
An official website of the United States government
The .gov means it’s official. Federal government websites often end in .gov or .mil. Before sharing sensitive information, make sure you’re on a federal government site.
The site is secure. The https:// ensures that you are connecting to the official website and that any information you provide is encrypted and transmitted securely.
- Publications
- Account settings
Preview improvements coming to the PMC website in October 2024. Learn More or Try it out now .
- Advanced Search
- Journal List
- Am J Case Rep

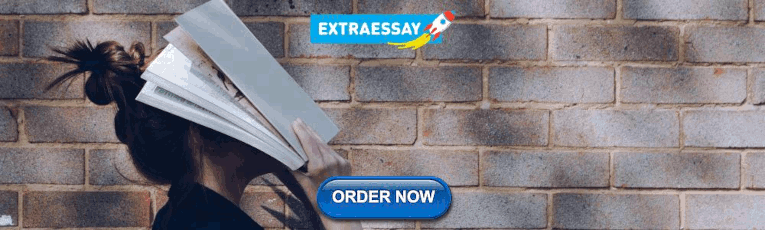
Non-Small Cell Lung Cancer in a Very Young Woman: A Case Report and Critical Review of the Literature
Valentina polo.
1 Department of Surgery, Oncology and Gastroenterology, University of Padova, Padova, Italy
2 Division of Medical Oncology 2, Istituto Oncologico Veneto IRCCS, Padova, Italy
Giulia Zago
Stefano frega, fabio canova, laura bonanno, adolfo favaretto, laura bonaldi.
3 Immunology and Molecular Oncology Unit, Istituto Oncologico Veneto IRCCS, Padova, Italy
Roberta Bertorelle
Pierfranco conte, giulia pasello.
Patient: Female, 19
Final Diagnosis: Lung adenocarcinoma
Symptoms: Chest pain
Medication: —
Clinical Procedure: Ct scan and pet-ct
Specialty: Oncology
Unusual clinical course
Background:
Lung cancer in young patients is quite uncommon; clinical presentation and outcome in this population compared to the older group are not yet well defined and data about this setting are mostly single-institutional retrospective analyses.
Case Report:
We report here a case of a very young woman with diagnosis of early-stage lung adenocarcinoma harboring EML4-ALK rearrangement; she underwent radical surgery and adjuvant chemotherapy according to the pathologic stage. Potential risk factors for lung cancer in our patient are discussed and clinico-pathologic features and outcomes of lung cancer in the young population compared to the elderly are reviewed through discussing studies with sample sizes larger than 100 patients.
Conclusions:
A wide clinical overview should be performed when lung cancer is diagnosed in a young patient. Large-population studies are required to define the molecular signature and clinical behavior of lung cancer in young patients.
According to the Surveillance, Epidemiology, and End Results Program (SEER) registry based on 2007–2011 new cases, lung cancer (LC) is more frequently diagnosed among people aged 65–74 with only 1.6% of all cases occurring in patients younger than 45 years [ 1 ]. Most published data about LC in young populations are single-institutional retrospective analyses and few report on very young patients specifically. Previous data suggest that LC in young adults may be an entity with distinct characteristics compared to LC in older patients; however, data are not always consistent among all series [ 2 – 11 ]. In addition, age limits ranging from 40 to 50 years have been variably chosen by different authors to define younger cohorts of patients. We report a case of a young woman with early-stage EML4-ALK rearranged lung adenocarcinoma who underwent surgery followed by adjuvant chemotherapy. We also consider possible susceptibility factors for LC in our patient and review the majority of clinical studies with a sample size larger than 100 patients, in order to highlight and discuss LC patterns in young versus old patients [ 2 – 11 ].
Case Report
In January 2014, a 19-year-old white, never-smoker woman experienced chest pain; a chest X-ray and a computed tomography (CT)-scan showed a cavitating right lung lesion in the upper lobe without enlargement of mediastinal lymph nodes ( Figure 1A ). A bronchoscopy was performed and the evaluation of cell block prepared from bronchial brushings led to the diagnosis of adenocarcinoma. A positron emission tomography/computerized tomography (PET/CT) scan excluded additional disease localizations ( Figure 1B ). In March 2014 the patient underwent right upper lobectomy with systematic lymphadenectomy by video-assisted thoracic surgery (VATS); a diagnosis of primary pulmonary adenocarcinoma with papillary predominant pattern was made. Immunohistochemistry showed that tumor cells were positive for TTF-1 and negative for p63; Ki67 was 70%. Molecular analysis showed no EGFR , KRAS , and BRAF gene mutations by Sanger’s direct sequencing, whereas fluorescent in situ hybridization (FISH) showed the presence of EML4-ALK rearrangement in 57% of cells ( Figure 2 ). The patient was then referred to our Institution in April 2014. Clinical examination showed Eastern Cooperative Oncology Group (ECOG) performance status 0, and no additional findings. As previous medical history, the patient referred a general discomfort occurring between May and October 2013, characterized by nausea, vomiting, diarrhea, and skin rash. Blood test results are reported in Table 1 . In November 2013, the patient underwent an esophagogastroduodenoscopy with multiple biopsies, leading to the diagnosis of celiac disease. A gluten-free diet induced symptoms regression. The cancer family history revealed that the patient’s father died of renal cell carcinoma in 2007. A genetic test on a blood sample did not show TP53 mutations and the constitutional karyotype was normal. According to the pathologic stage (pT2a N1, stage IIA), she received adjuvant chemotherapy with 4 cycles of cisplatin-pemetrexed from May to July 2014. Before starting chemotherapy, the patient underwent ovarian tissue cryopreservation and gonadotropin-releasing hormone analogue was administered during the adjuvant treatment. Because of persistence of elevated gamma-glutamyl-transpeptidase (GGT) and transaminases before and during chemotherapy, the patient had a specialist opinion, which resulted in the diagnosis of autoimmune hepatitis. Currently, the patient remains on oncological and hepatologic follow-up visits. At the last follow-up visit, in February 2015, a CT-scan showed no disease recurrence ( Figure 1C ).

Computed tomography (CT) scan at diagnosis and positron emission tomography and CT-scan (PET/CT) at diagnosis, before surgery ( A, B ). CT scan after surgery at last follow-up visit ( C ).

FISH analysis was performed with ALK dual-color break-apart probe labelled with SpectrumOrange (3’end) and SpectrumGreen (5’end) (Abbott Molecular). The predominant ALK-positive FISH pattern observed in the sample was isolated red signal.
Significant selection of blood tests performed before diagnosis of lung cancer.
Occurrence of LC in young adults is quite uncommon and is characterized by peculiar epidemiological, clinical, and prognostic features. To date, the pathogenesis of this disease in young people is still very unclear. None of the known risk factors for LC could explain the early onset of the malignancy and no specific genomic alteration has been detected in this subgroup of patients.
According to the SEER registry, the proportion of African-Americans, Asian, and Pacific Islanders was higher among younger than older patients [ 1 ]. This epidemiologic discrepancy may be due to differences in carcinogens exposure or to biologic differences such as inefficient cell cycle arrest and DNA damage accumulation or cytochrome polymorphisms [ 9 , 12 , 13 ]. Regarding the clinico-pathologic features of LC in the young population, most retrospective series with sample sizes of more than 100 patients ( Table 2 ) reported a higher proportion of women and adenocarcinoma in younger groups compared to older patients [ 3 , 4 , 6 – 11 ], but some cases of very young patients with squamous cell LC have also been reported in recent years [ 14 – 16 ]. Data on the proportion of asymptomatic patients at diagnosis in young and old groups are discordant. However, chest pain is definitively the most frequent symptom of younger patients in comparative analysis [ 6 , 8 ], such as in our patient. Finally, lower occurrence of early-stage disease in young people, described in most of the retrospective series, could be due to more aggressive disease or a delayed diagnosis due to a low degree of suspicion of cancer in young patients [ 4 , 9 – 11 ].
Studies comparing young lung cancer patients to older patients with sample size of more of 100 patients: data about clinico-pathologic features.
Our patient’s tumor sample tested positive for EML4-ALK rearrangement. To date, only a few studies have investigated molecular alterations in younger patients, with controversial results. Higher frequency of EML4-ALK rearrangement (11.6%) and EGFR mutation (20%) were shown in 53 non-small cell LC patients ≤50 years old compared with patients of all ages [ 17 ]. In contrast, Ye et al. showed no difference in terms of oncogenic mutations (P=0.396), but a higher prevalence of TP53 mutations (P<0.001) in 36 resected lung adenocarcinoma from patients younger than 40 years compared to their older counterparts [ 18 ]; similarly, Kim et al. did not find a statistically significant difference in EGFR and EML4-ALK status between young and old patients [ 19 ]. Further investigation is needed to address the issue of genetic derangements in young patients with LC.
Risk factors for the onset of LC specifically in young adults are still unknown. The increased frequency of adenocarcinoma and the long latency time between smoking exposure and cancer appearance suggest that LC among young people does not require as much carcinogen exposure, but rather genetic derangements. However, the smoking status is similar between younger and older patients in a few series collecting these data, with approximately 75–95% of young patients reporting smoking sometime. Whether the number of cigarettes smoked per day or the age at which smoking began lead to an early onset of LC is still unclear. These patients might have genetic susceptibility to develop LC or inherited sensitivity to smoking-related carcinogenesis. Recently, case-control studies have showed that gene mutations or polymorphisms involving xenobiotic metabolizing enzymes and DNA repair pathways are associated with increased risk of early-onset LC [ 20 – 23 ].
Our patient was a never-smoker female, and she did not report previous exposure to carcinogens; thus, when we investigated possible risk factors for LC, we focused on genetic, immunological, and/or infective predisposition. The hypothesis of a genetic component in the early onset of LC is also supported by a few series showing an increased family history among young patients. In a case-control study, the authors demonstrated the greatest contribution to LC risk (7-fold increase) among 40- to 59-year-old non-smoker subjects with a first-degree relative affected by LC [ 24 ]. Our patient did not report family history of LC among her first-degree relatives. Her father died because of a kidney cancer, which is not included in any genetic syndrome involving LC; moreover, germ-line mutations of TP53 gene or constitutional karyotype alterations were not observed. Hereditary LC syndromes are rare, and, while germline EGFR T790M mutation has been reported as a predisposing genetic feature, especially in non-smoker patients [ 25 ], no evidence of germline EML4-ALK rearrangement has been reported in LC.
The medical history of the patient included the diagnosis of celiac disease, which is associated with an increased risk of lymphoma. In a study from a large Swedish cohort, the authors found a neutral risk of LC in celiac disease, with a hazard ratio of 1.00 beyond the first year of follow-up after celiac diagnosis [ 26 ]. More recently, a large-population cohort study in Finland showed a decreased risk of LC among 32 439 celiac patients [ 27 ].
Additional significant findings in our case were the recent diagnosis of autoimmune hepatitis, anti-cytomegalovirus (CMV), immunoglobulin (Ig) G, and anti-Epstein-Barr virus (EBV) IgG and IgM positivity. Subsequent examinations excluded the positivity of CMV and EBV DNA and of all hepatitis viruses. The association between solid cancer and autoimmune systemic disease is uncommon and especially involves scleroderma and LC [ 28 ], even though increased risk for LC has also been reported in systemic lupus erythematosus (SLE) patients [ 29 ]. In a recent paper, a 4-fold risk of LC in patients with systemic sclerosis, discoid lupus erythematosus, and polymyositis/dermatomyositis has been described [ 30 ]. However, there are no data about a possible link between autoimmune hepatitis and risk of LC. Similarly, EBV and CMV infections seem to have a role in the pathogenesis of solid tumors, such as lymphoepithelioma-like carcinoma and glioblastoma, respectively, but no involvement in LC risk has been reported. Indeed, microRNA studies in LC did not support any role of EBV in LC [ 31 ]. To date, the only virus infections associated with LC are human immunodeficiency virus (HIV) and human papilloma virus (HPV) infections [ 32 , 33 ]. Thus, we have no data to support the hypothesis that LC risk in our patient had a genetic, immunological, or infective basis.
Data regarding clinical outcome of young LC patients have been presented in only a few retrospective studies [ 2 , 4 , 6 – 11 ] ( Table 3 ). According to Roviaro GC et al., no significant statistical differences were observed in terms of survival between patients younger or older than 45 years, in the whole population and according to type of treatment or disease [ 2 ]. This was also confirmed by another series in which the majority of examined patients were included in clinical trials, thus making the 2 groups well balanced in comorbidity patterns and treatment modalities [ 8 ]. On the other hand, other studies showed a longer survival in the young group, despite the higher frequency of advanced disease. This finding could be explained by the higher chance of receiving more aggressive treatments, including multimodality treatments and further lines of chemotherapy, due to the lower prevalence of comorbidities [ 4 , 6 , 7 , 9 – 11 ].
Studies comparing young lung cancer patients to older patients with sample size of more of 100 patients: data about patients’ outcome.
OS – overall survival; NA – not assessed; NS – not significant; NR – not reported.
In case of disease relapse, our patient could benefit from a first-generation ALK inhibitor, which has demonstrated remarkable clinical outcomes including better response rate and prolonged survival compared to standard chemotherapy [ 34 , 35 ]. However, despite an initial improvement, ALK -positive tumors inevitably develop several resistance mechanisms to the targeted drug, resulting in progression of the disease. Other strategies, including the development of new-generation ALK inhibitors, are currently under clinical evaluation to overcome the acquired resistance in these patients [ 36 ].
Conclusions
Despite a comprehensive review of the patient’s medical and family history, we did not identify any underlying risk factor for LC. Larger prospective studies are needed to define the molecular signature and clinical behavior of LC in young patients. To collect evidence of no-smoking related pathways involved in cancer risk, a wide clinical overview should be performed when LC diagnosis occurs in a young patient. Given the rarity of the disease in this setting, only an international multi-center study could address this issue.
Abbreviations:
References:.
- Introduction
- Conclusions
- Article Information
Evidence reviews for the US Preventive Services Task Force (USPSTF) use an analytic framework to visually display the key questions that the review will address to allow the USPSTF to evaluate the effectiveness and safety of a preventive service. The questions are depicted by linkages that relate interventions and outcomes. Additional details are provided in the USPSTF Procedure manual. 10 I-ELCAP indicates International Early Lung Cancer Action Program; NLST, National Lung Screening Trial; and SBRT, stereotactic body radiotherapy.
a The evaluation of evidence on treatment was limited to studies of surgical resection or SBRT for stage I non–small cell lung cancer.
ICTRP indicates International Clinical Trials Registry Platform; KQ, key question; SABR, stereotactic ablative radiation; SBRT, stereotactic body radiotherapy; USPSTF, US Preventive Services Task Force; WHO, World Health Organization.
a Because many articles contribute to 1 or more KQs, the number of articles listed per KQ in this section does not add up to 223.
DANTE indicates Detection and Screening of Early Lung Cancer by Novel Imaging Technology and Molecular Essays; DLCST, Danish Lung Cancer Screening Trial; IRR, incidence rate ratio; ITALUNG, Italian Lung Cancer Screening Trial; NELSON, Nederlands-Leuvens Longkanker Screenings Onderzoek; and NLST, National Lung Screening Trial.
DANTE indicates Detection and Screening of Early Lung Cancer by Novel Imaging Technology and Molecular Essays; DLCST, Danish Lung Cancer Screening Trial; IRR, incidence rate ratio; ITALUNG, Italian Lung Cancer Screening Trial; LDCT, low-dose computed tomography; LSS, Lung Screening Study; NELSON, Nederlands-Leuvens Longkanker Screenings Onderzoek; NLST, National Lung Screening Trial; NR, not reported.
eMethods. Literature Search Strategy
eContextual Questions
eDiscussion.
eTable 1. NSCLC Staging Overview, Typical 5-Year Survival, and Treatment Approaches
eTable 2. Eligibility Criteria
eTable 3. Risk of Bias and Overall Quality Assessment Ratings for Nonrandomized Studies
eTable 4. Risk of Bias and Overall Quality Assessment Ratings for Randomized Studies: Part 1
eTable 5. Risk of Bias and Overall Quality Assessment Ratings for Randomized Studies: Part 2
eTable 6. Risk of Bias and Overall Quality Assessment Ratings for Randomized Studies: Part 3
eTable 7. Risk of Bias and Overall Quality Assessment Ratings for Risk Prediction Model (KQ 2) Studies: Part 1
eTable 8. Risk of Bias and Overall Quality Assessment Ratings for Risk Prediction Model (KQ 2) Studies: Part 2
eTable 9. Risk of Bias and Overall Quality Assessment Ratings for Risk Prediction Model (KQ 2) Studies: Part 3
eTable 10. Risk of Bias and Overall Quality Assessment Ratings for Risk Prediction Model (KQ 2) Studies: Part 4
eTable 11. Risk of Bias and Overall Quality Assessment Ratings for Accuracy (KQ 3) Studies: Part 1
eTable 12. Risk of Bias and Overall Quality Assessment Ratings for Accuracy (KQ 3) Studies: Part 2
eTable 13. Risk of Bias and Overall Quality Assessment Ratings for Accuracy (KQ 3) Studies: Part 3
eTable 14. Predictors Used in Risk Prediction Models for Identifying Adults at Higher Risk of Lung Cancer Mortality and Model Applicability
eTable 15. PLCOm2012Model Estimated Benefits and Harms Over 6 Years Compared With USPSTF or NLST Criteria
eTable 16. Summary of Modeling Studies Evaluating Screen-Prevented Lung Cancer Deaths and NNS to Prevent One Lung Cancer Death
eTable 17. Accuracy of LDCT for Lung Cancer Screening in RCTs (KQ 3)
eTable 18. Accuracy of LDCT for Lung Cancer Screening in Nonrandomized Studies (KQ 3)
eTable 19. LDCT Parameters, by Study Type
eTable 20. Number and Percentage of False-Positive Results After Screening With LDCT
eTable 21. False-Positive Evaluations
eTable 22. Incidental Findings from KQ 4 Studies
eTable 23. KQs 6 and 7: Study and Patient Characteristics Table
eTable 24. KQs 6 & 7 Surgery Results
eTable 25. KQs 6 & 7 SBRT Results
eTable 26. KQs 6 and 7 Update Search Results: Study and Patient Characteristics
eTable 27. KQs 6 and 7 Update Search Results: Surgery Study Outcomes
eTable 28. KQs 6 and 7 Update Search Results: SBRT Study Outcomes
eTable 29. Summary of Multilevel Barriers to Effective Lung Cancer Screening
eTable 30. Summary of Randomized, Controlled Trials in the Systematic Review From 2019
eFigure. Trial Results for Lung Cancer Incidence (KQ 1)
eReferences
- USPSTF Modeling Study: Benefits and Harms of Lung Cancer Screening With LDCT JAMA US Preventive Services Task Force March 9, 2021 This simluation study models the benefits and harms associated with low-dose computed tomography (LDCT) screening for lung cancer based on a range of starting and stopping ages, screening frequencies, cumulative pack-years, and years since quitting smoking. Rafael Meza, PhD; Jihyoun Jeon, PhD; Iakovos Toumazis, PhD; Kevin ten Haaf, PhD; Pianpian Cao, MPH; Mehrad Bastani, PhD; Summer S. Han, PhD; Erik F. Blom, MD, PhD; Daniel E. Jonas, MD, MPH; Eric J. Feuer, PhD; Sylvia K. Plevritis, PhD; Harry J. de Koning, MD, PhD; Chung Yin Kong, PhD
- USPSTF Evidence Report: Screening for Lung Cancer JAMA US Preventive Services Task Force March 9, 2021 This US Preventive Services Task Force Recommendation Statement concludes with moderate certainty that annual screening for lung cancer with low-dose computed tomography has a moderate benefit in persons at high risk of lung cancer based on age, total cumulative exposure to tobacco smoke, and years since quitting smoking (Grade B). US Preventive Services Task Force; Alex H. Krist, MD, MPH; Karina W. Davidson, PhD, MASc; Carol M. Mangione, MD, MSPH; Michael J. Barry, MD; Michael Cabana, MD, MA, MPH; Aaron B. Caughey, MD, PhD; Esa M. Davis, MD, MPH; Katrina E. Donahue, MD, MPH; Chyke A. Doubeni, MD, MPH; Martha Kubik, PhD, RN; C. Seth Landefeld, MD; Li Li, MD, PhD, MPH; Gbenga Ogedegbe, MD, MPH; Douglas K. Owens, MD, MS; Lori Pbert, PhD; Michael Silverstein, MD, MPH; James Stevermer, MD, MSPH; Chien-Wen Tseng, MD, MPH, MSEE; John B. Wong, MD
- Broadened Eligibility for Lung Cancer Screening JAMA Editorial March 9, 2021 Louise M. Henderson, PhD; M. Patricia Rivera, MD; Ethan Basch, MD
- Patient Information: Screening for Lung Cancer JAMA JAMA Patient Page March 9, 2021 This JAMA Patient Page explains the US Preventive Services Task Force 2021 recommendation to screen for lung cancer in adults aged 50 to 80 years who have a 20-pack-year or greater smoking history and currently smoke or have quit within the past 15 years. Jill Jin, MD, MPH
- Expanded Access to Lung Cancer Screening—Implementing Wisely to Optimize Health JAMA Network Open Editorial March 9, 2021 Anne C. Melzer, MD, MS; Timothy J. Wilt, MD, MPH
- The 2021 USPSTF Recommendation on Lung Cancer Screening—the More Things Stay the Same… JAMA Oncology Editorial May 1, 2021 Mayuko Ito Fukunaga, MD; Renda Soylemez Wiener, MD, MPH; Christopher G. Slatore, MD, MS
- New USPSTF Guidelines for Lung Cancer Screening JAMA Surgery Editorial June 1, 2021 This Editorial discusses updated lung cancer screening recommendations from the US Preventive Services Task Force. Yolonda L. Colson, MD, PhD; Jo-Anne O. Shepard, MD; Inga T. Lennes, MD, MPH, MBA
See More About
Select your interests.
Customize your JAMA Network experience by selecting one or more topics from the list below.
- Academic Medicine
- Acid Base, Electrolytes, Fluids
- Allergy and Clinical Immunology
- American Indian or Alaska Natives
- Anesthesiology
- Anticoagulation
- Art and Images in Psychiatry
- Artificial Intelligence
- Assisted Reproduction
- Bleeding and Transfusion
- Caring for the Critically Ill Patient
- Challenges in Clinical Electrocardiography
- Climate and Health
- Climate Change
- Clinical Challenge
- Clinical Decision Support
- Clinical Implications of Basic Neuroscience
- Clinical Pharmacy and Pharmacology
- Complementary and Alternative Medicine
- Consensus Statements
- Coronavirus (COVID-19)
- Critical Care Medicine
- Cultural Competency
- Dental Medicine
- Dermatology
- Diabetes and Endocrinology
- Diagnostic Test Interpretation
- Drug Development
- Electronic Health Records
- Emergency Medicine
- End of Life, Hospice, Palliative Care
- Environmental Health
- Equity, Diversity, and Inclusion
- Facial Plastic Surgery
- Gastroenterology and Hepatology
- Genetics and Genomics
- Genomics and Precision Health
- Global Health
- Guide to Statistics and Methods
- Hair Disorders
- Health Care Delivery Models
- Health Care Economics, Insurance, Payment
- Health Care Quality
- Health Care Reform
- Health Care Safety
- Health Care Workforce
- Health Disparities
- Health Inequities
- Health Policy
- Health Systems Science
- History of Medicine
- Hypertension
- Images in Neurology
- Implementation Science
- Infectious Diseases
- Innovations in Health Care Delivery
- JAMA Infographic
- Law and Medicine
- Leading Change
- Less is More
- LGBTQIA Medicine
- Lifestyle Behaviors
- Medical Coding
- Medical Devices and Equipment
- Medical Education
- Medical Education and Training
- Medical Journals and Publishing
- Mobile Health and Telemedicine
- Narrative Medicine
- Neuroscience and Psychiatry
- Notable Notes
- Nutrition, Obesity, Exercise
- Obstetrics and Gynecology
- Occupational Health
- Ophthalmology
- Orthopedics
- Otolaryngology
- Pain Medicine
- Palliative Care
- Pathology and Laboratory Medicine
- Patient Care
- Patient Information
- Performance Improvement
- Performance Measures
- Perioperative Care and Consultation
- Pharmacoeconomics
- Pharmacoepidemiology
- Pharmacogenetics
- Pharmacy and Clinical Pharmacology
- Physical Medicine and Rehabilitation
- Physical Therapy
- Physician Leadership
- Population Health
- Primary Care
- Professional Well-being
- Professionalism
- Psychiatry and Behavioral Health
- Public Health
- Pulmonary Medicine
- Regulatory Agencies
- Reproductive Health
- Research, Methods, Statistics
- Resuscitation
- Rheumatology
- Risk Management
- Scientific Discovery and the Future of Medicine
- Shared Decision Making and Communication
- Sleep Medicine
- Sports Medicine
- Stem Cell Transplantation
- Substance Use and Addiction Medicine
- Surgical Innovation
- Surgical Pearls
- Teachable Moment
- Technology and Finance
- The Art of JAMA
- The Arts and Medicine
- The Rational Clinical Examination
- Tobacco and e-Cigarettes
- Translational Medicine
- Trauma and Injury
- Treatment Adherence
- Ultrasonography
- Users' Guide to the Medical Literature
- Vaccination
- Venous Thromboembolism
- Veterans Health
- Women's Health
- Workflow and Process
- Wound Care, Infection, Healing
Others Also Liked
- Download PDF
- X Facebook More LinkedIn
Jonas DE , Reuland DS , Reddy SM, et al. Screening for Lung Cancer With Low-Dose Computed Tomography : Updated Evidence Report and Systematic Review for the US Preventive Services Task Force . JAMA. 2021;325(10):971–987. doi:10.1001/jama.2021.0377
Manage citations:
© 2024
- Permissions
Screening for Lung Cancer With Low-Dose Computed Tomography : Updated Evidence Report and Systematic Review for the US Preventive Services Task Force
- 1 RTI International, University of North Carolina at Chapel Hill Evidence-based Practice Center
- 2 Department of Internal Medicine, The Ohio State University, Columbus
- 3 Department of Medicine, University of North Carolina at Chapel Hill
- 4 Cecil G. Sheps Center for Health Services Research, University of North Carolina at Chapel Hill
- 5 Lineberger Comprehensive Cancer Center, University of North Carolina at Chapel Hill
- 6 RTI International, Research Triangle Park, North Carolina
- 7 Michigan Medicine, University of Michigan, Ann Arbor
- 8 Department of Internal Medicine, Virginia Commonwealth University, Richmond
- 9 Department of Family Medicine, University of North Carolina at Chapel Hill
- Editorial Broadened Eligibility for Lung Cancer Screening Louise M. Henderson, PhD; M. Patricia Rivera, MD; Ethan Basch, MD JAMA
- Editorial Expanded Access to Lung Cancer Screening—Implementing Wisely to Optimize Health Anne C. Melzer, MD, MS; Timothy J. Wilt, MD, MPH JAMA Network Open
- Editorial The 2021 USPSTF Recommendation on Lung Cancer Screening—the More Things Stay the Same… Mayuko Ito Fukunaga, MD; Renda Soylemez Wiener, MD, MPH; Christopher G. Slatore, MD, MS JAMA Oncology
- Editorial New USPSTF Guidelines for Lung Cancer Screening Yolonda L. Colson, MD, PhD; Jo-Anne O. Shepard, MD; Inga T. Lennes, MD, MPH, MBA JAMA Surgery
- US Preventive Services Task Force USPSTF Modeling Study: Benefits and Harms of Lung Cancer Screening With LDCT Rafael Meza, PhD; Jihyoun Jeon, PhD; Iakovos Toumazis, PhD; Kevin ten Haaf, PhD; Pianpian Cao, MPH; Mehrad Bastani, PhD; Summer S. Han, PhD; Erik F. Blom, MD, PhD; Daniel E. Jonas, MD, MPH; Eric J. Feuer, PhD; Sylvia K. Plevritis, PhD; Harry J. de Koning, MD, PhD; Chung Yin Kong, PhD JAMA
- US Preventive Services Task Force USPSTF Evidence Report: Screening for Lung Cancer US Preventive Services Task Force; Alex H. Krist, MD, MPH; Karina W. Davidson, PhD, MASc; Carol M. Mangione, MD, MSPH; Michael J. Barry, MD; Michael Cabana, MD, MA, MPH; Aaron B. Caughey, MD, PhD; Esa M. Davis, MD, MPH; Katrina E. Donahue, MD, MPH; Chyke A. Doubeni, MD, MPH; Martha Kubik, PhD, RN; C. Seth Landefeld, MD; Li Li, MD, PhD, MPH; Gbenga Ogedegbe, MD, MPH; Douglas K. Owens, MD, MS; Lori Pbert, PhD; Michael Silverstein, MD, MPH; James Stevermer, MD, MSPH; Chien-Wen Tseng, MD, MPH, MSEE; John B. Wong, MD JAMA
- JAMA Patient Page Patient Information: Screening for Lung Cancer Jill Jin, MD, MPH JAMA
Importance Lung cancer is the leading cause of cancer-related death in the US.
Objective To review the evidence on screening for lung cancer with low-dose computed tomography (LDCT) to inform the US Preventive Services Task Force (USPSTF).
Data Sources MEDLINE, Cochrane Library, and trial registries through May 2019; references; experts; and literature surveillance through November 20, 2020.
Study Selection English-language studies of screening with LDCT, accuracy of LDCT, risk prediction models, or treatment for early-stage lung cancer.
Data Extraction and Synthesis Dual review of abstracts, full-text articles, and study quality; qualitative synthesis of findings. Data were not pooled because of heterogeneity of populations and screening protocols.
Main Outcomes and Measures Lung cancer incidence, lung cancer mortality, all-cause mortality, test accuracy, and harms.
Results This review included 223 publications. Seven randomized clinical trials (RCTs) (N = 86 486) evaluated lung cancer screening with LDCT; the National Lung Screening Trial (NLST, N = 53 454) and Nederlands-Leuvens Longkanker Screenings Onderzoek (NELSON, N = 15 792) were the largest RCTs. Participants were more likely to benefit than the US screening-eligible population (eg, based on life expectancy). The NLST found a reduction in lung cancer mortality (incidence rate ratio [IRR], 0.85 [95% CI, 0.75-0.96]; number needed to screen [NNS] to prevent 1 lung cancer death, 323 over 6.5 years of follow-up) with 3 rounds of annual LDCT screening compared with chest radiograph for high-risk current and former smokers aged 55 to 74 years. NELSON found a reduction in lung cancer mortality (IRR, 0.75 [95% CI, 0.61-0.90]; NNS to prevent 1 lung cancer death of 130 over 10 years of follow-up) with 4 rounds of LDCT screening with increasing intervals compared with no screening for high-risk current and former smokers aged 50 to 74 years. Harms of screening included radiation-induced cancer, false-positive results leading to unnecessary tests and invasive procedures, overdiagnosis, incidental findings, and increases in distress. For every 1000 persons screened in the NLST, false-positive results led to 17 invasive procedures (number needed to harm, 59) and fewer than 1 person having a major complication. Overdiagnosis estimates varied greatly (0%-67% chance that a lung cancer was overdiagnosed). Incidental findings were common, and estimates varied widely (4.4%-40.7% of persons screened).
Conclusions and Relevance Screening high-risk persons with LDCT can reduce lung cancer mortality but also causes false-positive results leading to unnecessary tests and invasive procedures, overdiagnosis, incidental findings, increases in distress, and, rarely, radiation-induced cancers. Most studies reviewed did not use current nodule evaluation protocols, which might reduce false-positive results and invasive procedures for false-positive results.
In 2020, lung cancer was the second most common cancer and the leading cause of cancer-related death in both men and women in the US. 1 Most patients diagnosed with lung cancer presented with distant or metastatic disease; less than 20% were diagnosed with localized (ie, stage 1) disease. 1 Lung cancer has traditionally been classified into 2 major categories: (1) non–small cell lung cancer (NSCLC) (eTable 1 in the Supplement ), which collectively comprises adenocarcinoma, squamous cell carcinoma, and large cell carcinoma, and (2) small cell lung cancer, which is more aggressive and has worse survival rates. 2 Approximately 85% of lung cancers are NSCLC. 3 The risk of developing lung cancer is largely driven by age and smoking status, with smoking estimated to account for nearly 90% of all lung cancers. 4 - 6 Other risk factors for lung cancer include environmental exposures, radiation therapy, other (noncancer) lung diseases, race/ethnicity, and family history. 7
In 2013, the US Preventive Services Task Force (USPSTF) recommended annual screening for lung cancer with low-dose computed tomography (LDCT) in adults aged 55 to 80 years who have a 30–pack-year smoking history and currently smoke or have quit within the past 15 years (B recommendation). 8 The USPSTF recommended that screening should be discontinued once a person has not smoked for 15 years or develops a health problem that substantially limits life expectancy or the ability or willingness to have curative lung surgery. 8 This updated review evaluates the current evidence on screening for lung cancer with LDCT for populations and settings relevant to primary care in the US to inform an updated recommendation by the USPSTF.
Figure 1 shows the analytic framework and key questions (KQs) that guided the review. Detailed methods are available in the full evidence report. 7
PubMed/MEDLINE and the Cochrane Library were searched for English-language articles published through May 2019. Search strategies are listed in the eMethods in the Supplement . Clinical trial registries were searched for unpublished studies. To supplement electronic searches, investigators reviewed reference lists of pertinent articles, studies suggested by reviewers, and comments received during public commenting periods. Since May 2019, ongoing surveillance was conducted through article alerts and targeted searches of journals to identify major studies published in the interim that may affect the conclusions or understanding of the evidence and the related USPSTF recommendation. The last surveillance was conducted on November 20, 2020.
Two investigators independently reviewed titles, abstracts, and full-text articles to determine eligibility using prespecified criteria (eTable 2 in the Supplement ). Disagreements were resolved by discussion and consensus. English-language studies of adults aged 18 years or older conducted in countries categorized as “very high” on the Human Development Index, 9 rated as fair or good quality, and published in or after 2001 were included. For all KQs, randomized clinical trials (RCTs) and nonrandomized controlled intervention studies were eligible. Cohort studies based on prospectively collected data that were intended to be used for evaluations relevant to this review were also eligible for KQs on harms of screening or workup (KQs 4 and 5) and treatment (KQs 6 and 7).
For KQ2 (on risk prediction), externally validated models aimed at identifying persons at increased risk of lung cancer using multiple variables, including at least age and smoking history, were included. Eligible risk prediction models had to be compared with either the 2013 USPSTF recommendations or criteria used by trials showing benefit. Eligible outcomes included estimated screen-preventable lung cancer deaths or all-cause mortality, estimated screening effectiveness (eg, number needed to screen [NNS]), and estimated screening harms.
For each included study, 1 investigator extracted pertinent information about the populations, tests or treatments, comparators, outcomes, settings, and designs, and a second investigator reviewed this information for completeness and accuracy. Two independent investigators assessed the quality of studies as good, fair, or poor, using predefined criteria developed by the USPSTF and adapted for this topic. 10 Disagreements were resolved by discussion.
Findings for each KQ were summarized in tabular and narrative format. The overall strength of the evidence for each KQ was assessed as high, moderate, low, or insufficient based on the overall quality of the studies, consistency of results between studies, precision of findings, risk of reporting bias, and limitations of the body of evidence, using methods developed for the USPSTF (and the Evidence-based Practice Center program). 10 Additionally, the applicability of the findings to US primary care populations and settings was assessed. Discrepancies were resolved through discussion.
To determine whether meta-analyses were appropriate, the clinical and methodological heterogeneity of the studies was assessed according to established guidance. 11 Meta-analyses were not conducted because of substantial clinical and methodological heterogeneity. For example, the trials of lung cancer screening differed in eligibility criteria (eg, age, pack-years of smoking, years since quitting), number of screening rounds (from 2 to 5), screening intervals (eg, annual, biennial, or escalating), thresholds for a positive screen (eg, 4 mm, 5 mm, or based on volume), and comparators (chest radiograph or no screening). For KQ1, forest plots were created to display the findings of each study by calculating incidence rate ratios (IRRs), using number of events and person-years of follow-up, for lung cancer incidence, lung cancer mortality, and all-cause mortality. Quantitative analyses were conducted using Stata version 14 (StataCorp).
A total of 223 publications were included ( Figure 2 ). Twenty-six articles addressed whether screening improves health outcomes. Most articles assessed accuracy, harms, or effectiveness of surgery or stereotactic body radiotherapy for early NSCLC. Results for KQs 6, 7, and 8 are in the eResults in the Supplement . Individual study quality ratings are reported in eTables 3 to 13 in the Supplement .
Key Question 1a. Does screening for lung cancer with LDCT change the incidence of lung cancer and the distribution of lung cancer types and stages (ie, stage shift)?
Key Question 1b. Does screening for lung cancer with LDCT change all-cause mortality, lung cancer mortality, or quality of life?
Key Question 1c. Does the effectiveness of screening for lung cancer with LDCT differ for subgroups defined by age, sex, race/ethnicity, presence of comorbid conditions, or other lung cancer risk factors?
Key Question 1d. Does the effectiveness of screening for lung cancer with LDCT differ by the number or frequency of LDCT scans (eg, annual screening for 3 years, the protocol used in the National Lung Screening Trial [NLST] vs other approaches)?
Seven RCTs (described in 26 articles) were included ( Table 1 ): NLST, Detection and Screening of Early Lung Cancer by Novel Imaging Technology and Molecular Essays (DANTE), Danish Lung Cancer Screening Trial (DLCST), Italian Lung Cancer Screening Trial (ITALUNG), Lung Screening Study (LSS), the German Lung Cancer Screening Intervention Trial (LUSI), and the Nederlands-Leuvens Longkanker Screenings Onderzoek (NELSON) study. 12 - 37 Two trials in the US compared LDCT with chest radiography (LSS and NLST), and 5 trials in Europe compared LDCT with no screening (DANTE, DLCST, ITALUNG, LUSI, and NELSON). Only the NLST (53 454 participants) and NELSON (15 792 participants) were adequately powered to assess for lung cancer mortality benefit. 24 , 31 The majority of participants were White in all trials; in the NLST, 91% were White, less than 5% were Black, and less than 2% were Hispanic or Latino.
Trials varied in their definition of a positive screen and in the follow-up evaluation process. NELSON was unique in using volumetric measurements of nodules and calculating volume doubling. Compared with the prior systematic review conducted for the USPSTF, 38 , 39 longer follow-up or more complete end point verification was available from DANTE, 12 DLCST, 16 LSS, 20 and the NLST, 33 , 37 and 3 additional trials—NELSON, 24 ITALUNG, 17 and LUSI 21 , 23 —reported data relevant to this KQ.
The cumulative incidence of lung cancer was higher in LDCT groups than in control groups for all studies except ITALUNG (eFigure in the Supplement ). Figure 3 shows the increases in early-stage (I-II) and decreases in late-stage (III-IV) lung cancer incidence.
Figure 4 shows the calculated IRRs for the trials that reported lung cancer mortality. Over almost 7 years of follow-up and more than 140 000 person-years of follow-up in each group, the NLST found a significant reduction in lung cancer mortality with 3 rounds of annual LDCT screening compared with chest radiography (calculated IRR, 0.85 [95% CI, 0.75-0.96]). These findings indicate an NNS to prevent 1 lung cancer death of 323 over 6.5 years of follow-up. Analysis of extended follow-up data of NLST participants at 12.3 years after randomization found a similar absolute difference between groups (1147 vs 1236 lung cancer deaths; risk ratio [RR], 0.92 [95% CI, 0.85-1.00]; absolute difference between groups of 3.3 [95% CI, −0.2 to 6.8] lung cancer deaths per 1000 participants). The NELSON trial reported a reduction in lung cancer mortality for 4 rounds of screening with increasing intervals between LDCTs (combining data for males and females, calculated IRR, 0.75 [95% CI, 0.61-0.90]; NNS to prevent 1 lung cancer death of 130 over 10 years of follow-up). Results of the other trials were very imprecise and did not show statistically significant differences between groups ( Figure 4 ).
The NLST found a reduction in all-cause mortality with LDCT screening compared with chest radiography (1912 vs 2039 deaths; 1141 per 100 000 person-years vs 1225 per 100 000 person-years; calculated IRR, 0.93 [95% CI, 0.88-0.99]). The other trials found no statistically significant differences between groups, but results were imprecise ( Figure 5 ).
All included trials enrolled participants at high risk for lung cancer (based on age and smoking history). Seven publications using DLCST, LUSI, NELSON, or NLST data described subgroup analyses for age, sex, race/ethnicity, smoking status and pack-years, history of chronic obstructive pulmonary disease (COPD), or other pulmonary conditions. 16 , 23 , 24 , 33 - 35 , 37 A post hoc analysis of NLST data reported that 88% of the benefit (lung cancer deaths averted) was achieved by screening the 60% of participants at highest risk for lung cancer death. 29 Other post hoc analyses of NLST data reported lung cancer mortality by sex (RR, 0.73 for women vs 0.92 for men; P = .08), age (RR, 0.82 for <65 years vs 0.87 for ≥65 years; P = .60), race/ethnicity (hazard ratio [HR], 0.61 for Black individuals vs 0.86 for White individuals; P = .29), and smoking status (RR, 0.81 for current smokers vs 0.91 for former smokers; P = .40), and did not identify statistically significant differences between groups. 33 - 35 A long-term follow-up of NLST participants at 12.3 years reported similar results for subgroups and did not identify statistically significant interactions by sex, age, or smoking status (sex: RR, 0.86 for women vs 0.97 for men, P = .17; age: RR, 0.86 for <65 years vs 1.01 for ≥65 years, P = .051; smoking status: RR, 0.88 for current smokers vs 1.01 for former smokers, P = .12). 37 Both LUSI and NELSON reported a similar pattern for subgroups by sex as found in the NLST that was not statistically significantly different between groups (LUSI: women, HR, 0.31 [95% CI, 0.10-0.96] vs men, HR, 0.94 [95% CI, 0.54-1.61], P = .09) or without reporting an interaction test (NELSON: women, RR, 0.67 [95% CI, 0.38-1.14] vs men, RR, 0.76 [95% CI, 0.61-0.94] at 10 years of follow-up). 23 , 24 NELSON reported analyses by age group among the men in the trial (not including the women in those analyses) but did not report interaction tests for subgroups defined by age (RRs ranged from 0.59 [95% CI, 0.35-0.98] for persons aged 65 to 69 years at randomization to 0.85 [95% CI, 0.48-1.50] for persons aged 50 to 54 years at randomization). 24
Key Question 2. Does the use of risk prediction models for identifying adults at higher risk of lung cancer mortality improve the balance of benefits and harms of screening compared with the use of trial eligibility criteria (eg, NLST criteria) or the 2013 USPSTF recommendations?
Detailed results for this KQ are in eResults and eTables 14-16 in the Supplement . In summary, 4 studies of 3 different risk prediction models (a modified version of a model developed from participants of the Prostate, Lung, Colorectal, and Ovarian Cancer Screening Trial [PLCOm2012], the Lung Cancer Death Risk Assessment Tool [LCDRAT], and Kovalchik model) estimating outcomes in 4 different cohorts reported increased screen-preventable deaths compared with the risk factor–based criteria used by the NLST or USPSTF (in the 2013 recommendations). Three studies demonstrated improved screening efficiency (determined by the NNS) of risk prediction models compared with risk factor−based screening, while 1 study showed mixed results. For harms, 8 studies of 13 different risk prediction models (PLCOm2012, simplified PLCOm2012, Bach, Liverpool Lung Project [LLP], simplified LLP, Knoke, Two-Stage Clonal Expansion [TSCE] incidence, TSCE Cancer Prevention Study death, TSCE Nurses’ Health Study/Health Professionals Follow-up Study, the HUNT Lung Cancer model, LCDRAT, COPD-LUCSS [Lung Cancer Screening Score], Kovalchik) estimating outcomes in 4 different cohorts reported similar numbers of false-positive selections from risk prediction (ie, the risk prediction model selected persons to be screened who did not have or develop lung cancer) and mixed findings for rates of false-positive selections when comparing risk prediction models with the risk factor–based criteria used by the NLST or USPSTF. In general, estimates were consistent but imprecise, primarily because of a lack of an established risk threshold to apply the model.
Key Question 3a. What is the accuracy of screening for lung cancer with LDCT?
Key Question 3b. Does the accuracy of screening for lung cancer with LDCT differ for subgroups defined by age, sex, race/ethnicity, presence of comorbid conditions, or other lung cancer risk factors?
Key Question 3c. Does the accuracy of screening for lung cancer with LDCT differ for various approaches to nodule classification (ie, those based on nodule size and characteristics)?
Detailed results for this KQ are in eResults and eTables 17 and 18 in the Supplement . Fifty-three articles were eligible for this KQ. 12 , 13 , 19 , 21 , 22 , 24 - 28 , 30 - 32 , 34 , 40 - 77 Of those, 24 publications with the most complete data are described. 12 , 21 , 24 , 34 , 41 , 43 - 45 , 47 - 49 , 52 , 53 , 56 , 58 , 60 , 64 , 66 - 68 , 71 , 72 , 75 , 76 Sensitivity of LDCT from 13 studies (76 856 total participants) ranged from 59% to 100%; all but 3 studies reported sensitivity greater than 80%. Specificity of LDCT from 13 studies (75 819 total participants) ranged from 26.4% to 99.7%; all but 3 reported specificity greater than 75%. Positive predictive value (14 studies, 77 840 participants) ranged from 3.3% to 43.5%. Negative predictive value (9 studies, 47 496 participants) ranged from 97.7% to 100%. Variability in accuracy was mainly attributed to heterogeneity of eligibility criteria, screening protocols (eg, number of screening rounds, screening intervals), follow-up length (eg, to identify false-negative screens), and definitions (eg, of positive tests, indeterminate tests). Three studies (73 404 participants) compared various approaches to nodule classification (Lung-RADS or International Early Lung Cancer Action Program [I-ELCAP]) and found that using Lung-RADS in the NLST would have increased specificity while decreasing sensitivity and that increases in positive predictive value are seen with increasing nodule size thresholds. 44 , 49 , 52
Key Question 4a. What are the harms associated with screening for lung cancer with LDCT?
Key Question 4b. Do the harms of screening for lung cancer with LDCT differ with the use of Lung-RADS, I-ELCAP, or similar approaches (eg, to reduce false-positive results)?
Key Question 4c. Do the harms of screening for lung cancer with LDCT differ for subgroups defined by age, sex, race/ethnicity, presence of comorbid conditions, or other lung cancer risk factors?
Key Question 5a. What are the harms associated with workup or surveillance of nodules?
Key Question 5b. Do the harms of workup or surveillance of nodules differ with the use of Lung-RADS, I-ELCAP, or similar approaches (eg, to reduce false-positive results)?
Key Question 5c. Do the harms of workup or surveillance of nodules differ for subgroups defined by age, sex, race/ethnicity, presence of comorbid conditions, or other lung cancer risk factors?
Detailed results are in eResults in the Supplement .
Nine publications reported on radiation associated with LDCT. 16 , 31 , 56 , 69 , 75 , 78 - 81 Most of those reported the radiation associated with 1 LDCT, with ranges from 0.65 mSv to 2.36 mSv (eTable 19 in the Supplement ). Two of the studies evaluated the cumulative radiation exposure for participants undergoing screening with LDCT 78 , 80 ; using those studies to estimate cumulative exposure for an individual from 25 years of annual screening (ie, from age 55 to 80 years as recommended by the USPSTF in 2013) yields 20.8 mSv to 32.5 mSv. One study estimated the lifetime risk of cancer from radiation of 10 annual LDCTs was 0.26 to 0.81 major cancers for every 1000 people screened. 80
Twenty-seven publications reported enough information to determine the rate of false-positives, defined as any result leading to additional evaluation (eg, repeat LDCT scan before the next annual screening, biopsy) that did not result in a diagnosis of cancer. 15 , 18 , 19 , 21 , 24 , 30 - 32 , 34 , 40 , 46 , 47 , 49 , 52 , 56 , 62 , 65 - 68 , 73 , 75 - 77 , 82 - 84 False-positive rates varied widely across studies, most likely because of differences in definitions of positive results, such as cutoffs for nodule size (eg, 4 mm vs 5 mm vs 6 mm), use of volume-doubling time, and nodule characteristics considered. The range of false-positive rates overall was 7.9% to 49.3% for baseline screening and 0.6% to 28.6% for individual incidence screening rounds, although rates for some subgroups were higher (eg, age ≥65 years) (eTable 20 in the Supplement ). False-positive rates generally declined with each screening round. 34 , 47 , 65 , 66 , 73 , 76
Among the trials that found lung cancer screening mortality benefit and cohort studies based in the US, false-positive rates were 9.6% to 28.9% for baseline and 5.0% to 28.6% for incidence rounds. The NLST reported false-positive rates for baseline, year 1, and year 2 of 26.3%, 27.2%, and 15.9%, respectively. 31 The NELSON trial noted false-positive rates of 19.8% at baseline, 7.1% at year 1, 9.0% for males at year 3, and 3.9% for males at year 5.5 of screening. 24 , 65 One study of 112 radiologists from 32 screening centers who each interpreted 100 or more NLST scans reported a mean (SD) false-positive rate of 28.7% (13.7) (range, 3.8%-69.0%). 46 Mean rates were similar for academic (n = 25) and nonacademic (n = 7) centers (27.9% vs 26.7%, respectively). 46 An implementation study through the Veterans Health Administration revealed a false-positive rate of 28.9% of veterans eligible for screening (58% of those who were actually screened) at baseline screening. 40 False-positive rates varied across 8 study sites, ranging from 12.6% to 45.8% of veterans eligible for screening. 40
Fourteen studies reported on the evaluation of false-positive results. 22 , 30 , 31 , 34 , 43 , 62 - 64 , 66 , 72 , 75 , 79 , 81 , 85 Among all patients screened, the percentage who had a needle biopsy for a false-positive result ranged from 0.09% to 0.56% (eTable 21 in the Supplement ). Surgical procedures for false-positive results were reported in 0.5% to 1.3% and surgical resections for false-positive results were reported in 0.1% to 0.5% of all screened participants.
In the NLST, false-positive results led to invasive procedures (needle biopsy, thoracotomy, thoracoscopy, mediastinoscopy, and bronchoscopy) in 1.7% of those screened (number needed to harm, 59). Complications occurred in 0.1% of those screened (number needed to harm, 1000), with major, intermediate, and minor complications occurring in 0.03%, 0.05%, and 0.01%, respectively, of those screened. Death in the 60 days following the most invasive procedure performed occurred in 0.007% of those screened. 31 One study using NLST data estimated that 117 invasive procedures for false-positive results (23.4% of all invasive procedures for false-positive results from the NLST) would be prevented by using Lung-RADS criteria. 44
Five studies specifically examined overdiagnosis, 81 , 86 - 89 and 7 additional trials were examined for differences in cancer incidence between LDCT and comparison groups. 14 , 17 , 19 , 24 , 31 , 90 , 91 Estimates of overdiagnosis ranged from 0% to 67.2% that a screen-detected lung cancer is overdiagnosed.
One RCT (DLCST; 4075 participants), studies of participants from RCTs (NELSON, NLST, LSS; 19 426 total participants), and 3 cohort studies (ELCAP, Mayo Lung Project, and Pittsburgh Lung Screening Study [PLuSS]; 5537 total participants) included evaluations of the effect of LDCT screening or screening results on smoking cessation and relapse. 91 - 100 Studies comparing LDCT vs controls (no screening or chest radiography) for smoking cessation or abstinence outcomes do not indicate that screening leads to false reassurance. Abnormal or indeterminate screening test results may increase cessation and continued abstinence, but normal screening test results had no influence. Regarding smoking intensity, evidence was minimal, and no study showed influence of screening or test result on smoking intensity.
Four RCTs (DLCST, NELSON, NLST, and UK Lung Cancer Screening [UKLS] trial; 12 096 total participants) reported in 6 publications, 62 , 101 - 105 1 uncontrolled cohort study (PLuSS, 400 participants), 106 and 2 studies of participants from the screening arm of an RCT (NELSON, 630 participants 107 ; UKLS, 1589 participants 108 ) included an evaluation of potential psychosocial consequences of LDCT screening. These studies evaluated general health-related quality of life (HRQoL; 3 studies), 101 , 104 , 107 anxiety (8 studies), 62 , 101 - 107 depression (2 studies), 62 , 102 distress (3 studies), 62 , 104 , 107 and other psychosocial consequences of LDCT screening (5 studies). 62 , 103 , 105 , 106 , 108 Taken together, there is moderate evidence to suggest that, compared with no screening, persons who receive LDCT screening do not have worse general HRQoL, anxiety, or distress over 2 years of follow-up. Some evidence suggests differential consequences by screening result such that general HRQoL and anxiety were worse, at least in the short-term, for individuals who received true-positive results compared with other screening results; distress was worse for participants who received an indeterminate screening result compared with other results. The strength of evidence is low for other psychosocial consequences, largely because of unknown consistency, imprecision, and only 1 or 2 studies assessed outcomes.
Studies reported a wide range of screening-related incidental findings (4.4% to 40.7%) that were deemed significant or requiring further evaluation (eResults and eTable 22 in the Supplement ). 34 , 40 , 62 , 82 , 109 - 112 Rates varied considerably in part because there was no consistent definition of what constitutes an incidental finding nor which findings were “actionable” or “clinically significant.” Older age was associated with a greater likelihood of incidental findings. Common incidental findings included coronary artery calcification, aortic aneurysms, emphysema, infectious and inflammatory processes, masses, nodules, or cysts of the kidney, breast, adrenal, liver, thyroid, pancreas, spine, and lymph nodes. Incidental findings led to downstream evaluation, including consultations, additional imaging, and invasive procedures with associated costs and burdens.
This evidence review evaluated screening for lung cancer with LDCT in populations and settings relevant to US primary care; a summary of the evidence is provided in Table 2 . Screening high-risk persons with LDCT can reduce lung cancer mortality but also causes a range of harms. For benefits of screening, the NLST demonstrated a reduction in lung cancer mortality and all-cause mortality with 3 rounds of annual LDCT screening compared with chest radiography, and the NELSON trial demonstrated a reduction in lung cancer mortality with 4 rounds of LDCT screening with increasing intervals. Harms of screening include false-positive results leading to unnecessary tests and invasive procedures, overdiagnosis, incidental findings, short-term increases in distress because of indeterminate results, and, rarely, radiation-induced cancer.
NLST and NELSON results are generally applicable to high-risk current and former smokers aged 50 to 74 years, but participants were younger, more highly educated, less likely to be current smokers than the US screening-eligible population, and had limited racial and ethnic diversity. The general US population eligible for lung cancer screening may be less likely to benefit from early detection compared with NLST and NELSON participants because they face a high risk of death from competing causes, such as heart disease and stroke. 113 Data from the 2012 Health and Retirement Study showed a lower 5-year survival rate and life expectancy in screening-eligible persons compared with NLST participants. 113 NELSON did not allow enrollment of persons with moderate or severe health problems and an inability to climb 2 flights of stairs; weight over 140 kg; or current or past kidney cancer, melanoma, or breast cancer.
The trials were mainly conducted at large academic centers, potentially limiting applicability to community-based practice (eg, because of challenges with implementation [eContextual Questions in the Supplement ], level of multidisciplinary expertise). Many of the trial centers are well recognized for expertise in thoracic radiology as well as cancer diagnosis and treatment. 31 The NLST noted that mortality associated with surgical resection was much lower in the trial than that reported for the US population (1% vs 4%). 31 , 114
Guidelines recommend that clinicians conduct a rigorous process of informed and shared decision-making about the benefits and harms of lung cancer screening before initiating screening. However, given the complex nature of benefits and harms associated with screening, there is some concern that robust shared decision-making is impractical to implement in actual practice. 115 - 117 eContextual question 1 in the Supplement describes the barriers to implementing lung cancer screening and surveillance in clinical practice in the US.
Most studies reviewed in this article (including the NLST) did not use current nodule evaluation protocols such as Lung-RADS (endorsed by the American College of Radiology). 118 A study included in this review estimated that Lung-RADS would reduce false-positive results compared with NLST criteria and that about 23% of all invasive procedures for false-positive results from the NLST would have been prevented by using Lung-RADS criteria. 44
Application of lung cancer screening with (1) current nodule management protocols and (2) the use of risk prediction models might improve the balance of benefits and harms, although the strength of evidence supporting this possibility was low. There remains considerable uncertainty about how such approaches would perform in actual practice because the evidence was largely derived from post hoc application of criteria to trial data (for Lung-RADS) and from modeling studies (for risk prediction) and does not include prospective clinical utility studies. Additional discussion of the evidence on risk prediction models is provided in the eDiscussion in the Supplement . When applied to current clinical practice, lung cancer screening programs have demonstrated significant variation, even within a single institution type. 40
This review has several limitations. First, non–English-language articles were excluded, as were studies with sample size less than 500 or 1000 for some KQs to focus on the best evidence. Doing so omitted some smaller studies that reported on harms of screening. For example, a study of 351 participants in the NELSON trial examined discomfort of LDCT scanning and waiting for the LDCT results. 119 Most participants (88%-99%) reported experiencing no discomfort related to the LDCT scan, but about half reported at least some discomfort from waiting for the result (46%) and dreading the result (51%). Second, the KQ on risk prediction models (KQ2) was limited to how well risk prediction models perform vs current recommended risk factor–based criteria for lung cancer screening. KQ2 complements the decision analysis report 120 by evaluating previously published studies that apply risk prediction models to cohorts or representative samples of the US population rather than simulated populations. Third, for accuracy, some included studies did not report accuracy metrics; rather, when sufficient data were reported, values were calculated from the study data. This approach introduces uncertainty and may account for variability.
Screening high-risk persons with LDCT can reduce lung cancer mortality but also causes false-positive results leading to unnecessary tests and invasive procedures, overdiagnosis, incidental findings, increases in distress, and, rarely, radiation-induced cancers. Most studies reviewed did not use current nodule evaluation protocols, which might reduce false-positive results and invasive procedures for false-positive results.
Corresponding Author: Daniel E. Jonas, MD, MPH, Department of Internal Medicine, Division of General Internal Medicine, The Ohio State University, 2050 Kenny Rd, Columbus, OH 43221 ( [email protected] ).
Accepted for Publication: January 19, 2021.
Author Contributions: Dr Jonas had full access to all of the data in the study and takes responsibility for the integrity of the data and the accuracy of the data analysis.
Concept and design: Jonas, Reuland, Nagle, Clark, Weber, Enyioha, Armstrong, Voisin.
Acquisition, analysis, or interpretation of data: Jonas, Reuland, Reddy, Nagle, Clark, Weber, Enyioha, Malo, Brenner, Armstrong, Coker-Schwimmer, Middleton, Harris.
Drafting of the manuscript: Jonas, Reuland, Reddy, Nagle, Clark, Enyioha, Malo, Brenner, Armstrong, Middleton, Voisin.
Critical revision of the manuscript for important intellectual content: Jonas, Reuland, Reddy, Weber, Brenner, Coker-Schwimmer, Harris.
Statistical analysis: Jonas, Reddy, Weber, Enyioha, Middleton.
Obtained funding: Jonas.
Administrative, technical, or material support: Jonas, Reddy, Clark, Weber, Armstrong, Middleton, Voisin.
Supervision: Jonas, Reuland, Armstrong, Harris.
Conflict of Interest Disclosures: Dr Jonas reported receiving a contract from the Agency for Healthcare Research and Quality (AHRQ) during the conduct of the study. Dr Reuland reported receiving a contract from the AHRQ to complete review during the conduct of the study. Dr Reddy reported receiving a contract from the AHRQ to complete review during the conduct of the study. Dr Clark reported receiving a contract from the AHRQ to complete review during the conduct of the study. Dr Weber reported receiving a contract from the AHRQ to conduct review during the conduct of the study. Dr Malo reported receiving a contract from the AHRQ to complete review during the conduct of the study. Dr Armstrong reported receiving a contract from the AHRQ to complete review during the conduct of the study. Dr Coker-Schwimmer reported receiving a contract from the AHRQ, which funded the research under contract No. HHSA-290-2015-00011-I, Task Order No. 11, during the conduct of the study. Dr Middleton reported receiving a contract from the AHRQ to complete review during the conduct of the study. Dr Voisin reported receiving a contract from the AHRQ to complete review during the conduct of the study. No other disclosures were reported.
Funding/Support: This research was funded under contract HHSA-290-2015-00011-I, Task Order 11, from the AHRQ, US Department of Health and Human Services, under a contract to support the US Preventive Services Task Force (USPSTF).
Role of the Funder/Sponsor: Investigators worked with USPSTF members and AHRQ staff to develop the scope, analytic framework, and key questions for this review. AHRQ had no role in study selection, quality assessment, or synthesis. AHRQ staff provided project oversight, reviewed the report to ensure that the analysis met methodological standards, and distributed the draft for public comment and review by federal partners. Otherwise, AHRQ had no role in the conduct of the study; collection, management, analysis, and interpretation of the data; and preparation, review, or approval of the manuscript findings. The opinions expressed in this document are those of the authors and do not reflect the official position of AHRQ or the US Department of Health and Human Services.
Additional Contributions: We gratefully acknowledge the following individuals for their contributions to this project, including AHRQ staff (Howard Tracer, MD, and Tracy Wolff, MD, MPH), RTI International–University of North Carolina at Chapel Hill Evidence-based Practice Center staff (Carol Woodell, BSPH; Sharon Barrell, MA; and Loraine Monroe), and expert consultant M. Patricia Rivera, MD, professor of medicine, Division of Pulmonary Diseases and Critical Care Medicine at University of North Carolina at Chapel Hill. The USPSTF members, expert consultants, peer reviewers, and federal partner reviewers did not receive financial compensation for their contributions. Ms Woodell, Ms Barrell, and Ms Monroe received compensation for their roles in this project.
Additional Information: A draft version of the full evidence report underwent external peer review from 5 content experts (Deni Aberle, MD, UCLA Medical Center; Peter Bach, MD, MAPP, Memorial Sloan-Kettering Cancer Center; Tanner Caverly, MD, University of Michigan; Michael Jaklitsch, MD, Brigham and Women’s Hospital; and Renda Soylemez Wiener, MD, MPH, US Department of Veteran’s Affairs, Boston University School of Medicine) and 3 federal partner reviewers (from the Centers for Disease Control and Prevention, the National Cancer Institute, and the National Institute of Nursing Research). Comments from reviewers were presented to the USPSTF during its deliberation of the evidence and were considered in preparing the final evidence review. USPSTF members and peer reviewers did not receive financial compensation for their contributions.
Editorial Disclaimer: This evidence report is presented as a document in support of the accompanying USPSTF Recommendation Statement. It did not undergo additional peer review after submission to JAMA .
- Register for email alerts with links to free full-text articles
- Access PDFs of free articles
- Manage your interests
- Save searches and receive search alerts
Advances in Lung Cancer Research
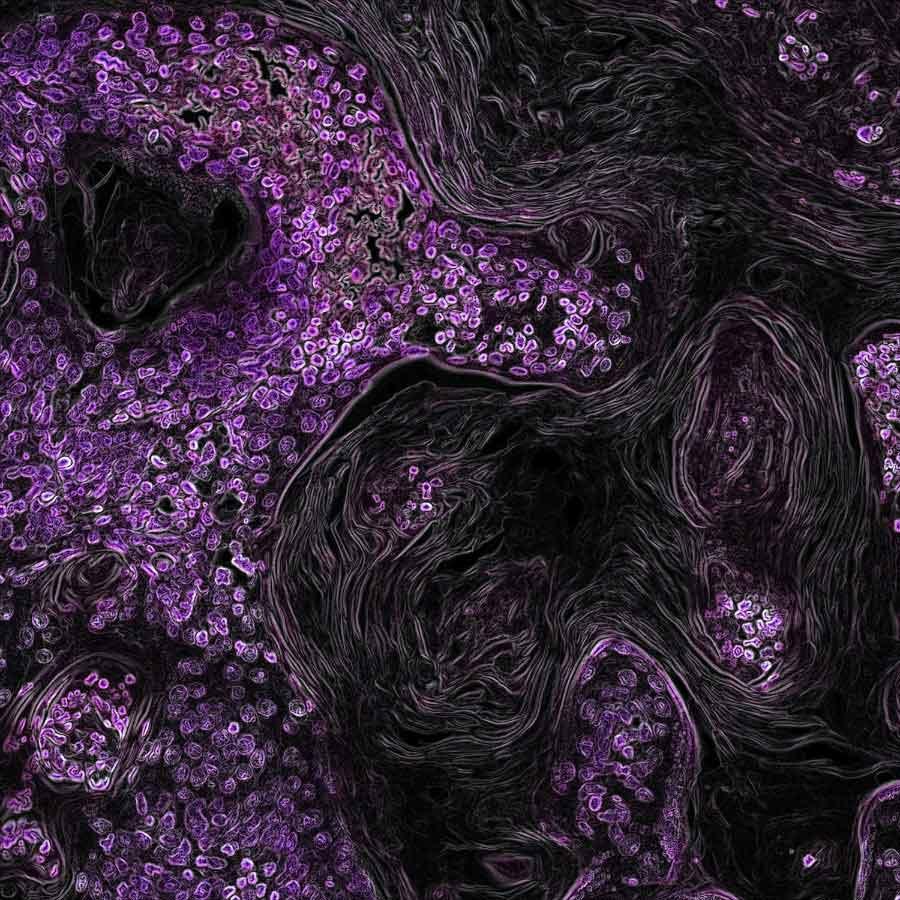
Lung cancer cells driven by the KRAS oncogene, which is highlighted in purple.
NCI-funded researchers are working to advance our understanding of how to prevent, detect, and treat lung cancer. In particular, scientists have made progress in identifying many different genetic alterations that can drive lung cancer growth.
This page highlights some of the latest research in non-small cell lung cancer (NSCLC), the most common form of lung cancer, including clinical advances that may soon translate into improved care, NCI-supported programs that are fueling progress, and research findings from recent studies.
Early Detection of Lung Cancer
A great deal of research has been conducted in ways to find lung cancer early. Several methods are currently being studied to see if they decrease the risk of dying from lung cancer.
The NCI-sponsored National Lung Screening Trial (NLST) showed that low-dose CT scans can be used to screen for lung cancer in people with a history of heavy smoking. Using this screening can decrease their risk of dying from lung cancer. Now researchers are looking for ways to refine CT screening to better predict whether cancer is present.
Markers in Blood and Sputum
Scientists are trying to develop or refine tests of sputum and blood that could be used to detect lung cancer early. Two active areas of research are:
- Analyzing blood samples to learn whether finding tumor cells or molecular markers in the blood will help diagnose lung cancer early.
- Examining sputum samples for the presence of abnormal cells or molecular markers that identify individuals who may need more follow-up.
Machine Learning
Machine learning is a method that allows computers to learn how to predict certain outcomes. In lung cancer, researchers are using computer algorithms to create computer-aided programs that are better able to identify cancer in CT scans than radiologists or pathologists. For example, in one artificial intelligence study , researchers trained a computer program to diagnose two types of lung cancer with 97% accuracy, as well as detect cancer-related genetic mutations.
Lung Cancer Treatment
Treatment options for lung cancer are surgery , radiation , chemotherapy , targeted therapy , immunotherapy , and combinations of these approaches. Researchers continue to look for new treatment options for all stages of lung cancer.
Treatments for early-stage lung cancer
Early-stage lung cancer can often be treated with surgery. Researchers are developing approaches to make surgery safer and more effective.
- When lung cancer is found early, people usually have surgery to remove an entire section ( lobe ) of the lung that contains the tumor. However, a recent clinical trial showed that, for certain people with early-stage NSCLC, removing a piece of the affected lobe is as effective as surgery to remove the whole lobe .
- The targeted therapy Osimertinib (Tagrisso ) was approved by the Food and Drug Administration (FDA) in 2021 to be given after surgery—that is, as adjuvant therapy —to people with early-stage NSCLC that has certain mutations in the EGFR gene.
- Two immunotherapy drugs, atezolizumab (Tecentriq) and pembrolizumab (Keytruda) have been approved by the FDA to be used as adjuvant treatments after surgery and chemotherapy, for some patients with early-stage NSCLC.
- The immunotherapy drug nivolumab (Opdivo) is approved to be used, together with chemotherapy, to treat patients with early-stage lung cancer before surgery (called neoadjuvant ). This approval, which came in 2022, was based on the results of the CheckMate 816 trial, which showed that patients at this stage who received neoadjuvant nivolumab plus chemotherapy lived longer than those who received chemotherapy alone .
- In another trial (Keynote-671), patients with early-stage NSCLC who received pembrolizumab plus chemotherapy before surgery and pembrolizumab after surgery had better outcomes than those who received just neoadjuvant or just adjuvant treatment.
Treatments for advanced lung cancer
Newer therapies are available for people with advanced lung cancer. These primarily include immunotherapies and targeted therapies, which continue to show benefits as research evolves.
Immunotherapy
Immunotherapies work with the body's immune system to help fight cancer. They are a major focus in lung cancer treatment research today. Clinical trials are ongoing to look at new combinations of immunotherapies with or without chemotherapy to treat lung cancer.
Immune checkpoint inhibitor s are drugs that block an interaction between proteins on immune cells and cancer cells which, in turn, lowers the immune response to the cancer. Several immune checkpoint inhibitors have been approved for advanced lung cancer, including p embrolizumab (Keytruda) , a tezolizumab (Tecentriq) , c emiplimab (Libtayo) , d urvalumab (Imfinzi) , and n ivolumab (Opdivo) .
A key issue with immunotherapies is deciding which patients are most likely to benefit. There is some evidence that patients whose tumor cells have high levels of an immune checkpoint protein called PD-L1 may be more responsive to immune checkpoint inhibitors. Another marker for immunotherapy response is tumor mutational burden , or TMB, which refers to the amount of mutations in the DNA of the cancer cells. In some lung cancer trials, positive responses to immune checkpoint inhibitors have been linked with a high TMB. However, these markers cannot always predict a response and there is ongoing work to find better markers.
To learn more, see Immunotherapy to Treat Cancer .
Targeted Therapies
Targeted treatments identify and attack certain types of cancer cells with less harm to normal cells. In recent years, many targeted therapies have become available for advanced lung cancer and more are in development. Targeted treatments for lung cancer include the below.
Anaplastic lymphoma kinase (ALK) Inhibitors
ALK inhibitors target cancer-causing rearrangements in a protein called ALK. These drugs continue to be refined for the 5% of NSCLC patients who have an ALK gene alteration. Approved treatments include ceritinib (Zykadia) , alectinib (Alecensa) , brigatinib (Alunbrig) , and lorlatinib (Lorbrena) .
These ALK inhibitors are improvements from previous ones in their enhanced ability to cross the blood–brain barrier. This progress is critical because, in non-small cell lung cancer patients with ALK alterations, disease progression tends to occur in the brain. Based on clinical trial results, in 2024 the FDA approved alectinib as adjuvant therapy for people with ALK-positive NSCLC .
EGFR Inhibitors
- Lung Cancer Trial of Osimertinib Draws Praise—and Some Criticism
The drug improved survival in a large clinical trial, but some question the trial’s design.
EGFR inhibitors block the activity of a protein called epidermal growth factor receptor (EGFR). Altered forms of EGFR are found at high levels in some lung cancers, causing them to grow rapidly. Osimertinib (Tagrisso) is the most effective and most widely used EGFR inhibitor. It is also used for adjuvant therapy after surgery for resectable NSCLC. Other drugs that target EGFR that are approved for treating NSCLC include afatinib (Gilotrif) , dacomitinib (Vizimpro) , erlotinib (Tarceva) , gefitinib (Iressa) . For people with Exon 20 mutations, amivantamab (Rybrevant) is an approved targeted therapy.
ROS1 Inhibitors
The ROS1 protein is involved in cell signaling and cell growth. A small percentage of people with NSCLC have rearranged forms of the ROS1 gene. Crizotinib (Xalkori) and entrectinib (Rozlytrek) are approved as treatments for patients with these alterations. In late 2023, the FDA approved repotrectinib (Augtyro) for advanced or metastatic NSCLC with ROS1 fusions as an initial treatment and as a second-line treatment in those who previously received a ROS1-targeted drug.
BRAF Inhibitors
The B-Raf protein is involved in sending signals in cells and cell growth. Certain changes in the B-Raf gene can increase the growth and spread of NSCLC cells.
The combination of the B-Raf-targeted drug dabrafenib (Tafinlar) and trametinib (Mekinist ), which targets a protein called MEK, has been approved as treatment for patients with NSCLC that has a specific mutation in the BRAF gene.
Encorafenib (Braftovi) combined with binimetinib (Mektovi) is approved for patients with metastatic NSCLC with a BRAF V600E mutation .
Other Inhibitors
Some NSCLCs have mutations in the genes NRTK-1 and NRTK-2 that can be treated with the targeted therapy larotrectinib (Vitrakvi). Those with certain mutations in the MET gene can be treated with tepotinib (Tepmetko) or capmatinib (Tabrecta) . And those with alterations in the RET gene are treated with selpercatinib (Retevmo) and pralsetinib (Gavreto) . A 2023 clinical trial showed that treatment with selpercatinib led to longer progression-free survival compared with people who received chemotherapy with or without pembrolizumab. Inhibitors of other targets that drive some lung cancers are being tested in clinical trials.
See a complete list of targeted therapies for lung cancer .
NCI-Supported Research Programs
Many NCI-funded researchers at the NIH campus, and across the United States and the world, are seeking ways to address lung cancer more effectively. Some research is basic, exploring questions as diverse as the biological underpinnings of cancer and the social factors that affect cancer risk. And some is more clinical, seeking to translate basic information into improved patient outcomes. The programs listed below are a small sampling of NCI’s research efforts in lung cancer.
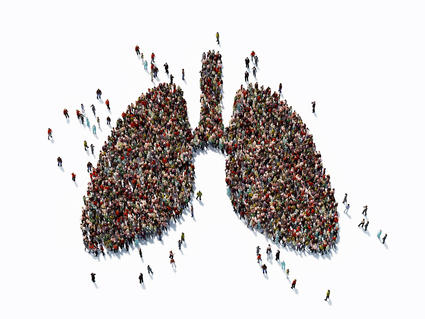
Pragmatica-Lung Study Enrolling Patients
The simplified trial may serve as a model for future cancer clinical trials.
- The Pragmatica-Lung Study is a randomized trial that will compare the combination of the targeted therapy ramucirumab (Cyramza) and the immunotherapy pembrolizumab (Keytruda) with standard chemotherapy in people with advanced NSCLC whose disease has progressed after previous treatment with immunotherapy and chemotherapy. In addition to looking at an important clinical question, the trial will serve as a model for future trials because it is designed to remove many of the barriers that prevent people from joining clinical trials.
- Begun in 2014, ALCHEMIST is a multicenter NCI trial for patients with early stage non-small cell lung cancer. It tests to see whether adding a targeted therapy after surgery, based on the genetics of a patient’s tumor, will improve survival.
- The Lung MAP trial is an ongoing multicenter trial for patients with advanced non-small cell lung cancer who have not responded to earlier treatment. Patients are assigned to specific targeted therapies based on their tumor’s genetic makeup.
- The Small Cell Lung Cancer Consortium was created to coordinate efforts and provide a network for investigators who focus on preclinical studies of small-cell lung cancer. The goal of the consortium is to accelerate progress on this disease through information exchange, data sharing and analysis, and face-to-face meetings.
- NCI funds eight lung cancer Specialized Programs of Research Excellence (Lung SPOREs) . These programs are designed to quickly move basic scientific findings into clinical settings. Each SPORE has multiple lung cancer projects underway.
Clinical Trials
NCI funds and oversees both early- and late-phase clinical trials to develop new treatments and improve patient care. Trials are available for both non-small cell lung cancer treatment and small cell lung cancer treatment .
Lung Cancer Research Results
The following are some of our latest news articles on lung cancer research:
- Alectinib Approved as an Adjuvant Treatment for Lung Cancer
- Repotrectinib Expands Treatment Options for Lung Cancers with ROS1 Fusions
- Tarlatamab Shows Promise for Some People with Small Cell Lung Cancer
- Selpercatinib Slows Progression of RET-Positive Lung, Medullary Thyroid Cancers
- Lung-Sparing Surgery Is Effective for Some with Early-Stage Lung Cancer
View the full list of Lung Cancer Research Results and Study Updates .
- Help & Support
- Lung Health & Diseases
- Lung Disease Lookup
- Lung Cancer
- Resource Library
Lung Cancer Reports
The American Lung Association produces detailed reports on lung health issues that inform the work of public health advocates, healthcare professionals and other stakeholders.
- State of Lung Cancer The American Lung Association's "State of Lung Cancer" report analyzes national and state lung cancer statistics to show how the toll of lung cancer varies across the country and how every state and the nation can do more to protect residents from lung cancer.
- Lung Cancer Trends Brief This publication reports on the burden of lung cancer, examining incidence, mortality and other important measures of disease magnitude.
- Addressing the Stigma of Lung Cancer This issue brief examines the stigma of lung cancer and makes recommendations for reducing it.
- Estimated Prevalence and Incidence of Lung Disease This publication examines several lung diseases, including lung cancer. It discusses the estimated number of new cases (incidence) of lung cancer. Use this report to find data for your county and state.
- Lung Health Barometer The annual Lung Health Barometer surveys over 1,000 American adult women and men to measure their awareness, knowledge and perceptions about lung cancer.
- State of the Air The "State of the Air" report grades cities and counties affected by the most widespread types of air pollution. The report also ranks both the cleanest and most polluted areas in the country. Breathing in polluted air can increase your risk of lung disease. Find out how your area ranks and what you can do to take action.
- State of Tobacco Control The "State of Tobacco Control" report tracks progress on key tobacco control policies. Published annually, the federal government, all 50 state governments and the District of Columbia are graded to determine if tobacco control laws are adequately protecting citizens from the enormous toll tobacco use takes on lives and the economy. Find out how your state ranks and learn how to take steps to improve tobacco control where you live. Reducing exposure to tobacco smoke is one way to protect your lungs.
Page last updated: November 17, 2022
A Breath of Fresh Air in Your Inbox
Join over 700,000 people who receive the latest news about lung health, including research, lung disease, air quality, quitting tobacco, inspiring stories and more!
You will now receive email updates from the American Lung Association.
Make a Donation
Your tax-deductible donation funds lung disease and lung cancer research, new treatments, lung health education, and more.
Become a Lung Health Insider
Thank you! You will now receive email updates from the American Lung Association.
Select Your Location
Select your location to view local American Lung Association events and news near you.
Change Language
Lung helpline.
Talk to our lung health experts at the American Lung Association. Our service is free and we are here to help you.
1-800-LUNG-USA
(1-800-586-4872)
Thank you for visiting nature.com. You are using a browser version with limited support for CSS. To obtain the best experience, we recommend you use a more up to date browser (or turn off compatibility mode in Internet Explorer). In the meantime, to ensure continued support, we are displaying the site without styles and JavaScript.
- View all journals
- My Account Login
- Explore content
- About the journal
- Publish with us
- Sign up for alerts
- Open access
- Published: 14 January 2022
Deep learning-based algorithm for lung cancer detection on chest radiographs using the segmentation method
- Akitoshi Shimazaki 1 ,
- Daiju Ueda 1 , 2 ,
- Antoine Choppin 3 ,
- Akira Yamamoto 1 ,
- Takashi Honjo 1 ,
- Yuki Shimahara 3 &
- Yukio Miki 1
Scientific Reports volume 12 , Article number: 727 ( 2022 ) Cite this article
21k Accesses
47 Citations
7 Altmetric
Metrics details
- Lung cancer
- Radiography
We developed and validated a deep learning (DL)-based model using the segmentation method and assessed its ability to detect lung cancer on chest radiographs. Chest radiographs for use as a training dataset and a test dataset were collected separately from January 2006 to June 2018 at our hospital. The training dataset was used to train and validate the DL-based model with five-fold cross-validation. The model sensitivity and mean false positive indications per image (mFPI) were assessed with the independent test dataset. The training dataset included 629 radiographs with 652 nodules/masses and the test dataset included 151 radiographs with 159 nodules/masses. The DL-based model had a sensitivity of 0.73 with 0.13 mFPI in the test dataset. Sensitivity was lower in lung cancers that overlapped with blind spots such as pulmonary apices, pulmonary hila, chest wall, heart, and sub-diaphragmatic space (0.50–0.64) compared with those in non-overlapped locations (0.87). The dice coefficient for the 159 malignant lesions was on average 0.52. The DL-based model was able to detect lung cancers on chest radiographs, with low mFPI.
Similar content being viewed by others
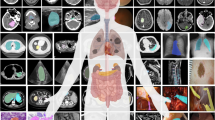
Segment anything in medical images
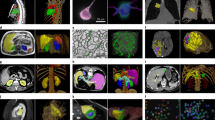
nnU-Net: a self-configuring method for deep learning-based biomedical image segmentation
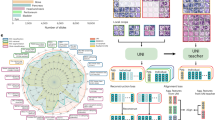
Towards a general-purpose foundation model for computational pathology
Introduction.
Lung cancer is the primary cause of cancer death worldwide, with 2.09 million new cases and 1.76 million people dying from lung cancer in 2018 1 . Four case-controlled studies from Japan reported in the early 2000s that the combined use of chest radiographs and sputum cytology in screening was effective for reducing lung cancer mortality 2 . In contrast, two randomized controlled trials conducted from 1980 to 1990 concluded that screening with chest radiographs was not effective in reducing mortality in lung cancer 3 , 4 . Although the efficacy of chest radiographs in lung cancer screening remains controversial, chest radiographs are more cost-effective, easier to access, and deliver lower radiation dose compared with low-dose computed tomography (CT). A further disadvantage of chest CT is excessive false positive (FP) results. It has been reported that 96% of nodules detected by low-dose CT screening are FPs, which commonly leads to unnecessary follow-up and invasive examinations 5 . Chest radiography is inferior to chest CT in terms of sensitivity but superior in terms of specificity. Taking these characteristics into consideration, the development of a computer-aided diagnosis (CAD) model for chest radiograph would have value by improving sensitivity while maintaining low FP results.
The recent application of convolutional neural networks (CNN), a field of deep learning (DL) 6 , 7 , has led to dramatic, state-of-the-art improvements in radiology 8 . DL-based models have also shown promise for nodule/mass detection on chest radiographs 9 , 10 , 11 , 12 , 13 , which have reported sensitivities in the range of 0.51–0.84 and mean number of FP indications per image (mFPI) of 0.02–0.34. In addition, radiologist performance for detecting nodules was better with these CAD models than without them 9 . In clinical practice, it is often challenging for radiologists to detect nodules and to differentiate between benign and malignant nodules. Normal anatomical structures often appear as if they are nodules, which is why radiologists must pay careful attention to the shape and marginal properties of nodules. As these problems are caused by the conditions rather than the ability of the radiologist, even skillful radiologists can misdiagnose 14 , 15 .
There are two main methods for detecting lesions using DL: detection and segmentation. The detection method is a region-level classification, whereas the segmentation method is a pixel-level classification. The segmentation method can provide more detailed information than the detection method. In clinical practice, classifying the size of a lesion at the pixel-level increases the likelihood of making a correct diagnosis. Pixel-level classification also makes it easier to follow up on changes in lesion size and shape, since the shape can be used as a reference during detection. It also makes it possible to consider not only the long and short diameters but also the area of the lesion when determining the effect of treatment 16 . However, to our knowledge, there are no studies using the segmentation method to detect pathologically proven lung cancer on chest radiographs.
The purpose of this study was to train and validate a DL-based model capable of detecting lung cancer on chest radiographs using the segmentation method, and to evaluate the characteristics of this DL-based model to improve sensitivity while maintaining low FP results.
The following points summarize the contributions of this article:
This study developed a deep learning-based model for detection and segmentation of lung cancer on chest radiographs.
Our dataset is high quality because all the nodules/masses were pathologically proven lung cancers, and these lesions were pixel-level annotated by two radiologists.
The segmentation method was more informative than the classification or detection methods, which is useful not only for the detection of lung cancer but also for follow-up and treatment efficacy.
Materials and methods
Study design.
We retrospectively collected consecutive chest radiographs from patients who had been pathologically diagnosed with lung cancer at our hospital. Radiologists annotated the lung cancer lesions on these chest radiographs. A DL-based model for detecting lung cancer on radiographs was trained and validated with the annotated radiographs. The model was then tested with an independent dataset for detecting lung cancers. The protocol for this study was comprehensively reviewed and approved by the Ethical Committee of Osaka City University Graduate School of Medicine (No. 4349). Because the radiographs had been acquired during daily clinical practice and informed consent for their use in research had been obtained from patients, the Ethical Committee of Osaka City University Graduate School of Medicine waived the need for further informed consent. All methods were performed in accordance with the relevant guidelines and regulations.
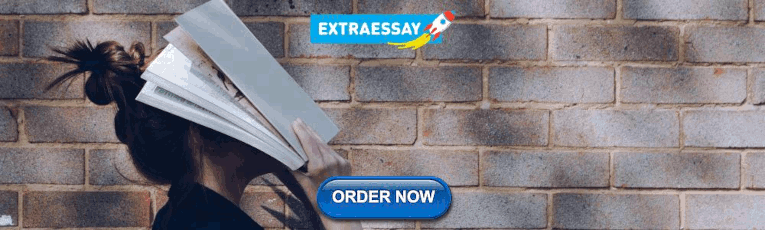
Eligibility and ground truth labelling
Two datasets were used to train and test the DL-based model, a training dataset and a test dataset. We retrospectively collected consecutive chest radiographs from patients pathologically diagnosed with lung cancer at our hospital. The training dataset was comprised of chest radiographs obtained between January 2006 and June 2017, and the test dataset contained those obtained between July 2017 and June 2018. The inclusion criteria were as follows: (a) pathologically proven lung cancer in a surgical specimen; (b) age > 40 years at the time of the preoperative chest radiograph; (c) chest CT performed within 1 month of the preoperative chest radiograph. If the patient had multiple chest radiographs that matched the above criteria, the latest radiograph was selected. Most of these chest radiographs were taken as per routine before hospitalization and were not intended to detect lung cancer. Chest radiographs on which radiologists could not identify the lesion, even with reference to CT, were excluded from analysis. For eligible radiographs, the lesions were annotated by two general radiologists (A.S. and D.U.), with 6 and 7 years of experience in chest radiography, using ITK-SNAP version 3.6.0 ( http://www.itksnap.org/ ) . These annotations were defined as ground truths. The radiologists had access to the chest CT and surgical reports and evaluated the lesion characteristics including size, location, and edge. If > 50% of the edge of the nodule was traceable, the nodule was considered to have a “traceable edge”; if not, it was termed an “untraceable edge”.
Model development
We adopted the CNN architecture using segmentation method. The segmentation method outputs more information than the detection method (which present a bounding box) or the classification method (which determine the malignancy from a single image). Maximal diameter of the tumor is particularly important in clinical practice. Since the largest diameter of the tumor often coincides with an oblique direction, not the horizontal nor the vertical direction, it is difficult to measure with detection methods which present a bounding box. Our CNN architecture was based on the encoder-decoder architecture to output segmentation 17 . The encoder-decoder architecture has a bottleneck structure, which reduces the resolution of the feature map and improves the model robustness to noise and overfitting 18 .
In addition, one characteristic of this DL-based model is that it used both a normal chest radiograph and a black-and-white inversion of a chest radiograph. This is an augmentation that makes use of the experience of radiologists 19 . It is known that black-and-white inversion makes it easier to confirm the presence of lung lesions overlapping blind spots. We considered that this augmentation could be effective for this model as well, so we applied a CNN architecture to each of the normal and inverted images and then an ensemble model using these two architectures 20 . Supplementary Fig. S1 online shows detailed information of the model.
Using chest radiographs from the training dataset, the model was trained and validated from scratch, utilizing five-fold cross-validation. The model when the value of the loss function was the smallest within 100 epochs using Adam (learning rate = 0.001, beta_1 = 0.9, beta_2 = 0.999, epsilon = 0.00000001, decay = 0.0) was adopted as the best-performing.
Model assessment
A detection performance test was performed on a per-lesion basis using the test dataset to evaluate whether the model could identify malignant lesions on radiographs. The model calculated the probability of malignancy in a lesion detected on chest radiographs as an integer between 0 and 255. If the center of output generated by the model was within the ground truth, it was considered true positive (TP). All other outputs were FPs. When two or more TPs were proposed by the model for one ground truth, they were considered as one TP. If there was no output from the model for one ground truth, it was one FN. Two radiologists (A.S. and D.U.) retrospectively referred to the radiograph and CT to evaluate what structures were detected by the FP output. The dice coefficient was also used to evaluate segmentation performance.
Statistical analysis
In the detection performance test, metrics were evaluated on a per-lesion basis. We used the free-response receiver-operating characteristic (FROC) curve to evaluate whether the bounding boxes proposed by the model accurately identified malignant cancers in radiographs 21 . The vertical axis of the FROC curve is sensitivity and the horizontal axis is mFPI. Sensitivity is the number of TPs that the model was able to identify divided by the number of ground truths. The mFPI is the number of FPs that the model mistakenly presented divided by the number of radiographs in the dataset. Thus, the FROC curve shows sensitivity as a function of the number of FPs shown on the image.
One of the authors (D.U.) performed all analyses, using R version 3.6.0 ( https://www.r-project.org/ ). The FROC curves were plotted by R software. All statistical inferences were performed with two-sided 5% significance level.
Figure 1 shows a flowchart of the eligibility criteria for the chest radiographs. For the training dataset, 629 radiographs with 652 nodules/masses were collected from 629 patients (age range 40–91 years, mean age 70 ± 9.0 years, 221 women). For the test dataset, 151 radiographs with 159 nodules/masses were collected from 151 patients (age range 43–84 years, mean age 70 ± 9.0 years, 57 women) (Table 1 ).
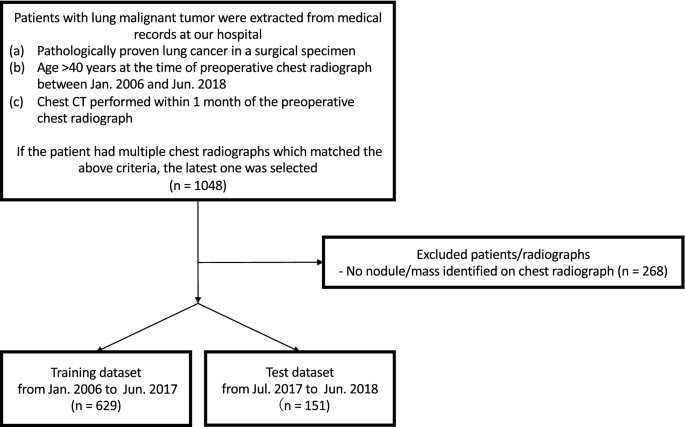
Flowchart of dataset selection.
The DL-based model had sensitivity of 0.73 with 0.13 mFPI in the test dataset (Table 2 ). The FROC curve is shown in Fig. 2 . The highest sensitivity the model attained was 1.00 for cancers with a diameter of 31–50 mm, and the second highest sensitivity was 0.85 for those with a diameter > 50 mm. For lung cancers that overlapped with blind spots such as the pulmonary apices, pulmonary hila, chest wall, heart, or sub-diaphragmatic space, sensitivity was 0.52, 0.64, 0.52, 0.56, and 0.50, respectively. The sensitivity of lesions with traceable edges on radiographs was 0.87, and that for untraceable edges was 0.21. Detailed results are shown in Table 2 .
The dice coefficient for all 159 lesions was on average 0.52 ± 0.37 (standard deviation, SD). For 116 lesions detected by the model, the dice coefficient was on average 0.71 ± 0.24 (SD). The dice coefficient for all 71 lesions overlapping blind spots was 0.34 ± 0.38 (SD). For 39 lesions detected by the model that overlapped with blind spots, the dice coefficient was 0.62 ± 0.29 (SD).
Of the 20 FPs, 19 could be identified as some kind of structure on the chest radiograph by radiologists (Table 3 ). In these 20 FPs, 13 overlapped with blind spots. There were 43 FNs, ranging in size from 9 to 72 mm (mean 21 ± 15 mm), 32 of which overlapped with blind spots (Table 4 ). There were four FNs > 50 mm, all of which overlapped with blind spots. Figure 3 shows representative cases of our model. Figure 4 shows overlapping of a FP output with normal anatomical structures and Fig. 5 shows a FN lung cancer that overlapped with a blind spot. Supplementary Fig. S2 online shows visualized images of the first and last layers. An ablation study to use black-and-white inversion images is shown in Supplementary Data online.
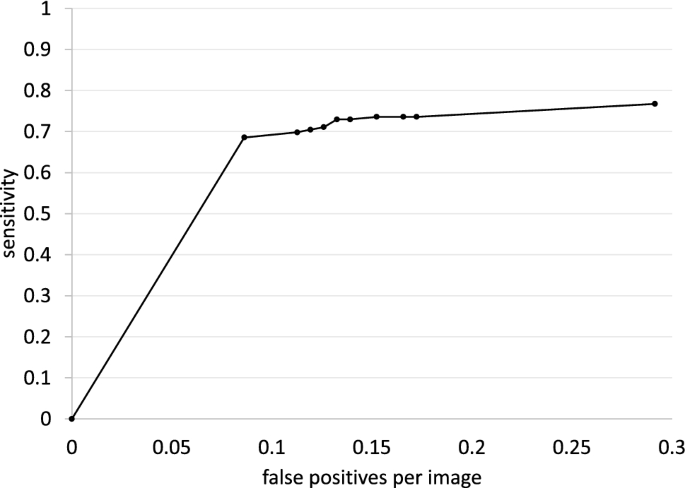
Free-response receiver-operating characteristic curve for the test dataset.
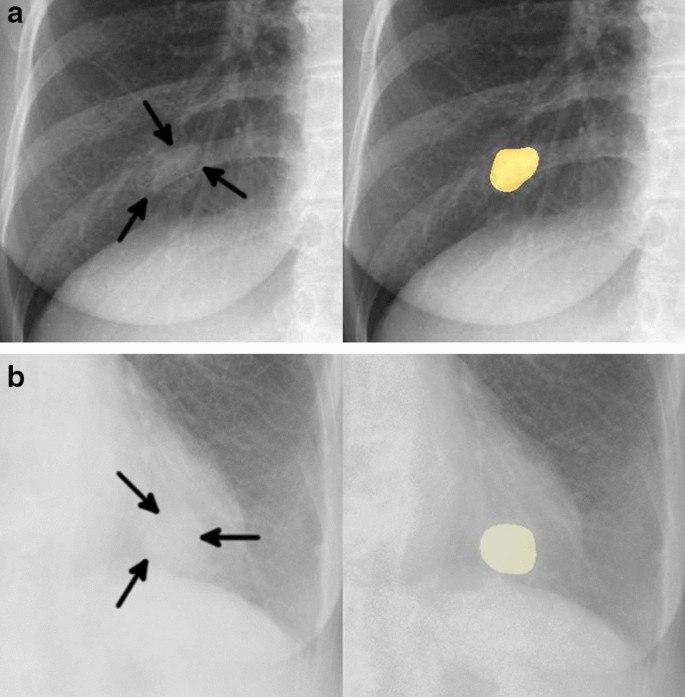
Two representative true positive cases. The images on the left are original images, and those on the right are images output by our model. ( a ) A 48-year-old woman with a nodule in the right lower lobe that was diagnosed as adenocarcinoma. The nodule was confused with rib and vessels (arrows). The model detected the nodule in the right middle lung field. ( b ) A 74-year-old woman with a nodule in the left lower lobe that was diagnosed as squamous cell carcinoma. The nodule overlapped with the heart (arrows). The lesion was identifiable by the model because its edges were traceable.
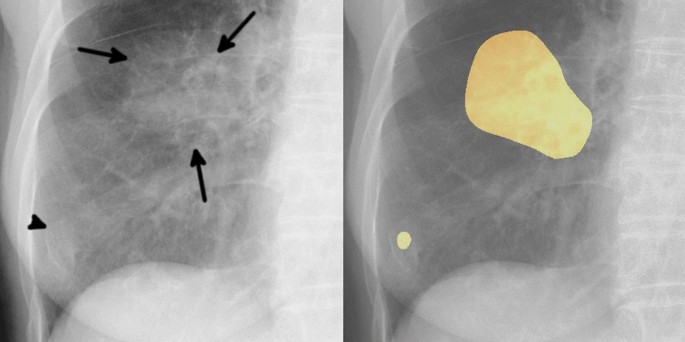
Example of one false positive case. The image on the left is an original image, and the image on the right is an image output by our model. An 81-year-old woman with a mass in the right lower lobe that was diagnosed as squamous cell carcinoma. The mass in the right middle lung field (arrows) was carcinoma. Our model detected this lesion, and also detected a slightly calcified nodule in the right lower lung field (arrowhead). This nodule was an old fracture of the right tenth rib, but was misidentified as a malignant lesion because its shape was obscured by overlap with the right eighth rib and breast.
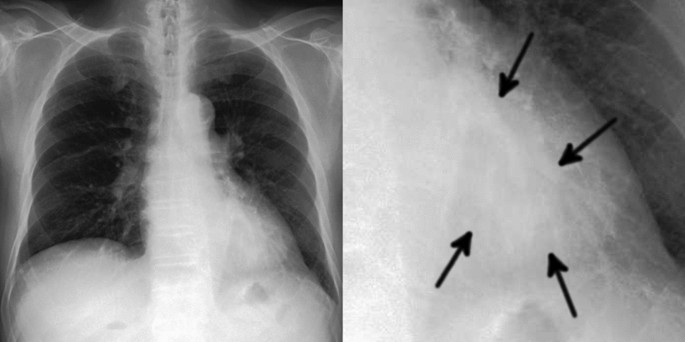
Example of one false negative case. The image on the left is a gross image, and the image on the right is an enlarged image of the lesion. A 68-year-old man with a mass in the left lower lobe that was diagnosed as adenocarcinoma. This lesion overlapped with the heart and is only faintly visible (arrows). Our model failed to detect the mass.
In this study, we developed a model for detecting lung cancer on chest radiographs and evaluated its performance. Adding pixel-level classification of lesions in the proposed DL-based model resulted in sensitivity of 0.73 with 0.13 mFPI in the test dataset.
To our knowledge, ours is the first study to use the segmentation method to detect pathologically proven lung cancer on chest radiographs. We found several studies that used classification or detection methods to detect lung cancer on chest radiographs, but not the segmentation method. Since the segmentation method has more information about the detected lesions than the classification or detection methods, it has advantages not only in the detection of lung cancer but also in follow-up and treatment efficacy. We achieved performance as high as that in similar previous studies 9 , 10 , 11 , 12 , 13 using DL-based lung nodule detection models, with fewer training data. It is particularly noteworthy that the present method achieved low mFPI. In previous studies, sensitivity and mFPI were 0.51–0.84 and 0.02–0.34, respectively, and used 3,500–13,326 radiographs with nodules or masses as the training data, compared with the 629 radiographs used in the present study. Although comparisons to these studies are difficult because the test datasets were different, our accuracy was similar to that of the detection models employed in most of the previous studies. We performed pixel-level classification of the lesions based on the segmentation method and included for analysis only lesions that were pathologically proven to be malignant, based on examination of surgically resected specimens. All previous studies 9 , 10 , 11 , 12 , 13 have included potentially benign lesions, clinically malignant lesions, or pathologically malignant lesions by biopsy in their training data. Therefore, our model may be able to analyze the features of the malignant lesions in more detail. In regard with the CNN, we created this model based on Inception-ResNet-v2 17 , which combines the Inception structure and the Residual connection. In the Inception-ResNet block, convolutional filters of multiple sizes are combined with residual connections. The use of residual connections not only avoids the degradation problem caused by deep structures but also reduces the training time. In theory, the combination of these features further improves the recognition accuracy and learning efficiency 17 . By using this model with combining normal and black-white-inversion images, our results achieved comparable or better performance with fewer training data than previous studies. In regard with the robustness of the model, we consider this model to be relatively robust against imaging conditions or body shape because we consecutively collected the dataset and did not set any exclusion criteria based on imaging conditions or body shape.
The dice coefficient for 159 malignant lesions was on average 0.52. On the other hand, for the 116 lesions detected by the model, the dice coefficient was on average 0.71. These values provide a benchmark for the segmentation performance of lung cancer on chest radiograph. The 71 lesions which overlapped with blind spots tended to have a low dice coefficient with an average of 0.34, but for 39 lesions detected by the model that overlapped with blind spots, the average dice coefficient was 0.62. This means that lesions overlapping blind spots were not only difficult to detect, but also had low accuracy in segmentation. On the other hand, the segmentation accuracy was relatively high for lesions that were detected by the model even if they overlapped with the blind spots.
Two interesting tendencies were found after retrospectively examining the characteristics of FP outputs. First, 95% (19/20) FPs could be visually recognized on chest radiographs as nodule/mass-like structures. The model identified some nodule-like structures (FPs), which overlapped with vascular shadows and ribs. This is also the case for radiologists in daily practice. Second, nodules with calcification overlapped with normal anatomical structures tended to be misdiagnosed by the model (FPs). Five FPs were non-malignant calcified lung nodules on CT and also overlapped with the heart, clavicle or ribs. As the model was trained only on malignant nodules without calcification in the training dataset, calcified nodules should not be identified in theory. Most calcified nodules are actually not identified by the model, however, this was not the case for calcified nodules that overlapped with normal anatomical structures. In other word, there is a possibility that the model could misidentify the lesion as a malignant if the features of calcification that should signal a benign lesion are masked by normal anatomical structures.
When we investigated FNs, we found that nodules in blind spots and metastatic nodules tended to be FNs. With regard to blind spots, our model showed a decrease in sensitivity for lesions that overlapped with normal anatomical structures. It was difficult for the model to identify lung cancers that overlapped with blind spots even when the tumor size was large (Fig. 5 ). In all FNs larger than 50 mm, there was wide overlap with normal anatomical structures, for the possible reason that it becomes difficult for the model to detect subtle density differences in lesions that overlapped with large structures such as the heart. With regard to metastatic nodules, 33% (14/43) metastatic lung cancers were FNs. These metastatic nodules ranged in size from 10 to 20 mm (mean 14 ± 3.8 mm) and were difficult to visually identify on radiographs, even with reference to CT. In fact, the radiologists had overlooked most of the small metastatic nodules at first and could only identify them retrospectively, with knowledge of the type of lung cancer and their locations.
There are some limitations of this study. The model was developed using a dataset collected from a single hospital. Although our model achieved high sensitivity with low FPs, the number of FPs may be higher in a screening cohort and the impact of this should be considered. Furthermore, an observer’s performance study is needed to evaluate the clinical utility of the model. In this study, we included only chest radiographs containing malignant nodules/masses. The fact that we used only pathologically proven lung cancers and pixel-level annotations by two radiologists in our dataset is a strength of our study, on the other hand, it may reduce the detection rate of benign nodules/masses. This is often not a problem in clinical practice. Technically, all areas other than the malignant nodules/masses could be trained as normal areas. However, normal images should be mixed in and tested to evaluate the model for detailed examination in clinical practice.
In conclusion, a DL-based model developed using the segmentation method showed high performance in the detection of lung cancer on chest radiographs. Compared with CT, chest radiographs have advantages in terms of accessibility, cost effectiveness, and low radiation dose. However, the known effectiveness of the model for lung cancer detection is limited. We believe that a CAD model with higher performance can support clinical detection and interpretation of malignant lesions on chest radiographs and offers additive value in lung cancer detection.
Bray, F. et al. Global cancer statistics 2018: GLOBOCAN estimates of incidence and mortality worldwide for 36 cancers in 185 countries. CA Cancer J. Clin. 68 , 394–424. https://doi.org/10.3322/caac.21492 (2018).
Article PubMed Google Scholar
Sagawa, M. et al. The efficacy of lung cancer screening conducted in 1990s: Four case–control studies in Japan. Lung Cancer 41 , 29–36. https://doi.org/10.1016/s0169-5002(03)00197-1 (2003).
Fontana, R. S. et al. Lung cancer screening: The Mayo program. J. Occup. Med. 28 , 746–750. https://doi.org/10.1097/00043764-198608000-00038 (1986).
Article CAS PubMed Google Scholar
Kubik, A. et al. Lack of benefit from semi-annual screening for cancer of the lung: Follow-up report of a randomized controlled trial on a population of high-risk males in Czechoslovakia. Int. J. Cancer 45 , 26–33. https://doi.org/10.1002/ijc.2910450107 (1990).
Raghu, V. K. et al. Feasibility of lung cancer prediction from low-dose CT scan and smoking factors using causal models. Thorax 74 , 643–649. https://doi.org/10.1136/thoraxjnl-2018-212638 (2019).
Hinton, G. Deep learning—a technology with the potential to transform health care. JAMA 320 , 1101–1102. https://doi.org/10.1001/jama.2018.11100 (2018).
LeCun, Y., Bengio, Y. & Hinton, G. Deep learning. Nature 521 , 436–444. https://doi.org/10.1038/nature14539 (2015).
Article ADS CAS PubMed Google Scholar
Ueda, D., Shimazaki, A. & Miki, Y. Technical and clinical overview of deep learning in radiology. Jpn. J. Radiol. 37 , 15–33. https://doi.org/10.1007/s11604-018-0795-3 (2019).
Nam, J. G. et al. Development and validation of deep learning–based automatic detection algorithm for malignant pulmonary nodules on chest radiographs. Radiology 290 , 218–228. https://doi.org/10.1148/radiol.2018180237 (2019).
Park, S. et al. Deep learning-based detection system for multiclass lesions on chest radiographs: Comparison with observer readings. Eur. Radiol. 30 , 1359–1368. https://doi.org/10.1007/s00330-019-06532-x (2020).
Yoo, H., Kim, K. H., Singh, R., Digumarthy, S. R. & Kalra, M. K. Validation of a deep learning algorithm for the detection of malignant pulmonary nodules in chest radiographs. JAMA Netw. Open 3 , e2017135. https://doi.org/10.1001/jamanetworkopen.2020.17135 (2020).
Article PubMed PubMed Central Google Scholar
Sim, Y. et al. Deep convolutional neural network–based software improves radiologist detection of malignant lung nodules on chest radiographs. Radiology 294 , 199–209. https://doi.org/10.1148/radiol.2019182465 (2020).
Hwang, E. J. et al. Development and validation of a deep learning-based automated detection algorithm for major thoracic diseases on chest radiographs. JAMA Netw. Open 2 , e191095. https://doi.org/10.1001/jamanetworkopen.2019.1095 (2019).
Manser, R. et al. Screening for lung cancer. Cochrane Database Syst Rev CD001991. https://doi.org/10.1002/14651858.CD001991.pub3 (2013).
Berlin, L. Radiologic errors, past, present and future. Diagnosis (Berl) 1 , 79–84. https://doi.org/10.1515/dx-2013-0012 (2014).
Article Google Scholar
From the RECIST committee. Schwartz, L.H. et al. RECIST 1.1-Update and clarification. Eur. J. Cancer. 62 , 132–137. https://doi.org/10.1016/j.ejca.2016.03.081 (2016).
Szegedy, C., Ioffe, S., Vanhoucke, V. & Alemi, A. A. Inception-v4, Inception-ResNet and the Impact of Residual Connections on Learning . In Proceedings of the Thirty-First AAAI Conference on Artificial Intelligence , 4278–4284 (AAAI Press, San Francisco, California, USA, 2017).
Matějka P. et al. Neural Network Bottleneck Features for Language Identification. In Proceedings of Odyssey 2014. vol. 2014. International Speech Communication Association , 299–304 (2014).
Sheline, M. E. et al. The diagnosis of pulmonary nodules: Comparison between standard and inverse digitized images and conventional chest radiographs. Am. J. Roentgenol. 152 (2), 261–263. https://doi.org/10.2214/ajr.152.2.261 (1989).
Article CAS Google Scholar
Wang, G., Hao, J., Ma, J. & Jiang, H. A comparative assessment of ensemble learning for credit scoring. Expert. Syst. Appl. 38 (1), 223–230 (2011).
Bunch, P., Hamilton, J., Sanderson, G. & Simmons, A. A free response approach to the measurement and characterization of radiographic observer performance. Proc. SPIE 127 , 124–135. https://doi.org/10.1117/12.955926 (1977).
Article ADS Google Scholar
Download references
Acknowledgements
We are grateful to LPIXEL Inc. for joining this study.
Author information
Authors and affiliations.
Department of Diagnostic and Interventional Radiology, Graduate School of Medicine, Osaka City University, Osaka, Japan
Akitoshi Shimazaki, Daiju Ueda, Akira Yamamoto, Takashi Honjo & Yukio Miki
Smart Life Science Lab, Center for Health Science Innovation, Osaka City University, Osaka, Japan
LPIXEL Inc, Tokyo, Japan
Antoine Choppin & Yuki Shimahara
You can also search for this author in PubMed Google Scholar
Contributions
All authors contributed to the study conception and design. Material preparation, data collection and analysis were performed by A.S., D.U., A.Y. and T.H. Model development was performed by A.C. and Y.S. The first draft of the manuscript was written by A.S. and all authors commented on previous versions of the manuscript. All authors read and approved the final manuscript.
Corresponding author
Correspondence to Daiju Ueda .
Ethics declarations
Competing interests.
Akitoshi Shimazaki has no relevant relationships to disclose. Daiju Ueda has no relevant relationships to disclose. Antoine Choppin is an employee of LPIXEL Inc. Akira Yamamoto has no relevant relationships to disclose. Takashi Honjo has no relevant relationships to disclose. Yuki Shimahara is the CEO of LPIXEL Inc. Yukio Miki has no relevant relationships to disclose.
Additional information
Publisher's note.
Springer Nature remains neutral with regard to jurisdictional claims in published maps and institutional affiliations.
Supplementary Information
Supplementary information., rights and permissions.
Open Access This article is licensed under a Creative Commons Attribution 4.0 International License, which permits use, sharing, adaptation, distribution and reproduction in any medium or format, as long as you give appropriate credit to the original author(s) and the source, provide a link to the Creative Commons licence, and indicate if changes were made. The images or other third party material in this article are included in the article's Creative Commons licence, unless indicated otherwise in a credit line to the material. If material is not included in the article's Creative Commons licence and your intended use is not permitted by statutory regulation or exceeds the permitted use, you will need to obtain permission directly from the copyright holder. To view a copy of this licence, visit http://creativecommons.org/licenses/by/4.0/ .
Reprints and permissions
About this article
Cite this article.
Shimazaki, A., Ueda, D., Choppin, A. et al. Deep learning-based algorithm for lung cancer detection on chest radiographs using the segmentation method. Sci Rep 12 , 727 (2022). https://doi.org/10.1038/s41598-021-04667-w
Download citation
Received : 04 August 2021
Accepted : 29 December 2021
Published : 14 January 2022
DOI : https://doi.org/10.1038/s41598-021-04667-w
Share this article
Anyone you share the following link with will be able to read this content:
Sorry, a shareable link is not currently available for this article.
Provided by the Springer Nature SharedIt content-sharing initiative
This article is cited by
Deep volcanic residual u-net for nodal metastasis (nmet) identification from lung cancer.
- M. Ramkumar
- K. Kalirajan
Biomedical Engineering Letters (2024)
ELCD-NSC2: a novel early lung cancer detection and non-small cell classification framework
- Hadeer A. Helaly
- Mahmoud Badawy
- Amira Y. Haikal
Neural Computing and Applications (2024)
Fairness of artificial intelligence in healthcare: review and recommendations
- Taichi Kakinuma
- Shinji Naganawa
Japanese Journal of Radiology (2024)
Lung tumor analysis using a thrice novelty block classification approach
- S. L. Soniya
- T. Ajith Bosco Raj
Signal, Image and Video Processing (2023)
Radon transform-based improved single seeded region growing segmentation for lung cancer detection using AMPWSVM classification approach
- K. Vijila Rani
- M. Eugine Prince
By submitting a comment you agree to abide by our Terms and Community Guidelines . If you find something abusive or that does not comply with our terms or guidelines please flag it as inappropriate.
Quick links
- Explore articles by subject
- Guide to authors
- Editorial policies
Sign up for the Nature Briefing: Cancer newsletter — what matters in cancer research, free to your inbox weekly.

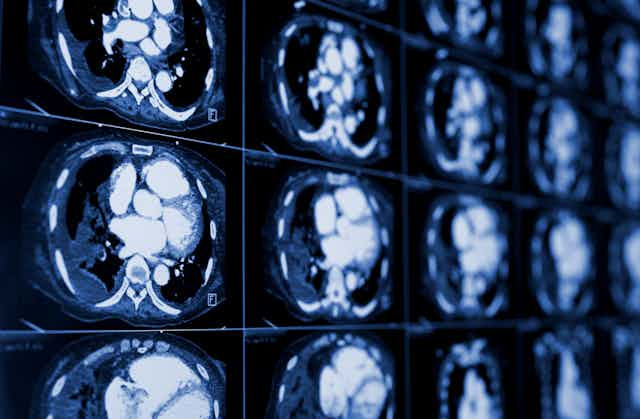
Lung cancer is the deadliest of all cancers, and screening could save many lives − if more people could access it
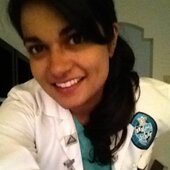
Assistant Professor of Pulmonary Sciences and Critical Care, University of Colorado Anschutz Medical Campus
Disclosure statement
Nina Thomas does not work for, consult, own shares in or receive funding from any company or organisation that would benefit from this article, and has disclosed no relevant affiliations beyond their academic appointment.
University of Colorado Anschutz Medical Campus provides funding as a member of The Conversation US.
View all partners
Many medical organizations have been recommending lung cancer screening for decades for those at high risk of developing the disease.
But in 2022, less than 6% of people in the U.S. eligible for screening actually got screened. Compared with other common cancer screenings, lung cancer screening rates fall terribly behind. For comparison, the screening rate in 2021 for colon cancer was 72% , and the rate for breast cancer was 76% . Why are lung cancer screening rates so poor?
I am a pulmonologist who specializes in screening and diagnosing lung cancer. In my research to improve early detection of lung cancer, I’ve found that numerous complex barriers hinder the widespread adoption of lung cancer screening.
Basics of lung cancer screening
Lung cancer is the leading cause of cancer-related death in the world. But early detection can significantly improve outcomes.
Survival rates for early stage lung cancer are nearly seven times higher than lung cancer that has spread in the body. Between 2015 and 2019, survival rates for lung cancer have improved by 22% , in part because of an increase in earlier diagnosis.
Most patients with early-stage lung cancer, however, have no symptoms and aren’t diagnosed until they reach more advanced stages that are harder to treat, underlining the need for effective lung cancer screening.
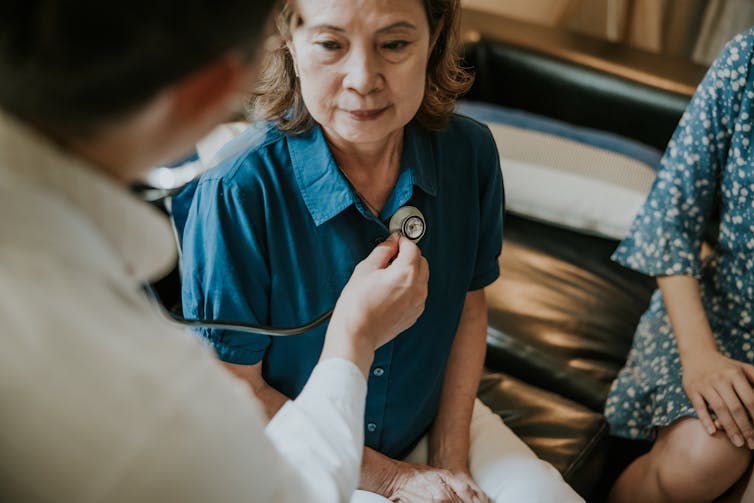
The U.S. Preventive Services Task Force recommends lung cancer screening for people 50-80 years old, people who currently smoke or previously smoked but quit within the past 15 years, and people who smoked at least 20 pack-years. Pack-years is an estimate of cigarette exposure in a person’s lifetime, calculated by multiplying the total number of years someone has smoked by the average number of packs smoked per day. For example, if someone smoked half a pack per day for 40 years, they would have smoked for 20 pack-years.
Low-dose CT scan for lung cancer
One of the primary barriers to lung cancer screening is lack of public awareness and understanding . Many people are unaware they qualify for lung cancer screening or have misconceptions around what to expect from the screening process.
Lung cancer screening involves a yearly low-dose computed tomography, or CT, scan , a diagnostic imaging tool that uses X-rays to create detailed cross sections of the inside of the body. Screening 250 patients can prevent one lung cancer death. In comparison, 645 people screened with a mammogram can prevent one breast cancer death.
Some people have reservations surrounding the scan itself because of radiation exposure . The radiation from a low-dose CT scan, however, is about 1.4 millisieverts – the scientific unit used to measure ionizing radiation – which is less than the 3 to 5 millisieverts of background radiation a person may be exposed to in a given year on average while living on Earth.
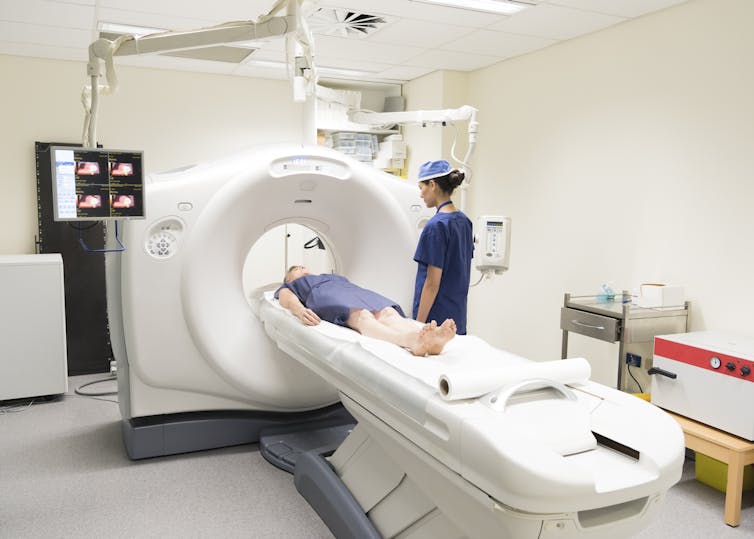
The whole process takes only a few minutes and does not require any needles, medications or infusions. During the scan, the patient lies on the scanner’s sliding table and raises their arms above their head. The technologist may ask them to hold their breath for 5 to 10 seconds as the table passes through the scanner.
A radiologist summarizes the results of the test for their doctor in a report. If the result is negative for findings in the lungs, the patient would return in one year for another scan. If the result is positive, it usually means the radiologist saw a lung nodule, or a spot on the lung. Not all lung nodules are cancer, and patients may require additional imaging or a biopsy to confirm.
Disparities in lung cancer screening
Public health campaigns and education initiatives work to engage the public and increase awareness of the availability and benefit of lung cancer screening. However, these efforts have been less effective in some of the communities most at risk for developing lung cancer.
African American men have the highest rates of developing and dying from lung cancer compared with other groups, even at younger ages and among those with less smoking history. The tobacco industry has explicitly targeted the Black community with pervasive marketing tactics in predominantly Black neighborhoods and stores. Since the 1970s, the tobacco industry has aggressively marketed menthol cigarettes to African Americans using tailored messaging and imagery. This compounds and exacerbates the socioeconomic disparities in health care and cancer care already seen in these underserved and underrepresented communities.
Rural communities face geographic barriers to lung cancer screening. About 80.5 million Americans live in counties with high rates of lung cancer and poor access to a quality screening center. Many of these counties are clustered in the Appalachian and southeastern regions of the U.S. where smoking rates are highest. Over 60% of people living in rural areas who are eligible for lung cancer screening have to travel over 20 miles to a screening facility.
Lung cancer screening may also be costly. While most private insurances and Medicare cover the cost of lung cancer screening, Medicaid policies vary state by state and may not cover the cost of lung cancer screening. In addition, while some plans may cover the initial low-dose CT scan, they might not cover follow-up testing, procedures and treatment. Supplementary costs such as transportation, time off from work, child care and other logistical issues can also add up to create additional socioeconomic barriers to screening.
Communities that are under- or uninsured are at the highest risk of developing lung cancer. They often lack access to primary care providers, let alone specialists who can facilitate screening.
Erasing smoking stigma
Because lung cancer is linked to smoking, stigma plays a significant role in preventing many people eligible for screening from pursuing it. Many people eligible for lung cancer screening fear being blamed for their previous or current tobacco use.
Stigma also discourages patients from discussing lung cancer screening with their health care providers or community. Clinicians can partner with patients and communities to destigmatize lung cancer and tobacco dependence, extricating the disease from blame. Framing screening as a proactive measure to help those at risk of developing lung cancer and as a collaboration with those who need help quitting smoking can empower people to actively engage in screening rather than dreading or avoiding it.
Fear is another barrier . Many people believe that a lung cancer diagnosis is a death sentence. When diagnosed early, however, doctors treat lung cancer with an intention to cure . Additionally, advances in lung cancer treatment over the past 10-15 years have led to remarkable improvements in survival for all stages of the disease. Reassurance and education around next steps and paths to treatment at the time of screening help diffuse some of the distress surrounding lung cancer.
Public health researchers are exploring how to minimize the significant and complex barriers to lung cancer screening for those who need it most.
- Public health
- Lung cancer
- Cancer screening
- Health disparities
- Racial health disparities
- Lung cancer screening

Case Management Specialist

Lecturer / Senior Lecturer - Marketing

Assistant Editor - 1 year cadetship

Executive Dean, Faculty of Health

Lecturer/Senior Lecturer, Earth System Science (School of Science)
- Cancer Home
- Clinical Trials
- Acknowledgements
A Case of Incidentally Discovered Congenital Complete Pericardial Defect during Lobectomy for Lung Cancer: A Case Report and Literature Review
Affiliation.
- 1 Department of Thoracic Surgery, Nagoya University Graduate School of Medicine, Nagoya, Aichi, Japan.
- PMID: 38749719
- DOI: 10.5761/atcs.cr.24-00041
An 82-year-old male patient underwent a left upper lobectomy with anterolateral thoracotomy for lung cancer. Although a complete left-pericardial defect was observed during surgery, the pericardial repair was not performed because the left lower lobe remained and the heart was considered stable. Postoperative pathological examination revealed primary synchronous double-lung squamous-cell carcinoma (pathological stage pT2a(2)N0M0 stage IB). He was discharged without complications on postoperative day 8. Leftward displacement of the heart and left diaphragmatic elevation, suspected of phrenic-nerve paralysis, were found in the chest X-ray after discharge. However, the patient's overall condition remained unaffected at the 5-month postoperative follow-up. To assess the need for pericardial repair, we compared cases of complete pericardial defects observed during lobectomy or pneumonectomy reported in the literature. Only one of 12 cases occurred postoperative death despite pericardial repair, and that case combined pectus excavatum and pericardial defects. Our assessment indicated that pericardial repair might not be necessary, excluding complex cases.
Keywords: congenital complete pericardial defect; lobectomy; pericardial repair.
Publication types
- Case Reports
- Aged, 80 and over
- Carcinoma, Squamous Cell* / diagnostic imaging
- Carcinoma, Squamous Cell* / pathology
- Carcinoma, Squamous Cell* / surgery
- Heart Defects, Congenital / diagnostic imaging
- Heart Defects, Congenital / surgery
- Incidental Findings*
- Lung Neoplasms* / diagnostic imaging
- Lung Neoplasms* / pathology
- Lung Neoplasms* / surgery
- Neoplasm Staging
- Pericardium* / transplantation
- Pneumonectomy* / adverse effects
- Thoracotomy
- Tomography, X-Ray Computed
- Treatment Outcome
FDA Approves New Drug for Deadly Lung Cancer
By Robin Foster HealthDay Reporter

FRIDAY, May, 17, 2024 (HealthDay News) -- The U.S. Food and Drug Administration on Thursday approved a new drug to treat patients with an advanced form of deadly lung cancer.
Importantly, tarlatamab (Imdelltra) is only for patients who have exhausted all other options to treat extensive-stage small cell lung cancer.
"The FDA's approval of Imdelltra marks a pivotal moment for patients battling [extensive-stage small cell lung cancer]," Dr. Jay Bradner , executive vice president of research and development and chief scientific officer at drug maker Amgen, said in a company news release . "Imdelltra offers these patients who are in urgent need of new innovative therapies hope, and we're proud to deliver this long-awaited effective treatment to them."
In a company trial, tarlatamab tripled patients’ life expectancy, giving them a median survival of 14 months. But not everyone benefited: Forty percent of those who got the drug responded.
U.S. Cities With the Most Homelessness

"After decades of minimal advancements in the [small cell lung cancer] treatment landscape, there is now an effective and innovative treatment option available," Laurie Fenton Ambrose , co-founder, president and CEO of GO2 for Lung Cancer, said in the Amgen news release.
Tarlatamab arrives after decades of no real treatment advances for this type of lung cancer, said Dr. Anish Thomas , a lung cancer specialist at the National Cancer Institute who was not involved in the trial.
“I feel it’s a light after a long time,” he told the New York Times .
Despite the drug's effectiveness, it does have a serious side effect called a cytokine release syndrome, the FDA said. That's when the immune system goes into overdrive, triggering symptoms like a rash, a rapid heartbeat and low blood pressure.
With small cell lung cancer, the disease has typically spread beyond the lung by the time it is diagnosed. The standard treatment is chemotherapy combined with immunotherapies that add about two months to patients’ lives, the Times reported.
Most patients live just eight to 13 months after their diagnosis, despite having chemotherapy and immunotherapy. The patients in the Amgen trial had already had two or even three rounds of chemotherapy, which is why their life expectancy without the drug was so short.
Patients in the clinical trial say they have a new lease on life.
Martha Warren, 65, of Westerly, R.I., found out last year that she had small cell lung cancer. After chemotherapy and immunotherapy, with her cancer still spreading rapidly, she was accepted into the Amgen study and began getting infusions of the drug.
Her cancer began shrinking almost immediately.
“I feel as normal as I did before I had cancer,” Warren told the Times . “There’s a lot of hope with this drug."
More information
Visit the National Cancer Institute for more on lung cancer .
SOURCE: U.S. Food and Drug Administration, news release, May 16, 2024; Amgen, news release, May 16, 2024; New York Times
Copyright © 2024 HealthDay . All rights reserved.
Join the Conversation
Tags: lung cancer
America 2024

Health News Bulletin
Stay informed on the latest news on health and COVID-19 from the editors at U.S. News & World Report.
Sign in to manage your newsletters »
Sign up to receive the latest updates from U.S News & World Report and our trusted partners and sponsors. By clicking submit, you are agreeing to our Terms and Conditions & Privacy Policy .
You May Also Like
The 10 worst presidents.
U.S. News Staff Feb. 23, 2024

Cartoons on President Donald Trump
Feb. 1, 2017, at 1:24 p.m.

Photos: Obama Behind the Scenes
April 8, 2022

Photos: Who Supports Joe Biden?
March 11, 2020
Flag Display Rattles SCOTUS Experts
Lauren Camera May 17, 2024

Will Trump Testify in His Own Trial?
Laura Mannweiler May 17, 2024

Viral House Spat Shows Chaotic Congress
Aneeta Mathur-Ashton May 17, 2024

QUOTES: Trump on Gun Control Policy
Cecelia Smith-Schoenwalder May 17, 2024

Leading Indicators: Economy Is Softening
Tim Smart May 17, 2024

Key Moments From Cohen Cross-Examination
Laura Mannweiler May 16, 2024

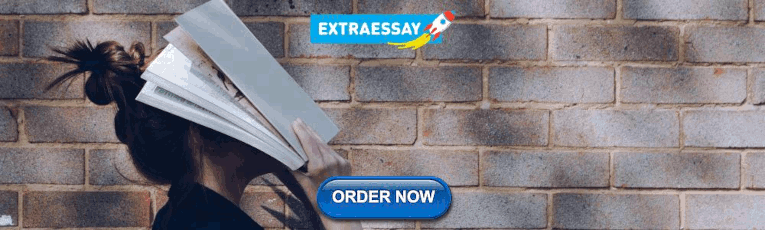
IMAGES
COMMENTS
Background. According to the Surveillance, Epidemiology, and End Results Program (SEER) registry based on 2007-2011 new cases, lung cancer (LC) is more frequently diagnosed among people aged 65-74 with only 1.6% of all cases occurring in patients younger than 45 years [].Most published data about LC in young populations are single-institutional retrospective analyses and few report on very ...
New Cases. More than 228,000 people will be diagnosed with lung cancer this year, with the rate of new cases varying by state. The report finds that Utah has the nation's best lung cancer rate while Kentucky has the worst at almost 2.5 times the incidence rate of Utah. Over the last five years, the rate of new cases decreased 9% nationally.
Dr. Mathew S. Lopes: A 65-year-old woman was transferred to this hospital because of chest pain. Six months before the current presentation, the patient presented to a hospital affiliated with ...
Lung cancer is broadly categorized into small-cell and non-small-cell histologies; non-small-cell lung cancer (NSCLC) comprises >85% of all cases and can be further classified by histological subtype.
The Lung Cancer Master Protocol, or Lung-MAP, is a precision medicine research study for people with advanced non-small cell lung cancer that has continued to grow after treatment. Patients are assigned to different study drug combinations based on the results of genomic profiling of their tumors.
Lung cancer is one of the most frequently diagnosed cancers and the leading cause of cancer-related deaths worldwide with an estimated 2 million new cases and 1·76 million deaths per year. Substantial improvements in our understanding of disease biology, application of predictive biomarkers, and refinements in treatment have led to remarkable progress in the past two decades and transformed ...
Close to 237,000 people will be diagnosed with lung cancer this year, with the rate of new cases varying by state. The report finds that Utah has the nation's best lung cancer rate while Kentucky has the worst at almost 2.3 times the incidence rate of Utah. Over the last five years, the rate of new cases decreased 11% nationally.
Lung cancer is one of the most frequently diagnosed cancers and the leading cause of cancer-related deaths worldwide with an estimated 2 million new cases and 1·76 million deaths per year. Substantial improvements in our understanding of disease biology, application of predictive biomarkers, and refinements in treatment have led to remarkable ...
Overdiagnosis. Five studies specifically examined overdiagnosis, 81, 86 - 89 and 7 additional trials were examined for differences in cancer incidence between LDCT and comparison groups. 14, 17, 19, 24, 31, 90, 91 Estimates of overdiagnosis ranged from 0% to 67.2% that a screen-detected lung cancer is overdiagnosed.
Lung cancer is a type of cancer that starts when abnormal cells grow in an uncontrolled way in the lungs. It is a serious health issue that can cause severe harm and death. Symptoms of lung cancer include a cough that does not go away, chest pain and shortness of breath. It is important to seek medical care early to avoid serious health effects.
The NCI-sponsored National Lung Screening Trial (NLST) showed that low-dose CT scans can be used to screen for lung cancer in people with a history of heavy smoking. Using this screening can decrease their risk of dying from lung cancer. Now researchers are looking for ways to refine CT screening to better predict whether cancer is present.
revealed a tumor on the left lower lobe with pleural effusion, and thoracic puncture cytology indicated lung adenocarcinoma. Interventions: Four cycles of carboplatin and docetaxel chemotherapy reduced the size of the tumor; however, it increased in size after 8 months, and re-challenge chemotherapy (RC) with the same drugs was performed. Repeated RC controlled disease activity for 6 years ...
Lung cancer is thought to be a genetic disease with a variety of unknown origins. Globocan2020 report tells in 2020 new cancer cases identified was 19.3 million and nearly 10.0 million died owed ...
The American Lung Association's "State of Lung Cancer" report analyzes national and state lung cancer statistics to show how the toll of lung cancer varies across the country and how every state and the nation can do more to protect residents from lung cancer. Lung Cancer Trends Brief. This publication reports on the burden of lung cancer ...
In India, lung cancer accounts for 5.9% of all cancers and 8.1% of all cancer-related deaths. 1. The prevalence of smoking in patients with lung cancer is nearly 80%. 2. Age-standardized incidence and mortality rates of lung cancer in India as compared with neighboring countries are depicted in Figure 1.
Kubik, A. et al. Lack of benefit from semi-annual screening for cancer of the lung: Follow-up report of a randomized controlled trial on a population of high-risk males in Czechoslovakia. Int. J.
Rural communities face geographic barriers to lung cancer screening. About 80.5 million Americans live in counties with high rates of lung cancer and poor access to a quality screening center ...
Asbestos-Related Lung Cancer: An Update. Edited by Nico van Zandwijk, Arthur Frank. 3 July 2023. View all special issues and article collections. View all issues. Read the latest articles of Lung Cancer at ScienceDirect.com, Elsevier's leading platform of peer-reviewed scholarly literature.
14. (MetaOpinion™) While lung cancer remains a very challenging cancer to treat, new treatments that capitalize on advances in our understanding of cancer biology are providing both patients and physicians with a reason for cautious optimism. Because cancer is a highly varied disease, one of the primary treatment challenges is in selecting ...
Background: This study aimed to systematically review case reports documenting rare adverse events in patients with small cell lung cancer (SCLC) following the administration of immune checkpoint inhibitors (ICIs). Methods: A systematic literature review was conducted to identify case reports detailing previously unreported adverse drug reactions to ICIs in patients with SCLC.
Precise recognition and delineation of tumors play a pivotal role in the radiotherapy of non-small cell lung cancer (NSCLC). The current manual delineation techniques used in clinical practice are both time-consuming and labor-intensive. While some studies have made progress in automating the segmentation of lung cancer targets, there is still room for further improvement in their effectiveness.
DOI: 10.1164/ajrccm-conference.2024.209.1_meetingabstracts.a6004 Corpus ID: 269546989; Case Report Emphasizing Importance of Lung Cancer Screening in Patients With Family History @article{Wong2024CaseRE, title={Case Report Emphasizing Importance of Lung Cancer Screening in Patients With Family History}, author={R.S.N. Wong and Y. Khalid and G. Maria and D.S. Cheah and M.Z. Haider}, journal={C57.
DOI: 10.1164/ajrccm-conference.2024.209.1_meetingabstracts.a6977 Corpus ID: 269541713; Anti-cancer Immune Activation Post Bronchoscopic Thermal Ablation in Non-small Cell Lung Cancer @article{Rangamuwa2024AnticancerIA, title={Anti-cancer Immune Activation Post Bronchoscopic Thermal Ablation in Non-small Cell Lung Cancer}, author={K. Rangamuwa and M. Christie and T. Leong and C. Aloe and Kenta ...
A Case of Incidentally Discovered Congenital Complete Pericardial Defect during Lobectomy for Lung Cancer: A Case Report and Literature Review Ann Thorac Cardiovasc Surg. 2024;30(1 ... An 82-year-old male patient underwent a left upper lobectomy with anterolateral thoracotomy for lung cancer. Although a complete left-pericardial defect was ...
FRIDAY, May, 17, 2024 (HealthDay News) -- The U.S. Food and Drug Administration on Thursday approved a new drug to treat patients with an advanced form of deadly lung cancer.
Background: Cancer surveillance data are essential to help understand where gaps exist and progress is being made in cancer control. We sought to summarize the expected impact of cancer in Canada in 2024, with projections of new cancer cases and deaths from cancer by sex and province or territory for all ages combined. Methods: We obtained data on new cancer cases (i.e., incidence, 1984-2019 ...
A775_G776insYVMA, the typical and predominant HER2 exon 20 insertion variant in non-small cell lung cancer, exhibits relative insensitivity to covalent HER2-targeted tyrosine kinase inhibitors. However, other less common insertions have shown better responses to HER2-targeted inhibitors. M774delinsWLV is a rare HER2 exon 20 insertion subtype and its clinical sensitivity to HER2-targeted ...