Log in using your username and password
- Search More Search for this keyword Advanced search
- Latest content
- Global health
- BMJ Journals More You are viewing from: Google Indexer
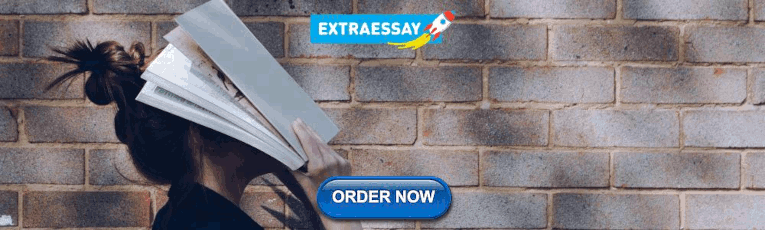
You are here
- Volume 13, Issue 8
- Clinical course of a 66-year-old man with an acute ischaemic stroke in the setting of a COVID-19 infection
- Article Text
- Article info
- Citation Tools
- Rapid Responses
- Article metrics

- http://orcid.org/0000-0002-7441-6952 Saajan Basi 1 , 2 ,
- Mohammad Hamdan 1 and
- Shuja Punekar 1
- 1 Department of Stroke and Acute Medicine , King's Mill Hospital , Sutton-in-Ashfield , UK
- 2 Department of Acute Medicine , University Hospitals of Derby and Burton , Derby , UK
- Correspondence to Dr Saajan Basi; saajan.basi{at}nhs.net
A 66-year-old man was admitted to hospital with a right frontal cerebral infarct producing left-sided weakness and a deterioration in his speech pattern. The cerebral infarct was confirmed with CT imaging. The only evidence of respiratory symptoms on admission was a 2 L oxygen requirement, maintaining oxygen saturations between 88% and 92%. In a matter of hours this patient developed a greater oxygen requirement, alongside reduced levels of consciousness. A positive COVID-19 throat swab, in addition to bilateral pneumonia on chest X-ray and lymphopaenia in his blood tests, confirmed a diagnosis of COVID-19 pneumonia. A proactive decision was made involving the patients’ family, ward and intensive care healthcare staff, to not escalate care above a ward-based ceiling of care. The patient died 5 days following admission under the palliative care provided by the medical team.
- respiratory medicine
- infectious diseases
- global health
This is an open access article distributed in accordance with the Creative Commons Attribution Non Commercial (CC BY-NC 4.0) license, which permits others to distribute, remix, adapt, build upon this work non-commercially, and license their derivative works on different terms, provided the original work is properly cited and the use is non-commercial. See: http://creativecommons.org/licenses/by-nc/4.0/ .
https://doi.org/10.1136/bcr-2020-235920
Statistics from Altmetric.com
Request permissions.
If you wish to reuse any or all of this article please use the link below which will take you to the Copyright Clearance Center’s RightsLink service. You will be able to get a quick price and instant permission to reuse the content in many different ways.
SARS-CoV-2 (Severe Acute Respiratory Syndrome Coronavirus 2) is a new strain of coronavirus that is thought to have originated in December 2019 in Wuhan, China. In a matter of months, it has erupted from non-existence to perhaps the greatest challenge to healthcare in modern times, grinding most societies globally to a sudden halt. Consequently, the study and research into SARS-CoV-2 is invaluable. Although coronaviruses are common, SARS-CoV-2 appears to be considerably more contagious. The WHO figures into the 2003 SARS-CoV-1 outbreak, from November 2002 to July 2003, indicate a total of 8439 confirmed cases globally. 1 In comparison, during a period of 4 months from December 2019 to July 2020, the number of global cases of COVID-19 reached 10 357 662, increasing exponentially, illustrating how much more contagious SARS-CoV-2 has been. 2
Previous literature has indicated infections, and influenza-like illness have been associated with an overall increase in the odds of stroke development. 3 There appears to be a growing correlation between COVID-19 positive patients presenting to hospital with ischaemic stroke; however, studies investigating this are in progress, with new data emerging daily. This patient report comments on and further characterises the link between COVID-19 pneumonia and the development of ischaemic stroke. At the time of this patients’ admission, there were 95 positive cases from 604 COVID-19 tests conducted in the local community, with a predicted population of 108 000. 4 Only 4 days later, when this patient died, the figure increased to 172 positive cases (81% increase), illustrating the rapid escalation towards the peak of the pandemic, and widespread transmission within the local community ( figure 1 ). As more cases of ischaemic stroke in COVID-19 pneumonia patients arise, the recognition and understanding of its presentation and aetiology can be deciphered. Considering the virulence of SARS-CoV-2 it is crucial as a global healthcare community, we develop this understanding, in order to intervene and reduce significant morbidity and mortality in stroke patients.
- Download figure
- Open in new tab
- Download powerpoint
A graph showing the number of patients with COVID-19 in the hospital and in the community over time.
Case presentation
A 66-year-old man presented to the hospital with signs of left-sided weakness. The patient had a background of chronic obstructive pulmonary disease (COPD), atrial fibrillation and had one previous ischaemic stroke, producing left-sided haemiparesis, which had completely resolved. He was a non-smoker and lived in a house. The patient was found slumped over on the sofa at home on 1 April 2020, by a relative at approximately 01:00, having been seen to have no acute medical illness at 22:00. The patients’ relative initially described disorientation and agitation with weakness noted in the left upper limb and dysarthria. At the time of presentation, neither the patient nor his relative identified any history of fever, cough, shortness of breath, loss of taste, smell or any other symptoms; however, the patient did have a prior admission 9 days earlier with shortness of breath.
The vague nature of symptoms, entwined with considerable concern over approaching the hospital, due to the risk of contracting COVID-19, created a delay in the patients’ attendance to the accident and emergency department. His primary survey conducted at 09:20 on 1 April 2020 demonstrated a patent airway, with spontaneous breathing and good perfusion. His Glasgow Coma Scale (GCS) score was 15 (a score of 15 is the highest level of consciousness), his blood glucose was 7.2, and he did not exhibit any signs of trauma. His abbreviated mental test score was 7 out of 10, indicating a degree of altered cognition. An ECG demonstrated atrial fibrillation with a normal heart rate. His admission weight measured 107 kg. At 09:57 the patient required 2 L of nasal cannula oxygen to maintain his oxygen saturations between 88% and 92%. He started to develop agitation associated with an increased respiratory rate at 36 breaths per minute. On auscultation of his chest, he demonstrated widespread coarse crepitation and bilateral wheeze. Throughout he was haemodynamically stable, with a systolic blood pressure between 143 mm Hg and 144 mm Hg and heart rate between 86 beats/min and 95 beats/min. From a neurological standpoint, he had a mild left facial droop, 2/5 power in both lower limbs, 2/5 power in his left upper limb and 5/5 power in his right upper limb. Tone in his left upper limb had increased. This patient was suspected of having COVID-19 pneumonia alongside an ischaemic stroke.
Investigations
A CT of his brain conducted at 11:38 on 1 April 2020 ( figure 2 ) illustrated an ill-defined hypodensity in the right frontal lobe medially, with sulcal effacement and loss of grey-white matter. This was highly likely to represent acute anterior cerebral artery territory infarction. Furthermore an oval low-density area in the right cerebellar hemisphere, that was also suspicious of an acute infarction. These vascular territories did not entirely correlate with his clinical picture, as limb weakness is not as prominent in anterior cerebral artery territory ischaemia. Therefore this left-sided weakness may have been an amalgamation of residual weakness from his previous stroke, in addition to his acute cerebral infarction. An erect AP chest X-ray with portable equipment ( figure 3 ) conducted on the same day demonstrated patchy peripheral consolidation bilaterally, with no evidence of significant pleural effusion. The pattern of lung involvement raised suspicion of COVID-19 infection, which at this stage was thought to have provoked the acute cerebral infarct. Clinically significant blood results from 1 April 2020 demonstrated a raised C-reactive protein (CRP) at 215 mg/L (normal 0–5 mg/L) and lymphopaenia at 0.5×10 9 (normal 1×10 9 to 3×10 9 ). Other routine blood results are provided in table 1 .
CT imaging of this patients’ brain demonstrating a wedge-shaped infarction of the anterior cerebral artery territory.
Chest X-ray demonstrating the bilateral COVID-19 pneumonia of this patient on admission.
- View inline
Clinical biochemistry and haematology blood results of the patient
Interestingly the patient, in this case, was clinically assessed in the accident and emergency department on 23 March 2020, 9 days prior to admission, with symptoms of shortness of breath. His blood results from this day showed a CRP of 22 mg/L and a greater lymphopaenia at 0.3×10 9 . He had a chest X-ray ( figure 4 ), which indicated mild radiopacification in the left mid zone. He was initially treated with intravenous co-amoxiclav and ciprofloxacin. The following day he had minimal symptoms (CURB 65 score 1 for being over 65 years). Given improving blood results (declining CRP), he was discharged home with a course of oral amoxicillin and clarithromycin. As national governmental restrictions due to COVID-19 had not been formally announced until 23 March 2020, and inconsistencies regarding personal protective equipment training and usage existed during the earlier stages of this rapidly evolving pandemic, it is possible that this patient contracted COVID-19 within the local community, or during his prior hospital admission. It could be argued that the patient had early COVID-19 signs and symptoms, having presented with shortness of breath, lymphopaenia, and having had subtle infective chest X-ray changes. The patient explained he developed a stagnant productive cough, which began 5 days prior to his attendance to hospital on 23 March 2020. He responded to antibiotics, making a full recovery following 7 days of treatment. This information does not assimilate with the typical features of a COVID-19 infection. A diagnosis of community-acquired pneumonia or infective exacerbation of COPD seem more likely. However, given the high incidence of COVID-19 infections during this patients’ illness, an exposure and early COVID-19 illness, prior to the 23 March 2020, cannot be completely ruled out.
Chest X-ray conducted on prior admission illustrating mild radiopacification in the left mid zone.
On the current admission, this patient was managed with nasal cannula oxygen at 2 L. By the end of the day, this had progressed to a venturi mask, requiring 8 L of oxygen to maintain oxygen saturation. He had also become increasingly drowsy and confused, his GCS declined from 15 to 12. However, the patient was still haemodynamically stable, as he had been in the morning. An arterial blood gas demonstrated a respiratory alkalosis (pH 7.55, pCO 2 3.1, pO 2 6.7 and HCO 3 24.9, lactate 1.8, base excess 0.5). He was commenced on intravenous co-amoxiclav and ciprofloxacin, to treat a potential exacerbation of COPD. This patient had a COVID-19 throat swab on 1 April 2020. Before the result of this swab, an early discussion was held with the intensive care unit staff, who decided at 17:00 on 1 April 2020 that given the patients presentation, rapid deterioration, comorbidities and likely COVID-19 diagnosis he would not be for escalation to the intensive care unit, and if he were to deteriorate further the end of life pathway would be most appropriate. The discussion was reiterated to the patients’ family, who were in agreement with this. Although he had evidence of an ischaemic stroke on CT of his brain, it was agreed by all clinicians that intervention for this was not as much of a priority as providing optimal palliative care, therefore, a minimally invasive method of treatment was advocated by the stroke team. The patient was given 300 mg of aspirin and was not a candidate for fibrinolysis.
Outcome and follow-up
The following day, before the throat swab result, had appeared the patient deteriorated further, requiring 15 L of oxygen through a non-rebreather face mask at 60% FiO 2 to maintain his oxygen saturation, at a maximum of 88% overnight. At this point, he was unresponsive to voice, with a GCS of 5. Although, he was still haemodynamically stable, with a blood pressure of 126/74 mm Hg and a heart rate of 98 beats/min. His respiratory rate was 30 breaths/min. His worsening respiratory condition, combined with his declining level of consciousness made it impossible to clinically assess progression of the neurological deficit generated by his cerebral infarction. Moreover, the patient was declining sharply while receiving the maximal ward-based treatment available. The senior respiratory physician overseeing the patients’ care decided that a palliative approach was in this his best interest, which was agreed on by all parties. The respiratory team completed the ‘recognising dying’ documentation, which signified that priorities of care had shifted from curative treatment to palliative care. Although the palliative team was not formally involved in the care of the patient, the patient received comfort measures without further attempts at supporting oxygenation, or conduction of regular clinical observations. The COVID-19 throat swab confirmed a positive result on 2 April 2020. The patient was treated by the medical team under jurisdiction of the hospital palliative care team. This included the prescribing of anticipatory medications and a syringe driver, which was established on 3 April 2020. His antibiotic treatment, non-essential medication and intravenous fluid treatment were discontinued. His comatose condition persisted throughout the admission. Once the patients’ GCS was 5, it did not improve. The patient was pronounced dead by doctors at 08:40 on 5 April 2020.
SARS-CoV-2 is a type of coronavirus that was first reported to have caused pneumonia-like infection in humans on 3 December 2019. 5 As a group, coronaviruses are a common cause of upper and lower respiratory tract infections (especially in children) and have been researched extensively since they were first characterised in the 1960s. 6 To date, there are seven coronaviruses that are known to cause infection in humans, including SARS-CoV-1, the first known zoonotic coronavirus outbreak in November 2002. 7 Coronavirus infections pass through communities during the winter months, causing small outbreaks in local communities, that do not cause significant mortality or morbidity.
SARS-CoV-2 strain of coronavirus is classed as a zoonotic coronavirus, meaning the virus pathogen is transmitted from non-humans to cause disease in humans. However the rapid spread of SARS-CoV-2 indicates human to human transmission is present. From previous research on the transmission of coronaviruses and that of SARS-CoV-2 it can be inferred that SARS-CoV-2 spreads via respiratory droplets, either from direct inhalation, or indirectly touching surfaces with the virus and exposing the eyes, nose or mouth. 8 Common signs and symptoms of the COVID-19 infection identified in patients include high fevers, severe fatigue, dry cough, acute breathing difficulties, bilateral pneumonia on radiological imaging and lymphopaenia. 9 Most of these features were identified in this case study. The significance of COVID-19 is illustrated by the speed of its global spread and the potential to cause severe clinical presentations, which as of April 2020 can only be treated symptomatically. In Italy, as of mid-March 2020, it was reported that 12% of the entire COVID-19 positive population and 16% of all hospitalised patients had an admission to the intensive care unit. 10
The patient, in this case, illustrates the clinical relevance of understanding COVID-19, as he presented with an ischaemic stroke underlined by minimal respiratory symptoms, which progressed expeditiously, resulting in acute respiratory distress syndrome and subsequent death.
Our case is an example of a new and ever-evolving clinical correlation, between patients who present with a radiological confirmed ischaemic stroke and severe COVID-19 pneumonia. As of April 2020, no comprehensive data of the relationship between ischaemic stroke and COVID-19 has been published, however early retrospective case series from three hospitals in Wuhan, China have indicated that up to 36% of COVID-19 patients had neurological manifestations, including stroke. 11 These studies have not yet undergone peer review, but they tell us a great deal about the relationship between COVID-19 and ischaemic stroke, and have been used to influence the American Heart Associations ‘Temporary Emergency Guidance to US Stroke Centres During the COVID-19 Pandemic’. 12
The relationship between similar coronaviruses and other viruses, such as influenza in the development of ischaemic stroke has previously been researched and provide a basis for further investigation, into the prominence of COVID-19 and its relation to ischaemic stroke. 3 Studies of SARS-CoV-2 indicate its receptor-binding region for entry into the host cell is the same as ACE2, which is present on endothelial cells throughout the body. It may be the case that SARS-CoV-2 alters the conventional ability of ACE2 to protect endothelial function in blood vessels, promoting atherosclerotic plaque displacement by producing an inflammatory response, thus increasing the risk of ischaemic stroke development. 13
Other hypothesised reasons for stroke development in COVID-19 patients are the development of hypercoagulability, as a result of critical illness or new onset of arrhythmias, caused by severe infection. Some case studies in Wuhan described immense inflammatory responses to COVID-19, including elevated acute phase reactants, such as CRP and D-dimer. Raised D-dimers are a non-specific marker of a prothrombotic state and have been associated with greater morbidity and mortality relating to stroke and other neurological features. 14
Arrhythmias such as atrial fibrillation had been identified in 17% of 138 COVID-19 patients, in a study conducted in Wuhan, China. 15 In this report, the patient was known to have atrial fibrillation and was treated with rivaroxaban. The acute inflammatory state COVID-19 is known to produce had the potential to create a prothrombotic environment, culminating in an ischaemic stroke.
Some early case studies produced in Wuhan describe patients in the sixth decade of life that had not been previously noted to have antiphospholipid antibodies, contain the antibodies in blood results. They are antibodies signify antiphospholipid syndrome; a prothrombotic condition. 16 This raises the hypothesis concerning the ability of COVID-19 to evoke the creation of these antibodies and potentiate thrombotic events, such as ischaemic stroke.
No peer-reviewed studies on the effects of COVID-19 and mechanism of stroke are published as of April 2020; therefore, it is difficult to evidence a specific reason as to why COVID-19 patients are developing neurological signs. It is suspected that a mixture of the factors mentioned above influence the development of ischaemic stroke.
If we delve further into this patients’ comorbid state exclusive to COVID-19 infection, it can be argued that this patient was already at a relatively higher risk of stroke development compared with the general population. The fact this patient had previously had an ischaemic stroke illustrates a prior susceptibility. This patient had a known background of hypertension and atrial fibrillation, which as mentioned previously, can influence blood clot or plaque propagation in the development of an acute ischaemic event. 15 Although the patient was prescribed rivaroxaban as an anticoagulant, true consistent compliance to rivaroxaban or other medications such as amlodipine, clopidogrel, candesartan and atorvastatin cannot be confirmed; all of which can contribute to the reduction of influential factors in the development of ischaemic stroke. Furthermore, the fear of contracting COVID-19, in addition to his vague symptoms, unlike his prior ischaemic stroke, which demonstrated dense left-sided haemiparesis, led to a delay in presentation to hospital. This made treatment options like fibrinolysis unachievable, although it can be argued that if he was already infected with COVID-19, he would have still developed life-threatening COVID-19 pneumonia, regardless of whether he underwent fibrinolysis. It is therefore important to consider that if this patient did not contract COVID-19 pneumonia, he still had many risk factors that made him prone to ischaemic stroke formation. Thus, we must consider whether similar patients would suffer from ischaemic stroke, regardless of COVID-19 infection and whether COVID-19 impacts on the severity of the stroke as an entity.
Having said this, the management of these patients is dependent on the likelihood of a positive outcome from the COVID-19 infection. Establishing the ceiling of care is crucial, as it prevents incredibly unwell or unfit patients’ from going through futile treatments, ensuring respect and dignity in death, if this is the likely outcome. It also allows for the provision of limited or intensive resources, such as intensive care beds or endotracheal intubation during the COVID-19 pandemic, to those who are assessed by the multidisciplinary team to benefit the most from their use. The way to establish this ceiling of care is through an early multidisciplinary discussion. In this case, the patient did not convey his wishes regarding his care to the medical team or his family; therefore it was decided among intensive care specialists, respiratory physicians, stroke physicians and the patients’ relatives. The patient was discussed with the intensive care team, who decided that as the patient sustained two acute life-threatening illnesses simultaneously and had rapidly deteriorated, ward-based care with a view to palliate if the further deterioration was in the patients’ best interests. These decisions were not easy to make, especially as it was on the first day of presentation. This decision was made in the context of the patients’ comorbidities, including COPD, the patients’ age, and the availability of intensive care beds during the steep rise in intensive care admissions, in the midst of the COVID-19 pandemic ( figure 1 ). Furthermore, the patients’ rapid and permanent decline in GCS, entwined with the severe stroke on CT imaging of the brain made it more unlikely that significant and permanent recovery could be achieved from mechanical intubation, especially as the damage caused by the stroke could not be significantly reversed. As hospitals manage patients with COVID-19 in many parts of the world, there may be tension between the need to provide higher levels of care for an individual patient and the need to preserve finite resources to maximise the benefits for most patients. This patient presented during a steep rise in intensive care admissions, which may have influenced the early decision not to treat the patient in an intensive care setting. Retrospective studies from Wuhan investigating mortality in patients with multiple organ failure, in the setting of COVID-19, requiring intubation have demonstrated mortality can be up to 61.5%. 17 The mortality risk is even higher in those over 65 years of age with respiratory comorbidities, indicating why this patient was unlikely to survive an admission to the intensive care unit. 18
Regularly updating the patients’ family ensured cooperation, empathy and sympathy. The patients’ stroke was not seen as a priority given the severity of his COVID-19 pneumonia, therefore the least invasive, but most appropriate treatment was provided for his stroke. The British Association of Stroke Physicians advocate this approach and also request the notification to their organisation of COVID-19-related stroke cases, in the UK. 19
Learning points
SARS-CoV-2 (Severe Acute Respiratory Syndrome Coronavirus 2) is one of seven known coronaviruses that commonly cause upper and lower respiratory tract infections. It is the cause of the 2019–2020 global coronavirus pandemic.
The significance of COVID-19 is illustrated by the rapid speed of its spread globally and the potential to cause severe clinical presentations, such as ischaemic stroke.
Early retrospective data has indicated that up to 36% of COVID-19 patients had neurological manifestations, including stroke.
Potential mechanisms behind stroke in COVID-19 patients include a plethora of hypercoagulability secondary to critical illness and systemic inflammation, the development of arrhythmia, alteration to the vascular endothelium resulting in atherosclerotic plaque displacement and dehydration.
It is vital that effective, open communication between the multidisciplinary team, patient and patients relatives is conducted early in order to firmly establish the most appropriate ceiling of care for the patient.
- Cannine M , et al
- Wunderink RG
- van Doremalen N ,
- Bushmaker T ,
- Morris DH , et al
- Wang X-G , et al
- Grasselli G ,
- Pesenti A ,
- Wang M , et al
- American Stroke Assocation, 2020
- Zhang Y-H ,
- Zhang Y-huan ,
- Dong X-F , et al
- Li X , et al
- Hu C , et al
- Zhang S , et al
- Jiang B , et al
- Xu J , et al
- British Association of Stroke Physicians
Contributors SB was involved in the collecting of information for the case, the initial written draft of the case and researching existing data on acute stroke and COVID-19. He also edited drafts of the report. MH was involved in reviewing and editing drafts of the report and contributing new data. SP oversaw the conduction of the project and contributed addition research papers.
Funding The authors have not declared a specific grant for this research from any funding agency in the public, commercial or not-for-profit sectors.
Competing interests None declared.
Patient consent for publication Next of kin consent obtained.
Provenance and peer review Not commissioned; externally peer reviewed.
Read the full text or download the PDF:
We have a new app!
Take the Access library with you wherever you go—easy access to books, videos, images, podcasts, personalized features, and more.
Download the Access App here: iOS and Android . Learn more here!
- Remote Access
- Save figures into PowerPoint
- Download tables as PDFs

Chapter 7: 10 Real Cases on Transient Ischemic Attack and Stroke: Diagnosis, Management, and Follow-Up
Jeirym Miranda; Fareeha S. Alavi; Muhammad Saad
- Download Chapter PDF
Disclaimer: These citations have been automatically generated based on the information we have and it may not be 100% accurate. Please consult the latest official manual style if you have any questions regarding the format accuracy.
Download citation file:
- Search Book
Jump to a Section
Case review, case discussion, clinical symptoms.
- Radiologic Findings
- Full Chapter
- Supplementary Content
Case 1: Management of Acute Thrombotic Cerebrovascular Accident Post Recombinant Tissue Plasminogen Activator Therapy
A 59-year-old Hispanic man presented with right upper and lower extremity weakness, associated with facial drop and slurred speech starting 2 hours before the presentation. He denied visual disturbance, headache, chest pain, palpitations, dyspnea, dysphagia, fever, dizziness, loss of consciousness, bowel or urinary incontinence, or trauma. His medical history was significant for uncontrolled type 2 diabetes mellitus, hypertension, hyperlipidemia, and benign prostatic hypertrophy. Social history included cigarette smoking (1 pack per day for 20 years) and alcohol intake of 3 to 4 beers daily. Family history was not significant, and he did not remember his medications. In the emergency department, his vital signs were stable. His physical examination was remarkable for right-sided facial droop, dysarthria, and right-sided hemiplegia. The rest of the examination findings were insignificant. His National Institutes of Health Stroke Scale (NIHSS) score was calculated as 7. Initial CT angiogram of head and neck reported no acute intracranial findings. The neurology team was consulted, and intravenous recombinant tissue plasminogen activator (t-PA) was administered along with high-intensity statin therapy. The patient was admitted to the intensive care unit where his hemodynamics were monitored for 24 hours and later transferred to the telemetry unit. MRI of the head revealed an acute 1.7-cm infarct of the left periventricular white matter and posterior left basal ganglia. How would you manage this case?
This case scenario presents a patient with acute ischemic cerebrovascular accident (CVA) requiring intravenous t-PA. Diagnosis was based on clinical neurologic symptoms and an NIHSS score of 7 and was later confirmed by neuroimaging. He had multiple comorbidities, including hypertension, diabetes, dyslipidemia, and smoking history, which put him at a higher risk for developing cardiovascular disease. Because his symptoms started within 4.5 hours of presentation, he was deemed to be a candidate for thrombolytics. The eligibility time line is estimated either by self-report or last witness of baseline status.
Ischemic strokes are caused by an obstruction of a blood vessel, which irrigates the brain mainly secondary to the development of atherosclerotic changes, leading to cerebral thrombosis and embolism. Diagnosis is made based on presenting symptoms and CT/MRI of the head, and the treatment is focused on cerebral reperfusion based on eligibility criteria and timing of presentation.
Symptoms include alteration of sensorium, numbness, decreased motor strength, facial drop, dysarthria, ataxia, visual disturbance, dizziness, and headache.
Sign in or create a free Access profile below to access even more exclusive content.
With an Access profile, you can save and manage favorites from your personal dashboard, complete case quizzes, review Q&A, and take these feature on the go with our Access app.
Pop-up div Successfully Displayed
This div only appears when the trigger link is hovered over. Otherwise it is hidden from view.
Please Wait
- Open access
- Published: 06 September 2022
Stroke in young adults, stroke types and risk factors: a case control study
- Priscilla Namaganda 1 ,
- Jane Nakibuuka 2 ,
- Mark Kaddumukasa 3 &
- Elly Katabira 4
BMC Neurology volume 22 , Article number: 335 ( 2022 ) Cite this article
10k Accesses
13 Citations
Metrics details
Stroke is the second leading cause of death above the age of 60 years, and the fifth leading cause in people aged 15 to 59 years old as reported by the World Health Organization global burden of diseases. Stroke in the young is particularly tragic because of the potential to create long-term disability, burden on the victims, their families, and the community at large. Despite this, there is limited data on stroke in young adults, and its risk factors in Uganda. Therefore, we determined the frequency and risk factors for stroke among young adults at Mulago hospital.
A case control study was conducted among patients presenting consecutively to the general medical wards with stroke during the study period September 2015 to March 2016. A brain Computerized Tomography scan was performed to confirm stroke and classify the stroke subtype. Controls were patients that presented to the surgical outpatient clinic with minor surgical conditions, matched for age and sex. Social demographic, clinical and laboratory characteristics were assessed for both cases and controls. Descriptive statistics including frequencies, percentages, means, and standard deviation were used to describe the social demographics of case and controls as well as the stroke types for cases. To determine risk factors for stroke, a conditional logistic regression, which accounts for matching (e.g., age and sex), was applied. Odds ratio (with 95% confidence interval) was used as a measure for associations.
Among 51 patients with stroke, 39(76.5%) had ischemic stroke and 12(23.5%) had hemorrhagic stroke. The mean age was 36.8 years (SD 7.4) for stroke patients (cases) and 36.8 years (SD 6.9) for controls. Female patients predominated in both groups 56.9% in cases and 52.9% in controls. Risk factors noted were HIV infection, OR 3.57 (95% CI 1.16–10.96), elevated waist to hip ratio, OR 11.59(95% CI 1.98–68.24) and sickle cell disease, OR 4.68 (95% CI 1.11–19.70). This study found a protective effect of oral contraceptive use for stroke OR 0.27 95% CI 0.08–0.87. There was no association between stroke and hypertension, diabetes, and hyperlipidemia.
Among young adults with stroke, ischemic stroke predominated over hemorrhagic stroke. Risk factors for stroke were HIV infection, elevated waist to hip ratio and sickle cell disease.
Peer Review reports
Stroke is the second leading cause of death above the age of 60 years, and the fifth leading cause in people aged 15 to 59 years old as reported by the World Health Organization (WHO) global burden of diseases [ 1 ]. The severity of stroke in the young is relatively low in developed countries ranging from 2 -7% in Italy and USA respectively [ 2 , 3 ]. In Africa, on the other hand the prevalence of stroke among young adults is 12.9% in Nigeria [ 4 ], 31% in South Africa [ 5 ], 28.9% in Morocco [ 6 ]. The incidence of ischemic stroke in the young has been increasing globally over the last 2–3 decades. From the Danish National Patient Register, the incidence rates of first‐time hospitalizations for ischemic stroke and transient ischemic attack (TIA) in young adults have increased substantially since the mid 1990s while the incidences of hospitalizations for intracerebral hemorrhage and subarachnoid hemorrhage remained stable during the study period [ 7 ].
In Uganda, literature on stroke in young adults is limited however results of a study done among acute stroke patients admitted to the national referral hospital (Mulago hospital) showed a 30-day mortality of 43.8%. Out of 133 patients, 32 patients (25%) were less than 51 years old. Out of the 56 patients that died, 13 patients (23%) were less than 51 years [ 8 ].
Rapid western cultural adaption (sedentary lifestyle, deleterious health behavior like consumption of tobacco and alcohol and high fat/cholesterol diet) and Human immunodeficiency syndrome/ Acquired immunodeficiency syndrome (HIV/AIDS) that is highly prevalent in Africa has accelerated risk factors and increased the burden of stroke [ 9 ].
Most literature indicates that the traditional risk factors i.e., hypertension, diabetes mellitus and dyslipidemia are still the commonest risk factors with hypertension having the highest frequency. Other risk factors common to the young include smoking, excessive alcohol intake, illicit drug use, oral contraceptive use and migraine [ 10 ].
Although stroke is predominantly a disease of the middle age and the elderly, its occurrence in younger age groups is not rare. Stroke in young adults seems to be increasing and is particularly tragic because of the potential to create long-term disability, burden on the victims, their families, and the community at large such as Uganda. Despite the huge socioeconomic impact of stroke in this age group, there is a scarcity of data regarding stroke in young adults in sub-Saharan Africa including Uganda. Effective stroke prevention strategies in the young require comprehensive information on risk factors and possible causes. Although case reports and etiologic investigations of possible causes of stroke in the young have been identified especially in developed countries, there is limited data on risk factors in Africa Uganda inclusive. Information obtained from this study will fill the knowledge gap in this area of stroke in the young which will inform institutional strategies on prevention and management of stroke in this age group. This study, therefore, seeks to determine the frequency of stroke types and risk factors for this population.
The aims of the study were:
To determine the frequency of stroke types among young adults on the general medical wards in Mulago hospital between September 2015 and March 2016.
To determine the risk factors for stroke (i.e., ischemic, and hemorrhagic stroke) among young adults on the general medical wards in Mulago hospital between September and March 2016.
This was a case control study. Cases were defined as patients with a confirmed diagnosis of stroke by brain computerized tomography (CT) scan that met the inclusion criteria. Controls were defined as patients with minor surgical conditions that met the inclusion criteria. The study was carried out in Mulago hospital which is the national referral hospital in Uganda as well as the teaching hospital of Makerere University College of health sciences. It has a bed capacity of 1500 beds and has both inpatient wards, outpatient departments both for medical and surgical specialties. It has a radiological department with CT scan and highly trained personnel and a well-equipped laboratory. Cases were recruited consecutively from the medical wards specifically on the neurology ward of Mulago hospital. Patients on the neurology ward are managed by physicians that have had additional training in the management of neurological conditions.
Controls were recruited from general surgical outpatient departments from Mulago hospital. They were matched for age and sex. Eligible patients were patients aged 15–45 years, confirmed diagnosis of stroke on brain CT scan and with a written informed consent or assent for patients less than 18 years. These included patients with intracranial hemorrhages and ischemic stroke, none had subarachnoid hemorrhage. Patients were excluded if they were unconscious and with no valid surrogate (next of kin) and HIV positive with opportunistic infections. Patients eligible as control were, patient aged 15–45 years, minor surgical condition, written informed consent or assent for patients less than 18 years. Patients with features of stroke secondary to non-vascular causes like trauma, tumors were excluded as controls. For controls, we chose patients with minor surgical conditions because we wanted controls to be hospital patients but with non-medical conditions that could confound our findings. Such conditions included lacerations, hernias, lipomas, ingrown toenails, circumcision.
Based on the catchment area of Mulago, patients with minor surgical conditions are likely to have similar social economic status and come from similar neighborhoods as would health controls living in the catchment areas as patients with stroke.
The best alternative would have been healthy controls from the neighborhoods of the patients with stroke, but this would have been resource consuming.
The sample size was calculated assuming a prevalence of 62.2% of hypertension among the stroke patients as was indicated in a similar study among the young Thai adults in Bangkok, Thailand (Bandasak et al., 2011) [ 11 ]. We also assumed that the risk for stroke is higher among the hypertensive with an OR of 3. With this sample size, we were powered to detect associations with other risk factors like smoking (OR 2.6) [ 12 ], diabetes (OR 13.2 for black men and 22.1 for black women) [ 13 ].
With these assumptions, a sample size of 51 cases and 51 controls was found sufficient with 80% power and 0.05 level of significance.
Sampling procedure
All young patients admitted on the general medical wards suspected of having stroke were screened and brain CT scan done. Once a diagnosis of stroke was confirmed on CT scan, participants who consented to participate in the study were recruited consecutively, a standardized questionnaire administered by the research team for those patients able to communicate. For patients not able to communicate, consent and information were obtained through the care givers. Controls were selected from the general surgical outpatient clinic using consecutive sampling method. This was done after we had obtained all the cases. These were matched for age and sex until the sample size was accrued.
Information was collected on:
Social demographic characteristics i.e., age, sex, level of education, occupation, religion, history of smoking and alcohol consumption, history of illicit drug use, history of oral contraceptive use.
Clinical examination included general physical examination, blood pressure using a digital blood pressure machine. For patients who were too weak to sit up, blood pressure measurement was taken in supine position. For those able to sit, it was taken in the sitting position. The two blood pressure measurements were taken at an interval of 5 min and the average blood pressure recorded as the final blood pressure.
Physical measurements for the weight and hip were taken using a stretchable tape measure. Waist measurements were taken at the narrowest point-umbilicus and hip measurements at the widest point- buttocks. A waist to hip ratio was obtained and recorded on the questionnaire.
Blood was drawn for laboratory tests; high density lipoprotein, low density lipoprotein (HDL/LDL), fasting blood sugar, full blood count, Hb electrophoresis, prothrombin time/ international normalization ratio (PT/INR), HIV serology, Treponema pallidum hemagglutination (TPHA).
Other information obtained was history and family history of diabetes and hypertension.
The general surgical outpatient clinic runs every Tuesday, and Thursday in Old Mulago hospital Participants were identified at the surgical outpatient clinic. Those matching the age and sex of the cases were recruited, written consent/assent obtained, and questionnaire was administered by the PI. The procedure as explained above was followed for the controls.
Data collection
A pre-tested and standardized questionnaire was used as a data collection tool. The principal investigator administered the questionnaire to the participants in data collection. Data on socio demographics and past medical history was collected.
Results from imaging and laboratory investigations were also recorded into the questionnaire.
Data collected was double entered into the computer using EPI-DATA (version 3.1) software to minimize data entry errors. Data was then backed up and archived in both soft and hard copy to avoid losses. Confidentiality was ensured using code numbers instead of patients’ names. Questionnaires were stored in a lockable cabinet for safety.
Data analysis
Data was analyzed using STATA Version 12 (StataCorp. 2011. Stata Statistical Software: Release 12 . College Station, TX: StataCorp LP). Descriptive statistics were used to describe characteristics of the study participants and the stroke subtypes which included frequencies, percentages, means and standard deviation. To determine factors associated with stroke, a conditional logistic regression, which accounts for matching (e.g., age and sex), was applied. Odds ratio (with 95% confidence interval) was used as a measure for associations. Factors with p -values < 0.2 at a bi-variable analysis were entered into a multiple conditional logistic regression to obtain the adjusted estimates. Factors whose 95% confidence interval for the odds ratio that excludes a 1 or whose p -value < 0.05, were considered statistically significant at the adjusted level. Post-hoc power calculation was performed for the adjusted analysis to check if there was enough power to detect a difference between cases and controls.
Quality control
To ensure quality of results several measures were undertaken, these included:
The questionnaires were pre-tested and standardized before study commenced.
The research team administered the structured, pre- coded and pre-tested questionnaire to enrolled participants on a face-to-face basis and brain CT scans were done by competent and well-trained radiology technicians and interpretation done by a specialist radiologist at the Radiology Department of Mulago hospital.
The questionnaires were checked for completeness at the end of every interview. The two files were compared, and any discordance corrected against data recorded with the questionnaire. The data were then backed up.
Ethical consideration
Written informed consent/ assent was obtained from all participants or their parent/guardian or legal authorized representative to participate in the study. Ethical approval was obtained from Makerere University, school of medicine research and ethics committee (SOMREC) (reference number #REC REF 2015–105).
Confidentiality was ensured using code numbers instead of patients’ names. Questionnaires were stored in a lockable cabinet for safety.
Profile of the study
Enrollment of study participants was carried out between September 2015 to March 2016 in Mulago hospital. The patient flow diagram for cases and controls is as shown in Fig. 1 .
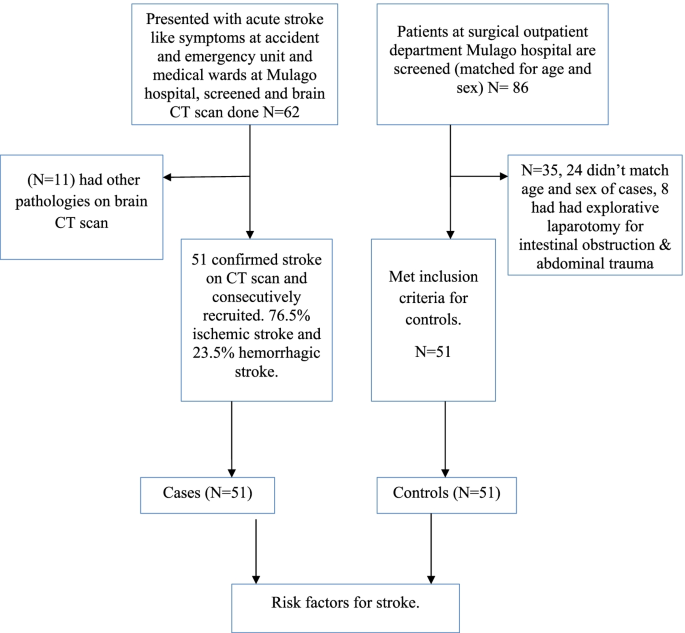
Patient flow diagram
Social demographic characteristics of the study population
A total of 51 cases aged 18 to 45 years and the same number of hospital control matched for age and sex were identified. The mean age of cases was 36.8 years (standard deviation (SD) 7.4) and the control was 36.8 years (SD 6.9). Females predominated in both groups with 56.9% in cases and 52.9% in controls. There was no significant difference in other baseline characteristics between cases and controls except in oral contraceptive use, waist to hip ratio, HIV status and sickle cell disease. Details of the social demographic characteristics are shown in Table 1 .
Clinical characteristics of the study participants
The mean fasting blood sugar was 6.6 (SD 3.9) for cases and 5.3 (SD 0.7) for controls. This was statistically significant with a p value of 0.015. Waist to hip ratio was also statistically significant with a p value of 0.007. Cases with an elevated wait to hip ratio were 14 (27.5%) and controls were 3 (5.9%). Table 2 shows the baseline clinical characteristics of the study participants.
Laboratory characteristics of the study participants
HIV serology and Hb electrophoresis were statistically significant with a p value of 0.076 and 0.023 respectively. 18 patients (35.3%) were reactive for HIV among cases and controls 10 (19.6%). 12 patients (23.5%) had abnormal Hb electrophoresis among cases controls 3 (5.9%). Table 3 shows the laboratory characteristics of the study participants.
Stroke types
Stroke types by social demographic characteristics of cases.
Among 62 patients, who had brain CT scan done, 11 patients had non stroke pathologies (4 had brain abscesses, 7 patients had ring enhancing lesions suggestive of toxoplasmosis). Among 51 patients with stroke confirmed on CT scan, the frequency of ischemic stroke was 76.5% and hemorrhagic stroke was 23.5%.
Most participants with ischemic or hemorrhagic stroke were in the age group 36–45 years. Females predominated in both ischemic and hemorrhagic stroke. Details of the social demographic characteristics by stroke types are shown in Table 4 .
Clinical and laboratory characteristics by stroke types
Majority of patients with hemorrhagic stroke were hypertensive (91.7%) compared to only 25.6% among patients with ischemic stroke. Details of the clinical and laboratory characteristics of the study participants by stroke subtypes are shown in Table 5 .
Risk factors for stroke at univariate analysis
Social demographic characteristics at univariate analysis.
Oral contraceptive use showed a significant difference with an unadjusted OR of 0.27 (95% CI 0.08–0.87) case subjects 23.3% and control subjects 56.5%. Belonging to other religion (seventh day advent, Pentecostal) was statistically significant with a p value of 0.009, OR 0.17. These findings are detailed in Table 6 below.
Clinical characteristics at univariate analysis
There was a significant difference in waist to hip ratio between cases (27.5%) and controls (5.9%), with unadjusted OR 6.85 (CI 1.70–27.62). HIV serology with an unadjusted OR of 2.64 (95% CI 1.03–6.82). Hb electrophoresis with an unadjusted OR of 4.31 (95% CI- 1.15–16.17). Fasting blood sugar with an unadjusted OR of 1.64 (95% CI 1.02–2.62). Details of the above findings are shown in Table 7 below.
Risk factors for stroke at multivariate analysis
At multivariate analysis, HIV serology (OR 3.72, 95% CI 1.16–10.96), waist to hip ratio (OR 11.26 95% CI 1.98–68.24) and sickle cell disease OR 4.78 95% CI 1.11–19.70) were independent risk factors for stroke in young adults. Table 8 shows risk factors at multivariate analysis. None of the patients with HIV met the definition of AIDS as defined by the occurrence of any of the more than 20 life-threatening cancers or “opportunistic infections”, by WHO.
This case–control study showed that the frequency of ischemic stroke was higher than that of hemorrhagic stroke in young Ugandan population. We showed that positive HIV serology, elevated waist to hip ratio and sickle cell disease were independent risk factors for stroke in this population.
This is consistent with several studies that have been done and found ischemic stroke to be more prevalent than hemorrhagic stroke. Studies done in Africa, in Libya reported 77% ischemic stroke and 23% hemorrhagic stroke (these included both intracerebral and subarachnoid hemorrhagic stroke) [ 14 ], in Morocco, 87.3% ischemic stroke and 12.7% hemorrhagic (study did not specify on the subtypes of hemorrhagic stroke) [ 6 ]. In a study from Bosnia and Herzegovina, Subarachnoid hemorrhage was more frequent in young adults compared with older patients (> 45 years of age) (22% vs. 3.5%), intracerebral hemorrhage (ICH) was similar in both groups (16.9% vs. 15.8%), but ischemic stroke (IS) was predominant stroke type in the older group (61% vs. 74%) [ 15 ]. On the other hand, studies focusing on all young stroke patients and including also subarachnoid hemorrhages have found much higher proportion of hemorrhagic strokes in younger vs. older individuals. Population-based studies have reported as low as 57% prevalence for ischemic stroke in those aged > 45, as reported by a recent narrative review [ 16 ]. This difference in occurrence of stroke subtypes could be due to the low prevalence of hypertension in this population in our setting given that hypertension has been reported to be the commonest risk factor for hemorrhagic stroke.
Most previous studies of HIV and stroke have been retrospective, but the prospective studies in Africa and East Africa have reported the importance of HIV as a risk factor for stroke [ 17 ]. A recently published study done in Malawi, with defined cases and population controls and 99% ascertainment of HIV status, reported HIV infection as an independent risk factor for stroke. This study further found that patients who had started standard HIV treatment in the previous six months had a higher risk of stroke (OR 15.6 95% CI 4.21–46.6). This was probably due to an immune reconstitution inflammatory syndrome (IRIS) like process [ 18 ]. A variety of mechanisms have been implicated in the association of HIV and stroke, these include HIV associated vasculopathy, vasculitis which causes abnormality of the intracranial or extracranial cerebral blood vessels and neoplastic involvement. Indirectly through cardioembolic, coagulopathy in association with protein C and protein S deficiency. Some infections are well established causes of stroke, such as Mycobacterium tuberculosi s , syphilis, and varicella zoster virus through increased susceptibility to acquisition or reactivation of these infections [ 19 , 20 ]. Combined antiretroviral therapy (cART) might unmask occult opportunistic infections that subsequently cause a stroke. This possibility should be considered in all patients who have had an acute stroke or have worsening of stroke symptoms after initiation of cART [ 21 ].
An elevated waist to hip ratio (WHR) was associated with 12 times increased risk of stroke among young adults in Mulago hospital compared to individuals with a normal waist to hip ratio. Abdominal obesity (measured as waist–hip ratio) is associated with an increased risk of myocardial infarction, stroke, and premature death [ 22 ]. This agrees with a few studies that have assessed the association of stroke with waist to hip ratio. Aaron et al. 1990, assessed the relation between body fat distribution, and the 2-year incidences of hypertension and stroke in a cohort of 41,837 women aged 55–69 years. Women who developed stroke were 2.1 (95% CI 1.5–2.9) times more likely to have an elevated ratio than those who did not [ 23 ]. Md Habib et al. 2011 assessed high waist to hip ratio as a risk factor for ischemic stroke for overall stroke and he found 64% of the ischemic stroke patient had abnormal WHR in Bangladesh [ 24 ]. Abdominal obesity measured with WHR was an independent risk factor for cryptogenic ischemic stroke (CIS) in young adults after rigorous adjustment for concomitant risk factors in the Revealing the Etiology, Triggers, and Outcome (SECRETO; NCT01934725) study, a prospective case–control study that included patients aged 18–49 years with a first ever CIS at 19 European university centers [ 25 ].
Sickle cell disease was also associated with increased risk of stroke among young adults in Mulago hospital. This agrees with several studies that have associated sickle cell disease with stroke. Ohene et al. 1998 assessed cerebrovascular accidents (CVA) in sickle cell disease, found the highest rates of prevalence of 4.01% and incidence of 0.61 per 100 patient-years. The incidence of hemorrhagic stroke was highest among patients aged 20 to 29 years [ 26 ].
In our study, the unadjusted OR for oral contraceptive use was 0.26 95% CI 0.08–0.87 with a p value of 0.028. This observation at the unadjusted level is significant but could be due to another variable which is a confounder to OC use such as higher socioeconomic status and better control of other possible risk factors.
In our study, we found no association between hypertension and stroke in young adults though it’s an independent risk factor for stroke in the older population. This finding is different from the multinational interstroke study which attributed most strokes among young adults in low- and middle-income countries to hypertension. In that study, only one fifth of the patients were from wealthier African countries where hypertension, diabetes and hypercholesterolemia are likely to occur with higher prevalence than in Mulago hospital [ 27 ]. Other studies have also reported the role of hypertension as a risk factor for stroke in young adults, low physical activity and hypertension were the most important risk factors, accounting for 59.7% and 27.1% of all strokes, respectively among a German nationwide case–control study based on patients enrolled in the SIFAP1 study (Stroke in Young Fabry Patients) 2007 to 2010 and controls from the population-based GEDA study (German Health Update) 2009 to 2010 [ 28 ]. A study that used population-based controls for hospitalized young patients with ischemic stroke demonstrated that independent risk factors for stroke were atrial fibrillation (OR 10.43; cardiovascular disease (OR, 8.01; type 1 diabetes mellitus (OR, 6.72; type 2 diabetes mellitus (OR, 2.31, low high‐density lipoprotein cholesterol (OR, 1.81; current smoking status (OR, 1.81; hypertension (OR, 1.43, and a family history of stroke (OR, 1.37) [ 29 ].
This finding could be explained by the high prevalence of hypertension in the general peri urban Ugandan population among young adults as reported by Kayima et al. 2015. He found a prevalence of 15% (95% CI 14.2 – 19.6%) % for young adults aged 18–44 years [ 30 ].
The study was conducted at Mulago hospital which is a national referral hospital in Uganda situated in central Uganda. Mulago hospital received patients both referred patients from all over Uganda and those from its catchment area. This is generally representative of the whole Ugandan population.
Uganda has a young population and with an HIV prevalence comparable to most countries in Sub-Saharan Africa, so the findings of this study are generalizable to other Sub-Saharan African populations.
Ischemic stroke is more prevalent than hemorrhagic stroke among young adults in Mulago hospital. Independent risk factors for stroke among young adults in Mulago hospital were HIV infection, elevated waist to hip ratio and sickle cell disease. Oral contraceptive use was found to be protective of stroke among young adults in Mulago hospital. There was no significant association between stroke among young adults and hypertension, diabetes, hyperlipidemia, smoking, alcohol use and illicit use.
Study limitations
The sample size was too small to detect all but the strongest associations with common exposures. When designing the study, we based on hypertension as a significant driver for strokes in this population based on other studies done to calculate the sample size, however based on our findings, hypertension was not a big driver of stroke in this population. Secondly the nature of stroke type associated with hypertension is hemorrhagic which were less common in this study. This was an unexpected finding and needs more evaluation.
Consecutive sampling methods has selection bias in which a variable that is associated with the outcome under investigation may occur more frequently or less in those sampled in this period as compared to the general population.
The use of a combined ischemic stroke and intracerebral hemorrhage group may have obscured relationships specific to one group, i.e., the risk factors for stroke were not stratified for type of stroke.
The best alternative for controls would have been healthy controls from the neighborhoods of the patients with stroke, but this would have been resource consuming hence the choice of hospital controls with different medical conditions from cases.
Availability of data and materials
The datasets used and/or analyzed during the current study are available from the corresponding author on reasonable request.
Go AS, Mozaffarian D, Roger VL, Benjamin EJ, Berry JD, Borden WB, Turner MB. Heart disease and stroke statistics—2013 update: a report from the American Heart Association. Circulation. 2013;127(1):e6–245.
PubMed Google Scholar
Griffiths D, Sturm J. Epidemiology and etiology of young stroke. Stroke research and treatment, 2011. 2011.
Google Scholar
Bevan HS, Sharma K, Bradley W. Stroke in young adults. Stroke. 1990;21(3):382–6.
Article CAS Google Scholar
Mustapha AF, Sanya EO, Bello TO. Stroke among young adults at the LAUTECH Teaching Hospital, Osogbo, Nigeria. Nig Q J Hosp Med. 2012;22(3):177–80.
CAS PubMed Google Scholar
Hoffmann M. Stroke in the young: the multiethnic prospective Durban stroke data bank results. J Stroke Cerebrovasc Dis. 1998;7(6):404–13.
Chraa M, Louhab N, Kissani N. Stroke in young adults: about 128 cases. Pan Afr Med J. 2014;17(37).
Tibæk M, Dehlendorff C, Jørgensen HS, Forchhammer HB, Johnsen SP, Kammersgaard LP. Increasing incidence of hospitalization for stroke and transient ischemic attack in young adults: a registry-based study. J Am Heart Assoc. 2016;5(5):e003158. https://doi.org/10.1161/JAHA.115.003158 .
Article PubMed PubMed Central Google Scholar
Kwarisima L, Mukisa R, Nakibuuka J, Matovu S, Katabira E. Thirty-day stroke mortality and associated clinical and laboratory factors among adult stroke patients admitted at Mulago Hospital (Uganda). Afr J Neurol Sci. 2014;33(1):79–86.
Feigin VL, Lawes CM, Bennett DA, Anderson CS. Stroke epidemiology: a review of population-based studies of incidence, prevalence, and case-fatality in the late 20th century. Lancet Neurol. 2003;2(1):43–53.
Article Google Scholar
Katsnelson MJ, Della-Morte D, Rundek T. Stroke in the young. Period Biol. 2012;114(3):347–53.
Bandasak R, Narksawat K, Tangkanakul C, Chinvarun Y, Siri S. Association between hypertension and stroke among young Thai adults in Bangkok, Thailand. Southeast Asian J Trop Med Public Health. 2011;42(5):1241–8 PMID: 22299451.
Bhat VM, Cole JW, Sorkin JD, Wozniak MA, Malarcher AM, Giles WH, Stern BJ, Kittner SJ. Dose-response relationship between cigarette smoking and risk of ischemic stroke in young women. Stroke. 2008;39(9):2439–43. https://doi.org/10.1161/STROKEAHA.107.510073 . Epub 2008 Aug 14. PMID: 18703815; PMCID: PMC3564048.
Rohr J, Kittner S, Feeser B, Hebel JR, Whyte MG, Weinstein A, Sherwin R. Traditional risk factors and ischemic stroke in young adults: the Baltimore-Washington Cooperative Young Stroke Study. Arch Neurol. 1996;53(7):603–7.
Radhakrishnan K, Ashok PP, Sridharan R, Mousa ME. Stroke in the young: incidence and pattern in Benghazi. Libya. 1986;73(4):434–8.
CAS Google Scholar
Smajlović D, Salihović D, Ibrahimagić OĆ, Sinanović O. Characteristics of stroke in young adults in Tuzla Canton Bosnia and Herzegovina. Coll Antropol. 2013;37(2):515–9.
Tatlisumak, et al. Nontraumatic intracerebral haemorrhage in young adults. Nat Rev Neurol. 2018;14:237–50. https://doi.org/10.1038/nrneurol.2018.17 .
Article PubMed Google Scholar
O’Donnell M, Yusuf S. Risk factors for stroke in Tanzania. Lancet Glob Health. 2013;1:e241–2.
Benjamin LA, Corbett EL, Connor MD, et al. HIV, antiretroviral treatment, hypertension, and stroke in Malawian adults, a case control study. Neurology. 2016;86:324–33. https://doi.org/10.1212/WNL.0000000000002278 .
Article CAS PubMed PubMed Central Google Scholar
Benjamin LA, Bryer A, Emsley HCA, Khoo S, Solomon T, Connor MD. HIV infection and stroke: current perspectives and future directions. Lancet Neurol. 2012;11(10):878–90. https://doi.org/10.1016/S1474-4422(12)70205-3 .
Lammie GA, Hewlett RH, Schoeman JF, Donald PR. Tuberculous cerebrovascular disease: a review. J Infect. 2009;59:156–66.
Berger JR. AIDS and stroke risk. Lancet Neurol. 2004;3:206–7 [PubMed: 15039031].
Nejtek V, Talari D. Novel Risk Factors for TIA and Stroke in Young Adults. 2016.
Folsom AR, Prineas RJ, Kaye SA, Munger RG. Incidence of hypertension and stroke in relation to body fat distribution and other risk factors in older women. Stroke. 1990;21:701–6.
Khan MH, Chakraborty SK, Biswas RSR. High Waist to Hip Ratio as a Risk Factor for Ischemic Stroke Patients Admitted in a Tertiary Care Hospital. Bangladesh J Anat. 2011;9(1):30–4.
JJaakonmäki N, Zedde M, Sarkanen T, Martinez-Majander N, Tuohinen S, Sinisalo J, SECRETO Study Group. Obesity and the risk of cryptogenic ischemic stroke in young adults. J Stroke Cerebrovasc Dis. 2022;31(5):106380.
Rothman SM, Fulling KH, Nelson JS. Sickle cell anemia and central nervous system infarction: a neuropathological study. Ann Neurol. 1986;20:684–90.
O’Donnell MJ, Xavier D, Liu L, et al. Risk factors for ischemic and intracerebral hemorrhagic stroke in 22 countries (the INTERSTROKE study): a case-control study. Lancet. 2010;376:112–23.
Aigner A, Grittner U, Rolfs A, Norrving B, Siegerink B, Busch MA. Contribution of established stroke risk factors to the burden of stroke in young adults. Stroke. 2017;48(7):1744–51.
Kivioja R, Pietilä A, Martinez-Majander N, Gordin D, Havulinna AS, Salomaa V, Putaala J. Risk factors for Early-Onset ischemic stroke: a Case-Control study. J Am Heart Assoc. 2018;7(21):e009774.
Kayima J, Nankabirwa J, Sinabulya I, Nakibuuka J, Zhu X, Rahman M, Kamya MR. Determinants of hypertension in a young adult Ugandan population in epidemiological transition—the MEPI-CVD survey. BMC Public Health. 2015;15(1):1–9.
Download references
Acknowledgements
We acknowledge the patients of Mulago hospital who gave us consent to obtain this information.
This study was funded with funds from the MEPI-Neurology program under Makerere University. The funding project had no role in the design of the study and collection, analysis, and interpretation of data and no role in writing the manuscript.
Author information
Authors and affiliations.
Kiruddu National Referral Hospital, P.O. Box 6553, Kampala, Uganda
Priscilla Namaganda
Mulago National Referral Hospital, Mulago Hospital Complex, P.O. Box 7272, Kampala, Uganda
Jane Nakibuuka
Department of Medicine, School of Medicine, College of Health Sciences, Makerere University, Kampala, Uganda
Mark Kaddumukasa
Infectious Diseases Institute, Makerere University, Kampala, Uganda
Elly Katabira
You can also search for this author in PubMed Google Scholar
Contributions
PN– conception, design of work, acquisition, analysis, interpretation of data, drafted and substantively revised the manuscript, JN– analysis, interpretation of data, drafted and substantively revised the manuscript, MK – analysis, interpretation of data, drafted and substantively revised the manuscript, EK– design of work, acquisition, analysis, interpretation of data, drafted and substantively revised the manuscript. All authors read and approved the final manuscript.
Corresponding author
Correspondence to Priscilla Namaganda .
Ethics declarations
Ethics approval and consent to participate.
Written informed consent/ assent was obtained from all participants or their parent/guardian or legal authorized representative to participate in the study. Ethical approval was obtained from Makerere University, school of medicine research and ethics committee (SOMREC) (reference number #REC REF 2015–105). All methods and procedures were carried out in accordance with relevant guidelines and regulations.
Consent for publication
Not applicable.
Competing interests
The authors declare that they have no competing interests.
Additional information
Publisher’s note.
Springer Nature remains neutral with regard to jurisdictional claims in published maps and institutional affiliations.
Rights and permissions
Open Access This article is licensed under a Creative Commons Attribution 4.0 International License, which permits use, sharing, adaptation, distribution and reproduction in any medium or format, as long as you give appropriate credit to the original author(s) and the source, provide a link to the Creative Commons licence, and indicate if changes were made. The images or other third party material in this article are included in the article's Creative Commons licence, unless indicated otherwise in a credit line to the material. If material is not included in the article's Creative Commons licence and your intended use is not permitted by statutory regulation or exceeds the permitted use, you will need to obtain permission directly from the copyright holder. To view a copy of this licence, visit http://creativecommons.org/licenses/by/4.0/ . The Creative Commons Public Domain Dedication waiver ( http://creativecommons.org/publicdomain/zero/1.0/ ) applies to the data made available in this article, unless otherwise stated in a credit line to the data.
Reprints and permissions
About this article
Cite this article.
Namaganda, P., Nakibuuka, J., Kaddumukasa, M. et al. Stroke in young adults, stroke types and risk factors: a case control study. BMC Neurol 22 , 335 (2022). https://doi.org/10.1186/s12883-022-02853-5
Download citation
Received : 18 March 2022
Accepted : 23 August 2022
Published : 06 September 2022
DOI : https://doi.org/10.1186/s12883-022-02853-5
Share this article
Anyone you share the following link with will be able to read this content:
Sorry, a shareable link is not currently available for this article.
Provided by the Springer Nature SharedIt content-sharing initiative
- Young adults
- Risk factors
BMC Neurology
ISSN: 1471-2377
- General enquiries: [email protected]

Case Presentation
Statement of ethics, conflict of interest statement, funding sources, author contributions, ischemic stroke in a 29-year-old patient with covid-19: a case report.

- Split-Screen
- Article contents
- Figures & tables
- Supplementary Data
- Peer Review
- Open the PDF for in another window
- Get Permissions
- Cite Icon Cite
- Search Site
Christian Avvantaggiato , Loredana Amoruso , Maria Pia Lo Muzio , Maria Assunta Mimmo , Michelina Delli Bergoli , Nicoletta Cinone , Luigi Santoro , Lucia Stuppiello , Antonio Turitto , Chiara Ciritella , Pietro Fiore , Andrea Santamato; Ischemic Stroke in a 29-Year-Old Patient with COVID-19: A Case Report. Case Rep Neurol 2 September 2021; 13 (2): 334–340. https://doi.org/10.1159/000515457
Download citation file:
- Ris (Zotero)
- Reference Manager
Increasing evidence reports a greater incidence of stroke among patients with Coronavirus disease 2019 (COVID-19) than the non-COVID-19 population and suggests that SARS-CoV-2 infection represents a risk factor for thromboembolic and acute ischemic stroke. Elderly people have higher risk factors associated with acute ischemic stroke or embolization vascular events, and advanced age is strongly associated with severe COVID-19 and death. We reported, instead, a case of an ischemic stroke in a young woman during her hospitalization for COVID-19-related pneumonia. A 29-year-old woman presented to the emergency department of our institution with progressive respiratory distress associated with a 2-day history of fever, nausea, and vomiting. The patient was transferred to the intensive care unit (ICU) where she underwent a tracheostomy for mechanical ventilation due to her severe clinical condition and her very low arterial partial pressure of oxygen. The nasopharyngeal swab test confirmed SARS-CoV-2 infection. Laboratory tests showed neutrophilic leucocytosis, a prolonged prothrombin time, and elevated D-dimer and fibrinogen levels. After 18 days, during her stay in the ICU after suspension of the medications used for sedation, left hemiplegia was reported. Central facial palsy on the left side, dysarthria, and facial drop were present, with complete paralysis of the ipsilateral upper and lower limbs. Computed tomography (CT) of the head and magnetic resonance imaging of the brain confirmed the presence of lesions in the right hemisphere affecting the territories of the anterior and middle cerebral arteries, consistent with ischemic stroke. Pulmonary and splenic infarcts were also found after CT of the chest. The age of the patient and the absence of serious concomitant cardiovascular diseases place the emphasis on the capacity of SARS-CoV-2 infection to be an independent cerebrovascular risk factor. Increased levels of D-dimer and positivity to β2-glycoprotein antibodies could confirm the theory of endothelial activation and hypercoagulability, but other mechanisms – still under discussion – should not be excluded.
Coronavirus disease 2019 (COVID-19), caused by the novel coronavirus SARS-CoV-2, is characterized by a wide range of symptoms, most of which cause acute respiratory distress syndrome [1, 2], associated with intensive care unit (ICU) admission and high mortality [3]. On March 11, 2020, the large global outbreak of the disease led the World Health Organization (WHO) to declare COVID-19 a pandemic, with 11,874,226 confirmed cases and 545,481 deaths worldwide (July 9, 2020) [4]. In many cases, the clinical manifestations of COVID-19 are characteristic of a mild disease that may, however, worsen to a critical lower respiratory infection [2]. At the onset of the disease, the most frequent symptoms are fever, dry cough, fatigue, and shortness of breath as the infection progresses may appear signs and symptoms of respiratory failure that require ICU admission [5, 6]. Although acute respiratory distress syndrome is the most important cause of ICU admission for COVID-19 patients, several studies have underlined the presence of neurological symptoms such as confusion, dizziness, impaired consciousness, ataxia, seizure, anosmia, ageusia, vision impairment, and stroke [7, 8]. In particular, the state of hypercoagulability in patients affected by COVID-19 favors the formation of small and/or large blood clots in multiple organs, including the brain, potentially leading to cerebrovascular disease (ischemic stroke but also intracranial hemorrhage) [9, 10 ].
We found an interesting case of stroke following a SARS-CoV-2 infection in a young patient. A 29-year-old woman, during her ICU hospitalization for COVID-19-related pneumonia, was diagnosed with ischemic stroke of the right hemisphere, without other cardiac/cerebrovascular risk factors except hypertension. The young age of the patient and the absence of higher cerebrovascular risk factors make the present case very interesting as it can help demonstrate that COVID-19 is an independent risk factor for acute ischemic stroke. In a case series of 214 patients with COVID-19 (mean [SD] age, 52.7 [15.5] years), neurologic symptoms were more common in patients with severe infection who were older than the others [ 11 ]. New-onset CVD was more common in COVID-19 patients who had underlying cerebrovascular risk factors, such as older age (>65 years) [ 12 ], and very few cases of stroke in patients younger than 50 years have been reported [ 12, 13 ]. Our case seems to be the only one younger than 30 years.
On the night between March 19 and 20, 2020, a 29-year-old woman was referred to our hospital “Policlinico Riuniti di Foggia” due to a progressive respiratory distress associated with a 2-day history of fever, nausea, and vomiting. At presentation, the heart rate was 128 bpm, the blood oxygen saturation measured by means of the pulse oximeter was 27%, the respiratory rate was 27 breaths per minute, and the blood pressure was 116/77 mm Hg. The arterial blood gas test showed a pH of 7.52, pO 2 20 mm Hg, and pCO 2 34 mm Hg. The patient was immediately transferred to the ICU where she underwent tracheostomy and endotracheal intubation for mechanical ventilation due to her severe clinical condition and deteriorated pulmonary gas exchange. The diagnosis of COVID-19 was confirmed by PCR on a nasopharyngeal swab.
The family medical history was normal, and the only known pre-existing medical conditions were polycystic ovary syndrome (diagnosed 3 years earlier), conversion disorder, and hypertension (both diagnosed 2 years earlier). Ramipril and nebivolol were prescribed for the high blood pressure treatment, and sertraline was prescribed for the conversion disorder treatment. Drug therapy adherence was inconstant. The patient had no history of diabetes, cardiac pathologies, strokes, transient ischemic attacks, thromboembolic, or other vascular pathologies.
Laboratory tests showed neutrophilic leukocytosis (white blood cell count 14.79 × 10 3 , neutrophil percentage 89.8%, and neutrophil count 13.29 × 10 3 ), a prolonged prothrombin time (15.3 s) with a slightly elevated international normalized ratio (1.38), and elevated D-dimer (6,912 ng/mL) and fibrinogen levels (766 mg/dL). Other findings are shown in Table 1 .
Laboratory test
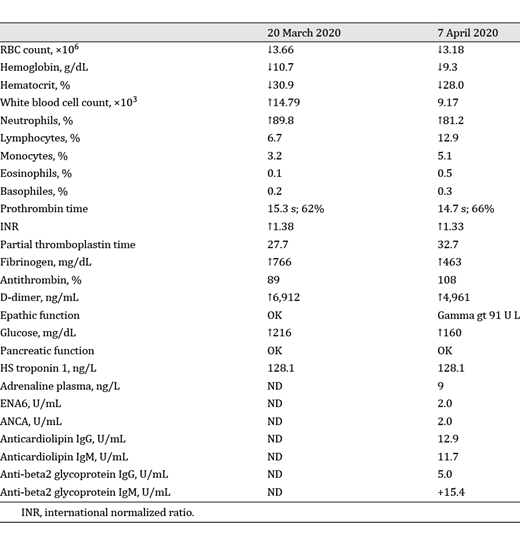
This pharmacological therapy was set as follows: enoxaparin 6,000 U.I. once a day, piperacillin 4 g/tazobactam 0.5 g twice a day; Kaletra, a combination of lopinavir and ritonavir indicated for human immunodeficiency virus (HIV) infection treatment, 2 tablets twice a day; hydroxychloroquine 200 mg once a day; and furosemide 250 mg, calcium gluconate, and aminophylline 240 mg 3 times a day. No adverse events were reported.
On April 7, 2020, during her stay in the ICU and after suspension of the medications used for sedation, left hemiplegia was reported. The same day, the patient underwent a computed tomography examination of the head, which showed areas of hypodensity in the right hemisphere due to recent cerebral ischemia.
On April 16, 2020, the patient was oriented to time, place, and person. Central facial palsy on the left side, dysarthria, and facial drop were present, with complete paralysis of the ipsilateral upper and lower limbs. The power of all the muscles of the left limbs was grade 0 according to the Medical Research Council (MRC) scale. Deep tendon reflexes were reduced on the left upper limb but hyperactive on the ipsilateral lower limb, with a slight increase in the muscle tonus. The senses of touch, vibration, and pain were reduced on the left side of the face and body.
On the same day, the patient underwent magnetic resonance imaging (MRI) of the brain (Fig. 1 a), showing lesions on the right hemisphere affecting the territories of the anterior and middle cerebral arteries. On May 5, 2020, magnetic resonance angiography showed an early duplication of the sphenoidal segment of the right middle cerebral artery, the branches of which are irregular with rosary bead-like aspects (Fig. 1 d, e); on the same day, the second MRI (Fig. 1 b) confirmed the lesions. Computed tomography of the chest (Fig. 1 c) and abdomen (Fig. 1 f), performed 5 days after the MRI of the brain, showed not only multifocal bilateral ground-glass opacities but also a basal subpleural area of increased density within the left lung (4 × 4 × 3 cm), consistent with a pulmonary infarction. In addition, a vascular lesion, consistent with a splenic infarct, was found in the inferior pole of the spleen. Doppler echocardiography of the hearth showed regular right chambers and left atrium and a slightly hypertrophic left ventricle with normal size and kinetics (ejection fraction: 55%). The age of the patient and the absence of serious concomitant cardiovascular diseases place the emphasis on the capacity of SARS-CoV-2 infection to be an independent cerebrovascular risk factor.
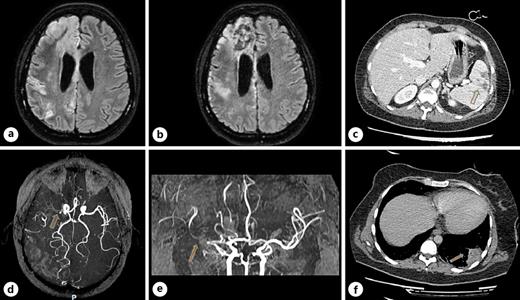
Imaging. a April 16, 2020; MRI of the brain: lesions in the right hemisphere affecting the territories of the anterior and the middle cerebral arteries. b May 5, 2020; MRI of the brain: same lesions in the right hemisphere shown in the previous image. d , e May 5, 2020; MRA showed an early duplication of the sphenoidal segment of the right middle cerebral artery, the branches of which are irregular with rosary bead-like aspect and reduction of blood flow in the middle cerebral artery. c April 20, 2020; CT of the abdomen: vascular lesion, consistent with a splenic infarct, found in the inferior pole of the spleen. f April 20, 2020; CT of the chest: basal subpleural area of increased density within the left lung (4 × 4 × 3 cm), consistent with a pulmonary infarction. MRA, magnetic resonance angiography; CT, computed tomography; MRI, magnetic resonance imaging.
The pandemic outbreak of novel SARS-CoV-2 infection has caused great concern among the services and authorities responsible for public health due to not only the mortality rate but also the danger of filling up hospital capacities in terms of ICU beds and acute non-ICU beds. In this regard, the nonrespiratory complications of COVID-19 should also be taken into great consideration, especially those that threaten patients’ lives and extend hospitalization times. Stroke is one of these complications, since a greater incidence of stroke among patients with COVID-19 than the non-COVID-19 population has been reported, and a preliminary case-control study demonstrated that SARS-CoV-2 infection represents a risk factor for acute ischemic stroke [ 14 ].
We found that the reported case is extremely interesting, since the woman is only 29 years old and considering how stroke in a young patient without other known risk factors is uncommon. Not only elderly people have higher risk factors associated with acute ischemic stroke or embolization vascular events [ 15 ], but it is also true that advanced age is strongly associated with severe COVID-19 and death. The severity of the disease is directly linked to immune dysregulation, cytokine storm, and acute inflammation state, which in turn are more common in patients who present immunosenescence [6].
Inflammation plays an important role in the occurrence of cardiovascular and cerebrovascular diseases since it favors atherosclerosis and affects plaque stability [ 16 ]. The ischemic stroke of the 29-year-old woman does not appear to be imputable to emboli originating a pre-existing atheromatous plaque, both for the age of the patient and for the absence of plaques at the Doppler ultrasound study of the supra-aortic trunks.
Most likely, COVID-19-associated hypercoagulability and endothelial dysfunction are the causes of ischemic stroke, as suggested by other studies and case reports [ 10, 13, 17 ]. Although the mechanisms by which SARS-CoV-2 infection leads to hypercoagulability are still being studied, current knowledge suggests that cross talk between inflammation and thrombosis has a crucial role [ 18 ]. The release of inflammatory cytokines leads to the activation of epithelial cells, monocytes, and macrophages. Direct infection of endothelial cells through the ACE2 receptor also leads to endothelial activation and dysfunction, expression of tissue factor, and platelet activation and increased levels of VWF and FVIII, all of which contribute to thrombin generation and fibrin clot formation [ 17 ]. The 29-year-old patient showed an increased level of D-dimer, which is a degradation product of cross-linked fibrin, indicating a global activation of hemostasis and fibrinolysis and conforming to the hypothesis of COVID-19-associated hypercoagulability. Endothelial activation and hypercoagulability are also confirmed by positivity to β2 glycoprotein antibodies. Anticardiolipin antibody and/or β2 glycoprotein antibody positivity has been reported in a few studies [ 17, 19, 20 ]. In addition, widespread thrombosis in SARS-CoV-2 infection could also be caused by neutrophil extracellular traps (NETs). Neutrophilia [ 21 ] and an elevated neutrophil-lymphocyte ratio [ 22 ] have been reported by numerous studies as predictive of worse disease outcomes, and recently, the contribution of NETs in the pathophysiology of COVID-19 was reported [ 23 ]. Thrombogenic involvement of NETs has been described in various settings of thrombosis, including stroke, myocardial infarction, and deep vein thrombosis [ 24 ]. The high neutrophil count found in our case does not exclude the hypothesis that NETs are involved in the pathogenesis of ischemic stroke.
Ischemic stroke in young patients without pre-existing cerebrovascular risk factors is very unusual. In this regard, our case of an ischemic stroke, reported in a 29-year-old woman, is very interesting. Although it is not possible to determine precisely when the thromboembolic event occurred, our case of stroke during COVID-19-related pneumonia seems to confirm that COVID-19 is an independent risk factor for acute ischemic stroke. The mechanisms by which coronavirus disease leads to stroke are still under study, but it is clear that hypercoagulability and endothelial activation play a key role. Testing for SARS-CoV-2 infection should be considered for patients who develop neurologic symptoms, but it is equally important to monitor COVID-19 patients during their hospitalization to find any neurological sign or symptom in a timely manner. Our case suggests that discovering neurological deficits in sedated patients promptly can be very difficult; for this reason, sedation in mechanically ventilated patients has to be considered only if strictly necessary. Performing serial laboratory testing and waking up the patient as soon as clinical conditions allow are strategies that should be taken into account.
Written informed consent was obtained from the patient for publication of this case report and any accompanying images. A copy of the written consent is available for review by the editor-in-chief of this journal.
The authors certify that there is no conflict of interest with any financial organization regarding the material discussed in the manuscript.
No funding was received for the publication of this case report.
All authors agree with the contents of the manuscript and were fully involved in the study and preparation of the manuscript. All authors read and approved the final version of the manuscript. M.A. Mimmo, M.P. Lo Muzio, M. Delli Bergoli, and L. Amoruso collected the data. C. Avvantaggiato wrote the manuscript with support of N. Cinone, L. Santoro, and C. Ciritella. C. Avvantaggiato, A. Turitto, and L. Stuppiello researched and discussed the neurophysiological principles of this study. P. Fiore and A. Santamato supervised the project.

Email alerts
Citing articles via, suggested reading.
- Online ISSN 1662-680X
INFORMATION
- Contact & Support
- Information & Downloads
- Rights & Permissions
- Terms & Conditions
- Catalogue & Pricing
- Policies & Information
- People & Organization
- Stay Up-to-Date
- Regional Offices
- Community Voice
SERVICES FOR
- Researchers
- Healthcare Professionals
- Patients & Supporters
- Health Sciences Industry
- Medical Societies
- Agents & Booksellers
Karger International
- S. Karger AG
- P.O Box, CH-4009 Basel (Switzerland)
- Allschwilerstrasse 10, CH-4055 Basel
- Tel: +41 61 306 11 11
- Fax: +41 61 306 12 34
- Contact: Front Office
- Experience Blog
- Privacy Policy
- Terms of Use
This Feature Is Available To Subscribers Only
Sign In or Create an Account
Stroke Helpline
Monday to Friday: 9 am to 5 pm
Saturday: 10 am to 1 pm
Sunday: Closed
Supporter Relations
Saturday: Closed
- Professionals
Our case studies
Michael pursey.
Former sports coach and teaching assistant Michael Pursey, from Wales, expected to be in a wheelchair forever after having a stroke in 2019 at the age of 24. Four years on, Michael can walk, talk, and drive again.
I returned home after six months in hospital. I felt lonely and depressed, without any belief that I could recover. When I got home, I cried my eyes out, thinking I’d be in a wheelchair forever.
My physiotherapist recommended that I attend a stroke group in Neath Port Talbot on World Stroke Day in 2019. The coordinators I met there then came to see me at home, talked to me about what I could access, supported me to do so and helped me to re-evaluate my goals. Driving, walking, it may not happen straight away but if I gave up at the first hurdle, then that was it. Every day I walked along my hallway, supported by the wall and a banister, filming myself at least once a week to visualise progression.
If you work hard, there’s no limit to your improvement. I needed that mentality, that push out of procrastination.
There’s life after stroke! Four years on, I can walk, talk, I’ve just passed my driving assessment and I’m helping other stroke survivors in their recovery.
Caroline and Matt Cooke
The lives of Caroline and Matt Cooke from Northern Ireland were turned upside down when Matt had a sudden stroke in August 2020.
At only 40 years old, Matt’s life had changed forever, his stroke left him with cognitive problems and left sided paralysis. He was in hospital for nearly a year and Caroline became his carer. She says, “the reality is everything has changed in my life as well as Matt’s life. A stroke has a huge impact on the person who has it but also on the family. We had shared roles but now everything falls on me and that is difficult.”
“When Matt had his stroke, I didn’t know anything about it. I was scared and worried about my husband, so I looked online and found the Stroke Association website. I called the Stroke Helpline, and they referred me to their local support service. It was really good and helped me with trying to deal with what was happening, especially as I had limited visits due to Covid. That emotional support for not only stroke survivors but carers as well is so important to helping to rebuild a life after stroke.”
“At the start, Matt couldn’t remember the days of the week, tell the time or even do one plus one. He was in a wheelchair when he came out of hospital, but he has regained movement in his left leg, so he’s come a long way. The journey hasn’t been easy but positivity, belief and having hope is so important - everyone’s life after a stroke is different”.
Dave and Jackie Parky n
Carpenter Dave Parkyn from Cornwall had a severe stroke in August 2020, aged just 28. This devastated his life, his mother’s life, and the lives of those closest to them. Dave is now blind in his right eye, partially sighted in his left and physically disabled.
For the first two years after his stroke, his family struggled with the huge emotional toll. Now, thanks to the emotional support received from Life After Stroke services, they have started to come to terms with what has happened.
Jackie (Dave’s Mum) told us “It has devastated Dave’s dreams. He has lost his income, his self-confidence, pride and ambition. He planned a future with a wife and family, was buying his own house, had just started his own business and absolutely everything changed.
“Dave and I were hesitant when we were offered a referral to the Stroke Association’s Cornwall Emotional Support Service, but being able to talk openly about our feelings has made a real difference.”
Dave said: “I didn’t think it would help and I was reluctant to go through with it but I’m very pleased that I did. I spoke to them about everything, my feelings of humiliation and worthlessness, my lack of confidence and my embarrassment at how I now felt I looked. It helped me think about how to let out my anger and frustration, which is a huge part of the healing process. It was just nice to be able to talk to someone and share my feelings and not feel judged.
Jackie said: “They enabled me to think and reflect and simply talk openly and honestly, never once telling me what or how to think or feel but helping me explore my feelings and come to some sort of acceptance of them. Dave will never fully be able to do the job he loved so much, but he’s recently returned to carpentry work.
“He is now beginning to accept some of what has happened and is trying to re-build a career and a life, albeit different to the one he planned.”
Julie Lomax
Mother, Julie Lomax had an ischaemic stroke in May of this year. For reasons unknown to us, Julie wasn’t referred to the community stroke team and felt left upstream without a paddle post-stroke.
“On the day of my stroke, I felt some tingling around my lips and my left cheek. It got worse down my left side the next morning and I lost all coordination. After 3 days under observation in my local hospital I was blue-lighted to Oldham to have an endarterectomy. When I first came out of the surgery, I felt great, and everything was going well. I was given my medication but then simply told to go home”.
“It was very odd getting home without any knowledge of what would happen next. I was sure this wasn’t a normal process. I felt left upstream without a paddle.”
The Bury Stroke Recovery Service received a referral for Julie, direct from Fairfield Hospital. For reasons unknown to us, Julie wasn’t referred to the community stroke team.
“Shortly after contact with our coordinator Jo I had appointments booked with coordinators, district nurses and physiotherapists.”
“Our coordinator, Jo did a home visit and told us to call anytime. She scooped me up and has been my safety net ever since. It’s as if her world revolves around my family. I had a bee’s nest at the front of my house and couldn’t get out. My daughter contacted Jo, who put me in touch with a local pest control and we got it sorted. It seems such a trivial issue, but not to Jo. She always goes the extra mile.”
Problems with memory and cognition are very common after a stroke, recovery can continue for months or even years. Life after stroke services help stroke survivors to increase confidence in decision making and execution of tasks.
“Without her emotional support, personability and guidance, I wouldn’t have the bright post-stroke outlook that I have today. I’ve got a life to keep living!”
Marwar Uddin
Marwar Uddin, 41, from Tower Hamlets, London, had a haemorrhagic stroke aged just 40 in August 2022. A Dad of three, his stroke not only changed his physical abilities but his role in family life. He now receives life after stroke support and regular care support.
Marwar said: “Stroke is one of the worst things because of the long-term impact it has on you as a person. I need help to go to the toilet. I can’t even dress myself. There is so much I can’t do anymore. My voice is different now, like I’m a different person. I cry myself to sleep most days. It’s difficult for me.
“My youngest daughter, she’s only five, came to the hospital to see me after about six weeks. When she walked in the room, she saw me and said, ‘That’s not my dad.’ This is my daughter who would come running into my arms when I picked her up from school and give me kisses. That was one of my most difficult days.
“After my stroke, I was contacted by several organisations. The Stroke Association was there right from the beginning and was with me throughout my whole journey. My Stroke Support Coordinator calls me regularly to see how I’m doing. They have sent me so much information, pointed me in the right direction for obtaining a disability lanyard and helped me to get my blue badge.
“I am also set to start a phased return to work later this year.
“Without life after stroke services, my life would be massively different. I have had so much encouragement from the Stroke Association and the community stroke teams. If I didn’t have any of this support, I think I would still be in a chair in my living room watching the world go by.
“It feels like the jigsaw puzzle pieces of my life are slowly taking shape again. I’m now registered disabled. I’m not the same person, and I probably never will be the same person again. But I’m determined to make things work.”

Ischemic Stroke
Author: Cynthia Leung MD PhD, The Ohio State University College of Medicine.
Editor: Rahul Patwari, MD, Rush University, Chicago, Illinois.
Last Update: November 2019
A 68-year-old female, with a history of hypertension and diabetes mellitus, presented to the ED after acute onset of speech difficulty and right-sided weakness. Her symptoms began 3 hours ago. On physical exam, the patient was found to have severe expressive aphasia, right hemiplegia, and right hemi-sensory loss.
Upon completion of this module, the student will be able to:
- Recognize signs and symptoms of stroke
- Identify clinical features suggestive of common stroke mimics
- Describe the initial management of acute stroke
- Discuss the treatment options for acute ischemic stroke
Introduction
Stroke is the fifth leading cause of death and the leading cause of disability in the US with estimated direct and indirect costs of roughly 70 billion dollars per year. Based on current estimates, the prevalence of stroke is expected to increase by twenty percent by the year 2030. Advancements in the diagnosis and treatment of stroke must continue to compensate for the increasing stroke burden on our society.
Stroke is characterized by the acute onset of neurologic deficit caused by disruption of cerebral blood flow to a localized region of the brain. The reversibility and extent of symptoms in stroke is critically dependent on the duration of this disruption. Therefore, early recognition and treatment is the key to reducing morbidity and mortality associated with stroke. As the first physician to see the patient with acute stroke, the actions of the Emergency Physician can have a profound impact on the outcome of stroke patients.
Acute stroke most commonly results from occlusion of an intracranial artery by thrombosis within the artery, thromboembolism from an extracranial source, or hemorrhage. Eighty seven percent of strokes are ischemic in etiology, with the remainder caused by intracerebral or subarachnoid hemorrhage. This module will focus exclusively on the evaluation and treatment of acute ischemic stroke. The evaluation and treatment of hemorrhagic stroke can be found in the intracranial hemorrhage module.
Patients with stroke may present with a variety of neurologic symptoms including changes in vision, changes in speech, focal numbness or weakness, disequilibrium or alteration in level of consciousness. There are many alternate diagnoses that can mimic the symptoms of stroke.
The differential diagnosis includes:
- Structural brain lesion (tumor, AVM, aneurysm, hemorrhage)
- Infection (cerebral abscess, septic emboli)
- Seizure Disorder and post-seizure neurologic deficit (Todd’s paralysis)
- Peripheral Neuropathy (Bell’s palsy)
- Complicated Migraine
- Toxic-metabolic disorders (Hypoglycemia and Hyponatremia)
- Conversion Disorder
Initial Actions and Primary Survey
The initial actions in the evaluation of a patient with suspected stroke begin with emergent stabilization of the patient. As with any emergent patient, the primary survey includes assessment of the patient’s airway, breathing and circulation. Hypoxemia and hypotension due to stroke or co-morbid conditions may worsen stroke symptoms and lead to death. Therefore, treatment of any critical conditions found on primary survey must be initiated prior to continuing the evaluation. Next, a focused H&P is performed to assess level of neurologic dysfunction, exclude alternate diagnoses, and determine the patient’s eligibility for therapy.
Presentation
The initial diagnosis of acute stroke is based on clinical findings. Part of the challenge in making the diagnosis is that there is no “textbook” presentation of stroke. The signs and symptoms of stroke are highly variable and depend not only on the particular blood vessel occluded, but also the extent of occlusion and amount of circulation provided by collateral vessels. Presentations may vary from multiple profound neurologic deficits in a large vessel occlusion to very subtle isolated deficits when smaller vessels are occluded.
The single most important component of the history is the exact time of onset of symptoms. This is defined as the time when the patient was last known to be symptom-free, commonly referred to as the “last known well”. In cases where the patient’s last known well time is unclear, focused questions should be deployed to help narrow down the time window as much as possible. For example, if the patient awakens from sleep with symptoms, questioning the patient about waking in the middle of the night to walk to the restroom or kitchen may help to determine a more accurate last known well time. In patients who were awake during symptom onset, asking about specific activities such as phone calls or television shows may help to further focus the timeframe of onset. Friends and family should also be asked to provide collateral information when possible.
The remainder of the history should focus on factors which may help differentiate a stroke mimic from a true stroke. The HPI should include a detailed history of the onset, time course and pattern of symptoms to help distinguish between stroke and alternate diagnoses. Symptoms which achieve maximal intensity within seconds to minutes of onset and simultaneously affect multiple different systems at once are typical of stroke. In contrast, symptoms which progress slowly over time or progress from one area of the body to another are more suggestive of stroke mimic. The past medical history should include assessment of stroke risk factors as well as risk factors for stroke mimics. Stroke risk factors include hypertension, diabetes, hyperlipidemia, tobacco abuse, advanced age, atrial fibrillation or prosthetic heart valve, and prior stroke. In patients receiving thrombolytic therapy, the most common stroke mimics include complicated migraine, seizure and conversion disorder. A past medical history which includes any of these disorders should heighten suspicion of these alternate diagnoses.
Once the primary survey is complete, a thorough neurologic exam should be performed. This should include assessment of level of consciousness, cranial nerves, strength, sensation, cerebellar function and gait.
Common Stroke Syndromes
Signs and symptoms of stroke should follow a vascular distribution of the brain. Knowledge of the functional areas supplied by each of the major intracranial blood vessels helps to predict signs and symptoms associated with occlusion of that particular vessel.
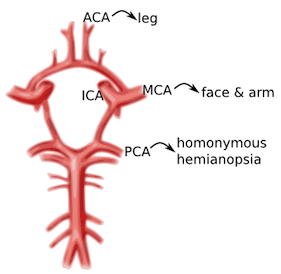
Image 1. Circle of Willis and the primary cerebral vessels. Labels added. Contect accessed from https://medlineplus.gov/ency/imagepages/18009.htm
Anterior Cerebral Artery (ACA): unilateral weakness and/or sensory loss of contralateral lower extremity greater than upper extremity
Middle Cerebral Artery (MCA): unilateral weakness and/or sensory loss of contralateral face and upper extremity greater than lower extremity with either aphasia (if dominant hemisphere) or neglect (if non-dominant hemisphere)
Posterior Cerebral Artery (PCA): unilateral visual field deficit in both eyes (homonymous hemianopsia).
Vertebrobasilar syndromes have multiple deficits which typically include contralateral weakness and/or sensory loss in combination with ipsilateral cranial nerve palsies. Suspicion for posterior circulation stroke is heightened if the patient exhibits one of these signs or symptoms beginning with “D”: diplopia, dysarthria, dysphagia, droopy face, dysequilibrium, dysmetria, and decreased level of consciousness. Nausea and vomiting are also frequently associated with brainstem stroke.
Lacunar infarcts are small strokes (measuring less than 1.5 cm) caused by occlusion of one of the deep perforating arteries which supplies the subcortical structures and brainstem. Lacunar infarcts can produce a large variety of clinical deficits depending on their location within the brainstem and have been characterized by more than 70 different clinical syndromes. However, the vast majority of lacunar strokes are described by the 5 most common lacunar syndromes: pure motor hemiparesis, sensorimotor stroke, ataxic hemiparesis, pure sensory stroke, and dysarthria-clumsy hand syndrome.
Diagnostic Testing
Rapid evaluation of patients with suspected stroke is critical because there is a very narrow time window in which stroke patients are eligible for treatment. A panel of experts convened by the National Institute of Neurological Disorders and Stroke (NINDS) has established several critical events in the identification, evaluation, and treatment of stroke patients in the ED. The most important of these time goals include a door to head CT time less than 25 minutes and a door to drug administration time of less than 60 minutes.
The diagnosis of stroke is based primarily on clinical presentation. The NIH Stroke Scale (NIHSS) provides a standardized clinical assessment which is generalizable from one physician to another and allows monitoring of the patient’s neurologic deficits over time. The NIHSS can serve as a measure of stroke severity and has been shown to be a predictor of both short and long term outcome of stroke patients. Many Emergency physicians find it convenient to keep an App on their phone to aid in rapidly calculating the NIHSS. There are also a variety of on-line NIHSS calculators available, such as the one found on MDcalc.com
The remainder of the diagnostic workup is focused on excluding alternative diagnoses, assessing for comorbid conditions and determining eligibility for therapy. The diagnostic workup includes:
Brain Imaging
Head CT without contrast should be performed on all patients to exclude intracranial hemorrhage. Unenhanced head CT is often able to identify other structural brain lesions and may detect early signs of stroke. Because radiologic changes associated with stroke are usually not visible on CT for several hours, the most common CT finding in acute ischemic stroke is normal brain. However, multiple subtle findings associated with acute ischemic stroke may be present in the first 3 hours after symptom onset. The earliest finding that may be seen on CT is hyperdensity representing acute thrombus or embolus in a major intracranial vessel. This is most frequently seen in the MCA (“hyperdense MCA sign”, see Image 2) and basilar arteries (“hyperdense basilar artery sign”). Subsequent findings include subtle hypo-attentuation causing obscuration of the nuclei in the basal ganglia and loss of gray/white differentiation in the cortex. Frank hypodensity on CT is indicative of completed stroke and may be a contraindication to thrombolytic therapy.
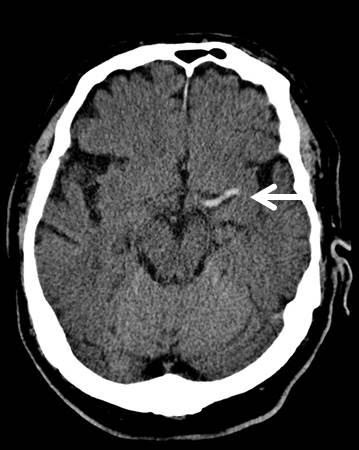
Image 2. MCA sign on CT head. Case courtesy of A.Prof Frank Gaillard, <a href=" https://radiopaedia.org/ ">Radiopaedia.org</a>. From the case <a href="https://radiopaedia.org/cases/7150">rID: 7150</a>
At specialized stroke centers, alternative testing such as diffusion weighted MRI (DWI) or CT angiography/CT perfusion studies may also be performed as these modalities are more sensitive for detecting early or evolving infarct and may help determine the most appropriate therapy.
Serum Glucose
Hypoglycemia may cause alteration in level of consciousness and any variety of neurologic symptoms. Point of care blood glucose level must be performed to exclude hypoglycemia prior to initiation of thrombolytic therapy.
EKG should be performed to exclude contemporaneous acute MI or atrial fibrillation as these conditions are frequently associated with thromboembolic stroke.
Additional laboratory studies
CBC, chemistries, PT/INR, aPTT, and cardiac markers are recommended to assess for serious comorbid conditions and aid in selection of therapy. Treatment should not be delayed for results of these additional studies unless a bleeding disorder is suspected.
The main goal of therapy in acute ischemic stroke is to remove occlusion from the involved vessel and restore blood flow to the affected area of the brain. The AHA/ASA currently recommends two forms of treatment for eligible patients with acute ischemic stroke: intravenous thrombolytic agents and mechanical thrombectomy.
Intravenous Thrombolytic Therapy
Intravenous recombinant Tissue Plasminogen Activator (rtPA) is a fibrinolytic agent that catalyzes the conversion of plasminogen to plasmin, the major enzyme responsible for clot breakdown. Treatment with IV rtPA has been shown to increase the percentage of patients with good functional outcome at 3 months and 1 year after stroke onset.
rtPA has been FDA approved for use in adult patients with symptoms attributable to ischemic stroke up to 3hrs after symptom onset. In addition, the American Heart Association has recommended rtPA for use up to 4.5 hours after symptom onset in a select subgroup of patients. Good functional outcomes are most likely to be achieved if rtPA is administered within 90 minutes of symptom onset. The likelihood of a good outcome decreases with increasing time from onset of symptoms. Therefore, every effort should be made to ensure that there are no delays in administration of thrombolytic therapy to eligible patients.
The major complication of rtPA administration in stroke is symptomatic intracranial hemorrhage. Careful selection of patients with an appropriate risk/benefit ratio is imperative to reduce the risk of symptomatic ICH. Exclusion criteria most commonly reflect factors that may increase likelihood of ICH including uncontrolled severe hypertension, coagulopathies, recent intracranial or spinal surgery, recent head trauma or stroke and history of prior ICH. The full list of inclusion and exclusion criteria for intravenous rtPA therapy can be found in the most recent version of the AHA Guidelines for the Early Management of Patients with Acute Ischemic Stroke (see references below).
In addition, strict adherence to the NINDS recommended protocol for administration of rtPA is critical to successful treatment in stroke patients. This protocol specifies important aspects of care during and after administration of rtPA. Admission to an ICU or stroke unit, frequent reassessment of the patient’s neurologic status and careful blood pressure monitoring are all vital in the first 24 hours after administration of rtPA. Most importantly, any patient who develops acute severe headache, acute severe hypertension, intractable nausea and vomiting, altered mental status or other evidence of neurologic deterioration during or after rtPA administration should have emergent noncontrast head CT to evaluate for ICH. In addition, rtPA infusion should be discontinued immediately if it has not already been completed.
Mechanical Thrombectomy
Mechanical thrombectomy is recommended for adult patients with ischemic stroke caused by occlusion of the internal carotid or proximal middle cerebral (M1) arteries and an NIHSS greater than 6, presenting within 6 hours of symptom onset. Thrombectomy is also recommended for a select group of patients presenting up to 16 hours after symptom onset if they have demonstrated perfusion mismatch on MRI or CTP and meet additional eligibility requirements. This recommendation was based on pooled analysis of 5 studies which demonstrated lower degree of disability at 3 months in patients undergoing mechanical thrombectomy compared to those undergoing thrombolytic therapy alone. This effect was most pronounced when the time from symptom onset to thrombectomy was under 2 hours, but persisted up to 7 hours after symptom onset.
Supportive Care
Unfortunately, only a small percentage of stroke patients present to the ED within the time limit to receive specialized therapy. In stroke patients not receiving rtPA or mechanical thrombectomy, the goal of care is to prevent or treat acute complications by providing supportive care. This includes ventilatory support and oxygenation if needed, prevention of hyperthermia, cardiac monitoring, and control of blood pressure and blood glucose.
Goals for Blood Pressure Control
In patients receiving intravenous rtPA, the rate of symptomatic ICH is directly related to increasing blood pressure. Therefore, strict guidelines for blood pressure control must be enforced in these patients to prevent ICH. Blood pressure should be maintained below 180/105 mm Hg in the first 24 hours after receiving thrombolytic therapy.
In contrast, the ideal blood pressure range for acute stroke patients not receiving thrombolytic therapy has not yet been determined. The current recommendations stress the importance of an individualized approach to blood pressure control with avoidance of hypotension or large fluctuations in blood pressure. For patients who do not have other medical conditions requiring aggressive blood pressure control, antihypertensive treatment should not be initiated unless blood pressure exceeds 220/120 mm Hg.
Antiplatelet Therapy
Administration of Aspirin within 48 hours after stroke has been shown to improve outcomes by reducing the rate of early recurrent stroke. In stroke patients not receiving rtPA, oral administration of aspirin within 24 – 48 hours of stroke onset is recommended. The safety of antiplatelet agents in combination with thrombolytic therapy has not been established. Therefore, aspirin should not be administered for at least 24 hours after administration of rtPA
Pearls and Pitfalls
- Use creative questioning to establish time of onset.
- Consider common conditions which may mimic the symptoms of stroke including seizure, complicated migraine, hypoglycemia, and conversion disorder. All adult patients presenting with neurologic deficit attributable to ischemic stroke within 3 hours of symptom onset should be considered for thrombolytic therapy.
- Minimum workup prior to thrombolytic therapy includes focused H&P, CT Head to exclude intracranial hemorrhage and point of care blood glucose level to exclude hypoglycemia.
- Time is brain! Do not delay administration of thrombolytic therapy to eligible patients.
- Adult patients presenting with acute ischemic stroke due to large vessel occlusion within 16 hours of symptom onset should be considered for mechanical thrombectomy.
- Patients that do not receive thrombolytic therapy should receive aspirin within 24 hours of symptom onset.
Case Study Resolution
The patient’s initial NIHSS was 11. Noncontrast CT of the head did not show any evidence of ICH. CT angiography revealed left M1 occlusion. The patient underwent mechanical thrombectomy with marked improvement in symptoms. Repeat NIHSS was 3. The patient was transferred to the neurologic critical care unit for further monitoring.
Guidelines for the Early Management of Patients with Acute Ischemic Stroke. Powers WJ, et al. Stroke 2018 Mar;49(3): e46-e99. PMID:29367334
Heart disease and StrokeStatistics—2018 Update: a report from the American Heart Association. Benjamin ES, et al. Circulation. 2018 Mar 1;137(12):e67-e493. PMID:29386200
Safety of thrombolysis in stroke mimics: results from a multicenter cohort study. Zinkstok SM, et al. Stroke. 2013 Apr;44(4):1080-4. PMID:23444310
Time to Treatment with Endovascular Thrombectomy and Outcomes from Ischemic Stroke: A Meta-analysis. Saver JL, et al. JAMA 2016; 316(12):1279-1288. PMID:
Time to treatment with intravenous alteplase and outcome in stroke: an updated pooled analysis of ECASS, ATLANTIS, NINDS, and EPITHET trials. Lees KR, et al. Lancet. 2010 May 15;375(9727):1695-1703. PMID:20472172

A .gov website belongs to an official government organization in the United States.
A lock ( ) or https:// means you've safely connected to the .gov website. Share sensitive information only on official, secure websites.
- Signs and Symptoms
- Risk Factors
- Stroke Facts
- Stroke Communications Toolkit
- About Heart Disease
- About High Blood Pressure
- About Cholesterol
Treatment and Intervention for Stroke
- If someone you know shows signs of stroke, call 9-1-1 right away.
If you have had a stroke, you are at high risk for another stroke.
If someone you know shows signs of stroke , call 9-1-1 right away.
Your stroke treatment begins the moment emergency medical services (EMS) arrive to take you to the hospital.
Once at the hospital, you may receive emergency care, treatment to prevent another stroke, rehabilitation to treat the side effects of stroke, and/or all three.
Stroke
A stroke, sometimes called a brain attack, occurs when something blocks blood supply to part of the brain or when a blood vessel in the brain bursts.
In either case, parts of the brain become damaged or die. A stroke can cause lasting brain damage, long-term disability, or even death.
How is stroke treated?
On the way to the hospital.
Calling 9-1-1 for an ambulance means that medical staff can begin life-saving treatment on the way to the emergency room.
Do not drive to the hospital or let someone else drive you. The key to stroke treatment and recovery is getting to the hospital quickly.
Stroke patients who are taken to the hospital in an ambulance may get diagnosed and treated more quickly than people who do not arrive in an ambulance. 1 This is because emergency treatment starts on the way to the hospital. The emergency workers may take you to a specialized stroke center to ensure that you receive the quickest possible diagnosis and treatment.
The emergency workers will also collect valuable information that guides treatment and alert hospital medical staff before you arrive at the emergency room, giving them time to prepare.
Learn more about the important role EMS plays in improving stroke care .

At the hospital
At the hospital, health professionals will ask about your medical history and the time your symptoms started.
Brain scans will show what type of stroke you had. You may also be seen by a neurologist who treats brain disorders, a neurosurgeon who performs surgery on the brain, or a specialist in another area of medicine.
Treating ischemic stroke
If you get to the hospital within 3 hours of the first symptoms of an ischemic stroke , you may get a type of medicine called a thrombolytic (a "clot-busting" drug) to break up blood clots. Tissue plasminogen activator (tPA) is a thrombolytic.
tPA improves the chances of recovering from a stroke. Studies show that patients with ischemic strokes who receive tPA are more likely to recover fully or have less disability than patients who do not receive the drug. 2 3 Patients treated with tPA are also less likely to need long-term care in a nursing home. 4
Recognizing the signs and symptoms and seeking immediate medical attention (by calling 9-1-1) is essential to prevent stroke related disability or death.
Doctors may also treat ischemic stroke with other medicines, such as blood thinners, as well as surgery to remove the clot.
Ischemic Stroke
Most strokes are ischemic strokes. 5 An ischemic stroke occurs when blood clots or other particles block the blood vessels to the brain.
Fatty deposits called plaque can also build up in the blood vessels causing blockages.
Treating hemorrhagic stroke
Other medicines, surgery, or procedures may be needed to stop the bleeding from hemorrhagic stroke and save brain tissue. For example:
- Endovascular procedures. Endovascular procedures, which can help repair a weak spot or break in a blood vessel, may be used to treat certain hemorrhagic strokes. Learn more about the procedure .
- Surgical treatment. Hemorrhagic strokes may be treated with surgery. If the bleeding is caused by a ruptured aneurysm, a metal clip may be put in place to stop the blood loss.
Learn more about how stroke is treated at the hospital from the Eunice Kennedy Shriver National Institute of Child Health and Human Development.
Hemorrhagic Stroke
A hemorrhagic stroke happens when an artery in the brain leaks blood or ruptures (breaks open). The leaked blood puts too much pressure on brain cells, which damages them.
High blood pressure and aneurysms—balloon-like bulges in an artery that can stretch and burst—are examples of conditions that can cause a hemorrhagic stroke.
What happens after stroke treatment?
- 1 in 4 stroke survivors has another stroke within 5 years. 5
- The risk of stroke within 90 days of a transient ischemic attack (TIA) may be as high as 17%, with the greatest risk during the first week. 6
Your doctor may give you medications or tell you to change your diet, exercise, or adopt other healthy lifestyle habits. Surgery may also be helpful in some cases. That's why it's important to treat the underlying causes of stroke.
If you have had a stroke, you are at high risk for another stroke. That's why it's important to treat the causes of stroke , including
- Heart disease .
- High blood pressure .
- Atrial fibrillation (fast, irregular heartbeat).
- High cholesterol .
You and your health care team can work together to prevent or treat the medical conditions that lead to stroke. Discuss your treatment plan regularly and bring a list of questions to your appointments. Your treatment plan will include medicine or surgery and lifestyle changes to lower your risk for another stroke. Be sure to take your medicine as directed and follow your doctor's instructions.
Learn how to find the right doctor for you from the "Live to the Beat" campaign.
Recovering from stroke: stroke rehabilitation
Rehabilitation after a stroke begins in the hospital, often within a day or 2 after the stroke. Rehab helps ease the transition from hospital to home and can help prevent another stroke.
Recovery time after a stroke is different for everyone—it can take weeks, months, or even years. Some people recover fully, but others have long-term or lifelong disabilities.
Learn more about stroke rehabilitation from the National Institute of Neurological Disorders and Stroke .
What to expect after a stroke
If you have had a stroke, you can make great progress in regaining your independence. However, some problems may continue:
- Paralysis (inability to move some parts of the body), weakness, or both on one side of the body.
- Trouble with thinking, awareness, attention, learning, judgment, and memory.
- Problems understanding or forming speech.
- Trouble controlling or expressing emotions.
- Numbness or strange sensations.
- Pain in the hands and feet that worsens with movement and temperature changes.
- Trouble with chewing and swallowing.
- Problems with bladder and bowel control.
- Depression.
You and your health care team can discuss what to expect after a stroke. Your health care team will work with you to prevent further strokes.
What is stroke rehabilitation?
Rehab can include working with speech, physical, and occupational therapists.
- Speech therapy helps people who have problems producing or understanding speech.
- Physical therapy uses exercises to help you relearn movement and coordination skills you may have lost because of the stroke.
- Occupational therapy focuses on improving daily activities, such as eating, drinking, dressing, bathing, reading, and writing.
Therapy and medicine may help with depression or other mental health conditions following a stroke. Joining a patient support group may help you adjust to life after a stroke. Talk with your health care team about local support groups, or check with an area medical center.
Support from family and friends can also help relieve fear and anxiety following a stroke. Let your loved ones know how you feel and what they can do to help you.
- Stroke Signs and Symptoms
- Heart Attack Symptoms, Risk, and Recovery
Other organizations
- National Institute of Neurological Disorders and Stroke: Stroke Information Page
- National Institutes of Health: Know Stroke Campaign
- National Institutes of Health: Mind Your Risks ®
- MedlinePlus: Stroke
- Ekundayo OJ, Saver JL, Fonarow GC, et al. Patterns of emergency medical services use and its association with timely stroke treatment: findings from Get With the Guidelines–Stroke. Circ Cardiovasc Qual Outcomes . 2013;6:262–269.
- National Institute of Neurological Disorders and Stroke rt-PA Stroke Study Group. Tissue plasminogen activator for acute ischemic stroke . N Engl J Med . 1995;333(24):1581–1587.
- Marler JR, Tilley BC, Lu M, et al. Early stroke treatment associated with better stroke outcome: the NINDS rt-PA stroke study . Neurology . 2000;55(11):1649–1655.
- National Institute of Neurological Disorders and Stroke. Stroke: Challenges, Progress, and Promise. National Institutes of Health; 2009. https://www.govinfo.gov/content/pkg/GOVPUB-HE20-PURL-gpo18272/pdf/GOVPUB-HE20-PURL-gpo18272.pdf
- Tsao CW, Aday AW, Almarzooq ZI, et al. Heart disease and stroke statistics—2023 update: a report from the American Heart Association. Circulation. 2023;147:e93–e621.
- Lambert M. Practice guidelines: AHA/ASA guidelines on prevention of recurrent stroke. Am Fam Physician . 2011;83(8):993–1001.
Stroke is a leading cause of death in the United States and is a major cause of serious disability for adults. It is also preventable and treatable.
For Everyone
Public health.
Ohio State nav bar
The Ohio State University
- BuckeyeLink
- Find People
- Search Ohio State
Stroke case study
- Sanaya Batcho
- Kara Kitzmiller
- Emma Overman
Our reason for choosing this disorder
Stroke is the leading cause of disability in the United States. As advanced practice nurses, we anticipate caring for those impacted by strokes in many healthcare settings including emergency rooms, acute care, rehab settings, extended care facilities, and in primary care. Early diagnosis and treatment are imperative in the treatment of a stroke in order to minimize permanent deficits so it is important for advanced practice nurses to be proficient in recognizing clinical manifestations of a stroke. There are also many modifiable risk factors for strokes so advanced practice nurses need to be able to educate patients and families on potential lifestyle changes that can decrease stroke risk.
Search form
In stroke response, speed is key; yale study reveals where delays are worst.

A map showing average community-level delays between stroke symptom onset and hospital arrival. Beige = less than three hours; orange = three to four and a half hours; red = four and a half to six hours; and maroon = more than six hours.
When it comes to responding to a stroke, speed is a crucial factor; the longer it takes for someone experiencing a stroke to get to a hospital, the worse the outcome will be. Yet across the United States, delays to treatment can be significant.
A Yale study uncovers new insights into factors associated with treatment delays and where in the United States patients are more likely to experience slower responses.
The findings, which were published May 24 in the journal Stroke , highlight where interventions should be focused in order to improve stroke outcomes across the country.
During acute stroke, a blood clot reaches the brain, blocking blood flow and essential oxygen to brain tissue. The longer it takes to restore blood flow, the more tissue will be damaged, leaving patients with reduced abilities in those functions for which the affected brain region is responsible.
Currently, there are two main treatments used in hospitals for patients experiencing a stroke; both aim to break the blood clot and restore blood flow. One involves a medication — a drug called tissue plasminogen activator (tPA) — and the other is a physical approach wherein doctors remove the clot with a tiny tube threaded through a patient’s blood vessels.
“ Both are time-dependent,” said Kevin Sheth , professor of neurology and neurosurgery at Yale School of Medicine and senior author of the new study. “The window to use tPA closes four and a half hours after the stroke. Physical removal is more complicated but can be done up to 12 to 16 hours after the stroke begins. But every minute counts , and even within those windows earlier is better.”
To better understand what factors contribute to treatment delays across the country, the researchers — in collaboration with researchers at Brigham and Women’s Hospital in Cambridge, Massachusetts — used data collected from the “ Get with the Guidelines-Stroke ” registry, a program launched in 2003 by the American Heart Association that distributes care guidelines and collects data from U.S. hospitals with the aim of improving patient outcomes.
Across nearly 150,000 patients who experienced a stroke between January 2015 and March 2017, 54% arrived at a hospital more than two hours after the onset of stroke symptoms, the researchers found. Compared with patients who arrived earlier, those who took longer were more likely to be older, female, Black, and to have had a mild stroke, findings that align with those of previous studies.
The researchers also looked at community-level social vulnerability. The U.S. Centers for Disease Control and Prevention calculates social vulnerability scores for every U.S. census tract based on socioeconomic factors, household characteristics and disability, racial and ethnic minority status, and housing type and transportation availability. The scores serve to track factors that adversely affect communities, with higher scores indicating greater vulnerability.
In the study, the researchers found that patients with longer delays to hospital arrival were more likely to live in communities with higher social vulnerability scores. Socioeconomic status, housing type, and transportation availability had the strongest associations with delay.
The researchers also created an interactive map that shows how long it took for patients to arrive at hospitals in different communities nationwide, which they say could be used for targeting interventions.
One important strategy to reduce delays, the researchers say, is the creation of education campaigns.
“ The most powerful motivator for going to the emergency department is pain,” said Sheth. “But stroke isn’t usually associated with pain. Plus, neurological symptoms can be weird, leaving people unsure what’s occurring. So informing people about the signs of stroke could help motivate people to seek treatment earlier.”
There have been large-scale education campaigns, Sheth added, but they haven’t made a sizable difference. Campaigns that target communities identified in this study as having longer delays, however, could be more effective.
More ideas for interventions are also needed, said Sheth.
“ For how big a problem this is, there hasn’t really been an effort to propose or test new interventions,” he said. “So we need new ideas because the single biggest problem in treating acute stroke is getting people to the hospital fast enough.”
Health & Medicine
Media Contact
Bess Connolly : [email protected] ,

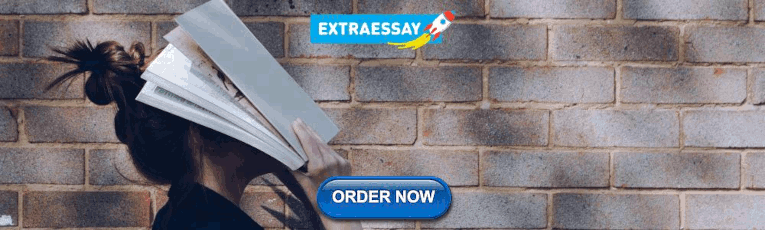
Maurie McInnis returns to Yale in a new role: President-elect

Screening room: Yale Law clinic offers legal guidance for documentaries

To avoid infection spread, how long a quarantine is sufficient? It depends

AI can write a more believable restaurant review than a human can
- Show More Articles
Thank you for visiting nature.com. You are using a browser version with limited support for CSS. To obtain the best experience, we recommend you use a more up to date browser (or turn off compatibility mode in Internet Explorer). In the meantime, to ensure continued support, we are displaying the site without styles and JavaScript.
- View all journals
- My Account Login
- Explore content
- About the journal
- Publish with us
- Sign up for alerts
- Open access
- Published: 20 May 2024
Predictive modelling and identification of key risk factors for stroke using machine learning
- Ahmad Hassan ORCID: orcid.org/0000-0001-6515-712X 1 ,
- Saima Gulzar Ahmad ORCID: orcid.org/0000-0002-8820-0570 1 ,
- Ehsan Ullah Munir ORCID: orcid.org/0000-0001-7838-0291 1 ,
- Imtiaz Ali Khan ORCID: orcid.org/0000-0001-7624-1319 2 &
- Naeem Ramzan ORCID: orcid.org/0000-0002-5088-1462 3
Scientific Reports volume 14 , Article number: 11498 ( 2024 ) Cite this article
409 Accesses
Metrics details
- Health care
Strokes are a leading global cause of mortality, underscoring the need for early detection and prevention strategies. However, addressing hidden risk factors and achieving accurate prediction become particularly challenging in the presence of imbalanced and missing data. This study encompasses three imputation techniques to deal with missing data. To tackle data imbalance, it employs the synthetic minority oversampling technique (SMOTE). The study initiates with a baseline model and subsequently employs an extensive range of advanced models. This study thoroughly evaluates the performance of these models by employing k-fold cross-validation on various imbalanced and balanced datasets. The findings reveal that age, body mass index (BMI), average glucose level, heart disease, hypertension, and marital status are the most influential features in predicting strokes. Furthermore, a Dense Stacking Ensemble (DSE) model is built upon previous advanced models after fine-tuning, with the best-performing model as a meta-classifier. The DSE model demonstrated over 96% accuracy across diverse datasets, with an AUC score of 83.94% on imbalanced imputed dataset and 98.92% on balanced one. This research underscores the remarkable performance of the DSE model, compared to the previous research on the same dataset. It highlights the model's potential for early stroke detection to improve patient outcomes.
Similar content being viewed by others
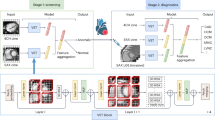
Screening and diagnosis of cardiovascular disease using artificial intelligence-enabled cardiac magnetic resonance imaging
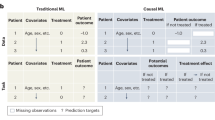
Causal machine learning for predicting treatment outcomes
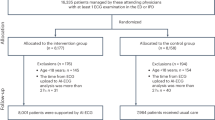
AI-enabled electrocardiography alert intervention and all-cause mortality: a pragmatic randomized clinical trial
Introduction.
Stroke, a devastating medical condition, is a leading cause of mortality worldwide. It occurs when the blood supply to the brain is interrupted or reduced, impairing brain functions 1 . As per the World Stroke Organization (WSO), there is a significant risk associated with strokes, with one in four individuals over the age of 25 facing the possibility of experiencing a stroke during their lifetime 2 . Stroke is a common condition that significantly affects the population. Stroke is the second most common cause of death and the third most prevalent reason for impairment in adults globally. It is a major factor in both death and disability 3 . The significant impact of chronic illness on people, families, and healthcare systems highlights the need for precise and timely prediction techniques to enhance patient outcomes 4 .
In the field of medicine, machine learning has become a powerful technology that has the potential to transform stroke prevention and prediction 5 , 6 , 7 . Machine learning models use large datasets and sophisticated algorithms to identify hidden risk factors, forecast outcomes, and offer tailored strategies for treatment 8 . Stroke prediction is a vital area of research in the medical field. However, there are several problems and issues that need to be resolved 9 , 10 . The accuracy of predictive models is one of the main issues. Machine learning models have shown potential in stroke prediction. Factors such as the data quality, the choice of features, and the choice of algorithm can impact how well models perform 11 . To ensure these model's dependability and efficacy in predicting strokes, it is crucial to assess and validate these factors 12 carefully. Another critical concern is the handling of missing data. Predictive prediction model performance can be severely impacted by incomplete data, producing erroneous or biased outcomes 13 . Appropriate data imputation approaches are needed to handle missing data and increase the precision of prediction models 14 .
The data imbalance is also a concern in stroke prediction 15 . Due to the rarity of pre-stroke datasets, they frequently contain imbalanced classifications, with most instances being non-stroke cases 16 . This imbalance can result in biased models that favour the majority and ignore the minority, resulting in low forecast accuracy. To solve this issue and increase the effectiveness of prediction models, several oversampling and undersampling methods are employed, the popular of which is the SMOTE 17 , 18 . Furthermore, due to ethical considerations, it is challenging to get stroke prediction datasets, especially regarding patient privacy 19 . Predictive models that employ sensitive health data must follow strong privacy standards and protect patients' rights and autonomy 20 , 21 , 22 .
Overall, stroke prediction is a complex and challenging area of study that demands careful evaluation of numerous challenges and concerns 23 . However, by developing innovative approaches and employing rigorous evaluation methods, the potential of machine learning in stroke prediction can be fully realized 24 . These approaches and methods can improve patient outcomes and lower the societal and individual burden of stroke 25 . Addressing stroke prediction difficulties such as accuracy, missing data, data imbalance, and interpretability is critical to reaching the full potential of machine learning in this domain 26 .
The alarming statistics and various issues highlight the urgent need for effective stroke prevention and prediction strategies. This research endeavour delves into the realm of advanced machine learning models to predict strokes and identify key risk factors. By harnessing the power of these models, it aims to enhance early detection, minimize the impact of strokes, and ultimately improve patient outcomes. This study uses a comprehensive analysis of various machine-learning models to predict strokes. It makes several contributions to stroke prediction and provides various previously unknown insights, including:
Exploring various data imputation techniques and addressing data imbalance issues in order to enhance the accuracy and robustness of stroke prediction models.
Identifying crucial features for stroke prediction and uncovering previously unknown risk factors, giving a comprehensive understanding of stroke risk assessment.
Creating an augmented dataset incorporating important key risk factor features using the imputed datasets, enhancing the effectiveness of stroke prediction models.
Assessing the effectiveness of advanced machine learning models across different datasets and creating a robust Dense Stacking Ensemble model for stroke prediction.
The key contribution is showcasing the enhanced predictive capabilities of the model in accurately identifying and testing strokes, surpassing the performance of prior studies that utilized the same dataset.
These contributions collectively enhance the overall understanding of stroke prediction and key contributing factors for stroke. It highlights the potential of machine learning models in accurately identifying individuals at risk of strokes. The literature review can be found in Section " Literature review ". Moving on to Section " Dataset and preprocessing ", which delves into examining the dataset used, the challenges that arise while data preparation, and the preprocessing strategies employed. Section " Data modelling " provides an overview of the main research workflow and outlines the approach to its execution. It examines many models for data modelling. Machine learning algorithms that are used for forecasting are discussed in Section " Machine learning algorithms ". Section " Results " presents an overview of the prediction results obtained through the utilization of different machine learning models and approaches, along with a discussion subsection. Meanwhile, Section " Conclusion " encompasses a conclusion of the findings along with recommendations for future research endeavours.
Literature review
The field of stroke prediction research has been the subject of numerous contributions by various authors over an extended period that uses various datasets. However, in this paper, recent contributions are focused that utilize the same dataset as these are also used for evaluation as well. Several machine learning models, including Naive Bayes, Support Vector Machine, Decision Tree, Random Forest, and Logistic Regression, are used to predict stroke. The authors also propose a Minimal Genetic Folding (MGF) model 27 for predicting the probability of stroke, achieving an accuracy of 83.2%. The MGF classification is the most accurate, surpassing the area under the curve (AUC) scores of the other specified kernels. The research supports the notion that a general MGF kernel could differentiate between various stages of stroke recovery, but more research is needed. The study's potential limitations include the oversampling method, which might have affected how well the MGF classifier performed.
The authors propose a strategy for predicting stroke using a Logistic Regression algorithm. The authors employ preprocessing techniques such as SMOTE, feature selection, and controlling outliers to enhance the model's performance 28 . By analyzing various factors such as blood pressure, body mass, heart conditions, age, previous smoking status, prior history of stroke, and glucose levels, the authors achieve an accuracy of 86% in stroke disease prediction, which outperforms other LR-based models. The research emphasizes the capacity of machine learning methods to reduce the adverse impacts of stroke and enable early detection. Multiple physiological attributes are used in various machine learning techniques to forecast strokes, that includes Logistic Regression, Decision Tree, Random Forest, K-Nearest Neighbours, Support Vector Machine, and Naive Bayes based 29 . The findings indicate that Naïve Bayes achieves the highest accuracy rate, reaching around 82%. These findings suggest that machine learning models can aid early stroke identification in the future.
To predict strokes and evaluate, the proposed model achieves 94% accuracy, and the model outperforms other algorithms, including Naive Bayes, Logistic Regression, Support Vector Machine, and Decision Tree 30 . The authors also used Ensembled Naive Bayes and Ensembled Decision Tree. Overall, the article's contributions to developing an integrated learning model and reorganizing the fixed structure of the developed algorithm.
The researchers employ machine learning algorithms for predicting stroke and evaluate their performance based on F1 score, recall, accuracy, and precision 31 . Preprocessing steps include handling missing values, one hot encoding, and feature scaling. The authors use three classifiers, Support Vector Machines, Decision Trees, and Logistic Regression, to train on the dataset and compare their results. The study emphasizes the value of early stroke prediction, and the paper's contribution lies in preparing the dataset using machine learning algorithms. The proposed model achieves an accuracy of 95.49% and can be used for early stroke prediction in real-world applications.
In another study, the authors put forth a predictive model for stroke detection using five different algorithms, i.e. K-Nearest Neighbours, Decision Tree, Random Forest, Support Vector Machine, and Logistic Regression 32 . A comparative analysis of the five models reveals that Random Forest has the highest accuracy of 95.5%. The authors conclude that they find that Random Forest is the model with the highest accuracy and fewest false negatives, and they use Tkinter to construct a Graphical User Interface (GUI) to make the application's use more convenient. The authors suggest that more medical attributes should be considered in future work for better performance of the model. Four distinct models are utilized, including Logistic Regression, Voting classifier, Decision Tree, and Random Forest 33 . Random Forest performs better by achieving the highest classification accuracy of 96%. The future scope of their research involves using more extensive datasets and different machine learning methods, such as AdaBoost, SVM, and bagging, to enhance prediction reliability further. The authors suggest that machine learning can aid patients in receiving early stroke treatment and enhance their quality of life.
In another article 34 , the authors explore the performance of Logistic Regression and Random Forest algorithms in predicting strokes using a preprocessed stroke dataset. The Random Forest algorithm outperforms Logistic Regression in terms of accuracy. The study also discusses the bias and variance of the models and their impact on the results. Although there are some limitations in the proposed work, such as only using two models are used however it provides valuable insight into stroke prediction research. A recent study suggests an ensemble RXLM model to predict stroke using Random Forest, XGBoost, and LightGBM 35 . The dataset is pre-processed using the KNN imputer technique, one-hot encoding, and SMOTE. The researchers fine-tune the hyperparameters of the ML algorithms by employing a random search technique to achieve optimal parameter values. The accuracy of the suggested ensemble RXLM model is 96.34%.
Authors in their study propose a machine learning model with K-Nearest Neighbours, Decision Tree, and Logistic Regression 36 . Exploratory data analysis is applied for preprocessing and uses the SMOTE technique to balance the dataset. Finally, a cloud-based mobile app is developed, which can gather user data for analysis and accurately warn a person of the likelihood of a stroke with an accuracy of 96%. Future work will focus on analyzing the dataset using deep learning methods to enhance accuracy. The authors explore ten machine-learning models to predict strokes 37 . The employed models included Gaussian Naive Bayes, Bernoulli Naive Bayes, Gradient Boosting, Stochastic Gradient Descent, K-nearest neighbours (KNN), support vector machine (SVM), Decision Tree, Random Forest, Logistic Regression, and MLP (Multi-Layer Perceptron). The study emphasizes the necessity of an early stroke diagnosis to lessen its effects with a 94% accuracy rate, the KNN algorithm outperforms the other models.
The literature review explores various machine learning models for stroke prediction that include Naive Bayes, Support Vector Machine, Decision Tree, Random Forest, and Logistic Regression. The studies also propose new models, highlighting the importance of early detection and achieving accuracy rates ranging from 82 to 96%. However, limitations such as limited model selection, feature selection/ engineering, and dataset size are identified. This research paper addresses these deficiencies by conducting a comprehensive analysis of advanced machine learning models, identifying key risk factors along with importance, and evaluating the performance on a larger augmented dataset.
Dataset and preprocessing
The stroke prediction dataset was created by McKinsey & Company and Kaggle is the source of the data used in this study 38 , 39 . The dataset is in comma separated values (CSV) format, including demographic and health-related information about individuals and whether or not they have had a stroke. The dataset was originally comprised a total of 29,072 records, while only 30% of the data is publicly accessible and the remaining 70% is designated as private data 40 . The source of the dataset is mentioned as confidential. The data originates from medical records associated with 5110 individuals residing in Bangladesh. The dataset has underwent preprocessing procedures, which involved modifications to the original dataset sourced from Electronic Health Records (EHR) managed by McKinsey & Company 41 . The data has some missing values, and there is an imbalance between the number of people who have had a stroke and those who have not. The aim is to address these issues using different data imputation techniques and oversampling methods.
Exploratory analysis
The dataset used for stroke prediction consists of 5110 observations, each containing 12 attributes. Out of these attributes, 10 are considered relevant for the prediction task. These attributes provide valuable patient information, including their identification number, age, gender, hypertension, marital status, occupation, residence type, presence of heart disease, average glucose level, BMI, smoking habits, and stroke status.
A detailed examination of stroke occurrences concerning different features is presented in Fig. 1 , with sub-figures. In sub-figure (Fig. 1 a), it is visible that there is a slight increase in the number of strokes among females when compared to males. Moving on to sub-figure (Fig. 1 b), a rising trend in stroke cases is observed as individuals age, with the highest incidence observed around the age of 80. Sub-figure (Fig. 1 c) reveals that individuals with heart disease are more vulnerable to experiencing strokes. Marital status is explored in sub-figure (Fig. 1 d), which suggests that married individuals may have a slightly higher incidence of strokes than unmarried individuals. The comparison between stroke occurrences in urban and rural areas is depicted in sub-figure (Fig. 1 e), indicating no significant difference between these groups regarding stroke risk. In sub-figure (Fig. 1 f), the relationship between average glucose levels and stroke risk is illustrated. It shows that individuals with average glucose levels falling within 60–120 and 190–230 are at an increased risk of experiencing strokes. Hypertension is emphasized in sub-figure (Fig. 1 g). It demonstrates a higher incidence of strokes among individuals diagnosed with hypertension.
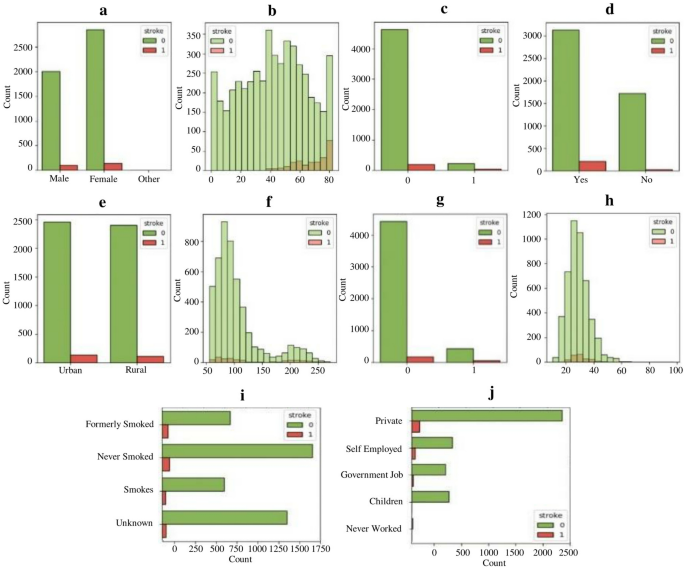
Distribution of features concerning stroke occurrence. ( a ) through ( j ) present diverse aspects of stroke occurrences, revealing nuanced patterns. ( a ) and ( b ) demonstrate gender and age-related trends. ( c ) associates strokes with heart disease, while ( d ) suggests marital status correlations. ( e ) explores urban–rural disparities. ( f ) and ( g ) show links to average glucose levels and hypertension. ( h ) relates BMI levels to stroke incidence. ( i ) emphasizes the role of smoking history, and ( j ) explores potential occupational influences on stroke likelihood.
The relationship between BMI and stroke occurrence is examined in sub-figure (Fig. 1 h). It reveals that individuals with a BMI ranging from 20 to 40 are more prone to strokes. Smoking habits are examined in sub-figure (Fig. 1 i), where it is observed that former or never smokers are more likely to suffer from strokes than current smokers. This finding highlights the importance of considering smoking history when assessing an individual's stroke risk. Lastly, shifting the focus to occupation, sub-figure (Fig. 1 j) indicates that individuals working in private or self-employed sectors may have a greater likelihood of experiencing strokes compared to those in other occupations. This observation may be attributed to various factors such as stress levels, working conditions, and lifestyle differences among different occupational groups. Overall, the comprehensive analysis of stroke occurrences concerning different features provides valuable insights into the dataset and aids in understanding the factors contributing to stroke risk.
The dataset used in this research contains three numerical features: average glucose level, BMI, and age, while the remaining features are categorical. To assess the presence of outliers in the numerical features, box plots have been constructed and displayed in Fig. 2 . The plots illustrate a notable presence of outliers in the average glucose level (Fig. 2 b) and BMI metrics (Fig. 2 c), emphasizing the need for meticulous data preprocessing. As depicted in Fig. 3 , the distributions of individual numerical attributes diverge notably between those with and without a stroke. The discernible non-uniformity in these distributions underscores the importance of these features as promising indicators for stroke prediction.

Box plots of numerical features to detect outliers. ( a ), ( b ) and ( c ) presents the boxt plots for age, BMI, and average glucose level to assess the presence of outliers.
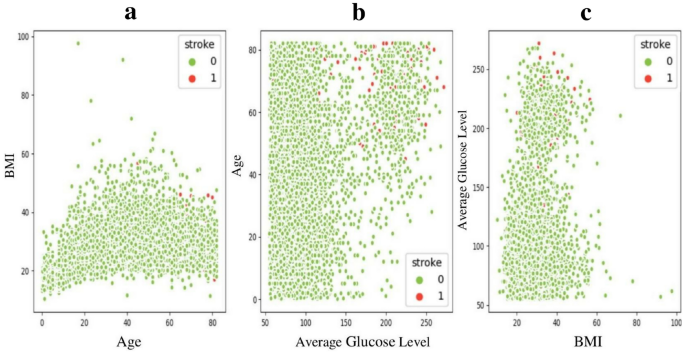
Distribution of numerical attributes with stroke and each other. ( a ), ( b ) and ( c ) presents the distribution plots for age, BMI, and average glucose level against eachother based on stroke occurences.
A more in-depth exploration of the mentioned numerical attributes holds the promise of unravelling their influence on stroke prediction, thus offering invaluable insights to enhance the accuracy and efficacy of the predictive models. To preprocess the data, outliers are removed using the robust scaler method and apply standard scaling for consistent feature ranges. One-hot encoding is also utilized to convert categorical variables into binary values. Figure 4 illustrates the visual representation of encoded features correlation, offering valuable insights into the relationships between variables. This analysis helps to uncover significant associations and dependencies among the features, enhancing the understanding of the underlying patterns and dynamics within the dataset.
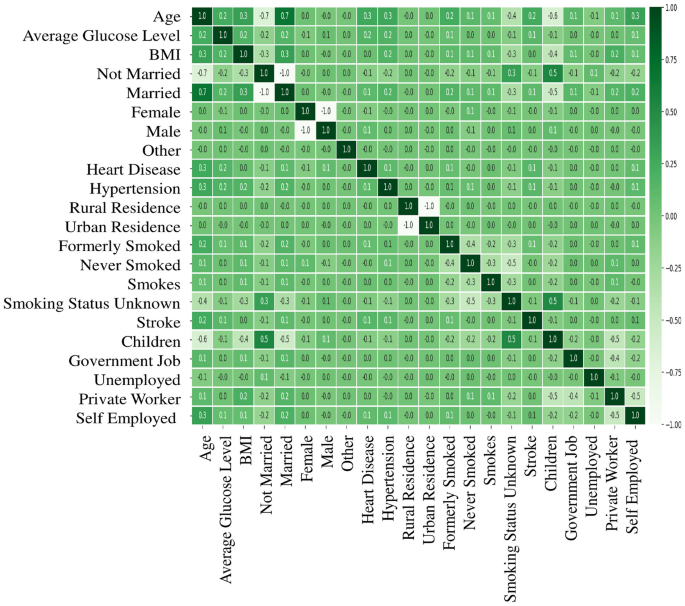
Features correlation heatmap for the dataset. Color intensity indicates the strength and direction of correlations, aiding in the identification of potential patterns and dependencies in the data.
Missing data
The dataset will now be analyzed to predict stroke while inspecting for any missing values. The sparsity/nullity matrix for the dataset is shown in Fig. 5 . It can be observed that the BMI feature has some missing values that need to be handled before proceeding with the analysis. As illustrated in Table 1 , the dataset overview provides insights into the total number of stroke cases and the count of entries with missing BMI values. The table showcases that out of the 5110 total cases, there are 201 cases with missing BMI values. Consequently, if rows with missing values were dropped, there would be a data loss percentage of 3.93%, making it almost 4% of the dataset which can lead to loss of valuable insights present in it.
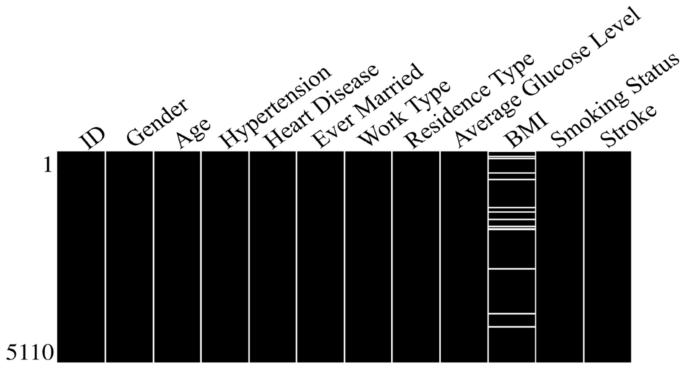
Sparsity matrix for the dataset. The empty spaces found in the corresponding column signify the presence of missing data values for the specific feature.
The naive response to missing values would be removing all those rows. But to avoid data loss through list-wise deletion, this study will use imputation techniques to fill in the missing values. Imputation is a method of replacing missing data with an appropriate approximation based on available information. However, if not chosen carefully, imputation can introduce assumptions or biases. Therefore, this study explores well-established techniques specifically chosen for their ability to mitigate these potential issues. Later, the performance of these imputation techniques will be compared with the standard approach of dropping incomplete observations. This study explores three different imputation techniques to maximize data utility while maintaining data integrity and minimizing bias.
Mean ımputation
A commonly employed technique in data preprocessing involves replacing missing values in a dataset with the mean value of the respective variables within the feature column. This approach helps maintain data integrity and ensures the resulting dataset is complete and ready for analysis. Imputing missing values with the mean minimizes the impact of incomplete data on subsequent analyses. Additionally, this method enables us to use as much available information as possible, contributing to more accurate and robust results.
Multivariate ımputation using chained equations (MICE)
It is an advanced approach that surpasses single imputations. It employs multiple imputations, allowing a more robust estimation of missing values. The process involves a sequential regression technique, where each variable's missing values are estimated using information from other variables that have complete data. MICE significantly improves the accuracy and reliability of imputations, providing a comprehensive solution for handling missing values in datasets.
Age group-based BMI ımputation
To enhance the analysis, the individuals are classified into four age groups: 0–20, 21–40, 41–60, and 61–80. The strategy of imputing the mean BMI for each respective age group is employed to address missing values. This approach allows us to account for missing data while maintaining the integrity of the analysis. Additionally, this division into age groups enables a more nuanced understanding of the relationship between age and BMI, contributing to the overall accuracy and reliability of the findings.
Data ımbalance
After addressing the missing data, the focus is shifted to the data imbalance problem. There are more non-stroke case instances than stroke case instances, making the stroke case instances a minority class. This data imbalance poses a challenge in developing accurate predictive models and warrants the need for specialized techniques to handle class imbalance effectively. An overview of the class label populations in the dataset is presented in Table 2 , revealing the presence of a minority class. This data imbalance can adversely affect model performance as the minority class is underrepresented. To mitigate the data imbalance issue, oversampling and undersampling techniques are commonly employed. Undersampling, which involves reducing the number of instances from the majority class, is not considered feasible. This approach may hinder the model's capacity to effectively learn patterns associated with stroke cases, potentially compromising its predictive accuracy. Oversampling is deemed feasible as it elevates the minority class distribution, so the predictive model performs well. However, if it is not implemented carefully, it may introduce the risk of overfitting. This study addresses this potential pitfall by investigating the efficacy of the models in predicting strokes using both balanced and imbalanced datasets. This approach will facilitates a rigorous evaluation of the predictive model's performance under diverse data conditions. To address this class imbalance issue, SMOTE is assessed as a potential remedy.
Synthetic minority oversampling technique
SMOTE is a widely recognized method for oversampling. It is employed to increase the representation of minority samples in a dataset. To understand how it operates. Consider a scenario where the training dataset consists of 's' samples and 'f' features. To enhance the representation of the minority class in the dataset, the methodology employed is known as oversampling. This technique involves selecting a sample from the minority class and identifying its k nearest neighbours in the feature space. Subsequently, it generates a new synthetic data point by combining the original data point with one of its closest neighbours. This combination is achieved by scaling the vector connecting the two points by a random number 'x' ranging from 0 to 1. Incorporating this synthetic data point into the existing dataset effectively addresses the class imbalance issue and generates a fresh, augmented data point. This process helps balance the classes and improve the overall representation of the minority class 42 .
Data modelling
A robust data modelling approach is essential to effectively analyze and predict stroke occurrences, encompassing raw data's systematic transformation and organization into a structured framework. Figure 6 depicts the data modelling pipeline utilized in this research, showcasing the various stages and methodologies employed. This pipeline enhances the data analysis and prediction approach's accuracy and efficiency.
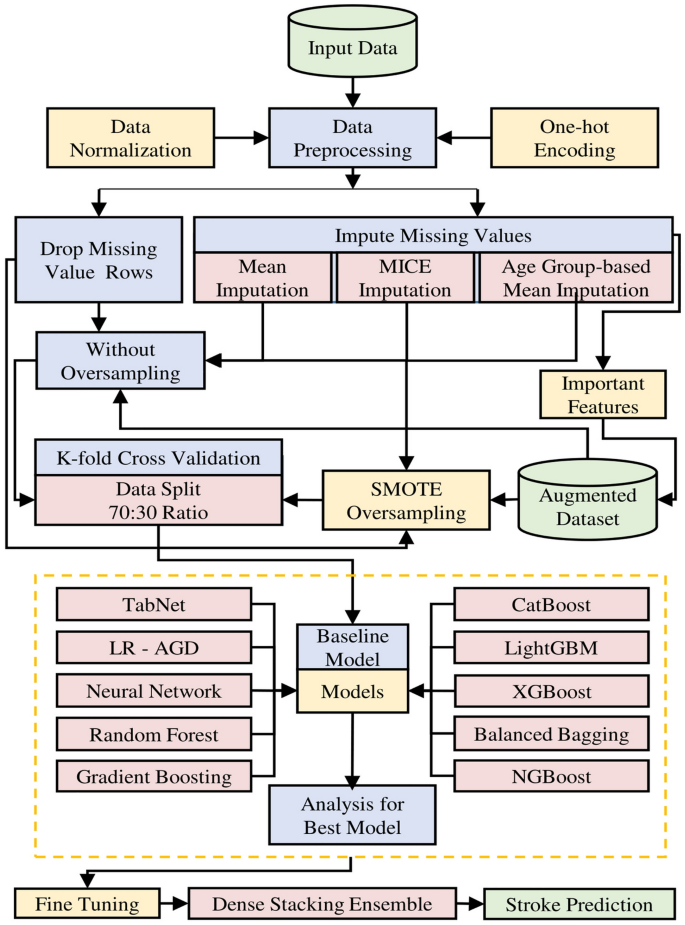
Pipeline for data modelling. Stroke prediction data modeling pipeline integrates techniques for missing and imbalanced data. Prediction models, from TabNet to NGBoost, undergo rigorous evaluation and testing, culminating in a Dense Stacking Ensemble (DSE) for enhanced and robust prediction results.
This research employs various techniques for stroke prediction to handle missing and imbalanced data issues. The researchers utilize mean, MICE, and age group-wise BMI mean imputation methods to handle missing values. To tackle the data imbalance issue, SMOTE is used to increase the representation of the minority class labels by generating synthetic samples. Additionally, the outliers are removed using the robust scaler method and apply standard scaling to ensure consistent feature ranges. Categorical variables are transformed into binary values through one-hot encoding. The predictive models encompass a baseline model, and then followed by advanced models including TabNet, Logistic Regression with AGD (LR-AGD), Neural Network, Random Forest, Gradient Boosting, CatBoost, LightGBM, XGBoost, Balanced Bagging, and NGBoost. The dataset is divided into training and testing data using a 70:30 ratio. The models are evaluated using k-fold cross-validation on both balanced and imbalanced imputed datasets and on the augmented dataset, generating multiple analyses for each model. Also, the trained models are tested on testing data to assess their generalization performance. The performance of each model is also compared to the standard approach of dropping incomplete observations on the original dataset. Consequently, a Dense Stacking Ensemble (DSE) model is built upon previous models after fine-tuning, with the best-performing model as a meta-classifier. Finally, all models are ranked and analyzed, including the DSE model, using various performance metrics.
Augmentation of dataset
The primary objective is to identify the key factors contributing to stroke prediction. To accomplish this, the authors highlight essential features in Fig. 7 that demonstrate a positive impact on stroke prediction and their corresponding importance factors. This analysis provides valuable insights into the factors that play a vital role in accurately predicting strokes. This study incorporates information from the previous three imputed datasets for dataset augmentation, resulting in a larger dataset with 10,421 distinct instances.
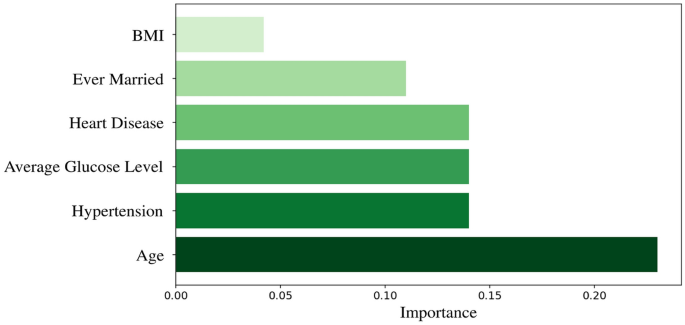
Features with positive importance factor for stroke prediction. Features of significant positive importance for stroke prediction include age, BMI, average glucose level, heart disease, hypertension, and marital status (ever-married) respectively.
The augmented dataset includes age, BMI, average glucose level, heart disease, hypertension, ever-married, and stroke label features. Interestingly, the findings align with another previously conducted comprehensive study that used the same dataset 43 , where the critical features identified for stroke prediction using the same dataset were the same, except for the inclusion of the "ever married" feature. It is noticed that the ever-married feature has a high frequency of stroke occurrences among those individuals who were or are married.
K-fold cross validation
To assess how well the models perform, it is required to divide the dataset into training, validation, and testing data. Since no separate "unlabeled" test dataset is available, this study adopts a tenfold validation method. This means that it divides the training dataset into K parts. During each iteration, a single part is designated as the validation dataset out of the K parts obtained by dividing the training dataset. The remaining parts are utilized for training the model. This process is repeated K times, allowing for a comprehensive evaluation of the model's performance. Performance metrics are recorded for each validation set. After all iterations, the metrics are averaged across all K iterations, ensuring that each bin served as a validation dataset at least once 44 .
Machine learning algorithms
The main objective is to develop an accurate and robust predictive model for stroke prediction. The authors begin by using a baseline model to establish a reference point for model performance. They then investigate different advanced classification models to determine the accuracy of these models in predicting stroke. After thorough fine-tuning, the authors construct a robust DSE model that leverages the best-performing model as a meta-classifier. The upcoming subsections will delve into the classification models, which are also used as base models, as well as the architecture of the DSE model.
Baseline model
This study employs Logistic Regression as the baseline model for stroke prediction. It is a statistical technique widely used for binary classification tasks. It estimates the probability of a binary outcome based on input features using the logistic function as given in Eq. ( 1 ).
where z represents the linear combination of input features weighted by corresponding coefficients. This model serves as an initial benchmark for evaluating the performance of more advanced classification models.
Advanced classification models
In the quest for creating a strong stroke prediction model, a variety of advanced contemporary classification models are carefully examined without fine-tuning and put to use. These models serve a dual purpose: first, they undergo rigorous evaluation for predictive accuracy, and second, they constitute the core elements of the DSE model, which employs a layered and efficient approach to predicting strokes.
TabNet is a supervised machine learning algorithm that operates on tabular data and employs a neural network architecture with attention-based feature selection and sequential decision steps. It is designed to handle structured data and can effectively capture complex relationships between input features to make accurate predictions for stroke classification.
In Eq. ( 2 ) and ( 3 ), let X be the input feature matrix, y be the binary target variable (0 or 1) representing the stroke, and \(\hat{y}\) be the predicted stroke probabilities. The TabNet algorithm aims to find the optimal parameters \(\theta\) that minimize the loss function L .
Logistic regression with AGD
Logistic Regression models the relationship between input variables and binary output. It utilizes the logistic function to estimate the probability of the outcome, making it suitable for binary classification tasks like stroke prediction. In this study, the logistic regression model is trained efficiently using the accelerated gradient descent (AGD) optimization technique. The model is limited to 100 maximum iterations during training.
In logistic regression with AGD, the model estimates the probability of stroke \(\hat{y}\) given the input features X using the logistic function, where \(\beta\) represents the model's coefficients as given in Eq. ( 4 ).
Neural network
Neural network is a powerful machine learning model that consists of interconnected nodes or "neurons" organized in layers. It is capable of learning complex patterns from data and making non-linear predictions. The neural network used in this research has five hidden layers with 24, 36, 48, 36, and 24 neurons, respectively. It is trained to recognize significant features related to stroke prediction and make accurate decisions based on them.
In Eq. ( 5 ), the neural network involves a series of calculations with weight matrices ( W ) biases ( b ), and activation functions ( \(\sigma\) ) in each layer. The output layer uses the sigmoid activation function to obtain the predicted stroke probabilities ( \(\widehat{y}\) ). The \({W}_{out}\) and \({b}_{out}\) are the weight matrix and bias vector of the output layer, respectively.
where in Eq. ( 6 ), the neural network aims to minimize the loss function L during training.
Random forest
The Random Forest model builds a collection of decision trees and combines their predictions to make final predictions. It utilizes random feature selection and bootstrapping to create diverse tree models. The number of estimators is denoted as N RF = 100.
Gradient boosting
The Gradient Boosting model is a powerful predictive model that utilizes a combination of weak prediction models, commonly decision trees, to generate accurate predictions. This model iteratively enhances its predictions by fitting new models to the residuals of previous models. In this study, N GB = 100 estimators are employed to optimize the performance of the Gradient Boosting model.
The category Boosting algorithm is specifically designed for categorical data. It utilizes gradient boosting and implements novel techniques to handle categorical variables effectively. The number of estimators for this model is N CB = 100.
A light Gradient Boosting Machine is a gradient boosting framework that aims to provide high efficiency and speed. It uses a histogram-based approach for gradient boosting and incorporates features like leaf-wise tree growth and data parallelism. N LGBM = 100 estimators are used.
Extreme Gradient Boosting is a gradient boosting algorithm known for its scalability and performance. It combines multiple weak prediction models and employs regularization techniques to prevent overfitting. For this model, N XGB = 100 estimators are used.
Balanced bagging
Balanced Bagging is an ensemble learning algorithm that combines multiple classifiers by training them on different subsets of the original dataset. It explicitly addresses class imbalance issues by using sampling techniques to balance the class distribution. Five Random Forest Classifiers as base estimators are used.
Natural gradient boosting is a gradient boosting algorithm that focuses on probabilistic prediction and uncertainty estimation. It utilizes natural gradient boosting and incorporates Bayesian methods for improved model calibration. For this model, N NGB = 100 estimators and a learning rate of 0.01 are used.
Dense stacking ensemble model
The cornerstone for robust stroke prediction system is the DSE model, as its high-level architecture is visually depicted in Fig. 8 . The DSE model is meticulously crafted to optimize predictive accuracy and robustness. The DSE architecture integrates a range of fine-tuned classification models, each playing a vital role as a base model.
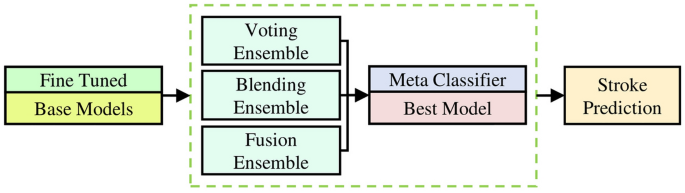
Architecture of the dense stacking ensemble model. The dense stacking ensemble integrates fine-tuned base models using three distinct approaches, each incorporating the best-performing model as a meta-classifier.
Within the this model, three distinct approaches are employed, with each utilizing the best-performing model as a meta-classifier. These approaches are strategically integrated as base models in the final DSE model to enhance predictive accuracy and reliability. These approaches are explained in the following.
Voting ensemble
The Voting ensemble approach operates by collecting predictions from multiple base models and making a collective prediction based on the most popular choice. This approach leverages the best-performing model as the meta-classifier, which means it gives more weight to the predictions of this model. By combining the insights from various models, the Voting ensemble aims to maximize the overall predictive power of the DSE model. It's like having a panel of experts vote on the most likely outcome, with the best expert's opinion carrying the most weight 45 .
Blending ensemble
In the Blending ensemble approach, a meta-classifier is trained using the predictions made by the base models. The best-performing model takes on the role of the meta-classifier in this approach. This model is skilled at blending the predictions of other models in a way that optimizes their collective predictive strength. The Blending ensemble essentially learns how to combine the different model outputs best, capitalizing on the unique strengths of each model to enhance the overall predictive accuracy of the DSE model 46 .
Fusion ensemble
The harmonious integration of both the base models and the best-performing model characterizes the Fusion ensemble approach. It doesn't just use the best-performing model as the meta-classifier; it collaboratively combines the strengths and insights of all models in a synergistic manner. This approach creates a final predictive model that benefits from the diverse perspectives and capabilities of the base models, thus producing a more robust and accurate prediction within the DSE framework 47 . It's like bringing together a team of experts to solve a complex problem, with each expert contributing their unique insights and skills.
In this section, a comprehensive analysis of results, along with various score plots, is provided. The datasets are generated using Mean, MICE, and Age Group-based imputation techniques to address missing values. The analysis also encompasses the score plots for the original dataset, in which missing values are managed through list-wise deletion. Furthermore, the results for the augmented dataset are presented in a similar manner. The results are presented in three subsections: The first and second subsections focuse on the performance of various classification models, with a particular emphasis on comparing their effectiveness against a baseline model. The second subsection is about the DSE model results.
To gauge the effectiveness of the models, a diverse set of metrics is employed. These metrics encompass the confusion matrix, which includes true positive (TP), false positive (FP), true negative (TN), and false negative (FN) values for actual and predicted data. The definition of these confusion matrix parameters can be found in Table 3 . Additional metrics, such as accuracy, precision, recall, F1 score, and AUC are utilized to provide a comprehensive evaluation of classifier performance 48 , 49 . Table 4 explains these metrics briefly. The findings highlight the effectiveness of the different imputation techniques in handling missing data and showcase the impact on model accuracy. The analysis of the augmented dataset demonstrates the improvement achieved by incorporating essential features. Overall, this section contributes to a comprehensive understanding of the various model's performance and insights for future research and model development.
Results of baseline model
The performance of the baseline model varied notably between the imbalanced and balanced datasets, as shown in Fig. 9 . On the imbalanced dataset, the highest F1 score achieved was only 23.09%, which is considerably lower than the F1 score of 87.04% observed on the balanced datasets. This discrepancy is consistent across all other metrics, including accuracy, precision, recall, and AUC. In contrast, the baseline model exhibited notably higher performance on the balanced dataset, both in terms of the original data and the imputed datasets. For instance, on the original dataset, the baseline model attained the highest accuracy of 87.12%, precision of 87.65%, recall of 86.44%, F1 score of 87.04%, and AUC of 87.12%. This level of performance was closely mirrored in the imputed datasets, with the MICE-imputed dataset showing an accuracy of 85.54%, precision of 85.95%, recall of 85.44%, F1 score of 85.46%, and AUC of 85.54%. These findings suggest that either dropping rows with missing values or employing imputation techniques can significantly enhance the usability of the dataset, likely due to the increased availability of data for training the models.
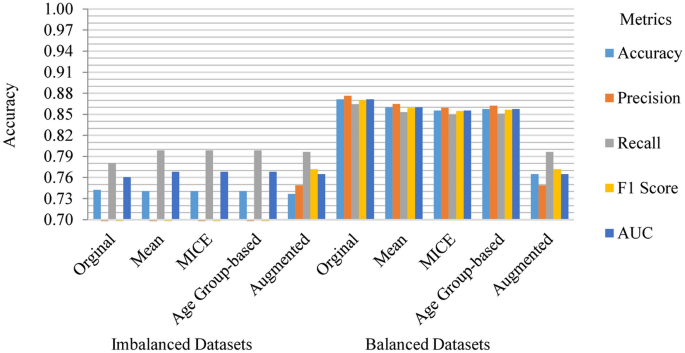
Baseline model performance across various datasets. The performance on imbalanced datasets is substantially lower than on the balanced datasets.
Results of advanced classification models
In this section, we present the outcomes of our rigorous evaluation of advanced classification models. These models have been extensively assessed to provide insights into their predictive performance for stroke occurrences.
Imbalanced and balanced ımputed datasets results
MICE imputation yields slightly better results than Mean and Age Group-based imputation among the imbalanced imputed datasets. The top-performing model across all imputed datasets is LR-AGD, followed by NGBoost and Balanced Bagging. LR-AGD achieves a k-fold mean accuracy of 94.94%. The precision, recall, and F1 score are 95.20%, 94.94%, and 92.50%, respectively. Figure 10 shows the k-fold mean accuracy for all models on the imbalanced imputed datasets.
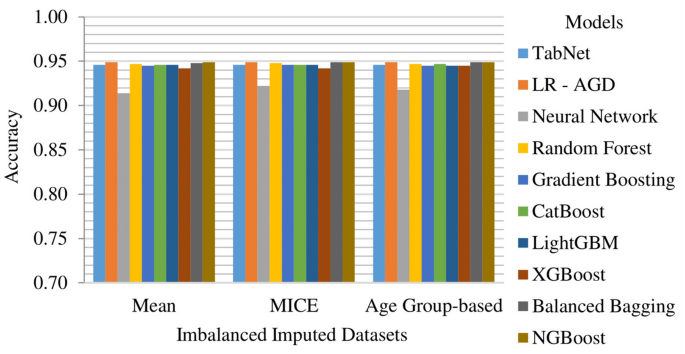
Models k-fold mean accuracy on imbalanced imputed datasets. The imputed imbalanced datasets are created using three different techniques namely as man, MICE, and age group-based.
The Age Group-based balanced imputed dataset performs slightly better than the Mean and MICE imputed balanced datasets. XGBoost is the top-performing model, followed closely by LightGBM and Random Forest. The k-folds mean accuracy for the top-performing model is 93.48%, with precision, recall, and F1 score of 94.03%, 93.77%, and 93.76%, respectively. Figure 11 presents the k-fold mean accuracy of all models on balanced imputed datasets.
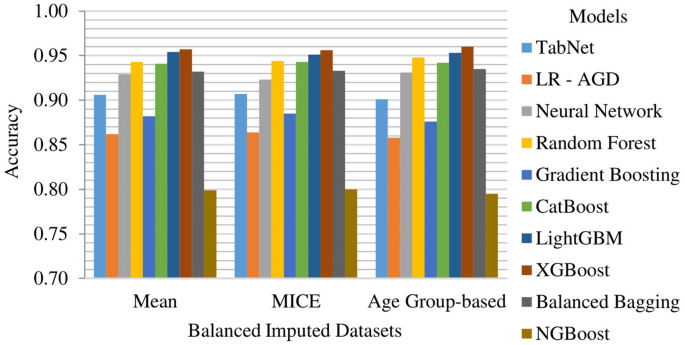
Models k-fold mean accuracy on balanced imputed datasets. The imputed balanced datasets are created using three different techniques namely as man, MICE, and age group-based.
On the imbalanced MICE imputed testing dataset, LR-AGD achieves an accuracy of 96.28%, precision of 100%, recall of 14.93%, and F1 score of 25.97%. Moreover, for XGBoost on balanced Age Group-Based imputed testing dataset, the testing accuracy, precision, recall, and F1 score are 96.37%, 96.62%, 96.09%, and 96.35% respectively. The confusion matrices for the LR-AGD and XGBoost model on the respective testing datasets are displayed in Fig. 12 . The matrix provides a visual representation of the model's performance. It allows for a comprehensive analysis of the model's accuracy and the distribution of correct and incorrect predictions across different classes.
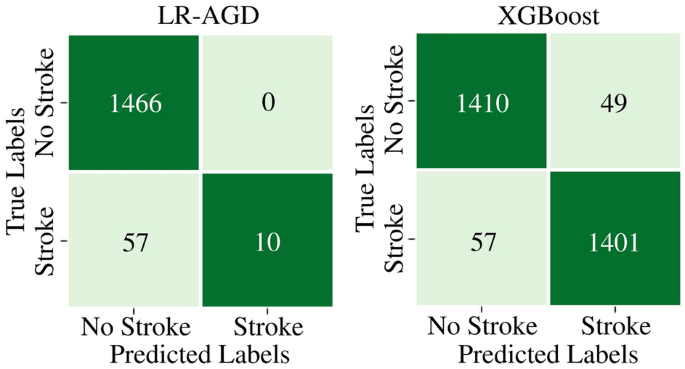
Confusion matrices of LR-AGD and XGBoost on imputed datasets. Confusion matrix illustrating the performance of LR-AGD (right) and XGBoost (left) models in stroke case classification on imbalanced and balanced imputed datasets, respectively.
The LR-AGD model consistently displayed the highest precision for the imbalanced datasets, with a value of 95.19%. It showcased its ability to predict positive instances accurately. On the other hand, when considering the balanced datasets, the XGBoost, LightGBM, and Random Forest models emerged as the top performers in terms of precision. The XGBoost model demonstrated the highest precision, ranging from 95.56% to 95.68%, followed closely by the LightGBM and Random Forest models with 95.53% and 94.96%, respectively. The k-fold mean precision of models on all imputed datasets is displayed in Fig. 13 .
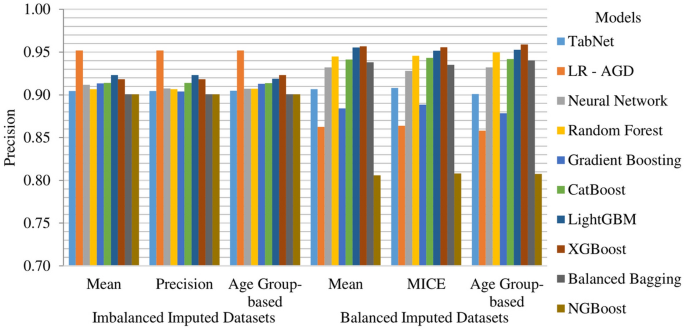
Models k-fold mean precision on all imputed datasets. The imputed datasets are created using three different techniques namely as man, MICE, and age group-based and divided into two categories named as imbalanced and balanced.
NGBoost, Balanced Bagging, and LR-AGD models consistently show high recall values, ranging from 94.91% to 94.94%, across different imputation techniques for the imbalanced datasets. In the case of balanced datasets, XGBoost, LightGBM, and Random Forest models exhibit higher recall values of 95.90%, 95.53%, and 94.84%, respectively. These results indicate that the models are generally effective in capturing actual positive instances and correctly identifying them. The k-fold mean recall of models on all imputed datasets is displayed in Fig. 14 .
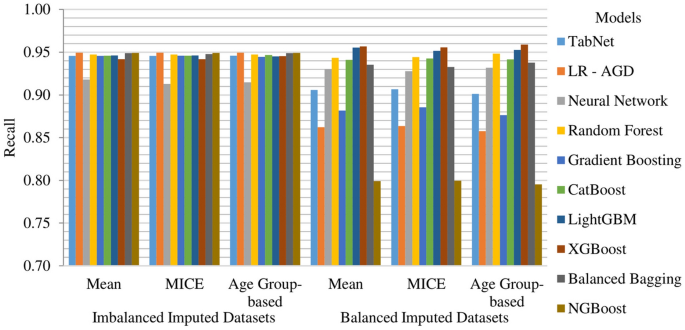
Models k-fold mean recall on all imputed datasets. The imputed datasets are created using three different techniques namely as man, MICE, and age group-based and divided into two categories named as imbalanced and balanced.
Among the models evaluated, surprisingly, the Random Forest model achieved the highest F1 score of 94.72% on the imbalanced MICE imputed dataset and consistently performed well across all imputation techniques. XGBoost and LightGBM also performed well. When considering the balanced datasets, XGBoost, LightGBM, and Random Forest models exhibited the highest F1 scores, with values of 95.90%, 95.53% and 94.82%, respectively. Overall, these results indicate that XGBoost, LightGBM, and Random Forest models are promising models for stroke prediction, showcasing their ability to achieve accurate classifications across different dataset characteristics. The k-fold mean F1 score of models on all imputed datasets is displayed in Fig. 15 .
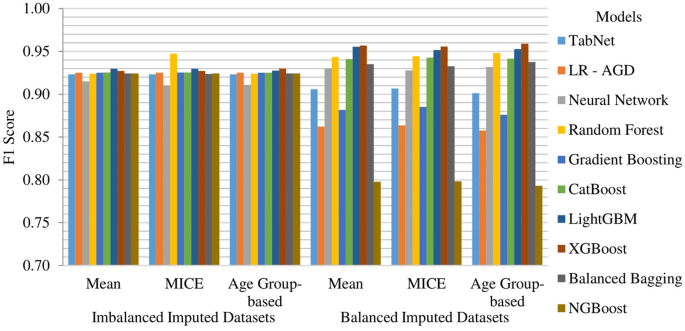
Models k-fold mean f1 score on all imputed datasets. The imputed datasets are created using three different techniques namely as man, MICE, and age group-based and divided into two categories named as imbalanced and balanced.
Imbalanced and balanced original datasets results
Among the models evaluated on the original dataset, which is created by removing the missing value rows, LR-AGD emerges as the top performer, closely followed by NGBoost and Balanced Bagging. LR-AGD achieves a k-fold mean accuracy of 95.46%, while NGBoost and Balanced Bagging achieve 95.46% and 95.43%, respectively. LR-AGD exhibits a precision, recall, and F1 score of 94.03%, 93.77%, and 93.76%, respectively. These exceptional results underscore the robustness and efficacy of LR-AGD in handling complex data scenarios. The k-fold mean accuracy for all models on imbalanced and balanced datasets is displayed in Fig. 16 . For a balanced dataset with removed missing value rows, XGBoost emerges as the top-performing model, achieving a k-fold mean accuracy of 96.14%. It also exhibits high precision, recall, and F1 score, all at 96.14%. LightGBM and Random Forest follow closely behind in terms of second and third best models.
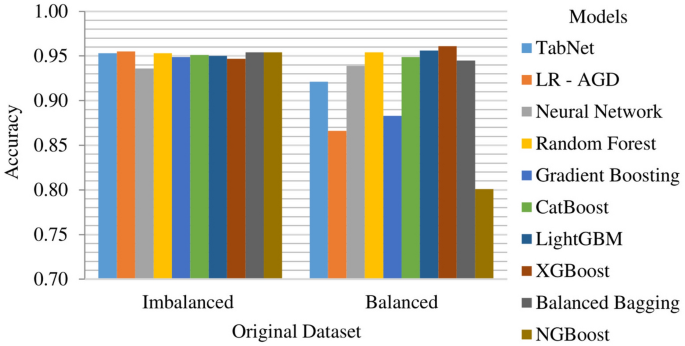
Models k-fold mean accuracy on original datasets. The dataset is categorized into two groups: imbalanced, reflecting its initial state, and balanced, achieved after employing oversampling technique.
LR-AGD on imbalanced testing original dataset achieves the testing accuracy of 96.81%, precision of 87.50%, recall of 13.21%, and F1 score of 22.95%, respectively. When tested on the balanced original dataset, XGBoost maintains its superior performance with a testing accuracy of 98.33% and F1 score of 98.86%, while precision of 98.95%, and recall of 98.77%. The confusion matrices for the LR-AGD and XGBoost models on the respective testing datasets are shown in Fig. 17 .
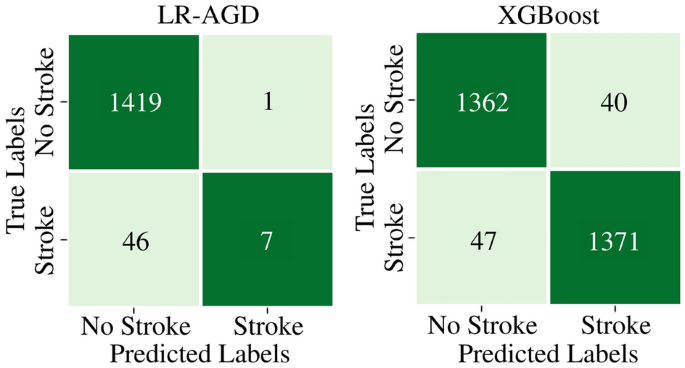
Confusion matrices of LR-AGD and XGBoost on original datasets. Confusion matrix illustrating the performance of LR-AGD (right) and XGBoost (left) models in stroke case classification on imbalanced and balanced original datasets, respectively.
On the imbalanced original dataset, LR-AGD consistently achieved the highest precision and recall scores, with values of 93.42% and 95.46%, respectively. Regarding precision, the second and third best models are Neural Network and Balanced Bagging. NGBoost and Balanced Bagging models also showed impressive recall, the same as the LR-AGD. When considering the F1 score, which provides a balanced measure of precision and recall, all models achieved similar scores of around 93.3% except Neural Network. On the other hand, on the balanced datasets, XGBoost, LightGBM, and Random Forest consistently outperformed the other models regarding precision, recall, and F1 score, with values of 96.14%, 95.78%, and 95.44%, respectively.
Above mentioned results highlight the effectiveness of LR-AGD, XGBoost, LightGBM, and Random Forest in accurately classifying strokes across imbalanced and balanced datasets depending on the nature of the balance in the dataset, emphasizing their potential for stroke prediction applications. Furthermore, the k-fold mean precision, recall, and F1 score of these models on both imbalanced and balanced original datasets are visually depicted in Fig. 18 .
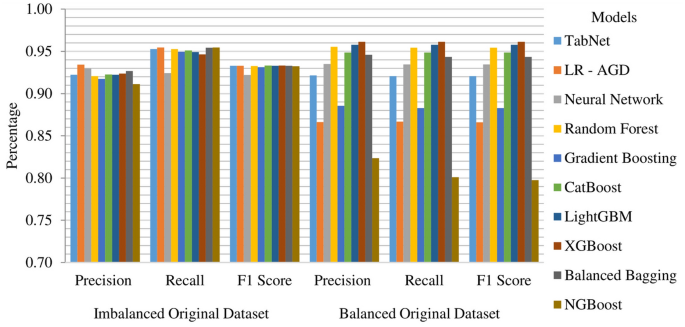
Models k-fold mean performance metrics on original datasets. The dataset is categorized into two groups: imbalanced, reflecting its initial state, and balanced, achieved after employing oversampling technique.
Imbalanced and balanced augmented datasets results
The Random Forest model demonstrates exceptional performance on the augmented dataset with imbalance, achieving a k-fold mean accuracy of 97.41%. It also exhibits high precision, recall, and F1 score, with values of 97.23%, 97.41%, and 97.14%, respectively. The remaining models display mean accuracies ranging from 95 to 96%. Figure 19 depicts the k-fold mean accuracy of all models on the augmented dataset, both imbalanced and balanced. The top performing model on the balanced augmented dataset is Random Forest, achieving a k-fold mean accuracy of 99.45% with a precision of 99.46% and 99.45% of recall and F1 score. It is closely followed by Balanced Bagging and XGBoost, with mean accuracies of 99.07% and 97.94%, respectively.
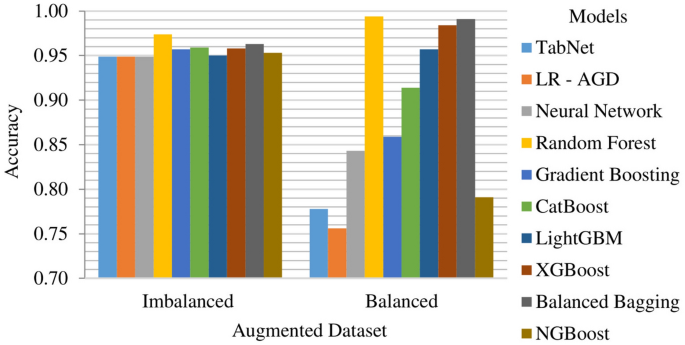
Models k-fold mean accuracy on augmented datasets. The dataset is categorized into two groups: imbalanced, and balanced, achieved after employing oversampling technique.
When evaluated on the imbalanced testing dataset, the Random Forest model maintains its strong performance with a testing accuracy of 97.57%, precision of 85.94%, recall of 65.48%, and F1 score of 74.32%. Random Forest also demonstrates impressive performance in testing on balanced dataset, with an accuracy of 99.61%, precision of 99.23%, recall of 100%, and an F1 score of 99.61%. The confusion matrices of the Random Forest model on imbalanced and balanced augmented testing datasets are depicted in Fig. 20 .
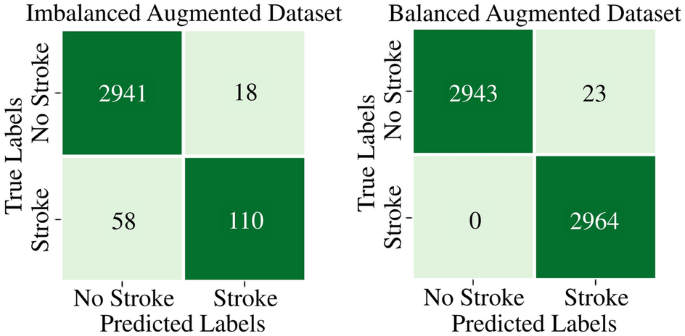
Confusion matrices of random forest on augmented datasets. Confusion matrix illustrating the performance of Random Forest model in stroke case classification on imbalanced (left) and balanced (right) augmented datasets, respectively.
The Random Forest model consistently demonstrates strong performance across all metrics on imbalanced and balanced augmented datasets. It achieves high precision scores of 97.23% on imbalanced data and 99.46% on balanced data, indicating its ability to identify true positive instances accurately. The Random Forest model also exhibits impressive recall scores of 97.41% on imbalanced data and 99.45% on balanced data, highlighting its capability to capture a high proportion of actual positive instances. In terms of F1 score, Random Forest Model achieves a balanced performance with scores of 97.14% on imbalanced data and 99.45% on balanced data. This indicates a harmonious balance between precision and recall, emphasizing its effectiveness in stroke prediction. The Balanced Bagging and XGBoost models also deliver competitive results across all metrics, showcasing their potential for accurate classification on balanced and imbalanced datasets. The k-fold mean precision, recall, and F1 score of models on all augmented datasets are shown in Fig. 21 .
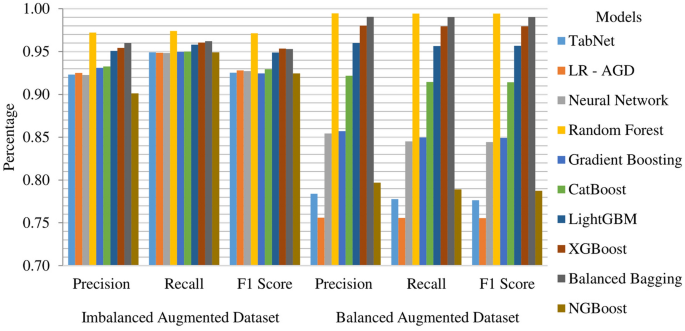
Models k-fold mean performance metrics on augmented datasets. The dataset is categorized into two groups: imbalanced, and balanced, achieved after employing oversampling technique.
Results of dense stacking ensemble model
In the analysis of base model results, it becomes apparent that the MICE-imputed datasets produce marginally superior outcomes. Notably, the Random Forest model stands out as a top performer. Within the DSE model, the Random Forest model assumes the role of the meta-classifier, while the remaining models serve as base models, highlighting the synergy derived from their collective strengths. The DSE model showcased remarkable performance on MICE-imputed datasets. For the imbalanced MICE-imputed datasets, the model yielded an accuracy of 96.13%, precision of 93.26%, recall of 96.18%, and an F1 score of 94.88%. Similarly, on balanced MICE-imputed datasets, the DSE model achieved an accuracy of 96.59%, with precision of 95.25%, recall of 96.27%, and F1 score of 95.79%. These results, also visualized in Fig. 22 , highlight the robust performance of the DSE model when tested on imputed datasets.
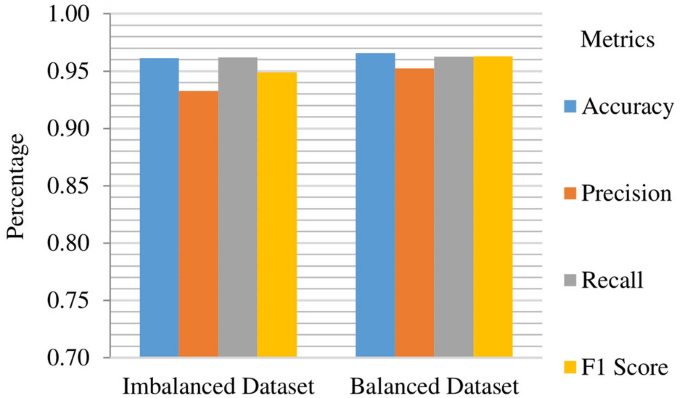
K-fold mean performance matrices of the proposed DSE model. The MICE imputed dataset is categorized into two groups: imbalanced, and balanced, achieved after employing oversampling technique.
The analysis of the AUC metric for the proposed DSE model reveals compelling insights into its predictive performance across different datasets. On the imbalanced dataset with MICE imputation, the DSE model achieves an AUC of 83.94%, showcasing its ability to discern between positive and negative instances despite the data's skewed distribution. Conversely, on the balanced dataset, the DSE model excels even further, attaining an impressive AUC of 98.92%. This substantial increase in AUC on the balanced dataset underscores the model's enhanced discriminatory power and robustness when trained on a more representative and balanced data distribution. The significant performance improvement achieved by the DSE model on the balanced dataset compared to the imbalanced one is visually represented in Fig. 23 .
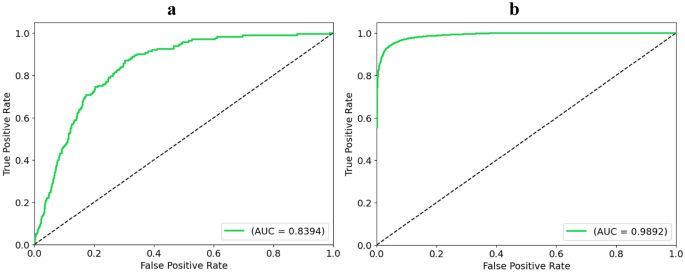
AUC results of the proposed DSE model. ( a ) represents the AUC results on imbalanced MICE imputed dataset and ( b ) on the balanced one.
Furthermore, on the imbalanced testing dataset, the model shows a testing accuracy of 99.15%, a precision of 84.93%, a recall of 98.88%, and an F1 score of 90.51%. For the balanced testing dataset, the model gives a testing accuracy of 97.19%, precision of 96.83%, recall of 97.38%, and an F1 score of 97.10%. The confusion matrices of the DSE model on imbalanced and balanced MICE-imputed testing datasets are depicted in Fig. 24 .
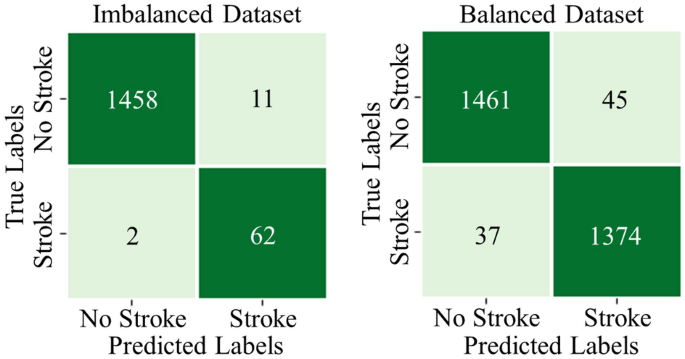
Confusion matrices of the proposed DSE model. Proposed DSE model’s confusion matrices on MICE imputed balanced and imbalanced datasets.
The analysis of feature importance is conducted to ascertain the influential factors in stroke prediction using the proposed DSE model. The analysis of feature importance revealed distinct patterns between the imbalanced and balanced datasets, as visualized in Fig. 25 . In both datasets, the top three features influencing stroke prediction were average glucose level, age, and BMI. However, notable differences were observed in their relative importance. In the imbalanced dataset, these top three features were relatively close in importance, with average glucose level slightly more influential than age and BMI. Conversely, in the balanced dataset, age emerged as the most important feature by a significant margin, followed by average glucose level and BMI. Additionally, the imbalanced dataset highlighted hypertension and heart disease as the 4th and 5th most important features, while the balanced dataset indicated that marital status (yes and no) played a more significant role in prediction. Interestingly, features such as work type (never worked and children) and gender (other) showed minimal contribution in both datasets, underscoring their limited impact on stroke prediction outcomes.
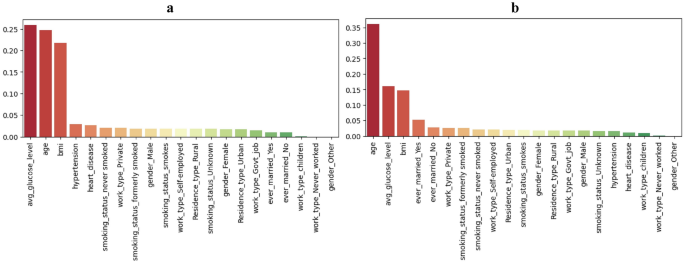
Feature importance comparison for the proposed DSE model. Feature importance graphs for imbalanced and balanced MICE-imputed datasets are displayed in ( a ) and ( b ) respectively.
While LR-AGD and XGBoost deliver accurate results with high accuracy, they both exhibit limitations. LR-AGD performs well when the data is imbalanced, but its performance significantly decreases when the dataset is balanced, behaving differently and yielding lower accuracy. Conversely, XGBoost performs exceptionally well on balanced datasets but struggles with imbalanced ones. Linear models excel in simpler, easily separable small data, while non-linear models perform better in complex and equally represented variables with intricate relationships. This highlights the importance of creating an appropriate model to handle these kinds of versatile dataset characteristics to yield optimal performance in stroke prediction. It is crucial to consider the balance between precision and recall to make informed decisions regarding model selection. Additionally, further research and development are needed to address the limitations of LR-AGD and XGBoost to enhance their performance across various dataset scenarios.
However, when an augmented dataset is created, incorporating crucial factors significantly contributing to stroke prediction, Random Forest emerges as the superior model. It consistently outperforms other models on both imbalanced and balanced augmented datasets with a mean accuracy of 97.409% and 99.068%, respectively. Random Forest also gives consistent and around 95% accurate results for non-augmented datasets. Ultimately, the Random Forest is then used as a meta-classifier in the DSE model. Tables 5 and 6 provide a comprehensive summary of the mean accuracy of advanced classification models and the DSE model across all imbalanced and balanced datasets, highlighting the superior performance of the DSE model. The DSE model achieves far more superior results when other models are incorporated within it as base models and Random Forest as meta-classifiers. The DSE achieves the highest accuracy ranging above 96% across all types of datasets, making it the most feasible and robust model for stroke prediction on diverse datasets.
Additionally, Table 7 compares stroke prediction results from the previous recent studies that utilized the same dataset. This comparative analysis provides valuable insights into the top-performing DSE machine learning model's performance on imbalanced and balanced datasets, showcasing its respective accuracies. The table serves as a comprehensive reference for understanding the effectiveness of these models in stroke prediction. The study 27 shows that the minimal genetic folding (mGF) model achieves an accuracy of 83.2% on the balanced dataset. Another study 28 utilizes Logistic Regression and achieves an accuracy of 86.00%. Naive Bayes 29 achieves an accuracy of 82.00%. Random Forest 30 achieves an impressive accuracy of 94.46% on the imbalanced dataset, while Support Vector Machine 31 reaches an accuracy of 95.49%. Additionally, Random Forest is studied 32 , 33 , 34 , 36 with accuracies ranging from 95.50% to 96.00%. The proposed RXLM 35 model achieves an accuracy of 96.34% on the balanced dataset. K-nearest Neighbours 37 model achieves accuracies of 94.00% on the balanced dataset. In this study, the proposed DSE model achieves an impressive accuracy of 96.13% on the imbalanced imputed dataset and 96.59% on the balanced dataset. The notable distinction in the DSE model's performance can be attributed to its unique ability to harness the strengths of multiple base models through ensemble techniques. By employing a strategic combination of Voting, Blending, and Fusion ensembles, the DSE model maximizes predictive accuracy by leveraging the diverse perspectives and capabilities of each individual model. This sophisticated integration of ensemble methods enables the DSE model to outperform standalone models, as seen in previous studies. Overall, Table 7 provides a comprehensive overview of stroke prediction results, showcasing the performance of previously used models on imbalanced and balanced datasets along with the performance of the proposed DSE model.
In the domain of practical application, the DSE model exhibits a seamless integration into a real-life scenario, as demonstrated in Fig. 26 . Users, whether they be individuals concerned about their health or medical professionals, can effortlessly input vital signs and demographic information through a user-friendly mobile or web application. This data is then securely transmitted to a cloud server where the pre-trained DSE model is deployed. The model processes the input information swiftly, with an average prediction time of 0.095 s per subject, showcasing its efficiency. Upon completion of the prediction, results are promptly relayed back to the user through the same cloud server, accessible via the mobile or web app. Crucially, if a subject is predicted to be at risk of a stroke, the system offers the option for immediate online consultation with a healthcare professional or assistance in locating a physical medical service through a third-party service. This innovative approach not only underscores the model's applicability in real-world scenarios but also highlights its potential to contribute significantly to proactive healthcare management.
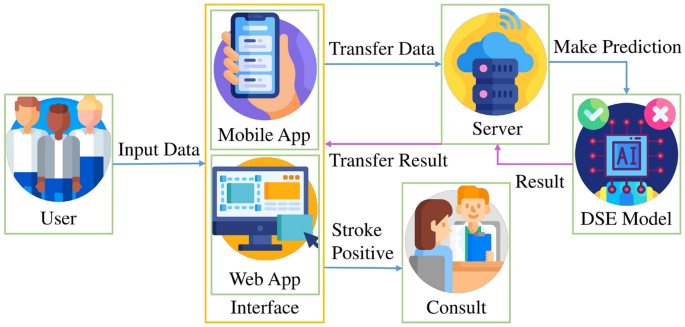
Integration of proposed DSE model in real-life scenario. The DSE model quickly processes vital signs on a user-friendly app, offering timely stroke risk predictions via a cloud server.
Given the substantial global impact of strokes on mortality rates, there is an urgent need for robust and generalizable early prediction methods. While stroke prediction models are pivotal in pinpointing high-risk individuals, they face obstacles such as missing data and data imbalance. This study aims to create an improved predictive model for stroke prediction and evaluate its performance across various imbalanced and balanced datasets. The comprehensive analysis of various advanced machine learning models for stroke prediction that are presented in this research paper sheds light on the efficacy of different techniques and models in handling missing data and data imbalance. The study reveals that most significant factors for stroke prediction are age, BMI, average glucose level, heart disease, hypertension, and ever-married status. Subsequently, an augmented dataset is created to incorporate these essential features, with the goal of enhancing the accuracy of stroke prediction models. The study uses an extensive range of advanced models such as TabNet, Logistic Regression, Neural Network, Random Forest, Gradient Boosting, CatBoost, LightGBM, XGBoost, Balanced Bagging, and NGBoost. The performance evaluation of predictive models is done by employing fivefold cross-validation.
The MICE imputation technique shows slightly better performance compared to two alternative methods. LR-AGD excels on imbalanced data with the highest accuracy of 96.46% and XGBoost performs well on balanced datasets with the highest accuracy of 96.14%. However, their effectiveness is limited by dataset characteristics. In contrast, Random Forest delivers consistent and generalizable results with an accuracy rate around 95% on all non-augmented datasets. This characteristic becomes particularly evident when using the augmented dataset, it gives highest accuracy above 97%. After thorough evaluation, a more robust Dense Stacking Ensemble (DSE) model is constructed. The Random Forest model acts as the meta-classifier within the DSE model, with other models serving as base models post fine-tuning. The DSE model exhibits robust performance across both imbalanced and balanced MICE-imputed datasets. In the case of imbalanced dataset, the model achieved an accuracy of 96.13%, precision of 93.26%, recall of 96.18%, and an F1 score of 94.88%. Similarly, on balanced dataset, the DSE model achieved an accuracy of 96.59%, precision of 95.25%, recall of 96.27%, and F1 score of 95.79%. In terms of AUC, the DSE model achieved an AUC of 83.94% on the imbalanced dataset. On the balanced dataset, the DSE model excelled further by reaching an impressive AUC of 98.92%. These AUC scores demonstrates the ability of DSE model to distinguish between positive and negative instances. In conclusion, the DSE model consistently delivers robust and stable results for stroke prediction across diverse datasets.
In the future, the validation scope can be expanded with larger datasets that will encompass more features. Additionally, it is intended to explore diverse data formats which will include images and hybrid datasets. Furthermore, more extensive and diverse datasets will provide valuable insights and facilitate the generalizability of the findings to a broader population. By conducting external validation studies on these diverse and independent datasets, the authors aim to evaluate and validate the performance of the proposed DSE model. The proposed DSE model can integrate seamlessly into daily life using mobile or web applications, allowing the users to input health data effortlessly. With swift processing and prediction time the results can be relayed promptly. This integration will help in making prompt healthcare intervention in cases of predicted stroke risk.
Data availability
The McKinsey & Company's stroke prediction dataset for healthcare analytics is used in this study. The dataset is available publicly at Analytics Vidhya and Kaggle website at: https://datahack.analyticsvidhya.com/contest/mckinsey-analytics-online-hackathon or https://www.kaggle.com/datasets/fedesoriano/stroke-prediction-dataset .
Bersano, A. & Gatti, L. Pathophysiology and treatment of stroke: Present status and future perspectives. Int. J. Mol. Sci. 24 , 14848 (2023).
Article PubMed PubMed Central Google Scholar
Feigin, V. L. et al. World stroke organization (wso): Global stroke fact sheet 2022. Int. J. Stroke 17 , 18–29 (2022).
Article PubMed Google Scholar
Feigin, V. L. et al. Global, regional, and national burden of stroke and its risk factors, 1990–2019: A systematic analysis for the global burden of disease study 2019. Lancet Neurol. 20 , 795–820 (2021).
Article CAS Google Scholar
Katan, M. & Luft, A. Global burden of stroke. Semin. Neurol. 38 , 208–211 (2018).
Pitchai, R. et al. An artificial intelligence-based bio-medical stroke prediction and analytical system using a machine learning approach. Comput. Intell. Neurosci. 2022 , 1–9 (2022).
Google Scholar
Amann, J. Machine learning in stroke medicine: Opportunities and challenges for risk prediction and prevention. Adv. Neuroethics https://doi.org/10.1007/978-3-030-74188-4_5 (2021).
Article Google Scholar
Moshawrab, M., Adda, M., Bouzouane, A., Ibrahim, H. & Raad, A. Reviewing multimodal machine learning and its use in cardiovascular diseases detection. Electronics 12 , 1558 (2023).
Javaid, M., Haleem, A., Pratap Singh, R., Suman, R. & Rab, S. Significance of machine learning in healthcare: Features, pillars and applications. Int. J. Intell. Netw. 3 , 58–73 (2022).
MacEachern, S. J. & Forkert, N. D. Machine learning for precision medicine. Genome 64 , 416–425 (2021).
Bonkhoff, A. K. & Grefkes, C. Precision medicine in stroke: Towards personalized outcome predictions using artificial intelligence. Brain 145 , 457–475 (2021).
Article PubMed Central Google Scholar
Sarker, I. H. Machine learning: Algorithms, real-world applications and research directions. SN Comput. Sci. 2 , 160 (2021).
Yu, J. et al. AI-based stroke disease prediction system using real-time electromyography signals. Appl. Sci. 10 , 6791 (2020).
Nijman, S. et al. Missing data is poorly handled and reported in prediction model studies using machine learning: A literature review. J. Clin. Epidemiol. 142 , 218–229 (2022).
Kumar, Y., Koul, A., Singla, R. & Ijaz, M. F. Artificial intelligence in disease diagnosis: A systematic literature review, synthesizing framework and future research agenda. J. Ambient Intell. Hum. Comput. 14 , 8459–8486 (2022).
Kokkotis, C. et al. An explainable machine learning pipeline for stroke prediction on imbalanced data. Diagnostics 12 , 2392 (2022).
Sirsat, M. S., Fermé, E. & Câmara, J. Machine learning for brain stroke: A review. J. Stroke Cerebrovasc. Dis. 29 , 105162 (2020).
Wongvorachan, T., He, S. & Bulut, O. A comparison of undersampling, oversampling, and smote methods for dealing with imbalanced classification in educational data mining. Information 14 , 54 (2023).
Sowjanya, A. M. & Mrudula, O. Effective treatment of imbalanced datasets in health care using modified smote coupled with stacked deep learning algorithms. Appl. Nanosci. 13 , 1829–1840 (2022).
Article ADS PubMed PubMed Central Google Scholar
Bernat, J. L. & Lukovits, T. G. Ethical issues in stroke management. Neurol. Clin. Pract. 11 , 3–5 (2021).
Murdoch, B. Privacy and artificial intelligence: Challenges for protecting health information in a new era. BMC Med. Ethics https://doi.org/10.1186/s12910-021-00687-3 (2021).
Martin, C. et al. The ethical considerations including inclusion and biases, data protection, and proper implementation among AI in radiology and potential implications. Intell. Based Med. 6 , 100073 (2022).
Wu, Y. & Fang, Y. Stroke prediction with machine learning methods among older Chinese. Int. J. Environ. Res. Public Health 17 , 1828 (2020).
Kaur, M., Sakhare, S. R., Wanjale, K. & Akter, F. Early stroke prediction methods for prevention of strokes. Behav. Neurol. 2022 , 1–9 (2022).
Alanazi, E. M., Abdou, A. & Luo, J. Predicting risk of stroke from lab tests using machine learning algorithms: Development and evaluation of prediction models. JMIR Format. Res. 5 , e23440 (2021).
Monteiro, M. et al. Using machine learning to improve the prediction of functional outcome in ischemic stroke patients. IEEE/ACM Trans. Comput. Biol. Bioinform. 15 , 1953–1959 (2018).
Shobayo, O., Zachariah, O., Odusami, M. O. & Ogunleye, B. Prediction of stroke disease with demographic and behavioural data using random forest algorithm. Analytics 2 , 604–617 (2023).
Mezher, M. A. Genetic folding (GF) algorithm with minimal kernel operators to predict stroke patients. Appl. Artif. Intell. https://doi.org/10.1080/08839514.2022.2151179 (2022).
Guhdar, M., Ismail Melhum, A. & Luqman Ibrahim, A. Optimizing accuracy of stroke prediction using logistic regression. J. Technol. Inform. (JoTI) 4 , 41–47 (2023).
Sailasya, G. & Kumari, G. L. Analyzing the performance of stroke prediction using ml classification algorithms. Int. J. Adv. Comput. Sci. Appl. https://doi.org/10.14569/IJACSA.2021.0120662 (2021).
Paul, D., Gain, G. & Orang, S. Advanced random forest ensemble for stroke prediction. Int. J. Adv. Res. Comput. Commun. Eng. https://doi.org/10.17148/IJARCCE.2022.11343 (2022).
Geethanjali, T. M., Divyashree, M. D., Monisha, S. K. & Sahana, M. K. Stroke prediction using machine learning. Int. J. Emerg. Technol. Innov. Res. 8 , 710–717 (2021).
Harshitha, K. V., Harshitha, P., Gupta, G., Vaishak, P. & Prajna, K. B. Stroke prediction using machine learning algorithms. Int. J. Innov. Res. Eng. Manag. https://doi.org/10.21276/ijirem.2021.8.4.2 (2021).
Tazin, T. et al. Stroke disease detection and prediction using robust learning approaches. J. Healthc. Eng. 2021 , 1–12 (2021).
Chen, Z. Stroke risk prediction based on machine learning algorithms. Highlights Sci. Eng. Technol. 38 , 932–941 (2023).
Alruily, M., El-Ghany, S. A., Mostafa, A. M., Ezz, M. & El-Aziz, A. A. A-tuning ensemble machine learning technique for cerebral stroke prediction. Appl. Sci. 13 , 5047 (2023).
Islam, Md. M. et al. Stroke prediction analysis using machine learning classifiers and feature technique. Int. J. Electron. Commun. Syst. 1 , 57–62 (2021).
Uma, S. K. & Rakshith, S. R. Stroke analysis using 10 ml comparison. Int. J. Res. Appl. Sci. Eng. Technol. 10 , 3857–3862 (2022).
Fedesoriano. Stroke prediction dataset. Kaggle . https://www.kaggle.com/datasets/fedesoriano/stroke-prediction-dataset/data (2021).
Mattas, P. S. Brain stroke prediction using machine learning. Int. J. Res. Publ. Rev. 3 , 711–722 (2022).
Pathan, M. S., Jianbiao, Z., John, D., Nag, A. & Dev, S. Identifying stroke indicators using rough sets. IEEE Access 8 , 210318–210327 (2020).
Emon, M. U. et al. Performance Analysis of Machine Learning Approaches in stroke prediction. In 2020 4th International Conference on Electronics, Communication and Aerospace Technology (ICECA) (2020).
Hassan, A. & Yousaf, N. Bankruptcy prediction using diverse machine learning algorithms. In 2022 International Conference on Frontiers of Information Technology (FIT) (2022).
Dev, S. et al. A predictive analytics approach for stroke prediction using machine learning and neural networks. Healthc. Anal. 2 , 100032 (2022).
Nguyen, L. P. et al. The utilization of machine learning algorithms for assisting physicians in the diagnosis of diabetes. Diagnostics 13 , 2087 (2023).
Article CAS PubMed PubMed Central Google Scholar
Mahajan, P., Uddin, S., Hajati, F. & Moni, M. A. Ensemble learning for disease prediction: A review. Healthcare 11 , 1808 (2023).
Jagan, S. et al. A meta-classification model for optimized zbot malware prediction using learning algorithms. Mathematics 11 , 2840 (2023).
Zhen, M. et al. Application of a fusion model based on machine learning in visibility prediction. Remote Sens. 15 , 1450 (2023).
Article ADS Google Scholar
Yuan, Q., Chen, K., Yu, Y., Le, N. Q. & Chua, M. C. Prediction of anticancer peptides based on an ensemble model of deep learning and machine learning using ordinal positional encoding. Brief. Bioinform. https://doi.org/10.1093/bib/bbac630 (2023).
Le, N.-Q.-K. & Ou, Y.-Y. Incorporating efficient radial basis function networks and significant amino acid pairs for predicting GTP binding sites in transport proteins. BMC Bioinform. https://doi.org/10.1186/s12859-016-1369-y (2016).
Download references
Acknowledgements
The authors express gratitude to the anonymous reviewers for their insightful comments and suggestions, contributing to the enhancement of this paper.
This research was partially supported by the SAFE-RH project under Grant No. ERASMUS + CBHE-619483 EPP-1-2020-1-UK-EPPKA2-CBHE.
Author information
Authors and affiliations.
Department of Computer Science, COMSATS University Islamabad, Wah Campus, Grand Trunk Road, Wah, 47010, Pakistan
Ahmad Hassan, Saima Gulzar Ahmad & Ehsan Ullah Munir
Department of Computer Science, Cardiff School of Technologies, Llandaff Campus, Western Avenue, Cardiff, CF5 2YB, UK
Imtiaz Ali Khan
School of Computing, Engineering and Physical Sciences, University of the West of Scotland, High Street, Paisley, PA1 2BE, UK
Naeem Ramzan
You can also search for this author in PubMed Google Scholar
Contributions
Ahmad Hassan wrote the main manuscript text, Saima Gulzar Ahmad and Ehsan Ullah Munir developed figures, and Naeem Ramzan contributed to the results section. Imtiaz Ali Khan conducted additional simulations and included supplementary results. All authors reviewed and enhanced the manuscript write-up. The final manuscript was read and approved by all authors.
Corresponding author
Correspondence to Naeem Ramzan .
Ethics declarations
Competing interests.
The authors declare no competing interests.
Additional information
Publisher's note.
Springer Nature remains neutral with regard to jurisdictional claims in published maps and institutional affiliations.
Rights and permissions
Open Access This article is licensed under a Creative Commons Attribution 4.0 International License, which permits use, sharing, adaptation, distribution and reproduction in any medium or format, as long as you give appropriate credit to the original author(s) and the source, provide a link to the Creative Commons licence, and indicate if changes were made. The images or other third party material in this article are included in the article's Creative Commons licence, unless indicated otherwise in a credit line to the material. If material is not included in the article's Creative Commons licence and your intended use is not permitted by statutory regulation or exceeds the permitted use, you will need to obtain permission directly from the copyright holder. To view a copy of this licence, visit http://creativecommons.org/licenses/by/4.0/ .
Reprints and permissions
About this article
Cite this article.
Hassan, A., Gulzar Ahmad, S., Ullah Munir, E. et al. Predictive modelling and identification of key risk factors for stroke using machine learning. Sci Rep 14 , 11498 (2024). https://doi.org/10.1038/s41598-024-61665-4
Download citation
Received : 26 February 2024
Accepted : 08 May 2024
Published : 20 May 2024
DOI : https://doi.org/10.1038/s41598-024-61665-4
Share this article
Anyone you share the following link with will be able to read this content:
Sorry, a shareable link is not currently available for this article.
Provided by the Springer Nature SharedIt content-sharing initiative
By submitting a comment you agree to abide by our Terms and Community Guidelines . If you find something abusive or that does not comply with our terms or guidelines please flag it as inappropriate.
Quick links
- Explore articles by subject
- Guide to authors
- Editorial policies
Sign up for the Nature Briefing newsletter — what matters in science, free to your inbox daily.

Click through the PLOS taxonomy to find articles in your field.
For more information about PLOS Subject Areas, click here .
Loading metrics
Open Access
Peer-reviewed
Research Article
Correlation between novel inflammatory markers and carotid atherosclerosis: A retrospective case-control study
Roles Conceptualization, Formal analysis, Investigation, Writing – original draft
Affiliation Department of General, Union Hospital, Tongji Medical College, Huazhong University of Science and Technology, Wuhan, Hubei Province, China
Roles Funding acquisition, Supervision
Roles Validation
Roles Conceptualization, Funding acquisition, Supervision, Writing – review & editing
* E-mail: [email protected] (YL); [email protected] (BQ)

- Man Liao,
- Lihua Liu,
- Lijuan Bai,
- Ruiyun Wang,
- Yun Liu,
- Liting Zhang,
- Jing Han,
- Yunqiao Li,
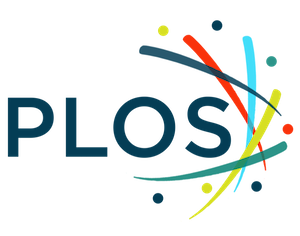
- Published: May 29, 2024
- https://doi.org/10.1371/journal.pone.0303869
- Peer Review
- Reader Comments
Carotid atherosclerosis is a chronic inflammatory disease, which is a major cause of ischemic stroke. The purpose of this study was to analyze the relationship between carotid atherosclerosis and novel inflammatory markers, including platelet to lymphocyte ratio (PLR), neutrophil to lymphocyte ratio (NLR), lymphocyte to monocyte ratio (LMR), platelet to neutrophil ratio (PNR), neutrophil to lymphocyte platelet ratio (NLPR), systemic immune-inflammation index (SII), systemic inflammation response index (SIRI), and aggregate index of systemic inflammation (AISI), in order to find the best inflammatory predictor of carotid atherosclerosis.
We included 10015 patients who underwent routine physical examinations at the physical examination center of our hospital from January 2016 to December 2019, among whom 1910 were diagnosed with carotid atherosclerosis. The relationship between novel inflammatory markers and carotid atherosclerosis was analyzed by logistic regression, and the effectiveness of each factor in predicting carotid atherosclerosis was evaluated by receiver operating characteristic (ROC) curve and area under the curve (AUC).
The level of PLR, LMR and PNR in the carotid atherosclerosis group were lower than those in the non-carotid atherosclerosis group, while NLR, NLPR, SII, SIRI and AISI in the carotid atherosclerosis group were significantly higher than those in the non-carotid atherosclerosis group. Logistic regression analysis showed that PLR, NLR, LMR, PNR, NLPR, SII, SIRI, AISI were all correlated with carotid atherosclerosis. The AUC value of NLPR was the highest, which was 0.67, the cut-off value was 0.78, the sensitivity was 65.8%, and the specificity was 57.3%. The prevalence rate of carotid atherosclerosis was 12.4% below the cut-off, 26.6% higher than the cut-off, and the prevalence rate increased by 114.5%.
New inflammatory markers were significantly correlated with carotid atherosclerosis, among which NLPR was the optimum inflammatory marker to predict the risk of carotid atherosclerosis.
Citation: Liao M, Liu L, Bai L, Wang R, Liu Y, Zhang L, et al. (2024) Correlation between novel inflammatory markers and carotid atherosclerosis: A retrospective case-control study. PLoS ONE 19(5): e0303869. https://doi.org/10.1371/journal.pone.0303869
Editor: Elvan Wiyarta, Universitas Indonesia Fakultas Kedokteran, INDONESIA
Received: January 11, 2024; Accepted: May 1, 2024; Published: May 29, 2024
Copyright: © 2024 Liao et al. This is an open access article distributed under the terms of the Creative Commons Attribution License , which permits unrestricted use, distribution, and reproduction in any medium, provided the original author and source are credited.
Data Availability: All relevant data are within the manuscript and its Supporting information files.
Funding: The study was supported by the National Natural Science Foundation of China (Grant No.81571373, No.81601217, No.82001491), Natural Science Foundation of Hubei Province of China (Grant No. 2017CFB627), Health Commission of Hubei Province scientific research project (Grant No. WJ2021M247) and Scientific Research Fund of Wuhan Union Hospital (Grant No.2019). The funders had no role in study design, data collection and analysis, decision to publish, or preparation of the manuscript.
Competing interests: The authors have declared that no competing interests exist.
Introduction
Cardiovascular was always the main cause of premature death and rising healthcare costs [ 1 , 2 ]. From 1990 to 2019, the total number cardiovascular disease cases has nearly doubled globally, while the number of cases and deaths from peripheral artery disease tripled [ 3 ]. Atherosclerosis, a major pathological process in most cardiovascular diseases, which may occuar as early as childhood and remain latent in the body for a long time [ 4 ]. Early detection of arterial disease in seemingly healthy individuals focuses on the peripheral arteries, especially the carotid arteries [ 5 ]. In clinical practice, carotid atherosclerosis (CAS) is the earlier and most easily detected form of atherosclerosis. Besides, carotid atherosclerotic plaque is an independent risk factor for stroke and coronary heart disease [ 6 ]. Atherosclerosis is widely recognized as a chronic inflammatory disease of the blood vessels caused by the accumulation of low density lipoprotein cholesterol [ 7 ]. Chronic inflammation is a low-grade, non-infectious, systemic inflammatory state that is associated with age, psychology, environment, lifestyle, and the resolution of acute inflammation [ 8 ]. Chronic inflammation is associated with endothelial dysfunction, leukocyte recruitment, transformation of monocytes into macrophages and eventually into foam cells, smooth muscle cell migration and other processes [ 7 ]. Chronic inflammation is involved in the whole process of the occurrence and development of atherosclerosis and is the core of atherosclerosis.
In clinical practice, peripheral blood cell count is often used as a predictor and evaluation factor for the severity of inflammation and treatment outcome of acute inflammatory diseases, such as lung infection and sepsis. Platelet to lymphocyte ratio (PLR), neutrophil to lymphocyte ratio (NLR), lymphocyte to monocyte ratio (LMR), platelet to neutrophil ratio (PNR), neutrophil to lymphocyte platelet ratio (NLPR), systemic immune-inflammation index (SII), systemic inflammation response index (SIRI), and aggregate index of systemic inflammation (AISI) are commonly used blood cell count derived values in clinical practice, also known as novel inflammatory markers. These novel inflammatory markers have repeatedly demonstrated their potential value in the early prediction and prognosis of cardiovascular disease [ 9 – 14 ]. However, the relationship between these readily available inflammation markers and atherosclerosis, especially carotid atherosclerosis, has not yet been clear, which nedds further research to be confirmed.
Therefore, this study aimed to explore the correlation between novel inflammatory markers and CAS, in order to find a better early warning indicator of clinical carotid atherosclerosis.
Study design
This is a retrospective case-control study. All methods were carried out in accordance with relevant guidelines and regulations and no important aspects of the study have been omitted. Patients’ personal information is being kept confidentially. The study complies with the Declaration of Helsinki and the ethical approval of the study was obtained from the Ethics Committee of Union Hospital, Tongji Medical College, Huazhong University of Science and Technology at Sep. 4 th 2023 ([2023]ID:0611). The ethics committee exempted the need for informed consent.
In this study, we included 14,115 patients who underwent routine physical examinations at our physical examination center from January 2016 to December 2019. The first time we accessed the data was on September 10, 2023. The following were the inclusion criteria for this study: (1) Age>18 years; (2) People who have underwent carotid vascular ultrasound. The exclusion criteria of participants were as follows: (1) Receiving or being receiving anti-inflammatory therapy within 6 months; (2) Critically ill patients with unstable vital signs; (3) Have received or are receiving glucocorticoid therapy within 6 months; (4) Incomplete clinical data or incomplete personal information. After screening, a total of 10015 patients were included and divided into CAS group and non-CAS group according to diagnosis.
Collected clinical data and laboratory indicators and definition of inflammatory markers
Age, gender, height, and weight of the patient at admission were obtained from the hospital electronic system, and BMI was calculated according to BMI = weight/height ^2. Proper amount of venous blood was extracted by professional nurses with nursing qualification during the fasting period and sent to the laboratory for analysis to obtain white blood cell count (WBC), neutrophil count (NC), lymphocyte count (LC), monocyte count (MC), platelet (PLT), neutrophil percentage (NP), lymphocyte percentage (LP), monocyte percentage (MP), PLR, NLR, LMR, PNR, NLPR, SII, SIRI, AISI and biochemical index, such as aspartate aminotransferase (AST), triglyceride (TG), total cholesterol (TC), high density lipoprotein cholesterol (HDL-C), low density lipoprotein cholesterol (LDL-C), uric acid (UA), urea nitrogen (BUN), creatinine. The estimated glomerular filtration rate (eGFR) was calculated based on the modified MDRD equation. SIRI was defined as neutrophil count*monocyte count/lymphocyte count. SII was calculated using the formula: NLR*platele. AISI was calculated using the formula: (neutrophil count*monocyte count*platelet)/lymphocyte count. NLPR was calculated using the formula: neutrophil count*100/lymphocyte count*platelet. Comorbidity, smoking history and drinking history were obtained from the patient’s personal history.
Diagnostic criteria for carotid atherosclerosis
Carotid atherosclerosis is diagnosed in one of the following situations: (1) A clear history of atherosclerosis or revascularization treatment; (2) Carotid artery ultrasound showed atherosclerotic plaque or carotid intima-media thickness of 1.0mm or more [ 5 ].
Statistical analysis
Shapiro-Wilkstest is used to test whether the continuous variables obey normal distribution, and the continuous variables with normal distribution are represented by mean ± standard deviation (SD). Continuous variables that are not normally distributed are expressed as medians with interquartile range (IQR). The categorical variable is represented by number with percentages (%). ANOVA tests (conforming to normal distribution) and Kruskal-Wallis tests (non-conforming to normal distribution) were used for the differences between of continuous data among CAS and non-CAS. Chi-square test was used to compare the difference of Categorical data between patients with CAS and non-CAS. Logistic regression analysis was used to evaluate the correlation between various inflammatory indicators and CAS after adjusting factors such as age, gender, BMI, serum biochemical indicators and clinical diagnosis and other factors. In Logistic regression analysis, forward selection method was used to identify CAS risk factors. Finally, the efficiency of each inflammatory markers to CAS was evaluated by receiver operating characteristic (ROC) curve. P<0.05 was considered to be statistically significant. All statistical analyses and diagrams were done using SPSS (version 23.0) or R language.
Clinical baseline data
A total of 10015 patients aged 18–94 were enrolled in the study, including 1910 (19.1%) in the CAS group. Table 1 shows the baseline characteristics between the two groups. There were no significant differences in BMI, PLR and dyshepatia between the two groups (P>0.05). While age, gender, WBC, NC, LC, MC, PLT, NP, LP, MP, NLR, LMR, PNR, NLPR, SII, SIRI, AISI, AST, TG, TC, HDL_C, LDL_C, BUN, creatinine, eGFR, UA, smoking history, drinking history, renaldysfuncyion, hyperuricemia, fatty liver, dyslipidemia, hypertension, diabetes and osteoporosis were significantly different between the two groups(P<0.05).
- PPT PowerPoint slide
- PNG larger image
- TIFF original image
https://doi.org/10.1371/journal.pone.0303869.t001
Relationship between novel inflammatory markers and CAS
The relationship between novel inflammation markers and CAS was observed by spline smoothing plot, shown in Fig 1 . LMR, PNR were negatively correlated with CAS, while PLR, NLR, NLPR, SII, SIRI, AISI, NLPR were positively correlated with CAS.
A non- linear relationship between novel inflammation markers and carotid atherosclerosis after adjusting for age, gender, body mass index, aspartate aminotransferase, triglyceride, total cholesterol, high density lipoprotein cholesterol, low density lipoprotein cholesterol, estimated glomerular filtration rate, uric acid, hypertension, diabetes, osteoporosis, fatty liver, smoking history, drinking history. CAS, carotid atherosclerosis; PLR, platelet to lymphocyte ratio; NLR, neutrophil to lymphocyte ratio; LMR, lymphocyte to monocyte ratio; PNR, platelet to neutrophil ratio; NLPR, neutrophil to lymphocyte platelet ratio; SII, systemic immune-inflammation index; SIRI, systemic inflammation response index; AISI, aggregate index of systemic inflammation.
https://doi.org/10.1371/journal.pone.0303869.g001
Logistic regression analysis of CAS
Univariate logistic regression analysis of the relationship between inflammatory markers and CAS showed the same difference as that between the two groups. Furthermore, after adjusting for many possible confounders such as age, sex, BMI, all inflammatory markers were statistically significant (P<0.05) with CAS. These infalmmatory markers have varying effects on CAS, and the risk value of NLPR is much higher than other markers (OR = 2.35). Their CAS risk value and forest plot were shown in Fig 2 .
Model 1 adjusted for none. Model 2 adjusted for age, sex, and body mass index. Model 3 adjusted for age, gender, body mass index, aspartate aminotransferase, triglyceride, total cholesterol, high density lipoprotein cholesterol, low density lipoprotein cholesterol, estimated glomerular filtration rate, uric acid, hypertension, diabetes, osteoporosis, smoking history, drinking history. Forest plot of novel inflammatory markers for carotid atherosclerosis risk after adjusting for Model 3. PLR, platelet to lymphocyte ratio; NLR, neutrophil to lymphocyte ratio; LMR, lymphocyte to monocyte ratio; PNR, platelet to neutrophil ratio; NLPR, neutrophil to lymphocyte platelet ratio; SII, systemic immune-inflammation index; SIRI, systemic inflammation response index; AISI, aggregate index of systemic inflammation.
https://doi.org/10.1371/journal.pone.0303869.g002
The efficacy of inflammatory markers to predict CAS was assessed by ROC curve. After adjusting for confounding factors, there were still statistical differences in all inflammatory markers. The ROC curve to evaluate the effectiveness of the above markers in CAS diagnosis is shown in Table 2 and Fig 3 . The AUC values of NLR, SII, SIRI, AISI and NLPR were all in the range of 0.5–0.7. The highest AUC value for NLPR recognition of CAS is 0.67. And NLPR uses the adjusted predictive value of Model 3 to identify the AUC value of CAS as 0.91. The cut-off value calculated by Youden index was that NLPR was 0.78, that the sensitivity was 65.8%, and that the specificity was 57.3%. The prevalence rate of carotid atherosclerosis was 12.4% below the cut-off, 26.6% higher than the cut-off, and the prevalence rate increased by 114.5%.
PLR, platelet to lymphocyte ratio; NLR, neutrophil to lymphocyte ratio; LMR, lymphocyte to monocyte ratio; PNR, platelet to neutrophil ratio; NLPR, neutrophil to lymphocyte platelet ratio; SII, systemic immune-inflammation index; SIRI, systemic inflammation response index; AISI, aggregate index of systemic inflammation.
https://doi.org/10.1371/journal.pone.0303869.g003
https://doi.org/10.1371/journal.pone.0303869.t002
Subgroup analyses between NLPR and CAS
In order to study the CAS risk and interaction of inflammatory markers in people with different clinicopathological characteristics. We further analyzed six subgroups of NLPR and CAS risk indicators (hyperuricemia, fatty liver, dyslipidemia, hypertension, diabetes, osteoporosis). The results showed that NLPR had the highest OR value in population without lipid metabolism abnormalities ( Table 3 ). CAS were significantly associated with NLPR in all subgroups. The interaction among subgroups revealed that NLPR had an interaction with hyperuricemia, fatty liver, dyslipidemia, hypertension, diabetes and osteoporosis, and all of these disease subgroups significantly weakened the risk of NLPR for CAS.
https://doi.org/10.1371/journal.pone.0303869.t003
Novel inflammatory markers have been proven to be closely related to various diseases, and their easy availability has important clinical value. In this cross-sectional study, we analyzed the effect of multiple inflammatory markers on CAS, where NLR, SII, SIRI, AISI, NLPR remained significant after adjusting for multiple confounding factors. At the same time, this study further evaluated the value of various inflammatory markers in predicting CAS, among which NLPR had the largest AUC value in identifying CAS risk. Compared with other inflammatory markers, NLPR has a higher value for predicting CAS which may be the best inflammatory indicator for identifying atherosclerosis.
Chronic inflammation leads to atherosclerosis and cardiovascular disease. Neutrophils are the most numerous type of white blood cell and play a major role in inflammation. Although neutrophil count is primarily used as a biomarker for acute infection and inflammation, it has also been shown to accelerate chronic inflammation [ 15 ]. Monocyte-derived macrophages are important mediators in the atherosclerotic cascade and are associated with plaque formation through infiltration into the subendothelial layer. Subsequently, uptake of LDL-C complexes leads to the formation of foam cells. In addition to plaque formation, macrophages are involved in extracellular matrix remodeling by secreting pro-inflammatory cytokines and chemokines [ 16 ]. In our study, the number of neutrophils and monocytes increased in patients with CAS compared with non-CAS patients, indicating that the higher the number of neutrophils and monocytes, the higher the incidence of CAS. In contrast, lymphocytes slow the progression of atherosclerosis [ 14 ]. Our findings were the same, with a reduced number of lymphocytes in patients with CAS, suggesting that the higher the number of lymphocytes, the lower the incidence of CAS. Platelets play two main roles in atherosclerosis: platelets directly adhere to the blood vessel wall to promote plaque formation, and platelets then release inflammatory mediators and chemokines to promote leukocyte recruitment [ 17 ]. In our results, platelet counts were reduced in patients with CAS compared to non-CAS patients. The reason for this opposite situation may be that platelets have multiple roles in the formation of atherosclerosis, and in our study, the number of platelets alone was not significantly associated with the development of carotid atherosclerosis. In subsequent binary logistic regression analysis, there was no statistical difference between platelet count and CAS risk after adjusting for confounders.
In previous studies, we found that NLR, MLR, and PLR increase the risk of arterial stiffness [ 18 ]. In recent years, LMR, PNR, NLPR, SII, SIRI, AISI and other indicators have also proved their clinical significance in cardiovascular diseases. A small sample observational study showed that the novel inflammatory markers SIRI, NLR, and LMR were associated with CAS risk in middle-aged and older men [ 9 ]. PNR have also been shown to predict mortality in patients with ischemic stroke [ 10 ]. SII was also found to be a strong independent predictor of adverse outcomes in patients with acute coronary syndromes [ 12 ]. To find the best predictors of CAS, we included these markers in a larger population study at the same time. The results showed that the NLPR had the highest accuracy in identifying the risk of CAS. NLPR is the ratio of neutrophil to lymphocyte*platelet. Initially, NLPR was found to have predictive value in tumor prognosis [ 19 , 20 ]. Subsequently, NLPR was also found to be associated with the prognosis of acute kidney injury, suppurative liver abscess, severe trauma, and COVID-19 [ 21 – 25 ]. The value of NLPR in cardiovascular disease is equally outstanding. Studies have shown that NLPR is an independent predictor of in-hospital mortality after acute type A aortic dissection [ 11 ]. A prospective cohort study found that NLPR was an independent predictor of adverse outcomes in patients with acute coronary syndrome, and patients with a higher NLRP had a higher incidence of major adverse cardiovascular events [ 26 ]. In this study, our results directly show that there is a significant correlation between NLPR and CAS, and the higher the level of NLPR, the higher the risk of CAS.
In statistical analyses of baseline characteristics, in addition to indicators of inflammation, we found a number of variables that were statistically different between the CAS and Non-CAS groups, including age, gender, AST, TG, TC, HDL_C, LDL_C, BUN, creatinine, eGFR, UA, smoking history, drinking history, renaldysfuncyion, hyperuricemia, fatty liver, dyslipidemia, hypertension, diabetes and osteoporosis. Undoubtedly, age is the number one risk factor for a wide range of chronic diseases and systemic chronic inflammatory states [ 8 ]. A significant increase in age in the CAS group was also found in our results. Some studies have shown that the incidence of atherosclerosis is higher in postmenopausal women compared to men [ 27 ]. Whereas, our results showed higher percentage of males in CAS group, which may be due to the uneven gender distribution in the total population of our medical examination, where males were significantly more than females. In addition to this, renaldysfuncyion, hyperuricaemia, fatty liver, dyslipidaemia, hypertension, diabetes, osteoporosis and history of smoking and alcohol consumption were all strongly associated with atherosclerosis [ 28 – 34 ]. And chronic inflammation can lead to cardiovascular disease, cancer, diabetes, chronic kidney disease, non-alcoholic fatty liver disease and many other diseases [ 8 ]. In our subsequent stratified analyses, we also analysed that there was a significant interaction between these diseases and NLPR, which together contributed to the development of CAS.
In this study, we evaluated the value of multiple inflammatory markers for the risk of CAS and found the best inflammatory marker that can be easily obtained to effectively identify the risk of CAS. In addition, the relatively large sample size ensures the accuracy and reliability of the results. Nevertheless, there are some limitations to this study. First, due to the cross-sectional design of the study, a causal relationship between inflammatory markers and CAS cannot be demonstrated, so more prospective studies are needed to confirm these findings. Second, because this study was a single-center study with a relatively narrow group of participants, the findings may not be well extrapolated to other populations. Finally, although we have carefully adjusted for potential confounding factors, it is difficult to rule out potential residual confounding.
Atherosclerosis is a chronic inflammatory disease of the walls of blood vessels. In this study, novel inflammatory markers have good predictive effects on carotid atherosclerosis, and NLPR has the highest predictive value. NLPR can be used as a potential predictor of carotid atherosclerosis.
Supporting information
S1 raw data..
https://doi.org/10.1371/journal.pone.0303869.s001
- View Article
- PubMed/NCBI
- Google Scholar

An official website of the United States government
The .gov means it’s official. Federal government websites often end in .gov or .mil. Before sharing sensitive information, make sure you’re on a federal government site.
The site is secure. The https:// ensures that you are connecting to the official website and that any information you provide is encrypted and transmitted securely.
- Publications
- Account settings
Preview improvements coming to the PMC website in October 2024. Learn More or Try it out now .
- Advanced Search
- Journal List

Breathing Exercises for Improving Cognitive Function in Patients with Stroke
Eui-soo kang.
1 Department of Sports Science Convergence-Graduate School, Dongguk University-Seoul, 30, Pildong-ro 1-gil, Jung-gu, Seoul 04620, Korea; moc.revan@08336426010
Jang Soo Yook
2 Center for Functional Connectomics, Brain Research Institute, Korea Institute of Science and Technology (KIST), Hwarang-ro 14-gil 5, Seongbuk-gu, Seoul 02792, Korea; moc.liamg@48kooyluos
Min-Seong Ha
3 Department of Sports Culture, College of the Arts, Dongguk University-Seoul, 30, Pildong-ro 1-gil, Jung-gu, Seoul 04620, Korea
Patients with stroke may experience a certain degree of cognitive decline during the period of recovery, and a considerable number of such patients have been reported to show permanent cognitive damage. Therefore, the period of recovery and rehabilitation following stroke is critical for rapid cognitive functional improvements. As dysfunctional breathing has been reported as one of the factors affecting the quality of life post stroke, a number of studies have focused on the need for improving the breathing function in these patients. Numerous breathing exercises have been reported to enhance the respiratory, pulmonary, cognitive, and psychological functions. However, scientific evidence on the underlying mechanisms by which these exercises improve cognitive function is scattered at best. Therefore, it has been difficult to establish a protocol of breathing exercises for patients with stroke. In this review, we summarize the psychological, vascular, sleep-related, and biochemical factors influencing cognition in patients and highlight the need for breathing exercises based on existing studies. Breathing exercises are expected to contribute to improvements in cognitive function in stroke based on a diverse array of supporting evidence. With relevant follow-up studies, a protocol of breathing exercises can be developed for improving the cognitive function in patients with stroke.
1. Introduction
Stroke is caused by a fall in blood supply to the brain or due to cerebral hemorrhage and is the most common cerebrovascular condition [ 1 ]. With the increase in the average life expectancy as a result of lifestyle improvements and advances in health care, the number of patients with stroke has seen an upward trend [ 2 ]. Furthermore, studies have shown a possible association between the coronavirus disease (COVID-19) and acute cerebrovascular diseases such as ischemic stroke, hemorrhagic stroke, and cerebral venous thrombosis [ 3 ]. With the continuing COVID-19 pandemic, a steep increase in stroke incidence is therefore likely.
Health-related quality of life (HRQoL), comprising physical, psychological, and cognitive functions, is substantially low among stroke survivors [ 4 , 5 , 6 ]. In particular, decline in attention, memory, and executive function is a serious problem in patients who have had a stroke [ 7 ]. Indeed, as high as 80% of patients with stroke are presumed to have cognitive decline [ 8 ] with more than 1 in 3 of them showing permanent decline [ 9 , 10 , 11 ]. Since cognitive function plays a critical role in the performance of most activities of daily living [ 12 , 13 ], rapid cognitive functional improvement is recognized as an important component of recovery and rehabilitation after stroke.
The following mechanism has been proposed for the pathophysiological role of breathing in stroke—hemiplegia causes an asymmetry in the body, which leads to inefficient movements, with a direct or indirect impact on the activation of respiratory muscles, ultimately affecting the respiratory cycle [ 14 ]. Muscles such as the diaphragm, transverse abdominis, and pelvic floor perform local stabilization, with dual roles in breathing and trunk stabilization [ 15 , 16 , 17 ]. When stroke adversely affects the activation of those muscles, trunk stabilization is impacted [ 18 , 19 ], which affects the muscles related to breathing [ 20 , 21 ]. Such pathological progression may lead to a vicious cycle, wherein the abnormal function of the muscles related to breathing affects trunk stabilization, which in turn affects breathing in patients with stroke [ 22 , 23 ]. This could be one of the factors deteriorating the HRQoL in these patients [ 24 , 25 ].
In addition, pulmonary complications, such as respiratory failure, pneumonia, pleural effusion, acute respiratory distress syndrome, pulmonary edema, and pulmonary embolism from venous thromboembolism, are commonly observed in patients with stroke [ 26 ]. Pulmonary function has been demonstrated to be significantly decreased in patients with stroke compared to that in healthy individuals [ 27 ]. In particular, the activity of the diaphragm has been shown to be reduced in patients with stroke with hemiplegia [ 19 ], which suggests that the respiratory muscle has a critical role in the recovery of pulmonary dysfunction after stroke. Previous studies have attempted to test interventions that improve the respiratory and pulmonary function of patients with stroke [ 14 , 28 , 29 , 30 ]. Furthermore, a meta-analysis reported that respiratory muscle exercises enhanced muscular strength and reduced the risk of respiratory complications in patients with stroke [ 31 ]. Despite adequate levels of chest wall expansion, the tidal ventilation, lung capacity, and lung volume should be maintained to improve the efficiency of breathing in patients with stroke [ 32 ]. However, the exact mechanisms of such effects have not been sufficiently studied, with a consequent lack of a suitable protocol related to breathing exercises for stroke.
Furthermore, the specific mechanism by which enhanced breathing improves cognitive function in patients with stroke is yet to be elucidated. However, the positive effects of breathing exercises on cognitive functional improvement have been reported in studies although not on patients with stroke. Multiple studies on healthy adults have reported a positive effect of breathing exercises on cognitive functional improvement [ 33 , 34 , 35 ]. Considering the synchronization of natural breathing and neuronal activity, breathing activates the cortex, hippocampus, and amygdala that are related to memory performance [ 36 ]. Abnormal breathing, such as mouth breathing in children, has been reported to reduce academic achievement and memory [ 37 ]. These studies suggest an association between normal breathing and cognitive function. Another study on patients with stroke indicated a potential interaction between breathing and cognitive function—walking activated the cortex to increase the respiratory demand, and thus, walking ability was reduced upon engagement of the respiratory cortex [ 38 ]. Therefore, it is necessary to investigate the influence of respiratory function and breathing exercises on cognitive function in patients with stroke. Herein, we review previous studies on the factors related to cognitive function in patients with stroke and the association between those factors and breathing ( Figure 1 ).

Relationship between cognitive function and breathing disorders in patients with stroke. Breathing pattern disorder and pulmonary dysfunction appearing after stroke not only cause various pulmonary complications but also affect the function of local core muscles directly related to breathing. Breathing pattern disorder has a negative effect on trunk stability and is thought to be related to cognitive decline in patients with stroke.
We performed the literature review using the terms “stroke”, “breathing pattern disorder”, “stroke vs. breathing pattern disorder”, “stroke vs. cognitive decline”, “stroke vs. depression vs. cognition”, “stroke vs. psychological factor vs. cognition”, “stroke vs. vascular factor vs. cognition”, “stroke vs. hypertension vs. cognition, “stroke vs. sleep factor vs. cognition”, “stroke vs. OSA vs. cognition”, “stroke vs. biochemical factor vs. cognition”, “stroke vs. IL-6 vs. cognition”, “psychological factor vs. breathing exercise”, “depression vs. breathing exercise”, “parasympathetic nerve vs. breathing exercise”, “vascular factor vs. breathing exercise”, “hypertension vs. breathing exercise”, “chemoreflex vs. breathing exercise”. “baroreflex vs. breathing exercise”, “sleep vs. breathing exercise”, “OSA vs. breathing exercise”, “biochemical factor vs. breathing exercise”, and “IL-6 vs. breathing exercise” along with the Medical Subject Headings (MESH) terms. The reference list of the articles was carefully reviewed as a potential source of information. The search was based on Pubmed , Scopus , and Google Scholar engines. Selected publications were analyzed, and their synthesis was used to write the review and support the hypothesis of the relationship between breathing exercise and stroke.
3. Common Factors of Cognitive Decline in Patients with Stroke
3.1. psychological factors involved in post-stroke cognitive impairment.
Cognitive function decline is a common occurrence in patients with stroke [ 8 , 39 ]. As a result of damage to the brain, approximately 80% of these patients exhibit a decline in cognitive function, and approximately 20–60% show depression [ 40 , 41 ]. The correlation between cognitive decline and post-stroke depression (PSD) is well-established [ 42 ]. The most consistent finding in systematic reviews, including the study by Mansur et al., on the predictive factors for depression after stroke is the association between PSD and post-stroke cognitive decline [ 43 , 44 ]. As such, numerous studies are in support of the association between cognitive decline in stroke and depression [ 45 , 46 ]. Of late, research interests surrounding this topic have increased remarkably.
3.2. Vascular Factors Involved in Post-Stroke Cognitive Impairment
Acute stroke and other types of vascular disease are well-known causes of cognitive impairment [ 47 , 48 , 49 , 50 ]. Asymptomatic cerebrovascular diseases, including silent brain infarcts and leukoaraiosis, manifest with cognitive impairment and are related to risk factors such as hypertension and atrial fibrillation [ 51 , 52 , 53 , 54 , 55 ]. Hypertension was observed in over 60% of patients with stroke [ 56 ], and approximately 25% of patients with stroke had atrial fibrillation [ 57 ]. Indeed, Waldstein et al. [ 58 ] reported that patients with peripheral arterial disease (PAD), as a common form of peripheral vascular disease, exhibited impairment of cognitive function. These previous studies suggest that vascular factors associated with cognitive impairment have been recognized as an important consequence of stroke [ 59 , 60 ].
3.3. Sleep-Related Factors Involved in Post-Stroke Cognitive Impairment
Sleep disorders occur frequently in patients with stroke. In a previous meta-analysis, over 50% of patients with stroke had a concomitant sleep disorder [ 61 , 62 ]. In addition, 68% of patients with acute ischemic stroke were estimated to have sleep disorders such as insomnia [ 63 ], and approximately 44% of patients with cerebral infarction were shown to have sleep disturbances at three months post-stroke [ 64 ]. Sleep disorder has a negative impact on various aspects of neurological recovery and quality of life [ 65 , 66 , 67 ]. Indeed, sleep disorder was shown to increase the risk of stroke recurrence [ 68 ] and had an undesirable effect on the cognitive function in patients with stroke [ 69 ].
Obstructive sleep apnea (OSA) is known to be an independent risk factor for stroke and is one of the common sleep disorders occurring in these patients. Approximately 30–70% of patients with stroke also have OSA [ 70 ]. In a recent study by Aaronson et al. [ 69 ], which was conducted on 80 patients with stroke with OSA and 67 without OSA, a higher decline in cognitive function was revealed in those with OSA. These results suggest that sleep disorder is a key factor influencing cognitive decline in patients with stroke as well as in healthy individuals [ 71 , 72 , 73 ].
3.4. Biochemical Factors Involved in Post-Stroke Cognitive Impairment
Blood lipid concentrations and abnormal neurotransmitter levels have been reported to be related to cognitive function decline in patients with stroke. In a study conducted on patients with acute ischemic stroke, the risk of cognitive decline was directly proportionate to non-high density lipoprotein cholesterol (non-HDL-C) levels [ 74 ]. Similarly, serum glutamate concentration was reported to be a critical predictor of the increased size of the cerebral infarct [ 75 ]. Zeydan et al. showed that a key factor in the cognitive decline in patients with stroke was the loss of glutamatergic neuron synaptic function [ 76 ]. Indeed, the preservation of glutamatergic neuron synaptic function was reported to help maintain the cognitive functions after stroke and prevent dementia [ 77 , 78 ]. The increase in the pleiotropic cytokine, interleukin-6 (IL-6), which induces an acute inflammatory response, was also reported to be related with cognitive decline in patients with stroke [ 79 ].
4. Associations between the Factors Influencing Cognitive Decline and Breathing in Stroke
Breathing exercises are known to improve the physiological, psychological, and cognitive function [ 80 , 81 , 82 , 83 ], and are currently suggested as a supplementary treatment for stress, anxiety, depression, asthma, and chronotropism [ 84 , 85 , 86 , 87 , 88 ]. Breathing can be either voluntary or involuntary. Voluntarily breathing is controlled by a complex feedback system involving the autonomous neural network, brain stem nucleus, limbic system, cortical areas, and the neuroendocrine system [ 80 , 84 ]. The voluntary control of breathing has been reported to exert a positive effect on autonomic nervous system functions, including variable heart rate, expiratory flow rate, and vagal tone [ 89 , 90 , 91 ]. Three months of slow breathing exercises have been shown to cause a decline in heart rate as well as an elevated sensitivity to cardiac response to standing in healthy individuals [ 92 ]. This study thus highlights the importance of breathing control as well as the method used for controlling one’s breathing. Furthermore, this study suggests future directions for research on breathing exercise methods to improve the breathing function in patients with stroke who frequently have breathing abnormalities.
4.1. Psychological Factors and Breathing in Stroke
PSD is the most common neuropsychiatric complication in patients with stroke [ 93 , 94 ]. Studies report that approximately one in three patients with stroke exhibit PSD [ 44 , 95 , 96 , 97 ]. In a study on healthy older adults, breathing exercises were shown to have a positive effect on psychological functions [ 98 , 99 , 100 ]. In a study by Brown and Gerbarg, breathing exercises were suggested to be beneficial for patients with PSD [ 80 ]. Breathing exercises restore the normal state of the autonomic system by regulating the movement of the respiratory system [ 92 , 101 ]. Moreover, enhanced parasympathetic nerve activity may lead to improvements in psychological as well as cognitive functions [ 101 , 102 ]. As the most significant positive effect of breathing exercises, enhanced parasympathetic nerve activity was shown to reduce the response to psychological stress and, consequently, exert positive effects on various domains across psychology, cognition, and behavior [ 80 , 84 ] ( Table 1 ).
Effects of breathing exercises on psychological factors.
4.2. Vascular Factors and Breathing in Stroke
Stroke-induced damage to areas of the brain associated with autonomous function has a substantial influence on the blood pressure control and cardiac function during the period of recovery [ 103 , 104 ]. In addition, patients showing post-stroke hemiplegia exhibited a markedly low level of residual blood flow in the paretic lower limb [ 105 , 106 , 107 ]. Such problems of vascular function can negatively affect the performance of activities of daily living (ADL) and the quality of life [ 108 , 109 ]. Reduced physical activity can in turn affect the blood flow velocity, endothelial function, and arterial diameter through secondary reduction in blood flow [ 108 , 110 ].
No study has yet directly investigated the effects of breathing exercise and respiratory function on vascular function in patients with stroke; however, several studies have been conducted on patients with hypertension, which is known to be the most serious risk factor for stroke incidence.
Breathing exercises are widely acknowledged as a non-pharmaceutical intervention for the control of hypertension, a risk factor of stroke [ 111 , 112 , 113 ]. The mechanism of action is as follows: the pressor receptor stimulating the autonomic nervous system during prolonged inhalation and exhalation increases the baroreflex sensitivity (BRS) and decreases the sympathetic activity and chemoreflex activation [ 114 , 115 ]. In numerous studies, slow breathing exercises have shown positive effects on BRS, blood pressure, and autonomic nervous system function [ 114 , 116 , 117 ]. Hypertension is a particularly important risk factor for hemorrhagic stroke although it contributes to atherosclerotic disease that can lead to ischemic stroke as well, increasing the risk of stroke by approximately 2.87 times [ 118 , 119 ]. The prevalence of stroke in hypertension patients aged 50 years or above was 20% of the total population, and the prevalence continuously increased with increasing age [ 120 ]. Based on the correlation between hypertension and stroke, further studies should be conducted to determine the effects of breathing exercises on the vascular function, blood pressure, and autonomic nervous system in patients with stroke ( Table 2 ).
Effects of breathing exercises on vascular factors.
4.3. Sleep and Breathing in Stroke
Sleep-related breathing disorders occur in more than half of patients with stroke [ 61 , 121 ]. They are also an independent risk factor for stroke [ 122 , 123 , 124 ] while being responsible for the risk of stroke recurrence, mortality, and deterioration of cognitive function [ 125 , 126 ]. Sleep-related breathing disorders include habitual snoring, upper respiratory tract resistance syndrome, aperiodic breathing, and sleep apnea syndrome. OSA refers to the partial or complete collapse of the upper airway during sleep, resulting in reduced or absent (or apnea) airflow lasting for 10 s [ 123 ]. Many OSA patients are highly likely to show cardiovascular or cerebrovascular diseases, as OSA is also associated with hypertension, a direct risk factor of stroke [ 120 , 127 , 128 , 129 ]. OSA is also associated with fibrinogen levels, a key independent risk factor for myocardial infarction and vascular diseases [ 130 , 131 ]. Elevated fibrinogen levels are correlated with increased risk of cardiovascular events in patients with stroke [ 130 , 131 , 132 ]. As OSA reduces the cerebral blood volume and decreases the blood supply to the brain, it is viewed as a risk factor for stroke [ 133 ].
In a study by Yaggi et al. conducted on 1022 adults with no history of stroke or myocardial infarction, the risk of stroke was shown to increase as the severity of OSA increased [ 134 ]. This result was verified in further follow-up studies and meta-analyses, where adults with OSA showed approximately a two-fold higher risk of stroke [ 135 , 136 , 137 , 138 , 139 ]. Additionally, untreated OSA after acute stroke increases long-term mortality and neurological outcomes [ 125 , 126 ], which highlights the importance of rapid treatment of post-stroke OSA.
The most well-known treatment of OSA in patients with stroke is continuous positive airway pressure (CPAP). While CPAP was shown to have positive effects on neuronal recovery, sleep, depression, and long-term survival [ 140 , 141 , 142 , 143 ], patients with stroke show a reduced long-term compliance to CPAP compared to healthy individuals [ 144 ]. This may be related to the difficulty in wearing and retaining the CPAP mask due to weak upper extremity and face as well as due to depression [ 144 ]. CPAP also has a role in causing phobia related to rhinocleisis [ 145 ], the arousal of the respiratory tract related to oral exposure and drying of mucous membranes [ 146 ]; therefore, there are challenges in its successful application in patients with stroke. This suggests the need for simple interventions or treatment strategies for OSA.
Among the interventions to improve OSA are a diversity of breathing re-education (BRE) approaches. These include the Buteyko method, inhalation resistance breathing training, and diaphragmatic breathing [ 147 , 148 , 149 , 150 ]. The BRE approach aims to improve the abnormal breathing pattern in patients with chronic hyperventilation. It involves exercises such as breath-holding and controlled breathing to restore the normal nasal/diaphragmatic pattern and treat dysfunctional breathing habits, such as abnormal mouth breathing and abnormal apical breathing or upper chest breathing [ 151 ].
Mouth breathing is associated with the severity of OSA [ 152 , 153 ], as it plays a part in snoring, OSA, apnea, and hypopnea [ 153 ]. In addition, patients with OSA were reported to show reduced strength of the diaphragm and muscles related to breathing compared with age- and sex-matched controls [ 147 ]. In a study by Courtney, the magnitude and stability of respiratory motor output for muscles of the upper airway was reported to be a key contributing factor across all forms of sleep apnea [ 147 ].
The BRE approach of Mckeown, which applies the Buteyko method, includes the conversion of mouth breathing to nasal breathing during rest, exercise, and sleep [ 151 ]. Such breathing exercises play a part in restoring nasal breathing, enhancing the function of the diaphragm, reducing breathing rate, and increasing the tolerance to changes in arterial carbon dioxide pressure [ 154 ]. Recent studies have shown that the BRE approach improves breathing patterns and can be beneficial for OSA patients [ 147 , 155 ]. However, no study has investigated the effects of breathing exercises or the re-education approaches for OSA and other sleep-related disorders in patients with stroke, suggesting the need for relevant further studies ( Table 3 ).
Effects of breathing exercises on sleep factors.
4.4. Biochemical Factors and Breathing in Stroke
The magnitude of cerebral infarction on CT increases with the level of IL-6. IL-6 is the most well-known biochemical marker of stroke and is associated with a poor 3-month and 12-month prognosis [ 157 , 158 ]. Respiratory failure caused by the SARS-CoV-2 virus or COVID-19 infection is correlated with the cytokine release syndrome that leads to the need for mechanical ventilation [ 159 ]. IL-6 is known as the main chemokine inducing the cytokine-release syndrome [ 159 , 160 , 161 ]. Moreover, COVID-19 severity was shown to be associated with the risk of acute stroke [ 162 , 163 ], suggesting that studies on the relationship between the level of IL-6 and breathing in patients with stroke are warranted.
In a recent study using a mouse model, the neurological damage in mice with lung damage could be reduced by suppressing levels of IL-6 [ 164 ]. Although only a few studies have reported on the relationship between breathing exercises and IL-6 in patients with stroke, several studies have been conducted on different sets of participants. In a study conducted on overweight or obese adult males, the practice of breathing exercises was reported to be associated with significantly reduced levels of plasma IL-6 [ 165 ]. Similarly, in another study conducted on healthy individuals, breathing exercises were reported to have reduced the levels of IL-6 [ 166 ].
In a study conducted on healthy mammals, the respiratory system was markedly affected by an increase in IL-6 levels, which increased the mechanical ventilation during exhalation [ 167 ]. These results suggest a possible correlation between the levels of IL-6 and breathing based on its role in the pathogenesis of respiratory diseases ( Table 4 ).
Effects of breathing exercises on biochemistry factors.
5. Rationale for the Development of Breathing Exercise Protocols to Attenuate Cognitive Decline in Patients with Stroke
Breathing exercises can restore the normal state of the autonomic nervous system [ 91 , 101 ] and increase the parasympathetic nervous system activity with the anticipated psychological changes and improved cognitive functions [ 101 , 102 ]. However, further research into the influence of breathing exercises on the vascular function, blood pressure, and autonomic nervous system changes in patients with stroke is necessary.
The importance of rapid treatment for post-stroke OSA has been recognized, and studies have reported on the positive effect of BRE based on the Buteyko method on breathing patterns in OSA patients [ 147 , 155 ]. However, no study has yet reported on the effects of general breathing exercises and BRE on OSA or other sleep-related breathing disorders in patients with stroke, and this is another gap in the literature that ought to be addressed.
Through literature review, we examined the factors related to cognitive decline in patients with stroke and their associations with normal natural breathing and breathing exercises. Breathing exercises were found to contribute to cognitive functional improvement in patients with stroke. In particular, it can be inferred that slow breathing exercise is the most helpful breathing exercise for improving cognitive function in stroke patients. However, there is a limitation in explaining the time, period, and intensity for breathing exercises; accordingly, the development of breathing exercise protocols to improve cognitive functions in patients with stroke is necessary.
The global COVID-19 pandemic that began in early 2020 has prompted focused efforts on the development of pulmonary rehabilitation using breathing exercise programs worldwide [ 168 ]. A similar high-quality breathing exercise protocol to suit the characteristics of patients with stroke would be useful in the current era. Finally, studies on the correlation between the effects of such protocols and cognitive decline that is most detected in patients with stroke would be highly beneficial.
Funding Statement
This work was supported by the Ministry of Education of the Republic of Korea and the National Research Foundation of Korea (NRF-2021S1A5A8069272).
Author Contributions
Conceptualization, E.-S.K. and M.-S.H.; methodology, E.-S.K. and M.-S.H.; validation, M.-S.H.; data curation, E.-S.K.; writing—original draft preparation, E.-S.K. and M.-S.H.; writing—review and editing, J.S.Y. and M.-S.H.; visualization, J.S.Y.; supervision, M.-S.H.; project administration, M.-S.H.; funding acquisition, M.-S.H. All authors critically revised the manuscript for important intellectual content, and approved the final version for publication. All authors significantly contributed to the research. All authors have read and agreed to the published version of the manuscript.
Conflicts of Interest
The authors declare no conflict of interest.
Publisher’s Note: MDPI stays neutral with regard to jurisdictional claims in published maps and institutional affiliations.
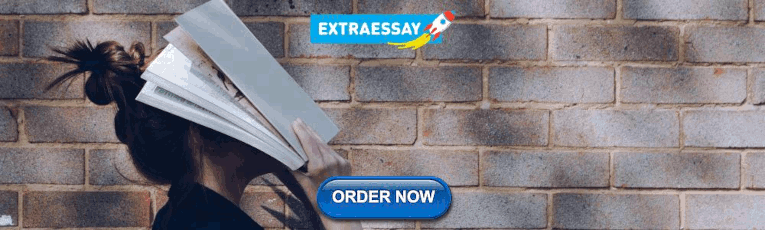
IMAGES
VIDEO
COMMENTS
CASE 1. A 20 year old man with no past medical history presented to a primary stroke center with sudden left sided weakness and imbalance followed by decreased level of consciousness. Head CT showed no hemorrhage, no acute ischemic changes, and a hyper-dense basilar artery. CT angiography showed a mid-basilar occlusion.
Some case studies in Wuhan described immense inflammatory responses to COVID-19, including elevated acute phase reactants, such as CRP and D-dimer. Raised D-dimers are a non-specific marker of a prothrombotic state and have been associated with greater morbidity and mortality relating to stroke and other neurological features. 14
Case 13-2016 — A ... the level is not commonly elevated after stroke in patients who do not have coronary heart disease. 33 In several small studies, the risk of stroke was up to 17% higher 34 ...
Read chapter 7 of Patient Management in the Telemetry/Cardiac Step-Down Unit: A Case-Based Approach online now, exclusively on AccessMedicine. AccessMedicine is a subscription-based resource from McGraw Hill that features trusted medical content from the best minds in medicine.
Acute Ischemic Stroke. •. Treatment for patients with acute ischemic stroke is guided by the time from the onset of stroke, the severity of neurologic deficit, and findings on neuroimaging. By ...
In one study, the five most frequent stroke mimics were seizure, syncope, sepsis, migraine and brain tumours 12; detailed reviews can be found in Practical Neurology. 13 14. ... Insufficient data, case-by-case risk decision with obstetric team. Unruptured intracranial aneurysm: Small (<10 mm) unsecured unruptured intracranial aneurysm should ...
A PFO has been demonstrated in 10%-26% of healthy adults. 14 In young patients who have had a cryptogenic stroke, however, the prevalence is thought to be much higher, for example, 40% in one study. 15 It is thought that a PFO allows microemboli to pass into the systemic circulation leading to a stroke.
The risk of both recurrent ischemic stroke and intracranial hemorrhage is highest in the first few days after acute ischemic stroke, and although studies and small randomized trials suggest that ...
This case-control study showed that the frequency of ischemic stroke was higher than that of hemorrhagic stroke in young Ugandan population. We showed that positive HIV serology, elevated waist to hip ratio and sickle cell disease were independent risk factors for stroke in this population.
Stroke is one of these complications, since a greater incidence of stroke among patients with COVID-19 than the non-COVID-19 population has been reported, and a preliminary case-control study demonstrated that SARS-CoV-2 infection represents a risk factor for acute ischemic stroke .
For this study, a stroke‐free control population, frequency matched for age (5‐year bands) and sex, was randomly selected from the southern Finland FINRISK survey participants from years 1997, 2002, and 2007, that is, from the same time period and the same geographic region as the case population.
Life after stroke support provides a lifeline to people affected by stroke, improves quality of life for both stroke survivors and carers, and means people can live well beyond stroke. ... Our case studies. Michael Pursey. Former sports coach and teaching assistant Michael Pursey, from Wales, expected to be in a wheelchair forever after having ...
IVT has been demonstrated to improve outcomes in patients with acute ischemic stroke within the first 4.5-h. 14, 15, 20-22 In 1996, the Food and Drug Administration (FDA) approved intravenous-tPA (IV-tPA) for treating acute ischemic stroke, based on successful outcomes in the National Institute of Neurological Disorders and Stroke study. 23, 24 ...
Case Study. A 68-year-old female, with a history of hypertension and diabetes mellitus, presented to the ED after acute onset of speech difficulty and right-sided weakness. Her symptoms began 3 hours ago. On physical exam, the patient was found to have severe expressive aphasia, right hemiplegia, and right hemi-sensory loss.
Pathophysiology and clinical presentation. (DiGiovanna, A. G; 1999) Cerebrovascular accidents, also known as strokes, happen when the blood supply becomes occluded in part of the brain. They can be classified as either ischemic, hemorrhagic, or cryptogenic. In ischemic strokes blood supply can be occluded for three different reasons, a vascular ...
In Uganda, literature on stroke in young adults is limited however results of a study done among acute stroke patients admitted to the national referral hospital (Mulago hospital) showed a 30-day mortality of 43.8%. Out of 133 patients, 32 patients (25%) were less than 51 years old. Out of the 56 patients that died, 13 patients (23%) were less ...
Selected references, frequently including the first description, are provided at the conclusion of each case. Drawing on the expertise of leading teachers and practitioners, and liberally illustrated, these case studies and the discussions that accompany them are an essential guide to learning the complexity of stroke diagnosis.
In either case, parts of the brain become damaged or die. A stroke can cause lasting brain damage, long-term disability, or even death. ... National Institute of Neurological Disorders and Stroke rt-PA Stroke Study Group. Tissue plasminogen activator for acute ischemic stroke. N Engl J Med. 1995;333(24):1581-1587.
Stroke case study Creators. Sanaya Batcho; Kara Kitzmiller; Emma Overman; Our reason for choosing this disorder. Stroke is the leading cause of disability in the United States. As advanced practice nurses, we anticipate caring for those impacted by strokes in many healthcare settings including emergency rooms, acute care, rehab settings ...
Some case studies in Wuhan described immense inflammatory responses to COVID-19, including elevated acute phase reactants, such as CRP and D-dimer. Raised D-dimers are a non-specific marker of a prothrombotic state and have been associated with greater morbidity and mortality relating to stroke and other neurological features. 14
A Yale study uncovers new insights into factors associated with treatment delays and where in the United States patients are more likely to experience slower responses. The findings, which were published May 24 in the journal Stroke, highlight where interventions should be focused in order to improve stroke outcomes across the country.
A case study conducted in the US showed that people with high financial status had better stroke treatment options than deprived individuals . ... Stroke studies should be conducted in both male and female subjects to exclude gender bias, and should take account of other confounders like hypertension, diabetes and obesity. ...
The stroke prediction dataset was created by McKinsey & Company and Kaggle is the source of the data used in this study 38,39. The dataset is in comma separated values (CSV) format, including ...
Objective Carotid atherosclerosis is a chronic inflammatory disease, which is a major cause of ischemic stroke. The purpose of this study was to analyze the relationship between carotid atherosclerosis and novel inflammatory markers, including platelet to lymphocyte ratio (PLR), neutrophil to lymphocyte ratio (NLR), lymphocyte to monocyte ratio (LMR), platelet to neutrophil ratio (PNR ...
Aims: We aimed to investigate the relationship between the tryptophan index and the risk of ischemic stroke. Methods: We performed a nested case-control study within a community-based cohort in eastern China over the period 2013 to 2018. The analysis included 321 cases of ischemic stroke and 321 controls matched by sex and date of birth.
This test showed a high validity in determining the presence of LMS in patients presenting with symptoms suggesting acute vestibular syndrome. 6 One study concluded that the HINTS detected the presence of stroke with 100% sensitivity and 96% specificity and, importantly, detected all patients with LMS. 10 Importantly, the maneuver revealed a ...
Stroke is a significant global cause of disability and death, and its burden has been on the rise, while ambient air pollution has been conclusively linked to stroke incidence. However, knowledge about effects of atmospheric oxidation on stroke and its interactions with fine particles (PM 2.5 ) are still limited. In this study, we investigated the short-term effects of ambient NO<SUB loc="post ...
1. Introduction. Stroke is caused by a fall in blood supply to the brain or due to cerebral hemorrhage and is the most common cerebrovascular condition [].With the increase in the average life expectancy as a result of lifestyle improvements and advances in health care, the number of patients with stroke has seen an upward trend [].Furthermore, studies have shown a possible association between ...