A Comprehensive Review of Speech Emotion Recognition Systems
Ieee account.
- Change Username/Password
- Update Address
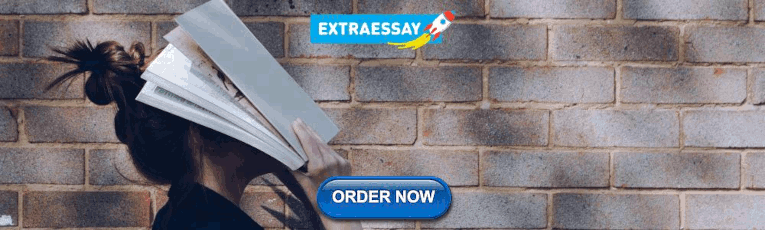
Purchase Details
- Payment Options
- Order History
- View Purchased Documents
Profile Information
- Communications Preferences
- Profession and Education
- Technical Interests
- US & Canada: +1 800 678 4333
- Worldwide: +1 732 981 0060
- Contact & Support
- About IEEE Xplore
- Accessibility
- Terms of Use
- Nondiscrimination Policy
- Privacy & Opting Out of Cookies
A not-for-profit organization, IEEE is the world's largest technical professional organization dedicated to advancing technology for the benefit of humanity. © Copyright 2024 IEEE - All rights reserved. Use of this web site signifies your agreement to the terms and conditions.
Academia.edu no longer supports Internet Explorer.
To browse Academia.edu and the wider internet faster and more securely, please take a few seconds to upgrade your browser .
Enter the email address you signed up with and we'll email you a reset link.
- We're Hiring!
- Help Center
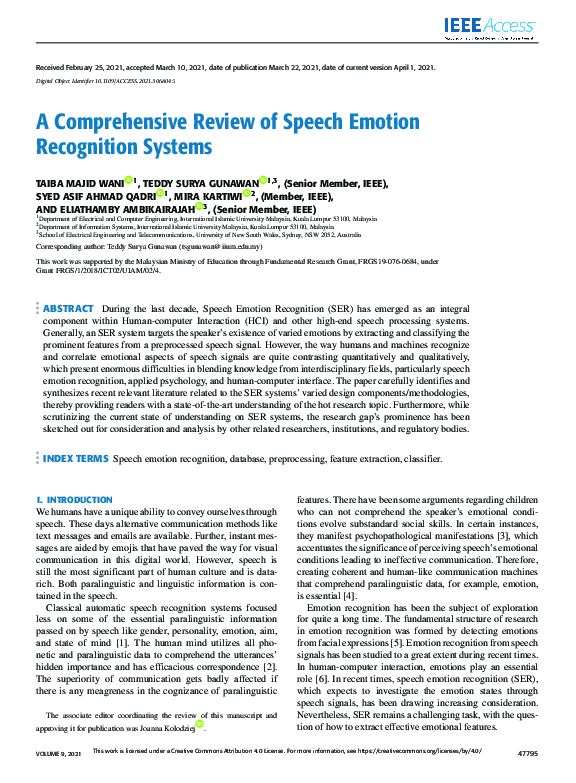
A Comprehensive Review of Speech Emotion Recognition Systems

IEEE Access
Related Papers
Katarzyna Klessa
This paper reports on the work directed at creating a framework for investigation of affective states and paralinguistic features in speech and their role in describing individual features of human voices. The work was carried out within a research-development project whose primary aim was to develop a speaker recognition and identification system mainly for forensic applications. The present paper describes the methods and preliminary results of examination of the choice of lexical means, vocal communication of affective states and voice quality features using "Paralingua‖ corpus, and introduces "Annotation System" - a novel tool designed specifically for annotation of paralinguistic features.
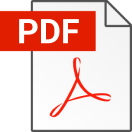
Lecture Notes in Computer Science
Ioannis Dologlou , N. Fragopanagos
Fatemeh Noroozi
Affective computing studies and develops systems capable of detecting humans affects. The search for universal well-performing features for speech-based emotion recognition is ongoing. In this paper, a small set of features with support vector machines as the classifier is evaluated on Surrey Audio-Visual Expressed Emotion database, Berlin Database of Emotional Speech, Polish Emotional Speech database and Serbian emotional speech database. It is shown that a set of 87 features can offer results on-par with state-of-the-art, yielding 80.21, 88.6, 75.42 and 93.41% average emotion recognition rate, respectively. In addition, an experiment is conducted to explore the significance of gender in emotion recognition using random forests. Two models, trained on the first and second database, respectively, and four speakers were used to determine the effects. It is seen that the feature set used in this work performs well for both male and female speakers, yielding approximately 27% average e...
David Sztahó
The human speech contains and reflects information about the emotional state of the speaker. The importance of research of emotions is increasing in telematics, information technologies and even in health services. The research of the mean acoustical parameters of the emotions is a very complicated task. The emotions are mainly characterized by suprasegmental parameters, but other segmental factors can contribute to the perception of the emotions as well. These parameters are varying within one language, according to speakers etc. In the first part of our research work, human emotion perception was examined. Steps of creating an emotional speech database are presented. The database contains recordings of 3 Hungarian sentences with 8 basic emotions pronounced by nonprofessional speakers. Comparison of perception test results obtained with database recorded by nonprofessional speakers showed similar recognition results as an earlier perception test obtained with professional actors/actresses. It was also made clear, that a neutral sentence before listening to the expression of the emotion pronounced by the same speakers cannot help the perception of the emotion in a great extent. In the second part of our research work, an automatic emotion recognition system was developed. Statistical methods (HMM) were used to train different emotional models. The optimization of the recognition was done by changing the acoustic preprocessing parameters and the number of states of the Markov models.
Speaker Classification II
Catherine Mathon
Computer Speech & Language
Anton Batliner
Asian Journal of Research in Computer Science
Zryan Rashid
Clients can adequately control PCs and create reports by speaking with the guide of innovation, discourse acknowledgment empowers records to be delivered all the more effectively in light of the fact that the program normally produces words as fast as they expressed, which is typically a lot faster than a human can compose. Discourse acknowledgment is an innovation that consequently finds the words and expressions that best match the contribution of human discourse. The most normal use of discourse acknowledgment is correspondence, where discourse acknowledgment can be utilized to create letters/messages and different reports. Point of discourse handling:-to comprehend discourse as a mechanism of correspondence, to reflect discourse for transmission and propagation;-to inspect discourse for robotized data discovery and extraction-to find some physiological highlights of the speaker. In discourse combination, there are two significant capacities. The first is the interpretation of voice to message. The second is for the content to be converted into human voice.
Constantine Kotropoulos , Dimitrios Ververidis
Antonio Bonafonte
International Journal of Advanced Research
RELATED PAPERS
Leyla Huseynova
International Journal of Health Sciences and Research
Revista Brasileira de Reumatologia
carlos alberto engelhorn
Neurobiology of aging
Ruslina Maesar
Anxiety, Stress, & Coping
Monique LELOUP
Ali Uromeihy
Octavia Sinta Wahyuningrum octaviasinta.2022
Journal of research in pharmacy practice
Pharmacognosy Research
muralidhar yegireddy
Wanida Caichompoo
The Proceedings of the Annual Convention of the Japanese Psychological Association
Sumire HIROTA
Journal of Medical Microbiology
Frederick Sirgel
ECS Meeting Abstracts
Arslan Abbas
Revista Brasileira De Pesquisa Em Educacao Em Ciencias
Geide Rosa Coelho
university of tehran
maryam nabizadeh
Journal of the American College of Cardiology
Statistics & Probability Letters
Arkady Tempelman
Valérie Audé
Jesús Alturo i Perucho
Francisco Beitia
- We're Hiring!
- Help Center
- Find new research papers in:
- Health Sciences
- Earth Sciences
- Cognitive Science
- Mathematics
- Computer Science
- Academia ©2024
A comprehensive review of speech emotion recognition systems
Wani, Taiba Majid and Gunawan, Teddy Surya and Ahmad Qadri, Syed Asif and Kartiwi, Mira and Ambikairajah, Eliathamby (2021) A comprehensive review of speech emotion recognition systems. IEEE Access, 9. pp. 47795-47814. E-ISSN 2169-3536
During the last decade, Speech Emotion Recognition (SER) has emerged as an integral component within Human-computer Interaction (HCI) and other high-end speech processing systems. Generally, an SER system targets the speaker’s existence of varied emotions by extracting and classifying the prominent features from a preprocessed speech signal. However, the way humans and machines recognize and correlate emotional aspects of speech signals are quite contrasting quantitatively and qualitatively, which present enormous difficulties in blending knowledge from interdisciplinary fields, particularly speech emotion recognition, applied psychology, and human-computer interface. The paper carefully identifies and synthesizes recent relevant literature related to the SER systems’ varied design components/methodologies, thereby providing readers with a state-of-the-art understanding of the hot research topic. Furthermore, while scrutinizing the current state of understanding on SER systems, the research gap’s prominence has been sketched out for consideration and analysis by other related researchers, institutions, and regulatory bodies.
Actions (login required)
Downloads per month over past year
View more statistics
- Create Account
Speech emotion recognition systems and their security aspects
- Open access
- Published: 21 May 2024
- Volume 57 , article number 148 , ( 2024 )
Cite this article
You have full access to this open access article
- Itzik Gurowiec 1 , 2 &
- Nir Nissim 1 , 2
Speech emotion recognition (SER) systems leverage information derived from sound waves produced by humans to identify the concealed emotions in utterances. Since 1996, researchers have placed effort on improving the accuracy of SER systems, their functionalities, and the diversity of emotions that can be identified by the system. Although SER systems have become very popular in a variety of domains in modern life and are highly connected to other systems and types of data, the security of SER systems has not been adequately explored. In this paper, we conduct a comprehensive analysis of potential cyber-attacks aimed at SER systems and the security mechanisms that may prevent such attacks. To do so, we first describe the core principles of SER systems and discuss prior work performed in this area, which was mainly aimed at expanding and improving the existing capabilities of SER systems. Then, we present the SER system ecosystem, describing the dataflow and interactions between each component and entity within SER systems and explore their vulnerabilities, which might be exploited by attackers. Based on the vulnerabilities we identified within the ecosystem, we then review existing cyber-attacks from different domains and discuss their relevance to SER systems. We also introduce potential cyber-attacks targeting SER systems that have not been proposed before. Our analysis showed that only 30% of the attacks can be addressed by existing security mechanisms, leaving SER systems unprotected in the face of the other 70% of potential attacks. Therefore, we also describe various concrete directions that could be explored in order to improve the security of SER systems.
Avoid common mistakes on your manuscript.
1 Introduction
Human beings can recognize emotions in the human voice (Blanton 1915 ). Even animals like dogs and horses can recognize and interpret the human voice, discerning tones of love, fear, anger, anxiety, and even depression. "The language of tones" is perhaps the most universal and oldest language known to human beings, animals, and all living creatures (Blanton 1915 ); in fact, this language is the basis of our communication.
Humans’ language of tones contains a range of personal information regarding the speaker 0. By understanding people's emotions, one can both better understand other people and be better understood by others. When interacting with others, we often provide clues that help them understand what we are feeling. These clues may involve tone changes, body language, facial expressions, etc. The emotional expressions of the people around us are a major aspect of our social communication. Being able to interpret and react to others' emotions is essential. The capability allows us to respond appropriately and build deeper connections with our surroundings and the people in them. By knowing how a person feels, we can therefore know the person's emotional state and current needs and react accordingly.
Charles Darwin believed that the emotions are adaptations that allow animals and humans to survive and reproduce. Footnote 1 The ability to identify emotions in human speech has existed since the late 1970s when John D. Williamson created a speech analyzer to analyze the pitch or frequency of human speech and determine the emotional state of the speaker (Williamson 1978 ). Since that time, verbal communication and interactions between humans and computerized systems have increased. Systems like Apple’s Siri, Footnote 2 Amazon’s Alexa, Footnote 3 and Microsoft’s Cortana Footnote 4 have become one of the basic functionalities in daily-used devices. The main question that arises, however, is whether such systems can truly recognize the speaker's emotions and react accordingly.
Emotion recognition systems, which are mainly based on analyzing facial expressions (Dzedzickis et al. 2020 ), learn to identify the link between an emotion and its external manifestation from large arrays of labeled data. This data may include audio or video recordings of TV shows, interviews, and experiments involving real people, clips of theatrical performances or movies, or dialogue delivered by professional actors.
Many areas can benefit from emotion recognition capabilities, including security (Utane and Nalbalwar 2013 ), customer-focused services (Utane and Nalbalwar 2013 ), and even the socialization of people with special needs (Rázuri et al. 2015 ). According to Gartner, Footnote 5 by the end of 2023, one in 10 gadgets (meaning 10% of technologies) will include emotion recognition technology.
The methods and sensors used to recognize emotions (Dzedzickis et al. 2020 ) can be categorized broadly as self-report techniques that are based on the examined person's assessment and machine assessment techniques that are based on physiologic and biologic parameters collected from the examined person, which include: electroencephalography (EEG) produced from the brain’s electrical system; electrocardiography (ECG) and heart rate variability (HRV) produced from the heart’s actions; skin temperature and skin response to different stimuli; respiration rate (RR); facial expression (FE); and speech analysis, which is known as speech emotion recognition (SER). Machine assessment techniques lie at the core of many emotion recognition systems, including SER systems, and their market share is projected to be valued at $37.1 billion by the end of 2026. Footnote 6 Figure 1 presents various domains in which SER systems have been implemented in recent years. As can be seen, SER systems are implemented in a wide range of domains. Figure 2 presents the number of papers pertaining to SER systems published over the last four decades (based on Google Scholar), with a forecast for the next decade (2020–2030) which was created using simple exponential regression (the red solid line in the graph), including the upper and lower bounds of the confidence interval using 5% statistical significance (the two dashed orange lines). Figure 2 includes papers relevant to SER systems, regardless of whether they pertain to the security of such systems. As can be seen, there has been exponential growth in the number of publications in the SER domain, with a forecast of over 230 publications per year by the end of 2030.
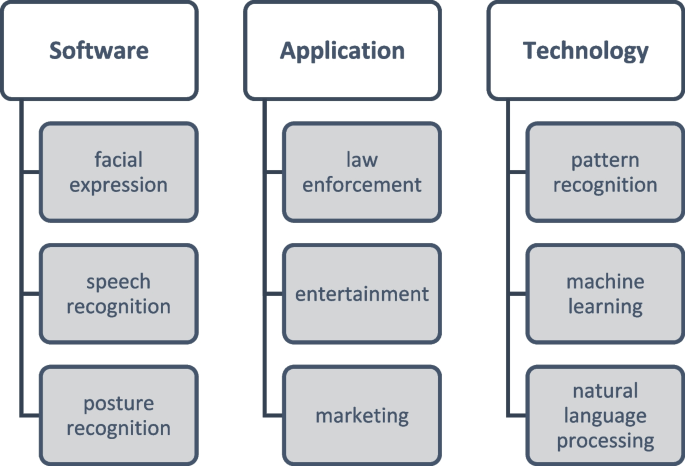
Domains in which SER systems have been implemented
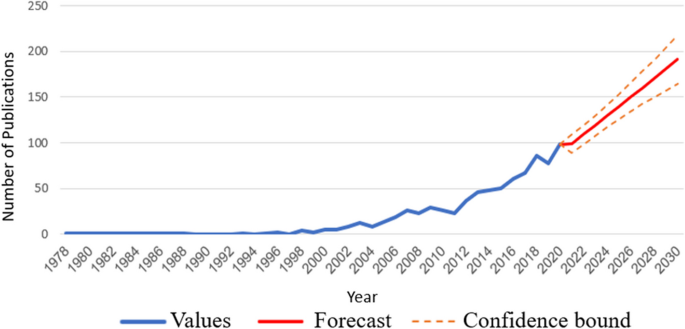
SER publications per year
The growing popularity and distribution of emotion recognition technologies (Dzedzickis et al. 2020 ; Garcia-Garcia et al. 2017 ), including SER systems, raises another important issue. Such technologies and systems can invade individuals’ personal cyber space and compromise their privacy and security. Therefore, they must be secured with mechanisms like user authentication and encryption. The General Data Protection Regulation Footnote 7 (GDPR) defines the rules for processing personal data in the European Union (EU). According to this regulation, voice and speech are not considered personal data, but voice or speech recordings are considered personal data if they are related to an identified person. According to the GDPR, sound data (voice/speech) can even be considered sensitive data (a special category of personal data benefitting from a higher level of protection because of its nature), since it may reveal ethnicity, political opinions, etc. SER systems, which rely on voice and speech recordings, must be secure in order to adhere to such regulations.
As voice-based systems have become more ubiquitous, they have become an attractive target for cyber-attacks. In March 2019, The Wall Street Journal (WSJ) Footnote 8 reported on a cyber-attack aimed at a British energy company. Attackers used an artificial intelligence (AI) algorithm to impersonate the CEO who called an employee and demanded a fraudulent transfer of $243,000. According to the WSJ , the attackers used publicly available sound recordings (such as those used in the SER system training phase) to perform the attack. In 2018, The New York Times Footnote 9 reported on the ease of performing a "dolphin attack" on voice assistant systems in which the attacker plays inaudible voice commands in order to exploit the ability of smartphones in the surrounding area that can be operated and controlled by sound gestures. The article reported that this capability can be used to switch a smartphone to airplane mode (preventing it from having Internet access) or visit a website (which could be malicious). In October 2021, a group of attackers used voice-based deep fake technology ("deep-voice" Footnote 10 ) to transfer $35 million from a company's bank account to several other accounts. Footnote 11 In the phone call to the bank, they mimicked the voice of a senior manager of the company. The use of a SER system by the bank may have prevented such an attack. For example, a SER system with synthetic sound as a neutral reference (Mdhaffar et al. 2021 ) can be used to detect fraudulent calls. Such systems can both detect synthetic sound (as used in "deep voice") and neutral emotion in the attacker's voice. The classification of the attacker's emotion during the attack as "neutral" could raise suspicion, since most attackers would probably feel nervous, excited, or stressed when performing the attack.
Although extensive research has been conducted in the area of SER systems (as shown in Fig. 2 ), and there is a wide range of potential attacks (as described above), not enough research focusing on the security of SER systems has been performed; while studies have been conducted on this topic (Latif et al. 2018 ; Zhang et al. 2017 ; Jati et al. 2020 ), there is a significant gap between the solutions they provide, the vulnerabilities we discovered (discussed in Sect. 6), and the potential attacks outlined in our paper.
It is essential to differentiate between direct cyber-attacks on SER systems and attacks focused on SER system model alteration or imitation. Cyber-attacks that directly compromise the integrity, availability, or confidentiality of SER systems are considered direct cyber-attacks. These include system breaches (Aloufi et al. 2019 ), data theft (McCormick 2008 ), denial-of-service attacks (McCormick 2008 ), and other malicious activities that target the core functionality and security of the system (McCormick 2008 ). On the other hand, attacks focused on the alteration or imitation of SER system models aim to manipulate the underlying machine learning (ML) models employed by SER systems. By modifying or injecting adversarial input into the training data, these attacks attempt to deceive or manipulate the SER system's decision-making process. Although both types of attacks pose significant threats to SER systems, they represent distinct categories of vulnerabilities (see Sect. 6.1). By examining both, we aim to provide a holistic and comprehensive overview of the diverse range of challenges and risks faced by SER systems. While we present a variety of cyber-attacks aimed at SER systems, due to the increased use of ML in diverse domains, most of the attacks are part of the second group of attacks, aimed at altering and imitating the SER model. Such attacks exploit existing vulnerabilities of SER systems (e.g., their use of external recording devices, training on publicly available datasets) enabling attackers to launch and initiate such attacks.
In addition to the existing cyber-attacks, new attacks can always be performed. This is exemplified by the COVID-19 pandemic, which created three kinds of worldwide crises: economic, healthcare, and mental health crises (Lotfian and Busso 2015 ). The periods of isolation and need to quarantine affected millions of people around the world, causing the depression rate to rise (27.8% of US adults reported suffering from depression during the pandemic, compared to just 8.5% before the pandemic, according to a study performed by the Boston University School of Public Health Footnote 12 ). Early detection of radical changes in a person's mood, especially during a pandemic, is crucial. Emotion recognition systems are a valuable tool in detecting changes in a person's mood, and just as the pandemic created new applications for SER systems, it also created new opportunities for attackers. The global shift to online work, learning, and other daily activities allowed people to apply filters to their voice (transferred via any online medium, e.g., Zoom), changing the way they sound. This ability can be utilized by cyber-attackers at any time.
Despite the wide range of existing and potential cyber-attacks, to the best of our knowledge, no studies have explored and analyzed the security aspects of SER systems, such as potential cyber-attacks on the systems and the systems’ vulnerabilities, which might have great impact on individuals, society, companies, the economy, and technology.
In this paper we address this gap. We provide the basic definitions required to understand SER systems and improve their security. We discuss the main studies performed in the SER field; the methods used in those studies include support vector machines (SVMs), hidden Markov models (HMMs), and deep learning algorithms (e.g., convolutional neural networks). We also present the SER system ecosystem and analyze potential cyber-attacks aimed at SER systems. In addition, we describe the existing security mechanisms aimed at providing protection against SER cyber-attacks and introduce two concrete directions that could be explored in order to improve the security of SER systems.
Although some of the studies performed a review of the SER domain (Joshi and Zalte 2013 ; Ayadi et al. 2011 ; Schuller 2018 ; Swain et al. 2018 ; Yan et al. 2022 ), they provided limited information regarding aspects of the SER process, focusing instead on the basic and fundamental information needed to develop such systems. For example, Joshi and Zalte ( 2013 ) focused mainly on the classifier selection and feature extraction and selection methods suitable for speech data, while Ayadi et. al. ( 2011 ) focused on the databases available for the task of classifying emotions, the recommended features to extract, and the existing classification schemes. However, none of the abovementioned papers provided detailed information on the sound wave itself or the data representation techniques used in SER systems. In 2022, Yan et. al. ( 2022 ) explored the security of voice assistant systems, providing a thorough survey of the attacks and countermeasures for voice assistants. Despite the study’s comprehensiveness, it did not discuss SER systems in particular or the SER system ecosystem, which is crucial for the analysis of potential cyber-attacks aimed directly at SER systems. Although the authors presented a wide range of cyber-attacks aimed at voice assistants, providing a comprehensive analysis of each attack, they only focused on existing cyber-attacks, without suggesting new attacks or security mechanisms, as we do in this paper. Our paper aims to address the abovementioned gaps identified in the previous studies, and its contributions are as follows:
We identify the different players (humans and components) within SER systems, analyze their interactions, and by doing so, create ecosystem diagrams for SER systems for the main domains and applications they are implemented in.
We discuss 10 possible attacks and vulnerabilities relevant for SER systems. Using the understanding derived from the ecosystem diagrams, we identify the vulnerable components and elements within SER systems that are exposed to cyber-attacks, as well as the attack vectors from which a cyber-attack can be initiated against SER systems.
We describe nine existing security mechanisms that can be used to secure SER systems against potential cyber-attacks and analyze the mechanisms’ ability to address the possible attacks; by doing so, we identify uncovered gaps regarding attacks and vulnerabilities.
We propose two security mechanisms for SER systems that can help address some of the attacks and vulnerabilities that are currently uncovered by an existing security mechanism.
2 Research methodology
In this section, we provide an overview of the structured methodology employed to explore the security aspects of SER systems, which is the main goal of our study. This methodology enabled our comprehensive analysis of SER systems. The methodology's six steps, which are presented in Fig. 3 , can be summarized as follows: In step 1, we perform a technical analysis of the foundations, principles, and building blocks of SER systems. In step 2, we explore the evolution of SER systems and the domains they are used in. In step 3, we analyze the SER system's ecosystem in the domains explored in step 2. In step 4, we perform a security analysis of SER systems in which we explore their vulnerabilities and identify potential cyber-attacks targeting them. In step 5, we assess the coverage of the existing security mechanisms against the cyber-attacks identified in step 4. The methodology concludes with step 6 in which we identify the security gaps associated with SER systems and propose security enhancements. This research methodology guided our investigation of the security aspects of SER systems, ensuring a systematic and comprehensive approach to the analysis of both the technical and security-related aspects of the SER system domain.
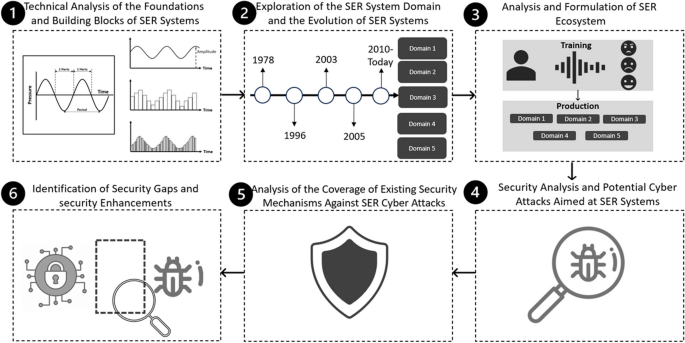
Overview of the research methodology employed in this study
2.1 Technical analysis of the foundations and building blocks of SER systems
To lay the groundwork for our study, we perform a comprehensive technical analysis of the principles of SER systems. This involves the in-depth exploration of sound waves, speech signals, signal processing techniques, feature extraction methods, and more.
2.2 Exploring of the SER system domain and the evolution of SER systems
A thorough literature review is performed, in which we cover the existing studies in the SER field and identify the main domains in which SER systems are used. This step served as a crucial step to the definition of the SER ecosystem.
2.3 Analysis and formulation of SER ecosystems
Building on the insights gained in the first two steps, we analyze and formulate the SER ecosystems in each domain. To do so, we identify all of the components in SER systems and the associated dataflow, starting from the development phase and continue to the diverse applications across various domains. The SER ecosystem provides a holistic framework for our subsequent security analysis.
2.4 Security analysis and potential cyber-attacks aimed at SER systems
To assess the security aspects of SER systems, we survey cyber-attacks targeting speech-based systems, with a particular focus on SER systems. By leveraging our understanding of the SER ecosystem, we analyze the vulnerabilities inherent in SER systems, examine the relevance of existing cyber attacks to the SER system domain, and present some new cyber attacks that could target SER systems.
2.5 Analysis of the coverage of existing security mechanisms against SER cyber attacks
In this step, we survey existing security mechanisms against cyber-attacks aimed at SER systems, reviewing the countermeasures designed to safeguard speech-based systems. This step results in an assessment of the current state of security measures and their efficacy in mitigating potential threats to SER systems.
2.6 Identification of security gaps and security enhancements
Based on our analysis of the potential cyber-attacks and existing security mechanisms, we first identify gaps in the security measure coverage against cyber-attacks aimed at SER systems. Then, we propose security enhancements for SER systems aimed at addressing the identified vulnerabilities and strengthening the overall resilience of these systems against potential threats.
3 Emotions and the principles of speech emotion recognition systems
To understand speech emotion recognition systems and design and develop proper security mechanisms for it, we must first provide several basic definitions. For example, what an emotion is and how it is manifested in sound waves. Thus, in this section we provide information regarding emotions, sound, sound waves, and how these abstract definitions can become data which can be used for emotion recognition or alternatively, can be exploited by an attacker. This section includes several sub-sections as follows: "Emotions" provide basic information of different emotional concepts, including types of emotions and sub-emotions and how they are classified; "Sound Waves" provide a brief explanation of what a sound wave is; Followed by that, in "Sound Data Representation" sub-section we present different representations of sound waves so that a digital system will be able to analyze it; Next, "Feature Extraction" sub-section provide information regarding different audio features' families that can be used to train a SER system; To conclude this section, in "Reflection of Emotions in Sound" sub-section we present how different emotions can be expressed in human's sound, and how each emotional state effects the sound humans produce.
3.1 Emotions
The scientific community has made numerous attempts to classify emotions and differentiate between emotions, mood, and affect; we now briefly explain some important terms.
An emotion is the response of a person to a specific stimulus; the stimulus can be another person, a real or virtual scenario, smell, taste, sound, image, or an event) (Wang et al. 2021 ). Usually the stimulus is intense with a brief experience, and the person is aware of it. Mood is the way a person feels at a particular time. It tends to be mild, long lasting, and less intense than a feeling (Dzedzickis et al. 2020 ). Affect is the result of the effect caused by a certain emotion (Dzedzickis et al. 2020 ; Wang et al. 2021 ) In other words, affect is the observable manifestation of a person's inner feelings.
In (Feidakis et al. 2011a ), the authors described 66 different emotions and divided them into two main groups: basic emotions, which include ten emotions (anger, anticipation, distrust, fear, happiness, joy, love, sadness, surprise, and trust) and secondary emotions, which include 56 semi-emotions. Classifying between different emotions is an extremely difficult task, especially when the classification process needs to be performed automatically. This is because the definition of emotion and its meaning have changed from one scientific era to another (Feidakis et al. 2011b ), therefore it is hard to define which emotional classes a SER system should include. It is also difficult to identify the relevant features to extract from the raw audio, since the different features selected could dramatically affect the performance of the SER system (Ayadi et al. 2011 ). Nevertheless, among the 66 emotions described by the authors, some emotions are considered "similar" (for example, calm and natural). To handle the issue of emotions' similarity in the classification process, researchers have focused on making classifications between the parameters of the emotions, including valence (negative/positive) and arousal (high/low), and analyzing just the basic emotions which can be defined more easily. Russell’s circumplex model of emotions (Dzedzickis et al. 2020 ) (presented in Fig. 4 ) provides a two-dimensional parameter space to differentiate between emotions with respect to the valence and arousal.
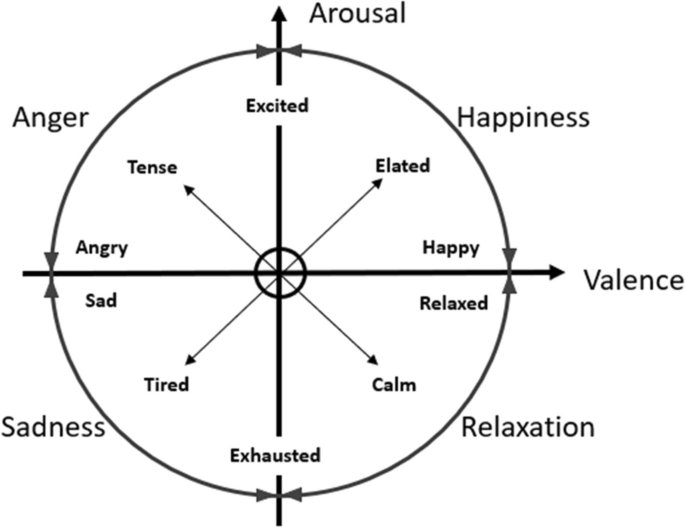
Russell's circumplex model of emotions
Using the abovementioned model, the classification of emotions becomes easier for a human expert, but still, as mentioned earlier, there are many challenges related to automated emotion recognition performed by a machine. To accomplish that, several measurable parameters for emotion assessment must be used, including heart rate, respiration rate, brain electric activity (measured in an electroencephalography), facial expression, natural speech, etc. Understanding the differences between emotions, especially when there are some emotions that are similar to one another, is crucial for developing a SER system. On the other hand, an attacker interested in creating bias and interfering with the accurate emotion classification process of a SER system could exploit the similarities that exist between emotions. Therefore, the detection of the appropriate attributes (level of arousal and valence) of each emotion is a basic step in the development of a proper security mechanism for SER.
3.2 Sound waves
Before discussing the representation of the sound by a machine, we need to have a basic understanding of sound waves and their attributes. Every sound we hear is the result of a sound source that has induced a vibration. The sound we hear is caused by vibrations that create fluctuations in the atmosphere. These fluctuations are called "sound waves." Fig. 5 illustrates invisible sound wave. The "pressure" axis in Fig. 5 represents the difference between the local atmospheric pressure and the ambient pressure.
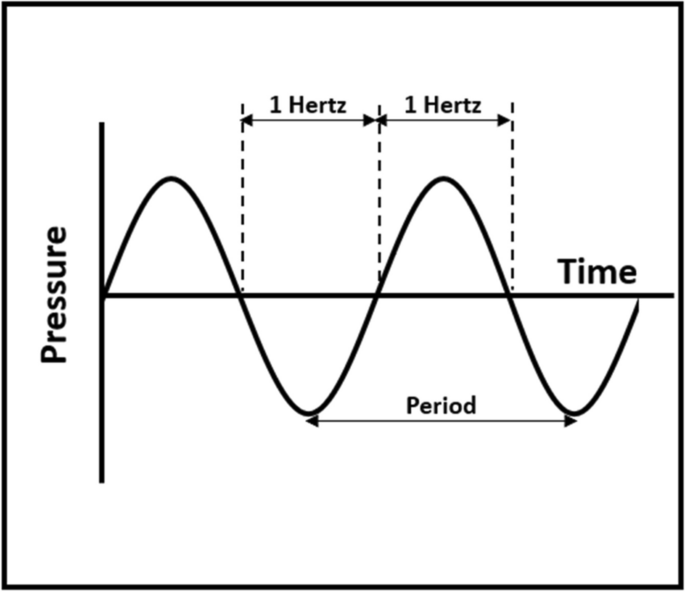
Invisible sound wave
Sound waves are nothing but organized vibrations that pass from molecule to molecule through almost every medium, including air, steel, concrete, wood, water, and metal. As a sound vibration is produced, the fluctuations are passed through these mediums, transferring energy from one particle of the medium to its neighboring particles. When air carries sound, waves contact our ear, and the eardrum vibrates at the same resonance as the sound wave. Tiny ear bones then stimulate nerves that deliver the energy we recognize as sound. While some sounds are pleasant and soothing to our brain, others are not, and this is considered noise. The loudness (amplitude) of a sound wave is measured in intensity by decibels (dB), while the pitch of the sound wave is measured in frequency by hertz (Hz). One hertz is equal to one sound wave cycle per second as illustrated in Fig. 5 . The hertz level does not decay over time or distance, but the decibel level does.
3.3 Sound data representation
In order to use algorithms that analyze and learn informative patterns in sound (as in SER systems), the sound waves should be converted into data types that can be read by a digital system. Sound waves can be represented in a variety of ways, depending on the conversion process applied. There are several algorithms used to convert sound waves, each of which utilizes different features (mainly frequency, amplitude, and time) of the sound wave and represents the sound differently. The main methods used for the conversion process are analog-to-digital conversion (ADC) and time–frequency conversion. Once the sound is converted to a digital format and saved in a computerized system, it becomes vulnerable to cyber-attacks; therefore, the process of representing the sound data in the computer for the task of SER must be done with the appropriate knowledge, to decrease the possibility of malicious usage of it.
A taxonomy of the main sound data representation methods (A/D conversion and time–frequency domain representations) is presented in Fig. 6 . It includes a layer for the various conversion techniques (with reference to the relevant paper), as well as a layer for the conversion algorithm sub-type, where we present several conversion sub-algorithms (with the year it was first presented), related to their higher-level main algorithm. Each method is suitable for a different task and provides different information regarding the original sound wave. Some of the methods (e.g., Mel frequency representation) are more suitable for learning tasks associated with the human perception of sound. Footnote 13 A description and comparison of the methods follows the taxonomy.
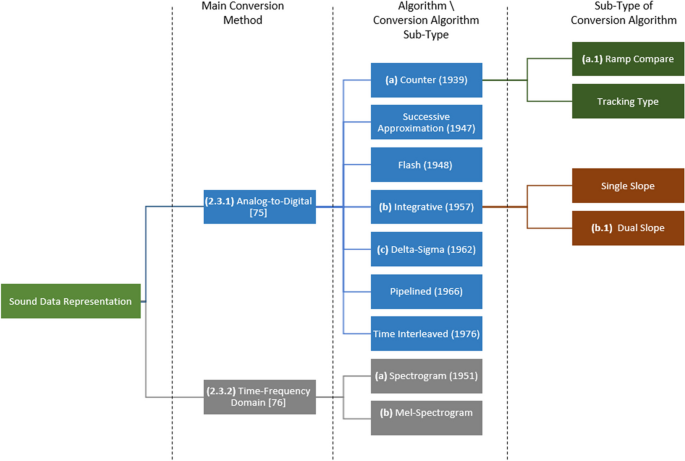
Taxonomy of raw sound data representation methods
3.3.1 Analog-to-digital conversion
Sound waves have a continuous analog form. Computer systems store data in a binary format using distinct values (sequences of 1 s and 0 s). Therefore, in order to be processed by computers, sound must be converted to a digital format. First, sound is recorded using a device (that can turn sound waves into an electrical signal). Following that, regular measurements of the signal's level (referred to as samples) are obtained. The samples are then converted into binary form. A computer can then process and store the digitized sound as a sequence of 1 s and 0 s. This method was invented by Bernard Marshall Gordon (Batliner et al. 2011 ), who is "the father of high-speed analog-to-digital conversion." During the conversion process, two main parameters need to be defined: the sampling frequency and the sample size. The Sampling frequency is the number of samples obtained per second, measured in hertz. An audio recording that was recorded with a high sampling frequency can be represented more accurately in its digital form. Figure 7 illustrates the effect of the sampling frequency on the representation accuracy. The upper plot shows the analog form of the sound, while the middle and bottom plots illustrate the effect of the sampling frequency on the sound wave; the middle plot shows a lower sampling frequency (and therefore a less accurate representation of the sound wave) than the bottom plot. The sample size is defined as the number of bits used to represent each sample. A larger sample size improves the quality of an audio recording; when more bits are available for each sample, more signal levels can be captured, resulting in more information in the recording, as illustrated in Fig. 8 . The top plot illustrates the use of one bit (1\0) per sample. The middle plot, which demonstrates the use of two bits per sample, which provides a more accurate representation of the sound. In the bottom plot, by using 16 bits to represent each sample, the accuracy of the digitized sound wave is almost identical to the analog form of the sound.
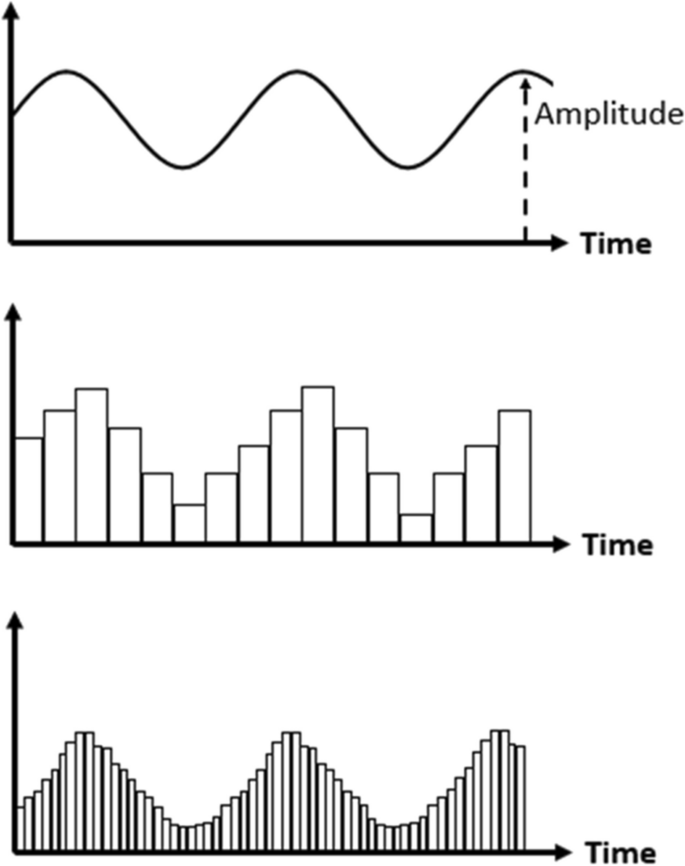
The effect of the sampling frequency on the representation accuracy
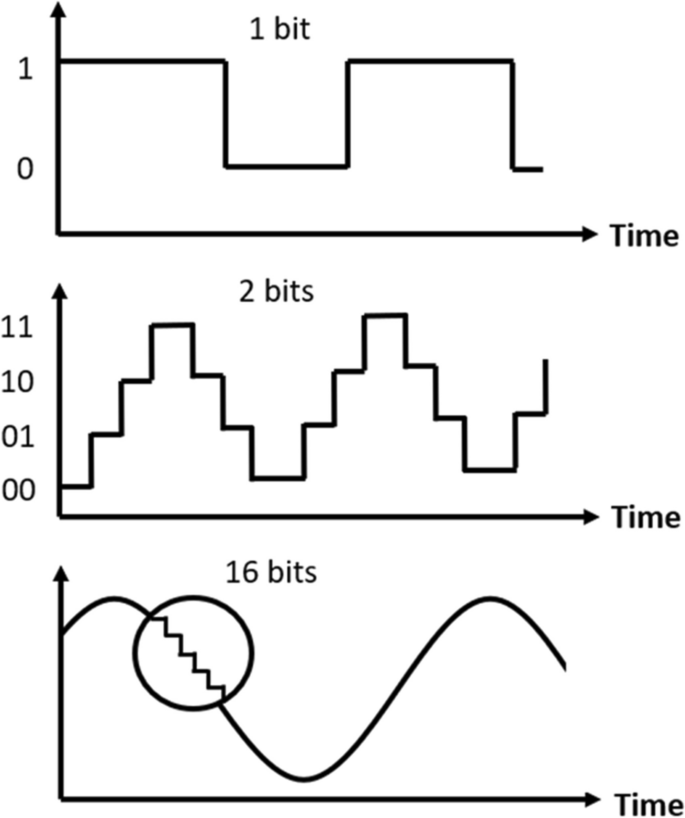
The effect of the sample size on the representation accuracy
To determine the size of a sound file, we need to multiply the sampling frequency by the sample size and the length of the sound recording in seconds:
Equation 1 . Calculation of a sound file size in bits.
Therefore, a sound file will become larger if the sampling frequency, sample size, or duration of the recording increase. When playing sound files over the Internet, the quality of the sound is affected by the bit rate, which is the number of bits transmitted over a network per second. The higher the bit rate, the more quickly a file can be transmitted and the better the sound quality of its playback. One should note that, in the case of securing SER systems, small-sized files are easier to transmit as part of a cyber-attack. That means that large sound files, which are more accurate in terms of the original sound recorded, are harder to utilize in order to perform a cyber-attack.
There are several types of analog-to-digital conversion algorithms used today to produce a digital form of a sound wave (Failed 2016 ), including the counter (which contains sub-types, e.g., ramp compare), ramp, integrative (which contains sub-converters, e.g., dual slope), and delta-sigma types.
3.3.2 Time-frequency domain
- Spectrogram
Visualizing the sound wave can be described in two domains: time domain and frequency domain. In order to convert the time domain to the frequency domain, we need to apply mathematical transformations. The time domain visualization shows the amplitude of a sound wave as it changes over with time. When the amplitude in the time domain is equal to zero, it represents silence. These amplitude values are not very informative, as they only refer to the loudness of an audio recording. To better understand the audio signal, it must be transformed into the frequency domain. The frequency domain representation of a signal tells us what different frequencies are present in the signal. Fourier transform (FT) is a mathematical transformation that can convert a sound wave (which is a continuous signal) from the time domain to the frequency domain.
Sound waves, which are audio signals, are complex signals. Sound waves travel in any medium as a combination of waves. Each sound wave has a specific frequency. As we record a sound, we can only record the resultant amplitudes of its constituent waves. By applying FT, a signal can be broken into its constituent frequencies. A Fourier transformation not only provides the frequency of each signal but also its magnitude. To use a sound wave as an input, we need to use fast Fourier transform (FFT) (Huzaifah 2017 ). FFT and FT differ in that FFT takes a continuous signal as input (like a sound wave, which is a sequence of amplitudes that were sampled from a continuous audio signal), while in FT the input is a discrete signal. Figures 9 and 10 illustrates the input audio signal and the output of the same audio signal after applying FFT. The original signal in Fig. 10 is a recording of the term "speech emotion recognition."
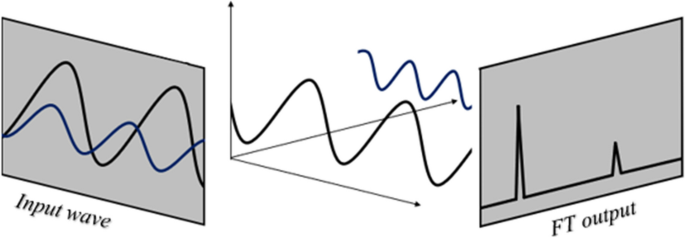
Fourier transform applied on a given sound wave
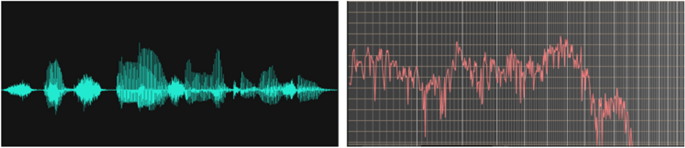
- Sound wave in the time domain (left) and frequency domain (right), after applying FFT
When we apply FFT to an audio file, we have the frequency values, but the time information is lost. In tasks such as speech recognition, the time information is critical to understand the flow of a sentence. Spectrograms are visual representations of the frequencies of a signal as they change in time. In a spectrogram representation plot one axis represents time, while the other axis represents frequencies. The colors represent the amplitude (in dB) of the observed frequency at a particular time. Figure 11 represents the spectrogram of the same audio signal shown above in Fig. 10 . Bright colors represent high frequencies. Similar to the FFT plot, lower frequencies ranging from 0–1 kHz are bright.
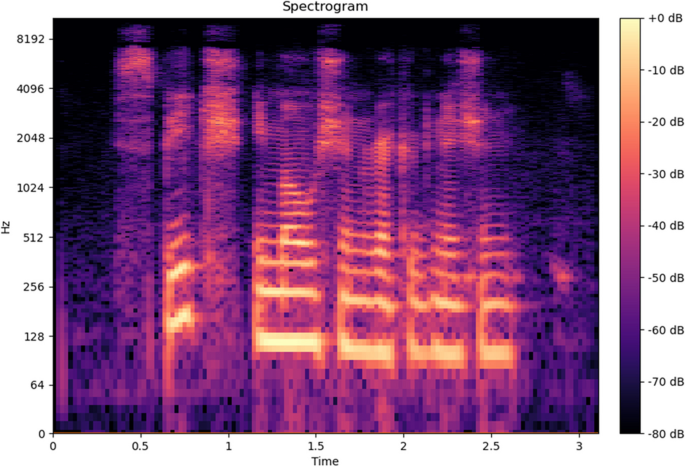
- Spectrogram of an audio signal
Mel spectrogram
The Mel Scale
The human brain does not perceive frequencies on a linear scale. The human brain is more sensitive to differences in low frequencies than high frequencies, meaning that we can detect the difference between 1000 and 1500 Hz, but we can barely tell the difference between 10,000 Hz and 10,500 Hz. Back in 1937, Stevens et al. ( 1937 ) proposed a new unit of pitch such that equal differences in pitch would sound equally distant to the listener. This unit is called Mel, which comes from the word "melody" and indicates that the scale is based on a pitch comparison. To convert frequencies (f in Hz) into Mel (m), we need to perform a mathematical operation:
Equation 2 . Frequency to Mel scale conversion formula.
Mel Spectrogram
A Mel spectrogram is a spectrogram where the frequencies (the y-axis) are converted to the Mel scale. Figure 12 presents the Mel-Spectrogram produced by the Librosa package Footnote 14 in Python. Note that in contrast to the spectrogram shown in Fig. 11 , the Mel-Spectrogram of the same sound signal has a different frequency which is directly affected by Eq. 1 .
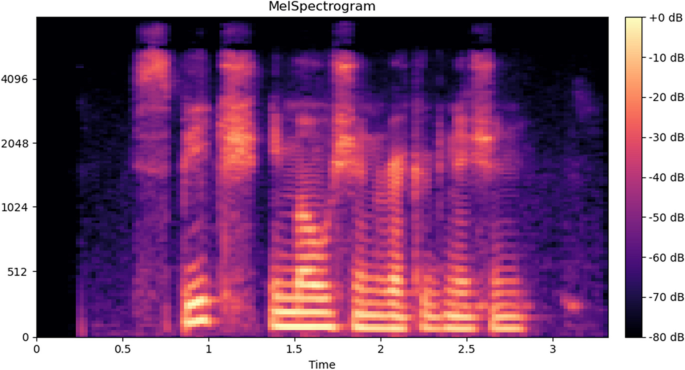
- Mel-Spectrogram of an audio signal
Given the above methods for the time–frequency domain, we can now compare the two representation methods (spectrograms and Mel-spectrograms). The frequency bins of a spectrogram are spaced at equal intervals based on a linear scaling. In contrast, Mel-scale frequency uses a logarithmical scheme similar to that of a human's auditory system.
Table 1 provides a comparison of the differences between abovementioned methods. As can be seen in the table, there are several main differences between analog-to-digital conversion and time–frequency domain representation methods. The parameters for comparison were chosen based on their relevance to audio analysis.
Based on Table 1 , digital representations and spectrograms, for example, are not well suited for human sound perception, meaning that those kinds of representations do not represent speech as a human hears it, a desired property in sound analysis tasks, as we wish the machine to "think," "hear," and "react" like a human being. Therefore, their use is not recommended in the SER task. Analog representation represents the sound in a way that suits human’s sound perception, however it poses analysis challenges (Batliner et al. 2011 ) (e.g., due to its inaccurate representation and high computational complexity). Spectrogram representation is widely used for text-to-speech tasks, as it can identify the difference in letters and words derived from the amplitudes of sound variance over time, however it is limited in its ability to identify informative changes in the same amplitude (it is more sensitive to variations in sound’s intonation), which are crucial for SER and categorization of sub-emotions. Therefore, and based on variety of recent successful studies, we find the Mel spectrogram as the ideal representation of sound for the task of SER mainly when using deep learning algorithms (Lech et al. 2018 ; Yao et al. 2020 ).
3.4 Feature extraction from the raw sound data
After converting the audio signals using one of the methods described in the previous section, a feature extraction phase needs to be performed. Anagnostopoulos et.al (Yan et al. 2022 ) described various features that can be extracted from the raw sound data. Swain et.al (Swain et al. 2018 ) surveyed a work performed in the speech emotion recognition field; they divided the features used in the SER domain into two main approaches. The first approach is based on prosodic features. This approach includes information regarding the flow of the speech signal and consists of features such as intonation, duration, intensity, and sound units correlated to pitch, energy, duration, glottal pulse, etc. (Rao and Yegnanarayana 2006 ). The second approach is based on vocal tract information, and these features are known as spectral features. To produce the spectral features, FT is applied on the speech frame, and the main features are the Mel frequency cepstral coefficient (MFCC), Footnote 15 perceptual linear prediction coefficient (PLPC), Footnote 16 and linear prediction cepstral coefficient (LPCC). Different studies used different features or a combination of the features described above. Each feature has its own advantages and disadvantages, but they all have one property in common: they contain the most sensitive information regarding the audio file, meaning that sabotaging the creation of the features will sabotage the entire SER system, including its accuracy and functionality. The related work section presents the studies that have leverage these kinds of features for the purpose of the SER task.
3.5 The reflection of emotions in human’s speech
Reliable acoustic correlates of emotion or affect in the audio signal's acoustic characteristics are required for the detection of emotions in speech. This has been investigated by several researchers (Harrington 1951 ; Cooley and Tukey 1965 ) who found that speech correlates are derived from physiological constraints and reflect a broad range of emotions. Their results are more controversial when examining the differences between fear and surprise, or boredom and sadness. Physical changes are often correlated with emotional states, and such changes in the physical environment produce mechanical effects on speech, especially on the pitch, timing, and voice quality. People who are angry, scared, or happy, for instance, experience an increase in heart rate and blood pressure, as well as a dry mouth. This results in higher frequency, louder, faster, and strongly enunciated speech. When a person is bored or sad, their parasympathetic nervous system is activated, their heart rate and blood pressure decrease, and their salivation increases. This results in monotonous, slow speech with little high-frequency energy. Based on this, we can classify four main emotions that have different effects on the human's speech (Pierre-Yves 2003 ). Table 2 compares different emotions in speech. Using the visualization of a sound wave described earlier, we can identify emotions in speech based on the specific physiological characteristics of each emotion.
4 The evolution of speech emotion recognition methods along the years
Understanding and categorizing the main methods by which an emotion is recognized by SER systems and how the systems analyze speech are important in identifying SER systems’ vulnerabilities and potential attacks that might exploit these vulnerabilities. Moreover, the ability to identify trends in the use of the methods could shed light on ways to mitigate potential attacks and address existing vulnerabilities.
The field of speech emotion recognition has engaged many researchers over the last three decades. At the beginning, the main challenges were determining how to analyze speech waves and which features could be extracted directly from sound waves. Later studies leveraged this knowledge and developed different algorithms for modern SER systems. The evolution of SER systems has been a continuous process of incorporating new components, algorithms, and databases to improve accuracy and efficiency. However, with each addition, the need for protection and security also increased. Therefore, previous studies on SER systems must be reviewed to identify potential vulnerabilities and develop measures to safeguard the system's integrity. By doing so, we can create more secure and reliable SER systems that can effectively recognize and respond to human emotions.
Although many studies have been performed in the field of SER, some of them has leveraged the knowledge in the SER domain by using novel techniques or unique methods, and some of them has focused on improving the system's performance by using existing methods. Table 3 presents the studies performed over the years that involved uniqueness, and the main methods and features proposed in those studies. The last column in Table 3 ("Uniqueness") presents the uniqueness of each study in a nutshell, while other studies that present similar work are mentioned in the text following Table 3 . As can be seen in Table 3 , the studies used different methods and techniques to detect the emotions in the speakers' utterances, with the usage of unique and \ or novel methods.
First analysis of emotions in speech
The first attempt to analyze speech was made in the 1970s, and the study’s main goal was to determine the emotional state of a person using a novel speech analyzer (Williamson 1978 ). In this study, Williamson used multiple processors to analyze pitch or frequency perturbations in a human speech to determine the emotional state of the speaker. This study was the first to analyze the existence of emotions in speech. Twenty years passed before another study proposed novel technologies in the context of modern SER; in the 1990s, with the increased use of machine learning algorithms and advancements in computational technology, different researchers began to use a variety of classic machine learning methods to detect emotions in speech.
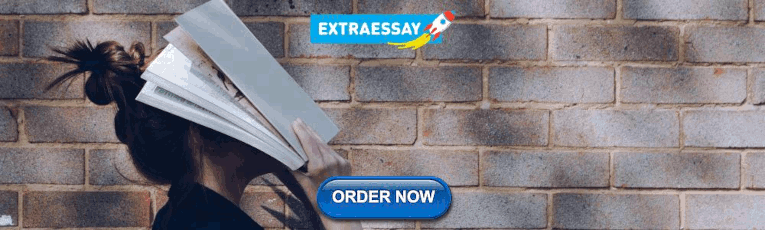
First use of ML algorithms and feature extraction methods
The early twenty-first century saw significant advances in artificial intelligence (AI) in general and in the machine learning domain in particular, and this was accompanied by the development of unique advanced machine learning algorithms and designated feature extraction methodologies. Dellaert et.al ( 1996 ) used both new features (based on a smoothing spline approximation of pitch contour) and three different ML algorithms (Kernel Regression, K-nearest neighbors and Maximum Likelihood Bayes classifier) for the task of SER. Until 2000, no large-scale study using the modern tools developed in the data mining and machine learning community has been conducted. Either one or two learning schemes were tested (Polzin and Waibel 2000 ; Slaney and McRoberts 1998 ), as a few or just simple features were used (Polzin and Waibel 2000 ; Slaney and McRoberts 1998 ; Whiteside 1998 ), which caused these statistical learning schemes to be inaccurate and unsatisfactory. In 2000, McGilloway et al. ( 2000 ) used the ASSESS system (Automatic Statistical Summary of Elementary Speech Structures) to extract features from a sound wave, which produced poor quality features resulting 55% accuracy with Linear Discriminants classification method.
First use of neural networks
Progress was made in 2003 when Pierre-Yves ( 2003 ) used neural networks, mainly radial basis function artificial neural networks (RBFNNs) (Orr 1996 ) for the task of SER for a human–robot interface. In this case, basic prosodic features, such as pitch and intensity extracted from audio recordings, served as input to the algorithm. Since then, many other studies have been conducted in the field of SER, in a variety of domains, ranging from single linguistic (Kryzhanovsky et al. 2018 ; Badshah et al. 2017 ; Lech et al. 2018 ; Lim et al. 2017 ; Bakir and Yuzkat 2018 ) to para-linguistic (Pierre-Yves 2003 ; Satt et al. 2017 ; Hajarolasvadi and Demirel 2019 ; Khanjani et al. 2021 ), from real-life utterances (Pierre-Yves 2003 ) to the recorded utterances of actors (Kryzhanovsky et al. 2018 ; Satt et al. 2017 ; Badshah et al. 2017 ; Lech et al. 2018 ; Hajarolasvadi and Demirel 2019 ), and from the use of digital data in the time domain (Williamson 1978 ) to the use of spectrograms in the frequency-time domain (Kryzhanovsky et al. 2018 ; Satt et al. 2017 ; Badshah et al. 2017 ; Lech et al. 2018 ; Hajarolasvadi and Demirel 2019 ).
Combining prosodic and spectral features
Between 2005 and 2010, several experiments were performed using prosodic features and/or spectral features (Luengo et al. 2005 ; Kao and Lee 2006 ; Zhu and Luo 2007 ; Zhang 2008 ; Iliou and Anagnostopoulos 2009 ; Pao et al. 2005 ; Neiberg et al. 2006 ; Khanjani et al. 2021 ). Those studies compared the performance of different machine learning and deep learning algorithms in the task of detecting the correct emotion in a specific utterance. Since then, many studies used a plethora of advanced data science methods for the task of SER. in Satt et al. ( 2017 ) for example, Satt et. al used an ensemble of neural networks (Convolutional and Recurrent neural networks) applied on spectrograms of the audio files to detect the emotions concealed in each recording. Moreover, they used harmonic analysis to remove non-speech components from the spectrograms. Later research (Badshah et al. 2017 ) used also deep neural networks on spectrogram, but with the usage of Transfer learning using the pre-trained AlexNet. In (Alshamsi et al. 2018 ) the researchers used cloud computing to classify real-time recordings from smartphones (using a SVM model stored in a cloud). In the last few years, many researchers have attempted to improve the accuracy of methods proposed in prior research by adjusting the algorithms (e.g., replacing layers in a neural network to adapt it for the SER task), creating new feature extraction methods, and modifying the algorithms for different types of technologies (e.g., robots, human–computer interface).
Figure 13 contains a timeline showing advancements in the SER domain and the most important milestones in the domain’s evolution. As can be seen below, SER has advanced significantly over the last three decades. The first attempt to detect emotions in speech in the 1970s in which prosodic features were proposed paved the way for the development of the advanced AI methodologies used in modern SER systems.
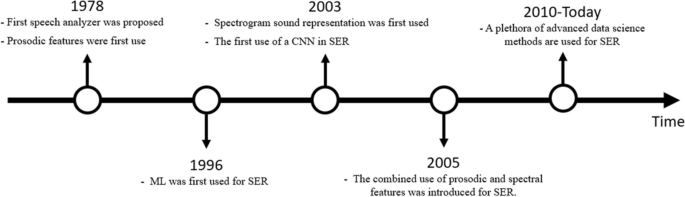
SER development timeline
5 Main domains and applications of SER systems
Understanding a human's emotions can be useful in many ways. As human beings, reacting appropriately to an emotion expressed in a conversation is part of our daily life. When interacting with a machine, we expect it to react like a human being. Therefore, emotion recognition systems were, and are still being developed to improve human–machine interfaces. Emotion recognition systems are used in many fields. In recent studies researchers have applied SER software code to real-life systems. For example, in 2015, Beherini et al. ( 2015 ) presented FILTWAM, a framework for improving learning through webcams and microphones. The system was developed to provide online feedback based on students’ vocal and facial expressions to improve the learning process. The data was collected in real time and inserted into a SER system to attempt to determine whether a student was satisfied with the learning, frustrated, depressed, etc. In another study, robots were programmed to recognize people's emotion in order to improve human–robot interaction (Rázuri et al. 2015 ). Such robots can react based on the emotional feedback of the person speaking. This could be a useful tool for understanding people with autism and the actual content of their speech. According to Utane and Nalbalwar ( 2013 ), SER systems can be used in a variety of different domains; for example, the use of SER systems at call centers could be helpful in identifying the best agent to respond to a specific customer's needs. Likewise, in airplane cockpits, SER systems can help recognize stress among pilots; conversations between criminals can be analyzed by SER systems to determine whether a crime is about to be performed; the accuracy of psychiatric diagnosis and the performance of lie detection systems could be improved with the use of a SER system; and in the field of cyber-security, the use of sound biometrics in authentication is being explored in another application of SER. Based on the studies mentioned above, we created a taxonomy of the various domains in which SER systems are used. The taxonomy is presented in Fig. 14 .
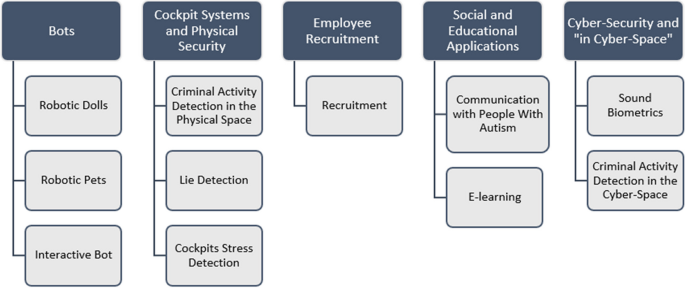
Taxonomy of SER domains and applications
As can be seen in the taxonomy, SER systems are used in diverse domains and applications. In the previous section, our overview of prior studies and the work performed in the SER field showed that despite their applications in many domains, no studies have focused on the security aspects of SER systems. Cyber-attacks, when aimed at SER systems, may damage a variety of different domains, regarding the fact that SER systems are useful in many daily actions. Attackers might attack customer service applications to sabotage a company's reputation. Moreover, attacks might be conducted on security systems using SER technology to disrupt investigations. Therefore, a secured system is required. As far as we could find, no work has been done regarding analyzing the security of SER systems. Since SER systems use actual voices of humans, they can be seen as a huge database of people's voices. For example, looking at a sound biometric system, which can use SER algorithm to accurately identify the individual in different emotional states, those systems are vulnerable to "Spoofing attack"- an attack in which a person or a program identifies as another. As written above, a huge amount of personal information is involved in the human's speech, especially when detecting the person's emotions. Cyber-attacks on SER systems may decrease drastically the demand of those systems, due to the privacy invasion latent in such systems. Therefore, on the following section we will elaborate and analyze the security aspects associated with SER systems.
6 Security analysis of speech emotion recognition systems
6.1 speech emotion recognition ecosystem.
Before we discuss the cyber-attacks aimed at SER systems, it is necessary to understand the SER ecosystem. An ecosystem (Kintzlinger and Nissim 2019 ; Landau et al. 2020 ; Eliash et al. 2020 ) is the combination of all of the players and devices contained in the main system and the interactions between them, which are crucial for the information flow in the system. This knowledge is important for understanding the SER system process, its existing vulnerabilities, and the potential cyber-attacks associated with it. Figure 15 below shows the SER ecosystem, and Table 4 contains the legend for Fig. 15 .
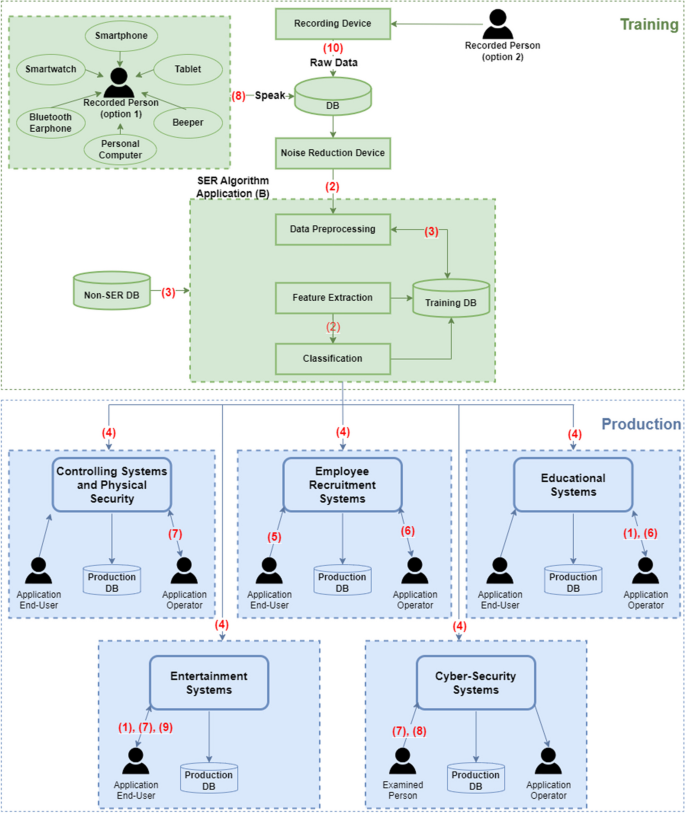
SER ecosystem
A SER system has two main phases, the training and production phases. In the training phase, the sound wave data is collected via a device in the (A) personal cyber space; such devices include external recording devices or various microphone-integrated devices, such as a smartphone, smartwatch, tablet, Bluetooth earphone, personal computer, beeper, or hearing aid device (Kintzlinger and Nissim 2019 ). The recordings collected in this phase are the raw data, which serves as input to the SER system. Note that to produce clear sound waves, without noise or background sounds, a noise reduction device is needed. Then, after recording the person, data processing and analysis is performed, in which features are extracted and a (B) classifier is induced and used to determine the emotion of the person when the recording was made. In addition, the SER system may use an external database (DB) that contains additional information, such as demographic information or gender, to improve its performance. After identifying the emotions expressed in the recorded utterances, it is possible to store both the original sound and its labels (the emotions) in a DB (i.e., the training DB in Fig. 15 ). Note that this training DB can also be used to train other classifiers or perform statistical analysis.
In the production phase, the SER system can be used in different applications, each of which has its own DB, end users, and operators. In some cases, the application’s end user and the application’s operator are the same person (for example, in entertainment applications), and in other cases, they are different people (for example, in employee recruitment applications). In addition, each end user or operator may be the recorded person for the SER algorithm. This usually occurs when the end user’s sounds are needed to continuously update (re-train and re-induce) SER classification models (e.g., cockpit controlling systems, entertainment SER-based systems). Note that in a SER system aimed at maintaining its updateability and relevance in the long term, these test DBs (after being verified and labeled) may be used to enrich the training DB and induce an updated SER classifier.
As can be seen in the SER ecosystem, in some cases, the end user receives and uses feedback from the SER system for his/her own benefit (e.g., entertainment systems), however there are other cases in which the operator is the only one who receives and uses the feedback, which serves as input to the SER system.
To better understand the importance of the end user and the operator to the ecosystem, we provide a brief description of their interaction in each of the domains shown in Fig. 15 (in the production phase).
Cockpit systems and physical security systems—the end user's utterances are fed to the SER system, and the operator analyzes the user’s emotional state based on the SER classification results. Since SER systems do not have the ability to fully understand human common sense in general, and particularly are unable to identify the context in which the utterances were said, intervention by a human operator is needed in some cases. For example, a soldier entering a battlefield wearing a helmet in which a SER system is embedded send signals to the operator (see the one-directional arrow in Fig. 15 ) who needs to decide whether to drill down (asking the end user questions and receiving answers for additional classification).
Employee recruitment systems—the operator (in this case, the human resources recruiter) uses the SER system to analyze the end user's (the candidate for the position) mental state during the job interview. The operator asks questions, and the SER system receives the answers from the end user, analyzes the user’s utterances, classifies his/her emotions, and provides feedback to the operator.
Educational systems—the operator, who may be a teacher, social worker, pedagogical director, etc., uses the SER system to better understand the emotions expressed by the end user (a student). For example, the end user may be a student with an autistic spectrum disorder who has difficulty expressing his/her emotions during a class or guidance session; the use of a SER system, which can automatically extract the student’s utterances and accurately classify them and provide additional information regarding the student’s mental and emotional state, may enable the human consultant (i.e., the operator) to better understand the student and meet his/her needs.
Entertainment systems—in this case, the same person acts as an operator and end user. An entertainment SER system allows the user to interact with the system, meaning that the user sends speech waves and receives the emotions classified during his/her leisure time. Virtual assistants (e.g., Siri, Alexa) in smartphones may contain a SER system that enables them to react properly in response to the end user's mood (see the two-directional arrow which reflects the interaction between the end user and the system in Fig. 15 ).
Cyber-security systems—the end user of SER systems in the cyber-security domain may be a person being queried by a lie detection system (e.g., polygraph). Lie detection systems that use an emotion-based approach (EBA) analyze the answers provided by the user in response to the operator's questions. Then the EBA system classifies the answers using its designated SER module, providing the operator with the classification decision regarding the emotions hidden in the user’s answers (in this case, a one-directional arrow reflects the interaction between the EBA system and the operator or the examined person in Fig. 15 ).
The SER ecosystem can suffer from vulnerabilities that leave it exposed to cyber-attacks; in some case, specific components may be vulnerable, and in others, the malicious use of the SER system can result in a cyber-attack. The different players (humans and components), and their interactions are presented in Fig. 15 , which describes the full SER ecosystem.
6.2 Potential cyber-attacks aimed at SER systems
Our analysis of the ecosystem presented in the previous subsection enabled us to identify vulnerabilities that can be exploited and compromised by adversaries to perform cyber-attacks. This analysis of the SER ecosystem, along with several review studies that focused on the cyber-security domain (Orr 1996 ; Chen et al. 2011 ), enabled us to further explore and identify potential attacks that can be performed on SER systems. In addition to the new attacks we suggest, there is a wide range of cyber-attacks aimed at voice-based systems (Orr 1996 ).
Given that SER systems incorporate an ML model and operate in the domain of voice-based technology, it is important to emphasize that any cyber-attack targeting voice-based or ML-based systems is also relevant to SER systems. While the core principles of cyber-security apply universally, SER systems' unique characteristics mean that attacks on these systems can have different, far-reaching consequences. Cyber-attacks aimed at SER systems can not only compromise model integrity and data privacy; they can also manipulate the interpretation of emotions and impact user experience. Therefore, understanding the threats pertaining to voice and ML-based systems is crucial. In this section we present the attacks that have the greatest impact on SER systems yet still lack a security mechanism capable of providing a defense against them.
Table 5 summarizes our analysis of 10 attacks aimed at SER systems, as well as the causes and impact of the attacks. For each attack, we indicate whether the attack is passive or active (in passive attacks there is no impact on the system’s resources, but the attacker can observe and/or copy content from the system, whereas in active attacks the attacker tries to modify the data and/or the system's functionality), the system phase in which the attack occurs (the training or production phase), the implications of the attack (meaning the impact the attack has on the system or its users), and the relevant application. For some attacks known in the cyber-security community (e.g., replay or poisoning attacks), we present the attack and its variation in the SER domain; for example, a replay attack, which is usually performed by replaying an original transaction for a different and malicious purpose, can be performed in the SER domain by combining parts of the original transaction (e.g., voice recording) in a different order, creating "new" content with the same voice (see attack #8 in Table 5 ).
The text that follows Table 5 provides more detailed information regarding each attack, particularly about the possible attack vectors (the path used by the attacker to access the system and exploit its vulnerabilities) and the attack's flow description.
6.2.1 Attack No. 1- data exfiltration
Possible attack vectors.
Malware is transmitted to the user’s device (e.g., smartphone, tablet, laptop) via the Web (e.g., Google Play) or a USB (e.g., using a malicious USB-based device).
Attack flow description
The compromised device uploads the recorded voice to a malicious third-party who exploits it for malicious purposes. Although this attack may be general and relevant to multiple systems, its execution in the SER domain is relatively easy, making the SER system extremely vulnerable to this kind of attack. Since SER systems (in production environments) use actual voice recordings of their users, which are usually collected via a user's personal cyber space devices, any malware distributed to such devices can compromise the SER system, allowing an attacker to exploit the voice recordings collected by the system and use them for their own malicious purposes.
6.2.2 Attack No. 2- malware distribution
An SER developer innocently downloads a malicious version of a common library for SER system development (e.g., Librosa, SoundFile, FFTPACK, from the Web in Python, Java, etc.).
SER system developers commonly use third-party libraries to streamline the development process. For example, SER systems usually rely on various libraries and software packages to perform audio processing, feature extraction, etc. Therefore, the integration of a malicious library can compromise the core functionality and security of a SER system. Moreover, since SER systems are usually implemented as part of larger systems, attackers might exploit SER systems to launch attacks on connected networks or devices. For example, after a malicious library has been imported to and compiled in the developed SER system, the system would become infected; the infected system could then be uploaded to application markets or Web applications and thereby get distributed to additional users, with the ability to compromise their systems for a variety of malicious purposes.
6.2.3 Attack No. 3- SER DB poisoning
An online SER DB used to train a SER-based model is maliciously manipulated by downloading an existing DB (e.g., RAVDESS Footnote 17 ) and uploading a new malicious one.
The attacker downloads an existing and widely used SER DB and adds some adversarial sound examples (e.g., mislabeled samples, misleading noise that will confuse the learning model) to it; this will result in an inaccurate induced SER model trained on a poisoned DB. Then the attacker uploads the poisoned DB to the Web in the form of a DB update or alternatively shares it as a new, publicly available SER DB.
6.2.4 Attack No. 4- malicious SER model distribution
Uploading an open-source malicious SER induced model to the Web, making it publicly available for use.
The trained malicious SER model is uploaded to the Web for public use (as a publicly available trained model, such as a VGG neural network, or ResNet model). The malicious model is intentionally trained using misleading data, which will result in incorrect emotion classification. The user may download a malicious SER model and adjust it for his/her SER system without being aware that it is a malicious model.
6.2.5 Attack No. 5- inaudible sound injection
The sound can be added via sound playback (playing high-frequency audio recordings) or ultrasound injection.
The attacker employs one of the following vectors: (1) the attacker is physically proximate to the target speaker; or (2) the attacker leverages a position-fixed speaker that produces ultrasounds (sound waves at a high frequency that can only be heard by a microphone and not human beings). The device that stores and executes the SER system listens to the ultrasounds, which may contain utterances with specific emotions (as desired by the attacker, such as calm, self-confident utterances), which differ from the victim's current mental state during the attack. By doing so, a candidate for a job position, for example, may be classified as calm and relaxed by the SER system, instead of nervous and anxious, and thus may be considered an appropriate candidate. Note that in this attack the attacker does not change the original voice recording but overrides the victim’s voice with an inaudible sound.
6.2.6 Attack No. 6- emotion removal
The emotion can be removed through the following procedures:
A generative adversarial network (GAN) is used to learn sensitive representations in speech and produce neutral emotion utterances.
Malware is transmitted to the user’s device, via either:
the Web (e.g., Google Play).
a malicious USB-based device.
The attacker uses an emotion removal ML model (based on CycleGAN-VC (Aloufi et al. 2019 )), which creates utterances with neutral emotion by removing the prosodic and spectral features from the original recording. Then the emotionless utterance is sent to the SER system which classifies every input as neutral.
6.2.7 Attack No. 7- adversarial sound
Playback of sound waves produced by a GAN.
An attacker uses a GAN to produce adversarial examples, which are samples aimed at distorting the model’s classification (e.g., producing a sample that expresses anger, which will be classified as joy). Using the classification of the discriminator (one of a GAN’s neural networks that differentiates genuine from artificial samples), the attacker can generate artificial samples (i.e. perturbed samples). After producing the adversarial samples, the attacker can fool the SER system by inserting the samples into the system, thereby misleading the classification process. For example, by producing those samples, an attacker could fool the SER system regarding his/her emotional state (e.g., the system could misclassify the attacker’s emotional state as a depressed state in order to allow the attacker to obtain a prescription for a specific medication).
6.2.8 Attack No. 8- voice impersonation replay attack
Audio samples collected via a spam call to a victim in which the person's voice is recorded, cloud servers that store audio files, online datasets used to train the SER system, and/or a malicious application uploaded to the market that exfiltrates a recording of a user.
An attacker who wishes to impersonate another person collects an audio sample of the victim via one of the attack vectors. A user authentication system (e.g., semi-autonomous car system that identifies the car owner's emotional state to determine his/her ability to drive or his/her emotional state while driving (Sini et al. 2020 )) can be misled by an attacker who uses recordings of the car owner in different emotional states to sabotage the user authentication system.
In a different scenario, the attacker can use the audio samples collected to produce new utterances with the same voice of the victim (by using the deep fake cut and paste method (Khanjani et al. 2021 ) that re-orders parts of the full utterance according to a text dependent system). By replaying the new utterances, an attacker can create fund transfers, smart home commands, etc.
6.2.9 Attack No. 9- induced classification model inference
Querying an online SER service via its API and inferring the model itself.
The SER model f is uploaded to an ML online-service (e.g., PredictionIO Footnote 18 ) for public use (mainly in the entertainment sector). The attacker, who obtains black-box access (accessible only via prediction queries) to the model via its API, queries the model as many times as needed to infer the learning procedure of the model itself (learning the decision boundaries) and then produces a model f ̂ that approximates f . By doing so, the black-box SER model becomes a nearly white-box model available to the attacker who can exploit or steal the model to meet his/her needs or otherwise profit from it. Once the attacker has constructed a model (f^) that approximates the original SER model, they effectively turn the black-box model into a nearly white-box model. This means that they can gain in-depth understanding of how the SER system functions, and this knowledge can be exploited for various purposes. For example, the attacker can manipulate the model to misclassify emotions, potentially causing the SER system to provide incorrect results. Such manipulation could have a variety of consequences, from affecting user experience to faulty decision-making. This cyber-attack, initially aimed at inferring and stealing any ML model, can be adapted to SER systems by accessing the SER model via its API. The consequences have various implications for both the SER application operator and its end users.
6.2.10 Attack No. 10- sound addition using embedded malicious filters
Concealing malicious components in a microphone during the manufacturing process or as part of a supply chain attack and selling them as benign components.
In the process of collecting data and recording the samples to train the SER system, the person being recorded can use a modified malicious recording device (e.g., microphone) rather than a benign device. The malicious microphone can add noise to the original sound wave (thereby distorting the recording process), producing perturbed samples which serve as the raw data used to train the SER model. Since a benign microphone also produces perturbations in some situations, it is impossible for the recorded person to know that he/she is using malicious hardware. Since there are companies developing AI solutions for SER systems (EMOSpeech, CrowdEmotion, deepAffects, etc.), an attacker could impair one of these services or degrade their quality or accuracy by marketing malicious recording equipment.
7 Security mechanisms for SER systems
To secure speech-based systems from cyber-attacks, there are several security mechanisms which were not specifically designed for SER attacks that can be utilized for that purpose. The main security mechanisms that are more tailored to SER systems are aimed at preventing adversarial ML attacks on SER systems, such as in Latif et al. ( 2018 ); Jati et al. 2020 ).
In this section, we first describe each of the relevant security mechanisms, and then in Table 6 , we map each security mechanism, indicating whether it covers the 10 attacks aimed at SER systems listed in Table 5 . For each security mechanism and attack, we calculated the percentage of attacks covered by each mechanism; as can be seen, some attacks remain unaddressed, leaving SER systems vulnerable to those attacks.
Latif et al. (Latif et al. 2018 ) suggested training the model with adversarial examples to defend against adversarial ML attacks on SER systems. In their paper, they trained a SER model with 10% adversarial samples and 90% benign samples to improve the model’s robustness against adversarial ML attacks. They also trained a different model based on a neural network on a dataset of samples with additional noise to generate a model that is robust to sound addition attacks. Another possible defense methodology that can be used against adversarial ML attacks described in Latif et al. ( 2018 ) involves the use of a GAN to clean the perturbed utterance before running the classifier on it. Their results show that training using samples with additional noise produces a higher error rate than training using adversarial samples. Moreover, the use of a GAN to clean the perturbed utterances before training produces the lowest error rate (37.18% error on average on two datasets), yet for training, GANs require precise information on the type and nature of adversarial examples.
In (Zhang et al. 2017 ), the authors described a simple yet efficient solution for preventing attacks using inaudible sound playback. The main reason for the success of an inaudible sound attack (attack #5 in Table 5 above) is that microphones sense sound waves at high frequencies (over 20 kHz). Most microphones implemented in smartphones (MEMS microphones) are built the same way. To prevent such an attack, the microphone should be enhanced and redesigned to block any sound waves in the ultrasound range (e.g., the iPhone 6 Plus microphone is designed to resist voice commands at high frequencies).
A security mechanism used to defend against an ML model theft attack via API querying was suggested by Lee et al. (Lee et al. 2018 ). As described in the previous section, during the attack the attacker will be able to steal an ML model if he/she obtains the outputs of the model for a certain input and the class probabilities (e.g., for a certain utterance the attacker obtains the emotion recognized in the utterance and its probability). The simplest method for avoiding the attack is for the SER system to only provide the final classification decision, without the classification probabilities. The authors suggested a different API query design that forces the attacker to discard the class probabilities when quering the model many times. Without the option for multiple queries, the attacker will be unable to restore the original model used for the SER task.
In (Blue et al. 2018 ), the authors proposed a method to defend sound-controlled systems against replay attacks and adversarial ML attacks by differentiating between sounds produced by humans and artificial sound (human vs artificial sound differentiation). Their strategy relies on the identification of the sound source of the received utterance. Utterances produced by playback devices will have a low frequency. Based on this property, the authors were able to determine whether the voice command came from a human being or a playback device. By leveraging this mechanism, one can differentiate between emotional utterances produced by a SER system’s end user and the utterances produced by a playback device or GAN (in the case of attack #7 in Table 5 ).
Another security mechanism for defending against replay attacks was suggested by Gong et al. ( 2019 ). In their study, the authors created a publicly available dataset containing genuine voice commands and replayed recordings of the same voice commands in various environmental conditions (noise, distance between the speaker and the recording device, etc.). By training on this dataset, an ML model can learn to differentiate between genuine audio samples and their replications, which may make the model robust to replay attacks.
Another countermeasure was proposed to prevent voice impersonation replay attacks (Li et al. 2000 ). Li et.al proposed automatic verbal information verification (VIV) for user authentication. In their method, spoken utterances of the speaker attempting to gain access are verified against a key piece of information in the speaker's registration information. During the authentication process, the speaker trying to gain access is asked a set of diverse questions, and his/her answers are compared to the answers stored for that speaker. Using this method, an attacker that uses voice recordings collected from a SER system's database will not necessarily have the correct answers to the verification questions, which will prevent the attacker from gaining unauthorized access. Although this countermeasure exists, it is important to note that it is only relevant for SER systems implemented in authentication systems.
Gui et al. (Gui et al. 2016 ) proposed a mechanism for defending against artificial input attacks, focusing on replay attacks. The scope of their study was brain print (EEG recordings) biometric systems, therefore the main data used was brain print data (EEG signals). To determine whether noise was added to the original data, the authors used an ensemble of classifiers. Although the study was conducted on brain print biometric systems, it can be utilized for SER systems, since brain prints are basically simple waves, like sound waves, and can be represented by a similar representation method.
To defend against malware distribution attacks, in 2019, an article was published by Veracode, Footnote 19 an American application security company, proposing a method for discovering malicious packages. A paper by Wysopal et al. (Wysopal et al. 2010 ) enabled the authors to identify the patterns commonly seen in malicious open-source libraries. Then, they implemented a malicious software package detector based on static analysis for each of the patterns described in Wysopal et al. ( 2010 ). Since malware distribution attacks in the form of malicious software packages are not well known in the programming community (meaning that a typical programmer would not be concerned with cyber-attacks when using an external software package in his/her code), the method proposed by Veracode is not widely used.
Regarding data exfiltration, in Ullah et al. ( 2018 ), the authors covered a wide range of defense mechanisms (not specially designed for SER systems but rather for data exfiltration attacks in general). The three main countermeasures mentioned are preventive, detective, and investigative countermeasures. Of these, the preventive countermeasures are the most relevant for SER systems; this group includes mechanisms such as data classification, encryption, and distributed storage. In (Kate et al. 2018 ), the authors presented a novel encryption method for voice data. Their method includes three main steps: receiving the audio file as a sequence of zeros and ones, increasing each sequence by two, and multiplying each sequence by 1e + 15 to create a 16-digit integer. After that, DNA encryption and a permutation function are applied. Note that the use of this method requires that the representation of the audio file be in a digital form, necessitating an ADC (as described in Section C).
As the results of our analysis on SER systems presented in the previous sections show, cyber-attacks that are unique to the SER domain are less common, and most of the attacks that can be performed on such systems are general attacks aimed at voice-controlled systems; only a few of the security mechanisms are specifically aimed at SER systems. Table 6 , which maps the security mechanisms’ coverage against the attacks aimed at SER systems, shows that many attacks, such as emotion removal, poisoning, and malicious SER model attacks, (30% of the attacks) remain unaddressed by the existing security mechanisms; these attacks pose a significant threat that must be considered, particularly when developing new SER systems. In addition, the security mechanism with the widest attack coverage, human vs artificial sound differentiation, covers only 30% of the attacks, meaning that even the best security mechanism is not relevant for 70% of the potential cyber-attacks aim at SER systems.
8 Directions for enhancing the security of SER systems
Given the potential cyber-attacks aimed at SER systems and the lack of sufficient defense mechanisms against such attacks, particularly emotion removal, poisoning, and malicious SER model attacks for which no security mechanism currently exists, there is a need to develop simple yet efficient defense solutions.
The first direction we present is aimed at improving SER systems’ defense against emotion removal attacks in which modified inputs are presented to the model which is unable to classify the emotions distorted in the modified input. Given a low-resolution audio sample, which has no emotion features in it, we suggest reconstructing the original audio sample (a.k.a. high-resolution sample) containing the emotion features. Then, the SER system will be able to classify the high-resolution audio sample based on the emotion expressed in it. Our suggestion can be illustrated using the following origami example. Imagine an origami bird constructed of folded paper. Unfolding the origami bird produces a piece of paper that contains traces of folding. The unfolded paper simulates the "modified input" of the origami bird. The goal is to reconstruct the original bird using the traces of the original folding that can be seen on the unfolded piece of paper. In our context of SER systems, we turn to a prior study (Kuleshov et al. 2017 ) that proposed a method for reconstructing a high-resolution audio sample from a low-resolution audio sample, using an artificial neural network trained on a large set of high- and low-resolution samples. While that research was not focused on enhancing the security of SER systems, it can be leveraged for this purpose as follows. For each time stamp, a speech signal has a duration and amplitude. The resolution of the speech signal is represented by the sampling rate; a higher sampling rate provides a higher resolution and vice versa. The high-resolution audio sample received will serve as the input for the SER system, which will now be able to identify the emotion it contains.
More formally, as described in Kuleshov et al. ( 2017 ), an audio sample is denoted as function \(s\left(t\right):[0,T]\to {\mathbb{R}}\) , where \(T\) is the duration of the sample in seconds and \(s\left(t\right)\) is the amplitude of the sample at time \(t\) . Then, to obtain the digital measurements of \(s\) , \(s\left(t\right)\) is discretized into a vector \(x\left(t\right)\) using parameter \(R\) which is the sampling rate of \(x\) , symbolizing the resolution of \(x\) . Based on (Kuleshov et al. 2017 ), the idea is to increase \(R\) by predicting \(x\) from a portion of its samples taken at any timestamp. The high-resolution version of \(x\) is \(y\) , where the sampling rate of \(y\) , denoted by \({R}_{2}\) , is larger than the sampling rate of \(x\) , denoted by \({R}_{1}\) . \(y\) is computed via a function \({f}_{\theta }\left(x\right)\) , where \(\theta\) is determined by training a fully convolutional neural network with parameter \(\theta\) on a set of samples \({x}_{i},{y}_{i}\) . After computing \(y\) , which is the reconstructed speech sample with emotion features, we can use it an input to the SER model, which will now be more robust to emotion removal and modified input attacks.
The above process, along with the origami bird example, are visualized in Fig. 16 . As can be seen, (A) is an unfolded piece of paper, containing the folding traces, while (B) is the bird produced by refolding the paper in a specific order. The inspiration and principle of the origami example in the sound domain follow, where (C) is a spectrogram of a low-resolution audio file containing no emotion features, and (D) is the spectrogram of the high-resolution audio reconstructed from the same audio sample shown in (C).
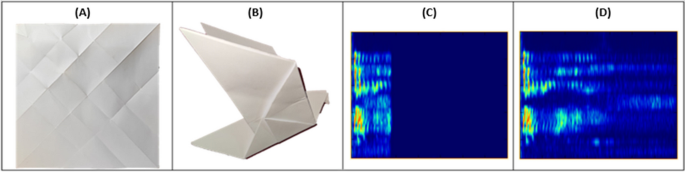
Origami bird and audio file reconstruction
Implementing the suggested concept as a defense mechanism in SER systems could protect the systems from attacks associated with modified inputs (like an emotion removal attack—attack #6 in Table 4 ), since the mechanism can also reconstruct the original audio sample in cases in which the sound has been modified.
Another direction for security enhancement aims at improving SER systems’ robustness against malicious model attacks (attack #4 in Table 5 ), by using natural language processing (NLP) algorithms (Batbaatar et al. 2019 ). We suggest using NLP algorithms to extract and understand the context of the words in utterances in the model’s training set samples. We suggest combining two methods for classifying the emotion expressed: (1) the spectral and prosodic features can be used to determine the emotion concealed in the speaker's voice (as in every SER system), and (2) the context of the utterance can be used to improve the classification’s accuracy. By applying NLP models to understand the meaning of every utterance said by a person, his/her mood and emotional state can be determined (negative words will indicate a bad mood, while positive words will indicate high spirits). This combination can help in situations where the context of the utterance is negative, but the pronunciation of it is positive, and vice versa. Moreover, implementing an NLP model in the SER system's training phase may improve the SER system's robustness against poisoning attacks. As described in Sect. 6.2, a poisoning attack is an attack in which the attacker downloads a publicly available dataset, which is used to train a SER model, replacing the correct labels with incorrect ones and uploading the dataset to the Web as a new dataset. In this way, the attacker can label an utterance as "happy," while its emotion is actually "sad." By using an NLP model, the SER system can combine the emotion features with the context of the words in every utterance to correctly classify the emotions expressed by a person.
Implementing the abovementioned mechanisms (all of which are based on machine learning algorithms) in a SER system can enhance the security of such systems, but in the long term, such machine learning mechanisms might suffer from limitations. For example, our voice changes over time (as a result of aging and lifestyle). Moreover, with technological developments, recording devices (which are used to collect the data) frequently change and are become better equipped with more functionalities and voice filters. Therefore, every SER system is exposed to the concept drift phenomenon (Žliobaitė 2010 ). Concept drift occurs when the statistical characteristics of the target variable that the model is trying to predict alter over time (the emotion concealed in the utterance, in the case of SER systems). Concept drift can result in a decrease in the generalization capability of the detection model as time passes. Therefore, there is a need to frequently update the learning model, both for the core models that are aimed at emotion recognition and for the models used in machine learning-based security mechanisms. An active learning approach can efficiently address the update gap that currently exists in SER systems. Applying active learning in the core SER model’s development and machine learning-based security mechanisms could reduce the effort and costs associated with the training phase. Active learning can reduce the number of samples required to train the model, by selecting a small yet informative set of samples. In addition, to add these informative samples to the training set, their true label must be determined (usually by a human expert); by reducing the number of samples needed, there will also be a reduction in the cost and time associated with the labeling procedure. In recent years, the use of active learning methods in the cyber-security domain has grown (Banse and Scherer 1996 ; Burkhardt and Sendlmeier 2000 ; Nissim et al. 2019 ; Nissim et al. 2017 ; Moskovitch et al. 2007 ), since it has been shown to enhance the detection model's detection capabilities over time and ensure that the model is up to date. Therefore, we suggest using active learning methods to cope with the concept drift phenomenon and further improve defense mechanisms’ detection capabilities. We also suggest considering relevant active learning methods presented in other domains, such as biomedical informatics (Moskovitch et al. 2010 ; Nissim et al. 2014 , 2015 ), for use in SER system security mechanisms.
9 Discussion and conclusions
In the last 10 years, speech emotion recognition systems have been widely implemented in various domains, allowing people to interact with products and services that utilize SER systems and companies to improve their services and interfaces. SER systems have been the subject of research for over four decades; most of the studies aimed at improving the accuracy and capabilities of the systems, while the security aspects of SER systems received little attention from researchers. This paper is the first to explore and analyze SER system security and contribute to the scientific community’s understanding of this underexplored area.
We started by providing information about the main principals of the SER domain and an overview of the work that has been performed in this domain over the years. This ranged from basic knowledge regarding emotions and the definition of sound waves to the main methods used to represent sound waves (formed as audio files) on the computer: analog-to-digital conversion, implemented with various mechanisms, and time–frequency domain conversion, which results in a spectrogram. We also analyzed how different emotions are expressed in a speaker's voice.
To better understand the vulnerabilities of SER systems, we analyzed the entire SER ecosystem, describing the data flow between each component and player in the ecosystem; the analysis performed enabled us to better understand the vulnerabilities in these systems. We identified 10 potential cyber-attacks targeting SER systems and the security mechanisms capable of preventing such attacks. Our analysis of the attacks shed light on the relevant attack vectors, possible attack scenarios, the phase in which the attack can be performed, the relevant domains, and the implications of the attack. This comprehensive analysis revealed major gaps in the existing protection against the attacks. We found that 30% of the attacks (including emotion removal, poisoning, and malicious SER model attacks) are not covered by any security mechanism, posing a very real threat to SER systems. We also found that voice impersonation replay attacks are the attacks best covered by the available defense mechanisms. From the security mechanism perspective, our analysis showed that the best mechanism available, human vs artificial sound differentiating, covers just 30% of the potential attacks, pointing out the need to develop improved security mechanisms.
The abovementioned insights raise some questions regarding the reason that three attacks we identified have no protection mechanism (i.e., no protection mechanism has been published so far). The first question is related to the effort required to address the attack. One may claim that the unaddressed attacks are too difficult to address, or alternatively, are trivial to address using existing tools, however the existing tools were not designed to specifically address these problems. Another reason why the three cyber-attacks have not been addressed is that they may not be considered important enough. From our perspective, the main reason these attacks have gone unaddressed stems from the limited use of SER systems in the past. Only in recent years have SER systems been more widely deployed and integrated in devices heavily used in modern life. As a result, many important aspects of these systems (such as their security and ethical concerns) have not been thoroughly examined.
Future research on the security of SER systems could explore the main security gap identified in this paper – the systems' vulnerability to emotion removal, malicious model, and poisoning attacks. We suggested a potential security mechanism for each of these attacks. For example, reconstruction of the sound algorithm could improve the robustness of SER systems against any type of modified input attacks, while combining NLP algorithms with SER algorithms could create an improved SER model, both in terms of the model’s classification accuracy and its ability to defend against poisoning and malicious SER model attacks. The use of these mechanisms in SER systems, which could dramatically improve the systems' robustness while preserving the users’ privacy of every SER system's user, is a direction for future research.
https://www.verywellmind.com/the-purpose-of-emotions-2795181
https://www.apple.com/siri/
https://www.alexa.com/
https://www.microsoft.com/en-us/cortana
https://www.gartner.com/en
https://www.alliedmarketresearch.com/emotion-detection-and-recognition-market
https://gdpr.eu/
https://www.wsj.com/
www.nytimes.com
http://research.baidu.com/Blog/index-view?id=91
https://www.forbes.com/sites/thomasbrewster/2021/10/14/huge-bank-fraud-uses-deep-fake-voice-tech-to-steal-millions/?sh=441c54157559
https://www.sciencedaily.com/
https://towardsdatascience.com/getting-to-know-the-mel-spectrogram-31bca3e2d9d0
https://librosa.org/doc/latest/index.html
http://practicalcryptography.com/miscellaneous/machine-learning/guide-mel-frequency-cepstral-coefficients-mfccs/
https://www.vocal.com/perceptual-filtering/perceptual-linear-prediction-cepstral-coefficients-in-speech/
https://smartlaboratory.org/ravdess/
https://predictionio.apache.org/
https://www.veracode.com/
Aloufi R, Haddadi H, Boyle D (2019) Emotionless: privacy-preserving speech analysis for voice assistants. arXiv preprint arXiv:1908.03632
Alshamsi H, Këpuska V, Alshamisi H (2018) Automated speech emotion recognition app development on smart phones using cloud computing. https://doi.org/10.9790/9622-0805027177
Badshah AM, Ahmad J, Rahim N, Baik SW (2017) Speech emotion recognition from spectrograms with deep convolutional neural network. 2017 international conference on platform technology and service, PlatCon 2017 - Proceedings, (July 2019). https://doi.org/10.1109/PlatCon.2017.7883728
Bahreini K, Nadolski R, Westera W (2015) Towards real-time speech emotion recognition for affective e-learning. Educ Inf Technol 1–20. https://doi.org/10.1007/s10639-015-9388-2
Bakir C, Yuzkat M (2018) Speech emotion classification and recognition with different methods for Turkish language. Balkan J Electr Comput Eng 6(2):54–60. https://doi.org/10.17694/bajece.419557
Article Google Scholar
Banse R, Scherer KR (1996) Acoustic profiles in vocal emotion expression. J Pers Soc Psychol 70(3):614. https://doi.org/10.1037/0022-3514.70.3.614
Bashir S, Ali S, Ahmed S, Kakkar V (2016) "Analog-to-digital converters: a comparative study and performance analysis," 2016 international conference on computing, communication and automation (ICCCA), Noida, pp 999–1001
Batbaatar E, Li M, Ryu KH (2019) Semantic-emotion neural network for emotion recognition from text. IEEE Access 7:111866–111878. https://doi.org/10.1109/ACCESS.2019.2934529
Batliner A, Steidl S, Schuller B, Seppi D, Vogt T, Wagner J, ... Amir N (2011) Whodunnit–searching for the most important feature types signalling emotion-related user states in speech. Comput Speech Lang 25(1):4–28
Blanton S (1915) The voice and the emotions. Q J Speech 1(2):154–172. https://doi.org/10.1145/3129340
Blue L, Vargas L, Traynor P (2018) Hello, is it me you're looking for? differentiating between human and electronic speakers for voice interface security. In Proceedings of the 11th ACM conference on security & privacy in wireless and mobile networks. pp 123–133. https://doi.org/10.1145/3212480.3212505
Burkhardt F, Sendlmeier WF (2000) Verification of acoustical correlates of emotional speech using formant-synthesis. In: ISCA Tutorial and Research Workshop (ITRW) on speech and emotion
Chen Y-T, Yeh J-H, Pao T-L (2011) Emotion recognition on mandarin speech: a comparative study and performance evaluation. VDM Verlag, Saarbrücken, DEU
Google Scholar
Cooley JW, Tukey JW (1965) An algorithm for the machine calculation of complex Fourier series. Math Comput 19(90):297–301
Article MathSciNet Google Scholar
Dellaert F, Polzin T, Waibel A (1996) Recognizing emotion in speech. In Proceedings of ICSLP 3, (Philadelphia, PA, 1996). IEEE, pp 1970–1973. https://doi.org/10.1109/ICSLP.1996.608022
Dzedzickis A, Kaklauskas A, Bucinskas V (2020) Human emotion recognition: review of sensors and methods. Sensors (Switzerland) 20(3):1–41. https://doi.org/10.3390/s20030592
El Ayadi M, Kamel MS, Karray F (2011) Survey on speech emotion recognition: Features, classification schemes, and databases. Pattern Recognit 44(3):572–587
Eliash C, Lazar I, Nissim N (2020) SEC-CU: the security of intensive care unit medical devices and their ecosystems. IEEE Access 8:64193–64224. https://doi.org/10.1109/ACCESS.2020.2984726
Farhi N, Nissim N, Elovici Y (2019) Malboard: a novel user keystroke impersonation attack and trusted detection framework based on side-channel analysis. Comput Secur 85:240–269. https://doi.org/10.1016/j.cose.2019.05.008
Feidakis M, Daradoumis T, Caballe S (2011a) "Emotion measurement in intelligent tutoring systems: what, when and how to measure," 2011 third international conference on intelligent networking and collaborative systems. pp 807-812. https://doi.org/10.1109/INCoS.2011.82
Feidakis M, Daradoumis T, Caballé S (2011b) Endowing e-learning systems with emotion awareness. In 2011 third international conference on intelligent networking and collaborative systems. IEEE, pp 68–75. https://doi.org/10.1109/INCoS.2011.83
Garcia-Garcia JM, Penichet VM, Lozano MD (2017) Emotion detection: a technology review. 1–8. https://doi.org/10.1145/3123818.3123852
Gong Y, Yang J, Huber J, MacKnight M, Poellabauer C (2019) ReMASC: realistic replay attack corpus for voice controlled systems. https://doi.org/10.21437/Interspeech.2019-1541 . arXiv preprint arXiv:1904.03365
Gui Q, Yang W, Jin Z, Ruiz-Blondet MV, Laszlo S (2016) A residual feature-based replay attack detection approach for brainprint biometric systems. In 2016 IEEE international workshop on information forensics and security (WIFS). IEEE, pp 1–6. https://doi.org/10.1109/WIFS.2016.7823907
Hajarolasvadi N, Demirel H (2019) 3D CNN-based speech emotion recognition using k-means clustering and spectrograms. Entropy 21(5). https://doi.org/10.3390/e21050479
Harrington DA (1951) An experimental study of the subjective and objective characteristics of sustained vowels at high pitches
Huzaifah M (2017) Comparison of time-frequency representations for environmental sound classification using convolutional neural networks. https://doi.org/10.48550/arXiv.1706.07156 . arXiv preprint arXiv:1706.07156
Iliou T, Anagnostopoulos CN (2009) Statistical evaluation of speech features for emotion recognition. In Fourth international conference on digital telecommunications, Colmar, France, pp 121–126. https://doi.org/10.1109/ICDT.2009.30
Jati A, Hsu CC, Pal M, Peri R, AbdAlmageed W, Narayanan S (2020) Adversarial attack and defense strategies for deep speaker recognition systems. https://doi.org/10.1016/j.csl.2021.101199 . arXiv preprint arXiv:2008.07685
Joshi DD, Zalte MB (2013) Speech emotion recognition: a review. IOSR J Electron Commun Eng (IOSR-JECE) 4(4):34–37
Kao YH, Lee LS (2006) Feature analysis for emotion recognition from Mandarin speech considering the special characteristics of Chinese language. In INTERSPEECH—ICSLP, Pittsburgh, Pennsylvania, pp 1814–1817. https://doi.org/10.21437/Interspeech.2006-501
Kate HK, Razmara J, Isazadeh A (2018) A novel fast and secure approach for voice encryption based on DNA computing. 3D Res 9(2):1–11. https://doi.org/10.1007/s13319-018-0167-x
Khanjani Z, Watson G, Janeja VP (2021) How deep are the fakes? Focusing on audio deepfake: a survey. arXiv preprint arXiv:2111.14203
Kintzlinger M, Nissim N (2019) Keep an eye on your personal belongings! The security of personal medical devices and their ecosystems. J Biomed Inform 95:103233. https://doi.org/10.1016/j.jbi.2019.103233
Kryzhanovsky B, Dunin-Barkowski W, Redko V (2018) Advances in neural computation, machine learning, and cognitive research: Selected papers from the XIX international conference on neuroinformatics, october 2–6, 2017, Moscow, Russia. Studies Comput Intell 736(October 2017):iii–iv. https://doi.org/10.1007/978-3-319-66604-4
Kuleshov V, Enam SZ, Ermon S (2017) Audio super-resolution using neural nets. In ICLR (Workshop Track). https://doi.org/10.48550/arXiv.1708.00853
Landau O, Puzis R, Nissim N (2020) Mind your mind: EEG-based brain-computer interfaces and their security in cyber space. ACM Comput Surv (CSUR) 53(1):1–38. https://doi.org/10.1145/3372043
Latif S, Rana R, Qadir J (2018) Adversarial machine learning and speech emotion recognition: Utilizing generative adversarial networks for robustness. arXiv preprint arXiv:1811.11402
Lech M, Stolar M, Bolia R, Skinner M (2018) Amplitude-frequency analysis of emotional speech using transfer learning and classification of spectrogram images. Adv Sci Technol Eng Syst 3(4):363–371. https://doi.org/10.25046/aj030437
Lee T, Edwards B, Molloy I, Su D (2018) Defending against machine learning model stealing attacks using deceptive perturbations. https://doi.org/10.48550/arXiv.1806.00054 . arXiv preprint arXiv:1806.00054
Li Q, Juang BH, Lee CH (2000) Automatic verbal information verification for user authentication. IEEE Trans Speech Audio Process 8(5):585–596. https://doi.org/10.1109/89.861378
Lim W, Jang D, Lee T (2017) Speech emotion recognition using convolutional and recurrent neural networks. 2016 Asia-pacific signal and information processing association annual summit and conference, APSIPA 2016. pp 1–4 https://doi.org/10.1109/APSIPA.2016.7820699
Liu Y, Ma S, Aafer Y, Lee WC, Zhai J, Wang W, Zhang X (2018) Trojaning attack on neural networks. In: 25th Annual Network And Distributed System Security Symposium (NDSS 2018). Internet Soc
Lotfian R, Busso C (2015) Emotion recognition using synthetic speech as neutral reference. In 2015 IEEE international conference on acoustics, speech and signal processing (ICASSP). IEEE, pp 4759–4763. https://doi.org/10.1109/ICASSP.2015.7178874
Luengo I, Navas E, Hernez I, Snchez J (2005) Automatic emotion recognition using prosodic parameters. In INTERSPEECH, Lisbon, Portugal, pp 493–496). https://doi.org/10.21437/Interspeech.2005-324
McCormick M (2008) Data theft: a prototypical insider threat. In Insider attack and cyber security: beyond the hacker. Springer US, Boston MA, pp 53–68 https://doi.org/10.1007/978-0-387-77322-3_4
McGilloway S, Cowie R, Douglas-Cowie E, Gielen S, Westerdijk M, Stroeve S (2000) Approaching automatic recognition of emotion from voice: A rough benchmark. In: ISCA Tutorial and Research Workshop (ITRW) on Speech and Emotion
Mdhaffar S, Bonastre JF, Tommasi M, Tomashenko N, Estève Y (2021) Retrieving speaker information from personalized acoustic models for speech recognition. https://doi.org/10.48550/arXiv.2111.04194 . arXiv preprint arXiv:2111.04194
Moskovitch R, Nissim N, Elovici Y (2007) “Malicious code detection and acquisition using active learning,” ISI 2007 2007 IEEE Intell Secur Informatics 372. https://doi.org/10.1109/ISI.2007.379505
Moskovitch R, Nissim N, Elovici Y (2010) Acquisition of malicious code using active learning. https://www.researchgate.net/publication/228953558
Neiberg D, Elenius K, Laskowski K (2006) Emotion recognition in spontaneous speech using GMMs. In Interspeech—ICSLP. Pittsburgh, Pennsylvania, pp 809–812. https://doi.org/10.21437/Interspeech.2006-277
Nissim N et al (2015) An active learning framework for efficient condition severity classification. Lect Notes Comput Sci (including Subser Lect Notes Artif Intell Lect Notes Bioinformatics) 9105:13–24. https://doi.org/10.1007/978-3-319-19551-3_3
Nissim N et al (2019) Sec-lib: protecting scholarly digital libraries from infected papers using active machine learning framework. IEEE Access 7:110050–110073. https://doi.org/10.1109/ACCESS.2019.2933197
Nissim N, Cohen A, Elovici Y (2017) ALDOCX: detection of unknown malicious Microsoft office documents using designated active learning methods based on new structural feature extraction methodology. IEEE Trans Inf Forensics Secur 12(3):631–646. https://doi.org/10.1109/TIFS.2016.2631905
Nissim N, Moskovitch R, Rokach L, Elovici Y (2014) Novel active learning methods for enhanced PC malware detection in windows OS. Expert Syst Appl 41(13):5843–5857
Oh SJ, Schiele B, Fritz M (2019) Towards reverse-engineering black-box neural networks. In explainable AI: interpreting, explaining and visualizing deep learning. Springer, Cham, pp 121–144. https://doi.org/10.1007/978-3-030-28954-6_7
Orr MJ (1996) Introduction to radial basis function networks
Pao TL, Chen YT, Yeh JH, Liao WY (2005) Combining acoustic features for improved emotion recognition in Mandarin speech. In Tao J, Tan T, Picard R (Eds.), LNCS. ACII, Berlin, Heidelberg (pp. 279–285), Berlin: Springer. https://doi.org/10.1007/11573548_36
Pierre-Yves O (2003) The production and recognition of emotions in speech: features and algorithms. Int J Hum Comput Stud 59(1–2):157–183. https://doi.org/10.1016/S1071-5819(02)00141-6
Polzin TS, Waibel A (2000) Emotion-sensitive human-computer interfaces. In: ISCA tutorial and research workshop (ITRW) on speech and emotion
Rao KS, Yegnanarayana B (2006) Prosody modification using instants of significant excitation. IEEE Trans Audio Speech Lang Process 14(3):972–980. https://doi.org/10.1109/TSA.2005.858051.DOI:10.1109/TSA.2005.858051
Rázuri JG, Sundgren D, Rahmani R, Moran A, Bonet I, Larsson A (2015) Speech emotion recognition in emotional feedback for human-robot interaction. Int J Adv Res Artif Intell (IJARAI) 4(2):20–27. https://doi.org/10.14569/IJARAI.2015.040204
Satt A, Rozenberg S, Hoory R (2017) Efficient emotion recognition from speech using deep learning on spectrograms. Proceedings of the Annual conference of the international speech communication association, interspeech, pp 1089–1093. https://doi.org/10.21437/Interspeech.2017-200
Schuller BW (2018) Speech emotion recognition: two decades in a nutshell, benchmarks, and ongoing trends. Commun ACM 61(5):90–99
Sini J, Marceddu AC, Violante M (2020) Automatic emotion recognition for the calibration of autonomous driving functions. Electronics 9(3):518. https://doi.org/10.3390/electronics9030518
Slaney M, McRoberts G (1998) Baby ears: a recognition system for affective vocalization. In: proceedings of ICASSP 1998. https://doi.org/10.1109/ICASSP.1998.675432
Song L, Mittal P (2017) POSTER: Inaudible voice commands. In Proceedings of the 2017 ACM SIGSAC conference on computer and communications security. pp 2583–2585. https://doi.org/10.1145/3133956.3138836
Stevens SS, Volkmann J, Newman EB (1937) A scale for the measurement of the psychological magnitude pitch. J Acoust Soc Am 8.3:185–190. https://doi.org/10.1121/1.1915893
Swain M, Routray A, Kabisatpathy P (2018) Databases, features and classifiers for speech emotion recognition: a review. Int J Speech Technol 21:93–120. https://doi.org/10.1007/s10772-018-9491-z.10.1007/s10772-018-9491-z
Tramèr F, Zhang F, Juels A, Reiter MK, Ristenpart T (2016) Stealing machine learning models via prediction {APIs}. In: 25th USENIX security symposium (USENIX Security 16), pp 601–618
Ullah F, Edwards M, Ramdhany R, Chitchyan R, Babar MA, Rashid A (2018) Data exfiltration: a review of external attack vectors and countermeasures. J Netw Comput Appl 101:18–54. https://doi.org/10.1016/j.jnca.2017.10.016
Utane AS, Nalbalwar SL (2013) Emotion recognition through Speech. Int J Appl Inf Syst (IJAIS) 5–8
Wang C, Wang D, Abbas J, Duan K, Mubeen R (2021) Global financial crisis, smart lockdown strategies, and the COVID-19 spillover impacts: A global perspective implications from Southeast Asia. Front Psychiatry 12:643783
Whiteside SP (1998) Simulated emotions: an acoustic study of voice and perturbation measures. In: Fifth International Conference on Spoken Language Processing
Williamson JD (1978) U.S. Patent No. 4,093,821. Washington, DC: U.S. Patent and Trademark Office.
Wysopal C, Eng C, Shields T (2010) Static detection of application backdoors. Datenschutz Und Datensicherheit-DuD 34(3):149–155. https://doi.org/10.1007/s11623-010-0024-4
Yan C, Ji X, Wang K, Jiang Q, Jin Z, Xu W (2022) A survey on voice assistant security: attacks and countermeasures. ACM Comput Surv (CSUR). https://doi.org/10.1145/3527153
Yao Z, Wang Z, Liu W, Liu Y, Pan J (2020) Speech emotion recognition using fusion of three multi-task learning-based classifiers: HSF-DNN, MS-CNN and LLD-RNN. Speech Commun. https://doi.org/10.1016/j.specom.2020.03.005
Zhang S (2008) Emotion recognition in Chinese natural speech by combining prosody and voice quality features. In Sun, et. al. (Eds.), Lecture notes in computer science. Advances in neural networks (pp. 457–464). Berlin: Springer. https://doi.org/10.1007/978-3-540-87734-9_52
Zhang G, Yan C, Ji X, Zhang T, Zhang T, Xu W (2017) Dolphinattack: inaudible voice commands. In Proceedings of the 2017 ACM SIGSAC conference on computer and communications security. pp 103–117. https://doi.org/10.1145/3133956.3134052
Zhu A, Luo Q (2007) Study on speech emotion recognition system in E-learning. In J. Jacko (Ed.), LNCS. Human computer interaction, Part III, HCII (pp. 544–552). Berlin: Springer
Žliobaitė I (2010) Learning under concept drift: an overview. arXiv preprint arXiv:1010.4784
Download references
Author information
Authors and affiliations.
Malware Lab, Cyber Security Research Center, Ben-Gurion University of the Negev, Beer-Sheva, Israel
Itzik Gurowiec & Nir Nissim
Department of Industrial Engineering and Management, Ben-Gurion University of the Negev, Beer-Sheva, Israel
You can also search for this author in PubMed Google Scholar
Contributions
Nir Nissim – Conceptualization, Investigation, Funding acquisition, Methodology, Supervision.
Nir Nissim and Itzik Gurowiec – Formal analysis, Resources, Visualization, Writing—original draft, Writing—review & editing.
Corresponding author
Correspondence to Nir Nissim .
Ethics declarations
Competing interests.
The authors declare no competing interests.
Additional information
Publisher's note.
Springer Nature remains neutral with regard to jurisdictional claims in published maps and institutional affiliations.
Rights and permissions
Open Access This article is licensed under a Creative Commons Attribution 4.0 International License, which permits use, sharing, adaptation, distribution and reproduction in any medium or format, as long as you give appropriate credit to the original author(s) and the source, provide a link to the Creative Commons licence, and indicate if changes were made. The images or other third party material in this article are included in the article's Creative Commons licence, unless indicated otherwise in a credit line to the material. If material is not included in the article's Creative Commons licence and your intended use is not permitted by statutory regulation or exceeds the permitted use, you will need to obtain permission directly from the copyright holder. To view a copy of this licence, visit http://creativecommons.org/licenses/by/4.0/ .
Reprints and permissions
About this article
Gurowiec, I., Nissim, N. Speech emotion recognition systems and their security aspects. Artif Intell Rev 57 , 148 (2024). https://doi.org/10.1007/s10462-024-10760-z
Download citation
Accepted : 07 April 2024
Published : 21 May 2024
DOI : https://doi.org/10.1007/s10462-024-10760-z
Share this article
Anyone you share the following link with will be able to read this content:
Sorry, a shareable link is not currently available for this article.
Provided by the Springer Nature SharedIt content-sharing initiative
- Recognition
- Cyber-attack
- Find a journal
- Publish with us
- Track your research
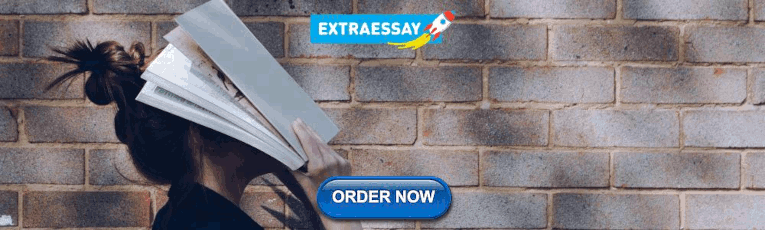
IMAGES
VIDEO
COMMENTS
During the last decade, Speech Emotion Recognition (SER) has emerged as an integral component within Human-computer Interaction (HCI) and other high-end speech processing systems. Generally, an SER system targets the speaker's existence of varied emotions by extracting and classifying the prominent features from a preprocessed speech signal. However, the way humans and machines recognize and ...
As humans, speech is the common as well as a natural way of expressing ourselves. Speech Emotion Recognition (SER) systems can be defined as an assortment of methods processes and classifies speech signals for the detection of associated emotions. Automatic emotion recognition is the technique of identification of human emotions from various signals like speech, facial expression and text ...
During the last decade, Speech Emotion Recognition (SER) has emerged as an integral component within Human-computer Interaction (HCI) and other high-end speech processing systems. Generally, an ...
The paper carefully identifies and synthesizes recent relevant literature related to the SER systems' varied design components/methodologies, thereby providing readers with a state-of-the-art understanding of the hot research topic. During the last decade, Speech Emotion Recognition (SER) has emerged as an integral component within Human-computer Interaction (HCI) and other high-end speech ...
The paper prudently aims at identifying and synthesizing contemporary pertinent literature associated to the SER systems with different methodologies or design components, thus providing the researchers with an up-to-date understanding of the research topic in the field of SER. As humans, speech is the common as well as a natural way of expressing ourselves. Speech Emotion Recognition (SER ...
Today, the speech emotion recognition (SER) system evaluates the emotional state of the speaker by investigating his/her speech signal. Emotion recognition is a challenging task for a machine.
These features are the ones passing on unique properties of emotional substance for speech emotion recognition. Energy, duration, and fundamental frequency are some characteristics on which broadly utilized prosodic features are based. 2) SPECTRAL FEATURES The vocal tract filters a sound when produced by an individual.
The speech-emotion recognition (SER) field became crucial in advanced Human-computer interaction (HCI). ... Gunawan et al. (2018) presented a comprehensive review of SER systems. They focused on feature extraction, classifier, and speech emotion database. However, all of them did not discuss the use of vocal burst features in SER.
A key source of emotional information is the spoken expression, which may be part of the interaction between the human and the machine. Speech emotion recognition (SER) is a very active area of research that involves the application of current machine learning and neural networks tools. This ongoing review covers recent and classical approaches ...
Speech Emotion Recognition Systems: A Comprehensive Review on… by proposing an ICNN technique. In order to creäte more discriminative representations of the emotion-related features, an interactive convolution method is used between feature maps of different scales. For being precise, MFCC chosen were categorized into two paral-
Abstract. Nowadays emotion recognition from speech (SER) is a demanding research area for researchers because of its wide real-life applications. There are many challenges for SER systems such as the availability of suitable emotional databases, identification of the relevant feature vector, and suitable classifiers.
A Comprehensive Review of Speech Emotion Recognition Systems. Taiba Majid Wani1, Teddy Surya Gunawan2, Senior Member, IEEE, Syed Asif Ahmad Qadri3, Mira Kartiwi4, Member, IEEE, Eliathamby ...
Speech Emotion Recognition (SER) systems can be defined as an assortment of methods processes and classifies speech signals for the detection of associated emotions. Automatic emotion recognition is the technique of identification of human emotions from various signals like speech, facial expression and text.
Abstract. During the last decade, Speech Emotion Recognition (SER) has emerged as an integral component within Human-computer Interaction (HCI) and other high-end speech processing systems. Generally, an SER system targets the speaker's existence of varied emotions by extracting and classifying the prominent features from a preprocessed ...
Automatic Speech Emotion Recognition (ASER) has recently garnered attention across various fields including artificial intelligence, pattern recognition, and human-computer interaction. However, ASER encounters numerous challenges such as a shortage of diverse datasets, appropriate feature selection, and suitable intelligent recognition techniques. To address these challenges, a systematic ...
Paper [8] provided a comprehensive review of emotional speech datasets, exploring the effect of emotions on acoustic features and examining classification techniques up to 2004. This analysis provides valuable insights into developing SER systems and the evolution of classification methods. ... Speech emotion recognition systems use pre ...
(DOI: 10.1109/ACCESS.2021.3068045) During the last decade, Speech Emotion Recognition (SER) has emerged as an integral component within Human-computer Interaction (HCI) and other high-end speech processing systems. Generally, an SER system targets the speaker's existence of varied emotions by extracting and classifying the prominent features from a preprocessed speech signal. However, the ...
Speech emotion recognition could be considered a new topic in speech processing where he plays that plays an essential role in human interaction. Emotions are a king of speech that recognizes the three significant aspects of designing the speech emotion recognition system. This article reviews the work on speech emotion recognition and is helpful for further research. Firstly, speech emotion ...
Speech emotion recognition (SER) as a Machine Learning (ML) problem continues to garner a significant amount of research interest, especially in the affective computing domain. This is due to its increasing potential, algorithmic advancements, and applications in real-world scenarios. Human speech contains para-linguistic information that can ...
Abstract. During the last decade, Speech Emotion Recognition (SER) has emerged as an integral component within Human-computer Interaction (HCI) and other high-end speech processing systems. Generally, an SER system targets the speaker's existence of varied emotions by extracting and classifying the prominent features from a preprocessed ...
Among the other modes of communication, such as text, body language, facial expressions, and so on, human beings employ speech as the most common. It contains a great deal of information, including the speaker's feelings. Detecting the speaker's emotions from his or her speech has shown to be quite useful in a variety of real-world applications. The dataset development, feature extraction ...
For speech-based emotion recognition, we have selected 28 journal articles. The summary of these articles used in the review analysis is shown in Table A.7. 10.1. Highlights of speech-based emotion recognition. As evident from the summary of Table A.7, one article each has been included from the years 2014, 2015, and 2017, respectively. The ...
Despite the recent progress in deep learning-based speech emotion recognition (SER), the performance of state-of-the-art systems significantly decreases in cross-language settings.
In this study, we delved deeply into a multimodal approach, consolidating speech and text, to develop a potent and efficient emotion-recognition system. The robust architecture, from convolutional neural networks for speech to the pre-trained BERT-based model for text, ensures a comprehensive analysis of inputs.
Speech emotion recognition (SER) systems leverage information derived from sound waves produced by humans to identify the concealed emotions in utterances. Since 1996, researchers have placed effort on improving the accuracy of SER systems, their functionalities, and the diversity of emotions that can be identified by the system. Although SER systems have become very popular in a variety of ...