- Open access
- Published: 23 May 2023
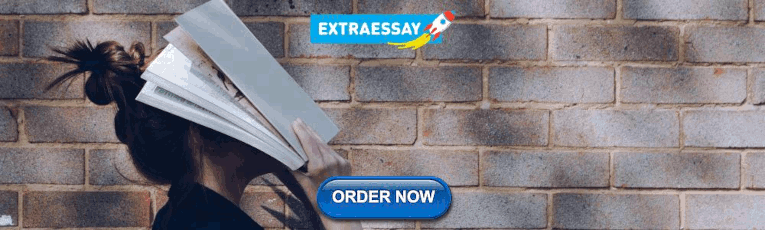
Covid-19 distance and online learning: a systematic literature review in pharmacy education
- Muhaimin Muhaimin 1 ,
- Akhmad Habibi 2 ,
- Yasir Riady 3 ,
- Turki Mesfer Alqahtani 4 ,
- Anis Yohana Chaerunisaa 1 ,
- Tommy Tanu Wijaya 5 ,
- Tiana Milanda 1 ,
- Farrah Dina Yusop 6 &
- Nour Awni Albelbisi 6
BMC Medical Education volume 23 , Article number: 367 ( 2023 ) Cite this article
3325 Accesses
2 Citations
3 Altmetric
Metrics details
The Covid-19 outbreak necessitated the implementation of social distancing mechanisms, such as the enforcement of lockdowns in numerous nations. The lockdown has disrupted many parts of everyday life, but this unusual event has particularly affected education. The temporary closure of educational institutions ushered in dozens of new reforms, including a shift into the distance and online learning. This study investigates the transition from traditional education in physical classrooms to online and distance and online learning in pharmacy education during Covid-19, especially about the challenges and benefits of distance and online learning. We did Preferred Reporting Items for Systematic Reviews and Meta-Analyses (PRISMA) for literature sources between 2020 and 2022 (n.14). The study elaborates on how the transition has influenced teachers and students of pharmacy education. The research also summarizes several recommendations, which may assist in minimizing the adverse impacts of lockdown and encourage streamlined processes to distance and online learning, particularly in pharmacy education.
Peer Review reports
Introduction
Covid-19, an infectious illness characterized by the SARS-CoV-2 virus, endangered the world quickly due to its highly infectious nature. As of 6 March 2023, there have been more than seven hudred million confirmed cases were documented, with over six million deaths [ 1 ]. The World Health Organization (WHO) labeled the virus a pandemic in March 2020. The pandemic caused havoc on a variety of activities of daily life, triggering governments worldwide to put in place a series of emergency response mechanisms [ 2 , 3 ]. Country leaders imposed temporary closure and enforced extended isolation time, disrupting educational activity around the globe, reducing infection, and flattening the curve to avoid overburdening healthcare services. This resulted in the temporary closure of educational institutions in various parts of the world. The situation affected teachers, students, and their families [ 4 , 5 , 6 ]. Some academic institutions facing closure gradually reopened and began working under distance and online learning methods to keep students on track academically while also taking steps to mitigate the effects of the present health crisis. In the past, infectious disease epidemics have resulted in widespread school closures, with variable levels of success [ 7 ]. At the most basic level, distance learning refers to taking classes away from the college. Although technically a type of distance learning, online learning is more frequently used to describe programs where the instructors are not present simultaneously as the students [ 8 ].
Institutions have been forced into quickly transitioning to distance and online learning approaches mainly based on technology. Many educational stakeholders, such as teachers, students, and school administration staff, have not prepared to face the transition because of the fast switch to distance and online learning [ 9 ]. This transition to remote learning happened in an unexpected situation, leaving little time for teachers, educational staff, and students to prepare, modify, and adjust the learning. The condition brought several problems to the economy and social life. According to UNESCO, the temporary school closures enacted in response to the Covid-19 pandemic have impacted more than a billion students worldwide. Owing to a lockdown in 2020, students from over 50 nations have been kept out of school, accounting for roughly 18% of total registered students [ 10 ]. Many studies have been conducted to understand the impacts of distance and online learning in education due to Covid-19 [ 11 , 12 , 13 ]. However, literature reviews in a specific field of study are still limited and important to understand the broad effects of distance and online learning [ 7 , 9 , 14 ]. This systematic literature review takes an in-depth look at the studies on the influence of the Covid-19 pandemic on a specific field of education. This research examines how the shift from traditional methods to distance and online learning has affected teachers and students in pharmacy education. The impact of the pandemic-based distance and online learning on pharmacy education should be investigated to improve didactical decisions in the future and bridge the gaps to more adaptable but effective online pedagogical approaches. Initially, the focus areas of the literature review were investigated within pharmacy education. Following the focus areas, the distance and online learning challenges and benefits were assessed and elaborated on. Finally, recommendations of the prior studies included in this meta-analysis were concluded.
Related work
Many governments were under pressure to prevent Covid-19 from spreading. This resulted in the temporary closures of many schools and universities [ 15 ]. Others switched to distance and online learning through technology. Viner et al. [ 16 ] did a systematic evaluation to determine the influence of school closures and other social distance techniques on disease rates and virus spread during crises. It was indicated that educational institution temporary closures play an insignificant role in virus transmission reduction. The minor advantages of such restrictions on the spread reduction might quickly be offset by the severe socio-economic implications [ 16 , 17 , 18 ]. The closure can have effects on individuals, families, and society. Therefore, any decision regarding school closures must carefully consider the potential trade-offs and aim to strike a balance between protecting public health and minimizing the adverse impacts on education, economy, and social well-being. As a result, many academic institutions have chosen the less drastic option of converting to distance and online learning [ 19 , 20 ].
Distance learning refers to online instruction systems to create educational materials, provide teaching, and manage programs [ 21 ]. There are two basic types of distance learning: synchronous and asynchronous [ 22 ]. The main goal of distance and online learning is to replicate regular classroom communication approaches. Live webinars and virtual classes are examples of synchronous distance and online learning. On the other hand, asynchronous learning allows for greater flexibility in terms of timing which does not require real-time engagement; materials are provided online. Video recordings and emails are instances of asynchronous learning.
A comprehensive review and meta-analysis of controlled studies on the efficiency and approval of distance and online learning in medical sciences published between January 2000 and March 2020 evaluated students’ understanding, abilities, and satisfaction levels [ 23 ]. The study reported insignificant differences between traditional and distance and online learning regarding usefulness and objective assessments. Distance and online learning obtained a better approval rating in subjective assessments, suggesting that it was preferred to some degree by learners [ 23 ]. Carrillo & Flores [ 24 ] also reviewed the literature on online teaching and learning practices in teacher development between January 2000 and April 2020 to investigate online learning in teacher development and explain its consequences in the sense of the disease outbreak. The review discussed sociological, intellectual, and pedagogical problems and a comprehensive representation of innovation utilized to enhance teaching and learning [ 24 ].
Daoud et al. [ 25 ] performed a comprehensive review that evaluated the academic benefits of providing internet access at home, focusing on equality surrounding household internet access. It discovered several favorable associations between household internet access and the value of education for qualification, personal character, and social life. However, the relationship was not apparent and did not prove causality. Variables affect the aspects of online behaviors, including how technology is integrated and determine the educational value of household internet use [ 25 ]. Di Pietro et al. [ 26 ] published a report in which they attempted to investigate the consequences of the Covid-19 pandemic on education. It generated projections regarding the influence and future of learning based on pre and during Covid-19 data. The following are the four critical conclusions drawn from the article: (a) learning is likely to experience a stumbling block; (b) the impact on student achievements is likely to differ with economic factors; (c) social-economic disparity expressed in extreme reactions, less-wealthy families are subjected to greater environmental strain; (d) the broadening social inequality could have long-lasting effects [ 26 ].
Some virtual cases of emergency learning methods have been chastised for failing to follow basic pedagogical principles and guidelines [ 27 ]. Several studies have raised concerns regarding the possible negative consequences of rushing to introduce educational technology changes without first assessing their impact [ 27 , 28 ]. Furthermore, the move to online education and distance and online learning technologies has sparked worries about spying and security and influenced students’ lifestyles [ 29 ]. In this research context, selected studies were diverse, from quantitative to qualitatitve approaches [ 30 ]. Because this phenomenon is still new, there is a lack of reflection on the pandemic digital revolution’s direct impact on postsecondary learning and its benefits, drawbacks, and future consequences.
The current research, a systematic literature review, follows the principles outlined in the preferred reporting items for systematic review and meta-analysis (PRISMA) procedures [ 31 , 32 ], which include five stages: search, screening, eligibility, initial inclusion, and inclusion. PRISMA is a standard approach to assist researchers in transparently informing the study, steps, and results within the context of the systematic literature review [ 33 ].
Research questions
This research investigates the effects of Covid-19 distance and online learning on pharmacy education. Four research questions were proposed: (1) what are the focus areas of the literature review? (2) what are the challenges of distance and online learning in pharmacy education? (3) what are the benefits of distance and online learning pharmacy education? (4) what recommendations were made?
The research questions were a basic guideline for determining the most popular search terms. The search includes terms synonymous with or closely linked to the main search phrases. The search was conducted using Science Direct, supported by Google Scholar search. The relevant search terms were used: “Distance learning in pharmacy education Covid 19,” “online education in pharmacy education Covid 19,” and “Technology integration in pharmacy education Covid-19.” The findings varied depending on the phrase combinations. However, in general, 17 to 81 papers (Table 1 ) were obtained each search, with the number growing relevant to the topics. Related phrases were gathered in all publications depending on the search. The terms sued in the search were determined through an in-depth discussion among the authors. We limited the terms so that future researchers can adapt this study for further investigation. The search limit provides narrow results for effective and efficient work for the most relevant answers to the research problems [ 34 ].
Articles published after 2020 were kept in the study. Only works from high-quality journals were included; we selected the articles from indexed journals in Web of Science or Scopus databases. We initially reviewed the selected papers against Elsevier’s abstract and reference repository, Scopus, to verify that they were of top standard and didn’t relate to fraudulent publications. We also double-checked that they were in the Scopus indexation for the SJR, a measure of academic journals’ scientific impact. Furthermore, the publications were evaluated using Beall’s List, a list comprising predatory accessible publications that do not conduct an adequate review process.
PRISMA procedures
A reference list of scholarly papers directly referencing Covid-19 online learning: A comprehensive literature review in pharmacy education was created after merging these lists. After the first search or first phase, 137 scholarly publications were presented (Table 1 ). By removing duplicated results, we were able to screen for them. Microsoft Word was used as a tool in the duplication removal procedure. We went through each repeated title and removed them one by one. The redundancy led to 54 academic papers being sent for additional review, with 83 being deleted. Further, the step included the following elimination process; the articles should address technology integration in pharmacy education during Covid-19 distance learning, inform findings in English, be empirical studies (research articles), and be published from 2020 to April 1st, 2022. From the process, 47 abstracts were dropped, and the remaining 36 articles were for eligibility and inclusion (Fig. 1 ).
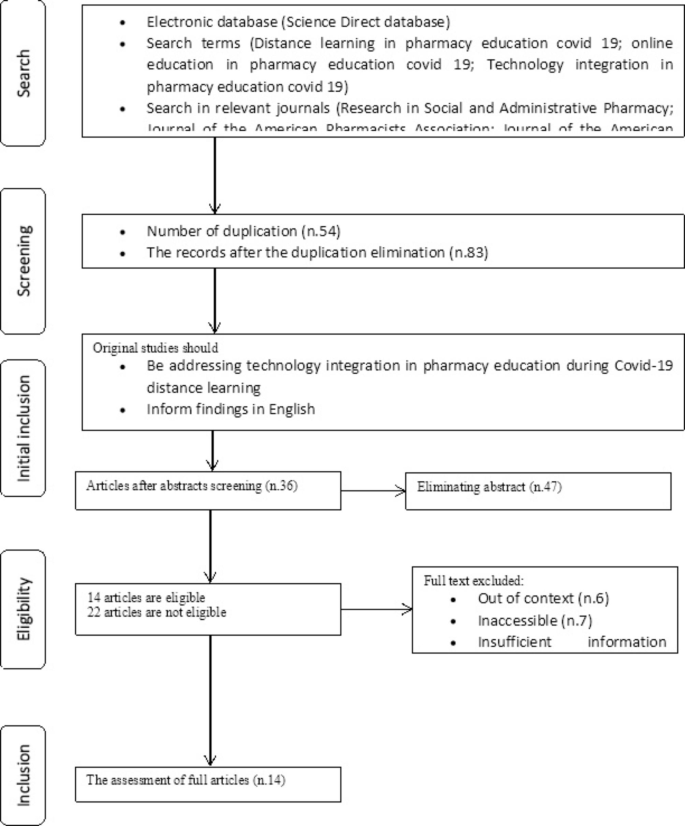
PRISMA flow diagram of the study
Following the initial screening, a review-coding method was performed using Macros in Microsoft Words for the abstracts, with the process documented by writing “included” 1st initial inclusion in the review box. After that, we added some information for every abstract, and the coding was done in a new draft where all the initial abstracts were included. The Macros [ 35 , 36 ] were used to encode and extract the selected papers [ 37 ]. Macros were chosen because of their efficiency and functionality [ 35 ]. Tables were created to manage the comments and metadata. The study’s aim, method, study site/ population, and findings are listed in the tables. Four authors discussed and did the coding and combined documents into one before extracting the comments for analysis. The Macros were obtained for free at http://www.thedoctools.com/index.php?show=mt_comments_extract .
In the end, 14 articles were collected, examined, and reviewed. The criteria for inclusion in this systematic review were accessible articles in the context of distance education during Covid-19 in the field of pharmacy education. Meanwhile, the exclusion criteria included articles that were not in the context of Covid-19 (n.6), pharmacy education, and distance and online learning. Besides, inaccessible articles (n.7) and articles with insufficient information (n.9) regarding the topic were also excluded.
Results and discussion
The results of this literature review are presented. The findings of every research topic are examined in depth. The focuse of the reviewed articles is presented in Table 2 .
In the selected investigations, most educational institutions moved to online learning. The quality requirements listed in Table 3 were used to construct 14 studies.
Area of focus
In this study, 14 publications considered the effect of COVID-19 on pharmacy education, specifically the technological change they sparked, distance and online learning challenges and benefits, and the recommendations for future studies. Eight papers discussed students’ and faculty’s experiences with remote learning and the participants’ perspectives on its possible benefits and drawbacks. Besides, four publications provided remote learning solutions or tested the performance of a specific technology. Three articles discussed educational policies considering the pandemic and examined the new approach to teaching and learning activities. Two papers investigated how the closure and subsequent transformation to technology-based education compounded achievement gaps. The gaps were revealed between students from lower-income households who lacked internet access and devices and those from higher-income families with devices and easy access to the Internet.
The key challenges can be summarized in the following points: disparity in accessibility, training insufficiency , lack of communication, technical issues, pressure, work, and confidence, and lack of student involvement, technical knowledge, and performance evaluatio n.
There is a disparity in accessibility for pharmacy students, typically linked to family income [ 42 , 45 , 46 , 51 ], discussed in four articles from the review sources. The shift to distance and online learning worsened the disparities between wealthy and disadvantaged pharmacy students. Students studying pharmacy in less affluent areas have little or no access to supporting devices and the Internet [ 42 , 45 ]. Students from low-income families were reported to have less skill and knowledge of technology than students from high-income families with strong economic backgrounds [ 38 , 41 ]. The inequality goes to institutions located in rural areas, which are under-equipped compared to institutions located in cities or urban areas [ 52 ], resulting in different challenges faced by each type of institution.
While technology can enhance the learning experience, it cannot completely replace it, especially in pharmacy professions requiring hands-on laboratory training that indeed produces training insufficiency [ 44 , 48 ]. The phenomenon is especially true in health-related fields, such as pharmacy. The papers on pharmacy education emphasized the importance of hands-on experience and how secondary knowledge derived through simulation, presentation recordings, or online meetings through video conferencing cannot replace the experience.
Because of the depreciation or lack of physical interaction and the intrinsic vagueness of textual exchanges, forming and maintaining connections and forging communication between students, their classmates, and their teachers became increasingly challenging [ 38 ]. With the inexistence of visible touch and the capacity to observe students in classrooms, teachers and instructors have a more challenging time explaining directions and evaluating student response, involvement, and participation. These lack of communication challenges have been revealed in three articles within this literature review [ 38 , 40 , 45 ].
Technical issues such as Internet or Wi-Fi access, tool malfunctions, and stream stability might obstruct communication [ 42 , 45 , 51 ]. As the pandemic spread over the globe, accessibility to a dependable internet connection became increasingly vital in the last year, and quite enough of day-to-day life shifted from in-person to online. Many students, however, have suffered from technological challenges since the start of Covid 19, and existing disparities have indeed been exacerbated by the lack of consistent accessibility [ 42 , 45 , 51 ].
Pressure, work, and confidence were all impacted by the students’ and teachers’ forced and quick transfer to remote learning. Many pharmacy students and faculty members faced financial and social anxiety due to the lockdown, which indirectly impacted their performance. Academic employees, for example, had to deal with increased or even quadrupled workloads. Extended time without face-to-face social interaction can also harm one’s mental health.
Technical knowledge is the next challenge of the current study [ 42 , 45 , 46 , 51 ]. Many educational institutions, schools, and universities were surprised by this rapid and forced digital change, giving educational leaders limited time to educate their professional personnel. The complex evidence and reality left non-tech-aware teachers and instructors unprepared and unequipped to work with complex technological-based activities. Teachers’ lack of technical expertise and prior experience using online tools are also challenges [ 42 , 51 ]. In many circumstances, the incapacity of faculty members to use technology hampered the success of distance and online learning.
Other difficulties include a lack of student involvement and performance evaluatio n [ 40 , 41 , 42 , 45 , 51 ]. Student engagement was occasionally weak due to dependency on recorded meetings, limitation of intention, and stress produced by using the devices. There was also weariness from staring at screens for long periods, isolated thoughts, and melancholy from a limited personal touch [ 40 , 42 ]. Teachers faced problems revising learning assessments to fairly record student academic performance and achievements [ 51 ], which is challenging during distance and online learning, especially for pharmacy students.
Other challenges might also be faced during distance and online learning due to Covid-19. The quality of online and distance learning in pharmacy education is one of them, and it can be a major issue. The government’s educational policy makes no explicit mention of distance and learning. Lack of quality control, development of e-resources, and content delivery can be present. This issue needs to be addressed in further work, especially in pharmacy education, so that all stakeholders can take advantage of the advantages of high-quality distance and online education. One should consider developing and improving the quality of learning for future pandemics.
This stage highlights the benefits of digital change in pharmacy education for more opportunities in the future of education. There are a number of benefits [ 39 , 41 , 42 , 45 , 47 , 49 , 50 ] informed by sources included in this systematic literature review, namely bridging the gap between time and place, communication effectiveness, information transition, and cost-effectiveness.
Distance and online learning bridge the gap between time and place , that gives pharmacy students and teachers the freedom to listen to academic lectures and speeches from the coziness of their living rooms or from anywhere else [ 42 , 47 , 50 ]. Due to the time, it also enables pupils to self-regulate their education and progress at their own pace. Distance and online learning give students the opportunities to listen to their lectures from the comfort of their own homes or from anywhere else. Because of the adaptability enabled by elements such as recording, distance and online learning also helps students to self-regulate their learning and continue at their speed. Online learning allows for a more modern and practical way of communication [ 39 , 41 , 47 , 49 , 50 ]. Significant debates might be addressed during courses, and participants can profit from these talks by observing or engaging in chat.
Distance and online learning facilitate communication effectiveness because participants shouldn’t have to talk face to face or deal with the anxiety that comes with talking in front of a live audience, which encourages more conversation. Parents of young children can also benefit from online learning by becoming more active in their children’s education [ 39 , 45 , 47 , 49 ]. The pressures of the pandemic to shift to digital and remote educational models in teaching revealed flaws in the approach and compelled lecturers to consider and evaluate present and prior instructional approaches, offering a glimpse into what educational technology could look like, encouraging didactical advancement and accelerating changes in technology-based education. The process can be considered a catalyst for curricular and classroom improvement [ 39 , 49 , 50 ].
The employment of simulations and other approaches for educational goals and the deployment of online learning are seen as beneficial and adequate, if not comprehensive, substitutes for traditional learning [ 39 , 41 , 42 , 45 , 47 , 49 , 50 ]. It met the goal of continuing to provide instruction in the face of the epidemic while also assisting pupils in meeting their expectations. Distance and online learning also help increase information transmission , with additional benefits of cost-effectiveness . Students are exposed to new and relevant technologies by integrating technology into education [ 39 , 45 , 49 ].
Recommendations and suggestions
The solution is raising and sustaining their motivation to promote morale and battle any lockdown-induced stress or worry. Accessible online learning portals are for institutions in pharmacy education. Generating and accepting feedback from learners to ensure the quality of online learning is another piece of advice made by the existing literature in pharmacy education [ 39 , 42 , 43 , 45 , 49 ]. They are examining the outcomes of distance and online learning and commenting on the distinctions between it and traditional education to identify which components are sustainable and fit the expectations placed on pharmacy education in general by the pandemic situation.
The current study also helps lecturers use effective instructional strategies and allows educational institutions to enhance online instructional resources continuously [ 53 , 54 ]. Pharmacy students comprehend the required courses and sense the connection of the study content to the actual world. Teachers must set clear expectations and establish course objectives and the value of the syllabus to accomplish this [ 39 , 49 ]. Early in the academic year, they must also define their roles and duties as instructors and facilitators [ 43 , 45 ]. Furthermore, authorities should aim to assess and prevent any dangers or disadvantages of economic or workload discrepancies because of this rapid transition from traditional learning to distance and online learning during crises like Covid-19 [ 55 ].
Another piece of advice is to reassess and rethink educational practices and formulate guidance to steer the shifts to online and distance learning and make necessary infrastructural improvements [ 56 , 57 ]. The activities are designed to familiarize students and professors with technology, develop their competence, and equip them to deal with technological challenges that may arise during online lectures [ 49 ]. This will also aid in the effective use of technology to fulfill its full potential in online education. Finally, it is critical to provide underequipped pupils with the essential tools to participate in online communications, such as devices and solid internet access [ 39 , 45 ].
Conclusion and future work
Covid-19 has a major effect on the world and how people arrange themselves in the actual world. It has revealed systemic flaws inside institutions and resulted in lengthy changes. This was also true in the educational system. This assessment aimed to examine and assess the impact of these developments on pharmacy education. In total, 14 articles regarding distance and online learning during Covid-19 were discussed. The current study uses the PRISMA approach to outline the findings through 5 steps (search, screening, eligibility, initial inclusion, and inclusion). To fill the gap of prior studies in pharmacy education, we examined the change in learning from traditional methods to distance and online learning, affecting all related stakeholders. The impact of pandemics on pharmacy education should be more elaborated for future research for the betterment of education, especially pharmacy education. In short, we focus the presentation of the study on the focus areas of the literature, benefits, and challenges of distance and online learning during Covid-19 in pharmacy education.
Availability of data and materials
The datasets used and/or analysed during the current study available from the corresponding author on reasonable request.
WHO. WHO Coronavirus (COVID-19) Dashboard. https://covid19.who.int/ . 2023.
Wang X, Wu W, Song P, He J. An international comparison analysis of reserve and supply system for emergency medical supplies between China, the United States, Australia, and Canada. Biosci Trends. 2020;14.
Djalante R, Shaw R, DeWit A. Building resilience against biological hazards and pandemics: COVID-19 and its implications for the Sendai Framework. Progress in Disaster Science. 2020;6.
Hammerstein S, König C, Dreisörner T, Frey A. Effects of COVID-19-Related School Closures on Student Achievement-A systematic review. Front Psychol. 2021;12.
Chaabane S, Doraiswamy S, Chaabna K, Mamtani R, Cheema S. The impact of covid-19 school closure on child and adolescent health: a rapid systematic review. Children. 2021;8.
Habibi A, Yaakob MFM, Al-Adwan AS. m-Learning Management System use during Covid-19. Inform Dev. 2023. https://doi.org/10.1177/02666669211035473 .
Article Google Scholar
Iwata K, Doi A, Miyakoshi C. Was school closure effective in mitigating coronavirus disease 2019 (COVID-19)? Time series analysis using bayesian inference. Int J Infect Dis. 2020;99.
Moore JL, Dickson-Deane C, Galyen K. E-Learning, online learning, and distance learning environments: Are they the same? Internet and Higher Education. 2011;14.
Maldonado JE, de Witte K. The effect of school closures on standardised student test outcomes. Br Educ Res J. 2022;48.
Aristovnik A, Keržič D, Ravšelj D, Tomaževič N, Umek L. Impacts of the COVID-19 pandemic on life of higher education students: a global perspective. Sustain (Switzerland). 2020;12.
Habibi A, Yaakob MFM, Sofwan M. Student use of digital libraries during COVID-19: structural equation modelling in indonesian and malaysian contexts. Electron Libr. 2022;40:472–85.
Nikou S, Maslov I. An analysis of students’ perspectives on e-learning participation – the case of COVID-19 pandemic. Int J Inform Learn Technol. 2021;38.
Wang XY, Li G, Malik S, Anwar A. Impact of COVID-19 on achieving the goal of sustainable development: E-learning and educational productivity. Economic Research-Ekonomska Istrazivanja. 2022;35.
Chavez Villegas C, Peirolo S, Rocca M, Ipince A, Bakrania S. Impacts of health-related school closures on child protection outcomes: a review of evidence from past pandemics and epidemics and lessons learned for COVID-19. Int J Educ Dev. 2021;84.
Shahzad A, Hassan R, Aremu AY, Hussain A, Lodhi RN. Effects of COVID-19 in E-learning on higher education institution students: the group comparison between male and female. Qual Quant. 2021;55:805–26.
Viner RM, Russell SJ, Croker H, Packer J, Ward J, Stansfield C et al. School closure and management practices during coronavirus outbreaks including COVID-19: a rapid systematic review. The Lancet Child and Adolescent Health. 2020;4.
Cohen J, Kupferschmidt K. Countries test tactics in “war” against COVID-19. Science. 2020;367.
van Lancker W, Parolin Z. COVID-19, school closures, and child poverty: a social crisis in the making. The Lancet Public Health. 2020;5.
Lewis D. What new COVID variants mean for schools is not yet clear. Nature. 2021;589.
Fauzi MA. E-learning in higher education institutions during COVID-19 pandemic: current and future trends through bibliometric analysis. Heliyon. 2022;8.
Fry K. E-learning markets and providers: some issues and prospects. Education + Training. 2001;43.
Turnbull D, Chugh R, Luck J. Transitioning to E-Learning during the COVID-19 pandemic: how have higher Education Institutions responded to the challenge? Educ Inf Technol (Dordr). 2021;26.
He L, Yang N, Xu L, Ping F, Li W, Sun Q et al. Synchronous distance education vs traditional education for health science students: a systematic review and meta-analysis. Med Educ. 2021;55.
Carrillo C, Flores MA. COVID-19 and teacher education: a literature review of online teaching and learning practices. Eur J Teacher Educ. 2020;43.
Daoud R, Starkey L, Eppel E, Vo TD, Sylvester A. The educational value of internet use in the home for school children: a systematic review of literature. J Res Technol Educ. 2020;53.
di Pietro G, Biagi F, Costa P, Karpiński Z, Mazza J. The likely impact of COVID-19 on education: Reflections based on the existing literature and recent international datasets. 2020.
Hodges C, Moore S, Lockee B, Trust T, Bond A. The Difference Between Emergency Remote Teaching and Online Learning. educause. 2020.
Selwyn N, Hillman T, Eynon R, Ferreira G, Knox J, Macgilchrist F et al. What’s next for Ed-Tech? Critical hopes and concerns for the 2020s. Learn Media Technol. 2020;45.
Brako K, Essel HB. Assessment of COVID-19 on Primary Education in. J Internet Bank Commer. 2020;25.
Rodrigues M, Franco M, Silva R. COVID-19 and disruption in management and education academics: bibliometric mapping and analysis. Sustain (Switzerland). 2020;12.
D’Amico S, Bodin P, Delpech M, Noteborn R. Prisma. In: Distributed Space Missions for Earth System Monitoring. 2013. p. 599–637.
Mateo S. A procedure for conduction of a successful literature review using the PRISMA method. Kinesitherapie. 2020;20.
Page MJ, McKenzie JE, Bossuyt PM, Boutron I, Hoffmann TC, Mulrow CD et al. The PRISMA 2020 statement: an updated guideline for reporting systematic reviews. The BMJ. 2021;372.
Bramer WM, de Jonge GB, Rethlefsen ML, Mast F, Kleijnen J. A systematic approach to searching: an efficient and complete method to develop literature searches. J Med Libr Association. 2018;106.
Ryan GW. Using a Word Processor to Tag and retrieve blocks of text. Field methods. 2004;16.
Habibi A, Yusop F, Razak D. RA. Factors affecting Indonesian pre-service teachers’ integration of ICT during teaching practices. In press. 2019.
la Pelle N. Simplifying Qualitative Data Analysis Using General Purpose Software Tools. Field methods. 2004;16.
Alqurshi A. Investigating the impact of COVID-19 lockdown on pharmaceutical education in Saudi Arabia – a call for a remote teaching contingency strategy. Saudi Pharm J. 2020;28.
Phillips CJ, Ford K. The next gen pharmacology classroom: a quality improvement approach to transformation. Teach Learn Nurs. 2021;16.
Attarabeen OF, Gresham-Dolby C, Broedel-Zaugg K. Pharmacy student stress with transition to online education during the COVID-19 pandemic. Curr Pharm Teach Learn. 2021;13.
Montepara CA, Schoen RR, Guarascio AJ, McConaha JL, Horn PJ. Health-system implementation of a collaborative core curriculum for advanced pharmacy experiential education during the COVID-19 pandemic. Am J Health Syst Pharm. 2021;78.
Ali M, Allihyani M, Abdulaziz A, Alansari S, Faqeh S, Kurdi A et al. What just happened? Impact of on-campus activities suspension on pharmacy education during COVID-19 lockdown – A students’ perspective. Saudi Pharm J. 2021;29.
Alzubaidi H, Jirjees FJ, Franson KL, Saidawi W, Othman AM, Rabeeah ZH et al. A global assessment of distance pharmacy education amid COVID-19: teaching, assessment and experiential training. Int J Pharm Pract. 2021;29.
Hamza MS, Badary OA, Elmazar MM. Cross-sectional study on awareness and knowledge of COVID-19 among senior pharmacy students. J Community Health. 2021;46.
Higbea A, Bald E, Isaacs AN, Richter SK, Stamm PL, Kassel LE. Forging ahead from adaptations of teaching during the COVID-19 pandemic: perspectives from multiple pharmacy programs. JACCP J Am Coll Clin Pharm. 2021;4.
Altwaijry N, Ibrahim A, Binsuwaidan R, Alnajjar LI, Alsfouk BA, Almutairi R. Distance education during covid-19 pandemic: a college of pharmacy experience. Risk Manag Healthc Policy. 2021;14.
Stone JK, Pate AN. The impact of COVID-19 through the eyes of a fourth-year pharmacy student. Am J Pharm Educ. 2020;84.
Elbeshbeshy R, Gim S, Quattrocchi E. Impact of COVID-19 on graduating U.S. pharmacy students in the early epicenter of the pandemic in New York City. Exploratory Res Clin Social Pharm. 2021;4.
Morgan K, Adams E, Elsobky T, Darr A, Brackbill M. Moving assessment online: experiences within a school of pharmacy. Online Learn J. 2021;25.
Reynolds PM, Rhein E, Nuffer M, Gleason SE. Educational Methods and Technological Innovations for Introductory Experiential Learning given the contact-related Limitations imposed by the SARS-CoV2/COVID-19 pandemic. Pharmacy. 2021;9.
Etando A, Amu AA, Haque M, Schellack N, Kurdi A, Alrasheedy AA, et al. Challenges and Innovations brought about by the COVID-19 pandemic regarding Medical and Pharmacy Education especially in Africa and Implications for the future. Aubrey Chichonyi Kalungia. 2021;20:24.
Google Scholar
Habibi A, Yaakob MFM, Mukminin A, Muhaimin M, Prasojo LD, Yusop FD et al. Teachers’ digital technology access to motivation, skills and use: a structural equation modeling study. Aslib Journal of Information Management. 2021;ahead-of-p ahead-of-print.
Dhawan S. Online learning: a panacea in the Time of COVID-19 Crisis. J Educational Technol Syst. 2020;49.
Eze SC, Chinedu-Eze VC, Bello AO. The utilisation of e-learning facilities in the educational delivery system of Nigeria: a study of M-University. Int J Educational Technol High Educ. 2018;15.
Aina AY, Ogegbo AA. Teaching and Assessment through Online Platforms during the COVID-19 pandemic: benefits and Challenges. J Educ Elearn Res. 2021;8.
Brindley JE. Learner support in online distance education: Essential and evolving. In: Online distance education: Towards a research agenda. 2014.
Rapanta C, Botturi L, Goodyear P, Guàrdia L, Koole M. Online University Teaching during and after the Covid-19 Crisis: refocusing Teacher Presence and Learning Activity. Postdigital Sci Educ. 2020;2.
Download references
Acknowledgements
We thank Universitas Padjadjaran, Universitas Jambi, Universitas Terbuka, Beijing Normal University, and Universiti Malaya to support the research.
This research is fully funded by Universitas Padjajaran.
Author information
Authors and affiliations.
Faculty of Pharmacy, Universitas Padjadjaran, Bandung, West Java, Indonesia
Muhaimin Muhaimin, Anis Yohana Chaerunisaa & Tiana Milanda
Universitas Jambi, Jambi, Indonesia
Akhmad Habibi
Universitas Terbuka, Banten, Indonesia
Yasir Riady
Jazan University, Jazan, Saudi Arabia
Turki Mesfer Alqahtani
Beijing Normal University, Beijing, China
Tommy Tanu Wijaya
Universiti Malaya, Kuala Lumpur, Malaysia
Farrah Dina Yusop & Nour Awni Albelbisi
You can also search for this author in PubMed Google Scholar
Contributions
Conceptualization—MM. contributed to conceptualization. Introduction—MM, TTW, and YR contributed to introduction. Methodology—AH, AC, and TM contributed to methodology. Literature Review—all authors contributed to literature review. Results—AH, TMA, MM, and YR contributed to the results. Data Curation— AH, MM, and YR contributed to data curation. Project Administration—MM, AC, and TM contributed to project administration. Writing (Original Draft)—MM, AH, TMA, TTW, FDY, NAA and YR contributed to writing the original data. AH, FDY, and NAA contirubuted to the revision of the writing.
Corresponding author
Correspondence to Muhaimin Muhaimin .
Ethics declarations
Competing interests.
The authors declare that they have no conflict of interest.
Ethics approval and consent to participate
Not applicable
Consent for publication
Additional information, publisher’s note.
Springer Nature remains neutral with regard to jurisdictional claims in published maps and institutional affiliations.
Rights and permissions
Open Access This article is licensed under a Creative Commons Attribution 4.0 International License, which permits use, sharing, adaptation, distribution and reproduction in any medium or format, as long as you give appropriate credit to the original author(s) and the source, provide a link to the Creative Commons licence, and indicate if changes were made. The images or other third party material in this article are included in the article’s Creative Commons licence, unless indicated otherwise in a credit line to the material. If material is not included in the article’s Creative Commons licence and your intended use is not permitted by statutory regulation or exceeds the permitted use, you will need to obtain permission directly from the copyright holder. To view a copy of this licence, visit http://creativecommons.org/licenses/by/4.0/ . The Creative Commons Public Domain Dedication waiver ( http://creativecommons.org/publicdomain/zero/1.0/ ) applies to the data made available in this article, unless otherwise stated in a credit line to the data.
Reprints and permissions
About this article
Cite this article.
Muhaimin, M., Habibi, A., Riady, Y. et al. Covid-19 distance and online learning: a systematic literature review in pharmacy education. BMC Med Educ 23 , 367 (2023). https://doi.org/10.1186/s12909-023-04346-6
Download citation
Received : 12 June 2022
Accepted : 10 May 2023
Published : 23 May 2023
DOI : https://doi.org/10.1186/s12909-023-04346-6
Share this article
Anyone you share the following link with will be able to read this content:
Sorry, a shareable link is not currently available for this article.
Provided by the Springer Nature SharedIt content-sharing initiative
- Distance and online learning
- Pharmacy Education
- Systematic literature review
BMC Medical Education
ISSN: 1472-6920
- Submission enquiries: [email protected]
- General enquiries: [email protected]
Thank you for visiting nature.com. You are using a browser version with limited support for CSS. To obtain the best experience, we recommend you use a more up to date browser (or turn off compatibility mode in Internet Explorer). In the meantime, to ensure continued support, we are displaying the site without styles and JavaScript.
- View all journals
- My Account Login
- Explore content
- About the journal
- Publish with us
- Sign up for alerts
- Open access
- Published: 09 May 2024
Looking back to move forward: comparison of instructors’ and undergraduates’ retrospection on the effectiveness of online learning using the nine-outcome influencing factors
- Yujie Su ORCID: orcid.org/0000-0003-1444-1598 1 ,
- Xiaoshu Xu ORCID: orcid.org/0000-0002-0667-4511 1 ,
- Yunfeng Zhang 2 ,
- Xinyu Xu 1 &
- Shanshan Hao 3
Humanities and Social Sciences Communications volume 11 , Article number: 594 ( 2024 ) Cite this article
97 Accesses
Metrics details
- Language and linguistics
This study delves into the retrospections of undergraduate students concerning their online learning experiences after the COVID-19 pandemic, using the nine key influencing factors: behavioral intention, instruction, engagement, interaction, motivation, self-efficacy, performance, satisfaction, and self-regulation. 46 Year 1 students from a comprehensive university in China were asked to maintain reflective diaries throughout an academic semester, providing first-person perspectives on the strengths and weaknesses of online learning. Meanwhile, 18 college teachers were interviewed with the same questions as the students. Using thematic analysis, the research identified 9 factors. The research revealed that instruction ranked highest among the 9 factors, followed by engagement, self-regulation, interaction, motivation, and others. Moreover, teachers and students had different attitudes toward instruction. Thirdly, teacher participants were different from student participants given self-efficacy and self-regulation due to their variant roles in online instruction. Lastly, the study reflected students were not independent learners, which explained why instruction ranked highest in their point of view. Findings offer valuable insights for educators, administrators, and policy-makers involved in higher education. Recommendations for future research include incorporating a more diverse sample, exploring relationships between the nine factors, and focusing on equipping students with skills for optimal online learning experiences.
Similar content being viewed by others
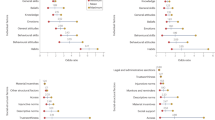
Determinants of behaviour and their efficacy as targets of behavioural change interventions
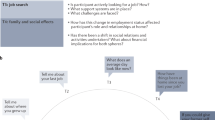
Interviews in the social sciences
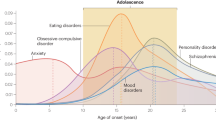
Mechanisms linking social media use to adolescent mental health vulnerability
Introduction.
The outbreak of the COVID-19 pandemic has had a profound impact on education worldwide, leading to the widescale adoption of online learning. According to the United Nations Educational, Scientific and Cultural Organization (UNESCO), at the peak of the pandemic, 192 countries had implemented nationwide closures, affecting approximately 99% of the world’s student population (UNESCO 2020 a). In response, educational institutions, teachers, and students quickly adapted to online learning platforms, leveraging digital technologies to continue education amidst the crisis (Marinoni et al. 2020 ).
The rapid and unexpected shift to online learning brought about a surge in research aiming to understand its impact, effectiveness, and challenges. Researchers across the globe have been investigating various dimensions of online learning. Some focus on students’ experiences and perspectives (Aristovnik et al. 2021 ), technological aspects (Bao 2020 ), pedagogical strategies (Hodges et al. 2020 ), and the socio-emotional aspect of learning (Ali 2020 ). Tan et al. ( 2021 ) found that motivation and satisfaction were mostly positively perceived by students, and lack of interaction was perceived as an unfavorable online instruction perception. Some center on teachers’ perceptions of the benefits and challenges (Lucas and Vicente, 2023 ; Mulla et al. 2023 ), post-pandemic pedagogisation (Rapanta et al. 2021 ), and post-pandemic further education (Kohnke et al. 2023 ; Torsani et al. 2023 ). It was worth noting that elements like interaction and engagement were central to the development and maintenance of the learning community (Lucas and Vincente 2023 ),
The rise of online learning has also posed unprecedented challenges. Studies have pointed out the digital divide and accessibility issues (Crawford et al. 2020 ), students’ motivation and engagement concerns (Martin and Bolliger 2018 ), and the need for effective online instructional practices (Trust and Whalen 2020 ). The rapid transition to online learning has highlighted the need for robust research to address these challenges and understand the effectiveness of online learning in this new educational paradigm.
Despite the extensive research on online learning during and after the COVID-19 pandemic, there remains a notable gap in understanding the retrospective perspectives of both undergraduates and teachers. Much of the current literature has focused on immediate response strategies to the transition to online learning, often overlooking the detailed insights that reflective retrospection can provide (Marinoni et al. 2020 ; Bao 2020 ). In addition, while many studies have examined isolated aspects of online learning, they have not often employed a comprehensive framework, leaving undergraduates’ voices, in particular, underrepresented in the discourse (Aristovnik et al. 2021 ; Crawford et al. 2020 ). This study, situated in the context of the COVID-19 pandemic’s impetus toward online learning, seeks to fill this crucial gap. By exploring online learning from the perspectives of both instructors and undergraduates, and analyzing nine key factors that include engagement, motivation, and self-efficacy, the research contributes vital insights into the dynamics of online education (Wang and Wang 2021 ). This exploration is especially pertinent as digital learning environments become increasingly prevalent worldwide (UNESCO 2020b ). The findings of our study are pivotal for shaping future educational policies and enhancing online education strategies in this continuously evolving educational landscape (Greenhow et al. 2021 ). Thus, three research questions were raised:
Q1: How do undergraduates and teachers in China retrospectively perceive the effectiveness of online learning after the COVID-19 pandemic?
Q2: Which of the nine outcome influencing factors had the most significant impact on online learning experiences after the pandemic, and why?
Q3: What recommendations can be proposed to enhance the effectiveness of online learning in the future?
The research takes place at a comprehensive university in China, with a sample of 46 Year 1 students and 18 experienced teachers. Their reflections on the effectiveness of online learning were captured through reflective diaries guided by four questions. These questions investigated the students’ online learning states and attitudes, identified issues and insufficiencies in online learning, analyzed the reasons behind these problems, and proposed improvements. By assessing their experiences and perceptions, we seek to explore the significant factors that shaped online learning outcomes after the pandemic and the means to enhance its effectiveness.
This paper first presents a review of the existing literature, focusing on the impact of the pandemic on online learning and discussing the nine significant factors influencing online learning outcomes. Following this, the methodology utilized for this study is detailed, setting the stage for a deeper understanding of the research process. Subsequently, we delve into the results of the thematic analysis conducted based on undergraduate students and teachers’ retrospections. Finally, the paper concludes by offering meaningful implications of the findings for various stakeholders and suggesting directions for future research in this critical area.
Literature review
Online learning application and evaluation in higher education.
Online learning, also known as e-learning or distance learning, refers to education that takes place over the Internet rather than in a traditional classroom setting. It has seen substantial growth over the past decade and has been accelerated due to the COVID-19 pandemic (Trust and Whalen 2020 ). Online learning allows for a flexible learning environment, breaking the temporal and spatial boundaries of traditional classroom settings (Bozkurt and Sharma 2020 ). In response to the COVID-19 pandemic, educational institutions globally have embraced online learning at an unprecedented scale. This has led to an immense surge in research focusing on the effects of the pandemic on online learning (Crawford et al. 2020 ; Marinoni et al. 2020 ).
Researchers were divided in their attitudes toward the effects of online learning, including positive, neutral, and negative. Research by Bahasoan et al. ( 2020 ), Bernard et al. ( 2004 ), Hernández-Lara and Serradell-López ( 2018 ), and Paechter and Maier ( 2010 ) indicated the effectiveness of online learning, including improved outcomes and engagement in online formats, providing flexibility and enhancing digital skills for instance. Research, including studies by Dolan Hancock and Wareing ( 2015 ) and Means et al. ( 2010 ), indicates that under equivalent conditions and with similar levels of support, there is frequently no substantial difference in learning outcomes between traditional face-to-face courses and completely online courses.
However, online learning was not without its challenges. Research showing less favorable results for specific student groups can be referenced in Dennen ( 2014 ), etc. The common problems faced by students included underdeveloped independent learning ability, lack of motivation, difficulties in self-regulation, student engagement and technical issues (Aristovnik et al. 2021 ; Martin and Bolliger 2018 ; Song et al. 2004 ; Zheng et al. 2022 ).
Moreover, factors like instructional strategies, course design, etc. were also linked to learning outcomes and successful online learning (Ali 2020 ; Hongsuchon et al. 2022 ). Careaga-Butter et al. ( 2020 ) critically analyze online education in pandemic and post-pandemic contexts, focusing on digital tools and resources for teaching in synchronous and asynchronous learning modalities. They discuss the swift adaptation to online learning during the pandemic, highlighting the importance of technological infrastructure, pedagogical strategies, and the challenges of digital divides. The article emphasizes the need for effective online learning environments and explores trends in post-pandemic education, providing insights into future educational strategies and practices.
Determinants of online learning outcomes
Online learning outcomes in this paper refer to the measurable educational results achieved through online learning methods, including knowledge acquisition, skill development, changes in attitudes or behaviors, and performance improvements (Chang 2016 ; Panigrahi et al. 2018 ). The literature review identified key factors influencing online learning outcomes, emphasizing their significant role in academic discourse. These factors, highlighted in scholarly literature, include student engagement, instructional design, technology infrastructure, student-teacher interaction, and student self-regulation.
Student Engagement: The level of a student’s engagement significantly impacts their learning outcomes. The more actively a student is engaged with the course content and activities, the better their performance tends to be. This underscores the importance of designing engaging course content and providing opportunities for active learning in an online environment (Martin and Bolliger 2018 ).
Instructional Design: How an online course is designed can greatly affect student outcomes. Key elements such as clarity of learning objectives, organization of course materials, and the use of diverse instructional strategies significantly impact student learning (Bozkurt and Sharma 2020 ).
Technology Infrastructure: The reliability and ease of use of the learning management system (LMS) also play a significant role in online learning outcomes. When students experience technical difficulties, it can lead to frustration, reduced engagement, and lower performance (Johnson et al. 2020 ).
Student-Teacher Interaction: Interaction between students and teachers in an online learning environment is a key determinant of successful outcomes. Regular, substantive feedback from instructors can promote student learning and motivation (Boling et al. 2012 ).
Student Self-Regulation: The autonomous nature of online learning requires students to be proficient in self-regulated learning, which involves setting learning goals, self-monitoring, and self-evaluation. Students who exhibit strong self-regulation skills are more likely to succeed in online learning (Broadbent 2017 ).
While many studies have investigated individual factors affecting online learning, there is a paucity of research offering a holistic view of these factors and their interrelationships, leading to a fragmented understanding of the influences on online learning outcomes. Given the multitude of experiences and variables encompassed by online learning, a comprehensive framework like is instrumental in ensuring a thorough investigation and interpretation of the breadth of students’ experiences.
Students’ perceptions of online learning
Understanding students’ perceptions of online learning is essential for enhancing its effectiveness and student satisfaction. Studies show students appreciate online learning for its flexibility and convenience, offering personalized learning paths and resource access (Händel et al. 2020 ; Johnson et al. 2020 ). Yet, challenges persist, notably in maintaining motivation and handling technical issues (Aristovnik et al. 2021 ; Händel et al. 2020 ). Aguilera-Hermida ( 2020 ) reported mixed feelings among students during the COVID-19 pandemic, including feelings of isolation and difficulty adjusting to online environments. Boling et al. ( 2012 ) emphasized students’ preferences for interactive and communicative online learning environments. Additionally, research indicates that students seek more engaging content and innovative teaching approaches, suggesting a gap between current online offerings and student expectations (Chakraborty and Muyia Nafukho 2014 ). Students also emphasize the importance of community and peer support in online settings, underlining the need for collaborative and social learning opportunities (Lai et al. 2019 ). These findings imply that while online learning offers significant benefits, addressing its shortcomings is critical for maximizing its potential.
The pandemic prompted a reconsideration of instructional modalities, with many students favoring face-to-face instruction due to the immediacy and focus issues (Aristovnik et al. 2021 ; Trust and Whalen 2020 ). Despite valuable insights, research gaps remain, particularly in long-term undergraduate reflections and the application of nine factors of comprehensive frameworks, indicating a need for more holistic research in online learning effectiveness.
Teachers’ perceptions of online learning
The pandemic has brought attention to how teachers manage instruction in virtual learning environments. Teachers and students are divided in terms of their attitudes toward online learning. Some teachers and students looked to the convenience and flexibility of online learning (Chuenyindee et al. 2022 ; Al-Emran and Shaalan 2021 ). They conceived that online learning provided opportunities to improve educational equality as well (Tenório et al. 2016 ). Even when COVID-19 was over, the dependence on online learning was likely here to stay, for some approaches of online learning were well-received by students and teachers (Al-Rahmi et al. 2019 ; Hongsuchon et al. 2022 ).
Teachers had shown great confidence in delivering instruction in an online environment in a satisfying manner. They also agreed that the difficulty of teaching was closely associated with course structures (Gavranović and Prodanović 2021 ).
Not all were optimistic about the effects of online learning. They sought out the challenges facing teachers and students during online learning.
A mixed-method study of K-12 teachers’ feelings, experiences, and perspectives that the major challenges faced by teachers during the COVID-19 pandemic were lack of student participation and engagement, technological support for online learning, lack of face-to-face interactions with students, no work-life balance and learning new technology.
The challenges to teachers’ online instruction included instruction technology (Maatuk et al. 2022 ; Rasheed et al. 2020 ), course design (Khojasteh et al. 2023 ), and teachers’ confidence (Gavranović and Prodanović 2021 ).
Self-regulation challenges and challenges in using technology were the key challenges to students, while the use of technology for teaching was the challenge facing teachers (Rasheed et al. 2020 ).
The quality of course design was another important factor in online learning. A research revealed the competency of the instructors and their expertise in content development contributed a lot to students’ satisfaction with the quality of e-contents.
Theoretical framework
The theoretical foundation of the research is deeply rooted in multifaceted framework for online learning, which provides a comprehensive and interwoven model encompassing nine critical factors that collectively shape the educational experience in online settings. This framework is instrumental in guiding our analysis and enhances the comparability and interpretability of our results within the context of existing literature.
Central to Yu’s framework is the concept of behavioral intention, which acts as a precursor to student engagement in online learning environments. This engagement, inherently linked to the students’ intentions and motivations, is significantly influenced by the quality of instruction they receive. Instruction, therefore, emerges as a pivotal element in this model, directly impacting not only student engagement but also fostering a sense of self-efficacy among learners. Such self-efficacy is crucial as it influences both the performance of students and their overall satisfaction with the learning process.
The framework posits that engagement, a derivative of both strong behavioral intention and effective instruction, plays a vital role in enhancing student performance. This engagement is tightly interlaced with self-regulation, an indispensable skill in the autonomous and often self-directed context of online learning. Interaction, encompassing various forms such as student-teacher and peer-to-peer communications, further enriches the learning experience. It significantly contributes to the development of motivation and self-efficacy, both of which are essential for sustaining engagement and fostering self-regulated learning.
Motivation, especially when intrinsically driven, acts as a catalyst, perpetuating engagement and self-regulation, which ultimately leads to increased satisfaction with the learning experience. In this framework, self-efficacy, nurtured through effective instruction and meaningful interactions, has a positive impact on students’ performance and satisfaction, thereby creating a reinforcing cycle of learning and achievement.
Performance in this model is viewed as a tangible measure of the synergistic interplay of engagement, instructional quality, and self-efficacy, while satisfaction reflects the culmination of the learning experience, shaped by the quality of instruction, the extent and nature of interactions, and the flexibility of the learning environment. This satisfaction, in turn, influences students’ future motivation and their continued engagement with online learning.
Yu’s model thus presents a dynamic ecosystem where changes in one factor can have ripple effects across the entire spectrum of online learning. It emphasizes the need for a holistic approach in the realm of online education, considering the complex interplay of these diverse yet interconnected elements to enhance both the effectiveness and the overall experience of online learning.
The current study employed a qualitative design to explore teachers’ and undergraduates’ retrospections on the effectiveness of online learning during the first semester of the 2022–2023 school year, which is in the post-pandemic period. Data were collected using reflective diaries, and thematic analysis was applied to understand the experiences based on the nine factors.
Sample and sampling
The study involved 18 teachers and 46 first-year students from a comprehensive university in China, selected through convenience sampling to ensure diverse representation across academic disciplines. To ensure a diverse range of experiences in online learning, the participant selection process involved an initial email inquiry about their prior engagement with online education. The first author of this study received ethics approval from the department research committee, and participants were informed of the study’s objectives two weeks before via email. Only those participants who provided written informed consent were included in the study and were free to withdraw at any time. Pseudonyms were used to protect participants’ identities during the data-coding process. For direct citations, acronyms of students’ names were used, while “T+number” was used for citations from teacher participants.
The 46 students are all first-year undergraduates, 9 females and 37 males majoring in English and non-English (see Table 1 ).
The 18 teachers are all experienced instructors with at least 5 years of teaching experience, 13 females and 5 male, majoring in English and Non-English (see Table 2 ).
Data collection
Students’ data were collected through reflective diaries in class during the first semester of the 2022–2023 school year. Each participant was asked to maintain a diary over the course of one academic semester, in which they responded to four questions.
The four questions include:
What was your state and attitude toward online learning?
What were the problems and shortcomings of online learning?
What do you think are the reasons for these problems?
What measures do you think should be taken to improve online learning?
This approach provided a first-person perspective on the participants’ online teaching or learning experiences, capturing the depth and complexity of their retrospections.
Teachers were interviewed separately by responding to the four questions the same as the students. Each interview was conducted in the office or the school canteen during the semester and lasted about 20 to 30 min.
Data analysis
We utilized thematic analysis to interpret the reflective diaries, guided initially by nine factors. This method involved extensive engagement with the data, from initial coding to the final report. While Yu’s factors provided a foundational structure, we remained attentive to new themes, ensuring a comprehensive analysis. Our approach was methodical: familiarizing ourselves with the data, identifying initial codes, systematically searching and reviewing themes, and then defining and naming them. To validate our findings, we incorporated peer debriefing, and member checking, and maintained an audit trail. This analysis method was chosen for its effectiveness in extracting in-depth insights from undergraduates’ retrospections on their online learning experiences post-pandemic, aligning with our research objectives.
According to the nine factors, the interviews of 18 teachers and 46 Year 1 undergraduates were catalogued and listed in Table 3 .
Behavioral intention towards online learning post-pandemic
Since the widespread of the COVID-19 pandemic, both teachers and students have experienced online learning. However, their online teaching or learning was forced rather than planned (Baber 2021 ; Bao 2020 ). Students more easily accepted online learning when they perceived the severity of COVID-19.
When entering the post-pandemic era, traditional teaching was resumed. Students often compared online learning with traditional learning by mentioning learning interests, eye contact, face-to-face learning and learning atmosphere.
“I don’t think online learning is a good form of learning because it is hard to focus on learning.” (DSY) “In unimportant courses, I would let the computer log to the platform and at the same time do other entertains such as watching movies, listening to the music, having snacks or do the cleaning.” (XYN) “Online learning makes it impossible to have eye contact between teachers and students and unable to create a face-to-face instructional environment, which greatly influences students’ initiative and engagement in classes.” (WRX)
They noted that positive attitudes toward online learning usually generated higher behavioral intention to use online learning than those with negative attitudes, as found in the research of Zhu et al. ( 2023 ). So they put more blame on distractions in the learning environment.
“Online learning relies on computers or cell phones which easily brings many distractions. … I can’t focus on studying, shifting constantly from study and games.” (YX) “When we talk about learning online, we are hit by an idea that we can take a rest in class. It’s because everyone believes that during online classes, the teacher is unable to see or know what we are doing.” (YM) “…I am easily disturbed by external factors, and I am not very active in class.” (WZB)
Teachers reported a majority of students reluctantly turning on their cameras during online instruction and concluded the possible reason for such behavior.
“One of the reasons why some students are unwilling to turn on the camera is that they are worried about their looks and clothing at home, or that they don’t want to become the focus.” (T4)
They also noticed students’ absent-mindedness and lazy attitude during online instruction.
“As for some students who are not self-regulated, they would not take online learning as seriously as offline learning. Whenever they are logged onto the online platform, they would be unable to stay focused and keep their attention.” (T1)
Challenges and opportunities in online instruction post-pandemic
Online teaching brought new challenges and opportunities for students during and after the pandemic. The distractions at home seemed to be significantly underestimated by teachers in an online learning environment (Radmer and Goodchild 2021 ). It might be the reason why students greatly expected and heavily relied on teachers’ supervision and management.
“The biggest problem of online learning is that online courses are as imperative as traditional classes, but not managed face to face the same as the traditional ones.” (PC) “It is unable to provide some necessary supervision.” (GJX) “It is incapable of giving timely attention to every student.” (GYC) “Teachers can’t understand students’ conditions in time in most cases so teachers can’t adjust their teaching plan.” (MZY) “Some courses are unable to reach the teaching objectives due to lack of experimental conduction and practical operation.” (YZH) “Insufficient teacher-student interaction and the use of cell phones make both groups unable to engage in classes. What’s more, though online learning doesn’t put a high requirement for places, its instructional environment may be crucial due to the possible distractions.” (YCY)
Teachers also viewed online instruction as an addition to face-to-face instruction.
“Online learning cannot run as smoothly as face-to-face instruction, but it can provide an in-time supplement to the practical teaching and students’ self-learning.” (T13, T17) “Online instruction is an essential way to ensure the normal function of school work during the special periods like the pandemic” (T1, T15)
Factors influencing student engagement in online learning
Learning engagement was found to contribute to gains in the study (Paul and Diana 2006 ). It was also referred to as a state closely intertwined with the three dimensions of learning, i.e., vigor, dedication, and absorption (Schaufeli et al. 2002 ). Previous studies have found that some key factors like learning interaction, self-regulation, and social presence could influence learning engagement and learning outcomes (Lowenthal and Dunlap 2020 ; Ng 2018 ). Due to the absence of face-to-face interaction like eye contact, facial expressions and body language, both groups of interviewees agreed that the students felt it hard to keep their attention and thus remain active in online classes.
“Students are unable to engage in study due to a lack of practical learning environment of online learning.” (ZMH, T12) “Online platforms may not provide the same level of engagement and interaction as in-person classrooms, making it harder for students to ask questions or engage in discussions.” (HCK) “The Internet is cold, lack of emotional clues and practical connections, which makes it unable to reproduce face-to-face offline learning so that teachers and students are unlikely to know each other’s true feelings or thoughts. In addition, different from the real-time learning supervision in offline learning, online learning leaves students more learning autonomy.” (XGH) “Lack of teachers’ supervision and practical learning environment, students are easily distracted.” (LMA, T9)
Just as Zhu et al. ( 2023 ) pointed out, we had been too optimistic about students’ engagement in online learning, because online learning relied more on students’ autonomy and efforts to complete online learning.
Challenges in teacher-student interaction in online learning
Online learning has a notable feature, i.e., a spatial and temporal separation among teachers and students. Thus, online teacher-student interactions, fundamentals of relationship formation, have more challenges for both teachers and students. The prior studies found that online interaction affected social presence and indirectly affected learning engagement through social presence (Miao and Ma 2022 ). In the present investigation, both teachers and students noted the striking disadvantage of online interaction.
“Online learning has many problems such as indirect teacher-student communication, inactive informative communication, late response of students and their inability to reflect their problems. For example, teachers cannot evaluate correctly whether the students have mastered or not.” (YYN) “Teachers and students are separated by screens. The students cannot make prompt responses to the teachers’ questions via loudspeakers or headphones. It is not convenient for students to participate in questioning and answering. …for most of the time, the students interact with teachers via typing.” (ZJY) “While learning online, students prefer texting the questions to answering them via the loudspeaker.”(T7)
Online learning interaction was also found closely related to online learning engagement, performance, and self-efficacy.
“Teachers and students are unable to have timely and effective communication, which reduces the learning atmosphere. Students are often distracted. While doing homework, the students are unable to give feedback to teachers.” (YR) “Students are liable to be distracted by many other side matters so that they can keep their attention to online learning.” (T15)
In the online learning environment, teachers need to make efforts to build rapport and personalizing interactions with students to help them perform better and achieve greater academic success (Harper 2018 ; Ong and Quek 2023 ) Meanwhile, teachers should also motivate students’ learning by designing the lessons, giving lectures and managing the processes of student interactions (Garrison 2003 ; Ong and Quek 2023 ).
Determinants of self-efficacy in online learning
Online learning self-efficacy refers to students’ perception of their abilities to fulfill specific tasks required in online learning (Calaguas and Consunji 2022 ; Zimmerman and Kulikowich 2016 ). Online learning self-efficacy was found to be influenced by various factors including task, learner, course, and technology level, among which task level was found to be most closely related (Xu et al. 2022 ). The responses from the 46 student participants reveal a shared concern, albeit without mentioning specific tasks; they highlight critical aspects influencing online learning: learner attributes, course structure, and technological infrastructure.
One unifying theme from the student feedback is the challenge of self-regulation and environmental distractions impacting learning efficacy. For instance, participant WSX notes the necessity for students to enhance time management skills due to deficiencies in self-regulation, which is crucial for successful online learning. Participant WY expands on this by pointing out the distractions outside traditional classroom settings, coupled with limited teacher-student interaction, which hampers idea exchange and independent thought, thereby undermining educational outcomes. These insights suggest a need for strategies that bolster students’ self-discipline and interactive opportunities in virtual learning environments.
On the technological front, participants WT and YCY address different but related issues. Participant WT emphasizes the importance of up-to-date course content and learning facilities, indicating that outdated materials and tools can significantly diminish the effectiveness of online education. Participant YCY adds to this by highlighting problems with online learning applications, such as subpar functionalities that can introduce additional barriers to learning.
Teacher participants, on the other hand, shed light on objective factors predominantly related to course content and technology. Participant T5’s response underscores the heavy dependency on technological advancement in online education and points out the current inability of platforms or apps to adequately monitor student engagement and progress. Participant T9 voices concerns about course content not being updated or aligned with contemporary trends and student interests, suggesting a disconnect between educational offerings and learner needs. Meanwhile, participant T8 identifies unstable network services as a significant hindrance to online teaching, highlighting infrastructure as a critical component of online education’s success.
Teachers also believed the insufficient mastery of facilities and unfamiliarity with online instruction posed difficulty.
“Most teachers and students are not familiar with online instruction. For example, some teachers are unable to manage online courses so they cannot design the courses well. Some students lack self-regulation, which leads to their distraction or avoidance in class.” (T9)
Influences on student performance in online learning
Students’ performance during online lessons is closely associated with their satisfaction and self-efficacy. Most of the student participants reflected on their distractions, confusion, and needs, which indicates their dissatisfaction with online learning.
“During online instruction, it is convenient for the students to make use of cell phones, but instead, cell phones bring lots of distraction.” (YSC) “Due to the limits of online learning, teachers are facing the computer screen and unable to know timely students’ needs and confusion. Meanwhile, it’s inconvenient for teachers to make clear explanations of the sample questions or problems.” (HZW)
They thought their low learning efficiency in performance was caused by external factors like the learning environment.
“The most obvious disadvantage of online learning goes to low efficiency. Students find it hard to keep attention to study outside the practical classroom or in a relaxing environment.” (WY) “Teachers are not strict enough with students, which leads to ineffective learning.” (WRX)
Teacher participants conceived students’ performance as closely related to valid online supervision and students’ self-regulation.
“Online instruction is unable to create a learning environment, which helps teachers know students’ instant reaction. Only when students well regulate themselves and stay focused during online learning can they achieve successful interactions and make good accomplishments in the class.” (T11) “Some students need teachers’ supervision and high self-regulation, or they were easily distracted.” (T16)
Student satisfaction and teaching effectiveness in online learning
Online learning satisfaction was found to be significantly and positively associated with online learning self-efficacy (Al-Nasa’h et al. 2021 ; Lashley et al. 2022 ). Around 46% of student participants were unsatisfied with teachers’ ways of teaching.
“Comparatively, bloggers are more interesting than teachers’ boring and dull voices in online learning.” (DSY) “Teachers’ voice sounds dull and boring through the internet, which may cause listeners to feel sleepy, and the teaching content is not interesting enough to the students.” (MFE)
It reflected partly that some teachers were not adapted to online teaching possibly due to a lack in experience of online teaching or learning (Zhu et al. 2022 ).
“Some teachers are not well-prepared for online learning. They are particularly unready for emergent technological problems when delivering the teaching.” (T1) “One of the critical reasons lies in the fact that teachers and students are not well trained before online learning. In addition, the online platform is not unified by the college administration, which has led to chaos and difficulty of online instruction.” (T17)
Teachers recognized their inadequate preparation and mastery of online learning as one of the reasons for dissatisfaction, but student participants exaggerated the role of teachers in online learning and ignored their responsibility in planning and managing their learning behavior, as in the research of (Xu et al. 2022 ).
The role of self-regulation in online learning success
In the context of online learning, self-regulation stands out as a crucial factor, necessitating heightened levels of student self-discipline and autonomy. This aspect, as Zhu et al. ( 2023 ) suggest, grants students significant control over their learning processes, making it a vital component for successful online education.
“Online learning requires learners to be of high discipline and self-regulation. Without good self-regulation, they are less likely to be effective in online learning.” (YZJ) “Most students lack self-control, unable to control the time of using electronic products. Some even use other electronic products during online learning, which greatly reduces their efficiency in learning.” (GPY) “Students are not well developed in self-control and easily distracted. Thus they are unable to engage fully in their study, which makes them unable to keep up with others” (XYN)
Both groups of participants had a clear idea of the positive role of self-regulation in successful learning, but they also admitted that students need to strengthen their self-regulation skills and it seemed they associated with the learning environment, learning efficiency and teachers’ supervision.
“If they are self-motivated, online learning can be conducted more easily and more efficiently. However, a majority are not strong in regulating themselves. Teachers’ direct supervision in offline learning can do better in motivating them to study hard…lack of interaction makes students less active and motivated.” (LY) “Students have a low level of self-discipline. Without teachers’ supervision, they find it hard to listen attentively or even quit listening. Moreover, in class, the students seldom think actively and independently.” (T13)
The analysis of participant responses, categorized into three distinct attitude groups – positive, neutral, and negative – reveals a multifaceted view of the disadvantages of online learning, as shown in Tables 4 and 5 . This classification provides a clearer understanding of how attitudes towards online learning influence perceptions of self-regulation and other related factors.
In Table 4 , the division among students is most pronounced in terms of interaction and self-efficacy. Those with neutral attitudes highlighted interaction as a primary concern, suggesting that it is less effective in an online setting. Participants with positive attitudes noted a lack of student motivation, while those with negative views emphasized the need for better self-efficacy. Across all attitudes, instruction, engagement, self-regulation, and behavior intention were consistently identified as areas needing improvement.
Table 5 sheds light on teachers’ perspectives, revealing a consensus on the significance and challenges of instruction, motivation, and self-efficacy in online learning. Teachers’ opinions vary most significantly on self-efficacy and engagement. Those with negative attitudes point to self-efficacy and instructional quality as critical areas needing attention, while neutral attitudes focus on the role of motivation.
Discussions
Using a qualitative and quantitative analysis of the questionnaire data showed that among the 18 college teachers and 46 year 1 undergraduate students of various majors taking part in the interview, about 38.9% of teachers and about 30.4% of students supported online learning. Only two teachers were neutral about online learning, and 50% of teachers did not support virtual learning. The percentages of students who expressed positive and neutral views on online learning were the same, i.e., 34.8%. This indicates that online learning could serve as a complementary approach to traditional education, yet it is not without challenges, particularly in terms of student engagement, self-regulation, and behavioral intention, which were often attributed to distractions inherent in online environments.
In analyzing nine factors, it was evident that both teachers and students did not perceive these factors uniformly. Instruction was a significant element for both groups, as validated by findings in Tables 3 and 5 . The absence of face-to-face interactions in online learning shifted the focus to online instruction quality. Teachers cited technological challenges as a central concern, while students criticized the lack of engaging content and teaching methods. This aligns with Miao and Ma ( 2022 ), who argued that direct online interaction does not necessarily influence learner engagement, thus underscoring the need for integrated approaches encompassing interactions, self-regulation, and social presence.
Furthermore, the role of technology acceptance in shaping self-efficacy was highlighted by Xu et al. ( 2022 ), suggesting that students with higher self-efficacy tend to challenge themselves more. Chen and Hsu ( 2022 ) noted the positive influence of using emojis in online lessons, emphasizing the importance of innovative pedagogical approaches in online settings.
The study revealed distinct priorities between teachers and students in online learning: teachers emphasized effective instruction delivery, while students valued learning outcomes, self-regulation, and engagement. This divergence highlights the unique challenges each group faces. Findings by Dennen et al. ( 2007 ) corroborate this, showing instructors focusing on content and guidance, while students prioritize interpersonal communication and individualized attention. Additionally, Lee et al. ( 2011 ) found that reduced transactional distance and increased student engagement led to enhanced perceptions of learning outcomes, aligning with students’ priorities in online courses. Understanding these differing perspectives is crucial for developing comprehensive online learning strategies that address the needs of both educators and learners.
Integrating these findings with broader contextual elements such as technological infrastructure, pedagogical strategies, socio-economic backgrounds, and environmental factors (Balanskat and Bingimlas 2006 ) further enriches our understanding. The interplay between these external factors and Yu’s nine key aspects forms a complex educational ecosystem. For example, government interventions and training programs have been shown to increase teachers’ enthusiasm for ICT and its routine use in education (Balanskat and Bingimlas 2006 ). Additionally, socioeconomic factors significantly impact students’ experiences with online learning, as the digital divide in connectivity and access to computers at home influences the ICT experience, an important factor for school achievement (OECD 2015 ; Punie et al. 2006 ).
In sum, the study advocates for a holistic approach to understanding and enhancing online education, recognizing the complex interplay between internal factors and external elements that shape the educational ecosystem in the digital age.
Conclusion and future research
This study offered a comprehensive exploration into the retrospective perceptions of college teachers and undergraduate students regarding their experiences with online learning following the COVID-19 pandemic. It was guided by a framework encompassing nine key factors that influence online learning outcomes. To delve into these perspectives, the research focused on three pivotal questions. These questions aimed to uncover how both undergraduates and teachers in China view the effectiveness of online learning post-pandemic, identify which of the nine influencing factors had the most significant impact, and propose recommendations for enhancing the future effectiveness of online learning.
In addressing the first research question concerning the retrospective perceptions of online learning’s effectiveness among undergraduates and teachers in China post-COVID-19 pandemic, the thematic analysis has delineated clear divergences in attitude between the two demographics. Participants were primarily divided into three categories based on their stance toward online learning: positive, neutral, and negative. The results highlighted a pronounced variance in attitude distribution between teachers and students, with a higher percentage of teachers expressing clear-cut opinions, either favorably or unfavorably, towards the effectiveness of online learning.
Conversely, students displayed a pronounced inclination towards neutrality, revealing a more cautious or mixed stance on the effectiveness of online learning. This prevalent neutrality within the student body could be attributed to a range of underlying reasons. It might signify students’ uncertainties or varied experiences with online platforms, differences in engagement levels, gaps in digital literacy, or fluctuating quality of online materials and teaching methods. Moreover, this neutral attitude may arise from the psychological and social repercussions of the pandemic, which have potentially altered students’ approaches to and perceptions of learning in an online context.
In the exploration of the nine influential factors in online learning, it was discovered that both teachers and students overwhelmingly identified instruction as the most critical aspect. This was closely followed by engagement, interaction, motivation, and other factors, while performance and satisfaction were perceived as less influential by both groups. However, the attitudes of teachers and students towards these factors revealed notable differences, particularly about instruction. Teachers often attributed challenges in online instruction to technological issues, whereas students perceived the quality of instruction as a major influence on their learning effectiveness. This dichotomy highlights the distinct perspectives arising from their different roles within the educational process.
A further divergence was observed in views on self-efficacy and self-regulation. Teachers, with a focus on delivering content, emphasized the importance of self-efficacy, while students, grappling with the demands of online learning, prioritized self-regulation. This reflects their respective positions in the online learning environment, with teachers concerned about the efficacy of their instructional strategies and students about managing their learning process. Interestingly, the study also illuminated that students did not always perceive themselves as independent learners, which contributed to the high priority they placed on instruction quality. This insight underlines a significant area for development in online learning strategies, emphasizing the need for fostering greater learner autonomy.
Notably, both teachers and students concurred that stimulating interest was a key factor in enhancing online learning. They proposed innovative approaches such as emulating popular online personalities, enhancing interactive elements, and contextualizing content to make it more relatable to students’ lives. Additionally, practical suggestions like issuing preview tasks and conducting in-class quizzes were highlighted as methods to boost student engagement and learning efficiency. The consensus on the importance of supervisory roles underscores the necessity for a balanced approach that integrates guidance and independence in the online learning environment.
The outcomes of our study highlight the multifaceted nature of online learning, accentuated by the varied perspectives and distinct needs of teachers and students. This complexity underscores the necessity of recognizing and addressing these nuances when designing and implementing online learning strategies. Furthermore, our findings offer a comprehensive overview of both the strengths and weaknesses of online learning during an unprecedented time, offering valuable insights for educators, administrators, and policy-makers involved in higher education. Moreover, it emphasized the intricate interplay of multiple factors—behavioral intention, instruction, engagement, interaction, motivation, self-efficacy, performance, satisfaction, and self-regulation—in shaping online learning outcomes. presents some limitations, notably its reliance on a single research method and a limited sample size.
However, the exclusive use of reflective diaries and interviews restricts the range of data collection methods, which might have been enriched by incorporating additional quantitative or mixed-method approaches. Furthermore, the sample, consisting only of students and teachers from one university, may not adequately represent the diverse experiences and perceptions of online learning across different educational contexts. These limitations suggest the need for a cautious interpretation of the findings and indicate areas for future research expansion. Future research could extend this study by incorporating a larger, more diverse sample to gain a broader understanding of undergraduate students’ retrospections across different contexts and cultures. Furthermore, research could also explore how to better equip students with the skills and strategies necessary to optimize their online learning experiences, especially in terms of the self-regulated learning and motivation aspects.
Data availability
The data supporting this study is available from https://doi.org/10.6084/m9.figshare.25583664.v1 . The data consists of reflective diaries from 46 Year 1 students from a comprehensive university in China and 18 college teachers. We utilized thematic analysis to interpret the reflective diaries, guided initially by nine factors. The results highlight the critical need for tailored online learning strategies and provide insights into its advantages and challenges for stakeholders in higher education.
Aguilera-Hermida AP (2020) College students’ use and acceptance of emergency online learning due to COVID-19. Int. J. Educ. Res. Open 1:100011. https://doi.org/10.1016/j.ijedro.2020.100011
Article Google Scholar
Al-Emran, M, & Shaalan, K (2021, October 27). E-podium technology: A medium of managing knowledge at Al Buraimi University College via M-learning. In Proceedings of the 2nd BCS International IT Conference, Abu Dhabi, United Arab Emirates 2014. Retrieved October 17, 2023, from https://dblp.uni-trier.de/rec/conf/bcsit/EmranS14.html
Ali W (2020) Online and remote learning in higher education institutes: A necessity in light of COVID-19 pandemic. High. Educ. Stud. 10(3):16–25. https://doi.org/10.5539/hes.v10n3p16
Al-Nasa’h M, Al-Tarawneh L, Awwad FMA, Ahmad I (2021) Estimating Students’ Online Learning Satisfaction during COVID-19: A Discriminant Analysis. Heliyon 7(12):1–7. https://doi.org/10.1016/j.helyon.2021.e08544
Al-Rahmi WM, Yahaya N, Aldraiweesh AA, Alamri MM, Aljarboa NA, Alturki U (2019) Integrating technology acceptance model with innovation diffusion theory: An empirical investigation on students’ intention to use E-learning systems. IEEE Access 7:26797–26809. https://doi.org/10.1109/ACCESS.2019.2899368
Aristovnik A, Keržič D, Ravšelj D, Tomaževič N, Umek L (2021) Impacts of the COVID-19 pandemic on life of higher education students: A global perspective. Sustainability 12(20):8438. https://doi.org/10.3390/su12208438
Article CAS Google Scholar
Baber H (2021) Modelling the Acceptance of E-learning during the Pandemic Of COVID-19-A Study of South Korea. Int. J. Manag. Educ. 19(2):1–15. https://doi.org/10.1016/j.ijme.2021.100503
Article MathSciNet Google Scholar
Bahasoan AN, Ayuandiani W, Mukhram M, Rahmat A (2020) Effectiveness of online learning in pandemic COVID-19. Int. J. Sci., Technol. Manag. 1(2):100–106
Google Scholar
Balanskat A, Bingimlas KA (2006) Barriers to the Successful Integration of ICT in Teaching and Learning Environments: A Review of the Literature. Eurasia J. Math. Sci. Technol. Educ. 5(3):235–245. https://doi.org/10.12973/ejmste/75275
Bao W (2020) COVID-19 and Online Teaching in Higher Education: A Case Study of Peking University. Hum. Behav. Emerg. Technol. 2(2):113–115. https://doi.org/10.1002/hbe2.191
Article PubMed PubMed Central Google Scholar
Bernard RM et al. (2004) How Does Distance Education Compare with Classroom Instruction? A Meta-Analysis of the Empirical Literature. Rev. Educ. Res. 74(3):379–439
Boling EC, Hough M, Krinsky H, Saleem H, Stevens M (2012) Cutting the distance in distance education: Perspectives on what promotes positive, online learning experiences. Internet High. Educ. 15:118–126. https://doi.org/10.1016/j.iheduc.2011.11.006
Bozkurt A, Sharma RC (2020) Emergency remote teaching in a time of global crisis due to Coronavirus pandemic. Asian J. Distance Educ. 15(1):i–vi
Broadbent J (2017) Comparing online and blended learner’s self-regulated learning strategies and academic performance. Internet High. Educ. 33:24–32. https://doi.org/10.1016/j.iheduc.2017.01.004
Calaguas NP, Consunji PMP (2022) A Structural Equation Model Predicting Adults’ Online Learning Self-efficacy. Educ. Inf. Technol. 27:6233–6249. https://doi.org/10.1007/s10639-021-10871-y
Careaga-Butter M, Quintana MGB, Fuentes-Henríquez C (2020) Critical and Prospective Analysis of Online Education in Pandemic and Post-pandemic Contexts: Digital Tools and Resources to Support Teaching in synchronous and Asynchronous Learning Modalities. Aloma: evista de. psicologia, ciències de. l’educació i de. l’esport Blanquerna 38(2):23–32
Chakraborty M, Muyia Nafukho F (2014) Strengthening Student Engagement: What do Students Want in Online Courses? Eur. J. Train. Dev. 38(9):782–802
Chang V (2016) Review and Discussion: E-learning for Academia and Industry. Int. J. Inf. Manag. 36(3):476–485. https://doi.org/10.1016/j.ijinfomgt.2015.12.007
Chen YJ, Hsu LW (2022) Enhancing EFL Learners’ Self-efficacy Beliefs of Learning English with emoji Feedbacks in Call: Why and How. Behav. Sci. 12(7):227. https://doi.org/10.3390/bs12070227
Chuenyindee T, Montenegro LD, Ong AKS, Prasetyo YT, Nadlifatin R, Ayuwati ID, Sittiwatethanasiri T, Robas KPE (2022) The Perceived Usability of the Learning Management System during the COVID-19 Pandemic: Integrating System Usability Scale, Technology Acceptance Model, and Task-technology Fit. Work 73(1):41–58. https://doi.org/10.3233/WOR-220015
Article PubMed Google Scholar
Crawford J, Butler-Henderson K, Rudolph J, Malkawi B, Glowatz M, Burton R, Lam S (2020) COVID-19: 20 countries’ higher education intra-period digital pedagogy responses. J. Appl. Teach. Learn. 3(1):120. https://doi.org/10.37074/jalt.2020.3.1.7
Dennen VP (2014) Becoming a blogger: Trajectories, norms, and activities in a community of practice. Computers Hum. Behav. 36:350–358. https://doi.org/10.1016/j.chb.2014.03.028
Dennen VP, Darabi AA, Smith LJ (2007) Instructor–learner Interaction in Online Courses: The Relative Perceived Importance of Particular Instructor Actions on Performance and Satisfaction. Distance Educ. 28(1):65–79
Dolan E, Hancock E, Wareing A (2015) An evaluation of online learning to teach practical competencies in undergraduate health science students. Internet High. Educ. 24:21–25
Garrison DR (2003) Cognitive Presence for Effective Asynchronous Online Learning: The Role of Reflective. Inq., Self-Direction Metacognition. Elem. Qual. Online Educ.: Pract. Direction 4(10):47–58
Gavranović V, Prodanović M (2021) ESP Teachers’ Perspectives on the Online Teaching Environment Imposed in the Covid-19 Era-A Case Study. N. Educ. Rev. 2:188–197. https://doi.org/10.15804/TNER.2021.64.2.15
Greenhow C, Lewin C, Staudt Willet KB (2021) The Educational Response to Covid-19 across Two Countries: a Critical Examination of Initial Digital Pedagogy Adoption. Technol., Pedagog. Educ. 30(1):7–25
Händel M, Stephan M, Gläser-Zikuda M, Kopp B, Bedenlier S, Ziegler A (2020) Digital readiness and its effects on higher education students’ socio-emotional perceptions in the context of the COVID-19 pandemic. J. Res. Technol. Educ. 53(2):1–13
Harper B (2018) Technology and Teacher-student Interactions: A Review of Empirical Research. J. Res. Technol. Educ. 50(3):214–225
Article ADS Google Scholar
Hernández-Lara AB, Serradell-López E (2018) Student interactions in online discussion forums: their perception on learning with business simulation games. Behav. Inf. Technol. 37(4):419–429
Hodges C, Moore S, Lockee B, Trust T, Bond A (2020) The difference between emergency remote teaching and online learning. Educause Rev. 27:1–12
Hongsuchon T, Emary IMME, Hariguna T, Qhal EMA (2022) Assessing the Impact of Online-learning Effectiveness and Benefits in Knowledge Management, the Antecedent of Online-learning Strategies and Motivations: An Empirical Study. Sustainability 14(5):1–16. https://doi.org/10.3390/su14052570 . (2020)
Johnson N, Veletsianos G, Seaman J (2020) US faculty and administrators’ experiences and approaches in the early weeks of the COVID-19 pandemic. Online Learn. 24(2):6–21
Khojasteh L, Karimian Z, Farahmandi AY, Nasiri E, Salehi N (2023) E-content Development of English Language Courses during COVID-19: A Comprehensive Analysis of Students’ Satisfaction. J. Computer Educ. 10(1):107–133. https://doi.org/10.1007/s40692-022-00224-0
Kohnke, L, & Foung, D (2023). Exploring Microlearning for Teacher Professional Development: Voices from Hong Kong. In Tafazoli, D, M Picard (Eds.). Handbook of CALL Teacher Education Professional Development (pp. 279-292). Singapore: Springer Nature Singapore Pte Ltd
Lai CH, Lin HW, Lin RM, Tho PD (2019) Effect of Peer Interaction among Online Learning Community on Learning Engagement and Achievement. Int. J. Distance Educ. Technol. (IJDET) 17(1):66–77
Lashley PM, Sobers NP, Campbell MH, Emmanuel MK, Greaves N, Gittens-St Hilaire M, Murphy MM, Majumder MAA (2022) Student Satisfaction and Self-Efficacy in a Novel Online Clinical Clerkship Curriculum Delivered during the COVID-19 Pandemic. Adv. Med. Educ. Pract. 13:1029–1038. https://doi.org/10.2147/AMEP.S374133
Lee SJ, Srinivasan S, Trail T, Lewis D, Lopez S (2011) Examining the Relationship among Student Perception of Support, Course Satisfaction, and Learning Outcomes in Online Learning. Internet High. Educ. 14(3):158–163
Lowenthal PR, Dunlap JC (2020) Social Presence and Online Discussions: A Mixed Method Investigation. Distance Educ. 41:490–514. https://doi.org/10.1080/01587919.2020.1821603
Lucas M, Vicente PN (2023) A Double-edged Sword: Teachers’ Perceptions of the Benefits and Challenges of Online Learning and Learning in Higher Education. Educ. Inf. Technol. 23:5083–5103. https://doi.org/10.1007/s10639-022-11363-3
Maatuk AM, Elberkawi EK, Aljawarneh S, Rashaideh H, Alharbi H (2022) The COVID-19 Pandemic and E-learning: Challenges and Opportunities from the Perspective of Students and Instructors. J. Comput. High. Educ. 34:21–38. https://doi.org/10.1007/s12528-021-09274-2
Marinoni G, Van’t Land H, Jensen T (2020) The Impact of Covid-19 on Higher Education around the World. IAU Glob. Surv. Rep. 23:1–17
Martin F, Bolliger DU (2018) Engagement matters: Student perceptions on the importance of engagement strategies in the online learning environment. Online Learn. 22(1):205–222
Means B, et al. (2010). Evaluation of Evidence-Based Practices in Online Learning: A Meta-Analysis and Review of Online Learning Studies. Washington, D. C.: U.S. Department of Education
Miao J, Ma L (2022) Students’ Online Interaction, Self-regulation, and Learning Engagement in Higher Education: The Importance of Social Presence to Online Learning. Front. Psychol. 13:1–9. https://doi.org/10.3389/fpsyg.2022.815220
Mulla T, Munir S, Mohan V (2023) An Exploratory Study to Understand Faculty Members’ Perceptions and Challenges in Online Teaching. Int. Rev. Educ. 69:73–99. https://doi.org/10.1007/s11159-023-100
Ng EW (2018) Integrating Self-regulation Principles with Flipped Classroom Pedagogy for First Year University Students. Computer Educ. 126:65–74. https://doi.org/10.1007/s11409-011-9082-8
Ong SGT, Quek GCL (2023) Enhancing teacher–student interactions and student online engagement in an online learning environment. Learn. Environ. Res. 26:681–707. https://doi.org/10.1007/s10984-022-09447-5
Organisation for Economic Co‑operation and Development. (2015). The g20 skills strategy for developing and using skills for the 21st century. Retrieved from https://www.oecd.org/g20/topics/employment-andsocial-policy/The-G20-Skills-Strategy-for-Developing-and-Using-Skills-for-the-21st-Century.pdf
Paechter M, Maier B (2010) Online or face-to-face? Students’ experiences and preferences in e-learning. internet High. Educ. 13(4):292–297
Panigrahi R, Srivastava PR, Sharma D (2018) Online Learning: Adoption, Continuance, and Learning Outcome—A Review of Literature. Int. J. Inf. Manag. 43:1–14
Paul C, Diana M (2006) Teaching Methods and Time on Task in Junior Classrooms. Educ. Res. 30:90–97
Punie, Y, Zinnbauer, D, & Cabrera, M (2006). A review of the impact of ICT on learning. European Commission, Brussels, 6(5), 635-650
Radmer F, Goodchild S (2021) Online Mathematics Teaching and Learning during the COVID-19 Pandemic: The Perspective of Lecturers and Students. Nord. J. STEM Educ. 5(1):1–5. https://doi.org/10.5324/njsteme.v5i1.3914
Rapanta C, Botturi L, Goodyear P, Guadia L, Koole M (2021) Balancing Technology, Pedagogy and the New Normal: Post-pandemic Challenges for Higher Education. Postdigital Sci. Educ. 3:715–742. https://doi.org/10.1007/s42438-021-00249-1
Rasheed, RA, Kamsin, A, & Abdullah, NA (2020) Challenges in the Online Component of Blended Learning: A Systematic Review. Computers & Education, 144. https://doi.org/10.1016/j.compedu.2019.103701
Schaufeli W, Salanova M, Gonzalez-Roma V (2002) The Measurement of Engagement and Burnout: A Two Sample Confirmatory Factor Analytic Approach. J. Happiness Stud. 3:71–92
Song L, Singleton ES, Hill JR, Koh MH (2004) Improving online learning: Student perceptions of useful and challenging characteristics. Internet High. Educ. 7(1):59–70. https://doi.org/10.1016/j.iheduc.2003.11.003
Tan HT, Chan PP, Said NM (2021) Higher Education Students’ Online Instruction Perceptions: A Quality Virtual Learning Environment. Sustainability 13:10840. https://doi.org/10.3390/su131910840
Tenório T, Bittencourt II, Isotani S, Silva AP (2016) Does peer assessment in online learning environments work? A systematic review of the literature. Computers Hum. Behav. 64:94–107. https://doi.org/10.1016/j.chb.2016.06.020
Torsani, S (2023) Teacher Education in Mobile Assisted Language Learning for Adult Migrants: A Study of Provincial Centers for Adult Education in Italy. In Tafazoli, D, & M Picard (eds.). Handbook of CALL Teacher Education Professional Development (pp. 179-192). Singapore: Springer Nature Singapore Pte Ltd
Trust T, Whalen J (2020) Should teachers be trained in emergency remote teaching? Lessons learned from the COVID-19 pandemic. J. Technol. Teach. Educ. 28(2):189–199
UNESCO (2020a) COVID-19 Impact on education. UNESCO. Retrieved from https://en.unesco.org/covid19/educationresponse
UNESCO (2020b) Education: From Disruption to Recovery. UNESCO. Retrieved from https://en.unesco.org/covid19/educationresponse
Wang M, & Wang F (2021, August) Comparative Analysis of University Education Effect under the Traditional Teaching and Online Teaching Mode. In The Sixth International Conference on Information Management and Technology (pp. 1-6)
Xu Q, Wu J, Peng HY (2022) Chinese EFL University Students’ Self-efficacy for Online Self-regulated Learning: Dynamic Features and Influencing Factors. Front. Psychol. 13:1–12. https://doi.org/10.3389/fpsyg.2022.912970
Zheng RK, Li F, Jiang L, Li SM (2022) Analysis of the Current Situation and Driving Factors of College Students’ Autonomous Learning in the Network Environment. Front. Humanit. Soc. Sci. 2(7):44–50
Zhu XM, Gong Q, Wang Q, He YJ, Sun ZQ, Liu FF (2023) Analysis of Students’ Online Learning Engagement during the COVID-19 Pandemic: A Case Study of a SPOC-Based Geography Education Undergraduate Course. Sustainability 15(5):4544. https://doi.org/10.3390/su15054544
Zhu Y, Geng G, Disney L, Pan Zihao (2023) Changes in University Students’ Behavioral intention to learn online throughout the COVID-19: Insights for Online Teaching in the Post-pandemic Era. Educ. Inf. Technol. 28:3859–3892. https://doi.org/10.1007/s10639-022-11320-0
Zhu YH, Xu YY, Wang XY, Yan SY, Zhao L (2022) The Selectivity and Suitability of Online Learning Resources as Predictor of the Effects of Self-efficacy on Teacher Satisfaction During the COVID-19 Lockdown. Front. Psychol. 13:1–11. https://doi.org/10.3389/fpsyg.2022.765832
Zimmerman WA, Kulikowich JM (2016) Online Learning Self-efficacy in Students with and Without Online Learning Experience. Am. J. Distance Educ. 30(3):180–190. https://doi.org/10.1080/08923647.2016.1193801
Download references
Acknowledgements
The Corresponding author received the National Social Science Foundation of China for Education General Program (BGA210054) for this work.
Author information
Authors and affiliations.
School of Foreign Studies, Wenzhou University, Wenzhou, China
Yujie Su, Xiaoshu Xu & Xinyu Xu
Faculty of Languages and Translation, Macao Polytechnic University, Macao, China
Yunfeng Zhang
Faculty of Applied Sciences, Macao Polytechnic University, Macao, China
Shanshan Hao
You can also search for this author in PubMed Google Scholar
Contributions
XSX was responsible for conceptualization and, alongside YFZ, for data curation. YJS and XYX conducted the formal analysis. Funding acquisition was managed by YFZ. The investigation was carried out by YJS and YFZ. Methodology development was a collaboration between YJS and XSX. XSX and YJS also managed project administration, with additional resource management by SSH and XYX. YJS handled the software aspect, and supervision was overseen by XSX. SSH and XYX were responsible for validation, and visualization was managed by YJS. The original draft was written by XSX and YJS, while the review and editing were conducted by YFZ and SSH.
Corresponding author
Correspondence to Xiaoshu Xu .
Ethics declarations
Competing interests.
The authors declare no competing interests.
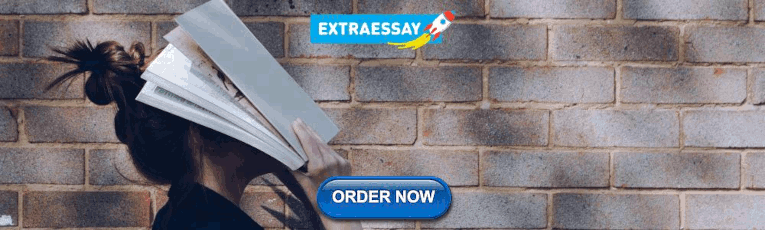
Ethical approval
The questionnaire and methodology for this study were approved by the Human Research Ethics Committee of Wenzhou University (Ethics approval number WGY202302).
Informed consent
Informed consent was diligently obtained from all participants involved in the study, ensuring adherence to ethical standards. Detailed consent forms, outlining the study’s scope and participants’ rights, were signed by participants. Documentation of this process is well-maintained and can be provided upon request during peer review or post-publication.
Additional information
Publisher’s note Springer Nature remains neutral with regard to jurisdictional claims in published maps and institutional affiliations.
Rights and permissions
Open Access This article is licensed under a Creative Commons Attribution 4.0 International License, which permits use, sharing, adaptation, distribution and reproduction in any medium or format, as long as you give appropriate credit to the original author(s) and the source, provide a link to the Creative Commons licence, and indicate if changes were made. The images or other third party material in this article are included in the article’s Creative Commons licence, unless indicated otherwise in a credit line to the material. If material is not included in the article’s Creative Commons licence and your intended use is not permitted by statutory regulation or exceeds the permitted use, you will need to obtain permission directly from the copyright holder. To view a copy of this licence, visit http://creativecommons.org/licenses/by/4.0/ .
Reprints and permissions
About this article
Cite this article.
Su, Y., Xu, X., Zhang, Y. et al. Looking back to move forward: comparison of instructors’ and undergraduates’ retrospection on the effectiveness of online learning using the nine-outcome influencing factors. Humanit Soc Sci Commun 11 , 594 (2024). https://doi.org/10.1057/s41599-024-03097-z
Download citation
Received : 24 October 2023
Accepted : 17 April 2024
Published : 09 May 2024
DOI : https://doi.org/10.1057/s41599-024-03097-z
Share this article
Anyone you share the following link with will be able to read this content:
Sorry, a shareable link is not currently available for this article.
Provided by the Springer Nature SharedIt content-sharing initiative
Quick links
- Explore articles by subject
- Guide to authors
- Editorial policies

- Research article
- Open access
- Published: 02 December 2020
Integrating students’ perspectives about online learning: a hierarchy of factors
- Montgomery Van Wart 1 ,
- Anna Ni 1 ,
- Pamela Medina 1 ,
- Jesus Canelon 1 ,
- Melika Kordrostami 1 ,
- Jing Zhang 1 &
International Journal of Educational Technology in Higher Education volume 17 , Article number: 53 ( 2020 ) Cite this article
148k Accesses
49 Citations
24 Altmetric
Metrics details
This article reports on a large-scale ( n = 987), exploratory factor analysis study incorporating various concepts identified in the literature as critical success factors for online learning from the students’ perspective, and then determines their hierarchical significance. Seven factors--Basic Online Modality, Instructional Support, Teaching Presence, Cognitive Presence, Online Social Comfort, Online Interactive Modality, and Social Presence--were identified as significant and reliable. Regression analysis indicates the minimal factors for enrollment in future classes—when students consider convenience and scheduling—were Basic Online Modality, Cognitive Presence, and Online Social Comfort. Students who accepted or embraced online courses on their own merits wanted a minimum of Basic Online Modality, Teaching Presence, Cognitive Presence, Online Social Comfort, and Social Presence. Students, who preferred face-to-face classes and demanded a comparable experience, valued Online Interactive Modality and Instructional Support more highly. Recommendations for online course design, policy, and future research are provided.
Introduction
While there are different perspectives of the learning process such as learning achievement and faculty perspectives, students’ perspectives are especially critical since they are ultimately the raison d’être of the educational endeavor (Chickering & Gamson, 1987 ). More pragmatically, students’ perspectives provide invaluable, first-hand insights into their experiences and expectations (Dawson et al., 2019 ). The student perspective is especially important when new teaching approaches are used and when new technologies are being introduced (Arthur, 2009 ; Crews & Butterfield, 2014 ; Van Wart, Ni, Ready, Shayo, & Court, 2020 ). With the renewed interest in “active” education in general (Arruabarrena, Sánchez, Blanco, et al., 2019 ; Kay, MacDonald, & DiGiuseppe, 2019 ; Nouri, 2016 ; Vlachopoulos & Makri, 2017 ) and the flipped classroom approach in particular (Flores, del-Arco, & Silva, 2016 ; Gong, Yang, & Cai, 2020 ; Lundin, et al., 2018 ; Maycock, 2019 ; McGivney-Burelle, 2013 ; O’Flaherty & Phillips, 2015 ; Tucker , 2012 ) along with extraordinary shifts in the technology, the student perspective on online education is profoundly important. What shapes students’ perceptions of quality integrate are their own sense of learning achievement, satisfaction with the support they receive, technical proficiency of the process, intellectual and emotional stimulation, comfort with the process, and sense of learning community. The factors that students perceive as quality online teaching, however, has not been as clear as it might be for at least two reasons.
First, it is important to note that the overall online learning experience for students is also composed of non-teaching factors which we briefly mention. Three such factors are (1) convenience, (2) learner characteristics and readiness, and (3) antecedent conditions that may foster teaching quality but are not directly responsible for it. (1) Convenience is an enormous non-quality factor for students (Artino, 2010 ) which has driven up online demand around the world (Fidalgo, Thormann, Kulyk, et al., 2020 ; Inside Higher Education and Gallup, 2019 ; Legon & Garrett, 2019 ; Ortagus, 2017 ). This is important since satisfaction with online classes is frequently somewhat lower than face-to-face classes (Macon, 2011 ). However, the literature generally supports the relative equivalence of face-to-face and online modes regarding learning achievement criteria (Bernard et al., 2004 ; Nguyen, 2015 ; Ni, 2013 ; Sitzmann, Kraiger, Stewart, & Wisher, 2006 ; see Xu & Jaggars, 2014 for an alternate perspective). These contrasts are exemplified in a recent study of business students, in which online students using a flipped classroom approach outperformed their face-to-face peers, but ironically rated instructor performance lower (Harjoto, 2017 ). (2) Learner characteristics also affect the experience related to self-regulation in an active learning model, comfort with technology, and age, among others,which affect both receptiveness and readiness of online instruction. (Alqurashi, 2016 ; Cohen & Baruth, 2017 ; Kintu, Zhu, & Kagambe, 2017 ; Kuo, Walker, Schroder, & Belland, 2013 ; Ventura & Moscoloni, 2015 ) (3) Finally, numerous antecedent factors may lead to improved instruction, but are not themselves directly perceived by students such as instructor training (Brinkley-Etzkorn, 2018 ), and the sources of faculty motivation (e.g., incentives, recognition, social influence, and voluntariness) (Wingo, Ivankova, & Moss, 2017 ). Important as these factors are, mixing them with the perceptions of quality tends to obfuscate the quality factors directly perceived by students.
Second, while student perceptions of quality are used in innumerable studies, our overall understanding still needs to integrate them more holistically. Many studies use student perceptions of quality and overall effectiveness of individual tools and strategies in online contexts such as mobile devices (Drew & Mann, 2018 ), small groups (Choi, Land, & Turgeon, 2005 ), journals (Nair, Tay, & Koh, 2013 ), simulations (Vlachopoulos & Makri, 2017 ), video (Lange & Costley, 2020 ), etc. Such studies, however, cannot provide the overall context and comparative importance. Some studies have examined the overall learning experience of students with exploratory lists, but have mixed non-quality factors with quality of teaching factors making it difficult to discern the instructor’s versus contextual roles in quality (e.g., Asoodar, Vaezi, & Izanloo, 2016 ; Bollinger & Martindale, 2004 ; Farrell & Brunton, 2020 ; Hong, 2002 ; Song, Singleton, Hill, & Koh, 2004 ; Sun, Tsai, Finger, Chen, & Yeh, 2008 ). The application of technology adoption studies also fall into this category by essentially aggregating all teaching quality in the single category of performance ( Al-Gahtani, 2016 ; Artino, 2010 ). Some studies have used high-level teaching-oriented models, primarily the Community of Inquiry model (le Roux & Nagel, 2018 ), but empirical support has been mixed (Arbaugh et al., 2008 ); and its elegance (i.e., relying on only three factors) has not provided much insight to practitioners (Anderson, 2016 ; Cleveland-Innes & Campbell, 2012 ).
Research questions
Integration of studies and concepts explored continues to be fragmented and confusing despite the fact that the number of empirical studies related to student perceptions of quality factors has increased. It is important to have an empirical view of what students’ value in a single comprehensive study and, also, to know if there is a hierarchy of factors, ranging from students who are least to most critical of the online learning experience. This research study has two research questions.
The first research question is: What are the significant factors in creating a high-quality online learning experience from students’ perspectives? That is important to know because it should have a significant effect on the instructor’s design of online classes. The goal of this research question is identify a more articulated and empirically-supported set of factors capturing the full range of student expectations.
The second research question is: Is there a priority or hierarchy of factors related to students’ perceptions of online teaching quality that relate to their decisions to enroll in online classes? For example, is it possible to distinguish which factors are critical for enrollment decisions when students are primarily motivated by convenience and scheduling flexibility (minimum threshold)? Do these factors differ from students with a genuine acceptance of the general quality of online courses (a moderate threshold)? What are the factors that are important for the students who are the most critical of online course delivery (highest threshold)?
This article next reviews the literature on online education quality, focusing on the student perspective and reviews eight factors derived from it. The research methods section discusses the study structure and methods. Demographic data related to the sample are next, followed by the results, discussion, and conclusion.
Literature review
Online education is much discussed (Prinsloo, 2016 ; Van Wart et al., 2019 ; Zawacki-Richter & Naidu, 2016 ), but its perception is substantially influenced by where you stand and what you value (Otter et al., 2013 ; Tanner, Noser, & Totaro, 2009 ). Accrediting bodies care about meeting technical standards, proof of effectiveness, and consistency (Grandzol & Grandzol, 2006 ). Institutions care about reputation, rigor, student satisfaction, and institutional efficiency (Jung, 2011 ). Faculty care about subject coverage, student participation, faculty satisfaction, and faculty workload (Horvitz, Beach, Anderson, & Xia, 2015 ; Mansbach & Austin, 2018 ). For their part, students care about learning achievement (Marks, Sibley, & Arbaugh, 2005 ; O’Neill & Sai, 2014 ; Shen, Cho, Tsai, & Marra, 2013 ), but also view online education as a function of their enjoyment of classes, instructor capability and responsiveness, and comfort in the learning environment (e.g., Asoodar et al., 2016 ; Sebastianelli, Swift, & Tamimi, 2015 ). It is this last perspective, of students, upon which we focus.
It is important to note students do not sign up for online classes solely based on perceived quality. Perceptions of quality derive from notions of the capacity of online learning when ideal—relative to both learning achievement and satisfaction/enjoyment, and perceptions about the likelihood and experience of classes living up to expectations. Students also sign up because of convenience and flexibility, and personal notions of suitability about learning. Convenience and flexibility are enormous drivers of online registration (Lee, Stringer, & Du, 2017 ; Mann & Henneberry, 2012 ). Even when students say they prefer face-to-face classes to online, many enroll in online classes and re-enroll in the future if the experience meets minimum expectations. This study examines the threshold expectations of students when they are considering taking online classes.
When discussing students’ perceptions of quality, there is little clarity about the actual range of concepts because no integrated empirical studies exist comparing major factors found throughout the literature. Rather, there are practitioner-generated lists of micro-competencies such as the Quality Matters consortium for higher education (Quality Matters, 2018 ), or broad frameworks encompassing many aspects of quality beyond teaching (Open and Distant Learning Quality Council, 2012 ). While checklists are useful for practitioners and accreditation processes, they do not provide robust, theoretical bases for scholarly development. Overarching frameworks are heuristically useful, but not for pragmatic purposes or theory building arenas. The most prominent theoretical framework used in online literature is the Community of Inquiry (CoI) model (Arbaugh et al., 2008 ; Garrison, Anderson, & Archer, 2003 ), which divides instruction into teaching, cognitive, and social presence. Like deductive theories, however, the supportive evidence is mixed (Rourke & Kanuka, 2009 ), especially regarding the importance of social presence (Annand, 2011 ; Armellini and De Stefani, 2016 ). Conceptually, the problem is not so much with the narrow articulation of cognitive or social presence; cognitive presence is how the instructor provides opportunities for students to interact with material in robust, thought-provoking ways, and social presence refers to building a community of learning that incorporates student-to-student interactions. However, teaching presence includes everything else the instructor does—structuring the course, providing lectures, explaining assignments, creating rehearsal opportunities, supplying tests, grading, answering questions, and so on. These challenges become even more prominent in the online context. While the lecture as a single medium is paramount in face-to-face classes, it fades as the primary vehicle in online classes with increased use of detailed syllabi, electronic announcements, recorded and synchronous lectures, 24/7 communications related to student questions, etc. Amassing the pedagogical and technological elements related to teaching under a single concept provides little insight.
In addition to the CoI model, numerous concepts are suggested in single-factor empirical studies when focusing on quality from a student’s perspective, with overlapping conceptualizations and nonstandardized naming conventions. Seven distinct factors are derived here from the literature of student perceptions of online quality: Instructional Support, Teaching Presence, Basic Online Modality, Social Presence, Online Social Comfort, cognitive Presence, and Interactive Online Modality.
Instructional support
Instructional Support refers to students’ perceptions of techniques by the instructor used for input, rehearsal, feedback, and evaluation. Specifically, this entails providing detailed instructions, designed use of multimedia, and the balance between repetitive class features for ease of use, and techniques to prevent boredom. Instructional Support is often included as an element of Teaching Presence, but is also labeled “structure” (Lee & Rha, 2009 ; So & Brush, 2008 ) and instructor facilitation (Eom, Wen, & Ashill, 2006 ). A prime example of the difference between face-to-face and online education is the extensive use of the “flipped classroom” (Maycock, 2019 ; Wang, Huang, & Schunn, 2019 ) in which students move to rehearsal activities faster and more frequently than traditional classrooms, with less instructor lecture (Jung, 2011 ; Martin, Wang, & Sadaf, 2018 ). It has been consistently supported as an element of student perceptions of quality (Espasa & Meneses, 2010 ).
- Teaching presence
Teaching Presence refers to students’ perceptions about the quality of communication in lectures, directions, and individual feedback including encouragement (Jaggars & Xu, 2016 ; Marks et al., 2005 ). Specifically, instructor communication is clear, focused, and encouraging, and instructor feedback is customized and timely. If Instructional Support is what an instructor does before the course begins and in carrying out those plans, then Teaching Presence is what the instructor does while the class is conducted and in response to specific circumstances. For example, a course could be well designed but poorly delivered because the instructor is distracted; or a course could be poorly designed but an instructor might make up for the deficit by spending time and energy in elaborate communications and ad hoc teaching techniques. It is especially important in student satisfaction (Sebastianelli et al., 2015 ; Young, 2006 ) and also referred to as instructor presence (Asoodar et al., 2016 ), learner-instructor interaction (Marks et al., 2005 ), and staff support (Jung, 2011 ). As with Instructional Support, it has been consistently supported as an element of student perceptions of quality.
Basic online modality
Basic Online Modality refers to the competent use of basic online class tools—online grading, navigation methods, online grade book, and the announcements function. It is frequently clumped with instructional quality (Artino, 2010 ), service quality (Mohammadi, 2015 ), instructor expertise in e-teaching (Paechter, Maier, & Macher, 2010 ), and similar terms. As a narrowly defined concept, it is sometimes called technology (Asoodar et al., 2016 ; Bollinger & Martindale, 2004 ; Sun et al., 2008 ). The only empirical study that did not find Basic Online Modality significant, as technology, was Sun et al. ( 2008 ). Because Basic Online Modality is addressed with basic instructor training, some studies assert the importance of training (e.g., Asoodar et al., 2016 ).
Social presence
Social Presence refers to students’ perceptions of the quality of student-to-student interaction. Social Presence focuses on the quality of shared learning and collaboration among students, such as in threaded discussion responses (Garrison et al., 2003 ; Kehrwald, 2008 ). Much emphasized but challenged in the CoI literature (Rourke & Kanuka, 2009 ), it has mixed support in the online literature. While some studies found Social Presence or related concepts to be significant (e.g., Asoodar et al., 2016 ; Bollinger & Martindale, 2004 ; Eom et al., 2006 ; Richardson, Maeda, Lv, & Caskurlu, 2017 ), others found Social Presence insignificant (Joo, Lim, & Kim, 2011 ; So & Brush, 2008 ; Sun et al., 2008 ).
Online social comfort
Online Social Comfort refers to the instructor’s ability to provide an environment in which anxiety is low, and students feel comfortable interacting even when expressing opposing viewpoints. While numerous studies have examined anxiety (e.g., Liaw & Huang, 2013 ; Otter et al., 2013 ; Sun et al., 2008 ), only one found anxiety insignificant (Asoodar et al., 2016 ); many others have not examined the concept.
- Cognitive presence
Cognitive Presence refers to the engagement of students such that they perceive they are stimulated by the material and instructor to reflect deeply and critically, and seek to understand different perspectives (Garrison et al., 2003 ). The instructor provides instructional materials and facilitates an environment that piques interest, is reflective, and enhances inclusiveness of perspectives (Durabi, Arrastia, Nelson, Cornille, & Liang, 2011 ). Cognitive Presence includes enhancing the applicability of material for student’s potential or current careers. Cognitive Presence is supported as significant in many online studies (e.g., Artino, 2010 ; Asoodar et al., 2016 ; Joo et al., 2011 ; Marks et al., 2005 ; Sebastianelli et al., 2015 ; Sun et al., 2008 ). Further, while many instructors perceive that cognitive presence is diminished in online settings, neuroscientific studies indicate this need not be the case (Takamine, 2017 ). While numerous studies failed to examine Cognitive Presence, this review found no studies that lessened its significance for students.
Interactive online modality
Interactive Online Modality refers to the “high-end” usage of online functionality. That is, the instructor uses interactive online class tools—video lectures, videoconferencing, and small group discussions—well. It is often included in concepts such as instructional quality (Artino, 2010 ; Asoodar et al., 2016 ; Mohammadi, 2015 ; Otter et al., 2013 ; Paechter et al., 2010 ) or engagement (Clayton, Blumberg, & Anthony, 2018 ). While individual methods have been investigated (e.g. Durabi et al., 2011 ), high-end engagement methods have not.
Other independent variables affecting perceptions of quality include age, undergraduate versus graduate status, gender, ethnicity/race, discipline, educational motivation of students, and previous online experience. While age has been found to be small or insignificant, more notable effects have been reported at the level-of-study, with graduate students reporting higher “success” (Macon, 2011 ), and community college students having greater difficulty with online classes (Legon & Garrett, 2019 ; Xu & Jaggars, 2014 ). Ethnicity and race have also been small or insignificant. Some situational variations and student preferences can be captured by paying attention to disciplinary differences (Arbaugh, 2005 ; Macon, 2011 ). Motivation levels of students have been reported to be significant in completion and achievement, with better students doing as well across face-to-face and online modes, and weaker students having greater completion and achievement challenges (Clayton et al., 2018 ; Lu & Lemonde, 2013 ).
Research methods
To examine the various quality factors, we apply a critical success factor methodology, initially introduced to schools of business research in the 1970s. In 1981, Rockhart and Bullen codified an approach embodying principles of critical success factors (CSFs) as a way to identify the information needs of executives, detailing steps for the collection and analyzation of data to create a set of organizational CSFs (Rockhart & Bullen, 1981 ). CSFs describe the underlying or guiding principles which must be incorporated to ensure success.
Utilizing this methodology, CSFs in the context of this paper define key areas of instruction and design essential for an online class to be successful from a student’s perspective. Instructors implicitly know and consider these areas when setting up an online class and designing and directing activities and tasks important to achieving learning goals. CSFs make explicit those things good instructors may intuitively know and (should) do to enhance student learning. When made explicit, CSFs not only confirm the knowledge of successful instructors, but tap their intuition to guide and direct the accomplishment of quality instruction for entire programs. In addition, CSFs are linked with goals and objectives, helping generate a small number of truly important matters an instructor should focus attention on to achieve different thresholds of online success.
After a comprehensive literature review, an instrument was created to measure students’ perceptions about the importance of techniques and indicators leading to quality online classes. Items were designed to capture the major factors in the literature. The instrument was pilot studied during academic year 2017–18 with a 397 student sample, facilitating an exploratory factor analysis leading to important preliminary findings (reference withheld for review). Based on the pilot, survey items were added and refined to include seven groups of quality teaching factors and two groups of items related to students’ overall acceptance of online classes as well as a variable on their future online class enrollment. Demographic information was gathered to determine their effects on students’ levels of acceptance of online classes based on age, year in program, major, distance from university, number of online classes taken, high school experience with online classes, and communication preferences.
This paper draws evidence from a sample of students enrolled in educational programs at Jack H. Brown College of Business and Public Administration (JHBC), California State University San Bernardino (CSUSB). The JHBC offers a wide range of online courses for undergraduate and graduate programs. To ensure comparable learning outcomes, online classes and face-to-face classes of a certain subject are similar in size—undergraduate classes are generally capped at 60 and graduate classes at 30, and often taught by the same instructors. Students sometimes have the option to choose between both face-to-face and online modes of learning.
A Qualtrics survey link was sent out by 11 instructors to students who were unlikely to be cross-enrolled in classes during the 2018–19 academic year. 1 Approximately 2500 students were contacted, with some instructors providing class time to complete the anonymous survey. All students, whether they had taken an online class or not, were encouraged to respond. Nine hundred eighty-seven students responded, representing a 40% response rate. Although drawn from a single business school, it is a broad sample representing students from several disciplines—management, accounting and finance, marketing, information decision sciences, and public administration, as well as both graduate and undergraduate programs of study.
The sample age of students is young, with 78% being under 30. The sample has almost no lower division students (i.e., freshman and sophomore), 73% upper division students (i.e., junior and senior) and 24% graduate students (master’s level). Only 17% reported having taken a hybrid or online class in high school. There was a wide range of exposure to university level online courses, with 47% reporting having taken 1 to 4 classes, and 21% reporting no online class experience. As a Hispanic-serving institution, 54% self-identified as Latino, 18% White, and 13% Asian and Pacific Islander. The five largest majors were accounting & finance (25%), management (21%), master of public administration (16%), marketing (12%), and information decision sciences (10%). Seventy-four percent work full- or part-time. See Table 1 for demographic data.
Measures and procedure
To increase the reliability of evaluation scores, composite evaluation variables are formed after an exploratory factor analysis of individual evaluation items. A principle component method with Quartimin (oblique) rotation was applied to explore the factor construct of student perceptions of online teaching CSFs. The item correlations for student perceptions of importance coefficients greater than .30 were included, a commonly acceptable ratio in factor analysis. A simple least-squares regression analysis was applied to test the significance levels of factors on students’ impression of online classes.
Exploratory factor constructs
Using a threshold loading of 0.3 for items, 37 items loaded on seven factors. All factors were logically consistent. The first factor, with eight items, was labeled Teaching Presence. Items included providing clear instructions, staying on task, clear deadlines, and customized feedback on strengths and weaknesses. Teaching Presence items all related to instructor involvement during the course as a director, monitor, and learning facilitator. The second factor, with seven items, aligned with Cognitive Presence. Items included stimulating curiosity, opportunities for reflection, helping students construct explanations posed in online courses, and the applicability of material. The third factor, with six items, aligned with Social Presence defined as providing student-to-student learning opportunities. Items included getting to know course participants for sense of belonging, forming impressions of other students, and interacting with others. The fourth factor, with six new items as well as two (“interaction with other students” and “a sense of community in the class”) shared with the third factor, was Instructional Support which related to the instructor’s roles in providing students a cohesive learning experience. They included providing sufficient rehearsal, structured feedback, techniques for communication, navigation guide, detailed syllabus, and coordinating student interaction and creating a sense of online community. This factor also included enthusiasm which students generally interpreted as a robustly designed course, rather than animation in a traditional lecture. The fifth factor was labeled Basic Online Modality and focused on the basic technological requirements for a functional online course. Three items included allowing students to make online submissions, use of online gradebooks, and online grading. A fourth item is the use of online quizzes, viewed by students as mechanical practice opportunities rather than small tests and a fifth is navigation, a key component of Online Modality. The sixth factor, loaded on four items, was labeled Online Social Comfort. Items here included comfort discussing ideas online, comfort disagreeing, developing a sense of collaboration via discussion, and considering online communication as an excellent medium for social interaction. The final factor was called Interactive Online Modality because it included items for “richer” communications or interactions, no matter whether one- or two-way. Items included videoconferencing, instructor-generated videos, and small group discussions. Taken together, these seven explained 67% of the variance which is considered in the acceptable range in social science research for a robust model (Hair, Black, Babin, & Anderson, 2014 ). See Table 2 for the full list.
To test for factor reliability, the Cronbach alpha of variables were calculated. All produced values greater than 0.7, the standard threshold used for reliability, except for system trust which was therefore dropped. To gauge students’ sense of factor importance, all items were means averaged. Factor means (lower means indicating higher importance to students), ranged from 1.5 to 2.6 on a 5-point scale. Basic Online Modality was most important, followed by Instructional Support and Teaching Presence. Students deemed Cognitive Presence, Social Online Comfort, and Online Interactive Modality less important. The least important for this sample was Social Presence. Table 3 arrays the critical success factor means, standard deviations, and Cronbach alpha.
To determine whether particular subgroups of respondents viewed factors differently, a series of ANOVAs were conducted using factor means as dependent variables. Six demographic variables were used as independent variables: graduate vs. undergraduate, age, work status, ethnicity, discipline, and past online experience. To determine strength of association of the independent variables to each of the seven CSFs, eta squared was calculated for each ANOVA. Eta squared indicates the proportion of variance in the dependent variable explained by the independent variable. Eta squared values greater than .01, .06, and .14 are conventionally interpreted as small, medium, and large effect sizes, respectively (Green & Salkind, 2003 ). Table 4 summarizes the eta squared values for the ANOVA tests with Eta squared values less than .01 omitted.
While no significant differences in factor means among students in different disciplines in the College occur, all five other independent variables have some small effect on some or all CSFs. Graduate students tend to rate Online Interactive Modality, Instructional Support, Teaching Presence, and Cognitive Presence higher than undergraduates. Elder students value more Online Interactive Modality. Full-time working students rate all factors, except Social Online Comfort, slightly higher than part-timers and non-working students. Latino and White rate Basic Online Modality and Instructional Support higher; Asian and Pacific Islanders rate Social Presence higher. Students who have taken more online classes rate all factors higher.
In addition to factor scores, two variables are constructed to identify the resultant impressions labeled online experience. Both were logically consistent with a Cronbach’s α greater than 0.75. The first variable, with six items, labeled “online acceptance,” included items such as “I enjoy online learning,” “My overall impression of hybrid/online learning is very good,” and “the instructors of online/hybrid classes are generally responsive.” The second variable was labeled “face-to-face preference” and combines four items, including enjoying, learning, and communicating more in face-to-face classes, as well as perceiving greater fairness and equity. In addition to these two constructed variables, a one-item variable was also used subsequently in the regression analysis: “online enrollment.” That question asked: if hybrid/online classes are well taught and available, how much would online education make up your entire course selection going forward?
Regression results
As noted above, two constructed variables and one item were used as dependent variables for purposes of regression analysis. They were online acceptance, F2F preference, and the selection of online classes. In addition to seven quality-of-teaching factors identified by factor analysis, control variables included level of education (graduate versus undergraduate), age, ethnicity, work status, distance to university, and number of online/hybrid classes taken in the past. See Table 5 .
When the ETA squared values for ANOVA significance were measured for control factors, only one was close to a medium effect. Graduate versus undergraduate status had a .05 effect (considered medium) related to Online Interactive Modality, meaning graduate students were more sensitive to interactive modality than undergraduates. Multiple regression analysis of critical success factors and online impressions were conducted to compare under what conditions factors were significant. The only consistently significant control factor was number of online classes taken. The more classes students had taken online, the more inclined they were to take future classes. Level of program, age, ethnicity, and working status do not significantly affect students’ choice or overall acceptance of online classes.
The least restrictive condition was online enrollment (Table 6 ). That is, students might not feel online courses were ideal, but because of convenience and scheduling might enroll in them if minimum threshold expectations were met. When considering online enrollment three factors were significant and positive (at the 0.1 level): Basic Online Modality, Cognitive Presence, and Online Social Comfort. These least-demanding students expected classes to have basic technological functionality, provide good opportunities for knowledge acquisition, and provide comfortable interaction in small groups. Students who demand good Instructional Support (e.g., rehearsal opportunities, standardized feedback, clear syllabus) are less likely to enroll.
Online acceptance was more restrictive (see Table 7 ). This variable captured the idea that students not only enrolled in online classes out of necessity, but with an appreciation of the positive attributes of online instruction, which balanced the negative aspects. When this standard was applied, students expected not only Basic Online Modality, Cognitive Presence, and Online Social Comfort, but expected their instructors to be highly engaged virtually as the course progressed (Teaching Presence), and to create strong student-to-student dynamics (Social Presence). Students who rated Instructional Support higher are less accepting of online classes.
Another restrictive condition was catering to the needs of students who preferred face-to-face classes (see Table 8 ). That is, they preferred face-to-face classes even when online classes were well taught. Unlike students more accepting of, or more likely to enroll in, online classes, this group rates Instructional Support as critical to enrolling, rather than a negative factor when absent. Again different from the other two groups, these students demand appropriate interactive mechanisms (Online Interactive Modality) to enable richer communication (e.g., videoconferencing). Student-to-student collaboration (Social Presence) was also significant. This group also rated Cognitive Presence and Online Social Comfort as significant, but only in their absence. That is, these students were most attached to direct interaction with the instructor and other students rather than specific teaching methods. Interestingly, Basic Online Modality and Teaching Presence were not significant. Our interpretation here is this student group, most critical of online classes for its loss of physical interaction, are beyond being concerned with mechanical technical interaction and demand higher levels of interactivity and instructional sophistication.
Discussion and study limitations
Some past studies have used robust empirical methods to identify a single factor or a small number of factors related to quality from a student’s perspective, but have not sought to be relatively comprehensive. Others have used a longer series of itemized factors, but have less used less robust methods, and have not tied those factors back to the literature. This study has used the literature to develop a relatively comprehensive list of items focused on quality teaching in a single rigorous protocol. That is, while a Beta test had identified five coherent factors, substantial changes to the current survey that sharpened the focus on quality factors rather than antecedent factors, as well as better articulating the array of factors often lumped under the mantle of “teaching presence.” In addition, it has also examined them based on threshold expectations: from minimal, such as when flexibility is the driving consideration, to modest, such as when students want a “good” online class, to high, when students demand an interactive virtual experience equivalent to face-to-face.
Exploratory factor analysis identified seven factors that were reliable, coherent, and significant under different conditions. When considering students’ overall sense of importance, they are, in order: Basic Online Modality, Instructional Support, Teaching Presence, Cognitive Presence, Social Online Comfort, Interactive Online Modality, and Social Presence. Students are most concerned with the basics of a course first, that is the technological and instructor competence. Next they want engagement and virtual comfort. Social Presence, while valued, is the least critical from this overall perspective.
The factor analysis is quite consistent with the range of factors identified in the literature, pointing to the fact that students can differentiate among different aspects of what have been clumped as larger concepts, such as teaching presence. Essentially, the instructor’s role in quality can be divided into her/his command of basic online functionality, good design, and good presence during the class. The instructor’s command of basic functionality is paramount. Because so much of online classes must be built in advance of the class, quality of the class design is rated more highly than the instructor’s role in facilitating the class. Taken as a whole, the instructor’s role in traditional teaching elements is primary, as we would expect it to be. Cognitive presence, especially as pertinence of the instructional material and its applicability to student interests, has always been found significant when studied, and was highly rated as well in a single factor. Finally, the degree to which students feel comfortable with the online environment and enjoy the learner-learner aspect has been less supported in empirical studies, was found significant here, but rated the lowest among the factors of quality to students.
Regression analysis paints a more nuanced picture, depending on student focus. It also helps explain some of the heterogeneity of previous studies, depending on what the dependent variables were. If convenience and scheduling are critical and students are less demanding, minimum requirements are Basic Online Modality, Cognitive Presence, and Online Social Comfort. That is, students’ expect an instructor who knows how to use an online platform, delivers useful information, and who provides a comfortable learning environment. However, they do not expect to get poor design. They do not expect much in terms of the quality teaching presence, learner-to-learner interaction, or interactive teaching.
When students are signing up for critical classes, or they have both F2F and online options, they have a higher standard. That is, they not only expect the factors for decisions about enrolling in noncritical classes, but they also expect good Teaching and Social Presence. Students who simply need a class may be willing to teach themselves a bit more, but students who want a good class expect a highly present instructor in terms responsiveness and immediacy. “Good” classes must not only create a comfortable atmosphere, but in social science classes at least, must provide strong learner-to-learner interactions as well. At the time of the research, most students believe that you can have a good class without high interactivity via pre-recorded video and videoconference. That may, or may not, change over time as technology thresholds of various video media become easier to use, more reliable, and more commonplace.
The most demanding students are those who prefer F2F classes because of learning style preferences, poor past experiences, or both. Such students (seem to) assume that a worthwhile online class has basic functionality and that the instructor provides a strong presence. They are also critical of the absence of Cognitive Presence and Online Social Comfort. They want strong Instructional Support and Social Presence. But in addition, and uniquely, they expect Online Interactive Modality which provides the greatest verisimilitude to the traditional classroom as possible. More than the other two groups, these students crave human interaction in the learning process, both with the instructor and other students.
These findings shed light on the possible ramifications of the COVID-19 aftermath. Many universities around the world jumped from relatively low levels of online instruction in the beginning of spring 2020 to nearly 100% by mandate by the end of the spring term. The question becomes, what will happen after the mandate is removed? Will demand resume pre-crisis levels, will it increase modestly, or will it skyrocket? Time will be the best judge, but the findings here would suggest that the ability/interest of instructors and institutions to “rise to the occasion” with quality teaching will have as much effect on demand as students becoming more acclimated to online learning. If in the rush to get classes online many students experience shoddy basic functional competence, poor instructional design, sporadic teaching presence, and poorly implemented cognitive and social aspects, they may be quite willing to return to the traditional classroom. If faculty and institutions supporting them are able to increase the quality of classes despite time pressures, then most students may be interested in more hybrid and fully online classes. If instructors are able to introduce high quality interactive teaching, nearly the entire student population will be interested in more online classes. Of course students will have a variety of experiences, but this analysis suggests that those instructors, departments, and institutions that put greater effort into the temporary adjustment (and who resist less), will be substantially more likely to have increases in demand beyond what the modest national trajectory has been for the last decade or so.
There are several study limitations. First, the study does not include a sample of non-respondents. Non-responders may have a somewhat different profile. Second, the study draws from a single college and university. The profile derived here may vary significantly by type of student. Third, some survey statements may have led respondents to rate quality based upon experience rather than assess the general importance of online course elements. “I felt comfortable participating in the course discussions,” could be revised to “comfort in participating in course discussions.” The authors weighed differences among subgroups (e.g., among majors) as small and statistically insignificant. However, it is possible differences between biology and marketing students would be significant, leading factors to be differently ordered. Emphasis and ordering might vary at a community college versus research-oriented university (Gonzalez, 2009 ).
Availability of data and materials
We will make the data available.
Al-Gahtani, S. S. (2016). Empirical investigation of e-learning acceptance and assimilation: A structural equation model. Applied Comput Information , 12 , 27–50.
Google Scholar
Alqurashi, E. (2016). Self-efficacy in online learning environments: A literature review. Contemporary Issues Educ Res (CIER) , 9 (1), 45–52.
Anderson, T. (2016). A fourth presence for the Community of Inquiry model? Retrieved from https://virtualcanuck.ca/2016/01/04/a-fourth-presence-for-the-community-of-inquiry-model/ .
Annand, D. (2011). Social presence within the community of inquiry framework. The International Review of Research in Open and Distributed Learning , 12 (5), 40.
Arbaugh, J. B. (2005). How much does “subject matter” matter? A study of disciplinary effects in on-line MBA courses. Academy of Management Learning & Education , 4 (1), 57–73.
Arbaugh, J. B., Cleveland-Innes, M., Diaz, S. R., Garrison, D. R., Ice, P., Richardson, J. C., & Swan, K. P. (2008). Developing a community of inquiry instrument: Testing a measure of the Community of Inquiry framework using a multi-institutional sample. Internet and Higher Education , 11 , 133–136.
Armellini, A., & De Stefani, M. (2016). Social presence in the 21st century: An adjustment to the Community of Inquiry framework. British Journal of Educational Technology , 47 (6), 1202–1216.
Arruabarrena, R., Sánchez, A., Blanco, J. M., et al. (2019). Integration of good practices of active methodologies with the reuse of student-generated content. International Journal of Educational Technology in Higher Education , 16 , #10.
Arthur, L. (2009). From performativity to professionalism: Lecturers’ responses to student feedback. Teaching in Higher Education , 14 (4), 441–454.
Artino, A. R. (2010). Online or face-to-face learning? Exploring the personal factors that predict students’ choice of instructional format. Internet and Higher Education , 13 , 272–276.
Asoodar, M., Vaezi, S., & Izanloo, B. (2016). Framework to improve e-learner satisfaction and further strengthen e-learning implementation. Computers in Human Behavior , 63 , 704–716.
Bernard, R. M., et al. (2004). How does distance education compare with classroom instruction? A meta-analysis of the empirical literature. Review of Educational Research , 74 (3), 379–439.
Bollinger, D., & Martindale, T. (2004). Key factors for determining student satisfaction in online courses. Int J E-learning , 3 (1), 61–67.
Brinkley-Etzkorn, K. E. (2018). Learning to teach online: Measuring the influence of faculty development training on teaching effectiveness through a TPACK lens. The Internet and Higher Education , 38 , 28–35.
Chickering, A. W., & Gamson, Z. F. (1987). Seven principles for good practice in undergraduate education. AAHE Bulletin , 3 , 7.
Choi, I., Land, S. M., & Turgeon, A. J. (2005). Scaffolding peer-questioning strategies to facilitate metacognition during online small group discussion. Instructional Science , 33 , 483–511.
Clayton, K. E., Blumberg, F. C., & Anthony, J. A. (2018). Linkages between course status, perceived course value, and students’ preferences for traditional versus non-traditional learning environments. Computers & Education , 125 , 175–181.
Cleveland-Innes, M., & Campbell, P. (2012). Emotional presence, learning, and the online learning environment. The International Review of Research in Open and Distributed Learning , 13 (4), 269–292.
Cohen, A., & Baruth, O. (2017). Personality, learning, and satisfaction in fully online academic courses. Computers in Human Behavior , 72 , 1–12.
Crews, T., & Butterfield, J. (2014). Data for flipped classroom design: Using student feedback to identify the best components from online and face-to-face classes. Higher Education Studies , 4 (3), 38–47.
Dawson, P., Henderson, M., Mahoney, P., Phillips, M., Ryan, T., Boud, D., & Molloy, E. (2019). What makes for effective feedback: Staff and student perspectives. Assessment & Evaluation in Higher Education , 44 (1), 25–36.
Drew, C., & Mann, A. (2018). Unfitting, uncomfortable, unacademic: A sociological reading of an interactive mobile phone app in university lectures. International Journal of Educational Technology in Higher Education , 15 , #43.
Durabi, A., Arrastia, M., Nelson, D., Cornille, T., & Liang, X. (2011). Cognitive presence in asynchronous online learning: A comparison of four discussion strategies. Journal of Computer Assisted Learning , 27 (3), 216–227.
Eom, S. B., Wen, H. J., & Ashill, N. (2006). The determinants of students’ perceived learning outcomes and satisfaction in university online education: An empirical investigation. Decision Sciences Journal of Innovative Education , 4 (2), 215–235.
Espasa, A., & Meneses, J. (2010). Analysing feedback processes in an online teaching and learning environment: An exploratory study. Higher Education , 59 (3), 277–292.
Farrell, O., & Brunton, J. (2020). A balancing act: A window into online student engagement experiences. International Journal of Educational Technology in High Education , 17 , #25.
Fidalgo, P., Thormann, J., Kulyk, O., et al. (2020). Students’ perceptions on distance education: A multinational study. International Journal of Educational Technology in High Education , 17 , #18.
Flores, Ò., del-Arco, I., & Silva, P. (2016). The flipped classroom model at the university: Analysis based on professors’ and students’ assessment in the educational field. International Journal of Educational Technology in Higher Education , 13 , #21.
Garrison, D. R., Anderson, T., & Archer, W. (2003). A theory of critical inquiry in online distance education. Handbook of Distance Education , 1 , 113–127.
Gong, D., Yang, H. H., & Cai, J. (2020). Exploring the key influencing factors on college students’ computational thinking skills through flipped-classroom instruction. International Journal of Educational Technology in Higher Education , 17 , #19.
Gonzalez, C. (2009). Conceptions of, and approaches to, teaching online: A study of lecturers teaching postgraduate distance courses. Higher Education , 57 (3), 299–314.
Grandzol, J. R., & Grandzol, C. J. (2006). Best practices for online business Education. International Review of Research in Open and Distance Learning , 7 (1), 1–18.
Green, S. B., & Salkind, N. J. (2003). Using SPSS: Analyzing and understanding data , (3rd ed., ). Upper Saddle River: Prentice Hall.
Hair, J. F., Black, W. C., Babin, B. J., & Anderson, R. E. (2014). Multivariate data analysis: Pearson new international edition . Essex: Pearson Education Limited.
Harjoto, M. A. (2017). Blended versus face-to-face: Evidence from a graduate corporate finance class. Journal of Education for Business , 92 (3), 129–137.
Hong, K.-S. (2002). Relationships between students’ instructional variables with satisfaction and learning from a web-based course. The Internet and Higher Education , 5 , 267–281.
Horvitz, B. S., Beach, A. L., Anderson, M. L., & Xia, J. (2015). Examination of faculty self-efficacy related to online teaching. Innovation Higher Education , 40 , 305–316.
Inside Higher Education and Gallup. (2019). The 2019 survey of faculty attitudes on technology. Author .
Jaggars, S. S., & Xu, D. (2016). How do online course design features influence student performance? Computers and Education , 95 , 270–284.
Joo, Y. J., Lim, K. Y., & Kim, E. K. (2011). Online university students’ satisfaction and persistence: Examining perceived level of presence, usefulness and ease of use as predictor in a structural model. Computers & Education , 57 (2), 1654–1664.
Jung, I. (2011). The dimensions of e-learning quality: From the learner’s perspective. Educational Technology Research and Development , 59 (4), 445–464.
Kay, R., MacDonald, T., & DiGiuseppe, M. (2019). A comparison of lecture-based, active, and flipped classroom teaching approaches in higher education. Journal of Computing in Higher Education , 31 , 449–471.
Kehrwald, B. (2008). Understanding social presence in text-based online learning environments. Distance Education , 29 (1), 89–106.
Kintu, M. J., Zhu, C., & Kagambe, E. (2017). Blended learning effectiveness: The relationship between student characteristics, design features and outcomes. International Journal of Educational Technology in Higher Education , 14 , #7.
Kuo, Y.-C., Walker, A. E., Schroder, K. E., & Belland, B. R. (2013). Interaction, internet self-efficacy, and self-regulated learning as predictors of student satisfaction in online education courses. Internet and Education , 20 , 35–50.
Lange, C., & Costley, J. (2020). Improving online video lectures: Learning challenges created by media. International Journal of Educational Technology in Higher Education , 17 , #16.
le Roux, I., & Nagel, L. (2018). Seeking the best blend for deep learning in a flipped classroom – Viewing student perceptions through the Community of Inquiry lens. International Journal of Educational Technology in High Education , 15 , #16.
Lee, H.-J., & Rha, I. (2009). Influence of structure and interaction on student achievement and satisfaction in web-based distance learning. Educational Technology & Society , 12 (4), 372–382.
Lee, Y., Stringer, D., & Du, J. (2017). What determines students’ preference of online to F2F class? Business Education Innovation Journal , 9 (2), 97–102.
Legon, R., & Garrett, R. (2019). CHLOE 3: Behind the numbers . Published online by Quality Matters and Eduventures. https://www.qualitymatters.org/sites/default/files/research-docs-pdfs/CHLOE-3-Report-2019-Behind-the-Numbers.pdf
Liaw, S.-S., & Huang, H.-M. (2013). Perceived satisfaction, perceived usefulness and interactive learning environments as predictors of self-regulation in e-learning environments. Computers & Education , 60 (1), 14–24.
Lu, F., & Lemonde, M. (2013). A comparison of online versus face-to-face students teaching delivery in statistics instruction for undergraduate health science students. Advances in Health Science Education , 18 , 963–973.
Lundin, M., Bergviken Rensfeldt, A., Hillman, T., Lantz-Andersson, A., & Peterson, L. (2018). Higher education dominance and siloed knowledge: a systematic review of flipped classroom research. International Journal of Educational Technology in Higher Education , 15 (1).
Macon, D. K. (2011). Student satisfaction with online courses versus traditional courses: A meta-analysis . Disssertation: Northcentral University, CA.
Mann, J., & Henneberry, S. (2012). What characteristics of college students influence their decisions to select online courses? Online Journal of Distance Learning Administration , 15 (5), 1–14.
Mansbach, J., & Austin, A. E. (2018). Nuanced perspectives about online teaching: Mid-career senior faculty voices reflecting on academic work in the digital age. Innovative Higher Education , 43 (4), 257–272.
Marks, R. B., Sibley, S. D., & Arbaugh, J. B. (2005). A structural equation model of predictors for effective online learning. Journal of Management Education , 29 (4), 531–563.
Martin, F., Wang, C., & Sadaf, A. (2018). Student perception of facilitation strategies that enhance instructor presence, connectedness, engagement and learning in online courses. Internet and Higher Education , 37 , 52–65.
Maycock, K. W. (2019). Chalk and talk versus flipped learning: A case study. Journal of Computer Assisted Learning , 35 , 121–126.
McGivney-Burelle, J. (2013). Flipping Calculus. PRIMUS Problems, Resources, and Issues in Mathematics Undergraduate . Studies , 23 (5), 477–486.
Mohammadi, H. (2015). Investigating users’ perspectives on e-learning: An integration of TAM and IS success model. Computers in Human Behavior , 45 , 359–374.
Nair, S. S., Tay, L. Y., & Koh, J. H. L. (2013). Students’ motivation and teachers’ teaching practices towards the use of blogs for writing of online journals. Educational Media International , 50 (2), 108–119.
Nguyen, T. (2015). The effectiveness of online learning: Beyond no significant difference and future horizons. MERLOT Journal of Online Learning and Teaching , 11 (2), 309–319.
Ni, A. Y. (2013). Comparing the effectiveness of classroom and online learning: Teaching research methods. Journal of Public Affairs Education , 19 (2), 199–215.
Nouri, J. (2016). The flipped classroom: For active, effective and increased learning – Especially for low achievers. International Journal of Educational Technology in Higher Education , 13 , #33.
O’Neill, D. K., & Sai, T. H. (2014). Why not? Examining college students’ reasons for avoiding an online course. Higher Education , 68 (1), 1–14.
O'Flaherty, J., & Phillips, C. (2015). The use of flipped classrooms in higher education: A scoping review. The Internet and Higher Education , 25 , 85–95.
Open & Distant Learning Quality Council (2012). ODLQC standards . England: Author https://www.odlqc.org.uk/odlqc-standards .
Ortagus, J. C. (2017). From the periphery to prominence: An examination of the changing profile of online students in American higher education. Internet and Higher Education , 32 , 47–57.
Otter, R. R., Seipel, S., Graef, T., Alexander, B., Boraiko, C., Gray, J., … Sadler, K. (2013). Comparing student and faculty perceptions of online and traditional courses. Internet and Higher Education , 19 , 27–35.
Paechter, M., Maier, B., & Macher, D. (2010). Online or face-to-face? Students’ experiences and preferences in e-learning. Internet and Higher Education , 13 , 292–329.
Prinsloo, P. (2016). (re)considering distance education: Exploring its relevance, sustainability and value contribution. Distance Education , 37 (2), 139–145.
Quality Matters (2018). Specific review standards from the QM higher Education rubric , (6th ed., ). MD: MarylandOnline.
Richardson, J. C., Maeda, Y., Lv, J., & Caskurlu, S. (2017). Social presence in relation to students’ satisfaction and learning in the online environment: A meta-analysis. Computers in Human Behavior , 71 , 402–417.
Rockhart, J. F., & Bullen, C. V. (1981). A primer on critical success factors . Cambridge: Center for Information Systems Research, Massachusetts Institute of Technology.
Rourke, L., & Kanuka, H. (2009). Learning in Communities of Inquiry: A Review of the Literature. The Journal of Distance Education / Revue de l'ducation Distance , 23 (1), 19–48 Athabasca University Press. Retrieved August 2, 2020 from https://www.learntechlib.org/p/105542/ .
Sebastianelli, R., Swift, C., & Tamimi, N. (2015). Factors affecting perceived learning, satisfaction, and quality in the online MBA: A structural equation modeling approach. Journal of Education for Business , 90 (6), 296–305.
Shen, D., Cho, M.-H., Tsai, C.-L., & Marra, R. (2013). Unpacking online learning experiences: Online learning self-efficacy and learning satisfaction. Internet and Higher Education , 19 , 10–17.
Sitzmann, T., Kraiger, K., Stewart, D., & Wisher, R. (2006). The comparative effectiveness of web-based and classroom instruction: A meta-analysis. Personnel Psychology , 59 (3), 623–664.
So, H. J., & Brush, T. A. (2008). Student perceptions of collaborative learning, social presence and satisfaction in a blended learning environment: Relationships and critical factors. Computers & Education , 51 (1), 318–336.
Song, L., Singleton, E. S., Hill, J. R., & Koh, M. H. (2004). Improving online learning: Student perceptions of useful and challenging characteristics. The Internet and Higher Education , 7 (1), 59–70.
Sun, P. C., Tsai, R. J., Finger, G., Chen, Y. Y., & Yeh, D. (2008). What drives a successful e-learning? An empirical investigation of the critical factors influencing learner satisfaction. Computers & Education , 50 (4), 1183–1202.
Takamine, K. (2017). Michelle D. miller: Minds online: Teaching effectively with technology. Higher Education , 73 , 789–791.
Tanner, J. R., Noser, T. C., & Totaro, M. W. (2009). Business faculty and undergraduate students’ perceptions of online learning: A comparative study. Journal of Information Systems Education , 20 (1), 29.
Tucker, B. (2012). The flipped classroom. Education Next , 12 (1), 82–83.
Van Wart, M., Ni, A., Ready, D., Shayo, C., & Court, J. (2020). Factors leading to online learner satisfaction. Business Educational Innovation Journal , 12 (1), 15–24.
Van Wart, M., Ni, A., Rose, L., McWeeney, T., & Worrell, R. A. (2019). Literature review and model of online teaching effectiveness integrating concerns for learning achievement, student satisfaction, faculty satisfaction, and institutional results. Pan-Pacific . Journal of Business Research , 10 (1), 1–22.
Ventura, A. C., & Moscoloni, N. (2015). Learning styles and disciplinary differences: A cross-sectional study of undergraduate students. International Journal of Learning and Teaching , 1 (2), 88–93.
Vlachopoulos, D., & Makri, A. (2017). The effect of games and simulations on higher education: A systematic literature review. International Journal of Educational Technology in Higher Education , 14 , #22.
Wang, Y., Huang, X., & Schunn, C. D. (2019). Redesigning flipped classrooms: A learning model and its effects on student perceptions. Higher Education , 78 , 711–728.
Wingo, N. P., Ivankova, N. V., & Moss, J. A. (2017). Faculty perceptions about teaching online: Exploring the literature using the technology acceptance model as an organizing framework. Online Learning , 21 (1), 15–35.
Xu, D., & Jaggars, S. S. (2014). Performance gaps between online and face-to-face courses: Differences across types of students and academic subject areas. Journal of Higher Education , 85 (5), 633–659.
Young, S. (2006). Student views of effective online teaching in higher education. American Journal of Distance Education , 20 (2), 65–77.
Zawacki-Richter, O., & Naidu, S. (2016). Mapping research trends from 35 years of publications in distance Education. Distance Education , 37 (3), 245–269.
Download references
Acknowledgements
No external funding/ NA.
Author information
Authors and affiliations.
Development for the JHB College of Business and Public Administration, 5500 University Parkway, San Bernardino, California, 92407, USA
Montgomery Van Wart, Anna Ni, Pamela Medina, Jesus Canelon, Melika Kordrostami, Jing Zhang & Yu Liu
You can also search for this author in PubMed Google Scholar
Contributions
Equal. The author(s) read and approved the final manuscript.
Corresponding author
Correspondence to Montgomery Van Wart .
Ethics declarations
Competing interests.
We have no competing interests.
Additional information
Publisher’s note.
Springer Nature remains neutral with regard to jurisdictional claims in published maps and institutional affiliations.
Rights and permissions
Open Access This article is licensed under a Creative Commons Attribution 4.0 International License, which permits use, sharing, adaptation, distribution and reproduction in any medium or format, as long as you give appropriate credit to the original author(s) and the source, provide a link to the Creative Commons licence, and indicate if changes were made. The images or other third party material in this article are included in the article's Creative Commons licence, unless indicated otherwise in a credit line to the material. If material is not included in the article's Creative Commons licence and your intended use is not permitted by statutory regulation or exceeds the permitted use, you will need to obtain permission directly from the copyright holder. To view a copy of this licence, visit http://creativecommons.org/licenses/by/4.0/ .
Reprints and permissions
About this article
Cite this article.
Van Wart, M., Ni, A., Medina, P. et al. Integrating students’ perspectives about online learning: a hierarchy of factors. Int J Educ Technol High Educ 17 , 53 (2020). https://doi.org/10.1186/s41239-020-00229-8
Download citation
Received : 29 April 2020
Accepted : 30 July 2020
Published : 02 December 2020
DOI : https://doi.org/10.1186/s41239-020-00229-8
Share this article
Anyone you share the following link with will be able to read this content:
Sorry, a shareable link is not currently available for this article.
Provided by the Springer Nature SharedIt content-sharing initiative
- Online education
- Online teaching
- Student perceptions
- Online quality
- Student presence
Purdue Online Writing Lab Purdue OWL® College of Liberal Arts
Welcome to the Purdue Online Writing Lab
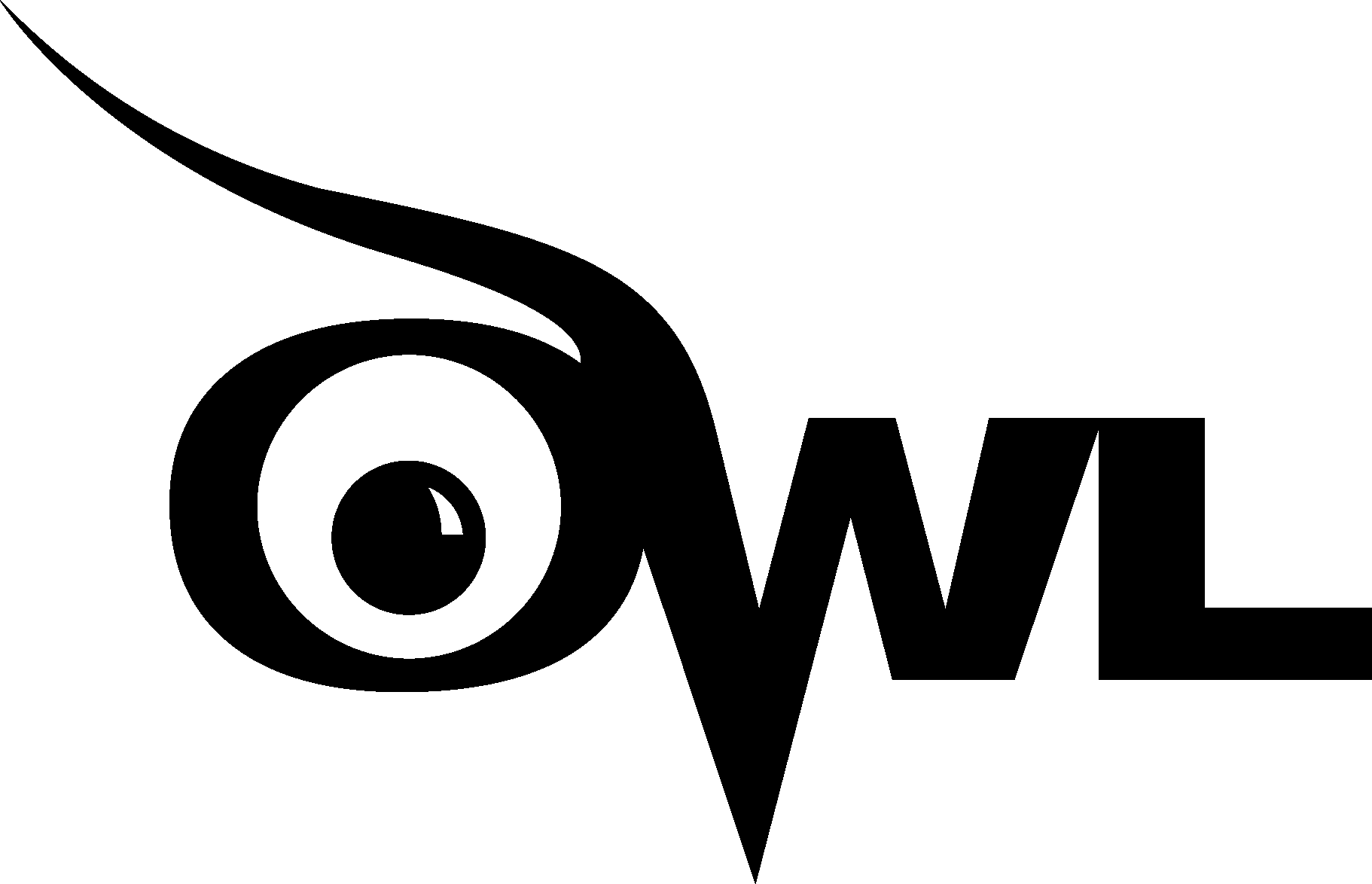
Welcome to the Purdue OWL
This page is brought to you by the OWL at Purdue University. When printing this page, you must include the entire legal notice.
Copyright ©1995-2018 by The Writing Lab & The OWL at Purdue and Purdue University. All rights reserved. This material may not be published, reproduced, broadcast, rewritten, or redistributed without permission. Use of this site constitutes acceptance of our terms and conditions of fair use.
The Online Writing Lab at Purdue University houses writing resources and instructional material, and we provide these as a free service of the Writing Lab at Purdue. Students, members of the community, and users worldwide will find information to assist with many writing projects. Teachers and trainers may use this material for in-class and out-of-class instruction.
The Purdue On-Campus Writing Lab and Purdue Online Writing Lab assist clients in their development as writers—no matter what their skill level—with on-campus consultations, online participation, and community engagement. The Purdue Writing Lab serves the Purdue, West Lafayette, campus and coordinates with local literacy initiatives. The Purdue OWL offers global support through online reference materials and services.
A Message From the Assistant Director of Content Development
The Purdue OWL® is committed to supporting students, instructors, and writers by offering a wide range of resources that are developed and revised with them in mind. To do this, the OWL team is always exploring possibilties for a better design, allowing accessibility and user experience to guide our process. As the OWL undergoes some changes, we welcome your feedback and suggestions by email at any time.
Please don't hesitate to contact us via our contact page if you have any questions or comments.
All the best,
Social Media
Facebook twitter.
Deep learning based active image steganalysis: a review
- REVIEW PAPERS
- Published: 27 November 2023
- Volume 15 , pages 786–799, ( 2024 )
Cite this article
- Punam Bedi 1 ,
- Anuradha Singhal ORCID: orcid.org/0000-0002-0818-6597 1 &
- Veenu Bhasin 1
224 Accesses
Explore all metrics
Steganalysis plays a vital role in cybersecurity in today’s digital era where exchange of malicious information can be done easily across web pages. Steganography techniques are used to hide data in an object where the existence of hidden information is also obscured. Steganalysis is the process for detection of steganography within an object and can be categorized as active and passive steganalysis. Passive steganalysis tries to classify a given object as a clean or modified object. Active steganalysis aims to extract more details about hidden contents such as length of embedded message, region of inserted message, key used for embedding, required by cybersecurity experts for comprehensive analysis. Images being a viable source of exchange of information in the era of internet, social media are the most susceptible source for such transmission. Many researchers have worked and developed techniques required to detect and alert about such counterfeit exchanges over the internet. Literature present in passive and active image steganalysis techniques, addresses these issues by detecting and unveiling details of such obscured communication respectively. This paper provides a systematic and comprehensive review of work done on active image steganalysis techniques using deep learning techniques. This review will be helpful to the new researchers to become aware and build a strong foundation of literature present in active image steganalysis using deep learning techniques. The paper also includes various steganographic algorithms, dataset and performance evaluation metrics used in literature. Open research challenges and possible future research directions are also discussed in the paper.
This is a preview of subscription content, log in via an institution to check access.
Access this article
Price includes VAT (Russian Federation)
Instant access to the full article PDF.
Rent this article via DeepDyve
Institutional subscriptions
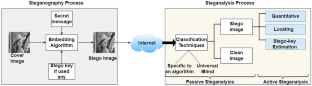
Similar content being viewed by others
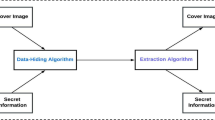
Image steganalysis using deep learning: a systematic review and open research challenges
Studies on Steganography Images and Videos Using Deep Learning Techniques
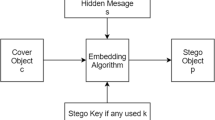
Multi-class blind steganalysis using deep residual networks
Data availability.
Not Applicable.
Ambalavanan A and Chandramouli R (2006) A Bayesian image steganalysis approach to estimate the embedded secret message. In: proceedings of the 7th multimedia and security workshop 2005, MM and Sec’05, vol 2006, pp 33–38. doi: https://doi.org/10.1145/1073170.1073177
Bagnall RJ (2002) Reversing the steganography myth in terrorist operations: the asymmetrical threat of simple intelligence dissemination techniques using common tools. Sans Inf Secur Read Room 19:1–16
Google Scholar
Bas P, Filler T, Pevný T (2011) Break our steganographic system: the ins and outs of organizing BOSS. In: lecture notes in computer science (including subseries lecture notes in artificial intelligence and lecture notes in bioinformatics), vol 6958 LNCS, pp 59–70. doi: https://doi.org/10.1007/978-3-642-24178-9_5
Bedi P, Singhal A (2022) Estimating cover image for Universal payload region detection in Stego Images. J King Saud Univ Comput Inf Sci. https://doi.org/10.1016/J.JKSUCI.2022.01.010
Article Google Scholar
Bengio Y, Louradour J, Collobert R, and Weston J (2009) Curriculum learning. In: proceedings of the 26th annual international conference on machine learning. vol 382, pp 41–48. doi: https://doi.org/10.1145/1553374.1553380
Bhatt C, Kumar I, Vijayakumar V, Singh KU, Kumar A (2021) The state of the art of deep learning models in medical science and their challenges. Multimed Syst 27(4):599–613. https://doi.org/10.1007/S00530-020-00694-1/FIGURES/10
Boroumand M, Chen M, Fridrich J (2018) Deep residual network for steganalysis of digital images. IEEE Trans Inf Forensics Secur 14(5):1181–1193. https://doi.org/10.1109/TIFS.2018.2871749
Chaumont M (2020) Deep learning in steganography and steganalysis. Digital media steganography: principles, algorithms, and advances. Elsevier, New York, pp 321–349. https://doi.org/10.1016/B978-0-12-819438-6.00022-0
Chapter Google Scholar
Chen C and Shi YQ (2008) JPEG image steganalysis utilizing both intrablock and interblock correlations. In: proceedings-IEEE international symposium on circuits and systems , pp 3029–3032. doi: https://doi.org/10.1109/ISCAS.2008.4542096
Chen M, Sedighi V, Boroumand M, and Fridrich J (2017) JPEG-phase-aware convolutional neural network for steganalysis of JPEG images. In: IH and MMSec 2017-proceedings of the 2017 ACM workshop on information hiding and multimedia security , pp 75–84. doi: https://doi.org/10.1145/3082031.3083248 .
Chen M, Boroumand M, and Fridrich J (2018) Deep learning regressors for quantitative steganalysis. In: is and t international symposium on electronic imaging science and technology, pp 160–167. doi: https://doi.org/10.2352/ISSN.2470-1173.2018.07.MWSF-160
Chutani S, Goyal A (2018) Improved universal quantitative steganalysis in spatial domain using ELM ensemble. Multimed Tools Appl 77(6):7447–7468. https://doi.org/10.1007/S11042-017-4656-3/FIGURES/7
Clark E (2019) GE Engineer Charged in Elaborate Theft of Trade Secrets. https://blog.twinstate.com/news/ge-trade-secrets-theft (Accessed Jan. 20, 2022)
Farooq N, Selwal A (2023) Image steganalysis using deep learning: a systematic review and open research challenges. J Ambient Intell Humaniz Comput 14(6):7761–7793. https://doi.org/10.1007/S12652-023-04591-Z
Fridrich J, Goljan M (2004) On estimation of secret message length in LSB steganography in spatial domain. Steganography Watermarking Multimedia Contents 5306:23. https://doi.org/10.1117/12.521350
Article ADS Google Scholar
Fridrich J, Kodovsky J (2012) Rich models for steganalysis of digital images. IEEE Trans Inf Forensics Secur 7(3):868–882. https://doi.org/10.1109/TIFS.2012.2190402
Fridrich J, Goljan M, Du R (2001) Steganalysis based on JPEG compatibility. Multimed Syst Appl IV 4518:275–280. https://doi.org/10.1117/12.448213
Fridrich J, Goljan M, Soukal D, Holotyak T (2005) Forensic steganalysis: determining the stego key in spatial domain steganography. Steganography Watermarking of Multimedia Contents 5681:631. https://doi.org/10.1117/12.585987
Fridrich J, Goljan M, and Soukal D (2004) Searching for the stego-key. In: security, steganography, and watermarking of multimedia contents VI, vol 5306, p. 70. doi: https://doi.org/10.1117/12.521353
Goodfellow I, Bengio Y, and Courville A (2016) Deep learning . MIT Press
Guan Q, Dong J, and Tan T, Blind Quantitative steganalysis based on feature fusion and gradient boosting. In: lecture notes in computer science (including subseries lecture notes in artificial intelligence and lecture notes in bioinformatics), 2010, vol 6526 LNCS, pp 266–279. doi: https://doi.org/10.1007/978-3-642-18405-5_22
Gui X, Li X, and Yang B (2012) Improved payload location for LSB matching steganography. In: proceedings-international conference on image processing, ICIP, pp 1125–1128. doi: https://doi.org/10.1109/ICIP.2012.6467062
Gul G, Kurugollu F (2010) Svd-based universal spatial domain image steganalysis. IEEE Trans Inf Forensics Secur 5(2):349–353. https://doi.org/10.1109/TIFS.2010.2041826
Hu J, Shen L, and Sun G (2018) Squeeze-and-excitation networks. In: proceedings of the IEEE conference on computer vision and pattern recognition, pp 7132–7141
Hussain I, Zeng J, Xinhong X, Tan S (2020) A survey on deep convolutional neural networks for image steganography and steganalysis. KSII Trans Int Inf Syst 14(3):1228–1248. https://doi.org/10.3837/TIIS.2020.03.017
Ker AD (2008) Locating Steganographic Payload via WS Residuals. In: proceedings of the 10th ACM workshop on multimedia and security, pp 27–32
Ker AD and Lubenko (2009) Feature reduction and payload location with WAM steganalysis. In: media forensics and security, international society for optics and photonics, p 72540A
Ker AD, Böhme R (2008) Revisiting weighted stego-image steganalysis. Forensics Steganography Watermarking Multimedia Contents 6819:681905. https://doi.org/10.1117/12.766820
Kodovský J, Fridrich J, Holub V (2012) Ensemble classifiers for steganalysis of digital media. IEEE Trans Inf Forensics Secur 7(2):432–444. https://doi.org/10.1109/TIFS.2011.2175919
Kodovský J and Fridrich J (2010) Quantitative steganalysis of LSB embedding in JPEG domain. In: MM and sec’10-proceedings of the 2010 ACM SIGMM multimedia and security workshop, pp 187–197. doi: https://doi.org/10.1145/1854229.1854265
Kodovský J andx Fridrich J (2013) Quantitative steganalysis using rich models. In: media watermarking, security, and forensics, vol 8665: 86650. doi: https://doi.org/10.1117/12.2001563
Kodovsky J, Fridrich J (2010) Quantitative structural steganalysis of Jsteg. IEEE Trans Inf Forensics Secur 5(4):681–693. https://doi.org/10.1109/TIFS.2010.2056684
Kong XW, Liu WF, and You XG (2005) Secret message location steganalysis based on local coherences of hue. In: lecture notes in computer science (including subseries lecture notes in artificial intelligence and lecture notes in bioinformatics), vol 3768 LNCS, pp 301–311. doi: https://doi.org/10.1007/11582267_27
Kumar V, Sharma S, Kumar C, Vishwa A, Sahu AK (2023) Latest trends in deep learning techniques for image steganography. Int J Digit Crime Forensics 15(1):1–14. https://doi.org/10.4018/IJDCF.318666
Lie WN, Lin GS (2005) A feature-based classification technique for blind image steganalysis. IEEE Trans Multimed 7(6):1007–1020. https://doi.org/10.1109/TMM.2005.858377
Liu J, Tang G (2012) Stego key estimation in LSB steganography. J Multimed 7(4):309. https://doi.org/10.4304/jmm.7.4.277-278
Liu J, Tian Y, Han T, Yang C, Liu W (2015) LSB steganographic payload location for JPEG-decompressed images. Digit Signal Process 38:66–76. https://doi.org/10.1016/j.dsp.2014.12.004
Article CAS Google Scholar
Liu J, Tian Y, Han T, Wang J, Luo X (2016) Stego key searching for LSB steganography on JPEG decompressed image. Sci China Inf Sci 59(3):1–15. https://doi.org/10.1007/S11432-015-5367-X
Liu J, Yang C, Wang J, Shi Y (2020) Stego key recovery method for F5 steganography with matrix encoding. Eurasip J Image Video Process 2020(1):1–17. https://doi.org/10.1186/S13640-020-00526-2
Article ADS CAS Google Scholar
Lou DC, Chou CL, Tso HK, Chiu CC (2012) Active steganalysis for histogram-shifting based reversible data hiding. Opt Commun 285(10–11):2510–2518. https://doi.org/10.1016/j.optcom.2012.01.021
Lu J, Yang C, Liu F, Luo X (2018) Quantitative steganalysis of JPEG additive steganography based on boundary effect. J Electron Imaging 27(02):1. https://doi.org/10.1117/1.jei.27.2.023004
Lyu S, Farid H (2006) Steganalysis using higher-order image statistics. IEEE Trans Inf Forensics Secur 1(1):111–119. https://doi.org/10.1109/TIFS.2005.863485
Malhotra R, Singh P (2023) Recent advances in deep learning models: a systematic literature review. Multimed Tools Appl 2023:1–84. https://doi.org/10.1007/S11042-023-15295-Z
Mandal PC, Mukherjee I, Paul G, Chatterji BN (2022) Digital image steganography: a literature survey. Inf Sci (ny) 609:1451–1488. https://doi.org/10.1016/J.INS.2022.07.120
Mathew A, Amudha P, Sivakumari S (2021) Deep learning techniques: an overview. Adv Intell Syst Comput 1141:599–608. https://doi.org/10.1007/978-981-15-3383-9_54/TABLES/2
Mo X, Tan S, Tang W, Li B, Huang J (2023) ReLOAD: using reinforcement learning to optimize asymmetric distortion for additive steganography. IEEE Trans Inf Forensics Secur 18:1524–1538. https://doi.org/10.1109/TIFS.2023.3244094
Muralidharan T, Cohen A, Cohen A, Processing NN-S, and undefined 2022 (2022) “The infinite race between steganography and steganalysis in images,” Signal Processing , vol 201, p 108711, Accessed: Jan 25, 2023. [Online]. Available: https://www.sciencedirect.com/science/article/pii/S016516842200250X?casa_token=t0S5VoPVAi8AAAAA:s5gDY44ig26t6_iLJ8FPDrEAjEZOM4j76RKqUlp2Dck4hmFAYL7osHZtdruhqny1zjVXT_2sCw
Nissar A, Mir AH (2010) Classification of steganalysis techniques: a study. Digit Signal Process A Rev J 20(6):1758–1770. https://doi.org/10.1016/j.dsp.2010.02.003
Pevný T, Fridrich J, Ker AD (2012) From blind to quantitative steganalysis. IEEE Trans Inf Forensics Secur 7(2):445–454. https://doi.org/10.1109/TIFS.2011.2175918
Pevný T and Ker AD (2014) Steganographic key leakage through payload metadata. In: IH and MMSec 2014-proceedings of the 2014 ACM information hiding and multimedia security workshop. pp 109–114. doi: https://doi.org/10.1145/2600918.2600921 .
Provos N and Honeyman P, Detecting steganographic content on the internet. In: USA Today, vol. 1001, no. 01–11, pp. 48103–4943, Aug. 2001, Accessed: Jan. 23, 2022. [Online]. Available: http://scholar.google.com/scholar?hl=en&btnG=Search&q=intitle:Detecting+Steganographic+Content+on+the+Internet#0
Qian Y, Dong J, Wang W, and Tan T (2016) Learning and transferring representations for image steganalysis using convolutional neural network. In: proceedings-international conference on image processing, ICIP , vol 2016 pp 2752–2756. doi: https://doi.org/10.1109/ICIP.2016.7532860 .
Quach TT (2011) Optimal cover estimation methods and steganographic payload location. IEEE Trans Inf Forensics Secur 6(4):1214–1222. https://doi.org/10.1109/TIFS.2011.2160855
Quach T-T (2014) Cover estimation and payload location using Markov random fields. In: Media Watermarking, Security, and Forensics 2014, vol 9028, p 90280H. doi: https://doi.org/10.1117/12.2032711
Ruan F, Zhang X, Zhu D, Xu Z, Wan S, Qi L (2020) Deep learning for real-time image steganalysis: a survey. J Real-Time Image Process 17(1):149–160. https://doi.org/10.1007/S11554-019-00915-5/TABLES/6
Schaefer G and Stich M (2003) UCID: an uncompressed color image database. In: storage and retrieval methods and applications for multimedia 2004, vol 5307, pp 472–480. doi: https://doi.org/10.1117/12.525375
Selvaraj A, Ezhilarasan A, Wellington SLJ, Sam AR (2021) Digital image steganalysis: a survey on paradigm shift from machine learning to deep learning based techniques. IET Image Process 15(2):504–522. https://doi.org/10.1049/ipr2.12043
Simmons GJ (1984) The prisoners problem. Advances in cryptology: proceedings of crypto , 83, pp 51–67. [Online]. Available: http://www.cs.nccu.edu.tw/~raylin/UndergraduateCourse/ComtenporaryCryptography/Spring2009/ThePrisonerProblem.pdf
Singh P, Gupta S, Gupta V (2023) Multi-objective hyperparameter optimization on gradient-boosting for breast cancer detection. Int J Syst Assur Eng Manag 1:1–11. https://doi.org/10.1007/S13198-023-01955-8/TABLES/8
Singhal A and Bedi P, Blind Quantitative Steganalysis using SVD Features. In: 2018 international conference on advances in computing, communications and informatics, ICACCI 2018, 2018, pp. 369–374. doi: https://doi.org/10.1109/ICACCI.2018.8554947 .
Singhal A and Bedi P, “Blind quantitative steganalysis using CNN–long short-term memory architecture. In: strategic system assurance and business analytics. Asset analytics (performance and safety management), pp 175–186. doi: https://doi.org/10.1007/978-981-15-3647-2_14
Singhal A and Bedi P (2022) Universal Quantitative steganalysis using deep residual networks. In: international conference on innovative computing and communications. Advances in intelligent systems and computing, pp 465–475. doi: https://doi.org/10.1007/978-981-16-3071-2_37
Singhal A, Bedi P (2021) Multi-class blind steganalysis using deep residual networks. Multimed Tools Appl 80(9):13931–13956. https://doi.org/10.1007/s11042-020-10353-2
Stier C (2010) Russian spy ring hid secret messages on the web. New Sci . https://www.newscientist.com/article/dn19126-russian-spy-ring-hid-secret-messages-on-the-web/ (Accessed Jan. 19, 2022).
Sun Y, Zhang H, Zhang T, Wang R (2019) Deep neural networks for efficient steganographic payload location. J Real-Time Image Process 16(3):635–647. https://doi.org/10.1007/S11554-019-00849-Y/TABLES/7
Sun Y and Li T (2019) A Method for Quantitative steganalysis based on deep learning. In: 2019 2nd international conference on information systems and computer aided education, ICISCAE 2019, pp 302–309. doi: https://doi.org/10.1109/ICISCAE48440.2019.221640 .
Tabares-Soto R, Raúl RP, Gustavo I (2019) Deep learning applied to steganalysis of digital images: a systematic review. IEEE Access 7:68970–68990. https://doi.org/10.1109/ACCESS.2019.2918086
Tang W, Li B, Barni M, Li J, Huang J (2021) An automatic cost learning framework for image steganography using deep reinforcement learning. IEEE Trans Inf Forensics Secur 16:952–967. https://doi.org/10.1109/TIFS.2020.3025438
Trivedi S, Chandramouli R (2005) Secret key estimation in sequential steganography. IEEE Trans Signal Process 53(2):746–757. https://doi.org/10.1109/TSP.2004.839925
Article ADS MathSciNet Google Scholar
Veena ST, Arivazhagan S (2018) Quantitative steganalysis of spatial LSB based stego images using reduced instances and features. Patt Recognit Lett 105:39–49. https://doi.org/10.1016/j.patrec.2017.08.016
Veena ST, Arivazhagan S (2019) Universal secret payload location identification in spatial LSB stego images. Ann Des Telecommun Telecommun 74(5–6):273–286. https://doi.org/10.1007/S12243-018-0676-X/TABLES/3
Wang Y, Moulin P (2007) Optimized feature extraction for learning-based image steganalysis. IEEE Trans Inf Forensics Secur 2(1):31–45. https://doi.org/10.1109/TIFS.2006.890517
Wang J, Yang C, Wang P, Song X, Lu J (2020) Payload location for JPEG image steganography based on co-frequency sub-image filtering. Int J Distrib Sens Netw 16(1):1550147719899569. https://doi.org/10.1177/1550147719899569
Wang J, Yang C, Zhu M, Song X, Liu Y, Lian Y (2021) JPEG image steganography payload location based on optimal estimation of cover co-frequency sub-image. Eurasip J Image Video Process 2021(1):1–14. https://doi.org/10.1186/S13640-020-00542-2/FIGURES/7
Wu S, Zhong S, Liu Y (2018) Deep residual learning for image steganalysis. Multimed Tools Appl 77(9):10437–10453. https://doi.org/10.1007/s11042-017-4440-4
Xu G, Wu HZ, Shi YQ (2016a) Structural design of convolutional neural networks for steganalysis. IEEE Signal Process Lett 23(5):708–712. https://doi.org/10.1109/LSP.2016.2548421
Xu C, Liu J, Gan J, Luo X (2018) Stego key recovery based on the optimal hypothesis test. Multimed Tools Appl 77(14):17973–17992. https://doi.org/10.1007/s11042-017-4878-4
Xu G, Wu HZ, and Shi YQ (2016) Ensemble of CNNs for steganalysis: an empirical study. In: IH and MMSec 2016-proceedings of the 2016 ACM information hiding and multimedia security workshop , pp 103–107. doi: https://doi.org/10.1145/2909827.2930798 .
Yang C, Luo X, Lu J, Liu F (2018) Extracting hidden messages of MLSB steganography based on optimal stego subset. Sci China Inf Sci 61(11):3. https://doi.org/10.1007/s11432-017-9328-2
Yang C, Liu F, Ge S, Lu J, Huang J (2019) Locating secret messages based on quantitative steganalysis. Math Biosci Eng 16(5):4908–4922. https://doi.org/10.3934/mbe.2019247
Article PubMed Google Scholar
Ye J, Ni J, Yi Y (2017) Deep learning hierarchical representations for image steganalysis. IEEE Trans Inf Forensics Secur 12(11):2545–2557. https://doi.org/10.1109/TIFS.2017.2710946
Yedroudj M, Comby F, and Chaumont M (2018) Yedroudj-net: an efficient CNN for spatial steganalysis. In: ICASSP, IEEE international conference on acoustics, speech and signal processing-proceedings , 2018, pp 2092–2096. doi: https://doi.org/10.1109/ICASSP.2018.8461438 .
You W, Zhang H, Zhao X (2021) A siamese CNN for image steganalysis. IEEE Trans Inf Forensics Secur 16:291–306. https://doi.org/10.1109/TIFS.2020.3013204
Zeng J, Tan S, Li B, Huang J (2017) Large-scale JPEG image steganalysis using hybrid deep-learning framework. IEEE Trans Inf Forensics Secur 13(5):1200–1214. https://doi.org/10.1109/TIFS.2017.2779446
Zou Y, Zhang G, Liu L (2019) Research on image steganography analysis based on deep learning. J vis Commun Image Represent 60:266–275. https://doi.org/10.1016/J.JVCIR.2019.02.034
Download references
Acknowledgements
We seriously thank the editor and anonymous reviewers for their valuable comments and suggestions which have immensely helped in improving the quality of our paper.
No funding was received to assist with the preparation of this manuscript.
Author information
Authors and affiliations.
Department of Computer Science, University of Delhi, Delhi, India
Punam Bedi, Anuradha Singhal & Veenu Bhasin
You can also search for this author in PubMed Google Scholar
Corresponding author
Correspondence to Anuradha Singhal .
Ethics declarations
Conflict of interest.
The authors declare that they have no known competing financial interests or personal relationships that could have appeared to influence the work reported in this paper.
Additional information
Publisher's note.
Springer Nature remains neutral with regard to jurisdictional claims in published maps and institutional affiliations.
List of acronyms used in paper is tabulated in Table 3 .
Rights and permissions
Springer Nature or its licensor (e.g. a society or other partner) holds exclusive rights to this article under a publishing agreement with the author(s) or other rightsholder(s); author self-archiving of the accepted manuscript version of this article is solely governed by the terms of such publishing agreement and applicable law.
Reprints and permissions
About this article
Bedi, P., Singhal, A. & Bhasin, V. Deep learning based active image steganalysis: a review. Int J Syst Assur Eng Manag 15 , 786–799 (2024). https://doi.org/10.1007/s13198-023-02203-9
Download citation
Received : 25 June 2023
Revised : 02 October 2023
Accepted : 04 November 2023
Published : 27 November 2023
Issue Date : March 2024
DOI : https://doi.org/10.1007/s13198-023-02203-9
Share this article
Anyone you share the following link with will be able to read this content:
Sorry, a shareable link is not currently available for this article.
Provided by the Springer Nature SharedIt content-sharing initiative
- Information security
- Cybersecurity
- Steganalysis
- Machine learning
- Deep learning
- Active image steganalysis
- Find a journal
- Publish with us
- Track your research
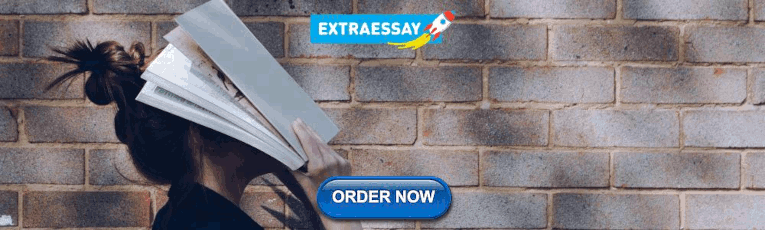
IMAGES
VIDEO
COMMENTS
This study is a literature review using meta-analysis. Meta-analysis is a review of research results systematic, especially on the results of research empirically related to online learning efficacy for designing and developing instructional materials that can provide wider access to quality higher education.
Online learning has provided the opportunity to teach and learn in innovative ways unlike the teaching and learning experiences in the normal classroom setting. Discussion As of July 2020, 98.6% of learners worldwide were affected by the pandemic, representing 1.725 billion children and youth, from pre-primary to higher education, in 200 ...
In this review of the literature on e-learning, we present and discuss definitions of e-learning, hybrid learning and blended learning, and we review the literature comparing different online teaching formats with traditional on-campus/face-to-face teaching. With this point of departure, we explore which factors affect
This review enabled us to identify the online learning research themes examined from 2009 to 2018. In the section below, we review the most studied research themes, engagement and learner characteristics along with implications, limitations, and directions for future research. 5.1. Most studied research themes.
The literature on online learning has long emphasised the role of effective interaction for the success of student learning. According to Muirhead and Juwah ... Interactivity in computer‐mediated college and university education: A recent review of the literature. Journal of Educational Technology & Society, 7 (1), 12-20.
The debate in the literature surrounding online learning versus F2F teaching continues to be a contentious one. A review of the literature reveals mixed findings when comparing the efficacy of online learning on student performance in relation to the traditional F2F medium of instruction (Lundberg et al., 2008; Nguyen, 2015).
This study compares university students' performance in traditional learning to that of online learning during the pandemic, ... Literature Review. Inevitable crises and disasters can profoundly affect the educational sector. Previously, the emergency procedure was to stop the educational process completely. However, today's technological ...
This systematic review of the research literature on online and blended learning from schools starts by outlining recent perspectives on emergency remote learning, as occurred during the Covid-19 pandemic. ... In this review, online or blended learning may have taken place for an entire programme of learning, or it may only have taken place for ...
The COVID-19 pandemic has created the largest disruption of education systems in human history, affecting nearly 1.6 billion learners in more than 200 countries. Closures of schools, institutions and other learning spaces have impacted more than 94% of the world's student population. This has brought far-reaching changes in all aspects of our ...
ABSTRACT. Online learning as a concept and as a keyword has consistently been a focus of education research for over two decades. In this paper, we present results from a systematic literature review for the definitions of online learning because the concept of online learning, though often defined, has a range of meanings attached to it.
This paper provides a review of the literature on online teaching and learning practices in teacher education. In total, 134 empirical studies were analysed. Online teaching and learning practices related to social, cognitive and teaching presence were identified. The findings highlighted the need for a comprehensive view of the pedagogy of ...
A reference list of scholarly papers directly referencing Covid-19 online learning: A comprehensive literature review in pharmacy education was created after merging these lists. After the first search or first phase, 137 scholarly publications were presented (Table 1). By removing duplicated results, we were able to screen for them.
The literature review identified key factors influencing online learning outcomes, emphasizing their significant role in academic discourse. ... (2010). Evaluation of Evidence-Based Practices in ...
This systematic literature review of 36 peer-reviewed empirical articles outlines eight strategies used by higher education lecturers and students to maintain educational continuity during the COVID-19 pandemic since January 2020. The findings show that students' online access and positive coping strategies could not eradicate their infrastructure and home environment challenges.
This article reports on a large-scale (n = 987), exploratory factor analysis study incorporating various concepts identified in the literature as critical success factors for online learning from the students' perspective, and then determines their hierarchical significance. Seven factors--Basic Online Modality, Instructional Support, Teaching Presence, Cognitive Presence, Online Social ...
The literature on online learning in ILI in academic libraries represents a variety of disciplinary concentrations. Twenty-six of the reviewed articles (47.3%) focused on instruction for a general audience, while the remaining 29 (52.7%) made reference to a specific discipline (see Fig. 2.1).Of these, 19 (34.6%) referenced instruction intended for the entire campus community, whether through a ...
This paper reviews the online learning literature to address the attrition issue and increase the learning outcomes. The review is classified into three categories, technology adoption, continuation of technology use, and learning outcomes. Antecedents of adoption, continuance, and learning outcome are classified according to social cognitive ...
Through a systematic literature review, 37 related literatures sourced from Science Direct, Taylor & Francis, and Emerald Insight were obtained. A ten-year timeframe between 2012 and 2022 was used ...
We present a brief literature review for how the shift to virtual instruction in the middle of the semester may introduce unique challenges for students, drawing on research that has investigated factors inhibiting or promoting success in online education. ... A randomized assessment of online learning. American Economic Review, 106(5), 378 ...
A guest post by Dr John L. Taylor, Director of Learning, Teaching and Innovation at Cranleigh School, offering a literature review on online distance learning from four sections: efficacy, community, success and self-regulation. The review covers the key terms, definitions and research evidence on online learning, as well as the challenges and opportunities of this mode of education.
This literature review develops a comprehensive de finition of distraction, summarizes three main types of distraction (multitasking, mind-wandering, and using digital devices), and proposes two ...
The literature is reviewed to determine factors influencing student persistence in online programs and solutions to reduce attrition rates and creates a foundation for further research on the issue of student retention in online programs.
The COVID-19 pandemic caused a sudden shift to virtual platforms. Physical distance and limited experience with both synchronous and asynchronous teamwork at work and school hampered problem-solving and the development of critical thinking skills. Under these circumstances, the implementation of team-based and problem-based learning (TBL, PBL, respectively) required a reevaluation of how teams ...
Anyway, the present review reveals the presence of high levels of stress and burnout among healthcare students and shows the possible consequences on the quality of their life. The scientific literature confirms these results on how stress may influence sleep quality and QT interval changes (Itagi et al., 2021).
Literature search and identification. In this study, we followed the principles and guidelines of the PRISMA (Preferred Reporting Items for Systematic Reviews and Meta-Analyses) 2020 statement (Page et al., Citation 2021).The literature search was conducted on CNKI.net, and we used the following methods and keywords for the search: title, abstract, and keywords including vocational education ...
The literature review has presented the results of a systematic review on the factors affecting the efficiency of online learning, and how it impacts learners' satisfaction and commitment. To conduct the literature review, approximately 40 empirical studies were reviewed and analysed.
Mission. The Purdue On-Campus Writing Lab and Purdue Online Writing Lab assist clients in their development as writers—no matter what their skill level—with on-campus consultations, online participation, and community engagement. The Purdue Writing Lab serves the Purdue, West Lafayette, campus and coordinates with local literacy initiatives.
Through this paper we provide comprehensive review of literature present in active image steganalysis using deep learning techniques. Presented review manuscript involves the approach for selecting articles as depicted in Fig. 7. The steps involved are identification of research questions, conducting inclusion and exclusion criteria, performing ...