
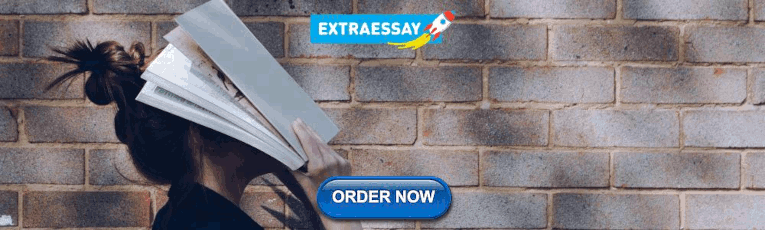
Federal Statistics, Multiple Data Sources, and Privacy Protection: Next Steps (2017)
Chapter: 6 quality frameworks for statistics using multiple data sources, 6 quality frameworks for statistics using multiple data sources.
A recurrent theme in the panel’s first report ( National Academies of Sciences, Engineering, and Medicine, 2017b ), as well as the previous chapters of this report, is that the quality of administrative and private-sector data sources needs careful examination before being used for federal statistics. The theme and the caution have been driven by the relatively recent novelty of the simultaneous use of multiple data sources and the fact that some potential new sources of data present new issues of data quality.
We begin this chapter with a discussion of quality frameworks for survey data and then briefly review additional quality features and some extensions of these frameworks for administrative and private-sector data sources. We then consider how different data sources have been or could be combined to produce federal statistics, with examples to illustrate some of the quality issues in using new data sources.
A QUALITY FRAMEWORK FOR SURVEY RESEARCH
The science of survey research has its origins in the provision of quantitative descriptive data for the use of the state beginning in the 17th century. In general, the approach was to enumerate the whole population (censuses) to provide a description of the population to rulers and administrators. Over time, the increasing demands for information by states for both planning and administrative functions placed greater strains on the capacity of national statistical offices to provide the data, particularly in a timely manner.
The International Statistical Institute, founded in the second half of the 19th century, brought together the chief statistical officers of developed countries, including some leading academic scholars. At its convention in 1895, the chief statistician of Norway, Anders Kiaer, proposed a radical innovation—to collect information on only a subset of the population, a representative sample. Over a period of 30 years, statisticians refined the ideas he presented and developed the form of the sample survey that remained the foundation of the collection of policy-related statistical data throughout the 20th century.
Beginning in the 1930s, statisticians worked on identifying sources of error in survey estimates, and when possible, measuring their effects. All these components are combined in the total survey error framework shown in Figure 6-1 . The basic inferential structure of the sample survey involves two separate processes. The first is a measurement inference, in which the questions answered or items sought from a sample unit are viewed as proxies for the true underlying phenomenon of interest. For example, every month interviewers asked a sample person: “what were you doing
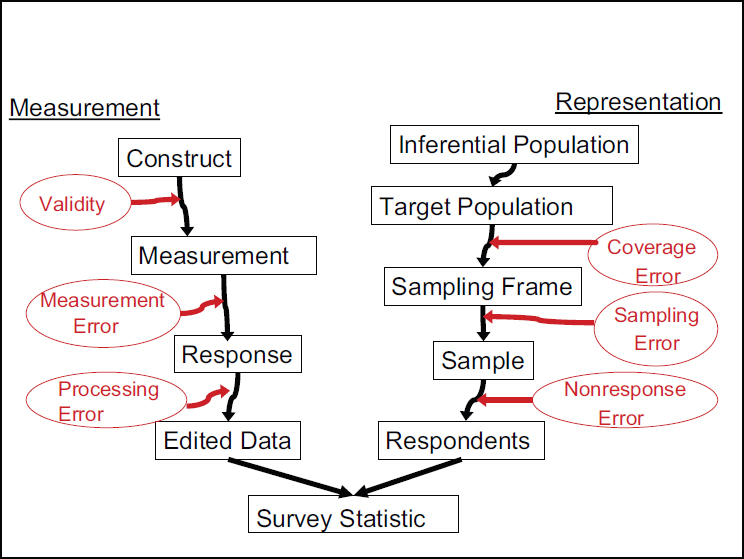
last week (the week of the 12th)?” as a key item for the statistic that is labeled as the monthly employment rate. There is a difference between the measurement of behavior for the week of the 12th and the concept of an unemployment rate for the whole month. The inference being made is complicated and threatened in months in which labor strikes occur in the middle of the month as the status of that week is unlike that of other weeks in some other way.
Another example, and one that provides a clearer notion of measurement inference, can be seen when the underlying concept is clearly unobservable without a self-report. Various attributes of human knowledge fit this case well. For example, the Trends in International Mathematics and Science Study (TIMSS) assesses mathematics knowledge among students in grades 4 and 8. Knowledge of mathematics is often measured through problems that can be solved only by those people with the requisite knowledge. There are many types of mathematics problems and thousands of instances of every type of mathematical knowledge. In some cases, there are an infinite number of ways to measure a type of mathematics knowledge. Each problem, therefore, might be viewed as one sample from that infinite population. And since each problem exhibits its own variability over conceptually repeated administrations, measuring mathematics knowledge with multiple problems increases the stability of an estimate. In this case, an inference is drawn from a question or set of questions to the underlying unobservable mathematics knowledge.
The second inferential step concerns the measurement of a subset of units of the target population. In this case, inference based on probability sampling is the foundation of government statistical agencies throughout the world. The inference, however, needs careful descriptions of the target population and the frame population: in an ideal situation, the frame has a one-to-one correspondence to the full population of interest, the target population. Government statistical agencies strive to create and maintain such universal frames for households and businesses. Some countries attempt to construct frames of people through population registers.
For each error source shown in Figure 6-1 , one can further distinguish two kinds of errors: (1) biases, which represent systematic errors that are integral to the process and would therefore not be ameliorated without a change in the process; and (2) variances, which represent instability in the estimates and depend on the number of units of a particular kind included in the data collection. Variance can be reduced by increasing the number of units of that kind.
Federal statistical agencies are very sensitive to the potential errors in their surveys. Their primary focus has been on the fact that data are collected on only a sample of the population, as it is this feature that distinguishes surveys from a complete population census. Many of the most
widely used sampling and estimation procedures for surveys were developed at the U.S. Census Bureau in the 1930s and 1940s. Other statistical agencies have also been leaders in the provision of detailed information on sampling errors (measures of precision for survey estimates), and most federal surveys produce detailed tables of standard errors for their estimates. Other aspects of data quality have been given less emphasis, though agencies have conducted important investigations of some nonsampling errors in major surveys.
There has also been some work to apply the same ideas of statistical inference to some other forms of measurement error (response error in surveys) particularly, models of interviewer effects (interviewer variance), using the concepts of simple and correlated response variance (by analogy with simple and correlated sampling variance), and looking at instability in responses (reliability). These models were refined by U.S. and other statistical agencies in the 1960s, 1970s, and 1980s. A major conceptual push toward the examination of measurement error occurred as a result of work on cognitive aspects of survey methods, which examined the psychological mechanisms that influence respondents’ understanding of questions and retrieval of information (see National Research Council, 1984 ). The major statistical agencies set up cognitive laboratories to incorporate these principles into the design of questions used in their surveys (see Tanur, 1999 ).
Concern about nonresponse has also been a feature of quality control in statistical agencies, and the emphasis has most often been on maximizing the response rate. The standards and guidelines for statistical surveys issued by the U.S. Office of Management and Budget (2006) stipulated standards for the collection of statistical information that included a high threshold for response rates (80 percent) and a detailed protocol for evaluating errors if the response rate is below this threshold. However, there is generally no reporting mechanism that quantifies the effect of nonresponse in a way that corresponds to the routine publication of standard errors. This reflects a general emphasis on variances (stability) rather than biases, as well as the ability to calculate standard errors directly from the sample, while determining nonresponse bias requires information external to the survey.
Some federal statistical agencies have created “quality profiles” for some major surveys to bring together what is known about the various sources of error in a survey. This was first done for the Current Population Survey in 1978 ( Federal Committee on Statistical Methodology, 1978 ) and was also done for other surveys (see, e.g., National Center for Education Statistics, 1994 ; U.S. Census Bureau, 1996 , 1998 , 2014b ). The Census Bureau currently includes this kind of information in design and methodology reports for the Current Population Survey and American Community Survey (see U.S. Census Bureau, 2006 , 2014a ).
CONCLUSION 6-1 Survey researchers and federal statistical agencies have developed useful frameworks for classifying and examining different potential sources of error in surveys, and the agencies have developed careful protocols for understanding and reporting potential errors in their survey data.
Effectively using frameworks for the different sources of error in surveys requires agencies to provide information or metrics for reporting on survey quality. A subcommittee of the Federal Committee on Statistical Methodology (2001) reviewed the different kinds of reports produced by statistical agencies and the amount of information provided about the different sources of error in each and further provided guidance to agencies on measuring and reporting sources of error in surveys. It recommended the minimum amount of information that should be provided, which depended on the length and detail of the report.
Measures of sampling error (estimates of the variability in the estimates due to sampling) are the most commonly reported metric of quality across all agency reports (see Federal Committee on Statistical Methodology, 2001 ). These measures include standard errors or coefficients of variation, and they are often the only quantitative indicator of quality reported by the agency. Other error sources are noted in more general narrative form, such as statements that surveys are also subject to nonsampling errors. Since standard errors are typically the only quantitative metric available at the estimate level, it is easy for users to conclude that this measure conveys the overall quality of the estimate.
The second principal metric of quality often used in official statistics is the response rate, which is also a very imperfect metric of quality. Low response rates do not, by themselves, indicate poor-quality statistics. Instead, lower rates indicate a higher risk of nonresponse error. Similarly, high response rates provide important protection against the potential for nonresponse bias. However, this is an area in which the direct collection of ancillary data (paradata) could facilitate a more comprehensive assessment of the danger of the vulnerability of survey results to bias arising from nonresponse. Furthermore, access to other data sources (administrative or private sector) could also provide validating (or invalidating) evidence. And in some cases paradata could provide a basis for imputing data when a survey failed to obtain information directly. In this instance, the incorporation of additional data would supplement or validate, rather than replace, the survey data.
CONCLUSION 6-2 Commonly used existing metrics for reporting survey quality may fall short in providing sufficient information for evaluating survey quality.
BROADER FRAMEWORKS FOR ASSESSING QUALITY
There is considerable inertia in any long-established system. Consequently, the evaluation of the quality of any statistic tends to be seen through the lens of the current dimensions of focus and concern. In the new environment in which emerging data sources with quite different provenance and characteristics provide alternative ways of measuring the underlying phenomena, it may be necessary to rethink the weight that is placed on traditional measures of quality relative to other quality measures.
There are broader quality frameworks that emphasize the granularity of the data and the estimates in ways that were previously not possible. An influential quality framework has been developed by Eurostat, the Statistical Office of the European Union ( European Statistical System Committee, 2013 ) (see Box 6-1 ). This framework has five major output quality components:
- Accuracy and Reliability
- Timeliness and Punctuality
- Accessibility and Clarity
- Coherence and Comparability
It is worth noting that all the technical aspects of the total survey error model discussed above are encompassed by just one of the above five output quality components—accuracy and reliability. Of course, many of the others are critical in determining what information is collected and disseminated, in particular, relevance. The criterion of coherence also makes important demands on the process by requiring that the estimates are consistent internally and comparable across subsets of a population. Maintaining relevance and coherence may be a particular challenge in moving from one system or configuration to another. This challenge is exacerbated in the case of surveys by the length of time it takes to develop and test survey components, leading to a lack of nimbleness in response to changes in circumstances and policy requirements.
Two aspects of data quality in particular warrant emphasis here: timeliness and spatial and subgroup granularity.
Though timeliness is described in the European Statistical System Committee’s quality framework in terms of the timing and punctuality of reports, it is important to recognize that existing systems have tailored their reporting mechanisms and their reporting requirements to the practical constraints and limitations in place at the time the systems were established. For example, the unemployment rate, calculated from the Current Population Survey (CPS), is published monthly based on information collected about a single week in the preceding month. When the system was established in the 1940s, this schedule (and basis) was at the outer limits of feasibility and practicality for a national survey. Changes in the data environment have made the range of possibilities much broader, and, therefore, the assessment of quality should now incorporate this new context.
For policy purposes, it is particularly important that information be available to decision makers in time to incorporate it into the decision-making process. Data collected through surveys tend to have a minimum interval between the beginning of data collection and the production of the estimate. Even with the CPS, there is a lag of about 3 weeks between the reference period for the survey responses and the production of the estimate. With some private-sector data that is captured electronically, the time lapse between the event being measured and the availability of the data is, in principle, negligible (though in practice this may not be the case).
In this context, one needs to think about the value of this aspect of statistical estimates and the particular use to be made of the estimate. With prices (see the discussion in Chapter 4 of the panel’s previous report), the current (old-fashioned) process of visiting stores to collect price information has the virtue of providing almost comprehensive coverage (through
probability sampling) of the population of stores, but it suffers from a considerable time lag in reporting. Data from the Internet, in contrast, can be harvested in a timely manner but may miss differentially key sectors of the population of stores. Researchers and analysts need to be able to evaluate the relative importance of timeliness and coverage for each particular purpose of a statistic. If the purpose is to provide early warning of price changes, one might argue that the Internet data, though deficient in coverage terms, would function better than the more comprehensively representative survey data. If the purpose is to provide an estimate that gives an unbiased measure of change in prices for the population as a whole, the argument would swing in favor of the probability sample of stores. Combining the sources offers the potential to improve the timeliness of estimates from probability samples and reduce the bias present in Internet data.
Spatial and Subgroup Granularity
A second important aspect of quality is the degree to which estimates can be obtained for small subdivisions of the population, such as spatial subdivisions or different socioeconomic status categories. In census and survey statistical terminology, these estimates are usually referred to as small-area statistics. The practical limitation on the size of the sample that can realistically be afforded for traditional surveys places a severe limit on the ability to provide reliable small-area statistics. With private-sector data, such refinement of the estimates may be accomplished at low marginal cost once the system processes have been put in place. The value to policy makers at a national level of having information at this more detailed level can be considerable and could compensate for some loss of quality on the dimensions of accuracy and reliability.
All of these considerations point to the importance of recognizing the need to evaluate quality in a more broad-based way. By combining data sources (as described in Chapter 2 and in the examples in this chapter, below), hybrid estimates can be produced that come close to possessing the positive quality aspects of the components used to construct them.
CONCLUSION 6-3 Timeliness and other dimensions of granularity have often been undervalued as indicators of quality; they are increasingly more relevant with statistics based on multiple data sources.
RECOMMENDATION 6-1 Federal statistical agencies should adopt a broader framework for statistical information than total survey error to include additional dimensions that better capture user needs, such as timeliness, relevance, accuracy, accessibility, coherence, integrity, privacy, transparency, and interpretability.
ASSESSING THE QUALITY OF ADMINISTRATIVE AND PRIVATE-SECTOR DATA
Administrative data.
Administrative and private-sector data have their own challenges and errors. These errors arise for multiple reasons, such as mistakes in understanding or interpreting metadata, errors in entity linkage, and incomplete or missing information. Unlike handling data from traditional surveys, these errors usually need to be dealt with after the data have been obtained and been through cleaning and processing (see Chapter 3 ). That is, the errors can usually not be avoided during data gathering or generation because the processes that generate the data have their own purposes: that is, unlike surveys, the production of the statistic is secondary to another objective. In contrast, survey designers spend a great deal of time and effort developing and pretesting survey instruments to ensure they are obtaining the information they want from respondents and minimizing measurement errors. Electronic survey instruments often include consistency checks and acceptable ranges of responses to further ensure that potential problems with data entry or responses are resolved at the point of collection.
Data Linkage and Integration
The use of administrative and private-sector data not only shifts the focus of reducing errors into the postdata gathering stage, it also adds a new error source that is not usually encountered in surveys: linkage errors. In many instances, it is necessary to match data related to the same real-world entities, even if they are identified in different ways. As we note in Chapter 2 , many linkage variables have variants: the same person might be listed in different data sources as “Susan Johnson Wright,” “Suzy Johnson,” “Sue Wright,” or “S.J. Wright.” Failure to recognize these as belonging to the same person will result in records being declared distinct when they ought to be linked—a missed link . Conversely, there may be multiple Susan Wrights in the population, and two records may match exactly on the identifying variables yet represent two different people—a false link . Missed links and false links can distort relationships among variables in the data sources or result in inaccurate measures of the population size when linkage is used to augment the number of records available for study.
In the case of a potential false link, one would need to look at other evidence, such as an address, to determine whether it is the same person. However, this is complicated, because people do move, so one would have to distinguish between the same person who has changed addresses and a different person who just happens to have the same name. And in the case
of matching individuals to themselves, there is the complication of the difficulty to match an individual after a name change, say, due to marriage or simply an interest in changing one’s name. Additional difficulties of linking individuals could also include joint bank accounts, accounts that have only one spouse’s name, and parents or others paying for a service for a child or other relative. 1 In short, good entity matching is very hard. However, there is much active research work in this area and a significant body of technology that can be exploited for this work.
Most data of interest to statistical agencies is likely to have a temporal component. Each fact will represent either an instant in time or a period of time. It is likely that the time frames for data reporting will not perfectly line up when integrating data sources. In such circumstances, techniques are needed to proceed with integration and, if at all possible, without introducing too much error. For instance, where reported aggregates change smoothly over time, some type of interpolation may be appropriate. For example, where certain secondary information, such as North American Industry Classification System codes or dictionary terms, is largely static, one may reasonably assume it has the same value at the time of interest as at another time.
Similar issues also arise for geographical alignment: if some data are reported by ZIP code and other data by county, one can integrate them only if one can transform one of the two datasets into a report by the other. Such transformation is complicated by the fact that ZIP code to county is a many-to-many relationship: many ZIP codes can include more than one county and vice versa. Techniques for small-area estimation (see Chapter 2 ) may be helpful in achieving such temporal and spatial alignment.
CONCLUSION 6-4 Quality frameworks for multiple data sources need to include well-developed treatments of data linkage errors and their potential effects on the resulting statistics.
Concepts of Interest and Other Quality Features
The kinds of statistical models discussed in Chapter 2 when using multiple data sources underscore the need for more attention to quality features that have not received as much attention in traditional statistics (see also Groves and Schoeffel, in press). We note here several issues with regard to administrative data that will need attention.
One core difference with respect to quality for administrative and private-sector data is tied to the concept of interest. Since data are being
___________________
1 Additional concerns arise if consent is required in order to link different datasets (see Chapter 4 ).
repurposed, the meanings of particular values are likely to be subtly different. A precise understanding of those differences is critical for correct interpretation and difficult due to varying metadata recording standards across data sources. Similarly, population coverage and sampling bias are also of particular concern when repurposing data. Understanding exactly what has been done is a necessary step to ensuring correctness. Finally, repurposing data will often require considerable manipulation. Therefore, recording the editing and cleaning processes applied to the data, the statistical transformations used, and the version of software run become particularly important (see Chapter 3 ).
It is likely that the population covered by one dataset will not match the population covered by another. Administrative records exist for program participants but not for nonparticipants, and some people may not be in any record system. Some administrative records contain people outside of the population of inference of a survey dataset to which records are to be linked (e.g., voter records may contain dead people formerly eligible to vote). There may be duplicate records in some sources, and it is possible that records from multiple datasets simply cannot be linked. Moreover, it will not be possible to separate linkage errors with coverage errors.
The level of measurement in the multiple data sources may vary. One dataset may be measured on the person level, another on the household level, another on the consumer unit level, another on the address level, and another on the tax unit level, and other data, such as credit card purchases, may be at a transaction level. Errors in statistics can arise due to mismatches when combining data from such different datasets.
The temporal extent of the data may vary. Some administrative data systems are updated on a relatively haphazard schedule, depending on interaction with the client. The result is that time can be variable over records in the same administrative dataset. The value reflects the “latest” version, sometimes with no metadata on the date the value was entered. The data may also contain information on an individual only for the time during which the individual was in a program. As individuals leave the program, they will not have new data recorded about them, but they may also not be removed from the system. Combining records to produce statistics that are designed to describe a population at a given time point is thus problematic.
The underlying measurement construct may differ across datasets. In the total survey error framework, this occurrence is sometimes labeled as an issue of validity or a gap between concept and measurement. For example, in one dataset the value of an economic transaction may capture only the goods or service provided, but in another it may also involve cash given to the customer by the provider but charged to the account of the customer.
The nature of missing data in records may vary across surveys and other sources. In survey data, questions may be skipped for several rea-
sons: because a respondent chooses to terminate participation before the question is posed; because of a refusal to answer the question because the respondent does not want to answer it; or because the respondent does not know the answer.
In addition, in administrative record systems, some data fields may be empty because of their lack of importance to the program: for example, a service clerk did not need the data to execute the task at hand. A field may also be empty because new processes fail to capture the datum. And less important information may not be captured as accurately or as carefully as information that is critical to the immediate administrative task.
As with surveys, some recorded items may be inaccurate because they are misunderstood by the clerk entering the information or by the respondent providing the information. To compensate for missing data, survey-based imputation schemes use patterns of correlations of known attributes to estimate values missing in the records. Those kinds of techniques may need some reassessment for administrative data. For example, unstructured text data appear in many medical record systems. Does the absence of the mention of some attribute mean that the attribute is nonexistent for the patient or that the health provider failed to record the attribute?
Frameworks for Assessing Quality of Administrative Data
In the panel’s first report ( National Academies of Sciences, Engineering, and Medicine, 2017b ) we concluded that “administrative records have demonstrated potential to enhance the quality, scope and cost efficiency of statistical products” (p. 35), and that “not enough is yet known about the fitness for use of administrative data in federal statistics” (p. 48). Therefore, we made the following recommendation:
Federal statistical agencies should systematically review their statistical portfolios and evaluate the potential benefits and risks of using administrative data. To this end, federal statistical agencies should create collaborative research programs to address the many challenges in using administrative data for federal statistics. ( National Academies of Sciences, Engineering, and Medicine, 2017b , Recommendation 3-1, p. 48).
As part of their review of administrative data, agencies need to assess the quality of the data and whether the data are fit for use for their intended statistical purposes (see, e.g., Iwig et al., 2013 ). Brackstone (1987 , p. 32) notes that the quality of administrative records for statistical purposes depends on at least three factors:
- the definitions used in the administrative system;
- the intended coverage of the administrative system;
- the quality with which data are reported and processed in the administrative system.
In the United States, Iwig and his colleagues (2013) created a tool for assessing the quality of administrative data for statistical uses to help statistical agencies systematically obtain the relevant information they need at each stage of the data-sharing process (see Box 6-2 ). Although the tool is based on quality frameworks from a number of national statistical offices, it is intended to help guide the conversation between a statistical agency and a program agency, rather than provide a comprehensive framework itself.
Administrative records are used by many national statistical offices for producing statistics; however, there have not been statistical theories developed for assessing the uncertainty from administrative data as there have been for surveys ( Holt, 2007 ). There have been efforts to examine the processes that generate administrative data and population registers (see Wallgren and Wallgren, 2007 ), as well as examinations of measurement errors in both administrative records and survey reports (e.g., see Oberski et al., 2017 ; Abowd and Stinson, 2013 ; Groen, 2012 ). Recently, Zhang (2012) has extended the Groves et al. (2009) model of survey error sources (shown in Figure 6-1 ) to create a two-phase (primary and secondary) life cycle of integrated statistical microdata. In this model, the first phase covers the data from each individual source, while the second phase concerns the integration of data from different sources, which typically involves some transformation of the original data (see Zhang [2012] for a complete discussion of the model).
Private-Sector Data
Different types of data.
In our first report ( National Academies of Sciences, Engineering, and Medicine, 2017b , p. 57) we noted the enormous amounts of private-sector data that are being generated constantly from a wide variety of sources. We distinguished between structured data, semi-structured data, and unstructured data:
- Structured data, such as mobile phone location sensors, or commercial transactions, are highly organized and can easily be placed in a database or spreadsheet, though they may still require substantial scrubbing and transformation for modeling and analysis.
- Semi-structured data, such as text messages or emails, have structure but also permit flexibility so that they cannot be placed in a relational database or spreadsheet; the scrubbing and transformation for modeling and analysis is usually more difficult than for structured data.
- Unstructured data, such as in images and videos, do not have any structure so that the information of value must first be extracted and then placed in a structured form for further processing and analysis.
The challenges with the quality of these different data sources led to a conclusion and recommendation:
The data from private-sector sources vary in their fitness for use in national statistics. Systematic research is necessary to evaluate the quality, stability, and reliability of data from each of these alternative sources currently held by private entities for their intended use. ( National Academies of Sciences, Engineering, and Medicine, 2017b , Conclusion 4-2, p. 70)
The Federal Interagency Council on Statistical Policy should urge the study of private-sector data and evaluate both their potential to enhance the quality of statistical products and the risks of their use. Federal statistical agencies should provide annual public reports of these activities. ( National Academies of Sciences, Engineering, and Medicine, 2017b , Recommendation 4-2, p. 70)
The panel thinks it likely that the data to be combined with traditional survey and census data will increasingly come from unstructured text, such as web-scraped data. Converting such data to a more structured form presents a range of challenges. Although errors arise in coding open-ended responses to surveys, the issues with unstructured text compound those errors given the ambiguity of the context and the development and use of coding algorithms, which can result in a special type of processing error. The ambiguity of words—does “lost work” refer to a job termination or a hard disk crash?—will be a constant challenge. The possibility of coding words on multiple dimensions (e.g., meaning and effect) arises. From a quality or error standpoint, a coding error might create a new source of bias if one coding algorithm is used, or a new source of variance in statistics if multiple coding algorithms are used. Training these algorithms using human-coded data essentially builds in any biases that were present in the original human coding. The emergence of computational linguistics since the early days of survey coding may offer help in this aspect of big data quality.
Some of the data used in federal statistics could be combinations of data arising from sensors. For example, traffic sensor data are useful in traffic volume statistics. From time to time, sensors fail, creating missing data for a period of time until the sensor is repaired or replaced. If the probability that the sensor fails is related to volume of traffic or traffic conditions (e.g., when heavy rain or snow occurs), then the existence of missing data can be correlated with the very statistic of interest, creating what survey researchers would label a type of item nonresponse error.
The panel’s first report also discussed the possibility that social media
data might be combined in certain circumstances with survey or census data. Social media data have an error source uncommon in surveys: the possibility that data are generated by actors outside the population of inference. For example, software bots are known to create Twitter posts. The software bots might have handles and profiles that appear to be people, with revealed geographic positions, under the Twitter protocols. However, the data generated by the software bots are not a person- or business-measurement unit eligible for a survey or census measurement. Although this might be viewed as a type of coverage error in traditional total survey error terms, it has such a distinct source that it needs its own attention.
Risks of Private-Sector Data
Although there is considerable potential in the enormous volume of private-sector data, these data carry considerable risks. First, as they are primarily administrative or transactional data collected for purposes related to the transactions they cover, they tend to be less stable in definition and form than federal survey data that are collected specifically for statistical purposes. Consequently, statistical agencies need to be cautious in relying on these data as a primary (or, especially, sole) source of information; private-sector data are vulnerable to being changed or discontinued without notice. Second, statistical agencies do not have control over the creation and curation of the data; there is the possibility of deliberate manipulation or “front-running” by private-sector data companies providing data to government agencies: that is, if the data supplied by a private-sector entity constituted a sufficiently influential portion of a statistic so that the entity itself could predict the agency’s results, the entity could profit by selling this information to others or by acting on it directly. Third, the data themselves could be subject to manipulation for financial gain.
Quality Frameworks
There have been some recent efforts to extend the total survey error framework to include big data (see Biemer, 2016 ; Japec et al., 2015 ; U.N. Economic and Social Council, 2014 ; U.N. Economic Commission for Europe [UNECE], 2014 ). The UNECE model is a multidimensional hierarchical framework that describes quality at the input, throughput and output phases of the business process (see Box 6-3 ).
Biemer (2016) and Japec et al. (2015) similarly distinguish between the phases of generation of the data, the extraction/transformation/load of the data, and the analysis of the data. In this scheme, data generation errors are analogous to survey data collection errors and may result in erroneous, incomplete, or missing data and metadata. Extraction/transformation/
load errors are similar to survey processing errors and include errors in specification, linking, coding, editing, and integration. Analysis errors are analogous to modeling and estimation errors in surveys and also include errors in adjustments and weighting, which may reflect errors in the original data or in filtering and sampling the data. Both models attempt to provide an overall conceptual view of errors in a broad array of data sources and a language to describe these errors; however, neither of the models has yet been applied to a variety of sources by researchers or analysts.
Hsieh and Murphy (2017) have taken a more specific approach and described error sources for a specific social media data source, creating a “total Twitter error.” The authors posit that major classes of errors occur during the data extraction and the analysis process.
CONCLUSION 6-5 New data sources require expanding and further development of existing quality frameworks to include new components and to emphasize different aspects of quality.
CONCLUSION 6-6 All data sources have strengths and weaknesses, and they need to be carefully examined to assess their usefulness for a given statistical purpose.
RECOMMENDATION 6-2 Federal statistical agencies should outline and evaluate the strengths and weaknesses of alternative data sources on the basis of a comprehensive quality framework and, if possible, quantify the quality attributes and make them transparent to users. Agencies should focus more attention on the tradeoffs between different quality aspects, such as trading precision for timeliness and granularity, rather than focusing primarily on accuracy.
As we note in previous chapters, expanding the use of data sources for federal statistics also requires expanding the skills of the statistical agency staff to address the new issues that arise when using these new data sources. Researchers and analysts who are trained in survey methodology have a solid foundation for conceptualizing and measuring different error sources, but expertise and training is also needed in computer science for processing, cleaning, and linking datasets and the errors that can arise in these operations. In addition, specific expertise is needed in the data generating mechanisms and current uses of different administrative and private-data sources that an agency is considering for use for statistical purposes.
RECOMMENDATION 6-3 Federal statistical agencies should ensure their statistical and methodological staff receive appropriate training in various aspects of quality and the appropriate metrics and methods for examining the quality of data from different sources.
THE QUALITY OF ALTERNATIVE DATA SOURCES: TWO ILLUSTRATIONS
In this section we provide two examples of the current approach for generating federal statistics and of existing different data sources that could be used. The two examples are measuring crime and measuring inflation. We discuss how some key quality characteristics of these sources might interact and permit enhanced federal statistics if these sources were combined. Our goal here is illustrative rather than prescriptive, and the responsible federal statistical agencies would need to conduct a much more in-depth review of the alternative sources and the methods for combining them than we can do here.
Measuring Crime
Current approach.
The National Crime Victimization Survey (NCVS) of the Bureau of Justice Statistics (BJS) is the nation’s primary source of information on
criminal victimization. 2 Since 1973, data have been obtained annually from a nationally representative sample of about 90,000 households, comprising nearly 160,000 people, on the frequency, characteristics, and consequences of criminal victimization in the United States. The NCVS screens respondents to identify people who have been victims of nonfatal personal crimes (rape or sexual assault, robbery, aggravated and simple assault, and personal larceny) and property crimes (burglary, motor vehicle theft, and other theft), and it includes crimes whether or not they have been reported to the police. For each crime incident, the victim provides information about the offender, characteristics of the crime, whether it was reported to the police, and the consequences of the crime, including the victim’s experiences with the criminal justice, victim services, and health care systems. In addition, there is a wealth of additional information collected—including a victim’s demographic characteristics, educational attainment, labor force participation, household composition, and housing structure—that is related to risk of victimization (see Langton et al., 2017 ; National Academies of Sciences, Engineering, and Medicine, 2016a ).
Despite the appearance of being comprehensive, the NCVS has its quality challenges:
- Not everyone can be reached for an interview, and if people who spend large amounts of time outside their homes are more likely to be at risk for victimization, crimes can be missed.
- Of the people who are reached, not all are willing to participate in the survey, introducing the possibility for bias if those who choose not to participate differ from those who do.
- Not everyone is willing to report crimes that happen to them.
- Some respondents may misunderstand the questions asked.
- Careful testing of measurement instruments and long redesign processes lead to lags in capturing emerging crimes, such as identity theft.
- Data collection takes considerable time, so that annual estimates are only available months after the end of the year in which the crimes occurred.
Alternative Approaches
Alternative data sources on crime include the Uniform Crime Reports (UCR), which is based on police administrative records. The UCR Summary System covers jurisdiction-level counts of seven index crimes that are collated and published by the FBI. The scope of this collection has remained
2 See https://www.bjs.gov/index.cfm?ty=dcdetail&iid=245#Collection_period [August 2017].
essentially constant since 1929. Several features make the UCR an attractive alternative. The UCR is intended to be a census of about 18,000 police departments covering the whole country; however, there are lots of missing data. It provides consistent classification over time, with only modest periodic changes: arson was added in 1978, and hate crimes in the 1980s. The UCR also can provide monthly data, allowing for finer granularity in time, as well as granularity in geography because of the jurisdiction-level counts of the major crime categories.
However, the UCR also has its own errors and limitations ( Biderman and Lynch, 1991 ; Lynch and Addington, 2007 ):
- It is restricted to crimes reported to the police, and the NCVS has found that more than 50 percent of crimes reported by victims are not known to the police.
- The data are reported at the jurisdiction level and cannot be disaggregated. That is, one cannot obtain counts of offenses or rates of crimes for a subjurisdictional area or any subpopulation in the jurisdiction.
- There is virtually no information provided on the characteristics of victims or offenders or the circumstances of the incident.
- Police jurisdictions map poorly onto census classifications of places that serve as the denominator of crime rates.
- There is little enforcement of standards of reporting. Although the FBI provides training and audits to check for appropriate counting and classification of offense, there is no way to ensure that all eligible crimes are included. (The FBI collates the reported data but does not provide a warrant of quality.) Occasional audits are performed on a small number of agencies annually, but they focus on counting rules and proper classification and not on the completeness with which eligible incidents are reported. Police agencies may also misreport or misclassify crimes: for example, the Los Angeles Times found that nearly 1,200 violent crimes had been misclassified by the Los Angeles Police Department as minor offenses, resulting in a lower reported violent crime rate. 3
- The system is voluntary at the national level. There are mandatory reporting laws in states that require that local police report to the states, but there are no requirements to report to the UCR. There are also few instances in which a state mandatory reporting law has been invoked for a jurisdiction’s failure to report. In addition, unit missing data is assessed on the basis of jurisdictions that have
3 See http://www.latimes.com/local/la-me-crimestats-lapd-20140810-story.html [August 2017].
previously reported to the UCR, so units that have never participated are not counted as missing.
In addition to the data available in the Summary System, the UCR also includes the National Incident Based Reporting System (NIBRS), which contains data on crime incidents rather than jurisdiction-level counts. Currently, however, this data system is available only in a relatively small proportion of police agencies, and it substantially underrepresents the larger jurisdictions that contain most of the crime.
This would appear to be a situation in which combining data from the different sources (see Chapter 2 ) could greatly enhance the value of the estimates. BJS’s small-area estimation program (see National Academies of Sciences, Engineering, and Medicine, 2017b , Ch. 2) illustrates how the UCR Summary System and the NCVS could be used jointly to increase understanding of crime in states and other subnational areas (see also Li et al., 2017 ). As NIBRS, through the National Crime Statistics Exchange (NCS-X), 4 is implemented more broadly across the nation, the blending of NCVS and NCS-X data could be done at the incident level, which would substantially increase the ability to leverage the two data sources. The fact that both NCS-X and NCVS are sample based may pose problems for such estimates, but the emphasis on large cities in both data collections may afford sufficient overlap for useful estimation.
Measuring Inflation
The Consumer Price Index (CPI), produced by the Bureau of Labor Statistics (BLS), provides monthly data on changes in the prices paid by urban consumers for a representative basket of goods and services. The CPI has three major uses. First, the CPI is an economic indicator and the most widely used measure of inflation. Second, the CPI is a deflator of other economic series: the CPI and its components are used to adjust other economic series for price inflation and to translate them into inflation-adjusted dollars. Third, the CPI is used to adjust wages: more than 2 million workers are covered by collective bargaining agreements that tie wages to inflation. 5
BLS produces thousands of component indexes, by areas of the country,
4 The NCS-X program is designed to generate nationally representative incident-based data on crimes reported to law enforcement agencies. It comprises a sample of 400 law enforcement agencies to supplement the existing NIBRS data by providing their incident data to their state or the federal NIBRS program. See https://www.bjs.gov/content/ncsx.cfm [September 2017].
5 See https://www.bls.gov/cpi/cpifaq.htm#Question_6 [August 2017].
by major groups of consumer expenditures, and for special categories, such as services. In each case the index is based on two components—the “representative basket of goods” and the price changes in that basket of goods. The market basket of goods is based on the Consumer Expenditure Survey (CE), which is a household survey conducted each year in a probability sample of households selected from all urban areas in the United States. In one part of the survey, 7,000 households are interviewed each quarter on their spending habits; an additional 7,000 families complete diaries for a 2-week period on everything they bought during those 2 weeks. The CE produces a picture of the expenditure patterns of households and selected subgroups, in terms of the quantities of different products and services they bought during the period. These are combined across the households in the sample to provide a picture of the total expenditure patterns of the U.S. household population and for subsets of that population. The weight for an item is derived from reported expenditures on that item divided by the total of all expenditures. The most recent CPI market basket is based on data from CE for 2013 and 2014.
Separate surveys and visits to retail stores are conducted by BLS to obtain prices for goods and services used to calculate the CPI, which are collected in 87 urban areas throughout the country, covering about 89 percent of the total U.S. population and about 23,000 retail and service establishments. Data on rents are collected from about 50,000 landlords or tenants.
These massive data collection efforts are facing challenges. As we described in our first report, response rates for the Consumer Expenditure interview and diary surveys have declined ( National Academies of Sciences, Engineering, and Medicine, 2017b , Ch. 2). Another major source of error in the CE is the quality of the measurement of expenditures, given that respondents are exposed to very lengthy questionnaires with many recall tasks. Facing many questions in a row about their expenditures and details about each expenditure item, respondents are likely to underreport and so shorten the interview (or skip recording items in the diary) in order to reduce the reporting burden (see National Research Council, 2013a ). And, as for the NCVS, some people are not willing to participate in the survey, reducing the precision of the resulting estimates and possibly introducing bias ( National Research Council, 2013b ).
The explosion in the availability of online consumer data suggests that this is an area in which there is enormous potential to use alternative data to supplement or replace the current measurement of inflation. One example of such an effort is the Billion Prices Project (BPP): it was initi-
ated in 2007 to provide an alternative inflation index for Argentina, given widespread distrust of the official level reported by the national statistical office, and has since expanded to cover almost 80 countries ( Cavallo and Rigobon, 2016 ). The objective of BPP was to substitute the collection of prices using web-scraping instead of visiting retail stores in person to collect prices. Although the data are dispersed across hundreds of websites and thousands of web pages, advances in automated scraping software now allow design and implementation of large-scale data collections on the web.
The main advantage of web-scraping is that sampling and nonresponse errors are minimized—and in some circumstances they might go to zero. 6 Furthermore, detailed information can be collected for each good, and new and disappearing products can be quickly detected and accounted for. Online data collection is cheap, fast, and accurate, making it an ideal complement to traditional methods of collecting prices, particularly in categories of goods that are well represented online, such as food, personal care, electronics, clothing, air travel, hotels, and transportation. In addition to being well-represented online, these sectors reflect prices that are close to the prices from all transactions (i.e., offline transactions are the same prices), making the data of high quality. In some sectors the data quality is less desirable. Gasoline is an example: gasoline is not bought online, and the prices are collected by third parties and then shown online. The quality of this procedure depends dramatically on how the data are collected and curated before they are shown. Because information about prices on the web may be very good in some sectors but seriously deficient in others, there is a continuing challenge to develop a described, researched error structure for a hybrid approach, which would combine information from different sources to calculate an index of inflation.
To create an inflation index, consumption quantities are needed in addition to prices. Information on quantities is essentially nonexistent online (at least not accessible through web-scraping). For the BPP, the consumption quantities (i.e., the data to construct the weights) come from a combination of the CE and inferences on how the web pages are organized. Therefore, the construction of online-based inflation indexes uses a hybrid approach of survey and alternative data sources, both the prices and quantities.
6 Nonresponse errors would be zero if all selected websites were able to be scraped. Sampling errors would be zero if the relevant universe was completely covered.
The environment for obtaining information and providing statistical data for policy makers and the public has changed significantly in the past decade, raising questions about the fundamental survey paradigm that underlies federal statistics. New data sources provide opportunities to develop a new paradigm that can improve timeliness, geographic or subpopulation detail, and statistical efficiency. It also has the potential to reduce the costs of producing federal statistics.
The panel's first report described federal statistical agencies' current paradigm, which relies heavily on sample surveys for producing national statistics, and challenges agencies are facing; the legal frameworks and mechanisms for protecting the privacy and confidentiality of statistical data and for providing researchers access to data, and challenges to those frameworks and mechanisms; and statistical agencies access to alternative sources of data. The panel recommended a new approach for federal statistical programs that would combine diverse data sources from government and private sector sources and the creation of a new entity that would provide the foundational elements needed for this new approach, including legal authority to access data and protect privacy.
This second of the panel's two reports builds on the analysis, conclusions, and recommendations in the first one. This report assesses alternative methods for implementing a new approach that would combine diverse data sources from government and private sector sources, including describing statistical models for combining data from multiple sources; examining statistical and computer science approaches that foster privacy protections; evaluating frameworks for assessing the quality and utility of alternative data sources; and various models for implementing the recommended new entity. Together, the two reports offer ideas and recommendations to help federal statistical agencies examine and evaluate data from alternative sources and then combine them as appropriate to provide the country with more timely, actionable, and useful information for policy makers, businesses, and individuals.
READ FREE ONLINE
Welcome to OpenBook!
You're looking at OpenBook, NAP.edu's online reading room since 1999. Based on feedback from you, our users, we've made some improvements that make it easier than ever to read thousands of publications on our website.
Do you want to take a quick tour of the OpenBook's features?
Show this book's table of contents , where you can jump to any chapter by name.
...or use these buttons to go back to the previous chapter or skip to the next one.
Jump up to the previous page or down to the next one. Also, you can type in a page number and press Enter to go directly to that page in the book.
Switch between the Original Pages , where you can read the report as it appeared in print, and Text Pages for the web version, where you can highlight and search the text.
To search the entire text of this book, type in your search term here and press Enter .
Share a link to this book page on your preferred social network or via email.
View our suggested citation for this chapter.
Ready to take your reading offline? Click here to buy this book in print or download it as a free PDF, if available.
Get Email Updates
Do you enjoy reading reports from the Academies online for free ? Sign up for email notifications and we'll let you know about new publications in your areas of interest when they're released.

Research Writing and Analysis
- NVivo Group and Study Sessions
- SPSS This link opens in a new window
- Statistical Analysis Group sessions
- Using Qualtrics
- Dissertation and Data Analysis Group Sessions
- Defense Schedule - Commons Calendar This link opens in a new window
- Research Process Flow Chart
- Research Alignment Chapter 1 This link opens in a new window
- Step 1: Seek Out Evidence
- Step 2: Explain
- Step 3: The Big Picture
- Step 4: Own It
- Step 5: Illustrate
- Annotated Bibliography
- Literature Review This link opens in a new window
- Systematic Reviews & Meta-Analyses
- How to Synthesize and Analyze
- Synthesis and Analysis Practice
- Synthesis and Analysis Group Sessions
- Problem Statement
- Purpose Statement
- Conceptual Framework
- Theoretical Framework
- Quantitative Research Questions
- Qualitative Research Questions
- Trustworthiness of Qualitative Data
- Analysis and Coding Example- Qualitative Data
- Thematic Data Analysis in Qualitative Design
- Dissertation to Journal Article This link opens in a new window
- International Journal of Online Graduate Education (IJOGE) This link opens in a new window
- Journal of Research in Innovative Teaching & Learning (JRIT&L) This link opens in a new window
Defining The Conceptual Framework
What is it.
- The researcher’s understanding/hypothesis/exploration of either an existing framework/model or how existing concepts come together to inform a particular problem. Shows the reader how different elements come together to facilitate research and a clear understanding of results.
- Informs the research questions/methodology (problem statement drives framework drives RQs drives methodology)
- A tool (linked concepts) to help facilitate the understanding of the relationship among concepts or variables in relation to the real-world. Each concept is linked to frame the project in question.
- Falls inside of a larger theoretical framework (theoretical framework = explains the why and how of a particular phenomenon within a particular body of literature).
- Can be a graphic or a narrative – but should always be explained and cited
- Can be made up of theories and concepts
What does it do?
- Explains or predicts the way key concepts/variables will come together to inform the problem/phenomenon
- Gives the study direction/parameters
- Helps the researcher organize ideas and clarify concepts
- Introduces your research and how it will advance your field of practice. A conceptual framework should include concepts applicable to the field of study. These can be in the field or neighboring fields – as long as important details are captured and the framework is relevant to the problem. (alignment)
What should be in it?
- Variables, concepts, theories, and/or parts of other existing frameworks
Making a Conceptual Framework
How to make a conceptual framework.
- With a topic in mind, go to the body of literature and start identifying the key concepts used by other studies. Figure out what’s been done by other researchers, and what needs to be done (either find a specific call to action outlined in the literature or make sure your proposed problem has yet to be studied in your specific setting). Use what you find needs to be done to either support a pre-identified problem or craft a general problem for study. Only rely on scholarly sources for this part of your research.
- Begin to pull out variables, concepts, theories, and existing frameworks explained in the relevant literature.
- If you’re building a framework, start thinking about how some of those variables, concepts, theories, and facets of existing frameworks come together to shape your problem. The problem could be a situational condition that requires a scholar-practitioner approach, the result of a practical need, or an opportunity to further an applicational study, project, or research. Remember, if the answer to your specific problem exists, you don’t need to conduct the study.
- The actionable research you’d like to conduct will help shape what you include in your framework. Sketch the flow of your Applied Doctoral Project from start to finish and decide which variables are truly the best fit for your research.
- Create a graphic representation of your framework (this part is optional, but often helps readers understand the flow of your research) Even if you do a graphic, first write out how the variables could influence your Applied Doctoral Project and introduce your methodology. Remember to use APA formatting in separating the sections of your framework to create a clear understanding of the framework for your reader.
- As you move through your study, you may need to revise your framework.
- Note for qualitative/quantitative research: If doing qualitative, make sure your framework doesn’t include arrow lines, which could imply causal or correlational linkages.
Conceptual Framework for DMFT Students
- Conceptural and Theoretical Framework for DMFT Students This document is specific to DMFT students working on a conceptual or theoretical framework for their applied project.
Conceptual Framework Guide
- Conceptual Framework Guide Use this guide to determine the guiding framework for your applied dissertation research.
Example Frameworks
Let’s say I’ve just taken a job as manager of a failing restaurant. Throughout first week, I notice the few customers they have are leaving unsatisfied. I need to figure out why and turn the establishment into a thriving restaurant. I get permission from the owner to do a study to figure out exactly what we need to do to raise levels of customer satisfaction. Since I have a specific problem and want to make sure my research produces valid results, I go to the literature to find out what others are finding about customer satisfaction in the food service industry. This particular restaurant is vegan focused – and my search of the literature doesn’t say anything specific about how to increase customer service in a vegan atmosphere, so I know this research needs to be done.
I find out there are different types of satisfaction across other genres of the food service industry, and the one I’m interested in is cumulative customer satisfaction. I then decide based on what I’m seeing in the literature that my definition of customer satisfaction is the way perception, evaluation, and psychological reaction to perception and evaluation of both tangible and intangible elements of the dining experience come together to inform customer expectations. Essentially, customer expectations inform customer satisfaction.
I then find across the literature many variables could be significant in determining customer satisfaction. Because the following keep appearing, they are the ones I choose to include in my framework: price, service, branding (branched out to include physical environment and promotion), and taste. I also learn by reading the literature, satisfaction can vary between genders – so I want to make sure to also collect demographic information in my survey. Gender, age, profession, and number of children are a few demographic variables I understand would be helpful to include based on my extensive literature review.
Note: this is a quantitative study. I’m including all variables in this study, and the variables I am testing are my independent variables. Here I’m working to see how each of the independent variables influences (or not) my dependent variable, customer satisfaction. If you are interested in qualitative study, read on for an example of how to make the same framework qualitative in nature.
Also note: when you create your framework, you’ll need to cite each facet of your framework. Tell the reader where you got everything you’re including. Not only is it in compliance with APA formatting, but also it raises your credibility as a researcher. Once you’ve built the narrative around your framework, you may also want to create a visual for your reader.
See below for one example of how to illustrate your framework:

If you’re interested in a qualitative study, be sure to omit arrows and other notations inferring statistical analysis. The only time it would be inappropriate to include a framework in qualitative study is in a grounded theory study, which is not something you’ll do in an applied doctoral study.
A visual example of a qualitative framework is below:

Additional Framework Resources
Some additional helpful resources in constructing a conceptual framework for study:.
- Problem Statement, Conceptual Framework, and Research Question. McGaghie, W. C.; Bordage, G.; and J. A. Shea (2001). Problem Statement, Conceptual Framework, and Research Question. Retrieved on January 5, 2015 from http://goo.gl/qLIUFg
- Building a Conceptual Framework: Philosophy, Definitions, and Procedure
- https://www.scribbr.com/dissertation/conceptual-framework/
- https://www.projectguru.in/developing-conceptual-framework-in-a-research-paper/
Conceptual Framework Research
A conceptual framework is a synthetization of interrelated components and variables which help in solving a real-world problem. It is the final lens used for viewing the deductive resolution of an identified issue (Imenda, 2014). The development of a conceptual framework begins with a deductive assumption that a problem exists, and the application of processes, procedures, functional approach, models, or theory may be used for problem resolution (Zackoff et al., 2019). The application of theory in traditional theoretical research is to understand, explain, and predict phenomena (Swanson, 2013). In applied research the application of theory in problem solving focuses on how theory in conjunction with practice (applied action) and procedures (functional approach) frames vision, thinking, and action towards problem resolution. The inclusion of theory in a conceptual framework is not focused on validation or devaluation of applied theories. A concise way of viewing the conceptual framework is a list of understood fact-based conditions that presents the researcher’s prescribed thinking for solving the identified problem. These conditions provide a methodological rationale of interrelated ideas and approaches for beginning, executing, and defining the outcome of problem resolution efforts (Leshem & Trafford, 2007).
The term conceptual framework and theoretical framework are often and erroneously used interchangeably (Grant & Osanloo, 2014). Just as with traditional research, a theory does not or cannot be expected to explain all phenomenal conditions, a conceptual framework is not a random identification of disparate ideas meant to incase a problem. Instead it is a means of identifying and constructing for the researcher and reader alike an epistemological mindset and a functional worldview approach to the identified problem.
Grant, C., & Osanloo, A. (2014). Understanding, Selecting, and Integrating a Theoretical Framework in Dissertation Research: Creating the Blueprint for Your “House. ” Administrative Issues Journal: Connecting Education, Practice, and Research, 4(2), 12–26
Imenda, S. (2014). Is There a Conceptual Difference between Theoretical and Conceptual Frameworks? Sosyal Bilimler Dergisi/Journal of Social Sciences, 38(2), 185.
Leshem, S., & Trafford, V. (2007). Overlooking the conceptual framework. Innovations in Education & Teaching International, 44(1), 93–105. https://doi-org.proxy1.ncu.edu/10.1080/14703290601081407
Swanson, R. (2013). Theory building in applied disciplines . San Francisco: Berrett-Koehler Publishers.
Zackoff, M. W., Real, F. J., Klein, M. D., Abramson, E. L., Li, S.-T. T., & Gusic, M. E. (2019). Enhancing Educational Scholarship Through Conceptual Frameworks: A Challenge and Roadmap for Medical Educators . Academic Pediatrics, 19(2), 135–141. https://doi-org.proxy1.ncu.edu/10.1016/j.acap.2018.08.003
Student Experience Feedback Buttons
Was this resource helpful.
- << Previous: Purpose Statement
- Next: Theoretical Framework >>
- Last Updated: Apr 29, 2024 1:16 PM
- URL: https://resources.nu.edu/researchtools

- ns-3
- Manual
- 3. Additional Tools
3.5. Statistical Framework ¶
This chapter outlines work on simulation data collection and the statistical framework for ns-3.
The source code for the statistical framework lives in the directory src/stats .
3.5.1. Goals ¶
Primary objectives for this effort are the following:
- Provide functionality to record, calculate, and present data and statistics for analysis of network simulations.
- Boost simulation performance by reducing the need to generate extensive trace logs in order to collect data.
- Enable simulation control via online statistics, e.g. terminating simulations or repeating trials.
Derived sub-goals and other target features include the following:
- Integration with the existing ns-3 tracing system as the basic instrumentation framework of the internal simulation engine, e.g. network stacks, net devices, and channels.
- Enabling users to utilize the statistics framework without requiring use of the tracing system.
- Helping users create, aggregate, and analyze data over multiple trials.
- Support for user created instrumentation, e.g. of application specific events and measures.
- Low memory and CPU overhead when the package is not in use.
- Leveraging existing analysis and output tools as much as possible. The framework may provide some basic statistics, but the focus is on collecting data and making it accessible for manipulation in established tools.
- Eventual support for distributing independent replications is important but not included in the first round of features.
3.5.2. Overview ¶
The statistics framework includes the following features:
- The core framework and two basic data collectors: A counter, and a min/max/avg/total observer.
- Extensions of those to easily work with times and packets.
- Plaintext output formatted for OMNet++ .
- Database output using SQLite , a standalone, lightweight, high performance SQL engine.
- Mandatory and open ended metadata for describing and working with runs.
- Constructs of a two node ad hoc WiFi network, with the nodes a parameterized distance apart.
- UDP traffic source and sink applications with slightly different behavior and measurement hooks than the stock classes.
- Data collection from the NS-3 core via existing trace signals, in particular data on frames transmitted and received by the WiFi MAC objects.
- Instrumentation of custom applications by connecting new trace signals to the stat framework, as well as via direct updates. Information is recorded about total packets sent and received, bytes transmitted, and end-to-end delay.
- An example of using packet tags to track end-to-end delay.
- A simple control script which runs a number of trials of the experiment at varying distances and queries the resulting database to produce a graph using GNUPlot.
3.5.3. To-Do ¶
High priority items include:
- Inclusion of online statistics code, e.g. for memory efficient confidence intervals.
- Provisions in the data collectors for terminating runs, i.e. when a threshold or confidence is met.
- Data collectors for logging samples over time, and output to the various formats.
- Demonstrate writing simple cyclic event glue to regularly poll some value.
Each of those should prove straightforward to incorporate in the current framework.
3.5.4. Approach ¶
The framework is based around the following core principles:
- One experiment trial is conducted by one instance of a simulation program, whether in parallel or serially.
- A control script executes instances of the simulation, varying parameters as necessary.
- Data is collected and stored for plotting and analysis using external scripts and existing tools.
- Measures within the ns-3 core are taken by connecting the stat framework to existing trace signals.
- Trace signals or direct manipulation of the framework may be used to instrument custom simulation code.
Those basic components of the framework and their interactions are depicted in the following figure.

3.5.5. Example ¶
This section goes through the process of constructing an experiment in the framework and producing data for analysis (graphs) from it, demonstrating the structure and API along the way.
3.5.5.1. Question ¶
‘’What is the (simulated) performance of ns-3’s WiFi NetDevices (using the default settings)? How far apart can wireless nodes be in a simulation before they cannot communicate reliably?’‘
- Hypothesis: Based on knowledge of real life performance, the nodes should communicate reasonably well to at least 100m apart. Communication beyond 200m shouldn’t be feasible.
Although not a very common question in simulation contexts, this is an important property of which simulation developers should have a basic understanding. It is also a common study done on live hardware.
3.5.5.2. Simulation Program ¶
The first thing to do in implementing this experiment is developing the simulation program. The code for this example can be found in examples/stats/wifi-example-sim.cc . It does the following main steps.
Declaring parameters and parsing the command line using ns3::CommandLine .
Creating nodes and network stacks using ns3::NodeContainer , ns3::WiFiHelper , and ns3::InternetStackHelper .
Positioning the nodes using ns3::MobilityHelper . By default the nodes have static mobility and won’t move, but must be positioned the given distance apart. There are several ways to do this; it is done here using ns3::ListPositionAllocator , which draws positions from a given list.
Installing a traffic generator and a traffic sink. The stock Applications could be used, but the example includes custom objects in src/test/test02-apps.(cc|h) . These have a simple behavior, generating a given number of packets spaced at a given interval. As there is only one of each they are installed manually; for a larger set the ns3::ApplicationHelper class could be used. The commented-out Config::Set line changes the destination of the packets, set to broadcast by default in this example. Note that in general WiFi may have different performance for broadcast and unicast frames due to different rate control and MAC retransmission policies.
Configuring the data and statistics to be collected. The basic paradigm is that an ns3::DataCollector object is created to hold information about this particular run, to which observers and calculators are attached to actually generate data. Importantly, run information includes labels for the ‘’experiment’‘, ‘’strategy’‘, ‘’input’‘, and ‘’run’‘. These are used to later identify and easily group data from multiple trials.
- The experiment is the study of which this trial is a member. Here it is on WiFi performance and distance.
- The strategy is the code or parameters being examined in this trial. In this example it is fixed, but an obvious extension would be to investigate different WiFi bit rates, each of which would be a different strategy.
- The input is the particular problem given to this trial. Here it is simply the distance between the two nodes.
- The runID is a unique identifier for this trial with which it’s information is tagged for identification in later analysis. If no run ID is given the example program makes a (weak) run ID using the current time.
Those four pieces of metadata are required, but more may be desired. They may be added to the record using the ns3::DataCollector::AddMetadata() method.
Actual observation and calculating is done by ns3::DataCalculator objects, of which several different types exist. These are created by the simulation program, attached to reporting or sampling code, and then registered with the ns3::DataCollector so they will be queried later for their output. One easy observation mechanism is to use existing trace sources, for example to instrument objects in the ns-3 core without changing their code. Here a counter is attached directly to a trace signal in the WiFi MAC layer on the target node.
Calculators may also be manipulated directly. In this example, a counter is created and passed to the traffic sink application to be updated when packets are received.
To increment the count, the sink’s packet processing code then calls one of the calculator’s update methods.
The program includes several other examples as well, using both the primitive calculators such as ns3::CounterCalculator and those adapted for observing packets and times. In src/test/test02-apps.(cc|h) it also creates a simple custom tag which it uses to track end-to-end delay for generated packets, reporting results to a ns3::TimeMinMaxAvgTotalCalculator data calculator.
Running the simulation, which is very straightforward once constructed.
Generating either OMNet++ or SQLite output, depending on the command line arguments. To do this a ns3::DataOutputInterface object is created and configured. The specific type of this will determine the output format. This object is then given the ns3::DataCollector object which it interrogates to produce the output.
Freeing any memory used by the simulation. This should come at the end of the main function for the example.
3.5.5.2.1. Logging ¶
To see what the example program, applications, and stat framework are doing in detail, set the NS_LOG variable appropriately. The following will provide copious output from all three.
Note that this slows down the simulation extraordinarily.
3.5.5.2.2. Sample Output ¶
Compiling and simply running the test program will append OMNet++ formatted output such as the following to data.sca .
3.5.5.3. Control Script ¶
In order to automate data collection at a variety of inputs (distances), a simple Bash script is used to execute a series of simulations. It can be found at examples/stats/wifi-example-db.sh . The script is meant to be run from the examples/stats/ directory.
The script runs through a set of distances, collecting the results into an SQLite database. At each distance five trials are conducted to give a better picture of expected performance. The entire experiment takes only a few dozen seconds to run on a low end machine as there is no output during the simulation and little traffic is generated.
3.5.5.4. Analysis and Conclusion ¶
Once all trials have been conducted, the script executes a simple SQL query over the database using the SQLite command line program. The query computes average packet loss in each set of trials associated with each distance. It does not take into account different strategies, but the information is present in the database to make some simple extensions and do so. The collected data is then passed to GNUPlot for graphing.
The GNUPlot script found at examples/stats/wifi-example.gnuplot simply defines the output format and some basic formatting for the graph.
3.5.5.4.1. End Result ¶
The resulting graph provides no evidence that the default WiFi model’s performance is necessarily unreasonable and lends some confidence to an at least token faithfulness to reality. More importantly, this simple investigation has been carried all the way through using the statistical framework. Success!

Table of Contents
- 3.5.1. Goals
- 3.5.2. Overview
- 3.5.3. To-Do
- 3.5.4. Approach
- 3.5.5.1. Question
- 3.5.5.2.1. Logging
- 3.5.5.2.2. Sample Output
- 3.5.5.3. Control Script
- 3.5.5.4.1. End Result
Previous topic
3.4.7. Scope/Limitations
3.6. Helpers
- Show Source
Quick search

An official website of the United States government
Here’s how you know
Official websites use .gov A .gov website belongs to an official government organization in the United States.
Secure .gov websites use HTTPS A lock ( Lock A locked padlock ) or https:// means you’ve safely connected to the .gov website. Share sensitive information only on official, secure websites.
2024 National Strategy for Suicide Prevention
Suicide is an urgent and growing public health crisis. More than 49,000 people in the United States died by suicide in 2022. That’s one death every 11 minutes.
National Strategy for Suicide Prevention
The 2024 National Strategy for Suicide Prevention is a bold new 10-year, comprehensive, whole-of-society approach to suicide prevention that provides concrete recommendations for addressing gaps in the suicide prevention field. This coordinated and comprehensive approach to suicide prevention at the national, state, tribal, local, and territorial levels relies upon critical partnerships across the public and private sectors. People with lived experience are critical to the success of this work.
The National Strategy seeks to prevent suicide risk in the first place; identify and support people with increased risk through treatment and crisis intervention; prevent reattempts; promote long-term recovery; and support survivors of suicide loss.
Four strategic directions guide the National Strategy:
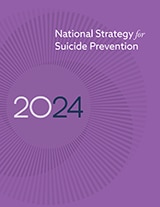
Strategic Direction 1: Community-Based Suicide Prevention
Goal 1: Establish effective, broad-based, collaborative, and sustainable suicide prevention partnerships.
Goal 2: Support upstream comprehensive community-based suicide prevention.
Goal 3: Reduce access to lethal means among people at risk of suicide.
Goal 4: Conduct postvention and support people with suicide-centered lived experience.
Goal 5: Integrate suicide prevention into the culture of the workplace and into other community settings.
Goal 6: Build and sustain suicide prevention infrastructure at the state, tribal, local, and territorial levels.
Goal 7: Implement research-informed suicide prevention communication activities in diverse populations using best practices from communication science.
Strategic Direction 2: Treatment and Crisis Services
Goal 8: Implement effective suicide prevention services as a core component of health care.
Goal 9: Improve the quality and accessibility of crisis care services across all communities.
Strategic Direction 3: Surveillance, Quality Improvement, and Research
Goal 10: Improve the quality, timeliness, scope, usefulness, and accessibility of data needed for suicide-related surveillance, research, evaluation, and quality improvement.
Goal 11: Promote and support research on suicide prevention.
Strategic Direction 4: Health Equity in Suicide Prevention
Goal 12: Embed health equity into all comprehensive suicide prevention activities.
Goal 13: Implement comprehensive suicide prevention strategies for populations disproportionately affected by suicide, with a focus on historically marginalized communities, persons with suicide-centered lived experience, and youth.
Goal 14: Create an equitable and diverse suicide prevention workforce that is equipped and supported to address the needs of the communities they serve.
Goal 15: Improve and expand effective suicide prevention programs for populations disproportionately impacted by suicide across the life span through improved data, research, and evaluation.
Federal Action Plan
The Federal Action Plan identifies more than 200 actions across the federal government to be taken over the next three years in support of those goals. These actions include:
- Evaluating promising community-based suicide prevention strategies
- Identifying ways to address substance use/overdose and suicide risk together in the clinical setting
- Funding a mobile crisis locator for use by 988 crisis centers
- Increasing support for survivors of suicide loss and others whose lives have been impacted by suicide
These actions will be monitored and evaluated regularly to determine progress and success, and to further identify barriers to suicide prevention.
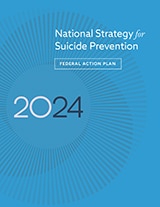
Get Involved
Join the conversation. Everyone has a role to play in preventing the tragedy of suicide. Find social media material, templates, and other resources to support and participate in the shared effort.
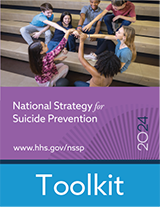
Read the press release
* This content is undergoing Section 508 remediation. For immediate assistance, contact [email protected] .
- Skip to content (access key: 1)
- Skip to Search (access key: 2)
Institutes, schools, other departments, and programs create their own web content and menus.
To help you better navigate the site, see here where you are at the moment.
Research Seminar at the Institute of Applied Statistics
April 25 th :
Prof. Asger Hobolth, Department of Mathematics, Aarhus University, Denmark: Phase-type distributions in mathematical population genetics: An emerging framework. (The talk is based on joint work with Iker Rivas-Gonzalez (Leipzig), Mogens Bladt (Copenhagen) and Andreas Futschik (Linz).)
![[Translate to Englisch:] [Translate to Englisch:]](https://www.jku.at/fileadmin/_processed_/a/6/csm_asger_hobolth_921x630_02e03ac6a0.jpg)
zoom link , opens an external URL in a new window
Meeting-ID: 280 519 2121 Passwort: 584190
A phase-type distribution is the time to absorption in a continuous- or discrete-time Markov chain. Phase-type distributions can be used as a general framework to calculate key properties of the standard coalescent model and many of its extensions. Here, the ‘phases’ in the phase-type distribution correspond to states in the ancestral process. For example, the time to the most recent common ancestor and the total branch length are phase-type distributed. Furthermore, the site frequency spectrum follows a multivariate discrete phase-type distribution and the joint distribution of total branch lengths in the two-locus coalescent-with-recombination model is multivariate phase-type distributed. In general, phase-type distributions provide a powerful mathematical framework for coalescent theory because they are analytically tractable using matrix manipulations. The purpose of this talk is to explain the phase-type theory and demonstrate how the theory can be applied to derive basic properties of coalescent models. These properties can then be used to obtain insight into the ancestral process, or they can be applied for statistical inference.
Add to my calendar
Johannes Kepler University Linz
Altenberger Straße 69
4040 Linz, Austria
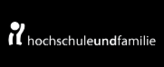
Use of cookies
Our website uses cookies to ensure you get the best experience on our website, for analytical purposes, to provide social media features, and for targeted advertising. This it is necessary in order to pass information on to respective service providers. If you would like additional information about cookies on this website, please see our data privacy policy .
Required cookies
These cookies are required to help our website run smoothly.
Web statistics cookies
These cookies help us to continuously improve our services and adapt our website to your needs. We statistically evaluate the pseudonymized data collected from our website.
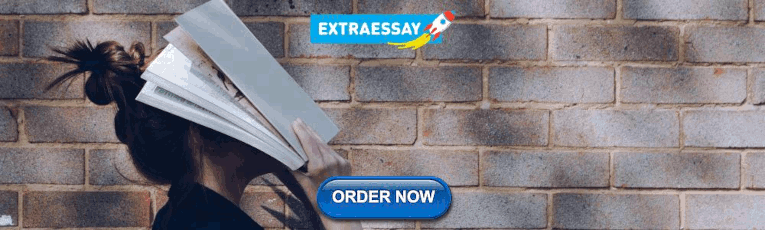
Marketing cookies
These cookies help us make our services more attractive to you as well as optimize our advertising and website content. We analyze and evaluate pseudonymised data collected from our website.
- COVID Information
- Patient Care
- Referring Providers
- Price Transparency
- Employee Resources
Fei Ye selected as 2024 Fellow of the American Statistical Association
We are thrilled to share the news that Fei Ye , professor of biostatistics and medicine on the Basic Science Educator track , has been selected as a 2024 Fellow of the American Statistical Association. The designation of ASA Fellow honors members of the association who have made outstanding contributions to statistical science. The process of being considered for ASA fellowship is exceptionally competitive, with very few honorees chosen among many highly qualified nominees. Dr. Ye, whose induction will take place during the Joint Statistical Meetings in Portland, Oregon, this August, is being recognized "for pioneering contributions to biomedical research, including statistical and bioinformatic methods, innovations in clinical trial design and analysis, and a deeper understanding of biomarkers, plus impactful collaborations and mentoring."
Dr. Ye earned her MSPH and PhD from the University of South Carolina and joined Vanderbilt University Medical Center as a staff biostatistician in 2006. Her current roles include serving as vice chair of collaborative studies for the department of biostatistics, and assistant editor for statistics with JAMA Oncology. Her statistical prowess has been a game-changer for dozens of research programs, both in terms of securing large grants and providing predictive models, diagnostic algorithms, and sophisticated data analyses to the investigations in question. In the words of Dr. Vivian Weiss, head of the high-impact translational research Weiss Lab , Dr. Ye's contributions "are paving the way for dramatic changes in the way we take care of thyroid cancer patients"; these include validating a commercial molecular diagnostic assay for children, developing a molecular assay for outcome prediction in adults, and setting in motion other novel strategies for assessing malignancies and their potential origins. Dr. Ye has also been at the forefront of discussions on liver allocation, with an eye toward addressing health inequity concerns in organ transplant policy and practice, as well as a go-to collaborator for a wide range of topics and conditions, from original research on atherosclerosis, atrial fibrillation, diverticulitis, multiple sclerosis, and more, to observations on surgical practice and ethics. She is also a trusted expert on statistical considerations for the design and implementation of clinical trials.
With Vanderbilt epidemiologist Dr. Jirong Long , Dr. Ye is Multiple Principal Investigator (MPI) of a pioneering investigation—the first large multi-racial methylation-wide association study (MeWAS)—that combines integrative bioinformatics, functional experiments, and high statistical power to expand and refine our understanding of the role DNA methylation plays in cancer development. Dr. Ye has also designed advanced modules for Vanderbilt’s Epidemiology PhD program, and she is on the faculty of the Vanderbilt Molecular and Genetic Epidemiology of Cancer (MAGEC) Training Program. International initiatives that have called on Dr. Ye’s experience include the Vietnam National Cancer Institute (VNCI) and Vinmec International Hospital, as well as the Vanderbilt-Zambia Cancer Research Training Program. A past president of the ASA's Middle Tennessee chapter, Dr. Ye is currently program chair of the ASA's Statistics in Epidemiology Section and poster chair for the 2024 International Chinese Statistical Association's Applied Statistics Symposium , which will convene in Nashville this June.
Fei Ye (center), flanked by lead biostatistician Amy Perkins and application developer Hui Wu at the department's January 2024 winter celebration.
- First-authored paper in Journal of Molecular Biology by Hua-Chang Chen
Help | Advanced Search
Computer Science > Machine Learning
Title: hippocrates: an open-source framework for advancing large language models in healthcare.
Abstract: The integration of Large Language Models (LLMs) into healthcare promises to transform medical diagnostics, research, and patient care. Yet, the progression of medical LLMs faces obstacles such as complex training requirements, rigorous evaluation demands, and the dominance of proprietary models that restrict academic exploration. Transparent, comprehensive access to LLM resources is essential for advancing the field, fostering reproducibility, and encouraging innovation in healthcare AI. We present Hippocrates, an open-source LLM framework specifically developed for the medical domain. In stark contrast to previous efforts, it offers unrestricted access to its training datasets, codebase, checkpoints, and evaluation protocols. This open approach is designed to stimulate collaborative research, allowing the community to build upon, refine, and rigorously evaluate medical LLMs within a transparent ecosystem. Also, we introduce Hippo, a family of 7B models tailored for the medical domain, fine-tuned from Mistral and LLaMA2 through continual pre-training, instruction tuning, and reinforcement learning from human and AI feedback. Our models outperform existing open medical LLMs models by a large-margin, even surpassing models with 70B parameters. Through Hippocrates, we aspire to unlock the full potential of LLMs not just to advance medical knowledge and patient care but also to democratize the benefits of AI research in healthcare, making them available across the globe.
Submission history
Access paper:.
- Other Formats

References & Citations
- Google Scholar
- Semantic Scholar
BibTeX formatted citation

Bibliographic and Citation Tools
Code, data and media associated with this article, recommenders and search tools.
- Institution
arXivLabs: experimental projects with community collaborators
arXivLabs is a framework that allows collaborators to develop and share new arXiv features directly on our website.
Both individuals and organizations that work with arXivLabs have embraced and accepted our values of openness, community, excellence, and user data privacy. arXiv is committed to these values and only works with partners that adhere to them.
Have an idea for a project that will add value for arXiv's community? Learn more about arXivLabs .
- Share full article
Advertisement
Supported by
Physical Fitness Linked to Better Mental Health in Young People
A new study bolsters existing research suggesting that exercise can protect against anxiety, depression and attention challenges.

By Matt Richtel
Physical fitness among children and adolescents may protect against developing depressive symptoms, anxiety and attention deficit hyperactivity disorder, according to a study published on Monday in JAMA Pediatrics.
The study also found that better performance in cardiovascular activities, strength and muscular endurance were each associated with greater protection against such mental health conditions. The researchers deemed this linkage “dose-dependent”, suggesting that a child or adolescent who is more fit may be accordingly less likely to experience the onset of a mental health disorder.
These findings come amid a surge of mental health diagnoses among children and adolescents, in the United States and abroad, that have prompted efforts to understand and curb the problem.

The new study, conducted by researchers in Taiwan, compared data from two large data sets: the Taiwan National Student Fitness Tests, which measures student fitness performance in schools, and the National Insurance Research Databases, which records medical claims, diagnoses prescriptions and other medical information. The researchers did not have access to the students’ names but were able to use the anonymized data to compare the students’ physical fitness and mental health results.
The risk of mental health disorder was weighted against three metrics for physical fitness: cardio fitness, as measured by a student’s time in an 800-meter run; muscle endurance, indicated by the number of situps performed; and muscle power, measured by the standing broad jump.
Improved performance in each activity was linked with a lower risk of mental health disorder. For instance, a 30-second decrease in 800-meter time was associated, in girls, with a lower risk of anxiety, depression and A.D.H.D. In boys, it was associated with lower anxiety and risk of the disorder.
An increase of five situps per minute was associated with lower anxiety and risk of the disorder in boys, and with decreased risk of depression and anxiety in girls.
“These findings suggest the potential of cardiorespiratory and muscular fitness as protective factors in mitigating the onset of mental health disorders among children and adolescents,” the researchers wrote in the journal article.
Physical and mental health were already assumed to be linked , they added, but previous research had relied largely on questionnaires and self-reports, whereas the new study drew from independent assessments and objective standards.
The Big Picture
The surgeon general, Dr. Vivek H. Murthy, has called mental health “the defining public health crisis of our time,” and he has made adolescent mental health central to his mission. In 2021 he issued a rare public advisory on the topic. Statistics at the time revealed alarming trends: From 2001 to 2019, the suicide rate for Americans ages 10 to 19 rose 40 percent, and emergency visits related to self-harm rose 88 percent.
Some policymakers and researchers have blamed the sharp increase on the heavy use of social media, but research has been limited and the findings sometimes contradictory. Other experts theorize that heavy screen use has affected adolescent mental health by displacing sleep, exercise and in-person activity, all of which are considered vital to healthy development. The new study appeared to support the link between physical fitness and mental health.
“The finding underscores the need for further research into targeted physical fitness programs,” its authors concluded. Such programs, they added, “hold significant potential as primary preventative interventions against mental disorders in children and adolescents.”
Matt Richtel is a health and science reporter for The Times, based in Boulder, Colo. More about Matt Richtel
Understanding A.D.H.D.
The challenges faced by those with attention deficit hyperactivity disorder can be daunting. but people who are diagnosed with it can still thrive..
Millions of children in the United States have received a diagnosis of A.D.H.D . Here is how their families can support them .
The condition is also being recognized more in adults . These are some of the behaviors that might be associated with adult A.D.H.D.
Since a nationwide Adderall shortage started, some people with A.D.H.D. have said their medication no longer helps with their symptoms. But there could be other factors at play .
Everyone has bouts of distraction and forgetfulness. Here is when psychiatrists diagnose it as something clinical .
The disorder can put a strain on relationships. But there are ways to cope .
Though meditation can be beneficial to those with A.D.H.D., sitting still and focusing on breathing can be hard for them. These tips can help .
Numbers, Facts and Trends Shaping Your World
Read our research on:
Full Topic List
Regions & Countries
- Publications
- Our Methods
- Short Reads
- Tools & Resources
Read Our Research On:
What the data says about crime in the U.S.
A growing share of Americans say reducing crime should be a top priority for the president and Congress to address this year. Around six-in-ten U.S. adults (58%) hold that view today, up from 47% at the beginning of Joe Biden’s presidency in 2021.
We conducted this analysis to learn more about U.S. crime patterns and how those patterns have changed over time.
The analysis relies on statistics published by the FBI, which we accessed through the Crime Data Explorer , and the Bureau of Justice Statistics (BJS), which we accessed through the National Crime Victimization Survey data analysis tool .
To measure public attitudes about crime in the U.S., we relied on survey data from Pew Research Center and Gallup.
Additional details about each data source, including survey methodologies, are available by following the links in the text of this analysis.
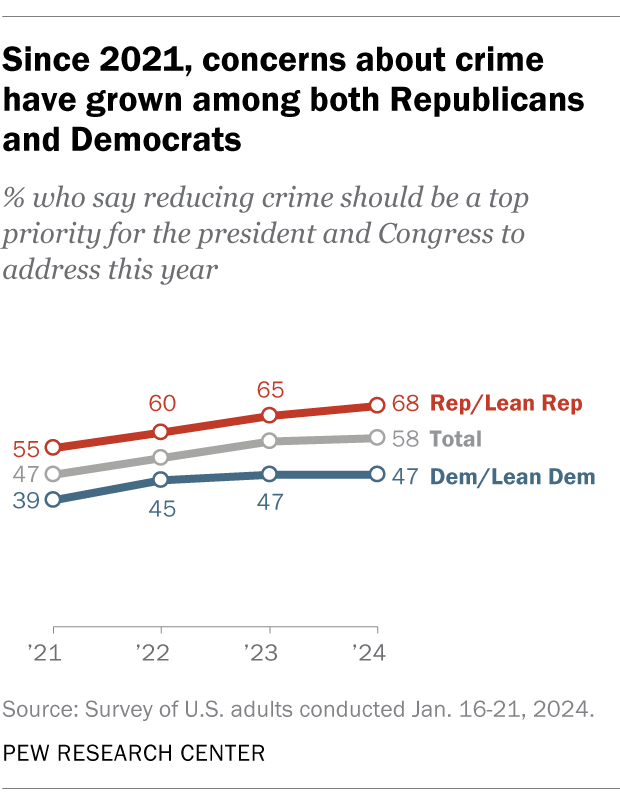
With the issue likely to come up in this year’s presidential election, here’s what we know about crime in the United States, based on the latest available data from the federal government and other sources.
How much crime is there in the U.S.?
It’s difficult to say for certain. The two primary sources of government crime statistics – the Federal Bureau of Investigation (FBI) and the Bureau of Justice Statistics (BJS) – paint an incomplete picture.
The FBI publishes annual data on crimes that have been reported to law enforcement, but not crimes that haven’t been reported. Historically, the FBI has also only published statistics about a handful of specific violent and property crimes, but not many other types of crime, such as drug crime. And while the FBI’s data is based on information from thousands of federal, state, county, city and other police departments, not all law enforcement agencies participate every year. In 2022, the most recent full year with available statistics, the FBI received data from 83% of participating agencies .
BJS, for its part, tracks crime by fielding a large annual survey of Americans ages 12 and older and asking them whether they were the victim of certain types of crime in the past six months. One advantage of this approach is that it captures both reported and unreported crimes. But the BJS survey has limitations of its own. Like the FBI, it focuses mainly on a handful of violent and property crimes. And since the BJS data is based on after-the-fact interviews with crime victims, it cannot provide information about one especially high-profile type of offense: murder.
All those caveats aside, looking at the FBI and BJS statistics side-by-side does give researchers a good picture of U.S. violent and property crime rates and how they have changed over time. In addition, the FBI is transitioning to a new data collection system – known as the National Incident-Based Reporting System – that eventually will provide national information on a much larger set of crimes , as well as details such as the time and place they occur and the types of weapons involved, if applicable.
Which kinds of crime are most and least common?
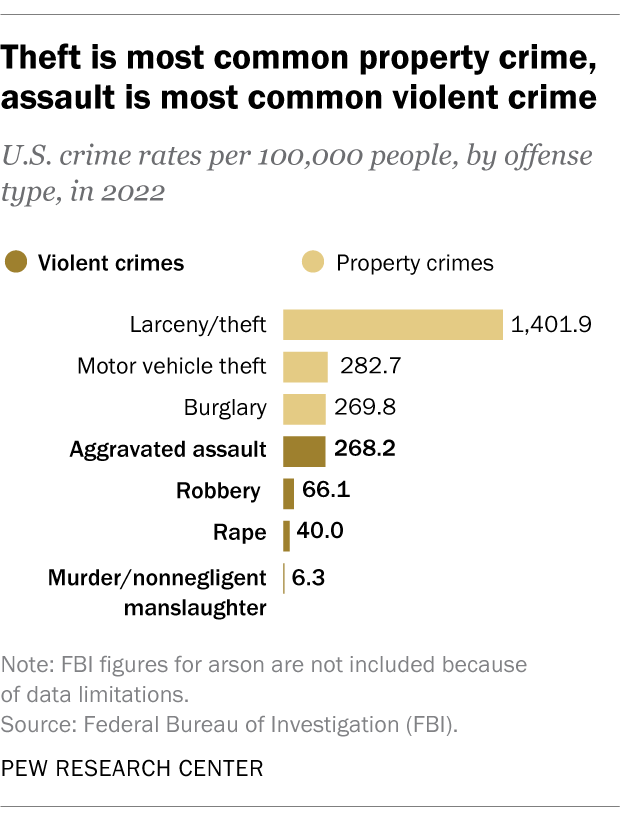
Property crime in the U.S. is much more common than violent crime. In 2022, the FBI reported a total of 1,954.4 property crimes per 100,000 people, compared with 380.7 violent crimes per 100,000 people.
By far the most common form of property crime in 2022 was larceny/theft, followed by motor vehicle theft and burglary. Among violent crimes, aggravated assault was the most common offense, followed by robbery, rape, and murder/nonnegligent manslaughter.
BJS tracks a slightly different set of offenses from the FBI, but it finds the same overall patterns, with theft the most common form of property crime in 2022 and assault the most common form of violent crime.
How have crime rates in the U.S. changed over time?
Both the FBI and BJS data show dramatic declines in U.S. violent and property crime rates since the early 1990s, when crime spiked across much of the nation.
Using the FBI data, the violent crime rate fell 49% between 1993 and 2022, with large decreases in the rates of robbery (-74%), aggravated assault (-39%) and murder/nonnegligent manslaughter (-34%). It’s not possible to calculate the change in the rape rate during this period because the FBI revised its definition of the offense in 2013 .
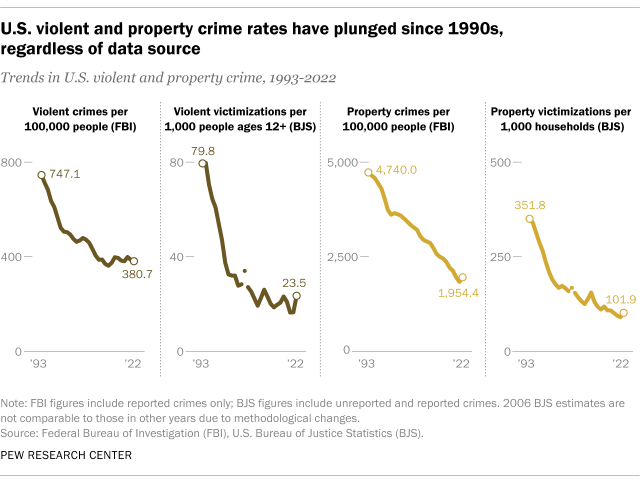
The FBI data also shows a 59% reduction in the U.S. property crime rate between 1993 and 2022, with big declines in the rates of burglary (-75%), larceny/theft (-54%) and motor vehicle theft (-53%).
Using the BJS statistics, the declines in the violent and property crime rates are even steeper than those captured in the FBI data. Per BJS, the U.S. violent and property crime rates each fell 71% between 1993 and 2022.
While crime rates have fallen sharply over the long term, the decline hasn’t always been steady. There have been notable increases in certain kinds of crime in some years, including recently.
In 2020, for example, the U.S. murder rate saw its largest single-year increase on record – and by 2022, it remained considerably higher than before the coronavirus pandemic. Preliminary data for 2023, however, suggests that the murder rate fell substantially last year .
How do Americans perceive crime in their country?
Americans tend to believe crime is up, even when official data shows it is down.
In 23 of 27 Gallup surveys conducted since 1993 , at least 60% of U.S. adults have said there is more crime nationally than there was the year before, despite the downward trend in crime rates during most of that period.
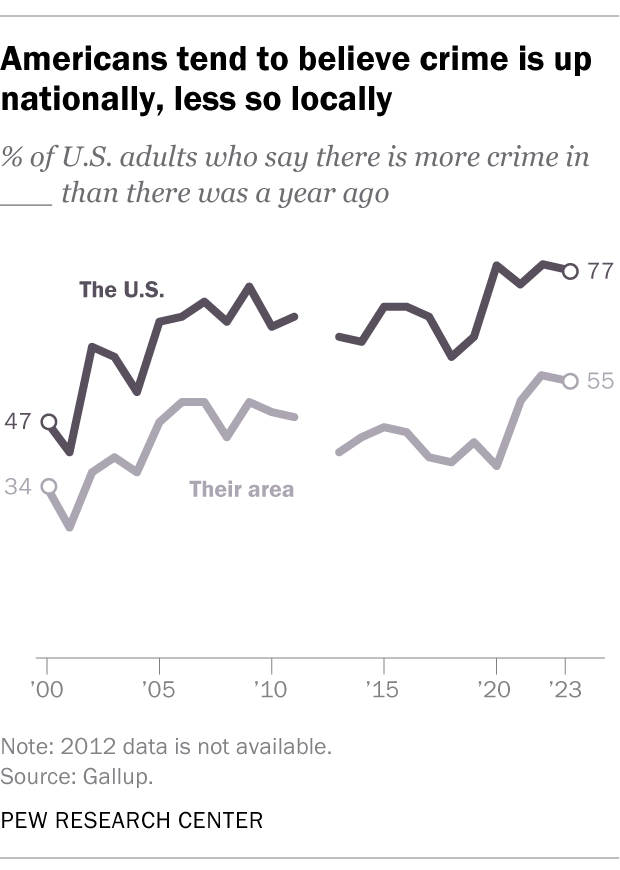
While perceptions of rising crime at the national level are common, fewer Americans believe crime is up in their own communities. In every Gallup crime survey since the 1990s, Americans have been much less likely to say crime is up in their area than to say the same about crime nationally.
Public attitudes about crime differ widely by Americans’ party affiliation, race and ethnicity, and other factors . For example, Republicans and Republican-leaning independents are much more likely than Democrats and Democratic leaners to say reducing crime should be a top priority for the president and Congress this year (68% vs. 47%), according to a recent Pew Research Center survey.
How does crime in the U.S. differ by demographic characteristics?
Some groups of Americans are more likely than others to be victims of crime. In the 2022 BJS survey , for example, younger people and those with lower incomes were far more likely to report being the victim of a violent crime than older and higher-income people.
There were no major differences in violent crime victimization rates between male and female respondents or between those who identified as White, Black or Hispanic. But the victimization rate among Asian Americans (a category that includes Native Hawaiians and other Pacific Islanders) was substantially lower than among other racial and ethnic groups.
The same BJS survey asks victims about the demographic characteristics of the offenders in the incidents they experienced.
In 2022, those who are male, younger people and those who are Black accounted for considerably larger shares of perceived offenders in violent incidents than their respective shares of the U.S. population. Men, for instance, accounted for 79% of perceived offenders in violent incidents, compared with 49% of the nation’s 12-and-older population that year. Black Americans accounted for 25% of perceived offenders in violent incidents, about twice their share of the 12-and-older population (12%).
As with all surveys, however, there are several potential sources of error, including the possibility that crime victims’ perceptions about offenders are incorrect.
How does crime in the U.S. differ geographically?
There are big geographic differences in violent and property crime rates.
For example, in 2022, there were more than 700 violent crimes per 100,000 residents in New Mexico and Alaska. That compares with fewer than 200 per 100,000 people in Rhode Island, Connecticut, New Hampshire and Maine, according to the FBI.
The FBI notes that various factors might influence an area’s crime rate, including its population density and economic conditions.
What percentage of crimes are reported to police? What percentage are solved?
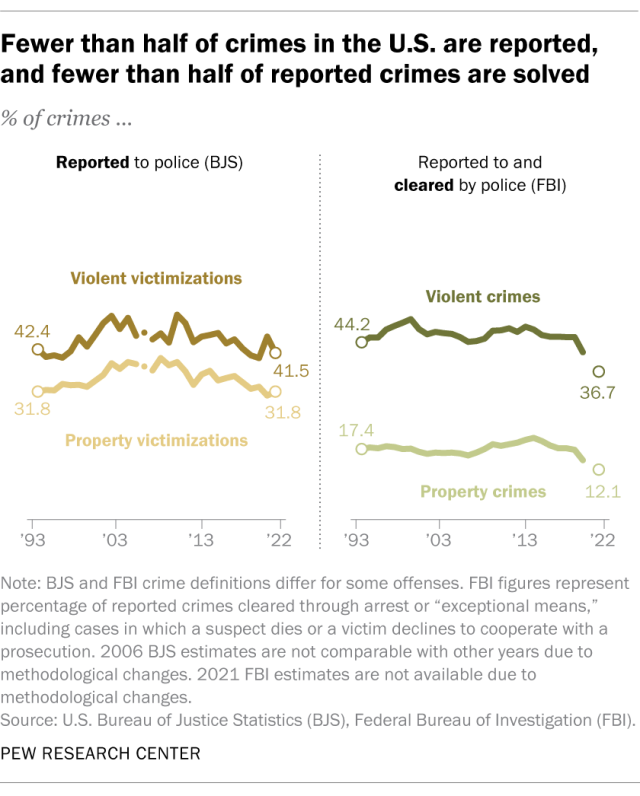
Most violent and property crimes in the U.S. are not reported to police, and most of the crimes that are reported are not solved.
In its annual survey, BJS asks crime victims whether they reported their crime to police. It found that in 2022, only 41.5% of violent crimes and 31.8% of household property crimes were reported to authorities. BJS notes that there are many reasons why crime might not be reported, including fear of reprisal or of “getting the offender in trouble,” a feeling that police “would not or could not do anything to help,” or a belief that the crime is “a personal issue or too trivial to report.”
Most of the crimes that are reported to police, meanwhile, are not solved , at least based on an FBI measure known as the clearance rate . That’s the share of cases each year that are closed, or “cleared,” through the arrest, charging and referral of a suspect for prosecution, or due to “exceptional” circumstances such as the death of a suspect or a victim’s refusal to cooperate with a prosecution. In 2022, police nationwide cleared 36.7% of violent crimes that were reported to them and 12.1% of the property crimes that came to their attention.
Which crimes are most likely to be reported to police? Which are most likely to be solved?
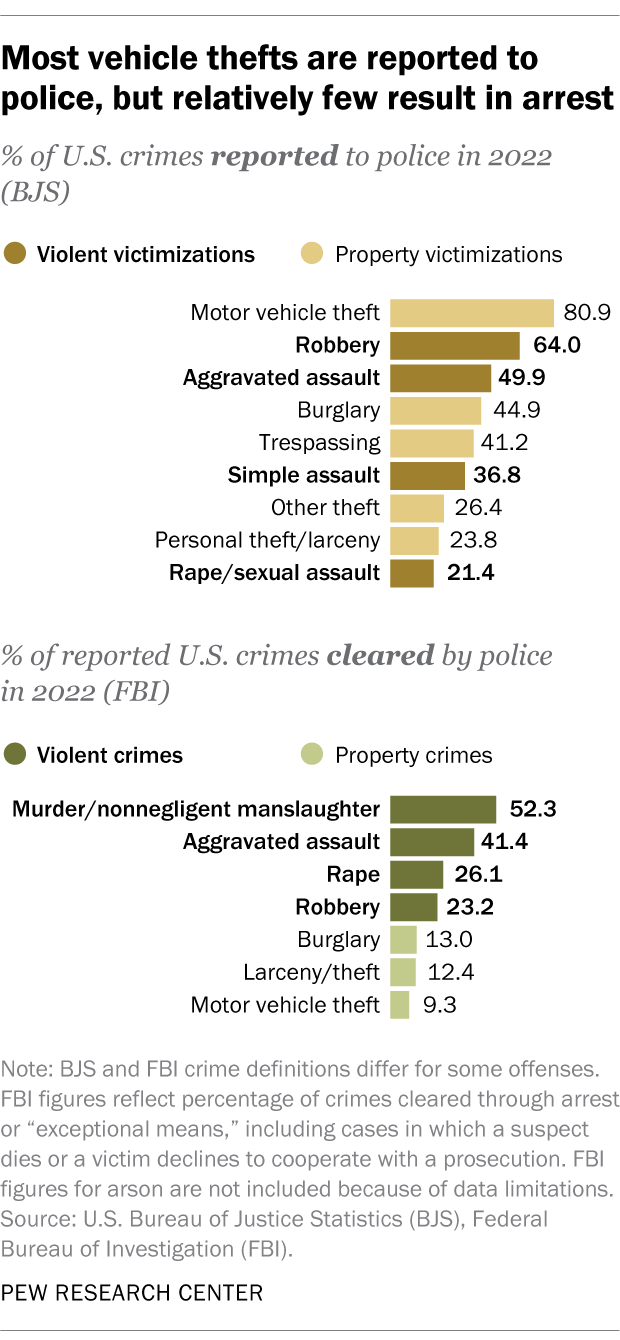
Around eight-in-ten motor vehicle thefts (80.9%) were reported to police in 2022, making them by far the most commonly reported property crime tracked by BJS. Household burglaries and trespassing offenses were reported to police at much lower rates (44.9% and 41.2%, respectively), while personal theft/larceny and other types of theft were only reported around a quarter of the time.
Among violent crimes – excluding homicide, which BJS doesn’t track – robbery was the most likely to be reported to law enforcement in 2022 (64.0%). It was followed by aggravated assault (49.9%), simple assault (36.8%) and rape/sexual assault (21.4%).
The list of crimes cleared by police in 2022 looks different from the list of crimes reported. Law enforcement officers were generally much more likely to solve violent crimes than property crimes, according to the FBI.
The most frequently solved violent crime tends to be homicide. Police cleared around half of murders and nonnegligent manslaughters (52.3%) in 2022. The clearance rates were lower for aggravated assault (41.4%), rape (26.1%) and robbery (23.2%).
When it comes to property crime, law enforcement agencies cleared 13.0% of burglaries, 12.4% of larcenies/thefts and 9.3% of motor vehicle thefts in 2022.
Are police solving more or fewer crimes than they used to?
Nationwide clearance rates for both violent and property crime are at their lowest levels since at least 1993, the FBI data shows.
Police cleared a little over a third (36.7%) of the violent crimes that came to their attention in 2022, down from nearly half (48.1%) as recently as 2013. During the same period, there were decreases for each of the four types of violent crime the FBI tracks:
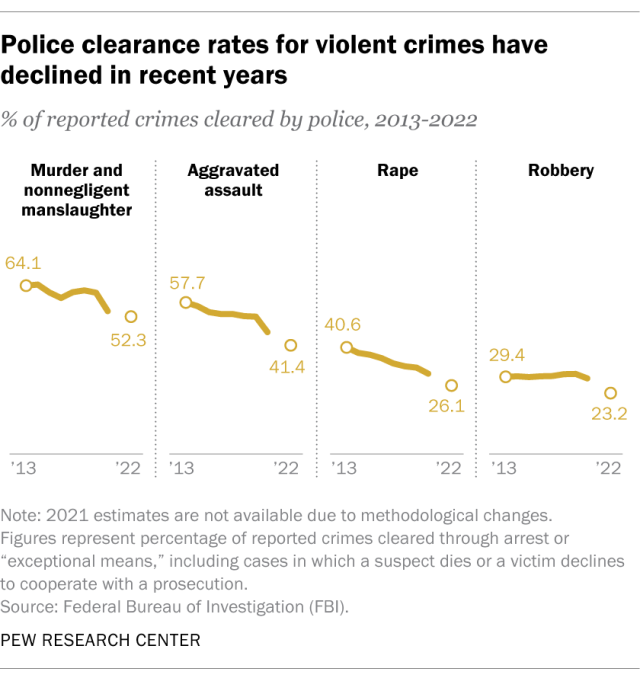
- Police cleared 52.3% of reported murders and nonnegligent homicides in 2022, down from 64.1% in 2013.
- They cleared 41.4% of aggravated assaults, down from 57.7%.
- They cleared 26.1% of rapes, down from 40.6%.
- They cleared 23.2% of robberies, down from 29.4%.
The pattern is less pronounced for property crime. Overall, law enforcement agencies cleared 12.1% of reported property crimes in 2022, down from 19.7% in 2013. The clearance rate for burglary didn’t change much, but it fell for larceny/theft (to 12.4% in 2022 from 22.4% in 2013) and motor vehicle theft (to 9.3% from 14.2%).
Note: This is an update of a post originally published on Nov. 20, 2020.
- Criminal Justice
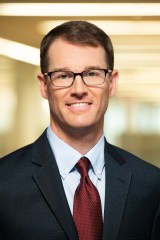
John Gramlich is an associate director at Pew Research Center
8 facts about Black Lives Matter
#blacklivesmatter turns 10, support for the black lives matter movement has dropped considerably from its peak in 2020, fewer than 1% of federal criminal defendants were acquitted in 2022, before release of video showing tyre nichols’ beating, public views of police conduct had improved modestly, most popular.
1615 L St. NW, Suite 800 Washington, DC 20036 USA (+1) 202-419-4300 | Main (+1) 202-857-8562 | Fax (+1) 202-419-4372 | Media Inquiries
Research Topics
- Age & Generations
- Coronavirus (COVID-19)
- Economy & Work
- Family & Relationships
- Gender & LGBTQ
- Immigration & Migration
- International Affairs
- Internet & Technology
- Methodological Research
- News Habits & Media
- Non-U.S. Governments
- Other Topics
- Politics & Policy
- Race & Ethnicity
- Email Newsletters
ABOUT PEW RESEARCH CENTER Pew Research Center is a nonpartisan fact tank that informs the public about the issues, attitudes and trends shaping the world. It conducts public opinion polling, demographic research, media content analysis and other empirical social science research. Pew Research Center does not take policy positions. It is a subsidiary of The Pew Charitable Trusts .
Copyright 2024 Pew Research Center
Terms & Conditions
Privacy Policy
Cookie Settings
Reprints, Permissions & Use Policy
Evaluating medical providers in terms of patient health disparities: a statistical framework
- Published: 15 February 2024
Cite this article
- Nicholas Hartman 1 , 2 &
- Claudia Dahlerus 2 , 3
83 Accesses
Explore all metrics
Statistical methods for provider profiling are designed to measure healthcare quality and create incentives for low-quality providers to improve. While most existing healthcare quality metrics assess the overall treatment delivered to providers’ entire patient populations, widespread racial and sociodemographic disparities in health outcomes highlight the need to evaluate providers’ healthcare quality across patient subgroups. Disparity measure development at the healthcare provider level is a nascent research area, and existing measures have lacked the statistical rigor and clear definitions of performance benchmarks that are characteristic of traditional provider profiling methodology. In response to these gaps, we propose a formal disparity model for provider evaluations, which can be used to construct null hypotheses and test statistics for concepts such as systemic disparities, equality in performance, and equity in performance. For each of these topics, we define the appropriate performance benchmark in terms of statistical parameters, and describe its relationship with the existing literature. These arguments also shed light on the long standing debate regarding social risk adjustments in assessments of overall healthcare quality. Finally, we develop an assessment chart to easily visualize disparity patterns and identify the most culpable providers. These methods are demonstrated through analyses of racial disparities in access to transplantation among End-Stage Renal Disease patients.
This is a preview of subscription content, log in via an institution to check access.
Access this article
Price includes VAT (Russian Federation)
Instant access to the full article PDF.
Rent this article via DeepDyve
Institutional subscriptions
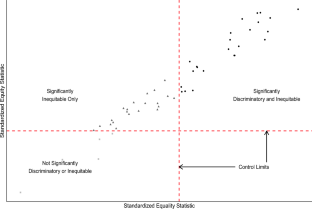
Similar content being viewed by others
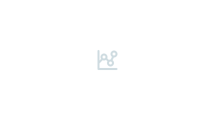
Could Pay-for-Performance Worsen Health Disparities?
Quality of Quality Measures
The impact of reimbursement systems on equity in access and quality of primary care: a systematic literature review, availability of data and materials.
The data are available upon request from the OPTN at https://optn.transplant.hrsa.gov/data/view-data-reports/request-data/ .
Acevedo-Garcia, D., Lochner, K., Osypuk, T., Subramanian, S.: Future directions in residential segregation and health research: a multilevel approach. Am. J. Public Health 93 (2), 215–221 (2003). https://doi.org/10.2105/ajph.93.2.215
Article PubMed PubMed Central Google Scholar
Agency for Healthcare Research and Quality: National healthcare disparities report. Rockville, MD (2022)
Binger, T., Chen, H., Harder, B.: Hospital rankings and health equity. J. Am. Med. Assoc. 328 (18), 1805–1806 (2022). https://doi.org/10.1001/jama.2022.19001
Article Google Scholar
Braveman, P.: Health disparities and health equity: concepts and measurement. Annu. Rev. Public Health 27 , 167–194 (2006). https://doi.org/10.1146/annurev.publhealth.27.021405.102103
Article PubMed Google Scholar
Daignault, K., Lawson, K., Finelli, A., Saarela, O.: Causal mediation analysis for standardized mortality ratios. Epidemiology 30 (4), 532–540 (2019). https://doi.org/10.1097/EDE.0000000000001015
Downing, N.S., Wang, C., Gupta, A., Wang, Y., Nuti, S.V., Ross, J.S., Krumholz, H.M.: Association of racial and socioeconomic disparities with outcomes among patients hospitalized with acute myocardial infarction, heart failure, and pneumonia: an analysis of within- and between-hospital variation. J. Am. Med. Assoc. Netw. Open 1 (5), e182044–e182044 (2018). https://doi.org/10.1001/jamanetworkopen.2018.2044
Eggers, P.W.: Racial disparities in access to transplantation: a tough nut to crack. Kidney Int. 76 (6), 589–590 (2009). https://doi.org/10.1038/ki.2009.256
Fiscella, K., Franks, P., Gold, M., Clancy, C.: Inequality in quality: addressing socioeconomic, racial, and ethnic disparities in health care. J. Am. Med. Assoc. 289 (19), 2579–2584 (2000). https://doi.org/10.1001/jama.283.19.2579
He, K., Kalbfleisch, J., Li, Y., Li, Y.: Evaluating hospital readmission rates in dialysis facilities; adjusting for hospital effects. Lifetime Data Anal. 19 (4), 490–512 (2013). https://doi.org/10.1007/s10985-013-9264-6
Article MathSciNet PubMed Google Scholar
Howard, R., Cornell, D., Schold, J.: CMS oversight, OPOs and transplant centers and the law of unintended consequences. Clin. Transplant. 23 (6), 778–783 (2009). https://doi.org/10.1111/j.1399-0012.2009.01157.x
Jackson, J., Williams, D., VanderWeele, T.: Disparities at the intersection of marginalized groups. Soc. Psychiatry Psychiatr. Epidemiol. 51 (10), 1349–1359 (2016). https://doi.org/10.1007/s00127-016-1276-6
Jay, C., Schold, J.D.: Measuring transplant center performance: the goals are not controversial but the methods and consequences can be. Curr. Transpl. Rep. 4 (1), 52–58 (2017). https://doi.org/10.1007/s40472-017-0138-9
Jones, H., Spiegelhalter, D.: The identification of “unusual” health-care providers from a hierarchical model. Am. Stat. 65 (3), 154–163 (2011). https://doi.org/10.1198/tast.2011.10190
Article MathSciNet Google Scholar
Kalbfleisch, J., Wolfe, R.: On monitoring outcomes of medical providers. Stat. Biosci. 5 (2), 286–302 (2013). https://doi.org/10.1007/s12561-013-9093-x
Kalbfleisch, J., Wolfe, R., Bell, S., Sun, R., Messana, J., Shearon, T., Li, Y.: Risk adjustment and the assessment of disparities in dialysis mortality outcomes. J. Am. Soc. Nephrol. 26 (11), 2641–2645 (2015). https://doi.org/10.1681/ASN.2014050512
Kalbfleisch, J., He, K., Xia, L., Li, Y.: Does the inter-unit reliability (IUR) measure reliability? Health Serv. Oucomes Res. Methodol. 18 , 215–225 (2018). https://doi.org/10.1007/s10742-018-0185-4
Keele, L., Stevenson, R.: Causal interaction and effect modification: same model, different concepts. Polit. Sci. Res. Methods 9 , 641–649 (2021). https://doi.org/10.1017/psrm.2020.12
Ku, E., Lee, B., McCulloch, C., Roll, G., Grimes, B., Adey, D.: Racial and ethnic disparities in kidney transplant access within a theoretical context of medical eligibility. Transplantation 104 (7), 1437–1444 (2020). https://doi.org/10.1097/TP.0000000000002962
Kulkarni, S., Ladin, K., Haakinson, D., Greene, E., Li, L., Deng, Y.: Association of racial disparities with access to kidney transplant after the implementation of the new kidney allocation system. J. Am. Med. Assoc. 154 (7), 618–625 (2019). https://doi.org/10.1001/jamasurg.2019.0512
Lloren, A., Liu, S., Herrin, J., Lin, Z., Zhou, G., Wang, Y., Bernheim, S.: Measuring hospital-specific disparities by dual eligibility and race to reduce health inequities. Health Serv. Res. 54 (Suppl 1), 243–254 (2019). https://doi.org/10.1111/1475-6773.13108
Mentias, A., Peterson, E., Keshvani, N., Kumbhani, D., Yancy, C., Morris, A., Pandey, A.: Achieving equity in hospital performance assessments using composite race-specific measures of risk-standardized readmission and mortality rates for heart failure. Circulation 147 (15), 1121–1133 (2023). https://doi.org/10.1161/CIRCULATIONAHA.122.061995
National Quality Forum: Risk adjustment for socioeconomic status or other sociodemographic factors. (2014). https://www.qualityforum.org/Publications/2014/08/Risk_Adjustment_for_Socioeconomic_Status_or_Other_Sociodemographic_Factors.aspx
National Quality Forum: A roadmap for promoting health equity and eliminating disparities: the four I’s for health equity. (2017). https://www.qualityforum.org/Publications/2017/09/A_Roadmap_for_Promoting_Health_Equity_and_Eliminating_Disparities__The_Four_I_s_for_Health_Equity.aspx
National Research Council: Realizing the promise of equity in the organ transplantation system. The National Academies Press, Washington, DC (2022)
Normand, S.L.T., Shahian, D.M.: Statistical and clinical aspects of hospital outcomes profiling. Stat. Sci. 22 (2), 206–226 (2007). https://doi.org/10.1214/088342307000000096
Organ Procurement and Transplantation Network: OPTN board approves elimination of race-based calculation for transplant candidate listing. (2022). https://optn.transplant.hrsa.gov/news/optn-board-approves-elimination-of-race-based-calculation-for-transplant-candidate-listing/
Park, C., Jones, M., Kaplan, S., Koller, F., Wilder, J., Boulware, L., McElroy, L.M.: A scoping review of inequities in access to organ transplant in the United States. Int. J. Equity Health (2022). https://doi.org/10.1186/s12939-021-01616-x
Rathore, S., Krumholz, H.: Differences, disparities, and biases: clarifying racial variations in health care use. Ann. Intern. Med. 141 (8), 635–638 (2004). https://doi.org/10.7326/0003-4819-141-8-200410190-00011
Scientific Registry of Transplant Recipients: Technical methods for the program-specific reports. (2022). https://www.srtr.org/about-the-data/technical-methods-for-the-program-specific-reports/
Silber, J., Rosenbaum, P., Ross, R., Ludwig, J., Wang, W., Niknam, B.A., Fleisher, L.A.: A hospital-specific template for benchmarking its cost and quality. Health Serv. Res. 49 (5), 1475–1497 (2014). https://doi.org/10.1111/1475-6773.12226
Smith, D.: The racial segregation of hospital care revisited: medicare discharge patterns and their implications. Am. J. Public Health 88 (3), 461–463 (1998). https://doi.org/10.2105/ajph.88.3.461
Article CAS PubMed PubMed Central Google Scholar
Spiegelhalter, D., Sherlaw-Johnson, C., Bardsley, M., Blunt, I., Wood, C., Grigg, O.: Statistical methods for healthcare regulation: rating, screening and surveillance. J. Roy. Stat. Soc. 175 (1), 1–47 (2012). https://doi.org/10.1111/j.1467-985X.2011.01010.x
Tang, T., Austin, P., Lawson, K., Finelli, A., Saarela, O.: Constructing inverse probability weights for institutional comparisons in healthcare. Stat. Med. 39 (23), 3156–3172 (2020). https://doi.org/10.1002/sim.8657
The Centers for Medicare and Medicaid Services: CMS Disparity Methods Confidential Reporting Methodology. (2023). https://qualitynet.cms.gov/inpatient/measures/disparity-methods/methodology
Trivedi, A.N., Nsa, W., Hausmann, L.R., Lee, J.S., Ma, A., Bratzler, D.W., Fine, M.J.: Quality and equity of care in U.S. hospitals. N. Engl. J. Med. 371 (24), 2298–2308 (2014). https://doi.org/10.1056/NEJMsa1405003
Article CAS PubMed Google Scholar
Valeri, L., Proust-Lima, C., Fan, W., Chen, J., Jacqmin-Gadda, H.: A multistate approach for the study of interventions on an intermediate time-to-event in health disparities research. Stat. Methods Med. Res. 32 (8), 1445–1460 (2023). https://doi.org/10.1177/09622802231163331
VanderWeele, T.: On the distinction between interaction and effect modification. Epidemiology 20 (6), 863–871 (2009)
Varewyck, M., Goetghebeur, E., Eriksson, M., Vansteelandt, S.: On shrinkage and model extrapolation in the evaluation of clinical center performance. Biostatistics 15 (4), 651–654 (2014). https://doi.org/10.1093/biostatistics/kxu019
Varewyck, M., Vansteelandt, S., Eriksson, M., Goetghebeur, E.: On the practice of ignoring center-patient interactions in evaluating hospital performance. Stat. Med. 35 (2), 227–238 (2016). https://doi.org/10.1002/sim.6634
White, K., Haas, J., Williams, D.: Elucidating the role of place in health care disparities: the example of racial/ethnic residential segregation. Health Serv. Res. 47 (3pt2), 1278–1299 (2012). https://doi.org/10.1111/j.1475-6773.2012.01410.x
Williams, D., Rucker, T.: Understanding and addressing racial disparities in health care. Health Care Financ. Rev. 21 (4), 75–90 (2000)
CAS PubMed PubMed Central Google Scholar
Wolfe, R., Ashby, V., Milford, E., Ojo, A., Ettenger, R., Agodoa, L., Port, F.: Comparison of mortality in all patients on dialysis, patients on dialysis awaiting transplantation, and recipients of a first cadaveric transplant. N. Engl. J. Med. 341 , 1725–1730 (1999). https://doi.org/10.1056/NEJM199912023412303
Xia, L., He, K., Li, Y., Kalbfleisch, J.: Accounting for total variation and robustness in profiling health care providers. Biostatistics 23 , 257–273 (2022). https://doi.org/10.1093/biostatistics/kxaa024
Download references
Acknowledgements
The authors thank Dr. Kevin He for his helpful comments and for providing the OPTN dataset.
This work was supported in part by Health Resources and Services Administration contract HHSH250-2019-00001C. The content is the responsibility of the authors alone and does not necessarily reflect the views or policies of the Department of Health and Human Services, nor does mention of trade names, commercial products, or organizations imply endorsement by the U.S. Government.
Author information
Authors and affiliations.
Department of Biostatistics, University of Michigan, 1415 Washington Heights, Ann Arbor, 48109, MI, USA
Nicholas Hartman
Kidney Epidemiology and Cost Center, University of Michigan, 1415 Washington Heights, Ann Arbor, 48109, MI, USA
Nicholas Hartman & Claudia Dahlerus
Division of Nephrology, University of Michigan, 1500 East Medical Center Drive, Ann Arbor, 48109, MI, USA
Claudia Dahlerus
You can also search for this author in PubMed Google Scholar
Contributions
NH conceived of the statistical concepts and performed the analyses. NH and CD developed the interpretation and discussion of the proposed framework, and approved of the final written manuscript.
Corresponding author
Correspondence to Nicholas Hartman .
Ethics declarations
Conflict of interest.
The authors have no competing interests to declare that are relevant to the content of this article.
Ethics approval
Not applicable.
Consent to participate
Consent for publication, code availability.
R codes are available online at https://github.com/nhart985/Healthcare_Disparities
Additional information
Publisher's note.
Springer Nature remains neutral with regard to jurisdictional claims in published maps and institutional affiliations.
Rights and permissions
Springer Nature or its licensor (e.g. a society or other partner) holds exclusive rights to this article under a publishing agreement with the author(s) or other rightsholder(s); author self-archiving of the accepted manuscript version of this article is solely governed by the terms of such publishing agreement and applicable law.
Reprints and permissions
About this article
Hartman, N., Dahlerus, C. Evaluating medical providers in terms of patient health disparities: a statistical framework. Health Serv Outcomes Res Method (2024). https://doi.org/10.1007/s10742-024-00323-8
Download citation
Received : 03 July 2023
Accepted : 18 January 2024
Published : 15 February 2024
DOI : https://doi.org/10.1007/s10742-024-00323-8
Share this article
Anyone you share the following link with will be able to read this content:
Sorry, a shareable link is not currently available for this article.
Provided by the Springer Nature SharedIt content-sharing initiative
- Healthcare quality measures
- Provider profiling
- Find a journal
- Publish with us
- Track your research

An official website of the United States government
The .gov means it’s official. Federal government websites often end in .gov or .mil. Before sharing sensitive information, make sure you’re on a federal government site.
The site is secure. The https:// ensures that you are connecting to the official website and that any information you provide is encrypted and transmitted securely.
- Publications
- Account settings
Preview improvements coming to the PMC website in October 2024. Learn More or Try it out now .
- Advanced Search
- Journal List
- J Korean Med Sci
- v.37(16); 2022 Apr 25

A Practical Guide to Writing Quantitative and Qualitative Research Questions and Hypotheses in Scholarly Articles
Edward barroga.
1 Department of General Education, Graduate School of Nursing Science, St. Luke’s International University, Tokyo, Japan.
Glafera Janet Matanguihan
2 Department of Biological Sciences, Messiah University, Mechanicsburg, PA, USA.
The development of research questions and the subsequent hypotheses are prerequisites to defining the main research purpose and specific objectives of a study. Consequently, these objectives determine the study design and research outcome. The development of research questions is a process based on knowledge of current trends, cutting-edge studies, and technological advances in the research field. Excellent research questions are focused and require a comprehensive literature search and in-depth understanding of the problem being investigated. Initially, research questions may be written as descriptive questions which could be developed into inferential questions. These questions must be specific and concise to provide a clear foundation for developing hypotheses. Hypotheses are more formal predictions about the research outcomes. These specify the possible results that may or may not be expected regarding the relationship between groups. Thus, research questions and hypotheses clarify the main purpose and specific objectives of the study, which in turn dictate the design of the study, its direction, and outcome. Studies developed from good research questions and hypotheses will have trustworthy outcomes with wide-ranging social and health implications.
INTRODUCTION
Scientific research is usually initiated by posing evidenced-based research questions which are then explicitly restated as hypotheses. 1 , 2 The hypotheses provide directions to guide the study, solutions, explanations, and expected results. 3 , 4 Both research questions and hypotheses are essentially formulated based on conventional theories and real-world processes, which allow the inception of novel studies and the ethical testing of ideas. 5 , 6
It is crucial to have knowledge of both quantitative and qualitative research 2 as both types of research involve writing research questions and hypotheses. 7 However, these crucial elements of research are sometimes overlooked; if not overlooked, then framed without the forethought and meticulous attention it needs. Planning and careful consideration are needed when developing quantitative or qualitative research, particularly when conceptualizing research questions and hypotheses. 4
There is a continuing need to support researchers in the creation of innovative research questions and hypotheses, as well as for journal articles that carefully review these elements. 1 When research questions and hypotheses are not carefully thought of, unethical studies and poor outcomes usually ensue. Carefully formulated research questions and hypotheses define well-founded objectives, which in turn determine the appropriate design, course, and outcome of the study. This article then aims to discuss in detail the various aspects of crafting research questions and hypotheses, with the goal of guiding researchers as they develop their own. Examples from the authors and peer-reviewed scientific articles in the healthcare field are provided to illustrate key points.
DEFINITIONS AND RELATIONSHIP OF RESEARCH QUESTIONS AND HYPOTHESES
A research question is what a study aims to answer after data analysis and interpretation. The answer is written in length in the discussion section of the paper. Thus, the research question gives a preview of the different parts and variables of the study meant to address the problem posed in the research question. 1 An excellent research question clarifies the research writing while facilitating understanding of the research topic, objective, scope, and limitations of the study. 5
On the other hand, a research hypothesis is an educated statement of an expected outcome. This statement is based on background research and current knowledge. 8 , 9 The research hypothesis makes a specific prediction about a new phenomenon 10 or a formal statement on the expected relationship between an independent variable and a dependent variable. 3 , 11 It provides a tentative answer to the research question to be tested or explored. 4
Hypotheses employ reasoning to predict a theory-based outcome. 10 These can also be developed from theories by focusing on components of theories that have not yet been observed. 10 The validity of hypotheses is often based on the testability of the prediction made in a reproducible experiment. 8
Conversely, hypotheses can also be rephrased as research questions. Several hypotheses based on existing theories and knowledge may be needed to answer a research question. Developing ethical research questions and hypotheses creates a research design that has logical relationships among variables. These relationships serve as a solid foundation for the conduct of the study. 4 , 11 Haphazardly constructed research questions can result in poorly formulated hypotheses and improper study designs, leading to unreliable results. Thus, the formulations of relevant research questions and verifiable hypotheses are crucial when beginning research. 12
CHARACTERISTICS OF GOOD RESEARCH QUESTIONS AND HYPOTHESES
Excellent research questions are specific and focused. These integrate collective data and observations to confirm or refute the subsequent hypotheses. Well-constructed hypotheses are based on previous reports and verify the research context. These are realistic, in-depth, sufficiently complex, and reproducible. More importantly, these hypotheses can be addressed and tested. 13
There are several characteristics of well-developed hypotheses. Good hypotheses are 1) empirically testable 7 , 10 , 11 , 13 ; 2) backed by preliminary evidence 9 ; 3) testable by ethical research 7 , 9 ; 4) based on original ideas 9 ; 5) have evidenced-based logical reasoning 10 ; and 6) can be predicted. 11 Good hypotheses can infer ethical and positive implications, indicating the presence of a relationship or effect relevant to the research theme. 7 , 11 These are initially developed from a general theory and branch into specific hypotheses by deductive reasoning. In the absence of a theory to base the hypotheses, inductive reasoning based on specific observations or findings form more general hypotheses. 10
TYPES OF RESEARCH QUESTIONS AND HYPOTHESES
Research questions and hypotheses are developed according to the type of research, which can be broadly classified into quantitative and qualitative research. We provide a summary of the types of research questions and hypotheses under quantitative and qualitative research categories in Table 1 .
Research questions in quantitative research
In quantitative research, research questions inquire about the relationships among variables being investigated and are usually framed at the start of the study. These are precise and typically linked to the subject population, dependent and independent variables, and research design. 1 Research questions may also attempt to describe the behavior of a population in relation to one or more variables, or describe the characteristics of variables to be measured ( descriptive research questions ). 1 , 5 , 14 These questions may also aim to discover differences between groups within the context of an outcome variable ( comparative research questions ), 1 , 5 , 14 or elucidate trends and interactions among variables ( relationship research questions ). 1 , 5 We provide examples of descriptive, comparative, and relationship research questions in quantitative research in Table 2 .
Hypotheses in quantitative research
In quantitative research, hypotheses predict the expected relationships among variables. 15 Relationships among variables that can be predicted include 1) between a single dependent variable and a single independent variable ( simple hypothesis ) or 2) between two or more independent and dependent variables ( complex hypothesis ). 4 , 11 Hypotheses may also specify the expected direction to be followed and imply an intellectual commitment to a particular outcome ( directional hypothesis ) 4 . On the other hand, hypotheses may not predict the exact direction and are used in the absence of a theory, or when findings contradict previous studies ( non-directional hypothesis ). 4 In addition, hypotheses can 1) define interdependency between variables ( associative hypothesis ), 4 2) propose an effect on the dependent variable from manipulation of the independent variable ( causal hypothesis ), 4 3) state a negative relationship between two variables ( null hypothesis ), 4 , 11 , 15 4) replace the working hypothesis if rejected ( alternative hypothesis ), 15 explain the relationship of phenomena to possibly generate a theory ( working hypothesis ), 11 5) involve quantifiable variables that can be tested statistically ( statistical hypothesis ), 11 6) or express a relationship whose interlinks can be verified logically ( logical hypothesis ). 11 We provide examples of simple, complex, directional, non-directional, associative, causal, null, alternative, working, statistical, and logical hypotheses in quantitative research, as well as the definition of quantitative hypothesis-testing research in Table 3 .
Research questions in qualitative research
Unlike research questions in quantitative research, research questions in qualitative research are usually continuously reviewed and reformulated. The central question and associated subquestions are stated more than the hypotheses. 15 The central question broadly explores a complex set of factors surrounding the central phenomenon, aiming to present the varied perspectives of participants. 15
There are varied goals for which qualitative research questions are developed. These questions can function in several ways, such as to 1) identify and describe existing conditions ( contextual research question s); 2) describe a phenomenon ( descriptive research questions ); 3) assess the effectiveness of existing methods, protocols, theories, or procedures ( evaluation research questions ); 4) examine a phenomenon or analyze the reasons or relationships between subjects or phenomena ( explanatory research questions ); or 5) focus on unknown aspects of a particular topic ( exploratory research questions ). 5 In addition, some qualitative research questions provide new ideas for the development of theories and actions ( generative research questions ) or advance specific ideologies of a position ( ideological research questions ). 1 Other qualitative research questions may build on a body of existing literature and become working guidelines ( ethnographic research questions ). Research questions may also be broadly stated without specific reference to the existing literature or a typology of questions ( phenomenological research questions ), may be directed towards generating a theory of some process ( grounded theory questions ), or may address a description of the case and the emerging themes ( qualitative case study questions ). 15 We provide examples of contextual, descriptive, evaluation, explanatory, exploratory, generative, ideological, ethnographic, phenomenological, grounded theory, and qualitative case study research questions in qualitative research in Table 4 , and the definition of qualitative hypothesis-generating research in Table 5 .
Qualitative studies usually pose at least one central research question and several subquestions starting with How or What . These research questions use exploratory verbs such as explore or describe . These also focus on one central phenomenon of interest, and may mention the participants and research site. 15
Hypotheses in qualitative research
Hypotheses in qualitative research are stated in the form of a clear statement concerning the problem to be investigated. Unlike in quantitative research where hypotheses are usually developed to be tested, qualitative research can lead to both hypothesis-testing and hypothesis-generating outcomes. 2 When studies require both quantitative and qualitative research questions, this suggests an integrative process between both research methods wherein a single mixed-methods research question can be developed. 1
FRAMEWORKS FOR DEVELOPING RESEARCH QUESTIONS AND HYPOTHESES
Research questions followed by hypotheses should be developed before the start of the study. 1 , 12 , 14 It is crucial to develop feasible research questions on a topic that is interesting to both the researcher and the scientific community. This can be achieved by a meticulous review of previous and current studies to establish a novel topic. Specific areas are subsequently focused on to generate ethical research questions. The relevance of the research questions is evaluated in terms of clarity of the resulting data, specificity of the methodology, objectivity of the outcome, depth of the research, and impact of the study. 1 , 5 These aspects constitute the FINER criteria (i.e., Feasible, Interesting, Novel, Ethical, and Relevant). 1 Clarity and effectiveness are achieved if research questions meet the FINER criteria. In addition to the FINER criteria, Ratan et al. described focus, complexity, novelty, feasibility, and measurability for evaluating the effectiveness of research questions. 14
The PICOT and PEO frameworks are also used when developing research questions. 1 The following elements are addressed in these frameworks, PICOT: P-population/patients/problem, I-intervention or indicator being studied, C-comparison group, O-outcome of interest, and T-timeframe of the study; PEO: P-population being studied, E-exposure to preexisting conditions, and O-outcome of interest. 1 Research questions are also considered good if these meet the “FINERMAPS” framework: Feasible, Interesting, Novel, Ethical, Relevant, Manageable, Appropriate, Potential value/publishable, and Systematic. 14
As we indicated earlier, research questions and hypotheses that are not carefully formulated result in unethical studies or poor outcomes. To illustrate this, we provide some examples of ambiguous research question and hypotheses that result in unclear and weak research objectives in quantitative research ( Table 6 ) 16 and qualitative research ( Table 7 ) 17 , and how to transform these ambiguous research question(s) and hypothesis(es) into clear and good statements.
a These statements were composed for comparison and illustrative purposes only.
b These statements are direct quotes from Higashihara and Horiuchi. 16
a This statement is a direct quote from Shimoda et al. 17
The other statements were composed for comparison and illustrative purposes only.
CONSTRUCTING RESEARCH QUESTIONS AND HYPOTHESES
To construct effective research questions and hypotheses, it is very important to 1) clarify the background and 2) identify the research problem at the outset of the research, within a specific timeframe. 9 Then, 3) review or conduct preliminary research to collect all available knowledge about the possible research questions by studying theories and previous studies. 18 Afterwards, 4) construct research questions to investigate the research problem. Identify variables to be accessed from the research questions 4 and make operational definitions of constructs from the research problem and questions. Thereafter, 5) construct specific deductive or inductive predictions in the form of hypotheses. 4 Finally, 6) state the study aims . This general flow for constructing effective research questions and hypotheses prior to conducting research is shown in Fig. 1 .

Research questions are used more frequently in qualitative research than objectives or hypotheses. 3 These questions seek to discover, understand, explore or describe experiences by asking “What” or “How.” The questions are open-ended to elicit a description rather than to relate variables or compare groups. The questions are continually reviewed, reformulated, and changed during the qualitative study. 3 Research questions are also used more frequently in survey projects than hypotheses in experiments in quantitative research to compare variables and their relationships.
Hypotheses are constructed based on the variables identified and as an if-then statement, following the template, ‘If a specific action is taken, then a certain outcome is expected.’ At this stage, some ideas regarding expectations from the research to be conducted must be drawn. 18 Then, the variables to be manipulated (independent) and influenced (dependent) are defined. 4 Thereafter, the hypothesis is stated and refined, and reproducible data tailored to the hypothesis are identified, collected, and analyzed. 4 The hypotheses must be testable and specific, 18 and should describe the variables and their relationships, the specific group being studied, and the predicted research outcome. 18 Hypotheses construction involves a testable proposition to be deduced from theory, and independent and dependent variables to be separated and measured separately. 3 Therefore, good hypotheses must be based on good research questions constructed at the start of a study or trial. 12
In summary, research questions are constructed after establishing the background of the study. Hypotheses are then developed based on the research questions. Thus, it is crucial to have excellent research questions to generate superior hypotheses. In turn, these would determine the research objectives and the design of the study, and ultimately, the outcome of the research. 12 Algorithms for building research questions and hypotheses are shown in Fig. 2 for quantitative research and in Fig. 3 for qualitative research.

EXAMPLES OF RESEARCH QUESTIONS FROM PUBLISHED ARTICLES
- EXAMPLE 1. Descriptive research question (quantitative research)
- - Presents research variables to be assessed (distinct phenotypes and subphenotypes)
- “BACKGROUND: Since COVID-19 was identified, its clinical and biological heterogeneity has been recognized. Identifying COVID-19 phenotypes might help guide basic, clinical, and translational research efforts.
- RESEARCH QUESTION: Does the clinical spectrum of patients with COVID-19 contain distinct phenotypes and subphenotypes? ” 19
- EXAMPLE 2. Relationship research question (quantitative research)
- - Shows interactions between dependent variable (static postural control) and independent variable (peripheral visual field loss)
- “Background: Integration of visual, vestibular, and proprioceptive sensations contributes to postural control. People with peripheral visual field loss have serious postural instability. However, the directional specificity of postural stability and sensory reweighting caused by gradual peripheral visual field loss remain unclear.
- Research question: What are the effects of peripheral visual field loss on static postural control ?” 20
- EXAMPLE 3. Comparative research question (quantitative research)
- - Clarifies the difference among groups with an outcome variable (patients enrolled in COMPERA with moderate PH or severe PH in COPD) and another group without the outcome variable (patients with idiopathic pulmonary arterial hypertension (IPAH))
- “BACKGROUND: Pulmonary hypertension (PH) in COPD is a poorly investigated clinical condition.
- RESEARCH QUESTION: Which factors determine the outcome of PH in COPD?
- STUDY DESIGN AND METHODS: We analyzed the characteristics and outcome of patients enrolled in the Comparative, Prospective Registry of Newly Initiated Therapies for Pulmonary Hypertension (COMPERA) with moderate or severe PH in COPD as defined during the 6th PH World Symposium who received medical therapy for PH and compared them with patients with idiopathic pulmonary arterial hypertension (IPAH) .” 21
- EXAMPLE 4. Exploratory research question (qualitative research)
- - Explores areas that have not been fully investigated (perspectives of families and children who receive care in clinic-based child obesity treatment) to have a deeper understanding of the research problem
- “Problem: Interventions for children with obesity lead to only modest improvements in BMI and long-term outcomes, and data are limited on the perspectives of families of children with obesity in clinic-based treatment. This scoping review seeks to answer the question: What is known about the perspectives of families and children who receive care in clinic-based child obesity treatment? This review aims to explore the scope of perspectives reported by families of children with obesity who have received individualized outpatient clinic-based obesity treatment.” 22
- EXAMPLE 5. Relationship research question (quantitative research)
- - Defines interactions between dependent variable (use of ankle strategies) and independent variable (changes in muscle tone)
- “Background: To maintain an upright standing posture against external disturbances, the human body mainly employs two types of postural control strategies: “ankle strategy” and “hip strategy.” While it has been reported that the magnitude of the disturbance alters the use of postural control strategies, it has not been elucidated how the level of muscle tone, one of the crucial parameters of bodily function, determines the use of each strategy. We have previously confirmed using forward dynamics simulations of human musculoskeletal models that an increased muscle tone promotes the use of ankle strategies. The objective of the present study was to experimentally evaluate a hypothesis: an increased muscle tone promotes the use of ankle strategies. Research question: Do changes in the muscle tone affect the use of ankle strategies ?” 23
EXAMPLES OF HYPOTHESES IN PUBLISHED ARTICLES
- EXAMPLE 1. Working hypothesis (quantitative research)
- - A hypothesis that is initially accepted for further research to produce a feasible theory
- “As fever may have benefit in shortening the duration of viral illness, it is plausible to hypothesize that the antipyretic efficacy of ibuprofen may be hindering the benefits of a fever response when taken during the early stages of COVID-19 illness .” 24
- “In conclusion, it is plausible to hypothesize that the antipyretic efficacy of ibuprofen may be hindering the benefits of a fever response . The difference in perceived safety of these agents in COVID-19 illness could be related to the more potent efficacy to reduce fever with ibuprofen compared to acetaminophen. Compelling data on the benefit of fever warrant further research and review to determine when to treat or withhold ibuprofen for early stage fever for COVID-19 and other related viral illnesses .” 24
- EXAMPLE 2. Exploratory hypothesis (qualitative research)
- - Explores particular areas deeper to clarify subjective experience and develop a formal hypothesis potentially testable in a future quantitative approach
- “We hypothesized that when thinking about a past experience of help-seeking, a self distancing prompt would cause increased help-seeking intentions and more favorable help-seeking outcome expectations .” 25
- “Conclusion
- Although a priori hypotheses were not supported, further research is warranted as results indicate the potential for using self-distancing approaches to increasing help-seeking among some people with depressive symptomatology.” 25
- EXAMPLE 3. Hypothesis-generating research to establish a framework for hypothesis testing (qualitative research)
- “We hypothesize that compassionate care is beneficial for patients (better outcomes), healthcare systems and payers (lower costs), and healthcare providers (lower burnout). ” 26
- Compassionomics is the branch of knowledge and scientific study of the effects of compassionate healthcare. Our main hypotheses are that compassionate healthcare is beneficial for (1) patients, by improving clinical outcomes, (2) healthcare systems and payers, by supporting financial sustainability, and (3) HCPs, by lowering burnout and promoting resilience and well-being. The purpose of this paper is to establish a scientific framework for testing the hypotheses above . If these hypotheses are confirmed through rigorous research, compassionomics will belong in the science of evidence-based medicine, with major implications for all healthcare domains.” 26
- EXAMPLE 4. Statistical hypothesis (quantitative research)
- - An assumption is made about the relationship among several population characteristics ( gender differences in sociodemographic and clinical characteristics of adults with ADHD ). Validity is tested by statistical experiment or analysis ( chi-square test, Students t-test, and logistic regression analysis)
- “Our research investigated gender differences in sociodemographic and clinical characteristics of adults with ADHD in a Japanese clinical sample. Due to unique Japanese cultural ideals and expectations of women's behavior that are in opposition to ADHD symptoms, we hypothesized that women with ADHD experience more difficulties and present more dysfunctions than men . We tested the following hypotheses: first, women with ADHD have more comorbidities than men with ADHD; second, women with ADHD experience more social hardships than men, such as having less full-time employment and being more likely to be divorced.” 27
- “Statistical Analysis
- ( text omitted ) Between-gender comparisons were made using the chi-squared test for categorical variables and Students t-test for continuous variables…( text omitted ). A logistic regression analysis was performed for employment status, marital status, and comorbidity to evaluate the independent effects of gender on these dependent variables.” 27
EXAMPLES OF HYPOTHESIS AS WRITTEN IN PUBLISHED ARTICLES IN RELATION TO OTHER PARTS
- EXAMPLE 1. Background, hypotheses, and aims are provided
- “Pregnant women need skilled care during pregnancy and childbirth, but that skilled care is often delayed in some countries …( text omitted ). The focused antenatal care (FANC) model of WHO recommends that nurses provide information or counseling to all pregnant women …( text omitted ). Job aids are visual support materials that provide the right kind of information using graphics and words in a simple and yet effective manner. When nurses are not highly trained or have many work details to attend to, these job aids can serve as a content reminder for the nurses and can be used for educating their patients (Jennings, Yebadokpo, Affo, & Agbogbe, 2010) ( text omitted ). Importantly, additional evidence is needed to confirm how job aids can further improve the quality of ANC counseling by health workers in maternal care …( text omitted )” 28
- “ This has led us to hypothesize that the quality of ANC counseling would be better if supported by job aids. Consequently, a better quality of ANC counseling is expected to produce higher levels of awareness concerning the danger signs of pregnancy and a more favorable impression of the caring behavior of nurses .” 28
- “This study aimed to examine the differences in the responses of pregnant women to a job aid-supported intervention during ANC visit in terms of 1) their understanding of the danger signs of pregnancy and 2) their impression of the caring behaviors of nurses to pregnant women in rural Tanzania.” 28
- EXAMPLE 2. Background, hypotheses, and aims are provided
- “We conducted a two-arm randomized controlled trial (RCT) to evaluate and compare changes in salivary cortisol and oxytocin levels of first-time pregnant women between experimental and control groups. The women in the experimental group touched and held an infant for 30 min (experimental intervention protocol), whereas those in the control group watched a DVD movie of an infant (control intervention protocol). The primary outcome was salivary cortisol level and the secondary outcome was salivary oxytocin level.” 29
- “ We hypothesize that at 30 min after touching and holding an infant, the salivary cortisol level will significantly decrease and the salivary oxytocin level will increase in the experimental group compared with the control group .” 29
- EXAMPLE 3. Background, aim, and hypothesis are provided
- “In countries where the maternal mortality ratio remains high, antenatal education to increase Birth Preparedness and Complication Readiness (BPCR) is considered one of the top priorities [1]. BPCR includes birth plans during the antenatal period, such as the birthplace, birth attendant, transportation, health facility for complications, expenses, and birth materials, as well as family coordination to achieve such birth plans. In Tanzania, although increasing, only about half of all pregnant women attend an antenatal clinic more than four times [4]. Moreover, the information provided during antenatal care (ANC) is insufficient. In the resource-poor settings, antenatal group education is a potential approach because of the limited time for individual counseling at antenatal clinics.” 30
- “This study aimed to evaluate an antenatal group education program among pregnant women and their families with respect to birth-preparedness and maternal and infant outcomes in rural villages of Tanzania.” 30
- “ The study hypothesis was if Tanzanian pregnant women and their families received a family-oriented antenatal group education, they would (1) have a higher level of BPCR, (2) attend antenatal clinic four or more times, (3) give birth in a health facility, (4) have less complications of women at birth, and (5) have less complications and deaths of infants than those who did not receive the education .” 30
Research questions and hypotheses are crucial components to any type of research, whether quantitative or qualitative. These questions should be developed at the very beginning of the study. Excellent research questions lead to superior hypotheses, which, like a compass, set the direction of research, and can often determine the successful conduct of the study. Many research studies have floundered because the development of research questions and subsequent hypotheses was not given the thought and meticulous attention needed. The development of research questions and hypotheses is an iterative process based on extensive knowledge of the literature and insightful grasp of the knowledge gap. Focused, concise, and specific research questions provide a strong foundation for constructing hypotheses which serve as formal predictions about the research outcomes. Research questions and hypotheses are crucial elements of research that should not be overlooked. They should be carefully thought of and constructed when planning research. This avoids unethical studies and poor outcomes by defining well-founded objectives that determine the design, course, and outcome of the study.
Disclosure: The authors have no potential conflicts of interest to disclose.
Author Contributions:
- Conceptualization: Barroga E, Matanguihan GJ.
- Methodology: Barroga E, Matanguihan GJ.
- Writing - original draft: Barroga E, Matanguihan GJ.
- Writing - review & editing: Barroga E, Matanguihan GJ.
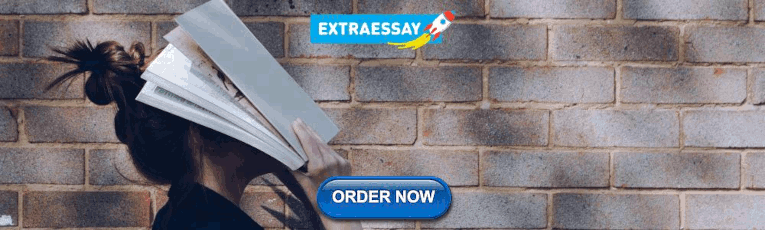
COMMENTS
Introduction. Statistical analysis is necessary for any research project seeking to make quantitative conclusions. The following is a primer for research-based statistical analysis. It is intended to be a high-level overview of appropriate statistical testing, while not diving too deep into any specific methodology.
Statistical analysis means investigating trends, patterns, and relationships using quantitative data. It is an important research tool used by scientists, governments, businesses, and other organizations. To draw valid conclusions, statistical analysis requires careful planning from the very start of the research process. You need to specify ...
This chapter concerns the characteristics of research questions, data designs, and the more common multivariate statistical methods. Data. Details are provided on different data formats and types that feature in the case studies. Properties of variables, including measurement levels, are also discussed. Designs.
The SAMBR checklists have three parts as follows: Part (A) focuses on the reporting elements of common biomedical research designs, part (B) shows the essential steps to be followed in common studies for quality assessment of statistical analysis, and part (C) comprises the steps for data analysis and related essential and preferred choices of ...
Bayesian and Frequentist statistical frameworks: Most of the current clinical research reporting is based on the frequentist approach and hypotheses testing p values and confidence intervals. The frequentist approach assumes the acquired data are random, attained by random sampling, through randomized experiments or influences, and with random ...
statistical methods. 1 Statistical Framework of Causal Inference What do we exactly mean when we say "An event A causes another event B"? Whether explicitly or implicitly, this question is asked and answered all the time in political science research. The most commonly used statistical framework of causality is based on the notion of ...
Developing a conceptual framework in research. A conceptual framework is a representation of the relationship you expect to see between your variables, or the characteristics or properties that you want to study. Conceptual frameworks can be written or visual and are generally developed based on a literature review of existing studies about ...
Abstract. This chapter introduces the reader to the theoretical foundations of statistical analysis by presenting a rigorous, multivariate, set-based approach to probability and statistical theory. The foundation is laid with consideration of the key statistical axioms and definitions, which formalise many of the concepts introduced in Chap. 1.
There are broader quality frameworks that emphasize the granularity of the data and the estimates in ways that were previously not possible. An influential quality framework has been developed by Eurostat, the Statistical Office of the European Union (European Statistical System Committee, 2013) (see Box 6-1).This framework has five major output quality components:
Abstract. This section presents the research design, provides a description and justification of the methodological approach and methods used, and details the research framework for the study. In addition, it presents the research objectives and highlights the research hypothesis; discusses about the research area, sampling techniques used, and ...
Statistical framework extended ... Depending on the research questions, we have to make one or more decisions about the appropriate way to analyse such data formats with completely missing rows and/or columns. Fig. 4.9. Data arrangements. Five publishers (A,..,E) by fifteen book types with two classes 1,...,10 and 11,...,15, respectively.
Statistical literacy frameworks. Despite the challenges of the terminology and definitions, it is generally accepted that statistical literacy is an important component of statistics education (Doyle, Citation 2008; Watson, Citation 2006).This section considers three frameworks or models that attempt to represent the features of statistical literacy discussed in the previous section.
A research design is a strategy for answering your research question using empirical data. Creating a research design means making decisions about: Your overall research objectives and approach. Whether you'll rely on primary research or secondary research. Your sampling methods or criteria for selecting subjects. Your data collection methods.
A simple statistical framework for small sample studies. D. S. Schwarzkopf, Zien Huang. Published in bioRxiv 22 September 2023. Psychology. Most studies in psychology, neuroscience, and life science research make inferences about how strong an effect is on average in the population. Yet, many research questions could instead be answered by ...
This manuscript is careful to distinguish between y t and x t, providing a framework that explicitly incorporates each of these critical elements of EC. Here we approach ECs from a hierarchical statistical modeling perspective (e.g., Cressie & Wikle, 2011). The relationship between observations, states or processes, and parameters, is related ...
Here we present such a framework, based on the ratio of binomial probabilities between a model assuming the universality of the phenomenon versus the null hypothesis that any incidence of the effect is sporadic. We demonstrate the benefits of this approach, which permits strong conclusions from samples as small as 2-5 participants and the ...
Indeed, for some research the mindless use of group statistics [11] can even hinder the interpretation of results [12]. The purpose of psychology and neuroscience research is to discover the mechanisms of the mind and brain. To answer many research questions, the average population effect is at best uninformative, or even irrelevant.
Conceptual statistical framework to measure corruption This approach considers the complexity of corruption by looking at the different dimensions of corruption together with elements that can describe it. The Framework is constructed through a table with different dimensions: • types of corruption (based on the United ...
Abstract. Although statistical models serve as the foundation of data analysis in clinical studies, their interpretation requires sufficient understanding of the underlying statistical framework. Statistical modeling is inherently a difficult task because of the general lack of information of the nature of observable data.
If you're interested in a qualitative study, be sure to omit arrows and other notations inferring statistical analysis. The only time it would be inappropriate to include a framework in qualitative study is in a grounded theory study, which is not something you'll do in an applied doctoral study. ... Selecting, and Integrating a Theoretical ...
The source code for the statistical framework lives in the directory src/stats. 3.5.1. Goals ¶. Primary objectives for this effort are the following: Provide functionality to record, calculate, and present data and statistics for analysis of network simulations. Boost simulation performance by reducing the need to generate extensive trace logs ...
Goal 7: Implement research-informed suicide prevention communication activities in diverse populations using best practices from communication science. Strategic Direction 2: Treatment and Crisis Services. Goal 8: Implement effective suicide prevention services as a core component of health care.
In general, phase-type distributions provide a powerful mathematical framework for coalescent theory because they are analytically tractable using matrix manipulations. The purpose of this talk is to explain the phase-type theory and demonstrate how the theory can be applied to derive basic properties of coalescent models.
View a PDF of the paper titled Research on Splicing Image Detection Algorithms Based on Natural Image Statistical Characteristics, by Ao Xiang and 4 other authors ... we have developed a detection framework that integrates advanced statistical analysis techniques and machine learning methods. The algorithm has been validated using multiple ...
Ye, whose induction will take place during the Joint Statistical Meetings in Portland, Oregon, this August, is being recognized "for pioneering contributions to biomedical research, including statistical and bioinformatic methods, innovations in clinical trial design and analysis, and a deeper understanding of biomarkers, plus impactful ...
The integration of Large Language Models (LLMs) into healthcare promises to transform medical diagnostics, research, and patient care. Yet, the progression of medical LLMs faces obstacles such as complex training requirements, rigorous evaluation demands, and the dominance of proprietary models that restrict academic exploration. Transparent, comprehensive access to LLM resources is essential ...
Statistics at the time revealed alarming trends: From 2001 to 2019, the suicide rate for Americans ages 10 to 19 rose 40 percent, and emergency visits related to self-harm rose 88 percent.
The analysis relies on statistics published by the FBI, which we accessed through the Crime Data Explorer, and the Bureau of Justice Statistics (BJS), which we accessed through the National Crime Victimization Survey data analysis tool. To measure public attitudes about crime in the U.S., we relied on survey data from Pew Research Center and ...
Statistical methods for provider profiling are designed to measure healthcare quality and create incentives for low-quality providers to improve. While most existing healthcare quality metrics assess the overall treatment delivered to providers' entire patient populations, widespread racial and sociodemographic disparities in health outcomes highlight the need to evaluate providers ...
INTRODUCTION. Scientific research is usually initiated by posing evidenced-based research questions which are then explicitly restated as hypotheses.1,2 The hypotheses provide directions to guide the study, solutions, explanations, and expected results.3,4 Both research questions and hypotheses are essentially formulated based on conventional theories and real-world processes, which allow the ...