
An official website of the United States government
The .gov means it’s official. Federal government websites often end in .gov or .mil. Before sharing sensitive information, make sure you’re on a federal government site.
The site is secure. The https:// ensures that you are connecting to the official website and that any information you provide is encrypted and transmitted securely.
- Publications
- Account settings
Preview improvements coming to the PMC website in October 2024. Learn More or Try it out now .
- Advanced Search
- Journal List
- Afr J Emerg Med
- v.7(3); 2017 Sep
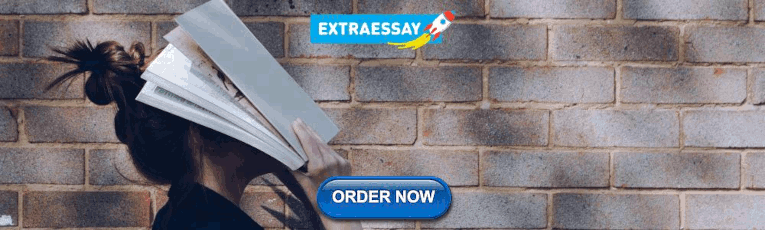
A hands-on guide to doing content analysis
Christen erlingsson.
a Department of Health and Caring Sciences, Linnaeus University, Kalmar 391 82, Sweden
Petra Brysiewicz
b School of Nursing & Public Health, University of KwaZulu-Natal, Durban 4041, South Africa
Associated Data
There is a growing recognition for the important role played by qualitative research and its usefulness in many fields, including the emergency care context in Africa. Novice qualitative researchers are often daunted by the prospect of qualitative data analysis and thus may experience much difficulty in the data analysis process. Our objective with this manuscript is to provide a practical hands-on example of qualitative content analysis to aid novice qualitative researchers in their task.
African relevance
- • Qualitative research is useful to deepen the understanding of the human experience.
- • Novice qualitative researchers may benefit from this hands-on guide to content analysis.
- • Practical tips and data analysis templates are provided to assist in the analysis process.
Introduction
There is a growing recognition for the important role played by qualitative research and its usefulness in many fields, including emergency care research. An increasing number of health researchers are currently opting to use various qualitative research approaches in exploring and describing complex phenomena, providing textual accounts of individuals’ “life worlds”, and giving voice to vulnerable populations our patients so often represent. Many articles and books are available that describe qualitative research methods and provide overviews of content analysis procedures [1] , [2] , [3] , [4] , [5] , [6] , [7] , [8] , [9] , [10] . Some articles include step-by-step directions intended to clarify content analysis methodology. What we have found in our teaching experience is that these directions are indeed very useful. However, qualitative researchers, especially novice researchers, often struggle to understand what is happening on and between steps, i.e., how the steps are taken.
As research supervisors of postgraduate health professionals, we often meet students who present brilliant ideas for qualitative studies that have potential to fill current gaps in the literature. Typically, the suggested studies aim to explore human experience. Research questions exploring human experience are expediently studied through analysing textual data e.g., collected in individual interviews, focus groups, documents, or documented participant observation. When reflecting on the proposed study aim together with the student, we often suggest content analysis methodology as the best fit for the study and the student, especially the novice researcher. The interview data are collected and the content analysis adventure begins. Students soon realise that data based on human experiences are complex, multifaceted and often carry meaning on multiple levels.
For many novice researchers, analysing qualitative data is found to be unexpectedly challenging and time-consuming. As they soon discover, there is no step-wise analysis process that can be applied to the data like a pattern cutter at a textile factory. They may become extremely annoyed and frustrated during the hands-on enterprise of qualitative content analysis.
The novice researcher may lament, “I’ve read all the methodology but don’t really know how to start and exactly what to do with my data!” They grapple with qualitative research terms and concepts, for example; differences between meaning units, codes, categories and themes, and regarding increasing levels of abstraction from raw data to categories or themes. The content analysis adventure may now seem to be a chaotic undertaking. But, life is messy, complex and utterly fascinating. Experiencing chaos during analysis is normal. Good advice for the qualitative researcher is to be open to the complexity in the data and utilise one’s flow of creativity.
Inspired primarily by descriptions of “conventional content analysis” in Hsieh and Shannon [3] , “inductive content analysis” in Elo and Kyngäs [5] and “qualitative content analysis of an interview text” in Graneheim and Lundman [1] , we have written this paper to help the novice qualitative researcher navigate the uncertainty in-between the steps of qualitative content analysis. We will provide advice and practical tips, as well as data analysis templates, to attempt to ease frustration and hopefully, inspire readers to discover how this exciting methodology contributes to developing a deeper understanding of human experience and our professional contexts.
Overview of qualitative content analysis
Synopsis of content analysis.
A common starting point for qualitative content analysis is often transcribed interview texts. The objective in qualitative content analysis is to systematically transform a large amount of text into a highly organised and concise summary of key results. Analysis of the raw data from verbatim transcribed interviews to form categories or themes is a process of further abstraction of data at each step of the analysis; from the manifest and literal content to latent meanings ( Fig. 1 and Table 1 ).

Example of analysis leading to higher levels of abstraction; from manifest to latent content.
Glossary of terms as used in this hands-on guide to doing content analysis. *
The initial step is to read and re-read the interviews to get a sense of the whole, i.e., to gain a general understanding of what your participants are talking about. At this point you may already start to get ideas of what the main points or ideas are that your participants are expressing. Then one needs to start dividing up the text into smaller parts, namely, into meaning units. One then condenses these meaning units further. While doing this, you need to ensure that the core meaning is still retained. The next step is to label condensed meaning units by formulating codes and then grouping these codes into categories. Depending on the study’s aim and quality of the collected data, one may choose categories as the highest level of abstraction for reporting results or you can go further and create themes [1] , [2] , [3] , [5] , [8] .
Content analysis as a reflective process
You must mould the clay of the data , tapping into your intuition while maintaining a reflective understanding of how your own previous knowledge is influencing your analysis, i.e., your pre-understanding. In qualitative methodology, it is imperative to vigilantly maintain an awareness of one’s pre-understanding so that this does not influence analysis and/or results. This is the difficult balancing task of keeping a firm grip on one’s assumptions, opinions, and personal beliefs, and not letting them unconsciously steer your analysis process while simultaneously, and knowingly, utilising one’s pre-understanding to facilitate a deeper understanding of the data.
Content analysis, as in all qualitative analysis, is a reflective process. There is no “step 1, 2, 3, done!” linear progression in the analysis. This means that identifying and condensing meaning units, coding, and categorising are not one-time events. It is a continuous process of coding and categorising then returning to the raw data to reflect on your initial analysis. Are you still satisfied with the length of meaning units? Do the condensed meaning units and codes still “fit” with each other? Do the codes still fit into this particular category? Typically, a fair amount of adjusting is needed after the first analysis endeavour. For example: a meaning unit might need to be split into two meaning units in order to capture an additional core meaning; a code modified to more closely match the core meaning of the condensed meaning unit; or a category name tweaked to most accurately describe the included codes. In other words, analysis is a flexible reflective process of working and re-working your data that reveals connections and relationships. Once condensed meaning units are coded it is easier to get a bigger picture and see patterns in your codes and organise codes in categories.
Content analysis exercise
The synopsis above is representative of analysis descriptions in many content analysis articles. Although correct, such method descriptions still do not provide much support for the novice researcher during the actual analysis process. Aspiring to provide guidance and direction to support the novice, a practical example of doing the actual work of content analysis is provided in the following sections. This practical example is based on a transcribed interview excerpt that was part of a study that aimed to explore patients’ experiences of being admitted into the emergency centre ( Fig. 2 ).

Excerpt from interview text exploring “Patient’s experience of being admitted into the emergency centre”
This content analysis exercise provides instructions, tips, and advice to support the content analysis novice in a) familiarising oneself with the data and the hermeneutic spiral, b) dividing up the text into meaning units and subsequently condensing these meaning units, c) formulating codes, and d) developing categories and themes.
Familiarising oneself with the data and the hermeneutic spiral
An important initial phase in the data analysis process is to read and re-read the transcribed interview while keeping your aim in focus. Write down your initial impressions. Embrace your intuition. What is the text talking about? What stands out? How did you react while reading the text? What message did the text leave you with? In this analysis phase, you are gaining a sense of the text as a whole.
You may ask why this is important. During analysis, you will be breaking down the whole text into smaller parts. Returning to your notes with your initial impressions will help you see if your “parts” analysis is matching up with your first impressions of the “whole” text. Are your initial impressions visible in your analysis of the parts? Perhaps you need to go back and check for different perspectives. This is what is referred to as the hermeneutic spiral or hermeneutic circle. It is the process of comparing the parts to the whole to determine whether impressions of the whole verify the analysis of the parts in all phases of analysis. Each part should reflect the whole and the whole should be reflected in each part. This concept will become clearer as you start working with your data.
Dividing up the text into meaning units and condensing meaning units
You have now read the interview a number of times. Keeping your research aim and question clearly in focus, divide up the text into meaning units. Located meaning units are then condensed further while keeping the central meaning intact ( Table 2 ). The condensation should be a shortened version of the same text that still conveys the essential message of the meaning unit. Sometimes the meaning unit is already so compact that no further condensation is required. Some content analysis sources warn researchers against short meaning units, claiming that this can lead to fragmentation [1] . However, our personal experience as research supervisors has shown us that a greater problem for the novice is basing analysis on meaning units that are too large and include many meanings which are then lost in the condensation process.
Suggestion for how the exemplar interview text can be divided into meaning units and condensed meaning units ( condensations are in parentheses ).
Formulating codes
The next step is to develop codes that are descriptive labels for the condensed meaning units ( Table 3 ). Codes concisely describe the condensed meaning unit and are tools to help researchers reflect on the data in new ways. Codes make it easier to identify connections between meaning units. At this stage of analysis you are still keeping very close to your data with very limited interpretation of content. You may adjust, re-do, re-think, and re-code until you get to the point where you are satisfied that your choices are reasonable. Just as in the initial phase of getting to know your data as a whole, it is also good to write notes during coding on your impressions and reactions to the text.
Suggestions for coding of condensed meaning units.
Developing categories and themes
The next step is to sort codes into categories that answer the questions who , what , when or where? One does this by comparing codes and appraising them to determine which codes seem to belong together, thereby forming a category. In other words, a category consists of codes that appear to deal with the same issue, i.e., manifest content visible in the data with limited interpretation on the part of the researcher. Category names are most often short and factual sounding.
In data that is rich with latent meaning, analysis can be carried on to create themes. In our practical example, we have continued the process of abstracting data to a higher level, from category to theme level, and developed three themes as well as an overarching theme ( Table 4 ). Themes express underlying meaning, i.e., latent content, and are formed by grouping two or more categories together. Themes are answering questions such as why , how , in what way or by what means? Therefore, theme names include verbs, adverbs and adjectives and are very descriptive or even poetic.
Suggestion for organisation of coded meaning units into categories and themes.
Some reflections and helpful tips
Understand your pre-understandings.
While conducting qualitative research, it is paramount that the researcher maintains a vigilance of non-bias during analysis. In other words, did you remain aware of your pre-understandings, i.e., your own personal assumptions, professional background, and previous experiences and knowledge? For example, did you zero in on particular aspects of the interview on account of your profession (as an emergency doctor, emergency nurse, pre-hospital professional, etc.)? Did you assume the patient’s gender? Did your assumptions affect your analysis? How about aspects of culpability; did you assume that this patient was at fault or that this patient was a victim in the crash? Did this affect how you analysed the text?
Staying aware of one’s pre-understandings is exactly as difficult as it sounds. But, it is possible and it is requisite. Focus on putting yourself and your pre-understandings in a holding pattern while you approach your data with an openness and expectation of finding new perspectives. That is the key: expect the new and be prepared to be surprised. If something in your data feels unusual, is different from what you know, atypical, or even odd – don’t by-pass it as “wrong”. Your reactions and intuitive responses are letting you know that here is something to pay extra attention to, besides the more comfortable condensing and coding of more easily recognisable meaning units.
Use your intuition
Intuition is a great asset in qualitative analysis and not to be dismissed as “unscientific”. Intuition results from tacit knowledge. Just as tacit knowledge is a hallmark of great clinicians [11] , [12] ; it is also an invaluable tool in analysis work [13] . Literally, take note of your gut reactions and intuitive guidance and remember to write these down! These notes often form a framework of possible avenues for further analysis and are especially helpful as you lift the analysis to higher levels of abstraction; from meaning units to condensed meaning units, to codes, to categories and then to the highest level of abstraction in content analysis, themes.
Aspects of coding and categorising hard to place data
All too often, the novice gets overwhelmed by interview material that deals with the general subject matter of the interview, but doesn’t seem to answer the research question. Don’t be too quick to consider such text as off topic or dross [6] . There is often data that, although not seeming to match the study aim precisely, is still important for illuminating the problem area. This can be seen in our practical example about exploring patients’ experiences of being admitted into the emergency centre. Initially the participant is describing the accident itself. While not directly answering the research question, the description is important for understanding the context of the experience of being admitted into the emergency centre. It is very common that participants will “begin at the beginning” and prologue their narratives in order to create a context that sets the scene. This type of contextual data is vital for gaining a deepened understanding of participants’ experiences.
In our practical example, the participant begins by describing the crash and the rescue, i.e., experiences leading up to and prior to admission to the emergency centre. That is why we have chosen in our analysis to code the condensed meaning unit “Ambulance staff looked worried about all the blood” as “In the ambulance” and place it in the category “Reliving the rescue”. We did not choose to include this meaning unit in the categories specifically about admission to the emergency centre itself. Do you agree with our coding choice? Would you have chosen differently?
Another common problem for the novice is deciding how to code condensed meaning units when the unit can be labelled in several different ways. At this point researchers usually groan and wish they had thought to ask one of those classic follow-up questions like “Can you tell me a little bit more about that?” We have examples of two such coding conundrums in the exemplar, as can be seen in Table 3 (codes we conferred on) and Table 4 (codes we reached consensus on). Do you agree with our choices or would you have chosen different codes? Our best advice is to go back to your impressions of the whole and lean into your intuition when choosing codes that are most reasonable and best fit your data.
A typical problem area during categorisation, especially for the novice researcher, is overlap between content in more than one initial category, i.e., codes included in one category also seem to be a fit for another category. Overlap between initial categories is very likely an indication that the jump from code to category was too big, a problem not uncommon when the data is voluminous and/or very complex. In such cases, it can be helpful to first sort codes into narrower categories, so-called subcategories. Subcategories can then be reviewed for possibilities of further aggregation into categories. In the case of a problematic coding, it is advantageous to return to the meaning unit and check if the meaning unit itself fits the category or if you need to reconsider your preliminary coding.
It is not uncommon to be faced by thorny problems such as these during coding and categorisation. Here we would like to reiterate how valuable it is to have fellow researchers with whom you can discuss and reflect together with, in order to reach consensus on the best way forward in your data analysis. It is really advantageous to compare your analysis with meaning units, condensations, coding and categorisations done by another researcher on the same text. Have you identified the same meaning units? Do you agree on coding? See similar patterns in the data? Concur on categories? Sometimes referred to as “researcher triangulation,” this is actually a key element in qualitative analysis and an important component when striving to ensure trustworthiness in your study [14] . Qualitative research is about seeking out variations and not controlling variables, as in quantitative research. Collaborating with others during analysis lets you tap into multiple perspectives and often makes it easier to see variations in the data, thereby enhancing the quality of your results as well as contributing to the rigor of your study. It is important to note that it is not necessary to force consensus in the findings but one can embrace these variations in interpretation and use that to capture the richness in the data.
Yet there are times when neither openness, pre-understanding, intuition, nor researcher triangulation does the job; for example, when analysing an interview and one is simply confused on how to code certain meaning units. At such times, there are a variety of options. A good starting place is to re-read all the interviews through the lens of this specific issue and actively search for other similar types of meaning units you might have missed. Another way to handle this is to conduct further interviews with specific queries that hopefully shed light on the issue. A third option is to have a follow-up interview with the same person and ask them to explain.
Additional tips
It is important to remember that in a typical project there are several interviews to analyse. Codes found in a single interview serve as a starting point as you then work through the remaining interviews coding all material. Form your categories and themes when all project interviews have been coded.
When submitting an article with your study results, it is a good idea to create a table or figure providing a few key examples of how you progressed from the raw data of meaning units, to condensed meaning units, coding, categorisation, and, if included, themes. Providing such a table or figure supports the rigor of your study [1] and is an element greatly appreciated by reviewers and research consumers.
During the analysis process, it can be advantageous to write down your research aim and questions on a sheet of paper that you keep nearby as you work. Frequently referring to your aim can help you keep focused and on track during analysis. Many find it helpful to colour code their transcriptions and write notes in the margins.
Having access to qualitative analysis software can be greatly helpful in organising and retrieving analysed data. Just remember, a computer does not analyse the data. As Jennings [15] has stated, “… it is ‘peopleware,’ not software, that analyses.” A major drawback is that qualitative analysis software can be prohibitively expensive. One way forward is to use table templates such as we have used in this article. (Three analysis templates, Templates A, B, and C, are provided as supplementary online material ). Additionally, the “find” function in word processing programmes such as Microsoft Word (Redmond, WA USA) facilitates locating key words, e.g., in transcribed interviews, meaning units, and codes.
Lessons learnt/key points
From our experience with content analysis we have learnt a number of important lessons that may be useful for the novice researcher. They are:
- • A method description is a guideline supporting analysis and trustworthiness. Don’t get caught up too rigidly following steps. Reflexivity and flexibility are just as important. Remember that a method description is a tool helping you in the process of making sense of your data by reducing a large amount of text to distil key results.
- • It is important to maintain a vigilant awareness of one’s own pre-understandings in order to avoid bias during analysis and in results.
- • Use and trust your own intuition during the analysis process.
- • If possible, discuss and reflect together with other researchers who have analysed the same data. Be open and receptive to new perspectives.
- • Understand that it is going to take time. Even if you are quite experienced, each set of data is different and all require time to analyse. Don’t expect to have all the data analysis done over a weekend. It may take weeks. You need time to think, reflect and then review your analysis.
- • Keep reminding yourself how excited you have felt about this area of research and how interesting it is. Embrace it with enthusiasm!
- • Let it be chaotic – have faith that some sense will start to surface. Don’t be afraid and think you will never get to the end – you will… eventually!
Peer review under responsibility of African Federation for Emergency Medicine.
Appendix A Supplementary data associated with this article can be found, in the online version, at http://dx.doi.org/10.1016/j.afjem.2017.08.001 .
Appendix A. Supplementary data
Skip to content
Read the latest news stories about Mailman faculty, research, and events.
Departments
We integrate an innovative skills-based curriculum, research collaborations, and hands-on field experience to prepare students.
Learn more about our research centers, which focus on critical issues in public health.
Our Faculty
Meet the faculty of the Mailman School of Public Health.
Become a Student
Life and community, how to apply.
Learn how to apply to the Mailman School of Public Health.
Content Analysis
Content analysis is a research tool used to determine the presence of certain words, themes, or concepts within some given qualitative data (i.e. text). Using content analysis, researchers can quantify and analyze the presence, meanings, and relationships of such certain words, themes, or concepts. As an example, researchers can evaluate language used within a news article to search for bias or partiality. Researchers can then make inferences about the messages within the texts, the writer(s), the audience, and even the culture and time of surrounding the text.
Description
Sources of data could be from interviews, open-ended questions, field research notes, conversations, or literally any occurrence of communicative language (such as books, essays, discussions, newspaper headlines, speeches, media, historical documents). A single study may analyze various forms of text in its analysis. To analyze the text using content analysis, the text must be coded, or broken down, into manageable code categories for analysis (i.e. “codes”). Once the text is coded into code categories, the codes can then be further categorized into “code categories” to summarize data even further.
Three different definitions of content analysis are provided below.
Definition 1: “Any technique for making inferences by systematically and objectively identifying special characteristics of messages.” (from Holsti, 1968)
Definition 2: “An interpretive and naturalistic approach. It is both observational and narrative in nature and relies less on the experimental elements normally associated with scientific research (reliability, validity, and generalizability) (from Ethnography, Observational Research, and Narrative Inquiry, 1994-2012).
Definition 3: “A research technique for the objective, systematic and quantitative description of the manifest content of communication.” (from Berelson, 1952)
Uses of Content Analysis
Identify the intentions, focus or communication trends of an individual, group or institution
Describe attitudinal and behavioral responses to communications
Determine the psychological or emotional state of persons or groups
Reveal international differences in communication content
Reveal patterns in communication content
Pre-test and improve an intervention or survey prior to launch
Analyze focus group interviews and open-ended questions to complement quantitative data
Types of Content Analysis
There are two general types of content analysis: conceptual analysis and relational analysis. Conceptual analysis determines the existence and frequency of concepts in a text. Relational analysis develops the conceptual analysis further by examining the relationships among concepts in a text. Each type of analysis may lead to different results, conclusions, interpretations and meanings.
Conceptual Analysis
Typically people think of conceptual analysis when they think of content analysis. In conceptual analysis, a concept is chosen for examination and the analysis involves quantifying and counting its presence. The main goal is to examine the occurrence of selected terms in the data. Terms may be explicit or implicit. Explicit terms are easy to identify. Coding of implicit terms is more complicated: you need to decide the level of implication and base judgments on subjectivity (an issue for reliability and validity). Therefore, coding of implicit terms involves using a dictionary or contextual translation rules or both.
To begin a conceptual content analysis, first identify the research question and choose a sample or samples for analysis. Next, the text must be coded into manageable content categories. This is basically a process of selective reduction. By reducing the text to categories, the researcher can focus on and code for specific words or patterns that inform the research question.
General steps for conducting a conceptual content analysis:
1. Decide the level of analysis: word, word sense, phrase, sentence, themes
2. Decide how many concepts to code for: develop a pre-defined or interactive set of categories or concepts. Decide either: A. to allow flexibility to add categories through the coding process, or B. to stick with the pre-defined set of categories.
Option A allows for the introduction and analysis of new and important material that could have significant implications to one’s research question.
Option B allows the researcher to stay focused and examine the data for specific concepts.
3. Decide whether to code for existence or frequency of a concept. The decision changes the coding process.
When coding for the existence of a concept, the researcher would count a concept only once if it appeared at least once in the data and no matter how many times it appeared.
When coding for the frequency of a concept, the researcher would count the number of times a concept appears in a text.
4. Decide on how you will distinguish among concepts:
Should text be coded exactly as they appear or coded as the same when they appear in different forms? For example, “dangerous” vs. “dangerousness”. The point here is to create coding rules so that these word segments are transparently categorized in a logical fashion. The rules could make all of these word segments fall into the same category, or perhaps the rules can be formulated so that the researcher can distinguish these word segments into separate codes.
What level of implication is to be allowed? Words that imply the concept or words that explicitly state the concept? For example, “dangerous” vs. “the person is scary” vs. “that person could cause harm to me”. These word segments may not merit separate categories, due the implicit meaning of “dangerous”.
5. Develop rules for coding your texts. After decisions of steps 1-4 are complete, a researcher can begin developing rules for translation of text into codes. This will keep the coding process organized and consistent. The researcher can code for exactly what he/she wants to code. Validity of the coding process is ensured when the researcher is consistent and coherent in their codes, meaning that they follow their translation rules. In content analysis, obeying by the translation rules is equivalent to validity.
6. Decide what to do with irrelevant information: should this be ignored (e.g. common English words like “the” and “and”), or used to reexamine the coding scheme in the case that it would add to the outcome of coding?
7. Code the text: This can be done by hand or by using software. By using software, researchers can input categories and have coding done automatically, quickly and efficiently, by the software program. When coding is done by hand, a researcher can recognize errors far more easily (e.g. typos, misspelling). If using computer coding, text could be cleaned of errors to include all available data. This decision of hand vs. computer coding is most relevant for implicit information where category preparation is essential for accurate coding.
8. Analyze your results: Draw conclusions and generalizations where possible. Determine what to do with irrelevant, unwanted, or unused text: reexamine, ignore, or reassess the coding scheme. Interpret results carefully as conceptual content analysis can only quantify the information. Typically, general trends and patterns can be identified.
Relational Analysis
Relational analysis begins like conceptual analysis, where a concept is chosen for examination. However, the analysis involves exploring the relationships between concepts. Individual concepts are viewed as having no inherent meaning and rather the meaning is a product of the relationships among concepts.
To begin a relational content analysis, first identify a research question and choose a sample or samples for analysis. The research question must be focused so the concept types are not open to interpretation and can be summarized. Next, select text for analysis. Select text for analysis carefully by balancing having enough information for a thorough analysis so results are not limited with having information that is too extensive so that the coding process becomes too arduous and heavy to supply meaningful and worthwhile results.
There are three subcategories of relational analysis to choose from prior to going on to the general steps.
Affect extraction: an emotional evaluation of concepts explicit in a text. A challenge to this method is that emotions can vary across time, populations, and space. However, it could be effective at capturing the emotional and psychological state of the speaker or writer of the text.
Proximity analysis: an evaluation of the co-occurrence of explicit concepts in the text. Text is defined as a string of words called a “window” that is scanned for the co-occurrence of concepts. The result is the creation of a “concept matrix”, or a group of interrelated co-occurring concepts that would suggest an overall meaning.
Cognitive mapping: a visualization technique for either affect extraction or proximity analysis. Cognitive mapping attempts to create a model of the overall meaning of the text such as a graphic map that represents the relationships between concepts.
General steps for conducting a relational content analysis:
1. Determine the type of analysis: Once the sample has been selected, the researcher needs to determine what types of relationships to examine and the level of analysis: word, word sense, phrase, sentence, themes. 2. Reduce the text to categories and code for words or patterns. A researcher can code for existence of meanings or words. 3. Explore the relationship between concepts: once the words are coded, the text can be analyzed for the following:
Strength of relationship: degree to which two or more concepts are related.
Sign of relationship: are concepts positively or negatively related to each other?
Direction of relationship: the types of relationship that categories exhibit. For example, “X implies Y” or “X occurs before Y” or “if X then Y” or if X is the primary motivator of Y.
4. Code the relationships: a difference between conceptual and relational analysis is that the statements or relationships between concepts are coded. 5. Perform statistical analyses: explore differences or look for relationships among the identified variables during coding. 6. Map out representations: such as decision mapping and mental models.
Reliability and Validity
Reliability : Because of the human nature of researchers, coding errors can never be eliminated but only minimized. Generally, 80% is an acceptable margin for reliability. Three criteria comprise the reliability of a content analysis:
Stability: the tendency for coders to consistently re-code the same data in the same way over a period of time.
Reproducibility: tendency for a group of coders to classify categories membership in the same way.
Accuracy: extent to which the classification of text corresponds to a standard or norm statistically.
Validity : Three criteria comprise the validity of a content analysis:
Closeness of categories: this can be achieved by utilizing multiple classifiers to arrive at an agreed upon definition of each specific category. Using multiple classifiers, a concept category that may be an explicit variable can be broadened to include synonyms or implicit variables.
Conclusions: What level of implication is allowable? Do conclusions correctly follow the data? Are results explainable by other phenomena? This becomes especially problematic when using computer software for analysis and distinguishing between synonyms. For example, the word “mine,” variously denotes a personal pronoun, an explosive device, and a deep hole in the ground from which ore is extracted. Software can obtain an accurate count of that word’s occurrence and frequency, but not be able to produce an accurate accounting of the meaning inherent in each particular usage. This problem could throw off one’s results and make any conclusion invalid.
Generalizability of the results to a theory: dependent on the clear definitions of concept categories, how they are determined and how reliable they are at measuring the idea one is seeking to measure. Generalizability parallels reliability as much of it depends on the three criteria for reliability.
Advantages of Content Analysis
Directly examines communication using text
Allows for both qualitative and quantitative analysis
Provides valuable historical and cultural insights over time
Allows a closeness to data
Coded form of the text can be statistically analyzed
Unobtrusive means of analyzing interactions
Provides insight into complex models of human thought and language use
When done well, is considered a relatively “exact” research method
Content analysis is a readily-understood and an inexpensive research method
A more powerful tool when combined with other research methods such as interviews, observation, and use of archival records. It is very useful for analyzing historical material, especially for documenting trends over time.
Disadvantages of Content Analysis
Can be extremely time consuming
Is subject to increased error, particularly when relational analysis is used to attain a higher level of interpretation
Is often devoid of theoretical base, or attempts too liberally to draw meaningful inferences about the relationships and impacts implied in a study
Is inherently reductive, particularly when dealing with complex texts
Tends too often to simply consist of word counts
Often disregards the context that produced the text, as well as the state of things after the text is produced
Can be difficult to automate or computerize
Textbooks & Chapters
Berelson, Bernard. Content Analysis in Communication Research.New York: Free Press, 1952.
Busha, Charles H. and Stephen P. Harter. Research Methods in Librarianship: Techniques and Interpretation.New York: Academic Press, 1980.
de Sola Pool, Ithiel. Trends in Content Analysis. Urbana: University of Illinois Press, 1959.
Krippendorff, Klaus. Content Analysis: An Introduction to its Methodology. Beverly Hills: Sage Publications, 1980.
Fielding, NG & Lee, RM. Using Computers in Qualitative Research. SAGE Publications, 1991. (Refer to Chapter by Seidel, J. ‘Method and Madness in the Application of Computer Technology to Qualitative Data Analysis’.)
Methodological Articles
Hsieh HF & Shannon SE. (2005). Three Approaches to Qualitative Content Analysis.Qualitative Health Research. 15(9): 1277-1288.
Elo S, Kaarianinen M, Kanste O, Polkki R, Utriainen K, & Kyngas H. (2014). Qualitative Content Analysis: A focus on trustworthiness. Sage Open. 4:1-10.
Application Articles
Abroms LC, Padmanabhan N, Thaweethai L, & Phillips T. (2011). iPhone Apps for Smoking Cessation: A content analysis. American Journal of Preventive Medicine. 40(3):279-285.
Ullstrom S. Sachs MA, Hansson J, Ovretveit J, & Brommels M. (2014). Suffering in Silence: a qualitative study of second victims of adverse events. British Medical Journal, Quality & Safety Issue. 23:325-331.
Owen P. (2012).Portrayals of Schizophrenia by Entertainment Media: A Content Analysis of Contemporary Movies. Psychiatric Services. 63:655-659.
Choosing whether to conduct a content analysis by hand or by using computer software can be difficult. Refer to ‘Method and Madness in the Application of Computer Technology to Qualitative Data Analysis’ listed above in “Textbooks and Chapters” for a discussion of the issue.
QSR NVivo: http://www.qsrinternational.com/products.aspx
Atlas.ti: http://www.atlasti.com/webinars.html
R- RQDA package: http://rqda.r-forge.r-project.org/
Rolly Constable, Marla Cowell, Sarita Zornek Crawford, David Golden, Jake Hartvigsen, Kathryn Morgan, Anne Mudgett, Kris Parrish, Laura Thomas, Erika Yolanda Thompson, Rosie Turner, and Mike Palmquist. (1994-2012). Ethnography, Observational Research, and Narrative Inquiry. Writing@CSU. Colorado State University. Available at: https://writing.colostate.edu/guides/guide.cfm?guideid=63 .
As an introduction to Content Analysis by Michael Palmquist, this is the main resource on Content Analysis on the Web. It is comprehensive, yet succinct. It includes examples and an annotated bibliography. The information contained in the narrative above draws heavily from and summarizes Michael Palmquist’s excellent resource on Content Analysis but was streamlined for the purpose of doctoral students and junior researchers in epidemiology.
At Columbia University Mailman School of Public Health, more detailed training is available through the Department of Sociomedical Sciences- P8785 Qualitative Research Methods.
Join the Conversation
Have a question about methods? Join us on Facebook
- Technical Support
- Find My Rep
You are here
Content Analysis An Introduction to Its Methodology
- Klaus Krippendorff - The Annenberg School for Communication, University of Pennsylvania
- Description
What matters in people’s social lives? What motivates and inspires our society? How do we enact what we know?
Since the first edition published in 1980, Content Analysis has helped shape and define the field. In the highly anticipated Fourth Edition, award-winning scholar and author Klaus Krippendorff introduces readers to the most current method of analyzing the textual fabric of contemporary society. Students and scholars will learn to treat data not as physical events but as communications that are created and disseminated to be seen, read, interpreted, enacted, and reflected upon according to the meanings they have for their recipients. Interpreting communications as texts in the contexts of their social uses distinguishes content analysis from other empirical methods of inquiry.
Organized into three parts, Content Analysis first examines the conceptual aspects of content analysis, then discusses components such as unitizing and sampling, and concludes by showing readers how to trace the analytical paths and apply evaluative techniques. The Fourth Edition has been completely revised to offer readers the most current techniques and research on content analysis, including new information on reliability and social media. Readers will also gain practical advice and experience for teaching academic and commercial researchers how to conduct content analysis.
Available with Perusall —an eBook that makes it easier to prepare for class Perusall is an award-winning eBook platform featuring social annotation tools that allow students and instructors to collaboratively mark up and discuss their SAGE textbook. Backed by research and supported by technological innovations developed at Harvard University, this process of learning through collaborative annotation keeps your students engaged and makes teaching easier and more effective. Learn more .
See what’s new to this edition by selecting the Features tab on this page. Should you need additional information or have questions regarding the HEOA information provided for this title, including what is new to this edition, please email [email protected] . Please include your name, contact information, and the name of the title for which you would like more information. For information on the HEOA, please go to http://ed.gov/policy/highered/leg/hea08/index.html .
For assistance with your order: Please email us at [email protected] or connect with your SAGE representative.
SAGE 2455 Teller Road Thousand Oaks, CA 91320 www.sagepub.com
Solid overview of various types of content analyses and practical tools
This is perhaps a little 'heavy going' for our students, who will usually be completely new to research methods, and won't have come across content analysis before. However, it's a really useful text for anyone wanting to explore content analysis techniques in more depth. I would recommend it as follow-up reading to a more 'entry-level' text.
This is a comprehensive and well structured book, and one that is easy for students to refer to when they need specific information on different topics.
This book and its previous editions is one of the fundamental readings in all of my courses. It covers all important aspects of qualitative and quantitative content analysis.
- An updated formula for computing the reliability of unitizations helps researchers standardize the units of text on which coders work and reduce coding errors.
- A new section on crowdsourcing shows readers how to measure the accuracy, surrogacy, and reliability of crowds.
- New discussions around social media show readers how computer-aided text has impacted the ways society examines itself.
- Several dimensions of Krippendorff’s Alpha have been expanded for assessing the reliability of crowdcoding, multi-valued coding of predefined units, and unitized textual continua.
- During the 38 years it has been in print, this award-winning text has pioneered the development of content analysis and continues to shape the field with each new edition. It has been widely adopted as a text in social science, humanities, and business curricula, served researchers as a guide for the design and execution of large and small content analyses, and provided epistemologically sound standards for justifying as well as critically evaluating content analysis findings.
- Winner of the International Communication Association’s Fellows Book Award for its lasting contribution to the advancement of communication studies , this market leader has taught generations of social scientists about the methodology of the research and has been translated into seven different languages.
- Gaining an enormous following, author Klaus Krippendorff’s work on content analysis was honored with the first methodological innovation award from the Mass Communication Division of International Communication Association.
- References to The Content Analysis Reader provide easy access to canonical examples to enrich the reader's experience.
- Krippendorff condenses the methodological requirements and conceptual opportunities of content and text analysis into a practical guide , incorporating his experiences as researcher, teacher, and consultant to academic and commercial projects, and providing readers with a useful entry into the use of the research method.
- A glossary of technical terms provides a further understanding of relevant concepts.
Sample Materials & Chapters
Chapter 1. History
Chapter 3. Uses and Inferences
For instructors
Select a purchasing option, related products.

This title is also available on SAGE Research Methods , the ultimate digital methods library. If your library doesn’t have access, ask your librarian to start a trial .
- Search Menu
Sign in through your institution
- Browse content in Arts and Humanities
- Browse content in Archaeology
- Anglo-Saxon and Medieval Archaeology
- Archaeological Methodology and Techniques
- Archaeology by Region
- Archaeology of Religion
- Archaeology of Trade and Exchange
- Biblical Archaeology
- Contemporary and Public Archaeology
- Environmental Archaeology
- Historical Archaeology
- History and Theory of Archaeology
- Industrial Archaeology
- Landscape Archaeology
- Mortuary Archaeology
- Prehistoric Archaeology
- Underwater Archaeology
- Urban Archaeology
- Zooarchaeology
- Browse content in Architecture
- Architectural Structure and Design
- History of Architecture
- Residential and Domestic Buildings
- Theory of Architecture
- Browse content in Art
- Art Subjects and Themes
- History of Art
- Industrial and Commercial Art
- Theory of Art
- Biographical Studies
- Byzantine Studies
- Browse content in Classical Studies
- Classical Literature
- Classical Reception
- Classical History
- Classical Philosophy
- Classical Mythology
- Classical Art and Architecture
- Classical Oratory and Rhetoric
- Greek and Roman Papyrology
- Greek and Roman Archaeology
- Greek and Roman Epigraphy
- Greek and Roman Law
- Late Antiquity
- Religion in the Ancient World
- Digital Humanities
- Browse content in History
- Colonialism and Imperialism
- Diplomatic History
- Environmental History
- Genealogy, Heraldry, Names, and Honours
- Genocide and Ethnic Cleansing
- Historical Geography
- History by Period
- History of Emotions
- History of Agriculture
- History of Education
- History of Gender and Sexuality
- Industrial History
- Intellectual History
- International History
- Labour History
- Legal and Constitutional History
- Local and Family History
- Maritime History
- Military History
- National Liberation and Post-Colonialism
- Oral History
- Political History
- Public History
- Regional and National History
- Revolutions and Rebellions
- Slavery and Abolition of Slavery
- Social and Cultural History
- Theory, Methods, and Historiography
- Urban History
- World History
- Browse content in Language Teaching and Learning
- Language Learning (Specific Skills)
- Language Teaching Theory and Methods
- Browse content in Linguistics
- Applied Linguistics
- Cognitive Linguistics
- Computational Linguistics
- Forensic Linguistics
- Grammar, Syntax and Morphology
- Historical and Diachronic Linguistics
- History of English
- Language Evolution
- Language Reference
- Language Variation
- Language Families
- Language Acquisition
- Lexicography
- Linguistic Anthropology
- Linguistic Theories
- Linguistic Typology
- Phonetics and Phonology
- Psycholinguistics
- Sociolinguistics
- Translation and Interpretation
- Writing Systems
- Browse content in Literature
- Bibliography
- Children's Literature Studies
- Literary Studies (Romanticism)
- Literary Studies (American)
- Literary Studies (Modernism)
- Literary Studies (Asian)
- Literary Studies (European)
- Literary Studies (Eco-criticism)
- Literary Studies - World
- Literary Studies (1500 to 1800)
- Literary Studies (19th Century)
- Literary Studies (20th Century onwards)
- Literary Studies (African American Literature)
- Literary Studies (British and Irish)
- Literary Studies (Early and Medieval)
- Literary Studies (Fiction, Novelists, and Prose Writers)
- Literary Studies (Gender Studies)
- Literary Studies (Graphic Novels)
- Literary Studies (History of the Book)
- Literary Studies (Plays and Playwrights)
- Literary Studies (Poetry and Poets)
- Literary Studies (Postcolonial Literature)
- Literary Studies (Queer Studies)
- Literary Studies (Science Fiction)
- Literary Studies (Travel Literature)
- Literary Studies (War Literature)
- Literary Studies (Women's Writing)
- Literary Theory and Cultural Studies
- Mythology and Folklore
- Shakespeare Studies and Criticism
- Browse content in Media Studies
- Browse content in Music
- Applied Music
- Dance and Music
- Ethics in Music
- Ethnomusicology
- Gender and Sexuality in Music
- Medicine and Music
- Music Cultures
- Music and Media
- Music and Culture
- Music and Religion
- Music Education and Pedagogy
- Music Theory and Analysis
- Musical Scores, Lyrics, and Libretti
- Musical Structures, Styles, and Techniques
- Musicology and Music History
- Performance Practice and Studies
- Race and Ethnicity in Music
- Sound Studies
- Browse content in Performing Arts
- Browse content in Philosophy
- Aesthetics and Philosophy of Art
- Epistemology
- Feminist Philosophy
- History of Western Philosophy
- Metaphysics
- Moral Philosophy
- Non-Western Philosophy
- Philosophy of Language
- Philosophy of Mind
- Philosophy of Perception
- Philosophy of Action
- Philosophy of Law
- Philosophy of Religion
- Philosophy of Science
- Philosophy of Mathematics and Logic
- Practical Ethics
- Social and Political Philosophy
- Browse content in Religion
- Biblical Studies
- Christianity
- East Asian Religions
- History of Religion
- Judaism and Jewish Studies
- Qumran Studies
- Religion and Education
- Religion and Health
- Religion and Politics
- Religion and Science
- Religion and Law
- Religion and Art, Literature, and Music
- Religious Studies
- Browse content in Society and Culture
- Cookery, Food, and Drink
- Cultural Studies
- Customs and Traditions
- Ethical Issues and Debates
- Hobbies, Games, Arts and Crafts
- Natural world, Country Life, and Pets
- Popular Beliefs and Controversial Knowledge
- Sports and Outdoor Recreation
- Technology and Society
- Travel and Holiday
- Visual Culture
- Browse content in Law
- Arbitration
- Browse content in Company and Commercial Law
- Commercial Law
- Company Law
- Browse content in Comparative Law
- Systems of Law
- Competition Law
- Browse content in Constitutional and Administrative Law
- Government Powers
- Judicial Review
- Local Government Law
- Military and Defence Law
- Parliamentary and Legislative Practice
- Construction Law
- Contract Law
- Browse content in Criminal Law
- Criminal Procedure
- Criminal Evidence Law
- Sentencing and Punishment
- Employment and Labour Law
- Environment and Energy Law
- Browse content in Financial Law
- Banking Law
- Insolvency Law
- History of Law
- Human Rights and Immigration
- Intellectual Property Law
- Browse content in International Law
- Private International Law and Conflict of Laws
- Public International Law
- IT and Communications Law
- Jurisprudence and Philosophy of Law
- Law and Society
- Law and Politics
- Browse content in Legal System and Practice
- Courts and Procedure
- Legal Skills and Practice
- Primary Sources of Law
- Regulation of Legal Profession
- Medical and Healthcare Law
- Browse content in Policing
- Criminal Investigation and Detection
- Police and Security Services
- Police Procedure and Law
- Police Regional Planning
- Browse content in Property Law
- Personal Property Law
- Study and Revision
- Terrorism and National Security Law
- Browse content in Trusts Law
- Wills and Probate or Succession
- Browse content in Medicine and Health
- Browse content in Allied Health Professions
- Arts Therapies
- Clinical Science
- Dietetics and Nutrition
- Occupational Therapy
- Operating Department Practice
- Physiotherapy
- Radiography
- Speech and Language Therapy
- Browse content in Anaesthetics
- General Anaesthesia
- Neuroanaesthesia
- Clinical Neuroscience
- Browse content in Clinical Medicine
- Acute Medicine
- Cardiovascular Medicine
- Clinical Genetics
- Clinical Pharmacology and Therapeutics
- Dermatology
- Endocrinology and Diabetes
- Gastroenterology
- Genito-urinary Medicine
- Geriatric Medicine
- Infectious Diseases
- Medical Toxicology
- Medical Oncology
- Pain Medicine
- Palliative Medicine
- Rehabilitation Medicine
- Respiratory Medicine and Pulmonology
- Rheumatology
- Sleep Medicine
- Sports and Exercise Medicine
- Community Medical Services
- Critical Care
- Emergency Medicine
- Forensic Medicine
- Haematology
- History of Medicine
- Browse content in Medical Skills
- Clinical Skills
- Communication Skills
- Nursing Skills
- Surgical Skills
- Medical Ethics
- Browse content in Medical Dentistry
- Oral and Maxillofacial Surgery
- Paediatric Dentistry
- Restorative Dentistry and Orthodontics
- Surgical Dentistry
- Medical Statistics and Methodology
- Browse content in Neurology
- Clinical Neurophysiology
- Neuropathology
- Nursing Studies
- Browse content in Obstetrics and Gynaecology
- Gynaecology
- Occupational Medicine
- Ophthalmology
- Otolaryngology (ENT)
- Browse content in Paediatrics
- Neonatology
- Browse content in Pathology
- Chemical Pathology
- Clinical Cytogenetics and Molecular Genetics
- Histopathology
- Medical Microbiology and Virology
- Patient Education and Information
- Browse content in Pharmacology
- Psychopharmacology
- Browse content in Popular Health
- Caring for Others
- Complementary and Alternative Medicine
- Self-help and Personal Development
- Browse content in Preclinical Medicine
- Cell Biology
- Molecular Biology and Genetics
- Reproduction, Growth and Development
- Primary Care
- Professional Development in Medicine
- Browse content in Psychiatry
- Addiction Medicine
- Child and Adolescent Psychiatry
- Forensic Psychiatry
- Learning Disabilities
- Old Age Psychiatry
- Psychotherapy
- Browse content in Public Health and Epidemiology
- Epidemiology
- Public Health
- Browse content in Radiology
- Clinical Radiology
- Interventional Radiology
- Nuclear Medicine
- Radiation Oncology
- Reproductive Medicine
- Browse content in Surgery
- Cardiothoracic Surgery
- Gastro-intestinal and Colorectal Surgery
- General Surgery
- Neurosurgery
- Paediatric Surgery
- Peri-operative Care
- Plastic and Reconstructive Surgery
- Surgical Oncology
- Transplant Surgery
- Trauma and Orthopaedic Surgery
- Vascular Surgery
- Browse content in Science and Mathematics
- Browse content in Biological Sciences
- Aquatic Biology
- Biochemistry
- Bioinformatics and Computational Biology
- Developmental Biology
- Ecology and Conservation
- Evolutionary Biology
- Genetics and Genomics
- Microbiology
- Molecular and Cell Biology
- Natural History
- Plant Sciences and Forestry
- Research Methods in Life Sciences
- Structural Biology
- Systems Biology
- Zoology and Animal Sciences
- Browse content in Chemistry
- Analytical Chemistry
- Computational Chemistry
- Crystallography
- Environmental Chemistry
- Industrial Chemistry
- Inorganic Chemistry
- Materials Chemistry
- Medicinal Chemistry
- Mineralogy and Gems
- Organic Chemistry
- Physical Chemistry
- Polymer Chemistry
- Study and Communication Skills in Chemistry
- Theoretical Chemistry
- Browse content in Computer Science
- Artificial Intelligence
- Computer Architecture and Logic Design
- Game Studies
- Human-Computer Interaction
- Mathematical Theory of Computation
- Programming Languages
- Software Engineering
- Systems Analysis and Design
- Virtual Reality
- Browse content in Computing
- Business Applications
- Computer Games
- Computer Security
- Computer Networking and Communications
- Digital Lifestyle
- Graphical and Digital Media Applications
- Operating Systems
- Browse content in Earth Sciences and Geography
- Atmospheric Sciences
- Environmental Geography
- Geology and the Lithosphere
- Maps and Map-making
- Meteorology and Climatology
- Oceanography and Hydrology
- Palaeontology
- Physical Geography and Topography
- Regional Geography
- Soil Science
- Urban Geography
- Browse content in Engineering and Technology
- Agriculture and Farming
- Biological Engineering
- Civil Engineering, Surveying, and Building
- Electronics and Communications Engineering
- Energy Technology
- Engineering (General)
- Environmental Science, Engineering, and Technology
- History of Engineering and Technology
- Mechanical Engineering and Materials
- Technology of Industrial Chemistry
- Transport Technology and Trades
- Browse content in Environmental Science
- Applied Ecology (Environmental Science)
- Conservation of the Environment (Environmental Science)
- Environmental Sustainability
- Environmentalist Thought and Ideology (Environmental Science)
- Management of Land and Natural Resources (Environmental Science)
- Natural Disasters (Environmental Science)
- Nuclear Issues (Environmental Science)
- Pollution and Threats to the Environment (Environmental Science)
- Social Impact of Environmental Issues (Environmental Science)
- History of Science and Technology
- Browse content in Materials Science
- Ceramics and Glasses
- Composite Materials
- Metals, Alloying, and Corrosion
- Nanotechnology
- Browse content in Mathematics
- Applied Mathematics
- Biomathematics and Statistics
- History of Mathematics
- Mathematical Education
- Mathematical Finance
- Mathematical Analysis
- Numerical and Computational Mathematics
- Probability and Statistics
- Pure Mathematics
- Browse content in Neuroscience
- Cognition and Behavioural Neuroscience
- Development of the Nervous System
- Disorders of the Nervous System
- History of Neuroscience
- Invertebrate Neurobiology
- Molecular and Cellular Systems
- Neuroendocrinology and Autonomic Nervous System
- Neuroscientific Techniques
- Sensory and Motor Systems
- Browse content in Physics
- Astronomy and Astrophysics
- Atomic, Molecular, and Optical Physics
- Biological and Medical Physics
- Classical Mechanics
- Computational Physics
- Condensed Matter Physics
- Electromagnetism, Optics, and Acoustics
- History of Physics
- Mathematical and Statistical Physics
- Measurement Science
- Nuclear Physics
- Particles and Fields
- Plasma Physics
- Quantum Physics
- Relativity and Gravitation
- Semiconductor and Mesoscopic Physics
- Browse content in Psychology
- Affective Sciences
- Clinical Psychology
- Cognitive Psychology
- Cognitive Neuroscience
- Criminal and Forensic Psychology
- Developmental Psychology
- Educational Psychology
- Evolutionary Psychology
- Health Psychology
- History and Systems in Psychology
- Music Psychology
- Neuropsychology
- Organizational Psychology
- Psychological Assessment and Testing
- Psychology of Human-Technology Interaction
- Psychology Professional Development and Training
- Research Methods in Psychology
- Social Psychology
- Browse content in Social Sciences
- Browse content in Anthropology
- Anthropology of Religion
- Human Evolution
- Medical Anthropology
- Physical Anthropology
- Regional Anthropology
- Social and Cultural Anthropology
- Theory and Practice of Anthropology
- Browse content in Business and Management
- Business Ethics
- Business History
- Business Strategy
- Business and Technology
- Business and Government
- Business and the Environment
- Comparative Management
- Corporate Governance
- Corporate Social Responsibility
- Entrepreneurship
- Health Management
- Human Resource Management
- Industrial and Employment Relations
- Industry Studies
- Information and Communication Technologies
- International Business
- Knowledge Management
- Management and Management Techniques
- Operations Management
- Organizational Theory and Behaviour
- Pensions and Pension Management
- Public and Nonprofit Management
- Strategic Management
- Supply Chain Management
- Browse content in Criminology and Criminal Justice
- Criminal Justice
- Criminology
- Forms of Crime
- International and Comparative Criminology
- Youth Violence and Juvenile Justice
- Development Studies
- Browse content in Economics
- Agricultural, Environmental, and Natural Resource Economics
- Asian Economics
- Behavioural Finance
- Behavioural Economics and Neuroeconomics
- Econometrics and Mathematical Economics
- Economic History
- Economic Methodology
- Economic Systems
- Economic Development and Growth
- Financial Markets
- Financial Institutions and Services
- General Economics and Teaching
- Health, Education, and Welfare
- History of Economic Thought
- International Economics
- Labour and Demographic Economics
- Law and Economics
- Macroeconomics and Monetary Economics
- Microeconomics
- Public Economics
- Urban, Rural, and Regional Economics
- Welfare Economics
- Browse content in Education
- Adult Education and Continuous Learning
- Care and Counselling of Students
- Early Childhood and Elementary Education
- Educational Equipment and Technology
- Educational Strategies and Policy
- Higher and Further Education
- Organization and Management of Education
- Philosophy and Theory of Education
- Schools Studies
- Secondary Education
- Teaching of a Specific Subject
- Teaching of Specific Groups and Special Educational Needs
- Teaching Skills and Techniques
- Browse content in Environment
- Applied Ecology (Social Science)
- Climate Change
- Conservation of the Environment (Social Science)
- Environmentalist Thought and Ideology (Social Science)
- Natural Disasters (Environment)
- Social Impact of Environmental Issues (Social Science)
- Browse content in Human Geography
- Cultural Geography
- Economic Geography
- Political Geography
- Browse content in Interdisciplinary Studies
- Communication Studies
- Museums, Libraries, and Information Sciences
- Browse content in Politics
- African Politics
- Asian Politics
- Chinese Politics
- Comparative Politics
- Conflict Politics
- Elections and Electoral Studies
- Environmental Politics
- European Union
- Foreign Policy
- Gender and Politics
- Human Rights and Politics
- Indian Politics
- International Relations
- International Organization (Politics)
- International Political Economy
- Irish Politics
- Latin American Politics
- Middle Eastern Politics
- Political Behaviour
- Political Economy
- Political Institutions
- Political Theory
- Political Methodology
- Political Communication
- Political Philosophy
- Political Sociology
- Politics and Law
- Politics of Development
- Public Policy
- Public Administration
- Quantitative Political Methodology
- Regional Political Studies
- Russian Politics
- Security Studies
- State and Local Government
- UK Politics
- US Politics
- Browse content in Regional and Area Studies
- African Studies
- Asian Studies
- East Asian Studies
- Japanese Studies
- Latin American Studies
- Middle Eastern Studies
- Native American Studies
- Scottish Studies
- Browse content in Research and Information
- Research Methods
- Browse content in Social Work
- Addictions and Substance Misuse
- Adoption and Fostering
- Care of the Elderly
- Child and Adolescent Social Work
- Couple and Family Social Work
- Direct Practice and Clinical Social Work
- Emergency Services
- Human Behaviour and the Social Environment
- International and Global Issues in Social Work
- Mental and Behavioural Health
- Social Justice and Human Rights
- Social Policy and Advocacy
- Social Work and Crime and Justice
- Social Work Macro Practice
- Social Work Practice Settings
- Social Work Research and Evidence-based Practice
- Welfare and Benefit Systems
- Browse content in Sociology
- Childhood Studies
- Community Development
- Comparative and Historical Sociology
- Economic Sociology
- Gender and Sexuality
- Gerontology and Ageing
- Health, Illness, and Medicine
- Marriage and the Family
- Migration Studies
- Occupations, Professions, and Work
- Organizations
- Population and Demography
- Race and Ethnicity
- Social Theory
- Social Movements and Social Change
- Social Research and Statistics
- Social Stratification, Inequality, and Mobility
- Sociology of Religion
- Sociology of Education
- Sport and Leisure
- Urban and Rural Studies
- Browse content in Warfare and Defence
- Defence Strategy, Planning, and Research
- Land Forces and Warfare
- Military Administration
- Military Life and Institutions
- Naval Forces and Warfare
- Other Warfare and Defence Issues
- Peace Studies and Conflict Resolution
- Weapons and Equipment

- < Previous chapter
- Next chapter >
4 Qualitative Content Analysis
- Published: November 2015
- Cite Icon Cite
- Permissions Icon Permissions
This chapter examines qualitative content analysis, a recent methodological innovation. Qualitative content analysis is defined and distinguished here from basic and interpretive approaches to content analysis. Qualitative content analysis is also distinguished from other qualitative research methods, though features and techniques overlap with other qualitative methods. Key differences in the predominant use of newly collected data and use of non-quantitative analysis techniques are detailed. Differences in epistemology and the role of researcher self-awareness and reflexivity are also discussed. Methods of graphic data presentation are illustrated. Three short exemplar studies using qualitative content analysis are described and examined. Qualitative content analysis is explored in detail in terms of its characteristic components: (1) the research purposes of content analysis, (2) target audiences, (3) epistemological issues, (4) ethical issues, (5) research designs, (6) sampling issues and methods, (7) collecting data, (8) coding and categorization methods, (9) data analysis methods, and (10) the role of researcher reflection.
Signed in as
Institutional accounts.
- Google Scholar Indexing
- GoogleCrawler [DO NOT DELETE]
Personal account
- Sign in with email/username & password
- Get email alerts
- Save searches
- Purchase content
- Activate your purchase/trial code
- Add your ORCID iD
Institutional access
Sign in with a library card.
- Sign in with username/password
- Recommend to your librarian
- Institutional account management
- Get help with access
Access to content on Oxford Academic is often provided through institutional subscriptions and purchases. If you are a member of an institution with an active account, you may be able to access content in one of the following ways:
IP based access
Typically, access is provided across an institutional network to a range of IP addresses. This authentication occurs automatically, and it is not possible to sign out of an IP authenticated account.
Choose this option to get remote access when outside your institution. Shibboleth/Open Athens technology is used to provide single sign-on between your institution’s website and Oxford Academic.
- Click Sign in through your institution.
- Select your institution from the list provided, which will take you to your institution's website to sign in.
- When on the institution site, please use the credentials provided by your institution. Do not use an Oxford Academic personal account.
- Following successful sign in, you will be returned to Oxford Academic.
If your institution is not listed or you cannot sign in to your institution’s website, please contact your librarian or administrator.
Enter your library card number to sign in. If you cannot sign in, please contact your librarian.
Society Members
Society member access to a journal is achieved in one of the following ways:
Sign in through society site
Many societies offer single sign-on between the society website and Oxford Academic. If you see ‘Sign in through society site’ in the sign in pane within a journal:
- Click Sign in through society site.
- When on the society site, please use the credentials provided by that society. Do not use an Oxford Academic personal account.
If you do not have a society account or have forgotten your username or password, please contact your society.
Sign in using a personal account
Some societies use Oxford Academic personal accounts to provide access to their members. See below.
A personal account can be used to get email alerts, save searches, purchase content, and activate subscriptions.
Some societies use Oxford Academic personal accounts to provide access to their members.
Viewing your signed in accounts
Click the account icon in the top right to:
- View your signed in personal account and access account management features.
- View the institutional accounts that are providing access.
Signed in but can't access content
Oxford Academic is home to a wide variety of products. The institutional subscription may not cover the content that you are trying to access. If you believe you should have access to that content, please contact your librarian.
For librarians and administrators, your personal account also provides access to institutional account management. Here you will find options to view and activate subscriptions, manage institutional settings and access options, access usage statistics, and more.
Our books are available by subscription or purchase to libraries and institutions.
- About Oxford Academic
- Publish journals with us
- University press partners
- What we publish
- New features
- Open access
- Rights and permissions
- Accessibility
- Advertising
- Media enquiries
- Oxford University Press
- Oxford Languages
- University of Oxford
Oxford University Press is a department of the University of Oxford. It furthers the University's objective of excellence in research, scholarship, and education by publishing worldwide
- Copyright © 2024 Oxford University Press
- Cookie settings
- Cookie policy
- Privacy policy
- Legal notice
This Feature Is Available To Subscribers Only
Sign In or Create an Account
This PDF is available to Subscribers Only
For full access to this pdf, sign in to an existing account, or purchase an annual subscription.
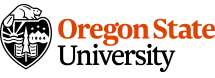
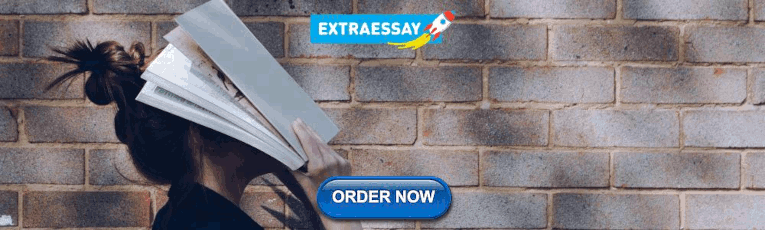
Chapter 17. Content Analysis
Introduction.
Content analysis is a term that is used to mean both a method of data collection and a method of data analysis. Archival and historical works can be the source of content analysis, but so too can the contemporary media coverage of a story, blogs, comment posts, films, cartoons, advertisements, brand packaging, and photographs posted on Instagram or Facebook. Really, almost anything can be the “content” to be analyzed. This is a qualitative research method because the focus is on the meanings and interpretations of that content rather than strictly numerical counts or variables-based causal modeling. [1] Qualitative content analysis (sometimes referred to as QCA) is particularly useful when attempting to define and understand prevalent stories or communication about a topic of interest—in other words, when we are less interested in what particular people (our defined sample) are doing or believing and more interested in what general narratives exist about a particular topic or issue. This chapter will explore different approaches to content analysis and provide helpful tips on how to collect data, how to turn that data into codes for analysis, and how to go about presenting what is found through analysis. It is also a nice segue between our data collection methods (e.g., interviewing, observation) chapters and chapters 18 and 19, whose focus is on coding, the primary means of data analysis for most qualitative data. In many ways, the methods of content analysis are quite similar to the method of coding.
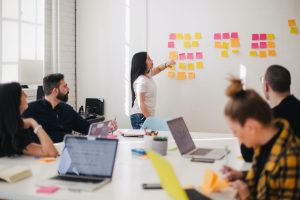
Although the body of material (“content”) to be collected and analyzed can be nearly anything, most qualitative content analysis is applied to forms of human communication (e.g., media posts, news stories, campaign speeches, advertising jingles). The point of the analysis is to understand this communication, to systematically and rigorously explore its meanings, assumptions, themes, and patterns. Historical and archival sources may be the subject of content analysis, but there are other ways to analyze (“code”) this data when not overly concerned with the communicative aspect (see chapters 18 and 19). This is why we tend to consider content analysis its own method of data collection as well as a method of data analysis. Still, many of the techniques you learn in this chapter will be helpful to any “coding” scheme you develop for other kinds of qualitative data. Just remember that content analysis is a particular form with distinct aims and goals and traditions.
An Overview of the Content Analysis Process
The first step: selecting content.
Figure 17.2 is a display of possible content for content analysis. The first step in content analysis is making smart decisions about what content you will want to analyze and to clearly connect this content to your research question or general focus of research. Why are you interested in the messages conveyed in this particular content? What will the identification of patterns here help you understand? Content analysis can be fun to do, but in order to make it research, you need to fit it into a research plan.
Figure 17.1. A Non-exhaustive List of "Content" for Content Analysis
To take one example, let us imagine you are interested in gender presentations in society and how presentations of gender have changed over time. There are various forms of content out there that might help you document changes. You could, for example, begin by creating a list of magazines that are coded as being for “women” (e.g., Women’s Daily Journal ) and magazines that are coded as being for “men” (e.g., Men’s Health ). You could then select a date range that is relevant to your research question (e.g., 1950s–1970s) and collect magazines from that era. You might create a “sample” by deciding to look at three issues for each year in the date range and a systematic plan for what to look at in those issues (e.g., advertisements? Cartoons? Titles of articles? Whole articles?). You are not just going to look at some magazines willy-nilly. That would not be systematic enough to allow anyone to replicate or check your findings later on. Once you have a clear plan of what content is of interest to you and what you will be looking at, you can begin, creating a record of everything you are including as your content. This might mean a list of each advertisement you look at or each title of stories in those magazines along with its publication date. You may decide to have multiple “content” in your research plan. For each content, you want a clear plan for collecting, sampling, and documenting.
The Second Step: Collecting and Storing
Once you have a plan, you are ready to collect your data. This may entail downloading from the internet, creating a Word document or PDF of each article or picture, and storing these in a folder designated by the source and date (e.g., “ Men’s Health advertisements, 1950s”). Sølvberg ( 2021 ), for example, collected posted job advertisements for three kinds of elite jobs (economic, cultural, professional) in Sweden. But collecting might also mean going out and taking photographs yourself, as in the case of graffiti, street signs, or even what people are wearing. Chaise LaDousa, an anthropologist and linguist, took photos of “house signs,” which are signs, often creative and sometimes offensive, hung by college students living in communal off-campus houses. These signs were a focal point of college culture, sending messages about the values of the students living in them. Some of the names will give you an idea: “Boot ’n Rally,” “The Plantation,” “Crib of the Rib.” The students might find these signs funny and benign, but LaDousa ( 2011 ) argued convincingly that they also reproduced racial and gender inequalities. The data here already existed—they were big signs on houses—but the researcher had to collect the data by taking photographs.
In some cases, your content will be in physical form but not amenable to photographing, as in the case of films or unwieldy physical artifacts you find in the archives (e.g., undigitized meeting minutes or scrapbooks). In this case, you need to create some kind of detailed log (fieldnotes even) of the content that you can reference. In the case of films, this might mean watching the film and writing down details for key scenes that become your data. [2] For scrapbooks, it might mean taking notes on what you are seeing, quoting key passages, describing colors or presentation style. As you might imagine, this can take a lot of time. Be sure you budget this time into your research plan.
Researcher Note
A note on data scraping : Data scraping, sometimes known as screen scraping or frame grabbing, is a way of extracting data generated by another program, as when a scraping tool grabs information from a website. This may help you collect data that is on the internet, but you need to be ethical in how to employ the scraper. A student once helped me scrape thousands of stories from the Time magazine archives at once (although it took several hours for the scraping process to complete). These stories were freely available, so the scraping process simply sped up the laborious process of copying each article of interest and saving it to my research folder. Scraping tools can sometimes be used to circumvent paywalls. Be careful here!
The Third Step: Analysis
There is often an assumption among novice researchers that once you have collected your data, you are ready to write about what you have found. Actually, you haven’t yet found anything, and if you try to write up your results, you will probably be staring sadly at a blank page. Between the collection and the writing comes the difficult task of systematically and repeatedly reviewing the data in search of patterns and themes that will help you interpret the data, particularly its communicative aspect (e.g., What is it that is being communicated here, with these “house signs” or in the pages of Men’s Health ?).
The first time you go through the data, keep an open mind on what you are seeing (or hearing), and take notes about your observations that link up to your research question. In the beginning, it can be difficult to know what is relevant and what is extraneous. Sometimes, your research question changes based on what emerges from the data. Use the first round of review to consider this possibility, but then commit yourself to following a particular focus or path. If you are looking at how gender gets made or re-created, don’t follow the white rabbit down a hole about environmental injustice unless you decide that this really should be the focus of your study or that issues of environmental injustice are linked to gender presentation. In the second round of review, be very clear about emerging themes and patterns. Create codes (more on these in chapters 18 and 19) that will help you simplify what you are noticing. For example, “men as outdoorsy” might be a common trope you see in advertisements. Whenever you see this, mark the passage or picture. In your third (or fourth or fifth) round of review, begin to link up the tropes you’ve identified, looking for particular patterns and assumptions. You’ve drilled down to the details, and now you are building back up to figure out what they all mean. Start thinking about theory—either theories you have read about and are using as a frame of your study (e.g., gender as performance theory) or theories you are building yourself, as in the Grounded Theory tradition. Once you have a good idea of what is being communicated and how, go back to the data at least one more time to look for disconfirming evidence. Maybe you thought “men as outdoorsy” was of importance, but when you look hard, you note that women are presented as outdoorsy just as often. You just hadn’t paid attention. It is very important, as any kind of researcher but particularly as a qualitative researcher, to test yourself and your emerging interpretations in this way.
The Fourth and Final Step: The Write-Up
Only after you have fully completed analysis, with its many rounds of review and analysis, will you be able to write about what you found. The interpretation exists not in the data but in your analysis of the data. Before writing your results, you will want to very clearly describe how you chose the data here and all the possible limitations of this data (e.g., historical-trace problem or power problem; see chapter 16). Acknowledge any limitations of your sample. Describe the audience for the content, and discuss the implications of this. Once you have done all of this, you can put forth your interpretation of the communication of the content, linking to theory where doing so would help your readers understand your findings and what they mean more generally for our understanding of how the social world works. [3]
Analyzing Content: Helpful Hints and Pointers
Although every data set is unique and each researcher will have a different and unique research question to address with that data set, there are some common practices and conventions. When reviewing your data, what do you look at exactly? How will you know if you have seen a pattern? How do you note or mark your data?
Let’s start with the last question first. If your data is stored digitally, there are various ways you can highlight or mark up passages. You can, of course, do this with literal highlighters, pens, and pencils if you have print copies. But there are also qualitative software programs to help you store the data, retrieve the data, and mark the data. This can simplify the process, although it cannot do the work of analysis for you.
Qualitative software can be very expensive, so the first thing to do is to find out if your institution (or program) has a universal license its students can use. If they do not, most programs have special student licenses that are less expensive. The two most used programs at this moment are probably ATLAS.ti and NVivo. Both can cost more than $500 [4] but provide everything you could possibly need for storing data, content analysis, and coding. They also have a lot of customer support, and you can find many official and unofficial tutorials on how to use the programs’ features on the web. Dedoose, created by academic researchers at UCLA, is a decent program that lacks many of the bells and whistles of the two big programs. Instead of paying all at once, you pay monthly, as you use the program. The monthly fee is relatively affordable (less than $15), so this might be a good option for a small project. HyperRESEARCH is another basic program created by academic researchers, and it is free for small projects (those that have limited cases and material to import). You can pay a monthly fee if your project expands past the free limits. I have personally used all four of these programs, and they each have their pluses and minuses.
Regardless of which program you choose, you should know that none of them will actually do the hard work of analysis for you. They are incredibly useful for helping you store and organize your data, and they provide abundant tools for marking, comparing, and coding your data so you can make sense of it. But making sense of it will always be your job alone.
So let’s say you have some software, and you have uploaded all of your content into the program: video clips, photographs, transcripts of news stories, articles from magazines, even digital copies of college scrapbooks. Now what do you do? What are you looking for? How do you see a pattern? The answers to these questions will depend partially on the particular research question you have, or at least the motivation behind your research. Let’s go back to the idea of looking at gender presentations in magazines from the 1950s to the 1970s. Here are some things you can look at and code in the content: (1) actions and behaviors, (2) events or conditions, (3) activities, (4) strategies and tactics, (5) states or general conditions, (6) meanings or symbols, (7) relationships/interactions, (8) consequences, and (9) settings. Table 17.1 lists these with examples from our gender presentation study.
Table 17.1. Examples of What to Note During Content Analysis
One thing to note about the examples in table 17.1: sometimes we note (mark, record, code) a single example, while other times, as in “settings,” we are recording a recurrent pattern. To help you spot patterns, it is useful to mark every setting, including a notation on gender. Using software can help you do this efficiently. You can then call up “setting by gender” and note this emerging pattern. There’s an element of counting here, which we normally think of as quantitative data analysis, but we are using the count to identify a pattern that will be used to help us interpret the communication. Content analyses often include counting as part of the interpretive (qualitative) process.
In your own study, you may not need or want to look at all of the elements listed in table 17.1. Even in our imagined example, some are more useful than others. For example, “strategies and tactics” is a bit of a stretch here. In studies that are looking specifically at, say, policy implementation or social movements, this category will prove much more salient.
Another way to think about “what to look at” is to consider aspects of your content in terms of units of analysis. You can drill down to the specific words used (e.g., the adjectives commonly used to describe “men” and “women” in your magazine sample) or move up to the more abstract level of concepts used (e.g., the idea that men are more rational than women). Counting for the purpose of identifying patterns is particularly useful here. How many times is that idea of women’s irrationality communicated? How is it is communicated (in comic strips, fictional stories, editorials, etc.)? Does the incidence of the concept change over time? Perhaps the “irrational woman” was everywhere in the 1950s, but by the 1970s, it is no longer showing up in stories and comics. By tracing its usage and prevalence over time, you might come up with a theory or story about gender presentation during the period. Table 17.2 provides more examples of using different units of analysis for this work along with suggestions for effective use.
Table 17.2. Examples of Unit of Analysis in Content Analysis
Every qualitative content analysis is unique in its particular focus and particular data used, so there is no single correct way to approach analysis. You should have a better idea, however, of what kinds of things to look for and what to look for. The next two chapters will take you further into the coding process, the primary analytical tool for qualitative research in general.
Further Readings
Cidell, Julie. 2010. “Content Clouds as Exploratory Qualitative Data Analysis.” Area 42(4):514–523. A demonstration of using visual “content clouds” as a form of exploratory qualitative data analysis using transcripts of public meetings and content of newspaper articles.
Hsieh, Hsiu-Fang, and Sarah E. Shannon. 2005. “Three Approaches to Qualitative Content Analysis.” Qualitative Health Research 15(9):1277–1288. Distinguishes three distinct approaches to QCA: conventional, directed, and summative. Uses hypothetical examples from end-of-life care research.
Jackson, Romeo, Alex C. Lange, and Antonio Duran. 2021. “A Whitened Rainbow: The In/Visibility of Race and Racism in LGBTQ Higher Education Scholarship.” Journal Committed to Social Change on Race and Ethnicity (JCSCORE) 7(2):174–206.* Using a “critical summative content analysis” approach, examines research published on LGBTQ people between 2009 and 2019.
Krippendorff, Klaus. 2018. Content Analysis: An Introduction to Its Methodology . 4th ed. Thousand Oaks, CA: SAGE. A very comprehensive textbook on both quantitative and qualitative forms of content analysis.
Mayring, Philipp. 2022. Qualitative Content Analysis: A Step-by-Step Guide . Thousand Oaks, CA: SAGE. Formulates an eight-step approach to QCA.
Messinger, Adam M. 2012. “Teaching Content Analysis through ‘Harry Potter.’” Teaching Sociology 40(4):360–367. This is a fun example of a relatively brief foray into content analysis using the music found in Harry Potter films.
Neuendorft, Kimberly A. 2002. The Content Analysis Guidebook . Thousand Oaks, CA: SAGE. Although a helpful guide to content analysis in general, be warned that this textbook definitely favors quantitative over qualitative approaches to content analysis.
Schrier, Margrit. 2012. Qualitative Content Analysis in Practice . Thousand Okas, CA: SAGE. Arguably the most accessible guidebook for QCA, written by a professor based in Germany.
Weber, Matthew A., Shannon Caplan, Paul Ringold, and Karen Blocksom. 2017. “Rivers and Streams in the Media: A Content Analysis of Ecosystem Services.” Ecology and Society 22(3).* Examines the content of a blog hosted by National Geographic and articles published in The New York Times and the Wall Street Journal for stories on rivers and streams (e.g., water-quality flooding).
- There are ways of handling content analysis quantitatively, however. Some practitioners therefore specify qualitative content analysis (QCA). In this chapter, all content analysis is QCA unless otherwise noted. ↵
- Note that some qualitative software allows you to upload whole films or film clips for coding. You will still have to get access to the film, of course. ↵
- See chapter 20 for more on the final presentation of research. ↵
- . Actually, ATLAS.ti is an annual license, while NVivo is a perpetual license, but both are going to cost you at least $500 to use. Student rates may be lower. And don’t forget to ask your institution or program if they already have a software license you can use. ↵
A method of both data collection and data analysis in which a given content (textual, visual, graphic) is examined systematically and rigorously to identify meanings, themes, patterns and assumptions. Qualitative content analysis (QCA) is concerned with gathering and interpreting an existing body of material.
Introduction to Qualitative Research Methods Copyright © 2023 by Allison Hurst is licensed under a Creative Commons Attribution-ShareAlike 4.0 International License , except where otherwise noted.
Communication
iResearchNet
Custom Writing Services
Quantitative content analysis.
Quantitative content analysis is an empirical method used in the social sciences primarily for analyzing recorded human communication in a quantitative, systematic, and intersubjective way. This material can include newspaper articles, films, advertisements, interview transcripts, or observational protocols, for instance. Thus, a quantitative content analysis can be applied to verbal material, and also to visual material like the evening news or television entertainment. Surveys, observations, and quantitative content analysis are the main three methods of data collection in empirical communication research, with quantitative content analysis the most prominent in the field. In other disciplines like psychology or sociology quantitative content analysis is not used as widely.
Quantitative And Qualitative Content Analysis
Ole R. Holsti (1969) defines quantitative content analysis as “any technique for making inferences by objectively and systematically identifying specified characteristics of messages.” Bernhard Berelson (1952) speaks of “a research technique for the objective, systematic and quantitative description of the manifest content of communication.” There has been much debate on this classical definition of quantitative content analysis: what does the word “manifest” mean, and is it possible to analyze latent structures of human communication beyond the surface of the manifest text, i.e., the “black marks on white”? In a more practical sense, the word “manifest” should be interpreted in terms of “making it manifest.” For example, if one were to look for irony in political commentaries, the construct of “irony” is not manifest in the sense of being directly identifiable from “black marks on white.” Whether or not there is irony in a commentary has to be interpreted. Thus, before a commentary is coded, it has to be precisely determined what words, phrases, key words, or arguments should serve as indicators for the category “irony.” In other words, this “latent” aspect of communication is made manifest by its definition.
With newspaper articles, the logic of quantitative content analysis can be described as a systematic form of “newspaper reading.” Here, the reader, i.e., the person charged with coding, assigns numeric codes (taken from a category system) to certain elements of the articles (e.g., issues or politicans mentioned in articles) by following a fixed plan (written down in a codebook). Of course, one does not analyze only one but many articles, reports, or commentaries from different newspapers. In this respect, quantitative content analysis differs from qualitative content analysis. While qualitative content analysis is limited to a number of roughly 50 to 100 sample elements (e.g., newspaper reports, interview transcripts, or observational protocols), quantitative content analysis can deal with a huge number of sample elements. Qualitative content analysis works rather inductively by summarizing and classifying elements or parts of the text material and by assigning labels or categories to them. Quantitative content analysis, however, works deductively and measures quantitatively by assigning numeric codes to parts of the material to be coded – which is called coding in quantitative content analysis.
Fields Of Application
Originally, quantitative content analysis was linked to propaganda research, for instance, propaganda material in World War II. Nowadays, quantitative content analysis is applied to many different forms of human communication like print and television coverage, public relations, entertainment, advertisements, photographs, pictures, or films. For instance, subjects of analysis can include national images in media coverage about foreign affairs, the role models presented in television advertisements, the kind and quality of arguments in company press releases, or the features of actors in modern Asian film. In theoretical terms, quantitative content analysis is being applied to many different fields of research in communication science, for example, in analyzing the kind of issues covered by the media in the context of agenda-setting. Similarly, quantitative content analysis is a key method in the cultivation approach, where it is called message system analysis. In both these approaches, content analysis is combined with a survey in field design. Further applications of quantitative content analysis are in: election studies, where it is used to examine election campaigns themselves or media coverage about the candidates, parties, and campaigns; and in media coverage of or public discourses on social problems and issues like racism, social movements, or collective violence.
Purposes Of Quantitative Content Analysis
Generally speaking, there are three major purposes and thus three basic types of quantitative content analysis which follow the popular “Lasswell formula,” which asks who says what, to whom, why, to what extent, and with what effect. The first purpose is to make inferences about the antecedents of communication, for example, examining the coverage of a liberal and a conservative newspaper (who). If there is a political bias in the commentaries or in the news reports (what), the bias would be explained (why), e.g., differences between the newspapers in their editorial lines or political orientation.
The second purpose is merely to describe communication (what, to what extent). Here, different techniques can be applied (which are described below). The third purpose is to draw inferences about the effects of communication (to whom, with what effect). Agendasetting and cultivation studies are good examples for this basic type of quantitative content analysis. Yet, strictly speaking, such an inference is not possible when nothing but the message is examined. Additionally, a survey should be carried out, for instance, before a statement is made about mass media coverage influencing people’s thoughts and perceptions of the world, as in the cultivation approach. The reason for this can be explained by constructivism. From the constructivist point of view, quantitative content analysis is a reactive method, like surveys for example, since the message to be coded is not fixed in an objective sense. Several people reading the same message may interpret it differently due to their individual schemata, beliefs, and attitudes. Thus, when making inferences about message effects, one should carry out not only a quantitative content analysis but also a reception study or an effects study.
Types Of Quantitative Content Analysis
There are different types of quantitative content analysis, i.e., different techniques of describing human communication. The first focuses on frequencies, where one merely counts, e.g., the appearance of issues, persons, or arguments in newspaper coverage. Many studies using the agenda-setting approach are such simple frequency content analyses, and most rely only on media archives. Thus, they do not examine the content or text, e.g. of a newspaper article, but focus only on the headline. This is a simplified version of quantitative content analysis.
The second technique focuses on tendencies. The above-mentioned example of political bias in the commentaries of a liberal and a conservative newspaper is a good example. In another example, the analysis would not only measure the number of articles on nuclear energy, but also their viewpoint on the issue, e.g., by noting the advantages and disadvantages mentioned. If the advantages reported exceed the disadvantages, the article would be observed to be “in favor” of nuclear energy, while if the disadvantages reported exceed the advantages, it would be considered to show “disapproval” or a “negative tendency.”
The third technique does not only focus on tendencies, but also on intensities. Here, one would not just code on an ordinal scale (using, e.g., “positive,” “ambivalent,” and “negative”), but use interval measurement (e.g., “strongly positive,” “positive,” “ambivalent,” “negative,” and “strongly negative”).
Finally, there are also several techniques that meet the popular objection that quantitative content analysis “disassembles” communication. Critics of quantitative content analysis (e.g., Siegfried Kracauer) claim that quantitative content analysis cannot examine relations between elements of communication, i.e., the semantic and syntactical structures of communication. They argue, for instance, that when arguments and persons in newspaper reports are counted separately, the fact that arguments are raised by persons is neglected; or that when statements are separately coded, the fact that a statement can refer to another statement, e.g., by supporting it, is not taken into account. The latter objection may apply with reference to most agenda-setting studies, but it is not a justified objection against quantitative content analysis in general.
A good example of a technique that meets the semantic and syntactical structures of communication is the Semantische Strukturund Inhaltsanalyse (Semantic Structure and Content Analysis) developed by Werner Früh. Without going into detail, the technique considers various elements of communication as well as the relations between them; for instance, it analyzes persons and roles mentioned in newspaper articles, but it also examines time aspects like anteriority and so-called “modifications” like persons’ features or local specifications. In addition, it looks for so-called “k-relations,” such as causal, intentional, or conditional relations as well as for “R-relations” mentioned in, e.g., news reports. Thus, newspaper articles are deconstructed into micro-propositions, but the semantic structure and content analysis reconstructs all relations between these micropropositions.
Most quantitative content analyses examine text or verbal material, i.e., transcribed or recorded human communication. Studies analyzing visual material like films, television advertisements, or televised debates between presidential candidates are comparably rare. There are three major reasons for this. First, copies of newspaper articles are easier to access than copies of the evening news, for instance; in retrospective studies (which most quantitative content analyses are), especially, visual material is often no longer available. Second, visual material is more complex than verbal or text material. For example, television news not only provides information via the audio channel but also via the visual channel. Since verbal and visual information can deliver different messages, one has to code both streams of information. This is more expensive than just coding print news. Finally, coding visual material like evening news on television requires more detailed coding instructions and more complex category definitions than a codebook for analyzing newspaper coverage.
A more or less recent development in quantitative content analysis is automatic, that is, computer-assisted, content analysis, where a computer program counts keywords and searches for related words in the same paragraph, for example. Before the coding process begins, all the relevant keywords or phrases in a so-called coding dictionary – an equivalent of the codebook of a conventional content analysis – have to be listed. While there has been some progress in this technique, it will be a while before the human coder becomes redundant.
Current challenges for quantitative content analysis stem from the world wide web, where the content of a private weblog, arguments in online chat, or the pictures in an online gallery can be subject to analysis. Compared to newspaper coverage, for example, a content analysis of online communication can be quite a problem. Here, the population from which a sample is taken for analysis is not fixed but changes from day to day, or even more quickly. It is therefore important to store all relevant communication for a specific study. But even if this were possible, one seldom has a view of the complete population since the world wide web, or the Internet, as a whole is not easy to grasp. Thus most studies that analyze online communication work with samples that are more or less clearly defined.
Like any other method in the social sciences, quantitative content analysis has to meet certain standards of quantitative empirical research. The first criterion of intersubjectivity calls for transparent research. This means that all the details of a quantitative content analysis have to be described and explained so that exactly what has been done is clear. The second criterion of systematics requires that the coding rules and sampling criteria are invariantly applied to all material. The third criterion of reliability calls for the codebook to be dependable. Different coders do not always agree on coding. For example, one coder may identify an argument in a newspaper article as argument 13 from the argument list in the codebook, while another coder will choose argument 15, with the result that the numeric codes assigned to the argument in the newspaper article do not match. Yet, in other cases the two coders may agree. Using all codings of both coders, one can divide the doubled number of matching pairs (e.g., 17 identical codings) by the number of all codings of the first coder and the number of all codings of the second coder (e.g., 20 codings each), to obtain a ratio called the Holsti-formula, which is a simple reliability coefficient. In this example we would find R = 17 * 2 / (20 + 20) = 0.85. The values of all reliability coefficients range from 0 (no matching at all) to 1 (perfect matching). Another popular reliability coefficient – better known from index calculation – is Cronbach’s alpha α.
The fourth standard for a quantitative content analysis is validity. An instrument of empirical research, i.e., the codebook with reference to CA, can claim to be valid when it measures what it intends to measure. For instance, if the codebook contains a category “stereotype,” then the coders should not measure political bias or irony when applying the coding rules for this category, but code stereotypes. According to Klaus Krippendorff, there are different forms of validity. The type of validity in the example can be called face validity. Predictive validity and concurrent validity both refer to an external criterion measure for validating data obtained by a quantitative CA. Such a measure may be another quantitative content analysis or may be statistical data from governmental sources. With concurrent validity the validity test is administered at the same time as the criterion is collected. With predictive validity, scores are predicted on some criterion measure.
Content Analysis As Research Practice
The research process using quantitative content analysis comprises six steps. It usually begins with theoretical considerations, literature review, and deducing empirical hypotheses. In the second step, the sample material that is to be coded, i.e., examined with the codebook, is defined. In the third step, the coding units (e.g., articles or arguments) are described. In the fourth step, the codebook with the category system is developed and pre-tested. The actual measurement, i.e., the process of coding, represents the fifth step of a CA. The final step is data analysis and data interpretation.
Most quantitative content analysis requires multilevel sampling, for instance, analysis of campaign coverage would involve the choice of a limited number of national newspapers which represent diverse political standpoints (e.g., from liberal to conservative). On the next level the time span to be analyzed (e.g., every day in the critical phase of the election campaign) is set. On the next level, the articles to be coded are determined (e.g., all articles on the front page). Usually the sample is called the unit of analysis. The coding unit, however, is the most important unit in quantitative CA. It defines the level of measurement. For example, if the features of an article (e.g., the main issue of the article) are examined, the single article is the coding unit, but if the attributes of an argument (e.g., the issues mentioned in an argument) are examined, then the single argument is the coding unit. In the first case, 100 articles (with 5 arguments per article) will lead to 100 codes, in the second case the same number of articles will produce 500 codes. The level of coding depends on the sample size as well as on the research question. If the the study focuses on argumentation structures, the article will not be chosen as the coding unit. If political coverage in 10 newspapers in the last 50 years is the focus of the study, the argument or statement will not be chosen as a coding unit, otherwise a vast number of cases will have to be coded.
References:
- Berelson, B. (1952). Content analysis in communication research. Glencoe, IL: Free Press.
- Früh, W. (1998). Inhaltsanalyse: Theorie und Praxis, 4th edn. [Content analysis: Theory and practice]. Constance: UVK.
- Holsti, O. R. (1969). Content analysis for the social sciences and humanities. Reading, MA: AddisonWesley.
- Krippendorff, K. (2004). Content analysis: An introduction to its methodology. Beverly Hills, CA: Sage.
- Neuendorf, K. A. (2002). The content analysis guidebook. Thousand Oaks, CA: Sage.
- Popping, R. (2000). Computer-assisted text analysis. Thousand Oaks, CA: Sage.
- Riffe, D., Lacy, S., & Fico, F. G. (2005). Analyzing media messages: Using quantitative content analysis in research. Mahwah, NJ: LEA.
- Weber, R. P. (1990). Basic content analysis, 2nd edn. Newbury Park, London, and New Delhi: Sage.
To read this content please select one of the options below:
Please note you do not have access to teaching notes, qualitative content analysis.
Empirical Methods for Bioethics: A Primer
ISBN : 978-0-7623-1266-5 , eISBN : 978-1-84950-383-9
Publication date: 5 December 2007
Content analysis is a family of systematic, rule-guided techniques used to analyze the informational contents of textual data (Mayring, 2000). It is used frequently in nursing research, and is rapidly becoming more prominent in the medical and bioethics literature. There are several types of content analysis including quantitative and qualitative methods all sharing the central feature of systematically categorizing textual data in order to make sense of it (Miles & Huberman, 1994). They differ, however, in the ways they generate categories and apply them to the data, and how they analyze the resulting data. In this chapter, we describe a type of qualitative content analysis in which categories are largely derived from the data, applied to the data through close reading, and analyzed solely qualitatively. The generation and application of categories that we describe can also be used in studies that include quantitative analysis.
Forman, J. and Damschroder, L. (2007), "Qualitative Content Analysis", Jacoby, L. and Siminoff, L.A. (Ed.) Empirical Methods for Bioethics: A Primer ( Advances in Bioethics, Vol. 11 ), Emerald Group Publishing Limited, Leeds, pp. 39-62. https://doi.org/10.1016/S1479-3709(07)11003-7
Emerald Group Publishing Limited
Copyright © 2007, Emerald Group Publishing Limited
We’re listening — tell us what you think
Something didn’t work….
Report bugs here
All feedback is valuable
Please share your general feedback
Join us on our journey
Platform update page.
Visit emeraldpublishing.com/platformupdate to discover the latest news and updates
Questions & More Information
Answers to the most commonly asked questions here

The Potential of Qualitative Content Analysis for Empirical Educational Research
- Michaela Gläser-Zikuda University of Erlangen-Nuremberg
- Gerda Hagenauer University of Salzburg
- Melanie Stephan University of Erlangen-Nuremberg
Researchers carrying out empirical studies in education are faced with complex and multifaceted phenomena that need to be investigated from different perspectives and with various methodological approaches. A suitable, often-applied method in empirical educational research is qualitative content analysis (QCA), developed by Philipp MAYRING (1983, 2015). This method can be used for inductive and deductive strategies of analysis, and is appropriate for combining qualitative and quantitative analyses, especially for research strategies based on mixed methods approaches (CRESWELL, 2015), which have for several years now been extensively discussed in empirical educational research (GLÄSER-ZIKUDA, SEIDEL, ROHLFS, GRÖSCHNER & ZIEGELBAUER, 2012; HAGENAUER & GLÄSER-ZIKUDA, 2019; MAYRING & GLÄSER-ZIKUDA, 2008). In this article, we discuss the potential of QCA for empirical educational research by giving insights into the basics of analysis and by providing research examples. We also briefly address the relevance of digitally supported analysis and describe specific software packages. Finally, we discuss the potential and challenges of applying QCA within mixed methods designs in the field of empirical educational research.
Author Biographies
Michaela gläser-zikuda, university of erlangen-nuremberg.
Michaela GLÄSER-ZIKUDA is a full professor at the Institute for Educational Science, Research and Teaching Unit School Education and Instructional Research at the University of Erlangen-Nuremberg. Her main research interests are emotions and self-regulation in school and higher education, instructional quality, and teacher education. She is also interested in qualitative research methods and mixed methods research.
Gerda Hagenauer, University of Salzburg
Gerda HAGENAUER is a full professor in the School of Education at the University of Salzburg. Her main research interests are emotions, motivation and social relationships in teaching and learning at school, in teacher education and in higher education. She is also interested in mixed methods research.
Melanie Stephan, University of Erlangen-Nuremberg
Melanie STEPHAN is a PhD student at the Institute for Educational Science, Research Unit for Media Education at the University of Erlangen-Nuremberg. In her PhD she focuses on technology-based teacher education. Her research interests are emotions in learning and instruction, media education, and teacher education.
How to Cite
- Endnote/Zotero/Mendeley (RIS)
Copyright (c) 2020 Michaela Gläser-Zikuda, Gerda Hagenauer, Melanie Stephan

This work is licensed under a Creative Commons Attribution 4.0 International License .
Most read articles by the same author(s)
- Christian Spannagel, Michaela Gläser-Zikuda, Ulrik Schroeder, Application of Qualitative Content Analysis in User-Program Interaction Research , Forum Qualitative Sozialforschung / Forum: Qualitative Social Research: Vol. 6 No. 2 (2005): Qualitative Inquiry: Research, Archiving, and Reuse
- Mathias Mejeh, Gerda Hagenauer, Michaela Gläser-Zikuda, Mixed Methods Research on Learning and Instruction—Meeting the Challenges of Multiple Perspectives and Levels Within a Complex Field , Forum Qualitative Sozialforschung / Forum: Qualitative Social Research: Vol. 24 No. 1 (2023): Mixed Methods and Multimethod Social Research—Current Applications and Future Directions
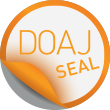
Make a Submission
Current issue, information.
- For Readers
- For Authors
- For Librarians
Usage Statistics Information
We log anonymous usage statistics. Please read the privacy information for details.
Developed By
2000-2024 Forum Qualitative Sozialforschung / Forum: Qualitative Social Research (ISSN 1438-5627) Institut für Qualitative Forschung , Internationale Akademie Berlin gGmbH
Hosting: Center for Digital Systems , Freie Universität Berlin Funding 2023-2025 by the KOALA project
Privacy Statement Accessibility Statement
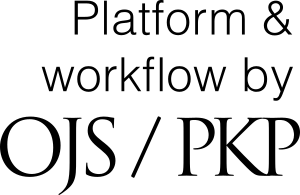
Content Analysis in the Research Field of Environmental & Climate Change Coverage
- Open Access
- First Online: 25 September 2022
Cite this chapter
You have full access to this open access chapter
- Daniela Mahl 6 &
- Lars Guenther 7
11k Accesses
In the study of climate and environmental communication, content analysis—in all its many facets—has been used numerous times and in various ways. This chapter provides an overview of common research designs and (combinations of) methods, showing that a variety of approaches have been applied, ranging from qualitative, to quantitative, to automated content analyses. In addition, we identify the main constructs employed in media content analyses—with issue attention, actors and sources, framing, uncertainty, or visual representations being of primary concern. Finally, we outline research desiderata that should be considered in future studies.
You have full access to this open access chapter, Download chapter PDF
Similar content being viewed by others
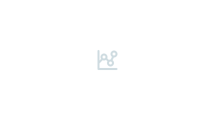
The use of qualitative research to better understand public opinions on climate change
Reconciling climate-conflict meta-analyses: reply to buhaug et al., systematic review approaches for climate change adaptation research.
- Climate Change
- Climate Communication
- Environmental Communication
- Coverage about Uncertainty of Climate Research
1 Introduction
Environmental communication has been defined as the “communication about the natural environment and ecosystem, commonly focusing on the relationships that human beings and their institutions maintain with the nonhuman natural environment” (Griffin and Dunwoody 2008 , p. 1; cf. Hansen 2017 , 2018 ). A large part of this communication—and especially media representations of the environment—deals with various environmental problems such as energy, pollution, extinction of species, the ozone hole, or population growth. In recent years, anthropogenic climate change in particular has become one of the defining topics in environmental communication (Hansen 2011 , 2018 ; Moser and Dilling 2008 ). Climate change-related communication and media coverage is decisive for how people perceive this “unobtrusive” but highly pressing issue (Carvalho 2010 , p. 172; Corbett and Durfee 2004 ). Most people do not experience climate change directly and its causes and effects lie beyond their everyday lives. However, media provide important access to scientific information, political and societal debates, risks of, and possible solutions to tackle climate change. As central arenas and actors “in the production, reproduction, and transformation of the meanings” (Carvalho 2010 , p. 172) of climate change, news coverage has significantly increased people’s awareness of the problem over the years (Sampei and Aoyagi-Usui 2009 ). Both environmental and climate change-related communication and media coverage show intersections with various other research fields, such as health communication , communication focusing on controversial technologies such as genetic engineering or nanotechnology, risk communication , and, ultimately, science communication (Hansen 2011 ; Schäfer and Bonfadelli 2017 ).
In the late 1980s and early 1990s, media and communication research on the environment emerged from various research strands such as environmental psychology, environmental sociology, and environmental communication (for an overview, see Cox 2010 ; Hansen 2017 , 2018 ; Hansen and Cox 2015 ). These analyses covered environmental crises and disasters as well as environmental journalists and their relationship to sources (e.g., Dunwoody 2019 ; Hansen 2018 ). In contrast, research on climate-related communication and media reporting has started in the early 1990s and increased significantly since the mid-2000s (Schäfer and Schlichting 2014 ). Over the course of the last two decades, research in the field of environmental communication has developed and diversified considerably (Hansen 2011 ): Whereas in the early years, studies of environmental coverage had a relatively narrow focus on specific environmental issues, disasters, or events, today research covers diverse topics from the fields of science, medicine, and health —with climate change being the most intensively researched area recently (e.g., Moser and Dilling 2008 ). Studies assessing media representations of climate change typically deal with questions such as whether media coverage differs between countries, how it develops over time, which topics are embedded in the climate discourse, which perspectives on climate change are covered, and how climate change is presented in textual or visual form (for an overview, see Metag 2016 ). Various meta-analyses and literature reviews outlined important trends synthesizing the development of the research field (e.g., Anderson 2009 ; Carvalho 2010 ; Metag 2016 ; Moser 2010 ; Schäfer 2012 ; Schäfer and Schlichting 2014 ). Results of these studies show three main trends. First, media coverage of climate change has increased over the last decades (Liu et al. 2011 ; Schäfer and Schlichting 2014 ; Schmidt et al. 2013 )—albeit there are significant differences in media attention across countries as well as differences in the amount of climate change-related coverage between media outlets (e.g., Hase et al. 2021 ; Schmidt et al. 2013 ). Second, the field of research has diversified: Content analyses have examined numerous countries and diverse continents (however with a clear bias towards Western, Anglophone countries; for an overview, see Eide and Kunelis 2012 ; Eide et al. 2010 ; Schäfer and Schlichting 2014 ); also more and more different media types were considered (Metag 2016 ). Thirdly, the thematic focus of climate change communication has shifted. While in the early 1990s anthropogenic climate change was mainly discussed from a scientific perspective, today political, economic, and social aspects are important issues in media coverage (Anderson 2011 ; Ivanova 2017 ). Since most research in environmental communication is dominated by research into climate change communication, this chapter will put emphasis on the latter, implying that climate change communication provides a good proxy for environmental communication overall (see Hansen 2011 , 2018 ; Moser and Dilling 2008 ).
2 Common Research Designs and Combinations of Methods
Literature reviews of climate change-related media coverage show that studies apply a variety of methods and research designs, including qualitative and quantitative content analysis—both are equally well represented in the literature and remain constant over time. Only few studies combine both research strategies (Schäfer and Schlichting 2014 ). Case studies, typically focusing on media coverage in a specific national context, have increasingly been replaced by cross-sectional studies that compare different countries or media, as well as longitudinal studies that analyze the development of media coverage over time (Guenther et al. 2022 ; Metag 2016 ). Only a few publications combine cross-sectional and longitudinal elements in one study (Schäfer and Schlichting 2014 ).
More recently, studies have investigated online climate change communication, for example in social networks (e.g., Kirilenko and Stepchenkova 2014 ) or blogs (e.g., Fløttum et al. 2014 ). In order to analyze climate-related online communication, automated content analysis is often used, due to the large amounts of available data. Typical approaches of computational content analysis are text mining and dictionary approaches (e.g., Ivanova 2017 ). Recently, probabilistic topic modelling with latent Dirichlet allocation (LDA) has also been applied as a form of unsupervised machine learning. LDA models use algorithms to identify latent thematic structures in large text corpora based on word occurrence and distribution (e.g., Hase et al. 2021 ; Keller et al. 2020 ; Kirilenko and Stepchenkova 2014 ). Most content analyses, however, still employ human coding (Metag 2016 ).
Various researchers use content analysis in combination with other methods. For example, studies combine manual content analysis with representative survey data (e.g., Feldman et al. 2012 ) to understand the relationship between the content of climate change coverage and the beliefs about global warming of the recipients. Furthermore, studies have combined manual content and social network analysis in order to investigate online communication about climate change between polarized groups (e.g., Williams et al. 2015 ).
3 Main Constructs Employed in Media Content Analyses
Existing research on media representations of climate change reveals diverse research subjects and issues, thus demonstrating that the analytical spectrum has expanded compared to early research. First, the studies have analyzed more and more different countries. Overall, European countries such as Germany (e.g., Schäfer 2016 ), Denmark (e.g., Eskjær 2016 ) or Switzerland (e.g., Bonfadelli 2016 ) received the largest share of scholarly attention. North American countries’ media coverage of climate change is analyzed almost as frequently as that of European countries (for the United States, see Boykoff and Boykoff 2004 ; for Canada, see Young and Dugas 2012 ). In contrast, Asian countries—India, the Middle East, China, Japan—received as little scholarly attention as Latin American or African countries. As countries of the Global South are significantly under-researched but often more affected by the impacts of climate change, their media coverage has recently been increasingly analyzed (for India, see Billett 2010 ; for Brazil, see Painter and Ashe 2012 ). Second, researchers have studied more and more media, such as climate change coverage in print media (for elite press coverage, see Billett 2010 ; for tabloids, see Boykoff 2008 ) or on TV (e.g., Boykoff 2007 ; Painter 2011 ) as well as social media depictions of climate change (e.g., Tandoc and Eng 2016 ).
Despite this diversity of the research field, the following common analytical constructs can be identified:
The overall amount of climate change-related coverage: Several studies, mostly single-country case studies, focusing almost exclusively on industrialized countries, have explored the amount of climate change-related coverage. Individual studies have examined media attention for climate change in countries such as the United States (e.g., Boykoff and Boykoff 2007 ), Australia (e.g., Farbotko 2005 ), Canada (e.g., Ahchong and Dodds 2012 ), Germany (e.g., Weingart et al. 2000 ), France (e.g., Brossard et al. 2004 ), the UK (e.g., Carvalho and Burgess 2005 ), and China (e.g., Yang 2010 ). Comparative studies mostly cover industrialized nations, though emerging economies or non-industrialized countries are also examined (e.g., Corfee-Morlot et al. 2007 ; Hase et al. 2021 ; Schmidt et al. 2013 ). Both individual and comparative studies have shown that media attention for climate change has increased in many countries since the mid-2000s.
The representation of different actors or sources in media reporting: Research so far has identified the common actors in reporting on climate change. Much focus has been on climate change advocates compared to climate skeptics (e.g., Metag 2016 ; Painter 2011 ). The discourse usually involves scientists, (transnational) institutions such as the Intergovernmental Panel on Climate Change (IPCC) and the United Nations Framework Convention on Climate Change (UNFCCC), politicians and especially green parties, (conservative) think tanks, non-governmental organizations (NGOs) such as Greenpeace, celebrities or prominent activists, news agencies, but also industrial actors like Exxon Mobil or Shell (e.g., Anderson 2009 ; Schäfer 2015 ; Schäfer and O'Neill 2016 ; Schlichting 2013 ; Schmidt et al. 2013 ). In early years of the debate, the lobbyist group Global Climate Coalition also appeared frequently. Researching different actors and their viewpoints is important as they all actively seek to establish their particular perspectives on the issue. Media attention for climate change has strongly fluctuated over time and peaked around specific events (e.g., Hase et al. 2021 ; Schäfer 2015 ). Hence, research regarding the sources of media reporting has extensively tried to identify what triggers media coverage about climate change. Scholars have concluded that international events (climate summits, such as the Conferences of the Parties (COPs) to the UNFCCC), scientific reports such as the IPCC assessment reports, NGO public relations efforts, extreme weather events, but also concerts and movies (e.g., An Inconvenient Truth) are substantial drivers of media attention (e.g., Anderson 2009 ; Moser 2010 ; Schäfer 2012 ; Schäfer et al. 2014 ; Schäfer & Schlichting, 2014). Peaks during COPs are probably related to the high stakes and the prominent political actors involved in the international negotiations (Schmidt et al. 2013 ).
The framing of climate change: Respective studies have analyzed how climate change is presented in news coverage or policy papers, which aspects are emphasized, or which responsibilities and possible solutions are derived. Studies have examined, for example, how stakeholders—scientists, industry, policymakers, non-governmental organizations (NGOs)—communicate their positions on climate change or which aspects and perspectives of climate change journalists select for media coverage (for an overview, see Schäfer 2016 ; Schäfer and O'Neill 2016 ; Schlichting 2013 ). In her meta-analysis of industry actors’ climate change communication, Schlichting ( 2013 ) identified three successive phases over time, each characterized by a dominant master frame: scientific uncertainty of climate change (early to mid-1990s), socio-economic consequences of mandatory emission reductions (1997 to early 2000s), and industrial leadership in climate protection (since mid-2000s). Engesser and Brüggemann ( 2016 ), in turn, focused on journalists’ framing of climate change and identified five frames: industrialized countries’ economic policies, sustainability, technological optimism, emerging economies’ responsibility, and global ecological discourse. In contrast to studies that have investigated the framing of climate change by stakeholders and journalists, a larger number of framing analyses focused on media coverage; hence, media frames. In addition to formal-stylistic frames, most scholars have examined issue-specific or topical content-oriented frames in climate change reporting, such as the generic frames conflict, human interest, responsibility, morality, Pandora’s box and economic consequences (e.g., Dirikx and Gelders 2010 ), or they have identified issue-specific frames (Billett 2010 ; Engesser and Brüggemann 2016 ; for an overview, see Schäfer and O'Neill 2016 ).
Uncertainty in climate change coverage: Although scientific uncertainty accompanies all scientific issues and thus also climate change, in climate change communication uncertainty deserves special notice. Discussing the uncertainty of climate change research, sometimes even referred to as the ‘scientific uncertainty frame’ (Schlichting 2013 ), was sponsored by industrial actors such as those from fossil fuel companies, conservative parties and organizations to highlight points where scientists disagree and to cast doubt (Metag 2016 ). As a consequence, the existence and the anthropogenic origin of global warming were (and in some cases are still) publicly questioned. Based on the journalistic norm of balance , different viewpoints were often represented in the media as if both sides were equally valid (Boykoff and Boykoff 2004 ) and accordingly journalistic reporting amplified uncertainty even more and encouraged political inaction (Anderson 2009 ; Moser 2010 ). Recent studies do not find this kind of balanced reporting anymore.
Visual representations of climate change: Scholarly interest in the visualization of climate change has grown over the last years (e.g., Guenther et al. 2022 ). These studies focused mainly on traditional media coverage in newspapers, newsmagazines and on television. Online content or fictional and entertainment formats, however, are rarely examined (for an overview, see Metag et al. 2016 ; O'Neill and Smith 2014 ). Content analysis studies of climate change imagery have explored a variety of visual themes, such as visual representations of impacts, threats, and causes of climate change (e.g., Lester and Cottle 2009 ), (untouched) nature (e.g., Rebich-Hespanha et al. 2015 ), (well-known) individuals (e.g., O'Neill et al. 2013 ), graphics and models (e.g., Schneider 2012 ), and carbon emissions and energy issues (e.g., Rebich-Hespanha et al. 2015 ). Visual representations of climate change have been analyzed for different countries (for Canada, see DiFrancesco and Young 2011 ; for comparative analysis of newspaper imagery, see O'Neill 2013 ; for the UK, see Smith and Joffe 2009 ). These studies have revealed that similar images are often used in climate change coverage across different countries and media outlets.
4 Research Desiderata
Future researchers interested in climate change coverage could work on the following common points of criticism regarding recent research in this area (e.g., Anderson 2009 ; Metag 2016 ; Moser 2010 ; Schäfer 2012 , 2015 ; Schäfer and O'Neill 2016 ): First, the scope of comparative studies needs to be expanded, especially in terms of countries (e.g., the Global South), media (e.g., there is a lack of research on television as well as fictional media and popular culture), time frames, focus (text vs. visuals, multimodality), and respective indicators; second, there needs to be more research on social media (e.g., not just Twitter) and online media such as blogs; third, methodologically, automated content analyses will allow to work with larger text corpora; lastly, fourth, more mixed-methods research is recommended to include more points of the climate change communication cycle, for instance, combining content analysis with audience research (Feldman et al. 2012 ), and especially regarding long-term effects of media exposure. So far, research on environmental communication has primarily focused on the sub-theme of climate change, therefore future research should address other environmental problems, such as energy or pollution, as well (Hansen 2018 ; Moser and Dilling 2008 ).
Relevant Variables in DOCA – Database of Variables for Content Analysis
Balance: https://doi.org/10.34778/2o
Generic frames: https://doi.org/10.34778/2p
Issue attention: https://doi.org/10.34778/2q
Ahchong, K., & Dodds, R. (2012). Anthropogenic climate change coverage in two Canadian newspapers, the Toronto Star and the Globe and Mail, from 1988 to 2007. Environmental Science & Policy, 15 (1), 48–59.
Article Google Scholar
Anderson, A. (2009). Media, politics and climate change: Towards a new research agenda. Sociology Compass, 3 (2), 166–182.
Anderson, A. (2011). Sources, media, and modes of climate change communication: The role of celebrities. Wiley Interdisciplinary Reviews: Climate Change, 2 (4), 535–546.
Google Scholar
Billett, S. (2010). Dividing climate change: global warming in the Indian mass media. Climatic Change, 99 (1–2), 1–16.
Bonfadelli, H. (2016). Climate change communication in Switzerland. In E. C. Tandoc & N. Eng (Eds.), Oxford research encyclopedias. Oxford: Oxford University Press.
Boykoff, M. T. (2007). From convergence to contention: United States mass media representations of anthropogenic climate change science. Transactions of the Institute of British Geographers, 32 (4), 477–489.
Boykoff, M. T. (2008). The cultural politics of climate change discourse in UK tabloids. Political Geography, 27 (5), 549–569.
Boykoff, M. T., & Boykoff, J. M. (2004). Balance as bias: Global warming and the US prestige press. Global Environmental Change, 14 (2), 125–136.
Boykoff, M. T., & Boykoff, J. M. (2007). Climate change and journalistic norms: A case-study of US mass-media coverage. Geoforum, 38 (6), 1190–1204.
Brossard, D., Shanahan, J., & McComas, K. (2004). Are issue-cycles culturally constructed? A comparison of French and American coverage of global climate change. Mass Communication and Society, 7 (3), 359–377.
Carvalho, A. (2010). Media(ted)discourses and climate change: A focus on political subjectivity and (dis)engagement. Wiley Interdisciplinary Reviews: Climate Change, 1 (2), 172–179.
Carvalho, A., & Burgess, J. (2005). Cultural circuits of climate change in U.K. Broadsheet newspapers, 1985–2003. Risk Analysis: An Official Publication of the Society for Risk Analysis, 25 (6), 1457–1469.
Corbett, J. B., & Durfee, J. L. (2004). Testing public (un)certainty of science. Science Communication, 26 (2), 129–151.
Corfee-Morlot, J., Maslin, M., & Burgess, J. (2007). Global warming in the public sphere. Philosophical transactions. Series A, Mathematical, Physical, and Engineering Sciences, 365 (1860), 2741–2776.
Cox, R. (2010). Environmental communication and the public sphere . Thousand Oaks, Calif.: Sage Publ.
DiFrancesco, A. D., & Young, N. (2011). Seeing climate change: The visual construction of global warming in Canadian national print media . Cultural Geographies, 18 (4), 517–536.
Dirikx, A., & Gelders, D. (2010). To frame is to explain: A deductive frame-analysis of Dutch and French climate change coverage during the annual UN conferences of the parties. Public Understanding of Science, 19(6), 732–742.
Dunwoody, S. (2019). Environment, health, and science coverage. In T. P. Vos & F. Hanusch (Eds.), Wiley Blackwell-ICA international encyclopedias of communication. The international encyclopedia of journalism studies (Vol. 19, pp. 1–9). Hoboken, NJ: Wiley-Blackwell.
Eide, E., & Kunelis, R. (Eds.) (2012). Media meets climate: The global challenge for journalism. Göteborg: Nordicom.
Eide, E., Kunelius, R., & Kumpu, V. (Eds.) (2010). Global journalism research series: Vol. 3. Global climate - local journalisms: A transnational study of how media make sense of climate summits. Bochum: Projekt-Verl.
Engesser, S., & Brüggemann, M. (2016). Mapping the minds of the mediators: The cognitive frames of climate journalists from five countries. Public Understanding of Science , 25(7).
Eskjær, M. F. (2016). Climate change communication in Denmark. In E. C. Tandoc & N. Eng (Eds.), Oxford research encyclopedias. Oxford: Oxford University Press.
Farbotko, C. (2005). Tuvalu and climate change: Constructions of environmental displacement in the Sydney morning herald. Human Geography, 87 (4), 279–293.
Feldman, L., Maibach, E. W., Roser-Renouf, C., & Leiserowitz, A. (2012). Climate on cable: The nature and impact of global warming coverage on Fox News, CNN, and MSNBC. The International Journal of Press/Politics, 17 (1), 3–31.
Fløttum, K., Gjesdal, A. M., Gjerstad, Ø., Koteyko, N., & Salway, A. (2014). Representations of the future in English language blogs on climate change. Global Environmental Change, 29, 213–222.
Griffin, R. J., & Dunwoody, S. (2008). Environmental communication. In W. Donsbach (Ed.), The international encyclopedia of communication (Vol. 38, p. 173). John Wiley & Sons Incorporated.
Guenther, L., Brüggemann, M., & Elkobros, S. (2022). From global doom to sustainable solutions: International news magazines’ multimodal framing of our future with climate change. Journalism Studies, 23 (1) 131–148.
Hansen, A. (2011). Communication, media and environment: Towards reconnecting research on the production, content and social implications of environmental communication. International Communication Gazette, 73 (1–2), 7–25.
Hansen, A. (2017). Media representation: Environment. In P. Rössler, C. A. Hoffner, & L. van Zoonen (Eds.), The Wiley Blackwell-ICA international encyclopedias of communication. The international encyclopedia of media effects (Vol. 7, pp. 1–12). Chichester, West Sussex, Malden, MA: John Wiley & Sons, Inc.
Hansen, A. (2018). Environmental communication. In R. L. Heath, W. Johansen, J. Falkheimer, K. Hallahan, J. Raupp, & B. Steyn (Eds.), The Wiley Blackwell-ICA international encyclopedias of communication. The international encyclopedia of strategic communication (pp. 1–10). Hoboken, NJ: Wiley.
Hansen, A., & Cox, R. (2015). The Routledge handbook of environment and communication. London: Routledge.
Book Google Scholar
Hase, V., Mahl, D., Schäfer, M. S., & Keller, T. R. (2021). Climate change in news media across the globe: An automated analysis of issue attention and themes in climate change coverage in 10 countries (2006–2018). Global Environmental Change https://doi.org/70102353-10.1016/j.gloenvcha.2021.102353 .
Ivanova, A. (2017). Transnationalisierung von Öffentlichkeiten [Transnationalization of public spheres]. Wiesbaden: Springer Fachmedien Wiesbaden.
Keller, T. R., Hase, V., Thaker, J., Mahl, D., & Schäfer, M. S. (2020). News media coverage of climate change in India 1997–2016: Using automated content analysis to assess themes and topics. Environmental Communication, 14 (2), 219–235.
Kirilenko, A. P., & Stepchenkova, S. O. (2014). Public microblogging on climate change: One year of Twitter worldwide. Global Environmental Change, 26, 171–182.
Lester, L., & Cottle, S. (2009). Visualizing climate change: Television news and ecological citizenship. International Journal of Communication, 3 , 920–936.
Liu, X., Lindquist, E., & Vedlitz, A. (2011). Explaining media and congressional attention to global climate change, 1969-2005: An empirical test of agenda-setting theory. Political Research Quarterly, 64 (2), 405–419.
Metag, J. (2016). Content analysis in climate change communication. In E. C. Tandoc & N. Eng (Eds.), Oxford research encyclopedias. Oxford: Oxford University Press.
Metag, J., Schäfer, M. S., Füchslin, T., Barsuhn, T., & Kleinen-von Königslöw, K. (2016). Perceptions of climate change imagery. Science Communication, 38 (2), 197–227.
Moser, S. C. (2010). Communicating climate change: History, challenges, process and future directions. Wiley Interdisciplinary Reviews: Climate Change, 1 (1), 31–53.
Moser, S. C., & Dilling, L. (Eds.) (2008). Creating a climate for change: Communicating climate change and facilitating social change. Cambridge: Cambridge Univ. Press.
O'Neill, S. J. (2013). Image matters: Climate change imagery in US, UK and Australian newspapers. Geoforum, 49, 10–19.
O'Neill, S. J., Boykoff, M., Niemeyer, S., & Day, S. A. (2013). On the use of imagery for climate change engagement. Global Environmental Change, 23 (2), 413–421.
O'Neill, S. J., & Smith, N. (2014). Climate change and visual imagery. Wiley Interdisciplinary Reviews: Climate Change, 5 (1), 73–87.
Painter, J. (2011). Poles apart. The international reporting of climate scepticism: Reuters Institute for the Study of Journalism.
Painter, J., & Ashe, T. (2012). Cross-national comparison of the presence of climate scepticism in the print media in six countries, 2007–10. Environmental Research Letters, 7 (4).
Rebich-Hespanha, S., Rice, R. E., Montello, D. R., Retzloff, S., Tien, S., & Hespanha, J. P. (2015). Image themes and frames in US print news stories about climate change. Environmental Communication, 9 (4), 491–519.
Sampei, Y., & Aoyagi-Usui, M. (2009). Mass-media coverage, its influence on public awareness of climate-change issues, and implications for Japan’s national campaign to reduce greenhouse gas emissions. Global Environmental Change, 1 9(2), 203–212.
Schäfer, M. S. (2012). Online communication on climate change and climate politics: a literature review. Wiley Interdisciplinary Reviews: Climate Change, 3 (6), 527–543.
Schäfer, M. S. (2015). Climate change and the media. In J. D. Wright (Ed.), International Encyclopedia of the Social & Behavioral Sciences (2nd ed., pp. 853–859). Amsterdam: Elsevier.
Chapter Google Scholar
Schäfer, M. S. (2016). Climate change communication in Germany. In E. C. Tandoc & N. Eng (Eds.), Oxford research encyclopedias. Oxford: Oxford University Press.
Schäfer, M. S., & Bonfadelli, H. (2017). Umwelt- und Klimawandelkommunikation [Environmental and climate change communication]. In H. Bonfadelli (Ed.), Forschungsfeld Wissenschaftskommunikation [The field of science communication] (pp. 315–338). Wiesbaden: Springer VS.
Schäfer, M. S., Ivanova, A., & Schmidt, A. (2014). What drives media attention for climate change? Explaining issue attention in Australian, German and Indian print media from 1996 to 2010. International Communication Gazette, 76 (2), 152–176.
Schäfer, M. S., & O'Neill, S. (2016). Frame analysis in climate change communication. In E. C. Tandoc & N. Eng (Eds.), Oxford research encyclopedias. Oxford: Oxford University Press.
Schäfer, M. S., & Schlichting, I. (2014). Media representations of climate change: A meta-analysis of the research field. Environmental Communication, 8 (2), 142–160.
Schlichting, I. (2013). Strategic framing of climate change by industry actors: A meta-analysis. Environmental Communication, 7 (4), 493–511.
Schmidt, A., Ivanova, A., & Schäfer, M. S. (2013). Media attention for climate change around the world: A comparative analysis of newspaper coverage in 27 countries. Global Environmental Change, 23 (5), 1233–1248.
Schneider, B. (2012). Climate model simulation visualization from a visual studies perspective. Wiley Interdisciplinary Reviews: Climate Change, 3 (2), 185–193.
Smith, N. W., & Joffe, H. (2009). Climate change in the British press: The role of the visual. Journal of Risk Research, 12 (5), 647–663.
Tandoc, E. C., & Eng, N. (2016). Climate change communication on Facebook, Twitter, Sina Weibo, and other social media platforms. In E. C. Tandoc & N. Eng (Eds.) , Oxford research encyclopedias . Oxford: Oxford University Press.
Weingart, P., Engels, A., & Pansegrau, P. (2000). Risks of communication: Discourses on climate change in science, politics, and the mass media. Public Understanding of Science, 9 (3), 261–283.
Williams, H. T.P., McMurray, J. R., Kurz, T., & Hugo Lambert, F. (2015). Network analysis reveals open forums and echo chambers in social media discussions of climate change. Global Environmental Change, 32 , 126–138.
Yang, G. (2010). Brokering environment and health in China: Issue entrepreneurs of the public sphere. Journal of Contemporary China, 19 (63), 101–118.
Young, N., & Dugas, E. (2012). Comparing climate change coverage in Canadian, English and French-language print media: Environmental values, media cultures, and the narration of global warming. Canadian Journal of Sociology, 37 (1), 25–54.
Download references
Author information
Authors and affiliations.
Universität Zürich, IKMZ - Institut für Kommunikationswissenschaft und Medienforschung, Zürich, Schweiz
Daniela Mahl
Universität Hamburg, JKW, Hamburg, Deutschland
Lars Guenther
You can also search for this author in PubMed Google Scholar
Corresponding author
Correspondence to Daniela Mahl .
Editor information
Editors and affiliations.
Fachhochschule Graubünden, Chur, Schweiz
Franziska Oehmer-Pedrazzi
IKMZ - Institut für Kommunikationswissenschaft und Medienforschung, Universität Zürich, Zürich, Schweiz
Sabrina Heike Kessler
Edda Humprecht
Zürcher Hochschule für angewandte Wissenschaft (ZHAW), Zürich, Schweiz
Katharina Sommer
Laia Castro
Rights and permissions
Open Access Dieses Kapitel wird unter der Creative Commons Namensnennung 4.0 International Lizenz ( http://creativecommons.org/licenses/by/4.0/deed.de ) veröffentlicht, welche die Nutzung, Vervielfältigung, Bearbeitung, Verbreitung und Wiedergabe in jeglichem Medium und Format erlaubt, sofern Sie den/die ursprünglichen Autor(en) und die Quelle ordnungsgemäß nennen, einen Link zur Creative Commons Lizenz beifügen und angeben, ob Änderungen vorgenommen wurden.
Die in diesem Kapitel enthaltenen Bilder und sonstiges Drittmaterial unterliegen ebenfalls der genannten Creative Commons Lizenz, sofern sich aus der Abbildungslegende nichts anderes ergibt. Sofern das betreffende Material nicht unter der genannten Creative Commons Lizenz steht und die betreffende Handlung nicht nach gesetzlichen Vorschriften erlaubt ist, ist für die oben aufgeführten Weiterverwendungen des Materials die Einwilligung des jeweiligen Rechteinhabers einzuholen.
Reprints and permissions
Copyright information
© 2023 Der/die Autor(en)
About this chapter
Mahl, D., Guenther, L. (2023). Content Analysis in the Research Field of Environmental & Climate Change Coverage. In: Oehmer-Pedrazzi, F., Kessler, S.H., Humprecht, E., Sommer, K., Castro, L. (eds) Standardisierte Inhaltsanalyse in der Kommunikationswissenschaft – Standardized Content Analysis in Communication Research. Springer VS, Wiesbaden. https://doi.org/10.1007/978-3-658-36179-2_18
Download citation
DOI : https://doi.org/10.1007/978-3-658-36179-2_18
Published : 25 September 2022
Publisher Name : Springer VS, Wiesbaden
Print ISBN : 978-3-658-36178-5
Online ISBN : 978-3-658-36179-2
eBook Packages : Social Science and Law (German Language)
Share this chapter
Anyone you share the following link with will be able to read this content:
Sorry, a shareable link is not currently available for this article.
Provided by the Springer Nature SharedIt content-sharing initiative
- Publish with us
Policies and ethics
- Find a journal
- Track your research
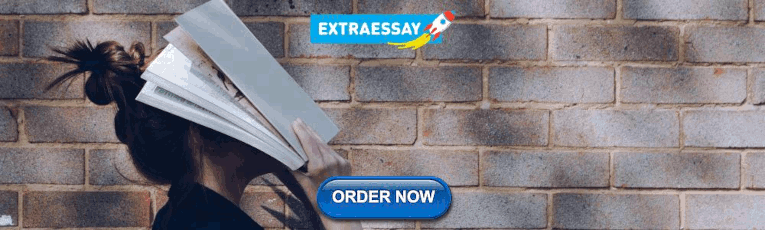
IMAGES
VIDEO
COMMENTS
Content analysis, as in all qualitative analysis, is a reflective process. There is no "step 1, 2, 3, done!" linear progression in the analysis. ... Graneheim U.H., Lundman B. Qualitative content analysis in nursing research: concepts, procedures, and measures to achieve trustworthiness. Nurse Educ Today. 2004; 24:105-112.
Content analysis is a research tool used to determine the presence of certain words, themes, or concepts within some given qualitative data (i.e. text). Using content analysis, researchers can quantify and analyze the presence, meanings, and relationships of such certain words, themes, or concepts.
ciated with content analysis, it is useful to assess the nature of past applications. Using the directives that Kassarjian offered for content-analysis research, the current study investigated (1) whether researchers con-ducted and reported studies in accordance with the critical method areas for content analysis, (2) whether
Content analysis is a research method that has been used increasingly in social and health research, including quality of life and well-being. ... Its aim was to examine research questions based on existing theories or previous empirical studies (Zhang & Wildemuth, 2009). The quantitative approach produces numbers that can be manipulated with ...
In his 1952 text on the subject of content analysis, Bernard Berelson traced the origins of the method to communication research and then listed what he called six distinguishing features of the approach. As one might expect, the six defining features reflect the concerns of social science as taught in the 1950s, an age in which the calls for an "objective," "systematic," and ...
Abstract. An overview of the development of empirical content analysis is presented. Its development relevant to educational research from classical to computer-aided content analysis is traced. The history of hermeneutic text interpretation is included as well as the early developments in the analysis of manifest content of communication.
Content analysis is a research method used to identify patterns in recorded communication. To conduct content analysis, you systematically collect data from a set of texts, which can be written, oral, or visual: Books, newspapers and magazines. Speeches and interviews. Web content and social media posts. Photographs and films.
Interpreting communications as texts in the contexts of their social uses distinguishes content analysis from other empirical methods of inquiry. ... The Fourth Edition has been completely revised to offer readers the most current techniques and research on content analysis, including new information on reliability and social media. Readers ...
It is a flexible research method ( Anastas, 1999 ). Qualitative content analysis may use either newly collected data, existing texts and materials, or a combination of both. It may be used in exploratory, descriptive, comparative, or explanatory research designs, though its primary use is descriptive.
quantitative content analysis is deductive, intended to test hypotheses or address questions generated from theories or previous empirical research. By contrast, qualitative content analysis is mainly inductive, grounding the examination of topics and themes, as well as the inferences drawn from them, in the data. In some cases, qualitative content
Chapter 17. Content Analysis Introduction. Content analysis is a term that is used to mean both a method of data collection and a method of data analysis. Archival and historical works can be the source of content analysis, but so too can the contemporary media coverage of a story, blogs, comment posts, films, cartoons, advertisements, brand packaging, and photographs posted on Instagram or ...
Outlining the technical steps of empirical analysis, this chapter operationalizes content analysisContent analysis as a tool to assess meaning-construction of emerging technologies through framing. ... The method used in this thesis to answer the research question is qualitative content analysis following Mayring (2010, 2015, 2014) and Früh ...
Quantitative content analysis is an empirical method used in the social sciences primarily for analyzing recorded human communication in a quantitative, systematic, and intersubjective way. This material can include newspaper articles, films, advertisements, interview transcripts, or observational protocols, for instance.
Content analysis is a family of systematic, rule-guided techniques used to analyze the informational contents of textual data (Mayring, 2000). It is used frequently in nursing research, and is rapidly becoming more prominent in the medical and bioethics literature. ... Empirical Methods for Bioethics: A Primer. ISBN: 978--7623-1266-5 ...
Abstract. An overview of the development of empirical content analysis is presented. Its development relevant to educational research from classical to computer-aided content analysis is traced. The history of hermeneutic text interpretation is included as well as the early developments in the analysis of manifest content of communication.
A suitable, often-applied method in empirical educational research is qualitative content analysis (QCA), developed by Philipp MAYRING (1983, 2015). This method can be used for inductive and deductive strategies of analysis, and is appropriate for combining qualitative and quantitative analyses, especially for research strategies based on mixed ...
Thematic Proximity in Content Analysis. Andreas Armborst. Business. 2017. TLDR. A novel theme-correlation coefficient is introduced that adds valuable information to traditional theme relation metrics and enables researchers to make new empirical observations in text data. Expand. 39. PDF.
Accordingly, we completed a content analysis of empirical studies on this topic. We utilized the Table of Contents of several psychology journals, psychological databases, and search engines to identify relevant literature. ... Moreno M. V. (2007). Multicultural counseling competencies research: A 20-year content analysis. Journal of Counseling ...
A common starting point for qualitative content analysis is often transcribed interview texts. The objective in qualitative content analysis is to systematically transform a large amount of text into a highly organised and concise summary of key results. Analysis of the raw data from verbatim transcribed interviews to form categories or themes ...
Qualitative content analysis is a research method attempt to identify core consistencies and meanings through the systematic classification process of coding and identifying themes or patterns (Hsieh & Shannon, 2005; ... In the exploratory stage of empirical research, a qualitative approach contributes to a richer analysis of phenomena referred ...
Abstract. In the study of climate and environmental communication, content analysis—in all its many facets—has been used numerous times and in various ways. This chapter provides an overview of common research designs and (combinations of) methods, showing that a variety of approaches have been applied, ranging from qualitative, to ...
To explore how gender role research has been conducted among Latinas/os in the psychological literature, we conducted a content analysis of empirical studies in this area, emphasizing content areas, sample characteristics, and research methods. We incorporated database searches and reviews of relevant publication reference lists and tables of contents of journals that typically publish ...
A total of 80 empirical studies on IMC published between 2000 and 2015 are examined and systematically presented and discussed. IMC implementation, impact, perception, measurement and its relationship with technology emerge as five main research topics. Researchers seem to prefer quantitative research over qualitative and descriptive statistics ...
The entertainment industry is a vast and ever-changing area that encompasses a wide range of media and content creation. In detail, the phrase "entertainment industry" refers to the production of theater, film, visual art, dance, opera, music, book publishing, television, and radio [].Nowadays, technological improvements have played a key influence in the innovation of the entertainment ...
The article relies on empirical material, consisting of the statements published by shipping companies under the UK Modern Slavery Act [MSA]. In addition to the descriptive observations on compliance, the study carries out a content analysis of the statements, seeking to identify the patterns of reporting and industry best practices.