- Skip to main content
- Skip to primary sidebar
- Skip to footer
- QuestionPro

- Solutions Industries Gaming Automotive Sports and events Education Government Travel & Hospitality Financial Services Healthcare Cannabis Technology Use Case NPS+ Communities Audience Contactless surveys Mobile LivePolls Member Experience GDPR Positive People Science 360 Feedback Surveys
- Resources Blog eBooks Survey Templates Case Studies Training Help center

Home Market Research
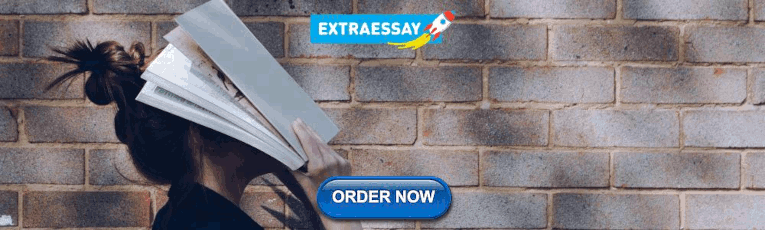
Qualitative Data Analysis: What is it, Methods + Examples
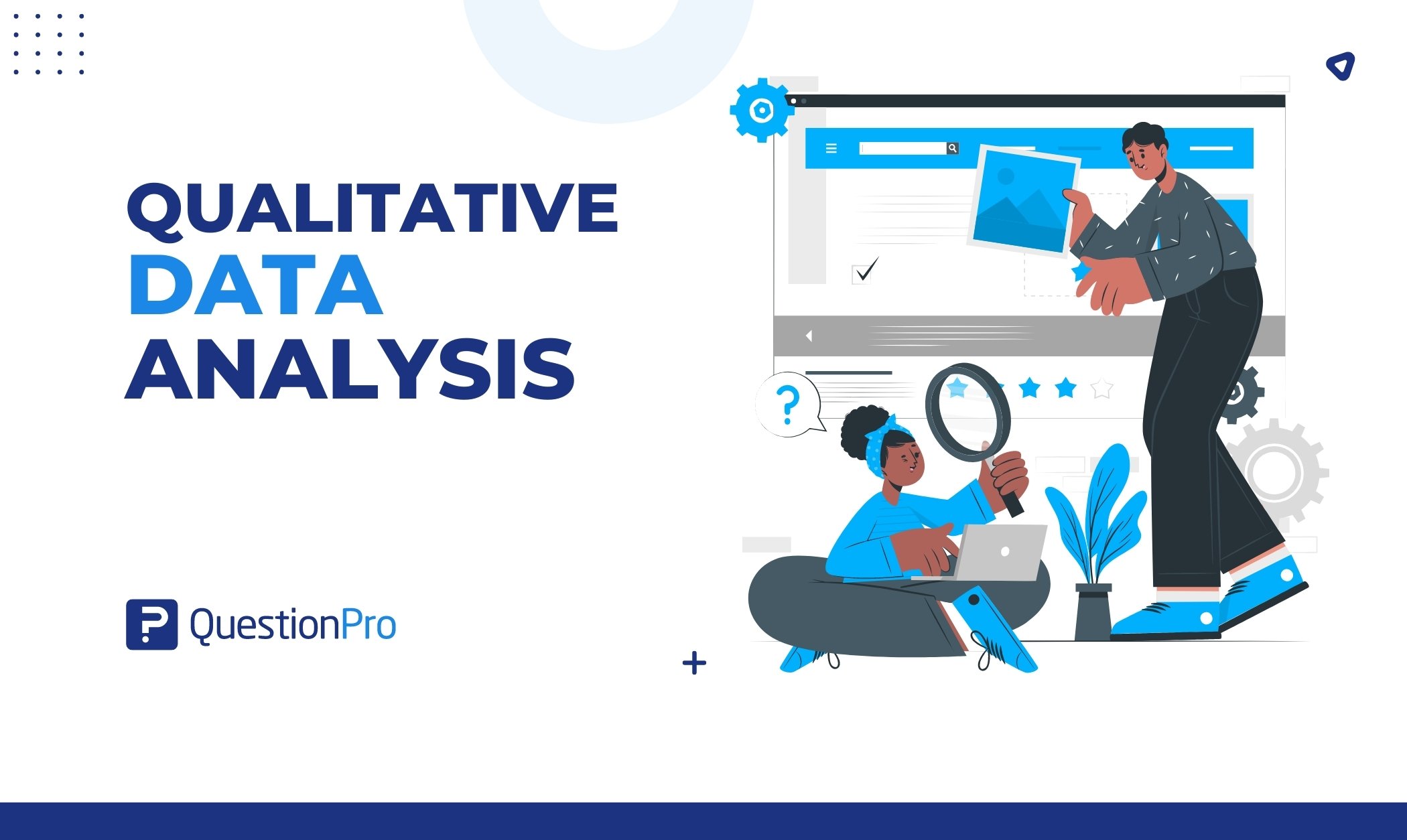
In a world rich with information and narrative, understanding the deeper layers of human experiences requires a unique vision that goes beyond numbers and figures. This is where the power of qualitative data analysis comes to light.
In this blog, we’ll learn about qualitative data analysis, explore its methods, and provide real-life examples showcasing its power in uncovering insights.
What is Qualitative Data Analysis?
Qualitative data analysis is a systematic process of examining non-numerical data to extract meaning, patterns, and insights.
In contrast to quantitative analysis, which focuses on numbers and statistical metrics, the qualitative study focuses on the qualitative aspects of data, such as text, images, audio, and videos. It seeks to understand every aspect of human experiences, perceptions, and behaviors by examining the data’s richness.
Companies frequently conduct this analysis on customer feedback. You can collect qualitative data from reviews, complaints, chat messages, interactions with support centers, customer interviews, case notes, or even social media comments. This kind of data holds the key to understanding customer sentiments and preferences in a way that goes beyond mere numbers.
Importance of Qualitative Data Analysis
Qualitative data analysis plays a crucial role in your research and decision-making process across various disciplines. Let’s explore some key reasons that underline the significance of this analysis:
In-Depth Understanding
It enables you to explore complex and nuanced aspects of a phenomenon, delving into the ‘how’ and ‘why’ questions. This method provides you with a deeper understanding of human behavior, experiences, and contexts that quantitative approaches might not capture fully.
Contextual Insight
You can use this analysis to give context to numerical data. It will help you understand the circumstances and conditions that influence participants’ thoughts, feelings, and actions. This contextual insight becomes essential for generating comprehensive explanations.
Theory Development
You can generate or refine hypotheses via qualitative data analysis. As you analyze the data attentively, you can form hypotheses, concepts, and frameworks that will drive your future research and contribute to theoretical advances.
Participant Perspectives
When performing qualitative research, you can highlight participant voices and opinions. This approach is especially useful for understanding marginalized or underrepresented people, as it allows them to communicate their experiences and points of view.
Exploratory Research
The analysis is frequently used at the exploratory stage of your project. It assists you in identifying important variables, developing research questions, and designing quantitative studies that will follow.
Types of Qualitative Data
When conducting qualitative research, you can use several qualitative data collection methods , and here you will come across many sorts of qualitative data that can provide you with unique insights into your study topic. These data kinds add new views and angles to your understanding and analysis.
Interviews and Focus Groups
Interviews and focus groups will be among your key methods for gathering qualitative data. Interviews are one-on-one talks in which participants can freely share their thoughts, experiences, and opinions.
Focus groups, on the other hand, are discussions in which members interact with one another, resulting in dynamic exchanges of ideas. Both methods provide rich qualitative data and direct access to participant perspectives.
Observations and Field Notes
Observations and field notes are another useful sort of qualitative data. You can immerse yourself in the research environment through direct observation, carefully documenting behaviors, interactions, and contextual factors.
These observations will be recorded in your field notes, providing a complete picture of the environment and the behaviors you’re researching. This data type is especially important for comprehending behavior in their natural setting.
Textual and Visual Data
Textual and visual data include a wide range of resources that can be qualitatively analyzed. Documents, written narratives, and transcripts from various sources, such as interviews or speeches, are examples of textual data.
Photographs, films, and even artwork provide a visual layer to your research. These forms of data allow you to investigate what is spoken and the underlying emotions, details, and symbols expressed by language or pictures.
When to Choose Qualitative Data Analysis over Quantitative Data Analysis
As you begin your research journey, understanding why the analysis of qualitative data is important will guide your approach to understanding complex events. If you analyze qualitative data, it will provide new insights that complement quantitative methodologies, which will give you a broader understanding of your study topic.
It is critical to know when to use qualitative analysis over quantitative procedures. You can prefer qualitative data analysis when:
- Complexity Reigns: When your research questions involve deep human experiences, motivations, or emotions, qualitative research excels at revealing these complexities.
- Exploration is Key: Qualitative analysis is ideal for exploratory research. It will assist you in understanding a new or poorly understood topic before formulating quantitative hypotheses.
- Context Matters: If you want to understand how context affects behaviors or results, qualitative data analysis provides the depth needed to grasp these relationships.
- Unanticipated Findings: When your study provides surprising new viewpoints or ideas, qualitative analysis helps you to delve deeply into these emerging themes.
- Subjective Interpretation is Vital: When it comes to understanding people’s subjective experiences and interpretations, qualitative data analysis is the way to go.
You can make informed decisions regarding the right approach for your research objectives if you understand the importance of qualitative analysis and recognize the situations where it shines.
Qualitative Data Analysis Methods and Examples
Exploring various qualitative data analysis methods will provide you with a wide collection for making sense of your research findings. Once the data has been collected, you can choose from several analysis methods based on your research objectives and the data type you’ve collected.
There are five main methods for analyzing qualitative data. Each method takes a distinct approach to identifying patterns, themes, and insights within your qualitative data. They are:
Method 1: Content Analysis
Content analysis is a methodical technique for analyzing textual or visual data in a structured manner. In this method, you will categorize qualitative data by splitting it into manageable pieces and assigning the manual coding process to these units.
As you go, you’ll notice ongoing codes and designs that will allow you to conclude the content. This method is very beneficial for detecting common ideas, concepts, or themes in your data without losing the context.
Steps to Do Content Analysis
Follow these steps when conducting content analysis:
- Collect and Immerse: Begin by collecting the necessary textual or visual data. Immerse yourself in this data to fully understand its content, context, and complexities.
- Assign Codes and Categories: Assign codes to relevant data sections that systematically represent major ideas or themes. Arrange comparable codes into groups that cover the major themes.
- Analyze and Interpret: Develop a structured framework from the categories and codes. Then, evaluate the data in the context of your research question, investigate relationships between categories, discover patterns, and draw meaning from these connections.
Benefits & Challenges
There are various advantages to using content analysis:
- Structured Approach: It offers a systematic approach to dealing with large data sets and ensures consistency throughout the research.
- Objective Insights: This method promotes objectivity, which helps to reduce potential biases in your study.
- Pattern Discovery: Content analysis can help uncover hidden trends, themes, and patterns that are not always obvious.
- Versatility: You can apply content analysis to various data formats, including text, internet content, images, etc.
However, keep in mind the challenges that arise:
- Subjectivity: Even with the best attempts, a certain bias may remain in coding and interpretation.
- Complexity: Analyzing huge data sets requires time and great attention to detail.
- Contextual Nuances: Content analysis may not capture all of the contextual richness that qualitative data analysis highlights.
Example of Content Analysis
Suppose you’re conducting market research and looking at customer feedback on a product. As you collect relevant data and analyze feedback, you’ll see repeating codes like “price,” “quality,” “customer service,” and “features.” These codes are organized into categories such as “positive reviews,” “negative reviews,” and “suggestions for improvement.”
According to your findings, themes such as “price” and “customer service” stand out and show that pricing and customer service greatly impact customer satisfaction. This example highlights the power of content analysis for obtaining significant insights from large textual data collections.
Method 2: Thematic Analysis
Thematic analysis is a well-structured procedure for identifying and analyzing recurring themes in your data. As you become more engaged in the data, you’ll generate codes or short labels representing key concepts. These codes are then organized into themes, providing a consistent framework for organizing and comprehending the substance of the data.
The analysis allows you to organize complex narratives and perspectives into meaningful categories, which will allow you to identify connections and patterns that may not be visible at first.
Steps to Do Thematic Analysis
Follow these steps when conducting a thematic analysis:
- Code and Group: Start by thoroughly examining the data and giving initial codes that identify the segments. To create initial themes, combine relevant codes.
- Code and Group: Begin by engaging yourself in the data, assigning first codes to notable segments. To construct basic themes, group comparable codes together.
- Analyze and Report: Analyze the data within each theme to derive relevant insights. Organize the topics into a consistent structure and explain your findings, along with data extracts that represent each theme.
Thematic analysis has various benefits:
- Structured Exploration: It is a method for identifying patterns and themes in complex qualitative data.
- Comprehensive knowledge: Thematic analysis promotes an in-depth understanding of the complications and meanings of the data.
- Application Flexibility: This method can be customized to various research situations and data kinds.
However, challenges may arise, such as:
- Interpretive Nature: Interpreting qualitative data in thematic analysis is vital, and it is critical to manage researcher bias.
- Time-consuming: The study can be time-consuming, especially with large data sets.
- Subjectivity: The selection of codes and topics might be subjective.
Example of Thematic Analysis
Assume you’re conducting a thematic analysis on job satisfaction interviews. Following your immersion in the data, you assign initial codes such as “work-life balance,” “career growth,” and “colleague relationships.” As you organize these codes, you’ll notice themes develop, such as “Factors Influencing Job Satisfaction” and “Impact on Work Engagement.”
Further investigation reveals the tales and experiences included within these themes and provides insights into how various elements influence job satisfaction. This example demonstrates how thematic analysis can reveal meaningful patterns and insights in qualitative data.
Method 3: Narrative Analysis
The narrative analysis involves the narratives that people share. You’ll investigate the histories in your data, looking at how stories are created and the meanings they express. This method is excellent for learning how people make sense of their experiences through narrative.
Steps to Do Narrative Analysis
The following steps are involved in narrative analysis:
- Gather and Analyze: Start by collecting narratives, such as first-person tales, interviews, or written accounts. Analyze the stories, focusing on the plot, feelings, and characters.
- Find Themes: Look for recurring themes or patterns in various narratives. Think about the similarities and differences between these topics and personal experiences.
- Interpret and Extract Insights: Contextualize the narratives within their larger context. Accept the subjective nature of each narrative and analyze the narrator’s voice and style. Extract insights from the tales by diving into the emotions, motivations, and implications communicated by the stories.
There are various advantages to narrative analysis:
- Deep Exploration: It lets you look deeply into people’s personal experiences and perspectives.
- Human-Centered: This method prioritizes the human perspective, allowing individuals to express themselves.
However, difficulties may arise, such as:
- Interpretive Complexity: Analyzing narratives requires dealing with the complexities of meaning and interpretation.
- Time-consuming: Because of the richness and complexities of tales, working with them can be time-consuming.
Example of Narrative Analysis
Assume you’re conducting narrative analysis on refugee interviews. As you read the stories, you’ll notice common themes of toughness, loss, and hope. The narratives provide insight into the obstacles that refugees face, their strengths, and the dreams that guide them.
The analysis can provide a deeper insight into the refugees’ experiences and the broader social context they navigate by examining the narratives’ emotional subtleties and underlying meanings. This example highlights how narrative analysis can reveal important insights into human stories.
Method 4: Grounded Theory Analysis
Grounded theory analysis is an iterative and systematic approach that allows you to create theories directly from data without being limited by pre-existing hypotheses. With an open mind, you collect data and generate early codes and labels that capture essential ideas or concepts within the data.
As you progress, you refine these codes and increasingly connect them, eventually developing a theory based on the data. Grounded theory analysis is a dynamic process for developing new insights and hypotheses based on details in your data.
Steps to Do Grounded Theory Analysis
Grounded theory analysis requires the following steps:
- Initial Coding: First, immerse yourself in the data, producing initial codes that represent major concepts or patterns.
- Categorize and Connect: Using axial coding, organize the initial codes, which establish relationships and connections between topics.
- Build the Theory: Focus on creating a core category that connects the codes and themes. Regularly refine the theory by comparing and integrating new data, ensuring that it evolves organically from the data.
Grounded theory analysis has various benefits:
- Theory Generation: It provides a one-of-a-kind opportunity to generate hypotheses straight from data and promotes new insights.
- In-depth Understanding: The analysis allows you to deeply analyze the data and reveal complex relationships and patterns.
- Flexible Process: This method is customizable and ongoing, which allows you to enhance your research as you collect additional data.
However, challenges might arise with:
- Time and Resources: Because grounded theory analysis is a continuous process, it requires a large commitment of time and resources.
- Theoretical Development: Creating a grounded theory involves a thorough understanding of qualitative data analysis software and theoretical concepts.
- Interpretation of Complexity: Interpreting and incorporating a newly developed theory into existing literature can be intellectually hard.
Example of Grounded Theory Analysis
Assume you’re performing a grounded theory analysis on workplace collaboration interviews. As you open code the data, you will discover notions such as “communication barriers,” “team dynamics,” and “leadership roles.” Axial coding demonstrates links between these notions, emphasizing the significance of efficient communication in developing collaboration.
You create the core “Integrated Communication Strategies” category through selective coding, which unifies new topics.
This theory-driven category serves as the framework for understanding how numerous aspects contribute to effective team collaboration. This example shows how grounded theory analysis allows you to generate a theory directly from the inherent nature of the data.
Method 5: Discourse Analysis
Discourse analysis focuses on language and communication. You’ll look at how language produces meaning and how it reflects power relations, identities, and cultural influences. This strategy examines what is said and how it is said; the words, phrasing, and larger context of communication.
The analysis is precious when investigating power dynamics, identities, and cultural influences encoded in language. By evaluating the language used in your data, you can identify underlying assumptions, cultural standards, and how individuals negotiate meaning through communication.
Steps to Do Discourse Analysis
Conducting discourse analysis entails the following steps:
- Select Discourse: For analysis, choose language-based data such as texts, speeches, or media content.
- Analyze Language: Immerse yourself in the conversation, examining language choices, metaphors, and underlying assumptions.
- Discover Patterns: Recognize the dialogue’s reoccurring themes, ideologies, and power dynamics. To fully understand the effects of these patterns, put them in their larger context.
There are various advantages of using discourse analysis:
- Understanding Language: It provides an extensive understanding of how language builds meaning and influences perceptions.
- Uncovering Power Dynamics: The analysis reveals how power dynamics appear via language.
- Cultural Insights: This method identifies cultural norms, beliefs, and ideologies stored in communication.
However, the following challenges may arise:
- Complexity of Interpretation: Language analysis involves navigating multiple levels of nuance and interpretation.
- Subjectivity: Interpretation can be subjective, so controlling researcher bias is important.
- Time-Intensive: Discourse analysis can take a lot of time because careful linguistic study is required in this analysis.
Example of Discourse Analysis
Consider doing discourse analysis on media coverage of a political event. You notice repeating linguistic patterns in news articles that depict the event as a conflict between opposing parties. Through deconstruction, you can expose how this framing supports particular ideologies and power relations.
You can illustrate how language choices influence public perceptions and contribute to building the narrative around the event by analyzing the speech within the broader political and social context. This example shows how discourse analysis can reveal hidden power dynamics and cultural influences on communication.
How to do Qualitative Data Analysis with the QuestionPro Research suite?
QuestionPro is a popular survey and research platform that offers tools for collecting and analyzing qualitative and quantitative data. Follow these general steps for conducting qualitative data analysis using the QuestionPro Research Suite:
- Collect Qualitative Data: Set up your survey to capture qualitative responses. It might involve open-ended questions, text boxes, or comment sections where participants can provide detailed responses.
- Export Qualitative Responses: Export the responses once you’ve collected qualitative data through your survey. QuestionPro typically allows you to export survey data in various formats, such as Excel or CSV.
- Prepare Data for Analysis: Review the exported data and clean it if necessary. Remove irrelevant or duplicate entries to ensure your data is ready for analysis.
- Code and Categorize Responses: Segment and label data, letting new patterns emerge naturally, then develop categories through axial coding to structure the analysis.
- Identify Themes: Analyze the coded responses to identify recurring themes, patterns, and insights. Look for similarities and differences in participants’ responses.
- Generate Reports and Visualizations: Utilize the reporting features of QuestionPro to create visualizations, charts, and graphs that help communicate the themes and findings from your qualitative research.
- Interpret and Draw Conclusions: Interpret the themes and patterns you’ve identified in the qualitative data. Consider how these findings answer your research questions or provide insights into your study topic.
- Integrate with Quantitative Data (if applicable): If you’re also conducting quantitative research using QuestionPro, consider integrating your qualitative findings with quantitative results to provide a more comprehensive understanding.
Qualitative data analysis is vital in uncovering various human experiences, views, and stories. If you’re ready to transform your research journey and apply the power of qualitative analysis, now is the moment to do it. Book a demo with QuestionPro today and begin your journey of exploration.
LEARN MORE FREE TRIAL
MORE LIKE THIS
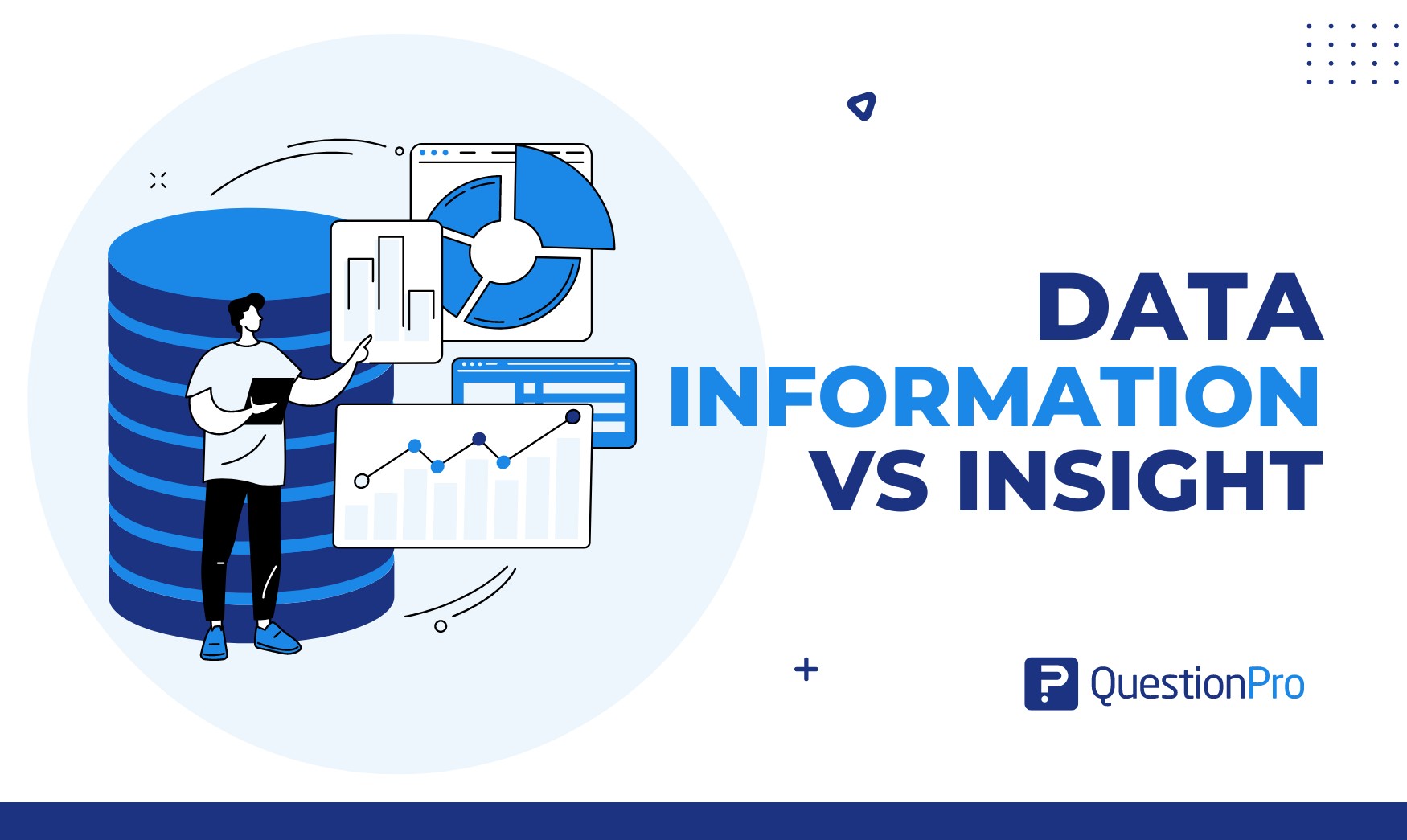
Data Information vs Insight: Essential differences
May 14, 2024
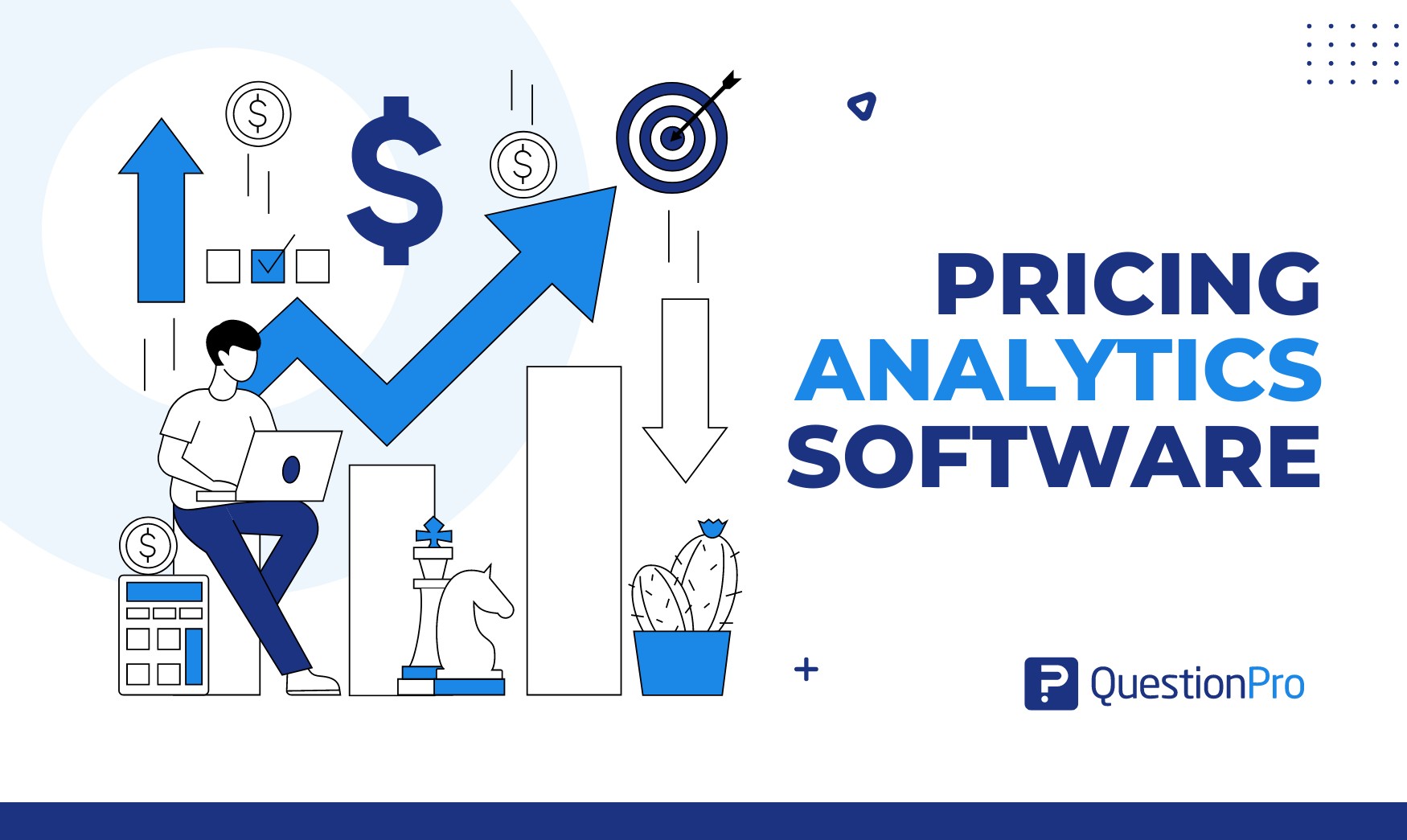
Pricing Analytics Software: Optimize Your Pricing Strategy
May 13, 2024
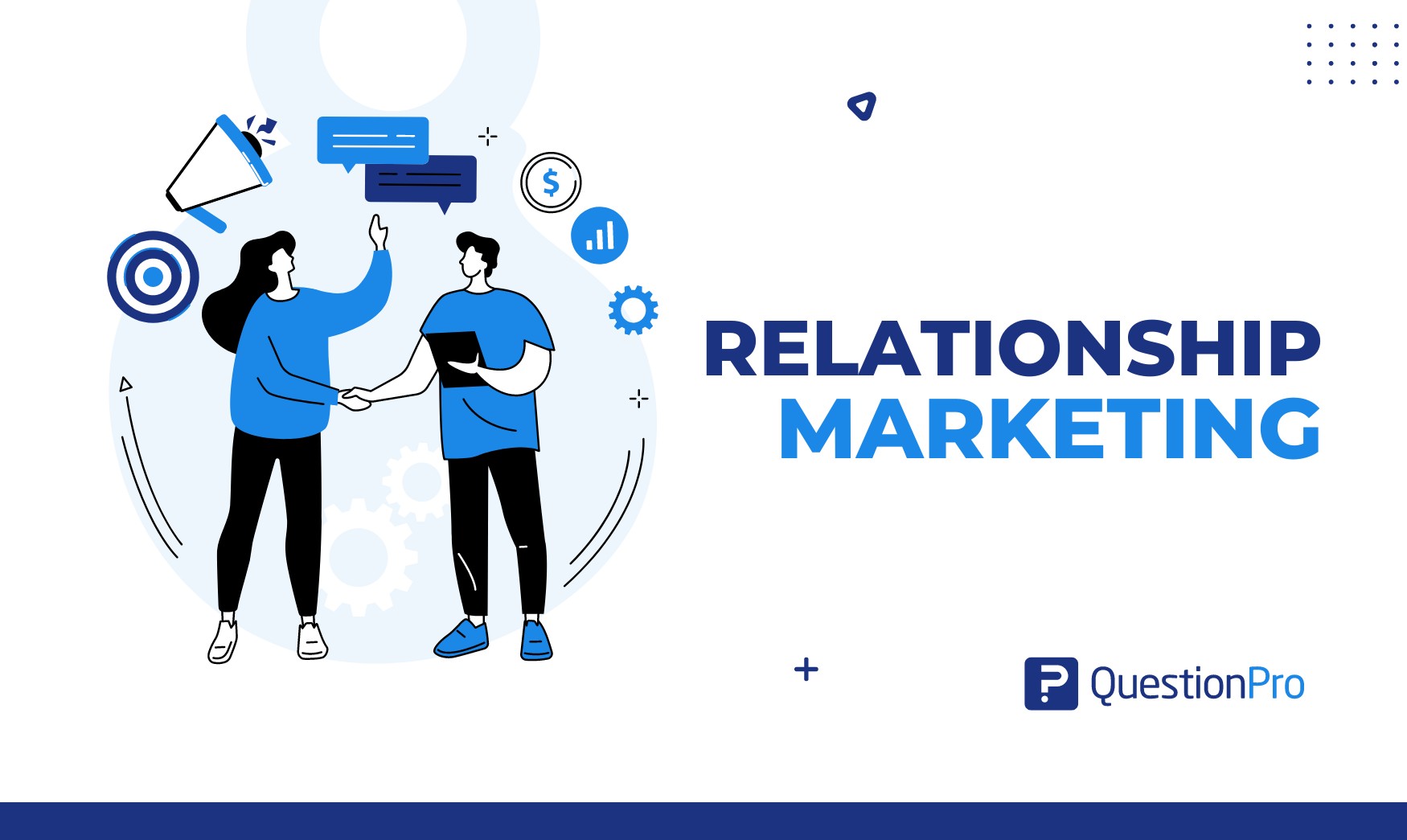
Relationship Marketing: What It Is, Examples & Top 7 Benefits
May 8, 2024
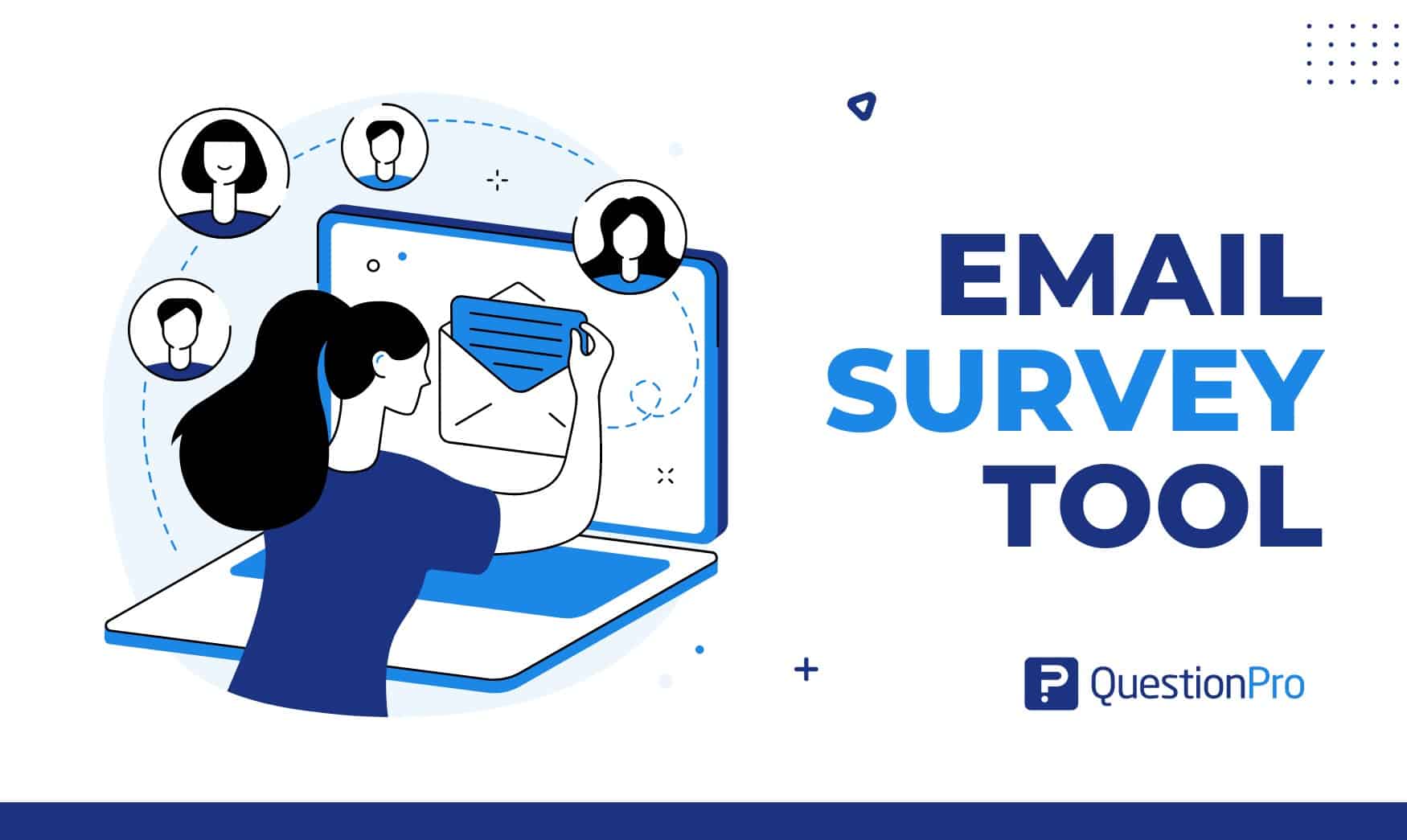
The Best Email Survey Tool to Boost Your Feedback Game
May 7, 2024
Other categories
- Academic Research
- Artificial Intelligence
- Assessments
- Brand Awareness
- Case Studies
- Communities
- Consumer Insights
- Customer effort score
- Customer Engagement
- Customer Experience
- Customer Loyalty
- Customer Research
- Customer Satisfaction
- Employee Benefits
- Employee Engagement
- Employee Retention
- Friday Five
- General Data Protection Regulation
- Insights Hub
- Life@QuestionPro
- Market Research
- Mobile diaries
- Mobile Surveys
- New Features
- Online Communities
- Question Types
- Questionnaire
- QuestionPro Products
- Release Notes
- Research Tools and Apps
- Revenue at Risk
- Survey Templates
- Training Tips
- Uncategorized
- Video Learning Series
- What’s Coming Up
- Workforce Intelligence
- Search Menu
- Browse content in Arts and Humanities
- Browse content in Archaeology
- Anglo-Saxon and Medieval Archaeology
- Archaeological Methodology and Techniques
- Archaeology by Region
- Archaeology of Religion
- Archaeology of Trade and Exchange
- Biblical Archaeology
- Contemporary and Public Archaeology
- Environmental Archaeology
- Historical Archaeology
- History and Theory of Archaeology
- Industrial Archaeology
- Landscape Archaeology
- Mortuary Archaeology
- Prehistoric Archaeology
- Underwater Archaeology
- Urban Archaeology
- Zooarchaeology
- Browse content in Architecture
- Architectural Structure and Design
- History of Architecture
- Residential and Domestic Buildings
- Theory of Architecture
- Browse content in Art
- Art Subjects and Themes
- History of Art
- Industrial and Commercial Art
- Theory of Art
- Biographical Studies
- Byzantine Studies
- Browse content in Classical Studies
- Classical History
- Classical Philosophy
- Classical Mythology
- Classical Literature
- Classical Reception
- Classical Art and Architecture
- Classical Oratory and Rhetoric
- Greek and Roman Epigraphy
- Greek and Roman Law
- Greek and Roman Papyrology
- Greek and Roman Archaeology
- Late Antiquity
- Religion in the Ancient World
- Digital Humanities
- Browse content in History
- Colonialism and Imperialism
- Diplomatic History
- Environmental History
- Genealogy, Heraldry, Names, and Honours
- Genocide and Ethnic Cleansing
- Historical Geography
- History by Period
- History of Emotions
- History of Agriculture
- History of Education
- History of Gender and Sexuality
- Industrial History
- Intellectual History
- International History
- Labour History
- Legal and Constitutional History
- Local and Family History
- Maritime History
- Military History
- National Liberation and Post-Colonialism
- Oral History
- Political History
- Public History
- Regional and National History
- Revolutions and Rebellions
- Slavery and Abolition of Slavery
- Social and Cultural History
- Theory, Methods, and Historiography
- Urban History
- World History
- Browse content in Language Teaching and Learning
- Language Learning (Specific Skills)
- Language Teaching Theory and Methods
- Browse content in Linguistics
- Applied Linguistics
- Cognitive Linguistics
- Computational Linguistics
- Forensic Linguistics
- Grammar, Syntax and Morphology
- Historical and Diachronic Linguistics
- History of English
- Language Acquisition
- Language Evolution
- Language Reference
- Language Variation
- Language Families
- Lexicography
- Linguistic Anthropology
- Linguistic Theories
- Linguistic Typology
- Phonetics and Phonology
- Psycholinguistics
- Sociolinguistics
- Translation and Interpretation
- Writing Systems
- Browse content in Literature
- Bibliography
- Children's Literature Studies
- Literary Studies (Asian)
- Literary Studies (European)
- Literary Studies (Eco-criticism)
- Literary Studies (Romanticism)
- Literary Studies (American)
- Literary Studies (Modernism)
- Literary Studies - World
- Literary Studies (1500 to 1800)
- Literary Studies (19th Century)
- Literary Studies (20th Century onwards)
- Literary Studies (African American Literature)
- Literary Studies (British and Irish)
- Literary Studies (Early and Medieval)
- Literary Studies (Fiction, Novelists, and Prose Writers)
- Literary Studies (Gender Studies)
- Literary Studies (Graphic Novels)
- Literary Studies (History of the Book)
- Literary Studies (Plays and Playwrights)
- Literary Studies (Poetry and Poets)
- Literary Studies (Postcolonial Literature)
- Literary Studies (Queer Studies)
- Literary Studies (Science Fiction)
- Literary Studies (Travel Literature)
- Literary Studies (War Literature)
- Literary Studies (Women's Writing)
- Literary Theory and Cultural Studies
- Mythology and Folklore
- Shakespeare Studies and Criticism
- Browse content in Media Studies
- Browse content in Music
- Applied Music
- Dance and Music
- Ethics in Music
- Ethnomusicology
- Gender and Sexuality in Music
- Medicine and Music
- Music Cultures
- Music and Religion
- Music and Media
- Music and Culture
- Music Education and Pedagogy
- Music Theory and Analysis
- Musical Scores, Lyrics, and Libretti
- Musical Structures, Styles, and Techniques
- Musicology and Music History
- Performance Practice and Studies
- Race and Ethnicity in Music
- Sound Studies
- Browse content in Performing Arts
- Browse content in Philosophy
- Aesthetics and Philosophy of Art
- Epistemology
- Feminist Philosophy
- History of Western Philosophy
- Metaphysics
- Moral Philosophy
- Non-Western Philosophy
- Philosophy of Science
- Philosophy of Language
- Philosophy of Mind
- Philosophy of Perception
- Philosophy of Action
- Philosophy of Law
- Philosophy of Religion
- Philosophy of Mathematics and Logic
- Practical Ethics
- Social and Political Philosophy
- Browse content in Religion
- Biblical Studies
- Christianity
- East Asian Religions
- History of Religion
- Judaism and Jewish Studies
- Qumran Studies
- Religion and Education
- Religion and Health
- Religion and Politics
- Religion and Science
- Religion and Law
- Religion and Art, Literature, and Music
- Religious Studies
- Browse content in Society and Culture
- Cookery, Food, and Drink
- Cultural Studies
- Customs and Traditions
- Ethical Issues and Debates
- Hobbies, Games, Arts and Crafts
- Lifestyle, Home, and Garden
- Natural world, Country Life, and Pets
- Popular Beliefs and Controversial Knowledge
- Sports and Outdoor Recreation
- Technology and Society
- Travel and Holiday
- Visual Culture
- Browse content in Law
- Arbitration
- Browse content in Company and Commercial Law
- Commercial Law
- Company Law
- Browse content in Comparative Law
- Systems of Law
- Competition Law
- Browse content in Constitutional and Administrative Law
- Government Powers
- Judicial Review
- Local Government Law
- Military and Defence Law
- Parliamentary and Legislative Practice
- Construction Law
- Contract Law
- Browse content in Criminal Law
- Criminal Procedure
- Criminal Evidence Law
- Sentencing and Punishment
- Employment and Labour Law
- Environment and Energy Law
- Browse content in Financial Law
- Banking Law
- Insolvency Law
- History of Law
- Human Rights and Immigration
- Intellectual Property Law
- Browse content in International Law
- Private International Law and Conflict of Laws
- Public International Law
- IT and Communications Law
- Jurisprudence and Philosophy of Law
- Law and Politics
- Law and Society
- Browse content in Legal System and Practice
- Courts and Procedure
- Legal Skills and Practice
- Primary Sources of Law
- Regulation of Legal Profession
- Medical and Healthcare Law
- Browse content in Policing
- Criminal Investigation and Detection
- Police and Security Services
- Police Procedure and Law
- Police Regional Planning
- Browse content in Property Law
- Personal Property Law
- Study and Revision
- Terrorism and National Security Law
- Browse content in Trusts Law
- Wills and Probate or Succession
- Browse content in Medicine and Health
- Browse content in Allied Health Professions
- Arts Therapies
- Clinical Science
- Dietetics and Nutrition
- Occupational Therapy
- Operating Department Practice
- Physiotherapy
- Radiography
- Speech and Language Therapy
- Browse content in Anaesthetics
- General Anaesthesia
- Neuroanaesthesia
- Browse content in Clinical Medicine
- Acute Medicine
- Cardiovascular Medicine
- Clinical Genetics
- Clinical Pharmacology and Therapeutics
- Dermatology
- Endocrinology and Diabetes
- Gastroenterology
- Genito-urinary Medicine
- Geriatric Medicine
- Infectious Diseases
- Medical Toxicology
- Medical Oncology
- Pain Medicine
- Palliative Medicine
- Rehabilitation Medicine
- Respiratory Medicine and Pulmonology
- Rheumatology
- Sleep Medicine
- Sports and Exercise Medicine
- Clinical Neuroscience
- Community Medical Services
- Critical Care
- Emergency Medicine
- Forensic Medicine
- Haematology
- History of Medicine
- Browse content in Medical Dentistry
- Oral and Maxillofacial Surgery
- Paediatric Dentistry
- Restorative Dentistry and Orthodontics
- Surgical Dentistry
- Browse content in Medical Skills
- Clinical Skills
- Communication Skills
- Nursing Skills
- Surgical Skills
- Medical Ethics
- Medical Statistics and Methodology
- Browse content in Neurology
- Clinical Neurophysiology
- Neuropathology
- Nursing Studies
- Browse content in Obstetrics and Gynaecology
- Gynaecology
- Occupational Medicine
- Ophthalmology
- Otolaryngology (ENT)
- Browse content in Paediatrics
- Neonatology
- Browse content in Pathology
- Chemical Pathology
- Clinical Cytogenetics and Molecular Genetics
- Histopathology
- Medical Microbiology and Virology
- Patient Education and Information
- Browse content in Pharmacology
- Psychopharmacology
- Browse content in Popular Health
- Caring for Others
- Complementary and Alternative Medicine
- Self-help and Personal Development
- Browse content in Preclinical Medicine
- Cell Biology
- Molecular Biology and Genetics
- Reproduction, Growth and Development
- Primary Care
- Professional Development in Medicine
- Browse content in Psychiatry
- Addiction Medicine
- Child and Adolescent Psychiatry
- Forensic Psychiatry
- Learning Disabilities
- Old Age Psychiatry
- Psychotherapy
- Browse content in Public Health and Epidemiology
- Epidemiology
- Public Health
- Browse content in Radiology
- Clinical Radiology
- Interventional Radiology
- Nuclear Medicine
- Radiation Oncology
- Reproductive Medicine
- Browse content in Surgery
- Cardiothoracic Surgery
- Gastro-intestinal and Colorectal Surgery
- General Surgery
- Neurosurgery
- Paediatric Surgery
- Peri-operative Care
- Plastic and Reconstructive Surgery
- Surgical Oncology
- Transplant Surgery
- Trauma and Orthopaedic Surgery
- Vascular Surgery
- Browse content in Science and Mathematics
- Browse content in Biological Sciences
- Aquatic Biology
- Biochemistry
- Bioinformatics and Computational Biology
- Developmental Biology
- Ecology and Conservation
- Evolutionary Biology
- Genetics and Genomics
- Microbiology
- Molecular and Cell Biology
- Natural History
- Plant Sciences and Forestry
- Research Methods in Life Sciences
- Structural Biology
- Systems Biology
- Zoology and Animal Sciences
- Browse content in Chemistry
- Analytical Chemistry
- Computational Chemistry
- Crystallography
- Environmental Chemistry
- Industrial Chemistry
- Inorganic Chemistry
- Materials Chemistry
- Medicinal Chemistry
- Mineralogy and Gems
- Organic Chemistry
- Physical Chemistry
- Polymer Chemistry
- Study and Communication Skills in Chemistry
- Theoretical Chemistry
- Browse content in Computer Science
- Artificial Intelligence
- Computer Architecture and Logic Design
- Game Studies
- Human-Computer Interaction
- Mathematical Theory of Computation
- Programming Languages
- Software Engineering
- Systems Analysis and Design
- Virtual Reality
- Browse content in Computing
- Business Applications
- Computer Security
- Computer Games
- Computer Networking and Communications
- Digital Lifestyle
- Graphical and Digital Media Applications
- Operating Systems
- Browse content in Earth Sciences and Geography
- Atmospheric Sciences
- Environmental Geography
- Geology and the Lithosphere
- Maps and Map-making
- Meteorology and Climatology
- Oceanography and Hydrology
- Palaeontology
- Physical Geography and Topography
- Regional Geography
- Soil Science
- Urban Geography
- Browse content in Engineering and Technology
- Agriculture and Farming
- Biological Engineering
- Civil Engineering, Surveying, and Building
- Electronics and Communications Engineering
- Energy Technology
- Engineering (General)
- Environmental Science, Engineering, and Technology
- History of Engineering and Technology
- Mechanical Engineering and Materials
- Technology of Industrial Chemistry
- Transport Technology and Trades
- Browse content in Environmental Science
- Applied Ecology (Environmental Science)
- Conservation of the Environment (Environmental Science)
- Environmental Sustainability
- Environmentalist Thought and Ideology (Environmental Science)
- Management of Land and Natural Resources (Environmental Science)
- Natural Disasters (Environmental Science)
- Nuclear Issues (Environmental Science)
- Pollution and Threats to the Environment (Environmental Science)
- Social Impact of Environmental Issues (Environmental Science)
- History of Science and Technology
- Browse content in Materials Science
- Ceramics and Glasses
- Composite Materials
- Metals, Alloying, and Corrosion
- Nanotechnology
- Browse content in Mathematics
- Applied Mathematics
- Biomathematics and Statistics
- History of Mathematics
- Mathematical Education
- Mathematical Finance
- Mathematical Analysis
- Numerical and Computational Mathematics
- Probability and Statistics
- Pure Mathematics
- Browse content in Neuroscience
- Cognition and Behavioural Neuroscience
- Development of the Nervous System
- Disorders of the Nervous System
- History of Neuroscience
- Invertebrate Neurobiology
- Molecular and Cellular Systems
- Neuroendocrinology and Autonomic Nervous System
- Neuroscientific Techniques
- Sensory and Motor Systems
- Browse content in Physics
- Astronomy and Astrophysics
- Atomic, Molecular, and Optical Physics
- Biological and Medical Physics
- Classical Mechanics
- Computational Physics
- Condensed Matter Physics
- Electromagnetism, Optics, and Acoustics
- History of Physics
- Mathematical and Statistical Physics
- Measurement Science
- Nuclear Physics
- Particles and Fields
- Plasma Physics
- Quantum Physics
- Relativity and Gravitation
- Semiconductor and Mesoscopic Physics
- Browse content in Psychology
- Affective Sciences
- Clinical Psychology
- Cognitive Psychology
- Cognitive Neuroscience
- Criminal and Forensic Psychology
- Developmental Psychology
- Educational Psychology
- Evolutionary Psychology
- Health Psychology
- History and Systems in Psychology
- Music Psychology
- Neuropsychology
- Organizational Psychology
- Psychological Assessment and Testing
- Psychology of Human-Technology Interaction
- Psychology Professional Development and Training
- Research Methods in Psychology
- Social Psychology
- Browse content in Social Sciences
- Browse content in Anthropology
- Anthropology of Religion
- Human Evolution
- Medical Anthropology
- Physical Anthropology
- Regional Anthropology
- Social and Cultural Anthropology
- Theory and Practice of Anthropology
- Browse content in Business and Management
- Business Strategy
- Business Ethics
- Business History
- Business and Government
- Business and Technology
- Business and the Environment
- Comparative Management
- Corporate Governance
- Corporate Social Responsibility
- Entrepreneurship
- Health Management
- Human Resource Management
- Industrial and Employment Relations
- Industry Studies
- Information and Communication Technologies
- International Business
- Knowledge Management
- Management and Management Techniques
- Operations Management
- Organizational Theory and Behaviour
- Pensions and Pension Management
- Public and Nonprofit Management
- Strategic Management
- Supply Chain Management
- Browse content in Criminology and Criminal Justice
- Criminal Justice
- Criminology
- Forms of Crime
- International and Comparative Criminology
- Youth Violence and Juvenile Justice
- Development Studies
- Browse content in Economics
- Agricultural, Environmental, and Natural Resource Economics
- Asian Economics
- Behavioural Finance
- Behavioural Economics and Neuroeconomics
- Econometrics and Mathematical Economics
- Economic Systems
- Economic History
- Economic Methodology
- Economic Development and Growth
- Financial Markets
- Financial Institutions and Services
- General Economics and Teaching
- Health, Education, and Welfare
- History of Economic Thought
- International Economics
- Labour and Demographic Economics
- Law and Economics
- Macroeconomics and Monetary Economics
- Microeconomics
- Public Economics
- Urban, Rural, and Regional Economics
- Welfare Economics
- Browse content in Education
- Adult Education and Continuous Learning
- Care and Counselling of Students
- Early Childhood and Elementary Education
- Educational Equipment and Technology
- Educational Strategies and Policy
- Higher and Further Education
- Organization and Management of Education
- Philosophy and Theory of Education
- Schools Studies
- Secondary Education
- Teaching of a Specific Subject
- Teaching of Specific Groups and Special Educational Needs
- Teaching Skills and Techniques
- Browse content in Environment
- Applied Ecology (Social Science)
- Climate Change
- Conservation of the Environment (Social Science)
- Environmentalist Thought and Ideology (Social Science)
- Natural Disasters (Environment)
- Social Impact of Environmental Issues (Social Science)
- Browse content in Human Geography
- Cultural Geography
- Economic Geography
- Political Geography
- Browse content in Interdisciplinary Studies
- Communication Studies
- Museums, Libraries, and Information Sciences
- Browse content in Politics
- African Politics
- Asian Politics
- Chinese Politics
- Comparative Politics
- Conflict Politics
- Elections and Electoral Studies
- Environmental Politics
- European Union
- Foreign Policy
- Gender and Politics
- Human Rights and Politics
- Indian Politics
- International Relations
- International Organization (Politics)
- International Political Economy
- Irish Politics
- Latin American Politics
- Middle Eastern Politics
- Political Methodology
- Political Communication
- Political Philosophy
- Political Sociology
- Political Behaviour
- Political Economy
- Political Institutions
- Political Theory
- Politics and Law
- Public Administration
- Public Policy
- Quantitative Political Methodology
- Regional Political Studies
- Russian Politics
- Security Studies
- State and Local Government
- UK Politics
- US Politics
- Browse content in Regional and Area Studies
- African Studies
- Asian Studies
- East Asian Studies
- Japanese Studies
- Latin American Studies
- Middle Eastern Studies
- Native American Studies
- Scottish Studies
- Browse content in Research and Information
- Research Methods
- Browse content in Social Work
- Addictions and Substance Misuse
- Adoption and Fostering
- Care of the Elderly
- Child and Adolescent Social Work
- Couple and Family Social Work
- Developmental and Physical Disabilities Social Work
- Direct Practice and Clinical Social Work
- Emergency Services
- Human Behaviour and the Social Environment
- International and Global Issues in Social Work
- Mental and Behavioural Health
- Social Justice and Human Rights
- Social Policy and Advocacy
- Social Work and Crime and Justice
- Social Work Macro Practice
- Social Work Practice Settings
- Social Work Research and Evidence-based Practice
- Welfare and Benefit Systems
- Browse content in Sociology
- Childhood Studies
- Community Development
- Comparative and Historical Sociology
- Economic Sociology
- Gender and Sexuality
- Gerontology and Ageing
- Health, Illness, and Medicine
- Marriage and the Family
- Migration Studies
- Occupations, Professions, and Work
- Organizations
- Population and Demography
- Race and Ethnicity
- Social Theory
- Social Movements and Social Change
- Social Research and Statistics
- Social Stratification, Inequality, and Mobility
- Sociology of Religion
- Sociology of Education
- Sport and Leisure
- Urban and Rural Studies
- Browse content in Warfare and Defence
- Defence Strategy, Planning, and Research
- Land Forces and Warfare
- Military Administration
- Military Life and Institutions
- Naval Forces and Warfare
- Other Warfare and Defence Issues
- Peace Studies and Conflict Resolution
- Weapons and Equipment

- < Previous chapter
- Next chapter >

29 Qualitative Data Analysis Strategies
Johnny Saldaña, School of Theatre and Film, Arizona State University
- Published: 02 September 2020
- Cite Icon Cite
- Permissions Icon Permissions
This chapter provides an overview of selected qualitative data analysis strategies with a particular focus on codes and coding. Preparatory strategies for a qualitative research study and data management are first outlined. Six coding methods are then profiled using comparable interview data: process coding, in vivo coding, descriptive coding, values coding, dramaturgical coding, and versus coding. Strategies for constructing themes and assertions from the data follow. Analytic memo writing is woven throughout as a method for generating additional analytic insight. Next, display and arts-based strategies are provided, followed by recommended qualitative data analytic software programs and a discussion on verifying the researcher’s analytic findings.
Qualitative Data Analysis Strategies
Anthropologist Clifford Geertz ( 1983 ) charmingly mused, “Life is just a bowl of strategies” (p. 25). Strategy , as I use it here, refers to a carefully considered plan or method to achieve a particular goal. The goal in this case is to develop a write-up of your analytic work with the qualitative data you have been given and collected as part of a study. The plans and methods you might employ to achieve that goal are what this article profiles.
Some may perceive strategy as an inappropriate, if not manipulative, word, suggesting formulaic or regimented approaches to inquiry. I assure you that is not my intent. My use of strategy is dramaturgical in nature: Strategies are actions that characters in plays take to overcome obstacles to achieve their objectives. Actors portraying these characters rely on action verbs to generate belief within themselves and to motivate them as they interpret their lines and move appropriately on stage.
What I offer is a qualitative researcher’s array of actions from which to draw to overcome the obstacles to thinking to achieve an analysis of your data. But unlike the prescripted text of a play in which the obstacles, strategies, and outcomes have been predetermined by the playwright, your work must be improvisational—acting, reacting, and interacting with data on a moment-by-moment basis to determine what obstacles stand in your way and thus what strategies you should take to reach your goals.
Another intriguing quote to keep in mind comes from research methodologist Robert E. Stake ( 1995 ), who posited, “Good research is not about good methods as much as it is about good thinking” (p. 19). In other words, strategies can take you only so far. You can have a box full of tools, but if you do not know how to use them well or use them creatively, the collection seems rather purposeless. One of the best ways we learn is by doing . So, pick up one or more of these strategies (in the form of verbs) and take analytic action with your data. Also keep in mind that these are discussed in the order in which they may typically occur, although humans think cyclically, iteratively, and reverberatively, and each research project has its unique contexts and needs. Be prepared for your mind to jump purposefully and/or idiosyncratically from one strategy to another throughout the study.
Qualitative Data Analysis Strategy: To Foresee
To foresee in qualitative data analysis (QDA) is to reflect beforehand on what forms of data you will most likely need and collect, which thus informs what types of data analytic strategies you anticipate using. Analysis, in a way, begins even before you collect data (Saldaña & Omasta, 2018 ). As you design your research study in your mind and on a text editing page, one strategy is to consider what types of data you may need to help inform and answer your central and related research questions. Interview transcripts, participant observation field notes, documents, artifacts, photographs, video recordings, and so on are not only forms of data but also foundations for how you may plan to analyze them. A participant interview, for example, suggests that you will transcribe all or relevant portions of the recording and use both the transcription and the recording itself as sources for data analysis. Any analytic memos (discussed later) you make about your impressions of the interview also become data to analyze. Even the computing software you plan to employ will be relevant to data analysis because it may help or hinder your efforts.
As your research design formulates, compose one to two paragraphs that outline how your QDA may proceed. This will necessitate that you have some background knowledge of the vast array of methods available to you. Thus, surveying the literature is vital preparatory work.
Qualitative Data Analysis Strategy: To Survey
To survey in QDA is to look for and consider the applicability of the QDA literature in your field that may provide useful guidance for your forthcoming data analytic work. General sources in QDA will provide a good starting point for acquainting you with the data analysis strategies available for the variety of methodologies or genres in qualitative inquiry (e.g., ethnography, phenomenology, case study, arts-based research, mixed methods). One of the most accessible (and humorous) is Galman’s ( 2013 ) The Good, the Bad, and the Data , and one of the most richly detailed is Frederick J. Wertz et al.’s ( 2011 ) Five Ways of Doing Qualitative Analysis . The author’s core texts for this chapter come from The Coding Manual for Qualitative Researchers (Saldaña, 2016 ) and Qualitative Research: Analyzing Life (Saldaña & Omasta, 2018 ).
If your study’s methodology or approach is grounded theory, for example, then a survey of methods works by authors such as Barney G. Glaser, Anselm L. Strauss, Juliet Corbin, and, in particular, the prolific Kathy Charmaz ( 2014 ) may be expected. But there has been a recent outpouring of additional book publications in grounded theory by Birks and Mills ( 2015 ), Bryant ( 2017 ), Bryant and Charmaz ( 2019 ), and Stern and Porr ( 2011 ), plus the legacy of thousands of articles and chapters across many disciplines that have addressed grounded theory in their studies.
Fields such as education, psychology, social work, healthcare, and others also have their own QDA methods literature in the form of texts and journals, as well as international conferences and workshops for members of the profession. It is important to have had some university coursework and/or mentorship in qualitative research to suitably prepare you for the intricacies of QDA, and you must acknowledge that the emergent nature of qualitative inquiry may require you to adopt analysis strategies that differ from what you originally planned.
Qualitative Data Analysis Strategy: To Collect
To collect in QDA is to receive the data given to you by participants and those data you actively gather to inform your study. Qualitative data analysis is concurrent with data collection and management. As interviews are transcribed, field notes are fleshed out, and documents are filed, the researcher uses opportunities to carefully read the corpus and make preliminary notations directly on the data documents by highlighting, bolding, italicizing, or noting in some way any particularly interesting or salient portions. As these data are initially reviewed, the researcher also composes supplemental analytic memos that include first impressions, reminders for follow-up, preliminary connections, and other thinking matters about the phenomena at work.
Some of the most common fieldwork tools you might use to collect data are notepads, pens and pencils; file folders for hard-copy documents; a laptop, tablet, or desktop with text editing software (Microsoft Word and Excel are most useful) and Internet access; and a digital camera and voice recorder (functions available on many electronic devices such as smartphones). Some fieldworkers may even employ a digital video camera to record social action, as long as participant permissions have been secured. But everything originates from the researcher. Your senses are immersed in the cultural milieu you study, taking in and holding onto relevant details, or significant trivia , as I call them. You become a human camera, zooming out to capture the broad landscape of your field site one day and then zooming in on a particularly interesting individual or phenomenon the next. Your analysis is only as good as the data you collect.
Fieldwork can be an overwhelming experience because so many details of social life are happening in front of you. Take a holistic approach to your entrée, but as you become more familiar with the setting and participants, actively focus on things that relate to your research topic and questions. Keep yourself open to the intriguing, surprising, and disturbing (Sunstein & Chiseri-Strater, 2012 , p. 115), because these facets enrich your study by making you aware of the unexpected.
Qualitative Data Analysis Strategy: To Feel
To feel in QDA is to gain deep emotional insight into the social worlds you study and what it means to be human. Virtually everything we do has an accompanying emotion(s), and feelings are both reactions and stimuli for action. Others’ emotions clue you to their motives, values, attitudes, beliefs, worldviews, identities, and other subjective perceptions and interpretations. Acknowledge that emotional detachment is not possible in field research. Attunement to the emotional experiences of your participants plus sympathetic and empathetic responses to the actions around you are necessary in qualitative endeavors. Your own emotional responses during fieldwork are also data because they document the tacit and visceral. It is important during such analytic reflection to assess why your emotional reactions were as they were. But it is equally important not to let emotions alone steer the course of your study. A proper balance must be found between feelings and facts.
Qualitative Data Analysis Strategy: To Organize
To organize in QDA is to maintain an orderly repository of data for easy access and analysis. Even in the smallest of qualitative studies, a large amount of data will be collected across time. Prepare both a hard drive and hard-copy folders for digital data and paperwork, and back up all materials for security from loss. I recommend that each data unit (e.g., one interview transcript, one document, one day’s worth of field notes) have its own file, with subfolders specifying the data forms and research study logistics (e.g., interviews, field notes, documents, institutional review board correspondence, calendar).
For small-scale qualitative studies, I have found it quite useful to maintain one large master file with all participant and field site data copied and combined with the literature review and accompanying researcher analytic memos. This master file is used to cut and paste related passages together, deleting what seems unnecessary as the study proceeds and eventually transforming the document into the final report itself. Cosmetic devices such as font style, font size, rich text (italicizing, bolding, underlining, etc.), and color can help you distinguish between different data forms and highlight significant passages. For example, descriptive, narrative passages of field notes are logged in regular font. “Quotations, things spoken by participants, are logged in bold font.” Observer’s comments, such as the researcher’s subjective impressions or analytic jottings, are set in italics.
Qualitative Data Analysis Strategy: To Jot
To jot in QDA is to write occasional, brief notes about your thinking or reminders for follow-up. A jot is a phrase or brief sentence that will fit on a standard-size sticky note. As data are brought and documented together, take some initial time to review their contents and jot some notes about preliminary patterns, participant quotes that seem vivid, anomalies in the data, and so forth.
As you work on a project, keep something to write with or to voice record with you at all times to capture your fleeting thoughts. You will most likely find yourself thinking about your research when you are not working exclusively on the project, and a “mental jot” may occur to you as you ruminate on logistical or analytic matters. Document the thought in some way for later retrieval and elaboration as an analytic memo.
Qualitative Data Analysis Strategy: To Prioritize
To prioritize in QDA is to determine which data are most significant in your corpus and which tasks are most necessary. During fieldwork, massive amounts of data in various forms may be collected, and your mind can be easily overwhelmed by the magnitude of the quantity, its richness, and its management. Decisions will need to be made about the most pertinent data because they help answer your research questions or emerge as salient pieces of evidence. As a sweeping generalization, approximately one half to two thirds of what you collect may become unnecessary as you proceed toward the more formal stages of QDA.
To prioritize in QDA is also to determine what matters most in your assembly of codes, categories, patterns, themes, assertions, propositions, and concepts. Return to your research purpose and questions to keep you framed for what the focus should be.
Qualitative Data Analysis Strategy: To Analyze
To analyze in QDA is to observe and discern patterns within data and to construct meanings that seem to capture their essences and essentials. Just as there are a variety of genres, elements, and styles of qualitative research, so too are there a variety of methods available for QDA. Analytic choices are most often based on what methods will harmonize with your genre selection and conceptual framework, what will generate the most sufficient answers to your research questions, and what will best represent and present the project’s findings.
Analysis can range from the factual to the conceptual to the interpretive. Analysis can also range from a straightforward descriptive account to an emergently constructed grounded theory to an evocatively composed short story. A qualitative research project’s outcomes may range from rigorously achieved, insightful answers to open-ended, evocative questions; from rich descriptive detail to a bullet-point list of themes; and from third-person, objective reportage to first-person, emotion-laden poetry. Just as there are multiple destinations in qualitative research, there are multiple pathways and journeys along the way.
Analysis is accelerated as you take cognitive ownership of your data. By reading and rereading the corpus, you gain intimate familiarity with its contents and begin to notice significant details as well as make new connections and insights about their meanings. Patterns, categories, themes, and their interrelationships become more evident the more you know the subtleties of the database.
Since qualitative research’s design, fieldwork, and data collection are most often provisional, emergent, and evolutionary processes, you reflect on and analyze the data as you gather them and proceed through the project. If preplanned methods are not working, you change them to secure the data you need. There is generally a postfieldwork period when continued reflection and more systematic data analysis occur, concurrent with or followed by additional data collection, if needed, and the more formal write-up of the study, which is in itself an analytic act. Through field note writing, interview transcribing, analytic memo writing, and other documentation processes, you gain cognitive ownership of your data; and the intuitive, tacit, synthesizing capabilities of your brain begin sensing patterns, making connections, and seeing the bigger picture. The purpose and outcome of data analysis is to reveal to others through fresh insights what we have observed and discovered about the human condition. Fortunately, there are heuristics for reorganizing and reflecting on your qualitative data to help you achieve that goal.
Qualitative Data Analysis Strategy: To Pattern
To pattern in QDA is to detect similarities within and regularities among the data you have collected. The natural world is filled with patterns because we, as humans, have constructed them as such. Stars in the night sky are not just a random assembly; our ancestors pieced them together to form constellations like the Big Dipper. A collection of flowers growing wild in a field has a pattern, as does an individual flower’s patterns of leaves and petals. Look at the physical objects humans have created and notice how pattern oriented we are in our construction, organization, and decoration. Look around you in your environment and notice how many patterns are evident on your clothing, in a room, and on most objects themselves. Even our sometimes mundane daily and long-term human actions are reproduced patterns in the form of routines, rituals, rules, roles, and relationships (Saldaña & Omasta, 2018 ).
This human propensity for pattern-making follows us into QDA. From the vast array of interview transcripts, field notes, documents, and other forms of data, there is this instinctive, hardwired need to bring order to the collection—not just to reorganize it but to look for and construct patterns out of it. The discernment of patterns is one of the first steps in the data analytic process, and the methods described next are recommended ways to construct them.
Qualitative Data Analysis Strategy: To Code
To code in QDA is to assign a truncated, symbolic meaning to each datum for purposes of qualitative analysis—primarily patterning and categorizing. Coding is a heuristic—a method of discovery—to the meanings of individual sections of data. These codes function as a way of patterning, classifying, and later reorganizing them into emergent categories for further analysis. Different types of codes exist for different types of research genres and qualitative data analytic approaches, but this chapter will focus on only a few selected methods. First, a code can be defined as follows:
A code in qualitative data analysis is most often a word or short phrase that symbolically assigns a summative, salient, essence-capturing, and/or evocative attribute for a portion of language-based or visual data. The data can consist of interview transcripts, participant observation field notes, journals, documents, open-ended survey responses, drawings, artifacts, photographs, video, Internet sites, e-mail correspondence, academic and fictional literature, and so on. The portion of data coded … can range in magnitude from a single word to a full paragraph, an entire page of text or a stream of moving images.… Just as a title represents and captures a book or film or poem’s primary content and essence, so does a code represent and capture a datum’s primary content and essence. (Saldaña, 2016 , p. 4)
One helpful precoding task is to divide or parse long selections of field note or interview transcript data into shorter stanzas . Stanza division unitizes or “chunks” the corpus into more manageable paragraph-like units for coding assignments and analysis. The transcript sample that follows illustrates one possible way of inserting line breaks between self-standing passages of interview text for easier readability.
Process Coding
As a first coding example, the following interview excerpt about an employed, single, lower middle-class adult male’s spending habits during a difficult economic period in the United States is coded in the right-hand margin in capital letters. The superscript numbers match the beginning of the datum unit with its corresponding code. This method is called process coding (Charmaz, 2014 ), and it uses gerunds (“-ing” words) exclusively to represent action suggested by the data. Processes can consist of observable human actions (e.g., BUYING BARGAINS), mental or internal processes (e.g., THINKING TWICE), and more conceptual ideas (e.g., APPRECIATING WHAT YOU’VE GOT). Notice that the interviewer’s (I) portions are not coded, just the participant’s (P). A code is applied each time the subtopic of the interview shifts—even within a stanza—and the same codes can (and should) be used more than once if the subtopics are similar. The central research question driving this qualitative study is, “In what ways are middle-class Americans influenced and affected by an economic recession?”
Different researchers analyzing this same piece of data may develop completely different codes, depending on their personal lenses, filters, and angles. The previous codes are only one person’s interpretation of what is happening in the data, not a definitive list. The process codes have transformed the raw data units into new symbolic representations for analysis. A listing of the codes applied to this interview transcript, in the order they appear, reads:
BUYING BARGAINS
QUESTIONING A PURCHASE
THINKING TWICE
STOCKING UP
REFUSING SACRIFICE
PRIORITIZING
FINDING ALTERNATIVES
LIVING CHEAPLY
NOTICING CHANGES
STAYING INFORMED
MAINTAINING HEALTH
PICKING UP THE TAB
APPRECIATING WHAT YOU’VE GOT
Coding the data is the first step in this approach to QDA, and categorization is just one of the next possible steps.
Qualitative Data Analysis Strategy: To Categorize
To categorize in QDA is to cluster similar or comparable codes into groups for pattern construction and further analysis. Humans categorize things in innumerable ways. Think of an average apartment or house’s layout. The rooms of a dwelling have been constructed or categorized by their builders and occupants according to function. A kitchen is designated as an area to store and prepare food and to store the cooking and dining materials, such as pots, pans, and utensils. A bedroom is designated for sleeping, a closet for clothing storage, a bathroom for bodily functions and hygiene, and so on. Each room is like a category in which related and relevant patterns of human action occur. There are exceptions now and then, such as eating breakfast in bed rather than in a dining area or living in a small studio apartment in which most possessions are contained within one large room (but nonetheless are most often organized and clustered into subcategories according to function and optimal use of space).
The point is that the patterns of social action we designate into categories during QDA are not perfectly bounded. Category construction is our best attempt to cluster the most seemingly alike things into the most seemingly appropriate groups. Categorizing is reorganizing and reordering the vast array of data from a study because it is from these smaller, larger, and meaning-rich units that we can better grasp the particular features of each one and the categories’ possible interrelationships with one another.
One analytic strategy with a list of codes is to classify them into similar clusters. The same codes share the same category, but it is also possible that a single code can merit its own group if you feel it is unique enough. After the codes have been classified, a category label is applied to each grouping. Sometimes a code can also double as a category name if you feel it best summarizes the totality of the cluster. Like coding, categorizing is an interpretive act, because there can be different ways of separating and collecting codes that seem to belong together. The cut-and-paste functions of text editing software are most useful for exploring which codes share something in common.
Below is my categorization of the 15 codes generated from the interview transcript presented earlier. Like the gerunds for process codes, the categories have also been labeled as “-ing” words to connote action. And there was no particular reason why 15 codes resulted in three categories—there could have been less or even more, but this is how the array came together after my reflections on which codes seemed to belong together. The category labels are ways of answering why they belong together. For at-a-glance differentiation, I place codes in CAPITAL LETTERS and categories in upper- and lowercase Bold Font :
Category 1: Thinking Strategically
Category 2: Spending Strategically
Category 3: Living Strategically
Notice that the three category labels share a common word: strategically . Where did this word come from? It came from analytic reflection on the original data, the codes, and the process of categorizing the codes and generating their category labels. It was the analyst’s choice based on the interpretation of what primary action was happening. Your categories generated from your coded data do not need to share a common word or phrase, but I find that this technique, when appropriate, helps build a sense of unity to the initial analytic scheme.
The three categories— Thinking Strategically, Spending Strategically , and Living Strategically —are then reflected on for how they might interact and interplay. This is where the next major facet of data analysis, analytic memos, enters the scheme. But a necessary section on the basic principles of interrelationship and analytic reasoning must precede that discussion.
Qualitative Data Analysis Strategy: To Interrelate
To interrelate in QDA is to propose connections within, between, and among the constituent elements of analyzed data. One task of QDA is to explore the ways our patterns and categories interact and interplay. I use these terms to suggest the qualitative equivalent of statistical correlation, but interaction and interplay are much more than a simple relationship. They imply interrelationship . Interaction refers to reverberative connections—for example, how one or more categories might influence and affect the others, how categories operate concurrently, or whether there is some kind of domino effect to them. Interplay refers to the structural and processual nature of categories—for example, whether some type of sequential order, hierarchy, or taxonomy exists; whether any overlaps occur; whether there is superordinate and subordinate arrangement; and what types of organizational frameworks or networks might exist among them. The positivist construct of cause and effect becomes influences and affects in QDA.
There can even be patterns of patterns and categories of categories if your mind thinks conceptually and abstractly enough. Our minds can intricately connect multiple phenomena, but only if the data and their analyses support the constructions. We can speculate about interaction and interplay all we want, but it is only through a more systematic investigation of the data—in other words, good thinking—that we can plausibly establish any possible interrelationships.
Qualitative Data Analysis Strategy: To Reason
To reason in QDA is to think in ways that lead to summative findings, causal probabilities, and evaluative conclusions. Unlike quantitative research, with its statistical formulas and established hypothesis-testing protocols, qualitative research has no standardized methods of data analysis. Rest assured, there are recommended guidelines from the field’s scholars and a legacy of analysis strategies from which to draw. But the primary heuristics (or methods of discovery) you apply during a study are retroductive, inductive, substructive, abductive , and deductive reasoning.
Retroduction is historic reconstruction, working backward to figure out how the current conditions came to exist. Induction is what we experientially explore and infer to be transferable from the particular to the general, based on an examination of the evidence and an accumulation of knowledge. Substruction takes things apart to more carefully examine the constituent elements of the whole. Abduction is surmising from a range of possibilities that which is most likely, those explanatory hunches of plausibility based on clues. Deduction is what we generally draw and conclude from established facts and evidence.
It is not always necessary to know the names of these five ways of reasoning as you proceed through analysis. In fact, you will more than likely reverberate quickly from one to another depending on the task at hand. But what is important to remember about reasoning is:
to examine the evidence carefully and make reasonable inferences;
to base your conclusions primarily on the participants’ experiences, not just your own;
not to take the obvious for granted, because sometimes the expected will not happen;
your hunches can be right and, at other times, quite wrong; and
to logically yet imaginatively think about what is going on and how it all comes together.
Futurists and inventors propose three questions when they think about creating new visions for the world: What is possible (induction)? What is plausible (abduction)? What is preferable (deduction)? These same three questions might be posed as you proceed through QDA and particularly through analytic memo writing, which is substructive and retroductive reflection on your analytic work thus far.
Qualitative Data Analysis Strategy: To Memo
To memo in QDA is to reflect in writing on the nuances, inferences, meanings, and transfer of coded and categorized data plus your analytic processes. Like field note writing, perspectives vary among practitioners as to the methods for documenting the researcher’s analytic insights and subjective experiences. Some advise that such reflections should be included in field notes as relevant to the data. Others advise that a separate researcher’s journal should be maintained for recording these impressions. And still others advise that these thoughts be documented as separate analytic memos. I prescribe the latter as a method because it is generated by and directly connected to the data themselves.
An analytic memo is a “think piece” of reflective free writing, a narrative that sets in words your interpretations of the data. Coding and categorizing are heuristics to detect some of the possible patterns and interrelationships at work within the corpus, and an analytic memo further articulates your retroductive, inductive, substructive, abductive, and deductive thinking processes on what things may mean. Though the metaphor is a bit flawed and limiting, think of codes and their consequent categories as separate jigsaw puzzle pieces and their integration into an analytic memo as the trial assembly of the complete picture.
What follows is an example of an analytic memo based on the earlier process coded and categorized interview transcript. It is intended not as the final write-up for a publication, but as an open-ended reflection on the phenomena and processes suggested by the data and their analysis thus far. As the study proceeds, however, initial and substantive analytic memos can be revisited and revised for eventual integration into the final report. Note how the memo is dated and given a title for future and further categorization, how participant quotes are occasionally included for evidentiary support, and how the category names are bolded and the codes kept in capital letters to show how they integrate or weave into the thinking:
April 14, 2017 EMERGENT CATEGORIES: A STRATEGIC AMALGAM There’s a popular saying: “Smart is the new rich.” This participant is Thinking Strategically about his spending through such tactics as THINKING TWICE and QUESTIONING A PURCHASE before he decides to invest in a product. There’s a heightened awareness of both immediate trends and forthcoming economic bad news that positively affects his Spending Strategically . However, he seems unaware that there are even more ways of LIVING CHEAPLY by FINDING ALTERNATIVES. He dines at all-you-can-eat restaurants as a way of STOCKING UP on meals, but doesn’t state that he could bring lunch from home to work, possibly saving even more money. One of his “bad habits” is cigarettes, which he refuses to give up; but he doesn’t seem to realize that by quitting smoking he could save even more money, not to mention possible health care costs. He balks at the idea of paying $2.00 for a soft drink, but doesn’t mind paying $6.00–$7.00 for a pack of cigarettes. Penny-wise and pound-foolish. Addictions skew priorities. Living Strategically , for this participant during “scary times,” appears to be a combination of PRIORITIZING those things which cannot be helped, such as pet care and personal dental care; REFUSING SACRIFICE for maintaining personal creature-comforts; and FINDING ALTERNATIVES to high costs and excessive spending. Living Strategically is an amalgam of thinking and action-oriented strategies.
There are several recommended topics for analytic memo writing throughout the qualitative study. Memos are opportunities to reflect on and write about:
A descriptive summary of the data;
How the researcher personally relates to the participants and/or the phenomenon;
The participants’ actions, reactions, and interactions;
The participants’ routines, rituals, rules, roles, and relationships;
What is surprising, intriguing, or disturbing (Sunstein & Chiseri-Strater, 2012 , p. 115);
Code choices and their operational definitions;
Emergent patterns, categories, themes, concepts, assertions, and propositions;
The possible networks and processes (links, connections, overlaps, flows) among the codes, patterns, categories, themes, concepts, assertions, and propositions;
An emergent or related existent theory;
Any problems with the study;
Any personal or ethical dilemmas with the study;
Future directions for the study;
The analytic memos generated thus far (i.e., metamemos);
Tentative answers to the study’s research questions; and
The final report for the study. (adapted from Saldaña & Omasta, 2018 , p. 54)
Since writing is analysis, analytic memos expand on the inferential meanings of the truncated codes, categories, and patterns as a transitional stage into a more coherent narrative with hopefully rich social insight.
Qualitative Data Analysis Strategy: To Code—A Different Way
The first example of coding illustrated process coding, a way of exploring general social action among humans. But sometimes a researcher works with an individual case study in which the language is unique or with someone the researcher wishes to honor by maintaining the authenticity of his or her speech in the analysis. These reasons suggest that a more participant-centered form of coding may be more appropriate.
In Vivo Coding
A second frequently applied method of coding is called in vivo coding. The root meaning of in vivo is “in that which is alive”; it refers to a code based on the actual language used by the participant (Strauss, 1987 ). The words or phrases in the data record you select as codes are those that seem to stand out as significant or summative of what is being said.
Using the same transcript of the male participant living in difficult economic times, in vivo codes are listed in the right-hand column. I recommend that in vivo codes be placed in quotation marks as a way of designating that the code is extracted directly from the data record. Note that instead of 15 codes generated from process coding, the total number of in vivo codes is 30. This is not to suggest that there should be specific numbers or ranges of codes used for particular methods. In vivo codes, however, tend to be applied more frequently to data. Again, the interviewer’s questions and prompts are not coded, just the participant’s responses:
The 30 in vivo codes are then extracted from the transcript and could be listed in the order they appear, but this time they are placed in alphabetical order as a heuristic to prepare them for analytic action and reflection:
“ALL-YOU-CAN-EAT”
“ANOTHER DING IN MY WALLET”
“BAD HABITS”
“CHEAP AND FILLING”
“COUPLE OF THOUSAND”
“DON’T REALLY NEED”
“HAVEN’T CHANGED MY HABITS”
“HIGH MAINTENANCE”
“INSURANCE IS JUST WORTHLESS”
“IT ALL ADDS UP”
“LIVED KIND OF CHEAP”
“NOT A BIG SPENDER”
“NOT AS BAD OFF”
“NOT PUTTING AS MUCH INTO SAVINGS”
“PICK UP THE TAB”
“SCARY TIMES”
“SKYROCKETED”
“SPENDING MORE”
“THE LITTLE THINGS”
“THINK TWICE”
“TWO-FOR-ONE”
Even though no systematic categorization has been conducted with the codes thus far, an analytic memo of first impressions can still be composed:
March 19, 2017 CODE CHOICES: THE EVERYDAY LANGUAGE OF ECONOMICS After eyeballing the in vivo codes list, I noticed that variants of “CHEAP” appear most often. I recall a running joke between me and a friend of mine when we were shopping for sales. We’d say, “We’re not ‘cheap,’ we’re frugal .” There’s no formal economic or business language in this transcript—no terms such as “recession” or “downsizing”—just the everyday language of one person trying to cope during “SCARY TIMES” with “ANOTHER DING IN MY WALLET.” The participant notes that he’s always “LIVED KIND OF CHEAP” and is “NOT A BIG SPENDER” and, due to his employment, “NOT AS BAD OFF” as others in the country. Yet even with his middle class status, he’s still feeling the monetary pinch, dining at inexpensive “ALL-YOU-CAN-EAT” restaurants and worried about the rising price of peanut butter, observing that he’s “NOT PUTTING AS MUCH INTO SAVINGS” as he used to. Of all the codes, “ANOTHER DING IN MY WALLET” stands out to me, particularly because on the audio recording he sounded bitter and frustrated. It seems that he’s so concerned about “THE LITTLE THINGS” because of high veterinary and dental charges. The only way to cope with a “COUPLE OF THOUSAND” dollars worth of medical expenses is to find ways of trimming the excess in everyday facets of living: “IT ALL ADDS UP.”
Like process coding, in vivo codes could be clustered into similar categories, but another simple data analytic strategy is also possible.
Qualitative Data Analysis Strategy: To Outline
To outline in QDA is to hierarchically, processually, and/or temporally assemble such things as codes, categories, themes, assertions, propositions, and concepts into a coherent, text-based display. Traditional outlining formats and content provide not only templates for writing a report but also templates for analytic organization. This principle can be found in several computer-assisted qualitative data analysis software (CAQDAS) programs through their use of such functions as “hierarchies,” “trees,” and “nodes,” for example. Basic outlining is simply a way of arranging primary, secondary, and subsecondary items into a patterned display. For example, an organized listing of things in a home might consist of the following:
Large appliances
Refrigerator
Stove-top oven
Microwave oven
Small appliances
Coffee maker
Dining room
In QDA, outlining may include descriptive nouns or topics but, depending on the study, it may also involve processes or phenomena in extended passages, such as in vivo codes or themes.
The complexity of what we learn in the field can be overwhelming, and outlining is a way of organizing and ordering that complexity so that it does not become complicated. The cut-and-paste and tab functions of a text editing page enable you to arrange and rearrange the salient items from your preliminary coded analytic work into a more streamlined flow. By no means do I suggest that the intricate messiness of life can always be organized into neatly formatted arrangements, but outlining is an analytic act that stimulates deep reflection on both the interconnectedness and the interrelationships of what we study. As an example, here are the 30 in vivo codes generated from the initial transcript analysis, arranged in such a way as to construct five major categories:
Now that the codes have been rearranged into an outline format, an analytic memo is composed to expand on the rationale and constructed meanings in progress:
March 19, 2017 NETWORKS: EMERGENT CATEGORIES The five major categories I constructed from the in vivo codes are: “SCARY TIMES,” “PRIORTY,” “ANOTHER DING IN MY WALLET,” “THE LITTLE THINGS,” and “LIVED KIND OF CHEAP.” One of the things that hit me today was that the reason he may be pinching pennies on smaller purchases is that he cannot control the larger ones he has to deal with. Perhaps the only way we can cope with or seem to have some sense of agency over major expenses is to cut back on the smaller ones that we can control. $1,000 for a dental bill? Skip lunch for a few days a week. Insulin medication to buy for a pet? Don’t buy a soft drink from a vending machine. Using this reasoning, let me try to interrelate and weave the categories together as they relate to this particular participant: During these scary economic times, he prioritizes his spending because there seems to be just one ding after another to his wallet. A general lifestyle of living cheaply and keeping an eye out for how to save money on the little things compensates for those major expenses beyond his control.
Qualitative Data Analysis Strategy: To Code—In Even More Ways
The process and in vivo coding examples thus far have demonstrated only two specific methods of 33 documented approaches (Saldaña, 2016 ). Which one(s) you choose for your analysis depends on such factors as your conceptual framework, the genre of qualitative research for your project, the types of data you collect, and so on. The following sections present four additional approaches available for coding qualitative data that you may find useful as starting points.
Descriptive Coding
Descriptive codes are primarily nouns that simply summarize the topic of a datum. This coding approach is particularly useful when you have different types of data gathered for one study, such as interview transcripts, field notes, open-ended survey responses, documents, and visual materials such as photographs. Descriptive codes not only help categorize but also index the data corpus’s basic contents for further analytic work. An example of an interview portion coded descriptively, taken from the participant living in tough economic times, follows to illustrate how the same data can be coded in multiple ways:
For initial analysis, descriptive codes are clustered into similar categories to detect such patterns as frequency (i.e., categories with the largest number of codes) and interrelationship (i.e., categories that seem to connect in some way). Keep in mind that descriptive coding should be used sparingly with interview transcript data because other coding methods will reveal richer participant dynamics.
Values Coding
Values coding identifies the values, attitudes, and beliefs of a participant, as shared by the individual and/or interpreted by the analyst. This coding method infers the “heart and mind” of an individual or group’s worldview as to what is important, perceived as true, maintained as opinion, and felt strongly. The three constructs are coded separately but are part of a complex interconnected system.
Briefly, a value (V) is what we attribute as important, be it a person, thing, or idea. An attitude (A) is the evaluative way we think and feel about ourselves, others, things, or ideas. A belief (B) is what we think and feel as true or necessary, formed from our “personal knowledge, experiences, opinions, prejudices, morals, and other interpretive perceptions of the social world” (Saldaña, 2016 , p. 132). Values coding explores intrapersonal, interpersonal, and cultural constructs, or ethos . It is an admittedly slippery task to code this way because it is sometimes difficult to discern what is a value, attitude, or belief since they are intricately interrelated. But the depth you can potentially obtain is rich. An example of values coding follows:
For analysis, categorize the codes for each of the three different constructs together (i.e., all values in one group, attitudes in a second group, and beliefs in a third group). Analytic memo writing about the patterns and possible interrelationships may reveal a more detailed and intricate worldview of the participant.
Dramaturgical Coding
Dramaturgical coding perceives life as performance and its participants as characters in a social drama. Codes are assigned to the data (i.e., a “play script”) that analyze the characters in action, reaction, and interaction. Dramaturgical coding of participants examines their objectives (OBJ) or wants, needs, and motives; the conflicts (CON) or obstacles they face as they try to achieve their objectives; the tactics (TAC) or strategies they employ to reach their objectives; their attitudes (ATT) toward others and their given circumstances; the particular emotions (EMO) they experience throughout; and their subtexts (SUB), or underlying and unspoken thoughts. The following is an example of dramaturgically coded data:
Not included in this particular interview excerpt are the emotions the participant may have experienced or talked about. His later line, “that’s another ding in my wallet,” would have been coded EMO: BITTER. A reader may not have inferred that specific emotion from seeing the line in print. But the interviewer, present during the event and listening carefully to the audio recording during transcription, noted that feeling in his tone of voice.
For analysis, group similar codes together (e.g., all objectives in one group, all conflicts in another group, all tactics in a third group) or string together chains of how participants deal with their circumstances to overcome their obstacles through tactics:
OBJ: SAVING MEAL MONEY → TAC: SKIPPING MEALS + COUPONS
Dramaturgical coding is particularly useful as preliminary work for narrative inquiry story development or arts-based research representations such as performance ethnography. The method explores how the individuals or groups manage problem solving in their daily lives.
Versus Coding
Versus (VS) coding identifies the conflicts, struggles, and power issues observed in social action, reaction, and interaction as an X VS Y code, such as MEN VS WOMEN, CONSERVATIVES VS LIBERALS, FAITH VS LOGIC, and so on. Conflicts are rarely this dichotomous; they are typically nuanced and much more complex. But humans tend to perceive these struggles with an US VS THEM mindset. The codes can range from the observable to the conceptual and can be applied to data that show humans in tension with others, themselves, or ideologies.
What follows are examples of versus codes applied to the case study participant’s descriptions of his major medical expenses:
As an initial analytic tactic, group the versus codes into one of three categories: the Stakeholders , their Perceptions and/or Actions , and the Issues at stake. Examine how the three interrelate and identify the central ideological conflict at work as an X VS Y category. Analytic memos and the final write-up can detail the nuances of the issues.
Remember that what has been profiled in this section is a broad brushstroke description of just a few basic coding processes, several of which can be compatibly mixed and matched within a single analysis (see Saldaña’s 2016 The Coding Manual for Qualitative Researchers for a complete discussion). Certainly with additional data, more in-depth analysis can occur, but coding is only one approach to extracting and constructing preliminary meanings from the data corpus. What now follows are additional methods for qualitative analysis.
Qualitative Data Analysis Strategy: To Theme
To theme in QDA is to construct summative, phenomenological meanings from data through extended passages of text. Unlike codes, which are most often single words or short phrases that symbolically represent a datum, themes are extended phrases or sentences that summarize the manifest (apparent) and latent (underlying) meanings of data (Auerbach & Silverstein, 2003 ; Boyatzis, 1998 ). Themes, intended to represent the essences and essentials of humans’ lived experiences, can also be categorized or listed in superordinate and subordinate outline formats as an analytic tactic.
Below is the interview transcript example used in the previous coding sections. (Hopefully you are not too fatigued at this point with the transcript, but it is important to know how inquiry with the same data set can be approached in several different ways.) During the investigation of the ways middle-class Americans are influenced and affected by an economic recession, the researcher noticed that participants’ stories exhibited facets of what he labeled economic intelligence , or EI (based on the formerly developed theories of Howard Gardner’s multiple intelligences and Daniel Goleman’s emotional intelligence). Notice how theming interprets what is happening through the use of two distinct phrases—ECONOMIC INTELLIGENCE IS (i.e., manifest or apparent meanings) and ECONOMIC INTELLIGENCE MEANS (i.e., latent or underlying meanings):
Unlike the 15 process codes and 30 in vivo codes in the previous examples, there are now 14 themes to work with. They could be listed in the order they appear, but one initial heuristic is to group them separately by “is” and “means” statements to detect any possible patterns (discussed later):
EI IS TAKING ADVANTAGE OF UNEXPECTED OPPORTUNITY
EI IS BUYING CHEAP
EI IS SAVING A FEW DOLLARS NOW AND THEN
EI IS SETTING PRIORITIES
EI IS FINDING CHEAPER FORMS OF ENTERTAINMENT
EI IS NOTICING PERSONAL AND NATIONAL ECONOMIC TRENDS
EI IS TAKING CARE OF ONE’S OWN HEALTH
EI MEANS THINKING BEFORE YOU ACT
EI MEANS SACRIFICE
EI MEANS KNOWING YOUR FLAWS
EI MEANS LIVING AN INEXPENSIVE LIFESTYLE
EI MEANS YOU CANNOT CONTROL EVERYTHING
EI MEANS KNOWING YOUR LUCK
There are several ways to categorize the themes as preparation for analytic memo writing. The first is to arrange them in outline format with superordinate and subordinate levels, based on how the themes seem to take organizational shape and structure. Simply cutting and pasting the themes in multiple arrangements on a text editing page eventually develops a sense of order to them. For example:
A second approach is to categorize the themes into similar clusters and to develop different category labels or theoretical constructs . A theoretical construct is an abstraction that transforms the central phenomenon’s themes into broader applications but can still use “is” and “means” as prompts to capture the bigger picture at work:
Theoretical Construct 1: EI Means Knowing the Unfortunate Present
Supporting Themes:
Theoretical Construct 2: EI Is Cultivating a Small Fortune
Theoretical Construct 3: EI Means a Fortunate Future
What follows is an analytic memo generated from the cut-and-paste arrangement of themes into “is” and “means” statements, into an outline, and into theoretical constructs:
March 19, 2017 EMERGENT THEMES: FORTUNE/FORTUNATELY/UNFORTUNATELY I first reorganized the themes by listing them in two groups: “is” and “means.” The “is” statements seemed to contain positive actions and constructive strategies for economic intelligence. The “means” statements held primarily a sense of caution and restriction with a touch of negativity thrown in. The first outline with two major themes, LIVING AN INEXPENSIVE LIFESTYLE and YOU CANNOT CONTROL EVERYTHING also had this same tone. This reminded me of the old children’s picture book, Fortunately/Unfortunately , and the themes of “fortune” as a motif for the three theoretical constructs came to mind. Knowing the Unfortunate Present means knowing what’s (most) important and what’s (mostly) uncontrollable in one’s personal economic life. Cultivating a Small Fortune consists of those small money-saving actions that, over time, become part of one’s lifestyle. A Fortunate Future consists of heightened awareness of trends and opportunities at micro and macro levels, with the understanding that health matters can idiosyncratically affect one’s fortune. These three constructs comprise this particular individual’s EI—economic intelligence.
Again, keep in mind that the examples for coding and theming were from one small interview transcript excerpt. The number of codes and their categorization would increase, given a longer interview and/or multiple interviews to analyze. But the same basic principles apply: codes and themes relegated into patterned and categorized forms are heuristics—stimuli for good thinking through the analytic memo-writing process on how everything plausibly interrelates. Methodologists vary in the number of recommended final categories that result from analysis, ranging anywhere from three to seven, with traditional grounded theorists prescribing one central or core category from coded work.
Qualitative Data Analysis Strategy: To Assert
To assert in QDA is to put forward statements that summarize particular fieldwork and analytic observations that the researcher believes credibly represent and transcend the experiences. Educational anthropologist Frederick Erickson ( 1986 ) wrote a significant and influential chapter on qualitative methods that outlined heuristics for assertion development . Assertions are declarative statements of summative synthesis, supported by confirming evidence from the data and revised when disconfirming evidence or discrepant cases require modification of the assertions. These summative statements are generated from an interpretive review of the data corpus and then supported and illustrated through narrative vignettes—reconstructed stories from field notes, interview transcripts, or other data sources that provide a vivid profile as part of the evidentiary warrant.
Coding or theming data can certainly precede assertion development as a way of gaining intimate familiarity with the data, but Erickson’s ( 1986 ) methods are a more admittedly intuitive yet systematic heuristic for analysis. Erickson promotes analytic induction and exploration of and inferences about the data, based on an examination of the evidence and an accumulation of knowledge. The goal is not to look for “proof” to support the assertions, but to look for plausibility of inference-laden observations about the local and particular social world under investigation.
Assertion development is the writing of general statements, plus subordinate yet related ones called subassertions and a major statement called a key assertion that represents the totality of the data. One also looks for key linkages between them, meaning that the key assertion links to its related assertions, which then link to their respective subassertions. Subassertions can include particulars about any discrepant related cases or specify components of their parent assertions.
Excerpts from the interview transcript of our case study will be used to illustrate assertion development at work. By now, you should be quite familiar with the contents, so I will proceed directly to the analytic example. First, there is a series of thematically related statements the participant makes:
“Buy one package of chicken, get the second one free. Now that was a bargain. And I got some.”
“With Sweet Tomatoes I get those coupons for a few bucks off for lunch, so that really helps.”
“I don’t go to movies anymore. I rent DVDs from Netflix or Redbox or watch movies online—so much cheaper than paying over ten or twelve bucks for a movie ticket.”
Assertions can be categorized into low-level and high-level inferences . Low-level inferences address and summarize what is happening within the particulars of the case or field site—the micro . High-level inferences extend beyond the particulars to speculate on what it means in the more general social scheme of things—the meso or macro . A reasonable low-level assertion about the three statements above collectively might read, The participant finds several small ways to save money during a difficult economic period . A high-level inference that transcends the case to the meso level might read, Selected businesses provide alternatives and opportunities to buy products and services at reduced rates during a recession to maintain consumer spending.
Assertions are instantiated (i.e., supported) by concrete instances of action or participant testimony, whose patterns lead to more general description outside the specific field site. The author’s interpretive commentary can be interspersed throughout the report, but the assertions should be supported with the evidentiary warrant . A few assertions and subassertions based on the case interview transcript might read as follows (and notice how high-level assertions serve as the paragraphs’ topic sentences):
Selected businesses provide alternatives and opportunities to buy products and services at reduced rates during a recession to maintain consumer spending. Restaurants, for example, need to find ways during difficult economic periods when potential customers may be opting to eat inexpensively at home rather than spending more money by dining out. Special offers can motivate cash-strapped clientele to patronize restaurants more frequently. An adult male dealing with such major expenses as underinsured dental care offers: “With Sweet Tomatoes I get those coupons for a few bucks off for lunch, so that really helps.” The film and video industries also seem to be suffering from a double-whammy during the current recession: less consumer spending on higher-priced entertainment, resulting in a reduced rate of movie theater attendance (recently 39 percent of the American population, according to a CNN report); coupled with a media technology and business revolution that provides consumers less costly alternatives through video rentals and Internet viewing: “I don’t go to movies anymore. I rent DVDs from Netflix or Redbox or watch movies online—so much cheaper than paying over ten or twelve bucks for a movie ticket.”
To clarify terminology, any assertion that has an if–then or predictive structure to it is called a proposition since it proposes a conditional event. For example, this assertion is also a proposition: “Special offers can motivate cash-strapped clientele to patronize restaurants more frequently.” Propositions are the building blocks of hypothesis testing in the field and for later theory construction. Research not only documents human action but also can sometimes formulate statements that predict it. This provides a transferable and generalizable quality in our findings to other comparable settings and contexts. And to clarify terminology further, all propositions are assertions, but not all assertions are propositions.
Particularizability —the search for specific and unique dimensions of action at a site and/or the specific and unique perspectives of an individual participant—is not intended to filter out trivial excess but to magnify the salient characteristics of local meaning. Although generalizable knowledge is difficult to formulate in qualitative inquiry since each naturalistic setting will contain its own unique set of social and cultural conditions, there will be some aspects of social action that are plausibly universal or “generic” across settings and perhaps even across time.
To work toward this, Erickson advocates that the interpretive researcher look for “concrete universals” by studying actions at a particular site in detail and then comparing those actions to actions at other sites that have also been studied in detail. The exhibit or display of these generalizable features is to provide a synoptic representation, or a view of the whole. What the researcher attempts to uncover is what is both particular and general at the site of interest, preferably from the perspective of the participants. It is from the detailed analysis of actions at a specific site that these universals can be concretely discerned, rather than abstractly constructed as in grounded theory.
In sum, assertion development is a qualitative data analytic strategy that relies on the researcher’s intense review of interview transcripts, field notes, documents, and other data to inductively formulate, with reasonable certainty, composite statements that credibly summarize and interpret participant actions and meanings and their possible representation of and transfer into broader social contexts and issues.
Qualitative Data Analysis Strategy: To Display
To display in QDA is to visually present the processes and dynamics of human or conceptual action represented in the data. Qualitative researchers use not only language but also illustrations to both analyze and display the phenomena and processes at work in the data. Tables, charts, matrices, flow diagrams, and other models and graphics help both you and your readers cognitively and conceptually grasp the essence and essentials of your findings. As you have seen thus far, even simple outlining of codes, categories, and themes is one visual tactic for organizing the scope of the data. Rich text, font, and format features such as italicizing, bolding, capitalizing, indenting, and bullet pointing provide simple emphasis to selected words and phrases within the longer narrative.
Think display was a phrase coined by methodologists Miles and Huberman ( 1994 ) to encourage the researcher to think visually as data were collected and analyzed. The magnitude of text can be essentialized into graphics for at-a-glance review. Bins in various shapes and lines of various thicknesses, along with arrows suggesting pathways and direction, render the study a portrait of action. Bins can include the names of codes, categories, concepts, processes, key participants, and/or groups.
As a simple example, Figure 29.1 illustrates the three categories’ interrelationship derived from process coding. It displays what could be the apex of this interaction, LIVING STRATEGICALLY, and its connections to THINKING STRATEGICALLY, which influences and affects SPENDING STRATEGICALLY.
Three categories’ interrelationship derived from process coding.
Figure 29.2 represents a slightly more complex (if not playful) model, based on the five major in vivo codes/categories generated from analysis. The graphic is used as a way of initially exploring the interrelationship and flow from one category to another. The use of different font styles, font sizes, and line and arrow thicknesses is intended to suggest the visual qualities of the participant’s language and his dilemmas—a way of heightening in vivo coding even further.
In vivo categories in rich text display
Accompanying graphics are not always necessary for a qualitative report. They can be very helpful for the researcher during the analytic stage as a heuristic for exploring how major ideas interrelate, but illustrations are generally included in published work when they will help supplement and clarify complex processes for readers. Photographs of the field setting or the participants (and only with their written permission) also provide evidentiary reality to the write-up and help your readers get a sense of being there.
Qualitative Data Analysis Strategy: To Narrate
To narrate in QDA is to create an evocative literary representation and presentation of the data in the form of creative nonfiction. All research reports are stories of one kind or another. But there is yet another approach to QDA that intentionally documents the research experience as story, in its traditional literary sense. Narrative inquiry serves to plot and story-line the participant’s experiences into what might be initially perceived as a fictional short story or novel. But the story is carefully crafted and creatively written to provide readers with an almost omniscient perspective about the participants’ worldview. The transformation of the corpus from database to creative nonfiction ranges from systematic transcript analysis to open-ended literary composition. The narrative, however, should be solidly grounded in and emerge from the data as a plausible rendering of social life.
The following is a narrative vignette based on interview transcript selections from the participant living through tough economic times:
Jack stood in front of the soft drink vending machine at work and looked almost worriedly at the selections. With both hands in his pants pockets, his fingers jingled the few coins he had inside them as he contemplated whether he could afford the purchase. Two dollars for a twenty-ounce bottle of Diet Coke. Two dollars. “I can practically get a two-liter bottle for that same price at the grocery store,” he thought. Then Jack remembered the upcoming dental surgery he needed—that would cost one thousand dollars—and the bottle of insulin and syringes he needed to buy for his diabetic, high maintenance cat—almost two hundred dollars. He sighed, took his hands out of his pockets, and walked away from the vending machine. He was skipping lunch that day anyway so he could stock up on dinner later at the cheap-but-filling all-you-can-eat Chinese buffet. He could get his Diet Coke there.
Narrative inquiry representations, like literature, vary in tone, style, and point of view. The common goal, however, is to create an evocative portrait of participants through the aesthetic power of literary form. A story does not always have to have a moral explicitly stated by its author. The reader reflects on personal meanings derived from the piece and how the specific tale relates to one’s self and the social world.
Qualitative Data Analysis Strategy: To Poeticize
To poeticize in QDA is to create an evocative literary representation and presentation of the data in poetic form. One approach to analyzing or documenting analytic findings is to strategically truncate interview transcripts, field notes, and other pertinent data into poetic structures. Like coding, poetic constructions capture the essence and essentials of data in a creative, evocative way. The elegance of the format attests to the power of carefully chosen language to represent and convey complex human experience.
In vivo codes (codes based on the actual words used by participants themselves) can provide imagery, symbols, and metaphors for rich category, theme, concept, and assertion development, in addition to evocative content for arts-based interpretations of the data. Poetic inquiry takes note of what words and phrases seem to stand out from the data corpus as rich material for reinterpretation. Using some of the participant’s own language from the interview transcript illustrated previously, a poetic reconstruction or “found poetry” might read as follows:
Scary Times Scary times … spending more (another ding in my wallet) a couple of thousand (another ding in my wallet) insurance is just worthless (another ding in my wallet) pick up the tab (another ding in my wallet) not putting as much into savings (another ding in my wallet) It all adds up. Think twice: don’t really need skip Think twice, think cheap: coupons bargains two-for-one free Think twice, think cheaper: stock up all-you-can-eat (cheap—and filling) It all adds up.
Anna Deavere Smith, a verbatim theatre performer, attests that people speak in forms of “organic poetry” in everyday life. Thus, in vivo codes can provide core material for poetic representation and presentation of lived experiences, potentially transforming the routine and mundane into the epic. Some researchers also find the genre of poetry to be the most effective way to compose original work that reflects their own fieldwork experiences and autoethnographic stories.
Qualitative Data Analysis Strategy: To Compute
To compute in QDA is to employ specialized software programs for qualitative data management and analysis. The acronym for computer-assisted qualitative data analysis software is CAQDAS. There are diverse opinions among practitioners in the field about the utility of such specialized programs for qualitative data management and analysis. The software, unlike statistical computation, does not actually analyze data for you at higher conceptual levels. These CAQDAS software packages serve primarily as a repository for your data (both textual and visual) that enables you to code them, and they can perform such functions as calculating the number of times a particular word or phrase appears in the data corpus (a particularly useful function for content analysis) and can display selected facets after coding, such as possible interrelationships. Basic software such as Microsoft Word and Excel provides utilities that can store and, with some preformatting and strategic entry, organize qualitative data to enable the researcher’s analytic review. The following Internet addresses are listed to help in exploring selected CAQDAS packages and obtaining demonstration/trial software; video tutorials are available on the companies’ websites and on YouTube:
ATLAS.ti: http://www.atlasti.com
Dedoose: http://www.dedoose.com
HyperRESEARCH: http://www.researchware.com
MAXQDA: http://www.maxqda.com
NVivo: http://www.qsrinternational.com
QDA Miner: http://www.provalisresearch.com
Quirkos: http://www.quirkos.com
Transana: http://www.transana.com
V-Note: http://www.v-note.org
Some qualitative researchers attest that the software is indispensable for qualitative data management, especially for large-scale studies. Others feel that the learning curve of most CAQDAS programs is too lengthy to be of pragmatic value, especially for small-scale studies. From my own experience, if you have an aptitude for picking up quickly on the scripts and syntax of software programs, explore one or more of the packages listed. If you are a novice to qualitative research, though, I recommend working manually or “by hand” for your first project so you can focus exclusively on the data and not on the software.
Qualitative Data Analysis Strategy: To Verify
To verify in QDA is to administer an audit of “quality control” to your analysis. After your data analysis and the development of key findings, you may be thinking to yourself, “Did I get it right?” “Did I learn anything new?” Reliability and validity are terms and constructs of the positivist quantitative paradigm that refer to the replicability and accuracy of measures. But in the qualitative paradigm, other constructs are more appropriate.
Credibility and trustworthiness (Lincoln & Guba, 1985 ) are two factors to consider when collecting and analyzing the data and presenting your findings. In our qualitative research projects, we must present a convincing story to our audiences that we “got it right” methodologically. In other words, the amount of time we spent in the field, the number of participants we interviewed, the analytic methods we used, the thinking processes evident to reach our conclusions, and so on should be “just right” to assure the reader that we have conducted our jobs soundly. But remember that we can never conclusively prove something; we can only, at best, convincingly suggest. Research is an act of persuasion.
Credibility in a qualitative research report can be established in several ways. First, citing the key writers of related works in your literature review is essential. Seasoned researchers will sometimes assess whether a novice has “done her homework” by reviewing the bibliography or references. You need not list everything that seminal writers have published about a topic, but their names should appear at least once as evidence that you know the field’s key figures and their work.
Credibility can also be established by specifying the particular data analysis methods you employed (e.g., “Interview transcripts were taken through two cycles of process coding, resulting in three primary categories”), through corroboration of data analysis with the participants themselves (e.g., “I asked my participants to read and respond to a draft of this report for their confirmation of accuracy and recommendations for revision”), or through your description of how data and findings were substantiated (e.g., “Data sources included interview transcripts, participant observation field notes, and participant response journals to gather multiple perspectives about the phenomenon”).
Data scientist W. Edwards Deming is attributed with offering this cautionary advice about making a convincing argument: “Without data, you’re just another person with an opinion.” Thus, researchers can also support their findings with relevant, specific evidence by quoting participants directly and/or including field note excerpts from the data corpus. These serve both as illustrative examples for readers and to present more credible testimony of what happened in the field.
Trustworthiness, or providing credibility to the writing, is when we inform the reader of our research processes. Some make the case by stating the duration of fieldwork (e.g., “Forty-five clock hours were spent in the field”; “The study extended over a 10-month period”). Others put forth the amounts of data they gathered (e.g., “Sixteen individuals were interviewed”; “My field notes totaled 157 pages”). Sometimes trustworthiness is established when we are up front or confessional with the analytic or ethical dilemmas we encountered (e.g., “It was difficult to watch the participant’s teaching effectiveness erode during fieldwork”; “Analysis was stalled until I recoded the entire data corpus with a new perspective”).
The bottom line is that credibility and trustworthiness are matters of researcher honesty and integrity . Anyone can write that he worked ethically, rigorously, and reflexively, but only the writer will ever know the truth. There is no shame if something goes wrong with your research. In fact, it is more than likely the rule, not the exception. Work and write transparently to achieve credibility and trustworthiness with your readers.
The length of this chapter does not enable me to expand on other QDA strategies such as to conceptualize, theorize, and write. Yet there are even more subtle thinking strategies to employ throughout the research enterprise, such as to synthesize, problematize, and create. Each researcher has his or her own ways of working, and deep reflexivity (another strategy) on your own methodology and methods as a qualitative inquirer throughout fieldwork and writing provides you with metacognitive awareness of data analysis processes and possibilities.
Data analysis is one of the most elusive practices in qualitative research, perhaps because it is a backstage, behind-the-scenes, in-your-head enterprise. It is not that there are no models to follow. It is just that each project is contextual and case specific. The unique data you collect from your unique research design must be approached with your unique analytic signature. It truly is a learning-by-doing process, so accept that and leave yourself open to discovery and insight as you carefully scrutinize the data corpus for patterns, categories, themes, concepts, assertions, propositions, and possibly new theories through strategic analysis.
Auerbach, C. F. , & Silverstein, L. B. ( 2003 ). Qualitative data: An introduction to coding and analysis . New York, NY: New York University Press.
Google Scholar
Google Preview
Birks, M. , & Mills, J. ( 2015 ). Grounded theory: A practical guide (2nd ed.). London, England: Sage.
Boyatzis, R. E. ( 1998 ). Transforming qualitative information: Thematic analysis and code development . Thousand Oaks, CA: Sage.
Bryant, A. ( 2017 ). Grounded theory and grounded theorizing: Pragmatism in research practice. New York, NY: Oxford.
Bryant, A. , & Charmaz, K. (Eds.). ( 2019 ). The Sage handbook of current developments in grounded theory . London, England: Sage.
Charmaz, K. ( 2014 ). Constructing grounded theory: A practical guide through qualitative analysis (2nd ed.). London, England: Sage.
Erickson, F. ( 1986 ). Qualitative methods in research on teaching. In M. C. Wittrock (Ed.), Handbook of research on teaching (3rd ed., pp. 119–161). New York, NY: Macmillan.
Galman, S. C. ( 2013 ). The good, the bad, and the data: Shane the lone ethnographer’s basic guide to qualitative data analysis. Walnut Creek, CA: Left Coast Press.
Geertz, C. ( 1983 ). Local knowledge: Further essays in interpretive anthropology . New York, NY: Basic Books.
Lincoln, Y. S. , & Guba, E. G. ( 1985 ). Naturalistic inquiry . Newbury Park, CA: Sage.
Miles, M. B. , & Huberman, A. M. ( 1994 ). Qualitative data analysis (2nd ed.). Thousand Oaks, CA: Sage.
Saldaña, J. ( 2016 ). The coding manual for qualitative researchers (3rd ed.). London, England: Sage.
Saldaña, J. , & Omasta, M. ( 2018 ). Qualitative research: Analyzing life . Thousand Oaks, CA: Sage.
Stake, R. E. ( 1995 ). The art of case study research . Thousand Oaks, CA: Sage.
Stern, P. N. , & Porr, C. J. ( 2011 ). Essentials of accessible grounded theory . Walnut Creek, CA: Left Coast Press.
Strauss, A. L. ( 1987 ). Qualitative analysis for social scientists . Cambridge, England: Cambridge University Press.
Sunstein, B. S. , & Chiseri-Strater, E. ( 2012 ). FieldWorking: Reading and writing research (4th ed.). Boston, MA: Bedford/St. Martin’s.
Wertz, F. J. , Charmaz, K. , McMullen, L. M. , Josselson, R. , Anderson, R. , & McSpadden, E. ( 2011 ). Five ways of doing qualitative analysis: Phenomenological psychology, grounded theory, discourse analysis, narrative research, and intuitive inquiry . New York, NY: Guilford Press.
- About Oxford Academic
- Publish journals with us
- University press partners
- What we publish
- New features
- Open access
- Institutional account management
- Rights and permissions
- Get help with access
- Accessibility
- Advertising
- Media enquiries
- Oxford University Press
- Oxford Languages
- University of Oxford
Oxford University Press is a department of the University of Oxford. It furthers the University's objective of excellence in research, scholarship, and education by publishing worldwide
- Copyright © 2024 Oxford University Press
- Cookie settings
- Cookie policy
- Privacy policy
- Legal notice
This Feature Is Available To Subscribers Only
Sign In or Create an Account
This PDF is available to Subscribers Only
For full access to this pdf, sign in to an existing account, or purchase an annual subscription.
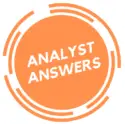
Data & Finance for Work & Life

Data Analysis for Qualitative Research: 6 Step Guide
Data analysis for qualitative research is not intuitive. This is because qualitative data stands in opposition to traditional data analysis methodologies: while data analysis is concerned with quantities, qualitative data is by definition unquantified . But there is an easy, methodical approach that anyone can take use to get reliable results when performing data analysis for qualitative research. The process consists of 6 steps that I’ll break down in this article:
- Perform interviews(if necessary )
- Gather all documents and transcribe any non-paper records
- Decide whether to either code analytical data, analyze word frequencies, or both
- Decide what interpretive angle you want to take: content analysis , narrative analysis, discourse analysis, framework analysis, and/or grounded theory
- Compile your data in a spreadsheet using document saving techniques (windows and mac)
- Identify trends in words, themes, metaphors, natural patterns, and more
To complete these steps, you will need:
- Microsoft word
- Microsoft excel
- Internet access
You can get the free Intro to Data Analysis eBook to cover the fundamentals and ensure strong progression in all your data endeavors.
What is qualitative research?
Qualitative research is not the same as quantitative research. In short, qualitative research is the interpretation of non-numeric data. It usually aims at drawing conclusions that explain why a phenomenon occurs, rather than that one does occur. Here’s a great quote from a nursing magazine about quantitative vs qualitative research:
“A traditional quantitative study… uses a predetermined (and auditable) set of steps to confirm or refute [a] hypothesis. “In contrast, qualitative research often takes the position that an interpretive understanding is only possible by way of uncovering or deconstructing the meanings of a phenomenon. Thus, a distinction between explaining how something operates (explanation) and why it operates in the manner that it does (interpretation) may be [an] effective way to distinguish quantitative from qualitative analytic processes involved in any particular study.” (bold added) (( EBN ))
Learn to Interpret Your Qualitative Data
This article explain what data analysis is and how to do it. To learn how to interpret the results, visualize, and write an insightful report, sign up for our handbook below.

Step 1a: Data collection methods and techniques in qualitative research: interviews and focus groups
Step 1 is collecting the data that you will need for the analysis. If you are not performing any interviews or focus groups to gather data, then you can skip this step. It’s for people who need to go into the field and collect raw information as part of their qualitative analysis.
Since the whole point of an interview and of qualitative analysis in general is to understand a research question better, you should start by making sure you have a specific, refined research question . Whether you’re a researcher by trade or a data analyst working on one-time project, you must know specifically what you want to understand in order to get results.
Good research questions are specific enough to guide action but open enough to leave room for insight and growth. Examples of good research questions include:
- Good : To what degree does living in a city impact the quality of a person’s life? (open-ended, complex)
- Bad : Does living in a city impact the quality of a person’s life? (closed, simple)
Once you understand the research question, you need to develop a list of interview questions. These questions should likewise be open-ended and provide liberty of expression to the responder. They should support the research question in an active way without prejudicing the response. Examples of good interview questions include:
- Good : Tell me what it’s like to live in a city versus in the country. (open, not leading)
- Bad : Don’t you prefer the city to the country because there are more people? (closed, leading)
Some additional helpful tips include:
- Begin each interview with a neutral question to get the person relaxed
- Limit each question to a single idea
- If you don’t understand, ask for clarity
- Do not pass any judgements
- Do not spend more than 15m on an interview, lest the quality of responses drop
Focus groups
The alternative to interviews is focus groups. Focus groups are a great way for you to get an idea for how people communicate their opinions in a group setting, rather than a one-on-one setting as in interviews.
In short, focus groups are gatherings of small groups of people from representative backgrounds who receive instruction, or “facilitation,” from a focus group leader. Typically, the leader will ask questions to stimulate conversation, reformulate questions to bring the discussion back to focus, and prevent the discussion from turning sour or giving way to bad faith.
Focus group questions should be open-ended like their interview neighbors, and they should stimulate some degree of disagreement. Disagreement often leads to valuable information about differing opinions, as people tend to say what they mean if contradicted.
However, focus group leaders must be careful not to let disagreements escalate, as anger can make people lie to be hurtful or simply to win an argument. And lies are not helpful in data analysis for qualitative research.
Step 1b: Tools for qualitative data collection
When it comes to data analysis for qualitative analysis, the tools you use to collect data should align to some degree with the tools you will use to analyze the data.
As mentioned in the intro, you will be focusing on analysis techniques that only require the traditional Microsoft suite programs: Microsoft Excel and Microsoft Word . At the same time, you can source supplementary tools from various websites, like Text Analyzer and WordCounter.
In short, the tools for qualitative data collection that you need are Excel and Word , as well as web-based free tools like Text Analyzer and WordCounter . These online tools are helpful in the quantitative part of your qualitative research.
Step 2: Gather all documents & transcribe non-written docs
Once you have your interviews and/or focus group transcripts, it’s time to decide if you need other documentation. If you do, you’ll need to gather it all into one place first, then develop a strategy for how to transcribe any non-written documents.
When do you need documentation other than interviews and focus groups? Two situations usually call for documentation. First , if you have little funding , then you can’t afford to run expensive interviews and focus groups.
Second , social science researchers typically focus on documents since their research questions are less concerned with subject-oriented data, while hard science and business researchers typically focus on interviews and focus groups because they want to know what people think, and they want to know today.
Non-written records
Other factors at play include the type of research, the field, and specific research goal. For those who need documentation and to describe non-written records, there are some steps to follow:
- Put all hard copy source documents into a sealed binder (I use plastic paper holders with elastic seals ).
- If you are sourcing directly from printed books or journals, then you will need to digitalize them by scanning them and making them text readable by the computer. To do so, turn all PDFs into Word documents using online tools such as PDF to Word Converter . This process is never full-proof, and it may be a source of error in the data collection, but it’s part of the process.
- If you are sourcing online documents, try as often as possible to get computer-readable PDF documents that you can easily copy/paste or convert. Locked PDFs are essentially a lost cause .
- Transcribe any audio files into written documents. There are free online tools available to help with this, such as 360converter . If you run a test through the system, you’ll see that the output is not 100%. The best way to use this tool is as a first draft generator. You can then correct and complete it with old fashioned, direct transcription.
Step 3: Decide on the type of qualitative research
Before step 3 you should have collected your data, transcribed it all into written-word documents, and compiled it in one place. Now comes the interesting part. You need to decide what you want to get out of your research by choosing an analytic angle, or type of qualitative research.
The available types of qualitative research are as follows. Each of them takes a unique angle that you must choose to get what information you want from the analysis . In addition, each of them has a different impact on the data analysis for qualitative research (coding vs word frequency) that we use.
Content analysis
Narrative analysis, discourse analysis.
- Framework analysis, and/or
Grounded theory
From a high level, content, narrative, and discourse analysis are actionable independent tactics, whereas framework analysis and grounded theory are ways of honing and applying the first three.
- Definition : Content analysis is identify and labelling themes of any kind within a text.
- Focus : Identifying any kind of pattern in written text, transcribed audio, or transcribed video. This could be thematic, word repetition, idea repetition. Most often, the patterns we find are idea that make up an argument.
- Goal : To simplify, standardize, and quickly reference ideas from any given text. Content analysis is a way to pull the main ideas from huge documents for comparison. In this way, it’s more a means to an end.
- Pros : The huge advantage of doing content analysis is that you can quickly process huge amounts of texts using simple coding and word frequency techniques we will look at below. To use a metaphore, it is to qualitative analysis documents what Spark notes are to books.
- Cons : The downside to content analysis is that it’s quite general. If you have a very specific, narrative research question, then tracing “any and all ideas” will not be very helpful to you.
- Definition : Narrative analysis is the reformulation and simplification of interview answers or documentation into small narrative components to identify story-like patterns.
- Focus : Understanding the text based on its narrative components as opposed to themes or other qualities.
- Goal : To reference the text from an angle closer to the nature of texts in order to obtain further insights.
- Pros : Narrative analysis is very useful for getting perspective on a topic in which you’re extremely limited. It can be easy to get tunnel vision when you’re digging for themes and ideas from a reason-centric perspective. Turning to a narrative approach will help you stay grounded. More importantly, it helps reveal different kinds of trends.
- Cons : Narrative analysis adds another layer of subjectivity to the instinctive nature of qualitative research. Many see it as too dependent on the researcher to hold any critical value.
- Definition : Discourse analysis is the textual analysis of naturally occurring speech. Any oral expression must be transcribed before undergoing legitimate discourse analysis.
- Focus : Understanding ideas and themes through language communicated orally rather than pre-processed on paper.
- Goal : To obtain insights from an angle outside the traditional content analysis on text.
- Pros : Provides a considerable advantage in some areas of study in order to understand how people communicate an idea, versus the idea itself. For example, discourse analysis is important in political campaigning. People rarely vote for the candidate who most closely corresponds to his/her beliefs, but rather for the person they like the most.
- Cons : As with narrative analysis, discourse analysis is more subjective in nature than content analysis, which focuses on ideas and patterns. Some do not consider it rigorous enough to be considered a legitimate subset of qualitative analysis, but these people are few.
Framework analysis
- Definition : Framework analysis is a kind of qualitative analysis that includes 5 ordered steps: coding, indexing, charting, mapping, and interpreting . In most ways, framework analysis is a synonym for qualitative analysis — the same thing. The significant difference is the importance it places on the perspective used in the analysis.
- Focus : Understanding patterns in themes and ideas.
- Goal : Creating one specific framework for looking at a text.
- Pros : Framework analysis is helpful when the researcher clearly understands what he/she wants from the project, as it’s a limitation approach. Since each of its step has defined parameters, framework analysis is very useful for teamwork.
- Cons : It can lead to tunnel vision.
- Definition : The use of content, narrative, and discourse analysis to examine a single case, in the hopes that discoveries from that case will lead to a foundational theory used to examine other like cases.
- Focus : A vast approach using multiple techniques in order to establish patterns.
- Goal : To develop a foundational theory.
- Pros : When successful, grounded theories can revolutionize entire fields of study.
- Cons : It’s very difficult to establish ground theories, and there’s an enormous amount of risk involved.
Step 4: Coding, word frequency, or both
Coding in data analysis for qualitative research is the process of writing 2-5 word codes that summarize at least 1 paragraphs of text (not writing computer code). This allows researchers to keep track of and analyze those codes. On the other hand, word frequency is the process of counting the presence and orientation of words within a text, which makes it the quantitative element in qualitative data analysis.
Video example of coding for data analysis in qualitative research
In short, coding in the context of data analysis for qualitative research follows 2 steps (video below):
- Reading through the text one time
- Adding 2-5 word summaries each time a significant theme or idea appears
Let’s look at a brief example of how to code for qualitative research in this video:
Click here for a link to the source text. 1
Example of word frequency processing
And word frequency is the process of finding a specific word or identifying the most common words through 3 steps:
- Decide if you want to find 1 word or identify the most common ones
- Use word’s “Replace” function to find a word or phrase
- Use Text Analyzer to find the most common terms
Here’s another look at word frequency processing and how you to do it. Let’s look at the same example above, but from a quantitative perspective.
Imagine we are already familiar with melanoma and KITs , and we want to analyze the text based on these keywords. One thing we can do is look for these words using the Replace function in word
- Locate the search bar
- Click replace
- Type in the word
- See the total results
Here’s a brief video example:
Another option is to use an online Text Analyzer. This methodology won’t help us find a specific word, but it will help us discover the top performing phrases and words. All you need to do it put in a link to a target page or paste a text. I pasted the abstract from our source text, and what turns up is as expected. Here’s a picture:

Step 5: Compile your data in a spreadsheet
After you have some coded data in the word document, you need to get it into excel for analysis. This process requires saving the word doc as an .htm extension, which makes it a website. Once you have the website, it’s as simple as opening that page, scrolling to the bottom, and copying/pasting the comments, or codes, into an excel document.
You will need to wrangle the data slightly in order to make it readable in excel. I’ve made a video to explain this process and places it below.
Step 6: Identify trends & analyze!
There are literally thousands of different ways to analyze qualitative data, and in most situations, the best technique depends on the information you want to get out of the research.
Nevertheless, there are a few go-to techniques. The most important of this is occurrences . In this short video, we finish the example from above by counting the number of times our codes appear. In this way, it’s very similar to word frequency (discussed above).
A few other options include:
- Ranking each code on a set of relevant criteria and clustering
- Pure cluster analysis
- Causal analysis
We cover different types of analysis like this on the website, so be sure to check out other articles on the home page .
How to analyze qualitative data from an interview
To analyze qualitative data from an interview , follow the same 6 steps for quantitative data analysis:
- Perform the interviews
- Transcribe the interviews onto paper
- Decide whether to either code analytical data (open, axial, selective), analyze word frequencies, or both
- Compile your data in a spreadsheet using document saving techniques (for windows and mac)
- Source text [ ↩ ]
About the Author
Noah is the founder & Editor-in-Chief at AnalystAnswers. He is a transatlantic professional and entrepreneur with 5+ years of corporate finance and data analytics experience, as well as 3+ years in consumer financial products and business software. He started AnalystAnswers to provide aspiring professionals with accessible explanations of otherwise dense finance and data concepts. Noah believes everyone can benefit from an analytical mindset in growing digital world. When he's not busy at work, Noah likes to explore new European cities, exercise, and spend time with friends and family.
File available immediately.
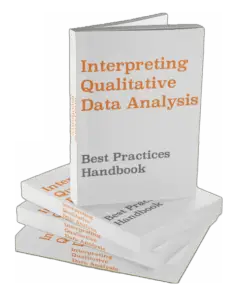
Notice: JavaScript is required for this content.


Qualitative Data Analysis Methods 101:
The “big 6” methods + examples.
By: Kerryn Warren (PhD) | Reviewed By: Eunice Rautenbach (D.Tech) | May 2020 (Updated April 2023)
Qualitative data analysis methods. Wow, that’s a mouthful.
If you’re new to the world of research, qualitative data analysis can look rather intimidating. So much bulky terminology and so many abstract, fluffy concepts. It certainly can be a minefield!
Don’t worry – in this post, we’ll unpack the most popular analysis methods , one at a time, so that you can approach your analysis with confidence and competence – whether that’s for a dissertation, thesis or really any kind of research project.
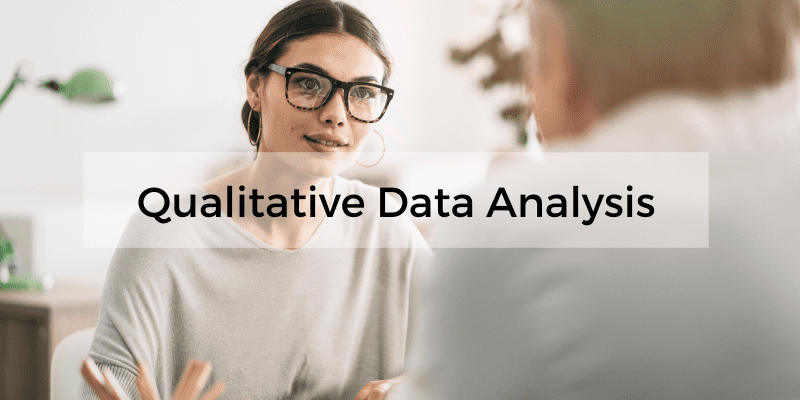
What (exactly) is qualitative data analysis?
To understand qualitative data analysis, we need to first understand qualitative data – so let’s step back and ask the question, “what exactly is qualitative data?”.
Qualitative data refers to pretty much any data that’s “not numbers” . In other words, it’s not the stuff you measure using a fixed scale or complex equipment, nor do you analyse it using complex statistics or mathematics.
So, if it’s not numbers, what is it?
Words, you guessed? Well… sometimes , yes. Qualitative data can, and often does, take the form of interview transcripts, documents and open-ended survey responses – but it can also involve the interpretation of images and videos. In other words, qualitative isn’t just limited to text-based data.
So, how’s that different from quantitative data, you ask?
Simply put, qualitative research focuses on words, descriptions, concepts or ideas – while quantitative research focuses on numbers and statistics . Qualitative research investigates the “softer side” of things to explore and describe , while quantitative research focuses on the “hard numbers”, to measure differences between variables and the relationships between them. If you’re keen to learn more about the differences between qual and quant, we’ve got a detailed post over here .
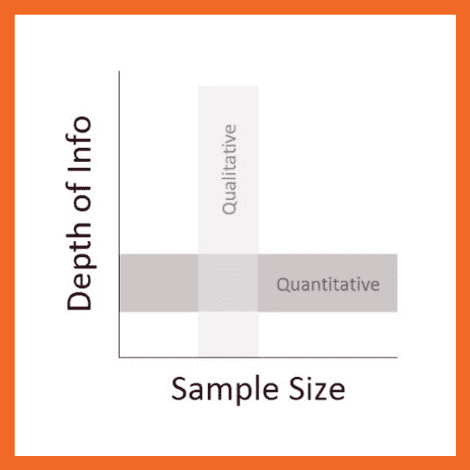
So, qualitative analysis is easier than quantitative, right?
Not quite. In many ways, qualitative data can be challenging and time-consuming to analyse and interpret. At the end of your data collection phase (which itself takes a lot of time), you’ll likely have many pages of text-based data or hours upon hours of audio to work through. You might also have subtle nuances of interactions or discussions that have danced around in your mind, or that you scribbled down in messy field notes. All of this needs to work its way into your analysis.
Making sense of all of this is no small task and you shouldn’t underestimate it. Long story short – qualitative analysis can be a lot of work! Of course, quantitative analysis is no piece of cake either, but it’s important to recognise that qualitative analysis still requires a significant investment in terms of time and effort.
Need a helping hand?
In this post, we’ll explore qualitative data analysis by looking at some of the most common analysis methods we encounter. We’re not going to cover every possible qualitative method and we’re not going to go into heavy detail – we’re just going to give you the big picture. That said, we will of course includes links to loads of extra resources so that you can learn more about whichever analysis method interests you.
Without further delay, let’s get into it.
The “Big 6” Qualitative Analysis Methods
There are many different types of qualitative data analysis, all of which serve different purposes and have unique strengths and weaknesses . We’ll start by outlining the analysis methods and then we’ll dive into the details for each.
The 6 most popular methods (or at least the ones we see at Grad Coach) are:
- Content analysis
- Narrative analysis
- Discourse analysis
- Thematic analysis
- Grounded theory (GT)
- Interpretive phenomenological analysis (IPA)
Let’s take a look at each of them…
QDA Method #1: Qualitative Content Analysis
Content analysis is possibly the most common and straightforward QDA method. At the simplest level, content analysis is used to evaluate patterns within a piece of content (for example, words, phrases or images) or across multiple pieces of content or sources of communication. For example, a collection of newspaper articles or political speeches.
With content analysis, you could, for instance, identify the frequency with which an idea is shared or spoken about – like the number of times a Kardashian is mentioned on Twitter. Or you could identify patterns of deeper underlying interpretations – for instance, by identifying phrases or words in tourist pamphlets that highlight India as an ancient country.
Because content analysis can be used in such a wide variety of ways, it’s important to go into your analysis with a very specific question and goal, or you’ll get lost in the fog. With content analysis, you’ll group large amounts of text into codes , summarise these into categories, and possibly even tabulate the data to calculate the frequency of certain concepts or variables. Because of this, content analysis provides a small splash of quantitative thinking within a qualitative method.
Naturally, while content analysis is widely useful, it’s not without its drawbacks . One of the main issues with content analysis is that it can be very time-consuming , as it requires lots of reading and re-reading of the texts. Also, because of its multidimensional focus on both qualitative and quantitative aspects, it is sometimes accused of losing important nuances in communication.
Content analysis also tends to concentrate on a very specific timeline and doesn’t take into account what happened before or after that timeline. This isn’t necessarily a bad thing though – just something to be aware of. So, keep these factors in mind if you’re considering content analysis. Every analysis method has its limitations , so don’t be put off by these – just be aware of them ! If you’re interested in learning more about content analysis, the video below provides a good starting point.
QDA Method #2: Narrative Analysis
As the name suggests, narrative analysis is all about listening to people telling stories and analysing what that means . Since stories serve a functional purpose of helping us make sense of the world, we can gain insights into the ways that people deal with and make sense of reality by analysing their stories and the ways they’re told.
You could, for example, use narrative analysis to explore whether how something is being said is important. For instance, the narrative of a prisoner trying to justify their crime could provide insight into their view of the world and the justice system. Similarly, analysing the ways entrepreneurs talk about the struggles in their careers or cancer patients telling stories of hope could provide powerful insights into their mindsets and perspectives . Simply put, narrative analysis is about paying attention to the stories that people tell – and more importantly, the way they tell them.
Of course, the narrative approach has its weaknesses , too. Sample sizes are generally quite small due to the time-consuming process of capturing narratives. Because of this, along with the multitude of social and lifestyle factors which can influence a subject, narrative analysis can be quite difficult to reproduce in subsequent research. This means that it’s difficult to test the findings of some of this research.
Similarly, researcher bias can have a strong influence on the results here, so you need to be particularly careful about the potential biases you can bring into your analysis when using this method. Nevertheless, narrative analysis is still a very useful qualitative analysis method – just keep these limitations in mind and be careful not to draw broad conclusions . If you’re keen to learn more about narrative analysis, the video below provides a great introduction to this qualitative analysis method.
QDA Method #3: Discourse Analysis
Discourse is simply a fancy word for written or spoken language or debate . So, discourse analysis is all about analysing language within its social context. In other words, analysing language – such as a conversation, a speech, etc – within the culture and society it takes place. For example, you could analyse how a janitor speaks to a CEO, or how politicians speak about terrorism.
To truly understand these conversations or speeches, the culture and history of those involved in the communication are important factors to consider. For example, a janitor might speak more casually with a CEO in a company that emphasises equality among workers. Similarly, a politician might speak more about terrorism if there was a recent terrorist incident in the country.
So, as you can see, by using discourse analysis, you can identify how culture , history or power dynamics (to name a few) have an effect on the way concepts are spoken about. So, if your research aims and objectives involve understanding culture or power dynamics, discourse analysis can be a powerful method.
Because there are many social influences in terms of how we speak to each other, the potential use of discourse analysis is vast . Of course, this also means it’s important to have a very specific research question (or questions) in mind when analysing your data and looking for patterns and themes, or you might land up going down a winding rabbit hole.
Discourse analysis can also be very time-consuming as you need to sample the data to the point of saturation – in other words, until no new information and insights emerge. But this is, of course, part of what makes discourse analysis such a powerful technique. So, keep these factors in mind when considering this QDA method. Again, if you’re keen to learn more, the video below presents a good starting point.
QDA Method #4: Thematic Analysis
Thematic analysis looks at patterns of meaning in a data set – for example, a set of interviews or focus group transcripts. But what exactly does that… mean? Well, a thematic analysis takes bodies of data (which are often quite large) and groups them according to similarities – in other words, themes . These themes help us make sense of the content and derive meaning from it.
Let’s take a look at an example.
With thematic analysis, you could analyse 100 online reviews of a popular sushi restaurant to find out what patrons think about the place. By reviewing the data, you would then identify the themes that crop up repeatedly within the data – for example, “fresh ingredients” or “friendly wait staff”.
So, as you can see, thematic analysis can be pretty useful for finding out about people’s experiences , views, and opinions . Therefore, if your research aims and objectives involve understanding people’s experience or view of something, thematic analysis can be a great choice.
Since thematic analysis is a bit of an exploratory process, it’s not unusual for your research questions to develop , or even change as you progress through the analysis. While this is somewhat natural in exploratory research, it can also be seen as a disadvantage as it means that data needs to be re-reviewed each time a research question is adjusted. In other words, thematic analysis can be quite time-consuming – but for a good reason. So, keep this in mind if you choose to use thematic analysis for your project and budget extra time for unexpected adjustments.
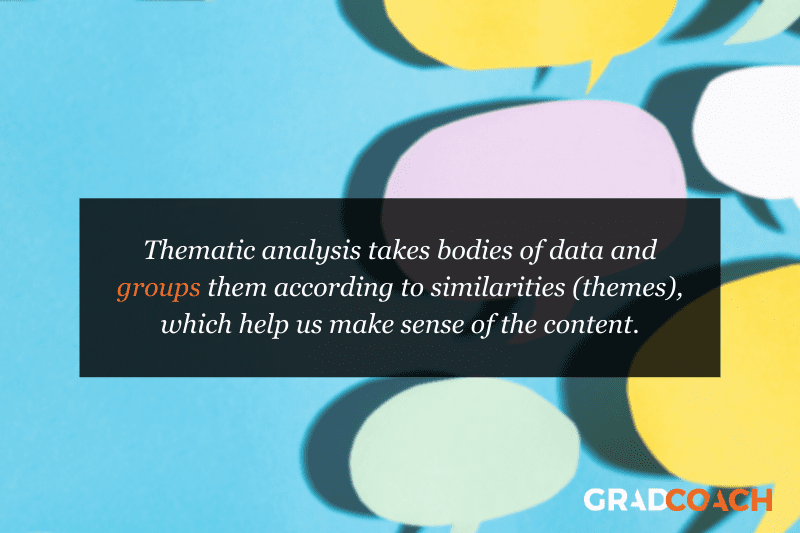
QDA Method #5: Grounded theory (GT)
Grounded theory is a powerful qualitative analysis method where the intention is to create a new theory (or theories) using the data at hand, through a series of “ tests ” and “ revisions ”. Strictly speaking, GT is more a research design type than an analysis method, but we’ve included it here as it’s often referred to as a method.
What’s most important with grounded theory is that you go into the analysis with an open mind and let the data speak for itself – rather than dragging existing hypotheses or theories into your analysis. In other words, your analysis must develop from the ground up (hence the name).
Let’s look at an example of GT in action.
Assume you’re interested in developing a theory about what factors influence students to watch a YouTube video about qualitative analysis. Using Grounded theory , you’d start with this general overarching question about the given population (i.e., graduate students). First, you’d approach a small sample – for example, five graduate students in a department at a university. Ideally, this sample would be reasonably representative of the broader population. You’d interview these students to identify what factors lead them to watch the video.
After analysing the interview data, a general pattern could emerge. For example, you might notice that graduate students are more likely to read a post about qualitative methods if they are just starting on their dissertation journey, or if they have an upcoming test about research methods.
From here, you’ll look for another small sample – for example, five more graduate students in a different department – and see whether this pattern holds true for them. If not, you’ll look for commonalities and adapt your theory accordingly. As this process continues, the theory would develop . As we mentioned earlier, what’s important with grounded theory is that the theory develops from the data – not from some preconceived idea.
So, what are the drawbacks of grounded theory? Well, some argue that there’s a tricky circularity to grounded theory. For it to work, in principle, you should know as little as possible regarding the research question and population, so that you reduce the bias in your interpretation. However, in many circumstances, it’s also thought to be unwise to approach a research question without knowledge of the current literature . In other words, it’s a bit of a “chicken or the egg” situation.
Regardless, grounded theory remains a popular (and powerful) option. Naturally, it’s a very useful method when you’re researching a topic that is completely new or has very little existing research about it, as it allows you to start from scratch and work your way from the ground up .
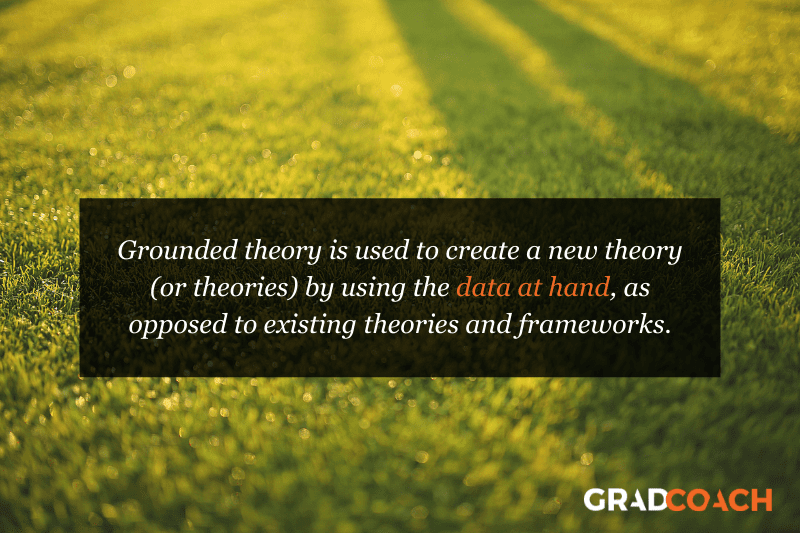
QDA Method #6: Interpretive Phenomenological Analysis (IPA)
Interpretive. Phenomenological. Analysis. IPA . Try saying that three times fast…
Let’s just stick with IPA, okay?
IPA is designed to help you understand the personal experiences of a subject (for example, a person or group of people) concerning a major life event, an experience or a situation . This event or experience is the “phenomenon” that makes up the “P” in IPA. Such phenomena may range from relatively common events – such as motherhood, or being involved in a car accident – to those which are extremely rare – for example, someone’s personal experience in a refugee camp. So, IPA is a great choice if your research involves analysing people’s personal experiences of something that happened to them.
It’s important to remember that IPA is subject – centred . In other words, it’s focused on the experiencer . This means that, while you’ll likely use a coding system to identify commonalities, it’s important not to lose the depth of experience or meaning by trying to reduce everything to codes. Also, keep in mind that since your sample size will generally be very small with IPA, you often won’t be able to draw broad conclusions about the generalisability of your findings. But that’s okay as long as it aligns with your research aims and objectives.
Another thing to be aware of with IPA is personal bias . While researcher bias can creep into all forms of research, self-awareness is critically important with IPA, as it can have a major impact on the results. For example, a researcher who was a victim of a crime himself could insert his own feelings of frustration and anger into the way he interprets the experience of someone who was kidnapped. So, if you’re going to undertake IPA, you need to be very self-aware or you could muddy the analysis.
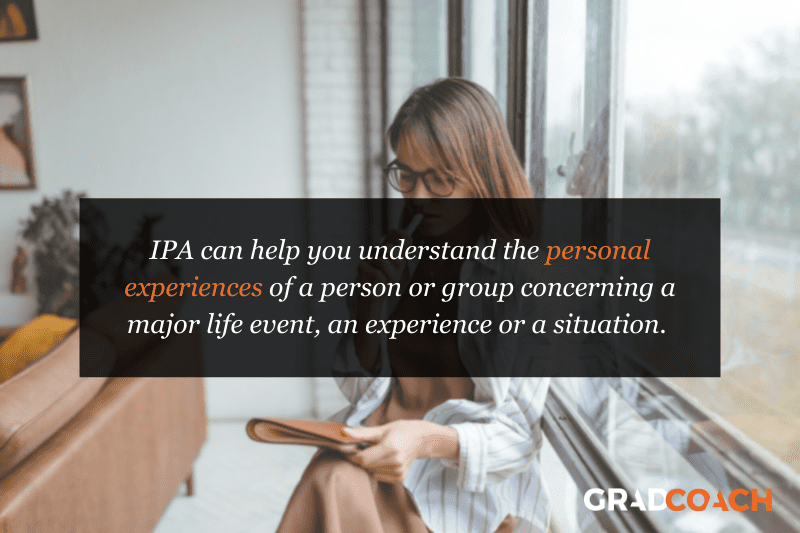
How to choose the right analysis method
In light of all of the qualitative analysis methods we’ve covered so far, you’re probably asking yourself the question, “ How do I choose the right one? ”
Much like all the other methodological decisions you’ll need to make, selecting the right qualitative analysis method largely depends on your research aims, objectives and questions . In other words, the best tool for the job depends on what you’re trying to build. For example:
- Perhaps your research aims to analyse the use of words and what they reveal about the intention of the storyteller and the cultural context of the time.
- Perhaps your research aims to develop an understanding of the unique personal experiences of people that have experienced a certain event, or
- Perhaps your research aims to develop insight regarding the influence of a certain culture on its members.
As you can probably see, each of these research aims are distinctly different , and therefore different analysis methods would be suitable for each one. For example, narrative analysis would likely be a good option for the first aim, while grounded theory wouldn’t be as relevant.
It’s also important to remember that each method has its own set of strengths, weaknesses and general limitations. No single analysis method is perfect . So, depending on the nature of your research, it may make sense to adopt more than one method (this is called triangulation ). Keep in mind though that this will of course be quite time-consuming.
As we’ve seen, all of the qualitative analysis methods we’ve discussed make use of coding and theme-generating techniques, but the intent and approach of each analysis method differ quite substantially. So, it’s very important to come into your research with a clear intention before you decide which analysis method (or methods) to use.
Start by reviewing your research aims , objectives and research questions to assess what exactly you’re trying to find out – then select a qualitative analysis method that fits. Never pick a method just because you like it or have experience using it – your analysis method (or methods) must align with your broader research aims and objectives.
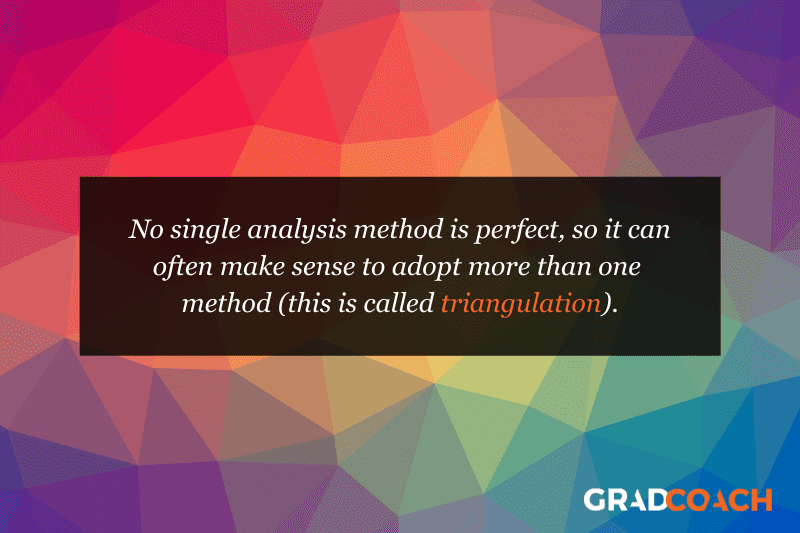
Let’s recap on QDA methods…
In this post, we looked at six popular qualitative data analysis methods:
- First, we looked at content analysis , a straightforward method that blends a little bit of quant into a primarily qualitative analysis.
- Then we looked at narrative analysis , which is about analysing how stories are told.
- Next up was discourse analysis – which is about analysing conversations and interactions.
- Then we moved on to thematic analysis – which is about identifying themes and patterns.
- From there, we went south with grounded theory – which is about starting from scratch with a specific question and using the data alone to build a theory in response to that question.
- And finally, we looked at IPA – which is about understanding people’s unique experiences of a phenomenon.
Of course, these aren’t the only options when it comes to qualitative data analysis, but they’re a great starting point if you’re dipping your toes into qualitative research for the first time.
If you’re still feeling a bit confused, consider our private coaching service , where we hold your hand through the research process to help you develop your best work.
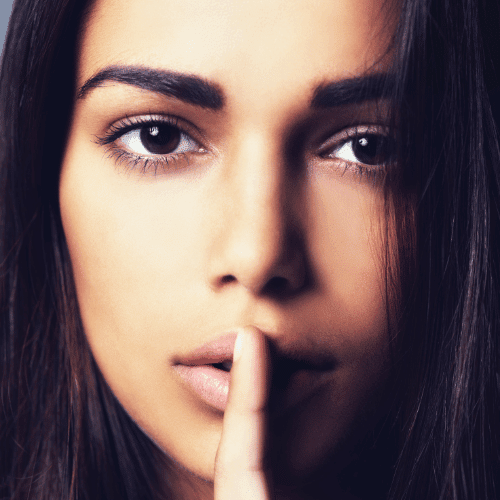
Psst... there’s more!
This post was based on one of our popular Research Bootcamps . If you're working on a research project, you'll definitely want to check this out ...
You Might Also Like:
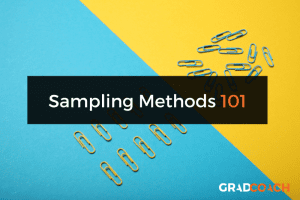
84 Comments
This has been very helpful. Thank you.
Thank you madam,
Thank you so much for this information
I wonder it so clear for understand and good for me. can I ask additional query?
Very insightful and useful
Good work done with clear explanations. Thank you.
Thanks so much for the write-up, it’s really good.
Thanks madam . It is very important .
thank you very good
This has been very well explained in simple language . It is useful even for a new researcher.
Great to hear that. Good luck with your qualitative data analysis, Pramod!
This is very useful information. And it was very a clear language structured presentation. Thanks a lot.
Thank you so much.
very informative sequential presentation
Precise explanation of method.
Hi, may we use 2 data analysis methods in our qualitative research?
Thanks for your comment. Most commonly, one would use one type of analysis method, but it depends on your research aims and objectives.
You explained it in very simple language, everyone can understand it. Thanks so much.
Thank you very much, this is very helpful. It has been explained in a very simple manner that even a layman understands
Thank nicely explained can I ask is Qualitative content analysis the same as thematic analysis?
Thanks for your comment. No, QCA and thematic are two different types of analysis. This article might help clarify – https://onlinelibrary.wiley.com/doi/10.1111/nhs.12048
This is my first time to come across a well explained data analysis. so helpful.
I have thoroughly enjoyed your explanation of the six qualitative analysis methods. This is very helpful. Thank you!
Thank you very much, this is well explained and useful
i need a citation of your book.
Thanks a lot , remarkable indeed, enlighting to the best
Hi Derek, What other theories/methods would you recommend when the data is a whole speech?
Keep writing useful artikel.
It is important concept about QDA and also the way to express is easily understandable, so thanks for all.
Thank you, this is well explained and very useful.
Very helpful .Thanks.
Hi there! Very well explained. Simple but very useful style of writing. Please provide the citation of the text. warm regards
The session was very helpful and insightful. Thank you
This was very helpful and insightful. Easy to read and understand
As a professional academic writer, this has been so informative and educative. Keep up the good work Grad Coach you are unmatched with quality content for sure.
Keep up the good work Grad Coach you are unmatched with quality content for sure.
Its Great and help me the most. A Million Thanks you Dr.
It is a very nice work
Very insightful. Please, which of this approach could be used for a research that one is trying to elicit students’ misconceptions in a particular concept ?
This is Amazing and well explained, thanks
great overview
What do we call a research data analysis method that one use to advise or determining the best accounting tool or techniques that should be adopted in a company.
Informative video, explained in a clear and simple way. Kudos
Waoo! I have chosen method wrong for my data analysis. But I can revise my work according to this guide. Thank you so much for this helpful lecture.
This has been very helpful. It gave me a good view of my research objectives and how to choose the best method. Thematic analysis it is.
Very helpful indeed. Thanku so much for the insight.
This was incredibly helpful.
Very helpful.
very educative
Nicely written especially for novice academic researchers like me! Thank you.
choosing a right method for a paper is always a hard job for a student, this is a useful information, but it would be more useful personally for me, if the author provide me with a little bit more information about the data analysis techniques in type of explanatory research. Can we use qualitative content analysis technique for explanatory research ? or what is the suitable data analysis method for explanatory research in social studies?
that was very helpful for me. because these details are so important to my research. thank you very much
I learnt a lot. Thank you
Relevant and Informative, thanks !
Well-planned and organized, thanks much! 🙂
I have reviewed qualitative data analysis in a simplest way possible. The content will highly be useful for developing my book on qualitative data analysis methods. Cheers!
Clear explanation on qualitative and how about Case study
This was helpful. Thank you
This was really of great assistance, it was just the right information needed. Explanation very clear and follow.
Wow, Thanks for making my life easy
This was helpful thanks .
Very helpful…. clear and written in an easily understandable manner. Thank you.
This was so helpful as it was easy to understand. I’m a new to research thank you so much.
so educative…. but Ijust want to know which method is coding of the qualitative or tallying done?
Thank you for the great content, I have learnt a lot. So helpful
precise and clear presentation with simple language and thank you for that.
very informative content, thank you.
You guys are amazing on YouTube on this platform. Your teachings are great, educative, and informative. kudos!
Brilliant Delivery. You made a complex subject seem so easy. Well done.
Beautifully explained.
Thanks a lot
Is there a video the captures the practical process of coding using automated applications?
Thanks for the comment. We don’t recommend using automated applications for coding, as they are not sufficiently accurate in our experience.
content analysis can be qualitative research?
THANK YOU VERY MUCH.
Thank you very much for such a wonderful content
do you have any material on Data collection
What a powerful explanation of the QDA methods. Thank you.
Great explanation both written and Video. i have been using of it on a day to day working of my thesis project in accounting and finance. Thank you very much for your support.
very helpful, thank you so much
Submit a Comment Cancel reply
Your email address will not be published. Required fields are marked *
Save my name, email, and website in this browser for the next time I comment.
- Print Friendly
- AI & NLP
- Churn & Loyalty
- Customer Experience
- Customer Journeys
- Customer Metrics
- Feedback Analysis
- Product Experience
- Product Updates
- Sentiment Analysis
- Surveys & Feedback Collection
- Try Thematic
Welcome to the community
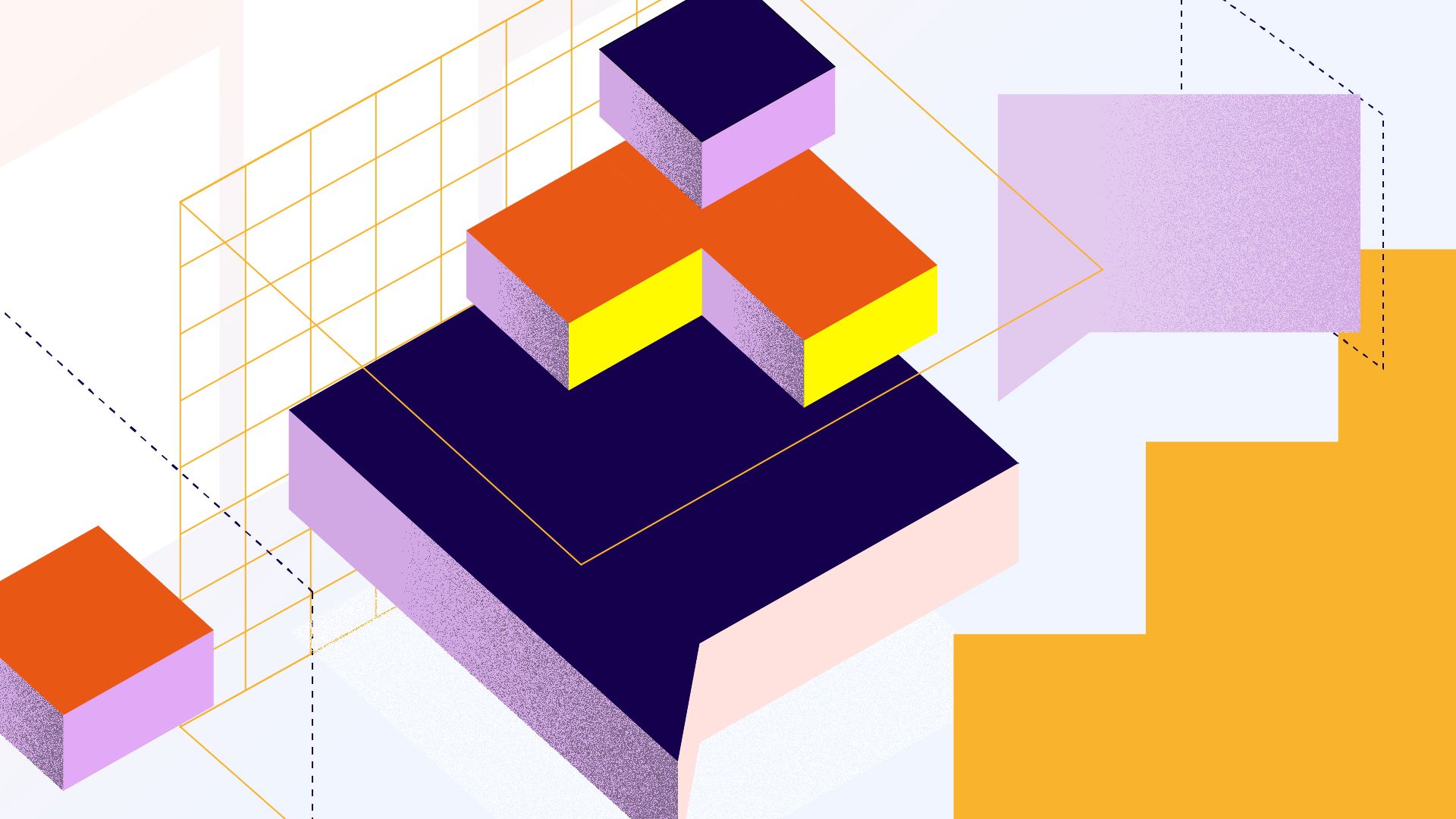
Qualitative Data Analysis: Step-by-Step Guide (Manual vs. Automatic)
When we conduct qualitative methods of research, need to explain changes in metrics or understand people's opinions, we always turn to qualitative data. Qualitative data is typically generated through:
- Interview transcripts
- Surveys with open-ended questions
- Contact center transcripts
- Texts and documents
- Audio and video recordings
- Observational notes
Compared to quantitative data, which captures structured information, qualitative data is unstructured and has more depth. It can answer our questions, can help formulate hypotheses and build understanding.
It's important to understand the differences between quantitative data & qualitative data . But unfortunately, analyzing qualitative data is difficult. While tools like Excel, Tableau and PowerBI crunch and visualize quantitative data with ease, there are a limited number of mainstream tools for analyzing qualitative data . The majority of qualitative data analysis still happens manually.
That said, there are two new trends that are changing this. First, there are advances in natural language processing (NLP) which is focused on understanding human language. Second, there is an explosion of user-friendly software designed for both researchers and businesses. Both help automate the qualitative data analysis process.
In this post we want to teach you how to conduct a successful qualitative data analysis. There are two primary qualitative data analysis methods; manual & automatic. We will teach you how to conduct the analysis manually, and also, automatically using software solutions powered by NLP. We’ll guide you through the steps to conduct a manual analysis, and look at what is involved and the role technology can play in automating this process.
More businesses are switching to fully-automated analysis of qualitative customer data because it is cheaper, faster, and just as accurate. Primarily, businesses purchase subscriptions to feedback analytics platforms so that they can understand customer pain points and sentiment.
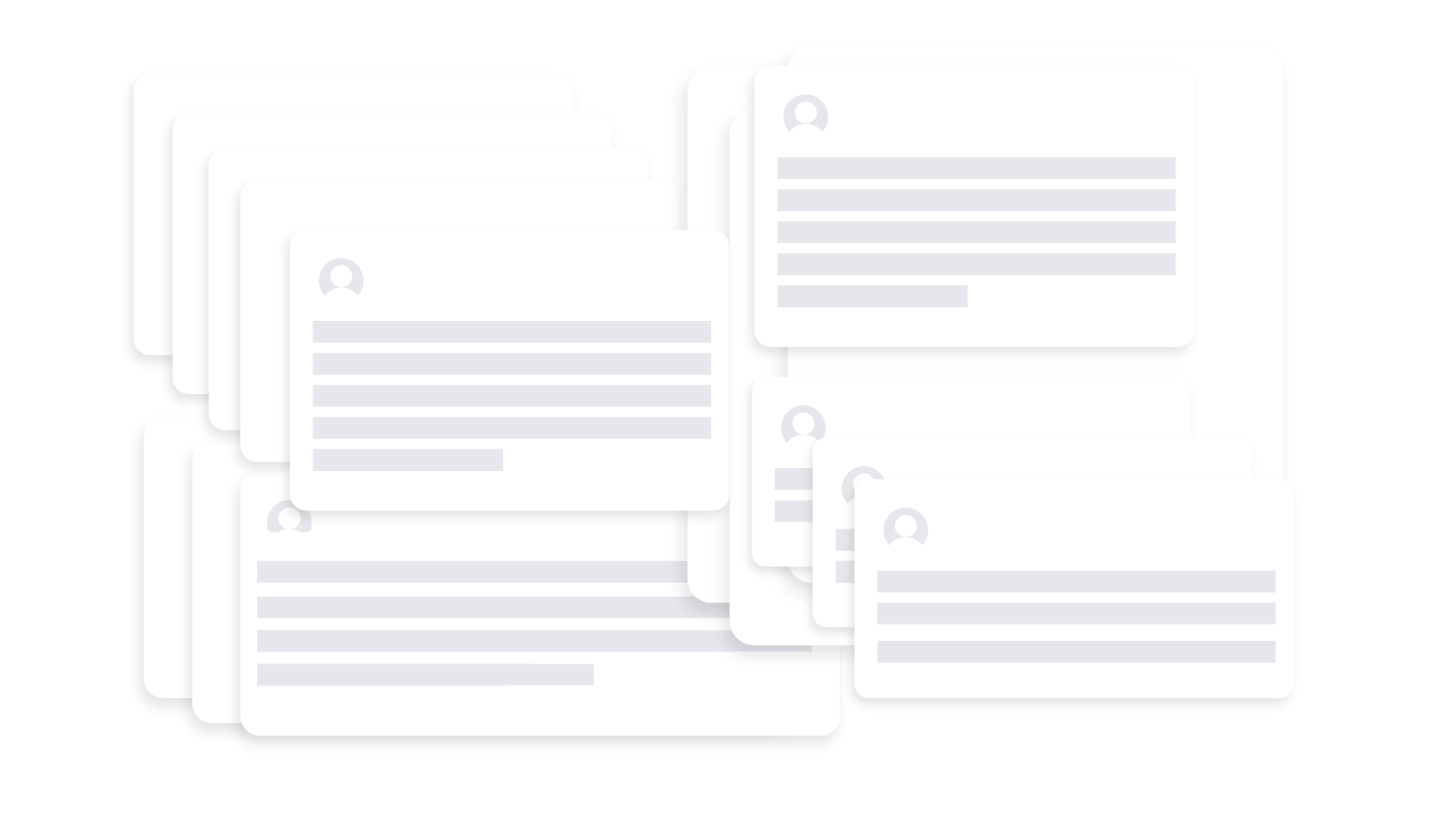
We’ll take you through 5 steps to conduct a successful qualitative data analysis. Within each step we will highlight the key difference between the manual, and automated approach of qualitative researchers. Here's an overview of the steps:
The 5 steps to doing qualitative data analysis
- Gathering and collecting your qualitative data
- Organizing and connecting into your qualitative data
- Coding your qualitative data
- Analyzing the qualitative data for insights
- Reporting on the insights derived from your analysis
What is Qualitative Data Analysis?
Qualitative data analysis is a process of gathering, structuring and interpreting qualitative data to understand what it represents.
Qualitative data is non-numerical and unstructured. Qualitative data generally refers to text, such as open-ended responses to survey questions or user interviews, but also includes audio, photos and video.
Businesses often perform qualitative data analysis on customer feedback. And within this context, qualitative data generally refers to verbatim text data collected from sources such as reviews, complaints, chat messages, support centre interactions, customer interviews, case notes or social media comments.
How is qualitative data analysis different from quantitative data analysis?
Understanding the differences between quantitative & qualitative data is important. When it comes to analyzing data, Qualitative Data Analysis serves a very different role to Quantitative Data Analysis. But what sets them apart?
Qualitative Data Analysis dives into the stories hidden in non-numerical data such as interviews, open-ended survey answers, or notes from observations. It uncovers the ‘whys’ and ‘hows’ giving a deep understanding of people’s experiences and emotions.
Quantitative Data Analysis on the other hand deals with numerical data, using statistics to measure differences, identify preferred options, and pinpoint root causes of issues. It steps back to address questions like "how many" or "what percentage" to offer broad insights we can apply to larger groups.
In short, Qualitative Data Analysis is like a microscope, helping us understand specific detail. Quantitative Data Analysis is like the telescope, giving us a broader perspective. Both are important, working together to decode data for different objectives.
Qualitative Data Analysis methods
Once all the data has been captured, there are a variety of analysis techniques available and the choice is determined by your specific research objectives and the kind of data you’ve gathered. Common qualitative data analysis methods include:
Content Analysis
This is a popular approach to qualitative data analysis. Other qualitative analysis techniques may fit within the broad scope of content analysis. Thematic analysis is a part of the content analysis. Content analysis is used to identify the patterns that emerge from text, by grouping content into words, concepts, and themes. Content analysis is useful to quantify the relationship between all of the grouped content. The Columbia School of Public Health has a detailed breakdown of content analysis .
Narrative Analysis
Narrative analysis focuses on the stories people tell and the language they use to make sense of them. It is particularly useful in qualitative research methods where customer stories are used to get a deep understanding of customers’ perspectives on a specific issue. A narrative analysis might enable us to summarize the outcomes of a focused case study.
Discourse Analysis
Discourse analysis is used to get a thorough understanding of the political, cultural and power dynamics that exist in specific situations. The focus of discourse analysis here is on the way people express themselves in different social contexts. Discourse analysis is commonly used by brand strategists who hope to understand why a group of people feel the way they do about a brand or product.
Thematic Analysis
Thematic analysis is used to deduce the meaning behind the words people use. This is accomplished by discovering repeating themes in text. These meaningful themes reveal key insights into data and can be quantified, particularly when paired with sentiment analysis . Often, the outcome of thematic analysis is a code frame that captures themes in terms of codes, also called categories. So the process of thematic analysis is also referred to as “coding”. A common use-case for thematic analysis in companies is analysis of customer feedback.
Grounded Theory
Grounded theory is a useful approach when little is known about a subject. Grounded theory starts by formulating a theory around a single data case. This means that the theory is “grounded”. Grounded theory analysis is based on actual data, and not entirely speculative. Then additional cases can be examined to see if they are relevant and can add to the original grounded theory.
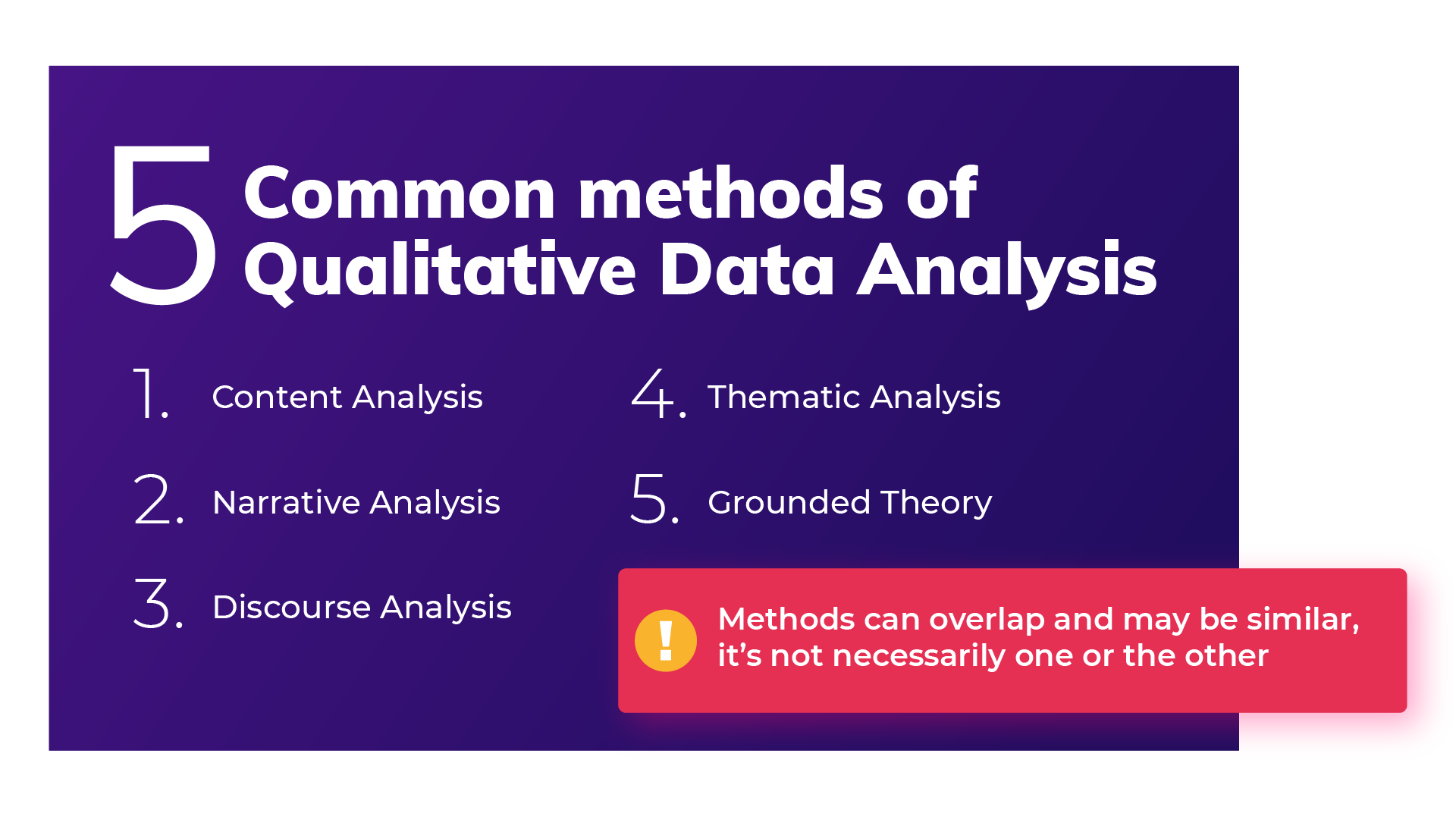
Challenges of Qualitative Data Analysis
While Qualitative Data Analysis offers rich insights, it comes with its challenges. Each unique QDA method has its unique hurdles. Let’s take a look at the challenges researchers and analysts might face, depending on the chosen method.
- Time and Effort (Narrative Analysis): Narrative analysis, which focuses on personal stories, demands patience. Sifting through lengthy narratives to find meaningful insights can be time-consuming, requires dedicated effort.
- Being Objective (Grounded Theory): Grounded theory, building theories from data, faces the challenges of personal biases. Staying objective while interpreting data is crucial, ensuring conclusions are rooted in the data itself.
- Complexity (Thematic Analysis): Thematic analysis involves identifying themes within data, a process that can be intricate. Categorizing and understanding themes can be complex, especially when each piece of data varies in context and structure. Thematic Analysis software can simplify this process.
- Generalizing Findings (Narrative Analysis): Narrative analysis, dealing with individual stories, makes drawing broad challenging. Extending findings from a single narrative to a broader context requires careful consideration.
- Managing Data (Thematic Analysis): Thematic analysis involves organizing and managing vast amounts of unstructured data, like interview transcripts. Managing this can be a hefty task, requiring effective data management strategies.
- Skill Level (Grounded Theory): Grounded theory demands specific skills to build theories from the ground up. Finding or training analysts with these skills poses a challenge, requiring investment in building expertise.
Benefits of qualitative data analysis
Qualitative Data Analysis (QDA) is like a versatile toolkit, offering a tailored approach to understanding your data. The benefits it offers are as diverse as the methods. Let’s explore why choosing the right method matters.
- Tailored Methods for Specific Needs: QDA isn't one-size-fits-all. Depending on your research objectives and the type of data at hand, different methods offer unique benefits. If you want emotive customer stories, narrative analysis paints a strong picture. When you want to explain a score, thematic analysis reveals insightful patterns
- Flexibility with Thematic Analysis: thematic analysis is like a chameleon in the toolkit of QDA. It adapts well to different types of data and research objectives, making it a top choice for any qualitative analysis.
- Deeper Understanding, Better Products: QDA helps you dive into people's thoughts and feelings. This deep understanding helps you build products and services that truly matches what people want, ensuring satisfied customers
- Finding the Unexpected: Qualitative data often reveals surprises that we miss in quantitative data. QDA offers us new ideas and perspectives, for insights we might otherwise miss.
- Building Effective Strategies: Insights from QDA are like strategic guides. They help businesses in crafting plans that match people’s desires.
- Creating Genuine Connections: Understanding people’s experiences lets businesses connect on a real level. This genuine connection helps build trust and loyalty, priceless for any business.
How to do Qualitative Data Analysis: 5 steps
Now we are going to show how you can do your own qualitative data analysis. We will guide you through this process step by step. As mentioned earlier, you will learn how to do qualitative data analysis manually , and also automatically using modern qualitative data and thematic analysis software.
To get best value from the analysis process and research process, it’s important to be super clear about the nature and scope of the question that’s being researched. This will help you select the research collection channels that are most likely to help you answer your question.
Depending on if you are a business looking to understand customer sentiment, or an academic surveying a school, your approach to qualitative data analysis will be unique.
Once you’re clear, there’s a sequence to follow. And, though there are differences in the manual and automatic approaches, the process steps are mostly the same.
The use case for our step-by-step guide is a company looking to collect data (customer feedback data), and analyze the customer feedback - in order to improve customer experience. By analyzing the customer feedback the company derives insights about their business and their customers. You can follow these same steps regardless of the nature of your research. Let’s get started.
Step 1: Gather your qualitative data and conduct research (Conduct qualitative research)
The first step of qualitative research is to do data collection. Put simply, data collection is gathering all of your data for analysis. A common situation is when qualitative data is spread across various sources.
Classic methods of gathering qualitative data
Most companies use traditional methods for gathering qualitative data: conducting interviews with research participants, running surveys, and running focus groups. This data is typically stored in documents, CRMs, databases and knowledge bases. It’s important to examine which data is available and needs to be included in your research project, based on its scope.
Using your existing qualitative feedback
As it becomes easier for customers to engage across a range of different channels, companies are gathering increasingly large amounts of both solicited and unsolicited qualitative feedback.
Most organizations have now invested in Voice of Customer programs , support ticketing systems, chatbot and support conversations, emails and even customer Slack chats.
These new channels provide companies with new ways of getting feedback, and also allow the collection of unstructured feedback data at scale.
The great thing about this data is that it contains a wealth of valubale insights and that it’s already there! When you have a new question about user behavior or your customers, you don’t need to create a new research study or set up a focus group. You can find most answers in the data you already have.
Typically, this data is stored in third-party solutions or a central database, but there are ways to export it or connect to a feedback analysis solution through integrations or an API.
Utilize untapped qualitative data channels
There are many online qualitative data sources you may not have considered. For example, you can find useful qualitative data in social media channels like Twitter or Facebook. Online forums, review sites, and online communities such as Discourse or Reddit also contain valuable data about your customers, or research questions.
If you are considering performing a qualitative benchmark analysis against competitors - the internet is your best friend. Gathering feedback in competitor reviews on sites like Trustpilot, G2, Capterra, Better Business Bureau or on app stores is a great way to perform a competitor benchmark analysis.
Customer feedback analysis software often has integrations into social media and review sites, or you could use a solution like DataMiner to scrape the reviews.
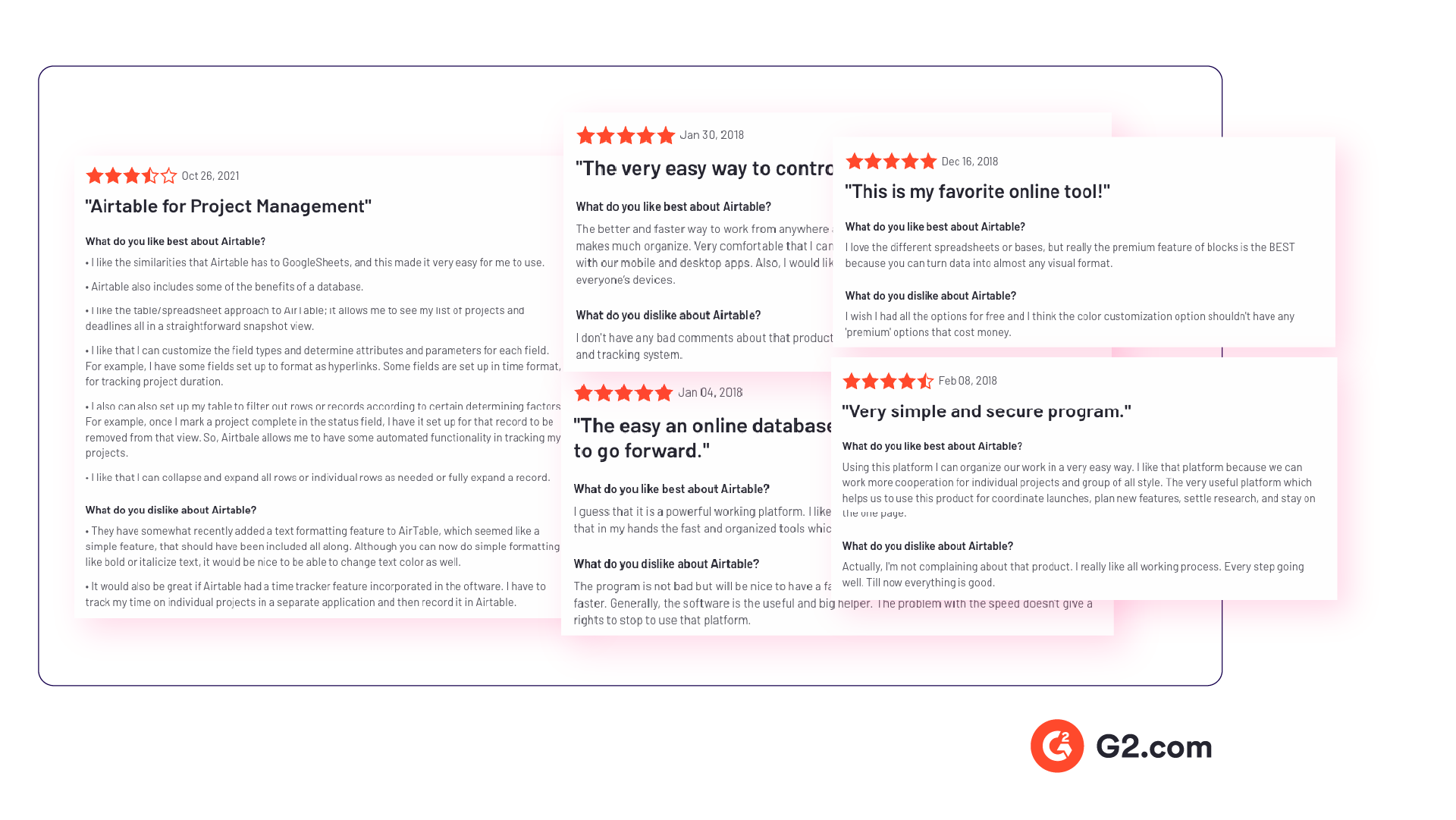
Step 2: Connect & organize all your qualitative data
Now you all have this qualitative data but there’s a problem, the data is unstructured. Before feedback can be analyzed and assigned any value, it needs to be organized in a single place. Why is this important? Consistency!
If all data is easily accessible in one place and analyzed in a consistent manner, you will have an easier time summarizing and making decisions based on this data.
The manual approach to organizing your data
The classic method of structuring qualitative data is to plot all the raw data you’ve gathered into a spreadsheet.
Typically, research and support teams would share large Excel sheets and different business units would make sense of the qualitative feedback data on their own. Each team collects and organizes the data in a way that best suits them, which means the feedback tends to be kept in separate silos.
An alternative and a more robust solution is to store feedback in a central database, like Snowflake or Amazon Redshift .
Keep in mind that when you organize your data in this way, you are often preparing it to be imported into another software. If you go the route of a database, you would need to use an API to push the feedback into a third-party software.
Computer-assisted qualitative data analysis software (CAQDAS)
Traditionally within the manual analysis approach (but not always), qualitative data is imported into CAQDAS software for coding.
In the early 2000s, CAQDAS software was popularised by developers such as ATLAS.ti, NVivo and MAXQDA and eagerly adopted by researchers to assist with the organizing and coding of data.
The benefits of using computer-assisted qualitative data analysis software:
- Assists in the organizing of your data
- Opens you up to exploring different interpretations of your data analysis
- Allows you to share your dataset easier and allows group collaboration (allows for secondary analysis)
However you still need to code the data, uncover the themes and do the analysis yourself. Therefore it is still a manual approach.
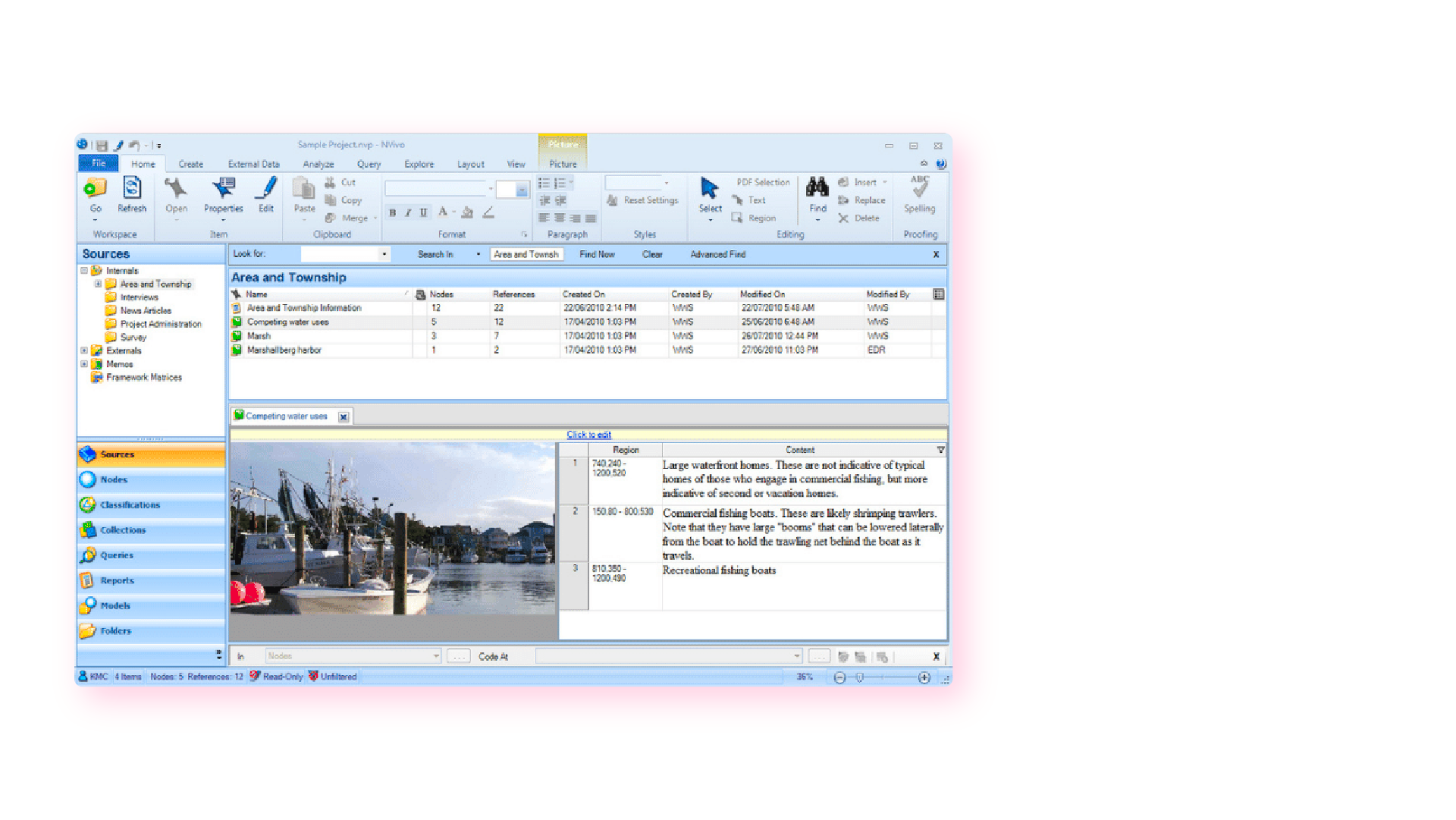
Organizing your qualitative data in a feedback repository
Another solution to organizing your qualitative data is to upload it into a feedback repository where it can be unified with your other data , and easily searchable and taggable. There are a number of software solutions that act as a central repository for your qualitative research data. Here are a couple solutions that you could investigate:
- Dovetail: Dovetail is a research repository with a focus on video and audio transcriptions. You can tag your transcriptions within the platform for theme analysis. You can also upload your other qualitative data such as research reports, survey responses, support conversations, and customer interviews. Dovetail acts as a single, searchable repository. And makes it easier to collaborate with other people around your qualitative research.
- EnjoyHQ: EnjoyHQ is another research repository with similar functionality to Dovetail. It boasts a more sophisticated search engine, but it has a higher starting subscription cost.
Organizing your qualitative data in a feedback analytics platform
If you have a lot of qualitative customer or employee feedback, from the likes of customer surveys or employee surveys, you will benefit from a feedback analytics platform. A feedback analytics platform is a software that automates the process of both sentiment analysis and thematic analysis . Companies use the integrations offered by these platforms to directly tap into their qualitative data sources (review sites, social media, survey responses, etc.). The data collected is then organized and analyzed consistently within the platform.
If you have data prepared in a spreadsheet, it can also be imported into feedback analytics platforms.
Once all this rich data has been organized within the feedback analytics platform, it is ready to be coded and themed, within the same platform. Thematic is a feedback analytics platform that offers one of the largest libraries of integrations with qualitative data sources.
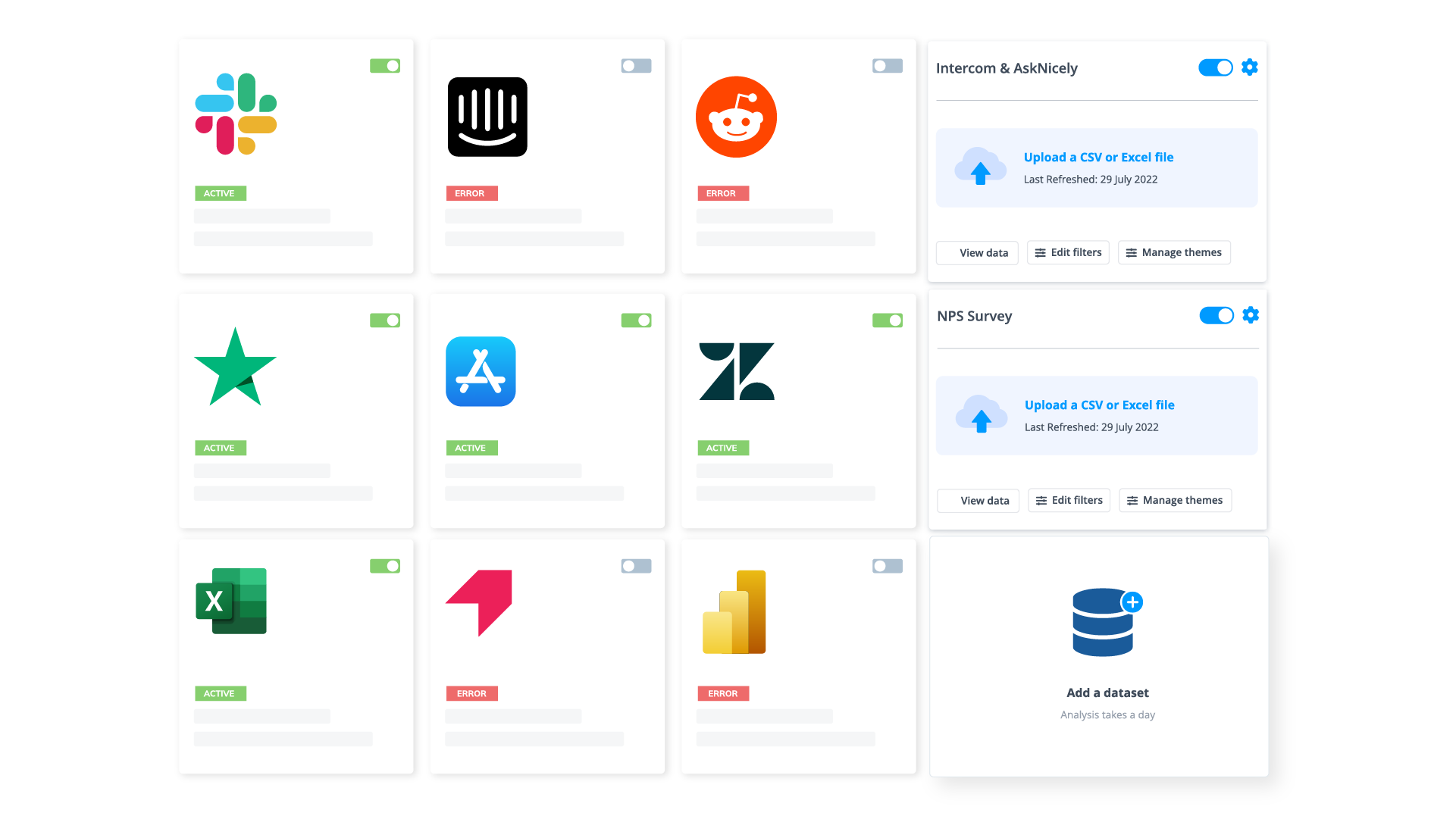
Step 3: Coding your qualitative data
Your feedback data is now organized in one place. Either within your spreadsheet, CAQDAS, feedback repository or within your feedback analytics platform. The next step is to code your feedback data so we can extract meaningful insights in the next step.
Coding is the process of labelling and organizing your data in such a way that you can then identify themes in the data, and the relationships between these themes.
To simplify the coding process, you will take small samples of your customer feedback data, come up with a set of codes, or categories capturing themes, and label each piece of feedback, systematically, for patterns and meaning. Then you will take a larger sample of data, revising and refining the codes for greater accuracy and consistency as you go.
If you choose to use a feedback analytics platform, much of this process will be automated and accomplished for you.
The terms to describe different categories of meaning (‘theme’, ‘code’, ‘tag’, ‘category’ etc) can be confusing as they are often used interchangeably. For clarity, this article will use the term ‘code’.
To code means to identify key words or phrases and assign them to a category of meaning. “I really hate the customer service of this computer software company” would be coded as “poor customer service”.
How to manually code your qualitative data
- Decide whether you will use deductive or inductive coding. Deductive coding is when you create a list of predefined codes, and then assign them to the qualitative data. Inductive coding is the opposite of this, you create codes based on the data itself. Codes arise directly from the data and you label them as you go. You need to weigh up the pros and cons of each coding method and select the most appropriate.
- Read through the feedback data to get a broad sense of what it reveals. Now it’s time to start assigning your first set of codes to statements and sections of text.
- Keep repeating step 2, adding new codes and revising the code description as often as necessary. Once it has all been coded, go through everything again, to be sure there are no inconsistencies and that nothing has been overlooked.
- Create a code frame to group your codes. The coding frame is the organizational structure of all your codes. And there are two commonly used types of coding frames, flat, or hierarchical. A hierarchical code frame will make it easier for you to derive insights from your analysis.
- Based on the number of times a particular code occurs, you can now see the common themes in your feedback data. This is insightful! If ‘bad customer service’ is a common code, it’s time to take action.
We have a detailed guide dedicated to manually coding your qualitative data .
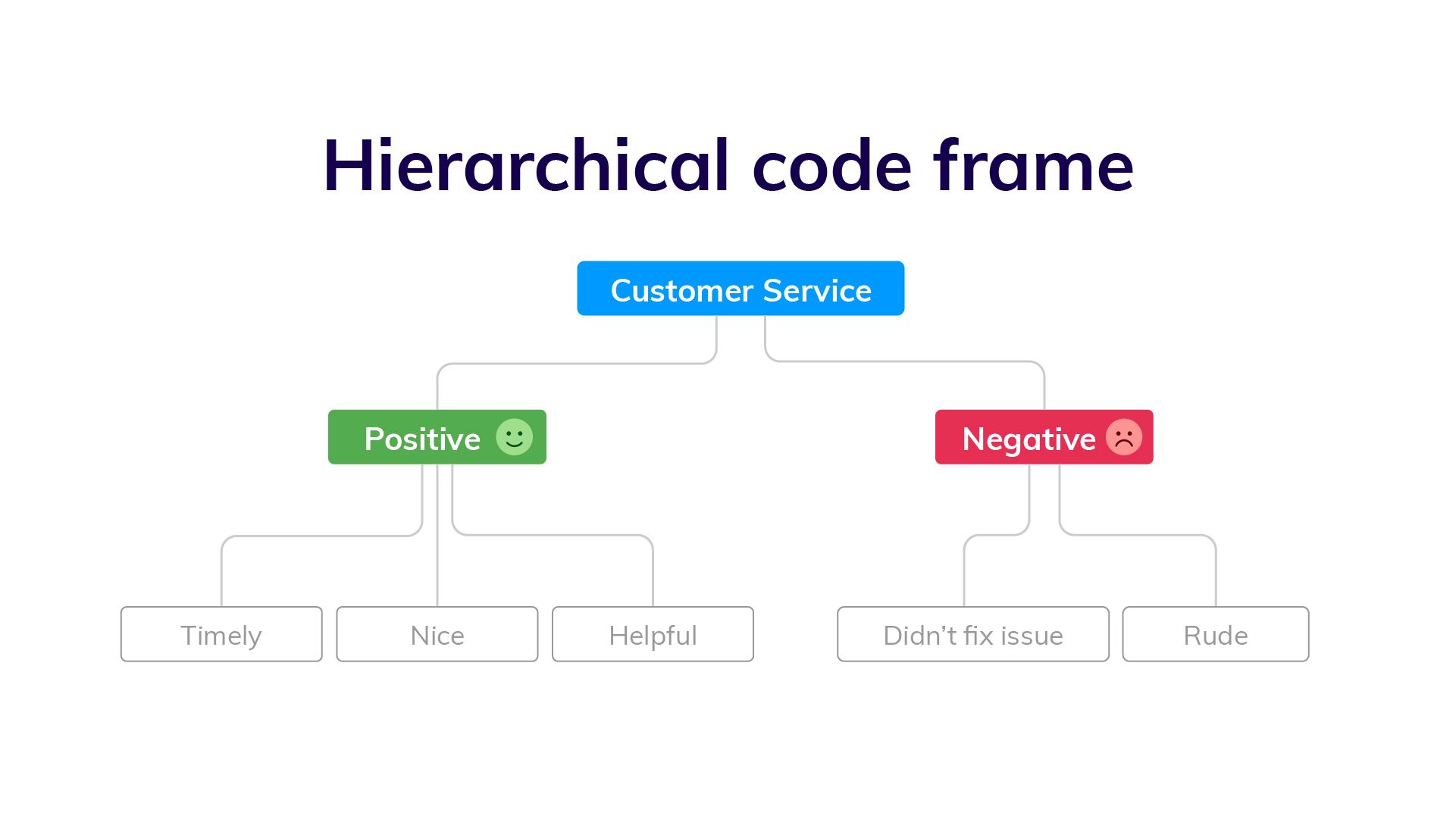
Using software to speed up manual coding of qualitative data
An Excel spreadsheet is still a popular method for coding. But various software solutions can help speed up this process. Here are some examples.
- CAQDAS / NVivo - CAQDAS software has built-in functionality that allows you to code text within their software. You may find the interface the software offers easier for managing codes than a spreadsheet.
- Dovetail/EnjoyHQ - You can tag transcripts and other textual data within these solutions. As they are also repositories you may find it simpler to keep the coding in one platform.
- IBM SPSS - SPSS is a statistical analysis software that may make coding easier than in a spreadsheet.
- Ascribe - Ascribe’s ‘Coder’ is a coding management system. Its user interface will make it easier for you to manage your codes.
Automating the qualitative coding process using thematic analysis software
In solutions which speed up the manual coding process, you still have to come up with valid codes and often apply codes manually to pieces of feedback. But there are also solutions that automate both the discovery and the application of codes.
Advances in machine learning have now made it possible to read, code and structure qualitative data automatically. This type of automated coding is offered by thematic analysis software .
Automation makes it far simpler and faster to code the feedback and group it into themes. By incorporating natural language processing (NLP) into the software, the AI looks across sentences and phrases to identify common themes meaningful statements. Some automated solutions detect repeating patterns and assign codes to them, others make you train the AI by providing examples. You could say that the AI learns the meaning of the feedback on its own.
Thematic automates the coding of qualitative feedback regardless of source. There’s no need to set up themes or categories in advance. Simply upload your data and wait a few minutes. You can also manually edit the codes to further refine their accuracy. Experiments conducted indicate that Thematic’s automated coding is just as accurate as manual coding .
Paired with sentiment analysis and advanced text analytics - these automated solutions become powerful for deriving quality business or research insights.
You could also build your own , if you have the resources!
The key benefits of using an automated coding solution
Automated analysis can often be set up fast and there’s the potential to uncover things that would never have been revealed if you had given the software a prescribed list of themes to look for.
Because the model applies a consistent rule to the data, it captures phrases or statements that a human eye might have missed.
Complete and consistent analysis of customer feedback enables more meaningful findings. Leading us into step 4.
Step 4: Analyze your data: Find meaningful insights
Now we are going to analyze our data to find insights. This is where we start to answer our research questions. Keep in mind that step 4 and step 5 (tell the story) have some overlap . This is because creating visualizations is both part of analysis process and reporting.
The task of uncovering insights is to scour through the codes that emerge from the data and draw meaningful correlations from them. It is also about making sure each insight is distinct and has enough data to support it.
Part of the analysis is to establish how much each code relates to different demographics and customer profiles, and identify whether there’s any relationship between these data points.
Manually create sub-codes to improve the quality of insights
If your code frame only has one level, you may find that your codes are too broad to be able to extract meaningful insights. This is where it is valuable to create sub-codes to your primary codes. This process is sometimes referred to as meta coding.
Note: If you take an inductive coding approach, you can create sub-codes as you are reading through your feedback data and coding it.
While time-consuming, this exercise will improve the quality of your analysis. Here is an example of what sub-codes could look like.
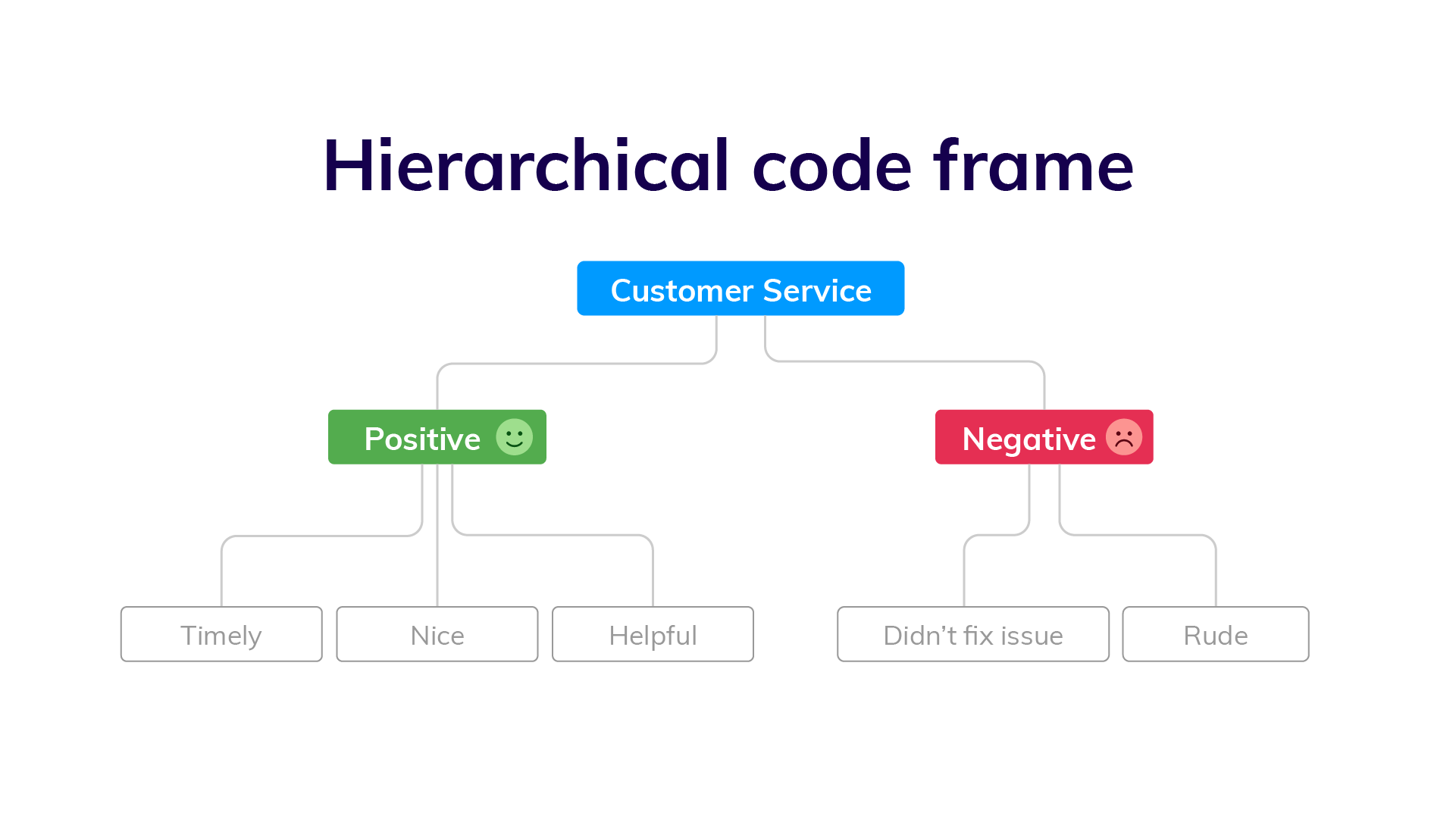
You need to carefully read your qualitative data to create quality sub-codes. But as you can see, the depth of analysis is greatly improved. By calculating the frequency of these sub-codes you can get insight into which customer service problems you can immediately address.
Correlate the frequency of codes to customer segments
Many businesses use customer segmentation . And you may have your own respondent segments that you can apply to your qualitative analysis. Segmentation is the practise of dividing customers or research respondents into subgroups.
Segments can be based on:
- Demographic
- And any other data type that you care to segment by
It is particularly useful to see the occurrence of codes within your segments. If one of your customer segments is considered unimportant to your business, but they are the cause of nearly all customer service complaints, it may be in your best interest to focus attention elsewhere. This is a useful insight!
Manually visualizing coded qualitative data
There are formulas you can use to visualize key insights in your data. The formulas we will suggest are imperative if you are measuring a score alongside your feedback.
If you are collecting a metric alongside your qualitative data this is a key visualization. Impact answers the question: “What’s the impact of a code on my overall score?”. Using Net Promoter Score (NPS) as an example, first you need to:
- Calculate overall NPS
- Calculate NPS in the subset of responses that do not contain that theme
- Subtract B from A
Then you can use this simple formula to calculate code impact on NPS .
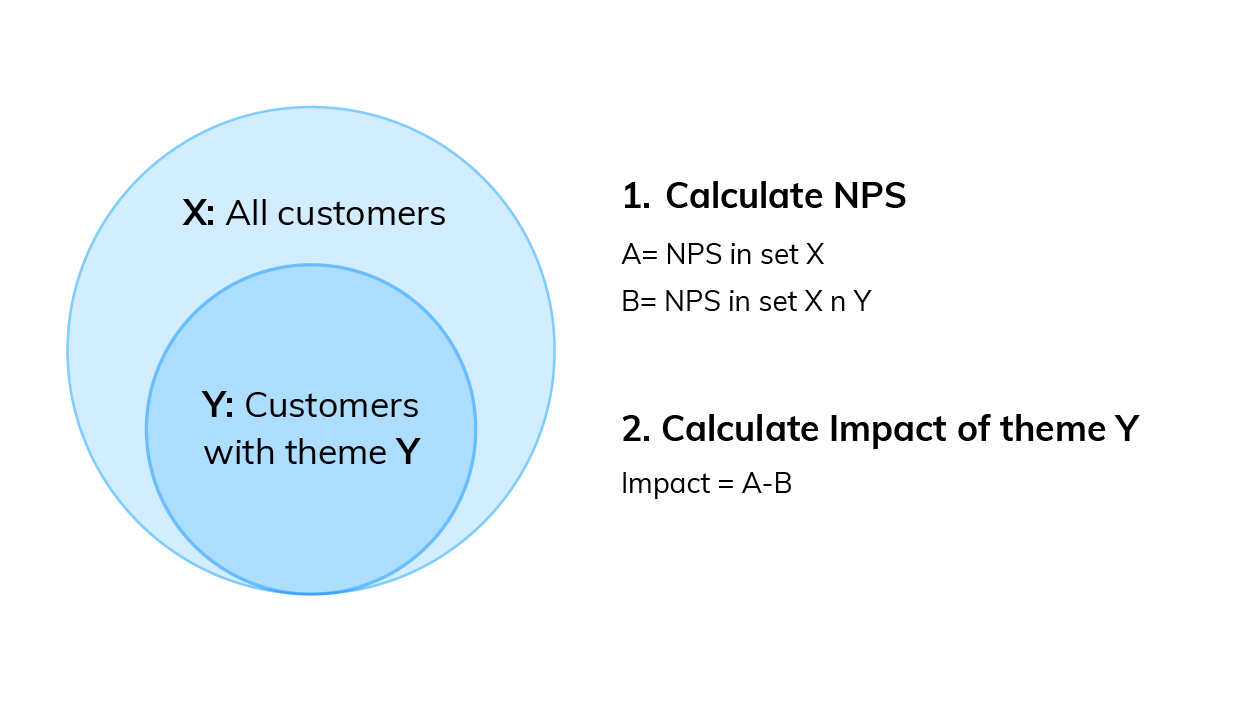
You can then visualize this data using a bar chart.
You can download our CX toolkit - it includes a template to recreate this.
Trends over time
This analysis can help you answer questions like: “Which codes are linked to decreases or increases in my score over time?”
We need to compare two sequences of numbers: NPS over time and code frequency over time . Using Excel, calculate the correlation between the two sequences, which can be either positive (the more codes the higher the NPS, see picture below), or negative (the more codes the lower the NPS).
Now you need to plot code frequency against the absolute value of code correlation with NPS. Here is the formula:
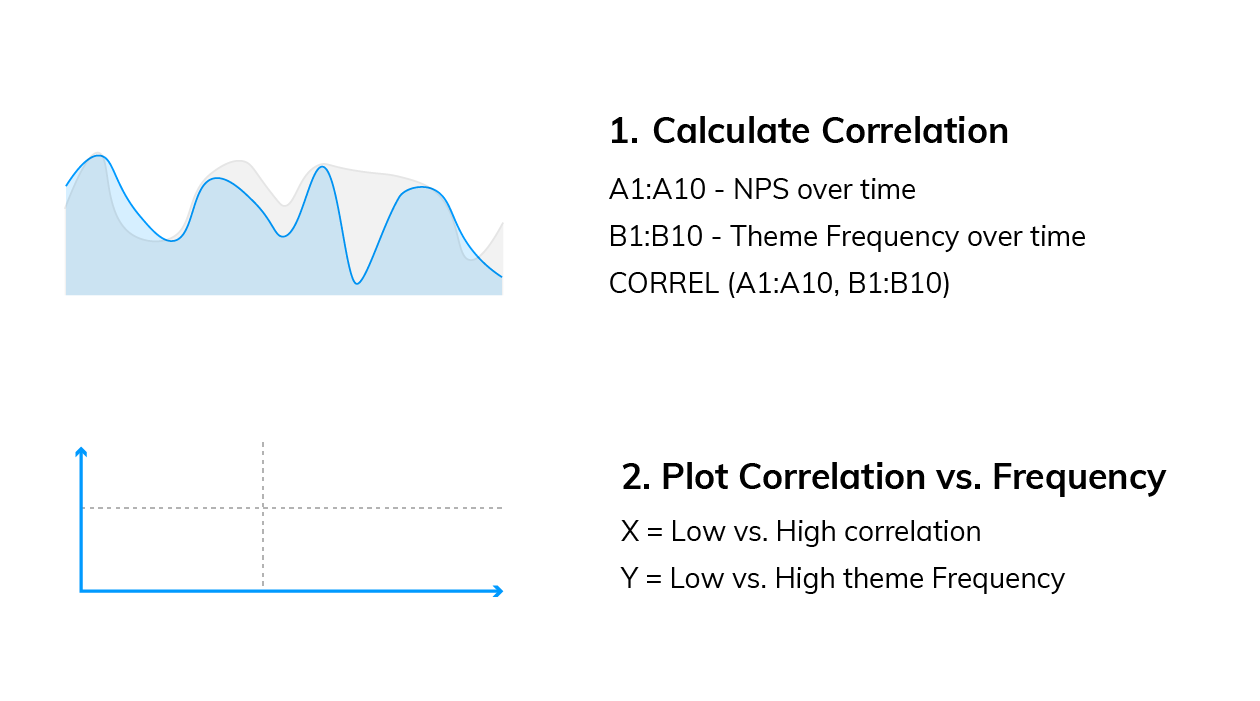
The visualization could look like this:
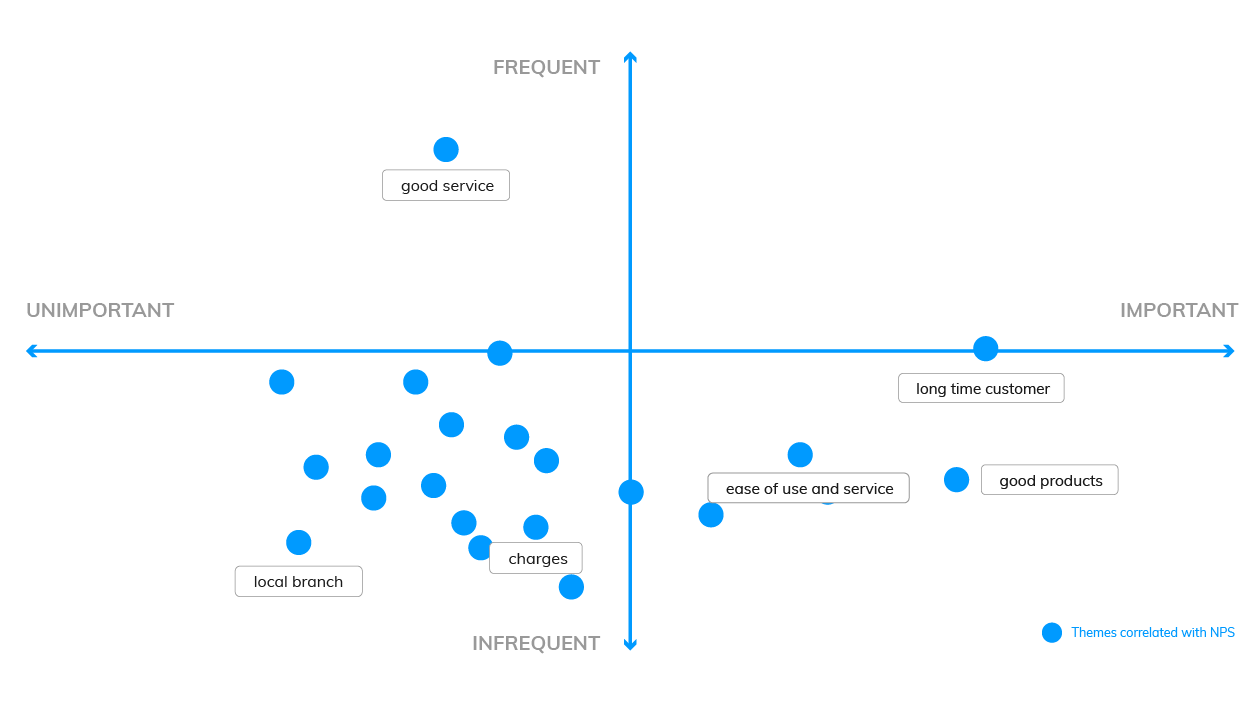
These are two examples, but there are more. For a third manual formula, and to learn why word clouds are not an insightful form of analysis, read our visualizations article .
Using a text analytics solution to automate analysis
Automated text analytics solutions enable codes and sub-codes to be pulled out of the data automatically. This makes it far faster and easier to identify what’s driving negative or positive results. And to pick up emerging trends and find all manner of rich insights in the data.
Another benefit of AI-driven text analytics software is its built-in capability for sentiment analysis, which provides the emotive context behind your feedback and other qualitative textual data therein.
Thematic provides text analytics that goes further by allowing users to apply their expertise on business context to edit or augment the AI-generated outputs.
Since the move away from manual research is generally about reducing the human element, adding human input to the technology might sound counter-intuitive. However, this is mostly to make sure important business nuances in the feedback aren’t missed during coding. The result is a higher accuracy of analysis. This is sometimes referred to as augmented intelligence .
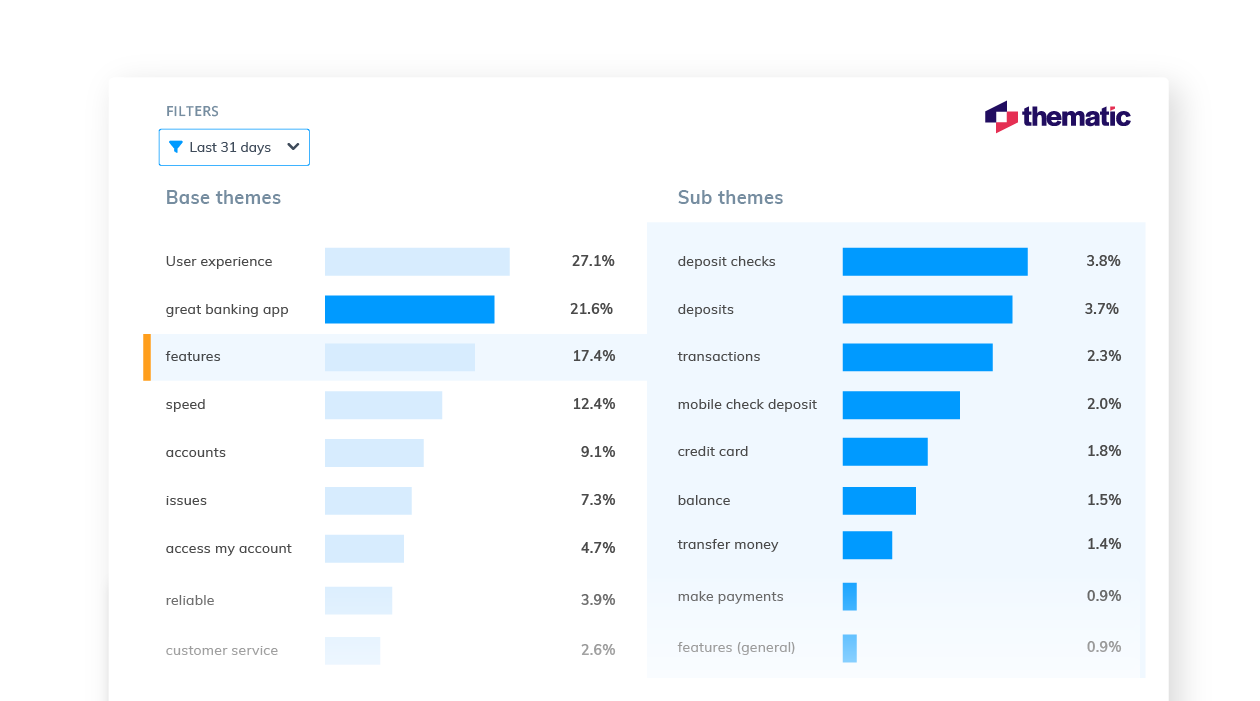
Step 5: Report on your data: Tell the story
The last step of analyzing your qualitative data is to report on it, to tell the story. At this point, the codes are fully developed and the focus is on communicating the narrative to the audience.
A coherent outline of the qualitative research, the findings and the insights is vital for stakeholders to discuss and debate before they can devise a meaningful course of action.
Creating graphs and reporting in Powerpoint
Typically, qualitative researchers take the tried and tested approach of distilling their report into a series of charts, tables and other visuals which are woven into a narrative for presentation in Powerpoint.
Using visualization software for reporting
With data transformation and APIs, the analyzed data can be shared with data visualisation software, such as Power BI or Tableau , Google Studio or Looker. Power BI and Tableau are among the most preferred options.
Visualizing your insights inside a feedback analytics platform
Feedback analytics platforms, like Thematic, incorporate visualisation tools that intuitively turn key data and insights into graphs. This removes the time consuming work of constructing charts to visually identify patterns and creates more time to focus on building a compelling narrative that highlights the insights, in bite-size chunks, for executive teams to review.
Using a feedback analytics platform with visualization tools means you don’t have to use a separate product for visualizations. You can export graphs into Powerpoints straight from the platforms.
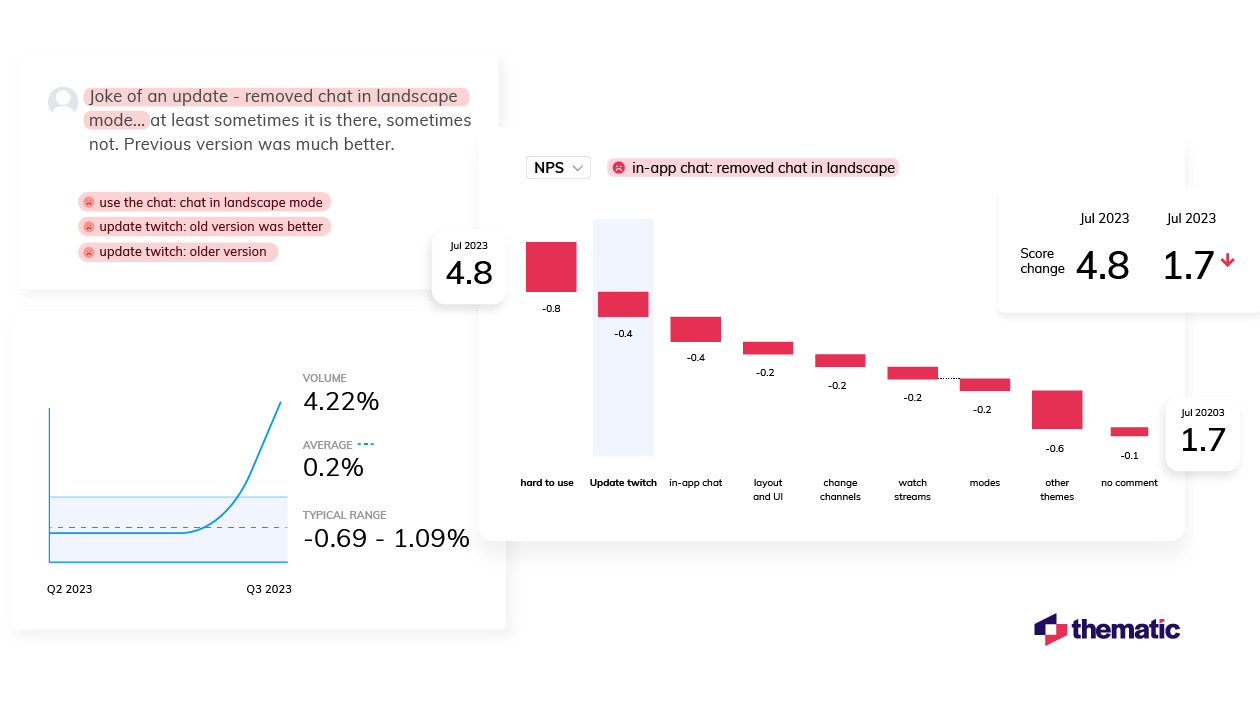
Conclusion - Manual or Automated?
There are those who remain deeply invested in the manual approach - because it’s familiar, because they’re reluctant to spend money and time learning new software, or because they’ve been burned by the overpromises of AI.
For projects that involve small datasets, manual analysis makes sense. For example, if the objective is simply to quantify a simple question like “Do customers prefer X concepts to Y?”. If the findings are being extracted from a small set of focus groups and interviews, sometimes it’s easier to just read them
However, as new generations come into the workplace, it’s technology-driven solutions that feel more comfortable and practical. And the merits are undeniable. Especially if the objective is to go deeper and understand the ‘why’ behind customers’ preference for X or Y. And even more especially if time and money are considerations.
The ability to collect a free flow of qualitative feedback data at the same time as the metric means AI can cost-effectively scan, crunch, score and analyze a ton of feedback from one system in one go. And time-intensive processes like focus groups, or coding, that used to take weeks, can now be completed in a matter of hours or days.
But aside from the ever-present business case to speed things up and keep costs down, there are also powerful research imperatives for automated analysis of qualitative data: namely, accuracy and consistency.
Finding insights hidden in feedback requires consistency, especially in coding. Not to mention catching all the ‘unknown unknowns’ that can skew research findings and steering clear of cognitive bias.
Some say without manual data analysis researchers won’t get an accurate “feel” for the insights. However, the larger data sets are, the harder it is to sort through the feedback and organize feedback that has been pulled from different places. And, the more difficult it is to stay on course, the greater the risk of drawing incorrect, or incomplete, conclusions grows.
Though the process steps for qualitative data analysis have remained pretty much unchanged since psychologist Paul Felix Lazarsfeld paved the path a hundred years ago, the impact digital technology has had on types of qualitative feedback data and the approach to the analysis are profound.
If you want to try an automated feedback analysis solution on your own qualitative data, you can get started with Thematic .
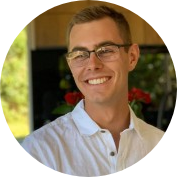
Community & Marketing
Tyler manages our community of CX, insights & analytics professionals. Tyler's goal is to help unite insights professionals around common challenges.
We make it easy to discover the customer and product issues that matter.
Unlock the value of feedback at scale, in one platform. Try it for free now!
- Questions to ask your Feedback Analytics vendor
- How to end customer churn for good
- Scalable analysis of NPS verbatims
- 5 Text analytics approaches
- How to calculate the ROI of CX
Our experts will show you how Thematic works, how to discover pain points and track the ROI of decisions. To access your free trial, book a personal demo today.
Recent posts
Watercare is New Zealand's largest water and wastewater service provider. They are responsible for bringing clean water to 1.7 million people in Tamaki Makaurau (Auckland) and safeguarding the wastewater network to minimize impact on the environment. Water is a sector that often gets taken for granted, with drainage and
Become a qualitative theming pro! Creating a perfect code frame is hard, but thematic analysis software makes the process much easier.
Qualtrics is one of the most well-known and powerful Customer Feedback Management platforms. But even so, it has limitations. We recently hosted a live panel where data analysts from two well-known brands shared their experiences with Qualtrics, and how they extended this platform’s capabilities. Below, we’ll share the
- Tools and Resources
- Customer Services
- Original Language Spotlight
- Alternative and Non-formal Education
- Cognition, Emotion, and Learning
- Curriculum and Pedagogy
- Education and Society
- Education, Change, and Development
- Education, Cultures, and Ethnicities
- Education, Gender, and Sexualities
- Education, Health, and Social Services
- Educational Administration and Leadership
- Educational History
- Educational Politics and Policy
- Educational Purposes and Ideals
- Educational Systems
- Educational Theories and Philosophies
- Globalization, Economics, and Education
- Languages and Literacies
- Professional Learning and Development
- Research and Assessment Methods
- Technology and Education
- Share This Facebook LinkedIn Twitter
Article contents
Qualitative data analysis and the use of theory.
- Carol Grbich Carol Grbich Flinders University
- https://doi.org/10.1093/acrefore/9780190264093.013.554
- Published online: 23 May 2019
The role of theory in qualitative data analysis is continually shifting and offers researchers many choices. The dynamic and inclusive nature of qualitative research has encouraged the entry of a number of interested disciplines into the field. These discipline groups have introduced new theoretical practices that have influenced and diversified methodological approaches. To add to these, broader shifts in chronological theoretical orientations in qualitative research can be seen in the four waves of paradigmatic change; the first wave showed a developing concern with the limitations of researcher objectivity, and empirical observation of evidence based data, leading to the second wave with its focus on realities - mutually constructed by researcher and researched, participant subjectivity, and the remedying of societal inequalities and mal-distributed power. The third wave was prompted by the advent of Postmodernism and Post- structuralism with their emphasis on chaos, complexity, intertextuality and multiple realities; and most recently the fourth wave brought a focus on visual images, performance, both an active researcher and an interactive audience, and the crossing of the theoretical divide between social science and classical physics. The methods and methodological changes, which have evolved from these paradigm shifts, can be seen to have followed a similar pattern of change. The researcher now has multiple paradigms, co-methodologies, diverse methods and a variety of theoretical choices, to consider. This continuum of change has shifted the field of qualitative research dramatically from limited choices to multiple options, requiring clarification of researcher decisions and transparency of process. However, there still remains the difficult question of the role that theory will now play in such a high level of complex design and critical researcher reflexivity.
- qualitative research
- data analysis
- methodologies
Theory and Qualitative Data Analysis
Researchers new to qualitative research, and particularly those coming from the quantitative tradition, have often expressed frustration at the need for what appears to be an additional and perhaps unnecessary process—that of the theoretical interpretation of their carefully designed, collected, and analyzed data. The justifications for this process have tended to fall into one of two areas: the need to lift data to a broader interpretation beyond the Monty Pythonesque “this is my theory and it’s my very own,” to illumination of findings from another perspective—by placing the data in its relevant discipline field for comparison with previous theoretical data interpretations, while possibly adding something original to the field.
“Theory” is broadly seen as a set of assumptions or propositions, developed from observation or investigation of perceived realties, that attempt to provide an explanation of relationships or phenomena. The framing of data via theoretical imposition can occur at different levels. At the lowest level, various concepts such as “role,” “power,” “socialization,” “evaluation,” or “learning styles” refer to limited aspects of social organization and are usually applied to a specific group of people.
At a more complex level, theories of the Middle Range, identified by Robert Merton to link theory and practice, are used to build theory from empirical data. These tend to be discipline specific and incorporate concepts plus variables such as “gender,” “race,” or “class.” Concepts and variables are then combined into meaningful statements, which can be applied to more diverse social groups. For example, in education an investigation of student performance could emphasize such concepts as “safety,” “zero bullying,” “communication,” and “tolerance,” with variables such as “race” and “gender” to lead to a statement that good microsystems and a focus on individual needs are necessary for optimal student performance.
The third and most complex level uses the established or grand theories such as those of Sigmund Freud’s stages of children’s development, Jean Piaget’s theory of cognitive development, or Urie Bronfenbrenner’s ecological systems, which have been widely accepted as meaningful across a number of disciplines and provide abstract explanations of the uniformity of aspects of social organization, social behavior, and social change.
The trend in qualitative research regarding the application of chosen levels of theory has been generally either toward theory direction/verification or theory generation, although the two are often intertwined. In the first, a relevant existing theory is chosen early and acts as a point of critical comparison for the data to be collected. This approach requires the researcher to think theoretically as s/he designs the study, collects data, and collates it into analytical groupings. The danger of theory direction is that an over focus on a chosen theoretical orientation may limit what the researcher can access or “see” in the data, but on the upside, this approach can also enable the generation of new theoretical aspects, as it is rare that findings will fall precisely within the implications of existing statements. Theory generation is a much looser approach and involves either one or a range of relevant levels of theory being identified at any point in the research process, and from which, in conjunction with data findings, some new combination or distillation can enhance interpretation.
The question of whether a well-designed study should negate the need for theoretical interpretation has been minimally debated. Mehdi and Mansor ( 2010 ) identified three trends in the literature on this topic: that theory in qualitative research relates to integrated methodology and epistemology; that theory is a separate and additional element to any methodological underpinnings; and that theory has no solid relationship with qualitative research. No clear agreement on any of these is evident. Overall, there appears to be general acceptance that the process of using theory, albeit etically (imposed) or emically (integrated), enhances outcomes, and moves research away from being a-theoretical or unilluminated by other ideas. However, regarding praxis, a closer look at the issue of the use of theory and data may be in order. Theoretical interpretation, as currently practiced, has limits. To begin with, the playing field is not level. In the grounded theory tradition, Glaser and Strauss ( 1967 ) were initially clear that in order to prevent undue influence on design and interpretation, the researcher should avoid reviewing the literature on a topic until after some data collection and analysis had been undertaken. The presumption that most researchers would already be well versed in theory/ies and would have a broad spectrum to draw on in order to facilitate the constant comparative process from which data-based concepts could be generated was found to be incorrect. Glaser ( 1978 ) suggested this lack could be improved at the conceptual level via personal and professional reflexivity.
This issue became even more of a problem with the advent of practice-led disciplines such as education and health into the field of qualitative research. These groups had not been widely exposed to the theories of the traditional social sciences such as sociology, psychology, and philosophy, although in education they would have been familiar with John Dewey’s concept of “pragmatism” linking learning with hands-on activity, and were more used to developing and using models of practice for comparison with current realities. By the mid- 20th century , Education was more established in research and had moved toward the use of middle range theories and the late 20th-century grand theorists: Michel Foucault, with his emphasis on power and knowledge control, and Jurgen Habermas, with his focus on pragmatism, communication, and knowledge management.
In addition to addictive identification with particular levels of theory and discipline-preferred theories and methods, activity across qualitative research seems to fall between two extremes. At one end it involves separate processes of data collection and analysis before searching for a theoretical framework within which to discuss the findings—often choosing a framework that has gained traction in a specific discipline. This “best/most acceptable fit” approach often adds little to the relevant field beyond repetition and appears somewhat forced. At the other extreme there are those who weave methods, methodologies, data, and theory throughout the whole research process, actively critiquing and modifying it as they go, usually with the outcome of creating some new direction for both theory and practice. The majority of qualitative research practice, however, tends to fall somewhere between these two.
The final aspect of framing data lies in the impact of researchers themselves, and the early- 21st-century emphasis is on exposing relevant personal frames, particularly those of culture, gender, socioeconomic class, life experiences such as education, work, and socialization, and the researcher’s own values and beliefs. The twin purposes of this exposure are to create researcher awareness and encourage accountability for their impact on the data, as well as allowing the reader to assess the value of research outcomes in terms of potential researcher bias or prejudice. This critical reflexivity is supposed to be undertaken at all stages of the research but it is not always clear that it has occurred.
Paradigms: From Interactionism to Performativity
It appears that there are potentially five sources of theory: that which is generally available and can be sourced from different disciplines; that which is imbedded in the chosen paradigm/s; that which underpins particular methodologies; that which the researcher brings, and that which the researched incorporate within their stories. Of these, the paradigm/s chosen are probably the most influential in terms of researcher position and design. The variety of the sets of assumptions, beliefs, and researcher practices that comprise the theoretical paradigms, perspectives, or broad world views available to researchers, and within which they are expected to locate their individual position and their research approach, has shifted dramatically since the 1930s. The changes have been distinct and identifiable, with their roots located in the societal shifts prompted by political, social, and economic change.
The First Wave
The Positivist paradigm dominated research, largely unquestioned, prior to the early 20th century . It emphasized the distancing of the researcher from his/her subjects; researcher objectivity; a focus on objective, cause–effect, evidence-based data derived from empirical observation of external realities; experimental quantitative methods involving testing hypotheses; and the provision of finite answers and unassailable future predictions. From the 1930s, concerns about the limitations of findings and the veracity of research outcomes, together with improved communication and exposure to the worldviews of other cultures, led to the advent of the realist/post-positivist paradigm. Post-positivism, or critical realism, recognized that certainty in proving the truth of a hypothesis was unachievable and that outcomes were probably limited to falsification (Popper, 1963 ), that true objectivity was unattainable and that the researcher was most likely to impact on or to contaminate data, that both qualitative and quantitative approaches were valuable, and that methodological pluralism was desirable.
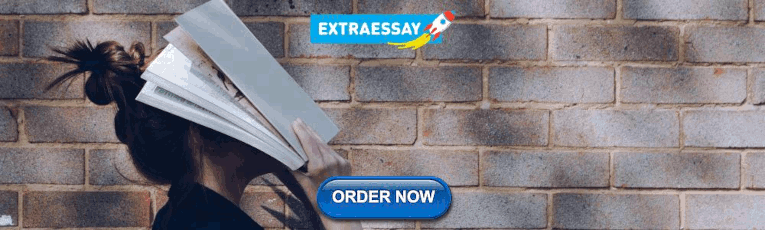
The Second Wave
Alongside the worldwide political shifts toward “people power” in the 1960s and 1970s, two other paradigms emerged. The first, the Interpretivist/Constructivist, focused on the social situations in which we as humans develop and how our construction of knowledge occurs through interactions with others in these contexts. This paradigm also emphasized the gaining of an understanding of the subjective views or experiences of the participants being researched, and recognized the impact of the researcher on researcher–researched mutually constructed realities. Here, theory generation is the preferred outcome to explain the what, how, and why of the findings. This usually involves the development of a conceptual model, forged from both the data gained and from the application/integration of relevant theory, to provide explanations for and interpretations of findings, together with a new perspective for the field/discipline.
The second paradigm, termed the Critical/Emancipatory, focused on locating, critiquing, and changing inequalities in society. The identification of the location of systemic power discrepancies or systematic power misuse in situations involving gender, sexuality, class, and race is expected to be followed by moves to right any oppression discovered. Here, the use of theory has been focused more on predetermined concept application for “fit.” This is because the very strong notion of problematic societal structures and power inappropriately wielded have been the dominant underpinnings.
In both the Interpretive and Critical paradigms, researcher position shifted from the elevated and distant position of positivism, to one of becoming equal with those being researched, and the notion of researcher framing emerged to cover this shift and help us—the readers—to “see” (and judge) the researcher and her/his processes of data management more clearly.
The Third Wave
In the 1980s, the next wave of paradigmatic options—postmodernism and poststructuralism—emerged. Postmodernism, with its overarching cultural implications, and poststructuralism, with its focus on language, severely challenged the construction, limitations, and claims to veracity of all knowledge and in particular the use of theory derived from siloed disciplines and confined research methods. Regardless of whether the postmodern/poststructural label is attached to grounded theory, ethnography, phenomenology, action, or evaluative designs, one general aspect that prevails is a focus on language. Language has become viewed as dubious, with notions of “slippage”—the multiple meanings of individual words, and “difference”—the difference and deferral of textual meaning (Derrida, 1970 , 1972 ), adding complexity. Double coding, irony, and juxtaposition are encouraged to further identify meaning, and to uncover aspects of social organization and behavior that have been previously marginalized or made invisible by existing discourses and discursive practices. Texts are seen as complex constructions, and intertextuality is favored, resulting in multiply constructed texts. The world is viewed as chaotic and unknowable; individuals are no longer seen as two dimensional—they are viewed as multifaceted with multiple realities. Complex “truths” are perceived as limited by time and context, requiring multiple data sets and many voices to illuminate them, and small-scale focused local research is seen as desirable. The role of researcher also changed: the politics of position and self-reflexivity dominate and the researcher needs to clearly expose past influences and formerly hidden aspects of his/her life. S/he inhabits the position of an offstage or decentered facilitator, presenting data for the reader to judge.
Theory is used mainly at the conceptual level with no particular approach being privileged. The researcher has become a “bricoleur” (Levi-Strauss, 1962 ) or handyman, using whatever methods or theories that are within reach, to adapt, craft, and meld technological skills with mythical intellectual reflection in order to create unique perspectives on the topic. Transitional interpretations dominate, awaiting further challenges and deconstruction by the next researcher in the field.
The need for multifaceted data sets in the 1990s led inevitably to a search for other research structures, and mixed and multiple methods have become topical. In crossing the divide between qualitative and quantitative approaches, the former initially developed its own sub-paradigms: pragmatist (complimentary communication and shared meanings) and transformative/emancipatory (inequalities in race, class, gender, and disability, to be righted). An increasing focus on multiple methods led to the advent of dialectics (multiple paradigm use) and critical realism (the acceptance of divergent results) (Shannon-Baker, 2016 ). The dilemmas of theory use raised by these changes include whether to segregate data sets and try to explain disparate outcomes in terms of diversity using different theories; whether to integrate them through a homogeneous “smoothing” process—one theory fits all, in order to promote a singular interpretation; or whether to let the strongest paradigm—in terms of data—dominate the theoretical findings.
The Fourth Wave
During the early 21st century , as the third wave was becoming firmly established, the Performative paradigm emerged. The incorporation of fine art–based courses into universities has challenged the prescribed rules of the doctoral thesis, initially resulting in a debate—with echoes of Glaser and Strauss—as to whether theory, if used initially, is too directive, thereby potentially contaminating the performance, or whether theory application should be an outcome to enhance performances, or even whether academic guidelines regarding theory use need to be changed to accommodate these disciplines (Bolt, 2004 ; Freeman, 2010 ; Riley & Hunter, 2009 ). Performativity is seen in terms of “effect,” a notion derived from John Austin’s ( 1962 ) assertion that words and speech utterances do not just act as descriptors of content, they have social force and impact on reality. Following this, a productive work is seen as capable of transforming reality (Bolt, 2016 ). The issue most heard here is the problem of how to judge this form of research when traditional guidelines of dependability, transformability, and trustworthiness appear to be irrelevant. Barbara Bolt suggests that drawing on Austin’s ( 1962 ) terms “locutionary” (semantic meaning), “illocutionary” (force), and “perlocutionary” (effect achieved on receivers), together with the mapping of these effects in material, effective, and discursive domains, may be useful, despite the fact that mapping transformation may be difficult to track in the short term.
During the second decade of the 21st century , however, discussions relating to the use of theory have increased dramatically in academic performative research and a variety of theoreticians are now cited apart from John Austin. These include Maurice Merleu-Ponty ( 1945 and the spatiality of lived events; Jacques Derrida ( 1982 ) on iterability, simultaneous sameness, and difference; Giles Deleuze and Felix Guatarri ( 1987 ) on rituals of material objects and transformative potential; Jean-Francois Lyotard ( 1988 ) on plurality of micro narratives, “affect,” and its silent disruption of discourse; and Bruno Latour ( 2005 ) with regard to actor network theory—where theory is used to engage with rather than to explain the world in a reflective political manner.
In performative doctoral theses, qualitative theory and methods are being creatively challenged. For example, from the discipline of theater and performance Lee Miller and Joanne/Bob Whalley ( 2010 ) disrupt the notion of usual spaces for sincere events by taking their six-hour-long performance Partly Cloudy, Chance of Rain , involving a public reaffirmation of their marriage vows, out of the usual habitats to a service station on a highway. The performance involves a choir, a band, a pianist, 20 performers dressed as brides and grooms, photographers, a TV crew, an Anglican priest, plus 50 guests. The theories applied to this event include an exploration of Marc Auge’s ( 1992 ) conception of the “non-place”; Mikhail Bakhtin’s ( 1992 ) concepts of “dialogism” (many voices) together with “heteroglossia” (juxtaposition of many voices in a dialogue); and Ludwig Wittgenstein’s ( 1953 ) discussion of the “duck rabbit”—once the rabbit is seen (participatory experience) the duck (audience) is always infected by its presence. This couple further challenged the guidelines of traditional doctoral theses by successfully negotiating two doctoral awards for a joint piece of research
A more formal example of a doctoral thesis (Reik, 2014 ) using traditional qualitative approaches has examined at school level the clash of paradigms of performative creative styles of teaching with the neoliberalist focus on testing, curriculum standardization, and student outcomes.
Leah Mercer ( 2012 ), an academic in performative studies, used the performative paradigm in her doctoral thesis to challenge and breach not only the methodological but also the theoretical silos of the quantitative–qualitative divide. The physics project is an original work using live performances of personal storytelling with video and web streaming to depict the memories, preoccupations, and the formative relationship of two women, an Australian and an American, living in contemporary mediatized society. Using scientific theory, Mercer explores personal identity by reframing the principles of contemporary physics (quantum mechanics and uncertainty principle) as aesthetic principles (uncertainty and light) with the physics of space (self), time (memory), light (inspiration), and complementarity (the reconciliation of opposites) to illuminate these experiences.
The performative paradigm has also shifted the focus on the reader, developed in postmodernism, to a broader group—an active audience. Multi-methods have been increased to include symbolic imagery, in particular visual images, as well as sound and live action. The researcher’s role here is often that of performer within a cultural frame, creating and investigating multiple realities and providing the link between the text/script and the audience/public. Theory is either minimized to the level of concepts or used to break through the silos of different disciplines to integrate and reconcile aspects from long-lasting theoretical divides.
In these chronological lines of paradigm shifts, changes in researcher position and changes in the application of theory can clearly be seen. The researcher has moved out of the shadows and into the mainstream; her/his role has shifted from an authoritarian collector and presenter of finite “truths” to a creator and often performer of multiple and disparate data images for the audience to respond to. Theory options have shifted from direction and generation within existing perspectives to creative amalgamations of concepts from disciplines previously rarely combined.
Methodologies: From Anthropology to Fine Arts
It would be a simple matter if all the researcher had to contend with was siting oneself in a particular paradigm/s. Unfortunately, not only have paradigms shifted in terms of researcher position and theoretical usage but so also have methodological choices and research design. One of the most popular methodologies, ethnography, with its roots in classical anthropology and its fieldwork-based observations of action and interaction in cultural contexts, can illustrate the process of methodological change following paradigm shift. If a researcher indicates that he/she has undertaken an ethnographic study, the reader will be most likely to query “which form?”: classical?, critical?, auto?, visual?, ethno drama?, cyber/net?, or performative? The following examples from this methodology should indicate how paradigm shifts have resulted in increasing complexity of design, methods, and interpretive options.
In c lassical ethnography the greatest borrowing is from traditional anthropology in terms of process and tools, and this can be seen with the inclusion of initial time spent in the setting to learn the language of the culture and to generally “bathe” oneself in the environment, often with minimal data collection. This process is supposed to help increase researcher understanding of the culture and minimize the problem of “othering” (treating as a different species/alien). Then a fairly lengthy amount of time is usually spent in the cultural setting either as an observer or as a participant observer to collect as much data as is relevant to answer the research question. This is followed by a return to post-check whether the findings previously gathered have stood the test of time. The analytical toolkit can involve domain analysis, freelists, pilesorts, triads and taxonomies, frame and social network, and event analysis. Truncated mini-ethnographies became more common as time became an issue, but these can still involve years of managing descriptive data, often collected by several participating researchers as seen in Douglas, Rasmussen, and Flanagan’s ( 1977 ) study of the culture of a nudist beach. Shorter versions undertaken by one researcher, for example Sohn ( 2015 ), have explored strategies of teacher and student learning in a science classroom. Theoretical interpretation can be by conceptual application for testing, such as Margaret Mead’s ( 1931 ) testing of the concept of “adolescence”—derived from American culture—in Samoan culture, or, more generally, by concept generation. The latter can be seen in David Rozenhan’s ( 1973 ) investigation of the experience of a group of researcher pseudo-patients admitted to hospitals for the mentally ill in the United States. The main concepts generated were labeling, powerlessness, and depersonalization.
De-colonial ethnography recognizes the “othering” frames of colonial and postcolonial research and takes a position that past colonial supremacy over Third World countries persists in political, economic, educational, and social constructions. Decolonizing requires a critical examination of language, attitudes, and research methods. Kakal Battacharya ( 2016 ) has exposed the micro-discourses of the continuing manifestation of colonial power in a parallel narrative written by a South Asian woman and a white American male. Concepts of colonialism and patriarchy, displayed through the discourses exposed, provide a theoretical critique.
Within critical ethnography , with its focus on power location and alleviation of oppression, Dale Spender ( 1980 ) used structured and timed observations of the styles, quality, and quantity of interaction between staff and students in a range of English classrooms. The theory-directive methodological frames of feminism and gender inequality were applied to identify and expose the lesser time and lesser quality of interaction that teachers had with female students in comparison with that assigned to male students. Widespread distribution of these results alerted education authorities and led to change, in some environments, toward introducing single-sex classrooms for certain topics. This was seen as progress toward alleviating oppressive behaviors. This approach has produced many excellent educational studies, including Peter Willis ( 1977 ) on the preparation of working-class kids for working-class jobs; Michele Fine ( 1991 ) on African American and Latino students who dropped out of a New York high school; Angela Valenzuela ( 1999 ) on emigrant and other under-achievers in American schools; Lisa Patel ( 2013 ) on inclusion and exclusion of immigrants into education; and Jean Anyon ( 1981 ) on social stratification of identical curriculum knowledge in different classrooms
A less concept-driven and more descriptive approach to critical ethnography was emphasized by Phil Carspecken’s hermeneutic approach ( 1996 ), which triggered a move toward data-generated theoretical concepts that could then be used to challenge mainstream theoretical positions.
Post-critical ethnography emphasizes power and ideology and the social practices that contribute to oppression, in particular objectivity, positionality, representation and reflexivity, and critical insufficiency or “antipower.”
Responsibility is shifted to the researcher for the world they create and critique when they interpret their research contexts (Noblit, Flores, & Murillo, 2004 ).
Autoethnography emerged from the postmodern paradigm, with its search for different “truths” and different relationships with readers, and prompted an emphasis on personal experience and documentation of the self in a particular cultural context (Ellis, 2004 ). In order to achieve this, the researcher has to inhabit the dual positions of being the focus of activities, feelings, and emotions experienced in the setting while at the same time being positioned distantly—observing and recording the behaviors of the self in that culture. Well-developed skills of critical reflexivity are required. The rejection of the power-laden discourses/grand theories of the past and the emphasis on transitional explanations has resulted in minimal theorizing and an emphasis on data display, the reader, and the reader’s response. Open presentations of data can be seen in the form of narrative storytelling, or re-presentations in the form of fiction, dramatic performances, and poetry. Carolyn Ellis ( 2004 ) has argued that “story is theory and theory is story” and our “making sense of stories” involves contributing to a broader understanding of human existence. Application/generation of concepts may also occur, and the term “Critical Autoethnography” has been used (Hughes & Pennington, 2017 ), particularly where experiences of race, class, or gender inequality are being experienced. Jennifer Potter ( 2015 ) used the concept “whiteness of silence” to introduce a critical race element into her autoethnographic experience of black–white racial hatred experiences within a university class on African American communication in which she was a student.
Visual ethnography uses a variety of tools, including photography, sketches, movies, social media, the Web and virtual reality, body art, clothing, painting, and sculpture, to demonstrate and track culture. This approach has been available for some time both as a methodology in its own right and as a method of data collection. An example of this approach, which mixes classical and visual ethnography, is Philippe Bourgois and Jeff Schonberg’s 12-year study of two dozen homeless heroin injectors and crack smokers living under a freeway overpass in San Francisco ( 2009 ). Their data comprised extensive black and white photos, dialogue, taped conversations, and fieldwork observation notes. The themes of violence, race relations, family trauma, power relations, and suffering were theoretically interpreted through reworked notions of “power” that incorporated Pierre Bourdieu’s ( 1977 , 1999 ) concepts of “symbolic violence”—linking observed practices to social domination, and “habitus”—an individual’s personal disposition comprising unique feelings and actions grounded in biography and history; Karl Marx’s “lumpen” from “lumpenproletariat” ( 1848 ), the residual class—the vagrants and beggars together with criminal elements that lie beneath the labor force; and Michel Foucault’s “biopower” ( 1978 , 2008 )—the techniques of subjugation used by the state on the population, and “governmentality” ( 1991 )—where individuals are disciplined through institutions and the “knowledge–power” nexus. The ideas of these three theorists were used to create and weave a theory of “lumpen abuse” to interpret the lives of the participants.
Ethno Drama involves transforming the results from an ethnographic study into a performance to be shared, for example the educational experiences of children and youth (Gabriel & Lester, 2013 ). The performance medium can vary from a film (Woo, 2008 ), an article presented in dramatic form (Carter, 2014 ), or more usually a play script to be staged for an audience in a theater (Ethno Theater). One of the main purposes is to provide a hearing space for voices that have been marginalized or previously silenced. These voices and their contexts can be presented by research participants, actors, or the research team, and are often directed at professionals from the field. Audience-based meetings to devise recommendations for further action may follow a performance. Because of the focus on inequality, critical theory has been the major theoretical orientation for this approach. The structure of the presentation invites audiences to identify situations of oppression, in the hope that this will inform them sufficiently to enable modification of their own practices or to be part of the development of recommendations for future change.
Lesnick and Humphrie ( 2018 ) explored the views of identity of LGBTQ+ youth between 14 and 24 years of age via interviews and online questionnaires, the transcriptions of which were woven into a script that was performed by actors presenting stories not congruent with their own racial/gender scripts in order to challenge audience expectations and labels. The research group encouraged the schools where they performed to structure discussion groups to follow the school-located performances. The scripts and discussions revealed and were lightly interpreted through concepts of homelessness, racism, and “oppression Olympics”—the way oppressed people sometimes view one another in competition rather than in solidarity. These issues were found to be relevant to both school and online communities. Support for these young people was discovered to be mostly from virtual sources, being provided by dialogues within Facebook groups.
Cyber/net or/virtual ethnographies involve the study of online communities within particular cultures. Problems which have emerged from the practice of this approach include; discovery of the researcher lurking without permission on sites, gaining prior permission which often disturbs the threads of interaction, gaining permission post–data collection but having many furious people decline participation, the “facelessness” of individuals who may have uncheckable multiple personas, and trying to make sense of very disparate data in incomplete and non-chronological order.. There has been acceptance that online and offline situations can influence each other. Dibbell ( 1993 ) demonstrated that online sexual violence toward another user’s avatar in a text-based “living room” reduced the violated person to tears as she posted pleas for the violator to be removed from the site. Theoretical interpretation at the conceptual level is common; Michel Foucault’s concept of heterotopia ( 1967 , 1984 ) was used to explain such spatio-temporal prisons as online rooms. Heterotropic spaces are seen as having the capacity to reflect and distort real and imagined experiences.
Poststructural ethnography tracks the instability of concepts both culturally and linguistically. This can be demonstrated in the deconstruction of language in education (Lather, 2001 ), particularly the contradictions and paradoxes of sexism, gender, and racism both in texts and in the classroom. These discourses are implicated in relations of power that are dynamic and within which resistance can be observed. Poststructuralism accepts that texts are multiple, as are the personas of those who created them, and that talk such as that which occurs in a classroom can be linked with knowledge control. Walter Humes ( 2000 ) discovered that the educational management discourses of “community,” “leadership,” and “participation” could be disguised by such terms as “learning communities” and “transformational leadership.” He analyzed the results with a conceptual framework derived from management theory and policy studies and linked the findings with political power.
Performative ethnography , from the post-postmodern paradigm, integrates the performances of art and theater with the focus on culture of ethnography (Denzin, 2003 ). A collaborative performance ethnography (van Katwyk & Seko, 2017 ) used a poem re-presenting themes from a previous research study on youth self-harming to form the basis of the creation of a performative dance piece. This process enabled the researcher participants to explore less dominant ways of knowing through co-learning and through the discovery of self-vulnerability. The research was driven by a social justice-derived concern that Foucault’s notion of “sovereignty” was being implemented through a web of relations that commodified and limited knowledge, and sanctioned the exploitation of individuals and communities.
This exploration of the diversity in ethnographic methods, methodologies, and interpretive strategies would be repeated in a similar trek through the interpretive, critical, postmodern, and post-postmodern approaches currently available for undertaking the various versions of grounded theory, phenomenology, feminist research, evaluation, action, or performative research.
Implications of Changes for the Researcher
The onus is now less on finding the “right” (or most familiar in a field) research approaches and following them meticulously, and much more on researchers making their own individual decisions as to which aspects of which methodologies, methods and theoretical explanations will best answer their research question. Ideally this should not be constrained by the state of the discipline they are part of; it should be equally as easy for a fine arts researcher to carry out a classical ethnography with a detailed theoretical interpretation derived from a grand theorist/s as it would be for a researcher in law to undertake a performative study with the minimum of conceptual insights and the maximum of visual and theoretical performances. Unfortunately, the reality is that trends within disciplines dictate publication access, thereby reinforcing the prevailing boundaries of knowledge.
However, the current diversity of choice has indeed shifted the field of qualitative research dramatically away from the position it was in several decades ago. The moves toward visual and performative displays may challenge certain disciplines but these approaches have now become well entrenched in others, and in qualitative research publishing. The creativity of the performative paradigm in daring to scale the siloed and well-protected boundaries of science in order to combine theoretical physics with the theories of social science, and to re-present data in a variety of newer ways from fiction to poetry to researcher performances, is exciting.
Given that theoretical as well as methodological and methods’ domains are now wide open to researchers to pick and choose from, two important aspects—justification and transparency of process—have become essential elements in the process of convincing the reader.
Justification incorporates the why of decision-making. Why was the research question chosen? Why was the particular paradigm, or paradigms, chosen best for the question? Why were the methodology and methods chosen most appropriate for both the paradigm/s and research question/s? And why were the concepts used the most appropriate and illuminating for the study?
Transparency of process not only requires that the researcher clarifies who they are in the field with relation to the research question and the participants chosen, but demands an assessment of what impact their background and personal and professional frames have had on research decisions at all stages from topic choice to theoretical analysis. Problems faced in the research process and how they were managed or overcome also requires exposition as does the chronology of decisions made and changed at all points of the research process.
Now to the issue of theory and the question of “where to?” This brief walk through the paradigmatic, methodological, and theoretical changes has demonstrated a significant move from the use of confined paradigms with limited methodological options to the availability of multiple paradigms, co-methodologies, and methods of many shades, for the researcher to select among Regarding theory use, there has been a clear move away from grand and middle range theories toward the application of individual concepts drawn from a variety of established and minor theoreticians and disciplines, which can be amalgamated into transitory explanations. The examples of theoretical interpretation presented in this article, in my view, very considerably extend, frame, and often shed new light on the themes that have been drawn out via analytical processes. Well-argued theory at any level is a great enhancer, lifting data to heights of illumination and comparison, but it could equally be argued that in the presence of critical researcher reflexivity, complex, layered, longitudinal, and well-justified design, meticulous analysis, and monitored audience response, it may no longer be essential.
Bibliography
- Eco, U. (1979). The role of the reader . Bloomington: Indiana University Press.
- Gadamer, H. (1989). Truth and method ( J. Weinheimer & D. Marshall , Trans.). NY: Crossroad.
- Grbich, C. (2004). New approaches in social Research . London, U.K.: SAGE.
- Grbich, C. (2013). Qualitative data analysis: An introduction . London, U.K.: SAGE.
- Lincoln, Y. , & Denzin, N. (2000). Paradigmatic controversies: Contradictions and emerging confluences. In N. Denzin and Y. Lincoln , Handbook of qualitative research (2nd ed.). Thousand Oaks, CA: SAGE.
- Lyotard, J. (1983). Answering the question: What is post modernism? In E. Hassan & S. Hassan (Eds.), Innovation and renovation . Madison: University of Wisconsin Press.
- Pink, S. (2012). Advances in visual methodology . Thousand Oaks, CA: SAGE.
- Riley, S. , & Hunter, L. (Eds.). (2009). Mapping landscapes for performance as research . London: Palgrave Macmillan.
- Tinkler, P. (2011). Using photography in social and historic research . New Dehli, India: SAGE.
- Vis, F. , & Thelwall, M. (2012). Researching social media . New Dehli, India: SAGE.
- Von Hantelmann, D. (2010). How to do things with art . Zurich, Switzerland: JRP Ringier.
- Anyon, J. (1981). Social class and school knowledge . Curriculum Inquiry , 11 (1), 3–42.
- Auge, M. (1992). Non-Places: An introduction to supermodernity ( John Howe ). London, U.K.: Verso.
- Augé, M. (1995). Non-places: An introduction to anthropology of supermodernity . London, U.K.: Verso.
- Austin, J. (1962). How to do things with words [The William James Lectures, 1955]. Oxford, U.K.: Oxford University Press.
- Bakhtin, M. (1992). The dialogic imagination: Four essays . Austin: University of Texas Press.
- Battacharya, K. , & Gillen, N. (2016). Power, race and higher education: A cross-cultural parallel narrative . Rotterdam, The Netherlands: Sense.
- Bolt, B. (2004). Art beyond representation: The performative power of the image . London, U.K.: I.B Tauris.
- Bolt, B. (2016). Artistic research: A performative paradigm? Parse. #3 Repetitions and Reneges . Gothenburg, Sweden: University of Gothenburg.
- Bourdieu, P. (1977). Outline of a theory of practice . Cambridge, U.K.: Cambridge University Press.
- Bourdieu, P. (1999) The Weight of the world: Social suffering in contemporary society . Cambridge, U.K.: Polity Press.
- Bourgois, P. , & Schonberg, J. (2009). Righteous dopefiend: Homelessness, addiction, and poverty in urban America . Berkeley: University of California Press.
- Bronfenbrenner, U. (1979). The ecology of human development: Experiments by nature and design . Cambridge, MA: Harvard University Press.
- Carspecken, P. (1996). Critical ethnography in educational research . New York: Routledge.
- Carter, M. (2014). The teacher monologues: Exploring the identities and experiences of artist-teachers . Rotterdam, The Netherlands: Sense.
- Deleuze, G. , & Guattari, F. (1987). A thousand Plateaus: Capitalism and schizophrenia ( B. Massumi , Trans.). Minneapolis: University of Minnesota Press.
- Denzin, N. (2003). Performance ethnography : Critical pedagogy and the politics of culture . Thousand Oaks, CA: SAGE.
- Derrida, J. (1970). Structure, sign and play in the discourse of human sciences lecture presented at johns hopkins university , October 21, 1966.
- Derrida, J. (1972). Margins of philosophy: Plato to footnotes ( A. Bass , Trans.). Sussex, U.K.: Brighton and Harvester Press.
- Derrida, J. (1982). Sending: On representation . Social Research , 49 (2), 294–326.
- Dewey, J. (1998). The essential Dewey ( L. Hickman & T. Alexander , Eds.). (2 vols.) Bloomington: Indiana University Press.
- Dibbell, J. (1993). A rape in cyberspace from chapter 1 of My Tiny Life . Published in The Village Voice .
- Douglas, J. , Rasmussen, P. , & Fanagan, C. (1977). The nude beach . Thousand Oaks, CA: SAGE.
- Ellis, C. (2004). The ethnographic I: A methodological novel about autoethnography . Walnut Creek, CA: AltaMira.
- Fine, M. (1991). Framing dropouts: Notes on the politics of an urban high school . New York, NY: SUNY Press.
- Foucault, M. (1967, 1984). Des espaces autres (J. Miscowiec, Trans.). [ Of other spaces: Utopias and heterotropias]. Archtecture/Mouvement/Continuite , October.
- Foucault, M. (1978). Security, territory, population. Lectures at the Collège de France 1977–1978 ( A. Davidson , Ed.). New York, NY: Palgrave Macmillan.
- Foucault, M. (1991). Studies in governmentality: Two lectures by and an interview with Michel Foucault ( G. Burchell , C. Gordon , & and P. Miller , Eds.). Chicago: University of Chicago Press.
- Foucault, M. (2008). The birth of biopolitics . Lectures at the Collège de France 1978–1979 ( M. Senellart , Ed, Trans.; G. Burchell , Trans.). New York, NY: Palgrave Macmillan. (First published as Naissance de la Biopolitique 2004).
- Freeman, J. (2010). Blood, sweat and theory: Research through practice in performance . Faringdon, U.K.: Libri.
- Freud, S. (1953–1974). The standard edition of the complete psychological works of Sigmund Freud ( J. Strachey , Trans. and Ed.) (24 Vols). London, U.K.: Hogarth and the Institute of Psycho-Analysis.
- Gabriel, R. , & Lester, J. (2013). Performances of research: Critical issues in K–12 education . New York, NY: Peter Lang.
- Glaser, B. (1978). Theoretical sensitivity: Advances in the theory of grounded theory . Mill Valley, CA: Sociology Press.
- Glaser, B. , & Strauss, A. (1967). The discovery of grounded theory: Strategies for qualitative research . London: Aldine.
- Hughes, S. , & Pennington, J. (2017). Autoethnography: Process, product and possibility for critical social research . Thousand Oaks, CA: SAGE.
- Humes, W. (2000). The discourses of educational management . Journal of Educational Enquiry , 1 (1).
- Lather, P. (2001). Postmodernism, post-structuralism and post(critical) ethnography: Of ruins, aporias and angels. In P. Atkinson , A. Coffey , S. Delamont , J. Lofland , & L. Lofland (Eds.), Handbook of ethnography . Thousand Oaks, CA: SAGE.
- Latour, B. (2005). Reassembling the social: An introduction to actor–network theory . Oxford, U.K.: Oxford University Press.
- Lesnick, E. , & Humphrie, J. (2018). Amplifying youth voices through ethnodrama research . Washington, DC: National Association of Independent Schools.
- Levi-Strauss, C. (1962). The savage mind ( La pensee sauvage) ( G. Weidenfield & Nicholson Ltd. , Trans.). Chicago, IL: University of Chicago Press.
- Lyotard, J. (1988). Peregrinations: Law, form, event . The Wellek Library lectures at the University of California, Irvine. New York, NY.: Columbia University Press.
- Marx, K. , & Engels, F. (1848). The Communist Manifesto ( S. Moore , Trans.).
- Mead, M. (1931). The life of a Samoan girl: ALL TRUE. The record of actual adventures that have happened to ten women of today . New York, NY.: Brewer, Warren and Putnam.
- Mehdi, T. , & Mansor, A. (2010). A General perspective on the role of theory in qualitative research . Journal of International Social Research , 3 (11).
- Mercer, L. (2012). The Physics Project. In L. Mercer , J. Robson , & D. Fenton (Eds.), Live research: Methods of practice led inquiry in research . Nerang: Queensland, Ladyfinger.
- Merleau-Ponty, M. (1945). Phénoménologie de la Perception . Paris, France: Editions Gallimard.
- Merleau-Ponty, M. (2005). Phenomenology of perception ( D. Landes , Trans.). London, U.K.: Routledge.
- Merton, R. (1968). Social theory and social structure . New York, NY: Free Press.
- Miller, L. , & Whalley, J. (2010). Case Study 11: Partly cloudy, chance of rain. In J. Freeman (Ed.), Blood, sweat and theory: Research through practice in performance . Faringdon, U.K.: Libri.
- Noblit, G. , Flores, S. , & Nurillo, E. (2004). Postcritical ethnography: Reinscribing critique . New York: Hampton Press.
- Patel, L. (2013). Youth held at the border: Immigration, education and the politics of inclusion . New York, NY: Columbia University Teacher’s College Press.
- Piaget, J. (1936). Origins of intelligence in the child . London, U.K.: Routledge and Kegan Paul.
- Popper, K. (1963). Science: Conjectures and refutations. Online lecture transcript .
- Potter, J. (2015). The whiteness of silence: A critical autoethnographic tale of a strategic rhetoric . Qualitative Report , 20 (9), 7.
- Reik, R. (2014). Arts education in a culture of performativity: A case study of what is valued in one Queensland school community . (Doctoral dissertation), Griffith University, Brisbane.
- Riley, S. R. , & Hunter, L. (Eds.). (2009). Mapping landscapes for performance as research . London, U.K.: Palgrave Macmillan.
- Rosenhan, D. (1973). On being sane in insane places . Science , 17 (January), 250–258.
- Shannon-Baker, P. (2016). Making paradigms meaningful in mixed methods research . Journal of Mixed Methods Research , 10 (4).
- Sohn, L. (2015). Ethnographic case study of a high school science classroom: Strategies in STEM education . (Doctoral dissertation). Texas A&M University, Corpus Christi, Texas.
- Spender, D. (1980). Talking in class. In D. Spender & E. Sarah (Eds.), Leaming to lose: Sexism and education . London, U.K.: Women’s Press.
- Valenzuela, A. (1999). Subtractive schooling: U.S.–Mexican youth and the politics of caring . Albany: SUNY.
- van Katwyk, T. , & Seko, Y. (2017). Knowing through improvisational dance: A collaborative autoethnography . Forum: Qualitative Social Research , 18 (2), 1.
- Willis, P. (1977). Learning to labour . New York, NY: Columbia University Press.
- Wittgenstein, L. (1953). Philosophical investigations ( G. Anscombe , Trans.). Oxford, U.K.: Blackwell.
- Woo, Y. (2008). Engaging new audiences: Translating research into popular media. Educational Researcher , 37 (6), 321–329.
Related Articles
- Autoethnography
- Risky Truth-Making in Qualitative Inquiry
- Ethnography and Education
- Qualitative Design Research Methods
- Qualitative Approaches to Studying Marginalized Communities
- Arts Education Research
- Poststructural Temporalities in School Ethnography
- Détournement as a Qualitative Method
- Ethnographies of Education and Anthropological Knowledge Production
- Network Ethnography as an Approach for the Study of New Governance Structures in Education
- Black Feminist Thought and Qualitative Research in Education
- Poetic Approaches to Qualitative Data Analysis
- Performance-Based Ethnography
- Complexity Theory as a Guide to Qualitative Methodology in Teacher Education
- Observing Schools and Classrooms
Printed from Oxford Research Encyclopedias, Education. Under the terms of the licence agreement, an individual user may print out a single article for personal use (for details see Privacy Policy and Legal Notice).
date: 18 May 2024
- Cookie Policy
- Privacy Policy
- Legal Notice
- Accessibility
- [66.249.64.20|109.248.223.228]
- 109.248.223.228
Character limit 500 /500
Log in using your username and password
- Search More Search for this keyword Advanced search
- Latest content
- Current issue
- Write for Us
- BMJ Journals More You are viewing from: Google Indexer
You are here
- Volume 3, Issue 3
- Data analysis in qualitative research
- Article Text
- Article info
- Citation Tools
- Rapid Responses
- Article metrics

- Sally Thorne , RN, PhD
- School of Nursing, University of British Columbia Vancouver, British Columbia, Canada
https://doi.org/10.1136/ebn.3.3.68
Statistics from Altmetric.com
Request permissions.
If you wish to reuse any or all of this article please use the link below which will take you to the Copyright Clearance Center’s RightsLink service. You will be able to get a quick price and instant permission to reuse the content in many different ways.
Unquestionably, data analysis is the most complex and mysterious of all of the phases of a qualitative project, and the one that receives the least thoughtful discussion in the literature. For neophyte nurse researchers, many of the data collection strategies involved in a qualitative project may feel familiar and comfortable. After all, nurses have always based their clinical practice on learning as much as possible about the people they work with, and detecting commonalities and variations among and between them in order to provide individualised care. However, creating a database is not sufficient to conduct a qualitative study. In order to generate findings that transform raw data into new knowledge, a qualitative researcher must engage in active and demanding analytic processes throughout all phases of the research. Understanding these processes is therefore an important aspect not only of doing qualitative research, but also of reading, understanding, and interpreting it.
For readers of qualitative studies, the language of analysis can be confusing. It is sometimes difficult to know what the researchers actually did during this phase and to understand how their findings evolved out of the data that were collected or constructed. Furthermore, in describing their processes, some authors use language that accentuates this sense of mystery and magic. For example, they may claim that their conceptual categories “emerged” from the data 1 —almost as if they left the raw data out overnight and awoke to find that the data analysis fairies had organised the data into a coherent new structure that explained everything! In this EBN notebook, I will try to help readers make sense of some of the assertions that are made about qualitative data analysis so that they can develop a critical eye for when an analytical claim is convincing and when it is not.
Qualitative data
Qualitative data come in various forms. In many qualitative nursing studies, the database consists of interview transcripts from open ended, focused, but exploratory interviews. However, there is no limit to what might possibly constitute a qualitative database, and increasingly we are seeing more and more creative use of such sources as recorded observations (both video and participatory), focus groups, texts and documents, multi-media or public domain sources, policy manuals, photographs, and lay autobiographical accounts.
Qualitative analytic reasoning processes
What makes a study qualitative is that it usually relies on inductive reasoning processes to interpret and structure the meanings that can be derived from data. Distinguishing inductive from deductive inquiry processes is an important step in identifying what counts as qualitative research. Generally, inductive reasoning uses the data to generate ideas (hypothesis generating), whereas deductive reasoning begins with the idea and uses the data to confirm or negate the idea (hypothesis testing). 2 In actual practice, however, many quantitative studies involve much inductive reasoning, whereas good qualitative analysis often requires access to a full range of strategies. 3 A traditional quantitative study in the health sciences typically begins with a theoretical grounding, takes direction from hypotheses or explicit study questions, and uses a predetermined (and auditable) set of steps to confirm or refute the hypothesis. It does this to add evidence to the development of specific, causal, and theoretical explanations of phenomena. 3 In contrast, qualitative research often takes the position that an interpretive understanding is only possible by way of uncovering or deconstructing the meanings of a phenomenon. Thus, a distinction between explaining how something operates (explanation) and why it operates in the manner that it does (interpretation) may be a more effective way to distinguish quantitative from qualitative analytic processes involved in any particular study.
Because data collection and analysis processes tend to be concurrent, with new analytic steps informing the process of additional data collection and new data informing the analytic processes, it is important to recognise that qualitative data analysis processes are not entirely distinguishable from the actual data. The theoretical lens from which the researcher approaches the phenomenon, the strategies that the researcher uses to collect or construct data, and the understandings that the researcher has about what might count as relevant or important data in answering the research question are all analytic processes that influence the data. Analysis also occurs as an explicit step in conceptually interpreting the data set as a whole, using specific analytic strategies to transform the raw data into a new and coherent depiction of the thing being studied. Although there are many qualitative data analysis computer programs available on the market today, these are essentially aids to sorting and organising sets of qualitative data, and none are capable of the intellectual and conceptualising processes required to transform data into meaningful findings.
Specific analytic strategies
Although a description of the actual procedural details and nuances of every qualitative data analysis strategy is well beyond the scope of a short paper, a general appreciation of the theoretical assumptions underlying some of the more common approaches can be helpful in understanding what a researcher is trying to say about how data were sorted, organised, conceptualised, refined, and interpreted.
CONSTANT COMPARATIVE ANALYSIS
Many qualitative analytic strategies rely on a general approach called “constant comparative analysis”. Originally developed for use in the grounded theory methodology of Glaser and Strauss, 4 which itself evolved out of the sociological theory of symbolic interactionism, this strategy involves taking one piece of data (one interview, one statement, one theme) and comparing it with all others that may be similar or different in order to develop conceptualisations of the possible relations between various pieces of data. For example, by comparing the accounts of 2 different people who had a similar experience, a researcher might pose analytical questions like: why is this different from that? and how are these 2 related? In many qualitative studies whose purpose it is to generate knowledge about common patterns and themes within human experience, this process continues with the comparison of each new interview or account until all have been compared with each other. A good example of this process is reported in a grounded theory study of how adults with brain injury cope with the social attitudes they face (see Evidence-Based Nursing , April 1999, p64).
Constant comparison analysis is well suited to grounded theory because this design is specifically used to study those human phenomena for which the researcher assumes that fundamental social processes explain something of human behaviour and experience, such as stages of grieving or processes of recovery. However, many other methodologies draw from this analytical strategy to create knowledge that is more generally descriptive or interpretive, such as coping with cancer, or living with illness. Naturalistic inquiry, thematic analysis, and interpretive description are methods that depend on constant comparative analysis processes to develop ways of understanding human phenomena within the context in which they are experienced.
PHENOMENOLOGICAL APPROACHES
Constant comparative analysis is not the only approach in qualitative research. Some qualitative methods are not oriented toward finding patterns and commonalities within human experience, but instead seek to discover some of the underlying structure or essence of that experience through the intensive study of individual cases. For example, rather than explain the stages and transitions within grieving that are common to people in various circumstances, a phenomenological study might attempt to uncover and describe the essential nature of grieving and represent it in such a manner that a person who had not grieved might begin to appreciate the phenomenon. The analytic methods that would be employed in these studies explicitly avoid cross comparisons and instead orient the researcher toward the depth and detail that can be appreciated only through an exhaustive, systematic, and reflective study of experiences as they are lived.
Although constant comparative methods might well permit the analyst to use some pre-existing or emergent theory against which to test all new pieces of data that are collected, these more phenomenological approaches typically challenge the researcher to set aside or “bracket” all such preconceptions so that they can work inductively with the data to generate entirely new descriptions and conceptualisations. There are numerous forms of phenomenological research; however, many of the most popular approaches used by nurses derive from the philosophical work of Husserl on modes of awareness (epistemology) and the hermeneutic tradition of Heidegger, which emphasises modes of being (ontology). 5 These approaches differ from one another in the degree to which interpretation is acceptable, but both represent strategies for immersing oneself in data, engaging with data reflectively, and generating a rich description that will enlighten a reader as to the deeper essential structures underlying a particular human experience. Examples of the kinds of human experience that are amenable to this type of inquiry are the suffering experienced by individuals who have a drinking problem (see Evidence-Based Nursing , October 1998, p134) and the emotional experiences of parents of terminally ill adolescents (see Evidence-Based Nursing , October 1999, p132). Sometimes authors explain their approaches not by the phenomenological position they have adopted, but by naming the theorist whose specific techniques they are borrowing. Colaizzi and Giorgi are phenomenologists who have rendered the phenomenological attitude into a set of manageable steps and processes for working with such data and have therefore become popular reference sources among phenomenological nurse researchers.
ETHNOGRAPHIC METHODS
Ethnographic research methods derive from anthropology's tradition of interpreting the processes and products of cultural behaviour. Ethnographers documented such aspects of human experience as beliefs, kinship patterns and ways of living. In the healthcare field, nurses and others have used ethnographic methods to uncover and record variations in how different social and cultural groups understand and enact health and illness. An example of this kind of study is an investigation of how older adults adjust to living in a nursing home environment (see Evidence-Based Nursing , October 1999, p136). When a researcher claims to have used ethnographic methods, we can assume that he or she has come to know a culture or group through immersion and engagement in fieldwork or participant observation and has also undertaken to portray that culture through text. 6 Ethnographic analysis uses an iterative process in which cultural ideas that arise during active involvement “in the field” are transformed, translated, or represented in a written document. It involves sifting and sorting through pieces of data to detect and interpret thematic categorisations, search for inconsistencies and contradictions, and generate conclusions about what is happening and why.
NARRATIVE ANALYSIS AND DISCOURSE ANALYSIS
Many qualitative nurse researchers have discovered the extent to which human experience is shaped, transformed, and understood through linguistic representation. The vague and subjective sensations that characterise cognitively unstructured life experiences take on meaning and order when we try to articulate them in communication. Putting experience into words, whether we do this verbally, in writing, or in thought, transforms the actual experience into a communicable representation of it. Thus, speech forms are not the experiences themselves, but a socially and culturally constructed device for creating shared understandings about them. Narrative analysis is a strategy that recognises the extent to which the stories we tell provide insights about our lived experiences. 7 For example, it was used as a strategy to learn more about the experiences of women who discover that they have a breast lump (see Evidence-Based Nursing , July 1999, p93). Through analytic processes that help us detect the main narrative themes within the accounts people give about their lives, we discover how they understand and make sense of their lives.
By contrast, discourse analysis recognises speech not as a direct representation of human experience, but as an explicit linguistic tool constructed and shaped by numerous social or ideological influences. Discourse analysis strategies draw heavily upon theories developed in such fields as sociolinguistics and cognitive psychology to try to understand what is represented by the various ways in which people communicate ideas. They capitalise on critical inquiry into the language that is used and the way that it is used to uncover the societal influences underlying our behaviours and thoughts. 8 Thus, although discourse analysis and narrative analysis both rely heavily on speech as the most relevant data form, their reasons for analysing speech differ. The table ⇓ illustrates the distinctions among the analytic strategies described above using breast cancer research as an example.
- View inline
General distinctions between selected qualitative research approaches: an illustration using breast cancer research
Cognitive processes inherent in qualitative analysis
The term “qualitative research” encompasses a wide range of philosophical positions, methodological strategies, and analytical procedures. Morse 1 has summarised the cognitive processes involved in qualitative research in a way that can help us to better understand how the researcher's cognitive processes interact with qualitative data to bring about findings and generate new knowledge. Morse believes that all qualitative analysis, regardless of the specific approach, involves:
comprehending the phenomenon under study
synthesising a portrait of the phenomenon that accounts for relations and linkages within its aspects
theorising about how and why these relations appear as they do, and
recontextualising , or putting the new knowledge about phenomena and relations back into the context of how others have articulated the evolving knowledge.
Although the form that each of these steps will take may vary according to such factors as the research question, the researcher's orientation to the inquiry, or the setting and context of the study, this set of steps helps to depict a series of intellectual processes by which data in their raw form are considered, examined, and reformulated to become a research product.
Quality measures in qualitative analysis
It used to be a tradition among qualitative nurse researchers to claim that such issues as reliability and validity were irrelevant to the qualitative enterprise. Instead, they might say that the proof of the quality of the work rested entirely on the reader's acceptance or rejection of the claims that were made. If the findings “rang true” to the intended audience, then the qualitative study was considered successful. More recently, nurse researchers have taken a lead among their colleagues in other disciplines in trying to work out more formally how the quality of a piece of qualitative research might be judged. Many of these researchers have concluded that systematic, rigorous, and auditable analytical processes are among the most significant factors distinguishing good from poor quality research. 9 Researchers are therefore encouraged to articulate their findings in such a manner that the logical processes by which they were developed are accessible to a critical reader, the relation between the actual data and the conclusions about data is explicit, and the claims made in relation to the data set are rendered credible and believable. Through this short description of analytical approaches, readers will be in a better position to critically evaluate individual qualitative studies, and decide whether and when to apply the findings of such studies to their nursing practice.
- ↵ Morse JM. “Emerging from the data”: the cognitive processes of analysis in qualitative inquiry. In: JM Morse, editor. Critical issues in qualitative research methods . Thousand Oaks, CA: Sage, 1994:23–43.
- ↵ Holloway I. Basic concepts for qualitative research . Oxford: Blackwell Science, 1997.
- ↵ Schwandt TA. Qualitative inquiry: a dictionary of terms . Thousand Oaks, CA: Sage, 1997.
- ↵ Glaser BG, Strauss AL. The discovery of grounded theory . Hawthorne, NY: Aldine, 1967.
- ↵ Ray MA. The richness of phenomenology: philosophic, theoretic, and methodologic concerns. In: J M Morse, editor. Critical issues in qualitative research methods . Thousand Oaks, CA: Sage, 1994:117–33.
- ↵ Boyle JS. Styles of ethnography. In: JM Morse, editor. Critical issues in qualitative research methods .. Thousand Oaks, CA: Sage, 1994:159–85.
- ↵ Sandelowski M. We are the stories we tell: narrative knowing in nursing practice. J Holist Nurs 1994 ; 12 : 23 –33. OpenUrl CrossRef PubMed
- ↵ Boutain DM. Critical language and discourse study: their transformative relevance for critical nursing inquiry. ANS Adv Nurs Sci 1999 ; 21 : 1 –8.
- ↵ Thorne S. The art (and science) of critiquing qualitative research. In: JM Morse, editor. Completing a qualitative project: details and dialogue . Thousand Oaks, CA: Sage, 1997:117–32.
Read the full text or download the PDF:
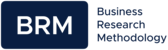
Qualitative Data Analysis
Qualitative data refers to non-numeric information such as interview transcripts, notes, video and audio recordings, images and text documents. Qualitative data analysis can be divided into the following five categories:
1. Content analysis . This refers to the process of categorizing verbal or behavioural data to classify, summarize and tabulate the data.
2. Narrative analysis . This method involves the reformulation of stories presented by respondents taking into account context of each case and different experiences of each respondent. In other words, narrative analysis is the revision of primary qualitative data by researcher.
3. Discourse analysis . A method of analysis of naturally occurring talk and all types of written text.
4. Framework analysis . This is more advanced method that consists of several stages such as familiarization, identifying a thematic framework, coding, charting, mapping and interpretation.
5. Grounded theory . This method of qualitative data analysis starts with an analysis of a single case to formulate a theory. Then, additional cases are examined to see if they contribute to the theory.
Qualitative data analysis can be conducted through the following three steps:
Step 1: Developing and Applying Codes . Coding can be explained as categorization of data. A ‘code’ can be a word or a short phrase that represents a theme or an idea. All codes need to be assigned meaningful titles. A wide range of non-quantifiable elements such as events, behaviours, activities, meanings etc. can be coded.
There are three types of coding:
- Open coding . The initial organization of raw data to try to make sense of it.
- Axial coding . Interconnecting and linking the categories of codes.
- Selective coding . Formulating the story through connecting the categories.
Coding can be done manually or using qualitative data analysis software such as
NVivo, Atlas ti 6.0, HyperRESEARCH 2.8, Max QDA and others.
When using manual coding you can use folders, filing cabinets, wallets etc. to gather together materials that are examples of similar themes or analytic ideas. Manual method of coding in qualitative data analysis is rightly considered as labour-intensive, time-consuming and outdated.
In computer-based coding, on the other hand, physical files and cabinets are replaced with computer based directories and files. When choosing software for qualitative data analysis you need to consider a wide range of factors such as the type and amount of data you need to analyse, time required to master the software and cost considerations.
Moreover, it is important to get confirmation from your dissertation supervisor prior to application of any specific qualitative data analysis software.
The following table contains examples of research titles, elements to be coded and identification of relevant codes:
Qualitative data coding
Step 2: Identifying themes, patterns and relationships . Unlike quantitative methods , in qualitative data analysis there are no universally applicable techniques that can be applied to generate findings. Analytical and critical thinking skills of researcher plays significant role in data analysis in qualitative studies. Therefore, no qualitative study can be repeated to generate the same results.
Nevertheless, there is a set of techniques that you can use to identify common themes, patterns and relationships within responses of sample group members in relation to codes that have been specified in the previous stage.
Specifically, the most popular and effective methods of qualitative data interpretation include the following:
- Word and phrase repetitions – scanning primary data for words and phrases most commonly used by respondents, as well as, words and phrases used with unusual emotions;
- Primary and secondary data comparisons – comparing the findings of interview/focus group/observation/any other qualitative data collection method with the findings of literature review and discussing differences between them;
- Search for missing information – discussions about which aspects of the issue was not mentioned by respondents, although you expected them to be mentioned;
- Metaphors and analogues – comparing primary research findings to phenomena from a different area and discussing similarities and differences.
Step 3: Summarizing the data . At this last stage you need to link research findings to hypotheses or research aim and objectives. When writing data analysis chapter, you can use noteworthy quotations from the transcript in order to highlight major themes within findings and possible contradictions.
It is important to note that the process of qualitative data analysis described above is general and different types of qualitative studies may require slightly different methods of data analysis.
My e-book, The Ultimate Guide to Writing a Dissertation in Business Studies: a step by step approach contains a detailed, yet simple explanation of qualitative data analysis methods . The e-book explains all stages of the research process starting from the selection of the research area to writing personal reflection. Important elements of dissertations such as research philosophy, research approach, research design, methods of data collection and data analysis are explained in simple words. John Dudovskiy
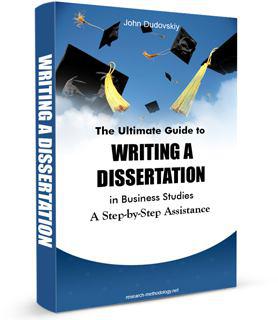

The world’s most powerful AI-based qualitative data analysis solution.
QualAI utilizes advanced AI technology to increase researcher efficiency, enhance data reliability, and mitigate bias.
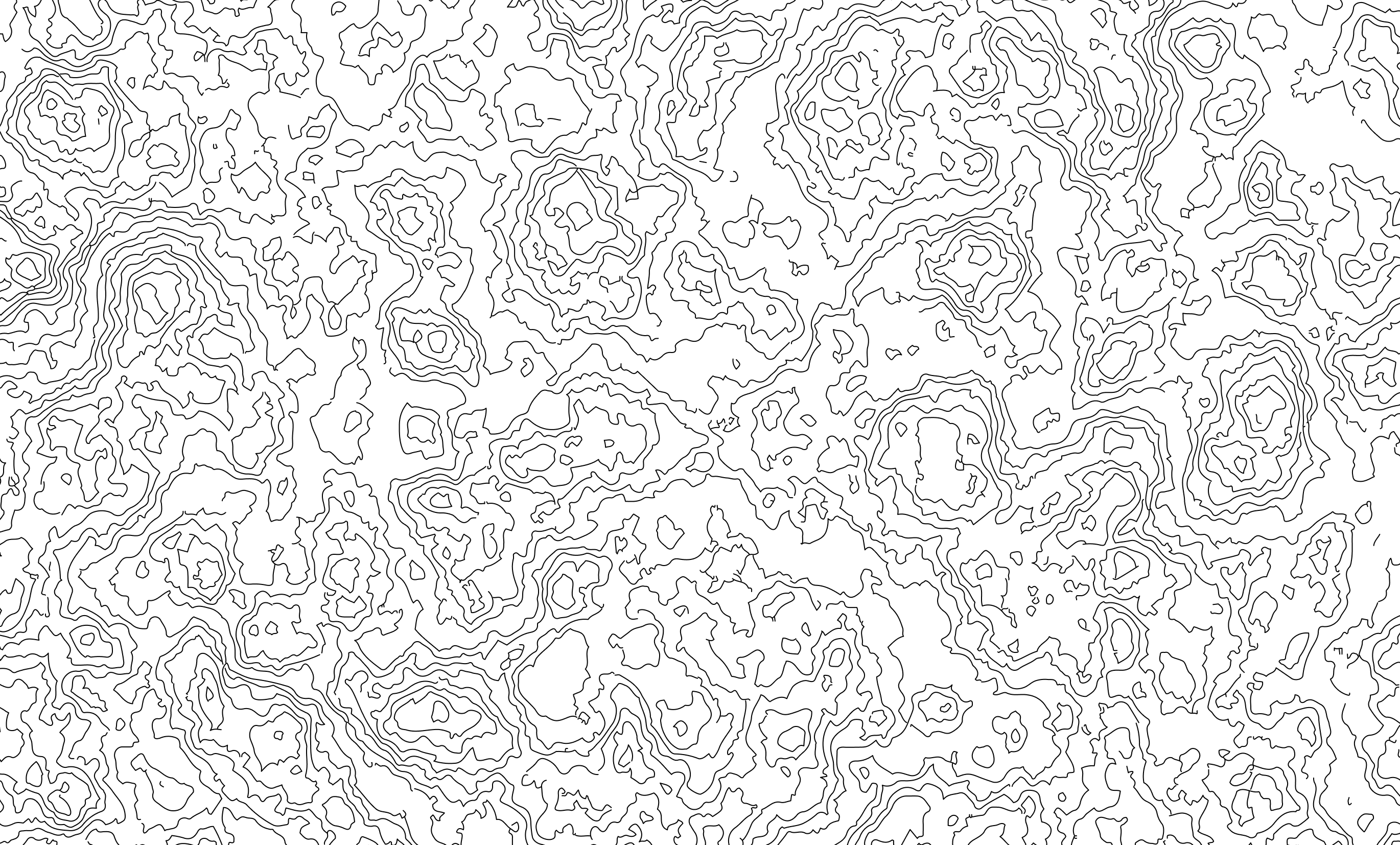
researchers
QualAI aids researchers with data codification, thematic analyses, and content summaries to increase data reliability and mitigate bias.
organizations
QualAI helps organizations with market research, consumer analysis, business development, data aggregation and interpretation.
See how QualAI helps students analyze large-scale qualitative data sets, codify transcripts, and generate themes to reduce bias and increase efficiency.

ERIK ALANSON, Ph.d.
Co-Founder, QualAI
Academic Researcher
University Professor
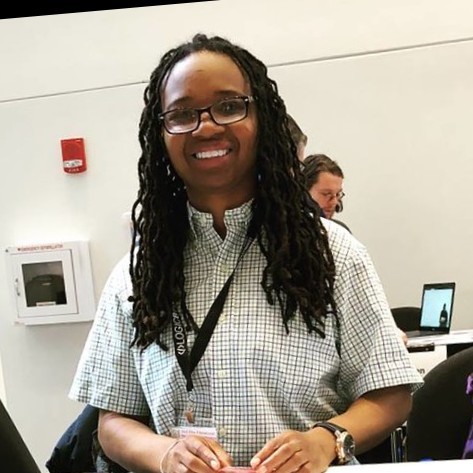
tonkia bridges, ed.d.
Powered by WordPress.com .
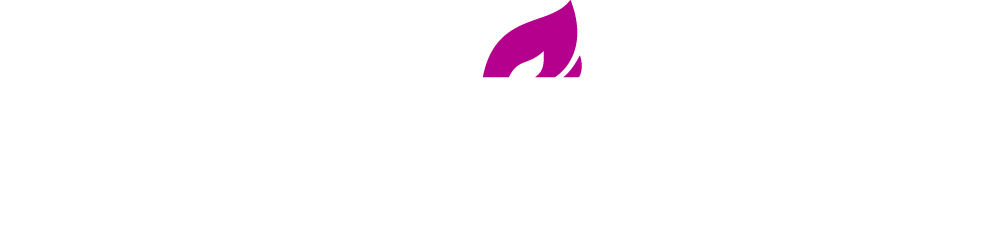
No products in the cart.
What is Qualitative Data Analysis Software (QDA Software)?
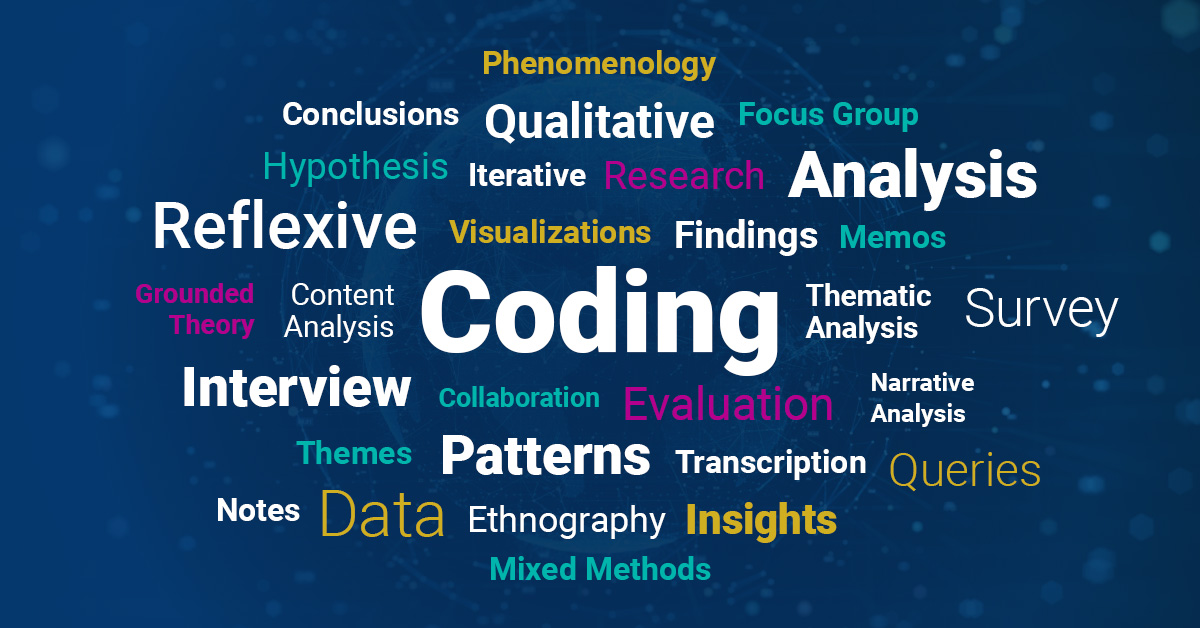
Qualitative Data Analysis Software (QDA software) allows researchers to organize, analyze and visualize their data, finding the patterns in qualitative data or unstructured data: interviews, surveys, field notes, videos, audio files, images, journal articles interviews, web content etc.
Quantitative vs. Qualitative Data Analysis
What is the difference between quantitative and qualitative data analysis. As the name implies, quantitative data analysis has to do with numbers. For example, any time you are doing statistical analysis, you are doing quantitative data analysis. Some examples of quantitative data analysis software are SPSS, STATA, SAS, and Lumivero’s own powerful statistics software, XLSTAT .
In contrast, qualitative analysis "helps you understand people’s perceptions and experiences by systematically coding and analyzing the data", as described in Qualitative vs Quantitative Research 101 . It tends to deal more with words than numbers. It can be useful when working with a lot of rich and deep data and when you aren’t trying to test something very specific. Some examples of qualitative data analysis software are MAXQDA, ATLAS.ti, Quirkos, and Lumivero’s NVivo, the leading tool for qualitative data analysis .
When would you use each one? Well, qualitative data analysis is often used for exploratory research or developing a theory, whereas quantitative is better if you want to test a hypothesis, find averages, and determine relationships between variables. With quantitative research you often want a large sample size to get relevant statistics. In contrast, qualitative research, because so much data in the form of text is involved, can have much smaller sample sizes and still yield valuable insights.
Of course, it’s not always so cut and dry, and many researchers end up taking a «mixed methods» approach, meaning that they combine both types of research. In this case they might use a combination of both types of software programs.
Learn how some qualitative researchers use QDA software for text analysis in the on-demand webinar Twenty-Five Qualitative Researchers Share How-To's for Data Analysis .

How is Qualitative Data Analysis Software Used for Research?
Qualitative Data Analysis Software works with any qualitative research methodology used by a researcher For example, software for qualitative data analysis can be used by a social scientist wanting to develop new concepts or theories may take a ‘grounded theory’ approach. Or a researcher looking for ways to improve health policy or program design might use ‘evaluation methods’. QDA software analysis tools don't favor a particular methodology — they're designed to facilitate common qualitative techniques no matter what method you use.
NVivo can help you to manage, explore and find patterns in your data and conduct thematic and sentiment analysis, but it cannot replace your analytical expertise.
Qualitative Research as an Iterative Process
Handling qualitative and mixed methods data is not usually a step-by-step process. Instead, it tends to be an iterative process where you explore, code, reflect, memo, code some more, query and so on. For example, this picture shows a path you might take to investigate an interesting theme using QDA software, like NVivo, to analyze data:
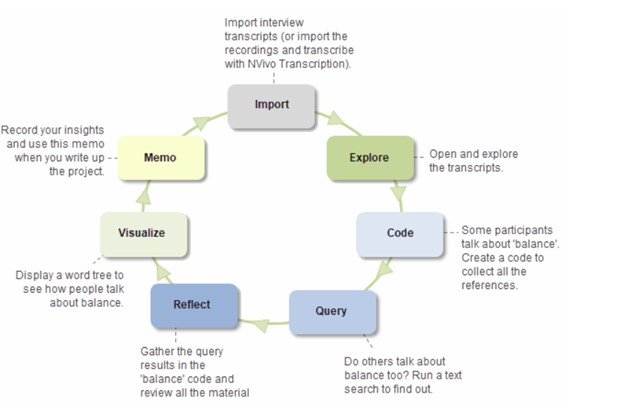
How Do I Choose the Best Approach for My Research Project with QDA Software?
Every research project is unique — the way you organize and analyze the material depends on your methodology, data and research design.
Here are some example scenarios for handling different types of research projects in QDA software — these are just suggestions to get you up and running.
A study with interviews exploring stakeholder perception of a community arts program
Your files consist of unstructured interview documents. You would set up a case for each interview participant, then code to codes and cases. You could then explore your data with simple queries or charts and use memos to record your discoveries.
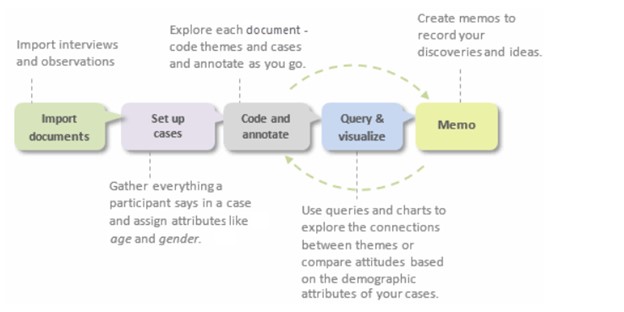
A study exploring community perceptions about climate change using autocoding with AI
Your files consist of structured, consistently formatted interviews (where each participant is asked the same set of questions). With AI, you could autocode the interviews and set up cases for each participant. Then code themes to query and visualize your data.
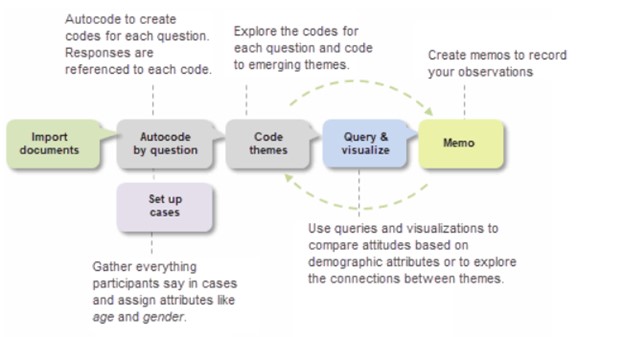
A literature review on adolescent depression
Your files consist of journal articles, books and web pages. You would classify your files before coding and querying them; and then you could critique each file in a memo. With Citavi integration in NVivo, you can import your Citavi references into NVivo.
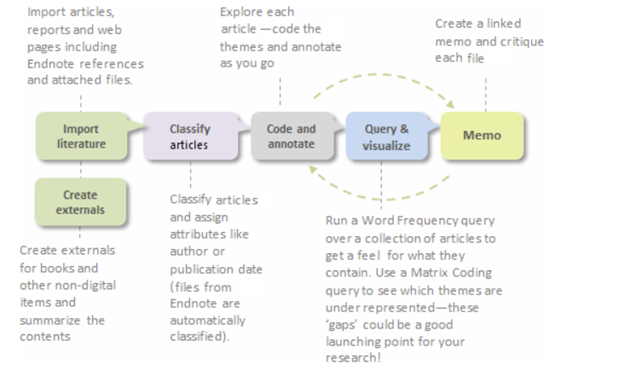
A social media study of the language used by members of an online community
Your files consist of Facebook data captured with NCapture. You would import it as a dataset ready to code and query. Use memos to record your insights.
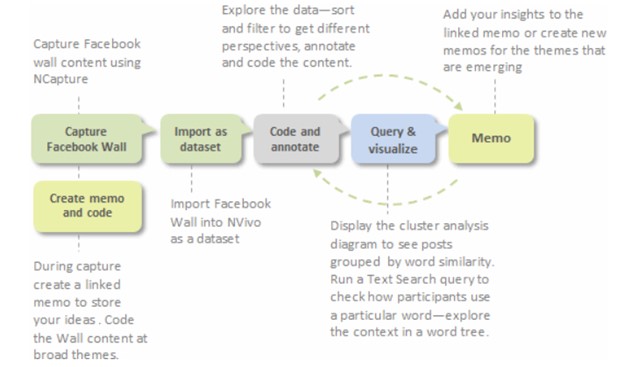
A quick analysis of a local government budget survey
Your file is a large dataset of survey responses. You would import it using the Survey Import Wizard, which prepares your data for analysis. As part of the import, choose to run automated insights with AI to identify and code to themes and sentiment so that you can quickly review results and report broad findings.
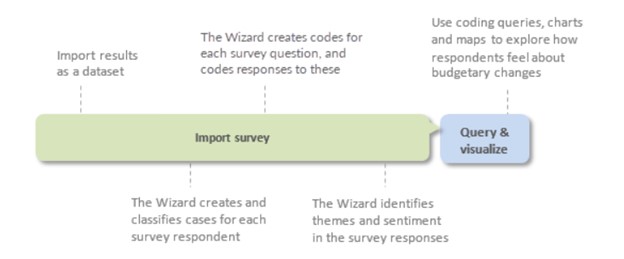
Ways to Get Started with Your Project with Qualitative Analysis Software
Since projects (and researchers) are unique there is no one 'best practice' approach to organizing and analyzing your data but there are some useful strategies to help you get up and running:
- Start now - don't wait until you have collected all the data. Import your research design, grant application or thesis proposal.
- Make a project journa l and state your research questions and record your goals. Why are you doing the project? What is it about? What do you expect to find and why?
- Make a mind map for your preliminary ideas. Show the relationships or patterns you expect to find in your data based on prior experience or preliminary reading.
- Import your interviews, field notes, focus groups —organize these files into folders for easy access.
- Set up an initial code structure based on your early reading and ideas—you could run a Word Frequency query over your data to tease out the common themes for creating your code structure.
- Set up cases for the people, places or other cases in your project.
- Explore your material and code themes as they emerge in your data mining —creating memos and describing your discoveries and interpretations.
- To protect your work, get in the habit of making regular back-ups.
QDA Analysis Tools Help You Work Toward Outcomes that are Robust and Transparent
Using QDA software to organize and analyze your data also increases the 'transparency' of your research outcomes—for example, you can:
- Demonstrate the evolution of your ideas in memos and maps.
- Document your early preconceptions and biases (in a memo or map) and demonstrate how these have been acknowledged and tested.
- Easily find illustrative quotes.
- Always return to the original context of your coded material.
- Save and revisit the queries and visualizations that helped you to arrive at your conclusions.
QDA software, like NVivo, can demonstrate the credibility of your findings in the following ways:
- If you used NVivo for your literature review, run a query or create a chart to demonstrate how your findings compare with the views of other authors.
- Was an issue or theme reported by more than one participant? Run a Matrix Coding query to see how many participants talked about a theme.
- Were multiple methods used to collect the data (interviews, observations, surveys)—and are the findings supported across these text data and video data files? Run a Matrix Coding query to see how often a theme is reported across all your files.

- If multiple researchers analyzed the material — were their findings consistent? Use coding stripes (or filter the contents in a code) to see how various team members have coded the material and run a Coding Comparison query to assess the level of agreement.
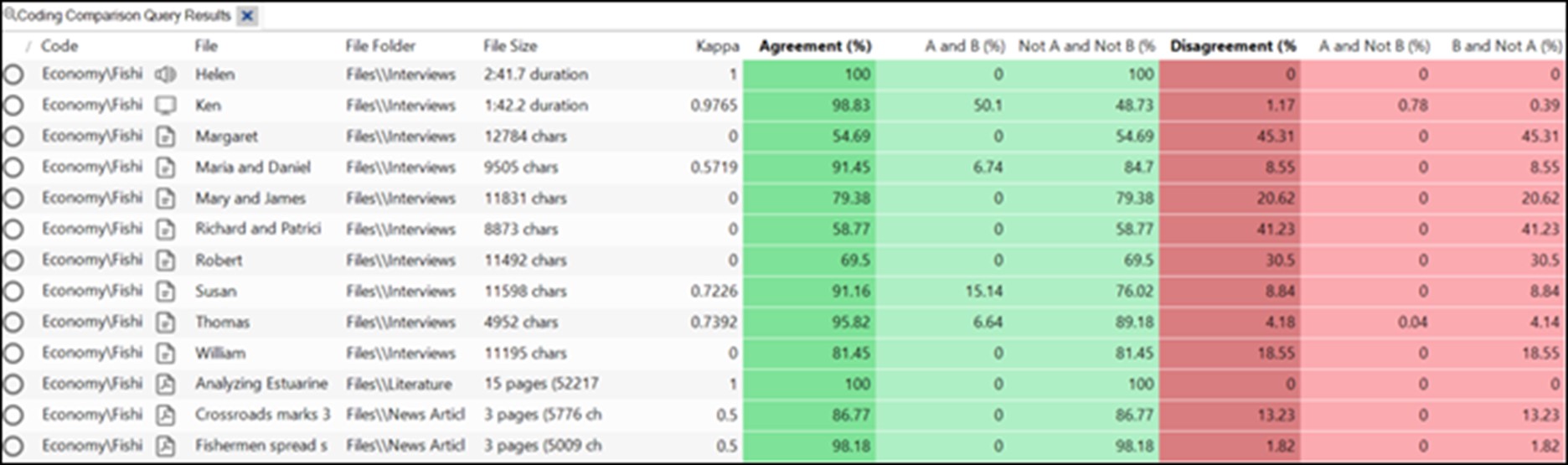
QDA Software Integrations
Many qualitative analysis software options have integration with other software to enhance your research process. NVivo integrates or can be used with the following software:
- NVivo Transcription to save you time and jump start your qualitative data analysis. Learn how in the on-demand webinar Transcription – Go Beyond the Words .
- Reference management software, like Lumivero’s Citavi, for reference management and writing. By combining Citavi and NVivo, you can create complicated searches for certain keywords, terms, and categories and make advanced search syntax, like wildcards, boolean operators, and regular expressions. This integration allows you to take your analyses beyond reference management by developing a central location to collect references and thoughts, analyze literature, and connect empirical data.
- Statistical software, like Lumivero’s XLSTAT , SPSS, or STATA to export your queries from NVivo to run statistical analysis
- Qualtrics, SurveyMonkey to import your survey results into NVivo to start analyzing.
Make Choosing QDA Software Easy — Try NVivo Today!
It's tough choosing QDA software! Test out NVivo, the most cited qualitative data analysis tool, by requesting a free 14-day trial of NVivo to start improving your qualitative and mixed methods research today.
Recent Articles
- Open access
- Published: 13 May 2024
Patient medication management, understanding and adherence during the transition from hospital to outpatient care - a qualitative longitudinal study in polymorbid patients with type 2 diabetes
- Léa Solh Dost ORCID: orcid.org/0000-0001-5767-1305 1 , 2 ,
- Giacomo Gastaldi ORCID: orcid.org/0000-0001-6327-7451 3 &
- Marie P. Schneider ORCID: orcid.org/0000-0002-7557-9278 1 , 2
BMC Health Services Research volume 24 , Article number: 620 ( 2024 ) Cite this article
131 Accesses
Metrics details
Continuity of care is under great pressure during the transition from hospital to outpatient care. Medication changes during hospitalization may be poorly communicated and understood, compromising patient safety during the transition from hospital to home. The main aims of this study were to investigate the perspectives of patients with type 2 diabetes and multimorbidities on their medications from hospital discharge to outpatient care, and their healthcare journey through the outpatient healthcare system. In this article, we present the results focusing on patients’ perspectives of their medications from hospital to two months after discharge.
Patients with type 2 diabetes, with at least two comorbidities and who returned home after discharge, were recruited during their hospitalization. A descriptive qualitative longitudinal research approach was adopted, with four in-depth semi-structured interviews per participant over a period of two months after discharge. Interviews were based on semi-structured guides, transcribed verbatim, and a thematic analysis was conducted.
Twenty-one participants were included from October 2020 to July 2021. Seventy-five interviews were conducted. Three main themes were identified: (A) Medication management, (B) Medication understanding, and (C) Medication adherence, during three periods: (1) Hospitalization, (2) Care transition, and (3) Outpatient care. Participants had varying levels of need for medication information and involvement in medication management during hospitalization and in outpatient care. The transition from hospital to autonomous medication management was difficult for most participants, who quickly returned to their routines with some participants experiencing difficulties in medication adherence.
Conclusions
The transition from hospital to outpatient care is a challenging process during which discharged patients are vulnerable and are willing to take steps to better manage, understand, and adhere to their medications. The resulting tension between patients’ difficulties with their medications and lack of standardized healthcare support calls for interprofessional guidelines to better address patients’ needs, increase their safety, and standardize physicians’, pharmacists’, and nurses’ roles and responsibilities.
Peer Review reports
Introduction
Continuity of patient care is characterized as the collaborative engagement between the patient and their physician-led care team in the ongoing management of healthcare, with the mutual objective of delivering high-quality and cost-effective medical care [ 1 ]. Continuity of care is under great pressure during the transition of care from hospital to outpatient care, with a risk of compromising patients’ safety [ 2 , 3 ]. The early post-discharge period is a high-risk and fragile transition: once discharged, one in five patients experience at least one adverse event during the first three weeks following discharge, and more than half of these adverse events are drug-related [ 4 , 5 ]. A retrospective study examining all discharged patients showed that adverse drug events (ADEs) account for up to 20% of 30-day hospital emergency readmissions [ 6 ]. During hospitalization, patients’ medications are generally modified, with an average of nearly four medication changes per patient [ 7 ]. Information regarding medications such as medication changes, the expected effect, side effects, and instructions for use are frequently poorly communicated to patients during hospitalization and at discharge [ 8 , 9 , 10 , 11 ]. Between 20 and 60% of discharged patients lack knowledge of their medications [ 12 , 13 ]. Consideration of patients’ needs and their active engagement in decision-making during hospitalization regarding their medications are often lacking [ 11 , 14 , 15 ]. This can lead to unsafe discharge and contribute to medication adherence difficulties, such as non-implementation of newly prescribed medications [ 16 , 17 ].
Patients with multiple comorbidities and polypharmacy are at higher risk of ADE [ 18 ]. Type 2 diabetes is one of the chronic health conditions most frequently associated with comorbidities and patients with type 2 diabetes often lack care continuum [ 19 , 20 , 21 ]. The prevalence of patients hospitalized with type 2 diabetes can exceed 40% [ 22 ] and these patients are at higher risk for readmission due to their comorbidities and their medications, such as insulin and oral hypoglycemic agents [ 23 , 24 , 25 ].
Interventions and strategies to improve patient care and safety at transition have shown mixed results worldwide in reducing cost, rehospitalization, ADE, and non-adherence [ 26 , 27 , 28 , 29 , 30 , 31 , 32 , 33 , 34 , 35 ]. However, interventions that are patient-centered, with a patient follow-up and led by interprofessional healthcare teams showed promising results [ 34 , 35 , 36 ]. Most of these interventions have not been implemented routinely due to the extensive time to translate research into practice and the lack of hybrid implementation studies [ 37 , 38 , 39 , 40 , 41 ]. In addition, patient-reported outcomes and perspectives have rarely been considered, yet patients’ involvement is essential for seamless and integrated care [ 42 , 43 ]. Interprofessional collaboration in which patients are full members of the interprofessional team, is still in its infancy in outpatient care [ 44 ]. Barriers and facilitators regarding medications at the transition of care have been explored in multiple qualitative studies at one given time in a given setting (e.g., at discharge, one-month post-discharge) [ 8 , 45 , 46 , 47 , 48 ]. However, few studies have adopted a holistic methodology from the hospital to the outpatient setting to explore changes in patients’ perspectives over time [ 49 , 50 , 51 ]. Finally, little is known about whether, how, and when patients return to their daily routine following hospitalization and the impact of hospitalization weeks after discharge.
In Switzerland, continuity of care after hospital discharge is still poorly documented, both in terms of contextual analysis and interventional studies, and is mainly conducted in the hospital setting [ 31 , 35 , 52 , 53 , 54 , 55 , 56 ]. The first step of an implementation science approach is to perform a contextual analysis to set up effective interventions adapted to patients’ needs and aligned to healthcare professionals’ activities in a specific context [ 41 , 57 ]. Therefore, the main aims of this study were to investigate the perspectives of patients with type 2 diabetes and multimorbidities on their medications from hospital discharge to outpatient care, and on their healthcare journey through the outpatient healthcare system. In this article, we present the results focusing on patients’ perspectives of their medications from hospital to two months after discharge.
Study design
This qualitative longitudinal study, conducted from October 2020 to July 2021, used a qualitative descriptive methodology through four consecutive in-depth semi-structured interviews per participant at three, 10-, 30- and 60-days post-discharge, as illustrated in Fig. 1 . Longitudinal qualitative research is characterized by qualitative data collection at different points in time and focuses on temporality, such as time and change [ 58 , 59 ]. Qualitative descriptive studies aim to explore and describe the depth and complexity of human experiences or phenomena [ 60 , 61 , 62 ]. We focused our qualitative study on the 60 first days after discharge as this period is considered highly vulnerable and because studies often use 30- or 60-days readmission as an outcome measure [ 5 , 63 ].
This qualitative study follows the Consolidated Criteria for Reporting Qualitative Research (COREQ). Ethics committee approval was sought and granted by the Cantonal Research Ethics Commission, Geneva (CCER) (2020 − 01779).
Recruitment took place during participants’ hospitalization in the general internal medicine divisions at the Geneva University Hospitals in the canton of Geneva (500 000 inhabitants), Switzerland. Interviews took place at participants’ homes, in a private office at the University of Geneva, by telephone or by secure video call, according to participants’ preference. Informal caregivers could also participate alongside the participants.
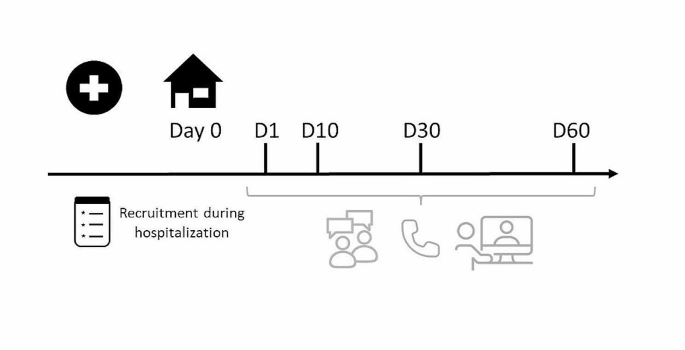
Study flowchart
Researcher characteristics
All the researchers were trained in qualitative studies. The diabetologist and researcher (GG) who enrolled the patients in the study was involved directly or indirectly (advice asked to the Geneva University Hospital diabetes team of which he was a part) for most participants’ care during hospitalization. LS (Ph.D. student and community pharmacist) was unknown to participants and presented herself during hospitalization as a “researcher” and not as a healthcare professional to avoid any risk of influencing participants’ answers. This study was not interventional, and the interviewer (LS) invited participants to contact a healthcare professional for any questions related to their medication or medical issues.
Population and sampling strategy
Patients with type 2 diabetes were chosen as an example population to describe polypharmacy patients as these patients usually have several health issues and polypharmacy [ 20 , 22 , 25 ]. Inclusions criteria for the study were: adult patients with type 2 diabetes, with at least two other comorbidities, hospitalized for at least three days in a general internal medicine ward, with a minimum of one medication change during hospital stay, and who self-managed their medications once discharged home. Exclusion criteria were patients not reachable by telephone following discharge, unable to give consent (patients with schizophrenia, dementia, brain damage, or drug/alcohol misuse), and who could not communicate in French. A purposive sampling methodology was applied aiming to include participants with different ages, genders, types, and numbers of health conditions by listing participants’ characteristics in a double-entry table, available in Supplementary Material 1 , until thematic saturation was reached. Thematic saturation was considered achieved when no new code or theme emerged and new data repeated previously coded information [ 64 ]. The participants were identified if they were hospitalized in the ward dedicated to diabetes care or when the diabetes team was contacted for advice. The senior ward physician (GG) screened eligible patients and the interviewer (LS) obtained written consent before hospital discharge.
Data collection and instruments
Sociodemographic (age, gender, educational level, living arrangement) and clinical characteristics (reason for hospitalization, date of admission, health conditions, diabetes diagnosis, medications before and during hospitalization) were collected by interviewing participants before their discharge and by extracting participants’ data from electronic hospital files by GG and LS. Participants’ pharmacies were contacted with the participant’s consent to obtain medication records from the last three months if information regarding medications before hospitalization was missing in the hospital files.
Semi-structured interview guides for each interview (at three, 10-, 30- and 60-days post-discharge) were developed based on different theories and components of health behavior and medication adherence: the World Health Organization’s (WHO) five dimensions for adherence, the Information-Motivation-Behavioral skills model and the Social Cognitive Theory [ 65 , 66 , 67 ]. Each interview explored participants’ itinerary in the healthcare system and their perspectives on their medications. Regarding medications, the following themes were mentioned at each interview: changes in medications, patients’ understanding and implication; information on their medications, self-management of their medications, and patients’ medication adherence. Other aspects were mentioned in specific interviews: patients’ hospitalization and experience on their return home (interview 1), motivation (interviews 2 and 4), and patient’s feedback on the past two months (interview 4). Interview guides translated from French are available in Supplementary Material 2 . The participants completed self-reported and self-administrated questionnaires at different interviews to obtain descriptive information on different factors that may affect medication management and adherence: self-report questionnaires on quality of life (EQ-5D-5 L) [ 68 ], literacy (Schooling-Opinion-Support questionnaire) [ 69 ], medication adherence (Adherence Visual Analogue Scale, A-VAS) [ 70 ] and Belief in Medication Questionnaire (BMQ) [ 71 ] were administered to each participant at the end of selected interviews to address the different factors that may affect medication management and adherence as well as to determine a trend of determinants over time. The BMQ contains two subscores: Specific-Necessity and Specific-Concerns, addressing respectively their perceived needs for their medications, and their concerns about adverse consequences associated with taking their medication [ 72 ].
Data management
Informed consent forms, including consent to obtain health data, were securely stored in a private office at the University of Geneva. The participants’ identification key was protected by a password known only by MS and LS. Confidentiality was guaranteed by pseudonymization of participants’ information and audio-recordings were destroyed once analyzed. Sociodemographic and clinical characteristics, medication changes, and answers to questionnaires were securely collected by electronic case report forms (eCRFs) on RedCap®. Interviews were double audio-recorded and field notes were taken during interviews. Recorded interviews were manually transcribed verbatim in MAXQDA® (2018.2) by research assistants and LS and transcripts were validated for accuracy by LS. A random sample of 20% of questionnaires was checked for accuracy for the transcription from the paper questionnaires to the eCRFs. Recorded sequences with no link to the discussed topics were not transcribed and this was noted in the transcripts.
Data analysis
A descriptive statistical analysis of sociodemographic, clinical characteristics and self-reported questionnaire data was carried out. A thematic analysis of transcripts was performed, as described by Braun and Clarke [ 73 ], by following six steps: raw data was read, text segments related to the study objectives were identified, text segments to create new categories were identified, similar or redundant categories were reduced and a model that integrated all significant categories was created. The analysis was conducted in parallel with patient enrolment to ensure data saturation. To ensure the validity of the coding method, transcripts were double coded independently and discussed by the research team until similar themes were obtained. The research group developed and validated an analysis grid, with which LS coded systematically the transcriptions and met regularly with the research team to discuss questions on data analysis and to ensure the quality of coding. The analysis was carried out in French, and the verbatims of interest cited in the manuscript were translated and validated by a native English-speaking researcher to preserve the meaning.
In this analysis, we used the term “healthcare professionals” when more than one profession could be involved in participants’ medication management. Otherwise, when a specific healthcare professional was involved, we used the designated profession (e.g. physicians, pharmacists).
Patient and public involvement
During the development phase of the study, interview guides and questionnaires were reviewed for clarity and validity and adapted by two patient partners, with multiple health conditions and who experienced previously a hospital discharge. They are part of the HUG Patients Partners + 3P platform for research and patient and public involvement.
Interviews and participants’ descriptions
A total of 75 interviews were conducted with 21 participants. In total, 31 patients were contacted, seven refused to participate (four at the project presentation and three at consent), two did not enter the selection criteria at discharge and one was unreachable after discharge. Among the 21 participants, 15 participated in all interviews, four in three interviews, one in two interviews, and one in one interview, due to scheduling constraints. Details regarding interviews and participants characteristics are presented in Tables 1 and 2 .
The median length of time between hospital discharge and interviews 1,2,3 and 4 was 5 (IQR: 4–7), 14 (13-20), 35 (22-38), and 63 days (61-68), respectively. On average, by comparing medications at hospital admission and discharge, a median of 7 medication changes (IQR: 6–9, range:2;17) occurred per participant during hospitalization and a median of 7 changes (5–12) during the two months following discharge. Details regarding participants’ medications are described in Table 3 .
Patient self-reported adherence over the past week for their three most challenging medications are available in Supplementary Material 3 .
Qualitative analysis
We defined care transition as the period from discharge until the first medical appointment post-discharge, and outpatient care as the period starting after the first medical appointment. Data was organized into three key themes (A. Medication management, B. Medication understanding, and C. Medication adherence) divided into subthemes at three time points (1. Hospitalization, 2. Care transition and 3. Outpatient care). Figure 2 summarizes and illustrates the themes and subthemes with their influencing factors as bullet points.
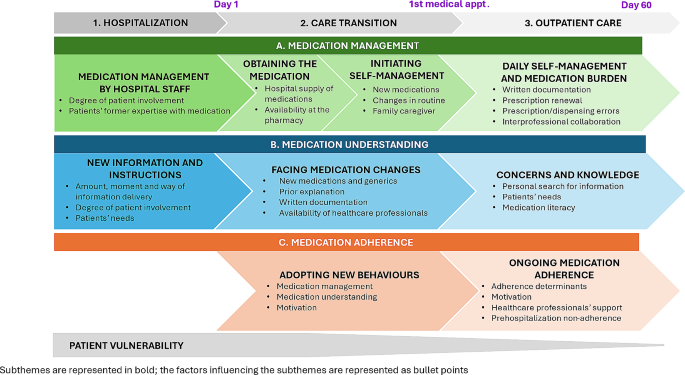
Participants’ medication management, understanding and adherence during hospitalization, care transition and outpatient care
A. Medication management
A.1 medication management during hospitalization: medication management by hospital staff.
Medications during hospitalization were mainly managed by hospital healthcare professionals (i.e. nurses and physicians) with varying degrees of patient involvement: “At the hospital, they prepared the medications for me. […] I didn’t even know what the packages looked like.” Participant 22; interview 1 (P22.1) Some participants reported having therapeutic education sessions with specialized nurses and physicians, such as the explanation and demonstration of insulin injection and glucose monitoring. A patient reported that he was given the choice of several treatments and was involved in shared decision-making. Other participants had an active role in managing and optimizing dosages, such as rapid insulin, due to prior knowledge and use of medications before hospitalization.
A.2 Medication management at transition: obtaining the medication and initiating self-management
Once discharged, some participants had difficulties obtaining their medications at the pharmacy because some medications were not stored and had to be ordered, delaying medication initiation. To counter this problem upstream, a few participants were provided a 24-to-48-hour supply of medications at discharge. It was sometimes requested by the patient or suggested by the healthcare professionals but was not systematic. The transition from medication management by hospital staff to self-management was exhausting for most participants who were faced with a large amount of new information and changes in their medications: “ When I was in the hospital, I didn’t even realize all the changes. When I came back home, I took away the old medication packages and got out the new ones. And then I thought : « my God, all this…I didn’t know I had all these changes » ” P2.1 Written documentation, such as the discharge prescription or dosage labels on medication packages, was helpful in managing their medication at home. Most participants used weekly pill organizers to manage their medications, which were either already used before hospitalization or were introduced post-discharge. The help of a family caregiver in managing and obtaining medications was reported as a facilitator.
A.3 Medication management in outpatient care: daily self-management and medication burden
A couple of days or weeks after discharge, most participants had acquired a routine so that medication management was less demanding, but the medication burden varied depending on the participants. For some, medication management became a simple action well implemented in their routine (“It has become automatic” , P23.4), while for others, the number of medications and the fact that the medications reminded them of the disease was a heavy burden to bear on a daily basis (“ During the first few days after getting out of the hospital, I thought I was going to do everything right. In the end, well [laughs] it’s complicated. I ended up not always taking the medication, not monitoring the blood sugar” P12.2) To support medication self-management, some participants had written documentation such as treatment plans, medication lists, and pictures of their medication packages on their phones. Some participants had difficulties obtaining medications weeks after discharge as discharge prescriptions were not renewable and participants did not see their physician in time. Others had to visit multiple physicians to have their prescriptions updated. A few participants were faced with prescription or dispensing errors, such as prescribing or dispensing the wrong dosage, which affected medication management and decreased trust in healthcare professionals. In most cases, according to participants, the pharmacy staff worked in an interprofessional collaboration with physicians to provide new and updated prescriptions.
B. Medication understanding
B.1 medication understanding during hospitalization: new information and instructions.
The amount of information received during hospitalization varied considerably among participants with some reporting having received too much, while others saying they received too little information regarding medication changes, the reason for changes, or for introducing new medications: “They told me I had to take this medication all my life, but they didn’t tell me what the effects were or why I was taking it.” P5.3
Hospitalization was seen by some participants as a vulnerable and tiring period during which they were less receptive to information. Information and explanations were generally given verbally, making it complicated for most participants to recall it. Some participants reported that hospital staff was attentive to their needs for information and used communication techniques such as teach-back (a way of checking understanding by asking participants to say in their own words what they need to know or do about their health or medications). Some participants were willing to be proactive in the understanding of their medications while others were more passive, had no specific needs for information, and did not see how they could be engaged more.
B.2 Medication understanding at transition: facing medication changes
At hospital discharge, the most challenging difficulty for participants was to understand the changes made regarding their medications. For newly diagnosed participants, the addition of new medications was more difficult to understand, whereas, for experienced participants, changes in known medications such as dosage modification, changes within a therapeutic class, and generic substitutions were the most difficult to understand. Not having been informed about changes caused confusion and misunderstanding. Therefore, medication reconciliation done by the patient was time-consuming, especially for participants with multiple medications: “ They didn’t tell me at all that they had changed my treatment completely. They just told me : « We’ve changed a few things. But it was the whole treatment ». ” P2.3 Written information, such as the discharge prescription, the discharge report (brief letter summarizing information about the hospitalization, given to the patient at discharge), or the label on the medication box (written by the pharmacist with instructions on dosage) helped them find or recall information about their medications and diagnoses. However, technical terms were used in hospital documentations and were not always understandable. For example, this participant said: “ On the prescription of valsartan, they wrote: ‘resume in the morning once profile…’[once hypertension profile allows]… I don’t know what that means.” P8.1 In addition, some documents were incomplete, as mentioned by a patient who did not have the insulin dosage mentioned on the hospital prescription. Some participants sought help from healthcare professionals, such as pharmacists, hospital physicians, or general practitioners a few days after discharge to review medications, answer questions, or obtain additional information.
B.3 Medication understanding in the outpatient care: concerns and knowledge
Weeks after discharge, most participants had concerns about the long-term use of their medications, their usefulness, and the possible risk of interactions or side effects. Some participants also reported having some lack of knowledge regarding indications, names, or how the medication worked: “I don’t even know what Brilique® [ticagrelor, antiplatelet agent] is for. It’s for blood pressure, isn’t it?. I don’t know.” P11.4 According to participants, the main reasons for the lack of understanding were the lack of information at the time of prescribing and the large number of medications, making it difficult to search for information and remember it. Participants sought information from different healthcare professionals or by themselves, on package inserts, through the internet, or from family and friends. Others reported having had all the information needed or were not interested in having more information. In addition, participants with low medication literacy, such as non-native speakers or elderly people, struggled more with medication understanding and sought help from family caregivers or healthcare professionals, even weeks after discharge: “ I don’t understand French very well […] [The doctor] explained it very quickly…[…] I didn’t understand everything he was saying” P16.2
C. Medication adherence
C.2 medication adherence at transition: adopting new behaviors.
Medication adherence was not mentioned as a concern during hospitalization and a few participants reported difficulties in medication initiation once back home: “I have an injection of Lantus® [insulin] in the morning, but obviously, the first day [after discharge], I forgot to do it because I was not used to it.” P23.1 Participants had to quickly adopt new behaviors in the first few days after discharge, especially for participants with few medications pre-hospitalization. The use of weekly pill organizers, alarms and specific storage space were reported as facilitators to support adherence. One patient did not initiate one of his medications because he did not understand the medication indication, and another patient took her old medications because she was used to them. Moreover, most participants experienced their hospitalization as a turning point, a time when they focused on their health, thought about the importance of their medications, and discussed any new lifestyle or dietary measures that might be implemented.
C.3 Medication adherence in outpatient care: ongoing medication adherence
More medication adherence difficulties appeared a few weeks after hospital discharge when most participants reported nonadherence behaviors, such as difficulties implementing the dosage regimen, or intentionally discontinuing the medication and modifying the medication regimen on their initiative. Determinants positively influencing medication adherence were the establishment of a routine; organizing medications in weekly pill-organizers; organizing pocket doses (medications for a short period that participants take with them when away from home); seeking support from family caregivers; using alarm clocks; and using specific storage places. Reasons for nonadherence were changes in daily routine; intake times that were not convenient for the patient; the large number of medications; and poor knowledge of the medication or side effects. Healthcare professionals’ assistance for medication management, such as the help of home nurses or pharmacists for the preparation of weekly pill-organizers, was requested by participants or offered by healthcare professionals to support medication adherence: “ I needed [a home nurse] to put my pills in the pillbox. […] I felt really weak […] and I was making mistakes. So, I’m very happy [the doctor] offered me [home care]. […] I have so many medications.” P22.3 Some participants who experienced prehospitalization non-adherence were more aware of their non-adherence and implemented strategies, such as modifying the timing of intake: “I said to my doctor : « I forget one time out of two […], can I take them in the morning? » We looked it up and yes, I can take it in the morning.” P11.2 In contrast, some participants were still struggling with adherence difficulties that they had before hospitalization. Motivations for taking medications two months after discharge were to improve health, avoid complications, reduce symptoms, reduce the number of medications in the future or out of obligation: “ I force myself to take them because I want to get to the end of my diabetes, I want to reduce the number of pills as much as possible.” P14.2 After a few weeks post-hospitalization, for some participants, health and illness were no longer the priority because of other life imperatives (e.g., family or financial situation).
This longitudinal study provided a multi-faceted representation of how patients manage, understand, and adhere to their medications from hospital discharge to two months after discharge. Our findings highlighted the varying degree of participants’ involvement in managing their medications during their hospitalization, the individualized needs for information during and after hospitalization, the complicated transition from hospital to autonomous medication management, the adaptation of daily routines around medication once back home, and the adherence difficulties that surfaced in the outpatient care, with nonadherence prior to hospitalization being an indicator of the behavior after discharge. Finally, our results confirmed the lack of continuity in care and showed the lack of patient care standardization experienced by the participants during the transition from hospital to outpatient care.
This in-depth analysis of patients’ experiences reinforces common challenges identified in the existing literature such as the lack of personalized information [ 9 , 10 , 11 ], loss of autonomy during hospitalization [ 14 , 74 , 75 ], difficulties in obtaining medication at discharge [ 11 , 45 , 76 ] and challenges in understanding treatment modifications and generics substitution [ 11 , 32 , 77 , 78 ]. Some of these studies were conducted during patients’ hospitalization [ 10 , 75 , 79 ] or up to 12 months after discharge [ 80 , 81 ], but most studies focused on the few days following hospital discharge [ 9 , 11 , 14 , 82 ]. Qualitative studies on medications at transition often focused on a specific topic, such as medication information, or a specific moment in time, and often included healthcare professionals, which muted patients’ voices [ 9 , 10 , 11 , 47 , 49 ]. Our qualitative longitudinal methodology was interested in capturing the temporal dynamics, in-depth narratives, and contextual nuances of patients’ medication experiences during transitions of care [ 59 , 83 ]. This approach provided a comprehensive understanding of how patients’ perspectives and behaviors evolved over time, offering insights into the complex interactions of medication management, understanding and adherence, and turning points within their medication journeys. A qualitative longitudinal design was used by Fylan et al. to underline patients’ resilience in medication management during and after discharge, by Brandberg et al. to show the dynamic process of self-management during the 4 weeks post-discharge and by Lawton et al. to examine how patients with type 2 diabetes perceived their care after discharge over a period of four years [ 49 , 50 , 51 ]. Our study focused on the first two months following hospitalization and future studies should focus on following discharged and at-risk patients over a longer period, as “transitions of care do not comprise linear trajectories of patients’ movements, with a starting and finishing point. Instead, they are endless loops of movements” [ 47 ].
Our results provide a particularly thorough description of how participants move from a state of total dependency during hospitalization regarding their medication management to a sudden and complete autonomy after hospital discharge impacting medication management, understanding, and adherence in the first days after discharge for some participants. Several qualitative studies have described the lack of shared decision-making and the loss of patient autonomy during hospitalization, which had an impact on self-management and created conflicts with healthcare professionals [ 75 , 81 , 84 ]. Our study also highlights nuanced patient experiences, including varying levels of patient needs, involvement, and proactivity during hospitalization and outpatient care, and our results contribute to capturing different perspectives that contrast with some literature that often portrays patients as more passive recipients of care [ 14 , 15 , 74 , 75 ]. Shared decision-making and proactive medication are key elements as they contribute to a smoother transition and better outcomes for patients post-discharge [ 85 , 86 , 87 ].
Consistent with the literature, the study identifies some challenges in medication initiation post-discharge [ 16 , 17 , 88 ] but our results also describe how daily routine rapidly takes over, either solidifying adherence behavior or generating barriers to medication adherence. Participants’ nonadherence prior to hospitalization was a factor influencing participants’ adherence post-hospitalization and this association should be further investigated, as literature showed that hospitalized patients have high scores of non-adherence [ 89 ]. Mortel et al. showed that more than 20% of discharged patients stopped their medications earlier than agreed with the physician and 25% adapted their medication intake [ 90 ]. Furthermore, patients who self-managed their medications had a lower perception of the necessity of their medication than patients who received help, which could negatively impact medication adherence [ 91 ]. Although participants in our study had high BMQ scores for necessity and lower scores for concerns, some participants expressed doubts about the need for their medications and a lack of motivation a few weeks after discharge. Targeted pharmacy interventions for newly prescribed medications have been shown to improve medication adherence, and hospital discharge is an opportune moment to implement this service [ 92 , 93 ].
Many medication changes were made during the transition of care (a median number of 7 changes during hospitalization and 7 changes during the two months after discharge), especially medication additions during hospitalization and interruptions after hospitalization. While medication changes during hospitalization are well described, the many changes following discharge are less discussed [ 7 , 94 ]. A Danish study showed that approximately 65% of changes made during hospitalization were accepted by primary healthcare professionals but only 43% of new medications initiated during hospitalization were continued after discharge [ 95 ]. The numerous changes after discharge may be caused by unnecessary intensification of medications during hospitalization, delayed discharge letters, lack of standardized procedures, miscommunication, patient self-management difficulties, or in response to an acute situation [ 96 , 97 , 98 ]. During the transition of care, in our study, both new and experienced participants were faced with difficulties in managing and understanding medication changes, either for newly prescribed medication or changes in previous medications. Such difficulties corroborate the findings of the literature [ 9 , 10 , 47 ] and our results showed that the lack of understanding during hospitalization led to participants having questions about their medications, even weeks after discharge. Particular attention should be given to patients’ understanding of medication changes jointly by physicians, nurses and pharmacists during the transition of care and in the months that follow as medications are likely to undergo as many changes as during hospitalization.
Implication for practice and future research
The patients’ perspectives in this study showed, at a system level, that there was a lack of standardization in healthcare professional practices regarding medication dispensing and follow-up. For now, in Switzerland, there are no official guidelines on medication prescription and dispensation during the transition of care although some international guidelines have been developed for outpatient healthcare professionals [ 3 , 99 , 100 , 101 , 102 ]. Here are some suggestions for improvement arising from our results. Patients should be included as partners and healthcare professionals should systematically assess (i) previous medication adherence, (ii) patients’ desired level of involvement and (iii) their needs for information during hospitalization. Hospital discharge processes should be routinely implemented to standardize hospital discharge preparation, medication prescribing, and dispensing. Discharge from the hospital should be planned with community pharmacies to ensure that all medications are available and, if necessary, doses of medications should be supplied by the hospital to bridge the gap. A partnership with outpatient healthcare professionals, such as general practitioners, community pharmacists, and homecare nurses, should be set up for effective asynchronous interprofessional collaboration to consolidate patients’ medication management, knowledge, and adherence, as well as to monitor signs of deterioration or adverse drug events.
Future research should consolidate our first attempt to develop a framework to better characterize medication at the transition of care, using Fig. 2 as a starting point. Contextualized interventions, co-designed by health professionals, patients and stakeholders, should be tested in a hybrid implementation study to test the implementation and effectiveness of the intervention for the health system [ 103 ].
Limitations
This study has some limitations. First, the transcripts were validated for accuracy by the interviewer but not by a third party, which could have increased the robustness of the transcription. Nevertheless, the interviewer followed all methodological recommendations for transcription. Second, patient inclusion took place during the COVID-19 pandemic, which may have had an impact on patient care and the availability of healthcare professionals. Third, we cannot guarantee the accuracy of some participants’ medication history before hospitalization, even though we contacted the participants’ main pharmacy, as participants could have gone to different pharmacies to obtain their medications. Fourth, our findings may not be generalizable to other populations and other healthcare systems because some issues may be specific to multimorbid patients with type 2 diabetes or to the Swiss healthcare setting. Nevertheless, issues encountered by our participants regarding their medications correlate with findings in the literature. Fifth, only 15 out of 21 participants took part in all the interviews, but most participants took part in at least three interviews and data saturation was reached. Lastly, by its qualitative and longitudinal design, it is possible that the discussion during interviews and participants’ reflections between interviews influenced participants’ management, knowledge, and adherence, even though this study was observational, and no advice or recommendations were given by the interviewer during interviews.
Discharged patients are willing to take steps to better manage, understand, and adhere to their medications, yet they are also faced with difficulties in the hospital and outpatient care. Furthermore, extensive changes in medications not only occur during hospitalization but also during the two months following hospital discharge, for which healthcare professionals should give particular attention. The different degrees of patients’ involvement, needs and resources should be carefully considered to enable them to better manage, understand and adhere to their medications. At a system level, patients’ experiences revealed a lack of standardization of medication practices during the transition of care. The healthcare system should provide the ecosystem needed for healthcare professionals responsible for or involved in the management of patients’ medications during the hospital stay, discharge, and outpatient care to standardize their practices while considering the patient as an active partner.
Data availability
The anonymized quantitative survey datasets and the qualitative codes are available in French from the corresponding author on reasonable request.
Abbreviations
adverse drug events
Adherence Visual Analogue Scale
Belief in Medication Questionnaire
Consolidated Criteria for Reporting Qualitative Research
case report form
standard deviation
World Health Organization
American Academy of Family Physician. Continuity of Care, Definition of 2020. Accessed 10 July 2022 https://www.aafp.org/about/policies/all/continuity-of-care-definition.html
Kripalani S, LeFevre F, Phillips CO, Williams MV, Basaviah P, Baker DW. Deficits in communication and information transfer between hospital-based and primary care physicians: implications for patient safety and continuity of care. JAMA. 2007;297(8):831–41.
Article CAS PubMed Google Scholar
World Health Organization (WHO). Medication Safety in Transitions of Care. 2019.
Forster AJ, Murff HJ, Peterson JF, Gandhi TK, Bates DW. The incidence and severity of adverse events affecting patients after discharge from the hospital. Ann Intern Med. 2003;138(3):161–7.
Article PubMed Google Scholar
Krumholz HM. Post-hospital syndrome–an acquired, transient condition of generalized risk. N Engl J Med. 2013;368(2):100–2.
Article CAS PubMed PubMed Central Google Scholar
Banholzer S, Dunkelmann L, Haschke M, Derungs A, Exadaktylos A, Krähenbühl S, et al. Retrospective analysis of adverse drug reactions leading to short-term emergency hospital readmission. Swiss Med Wkly. 2021;151:w20400.
Blozik E, Signorell A, Reich O. How does hospitalization affect continuity of drug therapy: an exploratory study. Ther Clin Risk Manag. 2016;12:1277–83.
Article PubMed PubMed Central Google Scholar
Allen J, Hutchinson AM, Brown R, Livingston PM. User experience and care for older people transitioning from hospital to home: patients’ and carers’ perspectives. Health Expect. 2018;21(2):518–27.
Daliri S, Bekker CL, Buurman BM, Scholte Op Reimer WJM, van den Bemt BJF, Karapinar-Çarkit F. Barriers and facilitators with medication use during the transition from hospital to home: a qualitative study among patients. BMC Health Serv Res. 2019;19(1):204.
Bekker CL, Mohsenian Naghani S, Natsch S, Wartenberg NS, van den Bemt BJF. Information needs and patient perceptions of the quality of medication information available in hospitals: a mixed method study. Int J Clin Pharm. 2020;42(6):1396–404.
Foulon V, Wuyts J, Desplenter F, Spinewine A, Lacour V, Paulus D, et al. Problems in continuity of medication management upon transition between primary and secondary care: patients’ and professionals’ experiences. Acta Clin Belgica: Int J Clin Lab Med. 2019;74(4):263–71.
Article Google Scholar
Micheli P, Kossovsky MP, Gerstel E, Louis-Simonet M, Sigaud P, Perneger TV, et al. Patients’ knowledge of drug treatments after hospitalisation: the key role of information. Swiss Med Wkly. 2007;137(43–44):614–20.
PubMed Google Scholar
Ziaeian B, Araujo KL, Van Ness PH, Horwitz LI. Medication reconciliation accuracy and patient understanding of intended medication changes on hospital discharge. J Gen Intern Med. 2012;27(11):1513–20.
Allen J, Hutchinson AM, Brown R, Livingston PM. User experience and care integration in Transitional Care for older people from hospital to home: a Meta-synthesis. Qual Health Res. 2016;27(1):24–36.
Mackridge AJ, Rodgers R, Lee D, Morecroft CW, Krska J. Cross-sectional survey of patients’ need for information and support with medicines after discharge from hospital. Int J Pharm Pract. 2018;26(5):433–41.
Mulhem E, Lick D, Varughese J, Barton E, Ripley T, Haveman J. Adherence to medications after hospital discharge in the elderly. Int J Family Med. 2013;2013:901845.
Fallis BA, Dhalla IA, Klemensberg J, Bell CM. Primary medication non-adherence after discharge from a general internal medicine service. PLoS ONE. 2013;8(5):e61735.
Zhou L, Rupa AP. Categorization and association analysis of risk factors for adverse drug events. Eur J Clin Pharmacol. 2018;74(4):389–404.
Moreau-Gruet F. La multimorbidité chez les personnes de 50 ans et plus. Résultats basés sur l’enqête SHARE (Survey of Health, Ageing and Retirement in Europe. Obsan Bulletin 4/2013. 2013(Neuchâtel: OBservatoire suisse de la santé).
Iglay K, Hannachi H, Joseph Howie P, Xu J, Li X, Engel SS, et al. Prevalence and co-prevalence of comorbidities among patients with type 2 diabetes mellitus. Curr Med Res Opin. 2016;32(7):1243–52.
Sibounheuang P, Olson PS, Kittiboonyakun P. Patients’ and healthcare providers’ perspectives on diabetes management: a systematic review of qualitative studies. Res Social Adm Pharm. 2020;16(7):854–74.
Müller-Wieland D, Merkel M, Hamann A, Siegel E, Ottillinger B, Woker R, et al. Survey to estimate the prevalence of type 2 diabetes mellitus in hospital patients in Germany by systematic HbA1c measurement upon admission. Int J Clin Pract. 2018;72(12):e13273.
Blanc AL, Fumeaux T, Stirnemann J, Dupuis Lozeron E, Ourhamoune A, Desmeules J, et al. Development of a predictive score for potentially avoidable hospital readmissions for general internal medicine patients. PLoS ONE. 2019;14(7):e0219348.
Hansen LO, Greenwald JL, Budnitz T, Howell E, Halasyamani L, Maynard G, et al. Project BOOST: effectiveness of a multihospital effort to reduce rehospitalization. J Hosp Med. 2013;8(8):421–7.
Khalid JM, Raluy-Callado M, Curtis BH, Boye KS, Maguire A, Reaney M. Rates and risk of hospitalisation among patients with type 2 diabetes: retrospective cohort study using the UK General Practice Research Database linked to English Hospital Episode statistics. Int J Clin Pract. 2014;68(1):40–8.
Lussier ME, Evans HJ, Wright EA, Gionfriddo MR. The impact of community pharmacist involvement on transitions of care: a systematic review and meta-analysis. J Am Pharm Assoc. 2020;60(1):153–.
van der Heijden A, de Bruijne MC, Nijpels G, Hugtenburg JG. Cost-effectiveness of a clinical medication review in vulnerable older patients at hospital discharge, a randomized controlled trial. Int J Clin Pharm. 2019;41(4):963–71.
Bingham J, Campbell P, Schussel K, Taylor AM, Boesen K, Harrington A, et al. The Discharge Companion Program: an interprofessional collaboration in Transitional Care Model Delivery. Pharm (Basel). 2019;7(2):68.
Google Scholar
Farris KB, Carter BL, Xu Y, Dawson JD, Shelsky C, Weetman DB, et al. Effect of a care transition intervention by pharmacists: an RCT. BMC Health Serv Res. 2014;14:406.
Meslot C, Gauchet A, Hagger MS, Chatzisarantis N, Lehmann A, Allenet B. A Randomised Controlled Trial to test the effectiveness of planning strategies to improve Medication Adherence in patients with Cardiovascular Disease. Appl Psychol Health Well Being. 2017;9(1):106–29.
Garnier A, Rouiller N, Gachoud D, Nachar C, Voirol P, Griesser AC, et al. Effectiveness of a transition plan at discharge of patients hospitalized with heart failure: a before-and-after study. ESC Heart Fail. 2018;5(4):657–67.
Daliri S, Bekker CL, Buurman BM, Scholte Op Reimer WJM, van den Bemt BJF, Karapinar-Çarkit F. Medication management during transitions from hospital to home: a focus group study with hospital and primary healthcare providers in the Netherlands. Int J Clin Pharm. 2020.
Hansen LO, Young RS, Hinami K, Leung A, Williams MV. Interventions to reduce 30-day rehospitalization: a systematic review. Ann Intern Med. 2011;155(8):520–8.
Leppin AL, Gionfriddo MR, Kessler M, Brito JP, Mair FS, Gallacher K, et al. Preventing 30-day hospital readmissions: a systematic review and meta-analysis of randomized trials. JAMA Intern Med. 2014;174(7):1095–107.
Donzé J, John G, Genné D, Mancinetti M, Gouveia A, Méan M et al. Effects of a Multimodal Transitional Care Intervention in patients at high risk of readmission: the TARGET-READ Randomized Clinical Trial. JAMA Intern Med. 2023.
Rodrigues CR, Harrington AR, Murdock N, Holmes JT, Borzadek EZ, Calabro K, et al. Effect of pharmacy-supported transition-of-care interventions on 30-Day readmissions: a systematic review and Meta-analysis. Ann Pharmacother. 2017;51(10):866–89.
Lam MYY, Dodds LJ, Corlett SA. Engaging patients to access the community pharmacy medicine review service after discharge from hospital: a cross-sectional study in England. Int J Clin Pharm. 2019;41(4):1110–7.
Hossain LN, Fernandez-Llimos F, Luckett T, Moullin JC, Durks D, Franco-Trigo L, et al. Qualitative meta-synthesis of barriers and facilitators that influence the implementation of community pharmacy services: perspectives of patients, nurses and general medical practitioners. BMJ Open. 2017;7(9):e015471.
En-Nasery-de Heer S, Uitvlugt EB, Bet PM, van den Bemt BJF, Alai A, van den Bemt P et al. Implementation of a pharmacist-led transitional pharmaceutical care programme: process evaluation of medication actions to reduce hospital admissions through a collaboration between Community and Hospital pharmacists (MARCH). J Clin Pharm Ther. 2022.
Morris ZS, Wooding S, Grant J. The answer is 17 years, what is the question: understanding time lags in translational research. J R Soc Med. 2011;104(12):510–20.
De Geest S, Zúñiga F, Brunkert T, Deschodt M, Zullig LL, Wyss K, et al. Powering Swiss health care for the future: implementation science to bridge the valley of death. Swiss Med Wkly. 2020;150:w20323.
Noonan VK, Lyddiatt A, Ware P, Jaglal SB, Riopelle RJ, Bingham CO 3, et al. Montreal Accord on patient-reported outcomes (PROs) use series - paper 3: patient-reported outcomes can facilitate shared decision-making and guide self-management. J Clin Epidemiol. 2017;89:125–35.
Hesselink G, Schoonhoven L, Barach P, Spijker A, Gademan P, Kalkman C, et al. Improving patient handovers from hospital to primary care: a systematic review. Ann Intern Med. 2012;157(6):417–28.
(OFSP) Interprofessionnalité dans le domaine de la santé Soins ambulatoire. Accessed 4 January 2024. https://www.bag.admin.ch/bag/fr/home/strategie-und-politik/nationale-gesundheitspolitik/foerderprogramme-der-fachkraefteinitiative-plus/foerderprogramme-interprofessionalitaet.html
Mitchell SE, Laurens V, Weigel GM, Hirschman KB, Scott AM, Nguyen HQ, et al. Care transitions from patient and caregiver perspectives. Ann Fam Med. 2018;16(3):225–31.
Davoody N, Koch S, Krakau I, Hägglund M. Post-discharge stroke patients’ information needs as input to proposing patient-centred eHealth services. BMC Med Inf Decis Mak. 2016;16:66.
Ozavci G, Bucknall T, Woodward-Kron R, Hughes C, Jorm C, Joseph K, et al. A systematic review of older patients’ experiences and perceptions of communication about managing medication across transitions of care. Res Social Adm Pharm. 2021;17(2):273–91.
Fylan B, Armitage G, Naylor D, Blenkinsopp A. A qualitative study of patient involvement in medicines management after hospital discharge: an under-recognised source of systems resilience. BMJ Qual Saf. 2018;27(7):539–46.
Fylan B, Marques I, Ismail H, Breen L, Gardner P, Armitage G, et al. Gaps, traps, bridges and props: a mixed-methods study of resilience in the medicines management system for patients with heart failure at hospital discharge. BMJ Open. 2019;9(2):e023440.
Brandberg C, Ekstedt M, Flink M. Self-management challenges following hospital discharge for patients with multimorbidity: a longitudinal qualitative study of a motivational interviewing intervention. BMJ Open. 2021;11(7):e046896.
Lawton J, Rankin D, Peel E, Douglas M. Patients’ perceptions and experiences of transitions in diabetes care: a longitudinal qualitative study. Health Expect. 2009;12(2):138–48.
Mabire C, Bachnick S, Ausserhofer D, Simon M. Patient readiness for hospital discharge and its relationship to discharge preparation and structural factors: a cross-sectional study. Int J Nurs Stud. 2019;90:13–20.
Meyers DC, Durlak JA, Wandersman A. The quality implementation framework: a synthesis of critical steps in the implementation process. Am J Community Psychol. 2012;50(3–4):462–80.
Meyer-Massetti C, Hofstetter V, Hedinger-Grogg B, Meier CR, Guglielmo BJ. Medication-related problems during transfer from hospital to home care: baseline data from Switzerland. Int J Clin Pharm. 2018;40(6):1614–20.
Neeman M, Dobrinas M, Maurer S, Tagan D, Sautebin A, Blanc AL, et al. Transition of care: a set of pharmaceutical interventions improves hospital discharge prescriptions from an internal medicine ward. Eur J Intern Med. 2017;38:30–7.
Geese F, Schmitt KU. Interprofessional Collaboration in Complex Patient Care Transition: a qualitative multi-perspective analysis. Healthc (Basel). 2023;11(3).
Craig P, Dieppe P, Macintyre S, Michie S, Nazareth I, Petticrew M. Developing and evaluating complex interventions: the new Medical Research Council guidance. Int J Nurs Stud. 2013;50(5):587–92.
Thomson R, Plumridge L, Holland J, Editorial. Int J Soc Res Methodol. 2003;6(3):185–7.
Audulv Å, Hall EOC, Kneck Å, Westergren T, Fegran L, Pedersen MK, et al. Qualitative longitudinal research in health research: a method study. BMC Med Res Methodol. 2022;22(1):255.
Kim H, Sefcik JS, Bradway C. Characteristics of qualitative descriptive studies: a systematic review. Res Nurs Health. 2017;40(1):23–42.
Sandelowski M. Whatever happened to qualitative description? Res Nurs Health. 2000;23(4):334–40.
Bradshaw C, Atkinson S, Doody O. Employing a qualitative description Approach in Health Care Research. Glob Qual Nurs Res. 2017;4:2333393617742282.
PubMed PubMed Central Google Scholar
Bellone JM, Barner JC, Lopez DA. Postdischarge interventions by pharmacists and impact on hospital readmission rates. J Am Pharm Assoc (2003). 2012;52(3):358–62.
Hennink MM, Kaiser BN, Marconi VC. Code saturation versus meaning saturation: how many interviews are Enough? Qual Health Res. 2016;27(4):591–608.
World Health Organization. Adherence to long-term therapies: evidence for action. 2003.
Fisher JD, Fisher WA, Amico KR, Harman JJ. An information-motivation-behavioral skills model of adherence to antiretroviral therapy. Health Psychol. 2006;25(4):462–73.
Bandura A. Health promotion from the perspective of social cognitive theory. Psychol Health. 1998;13(4):623–49.
ShiftEUROQOL Research FOndation EQ 5D Instruments. Accessed 30 July 2022 https://euroqol.org/eq-5d-instruments/sample-demo/
Jeppesen KM, Coyle JD, Miser WF. Screening questions to predict limited health literacy: a cross-sectional study of patients with diabetes mellitus. Ann Fam Med. 2009;7(1):24–31.
Giordano TP, Guzman D, Clark R, Charlebois ED, Bangsberg DR. Measuring adherence to antiretroviral therapy in a diverse population using a visual analogue scale. HIV Clin Trials. 2004;5(2):74–9.
Horne R, Weinman J, Hankins M. The beliefs about medicines questionnaire: the development and evaluation of a new method for assessing the cognitive representation of medication. Psychol Health. 1999;14(1):1–24.
Horne R, Chapman SC, Parham R, Freemantle N, Forbes A, Cooper V. Understanding patients’ adherence-related beliefs about medicines prescribed for long-term conditions: a meta-analytic review of the necessity-concerns Framework. PLoS ONE. 2013;8(12):e80633.
Braun V, Clarke V. Using thematic analysis in psychology. Qualitative Res Psychol. 2006;3(2):77–101.
Waibel S, Henao D, Aller M-B, Vargas I, Vázquez M-L. What do we know about patients’ perceptions of continuity of care? A meta-synthesis of qualitative studies. Int J Qual Health Care. 2011;24(1):39–48.
Rognan SE, Jørgensen MJ, Mathiesen L, Druedahl LC, Lie HB, Bengtsson K, et al. The way you talk, do I have a choice?’ Patient narratives of medication decision-making during hospitalization. Int J Qualitative Stud Health Well-being. 2023;18(1):2250084.
Michel B, Hemery M, Rybarczyk-Vigouret MC, Wehrle P, Beck M. Drug-dispensing problems community pharmacists face when patients are discharged from hospitals: a study about 537 prescriptions in Alsace. Int J Qual Health Care. 2016;28(6):779–84.
Bruhwiler LD, Hersberger KE, Lutters M. Hospital discharge: what are the problems, information needs and objectives of community pharmacists? A mixed method approach. Pharm Pract (Granada). 2017;15(3):1046.
Knight DA, Thompson D, Mathie E, Dickinson A. Seamless care? Just a list would have helped!’ Older people and their carer’s experiences of support with medication on discharge home from hospital. Health Expect. 2013;16(3):277–91.
Gualandi R, Masella C, Viglione D, Tartaglini D. Exploring the hospital patient journey: what does the patient experience? PLoS ONE. 2019;14(12):e0224899.
Norberg H, Håkansson Lindqvist M, Gustafsson M. Older individuals’ experiences of Medication Management and Care after Discharge from Hospital: an interview study. Patient Prefer Adherence. 2023;17:781–92.
Jones KC, Austad K, Silver S, Cordova-Ramos EG, Fantasia KL, Perez DC, et al. Patient perspectives of the hospital discharge process: a qualitative study. J Patient Exp. 2023;10:23743735231171564.
Hesselink G, Flink M, Olsson M, Barach P, Dudzik-Urbaniak E, Orrego C, et al. Are patients discharged with care? A qualitative study of perceptions and experiences of patients, family members and care providers. BMJ Qual Saf. 2012;21(Suppl 1):i39–49.
Murray SA, Kendall M, Carduff E, Worth A, Harris FM, Lloyd A, et al. Use of serial qualitative interviews to understand patients’ evolving experiences and needs. BMJ. 2009;339:b3702.
Berger ZD, Boss EF, Beach MC. Communication behaviors and patient autonomy in hospital care: a qualitative study. Patient Educ Couns. 2017;100(8):1473–81.
Davis RE, Jacklin R, Sevdalis N, Vincent CA. Patient involvement in patient safety: what factors influence patient participation and engagement? Health Expect. 2007;10(3):259–67.
Greene J, Hibbard JH. Why does patient activation matter? An examination of the relationships between patient activation and health-related outcomes. J Gen Intern Med. 2012;27(5):520–6.
Mitchell SE, Gardiner PM, Sadikova E, Martin JM, Jack BW, Hibbard JH, et al. Patient activation and 30-day post-discharge hospital utilization. J Gen Intern Med. 2014;29(2):349–55.
Weir DL, Motulsky A, Abrahamowicz M, Lee TC, Morgan S, Buckeridge DL, et al. Failure to follow medication changes made at hospital discharge is associated with adverse events in 30 days. Health Serv Res. 2020;55(4):512–23.
Kripalani S, Goggins K, Nwosu S, Schildcrout J, Mixon AS, McNaughton C, et al. Medication nonadherence before hospitalization for Acute Cardiac events. J Health Commun. 2015;20(Suppl 2):34–42.
Mortelmans L, De Baetselier E, Goossens E, Dilles T. What happens after Hospital Discharge? Deficiencies in Medication Management encountered by geriatric patients with polypharmacy. Int J Environ Res Public Health. 2021;18(13).
Mortelmans L, Goossens E, Dilles T. Beliefs about medication after hospital discharge in geriatric patients with polypharmacy. Geriatr Nurs. 2022;43:280–7.
Bandiera C, Ribaut J, Dima AL, Allemann SS, Molesworth K, Kalumiya K et al. Swiss Priority setting on implementing Medication Adherence interventions as Part of the European ENABLE COST action. Int J Public Health. 2022;67.
Elliott R, Boyd M, Nde S. at e. Supporting adherence for people starting a new medication for a long-term condition through community pharmacies: a pragmaticrandomised controlled trial of the New Medicine Service. 2015.
Grimmsmann T, Schwabe U, Himmel W. The influence of hospitalisation on drug prescription in primary care–a large-scale follow-up study. Eur J Clin Pharmacol. 2007;63(8):783–90.
Larsen MD, Rosholm JU, Hallas J. The influence of comprehensive geriatric assessment on drug therapy in elderly patients. Eur J Clin Pharmacol. 2014;70(2):233–9.
Viktil KK, Blix HS, Eek AK, Davies MN, Moger TA, Reikvam A. How are drug regimen changes during hospitalisation handled after discharge: a cohort study. BMJ Open. 2012;2(6):e001461.
Strehlau AG, Larsen MD, Søndergaard J, Almarsdóttir AB, Rosholm J-U. General practitioners’ continuation and acceptance of medication changes at sectorial transitions of geriatric patients - a qualitative interview study. BMC Fam Pract. 2018;19(1):168.
Anderson TS, Lee S, Jing B, Fung K, Ngo S, Silvestrini M, et al. Prevalence of diabetes medication intensifications in older adults discharged from US Veterans Health Administration Hospitals. JAMA Netw Open. 2020;3(3):e201511.
Royal Pharmaceutical Society. Keeping patients safewhen they transfer between care providers– getting the medicines right June 2012. Accessed 27 October 2023 https://www.rpharms.com/Portals/0/RPS%20document%20library/Open%20access/Publications/Keeping%20patients%20safe%20transfer%20of%20care%20report.pdf
International Pharmaceutical Federation (FIP). Medicines reconciliation: A toolkit for pharmacists. Accessed 23 September 2023 https://www.fip.org/file/4949
Californian Pharmacist Assiociation Transitions of Care Resource Guide. https://cdn.ymaws.com/www.cshp.org/resource/resmgr/Files/Practice-Policy/For_Pharmacists/transitions_of_care_final_10.pdf
Royal Collegue of Physicians. Medication safety at hospital discharge: Improvement guide and resource. Accessed 18 September 2023 https://www.rcplondon.ac.uk/file/33421/download
Douglas N, Campbell W, Hinckley J. Implementation science: buzzword or game changer. J Speech Lang Hear Res. 2015;58.
Download references
Acknowledgements
The authors would like to thank all the patients who took part in this study. We would also like to thank the Geneva University Hospitals Patients Partners + 3P platform as well as Mrs. Tourane Corbière and Mr. Joël Mermoud, patient partners, who reviewed interview guides for clarity and significance. We would like to thank Samuel Fabbi, Vitcoryavarman Koh, and Pierre Repiton for the transcriptions of the audio recordings.
This research did not receive any specific grant from funding agencies in the public, commercial, or not-for-profit sectors.
Open access funding provided by University of Geneva
Author information
Authors and affiliations.
School of Pharmaceutical Sciences, University of Geneva, Geneva, Switzerland
Léa Solh Dost & Marie P. Schneider
Institute of Pharmaceutical Sciences of Western Switzerland, University of Geneva, Geneva, Switzerland
Division of Endocrinology, Diabetes, Hypertension and Nutrition, Department of Medicine, Geneva University Hospitals, Geneva, Switzerland
Giacomo Gastaldi
You can also search for this author in PubMed Google Scholar
Contributions
LS, GG, and MS conceptualized and designed the study. LS and GG screened and recruited participants. LS conducted the interviews. LS, GG, and MS performed data analysis and interpretation. LS drafted the manuscript and LS and MS worked on the different versions. MS and GG approved the final manuscript.
Corresponding authors
Correspondence to Léa Solh Dost or Marie P. Schneider .
Ethics declarations
Ethics approval and consent to participate.
Ethics approval was sought and granted by the Cantonal Research Ethics Commission, Geneva (CCER) (2020 − 01779), and informed consent to participate was obtained from all participants.
Consent for publication
Informed consent for publication was obtained from all participants.
Competing interests
The authors declare no competing interests.
Additional information
Publisher’s note.
Springer Nature remains neutral with regard to jurisdictional claims in published maps and institutional affiliations.
Electronic supplementary material
Below is the link to the electronic supplementary material.
Supplementary Material 1
Supplementary material 2, supplementary material 3, rights and permissions.
Open Access This article is licensed under a Creative Commons Attribution 4.0 International License, which permits use, sharing, adaptation, distribution and reproduction in any medium or format, as long as you give appropriate credit to the original author(s) and the source, provide a link to the Creative Commons licence, and indicate if changes were made. The images or other third party material in this article are included in the article’s Creative Commons licence, unless indicated otherwise in a credit line to the material. If material is not included in the article’s Creative Commons licence and your intended use is not permitted by statutory regulation or exceeds the permitted use, you will need to obtain permission directly from the copyright holder. To view a copy of this licence, visit http://creativecommons.org/licenses/by/4.0/ . The Creative Commons Public Domain Dedication waiver ( http://creativecommons.org/publicdomain/zero/1.0/ ) applies to the data made available in this article, unless otherwise stated in a credit line to the data.
Reprints and permissions
About this article
Cite this article.
Solh Dost, L., Gastaldi, G. & Schneider, M. Patient medication management, understanding and adherence during the transition from hospital to outpatient care - a qualitative longitudinal study in polymorbid patients with type 2 diabetes. BMC Health Serv Res 24 , 620 (2024). https://doi.org/10.1186/s12913-024-10784-9
Download citation
Received : 28 June 2023
Accepted : 26 February 2024
Published : 13 May 2024
DOI : https://doi.org/10.1186/s12913-024-10784-9
Share this article
Anyone you share the following link with will be able to read this content:
Sorry, a shareable link is not currently available for this article.
Provided by the Springer Nature SharedIt content-sharing initiative
- Continuity of care
- Transition of care
- Patient discharge
- Medication management
- Medication adherence
- Qualitative research
- Longitudinal studies
- Patient-centered care
- Interprofessional collaboration
- Type 2 diabetes
BMC Health Services Research
ISSN: 1472-6963
- General enquiries: [email protected]
- Python For Data Analysis
- Data Science
- Data Analysis with R
- Data Analysis with Python
- Data Visualization with Python
- Data Analysis Examples
- Math for Data Analysis
- Data Analysis Interview questions
- Artificial Intelligence
- Data Analysis Projects
- Machine Learning
- Deep Learning
- Computer Vision
- What is Data Analytics?
- What is Statistical Analysis in Data Science?
- What Is Spatial Analysis, and How Does It Work
- What is Data Analysis?
- What is Data Munging in Analysis?
- What is Geospatial Data Analysis?
- What is Exploratory Data Analysis ?
- Qualitative and Quantitative Data
- What are Descriptive Analytics?
- What is Prescriptive Analytics in Data Science?
- Qualitative Data
- Data Analysis Tutorial
- Why Data Visualization Matters in Data Analytics?
- What is Data Lineage?
- Data analysis using R
- What is Data Organization?
- What is a Data Science Platform?
What is Qualitative Data Analysis?
Understanding qualitative information analysis is important for researchers searching for to uncover nuanced insights from non-numerical statistics. By exploring qualitative statistics evaluation, you can still draw close its importance in studies, understand its methodologies, and determine while and the way to apply it successfully to extract meaningful insights from qualitative records.
The article goals to provide a complete manual to expertise qualitative records evaluation, masking its significance, methodologies, steps, advantages, disadvantages, and applications.
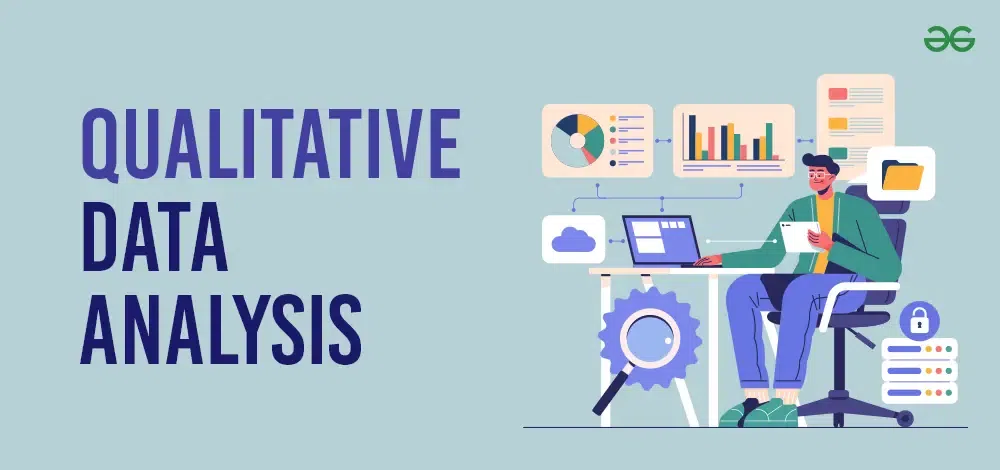
Table of Content
Understanding Qualitative Data Analysis
Importance of qualitative data analysis, steps to perform qualitative data analysis, 1. craft clear research questions, 2. gather rich customer insights, 3. organize and categorize data, 4. uncover themes and patterns : coding, 5. make hypotheses and validating, methodologies in qualitative data analysis, advantages of qualitative data analysis, disadvantages of qualitative data analysis, when qualitative data analysis is used, applications of qualitative data analysis.
Qualitative data analysis is the process of systematically examining and deciphering qualitative facts (such as textual content, pix, motion pictures, or observations) to discover patterns, themes, and meanings inside the statistics· Unlike quantitative statistics evaluation, which focuses on numerical measurements and statistical strategies, qualitative statistics analysis emphasizes know-how the context, nuances, and subjective views embedded inside the information.
Qualitative facts evaluation is crucial because it is going past the bloodless hard information and numbers to provide a richer expertise of why and the way things appear. Qualitative statistics analysis is important for numerous motives:
- Understanding Complexity and unveils the “Why” : Quantitative facts tells you “what” came about (e· g·, sales figures), however qualitative evaluation sheds light on the motives in the back of it (e·g·, consumer comments on product features).
- Contextual Insight : Numbers don’t exist in a vacuum. Qualitative information affords context to quantitative findings, making the bigger photo clearer· Imagine high customer churn – interviews would possibly monitor lacking functionalities or perplexing interfaces.
- Uncovers Emotions and Opinions: Qualitative records faucets into the human element· Surveys with open ended questions or awareness companies can display emotions, critiques, and motivations that can’t be captured by using numbers on my own.
- Informs Better Decisions: By understanding the “why” and the “how” at the back of customer behavior or employee sentiment, companies can make greater knowledgeable decisions about product improvement, advertising techniques, and internal techniques.
- Generates New Ideas : Qualitative analysis can spark clean thoughts and hypotheses· For example, via analyzing consumer interviews, commonplace subject matters may emerge that cause totally new product features.
- Complements Quantitative Data : While each facts sorts are precious, they paintings quality collectively· Imagine combining website site visitors records (quantitative) with person comments (qualitative) to apprehend user revel in on a particular webpage.
In essence, qualitative data evaluation bridges the gap among the what and the why, providing a nuanced know-how that empowers better choice making·
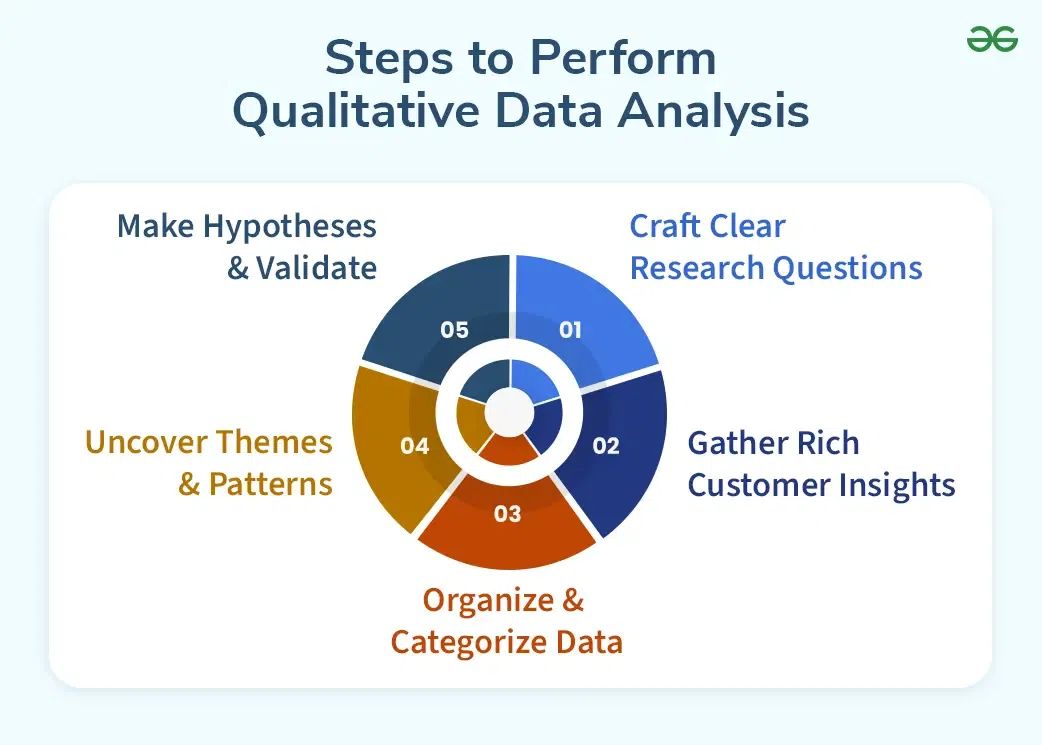
Qualitative data analysis process, follow the structure in below steps:
Qualitative information evaluation procedure, comply with the shape in underneath steps:
Before diving into evaluation, it is critical to outline clear and particular studies questions. These questions ought to articulate what you want to study from the data and manual your analysis towards actionable insights. For instance, asking “How do employees understand the organizational culture inside our agency?” helps makes a speciality of know-how personnel’ perceptions of the organizational subculture inside a selected business enterprise. By exploring employees’ perspectives, attitudes, and stories related to organizational tradition, researchers can find valuable insights into workplace dynamics, communication patterns, management patterns, and worker delight degrees.
There are numerous methods to acquire qualitative information, each offering specific insights into client perceptions and reviews.
- User Feedback: In-app surveys, app rankings, and social media feedback provide direct remarks from users approximately their studies with the products or services.
- In-Depth Interviews : One-on-one interviews allow for deeper exploration of particular topics and offer wealthy, special insights into individuals’ views and behaviors.
- Focus Groups : Facilitating group discussions allows the exploration of numerous viewpoints and permits individuals to construct upon every different’s ideas.
- Review Sites : Analyzing purchaser critiques on systems like Amazon, Yelp, or app shops can monitor not unusual pain points, pride levels, and areas for improvement.
- NPS Follow-Up Questions : Following up on Net Promoter Score (NPS) surveys with open-ended questions allows customers to elaborate on their rankings and provides qualitative context to quantitative ratings.
Efficient facts below is crucial for powerful analysis and interpretation.
- Centralize: Gather all qualitative statistics, along with recordings, notes, and transcripts, right into a valuable repository for smooth get admission to and control.
- Categorize through Research Question : Group facts primarily based at the specific studies questions they deal with. This organizational structure allows maintain consciousness in the course of analysis and guarantees that insights are aligned with the research objectives.
Coding is a scientific manner of assigning labels or categories to segments of qualitative statistics to uncover underlying issues and patterns.
- Theme Identification : Themes are overarching principles or ideas that emerge from the records· During coding, researchers perceive and label segments of statistics that relate to those themes, bearing in mind the identification of vital principles in the dataset.
- Pattern Detection : Patterns seek advice from relationships or connections between exceptional elements in the statistics. By reading coded segments, researchers can locate trends, repetitions, or cause-and-effect relationships, imparting deeper insights into patron perceptions and behaviors.
Based on the identified topics and styles, researchers can formulate hypotheses and draw conclusions about patron experiences and choices.
- Hypothesis Formulation: Hypotheses are tentative causes or predictions based on found styles within the information. Researchers formulate hypotheses to provide an explanation for why certain themes or styles emerge and make predictions approximately their effect on patron behavior.
- Validation : Researchers validate hypotheses by means of segmenting the facts based on one-of-a-kind standards (e.g., demographic elements, usage patterns) and analyzing variations or relationships inside the records. This procedure enables enhance the validity of findings and offers proof to assist conclusions drawn from qualitative evaluation.
There are five common methodologies utilized in Qualitative Data Analysis·
- Thematic Analysis : Thematic Analysis involves systematically figuring out and reading habitual subject matters or styles within qualitative statistics. Researchers begin with the aid of coding the facts, breaking it down into significant segments, and then categorizing these segments based on shared traits. Through iterative analysis, themes are advanced and refined, permitting researchers to benefit insight into the underlying phenomena being studied.
- Content Analysis: Content Analysis focuses on reading textual information to pick out and quantify particular styles or issues. Researchers code the statistics primarily based on predefined classes or subject matters, taking into consideration systematic agency and interpretation of the content. By analyzing how frequently positive themes occur and the way they’re represented inside the data, researchers can draw conclusions and insights relevant to their research objectives.
- Narrative Analysis: Narrative Analysis delves into the narrative or story within qualitative statistics, that specialize in its structure, content, and meaning. Researchers examine the narrative to understand its context and attitude, exploring how individuals assemble and speak their reports thru storytelling. By analyzing the nuances and intricacies of the narrative, researchers can find underlying issues and advantage a deeper know-how of the phenomena being studied.
- Grounded Theory : Grounded Theory is an iterative technique to growing and checking out theoretical frameworks primarily based on empirical facts. Researchers gather, code, and examine information without preconceived hypotheses, permitting theories to emerge from the information itself. Through constant assessment and theoretical sampling, researchers validate and refine theories, main to a deeper knowledge of the phenomenon under investigation.
- Phenomenological Analysis : Phenomenological Analysis objectives to discover and recognize the lived stories and views of people. Researchers analyze and interpret the meanings, essences, and systems of these reviews, figuring out not unusual topics and styles across individual debts. By immersing themselves in members’ subjective stories, researchers advantage perception into the underlying phenomena from the individuals’ perspectives, enriching our expertise of human behavior and phenomena.
- Richness and Depth: Qualitative records evaluation lets in researchers to discover complex phenomena intensive, shooting the richness and complexity of human stories, behaviors, and social processes.
- Flexibility : Qualitative techniques offer flexibility in statistics collection and evaluation, allowing researchers to conform their method based on emergent topics and evolving studies questions.
- Contextual Understanding: Qualitative evaluation presents perception into the context and meaning of information, helping researchers recognize the social, cultural, and historic elements that form human conduct and interactions.
- Subjective Perspectives : Qualitative methods allow researchers to explore subjective perspectives, beliefs, and reviews, offering a nuanced know-how of people’ mind, emotions, and motivations.
- Theory Generation : Qualitative information analysis can cause the generation of recent theories or hypotheses, as researchers uncover patterns, themes, and relationships in the records that might not were formerly recognized.
- Subjectivity: Qualitative records evaluation is inherently subjective, as interpretations can be stimulated with the aid of researchers’ biases, views, and preconceptions .
- Time-Intensive : Qualitative records analysis may be time-consuming, requiring giant data collection, transcription, coding, and interpretation.
- Generalizability: Findings from qualitative studies might not be effortlessly generalizable to larger populations, as the focus is often on know-how unique contexts and reviews in preference to making statistical inferences.
- Validity and Reliability : Ensuring the validity and reliability of qualitative findings may be difficult, as there are fewer standardized methods for assessing and establishing rigor in comparison to quantitative studies.
- Data Management : Managing and organizing qualitative information, together with transcripts, subject notes, and multimedia recordings, can be complicated and require careful documentation and garage.
- Exploratory Research: Qualitative records evaluation is nicely-suited for exploratory studies, wherein the aim is to generate hypotheses, theories, or insights into complex phenomena.
- Understanding Context : Qualitative techniques are precious for knowledge the context and which means of statistics, in particular in studies wherein social, cultural, or ancient factors are vital.
- Subjective Experiences : Qualitative evaluation is good for exploring subjective stories, beliefs, and views, providing a deeper knowledge of people’ mind, feelings, and behaviors.
- Complex Phenomena: Qualitative strategies are effective for studying complex phenomena that can not be effortlessly quantified or measured, allowing researchers to seize the richness and depth of human stories and interactions.
- Complementary to Quantitative Data: Qualitative information analysis can complement quantitative research by means of offering context, intensity, and insight into the meanings at the back of numerical statistics, enriching our knowledge of studies findings.
- Social Sciences: Qualitative information analysis is widely utilized in social sciences to apprehend human conduct, attitudes, and perceptions. Researchers employ qualitative methods to delve into the complexities of social interactions, cultural dynamics, and societal norms. By analyzing qualitative records which include interviews, observations, and textual resources, social scientists benefit insights into the elaborate nuances of human relationships, identity formation, and societal structures.
- Psychology : In psychology, qualitative data evaluation is instrumental in exploring and deciphering person reports, emotions, and motivations. Qualitative methods along with in-depth interviews, cognizance businesses, and narrative evaluation allow psychologists to delve deep into the subjective stories of individuals. This approach facilitates discover underlying meanings, beliefs, and emotions, dropping light on psychological processes, coping mechanisms, and personal narratives.
- Anthropology : Anthropologists use qualitative records evaluation to look at cultural practices, ideals, and social interactions inside various groups and societies. Through ethnographic research strategies such as player statement and interviews, anthropologists immerse themselves within the cultural contexts of different agencies. Qualitative analysis permits them to find the symbolic meanings, rituals, and social systems that form cultural identification and behavior.
- Qualitative Market Research : In the sphere of marketplace research, qualitative statistics analysis is vital for exploring consumer options, perceptions, and behaviors. Qualitative techniques which include consciousness groups, in-depth interviews, and ethnographic research permit marketplace researchers to gain a deeper understanding of customer motivations, choice-making methods, and logo perceptions· By analyzing qualitative facts, entrepreneurs can identify emerging developments, discover unmet wishes, and tell product development and advertising and marketing techniques.
- Healthcare: Qualitative statistics analysis plays a important function in healthcare studies via investigating patient experiences, delight, and healthcare practices. Researchers use qualitative techniques which includes interviews, observations, and patient narratives to explore the subjective reviews of people inside healthcare settings. Qualitative evaluation helps find affected person perspectives on healthcare services, treatment consequences, and pleasant of care, facilitating enhancements in patient-targeted care delivery and healthcare policy.
Qualitative data evaluation offers intensity, context, and know-how to investigate endeavors, enabling researchers to find wealthy insights and discover complicated phenomena via systematic examination of non-numerical information.
Please Login to comment...
Similar reads.
- Data Analysis
Improve your Coding Skills with Practice
What kind of Experience do you want to share?
- Open access
- Published: 13 May 2024
What are the strengths and limitations to utilising creative methods in public and patient involvement in health and social care research? A qualitative systematic review
- Olivia R. Phillips 1 , 2 na1 ,
- Cerian Harries 2 , 3 na1 ,
- Jo Leonardi-Bee 1 , 2 , 4 na1 ,
- Holly Knight 1 , 2 ,
- Lauren B. Sherar 2 , 3 ,
- Veronica Varela-Mato 2 , 3 &
- Joanne R. Morling 1 , 2 , 5
Research Involvement and Engagement volume 10 , Article number: 48 ( 2024 ) Cite this article
103 Accesses
2 Altmetric
Metrics details
There is increasing interest in using patient and public involvement (PPI) in research to improve the quality of healthcare. Ordinarily, traditional methods have been used such as interviews or focus groups. However, these methods tend to engage a similar demographic of people. Thus, creative methods are being developed to involve patients for whom traditional methods are inaccessible or non-engaging.
To determine the strengths and limitations to using creative PPI methods in health and social care research.
Electronic searches were conducted over five databases on 14th April 2023 (Web of Science, PubMed, ASSIA, CINAHL, Cochrane Library). Studies that involved traditional, non-creative PPI methods were excluded. Creative PPI methods were used to engage with people as research advisors, rather than study participants. Only primary data published in English from 2009 were accepted. Title, abstract and full text screening was undertaken by two independent reviewers before inductive thematic analysis was used to generate themes.
Twelve papers met the inclusion criteria. The creative methods used included songs, poems, drawings, photograph elicitation, drama performance, visualisations, social media, photography, prototype development, cultural animation, card sorting and persona development. Analysis identified four limitations and five strengths to the creative approaches. Limitations included the time and resource intensive nature of creative PPI, the lack of generalisation to wider populations and ethical issues. External factors, such as the lack of infrastructure to support creative PPI, also affected their implementation. Strengths included the disruption of power hierarchies and the creation of a safe space for people to express mundane or “taboo” topics. Creative methods are also engaging, inclusive of people who struggle to participate in traditional PPI and can also be cost and time efficient.
‘Creative PPI’ is an umbrella term encapsulating many different methods of engagement and there are strengths and limitations to each. The choice of which should be determined by the aims and requirements of the research, as well as the characteristics of the PPI group and practical limitations. Creative PPI can be advantageous over more traditional methods, however a hybrid approach could be considered to reap the benefits of both. Creative PPI methods are not widely used; however, this could change over time as PPI becomes embedded even more into research.
Plain English Summary
It is important that patients and public are included in the research process from initial brainstorming, through design to delivery. This is known as public and patient involvement (PPI). Their input means that research closely aligns with their wants and needs. Traditionally to get this input, interviews and group discussions are held, but this can exclude people who find these activities non-engaging or inaccessible, for example those with language challenges, learning disabilities or memory issues. Creative methods of PPI can overcome this. This is a broad term describing different (non-traditional) ways of engaging patients and public in research, such as through the use or art, animation or performance. This review investigated the reasons why creative approaches to PPI could be difficult (limitations) or helpful (strengths) in health and social care research. After searching 5 online databases, 12 studies were included in the review. PPI groups included adults, children and people with language and memory impairments. Creative methods included songs, poems, drawings, the use of photos and drama, visualisations, Facebook, creating prototypes, personas and card sorting. Limitations included the time, cost and effort associated with creative methods, the lack of application to other populations, ethical issues and buy-in from the wider research community. Strengths included the feeling of equality between academics and the public, creation of a safe space for people to express themselves, inclusivity, and that creative PPI can be cost and time efficient. Overall, this review suggests that creative PPI is worthwhile, however each method has its own strengths and limitations and the choice of which will depend on the research project, PPI group characteristics and other practical limitations, such as time and financial constraints.
Peer Review reports
Introduction
Patient and public involvement (PPI) is the term used to describe the partnership between patients (including caregivers, potential patients, healthcare users etc.) or the public (a community member with no known interest in the topic) with researchers. It describes research that is done “‘with’ or ‘by’ the public, rather than ‘to,’ ‘about’ or ‘for’ them” [ 1 ]. In 2009, it became a legislative requirement for certain health and social care organisations to include patients, families, carers and communities in not only the planning of health and social care services, but the commissioning, delivery and evaluation of them too [ 2 ]. For example, funding applications for the National Institute of Health and Care Research (NIHR), a UK funding body, mandates a demonstration of how researchers plan to include patients/service users, the public and carers at each stage of the project [ 3 ]. However, this should not simply be a tokenistic, tick-box exercise. PPI should help formulate initial ideas and should be an instrumental, continuous part of the research process. Input from PPI can provide unique insights not yet considered and can ensure that research and health services are closely aligned to the needs and requirements of service users PPI also generally makes research more relevant with clearer outcomes and impacts [ 4 ]. Although this review refers to both patients and the public using the umbrella term ‘PPI’, it is important to acknowledge that these are two different groups with different motivations, needs and interests when it comes to health research and service delivery [ 5 ].
Despite continuing recognition of the need of PPI to improve quality of healthcare, researchers have also recognised that there is no ‘one size fits all’ method for involving patients [ 4 ]. Traditionally, PPI methods invite people to take part in interviews or focus groups to facilitate discussion, or surveys and questionnaires. However, these can sometimes be inaccessible or non-engaging for certain populations. For example, someone with communication difficulties may find it difficult to engage in focus groups or interviews. If individuals lack the appropriate skills to interact in these types of scenarios, they cannot take advantage of the participation opportunities it can provide [ 6 ]. Creative methods, however, aim to resolve these issues. These are a relatively new concept whereby researchers use creative methods (e.g., artwork, animations, Lego), to make PPI more accessible and engaging for those whose voices would otherwise go unheard. They ensure that all populations can engage in research, regardless of their background or skills. Seminal work has previously been conducted in this area, which brought to light the use of creative methodologies in research. Leavy (2008) [ 7 ] discussed how traditional interviews had limits on what could be expressed due to their sterile, jargon-filled and formulaic structure, read by only a few specialised academics. It was this that called for more creative approaches, which included narrative enquiry, fiction-based research, poetry, music, dance, art, theatre, film and visual art. These practices, which can be used in any stage of the research cycle, supported greater empathy, self-reflection and longer-lasting learning experiences compared to interviews [ 7 ]. They also pushed traditional academic boundaries, which made the research accessible not only to researchers, but the public too. Leavy explains that there are similarities between arts-based approaches and scientific approaches: both attempts to investigate what it means to be human through exploration, and used together, these complimentary approaches can progress our understanding of the human experience [ 7 ]. Further, it is important to acknowledge the parallels and nuances between creative and inclusive methods of PPI. Although creative methods aim to be inclusive (this should underlie any PPI activity, whether creative or not), they do not incorporate all types of accessible, inclusive methodologies e.g., using sign language for people with hearing impairments or audio recordings for people who cannot read. Given that there was not enough scope to include an evaluation of all possible inclusive methodologies, this review will focus on creative methods of PPI only.
We aimed to conduct a qualitative systematic review to highlight the strengths of creative PPI in health and social care research, as well as the limitations, which might act as a barrier to their implementation. A qualitative systematic review “brings together research on a topic, systematically searching for research evidence from primary qualitative studies and drawing the findings together” [ 8 ]. This review can then advise researchers of the best practices when designing PPI.
Public involvement
The PHIRST-LIGHT Public Advisory Group (PAG) consists of a team of experienced public contributors with a diverse range of characteristics from across the UK. The PAG was involved in the initial question setting and study design for this review.
Search strategy
For the purpose of this review, the JBI approach for conducting qualitative systematic reviews was followed [ 9 ]. The search terms were (“creativ*” OR “innovat*” OR “authentic” OR “original” OR “inclu*”) AND (“public and patient involvement” OR “patient and public involvement” OR “public and patient involvement and engagement” OR “patient and public involvement and engagement” OR “PPI” OR “PPIE” OR “co-produc*” OR “co-creat*” OR “co-design*” OR “cooperat*” OR “co-operat*”). This search string was modified according to the requirements of each database. Papers were filtered by title, abstract and keywords (see Additional file 1 for search strings). The databases searched included Web of Science (WoS), PubMed, ASSIA and CINAHL. The Cochrane Library was also searched to identify relevant reviews which could lead to the identification of primary research. The search was conducted on 14/04/23. As our aim was to report on the use of creative PPI in research, rather than more generic public engagement, we used electronic databases of scholarly peer-reviewed literature, which represent a wide range of recognised databases. These identified studies published in general international journals (WoS, PubMed), those in social sciences journals (ASSIA), those in nursing and allied health journals (CINAHL), and trials of interventions (Cochrane Library).
Inclusion criteria
Only full-text, English language, primary research papers from 2009 to 2023 were included. This was the chosen timeframe as in 2009 the Health and Social Reform Act made it mandatory for certain Health and Social Care organisations to involve the public and patients in planning, delivering, and evaluating services [ 2 ]. Only creative methods of PPI were accepted, rather than traditional methods, such as interviews or focus groups. For the purposes of this paper, creative PPI included creative art or arts-based approaches (e.g., e.g. stories, songs, drama, drawing, painting, poetry, photography) to enhance engagement. Titles were related to health and social care and the creative PPI was used to engage with people as research advisors, not as study participants. Meta-analyses, conference abstracts, book chapters, commentaries and reviews were excluded. There were no limits concerning study location or the demographic characteristics of the PPI groups. Only qualitative data were accepted.
Quality appraisal
Quality appraisal using the Critical Appraisal Skills Programme (CASP) checklist [ 10 ] was conducted by the primary authors (ORP and CH). This was done independently, and discrepancies were discussed and resolved. If a consensus could not be reached, a third independent reviewer was consulted (JRM). The full list of quality appraisal questions can be found in Additional file 2 .
Data extraction
ORP extracted the study characteristics and a subset of these were checked by CH. Discrepancies were discussed and amendments made. Extracted data included author, title, location, year of publication, year study was carried out, research question/aim, creative methods used, number of participants, mean age, gender, ethnicity of participants, setting, limitations and strengths of creative PPI and main findings.
Data analysis
The included studies were analysed using inductive thematic analysis [ 11 ], where themes were determined by the data. The familiarisation stage took place during full-text reading of the included articles. Anything identified as a strength or limitation to creative PPI methods was extracted verbatim as an initial code and inputted into the data extraction Excel sheet. Similar codes were sorted into broader themes, either under ‘strengths’ or ‘limitations’ and reviewed. Themes were then assigned a name according to the codes.
The search yielded 9978 titles across the 5 databases: Web of Science (1480 results), PubMed (94 results), ASSIA (2454 results), CINAHL (5948 results) and Cochrane Library (2 results), resulting in 8553 different studies after deduplication. ORP and CH independently screened their titles and abstracts, excluding those that did not meet the criteria. After assessment, 12 studies were included (see Fig. 1 ).
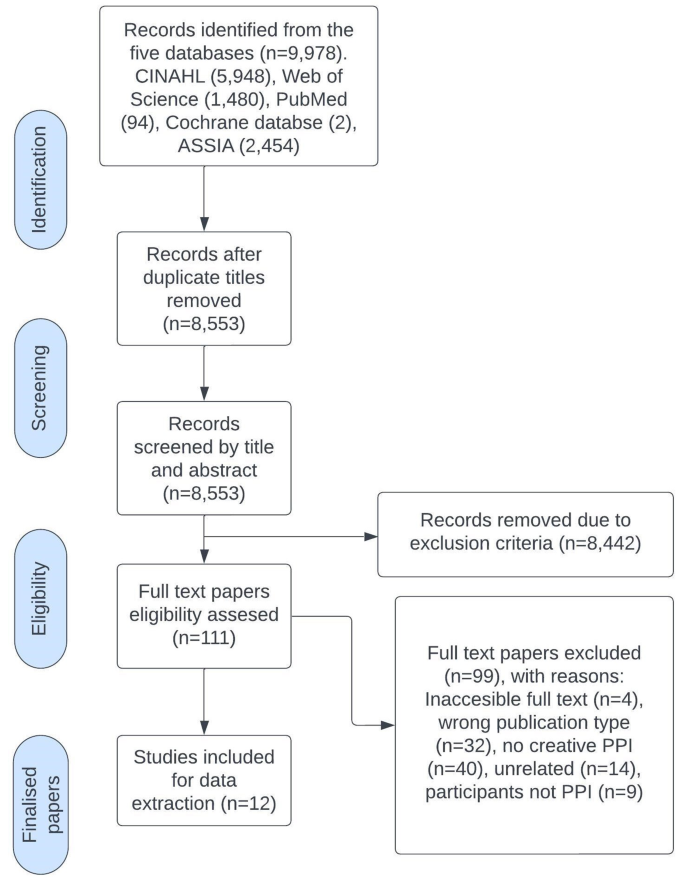
PRISMA flowchart of the study selection process
Study characteristics
The included studies were published between 2018 and 2022. Seven were conducted in the UK [ 12 , 14 , 15 , 17 , 18 , 19 , 23 ], two in Canada [ 21 , 22 ], one in Australia [ 13 ], one in Norway [ 16 ] and one in Ireland [ 20 ]. The PPI activities occurred across various settings, including a school [ 12 ], social club [ 12 ], hospital [ 17 ], university [ 22 ], theatre [ 19 ], hotel [ 20 ], or online [ 15 , 21 ], however this information was omitted in 5 studies [ 13 , 14 , 16 , 18 , 23 ]. The number of people attending the PPI sessions varied, ranging from 6 to 289, however the majority (ten studies) had less than 70 participants [ 13 , 14 , 16 , 17 , 18 , 19 , 20 , 21 , 22 , 23 ]. Seven studies did not provide information on the age or gender of the PPI groups. Of those that did, ages ranged from 8 to 76 and were mostly female. The ethnicities of the PPI group members were also rarely recorded (see Additional file 3 for data extraction table).
Types of creative methods
The type of creative methods used to engage the PPI groups were varied. These included songs, poems, drawings, photograph elicitation, drama performance, visualisations, Facebook, photography, prototype development, cultural animation, card sorting and creating personas (see Table 1 ). These were sometimes accompanied by traditional methods of PPI such as interviews and focus group discussions.
The 12 included studies were all deemed to be of good methodological quality, with scores ranging from 6/10 to 10/10 with the CASP critical appraisal tool [ 10 ] (Table 2 ).
Thematic analysis
Analysis identified four limitations and five strengths to creative PPI (see Fig. 2 ). Limitations included the time and resource intensity of creative PPI methods, its lack of generalisation, ethical issues and external factors. Strengths included the disruption of power hierarchies, the engaging and inclusive nature of the methods and their long-term cost and time efficiency. Creative PPI methods also allowed mundane and “taboo” topics to be discussed within a safe space.
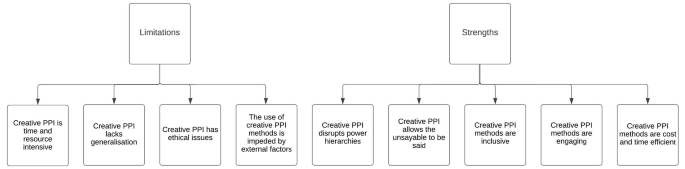
Theme map of strengths and limitations
Limitations of creative PPI
Creative ppi methods are time and resource intensive.
The time and resource intensive nature of creative PPI methods is a limitation, most notably for the persona-scenario methodology. Valaitis et al. [ 22 ] used 14 persona-scenario workshops with 70 participants to co-design a healthcare intervention, which aimed to promote optimal aging in Canada. Using the persona method, pairs composed of patients, healthcare providers, community service providers and volunteers developed a fictional character which they believed represented an ‘end-user’ of the healthcare intervention. Due to the depth and richness of the data produced the authors reported that it was time consuming to analyse. Further, they commented that the amount of information was difficult to disseminate to scientific leads and present at team meetings. Additionally, to ensure the production of high-quality data, to probe for details and lead group discussion there was a need for highly skilled facilitators. The resource intensive nature of the creative co-production was also noted in a study using the persona scenario and creative worksheets to develop a prototype decision support tool for individuals with malignant pleural effusion [ 17 ]. With approximately 50 people, this was also likely to yield a high volume of data to consider.
To prepare materials for populations who cannot engage in traditional methods of PPI was also timely. Kearns et al. [ 18 ] developed a feedback questionnaire for people with aphasia to evaluate ICT-delivered rehabilitation. To ensure people could participate effectively, the resources used during the workshops, such as PowerPoints, online images and photographs, had to be aphasia-accessible, which was labour and time intensive. The author warned that this time commitment should not be underestimated.
There are further practical limitations to implementing creative PPI, such as the costs of materials for activities as well as hiring a space for workshops. For example, the included studies in this review utilised pens, paper, worksheets, laptops, arts and craft supplies and magazines and took place in venues such as universities, a social club, and a hotel. Further, although not limited to creative PPI methods exclusively but rather most studies involving the public, a financial incentive was often offered for participation, as well as food, parking, transport and accommodation [ 21 , 22 ].
Creative PPI lacks generalisation
Another barrier to the use of creative PPI methods in health and social care research was the individual nature of its output. Those who participate, usually small in number, produce unique creative outputs specific to their own experiences, opinions and location. Craven et al. [ 13 ], used arts-based visualisations to develop a toolbox for adults with mental health difficulties. They commented, “such an approach might still not be worthwhile”, as the visualisations were individualised and highly personal. This indicates that the output may fail to meet the needs of its end-users. Further, these creative PPI groups were based in certain geographical regions such as Stoke-on-Trent [ 19 ] Sheffield [ 23 ], South Wales [ 12 ] or Ireland [ 20 ], which limits the extent the findings can be applied to wider populations, even within the same area due to individual nuances. Further, the study by Galler et al. [ 16 ], is specific to the Norwegian context and even then, maybe only a sub-group of the Norwegian population as the sample used was of higher socioeconomic status.
However, Grindell et al. [ 17 ], who used persona scenarios, creative worksheets and prototype development, pointed out that the purpose of this type of research is to improve a certain place, rather than apply findings across other populations and locations. Individualised output may, therefore, only be a limitation to research wanting to conduct PPI on a large scale.
If, however, greater generalisation within PPI is deemed necessary, then social media may offer a resolution. Fedorowicz et al. [ 15 ], used Facebook to gain feedback from the public on the use of video-recording methodology for an upcoming project. This had the benefit of including a more diverse range of people (289 people joined the closed group), who were spread geographically around the UK, as well as seven people from overseas.
Creative PPI has ethical issues
As with other research, ethical issues must be taken into consideration. Due to the nature of creative approaches, as well as the personal effort put into them, people often want to be recognised for their work. However, this compromises principles so heavily instilled in research such as anonymity and confidentiality. With the aim of exploring issues related to health and well-being in a town in South Wales, Byrne et al. [ 12 ], asked year 4/5 and year 10 pupils to create poems, songs, drawings and photographs. Community members also created a performance, mainly of monologues, to explore how poverty and inequalities are dealt with. Byrne noted the risks of these arts-based approaches, that being the possibility of over-disclosure and consequent emotional distress, as well as people’s desire to be named for their work. On one hand, the anonymity reduces the sense of ownership of the output as it does not portray a particular individual’s lived experience anymore. On the other hand, however, it could promote a more honest account of lived experience. Supporting this, Webber et al. [ 23 ], who used the persona method to co-design a back pain educational resource prototype, claimed that the anonymity provided by this creative technique allowed individuals to externalise and anonymise their own personal experience, thus creating a more authentic and genuine resource for future users. This implies that anonymity can be both a limitation and strength here.
The use of creative PPI methods is impeded by external factors
Despite the above limitations influencing the implementation of creative PPI techniques, perhaps the most influential is that creative methodologies are simply not mainstream [ 19 ]. This could be linked to the issues above, like time and resource intensity, generalisation and ethical issues but it is also likely to involve more systemic factors within the research community. Micsinszki et al. [ 21 ], who co-designed a hub for the health and well-being of vulnerable populations, commented that there is insufficient infrastructure to conduct meaningful co-design as well as a dominant medical model. Through a more holistic lens, there are “sociopolitical environments that privilege individualism over collectivism, self-sufficiency over collaboration, and scientific expertise over other ways of knowing based on lived experience” [ 21 ]. This, it could be suggested, renders creative co-design methodologies, which are based on the foundations of collectivism, collaboration and imagination an invalid technique in the research field, which is heavily dominated by more scientific methods offering reproducibility, objectivity and reliability.
Although we acknowledge that creative PPI techniques are not always appropriate, it may be that their main limitation is the lack of awareness of these methods or lack of willingness to use them. Further, there is always the risk that PPI, despite being a mandatory part of research, is used in a tokenistic or tick-box fashion [ 20 ], without considering the contribution that meaningful PPI could make to enhancing the research. It may be that PPI, let alone creative PPI, is not at the forefront of researchers’ minds when planning research.
Strengths of creative PPI
Creative ppi disrupts power hierarchies.
One of the main strengths of creative PPI techniques, cited most frequently in the included literature, was that they disrupt traditional power hierarchies [ 12 , 13 , 17 , 19 , 23 ]. For example, the use of theatre performance blurred the lines between professional and lay roles between the community and policy makers [ 12 ]. Individuals created a monologue to portray how poverty and inequality impact daily life and presented this to representatives of the National Assembly of Wales, Welsh Government, the Local Authority, Arts Council and Westminster. Byrne et al. [ 12 ], states how this medium allowed the community to engage with the people who make decisions about their lives in an environment of respect and understanding, where the hierarchies are not as visible as in other settings, e.g., political surgeries. Creative PPI methods have also removed traditional power hierarchies between researchers and adolescents. Cook et al. [ 13 ], used arts-based approaches to explore adolescents’ ideas about the “perfect” condom. They utilised the “Life Happens” resource, where adolescents drew and then decorated a person with their thoughts about sexual relationships, not too dissimilar from the persona-scenario method. This was then combined with hypothetical scenarios about sexuality. A condom-mapping exercise was then implemented, where groups shared the characteristics that make a condom “perfect” on large pieces of paper. Cook et al. [ 13 ], noted that usually power imbalances make it difficult to elicit information from adolescents, however these power imbalances were reduced due to the use of creative co-design techniques.
The same reduction in power hierarchies was noted by Grindell et al. [ 17 ], who used the person-scenario method and creative worksheets with individuals with malignant pleural effusion. This was with the aim of developing a prototype of a decision support tool for patients to help with treatment options. Although this process involved a variety of stakeholders, such as patients, carers and healthcare professionals, creative co-design was cited as a mechanism that worked to reduce power imbalances – a limitation of more traditional methods of research. Creative co-design blurred boundaries between end-users and clinical staff and enabled the sharing of ideas from multiple, valuable perspectives, meaning the prototype was able to suit user needs whilst addressing clinical problems.
Similarly, a specific creative method named cultural animation was also cited to dissolve hierarchies and encourage equal contributions from participants. Within this arts-based approach, Keleman et al. [ 19 ], explored the concept of “good health” with individuals from Stoke-on Trent. Members of the group created art installations using ribbons, buttons, cardboard and straws to depict their idea of a “healthy community”, which was accompanied by a poem. They also created a 3D Facebook page and produced another poem or song addressing the government to communicate their version of a “picture of health”. Public participants said that they found the process empowering, honest, democratic, valuable and practical.
This dissolving of hierarchies and levelling of power is beneficial as it increases the sense of ownership experienced by the creators/producers of the output [ 12 , 17 , 23 ]. This is advantageous as it has been suggested to improve its quality [ 23 ].
Creative PPI allows the unsayable to be said
Creative PPI fosters a safe space for mundane or taboo topics to be shared, which may be difficult to communicate using traditional methods of PPI. For example, the hypothetical nature of condom mapping and persona-scenarios meant that adolescents could discuss a personal topic without fear of discrimination, judgement or personal disclosure [ 13 ]. The safe space allowed a greater volume of ideas to be generated amongst peers where they might not have otherwise. Similarly, Webber et al. [ 23 ], , who used the persona method to co-design the prototype back pain educational resource, also noted how this method creates anonymity whilst allowing people the opportunity to externalise personal experiences, thoughts and feelings. Other creative methods were also used, such as drawing, collaging, role play and creating mood boards. A cardboard cube (labelled a “magic box”) was used to symbolise a physical representation of their final prototype. These creative methods levelled the playing field and made personal experiences accessible in a safe, open environment that fostered trust, as well as understanding from the researchers.
It is not only sensitive subjects that were made easier to articulate through creative PPI. The communication of mundane everyday experiences were also facilitated, which were deemed typically ‘unsayable’. This was specifically given in the context of describing intangible aspects of everyday health and wellbeing [ 11 ]. Graphic designers can also be used to visually represent the outputs of creative PPI. These captured the movement and fluidity of people and well as the relationships between them - things that cannot be spoken but can be depicted [ 21 ].
Creative PPI methods are inclusive
Another strength of creative PPI was that it is inclusive and accessible [ 17 , 19 , 21 ]. The safe space it fosters, as well as the dismantling of hierarchies, welcomed people from a diverse range of backgrounds and provided equal opportunities [ 21 ], especially for those with communication and memory difficulties who might be otherwise excluded from PPI. Kelemen et al. [ 19 ], who used creative methods to explore health and well-being in Stoke-on-Trent, discussed how people from different backgrounds came together and connected, discussed and reached a consensus over a topic which evoked strong emotions, that they all have in common. Individuals said that the techniques used “sets people to open up as they are not overwhelmed by words”. Similarly, creative activities, such as the persona method, have been stated to allow people to express themselves in an inclusive environment using a common language. Kearns et al. [ 18 ], who used aphasia-accessible material to develop a questionnaire with aphasic individuals, described how they felt comfortable in contributing to workshops (although this material was time-consuming to make, see ‘Limitations of creative PPI’ ).
Despite the general inclusivity of creative PPI, it can also be exclusive, particularly if online mediums are used. Fedorowicz et al. [ 15 ], used Facebook to create a PPI group, and although this may rectify previous drawbacks about lack of generalisation of creative methods (as Facebook can reach a greater number of people, globally), it excluded those who are not digitally active or have limited internet access or knowledge of technology. Online methods have other issues too. Maintaining the online group was cited as challenging and the volume of responses required researchers to interact outside of their working hours. Despite this, online methods like Facebook are very accessible for people who are physically disabled.
Creative PPI methods are engaging
The process of creative PPI is typically more engaging and produces more colourful data than traditional methods [ 13 ]. Individuals are permitted and encouraged to explore a creative self [ 19 ], which can lead to the exploration of new ideas and an overall increased enjoyment of the process. This increased engagement is particularly beneficial for younger PPI groups. For example, to involve children in the development of health food products, Galler et al. [ 16 ] asked 9-12-year-olds to take photos of their food and present it to other children in a “show and tell” fashion. They then created a newspaper article describing a new healthy snack. In this creative focus group, children were given lab coats to further their identity as inventors. Galler et al. [ 16 ], notes that the methods were highly engaging and facilitated teamwork and group learning. This collaborative nature of problem-solving was also observed in adults who used personas and creative worksheets to develop the resource for lower back pain [ 23 ]. Dementia patients too have been reported to enjoy the creative and informal approach to idea generation [ 20 ].
The use of cultural animation allowed people to connect with each other in a way that traditional methods do not [ 19 , 21 ]. These connections were held in place by boundary objects, such as ribbons, buttons, fabric and picture frames, which symbolised a shared meaning between people and an exchange of knowledge and emotion. Asking groups to create an art installation using these objects further fostered teamwork and collaboration, both at an individual and collective level. The exploration of a creative self increased energy levels and encouraged productive discussions and problem-solving [ 19 ]. Objects also encouraged a solution-focused approach and permitted people to think beyond their usual everyday scope [ 17 ]. They also allowed facilitators to probe deeper about the greater meanings carried by the object, which acted as a metaphor [ 21 ].
From the researcher’s point of view, co-creative methods gave rise to ideas they might not have initially considered. Valaitis et al. [ 22 ], found that over 40% of the creative outputs were novel ideas brought to light by patients, healthcare providers/community care providers, community service providers and volunteers. One researcher commented, “It [the creative methods] took me on a journey, in a way that when we do other pieces of research it can feel disconnected” [ 23 ]. Another researcher also stated they could not return to the way they used to do research, as they have learnt so much about their own health and community and how they are perceived [ 19 ]. This demonstrates that creative processes not only benefit the project outcomes and the PPI group, but also facilitators and researchers. However, although engaging, creative methods have been criticised for not demonstrating academic rigour [ 17 ]. Moreover, creative PPI may also be exclusive to people who do not like or enjoy creative activities.
Creative PPI methods are cost and time efficient
Creative PPI workshops can often produce output that is visible and tangible. This can save time and money in the long run as the output is either ready to be implemented in a healthcare setting or a first iteration has already been developed. This may also offset the time and costs it takes to implement creative PPI. For example, the prototype of the decision support tool for people with malignant pleural effusion was developed using personas and creative worksheets. The end result was two tangible prototypes to drive the initial idea forward as something to be used in practice [ 17 ]. The use of creative co-design in this case saved clinician time as well as the time it would take to develop this product without the help of its end-users. In the development of this particular prototype, analysis was iterative and informed the next stage of development, which again saved time. The same applies for the feedback questionnaire for the assessment of ICT delivered aphasia rehabilitation. The co-created questionnaire, designed with people with aphasia, was ready to be used in practice [ 18 ]. This suggests that to overcome time and resource barriers to creative PPI, researchers should aim for it to be engaging whilst also producing output.
That useable products are generated during creative workshops signals to participating patients and public members that they have been listened to and their thoughts and opinions acted upon [ 23 ]. For example, the development of the back pain resource based on patient experiences implies that their suggestions were valid and valuable. Further, those who participated in the cultural animation workshop reported that the process visualises change, and that it already feels as though the process of change has started [ 19 ].
The most cost and time efficient method of creative PPI in this review is most likely the use of Facebook to gather feedback on project methodology [ 15 ]. Although there were drawbacks to this, researchers could involve more people from a range of geographical areas at little to no cost. Feedback was instantaneous and no training was required. From the perspective of the PPI group, they could interact however much or little they wish with no time commitment.
This systematic review identified four limitations and five strengths to the use of creative PPI in health and social care research. Creative PPI is time and resource intensive, can raise ethical issues and lacks generalisability. It is also not accepted by the mainstream. These factors may act as barriers to the implementation of creative PPI. However, creative PPI disrupts traditional power hierarchies and creates a safe space for taboo or mundane topics. It is also engaging, inclusive and can be time and cost efficient in the long term.
Something that became apparent during data analysis was that these are not blanket strengths and limitations of creative PPI as a whole. The umbrella term ‘creative PPI’ is broad and encapsulates a wide range of activities, ranging from music and poems to prototype development and persona-scenarios, to more simplistic things like the use of sticky notes and ordering cards. Many different activities can be deemed ‘creative’ and the strengths and limitations of one does not necessarily apply to another. For example, cultural animation takes greater effort to prepare than the use of sticky notes and sorting cards, and the use of Facebook is cheaper and wider reaching than persona development. Researchers should use their discretion and weigh up the benefits and drawbacks of each method to decide on a technique which suits the project. What might be a limitation to creative PPI in one project may not be in another. In some cases, creative PPI may not be suitable at all.
Furthermore, the choice of creative PPI method also depends on the needs and characteristics of the PPI group. Children, adults and people living with dementia or language difficulties all have different engagement needs and capabilities. This indicates that creative PPI is not one size fits all and that the most appropriate method will change depending on the composition of the group. The choice of method will also be determined by the constraints of the research project, namely time, money and the research aim. For example, if there are time constraints, then a method which yields a lot of data and requires a lot of preparation may not be appropriate. If generalisation is important, then an online method is more suitable. Together this indicates that the choice of creative PPI method is highly individualised and dependent on multiple factors.
Although the limitations discussed in this review apply to creative PPI, they are not exclusive to creative PPI. Ethical issues are a consideration within general PPI research, especially when working with more vulnerable populations, such as children or adults living with a disability. It can also be the case that traditional PPI methods lack generalisability, as people who volunteer to be part of such a group are more likely be older, middle class and retired [ 24 ]. Most research is vulnerable to this type of bias, however, it is worth noting that generalisation is not always a goal and research remains valid and meaningful in its absence. Although online methods may somewhat combat issues related to generalisability, these methods still exclude people who do not have access to the internet/technology or who choose not to use it, implying that online PPI methods may not be wholly representative of the general population. Saying this, however, the accessibility of creative PPI techniques differs from person to person, and for some, online mediums may be more accessible (for example for those with a physical disability), and for others, this might be face-to-face. To combat this, a range of methods should be implemented. Planning multiple focus group and interviews for traditional PPI is also time and resource intensive, however the extra resources required to make this creative may be even greater. Although, the rich data provided may be worth the preparation and analysis time, which is also likely to depend on the number of participants and workshop sessions required. PPI, not just creative PPI, often requires the provision of a financial incentive, refreshments, parking and accommodation, which increase costs. These, however, are imperative and non-negotiable, as they increase the accessibility of research, especially to minority and lower-income groups less likely to participate. Adequate funding is also important for co-design studies where repeated engagement is required. One barrier to implementation, which appears to be exclusive to creative methods, however, is that creative methods are not mainstream. This cannot be said for traditional PPI as this is often a mandatory part of research applications.
Regarding the strengths of creative PPI, it could be argued that most appear to be exclusive to creative methodologies. These are inclusive by nature as multiple approaches can be taken to evoke ideas from different populations - approaches that do not necessarily rely on verbal or written communication like interviews and focus groups do. Given the anonymity provided by some creative methods, such as personas, people may be more likely to discuss their personal experiences under the guise of a general end-user, which might be more difficult to maintain when an interviewer is asking an individual questions directly. Additionally, creative methods are by nature more engaging and interactive than traditional methods, although this is a blanket statement and there may be people who find the question-and-answer/group discussion format more engaging. Creative methods have also been cited to eliminate power imbalances which exist in traditional research [ 12 , 13 , 17 , 19 , 23 ]. These imbalances exist between researchers and policy makers and adolescents, adults and the community. Lastly, although this may occur to a greater extent in creative methods like prototype development, it could be suggested that PPI in general – regardless of whether it is creative - is more time and cost efficient in the long-term than not using any PPI to guide or refine the research process. It must be noted that these are observations based on the literature. To be certain these differences exist between creative and traditional methods of PPI, direct empirical evaluation of both should be conducted.
To the best of our knowledge, this is the first review to identify the strengths and limitations to creative PPI, however, similar literature has identified barriers and facilitators to PPI in general. In the context of clinical trials, recruitment difficulties were cited as a barrier, as well as finding public contributors who were free during work/school hours. Trial managers reported finding group dynamics difficult to manage and the academic environment also made some public contributors feel nervous and lacking confidence to speak. Facilitators, however, included the shared ownership of the research – something that has been identified in the current review too. In addition, planning and the provision of knowledge, information and communication were also identified as facilitators [ 25 ]. Other research on the barriers to meaningful PPI in trial oversight committees included trialist confusion or scepticism over the PPI role and the difficulties in finding PPI members who had a basic understanding of research [ 26 ]. However, it could be argued that this is not representative of the average patient or public member. The formality of oversight meetings and the technical language used also acted as a barrier, which may imply that the informal nature of creative methods and its lack of dependency on literacy skills could overcome this. Further, a review of 42 reviews on PPI in health and social care identified financial compensation, resources, training and general support as necessary to conduct PPI, much like in the current review where the resource intensiveness of creative PPI was identified as a limitation. However, others were identified too, such as recruitment and representativeness of public contributors [ 27 ]. Like in the current review, power imbalances were also noted, however this was included as both a barrier and facilitator. Collaboration seemed to diminish hierarchies but not always, as sometimes these imbalances remained between public contributors and healthcare staff, described as a ‘them and us’ culture [ 27 ]. Although these studies compliment the findings of the current review, a direct comparison cannot be made as they do not concern creative methods. However, it does suggest that some strengths and weaknesses are shared between creative and traditional methods of PPI.
Strengths and limitations of this review
Although a general definition of creative PPI exists, it was up to our discretion to decide exactly which activities were deemed as such for this review. For example, we included sorting cards, the use of interactive whiteboards and sticky notes. Other researchers may have a more or less stringent criteria. However, two reviewers were involved in this decision which aids the reliability of the included articles. Further, it may be that some of the strengths and limitations cannot fully be attributed to the creative nature of the PPI process, but rather their co-created nature, however this is hard to disentangle as the included papers involved both these aspects.
During screening, it was difficult to decide whether the article was utilising creative qualitative methodology or creative PPI , as it was often not explicitly labelled as such. Regardless, both approaches involved the public/patients refining a healthcare product/service. This implies that if this review were to be replicated, others may do it differently. This may call for greater standardisation in the reporting of the public’s involvement in research. For example, the NIHR outlines different approaches to PPI, namely “consultation”, “collaboration”, “co-production” and “user-controlled”, which each signify an increased level of public power and influence [ 28 ]. Papers with elements of PPI could use these labels to clarify the extent of public involvement, or even explicitly state that there was no PPI. Further, given our decision to include only scholarly peer-reviewed literature, it is possible that data were missed within the grey literature. Similarly, the literature search will not have identified all papers relating to different types of accessible inclusion. However, the intent of the review was to focus solely on those within the definition of creative.
This review fills a gap in the literature and helps circulate and promote the concept of creative PPI. Each stage of this review, namely screening and quality appraisal, was conducted by two independent reviewers. However, four full texts could not be accessed during the full text reading stage, meaning there are missing data that could have altered or contributed to the findings of this review.
Research recommendations
Given that creative PPI can require effort to prepare, perform and analyse, sufficient time and funding should be allocated in the research protocol to enable meaningful and continuous PPI. This is worthwhile as PPI can significantly change the research output so that it aligns closely with the needs of the group it is to benefit. Researchers should also consider prototype development as a creative PPI activity as this might reduce future time/resource constraints. Shifting from a top-down approach within research to a bottom-up can be advantageous to all stakeholders and can help move creative PPI towards the mainstream. This, however, is the collective responsibility of funding bodies, universities and researchers, as well as committees who approve research bids.
A few of the included studies used creative techniques alongside traditional methods, such as interviews, which could also be used as a hybrid method of PPI, perhaps by researchers who are unfamiliar with creative techniques or to those who wish to reap the benefits of both. Often the characteristics of the PPI group were not included, including age, gender and ethnicity. It would be useful to include such information to assess how representative the PPI group is of the population of interest.
Creative PPI is a relatively novel approach of engaging the public and patients in research and it has both advantages and disadvantages compared to more traditional methods. There are many approaches to implementing creative PPI and the choice of technique will be unique to each piece of research and is reliant on several factors. These include the age and ability of the PPI group as well as the resource limitations of the project. Each method has benefits and drawbacks, which should be considered at the protocol-writing stage. However, given adequate funding, time and planning, creative PPI is a worthwhile and engaging method of generating ideas with end-users of research – ideas which may not be otherwise generated using traditional methods.
Data availability
No datasets were generated or analysed during the current study.
Abbreviations
Critical Appraisal Skills Programme
The Joanna Briggs Institute
National Institute of Health and Care Research
Public Advisory Group
Public and Patient Involvement
Web of Science
National Institute for Health and Care Research. What Is Patient and Public Involvement and Public Engagement? https://www.spcr.nihr.ac.uk/PPI/what-is-patient-and-public-involvement-and-engagement Accessed 01 Sept 2023.
Department of Health. Personal and Public Involvement (PPI) https://www.health-ni.gov.uk/topics/safety-and-quality-standards/personal-and-public-involvement-ppi#:~:text=The Health and Social Care Reform Act (NI) 2009 placed,delivery and evaluation of services . Accessed 01 Sept 2023.
National Institute for Health and Care Research. Policy Research Programme – Guidance for Stage 1 Applications https://www.nihr.ac.uk/documents/policy-research-programme-guidance-for-stage-1-applications-updated/26398 Accessed 01 Sept 2023.
Greenhalgh T, Hinton L, Finlay T, Macfarlane A, Fahy N, Clyde B, Chant A. Frameworks for supporting patient and public involvement in research: systematic review and co-design pilot. Health Expect. 2019. https://doi.org/10.1111/hex.12888
Article PubMed PubMed Central Google Scholar
Street JM, Stafinski T, Lopes E, Menon D. Defining the role of the public in health technology assessment (HTA) and HTA-informed decision-making processes. Int J Technol Assess Health Care. 2020. https://doi.org/10.1017/S0266462320000094
Article PubMed Google Scholar
Morrison C, Dearden A. Beyond tokenistic participation: using representational artefacts to enable meaningful public participation in health service design. Health Policy. 2013. https://doi.org/10.1016/j.healthpol.2013.05.008
Leavy P. Method meets art: arts-Based Research Practice. New York: Guilford; 2020.
Google Scholar
Seers K. Qualitative systematic reviews: their importance for our understanding of research relevant to pain. Br J Pain. 2015. https://doi.org/10.1177/2049463714549777
Lockwood C, Porritt K, Munn Z, Rittenmeyer L, Salmond S, Bjerrum M, Loveday H, Carrier J, Stannard D. Chapter 2: Systematic reviews of qualitative evidence. Aromataris E, Munn Z, editors. JBI Manual for Evidence Synthesis JBI. 2020. https://synthesismanual.jbi.global . https://doi.org/10.46658/JBIMES-20-03
CASP. CASP Checklists https://casp-uk.net/images/checklist/documents/CASP-Qualitative-Studies-Checklist/CASP-Qualitative-Checklist-2018_fillable_form.pdf (2022).
Braun V, Clarke V. Using thematic analysis in psychology. Qualitative Res Psychol. 2006. https://doi.org/10.1191/1478088706qp063oa
Article Google Scholar
Byrne E, Elliott E, Saltus R, Angharad J. The creative turn in evidence for public health: community and arts-based methodologies. J Public Health. 2018. https://doi.org/10.1093/pubmed/fdx151
Cook S, Grozdanovski L, Renda G, Santoso D, Gorkin R, Senior K. Can you design the perfect condom? Engaging young people to inform safe sexual health practice and innovation. Sex Educ. 2022. https://doi.org/10.1080/14681811.2021.1891040
Craven MP, Goodwin R, Rawsthorne M, Butler D, Waddingham P, Brown S, Jamieson M. Try to see it my way: exploring the co-design of visual presentations of wellbeing through a workshop process. Perspect Public Health. 2019. https://doi.org/10.1177/1757913919835231
Fedorowicz S, Riley V, Cowap L, Ellis NJ, Chambers R, Grogan S, Crone D, Cottrell E, Clark-Carter D, Roberts L, Gidlow CJ. Using social media for patient and public involvement and engagement in health research: the process and impact of a closed Facebook group. Health Expect. 2022. https://doi.org/10.1111/hex.13515
Galler M, Myhrer K, Ares G, Varela P. Listening to children voices in early stages of new product development through co-creation – creative focus group and online platform. Food Res Int. 2022. https://doi.org/10.1016/j.foodres.2022.111000
Grindell C, Tod A, Bec R, Wolstenholme D, Bhatnagar R, Sivakumar P, Morley A, Holme J, Lyons J, Ahmed M, Jackson S, Wallace D, Noorzad F, Kamalanathan M, Ahmed L, Evison M. Using creative co-design to develop a decision support tool for people with malignant pleural effusion. BMC Med Inf Decis Mak. 2020. https://doi.org/10.1186/s12911-020-01200-3
Kearns Á, Kelly H, Pitt I. Rating experience of ICT-delivered aphasia rehabilitation: co-design of a feedback questionnaire. Aphasiology. 2020. https://doi.org/10.1080/02687038.2019.1649913
Kelemen M, Surman E, Dikomitis L. Cultural animation in health research: an innovative methodology for patient and public involvement and engagement. Health Expect. 2018. https://doi.org/10.1111/hex.12677
Keogh F, Carney P, O’Shea E. Innovative methods for involving people with dementia and carers in the policymaking process. Health Expect. 2021. https://doi.org/10.1111/hex.13213
Micsinszki SK, Buettgen A, Mulvale G, Moll S, Wyndham-West M, Bruce E, Rogerson K, Murray-Leung L, Fleisig R, Park S, Phoenix M. Creative processes in co-designing a co-design hub: towards system change in health and social services in collaboration with structurally vulnerable populations. Evid Policy. 2022. https://doi.org/10.1332/174426421X16366319768599
Valaitis R, Longaphy J, Ploeg J, Agarwal G, Oliver D, Nair K, Kastner M, Avilla E, Dolovich L. Health TAPESTRY: co-designing interprofessional primary care programs for older adults using the persona-scenario method. BMC Fam Pract. 2019. https://doi.org/10.1186/s12875-019-1013-9
Webber R, Partridge R, Grindell C. The creative co-design of low back pain education resources. Evid Policy. 2022. https://doi.org/10.1332/174426421X16437342906266
National Institute for Health and Care Research. A Researcher’s Guide to Patient and Public Involvement. https://oxfordbrc.nihr.ac.uk/wp-content/uploads/2017/03/A-Researchers-Guide-to-PPI.pdf Accessed 01 Nov 2023.
Selman L, Clement C, Douglas M, Douglas K, Taylor J, Metcalfe C, Lane J, Horwood J. Patient and public involvement in randomised clinical trials: a mixed-methods study of a clinical trials unit to identify good practice, barriers and facilitators. Trials. 2021 https://doi.org/10.1186/s13063-021-05701-y
Coulman K, Nicholson A, Shaw A, Daykin A, Selman L, Macefield R, Shorter G, Cramer H, Sydes M, Gamble C, Pick M, Taylor G, Lane J. Understanding and optimising patient and public involvement in trial oversight: an ethnographic study of eight clinical trials. Trials. 2020. https://doi.org/10.1186/s13063-020-04495-9
Ocloo J, Garfield S, Franklin B, Dawson S. Exploring the theory, barriers and enablers for patient and public involvement across health, social care and patient safety: a systematic review of reviews. Health Res Policy Sys. 2021. https://doi.org/10.1186/s12961-020-00644-3
National Institute for Health and Care Research. Briefing notes for researchers - public involvement in NHS, health and social care research. https://www.nihr.ac.uk/documents/briefing-notes-for-researchers-public-involvement-in-nhs-health-and-social-care-research/27371 Accessed 01 Nov 2023.
Download references
Acknowledgements
With thanks to the PHIRST-LIGHT public advisory group and consortium for their thoughts and contributions to the design of this work.
The research team is supported by a National Institute for Health and Care Research grant (PHIRST-LIGHT Reference NIHR 135190).
Author information
Olivia R. Phillips and Cerian Harries share joint first authorship.
Authors and Affiliations
Nottingham Centre for Public Health and Epidemiology, Lifespan and Population Health, School of Medicine, University of Nottingham, Clinical Sciences Building, City Hospital Campus, Hucknall Road, Nottingham, NG5 1PB, UK
Olivia R. Phillips, Jo Leonardi-Bee, Holly Knight & Joanne R. Morling
National Institute for Health and Care Research (NIHR) PHIRST-LIGHT, Nottingham, UK
Olivia R. Phillips, Cerian Harries, Jo Leonardi-Bee, Holly Knight, Lauren B. Sherar, Veronica Varela-Mato & Joanne R. Morling
School of Sport, Exercise and Health Sciences, Loughborough University, Epinal Way, Loughborough, Leicestershire, LE11 3TU, UK
Cerian Harries, Lauren B. Sherar & Veronica Varela-Mato
Nottingham Centre for Evidence Based Healthcare, School of Medicine, University of Nottingham, Nottingham, UK
Jo Leonardi-Bee
NIHR Nottingham Biomedical Research Centre (BRC), Nottingham University Hospitals NHS Trust, University of Nottingham, Nottingham, NG7 2UH, UK
Joanne R. Morling
You can also search for this author in PubMed Google Scholar
Contributions
Author contributions: study design: ORP, CH, JRM, JLB, HK, LBS, VVM, literature searching and screening: ORP, CH, JRM, data curation: ORP, CH, analysis: ORP, CH, JRM, manuscript draft: ORP, CH, JRM, Plain English Summary: ORP, manuscript critical review and editing: ORP, CH, JRM, JLB, HK, LBS, VVM.
Corresponding author
Correspondence to Olivia R. Phillips .
Ethics declarations
Ethics approval and consent to participate.
The Ethics Committee of the Faculty of Medicine and Health Sciences, University of Nottingham advised that approval from the ethics committee and consent to participate was not required for systematic review studies.
Consent for publication
Not applicable.
Competing interests
The authors declare no competing interests.
Additional information
Publisher’s note.
Springer Nature remains neutral with regard to jurisdictional claims in published maps and institutional affiliations.
Electronic supplementary material
Below is the link to the electronic supplementary material.
40900_2024_580_MOESM1_ESM.docx
Additional file 1: Search strings: Description of data: the search strings and filters used in each of the 5 databases in this review
Additional file 2: Quality appraisal questions: Description of data: CASP quality appraisal questions
40900_2024_580_moesm3_esm.docx.
Additional file 3: Table 1: Description of data: elements of the data extraction table that are not in the main manuscript
Rights and permissions
Open Access This article is licensed under a Creative Commons Attribution 4.0 International License, which permits use, sharing, adaptation, distribution and reproduction in any medium or format, as long as you give appropriate credit to the original author(s) and the source, provide a link to the Creative Commons licence, and indicate if changes were made. The images or other third party material in this article are included in the article’s Creative Commons licence, unless indicated otherwise in a credit line to the material. If material is not included in the article’s Creative Commons licence and your intended use is not permitted by statutory regulation or exceeds the permitted use, you will need to obtain permission directly from the copyright holder. To view a copy of this licence, visit http://creativecommons.org/licenses/by/4.0/ . The Creative Commons Public Domain Dedication waiver ( http://creativecommons.org/publicdomain/zero/1.0/ ) applies to the data made available in this article, unless otherwise stated in a credit line to the data.
Reprints and permissions
About this article
Cite this article.
Phillips, O.R., Harries, C., Leonardi-Bee, J. et al. What are the strengths and limitations to utilising creative methods in public and patient involvement in health and social care research? A qualitative systematic review. Res Involv Engagem 10 , 48 (2024). https://doi.org/10.1186/s40900-024-00580-4
Download citation
Received : 28 November 2023
Accepted : 25 April 2024
Published : 13 May 2024
DOI : https://doi.org/10.1186/s40900-024-00580-4
Share this article
Anyone you share the following link with will be able to read this content:
Sorry, a shareable link is not currently available for this article.
Provided by the Springer Nature SharedIt content-sharing initiative
- Public and patient involvement
- Creative PPI
- Qualitative systematic review
Research Involvement and Engagement
ISSN: 2056-7529
- General enquiries: [email protected]

An official website of the United States government
The .gov means it’s official. Federal government websites often end in .gov or .mil. Before sharing sensitive information, make sure you’re on a federal government site.
The site is secure. The https:// ensures that you are connecting to the official website and that any information you provide is encrypted and transmitted securely.
- Publications
- Account settings
Preview improvements coming to the PMC website in October 2024. Learn More or Try it out now .
- Advanced Search
- Journal List
- J Prev Med Public Health
- v.56(2); 2023 Mar
- PMC10111102
Qualitative Research in Healthcare: Data Analysis
1 Department of Preventive Medicine, Ulsan University Hospital, University of Ulsan College of Medicine, Ulsan, Korea
2 Ulsan Metropolitan City Public Health Policy’s Institute, Ulsan, Korea
Hyeran Jung
3 Department of Preventive Medicine, University of Ulsan College of Medicine, Seoul, Korea
Qualitative research methodology has been applied with increasing frequency in various fields, including in healthcare research, where quantitative research methodology has traditionally dominated, with an empirically driven approach involving statistical analysis. Drawing upon artifacts and verbal data collected from in-depth interviews or participatory observations, qualitative research examines the comprehensive experiences of research participants who have experienced salient yet unappreciated phenomena. In this study, we review 6 representative qualitative research methodologies in terms of their characteristics and analysis methods: consensual qualitative research, phenomenological research, qualitative case study, grounded theory, photovoice, and content analysis. We mainly focus on specific aspects of data analysis and the description of results, while also providing a brief overview of each methodology’s philosophical background. Furthermore, since quantitative researchers have criticized qualitative research methodology for its perceived lack of validity, we examine various validation methods of qualitative research. This review article intends to assist researchers in employing an ideal qualitative research methodology and in reviewing and evaluating qualitative research with proper standards and criteria.
INTRODUCTION
Researchers should select the research methodology best suited for their study. Quantitative research, which is based on empiricism and positivism, has long been the mainstream research methodology in most scientific fields. In recent years, however, increasing attempts have been made to use qualitative research methodology in various research fields, either combined with quantitative research methodology or as a stand-alone research method. Unlike quantitative research, which performs statistical analyses using the results derived in numerical form through investigations or experiments, qualitative research uses various qualitative analysis methods based on verbal data obtained through participatory observations or in-depth interviews. Qualitative research is advantageous when researching topics that involve research participants’ in-depth experiences and perceptions, topics that are important but have not yet drawn sufficient attention, and topics that should be reviewed from a new perspective.
However, qualitative research remains relatively rare in healthcare research, with quantitative research still predominating as the mainstream research practice [ 1 ]. Consequently, there is a lack of understanding of qualitative research, its characteristics, and its procedures in healthcare research. The low level of awareness of qualitative research can lead to the denigration of its results. Therefore, it is essential not only for researchers conducting qualitative research to have a correct understanding of various qualitative research methods, but also for peer researchers who review research proposals, reports, and papers to properly understand the procedures and advantages/disadvantages of qualitative research.
In our previous review paper, we explored the characteristics of qualitative research in comparison to quantitative research and its usefulness in healthcare research [ 2 ]. Specifically, we conducted an in-depth review of the general qualitative research process, selection of research topics and problems, selection of theoretical frameworks and methods, literature analysis, and selection of research participants and data collection methods [ 2 ]. This review article is dedicated to data analysis and the description of results, which may be considered the core of qualitative research, in different qualitative research methods in greater detail, along with the criteria for evaluating the validity of qualitative research. This review article is expected to offer insights into selecting and implementing the qualitative research methodology best suited for a given research topic and evaluating the quality of research.
IN-DEPTH REVIEW OF QUALITATIVE RESEARCH METHODS
This section is devoted to the in-depth review of 6 qualitative research methodologies (consensual qualitative research, phenomenological research, qualitative case study, grounded theory, photovoice, and content analysis), focusing on their characteristics and concrete analysis processes. Table 1 summarizes the characteristics of each methodology.
Characteristics and key analytical approaches of each qualitative research methodology
Consensual Qualitative Research
Consensual qualitative research (CQR) was developed by Professor Clara Hill of the University of Maryland [ 3 ]. It emphasizes consensus within a research team (or analysis team) to address the problem of low objectivity being likely to occur when conducting qualitative research. This method seeks to maintain scientific rigor by deriving analysis results through team consensus, asserting the importance of ethical issues, trust, and the role of culture. In CQR, researchers are required to verify each conclusion whenever it is drawn by checking it against the original data.
Building a solid research team is the first step in conducting CQR. Most importantly, each team member should have resolute initiative and clear motivations for joining the research team. In general, at least 3 main team members are needed for data analysis, with 1 or 2 advisors (or auditors) reviewing their work. Researchers without experience in CQR should first receive prior education and training on its procedures and then team up with team members experienced in CQR. Furthermore, as is the case with other types of qualitative research, CQR attaches great importance to ensuring the objectivity of research by sharing prejudices, pre-understanding, and expectations of the research topic among the team members.
CQR is performed in 4 sequential steps: the initial stage, intra-case analysis stage, cross-analysis stage, and manuscript writing stage [ 4 ]. First, in the initial stage, the pre-formed team of researchers selects a research topic, performs a literature review, develops an interview guideline, and conducts pilot interviews. Research participants who fit the research topic are recruited using inclusion and exclusion criteria for selecting suitable participants. Then, interviews are conducted according to the interview guideline, recorded, and transcribed. The transcripts are sent to the interviewees for review. During this process, researchers could make slight modifications to explore the research topic better.
Second, in intra-case analysis stage, domains and subdomains are developed based on the initial interview guideline. The initial domains and subdomains are used to analyze 1 or 2 interviews, and afterward, the domains and subdomains are modified to reflect the analysis results. Core ideas are also created through interview analysis and are coded in domains and subdomains. The advisors review the domains, subdomains, and core ideas and provide suggestions for improvement. The remaining interviews are analyzed according to the revised domains, subdomains, and core ideas.
Third, in the cross-analysis stage, the core ideas from the interview analysis are categorized according to the domains and subdomains. In this process, repeated team discussions are encouraged to revise domains and subdomains and place the core ideas that do not lend themselves well to categorization into a miscellaneous category. The frequency of occurrence of each domain is then calculated for each interview case. In general, a domain is classified as a general category when it appears in all cases, a typical category when it appears in more than half of the cases, and a variant category when it appears in fewer than half of the cases [ 5 ]. However, the criteria for frequency counting may slightly differ from study to study. The advisors should also review the results of the cross-analysis stage, and the main analysis team revises the analysis results based on those comments.
Fourth, the intra-case analysis and cross-analysis results are described in the manuscript writing stage. It is essential to present a clear and convincing narrative to the audience [ 5 ], and it is thus recommended to revise and formulate the manuscript based on team discussions and advisor opinions. However, CQR does not guarantee that different research teams would reach similar conclusions, and the CQR research team dynamics strongly affect conflict-resolution issues during the consensus-building process [ 3 ].
As examined above, despite its limitations, the salient feature of CQR is its rigorous process for ensuring the objectivity of analysis results compared to other qualitative research methods. In addition, it is an accessible method for quantitative researchers because it explains the analysis results in terms of the frequency of domain occurrences. CQR can be a suitable research methodology to persuade researchers who are hesitant to accept the results of qualitative research. Although CQR is still rarely used in healthcare research, some studies have applied it to investigate topics of interest [ 6 , 7 ].
Phenomenological Research
Phenomenological research (PR) is, as its name suggests, qualitative research based on the phenomenological principle. The term “phenomenological principle” is based on Husserlian phenomenology, which seeks the essence (inner core) and the meaning of people’s lived experiences [ 8 ]. According to Husserl, it is necessary to go “back to the things themselves” (in German: zurück zu den Sachen selbst ) and accurately explore the essence of experience. Diverse reflective attitudes based on the phenomenological principle are required to understand “ Sachen ” without expectations and prejudices [ 9 ]. Thus, the purpose of PR using Husserl’s phenomenological principle can be understood as an inquiry into the essence of experience.
The process of PR aiming to fulfill this purpose differs among various schools and scholars. The Husserlian, Heideggerian, and Utrecht schools had major impacts on PR [ 10 ]. Representative Husserlian scholars who further developed the PR process include Amedeo Giorgi and Paul Colaizzi. Giorgi, who pioneered the field of phenomenological psychology, collected data through in-depth interviews and divided the analysis process into 4 steps [ 11 ]. Colaizzi, who was one of Giorgi’s students, proposed a more complex process from data collection to analysis [ 12 , 13 ]. Representative Heideggerian scholars are Patricia Benner, who introduced an interpretive phenomenological qualitative research method to the field of nursing on the subject of clinical placement of nursing students but did not fully clarify its specific procedure [ 14 ], and Nancy Diekelmann [ 15 ] and Nancy Diekelmann and David Allen [ 16 ], who emphasized the role of the team in the analysis process and proposed the 7-step method of analysis. Max Van Manen, a Dutch-born Canadian scholar, is a representative Utrecht School scholar who proposed a 6-step data collection and analysis process and emphasized the importance of phenomenological description [ 8 ]. As a scholar with no affiliation with any specific school, Adrian Van Kaam [ 17 ], an existentialist psychologist, developed an experiential PR method using descriptive texts. Despite differences in data collection and analysis processes, the common denominator of these approaches is a fundamentally phenomenological attitude and the goal of exploring the essence of experience.
In general, the process of phenomenological qualitative analysis can be divided into 5 steps based on the phenomenological attitude [ 18 ]: step 1, reading the data repeatedly to get a sense of the whole and gauge the meanings of the data; step 2, categorizing and clustering the data by meaning unit; step 3, writing analytically by meaning unit in a descriptive, reflective, and hermeneutic manner; step 4, deriving essential factors and thematizing while writing; and step 5, deriving the essential experiential structure by identifying the relationships between essential experiential factors. During the entire process, researchers must embrace the attitudes of “reduction” and “imaginative variation.” The term “reduction” reflects the thought of accepting the meaning of experience in the way it manifests itself [ 19 ]. An attitude of phenomenological reduction is required to recover freshness and curiosity about the research object through non-judgment, bracketing, and epoché , which assist to minimize the effects of researchers’ prejudices of research topic during the analysis process. An attitude of imaginative variation is required to diversify the meanings pertaining to data and view them as diametric opposites.
As described above, PR is characterized more by emphasizing the researcher’s constant reflection and interpretation/recording of the experience, seeking to explore its very essence, than by being conducted according to a concrete procedure. Based on these characteristics, PR in healthcare research has been applied to various topics, including research on the meaning of health behaviors such as drinking and smoking in various cultures since the 1970s [ 20 , 21 ], information and education needs of patients with diabetes [ 22 ], pain in cancer patients [ 23 ], and the experiences of healthcare students and professionals in patient safety activities [ 24 , 25 ].
Qualitative Case Study
Although case studies have long been conducted in various academic fields, in the 1980s [ 26 ], they began to be recognized as a qualitative research method with the case study publications by researchers such as Merriam [ 27 ], Stake [ 28 ], Yin [ 29 ], and Hays [ 30 ]. Case studies include both quantitative and qualitative strategies and can also be used with other qualitative research methods. In general, a qualitative case study (QCS) is a research method adopted to understand the complexity of a case, derive its meaning, and identify the process of change over time [ 27 ]. To achieve these goals, a QCS collects in-depth data using various information sources from rich contexts and explores one or more bounded systems [ 31 ].
A case, which is the core of a case study, has delimitation [ 28 ], contextuality [ 29 ], specificity [ 30 ], complexity [ 32 ], and newness [ 27 ]. The definition of a case study differs among scholars, but they agree that a case to be studied should have boundaries that distinguish it from other cases. Therefore, a case can be a person, a group, a program, or an event and can also be a single or complex case [ 28 ]. The types of QCSs are classified by the scale of the bounded system and the purpose of case analysis. From the latter perspective, Stake [ 28 ] divided case studies into intrinsic and instrumental case studies.
A QCS is conducted in 5 steps [ 33 ]. Stage 1 is the research design stage, where an overall plan is established for case selection, research question setting, research time and cost allocation, and the report format of research outcomes [ 28 ]. Yin [ 33 ] noted that 4 types of case studies could be designed based on the number of cases (single or multiple cases) and the number of analysis units (holistic design for a single unit or embedded design for multiple units). These types are called single holistic design, single embedded design, multiple holistic design, and multiple embedded design. Stage 2 is the preparation stage for data collection. The skills and qualifications required for the researcher are reviewed, prior training of researchers takes place, a protocol is developed, candidate cases are screened, and a pilot case study is conducted. Stage 3 is data collection. Data are collected from the data sources commonly used in case studies, such as documents, archival records, interviews, direct observations, participatory observations, and physical artifacts [ 33 ]. Other data sources for case studies include films, photos, videotapes, and life history studies [ 34 ]. The data collection period may vary depending on the research topic and the need for additional data collection during the analysis process. Stage 4 is the data analysis stage. The case is described in detail based on the collected data, and the data for concrete topics are analyzed [ 28 ]. With no prescribed method related to data collection and analysis for a case study, a general data analysis procedure is followed, and the choice of analysis method differs among researchers. In a multiple-case study, the meaning of the cases is interpreted by performing intra-case and inter-case analyses. The last stage is the interpretation stage, in which the researcher reports the meaning of the case—that is, the lessons learned from the case [ 35 ].
Compared to other qualitative research methods, QCSs have no prescribed procedure, which may prove challenging in the actual research process. However, when the researcher seeks an in-depth understanding of a bound system clearly distinguished from other cases, a QCS can be an appropriate approach. Based on the characteristics mentioned above, QCSs in healthcare research have been mainly conducted on unique cases or cases that should be known in detail, such as the experience of rare diseases [ 36 ], victims of medical malpractice [ 37 ], complications due to home birth [ 38 ], and post-stroke gender awareness of women of childbearing age [ 39 ].
Grounded Theory
Grounded theory (GT) is a research approach to gaining facts about an unfamiliar specific social phenomenon or a new understanding of a particular phenomenon [ 40 ]. GT involves the most systematic research process among all qualitative research methods [ 41 ]. Its most salient feature is generating a theory by collecting various data from research subjects and analyzing the relationship between the central phenomenon and each category through an elaborate analysis process. GT is adequate for understanding social and psychological structural phenomena regarding a specific object or social phenomenon, rather than framework or hypothesis testing [ 42 ].
GT was first introduced in 1967 by Strauss and Glaser. Their views subsequently diverged and each scholar separately developed different GT methods. Glaser’s GT focused on the natural emergence of categories and theories based on positivism [ 40 , 43 ]. Strauss, who was influenced by symbolic interactionism and pragmatism, teamed up with Corbin and systematically presented the techniques and procedures of the GT process [ 44 ]. Since then, various GT techniques have been developed [ 45 ]; Charmaz’s GT is based on constructivism [ 43 ].
Researchers using GT should collect data based on theoretical sampling and theoretical saturation. Theoretical sampling refers to selecting additional data using the theoretical concepts encountered in collecting and analyzing data, and theoretical saturation occurs when no new categories are expected to appear [ 40 ]. Researchers must also possess theoretical sensitivity—that is, the ability to react sensitively to the collected data and gain insight into them [ 40 ]. An analysis is performed through the constant comparative method, wherein researchers constantly compare the collected data and discover similarities and differences to understand the relationships between phenomena, concepts, and categories.
Among the different types of GT research designs, the one proposed by Strauss and Corbin is divided into 3 stages. Stage 1 is open coding; the concepts are derived from the data through a line-by-line data analysis, and the initial categorization occurs. Stage 2 is axial coding; the interrelationships among the categories derived from open coding are schematized in line with the structural framework defined as a paradigm. The major components of the paradigm are causal conditions, context, intervening conditions, action/interaction strategies, and consequences. Stage 3 is selective coding; the core category is first derived, the relationships between subcategories and concepts are identified, and the narrative outline is described. Lastly, the process is presented in a visual mode, whereupon a theoretical model is built and integrated. In contrast, Glaser’s analysis method involves theoretical coding that weaves practical concepts into hypotheses or theories instead of axial coding [ 46 ]. Currently, Strauss and Corbin’s GT method is the most widely used one [ 47 ], and given that different terms are used among scholars, it is crucial to accurately understand the meaning of a term in context instead of solely focusing on the term itself [ 48 ].
The most salient features of GT are that it seeks to generate a new theory from data based on the inductive principle through its analytical framework. This framework enables an understanding of the interaction experience and the structure of its performances [ 40 ]. Furthermore, the above-described characteristics of GT widen the pathway of quantitative researchers to apply GT more than other qualitative research methods [ 43 ], which has resulted in its broader application in healthcare research. GT has been used to explore a wide range of research topics, such as asthma patients’ experiences of disease management [ 48 ], the experiences of cancer patients or their families [ 49 , 50 ], and the experiences of caregivers of patients with cognitive disorders and dementia [ 51 ].
Photovoice, a research methodology initiated by Wang and Burris [ 52 ], has been used to highlight the experiences and perspectives of marginalized people using photos. In other words, photos and their narratives are at the heart of photovoice; this method is designed to make marginalized voices heard. Photovoice, which uses photos to bring to the fore the experiences of participants who have lived a marginalized life, requires the active engagement of the participants. In other research methods, the participants play an essential role in the data collection stage (interview, topic-related materials such as diary and doodle) and the research validation stage (participants’ review). In contrast, in photovoice research, which is classified as participatory action research, participants’ dynamic engagement is essential throughout the study process—from the data collection and analysis procedure to exhibition and policy development [ 53 ].
Specifically, the photovoice research design is as follows [ 54 , 55 ]: First, policymakers or community stakeholders, who will likely bring about practical improvements on the research topic, are recruited. Second, participants with a wealth of experience on a research topic are recruited. In this stage, it should be borne in mind that the drop-out rate is high because participants’ active involvement is required, and the process is relatively time-consuming. Third, the participants are provided with information on the purpose and process of photovoice research, and they are educated on research ethics and the potential risks. Fourth, consent is obtained from the participants for research participation and the use of their photos. Fifth, a brainstorming session is held to create a specific topic within the general research topic. Sixth, researchers select a type of camera and educate the participants on the camera and photo techniques. The characteristics of the camera function (e.g., autofocus and manual focus) should be considered when selecting a camera type (e.g., mobile phone camera, disposable camera, or digital camera). Seventh, participants are given time to take pictures for discussion. Eighth, a discussion is held on the photos provided by the participants. The collected data are managed and analyzed in 3 sub-steps: (1) participants’ photo selection (selecting a photo considered more meaningful or important than other photos); (2) contextualization (analyzing the selected photo and putting the meanings attached to the photo into context); and (3) codifying (categorizing similar photos and meanings among the data collected and summarizing them in writing). In sub-step 2, the “SHOWeD” question skill could be applied to facilitate the discussion [ 56 ]: “What do you S ee here? What’s really H appening here? How does this relate to O ur lives? W hy does this situation, concern, or strength E xist? What can we D o about it?” Ninth, the participants’ summarized experiences related to their respective photos are shared and presented. This process is significant because it provides the participants with an opportunity to exhibit their photos and improve the related topics’ conditions. It is recommended that policymakers or community stakeholders join the roundtable to reflect on the outcomes and discuss their potential involvement to improve the related topics.
Based on the characteristics described above, photovoice has been used in healthcare research since the early 2000s to reveal the experiences of marginalized people, such as the lives of Black lesbian, gay, bisexual, transgender and questioning people [ 57 ] and women with acquired immunodeficiency syndrome [ 58 ], and in studies on community health issues, such as the health status of indigenous women living in a remote community [ 59 ], the quality of life of breast cancer survivors living in rural areas [ 60 ], and healthy eating habits of rural youth [ 61 ].
Qualitative Content Analysis
Content analysis is a research method that can use both qualitative and quantitative methods to derive valid inferences from data [ 62 ]. It can use a wide range of data covering a long period and diverse fields [ 63 ]. It helps compare objects, identify a specific person’s characteristics or hidden intentions, or analyze a specific era’s characteristics [ 64 ]. Quantitative content analysis categorizes research data and analyzes the relationships between the derived categories using statistical methods [ 65 ]. In contrast, qualitative content analysis (QCA) uses data coding to identify categories’ extrinsic and intrinsic meanings. The parallelism of these aspects contributes to establishing the validity of conclusions in content analysis [ 63 ].
Historically, mass media, such as newspapers and news programs, played the role of the locomotive for the development of content analysis. As interest in mass media content dealing with particular events and issues increased, content analysis was increasingly used in research analyzing mass media. In particular, it was also used in various forms to analyze propaganda content during World War II. The subsequent emergence of computer technology led to the revival of various types of content analysis research [ 66 ].
QCA is largely divided into conventional, directed, and summative [ 67 ]. First, conventional content analysis is an inductive method for deriving categories from data without using perceived categories. Key concepts are derived via the coding process by repeatedly reading and analyzing the data collected through open-ended questions. Categorization is then performed by sorting the coded data while checking similarities and differences. Second, directed content analysis uses key concepts or categories extracted from existing theories or studies as the initial coding categories. Unlike conventional content analysis, directed content analysis is closer to a deductive method and is anchored in a more structured process. Summative content analysis, the third approach, not only counts the frequency of keywords or content, but also evaluates their contextual usage and provides qualitative interpretations. It is used to understand the context of a word, along with the frequency of its occurrence, and thus to find the range of meanings that a word can have.
Since there is no concrete set procedure, the content analysis procedure varies among researchers. Some of the typical processes are a 3-step process (preparation, organizing, reporting) proposed by Elo and Kyngäs [ 68 ], a 4-step process (formulating research questions, sampling, coding, analyzing) presented by White and Marsh [ 69 ], and a 6-step process proposed by Krippendorff [ 66 ].
The 6-step content analysis research process proposed by Krippendorff [ 66 ] is as follows: Step 1, unitizing, is a process in which the researcher selects a scheme for classifying the data of interest for data collection and analysis. Step 2, sampling, involves selecting a conceptually representative sample population. In Step 3, recording/coding, the researcher records materials that are difficult to preserve, such as verbal statements, in a way that allows repeated review. Step 4, reducing, refers to simplifying the data into a manageable format using statistical techniques or summaries. Step 5, abductively inferring, involves inferring a phenomenon in the context of a situation to understand the contextual phenomenon while analyzing the data. In Step 6, narrating, the research outcomes are presented in a narrative accessible to the audience. These 6 steps are not subject to a sequential order and may go through a cyclical or iterative process [ 63 ].
As examined above, content analysis is used in several fields due to its advantages of embracing both qualitative and quantitative aspects and processing comprehensive data [ 62 , 70 ]. In recognition of its research potential, the public health field is also increasingly using content analysis research, as exemplified by suicide-related social media content analysis [ 71 ], an analysis of children’s books in association with breast cancer [ 72 ], and an analysis of patients’ medical records [ 73 ].
VALIDATION OF QUALITATIVE RESEARCH
The validation of qualitative research begins when a researcher attempts to persuade others that the research results are worthy of attention [ 35 ]. Several researchers have advanced their arguments in many different ways, from the reason or justification for existence of the validity used in qualitative research to the assessment terms and their meanings [ 74 ]. We explain the validity of qualitative research, focusing on the argument advanced by Guba and Lincoln [ 75 ]. They emphasized that the evaluation of qualitative research is a socio-political process—namely, a researcher should assume the role of a mediator of the judgment process, not that of the judge [ 75 ]. Specifically, Lincoln and Guba [ 75 ] proposed trustworthiness as a validity criterion: credibility, transferability, dependability, and confirmability.
First, credibility is a concept that corresponds to internal validity in quantitative research. To enhance the credibility of qualitative research, a “member check” is used to directly assess whether the reality of the research participants is well-reflected in the raw data, transcripts, and analysis categories [ 76 , 77 ]. Second, transferability corresponds to external validity or generalizability in quantitative research. To enhance the transferability of qualitative research, researchers must describe the data collection and analysis processes in detail and provide thick data on the overall research process, including research participants and the context and culture of research [ 77 , 78 ]. Transferability can also be enhanced by checking whether the analysis results elicit similar feelings in those who have not participated in the study but share similar experiences. Third, dependability corresponds to reliability in quantitative research and is associated with data stability. To enhance the trustworthiness of qualitative research, it is common for multiple researchers to perform the analysis independently; alternatively, or if one researcher has performed the analysis, another researcher reviews the analysis results. Furthermore, a qualitative researcher must provide a detailed and transparent description of the entire research process so that other researchers, internal or external, can evaluate whether the researcher has adequately proceeded with the overall research process. Fourth, confirmability corresponds to objectivity in quantitative research. Bracketing, a process of disclosing and discussing the researcher’s pre-understanding that may affect the research process from the beginning to the end, is conducted to enhance the confirmability of qualitative research. The results of bracketing should be included in the study results so that readers can also track the possible influence [ 77 ].
However, regarding the validity of a qualitative study, it is necessary to consider the research topic, the target audience, and research costs. Caution should also be applied to the proposed theories because presentation methods vary among scholars and researchers. Apart from the methods discussed above, other methods are used to enhance the validity of qualitative research methods, such as prolonged involvement, persistent observation, triangulation, and peer debriefing. In prolonged involvement, a researcher depicts the core of a phenomenon while staying at the study site for a sufficient time to build rapport with the participants and pose a sufficient amount of questions. In persistent observation, a researcher repeatedly reviews and observes data resources until the factors closest to the research topic are identified, giving depth to the study. Triangulation is used to check whether the same results are drawn by a team of researchers who conduct a study using various resources, including individual interviews, talks, and field notes, and discuss their respective analysis processes and results. Lastly, in peer debriefing, research results are discussed with colleagues who have not participated in the study from the beginning to the end, but are well-informed about the research topic or phenomenon [ 76 , 78 ].
This review article examines the characteristics and analysis processes of 6 different qualitative research methodologies. Additionally, a detailed overview of various validation methods for qualitative research is provided. However, a few limitations should be considered when novice qualitative researchers follow the steps in this article. First, as each qualitative research methodology has extensive and unique research approaches and analysis procedures, it should be kept in mind that the priority of this article was to highlight each methodology’s most exclusive elements that essentially compromise the core of its identity. Its scope unfortunately does not include the inch-by-inch steps of individual methodologies—for this information, it would be necessary to review the references included in the section dedicated to each methodology. Another limitation is that this article does not concentrate on the direct comparison of each methodology, which might benefit novice researchers in the process of selecting an adequate methodology for their research topic. Instead, this review article emphasizes the advantages and limitations of each methodology. Nevertheless, this review article is expected to help researchers considering employing qualitative research methodologies in the field of healthcare select an optimal method and conduct a qualitative study properly. It is sincerely hoped that this review article, along with the previous one, will encourage many researchers in the healthcare domain to use qualitative research methodologies.
Ethics Statement
Approval from the institutional review board was not obtained as this study is a review article.
ACKNOWLEDGEMENTS
CONFLICT OF INTEREST
The authors have no conflicts of interest associated with the material presented in this paper.
AUTHOR CONTRIBUTIONS
Conceptualization: Ock M. Literature review: Im D, Pyo J, Lee H, Jung H, Ock M. Funding acquisition: None. Writing – original draft: Im D, Pyo J, Lee H, Jung H, Ock M. Writing – review & editing: Im D, Pyo J, Lee H, Jung H, Ock M.
This paper is in the following e-collection/theme issue:
Published on 17.5.2024 in Vol 26 (2024)
Reporting of Ethical Considerations in Qualitative Research Utilizing Social Media Data on Public Health Care: Scoping Review
Authors of this article:

- Yujie Zhang 1, 2 * , MD ;
- Jiaqi Fu 1, 2 * , MD ;
- Jie Lai 1, 2 * , MD ;
- Shisi Deng 1, 2 , MD ;
- Zihan Guo 1, 2 , MD ;
- Chuhan Zhong 1, 2 , MD ;
- Jianyao Tang 1, 2 , MD ;
- Wenqiong Cao 1 , BSc ;
- Yanni Wu 1 , PhD
1 Nanfang Hospital, Southern Medical University, Guangzhou, China
2 School of Nursing, Southern Medical University, Guangzhou, China
*these authors contributed equally
Corresponding Author:
Yanni Wu, PhD
Nanfang Hospital
Southern Medical University
No 1838 Guangzhou Avenue North
Baiyun District, Guangdong Province
Guangzhou, 510515
Phone: 86 02061641192
Email: [email protected]
Background: The internet community has become a significant source for researchers to conduct qualitative studies analyzing users’ views, attitudes, and experiences about public health. However, few studies have assessed the ethical issues in qualitative research using social media data.
Objective: This study aims to review the reportage of ethical considerations in qualitative research utilizing social media data on public health care.
Methods: We performed a scoping review of studies mining text from internet communities and published in peer-reviewed journals from 2010 to May 31, 2023. These studies, limited to the English language, were retrieved to evaluate the rates of reporting ethical approval, informed consent, and privacy issues. We searched 5 databases, that is, PubMed, Web of Science, CINAHL, Cochrane, and Embase. Gray literature was supplemented from Google Scholar and OpenGrey websites. Studies using qualitative methods mining text from the internet community focusing on health care topics were deemed eligible. Data extraction was performed using a standardized data extraction spreadsheet. Findings were reported using PRISMA-ScR (Preferred Reporting Items for Systematic Reviews and Meta-Analyses Extension for Scoping Reviews) guidelines.
Results: After 4674 titles, abstracts, and full texts were screened, 108 studies on mining text from the internet community were included. Nearly half of the studies were published in the United States, with more studies from 2019 to 2022. Only 59.3% (64/108) of the studies sought ethical approval, 45.3% (49/108) mentioned informed consent, and only 12.9% (14/108) of the studies explicitly obtained informed consent. Approximately 86% (12/14) of the studies that reported informed consent obtained digital informed consent from participants/administrators, while 14% (2/14) did not describe the method used to obtain informed consent. Notably, 70.3% (76/108) of the studies contained users’ written content or posts: 68% (52/76) contained verbatim quotes, while 32% (24/76) paraphrased the quotes to prevent traceability. However, 16% (4/24) of the studies that paraphrased the quotes did not report the paraphrasing methods. Moreover, 18.5% (20/108) of the studies used aggregated data analysis to protect users’ privacy. Furthermore, the rates of reporting ethical approval were different between different countries ( P =.02) and between papers that contained users’ written content (both direct and paraphrased quotes) and papers that did not contain users’ written content ( P <.001).
Conclusions: Our scoping review demonstrates that the reporting of ethical considerations is widely neglected in qualitative research studies using social media data; such studies should be more cautious in citing user quotes to maintain user privacy. Further, our review reveals the need for detailed information on the precautions of obtaining informed consent and paraphrasing to reduce the potential bias. A national consensus of ethical considerations such as ethical approval, informed consent, and privacy issues is needed for qualitative research of health care using social media data of internet communities.
Introduction
Social media are web-based computer-mediated tools to collaborate, share, or exchange information, ideas, pictures, or videos in virtual communities and networks such as message boards, communities, chat rooms, forums, Twitter, and Facebook [ 1 ]. Moreover, patients and researchers can use internet communities to provide health care and disseminate health information [ 2 , 3 ]. Health care refers to the efforts made to improve or maintain physical, mental, or emotional well-being, including prevention, diagnosis, treatment, recovery, and other physical and mental impairments [ 4 ]. Currently, with 57% of the global population’s access to social media, more than 40% of the patients and caregivers worldwide utilize the internet community for health care information needs [ 5 ]. With diverse populations accessing internet communities and sharing information about health care topics, researchers have the opportunity to collect and analyze text about health care from a diverse range of participants in the internet community, which was unavailable previously [ 6 ]. Usually, quantitative data are derived from information extraction, which can be analyzed statistically, and the summary results presented cannot be directly linked to individual participants. In contrast, qualitative research within internet community analysis posts and comments qualitatively or thematically involves a more detailed and in-depth analysis and understanding of the full written content [ 7 ]. However, a controversial ethical problem has been raised about conducting qualitative research containing internet users’ verbatim quotes that could lead to traceability of the original post, thereby causing a threat to an individual’s privacy [ 8 ]. Additionally, a previous study investigated public and patients’ views regarding ethics in research using social media data and reported that internet users were aggrieved if they found any of their quotes cited in a medical research paper without obtaining their informed consent [ 9 ]. Further, besides the privacy breach caused by posts being traced, there is greater harm for special groups or vulnerable groups if we do not highlight the importance of the technical standards for text mining and privacy protection in health care. For instance, some unusual postings, abnormal pictures, and interactions that were expressed by individuals with mental disorders in social media can be detected by researchers by using text mining tools without obtaining their consent [ 10 ]. The publication of research on mental disorders, including quotes in posts, can result in a high risk of information harm, which can lead to personal information being revealed and further stigmatization of the condition or disease [ 11 ]. Since 2001, ethical concerns have been debated for decades about ethical approval, informed consent, and how to ensure anonymity and preserve data privacy and confidentiality in qualitative research in the internet community [ 12 - 14 ].
With the rapid development of social media and internet research, some ethical guidelines or standards have been published to ensure that research based on internet communities is conducted ethically. The Association of Internet Researchers (internet research ethical guidelines 2.0 and 3.0) showed that researchers working without the direct approval of ethics review boards would have additional challenges to face, and obtaining informed consent is obviously impracticable in several big data projects. However, with the ethical issues about privacy breaches and harms of risk of discrimination, the Association of Internet Researchers recommended reserving the acquisition of informed consent to the dissemination stage by asking for informed consent from specific participants before publication of their quotes [ 15 , 16 ]. Furthermore, researchers should take responsibility for information confidentiality and anonymity according to the internet research ethics criteria prepared by the National Committee for Research Ethics in the Social Sciences and the Humanities guidelines, which recommend a basic research ethic norm for the analyses, reports, and evaluations that apply to all research [ 17 ]. Moreover, the National Committee for Research Ethics in the Social Sciences and the Humanities guidelines contain more details about the demand for legal consent and privacy standards imposed by the European Union’s General Data Protection Regulation. The General Data Protection Regulation is a European Union–wide regulation targeting the project of personal data processing. The General Data Protection Regulation defines personal data as any information relating to an identifiable person (data subject), including name, online identification number, location data, and other factors related to personal, physical, physiological, mental, or social identity [ 18 ]. The General Data Protection Regulation recommends using anonymous data and deleting identifiable information to ensure the confidentiality of the data. Consent should be obtained from the individual for use in scientific research [ 18 , 19 ]. The British Psychological Society guideline does not explicitly refer to the internet community but suggests that researchers may consider paraphrasing the verbatim quotes to reduce the risk of being traced or identified in qualitative research [ 20 ]. When paraphrasing, steps must be put into place to ensure that the original meaning of the message is maintained. Currently, there is no widespread consensus on ethical considerations by social media researchers.
Some researchers have tried to explore the reporting of existing ethical considerations in research papers using social media data. For instance, Sinnenberg et al [ 6 ] reported that only 32% and 12% of the papers mentioned acquiring ethical approval and informed consent, respectively, by utilizing multiple analysis methods, including surveillance, intervention, recruitment, engagement, content analysis, and network analysis with Twitter data before 2015. Thereafter, Takats et al [ 21 ] conducted an updated examination based on Sinnenberg et al’s [ 6 ] study. They found that of 367 studies using different methodological approaches, including sentiment mining, surveillance, and thematic exploration of public health research using Twitter data between 2010 to 2019, 17% of the studies included verbatim tweets and identifiable information about the internet users [ 21 ]. Similarly, Lathan et al [ 22 ] reviewed papers, including both qualitative and quantitative methods, by using Facebook data to explore public health issues and reported that only 48% and 10% of the papers obtained ethical approval and informed consent, respectively. Furthermore, in a study on research using YouTube data or comments, Tanner et al [ 23 ] found that only 26.1% of these studies sought ethical approval, only 1 paper (0.08%) sought informed consent, and 27.7% contained identifiable information. These findings indicate widespread neglect of ethical issues such as ethical approval, informed consent, and privacy issues in research papers using social media data.
Our study focuses on the ethical challenges of qualitative studies utilizing social media data. First, social media can be considered as sources for qualitative data collection because of the low cost, vast amount of available sources about health information, and users’ health behaviors, experiences, and attitudes. Second, qualitative research is context-dependent and mainly contains quotations and written content to support the viewpoint. It is acknowledged that quote materials from social media would potentially be traced back to the original posts and threaten the users’ privacy [ 24 ]. This is supported by findings reported by Ayers et al [ 25 ] who found that online searches of verbatim Twitter quotes in journal papers described as “content analyses” or “coded Twitter postings” can be traced back to individual internet users 84% of the time. Furthermore, Lathan et al [ 22 ] identified that 46% of the studies with verbatim or paraphrased quotes could be traced to the original posts in 10 minutes. Therefore, it is essential to investigate the extent to which ethical oversight is reported in qualitative studies using social media data. Moreover, qualitative research often involves personally sensitive data about health conditions and diseases; hence, anonymity and proper deidentification would be more important for researchers [ 26 , 27 ].
Previous studies have reviewed the ethical challenges and methodological use of social media platforms such as Twitter [ 6 , 21 ], Facebook [ 22 ], and YouTube [ 23 ] for health care research in both qualitative and quantitative studies. Although there is plenty of qualitative data pouring into social media such as blogs, Twitter, Facebook, and Weibo, evidence is lacking on the investigation of ethical considerations targeting qualitative data in different software and web-based discussion forums to provide a more comprehensive understanding of the ethical issues. To address the ethical considerations in qualitative research of different internet communities and draw the attention of researchers and publishers to ethical issues, we conducted this study to evaluate the ethical practices and ethical considerations of qualitative studies on health care by using data of internet communities. This review aims to (1) assess the rates of reporting institutional review board (IRB) approval and informed consent in studies focused on mining text in the internet community and social media, (2) compare these rates according to the year of publication, country conducting the research, website included in the study’s analysis, and journal’s guidelines about ethical approval for the type of study, and (3) describe whether the studies used anonymized/deidentified data.
Research Design
We conducted a scoping review to investigate how qualitative research mining social media data handles ethical approval, informed consent, and confidential issues. We performed this study according to the PRISMA-ScR (Preferred Reporting Items for Systematic Reviews and Meta-Analyses Extension for Scoping Reviews) guidelines. The completed PRISMA-ScR checklist is provided in Multimedia Appendix 1 .
Search Strategy
All published qualitative studies from 2010 to March 31, 2023, focusing on mining text from online community and social media sources about health care in the following databases were included in this study: PubMed, Web of Science, CINAHL, Cochrane, and Embase. A standardized search string containing Medical Subject Headings (MeSH) and non-MeSH entry terms was used in the search strategy. In addition, the reference lists of the retrieved papers and citation tracking were manually searched as a supplement to database searches to improve comprehensiveness. Gray literature was also identified through internet searches in Google Scholar and OpenGrey websites. The search strategies are represented in Multimedia Appendix 2 .
Inclusion and Exclusion Criteria
We divided the criteria into 2 parts. First, we limited the inclusion and exclusion criteria used at the title and abstract screening stage eligible for (1) studies mining existing text and posts from the internet community and social media data focusing on health care topics, (2) studies using qualitative methods or available qualitative parts in mixed methods studies to analyze data, and (3) studies only written in English. Ineligible studies were those related to investigating the use and dissemination of social media in health care, using social media or internet community as an intervention tool, and using social media to conduct web-based interviews, surveys, or focus groups. We also excluded studies published as reviews, case studies, conference abstracts, commentaries, policies, guidelines, and recommendations. Second, at the full screening stage, the specific eligible inclusion criteria were studies focused on mining text about health care topics with full-text papers. Studies that did not have the full text after contacting the authors and that were not originally in the English language were excluded.
Study Selection
All results of the searches were entered into the EndNote library, and duplicates were removed. Two researchers reviewed the titles and abstracts based on the inclusion and exclusion criteria independently. Those studies that were irrelevant to the study topic were discarded, and then the full text was screened to select eligible papers. Any disagreements were discussed and resolved by consensus or a third person.
Data Extraction
Data were extracted between April 2023 and May 2023. Two researchers independently read the full text carefully, and the results were extracted using a standardized data extraction spreadsheet, including research type, first author, study objective, sample size, publication time, country where the research was conducted or country of the first author, website or internet community the studies focus on, type of data collected from social media, language of collected posts or data, privacy level of data (public or privacy posts), study design, research results, published journal, and information about the ethical considerations. Disagreements were resolved by consensus of a third person. The information about ethical considerations was analyzed to investigate the rates of reporting ethical approval, informed consent, and privacy issues: whether IRB review was reported (IRB approval, IRB exemption, unnecessary, not mentioned) and the reason for not requiring IRB approval; whether informed consent was obtained from participants or the websites’ administrators, consent types (digitally informed consent or written informed consent, informed consent is not required, consent was waived by IRB), and the methods used to obtain consent in each study; and whether quoting a post in papers could lead to the identification of internet users in each study. The description of users’ posts (verbatim quote, paraphrase) was recorded. We also analyzed if posts were paraphrased to maintain the original meaning, if actions were taken to deidentify the internet users, and if the posts contained other identifying information (ie, usernames, photos, links, hashtags) attached to the post. As every journal would provide publication ethical considerations and requirements, we also searched the submission guidelines and editorial policies of each journal submission website to check whether the journal contained any ethical guidance targeting studies using data from internet community and social media platforms. Additional information was included about the details of ethical approval, informed consent, and privacy, for example, whether individuals can withdraw their quotes if they want to be excluded from the study at any time without any reprisal and whether the quotations were tested for deidentification via search engines. There was excellent agreement on the primary outcome between the 2 researchers (k>.95 for all).
Data Analysis
Data were analyzed using SPSS software (IBM Corp). The chi-square test or Fisher exact tests (when cell size was less than 5) were used to test for differences between the rates of informed consent and ethical approval according to publication year, website, and different countries. All P values were 2-sided, and P values <.05 indicated significance.
Study Selection for the Review
We reviewed 4674 papers after removing the duplicates. After screening the titles, abstracts, and full-texts, we reviewed 108 eligible papers ( Figure 1 ). The full list of the included papers and all the extracted information are incorporated in Multimedia Appendix 3 [ 28 - 135 ]. Of the 108 studies reviewed, 73 (67.6%) were qualitative studies and 35 (32.4%) were mixed methods studies. All papers had text mined from internet communities or social media for qualitative analysis. The sample size ranged from 32 to 392,962. Approximately 82.4% (89/108) of the studies were published after 2018, and there was a sharp increase in the number of studies from 2019 to 2022. Moreover, nearly half of the studies (55/108, 50.9%) were published in the United States. Regarding the websites for mining text, the most widely used social media platform was Twitter (42/108, 38.9%), followed by Facebook (17/108, 15.7%).

Ethics Approval in These Studies
Our results indicated that of the 108 studies, 78 (72.2%) reported ethics approval. Of the 78 studies, 31 (40%) explicitly stated that ethics approval was obtained before the study was undertaken, 33 (42%) reported that the ethics approval was granted through exemptions by the local IRB, and 14 (18%) explicitly demonstrated that approval by the ethics committee was not required because publicly available data were collected from internet communities and social media platforms. However, 30 (27.8%) of the 108 studies did not mention about obtaining IRB approval ( Table 1 ).
Based on our exploration of the ethical guidelines of each journal to determine whether there were ethical requirements for studies mining social media data, only 36.1% (39/108) of the studies were published in journals that required ethical considerations for studies gathering data from social media platforms by using internet and digital technologies. Of the 39 studies published in 19 journals, 27 (69%) were published in the Journal of Medical Internet Research and its sister journals. The submission guidelines of the Journal of Medical Internet Research state that authors of manuscripts describing studies of internet, digital tools, and technologies are required to verify that they have adhered to local, national, regional, and international laws and regulations, and are required to verify that they complied with informed consent guidelines. Moreover, 2 journals also provided a specific requirement, that is, when researchers interact with individuals or obtain privacy information gathered from social media platforms, they should obtain ethics approval prior to conducting the study and informed consent from anyone who could potentially be identified. Surprisingly, there were no significant differences in the ethics approval reportage between journals with ethics approval guidelines and those that did not have ethics guidelines for researchers gathering data from social media platforms ( P =.08). Notably, the rates of reporting ethics approval were different between different countries ( P =.02). However, there were no statistically significant differences between the rates of reporting ethical approval and different websites or publication years (all P >.05) ( Table 2 ).
Informed Consent
Of the 108 studies, 59 (54.7%) showed that they did not include any information about informed consent and 49 (45.3%) mentioned informed consent. Of the 49 studies that mentioned informed consent, 14 (13%) demonstrated that informed consent was waived by local institutional boards, and 21 (19%) reported that informed consent was not required because this information is publicly available in websites or did not involve human participants. We interpreted this as not seeking informed consent. Only 14 (12.9%) of the 108 studies explicitly indicated that informed consent was obtained ( Table 1 ). Among the 14 studies, 2 (14%) only provided a generic statement that informed consent was obtained but did not report the process of how the informed consent was obtained, while 12 (86%) received digital informed consent. Of the 12 studies that reported receiving digital informed consent, 6 reported that they sought permission from the communities’ or groups’ administrators and by posting a statement of the research objective on the group’s wall, while 5 studies contacted the participants privately via email, commenting below the posts and software to gain consent, and 1 study reported that it had sent a digital version of the informed consent book. Furthermore, among the studies that had obtained informed consent, 7 studies included the statement that the individuals’ posts would be removed if they wanted to be excluded from the study, and they could withdraw from the study whenever they wanted. In addition, the rates of reporting informed consent showed no statistical significance between publication years, different countries, and different websites (all P >.05) ( Table 2 ).
Confidentiality of the Information
All data sources were obtained from anonymous websites or communities, and the majority (104/108, 96.3%) of the data sources did not contain usernames. Notably, only 3.7% (4/108) of the studies contained the participants’ usernames or pseudonyms. One study reported that pseudonyms like Sasha had been used instead of the real name. The other 3 studies contained the expression for usernames but did not state whether pseudonyms were used. Except for 9 studies that used nonnative language quotes and 3 studies that were transcribed into text via video, among the 108 included studies, 76 (70.3%) quoted at least one native language post in their reports. Additionally, 20 studies presenting aggregated analysis or composite accounts did not include any quotation or written content. Of the 76 studies containing internet users’ written content, 52 (68%) contained just verbatim-quoted participants’ posts and 24 (32%) contained paraphrased posts ( Table 1 ). Among the 52 studies containing direct and verbatim quotations, which are likely to be traced to the original posts from users, only 17 (33%) studies took measures to deidentify the users. The 17 studies mentioned that all names or usernames were removed and personal identifying information was removed to maintain privacy, while 42% (22/52) of the studies did not mention any measures that were taken to deidentify the users and maintain confidentiality. Approximately 32% (24/76) of the studies described that they paraphrased posts and removed any explicitly identified personal information to maintain confidentiality to reduce the likelihood of users being identified via search engines. Of the 24 studies, 20 (83%) reported that the quotations were slightly modified or summarized for readability, the symbol information was removed using “…”, and key identifiable information was removed to protect privacy while maintaining the meaning of posts. Four of the 24 (17%) studies did not report the methods and details of paraphrasing. Notably, only 3% (2/76) of the studies containing users’ written content showed that researchers intentionally entered each quote into search engines to ensure that every quote did not lead to the original posts. Moreover, of the 76 studies containing written content, 62 (82%) did not contain other types of identity information attached to the posts, while 14 (18%) included other identifying data (hashtags, emojis, geolocation, photos, links, screenshots) attached to the original posts for analysis ( Table 1 ). Of the 14 studies including other identifying information, 4 (29%) contained photos and screenshots associated with the website pages. Of the 52 studies that disclosed verbatim quotes and other identifiable information, 26 (46%) studies reported informed consent consideration, and only 8 (15%) obtained explicitly informed consent. Additionally, of the 77% (40/52) of the studies that mentioned IRB or ethical review, 38% (15/40) received IRB approval, and 63% (25/40) of the studies were granted exemption. The proportion of reporting ethical approval in studies containing users’ written content was modestly higher than that in studies not containing users’ written content (60/76, 79% vs 14/32, 44%; P <.001) ( Table 2 ).
Principal Findings and Comparison to Prior Work
In this scoping review, we included 108 studies ( Multimedia Appendix 3 ; [ 28 - 135 ]) that focused on mining text from internet community and social media data for health care research, and we reviewed the ethical consideration reportage and outcome reports in these studies. We found that the rates of reporting IRB approval and informed consent in qualitative research on health care utilizing social media data were 59.3% (64/108) and 12.9% (14/108), respectively. Our findings demonstrate that the key ethical considerations for qualitative research in online communities are insufficiently discussed and described. However, the reporting rates of ethical considerations in the papers in our scoping review were much higher than those reported in systematic reviews including multiple analysis methodologies on only 1 social media platform. For example, ethics approval and informed consent were reported in 48% and 10% of research studies using only Facebook data [ 22 ], 32% and 0% from 2006 to 2019 [ 21 ], 40% and 0.9% (only 1 paper) from 2015 to 2016 in public health research using only Twitter data [ 25 ], and 26.1% and 0.8% (only 1 paper) in researches incorporating only YouTube data [ 23 ], respectively. In fact, previous studies were limited to only a few selected websites such as Twitter, Facebook, and YouTube. There is a lack of research that incorporates a variety of different social media data for comparisons. Differences in the reporting of ethical considerations may be attributed to the different methodologies adopted by studies. For example, Lathan et al [ 22 ] analyzed the ethical considerations in studies including predictive or model development, while our research focuses on the ethical considerations in qualitative studies.
Importantly, our findings indicate that there is a need to develop a standardized and apparent approach for the reporting of ethical considerations in qualitative research of data from social media and online communities. Our research demonstrates that the rates of reporting ethics approval are different in different countries ( P =.02). Specifically, a wide variety of national research ethics governing bodies and over 1000 laws, regulations, and standards provide oversight for human subjects research in 130 countries. Obviously, a guideline is needed for best ethical practices for qualitative research involving posts from social media platforms. Surprisingly, there were no significant differences between the rates of reporting ethical approval and those of journals specifying ethical requirements for studies involving text mining ( P =.08). This inconsistent result of publication guidelines and reports of ethical approval consent is similar to previous findings on the ethical standards in COVID-19 human studies [ 136 ]. Although there are journal publication guidelines for studies mining social media data, the reports of ethical approval and consent in the papers published in such journals do not exactly follow the guidelines. Consequently, this finding indicates that more ethical awareness is needed among researchers, editors, and reviewers for qualitative studies on data mining.
Besides the different legal and regulations in different countries, the inconsistency in the ethics approval in published papers may be because social media research is a highly interdisciplinary science, and computer science researchers may be less experienced or may pay less attention to the key ethical issues of protecting human subjects [ 137 ]. Medical and health science researchers may have considered some ethical concerns about gathering social media data but they may not be familiar with the relevant guidelines. For example, the Association of Internet Researchers has a detailed ethical guideline targeting social scientists conducting digital research, while it may be less popular and less well-known among medical and health care researchers. At the institute level, Ferretti et al [ 138 ] noticed that institutionalized review committees, especially the individual IRB institutes for universities and health care systems lack knowledge about the methodology, text mining technical standards, data security, and ethical harms for studies using big data and social media as sources. Because of this lack of knowledge, institutional ethics committees may have inconsistent ethical criteria and perspectives about web-based projects using social media data [ 139 ]. Therefore, some ethics review committees exclude research on internet communities from ethical oversights because their ethics standards are confined only to medical fields. Above all, it is additionally challenging for ethical approval institutions because of the continuous development and dynamic change of studies using social media data. Furthermore, it is necessary for ethics committee members to be trained about the ethical issues in studies mining text from social media. Inviting interdisciplinary researchers to join in the approval process would be an appropriate method to increase the awareness of ethical considerations [ 140 , 141 ].
Interestingly, the reporting rate of obtaining informed consent for mining social media data in qualitative studies was unexpected. The most influential ethical reports such as the Nuremberg Code [ 142 ], Declaration of Helsinki [ 143 ], and the Belmont Report [ 144 ] have demonstrated the principle of informed consent in research involving humans. Our review shows that only 12.9% (14/108) of the studies explicitly obtained informed consent and 32.4% (35/108) of the studies reported that informed consent was exempted by IRB or was not required, as the information was available publicly in websites or did not involve human participants. Our results are similar to those of Wongkoblap et al [ 145 ] who reported that only 16.7% of the studies received informed consent from participants prior to data analysis on data mining of social network data on mental health disorders.
There are multiple reasons for the challenges in obtaining informed consent in an internet setting. First, it is impractical for researchers to gain individual informed consent from a large number of users in an internet community [ 146 ]. Second, members of ethics review boards lack consensus about the need for informed consent from an internet community for qualitative research under the current legal definition [ 147 ]. Moreover, there has been a debate on the criteria of human subject research in using social media data. The federal regulation recommends that if data in the studies are obtained from public social media websites, where data are identifiable and do not require interaction with individuals, such studies do not constitute human subject research, while studies involving the identification of private information or interaction with the individual can be considered as human subject research [ 148 ]. In contrast, some researchers believe that social media and big data research are not ethically exceptional and should be treated in the same manner and with the same rules as those for traditional forms of research [ 149 ]. There is ambiguity as to what is appropriate or should be standard practice for obtaining informed consent.
Currently, it is challenging to maintain privacy and protect the traceability of individuals posting content in the internet community. Our findings indicated that 70.3% (76/108) of the studies contained internet users’ written content, of which 68% (52/76) included verbatim quotations of users’ posts that could lead to identification, and 18% (14/76) of the studies included other identifiable information such as links, screenshots, and emojis linked to original posts, which are similar to the findings of Ayers et al [ 25 ] and Lathan et al [ 22 ]. Usha Lawrance et al [ 150 ] and Wilkinson and Thelwall [ 151 ] argued that using direct quotes to support findings would lead to the identification of users and breach users’ confidentiality in internet community data. Moreover, quoting social media posts or disclosing usernames violate the International Committee of Medical Journal Editors’ ethics standards, which state that identifying information such as written descriptions and photos should not be published unless the information is essential for scientific purposes and the participants give written informed consent for publication [ 152 ]. Furthermore, our study demonstrates that the proportion of studies containing users’ written content (both direct and paraphrased quotations) is higher than that of studies that do not include any quotation or written content (60/76, 79% vs 14/32, 44%; P <.001)——a tentative explanation is that some researchers realized that ethical reportage should be stricter for qualitative papers with quotations from social media posts due to privacy and security issues. This is supported by Boyd and Crawford [ 153 ] who stated that rigorous thinking about the process of mining and anonymizing big data is required for ethics boards to ensure that people are protected. Our findings show that 32% (24/76) of the studies intentionally paraphrased the quotes to ensure that users could not locate them, and 20 studies used aggregated data interconnected with anonymity. Moreover, it is recommended by Wilkinson and Thelwall [ 151 ], Bond et al [ 154 ], and Markham et al [ 155 ] that researchers should not directly quote and work with aggregate data sets and separate texts from their original context, which is more acceptable to participants. In addition, the British Psychological Society guidelines recommend that researchers consider paraphrasing any verbatim quotes to reduce the risk of these being traced to the source [ 20 ]. Notably, 13 of the 25 papers in this study showed that they did not report the precautions taken for paraphrasing. This may be due to the lack of detailed methodology and consensus about paraphrasing quotes to reduce bias and maintaining the original meaning.
Limitations and Strengths
Our scoping review has several limitations. First, our research was limited to qualitative studies and the qualitative parts in mixed methods studies on text mining from social media, and it is unclear whether ethical considerations are critical in quantitative studies among internet communities. Second, we were restricted to studies published in English language and those with the full text available, and therefore, we could be underestimating the number of relevant papers published in other languages. Third, the rates of reporting ethical approval, informed consent, and privacy of this research relied on self-reported data. Thus, it is possible that although certain studies did not report the process of ethical considerations, such considerations may have been followed during the research. Conversely, some studies may have mentioned about the ethical considerations but may not have conducted them in practice. Hence, there is a bias because of the lack of accurate documentation that must be considered.
Social media text mining can be a useful tool for researchers to understand patient experiences of health conditions and health care. However, as illustrated by the absence of ethical discourse in publications, our analysis indicates significant gaps in the ethical considerations and governance of qualitative research of internet posts. Therefore, a complete and consistent consensus guideline of ethical considerations in qualitative research of internet posts is needed to protect users’ data. With the continued advancing development of text-mining techniques, qualitative studies mining text from social media should be more cautious while using user quotations to maintain user privacy and protect the traceability of the internet users posting content. We suggest that authors should report their results by using aggregated findings or deidentified ways like paraphrasing instead of verbatim quotations, which can prevent internet users from being identified through search engines. In addition, authors should provide more detailed information about the precautions taken for obtaining informed consent and paraphrasing to reduce the potential bias. Furthermore, journals and editors should pay more attention to the reporting standards of ethical consideration and privacy issues in qualitative research involving social media data.
Acknowledgments
This project was funded by the National Natural Science Foundation of China (72304131) and the Outstanding Youths Development Scheme of Nanfang Hospital, Southern Medical University (2023J005). The funders had no role in the study design, data collection and analysis, decision to publish, or preparation of this manuscript. We sincerely thank the funders of this study.
Data Availability
All data extracted and analyzed during this study are presented in this paper and in the multimedia appendices.
Authors' Contributions
YW was responsible for the protocol of the research and redrafted the paper critically. YZ and JF performed literature searches. YZ, JL, and WC performed study identification and screening. ZG, SD, CZ, and JT extracted and analyzed the data from the included journals. YZ and JL wrote the first draft of the paper. All authors read and approved the final manuscript.
Conflicts of Interest
None declared.
PRISMA-ScR (Preferred Reporting Items for Systematic Reviews and Meta-Analyses Extension for Scoping Reviews) checklist.
Search strategy for each database.
Summary of included literature.
- Obar JA, Wildman S. Social media definition and the governance challenge: An introduction to the special issue. Telecommunications Policy. Oct 2015;39(9):745-750. [ CrossRef ]
- Kaplan AM, Haenlein M. Users of the world, unite! The challenges and opportunities of social media. Business Horizons. Jan 2010;53(1):59-68. [ CrossRef ]
- VanDam C, Kanthawala S, Pratt W, Chai J, Huh J. Detecting clinically related content in online patient posts. J Biomed Inform. Nov 2017;75:96-106. [ FREE Full text ] [ CrossRef ] [ Medline ]
- Health care. Wikipedia. URL: https://en.wikipedia.org/wiki/Health_care [accessed 2023-06-01]
- Moorhead SA, Hazlett DE, Harrison L, Carroll JK, Irwin A, Hoving C. A new dimension of health care: systematic review of the uses, benefits, and limitations of social media for health communication. J Med Internet Res. Apr 23, 2013;15(4):e85. [ FREE Full text ] [ CrossRef ] [ Medline ]
- Sinnenberg L, Buttenheim AM, Padrez K, Mancheno C, Ungar L, Merchant RM. Twitter as a tool for health research: A systematic review. Am J Public Health. Jan 2017;107(1):e1-e8. [ CrossRef ] [ Medline ]
- Braun V, Clarke V. Using thematic analysis in psychology. Qualitative Research in Psychology. Jan 2006;3(2):77-101. [ CrossRef ]
- Hswen Y, Naslund JA, Brownstein JS, Hawkins JB. Monitoring online discussions about suicide among Twitter users with schizophrenia: exploratory study. JMIR Ment Health. Dec 13, 2018;5(4):e11483. [ FREE Full text ] [ CrossRef ] [ Medline ]
- Golder S, Ahmed S, Norman G, Booth A. Attitudes toward the ethics of research using social media: a systematic review. J Med Internet Res. Jun 06, 2017;19(6):e195. [ FREE Full text ] [ CrossRef ] [ Medline ]
- Athanasopoulou C, Sakellari E. Facebook and health information: content analysis of groups related to schizophrenia. Stud Health Technol Inform. 2015;213:255-258. [ Medline ]
- Conway M, O'Connor D. Social media, big data, and mental health: current advances and ethical implications. Curr Opin Psychol. Jun 2016;9:77-82. [ FREE Full text ] [ CrossRef ] [ Medline ]
- Eysenbach G, Till JE. Ethical issues in qualitative research on internet communities. BMJ. Nov 10, 2001;323(7321):1103-1105. [ FREE Full text ] [ CrossRef ] [ Medline ]
- Heilferty CM. Ethical considerations in the study of online illness narratives: a qualitative review. J Adv Nurs. May 2011;67(5):945-953. [ CrossRef ] [ Medline ]
- Roberts LD. Ethical issues in conducting qualitative research in online communities. Qualitative Research in Psychology. Apr 20, 2015;12(3):314-325. [ CrossRef ]
- Franzke A, Bechmann A, Zimmer M, Ess C, the Association of Internet Researchers. Internet research: ethical guidelines 3.0. Association of Internet Researchers. 2020. URL: https://aoir.org/reports/ethics3.pdf [accessed 2023-05-30]
- Markham A, Buchanan E. Ethical decision-making and internet research: recommendations from the AoIR ethics working committee (version 2.0). AoIR. 2012. URL: https://aoir.org/reports/ethics2.pdf [accessed 2023-06-05]
- The NCFREITSSH(. Ethical guidelines for internet research. 2022. URL: https://www.forskningsetikk.no/en/guidelines/social-sciences-humanities-law-and-theology/a-guide-to-internet-research-ethics [accessed 2023-06-13]
- Chapter 3: Rights of the data subject. General Data Protection Regulation (GDPR). European Commission. Official Journal of the European Union; 2018. URL: https://gdpr-info.eu/chapter-3/ [accessed 2023-11-29]
- Hand DJ. Aspects of data ethics in a changing world: where are we now? Big Data. Sep 01, 2018;6(3):176-190. [ FREE Full text ] [ CrossRef ] [ Medline ]
- British Psychological Society. Ethics guidelines for internet-mediated research. 2021. URL: https://explore.bps.org.uk/binary/bpsworks/64374754e0c1dd30/5a757d7c2d39a9837f0eedec1b9ba28fa5e9e38f0bffa9950b678ab727803959/rep155_2021.pdf [accessed 2023-05-30]
- Takats C, Kwan A, Wormer R, Goldman D, Jones HE, Romero D. Ethical and methodological considerations of Twitter data for public health research: systematic review. J Med Internet Res. Nov 29, 2022;24(11):e40380. [ FREE Full text ] [ CrossRef ] [ Medline ]
- Lathan HS, Kwan A, Takats C, Tanner JP, Wormer R, Romero D, et al. Ethical considerations and methodological uses of Facebook data in public health research: A systematic review. Soc Sci Med. Apr 2023;322:115807. [ FREE Full text ] [ CrossRef ] [ Medline ]
- Tanner J, Takats C, Lathan H, Kwan A, Wormer R, Romero D, et al. Approaches to research ethics in health research on YouTube: systematic review. J Med Internet Res. Oct 04, 2023;25:e43060. [ FREE Full text ] [ CrossRef ] [ Medline ]
- Stockdale J, Cassell J, Ford E. "Giving something back": A systematic review and ethical enquiry into public views on the use of patient data for research in the United Kingdom and the Republic of Ireland. Wellcome Open Res. 2018;3:6. [ FREE Full text ] [ CrossRef ] [ Medline ]
- Ayers JW, Caputi TL, Nebeker C, Dredze M. Don't quote me: reverse identification of research participants in social media studies. NPJ Digit Med. 2018;1:30. [ FREE Full text ] [ CrossRef ] [ Medline ]
- Aitken M, de St Jorre J, Pagliari C, Jepson R, Cunningham-Burley S. Public responses to the sharing and linkage of health data for research purposes: a systematic review and thematic synthesis of qualitative studies. BMC Med Ethics. Nov 10, 2016;17(1):73. [ FREE Full text ] [ CrossRef ] [ Medline ]
- Hunter RF, Gough A, O’Kane N, McKeown G, Fitzpatrick A, Walker T, et al. Ethical issues in social media research for public health. Am J Public Health. Mar 2018;108(3):343-348. [ CrossRef ]
- Ahmed OH, Sullivan SJ, Schneiders AG, McCrory P. iSupport: do social networking sites have a role to play in concussion awareness? Disabil Rehabil. 2010;32(22):1877-1883. [ CrossRef ] [ Medline ]
- Bender JL, Jimenez-Marroquin M, Jadad AR. Seeking support on Facebook: a content analysis of breast cancer groups. J Med Internet Res. Feb 04, 2011;13(1):e16. [ FREE Full text ] [ CrossRef ] [ Medline ]
- Gajaria A, Yeung E, Goodale T, Charach A. Beliefs about attention-deficit/hyperactivity disorder and response to stereotypes: youth postings in Facebook groups. J Adolesc Health. Jul 2011;49(1):15-20. [ CrossRef ] [ Medline ]
- Degroot JM. Maintaining relational continuity with the deceased on Facebook. Omega (Westport). Nov 01, 2012;65(3):195-212. [ CrossRef ]
- Donelle L, Booth RG. Health tweets: an exploration of health promotion on twitter. Online J Issues Nurs. Sep 30, 2012;17(3):4. [ FREE Full text ] [ Medline ]
- Rui JR, Chen Y, Damiano A. Health organizations providing and seeking social support: a Twitter-based content analysis. Cyberpsychol Behav Soc Netw. Sep 2013;16(9):669-673. [ CrossRef ] [ Medline ]
- Lyles CR, López A, Pasick R, Sarkar U. "5 mins of uncomfyness is better than dealing with cancer 4 a lifetime": an exploratory qualitative analysis of cervical and breast cancer screening dialogue on Twitter. J Cancer Educ. Mar 2013;28(1):127-133. [ CrossRef ] [ Medline ]
- Lee JL, DeCamp M, Dredze M, Chisolm MS, Berger ZD. What are health-related users tweeting? A qualitative content analysis of health-related users and their messages on twitter. J Med Internet Res. Oct 15, 2014;16(10):e237. [ FREE Full text ] [ CrossRef ] [ Medline ]
- Ahlwardt K, Heaivilin N, Gibbs J, Page J, Gerbert B, Tsoh JY. Tweeting about pain: comparing self-reported toothache experiences with those of backaches, earaches and headaches. J Am Dent Assoc. Jul 2014;145(7):737-743. [ FREE Full text ] [ CrossRef ] [ Medline ]
- Struik LL, Baskerville NB. The role of Facebook in Crush the Crave, a mobile- and social media-based smoking cessation intervention: qualitative framework analysis of posts. J Med Internet Res. Jul 11, 2014;16(7):e170. [ FREE Full text ] [ CrossRef ] [ Medline ]
- Abdel-Razig S, Anglade P, Ibrahim H. Impact of the COVID-19 pandemic on a physician group's WhatsApp chat: qualitative content analysis. JMIR Form Res. Dec 07, 2021;5(12):e31791. [ FREE Full text ] [ CrossRef ] [ Medline ]
- Reddy A. Skincare in social media: analyzing prominent themes in online dermatologic discussions. Cureus. May 7, 2021:e14890. [ CrossRef ] [ Medline ]
- van der Pijl MSG, Hollander MH, van der Linden T, Verweij R, Holten L, Kingma E, et al. Left powerless: A qualitative social media content analysis of the Dutch #breakthesilence campaign on negative and traumatic experiences of labour and birth. PLoS One. 2020;15(5):e0233114. [ FREE Full text ] [ CrossRef ] [ Medline ]
- Rath L, Vijiaratnam N, Skibina O. Alemtuzumab in multiple sclerosis: lessons from social media in enhancing patient care. Int J MS Care. 2017;19(6):323-328. [ CrossRef ] [ Medline ]
- Bloom R, Beck S, Chou W, Reblin M, Ellington L. In their own words: experiences of caregivers of adults with cancer as expressed on social media. ONF. Sep 1, 2019;46(5):617-630. [ CrossRef ]
- Grumme V, Gordon S. Social media use by transplant recipients for support and healing. Comput Inform Nurs. Dec 2016;34(12):570-577. [ CrossRef ] [ Medline ]
- Haug NA, Bielenberg J, Linder SH, Lembke A. Assessment of provider attitudes toward #naloxone on Twitter. Subst Abus. 2016;37(1):35-41. [ CrossRef ] [ Medline ]
- Oren E, Martinez L, Hensley RE, Jain P, Ahmed T, Purnajo I, et al. Twitter communication during an outbreak of hepatitis A in San Diego, 2016–2018. Am J Public Health. Oct 2020;110(S3):S348-S355. [ CrossRef ]
- Parker C, Zomer E, Liew D, Ayton D. Characterising experiences with acute myeloid leukaemia using an Instagram content analysis. PLoS One. 2021;16(5):e0250641. [ FREE Full text ] [ CrossRef ] [ Medline ]
- Kurko T, Linden K, Kolstela M, Pietilä K, Airaksinen M. Is nicotine replacement therapy overvalued in smoking cessation? Analysis of smokers' and quitters' communication in social media. Health Expect. Dec 2015;18(6):2962-2977. [ FREE Full text ] [ CrossRef ] [ Medline ]
- Reuter K, Lee D. Perspectives toward seeking treatment among patients with psoriasis: protocol for a Twitter content analysis. JMIR Res Protoc. Feb 18, 2021;10(2):e13731. [ FREE Full text ] [ CrossRef ] [ Medline ]
- Mollema L, Harmsen IA, Broekhuizen E, Clijnk R, De Melker H, Paulussen T, et al. Disease detection or public opinion reflection? Content analysis of tweets, other social media, and online newspapers during the measles outbreak in The Netherlands in 2013. J Med Internet Res. May 26, 2015;17(5):e128. [ FREE Full text ] [ CrossRef ] [ Medline ]
- O'Hagan ET, Traeger AC, Bunzli S, Leake HB, Schabrun SM, Wand BM, et al. What do people post on social media relative to low back pain? A content analysis of Australian data. Musculoskelet Sci Pract. Aug 2021;54:102402. [ CrossRef ] [ Medline ]
- Zhou F, Zhang W, Cai H, Cao Y. Portrayals of 2v, 4v and 9vHPV vaccines on Chinese social media: a content analysis of hot posts on Sina Weibo. Hum Vaccin Immunother. Nov 02, 2021;17(11):4433-4441. [ FREE Full text ] [ CrossRef ] [ Medline ]
- Golder S, Bach M, O'Connor K, Gross R, Hennessy S, Gonzalez Hernandez G. Public perspectives on anti-diabetic drugs: exploratory analysis of Twitter posts. JMIR Diabetes. Jan 26, 2021;6(1):e24681. [ FREE Full text ] [ CrossRef ] [ Medline ]
- Mercier R, Senter K, Webster R, Henderson RA. Instagram users? experiences of miscarriage. Obstet Gynecol 2020 Jan;? Jan 2020;135(1):166-173. [ CrossRef ]
- Alghamdi A, Abumelha K, Allarakia J, Al-Shehri A. Conversations and misconceptions about chemotherapy in Arabic tweets: content analysis. J Med Internet Res. Jul 29, 2020;22(7):e13979. [ FREE Full text ] [ CrossRef ] [ Medline ]
- Golder S, O'Connor K, Hennessy S, Gross R, Gonzalez-Hernandez G. Assessment of beliefs and attitudes about statins posted on Twitter: a qualitative study. JAMA Netw Open. Jun 01, 2020;3(6):e208953. [ FREE Full text ] [ CrossRef ] [ Medline ]
- Stekelenburg N, Horsham C, O'Hara M, Janda M. Using social media to determine the affective and cognitive components of tweets about sunburn. Dermatology. Feb 27, 2020;236(2):75-80. [ FREE Full text ] [ CrossRef ] [ Medline ]
- Meeking K. Patients' experiences of radiotherapy: Insights from Twitter. Radiography (Lond). Aug 2020;26(3):e146-e151. [ CrossRef ] [ Medline ]
- Shah S, Bradbury-Jones C, Taylor J. Using Facebook to tell stories of premature ageing and sexual and reproductive healthcare across the life course for women with cerebral palsy in the UK and USA. BMJ Open. Feb 17, 2020;10(2):e032172. [ FREE Full text ] [ CrossRef ] [ Medline ]
- Pretorius K, Choi E, Kang S, Mackert M. Sudden infant death syndrome on Facebook: qualitative descriptive content analysis to guide prevention efforts. J Med Internet Res. Jul 30, 2020;22(7):e18474. [ FREE Full text ] [ CrossRef ] [ Medline ]
- Karmegam D, Mapillairaju B. What people share about the COVID-19 outbreak on Twitter? An exploratory analysis. BMJ Health Care Inform. Nov 2020;27(3):e100133. [ FREE Full text ] [ CrossRef ] [ Medline ]
- Hairston TK, Links AR, Harris V, Tunkel DE, Walsh J, Beach MC, et al. Evaluation of parental perspectives and concerns about pediatric tonsillectomy in social media. JAMA Otolaryngol Head Neck Surg. Jan 01, 2019;145(1):45-52. [ FREE Full text ] [ CrossRef ] [ Medline ]
- Årsand E, Bradway M, Gabarron E. What are diabetes patients versus health care personnel discussing on social media? J Diabetes Sci Technol. Mar 2019;13(2):198-205. [ FREE Full text ] [ CrossRef ] [ Medline ]
- Jiang X, Jiang W, Cai J, Su Q, Zhou Z, He L, et al. Characterizing media content and effects of organ donation on a social media platform: content analysis. J Med Internet Res. Mar 12, 2019;21(3):e13058. [ FREE Full text ] [ CrossRef ] [ Medline ]
- Oser TK, Minnehan KA, Wong G, Parascando J, McGinley E, Radico J, et al. Using social media to broaden understanding of the barriers and facilitators to exercise in adults with type 1 diabetes. J Diabetes Sci Technol. May 2019;13(3):457-465. [ FREE Full text ] [ CrossRef ] [ Medline ]
- Sutton J, Vos SC, Olson MK, Woods C, Cohen E, Gibson CB, et al. Lung cancer messages on Twitter: content analysis and evaluation. J Am Coll Radiol. Jan 2018;15(1 Pt B):210-217. [ CrossRef ] [ Medline ]
- Thomas J, Prabhu AV, Heron DE, Beriwal S. Twitter and brachytherapy: An analysis of "tweets" over six years by patients and health care professionals. Brachytherapy. 2018;17(6):1004-1010. [ CrossRef ] [ Medline ]
- Kelly-Hedrick M, Grunberg PH, Brochu F, Zelkowitz P. "It's totally okay to be sad, but never lose hope": content analysis of infertility-related videos on YouTube in relation to viewer preferences. J Med Internet Res. May 23, 2018;20(5):e10199. [ FREE Full text ] [ CrossRef ] [ Medline ]
- Gage-Bouchard EA, LaValley S, Mollica M, Beaupin LK. Cancer communication on social media: examining how cancer caregivers use Facebook for cancer-related communication. Cancer Nurs. 2017;40(4):332-338. [ CrossRef ] [ Medline ]
- Rael CT, Pierre D, Frye V, Kessler D, Duffy L, Malos N, et al. Evaluating blood donor experiences and barriers/facilitators to blood donation in the United States using YouTube video content. Transfusion. Sep 2021;61(9):2650-2657. [ FREE Full text ] [ CrossRef ] [ Medline ]
- Fisher S, Jehassi A, Ziv M. Hidradenitis suppurativa on Facebook: thematic and content analyses of patient support group. Arch Dermatol Res. Aug 2020;312(6):421-426. [ CrossRef ] [ Medline ]
- Abdoli S, Hessler D, Vora A, Smither B, Stuckey H. Descriptions of diabetes burnout from individuals with Type 1 diabetes: an analysis of YouTube videos. Diabet Med. Aug 2020;37(8):1344-1351. [ CrossRef ] [ Medline ]
- Myneni S, Lewis B, Singh T, Paiva K, Kim SM, Cebula AV, et al. Diabetes self-management in the age of social media: large-scale analysis of peer interactions using semiautomated methods. JMIR Med Inform. Jun 30, 2020;8(6):e18441. [ FREE Full text ] [ CrossRef ] [ Medline ]
- Charlie AM, Gao Y, Heller SL. What do patients want to know? questions and concerns regarding mammography expressed through social media. J Am Coll Radiol. Oct 2018;15(10):1478-1486. [ CrossRef ] [ Medline ]
- Watts G, Christou P, Antonarakis G. Experiences of individuals concerning combined orthodontic and orthognathic surgical treatment: a qualitative Twitter analysis. Med Princ Pract. 2018;27(3):227-235. [ FREE Full text ] [ CrossRef ] [ Medline ]
- Pai RR, Alathur S. Assessing mobile health applications with Twitter analytics. Int J Med Inform. May 2018;113:72-84. [ CrossRef ] [ Medline ]
- Cheng TY, Liu L, Woo BK. Analyzing Twitter as a platform for Alzheimer-related dementia awareness: thematic analyses of tweets. JMIR Aging. Dec 10, 2018;1(2):e11542. [ FREE Full text ] [ CrossRef ] [ Medline ]
- Bridges N, Howell G, Schmied V. Exploring breastfeeding support on social media. Int Breastfeed J. 2018;13:22. [ FREE Full text ] [ CrossRef ] [ Medline ]
- Anderson JG, Hundt E, Dean M, Keim-Malpass J, Lopez RP. "The Church of Online Support": examining the use of blogs among family caregivers of persons with dementia. J Fam Nurs. Feb 2017;23(1):34-54. [ CrossRef ] [ Medline ]
- Kearney MD, Selvan P, Hauer MK, Leader AE, Massey PM. Characterizing HPV vaccine sentiments and content on Instagram. Health Educ Behav. Dec 2019;46(2_suppl):37-48. [ CrossRef ] [ Medline ]
- Davies SH, Langer MD, Klein A, Gonzalez-Hernandez G, Dowshen N. Adolescent perceptions of menstruation on Twitter: opportunities for advocacy and education. J Adolesc Health. Jul 2022;71(1):94-104. [ CrossRef ] [ Medline ]
- Van Diepen C, Rosales Valdes D. A content analysis on the perceptions of LGBTQ+ (centred) health care on Twitter. Health Expect. Dec 2022;25(6):3238-3245. [ FREE Full text ] [ CrossRef ] [ Medline ]
- Pleasure ZH, Frohwirth LF, Li N, Polis CB. A content analysis of Reddit users' posts about challenges to contraceptive care-seeking during COVID-19-related restrictions in the United States. J Health Commun. Oct 03, 2022;27(10):746-754. [ CrossRef ] [ Medline ]
- Du Y, Dennis B, Ramirez V, Li C, Wang J, Meireles CL. Experiences and disease self-management in individuals living with chronic kidney disease: qualitative analysis of the National Kidney Foundation's online community. BMC Nephrol. Mar 04, 2022;23(1):88. [ FREE Full text ] [ CrossRef ] [ Medline ]
- Sadek Attalla S, Ow NL, McNarry M, De Simoni A. Experiences of exercise in patients with asthma: a qualitative analysis of discussions in a UK asthma online community. BJGP Open. Apr 29, 2022;6(3):BJGPO.2021.0162. [ CrossRef ]
- Bartmess M, Talbot C, O'Dwyer ST, Lopez RP, Rose KM, Anderson JG. Using Twitter to understand perspectives and experiences of dementia and caregiving at the beginning of the COVID-19 pandemic. Dementia (London). Jul 2022;21(5):1734-1752. [ FREE Full text ] [ CrossRef ] [ Medline ]
- Brewer G, Centifanti L, Caicedo JC, Huxley G, Peddie C, Stratton K, et al. Experiences of mental distress during COVID-19: thematic analysis of discussion forum posts for anxiety, depression, and obsessive-compulsive disorder. Illn Crises Loss. Oct 2022;30(4):795-811. [ FREE Full text ] [ CrossRef ] [ Medline ]
- Castillo LIR, Hadjistavropoulos T, Beahm J. Social media discussions about long-term care and the COVID-19 pandemic. J Aging Stud. Dec 2022;63:101076. [ FREE Full text ] [ CrossRef ] [ Medline ]
- Colaceci S, Anderson G, Ricciuto V, Montinaro D, Alazraki G, Mena-Tudela D. Experiences of birth during COVID-19 pandemic in Italy and Spain: A thematic analysis. Int J Environ Res Public Health. Jun 18, 2022;19(12):7488. [ FREE Full text ] [ CrossRef ] [ Medline ]
- Tripathi SD, Parker PD, Prabhu AV, Thomas K, Rodriguez A. An examination of patients and caregivers on Reddit Navigating Brain Cancer: content analysis of the brain tumor Subreddit. JMIR Cancer. Jun 22, 2022;8(2):e35324. [ FREE Full text ] [ CrossRef ] [ Medline ]
- Jina-Pettersen N. Fear, neglect, coercion, and dehumanization: is inpatient psychiatric trauma contributing to a public health crisis? J Patient Exp. 2022;9:23743735221079138. [ FREE Full text ] [ CrossRef ] [ Medline ]
- Rossi NA, Devarajan K, Chokshi SN, Ochoa VJ, Benavidez M, Malaya LT, et al. Social media depictions of cochlear implants: An Instagram and TikTok analysis. Otol Neurotol. 2023;44(1):e13-e21. [ CrossRef ]
- Singh GK, Rego J, Chambers S, Fox J. Health professionals' perspectives of the role of palliative care during COVID-19: content analysis of articles and blogs posted on Twitter. Am J Hosp Palliat Care. Apr 2022;39(4):487-493. [ FREE Full text ] [ CrossRef ] [ Medline ]
- Koly KN, Tasnim Z, Ahmed S, Saba J, Mahmood R, Farin FT, et al. Mental healthcare-seeking behavior of women in Bangladesh: content analysis of a social media platform. BMC Psychiatry. Dec 19, 2022;22(1):797. [ FREE Full text ] [ CrossRef ] [ Medline ]
- Belcher R, Sim D, Meykler M, Owens-Walton J, Hassan N, Rubin R, et al. A qualitative analysis of female Reddit users' experiences with low libido: how do women perceive their changes in sexual desire? J Sex Med. Feb 27, 2023;20(3):287-297. [ CrossRef ] [ Medline ]
- Culp F, Wu Y, Wu D, Ren Y, Raynor P, Hung P, et al. Understanding alcohol use discourse and stigma patterns in perinatal care on Twitter. Healthcare (Basel). Nov 26, 2022;10(12):2375. [ FREE Full text ] [ CrossRef ] [ Medline ]
- Ferrey A, Ashworth G, Cabling M, Rundblad G, Ismail K. A thematic analysis of YouTube comments on a television documentary titled 'Diabulimia: The world's most dangerous eating disorder'. Diabet Med. May 2023;40(5):e15025. [ CrossRef ] [ Medline ]
- Watt S, Salway T, Gómez-Ramírez O, Ablona A, Barton L, Chang H, et al. Rumination, risk, and response: a qualitative analysis of sexual health anxiety among online sexual health chat service users. Sex. Health. May 23, 2022;19(3):182-191. [ CrossRef ]
- Naganathan G, Bilgen I, Cleland J, Reel E, Cil T. #COVID19 and #Breastcancer: a qualitative analysis of tweets. Curr Oncol. Nov 08, 2022;29(11):8483-8500. [ FREE Full text ] [ CrossRef ] [ Medline ]
- Potgieter I, Hoare DJ, Fackrell K. Hyperacusis in children: a thematic analysis of discussions in online forums. Am J Audiol. Mar 03, 2022;31(1):166-174. [ CrossRef ]
- Manning Hutson M, Hosking SM, Mantalvanos S, Berk M, Pasco J, Dunning T. What injured workers with complex claims look for in online communities: netnographic analysis. J Med Internet Res. Apr 07, 2022;24(4):e17180. [ FREE Full text ] [ CrossRef ] [ Medline ]
- Lawless MT, Hunter SC, Pinero de Plaza MA, Archibald MM, Kitson AL. "You Are By No Means Alone": a netnographic study of self-care support in an online community for older adults. Qual Health Res. Nov 2022;32(13):1935-1951. [ CrossRef ] [ Medline ]
- Keim-Malpass J, Mitchell EM, Sun E, Kennedy C. Using Twitter to understand public perceptions regarding the #HPV vaccine: opportunities for public health nurses to engage in social marketing. Public Health Nurs. Jul 2017;34(4):316-323. [ CrossRef ] [ Medline ]
- Yamada R, Rasmussen KM, Felice JP. "What is 'enough,' and how do I make it?": a qualitative examination of questions mothers ask on social media about pumping and providing an adequate amount of milk for their infants. Breastfeed Med. 2019;14(1):17-21. [ FREE Full text ] [ CrossRef ] [ Medline ]
- Osadchiy V, Mills JN, Eleswarapu SV. Understanding patient anxieties in the social media era: qualitative analysis and natural language processing of an online male infertility community. J Med Internet Res. Mar 10, 2020;22(3):e16728. [ FREE Full text ] [ CrossRef ] [ Medline ]
- Thomas TH, Nauth-Shelley K, Thompson MA, Attai DJ, Katz MS, Graham D, et al. The needs of women treated for ovarian cancer: results from a #gyncsm Twitter chat. J Patient Cent Res Rev. 2018;5(2):149-157. [ FREE Full text ] [ CrossRef ] [ Medline ]
- Mehta N, Zhu L, Lam K, Stall NM, Savage R, Read SH, et al. Health forums and Twitter for dementia research: opportunities and considerations. J Am Geriatr Soc. Dec 2020;68(12):2881-2889. [ CrossRef ] [ Medline ]
- Gupta R, Ariefdjohan M. Mental illness on Instagram: a mixed method study to characterize public content, sentiments, and trends of antidepressant use. J Ment Health. Aug 2021;30(4):518-525. [ CrossRef ] [ Medline ]
- He L, He C, Reynolds T, Bai Q, Huang Y, Li C, et al. Why do people oppose mask wearing? A comprehensive analysis of U.S. tweets during the COVID-19 pandemic. J Am Med Inform Assoc. Jul 14, 2021;28(7):1564-1573. [ FREE Full text ] [ CrossRef ] [ Medline ]
- Loeb S, Mihalcea R, Perez-Rosas V, Xu A, Taylor J, Byrne N, et al. Leveraging social media as a thermometer to gauge patient and caregiver concerns: COVID-19 and prostate cancer. Eur Urol Open Sci. Mar 2021;25:1-4. [ FREE Full text ] [ CrossRef ] [ Medline ]
- Graf I, Gerwing H, Hoefer K, Ehlebracht D, Christ H, Braumann B. Social media and orthodontics: A mixed-methods analysis of orthodontic-related posts on Twitter and Instagram. Am J Orthod Dentofacial Orthop. Aug 2020;158(2):221-228. [ CrossRef ] [ Medline ]
- Boon-Itt S, Skunkan Y. Public perception of the COVID-19 pandemic on Twitter: sentiment analysis and topic modeling study. JMIR Public Health Surveill. Nov 11, 2020;6(4):e21978. [ FREE Full text ] [ CrossRef ] [ Medline ]
- Wang J, Zhou Y, Zhang W, Evans R, Zhu C. Concerns expressed by Chinese social media users during the COVID-19 pandemic: content analysis of Sina Weibo microblogging data. J Med Internet Res. Nov 26, 2020;22(11):e22152. [ FREE Full text ] [ CrossRef ] [ Medline ]
- Wahbeh A, Nasralah T, Al-Ramahi M, El-Gayar O. Mining physicians' opinions on social media to obtain insights into COVID-19: mixed methods analysis. JMIR Public Health Surveill. Jun 18, 2020;6(2):e19276. [ FREE Full text ] [ CrossRef ] [ Medline ]
- Çınar S, Boztepe H, Prof. The use of social media among parents of infants with cleft lip and/or palate. J Pediatr Nurs. 2020;54:e91-e96. [ CrossRef ] [ Medline ]
- Jiang T, Osadchiy V, Mills JN, Eleswarapu SV. Is It all in my head? self-reported psychogenic erectile dysfunction and depression are common among young men seeking advice on social media. Urology. Aug 2020;142:133-140. [ CrossRef ] [ Medline ]
- Dzubur E, Khalil C, Almario CV, Noah B, Minhas D, Ishimori M, et al. Patient concerns and perceptions regarding biologic therapies in ankylosing spondylitis: insights from a large-scale survey of social media platforms. Arthritis Care Res (Hoboken). Feb 2019;71(2):323-330. [ FREE Full text ] [ CrossRef ] [ Medline ]
- Gonzalez G, Vaculik K, Khalil C, Zektser Y, Arnold C, Almario CV, et al. Women's experience with stress urinary incontinence: insights from social media analytics. Journal of Urology. May 2020;203(5):962-968. [ CrossRef ]
- Jha SR, McDonagh J, Prichard R, Newton PJ, Hickman LD, Fung E, et al. #Frailty: A snapshot Twitter report on frailty knowledge translation. Australas J Ageing. Dec 2018;37(4):309-312. [ CrossRef ] [ Medline ]
- Litchman ML, Snider C, Edelman LS, Wawrzynski SE, Gee PM. Diabetes online community user perceptions of successful aging with diabetes: analysis of a #DSMA Tweet chat. JMIR Aging. Jun 22, 2018;1(1):e10176. [ FREE Full text ] [ CrossRef ] [ Medline ]
- Jones J, Pradhan M, Hosseini M, Kulanthaivel A, Hosseini M. Novel approach to cluster patient-generated data into actionable topics: case study of a web-based breast cancer forum. JMIR Med Inform. Nov 29, 2018;6(4):e45. [ FREE Full text ] [ CrossRef ] [ Medline ]
- Gonzalez G, Vaculik K, Khalil C, Zektser Y, Arnold C, Almario CV, et al. Using large-scale social media analytics to understand patient perspectives about urinary tract infections: thematic analysis. J Med Internet Res. Jan 25, 2022;24(1):e26781. [ FREE Full text ] [ CrossRef ] [ Medline ]
- Sormunen T, Westerbotn M, Aanesen A, Fossum B, Karlgren K. Social media in the infertile community-using a text analysis tool to identify the topics of discussion on the multitude of infertility blogs. Womens Health (Lond). 2021;17:17455065211063280. [ FREE Full text ] [ CrossRef ] [ Medline ]
- Berkovic D, Ackerman IN, Briggs AM, Ayton D. Tweets by people with arthritis during the COVID-19 pandemic: content and sentiment analysis. J Med Internet Res. Dec 03, 2020;22(12):e24550. [ FREE Full text ] [ CrossRef ] [ Medline ]
- Della Rosa S, Sen F. Health topics on Facebook Groups: content analysis of posts in multiple sclerosis communities. Interact J Med Res. Feb 11, 2019;8(1):e10146. [ FREE Full text ] [ CrossRef ] [ Medline ]
- Awofeso N, Imam SA, Ahmed A. Content analysis of media coverage of childhood obesity topics in UAE newspapers and popular social media platforms, 2014-2017. Int J Health Policy Manag. Feb 01, 2019;8(2):81-89. [ FREE Full text ] [ CrossRef ] [ Medline ]
- Smith DJ, Mac VV, Hertzberg VS. Using Twitter for nursing research: a tweet analysis on heat illness and health. J Nurs Scholarsh. May 2021;53(3):343-350. [ CrossRef ] [ Medline ]
- Green BM, Van Horn KT, Gupte K, Evans M, Hayes S, Bhowmick A. Assessment of adaptive engagement and support model for people with chronic health conditions in online health communities: combined content analysis. J Med Internet Res. Jul 07, 2020;22(7):e17338. [ FREE Full text ] [ CrossRef ] [ Medline ]
- Osakwe ZT, Cortés YI. Impact of COVID-19: a text mining analysis of Twitter data in Spanish language. Hisp Health Care Int. Dec 2021;19(4):239-245. [ CrossRef ] [ Medline ]
- Sümeyye Yorulmaz D, Karadeniz H. Vaccination refusal debate on social media in Turkey: a content analysis of the comments on Instagram blogs. Iran J Public Health. Mar 2022;51(3):615-623. [ FREE Full text ] [ CrossRef ] [ Medline ]
- Choi E, Becker H, Kim S. A blog text analysis to explore psychosocial support in adolescents and young adults with cancer. Cancer Nurs. Dec 11, 2022;46(2):143-151. [ CrossRef ]
- Jun J, Wickersham K, Zain A, Ford R, Zhang N, Ciccarelli C, et al. Cancer and COVID-19 vaccines on Twitter: the voice and vaccine attitude of cancer community. J Health Commun. Jan 02, 2023;28(1):1-14. [ CrossRef ] [ Medline ]
- Yashpal S, Raghunath A, Gencerliler N, Burns LE. Exploring public perceptions of dental care affordability in the United States: mixed method analysis via Twitter. JMIR Form Res. Jul 01, 2022;6(7):e36315. [ FREE Full text ] [ CrossRef ] [ Medline ]
- Damier P, Henderson EJ, Romero-Imbroda J, Galimam L, Kronfeld N, Warnecke T. Impact of off-time on quality of life in Parkinson's patients and their caregivers: insights from social media. Parkinsons Dis. 2022;2022:1800567. [ FREE Full text ] [ CrossRef ] [ Medline ]
- Miller WR, Malloy C, Mravec M, Sposato MF, Groves D. Nursing in the spotlight: Talk about nurses and the nursing profession on Twitter during the early COVID-19 pandemic. Nurs Outlook. 2022;70(4):580-589. [ FREE Full text ] [ CrossRef ] [ Medline ]
- Hriberšek M, Eibensteiner F, Kapral L, Teufel A, Nawaz FA, Cenanovic M, et al. "Loved ones are not 'visitors' in a patient's life"-The importance of including loved ones in the patient's hospital stay: An international Twitter study of #HospitalsTalkToLovedOnes in times of COVID-19. Front Public Health. 2023;11:1100280. [ FREE Full text ] [ CrossRef ] [ Medline ]
- O'Sullivan L, Killeen RP, Doran P, Crowley RK. Adherence with reporting of ethical standards in COVID-19 human studies: a rapid review. BMC Med Ethics. Jun 28, 2021;22(1):80. [ FREE Full text ] [ CrossRef ] [ Medline ]
- Denecke K, Bamidis P, Bond C, Gabarron E, Househ M, Lau AYS, et al. Ethical issues of social media usage in healthcare. Yearb Med Inform. Aug 13, 2015;10(1):137-147. [ FREE Full text ] [ CrossRef ] [ Medline ]
- Ferretti A, Ienca M, Sheehan M, Blasimme A, Dove ES, Farsides B, et al. Ethics review of big data research: What should stay and what should be reformed? BMC Med Ethics. Apr 30, 2021;22(1):51. [ FREE Full text ] [ CrossRef ] [ Medline ]
- Kohn T, Shore C. The Ethics of University Ethics Committees: risk management and the research imagination. Death Public Univ Uncertain Futur High Educ Knowl Econ. May 2017:229-249. [ CrossRef ]
- Friesen P, Redman B, Caplan A. Of straws, camels, research regulation, and IRBs. Ther Innov Regul Sci. Jul 2019;53(4):526-534. [ CrossRef ] [ Medline ]
- Sellers C, Samuel G, Derrick G. Reasoning "uncharted territory": notions of expertise within ethics review panels assessing research use of social media. J Empir Res Hum Res Ethics. 2020;15(1-2):28-39. [ FREE Full text ] [ CrossRef ] [ Medline ]
- Kious B. The Nuremberg Code: its history and implications. Princet J Bioeth. 2001;4:7-19. [ Medline ]
- World Medical Association. World Medical Association Declaration of Helsinki: ethical principles for medical research involving human subjects. JAMA. Nov 27, 2013;310(20):2191-2194. [ CrossRef ] [ Medline ]
- Department of Health‚ Education‚Welfare, National Commission for the Protection of Human Subjects of BiomedicalBehavioral Research. The Belmont Report. Ethical principles and guidelines for the protection of human subjects of research. J Am Coll Dent. 2014;81(3):4-13. [ Medline ]
- Wongkoblap A, Vadillo MA, Curcin V. Researching mental health disorders in the era of social media: systematic review. J Med Internet Res. Jun 29, 2017;19(6):e228. [ FREE Full text ] [ CrossRef ] [ Medline ]
- Alim S. An initial exploration of ethical research practices regarding automated data extraction from online social media user profiles. First Monday. Jul 2014;19(7):105-127. [ CrossRef ] [ Medline ]
- Vitak J, Proferes N, Shilton K, Ashktorab Z. Ethics regulation in social computing research: examining the role of institutional review boards. J Empir Res Hum Res Ethics. Dec 2017;12(5):372-382. [ CrossRef ] [ Medline ]
- Moreno MA, Goniu N, Moreno PS, Diekema D. Ethics of social media research: common concerns and practical considerations. Cyberpsychol Behav Soc Netw. Sep 2013;16(9):708-713. [ FREE Full text ] [ CrossRef ] [ Medline ]
- Metcalf J, Crawford K. Where are human subjects in Big Data research? The emerging ethics divide. Big Data & Society. Jun 01, 2016;3(1):205395171665021. [ CrossRef ]
- Usha Lawrance J, Nayahi Jesudhasan JV. Privacy preserving parallel clustering based anonymization for big data using MapReduce framework. Applied Artificial Intelligence. Oct 17, 2021;35(15):1587-1620. [ CrossRef ]
- Wilkinson D, Thelwall M. Researching personal information on the public web. Social Science Computer Review. Aug 17, 2010;29(4):387-401. [ CrossRef ]
- International Committee of Medical Journal Editors. Uniform requirements for manuscripts submitted to biomedical journals: writing and editing for biomedical publication. Croat Med J. Dec 2003;44(6):770-783. [ FREE Full text ] [ Medline ]
- Boyd D, Crawford K. Critical questions for big data. Information, Communication & Society. Jun 2012;15(5):662-679. [ CrossRef ]
- Bond CS, Ahmed OH, Hind M, Thomas B, Hewitt-Taylor J. The conceptual and practical ethical dilemmas of using health discussion board posts as research data. J Med Internet Res. Jun 07, 2013;15(6):e112. [ FREE Full text ] [ CrossRef ] [ Medline ]
- Markham A, Tiidenberg K, Herman A. Ethics as methods: doing ethics in the era of big data research—introduction. Social Media + Society. Jul 19, 2018;4(3):205630511878450-205630511878416. [ CrossRef ]
Abbreviations
Edited by T Leung, T de Azevedo Cardoso; submitted 02.08.23; peer-reviewed by E Zibrowski, J Scheibner; comments to author 06.10.23; revised version received 29.11.23; accepted 16.04.24; published 17.05.24.
©Yujie Zhang, Jiaqi Fu, Jie Lai, Shisi Deng, Zihan Guo, Chuhan Zhong, Jianyao Tang, Wenqiong Cao, Yanni Wu. Originally published in the Journal of Medical Internet Research (https://www.jmir.org), 17.05.2024.
This is an open-access article distributed under the terms of the Creative Commons Attribution License (https://creativecommons.org/licenses/by/4.0/), which permits unrestricted use, distribution, and reproduction in any medium, provided the original work, first published in the Journal of Medical Internet Research, is properly cited. The complete bibliographic information, a link to the original publication on https://www.jmir.org/, as well as this copyright and license information must be included.
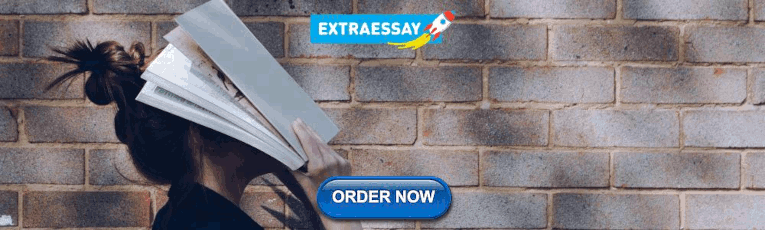
IMAGES
VIDEO
COMMENTS
For many researchers unfamiliar with qualitative research, determining how to conduct qualitative analyses is often quite challenging. Part of this challenge is due to the seemingly limitless approaches that a qualitative researcher might leverage, as well as simply learning to think like a qualitative researcher when analyzing data. From framework analysis (Ritchie & Spencer, 1994) to content ...
Qualitative data analysis is a systematic process of examining non-numerical data to extract meaning, patterns, and insights. In contrast to quantitative analysis, which focuses on numbers and statistical metrics, the qualitative study focuses on the qualitative aspects of data, such as text, images, audio, and videos.
The SAGE Handbook of. Qualitative Data Analysis. Uwe Flick. 00-Flick-Prelims.indd 5 29-Oct-13 2:00:39 PM. Data analysis is the central step in qualitative research. Whatever the data are, it is their analysis that, in a decisive way, forms the outcomes of the research. Sometimes, data collection is limited to recording and docu- menting ...
Data analysis in qualitative research is defined as the process of systematically searching and arranging the interview transcripts, observation notes, or other non-textual materials that the researcher accumulates to increase the understanding of the phenomenon.7 The process of analysing qualitative data predominantly involves coding or ...
Doing qualitative research is not easy and may require a complete rethink of how research is conducted, particularly for researchers who are more familiar with quantitative approaches. There are many ways of conducting qualitative research, and this paper has covered some of the practical issues regarding data collection, analysis, and management.
Learn what qualitative research is, how it differs from quantitative research, and what methods and approaches are used to collect and analyze non-numerical data. Find out the advantages and disadvantages of qualitative research and see examples of qualitative data analysis.
For data analysis, field-notes and audio-recordings are transcribed into protocols and transcripts, and coded using qualitative data management software. Criteria such as checklists, reflexivity, sampling strategies, piloting, co-coding, member-checking and stakeholder involvement can be used to enhance and assess the quality of the research ...
In this article, we take up this open question as a point of departure and offer the-matic analysis, an analytic method commonly used to identify patterns across lan-guage-based data (Braun & Clarke, 2006), as a useful starting point for learning about the qualitative analysis process.
Learn about various methods and techniques for analyzing qualitative data, such as codes, themes, memos, and software. This chapter covers preparatory, coding, interpretive, and display strategies with examples and references.
Step 1: Organizing the Data. "Valid analysis is immensely aided by data displays that are focused enough to permit viewing of a full data set in one location and are systematically arranged to answer the research question at hand." (Huberman and Miles, 1994, p. 432) The best way to organize your data is to go back to your interview guide.
Learn how to perform data analysis for qualitative research using Microsoft Excel and Word, and various online tools. Follow the steps to collect, transcribe, code, and interpret non-numeric data for your research question.
Learn the basics of six common qualitative data analysis methods, such as content analysis, narrative analysis, discourse analysis and more. See how to apply them to your research project and get extra resources to dive deeper.
Learn how to conduct qualitative data analysis using manual or automatic methods. Compare the steps, techniques, challenges and benefits of each approach, and see how software can help automate the process.
Qualitative data analysis is. concerned with transforming raw data by searching, evaluating, recogni sing, cod ing, mapping, exploring and describing patterns, trends, themes an d categories in ...
Theory and Qualitative Data Analysis. Researchers new to qualitative research, and particularly those coming from the quantitative tradition, have often expressed frustration at the need for what appears to be an additional and perhaps unnecessary process—that of the theoretical interpretation of their carefully designed, collected, and analyzed data.
Some have depicted qualitative analysis as craftsmanship, others as an art, and still others as a process of detective work (Patton, 2002).In any case, the qualitative data analyst is constantly on the hunt for concepts and themes that, when taken together, will provide the best explanation of "what's going on" in an inquiry.
However, in qualitative. research there are three strategies for the timing. of analysis. 1) After data collection is complete, the complete. set of data is focused upon, where analysis is a ...
Unquestionably, data analysis is the most complex and mysterious of all of the phases of a qualitative project, and the one that receives the least thoughtful discussion in the literature. For neophyte nurse researchers, many of the data collection strategies involved in a qualitative project may feel familiar and comfortable. After all, nurses have always based their clinical practice on ...
Learn about different methods and steps of qualitative data analysis, such as content analysis, narrative analysis, discourse analysis, framework analysis and grounded theory. Find examples of coding, identifying themes, patterns and relationships, and summarizing data.
When to use thematic analysis. Thematic analysis is a good approach to research where you're trying to find out something about people's views, opinions, knowledge, experiences or values from a set of qualitative data - for example, interview transcripts, social media profiles, or survey responses. Some types of research questions you might use thematic analysis to answer:
AI-Powered Qualitative Data Analysis Solutions. students. See how QualAI helps students analyze large-scale qualitative data sets, codify transcripts, and generate themes to reduce bias and increase efficiency.
Qualitative research is a type of research that explores and provides deeper insights into real-world problems.[1] Instead of collecting numerical data points or intervening or introducing treatments just like in quantitative research, qualitative research helps generate hypothenar to further investigate and understand quantitative data. Qualitative research gathers participants' experiences ...
Published: Oct. 23, 2023. Qualitative Data Analysis Software (QDA software) allows researchers to organize, analyze and visualize their data, finding the patterns in qualitative data or unstructured data: interviews, surveys, field notes, videos, audio files, images, journal articles interviews, web content etc.
Description: The workshop will review qualitative research data collection methods, specifically reviewing qualities of interview and focus group methods. Presenters will discuss data collection best practices, tips for developing data collection materials such as interview guides, and how to prepare for qualitative analysis.
Study design. This qualitative longitudinal study, conducted from October 2020 to July 2021, used a qualitative descriptive methodology through four consecutive in-depth semi-structured interviews per participant at three, 10-, 30- and 60-days post-discharge, as illustrated in Fig. 1.Longitudinal qualitative research is characterized by qualitative data collection at different points in time ...
Understanding Qualitative Data Analysis. Qualitative data analysis is the process of systematically examining and deciphering qualitative facts (such as textual content, pix, motion pictures, or observations) to discover patterns, themes, and meanings inside the statistics· Unlike quantitative statistics evaluation, which focuses on numerical measurements and statistical strategies ...
Trena M. Paulus, Ph.D., is a professor in the sociology and anthropology department at East Tennessee State University in Johnson City, Tennessee, USA.She specializes in the areas of qualitative research technologies, including the development of innovative methodologies for analyzing social media and online learning environments and is a certified professional trainer for ATLAS.ti qualitative ...
There is increasing interest in using patient and public involvement (PPI) in research to improve the quality of healthcare. Ordinarily, traditional methods have been used such as interviews or focus groups. However, these methods tend to engage a similar demographic of people. Thus, creative methods are being developed to involve patients for whom traditional methods are inaccessible or non ...
Qualitative research methodology has been applied with increasing frequency in various fields, including in healthcare research, where quantitative research methodology has traditionally dominated, with an empirically driven approach involving statistical analysis. Drawing upon artifacts and verbal data collected from in-depth interviews or ...
Background: The internet community has become a significant source for researchers to conduct qualitative studies analyzing users' views, attitudes, and experiences about public health. However, few studies have assessed the ethical issues in qualitative research using social media data. Objective: This study aims to review the reportage of ethical considerations in qualitative research ...