Data Security: A Systematic Literature Review and Critical Analysis
Ieee account.
- Change Username/Password
- Update Address
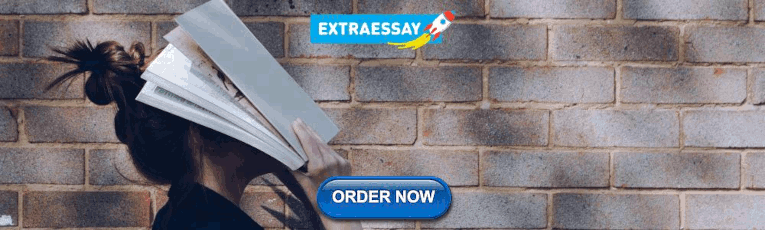
Purchase Details
- Payment Options
- Order History
- View Purchased Documents
Profile Information
- Communications Preferences
- Profession and Education
- Technical Interests
- US & Canada: +1 800 678 4333
- Worldwide: +1 732 981 0060
- Contact & Support
- About IEEE Xplore
- Accessibility
- Terms of Use
- Nondiscrimination Policy
- Privacy & Opting Out of Cookies
A not-for-profit organization, IEEE is the world's largest technical professional organization dedicated to advancing technology for the benefit of humanity. © Copyright 2024 IEEE - All rights reserved. Use of this web site signifies your agreement to the terms and conditions.
- Survey Paper
- Open access
- Published: 01 July 2020
Cybersecurity data science: an overview from machine learning perspective
- Iqbal H. Sarker ORCID: orcid.org/0000-0003-1740-5517 1 , 2 ,
- A. S. M. Kayes 3 ,
- Shahriar Badsha 4 ,
- Hamed Alqahtani 5 ,
- Paul Watters 3 &
- Alex Ng 3
Journal of Big Data volume 7 , Article number: 41 ( 2020 ) Cite this article
145k Accesses
244 Citations
51 Altmetric
Metrics details
In a computing context, cybersecurity is undergoing massive shifts in technology and its operations in recent days, and data science is driving the change. Extracting security incident patterns or insights from cybersecurity data and building corresponding data-driven model , is the key to make a security system automated and intelligent. To understand and analyze the actual phenomena with data, various scientific methods, machine learning techniques, processes, and systems are used, which is commonly known as data science. In this paper, we focus and briefly discuss on cybersecurity data science , where the data is being gathered from relevant cybersecurity sources, and the analytics complement the latest data-driven patterns for providing more effective security solutions. The concept of cybersecurity data science allows making the computing process more actionable and intelligent as compared to traditional ones in the domain of cybersecurity. We then discuss and summarize a number of associated research issues and future directions . Furthermore, we provide a machine learning based multi-layered framework for the purpose of cybersecurity modeling. Overall, our goal is not only to discuss cybersecurity data science and relevant methods but also to focus the applicability towards data-driven intelligent decision making for protecting the systems from cyber-attacks.
Introduction
Due to the increasing dependency on digitalization and Internet-of-Things (IoT) [ 1 ], various security incidents such as unauthorized access [ 2 ], malware attack [ 3 ], zero-day attack [ 4 ], data breach [ 5 ], denial of service (DoS) [ 2 ], social engineering or phishing [ 6 ] etc. have grown at an exponential rate in recent years. For instance, in 2010, there were less than 50 million unique malware executables known to the security community. By 2012, they were double around 100 million, and in 2019, there are more than 900 million malicious executables known to the security community, and this number is likely to grow, according to the statistics of AV-TEST institute in Germany [ 7 ]. Cybercrime and attacks can cause devastating financial losses and affect organizations and individuals as well. It’s estimated that, a data breach costs 8.19 million USD for the United States and 3.9 million USD on an average [ 8 ], and the annual cost to the global economy from cybercrime is 400 billion USD [ 9 ]. According to Juniper Research [ 10 ], the number of records breached each year to nearly triple over the next 5 years. Thus, it’s essential that organizations need to adopt and implement a strong cybersecurity approach to mitigate the loss. According to [ 11 ], the national security of a country depends on the business, government, and individual citizens having access to applications and tools which are highly secure, and the capability on detecting and eliminating such cyber-threats in a timely way. Therefore, to effectively identify various cyber incidents either previously seen or unseen, and intelligently protect the relevant systems from such cyber-attacks, is a key issue to be solved urgently.
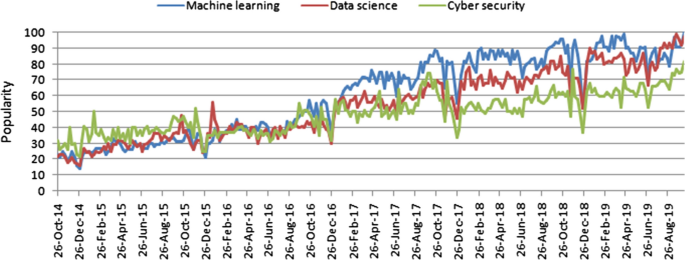
Popularity trends of data science, machine learning and cybersecurity over time, where x-axis represents the timestamp information and y axis represents the corresponding popularity values
Cybersecurity is a set of technologies and processes designed to protect computers, networks, programs and data from attack, damage, or unauthorized access [ 12 ]. In recent days, cybersecurity is undergoing massive shifts in technology and its operations in the context of computing, and data science (DS) is driving the change, where machine learning (ML), a core part of “Artificial Intelligence” (AI) can play a vital role to discover the insights from data. Machine learning can significantly change the cybersecurity landscape and data science is leading a new scientific paradigm [ 13 , 14 ]. The popularity of these related technologies is increasing day-by-day, which is shown in Fig. 1 , based on the data of the last five years collected from Google Trends [ 15 ]. The figure represents timestamp information in terms of a particular date in the x-axis and corresponding popularity in the range of 0 (minimum) to 100 (maximum) in the y-axis. As shown in Fig. 1 , the popularity indication values of these areas are less than 30 in 2014, while they exceed 70 in 2019, i.e., more than double in terms of increased popularity. In this paper, we focus on cybersecurity data science (CDS), which is broadly related to these areas in terms of security data processing techniques and intelligent decision making in real-world applications. Overall, CDS is security data-focused, applies machine learning methods to quantify cyber risks, and ultimately seeks to optimize cybersecurity operations. Thus, the purpose of this paper is for those academia and industry people who want to study and develop a data-driven smart cybersecurity model based on machine learning techniques. Therefore, great emphasis is placed on a thorough description of various types of machine learning methods, and their relations and usage in the context of cybersecurity. This paper does not describe all of the different techniques used in cybersecurity in detail; instead, it gives an overview of cybersecurity data science modeling based on artificial intelligence, particularly from machine learning perspective.
The ultimate goal of cybersecurity data science is data-driven intelligent decision making from security data for smart cybersecurity solutions. CDS represents a partial paradigm shift from traditional well-known security solutions such as firewalls, user authentication and access control, cryptography systems etc. that might not be effective according to today’s need in cyber industry [ 16 , 17 , 18 , 19 ]. The problems are these are typically handled statically by a few experienced security analysts, where data management is done in an ad-hoc manner [ 20 , 21 ]. However, as an increasing number of cybersecurity incidents in different formats mentioned above continuously appear over time, such conventional solutions have encountered limitations in mitigating such cyber risks. As a result, numerous advanced attacks are created and spread very quickly throughout the Internet. Although several researchers use various data analysis and learning techniques to build cybersecurity models that are summarized in “ Machine learning tasks in cybersecurity ” section, a comprehensive security model based on the effective discovery of security insights and latest security patterns could be more useful. To address this issue, we need to develop more flexible and efficient security mechanisms that can respond to threats and to update security policies to mitigate them intelligently in a timely manner. To achieve this goal, it is inherently required to analyze a massive amount of relevant cybersecurity data generated from various sources such as network and system sources, and to discover insights or proper security policies with minimal human intervention in an automated manner.
Analyzing cybersecurity data and building the right tools and processes to successfully protect against cybersecurity incidents goes beyond a simple set of functional requirements and knowledge about risks, threats or vulnerabilities. For effectively extracting the insights or the patterns of security incidents, several machine learning techniques, such as feature engineering, data clustering, classification, and association analysis, or neural network-based deep learning techniques can be used, which are briefly discussed in “ Machine learning tasks in cybersecurity ” section. These learning techniques are capable to find the anomalies or malicious behavior and data-driven patterns of associated security incidents to make an intelligent decision. Thus, based on the concept of data-driven decision making, we aim to focus on cybersecurity data science , where the data is being gathered from relevant cybersecurity sources such as network activity, database activity, application activity, or user activity, and the analytics complement the latest data-driven patterns for providing corresponding security solutions.
The contributions of this paper are summarized as follows.
We first make a brief discussion on the concept of cybersecurity data science and relevant methods to understand its applicability towards data-driven intelligent decision making in the domain of cybersecurity. For this purpose, we also make a review and brief discussion on different machine learning tasks in cybersecurity, and summarize various cybersecurity datasets highlighting their usage in different data-driven cyber applications.
We then discuss and summarize a number of associated research issues and future directions in the area of cybersecurity data science, that could help both the academia and industry people to further research and development in relevant application areas.
Finally, we provide a generic multi-layered framework of the cybersecurity data science model based on machine learning techniques. In this framework, we briefly discuss how the cybersecurity data science model can be used to discover useful insights from security data and making data-driven intelligent decisions to build smart cybersecurity systems.
The remainder of the paper is organized as follows. “ Background ” section summarizes background of our study and gives an overview of the related technologies of cybersecurity data science. “ Cybersecurity data science ” section defines and discusses briefly about cybersecurity data science including various categories of cyber incidents data. In “ Machine learning tasks in cybersecurity ” section, we briefly discuss various categories of machine learning techniques including their relations with cybersecurity tasks and summarize a number of machine learning based cybersecurity models in the field. “ Research issues and future directions ” section briefly discusses and highlights various research issues and future directions in the area of cybersecurity data science. In “ A multi-layered framework for smart cybersecurity services ” section, we suggest a machine learning-based framework to build cybersecurity data science model and discuss various layers with their roles. In “ Discussion ” section, we highlight several key points regarding our studies. Finally, “ Conclusion ” section concludes this paper.
In this section, we give an overview of the related technologies of cybersecurity data science including various types of cybersecurity incidents and defense strategies.
- Cybersecurity
Over the last half-century, the information and communication technology (ICT) industry has evolved greatly, which is ubiquitous and closely integrated with our modern society. Thus, protecting ICT systems and applications from cyber-attacks has been greatly concerned by the security policymakers in recent days [ 22 ]. The act of protecting ICT systems from various cyber-threats or attacks has come to be known as cybersecurity [ 9 ]. Several aspects are associated with cybersecurity: measures to protect information and communication technology; the raw data and information it contains and their processing and transmitting; associated virtual and physical elements of the systems; the degree of protection resulting from the application of those measures; and eventually the associated field of professional endeavor [ 23 ]. Craigen et al. defined “cybersecurity as a set of tools, practices, and guidelines that can be used to protect computer networks, software programs, and data from attack, damage, or unauthorized access” [ 24 ]. According to Aftergood et al. [ 12 ], “cybersecurity is a set of technologies and processes designed to protect computers, networks, programs and data from attacks and unauthorized access, alteration, or destruction”. Overall, cybersecurity concerns with the understanding of diverse cyber-attacks and devising corresponding defense strategies that preserve several properties defined as below [ 25 , 26 ].
Confidentiality is a property used to prevent the access and disclosure of information to unauthorized individuals, entities or systems.
Integrity is a property used to prevent any modification or destruction of information in an unauthorized manner.
Availability is a property used to ensure timely and reliable access of information assets and systems to an authorized entity.
The term cybersecurity applies in a variety of contexts, from business to mobile computing, and can be divided into several common categories. These are - network security that mainly focuses on securing a computer network from cyber attackers or intruders; application security that takes into account keeping the software and the devices free of risks or cyber-threats; information security that mainly considers security and the privacy of relevant data; operational security that includes the processes of handling and protecting data assets. Typical cybersecurity systems are composed of network security systems and computer security systems containing a firewall, antivirus software, or an intrusion detection system [ 27 ].
Cyberattacks and security risks
The risks typically associated with any attack, which considers three security factors, such as threats, i.e., who is attacking, vulnerabilities, i.e., the weaknesses they are attacking, and impacts, i.e., what the attack does [ 9 ]. A security incident is an act that threatens the confidentiality, integrity, or availability of information assets and systems. Several types of cybersecurity incidents that may result in security risks on an organization’s systems and networks or an individual [ 2 ]. These are:
Unauthorized access that describes the act of accessing information to network, systems or data without authorization that results in a violation of a security policy [ 2 ];
Malware known as malicious software, is any program or software that intentionally designed to cause damage to a computer, client, server, or computer network, e.g., botnets. Examples of different types of malware including computer viruses, worms, Trojan horses, adware, ransomware, spyware, malicious bots, etc. [ 3 , 26 ]; Ransom malware, or ransomware , is an emerging form of malware that prevents users from accessing their systems or personal files, or the devices, then demands an anonymous online payment in order to restore access.
Denial-of-Service is an attack meant to shut down a machine or network, making it inaccessible to its intended users by flooding the target with traffic that triggers a crash. The Denial-of-Service (DoS) attack typically uses one computer with an Internet connection, while distributed denial-of-service (DDoS) attack uses multiple computers and Internet connections to flood the targeted resource [ 2 ];
Phishing a type of social engineering , used for a broad range of malicious activities accomplished through human interactions, in which the fraudulent attempt takes part to obtain sensitive information such as banking and credit card details, login credentials, or personally identifiable information by disguising oneself as a trusted individual or entity via an electronic communication such as email, text, or instant message, etc. [ 26 ];
Zero-day attack is considered as the term that is used to describe the threat of an unknown security vulnerability for which either the patch has not been released or the application developers were unaware [ 4 , 28 ].
Beside these attacks mentioned above, privilege escalation [ 29 ], password attack [ 30 ], insider threat [ 31 ], man-in-the-middle [ 32 ], advanced persistent threat [ 33 ], SQL injection attack [ 34 ], cryptojacking attack [ 35 ], web application attack [ 30 ] etc. are well-known as security incidents in the field of cybersecurity. A data breach is another type of security incident, known as a data leak, which is involved in the unauthorized access of data by an individual, application, or service [ 5 ]. Thus, all data breaches are considered as security incidents, however, all the security incidents are not data breaches. Most data breaches occur in the banking industry involving the credit card numbers, personal information, followed by the healthcare sector and the public sector [ 36 ].
Cybersecurity defense strategies
Defense strategies are needed to protect data or information, information systems, and networks from cyber-attacks or intrusions. More granularly, they are responsible for preventing data breaches or security incidents and monitoring and reacting to intrusions, which can be defined as any kind of unauthorized activity that causes damage to an information system [ 37 ]. An intrusion detection system (IDS) is typically represented as “a device or software application that monitors a computer network or systems for malicious activity or policy violations” [ 38 ]. The traditional well-known security solutions such as anti-virus, firewalls, user authentication, access control, data encryption and cryptography systems, however might not be effective according to today’s need in the cyber industry
[ 16 , 17 , 18 , 19 ]. On the other hand, IDS resolves the issues by analyzing security data from several key points in a computer network or system [ 39 , 40 ]. Moreover, intrusion detection systems can be used to detect both internal and external attacks.
Intrusion detection systems are different categories according to the usage scope. For instance, a host-based intrusion detection system (HIDS), and network intrusion detection system (NIDS) are the most common types based on the scope of single computers to large networks. In a HIDS, the system monitors important files on an individual system, while it analyzes and monitors network connections for suspicious traffic in a NIDS. Similarly, based on methodologies, the signature-based IDS, and anomaly-based IDS are the most well-known variants [ 37 ].
Signature-based IDS : A signature can be a predefined string, pattern, or rule that corresponds to a known attack. A particular pattern is identified as the detection of corresponding attacks in a signature-based IDS. An example of a signature can be known patterns or a byte sequence in a network traffic, or sequences used by malware. To detect the attacks, anti-virus software uses such types of sequences or patterns as a signature while performing the matching operation. Signature-based IDS is also known as knowledge-based or misuse detection [ 41 ]. This technique can be efficient to process a high volume of network traffic, however, is strictly limited to the known attacks only. Thus, detecting new attacks or unseen attacks is one of the biggest challenges faced by this signature-based system.
Anomaly-based IDS : The concept of anomaly-based detection overcomes the issues of signature-based IDS discussed above. In an anomaly-based intrusion detection system, the behavior of the network is first examined to find dynamic patterns, to automatically create a data-driven model, to profile the normal behavior, and thus it detects deviations in the case of any anomalies [ 41 ]. Thus, anomaly-based IDS can be treated as a dynamic approach, which follows behavior-oriented detection. The main advantage of anomaly-based IDS is the ability to identify unknown or zero-day attacks [ 42 ]. However, the issue is that the identified anomaly or abnormal behavior is not always an indicator of intrusions. It sometimes may happen because of several factors such as policy changes or offering a new service.
In addition, a hybrid detection approach [ 43 , 44 ] that takes into account both the misuse and anomaly-based techniques discussed above can be used to detect intrusions. In a hybrid system, the misuse detection system is used for detecting known types of intrusions and anomaly detection system is used for novel attacks [ 45 ]. Beside these approaches, stateful protocol analysis can also be used to detect intrusions that identifies deviations of protocol state similarly to the anomaly-based method, however it uses predetermined universal profiles based on accepted definitions of benign activity [ 41 ]. In Table 1 , we have summarized these common approaches highlighting their pros and cons. Once the detecting has been completed, the intrusion prevention system (IPS) that is intended to prevent malicious events, can be used to mitigate the risks in different ways such as manual, providing notification, or automatic process [ 46 ]. Among these approaches, an automatic response system could be more effective as it does not involve a human interface between the detection and response systems.
- Data science
We are living in the age of data, advanced analytics, and data science, which are related to data-driven intelligent decision making. Although, the process of searching patterns or discovering hidden and interesting knowledge from data is known as data mining [ 47 ], in this paper, we use the broader term “data science” rather than data mining. The reason is that, data science, in its most fundamental form, is all about understanding of data. It involves studying, processing, and extracting valuable insights from a set of information. In addition to data mining, data analytics is also related to data science. The development of data mining, knowledge discovery, and machine learning that refers creating algorithms and program which learn on their own, together with the original data analysis and descriptive analytics from the statistical perspective, forms the general concept of “data analytics” [ 47 ]. Nowadays, many researchers use the term “data science” to describe the interdisciplinary field of data collection, preprocessing, inferring, or making decisions by analyzing the data. To understand and analyze the actual phenomena with data, various scientific methods, machine learning techniques, processes, and systems are used, which is commonly known as data science. According to Cao et al. [ 47 ] “data science is a new interdisciplinary field that synthesizes and builds on statistics, informatics, computing, communication, management, and sociology to study data and its environments, to transform data to insights and decisions by following a data-to-knowledge-to-wisdom thinking and methodology”. As a high-level statement in the context of cybersecurity, we can conclude that it is the study of security data to provide data-driven solutions for the given security problems, as known as “the science of cybersecurity data”. Figure 2 shows the typical data-to-insight-to-decision transfer at different periods and general analytic stages in data science, in terms of a variety of analytics goals (G) and approaches (A) to achieve the data-to-decision goal [ 47 ].
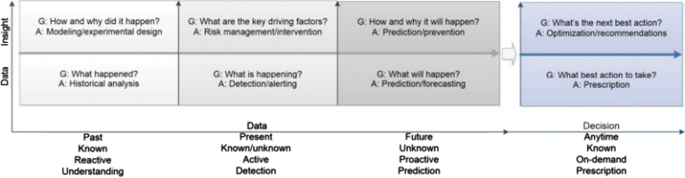
Data-to-insight-to-decision analytic stages in data science [ 47 ]
Based on the analytic power of data science including machine learning techniques, it can be a viable component of security strategies. By using data science techniques, security analysts can manipulate and analyze security data more effectively and efficiently, uncovering valuable insights from data. Thus, data science methodologies including machine learning techniques can be well utilized in the context of cybersecurity, in terms of problem understanding, gathering security data from diverse sources, preparing data to feed into the model, data-driven model building and updating, for providing smart security services, which motivates to define cybersecurity data science and to work in this research area.
Cybersecurity data science
In this section, we briefly discuss cybersecurity data science including various categories of cyber incidents data with the usage in different application areas, and the key terms and areas related to our study.
Understanding cybersecurity data
Data science is largely driven by the availability of data [ 48 ]. Datasets typically represent a collection of information records that consist of several attributes or features and related facts, in which cybersecurity data science is based on. Thus, it’s important to understand the nature of cybersecurity data containing various types of cyberattacks and relevant features. The reason is that raw security data collected from relevant cyber sources can be used to analyze the various patterns of security incidents or malicious behavior, to build a data-driven security model to achieve our goal. Several datasets exist in the area of cybersecurity including intrusion analysis, malware analysis, anomaly, fraud, or spam analysis that are used for various purposes. In Table 2 , we summarize several such datasets including their various features and attacks that are accessible on the Internet, and highlight their usage based on machine learning techniques in different cyber applications. Effectively analyzing and processing of these security features, building target machine learning-based security model according to the requirements, and eventually, data-driven decision making, could play a role to provide intelligent cybersecurity services that are discussed briefly in “ A multi-layered framework for smart cybersecurity services ” section.
Defining cybersecurity data science
Data science is transforming the world’s industries. It is critically important for the future of intelligent cybersecurity systems and services because of “security is all about data”. When we seek to detect cyber threats, we are analyzing the security data in the form of files, logs, network packets, or other relevant sources. Traditionally, security professionals didn’t use data science techniques to make detections based on these data sources. Instead, they used file hashes, custom-written rules like signatures, or manually defined heuristics [ 21 ]. Although these techniques have their own merits in several cases, it needs too much manual work to keep up with the changing cyber threat landscape. On the contrary, data science can make a massive shift in technology and its operations, where machine learning algorithms can be used to learn or extract insight of security incident patterns from the training data for their detection and prevention. For instance, to detect malware or suspicious trends, or to extract policy rules, these techniques can be used.
In recent days, the entire security industry is moving towards data science, because of its capability to transform raw data into decision making. To do this, several data-driven tasks can be associated, such as—(i) data engineering focusing practical applications of data gathering and analysis; (ii) reducing data volume that deals with filtering significant and relevant data to further analysis; (iii) discovery and detection that focuses on extracting insight or incident patterns or knowledge from data; (iv) automated models that focus on building data-driven intelligent security model; (v) targeted security alerts focusing on the generation of remarkable security alerts based on discovered knowledge that minimizes the false alerts, and (vi) resource optimization that deals with the available resources to achieve the target goals in a security system. While making data-driven decisions, behavioral analysis could also play a significant role in the domain of cybersecurity [ 81 ].
Thus, the concept of cybersecurity data science incorporates the methods and techniques of data science and machine learning as well as the behavioral analytics of various security incidents. The combination of these technologies has given birth to the term “cybersecurity data science”, which refers to collect a large amount of security event data from different sources and analyze it using machine learning technologies for detecting security risks or attacks either through the discovery of useful insights or the latest data-driven patterns. It is, however, worth remembering that cybersecurity data science is not just about a collection of machine learning algorithms, rather, a process that can help security professionals or analysts to scale and automate their security activities in a smart way and in a timely manner. Therefore, the formal definition can be as follows: “Cybersecurity data science is a research or working area existing at the intersection of cybersecurity, data science, and machine learning or artificial intelligence, which is mainly security data-focused, applies machine learning methods, attempts to quantify cyber-risks or incidents, and promotes inferential techniques to analyze behavioral patterns in security data. It also focuses on generating security response alerts, and eventually seeks for optimizing cybersecurity solutions, to build automated and intelligent cybersecurity systems.”
Table 3 highlights some key terms associated with cybersecurity data science. Overall, the outputs of cybersecurity data science are typically security data products, which can be a data-driven security model, policy rule discovery, risk or attack prediction, potential security service and recommendation, or the corresponding security system depending on the given security problem in the domain of cybersecurity. In the next section, we briefly discuss various machine learning tasks with examples within the scope of our study.
Machine learning tasks in cybersecurity
Machine learning (ML) is typically considered as a branch of “Artificial Intelligence”, which is closely related to computational statistics, data mining and analytics, data science, particularly focusing on making the computers to learn from data [ 82 , 83 ]. Thus, machine learning models typically comprise of a set of rules, methods, or complex “transfer functions” that can be applied to find interesting data patterns, or to recognize or predict behavior [ 84 ], which could play an important role in the area of cybersecurity. In the following, we discuss different methods that can be used to solve machine learning tasks and how they are related to cybersecurity tasks.
Supervised learning
Supervised learning is performed when specific targets are defined to reach from a certain set of inputs, i.e., task-driven approach. In the area of machine learning, the most popular supervised learning techniques are known as classification and regression methods [ 129 ]. These techniques are popular to classify or predict the future for a particular security problem. For instance, to predict denial-of-service attack (yes, no) or to identify different classes of network attacks such as scanning and spoofing, classification techniques can be used in the cybersecurity domain. ZeroR [ 83 ], OneR [ 130 ], Navies Bayes [ 131 ], Decision Tree [ 132 , 133 ], K-nearest neighbors [ 134 ], support vector machines [ 135 ], adaptive boosting [ 136 ], and logistic regression [ 137 ] are the well-known classification techniques. In addition, recently Sarker et al. have proposed BehavDT [ 133 ], and IntruDtree [ 106 ] classification techniques that are able to effectively build a data-driven predictive model. On the other hand, to predict the continuous or numeric value, e.g., total phishing attacks in a certain period or predicting the network packet parameters, regression techniques are useful. Regression analyses can also be used to detect the root causes of cybercrime and other types of fraud [ 138 ]. Linear regression [ 82 ], support vector regression [ 135 ] are the popular regression techniques. The main difference between classification and regression is that the output variable in the regression is numerical or continuous, while the predicted output for classification is categorical or discrete. Ensemble learning is an extension of supervised learning while mixing different simple models, e.g., Random Forest learning [ 139 ] that generates multiple decision trees to solve a particular security task.
Unsupervised learning
In unsupervised learning problems, the main task is to find patterns, structures, or knowledge in unlabeled data, i.e., data-driven approach [ 140 ]. In the area of cybersecurity, cyber-attacks like malware stays hidden in some ways, include changing their behavior dynamically and autonomously to avoid detection. Clustering techniques, a type of unsupervised learning, can help to uncover the hidden patterns and structures from the datasets, to identify indicators of such sophisticated attacks. Similarly, in identifying anomalies, policy violations, detecting, and eliminating noisy instances in data, clustering techniques can be useful. K-means [ 141 ], K-medoids [ 142 ] are the popular partitioning clustering algorithms, and single linkage [ 143 ] or complete linkage [ 144 ] are the well-known hierarchical clustering algorithms used in various application domains. Moreover, a bottom-up clustering approach proposed by Sarker et al. [ 145 ] can also be used by taking into account the data characteristics.
Besides, feature engineering tasks like optimal feature selection or extraction related to a particular security problem could be useful for further analysis [ 106 ]. Recently, Sarker et al. [ 106 ] have proposed an approach for selecting security features according to their importance score values. Moreover, Principal component analysis, linear discriminant analysis, pearson correlation analysis, or non-negative matrix factorization are the popular dimensionality reduction techniques to solve such issues [ 82 ]. Association rule learning is another example, where machine learning based policy rules can prevent cyber-attacks. In an expert system, the rules are usually manually defined by a knowledge engineer working in collaboration with a domain expert [ 37 , 140 , 146 ]. Association rule learning on the contrary, is the discovery of rules or relationships among a set of available security features or attributes in a given dataset [ 147 ]. To quantify the strength of relationships, correlation analysis can be used [ 138 ]. Many association rule mining algorithms have been proposed in the area of machine learning and data mining literature, such as logic-based [ 148 ], frequent pattern based [ 149 , 150 , 151 ], tree-based [ 152 ], etc. Recently, Sarker et al. [ 153 ] have proposed an association rule learning approach considering non-redundant generation, that can be used to discover a set of useful security policy rules. Moreover, AIS [ 147 ], Apriori [ 149 ], Apriori-TID and Apriori-Hybrid [ 149 ], FP-Tree [ 152 ], and RARM [ 154 ], and Eclat [ 155 ] are the well-known association rule learning algorithms that are capable to solve such problems by generating a set of policy rules in the domain of cybersecurity.
Neural networks and deep learning
Deep learning is a part of machine learning in the area of artificial intelligence, which is a computational model that is inspired by the biological neural networks in the human brain [ 82 ]. Artificial Neural Network (ANN) is frequently used in deep learning and the most popular neural network algorithm is backpropagation [ 82 ]. It performs learning on a multi-layer feed-forward neural network consists of an input layer, one or more hidden layers, and an output layer. The main difference between deep learning and classical machine learning is its performance on the amount of security data increases. Typically deep learning algorithms perform well when the data volumes are large, whereas machine learning algorithms perform comparatively better on small datasets [ 44 ]. In our earlier work, Sarker et al. [ 129 ], we have illustrated the effectiveness of these approaches considering contextual datasets. However, deep learning approaches mimic the human brain mechanism to interpret large amount of data or the complex data such as images, sounds and texts [ 44 , 129 ]. In terms of feature extraction to build models, deep learning reduces the effort of designing a feature extractor for each problem than the classical machine learning techniques. Beside these characteristics, deep learning typically takes a long time to train an algorithm than a machine learning algorithm, however, the test time is exactly the opposite [ 44 ]. Thus, deep learning relies more on high-performance machines with GPUs than classical machine-learning algorithms [ 44 , 156 ]. The most popular deep neural network learning models include multi-layer perceptron (MLP) [ 157 ], convolutional neural network (CNN) [ 158 ], recurrent neural network (RNN) or long-short term memory (LSTM) network [ 121 , 158 ]. In recent days, researchers use these deep learning techniques for different purposes such as detecting network intrusions, malware traffic detection and classification, etc. in the domain of cybersecurity [ 44 , 159 ].
Other learning techniques
Semi-supervised learning can be described as a hybridization of supervised and unsupervised techniques discussed above, as it works on both the labeled and unlabeled data. In the area of cybersecurity, it could be useful, when it requires to label data automatically without human intervention, to improve the performance of cybersecurity models. Reinforcement techniques are another type of machine learning that characterizes an agent by creating its own learning experiences through interacting directly with the environment, i.e., environment-driven approach, where the environment is typically formulated as a Markov decision process and take decision based on a reward function [ 160 ]. Monte Carlo learning, Q-learning, Deep Q Networks, are the most common reinforcement learning algorithms [ 161 ]. For instance, in a recent work [ 126 ], the authors present an approach for detecting botnet traffic or malicious cyber activities using reinforcement learning combining with neural network classifier. In another work [ 128 ], the authors discuss about the application of deep reinforcement learning to intrusion detection for supervised problems, where they received the best results for the Deep Q-Network algorithm. In the context of cybersecurity, genetic algorithms that use fitness, selection, crossover, and mutation for finding optimization, could also be used to solve a similar class of learning problems [ 119 ].
Various types of machine learning techniques discussed above can be useful in the domain of cybersecurity, to build an effective security model. In Table 4 , we have summarized several machine learning techniques that are used to build various types of security models for various purposes. Although these models typically represent a learning-based security model, in this paper, we aim to focus on a comprehensive cybersecurity data science model and relevant issues, in order to build a data-driven intelligent security system. In the next section, we highlight several research issues and potential solutions in the area of cybersecurity data science.
Research issues and future directions
Our study opens several research issues and challenges in the area of cybersecurity data science to extract insight from relevant data towards data-driven intelligent decision making for cybersecurity solutions. In the following, we summarize these challenges ranging from data collection to decision making.
Cybersecurity datasets : Source datasets are the primary component to work in the area of cybersecurity data science. Most of the existing datasets are old and might insufficient in terms of understanding the recent behavioral patterns of various cyber-attacks. Although the data can be transformed into a meaningful understanding level after performing several processing tasks, there is still a lack of understanding of the characteristics of recent attacks and their patterns of happening. Thus, further processing or machine learning algorithms may provide a low accuracy rate for making the target decisions. Therefore, establishing a large number of recent datasets for a particular problem domain like cyber risk prediction or intrusion detection is needed, which could be one of the major challenges in cybersecurity data science.
Handling quality problems in cybersecurity datasets : The cyber datasets might be noisy, incomplete, insignificant, imbalanced, or may contain inconsistency instances related to a particular security incident. Such problems in a data set may affect the quality of the learning process and degrade the performance of the machine learning-based models [ 162 ]. To make a data-driven intelligent decision for cybersecurity solutions, such problems in data is needed to deal effectively before building the cyber models. Therefore, understanding such problems in cyber data and effectively handling such problems using existing algorithms or newly proposed algorithm for a particular problem domain like malware analysis or intrusion detection and prevention is needed, which could be another research issue in cybersecurity data science.
Security policy rule generation : Security policy rules reference security zones and enable a user to allow, restrict, and track traffic on the network based on the corresponding user or user group, and service, or the application. The policy rules including the general and more specific rules are compared against the incoming traffic in sequence during the execution, and the rule that matches the traffic is applied. The policy rules used in most of the cybersecurity systems are static and generated by human expertise or ontology-based [ 163 , 164 ]. Although, association rule learning techniques produce rules from data, however, there is a problem of redundancy generation [ 153 ] that makes the policy rule-set complex. Therefore, understanding such problems in policy rule generation and effectively handling such problems using existing algorithms or newly proposed algorithm for a particular problem domain like access control [ 165 ] is needed, which could be another research issue in cybersecurity data science.
Hybrid learning method : Most commercial products in the cybersecurity domain contain signature-based intrusion detection techniques [ 41 ]. However, missing features or insufficient profiling can cause these techniques to miss unknown attacks. In that case, anomaly-based detection techniques or hybrid technique combining signature-based and anomaly-based can be used to overcome such issues. A hybrid technique combining multiple learning techniques or a combination of deep learning and machine-learning methods can be used to extract the target insight for a particular problem domain like intrusion detection, malware analysis, access control, etc. and make the intelligent decision for corresponding cybersecurity solutions.
Protecting the valuable security information : Another issue of a cyber data attack is the loss of extremely valuable data and information, which could be damaging for an organization. With the use of encryption or highly complex signatures, one can stop others from probing into a dataset. In such cases, cybersecurity data science can be used to build a data-driven impenetrable protocol to protect such security information. To achieve this goal, cyber analysts can develop algorithms by analyzing the history of cyberattacks to detect the most frequently targeted chunks of data. Thus, understanding such data protecting problems and designing corresponding algorithms to effectively handling these problems, could be another research issue in the area of cybersecurity data science.
Context-awareness in cybersecurity : Existing cybersecurity work mainly originates from the relevant cyber data containing several low-level features. When data mining and machine learning techniques are applied to such datasets, a related pattern can be identified that describes it properly. However, a broader contextual information [ 140 , 145 , 166 ] like temporal, spatial, relationship among events or connections, dependency can be used to decide whether there exists a suspicious activity or not. For instance, some approaches may consider individual connections as DoS attacks, while security experts might not treat them as malicious by themselves. Thus, a significant limitation of existing cybersecurity work is the lack of using the contextual information for predicting risks or attacks. Therefore, context-aware adaptive cybersecurity solutions could be another research issue in cybersecurity data science.
Feature engineering in cybersecurity : The efficiency and effectiveness of a machine learning-based security model has always been a major challenge due to the high volume of network data with a large number of traffic features. The large dimensionality of data has been addressed using several techniques such as principal component analysis (PCA) [ 167 ], singular value decomposition (SVD) [ 168 ] etc. In addition to low-level features in the datasets, the contextual relationships between suspicious activities might be relevant. Such contextual data can be stored in an ontology or taxonomy for further processing. Thus how to effectively select the optimal features or extract the significant features considering both the low-level features as well as the contextual features, for effective cybersecurity solutions could be another research issue in cybersecurity data science.
Remarkable security alert generation and prioritizing : In many cases, the cybersecurity system may not be well defined and may cause a substantial number of false alarms that are unexpected in an intelligent system. For instance, an IDS deployed in a real-world network generates around nine million alerts per day [ 169 ]. A network-based intrusion detection system typically looks at the incoming traffic for matching the associated patterns to detect risks, threats or vulnerabilities and generate security alerts. However, to respond to each such alert might not be effective as it consumes relatively huge amounts of time and resources, and consequently may result in a self-inflicted DoS. To overcome this problem, a high-level management is required that correlate the security alerts considering the current context and their logical relationship including their prioritization before reporting them to users, which could be another research issue in cybersecurity data science.
Recency analysis in cybersecurity solutions : Machine learning-based security models typically use a large amount of static data to generate data-driven decisions. Anomaly detection systems rely on constructing such a model considering normal behavior and anomaly, according to their patterns. However, normal behavior in a large and dynamic security system is not well defined and it may change over time, which can be considered as an incremental growing of dataset. The patterns in incremental datasets might be changed in several cases. This often results in a substantial number of false alarms known as false positives. Thus, a recent malicious behavioral pattern is more likely to be interesting and significant than older ones for predicting unknown attacks. Therefore, effectively using the concept of recency analysis [ 170 ] in cybersecurity solutions could be another issue in cybersecurity data science.
The most important work for an intelligent cybersecurity system is to develop an effective framework that supports data-driven decision making. In such a framework, we need to consider advanced data analysis based on machine learning techniques, so that the framework is capable to minimize these issues and to provide automated and intelligent security services. Thus, a well-designed security framework for cybersecurity data and the experimental evaluation is a very important direction and a big challenge as well. In the next section, we suggest and discuss a data-driven cybersecurity framework based on machine learning techniques considering multiple processing layers.
A multi-layered framework for smart cybersecurity services
As discussed earlier, cybersecurity data science is data-focused, applies machine learning methods, attempts to quantify cyber risks, promotes inferential techniques to analyze behavioral patterns, focuses on generating security response alerts, and eventually seeks for optimizing cybersecurity operations. Hence, we briefly discuss a multiple data processing layered framework that potentially can be used to discover security insights from the raw data to build smart cybersecurity systems, e.g., dynamic policy rule-based access control or intrusion detection and prevention system. To make a data-driven intelligent decision in the resultant cybersecurity system, understanding the security problems and the nature of corresponding security data and their vast analysis is needed. For this purpose, our suggested framework not only considers the machine learning techniques to build the security model but also takes into account the incremental learning and dynamism to keep the model up-to-date and corresponding response generation, which could be more effective and intelligent for providing the expected services. Figure 3 shows an overview of the framework, involving several processing layers, from raw security event data to services. In the following, we briefly discuss the working procedure of the framework.
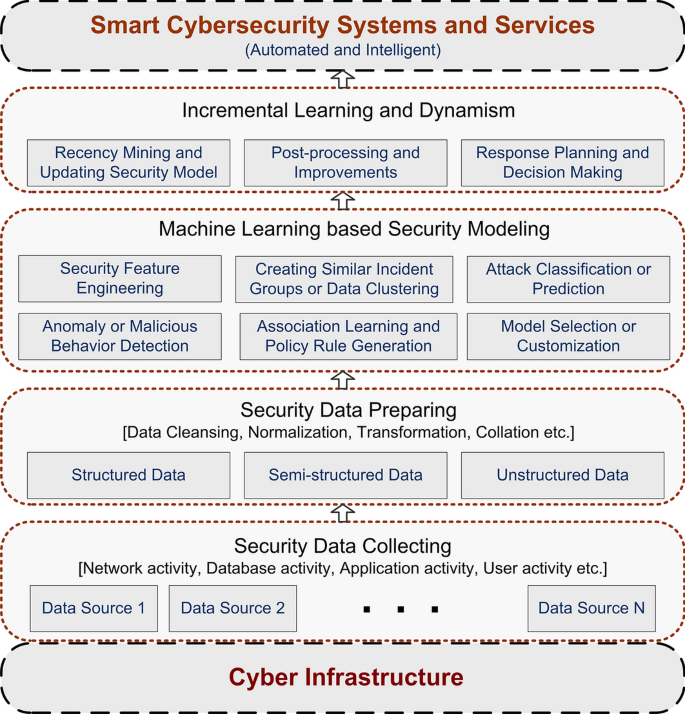
A generic multi-layered framework based on machine learning techniques for smart cybersecurity services
Security data collecting
Collecting valuable cybersecurity data is a crucial step, which forms a connecting link between security problems in cyberinfrastructure and corresponding data-driven solution steps in this framework, shown in Fig. 3 . The reason is that cyber data can serve as the source for setting up ground truth of the security model that affect the model performance. The quality and quantity of cyber data decide the feasibility and effectiveness of solving the security problem according to our goal. Thus, the concern is how to collect valuable and unique needs data for building the data-driven security models.
The general step to collect and manage security data from diverse data sources is based on a particular security problem and project within the enterprise. Data sources can be classified into several broad categories such as network, host, and hybrid [ 171 ]. Within the network infrastructure, the security system can leverage different types of security data such as IDS logs, firewall logs, network traffic data, packet data, and honeypot data, etc. for providing the target security services. For instance, a given IP is considered malicious or not, could be detected by performing data analysis utilizing the data of IP addresses and their cyber activities. In the domain of cybersecurity, the network source mentioned above is considered as the primary security event source to analyze. In the host category, it collects data from an organization’s host machines, where the data sources can be operating system logs, database access logs, web server logs, email logs, application logs, etc. Collecting data from both the network and host machines are considered a hybrid category. Overall, in a data collection layer the network activity, database activity, application activity, and user activity can be the possible security event sources in the context of cybersecurity data science.
Security data preparing
After collecting the raw security data from various sources according to the problem domain discussed above, this layer is responsible to prepare the raw data for building the model by applying various necessary processes. However, not all of the collected data contributes to the model building process in the domain of cybersecurity [ 172 ]. Therefore, the useless data should be removed from the rest of the data captured by the network sniffer. Moreover, data might be noisy, have missing or corrupted values, or have attributes of widely varying types and scales. High quality of data is necessary for achieving higher accuracy in a data-driven model, which is a process of learning a function that maps an input to an output based on example input-output pairs. Thus, it might require a procedure for data cleaning, handling missing or corrupted values. Moreover, security data features or attributes can be in different types, such as continuous, discrete, or symbolic [ 106 ]. Beyond a solid understanding of these types of data and attributes and their permissible operations, its need to preprocess the data and attributes to convert into the target type. Besides, the raw data can be in different types such as structured, semi-structured, or unstructured, etc. Thus, normalization, transformation, or collation can be useful to organize the data in a structured manner. In some cases, natural language processing techniques might be useful depending on data type and characteristics, e.g., textual contents. As both the quality and quantity of data decide the feasibility of solving the security problem, effectively pre-processing and management of data and their representation can play a significant role to build an effective security model for intelligent services.
Machine learning-based security modeling
This is the core step where insights and knowledge are extracted from data through the application of cybersecurity data science. In this section, we particularly focus on machine learning-based modeling as machine learning techniques can significantly change the cybersecurity landscape. The security features or attributes and their patterns in data are of high interest to be discovered and analyzed to extract security insights. To achieve the goal, a deeper understanding of data and machine learning-based analytical models utilizing a large number of cybersecurity data can be effective. Thus, various machine learning tasks can be involved in this model building layer according to the solution perspective. These are - security feature engineering that mainly responsible to transform raw security data into informative features that effectively represent the underlying security problem to the data-driven models. Thus, several data-processing tasks such as feature transformation and normalization, feature selection by taking into account a subset of available security features according to their correlations or importance in modeling, or feature generation and extraction by creating new brand principal components, may be involved in this module according to the security data characteristics. For instance, the chi-squared test, analysis of variance test, correlation coefficient analysis, feature importance, as well as discriminant and principal component analysis, or singular value decomposition, etc. can be used for analyzing the significance of the security features to perform the security feature engineering tasks [ 82 ].
Another significant module is security data clustering that uncovers hidden patterns and structures through huge volumes of security data, to identify where the new threats exist. It typically involves the grouping of security data with similar characteristics, which can be used to solve several cybersecurity problems such as detecting anomalies, policy violations, etc. Malicious behavior or anomaly detection module is typically responsible to identify a deviation to a known behavior, where clustering-based analysis and techniques can also be used to detect malicious behavior or anomaly detection. In the cybersecurity area, attack classification or prediction is treated as one of the most significant modules, which is responsible to build a prediction model to classify attacks or threats and to predict future for a particular security problem. To predict denial-of-service attack or a spam filter separating tasks from other messages, could be the relevant examples. Association learning or policy rule generation module can play a role to build an expert security system that comprises several IF-THEN rules that define attacks. Thus, in a problem of policy rule generation for rule-based access control system, association learning can be used as it discovers the associations or relationships among a set of available security features in a given security dataset. The popular machine learning algorithms in these categories are briefly discussed in “ Machine learning tasks in cybersecurity ” section. The module model selection or customization is responsible to choose whether it uses the existing machine learning model or needed to customize. Analyzing data and building models based on traditional machine learning or deep learning methods, could achieve acceptable results in certain cases in the domain of cybersecurity. However, in terms of effectiveness and efficiency or other performance measurements considering time complexity, generalization capacity, and most importantly the impact of the algorithm on the detection rate of a system, machine learning models are needed to customize for a specific security problem. Moreover, customizing the related techniques and data could improve the performance of the resultant security model and make it better applicable in a cybersecurity domain. The modules discussed above can work separately and combinedly depending on the target security problems.
Incremental learning and dynamism
In our framework, this layer is concerned with finalizing the resultant security model by incorporating additional intelligence according to the needs. This could be possible by further processing in several modules. For instance, the post-processing and improvement module in this layer could play a role to simplify the extracted knowledge according to the particular requirements by incorporating domain-specific knowledge. As the attack classification or prediction models based on machine learning techniques strongly rely on the training data, it can hardly be generalized to other datasets, which could be significant for some applications. To address such kind of limitations, this module is responsible to utilize the domain knowledge in the form of taxonomy or ontology to improve attack correlation in cybersecurity applications.
Another significant module recency mining and updating security model is responsible to keep the security model up-to-date for better performance by extracting the latest data-driven security patterns. The extracted knowledge discussed in the earlier layer is based on a static initial dataset considering the overall patterns in the datasets. However, such knowledge might not be guaranteed higher performance in several cases, because of incremental security data with recent patterns. In many cases, such incremental data may contain different patterns which could conflict with existing knowledge. Thus, the concept of RecencyMiner [ 170 ] on incremental security data and extracting new patterns can be more effective than the existing old patterns. The reason is that recent security patterns and rules are more likely to be significant than older ones for predicting cyber risks or attacks. Rather than processing the whole security data again, recency-based dynamic updating according to the new patterns would be more efficient in terms of processing and outcome. This could make the resultant cybersecurity model intelligent and dynamic. Finally, response planning and decision making module is responsible to make decisions based on the extracted insights and take necessary actions to prevent the system from the cyber-attacks to provide automated and intelligent services. The services might be different depending on particular requirements for a given security problem.
Overall, this framework is a generic description which potentially can be used to discover useful insights from security data, to build smart cybersecurity systems, to address complex security challenges, such as intrusion detection, access control management, detecting anomalies and fraud, or denial of service attacks, etc. in the area of cybersecurity data science.
Although several research efforts have been directed towards cybersecurity solutions, discussed in “ Background ” , “ Cybersecurity data science ”, and “ Machine learning tasks in cybersecurity ” sections in different directions, this paper presents a comprehensive view of cybersecurity data science. For this, we have conducted a literature review to understand cybersecurity data, various defense strategies including intrusion detection techniques, different types of machine learning techniques in cybersecurity tasks. Based on our discussion on existing work, several research issues related to security datasets, data quality problems, policy rule generation, learning methods, data protection, feature engineering, security alert generation, recency analysis etc. are identified that require further research attention in the domain of cybersecurity data science.
The scope of cybersecurity data science is broad. Several data-driven tasks such as intrusion detection and prevention, access control management, security policy generation, anomaly detection, spam filtering, fraud detection and prevention, various types of malware attack detection and defense strategies, etc. can be considered as the scope of cybersecurity data science. Such tasks based categorization could be helpful for security professionals including the researchers and practitioners who are interested in the domain-specific aspects of security systems [ 171 ]. The output of cybersecurity data science can be used in many application areas such as Internet of things (IoT) security [ 173 ], network security [ 174 ], cloud security [ 175 ], mobile and web applications [ 26 ], and other relevant cyber areas. Moreover, intelligent cybersecurity solutions are important for the banking industry, the healthcare sector, or the public sector, where data breaches typically occur [ 36 , 176 ]. Besides, the data-driven security solutions could also be effective in AI-based blockchain technology, where AI works with huge volumes of security event data to extract the useful insights using machine learning techniques, and block-chain as a trusted platform to store such data [ 177 ].
Although in this paper, we discuss cybersecurity data science focusing on examining raw security data to data-driven decision making for intelligent security solutions, it could also be related to big data analytics in terms of data processing and decision making. Big data deals with data sets that are too large or complex having characteristics of high data volume, velocity, and variety. Big data analytics mainly has two parts consisting of data management involving data storage, and analytics [ 178 ]. The analytics typically describe the process of analyzing such datasets to discover patterns, unknown correlations, rules, and other useful insights [ 179 ]. Thus, several advanced data analysis techniques such as AI, data mining, machine learning could play an important role in processing big data by converting big problems to small problems [ 180 ]. To do this, the potential strategies like parallelization, divide-and-conquer, incremental learning, sampling, granular computing, feature or instance selection, can be used to make better decisions, reducing costs, or enabling more efficient processing. In such cases, the concept of cybersecurity data science, particularly machine learning-based modeling could be helpful for process automation and decision making for intelligent security solutions. Moreover, researchers could consider modified algorithms or models for handing big data on parallel computing platforms like Hadoop, Storm, etc. [ 181 ].
Based on the concept of cybersecurity data science discussed in the paper, building a data-driven security model for a particular security problem and relevant empirical evaluation to measure the effectiveness and efficiency of the model, and to asses the usability in the real-world application domain could be a future work.
Motivated by the growing significance of cybersecurity and data science, and machine learning technologies, in this paper, we have discussed how cybersecurity data science applies to data-driven intelligent decision making in smart cybersecurity systems and services. We also have discussed how it can impact security data, both in terms of extracting insight of security incidents and the dataset itself. We aimed to work on cybersecurity data science by discussing the state of the art concerning security incidents data and corresponding security services. We also discussed how machine learning techniques can impact in the domain of cybersecurity, and examine the security challenges that remain. In terms of existing research, much focus has been provided on traditional security solutions, with less available work in machine learning technique based security systems. For each common technique, we have discussed relevant security research. The purpose of this article is to share an overview of the conceptualization, understanding, modeling, and thinking about cybersecurity data science.
We have further identified and discussed various key issues in security analysis to showcase the signpost of future research directions in the domain of cybersecurity data science. Based on the knowledge, we have also provided a generic multi-layered framework of cybersecurity data science model based on machine learning techniques, where the data is being gathered from diverse sources, and the analytics complement the latest data-driven patterns for providing intelligent security services. The framework consists of several main phases - security data collecting, data preparation, machine learning-based security modeling, and incremental learning and dynamism for smart cybersecurity systems and services. We specifically focused on extracting insights from security data, from setting a research design with particular attention to concepts for data-driven intelligent security solutions.
Overall, this paper aimed not only to discuss cybersecurity data science and relevant methods but also to discuss the applicability towards data-driven intelligent decision making in cybersecurity systems and services from machine learning perspectives. Our analysis and discussion can have several implications both for security researchers and practitioners. For researchers, we have highlighted several issues and directions for future research. Other areas for potential research include empirical evaluation of the suggested data-driven model, and comparative analysis with other security systems. For practitioners, the multi-layered machine learning-based model can be used as a reference in designing intelligent cybersecurity systems for organizations. We believe that our study on cybersecurity data science opens a promising path and can be used as a reference guide for both academia and industry for future research and applications in the area of cybersecurity.
Availability of data and materials
Not applicable.
Abbreviations
- Machine learning
Artificial Intelligence
Information and communication technology
Internet of Things
Distributed Denial of Service
Intrusion detection system
Intrusion prevention system
Host-based intrusion detection systems
Network Intrusion Detection Systems
Signature-based intrusion detection system
Anomaly-based intrusion detection system
Li S, Da Xu L, Zhao S. The internet of things: a survey. Inform Syst Front. 2015;17(2):243–59.
Google Scholar
Sun N, Zhang J, Rimba P, Gao S, Zhang LY, Xiang Y. Data-driven cybersecurity incident prediction: a survey. IEEE Commun Surv Tutor. 2018;21(2):1744–72.
McIntosh T, Jang-Jaccard J, Watters P, Susnjak T. The inadequacy of entropy-based ransomware detection. In: International conference on neural information processing. New York: Springer; 2019. p. 181–189
Alazab M, Venkatraman S, Watters P, Alazab M, et al. Zero-day malware detection based on supervised learning algorithms of api call signatures (2010)
Shaw A. Data breach: from notification to prevention using pci dss. Colum Soc Probs. 2009;43:517.
Gupta BB, Tewari A, Jain AK, Agrawal DP. Fighting against phishing attacks: state of the art and future challenges. Neural Comput Appl. 2017;28(12):3629–54.
Av-test institute, germany, https://www.av-test.org/en/statistics/malware/ . Accessed 20 Oct 2019.
Ibm security report, https://www.ibm.com/security/data-breach . Accessed on 20 Oct 2019.
Fischer EA. Cybersecurity issues and challenges: In brief. Congressional Research Service (2014)
Juniper research. https://www.juniperresearch.com/ . Accessed on 20 Oct 2019.
Papastergiou S, Mouratidis H, Kalogeraki E-M. Cyber security incident handling, warning and response system for the european critical information infrastructures (cybersane). In: International Conference on Engineering Applications of Neural Networks, p. 476–487 (2019). New York: Springer
Aftergood S. Cybersecurity: the cold war online. Nature. 2017;547(7661):30.
Hey AJ, Tansley S, Tolle KM, et al. The fourth paradigm: data-intensive scientific discovery. 2009;1:
Cukier K. Data, data everywhere: A special report on managing information, 2010.
Google trends. In: https://trends.google.com/trends/ , 2019.
Anwar S, Mohamad Zain J, Zolkipli MF, Inayat Z, Khan S, Anthony B, Chang V. From intrusion detection to an intrusion response system: fundamentals, requirements, and future directions. Algorithms. 2017;10(2):39.
MATH Google Scholar
Mohammadi S, Mirvaziri H, Ghazizadeh-Ahsaee M, Karimipour H. Cyber intrusion detection by combined feature selection algorithm. J Inform Sec Appl. 2019;44:80–8.
Tapiador JE, Orfila A, Ribagorda A, Ramos B. Key-recovery attacks on kids, a keyed anomaly detection system. IEEE Trans Depend Sec Comput. 2013;12(3):312–25.
Tavallaee M, Stakhanova N, Ghorbani AA. Toward credible evaluation of anomaly-based intrusion-detection methods. IEEE Transactions on Systems, Man, and Cybernetics, Part C (Applications and Reviews) 40(5), 516–524 (2010)
Foroughi F, Luksch P. Data science methodology for cybersecurity projects. arXiv preprint arXiv:1803.04219 , 2018.
Saxe J, Sanders H. Malware data science: Attack detection and attribution, 2018.
Rainie L, Anderson J, Connolly J. Cyber attacks likely to increase. Digital Life in. 2014, vol. 2025.
Fischer EA. Creating a national framework for cybersecurity: an analysis of issues and options. LIBRARY OF CONGRESS WASHINGTON DC CONGRESSIONAL RESEARCH SERVICE, 2005.
Craigen D, Diakun-Thibault N, Purse R. Defining cybersecurity. Technology Innovation. Manag Rev. 2014;4(10):13–21.
Council NR. et al. Toward a safer and more secure cyberspace, 2007.
Jang-Jaccard J, Nepal S. A survey of emerging threats in cybersecurity. J Comput Syst Sci. 2014;80(5):973–93.
MathSciNet MATH Google Scholar
Mukkamala S, Sung A, Abraham A. Cyber security challenges: Designing efficient intrusion detection systems and antivirus tools. Vemuri, V. Rao, Enhancing Computer Security with Smart Technology.(Auerbach, 2006), 125–163, 2005.
Bilge L, Dumitraş T. Before we knew it: an empirical study of zero-day attacks in the real world. In: Proceedings of the 2012 ACM conference on computer and communications security. ACM; 2012. p. 833–44.
Davi L, Dmitrienko A, Sadeghi A-R, Winandy M. Privilege escalation attacks on android. In: International conference on information security. New York: Springer; 2010. p. 346–60.
Jovičić B, Simić D. Common web application attack types and security using asp .net. ComSIS, 2006.
Warkentin M, Willison R. Behavioral and policy issues in information systems security: the insider threat. Eur J Inform Syst. 2009;18(2):101–5.
Kügler D. “man in the middle” attacks on bluetooth. In: International Conference on Financial Cryptography. New York: Springer; 2003, p. 149–61.
Virvilis N, Gritzalis D. The big four-what we did wrong in advanced persistent threat detection. In: 2013 International Conference on Availability, Reliability and Security. IEEE; 2013. p. 248–54.
Boyd SW, Keromytis AD. Sqlrand: Preventing sql injection attacks. In: International conference on applied cryptography and network security. New York: Springer; 2004. p. 292–302.
Sigler K. Crypto-jacking: how cyber-criminals are exploiting the crypto-currency boom. Comput Fraud Sec. 2018;2018(9):12–4.
2019 data breach investigations report, https://enterprise.verizon.com/resources/reports/dbir/ . Accessed 20 Oct 2019.
Khraisat A, Gondal I, Vamplew P, Kamruzzaman J. Survey of intrusion detection systems: techniques, datasets and challenges. Cybersecurity. 2019;2(1):20.
Johnson L. Computer incident response and forensics team management: conducting a successful incident response, 2013.
Brahmi I, Brahmi H, Yahia SB. A multi-agents intrusion detection system using ontology and clustering techniques. In: IFIP international conference on computer science and its applications. New York: Springer; 2015. p. 381–93.
Qu X, Yang L, Guo K, Ma L, Sun M, Ke M, Li M. A survey on the development of self-organizing maps for unsupervised intrusion detection. In: Mobile networks and applications. 2019;1–22.
Liao H-J, Lin C-HR, Lin Y-C, Tung K-Y. Intrusion detection system: a comprehensive review. J Netw Comput Appl. 2013;36(1):16–24.
Alazab A, Hobbs M, Abawajy J, Alazab M. Using feature selection for intrusion detection system. In: 2012 International symposium on communications and information technologies (ISCIT). IEEE; 2012. p. 296–301.
Viegas E, Santin AO, Franca A, Jasinski R, Pedroni VA, Oliveira LS. Towards an energy-efficient anomaly-based intrusion detection engine for embedded systems. IEEE Trans Comput. 2016;66(1):163–77.
Xin Y, Kong L, Liu Z, Chen Y, Li Y, Zhu H, Gao M, Hou H, Wang C. Machine learning and deep learning methods for cybersecurity. IEEE Access. 2018;6:35365–81.
Dutt I, Borah S, Maitra IK, Bhowmik K, Maity A, Das S. Real-time hybrid intrusion detection system using machine learning techniques. 2018, p. 885–94.
Ragsdale DJ, Carver C, Humphries JW, Pooch UW. Adaptation techniques for intrusion detection and intrusion response systems. In: Smc 2000 conference proceedings. 2000 IEEE international conference on systems, man and cybernetics.’cybernetics evolving to systems, humans, organizations, and their complex interactions’(cat. No. 0). IEEE; 2000. vol. 4, p. 2344–2349.
Cao L. Data science: challenges and directions. Commun ACM. 2017;60(8):59–68.
Rizk A, Elragal A. Data science: developing theoretical contributions in information systems via text analytics. J Big Data. 2020;7(1):1–26.
Lippmann RP, Fried DJ, Graf I, Haines JW, Kendall KR, McClung D, Weber D, Webster SE, Wyschogrod D, Cunningham RK, et al. Evaluating intrusion detection systems: The 1998 darpa off-line intrusion detection evaluation. In: Proceedings DARPA information survivability conference and exposition. DISCEX’00. IEEE; 2000. vol. 2, p. 12–26.
Kdd cup 99. http://kdd.ics.uci.edu/databases/kddcup99/kddcup99.html . Accessed 20 Oct 2019.
Tavallaee M, Bagheri E, Lu W, Ghorbani AA. A detailed analysis of the kdd cup 99 data set. In: 2009 IEEE symposium on computational intelligence for security and defense applications. IEEE; 2009. p. 1–6.
Caida ddos attack 2007 dataset. http://www.caida.org/data/ passive/ddos-20070804-dataset.xml/ . Accessed 20 Oct 2019.
Caida anonymized internet traces 2008 dataset. https://www.caida.org/data/passive/passive-2008-dataset . Accessed 20 Oct 2019.
Isot botnet dataset. https://www.uvic.ca/engineering/ece/isot/ datasets/index.php/ . Accessed 20 Oct 2019.
The honeynet project. http://www.honeynet.org/chapters/france/ . Accessed 20 Oct 2019.
Canadian institute of cybersecurity, university of new brunswick, iscx dataset, http://www.unb.ca/cic/datasets/index.html/ . Accessed 20 Oct 2019.
Shiravi A, Shiravi H, Tavallaee M, Ghorbani AA. Toward developing a systematic approach to generate benchmark datasets for intrusion detection. Comput Secur. 2012;31(3):357–74.
The ctu-13 dataset. https://stratosphereips.org/category/datasets-ctu13 . Accessed 20 Oct 2019.
Moustafa N, Slay J. Unsw-nb15: a comprehensive data set for network intrusion detection systems (unsw-nb15 network data set). In: 2015 Military Communications and Information Systems Conference (MilCIS). IEEE; 2015. p. 1–6.
Cse-cic-ids2018 [online]. available: https://www.unb.ca/cic/ datasets/ids-2018.html/ . Accessed 20 Oct 2019.
Cic-ddos2019 [online]. available: https://www.unb.ca/cic/datasets/ddos-2019.html/ . Accessed 28 Mar 2019.
Jing X, Yan Z, Jiang X, Pedrycz W. Network traffic fusion and analysis against ddos flooding attacks with a novel reversible sketch. Inform Fusion. 2019;51:100–13.
Xie M, Hu J, Yu X, Chang E. Evaluating host-based anomaly detection systems: application of the frequency-based algorithms to adfa-ld. In: International conference on network and system security. New York: Springer; 2015. p. 542–49.
Lindauer B, Glasser J, Rosen M, Wallnau KC, ExactData L. Generating test data for insider threat detectors. JoWUA. 2014;5(2):80–94.
Glasser J, Lindauer B. Bridging the gap: A pragmatic approach to generating insider threat data. In: 2013 IEEE Security and Privacy Workshops. IEEE; 2013. p. 98–104.
Enronspam. https://labs-repos.iit.demokritos.gr/skel/i-config/downloads/enron-spam/ . Accessed 20 Oct 2019.
Spamassassin. http://www.spamassassin.org/publiccorpus/ . Accessed 20 Oct 2019.
Lingspam. https://labs-repos.iit.demokritos.gr/skel/i-config/downloads/lingspampublic.tar.gz/ . Accessed 20 Oct 2019.
Alexa top sites. https://aws.amazon.com/alexa-top-sites/ . Accessed 20 Oct 2019.
Bambenek consulting—master feeds. available online: http://osint.bambenekconsulting.com/feeds/ . Accessed 20 Oct 2019.
Dgarchive. https://dgarchive.caad.fkie.fraunhofer.de/site/ . Accessed 20 Oct 2019.
Zago M, Pérez MG, Pérez GM. Umudga: A dataset for profiling algorithmically generated domain names in botnet detection. Data in Brief. 2020;105400.
Zhou Y, Jiang X. Dissecting android malware: characterization and evolution. In: 2012 IEEE Symposium on security and privacy. IEEE; 2012. p. 95–109.
Virusshare. http://virusshare.com/ . Accessed 20 Oct 2019.
Virustotal. https://virustotal.com/ . Accessed 20 Oct 2019.
Comodo. https://www.comodo.com/home/internet-security/updates/vdp/database . Accessed 20 Oct 2019.
Contagio. http://contagiodump.blogspot.com/ . Accessed 20 Oct 2019.
Kumar R, Xiaosong Z, Khan RU, Kumar J, Ahad I. Effective and explainable detection of android malware based on machine learning algorithms. In: Proceedings of the 2018 international conference on computing and artificial intelligence. ACM; 2018. p. 35–40.
Microsoft malware classification (big 2015). arXiv:org/abs/1802.10135/ . Accessed 20 Oct 2019.
Koroniotis N, Moustafa N, Sitnikova E, Turnbull B. Towards the development of realistic botnet dataset in the internet of things for network forensic analytics: bot-iot dataset. Future Gen Comput Syst. 2019;100:779–96.
McIntosh TR, Jang-Jaccard J, Watters PA. Large scale behavioral analysis of ransomware attacks. In: International conference on neural information processing. New York: Springer; 2018. p. 217–29.
Han J, Pei J, Kamber M. Data mining: concepts and techniques, 2011.
Witten IH, Frank E. Data mining: Practical machine learning tools and techniques, 2005.
Dua S, Du X. Data mining and machine learning in cybersecurity, 2016.
Kotpalliwar MV, Wajgi R. Classification of attacks using support vector machine (svm) on kddcup’99 ids database. In: 2015 Fifth international conference on communication systems and network technologies. IEEE; 2015. p. 987–90.
Pervez MS, Farid DM. Feature selection and intrusion classification in nsl-kdd cup 99 dataset employing svms. In: The 8th international conference on software, knowledge, information management and applications (SKIMA 2014). IEEE; 2014. p. 1–6.
Yan M, Liu Z. A new method of transductive svm-based network intrusion detection. In: International conference on computer and computing technologies in agriculture. New York: Springer; 2010. p. 87–95.
Li Y, Xia J, Zhang S, Yan J, Ai X, Dai K. An efficient intrusion detection system based on support vector machines and gradually feature removal method. Expert Syst Appl. 2012;39(1):424–30.
Raman MG, Somu N, Jagarapu S, Manghnani T, Selvam T, Krithivasan K, Sriram VS. An efficient intrusion detection technique based on support vector machine and improved binary gravitational search algorithm. Artificial Intelligence Review. 2019, p. 1–32.
Kokila R, Selvi ST, Govindarajan K. Ddos detection and analysis in sdn-based environment using support vector machine classifier. In: 2014 Sixth international conference on advanced computing (ICoAC). IEEE; 2014. p. 205–10.
Xie M, Hu J, Slay J. Evaluating host-based anomaly detection systems: Application of the one-class svm algorithm to adfa-ld. In: 2014 11th international conference on fuzzy systems and knowledge discovery (FSKD). IEEE; 2014. p. 978–82.
Saxena H, Richariya V. Intrusion detection in kdd99 dataset using svm-pso and feature reduction with information gain. Int J Comput Appl. 2014;98:6.
Chandrasekhar A, Raghuveer K. Confederation of fcm clustering, ann and svm techniques to implement hybrid nids using corrected kdd cup 99 dataset. In: 2014 international conference on communication and signal processing. IEEE; 2014. p. 672–76.
Shapoorifard H, Shamsinejad P. Intrusion detection using a novel hybrid method incorporating an improved knn. Int J Comput Appl. 2017;173(1):5–9.
Vishwakarma S, Sharma V, Tiwari A. An intrusion detection system using knn-aco algorithm. Int J Comput Appl. 2017;171(10):18–23.
Meng W, Li W, Kwok L-F. Design of intelligent knn-based alarm filter using knowledge-based alert verification in intrusion detection. Secur Commun Netw. 2015;8(18):3883–95.
Dada E. A hybridized svm-knn-pdapso approach to intrusion detection system. In: Proc. Fac. Seminar Ser., 2017, p. 14–21.
Sharifi AM, Amirgholipour SK, Pourebrahimi A. Intrusion detection based on joint of k-means and knn. J Converg Inform Technol. 2015;10(5):42.
Lin W-C, Ke S-W, Tsai C-F. Cann: an intrusion detection system based on combining cluster centers and nearest neighbors. Knowl Based Syst. 2015;78:13–21.
Koc L, Mazzuchi TA, Sarkani S. A network intrusion detection system based on a hidden naïve bayes multiclass classifier. Exp Syst Appl. 2012;39(18):13492–500.
Moon D, Im H, Kim I, Park JH. Dtb-ids: an intrusion detection system based on decision tree using behavior analysis for preventing apt attacks. J Supercomput. 2017;73(7):2881–95.
Ingre, B., Yadav, A., Soni, A.K.: Decision tree based intrusion detection system for nsl-kdd dataset. In: International conference on information and communication technology for intelligent systems. New York: Springer; 2017. p. 207–18.
Malik AJ, Khan FA. A hybrid technique using binary particle swarm optimization and decision tree pruning for network intrusion detection. Cluster Comput. 2018;21(1):667–80.
Relan NG, Patil DR. Implementation of network intrusion detection system using variant of decision tree algorithm. In: 2015 international conference on nascent technologies in the engineering field (ICNTE). IEEE; 2015. p. 1–5.
Rai K, Devi MS, Guleria A. Decision tree based algorithm for intrusion detection. Int J Adv Netw Appl. 2016;7(4):2828.
Sarker IH, Abushark YB, Alsolami F, Khan AI. Intrudtree: a machine learning based cyber security intrusion detection model. Symmetry. 2020;12(5):754.
Puthran S, Shah K. Intrusion detection using improved decision tree algorithm with binary and quad split. In: International symposium on security in computing and communication. New York: Springer; 2016. p. 427–438.
Balogun AO, Jimoh RG. Anomaly intrusion detection using an hybrid of decision tree and k-nearest neighbor, 2015.
Azad C, Jha VK. Genetic algorithm to solve the problem of small disjunct in the decision tree based intrusion detection system. Int J Comput Netw Inform Secur. 2015;7(8):56.
Jo S, Sung H, Ahn B. A comparative study on the performance of intrusion detection using decision tree and artificial neural network models. J Korea Soc Dig Indus Inform Manag. 2015;11(4):33–45.
Zhan J, Zulkernine M, Haque A. Random-forests-based network intrusion detection systems. IEEE Trans Syst Man Cybern C. 2008;38(5):649–59.
Tajbakhsh A, Rahmati M, Mirzaei A. Intrusion detection using fuzzy association rules. Appl Soft Comput. 2009;9(2):462–9.
Mitchell R, Chen R. Behavior rule specification-based intrusion detection for safety critical medical cyber physical systems. IEEE Trans Depend Secure Comput. 2014;12(1):16–30.
Alazab M, Venkataraman S, Watters P. Towards understanding malware behaviour by the extraction of api calls. In: 2010 second cybercrime and trustworthy computing Workshop. IEEE; 2010. p. 52–59.
Yuan Y, Kaklamanos G, Hogrefe D. A novel semi-supervised adaboost technique for network anomaly detection. In: Proceedings of the 19th ACM international conference on modeling, analysis and simulation of wireless and mobile systems. ACM; 2016. p. 111–14.
Ariu D, Tronci R, Giacinto G. Hmmpayl: an intrusion detection system based on hidden markov models. Comput Secur. 2011;30(4):221–41.
Årnes A, Valeur F, Vigna G, Kemmerer RA. Using hidden markov models to evaluate the risks of intrusions. In: International workshop on recent advances in intrusion detection. New York: Springer; 2006. p. 145–64.
Hansen JV, Lowry PB, Meservy RD, McDonald DM. Genetic programming for prevention of cyberterrorism through dynamic and evolving intrusion detection. Decis Supp Syst. 2007;43(4):1362–74.
Aslahi-Shahri B, Rahmani R, Chizari M, Maralani A, Eslami M, Golkar MJ, Ebrahimi A. A hybrid method consisting of ga and svm for intrusion detection system. Neural Comput Appl. 2016;27(6):1669–76.
Alrawashdeh K, Purdy C. Toward an online anomaly intrusion detection system based on deep learning. In: 2016 15th IEEE international conference on machine learning and applications (ICMLA). IEEE; 2016. p. 195–200.
Yin C, Zhu Y, Fei J, He X. A deep learning approach for intrusion detection using recurrent neural networks. IEEE Access. 2017;5:21954–61.
Kim J, Kim J, Thu HLT, Kim H. Long short term memory recurrent neural network classifier for intrusion detection. In: 2016 international conference on platform technology and service (PlatCon). IEEE; 2016. p. 1–5.
Almiani M, AbuGhazleh A, Al-Rahayfeh A, Atiewi S, Razaque A. Deep recurrent neural network for iot intrusion detection system. Simulation Modelling Practice and Theory. 2019;102031.
Kolosnjaji B, Zarras A, Webster G, Eckert C. Deep learning for classification of malware system call sequences. In: Australasian joint conference on artificial intelligence. New York: Springer; 2016. p. 137–49.
Wang W, Zhu M, Zeng X, Ye X, Sheng Y. Malware traffic classification using convolutional neural network for representation learning. In: 2017 international conference on information networking (ICOIN). IEEE; 2017. p. 712–17.
Alauthman M, Aslam N, Al-kasassbeh M, Khan S, Al-Qerem A, Choo K-KR. An efficient reinforcement learning-based botnet detection approach. J Netw Comput Appl. 2020;150:102479.
Blanco R, Cilla JJ, Briongos S, Malagón P, Moya JM. Applying cost-sensitive classifiers with reinforcement learning to ids. In: International conference on intelligent data engineering and automated learning. New York: Springer; 2018. p. 531–38.
Lopez-Martin M, Carro B, Sanchez-Esguevillas A. Application of deep reinforcement learning to intrusion detection for supervised problems. Exp Syst Appl. 2020;141:112963.
Sarker IH, Kayes A, Watters P. Effectiveness analysis of machine learning classification models for predicting personalized context-aware smartphone usage. J Big Data. 2019;6(1):1–28.
Holte RC. Very simple classification rules perform well on most commonly used datasets. Mach Learn. 1993;11(1):63–90.
John GH, Langley P. Estimating continuous distributions in bayesian classifiers. In: Proceedings of the eleventh conference on uncertainty in artificial intelligence. Morgan Kaufmann Publishers Inc.; 1995. p. 338–45.
Quinlan JR. C4.5: Programs for machine learning. Machine Learning, 1993.
Sarker IH, Colman A, Han J, Khan AI, Abushark YB, Salah K. Behavdt: a behavioral decision tree learning to build user-centric context-aware predictive model. Mobile Networks and Applications. 2019, p. 1–11.
Aha DW, Kibler D, Albert MK. Instance-based learning algorithms. Mach Learn. 1991;6(1):37–66.
Keerthi SS, Shevade SK, Bhattacharyya C, Murthy KRK. Improvements to platt’s smo algorithm for svm classifier design. Neural Comput. 2001;13(3):637–49.
Freund Y, Schapire RE, et al: Experiments with a new boosting algorithm. In: Icml, vol. 96, p. 148–156 (1996). Citeseer
Le Cessie S, Van Houwelingen JC. Ridge estimators in logistic regression. J Royal Stat Soc C. 1992;41(1):191–201.
Watters PA, McCombie S, Layton R, Pieprzyk J. Characterising and predicting cyber attacks using the cyber attacker model profile (camp). J Money Launder Control. 2012.
Breiman L. Random forests. Mach Learn. 2001;45(1):5–32.
Sarker IH. Context-aware rule learning from smartphone data: survey, challenges and future directions. J Big Data. 2019;6(1):95.
MacQueen J. Some methods for classification and analysis of multivariate observations. In: Fifth Berkeley symposium on mathematical statistics and probability, vol. 1, 1967.
Rokach L. A survey of clustering algorithms. In: Data Mining and Knowledge Discovery Handbook. New York: Springer; 2010. p. 269–98.
Sneath PH. The application of computers to taxonomy. J Gen Microbiol. 1957;17:1.
Sorensen T. method of establishing groups of equal amplitude in plant sociology based on similarity of species. Biol Skr. 1948;5.
Sarker IH, Colman A, Kabir MA, Han J. Individualized time-series segmentation for mining mobile phone user behavior. Comput J. 2018;61(3):349–68.
Kim G, Lee S, Kim S. A novel hybrid intrusion detection method integrating anomaly detection with misuse detection. Exp Syst Appl. 2014;41(4):1690–700.
MathSciNet Google Scholar
Agrawal R, Imieliński T, Swami A. Mining association rules between sets of items in large databases. In: ACM SIGMOD Record. ACM; 1993. vol. 22, p. 207–16.
Flach PA, Lachiche N. Confirmation-guided discovery of first-order rules with tertius. Mach Learn. 2001;42(1–2):61–95.
Agrawal R, Srikant R, et al: Fast algorithms for mining association rules. In: Proc. 20th Int. Conf. Very Large Data Bases, VLDB, 1994, vol. 1215, p. 487–99.
Houtsma M, Swami A. Set-oriented mining for association rules in relational databases. In: Proceedings of the eleventh international conference on data engineering. IEEE; 1995. p. 25–33.
Ma BLWHY. Integrating classification and association rule mining. In: Proceedings of the fourth international conference on knowledge discovery and data mining, 1998.
Han J, Pei J, Yin Y. Mining frequent patterns without candidate generation. In: ACM Sigmod Record. ACM; 2000. vol. 29, p. 1–12.
Sarker IH, Salim FD. Mining user behavioral rules from smartphone data through association analysis. In: Proceedings of the 22nd Pacific-Asia Conference on Knowledge Discovery and Data Mining (PAKDD), Melbourne, Australia. New York: Springer; 2018. p. 450–61.
Das A, Ng W-K, Woon Y-K. Rapid association rule mining. In: Proceedings of the tenth international conference on information and knowledge management. ACM; 2001. p. 474–81.
Zaki MJ. Scalable algorithms for association mining. IEEE Trans Knowl Data Eng. 2000;12(3):372–90.
Coelho IM, Coelho VN, Luz EJS, Ochi LS, Guimarães FG, Rios E. A gpu deep learning metaheuristic based model for time series forecasting. Appl Energy. 2017;201:412–8.
Van Efferen L, Ali-Eldin AM. A multi-layer perceptron approach for flow-based anomaly detection. In: 2017 International symposium on networks, computers and communications (ISNCC). IEEE; 2017. p. 1–6.
Liu H, Lang B, Liu M, Yan H. Cnn and rnn based payload classification methods for attack detection. Knowl Based Syst. 2019;163:332–41.
Berman DS, Buczak AL, Chavis JS, Corbett CL. A survey of deep learning methods for cyber security. Information. 2019;10(4):122.
Bellman R. A markovian decision process. J Math Mech. 1957;1:679–84.
Kaelbling LP, Littman ML, Moore AW. Reinforcement learning: a survey. J Artif Intell Res. 1996;4:237–85.
Sarker IH. A machine learning based robust prediction model for real-life mobile phone data. Internet of Things. 2019;5:180–93.
Kayes ASM, Han J, Colman A. OntCAAC: an ontology-based approach to context-aware access control for software services. Comput J. 2015;58(11):3000–34.
Kayes ASM, Rahayu W, Dillon T. An ontology-based approach to dynamic contextual role for pervasive access control. In: AINA 2018. IEEE Computer Society, 2018.
Colombo P, Ferrari E. Access control technologies for big data management systems: literature review and future trends. Cybersecurity. 2019;2(1):1–13.
Aleroud A, Karabatis G. Contextual information fusion for intrusion detection: a survey and taxonomy. Knowl Inform Syst. 2017;52(3):563–619.
Sarker IH, Abushark YB, Khan AI. Contextpca: Predicting context-aware smartphone apps usage based on machine learning techniques. Symmetry. 2020;12(4):499.
Madsen RE, Hansen LK, Winther O. Singular value decomposition and principal component analysis. Neural Netw. 2004;1:1–5.
Qiao L-B, Zhang B-F, Lai Z-Q, Su J-S. Mining of attack models in ids alerts from network backbone by a two-stage clustering method. In: 2012 IEEE 26th international parallel and distributed processing symposium workshops & Phd Forum. IEEE; 2012. p. 1263–9.
Sarker IH, Colman A, Han J. Recencyminer: mining recency-based personalized behavior from contextual smartphone data. J Big Data. 2019;6(1):49.
Ullah F, Babar MA. Architectural tactics for big data cybersecurity analytics systems: a review. J Syst Softw. 2019;151:81–118.
Zhao S, Leftwich K, Owens M, Magrone F, Schonemann J, Anderson B, Medhi D. I-can-mama: Integrated campus network monitoring and management. In: 2014 IEEE network operations and management symposium (NOMS). IEEE; 2014. p. 1–7.
Abomhara M, et al. Cyber security and the internet of things: vulnerabilities, threats, intruders and attacks. J Cyber Secur Mob. 2015;4(1):65–88.
Helali RGM. Data mining based network intrusion detection system: A survey. In: Novel algorithms and techniques in telecommunications and networking. New York: Springer; 2010. p. 501–505.
Ryoo J, Rizvi S, Aiken W, Kissell J. Cloud security auditing: challenges and emerging approaches. IEEE Secur Priv. 2013;12(6):68–74.
Densham B. Three cyber-security strategies to mitigate the impact of a data breach. Netw Secur. 2015;2015(1):5–8.
Salah K, Rehman MHU, Nizamuddin N, Al-Fuqaha A. Blockchain for ai: review and open research challenges. IEEE Access. 2019;7:10127–49.
Gandomi A, Haider M. Beyond the hype: big data concepts, methods, and analytics. Int J Inform Manag. 2015;35(2):137–44.
Golchha N. Big data-the information revolution. Int J Adv Res. 2015;1(12):791–4.
Hariri RH, Fredericks EM, Bowers KM. Uncertainty in big data analytics: survey, opportunities, and challenges. J Big Data. 2019;6(1):44.
Tsai C-W, Lai C-F, Chao H-C, Vasilakos AV. Big data analytics: a survey. J Big data. 2015;2(1):21.
Download references
Acknowledgements
The authors would like to thank all the reviewers for their rigorous review and comments in several revision rounds. The reviews are detailed and helpful to improve and finalize the manuscript. The authors are highly grateful to them.
Author information
Authors and affiliations.
Swinburne University of Technology, Melbourne, VIC, 3122, Australia
Iqbal H. Sarker
Chittagong University of Engineering and Technology, Chittagong, 4349, Bangladesh
La Trobe University, Melbourne, VIC, 3086, Australia
A. S. M. Kayes, Paul Watters & Alex Ng
University of Nevada, Reno, USA
Shahriar Badsha
Macquarie University, Sydney, NSW, 2109, Australia
Hamed Alqahtani
You can also search for this author in PubMed Google Scholar
Contributions
This article provides not only a discussion on cybersecurity data science and relevant methods but also to discuss the applicability towards data-driven intelligent decision making in cybersecurity systems and services. All authors read and approved the final manuscript.
Corresponding author
Correspondence to Iqbal H. Sarker .
Ethics declarations
Competing interests.
The authors declare that they have no competing interests.
Additional information
Publisher's note.
Springer Nature remains neutral with regard to jurisdictional claims in published maps and institutional affiliations.
Rights and permissions
Open Access This article is licensed under a Creative Commons Attribution 4.0 International License, which permits use, sharing, adaptation, distribution and reproduction in any medium or format, as long as you give appropriate credit to the original author(s) and the source, provide a link to the Creative Commons licence, and indicate if changes were made. The images or other third party material in this article are included in the article's Creative Commons licence, unless indicated otherwise in a credit line to the material. If material is not included in the article's Creative Commons licence and your intended use is not permitted by statutory regulation or exceeds the permitted use, you will need to obtain permission directly from the copyright holder. To view a copy of this licence, visit http://creativecommons.org/licenses/by/4.0/ .
Reprints and permissions
About this article
Cite this article.
Sarker, I.H., Kayes, A.S.M., Badsha, S. et al. Cybersecurity data science: an overview from machine learning perspective. J Big Data 7 , 41 (2020). https://doi.org/10.1186/s40537-020-00318-5
Download citation
Received : 26 October 2019
Accepted : 21 June 2020
Published : 01 July 2020
DOI : https://doi.org/10.1186/s40537-020-00318-5
Share this article
Anyone you share the following link with will be able to read this content:
Sorry, a shareable link is not currently available for this article.
Provided by the Springer Nature SharedIt content-sharing initiative
- Decision making
- Cyber-attack
- Security modeling
- Intrusion detection
- Cyber threat intelligence
The Impact of Artificial Intelligence on Data System Security: A Literature Review
Affiliations.
- 1 ISEC Lisboa, Instituto Superior de Educação e Ciências, 1750-142 Lisbon, Portugal.
- 2 Research Unit on Governance, Competitiveness and Public Policies (GOVCOPP), University of Aveiro, 3810-193 Aveiro, Portugal.
- PMID: 34770336
- PMCID: PMC8586986
- DOI: 10.3390/s21217029
Diverse forms of artificial intelligence (AI) are at the forefront of triggering digital security innovations based on the threats that are arising in this post-COVID world. On the one hand, companies are experiencing difficulty in dealing with security challenges with regard to a variety of issues ranging from system openness, decision making, quality control, and web domain, to mention a few. On the other hand, in the last decade, research has focused on security capabilities based on tools such as platform complacency, intelligent trees, modeling methods, and outage management systems in an effort to understand the interplay between AI and those issues. the dependence on the emergence of AI in running industries and shaping the education, transports, and health sectors is now well known in the literature. AI is increasingly employed in managing data security across economic sectors. Thus, a literature review of AI and system security within the current digital society is opportune. This paper aims at identifying research trends in the field through a systematic bibliometric literature review (LRSB) of research on AI and system security. the review entails 77 articles published in the Scopus ® database, presenting up-to-date knowledge on the topic. the LRSB results were synthesized across current research subthemes. Findings are presented. the originality of the paper relies on its LRSB method, together with an extant review of articles that have not been categorized so far. Implications for future research are suggested.
Keywords: artificial intelligence; security; security of data; security systems.
Publication types
- Artificial Intelligence*
- Computer Security
- Data Systems
data security Recently Published Documents
Total documents.
- Latest Documents
- Most Cited Documents
- Contributed Authors
- Related Sources
- Related Keywords
Big Data Security Management Countermeasures in the Prevention and Control of Computer Network Crime
This paper aims to study the Countermeasures of big data security management in the prevention and control of computer network crime in the absence of relevant legislation and judicial practice. Starting from the concepts and definitions of computer crime and network crime, this paper puts forward the comparison matrix, investigation and statistics method and characteristic measure of computer crime. Through the methods of crime scene investigation, network investigation and network tracking, this paper studies the big data security management countermeasures in the prevention and control of computer network crime from the perspective of criminology. The experimental results show that the phenomenon of low age is serious, and the number of Teenagers Participating in network crime is on the rise. In all kinds of cases, criminals under the age of 35 account for more than 50%.
Fog Computing with IoT Device’s Data Security Management Using Density Control Weighted Election and Extensible Authentication Protocol
Integration of blockchain with connected and autonomous vehicles: vision and challenge.
Connected and Autonomous Vehicles (CAVs) are introduced to improve individuals’ quality of life by offering a wide range of services. They collect a huge amount of data and exchange them with each other and the infrastructure. The collected data usually includes sensitive information about the users and the surrounding environment. Therefore, data security and privacy are among the main challenges in this industry. Blockchain, an emerging distributed ledger, has been considered by the research community as a potential solution for enhancing data security, integrity, and transparency in Intelligent Transportation Systems (ITS). However, despite the emphasis of governments on the transparency of personal data protection practices, CAV stakeholders have not been successful in communicating appropriate information with the end users regarding the procedure of collecting, storing, and processing their personal data, as well as the data ownership. This article provides a vision of the opportunities and challenges of adopting blockchain in ITS from the “data transparency” and “privacy” perspective. The main aim is to answer the following questions: (1) Considering the amount of personal data collected by the CAVs, such as location, how would the integration of blockchain technology affect transparency , fairness , and lawfulness of personal data processing concerning the data subjects (as this is one of the main principles in the existing data protection regulations)? (2) How can the trade-off between transparency and privacy be addressed in blockchain-based ITS use cases?
SecNVM: An Efficient and Write-Friendly Metadata Crash Consistency Scheme for Secure NVM
Data security is an indispensable part of non-volatile memory (NVM) systems. However, implementing data security efficiently on NVM is challenging, since we have to guarantee the consistency of user data and the related security metadata. Existing consistency schemes ignore the recoverability of the SGX style integrity tree (SIT) and the access correlation between metadata blocks, thereby generating unnecessary NVM write traffic. In this article, we propose SecNVM, an efficient and write-friendly metadata crash consistency scheme for secure NVM. SecNVM utilizes the observation that for a lazily updated SIT, the lost tree nodes after a crash can be recovered by the corresponding child nodes in NVM. It reduces the SIT persistency overhead through a restrained write-back metadata cache and exploits the SIT inter-layer dependency for recovery. Next, leveraging the strong access correlation between the counter and DMAC, SecNVM improves the efficiency of security metadata access through a novel collaborative counter-DMAC scheme. In addition, it adopts a lightweight address tracker to reduce the cost of address tracking for fast recovery. Experiments show that compared to the state-of-the-art schemes, SecNVM improves the performance and decreases write traffic a lot, and achieves an acceptable recovery time.
Review on Blockchain Technology
Abstract: Blockchain is a technology that has the potential to cause big changes in our corporate environment and will have a significant influence over the next few decades. It has the potential to alter our perception of business operations and revolutionise our economy. Blockchain is a decentralised and distributed ledger system that, since it cannot be tampered with or faked, attempts to assure transparency, data security, and integrity. Only a few studies have looked at the usage of Blockchain Technology in other contexts or sectors, with the majority of current Blockchain Technology research focusing on its use for cryptocurrencies like Bitcoin. Blockchain technology is more than simply bitcoin; it may be used in government, finance and banking, accounting, and business process managementAs a result, the goal of this study is to examine and investigate the advantages and drawbacks of Blockchain Technology for current and future applications. As a consequence, a large number of published studies were thoroughly assessed and analysed based on their contributions to the Blockchain body of knowledge. Keywords: Blockchain Technology, Bitcoin, Cryptocurrency, Digital currency
China’s Data Security Policies Leading to the Cyber Security Law
A novel framework of an iot-blockchain-based intelligent system.
With the growing need of technology into varied fields, dependency is getting directly proportional to ease of user-friendly smart systems. The advent of artificial intelligence in these smart systems has made our lives easier. Several Internet of Things- (IoT-) based smart refrigerator systems are emerging which support self-monitoring of contents, but the systems lack to achieve the optimized run time and data security. Therefore, in this research, a novel design is implemented with the hardware level of integration of equipment with a more sophisticated software design. It was attempted to design a new smart refrigerator system, which has the capability of automatic self-checking and self-purchasing, by integrating smart mobile device applications and IoT technology with minimal human intervention carried through Blynk application on a mobile phone. The proposed system automatically makes periodic checks and then waits for the owner’s decision to either allow the system to repurchase these products via Ethernet or reject the purchase option. The paper also discussed the machine level integration with artificial intelligence by considering several features and implemented state-of-the-art machine learning classifiers to give automatic decisions. The blockchain technology is cohesively combined to store and propagate data for the sake of data security and privacy concerns. In combination with IoT devices, machine learning, and blockchain technology, the proposed model of the paper can provide a more comprehensive and valuable feedback-driven system. The experiments have been performed and evaluated using several information retrieval metrics using visualization tools. Therefore, our proposed intelligent system will save effort, time, and money which helps us to have an easier, faster, and healthier lifestyle.
BARRIERS TO THE ADOPTION OF NEW SAFETY TECHNOLOGIES IN CONSTRUCTION: A DEVELOPING COUNTRY CONTEXT
The adoption rate of new technologies is still relatively low in the construction industry, particularly for mitigating occupational safety and health (OSH) risks, which is traditionally a largely labor-intensive activity in developing countries, occupying ill-afforded non-productive management resources. However, understanding why this is the case is a relatively unresearched area in developing countries such as Malaysia. In aiming to help redress this situation, this study explored the major barriers involved, firstly by a detailed literature review to identify the main barriers hampering the adoption of new technologies for safety science and management in construction. Then, a questionnaire survey of Malaysian construction practitioners was used to prioritize these barriers. A factor analysis further identified six major dimensions underlying the barriers, relating to the lack of OSH regulations and legislation, technological limitations, lack of genuine organizational commitment, prohibitive costs, poor safety culture within the construction industry, and privacy and data security concerns. Taken together, the findings provide a valuable reference to assist industry practitioners and researchers regarding the critical barriers to the adoption of new technologies for construction safety management in Malaysia and other similar developing countries, and bridge the identified knowledge gap concerning the dimensionality of the barriers.
Design and Development of Maritime Data Security Management Platform
Since the e-Navigation strategy was put forward, various countries and regions in the world have researched e-Navigation test platforms. However, the sources of navigation data are multi-source, and there are still difficulties in the unified acquisition, processing, analysis and application of multi-source data. Users often find it difficult to obtain the required comprehensive navigation information. The purpose of this paper is to use e-Navigation architecture to design and develop maritime data security management platform, strengthen navigation safety guarantee, strengthen Marine environment monitoring, share navigation and safety information, improve the ability of shipping transportation organizations in ports, and protect the marine environment. Therefore, this paper proposes a four-layer system architecture based on Java 2 Platform Enterprise Edition (J2EE) technology, and designs a unified maritime data storage, analysis and management platform, which realizes the intelligent, visualized and modular management of maritime data at shipside and the shore. This platform can provide comprehensive data resource services for ship navigation and support the analysis and mining of maritime big data. This paper expounds on the design, development scheme and demonstration operation scheme of the maritime data security management platform from the system structure and data exchange mode.
Mapping the quantity, quality and structural indicators of Asian (48 countries and 3 territories) research productivity on cloud computing
PurposeThe purpose of this study was to map the quantity (frequency), quality (impact) and structural indicators (correlations) of research produced on cloud computing in 48 countries and 3 territories in the Asia continent.Design/methodology/approachTo achieve the objectives of the study and scientifically map the indicators, data were extracted from the Scopus database. The extracted bibliographic data was first cleaned properly using Endnote and then analyzed using Biblioshiny and VosViewer application software. In the software, calculations include citations count; h, g and m indexes; Bradford's and Lotka's laws; and other scientific mappings.FindingsResults of the study indicate that China remained the most productive, impactful and collaborative country in Asia. All the top 20 impactful authors were also from China. The other most researched areas associated with cloud computing were revealed to be mobile cloud computing and data security in clouds. The most prominent journal currently publishing research studies on cloud computing was “Advances in Intelligent Systems and Computing.”Originality/valueThe study is the first of its kind which identified the quantity (frequencies), quality (impact) and structural indicators (correlations) of Asian (48 countries and 3 territories) research productivity on cloud computing. The results are of great importance for researchers and countries interested in further exploring, publishing and increasing cross country collaborations related to the phenomenon of cloud computing.
Export Citation Format
Share document.

An official website of the United States government
The .gov means it’s official. Federal government websites often end in .gov or .mil. Before sharing sensitive information, make sure you’re on a federal government site.
The site is secure. The https:// ensures that you are connecting to the official website and that any information you provide is encrypted and transmitted securely.
- Publications
- Account settings
Preview improvements coming to the PMC website in October 2024. Learn More or Try it out now .
- Advanced Search
- Journal List
- Int J Environ Res Public Health

A Comprehensive Survey on Security and Privacy for Electronic Health Data
1 Miro Corporation, Incheon 21988, Korea; moc.orimog@hors
Young-Duk Seo
2 Department of Computer Engineering, Inha University, Incheon 22212, Korea; rk.ca.ahni@88disym
Euijong Lee
3 Department of Computer Science, Chungbuk National University, Cheongju 28644, Korea; rk.ca.unbc@eagajjgnok
Young-Gab Kim
4 Department of Computer and Information Security, and Convergence Engineering for Intelligent Drone, Sejong University, Seoul 05006, Korea
Recently, the integration of state-of-the-art technologies, such as modern sensors, networks, and cloud computing, has revolutionized the conventional healthcare system. However, security concerns have increasingly been emerging due to the integration of technologies. Therefore, the security and privacy issues associated with e-health data must be properly explored. In this paper, to investigate the security and privacy of e-health systems, we identified major components of the modern e-health systems (i.e., e-health data, medical devices, medical networks and edge/fog/cloud). Then, we reviewed recent security and privacy studies that focus on each component of the e-health systems. Based on the review, we obtained research taxonomy, security concerns, requirements, solutions, research trends, and open challenges for the components with strengths and weaknesses of the analyzed studies. In particular, edge and fog computing studies for e-health security and privacy were reviewed since the studies had mostly not been analyzed in other survey papers.
1. Introduction
The advancement of modern technologies, such as sensors and cloud computing, has completely changed conventional healthcare systems. Such systems can demonstrate the strong potential of next-generation healthcare services after digitizing paper-based medical records. Individuals’ health conditions can be remotely sensed by medical devices, transmitted by medical networks, and processed by the edge, fog, and cloud computing. Innovative healthcare systems that can improve quality of life will become more essential for various smart healthcare services such as remote monitoring, diagnosis, treatment, and prescription based on personal electronic health (e-health) data. However, the modern e-healthcare system is a double-edged sword. While it gives us advanced healthcare services, security concerns have increasingly emerged.
E-health data are some of the most private information for individuals. Regulations for privacy protection such as the Health Insurance Portability and Accountability Act (HIPPA) [ 1 ] and General Data Protection Regulation (GDPR) [ 2 ] have been established to enhance the governance of healthcare data; however, e-health data has been frequently breached. In addition, as the accessibility and usability of e-health data increase, its security attack vectors have also been widening. Over the last decade, 1.5 million medical devices have been compromised due to software vulnerabilities and their wireless connection [ 3 ], and cloud computing services that store and process e-health data have become a target for big e-health data. According to the Protenus Breach Barometer, 41.4 million patients’ records were breached in 2019 [ 4 ].
Therefore, security and privacy issues must be explored to prevent breaches of e-health data. In particular, security concerns, requirements, and solutions must be identified to properly study how to secure e-healthcare systems. Consequently, the primary goal of this paper is to survey security and privacy studies to identify security concerns, requirements, and solutions. Specifically, because modern e-healthcare systems generally consist of several components (i.e., e-health data, medical devices, medical networks, and edge, fog, and cloud computing) that have their own characteristics, security concerns, requirements, and solutions are surveyed by component. In addition, this paper presents recent research trends and open challenges for each component.
During the last five years, many survey papers focusing on the security and privacy of e-health data have been published; however, there has been no comprehensive survey of an overall e-healthcare system, such as e-health data, medical devices, medical networks, and edge/fog/cloud computing that senses, transmits, stores, and processes e-health data. There have been some surveys focusing on specific components of e-healthcare systems, that is, e-health data security [ 5 , 6 , 7 , 8 ], medical device security [ 3 , 9 , 10 , 11 , 12 ], and medical network security [ 13 , 14 ]. Other studies [ 15 , 16 , 17 , 18 ] have aimed at more than one component of the e-healthcare system. However, the security and privacy issues for all components have not yet been surveyed. To the best of our knowledge, this is the first comprehensive survey paper to identify security concerns, requirements, solutions, research trends, and open challenges for each component of the e-health system consisting of e-health data, medical devices, medical networks, and edge, fog, and cloud computing. The main contributions of this paper are as follows:
- A comprehensive survey on the security and privacy issues for e-health data, medical devices, medical networks, edge, fog, and cloud computing;
- Identification and taxonomies of the security concerns, security requirements, and security solutions for e-health data, medical devices, medical networks, and edge/fog/cloud computing;
- Analysis and identification of the strengths and weaknesses of the surveyed studies;
- Identification of the research trends and open challenges for each component (i.e., e-health data, medical devices, medical networks, edge, fog, and cloud computing) of e-health systems.
In Section 2 , the background of this paper is described in terms of research questions, search strategy, target domains, and related works. Section 3 then provides security concerns, requirements, and solutions by reviewing recent security and privacy studies for e-health data. Similarly, for the medical device, medical network, and edge/fog/cloud computing, Section 4 , Section 5 and Section 6 , respectively, discuss security concerns, requirements, and solutions. Then, Section 7 discusses research trends and open challenges for the components of modern e-health systems. Finally, Section 8 concludes this survey.
2. Background
This section presents a method of searching and selecting security and privacy studies related to e-health data. Then, four main components (i.e., e-health data, medical devices, medical networks, and edge/fog/cloud) of modern e-health systems are identified as the target domains of this survey. Finally, related works, which are existing security and privacy surveys in the medical domains, are also analyzed.
2.1. Method
In this paper, we created and followed a method based on the systematic literature review (SLR) approach [ 19 ] to search and select studies that focus on security and privacy issues related to e-health data. Figure 1 denotes the literature review procedure.

Overview of the literature review procedure.
The primary goal of this survey is to highlight the security concerns, requirements, and solutions, research trends, and open challenges for e-health data. For a consistent and meaningful survey, we carefully formed the following key research questions (RQs). Note that the target domains of this paper are described in Section 2.2 based on the analysis of the selected studies.
- RQ 1: What are the representative security concerns, requirements, and solutions to protect e-health data for each target domain?
- RQ 2: What are the strengths and weaknesses of the surveyed studies for each target domain?
- RQ 3: What are the research trends and open challenges for each target domain?
To answer the questions, we selected general search keywords such as “security”, “privacy”, and “healthcare” as described in Figure 1 for a comprehensive survey. We compiled 831 studies from the international literature databases (i.e., IEEE Xplore, ACM Digital Library, ScienceDirect, SpringerLink, and PubMed). Then, following selection criteria were considered to select key studies for solving our questions.
- SC 1: Studies must have been published within five years;
- SC 2: Studies must use English;
- SC 3: Studies must focus on medical or healthcare domains. There were various security and privacy studies in diverse environments such as Internet of Things (IoT), edge, fog, and cloud; however, we excluded studies that did not focus on the medical or healthcare domains;
- SC 4: Studies must focus on technical research. we excluded some studies regarding medical policies, social sciences, etc.;
- SC 5: Journals should be ranked top 15% in Journal Citation Reports (JCR). If journals were not ranked in the JCR, it should have around 0.8 or higher SCImago Journal Rank (SJR). However, medical journals were selected even if they were ranked around the top 50% in JCR or had 0.4 or higher SJR because of their expertise.
In case of similar works, we compared their published date, originality, and overall quality. After selection, we finally obtained 96 studies that focus on security and privacy for e-health domains. Table 1 shows which journals published the surveyed studies; the impact factors (IFs) and SJRs in Table 1 correspond to 2020.
Journal sources.
2.2. Target Domain
Driven by diverse technical advancements, studies on the security and privacy of e-health data have been conducted with different target domains such as medical devices and networks. Therefore, to comprehensively survey the security and privacy issues of protecting data with the consideration of overall domains, we analyzed existing studies to identify the common components of modern e-health systems as the target domains of this survey. Then, we surveyed the studies according to the domains to identify security concerns, requirements, solutions, research trends, and open challenges for each domain. Figure 2 shows an overview of the e-health system and the target domains of this survey.

Overview of e-health system and the target domains.
In a modern e-health system, patients’ e-health data can be generated by medical devices, transmitted via medical networks, stored, and processed in edge/fog/cloud. Therefore, to comprehensively cover the security and privacy of e-health data, e-health data and the surrounding environments (i.e., medical devices, medical networks, and edge/fog/cloud computing) are the main target domains of this survey.
2.3. Related Work
Based on the searching and selection method described in Section 2.1 , we found 15 security and privacy survey papers in medical/healthcare domains. Table 2 shows the papers and their target domains.
Comparison of the survey papers in terms of the target domains.
Most surveys focused on one or two specific domains. Some of the surveys [ 5 , 6 , 7 , 8 ] studied the security and privacy of e-health data such as electronic health record (EHR) and genomic data, and some surveys [ 3 , 9 , 10 , 11 , 12 ] focused on the security and privacy of medical devices. In addition, two surveys [ 13 , 14 ] focused on the security and privacy of medical networks such as the Internet of Medical Things (IoMT) and Body Area Network (BAN), and there were security and privacy surveys on e-health challenges in the cloud, mobile healthcare (mHealth) systems, electronic health services, and the medical domain [ 15 , 16 , 17 , 18 ]. Here follows a brief summary of each survey.
Several studies investigated security and privacy factors for e-health data. Kruse et al. [ 5 ] collected 25 journals from PubMed, CINAHL, and ProQuest Nursing and Allied Health Source, and analyzed the journals to investigate security techniques for EHRs. The security techniques were analyzed and categorized into three themes: administrative safeguard (e.g., risk management and system security evaluation), physical safeguard (e.g., physical access control and workstation security), and technical safeguard (e.g., authentication, access control, audit, data encryption, and firewall). Abouelmehdi et al. [ 6 ] surveyed security and privacy challenges for big healthcare data. To accomplish the survey, several studies including security factors (i.e., authentication, data encryption, data masking, access control, de-identification, and identity-based anonymization) were analyzed. In addition, Mohammed et al. [ 7 ] and Aziz et al. [ 8 ] surveyed security and privacy for genomic data. Mohammed et al. identified three types of attacks (i.e., identity tracing, attribute disclosure, and completion attacks) to genome privacy. They also classified genome privacy-preserving solutions (e.g., differential privacy and homomorphic encryption) that is related to the attacks. Aziz et al. [ 8 ] discussed privacy problems on genome data and reviewed privacy-preserving solutions regarding homomorphic encryption, Garbled circuit, secure hardware, and differential privacy.
There are survey papers related to medical devices. Zheng et al. [ 9 ] surveyed challenges for securing wireless implantable medical devices (IMDs). In the paper, they discussed security requirements, security solutions supporting emergency access, and lightweight security schemes for access control. Wu et al. [ 10 ] specifically surveyed access control schemes for IMDs. They reviewed the existing studies for IMD access control and classified the IMD access control schemes into four groups (i.e., direct access control with preloaded keys, direct access control with temporary keys, indirect access control via a proxy, and anomaly detection-based schemes). Yaqoob et al. [ 3 ] surveyed studies for medical devices, but they focused on security vulnerabilities, attacks, and countermeasures of the networked medical devices. In the study, a network model and attack vector are described, then security vulnerabilities, attacks, and countermeasures were analyzed for the medical device products. In addition, Kintzlinger et al. [ 11 ] analyzed the security of personal medical devices (PMDs) and their ecosystems. They provided a specific attack flows in the PMDs and its ecosystem. They also surveyed possible attacks and mechanisms to protect the attacks. AlTawy et al. [ 12 ] also surveyed security attacks and threats of medical devices, but they focused on various types of security tradeoffs between security, safety, and availability.
Yaacoub et al. [ 13 ] and Sun et al. [ 14 ] surveyed security and privacy for IoMT. Yaacoub et al. [ 13 ] presented the components of IoMT (e.g., the types of IoMT, devices, and protocols), and analyzed the security issues, concerns, challenges, attacks, and countermeasures in the IoMT. Sun et al. [ 14 ] also surveyed security and privacy-related studies for IoMT. They identified 14 security and privacy requirements for the IoMT on several levels: data level, sensor level, personal server level, and medical server level.
Moreover, several studies were investigated the security and privacy of e-health challenges in the cloud environments, mobile healthcare system, electronic health services, and medical domain [ 15 , 16 , 17 , 18 ]. Chenthara et al. [ 15 ] reviewed security and privacy challenges and approaches of e-health solutions for electronic health records (EHR) in the cloud environment. In particular, they identified security and privacy requirements for e-health data and analyzed various studies focused on privacy-preserving approaches using cryptographic techniques (i.e., symmetric key encryption, public key encryption, and a few alternative cryptographic primitives) and non-cryptographic techniques (i.e., access control). In addition, Yüksel et al. [ 16 ] conducted a survey on the security and privacy for electronic health services (EHSs). They particularly categorized recent studies into six groups (i.e., architecture, access control, emergency, sharing, search, and anonymity), and presented analyzed results and open challenges based on the research groups. Wazid et al. [ 17 ] surveyed security protocols for mHealth. They discussed security requirements, issues, and threats for mHealth systems, and presented a taxonomy of security protocols for mHealth. They also performed a comparison of the protocols in terms of computation cost and communication cost. Razaque et al. [ 18 ] introduced a survey on security vulnerabilities and attacks for the medical domain. The security vulnerabilities and attacks were analyzed according to the dataflow (i.e., patient registration, data collection, storing and utilizing the data) in the medical domain.
We found more than 40 survey papers. However, only 15 surveys are analyzed in this paper because the others are not related to medical domains or not focused on security and privacy issues. Moreover, according to Table 2 , no surveys considered the overall components of modern e-health systems (i.e., e-health data, medical device, medical network, and edge/fog/cloud computing). Therefore, this survey focuses on the four major components of e-health systems to comprehensively identify the security concerns, requirements, solutions, research trends, and open challenges for each.
3. E-Health Data
This section presents security concerns, requirements, solutions, research trends, and open challenges for the security and privacy of e-health data.
3.1. Overview
The initial goal of this section was to explore various security and privacy studies on e-health data; however, there were no sufficient security or privacy studies focusing on e-health data itself. Most studies focused on proposing new security solutions such as cryptography and authentication required to protect the e-health data. Therefore, the contents of this section were collected and analyzed based on a few studies for e-health data and the various studies in diverse medical/healthcare domains such as medical devices and networks that mention e-health data security and privacy. Figure 3 shows a taxonomy for security concerns, requirements, and solutions for e-health data.

A taxonomy on the security and privacy for e-health data.
3.2. Security Concern, Requirement, and Solution
According to our survey, most studies focused on other target domains such as medical network and cloud computing rather than e-health data itself. Therefore, we collected and analyzed the security concerns, requirements, and solutions for e-health data from diverse studies in different domains that partially mentioned the security and privacy issues of e-health data. A few dedicated security and privacy studies on e-health data are also analyzed in this section.
3.2.1. Security Concern
E-health data are some of the most critical and private information in modern society. However, security concerns for the data have emerged because of insufficient security. For example, attackers can exploit some security vulnerabilities of e-health systems to breach the data and forge their identities to deceive the systems. Tampering e-health data becomes a critical issue since it can pose medical accidents. Four security concerns on e-health data, that were commonly mentioned in the surveyed studies are as follows.
Unauthorized access. Various security vulnerabilities in medical devices, networks, and platforms, such as edge, fog and cloud, are at risk of unauthorized access. By using their vulnerabilities, an attacker can access the system to capture sensitive e-health data.
Data disclosure. Data disclosure can occur throughout the e-healthcare system such as medical devices, networks, and edge/fog/cloud platforms because of their security vulnerabilities or an administrative mistake. E-health data are an attractive target for attackers since it is very valuable. According to AlTawy et al. [ 12 ], a personal health record (PHR) on the black market was priced at around $50 USD, while a social security number was priced at around $3 USD.
Data tampering. Data tampering denotes the modification of data without appropriate authentication and authorization. This attack, which is also known as data modification, could be a critical security concern because tampered e-health data may have strong implications for patients.
Data forgery. E-health data or user identities can be forged to deceive legitimate service providers or impersonate others. By forging data, an attacker can compromise e-health systems, or a user with a malicious purpose can take inappropriate profits.
3.2.2. Security Requirement
This section presents the six representative security requirements for e-health data. To securely protect e-health data with privacy preservation, security solutions for the data should consider proper security requirements. Data confidentiality, integrity, and availability are basic security requirements, and data anonymity is required for the patient’s privacy where the data are shared with someone who is not the data owner. Detailed descriptions of the security requirements are as follows.
Access restriction. Access restriction denotes the limitation of unauthorized access to assets such as e-health data, medical devices, and e-healthcare systems. This attack can be posed across entire medical domains; therefore, proper authentication and access control for each domain must be provided to prevent e-health data leaking.
Data confidentiality. In medical domains, the data confidentiality of e-health data is the most critical security requirement. An attacker can infringe data confidentiality by gathering data from various sources such as databases and networks. In particular, data can easily be captured from wireless medical networks such as IoMTs and wireless body area networks (WBANs). Data confidentiality is important; however, it can be breached when special cases happen in relation to critical patients.
Data integrity. Data integrity ensures that transmitted data are untampered with. This requirement is vital because doctors treat patients and prescribe medicine using the received data. The data integrity violation can directly influence patients’ health conditions. Therefore, receivers must verify whether transmitted data are untampered. Recently, Amato et al. [ 20 ] proposed a methodology for the validation of security and privacy policies in e-health systems.
Data availability. Databases and medical devices that store e-health data must be able to provide data regardless of time or location. Based on data availability, patients should be able to check their e-health data and medical staff should be able to use this data to treat their patients.
Data anonymity. Data anonymity should be provided by anonymizing e-health data to provide patient privacy when it has to be shared. In particular, there is a need to anonymize e-health data that is unrelated to a specific purpose and identity information about patients and medical staff that can be used to link anonymized data to identities.
Auditability and accountability. All information regarding e-health data such as generation time, owner, access records, and usage history must be recorded. By using recorded data (i.e., an audit trail), accountability can be satisfied to identify the person in charge when security incidents occur. These features, auditability and accountability, become critical if security attacks have implications for patients’ health conditions.
3.2.3. Security Solution
Six security solutions (i.e., access control, cryptography, anonymization, blockchain, steganography, and watermarking) for the security and privacy of e-health data are presented in this section. E-health system must adopt access control systems to restrict unauthorized access, and the data stored in the system must be encrypted or anonymized using cryptography and data anonymization techniques. In addition, steganography and watermarking have widely been used to achieve medical image security, and blockchain has also been studied recently to ensure the integrity of the e-health data.
Access control. Access control is an indispensable security solution to protect e-health data by restricting unauthorized access. Therefore, many studies focused on the security and privacy of e-health data based on access control.
Dankar et al. [ 21 ] proposed a risk-aware secure framework that controls access to medical data using contextual information related to data requests. In the framework, to store e-health data, a risk evaluation module identifies the risk of the data, and an access control module determines the proper data protection level based on the risk. After selecting the protection level, a protection level application module re-identifies the data to store the data. The constraints to access the data are also decided using the data protection level if the data are requested.
In addition, there are studies to design access control frameworks using blockchains for secure management in e-health data [ 22 , 23 , 24 ]. However, the frameworks ensure several security benefits such as data confidentiality, integrity, availability, and accountability. Rajput et al. [ 22 ] applied an emergency scenario into an access control framework by defining some access rules for the emergent scenario. In the scenario, conditional permissions are used for the authorization of emergent medical staff. Shahnaz et al. [ 23 ] proposed a role-based access control framework to protect EHRs and focused on solving the scalability problem of blockchain based on the off-chain scaling method. Xu et al. [ 24 ] proposed Healthchain that controls access from medical staff by sharing symmetric keys between a user and the staff.
Furthermore, Section 4 , Section 5 and Section 6 present other access control studies that focus on different target domains, that is, medical devices, networks, and edge/fog/cloud computing.
Cryptography. Cryptography has been widely used as an essential security solution to ensure several security requirements such as data confidentiality and integrity. Most studies adopted existing cryptography for basic purposes such as encryption and digital signatures, and only a few studies have proposed new cryptographic primitives, protocols, cryptosystems, and so forth. In general, well-known cryptosystems such as advanced encryption standard (AES) and Rivest–Shamir–Adleman (RSA) were utilized to protect e-health data in the studies, considering the different security requirements of the specific target domain. AES, developed by the National Institute of Standards and Technology (NIST) in 2001, is the most frequently used symmetric encryption technique [ 25 ]. Symmetric key techniques including AES are used in the medical/healthcare security research areas due to their fast encryption/decryption speed. On the other hand, RSA, developed in 1978 [ 26 ], is a public-key cryptosystem (PKC) that has two types of cryptographic key: a public key for encryption and a private key for decryption. RSA has been adopted for digital signatures rather than data encryption and decryption because it is neither a fast nor efficient cryptosystem.
Some studies focused on the security of digital image and communication on medicine (DICOM) [ 27 , 28 , 29 ]. Elhoseny et al. [ 27 ] simply applied AES and RSA to secure DICOM. Dzwonkowski et al. [ 28 ] and Parvees et al. [ 29 ] employed quaternion rotation and enhanced chaotic economic map (ECEM). They verified that the quaternion- and ECEM-based encryption schemes were more secure and efficient for medical image encryption than traditional cryptosystems such as AES.
In addition, Section 4 , Section 5 and Section 6 contain more studies based on diverse cryptography schemes such as elliptic curve cryptosystem (ECC), attribute-based encryption (ABE), and certificateless public-key cryptosystem (CL-PKC) that considered security concerns and requirements for medical devices, networks, and edge/fog/cloud computing.
Anonymization. Data anonymization is a process that eliminates, generalizes, or replaces identifiable information from personal information [ 30 ]. For data anonymization, four traditional models (i.e., k-anonymity, l-diversity, t-closeness, and differential privacy) have been widely adopted in medical research areas.
K-anonymity is an anonymization model proposed by Sweeney in 2002 [ 31 ] that reduces the possibility of specifying sensitive attributes by producing k or more records composed of the same quasi-identifier. Therefore, if k-anonymity is satisfied, the probability of identifiability for a specific person will be <1/k. The higher the k value, the better the data anonymity. However, if the k value is too high, data usability decreases because it becomes more difficult to explore the correlation between anonymized data. An optimal k value should be found to provide an appropriate trade-off between data anonymization and usability.
Although the individual is not identified when k-anonymity is achieved, the more the sensitive attributes remain the same, the more likely they are to be re-identified. L-diversity therefore proposed by Machanavajjhala et al. in 2007 [ 32 ] can be adopted to prevent the limitation of k-anonymity by diversifying sensitive attributes. This reduces the possibility of re-identifying a specific individual with one or more sensitive attributes. As with k-anonymity, the higher the l value, the better the data anonymity.
If there is bias or patterns in anonymized data, personal privacy may be exposed by means of these biases and patterns. In other words, even if k-anonymity and l-diversity are satisfied, privacy can be disclosed using the distribution of sensitive attributes. Therefore, T-closeness proposed by Li et al. in 2007 [ 33 ] measures data distributions to prevent data from being closed in a specific part.
K-anonymity and l-diversity have the limitation that sensitive attributes can be exposed if an attacker has experience exploiting vulnerabilities. Therefore, differential privacy was proposed by Dwork in 2006 [ 34 ] to mitigate the limitations of k-anonymity and l-diversity. This mathematical model prevents an attacker from inferring a specific individual with statistical data derived from multiple database queries by adding noise into the response to each query. The noise hinders the attacker from revealing the distribution of data that can be used for re-identification.
Blockchain. One critical threat to e-health data is data tampering, which can lead to patient medical accidents. Blockchain, which provides public and distributed ledger on a peer-to-peer network, was proposed by Nakamoto in 2008 [ 35 ] to ensure data integrity by recording all verified transactions in a ledger based on a consensus algorithm.
Some studies have taken advantage of blockchains for secure data preservation [ 36 ], secure data sharing [ 37 , 38 ], and the access control [ 21 , 22 , 23 , 24 ]. Li et al. [ 36 ] designed a reliable data storage for primitiveness and verifiability of e-health data based on the blockchain while preserving privacy. The anonymity of users and the data was also considered by using cryptographic algorithms such as AES. Fan et al. [ 37 ] and Patel [ 38 ] proposed e-health data sharing systems between the heterogeneous databases of hospitals (i.e., cross-domain). Because of the lack of standard data management and data sharing policy in the conventional EMR systems, Fan et al. [ 37 ] proposed MedBlock that is applied blockchain with public and distributed ledger. In the proposed system, hospitals can upload encrypted data to MedBlock, thus a user who has the right decryption key can retrieve and verify the data anywhere at any time. Patel [ 38 ] also designed a blockchain-based image sharing system. The blocks recorded a list of images and related patients, authorized entities by the patient to access the images, and the retrieval endpoint (i.e., URL) that actually has the images. Only authorized users by the patient can access the endpoint and retrieve images stored in the hospital database.
However, blockchain is not suitable for big data, thus network location information indicating the desired resource can be used instead of recording large data.
Steganography and watermarking. Steganography is a technology that hides secret information within other data, such as a medical image, whereas encryption converts original data into data that is unrecognizable without the proper key for decryption. This protects secret information and conceals its existence. Furthermore, steganography is generally categorized into spatial domain techniques (i.e., such as least significant bit (LSB), embedding, and spread technique) and transform domain techniques (i.e., discrete wavelet transform (DWT) and discrete cosine transform (DCT)). Spatial domain techniques are fast but vulnerable to compression and geometric distortion such as rotation, scaling, and cropping, whereas transform domain techniques require high computational power but have resistance to compression and geometric distortions [ 39 ].
Karakış et al. [ 39 ] proposed similarity-based LSB and fuzzy-logic-based LSB that select non-sequential LSBs of image pixels to insert secret messages. The proposed LSBs are compressed and encrypted in the message preprocessing stage in a cover image. An only authenticated user who has the right key can decrypt and read the hidden message including electroencephalogram (EEG) signal, doctor’s comment, and patient information. Mantos and Maglogiannis [ 40 ] also developed a new LSB-based steganography method. The method hides patient data and integrity hashes in the region of interest (ROI) and recovery data into the region of non-interest (RONI) that is required to recover the ROI. In the method, the patient data are protected by AES, and integrity is achieved as well with the hashes of the ROI part and the hidden data. Moreover, Elhoseny et al. [ 27 ] utilized 2D DWT to hide the secret data encrypted by AES for secure medical data transmission in IoT environments. It has a high-security level by applying AES, RSA, and 2D-DWT; however, they could not be suitable for the IoT environments that are resource-constrained in terms of network bandwidth, computational power, memory capacity, and so forth.
In addition, digital watermarking is a promising security solution that provides content authentication, integrity, and credibility of medical images [ 41 , 42 ]. Digital watermarking in e-healthcare services embeds sensitive information such as patient identity and diagnostic details into medical images by converting the gray level of pixels without any perceptible changes to the host image [ 43 ]. However, watermarking can distort medical images; this is a critical issue because it can lead doctors to misdiagnose patients. Therefore, Turuk et al. [ 41 ] proposed a reversible watermarking scheme based on quantized DWT, and the scheme supports watermark extraction from images and restoring the original medical image. The proposed scheme can also embed multiple watermarks by means of quantization function, and recover the original medical image using a tracking key which preserves sign change of the original image’s coefficient. Moreover, a fragile watermark was proposed by Walton in 2007 [ 44 ], and it has been studied to detect medical image tampering based on the sensitivity. With the fragile watermark, image tampering can easily be detected, because even a one-bit change can affect the verification results of integrity. Shehab et al. [ 42 ] proposed a scheme with the advantages of the fragile watermarking technique. The proposed scheme particularly used singular value decomposition (SVD) with the 4 × 4 size of image blocks for tamper localization that can localize attacked pixels and regions. The scheme can also be used for recovering the tampered region with Arnold transform.
Finally, Table 3 shows a summary of studies related to e-health data.
Summary for e-health data security and privacy studies.
4. Medical Device
With the advancement of sensors and network technologies, medical devices such as wearable devices and IMDs have been connected to networks to enable smart e-healthcare services, such as remote diagnosis and prescription. Medical devices play a role of sources that produce a huge amount of e-health data; therefore, the security of medical devices should be properly considered.
4.1. Overview
Figure 4 shows the taxonomy for security and privacy on medical devices. In a nutshell, physical and logical access control schemes, including proper authentication, must be adopted to prevent unauthorized access and network attacks on medical devices and cryptography should be required to protect the sensed e-health data and credentials stored in the device. In addition, secure hardware can be used to enhance the security of resource-constrained medical devices, and malware detection techniques are required since devices can be compromised by malware.

Taxonomy for security and privacy on medical device.
4.2. Security Concern, Requirement, and Solution
This section presents security concerns, requirements, and solutions for medical devices. Note that a limited number of studies are surveyed because of insufficient studies for medical devices that fulfill the selection criteria described in Section 2.1 .
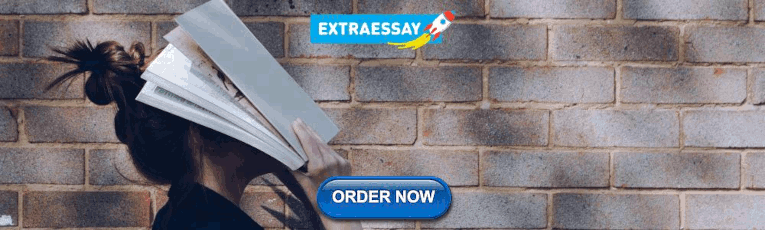
4.2.1. Security Concern
There are several security concerns that have implications for medical devices. Since the medical devices are networked, an attacker can access the device through the network to breach e-health data, or compromise the device using malware to make it follows some malicious operations which can affect patients’ health condition. In addition, depletion attacks can consume device resources such as computing power and battery to interrupt desired operations of the device so that it cannot provide e-health data to someone who needs the data. Detailed descriptions for the security concerns that are commonly described in several studies are as follows.
Unauthorized access. An attacker can access medical devices by means of some security holes in the devices. Unauthorized access from an attacker or user who has a malicious purpose can cause a wide range of concerns from data breach of patients to life threats. According to Yaqoob et al. [ 3 ], various attack methodologies such as reverse engineering and communication channel exploitation (e.g., lack of encryption, authentication, and access control) were used for the unauthorized access.
Data breach. As described in Section 3 , data including e-health data and credentials stored in medical devices can be leaked, tampered with, or deleted by unauthorized access. Protecting data stored in devices and transmitted via the Internet requires proper security solutions such as authentication, access control and cryptography. In addition, user identities should particularly be secured since the loss, theft, and disclosure of personally identifiable information accounts for one-fifth of all reported issues [ 45 ].
Network attack. As medical devices have been connected to medical networks and the Internet to support modern healthcare services, the network has become an entrance to medical devices [ 12 , 46 ]. In general, there are two types of network attack: passive and active. Passive attacks harm confidentiality by observing or copying network traffic and active attacks infringe on the integrity and availability by controlling the network traffic and modifying the messages in the traffic. Section 5 describes the network attacks in more detail.
Physical attack. A physical attack is one of the most representative security concerns for medical devices. Medical devices can be damaged by natural disasters or a malevolent person. In particular, someone can access or steal medical devices to capture patients’ private information.
Resource depletion attack. Most medical devices have limited resources such as computational power and battery life. An attacker can deplete medical device resources so that they no longer work properly. Since medical devices can directly affect patients’ health conditions, a resource depletion attack is very critical to the security of medical devices. A power-draining attack, which is a type of resource depletion attack, was demonstrated by Hei et al. [ 47 ].
Firmware Modification attack. This attack modifies the firmware stored in non-volatile memory that controls medical devices [ 3 ]. An attacker can inject malicious firmware into a device when it needs to be updated. By modifying or changing the original firmware, an attacker can control the medical devices as desired.
Malware. Malware, such as spyware, botnets and Trojans, are malicious software that can damage medical devices. Malware that controls devices are particularly critical because they can affect patients’ health condition and life [ 45 ]. Proper security solutions must check the data transmitted from other networks to detect or prevent malware injection. Since medical devices have constrained resources, a proxy could facilitate the solutions instead of the devices.
4.2.2. Security Requirement
As medical devices are networked, network attacks must be considered to secure the devices and the e-health data that are generated and stored in the devices. Security requirements that are generally mentioned in the medical device studies are as follows.
Access restriction. Unauthorized access to e-health data must be restricted appropriately. In other words, access must be authenticated and authorized to determine whether the user who requests data has proper permissions. This requirement includes the restriction of physical access and information access.
Confidentiality. E-health data and the credentials of medical devices must be confidential. In general, security by obscurity and cryptography are used to protect data confidentiality; however, security by obscurity is increasingly insufficient and strong cryptography has become important [ 48 ].
Integrity. Protecting the integrity of firmware and software and data integrity are critical issues for medical devices since compromised firmware and software can control devices. The violation of the integrity can affect patients’ health condition and, thus, it is one of the most important security requirements.
Availability. In addition to data availability, medical devices must also be available any time when the owner wants to use them or for medical staff in an emergency. Several attacks such as DoS and packet flooding attacks can infringe upon the availability of medical devices, similar to data availability. Fault tolerance is the one of primary functionalities that makes medical devices work consistently even when compromised.
Resistance to network attack. Several network attacks such as eavesdropping, replay, and impersonation can compromise medical devices. To design secure medical devices, network attacks must be considered since the devices have been connected to both medical networks and the Internet. This requirement is also important to protect against other attacks such as resource depletion and malware. Section 5 describes network attacks and related requirements in detail.
Reliability. A medical device has its own purpose and intrinsic features are important to a patient’s health condition. Malfunction of medical devices due to various causes such as software bugs, malware, and security attacks could damage patients. To protect patients’ safety, medical devices must provide reliability in terms of performing their intended function.
Lightweight. Security solutions adopted in medical devices should be lightweight because medical devices have resource constraints. Security solutions should work with limited resources to fulfill the minimum security requirements for medical devices and the data they hold.
Secure patch. Firmware and software are imperfect; they have hidden flaws and vulnerabilities including zero-day vulnerabilities [ 46 ]. Therefore, medical devices must have the ability to securely patch the firmware and software when vulnerabilities are uncovered and must be able to verify whether the firmware or software is untampered. This verification is required since the firmware and software downloaded via the network can be modified to compromise devices [ 48 ].
4.2.3. Security Solution
Representative security solutions to protect medical devices are authentication, access control, and cryptography. Since general medical devices are resource-constrained, the security solutions should be efficient and lightweight, and secure hardware can be used to enhance the security of the device. Detailed descriptions for the security solutions of the medical device are as follows.
Access control. Access control is an essential security solution to restrict unauthorized access. Access control for medical devices is crucial because it can be related to patients’ health condition and life. According to Wu et al. [ 10 ], there are two types of direct access control schemes: using a preloaded key and temporary key and an indirect access control scheme using a proxy. The direct access control schemes basically permit access by validating a key within a medical device, while the indirect access control scheme delegates the access control to a proxy server (e.g., smartphone and smartwatch) since the medical device has limited resources.
Authentication. Any user who accesses medical devices must be properly authenticated. In general, there are three factors in authentication schemes: ownership, knowledge and biometric.
Most medical devices authenticate a valid user based on their knowledge such as ID and password; however, biometric-based authentication schemes have recently emerged for the medical devices. Security by obscurity is not enough to secure medical devices [ 48 ]. Liu et al. [ 49 ] proposed local authentication and remote authentication for the cloud-assisted wearable devices. In the local authentication protocol, Hash-based selective disclosure mechanism and Chebyshev chaotic map are used to realize mutual authentication between a wearable device and a smartphone. After the local authentication, the cloud performs remote authentication of the device based on a yoking-proof. In addition, to make accessing the device challenging, multi-factor authentication [ 50 ], and biometric-based authentication schemes [ 51 , 52 ] can also be used. Zheng et al. [ 51 ] proposed a finger-to-heart (F2H) IMD authentication scheme that allows a doctor to access a patient’s device by scanning the fingerprint of the patient in an emergency. They emphasized that the proposed scheme is suitable for IMDs than ECG-based authentication scheme. Because the scheme requires only low resources because it is not required to capture or process biometric in every access. Belkhouja et al. [ 52 ] proposed a two-factor authentication scheme for IMDs using ECG signal and fingerprint.
ECG was used to authenticate medical staff in an emergency, and fingerprint was utilized as an assistance factor of the authentication.
Moreover, a lightweight and low power authentication scheme is required to solve the resource constraint problem of medical devices. For example, Halperin et al. [ 53 ] proposed a zero-power authentication method based on radio frequency (RF) power harvesting of an IMD programmer (i.e., a proxy device).
Cryptography. Cryptography is required to protect e-health data produced by medical devices and the devices’ credentials. In particular, strong encryption is required to protect highly sensitive e-health data [ 48 ]. Zheng et al. [ 54 ] proposed an ECG-based data encryption scheme for IMDs. The scheme used one-time pads (OTPs) generated from ECG signals as a key for encryption. In addition, the OTP-based keys are dynamically generated for each round of encryption, thus additional processes (i.e., require key distribution, storage, revocation, refreshment, and seed protection) are not required. In addition, lightweight and low-power cryptography are required for medical devices that are resource-constrained in terms of computation power, memory, and battery.
Secure hardware. Medical devices generally have constrained resources in terms of computing power and battery, which hinder their adoption of strong security. Therefore, secure hardware such as a hardware security module (HSM) and physical unclonable function (PUF), which would take care of security-related processes, can be used to enhance the security of medical devices. Diverse security solutions such as cryptography, authentication, and access control can be supported by secure hardware.
Malware detection. Malware detection techniques such as control-flow integrity verification and call stack monitoring are important for medical devices because malware remains unknown until detected [ 45 ]. The detection techniques are critical since undetected malware can consistently affect devices. In addition, hardware-based malware detection is a promising security solution because of the resource constraints of medical devices. Once the malware has been detected, it must be properly treated.
Table 4 shows a summary of the security and privacy studies for medical devices.
Summary for medical device studies on security and privacy.
5. Medical Network
This section presents security concerns, requirements, solutions, research trends and open challenges for security and privacy in medical networks. Since modern e-health systems are based on the network, the security and privacy for the network are must be considered to design secure e-health systems. Note that the term “medical networks” used in this paper includes diverse types of networks that transmit e-health data such as IoMT and WBAN.
5.1. Overview
We classified the security and privacy studies that focused on medical networks in terms of security concerns, requirements, and solutions. Figure 5 shows a taxonomy of these studies.

Taxonomy for security and privacy on medical network.
In a nutshell, as shown in Figure 5 , there were five security solutions for medical networks, 11 security requirements for the solutions, and eight security concerns remaining to be solved. In particular, cryptography, authentication, and access control were widely studied to provide data confidentiality, integrity, anonymity, authenticity, and non-repudiation against diverse security concerns such as eavesdropping attacks, denial of service (DoS), replay attacks, impersonation, man in the middle (MIMT) attacks, and spoofing attacks.
5.2. Security Concern, Requirement, and Solution
This section presents security concerns, requirements, and solutions for medical networks, such as WBANs and IoMTs, based on the medical network taxonomy.
5.2.1. Security Concerns
Similar to the conventional network, there are passive attacks and active attacks in medical networks. In other words, an attacker can eavesdrop the network communications and interrupt the communications to breach e-health data which is highly sensitive information. The six general security concerns that are the goals of the recent studies are as follows.
Eavesdropping. An adversary can eavesdrop on the traffic of medical networks to capture useful information such as patients’ e-health data. Even though the data in the air is generally anonymized or encrypted, this attack can be one of most critical because other attacks use the data captured by the eavesdropping attack; it becomes more serious if the data has not been properly anonymized or encrypted. Spoofing. Data such as nodes, identity information, and network addresses can be forged by an attacker in medical networks [ 55 , 56 ]. The attacker exploits a spoofing attack to deceive legitimate users or security systems for unauthorized access or further attacks.
Impersonation attack. An adversary can impersonate a legitimate entity on a medical network such as a user, device, or server by eavesdropping on some network traffic. The attacker can then perform other attacks using the impersonated identity [ 57 ]. This attack can be posed by weak authentication [ 58 ].
Resource depletion attack. Resource depletion in medical networks is an attack that threatens to exhaust network resources such as bandwidth and traffic. Medical networks such as IoMTs and WBANs are particularly lacking in resources; therefore, this type of attack can easily hinder the availability of medical services operated in medical networks. DoS is a typical resource depletion attack.
Replay attack. A replay attack can be done by capturing network packets and then transmitting them instead of the legitimate sender. This attack could be performed by an attacker to make a medical device or a server unavailable or to impersonate a valid user. To avoid replay attacks, random numbers or timestamps are generally included in packets.
Man in the middle attack. A man in the middle (MITM) attack can be done by intercepting and controlling the network communication between the two parties (e.g., medical devices and servers). It is difficult for the victims to detect the presence of an adversary, so they should believe that communication may be modified and transmitted by an adversary. If communication is related to remote treatment and prescription, this attack becomes very critical to patients.
Tracking attack. An attacker can track patient locations (e.g., their workplace or home) by monitoring medical networks to discover the identity of the patient and some additional related information [ 13 ]. The attacker can track several networked devices such as smartphones, smartwatches, medical devices, and RFID tags.
5.2.2. Security Requirements
Security concerns for the medical networks are similar to the conventional networks; however, the medical networks mostly transfer e-health data with the patient’s identity, which are highly sensitive. Therefore, security requirements for medical networks should be more rigorous than the conventional network [ 14 ]. In this section, the ten representative security requirements commonly mentioned in the medical network studies are presented.
Confidentiality, integrity, and availability. Data confidentiality, integrity, and availability were already discussed in Section 3 ; however, the requirements for these are more stringent when the data are transmitted via medical networks. E-health data must satisfy confidentiality and integrity since an adversary in a medical network can eavesdrop and modify the data. In addition, data availability is a prominent requirement in medical networks. Patients must be able to use their data whenever they want and medical staff must be able to use the data in a remote healthcare system, particularly in an emergency.
Authenticity. An adversary in medical networks can forge a message or impersonate a user. Therefore, the authenticity of each message’s origin [ 59 ] and a user’s identity [ 60 ] must be properly checked to prevent attacks on authenticity.
Non-repudiation. Non-repudiation denotes the ability that can assure that someone cannot deny the validity of something [ 61 ]. For example, non-repudiation could be provided for a doctor’s diagnosis in case of a medical incident [ 62 ].
Anonymity. Data anonymity is important; moreover, the identities of patients in medical networks must be anonymized. By making patients’ identities anonymized, an adversary who eavesdrops on network communications cannot obtain patients’ real identities.
Unlinkability. Even though e-health data or patient identity is anonymized, an adversary in medical networks must not be able to link captured data with a specific sender. If the data or identity of communications is linkable, the adversary may combine some data to obtain a personal health record by requesting different types of anonymized data for a person. Therefore, both anonymity and unlinkability are important in medical networks.
Traceability. In general, a user’s true identity must be anonymized to prevent a tracking attack for that identity by an adversary on medical networks. However, the true identity might be conditionally revealed when it is related to the adversary on the networks [ 60 , 63 ]. This requirement should be supported in special cases and must be carefully treated since it can also uncover a patient’s true identity.
Lightweight. Security solutions such as cryptography, authentication, and access control for medical networks should be lightweight since medical networks have limited resources in terms of bandwidth, traffic, and network nodes’ hardware specifications. Several studies have focused on lightweight security solutions [ 55 , 57 , 64 , 65 , 66 ], and the lightweight scheme becomes more important; however, strong security solutions that require high levels of resources are still needed to secure e-health data. Therefore, maintaining an appropriate tradeoff between efficiency and strength in security is a critical issue in medical network security.
Scalability. As the number of users, medical devices, and e-health data increases in medical networks, scalability for networks should be supported. Scalability is an important security requirement because it is related to availability, which is a very critical security requirement in medical domains. Based on scalability, medical services that use the networks can be continuously provided for patients.
5.2.3. Security Solution
This section describes five security solutions: cryptography, authentication, access control, compressive sensing, and traceback technique. Most studies were particularly focused on cryptography to protect the patients’ data and authentication schemes to check the true identity of network entities. In addition, since medical networks are resource-constrained, the studies in this area mainly aimed at efficient and lightweight security solutions.
Cryptography. There have been considerable studies on security and privacy that take advantage of diverse cryptography techniques in medical networks. A brief introduction to cryptography techniques and studies is as follows.
Advanced encryption standard. Lounis et al. [ 59 ] applied AES, and randomly generated symmetric key (RSK) to encrypt medical data for cloud-based scalable architecture, and the architecture can securely store and shares patient’s health data in wireless sensor networks (WSNs). The authors overcame the overhead of ABE by encrypting an AES key (i.e., RSK) rather than encrypting the whole of medical data. Guo et al. [ 55 ] also adopted AES for a lightweight encryption/decryption scheme in WBANs environment. They proposed a secure and privacy-preserving framework based on multi-level trust management with opportunistic computing [ 67 ]. The opportunistic computing allows an opportunistically contacted node to assist other WBAN node’s operations when the node has not enough energy and computing power. In the framework, different privacy protection strategies were applied for user’s privacy based on the groups which have different trust levels.
Elliptic curve cryptography. Elliptic curve cryptography (ECC) is a form of public-key cryptography using elliptic curves over finite fields. Compared with conventional public-key cryptosystem, ECC is faster and more efficient in terms of computational time, memory capacity, and bandwidth [ 57 ]. Therefore, most studies that use public-key cryptography were based on ECC for resource-constrained medical networks. Some studies [ 57 , 60 , 68 ] adopted ECC for design efficient authentication protocols, and Omala et al. [ 69 ] proposed a secure transmission scheme based on the ECC.
Attribute-based encryption. Attribute-based encryption (ABE), which is a type of public-key cryptosystem, was first proposed by Sahai and Waters in 2005 [ 70 ]. In many studies on medical network security, ABE was adopted to implement flexible and fine-grained access control systems [ 59 , 63 , 71 ] for e-health data, since the data can be encrypted based on diverse attributes such as patient name and treatment date. Conventional ABE is divided into two types: ciphertext-policy ABE (CP-ABE) and key-policy ABE (KP-ABE). The main difference between CP-ABE and KP-ABE is the position of the access policy. In CP-ABE, an access policy is encrypted with e-health data, whereas the policy is used to generate a decryption key in KP-ABE. Figure 6 shows the difference and overview of CP-ABE and KP-ABE.

Overview of ABE schemes.
In Figure 6 a, Doctor A, who has a decryption key and the attributes Doctor and Physician, can decrypt the ciphertext A, which includes the access policy consisting of Doctor AND Physician. Meanwhile, in Figure 6 b, Doctor B can decrypt the ciphertext B encrypted with the attributes Doctor and Surgeon if they have a decryption key generated using the access policy consisting of Doctor AND Surgeon. CP-ABE and KP-ABE are very promising cryptographic schemes for various applications; however, ABE is not suitable for resource-constrained medical networks such as WBANs and IoMTs because it requires high performance for cryptographic operations. Therefore, some methods have been proposed to overcome the resource limitations. To reduce computational overhead, Lounis et al. [ 59 ] encrypted secret keys for e-health data rather than encrypting the entire e-health data. On the other hand, Zheng et al. [ 71 ] used online/offline encryption techniques [ 72 ] to efficiently apply ABE into medical networks. In the offline phase, some cryptographic operations are performed in advance before the message to be encrypted is entered, which is required in the encryption phase. Then, based on the results of the online phase, encryption is performed in the online phase.
Homomorphic encryption. E-health data are highly sensitive. Even though the aggregation of e-health data could be very useful for various e-healthcare services, data confidentiality should be preserved when aggregators collect data from personal medical devices. With homomorphic encryption, e-health data collected by aggregators can be processed without decryption, thereby preserving privacy. In addition, data aggregation techniques are used to reduce the communication cost of medical networks (e.g., WBANs and IoMTs) in real-time data transmission.
Ara et al. [ 73 ] proposed a secure privacy-preserving data aggregation (SPPDA) scheme based on the bilinear ElGamal cryptosystem, which has the homomorphic property, for remote health monitoring systems.
To privately aggregate the e-health data from sensing nodes of patients, the aggregators adopt pairing-based homomorphic encryption and send the collected data to the medical server. In general, pairing operation requires high computation cost, however, this study executed heavy operations such as key generations and decryption in remote medical servers for efficiency. Huang et al. [ 74 ] collected e-health data from WBANs and transmitted the data to wireless personal area networks through WSNs by means of homomorphic encryption based on the matrix (HEBM), and Tang et al. [ 64 ] also proposed a privacy-preserving health data aggregation scheme that can securely collect health data from healthcare devices. In the study, Boneh–Goh–Nissim (BGN) cryptosystem was used which has some homomorphic features. In addition, Wang and Zhang [ 75 ] proposed a data division scheme using homomorphic encryption to prevent eavesdropping attacks in WSNs. By using the homomorphic encryption, e-health data was divided into three parts, sent to the central server separately, then merged and stored in the server after checking the integrity with the message authentication code (MAC) of the divided data. Wireless environments including WBANs and IoMTs are vulnerable to eavesdropping; however, patient’s privacy may not be fully disclosed since the data are divided.
Certificateless public-key cryptography. Due to the resource constraint problem in medical networks, traditional public-key infrastructure (PKI) is unsuitable for medical networks. Moreover, certificate management that needs a trusted third party, a certificate authority, is an obstacle. Therefore, an identity-based cryptosystem (IBC) [ 76 ] was proposed to remove certificates. IBC had a key escrow problem, but Al-Riyami and Paterson [ 77 ] solved this problem by proposing certificateless public-key cryptography (CL-PKC). In addition, signcryption [ 78 ], which is more efficient than a conventional sign-then-encrypt technique, has been widely adopted in WBANs.
To secure transmission between WBANs and servers, Omala et al. [ 69 ] designed an ECC-based certificateless signcryption (CLSC) scheme, and the scheme is lightweight and resistant to key escrow attack. Barbosa and Farshim (BF) [ 79 ] previously built a base scheme of this study using bilinear pairing, however, they improved the performance in terms of computation cost and energy consumption by means of ECC, and they utilized the proposed scheme to secure transmission from WBANs to a medical application provider. According to the evaluation results, the proposed scheme showed better performance in terms of energy consumption by 46% than BF’s scheme. Li et al. [ 80 ] also designed a CLSC scheme based on the identity-based signcryption (IBSC) scheme [ 81 ]. Based on CLSC, they solved key escrow and certificate management problems. Shen et al. [ 57 ] and Ji et al. [ 60 ] also adopted certificateless scheme to eliminate public key certificates in their authentication protocols.
Moreover, Zhang et al. [ 82 ] proposed a lightweight and secure device-to-device (D2D)-assisted data transmission protocol based on the CL-PKC in m-health systems. In general, there are three techniques of CL-PKC: certificateless signature, certificateless encryption, and certificate signcryption. This study particularly adopted certificateless generalized signcryption (CLGSC), which can support the three CL-PKC techniques, to provide data confidentiality, integrity, mutual authentication, contextual privacy. In addition, anonymity and unlinkability were also supported by using pseudo-identity and a random nonce value. They used D2D communications to transmit big health data collected by BAN instead of cellular networks that are already overburdened to transmit other data.
Authentication. Authentication is an essential security function for medical network security to authenticate unknown users or devices. In medical networks, authentication schemes/protocols have been widely studied considering various security requirements such as integrity, anonymity, unlinkability, authenticity, non-repudiation, and forward/backward secrecy to prevent several security concerns such as replay, impersonation, MITM, and spoofing attacks. According to our survey, authentication studies for medical networks generally considered efficiency and they were classified as mutual, anonymous, or certificateless authentication.
Some studies [ 62 , 83 , 84 ] designed a mutual authentication protocol. Li et al. [ 62 ] proposed a mutual authentication protocol and key agreement scheme based on Chebyshev chaotic maps and Diffie–Hellman key exchange. In the proposed medical system, only authorized doctors and medical staff can have permissions including access to patients’ health data collected from patients’ body sensors. In addition, a digital signature was utilized to provide non-repudiation for the doctor’s diagnosis. Cheng et al. [ 83 ] applied blockchain to avoid strong dependence on a trusted third party for a mutual authentication scheme. Ibrahim et al. [ 84 ] proposed a lightweight mutual authentication scheme for two-tier WBANs to ensure the originality and integrity of patient health data with anonymity between various body sensors. The proposed protocol only applied hash and XOR operations and required 480 bits memory on each WBAN nodes, and this characteristic makes the protocol is efficient for resource-constrained environments.
Certificateless authentication scheme is also researched. Shen et al. [ 57 ] presented an efficient multi-layer authentication protocol with a secure session key generation scheme and characteristics of WBANs. The proposed authentication protocols support two layers in WBANs. In the communication layer, sensors-to-personal digital assistance (PDA) group authentication protocol considering resource constraint of WBAN nodes was designed for performing between PDA and sensors. In the second layer, completely wireless environments are considered, and a non-pairing certificateless authentication protocol was designed to be used between PDA and application providers based on ECC that is an efficient scheme for WBANs. Ji et al. [ 60 ] also proposed an efficient and certificateless conditional privacy-preserving authentication scheme for WBANs based on ECC. They consisted that the traceability of real identity in anonymous environments is conditionally required because anonymity could be exploited by a malicious user. In an emergency, a trusted authority that acts as a key generation center (KGC) also can trace the real identity of a patient. In addition, to improve performance, the proposed scheme supported batch authentication which validates multiple WBAN clients at the same time.
In addition, there were authentication protocols for radio frequency identification (RFID). RFID is a promising identification technology to manage medical supplies, equipment, medications, and patients. In medical domains, RFID tags could contain sensitive information such as patients’ health data that require high security. Rahman et al. [ 85 ] proposed a privacy-preserving framework named PriSens-HSAC for RFID to support a group based anonymous authentication protocol. In order to authenticate a tag, a reader sends a challenge to a tag, and the tag responses to the reader by encrypting the challenge, identity of the tag, and a nonce with a group key. Jin et al. [ 68 ] proposed a secure ECC-based RFID mutual authentication scheme for patient medication safety. The proposed scheme consists of two phases: setup phase and authentication phase. In the setup phase, a back-end server creates public/private keys and the identity value of the tag (i.e., a random point on the elliptic curve), then the server sends the identity to the tag. Based on the setup parameters, the server and the tag can authenticate each other.
Fan et al. [ 65 ] presented a lightweight RFID medical privacy protection scheme in IoT. This study strongly depended on the proposed cross operation (i.e., the operation of bit cross) and index data table for an efficient RFID authentication scheme. However, Aghili et al. [ 66 ] identified several vulnerabilities of the authentication protocol proposed by Fan et al. [ 65 ] in terms of secret disclosure, reader impersonation, and tag traceability attack. Then, they proposed an improved mutual RFID authentication protocol, SecLAP, for secure communication and privacy protection in medical IoT. Recently, Attarian and Hashemi [ 86 ] researched an anonymity communication protocol based on blockchain and user datagram protocol (UDP) in mHealth environments. Their protocol was specifically designed to protect the data security and privacy of clients’ identities.
Access control. There were various access control studies in different target domains; therefore, this section specifically presents access control schemes focusing on medical networks. Lounis et al. [ 59 ] and Yang et al. [ 63 ] proposed a fine-grained access control framework based on ABE for the medical networks (i.e., WSNs and IoT). Lounis et al. [ 59 ] proposed an efficient fine-grained access control that supports complex and dynamic security policies using CP-ABE, and Yang et al. [ 63 ] also proposed a privacy-preserving e-healthcare system that provides fine-grained access control and flexible access policy update.
Since user identity is very sensitive information in medical networks, Li et al. [ 80 ] proposed an anonymous access control model based on the proposed certificateless signcryption (CLSC) scheme that is cost-effective for WBANs. Their proposed access control model has advantages that it does not have a key escrow problem and public key certificates that is required to be managed.
In addition, there was a study applied break-the-glass concept for the emergent situation. Maw et al. [ 87 ] proposed a flexible access control model, break-the-glass access control (BTG-AC), for medical data in wireless medical sensor networks. The model was mainly considered to solve the conflict between data privacy and availability using break-the-glass (BTG) concept. Unlike the conventional BTG-RBAC model, the proposed BTG-AC used BTG policy only in emergency situations with Ponder2 policy package, and it is designed to be lightweight for WSNs.
Compressive sensing. By using compressive sensing (CS), the effect of full sampling can be achieved with just a few sampling points [ 88 ]. Since the medical networks are resource-constrained, CS can be adopted to reduce communication costs while maintaining data confidentiality.
Peng et al. [ 58 ] proposed a secure and energy-efficient e-health data transmission system based on chaotic CS, which is energy-efficient and also has an encryption performance for the medical networks. Since conventional CS uses measurement matrices for both senders and receivers, they need huge storage space. Therefore, chaotic CS was adopted, which only requires partial parameters for matrix generation such as the chaotic parameter, initiation value, sampling initial position and distance as a key, to save the storage space. In addition, it is more secure than traditional CS techniques because of the sensitivity of chaos.
Traceback technique. A DDoS attack is a critical attack against the medical networks since it depletes the networks’ limited resources and thus hinders the transmission reliability of patients’ e-health data. Therefore, DDoS detection techniques represent an important research subject in this domain. According to Latif et al. [ 56 ], the probabilistic packet marking (PPM) traceback technique is widely used in IP-based networks to detect the source of a DDoS; however, it cannot be directly applied to a resource-constrained WBAN environment because of its high convergence time and overhead on sensor nodes in WBAN. Therefore, Latif et al. [ 56 ] presented a novel approach, efficient traceback technique (ETT), based on Dynamic Probabilistic Packet Marking (DPPM). In other words, they utilized variable marking probability based on the packet’s traveling distance with DPPM label in the MAC Protocol Data Unit (MPDU) to the target node.
Finally, Table 5 summarizes the study analysis.
Summary for security and privacy study analysis on medical network.
6. Edge, Fog, and Cloud
Recently, conventional healthcare systems have been combined with diverse technologies, such as big data, IoMT and WBAN, to provide more advanced e-health services. Cloud computing is a promising computing paradigm that is being used in medical research areas since it provides various advantages such as cost efficiency, scalability, availability, and flexibility. In addition, edge and fog computing have been studied to support time-sensitive medical operations. This section presents security concerns, requirements, solutions, research trends, and open challenges in edge, fog, and cloud computing.
6.1. Overview
Figure 7 shows the taxonomy for the security and privacy studies in edge, fog, and cloud computing. In a nutshell, the security and privacy studies that deploy edge, fog, and cloud computing generally applied cryptography, authentication, and access control to ensure various security requirements such as data confidentiality, integrity, availability, and public verifiability, that solve several security concerns such as unauthorized access, data breach, and single point of failure (SPoF). In particular, security solutions for cryptography, authentication, and access control have been studied to protect e-health data in the cloud since the cloud has mainly been utilized as secure data storage. Furthermore, provable data possession (PDP) and proofs of retrievability (PoR) that allow patients to verify the integrity and availability of outsourced e-health data have been studied since the edge, fog, and cloud cannot be fully trusted.

Taxonomy for security and privacy on edge, fog, cloud computing.
6.2. Security Concern, Requirement, and Solution
These computing paradigms improve accessibility, usability, and manageability for e-health data, meanwhile, responsibility for ensuring strong security and privacy of e-health data becomes increased because security attacks on the edge, fog, and cloud can affect huge number of patients. Therefore, security concerns, requirements, and solutions for the edge, fog, cloud computing for e-health data should be rigorously identified and discussed to securely protect the big e-health data.
6.2.1. Security Concern
Edge, fog, and cloud process and store diverse and various e-health data; hence, data breach is one of the most critical security concerns in the edge, fog, and cloud computing. Six security concerns that are generally mentioned in surveyed studies on the edge, fog, and cloud computing are as follows.
Unauthorized access. In addition to medical devices and networks, a secure storage that stores and processes e-health data based on edge, fog, cloud computing needs to restrict unauthorized access to the storage. Since the edge, fog and cloud are data aggregation points, robust access control is highly required to protect big e-health data by restricting unauthorized access.
Data breach. Data breaches, including data disclosure, tampering and forgery, present critical threats to e-health data in edge, fog, and cloud environments. Section 3 describes data breaches in more detail.
Denial of service attack. The cloud centrally provides various medical services; therefore, DoS attacks are a serious threat to the cloud, edge, and fog that can halt medical services. If medical services stop working, this can directly affect people’s lives.
Single point of failure. The major characteristic of the cloud environment is centralization. Although there are some advantages to centralization, SPoF has emerged as a main drawback.
Malicious insider. Even if all security solutions are well-designed and properly applied to the edge, fog, and cloud environments, malicious insiders who have the correct permissions can abuse or misuse systems for malicious purposes.
Network attack. The network is an essential component for using the edge, fog, and cloud; therefore, network attacks such as eavesdropping, replay, and impersonation can be used to attack the edge, fog, and cloud environments. For example, an adversary can eavesdrop on network traffic in those environments to capture useful information (e.g., users’ e-health and authentication data) for further attacks on the edge, fog and cloud.
6.2.2. Security Requirement
Data security is particularly important security requirement in edge, fog, and cloud computing and a user should be able to check the data status publicly because the edge, fog, and cloud are generally managed by semi-trusted party which cannot be fully trusted. In addition, efficiency and lightness are less important compared with other domains such as the medical device and network since the edge, fog and cloud have sufficient resources. More specifically, there are ten common security requirements that can solve the security concerns of the edge, fog and cloud computing.
Confidentiality. As described in Section 3 , data confidentiality is required in the edge, fog, and cloud environments. In particular, data confidentiality is more critical in the cloud than medical devices or networks because various and diverse e-health data are collected extensively from patients over long periods of time. If e-health data are disclosed when transmitted via medical networks, it shows very limited health information; however, if the data stored in the cloud is exposed, it can show the medical history of some or all patients in the cloud. Therefore, data confidentiality in the cloud, which is secure data storage, is particularly important compared with other target domains.
Integrity and public verifiability. Data integrity must be satisfied not only when transmitted over medical networks but also within the edge, fog, and cloud. The data stored on personal medical devices can easily be checked by the patients; however, outsourced data in the edge, fog, and cloud environments are difficult to check despite the patient being the data owner since the environments are managed by a service provider. Therefore, the patients should be able to check the integrity of the outsourced data stored in the edge, fog, and cloud environments.
Availability. E-health data in the edge, fog, and cloud environments must be available when the patient who owns are the data owner wants to use it. To this end, the edge, fog, and cloud environments must provide availability.
Anonymity. Anonymity of original e-health data in the cloud is achieved by means of encryption. However, the data must have been anonymized if it is required to be analyzed or shared for some reasons such as medical research.
Authenticity. To secure e-health data, both data authenticity and identity authenticity of a user must be provided. In particular, the authenticity of a user is required to authenticate them.
Accountability. Since e-health data are highly sensitive, the data processed and stored in edge, fog, and cloud environments should be accountable.
Resistance to network attack. Network attacks must be considered to secure communication between clients (e.g., users and devices) and edge, fog, and cloud servers. In other words, the edge, fog, and cloud servers must properly authenticate and authorize users and medical devices to protect against network attacks such as eavesdropping, replay, and impersonation.
Flexibility. There are diverse clients in various environments that use the services provided by the edge, fog, and cloud environments; therefore, the edge, fog, and cloud environments should flexibly accommodate different environments and their various requirements.
Scalability. As the data are increased and diversified, cloud storage must be scalable for the large volume of big e-health data. In addition, security solutions should provide scalability as clients such as medical devices and users have recently increased.
6.2.3. Security Solution
Existing strong security solutions can be adopted in edge, fog, and cloud computing based on the sufficient resources; therefore, studies in this research area focused on useful functionalities rather than efficient and lightweight security solutions. Six security solutions that were adopted by the surveyed studies to secure e-health data in the edge, fog, and cloud computing are as follows.
Cryptography. Cryptography is an essential security solution that has been used in across entire medical domains as well as in edge, fog, and cloud computing. Useful cryptographic schemes for the edge, fog, and cloud computing are as follows.
Proxy re-encryption. Permissions that allow a user to access e-health data could be changed according to the situations of a patient or medical staff. For example, if a patient’s family doctor has changed, the access permission for the patient’s e-health data must be transferred from the former doctor to the new doctor. In this context, proxy re-encryption (PRE), which was first introduced by Blaze et al. in 1998 [ 89 ], can be used as seen in Figure 8 .

Overview of proxy re-encryption.
PRE enables a proxy to generate new ciphertext that can be decrypted by the new doctor’s private key. The re-encrypted ciphertext is encrypted using the re-encryption key generated by the delegator (i.e., the former family doctor). PRE makes the delegation more easy, secure, and private because the re-encryption operates without any decryption of the ciphertext. There are two variations of PRE, unidirectional PRE and bidirectional PRE. Bidirectional PRE schemes have the advantage that they can convert ciphertext several times; however, this may cause a data breach because of the additional re-encryption capability. Therefore, since e-health data are critical information, the unidirectional PRE scheme that re-encrypts a ciphertext once is more suitable. In the medical/healthcare research area, an identity-based proxy re-encryption (IBPRE) scheme that was first proposed by Green and Ateniese in 2007 [ 90 ] was widely adopted since the identity-based encryption (IBE) scheme can help simplify certificate management.
Identity-based encryption. Public-key cryptography randomly generates the public key for a user. However, an IBE scheme, which is one type of public-key cryptography, generates the public key with a user’s identity information, for example, email address. Therefore, a sender who knows the receiver’s identity information can encrypt some messages without exchanging the receiver’s public key (i.e., public-key infrastructure; PKI). The concept of IBE was first introduced by Shamir in 1984 [ 76 ]; however, the first practical IBE scheme was proposed by Boneh and Franklink in 2001 [ 91 ].
With the identity-based cryptographic concept, Wang et al. [ 92 ] proposed a new IBE scheme and a new identity-based proxy re-encryption (IBPRE) scheme and adopted the proposed identity-based cryptographic techniques into an e-health cloud system to secure e-health data. In the proposed scheme, some randomness is added to the private key to resist an adversary who compromises the private key for information of the master key. They showed the advantages of IBE that authenticates public key implicitly and simplifies the certificate management. In the proposed system, the cloud acted as secure storage and medical service provider that supports the proposed encryption scheme.
Attribute-based Encryption. IBE generates a public key with a user’s identity information, whereas the private key or ciphertext in ABE is generated by attributes. ABE has been considered a more flexible encryption scheme than IBE schemes since the key can be generated using diverse attributes (e.g., subject, resource, action, and environmental attributes) including identity information. In general, CP-ABE has been widely used in cloud environments to provide secure data sharing because it is much more flexible and suitable for general applications [ 93 ].
There were several CP-ABE studies [ 93 , 94 , 95 , 96 , 97 ] to protect e-health data. Wang et al. [ 93 ] proposed an efficient file hierarchy CP-ABE (FH-CP-ABE) scheme in cloud computing since the existing CP-ABE has not considered the hierarchy structure of shared files. In the scheme, the hierarchical files are encrypted with an integrated access structure to efficiently reduce storage and time cost for encryption. On the other hand, Eom et al. [ 94 ] focused on the patient-centric CP-ABE scheme. They proposed a new CP-ABE scheme, patient-controlled ABE (PC-ABE), which enables patients to control access to their own e-health data. In PC-ABE, the decryption key for encrypted e-health data was generated based on a patient’s private key and attributes of the parties that want to access the data. Since the decryption key is not generated without the patient’s private key, the patient can control the access to the patient’s data consequently. In addition, Liu et al. [ 95 ] and Rao [ 96 ] proposed e-health data sharing scheme using CP-ABE signcryption (CP-ABSC). Liu et al. [ 95 ] proposed a CP-ABSC scheme for PHR system in cloud computing based on CP-ABE and attribute-based signature (ABS) which enables a patient to sign e-health data of the patient with the patient’s private key if the patient has proper a set of attributes for the data. The CP-ABSC is a promising cryptographic technology for fine-grained access control to share e-health data in cloud computing; however, Rao [ 96 ] claimed that the Liu et al.’s scheme cannot provide confidentiality because they did not adopt the standard Signcryption techniques (i.e., encrypt-then-sign and sign-then-encrypt). Therefore, Rao proposed a new CP-ABSC scheme based on previous studies [ 97 , 98 ], which is more secure and efficient. The proposed scheme also can provide signcryptor (e.g., a patient) privacy and public verifiability, which are important security requirements of e-health systems in cloud computing.
Homomorphic encryption. Homomorphic encryption is a promising cryptographic scheme in edge, fog, and cloud computing as well as medical networks, which need to securely collect e-health data with privacy preservation. Raisaro et al. [ 99 ] proposed MedCo which enables a group of medical service providers to federate and protect the e-health data for secure sharing using the homomorphic encryption scheme in a hybrid environment that includes central and decentral environments. In other words, the proposed framework, MedCo, allows the multiple sites that store e-health data to share their data by securely querying the data to the distributed sites without sharing their databases. It also provides differential privacy by adding dummy records into patients’ e-health data. Moreover, Alabdulatif et al. [ 100 ] adopted edge computing to aggregate and analyze the large-scale bio-signal data in real-time. In the proposed edge of things (EoT) framework, fully homomorphic encryption was performed in the edge IoT gateway, located between medical devices and the cloud, to protect sensitive e-health data including patients’ privacy.
Searchable encryption. According to Zhang et al. [ 101 ], outsourcing e-health data and data searching services to the cloud has been a promising trend since the cloud is usually employed as data storage. In this regard, searchable encryption (SE), which was first introduced by Song et al. [ 102 ] in 2000, can be used to share encrypted e-health data in the cloud. The SE, which is a cryptographic primitive, encrypts e-health data to be keyword-searchable over encrypted data as described in Figure 9 .

Overview of searchable encryption.
In a nutshell, if a patient (i.e., the data owner) first encrypts their e-health data to be searchable and uploads that encrypted data to the cloud, users (e.g., doctors and researchers) can then query the encrypted e-health data using desired keywords. Yang et al. [ 103 ], Xu et al. [ 104 ], and Chen et al. [ 105 ] adopted searchable encryption to share e-health data in the cloud environments. Yang et al. [ 103 ] proposed a new cryptographic primitive, conjunctive keyword search, with a proxy re-encryption function enabled by a designated tester (i.e., a server that can execute equality test function) and timing. Based on the proposed time-limited SE scheme, a patient can delegate access permissions to desired people so that they can search over the patient’s e-health data for a limited time. In addition, the time period to search and decrypt the patient’s data can be controlled, and the permissions are automatically revoked after the time period. Xu et al. [ 104 ] also proposed a privacy-preserving e-health data sharing scheme using SE with keyword range search and multiple keyword search. Moreover, the encrypted data can be searched by comparing different numeric types based on the proposed equality test function. In the proposed scheme, e-health data and keyword files for the data were encrypted using a symmetric key, and homomorphic encryption was used to protect the privacy of keyword in the equality test phase. Chen et al. [ 105 ] designed blockchain-based searchable encryption for e-health data sharing. They stored the indices for the data in blockchain as the form of complex logic expressions (e.g., “gender”: “male”) to make a user can use the indices for searching specific e-health data. Since the proposed scheme utilizes blockchain, it provides integrity, anti-tampering, and accountability. In addition, Yao et al. [ 106 ] proposed a multi-source order-preserving symmetric encryption (MOPSE) scheme. Compared with other searchable encryption schemes, the proposed scheme enables a data owner to efficiently query over multiple data providers’ encrypted e-health data. To this end, the cloud merges multiple encrypted indices from different data providers of the same data owner.
Authentication. Authentication is an indispensable security solution to prevent attackers and malicious users from accessing the data in the edge, fog, and cloud computing. The authentication becomes more important in cloud environments because the cloud stores patients’ big e-health data, which can show the patients’ medical history. There have been authentication studies focusing on mutual [ 107 , 108 , 109 , 110 ], anonymous [ 111 ] and traceable authentication [ 112 ].
First, mutual authentication schemes [ 107 , 108 , 109 , 110 ] were proposed for the edge, fog, and cloud environments. Li et al. [ 107 ] proposed a cloud-assisted mutual authentication scheme for telecare medical information systems (TMIS) by enhancing Mohit et al.’s authentication scheme [ 113 ] to be more secure and support anonymity using a dynamic pseudo-random nonce. In addition, Liu et al. proposed a novel privacy-preserving mutual authentication (NPMA) [ 108 ] and a blockchain-based privacy-preserving mutual authentication (MBPA) [ 109 ] for TMIS environments. The NPMA was designed for secure remote user authentication in the mobile edge-cloud network, which medical services are distributed in the most logical, nearby, and efficient place of the network [ 108 ]. In addition to the mutual authentication, the NPMA also provided anonymity of a patient and edge-cloud server and data confidentiality using anonyms and certificateless cryptography, respectively. On the other hand, in [ 109 ], a privacy-preserving mutual authentication was proposed for mobile medical cloud architecture based on blockchain to prevent data breach. They stored and managed the encrypted e-health data in a blockchain cloud. Each blockchain node shares the secret value for authentication. Especially, their sharing process is conducted without key negotiation rounds. Therefore, it only needs low computational cost between terminal and node rather than the traditional blockchain model. Last but not least, there was a mutual authentication study [ 110 ] designed for wearable devices using hybrid computing that consists of edge and cloud. In particular, mutual authentication was performed using the space-aware edge computing for allowing users to access the local services in a hospital.
Moreover, Mehmood et al. [ 111 ] proposed an anonymous authentication scheme based on cloud to provide complete privacy and anonymity to a user from the adversaries and the authentication server by utilizing a rotating group signature scheme based on ECC. In a group, all members share an expiration date and each of them updates their keys periodically to prevent the traceability. They also added an extra layer to provide anonymity on the network level by utilizing TOR. This can prevent traffic analysis attacks from an eavesdropper. Meanwhile, Liu et al. [ 112 ] proposed a traceable authentication protocol. They protected the privacy of patients and anonymity by means of randomized pseudonyms. The real identity of patients can also be extracted from the pseudonyms by the authentication server. The proposed authentication scheme is useful for resource-constrained mobile devices because it consumes low communication cost and energy.
Access control. Access control is another indispensable security solution with authentication for edge, fog, and cloud computing. Among various access control models, most studies were based on the ABE scheme to realize a fine-grained access control model [ 114 , 115 , 116 , 117 , 118 ] without a situation-based access control model [ 119 ]. In addition, there were access policy studies that focused on privacy preservation for access policy [ 120 ], dynamic access policy transformation [ 121 ], and updating access policies [ 122 ].
Since access control studies [ 114 , 115 , 116 , 117 , 118 ] are generally based on CP-ABE to provide a fine-grained access control model, the fundamental access control mechanism remains the same. E-health data are encrypted with a desired access policy using attributes and only authorized users who have proper attributes for the corresponding data’s access policy can access it. However, there are some differences in the details among the studies. Each study has an additional security solution (i.e., trust evaluation [ 114 ], dynamic auditing [ 115 ], online/offline CP-ABE [ 117 ], and unified access policy [ 118 ]) or a specific purpose (i.e., supporting multiple cloud servers [ 116 ]). The five access control studies are described in more detail below.
First, Yan et al. [ 114 ] proposed a flexible access control scheme based on ABE. Unlike the other ABE-based access control schemes, they adopted context-aware trust and reputation evaluation into the flexible access control scheme to support various data usage scenarios, for example, cloud data sharing with others. For example, data access can directly be determined by the data owner or reputation centers in an indirect way in case of the data owner is not available or cannot make an access decision. If a user has an adequate reputation, the reputation centers apply PRE to make a new ciphertext that the user can decrypt based on the pre-defined data owner’s access policy.
Second, Yeh et al. [ 115 ] proposed a cloud-based fine-grained access control framework. They controlled access using CP-ABE which enables a data owner to delegate access permissions to others by defining access policy. If a user has proper attributes for the access policy, a new ciphertext for the user is generated using PRE to make only authorized users can use the data. In addition, the proposed framework is suitable for resource-constrained IoT devices because only symmetric key encryption is used to encrypt the data when it is uploaded to the cloud. Dynamic data auditing was also used to verify data integrity using Merkle hash tree (MHT), which is a binary tree of hashes. Since a parent node’s hash is generated using the child nodes’ hashes, fast and efficient verifying integrity of e-health data can be done by checking a parent node’s hash.
Third, Roy et al. [ 116 ] designed a fine-grained access control for multi-server along with mutual authentication of users in mobile could computing environment. The proposed scheme guaranteed a low communication cost and lightweight authentication procedure because of no involvement of a registration server. It is also suitable for resource-constrained devices by mostly utilizing one-way hash function and bitwise XOR operations.
Fourth, Liu et al. [ 117 ] proposed a fine-grained access control scheme; however, they adopted online/offline CP-ABE to make resource-constrained devices in mobile cloud computing perform fine-grained data sharing. Based on the online/offline cryptography, a data owner can generate offline ciphertext before the data and access policy to be encrypted are known. The offline ciphertext which consumed a majority of computing power is then used to assemble the final ciphertext when the data and access policy are known.
Fifth, Li et al. [ 118 ] proposed a new ABE scheme for fine-grained access control framework based on unified access policy generated from multiple access policies of patients’ various e-health data. The proposed scheme improves the efficiency of encryption and decryption by combining encryption of different patients’ data that share common access policy to eliminate repetitive processes.
In addition to the ABE-based fine-grained access control schemes, Gope et al. [ 119 ] designed an access control model that can cover diverse situations including break-the-glass (i.e., emergency case) without compromising security to share e-health data based on RBAC and mandatory access control (MAC) policy. They specifically argued the access control model for e-health data should not compromise security even in an emergency because a user can misuse the break-the-glass situation for malicious purposes. To this end, this study considered the situations into the access control mechanism by proposing a situation controller that measures a patient’s situation according to the pre-defined situation types (i.e., normal, critical, emergency, and super emergency) so that it can control access depending on the situations.
Furthermore, three studies have focused on access policy in terms of privacy [ 120 ], transformation [ 121 ], and updating [ 122 ]. Ying et al. [ 120 ] designed a concealing algorithm of access policy, that can also recover hidden attributes to provide privacy regarding the access policy. To hide the access policy, they used a linear secret sharing scheme (LSSS) and proposed an element filter, Attribute Cuckoo Filter (ACF), to match whether given attributes are in the anonymized access policy. Rezaeibagha et al. [ 121 ] proposed a secure and privacy-preserving e-health data sharing scheme in hybrid cloud computing environments by transforming access policy from a private cloud to a public cloud. For the transformation, attribute-based proxy re-encryption was used. Lastly, Ying et al. [ 122 ] proposed a method to update access policy in the outsourced ciphertext of e-health data in cloud computing. Conventional CP-ABE needs to re-encrypt entire e-health data when an access policy for the data is changed, whereas the proposed scheme changes only a part of the ciphertext using LSSS if an access policy is updated.
Last but not least, Wang et al. [ 123 ] and Saha et al. [ 124 ] proposed fog computing-enabled access control schemes to protect e-health data. In more specific, Wang et al. [ 123 ] used an access controller that controls access based on the task types and pre-defined privacy levels, and Saha et al. [ 124 ] controlled access using identity token generated using the ABE scheme. They employed fog computing to reduce communication costs and response time between medical devices and the server.
Provable data possession and proofs of retrievability. Cloud storage is a semi-trust model, that is, an honest-but-curious model, so some security concerns have emerged for cloud computing. Generally, a user who outsources e-health data to the cloud cannot be aware of the data’s status. In other words, data stored in the cloud can be altered or deleted without the data owner’s consent. To solve the problem of public verifiability, provable data possession (PDP), which is a technique for checking the integrity of outsourced data, and proofs of retrievability (PoR), which is a technique that allows the owner to check the retrievability of the data, have been studied. Figure 10 shows a brief overview of PDP and PoR.

Overview of PDP and PoR.
In PDP ( Figure 10 a), a user requests a challenge to the cloud to check the integrity of their e-health data. The cloud that received the challenge request then computes the proof of possession (P) and sends this to the user. Finally, the user who requested the P can verify the integrity of their e-health data by comparing the P with the metadata that the user stored locally when they outsourced their data to the cloud [ 125 ]. PDP is an efficient scheme to verify the integrity of outsourced data, because the user does not need to store or verify all of their data. On the other hand, PoR ( Figure 10 b) encrypts data and randomly embeds a set of randomly valued check blocks (i.e., sentinels). The user challenges the cloud by specifying the positions of a set of sentinels and requesting that the cloud respond to the sentinel values [ 126 ]. Based on the sentinels, the user can check the availability and integrity of the outsourced e-health data without downloading all of their data.
There are studies that propose a scheme that supports public verifiability for the outsourced data in cloud-based on PDP and/or PoR. Wang et al. [ 127 ] proposed an identity-based data outsourcing (IBDO) to provide integrity and comprehensive auditing. In the scheme, a user or an authorized proxy can outsource data in the cloud with their identities. This is efficient for multi-user environments because it does not depend on the complex cryptographic certificates to identify the clients. In addition, the origin, type, and consistency of the outsourced data can be publicly verified using the proposed scheme. Fan et al. [ 128 ] then proposed a privacy-preserving identity-based auditing scheme. This scheme enables users to share e-health data with others while it keeps the private information invisible to the cloud and others including malicious cloud manager who has high privileges. Lastly, Shi et al. [ 129 ] proposed a certificateless provable data possession (CL-PDP) scheme that provides public verifiability and complete anonymity. In particular, the proposed scheme can prevent the key escrow problem since it is based on certificateless public-key cryptography, and it is efficient because it eliminates the bilinear pairing operations which need high computational cost.
Blockchain A blockchain ensures data integrity and accountability by recording every transaction in a distributed ledger; this has been widely adopted to securely store and share e-health data. To provide a secure sharing scheme, studies have combined a blockchain with other security solutions, that is, access control [ 130 ], searchable encryption [ 131 ], ECC [ 132 ], and Tor [ 133 ]. Details on these studies follow below.
Nguyen et al. [ 130 ] proposed a sharing framework for e-health data in mobile cloud computing by combining blockchain and decentralized interplanetary file system (IPFS) which is a solution to realize a file sharing platform in blockchain [ 134 ]. Especially, they designed an access control mechanism using smart contracts of blockchain to securely share e-health data. However, data confidentiality may not be ensured since EHR manager where manages the encryption and decryption keys of stored e-health data cannot be fully trusted. Then, Wang et al. [ 131 ] proposed a blockchain-based privacy-preserving e-health data sharing scheme using searchable encryption and proxy re-encryption. By using the proposed scheme, a user can search required data then receive the data under the owner’s authorization. In the study, the cloud is used to store ciphertext of e-health data and re-encrypt the ciphertext for sharing, and blockchain is used to store keyword ciphertext required to search and share the data. This scheme however cannot fully ensure the owner’s data ownership because of the data provider that uploads the data to the cloud server instead of the owner. Therefore, Omar et al. [ 132 ] proposed a user-centric e-health data management system that a user has full ownership of the data based on ECC. In other words, only the data owner can control access to the data since the owner manages the encryption key. Similarly, Rahman et al. [ 133 ] proposed a blockchain-based secure therapy framework that provides e-health data integrity, privacy, ownership, and sharing. However, compared with other works, they employed mobile edge computing (MEC) and Tor. The framework reduced network latency by means of MEC and supported anonymity using the Tor.
Decoy. A decoy technique, also known as a honeypot, can be used to lure intruders. If an intruder touches a decoy, it is closely monitored so that a security manager can detect the intrusion and prevent subsequent attacks. A good decoy should provide detectability, conspicuousness, believability, enticement, differentiability, and non-interference [ 135 ]. The decoy should first be easily detectable and accessible, and then seem authentic and attractive to attackers; at the same time, it should be differentiable and non-interfering to ensure that naive users do not use it. In a real scenario, a decoy can be e-health data in the cloud to detect intrusions and prevent attacks. Al Hamid et al. [ 136 ] proposed a security model utilizing a decoy technique to protect big medical data in the cloud using fog computing. In the proposed security model, the fog computing facility in front of the cloud generates a decoy e-health data then shows it to an attacker who accesses the system.
Table 6 shows a summary of the security and privacy studies on edge, fog, and cloud computing.
Summary for the security and privacy studies on edge, fog, and cloud computing.
7. Research Trend and Open Challenge
We reviewed recent security and privacy studies for the modern e-health systems in terms of data, device, network, and edge/fog/cloud computing. Based on the review, we identified recent research trends and open challenges for each component of the e-health systems. Therefore, we discuss the research trends and challenges in this section.
7.1. E-Health Data
Recent studies focused on designing a security solution that can protect the data. Most studies adopted cryptography and anonymization techniques for data confidentiality, integrity, anonymity, and secure sharing of e-health data. In addition, they proposed efficient security solutions to enhance the security and privacy of conventional e-health systems. Detailed descriptions of the research trends are as follows.
Fast and efficient encryption scheme with high security. Data confidentiality is the most important security requirement in the medical/healthcare research areas. Traditional cryptosystems such as AES and RSA have been widely utilized to design secure e-health systems; however, a faster and more efficient encryption/decryption scheme is particularly required when dealing with large volumes of e-health data. Since e-health data are highly sensitive, maintaining a tradeoff between the strength of encryption is a crucial issue. For example, several studies researched faster and more efficient cryptographic primitives or algorithms for medical image security [ 27 , 28 , 29 , 75 ].
Securing e-health data with a blockchain. E-health data can be lost, tampered, and deleted. A blockchain with a public and distributed ledger becomes a promising technology to secure e-health data since it records all transactions related to the data. In general, blockchains provide e-health data integrity with transparency, auditability, and accountability; however, authentication, access control, and other security applications such as secure data sharing have been studied based on smart contracts, which is a small program on a blockchain. In addition, studies have proposed efficient schemes to reduce blockchain transaction fees.
Privacy-preserving sharing of e-health data with data anonymization. Sharing e-health data is an emerging trend for several purposes such as remote care of individuals and studying big e-health data. To preserve privacy while sharing e-health data, data anonymization models such as k-anonymity, l-diversity, t-closeness, and differential privacy have been widely adopted in medical and healthcare research areas. Regarding this research topic, Zhang et al. [ 137 ] specifically studied on the security and privacy requirements and risks of medical data sharing based on blockchain.
Various studies for security and privacy of e-health data have been conducted; however, there are still some open challenges. In particular, re-identification prevention of anonymized data is an important research area since anonymized data can be re-identified, and data anonymization is the essential technique when e-health data should be shared. The detailed open challenges are as follows.
More efficient and faster cryptosystem. Though fast and efficient cryptosystems have been studied, more efficient and faster cryptosystems will be required as e-health data has become diversified and increased with emerging smart healthcare devices and services. Servers and aggregators of the e-health systems in particular need efficient, fast, and lightweight cryptosystems to provide data confidentiality with high scalability even in resource-constrained environments such as WBANs and IoMTs. In addition, considering medical imaging may become important because the medical imaging process accounts for 90% of all medical information processes [ 39 ].
Resistance to re-identification. Data anonymization is an essential technique to preserve privacy when e-health data are shared with someone. However, existing data anonymization techniques could be broken by some re-identification attacks. For example, Rocher et al. [ 138 ] recently proposed a model that can precisely estimate the re-identification likelihood of a specific person, even in an incomplete dataset. Data anonymization techniques will be evaluated on diverse datasets and attacks to prove that techniques can provide complete privacy preservation under any circumstances.
Emergent access to patient’s data. Access control for e-health data is an indispensable security solution to prevent unauthorized access to patients’ e-health data. Only authenticated and authorized users should be able to access the data by means of access control. However, medical staff may need to access patients’ data in an emergency, despite the data being highly confidential and the staff normally lacking access permission. This functionality is prominent since it can be directly related to a patient’s health condition and life in an emergency.
7.2. Medical Device
Conventional security solutions cannot be applied to medical devices because of the limited resources. Therefore, recent research trends for the security and privacy of medical devices are focused on efficient security solutions or a method that can alleviate the resource constraint problem. Three research trends for medical devices are as follows.
Online authentication. Online authentication is required when a doctor need to remotely access and monitor a medical device in an emergency situation. A secure channel should be established for the online authentication because it needs to access the device over the Internet. However, this scheme has the disadvantage that the Internet must be connected for authentication, which may not always be available [ 10 ].
Proxy-based security. A proxy server that supports security capabilities such as cryptography, authentication, and access control can be used between medical devices and external devices because medical devices lack the resources. Security solutions, such as IMD-Shield and IMDGuard, enhanced the security of existing medical devices in the middle of the communications [ 14 , 139 , 140 ] based on the proxy.
Low-power and zero-power security solutions. Security is not an essential part of the function of medical devices; however, it must be considered to secure medical devices and their e-health data. The problem is that security solutions have high energy demands for devices. Therefore, low-power and zero-power security solutions were studied to resolve the resource constraint problem of medical devices [ 10 ]. Based on the low-power or zero-power security solutions, a medical device can work securely for longer.
According to our survey, there are insufficient studies for the security and privacy of medical devices; therefore, huge effort to research the security and privacy for the modern medical devices is required in the near future. The open challenges are as follows.
Resource constraint of medical devices. Medical devices have limited resources such as low computing power, battery, and memory capacity; therefore, security solutions for medical devices should be designed with consideration of their constrained resources. To provide minimum security requirements for medical devices, efficient and lightweight security solutions for cryptographic primitives, encryption algorithms, authentication, and access control must be studied. As the demand for medical devices increases, efficient and lightweight security solutions for medical devices will become more important.
Security and privacy by design. Networked medical devices have been newly developed to support modern e-healthcare services. Therefore, the security concerns and requirements for medical devices have not been sufficiently studied and conventional security solutions are not suitable for medical devices because of their characteristics. To improve security and reliability, security and privacy by design are required to identify and adopt optimal security solutions for medical devices and their sensitive e-health data by studying their major security concerns and requirements.
Trust management. Medical devices that sense a patient’s health condition should manage the trust. It is important to provide a certain level of trust because doctors’ diagnoses that can affect patients’ health can differ depending on the sensed health information [ 3 ]. In particular, in the case of patients in critical condition, their medical devices must provide a high level of trust.
Emergent access to medical devices. One important challenge for medical devices is the capability of emergent access to medical devices [ 12 , 141 ]. Basically, strict authentication and authorization are required to protect the security of medical devices; however, medical staff should be able to access patients’ medical devices in an emergency if patients are unavailable or lose consciousness. This functionality is critical by dint of being directly related to the patients’ life.
7.3. Medical Network
To provide security and privacy for e-health data with limited resources, security solutions in the surveyed studies have generally been focused on efficiency and simplicity. Detailed research trends for the security and privacy of the medical networks are as follows.
Efficient and secure transmission. Most security and privacy studies applied cryptography to provide data confidentiality and integrity, which are the most important requirements when transmitting e-health data via a medical network. Meanwhile, efficiency should also be considered, since medical networks are resource-constrained in terms of computational costs, bandwidth, energy constraints, and so forth. Signcryption, online/offline encryption, compressive sensing, and batch operation are representative techniques to improve efficiency in the medical network research area.
Privacy-preserving data aggregation with homomorphic encryption. Once e-health data has been encrypted, only legitimate participants such as the data owner and medical staff must be able to decrypt the data. However, there is a case that needs to decrypt e-health data where the data has been aggregated and processed in the middle of the network. In this case, most studies adopted homomorphic encryption to protect data confidentiality by computing on ciphertext directly. In other words, sensitive e-health data can be processed without data decryption by means of homomorphic encryption. The studies utilized several cryptosystems that have the homomorphic property (e.g., ElGamal, Paillier, and Boneh–Goh–Nissim).
Certificateless cryptography techniques. Public-key cryptography is required to provide authenticity and non-repudiation; however, conventional public-key infrastructure has some shortcomings such as the key escrow problem and complex certificate management. Therefore, certificateless public-key cryptography has been utilized in various studies to eliminate both the key escrow problem and certificate management. In the surveyed studies, certificateless cryptography techniques were combined with diverse security solutions such as authentication, signature, and signcryption.
Mutual authentication. Mutual authentication is essential to ensure that e-health data are transmitted from the right patient and is received by the desired medical staff. For example, mutual authentication is required to ensure the integrity of a patient’s e-health data and the doctor’s prescription in a remote e-healthcare service. If the patient’s data or doctor’s prescription has been compromised, this can lead to a critical situation. According to our survey, authentication studies in the medical network research area were generally categorized into lightweight authentication schemes that are efficient for resource-constrained environments and anonymous authentication schemes that focus on privacy preservation during the authentication process.
Since medical networks transmit e-health data, strong security solutions should be applied. However, existing strong security solutions cannot be directly adopted because of the limited resources of medical networks. Maintaining a reasonable tradeoff between the strength and efficiency of the security solution, therefore, is the main challenge in the medical network research area. Open challenges for the security and privacy of the medical network areas are as follows.
Resource constraint. Conventional security solutions cannot be directly applied to medical networks such as WBANs and IoMTs since they are resource-constrained in terms of computing power, memory capacity, and bandwidth. Although resources are limited, security solutions such as cryptography and authentication are indispensable for network security. Efficient security solutions have been studied; however, it remains difficult to provide sufficient security and reliability for highly sensitive e-health data with limited resources compared to other environments. Making a better tradeoff between security and efficiency is a challenging problem.
Conditional privacy preservation. Patient privacy must be preserved. The identities of patients in medical networks are anonymous so that adversaries cannot specify patients’ real identities. However, a user’s real identity should be discernible in some cases so that a trusted provider can trace them. Based on this traceability, an adversary who has malicious purposes can be identified by a trusted server. To this end, Ji et al. [ 60 ] and Yang et al. [ 63 ] designed a conditional identity preservation scheme; however, some studies argued that identities must be anonymous in any circumstances to provide untraceability. Despite having the advantage that an adversary or malicious user can be traced, a conditional privacy-preserving scheme also has the disadvantage that an insider can abuse its functionality. In the near future, the tradeoff between the advantages and disadvantages should be studied in detail. In addition, an abuse prevention scheme should be studied for the conditional privacy-preserving function.
7.4. Edge, Fog, and Cloud
Based on the sufficient resources, security and privacy studies that deploy edge, fog, and cloud computing have focused on useful functionalities such as secure data outsourcing and sharing. Detailed descriptions for the research trends on the edge, fog and cloud computing are as follows.
Secure outsourcing. A cloud-based service provider is an honest-but-curious model. That is, although it follows the security protocols and solutions, it could also extract some private information during the process. Therefore, outsourcing e-health data to a cloud-based service provider must be secure and transparent. The data owner must be able to check the integrity of the outsourced data because it could be altered and deleted in the cloud. Efficient and comprehensive data auditing schemes that provide public verifiability such as PDP and PoR will become more important as the use of cloud-based healthcare services increases.
Secure sharing of e-health data. E-healthcare systems collect individuals’ e-health data. Nowadays, big e-health data are considered a valuable resource for diverse purposes. The e-health data both provide personalized healthcare services (e.g., remote health condition monitoring, diagnosis, and treatment) and can be used to study diseases. To this end, security and privacy must be required to share highly sensitive e-health data. The data requester (e.g., a patient) and receiver (e.g., a doctor) must be authenticated and authorized and certain cryptography schemes such as proxy re-encryption, attribute-based encryption, and searchable encryption were used to securely share data. In addition, blockchain is a promising security solution to ensure data integrity and accountability, which are important security requirements for e-health data sharing.
Fine-grained access control using attribute-based encryption. The cloud is mostly used to store e-health data; therefore, it is inevitable that the cloud will store big e-health data. In state-of-the-art studies, fine-grained access control schemes have been widely proposed to protect e-health data based on ABE. Since ABE encrypts and decrypts data with diverse attributes, it is very flexible in terms of realizing fine-grained access control. Moreover, recent studies have adopted additional security solutions such as blockchain, trust management, dynamic auditing, and online/offline cryptography schemes to support various security requirements.
Various studies have been conducted, however, there are some open challenges that should be solved in the near future. In particular, as e-health service providers increase, a need for secure data sharing and interoperability between the providers have been increasingly grown. The rest of this section presents the open challenges.
Improvement of usability for secure data sharing. Recently, secure data sharing studies have been conducted, and a service that shares e-health data has been launched. However, it still lacks usability because of security and privacy concerns. For example, the Healthcare Big Data Platform [ 4 ], a South Korean e-health data sharing service, has been launched for the public use of patients’ health data; however, it takes a long time to use the data because of complex request processes that consist of eight phases. In addition, researchers can request just limited data that is registered in the data catalog; therefore, enhancing the usability of sharing e-health data, while ensuring the security and privacy of data, is a challenging problem.
Secure interoperability among multiple e-health data providers. As e-healthcare services increase, patients’ e-health data have been widely distributed in various service providers. In a real scenario, data can be distributed despite belonging to the same patient because a patient can use various service providers. Therefore, secure interoperability among multiple data providers is required to provide user-centric data governance for distributed data. In other words, users should be able to search, use, and manage their distributed data across heterogeneous data providers based on secure interoperability. To this end, end-to-end security and mutual authentication must be established among providers and other security solutions including fine-grained access control and access policy translation should be studied with the consideration of newly emerging security concerns and requirements for secure interoperability.
Complete and conditional anonymity. E-health data and user identities should be anonymized depending on the situation. To this end, some studies provide complete anonymity in which data cannot be identified in any situation, while other studies provide conditional anonymity where data can be identified in a special situation. There is controversy among researchers over whether anonymity must not be breached in any case or conditional anonymity is required in a few cases to identity attackers. The two types of anonymization studies have different advantages: strong privacy, and conditional traceability, respectively; however, complete anonymity cannot provide traceability while conditional anonymity cannot provide a high level of privacy since identities can be revealed. Therefore, finding anonymization schemes that can provide high privacy while considering traceability is an open challenge.
In-depth security analysis for edge and fog computing. Storing and processing e-health data in cloud computing are prominent research trends; however, edge and fog computing paradigms have been emerging because of the latency-sensitive and context-awareness requirements [ 142 , 143 ]. In particular, edge and fog computing can be utilized to develop real-time medical services that support space- and time-awareness and can also preprocess and analyze e-health data in a secure and private manner to reduce the communication cost between medical devices and the cloud. Since the edge and fog computing paradigms have recently been integrated into modern e-healthcare systems, in-depth security analysis that considers real e-healthcare scenarios will be required in the near future to identify new types of security concerns and requirements.
A lack of open-source-based edge, fog, and cloud computing platforms. According to our survey, few studies have implemented the proposed security solutions based on real edge, fog, and cloud environments. The implementation of security solutions based on real environments would be valuable work to demonstrate their feasibility and real performance; however, it is difficult to build environments based on edge, fog, and cloud computing. Therefore, building open-source-based platforms for edge, fog, and cloud computing that can simply be used to implement and evaluate the proposed security solutions remains an open challenge.
8. Conclusions
Innovations in e-health systems present a double-edged sword. Although they provide advanced healthcare services, there are increasing security concerns with regard to e-health data, which is highly sensitive information. Therefore, we have surveyed recent studies on security and privacy issues related to e-health data according to the target domains, that is, e-health data, medical devices, medical networks, and edge/fog/cloud computing. In this survey, we identified the security concerns and requirements that are commonly mentioned in studies and provided promising security solutions. In particular, based on the literature review, we developed four taxonomies on the security concerns, requirements, and solutions for each component of modern e-health systems. Furthermore, we analyzed the strengths and weaknesses of the surveyed studies, and provided recent research trends and open challenges on security and privacy for the e-health systems. Compared to other surveys, we comprehensively reviewed the security and privacy issues for e-health data including the surrounding environments, that is, medical devices, medical networks, and edge/fog/cloud computing. Finally, as e-health systems become more complex across various layers and data have been exchanged among different domains, secure interoperability among heterogeneous e-health systems should be specifically researched in the near future.
Author Contributions
The authors contributed to this paper as follow: S.-R.O. wrote this article and and analyzed the research; E.L. and Y.-D.S. performed checks of the manuscripts; Y.-G.K. supervised and coordinated the investigation. All authors have read and agreed to the published version of the manuscript.
This research was supported by a grant from the Korea Health Technology R&D Project through the Korea Health Industry Development Institute (KHIDI), funded by the Ministry of Health & Welfare, Republic of Korea (grant number: HI18C1140).
Institutional Review Board Statement
Not applicable.
Informed Consent Statement
Conflicts of interest.
The authors declare no conflict of interest.
Publisher’s Note: MDPI stays neutral with regard to jurisdictional claims in published maps and institutional affiliations.
Secure Communications with THz Reconfigurable Intelligent Surfaces and Deep Learning in 6G Systems
- Published: 22 May 2024
Cite this article
- Ajmeera Kiran 1 ,
- Abhilash Sonker 2 ,
- Sachin Jadhav 3 ,
- Makarand Mohan Jadhav 4 ,
- Janjhyam Venkata Naga Ramesh 5 &
- Elangovan Muniyandy 6 , 7
In anticipation of the 6G era, this paper explores the integration of terahertz (THz) communications with Reconfigurable Intelligent Surfaces (RIS) and deep learning to establish a secure wireless network capable of ultra-high data rates. Addressing the non-convex challenge of maximizing secure energy efficiency, we introduce a novel deep learning framework that employs a variety of neural network architectures for optimizing RIS reflection and beamforming. Our simulations, set against scenarios with varying eavesdropper cooperation, confirm the efficacy of the proposed solution, achieving 97% of the optimal performance benchmarked against a genie-aided model. This research underlines a significant advancement in 6G network security, potentially influencing future standards and laying the groundwork for practical deployment, thereby marking a milestone in the convergence of THz technology, intelligent surfaces, and AI for future-proof secure communications.
This is a preview of subscription content, log in via an institution to check access.
Access this article
Price includes VAT (Russian Federation)
Instant access to the full article PDF.
Rent this article via DeepDyve
Institutional subscriptions
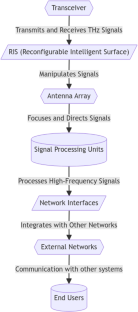
Data Availability
No datasets were generated or analysed during the current study.
Code Availability
No code is available for this manuscript.
Chaccour, C., Soorki, M., Saad, W., Bennis, M., Popovski, P., & Debbah, M. (2022). Seven defining features of terahertz (thz) wireless systems: A fellowship of communication and sensing. IEEE Communications Surveys & Tutorials , 24 (2), 967–993. https://doi.org/10.1109/comst.2022.3143454 .
Article Google Scholar
Chen, H., Padilla, W., Zide, J., Gossard, A., Taylor, A., & Averitt, R. (2006). Active terahertz metamaterial devices. Nature , 444 (7119), 597–600. https://doi.org/10.1038/nature05343 .
Gao, X., Zhang, T., Du, J., Weily, A., Guo, Y., & Foley, C. (2017). A wideband terahertz high-tcsuperconducting josephson-junction mixer: Electromagnetic design, analysis and characterization. Superconductor Science and Technology , 30 (9), 095011. https://doi.org/10.1088/1361-6668/aa7cc1 .
Gonzalez-Guerrero, L., Graham, C., George, J., Renaud, C., George, G., Hussain, B., & Porcel, M. (2021). Design and fabrication of sub-thz steerable photonic transmitter 1×4 array for short-distance wireless links. https://doi.org/10.1109/eucnc/6gsummit51104.2021.9482473 .
Rappaport, T., Xing, Y., Kanhere, O., Ju, S., Madanayake, A., Mandal, S., & Trichopoulos, G. (2019). Wireless communications and applications above 100 ghz: Opportunities and challenges for 6 g and beyond. IEEE Access , 7 , 78729–78757. https://doi.org/10.1109/access.2019.2921522 .
Rommel, S., Morales, A., Konstantinou, D., Raddo, T., & Monroy, I. (2018). Mm-wave and thz analog radio-over-fiber for 5 g, wireless communications and sensing. https://doi.org/10.1364/laop.2018.w3d.1 .
Shehata, M., Wang, K., Webber, J., Fujita, M., Nagatsuma, T., & Withayachumnankul, W. (2021). IEEE 802.15.3d-compliant waveforms for terahertz wireless communications. Journal of Lightwave Technology , 39 (24), 7748–7760. https://doi.org/10.1109/jlt.2021.3113310 .
Shin, D., Kim, B., Jang, H., Kim, Y., & Kim, S. (2023). Photonic comb-rooted synthesis of ultra-stable terahertz frequencies. Nature Communications , 14 (1). https://doi.org/10.1038/s41467-023-36507-y .
Yuan, X., Zhang, Y., Shi, Y., Yan, W., & Liu, H. (2020). Reconfigurable-intelligent-surface empowered wireless communications: challenges and opportunities. https://doi.org/10.48550/arxiv.2001.00364 .
Başar, E., Renzo, M., Rosny, J., Debbah, M., Alouini, M., & Zhang, R. (2019). Wireless communications through reconfigurable intelligent surfaces. IEEE Access , 7 , 116753–116773. https://doi.org/10.1109/access.2019.2935192 .
Dai, L., Wang, B., Wang, M., Yang, X., Tan, J., Bi, S., & Hanzo, L. (2020). Reconfigurable intelligent surface-based wireless communications: Antenna design, prototyping, and experimental results. IEEE Access , 8 , 45913–45923. https://doi.org/10.1109/access.2020.2977772 .
He, D., Quek, T., Chen, S., & Hanzo, L. (2021). Deep learning-assisted terahertz qpsk detection relying on single-bit quantization. IEEE Transactions on Communications , 69 (12), 8175–8187. https://doi.org/10.1109/tcomm.2021.3112216 .
Hou, T., Liu, Y., Song, Z., Sun, X., Chen, Y., & Hanzo, L. (2020). Reconfigurable intelligent surface aided noma networks. IEEE Journal on Selected Areas in Communications , 38 (11), 2575–2588. https://doi.org/10.1109/jsac.2020.3007039 .
Lallas, E. (2019). Key roles of plasmonics in wireless thz nanocommunications—a survey. Applied Sciences , 9 (24), 5488. https://doi.org/10.3390/app9245488 .
Pan, Y., Wang, K., Pan, C., Zhu, H., & Wang, J. (2022). Self-sustainable reconfigurable intelligent surface aided simultaneous terahertz information and power transfer (stipt). IEEE Transactions on Wireless Communications , 21 (7), 5420–5434. https://doi.org/10.1109/twc.2022.3140268 .
Petrov, V., Bodet, D., & Singh, A. (2023). Mobile near-field terahertz communications for 6 g and 7 g networks: Research challenges. Frontiers in Communications and Networks . https://doi.org/10.3389/frcmn.2023.1151324 . 4.
Renzo, M., Zappone, A., Debbah, M., Alouini, M., Yuen, C., Rosny, J., & Tretyakov, S. (2020). Smart radio environments empowered by reconfigurable intelligent surfaces: How it works, state of research, and the road ahead. IEEE Journal on Selected Areas in Communications , 38 (11), 2450–2525. https://doi.org/10.1109/jsac.2020.3007211 .
Xu, L., Yao, Y., Chang, J., Fang, H., & Li, X. (2021). Broadband hybrid precoding scheme based on cyclic delay in terahertz communications. IEEE Access , 9 , 141360–141366. https://doi.org/10.1109/access.2021.3120097 .
Download references
No funding has been provided to prepare the manuscript.
Author information
Authors and affiliations.
Department of Computer Science and Engineering, MLR Institute of Technology, Dundigal, Hyderabad, Telangana, 500043, India
Ajmeera Kiran
Department of IT, MITS Gwalior, Gwalior, Madhya Pradesh, India
Abhilash Sonker
Pimpri Chinchwad University, Pune, Maharashtra, India
Sachin Jadhav
Department of E & TC, N B N Sinhgad Technical Institutes Campus, Pune, India
Makarand Mohan Jadhav
Department of Computer Science and Engineering, Koneru Lakshmaiah Education Foundation, Guntur Dist, Vaddeswaram, Andhra Pradesh, 522302, India
Janjhyam Venkata Naga Ramesh
Department of R&D, Bond Marine Consultancy, London, EC1V 2NX, UK
Elangovan Muniyandy
Department of Biosciences, Saveetha School of Engineering, Saveetha Institute of Medical and Technical Sciences, Chennai, 602 105, India
You can also search for this author in PubMed Google Scholar
Contributions
A.K. developed the conceptual framework and methodology, performed review and editing of the manuscript.A.S. curated datasets, conducted formal analysis, and investigation of results.S.J. formulated methodology, developed software implementation, and validated findings.M.M.J. acquired resources, supervised experiments, and visualization.J.V.N.R. administered the project, and wrote the original draft.E.M. supplied resources, managed supervision, and verification of outcomes.All authors have approved the final manuscript.
Corresponding author
Correspondence to Janjhyam Venkata Naga Ramesh .
Ethics declarations
Ethical approval.
This article contains no studies with human participants or animals performed by any of the authors.
Competing Interests
The authors declare no competing interests.
Additional information
Publisher’s note.
Springer Nature remains neutral with regard to jurisdictional claims in published maps and institutional affiliations.
Rights and permissions
Springer Nature or its licensor (e.g. a society or other partner) holds exclusive rights to this article under a publishing agreement with the author(s) or other rightsholder(s); author self-archiving of the accepted manuscript version of this article is solely governed by the terms of such publishing agreement and applicable law.
Reprints and permissions
About this article
Kiran, A., Sonker, A., Jadhav, S. et al. Secure Communications with THz Reconfigurable Intelligent Surfaces and Deep Learning in 6G Systems. Wireless Pers Commun (2024). https://doi.org/10.1007/s11277-024-11163-7
Download citation
Accepted : 24 April 2024
Published : 22 May 2024
DOI : https://doi.org/10.1007/s11277-024-11163-7
Share this article
Anyone you share the following link with will be able to read this content:
Sorry, a shareable link is not currently available for this article.
Provided by the Springer Nature SharedIt content-sharing initiative
- 6G Networks
- Terahertz communication
- Millimetre wave
- Intelligent surfaces
- Reconfigurable metamaterials
- Physical layer security
Advertisement
- Find a journal
- Publish with us
- Track your research
Speedster jailed after cops tap in-car system in first case here

- Share on Twitter
- Share on WhatsApp
- E-mail this article
- 0 Engagements
In the first such case here, the police tapped into the infotainment system of a woman’s car to extract data, which they then used to nab her for speeding.
She was jailed for five days, and disqualified from driving for two years.
The police’s new vehicle forensics capability was revealed at the Police Workplan Seminar 2024 on May 24 at the Singapore University of Technology and Design in Upper Changi Road.
The police said they are preparing to roll it out fully in 2024.
The incident involving the speedster happened in 2022.
The police’s Cybercrime Command received a request from the Traffic Police in late 2022 to extract data from her vehicle’s infotainment system, to investigate a possible speeding offence.
No Buddhist elements in S. Korean DJ’s shows after police warning
Related stories, man stabs passengers on taiwan train on 10th anniversary of deadly subway stabbing spree, drunk man tries to seize submachine gun from cop at police station in penang, make-up, pens stolen as shop theft cases rise.
Officers used the tool to extract datasets including call logs, messages and other data.
The police said the data confirmed the woman’s identity, and she was prosecuted based on the evidence gathered. She was convicted in January 2023.
They declined to reveal further details, including the car’s make and model.
The police added that they are developing their capability to extract data from a vehicle’s On-Board Diagnostics (OBD) port.
A proof of concept of this was shown at the seminar, demonstrating how telemetry data could be extracted via the port to pinpoint a car’s location, braking and acceleration patterns.
The extracted data could then be used to reconstruct a video rendering of the scene to aid investigators.
In response to queries from The Straits Times, a police spokeswoman said the vehicle forensics capabilities will also apply to other vehicles.
These include motorcycles, as long as the vehicle system can be read or analysed or is compatible with the system the police will be using.
She said: “SPF developed the vehicle forensics capability as there are valuable datasets stored in the vehicle including the vehicle infotainment system and OBD port, which would be useful to aid investigations into road traffic incidents.”
Extraction of the data can take between several hours and days, depending on the vehicle make and model, she added.
Asked what safeguards there are to protect the privacy of vehicle users, the spokeswoman said only authorised officers can extract the vehicle data, and all extracted data will be for the purpose of criminal investigations.
She added SPF will work with the Home Team Science and Technology Agency to enhance its capabilities in response to technological advancements in the automotive industry, such as with electric vehicles.
She said: “The Vehicle Forensics Team will be continually developing the SPF’s vehicle forensics capabilities with petrol vehicles. The team will also be broadening their scope to include electric vehicles, which would be of a different make from the traditional petrol vehicles.”
Get The New Paper on your phone with the free TNP app. Download from the Apple App Store or Google Play Store now
- Share on Facebook
Microsoft Research Blog
Microsoft at chi 2024: innovations in human-centered design.
Published May 15, 2024
Share this page
- Share on Facebook
- Share on Twitter
- Share on LinkedIn
- Share on Reddit
- Subscribe to our RSS feed

The ways people engage with technology, through its design and functionality, determine its utility and acceptance in everyday use, setting the stage for widespread adoption. When computing tools and services respect the diversity of people’s experiences and abilities, technology is not only functional but also universally accessible. Human-computer interaction (HCI) plays a crucial role in this process, examining how technology integrates into our daily lives and exploring ways digital tools can be shaped to meet individual needs and enhance our interactions with the world.
The ACM CHI Conference on Human Factors in Computing Systems is a premier forum that brings together researchers and experts in the field, and Microsoft is honored to support CHI 2024 as a returning sponsor. We’re pleased to announce that 33 papers by Microsoft researchers and their collaborators have been accepted this year, with four winning the Best Paper Award and seven receiving honorable mentions.
This research aims to redefine how people work, collaborate, and play using technology, with a focus on design innovation to create more personalized, engaging, and effective interactions. Several projects emphasize customizing the user experience to better meet individual needs, such as exploring the potential of large language models (LLMs) to help reduce procrastination. Others investigate ways to boost realism in virtual and mixed reality environments, using touch to create a more immersive experience. There are also studies that address the challenges of understanding how people interact with technology. These include applying psychology and cognitive science to examine the use of generative AI and social media, with the goal of using the insights to guide future research and design directions. This post highlights these projects.
Microsoft Research Podcast

Collaborators: Holoportation™ communication technology with Spencer Fowers and Kwame Darko
Spencer Fowers and Kwame Darko break down how the technology behind Holoportation and the telecommunication device being built around it brings patients and doctors together when being in the same room isn’t an easy option and discuss the potential impact of the work.
Best Paper Award recipients
DynaVis: Dynamically Synthesized UI Widgets for Visualization Editing Priyan Vaithilingam, Elena L. Glassman, Jeevana Priya Inala , Chenglong Wang GUIs used for editing visualizations can overwhelm users or limit their interactions. To address this, the authors introduce DynaVis, which combines natural language interfaces with dynamically synthesized UI widgets, enabling people to initiate and refine edits using natural language.
Generative Echo Chamber? Effects of LLM-Powered Search Systems on Diverse Information Seeking Nikhil Sharma, Q. Vera Liao , Ziang Xiao Conversational search systems powered by LLMs potentially improve on traditional search methods, yet their influence on increasing selective exposure and fostering echo chambers remains underexplored. This research suggests that LLM-driven conversational search may enhance biased information querying, particularly when the LLM’s outputs reinforce user views, emphasizing significant implications for the development and regulation of these technologies.
Piet: Facilitating Color Authoring for Motion Graphics Video Xinyu Shi, Yinghou Wang, Yun Wang , Jian Zhao Motion graphic (MG) videos use animated visuals and color to effectively communicate complex ideas, yet existing color authoring tools are lacking. This work introduces Piet, a tool prototype that offers an interactive palette and support for quick theme changes and controlled focus, significantly streamlining the color design process.
The Metacognitive Demands and Opportunities of Generative AI Lev Tankelevitch , Viktor Kewenig, Auste Simkute, Ava Elizabeth Scott, Advait Sarkar , Abigail Sellen , Sean Rintel Generative AI systems offer unprecedented opportunities for transforming professional and personal work, yet they present challenges around prompting, evaluating and relying on outputs, and optimizing workflows. This paper shows that metacognition—the psychological ability to monitor and control one’s thoughts and behavior—offers a valuable lens through which to understand and design for these usability challenges.
Honorable Mentions
B ig or Small, It’s All in Your Head: Visuo-Haptic Illusion of Size-Change Using Finger-Repositioning Myung Jin Kim, Eyal Ofek, Michel Pahud , Mike J. Sinclair, Andrea Bianchi This research introduces a fixed-sized VR controller that uses finger repositioning to create a visuo-haptic illusion of dynamic size changes in handheld virtual objects, allowing users to perceive virtual objects as significantly smaller or larger than the actual device.
LLMR: Real-time Prompting of Interactive Worlds Using Large Language Models Fernanda De La Torre, Cathy Mengying Fang, Han Huang, Andrzej Banburski-Fahey, Judith Amores , Jaron Lanier Large Language Model for Mixed Reality (LLMR) is a framework for the real-time creation and modification of interactive mixed reality experiences using LLMs. It uses novel strategies to tackle difficult cases where ideal training data is scarce or where the design goal requires the synthesis of internal dynamics, intuitive analysis, or advanced interactivity.
Observer Effect in Social Media Use Koustuv Saha, Pranshu Gupta, Gloria Mark, Emre Kiciman , Munmun De Choudhury This work investigates the observer effect in behavioral assessments on social media use. The observer effect is a phenomenon in which individuals alter their behavior due to awareness of being monitored. Conducted over an average of 82 months (about 7 years) retrospectively and five months prospectively using Facebook data, the study found that deviations in expected behavior and language post-enrollment in the study reflected individual psychological traits. The authors recommend ways to mitigate the observer effect in these scenarios.
Reading Between the Lines: Modeling User Behavior and Costs in AI-Assisted Programming Hussein Mozannar, Gagan Bansal , Adam Fourney , Eric Horvitz By investigating how developers use GitHub Copilot, the authors created CUPS, a taxonomy of programmer activities during system interaction. This approach not only elucidates interaction patterns and inefficiencies but can also drive more effective metrics and UI design for code-recommendation systems with the goal of improving programmer productivity.
SharedNeRF: Leveraging Photorealistic and View-dependent Rendering for Real-time and Remote Collaboration Mose Sakashita, Bala Kumaravel, Nicolai Marquardt , Andrew D. Wilson SharedNeRF, a system for synchronous remote collaboration, utilizes neural radiance field (NeRF) technology to provide photorealistic, viewpoint-specific renderings that are seamlessly integrated with point clouds to capture dynamic movements and changes in a shared space. A preliminary study demonstrated its effectiveness, as participants used this high-fidelity, multi-perspective visualization to successfully complete a flower arrangement task.
Understanding the Role of Large Language Models in Personalizing and Scaffolding Strategies to Combat Academic Procrastination Ananya Bhattacharjee, Yuchen Zeng, Sarah Yi Xu, Dana Kulzhabayeva, Minyi Ma, Rachel Kornfield, Syed Ishtiaque Ahmed, Alex Mariakakis, Mary P. Czerwinski , Anastasia Kuzminykh, Michael Liut, Joseph Jay Williams In this study, the authors explore the potential of LLMs for customizing academic procrastination interventions, employing a technology probe to generate personalized advice. Their findings emphasize the need for LLMs to offer structured, deadline-oriented advice and adaptive questioning techniques, providing key design insights for LLM-based tools while highlighting cautions against their use for therapeutic guidance.
Where Are We So Far? Understanding Data Storytelling Tools from the Perspective of Human-AI Collaboration Haotian Li, Yun Wang , Huamin Qu This paper evaluates data storytelling tools using a dual framework to analyze the stages of the storytelling workflow—analysis, planning, implementation, communication—and the roles of humans and AI in each stage, such as creators, assistants, optimizers, and reviewers. The study identifies common collaboration patterns in existing tools, summarizes lessons from these patterns, and highlights future research opportunities for human-AI collaboration in data storytelling.
Learn more about our work and contributions to CHI 2024, including our full list of publications , on our conference webpage .
Related publications
Piet: facilitating color authoring for motion graphics video, dynavis: dynamically synthesized ui widgets for visualization editing, generative echo chamber effects of llm-powered search systems on diverse information seeking, understanding the role of large language models in personalizing and scaffolding strategies to combat academic procrastination, sharednerf: leveraging photorealistic and view-dependent rendering for real-time and remote collaboration, big or small, it’s all in your head: visuo-haptic illusion of size-change using finger-repositioning, llmr: real-time prompting of interactive worlds using large language models, reading between the lines: modeling user behavior and costs in ai-assisted programming, observer effect in social media use, where are we so far understanding data storytelling tools from the perspective of human-ai collaboration, the metacognitive demands and opportunities of generative ai, continue reading.
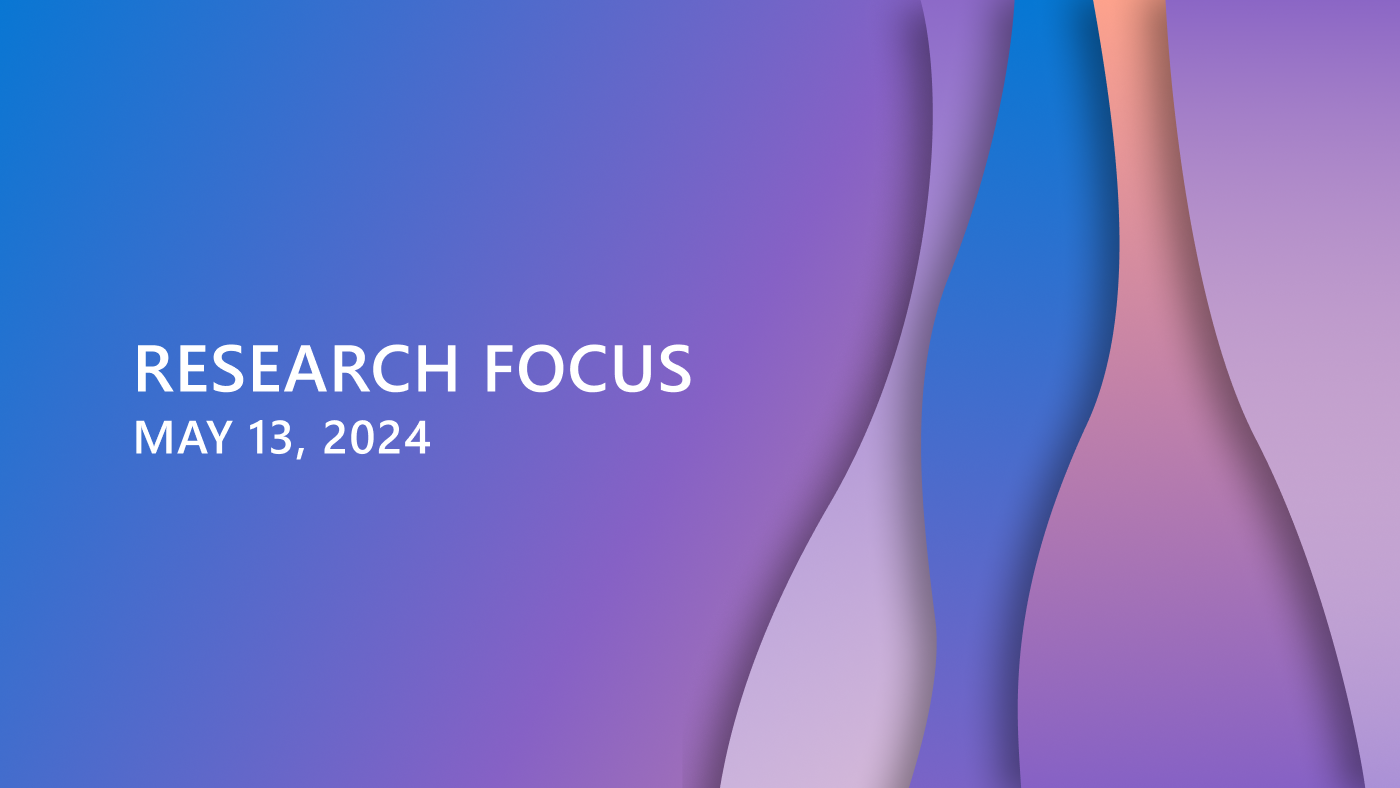
Research Focus: Week of May 13, 2024

Research Focus: Week of April 15, 2024

Research Focus: Week of March 18, 2024

Advancing human-centered AI: Updates on responsible AI research
Research areas.

Related events
- Microsoft at CHI 2024
Related labs
- AI Frontiers
- Microsoft Research Lab - Asia
- Microsoft Research Lab - Cambridge
- Microsoft Research Lab - Redmond
- Microsoft Research Lab – Montréal
- Follow on Twitter
- Like on Facebook
- Follow on LinkedIn
- Subscribe on Youtube
- Follow on Instagram
Share this page:
- Computer Vision
- Federated Learning
- Reinforcement Learning
- Natural Language Processing
- New Releases
- 100s of AI Courses
- Advisory Board Members
- 🐝 Partnership and Promotion
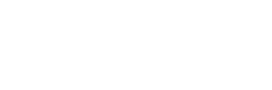
Key Advantages of Federated Learning
- Enhanced Privacy: Federated learning significantly reduces the risk of data breaches and misuse by keeping data on local devices. Sensitive information never leaves the device, ensuring user privacy is maintained.
- Improved Security: Since raw data is not transmitted over the network, the attack surface for potential breaches is minimized. Federated learning can incorporate secure aggregation techniques to protect model updates from being intercepted and reverse-engineered.
- Scalability: Federated learning leverages the computational power of edge devices, reducing the need for large-scale centralized infrastructure. This decentralized approach allows for scalable AI solutions that can operate efficiently across vast networks of devices.
Recent Advances in Federated Learning
- Local model training on each device and periodic averaging of model parameters across devices.
- Balances computational load and communication overhead.
- Secure aggregation protocols.
- Ensure model updates are aggregated without revealing individual updates.
- Use cryptographic methods for enhanced privacy and security.
- Methods proposed to handle data heterogeneity.
- Data sharing strategies and personalized federated learning approaches.
- Model compression techniques to reduce communication costs.
Applications of Federated Learning
- Collaborative medical research without compromising patient confidentiality.
- Example: Brain tumor segmentation across multiple hospitals without sharing patient data.
- Development of robust fraud detection systems while preserving user privacy.
- Financial institutions collaboratively train models on transaction data.
- Improvement of predictive text and personalized recommendations on smartphones.
- Models trained locally on user devices, maintaining privacy.
- Enhancing the capabilities of interconnected devices.
- Example: Smart home systems that learn user preferences locally.
Challenges for Federated Learning
Despite its advantages, federated learning faces several challenges that must be addressed for wider adoption. One of the primary challenges is the issue of non-IID (independent and identically distributed) data. In real-world scenarios, data across devices can be highly heterogeneous, which complicates the training process and may lead to biased models. Researchers have proposed methods to address data heterogeneity, such as data-sharing strategies and personalized federated learning approaches.
Another challenge is the high communication cost associated with transmitting model updates. Efficient communication protocols and model compression techniques are essential to mitigate this issue & ensure the feasibility of federated learning in resource-constrained environments. The integration of federated learning with other emerging technologies holds great potential. For instance, combining FL with blockchain can enhance security and transparency in decentralized AI systems. 5G networks will provide the bandwidth & low latency to support large-scale federated learning deployments.
Federated learning represents a paradigm shift in AI, offering a decentralized approach that enhances privacy and security. FL addresses critical concerns associated with traditional AI methods by enabling collaborative model training without centralized data collection. Despite the challenges, ongoing research paves the way for the broader adoption of federated learning across various industries. As this field continues to evolve, federated learning has the potential to become a cornerstone of secure and privacy-preserving AI systems.
- https://arxiv.org/abs/1806.00582
- https://arxiv.org/abs/1610.05492
- http://proceedings.mlr.press/v54/mcmahan17a.html
- https://dl.acm.org/doi/10.1145/3133956.3133982
- https://link.springer.com/chapter/10.1007/978-3-030-46640-4_34

Aswin AK is a consulting intern at MarkTechPost. He is pursuing his Dual Degree at the Indian Institute of Technology, Kharagpur. He is passionate about data science and machine learning, bringing a strong academic background and hands-on experience in solving real-life cross-domain challenges.
Exploring the Frontiers of Artificial Intelligence: A Comprehensive Analysis of Reinforcement Learning, Generative Adversarial Networks, and Ethical Implications in Modern AI Systems
- OpenRLHF: An Open-Source AI Framework Enabling Efficient Reinforcement Learning from Human Feedback RLHF Scaling
- Quantum Machine Learning for Accelerating EEG Signal Analysis
- TRANSMI: A Machine Learning Framework to Create Baseline Models Adapted for Transliterated Data from Existing Multilingual Pretrained Language Models mPLMs without Any Training
RELATED ARTICLES MORE FROM AUTHOR
This machine learning paper from stanford and the university of toronto proposes observational scaling laws: highlighting the surprising predictability of complex scaling phenomena, transformative applications of deep learning in regulatory genomics and biological imaging, ai wearables: transforming day-to-day life, cohere ai releases aya23 models: transformative multilingual nlp with 8b and 35b parameter models, theory of mind: how gpt-4 and llama-2 stack up against human intelligence, this machine learning paper from stanford and the university of toronto proposes observational scaling..., exploring the frontiers of artificial intelligence: a comprehensive analysis of reinforcement learning, generative adversarial....
- AI Magazine
- Privacy & TC
- Cookie Policy
🐝 🐝 Join the Fastest Growing AI Research Newsletter Read by Researchers from Google + NVIDIA + Meta + Stanford + MIT + Microsoft and many others...
Thank You 🙌
Privacy Overview
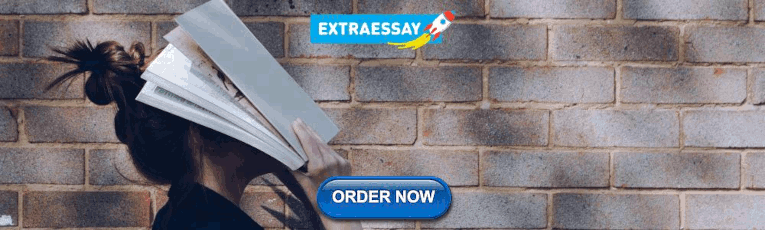
IMAGES
VIDEO
COMMENTS
This paper systematically reviews the existing literature on data security. This study aims to identify current research directions, identify the main challenges facing data security, and suggest future research directions in this field. The research method is a structured approach to searching and selecting relevant papers based on predefined inclusion and exclusion criteria. The selected ...
This paper aims at identifying research trends in the field through a systematic bibliometric literature review (LRSB) of research on AI and system security. the review entails 77 articles published in the Scopus ® database, presenting up-to-date knowledge on the topic. the LRSB results were synthesized across current research subthemes ...
The article is a full research paper (i.e., not a presentation or supplement to a poster). ... Security continuous monitoring is real-time monitoring of information systems and assets to gain a clear insight into their environment and detect security events. AI can be used to automate monitoring by providing security intelligence using a ...
In a computing context, cybersecurity is undergoing massive shifts in technology and its operations in recent days, and data science is driving the change. Extracting security incident patterns or insights from cybersecurity data and building corresponding data-driven model, is the key to make a security system automated and intelligent. To understand and analyze the actual phenomena with data ...
Abstract. This paper systematically reviews the existing literature on data security. This study aims to identify current research directions, identify the main challenges facing data security ...
Depending on the amount of data, the extent of the damage caused by a data breach can be significant, with the average cost being USD 392 million Footnote 1 (IBM Security 2020). This research paper reviews the existing literature and open data sources related to cybersecurity and cyber risk, focusing on the datasets used to improve academic ...
The term "policy" is used in a variety of areas related to cyber-security, and refers to information distribution rules and regulations, private sector goals for data conservation, system operations strategies for technology control. However, in the works of this field, the term cyber-security policy is used for different purposes.
This paper aims at identifying research trends in the field through a systematic bibliometric literature review (LRSB) of research on AI and system security. the review entails 77 articles ...
This paper aims at identifying research trends in the field through a systematic bibliometric literature review (LRSB) of research on AI and system security. the review entails 77 articles published in the Scopus ® database, presenting up-to-date knowledge on the topic. the LRSB results were synthesized across current research subthemes.
Against this background, this paper provides a summary of the present situation of global data security governance, points out the challenges, and then proceeds to raise solutions for further modernizing data security governance systems. ... government affairs data, and scientific research data that affect public society, governments worldwide ...
This research paper delves into the critical realm of database security, a pressing. concern for modern organizations with sensitive data. It explores v arious security. threats faced by database ...
This paper focuses on privacy and security concerns in Big Data. This paper also covers the encryption techniques by taking existing methods such as differential privacy, k-anonymity, T-closeness, and L-diversity.Several privacy-preserving techniques have been created to safeguard privacy at various phases of a large data life cycle.
Artificial intelligence (AI) is one of the key technologies of the Fourth Industrial Revolution (or Industry 4.0), which can be used for the protection of Internet-connected systems from cyber threats, attacks, damage, or unauthorized access. To intelligently solve today's various cybersecurity issues, popular AI techniques involving machine learning and deep learning methods, the concept of ...
As Figure 1 shows, this paper presents a comparative research analysis of the existing research work regarding the techniques used in the cloud computing through data security aspects including data integrity, confidentiality, and availability. Data privacy issues and technologies in the cloud are also studied, because data privacy is ...
This paper aims to study the Countermeasures of big data security management in the prevention and control of computer network crime in the absence of relevant legislation and judicial practice. Starting from the concepts and definitions of computer crime and network crime, this paper puts forward the comparison matrix, investigation and ...
The paper presents a comprehensive exploration of a novel image encryption and decryption methodology, leveraging finite state machines (FSM) for the secure transformation of visual data. The study meticulously evaluates the effectiveness of the proposed encryption algorithm using a diverse image dataset. The encryption algorithm demonstrates high proficiency in obfuscating the original ...
In addition, this paper presents recent research trends and open challenges for each component. During the last five years, many survey papers focusing on the security and privacy of e-health data have been published; however, there has been no comprehensive survey of an overall e-healthcare system, such as e-health data, medical devices ...
A new data encryption model has been designed. It is envisioned that the proposed data encryption model would be able to transfer IoT devices data securely over the open communication network. It also enhanced the data integrity of the model. The size of the generated cipher is unaffected if XORed with parity bits which makes it lightweight in nature. To make the cipher highly sensitive, a ...
Power information systems and physical systems are gradually being coupled and developed into power cyber-physical systems (CPS). A number of blackouts in recent years have shown that cyberspace cyber attacks on CPS can lead to the intensification and rapid spread of faults in the physical space of the power grid, and even system collapse.
In anticipation of the 6G era, this paper explores the integration of terahertz (THz) communications with Reconfigurable Intelligent Surfaces (RIS) and deep learning to establish a secure wireless network capable of ultra-high data rates. Addressing the non-convex challenge of maximizing secure energy efficiency, we introduce a novel deep learning framework that employs a variety of neural ...
Topic modeling of Information Systems Security research between 1990 and 2020. • Delphi study of CISOs to rank order important Information Systems Security concerns. • Explores the gap between what practitioners consider to be important and what researchers are currently studying. • Develop a research agenda in Information Systems Security.
More than three-quarters of researchers use some form of artificial intelligence (AI) tool in their research, despite having concerns about data security, intellectual property rights and AI's effectiveness, a new report finds. An Oxford University Press (OUP) survey released Thursday found that 76 percent of the 2,345 respondents use an AI tool when conducting their own research.
A black box system (BBS) in a car is crucial for recording and analyzing critical data to enhance safety, investigate accidents, and improve vehicle performance. This research presents a BBS developed using Arduino for cars, aimed at using the power of modern technology for comprehensive data capture and analysis in vehicular contexts. The BBS, or Event Data Recorder (EDR), is an essential ...
IET Information Security. About. Contribute. Alert. RSS Feeds. Calls for Papers. There are no open Special Issues for IET Information Security currently. You can read our published Special Issues here.
Officers used the tool to extract datasets including call logs, messages and other data. The police said the data confirmed the woman's identity, and she was prosecuted based on the evidence gathered. She was convicted in January 2023. They declined to reveal further details, including the car's make and model.
The ACM CHI Conference on Human Factors in Computing Systems is a premier forum that brings together researchers and experts in the field, and Microsoft is honored to support CHI 2024 as a returning sponsor. We're pleased to announce that 33 papers by Microsoft researchers and their collaborators have been accepted this year, with four ...
The rapid advancement of AI has revolutionized various industries, from healthcare to finance, by enabling sophisticated data analysis and predictive modeling. However, the traditional approach to AI, which involves centralizing vast amounts of data for training models, raises significant privacy and security concerns. Federated learning has emerged as a promising field that addresses these ...