are not necessarily the most important and interesting contexts, because they ultimately do not leave the comfort zone in terms of the openness of the possible question. Complicated problems can certainly be very complicated and pose large organizational and other challenges. Many cohort studies, for example, would initially fall into this category of massive undertakings, that while addressing complex questions, by design are “only” complicated. The distinction and its consequences are critical. Only once the data starts becoming available the efforts to untangle complexity within it can be started. This level has a different structure than the organization of the data acquisition. The genome project is an example of such project, in which the highly complicated endeavor led to a excessively complex data set.
If seen from the biological fact not the technological achievement, one would say that, conversely to common perception, complex situations might require approximations by means of complicated tools, whose inherent limitations have to be recognized and factored into the interpretation.
Boyle, Li and Pritchard have argued that for complex traits, the classical description as “polygenic” is not fully appropriate because the underlying causal networks extend to essentially the entire genome with minimal effect sizes from most genes, which in their entirety nevertheless explain most of the variance. They have coined the term “omnigenic” for this relationship (Boyle et al., 2017 ).
In addition, much of the more classical exploratory research (expeditions, field studies, etc.,) will be found in this category, but, again, not necessarily their results and the ensuing next questions. A consequential mistake would arise from the failure to realize that more than complicated research this is needed to address complexity.
Complex contexts
Although it is only one of the five contexts described by the Cynefin framework, complexity actually lies at the heart of the idea. The entire framework has been designed in order to identify this critical category correctly and allow decisions (actions) that are appropriate to the specific demands of these contexts, also in relation to the others. The complex contexts are those of the “known unknowns,” in which neither “best” nor “good” practice can be used, but “emergent practice” is needed. This description alone already suggests, why for the research context this category is fundamental.
As stated above, “complex” and “complicated” is not the same. In complex contexts we face a situation, in which key aspects are non-observable, latent or emergent. Here, (as well as the chaotic situation described next) action has to precede proper apprehension, in complex contexts in the form of probing the context. The complex situation is such that it has to be probed through (experimental) perturbations in order to reveal enough of the underlying causality structure to allow proper diagnosis and consequently insight and decision. That action is required before insight is possible is, fundamentally different from simple/obvious or complicated contexts, where a certain level understanding can or even must precede action. Higher-order biological functions or diseases like neurodegeneration or cancer obviously have these complex properties but the feature pervades seemingly much simpler contexts. Reducing complex processes of life, for example, to the addition of single-gene effects and accepting descriptive changes in individual signaling pathways (e.g., through KEGG) as sufficient approximation to complexity and as convincing “mechanism” might be deceiving and result in false explanations and simplifications (van Swinderen, 2005 ). The same is true, if we blindly assume that causalities uniformly act across scales, from molecules to behavioral and social. And finally, the same macroscopic outcome can be achieved through multiple, different mechanisms (Marder, 2011 ).
Figure Figure2 2 shows how the Cynefin framework can be applied to the central relationship between allele frequency and effect size in complex, polygenic traits (Manolio et al., 2009 ). It becomes obvious that different positions within the relationship depicted by that graph require very different approaches, decisions and actions. In some sense, the various fields of the graph are not comparable. It is not simple or even only complicated to close the gap between the calculated heritability of a trait and the total effect size of the known genetic variants (“missing heritability”).

The Cynefin scheme in complex genetics. Plotting effect size over allele frequency has been an important approach to visualize the complex situation underlying the noted “missing heritability,” the difference between overall heritability and the additive effect of identifiable gene loci (Manolio et al., 2009 ). Applying the Cynefin scheme to this pattern highlights the fact that contexts differ vastly across that scheme and that, thus, different consequences must arise. The statement that the situation is “complex” becomes enriched by details.
Chaotic contexts
Biological systems can be chaotic for various reasons: massive acute or late-stage disease, such as cancer are examples of chaotic breakdown of formerly orderly systems, but false security in dealing with biomedical phenomena are another, more subtle source of chaotic biomedical systems.
In the first cases, the reasons for chaos are immanent, in the seconds extrinsic or technical.
Many biological systems are said to be “poised at equilibrium,” which is often compared to a tight-rope walk and means that they border to chaos and fall (Mora and Bialek, 2011 ). Biological systems tend to push the extremes, and the resulting equilibria are consequently fragile. If they break down, the picture does not allow conclusions about the previous, orderly state and about the rules that governed it. Correctly diagnosing chaos and distinguishing it from complexity is crucial for understanding biological systems. A single-gene knockout might have reverberating consequences for large genetic networks, so that despite the defined molecular lesion the consequences become unintelligible. Despite being seemingly “simple” no assessable cause-consequence relationship remains detectable at the systems level. The mutation's effects dissolve in a flurry of loosely interacting changes across the system, often manifesting itself in high variance. But in many experimental settings, chaotic conditions might actually be hidden.
Complexity has to be distinguished from chaos because both require different actions. In chaotic systems, like in complex contexts, action is required, but in chaos this action first consists of crisis intervention to steer the system into calmer waters. This intervention goes beyond probing and, importantly, is not targeting insight and decision making but is an emergency measure for stabilization.
Practical medicine might run much greater danger than pure research to face chaotic situations that result in conditions which are much closer to the leadership issues that Snowden described in his original publication. An acute ischemic stroke, for example, calls for lysis treatment within the shortest possible time; all further diagnosis and therapy must wait.
Nevertheless, there have been only few direct applications of the Cynefin framework to Medicine. Pubmed as the main publication data base in this area currently (September 2017) lists a mere 10 reports with the keyword, mostly related to public health issues, not research or clinical practice. And none to decision making in medical emergencies.
In research, chaotically failing experiments might have to be abandoned with less dire consequences than the death of a patient but structurally, the problem is similar.
Chaotic contexts require “innovative practice” and cannot be dealt with the common toolbox. Note that the intervention might or must nevertheless follow simple protocols and that even the next steps might by necessity apply merely complicated routines, failing to address the underlying complexity. According to the logic of Cynefin, it thus becomes evident, in which respect such solutions are limited. The reference framework has the purpose to point to such critical limitations (as well as to the opportunities).
Disordered contexts
A key insight by Snowden and colleagues has been that many contexts cannot be categorized immediately and require a “wait and see” approach in order to appreciate into which of the other four causality categories they would fall. Especially complex contexts have a propensity to immanent change and development.
In the scientific context the “disordered” category is particularly interesting, because it includes many white spots on the map of knowledge. Being alert, sensitive and patient to recognize windows of opportunities in contexts that are not yet ready for mainstream creates opportunities. Applying Cynefin helps identifying contexts, which are worth further observation and careful probing. This does not implicate that the frontiers of knowledge necessarily have to present themselves as “disordered,” but only that “disordered” might be an indicator of novelty. The category will also include ballast, observations at the limits of technical resolution or plainly erroneous material (in which “wait and see” will ultimately not be fruitful).
In complex genetics, for example, common polymorphisms with small effect sizes test the limits of resolution and require distinction from mere stochasticity and noise in the system, before any incorporation into complex models of polygenic causality is possible.
The boundary between “simple” and “chaotic” contexts, in schematic drawings of the Cynefin framework often rendered as cliff, is dangerous, because a categorization as “simple” or “obvious” might lead to false security and premature conclusions. Because a straightforward causality is mistakenly assumed, the conclusions and resulting actions are inappropriate, leading to chaos. The context changes from seemingly simple to chaotic. In medicine this might relate to missing the chance for the appropriate surgical intervention because under an incorrect causality model symptoms have been treated too long with inadequate alternative medicine. In fundamental research similar constellations might underlie much of the irreproducibility crisis or whenever overly simplistic models of disease lead to the identification of pharmacological targets that fail to live up to the expectations in following clinical applications. A key insight from the Cynefin framework is that there is often no easy way back from the resulting chaotic conditions to simpler contexts.
There is a tendency in research (as in management as one learns) to move all phenomena to the complicated and simple fields, rather than to complexity, where, in fact, most issues will be and remain at home. Incorrectly identifying a context as complex, when it is only complicated, has little damaging consequence (except for the “impact” of the resulting paper), whereas missing the complexity of a context and mislabeling it as only complicated (or even simple) might have dire consequences. It is better to err on the more demanding side and re-categorize downwards than to “fall from the cliff.” The worst scenario would be the failure to recognize that this fall has happened.
In a small departure from the original Cynefin design one might with reference to biomedical research be inclined to extend the cliff also a distance into the complicated contexts.
Complex biomedical problems have aspects that are complicated or even simple/obvious
While most biomedical questions are complex, most biomedical problems have parts that are complicated or simple. This implies that to a certain extent, an action according to these categories will be appropriate. In Parkinson's disease (PD), treatment with dopamine precursor levodopa (L-dopa) usually is the initial therapy of choice and for basic diagnostics guidelines or SOPs can be developed at this level (Berardelli et al., 2013 ; Martí and Tolosa, 2013 ). At a later stage of the disease, to rely only on these SOPs will miserably fail and an extensive individual fine-tuning will become necessary. In systems biology, such basic, relatively self-contained units are called “modules” and although the concepts are not entirely congruent, to think of identifiable simple or moderately complicated relationships within complex contexts as modules will often be helpful and informative. But the disease (or other scientific problem) is more than the sum of the modules and any module points outside of itself. From a practical point of view, to break down complexity into manageable units is tempting and even necessary. But this approach might lead to the implicit belief that by working off the list of modules, the larger question can be answered. However, no additive bottom-up strategy can be successful in answering complex questions. This lies in the particular emergent properties of complex contexts. What is needed is a framework to make best use of modules to capture the complexity of their context. In research, individual classical experiments are such modules. But many publications fall short of highlighting (to say nothing of explaining) the gap between the sum of the experiments and their results and the greater answer that is actually sought. Raising awareness of the general properties of complex contexts, for example within a framework, such as Cynefin, can improve judgment and decision, put insight into perspective, facilitate realistic concrete translation and reduce the risk that complexity is simply ignored.
Decision making in research
In contrast to management, where Cynefin came from, biomedical research usually does not overtly emphasize decision making. This is different in the purely clinical setting, but a potentially damaging misconception lies in the implicit idea that in most other biomedical contexts decision making would not be a key issue. But this is not correct. While there are parts of medicine that are closer to management of the kind represented by “ Harvard Business Review ” than research (for example administering a hospital or managing a large clinical study), science itself is in fact crucially dependent on decision making and a particular kind of management as well. But this is rarely made explicit and is often left to intuition or neglected as soft factor with marginal impact. But research is action, organization, communication, and leadership as much as it is creativity, knowledge, persistence and luck. There is also a “market” for research results, spanning out between grant writing, collaborating, publishing and lobbying that sets the stage, on which researchers from their Ph.D. level onwards have to act. There is no such thing as pure science, and science does not naturally self-evolve. Science is led and managed, and whether we like it or not, science has consistently to be “sold” at every level. This is no digression from the elysian, innocent conditions in an ideal past but a fundamental property, because science itself is a complex human endeavor.
Every experiment requires decisions during every single phase. When experiments (and the questions they attempt to answer) are simple, this is no issue. The staged “experiment” that the physics teacher performs to demonstrate F = m × a is predictable down to the boredom of the pupils. Such experiments are replicative and “best practice,” not set up to push the borders of knowledge in general, but only in the audience. The experiments, as any application of “best practice,” are not used to inform larger decisions.
If a diabetic patient routinely measures blood glucose to decide on the required dose of insulin in the next injection, the underlying causality context is “simple.” Nevertheless, the same context is complex from a scientific perspective, resulting in different options and resulting decision-making processes. The practical level differs profoundly from the scientific level. And despite standard operating procedures of insulin measurement and injections schemes, this approach might fail, because the underlying complex reality cannot be captured with this approach.
As most real and novel experimental contexts are complicated or complex, various levels of management and decision making are necessary to initiate the research, perform it, interpret it, communicate it and thereby make it successful. This starts, of course, with the implicit and explicit decisions on the right questions.
In complex research contexts with their emergent properties causality cannot be fully predicted a priori but only approximated to guide the experimental process. The stringency of the hypotheses varies. Iterative processes with computational modeling and experimental verification lie at the heart of any “systems” approach as the accepted answer to biological and medical complexity. Initial questions here tend to be extremely open, so that they do not qualify as “hypothesis” anymore, although this might be a semantic issue and the decision lie in the eyes of the beholder. As a consequence, the answers deduced from the results of such research might be more concrete than the question that has originally been asked, invariably leading to a retrograde, reconstruction of a “storyline” that satisfies our desire for simplicity and a narrative structure. From a communication point of view this might be justifiable and even necessary, but it would require a wider appreciation that such reduced stories are punchlines of a generally complex world in order to avoid larger scale misunderstandings and inflated hopes for translation. Reductionism, simplification, narrative structures, etc., will always be necessary but need to be seen in the correct context of the real complexity (or chaos or disorderedness).
The Cynefin framework is one way of sensitizing at least the researchers, grant agencies, journalists and politicians (as the mainly involved managers) to the the complexity in biomedical research and its consequences to insight and decision making.
“Case manager” are an established solution to addressing (and reducing) clinical complexity in cancer, dementia and neurodegeneration and arrive at executable best solutions (Huston, 2002 ). The Cynefin scheme provides a straightforward framework to convey the necessity and benefits of such solutions to all stakeholders. Multi-disciplinary case management in science is still rare.
Case study: Parkinson's disease
Parkinson's disease (PD) is caused by the degeneration of dopaminergic neurons in the substantia nigra of the midbrain resulting in a characteristic pattern of motoric symptoms. This is a “simple,” monocausal description of the disease that is widely found in publications and is about the level of knowledge even of many health professionals. The obvious treatment option according to this description has been the extrinsic replacement of dopamine in the form of L-dopa, which indeed has the power of relieving the symptoms. But generally not quite. Some symptoms respond better than others, the effect might fluctuate over time and it wears off (Salat and Tolosa, 2013 ). There is also a massive inter-individual variation in the response. Treatment with L-dopa is initially the approach of choice in most patients, but over time inherent limits become apparent.
But there is a large variation in the clinical picture and the genetic causes anyway (Riess et al., 2000 ; Mullin and Schapira, 2015 ). The classical triade of tremor, rigor, and akinesia is found to a variable degree, there are non-motor symptoms, including dementia, the disease has a long clinically silent latency period, during which conditions, such as certain sleep disturbances might show up as first indicators and there are mixed forms with other types of neurodegeneration (Berardelli et al., 2013 ; Martí and Tolosa, 2013 ). Such situation obviously qualifies as “complicated” at the very least. Treatment requires expert knowledge in weighing symptoms and progression against treatment options. There is generally no single correct solution that fits all situations. Therapy belongs, at least temporally, into the hands of specialists, who can collect that information. We are at the upper-right quadrant of the Cynefin scheme.
But the disease is actually complex and while treating it as “complicated” is better than simplifying it, still has serious limitations. The genetics of PD reveal that the sporadic forms of the disease are polygenic with low effect sizes for individual loci (Riess et al., 2000 ; Mullin and Schapira, 2015 ). Most of these do not show any overt relationship to the dopaminergic system. The main pathogenic mechanism relates to the accumulation of alpha-synuclein, but mutations related to the alpha-synuclein gene account for only a very small subset of the disease (Lashuel et al., 2013 ). Polymorphisms in the Lrkk2 gene shows a high association with sporadic PD, but the effects are highly variable and no single mechanism has as yet emerged, how Lrkk2 might be causally involved in PD. It seems that no such single, unique relationship exists (Esteves et al., 2014 ).
In addition, environmental factors obviously play an important role, ranging from toxins, especially pesticides, to lifestyle risk and resilience factors (Checkoway and Nelson, 1999 ). The relative contribution and interaction of all of these is not really known and no comprehensive model of the disease exists. By all standards, PD qualifies as “complex” with all the consequences for insight and decision making. Sticking with a “simple” disease concept is prone to failure, with all the consequences of “falling over the cliff.” Concretely, the patient might be overwhelmed by side-effects, might not be treated for the full range of symptoms, and critical differential diagnoses are missed. Appreciating that the situation is complicated, in contrast, still misses the critical point that there are aspects which cannot be known in complex contexts and, most importantly, that finding solutions are approximations.
Organizational consequences
The original Cynefin framework emphasizes the social and cultural context. The approach has been designed to elucidate the relationship between individual, experience and context. The generalization to a tool of knowledge management allows the application to science and research at the level of contents, as outlined in this article, not just organization. But there are of course opportunities for combinations of the different domains, insight and knowledge on one side and decision-making and organization on the other. In fact they are closely related and will constantly inform and influence each other.
Some life-scientists might at first tend to find Cynefin rather common-sensical, but what they criticize in the concept might actually be an advantage. First in the sense of Ockham's razor, that the more simple description might actually the better one, but also by facilitating acceptance on the long run. Snowden characterized his tool as one whose outcome could be sketched on a napkin.
The point is not to invent a new layer of complexity that has to be dealt with in addition to the complexity that prevails anyway but to develop a culture in biomedical research that appreciates and accepts complexity and provides a reference framework and common language.
Many scientists experience the current organization of science and research as increasingly inadequate. Research in the life sciences is becoming more and more differentiated and specialized and takes place under an increasing numbers of preconditions that we are usually not aware of. These conditions includes opportunities and constraints and require additional efforts to decipher their impact and act accordingly.
Conclusions
The reproducibility crisis, the conceptual and practical challenges of multi-omics and big data, the discussions around personalized medicine, the heritability gap in genome wide association studies, the failure of clinical trials for cancer and neurodegeneration after successful animal experiments and the tremendous difficulties in defining criteria for satisfying “mechanistic” explanations of a biological observation are all examples of struggles with complexity in biomedicine. Living organism are complex, because they are adaptive, dynamic, self-organizing, developing, learning, etc., and have emergent properties, but in most scientific projects, from design to publication, and from Ph.D. theses over project reviews to institutional evaluations and research politics, consequences of complexity on results, insight and decisions are not considered systematically. The Cynefin scheme, originally published as tool for decision-making in management has developed into a broadly applicable approach for knowledge management, but remains to be discovered also by biologists and clinical researchers to conceptually and practically deal with complexity. Cynefin is no substitute for concrete scientific solutions to the consequences of complexity, but provides a common reference language and conceptual framework to talk about complexity and draw the appropriate conclusions for insight, decisions and actions. For biomedical research in general, Cynefin helps to capture the critical consequences of complexity at the place, where they arise, and prevents their sequestration into the specialist domains of “systems” biomedicine.
Author contributions
The author confirms being the sole contributor of this work and approved it for publication.
Conflict of interest statement
The author declares that the research was conducted in the absence of any commercial or financial relationships that could be construed as a potential conflict of interest.
Acknowledgments
I would like to thank the Helmholtz Academy and Dr. Oliver Mack (osb Vienna) for first exposing me to Cynefin, the participants of the 2017 Systems Genetics workshop (funded by Bundesministerium für Bildung und Forschung, BMBF) in Frauenchiemsee, Germany, for insightful discussion, and in particular Rob Williams for specific comments on the manuscript and for alerting me to a number of important references.
- Berardelli A., Wenning G. K., Antonini A., Berg D., Bloem B. R., Bonifati V., et al.. (2013). EFNS/MDS-ES/ENS [corrected] recommendations for the diagnosis of Parkinson's disease . Eur. J. Neurol. 20 , 16–34. 10.1111/ene.12022 [ PubMed ] [ CrossRef ] [ Google Scholar ]
- Boyle E. A., Li Y. I., Pritchard J. K. (2017). An expanded view of complex traits: from polygenic to omnigenic . Cell 169 , 1177–1186. 10.1016/j.cell.2017.05.038 [ PMC free article ] [ PubMed ] [ CrossRef ] [ Google Scholar ]
- Checkoway H., Nelson L. M. (1999). Epidemiologic approaches to the study of Parkinson's disease etiology . Epidemiology 10 , 327–336. 10.1097/00001648-199905000-00023 [ PubMed ] [ CrossRef ] [ Google Scholar ]
- Craver C. F., Darden L. (2013). In Search of Mechanisms: Discoveries Across the Life Sciences . Chicago, IL: University of Chicago Press. [ Google Scholar ]
- Esteves A. R., Swerdlow R. H., Cardoso S. M. (2014). LRRK2, a puzzling protein: insights into Parkinson's disease pathogenesis . Exp. Neurol. 261 , 206–216. 10.1016/j.expneurol.2014.05.025 [ PMC free article ] [ PubMed ] [ CrossRef ] [ Google Scholar ]
- Huston C. J. (2002). The role of the case manager in a disease management program . Lippincotts. Case Manage. 7 , 221–227. 10.1097/00129234-200211000-00003 [ PubMed ] [ CrossRef ] [ Google Scholar ]
- Lashuel H. A., Overk C. R., Oueslati A., Masliah E. (2013). The many faces of α-synuclein: from structure and toxicity to therapeutic target . Nat. Rev. Neurosci. 14 , 38–48. 10.1038/nrn3406 [ PMC free article ] [ PubMed ] [ CrossRef ] [ Google Scholar ]
- Manolio T. A., Collins F. S., Cox N. J., Goldstein D. B., Hindorff L. A., Hunter D. J., et al.. (2009). Finding the missing heritability of complex diseases . Nature 461 , 747–753. 10.1038/nature08494 [ PMC free article ] [ PubMed ] [ CrossRef ] [ Google Scholar ]
- Marder E. (2011). Variability, compensation, and modulation in neurons and circuits . Proc. Natl. Acad. Sci. U.S.A. 108 , 15542–15548. 10.1073/pnas.1010674108 [ PMC free article ] [ PubMed ] [ CrossRef ] [ Google Scholar ]
- Mark A. L. (2006). Notes from a small Island: researching organisational behaviour in healthcare from a UK perspective . J. Organ. Behav. 27 , 851–867. 10.1002/job.414 [ CrossRef ] [ Google Scholar ]
- Martí M. J., Tolosa E. (2013). Parkinson disease: new guidelines for diagnosis of Parkinson disease . Nat. Rev. Neurol. 9 , 190–191. 10.1038/nrneurol.2013.47 [ PubMed ] [ CrossRef ] [ Google Scholar ]
- Mora T., Bialek W. (2011). Are biological systems poised at criticality? J. Stat. Phys. 144 , 268–302. 10.1007/s10955-011-0229-4 [ CrossRef ] [ Google Scholar ]
- Mullin S., Schapira A. (2015). The genetics of Parkinson's disease . Br. Med. Bull. 114 , 39–52. 10.1093/bmb/ldv022 [ PubMed ] [ CrossRef ] [ Google Scholar ]
- ONeill L.-J. (2004). Faith and decision-making in the Bush presidency: the God elephant in the middle of America's living-room . Emerg. Complex. Organ. 6 , 149–156. [ Google Scholar ]
- Riess O., Kuhn W., Krüger R. (2000). Genetic influence on the development of Parkinson's disease . J. Neurol . 247 ( Suppl. 2 ), II69–II74. 10.1007/PL00007764 [ PubMed ] [ CrossRef ] [ Google Scholar ]
- Salat D., Tolosa E. (2013). Levodopa in the treatment of Parkinson's disease: current status and new developments . J. Parkinsons. Dis. 3 , 255–269. 10.3233/JPD-130186 [ PubMed ] [ CrossRef ] [ Google Scholar ]
- Sittig L. J., Carbonetto P., Engel K. A., Krauss K. S., Barrios-Camacho C. M., Palmer A. A. (2016). Genetic background limits generalizability of genotype-phenotype relationships . Neuron 91 , 1–8. 10.1016/j.neuron.2016.08.013 [ PMC free article ] [ PubMed ] [ CrossRef ] [ Google Scholar ]
- Snowden D. (2000). Cynefin: a sense of time and space, the social ecology of knowledge management , in Knowledge Horizons: The Present and the Promise of Knowledge Management , eds Despres C., Chauvel D. (Boston: Butterworth-Heinemann; ), 344. [ Google Scholar ]
- Snowden D. J., Boone M. E. (2007). A leader's framework for decision making . Harv. Bus. Rev . 85 , 68–76. [ PubMed ] [ Google Scholar ]
- Sturmberg J. P., Martin C. M. (2008). Knowing–in medicine . J. Eval. Clin. Pract. 14 , 767–770. 10.1111/j.1365-2753.2008.01011.x [ PubMed ] [ CrossRef ] [ Google Scholar ]
- Van Beurden E. K., Kia A. M., Zask A., Dietrich U., Rose L. (2013). Making sense in a complex landscape: how the cynefin framework from complex adaptive systems theory can inform health promotion practice . Health Promot. Int. 28 , 73–83. 10.1093/heapro/dar089 [ PubMed ] [ CrossRef ] [ Google Scholar ]
- van Swinderen B. (2005). Flexibility in a gene network affecting a simple behavior in Drosophila melanogaster . Genetics 169 , 2151–2163. 10.1534/genetics.104.032631 [ PMC free article ] [ PubMed ] [ CrossRef ] [ Google Scholar ]
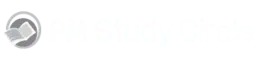
Cynefin Framework: Leaders Framework for Decision Making
Fahad Usmani, PMP
July 24, 2023
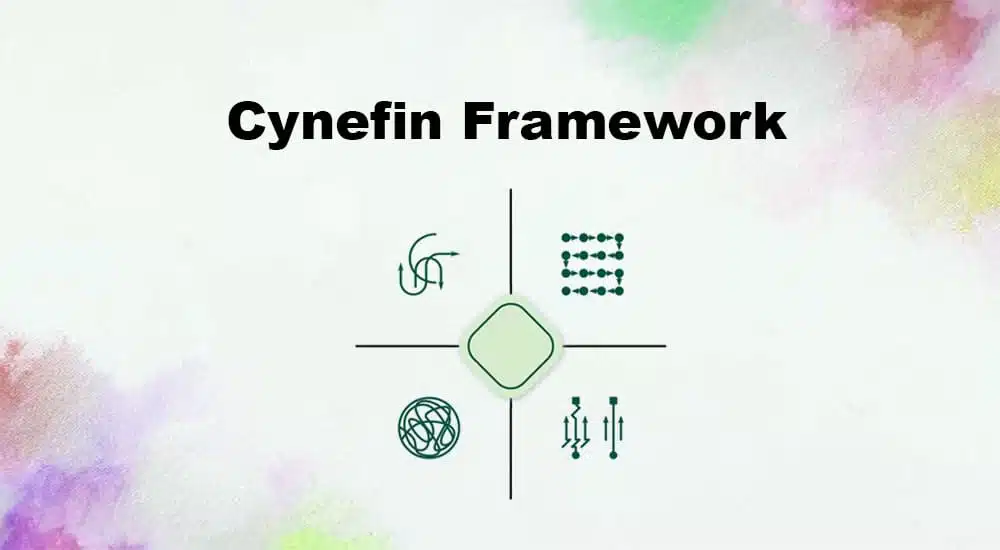
Leaders face many challenges and complexities in today’s dynamic and interconnected era. Understanding, categorizing, and addressing intricate issues has become fundamental for organizations that seek to thrive in uncertainty.
The Cynefin Framework emerges as an indispensable tool, empowering leaders or managers to embrace complexity and find clarity in ambiguity.
What is the Cynefin Framework?
The Cynefin Framework (pronounced as “ku-nev-in”) originated from the Welsh word “Cynefin,” meaning “habitat” or “place of being.” The Cynefin Framework is a sensemaking model that offers a comprehensive approach to understanding and managing diverse problem domains.
This sensemaking model was developed by Dave J. Snowden in 1999 to help leaders make decisions while working for IBM Global Services.
David Snowden described this framework using knowledge-management concepts and organizational strategies. He published the Cynefin Framework with his colleague Mary Boonein in the November 2007 issue of the Harvard Business Review .
The Cynefin Framework Domains
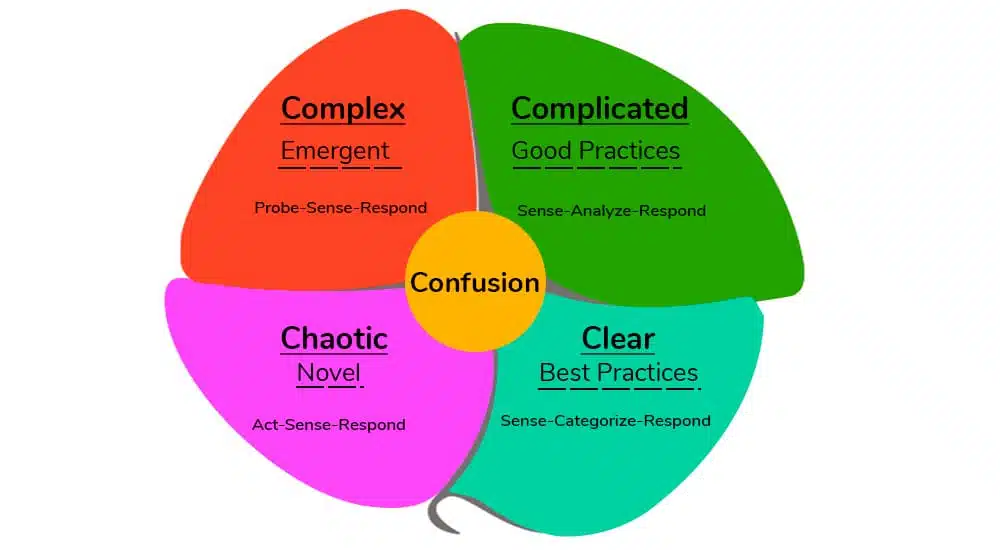
The Cynefin Framework defines domains by cause-and-effect relationships.
These domains are:
- Complicated
These domains help leaders understand that every situation is different and requires a unique approach to decision-making.
Each domain has a specific decision-making approach that helps leaders understand the situation better and choose the best way forward.
This is the domain of best practices for “known knowns,” meaning the situation is stable, rules are in place, and managers can see the clear relationship between cause and effect.
For example, if you do this, that will happen.
Here, you should “sense–categorize–respond.” Using sense, establish the facts, categorize the issues, and respond using best practices. The situation is clear, and you have clear choices. Cause-and-effect relationships are apparent to all stakeholders.
For example, an issue during a hot tap connection is normal, and supervisors have a solution. If some known issue occurs, they will apply the solution right away.
Here you will assess the situation, categorize its type and then base your response on the best practices. You will have the correct answer for the situation.
Sometimes, the gravity of the situation is ignored due to oversimplification and overconfidence. Therefore, communication must be simple, clear, and robust.
Often leaders are not receptive to new ideas as they are sure that the available best practice is the best and there is no possibility of improvement. Leaders must be open to new ideas.
#2. Complicated
This is the domain of experts for “known unknowns.” Managers need skills and expertise to identify the relationship between cause and effect. Leaders have a range of solutions.
In this domain, project managers should “sense–analyze–respond.”
The problem is complicated, and it has many solutions. The relationship between cause and effect is clear but only visible to experts, as the issue is complicated.
For example, you can have many routes to reach the destination, but experts know which route is the fastest and better to reach the destination with the least hassle.
You will assess the situation, analyze what is known, and decide the response using the best practice.
#3. Complex
This is the domain of emergence for “unknown unknowns.” Here, leaders can deduce the cause and effect in retrospect and have no answers. According to Snowden, “instructive pattern … can emerge.”
Here, you should “probe-sense-respond.”
Finding the correct solution or identifying the cause-and-effect relationship here is difficult. The best solution is to be patient, analyze the pattern, and then encourage a solution to emerge.
Communication is key here. Gather experts, seek their opinion, and devise an innovative solution to the complex problem using good practices.
Complicated and complex domains share many similarities, and sometimes it is difficult to identify from each other. However, if the information is incomplete, the domain is most likely the complex domain.
#4. Chaotic
Chaotic contexts are the domain of rapid response. Here, both cause and effect are not clear. Events are too confusing to develop a suitable logical knowledge base response.
Since it is chaos, your first objective is establishing order and stability. Emergency and crises fall into this domain.
Here, you should “act–sense–respond.” Act to establish order, sense it and then respond. The aim is to turn the chaotic situation into a complex and control it.
Project managers must be decisive enough to solve hot-button issues and respond quickly enough to move situations from chaos to complexity. Project managers must ensure that they have a comprehensive risk-and-crisis-management plan to deal with such situations.
#5. Confusion
This domain was known as “disorder” in an earlier version of the theory. In the confusion domain, total confusion reigns supreme, and leaders do not know the dominant domain.
Since you cannot determine the dominant domain, your goal will be to gather information so you can move to a known domain and act accordingly.
Snowden states, “Multiple perspectives jostle for prominence, factional leaders argue with one another, and cacophony rules.”
According to Snowden and Boone, “The way out of this realm is to break down the situation into constituent parts and assign each to one of the other four realms. Leaders can then make decisions and intervene in contextually appropriate ways.”
Applying the Cynefin Framework
The Cynefin Framework is a sensemaking model that helps businesses make decisions and understand the complexity of different situations.
Some applications of the Cynefin Framework are:
- Decision-Making: The Cynefin Framework can guide decision-making processes by categorizing situations into different domains. Project managers can use it to determine whether a problem is simple, complicated, complex, or chaotic and then suggest the appropriate approach for each domain.
- Problem-Solving: Project managers can use it to solve problems with a structured approach based on the nature of the problem. It encourages them to analyze and understand the complexity of a problem before selecting the appropriate methods, tools, and strategies for resolution.
- Strategy Development: The Cynefin Framework is useful for assessing context and determining suitable strategies for different domains. Leaders can use it to understand the nature of their environment, identify patterns, and make informed decisions on their direction and the actions they must take to get there.
- Organizational Change: When implementing organizational change, the Cynefin Framework assesses the complexity of the change effort. It helps change managers determine whether the change is simple, complicated, or complex, thus allowing organizations to tailor their change-management approaches accordingly.
- Innovation and Product Development: The Cynefin Framework can guide innovation and product development. By understanding market complexity, organizations can choose the appropriate methods to generate ideas, test prototypes, and adapt to changing circumstances.
- Project Management: The Cynefin Framework helps project managers assess the complexity and uncertainty involved. Project managers can use it to determine the appropriate project-management approach, communication strategies, and risk-management techniques based on the project’s context.
- Leadership and Team Dynamics: The Cynefin Framework can assist leaders with understanding their team’s complexity and adjusting their leadership style accordingly. It also encourages leaders to be flexible and adapt their approach based on the domain in which their teams operate.
Example of the Cynefin Framework
The technical-support agent must first assess the situation to determine whether it is clear, complicated, complex, or chaotic.
The agent can follow a standard process to resolve the issue if the situation is clear. For example, the agent might check the Knowledge Base to see if there is a known issue with the customer portal.
If the situation is complicated, the agent might need to gather more information from the customer to troubleshoot the issue. For example, the agent might need to ask the customer what steps they took before they noticed the slow response.
If the situation is complex, the agent might need to work with other teams to resolve the issue. For example, the agent might involve the development team to fix a bug in the customer portal.
If the situation is chaotic, the agent might need to act quickly and decisively to resolve the issue. For example, the agent might need to disable the customer portal until the issue is fixed.
Benefits of the Cynefin Framework
- Provides a structured framework to understand complex systems and problems
- Categories and makes sense of different types of problems and their solutions
- Enhances decision-making by promoting a more nuanced and context-specific approach
- Facilitates better communication and collaboration among team members by creating a shared understanding of the problem domain
- Enables organizations to identify and prioritize areas of improvement or intervention
- Reduces the risk of ill-informed decisions by considering a situation’s inherent complexity and/or uncertainty
- Encourages an adaptive and flexible mindset, thus promoting agility and resilience in the face of complex challenges
- Identifies patterns and trends within a system to help develop strategies and interventions
- Provides a learning and knowledge-management tool by capturing insights and lessons from different problem domains
- Explores multiple perspectives and encourages diverse thinking, thus leading to more innovative and effective solutions
The Cynefin Framework Vs the Theory of Constraints
The Cynefin Framework and the Theory of Constraints (ToC) are frameworks used to understand and address complex problems in different domains. Although both frameworks have distinct approaches, they can be complementary in some situations.
The Cynefin Framework was developed by Dave Snowden, and it categorizes problems into five different domains: (1) clear, (2) complicated, (3) complex, (4) chaotic, and (5) confusion. It identifies appropriate approaches to find solutions based on the problem. The Cynefin Framework emphasizes different management styles for different problems, thus encouraging leaders to adapt their decision-making processes accordingly.
The Theory of Constraints was formulated by Eliyahu Goldratt, and it focuses on identifying and removing bottlenecks within a system. It improves the system’s overall efficiency and productivity by pinpointing the constraints that limit the system’s performance. By systematically addressing these constraints, project managers can optimize their processes and improve efficiency.
While Cynefin provides a broader framework for understanding problem domains, ToC offers a specific methodology for improving system performance. In practice, these approaches intersect and complement each other.
For example, Cynefin can help determine whether a problem is best addressed through a ToC or requires a different management approach.
The Cynefin Framework helps leaders and managers understand their challenges and make decisions accordingly. It identifies the type of situations project managers face and ensures that their actions are based on reality and makes sense.
This framework is useful across all industries and sectors (e.g., business, project management, production, policymaking, healthcare, etc.).
Snowden, D., & Goh, Z. (2020). Cynefin—Weaving Sense-Making into the Fabric of Our World .
Snowden, D., & Boone, M. (2007). “A Leader’s Framework for Decision Making.” Harvard Business Review .
Snowden, D. (2010). “The Origins of Cynefin—Part 2.” Retrieved from: https://thecynefin.co/part-two-origins-of-cynefin/
Cognitive Edge: The Origins of Cynefin
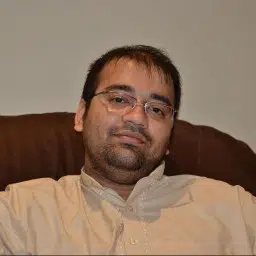
I am Mohammad Fahad Usmani, B.E. PMP, PMI-RMP. I have been blogging on project management topics since 2011. To date, thousands of professionals have passed the PMP exam using my resources.
PMP Question Bank
This is the most popular Question Bank for the PMP Exam. To date, it has helped over 10,000 PMP aspirants prepare for the exam.
PMP Training Program
This is a PMI-approved 35 contact hours training program and it is based on the latest exam content outline applicable in 2024.
Similar Posts
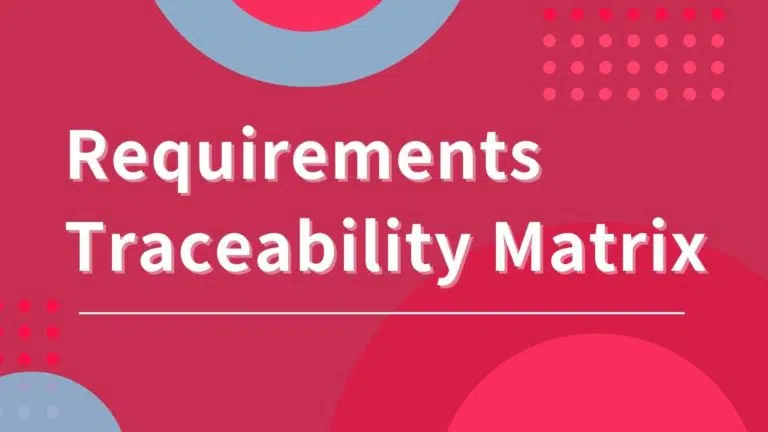
Requirements Traceability Matrix (RTM): Definition, Types, & Example
Definition: A requirement traceability matrix (RTM) shows the relationship between requirements and artifacts (e.g., test cases, defects, etc.). An RTM guarantees that all requirements are validated through test cases, and no functionality is skipped throughout the testing process. This results in an excellent finished product. A requirement traceability matrix documents requirements, tests, test results, and…
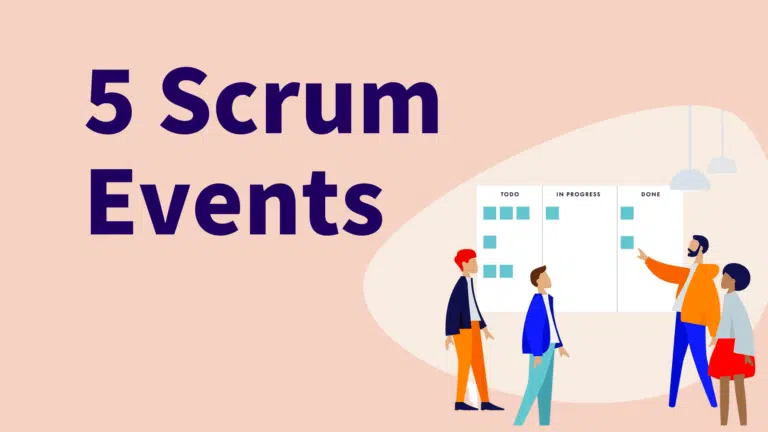
Five Scrum Events or Ceremonies for Beginners
In Agile methodology, collaboration and iterative product development are key to success. Implementing Five Scrum Events within this framework drives teams toward their product goals. These events, collectively known as the “Five Scrum Events,” provide a framework to plan, synchronize, inspect, adapt, and continuously improve the product. Each event has a distinct purpose and brings…

What is a Project Timeline and How to Write it?
When project objectives are accomplished and stakeholders are satisfied, we consider the project is successfully completed. The project objectives are at risk of being derailed in the absence of appropriate planning and tracking. The creation of a project timeline by project managers to monitor the status of a project’s performance and advancement is one way…
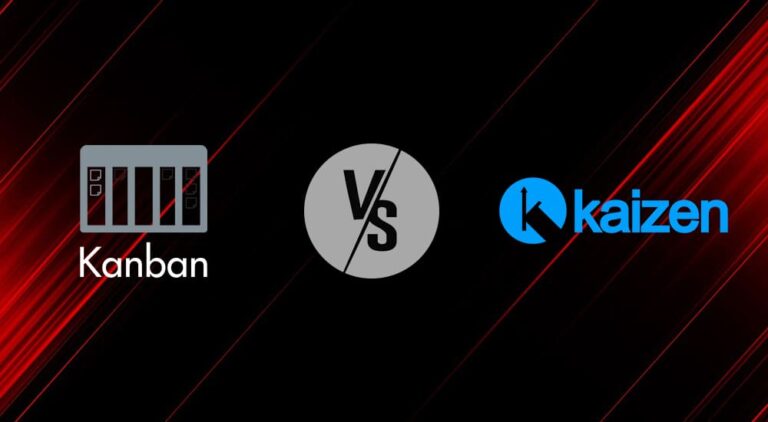
Kanban Vs Kaizen
Kanban and Kaizen are two approaches project managers use to consistently optimization in workflows and drive continuous improvement across various industries. From manufacturing to service–organizations use Kanban and Kaizen in their work environment to manage inventories, control manufacturing costs, etc., and in Agile project management. However, both methodologies differ from one another, and this blog…
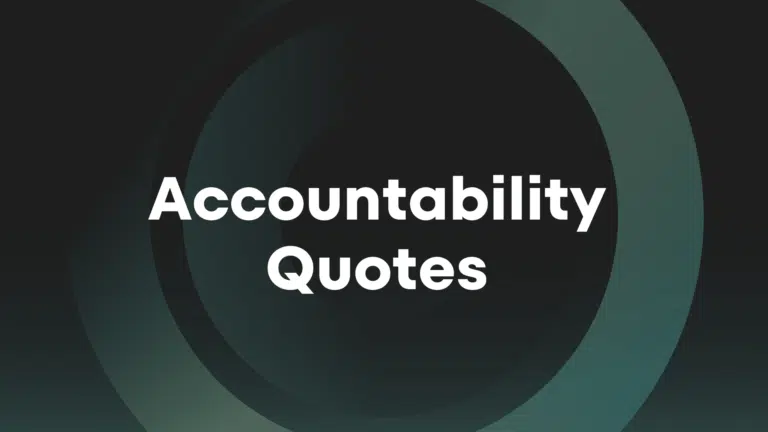
29 Best Accountability Quotes for the Workplace
When you work in a team, and your decisions affect your coworkers, you should understand the importance of taking responsibility for your actions. As a manager, apart from being responsible for your actions, you should also ensure that your team is responsible for their actions. The following accountability quote will help you encourage yourself and…
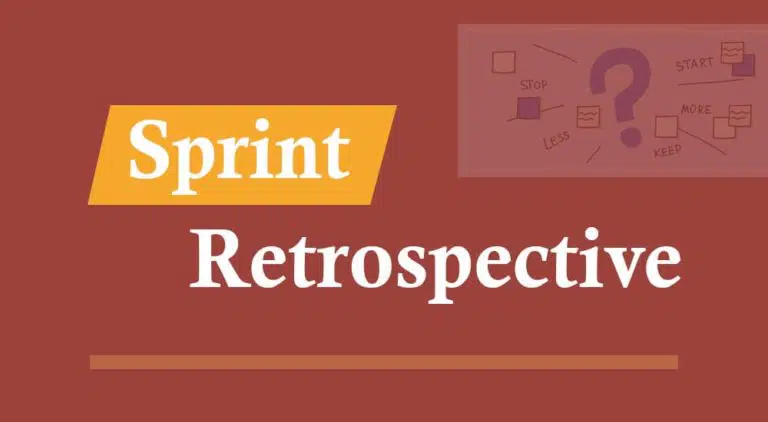
What is Sprint Retrospective & How to Run It
The sprint retrospective is a part of the scrum framework that aims to improve product quality and the scrum team’s effectiveness (source: Scrum Guide). During the Agile retrospective, the scrum team reviews the last sprint’s performance and discusses what went wrong and right. The scrum master discusses the issues, and the team solves the issues….
Leave a Reply Cancel reply
Your email address will not be published. Required fields are marked *

- Your Project
- Cynefin Framework
What is the Cynefin Framework?
The Cynefin Framework is a tool that guides professionals when making decisions by analyzing the context in which that decisions are made .
- Its name, Cynefin, is a Welsh word for “Habitat”.
It was created by Dave Snowden in 1999.
He defined 4 Common situations in which decisions are often made :
- Chaotic situations.
- Complex situations.
- Complicated situations.
- Clear situations.
Depending on the situation ( called Domains ) in which the decision must be made, the Cynefin Framework proposes one approach or another .
The Four Domains of the Cynefin Framework
The 4 Domains (contexts or situations) proposed by the Cynefin Framework are:
- Complicated .
- Clear (also called Simple).
Let’s see what this Tool proposes to make the best decision in each of these scenarios:
Chaotic Domain
What is a Chaotic situation?
- A situation where nobody knows what to expect .
- A situation where anything can happen .
- A situation where it is impossible to make future predictions .
How to act in a Chaotic Situation?
- First : Act differentiating the Urgent and the Important.
- Second : Use your Sense and evaluate the Result.
- Third : Respond to that Result and prepare to Act again.
Examples of Chaotic Domains
- New Innovative Products : It is almost impossible to know if people will love them or not.
- Anything that tries to predict people’s tastes or behaviors . People are unpredictable.
- Shocking New Events : Crisis, Revolutionary events, etc.
- Battle situations : Armies must adapt their operations to unexpected events.
Complex Domain
What is a complex situation?
- A situation in which many uncertain factors intervene, but not all .
- A situation in which many variables interven e .
- A situation that is not new but where it is very difficult to make predictions for the future.
How to act in a Complex situation.
- First : Probe what has proven to work in similar past situations.
- Second : Use your Sense and evaluate the Result.
- Third : Respond to that Result and decide if you need to Probe other things.
Examples of Complex Domains
- Weather predictions : There are lots of factors almost impossible to know.
- Stock exchange : You can have good results, but you also need some luck.
- Playing Poker : Certain strategies work better than others but luck plays a big Role.
- Epidemic control : There are certain things that work but there are many unknown factors.
Complicated Domain
What is a Complicated situation?
- A situation in which most of the variables involved are well known .
- A situation in which you know what you need but don’t know exactly how to achieve it .
- The best you can do is get a good result , but you cannot guarantee the best .
How to act in a Complicated situation?
- First : Use your Sense to list everything you need.
- Second : Analyze how you can maximize your Results.
- Third : Examine the Results and Respond the question: “Is the best result you can get?”.
Examples of Complicated Domains
- Improving an existing product : All variables are known but not how to improve them cost-effectively.
- Coaching a Team : You know how to do it but you have to adapt your method to the team.
- Adopting new methods : You know what to do but not exactly how to do it in the best way.
- Hiring Employees : You might know what to ask in the interview but nothing guarantees success.
Clear Domain
What is a Clear Situation?
- A situation in which all the steps to success are perfectly defined .
- All the variables involved are well known .
- It is possible and feasible to get the best possible result .
How to act in a Clear Situation?
- Second : Categorize all factors involved and follow the procedure to obtain the optimal result.
- Third : Examine the Results and Respond the question: “Did I follow the Steps adequately?”.
Examples of Clear Domains
- Mass producing the same product : you know all the equipment and steps necessary.
- Cooking : If you follow the exact procedure you’ll have a guaranteed success.
- Known Scientific Problems : If you calculate things correctly, you will get your answer.
- Lots of Legal issues : You only will have to follow the law.
Now, before we share some examples with you, we want to clarify one little thing:
- The Best Practice according to the Cynefin Framework .
Cynefin Best Practice
This Framework highlights that, in a Clear scenario, you can find the “Best practice” by:
- Categorize.
What does it mean?
It means that when all the variables are well known and perfectly “controllable”…
- That is when the best decisions can be made .
If there are no uncertainties, if everything is Clear, there is a “Best Practice” that can be applied .
Now, let’s look at some examples so you better understand When the Cynefin Framework can be applied and How:
Cynefin Framework examples
To better understand this Framework, we think that it is interesting to analyze the history of Aeronautics .
- How it started and How it is nowadays.
Why this industry?
Now, you’ll see why:
Chaotic Domain example - Cynefin Framework
At first, nobody knew “what to do to fly”.
- Everyone suspected that it could be possible by imitating birds, but really, no one had a clue.
Many people tried to develop a “flying machine” by building different designs.
- Sometimes, these designs looked weird but… Who knew…
It was a very good example of Chaotic situation.
How could people make good decisions?
- Just trying things out and learning what worked and what didn’t .
Complex Domain example - Cynefin Framework
As soon as the Wright brothers developed the first successful prototype airplane, everyone started to know where things were heading .
- Two wings, a front Propeller, etc.
A good stable and safe plane was still a long way off, but people had clues to follow .
How could engineers make the best decisions in this Complex scenario?
- By Testing what was already known and making small improvements .
Complicated Domain example - Cynefin Framework
Years passed and aircraft design began to improve.
However, there was a problem:
- Long and transoceanic flights were a real challenge.
Engineers knew what they needed, but not exactly how to get it :
- Isolated cabins.
- Resistant materials.
- Light fuselages.
- Good Turbines that could support freezing temperatures.
And most important: It had to be cost-efficient.
How could engineers make the best decisions in this Complicated scenario?
- By analyzing hundreds of materials, designs and Test them to get what they knew they needed .
Clear Domain - Cynefin Framework
Today, there are no mysteries for aircraft designers .
They know exactly how to design a safe, durable and fast airplane that can fly anywhere in the world.
There are still challenges in the aircraft industry? Of course.
But the development of a normal airplane that covers the basic needs of “flight” is well known in the aeronautical industries.
If a group of engineers want to develop a new plane in this Clear situation :
- They would apply all existing knowledge and follow the Steps necessary to build it .
Of course, with hundreds of engineers involved and millions of dollars invested.
- Clear or Simple doesn’t mean Easy .
Summarizing
The Cynefin Framework is a tool that guides professionals when making decisions by analyzing the context in which that decisions are made.
How to make the best decision depending on the context in which it is taken:
- Chaotic : Act, Sense, Respond.
- Complex : Probe, Sense, Respond.
- Complicated : Sense, Analyze, Respond.
- Clear : Sense, Categorize, Respond.
If there are no uncertainties, if everything is Clear, there is a “Best Practice” that can be applied.
Clear and Simple doesn’t necessarily mean Easy .
- Economies of Scale
- Business Plan for Beginners
- Business Plan Basics
- How to write a Business Plan
- Cash Flow Calculation
- Raising Funds for a Business
- 4 C’s of Credit
- Business Plan Templates
- Customer Insight
- Customer Experience
- Customer Pain Points
- 4C Marketing Model
- RATER Model
- Augmented Product
- Product Mix
- Unique Selling Proposition
- DAGMAR Model
- Marketing Storytelling
- Content Marketing
- Psychographics
- Barnum Effect
- Market Segmentation
- Market Research & Big Data
- Marketing to Generation Z
- 4P Marketing Mix
- 7P Marketing Mix
- Sales Funnel
- Loyalty Ladder
- RACE Planning
- Push and Pull Marketing
- Marketing Strategy
- Marketing Templates
- Starting your own business
- From Startup to a Business
- Entrepreneur FAQs
- Start your Business Idea
- Entrepreneur Golden Rules
- Innovate or Imitate?
- Design Thinking
- SCAMPER Model
- AAR Process
- Work From Home
- Growth strategies for Startups
- VMOST Analysis
- 3P Framework
- SOAR Analysis
- TELOS Analysis
- 5 C’s of Entrepreneurship
- Crowdfunding
- BATNA & ZOPA Negotiation
- Entrepreneur with no Money
- Entrepreneurship Templates
- Strategy vs Tactics
- Mission and Vision
- Business Values
- Value Chain
- Scenario Planning
- Porter 6 Forces
- Bowman’s Strategy Clock
- GE-McKinsey Matrix
- Delta Model
- PEST Analysis
- PESTEL Analysis
- SWOT Analysis
- VRIO Framework
- Strategy Canvas
- Competitive Advantages
- Porter’s Four Corners
- 5 Ps of Strategy
- Porter’s Generic Strategies
- Porter’s Diamond Model
- Wardley Map
- Core Competencies
- Resource Based View
- Bridges Transition Model
- CAGE Distance Framework
- McKinsey’s 3 Horizons
- Vertical Integration
- Horizontal Integration
- Blue Ocean Strategy
- Red Ocean Strategy
- Porter 5 Forces
- Ansoff Matrix
- McKinsey 7S Framework
- CATWOE Analysis
- Strategy Pyramid
- Bain’s RAPID Framework
- Balanced Scorecard
- Resources and Capabilities
- Strategy of Apple
- Strategy of Amazon
- Strategy of Starbucks
- Strategy Templates
- Communicate Effectively
- COIN Conversation Model
- SCARF Model
- SBI Feedback Model
- CEDAR Feedback Model
- How to behave at a meeting
- Gibbs’ Reflective Cycle
- Bloom’s Taxonomy
- 5E Learning Model
- 9-Box Performance Grid
- SEEDS Bias Model
- Halo Effect
- Pygmalion Rosenthal Effect
- Dunning-Kruger Effect
- How to be an Entrepreneur
- How to be a Leader
- Mintzberg Managerial Roles
- Cog’s Ladder
- The Peter Principle
- How to Negotiate
- Teamwork Skills and Profiles
- Gantt Chart
- RACI Matrix
- Eisenhower Matrix
- MoSCoW Method
- FMEA Process
- Problem Solving
- Ishikawa Fishbone diagram
- 5 Whys Method
- 8 Disciplines Method
- ADDIE Model
- ORAPAPA Method
- Just In Time
- SMART Goals
- KISS Principle
- Birkinshaw’s 4 Dimensions
- Parkinson’s Law
- OGSM Framework
- OKR Methodology
- APQP Framework
- Theory of Constraints
- Success through Organization
- ADKAR Model
- Lewin’s Change Model
- Kotter’s 8-Step Model
- The Greiner Curve
- GAP Analysis
- Planning Templates
- Mean, Median and Mode
- Define your Data
- Pareto Principle 80/20 Rule
- Decision Matrix
- Decision Tree
- TARA Framework
- Root Cause Analysis
- Simplex Process
- Forecasting Methods
- Product Life Cycle
- How to use Google Trends
- Correlation vs Causation
© 2024 - Consuunt .
We're not around right now. But you can send us an email and we'll get back to you, asap.
Log in with your credentials
Forgot your details.
- Share full article
Advertisement
Supported by
Study Suggests Genetics as a Cause, Not Just a Risk, for Some Alzheimer’s
People with two copies of the gene variant APOE4 are almost certain to get Alzheimer’s, say researchers, who proposed a framework under which such patients could be diagnosed years before symptoms.

By Pam Belluck
Scientists are proposing a new way of understanding the genetics of Alzheimer’s that would mean that up to a fifth of patients would be considered to have a genetically caused form of the disease.
Currently, the vast majority of Alzheimer’s cases do not have a clearly identified cause. The new designation, proposed in a study published Monday, could broaden the scope of efforts to develop treatments, including gene therapy, and affect the design of clinical trials.
It could also mean that hundreds of thousands of people in the United States alone could, if they chose, receive a diagnosis of Alzheimer’s before developing any symptoms of cognitive decline, although there currently are no treatments for people at that stage.
The new classification would make this type of Alzheimer’s one of the most common genetic disorders in the world, medical experts said.
“This reconceptualization that we’re proposing affects not a small minority of people,” said Dr. Juan Fortea, an author of the study and the director of the Sant Pau Memory Unit in Barcelona, Spain. “Sometimes we say that we don’t know the cause of Alzheimer’s disease,” but, he said, this would mean that about 15 to 20 percent of cases “can be tracked back to a cause, and the cause is in the genes.”
The idea involves a gene variant called APOE4. Scientists have long known that inheriting one copy of the variant increases the risk of developing Alzheimer’s, and that people with two copies, inherited from each parent, have vastly increased risk.
The new study , published in the journal Nature Medicine, analyzed data from over 500 people with two copies of APOE4, a significantly larger pool than in previous studies. The researchers found that almost all of those patients developed the biological pathology of Alzheimer’s, and the authors say that two copies of APOE4 should now be considered a cause of Alzheimer’s — not simply a risk factor.
The patients also developed Alzheimer’s pathology relatively young, the study found. By age 55, over 95 percent had biological markers associated with the disease. By 65, almost all had abnormal levels of a protein called amyloid that forms plaques in the brain, a hallmark of Alzheimer’s. And many started developing symptoms of cognitive decline at age 65, younger than most people without the APOE4 variant.
“The critical thing is that these individuals are often symptomatic 10 years earlier than other forms of Alzheimer’s disease,” said Dr. Reisa Sperling, a neurologist at Mass General Brigham in Boston and an author of the study.
She added, “By the time they are picked up and clinically diagnosed, because they’re often younger, they have more pathology.”
People with two copies, known as APOE4 homozygotes, make up 2 to 3 percent of the general population, but are an estimated 15 to 20 percent of people with Alzheimer’s dementia, experts said. People with one copy make up about 15 to 25 percent of the general population, and about 50 percent of Alzheimer’s dementia patients.
The most common variant is called APOE3, which seems to have a neutral effect on Alzheimer’s risk. About 75 percent of the general population has one copy of APOE3, and more than half of the general population has two copies.
Alzheimer’s experts not involved in the study said classifying the two-copy condition as genetically determined Alzheimer’s could have significant implications, including encouraging drug development beyond the field’s recent major focus on treatments that target and reduce amyloid.
Dr. Samuel Gandy, an Alzheimer’s researcher at Mount Sinai in New York, who was not involved in the study, said that patients with two copies of APOE4 faced much higher safety risks from anti-amyloid drugs.
When the Food and Drug Administration approved the anti-amyloid drug Leqembi last year, it required a black-box warning on the label saying that the medication can cause “serious and life-threatening events” such as swelling and bleeding in the brain, especially for people with two copies of APOE4. Some treatment centers decided not to offer Leqembi, an intravenous infusion, to such patients.
Dr. Gandy and other experts said that classifying these patients as having a distinct genetic form of Alzheimer’s would galvanize interest in developing drugs that are safe and effective for them and add urgency to current efforts to prevent cognitive decline in people who do not yet have symptoms.
“Rather than say we have nothing for you, let’s look for a trial,” Dr. Gandy said, adding that such patients should be included in trials at younger ages, given how early their pathology starts.
Besides trying to develop drugs, some researchers are exploring gene editing to transform APOE4 into a variant called APOE2, which appears to protect against Alzheimer’s. Another gene-therapy approach being studied involves injecting APOE2 into patients’ brains.
The new study had some limitations, including a lack of diversity that might make the findings less generalizable. Most patients in the study had European ancestry. While two copies of APOE4 also greatly increase Alzheimer’s risk in other ethnicities, the risk levels differ, said Dr. Michael Greicius, a neurologist at Stanford University School of Medicine who was not involved in the research.
“One important argument against their interpretation is that the risk of Alzheimer’s disease in APOE4 homozygotes varies substantially across different genetic ancestries,” said Dr. Greicius, who cowrote a study that found that white people with two copies of APOE4 had 13 times the risk of white people with two copies of APOE3, while Black people with two copies of APOE4 had 6.5 times the risk of Black people with two copies of APOE3.
“This has critical implications when counseling patients about their ancestry-informed genetic risk for Alzheimer’s disease,” he said, “and it also speaks to some yet-to-be-discovered genetics and biology that presumably drive this massive difference in risk.”
Under the current genetic understanding of Alzheimer’s, less than 2 percent of cases are considered genetically caused. Some of those patients inherited a mutation in one of three genes and can develop symptoms as early as their 30s or 40s. Others are people with Down syndrome, who have three copies of a chromosome containing a protein that often leads to what is called Down syndrome-associated Alzheimer’s disease .
Dr. Sperling said the genetic alterations in those cases are believed to fuel buildup of amyloid, while APOE4 is believed to interfere with clearing amyloid buildup.
Under the researchers’ proposal, having one copy of APOE4 would continue to be considered a risk factor, not enough to cause Alzheimer’s, Dr. Fortea said. It is unusual for diseases to follow that genetic pattern, called “semidominance,” with two copies of a variant causing the disease, but one copy only increasing risk, experts said.
The new recommendation will prompt questions about whether people should get tested to determine if they have the APOE4 variant.
Dr. Greicius said that until there were treatments for people with two copies of APOE4 or trials of therapies to prevent them from developing dementia, “My recommendation is if you don’t have symptoms, you should definitely not figure out your APOE status.”
He added, “It will only cause grief at this point.”
Finding ways to help these patients cannot come soon enough, Dr. Sperling said, adding, “These individuals are desperate, they’ve seen it in both of their parents often and really need therapies.”
Pam Belluck is a health and science reporter, covering a range of subjects, including reproductive health, long Covid, brain science, neurological disorders, mental health and genetics. More about Pam Belluck
The Fight Against Alzheimer’s Disease
Alzheimer’s is the most common form of dementia, but much remains unknown about this daunting disease..
How is Alzheimer’s diagnosed? What causes Alzheimer’s? We answered some common questions .
A study suggests that genetics can be a cause of Alzheimer’s , not just a risk, raising the prospect of diagnosis years before symptoms appear.
Determining whether someone has Alzheimer’s usually requires an extended diagnostic process . But new criteria could lead to a diagnosis on the basis of a simple blood test .
The F.D.A. has given full approval to the Alzheimer’s drug Leqembi. Here is what to know about i t.
Alzheimer’s can make communicating difficult. We asked experts for tips on how to talk to someone with the disease .
- How SenseMaker® works
- Get SenseMaker®
- QuickSenses & Pulses
- SenseMaker® Use Cases
- Citizens Engagement & Democratic Innovation
- Health & Care: making sense of the landscape
- Climate Change
- International Development & Human Rights
- Knowledge Mapping Quickstart
- Assessment & Learning
- Covid in Transition
- Our Thinking
- Our Products
- Learning & Training
- Memberships
- Method Kits
- Executive Briefings & Workshops
- Cynefin Wiki
- About The Cynefin Company
- Pioneers, Stewards & Partners
- Cynefin® Team
- The Cynefin® Framework
- Cynefin® Centre
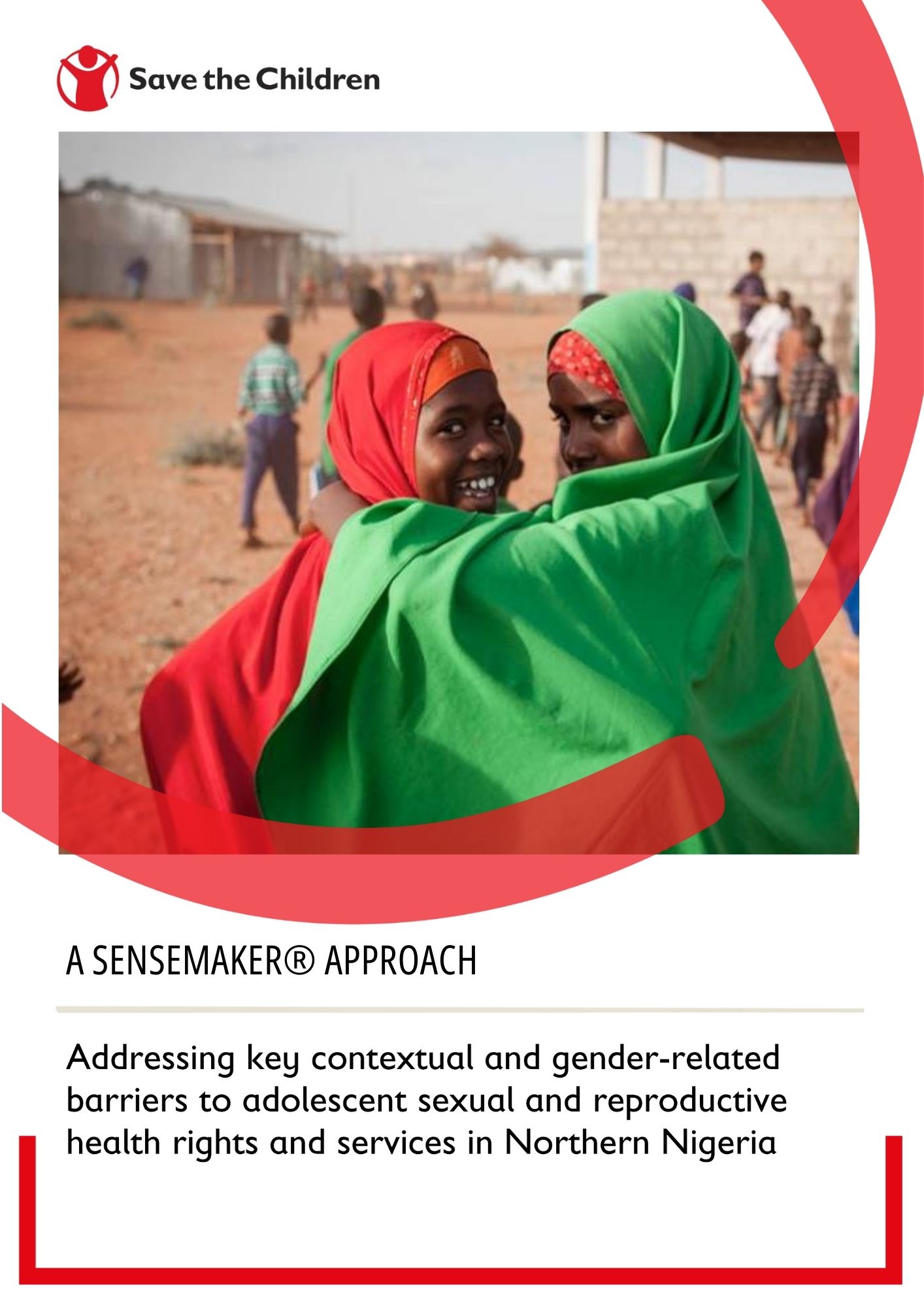
- Government (16)
- Research (13)
- Social Good (12)
- Business (9)
- International Development (9)
- Employment (3)
About the Cynefin Company
Cognitive Edge Ltd. & Cognitive Edge Pte. trading as The Cynefin Company and The Cynefin Centre.
© COPYRIGHT 2024
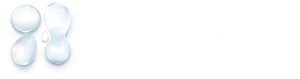
COOKIES ON OUR WEBSITE
Privacy overview.
- Open access
- Published: 15 May 2024
Learning together for better health using an evidence-based Learning Health System framework: a case study in stroke
- Helena Teede 1 , 2 na1 ,
- Dominique A. Cadilhac 3 , 4 na1 ,
- Tara Purvis 3 ,
- Monique F. Kilkenny 3 , 4 ,
- Bruce C.V. Campbell 4 , 5 , 6 ,
- Coralie English 7 ,
- Alison Johnson 2 ,
- Emily Callander 1 ,
- Rohan S. Grimley 8 , 9 ,
- Christopher Levi 10 ,
- Sandy Middleton 11 , 12 ,
- Kelvin Hill 13 &
- Joanne Enticott ORCID: orcid.org/0000-0002-4480-5690 1
BMC Medicine volume 22 , Article number: 198 ( 2024 ) Cite this article
192 Accesses
1 Altmetric
Metrics details
In the context of expanding digital health tools, the health system is ready for Learning Health System (LHS) models. These models, with proper governance and stakeholder engagement, enable the integration of digital infrastructure to provide feedback to all relevant parties including clinicians and consumers on performance against best practice standards, as well as fostering innovation and aligning healthcare with patient needs. The LHS literature primarily includes opinion or consensus-based frameworks and lacks validation or evidence of benefit. Our aim was to outline a rigorously codesigned, evidence-based LHS framework and present a national case study of an LHS-aligned national stroke program that has delivered clinical benefit.
Current core components of a LHS involve capturing evidence from communities and stakeholders (quadrant 1), integrating evidence from research findings (quadrant 2), leveraging evidence from data and practice (quadrant 3), and generating evidence from implementation (quadrant 4) for iterative system-level improvement. The Australian Stroke program was selected as the case study as it provides an exemplar of how an iterative LHS works in practice at a national level encompassing and integrating evidence from all four LHS quadrants. Using this case study, we demonstrate how to apply evidence-based processes to healthcare improvement and embed real-world research for optimising healthcare improvement. We emphasize the transition from research as an endpoint, to research as an enabler and a solution for impact in healthcare improvement.
Conclusions
The Australian Stroke program has nationally improved stroke care since 2007, showcasing the value of integrated LHS-aligned approaches for tangible impact on outcomes. This LHS case study is a practical example for other health conditions and settings to follow suit.
Peer Review reports
Internationally, health systems are facing a crisis, driven by an ageing population, increasing complexity, multi-morbidity, rapidly advancing health technology and rising costs that threaten sustainability and mandate transformation and improvement [ 1 , 2 ]. Although research has generated solutions to healthcare challenges, and the advent of big data and digital health holds great promise, entrenched siloes and poor integration of knowledge generation, knowledge implementation and healthcare delivery between stakeholders, curtails momentum towards, and consistent attainment of, evidence-and value-based care [ 3 ]. This is compounded by the short supply of research and innovation leadership within the healthcare sector, and poorly integrated and often inaccessible health data systems, which have crippled the potential to deliver on digital-driven innovation [ 4 ]. Current approaches to healthcare improvement are also often isolated with limited sustainability, scale-up and impact [ 5 ].
Evidence suggests that integration and partnership across academic and healthcare delivery stakeholders are key to progress, including those with lived experience and their families (referred to here as consumers and community), diverse disciplines (both research and clinical), policy makers and funders. Utilization of evidence from research and evidence from practice including data from routine care, supported by implementation research, are key to sustainably embedding improvement and optimising health care and outcomes. A strategy to achieve this integration is through the Learning Health System (LHS) (Fig. 1 ) [ 2 , 6 , 7 , 8 ]. Although there are numerous publications on LHS approaches [ 9 , 10 , 11 , 12 ], many focus on research perspectives and data, most do not demonstrate tangible healthcare improvement or better health outcomes. [ 6 ]
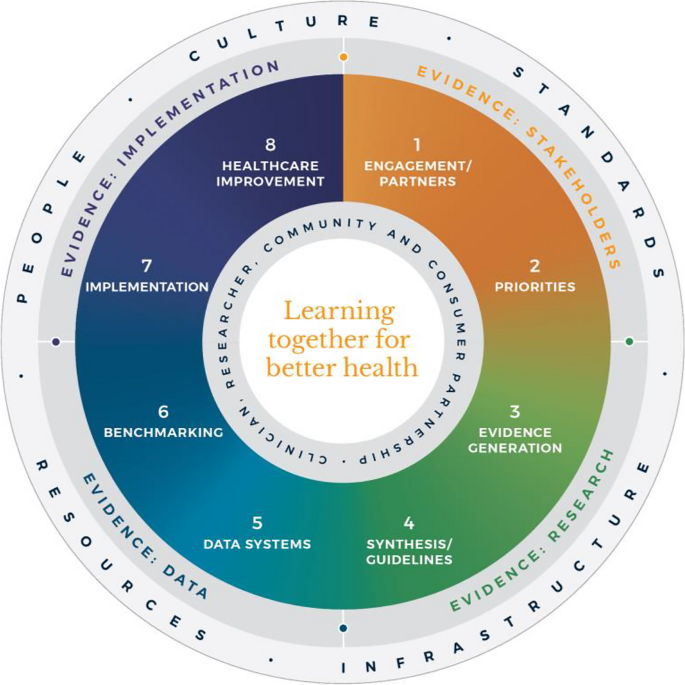
Monash Learning Health System: The Learn Together for Better Health Framework developed by Monash Partners and Monash University (from Enticott et al. 2021 [ 7 ]). Four evidence quadrants: Q1 (orange) is evidence from stakeholders; Q2 (green) is evidence from research; Q3 (light blue) is evidence from data; and, Q4 (dark blue) is evidence from implementation and healthcare improvement
In developed nations, it has been estimated that 60% of care provided aligns with the evidence base, 30% is low value and 10% is potentially harmful [ 13 ]. In some areas, clinical advances have been rapid and research and evidence have paved the way for dramatic improvement in outcomes, mandating rapid implementation of evidence into healthcare (e.g. polio and COVID-19 vaccines). However, healthcare improvement is challenging and slow [ 5 ]. Health systems are highly complex in their design, networks and interacting components, and change is difficult to enact, sustain and scale up. [ 3 ] New effective strategies are needed to meet community needs and deliver evidence-based and value-based care, which reorients care from serving the provider, services and system, towards serving community needs, based on evidence and quality. It goes beyond cost to encompass patient and provider experience, quality care and outcomes, efficiency and sustainability [ 2 , 6 ].
The costs of stroke care are expected to rise rapidly in the next decades, unless improvements in stroke care to reduce the disabling effects of strokes can be successfully developed and implemented [ 14 ]. Here, we briefly describe the Monash LHS framework (Fig. 1 ) [ 2 , 6 , 7 ] and outline an exemplar case in order to demonstrate how to apply evidence-based processes to healthcare improvement and embed real-world research for optimising healthcare. The Australian LHS exemplar in stroke care has driven nationwide improvement in stroke care since 2007.
An evidence-based Learning Health System framework
In Australia, members of this author group (HT, AJ, JE) have rigorously co-developed an evidence-based LHS framework, known simply as the Monash LHS [ 7 ]. The Monash LHS was designed to support sustainable, iterative and continuous robust benefit of improved clinical outcomes. It was created with national engagement in order to be applicable to Australian settings. Through this rigorous approach, core LHS principles and components have been established (Fig. 1 ). Evidence shows that people/workforce, culture, standards, governance and resources were all key to an effective LHS [ 2 , 6 ]. Culture is vital including trust, transparency, partnership and co-design. Key processes include legally compliant data sharing, linkage and governance, resources, and infrastructure [ 4 ]. The Monash LHS integrates disparate and often siloed stakeholders, infrastructure and expertise to ‘Learn Together for Better Health’ [ 7 ] (Fig. 1 ). This integrates (i) evidence from community and stakeholders including priority areas and outcomes; (ii) evidence from research and guidelines; (iii) evidence from practice (from data) with advanced analytics and benchmarking; and (iv) evidence from implementation science and health economics. Importantly, it starts with the problem and priorities of key stakeholders including the community, health professionals and services and creates an iterative learning system to address these. The following case study was chosen as it is an exemplar of how a Monash LHS-aligned national stroke program has delivered clinical benefit.
Australian Stroke Learning Health System
Internationally, the application of LHS approaches in stroke has resulted in improved stroke care and outcomes [ 12 ]. For example, in Canada a sustained decrease in 30-day in-hospital mortality has been found commensurate with an increase in resources to establish the multifactorial stroke system intervention for stroke treatment and prevention [ 15 ]. Arguably, with rapid advances in evidence and in the context of an ageing population with high cost and care burden and substantive impacts on quality of life, stroke is an area with a need for rapid research translation into evidence-based and value-based healthcare improvement. However, a recent systematic review found that the existing literature had few comprehensive examples of LHS adoption [ 12 ]. Although healthcare improvement systems and approaches were described, less is known about patient-clinician and stakeholder engagement, governance and culture, or embedding of data informatics into everyday practice to inform and drive improvement [ 12 ]. For example, in a recent review of quality improvement collaborations, it was found that although clinical processes in stroke care are improved, their short-term nature means there is uncertainty about sustainability and impacts on patient outcomes [ 16 ]. Table 1 provides the main features of the Australian Stroke LHS based on the four core domains and eight elements of the Learning Together for Better Health Framework described in Fig. 1 . The features are further expanded on in the following sections.
Evidence from stakeholders (LHS quadrant 1, Fig. 1 )
Engagement, partners and priorities.
Within the stroke field, there have been various support mechanisms to facilitate an LHS approach including partnership and broad stakeholder engagement that includes clinical networks and policy makers from different jurisdictions. Since 2008, the Australian Stroke Coalition has been co-led by the Stroke Foundation, a charitable consumer advocacy organisation, and Stroke Society of Australasia a professional society with membership covering academics and multidisciplinary clinician networks, that are collectively working to improve stroke care ( https://australianstrokecoalition.org.au/ ). Surveys, focus groups and workshops have been used for identifying priorities from stakeholders. Recent agreed priorities have been to improve stroke care and strengthen the voice for stroke care at a national ( https://strokefoundation.org.au/ ) and international level ( https://www.world-stroke.org/news-and-blog/news/world-stroke-organization-tackle-gaps-in-access-to-quality-stroke-care ), as well as reduce duplication amongst stakeholders. This activity is built on a foundation and culture of research and innovation embedded within the stroke ‘community of practice’. Consumers, as people with lived experience of stroke are important members of the Australian Stroke Coalition, as well as representatives from different clinical colleges. Consumers also provide critical input to a range of LHS activities via the Stroke Foundation Consumer Council, Stroke Living Guidelines committees, and the Australian Stroke Clinical Registry (AuSCR) Steering Committee (described below).
Evidence from research (LHS quadrant 2, Fig. 1 )
Advancement of the evidence for stroke interventions and synthesis into clinical guidelines.
To implement best practice, it is crucial to distil the large volume of scientific and trial literature into actionable recommendations for clinicians to use in practice [ 24 ]. The first Australian clinical guidelines for acute stroke were produced in 2003 following the increasing evidence emerging for prevention interventions (e.g. carotid endarterectomy, blood pressure lowering), acute medical treatments (intravenous thrombolysis, aspirin within 48 h of ischemic stroke), and optimised hospital management (care in dedicated stroke units by a specialised and coordinated multidisciplinary team) [ 25 ]. Importantly, a number of the innovations were developed, researched and proven effective by key opinion leaders embedded in the Australian stroke care community. In 2005, the clinical guidelines for Stroke Rehabilitation and Recovery [ 26 ] were produced, with subsequent merged guidelines periodically updated. However, the traditional process of periodic guideline updates is challenging for end users when new research can render recommendations redundant and this lack of currency erodes stakeholder trust [ 27 ]. In response to this challenge the Stroke Foundation and Cochrane Australia entered a pioneering project to produce the first electronic ‘living’ guidelines globally [ 20 ]. Major shifts in the evidence for reperfusion therapies (e.g. extended time-window intravenous thrombolysis and endovascular clot retrieval), among other advances, were able to be converted into new recommendations, approved by the Australian National Health and Medical Research Council within a few months of publication. Feedback on this process confirmed the increased use and trust in the guidelines by clinicians. The process informed other living guidelines programs, including the successful COVID-19 clinical guidelines [ 28 ].
However, best practice clinical guideline recommendations are necessary but insufficient for healthcare improvement and nesting these within an LHS with stakeholder partnership, enables implementation via a range of proven methods, including audit and feedback strategies [ 29 ].
Evidence from data and practice (LHS quadrant 3, Fig. 1 )
Data systems and benchmarking : revealing the disparities in care between health services. A national system for standardized stroke data collection was established as the National Stroke Audit program in 2007 by the Stroke Foundation [ 30 ] following various state-level programs (e.g. New South Wales Audit) [ 31 ] to identify evidence-practice gaps and prioritise improvement efforts to increase access to stroke units and other acute treatments [ 32 ]. The Audit program alternates each year between acute (commencing in 2007) and rehabilitation in-patient services (commencing in 2008). The Audit program provides a ‘deep dive’ on the majority of recommendations in the clinical guidelines whereby participating hospitals provide audits of up to 40 consecutive patient medical records and respond to a survey about organizational resources to manage stroke. In 2009, the AuSCR was established to provide information on patients managed in acute hospitals based on a small subset of quality processes of care linked to benchmarked reports of performance (Fig. 2 ) [ 33 ]. In this way, the continuous collection of high-priority processes of stroke care could be regularly collected and reviewed to guide improvement to care [ 34 ]. Plus clinical quality registry programs within Australia have shown a meaningful return on investment attributed to enhanced survival, improvements in quality of life and avoided costs of treatment or hospital stay [ 35 ].
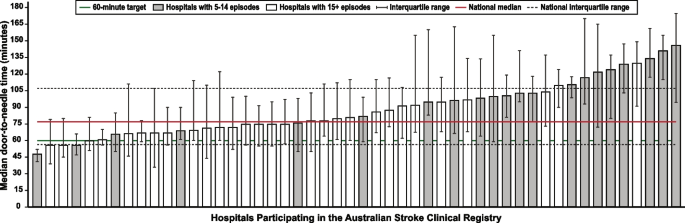
Example performance report from the Australian Stroke Clinical Registry: average door-to-needle time in providing intravenous thrombolysis by different hospitals in 2021 [ 36 ]. Each bar in the figure represents a single hospital
The Australian Stroke Coalition endorsed the creation of an integrated technological solution for collecting data through a single portal for multiple programs in 2013. In 2015, the Stroke Foundation, AuSCR consortium, and other relevant groups cooperated to design an integrated data management platform (the Australian Stroke Data Tool) to reduce duplication of effort for hospital staff in the collection of overlapping variables in the same patients [ 19 ]. Importantly, a national data dictionary then provided the common data definitions to facilitate standardized data capture. Another important feature of AuSCR is the collection of patient-reported outcome surveys between 90 and 180 days after stroke, and annual linkage with national death records to ascertain survival status [ 33 ]. To support a LHS approach, hospitals that participate in AuSCR have access to a range of real-time performance reports. In efforts to minimize the burden of data collection in the AuSCR, interoperability approaches to import data directly from hospital or state-level managed stroke databases have been established (Fig. 3 ); however, the application has been variable and 41% of hospitals still manually enter all their data.
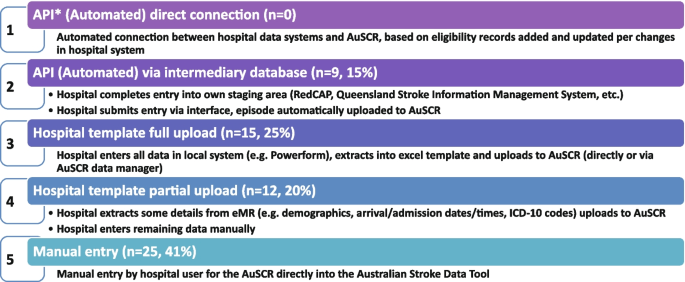
Current status of automated data importing solutions in the Australian Stroke Clinical Registry, 2022, with ‘ n ’ representing the number of hospitals. AuSCR, Australian Stroke Clinical Registry; AuSDaT, Australian Stroke Data Tool; API, Application Programming Interface; ICD, International Classification of Diseases; RedCAP, Research Electronic Data Capture; eMR, electronic medical records
For acute stroke care, the Australian Commission on Quality and Safety in Health Care facilitated the co-design (clinicians, academics, consumers) and publication of the national Acute Stroke Clinical Care Standard in 2015 [ 17 ], and subsequent review [ 18 ]. The indicator set for the Acute Stroke Standard then informed the expansion of the minimum dataset for AuSCR so that hospitals could routinely track their performance. The national Audit program enabled hospitals not involved in the AuSCR to assess their performance every two years against the Acute Stroke Standard. Complementing these efforts, the Stroke Foundation, working with the sector, developed the Acute and Rehabilitation Stroke Services Frameworks to outline the principles, essential elements, models of care and staffing recommendations for stroke services ( https://informme.org.au/guidelines/national-stroke-services-frameworks ). The Frameworks are intended to guide where stroke services should be developed, and monitor their uptake with the organizational survey component of the Audit program.
Evidence from implementation and healthcare improvement (LHS quadrant 4, Fig. 1 )
Research to better utilize and augment data from registries through linkage [ 37 , 38 , 39 , 40 ] and to ensure presentation of hospital or service level data are understood by clinicians has ensured advancement in the field for the Australian Stroke LHS [ 41 ]. Importantly, greater insights into whole patient journeys, before and after a stroke, can now enable exploration of value-based care. The LHS and stroke data platform have enabled focused and time-limited projects to create a better understanding of the quality of care in acute or rehabilitation settings [ 22 , 42 , 43 ]. Within stroke, all the elements of an LHS culminate into the ready availability of benchmarked performance data and support for implementation of strategies to address gaps in care.
Implementation research to grow the evidence base for effective improvement interventions has also been a key pillar in the Australian context. These include multi-component implementation interventions to achieve behaviour change for particular aspects of stroke care, [ 22 , 23 , 44 , 45 ] and real-world approaches to augmenting access to hyperacute interventions in stroke through the use of technology and telehealth [ 46 , 47 , 48 , 49 ]. The evidence from these studies feeds into the living guidelines program and the data collection systems, such as the Audit program or AuSCR, which are then amended to ensure data aligns to recommended care. For example, the use of ‘hyperacute aspirin within the first 48 h of ischemic stroke’ was modified to be ‘hyperacute antiplatelet…’ to incorporate new evidence that other medications or combinations are appropriate to use. Additionally, new datasets have been developed to align with evidence such as the Fever, Sugar, and Swallow variables [ 42 ]. Evidence on improvements in access to best practice care from the acute Audit program [ 50 ] and AuSCR is emerging [ 36 ]. For example, between 2007 and 2017, the odds of receiving intravenous thrombolysis after ischemic stroke increased by 16% 9OR 1.06 95% CI 1.13–1.18) and being managed in a stroke unit by 18% (OR 1.18 95% CI 1.17–1.20). Over this period, the median length of hospital stay for all patients decreased from 6.3 days in 2007 to 5.0 days in 2017 [ 51 ]. When considering the number of additional patients who would receive treatment in 2017 in comparison to 2007 it was estimated that without this additional treatment, over 17,000 healthy years of life would be lost in 2017 (17,786 disability-adjusted life years) [ 51 ]. There is evidence on the cost-effectiveness of different system-focussed strategies to augment treatment access for acute ischemic stroke (e.g. Victorian Stroke Telemedicine program [ 52 ] and Melbourne Mobile Stroke Unit ambulance [ 53 ]). Reciprocally, evidence from the national Rehabilitation Audit, where the LHS approach has been less complete or embedded, has shown fewer areas of healthcare improvement over time [ 51 , 54 ].
Within the field of stroke in Australia, there is indirect evidence that the collective efforts that align to establishing the components of a LHS have had an impact. Overall, the age-standardised rate of stroke events has reduced by 27% between 2001 and 2020, from 169 to 124 events per 100,000 population. Substantial declines in mortality rates have been reported since 1980. Commensurate with national clinical guidelines being updated in 2007 and the first National Stroke Audit being undertaken in 2007, the mortality rates for men (37.4 deaths per 100,000) and women (36.1 deaths per 100,0000 has declined to 23.8 and 23.9 per 100,000, respectively in 2021 [ 55 ].
Underpinning the LHS with the integration of the four quadrants of evidence from stakeholders, research and guidelines, practice and implementation, and core LHS principles have been addressed. Leadership and governance have been important, and programs have been established to augment workforce training and capacity building in best practice professional development. Medical practitioners are able to undertake courses and mentoring through the Australasian Stroke Academy ( http://www.strokeacademy.com.au/ ) while nurses (and other health professionals) can access teaching modules in stroke care from the Acute Stroke Nurses Education Network ( https://asnen.org/ ). The Association of Neurovascular Clinicians offers distance-accessible education and certification to develop stroke expertise for interdisciplinary professionals, including advanced stroke co-ordinator certification ( www.anvc.org ). Consumer initiative interventions are also used in the design of the AuSCR Public Summary Annual reports (available at https://auscr.com.au/about/annual-reports/ ) and consumer-related resources related to the Living Guidelines ( https://enableme.org.au/resources ).
The important success factors and lessons from stroke as a national exemplar LHS in Australia include leadership, culture, workforce and resources integrated with (1) established and broad partnerships across the academic-clinical sector divide and stakeholder engagement; (2) the living guidelines program; (3) national data infrastructure, including a national data dictionary that provides the common data framework to support standardized data capture; (4) various implementation strategies including benchmarking and feedback as well as engagement strategies targeting different levels of the health system; and (5) implementation and improvement research to advance stroke systems of care and reduce unwarranted variation in practice (Fig. 1 ). Priority opportunities now include the advancement of interoperability with electronic medical records as an area all clinical quality registry’s programs needs to be addressed, as well as providing more dynamic and interactive data dashboards tailored to the need of clinicians and health service executives.
There is a clear mandate to optimise healthcare improvement with big data offering major opportunities for change. However, we have lacked the approaches to capture evidence from the community and stakeholders, to integrate evidence from research, to capture and leverage data or evidence from practice and to generate and build on evidence from implementation using iterative system-level improvement. The LHS provides this opportunity and is shown to deliver impact. Here, we have outlined the process applied to generate an evidence-based LHS and provide a leading exemplar in stroke care. This highlights the value of moving from single-focus isolated approaches/initiatives to healthcare improvement and the benefit of integration to deliver demonstrable outcomes for our funders and key stakeholders — our community. This work provides insight into strategies that can both apply evidence-based processes to healthcare improvement as well as implementing evidence-based practices into care, moving beyond research as an endpoint, to research as an enabler, underpinning delivery of better healthcare.
Availability of data and materials
Not applicable
Abbreviations
Australian Stroke Clinical Registry
Confidence interval
- Learning Health System
World Health Organization. Delivering quality health services . OECD Publishing; 2018.
Enticott J, Braaf S, Johnson A, Jones A, Teede HJ. Leaders’ perspectives on learning health systems: A qualitative study. BMC Health Serv Res. 2020;20:1087.
Article PubMed PubMed Central Google Scholar
Melder A, Robinson T, McLoughlin I, Iedema R, Teede H. An overview of healthcare improvement: Unpacking the complexity for clinicians and managers in a learning health system. Intern Med J. 2020;50:1174–84.
Article PubMed Google Scholar
Alberto IRI, Alberto NRI, Ghosh AK, Jain B, Jayakumar S, Martinez-Martin N, et al. The impact of commercial health datasets on medical research and health-care algorithms. Lancet Digit Health. 2023;5:e288–94.
Article CAS PubMed PubMed Central Google Scholar
Dixon-Woods M. How to improve healthcare improvement—an essay by Mary Dixon-Woods. BMJ. 2019;367: l5514.
Enticott J, Johnson A, Teede H. Learning health systems using data to drive healthcare improvement and impact: A systematic review. BMC Health Serv Res. 2021;21:200.
Enticott JC, Melder A, Johnson A, Jones A, Shaw T, Keech W, et al. A learning health system framework to operationalize health data to improve quality care: An Australian perspective. Front Med (Lausanne). 2021;8:730021.
Dammery G, Ellis LA, Churruca K, Mahadeva J, Lopez F, Carrigan A, et al. The journey to a learning health system in primary care: A qualitative case study utilising an embedded research approach. BMC Prim Care. 2023;24:22.
Foley T, Horwitz L, Zahran R. The learning healthcare project: Realising the potential of learning health systems. 2021. Available from https://learninghealthcareproject.org/wp-content/uploads/2021/05/LHS2021report.pdf . Accessed Jan 2024.
Institute of Medicine. Best care at lower cost: The path to continuously learning health care in America. Washington: The National Academies Press; 2013.
Google Scholar
Zurynski Y, Smith CL, Vedovi A, Ellis LA, Knaggs G, Meulenbroeks I, et al. Mapping the learning health system: A scoping review of current evidence - a white paper. 2020:63
Cadilhac DA, Bravata DM, Bettger J, Mikulik R, Norrving B, Uvere E, et al. Stroke learning health systems: A topical narrative review with case examples. Stroke. 2023;54:1148–59.
Braithwaite J, Glasziou P, Westbrook J. The three numbers you need to know about healthcare: The 60–30-10 challenge. BMC Med. 2020;18:1–8.
Article Google Scholar
King D, Wittenberg R, Patel A, Quayyum Z, Berdunov V, Knapp M. The future incidence, prevalence and costs of stroke in the UK. Age Ageing. 2020;49:277–82.
Ganesh A, Lindsay P, Fang J, Kapral MK, Cote R, Joiner I, et al. Integrated systems of stroke care and reduction in 30-day mortality: A retrospective analysis. Neurology. 2016;86:898–904.
Lowther HJ, Harrison J, Hill JE, Gaskins NJ, Lazo KC, Clegg AJ, et al. The effectiveness of quality improvement collaboratives in improving stroke care and the facilitators and barriers to their implementation: A systematic review. Implement Sci. 2021;16:16.
Australian Commission on Safety and Quality in Health Care. Acute stroke clinical care standard. 2015. Available from https://www.safetyandquality.gov.au/our-work/clinical-care-standards/acute-stroke-clinical-care-standard . Accessed Jan 2024.
Australian Commission on Safety and Quality in Health Care. Acute stroke clinical care standard. Sydney: ACSQHC; 2019. Available from https://www.safetyandquality.gov.au/publications-and-resources/resource-library/acute-stroke-clinical-care-standard-evidence-sources . Accessed Jan 2024.
Ryan O, Ghuliani J, Grabsch B, Hill K, G CC, Breen S, et al. Development, implementation, and evaluation of the Australian Stroke Data Tool (AuSDaT): Comprehensive data capturing for multiple uses. Health Inf Manag. 2022:18333583221117184.
English C, Bayley M, Hill K, Langhorne P, Molag M, Ranta A, et al. Bringing stroke clinical guidelines to life. Int J Stroke. 2019;14:337–9.
English C, Hill K, Cadilhac DA, Hackett ML, Lannin NA, Middleton S, et al. Living clinical guidelines for stroke: Updates, challenges and opportunities. Med J Aust. 2022;216:510–4.
Cadilhac DA, Grimley R, Kilkenny MF, Andrew NE, Lannin NA, Hill K, et al. Multicenter, prospective, controlled, before-and-after, quality improvement study (Stroke123) of acute stroke care. Stroke. 2019;50:1525–30.
Cadilhac DA, Marion V, Andrew NE, Breen SJ, Grabsch B, Purvis T, et al. A stepped-wedge cluster-randomized trial to improve adherence to evidence-based practices for acute stroke management. Jt Comm J Qual Patient Saf. 2022.
Elliott J, Lawrence R, Minx JC, Oladapo OT, Ravaud P, Jeppesen BT, et al. Decision makers need constantly updated evidence synthesis. Nature. 2021;600:383–5.
Article CAS PubMed Google Scholar
National Stroke Foundation. National guidelines for acute stroke management. Melbourne: National Stroke Foundation; 2003.
National Stroke Foundation. Clinical guidelines for stroke rehabilitation and recovery. Melbourne: National Stroke Foundation; 2005.
Phan TG, Thrift A, Cadilhac D, Srikanth V. A plea for the use of systematic review methodology when writing guidelines and timely publication of guidelines. Intern Med J . 2012;42:1369–1371; author reply 1371–1362
Tendal B, Vogel JP, McDonald S, Norris S, Cumpston M, White H, et al. Weekly updates of national living evidence-based guidelines: Methods for the Australian living guidelines for care of people with COVID-19. J Clin Epidemiol. 2021;131:11–21.
Grimshaw JM, Eccles MP, Lavis JN, Hill SJ, Squires JE. Knowledge translation of research findings. Implement Sci. 2012;7:50.
Harris D, Cadilhac D, Hankey GJ, Hillier S, Kilkenny M, Lalor E. National stroke audit: The Australian experience. Clin Audit. 2010;2:25–31.
Cadilhac DA, Purvis T, Kilkenny MF, Longworth M, Mohr K, Pollack M, et al. Evaluation of rural stroke services: Does implementation of coordinators and pathways improve care in rural hospitals? Stroke. 2013;44:2848–53.
Cadilhac DA, Moss KM, Price CJ, Lannin NA, Lim JY, Anderson CS. Pathways to enhancing the quality of stroke care through national data monitoring systems for hospitals. Med J Aust. 2013;199:650–1.
Cadilhac DA, Lannin NA, Anderson CS, Levi CR, Faux S, Price C, et al. Protocol and pilot data for establishing the Australian Stroke Clinical Registry. Int J Stroke. 2010;5:217–26.
Ivers N, Jamtvedt G, Flottorp S, Young J, Odgaard-Jensen J, French S, et al. Audit and feedback: Effects on professional practice and healthcare outcomes. Cochrane Database Syst Rev . 2012
Australian Commission on Safety and Quality in Health Care. Economic evaluation of clinical quality registries. Final report. . 2016:79
Cadilhac DA, Dalli LL, Morrison J, Lester M, Paice K, Moss K, et al. The Australian Stroke Clinical Registry annual report 2021. Melbourne; 2022. Available from https://auscr.com.au/about/annual-reports/ . Accessed 6 May 2024.
Kilkenny MF, Kim J, Andrew NE, Sundararajan V, Thrift AG, Katzenellenbogen JM, et al. Maximising data value and avoiding data waste: A validation study in stroke research. Med J Aust. 2019;210:27–31.
Eliakundu AL, Smith K, Kilkenny MF, Kim J, Bagot KL, Andrew E, et al. Linking data from the Australian Stroke Clinical Registry with ambulance and emergency administrative data in Victoria. Inquiry. 2022;59:469580221102200.
PubMed Google Scholar
Andrew NE, Kim J, Cadilhac DA, Sundararajan V, Thrift AG, Churilov L, et al. Protocol for evaluation of enhanced models of primary care in the management of stroke and other chronic disease (PRECISE): A data linkage healthcare evaluation study. Int J Popul Data Sci. 2019;4:1097.
CAS PubMed PubMed Central Google Scholar
Mosalski S, Shiner CT, Lannin NA, Cadilhac DA, Faux SG, Kim J, et al. Increased relative functional gain and improved stroke outcomes: A linked registry study of the impact of rehabilitation. J Stroke Cerebrovasc Dis. 2021;30: 106015.
Ryan OF, Hancock SL, Marion V, Kelly P, Kilkenny MF, Clissold B, et al. Feedback of aggregate patient-reported outcomes (PROs) data to clinicians and hospital end users: Findings from an Australian codesign workshop process. BMJ Open. 2022;12:e055999.
Grimley RS, Rosbergen IC, Gustafsson L, Horton E, Green T, Cadigan G, et al. Dose and setting of rehabilitation received after stroke in Queensland, Australia: A prospective cohort study. Clin Rehabil. 2020;34:812–23.
Purvis T, Middleton S, Craig LE, Kilkenny MF, Dale S, Hill K, et al. Inclusion of a care bundle for fever, hyperglycaemia and swallow management in a national audit for acute stroke: Evidence of upscale and spread. Implement Sci. 2019;14:87.
Middleton S, McElduff P, Ward J, Grimshaw JM, Dale S, D’Este C, et al. Implementation of evidence-based treatment protocols to manage fever, hyperglycaemia, and swallowing dysfunction in acute stroke (QASC): A cluster randomised controlled trial. Lancet. 2011;378:1699–706.
Middleton S, Dale S, Cheung NW, Cadilhac DA, Grimshaw JM, Levi C, et al. Nurse-initiated acute stroke care in emergency departments. Stroke. 2019:STROKEAHA118020701.
Hood RJ, Maltby S, Keynes A, Kluge MG, Nalivaiko E, Ryan A, et al. Development and pilot implementation of TACTICS VR: A virtual reality-based stroke management workflow training application and training framework. Front Neurol. 2021;12:665808.
Bladin CF, Kim J, Bagot KL, Vu M, Moloczij N, Denisenko S, et al. Improving acute stroke care in regional hospitals: Clinical evaluation of the Victorian Stroke Telemedicine program. Med J Aust. 2020;212:371–7.
Bladin CF, Bagot KL, Vu M, Kim J, Bernard S, Smith K, et al. Real-world, feasibility study to investigate the use of a multidisciplinary app (Pulsara) to improve prehospital communication and timelines for acute stroke/STEMI care. BMJ Open. 2022;12:e052332.
Zhao H, Coote S, Easton D, Langenberg F, Stephenson M, Smith K, et al. Melbourne mobile stroke unit and reperfusion therapy: Greater clinical impact of thrombectomy than thrombolysis. Stroke. 2020;51:922–30.
Purvis T, Cadilhac DA, Hill K, Reyneke M, Olaiya MT, Dalli LL, et al. Twenty years of monitoring acute stroke care in Australia from the national stroke audit program (1999–2019): Achievements and areas of future focus. J Health Serv Res Policy. 2023.
Cadilhac DA, Purvis T, Reyneke M, Dalli LL, Kim J, Kilkenny MF. Evaluation of the national stroke audit program: 20-year report. Melbourne; 2019.
Kim J, Tan E, Gao L, Moodie M, Dewey HM, Bagot KL, et al. Cost-effectiveness of the Victorian Stroke Telemedicine program. Aust Health Rev. 2022;46:294–301.
Kim J, Easton D, Zhao H, Coote S, Sookram G, Smith K, et al. Economic evaluation of the Melbourne mobile stroke unit. Int J Stroke. 2021;16:466–75.
Stroke Foundation. National stroke audit – rehabilitation services report 2020. Melbourne; 2020.
Australian Institute of Health and Welfare. Heart, stroke and vascular disease: Australian facts. 2023. Webpage https://www.aihw.gov.au/reports/heart-stroke-vascular-diseases/hsvd-facts/contents/about (accessed Jan 2024).
Download references
Acknowledgements
The following authors hold National Health and Medical Research Council Research Fellowships: HT (#2009326), DAC (#1154273), SM (#1196352), MFK Future Leader Research Fellowship (National Heart Foundation #105737). The Funders of this work did not have any direct role in the design of the study, its execution, analyses, interpretation of the data, or decision to submit results for publication.
Author information
Helena Teede and Dominique A. Cadilhac contributed equally.
Authors and Affiliations
Monash Centre for Health Research and Implementation, 43-51 Kanooka Grove, Clayton, VIC, Australia
Helena Teede, Emily Callander & Joanne Enticott
Monash Partners Academic Health Science Centre, 43-51 Kanooka Grove, Clayton, VIC, Australia
Helena Teede & Alison Johnson
Stroke and Ageing Research, Department of Medicine, School of Clinical Sciences at Monash Health, Monash University, Level 2 Monash University Research, Victorian Heart Hospital, 631 Blackburn Rd, Clayton, VIC, Australia
Dominique A. Cadilhac, Tara Purvis & Monique F. Kilkenny
Stroke Theme, The Florey Institute of Neuroscience and Mental Health, University of Melbourne, Heidelberg, VIC, Australia
Dominique A. Cadilhac, Monique F. Kilkenny & Bruce C.V. Campbell
Department of Neurology, Melbourne Brain Centre, Royal Melbourne Hospital, Parkville, VIC, Australia
Bruce C.V. Campbell
Department of Medicine, Faculty of Medicine, Dentistry and Health Sciences, University of Melbourne, Victoria, Australia
School of Health Sciences, Heart and Stroke Program, University of Newcastle, Hunter Medical Research Institute, University Drive, Callaghan, NSW, Australia
Coralie English
School of Medicine and Dentistry, Griffith University, Birtinya, QLD, Australia
Rohan S. Grimley
Clinical Excellence Division, Queensland Health, Brisbane, Australia
John Hunter Hospital, Hunter New England Local Health District and University of Newcastle, Sydney, NSW, Australia
Christopher Levi
School of Nursing, Midwifery and Paramedicine, Australian Catholic University, Sydney, NSW, Australia
Sandy Middleton
Nursing Research Institute, St Vincent’s Health Network Sydney and and Australian Catholic University, Sydney, NSW, Australia
Stroke Foundation, Level 7, 461 Bourke St, Melbourne, VIC, Australia
Kelvin Hill
You can also search for this author in PubMed Google Scholar
Contributions
HT: conception, design and initial draft, developed the theoretical formalism for learning health system framework, approved the submitted version. DAC: conception, design and initial draft, provided essential literature and case study examples, approved the submitted version. TP: revised the manuscript critically for important intellectual content, approved the submitted version. MFK: revised the manuscript critically for important intellectual content, provided essential literature and case study examples, approved the submitted version. BC: revised the manuscript critically for important intellectual content, provided essential literature and case study examples, approved the submitted version. CE: revised the manuscript critically for important intellectual content, provided essential literature and case study examples, approved the submitted version. AJ: conception, design and initial draft, developed the theoretical formalism for learning health system framework, approved the submitted version. EC: revised the manuscript critically for important intellectual content, approved the submitted version. RSG: revised the manuscript critically for important intellectual content, provided essential literature and case study examples, approved the submitted version. CL: revised the manuscript critically for important intellectual content, provided essential literature and case study examples, approved the submitted version. SM: revised the manuscript critically for important intellectual content, provided essential literature and case study examples, approved the submitted version. KH: revised the manuscript critically for important intellectual content, provided essential literature and case study examples, approved the submitted version. JE: conception, design and initial draft, developed the theoretical formalism for learning health system framework, approved the submitted version. All authors read and approved the final manuscript.
Authors’ Twitter handles
@HelenaTeede
@DominiqueCad
@Coralie_English
@EmilyCallander
@EnticottJo
Corresponding authors
Correspondence to Helena Teede or Dominique A. Cadilhac .
Ethics declarations
Ethics approval and consent to participate, consent for publication, competing interests, additional information, publisher's note.
Springer Nature remains neutral with regard to jurisdictional claims in published maps and institutional affiliations.
Rights and permissions
Open Access This article is licensed under a Creative Commons Attribution 4.0 International License, which permits use, sharing, adaptation, distribution and reproduction in any medium or format, as long as you give appropriate credit to the original author(s) and the source, provide a link to the Creative Commons licence, and indicate if changes were made. The images or other third party material in this article are included in the article's Creative Commons licence, unless indicated otherwise in a credit line to the material. If material is not included in the article's Creative Commons licence and your intended use is not permitted by statutory regulation or exceeds the permitted use, you will need to obtain permission directly from the copyright holder. To view a copy of this licence, visit http://creativecommons.org/licenses/by/4.0/ . The Creative Commons Public Domain Dedication waiver ( http://creativecommons.org/publicdomain/zero/1.0/ ) applies to the data made available in this article, unless otherwise stated in a credit line to the data.
Reprints and permissions
About this article
Cite this article.
Teede, H., Cadilhac, D.A., Purvis, T. et al. Learning together for better health using an evidence-based Learning Health System framework: a case study in stroke. BMC Med 22 , 198 (2024). https://doi.org/10.1186/s12916-024-03416-w
Download citation
Received : 23 July 2023
Accepted : 30 April 2024
Published : 15 May 2024
DOI : https://doi.org/10.1186/s12916-024-03416-w
Share this article
Anyone you share the following link with will be able to read this content:
Sorry, a shareable link is not currently available for this article.
Provided by the Springer Nature SharedIt content-sharing initiative
- Evidence-based medicine
- Person-centred care
- Models of care
- Healthcare improvement
BMC Medicine
ISSN: 1741-7015
- Submission enquiries: [email protected]
- General enquiries: [email protected]
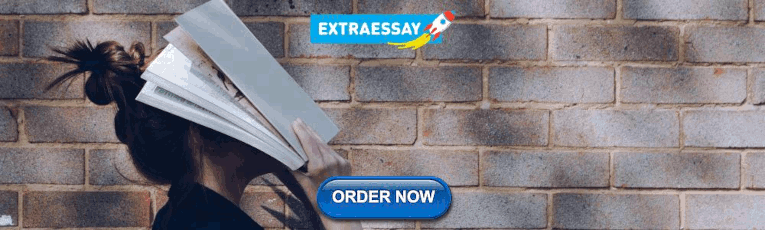
IMAGES
VIDEO
COMMENTS
Snowden and Boone have formed a new perspective on leadership and decision making that's based on complexity science. The result is the Cynefin framework, which helps executives sort issues into ...
Along with his colleague Mary Boone, he published the framework in the November 2007 issue of the Harvard Business Review. The Cynefin framework (Figure 1 below) is a problem-solving tool that helps you put situations into five "domains" defined by cause-and-effect relationships. This helps you assess your situation more accurately and respond ...
A Case Study Using the Cynefin Framework. Report this article Justyn Hornor Justyn Hornor ... The Cynefin Framework is a "sensing" model for decision-making: the situation can feel like chaos ...
Case Study: Applying the Cynefin Framework in a Team Decision-Making Process Imagine that you are leading a project team that is tasked with developing a new product for your company. As the project progresses, various challenges arise, and the team is struggling to make decisions effectively. By introducing the Cynefin Framework to the team ...
Academic Medicine: August 2022 - Volume 97 - Issue 8 - p 1137-1143 doi: 10.1097/ACM.0000000000004717 Use of the Cynefin Framework; Christine Wamsler, Gustav Osberg, Anna Panagiotou, Beth Smith, Peter Stanbridge, ... Anyone should feel free to write a case study, please do so as a separate article here and link ...
Beth's interview focused more on specific case study projects, some of which I've compiled as part of Citizen Engagement & Democratic Innovation programme's case studies. So dive into some diverse case studies to get some inspiration for what your own project could look like. This post is about my chat with Anna Panagiotou. Anna has been ...
The Cynefin framework, originating from knowledge management, is a heuristic tool for how people perceive and make sense of situations in order to make decisions ( Snowden, 2002 ). The framework has two domains, order and unorder. To categorize for order and unorder, one must discern the relationship between cause and effect of the problem.
David Snowden and the Cynefin Framework he has developed will help you in this regard. David Snowden of The Cognitive Edge has spent many years developing the concept of The Cynefin Framework which is a problem and decision-framing approach. You may have explored this concept in your other units. This complexity-framing model is one of the key ...
The Cynefin framework is one way of sensitizing at least the researchers, grant agencies, journalists and politicians (as the mainly involved managers) to the the complexity in biomedical research and its consequences to insight and decision making. ... Case Study: Parkinson's Disease.
The inside scope: Cynefin's case studies with Beth Smith (1 of 2) Linda Doyle. February 7, 2022. Cynefin Centre, News, relfections. From the valleys of Wales, to the streets of Malmö, and townships in Cape Town, The Cynefin Company has been on a journey through all kinds of wonderful citizen engagement projects and now we'd like to share ...
The Cynefin Framework (pronounced "kuh-nev-in") is a conceptual tool designed to help leaders, managers, and decision-makers navigate the complexity of real-world situations. It offers a typology of contexts that guides users in identifying the nature of the challenges they face and selecting appropriate strategies for action.
An introduction to Cynefin Framework. The Cynefin framework is a decision-making model that provides guidance for leaders to understand the different levels of complex situations they face and how ...
Sep 17, 2021. Cynefin was created in 1999 by Dave Snowden and was born with principles related to theories of how we perceive things. Cynefin (pronounced "kuh-nev-in") is a word of Welsh ...
The Cynefin Framework defines five domains that you can find yourself in. It's typically drawn in a diagram like I show here. ... This is the domain where short-cycle agile techniques or Deming's Plan-Do-Study-Act (also called Plan-Do-Check-Act) are useful. ... That's not really the case in the work of people. When dealing with systems of ...
The Cynefin framework distinguishes 5 different domains: the clear, the complicated, the complex, the chaotic and, in the middle - where you don't yet understand your context - the confused. Each of these domains, then, offers different types of constraints and different preferred reactions. For instance, while leaders in a chaotic context ...
The Cynefin framework is a sense-making tool used to help leaders think through a problem or situation to find a solution. It encourages decisions to be made based on circumstances and addresses the uncertainty of complex projects and systems. It breaks down problems to fit into four domains: Obvious, Complicated, Complex and Chaotic.
The Cynefin® Framework; Cynefin® Centre; close. Linda Doyle. Thinking. The inside scope: Cynefin's case studies with Anna Panagiotou (2 of 2) Linda Doyle. For the first blog in this mini-series in which I interview Beth Smith, click here. Beth's interview focused more on specific case study projects, some of which I've compiled as part ...
Given When Then - A Cynefin Case Study. By theitriskmanager. Dan and I created the "Given When Then" pattern on August 23rd 2004, or rather, that was the day we realised we needed the "Given" part. On November 30th, I wrote a series of blogs explaining the format that Dan and I had created in its more familiar form.
The Cynefin framework allows contextualization of situations that require a decision and response by providing a reference language ... Case study: Parkinson's disease. Parkinson's disease (PD) is caused by the degeneration of dopaminergic neurons in the substantia nigra of the midbrain resulting in a characteristic pattern of motoric symptoms ...
The Cynefin Framework is a sensemaking model that offers a comprehensive approach to understanding and managing diverse problem domains. This sensemaking model was developed by Dave J. Snowden in 1999 to help leaders make decisions while working for IBM Global Services.
The Cynefin Framework is a tool that guides professionals when making decisions by analyzing the context in which that decisions are made. Its name, Cynefin, is a Welsh word for "Habitat". It was created by Dave Snowden in 1999. He defined 4 Common situations in which decisions are often made: Chaotic situations. Complex situations.
The Cynefin framework addresses the need for experimentation and situational adaptability. The problem involving the hospital, staff reductions, and clinic closures in this case study is examined utilizing the Cynefin framework to address the more erratic elements, including stakeholder relationships, employee morale, the effect on the ...
Law document from University of the Free State, 2 pages, 1. Children Act (1989) - The Children Act 1989 sets out local authorities' duties and responsibilities regarding children's welfare. In this case, Children's Services became involved due to concerns about Jack and Leila's welfare following domestic incide
People with two copies of the gene variant APOE4 are almost certain to get Alzheimer's, say researchers, who proposed a framework under which such patients could be diagnosed years before symptoms.
The Cynefin Company (formerly known as Cognitive Edge) was founded in 2005 by Dave Snowden. We believe in praxis and focus on building methods, tools and capability that apply the wisdom from Complex Adaptive Systems theory and other scientific disciplines in social systems. We are the world leader in developing management approaches (in ...
Our aim was to outline a rigorously codesigned, evidence-based LHS framework and present a national case study of an LHS-aligned national stroke program that has delivered clinical benefit. Current core components of a LHS involve capturing evidence from communities and stakeholders (quadrant 1), integrating evidence from research findings ...
Land use and land cover change (LUCC) prediction of cities in Western China requires higher accuracy in quantitative demand and spatial layout because of complex challenges in balancing relationships between urban constructions and ecological developments. Considering city-level areas and various types of land use and land cover, existing LUCC models without constraint or with only loose ...
This research investigates environmental inequalities within Guangzhou's Huangpu District against the backdrop of rapid urbanisation and industrial expansion in Asia. This study identifies environmental hotspots, particularly in socially vulnerable areas characterised by high industrial density, using a vulnerability framework and analysing census and pollution data. Utilising satellite ...