
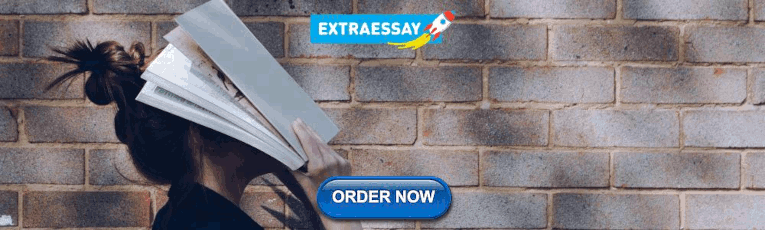
21 Action Research Examples (In Education)
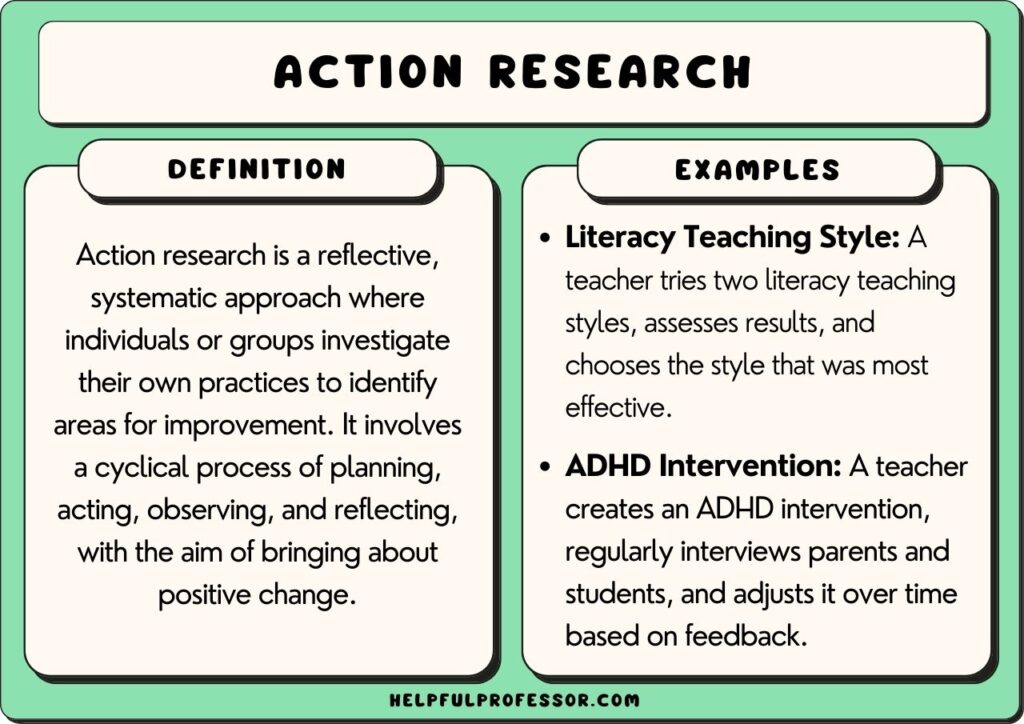
Action research is an example of qualitative research . It refers to a wide range of evaluative or investigative methods designed to analyze professional practices and take action for improvement.
Commonly used in education, those practices could be related to instructional methods, classroom practices, or school organizational matters.
The creation of action research is attributed to Kurt Lewin , a German-American psychologist also considered to be the father of social psychology.
Gillis and Jackson (2002) offer a very concise definition of action research: “systematic collection and analysis of data for the purpose of taking action and making change” (p.264).
The methods of action research in education include:
- conducting in-class observations
- taking field notes
- surveying or interviewing teachers, administrators, or parents
- using audio and video recordings.
The goal is to identify problematic issues, test possible solutions, or simply carry-out continuous improvement.
There are several steps in action research : identify a problem, design a plan to resolve, implement the plan, evaluate effectiveness, reflect on results, make necessary adjustment and repeat the process.
Action Research Examples
- Digital literacy assessment and training: The school’s IT department conducts a survey on students’ digital literacy skills. Based on the results, a tailored training program is designed for different age groups.
- Library resources utilization study: The school librarian tracks the frequency and type of books checked out by students. The data is then used to curate a more relevant collection and organize reading programs.
- Extracurricular activities and student well-being: A team of teachers and counselors assess the impact of extracurricular activities on student mental health through surveys and interviews. Adjustments are made based on findings.
- Parent-teacher communication channels: The school evaluates the effectiveness of current communication tools (e.g., newsletters, apps) between teachers and parents. Feedback is used to implement a more streamlined system.
- Homework load evaluation: Teachers across grade levels assess the amount and effectiveness of homework given. Adjustments are made to ensure a balance between academic rigor and student well-being.
- Classroom environment and learning: A group of teachers collaborates to study the impact of classroom layouts and decorations on student engagement and comprehension. Changes are made based on the findings.
- Student feedback on curriculum content: High school students are surveyed about the relevance and applicability of their current curriculum. The feedback is then used to make necessary curriculum adjustments.
- Teacher mentoring and support: New teachers are paired with experienced mentors. Both parties provide feedback on the effectiveness of the mentoring program, leading to continuous improvements.
- Assessment of school transportation: The school board evaluates the efficiency and safety of school buses through surveys with students and parents. Necessary changes are implemented based on the results.
- Cultural sensitivity training: After conducting a survey on students’ cultural backgrounds and experiences, the school organizes workshops for teachers to promote a more inclusive classroom environment.
- Environmental initiatives and student involvement: The school’s eco-club assesses the school’s carbon footprint and waste management. They then collaborate with the administration to implement greener practices and raise environmental awareness.
- Working with parents through research: A school’s admin staff conduct focus group sessions with parents to identify top concerns.Those concerns will then be addressed and another session conducted at the end of the school year.
- Peer teaching observations and improvements: Kindergarten teachers observe other teachers handling class transition techniques to share best practices.
- PTA surveys and resultant action: The PTA of a district conducts a survey of members regarding their satisfaction with remote learning classes.The results will be presented to the school board for further action.
- Recording and reflecting: A school administrator takes video recordings of playground behavior and then plays them for the teachers. The teachers work together to formulate a list of 10 playground safety guidelines.
- Pre/post testing of interventions: A school board conducts a district wide evaluation of a STEM program by conducting a pre/post-test of students’ skills in computer programming.
- Focus groups of practitioners : The professional development needs of teachers are determined from structured focus group sessions with teachers and admin.
- School lunch research and intervention: A nutrition expert is hired to evaluate and improve the quality of school lunches.
- School nurse systematic checklist and improvements: The school nurse implements a bathroom cleaning checklist to monitor cleanliness after the results of a recent teacher survey revealed several issues.
- Wearable technologies for pedagogical improvements; Students wear accelerometers attached to their hips to gain a baseline measure of physical activity.The results will identify if any issues exist.
- School counselor reflective practice : The school counselor conducts a student survey on antisocial behavior and then plans a series of workshops for both teachers and parents.
Detailed Examples
1. cooperation and leadership.
A science teacher has noticed that her 9 th grade students do not cooperate with each other when doing group projects. There is a lot of arguing and battles over whose ideas will be followed.
So, she decides to implement a simple action research project on the matter. First, she conducts a structured observation of the students’ behavior during meetings. She also has the students respond to a short questionnaire regarding their notions of leadership.
She then designs a two-week course on group dynamics and leadership styles. The course involves learning about leadership concepts and practices . In another element of the short course, students randomly select a leadership style and then engage in a role-play with other students.
At the end of the two weeks, she has the students work on a group project and conducts the same structured observation as before. She also gives the students a slightly different questionnaire on leadership as it relates to the group.
She plans to analyze the results and present the findings at a teachers’ meeting at the end of the term.
2. Professional Development Needs
Two high-school teachers have been selected to participate in a 1-year project in a third-world country. The project goal is to improve the classroom effectiveness of local teachers.
The two teachers arrive in the country and begin to plan their action research. First, they decide to conduct a survey of teachers in the nearby communities of the school they are assigned to.
The survey will assess their professional development needs by directly asking the teachers and administrators. After collecting the surveys, they analyze the results by grouping the teachers based on subject matter.
They discover that history and social science teachers would like professional development on integrating smartboards into classroom instruction. Math teachers would like to attend workshops on project-based learning, while chemistry teachers feel that they need equipment more than training.
The two teachers then get started on finding the necessary training experts for the workshops and applying for equipment grants for the science teachers.
3. Playground Accidents
The school nurse has noticed a lot of students coming in after having mild accidents on the playground. She’s not sure if this is just her perception or if there really is an unusual increase this year. So, she starts pulling data from the records over the last two years. She chooses the months carefully and only selects data from the first three months of each school year.
She creates a chart to make the data more easily understood. Sure enough, there seems to have been a dramatic increase in accidents this year compared to the same period of time from the previous two years.
She shows the data to the principal and teachers at the next meeting. They all agree that a field observation of the playground is needed.
Those observations reveal that the kids are not having accidents on the playground equipment as originally suspected. It turns out that the kids are tripping on the new sod that was installed over the summer.
They examine the sod and observe small gaps between the slabs. Each gap is approximately 1.5 inches wide and nearly two inches deep. The kids are tripping on this gap as they run.
They then discuss possible solutions.
4. Differentiated Learning
Trying to use the same content, methods, and processes for all students is a recipe for failure. This is why modifying each lesson to be flexible is highly recommended. Differentiated learning allows the teacher to adjust their teaching strategy based on all the different personalities and learning styles they see in their classroom.
Of course, differentiated learning should undergo the same rigorous assessment that all teaching techniques go through. So, a third-grade social science teacher asks his students to take a simple quiz on the industrial revolution. Then, he applies differentiated learning to the lesson.
By creating several different learning stations in his classroom, he gives his students a chance to learn about the industrial revolution in a way that captures their interests. The different stations contain: short videos, fact cards, PowerPoints, mini-chapters, and role-plays.
At the end of the lesson, students get to choose how they demonstrate their knowledge. They can take a test, construct a PPT, give an oral presentation, or conduct a simulated TV interview with different characters.
During this last phase of the lesson, the teacher is able to assess if they demonstrate the necessary knowledge and have achieved the defined learning outcomes. This analysis will allow him to make further adjustments to future lessons.
5. Healthy Habits Program
While looking at obesity rates of students, the school board of a large city is shocked by the dramatic increase in the weight of their students over the last five years. After consulting with three companies that specialize in student physical health, they offer the companies an opportunity to prove their value.
So, the board randomly assigns each company to a group of schools. Starting in the next academic year, each company will implement their healthy habits program in 5 middle schools.
Preliminary data is collected at each school at the beginning of the school year. Each and every student is weighed, their resting heart rate, blood pressure and cholesterol are also measured.
After analyzing the data, it is found that the schools assigned to each of the three companies are relatively similar on all of these measures.
At the end of the year, data for students at each school will be collected again. A simple comparison of pre- and post-program measurements will be conducted. The company with the best outcomes will be selected to implement their program city-wide.
Action research is a great way to collect data on a specific issue, implement a change, and then evaluate the effects of that change. It is perhaps the most practical of all types of primary research .
Most likely, the results will be mixed. Some aspects of the change were effective, while other elements were not. That’s okay. This just means that additional modifications to the change plan need to be made, which is usually quite easy to do.
There are many methods that can be utilized, such as surveys, field observations , and program evaluations.
The beauty of action research is based in its utility and flexibility. Just about anyone in a school setting is capable of conducting action research and the information can be incredibly useful.
Aronson, E., & Patnoe, S. (1997). The jigsaw classroom: Building cooperation in the classroom (2nd ed.). New York: Addison Wesley Longman.
Gillis, A., & Jackson, W. (2002). Research Methods for Nurses: Methods and Interpretation . Philadelphia: F.A. Davis Company.
Lewin, K. (1946). Action research and minority problems. Journal of SocialIssues, 2 (4), 34-46.
Macdonald, C. (2012). Understanding participatory action research: A qualitative research methodology option. Canadian Journal of Action Research, 13 , 34-50. https://doi.org/10.33524/cjar.v13i2.37 Mertler, C. A. (2008). Action Research: Teachers as Researchers in the Classroom . London: Sage.
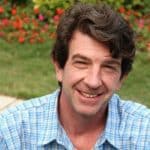
Dave Cornell (PhD)
Dr. Cornell has worked in education for more than 20 years. His work has involved designing teacher certification for Trinity College in London and in-service training for state governments in the United States. He has trained kindergarten teachers in 8 countries and helped businessmen and women open baby centers and kindergartens in 3 countries.
- Dave Cornell (PhD) https://helpfulprofessor.com/author/dave-cornell-phd/ 25 Positive Punishment Examples
- Dave Cornell (PhD) https://helpfulprofessor.com/author/dave-cornell-phd/ 25 Dissociation Examples (Psychology)
- Dave Cornell (PhD) https://helpfulprofessor.com/author/dave-cornell-phd/ 15 Zone of Proximal Development Examples
- Dave Cornell (PhD) https://helpfulprofessor.com/author/dave-cornell-phd/ Perception Checking: 15 Examples and Definition
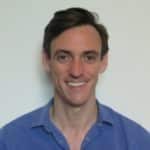
Chris Drew (PhD)
This article was peer-reviewed and edited by Chris Drew (PhD). The review process on Helpful Professor involves having a PhD level expert fact check, edit, and contribute to articles. Reviewers ensure all content reflects expert academic consensus and is backed up with reference to academic studies. Dr. Drew has published over 20 academic articles in scholarly journals. He is the former editor of the Journal of Learning Development in Higher Education and holds a PhD in Education from ACU.
- Chris Drew (PhD) #molongui-disabled-link 25 Positive Punishment Examples
- Chris Drew (PhD) #molongui-disabled-link 25 Dissociation Examples (Psychology)
- Chris Drew (PhD) #molongui-disabled-link 15 Zone of Proximal Development Examples
- Chris Drew (PhD) #molongui-disabled-link Perception Checking: 15 Examples and Definition
2 thoughts on “21 Action Research Examples (In Education)”
Where can I capture this article in a better user-friendly format, since I would like to provide it to my students in a Qualitative Methods course at the University of Prince Edward Island? It is a good article, however, it is visually disjointed in its current format. Thanks, Dr. Frank T. Lavandier
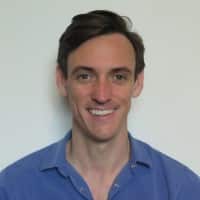
Hi Dr. Lavandier,
I’ve emailed you a word doc copy that you can use and edit with your class.
Best, Chris.
Leave a Comment Cancel Reply
Your email address will not be published. Required fields are marked *
- Technical Support
- Find My Rep
You are here
100 Questions (and Answers) About Action Research
- Luke Duesbery - San Diego State University, USA
- Todd Twyman - Pacific University, Oregon
- Description
See what’s new to this edition by selecting the Features tab on this page. Should you need additional information or have questions regarding the HEOA information provided for this title, including what is new to this edition, please email [email protected] . Please include your name, contact information, and the name of the title for which you would like more information. For information on the HEOA, please go to http://ed.gov/policy/highered/leg/hea08/index.html .
For assistance with your order: Please email us at [email protected] or connect with your SAGE representative.
SAGE 2455 Teller Road Thousand Oaks, CA 91320 www.sagepub.com
“100 Questions (and Answers) About Action Research provides a resource for professionals that want to learn and engage in action research. The question format helps a reader focus in on what information is going to be the most helpful and relevant to their topic.”
KEY FEATURES:
- The succinct and clear layperson’s language makes the information far easier to digest than traditional texts and increases access to important and complex action research concepts.
- In lieu of overwhelming citations within the text, an appendix is provided with books and articles worth pursuing.
Sample Materials & Chapters
Part 1, Questions 1 - 8
Part 6, Questions 44 - 51
For instructors
Select a purchasing option, related products.

This title is also available on SAGE Research Methods , the ultimate digital methods library. If your library doesn’t have access, ask your librarian to start a trial .
Module 3 > Topic 3-A > Developing Action Research Questions
- 3-A Activities
- Developing Action Research Questions
- 3-B Activities
- Determining Potential Data Sources
- 3-C Activities
- Developing an Action Research Plan
- 3-D Activities
- The Ethics of Access and Permissions
Action research is different from reflection in that it requires that we formulate research questions in advance. Those questions then are used to guide the action research process through the design and conduct of data collection, the analysis and validation (triangulation) of data, and the writing of the report.
Action research is an intentional act, and the research questions can be used to state our intentions.
When writing action research questions, you should keep the following criteria in mind:
- They should be broad in scope.
You likely will have 1-3 questions that guide your action research process. It is unlikely that you will have more than 6. These broad questions can then be subdivided into smaller questions, if you like. However, realize that it generally isn’t possible for one project to cover everything. If you spread yourself thin, you’ll learn a little about many things. If you focus, you’ll learn a lot about the things on which you focus. For future improvement, the latter is probably better.
- They should be research questions (questions about the action) rather than data collection questions (questions to be asked of participants in an interview or on a survey).
Your data collection questions will be derived from the research questions. For example, a research question might be "How do I get my students more involved in class discussion"? whereas in an interview you might ask a student "What would make you want to get more involved in a class discussion"?
- They should, when answered, have an impact on your practices.
This is the very essence of action research – that it involves practitioners on a data-driven journey through which they explore their own practices and develop action plans for improving those practices. Make the questions personal and reflective of your concerns as a practitioner.
- They should be answerable.
This point may seem obvious, but it is surprisingly easy to dream up questions that are too big or involve phenomena we can’t readily study through observations, asking questions of others, and reviewing records and documents.
Overview • Topic A • Topic B • Topic C • Topic D
© 2008 Performance Learning Systems
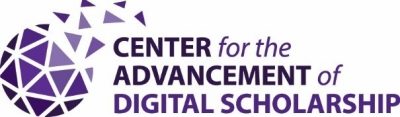
Want to create or adapt books like this? Learn more about how Pressbooks supports open publishing practices.
1 What is Action Research for Classroom Teachers?
ESSENTIAL QUESTIONS
- What is the nature of action research?
- How does action research develop in the classroom?
- What models of action research work best for your classroom?
- What are the epistemological, ontological, theoretical underpinnings of action research?
Educational research provides a vast landscape of knowledge on topics related to teaching and learning, curriculum and assessment, students’ cognitive and affective needs, cultural and socio-economic factors of schools, and many other factors considered viable to improving schools. Educational stakeholders rely on research to make informed decisions that ultimately affect the quality of schooling for their students. Accordingly, the purpose of educational research is to engage in disciplined inquiry to generate knowledge on topics significant to the students, teachers, administrators, schools, and other educational stakeholders. Just as the topics of educational research vary, so do the approaches to conducting educational research in the classroom. Your approach to research will be shaped by your context, your professional identity, and paradigm (set of beliefs and assumptions that guide your inquiry). These will all be key factors in how you generate knowledge related to your work as an educator.
Action research is an approach to educational research that is commonly used by educational practitioners and professionals to examine, and ultimately improve, their pedagogy and practice. In this way, action research represents an extension of the reflection and critical self-reflection that an educator employs on a daily basis in their classroom. When students are actively engaged in learning, the classroom can be dynamic and uncertain, demanding the constant attention of the educator. Considering these demands, educators are often only able to engage in reflection that is fleeting, and for the purpose of accommodation, modification, or formative assessment. Action research offers one path to more deliberate, substantial, and critical reflection that can be documented and analyzed to improve an educator’s practice.
Purpose of Action Research
As one of many approaches to educational research, it is important to distinguish the potential purposes of action research in the classroom. This book focuses on action research as a method to enable and support educators in pursuing effective pedagogical practices by transforming the quality of teaching decisions and actions, to subsequently enhance student engagement and learning. Being mindful of this purpose, the following aspects of action research are important to consider as you contemplate and engage with action research methodology in your classroom:
- Action research is a process for improving educational practice. Its methods involve action, evaluation, and reflection. It is a process to gather evidence to implement change in practices.
- Action research is participative and collaborative. It is undertaken by individuals with a common purpose.
- Action research is situation and context-based.
- Action research develops reflection practices based on the interpretations made by participants.
- Knowledge is created through action and application.
- Action research can be based in problem-solving, if the solution to the problem results in the improvement of practice.
- Action research is iterative; plans are created, implemented, revised, then implemented, lending itself to an ongoing process of reflection and revision.
- In action research, findings emerge as action develops and takes place; however, they are not conclusive or absolute, but ongoing (Koshy, 2010, pgs. 1-2).
In thinking about the purpose of action research, it is helpful to situate action research as a distinct paradigm of educational research. I like to think about action research as part of the larger concept of living knowledge. Living knowledge has been characterized as “a quest for life, to understand life and to create… knowledge which is valid for the people with whom I work and for myself” (Swantz, in Reason & Bradbury, 2001, pg. 1). Why should educators care about living knowledge as part of educational research? As mentioned above, action research is meant “to produce practical knowledge that is useful to people in the everyday conduct of their lives and to see that action research is about working towards practical outcomes” (Koshy, 2010, pg. 2). However, it is also about:
creating new forms of understanding, since action without reflection and understanding is blind, just as theory without action is meaningless. The participatory nature of action research makes it only possible with, for and by persons and communities, ideally involving all stakeholders both in the questioning and sense making that informs the research, and in the action, which is its focus. (Reason & Bradbury, 2001, pg. 2)
In an effort to further situate action research as living knowledge, Jean McNiff reminds us that “there is no such ‘thing’ as ‘action research’” (2013, pg. 24). In other words, action research is not static or finished, it defines itself as it proceeds. McNiff’s reminder characterizes action research as action-oriented, and a process that individuals go through to make their learning public to explain how it informs their practice. Action research does not derive its meaning from an abstract idea, or a self-contained discovery – action research’s meaning stems from the way educators negotiate the problems and successes of living and working in the classroom, school, and community.
While we can debate the idea of action research, there are people who are action researchers, and they use the idea of action research to develop principles and theories to guide their practice. Action research, then, refers to an organization of principles that guide action researchers as they act on shared beliefs, commitments, and expectations in their inquiry.
Reflection and the Process of Action Research
When an individual engages in reflection on their actions or experiences, it is typically for the purpose of better understanding those experiences, or the consequences of those actions to improve related action and experiences in the future. Reflection in this way develops knowledge around these actions and experiences to help us better regulate those actions in the future. The reflective process generates new knowledge regularly for classroom teachers and informs their classroom actions.
Unfortunately, the knowledge generated by educators through the reflective process is not always prioritized among the other sources of knowledge educators are expected to utilize in the classroom. Educators are expected to draw upon formal types of knowledge, such as textbooks, content standards, teaching standards, district curriculum and behavioral programs, etc., to gain new knowledge and make decisions in the classroom. While these forms of knowledge are important, the reflective knowledge that educators generate through their pedagogy is the amalgamation of these types of knowledge enacted in the classroom. Therefore, reflective knowledge is uniquely developed based on the action and implementation of an educator’s pedagogy in the classroom. Action research offers a way to formalize the knowledge generated by educators so that it can be utilized and disseminated throughout the teaching profession.
Research is concerned with the generation of knowledge, and typically creating knowledge related to a concept, idea, phenomenon, or topic. Action research generates knowledge around inquiry in practical educational contexts. Action research allows educators to learn through their actions with the purpose of developing personally or professionally. Due to its participatory nature, the process of action research is also distinct in educational research. There are many models for how the action research process takes shape. I will share a few of those here. Each model utilizes the following processes to some extent:
- Plan a change;
- Take action to enact the change;
- Observe the process and consequences of the change;
- Reflect on the process and consequences;
- Act, observe, & reflect again and so on.
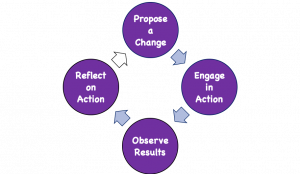
Figure 1.1 Basic action research cycle
There are many other models that supplement the basic process of action research with other aspects of the research process to consider. For example, figure 1.2 illustrates a spiral model of action research proposed by Kemmis and McTaggart (2004). The spiral model emphasizes the cyclical process that moves beyond the initial plan for change. The spiral model also emphasizes revisiting the initial plan and revising based on the initial cycle of research:
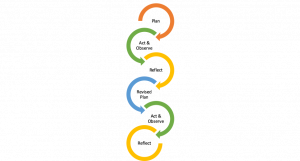
Figure 1.2 Interpretation of action research spiral, Kemmis and McTaggart (2004, p. 595)
Other models of action research reorganize the process to emphasize the distinct ways knowledge takes shape in the reflection process. O’Leary’s (2004, p. 141) model, for example, recognizes that the research may take shape in the classroom as knowledge emerges from the teacher’s observations. O’Leary highlights the need for action research to be focused on situational understanding and implementation of action, initiated organically from real-time issues:
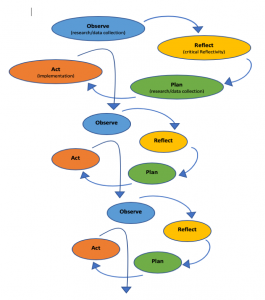
Figure 1.3 Interpretation of O’Leary’s cycles of research, O’Leary (2000, p. 141)
Lastly, Macintyre’s (2000, p. 1) model, offers a different characterization of the action research process. Macintyre emphasizes a messier process of research with the initial reflections and conclusions as the benchmarks for guiding the research process. Macintyre emphasizes the flexibility in planning, acting, and observing stages to allow the process to be naturalistic. Our interpretation of Macintyre process is below:
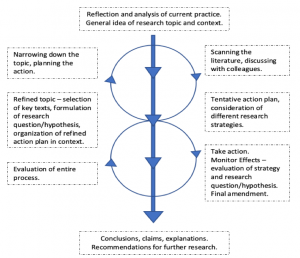
Figure 1.4 Interpretation of the action research cycle, Macintyre (2000, p. 1)
We believe it is important to prioritize the flexibility of the process, and encourage you to only use these models as basic guides for your process. Your process may look similar, or you may diverge from these models as you better understand your students, context, and data.
Definitions of Action Research and Examples
At this point, it may be helpful for readers to have a working definition of action research and some examples to illustrate the methodology in the classroom. Bassey (1998, p. 93) offers a very practical definition and describes “action research as an inquiry which is carried out in order to understand, to evaluate and then to change, in order to improve educational practice.” Cohen and Manion (1994, p. 192) situate action research differently, and describe action research as emergent, writing:
essentially an on-the-spot procedure designed to deal with a concrete problem located in an immediate situation. This means that ideally, the step-by-step process is constantly monitored over varying periods of time and by a variety of mechanisms (questionnaires, diaries, interviews and case studies, for example) so that the ensuing feedback may be translated into modifications, adjustment, directional changes, redefinitions, as necessary, so as to bring about lasting benefit to the ongoing process itself rather than to some future occasion.
Lastly, Koshy (2010, p. 9) describes action research as:
a constructive inquiry, during which the researcher constructs his or her knowledge of specific issues through planning, acting, evaluating, refining and learning from the experience. It is a continuous learning process in which the researcher learns and also shares the newly generated knowledge with those who may benefit from it.
These definitions highlight the distinct features of action research and emphasize the purposeful intent of action researchers to improve, refine, reform, and problem-solve issues in their educational context. To better understand the distinctness of action research, these are some examples of action research topics:
Examples of Action Research Topics
- Flexible seating in 4th grade classroom to increase effective collaborative learning.
- Structured homework protocols for increasing student achievement.
- Developing a system of formative feedback for 8th grade writing.
- Using music to stimulate creative writing.
- Weekly brown bag lunch sessions to improve responses to PD from staff.
- Using exercise balls as chairs for better classroom management.
Action Research in Theory
Action research-based inquiry in educational contexts and classrooms involves distinct participants – students, teachers, and other educational stakeholders within the system. All of these participants are engaged in activities to benefit the students, and subsequently society as a whole. Action research contributes to these activities and potentially enhances the participants’ roles in the education system. Participants’ roles are enhanced based on two underlying principles:
- communities, schools, and classrooms are sites of socially mediated actions, and action research provides a greater understanding of self and new knowledge of how to negotiate these socially mediated environments;
- communities, schools, and classrooms are part of social systems in which humans interact with many cultural tools, and action research provides a basis to construct and analyze these interactions.
In our quest for knowledge and understanding, we have consistently analyzed human experience over time and have distinguished between types of reality. Humans have constantly sought “facts” and “truth” about reality that can be empirically demonstrated or observed.
Social systems are based on beliefs, and generally, beliefs about what will benefit the greatest amount of people in that society. Beliefs, and more specifically the rationale or support for beliefs, are not always easy to demonstrate or observe as part of our reality. Take the example of an English Language Arts teacher who prioritizes argumentative writing in her class. She believes that argumentative writing demonstrates the mechanics of writing best among types of writing, while also providing students a skill they will need as citizens and professionals. While we can observe the students writing, and we can assess their ability to develop a written argument, it is difficult to observe the students’ understanding of argumentative writing and its purpose in their future. This relates to the teacher’s beliefs about argumentative writing; we cannot observe the real value of the teaching of argumentative writing. The teacher’s rationale and beliefs about teaching argumentative writing are bound to the social system and the skills their students will need to be active parts of that system. Therefore, our goal through action research is to demonstrate the best ways to teach argumentative writing to help all participants understand its value as part of a social system.
The knowledge that is conveyed in a classroom is bound to, and justified by, a social system. A postmodernist approach to understanding our world seeks knowledge within a social system, which is directly opposed to the empirical or positivist approach which demands evidence based on logic or science as rationale for beliefs. Action research does not rely on a positivist viewpoint to develop evidence and conclusions as part of the research process. Action research offers a postmodernist stance to epistemology (theory of knowledge) and supports developing questions and new inquiries during the research process. In this way action research is an emergent process that allows beliefs and decisions to be negotiated as reality and meaning are being constructed in the socially mediated space of the classroom.
Theorizing Action Research for the Classroom
All research, at its core, is for the purpose of generating new knowledge and contributing to the knowledge base of educational research. Action researchers in the classroom want to explore methods of improving their pedagogy and practice. The starting place of their inquiry stems from their pedagogy and practice, so by nature the knowledge created from their inquiry is often contextually specific to their classroom, school, or community. Therefore, we should examine the theoretical underpinnings of action research for the classroom. It is important to connect action research conceptually to experience; for example, Levin and Greenwood (2001, p. 105) make these connections:
- Action research is context bound and addresses real life problems.
- Action research is inquiry where participants and researchers cogenerate knowledge through collaborative communicative processes in which all participants’ contributions are taken seriously.
- The meanings constructed in the inquiry process lead to social action or these reflections and action lead to the construction of new meanings.
- The credibility/validity of action research knowledge is measured according to whether the actions that arise from it solve problems (workability) and increase participants’ control over their own situation.
Educators who engage in action research will generate new knowledge and beliefs based on their experiences in the classroom. Let us emphasize that these are all important to you and your work, as both an educator and researcher. It is these experiences, beliefs, and theories that are often discounted when more official forms of knowledge (e.g., textbooks, curriculum standards, districts standards) are prioritized. These beliefs and theories based on experiences should be valued and explored further, and this is one of the primary purposes of action research in the classroom. These beliefs and theories should be valued because they were meaningful aspects of knowledge constructed from teachers’ experiences. Developing meaning and knowledge in this way forms the basis of constructivist ideology, just as teachers often try to get their students to construct their own meanings and understandings when experiencing new ideas.
Classroom Teachers Constructing their Own Knowledge
Most of you are probably at least minimally familiar with constructivism, or the process of constructing knowledge. However, what is constructivism precisely, for the purposes of action research? Many scholars have theorized constructivism and have identified two key attributes (Koshy, 2010; von Glasersfeld, 1987):
- Knowledge is not passively received, but actively developed through an individual’s cognition;
- Human cognition is adaptive and finds purpose in organizing the new experiences of the world, instead of settling for absolute or objective truth.
Considering these two attributes, constructivism is distinct from conventional knowledge formation because people can develop a theory of knowledge that orders and organizes the world based on their experiences, instead of an objective or neutral reality. When individuals construct knowledge, there are interactions between an individual and their environment where communication, negotiation and meaning-making are collectively developing knowledge. For most educators, constructivism may be a natural inclination of their pedagogy. Action researchers have a similar relationship to constructivism because they are actively engaged in a process of constructing knowledge. However, their constructions may be more formal and based on the data they collect in the research process. Action researchers also are engaged in the meaning making process, making interpretations from their data. These aspects of the action research process situate them in the constructivist ideology. Just like constructivist educators, action researchers’ constructions of knowledge will be affected by their individual and professional ideas and values, as well as the ecological context in which they work (Biesta & Tedder, 2006). The relations between constructivist inquiry and action research is important, as Lincoln (2001, p. 130) states:
much of the epistemological, ontological, and axiological belief systems are the same or similar, and methodologically, constructivists and action researchers work in similar ways, relying on qualitative methods in face-to-face work, while buttressing information, data and background with quantitative method work when necessary or useful.
While there are many links between action research and educators in the classroom, constructivism offers the most familiar and practical threads to bind the beliefs of educators and action researchers.
Epistemology, Ontology, and Action Research
It is also important for educators to consider the philosophical stances related to action research to better situate it with their beliefs and reality. When researchers make decisions about the methodology they intend to use, they will consider their ontological and epistemological stances. It is vital that researchers clearly distinguish their philosophical stances and understand the implications of their stance in the research process, especially when collecting and analyzing their data. In what follows, we will discuss ontological and epistemological stances in relation to action research methodology.
Ontology, or the theory of being, is concerned with the claims or assumptions we make about ourselves within our social reality – what do we think exists, what does it look like, what entities are involved and how do these entities interact with each other (Blaikie, 2007). In relation to the discussion of constructivism, generally action researchers would consider their educational reality as socially constructed. Social construction of reality happens when individuals interact in a social system. Meaningful construction of concepts and representations of reality develop through an individual’s interpretations of others’ actions. These interpretations become agreed upon by members of a social system and become part of social fabric, reproduced as knowledge and beliefs to develop assumptions about reality. Researchers develop meaningful constructions based on their experiences and through communication. Educators as action researchers will be examining the socially constructed reality of schools. In the United States, many of our concepts, knowledge, and beliefs about schooling have been socially constructed over the last hundred years. For example, a group of teachers may look at why fewer female students enroll in upper-level science courses at their school. This question deals directly with the social construction of gender and specifically what careers females have been conditioned to pursue. We know this is a social construction in some school social systems because in other parts of the world, or even the United States, there are schools that have more females enrolled in upper level science courses than male students. Therefore, the educators conducting the research have to recognize the socially constructed reality of their school and consider this reality throughout the research process. Action researchers will use methods of data collection that support their ontological stance and clarify their theoretical stance throughout the research process.
Koshy (2010, p. 23-24) offers another example of addressing the ontological challenges in the classroom:
A teacher who was concerned with increasing her pupils’ motivation and enthusiasm for learning decided to introduce learning diaries which the children could take home. They were invited to record their reactions to the day’s lessons and what they had learnt. The teacher reported in her field diary that the learning diaries stimulated the children’s interest in her lessons, increased their capacity to learn, and generally improved their level of participation in lessons. The challenge for the teacher here is in the analysis and interpretation of the multiplicity of factors accompanying the use of diaries. The diaries were taken home so the entries may have been influenced by discussions with parents. Another possibility is that children felt the need to please their teacher. Another possible influence was that their increased motivation was as a result of the difference in style of teaching which included more discussions in the classroom based on the entries in the dairies.
Here you can see the challenge for the action researcher is working in a social context with multiple factors, values, and experiences that were outside of the teacher’s control. The teacher was only responsible for introducing the diaries as a new style of learning. The students’ engagement and interactions with this new style of learning were all based upon their socially constructed notions of learning inside and outside of the classroom. A researcher with a positivist ontological stance would not consider these factors, and instead might simply conclude that the dairies increased motivation and interest in the topic, as a result of introducing the diaries as a learning strategy.
Epistemology, or the theory of knowledge, signifies a philosophical view of what counts as knowledge – it justifies what is possible to be known and what criteria distinguishes knowledge from beliefs (Blaikie, 1993). Positivist researchers, for example, consider knowledge to be certain and discovered through scientific processes. Action researchers collect data that is more subjective and examine personal experience, insights, and beliefs.
Action researchers utilize interpretation as a means for knowledge creation. Action researchers have many epistemologies to choose from as means of situating the types of knowledge they will generate by interpreting the data from their research. For example, Koro-Ljungberg et al., (2009) identified several common epistemologies in their article that examined epistemological awareness in qualitative educational research, such as: objectivism, subjectivism, constructionism, contextualism, social epistemology, feminist epistemology, idealism, naturalized epistemology, externalism, relativism, skepticism, and pluralism. All of these epistemological stances have implications for the research process, especially data collection and analysis. Please see the table on pages 689-90, linked below for a sketch of these potential implications:
Again, Koshy (2010, p. 24) provides an excellent example to illustrate the epistemological challenges within action research:
A teacher of 11-year-old children decided to carry out an action research project which involved a change in style in teaching mathematics. Instead of giving children mathematical tasks displaying the subject as abstract principles, she made links with other subjects which she believed would encourage children to see mathematics as a discipline that could improve their understanding of the environment and historic events. At the conclusion of the project, the teacher reported that applicable mathematics generated greater enthusiasm and understanding of the subject.
The educator/researcher engaged in action research-based inquiry to improve an aspect of her pedagogy. She generated knowledge that indicated she had improved her students’ understanding of mathematics by integrating it with other subjects – specifically in the social and ecological context of her classroom, school, and community. She valued constructivism and students generating their own understanding of mathematics based on related topics in other subjects. Action researchers working in a social context do not generate certain knowledge, but knowledge that emerges and can be observed and researched again, building upon their knowledge each time.
Researcher Positionality in Action Research
In this first chapter, we have discussed a lot about the role of experiences in sparking the research process in the classroom. Your experiences as an educator will shape how you approach action research in your classroom. Your experiences as a person in general will also shape how you create knowledge from your research process. In particular, your experiences will shape how you make meaning from your findings. It is important to be clear about your experiences when developing your methodology too. This is referred to as researcher positionality. Maher and Tetreault (1993, p. 118) define positionality as:
Gender, race, class, and other aspects of our identities are markers of relational positions rather than essential qualities. Knowledge is valid when it includes an acknowledgment of the knower’s specific position in any context, because changing contextual and relational factors are crucial for defining identities and our knowledge in any given situation.
By presenting your positionality in the research process, you are signifying the type of socially constructed, and other types of, knowledge you will be using to make sense of the data. As Maher and Tetreault explain, this increases the trustworthiness of your conclusions about the data. This would not be possible with a positivist ontology. We will discuss positionality more in chapter 6, but we wanted to connect it to the overall theoretical underpinnings of action research.
Advantages of Engaging in Action Research in the Classroom
In the following chapters, we will discuss how action research takes shape in your classroom, and we wanted to briefly summarize the key advantages to action research methodology over other types of research methodology. As Koshy (2010, p. 25) notes, action research provides useful methodology for school and classroom research because:
Advantages of Action Research for the Classroom
- research can be set within a specific context or situation;
- researchers can be participants – they don’t have to be distant and detached from the situation;
- it involves continuous evaluation and modifications can be made easily as the project progresses;
- there are opportunities for theory to emerge from the research rather than always follow a previously formulated theory;
- the study can lead to open-ended outcomes;
- through action research, a researcher can bring a story to life.
Action Research Copyright © by J. Spencer Clark; Suzanne Porath; Julie Thiele; and Morgan Jobe is licensed under a Creative Commons Attribution-NonCommercial 4.0 International License , except where otherwise noted.
Share This Book
- Skip to main content
- Skip to primary sidebar
- Skip to footer
- QuestionPro

- Solutions Industries Gaming Automotive Sports and events Education Government Travel & Hospitality Financial Services Healthcare Cannabis Technology Use Case NPS+ Communities Audience Contactless surveys Mobile LivePolls Member Experience GDPR Positive People Science 360 Feedback Surveys
- Resources Blog eBooks Survey Templates Case Studies Training Help center

Home Market Research Research Tools and Apps
Action Research: What it is, Stages & Examples
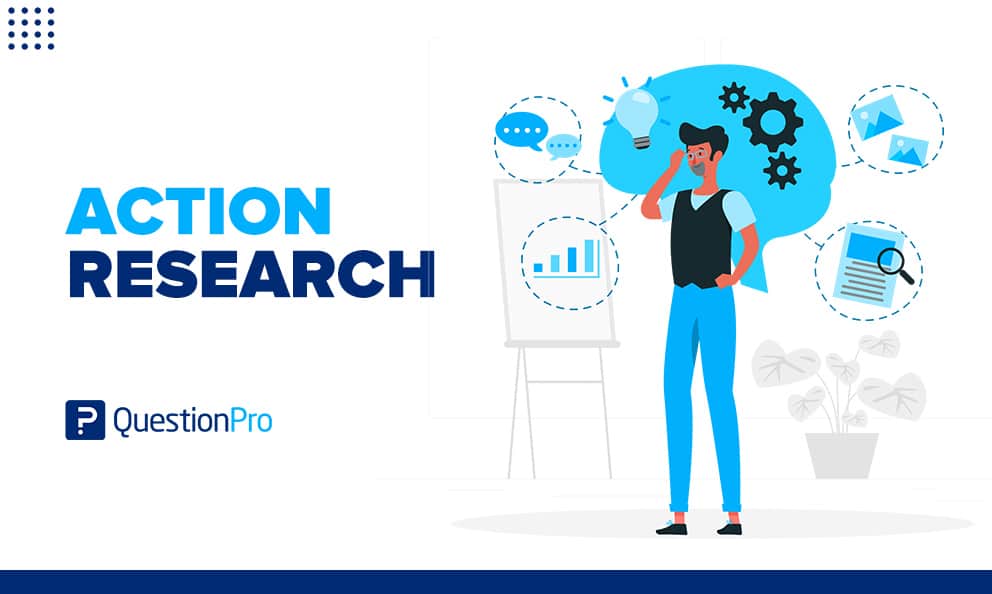
The best way to get things accomplished is to do it yourself. This statement is utilized in corporations, community projects, and national governments. These organizations are relying on action research to cope with their continuously changing and unstable environments as they function in a more interdependent world.
In practical educational contexts, this involves using systematic inquiry and reflective practice to address real-world challenges, improve teaching and learning, enhance student engagement, and drive positive changes within the educational system.
This post outlines the definition of action research, its stages, and some examples.
Content Index
What is action research?
Stages of action research, the steps to conducting action research, examples of action research, advantages and disadvantages of action research.
Action research is a strategy that tries to find realistic solutions to organizations’ difficulties and issues. It is similar to applied research.
Action research refers basically learning by doing. First, a problem is identified, then some actions are taken to address it, then how well the efforts worked are measured, and if the results are not satisfactory, the steps are applied again.
It can be put into three different groups:
- Positivist: This type of research is also called “classical action research.” It considers research a social experiment. This research is used to test theories in the actual world.
- Interpretive: This kind of research is called “contemporary action research.” It thinks that business reality is socially made, and when doing this research, it focuses on the details of local and organizational factors.
- Critical: This action research cycle takes a critical reflection approach to corporate systems and tries to enhance them.
All research is about learning new things. Collaborative action research contributes knowledge based on investigations in particular and frequently useful circumstances. It starts with identifying a problem. After that, the research process is followed by the below stages:
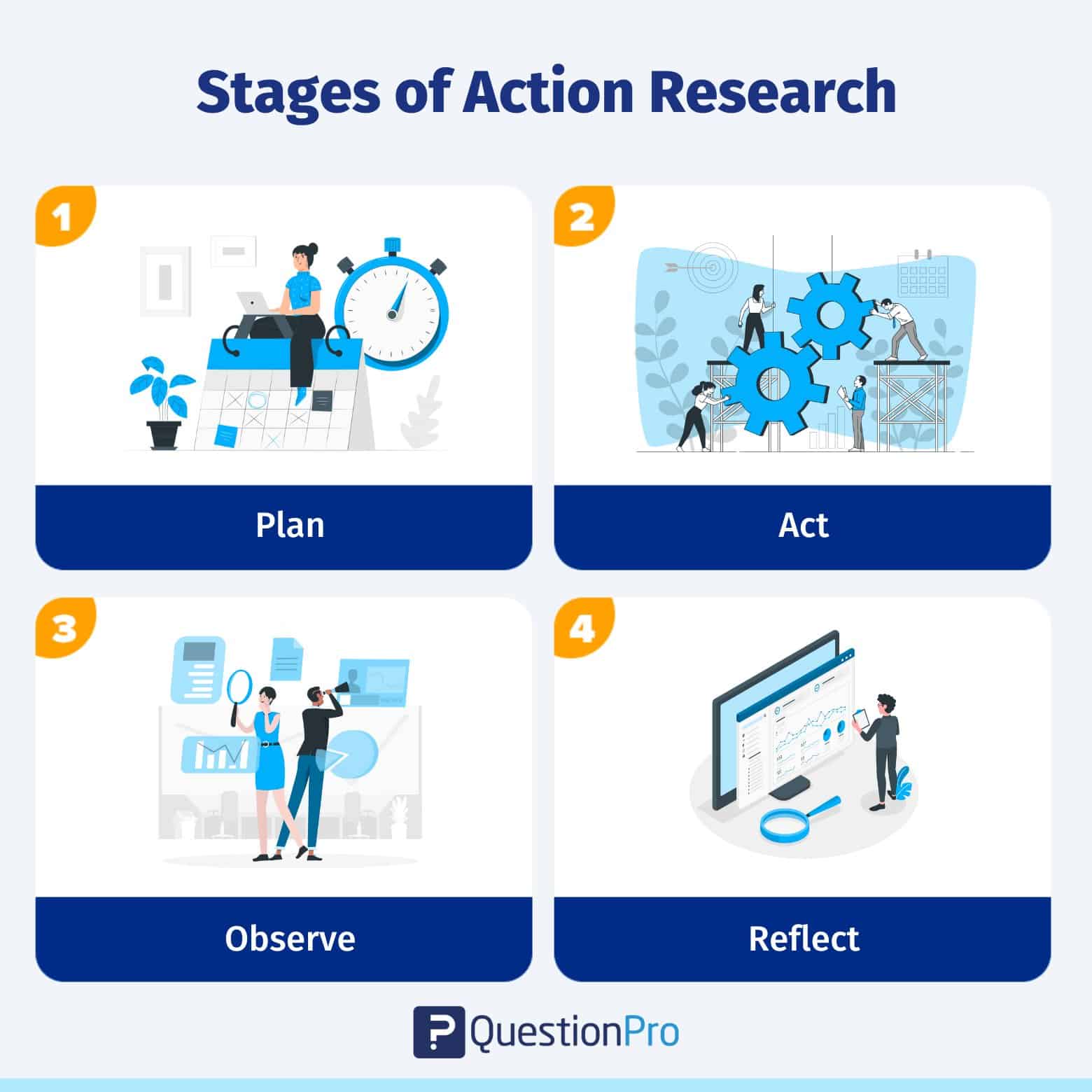
Stage 1: Plan
For an action research project to go well, the researcher needs to plan it well. After coming up with an educational research topic or question after a research study, the first step is to develop an action plan to guide the research process. The research design aims to address the study’s question. The research strategy outlines what to undertake, when, and how.
Stage 2: Act
The next step is implementing the plan and gathering data. At this point, the researcher must select how to collect and organize research data . The researcher also needs to examine all tools and equipment before collecting data to ensure they are relevant, valid, and comprehensive.
Stage 3: Observe
Data observation is vital to any investigation. The action researcher needs to review the project’s goals and expectations before data observation. This is the final step before drawing conclusions and taking action.
Different kinds of graphs, charts, and networks can be used to represent the data. It assists in making judgments or progressing to the next stage of observing.
Stage 4: Reflect
This step involves applying a prospective solution and observing the results. It’s essential to see if the possible solution found through research can really solve the problem being studied.
The researcher must explore alternative ideas when the action research project’s solutions fail to solve the problem.
Action research is a systematic approach researchers, educators, and practitioners use to identify and address problems or challenges within a specific context. It involves a cyclical process of planning, implementing, reflecting, and adjusting actions based on the data collected. Here are the general steps involved in conducting an action research process:
Identify the action research question or problem
Clearly define the issue or problem you want to address through your research. It should be specific, actionable, and relevant to your working context.
Review existing knowledge
Conduct a literature review to understand what research has already been done on the topic. This will help you gain insights, identify gaps, and inform your research design.
Plan the research
Develop a research plan outlining your study’s objectives, methods, data collection tools, and timeline. Determine the scope of your research and the participants or stakeholders involved.
Collect data
Implement your research plan by collecting relevant data. This can involve various methods such as surveys, interviews, observations, document analysis, or focus groups. Ensure that your data collection methods align with your research objectives and allow you to gather the necessary information.
Analyze the data
Once you have collected the data, analyze it using appropriate qualitative or quantitative techniques. Look for patterns, themes, or trends in the data that can help you understand the problem better.
Reflect on the findings
Reflect on the analyzed data and interpret the results in the context of your research question. Consider the implications and possible solutions that emerge from the data analysis. This reflection phase is crucial for generating insights and understanding the underlying factors contributing to the problem.
Develop an action plan
Based on your analysis and reflection, develop an action plan that outlines the steps you will take to address the identified problem. The plan should be specific, measurable, achievable, relevant, and time-bound (SMART goals). Consider involving relevant stakeholders in planning to ensure their buy-in and support.
Implement the action plan
Put your action plan into practice by implementing the identified strategies or interventions. This may involve making changes to existing practices, introducing new approaches, or testing alternative solutions. Document the implementation process and any modifications made along the way.
Evaluate and monitor progress
Continuously monitor and evaluate the impact of your actions. Collect additional data, assess the effectiveness of the interventions, and measure progress towards your goals. This evaluation will help you determine if your actions have the desired effects and inform any necessary adjustments.
Reflect and iterate
Reflect on the outcomes of your actions and the evaluation results. Consider what worked well, what did not, and why. Use this information to refine your approach, make necessary adjustments, and plan for the next cycle of action research if needed.
Remember that participatory action research is an iterative process, and multiple cycles may be required to achieve significant improvements or solutions to the identified problem. Each cycle builds on the insights gained from the previous one, fostering continuous learning and improvement.
Explore Insightfully Contextual Inquiry in Qualitative Research
Here are two real-life examples of action research.
Action research initiatives are frequently situation-specific. Still, other researchers can adapt the techniques. The example is from a researcher’s (Franklin, 1994) report about a project encouraging nature tourism in the Caribbean.
In 1991, this was launched to study how nature tourism may be implemented on the four Windward Islands in the Caribbean: St. Lucia, Grenada, Dominica, and St. Vincent.
For environmental protection, a government-led action study determined that the consultation process needs to involve numerous stakeholders, including commercial enterprises.
First, two researchers undertook the study and held search conferences on each island. The search conferences resulted in suggestions and action plans for local community nature tourism sub-projects.
Several islands formed advisory groups and launched national awareness and community projects. Regional project meetings were held to discuss experiences, self-evaluations, and strategies. Creating a documentary about a local initiative helped build community. And the study was a success, leading to a number of changes in the area.
Lau and Hayward (1997) employed action research to analyze Internet-based collaborative work groups.
Over two years, the researchers facilitated three action research problem -solving cycles with 15 teachers, project personnel, and 25 health practitioners from diverse areas. The goal was to see how Internet-based communications might affect their virtual workgroup.
First, expectations were defined, technology was provided, and a bespoke workgroup system was developed. Participants suggested shorter, more dispersed training sessions with project-specific instructions.
The second phase saw the system’s complete deployment. The final cycle witnessed system stability and virtual group formation. The key lesson was that the learning curve was poorly misjudged, with frustrations only marginally met by phone-based technical help. According to the researchers, the absence of high-quality online material about community healthcare was harmful.
Role clarity, connection building, knowledge sharing, resource assistance, and experiential learning are vital for virtual group growth. More study is required on how group support systems might assist groups in engaging with their external environment and boost group members’ learning.
Action research has both good and bad points.
- It is very flexible, so researchers can change their analyses to fit their needs and make individual changes.
- It offers a quick and easy way to solve problems that have been going on for a long time instead of complicated, long-term solutions based on complex facts.
- If It is done right, it can be very powerful because it can lead to social change and give people the tools to make that change in ways that are important to their communities.
Disadvantages
- These studies have a hard time being generalized and are hard to repeat because they are so flexible. Because the researcher has the power to draw conclusions, they are often not thought to be theoretically sound.
- Setting up an action study in an ethical way can be hard. People may feel like they have to take part or take part in a certain way.
- It is prone to research errors like selection bias , social desirability bias, and other cognitive biases.
LEARN ABOUT: Self-Selection Bias
This post discusses how action research generates knowledge, its steps, and real-life examples. It is very applicable to the field of research and has a high level of relevance. We can only state that the purpose of this research is to comprehend an issue and find a solution to it.
At QuestionPro, we give researchers tools for collecting data, like our survey software, and a library of insights for any long-term study. Go to the Insight Hub if you want to see a demo or learn more about it.
LEARN MORE FREE TRIAL
Frequently Asked Questions(FAQ’s)
Action research is a systematic approach to inquiry that involves identifying a problem or challenge in a practical context, implementing interventions or changes, collecting and analyzing data, and using the findings to inform decision-making and drive positive change.
Action research can be conducted by various individuals or groups, including teachers, administrators, researchers, and educational practitioners. It is often carried out by those directly involved in the educational setting where the research takes place.
The steps of action research typically include identifying a problem, reviewing relevant literature, designing interventions or changes, collecting and analyzing data, reflecting on findings, and implementing improvements based on the results.
MORE LIKE THIS
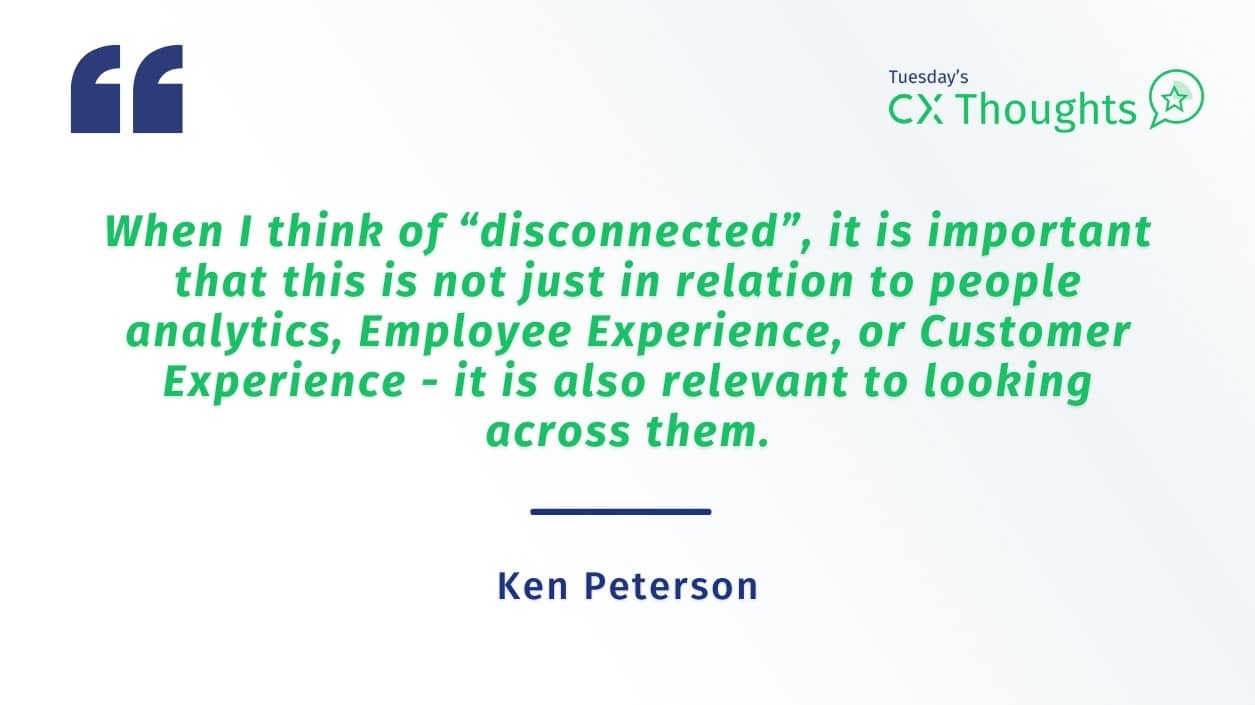
I Am Disconnected – Tuesday CX Thoughts
May 21, 2024
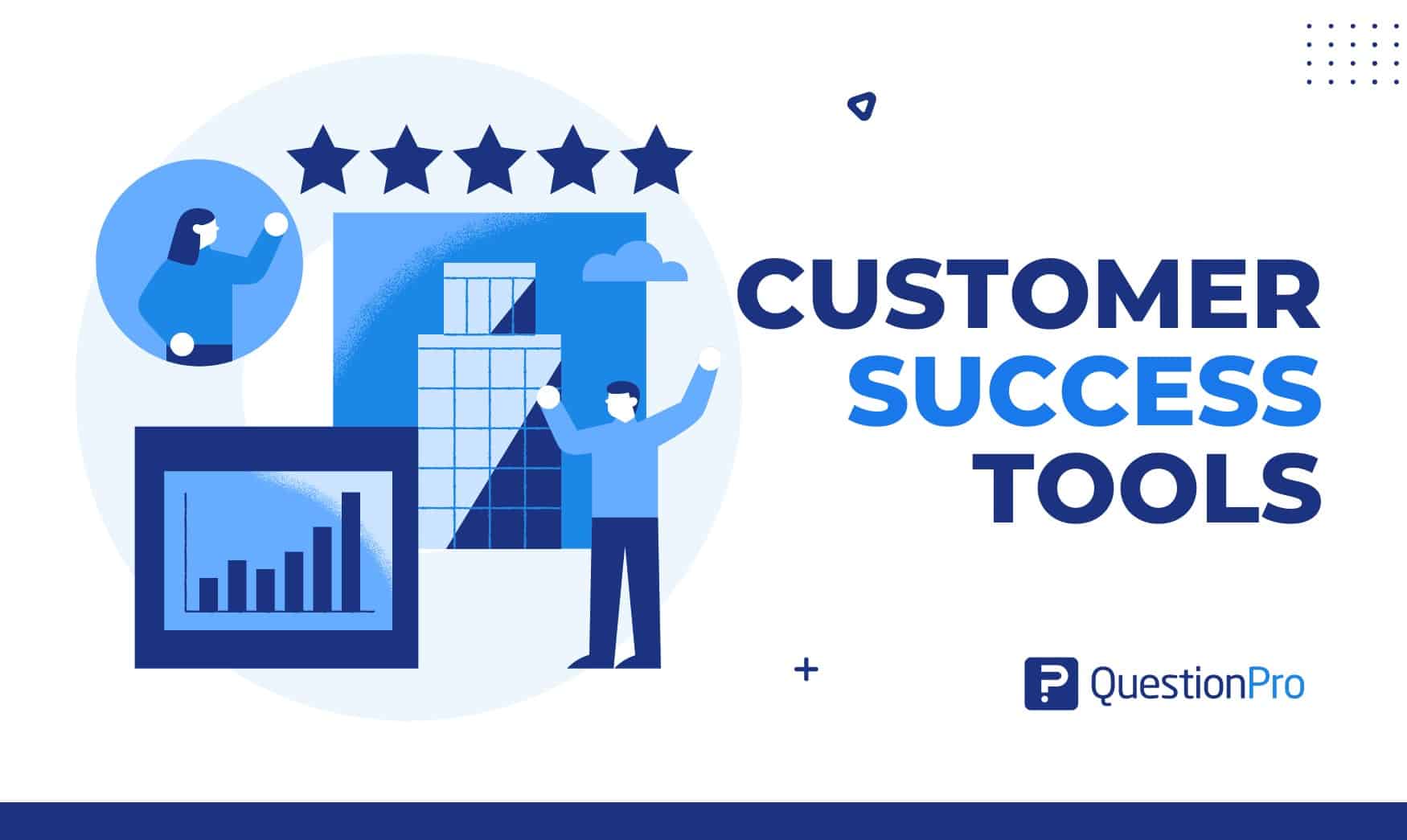
20 Best Customer Success Tools of 2024
May 20, 2024
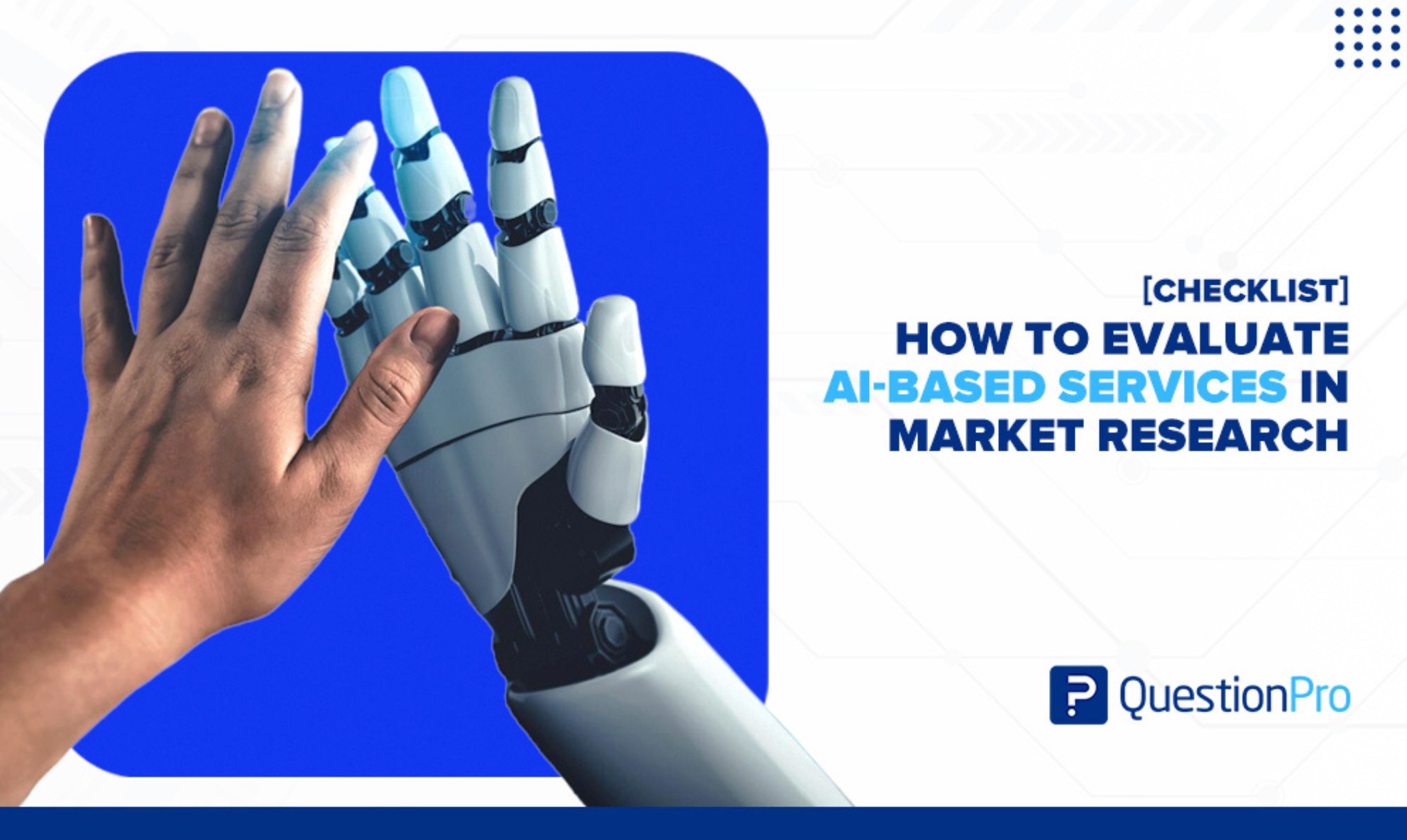
AI-Based Services Buying Guide for Market Research (based on ESOMAR’s 20 Questions)
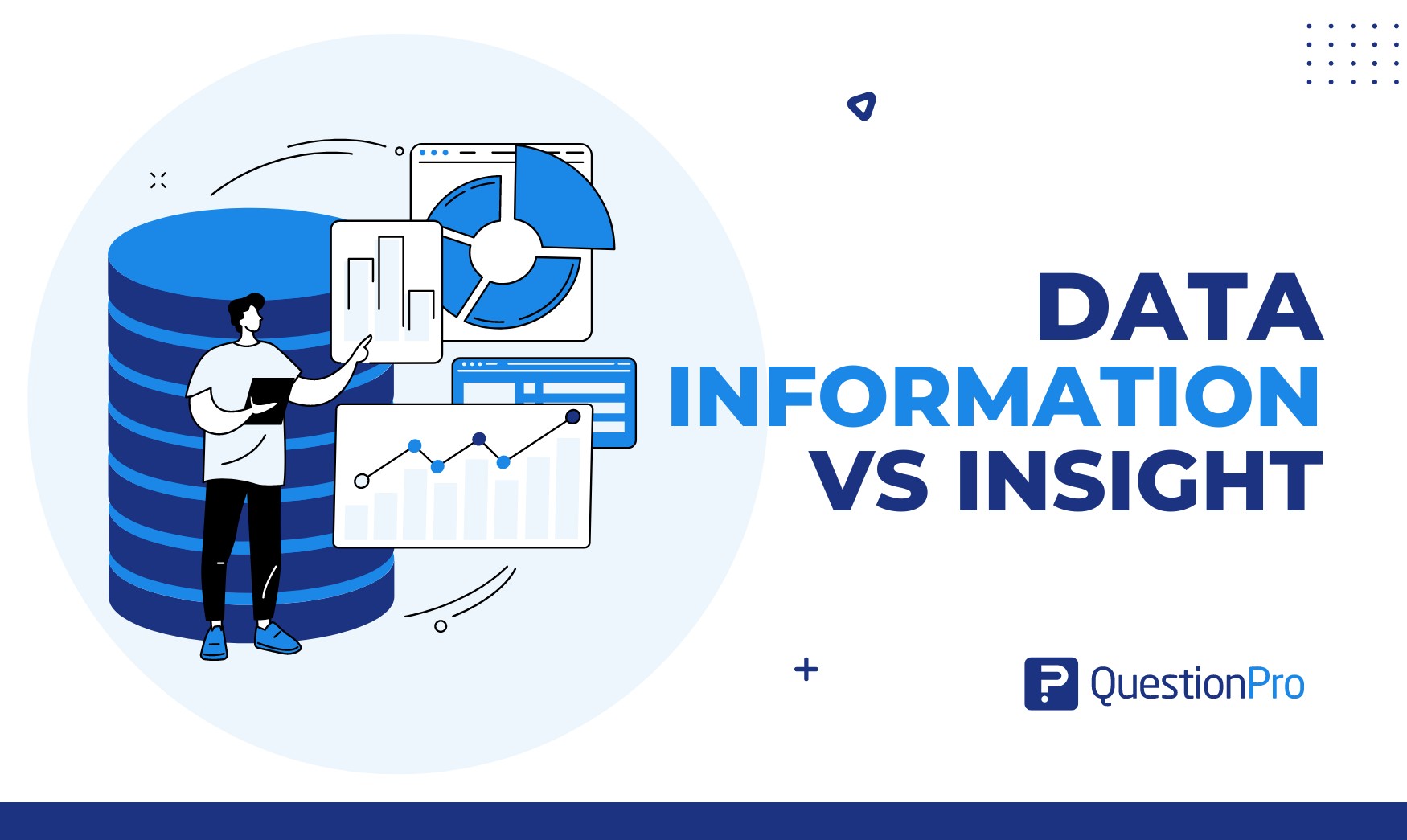
Data Information vs Insight: Essential differences
May 14, 2024
Other categories
- Academic Research
- Artificial Intelligence
- Assessments
- Brand Awareness
- Case Studies
- Communities
- Consumer Insights
- Customer effort score
- Customer Engagement
- Customer Experience
- Customer Loyalty
- Customer Research
- Customer Satisfaction
- Employee Benefits
- Employee Engagement
- Employee Retention
- Friday Five
- General Data Protection Regulation
- Insights Hub
- Life@QuestionPro
- Market Research
- Mobile diaries
- Mobile Surveys
- New Features
- Online Communities
- Question Types
- Questionnaire
- QuestionPro Products
- Release Notes
- Research Tools and Apps
- Revenue at Risk
- Survey Templates
- Training Tips
- Uncategorized
- Video Learning Series
- What’s Coming Up
- Workforce Intelligence
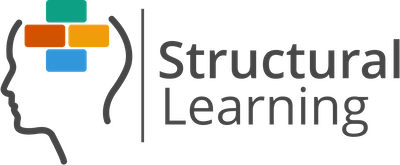
Action research in the classroom: A teacher's guide
November 26, 2021
Discover best practices for action research in the classroom, guiding teachers on implementing and facilitating impactful studies in schools.
Main, P (2021, November 26). Action research in the classroom: A teacher's guide. Retrieved from https://www.structural-learning.com/post/action-research-in-the-classroom-a-teachers-guide
What is action research?
Action research is a participatory process designed to empower educators to examine and improve their own practice. It is characterized by a cycle of planning , action, observation, and reflection, with the goal of achieving a deeper understanding of practice within educational contexts. This process encourages a wide range of approaches and can be adapted to various social contexts.
At its core, action research involves critical reflection on one's actions as a basis for improvement. Senior leaders and teachers are guided to reflect on their educational strategies , classroom management, and student engagement techniques. It's a collaborative effort that often involves not just the teachers but also the students and other stakeholders, fostering an inclusive process that values the input of all participants.
The action research process is iterative, with each cycle aiming to bring about a clearer understanding and improvement in practice. It typically begins with the identification of real-world problems within the school environment, followed by a circle of planning where strategies are developed to address these issues. The implementation of these strategies is then observed and documented, often through journals or participant observation, allowing for reflection and analysis.
The insights gained from action research contribute to Organization Development, enhancing the quality of teaching and learning. This approach is strongly aligned with the principles of Quality Assurance in Education, ensuring that the actions taken are effective and responsive to the needs of the school community.
Educators can share their findings in community forums or through publications in journals, contributing to the wider theory about practice . Tertiary education sector often draws on such studies to inform teacher training and curriculum development.
In summary, the significant parts of action research include:
- A continuous cycle of planning, action, observation, and reflection.
- A focus on reflective practice to achieve a deeper understanding of educational methodologies.
- A commitment to inclusive and participatory processes that engage the entire school community.
Creating an action research project
The action research process usually begins with a situation or issue that a teacher wants to change as part of school improvement initiatives .
Teachers get support in changing the ' interesting issue ' into a 'researchable question' and then taking to experiment. The teacher will draw on the outcomes of other researchers to help build actions and reveal the consequences .
Participatory action research is a strategy to the enquiry which has been utilised since the 1940s. Participatory action involves researchers and other participants taking informed action to gain knowledge of a problematic situation and change it to bring a positive effect. As an action researcher , a teacher carries out research . Enquiring into their practice would lead a teacher to question the norms and assumptions that are mostly overlooked in normal school life . Making a routine of inquiry can provide a commitment to learning and professional development . A teacher-researcher holds the responsibility for being the source and agent of change.
Examples of action research projects in education include a teacher working with students to improve their reading comprehension skills , a group of teachers collaborating to develop and implement a new curriculum, or a school administrator conducting a study on the effectiveness of a school-wide behavior management program.
In each of these cases, the research is aimed at improving the educational experience for students and addressing a specific issue or problem within the school community . Action research can be a powerful tool for educators to improve their practice and make a positive impact on their students' learning.
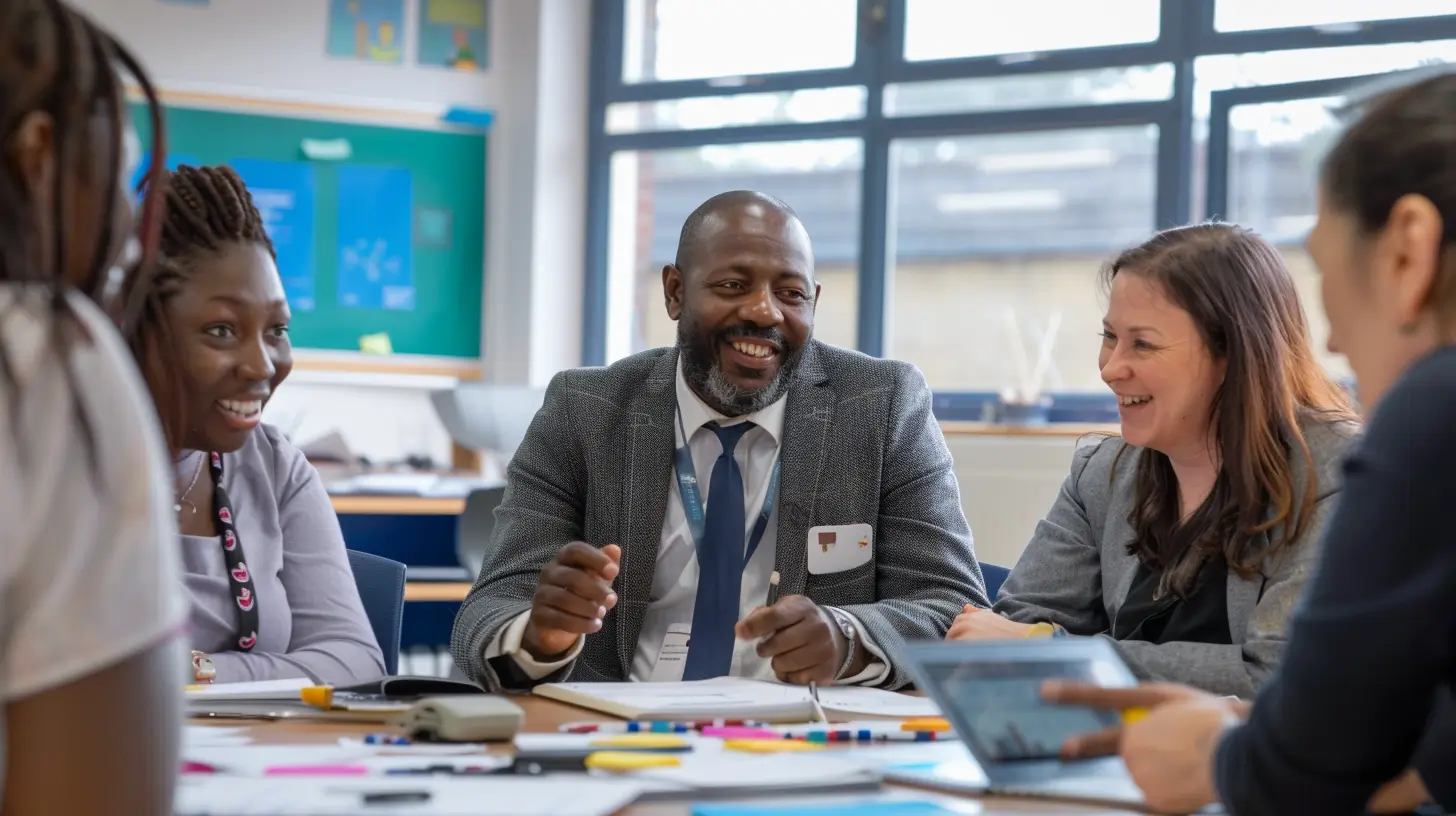
Potential research questions could include:
- How can dual-coding be used to improve my students memory ?
- Does mind-mapping lead to creativity?
- How does Oracy improve my classes writing?
- How can we advance critical thinking in year 10?
- How can graphic organisers be used for exam preparation?
Regardless of the types of action research your staff engage in, a solid cycle of inquiry is an essential aspect of the action research spiral. Building in the process of reflection will ensure that key points of learning can be extracted from the action research study.
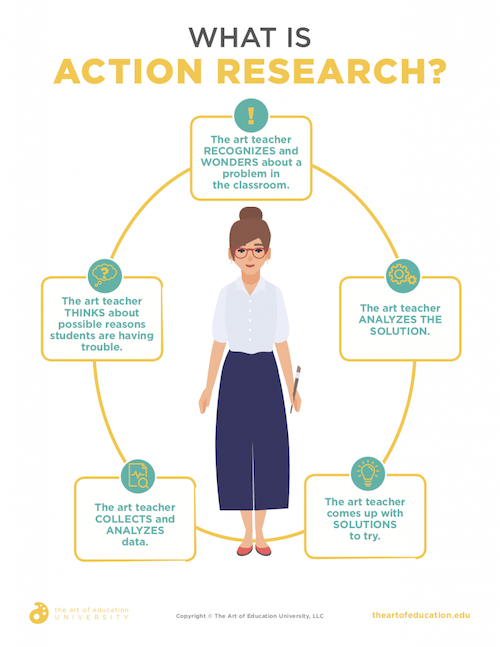
What is an action research cycle?
Action research in education is a cycle of reflection and action inquiry , which follows these steps:
1. Identifying the problem
It is the first stage of action research that starts when a teacher identifies a problem or question that they want to address. To make an a ction research approach successful, the teacher needs to ensure that the questions are the ones 'they' wish to solve. Their questions might involve social sciences, instructional strategies, everyday life and social management issues, guide for students analytical research methods for improving specific student performance or curriculum implementation etc. Teachers may seek help from a wide variety of existing literature , to find strategies and solutions that others have executed to solve any particular problem. It is also suggested to build a visual map or a table of problems, target performances, potential solutions and supporting references in the middle.
2. Developing an Action Plan
After identifying the problem, after r eviewing the relevant literature and describing the vision of how to solve the problem; the next step would be action planning which means to develop a plan of action . Action planning involves studying the literature and brainstorming can be used by the action research planner to create new techniques and strategies that can generate better results of both action learning and action research. One may go back to the visual map or table of contents and reorder or colour-code the potential outcomes. The items in the list can be ranked in order of significance and the amount of time needed for these strategies.
An action plan has the details of how to implement each idea and the factors that may keep them from their vision of success . Identify those factors that cannot be changed –these are the constants in an equation. The focus of action research at the planning stage must remain focused on the variables –the factors that can be changed using actions. An action plan must be how to implement a solution and how one's instruction, management style, and behaviour will affect each of the variables.
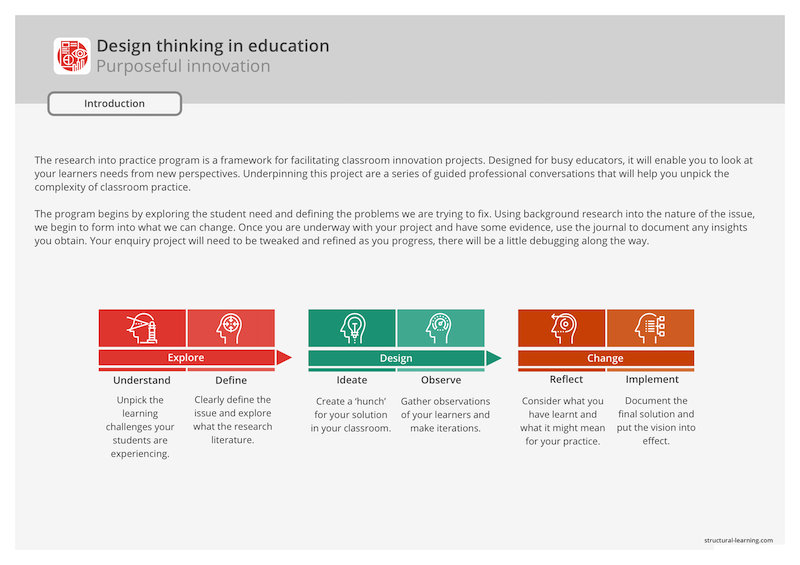
3. Data Collection
Before starting to implement a plan of action , the researcher must have a complete understanding of action research and must have knowledge of the type of data that may help in the success of the plan and must assess how to collect that data. For instance, if the goal is to improve class attendance, attendance records must be collected as useful data for the participatory action. If the goal is to improve time management, the data may include students and classroom observations . There are many options to choose from to collect data from. Selecting the most suitable methodology for data collection will provide more meaningful , accurate and valid data. Some sources of data are interviews and observation. Also, one may administer surveys , distribute questionnaires and watch videotapes of the classroom to collect data.
4. Data Analysis and Conclusions
At this action stage, an action researcher analyses the collected data and concludes. It is suggested to assess the data during the predefined process of data collection as it will help refine the action research agenda. If the collected data seems insufficient , the data collection plan must be revised. Data analysis also helps to reflect on what exactly happened. Did the action researcher perform the actions as planned? Were the study outcomes as expected? Which assumptions of the action researcher proved to be incorrect?
Adding details such as tables, opinions, and recommendations can help in identifying trends (correlations and relationships). One must share the findings while analysing data and drawing conclusions . Engaging in conversations for teacher growth is essential; hence, the action researcher would share the findings with other teachers through discussion of action research, who can yield useful feedback. One may also share the findings with students, as they can also provide additional insight . For example, if teachers and students agree with the conclusions of action research for educational change, it adds to the credibility of the data collection plan and analysis. If they don't seem to agree with the data collection plan and analysis , the action researchers may take informed action and refine the data collection plan and reevaluate conclusions .
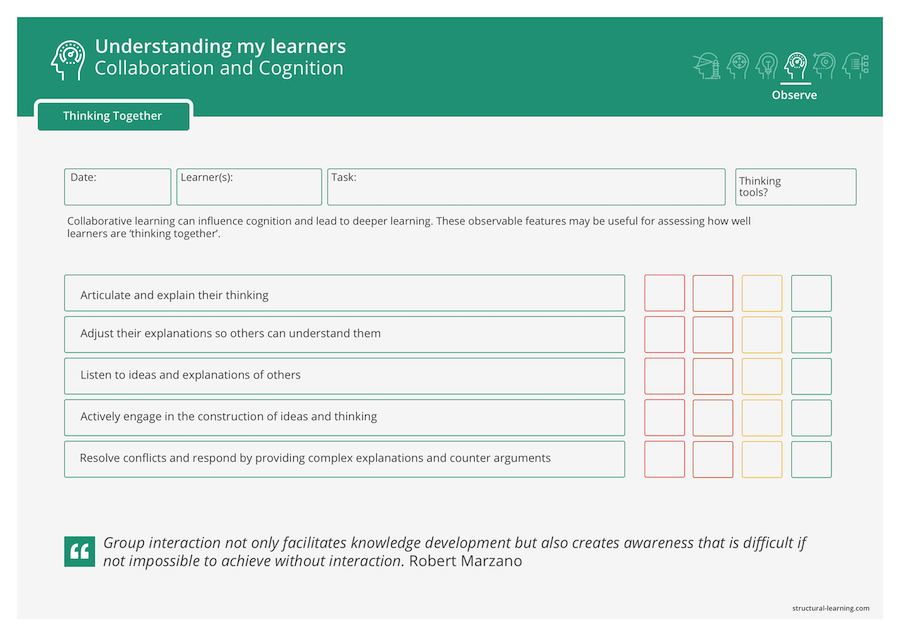
5. Modifying the Educational Theory and Repeat
After concluding, the process begins again. The teacher can adjust different aspects of the action research approach to theory or make it more specific according to the findings . Action research guides how to change the steps of action research development, how to modify the action plan , and provide better access to resources, start data collection once again, or prepare new questions to ask from the respondents.
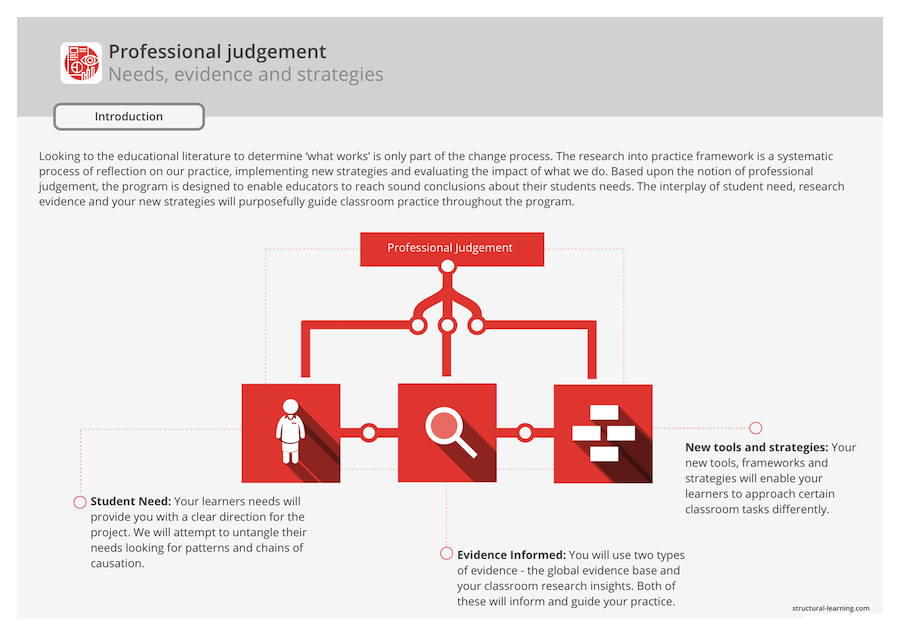
6. Report the Findings
Since the main approach to action research involves the informed action to introduce useful change into the classroom or schools, one must not forget to share the outcomes with others. Sharing the outcomes would help to further reflect on the problem and process, and it would help other teachers to use these findings to enhance their professional practice as an educator. One may print book and share the experience with the school leaders, principal, teachers and students as they served as guide to action research. Or, a community action researcher may present community-based action research at a conference so people from other areas can take advantage of this collaborative action. Also, teachers may use a digital storytelling tool to outline their results.
There are plenty of creative tools we can use to bring the research projects to life. We have seen videos, podcasts and research posters all being used to communicate the results of these programs. Community action research is a unique way to present details of the community-related adventures in the teacher profession, cultivate expertise and show how teachers think about education , so it is better to find unique ways to report the findings of community-led action research.
Final thoughts on action-research for teachers
As we have seen, action research can be an effective form of professional development, illuminating the path for teachers and school leaders seeking to refine their craft. This cyclical process of inquiry and reflection is not merely a methodological pursuit but a profound professional journey. The definition of action research, as a systematic inquiry conducted by teachers, administrators, and other stakeholders in the teaching/learning environment, emphasizes the collaborative nature of improving educational strategies and outcomes.
Action research transcends traditional disciplinary practices by immersing educators in the social contexts of their work, prompting them to question and adapt their methods to meet the evolving needs of their students . It is a form of reflective practice that demands critical thinking and flexibility, as one navigates through the iterative stages of planning, acting, observing, and reflecting.
The process of action research is inherently participatory, encouraging educators to engage with their learning communities to address key issues and social issues that impact educational settings. This method empowers professionals within universities and schools alike to take ownership of their learning and development, fostering a culture of continuous improvement and participatory approaches.
In summary, action research encapsulates the essence of what it means to be a learning professional in a dynamic educational landscape. It is the embodiment of a commitment to lifelong learning and a testament to the capacity of educators to enact change . The value of action research lies in its ability to transform practitioners into researchers, where the quest for knowledge becomes a powerful conduit for change and innovation. Thus, for educators at every level, embracing the rigorous yet rewarding path of action research can unveil potent insights and propel educational practice to new heights.
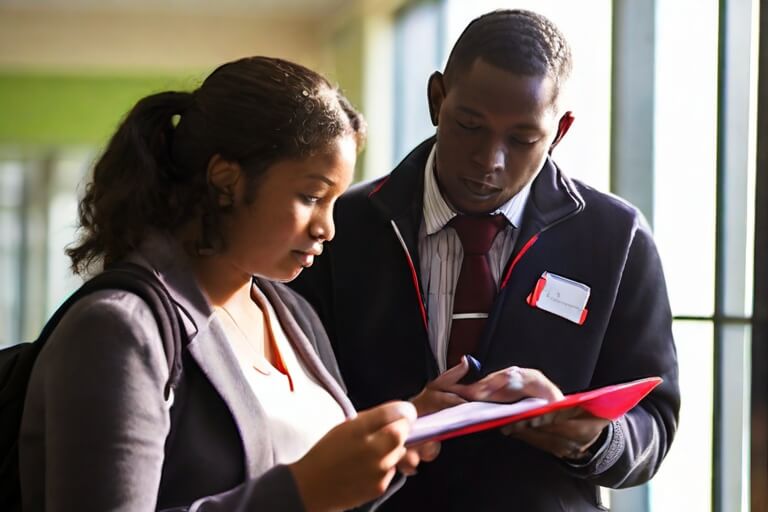
Key Papers on Action Research
- Utilizing Action Research During Student Teaching by James O. Barbre and Brenda J. Buckner (2013): This study explores how action research can be effectively utilized during student teaching to enhance professional pedagogical disposition through active reflection. It emphasizes developing a reflective habit of mind crucial for teachers to be effective in their classrooms and adaptive to the changing needs of their students.
- Repositioning T eacher Action Research in Science Teacher Education by B. Capobianco and A. Feldman (2010): This paper discusses the promotion of action research as a way for teachers to improve their practice and students' learning for over 50 years, focusing on science education. It highlights the importance of action research in advancing knowledge about teaching and learning in science.
- Action research and teacher leadership by K. Smeets and P. Ponte (2009): This article reports on a case study into the influence and impact of action research carried out by teachers in a special school. It found that action research not only helps teachers to get to grips with their work in the classroom but also has an impact on the work of others in the school.
- Teaching about the Nature of Science through History: Action Research in the Classroom by J. Solomon, Jon Duveen, Linda Scot, S. McCarthy (1992): This article reports on 18 months of action research monitoring British pupils' learning about the nature of science using historical aspects. It indicates areas of substantial progress in pupils' understanding of the nature of science.
- Action Research in the Classroom by V. Baumfield, E. Hall, K. Wall (2008): This comprehensive guide to conducting action research in the classroom covers various aspects, including deciding on a research question, choosing complementary research tools, collecting and interpreting data, and sharing findings. It aims to move classroom inquiry forward and contribute to professional development.
These studies highlight the significant role of action research in enhancing teacher effectiveness, student learning outcomes, and contributing to the broader educational community's knowledge and practices.
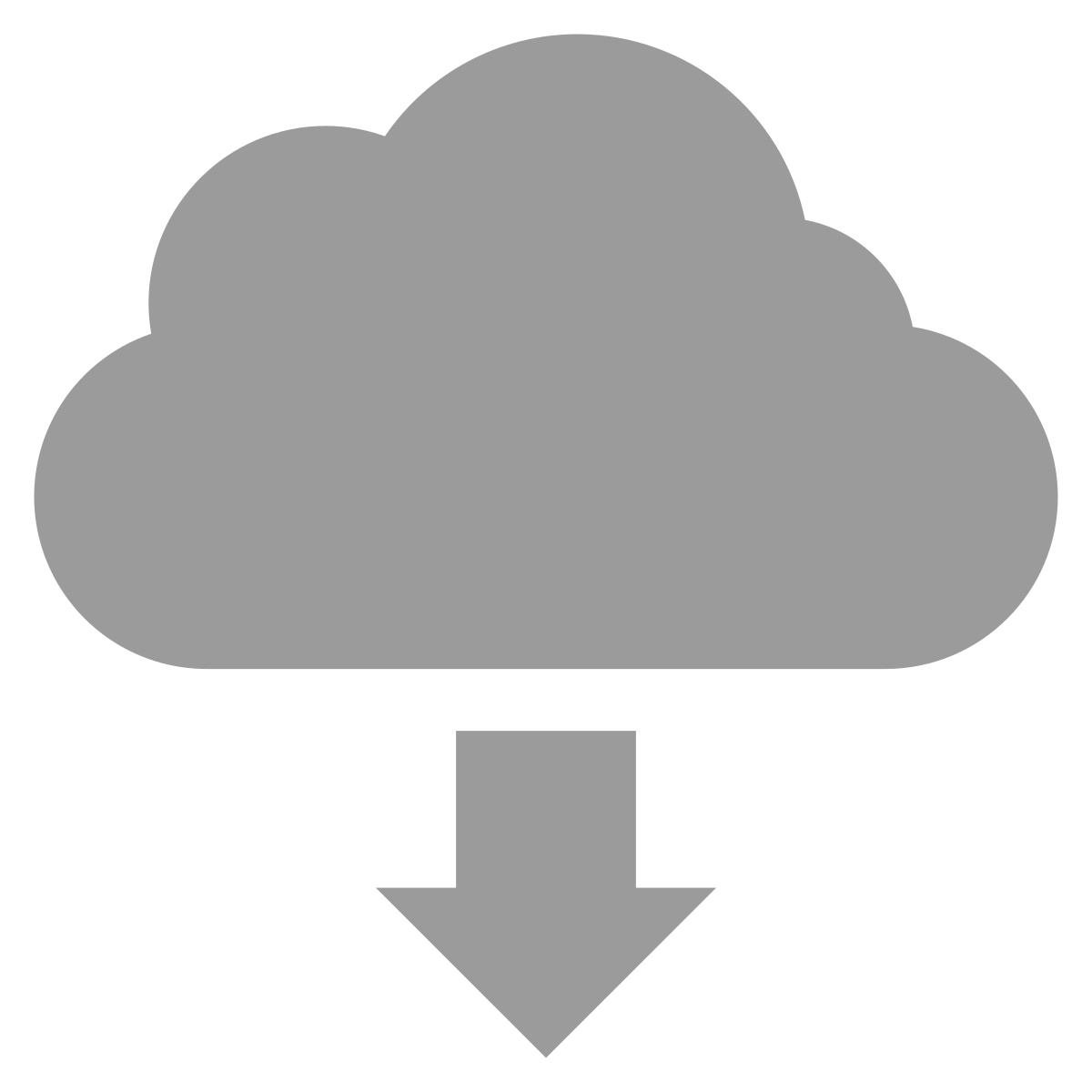
Enhance Learner Outcomes Across Your School
Download an Overview of our Support and Resources
We'll send it over now.
Please fill in the details so we can send over the resources.
What type of school are you?
We'll get you the right resource
Is your school involved in any staff development projects?
Are your colleagues running any research projects or courses?
Do you have any immediate school priorities?
Please check the ones that apply.
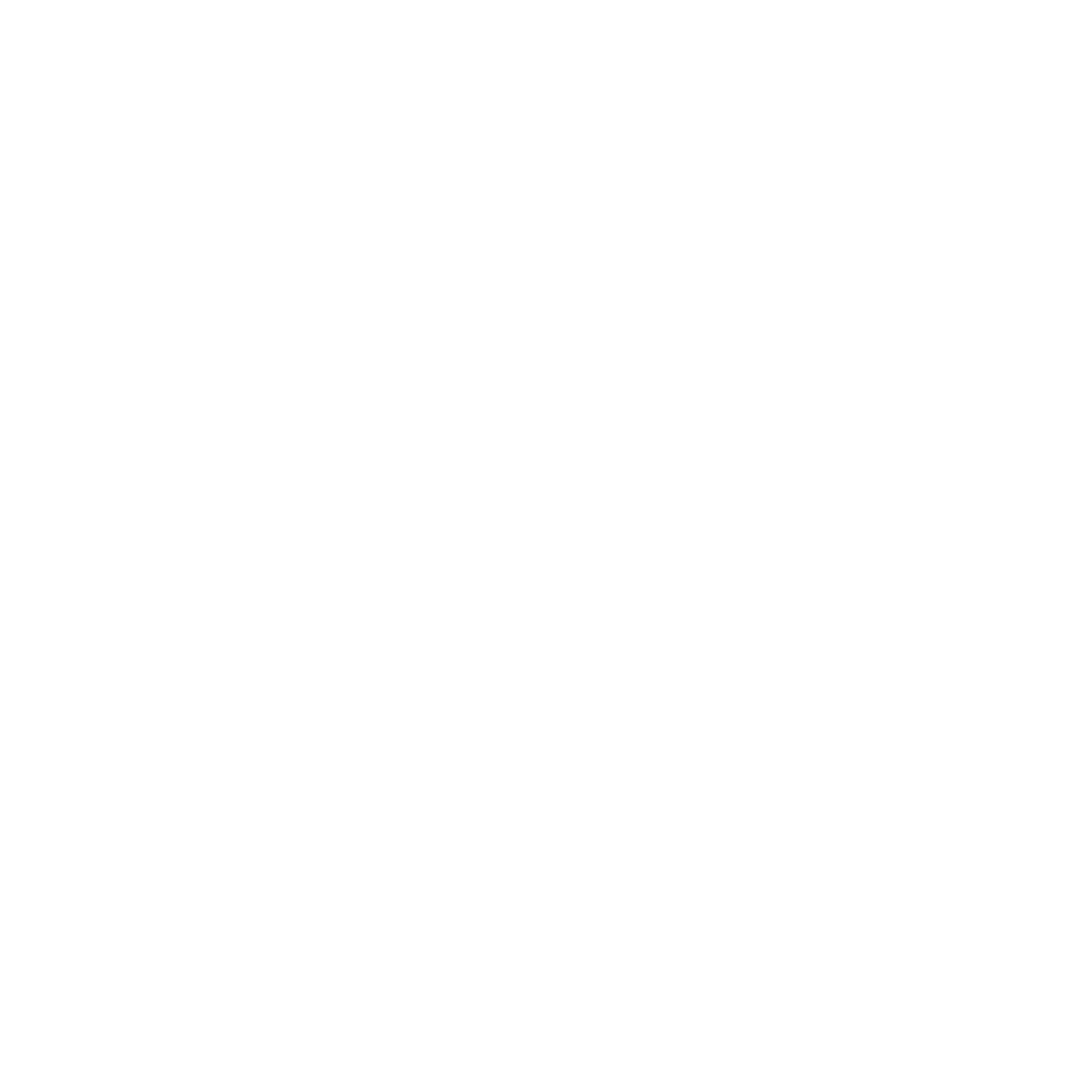
Download your resource
Thanks for taking the time to complete this form, submit the form to get the tool.
Classroom Practice

Research Aims, Objectives & Questions
The “Golden Thread” Explained Simply (+ Examples)
By: David Phair (PhD) and Alexandra Shaeffer (PhD) | June 2022
The research aims , objectives and research questions (collectively called the “golden thread”) are arguably the most important thing you need to get right when you’re crafting a research proposal , dissertation or thesis . We receive questions almost every day about this “holy trinity” of research and there’s certainly a lot of confusion out there, so we’ve crafted this post to help you navigate your way through the fog.
Overview: The Golden Thread
- What is the golden thread
- What are research aims ( examples )
- What are research objectives ( examples )
- What are research questions ( examples )
- The importance of alignment in the golden thread
What is the “golden thread”?
The golden thread simply refers to the collective research aims , research objectives , and research questions for any given project (i.e., a dissertation, thesis, or research paper ). These three elements are bundled together because it’s extremely important that they align with each other, and that the entire research project aligns with them.
Importantly, the golden thread needs to weave its way through the entirety of any research project , from start to end. In other words, it needs to be very clearly defined right at the beginning of the project (the topic ideation and proposal stage) and it needs to inform almost every decision throughout the rest of the project. For example, your research design and methodology will be heavily influenced by the golden thread (we’ll explain this in more detail later), as well as your literature review.
The research aims, objectives and research questions (the golden thread) define the focus and scope ( the delimitations ) of your research project. In other words, they help ringfence your dissertation or thesis to a relatively narrow domain, so that you can “go deep” and really dig into a specific problem or opportunity. They also help keep you on track , as they act as a litmus test for relevance. In other words, if you’re ever unsure whether to include something in your document, simply ask yourself the question, “does this contribute toward my research aims, objectives or questions?”. If it doesn’t, chances are you can drop it.
Alright, enough of the fluffy, conceptual stuff. Let’s get down to business and look at what exactly the research aims, objectives and questions are and outline a few examples to bring these concepts to life.
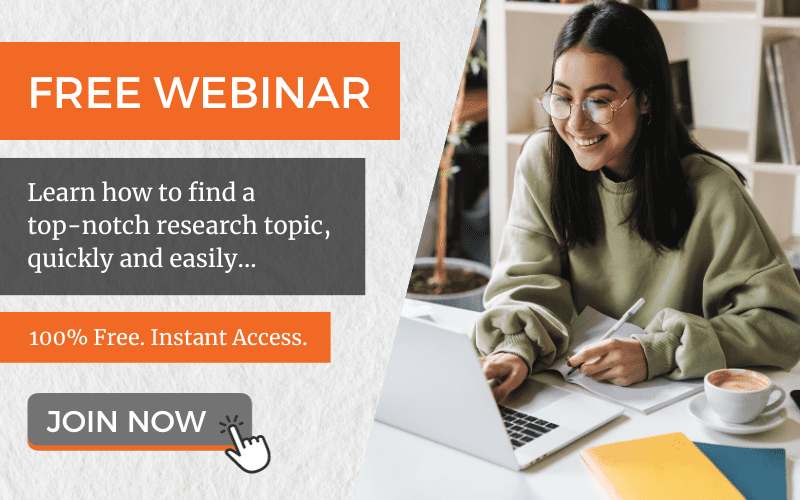
Research Aims: What are they?
Simply put, the research aim(s) is a statement that reflects the broad overarching goal (s) of the research project. Research aims are fairly high-level (low resolution) as they outline the general direction of the research and what it’s trying to achieve .
Research Aims: Examples
True to the name, research aims usually start with the wording “this research aims to…”, “this research seeks to…”, and so on. For example:
“This research aims to explore employee experiences of digital transformation in retail HR.” “This study sets out to assess the interaction between student support and self-care on well-being in engineering graduate students”
As you can see, these research aims provide a high-level description of what the study is about and what it seeks to achieve. They’re not hyper-specific or action-oriented, but they’re clear about what the study’s focus is and what is being investigated.
Need a helping hand?
Research Objectives: What are they?
The research objectives take the research aims and make them more practical and actionable . In other words, the research objectives showcase the steps that the researcher will take to achieve the research aims.
The research objectives need to be far more specific (higher resolution) and actionable than the research aims. In fact, it’s always a good idea to craft your research objectives using the “SMART” criteria. In other words, they should be specific, measurable, achievable, relevant and time-bound”.
Research Objectives: Examples
Let’s look at two examples of research objectives. We’ll stick with the topic and research aims we mentioned previously.
For the digital transformation topic:
To observe the retail HR employees throughout the digital transformation. To assess employee perceptions of digital transformation in retail HR. To identify the barriers and facilitators of digital transformation in retail HR.
And for the student wellness topic:
To determine whether student self-care predicts the well-being score of engineering graduate students. To determine whether student support predicts the well-being score of engineering students. To assess the interaction between student self-care and student support when predicting well-being in engineering graduate students.
As you can see, these research objectives clearly align with the previously mentioned research aims and effectively translate the low-resolution aims into (comparatively) higher-resolution objectives and action points . They give the research project a clear focus and present something that resembles a research-based “to-do” list.
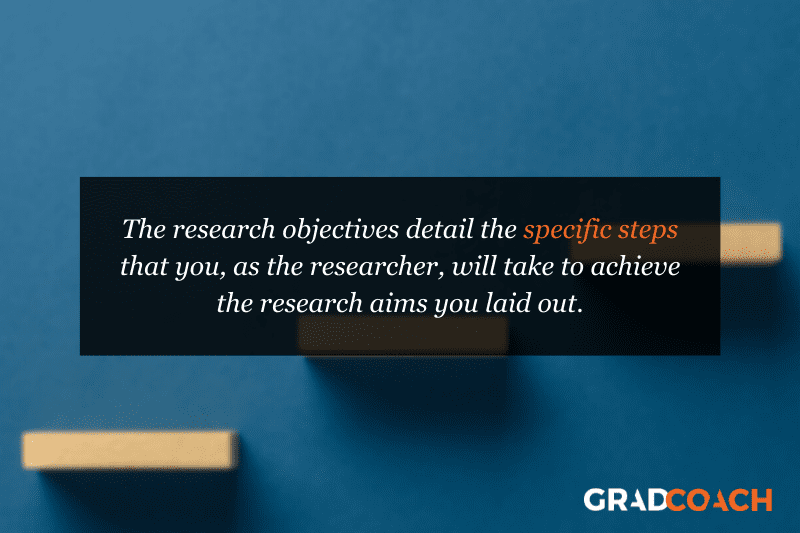
Research Questions: What are they?
Finally, we arrive at the all-important research questions. The research questions are, as the name suggests, the key questions that your study will seek to answer . Simply put, they are the core purpose of your dissertation, thesis, or research project. You’ll present them at the beginning of your document (either in the introduction chapter or literature review chapter) and you’ll answer them at the end of your document (typically in the discussion and conclusion chapters).
The research questions will be the driving force throughout the research process. For example, in the literature review chapter, you’ll assess the relevance of any given resource based on whether it helps you move towards answering your research questions. Similarly, your methodology and research design will be heavily influenced by the nature of your research questions. For instance, research questions that are exploratory in nature will usually make use of a qualitative approach, whereas questions that relate to measurement or relationship testing will make use of a quantitative approach.
Let’s look at some examples of research questions to make this more tangible.
Research Questions: Examples
Again, we’ll stick with the research aims and research objectives we mentioned previously.
For the digital transformation topic (which would be qualitative in nature):
How do employees perceive digital transformation in retail HR? What are the barriers and facilitators of digital transformation in retail HR?
And for the student wellness topic (which would be quantitative in nature):
Does student self-care predict the well-being scores of engineering graduate students? Does student support predict the well-being scores of engineering students? Do student self-care and student support interact when predicting well-being in engineering graduate students?
You’ll probably notice that there’s quite a formulaic approach to this. In other words, the research questions are basically the research objectives “converted” into question format. While that is true most of the time, it’s not always the case. For example, the first research objective for the digital transformation topic was more or less a step on the path toward the other objectives, and as such, it didn’t warrant its own research question.
So, don’t rush your research questions and sloppily reword your objectives as questions. Carefully think about what exactly you’re trying to achieve (i.e. your research aim) and the objectives you’ve set out, then craft a set of well-aligned research questions . Also, keep in mind that this can be a somewhat iterative process , where you go back and tweak research objectives and aims to ensure tight alignment throughout the golden thread.
The importance of strong alignment
Alignment is the keyword here and we have to stress its importance . Simply put, you need to make sure that there is a very tight alignment between all three pieces of the golden thread. If your research aims and research questions don’t align, for example, your project will be pulling in different directions and will lack focus . This is a common problem students face and can cause many headaches (and tears), so be warned.
Take the time to carefully craft your research aims, objectives and research questions before you run off down the research path. Ideally, get your research supervisor/advisor to review and comment on your golden thread before you invest significant time into your project, and certainly before you start collecting data .
Recap: The golden thread
In this post, we unpacked the golden thread of research, consisting of the research aims , research objectives and research questions . You can jump back to any section using the links below.
As always, feel free to leave a comment below – we always love to hear from you. Also, if you’re interested in 1-on-1 support, take a look at our private coaching service here.
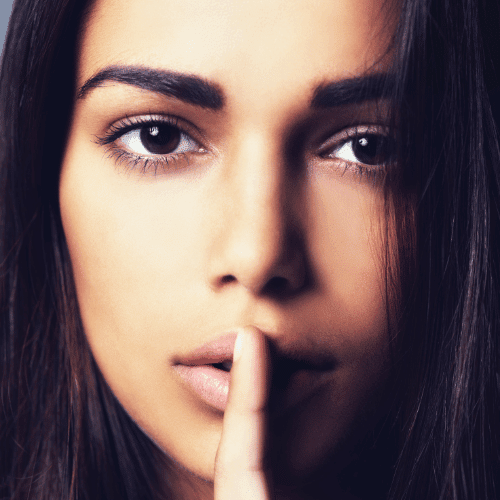
Psst... there’s more!
This post was based on one of our popular Research Bootcamps . If you're working on a research project, you'll definitely want to check this out ...
You Might Also Like:
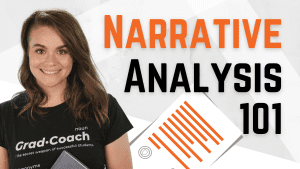
39 Comments
Thank you very much for your great effort put. As an Undergraduate taking Demographic Research & Methodology, I’ve been trying so hard to understand clearly what is a Research Question, Research Aim and the Objectives in a research and the relationship between them etc. But as for now I’m thankful that you’ve solved my problem.
Well appreciated. This has helped me greatly in doing my dissertation.
An so delighted with this wonderful information thank you a lot.
so impressive i have benefited a lot looking forward to learn more on research.
I am very happy to have carefully gone through this well researched article.
Infact,I used to be phobia about anything research, because of my poor understanding of the concepts.
Now,I get to know that my research question is the same as my research objective(s) rephrased in question format.
I please I would need a follow up on the subject,as I intends to join the team of researchers. Thanks once again.
Thanks so much. This was really helpful.
I know you pepole have tried to break things into more understandable and easy format. And God bless you. Keep it up
i found this document so useful towards my study in research methods. thanks so much.
This is my 2nd read topic in your course and I should commend the simplified explanations of each part. I’m beginning to understand and absorb the use of each part of a dissertation/thesis. I’ll keep on reading your free course and might be able to avail the training course! Kudos!
Thank you! Better put that my lecture and helped to easily understand the basics which I feel often get brushed over when beginning dissertation work.
This is quite helpful. I like how the Golden thread has been explained and the needed alignment.
This is quite helpful. I really appreciate!
The article made it simple for researcher students to differentiate between three concepts.
Very innovative and educational in approach to conducting research.
I am very impressed with all these terminology, as I am a fresh student for post graduate, I am highly guided and I promised to continue making consultation when the need arise. Thanks a lot.
A very helpful piece. thanks, I really appreciate it .
Very well explained, and it might be helpful to many people like me.
Wish i had found this (and other) resource(s) at the beginning of my PhD journey… not in my writing up year… 😩 Anyways… just a quick question as i’m having some issues ordering my “golden thread”…. does it matter in what order you mention them? i.e., is it always first aims, then objectives, and finally the questions? or can you first mention the research questions and then the aims and objectives?
Thank you for a very simple explanation that builds upon the concepts in a very logical manner. Just prior to this, I read the research hypothesis article, which was equally very good. This met my primary objective.
My secondary objective was to understand the difference between research questions and research hypothesis, and in which context to use which one. However, I am still not clear on this. Can you kindly please guide?
In research, a research question is a clear and specific inquiry that the researcher wants to answer, while a research hypothesis is a tentative statement or prediction about the relationship between variables or the expected outcome of the study. Research questions are broader and guide the overall study, while hypotheses are specific and testable statements used in quantitative research. Research questions identify the problem, while hypotheses provide a focus for testing in the study.
Exactly what I need in this research journey, I look forward to more of your coaching videos.
This helped a lot. Thanks so much for the effort put into explaining it.
What data source in writing dissertation/Thesis requires?
What is data source covers when writing dessertation/thesis
This is quite useful thanks
I’m excited and thankful. I got so much value which will help me progress in my thesis.
where are the locations of the reserch statement, research objective and research question in a reserach paper? Can you write an ouline that defines their places in the researh paper?
Very helpful and important tips on Aims, Objectives and Questions.
Thank you so much for making research aim, research objectives and research question so clear. This will be helpful to me as i continue with my thesis.
Thanks much for this content. I learned a lot. And I am inspired to learn more. I am still struggling with my preparation for dissertation outline/proposal. But I consistently follow contents and tutorials and the new FB of GRAD Coach. Hope to really become confident in writing my dissertation and successfully defend it.
As a researcher and lecturer, I find splitting research goals into research aims, objectives, and questions is unnecessarily bureaucratic and confusing for students. For most biomedical research projects, including ‘real research’, 1-3 research questions will suffice (numbers may differ by discipline).
Awesome! Very important resources and presented in an informative way to easily understand the golden thread. Indeed, thank you so much.
Well explained
The blog article on research aims, objectives, and questions by Grad Coach is a clear and insightful guide that aligns with my experiences in academic research. The article effectively breaks down the often complex concepts of research aims and objectives, providing a straightforward and accessible explanation. Drawing from my own research endeavors, I appreciate the practical tips offered, such as the need for specificity and clarity when formulating research questions. The article serves as a valuable resource for students and researchers, offering a concise roadmap for crafting well-defined research goals and objectives. Whether you’re a novice or an experienced researcher, this article provides practical insights that contribute to the foundational aspects of a successful research endeavor.
A great thanks for you. it is really amazing explanation. I grasp a lot and one step up to research knowledge.
I really found these tips helpful. Thank you very much Grad Coach.
I found this article helpful. Thanks for sharing this.
thank you so much, the explanation and examples are really helpful
Submit a Comment Cancel reply
Your email address will not be published. Required fields are marked *
Save my name, email, and website in this browser for the next time I comment.
- Print Friendly
- Privacy Policy

Home » Research Questions – Types, Examples and Writing Guide
Research Questions – Types, Examples and Writing Guide
Table of Contents

Research Questions
Definition:
Research questions are the specific questions that guide a research study or inquiry. These questions help to define the scope of the research and provide a clear focus for the study. Research questions are usually developed at the beginning of a research project and are designed to address a particular research problem or objective.
Types of Research Questions
Types of Research Questions are as follows:
Descriptive Research Questions
These aim to describe a particular phenomenon, group, or situation. For example:
- What are the characteristics of the target population?
- What is the prevalence of a particular disease in a specific region?
Exploratory Research Questions
These aim to explore a new area of research or generate new ideas or hypotheses. For example:
- What are the potential causes of a particular phenomenon?
- What are the possible outcomes of a specific intervention?
Explanatory Research Questions
These aim to understand the relationship between two or more variables or to explain why a particular phenomenon occurs. For example:
- What is the effect of a specific drug on the symptoms of a particular disease?
- What are the factors that contribute to employee turnover in a particular industry?
Predictive Research Questions
These aim to predict a future outcome or trend based on existing data or trends. For example :
- What will be the future demand for a particular product or service?
- What will be the future prevalence of a particular disease?
Evaluative Research Questions
These aim to evaluate the effectiveness of a particular intervention or program. For example:
- What is the impact of a specific educational program on student learning outcomes?
- What is the effectiveness of a particular policy or program in achieving its intended goals?
How to Choose Research Questions
Choosing research questions is an essential part of the research process and involves careful consideration of the research problem, objectives, and design. Here are some steps to consider when choosing research questions:
- Identify the research problem: Start by identifying the problem or issue that you want to study. This could be a gap in the literature, a social or economic issue, or a practical problem that needs to be addressed.
- Conduct a literature review: Conducting a literature review can help you identify existing research in your area of interest and can help you formulate research questions that address gaps or limitations in the existing literature.
- Define the research objectives : Clearly define the objectives of your research. What do you want to achieve with your study? What specific questions do you want to answer?
- Consider the research design : Consider the research design that you plan to use. This will help you determine the appropriate types of research questions to ask. For example, if you plan to use a qualitative approach, you may want to focus on exploratory or descriptive research questions.
- Ensure that the research questions are clear and answerable: Your research questions should be clear and specific, and should be answerable with the data that you plan to collect. Avoid asking questions that are too broad or vague.
- Get feedback : Get feedback from your supervisor, colleagues, or peers to ensure that your research questions are relevant, feasible, and meaningful.
How to Write Research Questions
Guide for Writing Research Questions:
- Start with a clear statement of the research problem: Begin by stating the problem or issue that your research aims to address. This will help you to formulate focused research questions.
- Use clear language : Write your research questions in clear and concise language that is easy to understand. Avoid using jargon or technical terms that may be unfamiliar to your readers.
- Be specific: Your research questions should be specific and focused. Avoid broad questions that are difficult to answer. For example, instead of asking “What is the impact of climate change on the environment?” ask “What are the effects of rising sea levels on coastal ecosystems?”
- Use appropriate question types: Choose the appropriate question types based on the research design and objectives. For example, if you are conducting a qualitative study, you may want to use open-ended questions that allow participants to provide detailed responses.
- Consider the feasibility of your questions : Ensure that your research questions are feasible and can be answered with the resources available. Consider the data sources and methods of data collection when writing your questions.
- Seek feedback: Get feedback from your supervisor, colleagues, or peers to ensure that your research questions are relevant, appropriate, and meaningful.
Examples of Research Questions
Some Examples of Research Questions with Research Titles:
Research Title: The Impact of Social Media on Mental Health
- Research Question : What is the relationship between social media use and mental health, and how does this impact individuals’ well-being?
Research Title: Factors Influencing Academic Success in High School
- Research Question: What are the primary factors that influence academic success in high school, and how do they contribute to student achievement?
Research Title: The Effects of Exercise on Physical and Mental Health
- Research Question: What is the relationship between exercise and physical and mental health, and how can exercise be used as a tool to improve overall well-being?
Research Title: Understanding the Factors that Influence Consumer Purchasing Decisions
- Research Question : What are the key factors that influence consumer purchasing decisions, and how do these factors vary across different demographics and products?
Research Title: The Impact of Technology on Communication
- Research Question : How has technology impacted communication patterns, and what are the effects of these changes on interpersonal relationships and society as a whole?
Research Title: Investigating the Relationship between Parenting Styles and Child Development
- Research Question: What is the relationship between different parenting styles and child development outcomes, and how do these outcomes vary across different ages and developmental stages?
Research Title: The Effectiveness of Cognitive-Behavioral Therapy in Treating Anxiety Disorders
- Research Question: How effective is cognitive-behavioral therapy in treating anxiety disorders, and what factors contribute to its success or failure in different patients?
Research Title: The Impact of Climate Change on Biodiversity
- Research Question : How is climate change affecting global biodiversity, and what can be done to mitigate the negative effects on natural ecosystems?
Research Title: Exploring the Relationship between Cultural Diversity and Workplace Productivity
- Research Question : How does cultural diversity impact workplace productivity, and what strategies can be employed to maximize the benefits of a diverse workforce?
Research Title: The Role of Artificial Intelligence in Healthcare
- Research Question: How can artificial intelligence be leveraged to improve healthcare outcomes, and what are the potential risks and ethical concerns associated with its use?
Applications of Research Questions
Here are some of the key applications of research questions:
- Defining the scope of the study : Research questions help researchers to narrow down the scope of their study and identify the specific issues they want to investigate.
- Developing hypotheses: Research questions often lead to the development of hypotheses, which are testable predictions about the relationship between variables. Hypotheses provide a clear and focused direction for the study.
- Designing the study : Research questions guide the design of the study, including the selection of participants, the collection of data, and the analysis of results.
- Collecting data : Research questions inform the selection of appropriate methods for collecting data, such as surveys, interviews, or experiments.
- Analyzing data : Research questions guide the analysis of data, including the selection of appropriate statistical tests and the interpretation of results.
- Communicating results : Research questions help researchers to communicate the results of their study in a clear and concise manner. The research questions provide a framework for discussing the findings and drawing conclusions.
Characteristics of Research Questions
Characteristics of Research Questions are as follows:
- Clear and Specific : A good research question should be clear and specific. It should clearly state what the research is trying to investigate and what kind of data is required.
- Relevant : The research question should be relevant to the study and should address a current issue or problem in the field of research.
- Testable : The research question should be testable through empirical evidence. It should be possible to collect data to answer the research question.
- Concise : The research question should be concise and focused. It should not be too broad or too narrow.
- Feasible : The research question should be feasible to answer within the constraints of the research design, time frame, and available resources.
- Original : The research question should be original and should contribute to the existing knowledge in the field of research.
- Significant : The research question should have significance and importance to the field of research. It should have the potential to provide new insights and knowledge to the field.
- Ethical : The research question should be ethical and should not cause harm to any individuals or groups involved in the study.
Purpose of Research Questions
Research questions are the foundation of any research study as they guide the research process and provide a clear direction to the researcher. The purpose of research questions is to identify the scope and boundaries of the study, and to establish the goals and objectives of the research.
The main purpose of research questions is to help the researcher to focus on the specific area or problem that needs to be investigated. They enable the researcher to develop a research design, select the appropriate methods and tools for data collection and analysis, and to organize the results in a meaningful way.
Research questions also help to establish the relevance and significance of the study. They define the research problem, and determine the research methodology that will be used to address the problem. Research questions also help to determine the type of data that will be collected, and how it will be analyzed and interpreted.
Finally, research questions provide a framework for evaluating the results of the research. They help to establish the validity and reliability of the data, and provide a basis for drawing conclusions and making recommendations based on the findings of the study.
Advantages of Research Questions
There are several advantages of research questions in the research process, including:
- Focus : Research questions help to focus the research by providing a clear direction for the study. They define the specific area of investigation and provide a framework for the research design.
- Clarity : Research questions help to clarify the purpose and objectives of the study, which can make it easier for the researcher to communicate the research aims to others.
- Relevance : Research questions help to ensure that the study is relevant and meaningful. By asking relevant and important questions, the researcher can ensure that the study will contribute to the existing body of knowledge and address important issues.
- Consistency : Research questions help to ensure consistency in the research process by providing a framework for the development of the research design, data collection, and analysis.
- Measurability : Research questions help to ensure that the study is measurable by defining the specific variables and outcomes that will be measured.
- Replication : Research questions help to ensure that the study can be replicated by providing a clear and detailed description of the research aims, methods, and outcomes. This makes it easier for other researchers to replicate the study and verify the results.
Limitations of Research Questions
Limitations of Research Questions are as follows:
- Subjectivity : Research questions are often subjective and can be influenced by personal biases and perspectives of the researcher. This can lead to a limited understanding of the research problem and may affect the validity and reliability of the study.
- Inadequate scope : Research questions that are too narrow in scope may limit the breadth of the study, while questions that are too broad may make it difficult to focus on specific research objectives.
- Unanswerable questions : Some research questions may not be answerable due to the lack of available data or limitations in research methods. In such cases, the research question may need to be rephrased or modified to make it more answerable.
- Lack of clarity : Research questions that are poorly worded or ambiguous can lead to confusion and misinterpretation. This can result in incomplete or inaccurate data, which may compromise the validity of the study.
- Difficulty in measuring variables : Some research questions may involve variables that are difficult to measure or quantify, making it challenging to draw meaningful conclusions from the data.
- Lack of generalizability: Research questions that are too specific or limited in scope may not be generalizable to other contexts or populations. This can limit the applicability of the study’s findings and restrict its broader implications.
About the author
Muhammad Hassan
Researcher, Academic Writer, Web developer
You may also like

Data Collection – Methods Types and Examples

Delimitations in Research – Types, Examples and...

Research Process – Steps, Examples and Tips

Research Design – Types, Methods and Examples

Institutional Review Board – Application Sample...

Evaluating Research – Process, Examples and...
The Research Questions
- The Research
Section: The Research
- The Participants
- The Setting
- The Findings
Exemplars of classroom practice were collected to address the following research questions:
- How do teacher-researchers reflect on and investigate their own classrooms in supporting intercultural practices?
- What elements of classroom pedagogy support participation, communication & respect, the intercultural capabilities of all learners as a ‘global child’?
- What are young children’s perspectives on participating, communicating & belonging in classrooms rich in cultural and linguistic diversity?
Dynamic Collective Action and the Power of Large Numbers
Collective action is a dynamic process where individuals in a group assess over time the benefits and costs of participating toward the success of a collective goal. Early participation improves the expectation of success and thus stimulates the subsequent participation of other individuals who might otherwise be unwilling to engage. On the other hand, a slow start can depress expectations and lead to failure for the group. Individuals have an incentive to procrastinate, not only in the hope of free riding, but also in order to observe the flow of participation by others, which allows them to better gauge whether their own participation will be useful or simply wasted. How do these phenomena affect the probability of success for a group? As the size of the group increases, will a “power of large numbers” prevail producing successful outcomes, or will a “curse of large numbers” lead to failure? In this paper, we address these questions by studying a dynamic collective action problem in which n individuals can achieve a collective goal if a share of them takes a costly action (e.g., participate in a protest, join a picket line, or sign an environmental agreement). Individuals have privately known participation costs and decide over time if and when to participate. We characterize the equilibria of this game and show that under general conditions the eventual success of collective action is necessarily probabilistic. The process starts for sure, and hence there is always a positive probability of success; however, the process “gets stuck” with positive probability, in the sense that participation stops short of the goal. Equilibrium outcomes have a simple characterization in large populations: welfare converges to either full efficiency or zero as n→∞ depending on a precise condition on the rate at which the share required for success converges to zero. Whether success is achievable or not, delays are always irrelevant: in the limit, success is achieved either instantly or never.
The paper has benefited from comments and suggestions by seminar audiences at Brown University, New York University, Northwestern University, Princeton University, Stanford University, and The University of Chicago. We also wish to thank Sandeep Baliga, Gary Cox, John Ferejohn, Emir Kamenica, Roger Myerson, and Andrea Prat for discussions and comments. We are responsible for any remaining shortcomings. The views expressed herein are those of the authors and do not necessarily reflect the views of the National Bureau of Economic Research.
MARC RIS BibTeΧ
Download Citation Data
More from NBER
In addition to working papers , the NBER disseminates affiliates’ latest findings through a range of free periodicals — the NBER Reporter , the NBER Digest , the Bulletin on Retirement and Disability , the Bulletin on Health , and the Bulletin on Entrepreneurship — as well as online conference reports , video lectures , and interviews .
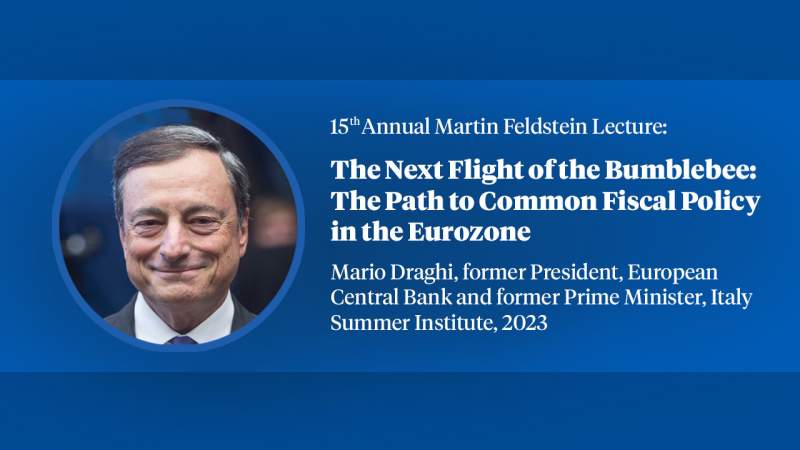

Research Question
Ai generator.
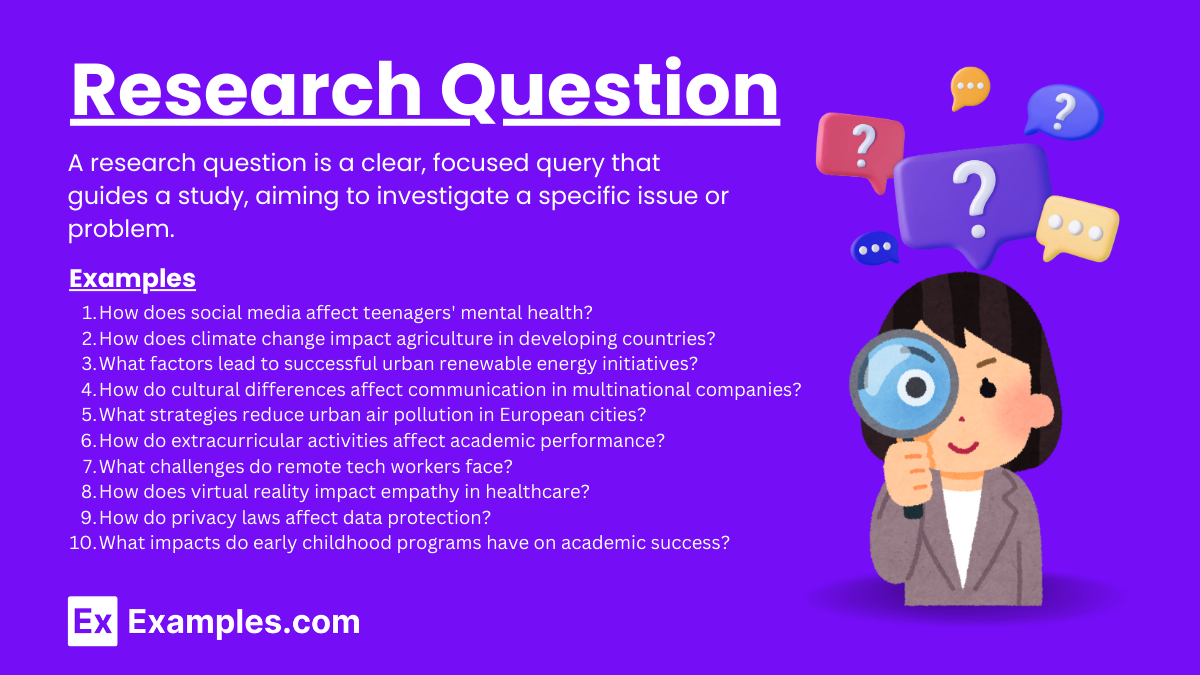
A research question serves as the foundation of any academic study, driving the investigation and framing the scope of inquiry. It focuses the research efforts, ensuring that the study addresses pertinent issues systematically. Crafting a strong research question is essential as it directs the methodology, data collection, and analysis, ultimately shaping the study’s conclusions and contributions to the field.
What is a Research Question?
A research question is the central query that guides a study, focusing on a specific problem or issue. It defines the purpose and direction of the research, influencing the methodology and analysis. A well-crafted research question ensures the study remains relevant, systematic, and contributes valuable insights to the field.
Types of Research Questions
Research questions are a crucial part of any research project. They guide the direction and focus of the study. Here are the main types of research questions:
1. Descriptive Research Questions
These questions aim to describe the characteristics or functions of a specific phenomenon or group. They often begin with “what,” “who,” “where,” “when,” or “how.”
- What are the common symptoms of depression in teenagers?
2. Comparative Research Questions
These questions compare two or more groups or variables to identify differences or similarities.
- How do the academic performances of students in private schools compare to those in public schools?
3. Correlational Research Questions
These questions seek to identify the relationships between two or more variables. They often use terms like “relationship,” “association,” or “correlation.”
- Is there a relationship between social media usage and self-esteem among adolescents?
4. Causal Research Questions
These questions aim to determine whether one variable causes or influences another. They are often used in experimental research.
- Does a new teaching method improve student engagement in the classroom?
5. Exploratory Research Questions
These questions are used when the researcher is exploring a new area or seeking to understand a complex phenomenon. They are often open-ended.
- What factors contribute to the success of start-up companies in the tech industry?
6. Predictive Research Questions
These questions aim to predict future occurrences based on current or past data. They often use terms like “predict,” “forecast,” or “expect.”
- Can high school GPA predict college success?
7. Evaluative Research Questions
These questions assess the effectiveness or impact of a program, intervention, or policy .
- How effective is the new community outreach program in reducing homelessness?
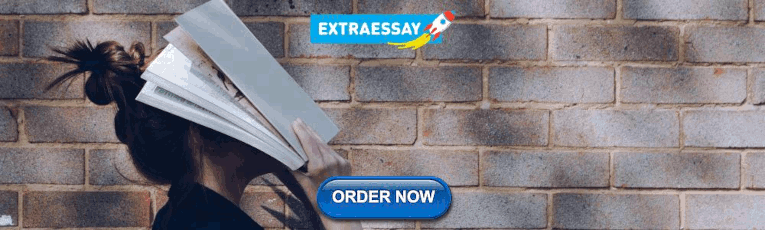
8. Ethnographic Research Questions
These questions are used in qualitative research to understand cultural phenomena from the perspective of the participants.
- How do cultural beliefs influence healthcare practices in rural communities?
9. Case Study Research Questions
These questions focus on an in-depth analysis of a specific case, event, or instance.
- What were the critical factors that led to the failure of Company X?
10. Phenomenological Research Questions
These questions explore the lived experiences of individuals to understand a particular phenomenon.
- What is the experience of living with chronic pain?
Research Question Format
A well-formulated research question is essential for guiding your study effectively. Follow this format to ensure clarity and precision:
- Begin with a broad subject area.
- Example: “Education technology”
- Define a specific aspect or variable.
- Example: “Impact of digital tools”
- Decide if you are describing, comparing, or investigating relationships.
- Example: “Effectiveness”
- Identify who or what is being studied.
- Example: “High school students”
- Formulate the complete question.
- Example: “How effective are digital tools in enhancing the learning experience of high school students?”
Sample Format: “How [specific aspect] affects [target population] in [context]?” Example: “How does the use of digital tools affect the academic performance of high school students in urban areas?”
Research Question Examples
Research questions in business.
- “What are the primary factors influencing customer loyalty in the retail industry?”
- “How does employee satisfaction differ between remote work and in-office work environments in tech companies?”
- “What is the relationship between social media marketing and brand awareness among small businesses?”
- “How does implementing a four-day workweek impact productivity in consulting firms?”
- “What are the emerging trends in consumer behavior post-COVID-19 in the e-commerce sector?”
- “Why do some startups succeed in attracting venture capital while others do not?”
- “How effective is corporate social responsibility in enhancing brand reputation for multinational companies?”
- “How do decision-making processes in family-owned businesses differ from those in publicly traded companies?”
- “What strategies do successful entrepreneurs use to scale their businesses in competitive markets?”
- “How does supply chain management affect the operational efficiency of manufacturing firms?”
Research Questions in Education
- “What are the most common challenges faced by first-year teachers in urban schools?”
- “How do student achievement levels differ between traditional classrooms and blended learning environments?”
- “What is the relationship between parental involvement and student academic performance in elementary schools?”
- “How does the implementation of project-based learning affect critical thinking skills in middle school students?”
- “What are the emerging trends in the use of artificial intelligence in education?”
- “Why do some students perform better in standardized tests than others despite similar instructional methods?”
- “How effective is the flipped classroom model in improving student engagement and learning outcomes in high school science classes?”
- “How do teachers’ professional development programs impact teaching practices and student outcomes in rural schools?”
- “What strategies can be employed to reduce the dropout rate among high school students in low-income areas?”
- “How does classroom size affect the quality of teaching and learning in elementary schools?”
Research Questions in Health Care
- “What are the most common barriers to accessing mental health services in rural areas?”
- “How does patient satisfaction differ between telemedicine and in-person consultations in primary care?”
- “What is the relationship between diet and the incidence of type 2 diabetes in adults?”
- “How does regular physical activity influence the recovery rate of patients with cardiovascular diseases?”
- “What are the emerging trends in the use of wearable technology for health monitoring?”
- “Why do some patients adhere to their medication regimen while others do not despite similar health conditions?”
- “How effective are community-based health interventions in reducing obesity rates among children?”
- “How do interdisciplinary team meetings impact patient care in hospitals?”
- “What strategies can be implemented to reduce the spread of infectious diseases in healthcare settings?”
- “How does nurse staffing level affect patient outcomes in intensive care units?”
Research Questions in Computer Science
- “What are the key features of successful machine learning algorithms used in natural language processing?”
- “How does the performance of quantum computing compare to classical computing in solving complex optimization problems?”
- “What is the relationship between software development methodologies and project success rates in large enterprises?”
- “How does the implementation of cybersecurity protocols impact the frequency of data breaches in financial institutions?”
- “What are the emerging trends in blockchain technology applications beyond cryptocurrency?”
- “Why do certain neural network architectures outperform others in image recognition tasks?”
- “How effective are different code review practices in reducing bugs in open-source software projects?”
- “How do agile development practices influence team productivity and product quality in software startups?”
- “What strategies can improve the scalability of distributed systems in cloud computing environments?”
- “How does the choice of programming language affect the performance and maintainability of enterprise-level software applications?”
Research Questions in Psychology
- “What are the most common symptoms of anxiety disorders among adolescents?”
- “How does the level of job satisfaction differ between remote workers and in-office workers?”
- “What is the relationship between social media use and self-esteem in teenagers?”
- “How does cognitive-behavioral therapy (CBT) affect the severity of depression symptoms in adults?”
- “What are the emerging trends in the treatment of post-traumatic stress disorder (PTSD)?”
- “Why do some individuals develop resilience in the face of adversity while others do not?”
- “How effective are mindfulness-based interventions in reducing stress levels among college students?”
- “How does group therapy influence the social skills development of children with autism spectrum disorder?”
- “What strategies can improve the early diagnosis of bipolar disorder in young adults?”
- “How do sleep patterns affect cognitive functioning and academic performance in high school students?”
More Research Question Examples
Research question examples for students.
- “What are the primary study habits of high-achieving college students?”
- “How do academic performances differ between students who participate in extracurricular activities and those who do not?”
- “What is the relationship between time management skills and academic success in high school students?”
- “How does the use of technology in the classroom affect students’ engagement and learning outcomes?”
- “What are the emerging trends in online learning platforms for high school students?”
- “Why do some students excel in standardized tests while others struggle despite similar study efforts?”
- “How effective are peer tutoring programs in improving students’ understanding of complex subjects?”
- “How do different teaching methods impact the learning process of students with learning disabilities?”
- “What strategies can help reduce test anxiety among middle school students?”
- “How does participation in group projects affect the development of collaboration skills in university students?”
Research Question Examples for College Students
- “What are the most common stressors faced by college students during final exams?”
- “How does academic performance differ between students who live on campus and those who commute?”
- “What is the relationship between part-time employment and GPA among college students?”
- “How does participation in study abroad programs impact cultural awareness and academic performance?”
- “What are the emerging trends in college students’ use of social media for academic purposes?”
- “Why do some college students engage in academic dishonesty despite awareness of the consequences?”
- “How effective are university mental health services in addressing students’ mental health issues?”
- “How do different learning styles affect the academic success of college students in online courses?”
- “What strategies can be employed to improve retention rates among first-year college students?”
- “How does participation in extracurricular activities influence leadership skills development in college students?”
Research Question Examples in Statistics
- “What are the most common statistical methods used in medical research?”
- “How does the accuracy of machine learning models compare to traditional statistical methods in predicting housing prices?”
- “What is the relationship between sample size and the power of a statistical test in clinical trials?”
- “How does the use of random sampling affect the validity of survey results in social science research?”
- “What are the emerging trends in the application of Bayesian statistics in data science?”
- “Why do some datasets require transformation before applying linear regression models?”
- “How effective are bootstrapping techniques in estimating the confidence intervals of small sample data?”
- “How do different imputation methods impact the results of analyses with missing data?”
- “What strategies can improve the interpretation of interaction effects in multiple regression analysis?”
- “How does the choice of statistical software affect the efficiency of data analysis in academic research?”
Research Question Examples in Socialogy
- “What are the primary social factors contributing to urban poverty in major cities?”
- “How does the level of social integration differ between immigrants and native-born citizens in urban areas?”
- “What is the relationship between educational attainment and social mobility in different socioeconomic classes?”
- “How does exposure to social media influence political participation among young adults?”
- “What are the emerging trends in family structures and their impact on child development?”
- “Why do certain communities exhibit higher levels of civic engagement than others?”
- “How effective are community policing strategies in reducing crime rates in diverse neighborhoods?”
- “How do socialization processes differ in single-parent households compared to two-parent households?”
- “What strategies can be implemented to reduce racial disparities in higher education enrollment?”
- “How does the implementation of public housing policies affect the quality of life for low-income families?”
Research Question Examples in Biology
- “What are the primary characteristics of the various stages of mitosis in eukaryotic cells?”
- “How do the reproductive strategies of amphibians compare to those of reptiles?”
- “What is the relationship between genetic diversity and the resilience of plant species to climate change?”
- “How does the presence of pollutants in freshwater ecosystems impact the growth and development of aquatic organisms?”
- “What are the emerging trends in the use of CRISPR technology for gene editing in agricultural crops?”
- “Why do certain bacteria develop antibiotic resistance more rapidly than others?”
- “How effective are different conservation strategies in protecting endangered species?”
- “How do various environmental factors influence the process of photosynthesis in marine algae?”
- “What strategies can enhance the effectiveness of reforestation programs in tropical rainforests?”
- “How does the method of seed dispersal affect the spatial distribution and genetic diversity of plant populations?”
Research Question Examples in History
- “What were the key social and economic factors that led to the Industrial Revolution in Britain?”
- “How did the political systems of ancient Athens and ancient Sparta differ in terms of governance and citizen participation?”
- “What is the relationship between the Renaissance and the subsequent scientific revolution in Europe?”
- “How did the Treaty of Versailles contribute to the rise of Adolf Hitler and the onset of World War II?”
- “What are the emerging perspectives on the causes and impacts of the American Civil Rights Movement?”
- “Why did the Roman Empire decline and eventually fall despite its extensive power and reach?”
- “How effective were the New Deal programs in alleviating the effects of the Great Depression in the United States?”
- “How did the processes of colonization and decolonization affect the political landscape of Africa in the 20th century?”
- “What strategies did the suffragette movement use to secure voting rights for women in the early 20th century?”
- “How did the logistics and strategies of the D-Day invasion contribute to the Allied victory in World War II?”
Importance of Research Questions
Research questions are fundamental to the success and integrity of any study. Their importance can be highlighted through several key aspects:
- Research questions provide a clear focus and direction for the study, ensuring that the researcher remains on track.
- Example: “How does online learning impact student engagement in higher education?”
- They establish the boundaries of the research, determining what will be included or excluded.
- Example: “What are the effects of air pollution on respiratory health in urban areas?”
- Research questions dictate the choice of research design, methodology, and data collection techniques.
- Example: “What is the relationship between physical activity and mental health in adolescents?”
- They make the objectives of the research explicit, providing clarity and precision to the study’s goals.
- Example: “Why do some startups succeed in securing venture capital while others fail?”
- Well-crafted research questions emphasize the significance and relevance of the study, justifying its importance.
- Example: “How effective are public health campaigns in increasing vaccination rates among young adults?”
- They enable a systematic approach to inquiry, ensuring that the study is coherent and logically structured.
- Example: “What are the social and economic impacts of remote work on urban communities?”
- Research questions offer a framework for analyzing and interpreting data, guiding the researcher in making sense of the findings.
- Example: “How does social media usage affect self-esteem among teenagers?”
- By addressing specific gaps or exploring new areas, research questions ensure that the study contributes meaningfully to the existing body of knowledge.
- Example: “What are the emerging trends in the use of artificial intelligence in healthcare?”
- Clear and precise research questions increase the credibility and reliability of the research by providing a focused approach.
- Example: “How do educational interventions impact literacy rates in low-income communities?”
- They help in clearly communicating the purpose and findings of the research to others, including stakeholders, peers, and the broader academic community.
- Example: “What strategies are most effective in reducing youth unemployment in developing countries?”
Research Question vs. Hypothesis
Chracteristics of research questions.
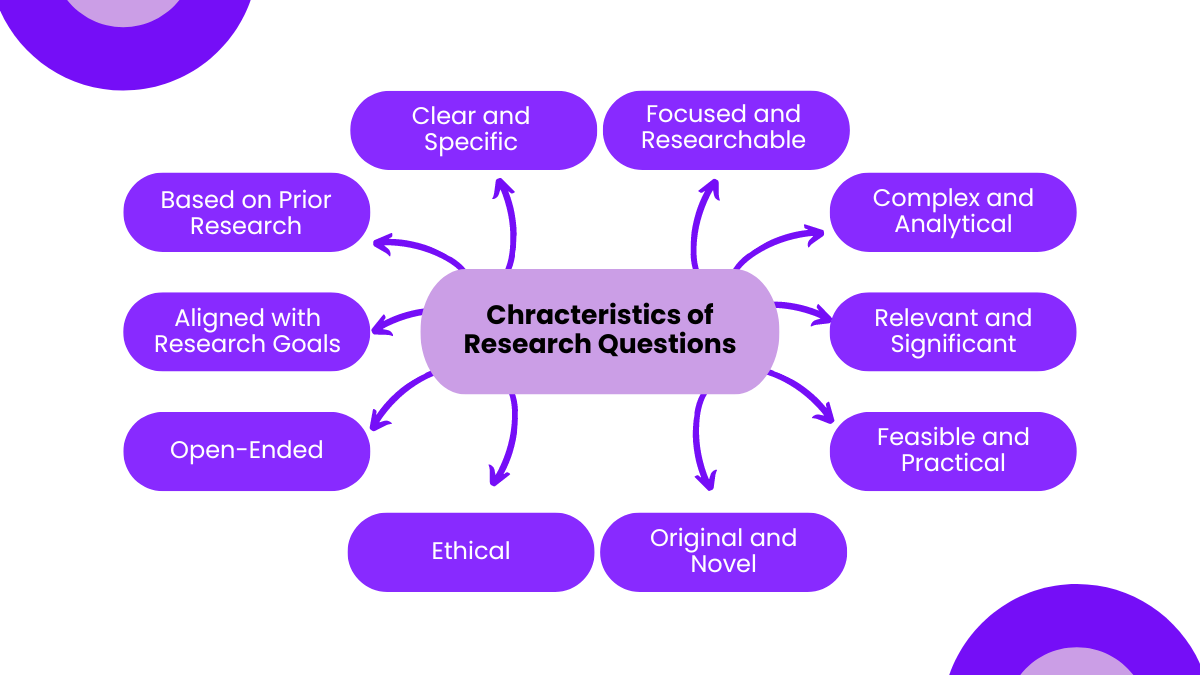
Research questions are fundamental to the research process as they guide the direction and focus of a study. Here are the key characteristics of effective research questions:
1. Clear and Specific
- The question should be clearly articulated and specific enough to be understood without ambiguity.
- Example: “What are the effects of social media on teenagers’ mental health?” rather than “How does social media affect people?”
2. Focused and Researchable
- The question should be narrow enough to be answerable through research and data collection.
- Example: “How does participation in extracurricular activities impact academic performance in high school students?” rather than “How do activities affect school performance?”
3. Complex and Analytical
- The question should require more than a simple yes or no answer and should invite analysis and discussion.
- Example: “What factors contribute to the success of renewable energy initiatives in urban areas?” rather than “Is renewable energy successful?”
4. Relevant and Significant
- The question should address an important issue or problem in the field of study and contribute to knowledge or practice.
- Example: “How does climate change affect agricultural productivity in developing countries?” rather than “What is climate change?”
5. Feasible and Practical
- The question should be feasible to answer within the constraints of time, resources, and access to information.
- Example: “What are the challenges faced by remote workers in the tech industry during the COVID-19 pandemic?” rather than “What are the challenges of remote work?”
6. Original and Novel
- The question should offer a new perspective or explore an area that has not been extensively studied.
- Example: “How do virtual reality technologies influence empathy in healthcare training?” rather than “What is virtual reality?”
- The question should be framed in a way that ensures the research can be conducted ethically.
- Example: “What are the impacts of privacy laws on consumer data protection in the digital age?” rather than “How can we collect personal data more effectively?”
8. Open-Ended
- The question should encourage detailed responses and exploration, rather than limiting answers to a simple yes or no.
- Example: “In what ways do cultural differences affect communication styles in multinational companies?” rather than “Do cultural differences affect communication?”
9. Aligned with Research Goals
- The question should align with the overall objectives of the research project or study.
- Example: “How do early childhood education programs influence long-term academic achievement?” if the goal is to understand educational impacts.
10. Based on Prior Research
- The question should build on existing literature and research, identifying gaps or new angles to explore.
- Example: “What strategies have proven effective in reducing urban air pollution in European cities?” after reviewing current studies on air pollution strategies.
Benefits of Research Question
Research questions are fundamental to the research process and offer numerous benefits, which include the following:
1. Guides the Research Process
A well-defined research question provides a clear focus and direction for your study. It helps in determining what data to collect, how to collect it, and how to analyze it.
Benefit: Ensures that the research stays on track and addresses the specific issue at hand.
2. Clarifies the Purpose of the Study
Research questions help to articulate the purpose and objectives of the study. They make it clear what the researcher intends to explore, describe, compare, or test.
Benefit: Helps in communicating the goals and significance of the research to others, including stakeholders and funding bodies.
3. Determines the Research Design
The type of research question informs the research design, including the choice of methodology, data collection methods, and analysis techniques.
Benefit: Ensures that the chosen research design is appropriate for answering the specific research question, enhancing the validity and reliability of the results.
4. Enhances Literature Review
A well-crafted research question provides a framework for conducting a thorough literature review. It helps in identifying relevant studies, theories, and gaps in existing knowledge.
Benefit: Facilitates a comprehensive understanding of the topic and ensures that the research is grounded in existing literature.
5. Focuses Data Collection
Research questions help in identifying the specific data needed to answer them. This focus prevents the collection of unnecessary data and ensures that all collected data is relevant to the study.
Benefit: Increases the efficiency of data collection and analysis, saving time and resources.
6. Improves Data Analysis
Having a clear research question aids in the selection of appropriate data analysis methods. It helps in determining how the data will be analyzed to draw meaningful conclusions.
Benefit: Enhances the accuracy and relevance of the findings, making them more impactful.
7. Facilitates Hypothesis Formation
In quantitative research, research questions often lead to the development of hypotheses that can be tested statistically.
Benefit: Provides a basis for hypothesis testing, which is essential for establishing cause-and-effect relationships.
8. Supports Result Interpretation
Research questions provide a lens through which the results of the study can be interpreted. They help in understanding what the findings mean in the context of the research objectives.
Benefit: Ensures that the conclusions drawn from the research are aligned with the original aims and objectives.
9. Enhances Reporting and Presentation
A clear research question makes it easier to organize and present the research findings. It helps in structuring the research report or presentation logically.
Benefit: Improves the clarity and coherence of the research report, making it more accessible and understandable to the audience.
10. Encourages Critical Thinking
Formulating research questions requires critical thinking and a deep understanding of the subject matter. It encourages researchers to think deeply about what they want to investigate and why.
Benefit: Promotes a more thoughtful and analytical approach to research, leading to more robust and meaningful findings.
How to Write a Research Question
Crafting a strong research question is crucial for guiding your study effectively. Follow these steps to write a clear and focused research question:
Identify a Broad Topic:
Start with a general area of interest that you are passionate about or that is relevant to your field. Example: “Climate change”
Conduct Preliminary Research:
Explore existing literature and studies to understand the current state of knowledge and identify gaps. Example: “Impact of climate change on agriculture”
Narrow Down the Topic:
Focus on a specific aspect or issue within the broad topic to make the research question more manageable. Example: “Effect of climate change on crop yields”
Consider the Scope:
Ensure the question is neither too broad nor too narrow. It should be specific enough to be answerable but broad enough to allow for thorough exploration. Example: “How does climate change affect corn crop yields in the Midwest United States?”
Determine the Research Type:
Decide whether your research will be descriptive, comparative, relational, or causal, as this will shape your question. Example: “How does climate change affect corn crop yields in the Midwest United States over the past decade?”
Formulate the Question:
Write a clear, concise question that specifies the variables, population, and context. Example: “What is the impact of increasing temperatures and changing precipitation patterns on corn crop yields in the Midwest United States from 2010 to 2020?”
Ensure Feasibility:
Make sure the question can be answered within the constraints of your resources, time, and data availability. Example: “How have corn crop yields in the Midwest United States been affected by climate change-related temperature increases and precipitation changes between 2010 and 2020?”
Review and Refine:
Evaluate the question for clarity, focus, and relevance. Revise as necessary to ensure it is well-defined and researchable. Example: “What are the specific impacts of temperature increases and changes in precipitation patterns on corn crop yields in the Midwest United States from 2010 to 2020?”
What is a research question?
A research question is a specific query guiding a study’s focus and objectives, shaping its methodology and analysis.
Why is a research question important?
It provides direction, defines scope, ensures relevance, and guides the methodology of the research.
How do you formulate a research question?
Identify a topic, narrow it down, conduct preliminary research, and ensure it is clear, focused, and researchable.
What makes a good research question?
Clarity, specificity, feasibility, relevance, and the ability to guide the research effectively.
Can a research question change?
Yes, it can evolve based on initial findings, further literature review, and the research process.
What is the difference between a research question and a hypothesis?
A research question guides the study; a hypothesis is a testable prediction about the relationship between variables.
How specific should a research question be?
It should be specific enough to provide clear direction but broad enough to allow for comprehensive investigation.
What are examples of good research questions?
Examples include: “How does social media affect academic performance?” and “What are the impacts of climate change on agriculture?”
Can a research question be too broad?
Yes, a too broad question can make the research unfocused and challenging to address comprehensively.
What role does a research question play in literature reviews?
It helps identify relevant studies, guides the search for literature, and frames the review’s focus.
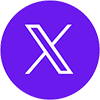
Text prompt
- Instructive
- Professional
10 Examples of Public speaking
20 Examples of Gas lighting
Loading metrics
Open Access
Peer-reviewed
Meta-Research Article
Meta-Research Articles feature data-driven examinations of the methods, reporting, verification, and evaluation of scientific research.
See Journal Information »
Assessing the evolution of research topics in a biological field using plant science as an example
Roles Conceptualization, Data curation, Formal analysis, Funding acquisition, Investigation, Methodology, Project administration, Resources, Software, Supervision, Validation, Visualization, Writing – original draft, Writing – review & editing
* E-mail: [email protected]
Affiliations Department of Plant Biology, Michigan State University, East Lansing, Michigan, United States of America, Department of Computational Mathematics, Science, and Engineering, Michigan State University, East Lansing, Michigan, United States of America, DOE-Great Lake Bioenergy Research Center, Michigan State University, East Lansing, Michigan, United States of America

Roles Conceptualization, Investigation, Project administration, Supervision, Writing – review & editing
Affiliation Department of Plant Biology, Michigan State University, East Lansing, Michigan, United States of America
- Shin-Han Shiu,
- Melissa D. Lehti-Shiu
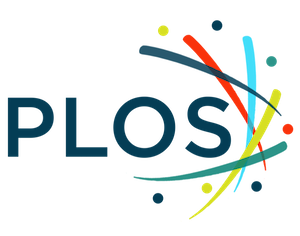
- Published: May 23, 2024
- https://doi.org/10.1371/journal.pbio.3002612
- Peer Review
- Reader Comments
Scientific advances due to conceptual or technological innovations can be revealed by examining how research topics have evolved. But such topical evolution is difficult to uncover and quantify because of the large body of literature and the need for expert knowledge in a wide range of areas in a field. Using plant biology as an example, we used machine learning and language models to classify plant science citations into topics representing interconnected, evolving subfields. The changes in prevalence of topical records over the last 50 years reflect shifts in major research trends and recent radiation of new topics, as well as turnover of model species and vastly different plant science research trajectories among countries. Our approaches readily summarize the topical diversity and evolution of a scientific field with hundreds of thousands of relevant papers, and they can be applied broadly to other fields.
Citation: Shiu S-H, Lehti-Shiu MD (2024) Assessing the evolution of research topics in a biological field using plant science as an example. PLoS Biol 22(5): e3002612. https://doi.org/10.1371/journal.pbio.3002612
Academic Editor: Ulrich Dirnagl, Charite Universitatsmedizin Berlin, GERMANY
Received: October 16, 2023; Accepted: April 4, 2024; Published: May 23, 2024
Copyright: © 2024 Shiu, Lehti-Shiu. This is an open access article distributed under the terms of the Creative Commons Attribution License , which permits unrestricted use, distribution, and reproduction in any medium, provided the original author and source are credited.
Data Availability: The plant science corpus data are available through Zenodo ( https://zenodo.org/records/10022686 ). The codes for the entire project are available through GitHub ( https://github.com/ShiuLab/plant_sci_hist ) and Zenodo ( https://doi.org/10.5281/zenodo.10894387 ).
Funding: This work was supported by the National Science Foundation (IOS-2107215 and MCB-2210431 to MDL and SHS; DGE-1828149 and IOS-2218206 to SHS), Department of Energy grant Great Lakes Bioenergy Research Center (DE-SC0018409 to SHS). The funders had no role in study design, data collection and analysis, decision to publish, or preparation of the manuscript.
Competing interests: The authors have declared that no competing interests exist.
Abbreviations: BERT, Bidirectional Encoder Representations from Transformers; br, brassinosteroid; ccTLD, country code Top Level Domain; c-Tf-Idf, class-based Tf-Idf; ChatGPT, Chat Generative Pretrained Transformer; ga, gibberellic acid; LOWESS, locally weighted scatterplot smoothing; MeSH, Medical Subject Heading; SHAP, SHapley Additive exPlanations; SJR, SCImago Journal Rank; Tf-Idf, Term frequency-Inverse document frequency; UMAP, Uniform Manifold Approximation and Projection
Introduction
The explosive growth of scientific data in recent years has been accompanied by a rapidly increasing volume of literature. These records represent a major component of our scientific knowledge and embody the history of conceptual and technological advances in various fields over time. Our ability to wade through these records is important for identifying relevant literature for specific topics, a crucial practice of any scientific pursuit [ 1 ]. Classifying the large body of literature into topics can provide a useful means to identify relevant literature. In addition, these topics offer an opportunity to assess how scientific fields have evolved and when major shifts in took place. However, such classification is challenging because the relevant articles in any topic or domain can number in the tens or hundreds of thousands, and the literature is in the form of natural language, which takes substantial effort and expertise to process [ 2 , 3 ]. In addition, even if one could digest all literature in a field, it would still be difficult to quantify such knowledge.
In the last several years, there has been a quantum leap in natural language processing approaches due to the feasibility of building complex deep learning models with highly flexible architectures [ 4 , 5 ]. The development of large language models such as Bidirectional Encoder Representations from Transformers (BERT; [ 6 ]) and Chat Generative Pretrained Transformer (ChatGPT; [ 7 ]) has enabled the analysis, generation, and modeling of natural language texts in a wide range of applications. The success of these applications is, in large part, due to the feasibility of considering how the same words are used in different contexts when modeling natural language [ 6 ]. One such application is topic modeling, the practice of establishing statistical models of semantic structures underlying a document collection. Topic modeling has been proposed for identifying scientific hot topics over time [ 1 ], for example, in synthetic biology [ 8 ], and it has also been applied to, for example, automatically identify topical scenes in images [ 9 ] and social network topics [ 10 ], discover gene programs highly correlated with cancer prognosis [ 11 ], capture “chromatin topics” that define cell-type differences [ 12 ], and investigate relationships between genetic variants and disease risk [ 13 ]. Here, we use topic modeling to ask how research topics in a scientific field have evolved and what major changes in the research trends have taken place, using plant science as an example.
Plant science corpora allow classification of major research topics
Plant science, broadly defined, is the study of photosynthetic species, their interactions with biotic/abiotic environments, and their applications. For modeling plant science topical evolution, we first identified a collection of plant science documents (i.e., corpus) using a text classification approach. To this end, we first collected over 30 million PubMed records and narrowed down candidate plant science records by searching for those with plant-related terms and taxon names (see Materials and methods ). Because there remained a substantial number of false positives (i.e., biomedical records mentioning plants in passing), a set of positive plant science examples from the 17 plant science journals with the highest numbers of plant science publications covering a wide range of subfields and a set of negative examples from journals with few candidate plant science records were used to train 4 types of text classification models (see Materials and methods ). The best text classification model performed well (F1 = 0.96, F1 of a naïve model = 0.5, perfect model = 1) where the positive and negative examples were clearly separated from each other based on prediction probability of the hold-out testing dataset (false negative rate = 2.6%, false positive rate = 5.2%, S1A and S1B Fig ). The false prediction rate for documents from the 17 plant science journals annotated with the Medical Subject Heading (MeSH) term “Plants” in NCBI was 11.7% (see Materials and methods ). The prediction probability distribution of positive instances with the MeSH term has an expected left-skew to lower values ( S1C Fig ) compared with the distributions of all positive instances ( S1A Fig ). Thus, this subset with the MeSH term is a skewed representation of articles from these 17 major plant science journals. To further benchmark the validity of the plant science records, we also conducted manual annotation of 100 records where the false positive and false negative rates were 14.6% and 10.6%, respectively (see Materials and methods ). Using 12 other plant science journals not included as positive examples as benchmarks, the false negative rate was 9.9% (see Materials and methods ). Considering the range of false prediction rate estimates with different benchmarks, we should emphasize that the model built with the top 17 plant science journals represents a substantial fraction of plant science publications but with biases. Applying the model to the candidate plant science record led to 421,658 positive predictions, hereafter referred to as “plant science records” ( S1D Fig and S1 Data ).
To better understand how the models classified plant science articles, we identified important terms from a more easily interpretable model (Term frequency-Inverse document frequency (Tf-Idf) model; F1 = 0.934) using Shapley Additive Explanations [ 14 ]; 136 terms contributed to predicting plant science records (e.g., Arabidopsis, xylem, seedling) and 138 terms contributed to non-plant science record predictions (e.g., patients, clinical, mice; Tf-Idf feature sheet, S1 Data ). Plant science records as well as PubMed articles grew exponentially from 1950 to 2020 ( Fig 1A ), highlighting the challenges of digesting the rapidly expanding literature. We used the plant science records to perform topic modeling, which consisted of 4 steps: representing each record as a BERT embedding, reducing dimensionality, clustering, and identifying the top terms by calculating class (i.e., topic)-based Tf-Idf (c-Tf-Idf; [ 15 ]). The c-Tf-Idf represents the frequency of a term in the context of how rare the term is to reduce the influence of common words. SciBERT [ 16 ] was the best model among those tested ( S2 Data ) and was used for building the final topic model, which classified 372,430 (88.3%) records into 90 topics defined by distinct combinations of terms ( S3 Data ). The topics contained 620 to 16,183 records and were named after the top 4 to 5 terms defining the topical areas ( Fig 1B and S3 Data ). For example, the top 5 terms representing the largest topic, topic 61 (16,183 records), are “qtl,” “resistance,” “wheat,” “markers,” and “traits,” which represent crop improvement studies using quantitative genetics.
- PPT PowerPoint slide
- PNG larger image
- TIFF original image
(A) Numbers of PubMed (magenta) and plant science (green) records between 1950 and 2020. (a, b, c) Coefficients of the exponential function, y = ae b . Data for the plot are in S1 Data . (B) Numbers of documents for the top 30 plant science topics. Each topic is designated by an index number (left) and the top 4–6 terms with the highest cTf-Idf values (right). Data for the plot are in S3 Data . (C) Two-dimensional representation of the relationships between plant science records generated by Uniform Manifold Approximation and Projection (UMAP, [ 17 ]) using SciBERT embeddings of plant science records. All topics panel: Different topics are assigned different colors. Outlier panel: UMAP representation of all records (gray) with outlier records in red. Blue dotted circles: areas with relatively high densities indicating topics that are below the threshold for inclusion in a topic. In the 8 UMAP representations on the right, records for example topics are in red and the remaining records in gray. Blue dotted circles indicate the relative position of topic 48.
https://doi.org/10.1371/journal.pbio.3002612.g001
Records with assigned topics clustered into distinct areas in a two-dimensional (2D) space ( Fig 1C , for all topics, see S4 Data ). The remaining 49,228 outlier records not assigned to any topic (11.7%, middle panel, Fig 1C ) have 3 potential sources. First, some outliers likely belong to unique topics but have fewer records than the threshold (>500, blue dotted circles, Fig 1C ). Second, some of the many outliers dispersed within the 2D space ( Fig 1C ) were not assigned to any single topic because they had relatively high prediction scores for multiple topics ( S2 Fig ). These likely represent studies across subdisciplines in plant science. Third, some outliers are likely interdisciplinary studies between plant science and other domains, such as chemistry, mathematics, and physics. Such connections can only be revealed if records from other domains are included in the analyses.
Topical clusters reveal closely related topics but with distinct key term usage
Related topics tend to be located close together in the 2D representation (e.g., topics 48 and 49, Fig 1C ). We further assessed intertopical relationships by determining the cosine similarities between topics using cTf-Idfs ( Figs 2A and S3 ). In this topic network, some topics are closely related and form topic clusters. For example, topics 25, 26, and 27 collectively represent a more general topic related to the field of plant development (cluster a , lower left in Fig 2A ). Other topic clusters represent studies of stress, ion transport, and heavy metals ( b ); photosynthesis, water, and UV-B ( c ); population and community biology (d); genomics, genetic mapping, and phylogenetics ( e , upper right); and enzyme biochemistry ( f , upper left in Fig 2A ).
(A) Graph depicting the degrees of similarity (edges) between topics (nodes). Between each topic pair, a cosine similarity value was calculated using the cTf-Idf values of all terms. A threshold similarity of 0.6 was applied to illustrate the most related topics. For the full matrix presented as a heatmap, see S4 Fig . The nodes are labeled with topic index numbers and the top 4–6 terms. The colors and width of the edges are defined based on cosine similarity. Example topic clusters are highlighted in yellow and labeled a through f (blue boxes). (B, C) Relationships between the cTf-Idf values (see S3 Data ) of the top terms for topics 26 and 27 (B) and for topics 25 and 27 (C) . Only terms with cTf-Idf ≥ 0.6 are labeled. Terms with cTf-Idf values beyond the x and y axis limit are indicated by pink arrows and cTf-Idf values. (D) The 2D representation in Fig 1C is partitioned into graphs for different years, and example plots for every 5-year period since 1975 are shown. Example topics discussed in the text are indicated. Blue arrows connect the areas occupied by records of example topics across time periods to indicate changes in document frequencies.
https://doi.org/10.1371/journal.pbio.3002612.g002
Topics differed in how well they were connected to each other, reflecting how general the research interests or needs are (see Materials and methods ). For example, topic 24 (stress mechanisms) is the most well connected with median cosine similarity = 0.36, potentially because researchers in many subfields consider aspects of plant stress even though it is not the focus. The least connected topics include topic 21 (clock biology, 0.12), which is surprising because of the importance of clocks in essentially all aspects of plant biology [ 18 ]. This may be attributed, in part, to the relatively recent attention in this area.
Examining topical relationships and the cTf-Idf values of terms also revealed how related topics differ. For example, topic 26 is closely related to topics 27 and 25 (cluster a on the lower left of Fig 2A ). Topics 26 and 27 both contain records of developmental process studies mainly in Arabidopsis ( Fig 2B ); however, topic 26 is focused on the impact of light, photoreceptors, and hormones such as gibberellic acids (ga) and brassinosteroids (br), whereas topic 27 is focused on flowering and floral development. Topic 25 is also focused on plant development but differs from topic 27 because it contains records of studies mainly focusing on signaling and auxin with less emphasis on Arabidopsis ( Fig 2C ). These examples also highlight the importance of using multiple top terms to represent the topics. The similarities in cTf-Idfs between topics were also useful for measuring the editorial scope (i.e., diverse, or narrow) of journals publishing plant science papers using a relative topic diversity measure (see Materials and methods ). For example, Proceedings of the National Academy of Sciences , USA has the highest diversity, while Theoretical and Applied Genetics has the lowest ( S4 Fig ). One surprise is the relatively low diversity of American Journal of Botany , which focuses on plant ecology, systematics, development, and genetics. The low diversity is likely due to the relatively larger number of cellular and molecular science records in PubMed, consistent with the identification of relatively few topical areas relevant to studies at the organismal, population, community, and ecosystem levels.
Investigation of the relative prevalence of topics over time reveals topical succession
We next asked whether relationships between topics reflect chronological progression of certain subfields. To address this, we assessed how prevalent topics were over time using dynamic topic modeling [ 19 ]. As shown in Fig 2D , there is substantial fluctuation in where the records are in the 2D space over time. For example, topic 44 (light, leaves, co, synthesis, photosynthesis) is among the topics that existed in 1975 but has diminished gradually since. In 1985, topic 39 (Agrobacterium-based transformation) became dense enough to be visualized. Additional examples include topics 79 (soil heavy metals), 42 (differential expression), and 82 (bacterial community metagenomics), which became prominent in approximately 2005, 2010, and 2020, respectively ( Fig 2D ). In addition, animating the document occupancy in the 2D space over time revealed a broad change in patterns over time: Some initially dense areas became sparse over time and a large number of topics in areas previously only loosely occupied at the turn of the century increased over time ( S5 Data ).
While the 2D representations reveal substantial details on the evolution of topics, comparison over time is challenging because the number of plant science records has grown exponentially ( Fig 1A ). To address this, the records were divided into 50 chronological bins each with approximately 8,400 records to make cross-bin comparisons feasible ( S6 Data ). We should emphasize that, because of the way the chronological bins were split, the number of records for each topic in each bin should be treated as a normalized value relative to all other topics during the same period. Examining this relative prevalence of topics across bins revealed a clear pattern of topic succession over time (one topic evolved into another) and the presence of 5 topical categories ( Fig 3 ). The topics were categorized based on their locally weighted scatterplot smoothing (LOWESS) fits and ordered according to timing of peak frequency ( S7 and S8 Data , see Materials and methods ). In Fig 3 , the relative decrease in document frequency does not mean that research output in a topic is dwindling. Because each row in the heatmap is normalized based on the minimum and maximum values within each topic, there still can be substantial research output in terms of numbers of publications even when the relative frequency is near zero. Thus, a reduced relative frequency of a topic reflects only a below-average growth rate compared with other topical areas.
(A-E) A heat map of relative topic frequency over time reveals 5 topical categories: (A) stable, (B) early, (C) transitional, (D) sigmoidal, and (E) rising. The x axis denotes different time bins with each bin containing a similar number of documents to account for the exponential growth of plant science records over time. The sizes of all bins except the first are drawn to scale based on the beginning and end dates. The y axis lists different topics denoted by the label and top 4 to 5 terms. In each cell, the prevalence of a topic in a time bin is colored according to the min-max normalized cTf-Idf values for that topic. Light blue dotted lines delineate different decades. The arrows left of a subset of topic labels indicate example relationships between topics in topic clusters. Blue boxes with labels a–f indicate topic clusters, which are the same as those in Fig 2 . Connecting lines indicate successional trends. Yellow circles/lines 1 – 3: 3 major transition patterns. The original data are in S5 Data .
https://doi.org/10.1371/journal.pbio.3002612.g003
The first topical category is a stable category with 7 topics mostly established before the 1980s that have since remained stable in terms of prevalence in the plant science records (top of Fig 3A ). These topics represent long-standing plant science research foci, including studies of plant physiology (topics 4, 58, and 81), genetics (topic 61), and medicinal plants (topic 53). The second category contains 8 topics established before the 1980s that have mostly decreased in prevalence since (the early category, Fig 3B ). Two examples are physiological and morphological studies of hormone action (topic 45, the second in the early category) and the characterization of protein, DNA, and RNA (topic 18, the second to last). Unlike other early topics, topic 78 (paleobotany and plant evolution studies, the last topic in Fig 3B ) experienced a resurgence in the early 2000s due to the development of new approaches and databases and changes in research foci [ 20 ].
The 33 topics in the third, transitional category became prominent in the 1980s, 1990s, or even 2000s but have clearly decreased in prevalence ( Fig 3C ). In some cases, the early and the transitional topics became less prevalent because of topical succession—refocusing of earlier topics led to newer ones that either show no clear sign of decrease (the sigmoidal category, Fig 3D ) or continue to increase in prevalence (the rising category, Fig 3E ). Consistent with the notion of topical succession, topics within each topic cluster ( Fig 2 ) were found across topic categories and/or were prominent at different time periods (indicated by colored lines linking topics, Fig 3 ). One example is topics in topic cluster b (connected with light green lines and arrows, compare Figs 2 and 3 ); the study of cation transport (topic 47, the third in the transitional category), prominent in the 1980s and early 1990s, is connected to 5 other topics, namely, another transitional topic 29 (cation channels and their expression) peaking in the 2000s and early 2010s, sigmoidal topics 24 and 28 (stress response, tolerance mechanisms) and 30 (heavy metal transport), which rose to prominence in mid-2000s, and the rising topic 42 (stress transcriptomic studies), which increased in prevalence in the mid-2010s.
The rise and fall of topics can be due to a combination of technological or conceptual breakthroughs, maturity of the field, funding constraints, or publicity. The study of transposable elements (topic 62) illustrates the effect of publicity; the rise in this field coincided with Barbara McClintock’s 1983 Nobel Prize but not with the publication of her studies in the 1950s [ 21 ]. The reduced prevalence in early 2000 likely occurred in part because analysis of transposons became a central component of genome sequencing and annotation studies, rather than dedicated studies. In addition, this example indicates that our approaches, while capable of capturing topical trends, cannot be used to directly infer major papers leading to the growth of a topic.
Three major topical transition patterns signify shifts in research trends
Beyond the succession of specific topics, 3 major transitions in the dynamic topic graph should be emphasized: (1) the relative decreasing trend of early topics in the late 1970s and early 1980s; (2) the rise of transitional topics in late 1980s; and (3) the relative decreasing trend of transitional topics in the late 1990s and early 2000s, which coincided with a radiation of sigmoidal and rising topics (yellow circles, Fig 3 ). The large numbers of topics involved in these transitions suggest major shifts in plant science research. In transition 1, early topics decreased in relative prevalence in the late 1970s to early 1980s, which coincided with the rise of transitional topics over the following decades (circle 1, Fig 3 ). For example, there was a shift from the study of purified proteins such as enzymes (early topic 48, S5A Fig ) to molecular genetic dissection of genes, proteins, and RNA (transitional topic 35, S5B Fig ) enabled by the wider adoption of recombinant DNA and molecular cloning technologies in late 1970s [ 22 ]. Transition 2 (circle 2, Fig 3 ) can be explained by the following breakthroughs in the late 1980s: better approaches to create transgenic plants and insertional mutants [ 23 ], more efficient creation of mutant plant libraries through chemical mutagenesis (e.g., [ 24 ]), and availability of gene reporter systems such as β-glucuronidase [ 25 ]. Because of these breakthroughs, molecular genetics studies shifted away from understanding the basic machinery to understanding the molecular underpinnings of specific processes, such as molecular mechanisms of flower and meristem development and the action of hormones such as auxin (topic 27, S5C Fig ); this type of research was discussed as a future trend in 1988 [ 26 ] and remains prevalent to this date. Another example is gene silencing (topic 12), which became a focal area of study along with the widespread use of transgenic plants [ 27 ].
Transition 3 is the most drastic: A large number of transitional, sigmoidal, and rising topics became prevalent nearly simultaneously at the turn of the century (circle 3, Fig 3 ). This period also coincides with a rapid increase in plant science citations ( Fig 1A ). The most notable breakthroughs included the availability of the first plant genome in 2000 [ 28 ], increasing ease and reduced cost of high-throughput sequencing [ 29 ], development of new mass spectrometry–based platforms for analyzing proteins [ 30 ], and advancements in microscopic and optical imaging approaches [ 31 ]. Advances in genomics and omics technology also led to an increase in stress transcriptomics studies (42, S5D Fig ) as well as studies in many other topics such as epigenetics (topic 11), noncoding RNA analysis (13), genomics and phylogenetics (80), breeding (41), genome sequencing and assembly (60), gene family analysis (23), and metagenomics (82 and 55).
In addition to the 3 major transitions across all topics, there were also transitions within topics revealed by examining the top terms for different time bins (heatmaps, S5 Fig ). Taken together, these observations demonstrate that knowledge about topical evolution can be readily revealed through topic modeling. Such knowledge is typically only available to experts in specific areas and is difficult to summarize manually, as no researcher has a command of the entire plant science literature.
Analysis of taxa studied reveals changes in research trends
Changes in research trends can also be illustrated by examining changes in the taxa being studied over time ( S9 Data ). There is a strong bias in the taxa studied, with the record dominated by research models and economically important taxa ( S6 Fig ). Flowering plants (Magnoliopsida) are found in 93% of records ( S6A Fig ), and the mustard family Brassicaceae dominates at the family level ( S6B Fig ) because the genus Arabidopsis contributes to 13% of plant science records ( Fig 4A ). When examining the prevalence of taxa being studied over time, clear patterns of turnover emerged similar to topical succession ( Figs 4B , S6C, and S6D ; Materials and methods ). Given that Arabidopsis is mentioned in more publications than other species we analyzed, we further examined the trends for Arabidopsis publications. The increase in the normalized number (i.e., relative to the entire plant science corpus) of Arabidopsis records coincided with advocacy of its use as a model system in the late 1980s [ 32 ]. While it remains a major plant model, there has been a decrease in overall Arabidopsis publications relative to all other plant science publications since 2011 (blue line, normalized total, Fig 4C ). Because the same chronological bins, each with same numbers of records, from the topic-over-time analysis ( Fig 3 ) were used, the decrease here does not mean that there were fewer Arabidopsis publications—in fact, the number of Arabidopsis papers has remained steady since 2011. This decrease means that Arabidopsis-related publications represent a relatively smaller proportion of plant science records. Interestingly, this decrease took place much earlier (approximately 2005) and was steeper in the United States (red line, Fig 4C ) than in all countries combined (blue line, Fig 4C ).
(A) Percentage of records mentioning specific genera. (B) Change in the prevalence of genera in plant science records over time. (C) Changes in the normalized numbers of all records (blue) and records from the US (red) mentioning Arabidopsis over time. The lines are LOWESS fits with fraction parameter = 0.2. (D) Topical over (red) and under (blue) representation among 5 genera with the most plant science records. LLR: log 2 likelihood ratios of each topic in each genus. Gray: topic-species combination not significantly enriched at the 5% level based on enrichment p -values adjusted for multiple testing with the Benjamini–Hochberg method [ 33 ]. The data used for plotting are in S9 Data . The statistics for all topics are in S10 Data .
https://doi.org/10.1371/journal.pbio.3002612.g004
Assuming that the normalized number of publications reflects the relative intensity of research activities, one hypothesis for the relative decrease in focus on Arabidopsis is that advances in, for example, plant transformation, genetic manipulation, and genome research have allowed the adoption of more previously nonmodel taxa. Consistent with this, there was a precipitous increase in the number of genera being published in the mid-90s to early 2000s during which approaches for plant transgenics became established [ 34 ], but the number has remained steady since then ( S7A Fig ). The decrease in the proportion of Arabidopsis papers is also negatively correlated with the timing of an increase in the number of draft genomes ( S7B Fig and S9 Data ). It is plausible that genome availability for other species may have contributed to a shift away from Arabidopsis. Strikingly, when we analyzed US National Science Foundation records, we found that the numbers of funded grants mentioning Arabidopsis ( S7C Fig ) have risen and fallen in near perfect synchrony with the normalized number of Arabidopsis publication records (red line, Fig 4C ). This finding likely illustrates the impact of funding on Arabidopsis research.
By considering both taxa information and research topics, we can identify clear differences in the topical areas preferred by researchers using different plant taxa ( Fig 4D and S10 Data ). For example, studies of auxin/light signaling, the circadian clock, and flowering tend to be carried out in Arabidopsis, while quantitative genetic studies of disease resistance tend to be done in wheat and rice, glyphosate research in soybean, and RNA virus research in tobacco. Taken together, joint analyses of topics and species revealed additional details about changes in preferred models over time, and the preferred topical areas for different taxa.
Countries differ in their contributions to plant science and topical preference
We next investigated whether there were geographical differences in topical preference among countries by inferring country information from 330,187 records (see Materials and methods ). The 10 countries with the most records account for 73% of the total, with China and the US contributing to approximately 18% each ( Fig 5A ). The exponential growth in plant science records (green line, Fig 1A ) was in large part due to the rapid rise in annual record numbers in China and India ( Fig 5B ). When we examined the publication growth rates using the top 17 plant science journals, the general patterns remained the same ( S7D Fig ). On the other hand, the US, Japan, Germany, France, and Great Britain had slower rates of growth compared with all non-top 10 countries. The rapid increase in records from China and India was accompanied by a rapid increase in metrics measuring journal impact ( Figs 5C and S8 and S9 Data ). For example, using citation score ( Fig 5C , see Materials and methods ), we found that during a 22-year period China (dark green) and India (light green) rapidly approached the global average (y = 0, yellow), whereas some of the other top 10 countries, particularly the US (red) and Japan (yellow green), showed signs of decrease ( Fig 5C ). It remains to be determined whether these geographical trends reflect changes in priority, investment, and/or interest in plant science research.
(A) Numbers of plant science records for countries with the 10 highest numbers. (B) Percentage of all records from each of the top 10 countries from 1980 to 2020. (C) Difference in citation scores from 1999 to 2020 for the top 10 countries. (D) Shown for each country is the relationship between the citation scores averaged from 1999 to 2020 and the slope of linear fit with year as the predictive variable and citation score as the response variable. The countries with >400 records and with <10% missing impact values are included. Data used for plots (A–D) are in S11 Data . (E) Correlation in topic enrichment scores between the top 10 countries. PCC, Pearson’s correlation coefficient, positive in red, negative in blue. Yellow rectangle: countries with more similar topical preferences. (F) Enrichment scores (LLR, log likelihood ratio) of selected topics among the top 10 countries. Red: overrepresentation, blue: underrepresentation. Gray: topic-country combination that is not significantly enriched at the 5% level based on enrichment p -values adjusted for multiple testing with the Benjamini–Hochberg method (for all topics and plotting data, see S12 Data ).
https://doi.org/10.1371/journal.pbio.3002612.g005
Interestingly, the relative growth/decline in citation scores over time (measured as the slope of linear fit of year versus citation score) was significantly and negatively correlated with average citation score ( Fig 5D ); i.e., countries with lower overall metrics tended to experience the strongest increase in citation scores over time. Thus, countries that did not originally have a strong influence on plant sciences now have increased impact. These patterns were also observed when using H-index or journal rank as metrics ( S8 Fig and S11 Data ) and were not due to increased publication volume, as the metrics were normalized against numbers of records from each country (see Materials and methods ). In addition, the fact that different metrics with different caveats and assumptions yielded consistent conclusions indicates the robustness of our observations. We hypothesize that this may be a consequence of the ease in scientific communication among geographically isolated research groups. It could also be because of the prevalence of online journals that are open access, which makes scientific information more readily accessible. Or it can be due to the increasing international collaboration. In any case, the causes for such regression toward the mean are not immediately clear and should be addressed in future studies.
We also assessed how the plant research foci of countries differ by comparing topical preference (i.e., the degree of enrichment of plant science records in different topics) between countries. For example, Italy and Spain cluster together (yellow rectangle, Fig 5E ) partly because of similar research focusing on allergens (topic 0) and mycotoxins (topic 54) and less emphasis on gene family (topic 23) and stress tolerance (topic 28) studies ( Fig 5F , for the fold enrichment and corrected p -values of all topics, see S12 Data ). There are substantial differences in topical focus between countries ( S9 Fig ). For example, research on new plant compounds associated with herbal medicine (topic 69) is a focus in China but not in the US, but the opposite is true for population genetics and evolution (topic 86) ( Fig 5F ). In addition to revealing how plant science research has evolved over time, topic modeling provides additional insights into differences in research foci among different countries, which are informative for science policy considerations.
In this study, topic modeling revealed clear transitions among research topics, which represent shifts in research trends in plant sciences. One limitation of our study is the bias in the PubMed-based corpus. The cellular, molecular, and physiological aspects of plant sciences are well represented, but there are many fewer records related to evolution, ecology, and systematics. Our use of titles/abstracts from the top 17 plant science journals as positive examples allowed us to identify papers we typically see in these journals, but this may have led to us missing “outlier” articles, which may be the most exciting. Another limitation is the need to assign only one topic to a record when a study is interdisciplinary and straddles multiple topics. Furthermore, a limited number of large, inherently heterogeneous topics were summarized to provide a more concise interpretation, which undoubtedly underrepresents the diversity of plant science research. Despite these limitations, dynamic topic modeling revealed changes in plant science research trends that coincide with major shifts in biological science. While we were interested in identifying conceptual advances, our approach can identify the trend but the underlying causes for such trends, particularly key records leading to the growth in certain topics, still need to be identified. It also remains to be determined which changes in research trends lead to paradigm shifts as defined by Kuhn [ 35 ].
The key terms defining the topics frequently describe various technologies (e.g., topic 38/39: transformation, 40: genome editing, 59: genetic markers, 65: mass spectrometry, 69: nuclear magnetic resonance) or are indicative of studies enabled through molecular genetics and omics technologies (e.g., topic 8/60: genome, 11: epigenetic modifications, 18: molecular biological studies of macromolecules, 13: small RNAs, 61: quantitative genetics, 82/84: metagenomics). Thus, this analysis highlights how technological innovation, particularly in the realm of omics, has contributed to a substantial number of research topics in the plant sciences, a finding that likely holds for other scientific disciplines. We also found that the pattern of topic evolution is similar to that of succession, where older topics have mostly decreased in relative prevalence but appear to have been superseded by newer ones. One example is the rise of transcriptome-related topics and the correlated, reduced focus on regulation at levels other than transcription. This raises the question of whether research driven by technology negatively impacts other areas of research where high-throughput studies remain challenging.
One observation on the overall trends in plant science research is the approximately 10-year cycle in major shifts. One hypothesis is related to not only scientific advances but also to the fashion-driven aspect of science. Nonetheless, given that there were only 3 major shifts and the sample size is small, it is difficult to speculate as to why they happened. By analyzing the country of origin, we found that China and India have been the 2 major contributors to the growth in the plant science records in the last 20 years. Our findings also show an equalizing trend in global plant science where countries without a strong plant science publication presence have had an increased impact over the last 20 years. In addition, we identified significant differences in research topics between countries reflecting potential differences in investment and priorities. Such information is important for discerning differences in research trends across countries and can be considered when making policy decisions about research directions.
Materials and methods
Collection and preprocessing of a candidate plant science corpus.
For reproducibility purposes, a random state value of 20220609 was used throughout the study. The PubMed baseline files containing citation information ( ftp://ftp.ncbi.nlm.nih.gov/pubmed/baseline/ ) were downloaded on November 11, 2021. To narrow down the records to plant science-related citations, a candidate citation was identified as having, within the titles and/or abstracts, at least one of the following words: “plant,” “plants,” “botany,” “botanical,” “planta,” and “plantarum” (and their corresponding upper case and plural forms), or plant taxon identifiers from NCBI Taxonomy ( https://www.ncbi.nlm.nih.gov/taxonomy ) or USDA PLANTS Database ( https://plants.sc.egov.usda.gov/home ). Note the search terms used here have nothing to do with the values of the keyword field in PubMed records. The taxon identifiers include all taxon names including and at taxonomic levels below “Viridiplantae” till the genus level (species names not used). This led to 51,395 search terms. After looking for the search terms, qualified entries were removed if they were duplicated, lacked titles and/or abstracts, or were corrections, errata, or withdrawn articles. This left 1,385,417 citations, which were considered the candidate plant science corpus (i.e., a collection of texts). For further analysis, the title and abstract for each citation were combined into a single entry. Text was preprocessed by lowercasing, removing stop-words (i.e., common words), removing non-alphanumeric and non-white space characters (except Greek letters, dashes, and commas), and applying lemmatization (i.e., grouping inflected forms of a word as a single word) for comparison. Because lemmatization led to truncated scientific terms, it was not included in the final preprocessing pipeline.
Definition of positive/negative examples
Upon closer examination, a large number of false positives were identified in the candidate plant science records. To further narrow down citations with a plant science focus, text classification was used to distinguish plant science and non-plant science articles (see next section). For the classification task, a negative set (i.e., non-plant science citations) was defined as entries from 7,360 journals that appeared <20 times in the filtered data (total = 43,329, journal candidate count, S1 Data ). For the positive examples (i.e., true plant science citations), 43,329 plant science citations (positive examples) were sampled from 17 established plant science journals each with >2,000 entries in the filtered dataset: “Plant physiology,” “Frontiers in plant science,” “Planta,” “The Plant journal: for cell and molecular biology,” “Journal of experimental botany,” “Plant molecular biology,” “The New phytologist,” “The Plant cell,” “Phytochemistry,” “Plant & cell physiology,” “American journal of botany,” “Annals of botany,” “BMC plant biology,” “Tree physiology,” “Molecular plant-microbe interactions: MPMI,” “Plant biology,” and “Plant biotechnology journal” (journal candidate count, S1 Data ). Plant biotechnology journal was included, but only 1,894 records remained after removal of duplicates, articles with missing info, and/or withdrawn articles. The positive and negative sets were randomly split into training and testing subsets (4:1) while maintaining a 1:1 positive-to-negative ratio.
Text classification based on Tf and Tf-Idf
Instead of using the preprocessed text as features for building classification models directly, text embeddings (i.e., representations of texts in vectors) were used as features. These embeddings were generated using 4 approaches (model summary, S1 Data ): Term-frequency (Tf), Tf-Idf [ 36 ], Word2Vec [ 37 ], and BERT [ 6 ]. The Tf- and Tf-Idf-based features were generated with CountVectorizer and TfidfVectorizer, respectively, from Scikit-Learn [ 38 ]. Different maximum features (1e4 to 1e5) and n-gram ranges (uni-, bi-, and tri-grams) were tested. The features were selected based on the p- value of chi-squared tests testing whether a feature had a higher-than-expected value among the positive or negative classes. Four different p- value thresholds were tested for feature selection. The selected features were then used to retrain vectorizers with the preprocessed training texts to generate feature values for classification. The classification model used was XGBoost [ 39 ] with 5 combinations of the following hyperparameters tested during 5-fold stratified cross-validation: min_child_weight = (1, 5, 10), gamma = (0.5, 1, 1.5, 2.5), subsample = (0.6, 0.8, 1.0), colsample_bytree = (0.6, 0.8, 1.0), and max_depth = (3, 4, 5). The rest of the hyperparameters were held constant: learning_rate = 0.2, n_estimators = 600, objective = binary:logistic. RandomizedSearchCV from Scikit-Learn was used for hyperparameter tuning and cross-validation with scoring = F1-score.
Because the Tf-Idf model had a relatively high model performance and was relatively easy to interpret (terms are frequency-based, instead of embedding-based like those generated by Word2Vec and BERT), the Tf-Idf model was selected as input to SHapley Additive exPlanations (SHAP; [ 14 ]) to assess the importance of terms. Because the Tf-Idf model was based on XGBoost, a tree-based algorithm, the TreeExplainer module in SHAP was used to determine a SHAP value for each entry in the training dataset for each Tf-Idf feature. The SHAP value indicates the degree to which a feature positively or negatively affects the underlying prediction. The importance of a Tf-Idf feature was calculated as the average SHAP value of that feature among all instances. Because a Tf-Idf feature is generated based on a specific term, the importance of the Tf-Idf feature indicates the importance of the associated term.
Text classification based on Word2Vec
The preprocessed texts were first split into train, validation, and test subsets (8:1:1). The texts in each subset were converted to 3 n-gram lists: a unigram list obtained by splitting tokens based on the space character, or bi- and tri-gram lists built with Gensim [ 40 ]. Each n-gram list of the training subset was next used to fit a Skip-gram Word2Vec model with vector_size = 300, window = 8, min_count = (5, 10, or 20), sg = 1, and epochs = 30. The Word2Vec model was used to generate word embeddings for train, validate, and test subsets. In the meantime, a tokenizer was trained with train subset unigrams using Tensorflow [ 41 ] and used to tokenize texts in each subset and turn each token into indices to use as features for training text classification models. To ensure all citations had the same number of features (500), longer texts were truncated, and shorter ones were zero-padded. A deep learning model was used to train a text classifier with an input layer the same size as the feature number, an attention layer incorporating embedding information for each feature, 2 bidirectional Long-Short-Term-Memory layers (15 units each), a dense layer (64 units), and a final, output layer with 2 units. During training, adam, accuracy, and sparse_categorical_crossentropy were used as the optimizer, evaluation metric, and loss function, respectively. The training process lasted 30 epochs with early stopping if validation loss did not improve in 5 epochs. An F1 score was calculated for each n-gram list and min_count parameter combination to select the best model (model summary, S1 Data ).
Text classification based on BERT models
Two pretrained models were used for BERT-based classification: DistilBERT (Hugging face repository [ 42 ] model name and version: distilbert-base-uncased [ 43 ]) and SciBERT (allenai/scibert-scivocab-uncased [ 16 ]). In both cases, tokenizers were retrained with the training data. BERT-based models had the following architecture: the token indices (512 values for each token) and associated masked values as input layers, pretrained BERT layer (512 × 768) excluding outputs, a 1D pooling layer (768 units), a dense layer (64 units), and an output layer (2 units). The rest of the training parameters were the same as those for Word2Vec-based models, except training lasted for 20 epochs. Cross-validation F1-scores for all models were compared and used to select the best model for each feature extraction method, hyperparameter combination, and modeling algorithm or architecture (model summary, S1 Data ). The best model was the Word2Vec-based model (min_count = 20, window = 8, ngram = 3), which was applied to the candidate plant science corpus to identify a set of plant science citations for further analysis. The candidate plant science records predicted as being in the positive class (421,658) by the model were collectively referred to as the “plant science corpus.”
Plant science record classification
In PubMed, 1,384,718 citations containing “plant” or any plant taxon names (from the phylum to genus level) were considered candidate plant science citations. To further distinguish plant science citations from those in other fields, text classification models were trained using titles and abstracts of positive examples consisting of citations from 17 plant science journals, each with >2,000 entries in PubMed, and negative examples consisting of records from journals with fewer than 20 entries in the candidate set. Among 4 models tested the best model (built with Word2Vec embeddings) had a cross validation F1 of 0.964 (random guess F1 = 0.5, perfect model F1 = 1, S1 Data ). When testing the model using 17,330 testing set citations independent from the training set, the F1 remained high at 0.961.
We also conducted another analysis attempting to use the MeSH term “Plants” as a benchmark. Records with the MeSH term “Plants” also include pharmaceutical studies of plants and plant metabolites or immunological studies of plants as allergens in journals that are not generally considered plant science journals (e.g., Acta astronautica , International journal for parasitology , Journal of chromatography ) or journals from local scientific societies (e.g., Acta pharmaceutica Hungarica , Huan jing ke xue , Izvestiia Akademii nauk . Seriia biologicheskaia ). Because we explicitly labeled papers from such journals as negative examples, we focused on 4,004 records with the “Plants” MeSH term published in the 17 plant science journals that were used as positive instances and found that 88.3% were predicted as the positive class. Thus, based on the MeSH term, there is an 11.7% false prediction rate.
We also enlisted 5 plant science colleagues (3 advanced graduate students in plant biology and genetic/genome science graduate programs, 1 postdoctoral breeder/quantitative biologist, and 1 postdoctoral biochemist/geneticist) to annotate 100 randomly selected abstracts as a reviewer suggested. Each record was annotated by 2 colleagues. Among 85 entries where the annotations are consistent between annotators, 48 were annotated as negative but with 7 predicted as positive (false positive rate = 14.6%) and 37 were annotated as positive but with 4 predicted as negative (false negative rate = 10.8%). To further benchmark the performance of the text classification model, we identified another 12 journals that focus on plant science studies to use as benchmarks: Current opinion in plant biology (number of articles: 1,806), Trends in plant science (1,723), Functional plant biology (1,717), Molecular plant pathology (1,573), Molecular plant (1,141), Journal of integrative plant biology (1,092), Journal of plant research (1,032), Physiology and molecular biology of plants (830), Nature plants (538), The plant pathology journal (443). Annual review of plant biology (417), and The plant genome (321). Among the 12,611 candidate plant science records, 11,386 were predicted as positive. Thus, there is a 9.9% false negative rate.
Global topic modeling
BERTopic [ 15 ] was used for preliminary topic modeling with n-grams = (1,2) and with an embedding initially generated by DistilBERT, SciBERT, or BioBERT (dmis-lab/biobert-base-cased-v1.2; [ 44 ]). The embedding models converted preprocessed texts to embeddings. The topics generated based on the 3 embeddings were similar ( S2 Data ). However, SciBERT-, BioBERT-, and distilBERT-based embedding models had different numbers of outlier records (268,848, 293,790, and 323,876, respectively) with topic index = −1. In addition to generating the fewest outliers, the SciBERT-based model led to the highest number of topics. Therefore, SciBERT was chosen as the embedding model for the final round of topic modeling. Modeling consisted of 3 steps. First, document embeddings were generated with SentenceTransformer [ 45 ]. Second, a clustering model to aggregate documents into clusters using hdbscan [ 46 ] was initialized with min_cluster_size = 500, metric = euclidean, cluster_selection_method = eom, min_samples = 5. Third, the embedding and the initialized hdbscan model were used in BERTopic to model topics with neighbors = 10, nr_topics = 500, ngram_range = (1,2). Using these parameters, 90 topics were identified. The initial topic assignments were conservative, and 241,567 records were considered outliers (i.e., documents not assigned to any of the 90 topics). After assessing the prediction scores of all records generated from the fitted topic models, the 95-percentile score was 0.0155. This score was used as the threshold for assigning outliers to topics: If the maximum prediction score was above the threshold and this maximum score was for topic t , then the outlier was assigned to t . After the reassignment, 49,228 records remained outliers. To assess if some of the outliers were not assigned because they could be assigned to multiple topics, the prediction scores of the records were used to put records into 100 clusters using k- means. Each cluster was then assessed to determine if the outlier records in a cluster tended to have higher prediction scores across multiple topics ( S2 Fig ).
Topics that are most and least well connected to other topics
The most well-connected topics in the network include topic 24 (stress mechanisms, median cosine similarity = 0.36), topic 42 (genes, stress, and transcriptomes, 0.34), and topic 35 (molecular genetics, 0.32, all t test p -values < 1 × 10 −22 ). The least connected topics include topic 0 (allergen research, median cosine similarity = 0.12), topic 21 (clock biology, 0.12), topic 1 (tissue culture, 0.15), and topic 69 (identification of compounds with spectroscopic methods, 0.15; all t test p- values < 1 × 10 −24 ). Topics 0, 1, and 69 are specialized topics; it is surprising that topic 21 is not as well connected as explained in the main text.
Analysis of documents based on the topic model
Topical diversity among top journals with the most plant science records
Using a relative topic diversity measure (ranging from 0 to 10), we found that there was a wide range of topical diversity among 20 journals with the largest numbers of plant science records ( S3 Fig ). The 4 journals with the highest relative topical diversities are Proceedings of the National Academy of Sciences , USA (9.6), Scientific Reports (7.1), Plant Physiology (6.7), and PLOS ONE (6.4). The high diversities are consistent with the broad, editorial scopes of these journals. The 4 journals with the lowest diversities are American Journal of Botany (1.6), Oecologia (0.7), Plant Disease (0.7), and Theoretical and Applied Genetics (0.3), which reflects their discipline-specific focus and audience of classical botanists, ecologists, plant pathologists, and specific groups of geneticists.
Dynamic topic modeling
The codes for dynamic modeling were based on _topic_over_time.py in BERTopics and modified to allow additional outputs for debugging and graphing purposes. The plant science citations were binned into 50 subsets chronologically (for timestamps of bins, see S5 Data ). Because the numbers of documents increased exponentially over time, instead of dividing them based on equal-sized time intervals, which would result in fewer records at earlier time points and introduce bias, we divided them into time bins of similar size (approximately 8,400 documents). Thus, the earlier time subsets had larger time spans compared with later time subsets. If equal-size time intervals were used, the numbers of documents between the intervals would differ greatly; the earlier time points would have many fewer records, which may introduce bias. Prior to binning the subsets, the publication dates were converted to UNIX time (timestamp) in seconds; the plant science records start in 1917-11-1 (timestamp = −1646247600.0) and end in 2021-1-1 (timestamp = 1609477201). The starting dates and corresponding timestamps for the 50 subsets including the end date are in S6 Data . The input data included the preprocessed texts, topic assignments of records from global topic modeling, and the binned timestamps of records. Three additional parameters were set for topics_over_time, namely, nr_bin = 50 (number of bins), evolution_tuning = True, and global_tuning = False. The evolution_tuning parameter specified that averaged c-Tf-Idf values for a topic be calculated in neighboring time bins to reduce fluctuation in c-Tf-Idf values. The global_tuning parameter was set to False because of the possibility that some nonexisting terms could have a high c-Tf-Idf for a time bin simply because there was a high global c-Tf-Idf value for that term.
The binning strategy based on similar document numbers per bin allowed us to increase signal particularly for publications prior to the 90s. This strategy, however, may introduce more noise for bins with smaller time durations (i.e., more recent bins) because of publication frequencies (there can be seasonal differences in the number of papers published, biased toward, e.g., the beginning of the year or the beginning of a quarter). To address this, we examined the relative frequencies of each topic over time ( S7 Data ), but we found that recent time bins had similar variances in relative frequencies as other time bins. We also moderated the impact of variation using LOWESS (10% to 30% of the data points were used for fitting the trend lines) to determine topical trends for Fig 3 . Thus, the influence of the noise introduced via our binning strategy is expected to be minimal.
Topic categories and ordering
The topics were classified into 5 categories with contrasting trends: stable, early, transitional, sigmoidal, and rising. To define which category a topic belongs to, the frequency of documents over time bins for each topic was analyzed using 3 regression methods. We first tried 2 forecasting methods: recursive autoregressor (the ForecasterAutoreg class in the skforecast package) and autoregressive integrated moving average (ARIMA implemented in the pmdarima package). In both cases, the forecasting results did not clearly follow the expected trend lines, likely due to the low numbers of data points (relative frequency values), which resulted in the need to extensively impute missing data. Thus, as a third approach, we sought to fit the trendlines with the data points using LOWESS (implemented in the statsmodels package) and applied additional criteria for assigning topics to categories. When fitting with LOWESS, 3 fraction parameters (frac, the fraction of the data used when estimating each y-value) were evaluated (0.1, 0.2, 0.3). While frac = 0.3 had the smallest errors for most topics, in situations where there were outliers, frac = 0.2 or 0.1 was chosen to minimize mean squared errors ( S7 Data ).
The topics were classified into 5 categories based on the slopes of the fitted line over time: (1) stable: topics with near 0 slopes over time; (2) early: topics with negative (<−0.5) slopes throughout (with the exception of topic 78, which declined early on but bounced back by the late 1990s); (3) transitional: early positive (>0.5) slopes followed by negative slopes at later time points; (4) sigmoidal: early positive slopes followed by zero slopes at later time points; and (5) rising: continuously positive slopes. For each topic, the LOWESS fits were also used to determine when the relative document frequency reached its peak, first reaching a threshold of 0.6 (chosen after trial and error for a range of 0.3 to 0.9), and the overall trend. The topics were then ordered based on (1) whether they belonged to the stable category or not; (2) whether the trends were decreasing, stable, or increasing; (3) the time the relative document frequency first reached 0.6; and (4) the time that the overall peak was reached ( S8 Data ).
Taxa information
To identify a taxon or taxa in all plant science records, NCBI Taxonomy taxdump datasets were downloaded from the NCBI FTP site ( https://ftp.ncbi.nlm.nih.gov/pub/taxonomy/new_taxdump/ ) on September 20, 2022. The highest-level taxon was Viridiplantae, and all its child taxa were parsed and used as queries in searches against the plant science corpus. In addition, a species-over-time analysis was conducted using the same time bins as used for dynamic topic models. The number of records in different time bins for top taxa are in the genus, family, order, and additional species level sheet in S9 Data . The degree of over-/underrepresentation of a taxon X in a research topic T was assessed using the p -value of a Fisher’s exact test for a 2 × 2 table consisting of the numbers of records in both X and T, in X but not T, in T but not X, and in neither ( S10 Data ).
For analysis of plant taxa with genome information, genome data of taxa in Viridiplantae were obtained from the NCBI Genome data-hub ( https://www.ncbi.nlm.nih.gov/data-hub/genome ) on October 28, 2022. There were 2,384 plant genome assemblies belonging to 1,231 species in 559 genera (genome assembly sheet, S9 Data ). The date of the assembly was used as a proxy for the time when a genome was sequenced. However, some species have updated assemblies and have more recent data than when the genome first became available.
Taxa being studied in the plant science records
Flowering plants (Magnoliopsida) are found in 93% of records, while most other lineages are discussed in <1% of records, with conifers and related species being exceptions (Acrogynomsopermae, 3.5%, S6A Fig ). At the family level, the mustard (Brassicaceae), grass (Poaceae), pea (Fabaceae), and nightshade (Solanaceae) families are in 51% of records ( S6B Fig ). The prominence of the mustard family in plant science research is due to the Brassica and Arabidopsis genera ( Fig 4A ). When examining the prevalence of taxa being studied over time, clear patterns of turnovers emerged ( Figs 4B , S6C, and S6D ). While the study of monocot species (Liliopsida) has remained steady, there was a significant uptick in the prevalence of eudicot (eudicotyledon) records in the late 90s ( S6C Fig ), which can be attributed to the increased number of studies in the mustard, myrtle (Myrtaceae), and mint (Lamiaceae) families among others ( S6D Fig ). At the genus level, records mentioning Gossypium (cotton), Phaseolus (bean), Hordeum (wheat), and Zea (corn), similar to the topics in the early category, were prevalent till the 1980s or 1990s but have mostly decreased in number since ( Fig 4B ). In contrast, Capsicum , Arabidopsis , Oryza , Vitus , and Solanum research has become more prevalent over the last 20 years.
Geographical information for the plant science corpus
The geographical information (country) of authors in the plant science corpus was obtained from the address (AD) fields of first authors in Medline XML records accessible through the NCBI EUtility API ( https://www.ncbi.nlm.nih.gov/books/NBK25501/ ). Because only first author affiliations are available for records published before December 2014, only the first author’s location was considered to ensure consistency between records before and after that date. Among the 421,658 records in the plant science corpus, 421,585 had Medline records and 421,276 had unique PMIDs. Among the records with unique PMIDs, 401,807 contained address fields. For each of the remaining records, the AD field content was split into tokens with a “,” delimiter, and the token likely containing geographical info (referred to as location tokens) was selected as either the last token or the second to last token if the last token contained “@” indicating the presence of an email address. Because of the inconsistency in how geographical information was described in the location tokens (e.g., country, state, city, zip code, name of institution, and different combinations of the above), the following 4 approaches were used to convert location tokens into countries.
The first approach was a brute force search where full names and alpha-3 codes of current countries (ISO 3166–1), current country subregions (ISO 3166–2), and historical country (i.e., country that no longer exists, ISO 3166–3) were used to search the address fields. To reduce false positives using alpha-3 codes, a space prior to each code was required for the match. The first approach allowed the identification of 361,242, 16,573, and 279,839 records with current country, historical country, and subregion information, respectively. The second method was the use of a heuristic based on common address field structures to identify “location strings” toward the end of address fields that likely represent countries, then the use of the Python pycountry module to confirm the presence of country information. This approach led to 329,025 records with country information. The third approach was to parse first author email addresses (90,799 records), recover top-level domain information, and use country code Top Level Domain (ccTLD) data from the ISO 3166 Wikipedia page to define countries (72,640 records). Only a subset of email addresses contains country information because some are from companies (.com), nonprofit organizations (.org), and others. Because a large number of records with address fields still did not have country information after taking the above 3 approaches, another approach was implemented to query address fields against a locally installed Nominatim server (v.4.2.3, https://github.com/mediagis/nominatim-docker ) using OpenStreetMap data from GEOFABRIK ( https://www.geofabrik.de/ ) to find locations. Initial testing indicated that the use of full address strings led to false positives, and the computing resource requirement for running the server was high. Thus, only location strings from the second approach that did not lead to country information were used as queries. Because multiple potential matches were returned for each query, the results were sorted based on their location importance values. The above steps led to an additional 72,401 records with country information.
Examining the overlap in country information between approaches revealed that brute force current country and pycountry searches were consistent 97.1% of the time. In addition, both approaches had high consistency with the email-based approach (92.4% and 93.9%). However, brute force subregion and Nominatim-based predictions had the lowest consistencies with the above 3 approaches (39.8% to 47.9%) and each other. Thus, a record’s country information was finalized if the information was consistent between any 2 approaches, except between the brute force subregion and Nominatim searches. This led to 330,328 records with country information.
Topical and country impact metrics
To determine annual country impact, impact scores were determined in the same way as that for annual topical impact, except that values for different countries were calculated instead of topics ( S8 Data ).
Topical preferences by country
To determine topical preference for a country C , a 2 × 2 table was established with the number of records in topic T from C , the number of records in T but not from C , the number of non- T records from C , and the number of non- T records not from C . A Fisher’s exact test was performed for each T and C combination, and the resulting p -values were corrected for multiple testing with the Bejamini–Hochberg method (see S12 Data ). The preference of T in C was defined as the degree of enrichment calculated as log likelihood ratio of values in the 2 × 2 table. Topic 5 was excluded because >50% of the countries did not have records for this topic.
The top 10 countries could be classified into a China–India cluster, an Italy–Spain cluster, and remaining countries (yellow rectangles, Fig 5E ). The clustering of Italy and Spain is partly due to similar research focusing on allergens (topic 0) and mycotoxins (topic 54) and less emphasis on gene family (topic 23) and stress tolerance (topic 28) studies ( Figs 5F and S9 ). There are also substantial differences in topical focus between countries. For example, plant science records from China tend to be enriched in hyperspectral imaging and modeling (topic 9), gene family studies (topic 23), stress biology (topic 28), and research on new plant compounds associated with herbal medicine (topic 69), but less emphasis on population genetics and evolution (topic 86, Fig 5F ). In the US, there is a strong focus on insect pest resistance (topic 75), climate, community, and diversity (topic 83), and population genetics and evolution but less focus on new plant compounds. In summary, in addition to revealing how plant science research has evolved over time, topic modeling provides additional insights into differences in research foci among different countries.
Supporting information
S1 fig. plant science record classification model performance..
(A–C) Distributions of prediction probabilities (y_prob) of (A) positive instances (plant science records), (B) negative instances (non-plant science records), and (C) positive instances with the Medical Subject Heading “Plants” (ID = D010944). The data are color coded in blue and orange if they are correctly and incorrectly predicted, respectively. The lower subfigures contain log10-transformed x axes for the same distributions as the top subfigure for better visualization of incorrect predictions. (D) Prediction probability distribution for candidate plant science records. Prediction probabilities plotted here are available in S13 Data .
https://doi.org/10.1371/journal.pbio.3002612.s001
S2 Fig. Relationships between outlier clusters and the 90 topics.
(A) Heatmap demonstrating that some outlier clusters tend to have high prediction scores for multiple topics. Each cell shows the average prediction score of a topic for records in an outlier cluster. (B) Size of outlier clusters.
https://doi.org/10.1371/journal.pbio.3002612.s002
S3 Fig. Cosine similarities between topics.
(A) Heatmap showing cosine similarities between topic pairs. Top-left: hierarchical clustering of the cosine similarity matrix using the Ward algorithm. The branches are colored to indicate groups of related topics. (B) Topic labels and names. The topic ordering was based on hierarchical clustering of topics. Colored rectangles: neighboring topics with >0.5 cosine similarities.
https://doi.org/10.1371/journal.pbio.3002612.s003
S4 Fig. Relative topical diversity for 20 journals.
The 20 journals with the most plant science records are shown. The journal names were taken from the journal list in PubMed ( https://www.nlm.nih.gov/bsd/serfile_addedinfo.html ).
https://doi.org/10.1371/journal.pbio.3002612.s004
S5 Fig. Topical frequency and top terms during different time periods.
(A-D) Different patterns of topical frequency distributions for example topics (A) 48, (B) 35, (C) 27, and (D) 42. For each topic, the top graph shows the frequency of topical records in each time bin, which are the same as those in Fig 3 (green line), and the end date for each bin is indicated. The heatmap below each line plot depicts whether a term is among the top terms in a time bin (yellow) or not (blue). Blue dotted lines delineate different decades (see S5 Data for the original frequencies, S6 Data for the LOWESS fitted frequencies and the top terms for different topics/time bins).
https://doi.org/10.1371/journal.pbio.3002612.s005
S6 Fig. Prevalence of records mentioning different taxonomic groups in Viridiplantae.
(A, B) Percentage of records mentioning specific taxa at the ( A) major lineage and (B) family levels. (C, D) The prevalence of taxon mentions over time at the (C) major lineage and (E) family levels. The data used for plotting are available in S9 Data .
https://doi.org/10.1371/journal.pbio.3002612.s006
S7 Fig. Changes over time.
(A) Number of genera being mentioned in plant science records during different time bins (the date indicates the end date of that bin, exclusive). (B) Numbers of genera (blue) and organisms (salmon) with draft genomes available from National Center of Biotechnology Information in different years. (C) Percentage of US National Science Foundation (NSF) grants mentioning the genus Arabidopsis over time with peak percentage and year indicated. The data for (A–C) are in S9 Data . (D) Number of plant science records in the top 17 plant science journals from the USA (red), Great Britain (GBR) (orange), India (IND) (light green), and China (CHN) (dark green) normalized against the total numbers of publications of each country over time in these 17 journals. The data used for plotting can be found in S11 Data .
https://doi.org/10.1371/journal.pbio.3002612.s007
S8 Fig. Change in country impact on plant science over time.
(A, B) Difference in 2 impact metrics from 1999 to 2020 for the 10 countries with the highest number of plant science records. (A) H-index. (B) SCImago Journal Rank (SJR). (C, D) Plots show the relationships between the impact metrics (H-index in (C) , SJR in (D) ) averaged from 1999 to 2020 and the slopes of linear fits with years as the predictive variable and impact metric as the response variable for different countries (A3 country codes shown). The countries with >400 records and with <10% missing impact values are included. The data used for plotting can be found in S11 Data .
https://doi.org/10.1371/journal.pbio.3002612.s008
S9 Fig. Country topical preference.
Enrichment scores (LLR, log likelihood ratio) of topics for each of the top 10 countries. Red: overrepresentation, blue: underrepresentation. The data for plotting can be found in S12 Data .
https://doi.org/10.1371/journal.pbio.3002612.s009
S1 Data. Summary of source journals for plant science records, prediction models, and top Tf-Idf features.
Sheet–Candidate plant sci record j counts: Number of records from each journal in the candidate plant science corpus (before classification). Sheet—Plant sci record j count: Number of records from each journal in the plant science corpus (after classification). Sheet–Model summary: Model type, text used (txt_flag), and model parameters used. Sheet—Model performance: Performance of different model and parameter combinations on the validation data set. Sheet–Tf-Idf features: The average SHAP values of Tf-Idf (Term frequency-Inverse document frequency) features associated with different terms. Sheet–PubMed number per year: The data for PubMed records in Fig 1A . Sheet–Plant sci record num per yr: The data for the plant science records in Fig 1A .
https://doi.org/10.1371/journal.pbio.3002612.s010
S2 Data. Numbers of records in topics identified from preliminary topic models.
Sheet–Topics generated with a model based on BioBERT embeddings. Sheet–Topics generated with a model based on distilBERT embeddings. Sheet–Topics generated with a model based on SciBERT embeddings.
https://doi.org/10.1371/journal.pbio.3002612.s011
S3 Data. Final topic model labels and top terms for topics.
Sheet–Topic label: The topic index and top 10 terms with the highest cTf-Idf values. Sheets– 0 to 89: The top 50 terms and their c-Tf-Idf values for topics 0 to 89.
https://doi.org/10.1371/journal.pbio.3002612.s012
S4 Data. UMAP representations of different topics.
For a topic T , records in the UMAP graph are colored red and records not in T are colored gray.
https://doi.org/10.1371/journal.pbio.3002612.s013
S5 Data. Temporal relationships between published documents projected onto 2D space.
The 2D embedding generated with UMAP was used to plot document relationships for each year. The plots from 1975 to 2020 were compiled into an animation.
https://doi.org/10.1371/journal.pbio.3002612.s014
S6 Data. Timestamps and dates for dynamic topic modeling.
Sheet–bin_timestamp: Columns are: (1) order index; (2) bin_idx–relative positions of bin labels; (3) bin_timestamp–UNIX time in seconds; and (4) bin_date–month/day/year. Sheet–Topic frequency per timestamp: The number of documents in each time bin for each topic. Sheets–LOWESS fit 0.1/0.2/0.3: Topic frequency per timestamp fitted with the fraction parameter of 0.1, 0.2, or 0.3. Sheet—Topic top terms: The top 5 terms for each topic in each time bin.
https://doi.org/10.1371/journal.pbio.3002612.s015
S7 Data. Locally weighted scatterplot smoothing (LOWESS) of topical document frequencies over time.
There are 90 scatter plots, one for each topic, where the x axis is time, and the y axis is the document frequency (blue dots). The LOWESS fit is shown as orange points connected with a green line. The category a topic belongs to and its order in Fig 3 are labeled on the top left corner. The data used for plotting are in S6 Data .
https://doi.org/10.1371/journal.pbio.3002612.s016
S8 Data. The 4 criteria used for sorting topics.
Peak: the time when the LOWESS fit of the frequencies of a topic reaches maximum. 1st_reach_thr: the time when the LOWESS fit first reaches a threshold of 60% maximal frequency (peak value). Trend: upward (1), no change (0), or downward (−1). Stable: whether a topic belongs to the stable category (1) or not (0).
https://doi.org/10.1371/journal.pbio.3002612.s017
S9 Data. Change in taxon record numbers and genome assemblies available over time.
Sheet–Genus: Number of records mentioning a genus during different time periods (in Unix timestamp) for the top 100 genera. Sheet–Genus: Number of records mentioning a family during different time periods (in Unix timestamp) for the top 100 families. Sheet–Genus: Number of records mentioning an order during different time periods (in Unix timestamp) for the top 20 orders. Sheet–Species levels: Number of records mentioning 12 selected taxonomic levels higher than the order level during different time periods (in Unix timestamp). Sheet–Genome assembly: Plant genome assemblies available from NCBI as of October 28, 2022. Sheet–Arabidopsis NSF: Absolute and normalized numbers of US National Science Foundation funded proposals mentioning Arabidopsis in proposal titles and/or abstracts.
https://doi.org/10.1371/journal.pbio.3002612.s018
S10 Data. Taxon topical preference.
Sheet– 5 genera LLR: The log likelihood ratio of each topic in each of the top 5 genera with the highest numbers of plant science records. Sheets– 5 genera: For each genus, the columns are: (1) topic; (2) the Fisher’s exact test p -value (Pvalue); (3–6) numbers of records in topic T and in genus X (n_inT_inX), in T but not in X (n_inT_niX), not in T but in X (n_niT_inX), and not in T and X (n_niT_niX) that were used to construct 2 × 2 tables for the tests; and (7) the log likelihood ratio generated with the 2 × 2 tables. Sheet–corrected p -value: The 4 values for generating LLRs were used to conduct Fisher’s exact test. The p -values obtained for each country were corrected for multiple testing.
https://doi.org/10.1371/journal.pbio.3002612.s019
S11 Data. Impact metrics of countries in different years.
Sheet–country_top25_year_count: number of total publications and publications per year from the top 25 countries with the most plant science records. Sheet—country_top25_year_top17j: number of total publications and publications per year from the top 25 countries with the highest numbers of plant science records in the 17 plant science journals used as positive examples. Sheet–prank: Journal percentile rank scores for countries (3-letter country codes following https://www.iban.com/country-codes ) in different years from 1999 to 2020. Sheet–sjr: Scimago Journal rank scores. Sheet–hidx: H-Index scores. Sheet–cite: Citation scores.
https://doi.org/10.1371/journal.pbio.3002612.s020
S12 Data. Topical enrichment for the top 10 countries with the highest numbers of plant science publications.
Sheet—Log likelihood ratio: For each country C and topic T, it is defined as log((a/b)/(c/d)) where a is the number of papers from C in T, b is the number from C but not in T, c is the number not from C but in T, d is the number not from C and not in T. Sheet: corrected p -value: The 4 values, a, b, c, and d, were used to conduct Fisher’s exact test. The p -values obtained for each country were corrected for multiple testing.
https://doi.org/10.1371/journal.pbio.3002612.s021
S13 Data. Text classification prediction probabilities.
This compressed file contains the PubMed ID (PMID) and the prediction probabilities (y_pred) of testing data with both positive and negative examples (pred_prob_testing), plant science candidate records with the MeSH term “Plants” (pred_prob_candidates_with_mesh), and all plant science candidate records (pred_prob_candidates_all). The prediction probability was generated using the Word2Vec text classification models for distinguishing positive (plant science) and negative (non-plant science) records.
https://doi.org/10.1371/journal.pbio.3002612.s022
Acknowledgments
We thank Maarten Grootendorst for discussions on topic modeling. We also thank Stacey Harmer, Eva Farre, Ning Jiang, and Robert Last for discussion on their respective research fields and input on how to improve this study and Rudiger Simon for the suggestion to examine differences between countries. We also thank Mae Milton, Christina King, Edmond Anderson, Jingyao Tang, Brianna Brown, Kenia Segura Abá, Eleanor Siler, Thilanka Ranaweera, Huan Chen, Rajneesh Singhal, Paulo Izquierdo, Jyothi Kumar, Daniel Shiu, Elliott Shiu, and Wiggler Catt for their good ideas, personal and professional support, collegiality, fun at parties, as well as the trouble they have caused, which helped us improve as researchers, teachers, mentors, and parents.
- View Article
- PubMed/NCBI
- Google Scholar
- 2. Blei DM, Lafferty JD. Topic Models. In: Srivastava A, Sahami M, editors. Text Mining. Cambridge: Chapman and Hall/CRC; 2009. pp. 71–93.
- 7. ChatGPT. [cited 2023 Aug 25]. Available from: https://chat.openai.com
- 9. Fei-Fei L, Perona P. A Bayesian hierarchical model for learning natural scene categories. 2005 IEEE Computer Society Conference on Computer Vision and Pattern Recognition (CVPR’05); 2005. pp. 524–531 vol. 2. https://doi.org/10.1109/CVPR.2005.16
- 19. Blei DM, Lafferty JD. Dynamic topic models. Proceedings of the 23rd International Conference on Machine learning. New York, NY, USA: Association for Computing Machinery; 2006. pp. 113–120. https://doi.org/10.1145/1143844.1143859
- 35. Kuhn T. The Structure of Scientific Revolution. Chicago: University of Chicago Press; 1962.
- 36. CiteSeer | Proceedings of the second international conference on Autonomous agents. [cited 2023 Aug 23]. Available from: https://dl.acm.org/doi/10.1145/280765.280786
- 39. Chen T, Guestrin C. XGBoost: A Scalable Tree Boosting System. Proceedings of the 22nd ACM SIGKDD International Conference on Knowledge Discovery and Data Mining. New York, NY, USA: ACM; 2016. pp. 785–794. https://doi.org/10.1145/2939672.2939785
- 40. Řehůřek R, Sojka P. Software Framework for Topic Modelling with Large Corpora. Proceedings of the LREC 2010 Workshop on New Challenges for NLP Frameworks. Valletta, Malta: ELRA; 2010. pp. 45–50.
- 42. Hugging Face–The AI community building the future. 2023 Aug 19 [cited 2023 Aug 25]. Available from: https://huggingface.co/

- SUGGESTED TOPICS
- The Magazine
- Newsletters
- Managing Yourself
- Managing Teams
- Work-life Balance
- The Big Idea
- Data & Visuals
- Reading Lists
- Case Selections
- HBR Learning
- Topic Feeds
- Account Settings
- Email Preferences
3 Ways to Make a Request That Doesn’t Feel Coercive
- Rachel Schlund,
- Roseanna Sommers,
- Vanessa Bohns

To get an authentic yes, give your employee room to say no.
Research shows that people feel more pressured to agree to requests than we realize, frequently agreeing to do things they would rather not do, such as taking on burdensome, low-promotability work tasks. As a manager, what can you do to ensure that your employees aren’t taking things on because they feel like they have to, but because they actually want to? In this article, the authors share three research-backed suggestions for how to elicit a more voluntary “yes” when making a request: 1) Give people time to respond. 2) Ask them to respond over email. 3) Share an example of how to say “no.”
When staffing a project, asking your team to work overtime, or finding someone for a last-minute task to meet a deadline, it can sometimes feel like you need to get your employees to say “yes” at any cost. But what is that cost? When employees feel pressured or guilted into agreeing to a request they personally find disagreeable it can lead to feelings of regret, frustration, and resentment. An employee who begrudgingly agrees to a request in the moment may provide lower-quality assistance or back out of their commitment at a less convenient time.

- Rachel Schlund is an incoming Principal Researcher at the University of Chicago, Booth School of Business. She is currently finishing her PhD in organizational behavior at Cornell University. You can learn more about her research here .
- Roseanna Sommers (JD/PhD) is an Assistant Professor of Law at the University of Michigan, where she directs the psychology and law studies lab. Her teaching and research interests revolve around the many ways in which the law misunderstands people and people misunderstand the law. You can learn more about her research on consent and related topics here .
- Vanessa Bohns is a Professor of Organizational Behavior at Cornell University and the author of You Have More Influence Than You Think . You can learn more about her research on social influence and persuasion here .
Partner Center

Research involvement of medical students in a medical school of India: exploring knowledge, attitude, practices, and perceived barriers
- Find this author on Google Scholar
- Find this author on PubMed
- Search for this author on this site
- ORCID record for Abhinav Jha
- ORCID record for Deepak Dhamnetiya
- For correspondence: [email protected]
- ORCID record for Ravi Prakash Jha
- Info/History
- Preview PDF
Introduction Research in the medical discipline significantly impacts society by improving the general well-being of the population, through improvements in diagnostic and treatment modalities. However, of 579 Indian medical colleges, 332 (57.3%) did not publish a single paper from the year 2005 to 2014," indicating a limited contribution from medical fraternity In order to probe in to the cause of this a study was conducted to assess the knowledge, attitude, practices (KAP) and perceived barriers to research among students of a medical school in Delhi, India.
Methods A cross-sectional study was conducted among medical students and the data on academic-cum-demographic information, assessment of knowledge, attitude, practices and barriers to research was collected using a pre-tested, semi-structured questionnaire. Chi-square test was used to check the association of various factors with the KAP of research. A p-value less than 0.05 was considered significant.
Results A total of 402 (N) subjects were enrolled in the study. Majority were male (79.6%) and from clinical professional years (57%). Majority (266, 66.2%) of the subjects had adequate knowledge. Of the study subjects (61,15%) having inadequate knowledge of research, sixty percent were from pre- and para-clinical years, while around 70 % of those having good knowledge were from clinical professional years. However, only 16.9% of the participants had participated in a research project, and only 4.72% had authored a publication. Sixty one percent of study subjects having a positive attitude towards research, were from pre- and para-clinical years. Among the study subjects having a positive attitude towards research, over 60% were from pre- and para-clinical years. The barriers for conducting research were mostly; lack of funds/laboratory equipment/infrastructure (85.1%), lack of exposure to opportunities for research in the medical (MBBS) curriculum (83.8%), and lack of time (83.3%). There was a statistically significant association between knowledge and attitude towards research with a professional year of study.
Conclusions The study revealed that while most of the students had a positive attitude towards research as well as an adequate knowledge of research, there was a poor level of participation in research. These challenges can be overcome by incorporating research as a part of the medical school curriculum from early years on, setting aside separate time for research, and establishing student research societies that can actively promote research.
Competing Interest Statement
The authors have declared no competing interest.
Funding Statement
This study did not receive any funding.
Author Declarations
I confirm all relevant ethical guidelines have been followed, and any necessary IRB and/or ethics committee approvals have been obtained.
The details of the IRB/oversight body that provided approval or exemption for the research described are given below:
The ethics committee of Dr Baba Saheb Ambedkar Medical College and Hospital, New Delhi gave ethical approval for this work.
I confirm that all necessary patient/participant consent has been obtained and the appropriate institutional forms have been archived, and that any patient/participant/sample identifiers included were not known to anyone (e.g., hospital staff, patients or participants themselves) outside the research group so cannot be used to identify individuals.
I understand that all clinical trials and any other prospective interventional studies must be registered with an ICMJE-approved registry, such as ClinicalTrials.gov. I confirm that any such study reported in the manuscript has been registered and the trial registration ID is provided (note: if posting a prospective study registered retrospectively, please provide a statement in the trial ID field explaining why the study was not registered in advance).
I have followed all appropriate research reporting guidelines, such as any relevant EQUATOR Network research reporting checklist(s) and other pertinent material, if applicable.
Email id: jhaabhinav677{at}gmail.com , manas.shah1999{at}gmail.com , Ritikgoyal152{at}gmail.com , drdeepakdhamnetiya{at}gmail.com , apoorv1729{at}gmail.com , raviprakashjha{at}gmail.com , dr.prachi.obg{at}bsamch.in
Data Availability
All data produced in the present study are available upon reasonable request to the authors.
View the discussion thread.
Thank you for your interest in spreading the word about medRxiv.
NOTE: Your email address is requested solely to identify you as the sender of this article.

Citation Manager Formats
- EndNote (tagged)
- EndNote 8 (xml)
- RefWorks Tagged
- Ref Manager
- Tweet Widget
- Facebook Like
- Google Plus One
Subject Area
- Medical Education
- Addiction Medicine (324)
- Allergy and Immunology (631)
- Anesthesia (167)
- Cardiovascular Medicine (2395)
- Dentistry and Oral Medicine (289)
- Dermatology (207)
- Emergency Medicine (380)
- Endocrinology (including Diabetes Mellitus and Metabolic Disease) (845)
- Epidemiology (11788)
- Forensic Medicine (10)
- Gastroenterology (704)
- Genetic and Genomic Medicine (3761)
- Geriatric Medicine (350)
- Health Economics (636)
- Health Informatics (2406)
- Health Policy (936)
- Health Systems and Quality Improvement (902)
- Hematology (342)
- HIV/AIDS (784)
- Infectious Diseases (except HIV/AIDS) (13336)
- Intensive Care and Critical Care Medicine (769)
- Medical Education (366)
- Medical Ethics (105)
- Nephrology (401)
- Neurology (3521)
- Nursing (199)
- Nutrition (528)
- Obstetrics and Gynecology (677)
- Occupational and Environmental Health (666)
- Oncology (1828)
- Ophthalmology (538)
- Orthopedics (219)
- Otolaryngology (287)
- Pain Medicine (234)
- Palliative Medicine (66)
- Pathology (447)
- Pediatrics (1035)
- Pharmacology and Therapeutics (426)
- Primary Care Research (424)
- Psychiatry and Clinical Psychology (3186)
- Public and Global Health (6168)
- Radiology and Imaging (1286)
- Rehabilitation Medicine and Physical Therapy (750)
- Respiratory Medicine (831)
- Rheumatology (379)
- Sexual and Reproductive Health (372)
- Sports Medicine (324)
- Surgery (403)
- Toxicology (50)
- Transplantation (172)
- Urology (147)
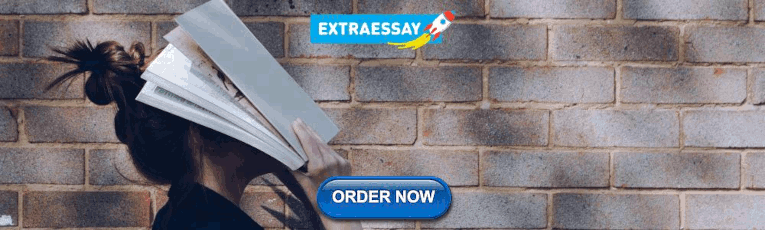
COMMENTS
The methods of action research in education include: conducting in-class observations. taking field notes. surveying or interviewing teachers, administrators, or parents. using audio and video recordings. The goal is to identify problematic issues, test possible solutions, or simply carry-out continuous improvement.
The first question asks for a ready-made solution, and is not focused or researchable. The second question is a clearer comparative question, but note that it may not be practically feasible. For a smaller research project or thesis, it could be narrowed down further to focus on the effectiveness of drunk driving laws in just one or two countries.
Action research is a research method that aims to simultaneously investigate and solve an issue. In other words, as its name suggests, action research conducts research and takes action at the same time. It was first coined as a term in 1944 by MIT professor Kurt Lewin.A highly interactive method, action research is often used in the social ...
Specific goals of this handbook are to help educators do the following: Define and explain Action Research. Demonstrate an understanding of how to use the recursive nature of Action Research to improve their teaching of instructional literacy. Provide examples of the Action Research process in action.
An action research project is a practical endeavor that will ultimately be shaped by your educational context and practice. Now that you have developed a literature review, you are ready to revise your initial plans and begin to plan your project. This chapter will provide some advice about your considerations when undertaking an action ...
A good research question is essential to guide your research paper, dissertation, or thesis. All research questions should be: Focused on a single problem or issue. Researchable using primary and/or secondary sources. Feasible to answer within the timeframe and practical constraints. Specific enough to answer thoroughly.
Those familiar with the action research model (see Kemmis et al., 2014) will recognise the value of these questions in helping teacher researchers to initiate work on the first major step of the cycle which is planning. In contrast to Jones' numerous questions, McNiff and Whitehead (2011: 134) focused more on one key question, claiming that ...
Passion is integral to doing action research and can be a resource for identifying a research question, as indicated by Dana and Yendol-Hoppey (2008, pp. 15-48). After analyzing more than 100 teacher class-room research studies, they identified eight passions as possibilities for finding a research question: 1.
Preview. 100 Questions (and Answers) About Action Research identifies and answers the essential questions on the process of systematically approaching your practice from an inquiry-oriented perspective, with a focus on improving that practice. This unique text offers progressive instructors an alternative to the research status quo and serves ...
Action research is an intentional act, and the research questions can be used to state our intentions. When writing action research questions, you should keep the following criteria in mind: They should be broad in scope. You likely will have 1-3 questions that guide your action research process. It is unlikely that you will have more than 6.
Action research is a process for improving educational practice. Its methods involve action, evaluation, and reflection. It is a process to gather evidence to implement change in practices. Action research is participative and collaborative. It is undertaken by individuals with a common purpose.
The cyclical and iterative action research process comprises four stages: planning, acting, develop- ing, and reflecting. 2. The planning stageconsists of the following four steps: • Identifying and limiting the topic • Gathering information • Reviewing the related literature • Developing a research plan 3.
Stage 1: Plan. For an action research project to go well, the researcher needs to plan it well. After coming up with an educational research topic or question after a research study, the first step is to develop an action plan to guide the research process. The research design aims to address the study's question.
Abstract. 100 Questions (and Answers) About Action Research identifies and answers the essential questions on the process of systematically approaching your practice from an inquiry-oriented ...
Creating an action research project. The action research process usually begins with a situation or issue that a teacher wants to change as part of school improvement initiatives.. Teachers get support in changing the 'interesting issue' into a 'researchable question' and then taking to experiment.The teacher will draw on the outcomes of other researchers to help build actions and reveal the ...
Introduction. Action research is a tool that is used to help teac hers and other educators uncover strategies to. improve teaching practices (Sagor, 2004); it is a viable and realistic endeavor ...
A well-crafted research question (or set of questions) sets the stage for a robust study and meaningful insights. But, if you're new to research, it's not always clear what exactly constitutes a good research question. In this post, we'll provide you with clear examples of quality research questions across various disciplines, so that you can approach your research project with confidence!
Research Aims: Examples. True to the name, research aims usually start with the wording "this research aims to…", "this research seeks to…", and so on. For example: "This research aims to explore employee experiences of digital transformation in retail HR.". "This study sets out to assess the interaction between student ...
The aims and objectives of this action research are to: To improve students' active participation in classroom teaching and learning. To explore the reasons why students hardly take part in ...
Definition: Research questions are the specific questions that guide a research study or inquiry. These questions help to define the scope of the research and provide a clear focus for the study. Research questions are usually developed at the beginning of a research project and are designed to address a particular research problem or objective.
<button>Click to continue</button>
Exemplars of classroom practice were collected to address the following research questions: How do teacher-researchers reflect on and investigate their own classrooms in supporting intercultural practices? What elements of classroom pedagogy support participation, communication & respect, the intercultural capabilities of all learners as a ...
DOI 10.3386/w32473. Issue Date May 2024. Collective action is a dynamic process where individuals in a group assess over time the benefits and costs of participating toward the success of a collective goal. Early participation improves the expectation of success and thus stimulates the subsequent participation of other individuals who might ...
A well-formulated research question is essential for guiding your study effectively. Follow this format to ensure clarity and precision: Specify the Topic: Begin with a broad subject area. Example: "Education technology". Narrow the Focus: Define a specific aspect or variable. Example: "Impact of digital tools".
Our ability to understand the progress of science through the evolution of research topics is limited by the need for specialist knowledge and the exponential growth of the literature. This study uses artificial intelligence and machine learning approaches to demonstrate how a biological field (plant science) has evolved, how the model systems have changed, and how countries differ in terms of ...
Step 1: Asking the right questions to guide your a Product/Market Fit study. In order to put together a solid product/market fit research process, you'll want to get to the bottom of three key types of questions: Your product market fit questions (obviously) "Getting to know you" questions — the higher level ways to better understand ...
2) Ask them to respond over email. 3) Share an example of how to say "no.". When staffing a project, asking your team to work overtime, or finding someone for a last-minute task to meet a ...
Introduction: Research in the medical discipline significantly impacts society by improving the general well-being of the population, through improvements in diagnostic and treatment modalities. However, of 579 Indian medical colleges, 332 (57.3%) did not publish a single paper from the year 2005 to 2014," indicating a limited contribution from medical fraternity In order to probe in to the ...
Relevant answer. James Leigh. Nov 21, 2023. Answer. Fundamental research is the creation of new knowledge for its own sake. Action research is seeking answers to a question with a specific goal in ...
To prepare for your interview and make a great first impression, you can explore this list of 10 common interview questions and plan your responses to them. 1. Tell me about yourself. This warm-up question is your chance to make an impactful first impression. Be prepared to describe yourself in a few sentences. You can mention: Your past ...