MBA Knowledge Base
Business • Management • Technology
Home » Management Case Studies » Case Study: Quality Management System at Coca Cola Company
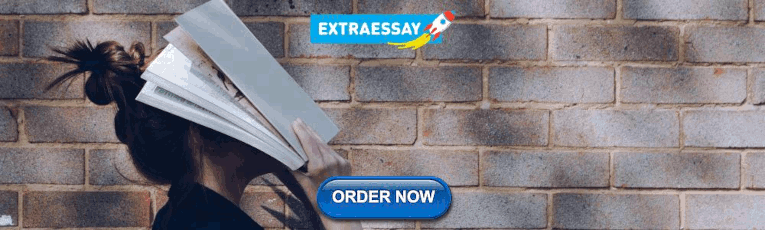
Case Study: Quality Management System at Coca Cola Company
Coca Cola’s history can be traced back to a man called Asa Candler, who bought a specific formula from a pharmacist named Smith Pemberton. Two years later, Asa founded his business and started production of soft drinks based on the formula he had bought. From then, the company grew to become the biggest producers of soft drinks with more than five hundred brands sold and consumed in more than two hundred nations worldwide.
Although the company is said to be the biggest bottler of soft drinks, they do not bottle much. Instead, Coca Cola Company manufactures a syrup concentrate, which is bought by bottlers all over the world. This distribution system ensures the soft drink is bottled by these smaller firms according to the company’s standards and guidelines. Although this franchised method of distribution is the primary method of distribution, the mother company has a key bottler in America, Coca Cola Refreshments.
In addition to soft drinks, which are Coca Cola’s main products, the company also produces diet soft drinks. These are variations of the original soft drinks with improvements in nutritional value, and reductions in sugar content. Saccharin replaced industrial sugar in 1963 so that the drinks could appeal to health-conscious consumers. A major cause for concern was the inter product competition which saw some sales dwindle in some products in favor of others.
Coca Cola started diversifying its products during the First World War when ‘Fanta’ was introduced. During World War 1, the heads of Coca Cola in Nazi Germany decided to establish a new soft drink into the market. During the ongoing war, America’s promotion in Germany was not acceptable. Therefore, he decided to use a new name and ‘Fanta’ was born. The creation was successful and production continued even after the war. ‘Sprite’ followed soon after.
In the 1990’s, health concerns among consumers of soft drinks forced their manufactures to consider altering the energy content of these products. ‘Minute Maid’ Juices, ‘PowerAde’ sports drinks, and a few flavored teas variants were Coca Cola’s initial reactions to this new interest. Although most of these new products were well received, some did not perform as well. An example of such was Coca Cola classic, dubbed C2.
Coca Cola Company has been a successful company for more than a century. This can be attributed partly to the nature of its products since soft drinks will always appeal to people. In addition to this, Coca Cola has one of the best commercial and public relations programs in the world. The company’s products can be found on adverts in virtually every corner of the globe. This success has led to its support for a wide range of sporting activities. Soccer, baseball, ice hockey, athletics and basketball are some of these sports, where Coca Cola is involved
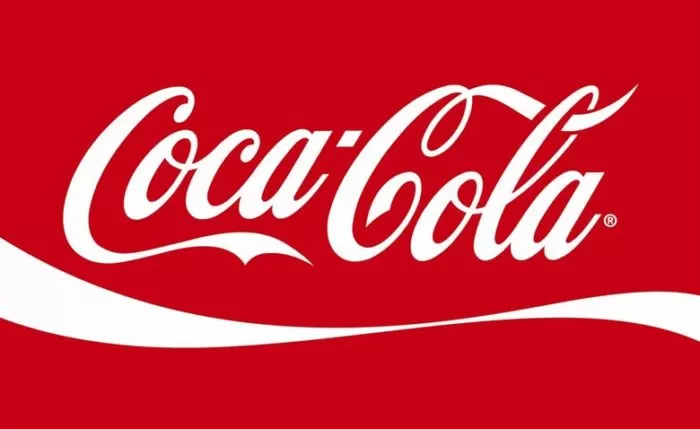
The Quality Management System at Coca Cola
It is very important that each product that Coca Cola produces is of a high quality standard to ensure that each product is exactly the same. This is important as the company wants to meet with customer requirements and expectations. With the brand having such a global presence, it is vital that these checks are continually consistent. The standardized bottle of Coca Cola has elements that need to be checked whilst on the production line to make sure that a high quality is being met. The most common checks include ingredients, packaging and distribution. Much of the testing being taken place is during the production process, as machines and a small team of employees monitor progress. It is the responsibility of all of Coca Colas staff to check quality from hygiene operators to product and packaging quality. This shows that these constant checks require staff to be on the lookout for problems and take responsibility for this, to ensure maintained quality.
Coca-cola uses inspection throughout its production process, especially in the testing of the Coca-Cola formula to ensure that each product meets specific requirements. Inspection is normally referred to as the sampling of a product after production in order to take corrective action to maintain the quality of products. Coca-Cola has incorporated this method into their organisational structure as it has the ability of eliminating mistakes and maintaining high quality standards, thus reducing the chance of product recall. It is also easy to implement and is cost effective.
Coca-cola uses both Quality Control (QC) and Quality Assurance (QA) throughout its production process. QC mainly focuses on the production line itself, whereas QA focuses on its entire operations process and related functions, addressing potential problems very quickly. In QC and QA, state of the art computers check all aspects of the production process, maintaining consistency and quality by checking the consistency of the formula, the creation of the bottle (blowing), fill levels of each bottle, labeling of each bottle, overall increasing the speed of production and quality checks, which ensures that product demands are met. QC and QA helps reduce the risk of defective products reaching a customer; problems are found and resolved in the production process, for example, bottles that are considered to be defective are placed in a waiting area for inspection. QA also focuses on the quality of supplied goods to Coca-cola, for example sugar, which is supplied by Tate and Lyle. Coca-cola informs that they have never had a problem with their suppliers. QA can also involve the training of staff ensuring that employees understand how to operate machinery. Coca-Cola ensures that all members of staff receive training prior to their employment, so that employees can operate machinery efficiently. Machinery is also under constant maintenance, which requires highly skilled engineers to fix problems, and help Coca-cola maintain high outputs.
Every bottle is also checked that it is at the correct fill level and has the correct label. This is done by a computer which every bottle passes through during the production process. Any faulty products are taken off the main production line. Should the quality control measures find any errors, the production line is frozen up to the last good check that was made. The Coca Cola bottling plant also checks the utilization level of each production line using a scorecard system. This shows the percentage of the line that is being utilized and allows managers to increase the production levels of a line if necessary.
Coca-Cola also uses Total Quality Management (TQM) , which involves the management of quality at every level of the organisation , including; suppliers, production, customers etc. This allows Coca-cola to retain/regain competitiveness to achieve increased customer satisfaction . Coca-cola uses this method to continuously improve the quality of their products. Teamwork is very important and Coca-cola ensures that every member of staff is involved in the production process, meaning that each employee understands their job/roles, thus improving morale and motivation , overall increasing productivity. TQM practices can also increase customer involvement as many organisations, including Coca-Cola relish the opportunity to receive feedback and information from their consumers. Overall, reducing waste and costs, provides Coca-cola with a competitive advantage .
The Production Process
Before production starts on the line cleaning quality tasks are performed to rinse internal pipelines, machines and equipment. This is often performed during a switch over of lines for example, changing Coke to Diet Coke to ensure that the taste is the same. This quality check is performed for both hygiene purposes and product quality. When these checks are performed the production process can begin.
Coca Cola uses a database system called Questar which enables them to perform checks on the line. For example, all materials are coded and each line is issued with a bill of materials before the process starts. This ensures that the correct materials are put on the line. This is a check that is designed to eliminate problems on the production line and is audited regularly. Without this system, product quality wouldn’t be assessed at this high level. Other quality checks on the line include packaging and carbonation which is monitored by an operator who notes down the values to ensure they are meeting standards.
To test product quality further lab technicians carry out over 2000 spot checks a day to ensure quality and consistency. This process can be prior to production or during production which can involve taking a sample of bottles off the production line. Quality tests include, the CO2 and sugar values, micro testing, packaging quality and cap tightness. These tests are designed so that total quality management ideas can be put forward. For example, one way in which Coca Cola has improved their production process is during the wrapping stage at the end of the line. The machine performed revolutions around the products wrapping it in plastic until the contents were secure. One initiative they adopted meant that one less revolution was needed. This idea however, did not impact on the quality of the packaging or the actual product therefore saving large amounts of money on packaging costs. This change has been beneficial to the organisation. Continuous improvement can also be used to adhere to environmental and social principles which the company has the responsibility to abide by. Continuous Improvement methods are sometimes easy to identify but could lead to a big changes within the organisation. The idea of continuous improvement is to reveal opportunities which could change the way something is performed. Any sources of waste, scrap or rework are potential projects which can be improved.
The successfulness of this system can be measured by assessing the consistency of the product quality. Coca Cola say that ‘Our Company’s Global Product Quality Index rating has consistently reached averages near 94 since 2007, with a 94.3 in 2010, while our Company Global Package Quality Index has steadily increased since 2007 to a 92.6 rating in 2010, our highest value to date’. This is an obvious indication this quality system is working well throughout the organisation. This increase of the index shows that the consistency of the products is being recognized by consumers.
Related posts:
- Case Study: The Coca-Cola Company Struggles with Ethical Crisis
- Case Study: Analysis of the Ethical Behavior of Coca Cola
- Case Study of Burger King: Achieving Competitive Advantage through Quality Management
- SWOT Analysis of Coca Cola
- Case Study: Marketing Strategy of Walt Disney Company
- Case Study of Papa John’s: Quality as a Core Business Strategy
- Case Study: Johnson & Johnson Company Analysis
- Case Study: Inventory Management Practices at Walmart
- Case Study: Analysis of Performance Management at British Petroleum
- Total Quality Management And Continuous Quality Improvement
Leave a Reply Cancel reply
Your email address will not be published. Required fields are marked *
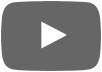
Sign up for the newsletter
Digital editions.
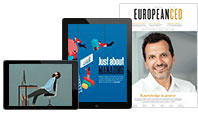
Total quality management: three case studies from around the world
With organisations to run and big orders to fill, it’s easy to see how some ceos inadvertently sacrifice quality for quantity. by integrating a system of total quality management it’s possible to have both.
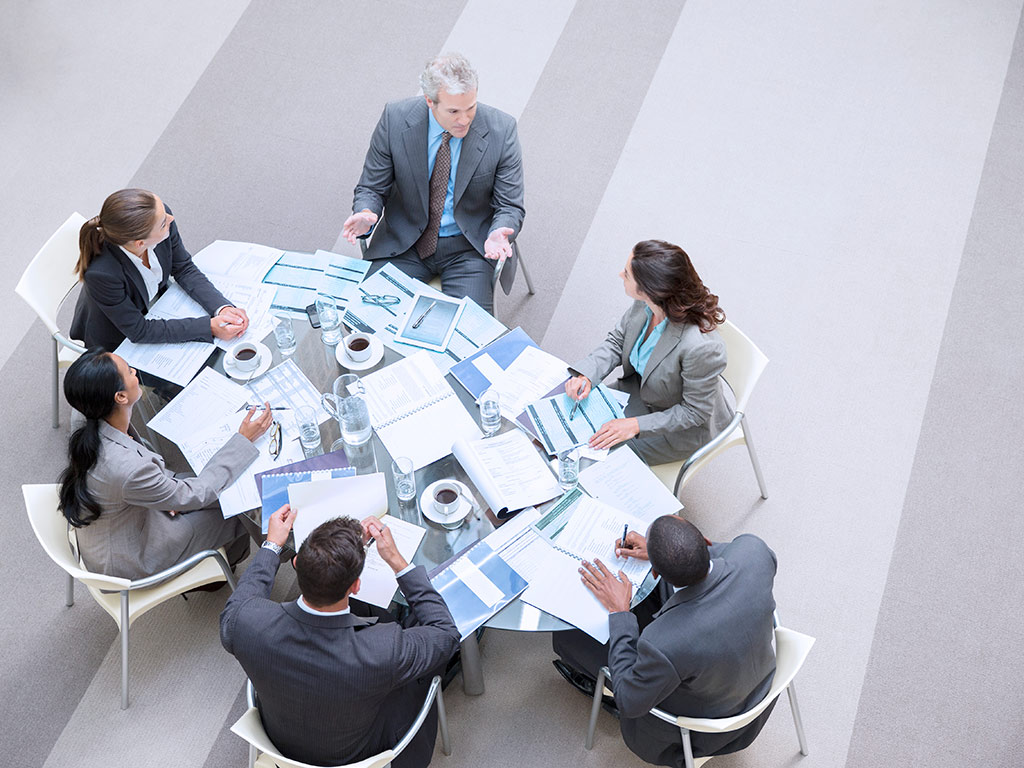
Top 5 ways to manage the board during turbulent times Top 5 ways to create a family-friendly work culture Top 5 tips for a successful joint venture Top 5 ways managers can support ethnic minority workers Top 5 ways to encourage gender diversity in the workplace Top 5 ways CEOs can create an ethical company culture Top 5 tips for going into business with your spouse Top 5 ways to promote a healthy workforce Top 5 ways to survive a recession Top 5 tips for avoiding the ‘conference vortex’ Top 5 ways to maximise new parents’ work-life balance with technology Top 5 ways to build psychological safety in the workplace Top 5 ways to prepare your workforce for the AI revolution Top 5 ways to tackle innovation stress in the workplace Top 5 tips for recruiting Millennials
There are few boardrooms in the world whose inhabitants don’t salivate at the thought of engaging in a little aggressive expansion. After all, there’s little room in a contemporary, fast-paced business environment for any firm whose leaders don’t subscribe to ambitions of bigger factories, healthier accounts and stronger turnarounds. Yet too often such tales of excess go hand-in-hand with complaints of a severe drop in quality.
Food and entertainment markets are riddled with cautionary tales, but service sectors such as health and education aren’t immune to the disappointing by-products of unsustainable growth either. As always, the first steps in avoiding a catastrophic forsaking of quality begins with good management.
There are plenty of methods and models geared at managing the quality of a particular company’s goods or services. Yet very few of those models take into consideration the widely held belief that any company is only as strong as its weakest link. With that in mind, management consultant William Deming developed an entirely new set of methods with which to address quality.
Deming, whose managerial work revolutionised the titanic Japanese manufacturing industry, perceived quality management to be more of a philosophy than anything else. Top-to-bottom improvement, he reckoned, required uninterrupted participation of all key employees and stakeholders. Thus, the total quality management (TQM) approach was born.
All in Similar to the Six Sigma improvement process, TQM ensures long-term success by enforcing all-encompassing internal guidelines and process standards to reduce errors. By way of serious, in-depth auditing – as well as some well-orchestrated soul-searching – TQM ensures firms meet stakeholder needs and expectations efficiently and effectively, without forsaking ethical values.
By opting to reframe the way employees think about the company’s goals and processes, TQM allows CEOs to make sure certain things are done right from day one. According to Teresa Whitacre, of international consulting firm ASQ , proper quality management also boosts a company’s profitability.
“Total quality management allows the company to look at their management system as a whole entity — not just an output of the quality department,” she says. “Total quality means the organisation looks at all inputs, human resources, engineering, production, service, distribution, sales, finance, all functions, and their impact on the quality of all products or services of the organisation. TQM can improve a company’s processes and bottom line.”
Embracing the entire process sees companies strive to improve in several core areas, including: customer focus, total employee involvement, process-centred thinking, systematic approaches, good communication and leadership and integrated systems. Yet Whitacre is quick to point out that companies stand to gain very little from TQM unless they’re willing to go all-in.
“Companies need to consider the inputs of each department and determine which inputs relate to its governance system. Then, the company needs to look at the same inputs and determine if those inputs are yielding the desired results,” she says. “For example, ISO 9001 requires management reviews occur at least annually. Aside from minimum standard requirements, the company is free to review what they feel is best for them. While implementing TQM, they can add to their management review the most critical metrics for their business, such as customer complaints, returns, cost of products, and more.”
The customer knows best: AtlantiCare TQM isn’t an easy management strategy to introduce into a business; in fact, many attempts tend to fall flat. More often than not, it’s because firms maintain natural barriers to full involvement. Middle managers, for example, tend to complain their authority is being challenged when boots on the ground are encouraged to speak up in the early stages of TQM. Yet in a culture of constant quality enhancement, the views of any given workforce are invaluable.
AtlantiCare in numbers
5,000 Employees
$280m Profits before quality improvement strategy was implemented
$650m Profits after quality improvement strategy
One firm that’s proven the merit of TQM is New Jersey-based healthcare provider AtlantiCare . Managing 5,000 employees at 25 locations, AtlantiCare is a serious business that’s boasted a respectable turnaround for nearly two decades. Yet in order to increase that margin further still, managers wanted to implement improvements across the board. Because patient satisfaction is the single-most important aspect of the healthcare industry, engaging in a renewed campaign of TQM proved a natural fit. The firm chose to adopt a ‘plan-do-check-act’ cycle, revealing gaps in staff communication – which subsequently meant longer patient waiting times and more complaints. To tackle this, managers explored a sideways method of internal communications. Instead of information trickling down from top-to-bottom, all of the company’s employees were given freedom to provide vital feedback at each and every level.
AtlantiCare decided to ensure all new employees understood this quality culture from the onset. At orientation, staff now receive a crash course in the company’s performance excellence framework – a management system that organises the firm’s processes into five key areas: quality, customer service, people and workplace, growth and financial performance. As employees rise through the ranks, this emphasis on improvement follows, so managers can operate within the company’s tight-loose-tight process management style.
After creating benchmark goals for employees to achieve at all levels – including better engagement at the point of delivery, increasing clinical communication and identifying and prioritising service opportunities – AtlantiCare was able to thrive. The number of repeat customers at the firm tripled, and its market share hit a six-year high. Profits unsurprisingly followed. The firm’s revenues shot up from $280m to $650m after implementing the quality improvement strategies, and the number of patients being serviced dwarfed state numbers.
Hitting the right notes: Santa Cruz Guitar Co For companies further removed from the long-term satisfaction of customers, it’s easier to let quality control slide. Yet there are plenty of ways in which growing manufacturers can pursue both quality and sales volumes simultaneously. Artisan instrument makers the Santa Cruz Guitar Co (SCGC) prove a salient example. Although the California-based company is still a small-scale manufacturing operation, SCGC has grown in recent years from a basement operation to a serious business.
SCGC in numbers
14 Craftsmen employed by SCGC
800 Custom guitars produced each year
Owner Dan Roberts now employs 14 expert craftsmen, who create over 800 custom guitars each year. In order to ensure the continued quality of his instruments, Roberts has created an environment that improves with each sale. To keep things efficient (as TQM must), the shop floor is divided into six workstations in which guitars are partially assembled and then moved to the next station. Each bench is manned by a senior craftsman, and no guitar leaves that builder’s station until he is 100 percent happy with its quality. This product quality is akin to a traditional assembly line; however, unlike a traditional, top-to-bottom factory, Roberts is intimately involved in all phases of instrument construction.
Utilising this doting method of quality management, it’s difficult to see how customers wouldn’t be satisfied with the artists’ work. Yet even if there were issues, Roberts and other senior management also spend much of their days personally answering web queries about the instruments. According to the managers, customers tend to be pleasantly surprised to find the company’s senior leaders are the ones answering their technical questions and concerns. While Roberts has no intentions of taking his manufacturing company to industrial heights, the quality of his instruments and high levels of customer satisfaction speak for themselves; the company currently boasts one lengthy backlog of orders.
A quality education: Ramaiah Institute of Management Studies Although it may appear easier to find success with TQM at a boutique-sized endeavour, the philosophy’s principles hold true in virtually every sector. Educational institutions, for example, have utilised quality management in much the same way – albeit to tackle decidedly different problems.
The global financial crisis hit higher education harder than many might have expected, and nowhere have the odds stacked higher than in India. The nation plays home to one of the world’s fastest-growing markets for business education. Yet over recent years, the relevance of business education in India has come into question. A report by one recruiter recently asserted just one in four Indian MBAs were adequately prepared for the business world.
RIMS in numbers
9% Increase in test scores post total quality management strategy
22% Increase in number of recruiters hiring from the school
20,000 Increase in the salary offered to graduates
50,000 Rise in placement revenue
At the Ramaiah Institute of Management Studies (RIMS) in Bangalore, recruiters and accreditation bodies specifically called into question the quality of students’ educations. Although the relatively small school has always struggled to compete with India’s renowned Xavier Labour Research Institute, the faculty finally began to notice clear hindrances in the success of graduates. The RIMS board decided it was time for a serious reassessment of quality management.
The school nominated Chief Academic Advisor Dr Krishnamurthy to head a volunteer team that would audit, analyse and implement process changes that would improve quality throughout (all in a particularly academic fashion). The team was tasked with looking at three key dimensions: assurance of learning, research and productivity, and quality of placements. Each member underwent extensive training to learn about action plans, quality auditing skills and continuous improvement tools – such as the ‘plan-do-study-act’ cycle.
Once faculty members were trained, the team’s first task was to identify the school’s key stakeholders, processes and their importance at the institute. Unsurprisingly, the most vital processes were identified as student intake, research, knowledge dissemination, outcomes evaluation and recruiter acceptance. From there, Krishnamurthy’s team used a fishbone diagram to help identify potential root causes of the issues plaguing these vital processes. To illustrate just how bad things were at the school, the team selected control groups and administered domain-based knowledge tests.
The deficits were disappointing. A RIMS students’ knowledge base was rated at just 36 percent, while students at Harvard rated 95 percent. Likewise, students’ critical thinking abilities rated nine percent, versus 93 percent at MIT. Worse yet, the mean salaries of graduating students averaged $36,000, versus $150,000 for students from Kellogg. Krishnamurthy’s team had their work cut out.
To tackle these issues, Krishnamurthy created an employability team, developed strategic architecture and designed pilot studies to improve the school’s curriculum and make it more competitive. In order to do so, he needed absolutely every employee and student on board – and there was some resistance at the onset. Yet the educator asserted it didn’t actually take long to convince the school’s stakeholders the changes were extremely beneficial.
“Once students started seeing the results, buy-in became complete and unconditional,” he says. Acceptance was also achieved by maintaining clearer levels of communication with stakeholders. The school actually started to provide shareholders with detailed plans and projections. Then, it proceeded with a variety of new methods, such as incorporating case studies into the curriculum, which increased general test scores by almost 10 percent. Administrators also introduced a mandate saying students must be certified in English by the British Council – increasing scores from 42 percent to 51 percent.
By improving those test scores, the perceived quality of RIMS skyrocketed. The number of top 100 businesses recruiting from the school shot up by 22 percent, while the average salary offers graduates were receiving increased by $20,000. Placement revenue rose by an impressive $50,000, and RIMS has since skyrocketed up domestic and international education tables.
No matter the business, total quality management can and will work. Yet this philosophical take on quality control will only impact firms that are in it for the long haul. Every employee must be in tune with the company’s ideologies and desires to improve, and customer satisfaction must reign supreme.
Contributors
- Industry Outlook
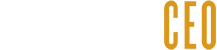

- SUGGESTED TOPICS
- The Magazine
- Newsletters
- Managing Yourself
- Managing Teams
- Work-life Balance
- The Big Idea
- Data & Visuals
- Reading Lists
- Case Selections
- HBR Learning
- Topic Feeds
- Account Settings
- Email Preferences
Quality management
- Business management
- Process management
- Project management
Fixing Health Care from the Inside, Today
- Steven J. Spear
- September 01, 2005
Creating a Culture of Quality
- Ashwin Srinivasan
- Bryan Kurey
- From the April 2014 Issue
Framing the Big Picture
- Scott D. Anthony
- March 31, 2011
Reign of Zero Tolerance (HBR Case Study)
- Janet Parker
- Eugene Volokh
- Jean Halloran
- Michael G. Cherkasky
- October 31, 2006
Manage Your Human Sigma
- John H. Fleming
- Curt Coffman
- James K. Harter
- From the July–August 2005 Issue
Strategies for Learning from Failure
- Amy C. Edmondson
- From the April 2011 Issue
Made in U.S.A.: A Renaissance in Quality
- Joseph M. Juran
- July 01, 1993
The Contradictions That Drive Toyota's Success
- Hirotaka Takeuchi
- Norihiko Shimizu
- From the June 2008 Issue
Making Mass Customization Work
- B. Joseph Pine II
- Bart Victor
- Andrew C. Boynton
- From the September–October 1993 Issue
Power of Internal Guarantees
- Christopher W.L. Hart
- From the January–February 1995 Issue
Why (and How) to Take a Plant Tour
- David M. Upton
- Stephen E. MacAdam
- From the May–June 1997 Issue

U.S. Health Care Reform Can't Wait for Quality Measures to Be Perfect
- Brian J Marcotte
- Annette Guarisco Fildes
- Michael Thompson
- Leah Binder
- October 04, 2017

Organizational Grit
- Thomas H. Lee
- Angela L. Duckworth
- From the September–October 2018 Issue
Selection Bias and the Perils of Benchmarking
- Jerker Denrell
- From the April 2005 Issue

4 Actions to Reduce Medical Errors in U.S. Hospitals
- John S. Toussaint
- Kenneth T Segel
- April 20, 2022

Teaching Smart People How to Learn
- Chris Argyris
- From the May–June 1991 Issue
Beyond Toyota: How to Root Out Waste and Pursue Perfection
- James P. Womack
- Daniel T. Jones
- From the September–October 1996 Issue

A Better Way to Onboard AI
- Boris Babic
- Daniel L. Chen
- Theodoros Evgeniou
- Anne-Laure Fayard
- From the July–August 2020 Issue

The CEO of Canada Goose on Creating a Homegrown Luxury Brand
- From the September–October 2019 Issue
Coming Commoditization of Processes
- Thomas H. Davenport
- From the June 2005 Issue

Solid as Steel: Production Planning at thyssenkrupp
- Karl Schmedders
- Markus Schulze
- February 11, 2016
Texas Instruments: Cost of Quality (A)
- Robert S. Kaplan
- Christopher D. Ittner
- August 18, 1988
Era of Quality at the Akshaya Patra Foundation
- Srujana H M
- Haritha Saranga
- Dinesh Kumar Unnikrishnan
- January 30, 2015
Pumping Iron at Cliffs & Associates: The Circored Iron Ore Reduction Plant in Trinidad
- Christoph H. Loch
- Christian Terwiesch
- December 06, 2002
Cost System Analysis
- December 01, 1994
Sky Deutschland (B): How Supply Chain Management Enabled a Dramatic Company Turnaround
- Ralf W. Seifert
- Katrin Siebenburger Hacki
- January 12, 2016
NEA Baptist Health System (A): Building a Management System One Experiment at a Time
- Sylvain Landry
- Valerie Belanger
- Martin Beaulieu
- Jean-Marc Legentil
- December 20, 2019
Lean as a Universal Model of Excellence: It Is Not Just a Manufacturing Tool!
- Elliott N. Weiss
- Donald Stevenson
- Austin English
- December 14, 2016
BPO, Incorporated
- Scott M. Shafer
- January 15, 2006
Eurasia International: Total Quality Management in the Shipping Industry
- Ali Farhoomand
- Amir Hoosain
- July 23, 2004
NEA Baptist Health System (B): Deployment of the Toyota Kata Practice and the Role of the Shepherding Group
- June Marques Fernandes
NovaStar Financial: A Short Seller's Battle
- Suraj Srinivasan
- March 13, 2013
Spin Master Toys (A): Finding a Manufacturer for E-Chargers
- John S. Haywood-Farmer
- January 19, 2001
John Smithers at Sigtek
- Todd D. Jick
- October 05, 1990
Philips: Redefining Telehealth
- Regina E. Herzlinger
- Alec Petersen
- Natalie Kindred
- Sara M McKinley
- March 24, 2021
Creating and Spreading New Knowledge at Hewlett-Packard
- Robert Cole
- Gwendolyn Lee
- August 01, 2004
Product Innovation at Aguas Danone
- Javier Jorge Silva
- Femando Zerboni
- Andres Chehtman
- Maria Alonso
- December 01, 2012
The Challenge Facing the U.S. Healthcare Delivery System
- Richard Bohmer
- Carin-Isabel Knoop
- June 06, 2006
Maestro Pizza (H): Making the Best of the Situation
- Ramon Casadesus-Masanell
- Fares Khrais
- May 26, 2022

Mastering the Dynamics of Innovation
- James M. Utterback
- August 16, 1996

Solid as Steel: Production Planning at thyssenkrupp, Teaching Note
- March 09, 2016
Solid as Steel: Production Planning at thyssenkrupp, Student Spreadsheet
- January 28, 2016
Managing Service Operations: The Managerial Research Design Process
- Frances X. Frei
- Dennis Campbell
- April 04, 2008

How to Prevent Your Customers from Failing
- Stephen S. Tax
- Mark Colgate
- David E. Bowen
- April 01, 2006
Popular Topics
Partner center.
Academia.edu no longer supports Internet Explorer.
To browse Academia.edu and the wider internet faster and more securely, please take a few seconds to upgrade your browser .
Enter the email address you signed up with and we'll email you a reset link.
- We're Hiring!
- Help Center
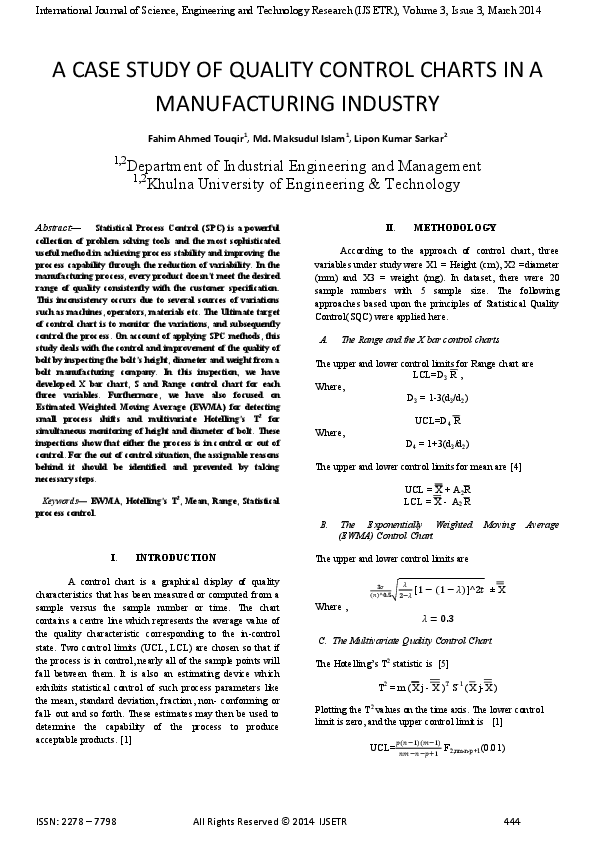
A CASE STUDY OF QUALITY CONTROL CHARTS IN A MANUFACTURING INDUSTRY

Related Papers
Eng Mohamed Hamdy
– Most of the modern industrial processes are naturally multivariate. Multivariate control charts are supplanted univariate control charts, as it takes into account the relationship between variables and identifies the real process changes, which are undetectable by univariate control charts. In practice, the basic assumption that the measurements are independently and identically distributed about a target value is not always valid. Violation of this assumption increases the False Alarm Rate (FAR) and deteriorates the separation of assignable causes from common causes. This paper presents the application of Multivariate Statistical Process Control (MSPC) charts (e. g., Hotelling , MEWMA) to monitor the flare making process in a straight fluorescent light bulb industry. Furthermore, it develops the appropriate procedure for monitoring a multivariate autocorrelated data variable (i. e., dynamic behavior) by using Autoregressive Integrated Moving Average (ARIMA) models. Univariate SPC charts and decomposition approach are used to identify the out-of-control signals that are generated from multivariate SPC charts. Software packages such as Minitab 17 and Statgraphics Centurion XVI are used to construct the control charts.
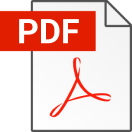
Jonathan Quiroz
cristian palacio
control estadistico de la calidad
Abidemi Adeniyi
This project is aimed at providing a stock price prediction system which can be used to forecast the future stock price of the Nigerian Stock Exchange using the artificial neural network. This study will attempt to reduce the stress people have in analyzing large amount of data in order to predict stock. This study will have to look into the problem areas of the stock market prediction and devise a method of proffering solutions to all these problems.
Seyed Taghi Akhavan Niaki
In this paper, two control charts based on the generalized linear test (GLT) and contingency table are proposed for Phase-II monitoring of multivariate categorical processes. The performances of the proposed methods are compared with the exponentially weighted moving average-generalized likelihood ratio test (EWMA-GLRT) control chart proposed in the literature. The results show the better performance of the proposed control charts under moderate and large shifts. Moreover, a new scheme is proposed to identify the parameter responsible for an out-of-control signal. The performance of the proposed diagnosing procedure is evaluated through some simulation experiments.
OLUMA URGESSA
Statistical Quality Control, 6th Edition
Regiz Faria
Anwar Shaker
sanika kamtekar
RELATED PAPERS
shayne cabrera
Journal of Materials in Civil Engineering
Barzin Mobasher
Shawn Dmello
Everardo Emmanuel Tovar
Jayesh Hirani
Russian Geology and Geophysics
Alexey Ariskin , Georgy Nikolaev
Loévanofski Hiribarnovitch
Alexey Ariskin
The Hymenoptera of Costa Rica
James M Carpenter
transstellar
TJPRC Publication
Mohammed Alami
Eli Bressert
Nature chemistry
Frank Neese
Ulrich Wienand , Michele Scagliarini , Napoli Nicola
Communications in Statistics - Theory and Methods
Nasir A R Syed , Raja Fawad Zafar
Ridwan Sanusi
Astronomy and Astrophysics
Quentin A Parker
Earth and Planetary Science Letters
Roberta Rudnick
Noorlisa Harun
Science Park Research Organization & Counselling
Amirhossein Amiri
Astronomical Journal
Ricardo Covarrubias
E. Nikogossian
Communications in Statistics - Simulation and Computation
Subha Chakraborti
T. Movsessian
Ridwan Sanusi , Nurudeen Adegoke
Environmental Science and Pollution Research
Pranvera Lazo
Economic Quality Control
Saddam Akber Abbasi
Dhaka University Institutional Repository
Md. Anwar Hossain
Arnoldo Sandoval
Hungarian Journal Of Industry And Chemistry Veszprém, Vol. 41(1) pp. 77-82 (2013)
Janos Abonyi
Héctor O Portillo Reyes, Fausto Elvir y Marcio Martínez
Héctor O R L A N D O Portillo Reyes
Michelle Cluver
Journal of Cellular Plastics
Nelson Oliveira
Jeh-Nan Pan
RELATED TOPICS
- We're Hiring!
- Help Center
- Find new research papers in:
- Health Sciences
- Earth Sciences
- Cognitive Science
- Mathematics
- Computer Science
- Academia ©2024
- Browse All Articles
- Newsletter Sign-Up
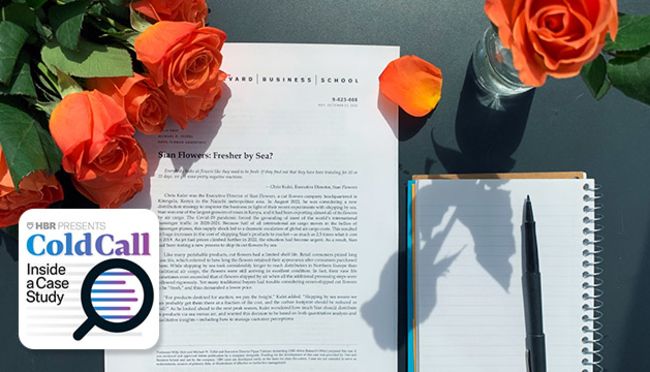
- 11 Apr 2023
- Cold Call Podcast
A Rose by Any Other Name: Supply Chains and Carbon Emissions in the Flower Industry
Headquartered in Kitengela, Kenya, Sian Flowers exports roses to Europe. Because cut flowers have a limited shelf life and consumers want them to retain their appearance for as long as possible, Sian and its distributors used international air cargo to transport them to Amsterdam, where they were sold at auction and trucked to markets across Europe. But when the Covid-19 pandemic caused huge increases in shipping costs, Sian launched experiments to ship roses by ocean using refrigerated containers. The company reduced its costs and cut its carbon emissions, but is a flower that travels halfway around the world truly a “low-carbon rose”? Harvard Business School professors Willy Shih and Mike Toffel debate these questions and more in their case, “Sian Flowers: Fresher by Sea?”
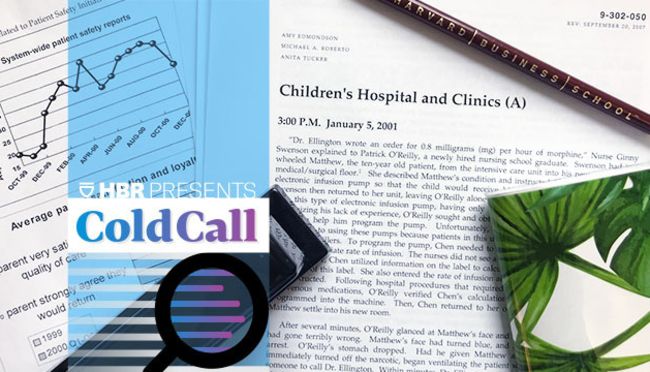
- 17 Sep 2019
How a New Leader Broke Through a Culture of Accuse, Blame, and Criticize
Children’s Hospital & Clinics COO Julie Morath sets out to change the culture by instituting a policy of blameless reporting, which encourages employees to report anything that goes wrong or seems substandard, without fear of reprisal. Professor Amy Edmondson discusses getting an organization into the “High Performance Zone.” Open for comment; 0 Comments.
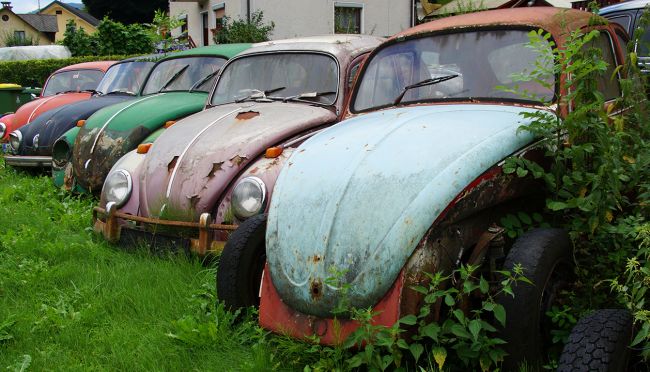
- 27 Feb 2019
- Research & Ideas
The Hidden Cost of a Product Recall
Product failures create managerial challenges for companies but market opportunities for competitors, says Ariel Dora Stern. The stakes have only grown higher. Open for comment; 0 Comments.
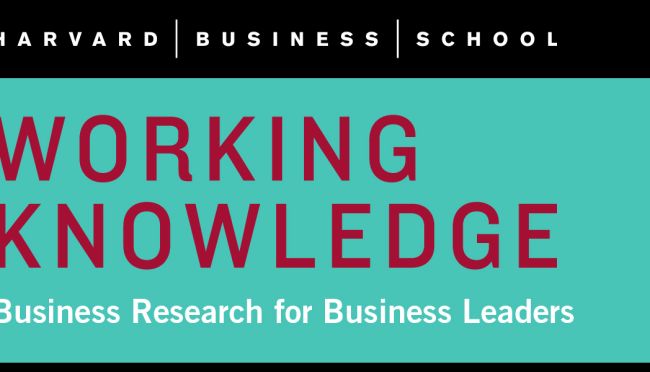
- 31 Mar 2018
- Working Paper Summaries
Expected Stock Returns Worldwide: A Log-Linear Present-Value Approach
Over the last 20 years, shortcomings of classical asset-pricing models have motivated research in developing alternative methods for measuring ex ante expected stock returns. This study evaluates the main paradigms for deriving firm-level expected return proxies (ERPs) and proposes a new framework for estimating them.
- 26 Apr 2017
Assessing the Quality of Quality Assessment: The Role of Scheduling
Accurate inspections enable companies to assess the quality, safety, and environmental practices of their business partners, and enable regulators to protect consumers, workers, and the environment. This study finds that inspectors are less stringent later in their workday and after visiting workplaces with fewer problems. Managers and regulators can improve inspection accuracy by mitigating these biases and their consequences.
- 23 Sep 2013
Status: When and Why It Matters
Status plays a key role in everything from the things we buy to the partnerships we make. Professor Daniel Malter explores when status matters most. Closed for comment; 0 Comments.
- 16 May 2011
What Loyalty? High-End Customers are First to Flee
Companies offering top-drawer customer service might have a nasty surprise awaiting them when a new competitor comes to town. Their best customers might be the first to defect. Research by Harvard Business School's Ryan W. Buell, Dennis Campbell, and Frances X. Frei. Key concepts include: Companies that offer high levels of customer service can't expect too much loyalty if a new competitor offers even better service. High-end businesses must avoid complacency and continue to proactively increase relative service levels when they're faced with even the potential threat of increased service competition. Even though high-end customers can be fickle, a company that sustains a superior service position in its local market can attract and retain customers who are more valuable over time. Firms rated lower in service quality are more or less immune from the high-end challenger. Closed for comment; 0 Comments.
- 08 Dec 2008
Thinking Twice About Supply-Chain Layoffs
Cutting the wrong employees can be counterproductive for retailers, according to research from Zeynep Ton. One suggestion: Pay special attention to staff who handle mundane tasks such as stocking and labeling. Your customers do. Closed for comment; 0 Comments.
- 01 Dec 2006
- What Do You Think?
How Important Is Quality of Labor? And How Is It Achieved?
A new book by Gregory Clark identifies "labor quality" as the major enticement for capital flows that lead to economic prosperity. By defining labor quality in terms of discipline and attitudes toward work, this argument minimizes the long-term threat of outsourcing to developed economies. By understanding labor quality, can we better confront anxieties about outsourcing and immigration? Closed for comment; 0 Comments.
- 20 Sep 2004
How Consumers Value Global Brands
What do consumers expect of global brands? Does it hurt to be an American brand? This Harvard Business Review excerpt co-written by HBS professor John A. Quelch identifies the three characteristics consumers look for to make purchase decisions. Closed for comment; 0 Comments.
What are you looking for?
Suggestions.
- Journalists
Pharma Quality Control Case Studies
BIOCAD’s Quest for a Reliable Microbiological Quantitative Reference Material
®</sup> 3D", "link" : "/content/biomerieux/corp/en/resource-hub/knowledge/case-studies/pharmaceutical-qc-case-studies/how-a-top-5-pharma-company-protects-production-an-increases-productivity-using-bact-alert-3d-media-statement.html", "type" : "Link"}}' href="/corp/en/resource-hub/knowledge/case-studies/pharmaceutical-qc-case-studies/how-a-top-5-pharma-company-protects-production-an-increases-productivity-using-bact-alert-3d-media-statement.html"> How a Top 5 Pharma Company Protects Production and Increases Productivity Using BACT/ALERT ® 3D
®</sup>", "link" : "/content/biomerieux/corp/en/resource-hub/knowledge/case-studies/pharmaceutical-qc-case-studies/how-thalgo-increased-productivity-with-chemunex-media-statement.html", "type" : "Link"}}' href="/corp/en/resource-hub/knowledge/case-studies/pharmaceutical-qc-case-studies/how-thalgo-increased-productivity-with-chemunex-media-statement.html"> How Thalgo Increased Productivity With CHEMUNEX ®
®</sup>", "link" : "/content/biomerieux/corp/en/resource-hub/knowledge/case-studies/pharmaceutical-qc-case-studies/how-shiseido-increased-the-efficiency-of-microbiological-controls-with-chemunex-case-study.html", "type" : "Link"}}' href="/corp/en/resource-hub/knowledge/case-studies/pharmaceutical-qc-case-studies/how-shiseido-increased-the-efficiency-of-microbiological-controls-with-chemunex-case-study.html"> How Shiseido Increased the Efficiency of Microbiological Controls With CHEMUNEX ®
®</sup> System?", "link" : "/content/biomerieux/corp/en/resource-hub/knowledge/case-studies/pharmaceutical-qc-case-studies/how-did-loreal-optimize-microbial-testing-with-the-chemunex-system-case-study.html", "type" : "Link"}}' href="/corp/en/resource-hub/knowledge/case-studies/pharmaceutical-qc-case-studies/how-did-loreal-optimize-microbial-testing-with-the-chemunex-system-case-study.html"> How did L’Oréal Optimize Microbial Testing With the CHEMUNEX ® System?
®</sup>?", "link" : "/content/biomerieux/corp/en/resource-hub/knowledge/case-studies/pharmaceutical-qc-case-studies/how-did-cosmebac-improve-microbiological-testing-process-with-chemunex-case-study.html", "type" : "Link"}}' href="/corp/en/resource-hub/knowledge/case-studies/pharmaceutical-qc-case-studies/how-did-cosmebac-improve-microbiological-testing-process-with-chemunex-case-study.html"> How did Cosmebac Improve Microbiological Testing Process With CHEMUNEX ® ?
Statistical quality control: A case study research
Ieee account.
- Change Username/Password
- Update Address
Purchase Details
- Payment Options
- Order History
- View Purchased Documents
Profile Information
- Communications Preferences
- Profession and Education
- Technical Interests
- US & Canada: +1 800 678 4333
- Worldwide: +1 732 981 0060
- Contact & Support
- About IEEE Xplore
- Accessibility
- Terms of Use
- Nondiscrimination Policy
- Privacy & Opting Out of Cookies
A not-for-profit organization, IEEE is the world's largest technical professional organization dedicated to advancing technology for the benefit of humanity. © Copyright 2024 IEEE - All rights reserved. Use of this web site signifies your agreement to the terms and conditions.
Recommended
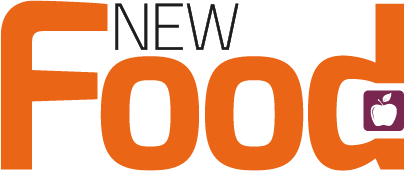
About us | Advertise with us | Contact us
Case Study: Nestle Nordic Quality management system audits
- Odnoklassniki
- Facebook Messenger
- LiveJournal
Posted: 6 November 2020 | Intertek | No comments yet
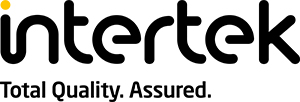
Nestlé is the world’s leading nutrition, health, and wellness company, with over 280,000 employees and over 450 factories globally.
The Challenge
Prior to obtaining ISO 9001 certification with Intertek, Nestlé used their own proprietary quality management system, However, in 2017, Nestlé’s global operations made the decision that the entire company would be converting to ISO standards. Being accredited to an international standard looked better from the perspective of customers. Since then, they have been audited to ISO 9001, ISO 14001, and ISO 45001 integrated into one management system.
Related content from this organisation
- Guide to Testing: The latest on lab techniques and research
- New Food Issue 4 2021
- Featured Partnership: Challenges in meat pathogen detection – improving your food safety plan
- Feature Partnership: Getting it right – the global allergy maze
- Food Integrity Supplement – April 2021
Related topics
Quality analysis & quality control (QA/QC)
Related organisations
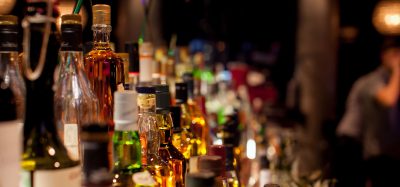
Scientists develop new method to detect fake alcoholic spirits
By Grace Galler
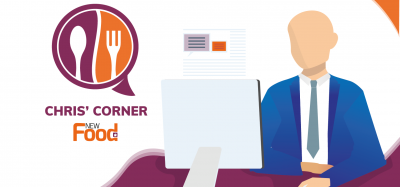
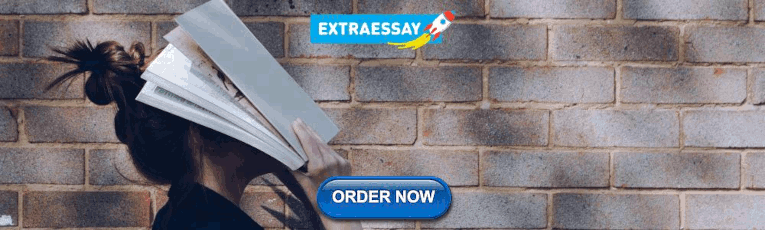
The Food Fortress: A story of success for others to follow?
By Professor Chris Elliott
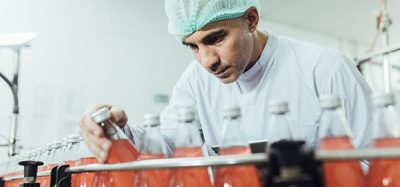
Staying ahead of the curve: Harnessing informatics to meet changing F&B consumer expectations
By STARLIMS Corporation
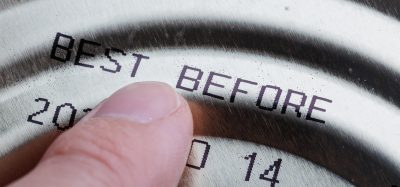
Researchers advocate for detection technology over best-before dates
Leave a reply cancel reply.
Your email address will not be published. Required fields are marked *
This site uses Akismet to reduce spam. Learn how your comment data is processed .
© Russell Publishing Limited , 2010-2024. All rights reserved. Terms & Conditions | Privacy Policy | Cookie Policy
Website design and development by e-Motive Media Limited .

Privacy Overview
This website uses cookies to improve your experience while you navigate through the website. Out of these cookies, the cookies that are categorised as "Necessary" are stored on your browser as they are as essential for the working of basic functionalities of the website. For our other types of cookies "Advertising & Targeting", "Analytics" and "Performance", these help us analyse and understand how you use this website. These cookies will be stored in your browser only with your consent. You also have the option to opt-out of these different types of cookies. But opting out of some of these cookies may have an effect on your browsing experience. You can adjust the available sliders to 'Enabled' or 'Disabled', then click 'Save and Accept'. View our Cookie Policy page.
Necessary cookies are absolutely essential for the website to function properly. This category only includes cookies that ensures basic functionalities and security features of the website. These cookies do not store any personal information.
Performance cookies are includes cookies that deliver enhanced functionalities of the website, such as caching. These cookies do not store any personal information.
Analytics cookies collect information about your use of the content, and in combination with previously collected information, are used to measure, understand, and report on your usage of this website.
Advertising and targeting cookies help us provide our visitors with relevant ads and marketing campaigns.
Analytical Instruments & Supplies
- Chromatography
- Mass Spectrometry
- Certified Pre-Owned Instruments
- Spectroscopy
- Capillary Electrophoresis
- Chromatography & Spectroscopy Lab Supplies
- Instrument Repair
- Sample Preparation
- Chemical Standards
Life Science
- Cell Analysis
- Automated Electrophoresis
- Microarray Solutions
- Mutagenesis & Cloning
- Next Generation Sequencing
- Research Flow Cytometry
- PCR/Real-Time PCR (qPCR)
- CRISPR/Cas9
- Microscopes and Microplate Instrumentation
- Oligo Pools & Oligo GMP Manufacturing
Clinical & Diagnostic Testing
- Immunohistochemistry
- Companion Diagnostics
- Hematoxylin & Eosin
- Special Stains
- In Situ Hybridization
- Clinical Flow Cytometry
- Specific Proteins
- Clinical Microplate Instrumentation
Lab Management & Consulting
- Lab Management
- Lab Consulting
Lab Software
- Software & Informatics
- Genomics Informatics
Lab Supplies
- Microplates
Dissolution Testing
- Dissolution
Lab Automation
- Automated Liquid Handling
Vacuum & Leak Detection
- Vacuum Technologies
- Leak Detection
- Applications & Industries
- Biopharma/Pharma
- Cancer Research
- Cannabis & Hemp Testing
- Clinical Diagnostics
- Clinical Research
- Infectious Disease
- Energy & Chemicals
- Environmental
- Food & Beverage Testing
- Materials Testing & Research
- Security, Defense & First Response
- Vacuum Solutions
- Lithium-Ion Battery Testing
- Oligonucleotide Therapeutics
- PFAS Testing in Water
- Training & Events
Mass spectrometry, chromatography, spectroscopy, software, dissolution, sample handling and vacuum technologies courses
On-demand continuing education
Instrument training and workshops
Live or on-demand webinars on product introductions, applications and software enhancements
Worldwide trade shows, conferences, local seminars and user group meetings
Lab Management Services
Service Plans, On Demand Repair, Preventive Maintenance, and Service Center Repair
Software to manage instrument access, sample processing, inventories, and more
Instrument/software qualifications, consulting, and data integrity validations
Learn essential lab skills and enhance your workflows
Instrument & equipment deinstallation, transportation, and reinstallation
Other Services Header1
CrossLab Connect services use laboratory data to improve control and decision-making
Advance lab operations with lab-wide services, asset management, relocation
Shorten the time it takes to start seeing the full value of your instrument investment
Other Services Header2
- Agilent Community
- Financial Solutions
- Agilent University
- Instrument Trade-In & BuyBack
Pathology Services
- Lab Solution Deployment Services
- Instrument & Solution Services
- Training & Application Services
- Workflow & Connectivity Services
Nucleic Acid Therapeutics
- Oligonucleotide GMP Manufacturing
Vacuum Product & Leak Detector Services
- Advance Exchange Service
- Repair Support Services & Spare Parts
- Support Services, Agreements & Training
- Technology Refresh & Upgrade
- Leak Detector Services
- Support & Resources
Technical Support
- Instrument Support Resources
- Columns, Supplies, & Standards
- Contact Support
- See All Technical Support
Purchase & Order Support
- Instrument Subscriptions
- Flexible Spend Plan
- eProcurement
- eCommerce Guides
Literature & Videos
- Application Notes
- Technical Overviews
- User Manuals
- Life Sciences Publication Database
- Electronic Instructions for Use (eIFU)
- Safety Data Sheets
- Technical Data Sheets
- Site Prep Checklist
E-Newsletters
- Solution Insights
- ICP-MS Journal
Certificates
- Certificate of Analysis
- Certificate of Conformance
- Certificate of Performance
- ISO Certificates
More Resources
- iOS & Android Apps
- QuikChange Primer Design Tools
- GC Calculators & Method Translation Software
- BioCalculators / Nucleic Acid Calculators
- Order Center
- Quick Order
- Request a Quote
- My Favorites
- Where to Buy
- Flex Spend Portal
- Genomics Applications and Solutions
- Sample Quality Control Solutions
- Sample Quality Control Case Studies

An official website of the United States government
Here’s how you know
Official websites use .gov A .gov website belongs to an official government organization in the United States.
Secure .gov websites use HTTPS A lock ( A locked padlock ) or https:// means you’ve safely connected to the .gov website. Share sensitive information only on official, secure websites.
- Heart-Healthy Living
- High Blood Pressure
- Sickle Cell Disease
- Sleep Apnea
- Information & Resources on COVID-19
- The Heart Truth®
- Learn More Breathe Better®
- Blood Diseases and Disorders Education Program
- Publications and Resources
- Blood Disorders and Blood Safety
- Sleep Science and Sleep Disorders
- Lung Diseases
- Health Disparities and Inequities
- Heart and Vascular Diseases
- Precision Medicine Activities
- Obesity, Nutrition, and Physical Activity
- Population and Epidemiology Studies
- Women’s Health
- Research Topics
- Clinical Trials
- All Science A-Z
- Grants and Training Home
- Policies and Guidelines
- Funding Opportunities and Contacts
- Training and Career Development
- Email Alerts
- NHLBI in the Press
- Research Features
- Past Events
- Upcoming Events
- Mission and Strategic Vision
- Divisions, Offices and Centers
- Advisory Committees
- Budget and Legislative Information
- Jobs and Working at the NHLBI
- Contact and FAQs
- NIH Sleep Research Plan
- < Back To Health Topics
Study Quality Assessment Tools
In 2013, NHLBI developed a set of tailored quality assessment tools to assist reviewers in focusing on concepts that are key to a study’s internal validity. The tools were specific to certain study designs and tested for potential flaws in study methods or implementation. Experts used the tools during the systematic evidence review process to update existing clinical guidelines, such as those on cholesterol, blood pressure, and obesity. Their findings are outlined in the following reports:
- Assessing Cardiovascular Risk: Systematic Evidence Review from the Risk Assessment Work Group
- Management of Blood Cholesterol in Adults: Systematic Evidence Review from the Cholesterol Expert Panel
- Management of Blood Pressure in Adults: Systematic Evidence Review from the Blood Pressure Expert Panel
- Managing Overweight and Obesity in Adults: Systematic Evidence Review from the Obesity Expert Panel
While these tools have not been independently published and would not be considered standardized, they may be useful to the research community. These reports describe how experts used the tools for the project. Researchers may want to use the tools for their own projects; however, they would need to determine their own parameters for making judgements. Details about the design and application of the tools are included in Appendix A of the reports.
Quality Assessment of Controlled Intervention Studies - Study Quality Assessment Tools
*CD, cannot determine; NA, not applicable; NR, not reported
Guidance for Assessing the Quality of Controlled Intervention Studies
The guidance document below is organized by question number from the tool for quality assessment of controlled intervention studies.
Question 1. Described as randomized
Was the study described as randomized? A study does not satisfy quality criteria as randomized simply because the authors call it randomized; however, it is a first step in determining if a study is randomized
Questions 2 and 3. Treatment allocation–two interrelated pieces
Adequate randomization: Randomization is adequate if it occurred according to the play of chance (e.g., computer generated sequence in more recent studies, or random number table in older studies). Inadequate randomization: Randomization is inadequate if there is a preset plan (e.g., alternation where every other subject is assigned to treatment arm or another method of allocation is used, such as time or day of hospital admission or clinic visit, ZIP Code, phone number, etc.). In fact, this is not randomization at all–it is another method of assignment to groups. If assignment is not by the play of chance, then the answer to this question is no. There may be some tricky scenarios that will need to be read carefully and considered for the role of chance in assignment. For example, randomization may occur at the site level, where all individuals at a particular site are assigned to receive treatment or no treatment. This scenario is used for group-randomized trials, which can be truly randomized, but often are "quasi-experimental" studies with comparison groups rather than true control groups. (Few, if any, group-randomized trials are anticipated for this evidence review.)
Allocation concealment: This means that one does not know in advance, or cannot guess accurately, to what group the next person eligible for randomization will be assigned. Methods include sequentially numbered opaque sealed envelopes, numbered or coded containers, central randomization by a coordinating center, computer-generated randomization that is not revealed ahead of time, etc. Questions 4 and 5. Blinding
Blinding means that one does not know to which group–intervention or control–the participant is assigned. It is also sometimes called "masking." The reviewer assessed whether each of the following was blinded to knowledge of treatment assignment: (1) the person assessing the primary outcome(s) for the study (e.g., taking the measurements such as blood pressure, examining health records for events such as myocardial infarction, reviewing and interpreting test results such as x ray or cardiac catheterization findings); (2) the person receiving the intervention (e.g., the patient or other study participant); and (3) the person providing the intervention (e.g., the physician, nurse, pharmacist, dietitian, or behavioral interventionist).
Generally placebo-controlled medication studies are blinded to patient, provider, and outcome assessors; behavioral, lifestyle, and surgical studies are examples of studies that are frequently blinded only to the outcome assessors because blinding of the persons providing and receiving the interventions is difficult in these situations. Sometimes the individual providing the intervention is the same person performing the outcome assessment. This was noted when it occurred.
Question 6. Similarity of groups at baseline
This question relates to whether the intervention and control groups have similar baseline characteristics on average especially those characteristics that may affect the intervention or outcomes. The point of randomized trials is to create groups that are as similar as possible except for the intervention(s) being studied in order to compare the effects of the interventions between groups. When reviewers abstracted baseline characteristics, they noted when there was a significant difference between groups. Baseline characteristics for intervention groups are usually presented in a table in the article (often Table 1).
Groups can differ at baseline without raising red flags if: (1) the differences would not be expected to have any bearing on the interventions and outcomes; or (2) the differences are not statistically significant. When concerned about baseline difference in groups, reviewers recorded them in the comments section and considered them in their overall determination of the study quality.
Questions 7 and 8. Dropout
"Dropouts" in a clinical trial are individuals for whom there are no end point measurements, often because they dropped out of the study and were lost to followup.
Generally, an acceptable overall dropout rate is considered 20 percent or less of participants who were randomized or allocated into each group. An acceptable differential dropout rate is an absolute difference between groups of 15 percentage points at most (calculated by subtracting the dropout rate of one group minus the dropout rate of the other group). However, these are general rates. Lower overall dropout rates are expected in shorter studies, whereas higher overall dropout rates may be acceptable for studies of longer duration. For example, a 6-month study of weight loss interventions should be expected to have nearly 100 percent followup (almost no dropouts–nearly everybody gets their weight measured regardless of whether or not they actually received the intervention), whereas a 10-year study testing the effects of intensive blood pressure lowering on heart attacks may be acceptable if there is a 20-25 percent dropout rate, especially if the dropout rate between groups was similar. The panels for the NHLBI systematic reviews may set different levels of dropout caps.
Conversely, differential dropout rates are not flexible; there should be a 15 percent cap. If there is a differential dropout rate of 15 percent or higher between arms, then there is a serious potential for bias. This constitutes a fatal flaw, resulting in a poor quality rating for the study.
Question 9. Adherence
Did participants in each treatment group adhere to the protocols for assigned interventions? For example, if Group 1 was assigned to 10 mg/day of Drug A, did most of them take 10 mg/day of Drug A? Another example is a study evaluating the difference between a 30-pound weight loss and a 10-pound weight loss on specific clinical outcomes (e.g., heart attacks), but the 30-pound weight loss group did not achieve its intended weight loss target (e.g., the group only lost 14 pounds on average). A third example is whether a large percentage of participants assigned to one group "crossed over" and got the intervention provided to the other group. A final example is when one group that was assigned to receive a particular drug at a particular dose had a large percentage of participants who did not end up taking the drug or the dose as designed in the protocol.
Question 10. Avoid other interventions
Changes that occur in the study outcomes being assessed should be attributable to the interventions being compared in the study. If study participants receive interventions that are not part of the study protocol and could affect the outcomes being assessed, and they receive these interventions differentially, then there is cause for concern because these interventions could bias results. The following scenario is another example of how bias can occur. In a study comparing two different dietary interventions on serum cholesterol, one group had a significantly higher percentage of participants taking statin drugs than the other group. In this situation, it would be impossible to know if a difference in outcome was due to the dietary intervention or the drugs.
Question 11. Outcome measures assessment
What tools or methods were used to measure the outcomes in the study? Were the tools and methods accurate and reliable–for example, have they been validated, or are they objective? This is important as it indicates the confidence you can have in the reported outcomes. Perhaps even more important is ascertaining that outcomes were assessed in the same manner within and between groups. One example of differing methods is self-report of dietary salt intake versus urine testing for sodium content (a more reliable and valid assessment method). Another example is using BP measurements taken by practitioners who use their usual methods versus using BP measurements done by individuals trained in a standard approach. Such an approach may include using the same instrument each time and taking an individual's BP multiple times. In each of these cases, the answer to this assessment question would be "no" for the former scenario and "yes" for the latter. In addition, a study in which an intervention group was seen more frequently than the control group, enabling more opportunities to report clinical events, would not be considered reliable and valid.
Question 12. Power calculation
Generally, a study's methods section will address the sample size needed to detect differences in primary outcomes. The current standard is at least 80 percent power to detect a clinically relevant difference in an outcome using a two-sided alpha of 0.05. Often, however, older studies will not report on power.
Question 13. Prespecified outcomes
Investigators should prespecify outcomes reported in a study for hypothesis testing–which is the reason for conducting an RCT. Without prespecified outcomes, the study may be reporting ad hoc analyses, simply looking for differences supporting desired findings. Investigators also should prespecify subgroups being examined. Most RCTs conduct numerous post hoc analyses as a way of exploring findings and generating additional hypotheses. The intent of this question is to give more weight to reports that are not simply exploratory in nature.
Question 14. Intention-to-treat analysis
Intention-to-treat (ITT) means everybody who was randomized is analyzed according to the original group to which they are assigned. This is an extremely important concept because conducting an ITT analysis preserves the whole reason for doing a randomized trial; that is, to compare groups that differ only in the intervention being tested. When the ITT philosophy is not followed, groups being compared may no longer be the same. In this situation, the study would likely be rated poor. However, if an investigator used another type of analysis that could be viewed as valid, this would be explained in the "other" box on the quality assessment form. Some researchers use a completers analysis (an analysis of only the participants who completed the intervention and the study), which introduces significant potential for bias. Characteristics of participants who do not complete the study are unlikely to be the same as those who do. The likely impact of participants withdrawing from a study treatment must be considered carefully. ITT analysis provides a more conservative (potentially less biased) estimate of effectiveness.
General Guidance for Determining the Overall Quality Rating of Controlled Intervention Studies
The questions on the assessment tool were designed to help reviewers focus on the key concepts for evaluating a study's internal validity. They are not intended to create a list that is simply tallied up to arrive at a summary judgment of quality.
Internal validity is the extent to which the results (effects) reported in a study can truly be attributed to the intervention being evaluated and not to flaws in the design or conduct of the study–in other words, the ability for the study to make causal conclusions about the effects of the intervention being tested. Such flaws can increase the risk of bias. Critical appraisal involves considering the risk of potential for allocation bias, measurement bias, or confounding (the mixture of exposures that one cannot tease out from each other). Examples of confounding include co-interventions, differences at baseline in patient characteristics, and other issues addressed in the questions above. High risk of bias translates to a rating of poor quality. Low risk of bias translates to a rating of good quality.
Fatal flaws: If a study has a "fatal flaw," then risk of bias is significant, and the study is of poor quality. Examples of fatal flaws in RCTs include high dropout rates, high differential dropout rates, no ITT analysis or other unsuitable statistical analysis (e.g., completers-only analysis).
Generally, when evaluating a study, one will not see a "fatal flaw;" however, one will find some risk of bias. During training, reviewers were instructed to look for the potential for bias in studies by focusing on the concepts underlying the questions in the tool. For any box checked "no," reviewers were told to ask: "What is the potential risk of bias that may be introduced by this flaw?" That is, does this factor cause one to doubt the results that were reported in the study?
NHLBI staff provided reviewers with background reading on critical appraisal, while emphasizing that the best approach to use is to think about the questions in the tool in determining the potential for bias in a study. The staff also emphasized that each study has specific nuances; therefore, reviewers should familiarize themselves with the key concepts.
Quality Assessment of Systematic Reviews and Meta-Analyses - Study Quality Assessment Tools
Guidance for Quality Assessment Tool for Systematic Reviews and Meta-Analyses
A systematic review is a study that attempts to answer a question by synthesizing the results of primary studies while using strategies to limit bias and random error.424 These strategies include a comprehensive search of all potentially relevant articles and the use of explicit, reproducible criteria in the selection of articles included in the review. Research designs and study characteristics are appraised, data are synthesized, and results are interpreted using a predefined systematic approach that adheres to evidence-based methodological principles.
Systematic reviews can be qualitative or quantitative. A qualitative systematic review summarizes the results of the primary studies but does not combine the results statistically. A quantitative systematic review, or meta-analysis, is a type of systematic review that employs statistical techniques to combine the results of the different studies into a single pooled estimate of effect, often given as an odds ratio. The guidance document below is organized by question number from the tool for quality assessment of systematic reviews and meta-analyses.
Question 1. Focused question
The review should be based on a question that is clearly stated and well-formulated. An example would be a question that uses the PICO (population, intervention, comparator, outcome) format, with all components clearly described.
Question 2. Eligibility criteria
The eligibility criteria used to determine whether studies were included or excluded should be clearly specified and predefined. It should be clear to the reader why studies were included or excluded.
Question 3. Literature search
The search strategy should employ a comprehensive, systematic approach in order to capture all of the evidence possible that pertains to the question of interest. At a minimum, a comprehensive review has the following attributes:
- Electronic searches were conducted using multiple scientific literature databases, such as MEDLINE, EMBASE, Cochrane Central Register of Controlled Trials, PsychLit, and others as appropriate for the subject matter.
- Manual searches of references found in articles and textbooks should supplement the electronic searches.
Additional search strategies that may be used to improve the yield include the following:
- Studies published in other countries
- Studies published in languages other than English
- Identification by experts in the field of studies and articles that may have been missed
- Search of grey literature, including technical reports and other papers from government agencies or scientific groups or committees; presentations and posters from scientific meetings, conference proceedings, unpublished manuscripts; and others. Searching the grey literature is important (whenever feasible) because sometimes only positive studies with significant findings are published in the peer-reviewed literature, which can bias the results of a review.
In their reviews, researchers described the literature search strategy clearly, and ascertained it could be reproducible by others with similar results.
Question 4. Dual review for determining which studies to include and exclude
Titles, abstracts, and full-text articles (when indicated) should be reviewed by two independent reviewers to determine which studies to include and exclude in the review. Reviewers resolved disagreements through discussion and consensus or with third parties. They clearly stated the review process, including methods for settling disagreements.
Question 5. Quality appraisal for internal validity
Each included study should be appraised for internal validity (study quality assessment) using a standardized approach for rating the quality of the individual studies. Ideally, this should be done by at least two independent reviewers appraised each study for internal validity. However, there is not one commonly accepted, standardized tool for rating the quality of studies. So, in the research papers, reviewers looked for an assessment of the quality of each study and a clear description of the process used.
Question 6. List and describe included studies
All included studies were listed in the review, along with descriptions of their key characteristics. This was presented either in narrative or table format.
Question 7. Publication bias
Publication bias is a term used when studies with positive results have a higher likelihood of being published, being published rapidly, being published in higher impact journals, being published in English, being published more than once, or being cited by others.425,426 Publication bias can be linked to favorable or unfavorable treatment of research findings due to investigators, editors, industry, commercial interests, or peer reviewers. To minimize the potential for publication bias, researchers can conduct a comprehensive literature search that includes the strategies discussed in Question 3.
A funnel plot–a scatter plot of component studies in a meta-analysis–is a commonly used graphical method for detecting publication bias. If there is no significant publication bias, the graph looks like a symmetrical inverted funnel.
Reviewers assessed and clearly described the likelihood of publication bias.
Question 8. Heterogeneity
Heterogeneity is used to describe important differences in studies included in a meta-analysis that may make it inappropriate to combine the studies.427 Heterogeneity can be clinical (e.g., important differences between study participants, baseline disease severity, and interventions); methodological (e.g., important differences in the design and conduct of the study); or statistical (e.g., important differences in the quantitative results or reported effects).
Researchers usually assess clinical or methodological heterogeneity qualitatively by determining whether it makes sense to combine studies. For example:
- Should a study evaluating the effects of an intervention on CVD risk that involves elderly male smokers with hypertension be combined with a study that involves healthy adults ages 18 to 40? (Clinical Heterogeneity)
- Should a study that uses a randomized controlled trial (RCT) design be combined with a study that uses a case-control study design? (Methodological Heterogeneity)
Statistical heterogeneity describes the degree of variation in the effect estimates from a set of studies; it is assessed quantitatively. The two most common methods used to assess statistical heterogeneity are the Q test (also known as the X2 or chi-square test) or I2 test.
Reviewers examined studies to determine if an assessment for heterogeneity was conducted and clearly described. If the studies are found to be heterogeneous, the investigators should explore and explain the causes of the heterogeneity, and determine what influence, if any, the study differences had on overall study results.
Quality Assessment Tool for Observational Cohort and Cross-Sectional Studies - Study Quality Assessment Tools
Guidance for Assessing the Quality of Observational Cohort and Cross-Sectional Studies
The guidance document below is organized by question number from the tool for quality assessment of observational cohort and cross-sectional studies.
Question 1. Research question
Did the authors describe their goal in conducting this research? Is it easy to understand what they were looking to find? This issue is important for any scientific paper of any type. Higher quality scientific research explicitly defines a research question.
Questions 2 and 3. Study population
Did the authors describe the group of people from which the study participants were selected or recruited, using demographics, location, and time period? If you were to conduct this study again, would you know who to recruit, from where, and from what time period? Is the cohort population free of the outcomes of interest at the time they were recruited?
An example would be men over 40 years old with type 2 diabetes who began seeking medical care at Phoenix Good Samaritan Hospital between January 1, 1990 and December 31, 1994. In this example, the population is clearly described as: (1) who (men over 40 years old with type 2 diabetes); (2) where (Phoenix Good Samaritan Hospital); and (3) when (between January 1, 1990 and December 31, 1994). Another example is women ages 34 to 59 years of age in 1980 who were in the nursing profession and had no known coronary disease, stroke, cancer, hypercholesterolemia, or diabetes, and were recruited from the 11 most populous States, with contact information obtained from State nursing boards.
In cohort studies, it is crucial that the population at baseline is free of the outcome of interest. For example, the nurses' population above would be an appropriate group in which to study incident coronary disease. This information is usually found either in descriptions of population recruitment, definitions of variables, or inclusion/exclusion criteria.
You may need to look at prior papers on methods in order to make the assessment for this question. Those papers are usually in the reference list.
If fewer than 50% of eligible persons participated in the study, then there is concern that the study population does not adequately represent the target population. This increases the risk of bias.
Question 4. Groups recruited from the same population and uniform eligibility criteria
Were the inclusion and exclusion criteria developed prior to recruitment or selection of the study population? Were the same underlying criteria used for all of the subjects involved? This issue is related to the description of the study population, above, and you may find the information for both of these questions in the same section of the paper.
Most cohort studies begin with the selection of the cohort; participants in this cohort are then measured or evaluated to determine their exposure status. However, some cohort studies may recruit or select exposed participants in a different time or place than unexposed participants, especially retrospective cohort studies–which is when data are obtained from the past (retrospectively), but the analysis examines exposures prior to outcomes. For example, one research question could be whether diabetic men with clinical depression are at higher risk for cardiovascular disease than those without clinical depression. So, diabetic men with depression might be selected from a mental health clinic, while diabetic men without depression might be selected from an internal medicine or endocrinology clinic. This study recruits groups from different clinic populations, so this example would get a "no."
However, the women nurses described in the question above were selected based on the same inclusion/exclusion criteria, so that example would get a "yes."
Question 5. Sample size justification
Did the authors present their reasons for selecting or recruiting the number of people included or analyzed? Do they note or discuss the statistical power of the study? This question is about whether or not the study had enough participants to detect an association if one truly existed.
A paragraph in the methods section of the article may explain the sample size needed to detect a hypothesized difference in outcomes. You may also find a discussion of power in the discussion section (such as the study had 85 percent power to detect a 20 percent increase in the rate of an outcome of interest, with a 2-sided alpha of 0.05). Sometimes estimates of variance and/or estimates of effect size are given, instead of sample size calculations. In any of these cases, the answer would be "yes."
However, observational cohort studies often do not report anything about power or sample sizes because the analyses are exploratory in nature. In this case, the answer would be "no." This is not a "fatal flaw." It just may indicate that attention was not paid to whether the study was sufficiently sized to answer a prespecified question–i.e., it may have been an exploratory, hypothesis-generating study.
Question 6. Exposure assessed prior to outcome measurement
This question is important because, in order to determine whether an exposure causes an outcome, the exposure must come before the outcome.
For some prospective cohort studies, the investigator enrolls the cohort and then determines the exposure status of various members of the cohort (large epidemiological studies like Framingham used this approach). However, for other cohort studies, the cohort is selected based on its exposure status, as in the example above of depressed diabetic men (the exposure being depression). Other examples include a cohort identified by its exposure to fluoridated drinking water and then compared to a cohort living in an area without fluoridated water, or a cohort of military personnel exposed to combat in the Gulf War compared to a cohort of military personnel not deployed in a combat zone.
With either of these types of cohort studies, the cohort is followed forward in time (i.e., prospectively) to assess the outcomes that occurred in the exposed members compared to nonexposed members of the cohort. Therefore, you begin the study in the present by looking at groups that were exposed (or not) to some biological or behavioral factor, intervention, etc., and then you follow them forward in time to examine outcomes. If a cohort study is conducted properly, the answer to this question should be "yes," since the exposure status of members of the cohort was determined at the beginning of the study before the outcomes occurred.
For retrospective cohort studies, the same principal applies. The difference is that, rather than identifying a cohort in the present and following them forward in time, the investigators go back in time (i.e., retrospectively) and select a cohort based on their exposure status in the past and then follow them forward to assess the outcomes that occurred in the exposed and nonexposed cohort members. Because in retrospective cohort studies the exposure and outcomes may have already occurred (it depends on how long they follow the cohort), it is important to make sure that the exposure preceded the outcome.
Sometimes cross-sectional studies are conducted (or cross-sectional analyses of cohort-study data), where the exposures and outcomes are measured during the same timeframe. As a result, cross-sectional analyses provide weaker evidence than regular cohort studies regarding a potential causal relationship between exposures and outcomes. For cross-sectional analyses, the answer to Question 6 should be "no."
Question 7. Sufficient timeframe to see an effect
Did the study allow enough time for a sufficient number of outcomes to occur or be observed, or enough time for an exposure to have a biological effect on an outcome? In the examples given above, if clinical depression has a biological effect on increasing risk for CVD, such an effect may take years. In the other example, if higher dietary sodium increases BP, a short timeframe may be sufficient to assess its association with BP, but a longer timeframe would be needed to examine its association with heart attacks.
The issue of timeframe is important to enable meaningful analysis of the relationships between exposures and outcomes to be conducted. This often requires at least several years, especially when looking at health outcomes, but it depends on the research question and outcomes being examined.
Cross-sectional analyses allow no time to see an effect, since the exposures and outcomes are assessed at the same time, so those would get a "no" response.
Question 8. Different levels of the exposure of interest
If the exposure can be defined as a range (examples: drug dosage, amount of physical activity, amount of sodium consumed), were multiple categories of that exposure assessed? (for example, for drugs: not on the medication, on a low dose, medium dose, high dose; for dietary sodium, higher than average U.S. consumption, lower than recommended consumption, between the two). Sometimes discrete categories of exposure are not used, but instead exposures are measured as continuous variables (for example, mg/day of dietary sodium or BP values).
In any case, studying different levels of exposure (where possible) enables investigators to assess trends or dose-response relationships between exposures and outcomes–e.g., the higher the exposure, the greater the rate of the health outcome. The presence of trends or dose-response relationships lends credibility to the hypothesis of causality between exposure and outcome.
For some exposures, however, this question may not be applicable (e.g., the exposure may be a dichotomous variable like living in a rural setting versus an urban setting, or vaccinated/not vaccinated with a one-time vaccine). If there are only two possible exposures (yes/no), then this question should be given an "NA," and it should not count negatively towards the quality rating.
Question 9. Exposure measures and assessment
Were the exposure measures defined in detail? Were the tools or methods used to measure exposure accurate and reliable–for example, have they been validated or are they objective? This issue is important as it influences confidence in the reported exposures. When exposures are measured with less accuracy or validity, it is harder to see an association between exposure and outcome even if one exists. Also as important is whether the exposures were assessed in the same manner within groups and between groups; if not, bias may result.
For example, retrospective self-report of dietary salt intake is not as valid and reliable as prospectively using a standardized dietary log plus testing participants' urine for sodium content. Another example is measurement of BP, where there may be quite a difference between usual care, where clinicians measure BP however it is done in their practice setting (which can vary considerably), and use of trained BP assessors using standardized equipment (e.g., the same BP device which has been tested and calibrated) and a standardized protocol (e.g., patient is seated for 5 minutes with feet flat on the floor, BP is taken twice in each arm, and all four measurements are averaged). In each of these cases, the former would get a "no" and the latter a "yes."
Here is a final example that illustrates the point about why it is important to assess exposures consistently across all groups: If people with higher BP (exposed cohort) are seen by their providers more frequently than those without elevated BP (nonexposed group), it also increases the chances of detecting and documenting changes in health outcomes, including CVD-related events. Therefore, it may lead to the conclusion that higher BP leads to more CVD events. This may be true, but it could also be due to the fact that the subjects with higher BP were seen more often; thus, more CVD-related events were detected and documented simply because they had more encounters with the health care system. Thus, it could bias the results and lead to an erroneous conclusion.
Question 10. Repeated exposure assessment
Was the exposure for each person measured more than once during the course of the study period? Multiple measurements with the same result increase our confidence that the exposure status was correctly classified. Also, multiple measurements enable investigators to look at changes in exposure over time, for example, people who ate high dietary sodium throughout the followup period, compared to those who started out high then reduced their intake, compared to those who ate low sodium throughout. Once again, this may not be applicable in all cases. In many older studies, exposure was measured only at baseline. However, multiple exposure measurements do result in a stronger study design.
Question 11. Outcome measures
Were the outcomes defined in detail? Were the tools or methods for measuring outcomes accurate and reliable–for example, have they been validated or are they objective? This issue is important because it influences confidence in the validity of study results. Also important is whether the outcomes were assessed in the same manner within groups and between groups.
An example of an outcome measure that is objective, accurate, and reliable is death–the outcome measured with more accuracy than any other. But even with a measure as objective as death, there can be differences in the accuracy and reliability of how death was assessed by the investigators. Did they base it on an autopsy report, death certificate, death registry, or report from a family member? Another example is a study of whether dietary fat intake is related to blood cholesterol level (cholesterol level being the outcome), and the cholesterol level is measured from fasting blood samples that are all sent to the same laboratory. These examples would get a "yes." An example of a "no" would be self-report by subjects that they had a heart attack, or self-report of how much they weigh (if body weight is the outcome of interest).
Similar to the example in Question 9, results may be biased if one group (e.g., people with high BP) is seen more frequently than another group (people with normal BP) because more frequent encounters with the health care system increases the chances of outcomes being detected and documented.
Question 12. Blinding of outcome assessors
Blinding means that outcome assessors did not know whether the participant was exposed or unexposed. It is also sometimes called "masking." The objective is to look for evidence in the article that the person(s) assessing the outcome(s) for the study (for example, examining medical records to determine the outcomes that occurred in the exposed and comparison groups) is masked to the exposure status of the participant. Sometimes the person measuring the exposure is the same person conducting the outcome assessment. In this case, the outcome assessor would most likely not be blinded to exposure status because they also took measurements of exposures. If so, make a note of that in the comments section.
As you assess this criterion, think about whether it is likely that the person(s) doing the outcome assessment would know (or be able to figure out) the exposure status of the study participants. If the answer is no, then blinding is adequate. An example of adequate blinding of the outcome assessors is to create a separate committee, whose members were not involved in the care of the patient and had no information about the study participants' exposure status. The committee would then be provided with copies of participants' medical records, which had been stripped of any potential exposure information or personally identifiable information. The committee would then review the records for prespecified outcomes according to the study protocol. If blinding was not possible, which is sometimes the case, mark "NA" and explain the potential for bias.
Question 13. Followup rate
Higher overall followup rates are always better than lower followup rates, even though higher rates are expected in shorter studies, whereas lower overall followup rates are often seen in studies of longer duration. Usually, an acceptable overall followup rate is considered 80 percent or more of participants whose exposures were measured at baseline. However, this is just a general guideline. For example, a 6-month cohort study examining the relationship between dietary sodium intake and BP level may have over 90 percent followup, but a 20-year cohort study examining effects of sodium intake on stroke may have only a 65 percent followup rate.
Question 14. Statistical analyses
Were key potential confounding variables measured and adjusted for, such as by statistical adjustment for baseline differences? Logistic regression or other regression methods are often used to account for the influence of variables not of interest.
This is a key issue in cohort studies, because statistical analyses need to control for potential confounders, in contrast to an RCT, where the randomization process controls for potential confounders. All key factors that may be associated both with the exposure of interest and the outcome–that are not of interest to the research question–should be controlled for in the analyses.
For example, in a study of the relationship between cardiorespiratory fitness and CVD events (heart attacks and strokes), the study should control for age, BP, blood cholesterol, and body weight, because all of these factors are associated both with low fitness and with CVD events. Well-done cohort studies control for multiple potential confounders.
Some general guidance for determining the overall quality rating of observational cohort and cross-sectional studies
The questions on the form are designed to help you focus on the key concepts for evaluating the internal validity of a study. They are not intended to create a list that you simply tally up to arrive at a summary judgment of quality.
Internal validity for cohort studies is the extent to which the results reported in the study can truly be attributed to the exposure being evaluated and not to flaws in the design or conduct of the study–in other words, the ability of the study to draw associative conclusions about the effects of the exposures being studied on outcomes. Any such flaws can increase the risk of bias.
Critical appraisal involves considering the risk of potential for selection bias, information bias, measurement bias, or confounding (the mixture of exposures that one cannot tease out from each other). Examples of confounding include co-interventions, differences at baseline in patient characteristics, and other issues throughout the questions above. High risk of bias translates to a rating of poor quality. Low risk of bias translates to a rating of good quality. (Thus, the greater the risk of bias, the lower the quality rating of the study.)
In addition, the more attention in the study design to issues that can help determine whether there is a causal relationship between the exposure and outcome, the higher quality the study. These include exposures occurring prior to outcomes, evaluation of a dose-response gradient, accuracy of measurement of both exposure and outcome, sufficient timeframe to see an effect, and appropriate control for confounding–all concepts reflected in the tool.
Generally, when you evaluate a study, you will not see a "fatal flaw," but you will find some risk of bias. By focusing on the concepts underlying the questions in the quality assessment tool, you should ask yourself about the potential for bias in the study you are critically appraising. For any box where you check "no" you should ask, "What is the potential risk of bias resulting from this flaw in study design or execution?" That is, does this factor cause you to doubt the results that are reported in the study or doubt the ability of the study to accurately assess an association between exposure and outcome?
The best approach is to think about the questions in the tool and how each one tells you something about the potential for bias in a study. The more you familiarize yourself with the key concepts, the more comfortable you will be with critical appraisal. Examples of studies rated good, fair, and poor are useful, but each study must be assessed on its own based on the details that are reported and consideration of the concepts for minimizing bias.
Quality Assessment of Case-Control Studies - Study Quality Assessment Tools
Guidance for Assessing the Quality of Case-Control Studies
The guidance document below is organized by question number from the tool for quality assessment of case-control studies.
Did the authors describe their goal in conducting this research? Is it easy to understand what they were looking to find? This issue is important for any scientific paper of any type. High quality scientific research explicitly defines a research question.
Question 2. Study population
Did the authors describe the group of individuals from which the cases and controls were selected or recruited, while using demographics, location, and time period? If the investigators conducted this study again, would they know exactly who to recruit, from where, and from what time period?
Investigators identify case-control study populations by location, time period, and inclusion criteria for cases (individuals with the disease, condition, or problem) and controls (individuals without the disease, condition, or problem). For example, the population for a study of lung cancer and chemical exposure would be all incident cases of lung cancer diagnosed in patients ages 35 to 79, from January 1, 2003 to December 31, 2008, living in Texas during that entire time period, as well as controls without lung cancer recruited from the same population during the same time period. The population is clearly described as: (1) who (men and women ages 35 to 79 with (cases) and without (controls) incident lung cancer); (2) where (living in Texas); and (3) when (between January 1, 2003 and December 31, 2008).
Other studies may use disease registries or data from cohort studies to identify cases. In these cases, the populations are individuals who live in the area covered by the disease registry or included in a cohort study (i.e., nested case-control or case-cohort). For example, a study of the relationship between vitamin D intake and myocardial infarction might use patients identified via the GRACE registry, a database of heart attack patients.
NHLBI staff encouraged reviewers to examine prior papers on methods (listed in the reference list) to make this assessment, if necessary.
Question 3. Target population and case representation
In order for a study to truly address the research question, the target population–the population from which the study population is drawn and to which study results are believed to apply–should be carefully defined. Some authors may compare characteristics of the study cases to characteristics of cases in the target population, either in text or in a table. When study cases are shown to be representative of cases in the appropriate target population, it increases the likelihood that the study was well-designed per the research question.
However, because these statistics are frequently difficult or impossible to measure, publications should not be penalized if case representation is not shown. For most papers, the response to question 3 will be "NR." Those subquestions are combined because the answer to the second subquestion–case representation–determines the response to this item. However, it cannot be determined without considering the response to the first subquestion. For example, if the answer to the first subquestion is "yes," and the second, "CD," then the response for item 3 is "CD."
Question 4. Sample size justification
Did the authors discuss their reasons for selecting or recruiting the number of individuals included? Did they discuss the statistical power of the study and provide a sample size calculation to ensure that the study is adequately powered to detect an association (if one exists)? This question does not refer to a description of the manner in which different groups were included or excluded using the inclusion/exclusion criteria (e.g., "Final study size was 1,378 participants after exclusion of 461 patients with missing data" is not considered a sample size justification for the purposes of this question).
An article's methods section usually contains information on sample size and the size needed to detect differences in exposures and on statistical power.
Question 5. Groups recruited from the same population
To determine whether cases and controls were recruited from the same population, one can ask hypothetically, "If a control was to develop the outcome of interest (the condition that was used to select cases), would that person have been eligible to become a case?" Case-control studies begin with the selection of the cases (those with the outcome of interest, e.g., lung cancer) and controls (those in whom the outcome is absent). Cases and controls are then evaluated and categorized by their exposure status. For the lung cancer example, cases and controls were recruited from hospitals in a given region. One may reasonably assume that controls in the catchment area for the hospitals, or those already in the hospitals for a different reason, would attend those hospitals if they became a case; therefore, the controls are drawn from the same population as the cases. If the controls were recruited or selected from a different region (e.g., a State other than Texas) or time period (e.g., 1991-2000), then the cases and controls were recruited from different populations, and the answer to this question would be "no."
The following example further explores selection of controls. In a study, eligible cases were men and women, ages 18 to 39, who were diagnosed with atherosclerosis at hospitals in Perth, Australia, between July 1, 2000 and December 31, 2007. Appropriate controls for these cases might be sampled using voter registration information for men and women ages 18 to 39, living in Perth (population-based controls); they also could be sampled from patients without atherosclerosis at the same hospitals (hospital-based controls). As long as the controls are individuals who would have been eligible to be included in the study as cases (if they had been diagnosed with atherosclerosis), then the controls were selected appropriately from the same source population as cases.
In a prospective case-control study, investigators may enroll individuals as cases at the time they are found to have the outcome of interest; the number of cases usually increases as time progresses. At this same time, they may recruit or select controls from the population without the outcome of interest. One way to identify or recruit cases is through a surveillance system. In turn, investigators can select controls from the population covered by that system. This is an example of population-based controls. Investigators also may identify and select cases from a cohort study population and identify controls from outcome-free individuals in the same cohort study. This is known as a nested case-control study.
Question 6. Inclusion and exclusion criteria prespecified and applied uniformly
Were the inclusion and exclusion criteria developed prior to recruitment or selection of the study population? Were the same underlying criteria used for all of the groups involved? To answer this question, reviewers determined if the investigators developed I/E criteria prior to recruitment or selection of the study population and if they used the same underlying criteria for all groups. The investigators should have used the same selection criteria, except for study participants who had the disease or condition, which would be different for cases and controls by definition. Therefore, the investigators use the same age (or age range), gender, race, and other characteristics to select cases and controls. Information on this topic is usually found in a paper's section on the description of the study population.
Question 7. Case and control definitions
For this question, reviewers looked for descriptions of the validity of case and control definitions and processes or tools used to identify study participants as such. Was a specific description of "case" and "control" provided? Is there a discussion of the validity of the case and control definitions and the processes or tools used to identify study participants as such? They determined if the tools or methods were accurate, reliable, and objective. For example, cases might be identified as "adult patients admitted to a VA hospital from January 1, 2000 to December 31, 2009, with an ICD-9 discharge diagnosis code of acute myocardial infarction and at least one of the two confirmatory findings in their medical records: at least 2mm of ST elevation changes in two or more ECG leads and an elevated troponin level. Investigators might also use ICD-9 or CPT codes to identify patients. All cases should be identified using the same methods. Unless the distinction between cases and controls is accurate and reliable, investigators cannot use study results to draw valid conclusions.
Question 8. Random selection of study participants
If a case-control study did not use 100 percent of eligible cases and/or controls (e.g., not all disease-free participants were included as controls), did the authors indicate that random sampling was used to select controls? When it is possible to identify the source population fairly explicitly (e.g., in a nested case-control study, or in a registry-based study), then random sampling of controls is preferred. When investigators used consecutive sampling, which is frequently done for cases in prospective studies, then study participants are not considered randomly selected. In this case, the reviewers would answer "no" to Question 8. However, this would not be considered a fatal flaw.
If investigators included all eligible cases and controls as study participants, then reviewers marked "NA" in the tool. If 100 percent of cases were included (e.g., NA for cases) but only 50 percent of eligible controls, then the response would be "yes" if the controls were randomly selected, and "no" if they were not. If this cannot be determined, the appropriate response is "CD."
Question 9. Concurrent controls
A concurrent control is a control selected at the time another person became a case, usually on the same day. This means that one or more controls are recruited or selected from the population without the outcome of interest at the time a case is diagnosed. Investigators can use this method in both prospective case-control studies and retrospective case-control studies. For example, in a retrospective study of adenocarcinoma of the colon using data from hospital records, if hospital records indicate that Person A was diagnosed with adenocarcinoma of the colon on June 22, 2002, then investigators would select one or more controls from the population of patients without adenocarcinoma of the colon on that same day. This assumes they conducted the study retrospectively, using data from hospital records. The investigators could have also conducted this study using patient records from a cohort study, in which case it would be a nested case-control study.
Investigators can use concurrent controls in the presence or absence of matching and vice versa. A study that uses matching does not necessarily mean that concurrent controls were used.
Question 10. Exposure assessed prior to outcome measurement
Investigators first determine case or control status (based on presence or absence of outcome of interest), and then assess exposure history of the case or control; therefore, reviewers ascertained that the exposure preceded the outcome. For example, if the investigators used tissue samples to determine exposure, did they collect them from patients prior to their diagnosis? If hospital records were used, did investigators verify that the date a patient was exposed (e.g., received medication for atherosclerosis) occurred prior to the date they became a case (e.g., was diagnosed with type 2 diabetes)? For an association between an exposure and an outcome to be considered causal, the exposure must have occurred prior to the outcome.
Question 11. Exposure measures and assessment
Were the exposure measures defined in detail? Were the tools or methods used to measure exposure accurate and reliable–for example, have they been validated or are they objective? This is important, as it influences confidence in the reported exposures. Equally important is whether the exposures were assessed in the same manner within groups and between groups. This question pertains to bias resulting from exposure misclassification (i.e., exposure ascertainment).
For example, a retrospective self-report of dietary salt intake is not as valid and reliable as prospectively using a standardized dietary log plus testing participants' urine for sodium content because participants' retrospective recall of dietary salt intake may be inaccurate and result in misclassification of exposure status. Similarly, BP results from practices that use an established protocol for measuring BP would be considered more valid and reliable than results from practices that did not use standard protocols. A protocol may include using trained BP assessors, standardized equipment (e.g., the same BP device which has been tested and calibrated), and a standardized procedure (e.g., patient is seated for 5 minutes with feet flat on the floor, BP is taken twice in each arm, and all four measurements are averaged).
Question 12. Blinding of exposure assessors
Blinding or masking means that outcome assessors did not know whether participants were exposed or unexposed. To answer this question, reviewers examined articles for evidence that the outcome assessor(s) was masked to the exposure status of the research participants. An outcome assessor, for example, may examine medical records to determine the outcomes that occurred in the exposed and comparison groups. Sometimes the person measuring the exposure is the same person conducting the outcome assessment. In this case, the outcome assessor would most likely not be blinded to exposure status. A reviewer would note such a finding in the comments section of the assessment tool.
One way to ensure good blinding of exposure assessment is to have a separate committee, whose members have no information about the study participants' status as cases or controls, review research participants' records. To help answer the question above, reviewers determined if it was likely that the outcome assessor knew whether the study participant was a case or control. If it was unlikely, then the reviewers marked "no" to Question 12. Outcome assessors who used medical records to assess exposure should not have been directly involved in the study participants' care, since they probably would have known about their patients' conditions. If the medical records contained information on the patient's condition that identified him/her as a case (which is likely), that information would have had to be removed before the exposure assessors reviewed the records.
If blinding was not possible, which sometimes happens, the reviewers marked "NA" in the assessment tool and explained the potential for bias.
Question 13. Statistical analysis
Were key potential confounding variables measured and adjusted for, such as by statistical adjustment for baseline differences? Investigators often use logistic regression or other regression methods to account for the influence of variables not of interest.
This is a key issue in case-controlled studies; statistical analyses need to control for potential confounders, in contrast to RCTs in which the randomization process controls for potential confounders. In the analysis, investigators need to control for all key factors that may be associated with both the exposure of interest and the outcome and are not of interest to the research question.
A study of the relationship between smoking and CVD events illustrates this point. Such a study needs to control for age, gender, and body weight; all are associated with smoking and CVD events. Well-done case-control studies control for multiple potential confounders.
Matching is a technique used to improve study efficiency and control for known confounders. For example, in the study of smoking and CVD events, an investigator might identify cases that have had a heart attack or stroke and then select controls of similar age, gender, and body weight to the cases. For case-control studies, it is important that if matching was performed during the selection or recruitment process, the variables used as matching criteria (e.g., age, gender, race) should be controlled for in the analysis.
General Guidance for Determining the Overall Quality Rating of Case-Controlled Studies
NHLBI designed the questions in the assessment tool to help reviewers focus on the key concepts for evaluating a study's internal validity, not to use as a list from which to add up items to judge a study's quality.
Internal validity for case-control studies is the extent to which the associations between disease and exposure reported in the study can truly be attributed to the exposure being evaluated rather than to flaws in the design or conduct of the study. In other words, what is ability of the study to draw associative conclusions about the effects of the exposures on outcomes? Any such flaws can increase the risk of bias.
In critical appraising a study, the following factors need to be considered: risk of potential for selection bias, information bias, measurement bias, or confounding (the mixture of exposures that one cannot tease out from each other). Examples of confounding include co-interventions, differences at baseline in patient characteristics, and other issues addressed in the questions above. High risk of bias translates to a poor quality rating; low risk of bias translates to a good quality rating. Again, the greater the risk of bias, the lower the quality rating of the study.
In addition, the more attention in the study design to issues that can help determine whether there is a causal relationship between the outcome and the exposure, the higher the quality of the study. These include exposures occurring prior to outcomes, evaluation of a dose-response gradient, accuracy of measurement of both exposure and outcome, sufficient timeframe to see an effect, and appropriate control for confounding–all concepts reflected in the tool.
If a study has a "fatal flaw," then risk of bias is significant; therefore, the study is deemed to be of poor quality. An example of a fatal flaw in case-control studies is a lack of a consistent standard process used to identify cases and controls.
Generally, when reviewers evaluated a study, they did not see a "fatal flaw," but instead found some risk of bias. By focusing on the concepts underlying the questions in the quality assessment tool, reviewers examined the potential for bias in the study. For any box checked "no," reviewers asked, "What is the potential risk of bias resulting from this flaw in study design or execution?" That is, did this factor lead to doubt about the results reported in the study or the ability of the study to accurately assess an association between exposure and outcome?
By examining questions in the assessment tool, reviewers were best able to assess the potential for bias in a study. Specific rules were not useful, as each study had specific nuances. In addition, being familiar with the key concepts helped reviewers assess the studies. Examples of studies rated good, fair, and poor were useful, yet each study had to be assessed on its own.
Quality Assessment Tool for Before-After (Pre-Post) Studies With No Control Group - Study Quality Assessment Tools
Guidance for Assessing the Quality of Before-After (Pre-Post) Studies With No Control Group
Question 1. Study question
Question 2. Eligibility criteria and study population
Did the authors describe the eligibility criteria applied to the individuals from whom the study participants were selected or recruited? In other words, if the investigators were to conduct this study again, would they know whom to recruit, from where, and from what time period?
Here is a sample description of a study population: men over age 40 with type 2 diabetes, who began seeking medical care at Phoenix Good Samaritan Hospital, between January 1, 2005 and December 31, 2007. The population is clearly described as: (1) who (men over age 40 with type 2 diabetes); (2) where (Phoenix Good Samaritan Hospital); and (3) when (between January 1, 2005 and December 31, 2007). Another sample description is women who were in the nursing profession, who were ages 34 to 59 in 1995, had no known CHD, stroke, cancer, hypercholesterolemia, or diabetes, and were recruited from the 11 most populous States, with contact information obtained from State nursing boards.
To assess this question, reviewers examined prior papers on study methods (listed in reference list) when necessary.
Question 3. Study participants representative of clinical populations of interest
The participants in the study should be generally representative of the population in which the intervention will be broadly applied. Studies on small demographic subgroups may raise concerns about how the intervention will affect broader populations of interest. For example, interventions that focus on very young or very old individuals may affect middle-aged adults differently. Similarly, researchers may not be able to extrapolate study results from patients with severe chronic diseases to healthy populations.
Question 4. All eligible participants enrolled
To further explore this question, reviewers may need to ask: Did the investigators develop the I/E criteria prior to recruiting or selecting study participants? Were the same underlying I/E criteria used for all research participants? Were all subjects who met the I/E criteria enrolled in the study?
Question 5. Sample size
Did the authors present their reasons for selecting or recruiting the number of individuals included or analyzed? Did they note or discuss the statistical power of the study? This question addresses whether there was a sufficient sample size to detect an association, if one did exist.
An article's methods section may provide information on the sample size needed to detect a hypothesized difference in outcomes and a discussion on statistical power (such as, the study had 85 percent power to detect a 20 percent increase in the rate of an outcome of interest, with a 2-sided alpha of 0.05). Sometimes estimates of variance and/or estimates of effect size are given, instead of sample size calculations. In any case, if the reviewers determined that the power was sufficient to detect the effects of interest, then they would answer "yes" to Question 5.
Question 6. Intervention clearly described
Another pertinent question regarding interventions is: Was the intervention clearly defined in detail in the study? Did the authors indicate that the intervention was consistently applied to the subjects? Did the research participants have a high level of adherence to the requirements of the intervention? For example, if the investigators assigned a group to 10 mg/day of Drug A, did most participants in this group take the specific dosage of Drug A? Or did a large percentage of participants end up not taking the specific dose of Drug A indicated in the study protocol?
Reviewers ascertained that changes in study outcomes could be attributed to study interventions. If participants received interventions that were not part of the study protocol and could affect the outcomes being assessed, the results could be biased.
Question 7. Outcome measures clearly described, valid, and reliable
Were the outcomes defined in detail? Were the tools or methods for measuring outcomes accurate and reliable–for example, have they been validated or are they objective? This question is important because the answer influences confidence in the validity of study results.
An example of an outcome measure that is objective, accurate, and reliable is death–the outcome measured with more accuracy than any other. But even with a measure as objective as death, differences can exist in the accuracy and reliability of how investigators assessed death. For example, did they base it on an autopsy report, death certificate, death registry, or report from a family member? Another example of a valid study is one whose objective is to determine if dietary fat intake affects blood cholesterol level (cholesterol level being the outcome) and in which the cholesterol level is measured from fasting blood samples that are all sent to the same laboratory. These examples would get a "yes."
An example of a "no" would be self-report by subjects that they had a heart attack, or self-report of how much they weight (if body weight is the outcome of interest).
Question 8. Blinding of outcome assessors
Blinding or masking means that the outcome assessors did not know whether the participants received the intervention or were exposed to the factor under study. To answer the question above, the reviewers examined articles for evidence that the person(s) assessing the outcome(s) was masked to the participants' intervention or exposure status. An outcome assessor, for example, may examine medical records to determine the outcomes that occurred in the exposed and comparison groups. Sometimes the person applying the intervention or measuring the exposure is the same person conducting the outcome assessment. In this case, the outcome assessor would not likely be blinded to the intervention or exposure status. A reviewer would note such a finding in the comments section of the assessment tool.
In assessing this criterion, the reviewers determined whether it was likely that the person(s) conducting the outcome assessment knew the exposure status of the study participants. If not, then blinding was adequate. An example of adequate blinding of the outcome assessors is to create a separate committee whose members were not involved in the care of the patient and had no information about the study participants' exposure status. Using a study protocol, committee members would review copies of participants' medical records, which would be stripped of any potential exposure information or personally identifiable information, for prespecified outcomes.
Question 9. Followup rate
Higher overall followup rates are always desirable to lower followup rates, although higher rates are expected in shorter studies, and lower overall followup rates are often seen in longer studies. Usually an acceptable overall followup rate is considered 80 percent or more of participants whose interventions or exposures were measured at baseline. However, this is a general guideline.
In accounting for those lost to followup, in the analysis, investigators may have imputed values of the outcome for those lost to followup or used other methods. For example, they may carry forward the baseline value or the last observed value of the outcome measure and use these as imputed values for the final outcome measure for research participants lost to followup.
Question 10. Statistical analysis
Were formal statistical tests used to assess the significance of the changes in the outcome measures between the before and after time periods? The reported study results should present values for statistical tests, such as p values, to document the statistical significance (or lack thereof) for the changes in the outcome measures found in the study.
Question 11. Multiple outcome measures
Were the outcome measures for each person measured more than once during the course of the before and after study periods? Multiple measurements with the same result increase confidence that the outcomes were accurately measured.
Question 12. Group-level interventions and individual-level outcome efforts
Group-level interventions are usually not relevant for clinical interventions such as bariatric surgery, in which the interventions are applied at the individual patient level. In those cases, the questions were coded as "NA" in the assessment tool.
General Guidance for Determining the Overall Quality Rating of Before-After Studies
The questions in the quality assessment tool were designed to help reviewers focus on the key concepts for evaluating the internal validity of a study. They are not intended to create a list from which to add up items to judge a study's quality.
Internal validity is the extent to which the outcome results reported in the study can truly be attributed to the intervention or exposure being evaluated, and not to biases, measurement errors, or other confounding factors that may result from flaws in the design or conduct of the study. In other words, what is the ability of the study to draw associative conclusions about the effects of the interventions or exposures on outcomes?
Critical appraisal of a study involves considering the risk of potential for selection bias, information bias, measurement bias, or confounding (the mixture of exposures that one cannot tease out from each other). Examples of confounding include co-interventions, differences at baseline in patient characteristics, and other issues throughout the questions above. High risk of bias translates to a rating of poor quality; low risk of bias translates to a rating of good quality. Again, the greater the risk of bias, the lower the quality rating of the study.
In addition, the more attention in the study design to issues that can help determine if there is a causal relationship between the exposure and outcome, the higher quality the study. These issues include exposures occurring prior to outcomes, evaluation of a dose-response gradient, accuracy of measurement of both exposure and outcome, and sufficient timeframe to see an effect.
Generally, when reviewers evaluate a study, they will not see a "fatal flaw," but instead will find some risk of bias. By focusing on the concepts underlying the questions in the quality assessment tool, reviewers should ask themselves about the potential for bias in the study they are critically appraising. For any box checked "no" reviewers should ask, "What is the potential risk of bias resulting from this flaw in study design or execution?" That is, does this factor lead to doubt about the results reported in the study or doubt about the ability of the study to accurately assess an association between the intervention or exposure and the outcome?
The best approach is to think about the questions in the assessment tool and how each one reveals something about the potential for bias in a study. Specific rules are not useful, as each study has specific nuances. In addition, being familiar with the key concepts will help reviewers be more comfortable with critical appraisal. Examples of studies rated good, fair, and poor are useful, but each study must be assessed on its own.
Quality Assessment Tool for Case Series Studies - Study Quality Assessment Tools
Background: development and use - study quality assessment tools.
Learn more about the development and use of Study Quality Assessment Tools.
Last updated: July, 2021
Integrating quality in resource-constrained time-cost trade-off optimization for civil construction projects using NSGA-III technique
- Published: 18 May 2024
Cite this article
- Ankit Shrivastava 1 &
- Mukesh Pandey 2
In the realm of civil construction projects, achieving an optimal balance between project time, cost, and quality is paramount for ensuring project success and stakeholder satisfaction. Traditional optimization approaches often focus solely on time and cost, potentially neglecting the critical aspect of quality. This study presents a novel framework aimed at integrating quality considerations into resource-constrained time-cost trade-off optimization process using Non-dominated Sorting Genetic Algorithm III (NSGA III) technique. The proposed framework addresses the inherent trade-offs among time, cost, and quality by simultaneously optimizing these objectives. By leveraging NSGA-III, a powerful multi-objective optimization algorithm, the framework generates a set of Pareto-optimal solutions that represent various trade-off options. This enables decision-makers to explore and select solutions that best align with project objectives and constraints. Through solving a real case study project, the effectiveness of the proposed framework is demonstrated in real-world civil construction projects. Results of the study indicate that integrating quality considerations into the time-cost trade-off optimization process leads to more informed decision-making, ultimately enhancing project outcomes and stakeholder satisfaction. This research contributes to advancing the field of project management in civil construction by providing a systematic approach for addressing the complex interplay of time, cost, and quality objectives.
This is a preview of subscription content, log in via an institution to check access.
Access this article
Price includes VAT (Russian Federation)
Instant access to the full article PDF.
Rent this article via DeepDyve
Institutional subscriptions
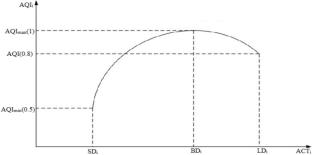
Similar content being viewed by others
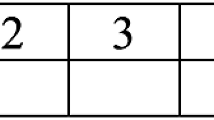
Optimizing construction time, cost, and quality: a hybrid AHP-NSGA-II optimization model
NSGA-III-Based Time–Cost–Environmental Impact Trade-Off Optimization Model for Construction Projects
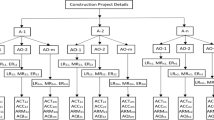
Construction time–cost–resources–quality trade-off optimization using NSGA-III
Data availability.
The related data is available from corresponding author upon reasonable request.
Afshar, A., Kaveh, A., & Shoghli, O. R. (2007). Multi-objective optimization of time-cost-quality using multi-colony ant algorithm. Fuzzy Sets and Systems, 8 (2), 113–124.
Google Scholar
Aminbakhsh, S., & Sonmez, R. (2016). Pareto front particle swarm optimizer for discrete time-cost trade-off problem. Journal of Computing in Civil Engineering . https://doi.org/10.1061/(ASCE)CP.1943-5487.0000606
Article Google Scholar
Babu, A. J. G., & Suresh, N. (1996). Project management with time, cost, and quality considerations. European Journal of Operational Research . https://doi.org/10.1016/0377-2217(94)00202-9
Das, I., & Dennis, J. E. (1998). Normal-boundary intersection: A new method for generating the Pareto surface in nonlinear multicriteria optimization problems. SIAM Journal on Optimization . https://doi.org/10.1137/S1052623496307510
Article MathSciNet Google Scholar
Deb, K., & Goyal, M. (1996). A combined genetic adaptive search (GeneAS) for engineering design. Computer Science and Informatics . http://citeseerx.ist.psu.edu/viewdoc/download?doi=10.1.1.27.767&rep=rep1&type=pdf
Deb, K., & Jain, H. (2014). An evolutionary many-objective optimization algorithm using reference-point-based nondominated sorting approach, part I: Solving problems with box constraints. IEEE Transactions on Evolutionary Computation . https://doi.org/10.1109/TEVC.2013.2281535
Eirgash, M. A., & Toğan, V. (2023). A novel oppositional teaching learning strategy based on the golden ratio to solve the time-cost-environmental impact trade-off optimization problems. Expert Systems with Applications . https://doi.org/10.1016/j.eswa.2023.119995
Elbeltagi, E., Ammar, M., Sanad, H., & Kassab, M. (2016). Overall multiobjective optimization of construction projects scheduling using particle swarm. Engineering, Construction and Architectural Management. https://doi.org/10.1108/ECAM-11-2014-0135
Eshtehardian, E., Afshar, A., & Abbasnia, R. (2009). Fuzzy-based MOGA approach to stochastic time-cost trade-off problem. Automation in Construction . https://doi.org/10.1016/j.autcon.2009.02.001
Ferreira, J. C., Fonseca, C. M., & Gaspar-Cunha, A. (2007). Methodology to select solutions from the Pareto-optimal set: A comparative study. In: Proceedings of GECCO 2007: Genetic and evolutionary computation conference . https://doi.org/10.1145/1276958.1277117
Huang, Y. S., Deng, J. J., & Zhang, Y. Y. (2008). TI time-cost-quality tradeoff optimization in construction project based on modified ant colony algorithm. In: Proceedings of the 7th international conference on machine learning and cybernetics, ICMLC , 2 (July), 1031–1035. https://doi.org/10.1109/ICMLC.2008.4620556
Kalhor, E., Khanzadi, M., Eshtehardian, E., & Afshar, A. (2011). Stochastic time-cost optimization using non-dominated archiving ant colony approach. Automation in Construction . https://doi.org/10.1016/j.autcon.2011.05.003
Kaveh, A. (2014). Advances in metaheuristic algorithms for optimal design of structures. In: Advances in metaheuristic algorithms for optimal design of structures (Vol. 9783319055). https://doi.org/10.1007/978-3-319-05549-7
Kaveh, A., Dadras, A., & Malek, N. G. (2018). Robust design optimization of laminated plates under uncertain bounded buckling loads. Struct Multidisc Optim , 59 , 877–891. https://doi.org/10.1007/s00158-018-2106-0
Kaveh, A., Fahimi-Farzam, M., & Kalateh-Ahani, M. (2015). Performance-based multi-objective optimal design of steel frame structures: Nonlinear dynamic procedure. Scientia Iranica, 22 (2), 373–387.
Kaveh, A., & Ilchi Ghazaan, M. (2020). A new VPS-based algorithm for multi-objective optimization problems. Engineering with Computers, 36 (3), 1029–1040. https://doi.org/10.1007/s00366-019-00747-8
Kaveh, A., Izadifard, R. A., & Mottaghi, L. (2020). Optimal design of planar RC frames considering CO2 emissions using ECBO, EVPS and PSO metaheuristic algorithms. Journal of Building Engineering, 28 , 101014. https://doi.org/10.1016/j.jobe.2019.101014
Kaveh, A., & Laknejadi, K. (2011). A novel hybrid charge system search and particle swarm optimization method for multi-objective optimization. Expert Systems with Applications, 38 (12), 15475–15488. https://doi.org/10.1016/j.eswa.2011.06.012
Kaveh, A., & Laknejadi, K. (2013). A new multi-swarm multi-objective optimization method for structural design. Advances in Engineering Software, 58 , 54–69. https://doi.org/10.1016/j.advengsoft.2013.01.004
Kaveh, A., Moghanni, R. M., & Javadi, S. M. (2019). Ground motion record selection using multi-objective optimization algorithms: A comparative study. Periodica Polytechnica Civil Engineering, 63 (3), 812–822. https://doi.org/10.3311/PPci.14354
Ke, H. (2014). A genetic algorithm-based optimizing approach for project time-cost trade-off with uncertain measure. Journal of Uncertainty Analysis and Applications . https://doi.org/10.1186/2195-5468-2-8
Kelley, J. E., & Walker, M. R. (1959). Critical-path planning and scheduling. Proceedings of the Eastern Joint Computer Conference, IRE-AIEE-ACM, 1959 , 160–173. https://doi.org/10.1145/1460299.1460318
Khalili-Damghani, K., Tavana, M., Abtahi, A. R., & Santos Arteaga, F. J. (2015). Solving multi-mode time-cost-quality trade-off problems under generalized precedence relations. Optimization Methods and Software . https://doi.org/10.1080/10556788.2015.1005838
Luong, D. L., Tran, D. H., & Nguyen, P. T. (2018). Optimizing multi-mode time-cost-quality trade-off of construction project using opposition multiple objective difference evolution. International Journal of Construction Management . https://doi.org/10.1080/15623599.2018.1526630
Mohamad Karimi, S., Jamshid Mousavi, S., Kaveh, A., & Afshar, A. (2007). Fuzzy optimization model for earthwork allocations with imprecise parameters. Journal of Construction Engineering and Management, 133 (2), 181–190. https://doi.org/10.1061/(asce)0733-9364(2007)133:2(181)
Narayanan, S., Kure, A. M., & Palaniappan, S. (2019). Study on time and cost overruns in mega infrastructure projects in India. Journal of the Institution of Engineers (india): Series A . https://doi.org/10.1007/s40030-018-0328-1
Panwar, A., Tripathi, K. K., & Jha, K. N. (2019). A qualitative framework for selection of optimization algorithm for multi-objective trade-off problem in construction projects. Engineering, Construction and Architectural Management. https://doi.org/10.1108/ECAM-06-2018-0246
Patil, A. S., Agarwal, A. K., Sharma, K., & Trivedi, M. K. (2024). Time-cost trade-off optimization model for retrofitting planning projects using MOGA. Asian Journal of Civil Engineering . https://doi.org/10.1007/s42107-024-01014-y
Sharma, K., & Trivedi, M. K. (2021). Development of multi-objective scheduling model for construction projects using opposition-based NSGA III. Journal of the Institution of Engineers (india): Series A . https://doi.org/10.1007/s40030-021-00529-w
Sharma, K., & Trivedi, M. K. (2022a). Artificial intelligence and sustainable computing: Proceedings of ICSISCET 2020. In H. M. Dubey, M. Pandit, L. Srivastava, & B. K. Panigrahi (Eds.), AHP and NSGA-II-based time–cost–quality trade-off optimization model for construction projects (pp. 45–63). Singapore: Springer. https://doi.org/10.1007/978-981-16-1220-6_5
Chapter Google Scholar
Sharma, K., & Trivedi, M. K. (2022b). Latin hypercube sampling-based NSGA-III optimization model for multimode resource constrained time–cost–quality–safety trade-off in construction projects. International Journal of Construction Management, 22 (16), 3158–3168. https://doi.org/10.1080/15623599.2020.1843769
Sharma, K., & Trivedi, M. K. (2023a). Discrete OBNSGA III method-based robust multi-objective scheduling model for civil construction projects. Asian Journal of Civil Engineering, 24 (7), 2247–2264. https://doi.org/10.1007/s42107-023-00638-w
Sharma, K., & Trivedi, M. K. (2023b). Modelling the resource constrained time-cost-quality-safety risk-environmental impact trade-off using opposition-based NSGA III. Asian Journal of Civil Engineering, 24 (8), 3083–3098. https://doi.org/10.1007/s42107-023-00696-0
Sharma, K., & Trivedi, M. K. (2023c). Statistical analysis of delay-causing factors in Indian highway construction projects under hybrid annuity model. Transportation Research Record, 2677 (10), 572–591. https://doi.org/10.1177/03611981231161594
Srinivas, N., & Deb, K. (1994). Muiltiobjective optimization using nondominated sorting in genetic algorithms. Evolutionary Computation . https://doi.org/10.1162/evco.1994.2.3.221
Tiwari, S., & Johari, S. (2015). Project scheduling by integration of time cost trade-off and constrained resource scheduling. Journal of the Institution of Engineers (india): Series A, 96 (1), 37–46. https://doi.org/10.1007/s40030-014-0099-2
Trivedi, M. K., & Sharma, K. (2023). Construction time–cost–resources–quality trade-off optimization using NSGA-III. Asian Journal of Civil Engineering, 24 (8), 3543–3555. https://doi.org/10.1007/s42107-023-00731-0
Wang, T., Abdallah, M., Clevenger, C., & Monghasemi, S. (2021). Time–cost–quality trade-off analysis for planning construction projects. Engineering, Construction and Architectural Management, 28 (1), 82–100. https://doi.org/10.1108/ECAM-12-2017-0271
Wang, X., Tang, Y., Song, R., & Li, J. (2023). A new model for investigating the effective factors in the development of modern clinical and health services in the time of COVID-19. Information Systems and E-Business Management . https://doi.org/10.1007/s10257-022-00616-w
Zheng, H. (2017). The bi-level optimization research for time-cost-quality-environment trade-off scheduling problem and its application to a construction project. Advances in Intelligent Systems and Computing . https://doi.org/10.1007/978-981-10-1837-4_62
Download references
Not applicable.
Author information
Authors and affiliations.
Civil Engineering Department, Institute of Technology and Management University, Gwalior, India
Ankit Shrivastava
Mukesh Pandey
You can also search for this author in PubMed Google Scholar
Contributions
Ankit Shrivastava wrote the main manuscript and Mukesh Pandey reviewed the manuscript.
Corresponding author
Correspondence to Ankit Shrivastava .
Ethics declarations
Conflict of interest.
The authors declare no competing interests.
Additional information
Publisher's note.
Springer Nature remains neutral with regard to jurisdictional claims in published maps and institutional affiliations.
Rights and permissions
Springer Nature or its licensor (e.g. a society or other partner) holds exclusive rights to this article under a publishing agreement with the author(s) or other rightsholder(s); author self-archiving of the accepted manuscript version of this article is solely governed by the terms of such publishing agreement and applicable law.
Reprints and permissions
About this article
Shrivastava, A., Pandey, M. Integrating quality in resource-constrained time-cost trade-off optimization for civil construction projects using NSGA-III technique. Asian J Civ Eng (2024). https://doi.org/10.1007/s42107-024-01068-y
Download citation
Received : 29 April 2024
Accepted : 02 May 2024
Published : 18 May 2024
DOI : https://doi.org/10.1007/s42107-024-01068-y
Share this article
Anyone you share the following link with will be able to read this content:
Sorry, a shareable link is not currently available for this article.
Provided by the Springer Nature SharedIt content-sharing initiative
- Construction projects
- Optimization
- Find a journal
- Publish with us
- Track your research

Many Chinese automotive companies have built comprehensive R&D systems that feature popular R&D tools and high-level informatization. But they are still facing problems such as lengthy R&D processes and difficulty in adapting to the new automotive lifecycle. R&D collaboration becomes critical in optimizing R&D processes and improving R&D efficiency. With changing customer needs and industry trends, innovation iteration and project delivery are running in parallel at a high speed, increasing the difficulty of quality management and leading automakers to set a higher bar for their suppliers’ quality traceability.
This presented a challenge for Yanfeng Electronic Technology, a subsidiary of leading global automotive supplier Yanfeng Auto. In a recent project, a leading automotive customer required Yanfeng Electronic Technology to acquire AutomotiveSPICE certification (a process evaluation model for the automotive industry jointly developed by major European automakers) and provide detailed traceability covering customer demands, including system, software, architecture design and test cases. Previously, Yanfeng Electronic Technology simply used spreadsheets, the granularity of which did not meet the customer’s demand, and content maintenance was also inefficient. In this light, Yanfeng Electronic Technology aimed to improve its own R&D capabilities and business operation, and it sought to quickly understand whether the coverage of customer needs and test coverage were being properly completed through visualization.
The IBM® Engineering Lifecycle Management (ELM) platform is the market-leading solution for efficient R&D management, consisting of systems and software engineering management tools that provide full lifecycle development management from early design to final vehicle inspection and certification. It also helps manage automotive compliance and compliance with regulatory requirements outlined by standards, including Automotive SPICE (ASPICE), ISO-26262, SOTIF, ISO/SAE 21434 and WP.29.
Impressed by the traceability of IBM ELM, Yanfeng Electronic Technology chose to work with IBM to create a more efficient ELM platform, and currently uses three of the modules to coordinate application development and monitor its quality and functionality:
- DOORS Next Requirement Management . It not only has the requirement management function to support demand collaboration and demand development technology, but also adds AI capabilities that guarantee the high quality of engineering requirements, reducing defect costs by up to 60% and manual review costs by 25% and accelerating time-to-market.
- Rhapsody Design . Covering architecture design, code generation, requirements model simulation and other functions, it also supports the full set of AUTOSAR frameworks that allow engineers to carry out complete AUTOSAR design and establish connections with requirements, tests, tasks, plans, defects, etc.
- Extended Warehouse Management (EMW) work items . As a workflow management module, EWM not only covers project management, plan management, task management and change management, but also supports problem management, issue management and defect management. It also supports code repository management and CICD, supporting DevOps and more development management modes through workflows, ensuring orderly, efficient and agile R&D workflow.
By deploying IBM ELM, Yanfeng Electronic Technology’s core goal is to comprehensively improve the quality of the company’s products. IBM ELM can help it quantitatively evaluate the quality of requirements management, design, test cases, and realize the data correlation of the whole process, improving the visibility of quality status of software products in real time.
Deng Xiaohui, Security and Sustainability Software Leader at IBM Greater China Group, says: “For traditional and emerging auto players alike, R&D efficiency is a battleground for value add. As a derivative and optimization of IBM Rational software, IBM ELM has been successfully adopted by many leading automotive companies. From Rational to ELM, IBM combines its expertise of automotive R&D with its technology prowess that help clients build an efficient, high-quality, and highly collaborative R&D management platform. In co-creating with Yanfeng Auto, we have worked closely in the fields of engineering lifecycle management and equipment lifecycle management to help it improve R&D efficiency and quality, and integrate agile development and rapid iteration into the whole process.”
ELM is in line with Yanfeng Auto’s pursuit of an efficient R&D and operation system. At present, Yanfeng Auto engineers can quickly integrate into work scenarios according to different product types, project levels and development standards in the ELM system, and complete role-based delivery, which greatly improves the delivery rate and quality.
It was difficult to understand the whole picture of quality management when Yanfeng Electronic Technology used tools such as Microsoft Word and Excel. Nowadays, issues such as requirements completion, design coverage and test coverage can be answered through the implementation of the IBM ELM tool chain, so as to achieve transparency of quality data at all stages, so that quality management can be transformed into forward control and prediction, rather than passive detection in the later stage.
Given the fierce competition, reducing costs and increasing efficiency is a major goal for auto players. According to a project leader at Yanfeng Electronic Technology, unlike what many have assumed, emphasizing traceability will not affect development efficiency and delivery speed; on the contrary, it improves efficiency. “If you only focus on delivery time, skip the part of requirements and design, and write code immediately, the quality cannot be guaranteed.”
In terms of IBM ELM empowerment, model reuse can save a lot of effort. For example, the Rhapsody model developed in previous projects can be inherited and easily updated according to new requirements, and the components and functions in it can be added or subtracted.
Thanks to IBM ELM, Yanfeng Electronic Technology was one of the two Chinese suppliers that passed Volkswagen’s AutomotiveSPICE Level 1 assessment in 2019. In 2016, Yanfeng Electronic Technology obtained the certification of AutomotiveSPICE Level 2 with the help of RQM (Rational Quality Management), the previous version of ELM, and passed the AutomotiveSPICE Level 3 assessment in 2020.
Li Fan, Deputy General Manager at Yanfeng Electronic Technology, adds: “As an end-to-end solution, ELM helped us achieve the integrated management of scalable R&D processes. Based on that, we are building a more integrated, sustainable and intelligent R&D platform to further reduce cost and increase efficiency. In the future, we hope to work closely with IBM in exporting Chinese models of manufacturing efficiency to the world for the benefit of the entire automotive industry, and making more progress in the field of Internet of Vehicles in addition to intelligent cockpit.”
Yanfeng (link resides outside of ibm.com) is a leading global automotive supplier focusing on interior, exterior, seating, cockpit electronics and passive safety. Yanfeng has more than 240 sites and approximately 57,000 employees worldwide. The technical team of 4,100 experts are based at 14 R&D centers and other regional offices, with complete capabilities including engineering and software development, design, as well as testing and validation. Focusing on smart cabin and lightweight technology, Yanfeng supports automakers as they explore future mobility spaces and provide leading cabin solutions.
Yanfeng Electronic Technology focuses on the field of intelligent cockpit, with the cockpit domain controller at its core, integrating the cockpit electronic system of interactive intelligence, scenario intelligence and personalized services, and collaborating with the innovation of user interface products such as interior, seat and safety, to provide consumers with a more intelligent and convenient interactive experience, and help automakers explore the future mobility space. Yanfeng Electronic Technology is committed to becoming an industry-leading provider of automotive cockpit electronic products and system solutions.
Discover more ways to design complex products efficiently and on time in highly regulated industries.
© Copyright IBM Corporation 2024. IBM and the IBM logo are trademarks or registered trademarks of IBM Corp., in the U.S. and/or other countries. This document is current as of the initial date of publication and may be changed by IBM at any time. Not all offerings are available in every country in which IBM operates.
Client examples are presented as illustrations of how those clients have used IBM products and the results they may have achieved. Actual performance, cost, savings or other results in other operating environments may vary.

2-way Texting
A better way to communicate with vehicle owners by text

Digital Vehicle Inspection
A better way to DVI with a best in class digital vehicle inspection
Streamline and standardize your process to automate your profits
Track technician efficiency in your bays with our digital work order.

Quality Control
Avoid costly mistakes with a digital quality control process
The easiest to boost your authentic online review score
CRM Marketing Module
Automate your customer follow-up and vehicle service reminders
Rewards & Referrals
Easy-to-use rewards program with a built-in innovative referral tool
Remote Payments
Text invoices to customers allowing them to pay from anywhere

Online scheduling for your website with calendar integration
Quality Control Case Study
Golden Rule Auto Care

- Is there grease on the hood, door, steering wheel, console, etc.?
- Are check engine or warning lights on?
- If an oil change was performed, are lights reset and sticker placed in windshield?
- Are tools left in the vehicle?
- Is the work completed and the vehicle fully reassembled?
- Are all caps put back on?
- Are all belts and hoses tightened?
- Are all fluids filled up?
- Are the tires properly inflated?
Chris found that 80% of the vehicles he inspected had one or more of these issues .. He presented the data to his team, and it was decided something had to change; a quality control process was to be implemented.

Quality control is a process followed by nearly all Fortune 500 companies to ensure that top quality work and performance are met on every single product and service. With his software engineering background, Chris applied the practice of quality control from the software industry to the auto repair industry. Software is not released until a separate team or person reviews and tests it for bugs.
Click here for a free quality control checklist 1
Click here for a free quality control checklist 2
Following is the plan that was implemented at Golden Rule Auto Care:
- Every vehicle was to be inspected and wiped down by someone at the front counter.
- If a vehicle had driveability work performed, a counter person would perform another test drive and complete the quality control inspection.
- The results were to be captured in a monthly report for employees to see the effectiveness of their new quality process.
- Golden Rule Auto Care wanted to celebrate the fact that they were finding the issues vs. the customer but also wanted to make sure the technicians were not using the quality control process as a crutch to not properly complete their jobs.
- The customer would be informed about the process as an added advantage to the shop and invited to observe or even participate in the quality control inspection.
Since initiating the quality control process, Chris has created a quality control checklist in Autoflow .
Note: There was push back from the counter people and technicians.
- Counter people said they didn’t have time but agreed change was needed with an 80% quality issue. It turned out, one average “QC” or quality control inspection took 5 to 10 minutes.
- Technicians felt they were being disrespected by someone reviewing their work. They were assured that mistakes are common and needed to be caught by the shop and not by customers.
- Customer Retention – Customers will keep coming back, knowing that they received top quality service the first time and every time they visit your shop. Loyal customers are worth 10 times their initial visit . It is 6-7 times more expensive to acquire a new customer than it is to keep an existing one.
- Positive Reputation – It takes 12 positive consumer experiences to make up for one bad consumer experience . Consumers are likely to tell 10-15 people about their bad experience.
- Add-on Sales – A service writer/counter person may notice something that was originally not written up, such as a past due oil change sticker that a technician missed, which can lead to add-on sales.
- Quality control time without drive time: 5 to 10 minutes
- Quality control time with drive time: 15 to 20 minutes
- Golden Rule Auto Care’s average: 12 minutes
- KPI or goal for Golden Rule Auto Care’s QC issues per month: 10% or less
- This year, they have run as high as 25% and as low as 9% per month.
- Most common issue found at Golden Rule Auto Care: grease on handle, door panel, seat, console, floor, kick plate, etc.
- Second most common issue found: fluids not full
- Intake boot under upper radiator hose was disconnected and pressed against exhaust manifold…melting & smoking.
- Vehicle started leaking a large amount of coolant due to incorrect installation.
- Positive battery terminal not fully tightened.
- Scanner still plugged in (these can get expensive to give away!)
Following is a snapshot of Golden Rule Auto Care’s monthly report:

Master data management: The key to getting more from your data
Picture this: a sales representative at a multibillion-dollar organization has an upcoming meeting with a prospective client. She searches for the client in the organization’s customer relationship management software and finds several accounts with the same name. She struggles to learn more about the products and services the client is already buying, the customer contacts that have already been engaged, and the relationships the contact may have with other sales representatives within the organization. As a result, the sales representative spends several hours manually pulling together information to get organized for the upcoming meeting.
About the authors
This article is a collaborative effort by Aziz Shaikh, Holger Harreis , Jorge Machado , and Kayvaun Rowshankish , with Rachit Saxena and Rajat Jain, representing views from McKinsey Digital.
This scenario is an example of poor master data management (MDM), which commonly results in suboptimal customer and employee experience, higher costs, and lost revenue opportunities. MDM is a critical component of any organization’s data strategy (see sidebar “About master data management”). These capabilities can make or break an organization’s efficiency and reliability—particularly in complex organizations with multiple business units, where data silos can lead to inefficiencies and errors.
About master data management
Typically, organizations have four types of data: transaction, reference, derived, and master. Of these, master data provides the most relevant, foundational information about entities and their attributes, unique identifiers, hierarchies, and relationships within an organization. This information is shared across business functions and systems to support business processes and decision making.
In 2023, McKinsey surveyed more than 80 large global organizations 1 Companies surveyed earned more than $100 million in annual revenue. across several industries to learn more about how they organize, use, and mature their master data. McKinsey’s Master Data Management Survey indicated that organizations have four top objectives in maturing their MDM capabilities: improving customer experience and satisfaction, enhancing revenue growth by presenting better cross- and up-selling opportunities, increasing sales productivity, and streamlining reporting (Exhibit 1).
MDM plays an important role with modern data architecture concepts and creates value in five ways:
- MDM cleans, enriches, and standardizes data for key functions, such as customer or product data, before it is loaded into the data lake. In this way, MDM ensures that data is accurate, complete, and consistent across an organization.
- In the context of data products, MDM provides a hub for high-quality data across entities, which improves the effectiveness, consistency, and reliability of data products for improved decision making, accurate reporting and analysis, and compliance with local regulations and standards.
- MDM standardizes data across entities to provide a unified view across various systems.
- MDM can act as a system of reference that shares data with applications and other domains via web services, typically representational state transfer application programming interfaces (REST APIs).
- MDM and artificial intelligence (AI) can benefit from each other. For instance, MDM can leverage AI algorithms to identify duplicate records and merge them intelligently, which can enhance the performance and reliability of generative AI systems.
But many organizations have not fully harnessed the potential of MDM. This article builds on the insights from our MDM survey, describes the common challenges companies face when integrating MDM capabilities, and highlights areas in which MDM could be optimized to help businesses gain a competitive advantage.
Common issues organizations face when implementing MDM
Small and large organizations alike can benefit from implementing MDM models, yet collecting and aggregating quality data can be difficult because of funding constraints, insufficient technological support, and low-caliber data. Based on our survey results, following are some of the most prevalent challenges to implementing MDM.
Difficulty of making a business case
Demonstrating potential savings through reduced data errors, enhanced operational efficiency, and improved decision making can provide a clear return on investment for MDM initiatives. However, this return is inherently difficult to quantify, so positioning MDM as a priority ahead of projects with more visible, immediate benefits can be challenging. Consequently, despite MDM’s potential to enhance an organization, leaders may have a difficult time building a business case for augmenting their MDM and investing in associated architecture and technology capabilities.

Creating value beyond the hype
Let’s deliver on the promise of technology from strategy to scale.
Organizational silos
Types of master data domains.
A variety of categories can serve as master data domains, and each serves a specific purpose. The most common categories include the following:
Customer data. Customer data includes key details such as customer contact information, purchasing history, preferences, and demographic data. Organizations can leverage customer data to optimize marketing strategies, personalize customer experiences, and foster long-term relationships.
Client data. Client data typically includes client names, contact information, billing and shipping addresses, payment terms, key decision makers, and other client-specific identifiers. Business-to-business (B2B) organizations can manage client data to tailor their strategies, personalize communications, and optimize sales and marketing efforts to better serve their clients’ needs and preferences.
Product data. Product data includes attributes such as product names, descriptions, SKUs, pricing, and specifications. Product data typically spans across R&D, supply chain, and sales.
Supplier data. Supplier data includes attributes such as vendor names, contact details, payment terms, tax information, and vendor-specific codes. Accurate supplier data helps to establish a single, complete, and consistent definition of vendors across the organization.
Financial data. Financial data typically includes information about legal or management entities (a company code, for instance), a chart of accounts, cost and profit centers, and financial hierarchies.
Employee data. Employee data includes attributes such as employee names, contact information, job titles, employee IDs, department assignments, and payroll information.
Asset data. Asset data includes attributes such as asset name, type, purchase date, installation date, manufacturer details, financial and depreciation details, and maintenance and repair details. Organizations can improve their operational performance by maintaining consistent, accurate, and efficient management of assets across an organization.
According to the McKinsey Master Data Management Survey 2023, 83 percent of organizations consider client and product data to be the most dominant domains.
Eighty percent of organizations responding to our survey reported that some of their divisions operate in silos, each with its own data management requirements, practices, source systems, and consumption behaviors. For example, a sales team may maintain client data in a customer relationship management (CRM) system, while a marketing team may use a client data platform (CDP) to create customer profiles and inform ad campaigns. Silos can lead to inconsistencies and errors, increasing the difficulty of making decisions related to business, data, and technology (see sidebar “Types of master data domains”).
Treating MDM as a technology discipline only
Organizations typically think of MDM as a technology discipline rather than as a differentiator that can drive enterprise value. According to our survey, only 16 percent of MDM programs are funded as organization-wide strategic programs, leaving IT or tech functions to carry the financial responsibility (Exhibit 2). Sixty-two percent of respondents reported that their organizations had no well-defined process for integrating new and existing data sources, which may hinder the effectiveness of MDM.
While technology plays a crucial role, the success of MDM initiatives requires significant business influence and sponsorship to set the strategic direction, understand data dependencies, improve the quality of data, enhance business processes, and, ultimately, support the organization in achieving its goals. It’s important for the role of data owner to be played by a business stakeholder—specifically, the head of the business unit that uses the data most, such as the head of sales and marketing for the client data domain. That leader can provide guidance for defining data requirements and data quality rules that are aligned with the business’s goals.
Poor data quality
Poor-quality data cannot deliver analytics-based insights without substantial manual adjustment. According to the MDM survey, 82 percent of respondents spent one or more days per week resolving master data quality issues, and 66 percent used manual review to assess, monitor, and manage the quality of their master data. Consequently, large, multidivisional organizations may be unable to efficiently generate KPIs or other metrics, and sales representatives may be unable to quickly generate a consistent, holistic view of prospective clients. According to the MDM survey, the most prevalent issues in organizations’ data quality were incompleteness, inconsistency, and inaccuracy (Exhibit 3).
In addition to incompleteness, inconsistency, and accuracy, many companies also contend with issues of uniqueness, or duplicate information, across systems. Traditionally, organizations classify data assets based on the stakeholders they interact with, but this approach can lead to duplication of information. For example, a supplier to an organization can also be its customer. These circumstances have led to the design of a “party” data domain that generalizes the characteristics of a person or organization and establishes the connection between them and their distinctive roles to the company.
Master data quality issues can cause customer dissatisfaction, operational inefficiencies, and poor decision making. Furthermore, companies handling private or sensitive consumer information have stricter compliance requirements and data quality, security, and privacy standards. Without good data, implementing MDM processes will be difficult.
Complex data integration requirements
Organizations may find it difficult to integrate MDM into their existing systems. Compatibility issues, data migration challenges, and system upgrades can hinder successful MDM implementation, and minimizing integration latency is crucial to provide timely and accurate data to the MDM system. Organizations may have to significantly model, map, and transform data systems so they can work with newer and older technologies.
How to effectively implement and optimize MDM capabilities
To overcome these challenges and successfully implement and optimize MDM capabilities, organizations must clearly identify the value they hope to create based on their priority business use cases such as operational efficiency and customer insights, which lead to cost savings and revenue growth. Organizations should measure the impact and effectiveness of MDM implementation using metrics such as ROI, total cost of ownership, and performance baselines. Organizations should maintain a forward-looking approach to adopt modern tools and technologies; create a robust data governance model backed by performance KPIs; and plan for capability building among stakeholders to ensure a uniform adoption of MDM principles.

The data dividend: Fueling generative AI
Build a ‘golden record’ that contains the most up-to-date information.
An MDM “golden record” is a repository that holds the most accurate information available in the organization’s data ecosystem. For example, a golden record of client data is a single, trusted source of truth that can be used by marketing and sales representatives to analyze customer preferences, trends, and behaviors; improve customer segmentation; offer personalized products and services; and increase cross-sales, interactions, customer experiences, and retention.
To build a golden record that contains the most up-to-date information, organizations integrate data from every business unit into the golden record and update it as more accurate information becomes available. Integrating information can be done with the help of AI and machine learning (ML) technology. Alternatively, organizations may establish one existing system as the golden record for a specific data domain to maintain consistency, precision, and timeliness across the enterprise.
Four common master data management design approaches
Organizations typically use one of four master data management design approaches, depending on the complexity of their data:
Registry MDM. This model aggregates data from multiple sources to spot duplicates in information. It is a simple, inexpensive approach that large, global organizations with many data sources often find helpful.
Consolidation MDM. This approach periodically sorts and matches information from multiple source systems to create or update the master data record. Simple and inexpensive to set up, it is a good option for organizations seeking to analyze large sets of data.
Centralized MDM. This approach establishes a single master repository to create, update, and maintain data, and shares it back with the respective source systems. This model is good for banks, insurance companies, government agencies, and hospital networks that require strict compliance to maintain integrity and control over their data.
Coexistence MDM. This approach creates and updates data in source systems, giving businesses the flexibility and autonomy to manage data attributes at the division or business-unit level while maintaining consistent core client data. This model is especially good for large, complex enterprises with many segments and business-unit structures that are frequently integrating new clients into their databases.
Organizations typically start by deploying more rudimentary MDM models, such as registry or consolidation, then evolve to more mature approaches, such as centralized or coexistence. These more mature models are more flexible but also more complex. When choosing an MDM deployment approach, organizations should consider the following questions, among others:
- How should the organization centralize and streamline master data across different systems and locations to maximize accessibility and usability?
- What methodologies should be used to manage the complexity of data relationships and structures to improve efficiency and interoperability across systems?
- What strategies need to be implemented to enable real-time master data updates and guarantee instant access to the most current and accurate information?
- How should the organization maintain consistent, high-quality data across all departments to support data-driven decision making?
- What initiatives need to be implemented to empower business units to increase autonomy and maturity, fostering innovation and agility throughout the organization?
- Which systems must be seamlessly integrated with the MDM strategy to establish a cohesive and unified data ecosystem?
- How should MDM support and enhance current and future business processes to drive sustainable growth and competitive advantage?
- What proactive measures should be in place to address regulatory and compliance requirements, ensuring risk mitigation and adherence to industry best practices?
There are four common MDM design approaches that can be used to update the golden record within the business unit data (see sidebar “Four common master data management design approaches”). Deploying a modular architecture enables fit-for-purpose consumption and integration patterns with various systems to manage the golden record. For example, every mastered client record could be linked back to the source systems and mapped to a hierarchy to show association in the MDM system. Alternatively, client data could be mastered and assigned a unique client ID within the golden record to stitch together data from all systems and create a single portfolio of a client.
Establish a robust data governance model to maintain integrity and reliability of MDM capabilities
Only 29 percent of companies responding to our survey had full upstream and downstream MDM integrations with source systems and business applications, as well as all governance or stewardship roles, in place. Organizations should clearly identify the single source of truth for data and properly train employees on handling integration failures to avoid saving stale information.
Data governance models for MDM should be designed with clear roles and responsibilities, be managed by a governance council with representatives from different business units and IT, and be shepherded by someone who can serve as an MDM liaison among business, data, and technology stakeholders. The structure should be complemented by a clearly defined policy framework and a tailored, business-backed, and IT-supported operating model for master data domains. These data governance processes will allow upstream system owners and a data governance council to address data quality issues—for example, when the MDM identifies new or updated information as conflicting with other information based on the survivorship strategy.
Choose an MDM tool that enhances data quality and accelerates transformation
MDM tools are becoming more intuitive and user-friendly, and recent innovations in AI, ML, cloud technologies, and federated architectures have opened new possibilities for data mastering and processing. For example, AI-enabled tools use pretrained AI and ML models to automate data quality, data matching, and entity resolution tasks with a higher degree of accuracy and greater efficiency. According to the survey, 69 percent of organizations are already using AI as part of their overall data management capabilities; however, only 31 percent are using advanced AI-based techniques to enhance match-and-merge capabilities and to improve master data quality more broadly.
Organizations should choose data management tools that align with their priorities and make the transition seamless. It’s also important to consider the return on investment and the incremental value that each MDM tool can bring to the organization. When choosing an MDM tool, relevant business stakeholders should understand data processes and requirements, including the data elements that affect business operations and the priority use cases, and then help determine the technology capabilities and workflows that are required to integrate new systems.
For example, stakeholders should assess the maturity of their organization’s capabilities, including its data quality, matching, and entity resolution, to determine how easily new systems will be able to integrate with existing systems and technologies. It is also important to consider these systems’ scalability and flexibility to accommodate future growth and evolving data management needs. Moreover, AI and ML capabilities should be considered to help the MDM tool automate tasks to improve data quality.
Plan for capability building and change management
Organizations that implement technology without changing their processes and the way people work with master data may not fully reap the benefits of MDM.
Change management is crucial to ensure that employees understand and embrace the changes brought about by MDM implementation. It typically includes securing executive sponsorship to demonstrate the importance of MDM to the organization; engaging with business and technology stakeholders to communicate the vision; setting expectations for accountability and processes; and rolling out comprehensive training programs to educate employees on MDM and data principles, processes, and tools.
Start with a pilot implementation
Organizations can start integrating MDM tools by first piloting MDM in one domain to validate its design, governance model, and workflows in a controlled environment. Organizations can then easily identify any potential issues or challenges and make the necessary adjustments before scaling up the implementation to other master data domains or to the entire organization. Piloting these tools also allows organizations to gather feedback from users and stakeholders to understand the user experience, identify areas for improvement, and make necessary changes to optimize the MDM tool and workflows.
Implementing and optimizing MDM capabilities can seem daunting, especially for large organizations with multiple complex systems. But once successfully deployed across master data domains—using an optimal design approach, an efficient governance structure, and sufficient change management efforts—MDM can ensure that high-quality data is available for strategic decision making, leading to cost savings and revenue opportunities across an organization.
Aziz Shaikh and Jorge Machado are partners in McKinsey’s New York office, where Kayvaun Rowshankish is a senior partner, Rachit Saxena is a consultant, and Rajat Jain is an associate partner. Holger Harreis is a senior partner in the Düsseldorf office.
The authors wish to thank Vladimir Alekseev for his contributions to this article.
Explore a career with us
Related articles.

How to unlock the full value of data? Manage it like a product

Realizing more value from data projects

Demystifying data mesh
- Skip to content
- Skip to search
- Skip to footer
Support & Downloads
- Worldwide - English
- Arabic - عربي
- Brazil - Português
- Canada - Français
- China - 简体中文
- China - 繁體中文 (臺灣)
- Germany - Deutsch
- Italy - Italiano
- Japan - 日本語
- Korea - 한국어
- Latin America - Español
- Netherlands - Nederlands">Netherlands - Nederlands
- Helpful Links
- Licensing Support
- Technology Support
- Support for Cisco Acquisitions
- Support Tools
- Cisco Community

To open or view a case, you need a service contract
Get instant updates on your TAC Case and more

Contact TAC by Phone
800-553-2447 US/Canada
866-606-1866 US/Canada
- Returns Portal
Products by Category
- Unified Communications
- Networking Software (IOS & NX-OS)
- Collaboration Endpoints and Phones
Status Tools
The Cisco Security portal provides actionable intelligence for security threats and vulnerabilities in Cisco products and services and third-party products.
Get to know any significant issues, other than security vulnerability-related issues, that directly involve Cisco products and typically require an upgrade, workaround, or other customer action.
Check the current status of services and components for Cisco's cloud-based Webex, Security and IoT offerings.
The Cisco Support Assistant (formerly TAC Connect Bot) provides a self-service experience for common case inquiries and basic transactions without waiting in a queue.
Suite of tools to assist you in the day to day operations of your Collaboration infrastructure.
The Cisco CLI Analyzer (formerly ASA CLI Analyzer) is a smart SSH client with internal TAC tools and knowledge integrated. It is designed to help troubleshoot and check the overall health of your Cisco supported software.
My Notifications allows an user to subscribe and receive notifications for Cisco Security Advisories, End of Life Announcements, Field Notices, and Software & Bug updates for specific Cisco products and technologies.
More Support
- Partner Support
- Small Business Product Support
- Business Critical Services
- Customer Experience
- DevNet Developer Support
- Cisco Trust Portal
Cisco Communities
Generate and manage PAK-based and other device licenses, including demo licenses.
Track and manage Smart Software Licenses.
Generate and manage licenses from Enterprise Agreements.
Solve common licensing issues on your own.
Software and Downloads
Find software bugs based on product, release and keyword.
View Cisco suggestions for supported products.
Use the Cisco Software Checker to search for Cisco Security Advisories that apply to specific Cisco IOS, IOS XE, NX-OS and NX-OS in ACI Mode software releases.
Get the latest updates, patches and releases of Cisco Software.

Protocol for the Enhanced Management of Multimorbid Patients with Chronic Pulmonary Diseases: Role of Indoor Air Quality
- Find this author on Google Scholar
- Find this author on PubMed
- Search for this author on this site
- ORCID record for Alba Gomez-Lopez
- ORCID record for Ebymar Arismendi
- For correspondence: [email protected]
- ORCID record for Isaac Cano
- ORCID record for Ramon Farre
- ORCID record for Carme Hernandez
- ORCID record for Nuria Sanchez-Ruano
- ORCID record for Benigno Sanchez
- ORCID record for Antoni Siso-Almirall
- ORCID record for Emili Vela
- ORCID record for Jose Fermoso
- ORCID record for Josep Roca
- ORCID record for Ruben Gonzalez-Colom
- Info/History
- Supplementary material
- Preview PDF
Introduction: Reducing unplanned hospital admissions in chronic patients at risk is a key area for action due to the high healthcare and societal burden of the phenomenon. The inconclusive results of preventive strategies in patients with chronic respiratory disorders and comorbidities are explainable by multifactorial but actionable factors. The current protocol (January 2024 to December 2025) relies on the hypothesis that intertwined actions in four dimensions: i) management change, ii) personalisation of the interventions based on early detection/treatment of acute episodes and enhanced management of comorbidities, iii) mature digital support, and iv) comprehensive assessment, can effectively overcome most of the limitations shown by previous preventive strategies. Accordingly, the main objective is to implement a novel integrated care preventive service for enhanced management of these patients, as well as to evaluate its potential for value generation. Methods and analysis: At the end of 2024, the specifics of the novel service will be defined through the articulation of its four main components: i) Enhanced lung function testing through oscillometry, ii) Continuous monitoring of indoor air quality as a potential triggering factor, iii) Digital support with an adaptive case management approach, and iv) Predictive modelling for early identification and management of exacerbations. During 2025, the novel service will be assessed using a Quintuple Aim approach. Moreover, the Consolidated Framework for Implementation Research will be applied to assess the implementation. The service components will be articulated through four sequential six-months Plan-Do-Study-Act cycles. Each cycle involves a targeted co-creation process following a mixed-methods approach with the active participation of patients, health professionals, managers, and digital experts. Ethics and dissemination: The Ethics Committee for Human Research at Hospital Clinic de Barcelona approved the protocol on June 29, 2023 (HCB/2023/0126). Before any procedure, all patients in the study must sign an informed consent form. Registration: NCT06421402 . Keywords: COPD, Severe Asthma, Digital Support, Integrated Care, Quintuple Aim, Service Assessment
Competing Interest Statement
Isaac Cano and Josep Roca hold shares of Health Circuit. All other authors declare no conflicts of interest.
Clinical Trial
NCT06421402
Funding Statement
The K-HEALTHinAIR project funded this study, Grant Agreement number 101057693, under a European Union Call on Environment and Health (HORIZON-HLTH-2021-ENVHLTH-02). Disclaimer: Views and opinions expressed are, however, those of the authors only and do not necessarily reflect those of the European Union or the European Health and Digital Executive Agency as granting authority. Neither the European Union nor the granting authority can be held responsible.
Author Declarations
I confirm all relevant ethical guidelines have been followed, and any necessary IRB and/or ethics committee approvals have been obtained.
The details of the IRB/oversight body that provided approval or exemption for the research described are given below:
The Ethical Committee for Human Research at Hospital Clinic de Barcelona approved the core study protocol of K-Health in Air on June 29, 2023 (HCB/2023/0126). The study design adheres to data minimisation principles, ensuring that only essential data are collected and utilised. The study will be conducted in compliance with the Helsinki Declaration (Stronghold Version, Brazil, October 2013) and in accordance with the protocol and the relevant legal requirements (Biomedical Research Act 14/2007 of July 3). All patients in the study must sign an informed consent form before any procedure. The participants can withdraw their consent at any time without altering their relationship with their doctor or harming their treatment. The One Beat watch does not hold medical device certification and will be utilized solely for data collection and exploring potential patterns informing exacerbations, not for decision-making within the study. Any future application derived from this research must ensure that the technology aligns with medical device regulations.
I confirm that all necessary patient/participant consent has been obtained and the appropriate institutional forms have been archived, and that any patient/participant/sample identifiers included were not known to anyone (e.g., hospital staff, patients or participants themselves) outside the research group so cannot be used to identify individuals.
I understand that all clinical trials and any other prospective interventional studies must be registered with an ICMJE-approved registry, such as ClinicalTrials.gov. I confirm that any such study reported in the manuscript has been registered and the trial registration ID is provided (note: if posting a prospective study registered retrospectively, please provide a statement in the trial ID field explaining why the study was not registered in advance).
I have followed all appropriate research reporting guidelines, such as any relevant EQUATOR Network research reporting checklist(s) and other pertinent material, if applicable.
Data Availability
Data availability does not apply to this article since it describes a protocol rather than presenting study results
View the discussion thread.
Supplementary Material
Thank you for your interest in spreading the word about medRxiv.
NOTE: Your email address is requested solely to identify you as the sender of this article.

Citation Manager Formats
- EndNote (tagged)
- EndNote 8 (xml)
- RefWorks Tagged
- Ref Manager
- Tweet Widget
- Facebook Like
- Google Plus One
- Addiction Medicine (324)
- Allergy and Immunology (628)
- Anesthesia (165)
- Cardiovascular Medicine (2379)
- Dentistry and Oral Medicine (289)
- Dermatology (207)
- Emergency Medicine (379)
- Endocrinology (including Diabetes Mellitus and Metabolic Disease) (837)
- Epidemiology (11775)
- Forensic Medicine (10)
- Gastroenterology (703)
- Genetic and Genomic Medicine (3747)
- Geriatric Medicine (350)
- Health Economics (634)
- Health Informatics (2399)
- Health Policy (933)
- Health Systems and Quality Improvement (898)
- Hematology (341)
- HIV/AIDS (782)
- Infectious Diseases (except HIV/AIDS) (13318)
- Intensive Care and Critical Care Medicine (768)
- Medical Education (365)
- Medical Ethics (105)
- Nephrology (398)
- Neurology (3508)
- Nursing (198)
- Nutrition (526)
- Obstetrics and Gynecology (675)
- Occupational and Environmental Health (664)
- Oncology (1825)
- Ophthalmology (538)
- Orthopedics (219)
- Otolaryngology (287)
- Pain Medicine (233)
- Palliative Medicine (66)
- Pathology (446)
- Pediatrics (1035)
- Pharmacology and Therapeutics (426)
- Primary Care Research (420)
- Psychiatry and Clinical Psychology (3178)
- Public and Global Health (6145)
- Radiology and Imaging (1280)
- Rehabilitation Medicine and Physical Therapy (747)
- Respiratory Medicine (828)
- Rheumatology (379)
- Sexual and Reproductive Health (372)
- Sports Medicine (323)
- Surgery (402)
- Toxicology (50)
- Transplantation (172)
- Urology (146)
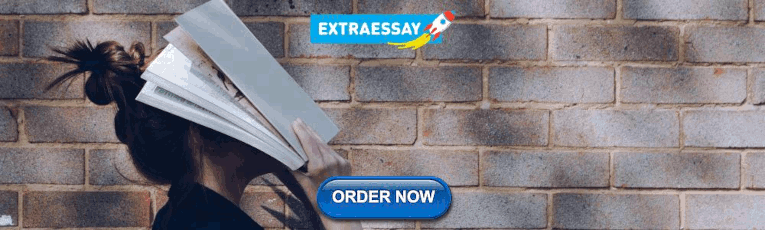
COMMENTS
Search more than 1,000 examples of case studies sharing quality solutions to real-world problems. Find more case studies Featured Case Studies Classic Case Studies ... The laboratory helps the U.S. Department of Defense establish quality control requirements when testing for chemical warfare agents (CWA). In preparation for accreditation, EML ...
The successfulness of this system can be measured by assessing the consistency of the product quality. Coca Cola say that 'Our Company's Global Product Quality Index rating has consistently reached averages near 94 since 2007, with a 94.3 in 2010, while our Company Global Package Quality Index has steadily increased since 2007 to a 92.6 rating in 2010, our highest value to date'.
Since publication in 2003 of a review 'Internal quality control: planning and implementation strategies,' 1 quality control (QC) has evolved as part of a comprehensive quality management system (QMS). The language of quality today is defined by International Standard Organization (ISO) in an effort to standardize terminology and quality management practices for world-wide applications.
outgoing quality control works for manufacturing product. There are t wo types of part was sel ected for. this case study which are huge and symmetrical parts. 85.06 seconds total inspection time ...
T oyota Quality System case study. Introduction. T oyota from the early 1960s alongside their supplier network consolidated the way in which. they were able to refine their production system ...
The smart quality approach allows pharma companies to deploy these technologies and to integrate their quality controls in development and manufacturing. 1 (see sidebar, "Smart quality at a glance"). Well-performing manufacturing facilities have started to create paperless labs, optimize testing, automate processes, and shift testing to the ...
Case study. Healthcare companies can use smart quality to redesign the quality management review process and see results quickly. At one pharmaceutical and medtech company, smart visualization of connected, cross-functional metrics significantly improved the effectiveness and efficiency of quality management review at all levels.
this single case study was to explore what quality improvement strategies senior ... The conceptual framework of this study was based on total quality management theory. Data collection was through face-to-face interviews and from a review of company documents. Yin's 5-step process was used to analyze the data.
According to Teresa Whitacre, of international consulting firm ASQ, proper quality management also boosts a company's profitability. "Total quality management allows the company to look at their management system as a whole entity — not just an output of the quality department," she says. "Total quality means the organisation looks at ...
Manage Your Human Sigma. Organizational Development Magazine Article. John H. Fleming. Curt Coffman. James K. Harter. If sales and service organizations are to improve, they must learn to measure ...
International Journal of Science, Engineering and Technology Research (IJSETR), Volume 3, Issue 3, March 2014 A CASE STUDY OF QUALITY CONTROL CHARTS IN A MANUFACTURING INDUSTRY Fahim Ahmed Touqir1, Md. Maksudul Islam1, Lipon Kumar Sarkar2 1,2 Department of Industrial Engineering and Management 1,2 Khulna University of Engineering & Technology II.
Shiseido is a globally-renowned manufacturer of cosmetics and fragrances. The company carries out intensive research and development activity on a continual basis, bringing multiple new product references to market every year. In this case study we discuss why Shiseido began using CHEMUNEX® for quality control of bulk raw materials and in ...
To elucidate the effectiveness of quality control methods, we embarked on a case study using the 7 QC tools and PDCA cycle on a data base of a sample bottle manufacturing unit.
by by Jim Heskett. A new book by Gregory Clark identifies "labor quality" as the major enticement for capital flows that lead to economic prosperity. By defining labor quality in terms of discipline and attitudes toward work, this argument minimizes the long-term threat of outsourcing to developed economies.
This paper studies a prediction problem using time series data and machine learning algorithms. The case study is related to the quality control of bumper beams in the automotive industry. These parts are milled during the production process, and the locations of the milled holes are subject to strict tolerance limits. Machine learning models are used to predict the location of milled holes in ...
Case study utilizes recognized quality risk management tools. Case study is appropriately simple and succinct to assure clear understanding. Case study provides areas for decreased and increased response actions. 7. Case study avoids excessive redundancy in subject and tools as compared to other planned models. 8.
A top 5 pharma company chose to implement a strong in-process testing routine strategically monitoring specific critical steps of their production process. This approach was designed to detect any microbial contamination at the earliest possible opportunity and has been key to maintaining superior control over their production processes.
In this paper, statistical quality control of a production line has been presented using the classical method Shewhart, cumulative sum method (CUSUM) and Exponentially Weighted Moving Average (EWMA). The Shewhart technique can be utilized in controlling the process in which there are big changes in mean. The cumulative sum method is more efficient in detecting small changes in the mean. The ...
Case Study: Nestle Nordic Quality management system audits. Nestlé is the world's leading nutrition, health, and wellness company, with over 280,000 employees and over 450 factories globally. The Challenge. Prior to obtaining ISO 9001 certification with Intertek, Nestlé used their own proprietary quality management system, However, in 2017 ...
Case Studies Highlight Why Researchers Use Automated Electrophoresis Solutions. Many scientists utilize Agilent automated electrophoresis systems for sample quality control (QC) in various application workflows, including next-generation sequencing (NGS), biobanking, fragment analysis, and nucleic acid vaccine development.
For case-control studies, it is important that if matching was performed during the selection or recruitment process, the variables used as matching criteria (e.g., age, gender, race) should be controlled for in the analysis. General Guidance for Determining the Overall Quality Rating of Case-Controlled Studies
In the realm of civil construction projects, achieving an optimal balance between project time, cost, and quality is paramount for ensuring project success and stakeholder satisfaction. Traditional optimization approaches often focus solely on time and cost, potentially neglecting the critical aspect of quality. This study presents a novel framework aimed at integrating quality considerations ...
By deploying IBM ELM, Yanfeng Electronic Technology's core goal is to comprehensively improve the quality of the company's products. IBM ELM can help it quantitatively evaluate the quality of requirements management, design, test cases, and realize the data correlation of the whole process, improving the visibility of quality status of software products in real time.
This study follows a combined approach including a systematic literature review to identify existing gaps in recent research and an expert panel to provide valuable insights and validation during the development of the framework. Additionally, a case study was conducted in an automotive manufacturing company to validate the findings.
Quality control time without drive time: 5 to 10 minutes; Quality control time with drive time: 15 to 20 minutes; Golden Rule Auto Care's average: 12 minutes; KPI or goal for Golden Rule Auto Care's QC issues per month: 10% or less; This year, they have run as high as 25% and as low as 9% per month. Most common issue found at Golden Rule Auto Care: grease on handle, door panel, seat ...
1. MDM plays an important role with modern data architecture concepts and creates value in five ways: MDM cleans, enriches, and standardizes data for key functions, such as customer or product data, before it is loaded into the data lake. In this way, MDM ensures that data is accurate, complete, and consistent across an organization.
Crop yield estimation plays a crucial role in agricultural production planning and risk management. Utilizing simultaneous localization and mapping (SLAM) technology for the three-dimensional reconstruction of crops allows for an intuitive understanding of their growth status and facilitates yield estimation. Therefore, this paper proposes a VINS-RGBD system incorporating a semantic ...
Check the current status of services and components for Cisco's cloud-based Webex, Security and IoT offerings. Cisco Support Assistant. The Cisco Support Assistant (formerly TAC Connect Bot) provides a self-service experience for common case inquiries and basic transactions without waiting in a queue.
The study design adheres to data minimisation principles, ensuring that only essential data are collected and utilised. The study will be conducted in compliance with the Helsinki Declaration (Stronghold Version, Brazil, October 2013) and in accordance with the protocol and the relevant legal requirements (Biomedical Research Act 14/2007 of ...