- Search Menu
- Advance articles
- Editor's Choice
- Original Articles
- Author Guidelines
- Submission Site
- Open Access
- About Forestry
- About the Institute of Chartered Foresters
- Editorial Board
- Advertising and Corporate Services
- Journals Career Network
- Self-Archiving Policy
- Dispatch Dates
- Journals on Oxford Academic
- Books on Oxford Academic
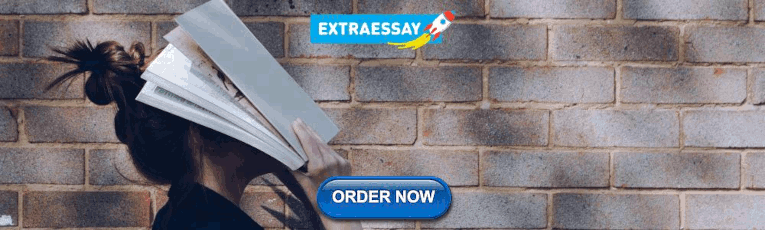
Article Contents
Introduction, acknowledgements, conflict of interest, data availability, predicting net growth rates in boreal forests using landsat time series and permanent sample plot data.

- Article contents
- Figures & tables
- Supplementary Data
Alexandre Morin-Bernard, Nicholas C Coops, Joanne C White, Alexis Achim, Predicting net growth rates in boreal forests using Landsat time series and permanent sample plot data, Forestry: An International Journal of Forest Research , 2023;, cpad055, https://doi.org/10.1093/forestry/cpad055
- Permissions Icon Permissions
Increasing temperature and changes in water dynamics are bringing uncertainty regarding the future productivity of boreal forests, even in the absence of stand-replacing disturbances. There is accumulating evidence that water deficits caused by warmer summer temperatures are linked to decreases in the growth rate of boreal tree species in some regions. In this context, it is essential to provide forest professionals with a means of monitoring net forest growth rates in undisturbed areas and at the scale of a management unit in order to determine where and when changes in growth are taking place. This is challenging using conventional forest inventory approaches. In this study, we use Landsat time series and data from permanent sample plots (PSP) to develop spatially explicit estimates of annual net basal area growth at a 30-m spatial resolution for a forest management unit in Canada. An ordinary least square regression model was developed using data from 120 PSPs and validated on an independent set of 60 PSPs, with R 2 values of 0.61 and 0.58, respectively. Applying the model over a 586 607-ha study area revealed considerable temporal and spatial variability in the predicted growth rates and their evolution through time. There was an overall decline in predicted growth rates over time, with this trend corroborated by the PSP data and attributed to the ageing demographics of the forests in the study area. This variability was related to forest development stage, species composition, and structural attributes derived from light detection and ranging (LiDAR). The information generated by the suggested approach can help to improve yield predictions, optimize rotation lengths, and allow for the identification of target areas where silvicultural interventions aimed at maintaining or enhancing growth could be conducted.
Boreal forests are amongst the largest ecosystems on earth ( Brandt et al. 2013 ) and contain a third of the global terrestrial biogenic carbon stock ( Pan et al. 2011 ). A large part of the boreal forest biome hosts management activities that account for a third of the lumber produced worldwide ( Gauthier et al. 2015 ). In Canada, ~70% of the forest extent lies within the boreal zone, with the contiguous boreal forest covering a total area of 270 Mha ( Brandt et al. 2013 ). This geographical extent makes it a particularly challenging ecosystem to manage, especially in the context of climate change. In particular, increasing rates of disturbances and tree mortality ( Peng et al. 2011 ; Seidl et al. 2017 ) as well as increasing temperature and changes in water dynamics will alter the growth response of boreal forests ( D’Orangeville et al. 2018 ; Girardin et al. 2016b ), bringing uncertainty regarding their future productivity.
Although boreal tree species can benefit from a lengthening of the growth season and increased CO 2 levels ( D’Orangeville et al. 2018 ; Subedi and Sharma 2013 ), the rapid changes in environmental conditions may be outpacing the ability of species to adapt, which could result in forested ecosystems where the species composition is no longer in equilibrium with the climate ( Huang et al. 2010 ). There is accumulating evidence that warmer summer temperatures are causing increased water deficits in some regions, linked to decreases in the growth rate of black spruce [ Picea mariana (Mill.) B.S.P.], the most abundant tree species in the boreal zone ( Chagnon et al. 2022 ; D’Orangeville et al. 2018 ; Girardin et al. 2016b ). The accumulation of drought events have already caused increased mortality rates in black spruce forests ( Peng et al. 2011 ; Sánchez-Pinillos et al. 2022 ). Jack pine ( Pinus banksiana Lamb), a fire-adapted species known for colonizing xeric sites, has also experienced a generalized growth decline in eastern Canada over the last decades, attributed to increased water stress ( Dietrich et al. 2016 ; Girardin et al. 2012 ).
Whilst the net aboveground biomass (AGB) of Canada’s forested ecosystems increased between 1984 and 2016, growth rates over the undisturbed persistent forests have been highly variable ( Wulder et al. 2020 ). Observed changes in growth are influenced by multiple factors such as species composition, stand age and development stage, structural attributes, and site characteristics ( Dietrich et al. 2016 ; D’Orangeville et al. 2018 ; Mamet et al. 2015 ; Marchand et al. 2019 ). Understanding what growth changes have already occurred and predicting future changes in this ecosystem is therefore very difficult, but crucial. Reduction in carbon assimilation rates could offset the effect of strategies aiming to increase the forest carbon sink ( Kurz et al. 2008 ; Seidl et al. 2014 ) and eventually lead to reductions in the timber supply ( Brecka et al. 2018 ; McDowell et al. 2020 ).
To manage risks associated with the uncertain growth response of trees across the landscape, forest practitioners will increasingly need to rely on adaptive silvicultural actions to promote more resistant and resilient forest stands ( Achim et al. 2022 ; Gardiner and Moore 2014 ; Moreau et al. 2022 ). In this context, it is essential to provide forest professionals with a means of monitoring net forest growth in undisturbed areas and at the scale of a management unit in order to determine where and when changes in growth are taking place, which is challenging using conventional forest inventory approaches ( Bowman et al. 2013 ; Gillis et al. 2005 ).
Remote sensing technologies can provide temporally continuous and spatially contiguous coverage of forest condition. With an analysis-ready image archive dating back to 1984 and a moderate spatial resolution (30 m), the high radiometric and geometric qualities of the Landsat TM, ETM+, and OLI sensors provide a robust basis for the characterization of change in boreal forests at both global and local scales ( Sulla-Menashe et al. 2018 ). These characteristics have made Landsat imagery a primary source of data for characterizing changes in forest condition ( Banskota et al. 2014 ; Wulder et al. 2022 ). Capitalizing on the known relationships between spectral indices and the physiological responses of trees, several studies have utilized Landsat data to investigate gradual changes in forest productivity (e.g. Vogelmann et al. 2016 ), model changes in forest biomass dynamics (e.g. Gomez et al. 2015 ), and identify declining stands ( Czerwinski et al. 2014 ). Whilst satellite imagery allows for the observation of changes over time, light detection and ranging (LiDAR) provides detailed wall-to-wall information on forest structural attributes and development stage ( Baltsavias 1999 ; Lefsky et al. 2002 ; Wulder et al. 2012 ). Using LiDAR in combination with satellite imagery has proven useful for extending the spatial and temporal coverage of LiDAR data sets ( Matasci et al. 2018 ; Zald et al. 2016 ), and offers the possibility to better understand patterns of growth across boreal forests in the last decades and relate them to the existing forest structure, generating useful information for the implementation of efficient, adaptive silvicultural practices ( Achim et al. 2022 ).
Our overall objective was to use Landsat time series data to develop spatially explicit estimates of net basal area growth at a 30-m spatial resolution for a forest management area in Ontario, Canada. We then examined the predicted growth rates in the context of forest development stage, species composition, and structural attributes derived from LiDAR and discuss the potential of the proposed approach to provide relevant information for the implementation of adapted forest management practices.
The current study comprises several methodological stages that involve relating field measurements of forest growth with variables derived from Landsat data. The annual net growth rate of conifer-dominated stands was first determined using repeated measurement data from permanent sample plots located in the boreal zone of eastern Canada. Subsequently, a model selection approach was used to identify the optimal set of Landsat-derived variables to construct a predictive model for net forest growth. This model was then applied to a forest management unit, which was subdivided into forest-type examples with unique structural attributes using LiDAR data. Model predictions were then examined in relation to forest composition and structure.
The Romeo Malette Forest (RMF), located in Ontario, Canada ( Fig. 1 ), covers 586 607 ha within the Lake Abitibi ecoregion in the Ontario Shield ecozone ( Wester et al. 2018 ). The main tree species in this forest management unit include black spruce, jack pine, white spruce [ Picea glauca (Moench) Voss], poplar ( Populus spp.) and white birch ( Betula papyrifera Marshall) with components of balsam fir [ Abies balsamea (L.) Mill], cedar ( Thuya spp.), Eastern larch [ Larix laricina (Du Roi) K. Koch], and white and red pines ( Pinus strobus L. and Pinus resinosa Soi ex. Aiton). The area is under active forest management by a sustainable forest license holder. The primary harvested species are black spruce, jack pine, balsam fir, and poplar, for a total harvested volume of 2 935 969 m 3 between 2012 and 2019. The RMF can be considered as a relatively young forest, with, as of 2023, a median age of 78 years, and only 5% of the forested area being older than 133 years of age ( Bilyk et al. 2021 ).
Characteristics of the permanent sample plots included in the development of the model at the time of the first and last field measurements.
Mean DBH corresponds to the root mean square DBH at the plot level.

(a) Location of the RMF in Canada. (b) Boundary of the RMF and location of the permanent sample plots used in model development.
The mean annual temperature in the area is 1.8°C and the area receives 773 mm of precipitation annually, based on the historical mean for the period 1981–2010 extracted from ClimateNA ( Wang et al. 2016 ). The disturbance regime in the study site is characterized by wildfire, windthrow, and insect defoliation mainly from the spruce budworm [ Choristoneura fumiferana (Clem.)] and forest tent caterpillar ( Malacosoma disstria Hübner) ( Urquizo et al. 2000 ). By 2050, according to projections made using a 13 global circulation model ensemble and averaged under four shared socioeconomic pathways (i.e. SSPs 1–4) scenarios ( Mahony et al. 2022 ), both the minimum and maximum temperatures are expected to increase by 2.9°C in this region. Warmer summers with no change in precipitation and warmer winters with less precipitation falling as snow could increase the risk of drought and vulnerability to forest pests ( Wotherspoon et al. 2022 ).
Data from permanent sample plots
Data on forest growth were gathered from permanent sample plots located within the RMF and in adjacent forest management units with high densities of PSP ( Fig. 1 ). The 11.28-m-radius (400 m 2 ) circular plots are part of the network of permanent field plots periodically measured within the growth and yield programme from the Ontario Ministry of Natural Resources and Forestry ( Hayden 1995 ). The current study focused on stands containing the dominant coniferous species in this region. We only selected plots in which >50% of the basal area at the time of the first measurement was composed of black spruce, jack pine, and balsam fir. We also excluded plots in which >20% of the basal area was composed of deciduous species. This threshold was set to avoid spectral inconsistencies due to changes in species composition during the measurement intervals, which are known to generate trends that are not related to forest growth and productivity, but rather to succession or disturbances ( Fiore et al. 2020 ). We initially selected 493 plots that were measured at least twice between 1993 and 2018, from which 169 were excluded due to the mixed species composition. The candidate plots were additionally filtered to exclude all plots that were disturbed during the measurement interval, due to harvesting, silvicultural treatments, or non-stand replacing disturbances. Finally, we ensured that the forest surrounding the plots was homogeneous within a radius of 80 m, resulting in a final number of 180 plots ( Fig. 1 ).
At each plot, the crown class and DBH of every tree with a minimum DBH of 2.5 cm were recorded in accordance with the Ontario growth and yield programme reference manual ( MNRF 2016 ). To derive an estimate of the current growth conditions, we used tree-level measurements from the earliest and latest measurement to calculate the plot-level annual net basal area increment, hereafter referred to as the net growth, in the interval between the visits, which ranged from 5 to 24 years (median of 10 years). The net growth consists of the basal area growth of trees that survived between the two measurements plus the basal area of trees that reached the DBH threshold of 2.5 cm during the interval, from which we deduce the basal area of trees that died in that same period. A net growth rate in m 2 ha −1 yr −1 was then calculated for each plot by dividing the net basal area growth by the number of growing seasons between the two field measurements. The median net growth rate measured in the selected plots was 0.52 m 2 ha −1 yr −1 (standard deviation = 0.38 m 2 ha −1 yr −1 ).
Landsat surface reflectance composite time series
We created annual, gap-free, best-available pixel surface reflectance composites (BAP, White et al. , 2014 ) over the entire study site for the period 1984–2021. BAPs are created from Landsat 5 TM, Landsat 7 ETM+, and Landsat 8 OLI orthorectified and atmospherically corrected surface reflectance images, by selecting the best pixel based on the proximity to a target date, distance to clouds and cloud shadows, atmospheric quality and acquisition sensor, and by subsequently filling data gaps if no valid observation was available for a given pixel ( Hermosilla et al. 2015 ; White et al. 2014 ). Only Landsat observations acquired between 1 July and 30 August were used to create the composites.
From the BAP composites, a time series of selected vegetation indices were extracted (VI, Table 2 ) and averaged over a 3 × 3 pixel window centred over each of the permanent sample plots, for the period corresponding to the interval between the first and last field measurements. This window size was chosen to account for the limited accuracy of the plots’ geolocation and ensured that the extracted spectral values would be representative of the actual canopy condition at the plot location. Among the selected vegetation indices for the current study was the normalized burn ratio (NBR), a normalized difference of the near-infrared (NIR) and second shortwave-infrared Landsat bands (SWIR2) and has been proven useful in monitoring post-disturbance recovery not only after fire but also after harvesting, even in the case of non-stand replacing disturbance events ( White et al. 2017 ). Photosynthetically active vegetation shows a higher reflectance in the near-infrared region and a lower reflectance in the shortwave infrared regions ( Asner 1998 ) compared to non-photosynthetic and unhealthy vegetation, making it an appropriate candidate for the monitoring of forest growth processes, which involves changes in the amount of photosynthetically active vegetation through the stages of development of a stand and in function of the mortality rates. Indices derived from the tasselled cap transformation (TCT, Crist and Cicone 1984 ) combine the information contained in six Landsat bands. TCT derived-indices, most notably the tasselled cap wetness (TCW) and tasselled cap angle (TCA), have been associated to the density of the forest cover and have been successfully used to measure subtle forest change and to retrospectively estimate forest structural attributes ( Matasci et al. 2018 ; Zald et al. 2016 ), making them relevant as tools to monitor growth even in the absence of disturbances. The normalized difference vegetation index (NDVI), relying on the NIR and red Landsat bands, is a well-known indicator of the amount of photosynthetically active vegetation, which is strongly absorptive in the red region of the spectrum ( Tucker 1979 ). Although the NDVI is subject to saturation and is sensitive to the presence of broadleaved vegetation in boreal environments ( Fiore et al. 2020 ; Sulla-Menashe et al. 2018 ), it has been used in several broad-scale studies on the effect of climate change on the growth of boreal forests ( Beck and Goetz 2011 ; Girardin et al. 2016a ) and was included in the current study to assess its performance for measuring the net basal area growth in this ecosystem.
Vegetation indices, equations, and references used in the current study.
Two categories of predictors were derived from the time series. The first category comprises the value of the VIs on the year of the first field measurement, hereafter called initial value. The second category represents the slope of the VIs over the interval between measurements and we used the Theil Sen slope estimator ( Sen 1968 ; Theil 1950 ) as it has been shown to be robust against outliers and noise and has been used previously in the analysis of time series of satellite images ( Bolton et al. 2020 ; Czerwinski et al. 2014 ; Rogers et al. 2018 ).
Modelling of the net basal area growth rate
We relied on a model selection procedure based on the second-order Akaike information criterion (AICc) to identify the best model and associated predictors from a set of candidates using the AICcmodavg package ( Mazerolle 2020 ) in the R programming environment. Candidate models comprised one to three of the Landsat-derived predictors ( Table 2 ), avoiding the inclusion of highly correlated variables (Pearson’s R ≥ 0.8). In the current study, model predictions were generated on a subset of the area where the training data were collected (i.e. geographic interpolation). To ensure the representativeness of the training data set, plot selection needs to be based on a form of random selection of the observations, in which all available observations had a greater than zero chance of being selected ( Hastie et al. 2009 ; Olofsson et al. 2014 ; Stehman 2001 ). Such an approach avoids restricting the predictor space for model training, which may occur when using methods based on spatial independence constraints ( Roberts et al. 2017 ).
We used a stratified random sampling approach based on the net growth rate measured at each plot to partition the data into training and validation data sets comprising two-thirds (120) and one-third (60) of the observations, respectively. This approach limits extrapolation when generating model predictions by ensuring a similar range of growth rates in the two data sets ( Olofsson et al. 2014 ; Roberts et al. 2017 ). We then used ordinary least square (OLS) regression to train the model on the training data set. For each candidate model, assumptions were verified graphically, through the Shapiro–Wilk normality test and Verbyla’s test for heteroscedasticity ( Shapiro and Wilk 1965 ; Verbyla 1993 ). We used Moran’s I ( Moran 1950 ) to test for spatial autocorrelation in models residuals. We then assessed the accuracy of the best-ranked model on the validation data set.
The presence of spatial dependence in the predictor space can lead to an overestimation of model performance when using a conventional validation approach ( Roberts et al. 2017 ). We therefore conducted an additional model performance assessment using k -fold spatial cross-validation. To conduct this step, we first tested for spatial dependence in the predictor space, using package BlockCV in the R programming environment ( Valavi et al. 2018 ). We determined the range of spatial autocorrelation in each of the three predictors included in the best-ranked model identified after the model-selection process. The size of the spatial blocks was set as the largest range of autocorrelation amongst all predictors raster layers (i.e. TCW slope, 6000 m). We then conducted a 5-fold spatial cross-validation repeated a hundred times in order to compare model performance with the first modelling process.
LiDAR-derived information on forest structure, composition, and site characteristics
Wall-to-wall information on forest structural attributes and site moisture was obtained from an aerial single-photon LiDAR (SPL) acquisition conducted in 2018 that only encompassed the RMF. Attributes derived from the SPL directly (at a 20-m resolution) included height, canopy cover, and the rumple index ( Queinnec et al. 2022b ). Height was the 95th percentile of SPL return heights, whereas canopy cover was the percentage of SPL returns above 2 m. The rumple index is the ratio of the canopy surface area on the ground surface area and is indicative of the canopy structural complexity ( Kane et al. 2008 ). Area-based predictions of stem density and quadratic mean DBH were also generated at a 20-m spatial resolution using a network of temporary sample plots measured in 2019 and the SPL data ( Queinnec et al. 2022b ). To get a general indication of site moisture, the topographic wetness index (TWI, Beven and Kirkby 1979 ), was calculated from the 2018 SPL DEM at a 1-m resolution and averaged over the 20-m footprint of the other layers’ cells. Finally, information on species composition was extracted from a species composition layer generated from the 2018 SPL data in combination with Sentinel-2 satellite imagery, indicating the dominant species in each 20-m pixel ( Queinnec et al. 2022a ).
Analysis of growth trends
The developed net basal area growth rate model was then applied over treed areas within the RMF management area. To delineate the area of model application, we used the aforementioned canopy cover and species composition layers to identify stands with similar attributes. The limited availability of LiDAR data, which was solely collected over the RMF, as well as the time gap between LiDAR acquisition and the most recent plot measurements, did not allow for the use of the LiDAR-derived attributes of the training plots for defining the model application area. Instead, we used the 20-m-resolution canopy cover layer derived from the SPL data to mask non-forested pixels, as well as areas with <50% canopy cover. A 50% canopy cover threshold was chosen to focus on stands developing as closed canopy forests, which are primarily targeted for forest management and harvest practices and to ensure the exclusion of treed bogs, frequent in the study area. We then used the species composition layer to remove areas that were dominated by species other than black spruce or jack pine. Stands that were harvested or severely disturbed between 1984 and 2021 were also excluded, using both information from the Ontario Provincial Forest Resources Inventory layer (FRI, Bilyk et al. 2021 ) and the stand-replacing disturbance history generated using the composite-to-change (C2C) approach ( Hermosilla et al. 2016 ). This resulted in the exclusion of four of the training plots from the model application area, three of which were excluded because a stand-replacing disturbance had occurred between the time of the last measurement and the LiDAR acquisition.
Growth trends over the entire forest management unit
The model was applied to a 10-year moving window of BAP composites, which corresponds to the median interval between the field measurements of the plots included in the model development. This resulted in a predicted growth rate for 26 consecutive 10-year windows. Predictions were averaged to obtain the predicted growth rate over the entire study area from 1984 to 2018. We then examined the range and variability of the predicted growth rate over the study area and analyzed its evolution through time. In an additional step, we investigated the predictor values across the model application area to determine the level of extrapolation by the model. To do so, we computed the 5th, 50th, and 95th percentile, as well as the minimum and maximum value of each predictor for all of the 26 moving windows for which growth predictions were generated.
Data from the PSPs located within the RMF was used to corroborate the model predicted trends in net growth rate over the study period. We identified 64 plots, measured at least three times between 1992 and 2019, allowing the calculation of the plot-level net annual basal area growth rate on a minimum of two consecutive intervals for each plot. This criterion resulted in a different sample of plots compared to the one used in model development and validation, since plots that were measured only twice had to be rejected. Only plots in which jack pine and black spruce were the dominant species, and in which no stand replacing disturbances occurred from 1984 to 2019 were selected. The time span between the first and last field measurement ranged from 8 to 18 years during which the plots were visited either three or four times. For each interval between field visits, the plot-level net annual basal area growth rate was calculated. Then, for each plot, the change in net growth rate was calculated as the difference in net growth rates between two consecutive intervals. This step resulted in positive net growth rate change values for plots in which the net growth rate increased over time, and negative values when the net growth rate decreased in the most recent interval. The growth rate change values were then averaged at the plot level, before calculating the average growth rate change for the 64 plots. The growth rate trends derived from these data were then compared to the growth rate trends derived from the model.
Growth trends in relation to forest structure and composition
To examine differences in growth trends in relation to forest composition and structure, we grouped all pixels into a predetermined number of eight structurally distinct forest type exemplars based on the information from the SPL-derived forest attribute and species composition layers, using a k -means clustering approach ( Hartigan et al. 1979 ). Instead of including all structural and compositional attributes in the clustering process, we first reduced the dimensionality of the data set by applying a principal component analysis (PCA), a well-established multivariate data reduction technique that allows summarizing several inter-correlated variables into a smaller number of uncorrelated variables, referred to as principal components ( Abdi and Williams 2010 ). Using PCA before applying k -means clustering is an effective approach that has the advantage of minimizing the sensitivity of k -means clustering to the initial positions of the cluster centres ( Celik 2009 ; Dash et al. 2010 ). The stem density, quadratic mean DBH (hereafter referred to as DBH), height, canopy cover, and value of the rumple index as well as the species dominance were all used for the PCA. The first three principal components respectively explained 45.0%, 28.3%, and 17.7% of the variance. The k -means algorithm was applied on the first three PCs since it captured a large percentage (i.e. 91.0%) of the variance.
For each of the resulting forest type exemplars, we extracted a 10 × 50 m profile from the 2018 SPL point cloud and summarized the average structural attributes to visualize differences between forest types. Using the forest attribute layer, areas corresponding to the average values (±5%) of the five structural attributes of each forest-type exemplars were located. We extracted the LiDAR point cloud over a 3 × 3, 20-m cells window at the locations that matched most closely to the desired structural attributes. The two-dimensional profile for each exemplar was then extracted after ensuring the forest cover was homogeneous within the point cloud. The evolution of the predicted growth through time was then plotted by extracting, for all pixels in each forest-type exemplar, the model-predicted growth rate and associated standard deviations for the 26 moving windows. Two descriptive statistics were calculated from the predicted growth time series to facilitate comparison between forest types: the average growth rate over the study period and the evolution of the growth rate through time, hereafter referred to as the growth trend. The average predicted growth was calculated from all the pixels included in each exemplar and corresponds to the average of the predicted growth for all the 26 moving windows. Analysis of variance and multiple comparisons with the Tukey honestly significant difference (HSD) test were conducted to verify if the average growth rate was significantly different amongst the forest-type exemplars. The growth trend corresponds to the linear slope of the predicted growth values calculated over the 26 consecutive moving windows. The significance of the trend was assessed using the t -test statistic and associated P -value of the OLS regression model built from the average predicted growth rate time series of each forest-type exemplar. The location of the eight forest-type exemplars was then mapped to allow relating their unique characteristics to the spatial variations in the predicted growth over time. We then examined the average predicted growth and its slope throughout the study period in relation to the unique structural characteristics associated with each exemplar, taking into account the available knowledge on the structural development of boreal stands in this region. To allow for better visualization of the spatial distribution of the forest type exemplars within the study area, we imposed a 500-m tessellation over the initial 20-m cells in order to display the most frequently occurring structural exemplar within each 500-m cell.
The best-performing model of net basal area growth rate, identified after the model-selection procedure conducted on the training data set ( n = 120), included the slope of the TCW index and the initial value of both the TCW and NBR indices as predictors ( Table 3 ). The slope of the TCW was the single predictor most strongly correlated to the net growth rate. The first-ranked model clearly stood out from the other candidates, with a model likelihood of 1 and a marked difference in AICc with the second-ranked. The spatial autocorrelation in the model residuals was not statistically significant (Moran’s I = 0.042, P -value = .12), therefore we did not integrate a spatial autocorrelation structure in the OLS model. The range of growth rates and predictor values was similar between the training and validation data sets ( Table 4 ).
Ranking of the candidate models for the prediction of the net growth rate.
AICc is the Akaike information criterion, corrected for small sample sizes, with deltaAICc (Δi), model likelihood (Mk), and AICc weight (Wt i ). The best-performing model is indicated in bold characters.
Values of the predictor and response variables from plots included in the training and validation data sets as well as across the entire model application area, considering all 26 moving windows.
The annual net growth rates reported for training and validation plots are observed growth rates, whilst growth rates reported for the model application area are predicted values.
The final model had an R 2 = 0.61 ( P < .01, RMSE = 0.23 m 2 ha −1 yr −1 ) with an R 2 = 0.58 ( P < .01, RMSE = 0.25 m 2 ha −1 yr −1 ) on the validation data ( n = 60, Fig. 2 ). The R 2 and RMSE (0.61, 25.20 m 2 ha −1 yr −1 ) obtained from the k -fold spatial cross-validation were similar. The median overall predicted growth rate for the PSPs included in the validation data set was 0.46 m 2 ha −1 yr −1 (standard deviation = 0.27 m 2 ha −1 yr −1 ) compared to 0.47 m 2 ha −1 yr −1 (0.32 m 2 ha −1 yr −1 ) calculated from the PSP data.

Actual vs predicted net growth rate (m 2 ha −1 yr −1 ) of the PSPs included in the validation data set.
Figure 3 shows the effects of the predictors included in the best-ranked model. An increase in the slope of the TCW led to an increase in the predicted net growth rate. A lower initial TCW value resulted in a higher predicted growth than for stands with a higher TCW initial value. The opposite relationship can be observed for NBR, for which a low initial value results in a lower predicted growth. The greatest predicted net growth occurs for pixels showing a highly positive TCW slope, a low initial TCW value, and a high initial NBR value.

Model predictions and unconditional 95% confidence intervals for the best-fit model parameters.
Growth rate from 1984 to 2018 over the entire study area
The application of the model over a total area of 185 939 ha revealed temporal and spatial variability in the predicted growth rates. Between 1984 and 2018, the median predicted net growth rate was 0.76 m 2 ha −1 yr −1 , with a standard deviation of 0.09 m 2 ha −1 yr −1 . There was a considerable range in predictions with a 5th percentile at 0.35 m 2 ha −1 yr −1 and a 95th percentile at 1.31 m 2 ha −1 yr −1 ( Figs 4a and 6a ). This was greater than the median net growth rate measured in the training and validation PSPs (0.47 m 2 ha −1 yr −1 . standard deviation = 0.38 m 2 ha −1 yr −1 ), but within the range of the growth rates measured in the PSPs, with minimum and maximum values of −0.39 and 1.90 m 2 ha −1 yr −1 , respectively ( Table 4 ). The median predicted growth rates were highly variable across the study site, as shown in Figure 6 .

(a) Distribution of the predicted growth rate (m 2 ha −1 yr −1 ) over the study area from 1984 to 2018 obtained by averaging the predictions computed on 26 10-year moving windows. Vertical dotted line indicates the median. (b) Evolution of the average predicted growth from 1984 to 2018 for the 26 10-year moving windows. The shaded region indicates the standard deviation.
Decomposing the time series in 10-year moving windows allowed for visualizing the evolution of the predicted growth rate over time ( Figs 4b and 6b ). In most of the model application area, predictions were made within the range of predictor values extracted over the plots included in the training data set. The range of predictor values across the entire model application area are presented in Table 4 . Since the growth rate statistics presented in Table 4 are derived from predictions across all 26 moving windows, they differ from the values averaged for the period 1984–2018.
Whilst the median net growth rate reached 0.92 m 2 ha −1 yr −1 for the 1984–93 period, it was considerably lower at the end of the study period, with a median predicted net growth of only 0.61 m 2 ha −1 yr −1 for the 2009–18 period, the lowest observed of all 26 moving windows. The highest median predicted growth was in the 1985–94 period (0.93 m 2 ha −1 yr −1 ). Between 1984 and 2018, the predicted growth averaged across the entire study site showed a significant negative linear trend ( P = .017), decreasing by an average of 0.007 m 2 ha −1 yr −1 from one moving window to the next.
The net growth rate also tended to decrease over time in the PSPs, with an average change of −0.048 m 2 ha −1 yr −1 (standard deviation = 0.59 m 2 ha −1 yr −1 ) between consecutive measurement intervals, although the growth rate increased over time in 41% of the plots ( Fig. 5 ). The median annual net growth rate observed in PSPs measured at least three times between 1992 and 2019 was 0.43 m 2 ha −1 yr −1 . This is slightly lower than the median growth rate measured in the selection of PSPs used for model development and validation (0.47 m 2 ha −1 yr −1 ), which also included plots measured only twice during the study period, but excluded plots that were located < 80 m away from roads, waterbodies, and stand boundaries.

Distribution of the plot-averaged changes in net growth rate (m 2 ha −1 yr −1 ) measured in the permanent sample plots located within the RMF between 1992 and 2019. Dotted line indicates the average.

(a) Predicted growth rate (m 2 ha −1 yr −1 ) over the study area from 1984 to 2018 obtained by averaging predictions computed on 26 10-year moving windows. (b) Predicted growth rate (m 2 ha −1 yr −1 ) over the study area from 1984 to 2018 decomposed in 26 10-year moving windows.
The eight forest-type exemplars identified after the clustering procedure were characterized by distinct structural and compositional attributes, described in Fig. 7 and Table 5 . These attributes are representative of the various development stages expected in boreal forests of this region ( Oliver and Larson 1996 ). The average predicted growth rate calculated over the entire study period was significantly different between all exemplars ( P < .001) and ranged from 0.60 to 0.90 m 2 ha −1 yr −1 (Table 6).
Average structural attributes, predicted growth rates, and slopes of the growth trend for the eight forest-type exemplars.
Values in parentheses indicate standard deviation. Asterisks indicate the level of significance of the linear slope of the growth time series calculated over the 26 10-year moving windows ( * * * 0.001, * * 0.01, * 0.05).

Characteristics of the eight forest-type exemplars. The first column shows the main structural parameters, extracted from the forest attributes layer generated using SPL data. The second column shows the normalized SPL profiles illustrating the typical forest structure associated with each of the forest-type exemplars. Each profile has a width of 10 m and a length of 50 m. The third column shows the model-predicted growth of each forest-type exemplar from 1984 to 2018, calculated over 26 10-year moving windows. The exemplars are presented in decreasing order of average predicted growth during the study period. The number on the left indicated the cluster number used in the text.
Exemplars 1, 2, and 4 were characterized by high mean growth rates over the entire study period, but the most pronounced and statistically significant negative growth trends amongst all exemplars ( Table 5 ). Exemplar 1, dominated by black spruce, was the fastest growing and also the most common, accounting for 21.4% (39 715.6 ha) of the area included in the analysis. Conversely, Exemplar 4, dominated by jack pine, was the least spatially extensive exemplar in the study area, accounting for only 9054.5 ha (4.9%). These two exemplars were characterized by the highest stem density values and high canopy cover, but low canopy complexity, as indicated by their low rumple index values. Representative of stands dominated by black spruce, Exemplar 2 accounted for 13.0% (25 151.5 ha) of the study area. This exemplar was characterized by very low height and DBH, average density and canopy cover values, and the second-highest TWI value. Accounting for almost 40% of the study area, Exemplars 1, 2, and 4 were mainly concentrated in the western and south-eastern regions of the study area ( Fig. 8 ). Their rapid growth at the beginning of the study period is followed by a gradual decrease, which is readily visible in Fig. 7 .

Location of the forest-type exemplars within the study site. For visualization, the 20-m pixels were aggregated into 500-m pixels showing the dominant forest-type exemplar.
Exemplars 3, 5, and 8 also showed significantly negative growth trends throughout the study period, although less pronounced than those of Exemplars 1, 2, and 4 ( Table 5 , Fig. 7 ). These exemplars had substantial differences in their mean growth rate during the study period. Dominated by black spruce, Exemplars 3 and 5 accounted for 14.7% (27 280.4 ha) and 15.4% (28 679.4 ha) of the area included in the study, respectively, and were relatively evenly distributed within the study area ( Fig. 8 ). They had average values of stem density, DBH, height, and rumple index, but a relatively high canopy cover, with Exemplar 3 being the one with the highest values for all structural attributes amongst both. Exemplar 8 was dominated by jack pine and occupied only 5.9% (11 003.5 ha) of the study area and was mostly concentrated in the eastern part of the study area ( Fig. 8 ). Exemplar 8 had the lowest average growth rate over the study period amongst all exemplars and had high rumple index, height, and DBH values, but a low stem density and canopy cover. The structural attributes of Exemplar 6 were similar to that of Exemplar 8, but Exemplar 6 was dominated by black spruce. Exemplar 6 occupied 11.2% (20 897.1 ha) of the area included in the analysis, showed a higher predicted growth than Exemplar 8 and did not show either a positive or negative trend in growth over the study period. Finally, Exemplar 7 was dominated by black spruce and accounted for 13.5% (25 157.0 ha) of the study area. This exemplar had the second-lowest average predicted growth, but did not show a significant trend in growth between 1984 and 2018. Exemplar 7 was characterized by a low stem density and the lowest canopy coverage, DBH, and height values, but showed a high rumple index value. This exemplar was concentrated on the northernmost part of the RMF ( Fig. 8 ) making it easily noticeable in Fig. 7 , since it displayed a slow growth rate throughout the entire 1984–2018 period.
In this study, we demonstrate how Landsat time series can be used to generate spatially explicit information on coniferous-dominated boreal forest net growth rate (annual basal area increment) in the absence of stand-replacing disturbances. Furthermore, airborne LiDAR data were used to indicate how predictions of growth rates are representative of differences in stand development stages, species composition, and structural attributes.
The field-calibrated and validated model provides wall-to-wall predictions of average annual net basal area growth rate over the past 36 years for undisturbed forests within a large forest management unit, which is critical information for silvicultural decision-making ( Forrester 2019 ). The developed net growth rate predictive model explained a substantial proportion of the variance in net growth rates measured from field data (58%, Fig. 2 ).
Amongst the tested predictors, the Theil Sen slope of the TCW is the best linked to net growth rate. The TCW is known to be correlated to various forest structural attributes such as average stand DBH, height, crown diameter, density, and basal area, making it a reliable indicator of stand development stages in closed canopy forests ( Cohen et al. 1995 ; Cohen and Spies 1992 ; Hansen et al. 2001 ). Landsat-derived TCW has been successfully used to detect forest growth and decline ( Czerwinski et al. 2014 ), and to map forest structural attributes and aboveground biomass ( Bolton et al. 2020 ; Matasci et al. 2018 ; Zald et al. 2016 ). These results confirm that spectral indices utilizing shortwave-infrared reflectance are the most appropriate Landsat-derived vegetation indices to monitor forest structural development through time. The poor correlation of NDVI with net growth brings additional evidence of the limited capacity of this index to capture processes of forest growth and decline in high canopy cover forest ( Fiore et al. 2020 ; Sulla-Menashe et al. 2018 ).
The initial condition of the forest was important in predicting subsequent growth. The first-ranked model included the initial value of both the TCW and the NBR. Indicative of earlier stages of stand development, low initial TCW values are associated with higher growth rates in the following period. This is consistent with the typical evolution of growth rates in boreal forests, being faster at early stages of development and gradually slowing down once canopy closure is reached ( Chen and Popadiouk 2002 ; Harper et al. 2005 ). Interestingly, lower initial NBR values are linked to subsequent slower growth. Low NBR values are associated with a higher proportion of non-photosynthetically active vegetation or more exposed soil when compared to areas with higher NBR values ( Asner 1998 ; Clevers 1988 ). In undisturbed forests, a low initial NBR may therefore suggest a sparse, less productive site, or stands that have previously experienced stress or mortality and are more likely to grow slowly in the future.
Changes in growth rate over the study period
The application of the predictive model on 10-year moving windows allowed for the visualization of the spatial and temporal variability in growth rates within the study site between 1984 and 2018 ( Figs 4b and 6b ). Figure 6 highlights this variability; while some areas of the RMF showed a sustained growth rate across all moving windows, a gradual decline in the predicted growth rate is observed in some regions. The generalized growth decline revealed by model predictions during the investigated period ( Fig. 4b ), corroborated by the decline in growth rates observed in the permanent sample plots located within the study site ( Fig. 5 ) is mainly attributable to an age-related decrease in growth rates. The analysis of growth trends was conducted exclusively on stands that were established before 1984, creating a bias due to the progressive reduction of the growth rate associated with stand age ( Chen et al. 2002 ). The high median growth rate observed in the first 10-year moving window (i.e. 1984–93) is related to the presence of young stands, regenerating consecutively to clear-cuts conducted from 1973 to 1983 on a total area of 8876 ha. The median growth rate predicted over this area went from 1.58 m 2 ha −1 yr −1 in the 1984–93 period, to only 0.73 m 2 ha −1 yr −1 in the 2009–18 period.
Fluctuations in the predicted growth rate within the study period may, however, be attributable to other factors, such as a growth response to environmental stressors. The predicted growth rate showed a substantial decrease in 1988–97 that lasted until the 1997–2006 period ( Fig. 4b ). In June, July, and August 2001, abnormally low amounts of precipitation accompanied by higher than normal temperatures resulted in 110 306 ha of visible drought damage in the Timmins District in Ontario, where our study site is located ( MNRF 2001 ). Examination of the values of the standardized precipitation evapotranspiration index (SPEI, Vicente-Serrano et al. 2010 ) during the study period also revealed abnormally low values in 1997, 2005, and 2011, which could have also impacted forest growth.
A widespread increase in mortality rates was observed in Canadian boreal forests between 1963 and 2008. Jack pine and black spruce, the most abundant species in our study area, both showed a significant increase in mortality rates during this period ( Peng et al. 2011 ). The mortality rates of these species were significantly and positively correlated to temperature, which increased continuously at our study site during the same period. Mean annual temperatures over the RMF, modelled using BioSim (Version 11; Régnière et al. 2017 ) followed a significantly increasing trend ( P = .01) between 1970 and 2018 with an average annual increase of 0.02°C. Marchand et al. (2019) also detected negative growth trends in black spruce and jack pine tree rings at several locations in the neighbouring province of Quebec between 1970 and 2005. The most widespread explanation for these growth declines in the boreal forest is related to an increase in temperatures that would cause some boreal stands to shift from temperature- to moisture-limited growth ( Chagnon et al. 2022 ; D’Orangeville et al. 2018 ; Girardin et al. 2016b ; Peng et al. 2011 ). Although small to moderate increases in temperature are expected to have positive effects on the growth of boreal species, higher increases in temperature that are not accompanied by higher amounts of precipitation can have detrimental effects, especially in sites were topography or a coarser deposit makes the water availability low ( Marchand et al. 2019 ). Soil water availability was found to be significantly correlated to the growth of black spruce forests in many regions, and is expected to become an increasingly important driver of productivity in the boreal zone ( Chagnon et al. 2022 ; Girardin et al. 2016b ; Peng et al. 2011 ). On the drier sites, jack pines would also be more likely to die prematurely, because of the species’ small physiological margin of safety from hydraulic failure during drought ( Mamet et al. 2015 ). The vulnerability of this species to pathogens has also been found to be higher on sites with low available water-holding capacities, by influencing carbon mobilization, allocation, and utilization for defence responses ( Hussain et al. 2020 ).
Growth trends in relation to forest structural attributes and composition
The model-predicted growth of the identified forest-type exemplars is consistent with previous research on the structural development of boreal stands in the same region ( Harper et al. 2005 ; Smirnova et al. 2008 ). The statistically significant decreasing slope of the predicted growth for Exemplars 1, 2, and 4 suggest that these stands are at an early stage of development. This is supported by the rumple index which is the lowest amongst all exemplars, indicating relatively homogeneous canopy surfaces and low structural complexity ( Kane et al. 2010 ). The high stem density and canopy cover of Exemplars 1 and 4 are typical of the transition from the stand initiation stage to the stem exclusion stage, where trees that established successfully and grew rapidly in open conditions are now occupying most of the available growing space, reaching canopy closure ( Harper et al. 2005 ; Oliver and Larson 1996 ; Smirnova et al. 2008 ). The moment at which trees start experiencing competition for light and other resources occurs faster in jack pine dominated stands than in black spruce dominated stands because of faster juvenile growth rates ( Harper et al. 2003 ). The jack pine-dominated Exemplar 4 indeed shows the highest predicted growth rate during the 1984–93 period (1.17 m 2 ha −1 yr −1 ), followed by the black spruce-dominated Exemplar 1 (1.09 m 2 ha −1 yr −1 ). Compared to the latter, Exemplar 2, which was also dominated by black spruce, has a lower density, canopy coverage and the lowest height, typical of the stand initiation stage or to forests developing on low productivity sites ( Harper et al. 2005 ; Oliver and Larson 1996 ). This exemplar had the second highest TWI value, indicative of hydric conditions. Development of boreal stands on organic and hydric sites occurs on a considerably longer timescale than on mesic sites and do not necessarily follow the development stages expected in managed boreal forests ( Oliver and Larson 1996 ), since they may never reach full canopy closure and experience self-thinning due to competition ( Harper et al. 2005 ). Although reliable information on stand age was not available over the entire study area, especially for older stands, data from the FRI on recent silvicultural interventions reveal that 82.4% of the area harvested from 1973 to 1983 (8876 ha) was classified either in Exemplars 1, 2, or 4, which is consistent with the observed patterns in model-predicted growth.
The structural attributes and growth patterns of Exemplars 3 and 5 suggest they have reached the stem exclusion stage ( Harper et al. 2005 ; Oliver and Larson 1996 ). The progressive decrease in growth rates associated with these exemplars, as indicated by a significant decreasing trend in the predicted growth, was less substantial than in the case of Exemplars 1, 2, and 4. Their lower stem density values, but relatively high height, DBH, and rumple index values are indicative of stands that entered the stem exclusion stage, where trees start experiencing the consequences of competition, leading to the mortality of trees with a less favourable position in the canopy, associated with a progressive reduction in stem density and growth at the stand level ( Oliver and Larson 1996 ). Differences in the predicted growth between these two exemplars may be attributable to differences in age or site productivity.
Exemplars 6, 7, and 8 have the lowest average predicted growth rate over the study period, consistent with their structural attributes that are indicative of older stands. These exemplars have the highest rumple index values, indicative of complex canopy surfaces, high DBH values, and low stem density. The low stem density of Exemplars 6 and 8 are likely due to a pronounced self-thinning due to mortality from competition, environmental, or age-related factors typical of the understory reinitiation stage ( Oliver and Larson 1996 ). Exemplar 7 is probably representative of an alternative stand development trajectory; with the highest TWI value, the lowest canopy coverage, and the third-lowest height, it possesses the characteristics of old black spruce stands located on hydric sites ( Harper et al. 2005 ). On these less productive sites, stands may never reach canopy closure and are growing slowly, but continuously for a period that can last until 200–250 years of age, whilst growth reaches a peak and decreases considerably earlier on more productive sites ( Harper et al. 2003 ). This is supported by a lack of a significant trend in the predicted growth of Exemplar 7 over the 26 moving windows. Conversely, Exemplar 8 showed the lowest average growth rate and a significant reduction in the predicted growth rate throughout the study period. This exemplar, characterized by the lowest TWI value, may be representative of the succession pathway of stands with high jack pine component on coarse deposits, where growth is sustained in the early development stages, but decreases more rapidly than black spruce dominated stands ( Harper et al. 2005 ). Because of their shorter longevity (i.e. 70–100 years), jack pine trees will eventually disappear, leaving space for the more shade tolerant black spruce and leading to an eventual decrease in forest canopy coverage and height ( Harper et al. 2002 ; Smirnova et al. 2008 ).
Implications for the management of boreal stands
The growth of boreal stands is influenced by numerous internal and external factors, such as stand age, structural attributes, and species composition, as well as site characteristics such as topography and surface deposits. For instance, a decline in growth rate is expected to occur earlier on productive sites dominated by jack pine than on less productive sites dominated by black spruce ( Harper et al. 2003 ). Changes in the growth rate can also happen in response to physiological stresses, caused notably by limited water availability due to topography or the nature of surficial deposits ( Johnstone et al. 2010 ; Marchand et al. 2019 ).
The availability of high-resolution, spatially explicit information on forest growth rates and the evolution of the growth rate over time offer the possibility of applying more precise and agile silviculture practices by discriminating actively growing stands from those that are stagnating or declining at the scale of a management unit. This information is crucial for efficient forest management ( Forrester 2019 ), but can be challenging to obtain using traditional inventory approaches that provide only a partial and intermittent picture of forest growth, since ground plots are spatially and temporally constrained, i.e. limited in number and measured only periodically ( Bowman et al. 2013 ; Gillis et al. 2005 ).
By combining information on forest stand structure derived from LiDAR data with growth information derived from Landsat time series, targeted silvicultural interventions could be carried out to stimulate the growth of stagnating stands, promote the resistance and resilience of vulnerable stands, or determine the best time for harvesting. Studying past growth rates and linking those with stand structure could enable the development of a library of representative growth curves for specific sites and species, thereby improving yield predictions ( Boisvenue and White 2019 ).
Limitations and future work
The approach developed in the current study is specifically designed for monitoring of gradual changes in undisturbed forests. A substantial proportion of boreal stands in Canada however will likely experience some form of disturbances be it from natural disturbances or silvicultural treatments. The relationships between the effect of these events on stand-level growth dynamics, structural attributes, and canopy spectral reflectance are complex and highly variable depending on the disturbance agent, making model-predicted growth rates more complex in this context. To be broadly applicable to boreal forests of Canada, the approach proposed in this study could be integrated within a continuous inventory framework, in which change detection algorithms are used to detect disturbances and stand attributes are updated accordingly (e.g. Coops et al. 2023 ). Combining these approaches would enable spatial and temporal delineation of the areas and periods for which model predictions could be generated reliably. Other studies have used similar approaches to exclude disturbed areas from productivity assessments ( Moan et al. 2023 ).
In the current study, growth rate predictions were generated using constant length, 10-year moving windows, which corresponds to the median interval between field measurements of the plots used for model development and validation. However, the interval between field measurements varied widely, ranging from 5 to 24 years. Despite such a large range, we could not find any patterns in the magnitude of errors in model predictions as a function of the length of the time between field measurements. Calculation of the predictions on shorter or longer intervals may, however, have produced slightly different results. The optimal window length should be investigated further and taken into account in the future development and application of such models, since related studies have concluded to the importance of this factor ( Bolton et al. 2020 ).
It is likely that the drought events and the continual rise in mean temperatures observed in the study area contributed to changes in moisture regimes, which played a role in the fluctuation of forest growth rates. The number of forest-type exemplars presented in this study was determined arbitrarily and do not likely encompass all of the variability in site conditions, forest structure, composition, and associated growth patterns within the study area. The clustering method employed focused exclusively on undisturbed forests and resulted in a fixed number of classes, which could have been expanded to capture more variability in growth conditions. Each forest-type exemplar represented between 9054 and 39 715 ha of forest land and did not consider differences in site conditions or stand age, both of which are important factors in growth response to environmental conditions. Accounting for these factors could have revealed more pronounced differences between forest types. As a result, the diversity in observed growth patterns was limited, with many exemplars showing trends that were similar to that of the entire forest management unit. Additionally, most exemplars exhibited large within-class variability in predicted growth rates, likely due to differences in site conditions, as evidenced by the high standard deviation of the TWI values within each exemplar. Nonetheless, differences in the structural and compositional attributes of the exemplars were sufficient to reveal dissimilarities in the average predicted growth and growth trends throughout the study period.
Future research will focus on evaluating the model's ability to capture differences in predicted growth rates amongst sites with varying levels of sensitivity to climate stressors using climate data and field-collected soil and growth data. This capacity to retrospectively assess the spatial manifestations of climate stressors on forest growth may also help inform the development of climate sensitive growth and yield models.
In the face of increasing uncertainty surrounding future growth and forest condition under changing environmental conditions, responsible and adapted forest management practices require the development of methods that enable spatially explicit monitoring of forest growth rates and their evolution over time. Our study demonstrates that a field-calibrated and -validated model, developed using Landsat time series, can predict the annual net basal area growth rate for undisturbed forests and reveal differences in growth rates that are consistent with stand development stage and species composition, as determined using airborne LiDAR and Sentinel-2 data. Linking a forest stand’s structural attributes with the evolution of growth over time has the potential to address several current challenges in the forest management domain. The proposed approach could eventually be used to create baseline growth trajectories for various forest types on different site conditions that could then be integrated into a near-real-time forest inventory framework, allowing for the updating of forest attribute layers in undisturbed forests. The information generated by this approach can improve yield predictions, optimize rotation lengths, and identify target areas where silvicultural interventions aimed at maintaining or enhancing growth could be conducted.
We would like to acknowledge our collaborators from the Ministry of Natural Resources and Forestry for sharing data from the Growth and Yield and Vegetation Sampling Network databases, and give special thanks to Dave Morris, Todd Little and Muhammad Waseem Ashiq for their help. We would also like to thank Chris McDonell from GreenFirst Forest Products and Grant McCartney from Forsite Consultants for their help in accessing and assembling the data required for this study.
None declared.
The NSERC Alliance project Silva21 (NSERC ALLRP 556265-20 to A.A.); the NSERC Alexander Graham Bell Graduate Scholarships-Doctoral Program (CGS-D to A.M.B.).
The data underlying this article were provided by the Ontario Ministry of Natural Resources and Forestry. Data will be shared on reasonable request to the corresponding author with permission of the Ontario Ministry of Natural Resources and Forestry.
Abdi H , Williams LJ . Principal component analysis . Wiley Interdiscip Rev Comput Stat 2010 ; 2 : 433 – 59 . https://doi.org/10.1002/wics.101 .
Google Scholar
Achim A , Moreau G , Coops NC . et al. The changing culture of silviculture . Forestry 2022 ; 95 : 143 – 52 . https://doi.org/10.1093/forestry/cpab047 .
Asner GP . Biophysical and biochemical sources of variability in canopy reflectance . Remote Sens Environ 1998 ; 64 : 234 – 53 . https://doi.org/10.1016/S0034-4257(98)00014-5 .
Baltsavias EP . Airborne laser scanning: basic relations and formulas . ISPRS J Photogramm Remote Sens 1999 ; 54 : 199 – 214 . https://doi.org/10.1016/S0924-2716(99)00015-5 .
Banskota A , Kayastha N , Falkowski MJ . et al. Forest monitoring using Landsat time series data: a review . Can J Remote Sens 2014 ; 40 : 362 – 84 . https://doi.org/10.1080/07038992.2014.987376 .
Beck PSA , Goetz SJ . Satellite observations of high northern latitude vegetation productivity changes between 1982 and 2008: ecological variability and regional differences . Environ Res Lett 2011 ; 6 :045501. https://doi.org/10.1088/1748-9326/6/4/045501 .
Beven KJ , Kirkby MJ . A physically based, variable contributing area model of basin hydrology/Un modèle à base physique de zone d’appel variable de l’hydrologie du bassin versant . Hydrol Sci J 1979 ; 24 : 43 – 69 . https://doi.org/10.1080/02626667909491834 .
Bilyk A , Pulkki R , Shahi C . et al. Development of the Ontario Forest Resources Inventory: a historical review . Can J For Res 2021 ; 51 : 198 – 209 . https://doi.org/10.1139/cjfr-2020-0234 .
Boisvenue C , White JC . Information needs of next-generation forest carbon models: opportunities for remote sensing science . Remote Sens 2019 ; 11 :463. https://doi.org/10.3390/rs11040463 .
Bolton DK , Tompalski P , Coops NC . et al. Optimizing Landsat time series length for regional mapping of LiDAR -derived forest structure . Remote Sens Environ 2020 ; 239 :111645. https://doi.org/10.1016/j.rse.2020.111645 .
Bowman DMJS , Brienen RJW , Gloor E . et al. Detecting trends in tree growth: not so simple . Trends Plant Sci 2013 ; 18 : 11 – 7 . https://doi.org/10.1016/j.tplants.2012.08.005 .
Brandt JP , Flannigan MD , Maynard DG . et al. An introduction to Canada’s boreal zone: ecosystem processes, health, sustainability, and environmental issues . Environ Rev 2013 ; 21 : 207 – 26 . https://doi.org/10.1139/er-2013-0040 .
Brecka AFJ , Shahi C , Chen HYH . Climate change impacts on boreal forest timber supply . Forest Policy Econ 2018 ; 92 : 11 – 21 . https://doi.org/10.1016/j.forpol.2018.03.010 .
Celik T . Unsupervised change detection in satellite images using principal component analysis and $k$-means clustering . IEEE Geosci Remote Sens Lett 2009 ; 6 : 772 – 6 . https://doi.org/10.1109/LGRS.2009.2025059 .
Chagnon C , Wotherspoon AR , Achim A . Deciphering the black spruce response to climate variation across eastern Canada using a meta-analysis approach . For Ecol Manage 2022 ; 520 :120375. https://doi.org/10.1016/j.foreco.2022.120375 .
Chen HY , Popadiouk RV . Dynamics of North American boreal mixedwoods . Environ Rev 2002 ; 10 : 137 – 66 . https://doi.org/10.1139/a02-007 .
Chen W , Chen JM , Price DT . et al. Effects of stand age on net primary productivity of boreal black spruce forests in Ontario, Canada . Can J For Res 2002 ; 32 : 833 – 42 . https://doi.org/10.1139/x01-165 .
Clevers JGPW . The derivation of a simplified reflectance model for the estimation of leaf area index . Remote Sens Environ 1988 ; 25 : 53 – 69 . https://doi.org/10.1016/0034-4257(88)90041-7 .
Cohen WB , Spies TA . Estimating structural attributes of Douglas-fir/western hemlock forest stands from Landsat and SPOT imagery . Remote Sens Environ 1992 ; 41 : 1 – 17 . https://doi.org/10.1016/0034-4257(92)90056-P .
Cohen WB , Spies TA , Fiorella M . Estimating the age and structure of forests in a multi-ownership landscape of western Oregon, U.S.A . Int J Remote Sens 1995 ; 16 : 721 – 46 . https://doi.org/10.1080/01431169508954436 .
Coops NC , Tompalski P , Goodbody TR. et al. Framework for near real-time forest inventory using multi source remote sensing data . Forestry 2023 ; 96 : 1 – 19 . https://doi.org/10.1093/forestry/cpac015 .
Crist EP . A TM tasseled cap equivalent transformation for reflectance factor data . Remote Sens Environ 1985 ; 17 : 301 – 6 . https://doi.org/10.1016/0034-4257(85)90102-6 .
Crist EP , Cicone RC . A physically-based transformation of thematic mapper data—the TM tasseled cap . IEEE Trans Geosci Remote Sens 1984 ; GE-22 : 256 – 63 . https://doi.org/10.1109/TGRS.1984.350619 .
Czerwinski CJ , King DJ , Mitchell SW . Mapping forest growth and decline in a temperate mixed forest using temporal trend analysis of Landsat imagery, 1987-2010 . Remote Sens Environ 2014 ; 141 : 188 – 200 . https://doi.org/10.1016/j.rse.2013.11.006 .
Dash B , Mishra D , Rath A . et al. A hybridized K-means clustering approach for high dimensional dataset . Int. J Eng Sci 2010 ; 2 :59–66. https://doi.org/10.4314/ijest.v2i2.59139 .
Dietrich R , Bell FW , Silva LCR . et al. Climatic sensitivity, water-use efficiency, and growth decline in boreal jack pine ( Pinus banksiana ) forests in Northern Ontario . J Geophys Res Biogeo 2016 ; 121 : 2761 – 74 . https://doi.org/10.1002/2016JG003440 .
D’Orangeville L , Houle D , Duchesne L . et al. Beneficial effects of climate warming on boreal tree growth may be transitory . Nat Commun 2018 ; 9 : 3213 . https://doi.org/10.1038/s41467-018-05705-4 .
Fiore NM , Goulden ML , Czimczik CI . et al. Do recent NDVI trends demonstrate boreal forest decline in Alaska? Environ Res Lett 2020 ; 15 :095007. https://doi.org/10.1088/1748-9326/ab9c4c .
Forrester DI . Linking forest growth with stand structure: tree size inequality, tree growth or resource partitioning and the asymmetry of competition . For Ecol Manage 2019 ; 447 : 139 – 57 . https://doi.org/10.1016/j.foreco.2019.05.053 .
Gardiner B , Moore J . Creating the wood supply of the future. In: Fenning T , ed. Challenges and Opportunities for the World’s Forests in the 21st Century . Netherlands : Springer , 2014 ; 677 – 704 .
Google Preview
Gauthier S , Bernier P , Kuuluvainen T . et al. Boreal forest health and global change . Science 2015 ; 349 : 819 – 22 . https://doi.org/10.1126/science.aaa9092 .
Gillis MD , Omule AY , Brierley T . Monitoring Canada’s forests: The National Forest Inventory . For Chron 2005 ; 81 : 214 – 21 . https://doi.org/10.5558/tfc81214-2 .
Girardin MP , Bouriaud O , Hogg EH . et al. No growth stimulation of Canada’s boreal forest under half-century of combined warming and CO 2 fertilization . Proc Natl Acad Sci U S A 2016a ; 113 : E8406 – 14 . https://doi.org/10.1073/pnas.1610156113 .
Girardin MP , Guo XJ , Bernier PY . et al. Changes in growth of pristine boreal North American forests from 1950 to 2005 driven by landscape demographics and species traits . Biogeosciences 2012 ; 9 : 2523 – 36 . https://doi.org/10.5194/bg-9-2523-2012 .
Girardin MP , Hogg EH , Bernier PY . et al. Negative impacts of high temperatures on growth of black spruce forests intensify with the anticipated climate warming . Glob Chang Biol 2016b ; 22 : 627 – 43 . https://doi.org/10.1111/gcb.13072 .
Gomez C , White J , Wulder M . et al. Integrated object-based spatiotemporal characterization of forest change from an annual time series of Landsat image composites . Can J Remote Sens 2015 ; 41 : 271 – 92 . https://doi.org/10.1080/07038992.2015.1089162 .
Hansen MJ , Franklin SE , Woudsma C . et al. Forest structure classification in the North Columbia mountains using the Landsat TM tasseled cap wetness component . Can J Remote Sens 2001 ; 27 : 20 – 32 . https://doi.org/10.1080/07038992.2001.10854916 .
Harper K , Bergeron Y , Drapeau P . et al. Structural development following fire in black spruce boreal forest . For Ecol Manage 2005 ; 206 : 293 – 306 . https://doi.org/10.1016/j.foreco.2004.11.008 .
Harper K , Bergeron Y , Gauthier S . et al. Post-fire development of canopy structure and composition in black spruce forests of Abitibi, Québec: a landscape scale study . Silva Fenn 2002 ; 36 :249–263. https://doi.org/10.14214/sf.561 .
Harper K , Boudreault C , DeGrandpré L . et al. Structure, composition, and diversity of old-growth black spruce boreal forest of the Clay Belt region in Quebec and Ontario . Environ Rev 2003 ; 11 : S79 – 98 . https://doi.org/10.1139/a03-013 .
Hartigan JA , Wong MA . et al. A k-means clustering algorithm . Appl Stat 1979 ; 28 : 100 – 8 . https://doi.org/10.2307/2346830 .
Hastie T , Tibshirani R , Friedman JH . et al. The Elements of Statistical Learning: Data Mining, Inference, and Prediction Vol 2 . New York, New York: Springer , 2009 .
Hayden , J. Ontario Forest Growth and Yield Program Field Manual for Establishing and Measuring Permanent Sample Plots . Ministry of Natural Resources , 1995 . Sault Ste. Marie, Ontario: Ontario Forest Research Institute. https://books.google.ca/books?id=gZKvAAAACAAJ .
Hermosilla T , Wulder MA , White JC . et al. An integrated Landsat time series protocol for change detection and generation of annual gap-free surface reflectance composites . Remote Sens Environ 2015 ; 158 : 220 – 34 . https://doi.org/10.1016/j.rse.2014.11.005 .
Hermosilla T , Wulder MA , White JC . et al. Mass data processing of time series Landsat imagery: pixels to data products for forest monitoring . Int J Digit Earth 2016 ; 9 : 1035 – 54 . https://doi.org/10.1080/17538947.2016.1187673 .
Huang J , Tardif JC , Bergeron Y . et al. Radial growth response of four dominant boreal tree species to climate along a latitudinal gradient in the eastern Canadian boreal forest . Glob Chang Biol 2010 ; 16 : 711 – 31 . https://doi.org/10.1111/j.1365-2486.2009.01990.x .
Hussain A , Classens G , Guevara-Rozo S . et al. Spatial variation in soil available water holding capacity alters carbon mobilization and allocation to chemical defenses along jack pine stems . Environ Exp Bot 2020 ; 171 :103902. https://doi.org/10.1016/j.envexpbot.2019.103902 .
Johnstone JF , McIntire EJB , Pedersen EJ . et al. A sensitive slope: estimating landscape patterns of forest resilience in a changing climate . Ecosphere 2010 ; 1 : 1 – 21 . https://doi.org/10.1890/ES10-00102.1 .
Kane VR , Gillespie AR , McGaughey R . et al. Interpretation and topographic compensation of conifer canopy self-shadowing . Remote Sens Environ 2008 ; 112 : 3820 – 32 . https://doi.org/10.1016/j.rse.2008.06.001 .
Kane VR , McGaughey RJ , Bakker JD . et al. Comparisons between field- and LiDAR-based measures of stand structural complexity . Can J For Res 2010 ; 40 : 761 – 73 . https://doi.org/10.1139/X10-024 .
Key CH , Benson NC . Landscape assessment (LA) sampling and analysis methods. In: Lutes DC , Keane RE , Caratti JF . et al. , eds. FIREMON: Fire Effects Monitoring and Inventory System . Ogden, UT : USDA Forest Service, Rocky Mountain Research Station , 2006 .
Kurz WA , Stinson G , Rampley GJ . et al. Risk of natural disturbances makes future contribution of Canada’s forests to the global carbon cycle highly uncertain . PNAS 2008 ; 105 : 1551 – 5 . https://doi.org/10.1073/pnas.0708133105 .
Lefsky MA , Cohen WB , Parker GG . et al. LiDAR Remote Sensing for Ecosystem Studies: LiDAR, an emerging remote sensing technology that directly measures the three-dimensional distribution of plant canopies, can accurately estimate vegetation structural attributes and should be of particular interest to forest, landscape, and global ecologists . Bioscience 2002 ; 52 : 19 – 30 . https://doi.org/10.1641/0006-3568(2002)052[0019:LRSFES]2.0.CO;2 .
Mahony CR , Wang T , Hamann A . et al. A global climate model ensemble for downscaled monthly climate normals over North America . Int J Climatol 2022 ; 42 : 5871 – 91 . https://doi.org/10.1002/joc.7566 .
Mamet SD , Chun KP , Metsaranta JM . et al. Tree rings provide early warning signals of jack pine mortality across a moisture gradient in the southern boreal forest . Environ Res Lett 2015 ; 10 :084021. https://doi.org/10.1088/1748-9326/10/8/084021 .
Marchand W , Girardin MP , Hartmann H . et al. Taxonomy, together with ontogeny and growing conditions, drives needleleaf species’ sensitivity to climate in boreal North America . Glob Chang Biol 2019 ; 25 : 2793 – 809 . https://doi.org/10.1111/gcb.14665 .
Matasci G , Hermosilla T , Wulder MA . et al. Large-area mapping of Canadian boreal forest cover, height, biomass and other structural attributes using Landsat composites and LiDAR plots . Remote Sens Environ 2018 ; 209 : 90 – 106 . https://doi.org/10.1016/j.rse.2017.12.020 .
Mazerolle MJ. AICcmodavg: Model selection and multimodel inference based on (Q)AIC(c). R package version 2.3–1 . 2020 . https://cran.r-project.org/package=AICcmodavg .
McDowell NG , Allen CD , Anderson-Teixeira K . et al. Pervasive shifts in forest dynamics in a changing world . Science 2020 ; 368 :eaaz9463.
MNRF . Forest Health Conditions in Ontario 2001 . Ottawa, Ontario: Ministry of Northern Development, Mines, Natural Resources and Forestry , 2001 , 20 . https://www.ontario.ca/files/2022-07/mnrf-srb-forest-health-conditions-ontario-2021-en-2022-07-20.pdf .
MNRF . Growth and Yield Program - PSP and PGP Reference Manual . Ontario Ministry of Natural Resources and Forestry , 2016 ; 677 . Ottawa, Ontario.
Moan MÅ , Noordermeer L , White JC. et al. Detecting and excluding disturbed forest areas improves site index determination using bitemporal airborne laser scanner data . Forestry 2023 . https://doi.org/10.1093/forestry/cpad025 .
Moran PA . Notes on continuous stochastic phenomena . Biometrika 1950 ; 37 : 17 – 23 . https://doi.org/10.1093/biomet/37.1-2.17 .
Moreau G , Chagnon C , Achim A . et al. Opportunities and limitations of thinning to increase resistance and resilience of trees and forests to global change . Forestry 2022 ; 95 : 595 – 615 .
Oliver CD , Larson BC . Forest Stand Dynamics . New York : Wiley , 1996 .
Olofsson P , Foody GM , Herold M . et al. Good practices for estimating area and assessing accuracy of land change . Remote Sens Environ 2014 ; 148 : 42 – 57 . https://doi.org/10.1016/j.rse.2014.02.015 .
Pan Y , Birdsey RA , Fang J . et al. A large and persistent carbon sink in the world’s forests . Science 2011 ; 333 : 988 – 93 . https://doi.org/10.1126/science.1201609 .
Peng C , Ma Z , Lei X . et al. A drought-induced pervasive increase in tree mortality across Canada’s boreal forests . Nature Clim Change 2011 ; 1 : 467 – 71 . https://doi.org/10.1038/nclimate1293 .
Powell SL , Cohen WB , Healey SP . et al. Quantification of live aboveground forest biomass dynamics with Landsat time-series and field inventory data: a comparison of empirical modeling approaches . Remote Sens Environ 2010 ; 114 : 1053 – 68 . https://doi.org/10.1016/j.rse.2009.12.018 .
Queinnec M , Coops NC , White JC . et al. Mapping dominant boreal tree species groups by combining area-based and individual tree crown LiDAR metrics with Sentinel-2 data . Can J Remote Sens 2022a ; 49 : 1 – 19 .
Queinnec M , Coops NC , White JC . et al. Developing a forest inventory approach using airborne single photon LiDAR data: from ground plot selection to forest attribute prediction . Forestry 2022b ; 95 : 347 – 62 . https://doi.org/10.1093/forestry/cpab051 .
Régnière J , Saint-Amant R , Béchard A . et al. BioSIM 11–Manuel d’utilisation . Quebec, QC : Natural Resources Canada, Canadian Forest Services, Laurentian Forestry Center , 2017 .
Roberts DR , Bahn V , Ciuti S . et al. Cross-validation strategies for data with temporal, spatial, hierarchical, or phylogenetic structure . Ecography 2017 ; 40 : 913 – 29 . https://doi.org/10.1111/ecog.02881 .
Rogers BM , Solvik K , Hogg EH . et al. Detecting early warning signals of tree mortality in boreal North America using multiscale satellite data . Glob Change Biol 2018 ; 24 : 2284 – 304 . https://doi.org/10.1111/gcb.14107 .
Sánchez-Pinillos M , D’Orangeville L , Boulanger Y . et al. Sequential droughts: a silent trigger of boreal forest mortality . Glob Chang Biol 2022 ; 28 : 542 – 56 . https://doi.org/10.1111/gcb.15913 .
Seidl R , Schelhaas M-J , Rammer W . et al. Increasing forest disturbances in Europe and their impact on carbon storage . Nature Clim Change 2014 ; 4 : 806 – 10 . https://doi.org/10.1038/nclimate2318 .
Seidl R , Thom D , Kautz M . et al. Forest disturbances under climate change . Nature Clim Change 2017 ; 7 : 395 – 402 . https://doi.org/10.1038/nclimate3303 .
Sen PK . Estimates of the regression coefficient based on Kendall’s tau . J Am Stat Assoc 1968 ; 63 : 1379 – 89 . https://doi.org/10.1080/01621459.1968.10480934 .
Shapiro SS , Wilk MB . An analysis of variance test for normality (complete samples) . Biometrika 1965 ; 52 : 591 – 611 . https://doi.org/10.1093/biomet/52.3-4.591 .
Smirnova E , Bergeron Y , Brais S . Influence of fire intensity on structure and composition of jack pine stands in the boreal forest of Quebec: Live trees, understory vegetation and dead wood dynamics . For Ecol Manage 2008 ; 255 : 2916 – 27 . https://doi.org/10.1016/j.foreco.2008.01.071 .
Stehman S . Statistical Rigor and Practical Utility in Thematic Map Accuracy Assessment . Photogramm Eng Remote Sensing 2001 ; 67 : 727 – 34 .
Subedi N , Sharma M . Climate-diameter growth relationships of black spruce and jack pine trees in boreal Ontario, Canada . Glob Chang Biol 2013 ; 19 : 505 – 16 . https://doi.org/10.1111/gcb.12033 .
Sulla-Menashe D , Woodcock CE , Friedl MA . Canadian boreal forest greening and browning trends: an analysis of biogeographic patterns and the relative roles of disturbance versus climate drivers . Environ Res Lett 2018 ; 13 :014007. https://doi.org/10.1088/1748-9326/aa9b88 .
Theil H . A rank-invariant method of linear and polynomial regression analysis . Indag Math 1950 ; 12 : 173 .
Tucker CJ . Red and photographic infrared linear combinations for monitoring vegetation . Remote Sens Environ 1979 ; 8 : 127 – 50 . https://doi.org/10.1016/0034-4257(79)90013-0 .
Urquizo N , Bastedo J , Brydges T. et al. Ecological Assessment of the Boreal Shield Ecozone . Ottawa, Ontario: Environment Canada , 2000 , 90 . https://publications.gc.ca/collections/collection_2014/ec/En40-600-2000-eng.pdf .
Valavi R , Elith J , Lahoz-Monfort JJ . et al. blockCV: An r package for generating spatially or environmentally separated folds for k-fold cross-validation of species distribution models . Methods Ecol Evol 2018 ; 10 :225–232.
Verbyla AP . Modelling variance heterogeneity: residual maximum likelihood and diagnostics . J R Stat Soc B Methodol 1993 ; 55 : 493 – 508 .
Vicente-Serrano SM , Beguería S , López-Moreno JI . A multiscalar drought index sensitive to global warming: the standardized precipitation evapotranspiration index . J Climate 2010 ; 23 : 1696 – 718 . https://doi.org/10.1175/2009JCLI2909.1 .
Vogelmann JE , Gallant AL , Shi H . et al. Perspectives on monitoring gradual change across the continuity of Landsat sensors using time-series data . Remote Sens Environ 2016 ; 185 : 258 – 70 . https://doi.org/10.1016/j.rse.2016.02.060 .
Wang T , Hamann A , Spittlehouse D . et al. Locally downscaled and spatially customizable climate data for historical and future periods for North America . PloS One 2016 ; 11 :e0156720. https://doi.org/10.1371/journal.pone.0156720 .
Wester M , Henson B , Crins W . et al. The Ecosystems of Ontario, Part 2: Ecodistricts . Peterborough, Ontario: Science and Research Technical Report-Ontario Ministry of Natural Resources and Forestry, TR-26 , 2018 .
White JC , Wulder MA , Hermosilla T . et al. A nationwide annual characterization of 25 years of forest disturbance and recovery for Canada using Landsat time series . Remote Sens Environ 2017 ; 194 : 303 – 21 . https://doi.org/10.1016/j.rse.2017.03.035 .
White JC , Wulder MA , Hobart GW . et al. Pixel-based image compositing for large-area dense time series applications and science . Can J Remote Sens 2014 ; 40 : 192 – 212 . https://doi.org/10.1080/07038992.2014.945827 .
Wotherspoon , A. , Burnett , M. , Bernard , A. , Achim , A. , Coops , N. C. Climate Scenarios for Canadian Forests . 2022 . https://doi.org/10.13140/RG.2.2.18449.92009 .
Wulder MA , Hermosilla T , White JC . et al. Biomass status and dynamics over Canada’s forests: disentangling disturbed area from associated aboveground biomass consequences . Environ Res Lett 2020 ; 15 :094093. https://doi.org/10.1088/1748-9326/ab8b11 .
Wulder MA , Roy DP , Radeloff VC . et al. Fifty years of Landsat science and impacts . Remote Sens Environ 2022 ; 280 :113195. https://doi.org/10.1016/j.rse.2022.113195 .
Wulder MA , White JC , Nelson RF . et al. LiDAR sampling for large-area forest characterization: a review . Remote Sens Environ 2012 ; 121 : 196 – 209 . https://doi.org/10.1016/j.rse.2012.02.001 .
Zald HSJ , Wulder MA , White JC . et al. Integrating Landsat pixel composites and change metrics with LiDAR plots to predictively map forest structure and aboveground biomass in Saskatchewan, Canada . Remote Sens Environ 2016 ; 176 : 188 – 201 . https://doi.org/10.1016/j.rse.2016.01.015 .
Email alerts
Citing articles via.
- Recommend to your Library
Affiliations
- Online ISSN 1464-3626
- Print ISSN 0015-752X
- Copyright © 2024 Institute of Chartered Foresters
- About Oxford Academic
- Publish journals with us
- University press partners
- What we publish
- New features
- Open access
- Institutional account management
- Rights and permissions
- Get help with access
- Accessibility
- Advertising
- Media enquiries
- Oxford University Press
- Oxford Languages
- University of Oxford
Oxford University Press is a department of the University of Oxford. It furthers the University's objective of excellence in research, scholarship, and education by publishing worldwide
- Copyright © 2024 Oxford University Press
- Cookie settings
- Cookie policy
- Privacy policy
- Legal notice
This Feature Is Available To Subscribers Only
Sign In or Create an Account
This PDF is available to Subscribers Only
For full access to this pdf, sign in to an existing account, or purchase an annual subscription.
Management of Boreal Forests
Theories and Applications for Ecosystem Services
- © 2022
- Seppo Kellomäki 0
School of Forest Sciences, University of Eastern Finland, Joensuu, Finland
You can also search for this author in PubMed Google Scholar
- Excellent textbook for forestry students in Europe, North America and Asia
- Offers management strategies to counteract climate change effects on boreal forests
- Explains the succession dynamics of boreal forest ecosystem and consequences for its management
7478 Accesses
2 Citations
This is a preview of subscription content, log in via an institution to check access.
Access this book
- Available as EPUB and PDF
- Read on any device
- Instant download
- Own it forever
- Compact, lightweight edition
- Dispatched in 3 to 5 business days
- Free shipping worldwide - see info
- Durable hardcover edition
Tax calculation will be finalised at checkout
Other ways to access
Licence this eBook for your library
Institutional subscriptions
Table of contents (20 chapters)
Front matter, background: management of forest for varying ecosystem services.
Seppo Kellomäki
Forest Environment of Boreal North and Forestry
Environmental conditions, site types, and climate change, structure and functioning of selected boreal trees, selected tree species of importance in boreal north, structure of selected tree species, regeneration biology of selected tree species, physiology, growth, and acclimatizing of boreal tree species to climate, long-term dynamics of boreal forest ecosystem, successional dynamics of boreal forest ecosystem, management vs ecosystem dynamics, management strategies, regimes, and operations, preparation of site for natural regeneration and planting in reforestation, natural regeneration in management for regrowth, planting in management for regrowth, management of spacing in pre-commercial phase, management of spacing and thinning in commercial phase, fertilization in management of site fertility, pruning of branches and management of timber quality.
- Adaptation to climate change
- Boreal forest
- Climate change
- Ecosystem services
- Ecosystem goods
- Ecosystem dynamics
- Forest succession
- Forest management
About this book
This comprehensive textbook explores the boreal forests of Northern Europe, Finland, Sweden and Norway. Students will gain an overview of the forest ecosystem and the services it provides for modern society. From the production of timber, to the supply of food products or their use as a recreational space for human wellbeing – our forests serve many needs. Accordingly, the respective chapters cover various types of ecosystem service, e.g. supporting, provisioning, regulating and cultural services.
The book’s main focus is on the management of boreal forests for the production of these ecosystem services. Addressing modern challenges, e.g. managing vulnerable boreal forests for adaptation to climate change, is an important aspect throughout the volume. Traditional forest management has to adapt and evolve in order to meet the increasing risk of abiotic and biotic damages to our forest biomass. Future forestry graduates will have to face more and more of these challenges; consequently, the book provides them with a wealth of scientific knowhow and possible counter-strategies.
Forestry students in the Northern Hemisphere, be it in Europe, North America or Asia, will find this book an excellent reference guide. To make the content more accessible, it has been enriched with a clear structure, numerous illustrations and learning objectives.
Authors and Affiliations
About the author.
The research career of Prof. Seppo Kellomäki has spanned from early 1970s to today. His research activities deal mainly with structure and functioning of boreal forests and sustainable management of boreal forests for varying ecosystem goods and services. His early studies had the focus on multiple use of forest, and management of Scots pine forest for high-quality timber. However, his main contribution in forest science deals with climate change impacts on the dynamics of boreal forest ecosystem in the conditions where forests and forest-based industry are important for the welfare of society. In this context, his focus has been in experimental studies on how the elevating temperature, atmospheric carbon dioxide and changes in precipitation likely effect on the physiology and growth of boreal tree species. These studies have provided a solid bases for modelling. This has made it possible to understand how climate change likely effects on the long-term dynamics of boreal forest ecosystem and how climate change effects on the future forest management. The main questions have been how management should be used and developed for avoiding detrimental effects of successional dynamics and management of boreal forest ecosystem for different ecosystem services. Many of his research papers further address the problems on how forest management and forests should be adapted to climate warming in the boreal conditions, and how management might be used to mitigate warming.
Bibliographic Information
Book Title : Management of Boreal Forests
Book Subtitle : Theories and Applications for Ecosystem Services
Authors : Seppo Kellomäki
DOI : https://doi.org/10.1007/978-3-030-88024-8
Publisher : Springer Cham
eBook Packages : Biomedical and Life Sciences , Biomedical and Life Sciences (R0)
Copyright Information : The Editor(s) (if applicable) and The Author(s), under exclusive license to Springer Nature Switzerland AG 2022
Hardcover ISBN : 978-3-030-88023-1 Published: 02 March 2022
Softcover ISBN : 978-3-030-88026-2 Published: 03 March 2023
eBook ISBN : 978-3-030-88024-8 Published: 01 March 2022
Edition Number : 1
Number of Pages : XXIII, 717
Number of Illustrations : 41 b/w illustrations, 18 illustrations in colour
Topics : Forestry , Ecosystems
- Publish with us
Policies and ethics
- Find a journal
- Track your research
Language selection
- Français fr
Boreal forest research
Series of articles assesses the health of canada’s boreal zone.
How do human activities, natural disturbances and climate change affect the boreal forest? What impact do non-native species have on the boreal zone?
To answer these questions and others, researchers from the Canadian Forest Service of Natural Resources Canada have analyzed thousands of scientific articles and studies. The 11 papers below summarize the findings of the analyses and assess the current health of ecosystems in Canada’s boreal zone.
Synthesizing a large body of scientific literature into this series makes the research more accessible to policy-makers and resource managers and enables them to make better-informed decisions to ensure Canada’s boreal forest remains healthy and sustainable.
SEE ALSO: 8 facts about Canada’s boreal forest
11 articles that review and synthesize boreal forest research
The first paper summarizes the current state of Canada’s boreal zone and puts the remaining articles in context.
- An introduction to Canada’s boreal zone: Ecosystem processes, health, sustainability, and environmental issues (2013)
- Anticipating the consequences of climate change for Canada’s boreal forest ecosystems (2013)
- Canadian boreal forests and climate change mitigation (2013)
- Carbon in Canada’s boreal forest – A synthesis (2013)
- Climate change vulnerability and adaptation in the managed Canadian boreal forest (2014)
- Effects of natural resource development on the terrestrial biodiversity of Canadian boreal forests (2014)
- How do natural disturbances and human activities affect soils and tree nutrition and growth in the Canadian boreal forest? (2014)
- Impacts and prognosis of natural resource development on aquatic biodiversity in Canada’s boreal zone (2013)
- Non-native species in Canada’s boreal zone: diversity, impacts, and risk (2014)
- Protected areas in boreal Canada: A baseline and considerations for the continued development of a representative and effective reserve network (2014)
- Impacts and prognosis of natural resource development on water and wetlands in Canada’s boreal zone (2015)
Learn about sustainable forestry and Canada’s boreal forest
How sustainable forest management ensures that Canada’s forests are healthy, safe and thriving.
- Facts about Canada’s boreal forest
- The State of Canada’s Forests report
- Climate change and Canada’s forests
- 7 facts on deforestation and disturbances
- Deforestation in Canada: Key myths and facts
Page details
You are using an outdated browser. Upgrade your browser today or install Google Chrome Frame to better experience this site.
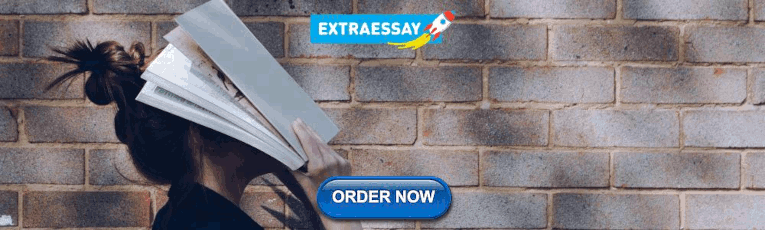
About IBFRA
About the boreal, the insight process, 60-second science, ibfra conference, the boreal forest.
The map is a hybrid product by IIASA © 2021, modified after Kraxner et al., 2017, Ogle et al., 2018 and NFIS Canada.
The circumboreal belt of boreal forest represents about 30% of the global forest area. Boreal forests provide critical services to local, regional, and global populations. Communities, including those of indigenous people, benefit from ecosystem services provided by the forest for fishing, hunting, leisure, spiritual activities, and economic opportunities. Internationally, more than 33% of the lumber and 25% of the paper on the export market originate from boreal regions. Globally, boreal forests help regulate climate through the exchange of energy and water. They are also a large reservoir of biogenic carbon.
- Open access
- Published: 11 May 2024
Conversion of boreal forests to agricultural systems: soil microbial responses along a land-conversion chronosequence
- Paul Benalcazar 1 ,
- Brent Seuradge 2 ,
- Amanda C. Diochon 3 ,
- Randall K. Kolka 4 &
- Lori A. Phillips 2
Environmental Microbiome volume 19 , Article number: 32 ( 2024 ) Cite this article
Metrics details
Boreal regions are warming at more than double the global average, creating opportunities for the northward expansion of agriculture. Expanding agricultural production in these regions will involve the conversion of boreal forests to agricultural fields, with cumulative impacts on soil microbial communities and associated biogeochemical cycling processes. Understanding the magnitude or rate of change that will occur with these biological processes will provide information that will enable these regions to be developed in a more sustainable manner, including managing carbon and nitrogen losses. This study, based in the southern boreal region of Canada where agricultural expansion has been occurring for decades, used a paired forest-adjacent agricultural field approach to quantify how soil microbial communities and functions were altered at three different stages post-conversion (< 10, > 10 and < 50, and > 50 years). Soil microbial functional capacity was assessed by quantitative PCR of genes associated with carbon (C), nitrogen, and phosphorous (P) cycling; microbial taxonomic diversity and community structure was assessed by amplicon sequencing.
Fungal alpha diversity did not change, but communities shifted from Basidiomycota to Ascomycota dominant within the first decade. Bacterial alpha diversity increased, with Gemmatimonadota groups generally increasing and Actinomycetota groups generally decreasing in agricultural soils. These altered communities led to altered functional capacity. Functional genes associated with nitrification and low molecular weight C cycling potential increased after conversion, while those associated with organic P mineralization potential decreased. Stable increases in most N cycling functions occurred within the first decade, but C cycling functions were still changing 50 years post conversion.
Conclusions
Microbial communities underwent a rapid shift in the first decade, followed by several decades of slower transition until stabilizing 50 years post conversion. Understanding how the microbial communities respond at different stages post-conversion improves our ability to predict C and N losses from emerging boreal agricultural systems, and provides insight into how best to manage these soils in a way that is sustainable at the local level and within a global context.
Introduction
Northern regions are warming at more than double the global average, with the growing season lengthening by an average of two days per decade since the 1950’s [ 1 ]. These climate change driven shifts in temperature and precipitation patterns are accelerating land use changes, including the northward expansion of agriculture [ 2 , 3 ]. Current models predict that, on average, 76% of boreal regions will have sufficient growing degree days to accommodate crop production systems by the end of the 21st century [ 2 ]. Expanding agricultural production in most of these regions will require the clearing of boreal forests, which typically involves clear-cutting trees then bulldozing and burning stumps and all other vegetation [ 1 , 4 ]. When forests are converted to cropping systems soil compaction, erosion, and salinization typically increase, and soil organic matter typically decreases [ 5 , 6 , 7 ]. In short, soils become degraded relative to their original state and their overall health and function is altered [ 8 ]. These cumulative impacts alter carbon (C), nitrogen (N), and phosphorus (P) cycling in the converted soils [ 9 , 10 , 11 ], including pathways associated with C- and N-based greenhouse gas (GHG) emissions [ 2 , 12 ]. The magnitude of change in these processes after conversion will be influenced by soil microbial community responses and, in particular, those groups that underpin biogeochemical cycling processes [ 13 , 14 , 15 ]. In order to develop northern regions in the most sustainable manner possible, we need a better understanding of the direction, rate, and extent of change that will occur with these functional groups after land conversion.
A global meta-analysis of forest disturbances found that land conversion from forest to agriculture decreased overall bacterial and fungal abundance, with total microbial carbon and nitrogen decreasing up to 56.7% and 54.5% (respectively), but increased both bacterial and fungal diversity [ 16 ]. Within this meta-analysis however, the only boreal site examined microbial responses to forest age and not to agricultural conversion [ 17 ]. A recent study in northern boreal regions in Canada [ 10 ] found that bacterial abundance was not impacted by land conversion to either grassland or cropland, while archaeal abundance increased and fungal abundance decreased. Regardless of the direction of change, any shift in microbial community structure will lead to altered microbial functionality, due in part to an altered abundance of taxa important for both C (e.g. Actinomycetota, Gemmatimonadota, Bacteroidota) and N cycling (e.g. Pseudomonadota, Bacillota, Nitrospirota) [ 7 , 18 , 19 ]. The Zhou et al. [ 16 ] meta-analysis found that forest conversion generally decreased the relative abundance of Actinomycetota, but overall impacts on Pseudomonadota and Bacteroidota varied according to the intensity of land use change.
The type of imposed agricultural management practice, in addition to land use change itself, will also alter microbial communities and their functional capacity [ 18 , 20 , 21 ]. The addition of organic amendments such as manures or composts may increase both fungal and bacterial abundance [ 18 , 21 , 22 ]. Increased inputs of mineral fertilizers however, may increase bacterial but not fungal growth, as was found with N and P inputs in European sub-arctic systems [ 23 , 24 ]. Shifts in bacterial populations under fertilization will be associated with direct changes in C and N cycling capacity [ 25 ]. When forests in Amazon regions were converted for agriculture, for example, microbial nitrification capacity increased by an order of magnitude [ 18 ]. Most, if not all, converted sites will also undergo tillage, which typically leads to increased rates of organic matter decomposition and subsequent nutrient release [ 26 ]. During forest conversion, the combination of tillage and generally altered carbon use efficiency associated with shifts in pH and nutrient balances [ 10 , 16 ] could accelerate total C loss rates. Conversely, N addition could increase microbial metabolic efficiency and promote the formation of stable mineral-associated soil organic carbon [ 9 , 10 , 27 ]. Although the overall outcome in any given region will be influenced by microbial communities and competition for available nutrients [ 24 ], both C and N loss rates are likely to be high in the initial years following conversion until soil communities equilibrate and a new stable state is reached [ 28 ].
Few studies have evaluated how microbial nutrient cycling processes are altered following land conversion in northern regions, in part because these types of land use changes were relatively rare in the past. Boreal forest soils in Alaska, USA [ 29 , 30 ], and Estonia [ 31 ] are known to have a high denitrifying capacity, which could be a significant N loss pathway following conversion. Seitz et al. [ 30 ] examined changes in diversity and associated functionality when vegetation and/or organic layers were removed from a permafrost site in Alaska. The researchers found that disturbance increased denitrification (nitrate reductase) and ligno-cellulose decomposition functions, and also increased the abundance of nitrifying taxa (Nitrosomonadaceae). Clearcutting in other northern forested systems has also been shown to increase nitrifier abundance [ 32 ], potentially due to a reduction in the deposition of volatile monoterpenes from conifers [ 33 ]. Boreal forest conversion for agricultural purposes, which will include both clear-cutting and the addition of fertilizer N, is likely to increase both nitrification and denitrification processes.
Climate change has expanded the northern boundaries of food production; however, a comprehensive understanding of how land conversion to agriculture in these regions will alter soil microbial communities and their biogeochemical cycling functions is currently incomplete and poorly understood [ 34 ]. Understanding how soil microbial systems are altered by land conversion at different stages post-conversion will provide information on how best to manage these expanding agricultural regions in a way that is sustainable at both the local level (e.g., enhanced fertility) and within a global context (e.g., reduced C and N losses). In this study, we investigated the soil microbiome at the current northern boundary of agriculture in Ontario Canada, using paired sites to compare baseline mature forest conditions with those of adjacent fields converted from forest to agriculture < 10 years ago, > 10 years ago but < 50 years ago, and > 50 years ago. Our objective was to evaluate post-land conversion changes in microbial functional potential, diversity, and community composition in a chronosequence according to time since conversion. We used qPCR to assess changes in microbial biogeochemical cycling functions (C, N, and P cycling) and amplicon sequencing to examine microbial community composition and diversity. We hypothesized that after land conversion (a) functions associated with N cycling would increase, (b) increased soil N and P fertility would lead to increased bacterial but decreased fungal abundance, and (c) overall diversity would increase due to a greater diversity of plants and plant litter inputs associated with agronomic cropping systems.
Materials and methods
Study sites and soil sampling.
Soil samples were collected from dairy farms in the Thunder Bay, Ontario region within the Murillo and Slate River area (45° 31’ to 48° 17’ N, 89° 30’ to 89° 22’ W; Supplementary Figure S1 ). The Murillo and Slate River regions were formed from water-laid alluvial silt, sand, and gravel deposits that vary depending on the basin and depth [ 35 ]. Thunder Bay is part of the ecoregion 3 W (Lake Nipigon), located on the Precambrian Shield with substantial basalt and volcanic rock formations with low to moderate buffering capacity [ 36 ]. These soils are characterized by high water retention capacity, low permeability, and poor drainage [ 35 ]. Each farm included a mature mixed-wood forest and fields that have been cultivated for less than ten years (< 10 y), between ten and 50 years (> 10 and < 50 y), and/or more than 50 years (> 50 y). Converted sites have been under conventional management systems with tillage, synthetic fertilizers, spring and fall manure applications, and crop rotations of alfalfa, barley, corn, canola, and spring and winter wheat [ 8 ].
Soil samples were collected from 30 separate sites, encompassing cropped fields and adjacent forests, from July to August 2019 using a soil split-core sampler (AMS Soil Sampler, Inc., American Fall, Idaho). Surface plant litter was removed prior to sampling, which in the forest sites included the LF (litter-fermented) horizons. Within each site a sampling area of approximately 16 ha ( ∼ 500 m x 320 m) was defined and 5 plots (20 m x 20 m) were established at the center and corners of this sampling area. Three soil cores were randomly collected within each plot for a total of 15 cores per site (Supplementary Figure S1 ). Soil cores were separated into two depths (0–5 and 5–15 cm) and composited to provide 2 depth-related samples per site (60 final composite samples). Soil samples were kept cool (4 °C) during transport to the laboratory, where they were sieved (field-moist) through 8 mm sieves to remove plant and other large material, and then through 4 mm sieves to homogenize the composite samples. Sub-samples of the field-moist sieved soils were immediately frozen at -40 °C for molecular analyses, transported frozen to the Agriculture and Agri-Food Canada (AAFC) Harrow Research and Development Center (Harrow, Ontario), and stored at -80 °C until analysis.
Soil physico-chemical properties were determined as previously described [ 8 ]. Briefly, available soil macro- and micronutrients (P, K, Mg, Fe, Mn, and Zn) were measured using inductively coupled plasma optical emission spectrometry analysis (SPECTRO Analytical Instrument Inc.) after extraction with a modified Morgan solution (pH 4.8). Soil pH was measured using a 1:1 soil:water ratio. Organic matter was determined by loss on ignition in a muffle furnace at 500 °C. Total N and total C were analyzed using a LECO CHN 628 Series total elemental analyzer (LECO Corporation, St. Joseph, Michigan).
Quantiative PCR evaluation of microbial function and abundance
DNA was extracted in duplicate (2 × 0.25 g) from soil samples using Qiagen DNeasy PowerSoil kits (Qiagen Inc., Canada), with an added 10 min incubation at 65 °C before bead-beating. DNA quality was first visualized on a 1.4% agarose gel, then quantity and quality were further evaluated using spectrophotometry (NanoDrop One, ThermoScientific). Once extraction reproducibility was confirmed, replicate extractions for each soil sample were pooled and DNA was re-quantified using fluorometric approaches (Quant-iT™ dsDNA BR kits; Life Technologies) then normalized to an initial working concentration of 10 ng µL − 1 . For qPCR analyses, DNA was further diluted to a working concentration of 3.0 ng µL − 1 and then re-quantified using a high-sensitivity fluorometric assay (Quant-iT™ dsDNA HS kits; Life Technologies, USA).
Soil microbial functional capacity was assessed by qPCR of 17 key genes associated with C, N, and P cycling (Supplementary Table S1 ). In addition, the relative abundances of bacteria, archaea, and fungi were assessed by qPCR of 16S rRNA and 18S rRNA gene regions. A complete list of assays and associated functions are provided in Supplementary Table S1 . Assays were performed in triplicate using the Bio-Rad CFX384 Touch Real-Time PCR Detection System (Bio-Rad, USA) in 5 µl reaction volumes containing 3.0 ng DNA (functional genes) or 0.3 ng DNA (taxonomic genes), and 0.12 µg of UltraPure™ BSA (Life Technologies, USA), with primer concentrations, commercial master mixes, and run conditions as listed in Supplementary Table S1 . Gene abundance was calculated with respect to a plasmid-based standard curve with a concentration range from 2 to 2 × 10 7 gene copies µl –1 DNA. Reaction efficiency ranged from 85 to 100%. Plasmids were generated by amplifying soil DNA in 50 µL PCR reactions using the appropriate primers (Table S1 ) and GoTaq master mix (Promega, USA), subcloning the cleaned (QIAquick PCR Purification Kit, Qiagen Inc., USA) PCR products into competent TOP10F’ One Shot E. coli (Invitrogen, USA), and extracting plasmids from positive clones (QIAprep Spin Miniprep Kit, Qiagen Inc., USA). Plasmids were sequenced (AAC, University of Guelph) and analyzed by blastn to confirm plasmid insert identity.
Microbial community sequencing
The amplicon sequencing strategy followed the approach developed under the Government of Canada’s Genomics Research and Development Initiative (GRDI), EcoBiomics Project [ 37 ]. The primers for bacteria and archaea target the V4-V5 hypervariable region of the 16 S rRNA gene (515FY/926R) [ 38 , 39 ] and the primers for fungi target the ITS2 region (ITS9F/ITS4R) [ 40 , 41 ]. A 10 ng µL − 1 aliquot of each composited DNA sample was shipped on ice to Genome Quebec for library preparation and paired-end sequencing (2 × 250 bp) on an Illumina NovaSeq6000 platform. Bioinformatic analysis was performed on the General Purpose Science Cluster in Dorval, Quebec [ 37 ] using a custom workflow as outlined in Pérez-Guzmán et al. [ 42 ] and available in the supplemental materials.
A total of 12,843,763 16S rRNA paired-end amplicon sequences were generated from 60 samples, with 32,013 operational taxonomic units (OTUs; 98% nucleotide identity) identified after quality filtering and clustering. A total of 14,478,951 ITS paired-end amplicon sequences were generated, with 16,082 OTUs (98% nucleotide identity) identified after quality filtering and clustering. Detailed information about sequencing QA/QC can be found in Supplementary Table S2 .
Statistical analysis
Unless otherwise specified, all tests were performed in R [ 43 ]. The impact of land conversion on functional genes associated with microbial nutrient cycling was evaluated using two-way ANOVAs, with time since conversion (forest, < 10 y in agriculture, > 10 and < 50 y in agriculture, and > 50 y in agriculture) and depth intervals (0–5 cm, and 5–15 cm) as fixed factors. Data was then analyzed using one-way ANOVAs followed by Tukey post hoc tests ( p < 0.05), to evaluate time groups within a depth increment. Data distribution and homoscedasticity was assessed using Shapiro-Wilk and Levene tests. Non-normal data were evaluated using the Kruskal-Wallis test followed by the Wilcoxon test using the p-value adjustment Bonferroni method. The qPCR data was analyzed on a copy number ng − 1 DNA basis (Supplementary Table S3 ); data provided on a per gram of soil basis is available in the Supplementary materials (Supplementary Tables S4 and S5 ).
To assess the influence of time since conversion and soil depth on overall community structure, Non-Parametric Multidimensional Scaling (NMDS) ordinations based on Bray-Curtis dissimilarities were constructed for each dataset (bacterial and fungal OTUs) using the vegan package in R (v2.6-2). The top 10 most abundant phyla were overlaid onto ordination space to assess overall phyla level shifts based on time since land conversion. Differences in time since conversion was assessed using PERMANOVA (“adonis” function; “vegan” package) on the overall bacterial and fungal community datasets as well as for each depth increment (Supplementary Table S6 ). Pairwise assessment of differences in groupings was done using the “pairwise.perm.manova” function in the “RVAideMemoire” package in R. P-values were adjusted for multiple comparisons using Benjamini-Hochberg corrections.
Changes in overall community diversity were evaluated using univariate approaches. Fungal and bacterial Shannon and Simpsons diversity indices were generated using QIIME 2 [ 44 ] and then evaluated using ANOVA or Kruskal-Wallis tests (normality assessed as indicated above), followed by Tukey HSD or pairwise Wilcoxon post hoc tests using the p-value adjustment Bonferroni method. Bacterial 16S rRNA and fungal ITS relative abundances were summarized at the class level and significant shifts were evaluated as outlined above. The relationship between soil microbial taxonomic and functional communities and soil physico-chemical properties (Supplementary Table S7 and [ 8 ]) was assessed using Spearman’s rank correlation.
Overall change in microbial abundance and functional potential
Microbial abundance and function differed both with time since conversion and by soil depth. Microbial molecular biomass generally decreased after land conversion from an average high of 30 µg DNA g −1 dry soil in forest soils to an average low of 20 µg DNA g −1 dry soil in agricultural soils (Fig. 1 A). Bacterial alpha diversity (Fig. 1 B) within this microbial biomass increased over time at both soil depths, but fungal alpha diversity (Fig. 1 C) was not significantly impacted. The increase in bacterial diversity was not accompanied by an increase in the relative abundance of bacteria 16S rRNA genes, which remained relatively constant at 1.8 × 10 7 16S rRNA copies ng −1 DNA (Fig. 1 D). In contrast, the relative abundance of archaea ( p < 0.05) and fungi ( p < 0.001) taxonomic genes increased more than 70% at both soil depths as the time since conversion increased (Fig. 1 E, F).
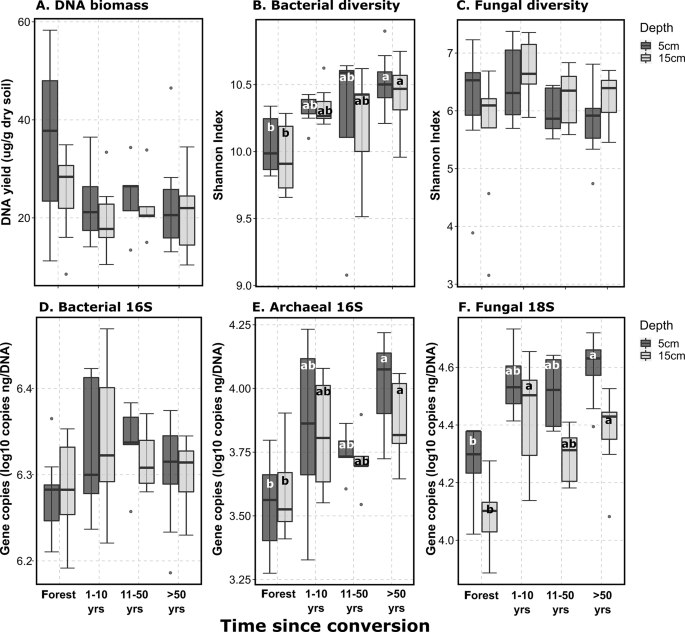
Abundance and diversity of soil microbial communities after forest conversion. A : Molecular microbial biomass (DNA yield); B - C : bacterial and fungal Shannon diversity indices; D - F : bacterial 16S rRNA, archaeal 16S rRNA, and fungal 18S rRNA gene copies ng -1 DNA. Significant differences ( p < 0.05) within an individual depth increment (5–15 cm) are denoted by different letters. Forest n = 9, 1–10 years n = 8, 11–50 years n = 5, > 50 years n = 8
The relative abundance of functions associated with complex organic matter decomposition (Fig. 2 A; laccase) did not change after conversion, but those associated with lower molecular weight C cycling increased. Genes associated with the breakdown of cellulolytic compounds (cellobiohydrolase; Fig. 2 B), hemicellulose and cellulose (glycoside hydrolase; Fig. 2 C), and oligosaccharides (β-glucosidase; Fig. 2 D) increased on average by 49, 103, and 33%, respectively, in the surface soils ( p < 0.05); glycoside hydrolase also showed significant increases in the lower soil depths (Fig. 2 C; average increase of 83%).
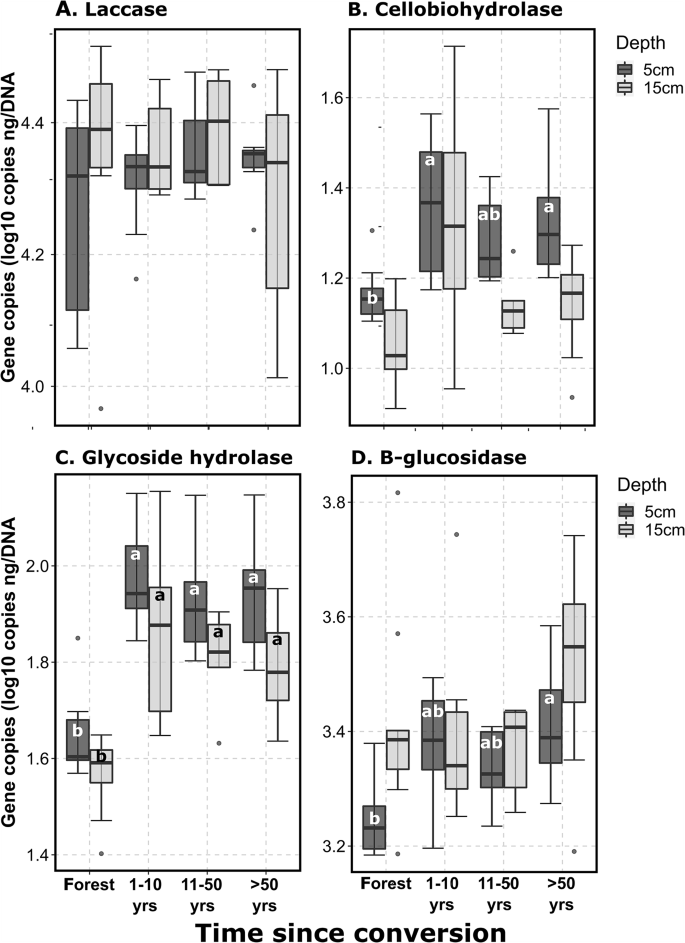
Shifts in bacterial and fungal carbon cycling functions after forest conversion. A : laccase (bacteria and fungi); B : Cellobiohydrolase (fungi); C : Glycoside hydrolase (fungi); D : β-glucosidase (bacteria). Quantitative PCR data shown as gene copies ng -1 DNA. Significant differences ( p < 0.05) within an individual depth increment (5–15 cm) are denoted by different letters. Forest n = 9, 1–10 years n = 8, 11–50 years n = 5, > 50 years n = 8
Similar to patterns seen with lower molecular weight C functions, bacterial and archaeal functional genes associated with nitrification (N cycling) all significantly increased after land conversion (Fig. 3 A-C; p < 0.001), with increases ranging from a low of 112% for archaeal ammonia monooxygenase genes (5–15 cm soil depth) to a high of 1310% for bacterial ammonia monooxygenase genes (0–5 cm soil depth). All other assessed N cycling genes, including those associated with ammonification (nitrate reductase; Fig. 3 D) and denitrification (nitrite reductase and nitrous oxide reductase; Fig. 3 E-F and Supplementary Tables S3 ) did not significantly change after land conversion.
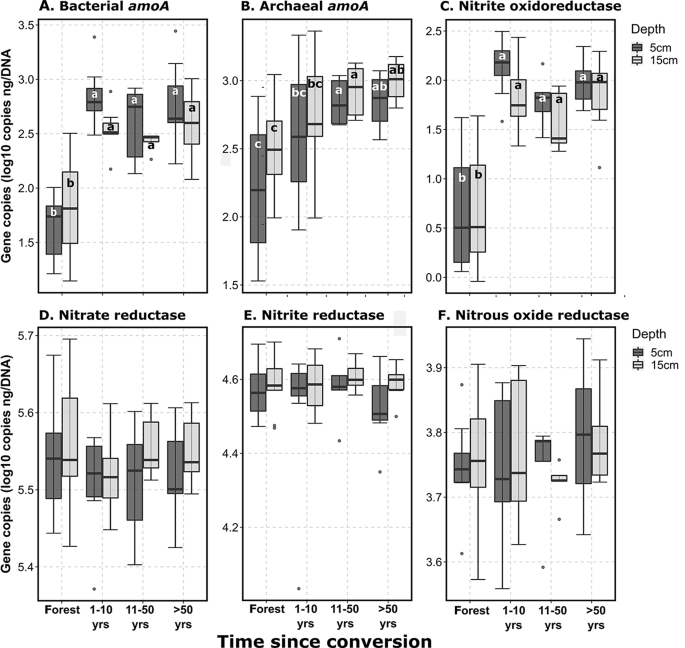
Shifts in bacterial and archaeal nitrogen cycling functions after forest conversion. Quantitative PCR data shown as gene copies ng -1 DNA. Significant differences ( p < 0.05) within an individual depth increment (5–15 cm) are denoted by different letters. amoA , ammonium monooxygenase. All assays except Archaeal amoA target bacterial functions. Forest n = 9, 1–10 years n = 8, 11–50 years n = 5, > 50 years n = 8
Finally, changes in genes associated with different P cycling functions were variable. The relative abundance of functional genes associated with P mineralization decreased after land conversion (Fig. 4 A, B). These changes were significant in the surface soils ( p < 0.001), with average decreases of 20 and 33%, respectively for alkaline and acid phosphatase. The other two assessed genes associated with P cycling, phosphono-acetaldehyde hydrolase (C-P bond cleavage) and pyrroloquinoline quinone (associated with P solubilization) were not affected by land clearing.
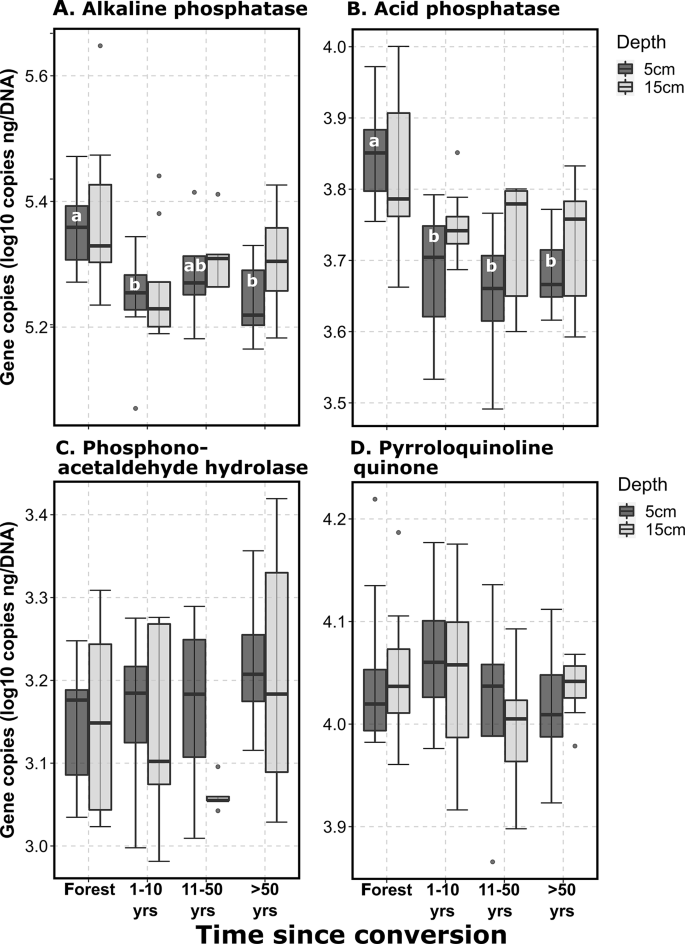
Shifts in bacterial phosphorous cycling functions after forest conversion. Quantitative PCR data shown as gene copies ng -1 DNA. Significant differences ( p < 0.05) within an individual depth increment (5–15 cm) are denoted by different letters. Forest n = 9, 1–10 years n = 8, 11–50 years n = 5, > 50 years n = 8
Shifts in bacterial and fungal communities following land conversion
Unique bacterial and fungal communities developed after land conversion (Fig. 5 ). Bacterial community structure in the reference forested sites was significantly different from the agricultural systems ( p < 0.042) in both surface and sub-surface soils. When examined across both depths, communities in the forest and > 50 years post-conversion sites each clustered separately than all other systems ( p < 0.025) while the 1–10 and 11–50 year systems were similar ( p = 0.116, Supplementary Table S6 ). The discrete clustering of forest bacterial communities at both depths was associated with increased Acidobacteriota, Actinomycetota, and Bacteroidota OTUs, while those of agriculture systems were associated with increased Gemmatimonadota OTUs (Fig. 5 ). Similarly, fungal community composition in the forested sites was significantly different from the other systems, both within each depth increment (Fig. 5 , p < 0.002) and when examined across both depths, where all systems were significantly different ( p < 0.027, Supplementary Table S6 ). The distinct clustering of forest fungal communities at both depths was associated with increased Basidiomycota and Mucoromycota OTUs, while those of agriculture systems were associated with increased Ascomycota OTUs (Fig. 5 ).
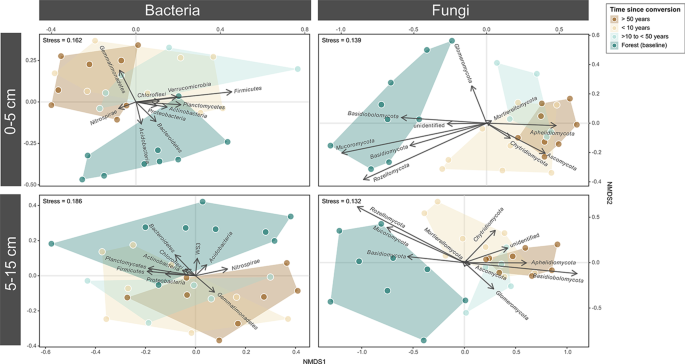
Overall shifts in bacterial and fungal communities after conversion from forest to agriculture. The prevalence of the top ten most abundant phyla for each depth subset are shown as vectors. NMDS ordinations were based on Bray-Curtis dissimilarities
Bacterial communities in the surface soils experienced more significant shifts at the class level than those in the sub-surface (Fig. 6 A). The relative abundance of the Bacillota class Clostridia more than doubled in the 0–5 cm agricultural soils relative to the forest soils. At both depths two Actinomycetota classes (Acidimicrobiia, MB.A2.108) decreased in relative abundance after conversion to agriculture, while two Gemmatimonadota classes (Gemmatimonadetes, Gemm.1) increased in relative abundance. In fungal communities, the clear shift towards an Ascomycota-dominated system (Fig. 5 ) was associated with highly significant increases in the relative abundance of Ascomycota classes Sordariomycetes and Dothideomycetes, which increased by 329% and 433% (respectively) in the deeper soils and by 182% and 198% (respectively) in the surface soils (Fig. 6 B). The relative abundance of the Basidiomycota class Agaricomycetes decreased after forest conversion by 84% and 52% in the surface and deeper soils, respectively (Fig. 6 B).
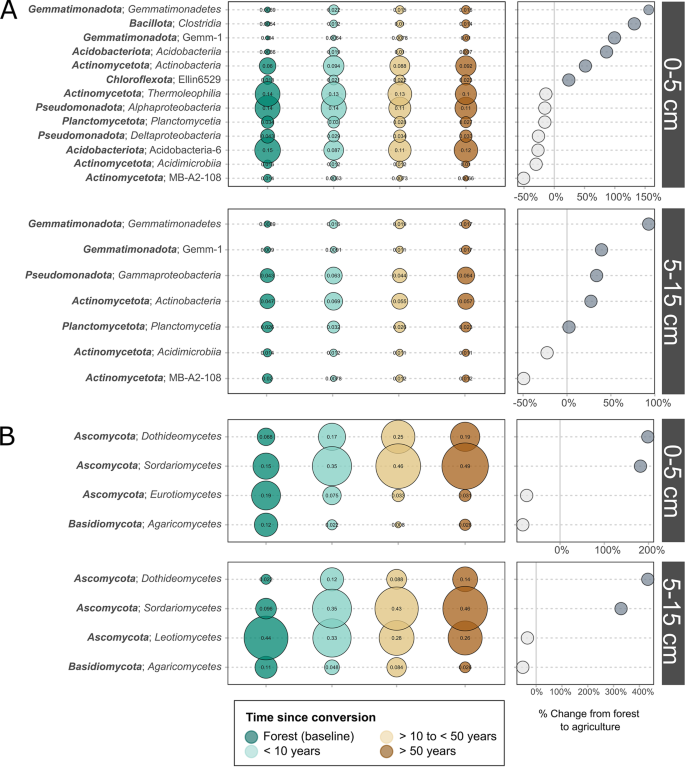
Change in the relative abundance of microbial taxonomic classes after conversion from forest to agriculture. ( A ) Bacteria; ( B ) Fungi. Only class level shifts showing significant differences based on time since conversion ( p < 0.05; ANOVA) within each depth increment are shown. The magnitude of the change in mean relative abundance from the forested sites to agricultural fields after greater than 50 years is shown in the right panel
Relationship between soil organic matter, soil chemical properties, and soil biology
Spearman’s rank correlation was used to assess the interactions between soil properties (Supplementary Table S7 and Benalcazar et al., 2022) and microbial taxonomic and functional gene abundance (Table 1 ). The overall functional potential of the soil microbial communities to break down low molecular weight carbon compounds (β-glucosidase) was negatively correlated with SOM and C + N pools, and positively correlated with pH. In contrast, the cellulolytic (cellobiohydrolase) potential decreased with increasing pH and both cellulolytic and hemicellulolytic (glycoside hydrolase) potential increased with increased P and K availability. Functions associated with nitrification (ammonium monooxygenase; nitrite oxidoreductase) were negatively correlated with SOM and total C while those associated with N immobilization (dissimilatory nitrite reductase) and P mineralization (acid phosphatase) were positively correlated with SOM and total C.
Within the bacterial communities, two Acidobacteriota classes (Acidimicrobiia, Acidobacteria.6), Actinomycetota class MB.A2.108, and Planctomycetia were positively correlated with SOM, with most also positively correlated with Total C and N (Table 2 ). A separate Acidobacteriota class, Acidobacteriia, and the Gemmatimonadota class Gemm.1 were negatively correlated with the same parameters. Nitrospira was not correlated with C and N pools, but was negatively associated with Fe, Mn, and Zn. In contrast Planctomycetia and Alphaproteobacteria were positively correlated with these micronutrients. The fungal class Sordariomycetes was negatively associated with SOM and total N, but positively associated with P and K, while class Agaricomycetes showed reverse patterns.
Land conversion to agriculture at the southern limit of the boreal region (Thunder Bay, Ontario) altered both the abundance and functionality of the soil microbial communities, with the magnitude and direction of change differing with time and with soil depth. Molecular microbial biomass, or the amount of extractable DNA, generally decreased by 31% in surface soils and 18% in subsurface soils, mirroring previously reported patterns of organic matter loss in this system [ 8 ]. Land conversion to agriculture across the globe has been shown to alter microbial biomass [ 16 ] as the clearing of vegetation, site preparation, and subsequent tillage typically depletes organic matter and increases compaction in surface soils [ 5 , 6 ].
The general decrease in microbial biomass after conversion was not accompanied by comparable decreases in either microbial diversity (assessed by sequencing) or relative abundance (assessed by quantitative PCR). Fungal diversity was maintained and the relative abundance of fungi and archaea within the microbial biomass increased, while bacterial diversity increased and the relative abundance of bacteria was maintained. A recent study on converted systems in the Yukon Territory similarly found that archaeal abundance increased, and bacterial abundance did not change [ 10 ]. In contrast to our findings however, Schroeder et al. [ 10 ] found that fungal abundance in both pasture- and crop-based agricultural systems was reduced relative to the adjacent forest. However, substantive difference in climate, agricultural practices, and soil type between the two studies, including the presence of permafrost in most of the forested Yukon sites and horticultural cropping practices in the Yukon cropland sites [ 9 , 10 ], makes direct comparisons challenging. Management practices in the converted Ontario agricultural systems included the use of manure and mineral fertilizers, crop rotation (commonly with alfalfa, corn, barley, and winter wheat), and conservation tillage [ 8 ]. These moderate intensity management practices, which alter the soil structure and nutrient balances of the initial system, have been shown to increase bacterial and fungal abundance [ 22 ] and diversity [ 11 , 13 , 45 ] in agricultural soils, in part because they generate increased niche and resource heterogeneity.
The overall microbial community structure was significantly different in the agricultural systems, and these shifts occurred rapidly after conversion. Fungal communities were rapidly altered, but significantly different communities were found in each of the post-conversion age increments as Ascomycota-Dothideomycetes and -Sordariomycetes continued to increase and Ascomycota-Eurotiomycetes and -Leotiomycetes continued to decrease in relative abundance. Basidiomycota, and in particular the class Agaricomycetes which includes many conifer-associated ectomycorrhizal fungi [ 46 ], also significantly decreased as the system shifted from forest dominant to crop dominant. These substantive shifts in fungal groups have implications both for carbon cycling as well as for agricultural soil health. For example, both Sordariomycetes and Dothideomycetes are diverse classes of fungal decomposers, like most Ascomycota, but both also include important crop pathogens such as Fusarium and Phaeosphaeria spp [ 47 ], which are known to generally increase in Ontario grain cropping systems [ 42 ].
Bacterial community structure in the surface soils was also altered within the first few years after land conversion, followed by several decades of transition before stabilizing more than 50 years post-conversion. At the phyla level, the relative abundance of Acidobacteriota, Actinomycetota, Bacteroidota, and Pseudomonadota decreased, while the abundance of Gemmatimonadota increased. Previous studies have shown that Acidobacteriota, Actinomycetota, Pseudomonadota are dominant phyla in boreal soils [ 27 ], and that increased pH due to the addition of wood ash and liming in agricultural fields reduces their abundance [ 48 ]. Within each phylum however, different shifts in the relative abundance of classes occurred. For example, Actinomycetota-Actinobacteria increased by up to 50% in surface and subsurface soils, while Actinomycetota-MB-A2-108 decreased by approximately the same amount. There was a significant relationship between pH and many of the bacterial classes that either increased (positive association) or decreased (negative association) post-conversion. Altered pH, which in general co-occurred with altered OM levels as found in other boreal systems [ 15 ], is well known to be a dominant factor influencing the composition of bacterial communities [ 14 ], but within a given system multiple complex factors come into play. For example, MB-A2-108 was positively associated with pH yet still decreased in converted systems, and its overall abundance was more strongly controlled by soil fertility (e.g., P levels), as has also been found in other agricultural systems [ 49 ]. The greatest shift in relative abundance occurred in Gemmatimonadota. This phylum has been shown to be more abundant in agricultural soils globally [ 50 ], with an increased abundance believed to be promoted due to generally higher nutrient status and more neutral pH, relative to forest soils. Since different Gemmatimonadota are known to have the genetic potential to reduce N 2 O to N 2 [ 50 ], their increase in northern agricultural soils could act to mitigate N-based GHG emissions as agriculture in these regions expands.
Although changes in overall bacterial and fungal communities were highly significant post-conversion, their functional complexity means that these taxonomic level changes may not be useful to evaluate how forest conversion will alter C and N cycling dynamics, as most bacterial and fungal taxa are involved in C and N cycling at some level [ 18 , 27 , 51 , 52 ]. Instead, co-associated shifts in different biogeochemical cycling functional potential may serve as better indicators of overall soil health change.
Land conversion had differing effects on the capacity of the soil communities to cycle different carbon compounds. Bölscher et al. [ 53 ] previously reported that microbial communities in forest soils were more efficient in using lower molecular weight (LMW) C substrates, but Zhou et al. [ 16 ] found that C-decomposition increased following forest disturbance. In the northern Ontario we found that the relative abundance of genes associated with complex OM decomposition (laccase) did not change, but the relative abundance of genes associated with the decomposition of LMW-C compounds increased with the switch to high inputs of bioavailable crop C (roots and crop residues) and nutrients (e.g. N and P). Functional shifts in fungal genes associated with C cycling stabilized within 10 years while bacterial genes associated with C cycling (B-glucosidase) were still in undergoing shifts decades later. Although we did not directly measure how different C-pools changed after land conversion, compounds like cellulose, hemicellulose, and sugars are common in agricultural fields [ 54 , 55 ], where C is more abundant as bio-available particulate organic matter relative to more stable mineral-associated organic matter [ 5 ]. Schroeder et al. [ 10 ] found that microbial carbon use efficiency (CUE) increased following forest conversion but suggested that increased CUE was primarily mediated through abiotic factors rather than altered microbial communities, as they found no difference in the relative abundance of bacteria or fungi post-conversion. In our study, fungal abundance clearly increased post-conversion, and the significant shifts in the capacity of both bacteria and fungi to cycle LMW-C would contribute to increased CUE.
Functions associated with N and P cycling also shifted after land conversion, as both mineral and organic fertilizer inputs altered soil nutrient status [ 8 ]. The relative abundance of genes associated with nitrification ( amoA and nxrA ) generally increased, however genes associated with denitrification were not impacted by forest to agricultural conversion. Soil management practices including tillage, organic amendments, and fertilizer N are well known to increase the abundance of both bacterial and archaeal nitrifiers [ 57 , 58 ]. Although archaeal ammonia oxidizers dominate nitrification when ammonia is produced by the mineralization of organic N rather than fertilizer N, these nitrifiers have also been shown to increase in response to N application in acidic soils [ 59 ]. In addition, nitrifier abundance increases in soils amended with cattle manure [ 60 ], which is a common practice among dairy farmers in the study region [ 8 ]. Functions associated with organic P mineralization potential (acid and alkaline phosphatase) followed opposite trends to those seen with nitrification functions and decreased after conversion. Increased stores of extractable P, which occur when converted forest soils are fertilized [ 6 , 8 ], have been shown to lead to reduced alkaline phosphatase abundance [ 61 ]. Although there was no direct relationship between phosphatase gene abundance and extractable P at our site, acid phosphatase abundance was positively associated with soil organic matter. This finding suggests that a shift from mainly organic P to mineral P contributed to the observed functional changes, as has been seen in other systems [ 62 , 63 ].
In this study, we found that forest conversion to agriculture in boreal soils affected microbial abundance, diversity, composition, and functional potential. Although substantive changes began to occur soon after land conversion to agriculture, microbial community structure and functional potential continued to change for decades. Bacterial nitrification processes increased within the first decade after which they stabilized, but C cycling processes were still changing 50 years post conversion. In general however, variability in both community structure and functional potential decreased after the first decade and new stable states [ 28 ] began to emerge. The type and timeline of changes are similar to those that occur when land use change occurs in the other direction, for example when previously cropped soils are converted to forests. In afforested systems, it similarly takes decades for microbial abundance and C cycling processes to stabilize [ 64 , 65 , 66 ]. Our findings highlight how C, N, and P cycling processes may be altered as agriculture expands northward into forested regions of Canada and provides insight into how better to manage these systems to ensure their sustainability.
Data availability
The sequence data supporting the conclusions of this article are available in NCBI Short Read Archive under Project number PRJNA1062837. The chemical and quantitative PCR datasets supporting the conclusions of this article are included within the article and its additional files.
Bush E, Lemmen DS, editors. Canada’s changing climate report. Ottawa: Government of Canada; 2019. p. 444.
Google Scholar
King M, Altdorff D, Li P, Glagedara L, Holden J, Unc A. Northward shift of the agricultural climate zone under 21st-century global climate change. Sci Rep. 2018;8:7904.
Article PubMed PubMed Central Google Scholar
Unc A, Altdorff D, Abakumov E, Adl S, Baldursson S, Bechtold M, et al. Expansion of agriculture in northern cold-climate regions: a cross-sectoral perspective on opportunities and challenges. Front Sustain Food Syst. 2021;5:663448.
Article Google Scholar
Altdorff D, Borchard N, Young EH, Galagedara L, Sorvali J, Quideau S, et al. Agriculture in boreal and Arctic regions requires an integrated global approach for research and policy. Agron Sustain Dev. 2021;41:23.
DeGryze S, Six J, Paustian K, Morris SJ, Paul EA, Merckx R. Soil organic carbon pool changes following land-use conversions. Glob Change Biol. 2004;10:1120–32.
Ellert B, Gregorich EG. Storage of carbon, nitrogen and phosphorus in cultivated and adjacent forest soils of Ontario. Soil Sci. 1996;161:587–603.
Article CAS Google Scholar
Reicosky D. Managing soil health for sustainable agriculture volume 1: fundamentals. 1st ed. Burleigh Dodds Science Publishing; 2018.
Benalcazar P, Diochon A, Kolka R, Schindelbeck R, Sahota T, McLaren BE. The impact of land conversion from boreal forest to agriculture on soil health indicators. Can J Soil Sci. 2022;87:403–15.
Peplau T, Schroeder J, Gregorich E, Poeplau C. Subarctic soil carbon losses after deforestation for agriculture depend on permafrost abundance. Glob Change Biol. 2022;28:5227–42.
Schroeder J, Peplau T, Pennekamp F, Gregorich E, Tebbe CC, Poeplau C. Deforestation for agriculture increases microbial carbon use efficiency in subarctic soils. Biol Fertil Soils. 2024;60:17–34.
Tardy V, Spor A, Mathieu O, Lévèque J, Terrat S, Plassart P, et al. Shifts in microbial diversity through land use intensity as drivers of carbon mineralization in soil. Soil Biol Biochem. 2015;90:204–13.
Mäkipää R, Abramoff R, Adamczyk B, Baldy V, Biryol C, Bosela M, et al. How does management affect soil C sequestration and greenhouse gas fluxes in boreal and temperate forests? – a review. Ecol Manag. 2023;529:120637.
de Graaff M-A, Hornslein N, Throop HL, Kardol P, van Diepen LTA. Effects of agricultural intensification on soil biodiversity and implications for ecosystem functioning: a meta-analysis. Adv Agron. 2019;155:1–44.
Fierer N. Embracing the unknown: disentangling the complexities of the soil microbiome. Nat Rev Microbiol. 2017;15:579–90.
Article CAS PubMed Google Scholar
Giguère-Tremblay R, Laperriere G, de Grandpré A, Morneault A, Bisson D, Chagnon P-L, et al. Boreal forest multifunctionality is promoted by low soil organic matter content and high regional bacterial biodiversity in Northeastern Canada. Forests. 2020;11:149.
Zhou Z, Wang C, Luo Y. Effects of forest degradation on microbial communities and soil carbon cycling: a global meta-analysis. Glob Ecol Biogeogr. 2018;27:110–24.
Bauhus J, Pare D, Côté L. Effects of tree species, stand age and soil type on soil microbial biomass and its activity in a southern boreal forest. Soil Biol Biochem. 1998;30:1077–89.
Merloti LF, Mendes LW, Pedrinho A, de Souza LF, Ferrari BM, Tsai SM. Forest-to-agriculture conversion in Amazon drives soil microbial communities and N-cycle. Soil Biol Biochem. 2019;137:107567.
Pankhurst CE, Doube BM. Biological indicators of soil health: synthesis. In: Pankhurst CE, Doube BM, Gupta VVSR, editors. Biological indicators of soil health. Oxon: CABI International; 1997. pp. 419–35.
Bevivino A, Paganin P, Bacci G, Florio A, Pellicer MS, Papaleo MC, et al. Soil bacterial community response to differences in agricultural management along with seasonal changes in a Mediterranean region. PLoS ONE. 2014;9:e105515.
Peltoniemi K, Velmala S, Fritze H, Lemola R, Pennanen T. Long-term impacts of organic and conventional farming on the soil microbiome in boreal arable soil. Eur J Soil Biol. 2021;104:103314.
Morugán-Coronado A, Pérez-Rodríguez P, Insolia E, Soto-Gómez D, Fernández-Calviño D, Zornoza R. The impact of crop diversification, tillage and fertilization type on soil total microbial, fungal and bacterial abundance: a worldwide meta-analysis of agricultural sites. Agric Ecosyst Environ. 2022;329:107867.
Hicks LC, Rousk K, Rinnan R, Rousk J. Soil microbial responses to 28 years of nutrient fertilization in a subarctic heath. Ecosystems. 2020;23:1107–19.
Neurauter M, Yuan M, Hicks LC, Rousk J. Soil microbial resource limitation along a subarctic ecotone from birch forest to tundra heath. Soil Biol Biochem. 2023;177:108919.
Allison SD, Czimczik CI, Treseder KK. Microbial activity and soil respiration under nitrogen addition in alaskan boreal forest. Glob Change Biol. 2008;14:1156–168.
Kibblewhite MG, Ritz K, Swift MJ. Soil health in agricultural systems. Philos Trans R Soc. 2008;363:685–701.
Lladó S, López-Mondéjar R, Baldrian P. Forest soil bacteria: diversity, involvement in ecosystem processes, and response to global change. Microbiol Mol Biol Rev. 2017;81:e00063–16.
Shade A, Peter H, Allison S, Baho D, Berga M, Buergmann H, et al. Fundamentals of microbial community resistance and resilience. Front Microbiol. 2012;3:417.
Burnett MS, Schütte UME, Harms TK. Widespread capacity for denitrification across a boreal forest landscape. Biogeochemistry. 2022;158:215–32.
Article CAS PubMed PubMed Central Google Scholar
Seitz TJ, Schütte UME, Drown DM. Unearthing shifts in microbial communities across a disturbance gradient. Front Microbiol. 2022;13:781051.
Truu M, Nõlvak H, Ostonen I, Oopkaup K, Maddison M, Ligi T, et al. Soil bacterial and archaeal communities and their potential to perform n-cycling processes in soils of boreal forests growing on well-drained peat. Front Microbiol. 2020;11:591358.
Paavolainen L, Smolander A. Nitrification and denitrification in soil from a clear-cut Norway spruce ( Picea abies ) stand. Soil Biol Biochem. 1998;30:775–81.
Paavolainen L, Kitunen V, Smolander A. Inhibition of nitrification in forest soil by monoterpenes. Plant Soil. 1998;205:147–54.
Li J, Delgado-Baquerizo M, Wang J-T, Hu H-W, Cai Z-J, Zhu Y-N, et al. Fungal richness contributes to multifunctionality in boreal forest soil. Soil Biol Biochem. 2019;136:107526.
Mollard DG, Mollard JD. Thunder Bay Area (NTS 52A/SW), District of Thunder Bay. In: Northern Ontario Engineering Geology Terrain Study 71. Ontario Geological Survey. 1983. http://www.geologyontario.mndmf.gov.on.ca/mndmfiles/pub/data/imaging/NOEGTS071TS071.pdf . Accessed 10 Nov 2023.
Wester MC, Henson BL, Crins WJ, Uhlig PWC, Gray PA. The ecosystems of Ontario, Part 2: Ecodistricts. Ontario Ministry of Natural Resources and Forestry, Science and Research Branch, Peterborough, ON. Science and Research Technical Report- TR-26. 2018.
Edge TA, Baird DJ, Bilodeau G, Gagné N, Greer C, Konkin D, et al. The Ecobiomics project: advancing metagenomics assessment of soil health and freshwater quality in Canada. Sci Total Environ. 2020;710:135906. https://www.ontario.ca/page/ecosystems-ontario-part-2-ecodistricts . Accessed 10 Nov 2023.
Parada AE, Needham DM, Fuhrman JA. Every base matters: assessing small subunit rRNA primers for marine microbiomes with mock communities, time series and global field samples. Environ Microbiol. 2016;18:1403–14.
Quince C, Lanzen A, Davenport RJ, Turnbaugh PJ. Removing noise from pyrosequenced amplicons. BMC Bioinform. 2011;12:38.
Ihrmark K, Bödeker ITM, Cruz-Martinez K, Friberg H, Kubartova A, Schenck J, et al. New primers to amplify the fungal ITS2 region–evaluation by 454-sequencing of artificial and natural communities. FEMS Microbiol Ecol. 2012;82:666–77.
White TJ, Bruns TD, Lee SB, Taylor J. Amplification and direct sequencing of fungal ribosomal RNA genes for phylogenetics. In: Innis MA, Gelfand DH, Sninsky JJ, White TJ, editors. PCR protocols: a guide to methods and applications. New York: Academic; 1990. pp. 315–22.
Pérez-Guzmán L, Phillips LA, Seuradge BJ, Agomoh I, Drury CF, Acosta‐Martínez V. An evaluation of biological soil health indicators in four long‐term continuous agroecosystems in Canada. Agric Ecosyst Environ. 2021;4:e20164.
R Core Team. R: A language and environment for statistical computing R. Foundation for Statistical Computing, Vienna, Austria. 2020. https://www.r-project.org/ .
Bolyen E, Rideout JR, Dillon MR, Bokulich NA, Abnet CC, Al-Ghalith GA, et al. Reproducible, interactive, scalable and extensible microbiome data science using QIIME 2. Nat Biotechnol. 2019;37:852–7.
Trivedi P, Delgado-Baquerizo M, Anderson IC, Singh BK. Response of soil properties and microbial communities to agriculture: implications for primary productivity and soil health indicators. Front Plant Sci. 2016;7:990.
McGuire KL, Allison SD, Fierer N, Treseder KK. Ectomycorrhizal-dominated boreal and tropical forests have distinct fungal communities, but analogous spatial patterns across soil horizons. PLoS ONE. 2013;8:e68278.
Termorshuizen AJ. Ecology of fungal plant pathogens. In: Heitman J, Howlet BJ, Crous PW, Stukenbrock EH, James TY, Gow NAR, editors. The fungal kingdom. ASM; 2017. pp. 387–97.
Reid C, Watmough SA. Evaluating the effects of liming and wood-ash treatment on forest ecosystems through systematic meta-analysis. Can J Res. 2014;44:867–85.
Megyes M, Borsodi AK, Árendás T, Márialigeti K. Variations in the diversity of soil bacterial and archaeal communities in response to different long-term fertilization regimes in maize fields. Appl Soil Ecol. 2021;168:104120.
Mujakić I, Piwosz K, Koblížek M. Phylum Gemmatimonadota and its role in the environment. Microorganisms. 2022;10:151.
López-Mondéjar R, Zühlke D, Becher D, Riedel K, Baldrian P. Cellulose and hemicellulose decomposition by forest soil bacteria proceeds by the action of structurally variable enzymatic systems. Sci Rep. 2016;6:25279.
van Insberghe D, Maas KR, Cardenas E, Strachan CR, Hallam SJ, Mohn WW. Non-symbiotic Bradyrhizobium ecotypes dominate north American forest soils. ISME J. 2015;9:2435–41.
Bölscher T, Wadsö L, Börjesson G, Herrmann AM. Differences in substrate use efficiency: impacts of microbial community composition, land use management, and substrate complexity. Biol Fertil Soils. 2016;52:547–59.
Cherubini F, Ulgiati S. Crop residues as raw materials for biorefinery systems–A LCA case study. Appl Energy. 2010;87:47–57.
Ginni G, Kavitha S, Kannah Y, Bhatia SK, Kumar A, Rajkumar M, et al. Valorization of agricultural residues: different biorefinery routes. J Environ Chem Eng. 2021;9:105435.
Attard E, Poly F, Commeaux C, Laurent F, Terada A, Smets BF, et al. Shifts between Nitrospira- and Nitrobacter-like nitrite oxidizers underlie the response of soil potential nitrite oxidation to changes in tillage practices. Environ Microbiol. 2010;12:315–26.
Banning NC, Maccarone LD, Fisk LM, Murphy DV. Ammonia-oxidising bacteria not archaea dominate nitrification activity in semi-arid agricultural soil. Sci Rep. 2015;5:11146.
Liang F, Wen Y, Dong X, Wang Y, Pan G, Jiang F, et al. Response of activity and community composition of nitrite-oxidizing bacteria to partial substitution of chemical fertilizer by organic fertilizer. Environ Sci Pollut Res. 2021;28:29332–43.
Gubry-Rangin C, Kratsch C, Williams TA, McHardy AC, Embley TM, Prosser JI, et al. Coupling of diversification and pH adaptation during the evolution of terrestrial Thaumarchaeota. PNAS. 2015;112:9370–5.
Tatti E, Goyer C, Chantigny M, Wertz S, Zebarth BJ, Burton DL, Filion M. Influences of over winter conditions on denitrification and nitrous oxide-producing microorganism abundance and structure in an agricultural soil amended with different nitrogen sources. Agric Ecosyst Environ. 2014;183:47–59.
Fraser TD, Lynch DH, Gaiero J, Khosla K, Dunfield KE. Quantification of bacterial non-specific acid ( phoC ) and alkaline ( phoD ) phosphatase genes in bulk and rhizosphere soil from organically managed soybean fields. Appl Soil Ecol. 2017;111:48–56.
Lang M, Zou W, Chen X, Zou C, Zhang W, Deng Y, et al. Soil microbial composition and phoD gene abundance are sensitive to phosphorus level in a long-term wheat-maize crop system. Front Microbiol. 2021;11:605955.
Ragot SA, Kertesz MA, Bünemann EK. PhoD alkaline phosphatase gene diversity in soil. Appl Environ Microbiol. 2015;81:7281–9.
Luo X, Hou E, Zhang L, Kuan Y, Wen D. Altered soil microbial properties and functions after afforestation increase soil carbon and nitrogen but not phosphorus accumulation. Biol Fertil Soils. 2023. https://doi.org/10.1007/s00374-023-01726-4 .
Zhang Q, Wu J, Yang F, Zhang Q, Cheng X. Alteration in soil microbial communities’ composition and biomass following agricultural land use change. Sci Rep. 2016;6:36587.
Zhao FZ, Ren CJ, Han XH, Yang GH, Wang J, Doughty R, et al. Trends in soil microbial communities in afforestation ecosystem modulated by aggradation phases. Ecol Manag. 2019;441:167–75.
Download references
Acknowledgements
Library preparation and sequencing was performed by Génome Québec, Montreal, Canada. Dr. Brian McLaren, Lakehead University, supervised PB during his core Ph.D. research.
PB was supported by the Canadian Queen Elizabeth II Diamond Jubilee Advanced Scholars Program, with financial support from the International Development Research Centre and the Social Sciences and Humanities Research Council. Financial support for the microbial work within this study was provided by Agriculture and Agri-Food Canada through the GRDI Ecobiomics (J-001263) and Genomic Indicators (J-002369) projects.
Author information
Authors and affiliations.
Faculty of Natural Resources Management, Lakehead University, Thunder Bay, ON, Canada
Paul Benalcazar
Agriculture and Agri-Food Canada, Harrow Research and Development Centre, Harrow, ON, Canada
Brent Seuradge & Lori A. Phillips
Department of Geology, Lakehead University, Thunder Bay, ON, Canada
Amanda C. Diochon
USDA Forest Services Northern Research Station, Grand Rapid, MN, 55744, USA
Randall K. Kolka
You can also search for this author in PubMed Google Scholar
Contributions
PB, ACD, and RKK conceived the overall study. PB collected samples, performed all chemical analyses, and prepared associated tables. PB, BS and LAP performed all molecular analyses. BS performed bioinformatic analyses and prepared associated figures. LAP performed qPCR analyses and prepared associated figures. ACD, RKK, and LAP were involved in funding acquisition, provided the resources, and supervised research activities. PB and LAP wrote the original draft. All authors edited the manuscript and approved the final draft.
Corresponding author
Correspondence to Lori A. Phillips .
Ethics declarations
Ethics approval and consent to participate.
Not applicable.
Consent for publication
Competing interests.
The authors declare that they have no competing interests.
Additional information
Publisher’s note.
Springer Nature remains neutral with regard to jurisdictional claims in published maps and institutional affiliations.
Electronic supplementary material
Below is the link to the electronic supplementary material.
Supplementary Material 1
Rights and permissions.
Open Access This article is licensed under a Creative Commons Attribution 4.0 International License, which permits use, sharing, adaptation, distribution and reproduction in any medium or format, as long as you give appropriate credit to the original author(s) and the source, provide a link to the Creative Commons licence, and indicate if changes were made. The images or other third party material in this article are included in the article’s Creative Commons licence, unless indicated otherwise in a credit line to the material. If material is not included in the article’s Creative Commons licence and your intended use is not permitted by statutory regulation or exceeds the permitted use, you will need to obtain permission directly from the copyright holder. To view a copy of this licence, visit http://creativecommons.org/licenses/by/4.0/ . The Creative Commons Public Domain Dedication waiver ( http://creativecommons.org/publicdomain/zero/1.0/ ) applies to the data made available in this article, unless otherwise stated in a credit line to the data.
Reprints and permissions
About this article
Cite this article.
Benalcazar, P., Seuradge, B., Diochon, A.C. et al. Conversion of boreal forests to agricultural systems: soil microbial responses along a land-conversion chronosequence. Environmental Microbiome 19 , 32 (2024). https://doi.org/10.1186/s40793-024-00576-3
Download citation
Received : 17 January 2024
Accepted : 02 May 2024
Published : 11 May 2024
DOI : https://doi.org/10.1186/s40793-024-00576-3
Share this article
Anyone you share the following link with will be able to read this content:
Sorry, a shareable link is not currently available for this article.
Provided by the Springer Nature SharedIt content-sharing initiative
- Climate change
- Northern agricultural expansion
- Soil microbiome
- Biogeochemical cycling
- Functional genes
- Amplicon sequencing
Environmental Microbiome
ISSN: 2524-6372
- Submission enquiries: [email protected]
A bird’s eye view of boreal forests: using satellite remote sensing to monitor biodiversity richness
Issue 610: Remote mapping shows promise as a cost-efficient way to roughly predict lichen communities in European boreal forests, finds a study of aspen trees in southern Finland.
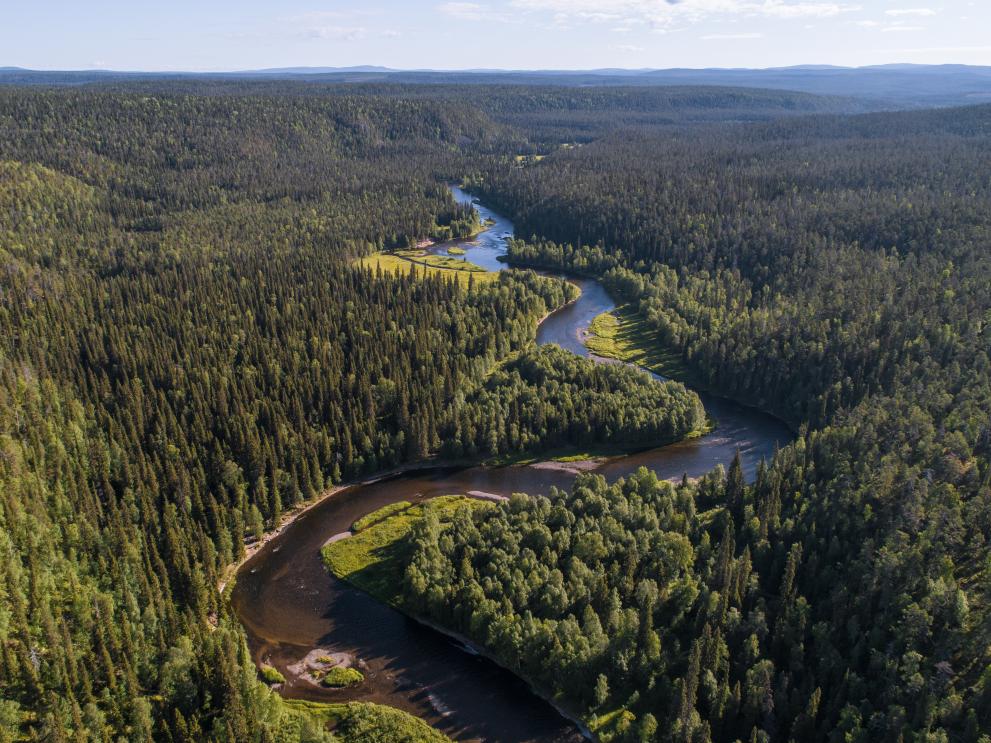
The European aspen ( Populus tremula L. ) is a keystone species in boreal forests and increases forest biodiversity by hosting accompanying species such as fungi, mosses, woodpeckers, small mammals and invertebrates. These species include lichens, which grow on the trees – many exclusively on aspen – but do not feed from them (‘epiphytic’ lichens). Because of this, the presence and abundance of aspen is a useful indicator of boreal forest biodiversity, and the species richness and community composition of epiphytic lichens can tell us much about the influencing factors in their environment (humidity, tree density and age, light availability and more). However, intensive forestry practices are causing aspen populations to decline, leading to a subsequent loss of lichen habitat.
To reliably detect patterns and trends in biodiversity, standardised measures and tools are required. The use of remote sensing methods for this purpose is increasing, with ‘light detection and ranging’ (LiDAR) and hyperspectral imaging being increasingly used to hunt for diversity in habitats, plant species and plant characteristics.
This study compares remote sensing methods (LiDAR and hyperspectral imaging) to in-field measurements to assess their predictive accuracy, using these two approaches to evaluate epiphytic lichen species richness and community composition on aspen in Finland (a region containing both protected and non-protected forest).
In-field measurements were taken of four substrate and habitat parameters – aspen diameter and height, bark acidity, and the depth of bark furrows on the trunk – while remote sensing measurements looked at nine factors including mean canopy height and area, surrounding tree species composition, number of trees and distance to the nearest water body.
The researchers sampled 230 aspens and identified 106 different epiphytic lichen species. They found that remote sensing measurements predicted the community composition of lichen species reasonably accurately – with several remote sensing measurements 1 outperforming those taken in the field – implying that airborne LiDAR may be an effective tool for surveying boreal forest biodiversity.
Communities were also predicted more accurately when a wider radius around the sampled aspen (30 metres (m) versus 10 m) was surveyed, implying that it is not only the host tree that defines the environment for lichens, but rather the properties of the surrounding stand. This highlights the cost-efficiency of remote sensing, say the researchers, when considering the expense of covering a similar area in the field.
While the make-up of lichen communities was affected most strongly by parameters indicating tree and forest maturity – tree and canopy height, tree diameter and deeper furrow depths – lichen species richness was directly connected to the pH of tree bark. Protected and non-protected areas differed from each other in species richness; however, bark pH was the only parameter affecting species richness regardless of whether the forest was protected or not, whereas the effects of tree diameter, height and furrow depth were only significant in protected areas. Protected areas hosted a higher tree-specific number of epiphytic lichen species and ‘red listed’ species , than non-protected groups 2 .
While a field survey is always required to confirm the presence or absence of lichen species, the results support the potential cost and efficiency benefits of using remote sensing to map potential high-biodiversity habitats, and highlight the importance of Europe’s protected areas . The remote sensing approach used in this study was more effective at predicting lichen communities than traditional field methods characterising the suitability of individual trees for lichen growth. The researchers suggest that, as global biodiversity loss accelerates, it is increasingly important to identify the most accurate, efficient ways to map possible biodiversity hotspots.
- Mean canopy height, tree density, dominant tree species and tree-stand species diversity.
- The researchers posit that this may be due to the older age of trees in protected areas, which have been able to enjoy longer, undisturbed histories that allow lichen to grow.
Palmroos, I., Norros, V., Keski-Saari, S., Mäyrä, J., Tanhuanpää, T., Kivinen, S., Pykälä, J., Kullberg, P., Kumpula, T., and Vihervaara, P. (2023) Remote sensing in mapping biodiversity – A case study of epiphytic lichen communities. Forest Ecology and Management 538: 120993. Available from: https://doi.org/10.1016/j.foreco.2023.120993
To cite this article/service:
“ Science for Environment Policy ”: European Commission DG Environment News Alert Service, edited by the Science Communication Unit, The University of the West of England, Bristol.
Notes on content:
The contents and views included in Science for Environment Policy are based on independent, peer reviewed research and do not necessarily reflect the position of the European Commission. Please note that this article is a summary of only one study. Other studies may come to other conclusions.
EU Environment newsletter
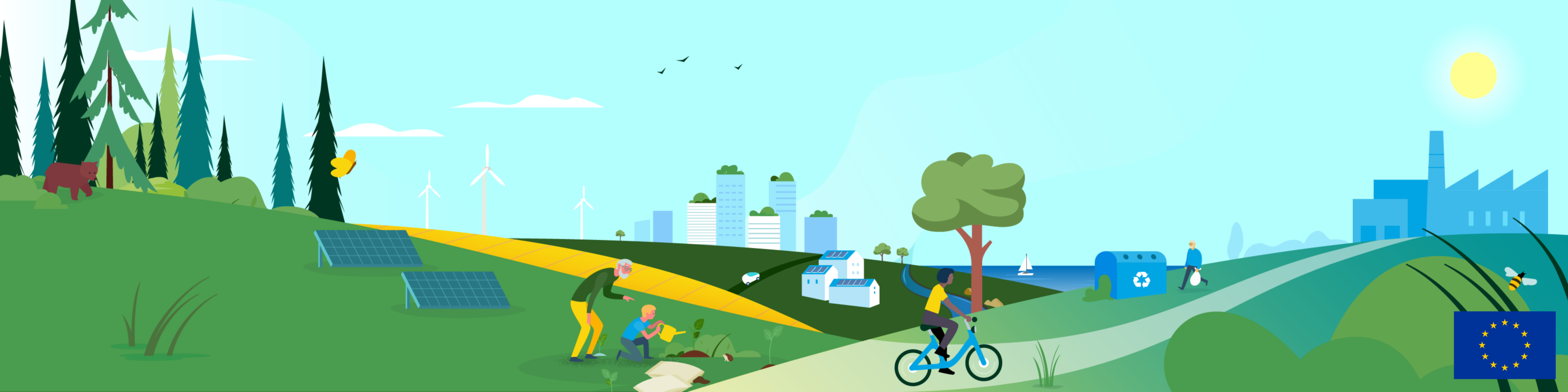
EU Environment newsletters deliver the latest updates about the European Commission’s environmental priorities straight to your inbox.
Share this page
Boreal Forest Provides Hope in the Face of an Insect Apocalypse

Watching Cliff Swallows dart from their gourd-shaped nests under the eaves of the barn searching for insects to feed their rapidly growing young is one of my favorite memories from being a teenager growing up in Maine. Although that same barn still stands in the same place, essentially the same as it was 40 years ago, the swallows are gone now. They’ve been gone for several decades in fact, and they are gone from most of their former nesting places in New England. Cliff Swallows are only one of a group of insect-eating birds in North America that have undergone major declines in the last half-century.
An article written by Brooke Jarvis for the New York Times Magazine several years ago entitled “Insect Apocalypse” helps explain why. In it, Jarvis describes the mounting evidence that the overall numbers of insects on the planet have drastically declined in recent decades. The first major awareness of this problem came from a 2016 academic paper that reported the overall abundance of insects had plummeted by more than 70% in 63 German nature reserves surveyed over the last 27 years.
These alarming drops have major implications for migratory birds and other species that depend on insects. Luckily, the research also points to a powerful solution: conserving large expanses of healthy lands and waterways where living things, including insects, can find refuge.
Places like the North American Boreal Forest (in Canada and Alaska) where there are still an estimated 1.2 billion acres of largely intact forests and wetlands interconnected with countless streams, rivers, lakes, and ponds are especially critical to protect. This massive expanse of vital habitat is estimated to support between one and three billion nesting birds—birds that rely upon an abundance of insects to survive and raise their young.
Insects are an essential part of a healthy planet. As the base of the food chain, abundant insects are required to support the billions of birds, fish, and other animals that rely on them. Bird researchers have independently discovered that some of the fastest declining birds in North America are birds that capture insects in flight—technically known as “aerial insectivores.” This group includes the aptly named flycatchers as well as swallows and nightjars (birds like nighthawks and whip-poor-wills). Sadly, Canada’s official list of federally threatened species now reads like a who’s who of aerial insectivores including the Bank and Barn swallows, Olive-sided Flycatcher, the Common Nighthawk, and the Eastern Whip-poor-will.
At a time when Canada, the U.S., and nations around the globe are striving to reach their international commitment to protect at least 30% of their lands and waters by 2030, moving proposed protected areas more quickly to the finish line needs to be a priority. In Canada, Indigenous governments and communities are leading the way with a multitude of land-use plans and Indigenous Protected Area proposals already on the table or in process.
When humanity is confronted with problems like the “insect apocalypse” and all its implications for the health and future of our world, all of us from individuals up to governments, need to express our support for expediting conservation. Let’s show our support and get behind the Indigenous conservation work happening in the Boreal Forest region of Canada and around the world.
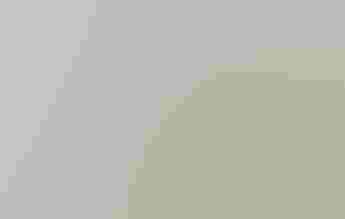
Pledge to stand with Audubon to call on elected officials to listen to science and work towards climate solutions.
May 7, 2024
Scientists Warn against Treating Forests as Carbon Commodities
Using forests to prop up carbon markets can lead to “perverse effects” on land management, such as cutting out local communities
By Chelsea Harvey & E&E News
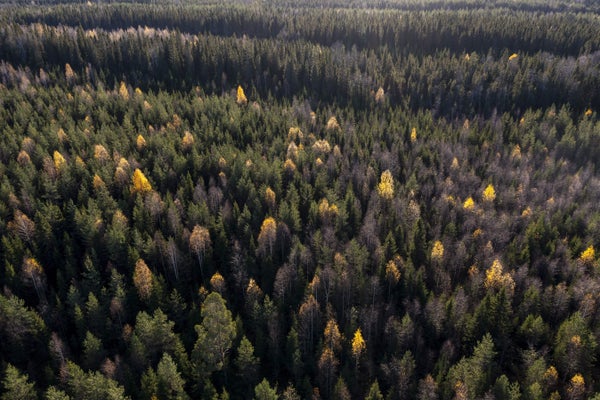
This aerial view shows the Boreal Forest, above The Arctic Circle in Finnish Lapland on October 6, 2022.
Oliver Morin/AFP via Getty Images
CLIMATEWIRE | Growing alarm over climate change has pushed world leaders in recent years to see Earth's forests as a critical resource in the fight against global warming.
But the newfound attention might not always be a good thing. The focus on forests and their value as carbon sinks could be contributing to an increase in global inequalities and create too much reliance on market-based solutions, such as carbon offsets.
The warning was included in a new report from the International Union of Forest Research Organizations, a nonprofit network of forest scientists. Published Monday, the report provides a scientific review of recent trends in global forest governance.
On supporting science journalism
If you're enjoying this article, consider supporting our award-winning journalism by subscribing . By purchasing a subscription you are helping to ensure the future of impactful stories about the discoveries and ideas shaping our world today.
The findings will be officially launched Friday at the U.N. Forum on Forests in New York.
There's been growing recognition about the role that forests play in the climate fight, the report notes. Trees naturally draw carbon dioxide out of the atmosphere and store it away. But cutting them down releases the carbon back into the air.
Their value as carbon sinks has fueled an increased urgency in global efforts to halt deforestation. That, in turn, has led to a flood of global and regional pledges and targets aimed at preserving the world’s trees.
But focusing entirely on their carbon value could risk neglecting the other benefits that forests provide, such as their cultural importance to Indigenous communities.
Most critiques of international forest governance and management are focused almost entirely on deforestation rates. But that shows “a limited awareness of the diversity of needs and demands connected to forests globally,” the report warns.
Forest managers should investigate other metrics of success or failure, such as the social impacts of forest policies on the communities that depend on them.
The focus on carbon also has contributed to the growing popularity of market-based governance techniques, such as forest carbon markets — a system in which corporations can pay forest managers to preserve trees in exchange for carbon offsets.
Yet experts have warned that many carbon offset schemes may not be as effective as they claim — both at reducing emissions and at protecting trees. One recent study looked at 26 carbon offset projects around the world and found that most have not significantly reduced deforestation rates.
In addition, forest carbon markets can shut local communities out of forest management discussions in their home regions. And they run the risk of prioritizing short-term financial gain over long-term advances in sustainable forest management, the report warns.
These kinds of market-based incentives “risk perpetuating inequalities and producing perverse effects on sustainable forest management,” said report co-author Constance McDermott, a social scientist and expert on forest governance at the University of Oxford, in a statement. “Non-market-based mechanisms such as state regulation and community-led initiatives offer important alternative pathways for just forest governance.”
So while combating climate change is a crucial component of global forest management, the report suggests, it shouldn’t be the only priority.
“Measuring governance has mainly been related to the deforestation rate as the main indicator,” said study co-author Daniela Kleinschmit, vice president of IUFRO, in a statement. “However, forests provide many goods and services essential for people, which is why the effectiveness of international forest governance should also be measured against these needs.”
Reprinted from E&E News with permission from POLITICO, LLC. Copyright 2024. E&E News provides essential news for energy and environment professionals.
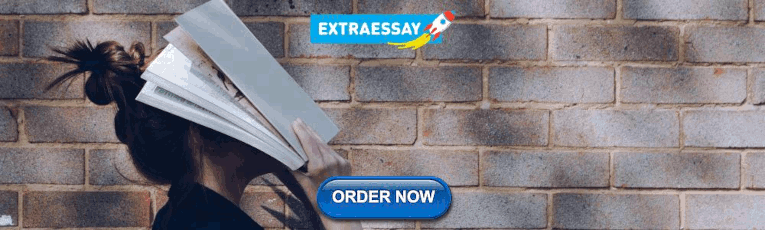
COMMENTS
Boreal forests are defined as forests growing in high-latitude environments where freezing temperatures occur for 6 to 8 months and in which trees are capable of reaching a minimum height of 5 m and a canopy cover of 10% ().Boreal forest ecosystems have evolved under the constraints imposed by a short growing season and severe winters during which snow cover may last for several months (2, 11).
Boreal forests or taiga (as it is called in Russia) is the earth's second largest land biome after desert and xeric shrub lands covering about 17 million square kilometers representing 29% of global forest area.The boreal forest covers most of inland Canada and Alaska; most of Sweden, Finland, and inland Norway; large area of Russia; and the northern parts of Kazakhstan, Mongolia, and Japan.
The sensitivity of forests to near-term warming and associated precipitation shifts remains uncertain 1,2,3,4,5,6,7,8,9.Herein, using a 5-year open-air experiment in southern boreal forest, we ...
1 INTRODUCTION. Boreal forests are expansive carbon (C) rich landscapes that serve as strong net C sinks, and therefore play a pivotal role in the global C cycle (Watts et al., 2023).They cover approximately 17% of the terrestrial land surface area, and account for somewhere between 20% to 40% of terrestrial C stocks (Anderson, 1991; Bradshaw & Warkentin, 2015; Lorenz et al., 2010; Tarnocai et ...
Map showing the global extent of the boreal zone. Darker green represents denser forest cover (Data source: Global Land Cover Facility, Tree Canopy Cover 2010; figure reused with permission from [5])
PDF | On Jan 1, 2020, Imrul Kayes and others published Boreal Forests: Distributions, Biodiversity, and Management | Find, read and cite all the research you need on ResearchGate
The boreal forest of the Northwest Territories, Canada comprises nearly 20% of the Canadian boreal forest and 5% of the global boreal forest and has carbon-rich soils that can store up to 75 ...
Abstract. Boreal forests are the northernmost forests on the planet, dominated by species of spruce, fir, larch, pine, birch, and aspen, with large tracts of intact primary forest, as well as industrial forests, frequently logged for timber and pulp for paper and packaging products. Natural disturbance regimes include large, high-severity crown ...
Deciduous tree cover is expected to increase in North American boreal forests with climate warming and wildfire. ... Ontario Forest Research ... R.M., S.J.G., B.M.R. and L.T.B. wrote the paper ...
Boreal forests, which cover some 16.6 million km 2 across the circumpolar region and contain roughly two-thirds of global forest carbon (5, 6), have the potential to play an outsized role in future fire-related emissions. Across the boreal biome, societies manage wildfires to protect human life and infrastructure; however, carbon and climate ...
These forests account for 33% of lumber and 25% of paper products within the global export market (Burton et al., 2010). ... Even if restoration represents a major challenge in boreal forests, the research effort in this field is limited relative to that in other biomes, e.g., tropical forests. ...
Introduction. Boreal forests are amongst the largest ecosystems on earth (Brandt et al. 2013) and contain a third of the global terrestrial biogenic carbon stock (Pan et al. 2011).A large part of the boreal forest biome hosts management activities that account for a third of the lumber produced worldwide (Gauthier et al. 2015).In Canada, ~70% of the forest extent lies within the boreal zone ...
From those identified by the keyword searches, we selected the research papers of most relevance to our study objectives, i.e., (i) those of conceptual relevance to the development of the field of forest succession, (ii) studies of North American boreal forest ecosystem successional dynamics, and (iii) studies focused on synthesizing methods ...
Addressing modern challenges, e.g. managing vulnerable boreal forests for adaptation to climate change, is an important aspect throughout the volume. ... Many of his research papers further address the problems on how forest management and forests should be adapted to climate warming in the boreal conditions, and how management might be used to ...
Boreal forests cover over 1 billion ha, an area larger than the total land area of China1. Based on data from the Food and Agriculture Organization of the UN (FAO), the deforestation ... Areas offer hope for biodiversity and advancing reconciliation in the Canadian boreal forest. NRC Research Press, 200-201. 13. UNESCO, 2021. Pimachiowin Aki ...
In 2021, boreal fires generated peak CO 2 emissions of 0.48 Gt C (0.17 Gt C from boreal North America and 0.31 Gt C from boreal Eurasia), which is 150% (equivalent to 0.29 Gt) greater than the annual mean emissions between 2000 and 2020, surpassing three standard deviations above the 2000 to 2020 average boreal fire emissions.
This paper reports on the boreal forest, a broad, circumpolar mixture of cool coniferous and deciduous tree species which covers over 14.7 million km{sup 2}, or 11%, of the earth's terrestrial ...
Introduction. Boreal forests account for approximately one-third of the world's forest cover (UNEP et al. 2009).These forests are a major source of timber products, but also provide a range of other goods and services that are essential to human well-being (Vanhanen et al. 2012; Brandt et al. 2013; Gauthier et al. 2015).In general, the multifunctional role of forests is widely recognized ...
11 articles that review and synthesize boreal forest research. The first paper summarizes the current state of Canada's boreal zone and puts the remaining articles in context. An introduction to Canada's boreal zone: Ecosystem processes, health, sustainability, and environmental issues (2013) Anticipating the consequences of climate change ...
Feature papers represent the most advanced research with significant potential for high impact in the field. A Feature Paper should be a substantial original Article that involves several techniques or approaches, provides an outlook for future research directions and describes possible research applications. ... In boreal forests, the pH and ...
The boreal forest is one of the world's largest terrestrial biome and plays crucial roles in global biogeochemical cycles, such as carbon (C) sequestration in vegetation and soil. However, the impacts of decades of N deposition on N-limited ecosystems, like the eastern Canadian boreal forest, remain unclear.
Boreal forests provide critical services to local, regional, and global populations. Communities, including those of indigenous people, benefit from ecosystem services provided by the forest for fishing, hunting, leisure, spiritual activities, and economic opportunities. Internationally, more than 33% of the lumber and 25% of the paper on the ...
Here, to understand whether and how bats respond to the presence of wind turbines in the boreal forests, we studied bat acoustic activity of the most common species ( Eptesicus nilssonii) and species group ( Myotis spp .) in Finland, at a landscape scale, up to 1,000 m from operating turbines. The study made use of passive recorders to sample ...
However, the value for 1990-2008 falls within the range reported by previous studies49,59. For North American boreal forests, our estimation was 57.1 Tg C year−1for 1986-2016 and 44.9 for ...
Background Boreal regions are warming at more than double the global average, creating opportunities for the northward expansion of agriculture. Expanding agricultural production in these regions will involve the conversion of boreal forests to agricultural fields, with cumulative impacts on soil microbial communities and associated biogeochemical cycling processes. Understanding the magnitude ...
Semantic Scholar extracted view of "Recent advances in studying vegetation at forest edges" by Christina L. Rinas et al. ... Comparison of edge effects from well pads and industrial roads on mixed upland boreal forest vegetation in Alberta. ... AI-powered research tool for scientific literature, based at the Allen Institute for AI. Learn More.
Alaska's boreal forest is facing unprecedented challenges under rapid climate warming (increasingly severe fires, droughts, pest/disease outbreaks) that may destabilize its function as a global carbon sink. Forests near Fairbanks may be especially vulnerable, impacting air quality and ecosystem services. We combined GT (ground truthing) with Airborne Visible InfraRed Imaging Spectrometer ...
The European aspen (Populus tremula L.) is a keystone species in boreal forests and increases forest biodiversity by hosting accompanying species such as fungi, mosses, woodpeckers, small mammals and invertebrates.These species include lichens, which grow on the trees - many exclusively on aspen - but do not feed from them ('epiphytic' lichens).
Luckily, the research also points to a powerful solution: conserving large expanses of healthy lands and waterways where living things, including insects, can find refuge. ... Places like the North American Boreal Forest (in Canada and Alaska) where there are still an estimated 1.2 billion acres of largely intact forests and wetlands ...
The warning was included in a new report from the International Union of Forest Research Organizations, a nonprofit network of forest scientists. Published Monday, the report provides a scientific ...