Reset password New user? Sign up
Existing user? Log in
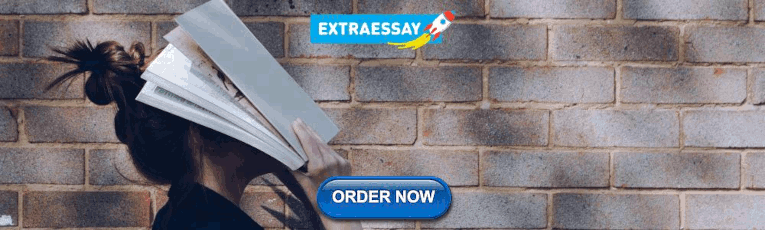
Hungarian Maximum Matching Algorithm
Already have an account? Log in here.
The Hungarian matching algorithm , also called the Kuhn-Munkres algorithm, is a \(O\big(|V|^3\big)\) algorithm that can be used to find maximum-weight matchings in bipartite graphs , which is sometimes called the assignment problem . A bipartite graph can easily be represented by an adjacency matrix , where the weights of edges are the entries. Thinking about the graph in terms of an adjacency matrix is useful for the Hungarian algorithm.
A matching corresponds to a choice of 1s in the adjacency matrix, with at most one 1 in each row and in each column.
The Hungarian algorithm solves the following problem:
In a complete bipartite graph \(G\), find the maximum-weight matching. (Recall that a maximum-weight matching is also a perfect matching.)
This can also be adapted to find the minimum-weight matching.
Say you are having a party and you want a musician to perform, a chef to prepare food, and a cleaning service to help clean up after the party. There are three companies that provide each of these three services, but one company can only provide one service at a time (i.e. Company B cannot provide both the cleaners and the chef). You are deciding which company you should purchase each service from in order to minimize the cost of the party. You realize that is an example of the assignment problem, and set out to make a graph out of the following information: \(\quad\) Company\(\quad\) \(\quad\) Cost for Musician\(\quad\) \(\quad\) Cost for Chef\(\quad\) \(\quad\) Cost for Cleaners\(\quad\) \(\quad\) Company A\(\quad\) \(\quad\) $108\(\quad\) \(\quad\) $125\(\quad\) \(\quad\) $150\(\quad\) \(\quad\) Company B\(\quad\) \(\quad\) $150\(\quad\) \(\quad\) $135\(\quad\) \(\quad\) $175\(\quad\) \(\quad\) Company C\(\quad\) \(\quad\) $122\(\quad\) \(\quad\) $148\(\quad\) \(\quad\) $250\(\quad\) Can you model this table as a graph? What are the nodes? What are the edges? Show Answer The nodes are the companies and the services. The edges are weighted by the price.
What are some ways to solve the problem above? Since the table above can be thought of as a \(3 \times 3\) matrix, one could certainly solve this problem using brute force, checking every combination and seeing what yields the lowest price. However, there are \(n!\) combinations to check, and for large \(n\), this method becomes very inefficient very quickly.
The Hungarian Algorithm Using an Adjacency Matrix
The hungarian algorithm using a graph.
With the cost matrix from the example above in mind, the Hungarian algorithm operates on this key idea: if a number is added to or subtracted from all of the entries of any one row or column of a cost matrix, then an optimal assignment for the resulting cost matrix is also an optimal assignment for the original cost matrix.
The Hungarian Method [1] Subtract the smallest entry in each row from all the other entries in the row. This will make the smallest entry in the row now equal to 0. Subtract the smallest entry in each column from all the other entries in the column. This will make the smallest entry in the column now equal to 0. Draw lines through the row and columns that have the 0 entries such that the fewest lines possible are drawn. If there are \(n\) lines drawn, an optimal assignment of zeros is possible and the algorithm is finished. If the number of lines is less than \(n\), then the optimal number of zeroes is not yet reached. Go to the next step. Find the smallest entry not covered by any line. Subtract this entry from each row that isn’t crossed out, and then add it to each column that is crossed out. Then, go back to Step 3.
Solve for the optimal solution for the example in the introduction using the Hungarian algorithm described above. Here is the initial adjacency matrix: Subtract the smallest value in each row from the other values in the row: Now, subtract the smallest value in each column from all other values in the column: Draw lines through the row and columns that have the 0 entries such that the fewest possible lines are drawn: There are 2 lines drawn, and 2 is less than 3, so there is not yet the optimal number of zeroes. Find the smallest entry not covered by any line. Subtract this entry from each row that isn’t crossed out, and then add it to each column that is crossed out. Then, go back to Step 3. 2 is the smallest entry. First, subtract from the uncovered rows: Now add to the covered columns: Now go back to step 3, drawing lines through the rows and columns that have 0 entries: There are 3 lines (which is \(n\)), so we are done. The assignment will be where the 0's are in the matrix such that only one 0 per row and column is part of the assignment. Replace the original values: The Hungarian algorithm tells us that it is cheapest to go with the musician from company C, the chef from company B, and the cleaners from company A. We can verify this by brute force. 108 + 135 + 250 = 493 108 + 148 + 175 = 431 150 + 125 + 250 = 525 150 + 148 + 150 = 448 122 + 125 + 175 = 422 122 + 135 + 150 = 407. We can see that 407 is the lowest price and matches the assignment the Hungarian algorithm determined. \(_\square\)
The Hungarian algorithm can also be executed by manipulating the weights of the bipartite graph in order to find a stable, maximum (or minimum) weight matching. This can be done by finding a feasible labeling of a graph that is perfectly matched, where a perfect matching is denoted as every vertex having exactly one edge of the matching.
How do we know that this creates a maximum-weight matching?
A feasible labeling on a perfect match returns a maximum-weighted matching. Suppose each edge \(e\) in the graph \(G\) connects two vertices, and every vertex \(v\) is covered exactly once. With this, we have the following inequality: \[w(M’) = \sum_{e\ \epsilon\ E} w(e) \leq \sum_{e\ \epsilon\ E } \big(l(e_x) + l(e_y)\big) = \sum_{v\ \epsilon\ V} l(v),\] where \(M’\) is any perfect matching in \(G\) created by a random assignment of vertices, and \(l(x)\) is a numeric label to node \(x\). This means that \(\sum_{v\ \epsilon\ V}\ l(v)\) is an upper bound on the cost of any perfect matching. Now let \(M\) be a perfect match in \(G\), then \[w(M) = \sum_{e\ \epsilon\ E} w(e) = \sum_{v\ \epsilon\ V}\ l(v).\] So \(w(M’) \leq w(M)\) and \(M\) is optimal. \(_\square\)
Start the algorithm by assigning any weight to each individual node in order to form a feasible labeling of the graph \(G\). This labeling will be improved upon by finding augmenting paths for the assignment until the optimal one is found.
A feasible labeling is a labeling such that
\(l(x) + l(y) \geq w(x,y)\ \forall x \in X, y \in Y\), where \(X\) is the set of nodes on one side of the bipartite graph, \(Y\) is the other set of nodes, \(l(x)\) is the label of \(x\), etc., and \(w(x,y)\) is the weight of the edge between \(x\) and \(y\).
A simple feasible labeling is just to label a node with the number of the largest weight from an edge going into the node. This is certain to be a feasible labeling because if \(A\) is a node connected to \(B\), the label of \(A\) plus the label of \(B\) is greater than or equal to the weight \(w(x,y)\) for all \(y\) and \(x\).
A feasible labeling of nodes, where labels are in red [2] .
Imagine there are four soccer players and each can play a few positions in the field. The team manager has quantified their skill level playing each position to make assignments easier.
How can players be assigned to positions in order to maximize the amount of skill points they provide?
The algorithm starts by labeling all nodes on one side of the graph with the maximum weight. This can be done by finding the maximum-weighted edge and labeling the adjacent node with it. Additionally, match the graph with those edges. If a node has two maximum edges, don’t connect them.
Although Eva is the best suited to play defense, she can't play defense and mid at the same time!
If the matching is perfect, the algorithm is done as there is a perfect matching of maximum weights. Otherwise, there will be two nodes that are not connected to any other node, like Tom and Defense. If this is the case, begin iterating.
Improve the labeling by finding the non-zero label vertex without a match, and try to find the best assignment for it. Formally, the Hungarian matching algorithm can be executed as defined below:
The Hungarian Algorithm for Graphs [3] Given: the labeling \(l\), an equality graph \(G_l = (V, E_l)\), an initial matching \(M\) in \(G_l\), and an unmatched vertex \(u \in V\) and \(u \notin M\) Augmenting the matching A path is augmenting for \(M\) in \(G_l\) if it alternates between edges in the matching and edges not in the matching, and the first and last vertices are free vertices , or unmatched, in \(M\). We will keep track of a candidate augmenting path starting at the vertex \(u\). If the algorithm finds an unmatched vertex \(v\), add on to the existing augmenting path \(p\) by adding the \(u\) to \(v\) segment. Flip the matching by replacing the edges in \(M\) with the edges in the augmenting path that are not in \(M\) \((\)in other words, the edges in \(E_l - M).\) Improving the labeling \(S \subseteq X\) and \(T \subseteq Y,\) where \(S\) and \(T\) represent the candidate augmenting alternating path between the matching and the edges not in the matching. Let \(N_l(S)\) be the neighbors to each node that is in \(S\) along edges in \(E_l\) such that \(N_l(S) = \{v|\forall u \in S: (u,v) \in E_l\}\). If \(N_l(S) = T\), then we cannot increase the size of the alternating path (and therefore can't further augment), so we need to improve the labeling. Let \(\delta_l\) be the minimum of \(l(u) + l(v) - w(u,v)\) over all of the \(u \in S\) and \(v \notin T\). Improve the labeling \(l\) to \(l'\): If \(r \in S,\) then \(l'(r) = l(r) - \delta_l,\) If \(r \in T,\) then \(l'(r) = l(r) + \delta_l.\) If \(r \notin S\) and \(r \notin T,\) then \(l'(r) = l(r).\) \(l'\) is a valid labeling and \(E_l \subset E_{l'}.\) Putting it all together: The Hungarian Algorithm Start with some matching \(M\), a valid labeling \(l\), where \(l\) is defined as the labelling \(\forall x \in X, y \in Y| l(y) = 0, l(x) = \text{ max}_{y \in Y}(w\big(x, y)\big)\). Do these steps until a perfect matching is found \((\)when \(M\) is perfect\():\) (a) Look for an augmenting path in \(M.\) (b) If an augmenting path does not exist, improve the labeling and then go back to step (a).
Each step will increase the size of the matching \(M\) or it will increase the size of the set of labeled edges, \(E_l\). This means that the process will eventually terminate since there are only so many edges in the graph \(G\). [4]
When the process terminates, \(M\) will be a perfect matching. By the Kuhn-Munkres theorem , this means that the matching is a maximum-weight matching.
The algorithm defined above can be implemented in the soccer scenario. First, the conflicting node is identified, implying that there is an alternating tree that must be reconfigured.
There is an alternating path between defense, Eva, mid, and Tom.
To find the best appropriate node, find the minimum \(\delta_l\), as defined in step 4 above, where \(l_u\) is the label for player \(u,\) \(l_v\) is the label for position \(v,\) and \(w_{u, v}\) is the weight on that edge.
The \(\delta_l\) of each unmatched node is computed, where the minimum is found to be a value of 2, between Tom playing mid \((8 + 0 – 6 = 2).\)
The labels are then augmented and the new edges are graphed in the example. Notice that defense and mid went down by 2 points, whereas Eva’s skillset got back two points. However, this is expected as Eva can't play in both positions at once.
Augmenting path leads to relabeling of nodes, which gives rise to the maximum-weighted path.
These new edges complete the perfect matching of the graph, which implies that a maximum-weighted graph has been found and the algorithm can terminate.
The complexity of the algorithm will be analyzed using the graph-based technique as a reference, yet the result is the same as for the matrix-based one.
Algorithm analysis [3] At each \(a\) or \(b\) step, the algorithm adds one edge to the matching and this happens \(O\big(|V|\big)\) times. It takes \(O\big(|V|\big)\) time to find the right vertex for the augmenting (if there is one at all), and it is \(O\big(|V|\big)\) time to flip the matching. Improving the labeling takes \(O\big(|V|\big)\) time to find \(\delta_l\) and to update the labelling accordingly. We might have to improve the labeling up to \(O\big(|V|\big)\) times if there is no augmenting path. This makes for a total of \(O\big(|V|^2\big)\) time. In all, there are \(O\big(|V|\big)\) iterations each taking \(O\big(|V|\big)\) work, leading to a total running time of \(O\big(|V|^3\big)\).
- Matching Algorithms
- Bruff, D. The Assignment Problem and the Hungarian Method . Retrieved June 26, 2016, from http://www.math.harvard.edu/archive/20_spring_05/handouts/assignment_overheads.pdf
- Golin, M. Bipartite Matching & the Hungarian Method . Retrieved Retrieved June 26th, 2016, from http://www.cse.ust.hk/~golin/COMP572/Notes/Matching.pdf
- Grinman, A. The Hungarian Algorithm for Weighted Bipartite Graphs . Retrieved June 26, 2016, from http://math.mit.edu/~rpeng/18434/hungarianAlgorithm.pdf
- Golin, M. Bipartite Matching & the Hungarian Method . Retrieved June 26, 2016, from http://www.cse.ust.hk/~golin/COMP572/Notes/Matching.pdf
Problem Loading...
Note Loading...
Set Loading...
- Data Structures
- Linked List
- Binary Tree
- Binary Search Tree
- Segment Tree
- Disjoint Set Union
- Fenwick Tree
- Red-Black Tree
- Advanced Data Structures
Hungarian Algorithm for Assignment Problem | Set 1 (Introduction)
- Hungarian Algorithm for Assignment Problem | Set 2 (Implementation)
- Introduction to Exact Cover Problem and Algorithm X
- Greedy Approximate Algorithm for Set Cover Problem
- Job Assignment Problem using Branch And Bound
- Implementation of Exhaustive Search Algorithm for Set Packing
- Channel Assignment Problem
- Chocolate Distribution Problem | Set 2
- Transportation Problem | Set 1 (Introduction)
- OLA Interview Experience | Set 11 ( For Internship)
- Top 20 Greedy Algorithms Interview Questions
- Job Sequencing Problem - Loss Minimization
- Prim's Algorithm (Simple Implementation for Adjacency Matrix Representation)
- Data Structures and Algorithms | Set 21
- Adobe Interview Experience | Set 55 (On-Campus Full Time for MTS profile)
- Amazon Interview Experience | Set 211 (On-Campus for Internship)
- OYO Rooms Interview Experience | Set 3 (For SDE-II, Gurgaon)
- C# Program for Dijkstra's shortest path algorithm | Greedy Algo-7
- Algorithms | Dynamic Programming | Question 7
- Amazon Interview | Set 46 (On-campus for Internship)
- Merge Sort - Data Structure and Algorithms Tutorials
- Must Do Coding Questions for Companies like Amazon, Microsoft, Adobe, ...
- QuickSort - Data Structure and Algorithm Tutorials
- Bubble Sort - Data Structure and Algorithm Tutorials
- Tree Traversal Techniques - Data Structure and Algorithm Tutorials
- Binary Search - Data Structure and Algorithm Tutorials
- Insertion Sort - Data Structure and Algorithm Tutorials
- Selection Sort – Data Structure and Algorithm Tutorials
- Understanding the basics of Linked List
- Breadth First Search or BFS for a Graph
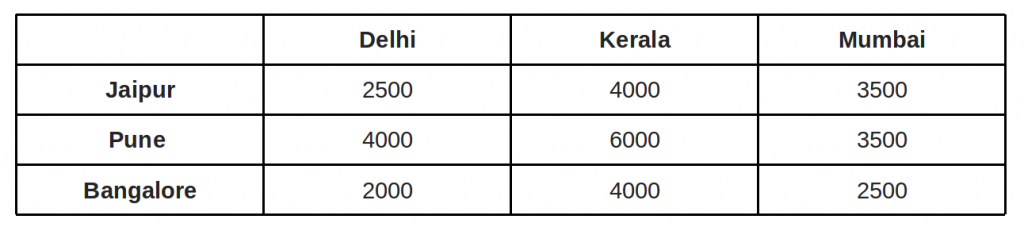
- For each row of the matrix, find the smallest element and subtract it from every element in its row.
- Do the same (as step 1) for all columns.
- Cover all zeros in the matrix using minimum number of horizontal and vertical lines.
- Test for Optimality: If the minimum number of covering lines is n, an optimal assignment is possible and we are finished. Else if lines are lesser than n, we haven’t found the optimal assignment, and must proceed to step 5.
- Determine the smallest entry not covered by any line. Subtract this entry from each uncovered row, and then add it to each covered column. Return to step 3.
Try it before moving to see the solution
Explanation for above simple example:
An example that doesn’t lead to optimal value in first attempt: In the above example, the first check for optimality did give us solution. What if we the number covering lines is less than n.
Time complexity : O(n^3), where n is the number of workers and jobs. This is because the algorithm implements the Hungarian algorithm, which is known to have a time complexity of O(n^3).
Space complexity : O(n^2), where n is the number of workers and jobs. This is because the algorithm uses a 2D cost matrix of size n x n to store the costs of assigning each worker to a job, and additional arrays of size n to store the labels, matches, and auxiliary information needed for the algorithm.
In the next post, we will be discussing implementation of the above algorithm. The implementation requires more steps as we need to find minimum number of lines to cover all 0’s using a program. References: http://www.math.harvard.edu/archive/20_spring_05/handouts/assignment_overheads.pdf https://www.youtube.com/watch?v=dQDZNHwuuOY
Please Login to comment...
Similar reads.
- Mathematical

Improve your Coding Skills with Practice
What kind of Experience do you want to share?
CodeForces Segment Trees, Part 2, 4.4
Problem statement.
https://codeforces.com/edu/course/2/lesson/5/4/practice/contest/280801/problem/D
This segment tree will support two operations:
- Add value d to segment [r, l) .
- For segment [r, l) find weighted sum: A[r]*1 + A[r+1]*2 + ...
We will keep in T two values (sum, weighted sum) . We will keep in L one value lazy update: d , now what we need to understand is how to propagate updates.
Standart compexities for segment tree.
we can use Segment problems 2.4 and 4.2 and combine them.
Instructors: Yihan Sun and Yan Gu
Winter 2023
Assignments & Projects
Please carefully read the Academic Integrity before you start working on the homework assignments.
In short, you can get help from the instructors, TAs, textbooks (or relevant books), the Internet, or discussions with your classmates, but you must cite them fully and completely (i.e., provide citations to the book or website link, acknowledge the other students that had discussions with you). Again, you are NOT allowed to :
- copy anything from the book or the Internet,
- read or look at anyone else’s solutions (write-up or code),
- share your solutions (write-up or code) with any other students, during or after the completion of the course.
When you write down your solution, it MUST be close-book . This is to make sure you truly understand and can recreate the solutions.
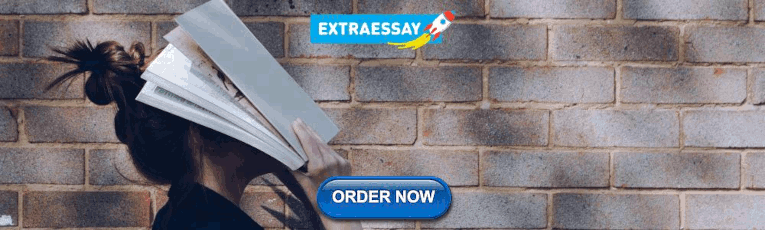
📃 Course Policy Test
We have a course policy test about academic integrity and course policy. It takes 1/100 point in your overall grade. This test is due on 01/12.
📃 Entrance Exam
There is an entrance exam for this course. There are 4 programming problems, covering several important algorithms you should have learned in prerequisite courses (simple programming, simple sorting, graph algorithms, and some algorithm design ideas). You need to finish them in the first week. They will take 9/100 points in your overall grade. The four problems will be 11 points in total, and the full mark is 9 points. If you get more than 9 points, they will count as bonus points. Ideally you should pass all the 4 problems. ⚠ We would not recommend anyone getting <= 6 points to take the course.
If you cannot finish them in the first week, then CS 142 can be very challenging for you. You should consider taking prerequi courses for algorithms and programming, including CS 10A/B/C, 11, 111 and 141.
Problem-Solving Assignments
All problems will be given on codeforces (including the entrance exam), so we do not post the problems here.
We will use CodeForces to submit and test codes. You also need to submit a short report to describe your algorithm and specify your submission id. Here is a guideline about using CodeForces. Here is a beginner’s cheatsheet for learning programming.
We run automatic code comparison programs on student solutions. These programs are very good at detecting similarity between code, even code that has been purposefully obfuscated. Such programs can compare a submitted assignment against all other submitted assignments, against all known previous solutions of a problem, etc. The signal-to-noise ratio of such comparisons is usually very distinctive, making it very clear what code is a student’s original creative work and what code is merely transcribed from some other source. Cheating is simply not worth the risk.
You need to submit a brief report on how you solved these problems, including:
- Your name, SID and/or NETID, and your codeforces ID;
- Submission ID (there is a unique id for each submission);
- Describe the algorithm you designed;
- Show cost analysis if necessary.
You will not get the point if you don’t provide the report to explain how your algorithm works.
Each programming assignment takes 10/100 points in your overall grade. In each assignment, there are six problems. The first three problems are basic problems, and are easier. Each of them is worth for 3 points. The last three problems are challenge problems and are haeder. Each of them is 1 point.
You can get 12 points at maximum for each assignment. If you get more than 10 points in one assignment, they will be counted as bonus bonus. Note that you need to finish at least 1 challenge problem to get full points.
For challenge programming assignments, you can work on them after the deadline - as long as you finish them before the end of the class, you can still get half of the credits.
Performance-Engineering Assignments
Performance-Engineering Assignments are mini projects that requires programming. Generally, your goal is to write parallel code to engineer a specific problem. You will test and run your code on a server at UCR, and the detailed instruction will be given in the assignment problem description.
Each performance-engineering assignment contributes 15/100 to your overall grade. Similar to the problem-solving training, you can get more than 15 points if you get the bonus points.
Written Assignments
There are two written assignmetns. Each takes 5/100 points in your overall grade. The written part is similar to other algorithm courses you have taken, and you must use LaTeX to prepare your solution. Here you can find sample code for writing solutions using LaTeX.
In grading, we will reward not only correctness but also clarity and simplicity . To avoid losing points for hard-to-understand solutions, you should make sure your solutions are either self-explanatory or contains good explanations. Please understand that grading your assignments is a lot of work for your TAs and readers, especially determining the correctness and cost bounds for your algorithms. We reserve the right to manually deduct points for solutions that are conceptually correct but does not show a sincere attempt to explain the ideas clearly.
Presentation
At the end of the class, you can choose to give a presentation in class to get bonus points. We encourage you to present one of your favorite bonus questions and your solution to the class. For bonus question presentation, you have to pass all the test cases before the presentation.
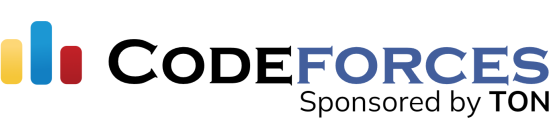
CodeChef Starters 133 Solution Discussion

- MikeMirzayanov
- Submissions
- Problemsetting
Navigation Menu
Search code, repositories, users, issues, pull requests..., provide feedback.
We read every piece of feedback, and take your input very seriously.
Saved searches
Use saved searches to filter your results more quickly.
To see all available qualifiers, see our documentation .
- Notifications
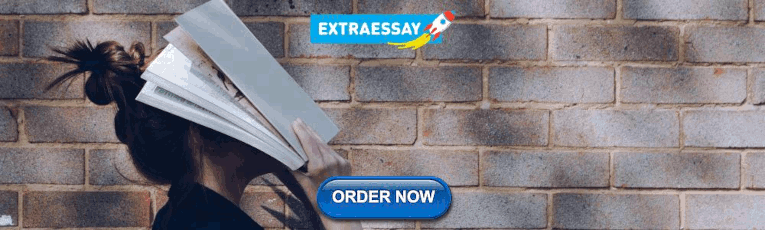
IMAGES
VIDEO
COMMENTS
The Hungarian matching algorithm, also called the Kuhn-Munkres algorithm, is a \(O\big(|V|^3\big)\) algorithm that can be used to find maximum-weight matchings in bipartite graphs, which is sometimes called the assignment problem.A bipartite graph can easily be represented by an adjacency matrix, where the weights of edges are the entries.Thinking about the graph in terms of an adjacency ...
Time complexity : O(n^3), where n is the number of workers and jobs. This is because the algorithm implements the Hungarian algorithm, which is known to have a time complexity of O(n^3). Space complexity : O(n^2), where n is the number of workers and jobs.This is because the algorithm uses a 2D cost matrix of size n x n to store the costs of assigning each worker to a job, and additional ...
But regarding the min cost max flow problem, a pre requisite to it is the classic Maximum Bipartite Matching. The one you linked in CSES is a bit more advanced. Codeforces. Programming competitions and contests, programming community.
Codeforces. Programming competitions and contests, programming community. Rating changes for last rounds are temporarily rolled back. They will be returned soon.
The bold parts above are what makes it different than the standard assignment problem. It's guaranteed that sum of machine power = sum of job request power. Which means all jobs can be completed. I want to minimize the number of assignments (machine, job). Example: Machine A (50) Machine B (30) Machine C (40) Job A (70) Job B (50) Minimum ...
Solution. This segment tree will support two operations: Add value d to segment [r, l). For segment [r, l) find weighted sum: A[r]*1 + A[r+1]*2 + ... We will keep in T two values (sum, weighted sum). We will keep in L one value lazy update: d, now what we need to understand is how to propagate updates.
Collection of my Codeforces problems as well as popular algorithms with easy to understand solutions and comments about ideas in problems - Ramez-/Codeforces-solutions-explained
My code solutions for competitive programming platform, codeforces - codeforces/C_Assignment_to_Segment.cpp at master · dzuizz/codeforces
All problems will be given on codeforces (including the entrance exam), so we do not post the problems here. ... You will test and run your code on a server at UCR, and the detailed instruction will be given in the assignment problem description. Each performance-engineering assignment contributes 15/100 to your overall grade. Similar to the ...
You signed in with another tab or window. Reload to refresh your session. You signed out in another tab or window. Reload to refresh your session. You switched accounts on another tab or window.
The answer of your post question (already given in Yuval comment) is that there is no greedy techniques providing you the optimal answer to an assignment problem. The commonly used solution is the Hungarian algorithm, see. Harold W. Kuhn, "The Hungarian Method for the assignment problem", Naval Research Logistics Quarterly, 2: 83-97, 1955.
Codeforces. Programming competitions and contests, programming community . ... An assignment problem. Please tell an efficient algorithm for the following problem: By shubhinanugullu ... This is the boolean satisfiability problem (SAT), which is famously NP-complete.
The "Custom invocation" tab does not know about the interactor for the problem, so you can't fully testing your solution. Sometimes on the Codeforces Rounds interactive problems will use. In this case the fromat of tests for hacks will described in the statements of the problems. Output endl in cout in C++ performs flush operation automatically.
Codeforces Round 943 (Div. 3) Finished: ... If you've seen these problems, a virtual contest is not for you - solve these problems in the archive. If you just want to solve some problem from a contest, a virtual contest is not for you - solve this problem in the archive. Never use someone else's code, read the tutorials or communicate with ...
Codeforces Round 733 (Div. 1 + Div. 2, based on VK Cup 2021 - Elimination (Engine)) ... If you've seen these problems, a virtual contest is not for you - solve these problems in the archive. If you just want to solve some problem from a contest, a virtual contest is not for you - solve this problem in the archive. ... The assignment is usually ...
You signed in with another tab or window. Reload to refresh your session. You signed out in another tab or window. Reload to refresh your session. You switched accounts on another tab or window.
Before contest Educational Codeforces Round 165 (Rated for Div. 2) 38:51:57 Register now » → Filter Problems Difficulty: — Add tag ...
To associate your repository with the codeforces-edu topic, visit your repo's landing page and select "manage topics." GitHub is where people build software. More than 100 million people use GitHub to discover, fork, and contribute to over 420 million projects.
Codeforces Round 941 (Div. 2) Finished: ... If you've seen these problems, a virtual contest is not for you - solve these problems in the archive. If you just want to solve some problem from a contest, a virtual contest is not for you - solve this problem in the archive. Never use someone else's code, read the tutorials or communicate with ...
Codeforces. Programming competitions and contests, programming community. The only programming contests Web 2.0 platform
The first line of the input contains integer n (1 ≤ n ≤ 200) — the number of reposts.Next follow the reposts in the order they were made. Each of them is written on a single line and looks as "name1 reposted name2".All the names in the input consist of lowercase or uppercase English letters and/or digits and have lengths from 2 to 24 characters, inclusive.
You have unweighted tree of n n vertices. You have to assign a positive weight to each edge so that the following condition would hold: For every two different leaves v1 v 1 and v2 v 2 of this tree, bitwise XOR of weights of all edges on the simple path between v1 v 1 and v2 v 2 has to be equal to 0 0. Note that you can put very large positive ...
You signed in with another tab or window. Reload to refresh your session. You signed out in another tab or window. Reload to refresh your session. You switched accounts on another tab or window.