Guidance for biostatisticians on their essential contributions to clinical and translational research protocol review
Affiliations.
- 1 Department of Preventive Medicine, Division of Biostatistics, Feinberg School of Medicine, Northwestern University, Chicago, IL, USA.
- 2 Department of Biostatistics, University of Michigan, Washington Heights, Ann Arbor, MI, USA.
- 3 Department of Biostatistics and Data Science, Division of Public Health Sciences, Wake Forest School of Medicine, Winston-Salem, NC, USA.
- 4 Michigan Institute for Clinical & Health Research (MICHR), University of Michigan, Ann Arbor, MI, USA.
- 5 Department of Public Health, Division of Biostatistics, University of Miami, Miami, FL, USA.
- 6 School of Public Health, Oregon Health & Sciences University, Portland, OR, USA.
- 7 Department of Public Health Sciences, Medical University of South Carolina, Charleston, SC, USA.
- 8 Department of Medicine, Division of Preventive Medicine, University of Alabama at Birmingham, Birmingham, AL, UK.
- 9 Department of Biostatistics, Indiana University, Indianapolis, IN, USA.
- 10 Department of Public Health Sciences, UC Davis School of Medicine, Davis, CA, USA.
- 11 Duke Biostatistics, Epidemiology and Research Design (BERD) Methods Core, Duke University, Durham, NC, USA.
- 12 Tufts Clinical and Translational Science Institute, Tufts University, Boston, MA, USA.
- 13 Institute of Clinical Research and Health Policy Studies, Tufts Medical Center, Boston, MA, USA.
- 14 Department of Medicine, Division of Allergy, Pulmonary, and Critical Care Medicine, Medical Director, Vanderbilt Human Research Protections Program, Vice-President for Clinical Trials Innovation and Operations, Nashville, TN, USA.
- 15 Department of Biomedical Data Science, Division of Biostatistics, Geisel School of Medicine at Dartmouth, Hanover, NH, USA.
- 16 Department of Biostatistics, Vanderbilt University Medical Center, Nashville, TN, USA.
- 17 Department of Preventive Medicine and Population Health, University of Texas Medical Branch, Galveston, TX, USA.
- PMID: 34527300
- PMCID: PMC8427547
- DOI: 10.1017/cts.2021.814
Rigorous scientific review of research protocols is critical to making funding decisions, and to the protection of both human and non-human research participants. Given the increasing complexity of research designs and data analysis methods, quantitative experts, such as biostatisticians, play an essential role in evaluating the rigor and reproducibility of proposed methods. However, there is a common misconception that a statistician's input is relevant only to sample size/power and statistical analysis sections of a protocol. The comprehensive nature of a biostatistical review coupled with limited guidance on key components of protocol review motived this work. Members of the Biostatistics, Epidemiology, and Research Design Special Interest Group of the Association for Clinical and Translational Science used a consensus approach to identify the elements of research protocols that a biostatistician should consider in a review, and provide specific guidance on how each element should be reviewed. We present the resulting review framework as an educational tool and guideline for biostatisticians navigating review boards and panels. We briefly describe the approach to developing the framework, and we provide a comprehensive checklist and guidance on review of each protocol element. We posit that the biostatistical reviewer, through their breadth of engagement across multiple disciplines and experience with a range of research designs, can and should contribute significantly beyond review of the statistical analysis plan and sample size justification. Through careful scientific review, we hope to prevent excess resource expenditure and risk to humans and animals on poorly planned studies.
Keywords: Biostatistician; Protocol; Review; Scientific rigor; Translational research.
© The Association for Clinical and Translational Science 2021.
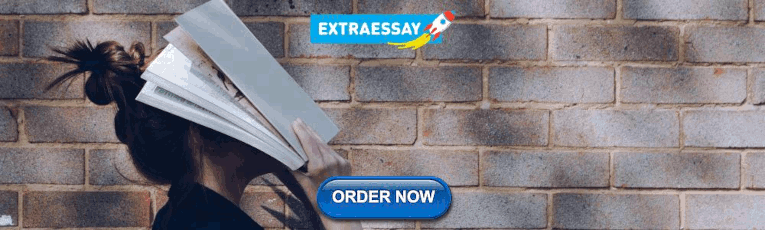
Publication types
Grants and funding.
- UL1 TR003096/TR/NCATS NIH HHS/United States
- P30 DK123704/DK/NIDDK NIH HHS/United States
- P30 CA023108/CA/NCI NIH HHS/United States
- P30 AR072582/AR/NIAMS NIH HHS/United States
- UL1 TR001420/TR/NCATS NIH HHS/United States
- UL1 TR001439/TR/NCATS NIH HHS/United States
- UL1 TR002243/TR/NCATS NIH HHS/United States
- UL1 TR001450/TR/NCATS NIH HHS/United States
- UL1 TR002544/TR/NCATS NIH HHS/United States
- UL1 TR002529/TR/NCATS NIH HHS/United States
- UL1 TR002736/TR/NCATS NIH HHS/United States
- UL1 TR001086/TR/NCATS NIH HHS/United States
- UL1 TR001422/TR/NCATS NIH HHS/United States
- U54 GM104941/GM/NIGMS NIH HHS/United States
- UL1 TR002553/TR/NCATS NIH HHS/United States
- UL1 TR000002/TR/NCATS NIH HHS/United States
- UL1 TR002240/TR/NCATS NIH HHS/United States
Biostatistics and Research Design for Clinicians
- First Online: 04 May 2018
Cite this chapter
- Tarsicio Uribe-Leitz 5 ,
- Alyssa Fitzpatrick Harlow 5 &
- Adil H. Haider 5
2045 Accesses
The objective of this chapter is to provide the reader with a basic understanding of core statistical concepts that will aid in the translation of meaningful research results. We emphasize the importance of a well-defined study design as a strong foundation that will lead to sound evidence. First, we define foundational statistical terminology; we then discuss the steps to generating and testing hypotheses through development of a solid research question and review how to choose an appropriate study design. Second, we describe a twofold approach to data analysis that uses descriptive statistics followed by inferential statistics. Finally, we cover essentials for sound evidence, based on a systematic point-based approach to judge the quality of data and strength of recommendations produced by research studies.
This is a preview of subscription content, log in via an institution to check access.
Access this chapter
- Available as PDF
- Read on any device
- Instant download
- Own it forever
- Available as EPUB and PDF
- Compact, lightweight edition
- Dispatched in 3 to 5 business days
- Free shipping worldwide - see info
- Durable hardcover edition
Tax calculation will be finalised at checkout
Purchases are for personal use only
Institutional subscriptions
Austin PC. An introduction to propensity score methods for reducing the effects of confounding in observational studies. Multivar Behav Res. 2011;46(3):399–424. https://doi.org/10.1080/00273171.2011.568786 .
Article Google Scholar
Biesecker LG. Hypothesis-generating research and predictive medicine. Genome Res. 2013;23(7):1051–3. https://doi.org/10.1101/gr.157826.113 .
Article CAS PubMed PubMed Central Google Scholar
Brian Haynes R. Forming research questions. J Clin Epidemiol. 2006;59(9):881–6. https://doi.org/10.1016/j.jclinepi.2006.06.006
Article CAS Google Scholar
Counsell C. Formulating questions and locating primary studies for inclusion in systematic reviews. Ann Intern Med. 1997;127(5):380–7.
Daniel WW, Cross CL. Biostatistics: a foundation for analysis in the health sciences. 10th ed. Hoboken: Wiley; 2013.
Google Scholar
Harris RP, Helfand M, Woolf SH, Lohr KN, Mulrow CD, Teutsch SM, Atkins D. Current methods of the U.S. Preventive Services Task Force: a review of the process. Am J Prev Med. 2001;20(3 Supplement):21–35. https://doi.org/10.1016/S0749-3797(01)00261-6
Himmelfarb Health Sciences Library. Study Design 101. Washington, DC: George Washington University. 2011. https://himmelfarb.gwu.edu/tutorials/studydesign101/ . Retrieved 24 Mar 2017.
Hulley SB, Cummings SR, Browner WS, Grady DG, Newman TB. Designing clinical research. 3rd ed. Philadelphia: Lippincott Williams and Wilkins; 2007.
Richardson WS, Wilson MC, Nishikawa J, Hayward RS. The well-built clinical question: a key to evidence-based decisions. ACP J Club. 1995;123(3):A12–3.
CAS PubMed Google Scholar
Simianu VV, Farjah F, Flum DR. Evidence-based surgery: critically assessing surgical literature. In: Sabiston textbook of surgery: the biological basis of modern surgical practice. 20th ed. Philadelphia: Elsevier; 2013.
Sullivan LM. Essentials of biostatistics in public health. 2nd ed. Sudbury: Jones & Bartlett Learning; 2012.
What is GRADE? BMJ Clinical Evidence. London. 2012. http://clinicalevidence.bmj.com/x/set/static/ebm/learn/665072.html . Retrieved 29 Mar 2017.
Download references
Acknowledgment
Dr. Haider would like to thank the Career Development Course of the Association for Academic Surgery where he has lectured on this topic over the past several years. Most of the concepts presented here have been discussed during the course of these presentations.
Author information
Authors and affiliations.
Center for Surgery and Public Health (CSPH), Brigham and Women’s Hospital, Boston, MA, USA
Tarsicio Uribe-Leitz, Alyssa Fitzpatrick Harlow & Adil H. Haider
You can also search for this author in PubMed Google Scholar
Corresponding author
Correspondence to Adil H. Haider .
Editor information
Editors and affiliations.
Brigham and Womens’s Hospital, Harvard Medical School, Boston, Massachusetts, USA
Dell Medical School, University of Texas at Austin, Austin, Texas, USA
Carlos Brown
Division of Trauma Surgery, Rm C5L100, University of Southern California, Los Angeles, California, USA
Kenji Inaba
Trauma and Emergency Surgery Service, Legacy Emanuel Medical Center, Portland, Oregon, USA
Matthew J. Martin
Rights and permissions
Reprints and permissions
Copyright information
© 2018 Springer International Publishing AG, part of Springer Nature
About this chapter
Uribe-Leitz, T., Harlow, A.F., Haider, A.H. (2018). Biostatistics and Research Design for Clinicians. In: Salim, A., Brown, C., Inaba, K., Martin, M. (eds) Surgical Critical Care Therapy . Springer, Cham. https://doi.org/10.1007/978-3-319-71712-8_60
Download citation
DOI : https://doi.org/10.1007/978-3-319-71712-8_60
Published : 04 May 2018
Publisher Name : Springer, Cham
Print ISBN : 978-3-319-71711-1
Online ISBN : 978-3-319-71712-8
eBook Packages : Medicine Medicine (R0)
Share this chapter
Anyone you share the following link with will be able to read this content:
Sorry, a shareable link is not currently available for this article.
Provided by the Springer Nature SharedIt content-sharing initiative
- Publish with us
Policies and ethics
- Find a journal
- Track your research
Thank you for visiting nature.com. You are using a browser version with limited support for CSS. To obtain the best experience, we recommend you use a more up to date browser (or turn off compatibility mode in Internet Explorer). In the meantime, to ensure continued support, we are displaying the site without styles and JavaScript.
- View all journals
Biostatistics articles from across Nature Portfolio
Biostatistics is the application of statistical methods in studies in biology, and encompasses the design of experiments, the collection of data from them, and the analysis and interpretation of data. The data come from a wide range of sources, including genomic studies, experiments with cells and organisms, and clinical trials.
Latest Research and Reviews
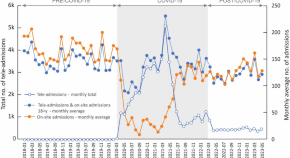
Impact of COVID-19 on antibiotic usage in primary care: a retrospective analysis
- Anna Romaszko-Wojtowicz
- K. Tokarczyk-Malesa
- K. Glińska-Lewczuk
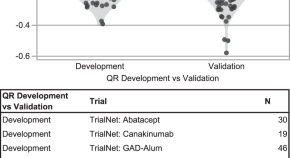
A standardized metric to enhance clinical trial design and outcome interpretation in type 1 diabetes
The use of a standardized outcome metric enhances clinical trial interpretation and cross-trial comparison. Here, the authors show the implementation of such a metric using type 1 diabetes trial data, reassess and compare results from these trials, and extend its use to define response to therapy.
- Alyssa Ylescupidez
- Henry T. Bahnson
- Carla J. Greenbaum
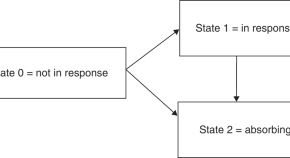
A novel approach to visualize clinical benefit of therapies for chronic graft versus host disease (cGvHD): the probability of being in response (PBR) applied to the REACH3 study
- Norbert Hollaender
- Ekkehard Glimm
- Robert Zeiser
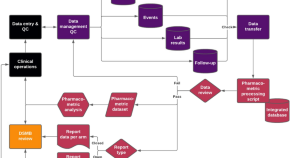
Reproducibility in pharmacometrics applied in a phase III trial of BCG-vaccination for COVID-19
- Rob C. van Wijk
- Laurynas Mockeliunas
- Ulrika S. H. Simonsson
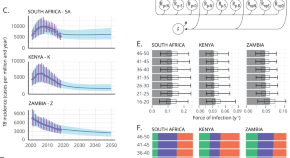
Addressing mechanism bias in model-based impact forecasts of new tuberculosis vaccines
The complex transmission chain of tuberculosis (TB) forces mathematical modelers to make mechanistic assumptions when modelling vaccine effects. Here, authors posit a Bayesian formalism that unlocks mechanism-agnostic impact forecasts for TB vaccines.
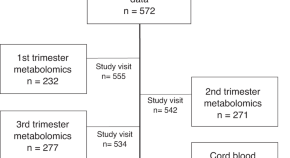
Early ascending growth is associated with maternal lipoprotein profile during mid and late pregnancy and in cord blood
- Elina Blanco Sequeiros
- Anna-Kaisa Tuomaala
- Emilia Huvinen
News and Comment
Mitigating immortal-time bias: exploring osteonecrosis and survival in pediatric all - aall0232 trial insights.
- Shyam Srinivasan
- Swaminathan Keerthivasagam
Response to Pfirrmann et al.’s comment on How should we interpret conclusions of TKI-stopping studies
- Junren Chen
- Robert Peter Gale
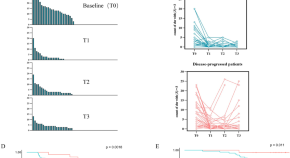
Cell-free DNA chromosome copy number variations predict outcomes in plasma cell myeloma
- Wanting Qiang
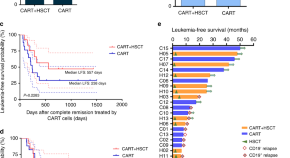
The role of allogeneic haematopoietic cell transplantation as consolidation after anti-CD19 CAR-T cell therapy in adults with relapsed/refractory acute lymphoblastic leukaemia: a prospective cohort study
- Lijuan Zhou
Clinical trials: design, endpoints and interpretation of outcomes
- Megan Othus
- Mei-Jie Zhang
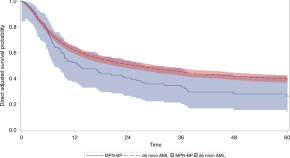
A SAS macro for estimating direct adjusted survival functions for time-to-event data with or without left truncation
- Zhen-Huan Hu
- Hai-Lin Wang
Quick links
- Explore articles by subject
- Guide to authors
- Editorial policies

- Article Information
See More About
Select your interests.
Customize your JAMA Network experience by selecting one or more topics from the list below.
- Academic Medicine
- Acid Base, Electrolytes, Fluids
- Allergy and Clinical Immunology
- American Indian or Alaska Natives
- Anesthesiology
- Anticoagulation
- Art and Images in Psychiatry
- Artificial Intelligence
- Assisted Reproduction
- Bleeding and Transfusion
- Caring for the Critically Ill Patient
- Challenges in Clinical Electrocardiography
- Climate and Health
- Climate Change
- Clinical Challenge
- Clinical Decision Support
- Clinical Implications of Basic Neuroscience
- Clinical Pharmacy and Pharmacology
- Complementary and Alternative Medicine
- Consensus Statements
- Coronavirus (COVID-19)
- Critical Care Medicine
- Cultural Competency
- Dental Medicine
- Dermatology
- Diabetes and Endocrinology
- Diagnostic Test Interpretation
- Drug Development
- Electronic Health Records
- Emergency Medicine
- End of Life, Hospice, Palliative Care
- Environmental Health
- Equity, Diversity, and Inclusion
- Facial Plastic Surgery
- Gastroenterology and Hepatology
- Genetics and Genomics
- Genomics and Precision Health
- Global Health
- Guide to Statistics and Methods
- Hair Disorders
- Health Care Delivery Models
- Health Care Economics, Insurance, Payment
- Health Care Quality
- Health Care Reform
- Health Care Safety
- Health Care Workforce
- Health Disparities
- Health Inequities
- Health Policy
- Health Systems Science
- History of Medicine
- Hypertension
- Images in Neurology
- Implementation Science
- Infectious Diseases
- Innovations in Health Care Delivery
- JAMA Infographic
- Law and Medicine
- Leading Change
- Less is More
- LGBTQIA Medicine
- Lifestyle Behaviors
- Medical Coding
- Medical Devices and Equipment
- Medical Education
- Medical Education and Training
- Medical Journals and Publishing
- Mobile Health and Telemedicine
- Narrative Medicine
- Neuroscience and Psychiatry
- Notable Notes
- Nutrition, Obesity, Exercise
- Obstetrics and Gynecology
- Occupational Health
- Ophthalmology
- Orthopedics
- Otolaryngology
- Pain Medicine
- Palliative Care
- Pathology and Laboratory Medicine
- Patient Care
- Patient Information
- Performance Improvement
- Performance Measures
- Perioperative Care and Consultation
- Pharmacoeconomics
- Pharmacoepidemiology
- Pharmacogenetics
- Pharmacy and Clinical Pharmacology
- Physical Medicine and Rehabilitation
- Physical Therapy
- Physician Leadership
- Population Health
- Primary Care
- Professional Well-being
- Professionalism
- Psychiatry and Behavioral Health
- Public Health
- Pulmonary Medicine
- Regulatory Agencies
- Reproductive Health
- Research, Methods, Statistics
- Resuscitation
- Rheumatology
- Risk Management
- Scientific Discovery and the Future of Medicine
- Shared Decision Making and Communication
- Sleep Medicine
- Sports Medicine
- Stem Cell Transplantation
- Substance Use and Addiction Medicine
- Surgical Innovation
- Surgical Pearls
- Teachable Moment
- Technology and Finance
- The Art of JAMA
- The Arts and Medicine
- The Rational Clinical Examination
- Tobacco and e-Cigarettes
- Translational Medicine
- Trauma and Injury
- Treatment Adherence
- Ultrasonography
- Users' Guide to the Medical Literature
- Vaccination
- Venous Thromboembolism
- Veterans Health
- Women's Health
- Workflow and Process
- Wound Care, Infection, Healing
Others Also Liked
- Download PDF
- X Facebook More LinkedIn
Windish DM , Huot SJ , Green ML. Medicine Residents' Understanding of the Biostatistics and Results in the Medical Literature. JAMA. 2007;298(9):1010–1022. doi:10.1001/jama.298.9.1010
Manage citations:
© 2024
- Permissions
Medicine Residents' Understanding of the Biostatistics and Results in the Medical Literature
Author Affiliations: Department of Internal Medicine, Yale University School of Medicine, New Haven, Connecticut.
Context Physicians depend on the medical literature to keep current with clinical information. Little is known about residents' ability to understand statistical methods or how to appropriately interpret research outcomes.
Objective To evaluate residents' understanding of biostatistics and interpretation of research results.
Design, Setting, and Participants Multiprogram cross-sectional survey of internal medicine residents.
Main Outcome Measure Percentage of questions correct on a biostatistics/study design multiple-choice knowledge test.
Results The survey was completed by 277 of 367 residents (75.5%) in 11 residency programs. The overall mean percentage correct on statistical knowledge and interpretation of results was 41.4% (95% confidence interval [CI], 39.7%-43.3%) vs 71.5% (95% CI, 57.5%-85.5%) for fellows and general medicine faculty with research training ( P < .001). Higher scores in residents were associated with additional advanced degrees (50.0% [95% CI, 44.5%-55.5%] vs 40.1% [95% CI, 38.3%-42.0%]; P < .001); prior biostatistics training (45.2% [95% CI, 42.7%-47.8%] vs 37.9% [95% CI, 35.4%-40.3%]; P = .001); enrollment in a university-based training program (43.0% [95% CI, 41.0%-45.1%] vs 36.3% [95% CI, 32.6%-40.0%]; P = .002); and male sex (44.0% [95% CI, 41.4%-46.7%] vs 38.8% [95% CI, 36.4%-41.1%]; P = .004). On individual knowledge questions, 81.6% correctly interpreted a relative risk. Residents were less likely to know how to interpret an adjusted odds ratio from a multivariate regression analysis (37.4%) or the results of a Kaplan-Meier analysis (10.5%). Seventy-five percent indicated they did not understand all of the statistics they encountered in journal articles, but 95% felt it was important to understand these concepts to be an intelligent reader of the literature.
Conclusions Most residents in this study lacked the knowledge in biostatistics needed to interpret many of the results in published clinical research. Residency programs should include more effective biostatistics training in their curricula to successfully prepare residents for this important lifelong learning skill.
Physicians must keep current with clinical information to practice evidence-based medicine (EBM). In doing so, most prefer to seek evidence-based summaries, which give the clinical bottom line, 1 or evidence-based practice guidelines. 1 - 3 Resources that maintain these information summaries, however, currently include a limited number of common conditions. 4 Thus, to answer many of their clinical questions, physicians need to access reports of original research. This requires the reader to critically appraise the design, conduct, and analysis of each study and subsequently interpret the results.
Several surveys in the 1980s demonstrated that practicing physicians, particularly those with no formal education in epidemiology and biostatistics, had a poor understanding of common statistical tests and limited ability to interpret study results. 5 - 7 Many physicians likely have increased difficulty today because more complicated statistical methods are being reported in the medical literature. 8 They may be able to understand the analysis and interpretation of results in only 21% of research articles. 8
Educators have responded by increasing training in critical appraisal and biostatistics throughout the continuum of medical education. Many medical schools currently provide some formal teaching of basic statistical concepts. 9 As part of the Accreditation Council for Graduate Medical Education's practice-based learning and improvement competency, residents must demonstrate ability in “locating, appraising, and assimilating evidence from scientific studies related to their patients' problems and apply knowledge of study designs and statistical methods to the appraisal of clinical studies.” 10 Most residency programs address this competency through EBM curricula or journal clubs. 11 - 13 In 2000, the majority of these programs included training in appraisal of studies and study conduct, but fewer specifically addressed the selection and interpretation of statistical tests. 11 , 14 In addition, the majority of published assessments of residents' knowledge and skills in EBM were performed at single programs, were conducted in the context of determining the impact of a specific curriculum, evaluated critical appraisal skills more commonly than biostatistics, and found that residents scored well below EBM “experts” on evaluation instruments. 15 We performed a multiprogram assessment of residents' biostatistics knowledge and interpretation of study results using a new instrument developed for this study.
We developed an instrument to reflect the statistical methods and results most commonly represented in contemporary research studies ( Appendix ). Thus, we reviewed all 239 original articles published from January to March of 2005 in each issue of 6 general medical journals ( American Journal of Medicine , Annals of Internal Medicine , BMJ , JAMA , Lancet , and New England Journal of Medicine ) and summarized the frequency of statistical methods described ( Table 1 ). From this review, we developed questions that focused on identifying and interpreting the results of the most frequently occurring simple statistical methods (eg, χ 2 , t test, analysis of variance) and multivariate analyses (eg, Cox proportional hazards regression, multiple logistic regression).
The survey ( Appendix ) contained 4 sets of questions: (1) 11 demographic questions that included age, sex, current training level, past training in biostatistics and EBM, and current journal-reading practices; (2) 5 attitude questions regarding statistics; (3) 4 confidence questions about interpreting and assessing statistical concepts; and (4) a 20-question biostatistics knowledge test that assessed understanding of statistical methods, study design, and interpretation of study results. Statistical attitudes and confidence questions were adapted from surveys on the Assessment Resource Tools for Improving Statistical Thinking (ARTIST) Web site, which is a resource for teaching statistical literacy, reasoning, and thinking. 16 Attitudes regarding statistics were rated on a 5-point Likert scale. Confidence questions were assessed using a 5-point scale in which 1 indicated no confidence and 5 indicated complete confidence. The remaining 20 knowledge test questions addressed understanding of statistical techniques, study design, and interpretation of study results most commonly represented in our journal review. These questions were multiple-choice, clinically oriented with a case vignette, and required no calculations. Two questions were adapted from a study of Danish physicians' statistical knowledge. 7 Seven questions were adapted from course materials used in statistics courses at the Johns Hopkins Bloomberg School of Public Health. 17 The remaining questions were developed by one of the study authors (D.M.W.). The knowledge questions addressed research variable types, statistical methods, confidence intervals, P values, sensitivity and specificity, power and sample size, study design, and interpretation of study results.
The original test contained 22 knowledge questions and was pilot tested with 5 internal medicine faculty with advanced training in epidemiology and biostatistics and 12 primary care internal medicine residents at 1 residency program. Faculty reviewed the instrument for content validity, completed the test, and provided feedback. Residents completed the test and provided written and oral feedback. Four of the 5 faculty answered 21 of 22 questions correctly and 1 faculty member correctly answered 19 questions. This resulted in an overall mean score of 94%. Incorrect responses did not favor any particular question. Residents answered 53% of questions correctly. Based on feedback, 1 question was modified to improve clarity, 3 questions were eliminated to avoid duplicating similar concepts, and 1 question was added to further assess interpretation of results. Therefore, the final version of the test consisted of 20 questions.
We conducted an anonymous cross-sectional survey from February through July 2006 of 11 internal medicine residency programs in Connecticut, including 7 traditional internal medicine programs, 2 primary care medicine programs, 1 medicine/pediatrics program, and 1 medicine/preventive medicine program. We initially contacted all 15 internal medicine residency programs in Connecticut to ask for their participation in the study. All programs were successfully contacted and expressed interest. However, 3 programs could not accommodate the study because of scheduling conflicts, and 1 program was not included because its residents (medicine/pediatrics) were distributed to different training sites and therefore were not present at the conferences used for the survey.
Included residencies were both university affiliated (7 programs) and community based (4 programs). Residents at all postgraduate levels of training were invited to participate. Oral consent was obtained from each participant after providing a description of the survey's purpose. The survey was administered during the first 25 minutes of an inpatient noon conference lecture for current residents. After all questionnaires were collected, the remainder of the time was devoted to a seminar in statistical methods and interpretation of the literature. Four residency programs also allowed us to survey their entering intern classes during their orientations. To provide data for validity testing, an additional 10 faculty and fellows trained in clinical investigation also completed the final survey. The Yale University human investigation committee approved the study protocol.
In addition to assessing the content validity, the psychometric properties of the 20-question knowledge test were determined by assessing internal consistency using Cronbach α. Discriminative validity was assessed by comparing the difference in mean scores obtained between residents and research-trained fellows and faculty using the t test.
The biostatistics knowledge test was scored by determining the percentage of questions correct, weighting each question equally. Missing values were counted as incorrect responses. The t test or a 1-way analysis of variance was used to compare survey scores by respondent characteristics. We calculated the percentage of residents who agreed or strongly agreed with each attitudinal question. We determined the percentage of respondents with fair to high confidence for each confidence question and the mean confidence score based on the sum of all 4 questions.
Correlation analyses were performed to test for multicollinearity between 3 sets of factors we hypothesized might be highly correlated (training outside of the United States and years since medical school graduation; training level and age; and past biostatistics training and past epidemiology training). Bivariate analyses were performed to identify factors that might be associated with knowledge scores. Candidate variables included sex, age, academic affiliation of residency program, advanced degrees, years since medical school graduation, training outside of the United States, current level of training, past biostatistics training, past epidemiology training, past EBM training, and currently reading medical journals. We also tested for effect modification for pairs of factors including past biostatistics training and past EBM training; past biostatistics training and past epidemiology training; and past biostatistics training and sex. The results of the correlation, bivariate, and effect modification analyses were used to determine which demographic variables to include in the multivariable model. Decisions to include factors in the multivariable regression analysis were based on the strength of correlated factors ( r <0.75) or a P value <.05 on bivariate analyses. Forward stepwise regression was subsequently used to identify which demographic factors were independently associated with biostatistics knowledge scores.
To adjust for multiple pairwise comparisons, a 2-sided level of statistical significance was set at P < .01 using a Bonferroni correction. With a sample size of 277 and a P value of .01, the study had 80% power to detect a 4.4% difference in mean knowledge scores. All analyses were performed using Stata release 8.2 (StataCorp, College Station, Texas).
The 11 targeted training programs had 532 residents, with a mean of 53.6 trainees (range, 12-118). 18 , 19 In comparison, the 388 internal medicine training programs in the United States have a total of 21 885 residents, with a mean of 56.4 trainees (range, 4-170) ( P = .76 compared to targeted programs). 20 The study programs had 41.9% women residents, compared with 42.1% nationally ( P = .96), and 49.9% of residents with training outside of the United States vs 52.3% nationally ( P = .51). 19 Comparing targeted programs with all internal medicine programs, no statistically significant differences were seen for postgraduate year 1 trainees in mean duty hours per week (61.9 vs 65.2, P = .13), mean consecutive work hours (30 vs 27.5, P = .09), and mean number of days off per week (1.3 vs 1.2, P = .31). 18 Targeted programs also did not differ in these characteristics from the remaining 4 Connecticut training programs.
Three hundred sixty-seven residents in the 11 targeted programs were on rotations that would make them available to attend their respective noon conferences on the day of the survey. Of these, 309 (84.2%) were in attendance. Of the total available residents, 277 (75.5%) completed the assessment. The response rate for individual programs ranged from 28.1% to 80%. No differences in response rates or attendance were seen based on sex, level of training, or past training outside of the United States. Table 2 lists the respondents' demographic characteristics. Approximately equal numbers of men and women were represented. Fifty-eight percent were enrolled in traditional internal medicine programs, 76.5% participated in university-based programs, 50.6% had some training outside of the United States, and 14.8% had advanced degrees. More than 68% of respondents had some training in biostatistics, with approximately 70% of this training occurring during medical school.
The survey instrument had high internal consistency (Cronbach α = 0.81). Fellows and general medicine faculty with advanced training in biostatistics had a significantly higher score than residents (mean percentage correct, 71.5% [95% confidence interval {CI}, 57.5%-85.5%] vs 41.1% [95% CI, 39.7%-43.3%]; P < .001), indicating good discriminative validity.
The overall mean resident knowledge score was 41.1% (SD, 15.2%; range, 10%-90%). Residents scored highest in recognition of double-blind studies (87.4% [95% CI, 83.5%-91.3%] answering correctly) and interpretation of relative risk (81.6% [95% CI, 77.0%-86.2%] answering correctly) ( Table 3 ). They were least able to interpret the results of a Kaplan-Meier analysis, with 10.5% (95% CI, 6.9%-14.1%) answering correctly. Only 37.4% (95% CI, 31.9%-43.3%) understood how to interpret an adjusted odds ratio from a multivariate regression analysis, while 58.8% (95% CI, 53.0%-64.6%) could interpret the meaning of a P value.
Training outside of the United States had moderate correlation with years since medical school graduation ( r = 0.59), as did past epidemiology training with past biostatistics training ( r = 0.53). Training level had a fair correlation with age ( r = 0.46). No effect modification was seen for the 3 sets of factors assessed. In bivariate analyses, differences in scores were seen based on residency program type, with medicine/pediatric residents scoring the highest ( Table 4 ). Residents with advanced degrees performed better than those without advanced training (50.0% [95% CI, 44.5%-55.5%] vs 40.1% [95% CI, 38.3%-42.0%]; P < .001). Statistically significant higher scores were also seen in residents who were just entering residency, had prior biostatistics training, were enrolled in a university-based training program, and were men ( Table 4 ).
Using forward stepwise regression, 5 factors were found to be independently associated with knowledge scores ( Table 4 ). An advanced degree was associated with an absolute increase of 9.2% questions correct after adjustment for other factors ( P < .001). Successive years since medical school graduation were associated with decreasing knowledge scores, with 11 years or more postgraduation associated with a 12.3% absolute decrease in score compared with less than 1 year postgraduation. Male sex, belonging to a university-based program, and past biostatistics training were all associated with higher scores.
The majority of residents agreed or strongly agreed that to be an intelligent reader of the literature it is necessary to know something about statistics (95%) and indicated they would like to learn more about statistics (77%). Seventy-five percent reported they did not understand all of the statistics they encountered in the literature, whereas only 15% felt that they do not trust statistics “because it is easy to lie.” More than 58% of respondents indicated that they use statistical information in forming opinions or when making decisions in medical care.
The mean confidence score in understanding certain statistical concepts was 11.4 (SD, 2.7) (maximum possible confidence score, 20). The majority of residents reported fair to complete confidence in understanding P values (88%). Fewer were confident in interpreting results of statistical methods used in research (68%), identifying factors influencing a study's power (55%), or assessing if a correct statistical procedure was used (38%).
Respondents with higher confidence in their statistical knowledge (a score higher than the mean confidence score) performed better on the knowledge questions than those with lower confidence (43.6% [95% CI, 40.8%-46.3%] vs 39.3% [95% CI, 37.0%-41.6%]; P = .02). Those who reported fair to high confidence in interpreting a P value were more likely to correctly interpret its meaning (62.8% [95% CI, 56.8%-67.2%] vs 38.2% [95% CI, 24.3%-51.7%]; P = .006). No differences were seen in a resident's ability to appropriately identify the correct statistical procedure used based on their confidence to do so.
In this multiprogram survey of internal medicine residents' confidence in, attitudes toward, and knowledge of statistical methods and interpretation of research results, 95% believed that it was important to understand these concepts to be an intelligent reader of the literature, yet three-fourths of residents acknowledged low confidence in understanding the statistics they encounter in the medical literature. This lack of confidence was validated by their low knowledge scores, in which on average only 8 of 20 questions were answered correctly. Although past instruction in biostatistics and advanced degrees were associated with better performance, knowledge scores appeared to decline with progression through training.
The poor knowledge in biostatistics and interpretation of study results among residents in our study likely reflects insufficient training. Nearly one-third of trainees indicated that they never received biostatistics teaching at any point in their career. When training did occur, the majority of instruction took place during undergraduate medical education and was not reinforced in residency. The most recent comprehensive survey of medical school biostatistics teaching was conducted in the 1990s and found that more than 90% of medical schools focused their biostatistics teaching in the preclinical years without later instruction and that the depth and breadth of this education varied greatly among schools. 21 That review reported that familiar concepts such as P values, t tests, and χ 2 analyses were frequently addressed (95%, 92%, and 88%, respectively), but advanced methods (such as Cox proportional hazards regression, multiple logistic regression, and Kaplan-Meier analyses) were not included in instruction. 21 If biostatistics teaching has continued at the same level in recent years, it would not be surprising that only a small percentage of residents in our survey (10.5%-37.6%) understood the results and use of these analyses.
The correlates of differences in knowledge scores might have been expected. Residents with prior biostatistical training and those with advanced instruction through a master's or PhD degree scored better than their counterparts. More senior residents performed worse than junior residents, potentially reflecting loss of knowledge over time, lack of reinforcement, or both. Although fourth-year residents were an exception to this pattern, these residents were part of a single medicine/pediatrics program that outperformed all other training programs. The higher scores in university-based residency programs may reflect exposure to faculty with more biostatistical training or teaching experience. In a survey study, community faculty considered EBM less important, were less confident in their EBM knowledge, and demonstrated poorer EBM skills than full-time faculty. 22
Although sex was associated with a difference in scores, this finding is not supported by other literature. Studies of evidence-based practice knowledge and skills rarely report analyses by sex. In 2 studies, investigators found no sex differences in critical appraisal skills among family physicians 23 or in use of online evidence databases among public health practitioners. 24 Six studies assessing the biostatistics and epidemiology knowledge of physicians and trainees did not conduct comparisons by sex. 5 - 7 , 25 - 27 Furthermore, our result was not a confirmation of an a priori hypothesis and so should be interpreted with caution.
Our final regression model found 5 predictors of knowledge scores: advanced degrees, academic affiliation, prior biostatistics training, sex, and years since medical school graduation. The proportion of explained variation for the model was small, with R 2 = 0.18. This likely reflects in part the low variance in resident scores.
Our results suggest the need for more effective training in biostatistics in residency education. Such training has proven difficult, with systematic reviews showing only limited effectiveness of many journal clubs and EBM curricula. 14 , 28 - 32 Thus, it is not surprising that prior EBM experience, which in the past has not included biostatistics training, 11 , 14 was not associated with higher scores in our multivariable analysis. Interactive, self-directed, and clinically instructional strategies seem to stand the best chance of success. 33 Involvement in hypothesis-driven research during training that requires comprehensive reading of the literature may also enhance residents' knowledge and understanding. 34
Faculty who are implementing biostatistics curricula can access several teaching resources. In internal medicine, the American College of Physicians' ACP Journal Club has presented a series of reports emphasizing basic study designs and statistics. 35 CMAJ has published a series of EBM “teaching tips” for learners and teachers. 36 A guide designed to help medical educators choose and interpret statistical tests when developing educational studies or when reading the medical literature is also available. 37
Limitations of this study should be considered. First, while our instrument showed good content validity, internal consistency, and discriminative validity, these psychometric properties were not known in advance but were established in the current study. Second, our survey was purposely kept brief, thus limiting our ability to assess understanding of all biostatistical concepts and research results. Nonetheless, our questions focused on the most commonly used methods and results found in the contemporary literature. Third, we attempted to survey only those residents who were present at the time of their inpatient conference. Residents who did not attend, either by choice or by chance, might have scored differently. However, since we found no differences in demographic characteristics between responders and nonresponders, this is less likely. Fourth, our study was confined to internal medicine residents, limiting generalizability to other resident physicians. Nevertheless, we were able to assess multiple types of internal medicine training programs and found similar results.
Despite these limitations, this study also has several strengths. First, it was a multiprogram study that captured information on a wide range of internal medicine residents at different types of residency programs. Second, the residents in our survey, although limited to 1 state, possessed characteristics similar to all other trainees in internal medicine programs across the United States. Third, the 11 residency programs were similar in size and composition to the average US internal medicine program, and thus our study appears to be generalizable to internal medicine trainees and training programs in the United States.
Higher levels of statistical methods are being used in contemporary medical literature, but basic concepts, frequently occurring tests, and interpretation of results are not well understood by resident physicians. This inadequate preparation demonstrates lack of competence in meeting part of the Accreditation Council for Graduate Medical Education's practice-based learning and improvement requirement. 10 If physicians cannot detect appropriate statistical analyses and accurately understand their results, the risk of incorrect interpretation may lead to erroneous applications of clinical research. Educators should reevaluate how this information is taught and reinforced in order to adequately prepare trainees for lifelong learning, and further research should examine the effectiveness of specific educational interventions.
Corresponding Author: Donna M. Windish, MD, MPH, Yale Primary Care Residency Program, 64 Robbins St, Waterbury, CT 06708 ( [email protected] ).
Author Contributions: Dr Windish had full access to all of the data in the study and takes responsibility for the integrity of the data and the accuracy of the data analysis.
Study concept and design : Windish, Huot, Green.
Acquisition of data : Windish.
Analysis and interpretation of data : Windish, Green.
Drafting of the manuscript : Windish, Green.
Critical revision of the manuscript for important intellectual content : Windish, Huot, Green.
Statistical analysis : Windish, Green.
Administrative, technical, or material support : Huot.
Study supervision : Green.
Financial Disclosures: None reported.
- Register for email alerts with links to free full-text articles
- Access PDFs of free articles
- Manage your interests
- Save searches and receive search alerts
Click through the PLOS taxonomy to find articles in your field.
For more information about PLOS Subject Areas, click here .
Loading metrics
Open Access
Peer-reviewed
Research Article
The incremental value of the contribution of a biostatistician to the reporting quality in health research—A retrospective, single center, observational cohort study
Contributed equally to this work with: Ulrike Held, Klaus Steigmiller
Roles Conceptualization, Data curation, Formal analysis, Methodology, Project administration, Resources, Software, Supervision, Validation, Visualization, Writing – original draft, Writing – review & editing
* E-mail: [email protected]
Affiliation Department of Biostatistics at Epidemiology, Biostatistics and Prevention Institute, University of Zurich, Zurich, Switzerland

Roles Conceptualization, Data curation, Formal analysis, Investigation, Methodology, Project administration, Resources, Software, Validation, Visualization, Writing – review & editing
Roles Investigation, Project administration, Validation, Writing – review & editing
Affiliation Institute of Mathematics, University of Zurich, Zurich, Switzerland
Roles Data curation, Investigation, Resources, Writing – review & editing
Affiliation Department of Cardiology, University Heart Center, University Hospital Zurich, University of Zurich, Zurich, Switzerland
Affiliation Department of Rheumatology, University Hospital Zurich, University of Zurich, Zurich, Switzerland
Affiliation Department of Trauma, University Hospital Zurich, University of Zurich, Zurich, Switzerland
Affiliation CTU Bern, University of Bern, Bern, Switzerland
Affiliation Department of Epidemiology at Epidemiology, Biostatistics and Prevention Institute, University of Zurich, Zurich, Switzerland
Roles Investigation, Methodology, Resources, Writing – review & editing
Affiliation Main Library, University of Zurich, Zurich, Switzerland
Roles Conceptualization, Data curation, Investigation, Methodology, Validation, Writing – review & editing
Roles Conceptualization, Investigation, Methodology, Validation, Writing – review & editing
Roles Conceptualization, Data curation, Formal analysis, Funding acquisition, Methodology, Project administration, Software, Supervision, Validation, Writing – review & editing
- Ulrike Held,
- Klaus Steigmiller,
- Michael Hediger,
- Victoria L. Cammann,
- Alexandru Garaiman,
- Sascha Halvachizadeh,
- Sylvain Losdat,
- Erin Ashley West,
- Martina Gosteli,
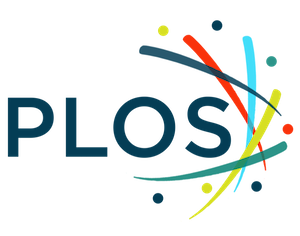
- Published: March 4, 2022
- https://doi.org/10.1371/journal.pone.0264819
- Peer Review
- Reader Comments
Registered Report Protocol
6 Nov 2020: Held U, Steigmiller K, Hediger M, Gosteli M, Reeve KA, et al. (2020) Is reporting quality in medical publications associated with biostatisticians as co-authors? A registered report protocol. PLOS ONE 15(11): e0241897. https://doi.org/10.1371/journal.pone.0241897 View registered report protocol
The reporting quality in medical research has recently been critically discussed. While reporting guidelines intend to maximize the value from funded research, and initiatives such as the EQUATOR network have been introduced to advance high quality reporting, the uptake of the guidelines by researchers could be improved. The aim of this study was to assess the contribution of a biostatistician to the reporting and methodological quality of health research, and to identify methodological knowledge gaps.
In a retrospective, single center, observational cohort study, two groups of publications were compared. The group of exposed publications had an academic biostatistician on the author list, whereas the group of non-exposed publications did not include a biostatistician of the evaluated group. Rating of reporting quality was done in blinded fashion and in duplicate. The primary outcome was a sum score based on six dimensions, ranging between 0 (worst) and 11 (best). The study protocol was reviewed and approved as a registered report.
There were 131 publications in the exposed group published between 2017 and 2018. Of these, 95 were either RCTs, observational, or prediction / prognostic studies. Corresponding matches in the group of non-exposed publications were identified in a reproducible manner. Comparison of reporting quality overall revealed a 1.60 (95%CI from 0.92 to 2.28, p <0.0001) units higher reporting quality for exposed publications. A subgroup analysis within study types showed higher reporting quality across all three study types.
Our study is the first to report an association of a higher reporting quality and methodological strength in health research publications with a biostatistician on the author list. The higher reporting quality persisted through subgroups of study types and dimensions. Methodological knowledge gaps were identified for prediction / prognostic studies, and for reporting on statistical methods in general and missing values, specifically.
Citation: Held U, Steigmiller K, Hediger M, Cammann VL, Garaiman A, Halvachizadeh S, et al. (2022) The incremental value of the contribution of a biostatistician to the reporting quality in health research—A retrospective, single center, observational cohort study. PLoS ONE 17(3): e0264819. https://doi.org/10.1371/journal.pone.0264819
Editor: Dylan A. Mordaunt, Illawarra Shoalhaven Local Health District, AUSTRALIA
Received: December 7, 2021; Accepted: February 18, 2022; Published: March 4, 2022
Copyright: © 2022 Held et al. This is an open access article distributed under the terms of the Creative Commons Attribution License , which permits unrestricted use, distribution, and reproduction in any medium, provided the original author and source are credited.
Data Availability: The data underlying the results presented in the study are available on the Open Science Framework ( https://osf.io/5egrn/ ).
Funding: The study was supported by the Center for Reproducible Science at the University of Zurich ( https://www.crs.uzh.ch/en.html ). The funder had no role in study design, data collection and analysis, decision to publish, or preparation of the manuscript.
Competing interests: I have read the journal’s policy and SL has the following competing interests: SL is employed by CTU Bern, University of Bern, which has a staff policy of not accepting honoraria or consultancy fees. However, CTU Bern is involved in design, conduct, or analysis of clinical studies funded by not-for profit and for-profit organizations. In particular, pharmaceutical and medical device companies provide direct funding to some of these studies. For an up-to-date list of CTU Bern’s conflicts of interest: http://www.ctu.unibe.ch/research/declaration of interest/index eng.html . UH, KS, MH, VLC, AG, SH, EAW, MG, KAR, SVF, and EF declare to have no conflict of interest. This does not alter our adherence to PLOS ONE policies on sharing data and materials.
Introduction
Despite measures to increase the reporting quality in the field of health research, for example, by introducing reporting guidelines and inclusion of such guidelines in recommendations for authors by many publishers, quality standards are still oftentimes not met. Recent evaluations of the literature showed that for observational studies, the corresponding STROBE guideline was not used by nearly 18% of the authors because the authors had not heard of the guideline before. An additional 19% of authors had heard of it but still did not use it [ 1 ]. Journals obviously play an important role, and a systematic evaluation showed that journal endorsement rates to the STROBE guidelines are only around 50% [ 2 ]. When it comes to the reporting of randomized trials, Dechartres et al. [ 3 ] have systematically evaluated reporting of more than 20’000 trials included in Cochrane reviews. They conclude that poor reporting has decreased over time, but that especially lower impact factor journals show room for improvement. Reporting quality of clinical prediction models has recently been evaluated systematically in the context of research on Severe acute respiratory syndrome coronavirus 2 (Sars-Cov-2) [ 4 ]. The authors concluded that almost all published models for predicting mortality were poorly reported, and that the corresponding Transparent reporting of a multivariable prediction model for individual prognosis or diagnosis (TRIPOD [ 5 ]) guideline was largely omitted.
In Switzerland, the government paid 22.9 billion Swiss francs for research and development, representing more than 3% of the gross domestic product in 2019. Publications in the field of “clinical medicine” represent 25% of all publications [ 6 ], and given the large amounts of resources, value from research and publications should be maximized.
The objectives of the current study were, first, to assess the contribution of a biostatistician as co-author on the quality of reporting and methodological strength in health research publications; second, to identify dimensions of reporting quality and study types with methodological knowledge gaps; and third, to promote the awareness of the importance of good reporting among clinical researchers and biostatisticians.
Materials and methods
Study design.
The study is a retrospective, single-center observational cohort study, conducted at the University of Zurich (UZH) and its University Hospital (USZ).
Selection of exposed and non-exposed publications
In this study, two groups of publications were compared. The group of “exposed” publications was defined according to their exposure to one or more of a set of 13 academic biostatisticians from the Epidemiology, Biostatistics and Prevention Institute, and the Institute of Mathematics, both localized at University of Zurich, as a co-author. The group will be referred to as biostatisticians in the following. The group of exposed publications was published between 2017 and 2018, and it was retrieved in a PubMed search, with a search string as specified in S1 Appendix on Dec 9, 2019. Methodological publications as well as non-English language publications were excluded.
To define the group of “non-exposed” publications for comparison, all medical research publications found in PubMed between 2017 and 2018, with the affiliation UZH or USZ or any of the affiliated university hospitals for the first and / or the second author were extracted on Dec 16, 2019. Details on the search string can again be found in S1 Appendix . The non-exposed publications have none of the defined set of biostatisticians on the author list. It cannot be excluded that a biostatistician from outside of the group was on the author list. The full list of affiliations considered can be found in S2 Appendix . Based on the full list, a list of affiliations relevant for this study was created, in which for example typographic errors were removed. The large number of non-exposed publications resulting from the affiliation list was used in a random but replicable order—aiming to remove potential chronological ordering or any other systematic ordering while adhering to high standards of reproducibility.
Categorization into study types
For each of the exposed publications, the study type was determined, and the subset of all RCTs, observational studies, and prediction / prognostic studies was evaluated further. Categorization into study types was performed by the set of biostatisticians. For most publications, the authors themselves determined the study type. For some publications, the biostatistician as co-author had left the department, and thus the study type was categorized independently and in duplicate by two authors (UH, EF). After consensus on study type was reached, record count for each study type for each publication year was obtained. The three study types RCT, observational study, and prediction / prognostic study were the most frequent types. Other types (e.g. systematic reviews) had been abandoned a priori.
The number of non-exposed publications was much larger than the number of exposed publications. For that reason, the categorization of the non-exposed publications into RCTs, observational studies and prediction / prognostic studies was performed in random but replicable order until the numbers of non-exposed publications of these study types matched the corresponding number of exposed publications per year. Categorization was performed independently and in duplicate by the authors UH and MH (for papers published in 2017) and by EF and MH (for 2018). Any discrepancies were resolved by discussion and third-party arbitration (KS). The final set of publications was considered the non-exposed group of publications.
Selection of items from reporting guideline
For each study type, a set of six items measuring reporting quality were identified by reaching group consensus among the set of biostatisticians. The quality criteria were based on the reporting guidelines CONSORT [ 7 ], STROBE, and TRIPOD, and they reflect characteristics of a publication that are especially important for judging the validity of the results and methodological strength.
Specification of the reporting quality items
The reporting guideline items chosen for the ratings represented the following general dimensions for all three study types: 1. variable specification; 2. how study size was arrived at; 3. missing data; 4. statistical methods; 5. precision of results; and 6. whether the corresponding reporting guideline was mentioned.
The rating of publications regarding these six items was operationalized and piloted, such that they could be used efficiently and robustly to rate each publication consistently. Each dimension had different possible answer categories, also dependent on study type, resulting in a rating varying between 0 (lowest) to 2 (highest) for dimensions 1 to 5, plus an additional point for mentioning the corresponding reporting guideline. Details of the operationalization can be found in S3 Appendix . The range of the total score was from 0 (lowest) to 11 (highest).
The primary outcome of this study was the sum score of reporting quality and methodological strength in exposed and non-exposed publications, with respect to the six dimensions. The primary outcome was assessed in blinded fashion and in duplicate by two independent raters. The raters were recruited from outside of the departments. Blinding to whether the publication belonged to the exposed or non-exposed group was guaranteed by removing author names, affiliation lists, journal name, corresponding author name, author contributions, date, acknowledgements, references, and DOI from every publication’s PDF. Discrepancies in the ratings between the two raters were resolved by a third rating and discussion until consensus was reached.
The secondary outcome of this study was the number of citations in the group of exposed and non-exposed publications at a fixed date (July 20, 2021).
Outcome rating and rater training
The outcome rating and its operationalization was developed by four authors (UH, KS, MH, EF). After operationalization was finalized the resulting questions for each study type were programmed to be evaluated through an R Shiny app [ 8 ], which underwent quality review and a testing period. The questionnaire can be found in S3 Appendix . To find raters, outside of the core study team and outside of the departments, PhD programs in health research across Switzerland, as well as groups of researchers interested in Research on Research were contacted. Each candidate rater could chose a study type, and received written instructions for the rating task. The candidate raters were instructed and trained by rating vignette publications for calibration. These vignette publications of all study types were similar publications as those under study, but they were published in 2019 and were rated with scrutiny by the study authors, including detailed explanation. Only upon successful completion of test ratings, the raters received sets of 11–12 papers of the same study type for rating. The raters were obliged to rate the reporting quality based on the blinded PDF’s alone, and not to use additional information from the internet while doing so. Ratings were performed in blinded fashion, meaning that the raters were unaware of the classification of publications as exposed or non-exposed, and of authors on the publications. The ratings were performed in duplicate, and any discrepancies were resolved by a third independent rating. The raters were reimbursed with vouchers for every set of 11–12 publications. Additionally, raters were asked for co-authorship after completion of 33 or more ratings. In total, 15 raters were recruited. The ratings were done between May and July 2021.
Sample size considerations
The sample size was justified a priori, based on the consideration that with 95 publications in the exposed group, and 95 publications in the non-exposed group at a significance level of 5% and with a power of 80% an effect size of 0.41 (Cohen’s d ) could be detected, using a 2-sided, 2-sample t-test with equal variance assumption. The effect size would be considered a medium effect size. The number of 95 publications corresponded to all publications in the exposed group in the years 2017 and 2018.
Data management
Data collection in the context of this study had to cover two different aspects. First, categorization of the exposed and non-exposed publications into the three study types was performed with the help of a specifically programmed R Shiny app, in which the title and abstract, as well as the link to the full text was provided, such that the categorization could be performed independently and in duplicate and that any discrepancies could be detected and resolved by discussion. Second, reporting quality rating was performed using another R Shiny app, implementing the operationalized quality dimensions. The electronic records of the two independent ratings, and the consensus rating were saved. The use of R Shiny apps in this research guaranteed highly reliable data entry.
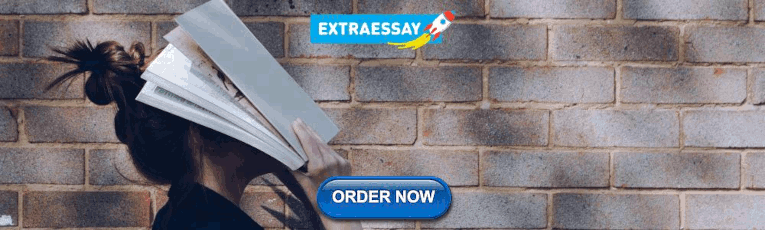
Risk of bias
The study was designed to compensate the following biases a priori. Risk of detection bias was addressed with blinded and duplicate outcome ratings by researchers not otherwise involved in the study. Risk of selection bias was addressed by considering all publications within two years for the exposed group and by reproducible random sub sampling of PubMed publications from medical publications with UZH / USZ affiliation for the group of non-exposed publications. The results of the study could be confounded by indication, if more complex research projects were brought to the group of biostatisticians’ attention whereas less complex projects were addressed by the clinicians without asking for help from an academic biostatistician. This bias was partially addressed by comparing the number of citations of exposed and non-exposed publications, under the hypothesis that equal citation numbers would indicate that less confounding by indication was present.
Statistical methods and programming
For assessing the level of agreement of reporting quality between the two independent ratings, squared-weighted Cohen’s κ values were estimated, and reported with 95% confidence intervals based on 1000 bootstrap samples. These analyses of agreement were reported overall and in subgroups of study type. Interpretation of the κ values were based on the categorization suggested by Altman [ 9 ].
Statistical methods for the primary outcome included visualization of the results with dot-plots (lollipop plots), in which the means of the outcome in the exposed and non-exposed publications are shown, overall (score 0 to 11), and in subgroups of study type (score 0 to 11) and reporting quality dimension (score 0 to 2). Besides that, the estimated between-group differences, overall and in subgroups of study type with 95% confidence intervals (CI) were reported. The two-sided, two-sample t-test under the assumption of equal variances was used to test the hypothesis of no difference in reporting quality between exposed and non-exposed publications. Corresponding Cohen’s d was calculated using pooled standard deviations assuming equal variances.
The number of citations was reported overall, and in subgroups of study type, with medians and interquartile ranges, as the distribution was right-skewed. The non-parametric exact Wilcoxon-Mann-Whitney method was used to test the hypothesis of no difference in number of citations between exposed and non-exposed publications, and to estimate a confidence interval. The between-group difference in location was estimated and reported with 95% CI, based on rank statistics.
The software used for statistical analysis was extracted across all publications. It was reported as number and percentage of total. In many publications, more than one software was used, and for that reason the percentages exceed 100%.
All analyses, including subgroup analyses described above were pre-specified in the registered report study protocol [ 10 ]. The unit of analysis was the individual publication, or the reporting quality dimension.
Statistical programming was performed with R 4.1.1 [ 11 ], in combination with dynamic reporting. Statistical programming included downloading all potential non-exposed publications, random reordering, development of an R Shiny app for categorization of the publications, development of an R Shiny app for the recording of reporting quality ratings, as well as statistical programming of the methods for data analysis and visualization. Results of the study were reported according to STROBE guidelines [ 12 ]. All anonymized data was uploaded in an OSF repository.
In total there were 131 exposed papers published in 2017 and 2018. Of these, 95 publications were of the study types RCT, observational study, or prediction / prognostic study. There were six RCTs, 77 observational studies, and 12 prediction / prognostic studies. The literature search for non-exposed publications with first and / or second author with suitable affiliation and year resulted in a total number of 3420 publications. Four hundred publications of these in random order were categorized into one of the three study types RCT, observational, or prediction / prognostic study, and the retrieved case numbers of the exposed papers could be frequency matched individually for 2017 and 2018. The corresponding flow-chart is shown in Fig 1 . All data was made available on OSF [ 13 ].
- PPT PowerPoint slide
- PNG larger image
- TIFF original image
Selection process for the exposed publications (left) and the non-exposed publications (right), including screening of affiliation lists of first and second author.
https://doi.org/10.1371/journal.pone.0264819.g001
Ten of the exposed publications and two of the non-exposed publications mentioned the corresponding reporting guideline. In 48 of the exposed publications, and in 14 of the non-exposed publications, the programming language R was used for the statistical analysis. All descriptive results can be found in Table 1 . There were no missing values in the data throughout.
https://doi.org/10.1371/journal.pone.0264819.t001
The agreement between the two ratings of each publication was 0.52 (95%CI from 0.46 to 0.57) overall, indicating moderate agreement, according to Altman [ 9 ].
For the three different study types, however, the agreement varied between 0.31 (95%CI from 0.05 to 0.52) for RCTs and 0.52 (95%CI from 0.46 to 0.59) and 0.53 (95%CI from 0.35 to 0.68) for observational and prediction studies, respectively. To reach consensus for all ratings with discrepancies a third blinded rater was involved.
Primary outcome
The estimated between-group difference for the primary outcome was 1.60 (95%CI from 0.92 to 2.28, p < 0.0001) in favor of the exposed publications. This result corresponds to a Cohen’s d of 0.67 (95%CI from 0.38 to 0.97). In the pre-specified subgroups of study type, the estimated between group difference was 3.33 (95%CI from -0.84 to 7.51), 1.39 (95%CI from 0.68 to 2.09) and 2.08 (95%CI from 0.12 to 4.04) for randomized, observational and prediction / prognostic studies, respectively ( Fig 2 ), showing higher reporting quality across all study types. In addition to the estimation of the between group difference, the representation of each subgroup’s mean values shows that generally for RCTs the reporting quality was higher than for observational and prediction / prognostic studies.
Unit of analysis is publication.
https://doi.org/10.1371/journal.pone.0264819.g002
Dimension-specific score values
For each of the five reporting dimensions, the between group difference was estimated. The corresponding range of values was between 0 (worst) and 2 (best). Again the results are shown in a graphical representation ( Fig 3 ). The dimension “Variables” had a smaller between group difference, and a higher reporting quality overall, whereas the “Missing data” and “Statistical methods” dimensions were generally reported with less detail. The mean reporting quality in the exposed publications was higher throughout, than that of the non-exposed publications.
Unit of analysis is dimension.
https://doi.org/10.1371/journal.pone.0264819.g003
Number of citations
The number of citations, extracted on July 20, 2021, had a non-normal, right-skewed distribution, and for that reason the non-parametric exact Wilcoxon-Mann-Whitney method was used for the estimation of the between group difference and its confidence interval. The estimate was -2 (95%CI from -4 to 0, p = 0.07) indicating weak evidence for higher citation numbers for the non-exposed publications. All descriptive statistics for number of citations can be found in Table 2 . It can be seen that the number of citations was relatively balanced for observational studies and prediction / prognostic studies, whereas in the RCTs the number of citations was much larger in the non-exposed group of publications as compared to the exposed publications.
Estimates show the median [IQR].
https://doi.org/10.1371/journal.pone.0264819.t002
Our study demonstrates that the associated effect of academic biostatisticians as co-authors is to increase reporting quality and methodological strength in health research publications, overall and in subgroups of study types. In addition to that, the subgroup analyses demonstrated that there was evidence for a higher reporting quality in the exposed publications for observational studies and prediction / prognostic studies. The CONSORT statement seems to have been taken up well, because reporting quality was highest generally, for both exposed and non-exposed publications in RCTs.
Citation numbers were comparable for exposed and non-exposed publications in the study types observational and prediction / prognostic studies, but the median number of citations for RCTs was higher in the non-exposed group of publications. The number of citations was evaluated to address the potential bias of confounding by indication. Our findings for observational and prediction / prognostic studies were reassuring, since balanced citation numbers showed that there was no evidence for confounding by indication. The imbalance in citation numbers for RCTs is not necessarily concerning since RCTs may anyhow be considered a special case. They are heavily regulated, CONSORT is generally well enforced by journals, they are expensive studies usually focused on “important” research questions, and they are often multi-center studies and hence likely to be including statisticians from other centers. RCTs are more frequently published in high-ranked medical journals and may therefore have higher citation numbers automatically. Together with the fact that only a low number of RCTs was assessed in this study we believe that there is a low risk of confounding by indication also in the case of RCTs.
Methodological knowledge gaps seemed to be more prominent in the areas of statistical methods, and missing values. Nevertheless the mean reporting quality was higher in the exposed publications, throughout all subgroups. While it seems reasonable to assume that in the exposed papers the biostatisticians knew the methods well, there was still sub optimal reporting of these. The rating of reporting quality was performed in duplicate, and the agreement between first and second ratings were moderate to good, overall. The difficulties in the rating tasks were an indicator of sub optimal reporting in itself. Our study is the first to our knowledge to develop and use a rating score that is usable across study types, and which allows the comparison of reporting quality across study types. Low citation numbers of corresponding reporting guidelines in both, the group of exposed and non-exposed publications may be an indication of lack of awareness among study authors.
Results in the light of the literature
Our findings are in line with research on research studies evaluating the reporting quality of RCTs, observational studies and prediction / prognostic studies. While the development of reporting guidelines has been ongoing over the last 20 years, and the use of CONSORT is well-established for RCTs, there seem to remain areas in which good reporting less frequently observed. The Cochrane collaboration has initiated the “Prognosis Methods Group” to encourage and facilitate the systematic review and meta analysis of prognostic models in clinical research. Similar to the many systematic evaluations of research questions addressed with RCTs in Cochrane, the field of prediction / prognostic research will benefit and reporting as well as methodological quality will likely increase. Currently, there are 12 prognostic model reviews being undertaken in different fields of clinical research, of which one has been published [ 14 ].
Observational studies were the most frequent study type in the sample at hand, and reporting as well as methodological quality was only moderately higher in the exposed publications than in the non-exposed publications. Although the STROBE reporting guidelines have been published in 2004, and taken up by many journals, study authors need continuing reminders as a recent publication in JAMA Surgery by Brooke et al. showed [ 15 ].
Limitations and strengths
Our study has several limitations. The sum score to assess reporting quality and methodological strength was derived and used for the first time in the context of this study. We took multiple means to propose a consistent and valid sum score by using items from the corresponding reporting guidelines CONSORT, STROBE, and TRIPOD directly, and thorough piloting and testing. The sum score addressed the reporting quality in dimensions in which biostatisticians play a relatively prominent role. However, methodological strength could not be rated explicitly. In our view, the assessment of methodological quality is hampered if the reporting quality is low, making reporting assessment and improvement a first important milestone in improving the quality of health research in general. In the selection process, reporting items were chosen that would partially allow the assessment of methodological quality. For example in the dimension “Study size’, post-hoc power calculations for observational studies were assigned zero points, and in “Statistical methods” for prediction / prognostic studies zero points were assigned if model performance measures like discrimination or calibration were not reported. Questions across study types addressed the question of “Missing values”, and explicitly asked for methods to address them if present. Risk of bias assessment could be facilitated if reporting quality was higher generally. Another limitation of our study was the low agreement between ratings for the RCTs, which turned out to be only fair. An explanation for this could have been the small number of RCTs being rated by only two different raters. Both raters were relatively consistent in their ratings: one of them being somewhat strict, and the other one relatively lenient. The discrepancies led to the fact that many questions had to be rated by a third rater to come to a consensus.
Our study has several strengths. First of all, we had written a clear study protocol, receiving an external review as a registered report. Upon review of the protocol, the study design and operationalization could be revised and improved. Second, several measures were taken to compensate for different sources of bias, as our study was observational and retrospective. These included double ratings of reporting quality, unbiased assessment of reporting quality through blinded PDFs, and highly reliable data entry through the specifically designed R Shiny app.
Implications
Our study has several implications for future research. First of all, the study design can repeatedly be applied for future assessments of reporting quality in our group or other academic centers over time. The continuing discussions about the assessment already had an impact on the awareness of the topic among the people involved. In addition, the setup can be generalized to address other documents, e.g., systematic reviews (based on PRISMA [ 16 ]), statistical analysis plans [ 17 ], or research proposals (SPIRIT [ 18 ]).
Academic biostatisticians should take more responsibility in the review of final manuscript versions, and verify the adherence to established reporting guidelines. For reporting of statistical methods and of results with precision, there should be left enough room in the publication. More emphasis should be put on adequate methods to deal with missing values and the reporting thereof.
Conclusions
Our study is the first to systematically assess the valuable impact of a biostatistician on reporting quality and methodological strength in health research. Higher reporting quality persisted through subgroups of study types and dimensions. The operationalization of the quality assessment allows the direct comparison across study types and dimensions. Methodological knowledge gaps were identified for prediction and prognostic studies, and for the reporting on statistical methods and missing values.
Supporting information
S1 appendix. search string..
Search string for the identification of potential control publications.
https://doi.org/10.1371/journal.pone.0264819.s001
S2 Appendix. Affiliation list.
Affiliation list for control publications.
https://doi.org/10.1371/journal.pone.0264819.s002
S3 Appendix. Questionnaire.
Questionnaires for the assessment of reporting quality.
https://doi.org/10.1371/journal.pone.0264819.s003
Acknowledgments
We would like to thank Julia Braun, Sarah Haile, and Leonhard Held for their support in extracting relevant items from the corresponding reporting guidelines, and Tina Wünn for support in data preparation and statistical programming of the Shiny apps. We would like to thank the raters involved in our study: Mattia Branca, Victoria Lucia Cammann, Sascha Halvachizadeh, Monika Hebeisen, Brigitta Gahl, Alexandru Garaiman, Andrea Götschi, Stefanie Hayoz, Nadja Hedrich, Arnaud Künzi, Sylvain Losdat, Vera Neumeier, Kelly A. Reeve, Mari Sasaki, Céline Steger, Erin West.
- View Article
- PubMed/NCBI
- Google Scholar
- 6. Schweizerische Eidgenosssenschaft. Forschung und Entwicklung (F+E) beim Bund | Steckbrief; 2019. Available from: https://www.bfs.admin.ch/bfs/de/home/statistiken/volkswirtschaft/forschung-entwicklung.gnpdetail.2021-0413.html#id_af0d2f9fa931c57a073be94d3e7a9017 .
- 8. Chang W, Cheng J, Allaire J, Sievert C, Schloerke B, Xie Y, et al. shiny: Web Application Framework for R; 2021. Available from: https://CRAN.R-project.org/package=shiny .
- 9. Altman D. Practical Statistics for Medical Research; 1991.
- 11. R Core Team. R: A Language and Environment for Statistical Computing; 2021. Available from: https://www.R-project.org/ .
- 13. Steigmiller K, Held U, Furrer E. BIQMR_Repository; 2021. Available from: https://osf.io/5egrn/ .
- Open access
- Published: 05 February 2020
Why do you need a biostatistician?
- Antonia Zapf ORCID: orcid.org/0000-0001-5339-2472 1 ,
- Geraldine Rauch 2 &
- Meinhard Kieser 3
BMC Medical Research Methodology volume 20 , Article number: 23 ( 2020 ) Cite this article
25k Accesses
15 Citations
23 Altmetric
Metrics details
The quality of medical research importantly depends, among other aspects, on a valid statistical planning of the study, analysis of the data, and reporting of the results, which is usually guaranteed by a biostatistician. However, there are several related professions next to the biostatistician, for example epidemiologists, medical informaticians and bioinformaticians. For medical experts, it is often not clear what the differences between these professions are and how the specific role of a biostatistician can be described. For physicians involved in medical research, this is problematic because false expectations often lead to frustration on both sides. Therefore, the aim of this article is to outline the tasks and responsibilities of biostatisticians in clinical trials as well as in other fields of application in medical research.
Peer Review reports
What is a biostatistician, what does he or she actually do and what distinguishes him or her from, for example, an epidemiologist? If we would ask this our main cooperation partners like physicians or biologists, they probably could not give a satisfying answer. This is problematic because false expectations often lead to frustration on both sides. Therefore, in this article we want to clarify the tasks and responsibilities of biostatisticians.
There are some expressions which are often used interchangeably to the term ‘biostatistician’. In here, we will use the expression ‘(medical) biostatistics’ as a synonym for ‘medical biometry’ and ‘medical statistics’, and analogously we will do for the term ‘biostatistician’.
In contrast to the clearly defined educational and professional career steps of a physician, there is no unique way of becoming a biostatistician. Only very few universities do indeed offer studies in biometry, which is why most people working as biostatisticians studied something related, subjects such as mathematics or statistics, or application subjects such as medicine, psychology, or biology. So a biostatistician cannot be defined by his or her education, but must be defined by his or her expertise and competencies [ 1 ]. This corresponds to our definition of a biostatistician in this article. The International Biometric Society (IBS) provides a definition of biometrics as a ‘field of development of statistical and mathematical methods applicable in the biological sciences’ [ 2 ]. In here, we will focus on (human) medicine as area of application, but the results can be easily transferred to the other biological sciences like, for example, agriculture or ecology. As mentioned above, there are some professions neighbouring biostatistics, and for many cooperation partners, the differences between biostatisticians, medical informaticians, bioinformaticians, and epidemiologists are not clear. According to the current representatives of these four disciplines within the German Association for Medical Informatics, Biometry and Epidemiology (GMDS) e. V.:
‘Medical biostatistics develops, implements, and uses statistical and mathematical methods to allow for a gain of knowledge from medical data.’ ‘Results are made accessible for the individual medical disciplines and for the public by statistically valid interpretations and suitable presentations’ (authors’ translation from [ 3 ]).
‘Medical informatics is the science of the systematic development, management, storage, processing, and provision of data, information and knowledge in medicine and healthcare’ (authors’ translation from [ 4 ]).
Bioinformatics is a science for ‘the research, development and application of computer-based methods used to answer biomolecular and biomedical research questions. Bioinformatics mainly focusses on models and algorithms for data on the molecular and cell-biological level’ [ 5 ].
‘Epidemiology deals with the spread and the course of diseases and the underlying factors in the public. Apart from conducting research into the causes of disease, epidemiology also investigates options of prevention’ (authors’ translation from [ 6 ]).
Another discipline is data science, which is a relatively new expression used in a multitude of different contexts. Often it is meant as a global summarizing term covering all of the above mentioned fields. As there is no common agreement on what data science is and as this term does not correspond to a uniquely defined profession, this expression will not be discussed in more detail.
The self-descriptions as stated above are rather general and not necessarily complete. Therefore, we will in the following describe the specific tasks and responsibilities of biostatisticians in different important application fields in more detail. This allows us to specify what cooperation partners may (or may not) expect from a biostatistician. Furthermore, clarification of the roles of all involved parties and their successful implementation in practice will overall lead to more efficient collaborations and higher quality.
Tasks and responsibilities of biostatisticians
There are many medical areas where biostatisticians can contribute to the general research progress. These fields of application and the related biostatistical methods are not strictly separated, but there are many overlaps and a classification of the related methodology can be done in various ways. We consider in the following the important application fields of clinical trials, systematic reviews and meta-analysis, observational and complex interventional studies, and statistical genetics to highlight the tasks and responsibilities of biostatisticians working in these areas.
Biostatisticians working in the area of clinical trials
The tasks of biostatisticians in clinical trials are not limited to the analysis of the data, but there are many more responsibilities. It is a quite misguided view that biostatisticians are only required after the data has been collected. According to Lewis et al. (1996), statistical considerations are not only relevant for the analysis of data but also for the design of the trial [ 7 ]. This is not a personal view, but general consensus. It is demanded by the ethics committee and confirmed by the principle investigator and / or the sponsor when stating that the clinical trial will be conducted according to Good Clinical Practice (GCP). The corresponding guideline E6 from the International Council for Harmonisation of Technical Requirements for Pharmaceuticals for Human Use (ICH) explicitly states that statistical expertise should be utilized throughout all stages [ 8 ]. In there, it is stated in Section 5.4.1: ‘The sponsor should utilize qualified individuals (e.g. biostatisticians, clinical pharmacologists, and physicians) as appropriate, throughout all stages of the trial process, from designing the protocol and CRFs [case report forms, AZ] and planning the analyses to analyzing and preparing interim and final clinical trial reports.’ Mansmann et al. [ 9 ] provided a more specific guidance about good biometrical practice in medical research and the responsibilities of a biostatistician. In there, the responsibility of a biostatistician is described as a person participating in the planning and the execution of a study, in the dissemination of the results and in statistical refereeing. These are very general descriptions of the tasks and responsibilities of biostatisticians. In the following, we will explain the biostatistician’s mission in more detail based on the guidance on good biometrical practice [ 9 ] and on the E9 guideline from the ICH about Statistical Principles for Clinical Trials [ 10 ].
In the initial phase of a medical research project, a biostatistician should actively participate in the assessment of the relevance and the feasibility of the study. During the planning phase, the biostatistician should already be involved in the discussion of general study aspects as outlined in more detail below. It is evident that the physician must provide the framework for this. However, the biostatistician can and should point out important biostatistical issues which will have important influence on the whole construct of the study. Therefore, an important part of the biostatistician’s work is to be done long before a study can start. For example, the appropriate study population (special subgroups or healthy subjects in early phases versus large representative samples of the targeted patient population in confirmatory trials) and reasonable primary and secondary endpoints (e.g. suitable to the study aim, objectively measurable, clearly and uniquely defined) need to be identified. He also should make the physician aware of the potential problems with multiple or composite primary endpoints and with surrogate or categorised (especially dichotomized) variables. Another very important topic related to the general study design is blinding and randomisation as techniques to avoid bias. Moreover, the comparators or treatment arms must be specified and it has to be defined how they are embedded in the general study design (for example parallel or crossover). It also has to be specified the aim in whether is to show superiority or non-inferiority of the new treatment and whether interim analyses are reasonable (group sequential designs). Moreover the procedures for data capture and processing have to be discussed at this point. Only after fixing all these planning aspects, the biostatistician can provide an elaborated sample size calculation.
During the ongoing study, main tasks and responsibilities consist of biostatistical monitoring (for example as part of a data safety monitoring board) and performing interim analyses (if planned). If any modifications of the study design are urgently required during the ongoing trial (for example changes within an adaptive designs, or early stopping after an interim analysis), the biostatistician has to be involved in the discussions and decisions as otherwise the integrity of the study can be damaged.
The main data analysis is performed after all patients were recruited and fully observed. However, the statistical methods applied within the data analysis must already be specified during the planning phase within the study protocol. The study protocol should already be as detailed as possible in particular with regard to the analysis of the primary endpoint(s). In addition, the statistical analysis plan (SAP), which must be finalized before start for the data analysis, provides a document which describes all details on the primary, secondary and safety analyses. It also covers possible data transformations, applied point and interval estimators, statistical tests, subgroup analyses, and the consideration of interactions and covariates. Furthermore, the used data sets (for example intention to treat or per protocol), the handling of missing values, and a possible adjustment for multiplicity should be described and discussed. Another important issue is how the integrity of the data and the validity of the statistical software can be guaranteed.
In a last step, after the finalization of the data analysis according to the SAP, the biostatistician contributes to reporting the results in the study report as well as in the related publications submitted to medical journals. He or she is responsible for the appropriate presentation and the correct interpretation of the results.
To sum up, in clinical studies, the tasks and responsibilities of biostatisticians thus extend from the planning phase, through the execution of the study to data analysis and publication of the results. In particular, a careful study planning, in which the contribution of a biostatistician is indispensable, is essential to obtain valid study results.
Biostatisticians working in the area of systematic reviews and meta-analysis
To judge the level of evidence of medical research, different systems of evidence grading were suggested. The recent grading system from the Oxford Centre for Evidence-Based Medicine (OCEBM) defines ten evidence levels. The highest level is a systematic review of high quality studies for the therapeutic as well as for the diagnostic and prognostic context [ 11 ]. The need for such reviews results from the huge amount of articles in the medical literature, which has to be aggregated appropriately [ 12 ]. As Gopalakrishnan and Ganeshkumar describe, the aim of a systematic review is to ‘systematically search, critically appraise, and synthesize on a specific issue’ [ 13 ]. A meta-analysis, which additionally provides a quantitative summary, can be part of a systematic review, if a reasonable number of individual studies are available. The task and responsibilities of biostatisticians in this field are described in the following. As in clinical trials, the biostatistician should already be involved during the planning phase of a systematic review/meta-analysis to discuss the design aspects and the feasibility. Beside the literature search and the collection of the study data (most often not available on an individual patient level), the assessment of the study quality and the risk of bias are important topics. There are different tools for the assessment, like the GRADE approach (Grading of Recommendations, Assessment, Development and Evaluation) [ 14 ] or the QUADAS-2 tool (Quality Assessment of Diagnostic Accuracy Studies) for diagnostic meta-analyses [ 15 ]. A general description of these approaches can be found in the Cochrane Handbook [ 16 ]. The main task of biostatisticians in the field of systematic reviews is then to perform the meta-analysis itself including the calculation of weighted summary measures, creation of graphs, and performing subgroup and sensitivity analyses. As a last step, the biostatistician should again support the physicians in interpreting und publishing the results.
In summary, the tasks and responsibilities of biostatisticians in the field of systematic reviews and meta-analyses relate to the proper planning, the evaluation of the quality of the individual studies, the meta-analysis itself and the publication of the results.
Biostatisticians working in the area of observational and complex interventional studies
In observational studies, where confounding plays a major role, statistical modelling aims at incorporating, investigating, and exploiting relationships between variables using mathematical equations. Other important examples for application of the related techniques are longitudinal data measured repeatedly in time for the same subject or data with an inherent hierarchical structure, for example data of patients observed in different departments within various clinics. Valid conclusions from the analysis are only obtained if the functional relationship between the variables is correctly taken into account [ 17 ]. Another prominent task of statistical modelling is prediction, for example to forecast a future outcome of patients. Frequently, the relationship between the involved variables is complex. For example, patients may undergo several states between start of observation and outcome and the transitions between these states as well as potential competing risks have to be adequately considered (see, for example, Hansen et al. [ 18 ]). Extrapolation is another field of growing interest where techniques of statistical modelling are indispensable. This process can be defined as ‘extending information and conclusions available from studies in one or more subgroups of the patient population (source population), or in related conditions or with related medicinal products, to make inferences for another subgroup of the population (target population), or condition or product’ [ 19 ]. For example, clinical trial data for adults may be used to assist the development of treatments for children [ 20 ]. Last but not least, statistical modelling may be of help in situations where data of different origin shall be synthesized to increase evidence, for example, from randomized clinical trials, observational studies, and registries. These examples are by far not exhaustive and illustrate the wide spectrum of potential data sources and applications. It is obvious that there are direct connections to the two working areas of biostatisticians described in the preceding subsections, and consequently there are substantial overlaps in the related tasks and responsibilities. As in the other working areas considered, the biostatistician is responsible for choosing a correct and efficient analysis method that includes all relevant information. Due to the complexity of statistical models, this point is especially challenging here. Furthermore, it is the task of biostatisticians to decide whether the mandatory data required to adequately map the underlying relationships are included in the available data set, whether data quality and completeness is sufficiently high to justify a reliable analysis, and to define appropriate methods dealing with missing values. It is highly recommended to prepare an SAP not only for clinical trials (see Biostatisticians working in the area of clinical trials section) but also for analyses using methods of statistical modelling.
Again, the biostatistician is responsible not only for a proper planning and conducting of the analyses but also for appropriate interpretation and presentation of the results. The particular challenge for biostatisticians in this area is to choose appropriate statistical models for the analysis of data with a complex structure.
Biostatisticians working in the area of statistical genetics
Biostatisticians working in the fields of genetics and genomics are often the responsible persons for the final integration of multidisciplinary expertise in mathematics, statistics, genetics, epidemiology, and bioinformatics to only cite some common ingredients. Planning tasks include the design of research studies, which may pursue exploratory and/or confirmatory objectives. There exist a broad range of possible study designs which make use of well-differentiated modelling techniques. Generated data are often pre-processed by bioinformaticians before it reaches the biostatistician. Pre-processing of sequencing data, for instance, usually comprises quality control of sequenced reads, alignment to the human reference genome and markup of duplicates previously to the identification of somatic mutations and indels. Good knowledge of the limitations of applied pre-processing techniques by the statistician is often very helpful. A strong background and a deep understanding of genetics and genomics as well as an interdisciplinary thinking are a must for biostatisticians working in this area. These competences will be even more important in future. For example, emerging fields of research like Mendelian randomization where genetic variants are used as instruments to predict causality will require an even stronger interaction between statistics and genetics.
In the field of statistical genetics, tasks and responsibilities relate in particular to study planning, critical review of pre-processing, and data analysis using appropriate statistical models.
Biostatistics mainly addresses the development, implementation, and application of statistical methods in the field of medical research [ 3 ]. Therefore, an understanding of the medical background and the clinical context of the research problem they are working on is essential for biostatisticians [ 21 ]. Furthermore, a specific professional expertise is inevitable, and also soft skill competencies are very important. Regarding the professional expertise, the ICH E9 guideline states that a trial statistician should be qualified and experienced [ 10 ]. Qualification, which means biostatistical expertise, covers methodological background (mathematics, statistics, and biostatistics), biostatistical application, medical background, medical documentation, and statistical programming. The experience relates to consulting, planning, conducting and analysing medical studies. Jaki et al. [ 22 ] gave a review of training provided by existing medical statistics programmes and made recommendations for a curriculum for biostatisticians working in drug development. Regarding the soft skills of a biostatistician, some literature exists (for example [ 23 ] or [ 24 ]). Furthermore, Zapf et al. [ 1 ] summarize the professional expertise and the needed soft skills of a biostatistician according to the CanMEDS framework [ 25 ], which was developed to describe the required abilities of physician (the original abbreviation ‘Canadian Medical Education Directions for Specialists’ is no longer in use).
In this article, we did not explicitly consider the recently upcoming field of biomedical data science which is applied in many different areas of medical research such as, for example, individualized medicine, omics research, big data analysis. The tasks and responsibilities of biostatisticians working in this domain are not different from those reported above but in fact include all mentioned aspects [ 26 ].
There is evidently an overlap between the tasks and responsibilities of medical biostatisticians and neighbouring professions. However, all disciplines have different focuses. Important application fields of biostatistics are clinical studies, systematic reviews / meta-analysis, observational and complex interventional studies, and statistical genetics.
In all fields of biostatistical activities, the working environment is diverse and multi-disciplinary. Therefore, it is essential for fruitful, efficient, and high-quality collaborations to clearly define the tasks and responsibilities of the cooperating partners. In summary, the tasks and responsibilities of a biostatistician across all application areas cover active participation in a proper planning, consultation during the entire study duration, data analysis using appropriate statistical methods as well as interpretation and suitable presentation of the results in reports and publications. These tasks are similarly formulated by the ICH E6 guideline concerning good clinical practice [ 8 ].
Availability of data and materials
Not applicable.
Abbreviations
Canadian Medical Education Directions for Specialists
Case report form
Good Clinical Practice
German Association for Medical Informatics, Biometry and Epidemiology
Grading of Recommendations, Assessment, Development and Evaluation
International Biometric Society
International Council for Harmonisation of Technical Requirements for Pharmaceuticals for Human Use
Oxford Centre for Evidence-Based Medicine
Quality Assessment of Diagnostic Accuracy Studies
Statistical analysis plan
Zapf A, Hübner M, Rauch G, Kieser M. What makes a biostatistician? Stat Med. 2018;38(4):695–701.
Article Google Scholar
Homepage of the International Biometric Society. http://www.biometricsociety.org/about/definition-of-biometrics/ . Accessed 11 Nov 2019.
Homepage of the German Society of Medical Informatics, Biometry and Epidemiology (GMDS), Section Medical Biometry. http://www.gmds.de/fachbereiche/biometrie/index.php . Accessed 11 Nov 2019.
Homepage of the German Society of Medical Informatics, Biometry and Epidemiology (GMDS), Section Medical Informatics. https://gmds.de/aktivitaeten/medizinische-informatik/ . Accessed 11 Nov 2019.
Homepage from the professional group bioinformatics (FaBI). https://www.bioinformatik.de/en/bioinformatics.html . Accessed 11 Nov 2019.
Homepage of the German Society of Medical Informatics, Biometry and Epidemiology (GMDS), Section Epidemiology. https://gmds.de/aktivitaeten/epidemiologie/ . Access 11 Nov 2019.
Lewis JA. Editorial: statistics and statisticians in the regulation of medicines. J R Stat Soc Ser A. 1996;159(3):359–62.
International Conference on Harmonisation of Technical Requirements for Registration of Pharmaceuticals for Human Use (1996). Guideline for good clinical practice E6 (R2). https://www.ema.europa.eu/en/documents/scientific-guideline/ich-e-6-r2-guideline-good-clinical-practice-step-5_en.pdf . Access 11 Nov 2019.
Google Scholar
Mansmann U, Jensen K, Dirschedl P. Good biometrical practice in medical research - guidelines and recommendations. Informatik, Biometrie und Epidemiologie in Medizin und Biologie. 2004;35:63–71.
International Conference on Harmonisation of Technical Requirements for Registration of Pharmaceuticals for Human Use (1998). Statistical principles for clinical trials E9. https://www.ema.europa.eu/en/documents/scientific-guideline/ich-e-9-statistical-principles-clinical-trials-step-5_en.pdf . Accessed 11 Nov 2019.
OCEBM. The Oxford 2011 levels of evidence: Oxford Centre for Evidence-Based Medicine; 2011. http://www.cebm.net/oxford-centre-evidence-based-medicine-levels-evidence-march-2009/ . Accessed 11 Nov 2019
Mulrow CD. Systematic reviews: rationale for systematic reviews. BMJ. 1994;309:597–9.
Article CAS Google Scholar
Gopalakrishnan S, Ganeshkumar P. Systematic reviews and meta-analysis: understanding the best evidence in primary healthcare. J Fam Med Prim Care. 2013;2(1):9–14.
Schünemann H, Brożek J, Guyatt G, Oxman A, editors. GRADE handbook for grading quality of evidence and strength of recommendations. Updated October 2013: The GRADE Working Group; 2013. Available from https://gdt.gradepro.org/app/handbook/handbook.html . Accessed 11 Nov 2019
Whiting PF, Rutjes AWS, Westwood ME, Mallett S, Deeks JJ, Reitsma JB, Leeflang MMG, Sterne JAC, Bossuyt PMM, the QUADAS-2 Group. QUADAS-2: a revised tool for the quality assessment of diagnostic accuracy studies. Ann Intern Med. 2011;155:529–36.
Higgins JPT, Green S, editors. Cochrane handbook for systematic reviews of interventions version 5.1.0 [updated March 2011]: The Cochrane Collaboration; 2011. Available from http://handbook.cochrane.org . Accessed 11 Nov 2019
Snijders AB, Bosker RJ. Multilevel analysis - an introduction to basic and advanced multilevel modeling. London: SAGE Publications; 1999.
Hansen BE, Thorogood J, Hermans J, Ploeg RJ, van Bockel JH, van Houwelingen JC. Multistate modelling of liver transplantation data. Stat Med. 1994;13:2517–29.
European Medicines Agency (2012). Concept paper on extrapolation of efficacy and safety in medicine development - EMA/129698/2012. http://www.ema.europa.eu/docs/en_GB/document_library/Scientific_guideline/2013/04/WC500142358.pdf . Accessed 11 Nov 2019.
Wadsworth I, Hampson LV, Jaki T. Extrapolation of efficacy and other data to support the development of new medicines for children: a systematic review of methods. Stat Meth Med Res. 2018;27(2):398–413.
Simon R. Challenges for biometry in 21st century oncology. In: Matsui S, Crowley J, editors. Frontiers of biostatistical methods and applications in clinical oncology. Singapore: Springer; 2017. Available from https://link.springer.com/chapter/10.1007%2F978-981-10-0126-0_1 . Accessed 25 Nov 2019.
Jaki T, Gordon A, Forster P, Bijnens L, Bornkamp B, Brannath W, Fontana R, Gasparini M, Hampson LV, Jacobs T, Jones B, Paoletti X, Posch M, Titman A, Vonk R, Koenig F. A proposal for a new PhD level curriculum on quantitative methods for drug development. Pharm Stat. 2018;17:593–606.
CAS PubMed PubMed Central Google Scholar
Lewis T. Statisticians in the pharmaceutical industry. In: Stonier PD, editor. Discovering new medicines. Chichester: Wiley; 1994. p. 153–63.
Chuang-Stein C, Bain R, Branson M, Burton C, Hoseyni C, Rockhold FW, Ruberg SJ, Zhang J. Statisticians in the pharmaceutical industry: the 21st century. Stat Biopharm Res. 2010;2(2):145–52.
Royal College of Physicians and Surgeons of Canada. CanMEDS: better standards, better physician, better care. http://www.royalcollege.ca/rcsite/canmeds/canmeds-framework-e . Accessed 11 Nov 2019.
Alarcón-Soto Y, Espasandín-Domínguez J, Guler I, Conde-Amboage M, Gude-Sampedro F, Langohr K, Cadarso-Suárez C, Gómez-Melis G (2019) Data Science in Biomedicine. arXiv:1909.04486v1. Available from https://arxiv.org/abs/1909.04486v1 . Accessed 25 Nov 2019.
Download references
Acknowledgements
There was no funding for this project.
Author information
Authors and affiliations.
Department of Medical Biometry and Epidemiology, University Medical Center Hamburg-Eppendorf, Martinistr. 52, 20246, Hamburg, Germany
Antonia Zapf
Charité - Universitätsmedizin Berlin, corporate member of Freie Universität Berlin, Humboldt-Universität zu Berlin, and Berlin Institute of Health, Institute of Biometry and Clinical Epidemiology, Charitéplatz 1, 10117, Berlin, Germany
Geraldine Rauch
Institute of Medical Biometry and Informatics, Heidelberg University Hospital, Im Neuenheimer Feld 130.3, 69120, Heidelberg, Germany
Meinhard Kieser
You can also search for this author in PubMed Google Scholar
Contributions
AZ drafted the work and all authors substantively revised it. All authors approved the final version and agreed both to be personally accountable for the author’s own contributions and to ensure that questions related to the accuracy or integrity of any part of the work are appropriately investigated, resolved, and the resolution documented in the literature.
Corresponding author
Correspondence to Antonia Zapf .
Ethics declarations
Ethics approval and consent to participate, consent for publication, competing interests.
The authors declare that they have no competing interests.
Additional information
Publisher’s note.
Springer Nature remains neutral with regard to jurisdictional claims in published maps and institutional affiliations.
Rights and permissions
Open Access This article is distributed under the terms of the Creative Commons Attribution 4.0 International License ( http://creativecommons.org/licenses/by/4.0/ ), which permits unrestricted use, distribution, and reproduction in any medium, provided you give appropriate credit to the original author(s) and the source, provide a link to the Creative Commons license, and indicate if changes were made. The Creative Commons Public Domain Dedication waiver ( http://creativecommons.org/publicdomain/zero/1.0/ ) applies to the data made available in this article, unless otherwise stated.
Reprints and permissions
About this article
Cite this article.
Zapf, A., Rauch, G. & Kieser, M. Why do you need a biostatistician?. BMC Med Res Methodol 20 , 23 (2020). https://doi.org/10.1186/s12874-020-0916-4
Download citation
Received : 21 March 2019
Accepted : 28 January 2020
Published : 05 February 2020
DOI : https://doi.org/10.1186/s12874-020-0916-4
Share this article
Anyone you share the following link with will be able to read this content:
Sorry, a shareable link is not currently available for this article.
Provided by the Springer Nature SharedIt content-sharing initiative
- Medical research
- Biostatistician
- Responsibilities
BMC Medical Research Methodology
ISSN: 1471-2288
- General enquiries: [email protected]

An official website of the United States government
The .gov means it's official. Federal government websites often end in .gov or .mil. Before sharing sensitive information, make sure you're on a federal government site.
The site is secure. The https:// ensures that you are connecting to the official website and that any information you provide is encrypted and transmitted securely.
- Publications
- Account settings
- Browse Titles
NCBI Bookshelf. A service of the National Library of Medicine, National Institutes of Health.
StatPearls [Internet]. Treasure Island (FL): StatPearls Publishing; 2024 Jan-.

StatPearls [Internet].
Understanding biostatistics interpretation.
Elizabeth Cash ; Sameh W. Boktor .
Affiliations
Last Update: March 13, 2023 .
- Introduction
A basic understanding of statistical concepts is necessary to effectively evaluate existing literature. Statistical results do not, however, allow one to determine the clinical applicability of published findings. Statistical results can be used to make inferences about the probability of an event among a given population. Careful interpretation by the clinician is required to determine the value of the data as it applies to an individual patient or group of patients. [1]
Good research studies will provide a clear, testable hypothesis, or prediction, about what they expect to find in the relationships being tested. [2] The hypothesis will be grounded in the empirical literature, based on clinical observations or expertise, and should be innovative in its tests of a novel relationship or confirmation of a prior study. There are at minimum two hypotheses in any study: (1) the null hypothesis assumes there is no difference or that there is no effect, and (2) the experimental or alternative hypothesis predicts an event or outcome will occur. Often the null hypothesis is not stated or is assumed. Hypotheses are tested by examining relationships between independent variables, or those thought to have some effect, and dependent variables, or those thought to be moved or affected by the independent variable. These also are called predictor and outcome variables, respectively.
Statistics are used to test a study’s alternative or experimental hypothesis. Statistical models are fitted based on the nature, type, and other characteristics of the dataset. Data typically involves levels of measurement, and these determine the type of statistical models that can be applied to test a hypothesis. [3] Nominal data are those variables containing two or more categories without underlying order or value. Examples of nominal data include indicators of group membership, such as male or female. Ordinal data is nominal data that includes an order or rank but has undefined spacing between groups or levels, such as faculty ranking, or educational level. Interval data is ordinal data with clearly defined spacing between the intervals and no absolute zero points. An example of interval data is the temperature scale, as the magnitude of the difference between intervals is consistent and measurable (one degree). Ratio data are interval data that include an absolute zero such as the amount of student loan debt. Nominal and ordinal data are categorical, where entities are divided into distinct groups, whereas, interval and ratio data are considered continuous such that each observation gets a distinct score. [4]
It is up to the researcher to appropriately apply statistical models when testing hypotheses. Several approaches can be used to analyze the same dataset, and how this is accomplished depends heavily on the nature of the wording in a researcher’s hypothesis. [5] There exist a variety of statistical software packages, some available for free while others charge annual license fees, that can be used to analyze data. Nearly all packages require the user to have a basic understanding of the types of data and appropriate application of statistical models for each type. More sophisticated packages require the user to use the program’s proprietary coding language to perform hypothesis tests. These can require a good amount of time to learn, and errors can easily slip past the untrained eye.
It is strongly recommended that unfamiliar users consult with a statistical analyst when designing and running statistical models. Biostatistician consultations can occur at any time during a study, but earlier consultations are wise to prevent the introduction of accidental bias into study data and to help ensure accuracy and collection methods that will be adequate to allow for tests of hypotheses.
- Issues of Concern
Statistical Significance
If the probability of obtaining a test statistic value by chance (p-value) is less than .05, then the experimental hypothesis is accepted as true. Another way of to think about p-values is the probability that the null hypothesis is true, which for a cutoff of p is less than .05 would mean there is a less than 5% chance that the difference observed is not a true difference. [4] However, when interpreting statistical results, the p-value alone is not enough. [6] Significant does not always equate to important. Very small, potentially unimportant effects can turn out to be statistically significant. [7]
- Clinical Significance
To evaluate the clinical relevance or importance of a significant result, one must be certain to consider the size of the effect. [8] Effect measures are standardized to allow application across different scales of measurement. [9] The following are some of the more common ways effect sizes can be estimated:
- Conducting a review of the literature and examining reported results,
- Conducting pilot studies to get an indication of effects that might be seen in larger studies,
- Making educated guesses based on what is clinically or practically meaningful and informed by experience
- Using conventional recommendations for effect size measures
One common measure of effect is the correlation coefficient, r. In general, small effects, or r=.10, indicate that the effect explains 1% of the total variance. Likewise, r=.30 is considered a medium effect, and r=.50 is considered large, explaining 25% of the variance and holding greater clinical relevance. The square of a correlational r-value indicates the proportion of variance explained by the relationship tested. Similarly, confidence intervals offer a way to determine the clinical strength or magnitude of observed effects. [10] A 95% confidence interval indicates a range of plausible values around another parameter (e.g., mean or odds ratio) where there is a 95% chance that the data within that interval truly captures the value observed in the population being studied. [4] Confidence intervals also provide information about accuracy, as smaller intervals suggest greater precision; whereas, larger intervals may suggest a high level of variability. It has been recommended that, at a minimum, studies should report estimates of effect and confidence intervals to allow for appropriate interpretation of their results. [9]
It is also important to note that although a study may be designed and statistically tested in a way that suggests inference and causation could be concluded (e.g., longitudinal observations of change over time), only studies that employ a randomized and/or controlled design will permit causative declarations to be made from their results. [11]
- Enhancing Healthcare Team Outcomes
Statistical analysis is essential for any clinical research. Of greater importance is to understand the clinical significance of reported results and to determine whether those results can be extrapolated to the general population. Understanding the definitions and methods described above should help in better understanding and usability for medical professionals and students.
- Review Questions
- Access free multiple choice questions on this topic.
- Comment on this article.
Disclosure: Elizabeth Cash declares no relevant financial relationships with ineligible companies.
Disclosure: Sameh Boktor declares no relevant financial relationships with ineligible companies.
This book is distributed under the terms of the Creative Commons Attribution-NonCommercial-NoDerivatives 4.0 International (CC BY-NC-ND 4.0) ( http://creativecommons.org/licenses/by-nc-nd/4.0/ ), which permits others to distribute the work, provided that the article is not altered or used commercially. You are not required to obtain permission to distribute this article, provided that you credit the author and journal.
- Cite this Page Cash E, Boktor SW. Understanding Biostatistics Interpretation. [Updated 2023 Mar 13]. In: StatPearls [Internet]. Treasure Island (FL): StatPearls Publishing; 2024 Jan-.
In this Page
Bulk download.
- Bulk download StatPearls data from FTP
Related information
- PMC PubMed Central citations
- PubMed Links to PubMed
Similar articles in PubMed
- Qualitative Study. [StatPearls. 2024] Qualitative Study. Tenny S, Brannan JM, Brannan GD. StatPearls. 2024 Jan
- Folic acid supplementation and malaria susceptibility and severity among people taking antifolate antimalarial drugs in endemic areas. [Cochrane Database Syst Rev. 2022] Folic acid supplementation and malaria susceptibility and severity among people taking antifolate antimalarial drugs in endemic areas. Crider K, Williams J, Qi YP, Gutman J, Yeung L, Mai C, Finkelstain J, Mehta S, Pons-Duran C, Menéndez C, et al. Cochrane Database Syst Rev. 2022 Feb 1; 2(2022). Epub 2022 Feb 1.
- Prescription of Controlled Substances: Benefits and Risks. [StatPearls. 2024] Prescription of Controlled Substances: Benefits and Risks. Preuss CV, Kalava A, King KC. StatPearls. 2024 Jan
- Review Translational Metabolomics of Head Injury: Exploring Dysfunctional Cerebral Metabolism with Ex Vivo NMR Spectroscopy-Based Metabolite Quantification. [Brain Neurotrauma: Molecular, ...] Review Translational Metabolomics of Head Injury: Exploring Dysfunctional Cerebral Metabolism with Ex Vivo NMR Spectroscopy-Based Metabolite Quantification. Wolahan SM, Hirt D, Glenn TC. Brain Neurotrauma: Molecular, Neuropsychological, and Rehabilitation Aspects. 2015
- Review Refinement of the HCUP Quality Indicators [ 2001] Review Refinement of the HCUP Quality Indicators Davies SM, Geppert J, McClellan M, McDonald KM, Romano PS, Shojania KG. 2001 May
Recent Activity
- Understanding Biostatistics Interpretation - StatPearls Understanding Biostatistics Interpretation - StatPearls
Your browsing activity is empty.
Activity recording is turned off.
Turn recording back on
Connect with NLM
National Library of Medicine 8600 Rockville Pike Bethesda, MD 20894
Web Policies FOIA HHS Vulnerability Disclosure
Help Accessibility Careers
Biostatistics and Research Methodology – B. Pharma 8th Semester Notes Pdf
Biostatistics and research methodology – b. pharma 8th semester notes pdf.

Scope: To understand the applications of Biostatistics and Research Methodology in Pharmacy. This subject deals with descriptive statistics, Graphics, Correlation, Regression, logistic regression Probability theory, Sampling technique, Parametric tests, Non Parametric tests, ANOVA, Introduction to Design of Experiments, Phases of Clinical trials and Observational and Experimental studies, SPSS, R and MINITAB statistical software’s, analyzing the statistical data using Excel.
Objectives: Upon completion of the course the student shall be able to
• Know the operation of M.S. Excel, SPSS, R and MINITAB®, DoE (Design of Experiment)
• Know the various statistical techniques to solve statistical problems
• Appreciate statistical techniques in solving the problems.
Course content:
Biostatistics and Research Methodology Unit-I
Introduction: Statistics, Biostatistics, Frequency distribution
Measures of central tendency: Mean, Median, Mode- Pharmaceutical examples Measures of dispersion: Dispersion, Range, standard deviation, Pharmaceutical problems
Correlation: Definition, Karl Pearson’s coefficient of correlation, Multiple correlation – Pharmaceuticals examples
Biostatistics and Research Methodology Unit-II
Regression: Curve fitting by the method of least squares, fitting the lines y= a + bx and x = a +by, Multiple regression, standard error of regression– Pharmaceutical Examples
Probability: Definition of probability, Binomial distribution, Normal distribution, Poisson’s distribution, properties – problems
Sample, Population, large sample, small sample, Null hypothesis, alternative hypothesis, sampling, essence of sampling, types of sampling, Error-I type, Error-II t ype, Standard error of mean (SEM) – Pharmaceutical examples
Parametric test: t-test (Sample, Pooled or Unpaired and Paired) , ANOVA, (One way and Two way), Least Significance difference
Biostatistics and Research Methodology Unit-III
Non Parametric tests: Wilcoxon Rank Sum Test, Mann-Whitney U test, Kruskal-Wallis test, Friedman Test
Introduction to Research: Need for research, Need for design of Experiments, Experiential Design Technique, plagiarism
Graphs: Histogram, Pie Chart, Cubic Graph, response surface plot, Counter Plot graph Designing the methodology: Sample size determination and Power of a study, Report writing and presentation of data, Protocol, Cohorts studies, Observational studies, Experimental studies, Designing clinical trial, various phases.
Biostatistics and Research Methodology Unit-IV
Blocking and confounding system for Two-level factorials
Regression modeling: Hypothesis testing in Simple and Multiple regressionmodels Introduction to Practical components of Industrial and Clinical Trials Problems: Statistical Analysis Using Excel, SPSS, MINITAB®, DESIGN OF EXPERIMENTS, R – Online Statistical Software’s to Industrial and Clinical trial approach
Biostatistics and Research Methodology Unit-V
Design and Analysis of experiments:
Factorial Design: Definition, 22, 23design. Advantage of factorial design
Response Surface methodology: Central composite design, Historical design, Optimization Techniques
Biostatistics and Research Methodology Recommended Books (Latest edition):
1. Pharmaceutical statistics- Practical and clinical applications, Sanford Bolton, publisher Marcel Dekker Inc. NewYork.
2. Fundamental of Statistics – Himalaya Publishing House- S.C.Guptha
3. Design and Analysis of Experiments –PHI Learning Private Limited, R. Pannerselvam,
4. Design and Analysis of Experiments – Wiley Students Edition, Douglas and C. Montgomery
Notes Coming Soon……………….
Also, Visit:
B. Pharma Notes | B. Pharma Notes | Study material Bachelor of Pharmacy pdf
B. Pharma Handwritten Notes
B. Pharma PDF Books
B. Pharma Lab Manual
D. Pharma Lab Manual
B. Pharma 8th Semester Previous Year Question Paper
D. Pharma Notes
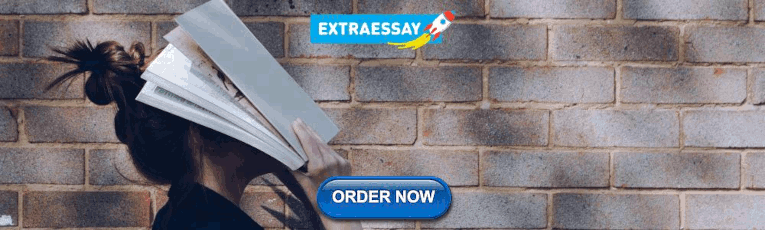
IMAGES
VIDEO
COMMENTS
Introduction. Rigorous scientific review of research protocols is critical to making funding decisions [1, 2], and to the protection of both human and non-human research participants [].Two pillars of ethical clinical and translational research include scientific validity and independent review of the proposed research [].As such, the review process often emphasizes the scientific approach and ...
Members of the Biostatistics, Epidemiology, and Research Design Special Interest Group of the Association for Clinical and Translational Science used a consensus approach to identify the elements of research protocols that a biostatistician should consider in a review, and provide specific guidance on how each element should be reviewed.
1 Core Concepts. By the term "biostatistics," we mean the application of the field of probability and statistics to a wide range of topics that pertain to the biological sciences. We focus our discussion on the practical applications of fundamental biostatistics in the domain of healthcare, including experimental and clinical medicine ...
Biostatistics plays an important role in the design, conduct, and reporting of clinical trials. In recent years, the recognition and appreciation of biostatistics have greatly improved. ... Chapter 4 of this book discusses observational studies and provides more details on the design types used for these studies. A research goal can overlap ...
basic study designs is shown in Fig. 60.1 ("Study Designs"). Descriptive studies describe what is happening on a popu-lation level. These include case reports, case series, qualita-tive studies, and cross-sectional survey studies. Analytic studies examine the effect of interventions/exposures on out-comes.
This is a course on Biostatistics & Research Methodology, taught by Dr. Puspendra. It covers topics related to frequency distributions, measures of central tendency, mean, weighted mean, geometric mean, harmonic mean, median, mode, measures of dispersion, range, standard deviation, correlation analysis, Karl Pearson's correlation coefficient, regression analysis, multiple regression analysis ...
Any research idea starts with identifying a gap in the present knowledge, i.e. framing a research question. This step is followed by proposing a hypothesis, designing a study to test the hypothesis, collecting and presenting research data, analysing the data, and interpreting the results obtained.
Biostatistics is the application of statistical methods in studies in biology, and encompasses the design of experiments, the collection of data from them, and the analysis and interpretation of data.
Biostatistics, the application of statistical methods to biological and medical data, plays a pivotal role in shaping the narrative of clinical research studies. Through meticulous analysis and ...
Research methodology & Biostatistics - Download as a PDF or view online for free. ... Study design in research . ... Inferences 10. Report Writing 11. Dissemination of Report 12/08/2012 Dr. Kusum Gaur 3 4. Process of Concluding 8 ...
Studies of evidence-based practice knowledge and skills rarely report analyses by sex. In 2 studies, investigators found no sex differences in critical appraisal skills among family physicians 23 or in use of online evidence databases among public health practitioners. 24 Six studies assessing the biostatistics and epidemiology knowledge of ...
This slide contains B.Pharm 8th Sem Biostatistics and research methodology, Unit-3. Topic covered: Designing the methodology, Sample size determination and Power of a study, Report writing and presentation of data, Protocol, Cohorts studies, Observational studies, Experimental studies, Designing clinical trial, various phases.
No matter how good a research study, and how meticulously the research study has been conducted, the findings of the research are of little value unless they are effectively documented and communicated to others. TYPES OF RESEARCH REPORT The research report is classified based on 2 things; Nature of research and Target audience.
Background The reporting quality in medical research has recently been critically discussed. While reporting guidelines intend to maximize the value from funded research, and initiatives such as the EQUATOR network have been introduced to advance high quality reporting, the uptake of the guidelines by researchers could be improved. The aim of this study was to assess the contribution of a ...
INTRODUCTION. Biostatistics is a branch of applied statistics and it must be taught with the focus being on its various applications in biomedical research.[] It is an essential tool for medical research, clinical decision making, and health management.[] Statisticians have long expressed concern about the slow uptake of statistical ideas by the medical profession and the frequent misuse of ...
Replication studies reinforce the need for clear written communication in manuscript writing, especially for the statistical methods sections and the results sections. To complete the replication, the students used real data that were collected as part of research studies.
The quality of medical research importantly depends, among other aspects, on a valid statistical planning of the study, analysis of the data, and reporting of the results, which is usually guaranteed by a biostatistician. However, there are several related professions next to the biostatistician, for example epidemiologists, medical informaticians and bioinformaticians. For medical experts, it ...
A basic understanding of statistical concepts is necessary to effectively evaluate existing literature. Statistical results do not, however, allow one to determine the clinical applicability of published findings. Statistical results can be used to make inferences about the probability of an event among a given population. Careful interpretation by the clinician is required to determine the ...
3. Definition • Research is a systematic and scientific method of finding solutions by obtaining various types of data and systematic analysis of the multiple aspects of the issues related. • The techniques or the specific procedure which helps to identify, choose, process, and analyze information about a subject is called Research Methodology.
Scope: To understand the applications of Biostatistics and Research Methodology in Pharmacy. This subject deals with descriptive statistics, Graphics, Correlation, Regression, logistic regression Probability theory, Sampling technique, Parametric tests, Non Parametric tests, ANOVA, Introduction to Design of Experiments, Phases of Clinical trials and Observational and Experimental studies, SPSS ...